text
stringlengths 7
328k
| id
stringlengths 14
166
| metadata
dict | __index_level_0__
int64 0
459
|
---|---|---|---|
# SE-ResNeXt
**SE ResNeXt** is a variant of a [ResNext](https://www.paperswithcode.com/method/resneXt) that employs [squeeze-and-excitation blocks](https://paperswithcode.com/method/squeeze-and-excitation-block) to enable the network to perform dynamic channel-wise feature recalibration.
## How do I use this model on an image?
To load a pretrained model:
```py
>>> import timm
>>> model = timm.create_model('seresnext26d_32x4d', pretrained=True)
>>> model.eval()
```
To load and preprocess the image:
```py
>>> import urllib
>>> from PIL import Image
>>> from timm.data import resolve_data_config
>>> from timm.data.transforms_factory import create_transform
>>> config = resolve_data_config({}, model=model)
>>> transform = create_transform(**config)
>>> url, filename = ("https://github.com/pytorch/hub/raw/master/images/dog.jpg", "dog.jpg")
>>> urllib.request.urlretrieve(url, filename)
>>> img = Image.open(filename).convert('RGB')
>>> tensor = transform(img).unsqueeze(0) # transform and add batch dimension
```
To get the model predictions:
```py
>>> import torch
>>> with torch.no_grad():
... out = model(tensor)
>>> probabilities = torch.nn.functional.softmax(out[0], dim=0)
>>> print(probabilities.shape)
>>> # prints: torch.Size([1000])
```
To get the top-5 predictions class names:
```py
>>> # Get imagenet class mappings
>>> url, filename = ("https://raw.githubusercontent.com/pytorch/hub/master/imagenet_classes.txt", "imagenet_classes.txt")
>>> urllib.request.urlretrieve(url, filename)
>>> with open("imagenet_classes.txt", "r") as f:
... categories = [s.strip() for s in f.readlines()]
>>> # Print top categories per image
>>> top5_prob, top5_catid = torch.topk(probabilities, 5)
>>> for i in range(top5_prob.size(0)):
... print(categories[top5_catid[i]], top5_prob[i].item())
>>> # prints class names and probabilities like:
>>> # [('Samoyed', 0.6425196528434753), ('Pomeranian', 0.04062102362513542), ('keeshond', 0.03186424449086189), ('white wolf', 0.01739676296710968), ('Eskimo dog', 0.011717947199940681)]
```
Replace the model name with the variant you want to use, e.g. `seresnext26d_32x4d`. You can find the IDs in the model summaries at the top of this page.
To extract image features with this model, follow the [timm feature extraction examples](../feature_extraction), just change the name of the model you want to use.
## How do I finetune this model?
You can finetune any of the pre-trained models just by changing the classifier (the last layer).
```py
>>> model = timm.create_model('seresnext26d_32x4d', pretrained=True, num_classes=NUM_FINETUNE_CLASSES)
```
To finetune on your own dataset, you have to write a training loop or adapt [timm's training
script](https://github.com/rwightman/pytorch-image-models/blob/master/train.py) to use your dataset.
## How do I train this model?
You can follow the [timm recipe scripts](../scripts) for training a new model afresh.
## Citation
```BibTeX
@misc{hu2019squeezeandexcitation,
title={Squeeze-and-Excitation Networks},
author={Jie Hu and Li Shen and Samuel Albanie and Gang Sun and Enhua Wu},
year={2019},
eprint={1709.01507},
archivePrefix={arXiv},
primaryClass={cs.CV}
}
```
<!--
Type: model-index
Collections:
- Name: SEResNeXt
Paper:
Title: Squeeze-and-Excitation Networks
URL: https://paperswithcode.com/paper/squeeze-and-excitation-networks
Models:
- Name: seresnext26d_32x4d
In Collection: SEResNeXt
Metadata:
FLOPs: 3507053024
Parameters: 16810000
File Size: 67425193
Architecture:
- 1x1 Convolution
- Batch Normalization
- Convolution
- Global Average Pooling
- Grouped Convolution
- Max Pooling
- ReLU
- ResNeXt Block
- Residual Connection
- Softmax
- Squeeze-and-Excitation Block
Tasks:
- Image Classification
Training Techniques:
- Label Smoothing
- SGD with Momentum
- Weight Decay
Training Data:
- ImageNet
Training Resources: 8x NVIDIA Titan X GPUs
ID: seresnext26d_32x4d
LR: 0.6
Epochs: 100
Layers: 26
Dropout: 0.2
Crop Pct: '0.875'
Momentum: 0.9
Batch Size: 1024
Image Size: '224'
Interpolation: bicubic
Code: https://github.com/rwightman/pytorch-image-models/blob/a7f95818e44b281137503bcf4b3e3e94d8ffa52f/timm/models/resnet.py#L1234
Weights: https://github.com/rwightman/pytorch-image-models/releases/download/v0.1-weights/seresnext26d_32x4d-80fa48a3.pth
Results:
- Task: Image Classification
Dataset: ImageNet
Metrics:
Top 1 Accuracy: 77.59%
Top 5 Accuracy: 93.61%
- Name: seresnext26t_32x4d
In Collection: SEResNeXt
Metadata:
FLOPs: 3466436448
Parameters: 16820000
File Size: 67414838
Architecture:
- 1x1 Convolution
- Batch Normalization
- Convolution
- Global Average Pooling
- Grouped Convolution
- Max Pooling
- ReLU
- ResNeXt Block
- Residual Connection
- Softmax
- Squeeze-and-Excitation Block
Tasks:
- Image Classification
Training Techniques:
- Label Smoothing
- SGD with Momentum
- Weight Decay
Training Data:
- ImageNet
Training Resources: 8x NVIDIA Titan X GPUs
ID: seresnext26t_32x4d
LR: 0.6
Epochs: 100
Layers: 26
Dropout: 0.2
Crop Pct: '0.875'
Momentum: 0.9
Batch Size: 1024
Image Size: '224'
Interpolation: bicubic
Code: https://github.com/rwightman/pytorch-image-models/blob/a7f95818e44b281137503bcf4b3e3e94d8ffa52f/timm/models/resnet.py#L1246
Weights: https://github.com/rwightman/pytorch-image-models/releases/download/v0.1-weights/seresnext26tn_32x4d-569cb627.pth
Results:
- Task: Image Classification
Dataset: ImageNet
Metrics:
Top 1 Accuracy: 77.99%
Top 5 Accuracy: 93.73%
- Name: seresnext50_32x4d
In Collection: SEResNeXt
Metadata:
FLOPs: 5475179184
Parameters: 27560000
File Size: 110569859
Architecture:
- 1x1 Convolution
- Batch Normalization
- Convolution
- Global Average Pooling
- Grouped Convolution
- Max Pooling
- ReLU
- ResNeXt Block
- Residual Connection
- Softmax
- Squeeze-and-Excitation Block
Tasks:
- Image Classification
Training Techniques:
- Label Smoothing
- SGD with Momentum
- Weight Decay
Training Data:
- ImageNet
Training Resources: 8x NVIDIA Titan X GPUs
ID: seresnext50_32x4d
LR: 0.6
Epochs: 100
Layers: 50
Dropout: 0.2
Crop Pct: '0.875'
Momentum: 0.9
Batch Size: 1024
Image Size: '224'
Interpolation: bicubic
Code: https://github.com/rwightman/pytorch-image-models/blob/a7f95818e44b281137503bcf4b3e3e94d8ffa52f/timm/models/resnet.py#L1267
Weights: https://github.com/rwightman/pytorch-image-models/releases/download/v0.1-weights/seresnext50_32x4d_racm-a304a460.pth
Results:
- Task: Image Classification
Dataset: ImageNet
Metrics:
Top 1 Accuracy: 81.27%
Top 5 Accuracy: 95.62%
--> | pytorch-image-models/hfdocs/source/models/seresnext.mdx/0 | {
"file_path": "pytorch-image-models/hfdocs/source/models/seresnext.mdx",
"repo_id": "pytorch-image-models",
"token_count": 2753
} | 187 |
# Quickstart
This quickstart is intended for developers who are ready to dive into the code and see an example of how to integrate `timm` into their model training workflow.
First, you'll need to install `timm`. For more information on installation, see [Installation](installation).
```bash
pip install timm
```
## Load a Pretrained Model
Pretrained models can be loaded using [`create_model`].
Here, we load the pretrained `mobilenetv3_large_100` model.
```py
>>> import timm
>>> m = timm.create_model('mobilenetv3_large_100', pretrained=True)
>>> m.eval()
```
<Tip>
Note: The returned PyTorch model is set to train mode by default, so you must call .eval() on it if you plan to use it for inference.
</Tip>
## List Models with Pretrained Weights
To list models packaged with `timm`, you can use [`list_models`]. If you specify `pretrained=True`, this function will only return model names that have associated pretrained weights available.
```py
>>> import timm
>>> from pprint import pprint
>>> model_names = timm.list_models(pretrained=True)
>>> pprint(model_names)
[
'adv_inception_v3',
'cspdarknet53',
'cspresnext50',
'densenet121',
'densenet161',
'densenet169',
'densenet201',
'densenetblur121d',
'dla34',
'dla46_c',
]
```
You can also list models with a specific pattern in their name.
```py
>>> import timm
>>> from pprint import pprint
>>> model_names = timm.list_models('*resne*t*')
>>> pprint(model_names)
[
'cspresnet50',
'cspresnet50d',
'cspresnet50w',
'cspresnext50',
...
]
```
## Fine-Tune a Pretrained Model
You can finetune any of the pre-trained models just by changing the classifier (the last layer).
```py
>>> model = timm.create_model('mobilenetv3_large_100', pretrained=True, num_classes=NUM_FINETUNE_CLASSES)
```
To fine-tune on your own dataset, you have to write a PyTorch training loop or adapt `timm`'s [training script](training_script) to use your dataset.
## Use a Pretrained Model for Feature Extraction
Without modifying the network, one can call model.forward_features(input) on any model instead of the usual model(input). This will bypass the head classifier and global pooling for networks.
For a more in depth guide to using `timm` for feature extraction, see [Feature Extraction](feature_extraction).
```py
>>> import timm
>>> import torch
>>> x = torch.randn(1, 3, 224, 224)
>>> model = timm.create_model('mobilenetv3_large_100', pretrained=True)
>>> features = model.forward_features(x)
>>> print(features.shape)
torch.Size([1, 960, 7, 7])
```
## Image Augmentation
To transform images into valid inputs for a model, you can use [`timm.data.create_transform`], providing the desired `input_size` that the model expects.
This will return a generic transform that uses reasonable defaults.
```py
>>> timm.data.create_transform((3, 224, 224))
Compose(
Resize(size=256, interpolation=bilinear, max_size=None, antialias=None)
CenterCrop(size=(224, 224))
ToTensor()
Normalize(mean=tensor([0.4850, 0.4560, 0.4060]), std=tensor([0.2290, 0.2240, 0.2250]))
)
```
Pretrained models have specific transforms that were applied to images fed into them while training. If you use the wrong transform on your image, the model won't understand what it's seeing!
To figure out which transformations were used for a given pretrained model, we can start by taking a look at its `pretrained_cfg`
```py
>>> model.pretrained_cfg
{'url': 'https://github.com/rwightman/pytorch-image-models/releases/download/v0.1-weights/mobilenetv3_large_100_ra-f55367f5.pth',
'num_classes': 1000,
'input_size': (3, 224, 224),
'pool_size': (7, 7),
'crop_pct': 0.875,
'interpolation': 'bicubic',
'mean': (0.485, 0.456, 0.406),
'std': (0.229, 0.224, 0.225),
'first_conv': 'conv_stem',
'classifier': 'classifier',
'architecture': 'mobilenetv3_large_100'}
```
We can then resolve only the data related configuration by using [`timm.data.resolve_data_config`].
```py
>>> timm.data.resolve_data_config(model.pretrained_cfg)
{'input_size': (3, 224, 224),
'interpolation': 'bicubic',
'mean': (0.485, 0.456, 0.406),
'std': (0.229, 0.224, 0.225),
'crop_pct': 0.875}
```
We can pass this data config to [`timm.data.create_transform`] to initialize the model's associated transform.
```py
>>> data_cfg = timm.data.resolve_data_config(model.pretrained_cfg)
>>> transform = timm.data.create_transform(**data_cfg)
>>> transform
Compose(
Resize(size=256, interpolation=bicubic, max_size=None, antialias=None)
CenterCrop(size=(224, 224))
ToTensor()
Normalize(mean=tensor([0.4850, 0.4560, 0.4060]), std=tensor([0.2290, 0.2240, 0.2250]))
)
```
<Tip>
Note: Here, the pretrained model's config happens to be the same as the generic config we made earlier. This is not always the case. So, it's safer to use the data config to create the transform as we did here instead of using the generic transform.
</Tip>
## Using Pretrained Models for Inference
Here, we will put together the above sections and use a pretrained model for inference.
First we'll need an image to do inference on. Here we load a picture of a leaf from the web:
```py
>>> import requests
>>> from PIL import Image
>>> from io import BytesIO
>>> url = 'https://datasets-server.huggingface.co/assets/imagenet-1k/--/default/test/12/image/image.jpg'
>>> image = Image.open(requests.get(url, stream=True).raw)
>>> image
```
Here's the image we loaded:
<img src="https://datasets-server.huggingface.co/assets/imagenet-1k/--/default/test/12/image/image.jpg" alt="An Image from a link" width="300"/>
Now, we'll create our model and transforms again. This time, we make sure to set our model in evaluation mode.
```py
>>> model = timm.create_model('mobilenetv3_large_100', pretrained=True).eval()
>>> transform = timm.data.create_transform(
**timm.data.resolve_data_config(model.pretrained_cfg)
)
```
We can prepare this image for the model by passing it to the transform.
```py
>>> image_tensor = transform(image)
>>> image_tensor.shape
torch.Size([3, 224, 224])
```
Now we can pass that image to the model to get the predictions. We use `unsqueeze(0)` in this case, as the model is expecting a batch dimension.
```py
>>> output = model(image_tensor.unsqueeze(0))
>>> output.shape
torch.Size([1, 1000])
```
To get the predicted probabilities, we apply softmax to the output. This leaves us with a tensor of shape `(num_classes,)`.
```py
>>> probabilities = torch.nn.functional.softmax(output[0], dim=0)
>>> probabilities.shape
torch.Size([1000])
```
Now we'll find the top 5 predicted class indexes and values using `torch.topk`.
```py
>>> values, indices = torch.topk(probabilities, 5)
>>> indices
tensor([162, 166, 161, 164, 167])
```
If we check the imagenet labels for the top index, we can see what the model predicted...
```py
>>> IMAGENET_1k_URL = 'https://storage.googleapis.com/bit_models/ilsvrc2012_wordnet_lemmas.txt'
>>> IMAGENET_1k_LABELS = requests.get(IMAGENET_1k_URL).text.strip().split('\n')
>>> [{'label': IMAGENET_1k_LABELS[idx], 'value': val.item()} for val, idx in zip(values, indices)]
[{'label': 'beagle', 'value': 0.8486220836639404},
{'label': 'Walker_hound, Walker_foxhound', 'value': 0.03753996267914772},
{'label': 'basset, basset_hound', 'value': 0.024628572165966034},
{'label': 'bluetick', 'value': 0.010317106731235981},
{'label': 'English_foxhound', 'value': 0.006958036217838526}]
``` | pytorch-image-models/hfdocs/source/quickstart.mdx/0 | {
"file_path": "pytorch-image-models/hfdocs/source/quickstart.mdx",
"repo_id": "pytorch-image-models",
"token_count": 2583
} | 188 |
# Validation and Benchmark Results
This folder contains validation and benchmark results for the models in this collection. Validation scores are currently only run for models with pretrained weights and ImageNet-1k heads, benchmark numbers are run for all.
## Datasets
There are currently results for the ImageNet validation set and 5 additional test / label sets.
The test set results include rank and top-1/top-5 differences from clean validation. For the "Real Labels", ImageNetV2, and Sketch test sets, the differences were calculated against the full 1000 class ImageNet-1k validation set. For both the Adversarial and Rendition sets, the differences were calculated against 'clean' runs on the ImageNet-1k validation set with the same 200 classes used in each test set respectively.
### ImageNet Validation - [`results-imagenet.csv`](results-imagenet.csv)
The standard 50,000 image ImageNet-1k validation set. Model selection during training utilizes this validation set, so it is not a true test set. Question: Does anyone have the official ImageNet-1k test set classification labels now that challenges are done?
* Source: http://image-net.org/challenges/LSVRC/2012/index
* Paper: "ImageNet Large Scale Visual Recognition Challenge" - https://arxiv.org/abs/1409.0575
### ImageNet-"Real Labels" - [`results-imagenet-real.csv`](results-imagenet-real.csv)
The usual ImageNet-1k validation set with a fresh new set of labels intended to improve on mistakes in the original annotation process.
* Source: https://github.com/google-research/reassessed-imagenet
* Paper: "Are we done with ImageNet?" - https://arxiv.org/abs/2006.07159
### ImageNetV2 Matched Frequency - [`results-imagenetv2-matched-frequency.csv`](results-imagenetv2-matched-frequency.csv)
An ImageNet test set of 10,000 images sampled from new images roughly 10 years after the original. Care was taken to replicate the original ImageNet curation/sampling process.
* Source: https://github.com/modestyachts/ImageNetV2
* Paper: "Do ImageNet Classifiers Generalize to ImageNet?" - https://arxiv.org/abs/1902.10811
### ImageNet-Sketch - [`results-sketch.csv`](results-sketch.csv)
50,000 non photographic (or photos of such) images (sketches, doodles, mostly monochromatic) covering all 1000 ImageNet classes.
* Source: https://github.com/HaohanWang/ImageNet-Sketch
* Paper: "Learning Robust Global Representations by Penalizing Local Predictive Power" - https://arxiv.org/abs/1905.13549
### ImageNet-Adversarial - [`results-imagenet-a.csv`](results-imagenet-a.csv)
A collection of 7500 images covering 200 of the 1000 ImageNet classes. Images are naturally occurring adversarial examples that confuse typical ImageNet classifiers. This is a challenging dataset, your typical ResNet-50 will score 0% top-1.
For clean validation with same 200 classes, see [`results-imagenet-a-clean.csv`](results-imagenet-a-clean.csv)
* Source: https://github.com/hendrycks/natural-adv-examples
* Paper: "Natural Adversarial Examples" - https://arxiv.org/abs/1907.07174
### ImageNet-Rendition - [`results-imagenet-r.csv`](results-imagenet-r.csv)
Renditions of 200 ImageNet classes resulting in 30,000 images for testing robustness.
For clean validation with same 200 classes, see [`results-imagenet-r-clean.csv`](results-imagenet-r-clean.csv)
* Source: https://github.com/hendrycks/imagenet-r
* Paper: "The Many Faces of Robustness" - https://arxiv.org/abs/2006.16241
### TODO
* Explore adding a reduced version of ImageNet-C (Corruptions) and ImageNet-P (Perturbations) from https://github.com/hendrycks/robustness. The originals are huge and image size specific.
## Benchmark
CSV files with a `model_benchmark` prefix include benchmark numbers for models on various accelerators with different precision. Currently only run on RTX 3090 w/ AMP for inference, I intend to add more in the future.
## Metadata
CSV files with `model_metadata` prefix contain extra information about the source training, currently the pretraining dataset and technique (ie distillation, SSL, WSL, etc). Eventually I'd like to have metadata about augmentation, regularization, etc. but that will be a challenge to source consistently.
| pytorch-image-models/results/README.md/0 | {
"file_path": "pytorch-image-models/results/README.md",
"repo_id": "pytorch-image-models",
"token_count": 1173
} | 189 |
from copy import deepcopy
__all__ = ['get_img_extensions', 'is_img_extension', 'set_img_extensions', 'add_img_extensions', 'del_img_extensions']
IMG_EXTENSIONS = ('.png', '.jpg', '.jpeg') # singleton, kept public for bwd compat use
_IMG_EXTENSIONS_SET = set(IMG_EXTENSIONS) # set version, private, kept in sync
def _set_extensions(extensions):
global IMG_EXTENSIONS
global _IMG_EXTENSIONS_SET
dedupe = set() # NOTE de-duping tuple while keeping original order
IMG_EXTENSIONS = tuple(x for x in extensions if x not in dedupe and not dedupe.add(x))
_IMG_EXTENSIONS_SET = set(extensions)
def _valid_extension(x: str):
return x and isinstance(x, str) and len(x) >= 2 and x.startswith('.')
def is_img_extension(ext):
return ext in _IMG_EXTENSIONS_SET
def get_img_extensions(as_set=False):
return deepcopy(_IMG_EXTENSIONS_SET if as_set else IMG_EXTENSIONS)
def set_img_extensions(extensions):
assert len(extensions)
for x in extensions:
assert _valid_extension(x)
_set_extensions(extensions)
def add_img_extensions(ext):
if not isinstance(ext, (list, tuple, set)):
ext = (ext,)
for x in ext:
assert _valid_extension(x)
extensions = IMG_EXTENSIONS + tuple(ext)
_set_extensions(extensions)
def del_img_extensions(ext):
if not isinstance(ext, (list, tuple, set)):
ext = (ext,)
extensions = tuple(x for x in IMG_EXTENSIONS if x not in ext)
_set_extensions(extensions)
| pytorch-image-models/timm/data/readers/img_extensions.py/0 | {
"file_path": "pytorch-image-models/timm/data/readers/img_extensions.py",
"repo_id": "pytorch-image-models",
"token_count": 582
} | 190 |
""" Activations
A collection of activations fn and modules with a common interface so that they can
easily be swapped. All have an `inplace` arg even if not used.
Hacked together by / Copyright 2020 Ross Wightman
"""
import torch
from torch import nn as nn
from torch.nn import functional as F
def swish(x, inplace: bool = False):
"""Swish - Described in: https://arxiv.org/abs/1710.05941
"""
return x.mul_(x.sigmoid()) if inplace else x.mul(x.sigmoid())
class Swish(nn.Module):
def __init__(self, inplace: bool = False):
super(Swish, self).__init__()
self.inplace = inplace
def forward(self, x):
return swish(x, self.inplace)
def mish(x, inplace: bool = False):
"""Mish: A Self Regularized Non-Monotonic Neural Activation Function - https://arxiv.org/abs/1908.08681
NOTE: I don't have a working inplace variant
"""
return x.mul(F.softplus(x).tanh())
class Mish(nn.Module):
"""Mish: A Self Regularized Non-Monotonic Neural Activation Function - https://arxiv.org/abs/1908.08681
"""
def __init__(self, inplace: bool = False):
super(Mish, self).__init__()
def forward(self, x):
return mish(x)
def sigmoid(x, inplace: bool = False):
return x.sigmoid_() if inplace else x.sigmoid()
# PyTorch has this, but not with a consistent inplace argmument interface
class Sigmoid(nn.Module):
def __init__(self, inplace: bool = False):
super(Sigmoid, self).__init__()
self.inplace = inplace
def forward(self, x):
return x.sigmoid_() if self.inplace else x.sigmoid()
def tanh(x, inplace: bool = False):
return x.tanh_() if inplace else x.tanh()
# PyTorch has this, but not with a consistent inplace argmument interface
class Tanh(nn.Module):
def __init__(self, inplace: bool = False):
super(Tanh, self).__init__()
self.inplace = inplace
def forward(self, x):
return x.tanh_() if self.inplace else x.tanh()
def hard_swish(x, inplace: bool = False):
inner = F.relu6(x + 3.).div_(6.)
return x.mul_(inner) if inplace else x.mul(inner)
class HardSwish(nn.Module):
def __init__(self, inplace: bool = False):
super(HardSwish, self).__init__()
self.inplace = inplace
def forward(self, x):
return hard_swish(x, self.inplace)
def hard_sigmoid(x, inplace: bool = False):
if inplace:
return x.add_(3.).clamp_(0., 6.).div_(6.)
else:
return F.relu6(x + 3.) / 6.
class HardSigmoid(nn.Module):
def __init__(self, inplace: bool = False):
super(HardSigmoid, self).__init__()
self.inplace = inplace
def forward(self, x):
return hard_sigmoid(x, self.inplace)
def hard_mish(x, inplace: bool = False):
""" Hard Mish
Experimental, based on notes by Mish author Diganta Misra at
https://github.com/digantamisra98/H-Mish/blob/0da20d4bc58e696b6803f2523c58d3c8a82782d0/README.md
"""
if inplace:
return x.mul_(0.5 * (x + 2).clamp(min=0, max=2))
else:
return 0.5 * x * (x + 2).clamp(min=0, max=2)
class HardMish(nn.Module):
def __init__(self, inplace: bool = False):
super(HardMish, self).__init__()
self.inplace = inplace
def forward(self, x):
return hard_mish(x, self.inplace)
class PReLU(nn.PReLU):
"""Applies PReLU (w/ dummy inplace arg)
"""
def __init__(self, num_parameters: int = 1, init: float = 0.25, inplace: bool = False) -> None:
super(PReLU, self).__init__(num_parameters=num_parameters, init=init)
def forward(self, input: torch.Tensor) -> torch.Tensor:
return F.prelu(input, self.weight)
def gelu(x: torch.Tensor, inplace: bool = False) -> torch.Tensor:
return F.gelu(x)
class GELU(nn.Module):
"""Applies the Gaussian Error Linear Units function (w/ dummy inplace arg)
"""
def __init__(self, inplace: bool = False):
super(GELU, self).__init__()
def forward(self, input: torch.Tensor) -> torch.Tensor:
return F.gelu(input)
def gelu_tanh(x: torch.Tensor, inplace: bool = False) -> torch.Tensor:
return F.gelu(x, approximate='tanh')
class GELUTanh(nn.Module):
"""Applies the Gaussian Error Linear Units function (w/ dummy inplace arg)
"""
def __init__(self, inplace: bool = False):
super(GELUTanh, self).__init__()
def forward(self, input: torch.Tensor) -> torch.Tensor:
return F.gelu(input, approximate='tanh')
def quick_gelu(x: torch.Tensor, inplace: bool = False) -> torch.Tensor:
return x * torch.sigmoid(1.702 * x)
class QuickGELU(nn.Module):
"""Applies the Gaussian Error Linear Units function (w/ dummy inplace arg)
"""
def __init__(self, inplace: bool = False):
super(QuickGELU, self).__init__()
def forward(self, input: torch.Tensor) -> torch.Tensor:
return quick_gelu(input)
| pytorch-image-models/timm/layers/activations.py/0 | {
"file_path": "pytorch-image-models/timm/layers/activations.py",
"repo_id": "pytorch-image-models",
"token_count": 2012
} | 191 |
""" Create Conv2d Factory Method
Hacked together by / Copyright 2020 Ross Wightman
"""
from .mixed_conv2d import MixedConv2d
from .cond_conv2d import CondConv2d
from .conv2d_same import create_conv2d_pad
def create_conv2d(in_channels, out_channels, kernel_size, **kwargs):
""" Select a 2d convolution implementation based on arguments
Creates and returns one of torch.nn.Conv2d, Conv2dSame, MixedConv2d, or CondConv2d.
Used extensively by EfficientNet, MobileNetv3 and related networks.
"""
if isinstance(kernel_size, list):
assert 'num_experts' not in kwargs # MixNet + CondConv combo not supported currently
if 'groups' in kwargs:
groups = kwargs.pop('groups')
if groups == in_channels:
kwargs['depthwise'] = True
else:
assert groups == 1
# We're going to use only lists for defining the MixedConv2d kernel groups,
# ints, tuples, other iterables will continue to pass to normal conv and specify h, w.
m = MixedConv2d(in_channels, out_channels, kernel_size, **kwargs)
else:
depthwise = kwargs.pop('depthwise', False)
# for DW out_channels must be multiple of in_channels as must have out_channels % groups == 0
groups = in_channels if depthwise else kwargs.pop('groups', 1)
if 'num_experts' in kwargs and kwargs['num_experts'] > 0:
m = CondConv2d(in_channels, out_channels, kernel_size, groups=groups, **kwargs)
else:
m = create_conv2d_pad(in_channels, out_channels, kernel_size, groups=groups, **kwargs)
return m
| pytorch-image-models/timm/layers/create_conv2d.py/0 | {
"file_path": "pytorch-image-models/timm/layers/create_conv2d.py",
"repo_id": "pytorch-image-models",
"token_count": 652
} | 192 |
""" Interpolation helpers for timm layers
RegularGridInterpolator from https://github.com/sbarratt/torch_interpolations
Copyright Shane Barratt, Apache 2.0 license
"""
import torch
from itertools import product
class RegularGridInterpolator:
""" Interpolate data defined on a rectilinear grid with even or uneven spacing.
Produces similar results to scipy RegularGridInterpolator or interp2d
in 'linear' mode.
Taken from https://github.com/sbarratt/torch_interpolations
"""
def __init__(self, points, values):
self.points = points
self.values = values
assert isinstance(self.points, tuple) or isinstance(self.points, list)
assert isinstance(self.values, torch.Tensor)
self.ms = list(self.values.shape)
self.n = len(self.points)
assert len(self.ms) == self.n
for i, p in enumerate(self.points):
assert isinstance(p, torch.Tensor)
assert p.shape[0] == self.values.shape[i]
def __call__(self, points_to_interp):
assert self.points is not None
assert self.values is not None
assert len(points_to_interp) == len(self.points)
K = points_to_interp[0].shape[0]
for x in points_to_interp:
assert x.shape[0] == K
idxs = []
dists = []
overalls = []
for p, x in zip(self.points, points_to_interp):
idx_right = torch.bucketize(x, p)
idx_right[idx_right >= p.shape[0]] = p.shape[0] - 1
idx_left = (idx_right - 1).clamp(0, p.shape[0] - 1)
dist_left = x - p[idx_left]
dist_right = p[idx_right] - x
dist_left[dist_left < 0] = 0.
dist_right[dist_right < 0] = 0.
both_zero = (dist_left == 0) & (dist_right == 0)
dist_left[both_zero] = dist_right[both_zero] = 1.
idxs.append((idx_left, idx_right))
dists.append((dist_left, dist_right))
overalls.append(dist_left + dist_right)
numerator = 0.
for indexer in product([0, 1], repeat=self.n):
as_s = [idx[onoff] for onoff, idx in zip(indexer, idxs)]
bs_s = [dist[1 - onoff] for onoff, dist in zip(indexer, dists)]
numerator += self.values[as_s] * \
torch.prod(torch.stack(bs_s), dim=0)
denominator = torch.prod(torch.stack(overalls), dim=0)
return numerator / denominator
| pytorch-image-models/timm/layers/interpolate.py/0 | {
"file_path": "pytorch-image-models/timm/layers/interpolate.py",
"repo_id": "pytorch-image-models",
"token_count": 1121
} | 193 |
""" Sin-cos, fourier, rotary position embedding modules and functions
Hacked together by / Copyright 2022 Ross Wightman
"""
import math
from typing import List, Tuple, Optional, Union
import torch
from torch import nn as nn
from .grid import ndgrid
from .trace_utils import _assert
def pixel_freq_bands(
num_bands: int,
max_freq: float = 224.,
linear_bands: bool = True,
device: Optional[torch.device] = None,
):
if linear_bands:
bands = torch.linspace(1.0, max_freq / 2, num_bands, dtype=torch.float32, device=device)
else:
bands = 2 ** torch.linspace(0, math.log(max_freq, 2) - 1, num_bands, dtype=torch.float32, device=device)
return bands * torch.pi
def freq_bands(
num_bands: int,
temperature: float = 10000.,
step: int = 2,
device: Optional[torch.device] = None,
) -> torch.Tensor:
exp = torch.arange(0, num_bands, step, dtype=torch.int64, device=device).to(torch.float32) / num_bands
bands = 1. / (temperature ** exp)
return bands
def build_sincos2d_pos_embed(
feat_shape: List[int],
dim: int = 64,
temperature: float = 10000.,
reverse_coord: bool = False,
interleave_sin_cos: bool = False,
dtype: torch.dtype = torch.float32,
device: Optional[torch.device] = None
) -> torch.Tensor:
"""
Args:
feat_shape:
dim:
temperature:
reverse_coord: stack grid order W, H instead of H, W
interleave_sin_cos: sin, cos, sin, cos stack instead of sin, sin, cos, cos
dtype:
device:
Returns:
"""
assert dim % 4 == 0, 'Embed dimension must be divisible by 4 for sin-cos 2D position embedding'
pos_dim = dim // 4
bands = freq_bands(pos_dim, temperature=temperature, step=1, device=device)
if reverse_coord:
feat_shape = feat_shape[::-1] # stack W, H instead of H, W
grid = torch.stack(ndgrid([
torch.arange(s, device=device, dtype=torch.int64).to(torch.float32)
for s in feat_shape
])).flatten(1).transpose(0, 1)
pos2 = grid.unsqueeze(-1) * bands.unsqueeze(0)
# FIXME add support for unflattened spatial dim?
stack_dim = 2 if interleave_sin_cos else 1 # stack sin, cos, sin, cos instead of sin sin cos cos
pos_emb = torch.stack([torch.sin(pos2), torch.cos(pos2)], dim=stack_dim).flatten(1)
return pos_emb.to(dtype=dtype)
def build_fourier_pos_embed(
feat_shape: List[int],
bands: Optional[torch.Tensor] = None,
num_bands: int = 64,
max_res: int = 224,
temperature: float = 10000.,
linear_bands: bool = False,
include_grid: bool = False,
in_pixels: bool = True,
ref_feat_shape: Optional[List[int]] = None,
dtype: torch.dtype = torch.float32,
device: Optional[torch.device] = None,
) -> List[torch.Tensor]:
"""
Args:
feat_shape: Feature shape for embedding.
bands: Pre-calculated frequency bands.
num_bands: Number of frequency bands (determines output dim).
max_res: Maximum resolution for pixel based freq.
temperature: Temperature for non-pixel freq.
linear_bands: Linear band spacing for pixel based freq.
include_grid: Include the spatial grid in output.
in_pixels: Output in pixel freq.
ref_feat_shape: Reference feature shape for resize / fine-tune.
dtype: Output dtype.
device: Output device.
Returns:
"""
if bands is None:
if in_pixels:
bands = pixel_freq_bands(
num_bands,
float(max_res),
linear_bands=linear_bands,
device=device,
)
else:
bands = freq_bands(
num_bands,
temperature=temperature,
step=1,
device=device,
)
else:
if device is None:
device = bands.device
if dtype is None:
dtype = bands.dtype
if in_pixels:
t = [torch.linspace(-1., 1., steps=s, device=device, dtype=torch.float32) for s in feat_shape]
else:
t = [torch.arange(s, device=device, dtype=torch.int64).to(torch.float32) for s in feat_shape]
if ref_feat_shape is not None:
# eva's scheme for resizing rope embeddings (ref shape = pretrain)
t = [x / f * r for x, f, r in zip(t, feat_shape, ref_feat_shape)]
grid = torch.stack(ndgrid(t), dim=-1)
grid = grid.unsqueeze(-1)
pos = grid * bands
pos_sin, pos_cos = pos.sin().to(dtype=dtype), pos.cos().to(dtype)
out = [grid, pos_sin, pos_cos] if include_grid else [pos_sin, pos_cos]
return out
class FourierEmbed(nn.Module):
def __init__(
self,
max_res: int = 224,
num_bands: int = 64,
concat_grid=True,
keep_spatial=False,
):
super().__init__()
self.max_res = max_res
self.num_bands = num_bands
self.concat_grid = concat_grid
self.keep_spatial = keep_spatial
self.register_buffer(
'bands',
pixel_freq_bands(max_res, num_bands),
persistent=False,
)
def forward(self, x):
B, C = x.shape[:2]
feat_shape = x.shape[2:]
emb = build_fourier_pos_embed(
feat_shape,
self.bands,
include_grid=self.concat_grid,
dtype=x.dtype,
device=x.device,
)
emb = torch.cat(emb, dim=-1)
emb = emb.transpose(-1, -2).flatten(len(feat_shape))
batch_expand = (B,) + (-1,) * (x.ndim - 1)
# FIXME support nD
if self.keep_spatial:
x = torch.cat([x, emb.unsqueeze(0).expand(batch_expand).permute(0, 3, 1, 2)], dim=1)
else:
x = torch.cat([x.permute(0, 2, 3, 1), emb.unsqueeze(0).expand(batch_expand)], dim=-1)
x = x.reshape(B, feat_shape.numel(), -1)
return x
def rot(x):
return torch.stack([-x[..., 1::2], x[..., ::2]], -1).reshape(x.shape)
def apply_rot_embed(x: torch.Tensor, sin_emb, cos_emb):
if sin_emb.ndim == 3:
return x * cos_emb.unsqueeze(1).expand_as(x) + rot(x) * sin_emb.unsqueeze(1).expand_as(x)
return x * cos_emb + rot(x) * sin_emb
def apply_rot_embed_list(x: List[torch.Tensor], sin_emb, cos_emb):
if isinstance(x, torch.Tensor):
x = [x]
return [t * cos_emb + rot(t) * sin_emb for t in x]
def apply_rot_embed_cat(x: torch.Tensor, emb):
sin_emb, cos_emb = emb.tensor_split(2, -1)
if sin_emb.ndim == 3:
return x * cos_emb.unsqueeze(1).expand_as(x) + rot(x) * sin_emb.unsqueeze(1).expand_as(x)
return x * cos_emb + rot(x) * sin_emb
def apply_keep_indices_nlc(x, pos_embed, keep_indices):
pos_embed = pos_embed.unsqueeze(0).expand(x.shape[0], -1, -1)
pos_embed = pos_embed.gather(1, keep_indices.unsqueeze(-1).expand(-1, -1, pos_embed.shape[-1]))
return pos_embed
def build_rotary_pos_embed(
feat_shape: List[int],
bands: Optional[torch.Tensor] = None,
dim: int = 64,
max_res: int = 224,
temperature: float = 10000.,
linear_bands: bool = False,
in_pixels: bool = True,
ref_feat_shape: Optional[List[int]] = None,
dtype: torch.dtype = torch.float32,
device: Optional[torch.device] = None,
):
"""
Args:
feat_shape: Spatial shape of the target tensor for embedding.
bands: Optional pre-generated frequency bands
dim: Output dimension of embedding tensor.
max_res: Maximum resolution for pixel mode.
temperature: Temperature (inv freq) for non-pixel mode
linear_bands: Linearly (instead of log) spaced bands for pixel mode
in_pixels: Pixel vs language (inv freq) mode.
dtype: Output dtype.
device: Output device.
Returns:
"""
sin_emb, cos_emb = build_fourier_pos_embed(
feat_shape,
bands=bands,
num_bands=dim // 4,
max_res=max_res,
temperature=temperature,
linear_bands=linear_bands,
in_pixels=in_pixels,
ref_feat_shape=ref_feat_shape,
device=device,
dtype=dtype,
)
num_spatial_dim = 1
# this would be much nicer as a .numel() call to torch.Size(), but torchscript sucks
for x in feat_shape:
num_spatial_dim *= x
sin_emb = sin_emb.reshape(num_spatial_dim, -1).repeat_interleave(2, -1)
cos_emb = cos_emb.reshape(num_spatial_dim, -1).repeat_interleave(2, -1)
return sin_emb, cos_emb
class RotaryEmbedding(nn.Module):
""" Rotary position embedding
NOTE: This is my initial attempt at impl rotary embedding for spatial use, it has not
been well tested, and will likely change. It will be moved to its own file.
The following impl/resources were referenced for this impl:
* https://github.com/lucidrains/vit-pytorch/blob/6f3a5fcf0bca1c5ec33a35ef48d97213709df4ba/vit_pytorch/rvt.py
* https://blog.eleuther.ai/rotary-embeddings/
"""
def __init__(
self,
dim,
max_res=224,
temperature=10000,
in_pixels=True,
linear_bands: bool = False,
feat_shape: Optional[List[int]] = None,
ref_feat_shape: Optional[List[int]] = None,
):
super().__init__()
self.dim = dim
self.max_res = max_res
self.temperature = temperature
self.in_pixels = in_pixels
self.feat_shape = feat_shape
self.ref_feat_shape = ref_feat_shape
if feat_shape is None:
# only cache bands
if in_pixels:
bands = pixel_freq_bands(
dim // 4,
float(max_res),
linear_bands=linear_bands,
)
else:
bands = freq_bands(
dim // 4,
temperature=temperature,
step=1,
)
print(bands)
self.register_buffer(
'bands',
bands,
persistent=False,
)
self.pos_embed_sin = None
self.pos_embed_cos = None
else:
# cache full sin/cos embeddings if shape provided up front
emb_sin, emb_cos = build_rotary_pos_embed(
feat_shape=feat_shape,
dim=dim,
max_res=max_res,
linear_bands=linear_bands,
in_pixels=in_pixels,
ref_feat_shape=self.ref_feat_shape,
)
self.bands = None
self.register_buffer(
'pos_embed_sin',
emb_sin,
persistent=False,
)
self.register_buffer(
'pos_embed_cos',
emb_cos,
persistent=False,
)
def get_embed(self, shape: Optional[List[int]] = None):
if self.bands is not None:
# rebuild embeddings every call, use if target shape changes
assert shape is not None
return build_rotary_pos_embed(
shape,
self.bands,
in_pixels=self.in_pixels,
)
else:
return self.pos_embed_sin, self.pos_embed_cos
def forward(self, x):
# assuming channel-first tensor where spatial dim are >= 2
sin_emb, cos_emb = self.get_embed(x.shape[2:])
return apply_rot_embed(x, sin_emb, cos_emb)
class RotaryEmbeddingCat(nn.Module):
""" Rotary position embedding w/ concatenatd sin & cos
The following impl/resources were referenced for this impl:
* https://github.com/lucidrains/vit-pytorch/blob/6f3a5fcf0bca1c5ec33a35ef48d97213709df4ba/vit_pytorch/rvt.py
* https://blog.eleuther.ai/rotary-embeddings/
"""
def __init__(
self,
dim,
max_res=224,
temperature=10000,
in_pixels=True,
linear_bands: bool = False,
feat_shape: Optional[List[int]] = None,
ref_feat_shape: Optional[List[int]] = None,
):
super().__init__()
self.dim = dim
self.max_res = max_res
self.temperature = temperature
self.in_pixels = in_pixels
self.feat_shape = feat_shape
self.ref_feat_shape = ref_feat_shape
if feat_shape is None:
# only cache bands
if in_pixels:
bands = pixel_freq_bands(
dim // 4,
float(max_res),
linear_bands=linear_bands,
)
else:
bands = freq_bands(
dim // 4,
temperature=temperature,
step=1,
)
self.register_buffer(
'bands',
bands,
persistent=False,
)
self.pos_embed = None
else:
# cache full sin/cos embeddings if shape provided up front
embeds = build_rotary_pos_embed(
feat_shape=feat_shape,
dim=dim,
max_res=max_res,
linear_bands=linear_bands,
in_pixels=in_pixels,
ref_feat_shape=self.ref_feat_shape,
)
self.bands = None
self.register_buffer(
'pos_embed',
torch.cat(embeds, -1),
persistent=False,
)
def get_embed(self, shape: Optional[List[int]] = None):
if self.bands is not None and shape is not None:
# rebuild embeddings every call, use if target shape changes
embeds = build_rotary_pos_embed(
shape,
self.bands,
in_pixels=self.in_pixels,
ref_feat_shape=self.ref_feat_shape,
)
return torch.cat(embeds, -1)
elif self.pos_embed is not None:
return self.pos_embed
else:
assert False, "get_embed() requires pre-computed pos_embed or valid shape w/ pre-computed bands"
def forward(self, x):
# assuming channel-first tensor where spatial dim are >= 2
pos_embed = self.get_embed(x.shape[2:])
return apply_rot_embed_cat(x, pos_embed)
| pytorch-image-models/timm/layers/pos_embed_sincos.py/0 | {
"file_path": "pytorch-image-models/timm/layers/pos_embed_sincos.py",
"repo_id": "pytorch-image-models",
"token_count": 7200
} | 194 |
import torch
import torch.nn as nn
import torch.nn.functional as F
from .cross_entropy import LabelSmoothingCrossEntropy
class JsdCrossEntropy(nn.Module):
""" Jensen-Shannon Divergence + Cross-Entropy Loss
Based on impl here: https://github.com/google-research/augmix/blob/master/imagenet.py
From paper: 'AugMix: A Simple Data Processing Method to Improve Robustness and Uncertainty -
https://arxiv.org/abs/1912.02781
Hacked together by / Copyright 2020 Ross Wightman
"""
def __init__(self, num_splits=3, alpha=12, smoothing=0.1):
super().__init__()
self.num_splits = num_splits
self.alpha = alpha
if smoothing is not None and smoothing > 0:
self.cross_entropy_loss = LabelSmoothingCrossEntropy(smoothing)
else:
self.cross_entropy_loss = torch.nn.CrossEntropyLoss()
def __call__(self, output, target):
split_size = output.shape[0] // self.num_splits
assert split_size * self.num_splits == output.shape[0]
logits_split = torch.split(output, split_size)
# Cross-entropy is only computed on clean images
loss = self.cross_entropy_loss(logits_split[0], target[:split_size])
probs = [F.softmax(logits, dim=1) for logits in logits_split]
# Clamp mixture distribution to avoid exploding KL divergence
logp_mixture = torch.clamp(torch.stack(probs).mean(axis=0), 1e-7, 1).log()
loss += self.alpha * sum([F.kl_div(
logp_mixture, p_split, reduction='batchmean') for p_split in probs]) / len(probs)
return loss
| pytorch-image-models/timm/loss/jsd.py/0 | {
"file_path": "pytorch-image-models/timm/loss/jsd.py",
"repo_id": "pytorch-image-models",
"token_count": 639
} | 195 |
""" Deep Layer Aggregation and DLA w/ Res2Net
DLA original adapted from Official Pytorch impl at: https://github.com/ucbdrive/dla
DLA Paper: `Deep Layer Aggregation` - https://arxiv.org/abs/1707.06484
Res2Net additions from: https://github.com/gasvn/Res2Net/
Res2Net Paper: `Res2Net: A New Multi-scale Backbone Architecture` - https://arxiv.org/abs/1904.01169
"""
import math
from typing import List, Optional
import torch
import torch.nn as nn
import torch.nn.functional as F
from timm.data import IMAGENET_DEFAULT_MEAN, IMAGENET_DEFAULT_STD
from timm.layers import create_classifier
from ._builder import build_model_with_cfg
from ._registry import register_model, generate_default_cfgs
__all__ = ['DLA']
class DlaBasic(nn.Module):
"""DLA Basic"""
def __init__(self, inplanes, planes, stride=1, dilation=1, **_):
super(DlaBasic, self).__init__()
self.conv1 = nn.Conv2d(
inplanes, planes, kernel_size=3,
stride=stride, padding=dilation, bias=False, dilation=dilation)
self.bn1 = nn.BatchNorm2d(planes)
self.relu = nn.ReLU(inplace=True)
self.conv2 = nn.Conv2d(
planes, planes, kernel_size=3,
stride=1, padding=dilation, bias=False, dilation=dilation)
self.bn2 = nn.BatchNorm2d(planes)
self.stride = stride
def forward(self, x, shortcut: Optional[torch.Tensor] = None, children: Optional[List[torch.Tensor]] = None):
if shortcut is None:
shortcut = x
out = self.conv1(x)
out = self.bn1(out)
out = self.relu(out)
out = self.conv2(out)
out = self.bn2(out)
out += shortcut
out = self.relu(out)
return out
class DlaBottleneck(nn.Module):
"""DLA/DLA-X Bottleneck"""
expansion = 2
def __init__(self, inplanes, outplanes, stride=1, dilation=1, cardinality=1, base_width=64):
super(DlaBottleneck, self).__init__()
self.stride = stride
mid_planes = int(math.floor(outplanes * (base_width / 64)) * cardinality)
mid_planes = mid_planes // self.expansion
self.conv1 = nn.Conv2d(inplanes, mid_planes, kernel_size=1, bias=False)
self.bn1 = nn.BatchNorm2d(mid_planes)
self.conv2 = nn.Conv2d(
mid_planes, mid_planes, kernel_size=3,
stride=stride, padding=dilation, bias=False, dilation=dilation, groups=cardinality)
self.bn2 = nn.BatchNorm2d(mid_planes)
self.conv3 = nn.Conv2d(mid_planes, outplanes, kernel_size=1, bias=False)
self.bn3 = nn.BatchNorm2d(outplanes)
self.relu = nn.ReLU(inplace=True)
def forward(self, x, shortcut: Optional[torch.Tensor] = None, children: Optional[List[torch.Tensor]] = None):
if shortcut is None:
shortcut = x
out = self.conv1(x)
out = self.bn1(out)
out = self.relu(out)
out = self.conv2(out)
out = self.bn2(out)
out = self.relu(out)
out = self.conv3(out)
out = self.bn3(out)
out += shortcut
out = self.relu(out)
return out
class DlaBottle2neck(nn.Module):
""" Res2Net/Res2NeXT DLA Bottleneck
Adapted from https://github.com/gasvn/Res2Net/blob/master/dla.py
"""
expansion = 2
def __init__(self, inplanes, outplanes, stride=1, dilation=1, scale=4, cardinality=8, base_width=4):
super(DlaBottle2neck, self).__init__()
self.is_first = stride > 1
self.scale = scale
mid_planes = int(math.floor(outplanes * (base_width / 64)) * cardinality)
mid_planes = mid_planes // self.expansion
self.width = mid_planes
self.conv1 = nn.Conv2d(inplanes, mid_planes * scale, kernel_size=1, bias=False)
self.bn1 = nn.BatchNorm2d(mid_planes * scale)
num_scale_convs = max(1, scale - 1)
convs = []
bns = []
for _ in range(num_scale_convs):
convs.append(nn.Conv2d(
mid_planes, mid_planes, kernel_size=3,
stride=stride, padding=dilation, dilation=dilation, groups=cardinality, bias=False))
bns.append(nn.BatchNorm2d(mid_planes))
self.convs = nn.ModuleList(convs)
self.bns = nn.ModuleList(bns)
self.pool = nn.AvgPool2d(kernel_size=3, stride=stride, padding=1) if self.is_first else None
self.conv3 = nn.Conv2d(mid_planes * scale, outplanes, kernel_size=1, bias=False)
self.bn3 = nn.BatchNorm2d(outplanes)
self.relu = nn.ReLU(inplace=True)
def forward(self, x, shortcut: Optional[torch.Tensor] = None, children: Optional[List[torch.Tensor]] = None):
if shortcut is None:
shortcut = x
out = self.conv1(x)
out = self.bn1(out)
out = self.relu(out)
spx = torch.split(out, self.width, 1)
spo = []
sp = spx[0] # redundant, for torchscript
for i, (conv, bn) in enumerate(zip(self.convs, self.bns)):
if i == 0 or self.is_first:
sp = spx[i]
else:
sp = sp + spx[i]
sp = conv(sp)
sp = bn(sp)
sp = self.relu(sp)
spo.append(sp)
if self.scale > 1:
if self.pool is not None: # self.is_first == True, None check for torchscript
spo.append(self.pool(spx[-1]))
else:
spo.append(spx[-1])
out = torch.cat(spo, 1)
out = self.conv3(out)
out = self.bn3(out)
out += shortcut
out = self.relu(out)
return out
class DlaRoot(nn.Module):
def __init__(self, in_channels, out_channels, kernel_size, shortcut):
super(DlaRoot, self).__init__()
self.conv = nn.Conv2d(
in_channels, out_channels, 1, stride=1, bias=False, padding=(kernel_size - 1) // 2)
self.bn = nn.BatchNorm2d(out_channels)
self.relu = nn.ReLU(inplace=True)
self.shortcut = shortcut
def forward(self, x_children: List[torch.Tensor]):
x = self.conv(torch.cat(x_children, 1))
x = self.bn(x)
if self.shortcut:
x += x_children[0]
x = self.relu(x)
return x
class DlaTree(nn.Module):
def __init__(
self,
levels,
block,
in_channels,
out_channels,
stride=1,
dilation=1,
cardinality=1,
base_width=64,
level_root=False,
root_dim=0,
root_kernel_size=1,
root_shortcut=False,
):
super(DlaTree, self).__init__()
if root_dim == 0:
root_dim = 2 * out_channels
if level_root:
root_dim += in_channels
self.downsample = nn.MaxPool2d(stride, stride=stride) if stride > 1 else nn.Identity()
self.project = nn.Identity()
cargs = dict(dilation=dilation, cardinality=cardinality, base_width=base_width)
if levels == 1:
self.tree1 = block(in_channels, out_channels, stride, **cargs)
self.tree2 = block(out_channels, out_channels, 1, **cargs)
if in_channels != out_channels:
# NOTE the official impl/weights have project layers in levels > 1 case that are never
# used, I've moved the project layer here to avoid wasted params but old checkpoints will
# need strict=False while loading.
self.project = nn.Sequential(
nn.Conv2d(in_channels, out_channels, kernel_size=1, stride=1, bias=False),
nn.BatchNorm2d(out_channels))
self.root = DlaRoot(root_dim, out_channels, root_kernel_size, root_shortcut)
else:
cargs.update(dict(root_kernel_size=root_kernel_size, root_shortcut=root_shortcut))
self.tree1 = DlaTree(
levels - 1,
block,
in_channels,
out_channels,
stride,
root_dim=0,
**cargs,
)
self.tree2 = DlaTree(
levels - 1,
block,
out_channels,
out_channels,
root_dim=root_dim + out_channels,
**cargs,
)
self.root = None
self.level_root = level_root
self.root_dim = root_dim
self.levels = levels
def forward(self, x, shortcut: Optional[torch.Tensor] = None, children: Optional[List[torch.Tensor]] = None):
if children is None:
children = []
bottom = self.downsample(x)
shortcut = self.project(bottom)
if self.level_root:
children.append(bottom)
x1 = self.tree1(x, shortcut)
if self.root is not None: # levels == 1
x2 = self.tree2(x1)
x = self.root([x2, x1] + children)
else:
children.append(x1)
x = self.tree2(x1, None, children)
return x
class DLA(nn.Module):
def __init__(
self,
levels,
channels,
output_stride=32,
num_classes=1000,
in_chans=3,
global_pool='avg',
cardinality=1,
base_width=64,
block=DlaBottle2neck,
shortcut_root=False,
drop_rate=0.0,
):
super(DLA, self).__init__()
self.channels = channels
self.num_classes = num_classes
self.cardinality = cardinality
self.base_width = base_width
assert output_stride == 32 # FIXME support dilation
self.base_layer = nn.Sequential(
nn.Conv2d(in_chans, channels[0], kernel_size=7, stride=1, padding=3, bias=False),
nn.BatchNorm2d(channels[0]),
nn.ReLU(inplace=True),
)
self.level0 = self._make_conv_level(channels[0], channels[0], levels[0])
self.level1 = self._make_conv_level(channels[0], channels[1], levels[1], stride=2)
cargs = dict(cardinality=cardinality, base_width=base_width, root_shortcut=shortcut_root)
self.level2 = DlaTree(levels[2], block, channels[1], channels[2], 2, level_root=False, **cargs)
self.level3 = DlaTree(levels[3], block, channels[2], channels[3], 2, level_root=True, **cargs)
self.level4 = DlaTree(levels[4], block, channels[3], channels[4], 2, level_root=True, **cargs)
self.level5 = DlaTree(levels[5], block, channels[4], channels[5], 2, level_root=True, **cargs)
self.feature_info = [
dict(num_chs=channels[0], reduction=1, module='level0'), # rare to have a meaningful stride 1 level
dict(num_chs=channels[1], reduction=2, module='level1'),
dict(num_chs=channels[2], reduction=4, module='level2'),
dict(num_chs=channels[3], reduction=8, module='level3'),
dict(num_chs=channels[4], reduction=16, module='level4'),
dict(num_chs=channels[5], reduction=32, module='level5'),
]
self.num_features = channels[-1]
self.global_pool, self.head_drop, self.fc = create_classifier(
self.num_features,
self.num_classes,
pool_type=global_pool,
use_conv=True,
drop_rate=drop_rate,
)
self.flatten = nn.Flatten(1) if global_pool else nn.Identity()
for m in self.modules():
if isinstance(m, nn.Conv2d):
n = m.kernel_size[0] * m.kernel_size[1] * m.out_channels
m.weight.data.normal_(0, math.sqrt(2. / n))
elif isinstance(m, nn.BatchNorm2d):
m.weight.data.fill_(1)
m.bias.data.zero_()
def _make_conv_level(self, inplanes, planes, convs, stride=1, dilation=1):
modules = []
for i in range(convs):
modules.extend([
nn.Conv2d(
inplanes, planes, kernel_size=3,
stride=stride if i == 0 else 1,
padding=dilation, bias=False, dilation=dilation),
nn.BatchNorm2d(planes),
nn.ReLU(inplace=True)])
inplanes = planes
return nn.Sequential(*modules)
@torch.jit.ignore
def group_matcher(self, coarse=False):
matcher = dict(
stem=r'^base_layer',
blocks=r'^level(\d+)' if coarse else [
# an unusual arch, this achieves somewhat more granularity without getting super messy
(r'^level(\d+)\.tree(\d+)', None),
(r'^level(\d+)\.root', (2,)),
(r'^level(\d+)', (1,))
]
)
return matcher
@torch.jit.ignore
def set_grad_checkpointing(self, enable=True):
assert not enable, 'gradient checkpointing not supported'
@torch.jit.ignore
def get_classifier(self):
return self.fc
def reset_classifier(self, num_classes, global_pool='avg'):
self.num_classes = num_classes
self.global_pool, self.fc = create_classifier(
self.num_features, self.num_classes, pool_type=global_pool, use_conv=True)
self.flatten = nn.Flatten(1) if global_pool else nn.Identity()
def forward_features(self, x):
x = self.base_layer(x)
x = self.level0(x)
x = self.level1(x)
x = self.level2(x)
x = self.level3(x)
x = self.level4(x)
x = self.level5(x)
return x
def forward_head(self, x, pre_logits: bool = False):
x = self.global_pool(x)
x = self.head_drop(x)
if pre_logits:
return self.flatten(x)
x = self.fc(x)
return self.flatten(x)
def forward(self, x):
x = self.forward_features(x)
x = self.forward_head(x)
return x
def _create_dla(variant, pretrained=False, **kwargs):
return build_model_with_cfg(
DLA,
variant,
pretrained,
pretrained_strict=False,
feature_cfg=dict(out_indices=(1, 2, 3, 4, 5)),
**kwargs,
)
def _cfg(url='', **kwargs):
return {
'url': url,
'num_classes': 1000, 'input_size': (3, 224, 224), 'pool_size': (7, 7),
'crop_pct': 0.875, 'interpolation': 'bilinear',
'mean': IMAGENET_DEFAULT_MEAN, 'std': IMAGENET_DEFAULT_STD,
'first_conv': 'base_layer.0', 'classifier': 'fc',
**kwargs
}
default_cfgs = generate_default_cfgs({
'dla34.in1k': _cfg(hf_hub_id='timm/'),
'dla46_c.in1k': _cfg(hf_hub_id='timm/'),
'dla46x_c.in1k': _cfg(hf_hub_id='timm/'),
'dla60x_c.in1k': _cfg(hf_hub_id='timm/'),
'dla60.in1k': _cfg(hf_hub_id='timm/'),
'dla60x.in1k': _cfg(hf_hub_id='timm/'),
'dla102.in1k': _cfg(hf_hub_id='timm/'),
'dla102x.in1k': _cfg(hf_hub_id='timm/'),
'dla102x2.in1k': _cfg(hf_hub_id='timm/'),
'dla169.in1k': _cfg(hf_hub_id='timm/'),
'dla60_res2net.in1k': _cfg(hf_hub_id='timm/'),
'dla60_res2next.in1k': _cfg(hf_hub_id='timm/'),
})
@register_model
def dla60_res2net(pretrained=False, **kwargs) -> DLA:
model_args = dict(
levels=(1, 1, 1, 2, 3, 1), channels=(16, 32, 128, 256, 512, 1024),
block=DlaBottle2neck, cardinality=1, base_width=28)
return _create_dla('dla60_res2net', pretrained, **dict(model_args, **kwargs))
@register_model
def dla60_res2next(pretrained=False,**kwargs):
model_args = dict(
levels=(1, 1, 1, 2, 3, 1), channels=(16, 32, 128, 256, 512, 1024),
block=DlaBottle2neck, cardinality=8, base_width=4)
return _create_dla('dla60_res2next', pretrained, **dict(model_args, **kwargs))
@register_model
def dla34(pretrained=False, **kwargs) -> DLA: # DLA-34
model_args = dict(
levels=[1, 1, 1, 2, 2, 1], channels=[16, 32, 64, 128, 256, 512], block=DlaBasic)
return _create_dla('dla34', pretrained, **dict(model_args, **kwargs))
@register_model
def dla46_c(pretrained=False, **kwargs) -> DLA: # DLA-46-C
model_args = dict(
levels=[1, 1, 1, 2, 2, 1], channels=[16, 32, 64, 64, 128, 256], block=DlaBottleneck)
return _create_dla('dla46_c', pretrained, **dict(model_args, **kwargs))
@register_model
def dla46x_c(pretrained=False, **kwargs) -> DLA: # DLA-X-46-C
model_args = dict(
levels=[1, 1, 1, 2, 2, 1], channels=[16, 32, 64, 64, 128, 256],
block=DlaBottleneck, cardinality=32, base_width=4)
return _create_dla('dla46x_c', pretrained, **dict(model_args, **kwargs))
@register_model
def dla60x_c(pretrained=False, **kwargs) -> DLA: # DLA-X-60-C
model_args = dict(
levels=[1, 1, 1, 2, 3, 1], channels=[16, 32, 64, 64, 128, 256],
block=DlaBottleneck, cardinality=32, base_width=4)
return _create_dla('dla60x_c', pretrained, **dict(model_args, **kwargs))
@register_model
def dla60(pretrained=False, **kwargs) -> DLA: # DLA-60
model_args = dict(
levels=[1, 1, 1, 2, 3, 1], channels=[16, 32, 128, 256, 512, 1024],
block=DlaBottleneck)
return _create_dla('dla60', pretrained, **dict(model_args, **kwargs))
@register_model
def dla60x(pretrained=False, **kwargs) -> DLA: # DLA-X-60
model_args = dict(
levels=[1, 1, 1, 2, 3, 1], channels=[16, 32, 128, 256, 512, 1024],
block=DlaBottleneck, cardinality=32, base_width=4)
return _create_dla('dla60x', pretrained, **dict(model_args, **kwargs))
@register_model
def dla102(pretrained=False, **kwargs) -> DLA: # DLA-102
model_args = dict(
levels=[1, 1, 1, 3, 4, 1], channels=[16, 32, 128, 256, 512, 1024],
block=DlaBottleneck, shortcut_root=True)
return _create_dla('dla102', pretrained, **dict(model_args, **kwargs))
@register_model
def dla102x(pretrained=False, **kwargs) -> DLA: # DLA-X-102
model_args = dict(
levels=[1, 1, 1, 3, 4, 1], channels=[16, 32, 128, 256, 512, 1024],
block=DlaBottleneck, cardinality=32, base_width=4, shortcut_root=True)
return _create_dla('dla102x', pretrained, **dict(model_args, **kwargs))
@register_model
def dla102x2(pretrained=False, **kwargs) -> DLA: # DLA-X-102 64
model_args = dict(
levels=[1, 1, 1, 3, 4, 1], channels=[16, 32, 128, 256, 512, 1024],
block=DlaBottleneck, cardinality=64, base_width=4, shortcut_root=True)
return _create_dla('dla102x2', pretrained, **dict(model_args, **kwargs))
@register_model
def dla169(pretrained=False, **kwargs) -> DLA: # DLA-169
model_args = dict(
levels=[1, 1, 2, 3, 5, 1], channels=[16, 32, 128, 256, 512, 1024],
block=DlaBottleneck, shortcut_root=True)
return _create_dla('dla169', pretrained, **dict(model_args, **kwargs))
| pytorch-image-models/timm/models/dla.py/0 | {
"file_path": "pytorch-image-models/timm/models/dla.py",
"repo_id": "pytorch-image-models",
"token_count": 9144
} | 196 |
from functools import partial
import torch.nn as nn
from timm.data import IMAGENET_DEFAULT_MEAN, IMAGENET_DEFAULT_STD
from ._builder import build_model_with_cfg
from ._builder import pretrained_cfg_for_features
from ._efficientnet_blocks import SqueezeExcite
from ._efficientnet_builder import decode_arch_def, resolve_act_layer, resolve_bn_args, round_channels
from ._registry import register_model, generate_default_cfgs
from .mobilenetv3 import MobileNetV3, MobileNetV3Features
__all__ = [] # model_registry will add each entrypoint fn to this
def _gen_hardcorenas(pretrained, variant, arch_def, **kwargs):
"""Creates a hardcorenas model
Ref impl: https://github.com/Alibaba-MIIL/HardCoReNAS
Paper: https://arxiv.org/abs/2102.11646
"""
num_features = 1280
se_layer = partial(SqueezeExcite, gate_layer='hard_sigmoid', force_act_layer=nn.ReLU, rd_round_fn=round_channels)
model_kwargs = dict(
block_args=decode_arch_def(arch_def),
num_features=num_features,
stem_size=32,
norm_layer=partial(nn.BatchNorm2d, **resolve_bn_args(kwargs)),
act_layer=resolve_act_layer(kwargs, 'hard_swish'),
se_layer=se_layer,
**kwargs,
)
features_only = False
model_cls = MobileNetV3
kwargs_filter = None
if model_kwargs.pop('features_only', False):
features_only = True
kwargs_filter = ('num_classes', 'num_features', 'global_pool', 'head_conv', 'head_bias', 'global_pool')
model_cls = MobileNetV3Features
model = build_model_with_cfg(
model_cls,
variant,
pretrained,
pretrained_strict=not features_only,
kwargs_filter=kwargs_filter,
**model_kwargs,
)
if features_only:
model.default_cfg = pretrained_cfg_for_features(model.default_cfg)
return model
def _cfg(url='', **kwargs):
return {
'url': url, 'num_classes': 1000, 'input_size': (3, 224, 224), 'pool_size': (7, 7),
'crop_pct': 0.875, 'interpolation': 'bilinear',
'mean': IMAGENET_DEFAULT_MEAN, 'std': IMAGENET_DEFAULT_STD,
'first_conv': 'conv_stem', 'classifier': 'classifier',
**kwargs
}
default_cfgs = generate_default_cfgs({
'hardcorenas_a.miil_green_in1k': _cfg(hf_hub_id='timm/'),
'hardcorenas_b.miil_green_in1k': _cfg(hf_hub_id='timm/'),
'hardcorenas_c.miil_green_in1k': _cfg(hf_hub_id='timm/'),
'hardcorenas_d.miil_green_in1k': _cfg(hf_hub_id='timm/'),
'hardcorenas_e.miil_green_in1k': _cfg(hf_hub_id='timm/'),
'hardcorenas_f.miil_green_in1k': _cfg(hf_hub_id='timm/'),
})
@register_model
def hardcorenas_a(pretrained=False, **kwargs) -> MobileNetV3:
""" hardcorenas_A """
arch_def = [['ds_r1_k3_s1_e1_c16_nre'], ['ir_r1_k5_s2_e3_c24_nre', 'ir_r1_k5_s1_e3_c24_nre_se0.25'],
['ir_r1_k5_s2_e3_c40_nre', 'ir_r1_k5_s1_e6_c40_nre_se0.25'],
['ir_r1_k5_s2_e6_c80_se0.25', 'ir_r1_k5_s1_e6_c80_se0.25'],
['ir_r1_k5_s1_e6_c112_se0.25', 'ir_r1_k5_s1_e6_c112_se0.25'],
['ir_r1_k5_s2_e6_c192_se0.25', 'ir_r1_k5_s1_e6_c192_se0.25'], ['cn_r1_k1_s1_c960']]
model = _gen_hardcorenas(pretrained=pretrained, variant='hardcorenas_a', arch_def=arch_def, **kwargs)
return model
@register_model
def hardcorenas_b(pretrained=False, **kwargs) -> MobileNetV3:
""" hardcorenas_B """
arch_def = [['ds_r1_k3_s1_e1_c16_nre'],
['ir_r1_k5_s2_e3_c24_nre', 'ir_r1_k5_s1_e3_c24_nre_se0.25', 'ir_r1_k3_s1_e3_c24_nre'],
['ir_r1_k5_s2_e3_c40_nre', 'ir_r1_k5_s1_e3_c40_nre', 'ir_r1_k5_s1_e3_c40_nre'],
['ir_r1_k5_s2_e3_c80', 'ir_r1_k5_s1_e3_c80', 'ir_r1_k3_s1_e3_c80', 'ir_r1_k3_s1_e3_c80'],
['ir_r1_k5_s1_e3_c112', 'ir_r1_k3_s1_e3_c112', 'ir_r1_k3_s1_e3_c112', 'ir_r1_k3_s1_e3_c112'],
['ir_r1_k5_s2_e6_c192_se0.25', 'ir_r1_k5_s1_e6_c192_se0.25', 'ir_r1_k3_s1_e3_c192_se0.25'],
['cn_r1_k1_s1_c960']]
model = _gen_hardcorenas(pretrained=pretrained, variant='hardcorenas_b', arch_def=arch_def, **kwargs)
return model
@register_model
def hardcorenas_c(pretrained=False, **kwargs) -> MobileNetV3:
""" hardcorenas_C """
arch_def = [['ds_r1_k3_s1_e1_c16_nre'], ['ir_r1_k5_s2_e3_c24_nre', 'ir_r1_k5_s1_e3_c24_nre_se0.25'],
['ir_r1_k5_s2_e3_c40_nre', 'ir_r1_k5_s1_e3_c40_nre', 'ir_r1_k5_s1_e3_c40_nre',
'ir_r1_k5_s1_e3_c40_nre'],
['ir_r1_k5_s2_e4_c80', 'ir_r1_k5_s1_e6_c80_se0.25', 'ir_r1_k3_s1_e3_c80', 'ir_r1_k3_s1_e3_c80'],
['ir_r1_k5_s1_e6_c112_se0.25', 'ir_r1_k3_s1_e3_c112', 'ir_r1_k3_s1_e3_c112', 'ir_r1_k3_s1_e3_c112'],
['ir_r1_k5_s2_e6_c192_se0.25', 'ir_r1_k5_s1_e6_c192_se0.25', 'ir_r1_k3_s1_e3_c192_se0.25'],
['cn_r1_k1_s1_c960']]
model = _gen_hardcorenas(pretrained=pretrained, variant='hardcorenas_c', arch_def=arch_def, **kwargs)
return model
@register_model
def hardcorenas_d(pretrained=False, **kwargs) -> MobileNetV3:
""" hardcorenas_D """
arch_def = [['ds_r1_k3_s1_e1_c16_nre'], ['ir_r1_k5_s2_e3_c24_nre_se0.25', 'ir_r1_k5_s1_e3_c24_nre_se0.25'],
['ir_r1_k5_s2_e3_c40_nre_se0.25', 'ir_r1_k5_s1_e4_c40_nre_se0.25', 'ir_r1_k3_s1_e3_c40_nre_se0.25'],
['ir_r1_k5_s2_e4_c80_se0.25', 'ir_r1_k3_s1_e3_c80_se0.25', 'ir_r1_k3_s1_e3_c80_se0.25',
'ir_r1_k3_s1_e3_c80_se0.25'],
['ir_r1_k3_s1_e4_c112_se0.25', 'ir_r1_k5_s1_e4_c112_se0.25', 'ir_r1_k3_s1_e3_c112_se0.25',
'ir_r1_k5_s1_e3_c112_se0.25'],
['ir_r1_k5_s2_e6_c192_se0.25', 'ir_r1_k5_s1_e6_c192_se0.25', 'ir_r1_k5_s1_e6_c192_se0.25',
'ir_r1_k3_s1_e6_c192_se0.25'], ['cn_r1_k1_s1_c960']]
model = _gen_hardcorenas(pretrained=pretrained, variant='hardcorenas_d', arch_def=arch_def, **kwargs)
return model
@register_model
def hardcorenas_e(pretrained=False, **kwargs) -> MobileNetV3:
""" hardcorenas_E """
arch_def = [['ds_r1_k3_s1_e1_c16_nre'], ['ir_r1_k5_s2_e3_c24_nre_se0.25', 'ir_r1_k5_s1_e3_c24_nre_se0.25'],
['ir_r1_k5_s2_e6_c40_nre_se0.25', 'ir_r1_k5_s1_e4_c40_nre_se0.25', 'ir_r1_k5_s1_e4_c40_nre_se0.25',
'ir_r1_k3_s1_e3_c40_nre_se0.25'], ['ir_r1_k5_s2_e4_c80_se0.25', 'ir_r1_k3_s1_e6_c80_se0.25'],
['ir_r1_k5_s1_e6_c112_se0.25', 'ir_r1_k5_s1_e6_c112_se0.25', 'ir_r1_k5_s1_e6_c112_se0.25',
'ir_r1_k5_s1_e3_c112_se0.25'],
['ir_r1_k5_s2_e6_c192_se0.25', 'ir_r1_k5_s1_e6_c192_se0.25', 'ir_r1_k5_s1_e6_c192_se0.25',
'ir_r1_k3_s1_e6_c192_se0.25'], ['cn_r1_k1_s1_c960']]
model = _gen_hardcorenas(pretrained=pretrained, variant='hardcorenas_e', arch_def=arch_def, **kwargs)
return model
@register_model
def hardcorenas_f(pretrained=False, **kwargs) -> MobileNetV3:
""" hardcorenas_F """
arch_def = [['ds_r1_k3_s1_e1_c16_nre'], ['ir_r1_k5_s2_e3_c24_nre_se0.25', 'ir_r1_k5_s1_e3_c24_nre_se0.25'],
['ir_r1_k5_s2_e6_c40_nre_se0.25', 'ir_r1_k5_s1_e6_c40_nre_se0.25'],
['ir_r1_k5_s2_e6_c80_se0.25', 'ir_r1_k5_s1_e6_c80_se0.25', 'ir_r1_k3_s1_e3_c80_se0.25',
'ir_r1_k3_s1_e3_c80_se0.25'],
['ir_r1_k3_s1_e6_c112_se0.25', 'ir_r1_k5_s1_e6_c112_se0.25', 'ir_r1_k5_s1_e6_c112_se0.25',
'ir_r1_k3_s1_e3_c112_se0.25'],
['ir_r1_k5_s2_e6_c192_se0.25', 'ir_r1_k5_s1_e6_c192_se0.25', 'ir_r1_k3_s1_e6_c192_se0.25',
'ir_r1_k3_s1_e6_c192_se0.25'], ['cn_r1_k1_s1_c960']]
model = _gen_hardcorenas(pretrained=pretrained, variant='hardcorenas_f', arch_def=arch_def, **kwargs)
return model
| pytorch-image-models/timm/models/hardcorenas.py/0 | {
"file_path": "pytorch-image-models/timm/models/hardcorenas.py",
"repo_id": "pytorch-image-models",
"token_count": 4629
} | 197 |
""" Multi-Scale Vision Transformer v2
@inproceedings{li2021improved,
title={MViTv2: Improved multiscale vision transformers for classification and detection},
author={Li, Yanghao and Wu, Chao-Yuan and Fan, Haoqi and Mangalam, Karttikeya and Xiong, Bo and Malik, Jitendra and Feichtenhofer, Christoph},
booktitle={CVPR},
year={2022}
}
Code adapted from original Apache 2.0 licensed impl at https://github.com/facebookresearch/mvit
Original copyright below.
Modifications and timm support by / Copyright 2022, Ross Wightman
"""
# Copyright (c) Meta Platforms, Inc. and affiliates. All Rights Reserved. All Rights Reserved.
import operator
from collections import OrderedDict
from dataclasses import dataclass
from functools import partial, reduce
from typing import Union, List, Tuple, Optional
import torch
import torch.utils.checkpoint as checkpoint
from torch import nn
from timm.data import IMAGENET_DEFAULT_MEAN, IMAGENET_DEFAULT_STD
from timm.layers import Mlp, DropPath, trunc_normal_tf_, get_norm_layer, to_2tuple
from ._builder import build_model_with_cfg
from ._features_fx import register_notrace_function
from ._registry import register_model, register_model_deprecations, generate_default_cfgs
__all__ = ['MultiScaleVit', 'MultiScaleVitCfg'] # model_registry will add each entrypoint fn to this
@dataclass
class MultiScaleVitCfg:
depths: Tuple[int, ...] = (2, 3, 16, 3)
embed_dim: Union[int, Tuple[int, ...]] = 96
num_heads: Union[int, Tuple[int, ...]] = 1
mlp_ratio: float = 4.
pool_first: bool = False
expand_attn: bool = True
qkv_bias: bool = True
use_cls_token: bool = False
use_abs_pos: bool = False
residual_pooling: bool = True
mode: str = 'conv'
kernel_qkv: Tuple[int, int] = (3, 3)
stride_q: Optional[Tuple[Tuple[int, int]]] = ((1, 1), (2, 2), (2, 2), (2, 2))
stride_kv: Optional[Tuple[Tuple[int, int]]] = None
stride_kv_adaptive: Optional[Tuple[int, int]] = (4, 4)
patch_kernel: Tuple[int, int] = (7, 7)
patch_stride: Tuple[int, int] = (4, 4)
patch_padding: Tuple[int, int] = (3, 3)
pool_type: str = 'max'
rel_pos_type: str = 'spatial'
act_layer: Union[str, Tuple[str, str]] = 'gelu'
norm_layer: Union[str, Tuple[str, str]] = 'layernorm'
norm_eps: float = 1e-6
def __post_init__(self):
num_stages = len(self.depths)
if not isinstance(self.embed_dim, (tuple, list)):
self.embed_dim = tuple(self.embed_dim * 2 ** i for i in range(num_stages))
assert len(self.embed_dim) == num_stages
if not isinstance(self.num_heads, (tuple, list)):
self.num_heads = tuple(self.num_heads * 2 ** i for i in range(num_stages))
assert len(self.num_heads) == num_stages
if self.stride_kv_adaptive is not None and self.stride_kv is None:
_stride_kv = self.stride_kv_adaptive
pool_kv_stride = []
for i in range(num_stages):
if min(self.stride_q[i]) > 1:
_stride_kv = [
max(_stride_kv[d] // self.stride_q[i][d], 1)
for d in range(len(_stride_kv))
]
pool_kv_stride.append(tuple(_stride_kv))
self.stride_kv = tuple(pool_kv_stride)
def prod(iterable):
return reduce(operator.mul, iterable, 1)
class PatchEmbed(nn.Module):
"""
PatchEmbed.
"""
def __init__(
self,
dim_in=3,
dim_out=768,
kernel=(7, 7),
stride=(4, 4),
padding=(3, 3),
):
super().__init__()
self.proj = nn.Conv2d(
dim_in,
dim_out,
kernel_size=kernel,
stride=stride,
padding=padding,
)
def forward(self, x) -> Tuple[torch.Tensor, List[int]]:
x = self.proj(x)
# B C H W -> B HW C
return x.flatten(2).transpose(1, 2), x.shape[-2:]
@register_notrace_function
def reshape_pre_pool(
x,
feat_size: List[int],
has_cls_token: bool = True
) -> Tuple[torch.Tensor, Optional[torch.Tensor]]:
H, W = feat_size
if has_cls_token:
cls_tok, x = x[:, :, :1, :], x[:, :, 1:, :]
else:
cls_tok = None
x = x.reshape(-1, H, W, x.shape[-1]).permute(0, 3, 1, 2).contiguous()
return x, cls_tok
@register_notrace_function
def reshape_post_pool(
x,
num_heads: int,
cls_tok: Optional[torch.Tensor] = None
) -> Tuple[torch.Tensor, List[int]]:
feat_size = [x.shape[2], x.shape[3]]
L_pooled = x.shape[2] * x.shape[3]
x = x.reshape(-1, num_heads, x.shape[1], L_pooled).transpose(2, 3)
if cls_tok is not None:
x = torch.cat((cls_tok, x), dim=2)
return x, feat_size
@register_notrace_function
def cal_rel_pos_type(
attn: torch.Tensor,
q: torch.Tensor,
has_cls_token: bool,
q_size: List[int],
k_size: List[int],
rel_pos_h: torch.Tensor,
rel_pos_w: torch.Tensor,
):
"""
Spatial Relative Positional Embeddings.
"""
sp_idx = 1 if has_cls_token else 0
q_h, q_w = q_size
k_h, k_w = k_size
# Scale up rel pos if shapes for q and k are different.
q_h_ratio = max(k_h / q_h, 1.0)
k_h_ratio = max(q_h / k_h, 1.0)
dist_h = (
torch.arange(q_h, device=q.device).unsqueeze(-1) * q_h_ratio -
torch.arange(k_h, device=q.device).unsqueeze(0) * k_h_ratio
)
dist_h += (k_h - 1) * k_h_ratio
q_w_ratio = max(k_w / q_w, 1.0)
k_w_ratio = max(q_w / k_w, 1.0)
dist_w = (
torch.arange(q_w, device=q.device).unsqueeze(-1) * q_w_ratio -
torch.arange(k_w, device=q.device).unsqueeze(0) * k_w_ratio
)
dist_w += (k_w - 1) * k_w_ratio
rel_h = rel_pos_h[dist_h.long()]
rel_w = rel_pos_w[dist_w.long()]
B, n_head, q_N, dim = q.shape
r_q = q[:, :, sp_idx:].reshape(B, n_head, q_h, q_w, dim)
rel_h = torch.einsum("byhwc,hkc->byhwk", r_q, rel_h)
rel_w = torch.einsum("byhwc,wkc->byhwk", r_q, rel_w)
attn[:, :, sp_idx:, sp_idx:] = (
attn[:, :, sp_idx:, sp_idx:].view(B, -1, q_h, q_w, k_h, k_w)
+ rel_h.unsqueeze(-1)
+ rel_w.unsqueeze(-2)
).view(B, -1, q_h * q_w, k_h * k_w)
return attn
class MultiScaleAttentionPoolFirst(nn.Module):
def __init__(
self,
dim,
dim_out,
feat_size,
num_heads=8,
qkv_bias=True,
mode="conv",
kernel_q=(1, 1),
kernel_kv=(1, 1),
stride_q=(1, 1),
stride_kv=(1, 1),
has_cls_token=True,
rel_pos_type='spatial',
residual_pooling=True,
norm_layer=nn.LayerNorm,
):
super().__init__()
self.num_heads = num_heads
self.dim_out = dim_out
self.head_dim = dim_out // num_heads
self.scale = self.head_dim ** -0.5
self.has_cls_token = has_cls_token
padding_q = tuple([int(q // 2) for q in kernel_q])
padding_kv = tuple([int(kv // 2) for kv in kernel_kv])
self.q = nn.Linear(dim, dim_out, bias=qkv_bias)
self.k = nn.Linear(dim, dim_out, bias=qkv_bias)
self.v = nn.Linear(dim, dim_out, bias=qkv_bias)
self.proj = nn.Linear(dim_out, dim_out)
# Skip pooling with kernel and stride size of (1, 1, 1).
if prod(kernel_q) == 1 and prod(stride_q) == 1:
kernel_q = None
if prod(kernel_kv) == 1 and prod(stride_kv) == 1:
kernel_kv = None
self.mode = mode
self.unshared = mode == 'conv_unshared'
self.pool_q, self.pool_k, self.pool_v = None, None, None
self.norm_q, self.norm_k, self.norm_v = None, None, None
if mode in ("avg", "max"):
pool_op = nn.MaxPool2d if mode == "max" else nn.AvgPool2d
if kernel_q:
self.pool_q = pool_op(kernel_q, stride_q, padding_q)
if kernel_kv:
self.pool_k = pool_op(kernel_kv, stride_kv, padding_kv)
self.pool_v = pool_op(kernel_kv, stride_kv, padding_kv)
elif mode == "conv" or mode == "conv_unshared":
dim_conv = dim // num_heads if mode == "conv" else dim
if kernel_q:
self.pool_q = nn.Conv2d(
dim_conv,
dim_conv,
kernel_q,
stride=stride_q,
padding=padding_q,
groups=dim_conv,
bias=False,
)
self.norm_q = norm_layer(dim_conv)
if kernel_kv:
self.pool_k = nn.Conv2d(
dim_conv,
dim_conv,
kernel_kv,
stride=stride_kv,
padding=padding_kv,
groups=dim_conv,
bias=False,
)
self.norm_k = norm_layer(dim_conv)
self.pool_v = nn.Conv2d(
dim_conv,
dim_conv,
kernel_kv,
stride=stride_kv,
padding=padding_kv,
groups=dim_conv,
bias=False,
)
self.norm_v = norm_layer(dim_conv)
else:
raise NotImplementedError(f"Unsupported model {mode}")
# relative pos embedding
self.rel_pos_type = rel_pos_type
if self.rel_pos_type == 'spatial':
assert feat_size[0] == feat_size[1]
size = feat_size[0]
q_size = size // stride_q[1] if len(stride_q) > 0 else size
kv_size = size // stride_kv[1] if len(stride_kv) > 0 else size
rel_sp_dim = 2 * max(q_size, kv_size) - 1
self.rel_pos_h = nn.Parameter(torch.zeros(rel_sp_dim, self.head_dim))
self.rel_pos_w = nn.Parameter(torch.zeros(rel_sp_dim, self.head_dim))
trunc_normal_tf_(self.rel_pos_h, std=0.02)
trunc_normal_tf_(self.rel_pos_w, std=0.02)
self.residual_pooling = residual_pooling
def forward(self, x, feat_size: List[int]):
B, N, _ = x.shape
fold_dim = 1 if self.unshared else self.num_heads
x = x.reshape(B, N, fold_dim, -1).permute(0, 2, 1, 3)
q = k = v = x
if self.pool_q is not None:
q, q_tok = reshape_pre_pool(q, feat_size, self.has_cls_token)
q = self.pool_q(q)
q, q_size = reshape_post_pool(q, self.num_heads, q_tok)
else:
q_size = feat_size
if self.norm_q is not None:
q = self.norm_q(q)
if self.pool_k is not None:
k, k_tok = reshape_pre_pool(k, feat_size, self.has_cls_token)
k = self.pool_k(k)
k, k_size = reshape_post_pool(k, self.num_heads, k_tok)
else:
k_size = feat_size
if self.norm_k is not None:
k = self.norm_k(k)
if self.pool_v is not None:
v, v_tok = reshape_pre_pool(v, feat_size, self.has_cls_token)
v = self.pool_v(v)
v, v_size = reshape_post_pool(v, self.num_heads, v_tok)
else:
v_size = feat_size
if self.norm_v is not None:
v = self.norm_v(v)
q_N = q_size[0] * q_size[1] + int(self.has_cls_token)
q = q.transpose(1, 2).reshape(B, q_N, -1)
q = self.q(q).reshape(B, q_N, self.num_heads, -1).transpose(1, 2)
k_N = k_size[0] * k_size[1] + int(self.has_cls_token)
k = k.transpose(1, 2).reshape(B, k_N, -1)
k = self.k(k).reshape(B, k_N, self.num_heads, -1)
v_N = v_size[0] * v_size[1] + int(self.has_cls_token)
v = v.transpose(1, 2).reshape(B, v_N, -1)
v = self.v(v).reshape(B, v_N, self.num_heads, -1).transpose(1, 2)
attn = (q * self.scale) @ k
if self.rel_pos_type == 'spatial':
attn = cal_rel_pos_type(
attn,
q,
self.has_cls_token,
q_size,
k_size,
self.rel_pos_h,
self.rel_pos_w,
)
attn = attn.softmax(dim=-1)
x = attn @ v
if self.residual_pooling:
x = x + q
x = x.transpose(1, 2).reshape(B, -1, self.dim_out)
x = self.proj(x)
return x, q_size
class MultiScaleAttention(nn.Module):
def __init__(
self,
dim,
dim_out,
feat_size,
num_heads=8,
qkv_bias=True,
mode="conv",
kernel_q=(1, 1),
kernel_kv=(1, 1),
stride_q=(1, 1),
stride_kv=(1, 1),
has_cls_token=True,
rel_pos_type='spatial',
residual_pooling=True,
norm_layer=nn.LayerNorm,
):
super().__init__()
self.num_heads = num_heads
self.dim_out = dim_out
self.head_dim = dim_out // num_heads
self.scale = self.head_dim ** -0.5
self.has_cls_token = has_cls_token
padding_q = tuple([int(q // 2) for q in kernel_q])
padding_kv = tuple([int(kv // 2) for kv in kernel_kv])
self.qkv = nn.Linear(dim, dim_out * 3, bias=qkv_bias)
self.proj = nn.Linear(dim_out, dim_out)
# Skip pooling with kernel and stride size of (1, 1, 1).
if prod(kernel_q) == 1 and prod(stride_q) == 1:
kernel_q = None
if prod(kernel_kv) == 1 and prod(stride_kv) == 1:
kernel_kv = None
self.mode = mode
self.unshared = mode == 'conv_unshared'
self.norm_q, self.norm_k, self.norm_v = None, None, None
self.pool_q, self.pool_k, self.pool_v = None, None, None
if mode in ("avg", "max"):
pool_op = nn.MaxPool2d if mode == "max" else nn.AvgPool2d
if kernel_q:
self.pool_q = pool_op(kernel_q, stride_q, padding_q)
if kernel_kv:
self.pool_k = pool_op(kernel_kv, stride_kv, padding_kv)
self.pool_v = pool_op(kernel_kv, stride_kv, padding_kv)
elif mode == "conv" or mode == "conv_unshared":
dim_conv = dim_out // num_heads if mode == "conv" else dim_out
if kernel_q:
self.pool_q = nn.Conv2d(
dim_conv,
dim_conv,
kernel_q,
stride=stride_q,
padding=padding_q,
groups=dim_conv,
bias=False,
)
self.norm_q = norm_layer(dim_conv)
if kernel_kv:
self.pool_k = nn.Conv2d(
dim_conv,
dim_conv,
kernel_kv,
stride=stride_kv,
padding=padding_kv,
groups=dim_conv,
bias=False,
)
self.norm_k = norm_layer(dim_conv)
self.pool_v = nn.Conv2d(
dim_conv,
dim_conv,
kernel_kv,
stride=stride_kv,
padding=padding_kv,
groups=dim_conv,
bias=False,
)
self.norm_v = norm_layer(dim_conv)
else:
raise NotImplementedError(f"Unsupported model {mode}")
# relative pos embedding
self.rel_pos_type = rel_pos_type
if self.rel_pos_type == 'spatial':
assert feat_size[0] == feat_size[1]
size = feat_size[0]
q_size = size // stride_q[1] if len(stride_q) > 0 else size
kv_size = size // stride_kv[1] if len(stride_kv) > 0 else size
rel_sp_dim = 2 * max(q_size, kv_size) - 1
self.rel_pos_h = nn.Parameter(torch.zeros(rel_sp_dim, self.head_dim))
self.rel_pos_w = nn.Parameter(torch.zeros(rel_sp_dim, self.head_dim))
trunc_normal_tf_(self.rel_pos_h, std=0.02)
trunc_normal_tf_(self.rel_pos_w, std=0.02)
self.residual_pooling = residual_pooling
def forward(self, x, feat_size: List[int]):
B, N, _ = x.shape
qkv = self.qkv(x).reshape(B, N, 3, self.num_heads, -1).permute(2, 0, 3, 1, 4)
q, k, v = qkv.unbind(dim=0)
if self.pool_q is not None:
q, q_tok = reshape_pre_pool(q, feat_size, self.has_cls_token)
q = self.pool_q(q)
q, q_size = reshape_post_pool(q, self.num_heads, q_tok)
else:
q_size = feat_size
if self.norm_q is not None:
q = self.norm_q(q)
if self.pool_k is not None:
k, k_tok = reshape_pre_pool(k, feat_size, self.has_cls_token)
k = self.pool_k(k)
k, k_size = reshape_post_pool(k, self.num_heads, k_tok)
else:
k_size = feat_size
if self.norm_k is not None:
k = self.norm_k(k)
if self.pool_v is not None:
v, v_tok = reshape_pre_pool(v, feat_size, self.has_cls_token)
v = self.pool_v(v)
v, _ = reshape_post_pool(v, self.num_heads, v_tok)
if self.norm_v is not None:
v = self.norm_v(v)
attn = (q * self.scale) @ k.transpose(-2, -1)
if self.rel_pos_type == 'spatial':
attn = cal_rel_pos_type(
attn,
q,
self.has_cls_token,
q_size,
k_size,
self.rel_pos_h,
self.rel_pos_w,
)
attn = attn.softmax(dim=-1)
x = attn @ v
if self.residual_pooling:
x = x + q
x = x.transpose(1, 2).reshape(B, -1, self.dim_out)
x = self.proj(x)
return x, q_size
class MultiScaleBlock(nn.Module):
def __init__(
self,
dim,
dim_out,
num_heads,
feat_size,
mlp_ratio=4.0,
qkv_bias=True,
drop_path=0.0,
norm_layer=nn.LayerNorm,
kernel_q=(1, 1),
kernel_kv=(1, 1),
stride_q=(1, 1),
stride_kv=(1, 1),
mode="conv",
has_cls_token=True,
expand_attn=False,
pool_first=False,
rel_pos_type='spatial',
residual_pooling=True,
):
super().__init__()
proj_needed = dim != dim_out
self.dim = dim
self.dim_out = dim_out
self.has_cls_token = has_cls_token
self.norm1 = norm_layer(dim)
self.shortcut_proj_attn = nn.Linear(dim, dim_out) if proj_needed and expand_attn else None
if stride_q and prod(stride_q) > 1:
kernel_skip = [s + 1 if s > 1 else s for s in stride_q]
stride_skip = stride_q
padding_skip = [int(skip // 2) for skip in kernel_skip]
self.shortcut_pool_attn = nn.MaxPool2d(kernel_skip, stride_skip, padding_skip)
else:
self.shortcut_pool_attn = None
att_dim = dim_out if expand_attn else dim
attn_layer = MultiScaleAttentionPoolFirst if pool_first else MultiScaleAttention
self.attn = attn_layer(
dim,
att_dim,
num_heads=num_heads,
feat_size=feat_size,
qkv_bias=qkv_bias,
kernel_q=kernel_q,
kernel_kv=kernel_kv,
stride_q=stride_q,
stride_kv=stride_kv,
norm_layer=norm_layer,
has_cls_token=has_cls_token,
mode=mode,
rel_pos_type=rel_pos_type,
residual_pooling=residual_pooling,
)
self.drop_path1 = DropPath(drop_path) if drop_path > 0.0 else nn.Identity()
self.norm2 = norm_layer(att_dim)
mlp_dim_out = dim_out
self.shortcut_proj_mlp = nn.Linear(dim, dim_out) if proj_needed and not expand_attn else None
self.mlp = Mlp(
in_features=att_dim,
hidden_features=int(att_dim * mlp_ratio),
out_features=mlp_dim_out,
)
self.drop_path2 = DropPath(drop_path) if drop_path > 0.0 else nn.Identity()
def _shortcut_pool(self, x, feat_size: List[int]):
if self.shortcut_pool_attn is None:
return x
if self.has_cls_token:
cls_tok, x = x[:, :1, :], x[:, 1:, :]
else:
cls_tok = None
B, L, C = x.shape
H, W = feat_size
x = x.reshape(B, H, W, C).permute(0, 3, 1, 2).contiguous()
x = self.shortcut_pool_attn(x)
x = x.reshape(B, C, -1).transpose(1, 2)
if cls_tok is not None:
x = torch.cat((cls_tok, x), dim=1)
return x
def forward(self, x, feat_size: List[int]):
x_norm = self.norm1(x)
# NOTE as per the original impl, this seems odd, but shortcut uses un-normalized input if no proj
x_shortcut = x if self.shortcut_proj_attn is None else self.shortcut_proj_attn(x_norm)
x_shortcut = self._shortcut_pool(x_shortcut, feat_size)
x, feat_size_new = self.attn(x_norm, feat_size)
x = x_shortcut + self.drop_path1(x)
x_norm = self.norm2(x)
x_shortcut = x if self.shortcut_proj_mlp is None else self.shortcut_proj_mlp(x_norm)
x = x_shortcut + self.drop_path2(self.mlp(x_norm))
return x, feat_size_new
class MultiScaleVitStage(nn.Module):
def __init__(
self,
dim,
dim_out,
depth,
num_heads,
feat_size,
mlp_ratio=4.0,
qkv_bias=True,
mode="conv",
kernel_q=(1, 1),
kernel_kv=(1, 1),
stride_q=(1, 1),
stride_kv=(1, 1),
has_cls_token=True,
expand_attn=False,
pool_first=False,
rel_pos_type='spatial',
residual_pooling=True,
norm_layer=nn.LayerNorm,
drop_path=0.0,
):
super().__init__()
self.grad_checkpointing = False
self.blocks = nn.ModuleList()
if expand_attn:
out_dims = (dim_out,) * depth
else:
out_dims = (dim,) * (depth - 1) + (dim_out,)
for i in range(depth):
attention_block = MultiScaleBlock(
dim=dim,
dim_out=out_dims[i],
num_heads=num_heads,
feat_size=feat_size,
mlp_ratio=mlp_ratio,
qkv_bias=qkv_bias,
kernel_q=kernel_q,
kernel_kv=kernel_kv,
stride_q=stride_q if i == 0 else (1, 1),
stride_kv=stride_kv,
mode=mode,
has_cls_token=has_cls_token,
pool_first=pool_first,
rel_pos_type=rel_pos_type,
residual_pooling=residual_pooling,
expand_attn=expand_attn,
norm_layer=norm_layer,
drop_path=drop_path[i] if isinstance(drop_path, (list, tuple)) else drop_path,
)
dim = out_dims[i]
self.blocks.append(attention_block)
if i == 0:
feat_size = tuple([size // stride for size, stride in zip(feat_size, stride_q)])
self.feat_size = feat_size
def forward(self, x, feat_size: List[int]):
for blk in self.blocks:
if self.grad_checkpointing and not torch.jit.is_scripting():
x, feat_size = checkpoint.checkpoint(blk, x, feat_size)
else:
x, feat_size = blk(x, feat_size)
return x, feat_size
class MultiScaleVit(nn.Module):
"""
Improved Multiscale Vision Transformers for Classification and Detection
Yanghao Li*, Chao-Yuan Wu*, Haoqi Fan, Karttikeya Mangalam, Bo Xiong, Jitendra Malik,
Christoph Feichtenhofer*
https://arxiv.org/abs/2112.01526
Multiscale Vision Transformers
Haoqi Fan*, Bo Xiong*, Karttikeya Mangalam*, Yanghao Li*, Zhicheng Yan, Jitendra Malik,
Christoph Feichtenhofer*
https://arxiv.org/abs/2104.11227
"""
def __init__(
self,
cfg: MultiScaleVitCfg,
img_size: Tuple[int, int] = (224, 224),
in_chans: int = 3,
global_pool: Optional[str] = None,
num_classes: int = 1000,
drop_path_rate: float = 0.,
drop_rate: float = 0.,
):
super().__init__()
img_size = to_2tuple(img_size)
norm_layer = partial(get_norm_layer(cfg.norm_layer), eps=cfg.norm_eps)
self.num_classes = num_classes
self.drop_rate = drop_rate
if global_pool is None:
global_pool = 'token' if cfg.use_cls_token else 'avg'
self.global_pool = global_pool
self.depths = tuple(cfg.depths)
self.expand_attn = cfg.expand_attn
embed_dim = cfg.embed_dim[0]
self.patch_embed = PatchEmbed(
dim_in=in_chans,
dim_out=embed_dim,
kernel=cfg.patch_kernel,
stride=cfg.patch_stride,
padding=cfg.patch_padding,
)
patch_dims = (img_size[0] // cfg.patch_stride[0], img_size[1] // cfg.patch_stride[1])
num_patches = prod(patch_dims)
if cfg.use_cls_token:
self.cls_token = nn.Parameter(torch.zeros(1, 1, embed_dim))
self.num_prefix_tokens = 1
pos_embed_dim = num_patches + 1
else:
self.num_prefix_tokens = 0
self.cls_token = None
pos_embed_dim = num_patches
if cfg.use_abs_pos:
self.pos_embed = nn.Parameter(torch.zeros(1, pos_embed_dim, embed_dim))
else:
self.pos_embed = None
num_stages = len(cfg.embed_dim)
feat_size = patch_dims
dpr = [x.tolist() for x in torch.linspace(0, drop_path_rate, sum(cfg.depths)).split(cfg.depths)]
self.stages = nn.ModuleList()
for i in range(num_stages):
if cfg.expand_attn:
dim_out = cfg.embed_dim[i]
else:
dim_out = cfg.embed_dim[min(i + 1, num_stages - 1)]
stage = MultiScaleVitStage(
dim=embed_dim,
dim_out=dim_out,
depth=cfg.depths[i],
num_heads=cfg.num_heads[i],
feat_size=feat_size,
mlp_ratio=cfg.mlp_ratio,
qkv_bias=cfg.qkv_bias,
mode=cfg.mode,
pool_first=cfg.pool_first,
expand_attn=cfg.expand_attn,
kernel_q=cfg.kernel_qkv,
kernel_kv=cfg.kernel_qkv,
stride_q=cfg.stride_q[i],
stride_kv=cfg.stride_kv[i],
has_cls_token=cfg.use_cls_token,
rel_pos_type=cfg.rel_pos_type,
residual_pooling=cfg.residual_pooling,
norm_layer=norm_layer,
drop_path=dpr[i],
)
embed_dim = dim_out
feat_size = stage.feat_size
self.stages.append(stage)
self.num_features = embed_dim
self.norm = norm_layer(embed_dim)
self.head = nn.Sequential(OrderedDict([
('drop', nn.Dropout(self.drop_rate)),
('fc', nn.Linear(self.num_features, num_classes) if num_classes > 0 else nn.Identity())
]))
if self.pos_embed is not None:
trunc_normal_tf_(self.pos_embed, std=0.02)
if self.cls_token is not None:
trunc_normal_tf_(self.cls_token, std=0.02)
self.apply(self._init_weights)
def _init_weights(self, m):
if isinstance(m, nn.Linear):
trunc_normal_tf_(m.weight, std=0.02)
if isinstance(m, nn.Linear) and m.bias is not None:
nn.init.constant_(m.bias, 0.0)
@torch.jit.ignore
def no_weight_decay(self):
return {k for k, _ in self.named_parameters()
if any(n in k for n in ["pos_embed", "rel_pos_h", "rel_pos_w", "cls_token"])}
@torch.jit.ignore
def group_matcher(self, coarse=False):
matcher = dict(
stem=r'^patch_embed', # stem and embed
blocks=[(r'^stages\.(\d+)', None), (r'^norm', (99999,))]
)
return matcher
@torch.jit.ignore
def set_grad_checkpointing(self, enable=True):
for s in self.stages:
s.grad_checkpointing = enable
@torch.jit.ignore
def get_classifier(self):
return self.head.fc
def reset_classifier(self, num_classes, global_pool=None):
self.num_classes = num_classes
if global_pool is not None:
self.global_pool = global_pool
self.head = nn.Sequential(OrderedDict([
('drop', nn.Dropout(self.drop_rate)),
('fc', nn.Linear(self.num_features, num_classes) if num_classes > 0 else nn.Identity())
]))
def forward_features(self, x):
x, feat_size = self.patch_embed(x)
B, N, C = x.shape
if self.cls_token is not None:
cls_tokens = self.cls_token.expand(B, -1, -1)
x = torch.cat((cls_tokens, x), dim=1)
if self.pos_embed is not None:
x = x + self.pos_embed
for stage in self.stages:
x, feat_size = stage(x, feat_size)
x = self.norm(x)
return x
def forward_head(self, x, pre_logits: bool = False):
if self.global_pool:
if self.global_pool == 'avg':
x = x[:, self.num_prefix_tokens:].mean(1)
else:
x = x[:, 0]
return x if pre_logits else self.head(x)
def forward(self, x):
x = self.forward_features(x)
x = self.forward_head(x)
return x
def checkpoint_filter_fn(state_dict, model):
if 'stages.0.blocks.0.norm1.weight' in state_dict:
return state_dict
import re
if 'model_state' in state_dict:
state_dict = state_dict['model_state']
depths = getattr(model, 'depths', None)
expand_attn = getattr(model, 'expand_attn', True)
assert depths is not None, 'model requires depth attribute to remap checkpoints'
depth_map = {}
block_idx = 0
for stage_idx, d in enumerate(depths):
depth_map.update({i: (stage_idx, i - block_idx) for i in range(block_idx, block_idx + d)})
block_idx += d
out_dict = {}
for k, v in state_dict.items():
k = re.sub(
r'blocks\.(\d+)',
lambda x: f'stages.{depth_map[int(x.group(1))][0]}.blocks.{depth_map[int(x.group(1))][1]}',
k)
if expand_attn:
k = re.sub(r'stages\.(\d+).blocks\.(\d+).proj', f'stages.\\1.blocks.\\2.shortcut_proj_attn', k)
else:
k = re.sub(r'stages\.(\d+).blocks\.(\d+).proj', f'stages.\\1.blocks.\\2.shortcut_proj_mlp', k)
if 'head' in k:
k = k.replace('head.projection', 'head.fc')
out_dict[k] = v
# for k, v in state_dict.items():
# if model.pos_embed is not None and k == 'pos_embed' and v.shape[1] != model.pos_embed.shape[1]:
# # To resize pos embedding when using model at different size from pretrained weights
# v = resize_pos_embed(
# v,
# model.pos_embed,
# 0 if getattr(model, 'no_embed_class') else getattr(model, 'num_prefix_tokens', 1),
# model.patch_embed.grid_size
# )
return out_dict
model_cfgs = dict(
mvitv2_tiny=MultiScaleVitCfg(
depths=(1, 2, 5, 2),
),
mvitv2_small=MultiScaleVitCfg(
depths=(1, 2, 11, 2),
),
mvitv2_base=MultiScaleVitCfg(
depths=(2, 3, 16, 3),
),
mvitv2_large=MultiScaleVitCfg(
depths=(2, 6, 36, 4),
embed_dim=144,
num_heads=2,
expand_attn=False,
),
mvitv2_small_cls=MultiScaleVitCfg(
depths=(1, 2, 11, 2),
use_cls_token=True,
),
mvitv2_base_cls=MultiScaleVitCfg(
depths=(2, 3, 16, 3),
use_cls_token=True,
),
mvitv2_large_cls=MultiScaleVitCfg(
depths=(2, 6, 36, 4),
embed_dim=144,
num_heads=2,
use_cls_token=True,
expand_attn=True,
),
mvitv2_huge_cls=MultiScaleVitCfg(
depths=(4, 8, 60, 8),
embed_dim=192,
num_heads=3,
use_cls_token=True,
expand_attn=True,
),
)
def _create_mvitv2(variant, cfg_variant=None, pretrained=False, **kwargs):
if kwargs.get('features_only', None):
raise RuntimeError('features_only not implemented for Multiscale Vision Transformer models.')
return build_model_with_cfg(
MultiScaleVit,
variant,
pretrained,
model_cfg=model_cfgs[variant] if not cfg_variant else model_cfgs[cfg_variant],
pretrained_filter_fn=checkpoint_filter_fn,
feature_cfg=dict(flatten_sequential=True),
**kwargs,
)
def _cfg(url='', **kwargs):
return {
'url': url,
'num_classes': 1000, 'input_size': (3, 224, 224), 'pool_size': None,
'crop_pct': .9, 'interpolation': 'bicubic',
'mean': IMAGENET_DEFAULT_MEAN, 'std': IMAGENET_DEFAULT_STD,
'first_conv': 'patch_embed.proj', 'classifier': 'head.fc',
'fixed_input_size': True,
**kwargs
}
default_cfgs = generate_default_cfgs({
'mvitv2_tiny.fb_in1k': _cfg(
url='https://dl.fbaipublicfiles.com/mvit/mvitv2_models/MViTv2_T_in1k.pyth',
hf_hub_id='timm/'),
'mvitv2_small.fb_in1k': _cfg(url='https://dl.fbaipublicfiles.com/mvit/mvitv2_models/MViTv2_S_in1k.pyth',
hf_hub_id='timm/'),
'mvitv2_base.fb_in1k': _cfg(url='https://dl.fbaipublicfiles.com/mvit/mvitv2_models/MViTv2_B_in1k.pyth',
hf_hub_id='timm/'),
'mvitv2_large.fb_in1k': _cfg(url='https://dl.fbaipublicfiles.com/mvit/mvitv2_models/MViTv2_L_in1k.pyth',
hf_hub_id='timm/'),
'mvitv2_small_cls': _cfg(url=''),
'mvitv2_base_cls.fb_inw21k': _cfg(
url='https://dl.fbaipublicfiles.com/mvit/mvitv2_models/MViTv2_B_in21k.pyth',
hf_hub_id='timm/',
num_classes=19168),
'mvitv2_large_cls.fb_inw21k': _cfg(
url='https://dl.fbaipublicfiles.com/mvit/mvitv2_models/MViTv2_L_in21k.pyth',
hf_hub_id='timm/',
num_classes=19168),
'mvitv2_huge_cls.fb_inw21k': _cfg(
url='https://dl.fbaipublicfiles.com/mvit/mvitv2_models/MViTv2_H_in21k.pyth',
hf_hub_id='timm/',
num_classes=19168),
})
@register_model
def mvitv2_tiny(pretrained=False, **kwargs) -> MultiScaleVit:
return _create_mvitv2('mvitv2_tiny', pretrained=pretrained, **kwargs)
@register_model
def mvitv2_small(pretrained=False, **kwargs) -> MultiScaleVit:
return _create_mvitv2('mvitv2_small', pretrained=pretrained, **kwargs)
@register_model
def mvitv2_base(pretrained=False, **kwargs) -> MultiScaleVit:
return _create_mvitv2('mvitv2_base', pretrained=pretrained, **kwargs)
@register_model
def mvitv2_large(pretrained=False, **kwargs) -> MultiScaleVit:
return _create_mvitv2('mvitv2_large', pretrained=pretrained, **kwargs)
@register_model
def mvitv2_small_cls(pretrained=False, **kwargs) -> MultiScaleVit:
return _create_mvitv2('mvitv2_small_cls', pretrained=pretrained, **kwargs)
@register_model
def mvitv2_base_cls(pretrained=False, **kwargs) -> MultiScaleVit:
return _create_mvitv2('mvitv2_base_cls', pretrained=pretrained, **kwargs)
@register_model
def mvitv2_large_cls(pretrained=False, **kwargs) -> MultiScaleVit:
return _create_mvitv2('mvitv2_large_cls', pretrained=pretrained, **kwargs)
@register_model
def mvitv2_huge_cls(pretrained=False, **kwargs) -> MultiScaleVit:
return _create_mvitv2('mvitv2_huge_cls', pretrained=pretrained, **kwargs)
| pytorch-image-models/timm/models/mvitv2.py/0 | {
"file_path": "pytorch-image-models/timm/models/mvitv2.py",
"repo_id": "pytorch-image-models",
"token_count": 19585
} | 198 |
""" ReXNet
A PyTorch impl of `ReXNet: Diminishing Representational Bottleneck on Convolutional Neural Network` -
https://arxiv.org/abs/2007.00992
Adapted from original impl at https://github.com/clovaai/rexnet
Copyright (c) 2020-present NAVER Corp. MIT license
Changes for timm, feature extraction, and rounded channel variant hacked together by Ross Wightman
Copyright 2020 Ross Wightman
"""
from functools import partial
from math import ceil
import torch
import torch.nn as nn
from timm.data import IMAGENET_DEFAULT_MEAN, IMAGENET_DEFAULT_STD
from timm.layers import ClassifierHead, create_act_layer, ConvNormAct, DropPath, make_divisible, SEModule
from ._builder import build_model_with_cfg
from ._efficientnet_builder import efficientnet_init_weights
from ._manipulate import checkpoint_seq
from ._registry import generate_default_cfgs, register_model
__all__ = ['RexNet'] # model_registry will add each entrypoint fn to this
SEWithNorm = partial(SEModule, norm_layer=nn.BatchNorm2d)
class LinearBottleneck(nn.Module):
def __init__(
self,
in_chs,
out_chs,
stride,
dilation=(1, 1),
exp_ratio=1.0,
se_ratio=0.,
ch_div=1,
act_layer='swish',
dw_act_layer='relu6',
drop_path=None,
):
super(LinearBottleneck, self).__init__()
self.use_shortcut = stride == 1 and dilation[0] == dilation[1] and in_chs <= out_chs
self.in_channels = in_chs
self.out_channels = out_chs
if exp_ratio != 1.:
dw_chs = make_divisible(round(in_chs * exp_ratio), divisor=ch_div)
self.conv_exp = ConvNormAct(in_chs, dw_chs, act_layer=act_layer)
else:
dw_chs = in_chs
self.conv_exp = None
self.conv_dw = ConvNormAct(
dw_chs,
dw_chs,
kernel_size=3,
stride=stride,
dilation=dilation[0],
groups=dw_chs,
apply_act=False,
)
if se_ratio > 0:
self.se = SEWithNorm(dw_chs, rd_channels=make_divisible(int(dw_chs * se_ratio), ch_div))
else:
self.se = None
self.act_dw = create_act_layer(dw_act_layer)
self.conv_pwl = ConvNormAct(dw_chs, out_chs, 1, apply_act=False)
self.drop_path = drop_path
def feat_channels(self, exp=False):
return self.conv_dw.out_channels if exp else self.out_channels
def forward(self, x):
shortcut = x
if self.conv_exp is not None:
x = self.conv_exp(x)
x = self.conv_dw(x)
if self.se is not None:
x = self.se(x)
x = self.act_dw(x)
x = self.conv_pwl(x)
if self.use_shortcut:
if self.drop_path is not None:
x = self.drop_path(x)
x = torch.cat([x[:, 0:self.in_channels] + shortcut, x[:, self.in_channels:]], dim=1)
return x
def _block_cfg(
width_mult=1.0,
depth_mult=1.0,
initial_chs=16,
final_chs=180,
se_ratio=0.,
ch_div=1,
):
layers = [1, 2, 2, 3, 3, 5]
strides = [1, 2, 2, 2, 1, 2]
layers = [ceil(element * depth_mult) for element in layers]
strides = sum([[element] + [1] * (layers[idx] - 1) for idx, element in enumerate(strides)], [])
exp_ratios = [1] * layers[0] + [6] * sum(layers[1:])
depth = sum(layers[:]) * 3
base_chs = initial_chs / width_mult if width_mult < 1.0 else initial_chs
# The following channel configuration is a simple instance to make each layer become an expand layer.
out_chs_list = []
for i in range(depth // 3):
out_chs_list.append(make_divisible(round(base_chs * width_mult), divisor=ch_div))
base_chs += final_chs / (depth // 3 * 1.0)
se_ratios = [0.] * (layers[0] + layers[1]) + [se_ratio] * sum(layers[2:])
return list(zip(out_chs_list, exp_ratios, strides, se_ratios))
def _build_blocks(
block_cfg,
prev_chs,
width_mult,
ch_div=1,
output_stride=32,
act_layer='swish',
dw_act_layer='relu6',
drop_path_rate=0.,
):
feat_chs = [prev_chs]
feature_info = []
curr_stride = 2
dilation = 1
features = []
num_blocks = len(block_cfg)
for block_idx, (chs, exp_ratio, stride, se_ratio) in enumerate(block_cfg):
next_dilation = dilation
if stride > 1:
fname = 'stem' if block_idx == 0 else f'features.{block_idx - 1}'
feature_info += [dict(num_chs=feat_chs[-1], reduction=curr_stride, module=fname)]
if curr_stride >= output_stride:
next_dilation = dilation * stride
stride = 1
block_dpr = drop_path_rate * block_idx / (num_blocks - 1) # stochastic depth linear decay rule
drop_path = DropPath(block_dpr) if block_dpr > 0. else None
features.append(LinearBottleneck(
in_chs=prev_chs,
out_chs=chs,
exp_ratio=exp_ratio,
stride=stride,
dilation=(dilation, next_dilation),
se_ratio=se_ratio,
ch_div=ch_div,
act_layer=act_layer,
dw_act_layer=dw_act_layer,
drop_path=drop_path,
))
curr_stride *= stride
dilation = next_dilation
prev_chs = chs
feat_chs += [features[-1].feat_channels()]
pen_chs = make_divisible(1280 * width_mult, divisor=ch_div)
feature_info += [dict(num_chs=feat_chs[-1], reduction=curr_stride, module=f'features.{len(features) - 1}')]
features.append(ConvNormAct(prev_chs, pen_chs, act_layer=act_layer))
return features, feature_info
class RexNet(nn.Module):
def __init__(
self,
in_chans=3,
num_classes=1000,
global_pool='avg',
output_stride=32,
initial_chs=16,
final_chs=180,
width_mult=1.0,
depth_mult=1.0,
se_ratio=1/12.,
ch_div=1,
act_layer='swish',
dw_act_layer='relu6',
drop_rate=0.2,
drop_path_rate=0.,
):
super(RexNet, self).__init__()
self.num_classes = num_classes
self.drop_rate = drop_rate
self.grad_checkpointing = False
assert output_stride in (32, 16, 8)
stem_base_chs = 32 / width_mult if width_mult < 1.0 else 32
stem_chs = make_divisible(round(stem_base_chs * width_mult), divisor=ch_div)
self.stem = ConvNormAct(in_chans, stem_chs, 3, stride=2, act_layer=act_layer)
block_cfg = _block_cfg(width_mult, depth_mult, initial_chs, final_chs, se_ratio, ch_div)
features, self.feature_info = _build_blocks(
block_cfg,
stem_chs,
width_mult,
ch_div,
output_stride,
act_layer,
dw_act_layer,
drop_path_rate,
)
self.num_features = features[-1].out_channels
self.features = nn.Sequential(*features)
self.head = ClassifierHead(self.num_features, num_classes, global_pool, drop_rate)
efficientnet_init_weights(self)
@torch.jit.ignore
def group_matcher(self, coarse=False):
matcher = dict(
stem=r'^stem',
blocks=r'^features\.(\d+)',
)
return matcher
@torch.jit.ignore
def set_grad_checkpointing(self, enable=True):
self.grad_checkpointing = enable
@torch.jit.ignore
def get_classifier(self):
return self.head.fc
def reset_classifier(self, num_classes, global_pool='avg'):
self.head = ClassifierHead(self.num_features, num_classes, pool_type=global_pool, drop_rate=self.drop_rate)
def forward_features(self, x):
x = self.stem(x)
if self.grad_checkpointing and not torch.jit.is_scripting():
x = checkpoint_seq(self.features, x, flatten=True)
else:
x = self.features(x)
return x
def forward_head(self, x, pre_logits: bool = False):
return self.head(x, pre_logits=pre_logits) if pre_logits else self.head(x)
def forward(self, x):
x = self.forward_features(x)
x = self.forward_head(x)
return x
def _create_rexnet(variant, pretrained, **kwargs):
feature_cfg = dict(flatten_sequential=True)
return build_model_with_cfg(
RexNet,
variant,
pretrained,
feature_cfg=feature_cfg,
**kwargs,
)
def _cfg(url='', **kwargs):
return {
'url': url, 'num_classes': 1000, 'input_size': (3, 224, 224), 'pool_size': (7, 7),
'crop_pct': 0.875, 'interpolation': 'bicubic',
'mean': IMAGENET_DEFAULT_MEAN, 'std': IMAGENET_DEFAULT_STD,
'first_conv': 'stem.conv', 'classifier': 'head.fc',
'license': 'mit', **kwargs
}
default_cfgs = generate_default_cfgs({
'rexnet_100.nav_in1k': _cfg(hf_hub_id='timm/'),
'rexnet_130.nav_in1k': _cfg(hf_hub_id='timm/'),
'rexnet_150.nav_in1k': _cfg(hf_hub_id='timm/'),
'rexnet_200.nav_in1k': _cfg(hf_hub_id='timm/'),
'rexnet_300.nav_in1k': _cfg(hf_hub_id='timm/'),
'rexnetr_100.untrained': _cfg(),
'rexnetr_130.untrained': _cfg(),
'rexnetr_150.untrained': _cfg(),
'rexnetr_200.sw_in12k_ft_in1k': _cfg(
hf_hub_id='timm/',
crop_pct=0.95, test_crop_pct=1.0, test_input_size=(3, 288, 288), license='apache-2.0'),
'rexnetr_300.sw_in12k_ft_in1k': _cfg(
hf_hub_id='timm/',
crop_pct=0.95, test_crop_pct=1.0, test_input_size=(3, 288, 288), license='apache-2.0'),
'rexnetr_200.sw_in12k': _cfg(
hf_hub_id='timm/',
num_classes=11821,
crop_pct=0.95, test_crop_pct=1.0, test_input_size=(3, 288, 288), license='apache-2.0'),
'rexnetr_300.sw_in12k': _cfg(
hf_hub_id='timm/',
num_classes=11821,
crop_pct=0.95, test_crop_pct=1.0, test_input_size=(3, 288, 288), license='apache-2.0'),
})
@register_model
def rexnet_100(pretrained=False, **kwargs) -> RexNet:
"""ReXNet V1 1.0x"""
return _create_rexnet('rexnet_100', pretrained, **kwargs)
@register_model
def rexnet_130(pretrained=False, **kwargs) -> RexNet:
"""ReXNet V1 1.3x"""
return _create_rexnet('rexnet_130', pretrained, width_mult=1.3, **kwargs)
@register_model
def rexnet_150(pretrained=False, **kwargs) -> RexNet:
"""ReXNet V1 1.5x"""
return _create_rexnet('rexnet_150', pretrained, width_mult=1.5, **kwargs)
@register_model
def rexnet_200(pretrained=False, **kwargs) -> RexNet:
"""ReXNet V1 2.0x"""
return _create_rexnet('rexnet_200', pretrained, width_mult=2.0, **kwargs)
@register_model
def rexnet_300(pretrained=False, **kwargs) -> RexNet:
"""ReXNet V1 3.0x"""
return _create_rexnet('rexnet_300', pretrained, width_mult=3.0, **kwargs)
@register_model
def rexnetr_100(pretrained=False, **kwargs) -> RexNet:
"""ReXNet V1 1.0x w/ rounded (mod 8) channels"""
return _create_rexnet('rexnetr_100', pretrained, ch_div=8, **kwargs)
@register_model
def rexnetr_130(pretrained=False, **kwargs) -> RexNet:
"""ReXNet V1 1.3x w/ rounded (mod 8) channels"""
return _create_rexnet('rexnetr_130', pretrained, width_mult=1.3, ch_div=8, **kwargs)
@register_model
def rexnetr_150(pretrained=False, **kwargs) -> RexNet:
"""ReXNet V1 1.5x w/ rounded (mod 8) channels"""
return _create_rexnet('rexnetr_150', pretrained, width_mult=1.5, ch_div=8, **kwargs)
@register_model
def rexnetr_200(pretrained=False, **kwargs) -> RexNet:
"""ReXNet V1 2.0x w/ rounded (mod 8) channels"""
return _create_rexnet('rexnetr_200', pretrained, width_mult=2.0, ch_div=8, **kwargs)
@register_model
def rexnetr_300(pretrained=False, **kwargs) -> RexNet:
"""ReXNet V1 3.0x w/ rounded (mod 16) channels"""
return _create_rexnet('rexnetr_300', pretrained, width_mult=3.0, ch_div=16, **kwargs)
| pytorch-image-models/timm/models/rexnet.py/0 | {
"file_path": "pytorch-image-models/timm/models/rexnet.py",
"repo_id": "pytorch-image-models",
"token_count": 5784
} | 199 |
""" Relative Position Vision Transformer (ViT) in PyTorch
NOTE: these models are experimental / WIP, expect changes
Hacked together by / Copyright 2022, Ross Wightman
"""
import logging
import math
from functools import partial
from typing import Optional, Tuple, Type, Union
try:
from typing import Literal
except ImportError:
from typing_extensions import Literal
import torch
import torch.nn as nn
from torch.jit import Final
from torch.utils.checkpoint import checkpoint
from timm.data import IMAGENET_INCEPTION_MEAN, IMAGENET_INCEPTION_STD
from timm.layers import PatchEmbed, Mlp, DropPath, RelPosMlp, RelPosBias, use_fused_attn, LayerType
from ._builder import build_model_with_cfg
from ._manipulate import named_apply
from ._registry import generate_default_cfgs, register_model
from .vision_transformer import get_init_weights_vit
__all__ = ['VisionTransformerRelPos'] # model_registry will add each entrypoint fn to this
_logger = logging.getLogger(__name__)
class RelPosAttention(nn.Module):
fused_attn: Final[bool]
def __init__(
self,
dim,
num_heads=8,
qkv_bias=False,
qk_norm=False,
rel_pos_cls=None,
attn_drop=0.,
proj_drop=0.,
norm_layer=nn.LayerNorm,
):
super().__init__()
assert dim % num_heads == 0, 'dim should be divisible by num_heads'
self.num_heads = num_heads
self.head_dim = dim // num_heads
self.scale = self.head_dim ** -0.5
self.fused_attn = use_fused_attn()
self.qkv = nn.Linear(dim, dim * 3, bias=qkv_bias)
self.q_norm = norm_layer(self.head_dim) if qk_norm else nn.Identity()
self.k_norm = norm_layer(self.head_dim) if qk_norm else nn.Identity()
self.rel_pos = rel_pos_cls(num_heads=num_heads) if rel_pos_cls else None
self.attn_drop = nn.Dropout(attn_drop)
self.proj = nn.Linear(dim, dim)
self.proj_drop = nn.Dropout(proj_drop)
def forward(self, x, shared_rel_pos: Optional[torch.Tensor] = None):
B, N, C = x.shape
qkv = self.qkv(x).reshape(B, N, 3, self.num_heads, self.head_dim).permute(2, 0, 3, 1, 4)
q, k, v = qkv.unbind(0)
q = self.q_norm(q)
k = self.k_norm(k)
if self.fused_attn:
if self.rel_pos is not None:
attn_bias = self.rel_pos.get_bias()
elif shared_rel_pos is not None:
attn_bias = shared_rel_pos
else:
attn_bias = None
x = torch.nn.functional.scaled_dot_product_attention(
q, k, v,
attn_mask=attn_bias,
dropout_p=self.attn_drop.p if self.training else 0.,
)
else:
q = q * self.scale
attn = q @ k.transpose(-2, -1)
if self.rel_pos is not None:
attn = self.rel_pos(attn, shared_rel_pos=shared_rel_pos)
elif shared_rel_pos is not None:
attn = attn + shared_rel_pos
attn = attn.softmax(dim=-1)
attn = self.attn_drop(attn)
x = attn @ v
x = x.transpose(1, 2).reshape(B, N, C)
x = self.proj(x)
x = self.proj_drop(x)
return x
class LayerScale(nn.Module):
def __init__(self, dim, init_values=1e-5, inplace=False):
super().__init__()
self.inplace = inplace
self.gamma = nn.Parameter(init_values * torch.ones(dim))
def forward(self, x):
return x.mul_(self.gamma) if self.inplace else x * self.gamma
class RelPosBlock(nn.Module):
def __init__(
self,
dim,
num_heads,
mlp_ratio=4.,
qkv_bias=False,
qk_norm=False,
rel_pos_cls=None,
init_values=None,
proj_drop=0.,
attn_drop=0.,
drop_path=0.,
act_layer=nn.GELU,
norm_layer=nn.LayerNorm,
):
super().__init__()
self.norm1 = norm_layer(dim)
self.attn = RelPosAttention(
dim,
num_heads,
qkv_bias=qkv_bias,
qk_norm=qk_norm,
rel_pos_cls=rel_pos_cls,
attn_drop=attn_drop,
proj_drop=proj_drop,
)
self.ls1 = LayerScale(dim, init_values=init_values) if init_values else nn.Identity()
# NOTE: drop path for stochastic depth, we shall see if this is better than dropout here
self.drop_path1 = DropPath(drop_path) if drop_path > 0. else nn.Identity()
self.norm2 = norm_layer(dim)
self.mlp = Mlp(
in_features=dim,
hidden_features=int(dim * mlp_ratio),
act_layer=act_layer,
drop=proj_drop,
)
self.ls2 = LayerScale(dim, init_values=init_values) if init_values else nn.Identity()
self.drop_path2 = DropPath(drop_path) if drop_path > 0. else nn.Identity()
def forward(self, x, shared_rel_pos: Optional[torch.Tensor] = None):
x = x + self.drop_path1(self.ls1(self.attn(self.norm1(x), shared_rel_pos=shared_rel_pos)))
x = x + self.drop_path2(self.ls2(self.mlp(self.norm2(x))))
return x
class ResPostRelPosBlock(nn.Module):
def __init__(
self,
dim,
num_heads,
mlp_ratio=4.,
qkv_bias=False,
qk_norm=False,
rel_pos_cls=None,
init_values=None,
proj_drop=0.,
attn_drop=0.,
drop_path=0.,
act_layer=nn.GELU,
norm_layer=nn.LayerNorm,
):
super().__init__()
self.init_values = init_values
self.attn = RelPosAttention(
dim,
num_heads,
qkv_bias=qkv_bias,
qk_norm=qk_norm,
rel_pos_cls=rel_pos_cls,
attn_drop=attn_drop,
proj_drop=proj_drop,
)
self.norm1 = norm_layer(dim)
self.drop_path1 = DropPath(drop_path) if drop_path > 0. else nn.Identity()
self.mlp = Mlp(
in_features=dim,
hidden_features=int(dim * mlp_ratio),
act_layer=act_layer,
drop=proj_drop,
)
self.norm2 = norm_layer(dim)
self.drop_path2 = DropPath(drop_path) if drop_path > 0. else nn.Identity()
self.init_weights()
def init_weights(self):
# NOTE this init overrides that base model init with specific changes for the block type
if self.init_values is not None:
nn.init.constant_(self.norm1.weight, self.init_values)
nn.init.constant_(self.norm2.weight, self.init_values)
def forward(self, x, shared_rel_pos: Optional[torch.Tensor] = None):
x = x + self.drop_path1(self.norm1(self.attn(x, shared_rel_pos=shared_rel_pos)))
x = x + self.drop_path2(self.norm2(self.mlp(x)))
return x
class VisionTransformerRelPos(nn.Module):
""" Vision Transformer w/ Relative Position Bias
Differing from classic vit, this impl
* uses relative position index (swin v1 / beit) or relative log coord + mlp (swin v2) pos embed
* defaults to no class token (can be enabled)
* defaults to global avg pool for head (can be changed)
* layer-scale (residual branch gain) enabled
"""
def __init__(
self,
img_size: Union[int, Tuple[int, int]] = 224,
patch_size: Union[int, Tuple[int, int]] = 16,
in_chans: int = 3,
num_classes: int = 1000,
global_pool: Literal['', 'avg', 'token', 'map'] = 'avg',
embed_dim: int = 768,
depth: int = 12,
num_heads: int = 12,
mlp_ratio: float = 4.,
qkv_bias: bool = True,
qk_norm: bool = False,
init_values: Optional[float] = 1e-6,
class_token: bool = False,
fc_norm: bool = False,
rel_pos_type: str = 'mlp',
rel_pos_dim: Optional[int] = None,
shared_rel_pos: bool = False,
drop_rate: float = 0.,
proj_drop_rate: float = 0.,
attn_drop_rate: float = 0.,
drop_path_rate: float = 0.,
weight_init: Literal['skip', 'jax', 'moco', ''] = 'skip',
fix_init: bool = False,
embed_layer: Type[nn.Module] = PatchEmbed,
norm_layer: Optional[LayerType] = None,
act_layer: Optional[LayerType] = None,
block_fn: Type[nn.Module] = RelPosBlock
):
"""
Args:
img_size: input image size
patch_size: patch size
in_chans: number of input channels
num_classes: number of classes for classification head
global_pool: type of global pooling for final sequence (default: 'avg')
embed_dim: embedding dimension
depth: depth of transformer
num_heads: number of attention heads
mlp_ratio: ratio of mlp hidden dim to embedding dim
qkv_bias: enable bias for qkv if True
qk_norm: Enable normalization of query and key in attention
init_values: layer-scale init values
class_token: use class token (default: False)
fc_norm: use pre classifier norm instead of pre-pool
rel_pos_type: type of relative position
shared_rel_pos: share relative pos across all blocks
drop_rate: dropout rate
proj_drop_rate: projection dropout rate
attn_drop_rate: attention dropout rate
drop_path_rate: stochastic depth rate
weight_init: weight init scheme
fix_init: apply weight initialization fix (scaling w/ layer index)
embed_layer: patch embedding layer
norm_layer: normalization layer
act_layer: MLP activation layer
"""
super().__init__()
assert global_pool in ('', 'avg', 'token')
assert class_token or global_pool != 'token'
norm_layer = norm_layer or partial(nn.LayerNorm, eps=1e-6)
act_layer = act_layer or nn.GELU
self.num_classes = num_classes
self.global_pool = global_pool
self.num_features = self.embed_dim = embed_dim # num_features for consistency with other models
self.num_prefix_tokens = 1 if class_token else 0
self.grad_checkpointing = False
self.patch_embed = embed_layer(
img_size=img_size,
patch_size=patch_size,
in_chans=in_chans,
embed_dim=embed_dim,
)
feat_size = self.patch_embed.grid_size
rel_pos_args = dict(window_size=feat_size, prefix_tokens=self.num_prefix_tokens)
if rel_pos_type.startswith('mlp'):
if rel_pos_dim:
rel_pos_args['hidden_dim'] = rel_pos_dim
if 'swin' in rel_pos_type:
rel_pos_args['mode'] = 'swin'
rel_pos_cls = partial(RelPosMlp, **rel_pos_args)
else:
rel_pos_cls = partial(RelPosBias, **rel_pos_args)
self.shared_rel_pos = None
if shared_rel_pos:
self.shared_rel_pos = rel_pos_cls(num_heads=num_heads)
# NOTE shared rel pos currently mutually exclusive w/ per-block, but could support both...
rel_pos_cls = None
self.cls_token = nn.Parameter(torch.zeros(1, self.num_prefix_tokens, embed_dim)) if class_token else None
dpr = [x.item() for x in torch.linspace(0, drop_path_rate, depth)] # stochastic depth decay rule
self.blocks = nn.ModuleList([
block_fn(
dim=embed_dim,
num_heads=num_heads,
mlp_ratio=mlp_ratio,
qkv_bias=qkv_bias,
qk_norm=qk_norm,
rel_pos_cls=rel_pos_cls,
init_values=init_values,
proj_drop=proj_drop_rate,
attn_drop=attn_drop_rate,
drop_path=dpr[i],
norm_layer=norm_layer,
act_layer=act_layer,
)
for i in range(depth)])
self.norm = norm_layer(embed_dim) if not fc_norm else nn.Identity()
# Classifier Head
self.fc_norm = norm_layer(embed_dim) if fc_norm else nn.Identity()
self.head_drop = nn.Dropout(drop_rate)
self.head = nn.Linear(self.embed_dim, num_classes) if num_classes > 0 else nn.Identity()
if weight_init != 'skip':
self.init_weights(weight_init)
if fix_init:
self.fix_init_weight()
def init_weights(self, mode=''):
assert mode in ('jax', 'moco', '')
if self.cls_token is not None:
nn.init.normal_(self.cls_token, std=1e-6)
named_apply(get_init_weights_vit(mode), self)
def fix_init_weight(self):
def rescale(param, _layer_id):
param.div_(math.sqrt(2.0 * _layer_id))
for layer_id, layer in enumerate(self.blocks):
rescale(layer.attn.proj.weight.data, layer_id + 1)
rescale(layer.mlp.fc2.weight.data, layer_id + 1)
@torch.jit.ignore
def no_weight_decay(self):
return {'cls_token'}
@torch.jit.ignore
def group_matcher(self, coarse=False):
return dict(
stem=r'^cls_token|patch_embed', # stem and embed
blocks=[(r'^blocks\.(\d+)', None), (r'^norm', (99999,))]
)
@torch.jit.ignore
def set_grad_checkpointing(self, enable=True):
self.grad_checkpointing = enable
@torch.jit.ignore
def get_classifier(self):
return self.head
def reset_classifier(self, num_classes: int, global_pool=None):
self.num_classes = num_classes
if global_pool is not None:
assert global_pool in ('', 'avg', 'token')
self.global_pool = global_pool
self.head = nn.Linear(self.embed_dim, num_classes) if num_classes > 0 else nn.Identity()
def forward_features(self, x):
x = self.patch_embed(x)
if self.cls_token is not None:
x = torch.cat((self.cls_token.expand(x.shape[0], -1, -1), x), dim=1)
shared_rel_pos = self.shared_rel_pos.get_bias() if self.shared_rel_pos is not None else None
for blk in self.blocks:
if self.grad_checkpointing and not torch.jit.is_scripting():
x = checkpoint(blk, x, shared_rel_pos=shared_rel_pos)
else:
x = blk(x, shared_rel_pos=shared_rel_pos)
x = self.norm(x)
return x
def forward_head(self, x, pre_logits: bool = False):
if self.global_pool:
x = x[:, self.num_prefix_tokens:].mean(dim=1) if self.global_pool == 'avg' else x[:, 0]
x = self.fc_norm(x)
x = self.head_drop(x)
return x if pre_logits else self.head(x)
def forward(self, x):
x = self.forward_features(x)
x = self.forward_head(x)
return x
def _create_vision_transformer_relpos(variant, pretrained=False, **kwargs):
if kwargs.get('features_only', None):
raise RuntimeError('features_only not implemented for Vision Transformer models.')
model = build_model_with_cfg(VisionTransformerRelPos, variant, pretrained, **kwargs)
return model
def _cfg(url='', **kwargs):
return {
'url': url,
'num_classes': 1000, 'input_size': (3, 224, 224), 'pool_size': None,
'crop_pct': .9, 'interpolation': 'bicubic', 'fixed_input_size': True,
'mean': IMAGENET_INCEPTION_MEAN, 'std': IMAGENET_INCEPTION_STD,
'first_conv': 'patch_embed.proj', 'classifier': 'head',
**kwargs
}
default_cfgs = generate_default_cfgs({
'vit_relpos_base_patch32_plus_rpn_256.sw_in1k': _cfg(
url='https://github.com/rwightman/pytorch-image-models/releases/download/v0.1-tpu-weights/vit_replos_base_patch32_plus_rpn_256-sw-dd486f51.pth',
hf_hub_id='timm/',
input_size=(3, 256, 256)),
'vit_relpos_base_patch16_plus_240.untrained': _cfg(url='', input_size=(3, 240, 240)),
'vit_relpos_small_patch16_224.sw_in1k': _cfg(
url='https://github.com/rwightman/pytorch-image-models/releases/download/v0.1-tpu-weights/vit_relpos_small_patch16_224-sw-ec2778b4.pth',
hf_hub_id='timm/'),
'vit_relpos_medium_patch16_224.sw_in1k': _cfg(
url='https://github.com/rwightman/pytorch-image-models/releases/download/v0.1-tpu-weights/vit_relpos_medium_patch16_224-sw-11c174af.pth',
hf_hub_id='timm/'),
'vit_relpos_base_patch16_224.sw_in1k': _cfg(
url='https://github.com/rwightman/pytorch-image-models/releases/download/v0.1-tpu-weights/vit_relpos_base_patch16_224-sw-49049aed.pth',
hf_hub_id='timm/'),
'vit_srelpos_small_patch16_224.sw_in1k': _cfg(
url='https://github.com/rwightman/pytorch-image-models/releases/download/v0.1-tpu-weights/vit_srelpos_small_patch16_224-sw-6cdb8849.pth',
hf_hub_id='timm/'),
'vit_srelpos_medium_patch16_224.sw_in1k': _cfg(
url='https://github.com/rwightman/pytorch-image-models/releases/download/v0.1-tpu-weights/vit_srelpos_medium_patch16_224-sw-ad702b8c.pth',
hf_hub_id='timm/'),
'vit_relpos_medium_patch16_cls_224.sw_in1k': _cfg(
url='https://github.com/rwightman/pytorch-image-models/releases/download/v0.1-tpu-weights/vit_relpos_medium_patch16_cls_224-sw-cfe8e259.pth',
hf_hub_id='timm/'),
'vit_relpos_base_patch16_cls_224.untrained': _cfg(),
'vit_relpos_base_patch16_clsgap_224.sw_in1k': _cfg(
url='https://github.com/rwightman/pytorch-image-models/releases/download/v0.1-tpu-weights/vit_relpos_base_patch16_gapcls_224-sw-1a341d6c.pth',
hf_hub_id='timm/'),
'vit_relpos_small_patch16_rpn_224.untrained': _cfg(),
'vit_relpos_medium_patch16_rpn_224.sw_in1k': _cfg(
url='https://github.com/rwightman/pytorch-image-models/releases/download/v0.1-tpu-weights/vit_relpos_medium_patch16_rpn_224-sw-5d2befd8.pth',
hf_hub_id='timm/'),
'vit_relpos_base_patch16_rpn_224.untrained': _cfg(),
})
@register_model
def vit_relpos_base_patch32_plus_rpn_256(pretrained=False, **kwargs) -> VisionTransformerRelPos:
""" ViT-Base (ViT-B/32+) w/ relative log-coord position and residual post-norm, no class token
"""
model_args = dict(patch_size=32, embed_dim=896, depth=12, num_heads=14, block_fn=ResPostRelPosBlock)
model = _create_vision_transformer_relpos(
'vit_relpos_base_patch32_plus_rpn_256', pretrained=pretrained, **dict(model_args, **kwargs))
return model
@register_model
def vit_relpos_base_patch16_plus_240(pretrained=False, **kwargs) -> VisionTransformerRelPos:
""" ViT-Base (ViT-B/16+) w/ relative log-coord position, no class token
"""
model_args = dict(patch_size=16, embed_dim=896, depth=12, num_heads=14)
model = _create_vision_transformer_relpos(
'vit_relpos_base_patch16_plus_240', pretrained=pretrained, **dict(model_args, **kwargs))
return model
@register_model
def vit_relpos_small_patch16_224(pretrained=False, **kwargs) -> VisionTransformerRelPos:
""" ViT-Base (ViT-B/16) w/ relative log-coord position, no class token
"""
model_args = dict(patch_size=16, embed_dim=384, depth=12, num_heads=6, qkv_bias=False, fc_norm=True)
model = _create_vision_transformer_relpos(
'vit_relpos_small_patch16_224', pretrained=pretrained, **dict(model_args, **kwargs))
return model
@register_model
def vit_relpos_medium_patch16_224(pretrained=False, **kwargs) -> VisionTransformerRelPos:
""" ViT-Base (ViT-B/16) w/ relative log-coord position, no class token
"""
model_args = dict(
patch_size=16, embed_dim=512, depth=12, num_heads=8, qkv_bias=False, fc_norm=True)
model = _create_vision_transformer_relpos(
'vit_relpos_medium_patch16_224', pretrained=pretrained, **dict(model_args, **kwargs))
return model
@register_model
def vit_relpos_base_patch16_224(pretrained=False, **kwargs) -> VisionTransformerRelPos:
""" ViT-Base (ViT-B/16) w/ relative log-coord position, no class token
"""
model_args = dict(
patch_size=16, embed_dim=768, depth=12, num_heads=12, qkv_bias=False, fc_norm=True)
model = _create_vision_transformer_relpos(
'vit_relpos_base_patch16_224', pretrained=pretrained, **dict(model_args, **kwargs))
return model
@register_model
def vit_srelpos_small_patch16_224(pretrained=False, **kwargs) -> VisionTransformerRelPos:
""" ViT-Base (ViT-B/16) w/ shared relative log-coord position, no class token
"""
model_args = dict(
patch_size=16, embed_dim=384, depth=12, num_heads=6, qkv_bias=False, fc_norm=False,
rel_pos_dim=384, shared_rel_pos=True)
model = _create_vision_transformer_relpos(
'vit_srelpos_small_patch16_224', pretrained=pretrained, **dict(model_args, **kwargs))
return model
@register_model
def vit_srelpos_medium_patch16_224(pretrained=False, **kwargs) -> VisionTransformerRelPos:
""" ViT-Base (ViT-B/16) w/ shared relative log-coord position, no class token
"""
model_args = dict(
patch_size=16, embed_dim=512, depth=12, num_heads=8, qkv_bias=False, fc_norm=False,
rel_pos_dim=512, shared_rel_pos=True)
model = _create_vision_transformer_relpos(
'vit_srelpos_medium_patch16_224', pretrained=pretrained, **dict(model_args, **kwargs))
return model
@register_model
def vit_relpos_medium_patch16_cls_224(pretrained=False, **kwargs) -> VisionTransformerRelPos:
""" ViT-Base (ViT-M/16) w/ relative log-coord position, class token present
"""
model_args = dict(
patch_size=16, embed_dim=512, depth=12, num_heads=8, qkv_bias=False, fc_norm=False,
rel_pos_dim=256, class_token=True, global_pool='token')
model = _create_vision_transformer_relpos(
'vit_relpos_medium_patch16_cls_224', pretrained=pretrained, **dict(model_args, **kwargs))
return model
@register_model
def vit_relpos_base_patch16_cls_224(pretrained=False, **kwargs) -> VisionTransformerRelPos:
""" ViT-Base (ViT-B/16) w/ relative log-coord position, class token present
"""
model_args = dict(
patch_size=16, embed_dim=768, depth=12, num_heads=12, qkv_bias=False, class_token=True, global_pool='token')
model = _create_vision_transformer_relpos(
'vit_relpos_base_patch16_cls_224', pretrained=pretrained, **dict(model_args, **kwargs))
return model
@register_model
def vit_relpos_base_patch16_clsgap_224(pretrained=False, **kwargs) -> VisionTransformerRelPos:
""" ViT-Base (ViT-B/16) w/ relative log-coord position, class token present
NOTE this config is a bit of a mistake, class token was enabled but global avg-pool w/ fc-norm was not disabled
Leaving here for comparisons w/ a future re-train as it performs quite well.
"""
model_args = dict(
patch_size=16, embed_dim=768, depth=12, num_heads=12, qkv_bias=False, fc_norm=True, class_token=True)
model = _create_vision_transformer_relpos(
'vit_relpos_base_patch16_clsgap_224', pretrained=pretrained, **dict(model_args, **kwargs))
return model
@register_model
def vit_relpos_small_patch16_rpn_224(pretrained=False, **kwargs) -> VisionTransformerRelPos:
""" ViT-Base (ViT-B/16) w/ relative log-coord position and residual post-norm, no class token
"""
model_args = dict(
patch_size=16, embed_dim=384, depth=12, num_heads=6, qkv_bias=False, block_fn=ResPostRelPosBlock)
model = _create_vision_transformer_relpos(
'vit_relpos_small_patch16_rpn_224', pretrained=pretrained, **dict(model_args, **kwargs))
return model
@register_model
def vit_relpos_medium_patch16_rpn_224(pretrained=False, **kwargs) -> VisionTransformerRelPos:
""" ViT-Base (ViT-B/16) w/ relative log-coord position and residual post-norm, no class token
"""
model_args = dict(
patch_size=16, embed_dim=512, depth=12, num_heads=8, qkv_bias=False, block_fn=ResPostRelPosBlock)
model = _create_vision_transformer_relpos(
'vit_relpos_medium_patch16_rpn_224', pretrained=pretrained, **dict(model_args, **kwargs))
return model
@register_model
def vit_relpos_base_patch16_rpn_224(pretrained=False, **kwargs) -> VisionTransformerRelPos:
""" ViT-Base (ViT-B/16) w/ relative log-coord position and residual post-norm, no class token
"""
model_args = dict(
patch_size=16, embed_dim=768, depth=12, num_heads=12, qkv_bias=False, block_fn=ResPostRelPosBlock)
model = _create_vision_transformer_relpos(
'vit_relpos_base_patch16_rpn_224', pretrained=pretrained, **dict(model_args, **kwargs))
return model
| pytorch-image-models/timm/models/vision_transformer_relpos.py/0 | {
"file_path": "pytorch-image-models/timm/models/vision_transformer_relpos.py",
"repo_id": "pytorch-image-models",
"token_count": 11608
} | 200 |
""" Lion Optimizer
Paper: `Symbolic Discovery of Optimization Algorithms` - https://arxiv.org/abs/2302.06675
Original Impl: https://github.com/google/automl/tree/master/lion
"""
# Copyright 2023 Google Research. All Rights Reserved.
#
# Licensed under the Apache License, Version 2.0 (the "License");
# you may not use this file except in compliance with the License.
# You may obtain a copy of the License at
#
# http://www.apache.org/licenses/LICENSE-2.0
#
# Unless required by applicable law or agreed to in writing, software
# distributed under the License is distributed on an "AS IS" BASIS,
# WITHOUT WARRANTIES OR CONDITIONS OF ANY KIND, either express or implied.
# See the License for the specific language governing permissions and
# limitations under the License.
# ==============================================================================
from typing import List
import torch
from torch.optim.optimizer import Optimizer
class Lion(Optimizer):
r"""Implements Lion algorithm."""
def __init__(
self,
params,
lr=1e-4,
betas=(0.9, 0.99),
weight_decay=0.0,
maximize=False,
foreach=None,
):
"""Initialize the hyperparameters.
Args:
params (iterable): iterable of parameters to optimize or dicts defining
parameter groups
lr (float, optional): learning rate (default: 1e-4)
betas (Tuple[float, float], optional): coefficients used for computing
running averages of gradient and its square (default: (0.9, 0.99))
weight_decay (float, optional): weight decay coefficient (default: 0)
"""
if not 0.0 <= lr:
raise ValueError('Invalid learning rate: {}'.format(lr))
if not 0.0 <= betas[0] < 1.0:
raise ValueError('Invalid beta parameter at index 0: {}'.format(betas[0]))
if not 0.0 <= betas[1] < 1.0:
raise ValueError('Invalid beta parameter at index 1: {}'.format(betas[1]))
defaults = dict(
lr=lr,
betas=betas,
weight_decay=weight_decay,
foreach=foreach,
maximize=maximize,
)
super().__init__(params, defaults)
def __setstate__(self, state):
super().__setstate__(state)
for group in self.param_groups:
group.setdefault('maximize', False)
group.setdefault('foreach', None)
@torch.no_grad()
def step(self, closure=None):
"""Performs a single optimization step.
Args:
closure (callable, optional): A closure that reevaluates the model
and returns the loss.
Returns:
the loss.
"""
loss = None
if closure is not None:
with torch.enable_grad():
loss = closure()
for group in self.param_groups:
params_with_grad = []
grads = []
exp_avgs = []
beta1, beta2 = group['betas']
for p in group['params']:
if p.grad is None:
continue
params_with_grad.append(p)
if p.grad.is_sparse:
raise RuntimeError('Lion does not support sparse gradients')
grads.append(p.grad)
state = self.state[p]
# State initialization
if len(state) == 0:
state['exp_avg'] = torch.zeros_like(p, memory_format=torch.preserve_format)
exp_avgs.append(state['exp_avg'])
lion(
params_with_grad,
grads,
exp_avgs,
beta1=beta1,
beta2=beta2,
lr=group['lr'],
weight_decay=group['weight_decay'],
maximize=group['maximize'],
foreach=group['foreach'],
)
return loss
def lion(
params: List[torch.Tensor],
grads: List[torch.Tensor],
exp_avgs: List[torch.Tensor],
# kwonly args with defaults are not supported by functions compiled with torchscript issue #70627
# setting this as kwarg for now as functional API is compiled by torch/distributed/optim
maximize: bool = False,
foreach: bool = None,
*,
beta1: float,
beta2: float,
lr: float,
weight_decay: float,
):
r"""Functional API that performs Lion algorithm computation.
"""
if foreach is None:
# Placeholder for more complex foreach logic to be added when value is not set
foreach = False
if foreach and torch.jit.is_scripting():
raise RuntimeError('torch.jit.script not supported with foreach optimizers')
if foreach and not torch.jit.is_scripting():
func = _multi_tensor_lion
else:
func = _single_tensor_lion
func(
params,
grads,
exp_avgs,
beta1=beta1,
beta2=beta2,
lr=lr,
weight_decay=weight_decay,
maximize=maximize,
)
def _single_tensor_lion(
params: List[torch.Tensor],
grads: List[torch.Tensor],
exp_avgs: List[torch.Tensor],
*,
beta1: float,
beta2: float,
lr: float,
weight_decay: float,
maximize: bool,
):
for i, param in enumerate(params):
grad = grads[i] if not maximize else -grads[i]
exp_avg = exp_avgs[i]
if torch.is_complex(param):
grad = torch.view_as_real(grad)
exp_avg = torch.view_as_real(exp_avg)
param = torch.view_as_real(param)
# Perform stepweight decay
param.mul_(1 - lr * weight_decay)
# Weight update
update = exp_avg.mul(beta1).add_(grad, alpha=1 - beta1)
param.add_(torch.sign(update), alpha=-lr)
# Decay the momentum running average coefficient
exp_avg.lerp_(grad, 1 - beta2)
def _multi_tensor_lion(
params: List[torch.Tensor],
grads: List[torch.Tensor],
exp_avgs: List[torch.Tensor],
*,
beta1: float,
beta2: float,
lr: float,
weight_decay: float,
maximize: bool,
):
if len(params) == 0:
return
if maximize:
grads = torch._foreach_neg(tuple(grads)) # type: ignore[assignment]
grads = [torch.view_as_real(x) if torch.is_complex(x) else x for x in grads]
exp_avgs = [torch.view_as_real(x) if torch.is_complex(x) else x for x in exp_avgs]
params = [torch.view_as_real(x) if torch.is_complex(x) else x for x in params]
# Perform stepweight decay
torch._foreach_mul_(params, 1 - lr * weight_decay)
# Weight update
updates = torch._foreach_mul(exp_avgs, beta1)
torch._foreach_add_(updates, grads, alpha=1 - beta1)
updates = [u.sign() for u in updates]
torch._foreach_add_(params, updates, alpha=-lr)
# Decay the momentum running average coefficient
torch._foreach_mul_(exp_avgs, beta2)
torch._foreach_add_(exp_avgs, grads, alpha=1 - beta2)
| pytorch-image-models/timm/optim/lion.py/0 | {
"file_path": "pytorch-image-models/timm/optim/lion.py",
"repo_id": "pytorch-image-models",
"token_count": 3257
} | 201 |
import abc
from abc import ABC
from typing import Any, Dict, Optional
import torch
class Scheduler(ABC):
""" Parameter Scheduler Base Class
A scheduler base class that can be used to schedule any optimizer parameter groups.
Unlike the builtin PyTorch schedulers, this is intended to be consistently called
* At the END of each epoch, before incrementing the epoch count, to calculate next epoch's value
* At the END of each optimizer update, after incrementing the update count, to calculate next update's value
The schedulers built on this should try to remain as stateless as possible (for simplicity).
This family of schedulers is attempting to avoid the confusion of the meaning of 'last_epoch'
and -1 values for special behaviour. All epoch and update counts must be tracked in the training
code and explicitly passed in to the schedulers on the corresponding step or step_update call.
Based on ideas from:
* https://github.com/pytorch/fairseq/tree/master/fairseq/optim/lr_scheduler
* https://github.com/allenai/allennlp/tree/master/allennlp/training/learning_rate_schedulers
"""
def __init__(
self,
optimizer: torch.optim.Optimizer,
param_group_field: str,
t_in_epochs: bool = True,
noise_range_t=None,
noise_type='normal',
noise_pct=0.67,
noise_std=1.0,
noise_seed=None,
initialize: bool = True,
) -> None:
self.optimizer = optimizer
self.param_group_field = param_group_field
self._initial_param_group_field = f"initial_{param_group_field}"
if initialize:
for i, group in enumerate(self.optimizer.param_groups):
if param_group_field not in group:
raise KeyError(f"{param_group_field} missing from param_groups[{i}]")
group.setdefault(self._initial_param_group_field, group[param_group_field])
else:
for i, group in enumerate(self.optimizer.param_groups):
if self._initial_param_group_field not in group:
raise KeyError(f"{self._initial_param_group_field} missing from param_groups[{i}]")
self.base_values = [group[self._initial_param_group_field] for group in self.optimizer.param_groups]
self.metric = None # any point to having this for all?
self.t_in_epochs = t_in_epochs
self.noise_range_t = noise_range_t
self.noise_pct = noise_pct
self.noise_type = noise_type
self.noise_std = noise_std
self.noise_seed = noise_seed if noise_seed is not None else 42
self.update_groups(self.base_values)
def state_dict(self) -> Dict[str, Any]:
return {key: value for key, value in self.__dict__.items() if key != 'optimizer'}
def load_state_dict(self, state_dict: Dict[str, Any]) -> None:
self.__dict__.update(state_dict)
@abc.abstractmethod
def _get_lr(self, t: int) -> float:
pass
def _get_values(self, t: int, on_epoch: bool = True) -> Optional[float]:
proceed = (on_epoch and self.t_in_epochs) or (not on_epoch and not self.t_in_epochs)
if not proceed:
return None
return self._get_lr(t)
def step(self, epoch: int, metric: float = None) -> None:
self.metric = metric
values = self._get_values(epoch, on_epoch=True)
if values is not None:
values = self._add_noise(values, epoch)
self.update_groups(values)
def step_update(self, num_updates: int, metric: float = None):
self.metric = metric
values = self._get_values(num_updates, on_epoch=False)
if values is not None:
values = self._add_noise(values, num_updates)
self.update_groups(values)
def update_groups(self, values):
if not isinstance(values, (list, tuple)):
values = [values] * len(self.optimizer.param_groups)
for param_group, value in zip(self.optimizer.param_groups, values):
if 'lr_scale' in param_group:
param_group[self.param_group_field] = value * param_group['lr_scale']
else:
param_group[self.param_group_field] = value
def _add_noise(self, lrs, t):
if self._is_apply_noise(t):
noise = self._calculate_noise(t)
lrs = [v + v * noise for v in lrs]
return lrs
def _is_apply_noise(self, t) -> bool:
"""Return True if scheduler in noise range."""
apply_noise = False
if self.noise_range_t is not None:
if isinstance(self.noise_range_t, (list, tuple)):
apply_noise = self.noise_range_t[0] <= t < self.noise_range_t[1]
else:
apply_noise = t >= self.noise_range_t
return apply_noise
def _calculate_noise(self, t) -> float:
g = torch.Generator()
g.manual_seed(self.noise_seed + t)
if self.noise_type == 'normal':
while True:
# resample if noise out of percent limit, brute force but shouldn't spin much
noise = torch.randn(1, generator=g).item()
if abs(noise) < self.noise_pct:
return noise
else:
noise = 2 * (torch.rand(1, generator=g).item() - 0.5) * self.noise_pct
return noise
| pytorch-image-models/timm/scheduler/scheduler.py/0 | {
"file_path": "pytorch-image-models/timm/scheduler/scheduler.py",
"repo_id": "pytorch-image-models",
"token_count": 2361
} | 202 |
""" Exponential Moving Average (EMA) of model updates
Hacked together by / Copyright 2020 Ross Wightman
"""
import logging
from collections import OrderedDict
from copy import deepcopy
from typing import Optional
import torch
import torch.nn as nn
_logger = logging.getLogger(__name__)
class ModelEma:
""" Model Exponential Moving Average (DEPRECATED)
Keep a moving average of everything in the model state_dict (parameters and buffers).
This version is deprecated, it does not work with scripted models. Will be removed eventually.
This is intended to allow functionality like
https://www.tensorflow.org/api_docs/python/tf/train/ExponentialMovingAverage
A smoothed version of the weights is necessary for some training schemes to perform well.
E.g. Google's hyper-params for training MNASNet, MobileNet-V3, EfficientNet, etc that use
RMSprop with a short 2.4-3 epoch decay period and slow LR decay rate of .96-.99 requires EMA
smoothing of weights to match results. Pay attention to the decay constant you are using
relative to your update count per epoch.
To keep EMA from using GPU resources, set device='cpu'. This will save a bit of memory but
disable validation of the EMA weights. Validation will have to be done manually in a separate
process, or after the training stops converging.
This class is sensitive where it is initialized in the sequence of model init,
GPU assignment and distributed training wrappers.
"""
def __init__(self, model, decay=0.9999, device='', resume=''):
# make a copy of the model for accumulating moving average of weights
self.ema = deepcopy(model)
self.ema.eval()
self.decay = decay
self.device = device # perform ema on different device from model if set
if device:
self.ema.to(device=device)
self.ema_has_module = hasattr(self.ema, 'module')
if resume:
self._load_checkpoint(resume)
for p in self.ema.parameters():
p.requires_grad_(False)
def _load_checkpoint(self, checkpoint_path):
checkpoint = torch.load(checkpoint_path, map_location='cpu')
assert isinstance(checkpoint, dict)
if 'state_dict_ema' in checkpoint:
new_state_dict = OrderedDict()
for k, v in checkpoint['state_dict_ema'].items():
# ema model may have been wrapped by DataParallel, and need module prefix
if self.ema_has_module:
name = 'module.' + k if not k.startswith('module') else k
else:
name = k
new_state_dict[name] = v
self.ema.load_state_dict(new_state_dict)
_logger.info("Loaded state_dict_ema")
else:
_logger.warning("Failed to find state_dict_ema, starting from loaded model weights")
def update(self, model):
# correct a mismatch in state dict keys
needs_module = hasattr(model, 'module') and not self.ema_has_module
with torch.no_grad():
msd = model.state_dict()
for k, ema_v in self.ema.state_dict().items():
if needs_module:
k = 'module.' + k
model_v = msd[k].detach()
if self.device:
model_v = model_v.to(device=self.device)
ema_v.copy_(ema_v * self.decay + (1. - self.decay) * model_v)
class ModelEmaV2(nn.Module):
""" Model Exponential Moving Average V2
Keep a moving average of everything in the model state_dict (parameters and buffers).
V2 of this module is simpler, it does not match params/buffers based on name but simply
iterates in order. It works with torchscript (JIT of full model).
This is intended to allow functionality like
https://www.tensorflow.org/api_docs/python/tf/train/ExponentialMovingAverage
A smoothed version of the weights is necessary for some training schemes to perform well.
E.g. Google's hyper-params for training MNASNet, MobileNet-V3, EfficientNet, etc that use
RMSprop with a short 2.4-3 epoch decay period and slow LR decay rate of .96-.99 requires EMA
smoothing of weights to match results. Pay attention to the decay constant you are using
relative to your update count per epoch.
To keep EMA from using GPU resources, set device='cpu'. This will save a bit of memory but
disable validation of the EMA weights. Validation will have to be done manually in a separate
process, or after the training stops converging.
This class is sensitive where it is initialized in the sequence of model init,
GPU assignment and distributed training wrappers.
"""
def __init__(self, model, decay=0.9999, device=None):
super().__init__()
# make a copy of the model for accumulating moving average of weights
self.module = deepcopy(model)
self.module.eval()
self.decay = decay
self.device = device # perform ema on different device from model if set
if self.device is not None:
self.module.to(device=device)
def _update(self, model, update_fn):
with torch.no_grad():
for ema_v, model_v in zip(self.module.state_dict().values(), model.state_dict().values()):
if self.device is not None:
model_v = model_v.to(device=self.device)
ema_v.copy_(update_fn(ema_v, model_v))
def update(self, model):
self._update(model, update_fn=lambda e, m: self.decay * e + (1. - self.decay) * m)
def set(self, model):
self._update(model, update_fn=lambda e, m: m)
def forward(self, *args, **kwargs):
return self.module(*args, **kwargs)
class ModelEmaV3(nn.Module):
""" Model Exponential Moving Average V3
Keep a moving average of everything in the model state_dict (parameters and buffers).
V3 of this module leverages for_each and in-place operations for faster performance.
Decay warmup based on code by @crowsonkb, her comments:
If inv_gamma=1 and power=1, implements a simple average. inv_gamma=1, power=2/3 are
good values for models you plan to train for a million or more steps (reaches decay
factor 0.999 at 31.6K steps, 0.9999 at 1M steps), inv_gamma=1, power=3/4 for models
you plan to train for less (reaches decay factor 0.999 at 10K steps, 0.9999 at
215.4k steps).
This is intended to allow functionality like
https://www.tensorflow.org/api_docs/python/tf/train/ExponentialMovingAverage
To keep EMA from using GPU resources, set device='cpu'. This will save a bit of memory but
disable validation of the EMA weights. Validation will have to be done manually in a separate
process, or after the training stops converging.
This class is sensitive where it is initialized in the sequence of model init,
GPU assignment and distributed training wrappers.
"""
def __init__(
self,
model,
decay: float = 0.9999,
min_decay: float = 0.0,
update_after_step: int = 0,
use_warmup: bool = False,
warmup_gamma: float = 1.0,
warmup_power: float = 2/3,
device: Optional[torch.device] = None,
foreach: bool = True,
exclude_buffers: bool = False,
):
super().__init__()
# make a copy of the model for accumulating moving average of weights
self.module = deepcopy(model)
self.module.eval()
self.decay = decay
self.min_decay = min_decay
self.update_after_step = update_after_step
self.use_warmup = use_warmup
self.warmup_gamma = warmup_gamma
self.warmup_power = warmup_power
self.foreach = foreach
self.device = device # perform ema on different device from model if set
self.exclude_buffers = exclude_buffers
if self.device is not None and device != next(model.parameters()).device:
self.foreach = False # cannot use foreach methods with different devices
self.module.to(device=device)
def get_decay(self, step: Optional[int] = None) -> float:
"""
Compute the decay factor for the exponential moving average.
"""
if step is None:
return self.decay
step = max(0, step - self.update_after_step - 1)
if step <= 0:
return 0.0
if self.use_warmup:
decay = 1 - (1 + step / self.warmup_gamma) ** -self.warmup_power
decay = max(min(decay, self.decay), self.min_decay)
else:
decay = self.decay
return decay
@torch.no_grad()
def update(self, model, step: Optional[int] = None):
decay = self.get_decay(step)
if self.exclude_buffers:
self.apply_update_no_buffers_(model, decay)
else:
self.apply_update_(model, decay)
def apply_update_(self, model, decay: float):
# interpolate parameters and buffers
if self.foreach:
ema_lerp_values = []
model_lerp_values = []
for ema_v, model_v in zip(self.module.state_dict().values(), model.state_dict().values()):
if ema_v.is_floating_point():
ema_lerp_values.append(ema_v)
model_lerp_values.append(model_v)
else:
ema_v.copy_(model_v)
if hasattr(torch, '_foreach_lerp_'):
torch._foreach_lerp_(ema_lerp_values, model_lerp_values, weight=1. - decay)
else:
torch._foreach_mul_(ema_lerp_values, scalar=decay)
torch._foreach_add_(ema_lerp_values, model_lerp_values, alpha=1. - decay)
else:
for ema_v, model_v in zip(self.module.state_dict().values(), model.state_dict().values()):
if ema_v.is_floating_point():
ema_v.lerp_(model_v, weight=1. - decay)
else:
ema_v.copy_(model_v)
def apply_update_no_buffers_(self, model, decay: float):
# interpolate parameters, copy buffers
ema_params = tuple(self.module.parameters())
model_params = tuple(model.parameters())
if self.foreach:
if hasattr(torch, '_foreach_lerp_'):
torch._foreach_lerp_(ema_params, model_params, weight=1. - decay)
else:
torch._foreach_mul_(ema_params, scalar=decay)
torch._foreach_add_(ema_params, model_params, alpha=1 - decay)
else:
for ema_p, model_p in zip(ema_params, model_params):
ema_p.lerp_(model_p, weight=1. - decay)
for ema_b, model_b in zip(self.module.buffers(), model.buffers()):
ema_b.copy_(model_b.to(device=self.device))
@torch.no_grad()
def set(self, model):
for ema_v, model_v in zip(self.module.state_dict().values(), model.state_dict().values()):
ema_v.copy_(model_v.to(device=self.device))
def forward(self, *args, **kwargs):
return self.module(*args, **kwargs) | pytorch-image-models/timm/utils/model_ema.py/0 | {
"file_path": "pytorch-image-models/timm/utils/model_ema.py",
"repo_id": "pytorch-image-models",
"token_count": 4590
} | 203 |
mod app;
mod event;
mod generation;
mod table;
mod utils;
use crate::app::App;
use crate::event::Event;
use crossterm::ExecutableCommand;
use std::io;
use text_generation_client::{GrammarType, NextTokenChooserParameters, ShardedClient};
use tokenizers::Tokenizer;
use tokio::sync::{broadcast, mpsc};
use tui::backend::CrosstermBackend;
use tui::Terminal;
/// Run benchmarking app
#[allow(clippy::too_many_arguments)]
pub async fn run(
tokenizer_name: String,
tokenizer: Tokenizer,
batch_size: Vec<u32>,
sequence_length: u32,
decode_length: u32,
top_n_tokens: Option<u32>,
n_runs: usize,
warmups: usize,
temperature: Option<f32>,
top_k: Option<u32>,
top_p: Option<f32>,
typical_p: Option<f32>,
repetition_penalty: Option<f32>,
frequency_penalty: Option<f32>,
watermark: bool,
do_sample: bool,
client: ShardedClient,
) -> Result<(), std::io::Error> {
let parameters = NextTokenChooserParameters {
temperature: temperature.unwrap_or(1.0),
top_k: top_k.unwrap_or(0),
top_p: top_p.unwrap_or(1.0),
typical_p: typical_p.unwrap_or(1.0),
do_sample,
seed: 0,
repetition_penalty: repetition_penalty.unwrap_or(1.0),
frequency_penalty: frequency_penalty.unwrap_or(0.0),
watermark,
grammar: String::new(),
grammar_type: GrammarType::None as i32,
};
// Initialize terminal properties
crossterm::terminal::enable_raw_mode()?;
io::stdout().execute(crossterm::terminal::EnterAlternateScreen)?;
io::stdout().execute(crossterm::cursor::Hide)?;
// Initialize terminal
let mut terminal = {
let backend = CrosstermBackend::new(io::stdout());
Terminal::new(backend)?
};
// Create message channel between generation_task and app
let (run_sender, run_receiver) = mpsc::channel(8);
// Crossterm event channel
let (event_sender, mut event_receiver) = mpsc::channel(8);
// Shutdown channel to terminate tasks
let (shutdown_sender, _) = broadcast::channel(1);
// Channel to check if tasks terminated
let (shutdown_guard_sender, mut shutdown_guard_receiver) = mpsc::channel(1);
// Create generation task
tokio::spawn(generation::generation_task(
tokenizer,
batch_size.clone(),
sequence_length,
decode_length,
top_n_tokens,
n_runs,
warmups,
parameters,
client,
run_sender,
shutdown_sender.subscribe(),
shutdown_guard_sender.clone(),
));
// Create event task
tokio::spawn(event::terminal_event_task(
250,
event_sender,
shutdown_sender.subscribe(),
shutdown_guard_sender.clone(),
));
// Drop our end of shutdown sender
drop(shutdown_guard_sender);
// Create App
let mut app = App::new(
run_receiver,
tokenizer_name.clone(),
sequence_length,
decode_length,
n_runs,
batch_size,
);
while app.running {
// Draw frame
terminal.draw(|frame| app.render(frame))?;
// Await a new event from event handling task
match event_receiver.recv().await {
None => break,
// Update app state
Some(event) => match event {
Event::Tick => app.tick(),
Event::Key(key_event) => app.handle_key_event(key_event),
_ => {}
},
}
}
// Ask tasks to shutdown
let _ = shutdown_sender.send(());
// Wait for tasks to shutdown
let _ = shutdown_guard_receiver.recv().await;
// Revert terminal to original view
io::stdout().execute(crossterm::terminal::LeaveAlternateScreen)?;
crossterm::terminal::disable_raw_mode()?;
io::stdout().execute(crossterm::cursor::Show)?;
let parameters_table = table::parameters_table(
tokenizer_name,
sequence_length,
decode_length,
top_n_tokens,
n_runs,
warmups,
temperature,
top_k,
top_p,
typical_p,
repetition_penalty,
frequency_penalty,
watermark,
do_sample,
);
println!("\n{parameters_table}\n");
let latency_table = table::latency_table(&app.data);
println!("\n{latency_table}\n");
let throughput_table = table::throughput_table(&app.data);
println!("\n{throughput_table}\n");
Ok(())
}
| text-generation-inference/benchmark/src/lib.rs/0 | {
"file_path": "text-generation-inference/benchmark/src/lib.rs",
"repo_id": "text-generation-inference",
"token_count": 1946
} | 204 |
from typing import Dict
# Text Generation Inference Errors
class ValidationError(Exception):
def __init__(self, message: str):
super().__init__(message)
class GenerationError(Exception):
def __init__(self, message: str):
super().__init__(message)
class OverloadedError(Exception):
def __init__(self, message: str):
super().__init__(message)
class IncompleteGenerationError(Exception):
def __init__(self, message: str):
super().__init__(message)
# API Inference Errors
class BadRequestError(Exception):
def __init__(self, message: str):
super().__init__(message)
class ShardNotReadyError(Exception):
def __init__(self, message: str):
super().__init__(message)
class ShardTimeoutError(Exception):
def __init__(self, message: str):
super().__init__(message)
class NotFoundError(Exception):
def __init__(self, message: str):
super().__init__(message)
class RateLimitExceededError(Exception):
def __init__(self, message: str):
super().__init__(message)
class NotSupportedError(Exception):
def __init__(self, model_id: str):
message = (
f"Model `{model_id}` is not available for inference with this client. \n"
"Use `huggingface_hub.inference_api.InferenceApi` instead."
)
super(NotSupportedError, self).__init__(message)
# Unknown error
class UnknownError(Exception):
def __init__(self, message: str):
super().__init__(message)
def parse_error(status_code: int, payload: Dict[str, str]) -> Exception:
"""
Parse error given an HTTP status code and a json payload
Args:
status_code (`int`):
HTTP status code
payload (`Dict[str, str]`):
Json payload
Returns:
Exception: parsed exception
"""
# Try to parse a Text Generation Inference error
message = payload["error"]
if "error_type" in payload:
error_type = payload["error_type"]
if error_type == "generation":
return GenerationError(message)
if error_type == "incomplete_generation":
return IncompleteGenerationError(message)
if error_type == "overloaded":
return OverloadedError(message)
if error_type == "validation":
return ValidationError(message)
# Try to parse a APIInference error
if status_code == 400:
return BadRequestError(message)
if status_code == 403 or status_code == 424:
return ShardNotReadyError(message)
if status_code == 504:
return ShardTimeoutError(message)
if status_code == 404:
return NotFoundError(message)
if status_code == 429:
return RateLimitExceededError(message)
# Fallback to an unknown error
return UnknownError(message)
| text-generation-inference/clients/python/text_generation/errors.py/0 | {
"file_path": "text-generation-inference/clients/python/text_generation/errors.py",
"repo_id": "text-generation-inference",
"token_count": 1080
} | 205 |
# Safetensors
Safetensors is a model serialization format for deep learning models. It is [faster](https://huggingface.co/docs/safetensors/speed) and safer compared to other serialization formats like pickle (which is used under the hood in many deep learning libraries).
TGI depends on safetensors format mainly to enable [tensor parallelism sharding](./tensor_parallelism). For a given model repository during serving, TGI looks for safetensors weights. If there are no safetensors weights, TGI converts the PyTorch weights to safetensors format.
You can learn more about safetensors by reading the [safetensors documentation](https://huggingface.co/docs/safetensors/index).
| text-generation-inference/docs/source/conceptual/safetensors.md/0 | {
"file_path": "text-generation-inference/docs/source/conceptual/safetensors.md",
"repo_id": "text-generation-inference",
"token_count": 184
} | 206 |
{
"details": {
"best_of_sequences": null,
"finish_reason": "length",
"generated_tokens": 10,
"prefill": [
{
"id": 1,
"logprob": null,
"text": "<s>"
},
{
"id": 338,
"logprob": -9.0859375,
"text": "is"
},
{
"id": 21784,
"logprob": -10.90625,
"text": "Deep"
},
{
"id": 29257,
"logprob": -2.65625,
"text": "Learning"
},
{
"id": 29973,
"logprob": -4.8085938,
"text": "?"
}
],
"seed": 0,
"tokens": [
{
"id": 13,
"logprob": -0.19958496,
"special": false,
"text": "\n"
},
{
"id": 4013,
"logprob": -2.203125,
"special": false,
"text": "This"
},
{
"id": 1139,
"logprob": -0.23693848,
"special": false,
"text": " question"
},
{
"id": 756,
"logprob": 0.0,
"special": false,
"text": " has"
},
{
"id": 1063,
"logprob": -0.076538086,
"special": false,
"text": " been"
},
{
"id": 4433,
"logprob": 0.0,
"special": false,
"text": " asked"
},
{
"id": 1784,
"logprob": -1.1367188,
"special": false,
"text": " many"
},
{
"id": 3064,
"logprob": 0.0,
"special": false,
"text": " times"
},
{
"id": 322,
"logprob": -1.7460938,
"special": false,
"text": " and"
},
{
"id": 306,
"logprob": 0.0,
"special": false,
"text": " I"
}
],
"top_tokens": null
},
"generated_text": "What is Deep Learning?\nThis question has been asked many times and I"
}
| text-generation-inference/integration-tests/models/__snapshots__/test_flash_awq/test_flash_llama_awq_all_params.json/0 | {
"file_path": "text-generation-inference/integration-tests/models/__snapshots__/test_flash_awq/test_flash_llama_awq_all_params.json",
"repo_id": "text-generation-inference",
"token_count": 1165
} | 207 |
{
"details": {
"best_of_sequences": null,
"finish_reason": "stop_sequence",
"generated_tokens": 5,
"prefill": [
{
"id": 1,
"logprob": null,
"text": "<s>"
},
{
"id": 4321,
"logprob": -8.6875,
"text": "Test"
},
{
"id": 2009,
"logprob": -11.546875,
"text": "request"
}
],
"seed": 0,
"tokens": [
{
"id": 5229,
"logprob": -2.5839844,
"special": false,
"text": " failed"
},
{
"id": 29901,
"logprob": -0.44970703,
"special": false,
"text": ":"
},
{
"id": 4829,
"logprob": -1.8339844,
"special": false,
"text": " Error"
},
{
"id": 297,
"logprob": -1.0556641,
"special": false,
"text": " in"
},
{
"id": 1243,
"logprob": 0.0,
"special": false,
"text": " test"
}
],
"top_tokens": null
},
"generated_text": "Test request failed: Error in test"
}
| text-generation-inference/integration-tests/models/__snapshots__/test_flash_llama/test_flash_llama_all_params.json/0 | {
"file_path": "text-generation-inference/integration-tests/models/__snapshots__/test_flash_llama/test_flash_llama_all_params.json",
"repo_id": "text-generation-inference",
"token_count": 669
} | 208 |
{
"details": {
"best_of_sequences": null,
"finish_reason": "stop_sequence",
"generated_tokens": 6,
"prefill": [
{
"id": 14402,
"logprob": null,
"text": "Test"
},
{
"id": 2581,
"logprob": -11.6171875,
"text": " request"
}
],
"seed": 0,
"tokens": [
{
"id": 284,
"logprob": -0.19421387,
"special": false,
"text": " to"
},
{
"id": 3758,
"logprob": -0.62597656,
"special": false,
"text": " send"
},
{
"id": 1366,
"logprob": -0.87060547,
"special": false,
"text": " data"
},
{
"id": 625,
"logprob": -0.88427734,
"special": false,
"text": " over"
},
{
"id": 257,
"logprob": -1.0830078,
"special": false,
"text": " a"
},
{
"id": 3127,
"logprob": -1.9462891,
"special": false,
"text": " network"
}
],
"top_tokens": null
},
"generated_text": "Test request to send data over a network"
}
| text-generation-inference/integration-tests/models/__snapshots__/test_flash_phi/test_flash_phi_all_params.json/0 | {
"file_path": "text-generation-inference/integration-tests/models/__snapshots__/test_flash_phi/test_flash_phi_all_params.json",
"repo_id": "text-generation-inference",
"token_count": 690
} | 209 |
import pytest
@pytest.fixture(scope="module")
def flash_mistral_handle(launcher):
with launcher("mistralai/Mistral-7B-Instruct-v0.1") as handle:
yield handle
@pytest.fixture(scope="module")
async def flash_mistral(flash_mistral_handle):
await flash_mistral_handle.health(300)
return flash_mistral_handle.client
@pytest.mark.asyncio
async def test_flash_mistral(flash_mistral, response_snapshot):
response = await flash_mistral.generate(
"Test request", max_new_tokens=10, decoder_input_details=True
)
assert response.details.generated_tokens == 10
assert response.generated_text == ": Let n = 10 - 1"
assert response == response_snapshot
@pytest.mark.asyncio
async def test_flash_mistral_all_params(flash_mistral, response_snapshot):
response = await flash_mistral.generate(
"Test request",
max_new_tokens=10,
repetition_penalty=1.2,
return_full_text=True,
stop_sequences=["test"],
temperature=0.5,
top_p=0.9,
top_k=10,
truncate=5,
typical_p=0.9,
watermark=True,
decoder_input_details=True,
seed=0,
)
assert response.details.generated_tokens == 10
assert response == response_snapshot
@pytest.mark.asyncio
async def test_flash_mistral_load(flash_mistral, generate_load, response_snapshot):
responses = await generate_load(
flash_mistral, "Test request", max_new_tokens=10, n=4
)
assert len(responses) == 4
assert all(
[r.generated_text == responses[0].generated_text for r in responses]
), f"{[r.generated_text for r in responses]}"
assert responses[0].generated_text == ": Let n = 10 - 1"
assert responses == response_snapshot
| text-generation-inference/integration-tests/models/test_flash_mistral.py/0 | {
"file_path": "text-generation-inference/integration-tests/models/test_flash_mistral.py",
"repo_id": "text-generation-inference",
"token_count": 714
} | 210 |
import pytest
@pytest.fixture(scope="module")
def t5_sharded_handle(launcher):
with launcher("google/flan-t5-xxl", num_shard=2) as handle:
yield handle
@pytest.fixture(scope="module")
async def t5_sharded(t5_sharded_handle):
await t5_sharded_handle.health(300)
return t5_sharded_handle.client
@pytest.mark.asyncio
async def test_t5_sharded(t5_sharded, response_snapshot):
response = await t5_sharded.generate(
"Please answer the following question. What is the boiling point of Nitrogen?",
max_new_tokens=10,
decoder_input_details=True,
)
assert response == response_snapshot
@pytest.mark.asyncio
async def test_t5_sharded_load(t5_sharded, generate_load, response_snapshot):
responses = await generate_load(
t5_sharded,
"Please answer the following question. What is the boiling point of Nitrogen?",
max_new_tokens=10,
n=4,
)
assert len(responses) == 4
assert all([r.generated_text == responses[0].generated_text for r in responses])
assert responses == response_snapshot
| text-generation-inference/integration-tests/models/test_t5_sharded.py/0 | {
"file_path": "text-generation-inference/integration-tests/models/test_t5_sharded.py",
"repo_id": "text-generation-inference",
"token_count": 427
} | 211 |
# Router
Also named `webserver` throughout the docs.
This router is handling most of the logic to handle the "batches" tell
when to pass new `prefill` requests and pausing `decode` requests, which ones etc...
It uses gRPC to communicate with the shards which can therefore be kept
much simpler and focus on having the most efficient forward passes as possible.
## Continuous batching
One important feature of `text-generation-inference` is enabled
by this `router`.
Continuous batching is the act of regularly running queries in the same
`forward` step of the LLM (a "batch") and also removing them when they are
finished.
In order for continuous batching to be useful, you need to have more compute available
with respect to the memory requirements of your model. This is essentially true for
LLMs and the larger the model, the truer it gets (since you have to pool multiple
GPUs to load the model, you effectively have a lot of compute power at your hands).
Static batching is the act of doing several queries at the same time, but usually
this is controlled by the client, and therefore the amount of batching is decided
beforehand.
For text-generation, and LLMs which are memory bound we can try to be much more
efficient with the available compute, by having client sending us single queries,
and let the router mix&match queries into or out of batches to make the use the
compute the most efficiently. This is possible because for LLMs the total compute
for running the model is much bigger than doing mix&match of the batches themselves.
### Simple continuous batching
text-generation works by feeding a prompt to a model, and iteratively calling
`forward` on the model to produce new text, 1 token at a time.
The first idea is simple, when a query arrives, we start working on it directly.
When new queries arrive, we simply wait for the current `forward` to be finished
then batch the current running prompt with the new query, and call `forward`.
Whenever either query is finished: either the model produce EOS (end of sentence) token
or the query reached the allowed limit. We simply drop it from the batch, remove
all the allocated memory and we can continue with the rest until nothing is left.
This simple idea generalizes very well and we could potentially stack many requests
in the same batch.
One thing to note, is that queries can be potentially run with different parameters
meaning different way to choose the next token (sampling, not sampling, temperature, top_k etc..). This is not problematic for the proposed approach we just need to do the sampling
independantly on each member of the batch.
### Prefill, decode and past key values
In order to make LLMs and text-generation efficient, there's actually a very powerful
trick that can be used, which is the "caching" of some attention matrices. [More on that
in the first part of this blog](https://huggingface.co/blog/accelerated-inference#getting-to-the-first-10x-speedup)
What this means, is that the first "pass" of a prompt is different from the subsequent
"forward" passes. Since for the first one we have to compute the entire attention matrix, whereas in the follow-ups only require to compute the new token attention.
The first pass is called `prefill` throughout this codebase where as the follow-ups are called `decode`.
Since `prefill` is much more expensive than `decode` we don't want to do it all the time,
but a currently running query is probably doing `decode`. If we want to do the continuous
batching as explained previously we need to run `prefill` at some point in order to create
the attention matrix required to be able to join the `decode` group.
`text-generation-inference` uses a bunch of different strategies and parameters in
order to enable you to find the sweet spot between exploiting the hardware and perceived latency.
With no continuous batching at all, latency is going to be super good, but throughput (meaning
the total number of requests allowed in a given timeframe) is going to be super bad (since it's essentially 1).
With static batching, you can probably reach the maximum throughput (by using the maximum total batch size applicable to your hardware), but the latency is super bad since in order to have maximum throughput you need to wait for requests to come in before processing.
With continuous batching you can find a sweet spot. In general latency is the most critical
parameter users care about. But a 2x latency slowdown for 10x more users on the same
hardware is an acceptable tradeoff.
## Token streaming
This is a very important aspect of client UX. As mentionned above, latency is the
most critical perceived quality of an LLM API.
With token streaming, the server can start answering after the first `prefill` pass
directly, without waiting for all the generation to be done. For extremely long queries
this means clients can start to see something happening orders of magnitude before
the work is done. Seeing something in progress allows them to cut short if it's not
what's wanted but also it "feels" better.
| text-generation-inference/router/README.md/0 | {
"file_path": "text-generation-inference/router/README.md",
"repo_id": "text-generation-inference",
"token_count": 1175
} | 212 |
/// Payload validation logic
use crate::validation::ValidationError::{BestOfSampling, BestOfSeed, EmptyInput};
use crate::{GenerateParameters, GenerateRequest, GrammarType};
use jsonschema::{Draft, JSONSchema};
use rand::{thread_rng, Rng};
use serde_json::Value;
use text_generation_client::{
GrammarType as ProtoGrammarType, NextTokenChooserParameters, StoppingCriteriaParameters,
};
use thiserror::Error;
use tokenizers::tokenizer::Tokenizer;
use tokenizers::TruncationDirection;
use tokio::sync::mpsc;
use tokio::sync::oneshot;
use tracing::{instrument, Span};
/// Validation
#[derive(Debug, Clone)]
pub struct Validation {
/// Validation parameters
max_best_of: usize,
max_stop_sequences: usize,
max_top_n_tokens: u32,
max_input_length: usize,
max_total_tokens: usize,
disable_grammar_support: bool,
/// Channel to communicate with the background tokenization task
sender: Option<mpsc::UnboundedSender<TokenizerRequest>>,
}
impl Validation {
#[allow(clippy::too_many_arguments)]
pub(crate) fn new(
workers: usize,
tokenizer: Option<Tokenizer>,
max_best_of: usize,
max_stop_sequences: usize,
max_top_n_tokens: u32,
max_input_length: usize,
max_total_tokens: usize,
disable_grammar_support: bool,
) -> Self {
// If we have a fast tokenizer
let sender = if let Some(tokenizer) = tokenizer {
// Create round robin channel
let (validation_sender, validation_round_robin_receiver) = mpsc::unbounded_channel();
let mut senders = Vec::with_capacity(workers);
// Create workers
for _ in 0..workers {
let tokenizer_clone = tokenizer.clone();
let (tokenizer_sender, tokenizer_receiver) = mpsc::unbounded_channel();
senders.push(tokenizer_sender);
// Spawn worker
tokio::task::spawn_blocking(move || {
tokenizer_worker(tokenizer_clone, tokenizer_receiver)
});
}
// Create tokenization round robin task
tokio::spawn(round_robin_task(validation_round_robin_receiver, senders));
Some(validation_sender)
} else {
None
};
Self {
max_best_of,
sender,
max_stop_sequences,
max_top_n_tokens,
max_input_length,
max_total_tokens,
disable_grammar_support,
}
}
#[instrument(skip(self, inputs))]
pub async fn tokenize(
&self,
inputs: String,
truncate: Option<usize>,
) -> Result<Option<(tokenizers::Encoding, String)>, ValidationError> {
// If we have a fast tokenizer
if let Some(sender) = &self.sender {
// Create response channel
let (response_sender, response_receiver) = oneshot::channel();
// Send request to the background validation task
// Unwrap is safe here
sender
.send(((inputs, truncate), response_sender, Span::current()))
.unwrap();
// Await on response channel
// Unwrap is safe here
let encoding = response_receiver.await.unwrap()?;
Ok(Some(encoding))
} else {
Ok(None)
}
}
#[instrument(skip(self, inputs))]
async fn validate_input(
&self,
inputs: String,
truncate: Option<usize>,
max_new_tokens: Option<u32>,
) -> Result<(String, usize, u32), ValidationError> {
// If we have a fast tokenizer
if let Some((encoding, inputs)) = self.tokenize(inputs.clone(), truncate).await? {
// Create response channel
let input_length = encoding.len();
// Get total tokens
let max_new_tokens: u32 = if let Some(max_new_tokens) = max_new_tokens {
max_new_tokens
} else {
self.max_total_tokens.saturating_sub(input_length) as u32
};
let total_tokens = input_length + max_new_tokens as usize;
// Validate MaxTotalTokens
if total_tokens > self.max_total_tokens {
return Err(ValidationError::MaxTotalTokens(
self.max_total_tokens,
input_length,
max_new_tokens,
));
}
// Validate InputLength
if input_length > self.max_input_length {
return Err(ValidationError::InputLength(
self.max_input_length,
input_length,
));
}
metrics::histogram!("tgi_request_input_length", input_length as f64);
Ok((inputs, input_length, max_new_tokens))
}
// Return inputs without validation
else {
// In this case, we don't know the real length in tokens of the inputs
// However, the inputs will be truncated by the python servers
// We make sure that truncate + max_new_tokens <= self.max_total_tokens
let max_new_tokens: u32 = if let Some(max_new_tokens) = max_new_tokens {
max_new_tokens
} else if let Some(truncate) = truncate {
self.max_total_tokens.saturating_sub(truncate) as u32
} else {
return Err(ValidationError::UnsetMaxNewTokens);
};
let input_length = truncate.unwrap_or(self.max_input_length);
// Validate MaxNewTokens
if (input_length as u32 + max_new_tokens) > self.max_total_tokens as u32 {
return Err(ValidationError::MaxNewTokens(
self.max_total_tokens - self.max_input_length,
max_new_tokens,
));
}
Ok((inputs, input_length, max_new_tokens))
}
}
/// Validate a payload and get the number of tokens in the input
#[instrument(skip_all)]
pub(crate) async fn validate(
&self,
request: GenerateRequest,
) -> Result<ValidGenerateRequest, ValidationError> {
let GenerateParameters {
best_of,
temperature,
repetition_penalty,
frequency_penalty,
top_k,
top_p,
typical_p,
do_sample,
max_new_tokens,
stop: stop_sequences,
truncate,
seed,
watermark,
decoder_input_details,
top_n_tokens,
grammar,
..
} = request.parameters;
// sampling must be true when best_of > 1
let best_of = best_of.unwrap_or(1);
let sampling = do_sample
|| temperature.is_some()
|| top_k.is_some()
|| top_p.is_some()
|| typical_p.is_some();
if best_of > 1 && !sampling {
return Err(BestOfSampling);
}
let temperature = temperature.unwrap_or(1.0);
if temperature <= 0.0 {
return Err(ValidationError::Temperature);
}
let repetition_penalty = repetition_penalty.unwrap_or(1.0);
if repetition_penalty <= 0.0 {
return Err(ValidationError::RepetitionPenalty);
}
let frequency_penalty = frequency_penalty.unwrap_or(0.0);
if !(-2.0..=2.0).contains(&frequency_penalty) {
return Err(ValidationError::FrequencyPenalty);
}
// Different because the proto default value is not a valid value
// for the user
let top_p = top_p
.map(|value| {
if value <= 0.0 || value >= 1.0 {
return Err(ValidationError::TopP);
}
Ok(value)
})
.unwrap_or(Ok(1.0))?;
let typical_p = typical_p
.map(|value| {
if value <= 0.0 || value >= 1.0 {
return Err(ValidationError::TypicalP);
}
Ok(value)
})
.unwrap_or(Ok(1.0))?;
let top_k: u32 = top_k
.map(|value| {
if value <= 0 {
return Err(ValidationError::TopK);
}
Ok(value as u32)
})
.unwrap_or(Ok(0))?;
if max_new_tokens == Some(0) {
return Err(ValidationError::NegativeMaxNewTokens);
}
if stop_sequences.len() > self.max_stop_sequences {
return Err(ValidationError::StopSequence(
self.max_stop_sequences,
stop_sequences.len(),
));
}
// If seed is None, assign a random one
let seed = match seed {
None => thread_rng().gen(),
Some(seed) => {
if best_of > 1 {
return Err(BestOfSeed);
}
seed
}
};
let top_n_tokens = top_n_tokens
.map(|value| {
if value > self.max_top_n_tokens {
return Err(ValidationError::TopNTokens(self.max_top_n_tokens, value));
}
Ok(value)
})
.unwrap_or(Ok(0))?;
// Check if inputs is empty
if request.inputs.is_empty() {
return Err(EmptyInput);
}
// Check if truncate is strictly positive and less than max_input_length
let truncate = truncate
.map(|value| {
if value == 0 || value > self.max_input_length {
return Err(ValidationError::Truncate(self.max_input_length, value));
}
Ok(Some(value))
})
.unwrap_or(Ok(None))?;
// Validate inputs
let (inputs, input_length, max_new_tokens) = self
.validate_input(request.inputs, truncate, max_new_tokens)
.await?;
// TODO: we should build the FSM here and pass the compiled FSM instead of the grammar
// NOTE: this is currently difficult because we need the tokenizer in Python to build
// the FSM and we'd have to load a copy of the tokenizer into our Pyo3 instance which
// may be slow and memory intensive. Best case is to have a Rust implementation of the FSM
// compiler and use that to build the FSM here.
// Validate grammar and unpack the grammar and type for the proto message
let (grammar, grammar_type) = match grammar {
Some(grammar) => {
// Ensure that grammar is not set if it's not supported
if self.disable_grammar_support {
return Err(ValidationError::Grammar);
}
match grammar {
GrammarType::Json(json) => {
let json = match json {
// if value is a string, we need to parse it again to make sure its
// a valid json
Value::String(s) => serde_json::from_str(&s)
.map_err(|e| ValidationError::InvalidGrammar(e.to_string())),
Value::Object(_) => Ok(json),
_ => Err(ValidationError::Grammar),
}?;
// Check if the json is a valid JSONSchema
JSONSchema::options()
.with_draft(Draft::Draft202012)
.compile(&json)
.map_err(|e| ValidationError::InvalidGrammar(e.to_string()))?;
(
// Serialize json to string
serde_json::to_string(&json)
.map_err(|e| ValidationError::InvalidGrammar(e.to_string()))?,
ProtoGrammarType::Json.into(),
)
}
GrammarType::Regex(regex) => (regex, ProtoGrammarType::Regex.into()),
}
}
None => (String::new(), ProtoGrammarType::None.into()),
};
let parameters = NextTokenChooserParameters {
temperature,
repetition_penalty,
frequency_penalty,
top_k,
top_p,
typical_p,
do_sample,
seed,
watermark,
grammar,
grammar_type,
};
let stopping_parameters = StoppingCriteriaParameters {
max_new_tokens,
stop_sequences,
ignore_eos_token: false,
};
metrics::histogram!("tgi_request_max_new_tokens", max_new_tokens as f64);
Ok(ValidGenerateRequest {
inputs,
decoder_input_details,
input_length: input_length as u32,
truncate: truncate.unwrap_or(self.max_input_length) as u32,
parameters,
stopping_parameters,
top_n_tokens,
})
}
/// Validate the best_of parameter
#[instrument(skip_all)]
pub(crate) fn validate_best_of(&self, best_of: usize) -> Result<usize, ValidationError> {
if self.max_best_of == 1 && best_of != 1 {
return Err(ValidationError::BestOfDisabled);
}
if best_of > self.max_best_of {
return Err(ValidationError::BestOf(self.max_best_of, best_of));
}
Ok(best_of)
}
}
/// Round robin tokenization task
async fn round_robin_task(
mut receiver: mpsc::UnboundedReceiver<TokenizerRequest>,
senders: Vec<mpsc::UnboundedSender<TokenizerRequest>>,
) {
loop {
for sender in &senders {
match receiver.recv().await {
None => return,
Some(request) => sender.send(request).unwrap(),
};
}
}
}
/// Start tokenization workers
fn tokenizer_worker(tokenizer: Tokenizer, mut receiver: mpsc::UnboundedReceiver<TokenizerRequest>) {
// Loop over requests
while let Some(((inputs, truncate), response_tx, parent_span)) = receiver.blocking_recv() {
parent_span.in_scope(|| {
response_tx
.send(prepare_input(inputs, truncate, &tokenizer))
.unwrap_or(())
})
}
}
/// Get input length and optionally truncate it
fn prepare_input(
mut inputs: String,
truncate: Option<usize>,
tokenizer: &Tokenizer,
) -> Result<(tokenizers::Encoding, String), ValidationError> {
// Get the number of tokens in the input
let mut encoding = tokenizer
.encode(inputs.clone(), true)
.map_err(|err| ValidationError::Tokenizer(err.to_string()))?;
// Optionally truncate
if let Some(truncate) = truncate {
if truncate < encoding.len() {
encoding.truncate(truncate, 0, TruncationDirection::Left);
inputs = tokenizer
.decode(encoding.get_ids(), false)
.map_err(|err| ValidationError::Tokenizer(err.to_string()))?;
}
}
Ok((encoding, inputs))
}
type TokenizerRequest = (
(String, Option<usize>),
oneshot::Sender<Result<(tokenizers::Encoding, String), ValidationError>>,
Span,
);
#[derive(Debug, Clone)]
pub(crate) struct ValidGenerateRequest {
pub inputs: String,
pub input_length: u32,
pub truncate: u32,
pub decoder_input_details: bool,
pub parameters: NextTokenChooserParameters,
pub stopping_parameters: StoppingCriteriaParameters,
pub top_n_tokens: u32,
}
#[derive(Error, Debug)]
pub enum ValidationError {
#[error("`best_of` must be > 0 and <= {0}. Given: {1}")]
BestOf(usize, usize),
#[error("`best_of` != 1 is not allowed for this endpoint")]
BestOfDisabled,
#[error("you must use sampling when `best_of` is > 1")]
BestOfSampling,
#[error("`seed` must not be set when `best_of` > 1")]
BestOfSeed,
#[error("`best_of` != 1 is not supported when streaming tokens")]
BestOfStream,
#[error("`top_n_tokens` must be >= 0 and <= {0}. Given: {1}")]
TopNTokens(u32, u32),
#[error("`top_n_tokens` != 0 is not allowed for this endpoint")]
TopNTokensDisabled,
#[error("`decoder_input_details` == true is not supported when streaming tokens")]
PrefillDetailsStream,
#[error("`temperature` must be strictly positive")]
Temperature,
#[error("`repetition_penalty` must be strictly positive")]
RepetitionPenalty,
#[error("`frequency_penalty` must be >= -2.0 and <= 2.0")]
FrequencyPenalty,
#[error("`top_p` must be > 0.0 and < 1.0")]
TopP,
#[error("`top_k` must be strictly positive")]
TopK,
#[error("`truncate` must be strictly positive and less than {0}. Given: {1}")]
Truncate(usize, usize),
#[error("`typical_p` must be > 0.0 and < 1.0")]
TypicalP,
#[error("one of `max_new_tokens` or `truncate` must be set if a fast tokenizer is not in use")]
UnsetMaxNewTokens,
#[error("`max_new_tokens` must be strictly positive")]
NegativeMaxNewTokens,
#[error("`max_new_tokens` must be <= {0}. Given: {1}")]
MaxNewTokens(usize, u32),
#[error("`inputs` tokens + `max_new_tokens` must be <= {0}. Given: {1} `inputs` tokens and {2} `max_new_tokens`")]
MaxTotalTokens(usize, usize, u32),
#[error("`inputs` must have less than {0} tokens. Given: {1}")]
InputLength(usize, usize),
#[error("`inputs` cannot be empty")]
EmptyInput,
#[error("`stop` supports up to {0} stop sequences. Given: {1}")]
StopSequence(usize, usize),
#[error("tokenizer error {0}")]
Tokenizer(String),
#[error("grammar is not supported")]
Grammar,
#[error("grammar is not valid: {0}")]
InvalidGrammar(String),
}
#[cfg(test)]
mod tests {
use super::*;
use crate::default_parameters;
use crate::tests::get_tokenizer;
#[tokio::test]
async fn test_validation_max_new_tokens() {
let tokenizer = None;
let max_best_of = 2;
let max_stop_sequence = 3;
let max_top_n_tokens = 4;
let max_input_length = 5;
let max_total_tokens = 6;
let workers = 1;
let disable_grammar_support = true;
let validation = Validation::new(
workers,
tokenizer,
max_best_of,
max_stop_sequence,
max_top_n_tokens,
max_input_length,
max_total_tokens,
disable_grammar_support,
);
let max_new_tokens = 10;
match validation
.validate_input("Hello".to_string(), None, Some(max_new_tokens))
.await
{
Err(ValidationError::MaxNewTokens(1, 10)) => (),
_ => panic!("Unexpected not max new tokens"),
}
}
#[tokio::test]
async fn test_validation_input_length() {
let tokenizer = Some(get_tokenizer().await);
let max_best_of = 2;
let max_stop_sequence = 3;
let max_top_n_tokens = 4;
let max_input_length = 5;
let max_total_tokens = 6;
let disable_grammar_support = true;
let workers = 1;
let validation = Validation::new(
workers,
tokenizer,
max_best_of,
max_stop_sequence,
max_top_n_tokens,
max_input_length,
max_total_tokens,
disable_grammar_support,
);
let max_new_tokens = 10;
match validation
.validate_input("Hello".to_string(), None, Some(max_new_tokens))
.await
{
Err(ValidationError::MaxTotalTokens(6, 1, 10)) => (),
_ => panic!("Unexpected not max new tokens"),
}
}
#[tokio::test]
async fn test_validation_best_of_sampling() {
let tokenizer = Some(get_tokenizer().await);
let max_best_of = 2;
let max_stop_sequence = 3;
let max_top_n_tokens = 4;
let max_input_length = 5;
let max_total_tokens = 6;
let workers = 1;
let disable_grammar_support = true;
let validation = Validation::new(
workers,
tokenizer,
max_best_of,
max_stop_sequence,
max_top_n_tokens,
max_input_length,
max_total_tokens,
disable_grammar_support,
);
match validation
.validate(GenerateRequest {
inputs: "Hello".to_string(),
parameters: GenerateParameters {
best_of: Some(2),
do_sample: false,
..default_parameters()
},
})
.await
{
Err(ValidationError::BestOfSampling) => (),
_ => panic!("Unexpected not best of sampling"),
}
}
#[tokio::test]
async fn test_validation_top_p() {
let tokenizer = Some(get_tokenizer().await);
let max_best_of = 2;
let max_stop_sequence = 3;
let max_top_n_tokens = 4;
let max_input_length = 5;
let max_total_tokens = 106;
let workers = 1;
let disable_grammar_support = true;
let validation = Validation::new(
workers,
tokenizer,
max_best_of,
max_stop_sequence,
max_top_n_tokens,
max_input_length,
max_total_tokens,
disable_grammar_support,
);
match validation
.validate(GenerateRequest {
inputs: "Hello".to_string(),
parameters: GenerateParameters {
top_p: Some(1.0),
max_new_tokens: Some(5),
..default_parameters()
},
})
.await
{
Err(ValidationError::TopP) => (),
_ => panic!("Unexpected top_p"),
}
match validation
.validate(GenerateRequest {
inputs: "Hello".to_string(),
parameters: GenerateParameters {
top_p: Some(0.99),
max_new_tokens: Some(5),
..default_parameters()
},
})
.await
{
Ok(_) => (),
_ => panic!("Unexpected top_p error"),
}
let valid_request = validation
.validate(GenerateRequest {
inputs: "Hello".to_string(),
parameters: GenerateParameters {
top_p: None,
max_new_tokens: Some(5),
..default_parameters()
},
})
.await
.unwrap();
// top_p == 1.0 is invalid for users to ask for but it's the default resolved value.
assert_eq!(valid_request.parameters.top_p, 1.0);
}
#[tokio::test]
async fn test_validation_top_n_tokens() {
let tokenizer = Some(get_tokenizer().await);
let max_best_of = 2;
let max_stop_sequences = 3;
let max_top_n_tokens = 4;
let max_input_length = 5;
let max_total_tokens = 106;
let workers = 1;
let disable_grammar_support = true;
let validation = Validation::new(
workers,
tokenizer,
max_best_of,
max_stop_sequences,
max_top_n_tokens,
max_input_length,
max_total_tokens,
disable_grammar_support,
);
match validation
.validate(GenerateRequest {
inputs: "Hello".to_string(),
parameters: GenerateParameters {
top_n_tokens: Some(5),
max_new_tokens: Some(5),
..default_parameters()
},
})
.await
{
Err(ValidationError::TopNTokens(4, 5)) => (),
_ => panic!("Unexpected top_n_tokens"),
}
validation
.validate(GenerateRequest {
inputs: "Hello".to_string(),
parameters: GenerateParameters {
top_n_tokens: Some(4),
max_new_tokens: Some(5),
..default_parameters()
},
})
.await
.unwrap();
validation
.validate(GenerateRequest {
inputs: "Hello".to_string(),
parameters: GenerateParameters {
top_n_tokens: Some(0),
max_new_tokens: Some(5),
..default_parameters()
},
})
.await
.unwrap();
let valid_request = validation
.validate(GenerateRequest {
inputs: "Hello".to_string(),
parameters: GenerateParameters {
top_n_tokens: None,
max_new_tokens: Some(5),
..default_parameters()
},
})
.await
.unwrap();
assert_eq!(valid_request.top_n_tokens, 0);
}
}
| text-generation-inference/router/src/validation.rs/0 | {
"file_path": "text-generation-inference/router/src/validation.rs",
"repo_id": "text-generation-inference",
"token_count": 13034
} | 213 |
// Adapted from turboderp exllama: https://github.com/turboderp/exllama
#define _cuda_buffers_cu
#include "cuda_buffers.cuh"
CudaBuffers* g_buffers[CUDA_MAX_DEVICES] = {NULL};
// __constant__ half2 q4_table[16][256];
// half2 q4_table_host[16][256];
// bool q4_table_init = false;
CudaBuffers::CudaBuffers
(
int _device,
half* _temp_state,
half* _temp_dq
) :
device(_device),
temp_state(_temp_state),
temp_dq(_temp_dq)
{
cudaSetDevice(_device);
cudaStreamCreate(&alt_stream_1);
cudaStreamCreate(&alt_stream_2);
cudaStreamCreate(&alt_stream_3);
cudaEventCreate(&alt_stream_1_done);
cudaEventCreate(&alt_stream_2_done);
cudaEventCreate(&alt_stream_3_done);
}
CudaBuffers::~CudaBuffers()
{
cudaStreamDestroy(alt_stream_1);
cudaStreamDestroy(alt_stream_2);
cudaStreamDestroy(alt_stream_3);
cudaEventDestroy(alt_stream_1_done);
cudaEventDestroy(alt_stream_2_done);
cudaEventDestroy(alt_stream_3_done);
}
CudaBuffers* get_buffers(const int device_index)
{
return g_buffers[device_index];
}
void prepare_buffers_cuda
(
int _device,
half* _temp_state,
half* _temp_dq
)
{
CudaBuffers* buffers = new CudaBuffers
(
_device,
_temp_state,
_temp_dq
);
g_buffers[_device] = buffers;
}
void cleanup_buffers_cuda()
{
for (int i = 0; i < CUDA_MAX_DEVICES; i++)
{
if (!g_buffers[i]) continue;
delete g_buffers[i];
g_buffers[i] = NULL;
}
}
| text-generation-inference/server/exllama_kernels/exllama_kernels/cuda_buffers.cu/0 | {
"file_path": "text-generation-inference/server/exllama_kernels/exllama_kernels/cuda_buffers.cu",
"repo_id": "text-generation-inference",
"token_count": 680
} | 214 |
#include <torch/extension.h>
#include <c10/cuda/CUDAGuard.h>
#include <ATen/cuda/CUDAContext.h>
#include <cuda_runtime.h>
#include <cuda_fp16.h>
#include <cstdint>
#include <cstdio>
#include "config.h"
#include "cuda/q_matrix.cuh"
#include "cuda/q_gemm.cuh"
#include "cpp/util.h"
// Some decluttering macros
#define TORCH_CHECK_DTYPE(__x, __dtype) TORCH_CHECK((__x).dtype() == torch::__dtype, #__x " is incorrect datatype, must be " #__dtype)
#define TORCH_CHECK_DTYPE_OPT(__x, __dtype) TORCH_CHECK((__x).device().is_meta() || (__x).dtype() == torch::__dtype, #__x " is incorrect datatype, must be " #__dtype)
#define TORCH_CHECK_SHAPES(__x, __dim_x, __y, __dim_y, __scale_y) TORCH_CHECK((__x).size(__dim_x) == (__y).size(__dim_y) * __scale_y, #__x " and " #__y " have incompatible shapes")
#define TORCH_CHECK_SHAPES_OPT(__x, __dim_x, __y, __dim_y, __scale_y) TORCH_CHECK((__x).device().is_meta() || (__x).size(__dim_x) == (__y).size(__dim_y) * __scale_y, #__x " and " #__y " have incompatible shapes")
// Quant matrix
uintptr_t make_q_matrix
(
torch::Tensor q_weight,
torch::Tensor q_perm,
torch::Tensor q_invperm,
torch::Tensor q_scale,
torch::Tensor q_scale_max,
torch::Tensor q_groups,
torch::Tensor q_group_map,
torch::Tensor gptq_qzeros,
torch::Tensor gptq_scales,
torch::Tensor gptq_g_idx,
torch::Tensor temp_dq
)
{
TORCH_CHECK_DTYPE(q_weight, kInt);
TORCH_CHECK_DTYPE_OPT(q_perm, kShort);
TORCH_CHECK_DTYPE_OPT(q_invperm, kShort);
TORCH_CHECK_DTYPE_OPT(q_scale, kInt);
TORCH_CHECK_DTYPE_OPT(q_scale_max, kHalf);
TORCH_CHECK_DTYPE_OPT(q_groups, kShort);
TORCH_CHECK_DTYPE_OPT(q_group_map, kShort);
TORCH_CHECK_DTYPE_OPT(gptq_qzeros, kInt);
TORCH_CHECK_DTYPE_OPT(gptq_scales, kHalf);
TORCH_CHECK_DTYPE_OPT(gptq_g_idx, kInt);
TORCH_CHECK_SHAPES(q_perm, 0, q_invperm, 0, 1);
int device = q_weight.device().index();
int width = q_weight.size(1);
int groups;
int height;
if (!q_scale.device().is_meta())
{
TORCH_CHECK_SHAPES(q_weight, 1, q_scale, 1, 8);
TORCH_CHECK_SHAPES(q_scale_max, 0, q_scale, 0, 1);
groups = q_scale.size(0);
height = q_invperm.size(0);
}
else
{
TORCH_CHECK_SHAPES(q_weight, 1, gptq_qzeros, 1, 8);
TORCH_CHECK_SHAPES(q_weight, 1, gptq_scales, 1, 1);
groups = gptq_qzeros.size(0);
height = q_weight.size(0) * 8;
}
TORCH_CHECK(temp_dq.size(0) >= width * height, "Insufficient size of temp_dq buffer")
QMatrix* m = new QMatrix
(
device,
height,
width,
groups,
(uint32_t*) q_weight.data_ptr(),
q_perm.device().is_meta() ? NULL : (uint16_t*) q_perm.data_ptr(),
q_invperm.device().is_meta() ? NULL : (uint16_t*) q_invperm.data_ptr(),
q_scale.device().is_meta() ? NULL : (uint32_t*) q_scale.data_ptr(),
q_scale_max.device().is_meta() ? NULL : (half*) q_scale_max.data_ptr(),
q_groups.device().is_meta() ? NULL : (uint16_t*) q_groups.data_ptr(),
q_group_map.device().is_meta() ? NULL : (uint16_t*) q_group_map.data_ptr(),
gptq_qzeros.device().is_meta() ? NULL : (uint32_t*) gptq_qzeros.data_ptr(),
gptq_scales.device().is_meta() ? NULL : (half*) gptq_scales.data_ptr(),
gptq_g_idx.device().is_meta() ? NULL : (uint32_t*) gptq_g_idx.data_ptr(),
(half*) temp_dq.data_ptr()
);
if (m->failed) throw std::runtime_error("CUDA out of memory");
return reinterpret_cast<uintptr_t> (m);
}
void gemm_half_q_half
(
torch::Tensor a,
uintptr_t b,
torch::Tensor c,
bool force_cuda
)
{
QMatrix* qm = reinterpret_cast<QMatrix*> (b);
TORCH_CHECK_DTYPE(a, kHalf);
TORCH_CHECK_DTYPE(c, kHalf);
TORCH_CHECK_SHAPES(a, 0, c, 0, 1);
TORCH_CHECK(qm->height == a.size(1), "a and b have incompatible shapes")
TORCH_CHECK(qm->width == c.size(1), "b and c have incompatible shapes")
const at::cuda::OptionalCUDAGuard device_guard(device_of(a));
gemm_half_q_half_cuda
(
at::cuda::getCurrentCUDABlasHandle(),
(const half*) a.data_ptr(),
qm,
(half*) c.data_ptr(),
c.size(0), // m
c.size(1), // n
a.size(1), // k
true,
NULL,
force_cuda
);
}
// Bindings
PYBIND11_MODULE(TORCH_EXTENSION_NAME, m)
{
m.def("make_q_matrix", &make_q_matrix, "make_q_matrix");
m.def("gemm_half_q_half", &gemm_half_q_half, "gemm_half_q_half");
}
| text-generation-inference/server/exllamav2_kernels/exllamav2_kernels/ext.cpp/0 | {
"file_path": "text-generation-inference/server/exllamav2_kernels/exllamav2_kernels/ext.cpp",
"repo_id": "text-generation-inference",
"token_count": 2184
} | 215 |
import torch
from text_generation_server.utils.tokens import (
StopSequenceCriteria,
StoppingCriteria,
FinishReason,
batch_top_tokens,
)
def test_stop_sequence_criteria():
criteria = StopSequenceCriteria("/test;")
assert not criteria("/")
assert not criteria("/test")
assert criteria("/test;")
assert not criteria("/test; ")
def test_stop_sequence_criteria_escape():
criteria = StopSequenceCriteria("<|stop|>")
assert not criteria("<")
assert not criteria("<|stop")
assert criteria("<|stop|>")
assert not criteria("<|stop|> ")
def test_stopping_criteria():
criteria = StoppingCriteria(0, [StopSequenceCriteria("/test;")], max_new_tokens=5)
assert criteria(65827, "/test") == (False, None)
assert criteria(30, ";") == (True, FinishReason.FINISH_REASON_STOP_SEQUENCE)
def test_stopping_criteria_eos():
criteria = StoppingCriteria(0, [StopSequenceCriteria("/test;")], max_new_tokens=5)
assert criteria(1, "") == (False, None)
assert criteria(0, "") == (True, FinishReason.FINISH_REASON_EOS_TOKEN)
def test_stopping_criteria_max():
criteria = StoppingCriteria(0, [StopSequenceCriteria("/test;")], max_new_tokens=5)
assert criteria(1, "") == (False, None)
assert criteria(1, "") == (False, None)
assert criteria(1, "") == (False, None)
assert criteria(1, "") == (False, None)
assert criteria(1, "") == (True, FinishReason.FINISH_REASON_LENGTH)
def test_batch_top_tokens():
top_n_tokens = [0, 2, 3, 4, 5]
top_n_tokens_tensor = torch.tensor(top_n_tokens)
inp_logprobs = torch.tensor([[-1.0, -3.0, -4.0, -2.0, -3.0]] * 5)
accepted_ids = torch.ones_like(top_n_tokens_tensor)
topn_tok_ids, topn_tok_logprobs = batch_top_tokens(
top_n_tokens, top_n_tokens_tensor, inp_logprobs, accepted_ids
)
assert topn_tok_ids[0] == [[]]
assert topn_tok_ids[1] == [[0, 3]]
assert topn_tok_ids[2] == [[0, 3, 1, 4]]
assert topn_tok_ids[3] == [[0, 3, 1, 4]]
assert topn_tok_ids[4] == [[0, 3, 1, 4, 2]]
assert topn_tok_logprobs[0] == [[]]
assert topn_tok_logprobs[1] == [[-1, -2]]
assert topn_tok_logprobs[2] == [[-1, -2, -3, -3]]
assert topn_tok_logprobs[3] == [[-1, -2, -3, -3]]
assert topn_tok_logprobs[4] == [[-1, -2, -3, -3, -4]]
# Now let's make second member of the batch be speculated
inp_logprobs = torch.tensor([[-1.0, -3.0, -4.0, -2.0, -3.0]] * 5 * 2)
accepted_ids[1] = 2
topn_tok_ids, topn_tok_logprobs = batch_top_tokens(
top_n_tokens, top_n_tokens_tensor, inp_logprobs, accepted_ids
)
assert topn_tok_ids[0] == [[]]
assert topn_tok_ids[1] == [[0, 3], [0, 3]]
assert topn_tok_ids[2] == [[0, 3, 1, 4]]
assert topn_tok_ids[3] == [[0, 3, 1, 4]]
assert topn_tok_ids[4] == [[0, 3, 1, 4, 2]]
assert topn_tok_logprobs[0] == [[]]
assert topn_tok_logprobs[1] == [[-1, -2], [-1, -2]]
assert topn_tok_logprobs[2] == [[-1, -2, -3, -3]]
assert topn_tok_logprobs[3] == [[-1, -2, -3, -3]]
assert topn_tok_logprobs[4] == [[-1, -2, -3, -3, -4]]
| text-generation-inference/server/tests/utils/test_tokens.py/0 | {
"file_path": "text-generation-inference/server/tests/utils/test_tokens.py",
"repo_id": "text-generation-inference",
"token_count": 1427
} | 216 |
# coding=utf-8
# Copyright 2022 EleutherAI and the HuggingFace Inc. team. All rights reserved.
#
# This code is based on EleutherAI's GPT-NeoX library and the GPT-NeoX
# and OPT implementations in this library. It has been modified from its
# original forms to accommodate minor architectural differences compared
# to GPT-NeoX and OPT used by the Meta AI team that trained the model.
#
# Licensed under the Apache License, Version 2.0 (the "License");
# you may not use this file except in compliance with the License.
# You may obtain a copy of the License at
#
# http://www.apache.org/licenses/LICENSE-2.0
#
# Unless required by applicable law or agreed to in writing, software
# distributed under the License is distributed on an "AS IS" BASIS,
# WITHOUT WARRANTIES OR CONDITIONS OF ANY KIND, either express or implied.
# See the License for the specific language governing permissions and
# limitations under the License.
import torch
import torch.distributed
from torch import nn
from transformers.activations import ACT2FN
from transformers.modeling_utils import PreTrainedModel
from transformers.models.gpt_neox import GPTNeoXConfig
from typing import Optional, List, Tuple
from text_generation_server.utils import paged_attention, flash_attn
from text_generation_server.utils.flash_attn import attention
from text_generation_server.utils.layers import (
TensorParallelRowLinear,
TensorParallelColumnLinear,
TensorParallelEmbedding,
SpeculativeHead,
FastLayerNorm,
PositionRotaryEmbedding,
get_linear,
)
def load_row(config, prefix: str, weights, bias: bool):
weight = weights.get_multi_weights_row(prefix, quantize=config.quantize)
if bias and weights.process_group.rank() == 0:
# Rank is only on the first rank process
bias = weights.get_tensor(f"{prefix}.bias")
else:
bias = None
linear = get_linear(weight, bias, config.quantize)
if config.use_parallel_residual:
return linear
else:
return TensorParallelRowLinear(linear, process_group=weights.process_group)
def load_qkv(config, prefix: str, weights, num_heads, head_size, hidden_size):
weight = weights.get_multi_weights_col([prefix], quantize=config.quantize, dim=0)
if isinstance(weight, torch.Tensor):
# Only on non quantized versions
weight = (
weight.view(
num_heads,
3,
head_size,
hidden_size,
)
.permute(1, 0, 2, 3)
.reshape(-1, hidden_size)
)
bias = weights.get_sharded(f"{prefix}.bias", dim=0)
bias = bias.view(num_heads, 3, head_size).permute(1, 0, 2).reshape(-1)
linear = get_linear(weight, bias, config.quantize)
if config.use_parallel_residual:
return linear
else:
return TensorParallelColumnLinear(linear)
class FlashNeoxAttention(torch.nn.Module):
def __init__(self, config, prefix, weights):
super().__init__()
num_heads = config.num_attention_heads
hidden_size = config.hidden_size
self.num_heads = num_heads
self.hidden_size = hidden_size
self.head_size = hidden_size // num_heads
self.rotary_dim = int(config.rotary_pct * self.head_size)
if self.num_heads % weights.process_group.size() != 0:
raise ValueError(
f"`num_heads` must be divisible by `num_shards` (got `num_heads`: {self.num_heads} "
f"and `num_shards`: {weights.process_group.size()}"
)
self.num_heads = self.num_heads // weights.process_group.size()
self.rotary_emb = PositionRotaryEmbedding.static(
config=config,
dim=self.rotary_dim,
base=config.rotary_emb_base,
device=weights.device,
)
self.softmax_scale = self.head_size ** (-0.5)
self.query_key_value = load_qkv(
config,
prefix=f"{prefix}.query_key_value",
weights=weights,
num_heads=self.num_heads,
head_size=self.head_size,
hidden_size=self.hidden_size,
)
self.dense = load_row(
config, prefix=f"{prefix}.dense", weights=weights, bias=True
)
self.kv_head_mapping = torch.arange(
0, self.num_heads, dtype=torch.int32, device=weights.device
)
def forward(
self,
hidden_states,
cos,
sin,
cu_seqlen_prefill,
kv_cache,
block_tables,
slots,
input_lengths,
max_s,
):
qkv = self.query_key_value(hidden_states)
qkv = qkv.view(-1, 3, self.num_heads, self.head_size)
# Inplace rotary
self.rotary_emb(qkv[:, 0], qkv[:, 1], cos, sin)
paged_attention.reshape_and_cache(
qkv[:, 1], qkv[:, 2], kv_cache[0], kv_cache[1], slots
)
# output tensor
attn_output = torch.empty_like(qkv[:, 0])
# Prefill
if cu_seqlen_prefill is not None:
# flash attention
flash_attn.attention(
qkv[:, 0],
qkv[:, 1],
qkv[:, 2],
attn_output,
cu_seqlen_prefill,
max_s,
self.softmax_scale,
)
# Decode
else:
paged_attention.attention(
attn_output,
qkv[:, 0],
kv_cache[0],
kv_cache[1],
self.kv_head_mapping,
self.softmax_scale,
block_tables,
input_lengths,
max_s,
)
return self.dense(attn_output.view(-1, self.num_heads * self.head_size))
class FlashMLP(nn.Module):
def __init__(self, config, prefix, weights):
super().__init__()
act = config.hidden_act
self.act = (
ACT2FN[act]
if "gelu" not in act
else lambda x: torch.nn.functional.gelu(
x,
approximate=(
"tanh" if act in ["gelu_fast", "gelu_pytorch_tanh"] else "none"
),
)
)
self.dense_h_to_4h = TensorParallelColumnLinear.load(
config, prefix=f"{prefix}.dense_h_to_4h", weights=weights, bias=True
)
self.dense_4h_to_h = load_row(
config, prefix=f"{prefix}.dense_4h_to_h", weights=weights, bias=True
)
def forward(self, hidden_states):
hidden_states = self.dense_h_to_4h(hidden_states)
hidden_states = self.act(hidden_states)
hidden_states = self.dense_4h_to_h(hidden_states)
return hidden_states
class FlashNeoXLayer(nn.Module):
def __init__(self, layer_id, config, weights):
super().__init__()
layer_norm_eps = config.layer_norm_eps
prefix = f"gpt_neox.layers.{layer_id}"
self.use_parallel_residual = config.use_parallel_residual
self.input_layernorm = FastLayerNorm.load(
prefix=f"{prefix}.input_layernorm", weights=weights, eps=layer_norm_eps
)
self.post_attention_layernorm = FastLayerNorm.load(
prefix=f"{prefix}.post_attention_layernorm",
weights=weights,
eps=layer_norm_eps,
)
self.attention = FlashNeoxAttention(
config, prefix=f"{prefix}.attention", weights=weights
)
self.mlp = FlashMLP(config, prefix=f"{prefix}.mlp", weights=weights)
self.process_group = weights.process_group
def forward(
self,
hidden_states,
residual,
cos,
sin,
cu_seqlen_prefill,
kv_cache,
block_tables,
slots,
input_lengths,
max_s,
):
if self.use_parallel_residual:
ln1_hidden_states, _ = self.input_layernorm(hidden_states)
attn_output = self.attention(
ln1_hidden_states,
cos,
sin,
cu_seqlen_prefill,
kv_cache,
block_tables,
slots,
input_lengths,
max_s,
)
ln2_hidden_states, _ = self.post_attention_layernorm(hidden_states)
mlp_output = self.mlp(ln2_hidden_states)
intermediate = mlp_output + attn_output
if self.process_group.size() > 1:
torch.distributed.all_reduce(intermediate, group=self.process_group)
return intermediate + hidden_states, None
else:
hidden_states, residual = self.input_layernorm(hidden_states, residual)
hidden_states = self.attention(
hidden_states,
cos,
sin,
cu_seqlen_prefill,
kv_cache,
block_tables,
slots,
input_lengths,
max_s,
)
hidden_states, residual = self.post_attention_layernorm(
hidden_states, residual
)
mlp_output = self.mlp(hidden_states)
return mlp_output, residual
class FlashGPTNeoXPreTrainedModel(PreTrainedModel):
config_class = GPTNeoXConfig
base_model_prefix = "gpt_neox"
supports_gradient_checkpointing = False
_no_split_modules = None
class FlashGPTNeoXModel(FlashGPTNeoXPreTrainedModel):
def __init__(self, config, weights):
super().__init__(config)
self.config = config
self.embed_in = TensorParallelEmbedding(
prefix="gpt_neox.embed_in", weights=weights
)
self.layers = nn.ModuleList(
[
FlashNeoXLayer(layer_id, config, weights)
for layer_id in range(config.num_hidden_layers)
]
)
self.final_layer_norm = FastLayerNorm.load(
prefix="gpt_neox.final_layer_norm",
weights=weights,
eps=config.layer_norm_eps,
)
self.gradient_checkpointing = False
self.head_size = self.layers[0].attention.head_size
self.num_heads = self.layers[0].attention.num_heads
def forward(
self,
input_ids: torch.Tensor,
position_ids: torch.Tensor,
cu_seqlen_prefill: Optional[torch.Tensor],
kv_cache: List[Tuple[torch.Tensor, torch.Tensor]],
block_tables: torch.Tensor,
slots: torch.Tensor,
input_lengths: torch.Tensor,
max_s: int,
) -> torch.Tensor:
hidden_states = self.embed_in(input_ids)
# Get rotary cos and sin for this forward
# Avoid to index in each layer
cos, sin = self.layers[0].attention.rotary_emb.get_cos_sin(
position_ids, max_s, hidden_states.dtype
)
residual = None
for i, layer in enumerate(self.layers):
hidden_states, residual = layer(
hidden_states,
residual,
cos,
sin,
cu_seqlen_prefill,
kv_cache[i],
block_tables,
slots,
input_lengths,
max_s,
)
hidden_states, _ = self.final_layer_norm(hidden_states, residual)
return hidden_states
class FlashGPTNeoXForCausalLM(FlashGPTNeoXPreTrainedModel):
def __init__(self, config, weights):
super().__init__(config)
self.gpt_neox = FlashGPTNeoXModel(config, weights)
self.embed_out = SpeculativeHead.load(
config, prefix="embed_out", weights=weights
)
def forward(
self,
input_ids: torch.Tensor,
position_ids: torch.Tensor,
cu_seqlen_prefill: Optional[torch.Tensor],
kv_cache: List[Tuple[torch.Tensor, torch.Tensor]],
block_tables: torch.Tensor,
slots: torch.Tensor,
input_lengths: torch.Tensor,
max_s: int,
lm_head_indices: Optional[torch.Tensor] = None,
) -> torch.Tensor:
hidden_states = self.gpt_neox(
input_ids,
position_ids,
cu_seqlen_prefill,
kv_cache,
block_tables,
slots,
input_lengths,
max_s,
)
if lm_head_indices is not None:
hidden_states = hidden_states[lm_head_indices]
logits = self.embed_out(hidden_states)
return logits
| text-generation-inference/server/text_generation_server/models/custom_modeling/flash_neox_modeling.py/0 | {
"file_path": "text-generation-inference/server/text_generation_server/models/custom_modeling/flash_neox_modeling.py",
"repo_id": "text-generation-inference",
"token_count": 6185
} | 217 |
# imlementation of the PhiModel and PhiForCausalLM classes
import torch
import torch.distributed
import math
from torch import nn
from typing import Optional, List, Tuple, Any
from transformers.configuration_utils import PretrainedConfig
from transformers.modeling_outputs import CausalLMOutputWithPast
from text_generation_server.utils.layers import (
TensorParallelRowLinear,
TensorParallelColumnLinear,
TensorParallelEmbedding,
SpeculativeHead,
FastLinear,
)
# PhiConfig is the configuration class for the PhiModel.
class PhiConfig(PretrainedConfig):
def __init__(
self,
vocab_size=51200,
n_positions=2048,
n_embd=2560,
n_layer=32,
n_inner=None,
n_head=32,
rotary_dim=32,
layer_norm_epsilon=1e-5,
tie_word_embeddings=False,
pad_vocab_size_multiple=64,
pad_token_id=0,
bos_token_id=1,
eos_token_id=2,
no_bias=False,
**kwargs,
):
self.vocab_size = vocab_size
self.n_positions = n_positions
self.n_embd = n_embd
self.n_layer = n_layer
self.n_inner = n_inner
self.n_head = n_head
self.rotary_dim = rotary_dim
self.layer_norm_epsilon = layer_norm_epsilon
self.tie_word_embeddings = tie_word_embeddings
self.pad_vocab_size_multiple = pad_vocab_size_multiple
self.pad_token_id = pad_token_id
self.bos_token_id = bos_token_id
self.eos_token_id = eos_token_id
self.no_bias = no_bias
super().__init__(
pad_token_id=pad_token_id,
bos_token_id=bos_token_id,
eos_token_id=eos_token_id,
tie_word_embeddings=tie_word_embeddings,
**kwargs,
)
# RotaryEmbedding is a class that implements the rotary embedding.
class RotaryEmbedding(nn.Module):
def __init__(self, dim, max_seq_len):
super().__init__()
inv_freq = [1.0 / 10000.0 ** (i / dim) for i in range(0, dim, 2)]
inv_freq_len = len(inv_freq)
inv_freq = torch.tensor(inv_freq).view(1, inv_freq_len)
t = torch.arange(0, max_seq_len, dtype=torch.float).view(max_seq_len, 1)
freqs = t.matmul(inv_freq)
self.sin = freqs.sin()
self.cos = freqs.cos()
def apply_rotary_emb_qkv(self, qkv, seqlen_offset):
b_size, seqlen, three, _, _headdim = qkv.shape
if three != 3:
raise Exception("unexpected shape for qkv")
_, rotary_dim = self.cos.shape
rotary_dim = rotary_dim * 2
q_rot = qkv[:, :, 0, :, :rotary_dim]
q_pass = qkv[:, :, 0, :, rotary_dim:]
k_rot = qkv[:, :, 1, :, :rotary_dim]
k_pass = qkv[:, :, 1, :, rotary_dim:]
q12 = torch.chunk(q_rot, 2, dim=-1)
k12 = torch.chunk(k_rot, 2, dim=-1)
q1, q2 = q12[0], q12[1]
k1, k2 = k12[0], k12[1]
c = self.cos.narrow(0, seqlen_offset, seqlen).unsqueeze(1)
s = self.sin.narrow(0, seqlen_offset, seqlen).unsqueeze(1)
q_rot = torch.cat(
[
q1 * c - q2 * s,
q1 * s + q2 * c,
],
dim=-1,
)
k_rot = torch.cat(
[
k1 * c - k2 * s,
k1 * s + k2 * c,
],
dim=-1,
)
q = torch.cat([q_rot, q_pass], dim=-1)
k = torch.cat([k_rot, k_pass], dim=-1)
v = qkv[:, :, 2]
return q, k, v
# PhiCausalLMHead is the head of the PhiModel. It is a linear layer with a layer norm.
class PhiCausalLMHead(nn.Module):
def __init__(self, config, weights):
super().__init__()
self.ln = nn.LayerNorm.load(
prefix="lm_head.ln",
weights=weights,
eps=config.layer_norm_epsilon,
)
self.linear = SpeculativeHead.load(
config=config, prefix="lm_head.linear", weights=weights
)
def forward(self, hidden_states):
hidden_states = self.ln(hidden_states)
hidden_states = self.linear(hidden_states)
return hidden_states
# PhiMHA is a multi-head attention layer. This layer uses an attention mask to prevent tokens from attending to subsequent tokens.
class PhiMHA(nn.Module):
def __init__(self, prefix, config, weights):
super().__init__()
self.Wqkv = TensorParallelColumnLinear.load(
config, prefix=f"{prefix}.Wqkv", weights=weights, bias=not config.no_bias
)
self.out_proj = TensorParallelRowLinear.load(
config,
prefix=f"{prefix}.out_proj",
weights=weights,
bias=not config.no_bias,
)
self.op_size = config.n_embd
self.head_dim = int(config.n_embd / config.n_head)
self.num_heads = config.n_head
self.rotary_emb = RotaryEmbedding(
config.rotary_dim,
config.n_positions,
)
self.softmax_scale = 1.0 / math.sqrt(self.head_dim)
def forward(
self,
hidden_states,
past_kv_cache,
attention_mask=None,
):
b_size, seq_len, _n_embd = hidden_states.shape
qkv = self.Wqkv(hidden_states)
qkv = qkv.view(b_size, seq_len, 3, self.num_heads, self.head_dim)
seqlen_offset = 0 if past_kv_cache is None else past_kv_cache[0].shape[1]
q, k, v = self.rotary_emb.apply_rotary_emb_qkv(qkv, seqlen_offset)
# if there is a kv_cache, then we need to concatenate
if past_kv_cache is not None:
prev_k, prev_v = past_kv_cache
k = torch.cat([prev_k, k], dim=1)
v = torch.cat([prev_v, v], dim=1)
past_kv_cache = [k, v]
attn_weights = torch.einsum("bthd,bshd->bhts", q, k * self.softmax_scale)
if attention_mask is not None:
seqlen_k = k.shape[1]
seqlen_q = q.shape[1]
causal_mask = torch.triu(
torch.full((seqlen_q, seqlen_k), -10000.0, device=attn_weights.device),
1,
)
attn_weights = attn_weights + causal_mask.to(dtype=attn_weights.dtype)
attn_weights = torch.nn.functional.softmax(attn_weights, dim=-1)
attn_output = attn_weights.matmul(v.transpose(1, 2)).squeeze(0)
attn_output = (
attn_output.view((b_size, self.num_heads, seq_len, self.head_dim))
.transpose(1, 2)
.flatten(-2)
)
return self.out_proj(attn_output), past_kv_cache
# PhiMLP is a multi-layer perceptron. It contains two linear layers with a gelu activation function.
class PhiMLP(nn.Module):
def __init__(self, prefix, config, weights):
super().__init__()
self.n_inner = config.n_inner
self.fc1 = FastLinear.load(
config=config,
prefix=f"{prefix}.fc1",
weights=weights,
bias=False,
)
self.fc2 = FastLinear.load(
config=config,
prefix=f"{prefix}.fc2",
weights=weights,
bias=False,
)
self.activation = torch.nn.functional.gelu
def forward(self, hidden_states):
hidden_states = self.fc1(hidden_states)
hidden_states = self.activation(hidden_states)
hidden_states = self.fc2(hidden_states)
return hidden_states
# PhiBlock is a single transformer block. It contains a layer norm, a multi-head attention layer and an multi-layer perceptron.
class PhiBlock(nn.Module):
def __init__(self, layer_id, config, weights):
super().__init__()
self.layer_id = layer_id
self.layer_norm = nn.LayerNorm.load(
prefix=f"{layer_id}.ln", weights=weights, eps=config.layer_norm_epsilon
)
self.mixer = PhiMHA(prefix=f"{layer_id}.mixer", config=config, weights=weights)
self.mlp = PhiMLP(prefix=f"{layer_id}.mlp", config=config, weights=weights)
def forward(
self,
hidden_states,
kv_cache,
attention_mask,
):
residual = hidden_states
hidden_states = self.layer_norm(hidden_states)
attn_outputs, past_kv_cache = self.mixer(
hidden_states, kv_cache, attention_mask
)
feed_forward_hidden_states = self.mlp(hidden_states)
out = attn_outputs + feed_forward_hidden_states + residual
return out, past_kv_cache
# PhiModel implements the embedding layer and the transformer blocks.
class PhiModel(nn.Module):
def __init__(self, config, weights):
super().__init__()
self.tp_rank = weights.process_group.rank()
self.tp_world_size = weights.process_group.size()
self.embed_tokens = TensorParallelEmbedding(
prefix="transformer.embd.wte", weights=weights
)
self.blocks = nn.ModuleList(
[
PhiBlock(f"transformer.h.{layer_id}", config, weights)
for layer_id in range(config.n_layer)
]
)
def forward(
self,
input_ids: torch.LongTensor,
past_key_values: Optional[List[Tuple[torch.FloatTensor]]] = None,
attention_mask: Optional[torch.ByteTensor] = None,
return_dict: Optional[bool] = None,
use_cache: Optional[bool] = None,
) -> Tuple[torch.Tensor, List[Tuple[torch.Tensor, torch.Tensor]]]:
hidden_states = self.embed_tokens(input_ids)
seq_len = hidden_states.shape[1]
mask = None if seq_len <= 1 else attention_mask
past_key_values = (
[None] * len(self.blocks) if past_key_values is None else past_key_values
)
for index, block in enumerate(self.blocks):
hidden_states, new_key_values = block(
hidden_states, past_key_values[index], mask
)
past_key_values[index] = new_key_values
return hidden_states, past_key_values
# PhiForCausalLM wraps the PhiModel and PhiCausalLMHead together and returns a CausalLMOutputWithPast object.
class PhiForCausalLM(torch.nn.Module):
def __init__(self, config, weights):
super().__init__()
self.model = PhiModel(config, weights)
self.lm_head = PhiCausalLMHead(config, weights)
def forward(
self,
input_ids: torch.LongTensor,
past_key_values: Optional[List[Tuple[torch.FloatTensor]]] = None,
attention_mask: Optional[torch.ByteTensor] = None,
return_dict: Optional[bool] = None,
use_cache: Optional[bool] = None,
labels: Optional[torch.LongTensor] = None,
) -> Tuple[torch.Tensor, List[Tuple[torch.Tensor, torch.Tensor]]]:
model_output = self.model(
input_ids, past_key_values, attention_mask, return_dict, use_cache
)
logits = self.lm_head(model_output[0])
loss = None
if labels is not None:
loss = nn.CrossEntropyLoss()(
logits[:, :-1].view(-1, logits.size(-1)), labels[:, 1:].view(-1)
)
if not return_dict:
return (
((loss,) + (logits,) + model_output[1:])
if loss is not None
else (logits,) + model_output[1:]
)
return CausalLMOutputWithPast(
loss=loss,
logits=logits,
past_key_values=model_output[1],
hidden_states=None,
attentions=None,
)
| text-generation-inference/server/text_generation_server/models/custom_modeling/phi_modeling.py/0 | {
"file_path": "text-generation-inference/server/text_generation_server/models/custom_modeling/phi_modeling.py",
"repo_id": "text-generation-inference",
"token_count": 5626
} | 218 |
import torch
import torch.distributed
from typing import List, Optional, Tuple
from transformers import (
AutoTokenizer,
AutoConfig,
AutoProcessor,
)
from text_generation_server.models.custom_modeling.idefics_config import IdeficsConfig
from text_generation_server.models.custom_modeling.idefics_processing import (
IdeficsProcessor,
)
from transformers import LlamaTokenizerFast
from text_generation_server.models.custom_modeling.idefics_modeling import (
IdeficsForVisionText2Text,
)
from text_generation_server.models.idefics_causal_lm import IdeficsCausalLM
from text_generation_server.utils import (
initialize_torch_distributed,
weight_files,
Weights,
)
class IDEFICSSharded(IdeficsCausalLM):
def __init__(
self,
model_id: str,
revision: Optional[str] = None,
quantize: Optional[str] = None,
use_medusa: Optional[str] = None,
dtype: Optional[torch.dtype] = None,
trust_remote_code: bool = False,
):
self.process_group, rank, world_size = initialize_torch_distributed()
if torch.cuda.is_available():
device = torch.device(f"cuda:{rank}")
# 9b seems to work correctly enough in float16, but 80b seems
# to be really saturating for f16.
dtype = torch.float16 if dtype is None else dtype
else:
device = torch.device("cpu")
dtype = torch.float32 if dtype is None else dtype
self.device, self.dtype = device, dtype
config = IdeficsConfig.from_pretrained(
model_id,
revision=revision,
trust_remote_code=trust_remote_code,
)
config.quantize = quantize
config.use_medusa = use_medusa
config.vision_config.quantize = quantize
tokenizer = LlamaTokenizerFast.from_pretrained(
model_id,
revision=revision,
padding_side="left",
truncation_side="left",
trust_remote_code=trust_remote_code,
)
self.processor = IdeficsProcessor.from_pretrained(
model_id,
revision=revision,
padding_side="left",
truncation_side="left",
trust_remote_code=trust_remote_code,
)
torch.distributed.barrier(group=self.process_group)
filenames = weight_files(model_id, revision=revision, extension=".safetensors")
weights = Weights(
filenames,
device=device,
dtype=dtype,
process_group=self.process_group,
)
model = IdeficsForVisionText2Text(config, weights)
torch.distributed.barrier(group=self.process_group)
super(IdeficsCausalLM, self).__init__(
model=model,
tokenizer=tokenizer,
requires_padding=True,
dtype=dtype,
device=device,
rank=rank,
world_size=world_size,
)
| text-generation-inference/server/text_generation_server/models/idefics.py/0 | {
"file_path": "text-generation-inference/server/text_generation_server/models/idefics.py",
"repo_id": "text-generation-inference",
"token_count": 1344
} | 219 |
import torch
from typing import List
AWQ_PACK_ORDER = [0, 2, 4, 6, 1, 3, 5, 7]
REVERSE_AWQ_PACK_ORDER = [0, 4, 1, 5, 2, 6, 3, 7]
def pack(imatrix: torch.Tensor, direction: str = "column"):
"""
Packs a 4-bit integer matrix into a packed 32-bit integer matrix.
Args:
imatrix (torch.Tensor): matrix of integers
direction (str): direction of packing, either "column" or "row"
Returns:
qmatrix (torch.Tensor): packed matrix of integers
"""
shifts = torch.arange(0, 32, 4, dtype=torch.int32, device=imatrix.device)
imatrix = imatrix.to(torch.int8) & 0x0F # eventually correct overflow
if direction == "column":
imatrix = imatrix.view(-1, imatrix.shape[1] // (32 // 4), (32 // 4))
qmatrix = torch.bitwise_left_shift(imatrix, shifts[None, None, :]).sum(dim=-1)
elif direction == "row":
imatrix = imatrix.view(imatrix.shape[0] // (32 // 4), (32 // 4), -1)
qmatrix = torch.bitwise_left_shift(imatrix, shifts[None, :, None]).sum(dim=1)
qmatrix = qmatrix.to(torch.int32)
return qmatrix
def unpack(qmatrix: torch.Tensor, direction: str = "column"):
"""
Unpacks a 32-bit packed integer matrix into a 4-bit integer matrix.
Args:
qmatrix (torch.Tensor): matrix of packed integers
direction (str): direction of unpacking, either "column" or "row"
Returns:
imatrix (torch.Tensor): matrix of integers
"""
shifts = torch.arange(0, 32, 4, device=qmatrix.device)
if direction == "column":
imatrix = torch.bitwise_right_shift(
qmatrix[:, :, None], shifts[None, None, :]
).view(qmatrix.shape[0], -1)
elif direction == "row":
imatrix = torch.bitwise_right_shift(
qmatrix[:, None, :], shifts[None, :, None]
).view(-1, qmatrix.shape[-1])
imatrix = imatrix.to(torch.int8) & 0x0F # eventually correct overflow
return imatrix
def apply_order(
imatrix: torch.Tensor,
direction: str = "column",
order: List[int] = AWQ_PACK_ORDER,
):
"""
Applies the order to a 4-bit integer matrix.
Args:
imatrix (torch.Tensor): matrix of integers
direction (str): direction of applying order, either "column" or "row"
order (List[int]): order to apply, default is AWQ_PACK_ORDER
Returns:
imatrix (torch.Tensor): matrix of integers
"""
if direction == "column":
imatrix = imatrix.view(-1, (32 // 4))[:, order].view(imatrix.shape)
elif direction == "row":
imatrix = imatrix.view((32 // 4), -1)[order, :].view(imatrix.shape)
return imatrix
def fast_awq_to_gptq(qweight, qzeros):
# awq uses column packing for both weights and zeros
izeros = unpack(qzeros, direction="column")
iweights = unpack(qweight, direction="column")
# Reverse the order of the iweight and izeros tensors
izeros = apply_order(izeros, direction="column", order=REVERSE_AWQ_PACK_ORDER)
iweights = apply_order(iweights, direction="column", order=REVERSE_AWQ_PACK_ORDER)
# Subtract 1 from the izeros tensor (gptq adds 1 to the zeros)
izeros = izeros - 1
# exllama uses row packing for weights and column packing for zeros
qzeros = pack(izeros, direction="column")
qweight = pack(iweights, direction="row")
return qweight, qzeros
| text-generation-inference/server/text_generation_server/utils/awq/conversion_utils.py/0 | {
"file_path": "text-generation-inference/server/text_generation_server/utils/awq/conversion_utils.py",
"repo_id": "text-generation-inference",
"token_count": 1384
} | 220 |
import os
import json
from loguru import logger
import torch
from transformers import AutoTokenizer
from peft import AutoPeftModelForCausalLM, AutoPeftModelForSeq2SeqLM
def download_and_unload_peft(model_id, revision, trust_remote_code):
torch_dtype = torch.float16
logger.info("Trying to load a Peft model. It might take a while without feedback")
try:
model = AutoPeftModelForCausalLM.from_pretrained(
model_id,
revision=revision,
torch_dtype=torch_dtype,
trust_remote_code=trust_remote_code,
low_cpu_mem_usage=True,
)
except Exception:
model = AutoPeftModelForSeq2SeqLM.from_pretrained(
model_id,
revision=revision,
torch_dtype=torch_dtype,
trust_remote_code=trust_remote_code,
low_cpu_mem_usage=True,
)
logger.info("Peft model detected.")
logger.info(f"Merging the lora weights.")
base_model_id = model.peft_config["default"].base_model_name_or_path
model = model.merge_and_unload()
os.makedirs(model_id, exist_ok=True)
cache_dir = model_id
logger.info(f"Saving the newly created merged model to {cache_dir}")
tokenizer = AutoTokenizer.from_pretrained(
base_model_id, trust_remote_code=trust_remote_code
)
model.save_pretrained(cache_dir, safe_serialization=True)
model.config.save_pretrained(cache_dir)
tokenizer.save_pretrained(cache_dir)
| text-generation-inference/server/text_generation_server/utils/peft.py/0 | {
"file_path": "text-generation-inference/server/text_generation_server/utils/peft.py",
"repo_id": "text-generation-inference",
"token_count": 629
} | 221 |
[package]
authors = ["Nicolas Patry <[email protected]>"]
edition = "2021"
name = "node"
version = "0.15.3-dev.0"
# See more keys and their definitions at https://doc.rust-lang.org/cargo/reference/manifest.html
[lib]
crate-type = ["cdylib"]
[dependencies]
napi = "2"
napi-derive = "2"
serde = { version = "1.0.163", features = ["derive"] }
tokenizers = { path = "../../tokenizers/" }
[build-dependencies]
napi-build = "2"
[profile.release]
lto = true
| tokenizers/bindings/node/Cargo.toml/0 | {
"file_path": "tokenizers/bindings/node/Cargo.toml",
"repo_id": "tokenizers",
"token_count": 200
} | 222 |
import { prependNormalizer, stripAccentsNormalizer, stripNormalizer } from '../../'
describe('stripNormalizer', () => {
it('instantiates with no parameters', () => {
const normalizer = stripNormalizer()
expect(normalizer.constructor.name).toEqual('Normalizer')
})
it('accepts `undefined` as first parameter', () => {
expect(stripNormalizer(undefined)).toBeDefined()
})
it('accepts `undefined` as second parameter', () => {
expect(stripNormalizer(false, undefined)).toBeDefined()
})
it('instantiates with one parameter', () => {
const normalizer = stripNormalizer(false)
expect(normalizer.constructor.name).toEqual('Normalizer')
})
it('instantiates with two parameters', () => {
const normalizer = stripNormalizer(false, true)
expect(normalizer.constructor.name).toEqual('Normalizer')
})
it('prepend instantiates with one parameter', () => {
const normalizer = prependNormalizer('_')
expect(normalizer.constructor.name).toEqual('Normalizer')
expect(normalizer.normalizeString('Hello')).toEqual('_Hello')
})
it('can normalize strings', () => {
const normalizer = stripNormalizer()
expect(normalizer.normalizeString(' Hello there ')).toEqual('Hello there')
})
})
describe('stripAccentsNormalizer', () => {
it('initialize', () => {
const normalizer = stripAccentsNormalizer()
expect(normalizer.constructor.name).toEqual('Normalizer')
})
})
| tokenizers/bindings/node/lib/bindings/normalizers.test.ts/0 | {
"file_path": "tokenizers/bindings/node/lib/bindings/normalizers.test.ts",
"repo_id": "tokenizers",
"token_count": 468
} | 223 |
{
"name": "tokenizers-linux-arm-gnueabihf",
"version": "0.13.4-rc1",
"os": [
"linux"
],
"cpu": [
"arm"
],
"main": "tokenizers.linux-arm-gnueabihf.node",
"files": [
"tokenizers.linux-arm-gnueabihf.node"
],
"description": "Tokenizers platform specific bindings",
"keywords": [
"napi-rs",
"NAPI",
"N-API",
"Rust",
"node-addon",
"node-addon-api"
],
"license": "MIT",
"engines": {
"node": ">= 10"
},
"publishConfig": {
"registry": "https://registry.npmjs.org/",
"access": "public"
},
"repository": "tokenizers"
} | tokenizers/bindings/node/npm/linux-arm-gnueabihf/package.json/0 | {
"file_path": "tokenizers/bindings/node/npm/linux-arm-gnueabihf/package.json",
"repo_id": "tokenizers",
"token_count": 278
} | 224 |
tab_spaces = 2
| tokenizers/bindings/node/rustfmt.toml/0 | {
"file_path": "tokenizers/bindings/node/rustfmt.toml",
"repo_id": "tokenizers",
"token_count": 7
} | 225 |
export type TextInputSequence = string
export type PreTokenizedInputSequence = string[]
export type InputSequence = TextInputSequence | PreTokenizedInputSequence
export type TextEncodeInput = TextInputSequence | [TextInputSequence, TextInputSequence]
export type PreTokenizedEncodeInput = PreTokenizedInputSequence | [PreTokenizedInputSequence, PreTokenizedInputSequence]
export type EncodeInput = TextEncodeInput | PreTokenizedEncodeInput
| tokenizers/bindings/node/types.ts/0 | {
"file_path": "tokenizers/bindings/node/types.ts",
"repo_id": "tokenizers",
"token_count": 114
} | 226 |
from enum import Enum
from typing import List, Tuple, Union
Offsets = Tuple[int, int]
TextInputSequence = str
"""A :obj:`str` that represents an input sequence """
PreTokenizedInputSequence = Union[List[str], Tuple[str]]
"""A pre-tokenized input sequence. Can be one of:
- A :obj:`List` of :obj:`str`
- A :obj:`Tuple` of :obj:`str`
"""
TextEncodeInput = Union[
TextInputSequence,
Tuple[TextInputSequence, TextInputSequence],
List[TextInputSequence],
]
"""Represents a textual input for encoding. Can be either:
- A single sequence: :data:`~tokenizers.TextInputSequence`
- A pair of sequences:
- A :obj:`Tuple` of :data:`~tokenizers.TextInputSequence`
- Or a :obj:`List` of :data:`~tokenizers.TextInputSequence` of size 2
"""
PreTokenizedEncodeInput = Union[
PreTokenizedInputSequence,
Tuple[PreTokenizedInputSequence, PreTokenizedInputSequence],
List[PreTokenizedInputSequence],
]
"""Represents a pre-tokenized input for encoding. Can be either:
- A single sequence: :data:`~tokenizers.PreTokenizedInputSequence`
- A pair of sequences:
- A :obj:`Tuple` of :data:`~tokenizers.PreTokenizedInputSequence`
- Or a :obj:`List` of :data:`~tokenizers.PreTokenizedInputSequence` of size 2
"""
InputSequence = Union[TextInputSequence, PreTokenizedInputSequence]
"""Represents all the possible types of input sequences for encoding. Can be:
- When ``is_pretokenized=False``: :data:`~TextInputSequence`
- When ``is_pretokenized=True``: :data:`~PreTokenizedInputSequence`
"""
EncodeInput = Union[TextEncodeInput, PreTokenizedEncodeInput]
"""Represents all the possible types of input for encoding. Can be:
- When ``is_pretokenized=False``: :data:`~TextEncodeInput`
- When ``is_pretokenized=True``: :data:`~PreTokenizedEncodeInput`
"""
class OffsetReferential(Enum):
ORIGINAL = "original"
NORMALIZED = "normalized"
class OffsetType(Enum):
BYTE = "byte"
CHAR = "char"
class SplitDelimiterBehavior(Enum):
REMOVED = "removed"
ISOLATED = "isolated"
MERGED_WITH_PREVIOUS = "merged_with_previous"
MERGED_WITH_NEXT = "merged_with_next"
CONTIGUOUS = "contiguous"
from .tokenizers import (
AddedToken,
Encoding,
NormalizedString,
PreTokenizedString,
Regex,
Token,
Tokenizer,
decoders,
models,
normalizers,
pre_tokenizers,
processors,
trainers,
__version__,
)
from .implementations import (
BertWordPieceTokenizer,
ByteLevelBPETokenizer,
CharBPETokenizer,
SentencePieceBPETokenizer,
SentencePieceUnigramTokenizer,
)
| tokenizers/bindings/python/py_src/tokenizers/__init__.py/0 | {
"file_path": "tokenizers/bindings/python/py_src/tokenizers/__init__.py",
"repo_id": "tokenizers",
"token_count": 984
} | 227 |
# Generated content DO NOT EDIT
class PreTokenizer:
"""
Base class for all pre-tokenizers
This class is not supposed to be instantiated directly. Instead, any implementation of a
PreTokenizer will return an instance of this class when instantiated.
"""
def pre_tokenize(self, pretok):
"""
Pre-tokenize a :class:`~tokenizers.PyPreTokenizedString` in-place
This method allows to modify a :class:`~tokenizers.PreTokenizedString` to
keep track of the pre-tokenization, and leverage the capabilities of the
:class:`~tokenizers.PreTokenizedString`. If you just want to see the result of
the pre-tokenization of a raw string, you can use
:meth:`~tokenizers.pre_tokenizers.PreTokenizer.pre_tokenize_str`
Args:
pretok (:class:`~tokenizers.PreTokenizedString):
The pre-tokenized string on which to apply this
:class:`~tokenizers.pre_tokenizers.PreTokenizer`
"""
pass
def pre_tokenize_str(self, sequence):
"""
Pre tokenize the given string
This method provides a way to visualize the effect of a
:class:`~tokenizers.pre_tokenizers.PreTokenizer` but it does not keep track of the
alignment, nor does it provide all the capabilities of the
:class:`~tokenizers.PreTokenizedString`. If you need some of these, you can use
:meth:`~tokenizers.pre_tokenizers.PreTokenizer.pre_tokenize`
Args:
sequence (:obj:`str`):
A string to pre-tokeize
Returns:
:obj:`List[Tuple[str, Offsets]]`:
A list of tuple with the pre-tokenized parts and their offsets
"""
pass
class BertPreTokenizer(PreTokenizer):
"""
BertPreTokenizer
This pre-tokenizer splits tokens on spaces, and also on punctuation.
Each occurence of a punctuation character will be treated separately.
"""
def __init__(self):
pass
def pre_tokenize(self, pretok):
"""
Pre-tokenize a :class:`~tokenizers.PyPreTokenizedString` in-place
This method allows to modify a :class:`~tokenizers.PreTokenizedString` to
keep track of the pre-tokenization, and leverage the capabilities of the
:class:`~tokenizers.PreTokenizedString`. If you just want to see the result of
the pre-tokenization of a raw string, you can use
:meth:`~tokenizers.pre_tokenizers.PreTokenizer.pre_tokenize_str`
Args:
pretok (:class:`~tokenizers.PreTokenizedString):
The pre-tokenized string on which to apply this
:class:`~tokenizers.pre_tokenizers.PreTokenizer`
"""
pass
def pre_tokenize_str(self, sequence):
"""
Pre tokenize the given string
This method provides a way to visualize the effect of a
:class:`~tokenizers.pre_tokenizers.PreTokenizer` but it does not keep track of the
alignment, nor does it provide all the capabilities of the
:class:`~tokenizers.PreTokenizedString`. If you need some of these, you can use
:meth:`~tokenizers.pre_tokenizers.PreTokenizer.pre_tokenize`
Args:
sequence (:obj:`str`):
A string to pre-tokeize
Returns:
:obj:`List[Tuple[str, Offsets]]`:
A list of tuple with the pre-tokenized parts and their offsets
"""
pass
class ByteLevel(PreTokenizer):
"""
ByteLevel PreTokenizer
This pre-tokenizer takes care of replacing all bytes of the given string
with a corresponding representation, as well as splitting into words.
Args:
add_prefix_space (:obj:`bool`, `optional`, defaults to :obj:`True`):
Whether to add a space to the first word if there isn't already one. This
lets us treat `hello` exactly like `say hello`.
use_regex (:obj:`bool`, `optional`, defaults to :obj:`True`):
Set this to :obj:`False` to prevent this `pre_tokenizer` from using
the GPT2 specific regexp for spliting on whitespace.
"""
def __init__(self, add_prefix_space=True, use_regex=True):
pass
@staticmethod
def alphabet():
"""
Returns the alphabet used by this PreTokenizer.
Since the ByteLevel works as its name suggests, at the byte level, it
encodes each byte value to a unique visible character. This means that there is a
total of 256 different characters composing this alphabet.
Returns:
:obj:`List[str]`: A list of characters that compose the alphabet
"""
pass
def pre_tokenize(self, pretok):
"""
Pre-tokenize a :class:`~tokenizers.PyPreTokenizedString` in-place
This method allows to modify a :class:`~tokenizers.PreTokenizedString` to
keep track of the pre-tokenization, and leverage the capabilities of the
:class:`~tokenizers.PreTokenizedString`. If you just want to see the result of
the pre-tokenization of a raw string, you can use
:meth:`~tokenizers.pre_tokenizers.PreTokenizer.pre_tokenize_str`
Args:
pretok (:class:`~tokenizers.PreTokenizedString):
The pre-tokenized string on which to apply this
:class:`~tokenizers.pre_tokenizers.PreTokenizer`
"""
pass
def pre_tokenize_str(self, sequence):
"""
Pre tokenize the given string
This method provides a way to visualize the effect of a
:class:`~tokenizers.pre_tokenizers.PreTokenizer` but it does not keep track of the
alignment, nor does it provide all the capabilities of the
:class:`~tokenizers.PreTokenizedString`. If you need some of these, you can use
:meth:`~tokenizers.pre_tokenizers.PreTokenizer.pre_tokenize`
Args:
sequence (:obj:`str`):
A string to pre-tokeize
Returns:
:obj:`List[Tuple[str, Offsets]]`:
A list of tuple with the pre-tokenized parts and their offsets
"""
pass
class CharDelimiterSplit(PreTokenizer):
"""
This pre-tokenizer simply splits on the provided char. Works like `.split(delimiter)`
Args:
delimiter: str:
The delimiter char that will be used to split input
"""
def pre_tokenize(self, pretok):
"""
Pre-tokenize a :class:`~tokenizers.PyPreTokenizedString` in-place
This method allows to modify a :class:`~tokenizers.PreTokenizedString` to
keep track of the pre-tokenization, and leverage the capabilities of the
:class:`~tokenizers.PreTokenizedString`. If you just want to see the result of
the pre-tokenization of a raw string, you can use
:meth:`~tokenizers.pre_tokenizers.PreTokenizer.pre_tokenize_str`
Args:
pretok (:class:`~tokenizers.PreTokenizedString):
The pre-tokenized string on which to apply this
:class:`~tokenizers.pre_tokenizers.PreTokenizer`
"""
pass
def pre_tokenize_str(self, sequence):
"""
Pre tokenize the given string
This method provides a way to visualize the effect of a
:class:`~tokenizers.pre_tokenizers.PreTokenizer` but it does not keep track of the
alignment, nor does it provide all the capabilities of the
:class:`~tokenizers.PreTokenizedString`. If you need some of these, you can use
:meth:`~tokenizers.pre_tokenizers.PreTokenizer.pre_tokenize`
Args:
sequence (:obj:`str`):
A string to pre-tokeize
Returns:
:obj:`List[Tuple[str, Offsets]]`:
A list of tuple with the pre-tokenized parts and their offsets
"""
pass
class Digits(PreTokenizer):
"""
This pre-tokenizer simply splits using the digits in separate tokens
Args:
individual_digits (:obj:`bool`, `optional`, defaults to :obj:`False`):
If set to True, digits will each be separated as follows::
"Call 123 please" -> "Call ", "1", "2", "3", " please"
If set to False, digits will grouped as follows::
"Call 123 please" -> "Call ", "123", " please"
"""
def __init__(self, individual_digits=False):
pass
def pre_tokenize(self, pretok):
"""
Pre-tokenize a :class:`~tokenizers.PyPreTokenizedString` in-place
This method allows to modify a :class:`~tokenizers.PreTokenizedString` to
keep track of the pre-tokenization, and leverage the capabilities of the
:class:`~tokenizers.PreTokenizedString`. If you just want to see the result of
the pre-tokenization of a raw string, you can use
:meth:`~tokenizers.pre_tokenizers.PreTokenizer.pre_tokenize_str`
Args:
pretok (:class:`~tokenizers.PreTokenizedString):
The pre-tokenized string on which to apply this
:class:`~tokenizers.pre_tokenizers.PreTokenizer`
"""
pass
def pre_tokenize_str(self, sequence):
"""
Pre tokenize the given string
This method provides a way to visualize the effect of a
:class:`~tokenizers.pre_tokenizers.PreTokenizer` but it does not keep track of the
alignment, nor does it provide all the capabilities of the
:class:`~tokenizers.PreTokenizedString`. If you need some of these, you can use
:meth:`~tokenizers.pre_tokenizers.PreTokenizer.pre_tokenize`
Args:
sequence (:obj:`str`):
A string to pre-tokeize
Returns:
:obj:`List[Tuple[str, Offsets]]`:
A list of tuple with the pre-tokenized parts and their offsets
"""
pass
class Metaspace(PreTokenizer):
"""
Metaspace pre-tokenizer
This pre-tokenizer replaces any whitespace by the provided replacement character.
It then tries to split on these spaces.
Args:
replacement (:obj:`str`, `optional`, defaults to :obj:`▁`):
The replacement character. Must be exactly one character. By default we
use the `▁` (U+2581) meta symbol (Same as in SentencePiece).
add_prefix_space (:obj:`bool`, `optional`, defaults to :obj:`True`):
Whether to add a space to the first word if there isn't already one. This
lets us treat `hello` exactly like `say hello`.
"""
def __init__(self, replacement="_", add_prefix_space=True):
pass
def pre_tokenize(self, pretok):
"""
Pre-tokenize a :class:`~tokenizers.PyPreTokenizedString` in-place
This method allows to modify a :class:`~tokenizers.PreTokenizedString` to
keep track of the pre-tokenization, and leverage the capabilities of the
:class:`~tokenizers.PreTokenizedString`. If you just want to see the result of
the pre-tokenization of a raw string, you can use
:meth:`~tokenizers.pre_tokenizers.PreTokenizer.pre_tokenize_str`
Args:
pretok (:class:`~tokenizers.PreTokenizedString):
The pre-tokenized string on which to apply this
:class:`~tokenizers.pre_tokenizers.PreTokenizer`
"""
pass
def pre_tokenize_str(self, sequence):
"""
Pre tokenize the given string
This method provides a way to visualize the effect of a
:class:`~tokenizers.pre_tokenizers.PreTokenizer` but it does not keep track of the
alignment, nor does it provide all the capabilities of the
:class:`~tokenizers.PreTokenizedString`. If you need some of these, you can use
:meth:`~tokenizers.pre_tokenizers.PreTokenizer.pre_tokenize`
Args:
sequence (:obj:`str`):
A string to pre-tokeize
Returns:
:obj:`List[Tuple[str, Offsets]]`:
A list of tuple with the pre-tokenized parts and their offsets
"""
pass
class Punctuation(PreTokenizer):
"""
This pre-tokenizer simply splits on punctuation as individual characters.
Args:
behavior (:class:`~tokenizers.SplitDelimiterBehavior`):
The behavior to use when splitting.
Choices: "removed", "isolated" (default), "merged_with_previous", "merged_with_next",
"contiguous"
"""
def __init__(self, behavior="isolated"):
pass
def pre_tokenize(self, pretok):
"""
Pre-tokenize a :class:`~tokenizers.PyPreTokenizedString` in-place
This method allows to modify a :class:`~tokenizers.PreTokenizedString` to
keep track of the pre-tokenization, and leverage the capabilities of the
:class:`~tokenizers.PreTokenizedString`. If you just want to see the result of
the pre-tokenization of a raw string, you can use
:meth:`~tokenizers.pre_tokenizers.PreTokenizer.pre_tokenize_str`
Args:
pretok (:class:`~tokenizers.PreTokenizedString):
The pre-tokenized string on which to apply this
:class:`~tokenizers.pre_tokenizers.PreTokenizer`
"""
pass
def pre_tokenize_str(self, sequence):
"""
Pre tokenize the given string
This method provides a way to visualize the effect of a
:class:`~tokenizers.pre_tokenizers.PreTokenizer` but it does not keep track of the
alignment, nor does it provide all the capabilities of the
:class:`~tokenizers.PreTokenizedString`. If you need some of these, you can use
:meth:`~tokenizers.pre_tokenizers.PreTokenizer.pre_tokenize`
Args:
sequence (:obj:`str`):
A string to pre-tokeize
Returns:
:obj:`List[Tuple[str, Offsets]]`:
A list of tuple with the pre-tokenized parts and their offsets
"""
pass
class Sequence(PreTokenizer):
"""
This pre-tokenizer composes other pre_tokenizers and applies them in sequence
"""
def __init__(self, pretokenizers):
pass
def pre_tokenize(self, pretok):
"""
Pre-tokenize a :class:`~tokenizers.PyPreTokenizedString` in-place
This method allows to modify a :class:`~tokenizers.PreTokenizedString` to
keep track of the pre-tokenization, and leverage the capabilities of the
:class:`~tokenizers.PreTokenizedString`. If you just want to see the result of
the pre-tokenization of a raw string, you can use
:meth:`~tokenizers.pre_tokenizers.PreTokenizer.pre_tokenize_str`
Args:
pretok (:class:`~tokenizers.PreTokenizedString):
The pre-tokenized string on which to apply this
:class:`~tokenizers.pre_tokenizers.PreTokenizer`
"""
pass
def pre_tokenize_str(self, sequence):
"""
Pre tokenize the given string
This method provides a way to visualize the effect of a
:class:`~tokenizers.pre_tokenizers.PreTokenizer` but it does not keep track of the
alignment, nor does it provide all the capabilities of the
:class:`~tokenizers.PreTokenizedString`. If you need some of these, you can use
:meth:`~tokenizers.pre_tokenizers.PreTokenizer.pre_tokenize`
Args:
sequence (:obj:`str`):
A string to pre-tokeize
Returns:
:obj:`List[Tuple[str, Offsets]]`:
A list of tuple with the pre-tokenized parts and their offsets
"""
pass
class Split(PreTokenizer):
"""
Split PreTokenizer
This versatile pre-tokenizer splits using the provided pattern and
according to the provided behavior. The pattern can be inverted by
making use of the invert flag.
Args:
pattern (:obj:`str` or :class:`~tokenizers.Regex`):
A pattern used to split the string. Usually a string or a a regex built with `tokenizers.Regex`
behavior (:class:`~tokenizers.SplitDelimiterBehavior`):
The behavior to use when splitting.
Choices: "removed", "isolated", "merged_with_previous", "merged_with_next",
"contiguous"
invert (:obj:`bool`, `optional`, defaults to :obj:`False`):
Whether to invert the pattern.
"""
def __init__(self, pattern, behavior, invert=False):
pass
def pre_tokenize(self, pretok):
"""
Pre-tokenize a :class:`~tokenizers.PyPreTokenizedString` in-place
This method allows to modify a :class:`~tokenizers.PreTokenizedString` to
keep track of the pre-tokenization, and leverage the capabilities of the
:class:`~tokenizers.PreTokenizedString`. If you just want to see the result of
the pre-tokenization of a raw string, you can use
:meth:`~tokenizers.pre_tokenizers.PreTokenizer.pre_tokenize_str`
Args:
pretok (:class:`~tokenizers.PreTokenizedString):
The pre-tokenized string on which to apply this
:class:`~tokenizers.pre_tokenizers.PreTokenizer`
"""
pass
def pre_tokenize_str(self, sequence):
"""
Pre tokenize the given string
This method provides a way to visualize the effect of a
:class:`~tokenizers.pre_tokenizers.PreTokenizer` but it does not keep track of the
alignment, nor does it provide all the capabilities of the
:class:`~tokenizers.PreTokenizedString`. If you need some of these, you can use
:meth:`~tokenizers.pre_tokenizers.PreTokenizer.pre_tokenize`
Args:
sequence (:obj:`str`):
A string to pre-tokeize
Returns:
:obj:`List[Tuple[str, Offsets]]`:
A list of tuple with the pre-tokenized parts and their offsets
"""
pass
class UnicodeScripts(PreTokenizer):
"""
This pre-tokenizer splits on characters that belong to different language family
It roughly follows https://github.com/google/sentencepiece/blob/master/data/Scripts.txt
Actually Hiragana and Katakana are fused with Han, and 0x30FC is Han too.
This mimicks SentencePiece Unigram implementation.
"""
def __init__(self):
pass
def pre_tokenize(self, pretok):
"""
Pre-tokenize a :class:`~tokenizers.PyPreTokenizedString` in-place
This method allows to modify a :class:`~tokenizers.PreTokenizedString` to
keep track of the pre-tokenization, and leverage the capabilities of the
:class:`~tokenizers.PreTokenizedString`. If you just want to see the result of
the pre-tokenization of a raw string, you can use
:meth:`~tokenizers.pre_tokenizers.PreTokenizer.pre_tokenize_str`
Args:
pretok (:class:`~tokenizers.PreTokenizedString):
The pre-tokenized string on which to apply this
:class:`~tokenizers.pre_tokenizers.PreTokenizer`
"""
pass
def pre_tokenize_str(self, sequence):
"""
Pre tokenize the given string
This method provides a way to visualize the effect of a
:class:`~tokenizers.pre_tokenizers.PreTokenizer` but it does not keep track of the
alignment, nor does it provide all the capabilities of the
:class:`~tokenizers.PreTokenizedString`. If you need some of these, you can use
:meth:`~tokenizers.pre_tokenizers.PreTokenizer.pre_tokenize`
Args:
sequence (:obj:`str`):
A string to pre-tokeize
Returns:
:obj:`List[Tuple[str, Offsets]]`:
A list of tuple with the pre-tokenized parts and their offsets
"""
pass
class Whitespace(PreTokenizer):
"""
This pre-tokenizer simply splits using the following regex: `\w+|[^\w\s]+`
"""
def __init__(self):
pass
def pre_tokenize(self, pretok):
"""
Pre-tokenize a :class:`~tokenizers.PyPreTokenizedString` in-place
This method allows to modify a :class:`~tokenizers.PreTokenizedString` to
keep track of the pre-tokenization, and leverage the capabilities of the
:class:`~tokenizers.PreTokenizedString`. If you just want to see the result of
the pre-tokenization of a raw string, you can use
:meth:`~tokenizers.pre_tokenizers.PreTokenizer.pre_tokenize_str`
Args:
pretok (:class:`~tokenizers.PreTokenizedString):
The pre-tokenized string on which to apply this
:class:`~tokenizers.pre_tokenizers.PreTokenizer`
"""
pass
def pre_tokenize_str(self, sequence):
"""
Pre tokenize the given string
This method provides a way to visualize the effect of a
:class:`~tokenizers.pre_tokenizers.PreTokenizer` but it does not keep track of the
alignment, nor does it provide all the capabilities of the
:class:`~tokenizers.PreTokenizedString`. If you need some of these, you can use
:meth:`~tokenizers.pre_tokenizers.PreTokenizer.pre_tokenize`
Args:
sequence (:obj:`str`):
A string to pre-tokeize
Returns:
:obj:`List[Tuple[str, Offsets]]`:
A list of tuple with the pre-tokenized parts and their offsets
"""
pass
class WhitespaceSplit(PreTokenizer):
"""
This pre-tokenizer simply splits on the whitespace. Works like `.split()`
"""
def __init__(self):
pass
def pre_tokenize(self, pretok):
"""
Pre-tokenize a :class:`~tokenizers.PyPreTokenizedString` in-place
This method allows to modify a :class:`~tokenizers.PreTokenizedString` to
keep track of the pre-tokenization, and leverage the capabilities of the
:class:`~tokenizers.PreTokenizedString`. If you just want to see the result of
the pre-tokenization of a raw string, you can use
:meth:`~tokenizers.pre_tokenizers.PreTokenizer.pre_tokenize_str`
Args:
pretok (:class:`~tokenizers.PreTokenizedString):
The pre-tokenized string on which to apply this
:class:`~tokenizers.pre_tokenizers.PreTokenizer`
"""
pass
def pre_tokenize_str(self, sequence):
"""
Pre tokenize the given string
This method provides a way to visualize the effect of a
:class:`~tokenizers.pre_tokenizers.PreTokenizer` but it does not keep track of the
alignment, nor does it provide all the capabilities of the
:class:`~tokenizers.PreTokenizedString`. If you need some of these, you can use
:meth:`~tokenizers.pre_tokenizers.PreTokenizer.pre_tokenize`
Args:
sequence (:obj:`str`):
A string to pre-tokeize
Returns:
:obj:`List[Tuple[str, Offsets]]`:
A list of tuple with the pre-tokenized parts and their offsets
"""
pass
| tokenizers/bindings/python/py_src/tokenizers/pre_tokenizers/__init__.pyi/0 | {
"file_path": "tokenizers/bindings/python/py_src/tokenizers/pre_tokenizers/__init__.pyi",
"repo_id": "tokenizers",
"token_count": 9461
} | 228 |
use pyo3::exceptions;
use pyo3::prelude::*;
use pyo3::type_object::PyTypeInfo;
use std::fmt::{Display, Formatter, Result as FmtResult};
use tokenizers::tokenizer::Result;
#[derive(Debug)]
pub struct PyError(pub String);
impl PyError {
#[allow(dead_code)]
pub fn from(s: &str) -> Self {
PyError(String::from(s))
}
pub fn into_pyerr<T: PyTypeInfo>(self) -> PyErr {
PyErr::new::<T, _>(format!("{}", self))
}
}
impl Display for PyError {
fn fmt(&self, fmt: &mut Formatter) -> FmtResult {
write!(fmt, "{}", self.0)
}
}
impl std::error::Error for PyError {}
pub struct ToPyResult<T>(pub Result<T>);
impl<T> From<ToPyResult<T>> for PyResult<T> {
fn from(v: ToPyResult<T>) -> Self {
v.0.map_err(|e| exceptions::PyException::new_err(format!("{}", e)))
}
}
impl<T> ToPyResult<T> {
pub fn into_py(self) -> PyResult<T> {
self.into()
}
}
pub(crate) fn deprecation_warning(py: Python<'_>, version: &str, message: &str) -> PyResult<()> {
let deprecation_warning = py.import("builtins")?.getattr("DeprecationWarning")?;
let full_message = format!("Deprecated in {}: {}", version, message);
pyo3::PyErr::warn(py, deprecation_warning, &full_message, 0)
}
| tokenizers/bindings/python/src/error.rs/0 | {
"file_path": "tokenizers/bindings/python/src/error.rs",
"repo_id": "tokenizers",
"token_count": 531
} | 229 |
from tokenizers import BertWordPieceTokenizer
from ..utils import bert_files, data_dir, multiprocessing_with_parallelism
class TestBertWordPieceTokenizer:
def test_basic_encode(self, bert_files):
tokenizer = BertWordPieceTokenizer.from_file(bert_files["vocab"])
# Encode with special tokens by default
output = tokenizer.encode("My name is John", "pair")
assert output.ids == [101, 2026, 2171, 2003, 2198, 102, 3940, 102]
assert output.tokens == [
"[CLS]",
"my",
"name",
"is",
"john",
"[SEP]",
"pair",
"[SEP]",
]
assert output.offsets == [
(0, 0),
(0, 2),
(3, 7),
(8, 10),
(11, 15),
(0, 0),
(0, 4),
(0, 0),
]
assert output.type_ids == [0, 0, 0, 0, 0, 0, 1, 1]
# Can encode without the special tokens
output = tokenizer.encode("My name is John", "pair", add_special_tokens=False)
assert output.ids == [2026, 2171, 2003, 2198, 3940]
assert output.tokens == ["my", "name", "is", "john", "pair"]
assert output.offsets == [(0, 2), (3, 7), (8, 10), (11, 15), (0, 4)]
assert output.type_ids == [0, 0, 0, 0, 1]
def test_multiprocessing_with_parallelism(self, bert_files):
tokenizer = BertWordPieceTokenizer.from_file(bert_files["vocab"])
multiprocessing_with_parallelism(tokenizer, False)
multiprocessing_with_parallelism(tokenizer, True)
def test_train_from_iterator(self):
text = ["A first sentence", "Another sentence", "And a last one"]
tokenizer = BertWordPieceTokenizer()
tokenizer.train_from_iterator(text, show_progress=False)
output = tokenizer.encode("A sentence")
assert output.tokens == ["a", "sentence"]
| tokenizers/bindings/python/tests/implementations/test_bert_wordpiece.py/0 | {
"file_path": "tokenizers/bindings/python/tests/implementations/test_bert_wordpiece.py",
"repo_id": "tokenizers",
"token_count": 914
} | 230 |
# Post-processors
<tokenizerslangcontent>
<python>
## BertProcessing
[[autodoc]] tokenizers.processors.BertProcessing
## ByteLevel
[[autodoc]] tokenizers.processors.ByteLevel
## RobertaProcessing
[[autodoc]] tokenizers.processors.RobertaProcessing
## TemplateProcessing
[[autodoc]] tokenizers.processors.TemplateProcessing
</python>
<rust>
The Rust API Reference is available directly on the [Docs.rs](https://docs.rs/tokenizers/latest/tokenizers/) website.
</rust>
<node>
The node API has not been documented yet.
</node>
</tokenizerslangcontent> | tokenizers/docs/source-doc-builder/api/post-processors.mdx/0 | {
"file_path": "tokenizers/docs/source-doc-builder/api/post-processors.mdx",
"repo_id": "tokenizers",
"token_count": 174
} | 231 |
Crates.io
----------------------------------------------------------------------------------------------------
🤗 Tokenizers is available on `crates.io <https://crates.io/crates/tokenizers>`__.
You just need to add it to your :obj:`Cargo.toml`::
tokenizers = "0.10"
| tokenizers/docs/source/installation/rust.inc/0 | {
"file_path": "tokenizers/docs/source/installation/rust.inc",
"repo_id": "tokenizers",
"token_count": 74
} | 232 |
{
"name": "create-wasm-app",
"version": "0.1.0",
"description": "create an app to consume rust-generated wasm packages",
"main": "index.js",
"bin": {
"create-wasm-app": ".bin/create-wasm-app.js"
},
"scripts": {
"build": "webpack --config webpack.config.js",
"start": "NODE_OPTIONS=--openssl-legacy-provider webpack-dev-server"
},
"repository": {
"type": "git",
"url": "git+https://github.com/rustwasm/create-wasm-app.git"
},
"keywords": ["webassembly", "wasm", "rust", "webpack"],
"author": "Ashley Williams <[email protected]>",
"license": "(MIT OR Apache-2.0)",
"bugs": {
"url": "https://github.com/rustwasm/create-wasm-app/issues"
},
"homepage": "https://github.com/rustwasm/create-wasm-app#readme",
"devDependencies": {
"copy-webpack-plugin": "^11.0.0",
"webpack": "^5.75.0",
"webpack-cli": "^5.0.1",
"webpack-dev-server": "^4.10.0"
},
"dependencies": {
"unstable_wasm": "file:../pkg"
}
}
| tokenizers/tokenizers/examples/unstable_wasm/www/package.json/0 | {
"file_path": "tokenizers/tokenizers/examples/unstable_wasm/www/package.json",
"repo_id": "tokenizers",
"token_count": 516
} | 233 |
use super::Pair;
use rand::{thread_rng, Rng};
use std::cmp::Ordering;
use std::collections::{BinaryHeap, HashMap};
#[derive(Debug, Eq)]
struct Merge {
pos: usize,
rank: u32,
new_id: u32,
}
impl PartialEq for Merge {
fn eq(&self, other: &Self) -> bool {
self.rank == other.rank && self.pos == other.pos
}
}
impl PartialOrd for Merge {
fn partial_cmp(&self, other: &Self) -> Option<Ordering> {
// By manually implementing this, we make the containing BinaryHeap a
// min-heap ordered first on the rank, and the pos otherwise
Some(self.cmp(other))
}
}
impl Ord for Merge {
fn cmp(&self, other: &Self) -> Ordering {
if self.rank != other.rank {
other.rank.cmp(&self.rank)
} else {
other.pos.cmp(&self.pos)
}
}
}
#[derive(Debug, Clone, Copy)]
struct Symbol {
c: u32,
prev: isize,
next: isize,
len: usize,
}
impl Symbol {
/// Merges the current Symbol with the other one.
/// In order to update prev/next, we consider Self to be the Symbol on the left,
/// and other to be the next one on the right.
pub fn merge_with(&mut self, other: &Self, new_c: u32) {
self.c = new_c;
self.len += other.len;
self.next = other.next;
}
}
#[derive(Clone, Default)]
pub(super) struct Word {
symbols: Vec<Symbol>,
}
impl std::fmt::Debug for Word {
fn fmt(&self, fmt: &mut std::fmt::Formatter) -> std::fmt::Result {
fmt.debug_struct("Word")
.field(
"chars",
&self
.symbols
.iter()
.map(|s| s.c.to_string())
.collect::<Vec<_>>()
.join(" "),
)
.field("symbols", &self.symbols)
.finish()
}
}
impl Word {
pub(super) fn new() -> Self {
Word { symbols: vec![] }
}
pub(super) fn with_capacity(capacity: usize) -> Self {
Self {
symbols: Vec::with_capacity(capacity),
}
}
pub(super) fn add(&mut self, c: u32, byte_len: usize) {
let (prev, next) = {
let len = self.symbols.len() as isize;
if let Some(last) = self.symbols.last_mut() {
// Update `next` on the previous one
last.next = len;
(len - 1, -1)
} else {
(-1, -1)
}
};
self.symbols.push(Symbol {
c,
prev,
next,
len: byte_len,
});
}
pub(super) fn merge(
&mut self,
c1: u32,
c2: u32,
replacement: u32,
max_length: usize,
) -> Vec<(Pair, i32)> {
let mut changes: Vec<(Pair, i32)> = vec![];
let mut i = 0;
loop {
if i >= self.symbols.len() {
break;
}
// Found a pair
if self.symbols[i].c == c1 && i + 1 < self.symbols.len() && self.symbols[i + 1].c == c2
{
let first = self.symbols[i];
let second = self.symbols[i + 1];
// Remove in place
let new_s = Symbol {
c: replacement,
prev: first.prev,
next: second.next,
len: first.len + second.len,
};
// If there are other characters before the pair
if i > 0 {
changes.push(((self.symbols[i - 1].c, first.c), -1));
if self.symbols[i - 1].len + new_s.len < max_length {
changes.push(((self.symbols[i - 1].c, replacement), 1));
}
}
self.symbols.insert(i, new_s); // Insert replacement before first char of pair
self.symbols.remove(i + 1); // Remove first char of pair
self.symbols.remove(i + 1); // And then the second
// If there are other characters after the pair
if i < self.symbols.len() - 1 {
changes.push(((second.c, self.symbols[i + 1].c), -1));
if self.symbols[i + 1].len + new_s.len < max_length {
changes.push(((replacement, self.symbols[i + 1].c), 1));
}
}
}
i += 1;
}
changes
}
pub(super) fn merge_all(&mut self, merges: &HashMap<Pair, (u32, u32)>, dropout: Option<f32>) {
let mut queue = BinaryHeap::with_capacity(self.symbols.len());
let mut skip = Vec::with_capacity(queue.len());
queue.extend(
self.symbols
.windows(2)
.enumerate()
.filter_map(|(index, window)| {
let pair = (window[0].c, window[1].c);
merges.get(&pair).map(|m| Merge {
pos: index,
rank: m.0,
new_id: m.1,
})
}),
);
while let Some(top) = queue.pop() {
if dropout
.map(|d| thread_rng().gen::<f32>() < d)
.unwrap_or(false)
{
skip.push(top);
} else {
// Re-insert the skipped elements
queue.extend(skip.drain(..));
if self.symbols[top.pos].len == 0 {
continue;
}
// Do nothing if we are the last symbol
if self.symbols[top.pos].next == -1 {
continue;
}
let next_pos = self.symbols[top.pos].next as usize;
let right = self.symbols[next_pos];
// Make sure we are not processing an expired queue entry
let target_new_pair = (self.symbols[top.pos].c, right.c);
if !merges
.get(&target_new_pair)
.map_or(false, |(_, new_id)| *new_id == top.new_id)
{
continue;
}
// Otherwise, let's merge
self.symbols[top.pos].merge_with(&right, top.new_id);
// Tag the right part as removed
self.symbols[next_pos].len = 0;
// Update `prev` on the new `next` to the current pos
if right.next > -1 && (right.next as usize) < self.symbols.len() {
self.symbols[right.next as usize].prev = top.pos as isize;
}
// Insert the new pair formed with the previous symbol
let current = &self.symbols[top.pos];
if current.prev >= 0 {
let prev = current.prev as usize;
let prev_symbol = self.symbols[prev];
let new_pair = (prev_symbol.c, current.c);
if let Some((rank, new_id)) = merges.get(&new_pair) {
queue.push(Merge {
pos: current.prev as usize,
rank: *rank,
new_id: *new_id,
});
}
}
// Insert the new pair formed with the next symbol
let next = current.next as usize;
if next < self.symbols.len() {
let next_symbol = self.symbols[next];
let new_pair = (current.c, next_symbol.c);
if let Some((rank, new_id)) = merges.get(&new_pair) {
queue.push(Merge {
pos: top.pos,
rank: *rank,
new_id: *new_id,
});
}
}
}
}
// Filter out the removed symbols
self.symbols.retain(|s| s.len != 0);
}
pub(super) fn get_chars(&self) -> Vec<u32> {
self.symbols.iter().map(|s| s.c).collect()
}
pub(super) fn get_chars_iter(&self) -> impl Iterator<Item = u32> + '_ {
self.symbols.iter().map(|s| s.c)
}
pub(super) fn get_offsets_iter(&self) -> impl Iterator<Item = (usize, usize)> + '_ {
let mut pos = 0;
self.symbols.iter().map(move |symbol| {
let new_pos = pos + symbol.len;
let offset = (pos, new_pos);
pos = new_pos;
offset
})
}
}
#[cfg(test)]
mod tests {
use super::*;
#[test]
fn test_merge() {
// Let's say we have the word 'hello' and a word-to-id vocab that looks
// like this: {'h': 0, 'e': 1, 'l': 2, 'o': 3}.
let mut word = Word::new();
word.add(0, 1); // 'h'
word.add(1, 1); // 'e'
word.add(2, 1); // 'l'
word.add(2, 1); // 'l'
word.add(3, 1); // 'o'
// We're going to perform a merge on the pair ('l', 'l') ~= (2, 2). Let's
// say that 'll' has the ID of 4 in the updated word-to-id vocab.
let changes = word.merge(2, 2, 4, usize::MAX);
// So the word should now look like this:
assert_eq!(
word.get_chars(),
&[
0u32, // 'h'
1u32, // 'e'
4u32, // 'll'
3u32, // 'o'
]
);
// The return value `changes` will be used to update the pair counts during
// training. This merge affects the counts for the pairs
// ('e', 'l') ~= (1, 2),
// ('e', 'll') ~= (1, 4),
// ('l', 'o') ~= (2, 3), and
// ('ll', 'o') ~= (4, 3).
// So the changes should reflect that:
assert_eq!(
changes,
&[
((1u32, 2u32), -1i32), // count for ('e', 'l') should be decreased by 1.
((1u32, 4u32), 1i32), // count for ('e', 'll') should be increased by 1.
((2u32, 3u32), -1i32), // count for ('l', 'o') should be decreased by 1.
((4u32, 3u32), 1i32), // count for ('ll', 'o') should be increased by 1.
]
);
}
#[test]
fn test_merge_max_length() {
// Let's say we have the word 'hello' and a word-to-id vocab that looks
// like this: {'h': 0, 'e': 1, 'l': 2, 'o': 3}.
let mut word = Word::new();
word.add(0, 1); // 'h'
word.add(1, 1); // 'e'
word.add(2, 1); // 'l'
word.add(2, 1); // 'l'
word.add(3, 1); // 'o'
// We're going to perform a merge on the pair ('l', 'l') ~= (2, 2). Let's
// say that 'll' has the ID of 4 in the updated word-to-id vocab.
let changes = word.merge(2, 2, 4, 2);
assert_eq!(
word.get_chars(),
&[
0u32, // 'h'
1u32, // 'e'
4u32, // 'll'
3u32, // 'o'
]
);
assert_eq!(
changes,
&[
((1u32, 2u32), -1i32), // count for ('e', 'l') should be decreased by 1.
// ((1u32, 4u32), 1i32), Missing since this would be larger than 2
((2u32, 3u32), -1i32), // count for ('l', 'o') should be decreased by 1.
// ((4u32, 3u32), 1i32), Missing since this would be larger than 2
]
);
}
}
| tokenizers/tokenizers/src/models/bpe/word.rs/0 | {
"file_path": "tokenizers/tokenizers/src/models/bpe/word.rs",
"repo_id": "tokenizers",
"token_count": 6488
} | 234 |
use crate::tokenizer::{NormalizedString, Normalizer, Result};
pub use spm_precompiled::Precompiled;
use std::cmp::Ordering;
use unicode_segmentation::UnicodeSegmentation;
fn replace(transformations: &mut Vec<(char, isize)>, old_part: &str, new_part: &str) {
let old_count = old_part.chars().count() as isize;
let new_count = new_part.chars().count() as isize;
let diff = new_count - old_count;
// If we are just replacing characters, all changes should be == 0
transformations.extend(new_part.chars().map(|c| (c, 0)));
match diff.cmp(&0) {
// If we are adding some characters, the last DIFF characters shoud be == 1
Ordering::Greater => {
transformations
.iter_mut()
.rev()
.take(diff as usize)
.for_each(|(_, cs)| *cs = 1);
}
// If we are removing some characters, the last one should include the diff
Ordering::Less => {
if let Some((_, cs)) = transformations.last_mut() {
*cs += diff;
}
}
_ => {}
}
}
impl Normalizer for Precompiled {
fn normalize(&self, normalized: &mut NormalizedString) -> Result<()> {
let mut transformations = Vec::with_capacity(normalized.get().len());
// Future reader. From @Narsil.
// Yes, this is weird,
// Yes, this seems broken
// No, I don't know why Google did this.
// If you question this code, check this normalizer against
// XNLI database (all languages) with Unigram model against
// Mbart, XLMRoberta *AND* Marian. If you don't get 100% or
// break a single test.
// You don't pass.
let mut modified = false;
normalized.get().graphemes(true).for_each(|grapheme| {
if grapheme.len() < 6 {
if let Some(norm) = self.transform(grapheme) {
modified = true;
replace(&mut transformations, grapheme, norm);
return;
}
}
for (char_index, c) in grapheme.char_indices() {
let part = &grapheme[char_index..char_index + c.len_utf8()];
if let Some(norm) = self.transform(part) {
modified = true;
replace(&mut transformations, part, norm);
} else {
transformations.push((c, 0));
}
}
});
if modified {
normalized.transform(transformations, 0);
}
Ok(())
}
}
#[cfg(test)]
mod tests {
use super::*;
#[test]
fn expansion_followed_by_removal() {
// Simulate transformations from "™\x1eg" to "TMg"
let mut transformations = vec![];
let mut n = NormalizedString::from("™\x1eg");
replace(&mut transformations, "™", "TM");
replace(&mut transformations, "\x1e", "");
transformations.push(('g', 0));
n.transform(transformations, 0);
assert_eq!(n.get(), "TMg");
}
}
| tokenizers/tokenizers/src/normalizers/precompiled.rs/0 | {
"file_path": "tokenizers/tokenizers/src/normalizers/precompiled.rs",
"repo_id": "tokenizers",
"token_count": 1432
} | 235 |
use crate::pre_tokenizers::unicode_scripts::scripts::{get_script, Script};
use crate::tokenizer::{normalizer::Range, PreTokenizedString, PreTokenizer, Result};
use crate::utils::macro_rules_attribute;
#[derive(Clone, Debug, PartialEq, Eq)]
#[macro_rules_attribute(impl_serde_type!)]
pub struct UnicodeScripts;
impl UnicodeScripts {
pub fn new() -> Self {
Self {}
}
}
impl Default for UnicodeScripts {
fn default() -> Self {
Self::new()
}
}
// This code exists in the Unigram default IsValidSentencePiece.
// It could be integrated directly within `get_script` but I
// think it's kind of tricky to see those modifications later
// I am guessing release mode will optimize this away anyway.
fn fixed_script(c: char) -> Script {
let raw_script = get_script(c);
if c as u32 == 0x30FC {
Script::Han
} else if c == ' ' {
Script::Any
} else {
match raw_script {
Script::Hiragana => Script::Han,
Script::Katakana => Script::Han,
script => script,
}
}
}
impl PreTokenizer for UnicodeScripts {
fn pre_tokenize(&self, pretokenized: &mut PreTokenizedString) -> Result<()> {
pretokenized.split(|_, normalized| {
let mut last_script = None;
let mut offset = 0;
let mut ranges: Vec<_> = normalized
.get()
.chars()
.filter_map(|c| {
let script = Some(fixed_script(c));
let result = if script != Some(Script::Any)
&& last_script != Some(Script::Any)
&& last_script != script
{
Some(offset)
} else {
None
};
offset += c.len_utf8();
if script != Some(Script::Any) {
last_script = script;
}
result
})
.collect();
ranges.push(normalized.get().len());
Ok(ranges
.windows(2)
.map(|item| {
normalized
.slice(Range::Normalized(item[0]..item[1]))
.expect("NormalizedString bad split")
})
.collect::<Vec<_>>())
})
}
}
#[cfg(test)]
mod tests {
use super::*;
use crate::OffsetReferential;
use crate::OffsetType;
#[test]
fn basic() {
let pretok = UnicodeScripts {};
let mut pretokenized = PreTokenizedString::from("どこで生れ。Yes");
pretok.pre_tokenize(&mut pretokenized).unwrap();
assert_eq!(
pretokenized
.get_splits(OffsetReferential::Normalized, OffsetType::Byte)
.into_iter()
.map(|(s, o, _)| (s, o))
.collect::<Vec<_>>(),
vec![("どこで生れ", (0, 15)), ("。", (15, 18)), ("Yes", (18, 21))]
);
assert_eq!(
pretokenized
.get_splits(OffsetReferential::Original, OffsetType::Byte)
.into_iter()
.map(|(s, o, _)| (s, o))
.collect::<Vec<_>>(),
vec![("どこで生れ", (0, 15)), ("。", (15, 18)), ("Yes", (18, 21))]
);
}
#[test]
fn spaces_are_included_in_every_script() {
let pretok = UnicodeScripts {};
let mut pretokenized = PreTokenizedString::from("Apples are りんご 林檎");
pretok.pre_tokenize(&mut pretokenized).unwrap();
assert_eq!(
pretokenized
.get_splits(OffsetReferential::Normalized, OffsetType::Byte)
.into_iter()
.map(|(s, o, _)| (s, o))
.collect::<Vec<_>>(),
vec![("Apples are ", (0, 11)), ("りんご 林檎", (11, 27))]
);
assert_eq!(
pretokenized
.get_splits(OffsetReferential::Original, OffsetType::Byte)
.into_iter()
.map(|(s, o, _)| (s, o))
.collect::<Vec<_>>(),
vec![("Apples are ", (0, 11)), ("りんご 林檎", (11, 27))]
);
}
#[test]
fn test_unicode_script() {
assert_eq!(Script::Han, fixed_script('京'));
assert_eq!(Script::Han, fixed_script('太'));
assert_eq!(Script::Han, fixed_script('い'));
assert_eq!(Script::Han, fixed_script('グ'));
assert_eq!(Script::Han, fixed_script('ー'));
assert_eq!(Script::Latin, fixed_script('a'));
assert_eq!(Script::Latin, fixed_script('A'));
assert_eq!(Script::Common, fixed_script('0'));
assert_eq!(Script::Common, fixed_script('$'));
assert_eq!(Script::Common, fixed_script('@'));
assert_eq!(Script::Common, fixed_script('-'));
assert_eq!(Script::Any, fixed_script(' '));
}
}
| tokenizers/tokenizers/src/pre_tokenizers/unicode_scripts/pre_tokenizer.rs/0 | {
"file_path": "tokenizers/tokenizers/src/pre_tokenizers/unicode_scripts/pre_tokenizer.rs",
"repo_id": "tokenizers",
"token_count": 2584
} | 236 |
use fancy_regex::Regex;
use std::error::Error;
#[derive(Debug)]
pub struct SysRegex {
regex: Regex,
}
impl SysRegex {
pub fn find_iter<'r, 't>(&'r self, inside: &'t str) -> Matches<'r, 't> {
Matches(self.regex.find_iter(inside))
}
pub fn new(regex_str: &str) -> Result<Self, Box<dyn Error + Send + Sync + 'static>> {
Ok(Self {
regex: Regex::new(regex_str)?,
})
}
}
pub struct Matches<'r, 't>(fancy_regex::Matches<'r, 't>);
impl<'r, 't> Iterator for Matches<'r, 't> {
type Item = (usize, usize);
fn next(&mut self) -> Option<Self::Item> {
match self.0.next() {
Some(Ok(mat)) => Some((mat.start(), mat.end())),
// stop if an error is encountered
None | Some(Err(_)) => None,
}
}
}
| tokenizers/tokenizers/src/utils/fancy.rs/0 | {
"file_path": "tokenizers/tokenizers/src/utils/fancy.rs",
"repo_id": "tokenizers",
"token_count": 396
} | 237 |
#[cfg(not(debug_assertions))]
use assert_approx_eq::assert_approx_eq;
use std::collections::HashMap;
use std::fs::read_to_string;
use std::path::Path;
#[cfg(not(debug_assertions))]
use tokenizers::models::unigram::Lattice;
use tokenizers::models::unigram::Unigram;
use tokenizers::models::unigram::UnigramTrainer;
use tokenizers::tokenizer::Model;
#[test]
fn test_unigram_from_file() {
let model = Unigram::load(Path::new("data/unigram.json")).unwrap();
let string = "吾輩《わがはい》は猫である。名前はまだ無い。";
assert_eq!(
model
.tokenize(string)
.unwrap()
.iter()
.map(|tok| tok.value.clone())
.collect::<Vec<_>>(),
vec![
"吾輩",
"《",
"わが",
"はい",
"》",
"は",
"猫",
"である",
"。",
"名前",
"はまだ",
"無い",
"。"
]
);
}
#[test]
fn test_train_unigram_from_file() {
let content = read_to_string("data/small.txt").unwrap();
let mut word_counts = HashMap::new();
content.split_whitespace().for_each(|word| {
// This is important for the test of char vs u8
let word = format!("▁{}", word);
*word_counts.entry(word).or_insert(0) += 1;
});
// println!("Words counts {:?}", word_counts);
let trainer = UnigramTrainer::builder()
.show_progress(false)
.unk_token(Some("<UNK>".into()))
.build()
.unwrap();
let mut model = Unigram::default();
let sentences: Vec<_> = word_counts
.iter()
.map(|(s, i)| (s.to_owned(), *i))
.collect();
trainer.do_train(sentences, &mut model).unwrap();
assert_eq!(model.get_vocab_size(), 719);
}
#[cfg(not(debug_assertions))]
#[test]
fn test_sample() {
let mut lattice = Lattice::from("ABC", 0, 2);
lattice.insert(0, 1, 1.0, 3); // A
lattice.insert(1, 1, 1.2, 4); // B
lattice.insert(2, 1, 1.5, 5); // C
lattice.insert(0, 2, 1.6, 6); // AB
lattice.insert(1, 2, 1.7, 7); // BC
lattice.insert(0, 3, 1.8, 8); // ABC
let thetas: Vec<f64> = vec![0.0, 0.01, 0.5, 0.7, 1.0];
for theta in thetas {
let mut probs: HashMap<String, f64> = HashMap::new();
probs.insert("A B C".to_string(), (theta * (1.0 + 1.2 + 1.5)).exp());
probs.insert("AB C".to_string(), (theta * (1.6 + 1.5)).exp());
probs.insert("A BC".to_string(), (theta * (1.0 + 1.7)).exp());
probs.insert("ABC".to_string(), (theta * (1.8)).exp());
// Computes expected probabilities.
let mut z = 0.0;
for (_, p) in probs.iter() {
z += p;
}
for (_, p) in probs.iter_mut() {
*p /= z;
}
let n_trials = 10_000;
let mut freq: HashMap<String, u32> = HashMap::new();
for _ in 0..n_trials {
let string = lattice.sample_token(theta).join(" ");
*freq.entry(string).or_insert(0) += 1;
}
assert_eq!(freq.len(), probs.len());
for (s, p) in probs.iter() {
assert_approx_eq!(1.0 * (freq[s] as f64) / (n_trials as f64), p, 0.03)
}
}
}
| tokenizers/tokenizers/tests/unigram.rs/0 | {
"file_path": "tokenizers/tokenizers/tests/unigram.rs",
"repo_id": "tokenizers",
"token_count": 1698
} | 238 |
FROM rocm/dev-ubuntu-20.04:5.6
# rocm/pytorch has no version with 2.1.0
LABEL maintainer="Hugging Face"
ARG DEBIAN_FRONTEND=noninteractive
ARG PYTORCH='2.1.0'
ARG TORCH_VISION='0.16.0'
ARG TORCH_AUDIO='2.1.0'
ARG ROCM='5.6'
RUN apt update && \
apt install -y --no-install-recommends git libsndfile1-dev tesseract-ocr espeak-ng python3 python3-dev python3-pip ffmpeg && \
apt clean && \
rm -rf /var/lib/apt/lists/*
RUN python3 -m pip install --no-cache-dir --upgrade pip
RUN python3 -m pip install torch==$PYTORCH torchvision==$TORCH_VISION torchaudio==$TORCH_AUDIO --index-url https://download.pytorch.org/whl/rocm$ROCM
RUN python3 -m pip install --no-cache-dir --upgrade pip setuptools ninja git+https://github.com/facebookresearch/detectron2.git pytesseract "itsdangerous<2.1.0"
ARG REF=main
WORKDIR /
# Invalidate docker cache from here if new commit is available.
ADD https://api.github.com/repos/huggingface/transformers/git/refs/heads/main version.json
RUN git clone https://github.com/huggingface/transformers && cd transformers && git checkout $REF
RUN python3 -m pip install --no-cache-dir -e ./transformers[dev-torch,testing,video]
RUN python3 -m pip uninstall -y tensorflow flax
# When installing in editable mode, `transformers` is not recognized as a package.
# this line must be added in order for python to be aware of transformers.
RUN cd transformers && python3 setup.py develop
| transformers/docker/transformers-pytorch-amd-gpu/Dockerfile/0 | {
"file_path": "transformers/docker/transformers-pytorch-amd-gpu/Dockerfile",
"repo_id": "transformers",
"token_count": 516
} | 239 |
<!--Copyright 2022 The HuggingFace Team. All rights reserved.
Licensed under the Apache License, Version 2.0 (the "License"); you may not use this file except in compliance with
the License. You may obtain a copy of the License at
http://www.apache.org/licenses/LICENSE-2.0
Unless required by applicable law or agreed to in writing, software distributed under the License is distributed on
an "AS IS" BASIS, WITHOUT WARRANTIES OR CONDITIONS OF ANY KIND, either express or implied. See the License for the
specific language governing permissions and limitations under the License.
⚠️ Note that this file is in Markdown but contain specific syntax for our doc-builder (similar to MDX) that may not be
rendered properly in your Markdown viewer.
-->
# Verteiltes Training mit 🤗 Accelerate
Da die Modelle immer größer werden, hat sich die Parallelität als Strategie zum Trainieren größerer Modelle auf begrenzter Hardware und zur Beschleunigung der Trainingsgeschwindigkeit um mehrere Größenordnungen erwiesen. Bei Hugging Face haben wir die Bibliothek [🤗 Accelerate](https://huggingface.co/docs/accelerate) entwickelt, um Nutzern zu helfen, ein 🤗 Transformers-Modell auf jeder Art von verteiltem Setup zu trainieren, egal ob es sich um mehrere GPUs auf einer Maschine oder mehrere GPUs auf mehreren Maschinen handelt. In diesem Tutorial lernen Sie, wie Sie Ihre native PyTorch-Trainingsschleife anpassen, um das Training in einer verteilten Umgebung zu ermöglichen.
## Einrichtung
Beginnen Sie mit der Installation von 🤗 Accelerate:
```bash
pip install accelerate
```
Dann importieren und erstellen Sie ein [`~accelerate.Accelerator`]-Objekt. Der [`~accelerate.Accelerator`] wird automatisch Ihre Art der verteilten Einrichtung erkennen und alle notwendigen Komponenten für das Training initialisieren. Sie müssen Ihr Modell nicht explizit auf einem Gerät platzieren.
```py
>>> from accelerate import Accelerator
>>> accelerator = Accelerator()
```
## Vorbereiten auf die Beschleunigung
Der nächste Schritt ist die Übergabe aller relevanten Trainingsobjekte an die Methode [`~accelerate.Accelerator.prepare`]. Dazu gehören Ihre Trainings- und Evaluierungs-DataLoader, ein Modell und ein Optimierer:
```py
>>> train_dataloader, eval_dataloader, model, optimizer = accelerator.prepare(
... train_dataloader, eval_dataloader, model, optimizer
... )
```
## Rückwärts
Die letzte Ergänzung besteht darin, das typische `loss.backward()` in der Trainingsschleife durch die 🤗 Accelerate-Methode [`~accelerate.Accelerator.backward`] zu ersetzen:
```py
>>> for epoch in range(num_epochs):
... for batch in train_dataloader:
... outputs = model(**batch)
... loss = outputs.loss
... accelerator.backward(loss)
... optimizer.step()
... lr_scheduler.step()
... optimizer.zero_grad()
... progress_bar.update(1)
```
Wie Sie im folgenden Code sehen können, müssen Sie nur vier zusätzliche Codezeilen zu Ihrer Trainingsschleife hinzufügen, um verteiltes Training zu ermöglichen!
```diff
+ from accelerate import Accelerator
from transformers import AdamW, AutoModelForSequenceClassification, get_scheduler
+ accelerator = Accelerator()
model = AutoModelForSequenceClassification.from_pretrained(checkpoint, num_labels=2)
optimizer = AdamW(model.parameters(), lr=3e-5)
- device = torch.device("cuda") if torch.cuda.is_available() else torch.device("cpu")
- model.to(device)
+ train_dataloader, eval_dataloader, model, optimizer = accelerator.prepare(
+ train_dataloader, eval_dataloader, model, optimizer
+ )
num_epochs = 3
num_training_steps = num_epochs * len(train_dataloader)
lr_scheduler = get_scheduler(
"linear",
optimizer=optimizer,
num_warmup_steps=0,
num_training_steps=num_training_steps
)
progress_bar = tqdm(range(num_training_steps))
model.train()
for epoch in range(num_epochs):
for batch in train_dataloader:
- batch = {k: v.to(device) for k, v in batch.items()}
outputs = model(**batch)
loss = outputs.loss
- loss.backward()
+ accelerator.backward(loss)
optimizer.step()
lr_scheduler.step()
optimizer.zero_grad()
progress_bar.update(1)
```
## Trainieren
Sobald Sie die entsprechenden Codezeilen hinzugefügt haben, starten Sie Ihr Training in einem Skript oder einem Notebook wie Colaboratory.
### Trainieren mit einem Skript
Wenn Sie Ihr Training mit einem Skript durchführen, führen Sie den folgenden Befehl aus, um eine Konfigurationsdatei zu erstellen und zu speichern:
```bash
accelerate config
```
Dann starten Sie Ihr Training mit:
```bash
accelerate launch train.py
```
### Trainieren mit einem Notebook
🤗 Accelerate kann auch in einem Notebook laufen, wenn Sie planen, die TPUs von Colaboratory zu verwenden. Verpacken Sie den gesamten Code, der für das Training verantwortlich ist, in eine Funktion und übergeben Sie diese an [`~accelerate.notebook_launcher`]:
```py
>>> from accelerate import notebook_launcher
>>> notebook_launcher(training_function)
```
Weitere Informationen über 🤗 Accelerate und seine umfangreichen Funktionen finden Sie in der [Dokumentation](https://huggingface.co/docs/accelerate). | transformers/docs/source/de/accelerate.md/0 | {
"file_path": "transformers/docs/source/de/accelerate.md",
"repo_id": "transformers",
"token_count": 1929
} | 240 |
<!--Copyright 2020 The HuggingFace Team. All rights reserved.
Licensed under the Apache License, Version 2.0 (the "License"); you may not use this file except in compliance with
the License. You may obtain a copy of the License at
http://www.apache.org/licenses/LICENSE-2.0
Unless required by applicable law or agreed to in writing, software distributed under the License is distributed on
an "AS IS" BASIS, WITHOUT WARRANTIES OR CONDITIONS OF ANY KIND, either express or implied. See the License for the
specific language governing permissions and limitations under the License.
⚠️ Note that this file is in Markdown but contain specific syntax for our doc-builder (similar to MDX) that may not be
rendered properly in your Markdown viewer.
-->
# Testen
Werfen wir einen Blick darauf, wie 🤗 Transformers-Modelle getestet werden und wie Sie neue Tests schreiben und die vorhandenen verbessern können.
Es gibt 2 Testsuiten im Repository:
1. `tests` -- Tests für die allgemeine API
2. `examples` -- Tests hauptsächlich für verschiedene Anwendungen, die nicht Teil der API sind
## Wie Transformatoren getestet werden
1. Sobald ein PR eingereicht wurde, wird er mit 9 CircleCi Jobs getestet. Jeder neue Commit zu diesem PR wird erneut getestet. Diese Aufträge
sind in dieser [Konfigurationsdatei](https://github.com/huggingface/transformers/tree/main/.circleci/config.yml) definiert, so dass Sie bei Bedarf die gleiche Umgebung auf Ihrem Rechner reproduzieren können.
Umgebung auf Ihrem Rechner reproduzieren können.
Diese CI-Jobs führen keine `@slow`-Tests durch.
2. Es gibt 3 Jobs, die von [github actions](https://github.com/huggingface/transformers/actions) ausgeführt werden:
- [torch hub integration](https://github.com/huggingface/transformers/tree/main/.github/workflows/github-torch-hub.yml): prüft, ob die torch hub
Integration funktioniert.
- [self-hosted (push)](https://github.com/huggingface/transformers/tree/main/.github/workflows/self-push.yml): führt schnelle Tests auf der GPU nur bei Commits auf
`main`. Es wird nur ausgeführt, wenn ein Commit auf `main` den Code in einem der folgenden Ordner aktualisiert hat: `src`,
`tests`, `.github` (um zu verhindern, dass er auf hinzugefügten Modellkarten, Notebooks usw. läuft)
- [self-hosted runner](https://github.com/huggingface/transformers/tree/main/.github/workflows/self-scheduled.yml): führt normale und langsame Tests auf GPU in
`tests` und `examples`:
```bash
RUN_SLOW=1 pytest tests/
RUN_SLOW=1 pytest examples/
```
Die Ergebnisse können Sie [hier](https://github.com/huggingface/transformers/actions) sehen.
## Tests ausführen
### Auswahl der auszuführenden Tests
In diesem Dokument wird ausführlich erläutert, wie Tests ausgeführt werden können. Wenn Sie nach der Lektüre noch mehr Details benötigen
finden Sie diese [hier](https://docs.pytest.org/en/latest/usage.html).
Hier sind einige der nützlichsten Möglichkeiten, Tests auszuführen.
Alle ausführen:
```console
pytest
```
oder:
```bash
make test
```
Beachten Sie, dass Letzteres wie folgt definiert ist:
```bash
python -m pytest -n auto --dist=loadfile -s -v ./tests/
```
was pytest anweist:
- so viele Testprozesse laufen zu lassen, wie es CPU-Kerne gibt (was zu viele sein könnten, wenn Sie nicht über eine Menge RAM verfügen!)
- sicherzustellen, dass alle Tests aus derselben Datei von demselben Testprozess ausgeführt werden
- Erfassen Sie keine Ausgaben
- im ausführlichen Modus laufen lassen
### Abrufen der Liste aller Tests
Alle Tests der Testsuite:
```bash
pytest --collect-only -q
```
Alle Tests einer bestimmten Testdatei:
```bash
pytest tests/test_optimization.py --collect-only -q
```
### Führen Sie ein bestimmtes Testmodul aus
Um ein einzelnes Testmodul auszuführen:
```bash
pytest tests/utils/test_logging.py
```
### Spezifische Tests ausführen
Da unittest in den meisten Tests verwendet wird, müssen Sie, um bestimmte Untertests auszuführen, den Namen der unittest
Klasse, die diese Tests enthält. Er könnte zum Beispiel lauten:
```bash
pytest tests/test_optimization.py::OptimizationTest::test_adam_w
```
Hier:
- `tests/test_optimization.py` - die Datei mit den Tests
- `OptimizationTest` - der Name der Klasse
- `test_adam_w` - der Name der spezifischen Testfunktion
Wenn die Datei mehrere Klassen enthält, können Sie auswählen, dass nur die Tests einer bestimmten Klasse ausgeführt werden sollen. Zum Beispiel:
```bash
pytest tests/test_optimization.py::OptimizationTest
```
führt alle Tests innerhalb dieser Klasse aus.
Wie bereits erwähnt, können Sie sehen, welche Tests in der Klasse `OptimizationTest` enthalten sind, indem Sie sie ausführen:
```bash
pytest tests/test_optimization.py::OptimizationTest --collect-only -q
```
Sie können Tests mit Hilfe von Schlüsselwortausdrücken ausführen.
Um nur Tests auszuführen, deren Name `adam` enthält:
```bash
pytest -k adam tests/test_optimization.py
```
Die logischen `und` und `oder` können verwendet werden, um anzugeben, ob alle Schlüsselwörter übereinstimmen sollen oder nur eines. `nicht` kann verwendet werden, um
negieren.
Um alle Tests auszuführen, außer denen, deren Name `adam` enthält:
```bash
pytest -k "not adam" tests/test_optimization.py
```
Und Sie können die beiden Muster in einem kombinieren:
```bash
pytest -k "ada and not adam" tests/test_optimization.py
```
Um zum Beispiel sowohl `test_adafactor` als auch `test_adam_w` auszuführen, können Sie verwenden:
```bash
pytest -k "test_adam_w or test_adam_w" tests/test_optimization.py
```
Beachten Sie, dass wir hier `oder` verwenden, da wir wollen, dass eines der Schlüsselwörter übereinstimmt, um beide einzuschließen.
Wenn Sie nur Tests einschließen möchten, die beide Muster enthalten, müssen Sie `und` verwenden:
```bash
pytest -k "test and ada" tests/test_optimization.py
```
### Führen Sie `accelerate` Tests durch
Manchmal müssen Sie `accelerate` Tests für Ihre Modelle ausführen. Dazu fügen Sie einfach `-m accelerate_tests` zu Ihrem Befehl hinzu, wenn Sie diese Tests bei einem `OPT`-Lauf ausführen möchten:
```bash
RUN_SLOW=1 pytest -m accelerate_tests tests/models/opt/test_modeling_opt.py
```
### Dokumentationstests ausführen
Um zu testen, ob die Dokumentationsbeispiele korrekt sind, sollten Sie überprüfen, ob die `doctests` erfolgreich sind.
Lassen Sie uns als Beispiel den docstring von [WhisperModel.forward](https://github.com/huggingface/transformers/blob/main/src/transformers/models/whisper/modeling_whisper.py#L1017-L1035) verwenden:
```python
r"""
Returns:
Example:
```python
>>> import torch
>>> from transformers import WhisperModel, WhisperFeatureExtractor
>>> from datasets import load_dataset
>>> model = WhisperModel.from_pretrained("openai/whisper-base")
>>> feature_extractor = WhisperFeatureExtractor.from_pretrained("openai/whisper-base")
>>> ds = load_dataset("hf-internal-testing/librispeech_asr_dummy", "clean", split="validation")
>>> inputs = feature_extractor(ds[0]["audio"]["array"], return_tensors="pt")
>>> input_features = inputs.input_features
>>> decoder_input_ids = torch.tensor([[1, 1]]) * model.config.decoder_start_token_id
>>> last_hidden_state = model(input_features, decoder_input_ids=decoder_input_ids).last_hidden_state
>>> list(last_hidden_state.shape)
[1, 2, 512]
```"""
```
Führen Sie einfach die folgende Zeile aus, um automatisch jedes docstring-Beispiel in der gewünschten Datei zu testen:
```bash
pytest --doctest-modules <path_to_file_or_dir>
```
Wenn die Datei eine Markdown-Erweiterung hat, sollten Sie das Argument `--doctest-glob="*.md"` hinzufügen.
### Nur geänderte Tests ausführen
Mit [pytest-picked](https://github.com/anapaulagomes/pytest-picked) können Sie die Tests ausführen, die sich auf die unstaged Dateien oder den aktuellen Zweig (gemäß Git) beziehen. Auf diese Weise können Sie schnell testen, ob Ihre Änderungen nichts kaputt gemacht haben.
nichts kaputt gemacht haben, da die Tests für Dateien, die Sie nicht verändert haben, nicht ausgeführt werden.
```bash
pip install pytest-picked
```
```bash
pytest --picked
```
Alle Tests werden von Dateien und Ordnern ausgeführt, die geändert, aber noch nicht übergeben wurden.
### Fehlgeschlagene Tests bei Änderung der Quelle automatisch wiederholen
[pytest-xdist](https://github.com/pytest-dev/pytest-xdist) bietet eine sehr nützliche Funktion zur Erkennung aller fehlgeschlagenen
Tests zu erkennen und dann darauf zu warten, dass Sie Dateien ändern, um die fehlgeschlagenen Tests so lange zu wiederholen, bis sie erfolgreich sind, während Sie die
sie reparieren. So müssen Sie pytest nicht erneut starten, nachdem Sie die Korrektur vorgenommen haben. Dies wird so lange wiederholt, bis alle Tests bestanden sind.
Danach wird erneut ein vollständiger Durchlauf durchgeführt.
```bash
pip install pytest-xdist
```
So rufen Sie den Modus auf: `pytest -f` oder `pytest --looponfail`
Datei-Änderungen werden erkannt, indem die Wurzelverzeichnisse von `looponfailroots` und alle ihre Inhalte (rekursiv) untersucht werden.
Wenn die Vorgabe für diesen Wert für Sie nicht funktioniert, können Sie ihn in Ihrem Projekt ändern, indem Sie eine Konfigurations
Option in der Datei `setup.cfg` ändern:
```ini
[tool:pytest]
looponfailroots = transformers tests
```
oder die Dateien `pytest.ini`/`tox.ini``:
```ini
[pytest]
looponfailroots = transformers tests
```
Dies würde dazu führen, dass nur nach Dateiänderungen in den jeweiligen Verzeichnissen gesucht wird, die relativ zum Verzeichnis der ini-Datei angegeben sind.
Verzeichnis.
[pytest-watch](https://github.com/joeyespo/pytest-watch) ist eine alternative Implementierung dieser Funktionalität.
### Überspringen eines Testmoduls
Wenn Sie alle Testmodule ausführen möchten, mit Ausnahme einiger weniger, können Sie diese ausschließen, indem Sie eine explizite Liste der auszuführenden Tests angeben. Für
Beispiel: Um alle Tests außer `test_modeling_*.py` auszuführen:
```bash
pytest *ls -1 tests/*py | grep -v test_modeling*
```
### Status leeren
CI-Builds und wenn Isolation wichtig ist (gegen Geschwindigkeit), sollte der Cache geleert werden:
```bash
pytest --cache-clear tests
```
### Tests parallel ausführen
Wie bereits erwähnt, führt `make test` über das Plugin `pytest-xdist` Tests parallel aus (Argument `-n X`, z.B. `-n 2`
um 2 Jobs parallel laufen zu lassen).
Mit der Option `--dist=` von `pytest-xdist` können Sie steuern, wie die Tests gruppiert werden. Mit `--dist=loadfile` werden die
Tests, die sich in einer Datei befinden, in denselben Prozess.
Da die Reihenfolge der ausgeführten Tests unterschiedlich und nicht vorhersehbar ist, kann die Ausführung der Testsuite mit `pytest-xdist`
zu Fehlern führt (was bedeutet, dass wir einige unentdeckte gekoppelte Tests haben), verwenden Sie [pytest-replay](https://github.com/ESSS/pytest-replay), um die Tests in der gleichen Reihenfolge abzuspielen, was dabei helfen sollte
diese fehlgeschlagene Sequenz auf ein Minimum zu reduzieren.
### Testreihenfolge und Wiederholung
Es ist gut, die Tests mehrmals zu wiederholen, nacheinander, zufällig oder in Gruppen, um mögliche
Abhängigkeiten und zustandsbezogene Fehler zu erkennen (Abriss). Und die einfache, mehrfache Wiederholung ist einfach gut, um
einige Probleme zu erkennen, die durch die Zufälligkeit von DL aufgedeckt werden.
#### Wiederholungstests
- [pytest-flakefinder](https://github.com/dropbox/pytest-flakefinder):
```bash
pip install pytest-flakefinder
```
Und führen Sie dann jeden Test mehrmals durch (standardmäßig 50):
```bash
pytest --flake-finder --flake-runs=5 tests/test_failing_test.py
```
<Tip>
Dieses Plugin funktioniert nicht mit dem `-n` Flag von `pytest-xdist`.
</Tip>
<Tip>
Es gibt noch ein anderes Plugin `pytest-repeat`, aber es funktioniert nicht mit `unittest`.
</Tip>
#### Run tests in a random order
```bash
pip install pytest-random-order
```
Wichtig: Das Vorhandensein von `pytest-random-order` sorgt für eine automatische Zufallsanordnung der Tests, es sind keine Konfigurationsänderungen oder
Befehlszeilenoptionen sind nicht erforderlich.
Wie bereits erläutert, ermöglicht dies die Erkennung von gekoppelten Tests - bei denen der Zustand eines Tests den Zustand eines anderen beeinflusst. Wenn
`pytest-random-order` installiert ist, gibt es den Zufallswert aus, der für diese Sitzung verwendet wurde, z.B:
```bash
pytest tests
[...]
Using --random-order-bucket=module
Using --random-order-seed=573663
```
Wenn eine bestimmte Sequenz fehlschlägt, können Sie sie reproduzieren, indem Sie genau diesen Seed hinzufügen, z.B:
```bash
pytest --random-order-seed=573663
[...]
Using --random-order-bucket=module
Using --random-order-seed=573663
```
Es wird nur dann die exakte Reihenfolge reproduzieren, wenn Sie genau dieselbe Liste von Tests (oder gar keine Liste) verwenden. Sobald Sie beginnen, die Liste
die Liste manuell einzugrenzen, können Sie sich nicht mehr auf den Seed verlassen, sondern müssen die Tests manuell in der genauen Reihenfolge auflisten
auflisten und pytest anweisen, sie nicht zu randomisieren, indem Sie `--random-order-bucket=none` verwenden, z.B.:
```bash
pytest --random-order-bucket=none tests/test_a.py tests/test_c.py tests/test_b.py
```
So deaktivieren Sie das Shuffling für alle Tests:
```bash
pytest --random-order-bucket=none
```
Standardmäßig ist `--random-order-bucket=module` impliziert, wodurch die Dateien auf den Modulebenen gemischt werden. Es kann auch
auf den Ebenen `class`, `package`, `global` und `none` mischen. Die vollständigen Details entnehmen Sie bitte der
[Dokumentation](https://github.com/jbasko/pytest-random-order).
Eine weitere Alternative zur Randomisierung ist: [`pytest-random`](https://github.com/pytest-dev/pytest-randomly). Dieses
Modul hat eine sehr ähnliche Funktionalität/Schnittstelle, aber es hat nicht die Eimermodi, die in
`pytest-random-order` zur Verfügung. Es hat das gleiche Problem, dass es sich nach der Installation aufdrängt.
### Variationen von Aussehen und Bedienung
#### pytest-zucker
[pytest-sugar](https://github.com/Frozenball/pytest-sugar) ist ein Plugin, das das Erscheinungsbild verbessert, eine
Fortschrittsbalken hinzufügt und Tests, die fehlschlagen, sowie die Bestätigung sofort anzeigt. Es wird bei der Installation automatisch aktiviert.
```bash
pip install pytest-sugar
```
Um Tests ohne sie durchzuführen, führen Sie aus:
```bash
pytest -p no:sugar
```
oder deinstallieren Sie es.
#### Melden Sie den Namen jedes Subtests und seinen Fortschritt
Für einen einzelnen oder eine Gruppe von Tests über `pytest` (nach `pip install pytest-pspec`):
```bash
pytest --pspec tests/test_optimization.py
```
#### Zeigt fehlgeschlagene Tests sofort an
[pytest-instafail](https://github.com/pytest-dev/pytest-instafail) zeigt Fehlschläge und Fehler sofort an, anstatt
bis zum Ende der Testsitzung zu warten.
```bash
pip install pytest-instafail
```
```bash
pytest --instafail
```
### Zu GPU oder nicht zu GPU
Bei einem GPU-aktivierten Setup fügen Sie zum Testen im reinen CPU-Modus `CUDA_VISIBLE_DEVICES=""` hinzu:
```bash
CUDA_VISIBLE_DEVICES="" pytest tests/utils/test_logging.py
```
oder wenn Sie mehrere Grafikprozessoren haben, können Sie angeben, welcher von `pytest` verwendet werden soll. Wenn Sie zum Beispiel nur den
zweiten Grafikkarte zu verwenden, wenn Sie die Grafikkarten `0` und `1` haben, können Sie folgendes ausführen:
```bash
CUDA_VISIBLE_DEVICES="1" pytest tests/utils/test_logging.py
```
Dies ist praktisch, wenn Sie verschiedene Aufgaben auf verschiedenen GPUs ausführen möchten.
Einige Tests müssen nur auf der CPU ausgeführt werden, andere entweder auf der CPU, der GPU oder der TPU und wieder andere auf mehreren GPUs. Die folgenden skip
Dekorateure werden verwendet, um die Anforderungen von Tests in Bezug auf CPU/GPU/TPU festzulegen:
- `require_torch` - dieser Test wird nur unter Torch ausgeführt
- `require_torch_gpu` - wie `require_torch` plus erfordert mindestens 1 GPU
- `require_torch_multi_gpu` - wie `require_torch` und zusätzlich mindestens 2 GPUs erforderlich
- `require_torch_non_multi_gpu` - wie `require_torch` plus benötigt 0 oder 1 GPUs
- `require_torch_up_to_2_gpus` - wie `require_torch` plus erfordert 0 oder 1 oder 2 GPUs
- `require_torch_xla` - wie `require_torch` plus erfordert mindestens 1 TPU
Lassen Sie uns die GPU-Anforderungen in der folgenden Tabelle darstellen:
| n gpus | decorator |
|--------|--------------------------------|
| `>= 0` | `@require_torch` |
| `>= 1` | `@require_torch_gpu` |
| `>= 2` | `@require_torch_multi_gpu` |
| `< 2` | `@require_torch_non_multi_gpu` |
| `< 3` | `@require_torch_up_to_2_gpus` |
Hier ist zum Beispiel ein Test, der nur ausgeführt werden muss, wenn 2 oder mehr GPUs verfügbar sind und pytorch installiert ist:
```python no-style
@require_torch_multi_gpu
def test_example_with_multi_gpu():
```
Wenn ein Test `tensorflow` benötigt, verwenden Sie den Dekorator `require_tf`. Zum Beispiel:
```python no-style
@require_tf
def test_tf_thing_with_tensorflow():
```
Diese Dekors können gestapelt werden. Wenn zum Beispiel ein Test langsam ist und mindestens eine GPU unter pytorch benötigt, können Sie
wie Sie ihn einrichten können:
```python no-style
@require_torch_gpu
@slow
def test_example_slow_on_gpu():
```
Einige Dekoratoren wie `@parametrized` schreiben Testnamen um, daher müssen `@require_*`-Sprungdekoratoren als letztes aufgeführt werden.
zuletzt aufgeführt werden, damit sie korrekt funktionieren. Hier ist ein Beispiel für die korrekte Verwendung:
```python no-style
@parameterized.expand(...)
@require_torch_multi_gpu
def test_integration_foo():
```
Dieses Problem mit der Reihenfolge gibt es bei `@pytest.mark.parametrize` nicht, Sie können es an den Anfang oder an den Schluss setzen und es wird trotzdem funktionieren.
funktionieren. Aber es funktioniert nur bei Nicht-Unittests.
Innerhalb von Tests:
- Wie viele GPUs sind verfügbar:
```python
from transformers.testing_utils import get_gpu_count
n_gpu = get_gpu_count() # works with torch and tf
```
### Testen mit einem bestimmten PyTorch-Backend oder Gerät
Um die Testsuite auf einem bestimmten Torch-Gerät auszuführen, fügen Sie `TRANSFORMERS_TEST_DEVICE="$Gerät"` hinzu, wobei `$Gerät` das Ziel-Backend ist. Zum Beispiel, um nur auf der CPU zu testen:
```bash
TRANSFORMERS_TEST_DEVICE="cpu" pytest tests/utils/test_logging.py
```
Diese Variable ist nützlich, um benutzerdefinierte oder weniger verbreitete PyTorch-Backends wie `mps` zu testen. Sie kann auch verwendet werden, um den gleichen Effekt wie `CUDA_VISIBLE_DEVICES` zu erzielen, indem Sie bestimmte GPUs anvisieren oder im reinen CPU-Modus testen.
Bestimmte Geräte erfordern einen zusätzlichen Import, nachdem Sie `torch` zum ersten Mal importiert haben. Dies kann über die Umgebungsvariable `TRANSFORMERS_TEST_BACKEND` festgelegt werden:
```bash
TRANSFORMERS_TEST_BACKEND="torch_npu" pytest tests/utils/test_logging.py
```
### Verteiltes Training
`pytest` kann nicht direkt mit verteiltem Training umgehen. Wenn dies versucht wird, tun die Unterprozesse nicht das Richtige
und denken am Ende, sie seien `pytest` und beginnen, die Testsuite in Schleifen auszuführen. Es funktioniert jedoch, wenn man
einen normalen Prozess erzeugt, der dann mehrere Worker erzeugt und die IO-Pipes verwaltet.
Hier sind einige Tests, die dies verwenden:
- [test_trainer_distributed.py](https://github.com/huggingface/transformers/tree/main/tests/trainer/test_trainer_distributed.py)
- [test_deepspeed.py](https://github.com/huggingface/transformers/tree/main/tests/deepspeed/test_deepspeed.py)
Um direkt mit der Ausführung zu beginnen, suchen Sie in diesen Tests nach dem Aufruf `execute_subprocess_async`.
Sie benötigen mindestens 2 GPUs, um diese Tests in Aktion zu sehen:
```bash
CUDA_VISIBLE_DEVICES=0,1 RUN_SLOW=1 pytest -sv tests/test_trainer_distributed.py
```
### Erfassung von Ausgaben
Während der Testausführung werden alle Ausgaben, die an `stdout` und `stderr` gesendet werden, aufgezeichnet. Wenn ein Test oder eine Setup-Methode fehlschlägt, wird die
wird die entsprechende aufgezeichnete Ausgabe in der Regel zusammen mit dem Fehler-Traceback angezeigt.
Um die Aufzeichnung von Ausgaben zu deaktivieren und `stdout` und `stderr` normal zu erhalten, verwenden Sie `-s` oder `--capture=no`:
```bash
pytest -s tests/utils/test_logging.py
```
So senden Sie Testergebnisse an die JUnit-Formatausgabe:
```bash
py.test tests --junitxml=result.xml
```
### Farbsteuerung
Keine Farbe zu haben (z.B. gelb auf weißem Hintergrund ist nicht lesbar):
```bash
pytest --color=no tests/utils/test_logging.py
```
### Testbericht an den Online-Dienst pastebin senden
Erstellen Sie eine URL für jeden Testfehler:
```bash
pytest --pastebin=failed tests/utils/test_logging.py
```
Dadurch werden Informationen über den Testlauf an einen entfernten Paste-Dienst übermittelt und eine URL für jeden Fehlschlag bereitgestellt. Sie können die
Tests wie gewohnt auswählen oder z.B. -x hinzufügen, wenn Sie nur einen bestimmten Fehler senden möchten.
Erstellen einer URL für ein ganzes Testsitzungsprotokoll:
```bash
pytest --pastebin=all tests/utils/test_logging.py
```
## Tests schreiben
🤗 Die Tests von Transformers basieren auf `unittest`, werden aber von `pytest` ausgeführt, so dass die meiste Zeit Funktionen aus beiden Systemen
verwendet werden können.
Sie können [hier](https://docs.pytest.org/en/stable/unittest.html) nachlesen, welche Funktionen unterstützt werden, aber das Wichtigste ist
Wichtig ist, dass die meisten `pytest`-Fixtures nicht funktionieren. Auch die Parametrisierung nicht, aber wir verwenden das Modul
`parametrisiert`, das auf ähnliche Weise funktioniert.
### Parametrisierung
Oft besteht die Notwendigkeit, denselben Test mehrmals auszuführen, aber mit unterschiedlichen Argumenten. Das könnte innerhalb des Tests geschehen
des Tests gemacht werden, aber dann gibt es keine Möglichkeit, den Test mit nur einem Satz von Argumenten auszuführen.
```python
# test_this1.py
import unittest
from parameterized import parameterized
class TestMathUnitTest(unittest.TestCase):
@parameterized.expand(
[
("negative", -1.5, -2.0),
("integer", 1, 1.0),
("large fraction", 1.6, 1),
]
)
def test_floor(self, name, input, expected):
assert_equal(math.floor(input), expected)
```
Nun wird dieser Test standardmäßig 3 Mal ausgeführt, wobei jedes Mal die letzten 3 Argumente von `test_floor` den entsprechenden Argumenten in der Parameterliste zugeordnet werden.
die entsprechenden Argumente in der Parameterliste.
Sie können auch nur die Parameter `negativ` und `ganzzahlig` mit ausführen:
```bash
pytest -k "negative and integer" tests/test_mytest.py
```
oder alle Untertests außer `negativ`, mit:
```bash
pytest -k "not negative" tests/test_mytest.py
```
Neben der Verwendung des gerade erwähnten Filters `-k` können Sie auch den genauen Namen jedes Untertests herausfinden und jeden
oder alle unter Verwendung ihrer genauen Namen ausführen.
```bash
pytest test_this1.py --collect-only -q
```
und es wird aufgelistet:
```bash
test_this1.py::TestMathUnitTest::test_floor_0_negative
test_this1.py::TestMathUnitTest::test_floor_1_integer
test_this1.py::TestMathUnitTest::test_floor_2_large_fraction
```
Jetzt können Sie also nur 2 spezifische Untertests durchführen:
```bash
pytest test_this1.py::TestMathUnitTest::test_floor_0_negative test_this1.py::TestMathUnitTest::test_floor_1_integer
```
Das Modul [parametrisiert](https://pypi.org/project/parameterized/), das sich bereits in den Entwickler-Abhängigkeiten befindet
von `transformers` befindet, funktioniert sowohl für `unittests` als auch für `pytest` Tests.
Wenn es sich bei dem Test jedoch nicht um einen `Unittest` handelt, können Sie `pytest.mark.parametrize` verwenden (oder Sie können sehen, dass es in
einigen bestehenden Tests verwendet wird, meist unter `Beispiele`).
Hier ist das gleiche Beispiel, diesmal unter Verwendung der `parametrize`-Markierung von `pytest`:
```python
# test_this2.py
import pytest
@pytest.mark.parametrize(
"name, input, expected",
[
("negative", -1.5, -2.0),
("integer", 1, 1.0),
("large fraction", 1.6, 1),
],
)
def test_floor(name, input, expected):
assert_equal(math.floor(input), expected)
```
Genau wie bei `parametrisiert` können Sie mit `pytest.mark.parametrize` genau steuern, welche Subtests ausgeführt werden
ausgeführt werden, wenn der Filter `-k` nicht ausreicht. Allerdings erzeugt diese Parametrisierungsfunktion einen etwas anderen Satz von
Namen für die Untertests. Sie sehen folgendermaßen aus:
```bash
pytest test_this2.py --collect-only -q
```
und es wird aufgelistet:
```bash
test_this2.py::test_floor[integer-1-1.0]
test_this2.py::test_floor[negative--1.5--2.0]
test_this2.py::test_floor[large fraction-1.6-1]
```
Jetzt können Sie also nur den spezifischen Test durchführen:
```bash
pytest test_this2.py::test_floor[negative--1.5--2.0] test_this2.py::test_floor[integer-1-1.0]
```
wie im vorherigen Beispiel.
### Dateien und Verzeichnisse
In Tests müssen wir oft wissen, wo sich Dinge relativ zur aktuellen Testdatei befinden, und das ist nicht trivial, da der Test
von mehreren Verzeichnissen aus aufgerufen werden kann oder sich in Unterverzeichnissen mit unterschiedlicher Tiefe befinden kann. Eine Hilfsklasse
`transformers.test_utils.TestCasePlus` löst dieses Problem, indem sie alle grundlegenden Pfade sortiert und einfache
Zugriffsmöglichkeiten auf sie bietet:
- `pathlib`-Objekte (alle vollständig aufgelöst):
- `test_file_path` - der aktuelle Testdateipfad, d.h. `__file__`
- `test_file_dir` - das Verzeichnis, das die aktuelle Testdatei enthält
- `tests_dir` - das Verzeichnis der `tests` Testreihe
- `examples_dir` - das Verzeichnis der `examples` Test-Suite
- `repo_root_dir` - das Verzeichnis des Repositorys
- `src_dir` - das Verzeichnis von `src` (d.h. wo sich das Unterverzeichnis `transformers` befindet)
- stringifizierte Pfade - wie oben, aber diese geben Pfade als Strings zurück, anstatt als `pathlib`-Objekte:
- `test_file_path_str`
- `test_file_dir_str`
- `tests_dir_str`
- `examples_dir_str`
- `repo_root_dir_str`
- `src_dir_str`
Um diese zu verwenden, müssen Sie lediglich sicherstellen, dass der Test in einer Unterklasse von
`transformers.test_utils.TestCasePlus` befindet. Zum Beispiel:
```python
from transformers.testing_utils import TestCasePlus
class PathExampleTest(TestCasePlus):
def test_something_involving_local_locations(self):
data_dir = self.tests_dir / "fixtures/tests_samples/wmt_en_ro"
```
Wenn Sie Pfade nicht über `pathlib` manipulieren müssen oder nur einen Pfad als String benötigen, können Sie jederzeit
`str()` auf das `pathlib`-Objekt anwenden oder die Accessoren mit der Endung `_str` verwenden. Zum Beispiel:
```python
from transformers.testing_utils import TestCasePlus
class PathExampleTest(TestCasePlus):
def test_something_involving_stringified_locations(self):
examples_dir = self.examples_dir_str
```
### Temporäre Dateien und Verzeichnisse
Die Verwendung eindeutiger temporärer Dateien und Verzeichnisse ist für die parallele Durchführung von Tests unerlässlich, damit sich die Tests nicht gegenseitig überschreiben.
Daten gegenseitig überschreiben. Außerdem möchten wir, dass die temporären Dateien und Verzeichnisse am Ende jedes Tests, der sie erstellt hat, gelöscht werden.
erstellt hat. Daher ist die Verwendung von Paketen wie `tempfile`, die diese Anforderungen erfüllen, unerlässlich.
Beim Debuggen von Tests müssen Sie jedoch sehen können, was in der temporären Datei oder dem temporären Verzeichnis gespeichert wird und Sie möchten
Sie müssen den genauen Pfad kennen und dürfen ihn nicht bei jedem neuen Testdurchlauf zufällig ändern.
Für solche Zwecke ist die Hilfsklasse `transformers.test_utils.TestCasePlus` am besten geeignet. Sie ist eine Unterklasse von
Unittest.TestCase`, so dass wir in den Testmodulen einfach von ihr erben können.
Hier ist ein Beispiel für die Verwendung dieser Klasse:
```python
from transformers.testing_utils import TestCasePlus
class ExamplesTests(TestCasePlus):
def test_whatever(self):
tmp_dir = self.get_auto_remove_tmp_dir()
```
Dieser Code erstellt ein eindeutiges temporäres Verzeichnis und setzt `tmp_dir` auf dessen Speicherort.
- Erstellen Sie ein eindeutiges temporäres Verzeichnis:
```python
def test_whatever(self):
tmp_dir = self.get_auto_remove_tmp_dir()
```
tmp_dir" enthält den Pfad zu dem erstellten temporären Verzeichnis. Es wird am Ende des Tests automatisch entfernt.
Tests entfernt.
- Erstellen Sie ein temporäres Verzeichnis meiner Wahl, stellen Sie sicher, dass es leer ist, bevor der Test beginnt, und leeren Sie es nach dem Test nicht.
```python
def test_whatever(self):
tmp_dir = self.get_auto_remove_tmp_dir("./xxx")
```
Dies ist nützlich für die Fehlersuche, wenn Sie ein bestimmtes Verzeichnis überwachen und sicherstellen möchten, dass die vorherigen Tests keine Daten darin hinterlassen haben.
keine Daten dort hinterlassen haben.
- Sie können das Standardverhalten außer Kraft setzen, indem Sie die Argumente `before` und `after` direkt überschreiben, was zu einem der folgenden Verhaltensweisen führt
folgenden Verhaltensweisen:
- `before=True`: das temporäre Verzeichnis wird immer zu Beginn des Tests gelöscht.
- `before=False`: wenn das temporäre Verzeichnis bereits existiert, bleiben alle vorhandenen Dateien dort erhalten.
- `after=True`: das temporäre Verzeichnis wird immer am Ende des Tests gelöscht.
- `after=False`: das temporäre Verzeichnis wird am Ende des Tests immer beibehalten.
<Tip>
Um das Äquivalent von `rm -r` sicher ausführen zu können, sind nur Unterverzeichnisse des Projektarchivs checkout erlaubt, wenn
ein explizites `tmp_dir` verwendet wird, so dass nicht versehentlich ein `/tmp` oder ein ähnlich wichtiger Teil des Dateisystems vernichtet wird.
d.h. geben Sie bitte immer Pfade an, die mit `./` beginnen.
</Tip>
<Tip>
Jeder Test kann mehrere temporäre Verzeichnisse registrieren, die alle automatisch entfernt werden, sofern nicht anders gewünscht.
anders.
</Tip>
### Temporäre Überschreibung von sys.path
Wenn Sie `sys.path` vorübergehend überschreiben müssen, um z.B. von einem anderen Test zu importieren, können Sie den
Kontextmanager `ExtendSysPath` verwenden. Beispiel:
```python
import os
from transformers.testing_utils import ExtendSysPath
bindir = os.path.abspath(os.path.dirname(__file__))
with ExtendSysPath(f"{bindir}/.."):
from test_trainer import TrainerIntegrationCommon # noqa
```
### Überspringen von Tests
Dies ist nützlich, wenn ein Fehler gefunden und ein neuer Test geschrieben wird, der Fehler aber noch nicht behoben ist. Damit wir ihn
in das Haupt-Repository zu übertragen, müssen wir sicherstellen, dass er bei `make test` übersprungen wird.
Methoden:
- Ein **Skip** bedeutet, dass Sie erwarten, dass Ihr Test nur dann erfolgreich ist, wenn einige Bedingungen erfüllt sind, andernfalls sollte pytest den Test überspringen.
die Ausführung des Tests ganz überspringen. Übliche Beispiele sind das Überspringen von Tests, die nur unter Windows laufen, auf Nicht-Windows-Plattformen oder das Überspringen von
Tests, die von einer externen Ressource abhängen, die im Moment nicht verfügbar ist (z.B. eine Datenbank).
- Ein **xfail** bedeutet, dass Sie erwarten, dass ein Test aus irgendeinem Grund fehlschlägt. Ein gängiges Beispiel ist ein Test für eine Funktion, die noch nicht
noch nicht implementiert oder ein noch nicht behobener Fehler. Wenn ein Test trotz eines erwarteten Fehlschlags bestanden wird (markiert mit
pytest.mark.xfail), ist dies ein xpass und wird in der Testzusammenfassung gemeldet.
Einer der wichtigsten Unterschiede zwischen den beiden ist, dass `skip` den Test nicht ausführt, während `xfail` dies tut. Wenn also der
Code, der fehlerhaft ist, einen schlechten Zustand verursacht, der sich auf andere Tests auswirkt, sollten Sie also nicht `xfail` verwenden.
#### Implementierung
- Hier sehen Sie, wie Sie einen ganzen Test bedingungslos überspringen können:
```python no-style
@unittest.skip("this bug needs to be fixed")
def test_feature_x():
```
oder mit pytest:
```python no-style
@pytest.mark.skip(reason="this bug needs to be fixed")
```
oder mit dem `xfail` Weg:
```python no-style
@pytest.mark.xfail
def test_feature_x():
```
- Hier erfahren Sie, wie Sie einen Test aufgrund einer internen Prüfung innerhalb des Tests auslassen können:
```python
def test_feature_x():
if not has_something():
pytest.skip("unsupported configuration")
```
oder das ganze Modul:
```python
import pytest
if not pytest.config.getoption("--custom-flag"):
pytest.skip("--custom-flag is missing, skipping tests", allow_module_level=True)
```
oder mit dem `xfail` Weg:
```python
def test_feature_x():
pytest.xfail("expected to fail until bug XYZ is fixed")
```
- Hier erfahren Sie, wie Sie alle Tests in einem Modul überspringen können, wenn ein Import fehlt:
```python
docutils = pytest.importorskip("docutils", minversion="0.3")
```
- Einen Test aufgrund einer Bedingung überspringen:
```python no-style
@pytest.mark.skipif(sys.version_info < (3,6), reason="requires python3.6 or higher")
def test_feature_x():
```
oder:
```python no-style
@unittest.skipIf(torch_device == "cpu", "Can't do half precision")
def test_feature_x():
```
oder überspringen Sie das ganze Modul:
```python no-style
@pytest.mark.skipif(sys.platform == 'win32', reason="does not run on windows")
class TestClass():
def test_feature_x(self):
```
Weitere Details, Beispiele und Möglichkeiten finden Sie [hier](https://docs.pytest.org/en/latest/skipping.html).
### Langsame Tests
Die Bibliothek der Tests wächst ständig, und einige der Tests brauchen Minuten, um ausgeführt zu werden, daher können wir es uns nicht leisten, eine Stunde zu warten, bis die
eine Stunde auf die Fertigstellung der Testsuite auf CI zu warten. Daher sollten langsame Tests, mit einigen Ausnahmen für wichtige Tests, wie im folgenden Beispiel
wie im folgenden Beispiel markiert werden:
```python no-style
from transformers.testing_utils import slow
@slow
def test_integration_foo():
```
Sobald ein Test als `@slow` markiert ist, setzen Sie die Umgebungsvariable `RUN_SLOW=1`, um solche Tests auszuführen, z.B:
```bash
RUN_SLOW=1 pytest tests
```
Einige Dekoratoren wie `@parameterized` schreiben Testnamen um, daher müssen `@slow` und die übrigen Skip-Dekoratoren
`@require_*` müssen als letztes aufgeführt werden, damit sie korrekt funktionieren. Hier ist ein Beispiel für die korrekte Verwendung:
```python no-style
@parameterized.expand(...)
@slow
def test_integration_foo():
```
Wie zu Beginn dieses Dokuments erläutert, werden langsame Tests nach einem Zeitplan ausgeführt und nicht in PRs CI
Prüfungen. Es ist also möglich, dass einige Probleme bei der Einreichung eines PRs übersehen werden und zusammengeführt werden. Solche Probleme werden
werden beim nächsten geplanten CI-Job abgefangen. Das bedeutet aber auch, dass es wichtig ist, die langsamen Tests auf Ihrem
Rechner auszuführen, bevor Sie den PR einreichen.
Hier ist ein grober Entscheidungsmechanismus für die Auswahl der Tests, die als langsam markiert werden sollen:
Wenn der Test auf eine der internen Komponenten der Bibliothek ausgerichtet ist (z.B. Modellierungsdateien, Tokenisierungsdateien,
Pipelines), dann sollten wir diesen Test in der nicht langsamen Testsuite ausführen. Wenn er sich auf einen anderen Aspekt der Bibliothek bezieht,
wie z.B. die Dokumentation oder die Beispiele, dann sollten wir diese Tests in der langsamen Testsuite durchführen. Und dann, zur Verfeinerung
Ansatz zu verfeinern, sollten wir Ausnahmen einführen:
- Alle Tests, die einen umfangreichen Satz von Gewichten oder einen Datensatz mit einer Größe von mehr als ~50MB herunterladen müssen (z.B. Modell- oder
Tokenizer-Integrationstests, Pipeline-Integrationstests) sollten auf langsam gesetzt werden. Wenn Sie ein neues Modell hinzufügen, sollten Sie
sollten Sie eine kleine Version des Modells (mit zufälligen Gewichtungen) für Integrationstests erstellen und in den Hub hochladen. Dies wird
wird in den folgenden Abschnitten erläutert.
- Alle Tests, die ein Training durchführen müssen, das nicht speziell auf Schnelligkeit optimiert ist, sollten auf langsam gesetzt werden.
- Wir können Ausnahmen einführen, wenn einige dieser Tests, die nicht langsam sein sollten, unerträglich langsam sind, und sie auf
`@slow`. Auto-Modellierungstests, die große Dateien auf der Festplatte speichern und laden, sind ein gutes Beispiel für Tests, die als
als `@slow` markiert sind.
- Wenn ein Test in weniger als 1 Sekunde auf CI abgeschlossen wird (einschließlich eventueller Downloads), sollte es sich trotzdem um einen normalen Test handeln.
Insgesamt müssen alle nicht langsamen Tests die verschiedenen Interna abdecken und dabei schnell bleiben. Zum Beispiel,
kann eine signifikante Abdeckung erreicht werden, indem Sie mit speziell erstellten kleinen Modellen mit zufälligen Gewichten testen. Solche Modelle
haben eine sehr geringe Anzahl von Schichten (z.B. 2), Vokabeln (z.B. 1000), usw. Dann können die `@slow`-Tests große
langsame Modelle verwenden, um qualitative Tests durchzuführen. Um die Verwendung dieser Modelle zu sehen, suchen Sie einfach nach *winzigen* Modellen mit:
```bash
grep tiny tests examples
```
Hier ist ein Beispiel für ein [Skript](https://github.com/huggingface/transformers/tree/main/scripts/fsmt/fsmt-make-tiny-model.py), das das winzige Modell erstellt hat
[stas/tiny-wmt19-en-de](https://huggingface.co/stas/tiny-wmt19-en-de). Sie können es ganz einfach an Ihre eigene
Architektur Ihres Modells anpassen.
Es ist leicht, die Laufzeit falsch zu messen, wenn zum Beispiel ein großes Modell heruntergeladen wird, aber wenn
Sie es lokal testen, würden die heruntergeladenen Dateien zwischengespeichert und somit die Download-Zeit nicht gemessen werden. Prüfen Sie daher den
Ausführungsgeschwindigkeitsbericht in den CI-Protokollen (die Ausgabe von `pytest --durations=0 tests`).
Dieser Bericht ist auch nützlich, um langsame Ausreißer zu finden, die nicht als solche gekennzeichnet sind oder die neu geschrieben werden müssen, um schnell zu sein.
Wenn Sie bemerken, dass die Testsuite beim CI langsam wird, zeigt die oberste Liste dieses Berichts die langsamsten
Tests.
### Testen der stdout/stderr-Ausgabe
Um Funktionen zu testen, die in `stdout` und/oder `stderr` schreiben, kann der Test auf diese Ströme zugreifen, indem er die
[capsys system](https://docs.pytest.org/en/latest/capture.html) von `pytest` zugreifen. So wird dies bewerkstelligt:
```python
import sys
def print_to_stdout(s):
print(s)
def print_to_stderr(s):
sys.stderr.write(s)
def test_result_and_stdout(capsys):
msg = "Hello"
print_to_stdout(msg)
print_to_stderr(msg)
out, err = capsys.readouterr() # consume the captured output streams
# optional: if you want to replay the consumed streams:
sys.stdout.write(out)
sys.stderr.write(err)
# test:
assert msg in out
assert msg in err
```
Und natürlich wird `stderr` in den meisten Fällen als Teil einer Ausnahme auftreten, so dass try/except in einem solchen Fall verwendet werden muss
Fall verwendet werden:
```python
def raise_exception(msg):
raise ValueError(msg)
def test_something_exception():
msg = "Not a good value"
error = ""
try:
raise_exception(msg)
except Exception as e:
error = str(e)
assert msg in error, f"{msg} is in the exception:\n{error}"
```
Ein anderer Ansatz zur Erfassung von stdout ist `contextlib.redirect_stdout`:
```python
from io import StringIO
from contextlib import redirect_stdout
def print_to_stdout(s):
print(s)
def test_result_and_stdout():
msg = "Hello"
buffer = StringIO()
with redirect_stdout(buffer):
print_to_stdout(msg)
out = buffer.getvalue()
# optional: if you want to replay the consumed streams:
sys.stdout.write(out)
# test:
assert msg in out
```
Ein wichtiges potenzielles Problem beim Erfassen von stdout ist, dass es `r` Zeichen enthalten kann, die bei normalem `print`
alles zurücksetzen, was bisher gedruckt wurde. Mit `pytest` gibt es kein Problem, aber mit `pytest -s` werden diese
werden diese Zeichen in den Puffer aufgenommen. Um den Test mit und ohne `-s` laufen zu lassen, müssen Sie also eine zusätzliche Bereinigung
zusätzliche Bereinigung der erfassten Ausgabe vornehmen, indem Sie `re.sub(r'~.*\r', '', buf, 0, re.M)` verwenden.
Aber dann haben wir einen Hilfskontextmanager-Wrapper, der sich automatisch um alles kümmert, unabhängig davon, ob er
einige "*.*.*.*" enthält oder nicht:
```python
from transformers.testing_utils import CaptureStdout
with CaptureStdout() as cs:
function_that_writes_to_stdout()
print(cs.out)
```
Hier ist ein vollständiges Testbeispiel:
```python
from transformers.testing_utils import CaptureStdout
msg = "Secret message\r"
final = "Hello World"
with CaptureStdout() as cs:
print(msg + final)
assert cs.out == final + "\n", f"captured: {cs.out}, expecting {final}"
```
Wenn Sie `stderr` aufzeichnen möchten, verwenden Sie stattdessen die Klasse `CaptureStderr`:
```python
from transformers.testing_utils import CaptureStderr
with CaptureStderr() as cs:
function_that_writes_to_stderr()
print(cs.err)
```
Wenn Sie beide Streams auf einmal erfassen müssen, verwenden Sie die übergeordnete Klasse `CaptureStd`:
```python
from transformers.testing_utils import CaptureStd
with CaptureStd() as cs:
function_that_writes_to_stdout_and_stderr()
print(cs.err, cs.out)
```
Um das Debuggen von Testproblemen zu erleichtern, geben diese Kontextmanager standardmäßig die aufgezeichneten Streams beim Verlassen
aus dem Kontext wieder.
### Erfassen von Logger-Streams
Wenn Sie die Ausgabe eines Loggers validieren müssen, können Sie `CaptureLogger` verwenden:
```python
from transformers import logging
from transformers.testing_utils import CaptureLogger
msg = "Testing 1, 2, 3"
logging.set_verbosity_info()
logger = logging.get_logger("transformers.models.bart.tokenization_bart")
with CaptureLogger(logger) as cl:
logger.info(msg)
assert cl.out, msg + "\n"
```
### Testen mit Umgebungsvariablen
Wenn Sie die Auswirkungen von Umgebungsvariablen für einen bestimmten Test testen möchten, können Sie einen Hilfsdekorator verwenden
`transformers.testing_utils.mockenv`
```python
from transformers.testing_utils import mockenv
class HfArgumentParserTest(unittest.TestCase):
@mockenv(TRANSFORMERS_VERBOSITY="error")
def test_env_override(self):
env_level_str = os.getenv("TRANSFORMERS_VERBOSITY", None)
```
Manchmal muss ein externes Programm aufgerufen werden, was die Einstellung von `PYTHONPATH` in `os.environ` erfordert, um mehrere lokale Pfade einzuschließen.
mehrere lokale Pfade. Eine Hilfsklasse `transformers.test_utils.TestCasePlus` hilft Ihnen dabei:
```python
from transformers.testing_utils import TestCasePlus
class EnvExampleTest(TestCasePlus):
def test_external_prog(self):
env = self.get_env()
# now call the external program, passing `env` to it
```
Je nachdem, ob die Testdatei in der Testsuite `tests` oder in `examples` war, wird sie korrekt eingerichtet
`env[PYTHONPATH]` eines dieser beiden Verzeichnisse und auch das `src` Verzeichnis, um sicherzustellen, dass der Test gegen das aktuelle
um sicherzustellen, dass der Test mit dem aktuellen Projektarchiv durchgeführt wird, und schließlich mit dem, was in `env[PYTHONPATH]` bereits eingestellt war, bevor der Test aufgerufen wurde.
wenn überhaupt.
Diese Hilfsmethode erstellt eine Kopie des Objekts `os.environ`, so dass das Original intakt bleibt.
### Reproduzierbare Ergebnisse erhalten
In manchen Situationen möchten Sie vielleicht die Zufälligkeit Ihrer Tests beseitigen. Um identische, reproduzierbare Ergebnisse zu erhalten, müssen Sie
müssen Sie den Seed festlegen:
```python
seed = 42
# python RNG
import random
random.seed(seed)
# pytorch RNGs
import torch
torch.manual_seed(seed)
torch.backends.cudnn.deterministic = True
if torch.cuda.is_available():
torch.cuda.manual_seed_all(seed)
# numpy RNG
import numpy as np
np.random.seed(seed)
# tf RNG
tf.random.set_seed(seed)
```
### Tests debuggen
Um einen Debugger an der Stelle zu starten, an der die Warnung auftritt, gehen Sie wie folgt vor:
```bash
pytest tests/utils/test_logging.py -W error::UserWarning --pdb
```
## Arbeiten mit Github-Aktionen-Workflows
Um einen CI-Job für einen Self-Push-Workflow auszulösen, müssen Sie:
1. Erstellen Sie einen neuen Zweig auf `transformers` Ursprung (keine Gabelung!).
2. Der Name der Verzweigung muss entweder mit `ci_` oder `ci-` beginnen (`main` löst ihn auch aus, aber wir können keine PRs auf
`main`). Es wird auch nur für bestimmte Pfade ausgelöst - Sie können die aktuelle Definition finden, falls sie
falls sie sich seit der Erstellung dieses Dokuments geändert hat [hier](https://github.com/huggingface/transformers/blob/main/.github/workflows/self-push.yml) unter *push:*
3. Erstellen Sie einen PR von diesem Zweig.
4. Dann können Sie sehen, wie der Job erscheint [hier](https://github.com/huggingface/transformers/actions/workflows/self-push.yml). Er wird möglicherweise nicht sofort ausgeführt, wenn es
ein Backlog vorhanden ist.
## Testen experimenteller CI-Funktionen
Das Testen von CI-Funktionen kann potenziell problematisch sein, da es die normale CI-Funktion beeinträchtigen kann. Wenn also eine
neue CI-Funktion hinzugefügt werden soll, sollte dies wie folgt geschehen.
1. Erstellen Sie einen neuen Auftrag, der die zu testende Funktion testet.
2. Der neue Job muss immer erfolgreich sein, so dass er uns ein grünes ✓ gibt (Details unten).
3. Lassen Sie ihn einige Tage lang laufen, um zu sehen, dass eine Vielzahl verschiedener PR-Typen darauf laufen (Benutzer-Gabelzweige,
nicht geforkte Zweige, Zweige, die von github.com UI direct file edit stammen, verschiedene erzwungene Pushes, etc. - es gibt
es gibt so viele), während Sie die Protokolle des experimentellen Jobs überwachen (nicht den gesamten Job grün, da er absichtlich immer
grün)
4. Wenn klar ist, dass alles in Ordnung ist, fügen Sie die neuen Änderungen in die bestehenden Jobs ein.
Auf diese Weise wird der normale Arbeitsablauf nicht durch Experimente mit der CI-Funktionalität selbst beeinträchtigt.
Wie können wir nun dafür sorgen, dass der Auftrag immer erfolgreich ist, während die neue CI-Funktion entwickelt wird?
Einige CIs, wie TravisCI, unterstützen ignore-step-failure und melden den gesamten Job als erfolgreich, aber CircleCI und
Github Actions unterstützen dies zum jetzigen Zeitpunkt nicht.
Sie können also die folgende Abhilfe verwenden:
1. Setzen Sie `set +euo pipefail` am Anfang des Ausführungsbefehls, um die meisten potenziellen Fehler im Bash-Skript zu unterdrücken.
2. Der letzte Befehl muss ein Erfolg sein: `echo "done"` oder einfach `true` reicht aus.
Hier ist ein Beispiel:
```yaml
- run:
name: run CI experiment
command: |
set +euo pipefail
echo "setting run-all-despite-any-errors-mode"
this_command_will_fail
echo "but bash continues to run"
# emulate another failure
false
# but the last command must be a success
echo "during experiment do not remove: reporting success to CI, even if there were failures"
```
Für einfache Befehle können Sie auch Folgendes tun:
```bash
cmd_that_may_fail || true
```
Wenn Sie mit den Ergebnissen zufrieden sind, integrieren Sie den experimentellen Schritt oder Job natürlich in den Rest der normalen Jobs,
Entfernen Sie dabei `set +euo pipefail` oder andere Dinge, die Sie eventuell hinzugefügt haben, um sicherzustellen, dass der experimentelle Auftrag nicht
den normalen CI-Betrieb nicht beeinträchtigt.
Dieser ganze Prozess wäre viel einfacher gewesen, wenn wir nur etwas wie `allow-failure` für den
experimentellen Schritt festlegen könnten und ihn scheitern lassen würden, ohne den Gesamtstatus der PRs zu beeinträchtigen. Aber wie bereits erwähnt, haben CircleCI und
Github Actions dies im Moment nicht unterstützen.
Sie können in diesen CI-spezifischen Threads für diese Funktion stimmen und sehen, wo sie steht:
- [Github Actions:](https://github.com/actions/toolkit/issues/399)
- [CircleCI:](https://ideas.circleci.com/ideas/CCI-I-344)
| transformers/docs/source/de/testing.md/0 | {
"file_path": "transformers/docs/source/de/testing.md",
"repo_id": "transformers",
"token_count": 19303
} | 241 |
<!--Copyright 2022 The HuggingFace Team. All rights reserved.
Licensed under the Apache License, Version 2.0 (the "License"); you may not use this file except in compliance with
the License. You may obtain a copy of the License at
http://www.apache.org/licenses/LICENSE-2.0
Unless required by applicable law or agreed to in writing, software distributed under the License is distributed on
an "AS IS" BASIS, WITHOUT WARRANTIES OR CONDITIONS OF ANY KIND, either express or implied. See the License for the
specific language governing permissions and limitations under the License.
⚠️ Note that this file is in Markdown but contain specific syntax for our doc-builder (similar to MDX) that may not be
rendered properly in your Markdown viewer.
-->
# AltCLIP
## Overview
The AltCLIP model was proposed in [AltCLIP: Altering the Language Encoder in CLIP for Extended Language Capabilities](https://arxiv.org/abs/2211.06679v2) by Zhongzhi Chen, Guang Liu, Bo-Wen Zhang, Fulong Ye, Qinghong Yang, Ledell Wu. AltCLIP
(Altering the Language Encoder in CLIP) is a neural network trained on a variety of image-text and text-text pairs. By switching CLIP's
text encoder with a pretrained multilingual text encoder XLM-R, we could obtain very close performances with CLIP on almost all tasks, and extended original CLIP's capabilities such as multilingual understanding.
The abstract from the paper is the following:
*In this work, we present a conceptually simple and effective method to train a strong bilingual multimodal representation model.
Starting from the pretrained multimodal representation model CLIP released by OpenAI, we switched its text encoder with a pretrained
multilingual text encoder XLM-R, and aligned both languages and image representations by a two-stage training schema consisting of
teacher learning and contrastive learning. We validate our method through evaluations of a wide range of tasks. We set new state-of-the-art
performances on a bunch of tasks including ImageNet-CN, Flicker30k- CN, and COCO-CN. Further, we obtain very close performances with
CLIP on almost all tasks, suggesting that one can simply alter the text encoder in CLIP for extended capabilities such as multilingual understanding.*
This model was contributed by [jongjyh](https://huggingface.co/jongjyh).
## Usage tips and example
The usage of AltCLIP is very similar to the CLIP. the difference between CLIP is the text encoder. Note that we use bidirectional attention instead of casual attention
and we take the [CLS] token in XLM-R to represent text embedding.
AltCLIP is a multi-modal vision and language model. It can be used for image-text similarity and for zero-shot image
classification. AltCLIP uses a ViT like transformer to get visual features and a bidirectional language model to get the text
features. Both the text and visual features are then projected to a latent space with identical dimension. The dot
product between the projected image and text features is then used as a similar score.
To feed images to the Transformer encoder, each image is split into a sequence of fixed-size non-overlapping patches,
which are then linearly embedded. A [CLS] token is added to serve as representation of an entire image. The authors
also add absolute position embeddings, and feed the resulting sequence of vectors to a standard Transformer encoder.
The [`CLIPImageProcessor`] can be used to resize (or rescale) and normalize images for the model.
The [`AltCLIPProcessor`] wraps a [`CLIPImageProcessor`] and a [`XLMRobertaTokenizer`] into a single instance to both
encode the text and prepare the images. The following example shows how to get the image-text similarity scores using
[`AltCLIPProcessor`] and [`AltCLIPModel`].
```python
>>> from PIL import Image
>>> import requests
>>> from transformers import AltCLIPModel, AltCLIPProcessor
>>> model = AltCLIPModel.from_pretrained("BAAI/AltCLIP")
>>> processor = AltCLIPProcessor.from_pretrained("BAAI/AltCLIP")
>>> url = "http://images.cocodataset.org/val2017/000000039769.jpg"
>>> image = Image.open(requests.get(url, stream=True).raw)
>>> inputs = processor(text=["a photo of a cat", "a photo of a dog"], images=image, return_tensors="pt", padding=True)
>>> outputs = model(**inputs)
>>> logits_per_image = outputs.logits_per_image # this is the image-text similarity score
>>> probs = logits_per_image.softmax(dim=1) # we can take the softmax to get the label probabilities
```
<Tip>
This model is based on `CLIPModel`, use it like you would use the original [CLIP](clip).
</Tip>
## AltCLIPConfig
[[autodoc]] AltCLIPConfig
- from_text_vision_configs
## AltCLIPTextConfig
[[autodoc]] AltCLIPTextConfig
## AltCLIPVisionConfig
[[autodoc]] AltCLIPVisionConfig
## AltCLIPProcessor
[[autodoc]] AltCLIPProcessor
## AltCLIPModel
[[autodoc]] AltCLIPModel
- forward
- get_text_features
- get_image_features
## AltCLIPTextModel
[[autodoc]] AltCLIPTextModel
- forward
## AltCLIPVisionModel
[[autodoc]] AltCLIPVisionModel
- forward | transformers/docs/source/en/model_doc/altclip.md/0 | {
"file_path": "transformers/docs/source/en/model_doc/altclip.md",
"repo_id": "transformers",
"token_count": 1400
} | 242 |
<!--Copyright 2022 The HuggingFace Team. All rights reserved.
Licensed under the Apache License, Version 2.0 (the "License"); you may not use this file except in compliance with
the License. You may obtain a copy of the License at
http://www.apache.org/licenses/LICENSE-2.0
Unless required by applicable law or agreed to in writing, software distributed under the License is distributed on
an "AS IS" BASIS, WITHOUT WARRANTIES OR CONDITIONS OF ANY KIND, either express or implied. See the License for the
specific language governing permissions and limitations under the License.
⚠️ Note that this file is in Markdown but contain specific syntax for our doc-builder (similar to MDX) that may not be
rendered properly in your Markdown viewer.
-->
# Big Transfer (BiT)
## Overview
The BiT model was proposed in [Big Transfer (BiT): General Visual Representation Learning](https://arxiv.org/abs/1912.11370) by Alexander Kolesnikov, Lucas Beyer, Xiaohua Zhai, Joan Puigcerver, Jessica Yung, Sylvain Gelly, Neil Houlsby.
BiT is a simple recipe for scaling up pre-training of [ResNet](resnet)-like architectures (specifically, ResNetv2). The method results in significant improvements for transfer learning.
The abstract from the paper is the following:
*Transfer of pre-trained representations improves sample efficiency and simplifies hyperparameter tuning when training deep neural networks for vision. We revisit the paradigm of pre-training on large supervised datasets and fine-tuning the model on a target task. We scale up pre-training, and propose a simple recipe that we call Big Transfer (BiT). By combining a few carefully selected components, and transferring using a simple heuristic, we achieve strong performance on over 20 datasets. BiT performs well across a surprisingly wide range of data regimes -- from 1 example per class to 1M total examples. BiT achieves 87.5% top-1 accuracy on ILSVRC-2012, 99.4% on CIFAR-10, and 76.3% on the 19 task Visual Task Adaptation Benchmark (VTAB). On small datasets, BiT attains 76.8% on ILSVRC-2012 with 10 examples per class, and 97.0% on CIFAR-10 with 10 examples per class. We conduct detailed analysis of the main components that lead to high transfer performance.*
This model was contributed by [nielsr](https://huggingface.co/nielsr).
The original code can be found [here](https://github.com/google-research/big_transfer).
## Usage tips
- BiT models are equivalent to ResNetv2 in terms of architecture, except that: 1) all batch normalization layers are replaced by [group normalization](https://arxiv.org/abs/1803.08494),
2) [weight standardization](https://arxiv.org/abs/1903.10520) is used for convolutional layers. The authors show that the combination of both is useful for training with large batch sizes, and has a significant
impact on transfer learning.
## Resources
A list of official Hugging Face and community (indicated by 🌎) resources to help you get started with BiT.
<PipelineTag pipeline="image-classification"/>
- [`BitForImageClassification`] is supported by this [example script](https://github.com/huggingface/transformers/tree/main/examples/pytorch/image-classification) and [notebook](https://colab.research.google.com/github/huggingface/notebooks/blob/main/examples/image_classification.ipynb).
- See also: [Image classification task guide](../tasks/image_classification)
If you're interested in submitting a resource to be included here, please feel free to open a Pull Request and we'll review it! The resource should ideally demonstrate something new instead of duplicating an existing resource.
## BitConfig
[[autodoc]] BitConfig
## BitImageProcessor
[[autodoc]] BitImageProcessor
- preprocess
## BitModel
[[autodoc]] BitModel
- forward
## BitForImageClassification
[[autodoc]] BitForImageClassification
- forward | transformers/docs/source/en/model_doc/bit.md/0 | {
"file_path": "transformers/docs/source/en/model_doc/bit.md",
"repo_id": "transformers",
"token_count": 1005
} | 243 |
<!--Copyright 2023 The HuggingFace Team. All rights reserved.
Licensed under the Apache License, Version 2.0 (the "License"); you may not use this file except in compliance with
the License. You may obtain a copy of the License at
http://www.apache.org/licenses/LICENSE-2.0
Unless required by applicable law or agreed to in writing, software distributed under the License is distributed on
an "AS IS" BASIS, WITHOUT WARRANTIES OR CONDITIONS OF ANY KIND, either express or implied. See the License for the
specific language governing permissions and limitations under the License.
⚠️ Note that this file is in Markdown but contain specific syntax for our doc-builder (similar to MDX) that may not be
rendered properly in your Markdown viewer.
-->
# CLVP
## Overview
The CLVP (Contrastive Language-Voice Pretrained Transformer) model was proposed in [Better speech synthesis through scaling](https://arxiv.org/abs/2305.07243) by James Betker.
The abstract from the paper is the following:
*In recent years, the field of image generation has been revolutionized by the application of autoregressive transformers and DDPMs. These approaches model the process of image generation as a step-wise probabilistic processes and leverage large amounts of compute and data to learn the image distribution. This methodology of improving performance need not be confined to images. This paper describes a way to apply advances in the image generative domain to speech synthesis. The result is TorToise - an expressive, multi-voice text-to-speech system.*
This model was contributed by [Susnato Dhar](https://huggingface.co/susnato).
The original code can be found [here](https://github.com/neonbjb/tortoise-tts).
## Usage tips
1. CLVP is an integral part of the Tortoise TTS model.
2. CLVP can be used to compare different generated speech candidates with the provided text, and the best speech tokens are forwarded to the diffusion model.
3. The use of the [`ClvpModelForConditionalGeneration.generate()`] method is strongly recommended for tortoise usage.
4. Note that the CLVP model expects the audio to be sampled at 22.05 kHz contrary to other audio models which expects 16 kHz.
## Brief Explanation:
- The [`ClvpTokenizer`] tokenizes the text input, and the [`ClvpFeatureExtractor`] extracts the log mel-spectrogram from the desired audio.
- [`ClvpConditioningEncoder`] takes those text tokens and audio representations and converts them into embeddings conditioned on the text and audio.
- The [`ClvpForCausalLM`] uses those embeddings to generate multiple speech candidates.
- Each speech candidate is passed through the speech encoder ([`ClvpEncoder`]) which converts them into a vector representation, and the text encoder ([`ClvpEncoder`]) converts the text tokens into the same latent space.
- At the end, we compare each speech vector with the text vector to see which speech vector is most similar to the text vector.
- [`ClvpModelForConditionalGeneration.generate()`] compresses all of the logic described above into a single method.
Example :
```python
>>> import datasets
>>> from transformers import ClvpProcessor, ClvpModelForConditionalGeneration
>>> # Define the Text and Load the Audio (We are taking an audio example from HuggingFace Hub using `datasets` library).
>>> text = "This is an example text."
>>> ds = datasets.load_dataset("hf-internal-testing/librispeech_asr_dummy", "clean", split="validation")
>>> ds = ds.cast_column("audio", datasets.Audio(sampling_rate=22050))
>>> sample = ds[0]["audio"]
>>> # Define processor and model.
>>> processor = ClvpProcessor.from_pretrained("susnato/clvp_dev")
>>> model = ClvpModelForConditionalGeneration.from_pretrained("susnato/clvp_dev")
>>> # Generate processor output and model output.
>>> processor_output = processor(raw_speech=sample["array"], sampling_rate=sample["sampling_rate"], text=text, return_tensors="pt")
>>> generated_output = model.generate(**processor_output)
```
## ClvpConfig
[[autodoc]] ClvpConfig
- from_sub_model_configs
## ClvpEncoderConfig
[[autodoc]] ClvpEncoderConfig
## ClvpDecoderConfig
[[autodoc]] ClvpDecoderConfig
## ClvpTokenizer
[[autodoc]] ClvpTokenizer
- save_vocabulary
## ClvpFeatureExtractor
[[autodoc]] ClvpFeatureExtractor
- __call__
## ClvpProcessor
[[autodoc]] ClvpProcessor
- __call__
- decode
- batch_decode
## ClvpModelForConditionalGeneration
[[autodoc]] ClvpModelForConditionalGeneration
- forward
- generate
- get_text_features
- get_speech_features
## ClvpForCausalLM
[[autodoc]] ClvpForCausalLM
## ClvpModel
[[autodoc]] ClvpModel
## ClvpEncoder
[[autodoc]] ClvpEncoder
## ClvpDecoder
[[autodoc]] ClvpDecoder
| transformers/docs/source/en/model_doc/clvp.md/0 | {
"file_path": "transformers/docs/source/en/model_doc/clvp.md",
"repo_id": "transformers",
"token_count": 1339
} | 244 |
<!--Copyright 2022 The HuggingFace Team. All rights reserved.
Licensed under the Apache License, Version 2.0 (the "License"); you may not use this file except in compliance with
the License. You may obtain a copy of the License at
http://www.apache.org/licenses/LICENSE-2.0
Unless required by applicable law or agreed to in writing, software distributed under the License is distributed on
an "AS IS" BASIS, WITHOUT WARRANTIES OR CONDITIONS OF ANY KIND, either express or implied. See the License for the
specific language governing permissions and limitations under the License.
⚠️ Note that this file is in Markdown but contain specific syntax for our doc-builder (similar to MDX) that may not be
rendered properly in your Markdown viewer.
-->
# Deformable DETR
## Overview
The Deformable DETR model was proposed in [Deformable DETR: Deformable Transformers for End-to-End Object Detection](https://arxiv.org/abs/2010.04159) by Xizhou Zhu, Weijie Su, Lewei Lu, Bin Li, Xiaogang Wang, Jifeng Dai.
Deformable DETR mitigates the slow convergence issues and limited feature spatial resolution of the original [DETR](detr) by leveraging a new deformable attention module which only attends to a small set of key sampling points around a reference.
The abstract from the paper is the following:
*DETR has been recently proposed to eliminate the need for many hand-designed components in object detection while demonstrating good performance. However, it suffers from slow convergence and limited feature spatial resolution, due to the limitation of Transformer attention modules in processing image feature maps. To mitigate these issues, we proposed Deformable DETR, whose attention modules only attend to a small set of key sampling points around a reference. Deformable DETR can achieve better performance than DETR (especially on small objects) with 10 times less training epochs. Extensive experiments on the COCO benchmark demonstrate the effectiveness of our approach.*
<img src="https://huggingface.co/datasets/huggingface/documentation-images/resolve/main/deformable_detr_architecture.png"
alt="drawing" width="600"/>
<small> Deformable DETR architecture. Taken from the <a href="https://arxiv.org/abs/2010.04159">original paper</a>.</small>
This model was contributed by [nielsr](https://huggingface.co/nielsr). The original code can be found [here](https://github.com/fundamentalvision/Deformable-DETR).
## Usage tips
- Training Deformable DETR is equivalent to training the original [DETR](detr) model. See the [resources](#resources) section below for demo notebooks.
## Resources
A list of official Hugging Face and community (indicated by 🌎) resources to help you get started with Deformable DETR.
<PipelineTag pipeline="object-detection"/>
- Demo notebooks regarding inference + fine-tuning on a custom dataset for [`DeformableDetrForObjectDetection`] can be found [here](https://github.com/NielsRogge/Transformers-Tutorials/tree/master/Deformable-DETR).
- See also: [Object detection task guide](../tasks/object_detection).
If you're interested in submitting a resource to be included here, please feel free to open a Pull Request and we'll review it! The resource should ideally demonstrate something new instead of duplicating an existing resource.
## DeformableDetrImageProcessor
[[autodoc]] DeformableDetrImageProcessor
- preprocess
- post_process_object_detection
## DeformableDetrFeatureExtractor
[[autodoc]] DeformableDetrFeatureExtractor
- __call__
- post_process_object_detection
## DeformableDetrConfig
[[autodoc]] DeformableDetrConfig
## DeformableDetrModel
[[autodoc]] DeformableDetrModel
- forward
## DeformableDetrForObjectDetection
[[autodoc]] DeformableDetrForObjectDetection
- forward
| transformers/docs/source/en/model_doc/deformable_detr.md/0 | {
"file_path": "transformers/docs/source/en/model_doc/deformable_detr.md",
"repo_id": "transformers",
"token_count": 1014
} | 245 |
<!--Copyright 2020 The HuggingFace Team. All rights reserved.
Licensed under the Apache License, Version 2.0 (the "License"); you may not use this file except in compliance with
the License. You may obtain a copy of the License at
http://www.apache.org/licenses/LICENSE-2.0
Unless required by applicable law or agreed to in writing, software distributed under the License is distributed on
an "AS IS" BASIS, WITHOUT WARRANTIES OR CONDITIONS OF ANY KIND, either express or implied. See the License for the
specific language governing permissions and limitations under the License.
⚠️ Note that this file is in Markdown but contain specific syntax for our doc-builder (similar to MDX) that may not be
rendered properly in your Markdown viewer.
-->
# ELECTRA
<div class="flex flex-wrap space-x-1">
<a href="https://huggingface.co/models?filter=electra">
<img alt="Models" src="https://img.shields.io/badge/All_model_pages-electra-blueviolet">
</a>
<a href="https://huggingface.co/spaces/docs-demos/electra_large_discriminator_squad2_512">
<img alt="Spaces" src="https://img.shields.io/badge/%F0%9F%A4%97%20Hugging%20Face-Spaces-blue">
</a>
</div>
## Overview
The ELECTRA model was proposed in the paper [ELECTRA: Pre-training Text Encoders as Discriminators Rather Than
Generators](https://openreview.net/pdf?id=r1xMH1BtvB). ELECTRA is a new pretraining approach which trains two
transformer models: the generator and the discriminator. The generator's role is to replace tokens in a sequence, and
is therefore trained as a masked language model. The discriminator, which is the model we're interested in, tries to
identify which tokens were replaced by the generator in the sequence.
The abstract from the paper is the following:
*Masked language modeling (MLM) pretraining methods such as BERT corrupt the input by replacing some tokens with [MASK]
and then train a model to reconstruct the original tokens. While they produce good results when transferred to
downstream NLP tasks, they generally require large amounts of compute to be effective. As an alternative, we propose a
more sample-efficient pretraining task called replaced token detection. Instead of masking the input, our approach
corrupts it by replacing some tokens with plausible alternatives sampled from a small generator network. Then, instead
of training a model that predicts the original identities of the corrupted tokens, we train a discriminative model that
predicts whether each token in the corrupted input was replaced by a generator sample or not. Thorough experiments
demonstrate this new pretraining task is more efficient than MLM because the task is defined over all input tokens
rather than just the small subset that was masked out. As a result, the contextual representations learned by our
approach substantially outperform the ones learned by BERT given the same model size, data, and compute. The gains are
particularly strong for small models; for example, we train a model on one GPU for 4 days that outperforms GPT (trained
using 30x more compute) on the GLUE natural language understanding benchmark. Our approach also works well at scale,
where it performs comparably to RoBERTa and XLNet while using less than 1/4 of their compute and outperforms them when
using the same amount of compute.*
This model was contributed by [lysandre](https://huggingface.co/lysandre). The original code can be found [here](https://github.com/google-research/electra).
## Usage tips
- ELECTRA is the pretraining approach, therefore there is nearly no changes done to the underlying model: BERT. The
only change is the separation of the embedding size and the hidden size: the embedding size is generally smaller,
while the hidden size is larger. An additional projection layer (linear) is used to project the embeddings from their
embedding size to the hidden size. In the case where the embedding size is the same as the hidden size, no projection
layer is used.
- ELECTRA is a transformer model pretrained with the use of another (small) masked language model. The inputs are corrupted by that language model, which takes an input text that is randomly masked and outputs a text in which ELECTRA has to predict which token is an original and which one has been replaced. Like for GAN training, the small language model is trained for a few steps (but with the original texts as objective, not to fool the ELECTRA model like in a traditional GAN setting) then the ELECTRA model is trained for a few steps.
- The ELECTRA checkpoints saved using [Google Research's implementation](https://github.com/google-research/electra)
contain both the generator and discriminator. The conversion script requires the user to name which model to export
into the correct architecture. Once converted to the HuggingFace format, these checkpoints may be loaded into all
available ELECTRA models, however. This means that the discriminator may be loaded in the
[`ElectraForMaskedLM`] model, and the generator may be loaded in the
[`ElectraForPreTraining`] model (the classification head will be randomly initialized as it
doesn't exist in the generator).
## Resources
- [Text classification task guide](../tasks/sequence_classification)
- [Token classification task guide](../tasks/token_classification)
- [Question answering task guide](../tasks/question_answering)
- [Causal language modeling task guide](../tasks/language_modeling)
- [Masked language modeling task guide](../tasks/masked_language_modeling)
- [Multiple choice task guide](../tasks/multiple_choice)
## ElectraConfig
[[autodoc]] ElectraConfig
## ElectraTokenizer
[[autodoc]] ElectraTokenizer
## ElectraTokenizerFast
[[autodoc]] ElectraTokenizerFast
## Electra specific outputs
[[autodoc]] models.electra.modeling_electra.ElectraForPreTrainingOutput
[[autodoc]] models.electra.modeling_tf_electra.TFElectraForPreTrainingOutput
<frameworkcontent>
<pt>
## ElectraModel
[[autodoc]] ElectraModel
- forward
## ElectraForPreTraining
[[autodoc]] ElectraForPreTraining
- forward
## ElectraForCausalLM
[[autodoc]] ElectraForCausalLM
- forward
## ElectraForMaskedLM
[[autodoc]] ElectraForMaskedLM
- forward
## ElectraForSequenceClassification
[[autodoc]] ElectraForSequenceClassification
- forward
## ElectraForMultipleChoice
[[autodoc]] ElectraForMultipleChoice
- forward
## ElectraForTokenClassification
[[autodoc]] ElectraForTokenClassification
- forward
## ElectraForQuestionAnswering
[[autodoc]] ElectraForQuestionAnswering
- forward
</pt>
<tf>
## TFElectraModel
[[autodoc]] TFElectraModel
- call
## TFElectraForPreTraining
[[autodoc]] TFElectraForPreTraining
- call
## TFElectraForMaskedLM
[[autodoc]] TFElectraForMaskedLM
- call
## TFElectraForSequenceClassification
[[autodoc]] TFElectraForSequenceClassification
- call
## TFElectraForMultipleChoice
[[autodoc]] TFElectraForMultipleChoice
- call
## TFElectraForTokenClassification
[[autodoc]] TFElectraForTokenClassification
- call
## TFElectraForQuestionAnswering
[[autodoc]] TFElectraForQuestionAnswering
- call
</tf>
<jax>
## FlaxElectraModel
[[autodoc]] FlaxElectraModel
- __call__
## FlaxElectraForPreTraining
[[autodoc]] FlaxElectraForPreTraining
- __call__
## FlaxElectraForCausalLM
[[autodoc]] FlaxElectraForCausalLM
- __call__
## FlaxElectraForMaskedLM
[[autodoc]] FlaxElectraForMaskedLM
- __call__
## FlaxElectraForSequenceClassification
[[autodoc]] FlaxElectraForSequenceClassification
- __call__
## FlaxElectraForMultipleChoice
[[autodoc]] FlaxElectraForMultipleChoice
- __call__
## FlaxElectraForTokenClassification
[[autodoc]] FlaxElectraForTokenClassification
- __call__
## FlaxElectraForQuestionAnswering
[[autodoc]] FlaxElectraForQuestionAnswering
- __call__
</jax>
</frameworkcontent>
| transformers/docs/source/en/model_doc/electra.md/0 | {
"file_path": "transformers/docs/source/en/model_doc/electra.md",
"repo_id": "transformers",
"token_count": 2211
} | 246 |
<!--Copyright 2023 The HuggingFace Team. All rights reserved.
Licensed under the Apache License, Version 2.0 (the "License"); you may not use this file except in compliance with
the License. You may obtain a copy of the License at
http://www.apache.org/licenses/LICENSE-2.0
Unless required by applicable law or agreed to in writing, software distributed under the License is distributed on
an "AS IS" BASIS, WITHOUT WARRANTIES OR CONDITIONS OF ANY KIND, either express or implied. See the License for the
specific language governing permissions and limitations under the License.
⚠️ Note that this file is in Markdown but contain specific syntax for our doc-builder (similar to MDX) that may not be
rendered properly in your Markdown viewer.
-->
# Fuyu
## Overview
The Fuyu model was created by [ADEPT](https://www.adept.ai/blog/fuyu-8b), and authored by Rohan Bavishi, Erich Elsen, Curtis Hawthorne, Maxwell Nye, Augustus Odena, Arushi Somani, Sağnak Taşırlar.
The authors introduced Fuyu-8B, a decoder-only multimodal model based on the classic transformers architecture, with query and key normalization. A linear encoder is added to create multimodal embeddings from image inputs.
By treating image tokens like text tokens and using a special image-newline character, the model knows when an image line ends. Image positional embeddings are removed. This avoids the need for different training phases for various image resolutions. With 8 billion parameters and licensed under CC-BY-NC, Fuyu-8B is notable for its ability to handle both text and images, its impressive context size of 16K, and its overall performance.
<Tip warning={true}>
The `Fuyu` models were trained using `bfloat16`, but the original inference uses `float16` The checkpoints uploaded on the hub use `torch_dtype = 'float16'` which will be
used by the `AutoModel` API to cast the checkpoints from `torch.float32` to `torch.float16`.
The `dtype` of the online weights is mostly irrelevant, unless you are using `torch_dtype="auto"` when initializing a model using `model = AutoModelForCausalLM.from_pretrained("path", torch_dtype = "auto")`. The reason is that the model will first be downloaded ( using the `dtype` of the checkpoints online) then it will be cast to the default `dtype` of `torch` (becomes `torch.float32`). Users should specify the `torch_dtype` they want, and if they don't it will be `torch.float32`.
Finetuning the model in `float16` is not recommended and known to produce `nan`, as such the model should be fine-tuned in `bfloat16`.
</Tip>
Tips:
- To convert the model, you need to clone the original repository using `git clone https://github.com/persimmon-ai-labs/adept-inference`, then get the checkpoints:
```bash
git clone https://github.com/persimmon-ai-labs/adept-inference
wget path/to/fuyu-8b-model-weights.tar
tar -xvf fuyu-8b-model-weights.tar
python src/transformers/models/fuyu/convert_fuyu_weights_to_hf.py --input_dir /path/to/downloaded/fuyu/weights/ --output_dir /output/path \
--pt_model_path /path/to/fuyu_8b_release/iter_0001251/mp_rank_00/model_optim_rng.pt
--ada_lib_path /path/to/adept-inference
```
For the chat model:
```bash
wget https://axtkn4xl5cip.objectstorage.us-phoenix-1.oci.customer-oci.com/n/axtkn4xl5cip/b/adept-public-data/o/8b_chat_model_release.tar
tar -xvf 8b_base_model_release.tar
```
Then, model can be loaded via:
```py
from transformers import FuyuConfig, FuyuForCausalLM
model_config = FuyuConfig()
model = FuyuForCausalLM(model_config).from_pretrained('/output/path')
```
Inputs need to be passed through a specific Processor to have the correct formats.
A processor requires an image_processor and a tokenizer. Hence, inputs can be loaded via:
```py
from PIL import Image
from transformers import AutoTokenizer
from transformers.models.fuyu.processing_fuyu import FuyuProcessor
from transformers.models.fuyu.image_processing_fuyu import FuyuImageProcessor
tokenizer = AutoTokenizer.from_pretrained('adept-hf-collab/fuyu-8b')
image_processor = FuyuImageProcessor()
processor = FuyuProcessor(image_processor=image_processor, tokenizer=tokenizer)
text_prompt = "Generate a coco-style caption.\\n"
bus_image_url = "https://huggingface.co/datasets/hf-internal-testing/fixtures-captioning/resolve/main/bus.png"
bus_image_pil = Image.open(io.BytesIO(requests.get(bus_image_url).content))
inputs_to_model = processor(text=text_prompt, images=bus_image_pil)
```
This model was contributed by [Molbap](https://huggingface.co/Molbap).
The original code can be found [here](https://github.com/persimmon-ai-labs/adept-inference).
- Fuyu uses a `sentencepiece` based tokenizer, with a `Unigram` model. It supports bytefallback, which is only available in `tokenizers==0.14.0` for the fast tokenizer.
The `LlamaTokenizer` is used as it is a standard wrapper around sentencepiece.
- The authors suggest to use the following prompt for image captioning: `f"Generate a coco-style caption.\\n"`
## FuyuConfig
[[autodoc]] FuyuConfig
## FuyuForCausalLM
[[autodoc]] FuyuForCausalLM
- forward
## FuyuImageProcessor
[[autodoc]] FuyuImageProcessor
- __call__
## FuyuProcessor
[[autodoc]] FuyuProcessor
- __call__
| transformers/docs/source/en/model_doc/fuyu.md/0 | {
"file_path": "transformers/docs/source/en/model_doc/fuyu.md",
"repo_id": "transformers",
"token_count": 1657
} | 247 |
<!--Copyright 2020 The HuggingFace Team. All rights reserved.
Licensed under the Apache License, Version 2.0 (the "License"); you may not use this file except in compliance with
the License. You may obtain a copy of the License at
http://www.apache.org/licenses/LICENSE-2.0
Unless required by applicable law or agreed to in writing, software distributed under the License is distributed on
an "AS IS" BASIS, WITHOUT WARRANTIES OR CONDITIONS OF ANY KIND, either express or implied. See the License for the
specific language governing permissions and limitations under the License.
⚠️ Note that this file is in Markdown but contain specific syntax for our doc-builder (similar to MDX) that may not be
rendered properly in your Markdown viewer.
-->
# I-BERT
## Overview
The I-BERT model was proposed in [I-BERT: Integer-only BERT Quantization](https://arxiv.org/abs/2101.01321) by
Sehoon Kim, Amir Gholami, Zhewei Yao, Michael W. Mahoney and Kurt Keutzer. It's a quantized version of RoBERTa running
inference up to four times faster.
The abstract from the paper is the following:
*Transformer based models, like BERT and RoBERTa, have achieved state-of-the-art results in many Natural Language
Processing tasks. However, their memory footprint, inference latency, and power consumption are prohibitive for
efficient inference at the edge, and even at the data center. While quantization can be a viable solution for this,
previous work on quantizing Transformer based models use floating-point arithmetic during inference, which cannot
efficiently utilize integer-only logical units such as the recent Turing Tensor Cores, or traditional integer-only ARM
processors. In this work, we propose I-BERT, a novel quantization scheme for Transformer based models that quantizes
the entire inference with integer-only arithmetic. Based on lightweight integer-only approximation methods for
nonlinear operations, e.g., GELU, Softmax, and Layer Normalization, I-BERT performs an end-to-end integer-only BERT
inference without any floating point calculation. We evaluate our approach on GLUE downstream tasks using
RoBERTa-Base/Large. We show that for both cases, I-BERT achieves similar (and slightly higher) accuracy as compared to
the full-precision baseline. Furthermore, our preliminary implementation of I-BERT shows a speedup of 2.4 - 4.0x for
INT8 inference on a T4 GPU system as compared to FP32 inference. The framework has been developed in PyTorch and has
been open-sourced.*
This model was contributed by [kssteven](https://huggingface.co/kssteven). The original code can be found [here](https://github.com/kssteven418/I-BERT).
## Resources
- [Text classification task guide](../tasks/sequence_classification)
- [Token classification task guide](../tasks/token_classification)
- [Question answering task guide](../tasks/question_answering)
- [Masked language modeling task guide](../tasks/masked_language_modeling)
- [Multiple choice task guide](../tasks/masked_language_modeling)
## IBertConfig
[[autodoc]] IBertConfig
## IBertModel
[[autodoc]] IBertModel
- forward
## IBertForMaskedLM
[[autodoc]] IBertForMaskedLM
- forward
## IBertForSequenceClassification
[[autodoc]] IBertForSequenceClassification
- forward
## IBertForMultipleChoice
[[autodoc]] IBertForMultipleChoice
- forward
## IBertForTokenClassification
[[autodoc]] IBertForTokenClassification
- forward
## IBertForQuestionAnswering
[[autodoc]] IBertForQuestionAnswering
- forward
| transformers/docs/source/en/model_doc/ibert.md/0 | {
"file_path": "transformers/docs/source/en/model_doc/ibert.md",
"repo_id": "transformers",
"token_count": 947
} | 248 |
<!--Copyright 2023 The HuggingFace Team. All rights reserved.
Licensed under the Apache License, Version 2.0 (the "License"); you may not use this file except in compliance with
the License. You may obtain a copy of the License at
http://www.apache.org/licenses/LICENSE-2.0
Unless required by applicable law or agreed to in writing, software distributed under the License is distributed on
an "AS IS" BASIS, WITHOUT WARRANTIES OR CONDITIONS OF ANY KIND, either express or implied. See the License for the
specific language governing permissions and limitations under the License.
⚠️ Note that this file is in Markdown but contain specific syntax for our doc-builder (similar to MDX) that may not be
rendered properly in your Markdown viewer.
-->
# LLaVa
## Overview
LLaVa is an open-source chatbot trained by fine-tuning LlamA/Vicuna on GPT-generated multimodal instruction-following data. It is an auto-regressive language model, based on the transformer architecture. In other words, it is an multi-modal version of LLMs fine-tuned for chat / instructions.
The LLaVa model was proposed in [Visual Instruction Tuning](https://arxiv.org/abs/2304.08485) and improved in [Improved Baselines with Visual Instruction Tuning](https://arxiv.org/pdf/2310.03744) by Haotian Liu, Chunyuan Li, Yuheng Li and Yong Jae Lee.
The abstract from the paper is the following:
*Large multimodal models (LMM) have recently shown encouraging progress with visual instruction tuning. In this note, we show that the fully-connected vision-language cross-modal connector in LLaVA is surprisingly powerful and data-efficient. With simple modifications to LLaVA, namely, using CLIP-ViT-L-336px with an MLP projection and adding academic-task-oriented VQA data with simple response formatting prompts, we establish stronger baselines that achieve state-of-the-art across 11 benchmarks. Our final 13B checkpoint uses merely 1.2M publicly available data, and finishes full training in ∼1 day on a single 8-A100 node. We hope this can make state-of-the-art LMM research more accessible. Code and model will be publicly available*
<img src="https://huggingface.co/datasets/huggingface/documentation-images/resolve/main/transformers/model_doc/llava_architecture.jpg"
alt="drawing" width="600"/>
<small> LLaVa architecture. Taken from the <a href="https://arxiv.org/abs/2304.08485">original paper.</a> </small>
This model was contributed by [ArthurZ](https://huggingface.co/ArthurZ) and [ybelkada](https://huggingface.co/ybelkada).
The original code can be found [here](https://github.com/haotian-liu/LLaVA/tree/main/llava).
## Usage tips
- We advise users to use `padding_side="left"` when computing batched generation as it leads to more accurate results. Simply make sure to call `processor.tokenizer.padding_side = "left"` before generating.
- Note the model has not been explicitly trained to process multiple images in the same prompt, although this is technically possible, you may experience inaccurate results.
- For better results, we recommend users to prompt the model with the correct prompt format:
```bash
"USER: <image>\n<prompt>ASSISTANT:"
```
For multiple turns conversation:
```bash
"USER: <image>\n<prompt1>ASSISTANT: <answer1>USER: <prompt2>ASSISTANT: <answer2>USER: <prompt3>ASSISTANT:"
```
### Using Flash Attention 2
Flash Attention 2 is an even faster, optimized version of the previous optimization, please refer to the [Flash Attention 2 section of performance docs](https://huggingface.co/docs/transformers/perf_infer_gpu_one).
## Resources
A list of official Hugging Face and community (indicated by 🌎) resources to help you get started with BEiT.
<PipelineTag pipeline="image-to-text"/>
- A [Google Colab demo](https://colab.research.google.com/drive/1qsl6cd2c8gGtEW1xV5io7S8NHh-Cp1TV?usp=sharing) on how to run Llava on a free-tier Google colab instance leveraging 4-bit inference.
- A [similar notebook](https://github.com/NielsRogge/Transformers-Tutorials/blob/master/LLaVa/Inference_with_LLaVa_for_multimodal_generation.ipynb) showcasing batched inference. 🌎
## LlavaConfig
[[autodoc]] LlavaConfig
## LlavaProcessor
[[autodoc]] LlavaProcessor
## LlavaForConditionalGeneration
[[autodoc]] LlavaForConditionalGeneration
- forward
| transformers/docs/source/en/model_doc/llava.md/0 | {
"file_path": "transformers/docs/source/en/model_doc/llava.md",
"repo_id": "transformers",
"token_count": 1228
} | 249 |
<!--Copyright 2020 The HuggingFace Team. All rights reserved.
Licensed under the Apache License, Version 2.0 (the "License"); you may not use this file except in compliance with
the License. You may obtain a copy of the License at
http://www.apache.org/licenses/LICENSE-2.0
Unless required by applicable law or agreed to in writing, software distributed under the License is distributed on
an "AS IS" BASIS, WITHOUT WARRANTIES OR CONDITIONS OF ANY KIND, either express or implied. See the License for the
specific language governing permissions and limitations under the License.
⚠️ Note that this file is in Markdown but contain specific syntax for our doc-builder (similar to MDX) that may not be
rendered properly in your Markdown viewer.
-->
# mT5
<div class="flex flex-wrap space-x-1">
<a href="https://huggingface.co/models?filter=mt5">
<img alt="Models" src="https://img.shields.io/badge/All_model_pages-mt5-blueviolet">
</a>
<a href="https://huggingface.co/spaces/docs-demos/mt5-small-finetuned-arxiv-cs-finetuned-arxiv-cs-full">
<img alt="Spaces" src="https://img.shields.io/badge/%F0%9F%A4%97%20Hugging%20Face-Spaces-blue">
</a>
</div>
## Overview
The mT5 model was presented in [mT5: A massively multilingual pre-trained text-to-text transformer](https://arxiv.org/abs/2010.11934) by Linting Xue, Noah Constant, Adam Roberts, Mihir Kale, Rami Al-Rfou, Aditya
Siddhant, Aditya Barua, Colin Raffel.
The abstract from the paper is the following:
*The recent "Text-to-Text Transfer Transformer" (T5) leveraged a unified text-to-text format and scale to attain
state-of-the-art results on a wide variety of English-language NLP tasks. In this paper, we introduce mT5, a
multilingual variant of T5 that was pre-trained on a new Common Crawl-based dataset covering 101 languages. We detail
the design and modified training of mT5 and demonstrate its state-of-the-art performance on many multilingual
benchmarks. We also describe a simple technique to prevent "accidental translation" in the zero-shot setting, where a
generative model chooses to (partially) translate its prediction into the wrong language. All of the code and model
checkpoints used in this work are publicly available.*
Note: mT5 was only pre-trained on [mC4](https://huggingface.co/datasets/mc4) excluding any supervised training.
Therefore, this model has to be fine-tuned before it is usable on a downstream task, unlike the original T5 model.
Since mT5 was pre-trained unsupervisedly, there's no real advantage to using a task prefix during single-task
fine-tuning. If you are doing multi-task fine-tuning, you should use a prefix.
Google has released the following variants:
- [google/mt5-small](https://huggingface.co/google/mt5-small)
- [google/mt5-base](https://huggingface.co/google/mt5-base)
- [google/mt5-large](https://huggingface.co/google/mt5-large)
- [google/mt5-xl](https://huggingface.co/google/mt5-xl)
- [google/mt5-xxl](https://huggingface.co/google/mt5-xxl).
This model was contributed by [patrickvonplaten](https://huggingface.co/patrickvonplaten). The original code can be
found [here](https://github.com/google-research/multilingual-t5).
## Resources
- [Translation task guide](../tasks/translation)
- [Summarization task guide](../tasks/summarization)
## MT5Config
[[autodoc]] MT5Config
## MT5Tokenizer
[[autodoc]] MT5Tokenizer
See [`T5Tokenizer`] for all details.
## MT5TokenizerFast
[[autodoc]] MT5TokenizerFast
See [`T5TokenizerFast`] for all details.
<frameworkcontent>
<pt>
## MT5Model
[[autodoc]] MT5Model
## MT5ForConditionalGeneration
[[autodoc]] MT5ForConditionalGeneration
## MT5EncoderModel
[[autodoc]] MT5EncoderModel
## MT5ForSequenceClassification
[[autodoc]] MT5ForSequenceClassification
## MT5ForTokenClassification
[[autodoc]] MT5ForTokenClassification
## MT5ForQuestionAnswering
[[autodoc]] MT5ForQuestionAnswering
</pt>
<tf>
## TFMT5Model
[[autodoc]] TFMT5Model
## TFMT5ForConditionalGeneration
[[autodoc]] TFMT5ForConditionalGeneration
## TFMT5EncoderModel
[[autodoc]] TFMT5EncoderModel
</tf>
<jax>
## FlaxMT5Model
[[autodoc]] FlaxMT5Model
## FlaxMT5ForConditionalGeneration
[[autodoc]] FlaxMT5ForConditionalGeneration
## FlaxMT5EncoderModel
[[autodoc]] FlaxMT5EncoderModel
</jax>
</frameworkcontent>
| transformers/docs/source/en/model_doc/mt5.md/0 | {
"file_path": "transformers/docs/source/en/model_doc/mt5.md",
"repo_id": "transformers",
"token_count": 1400
} | 250 |
<!--Copyright 2024 The Qwen Team and The HuggingFace Team. All rights reserved.
Licensed under the Apache License, Version 2.0 (the "License"); you may not use this file except in compliance with
the License. You may obtain a copy of the License at
http://www.apache.org/licenses/LICENSE-2.0
Unless required by applicable law or agreed to in writing, software distributed under the License is distributed on
an "AS IS" BASIS, WITHOUT WARRANTIES OR CONDITIONS OF ANY KIND, either express or implied. See the License for the
specific language governing permissions and limitations under the License.
⚠️ Note that this file is in Markdown but contain specific syntax for our doc-builder (similar to MDX) that may not be
rendered properly in your Markdown viewer.
-->
# Qwen2
## Overview
Qwen2 is the new model series of large language models from the Qwen team. Previously, we released the Qwen series, including Qwen-72B, Qwen-1.8B, Qwen-VL, Qwen-Audio, etc.
### Model Details
Qwen2 is a language model series including decoder language models of different model sizes. For each size, we release the base language model and the aligned chat model. It is based on the Transformer architecture with SwiGLU activation, attention QKV bias, group query attention, mixture of sliding window attention and full attention, etc. Additionally, we have an improved tokenizer adaptive to multiple natural languages and codes.
## Usage tips
`Qwen2-7B-beta` and `Qwen2-7B-Chat-beta` can be found on the [Huggingface Hub](https://huggingface.co/Qwen)
In the following, we demonstrate how to use `Qwen2-7B-Chat-beta` for the inference. Note that we have used the ChatML format for dialog, in this demo we show how to leverage `apply_chat_template` for this purpose.
```python
>>> from transformers import AutoModelForCausalLM, AutoTokenizer
>>> device = "cuda" # the device to load the model onto
>>> model = AutoModelForCausalLM.from_pretrained("Qwen/Qwen1.5-7B-Chat", device_map="auto")
>>> tokenizer = AutoTokenizer.from_pretrained("Qwen/Qwen1.5-7B-Chat")
>>> prompt = "Give me a short introduction to large language model."
>>> messages = [{"role": "user", "content": prompt}]
>>> text = tokenizer.apply_chat_template(messages, tokenize=False, add_generation_prompt=True)
>>> model_inputs = tokenizer([text], return_tensors="pt").to(device)
>>> generated_ids = model.generate(model_inputs.input_ids, max_new_tokens=512, do_sample=True)
>>> generated_ids = [output_ids[len(input_ids):] for input_ids, output_ids in zip(model_inputs.input_ids, generated_ids)]
>>> response = tokenizer.batch_decode(generated_ids, skip_special_tokens=True)[0]
```
## Qwen2Config
[[autodoc]] Qwen2Config
## Qwen2Tokenizer
[[autodoc]] Qwen2Tokenizer
- save_vocabulary
## Qwen2TokenizerFast
[[autodoc]] Qwen2TokenizerFast
## Qwen2Model
[[autodoc]] Qwen2Model
- forward
## Qwen2ForCausalLM
[[autodoc]] Qwen2ForCausalLM
- forward
## Qwen2ForSequenceClassification
[[autodoc]] Qwen2ForSequenceClassification
- forward
| transformers/docs/source/en/model_doc/qwen2.md/0 | {
"file_path": "transformers/docs/source/en/model_doc/qwen2.md",
"repo_id": "transformers",
"token_count": 918
} | 251 |
<!--Copyright 2021 The HuggingFace Team. All rights reserved.
Licensed under the Apache License, Version 2.0 (the "License"); you may not use this file except in compliance with
the License. You may obtain a copy of the License at
http://www.apache.org/licenses/LICENSE-2.0
Unless required by applicable law or agreed to in writing, software distributed under the License is distributed on
an "AS IS" BASIS, WITHOUT WARRANTIES OR CONDITIONS OF ANY KIND, either express or implied. See the License for the
specific language governing permissions and limitations under the License.
⚠️ Note that this file is in Markdown but contain specific syntax for our doc-builder (similar to MDX) that may not be
rendered properly in your Markdown viewer.
-->
# SegFormer
## Overview
The SegFormer model was proposed in [SegFormer: Simple and Efficient Design for Semantic Segmentation with Transformers](https://arxiv.org/abs/2105.15203) by Enze Xie, Wenhai Wang, Zhiding Yu, Anima Anandkumar, Jose M. Alvarez, Ping
Luo. The model consists of a hierarchical Transformer encoder and a lightweight all-MLP decode head to achieve great
results on image segmentation benchmarks such as ADE20K and Cityscapes.
The abstract from the paper is the following:
*We present SegFormer, a simple, efficient yet powerful semantic segmentation framework which unifies Transformers with
lightweight multilayer perception (MLP) decoders. SegFormer has two appealing features: 1) SegFormer comprises a novel
hierarchically structured Transformer encoder which outputs multiscale features. It does not need positional encoding,
thereby avoiding the interpolation of positional codes which leads to decreased performance when the testing resolution
differs from training. 2) SegFormer avoids complex decoders. The proposed MLP decoder aggregates information from
different layers, and thus combining both local attention and global attention to render powerful representations. We
show that this simple and lightweight design is the key to efficient segmentation on Transformers. We scale our
approach up to obtain a series of models from SegFormer-B0 to SegFormer-B5, reaching significantly better performance
and efficiency than previous counterparts. For example, SegFormer-B4 achieves 50.3% mIoU on ADE20K with 64M parameters,
being 5x smaller and 2.2% better than the previous best method. Our best model, SegFormer-B5, achieves 84.0% mIoU on
Cityscapes validation set and shows excellent zero-shot robustness on Cityscapes-C.*
The figure below illustrates the architecture of SegFormer. Taken from the [original paper](https://arxiv.org/abs/2105.15203).
<img width="600" src="https://huggingface.co/datasets/huggingface/documentation-images/resolve/main/segformer_architecture.png"/>
This model was contributed by [nielsr](https://huggingface.co/nielsr). The TensorFlow version
of the model was contributed by [sayakpaul](https://huggingface.co/sayakpaul). The original code can be found [here](https://github.com/NVlabs/SegFormer).
## Usage tips
- SegFormer consists of a hierarchical Transformer encoder, and a lightweight all-MLP decoder head.
[`SegformerModel`] is the hierarchical Transformer encoder (which in the paper is also referred to
as Mix Transformer or MiT). [`SegformerForSemanticSegmentation`] adds the all-MLP decoder head on
top to perform semantic segmentation of images. In addition, there's
[`SegformerForImageClassification`] which can be used to - you guessed it - classify images. The
authors of SegFormer first pre-trained the Transformer encoder on ImageNet-1k to classify images. Next, they throw
away the classification head, and replace it by the all-MLP decode head. Next, they fine-tune the model altogether on
ADE20K, Cityscapes and COCO-stuff, which are important benchmarks for semantic segmentation. All checkpoints can be
found on the [hub](https://huggingface.co/models?other=segformer).
- The quickest way to get started with SegFormer is by checking the [example notebooks](https://github.com/NielsRogge/Transformers-Tutorials/tree/master/SegFormer) (which showcase both inference and
fine-tuning on custom data). One can also check out the [blog post](https://huggingface.co/blog/fine-tune-segformer) introducing SegFormer and illustrating how it can be fine-tuned on custom data.
- TensorFlow users should refer to [this repository](https://github.com/deep-diver/segformer-tf-transformers) that shows off-the-shelf inference and fine-tuning.
- One can also check out [this interactive demo on Hugging Face Spaces](https://huggingface.co/spaces/chansung/segformer-tf-transformers)
to try out a SegFormer model on custom images.
- SegFormer works on any input size, as it pads the input to be divisible by `config.patch_sizes`.
- One can use [`SegformerImageProcessor`] to prepare images and corresponding segmentation maps
for the model. Note that this image processor is fairly basic and does not include all data augmentations used in
the original paper. The original preprocessing pipelines (for the ADE20k dataset for instance) can be found [here](https://github.com/NVlabs/SegFormer/blob/master/local_configs/_base_/datasets/ade20k_repeat.py). The most
important preprocessing step is that images and segmentation maps are randomly cropped and padded to the same size,
such as 512x512 or 640x640, after which they are normalized.
- One additional thing to keep in mind is that one can initialize [`SegformerImageProcessor`] with
`reduce_labels` set to `True` or `False`. In some datasets (like ADE20k), the 0 index is used in the annotated
segmentation maps for background. However, ADE20k doesn't include the "background" class in its 150 labels.
Therefore, `reduce_labels` is used to reduce all labels by 1, and to make sure no loss is computed for the
background class (i.e. it replaces 0 in the annotated maps by 255, which is the *ignore_index* of the loss function
used by [`SegformerForSemanticSegmentation`]). However, other datasets use the 0 index as
background class and include this class as part of all labels. In that case, `reduce_labels` should be set to
`False`, as loss should also be computed for the background class.
- As most models, SegFormer comes in different sizes, the details of which can be found in the table below
(taken from Table 7 of the [original paper](https://arxiv.org/abs/2105.15203)).
| **Model variant** | **Depths** | **Hidden sizes** | **Decoder hidden size** | **Params (M)** | **ImageNet-1k Top 1** |
| :---------------: | ------------- | ------------------- | :---------------------: | :------------: | :-------------------: |
| MiT-b0 | [2, 2, 2, 2] | [32, 64, 160, 256] | 256 | 3.7 | 70.5 |
| MiT-b1 | [2, 2, 2, 2] | [64, 128, 320, 512] | 256 | 14.0 | 78.7 |
| MiT-b2 | [3, 4, 6, 3] | [64, 128, 320, 512] | 768 | 25.4 | 81.6 |
| MiT-b3 | [3, 4, 18, 3] | [64, 128, 320, 512] | 768 | 45.2 | 83.1 |
| MiT-b4 | [3, 8, 27, 3] | [64, 128, 320, 512] | 768 | 62.6 | 83.6 |
| MiT-b5 | [3, 6, 40, 3] | [64, 128, 320, 512] | 768 | 82.0 | 83.8 |
Note that MiT in the above table refers to the Mix Transformer encoder backbone introduced in SegFormer. For
SegFormer's results on the segmentation datasets like ADE20k, refer to the [paper](https://arxiv.org/abs/2105.15203).
## Resources
A list of official Hugging Face and community (indicated by 🌎) resources to help you get started with SegFormer.
<PipelineTag pipeline="image-classification"/>
- [`SegformerForImageClassification`] is supported by this [example script](https://github.com/huggingface/transformers/tree/main/examples/pytorch/image-classification) and [notebook](https://colab.research.google.com/github/huggingface/notebooks/blob/main/examples/image_classification.ipynb).
- [Image classification task guide](../tasks/image_classification)
Semantic segmentation:
- [`SegformerForSemanticSegmentation`] is supported by this [example script](https://github.com/huggingface/transformers/tree/main/examples/pytorch/semantic-segmentation).
- A blog on fine-tuning SegFormer on a custom dataset can be found [here](https://huggingface.co/blog/fine-tune-segformer).
- More demo notebooks on SegFormer (both inference + fine-tuning on a custom dataset) can be found [here](https://github.com/NielsRogge/Transformers-Tutorials/tree/master/SegFormer).
- [`TFSegformerForSemanticSegmentation`] is supported by this [example notebook](https://github.com/huggingface/notebooks/blob/main/examples/semantic_segmentation-tf.ipynb).
- [Semantic segmentation task guide](../tasks/semantic_segmentation)
If you're interested in submitting a resource to be included here, please feel free to open a Pull Request and we'll review it! The resource should ideally demonstrate something new instead of duplicating an existing resource.
## SegformerConfig
[[autodoc]] SegformerConfig
## SegformerFeatureExtractor
[[autodoc]] SegformerFeatureExtractor
- __call__
- post_process_semantic_segmentation
## SegformerImageProcessor
[[autodoc]] SegformerImageProcessor
- preprocess
- post_process_semantic_segmentation
<frameworkcontent>
<pt>
## SegformerModel
[[autodoc]] SegformerModel
- forward
## SegformerDecodeHead
[[autodoc]] SegformerDecodeHead
- forward
## SegformerForImageClassification
[[autodoc]] SegformerForImageClassification
- forward
## SegformerForSemanticSegmentation
[[autodoc]] SegformerForSemanticSegmentation
- forward
</pt>
<tf>
## TFSegformerDecodeHead
[[autodoc]] TFSegformerDecodeHead
- call
## TFSegformerModel
[[autodoc]] TFSegformerModel
- call
## TFSegformerForImageClassification
[[autodoc]] TFSegformerForImageClassification
- call
## TFSegformerForSemanticSegmentation
[[autodoc]] TFSegformerForSemanticSegmentation
- call
</tf>
</frameworkcontent> | transformers/docs/source/en/model_doc/segformer.md/0 | {
"file_path": "transformers/docs/source/en/model_doc/segformer.md",
"repo_id": "transformers",
"token_count": 3177
} | 252 |
<!--Copyright 2022 The HuggingFace Team. All rights reserved.
Licensed under the Apache License, Version 2.0 (the "License"); you may not use this file except in compliance with
the License. You may obtain a copy of the License at
http://www.apache.org/licenses/LICENSE-2.0
Unless required by applicable law or agreed to in writing, software distributed under the License is distributed on
an "AS IS" BASIS, WITHOUT WARRANTIES OR CONDITIONS OF ANY KIND, either express or implied. See the License for the
specific language governing permissions and limitations under the License.
⚠️ Note that this file is in Markdown but contain specific syntax for our doc-builder (similar to MDX) that may not be
rendered properly in your Markdown viewer.
-->
# Swin2SR
## Overview
The Swin2SR model was proposed in [Swin2SR: SwinV2 Transformer for Compressed Image Super-Resolution and Restoration](https://arxiv.org/abs/2209.11345) by Marcos V. Conde, Ui-Jin Choi, Maxime Burchi, Radu Timofte.
Swin2R improves the [SwinIR](https://github.com/JingyunLiang/SwinIR/) model by incorporating [Swin Transformer v2](swinv2) layers which mitigates issues such as training instability, resolution gaps between pre-training
and fine-tuning, and hunger on data.
The abstract from the paper is the following:
*Compression plays an important role on the efficient transmission and storage of images and videos through band-limited systems such as streaming services, virtual reality or videogames. However, compression unavoidably leads to artifacts and the loss of the original information, which may severely degrade the visual quality. For these reasons, quality enhancement of compressed images has become a popular research topic. While most state-of-the-art image restoration methods are based on convolutional neural networks, other transformers-based methods such as SwinIR, show impressive performance on these tasks.
In this paper, we explore the novel Swin Transformer V2, to improve SwinIR for image super-resolution, and in particular, the compressed input scenario. Using this method we can tackle the major issues in training transformer vision models, such as training instability, resolution gaps between pre-training and fine-tuning, and hunger on data. We conduct experiments on three representative tasks: JPEG compression artifacts removal, image super-resolution (classical and lightweight), and compressed image super-resolution. Experimental results demonstrate that our method, Swin2SR, can improve the training convergence and performance of SwinIR, and is a top-5 solution at the "AIM 2022 Challenge on Super-Resolution of Compressed Image and Video".*
<img src="https://huggingface.co/datasets/huggingface/documentation-images/resolve/main/transformers/model_doc/swin2sr_architecture.png"
alt="drawing" width="600"/>
<small> Swin2SR architecture. Taken from the <a href="https://arxiv.org/abs/2209.11345">original paper.</a> </small>
This model was contributed by [nielsr](https://huggingface.co/nielsr).
The original code can be found [here](https://github.com/mv-lab/swin2sr).
## Resources
Demo notebooks for Swin2SR can be found [here](https://github.com/NielsRogge/Transformers-Tutorials/tree/master/Swin2SR).
A demo Space for image super-resolution with SwinSR can be found [here](https://huggingface.co/spaces/jjourney1125/swin2sr).
## Swin2SRImageProcessor
[[autodoc]] Swin2SRImageProcessor
- preprocess
## Swin2SRConfig
[[autodoc]] Swin2SRConfig
## Swin2SRModel
[[autodoc]] Swin2SRModel
- forward
## Swin2SRForImageSuperResolution
[[autodoc]] Swin2SRForImageSuperResolution
- forward
| transformers/docs/source/en/model_doc/swin2sr.md/0 | {
"file_path": "transformers/docs/source/en/model_doc/swin2sr.md",
"repo_id": "transformers",
"token_count": 979
} | 253 |
<!--Copyright 2022 The HuggingFace Team. All rights reserved.
Licensed under the Apache License, Version 2.0 (the "License"); you may not use this file except in compliance with
the License. You may obtain a copy of the License at
http://www.apache.org/licenses/LICENSE-2.0
Unless required by applicable law or agreed to in writing, software distributed under the License is distributed on
an "AS IS" BASIS, WITHOUT WARRANTIES OR CONDITIONS OF ANY KIND, either express or implied. See the License for the
specific language governing permissions and limitations under the License.
⚠️ Note that this file is in Markdown but contain specific syntax for our doc-builder (similar to MDX) that may not be
rendered properly in your Markdown viewer.
-->
# ViTMSN
## Overview
The ViTMSN model was proposed in [Masked Siamese Networks for Label-Efficient Learning](https://arxiv.org/abs/2204.07141) by Mahmoud Assran, Mathilde Caron, Ishan Misra, Piotr Bojanowski, Florian Bordes,
Pascal Vincent, Armand Joulin, Michael Rabbat, Nicolas Ballas. The paper presents a joint-embedding architecture to match the prototypes
of masked patches with that of the unmasked patches. With this setup, their method yields excellent performance in the low-shot and extreme low-shot
regimes.
The abstract from the paper is the following:
*We propose Masked Siamese Networks (MSN), a self-supervised learning framework for learning image representations. Our
approach matches the representation of an image view containing randomly masked patches to the representation of the original
unmasked image. This self-supervised pre-training strategy is particularly scalable when applied to Vision Transformers since only the
unmasked patches are processed by the network. As a result, MSNs improve the scalability of joint-embedding architectures,
while producing representations of a high semantic level that perform competitively on low-shot image classification. For instance,
on ImageNet-1K, with only 5,000 annotated images, our base MSN model achieves 72.4% top-1 accuracy,
and with 1% of ImageNet-1K labels, we achieve 75.7% top-1 accuracy, setting a new state-of-the-art for self-supervised learning on this benchmark.*
<img src="https://i.ibb.co/W6PQMdC/Screenshot-2022-09-13-at-9-08-40-AM.png" alt="drawing" width="600"/>
<small> MSN architecture. Taken from the <a href="https://arxiv.org/abs/2204.07141">original paper.</a> </small>
This model was contributed by [sayakpaul](https://huggingface.co/sayakpaul). The original code can be found [here](https://github.com/facebookresearch/msn).
## Usage tips
- MSN (masked siamese networks) is a method for self-supervised pre-training of Vision Transformers (ViTs). The pre-training
objective is to match the prototypes assigned to the unmasked views of the images to that of the masked views of the same images.
- The authors have only released pre-trained weights of the backbone (ImageNet-1k pre-training). So, to use that on your own image classification dataset,
use the [`ViTMSNForImageClassification`] class which is initialized from [`ViTMSNModel`]. Follow
[this notebook](https://github.com/huggingface/notebooks/blob/main/examples/image_classification.ipynb) for a detailed tutorial on fine-tuning.
- MSN is particularly useful in the low-shot and extreme low-shot regimes. Notably, it achieves 75.7% top-1 accuracy with only 1% of ImageNet-1K
labels when fine-tuned.
## Resources
A list of official Hugging Face and community (indicated by 🌎) resources to help you get started with ViT MSN.
<PipelineTag pipeline="image-classification"/>
- [`ViTMSNForImageClassification`] is supported by this [example script](https://github.com/huggingface/transformers/tree/main/examples/pytorch/image-classification) and [notebook](https://colab.research.google.com/github/huggingface/notebooks/blob/main/examples/image_classification.ipynb).
- See also: [Image classification task guide](../tasks/image_classification)
If you're interested in submitting a resource to be included here, please feel free to open a Pull Request and we'll review it! The resource should ideally demonstrate something new instead of duplicating an existing resource.
## ViTMSNConfig
[[autodoc]] ViTMSNConfig
## ViTMSNModel
[[autodoc]] ViTMSNModel
- forward
## ViTMSNForImageClassification
[[autodoc]] ViTMSNForImageClassification
- forward
| transformers/docs/source/en/model_doc/vit_msn.md/0 | {
"file_path": "transformers/docs/source/en/model_doc/vit_msn.md",
"repo_id": "transformers",
"token_count": 1191
} | 254 |
<!--Copyright 2023 The HuggingFace Team. All rights reserved.
Licensed under the Apache License, Version 2.0 (the "License"); you may not use this file except in compliance with
the License. You may obtain a copy of the License at
http://www.apache.org/licenses/LICENSE-2.0
Unless required by applicable law or agreed to in writing, software distributed under the License is distributed on
an "AS IS" BASIS, WITHOUT WARRANTIES OR CONDITIONS OF ANY KIND, either express or implied. See the License for the
specific language governing permissions and limitations under the License.
⚠️ Note that this file is in Markdown but contain specific syntax for our doc-builder (similar to MDX) that may not be
rendered properly in your Markdown viewer.
-->
# XLM-V
## Overview
XLM-V is multilingual language model with a one million token vocabulary trained on 2.5TB of data from Common Crawl (same as XLM-R).
It was introduced in the [XLM-V: Overcoming the Vocabulary Bottleneck in Multilingual Masked Language Models](https://arxiv.org/abs/2301.10472)
paper by Davis Liang, Hila Gonen, Yuning Mao, Rui Hou, Naman Goyal, Marjan Ghazvininejad, Luke Zettlemoyer and Madian Khabsa.
From the abstract of the XLM-V paper:
*Large multilingual language models typically rely on a single vocabulary shared across 100+ languages.
As these models have increased in parameter count and depth, vocabulary size has remained largely unchanged.
This vocabulary bottleneck limits the representational capabilities of multilingual models like XLM-R.
In this paper, we introduce a new approach for scaling to very large multilingual vocabularies by
de-emphasizing token sharing between languages with little lexical overlap and assigning vocabulary capacity
to achieve sufficient coverage for each individual language. Tokenizations using our vocabulary are typically
more semantically meaningful and shorter compared to XLM-R. Leveraging this improved vocabulary, we train XLM-V,
a multilingual language model with a one million token vocabulary. XLM-V outperforms XLM-R on every task we
tested on ranging from natural language inference (XNLI), question answering (MLQA, XQuAD, TyDiQA), and
named entity recognition (WikiAnn) to low-resource tasks (Americas NLI, MasakhaNER).*
This model was contributed by [stefan-it](https://huggingface.co/stefan-it), including detailed experiments with XLM-V on downstream tasks.
The experiments repository can be found [here](https://github.com/stefan-it/xlm-v-experiments).
## Usage tips
- XLM-V is compatible with the XLM-RoBERTa model architecture, only model weights from [`fairseq`](https://github.com/facebookresearch/fairseq)
library had to be converted.
- The `XLMTokenizer` implementation is used to load the vocab and performs tokenization.
A XLM-V (base size) model is available under the [`facebook/xlm-v-base`](https://huggingface.co/facebook/xlm-v-base) identifier.
<Tip>
XLM-V architecture is the same as XLM-RoBERTa, refer to [XLM-RoBERTa documentation](xlm-roberta) for API reference, and examples.
</Tip> | transformers/docs/source/en/model_doc/xlm-v.md/0 | {
"file_path": "transformers/docs/source/en/model_doc/xlm-v.md",
"repo_id": "transformers",
"token_count": 809
} | 255 |
<!--Copyright 2022 The HuggingFace Team. All rights reserved.
Licensed under the Apache License, Version 2.0 (the "License"); you may not use this file except in compliance with
the License. You may obtain a copy of the License at
http://www.apache.org/licenses/LICENSE-2.0
Unless required by applicable law or agreed to in writing, software distributed under the License is distributed on
an "AS IS" BASIS, WITHOUT WARRANTIES OR CONDITIONS OF ANY KIND, either express or implied. See the License for the
⚠️ Note that this file is in Markdown but contain specific syntax for our doc-builder (similar to MDX) that may not be
rendered properly in your Markdown viewer.
-->
# CPU inference
With some optimizations, it is possible to efficiently run large model inference on a CPU. One of these optimization techniques involves compiling the PyTorch code into an intermediate format for high-performance environments like C++. The other technique fuses multiple operations into one kernel to reduce the overhead of running each operation separately.
You'll learn how to use [BetterTransformer](https://pytorch.org/blog/a-better-transformer-for-fast-transformer-encoder-inference/) for faster inference, and how to convert your PyTorch code to [TorchScript](https://pytorch.org/tutorials/beginner/Intro_to_TorchScript_tutorial.html). If you're using an Intel CPU, you can also use [graph optimizations](https://intel.github.io/intel-extension-for-pytorch/cpu/latest/tutorials/features.html#graph-optimization) from [Intel Extension for PyTorch](https://intel.github.io/intel-extension-for-pytorch/cpu/latest/index.html) to boost inference speed even more. Finally, learn how to use 🤗 Optimum to accelerate inference with ONNX Runtime or OpenVINO (if you're using an Intel CPU).
## BetterTransformer
BetterTransformer accelerates inference with its fastpath (native PyTorch specialized implementation of Transformer functions) execution. The two optimizations in the fastpath execution are:
1. fusion, which combines multiple sequential operations into a single "kernel" to reduce the number of computation steps
2. skipping the inherent sparsity of padding tokens to avoid unnecessary computation with nested tensors
BetterTransformer also converts all attention operations to use the more memory-efficient [scaled dot product attention](https://pytorch.org/docs/master/generated/torch.nn.functional.scaled_dot_product_attention).
<Tip>
BetterTransformer is not supported for all models. Check this [list](https://huggingface.co/docs/optimum/bettertransformer/overview#supported-models) to see if a model supports BetterTransformer.
</Tip>
Before you start, make sure you have 🤗 Optimum [installed](https://huggingface.co/docs/optimum/installation).
Enable BetterTransformer with the [`PreTrainedModel.to_bettertransformer`] method:
```py
from transformers import AutoModelForCausalLM
model = AutoModelForCausalLM.from_pretrained("bigcode/starcoder")
model.to_bettertransformer()
```
## TorchScript
TorchScript is an intermediate PyTorch model representation that can be run in production environments where performance is important. You can train a model in PyTorch and then export it to TorchScript to free the model from Python performance constraints. PyTorch [traces](https://pytorch.org/docs/stable/generated/torch.jit.trace.html) a model to return a [`ScriptFunction`] that is optimized with just-in-time compilation (JIT). Compared to the default eager mode, JIT mode in PyTorch typically yields better performance for inference using optimization techniques like operator fusion.
For a gentle introduction to TorchScript, see the [Introduction to PyTorch TorchScript](https://pytorch.org/tutorials/beginner/Intro_to_TorchScript_tutorial.html) tutorial.
With the [`Trainer`] class, you can enable JIT mode for CPU inference by setting the `--jit_mode_eval` flag:
```bash
python run_qa.py \
--model_name_or_path csarron/bert-base-uncased-squad-v1 \
--dataset_name squad \
--do_eval \
--max_seq_length 384 \
--doc_stride 128 \
--output_dir /tmp/ \
--no_cuda \
--jit_mode_eval
```
<Tip warning={true}>
For PyTorch >= 1.14.0, JIT-mode could benefit any model for prediction and evaluation since the dict input is supported in `jit.trace`.
For PyTorch < 1.14.0, JIT-mode could benefit a model if its forward parameter order matches the tuple input order in `jit.trace`, such as a question-answering model. If the forward parameter order does not match the tuple input order in `jit.trace`, like a text classification model, `jit.trace` will fail and we are capturing this with the exception here to make it fallback. Logging is used to notify users.
</Tip>
## IPEX graph optimization
Intel® Extension for PyTorch (IPEX) provides further optimizations in JIT mode for Intel CPUs, and we recommend combining it with TorchScript for even faster performance. The IPEX [graph optimization](https://intel.github.io/intel-extension-for-pytorch/cpu/latest/tutorials/features/graph_optimization.html) fuses operations like Multi-head attention, Concat Linear, Linear + Add, Linear + Gelu, Add + LayerNorm, and more.
To take advantage of these graph optimizations, make sure you have IPEX [installed](https://intel.github.io/intel-extension-for-pytorch/cpu/latest/tutorials/installation.html):
```bash
pip install intel_extension_for_pytorch
```
Set the `--use_ipex` and `--jit_mode_eval` flags in the [`Trainer`] class to enable JIT mode with the graph optimizations:
```bash
python run_qa.py \
--model_name_or_path csarron/bert-base-uncased-squad-v1 \
--dataset_name squad \
--do_eval \
--max_seq_length 384 \
--doc_stride 128 \
--output_dir /tmp/ \
--no_cuda \
--use_ipex \
--jit_mode_eval
```
## 🤗 Optimum
<Tip>
Learn more details about using ORT with 🤗 Optimum in the [Optimum Inference with ONNX Runtime](https://huggingface.co/docs/optimum/onnxruntime/usage_guides/models) guide. This section only provides a brief and simple example.
</Tip>
ONNX Runtime (ORT) is a model accelerator that runs inference on CPUs by default. ORT is supported by 🤗 Optimum which can be used in 🤗 Transformers, without making too many changes to your code. You only need to replace the 🤗 Transformers `AutoClass` with its equivalent [`~optimum.onnxruntime.ORTModel`] for the task you're solving, and load a checkpoint in the ONNX format.
For example, if you're running inference on a question answering task, load the [optimum/roberta-base-squad2](https://huggingface.co/optimum/roberta-base-squad2) checkpoint which contains a `model.onnx` file:
```py
from transformers import AutoTokenizer, pipeline
from optimum.onnxruntime import ORTModelForQuestionAnswering
model = ORTModelForQuestionAnswering.from_pretrained("optimum/roberta-base-squad2")
tokenizer = AutoTokenizer.from_pretrained("deepset/roberta-base-squad2")
onnx_qa = pipeline("question-answering", model=model, tokenizer=tokenizer)
question = "What's my name?"
context = "My name is Philipp and I live in Nuremberg."
pred = onnx_qa(question, context)
```
If you have an Intel CPU, take a look at 🤗 [Optimum Intel](https://huggingface.co/docs/optimum/intel/index) which supports a variety of compression techniques (quantization, pruning, knowledge distillation) and tools for converting models to the [OpenVINO](https://huggingface.co/docs/optimum/intel/inference) format for higher performance inference.
| transformers/docs/source/en/perf_infer_cpu.md/0 | {
"file_path": "transformers/docs/source/en/perf_infer_cpu.md",
"repo_id": "transformers",
"token_count": 2080
} | 256 |
<!--Copyright 2023 The HuggingFace Team. All rights reserved.
Licensed under the Apache License, Version 2.0 (the "License"); you may not use this file except in compliance with
the License. You may obtain a copy of the License at
http://www.apache.org/licenses/LICENSE-2.0
Unless required by applicable law or agreed to in writing, software distributed under the License is distributed on
an "AS IS" BASIS, WITHOUT WARRANTIES OR CONDITIONS OF ANY KIND, either express or implied. See the License for the
specific language governing permissions and limitations under the License.
⚠️ Note that this file is in Markdown but contain specific syntax for our doc-builder (similar to MDX) that may not be
rendered properly in your Markdown viewer.
-->
# Quantization
Quantization techniques focus on representing data with less information while also trying to not lose too much accuracy. This often means converting a data type to represent the same information with fewer bits. For example, if your model weights are stored as 32-bit floating points and they're quantized to 16-bit floating points, this halves the model size which makes it easier to store and reduces memory-usage. Lower precision can also speedup inference because it takes less time to perform calculations with fewer bits.
Transformers supports several quantization schemes to help you run inference with large language models (LLMs) and finetune adapters on quantized models. This guide will show you how to use Activation-aware Weight Quantization (AWQ), AutoGPTQ, and bitsandbytes.
<Tip>
Interested in adding a new quantization method to Transformers? Read the [HfQuantizer](./hf_quantizer) guide to learn how!
</Tip>
## Quanto
<Tip>
Try Quanto + transformers with this [notebook](https://colab.research.google.com/drive/16CXfVmtdQvciSh9BopZUDYcmXCDpvgrT?usp=sharing)!
</Tip>
[🤗 Quanto](https://github.com/huggingface/quanto) library is a versatile pytorch quantization toolkit. The quantization method used is the linear quantization. Quanto provides several unique features such as:
- weights quantization (`float8`,`int8`,`int4`,`int2`)
- activation quantization (`float8`,`int8`)
- modality agnostic (e.g CV,LLM)
- device agnostic (e.g CUDA,MPS,CPU)
- compatibility with `torch.compile`
- easy to add custom kernel for specific device
- supports quantization aware training
<!-- Add link to the blogpost -->
Before you begin, make sure the following libraries are installed:
```bash
pip install quanto
pip install git+https://github.com/huggingface/accelerate.git
pip install git+https://github.com/huggingface/transformers.git
```
Now you can quantize a model by passing [`QuantoConfig`] object in the [`~PreTrainedModel.from_pretrained`] method. This works for any model in any modality, as long as it contains `torch.nn.Linear` layers.
The integration with transformers only supports weights quantization. For the more complex use case such as activation quantization, calibration and quantization aware training, you should use [quanto](https://github.com/huggingface/quanto) library instead.
```py
from transformers import AutoModelForCausalLM, AutoTokenizer, QuantoConfig
model_id = "facebook/opt-125m"
tokenizer = AutoTokenizer.from_pretrained(model_id)
quantization_config = QuantoConfig(weights="int8")
quantized_model = AutoModelForCausalLM.from_pretrained(model_id, device_map="cuda:0", quantization_config=quantization_config)
```
Note that serialization is not supported yet with transformers but it is coming soon! If you want to save the model, you can use quanto library instead.
Quanto library uses linear quantization algorithm for quantization. Even though this is a basic quantization technique, we get very good results! Have a look at the following becnhmark (llama-2-7b on perplexity metric). You can find more benchamarks [here](https://github.com/huggingface/quanto/tree/main/bench/generation)
<div class="flex gap-4">
<div>
<img class="rounded-xl" src="https://huggingface.co/datasets/huggingface/documentation-images/resolve/main/quantization/NousResearch-Llama-2-7b-hf_Perplexity.png" alt="llama-2-7b-quanto-perplexity" />
</div>
</div>
The library is versatible enough to be compatible with most PTQ optimization algorithms. The plan in the future is to integrate the most popular algorithms in the most seamless possible way (AWQ, Smoothquant).
## AQLM
Try AQLM on [Google Colab](https://colab.research.google.com/drive/1-xZmBRXT5Fm3Ghn4Mwa2KRypORXb855X?usp=sharing)!
Additive Quantization of Language Models ([AQLM](https://arxiv.org/abs/2401.06118)) is a Large Language Models compression method. It quantizes multiple weights together and take advantage of interdependencies between them. AQLM represents groups of 8-16 weights as a sum of multiple vector codes.
Inference support for AQLM is realised in the `aqlm` library. Make sure to install it to run the models (note aqlm works only with python>=3.10):
```bash
pip install aqlm[gpu,cpu]
```
The library provides efficient kernels for both GPU and CPU inference and training.
The instructions on how to quantize models yourself, as well as all the relevant code can be found in the corresponding GitHub [repository](https://github.com/Vahe1994/AQLM).
### PEFT
Starting with version `aqlm 1.0.2`, AQLM supports Parameter-Efficient Fine-Tuning in a form of [LoRA](https://huggingface.co/docs/peft/package_reference/lora) integrated into the [PEFT](https://huggingface.co/blog/peft) library.
### AQLM configurations
AQLM quantization setups vary mainly on the number of codebooks used as well as codebook sizes in bits. The most popular setups, as well as inference kernels they support are:
| Kernel | Number of codebooks | Codebook size, bits | Notation | Accuracy | Speedup | Fast GPU inference | Fast CPU inference |
|---|---------------------|---------------------|----------|-------------|-------------|--------------------|--------------------|
| Triton | K | N | KxN | - | Up to ~0.7x | ✅ | ❌ |
| CUDA | 1 | 16 | 1x16 | Best | Up to ~1.3x | ✅ | ❌ |
| CUDA | 2 | 8 | 2x8 | OK | Up to ~3.0x | ✅ | ❌ |
| Numba | K | 8 | Kx8 | Good | Up to ~4.0x | ❌ | ✅ |
## AWQ
<Tip>
Try AWQ quantization with this [notebook](https://colab.research.google.com/drive/1HzZH89yAXJaZgwJDhQj9LqSBux932BvY)!
</Tip>
[Activation-aware Weight Quantization (AWQ)](https://hf.co/papers/2306.00978) doesn't quantize all the weights in a model, and instead, it preserves a small percentage of weights that are important for LLM performance. This significantly reduces quantization loss such that you can run models in 4-bit precision without experiencing any performance degradation.
There are several libraries for quantizing models with the AWQ algorithm, such as [llm-awq](https://github.com/mit-han-lab/llm-awq), [autoawq](https://github.com/casper-hansen/AutoAWQ) or [optimum-intel](https://huggingface.co/docs/optimum/main/en/intel/optimization_inc). Transformers supports loading models quantized with the llm-awq and autoawq libraries. This guide will show you how to load models quantized with autoawq, but the process is similar for llm-awq quantized models.
Make sure you have autoawq installed:
```bash
pip install autoawq
```
AWQ-quantized models can be identified by checking the `quantization_config` attribute in the model's [config.json](https://huggingface.co/TheBloke/zephyr-7B-alpha-AWQ/blob/main/config.json) file:
```json
{
"_name_or_path": "/workspace/process/huggingfaceh4_zephyr-7b-alpha/source",
"architectures": [
"MistralForCausalLM"
],
...
...
...
"quantization_config": {
"quant_method": "awq",
"zero_point": true,
"group_size": 128,
"bits": 4,
"version": "gemm"
}
}
```
A quantized model is loaded with the [`~PreTrainedModel.from_pretrained`] method. If you loaded your model on the CPU, make sure to move it to a GPU device first. Use the `device_map` parameter to specify where to place the model:
```py
from transformers import AutoModelForCausalLM, AutoTokenizer
model_id = "TheBloke/zephyr-7B-alpha-AWQ"
model = AutoModelForCausalLM.from_pretrained(model_id, device_map="cuda:0")
```
Loading an AWQ-quantized model automatically sets other weights to fp16 by default for performance reasons. If you want to load these other weights in a different format, use the `torch_dtype` parameter:
```py
from transformers import AutoModelForCausalLM, AutoTokenizer
model_id = "TheBloke/zephyr-7B-alpha-AWQ"
model = AutoModelForCausalLM.from_pretrained(model_id, torch_dtype=torch.float32)
```
AWQ quantization can also be combined with [FlashAttention-2](perf_infer_gpu_one#flashattention-2) to further accelerate inference:
```py
from transformers import AutoModelForCausalLM, AutoTokenizer
model = AutoModelForCausalLM.from_pretrained("TheBloke/zephyr-7B-alpha-AWQ", attn_implementation="flash_attention_2", device_map="cuda:0")
```
### Fused modules
Fused modules offers improved accuracy and performance and it is supported out-of-the-box for AWQ modules for [Llama](https://huggingface.co/meta-llama) and [Mistral](https://huggingface.co/mistralai/Mistral-7B-v0.1) architectures, but you can also fuse AWQ modules for unsupported architectures.
<Tip warning={true}>
Fused modules cannot be combined with other optimization techniques such as FlashAttention-2.
</Tip>
<hfoptions id="fuse">
<hfoption id="supported architectures">
To enable fused modules for supported architectures, create an [`AwqConfig`] and set the parameters `fuse_max_seq_len` and `do_fuse=True`. The `fuse_max_seq_len` parameter is the total sequence length and it should include the context length and the expected generation length. You can set it to a larger value to be safe.
For example, to fuse the AWQ modules of the [TheBloke/Mistral-7B-OpenOrca-AWQ](https://huggingface.co/TheBloke/Mistral-7B-OpenOrca-AWQ) model.
```python
import torch
from transformers import AwqConfig, AutoModelForCausalLM
model_id = "TheBloke/Mistral-7B-OpenOrca-AWQ"
quantization_config = AwqConfig(
bits=4,
fuse_max_seq_len=512,
do_fuse=True,
)
model = AutoModelForCausalLM.from_pretrained(model_id, quantization_config=quantization_config).to(0)
```
</hfoption>
<hfoption id="unsupported architectures">
For architectures that don't support fused modules yet, you need to create a custom fusing mapping to define which modules need to be fused with the `modules_to_fuse` parameter. For example, to fuse the AWQ modules of the [TheBloke/Yi-34B-AWQ](https://huggingface.co/TheBloke/Yi-34B-AWQ) model.
```python
import torch
from transformers import AwqConfig, AutoModelForCausalLM
model_id = "TheBloke/Yi-34B-AWQ"
quantization_config = AwqConfig(
bits=4,
fuse_max_seq_len=512,
modules_to_fuse={
"attention": ["q_proj", "k_proj", "v_proj", "o_proj"],
"layernorm": ["ln1", "ln2", "norm"],
"mlp": ["gate_proj", "up_proj", "down_proj"],
"use_alibi": False,
"num_attention_heads": 56,
"num_key_value_heads": 8,
"hidden_size": 7168
}
)
model = AutoModelForCausalLM.from_pretrained(model_id, quantization_config=quantization_config).to(0)
```
The parameter `modules_to_fuse` should include:
- `"attention"`: The names of the attention layers to fuse in the following order: query, key, value and output projection layer. If you don't want to fuse these layers, pass an empty list.
- `"layernorm"`: The names of all the LayerNorm layers you want to replace with a custom fused LayerNorm. If you don't want to fuse these layers, pass an empty list.
- `"mlp"`: The names of the MLP layers you want to fuse into a single MLP layer in the order: (gate (dense, layer, post-attention) / up / down layers).
- `"use_alibi"`: If your model uses ALiBi positional embedding.
- `"num_attention_heads"`: The number of attention heads.
- `"num_key_value_heads"`: The number of key value heads that should be used to implement Grouped Query Attention (GQA). If `num_key_value_heads=num_attention_heads`, the model will use Multi Head Attention (MHA), if `num_key_value_heads=1` the model will use Multi Query Attention (MQA), otherwise GQA is used.
- `"hidden_size"`: The dimension of the hidden representations.
</hfoption>
</hfoptions>
### Exllama-v2 support
Recent versions of `autoawq` supports exllama-v2 kernels for faster prefill and decoding. To get started, first install the latest version of `autoawq` by running:
```bash
pip install git+https://github.com/casper-hansen/AutoAWQ.git
```
Get started by passing an `AwqConfig()` with `version="exllama"`.
```python
import torch
from transformers import AutoModelForCausalLM, AutoTokenizer, AwqConfig
quantization_config = AwqConfig(version="exllama")
model = AutoModelForCausalLM.from_pretrained(
"TheBloke/Mistral-7B-Instruct-v0.1-AWQ",
quantization_config=quantization_config,
device_map="auto",
)
input_ids = torch.randint(0, 100, (1, 128), dtype=torch.long, device="cuda")
output = model(input_ids)
print(output.logits)
tokenizer = AutoTokenizer.from_pretrained("TheBloke/Mistral-7B-Instruct-v0.1-AWQ")
input_ids = tokenizer.encode("How to make a cake", return_tensors="pt").to(model.device)
output = model.generate(input_ids, do_sample=True, max_length=50, pad_token_id=50256)
print(tokenizer.decode(output[0], skip_special_tokens=True))
```
<Tip warning={true}>
Note this feature is supported on AMD GPUs.
</Tip>
## AutoGPTQ
<Tip>
Try GPTQ quantization with PEFT in this [notebook](https://colab.research.google.com/drive/1_TIrmuKOFhuRRiTWN94iLKUFu6ZX4ceb?usp=sharing) and learn more about it's details in this [blog post](https://huggingface.co/blog/gptq-integration)!
</Tip>
The [AutoGPTQ](https://github.com/PanQiWei/AutoGPTQ) library implements the GPTQ algorithm, a post-training quantization technique where each row of the weight matrix is quantized independently to find a version of the weights that minimizes the error. These weights are quantized to int4, but they're restored to fp16 on the fly during inference. This can save your memory-usage by 4x because the int4 weights are dequantized in a fused kernel rather than a GPU's global memory, and you can also expect a speedup in inference because using a lower bitwidth takes less time to communicate.
Before you begin, make sure the following libraries are installed:
```bash
pip install auto-gptq
pip install git+https://github.com/huggingface/optimum.git
pip install git+https://github.com/huggingface/transformers.git
pip install --upgrade accelerate
```
To quantize a model (currently only supported for text models), you need to create a [`GPTQConfig`] class and set the number of bits to quantize to, a dataset to calibrate the weights for quantization, and a tokenizer to prepare the dataset.
```py
from transformers import AutoModelForCausalLM, AutoTokenizer, GPTQConfig
model_id = "facebook/opt-125m"
tokenizer = AutoTokenizer.from_pretrained(model_id)
gptq_config = GPTQConfig(bits=4, dataset="c4", tokenizer=tokenizer)
```
You could also pass your own dataset as a list of strings, but it is highly recommended to use the same dataset from the GPTQ paper.
```py
dataset = ["auto-gptq is an easy-to-use model quantization library with user-friendly apis, based on GPTQ algorithm."]
gptq_config = GPTQConfig(bits=4, dataset=dataset, tokenizer=tokenizer)
```
Load a model to quantize and pass the `gptq_config` to the [`~AutoModelForCausalLM.from_pretrained`] method. Set `device_map="auto"` to automatically offload the model to a CPU to help fit the model in memory, and allow the model modules to be moved between the CPU and GPU for quantization.
```py
quantized_model = AutoModelForCausalLM.from_pretrained(model_id, device_map="auto", quantization_config=gptq_config)
```
If you're running out of memory because a dataset is too large, disk offloading is not supported. If this is the case, try passing the `max_memory` parameter to allocate the amount of memory to use on your device (GPU and CPU):
```py
quantized_model = AutoModelForCausalLM.from_pretrained(model_id, device_map="auto", max_memory={0: "30GiB", 1: "46GiB", "cpu": "30GiB"}, quantization_config=gptq_config)
```
<Tip warning={true}>
Depending on your hardware, it can take some time to quantize a model from scratch. It can take ~5 minutes to quantize the [facebook/opt-350m](https://huggingface.co/facebook/opt-350m) model on a free-tier Google Colab GPU, but it'll take ~4 hours to quantize a 175B parameter model on a NVIDIA A100. Before you quantize a model, it is a good idea to check the Hub if a GPTQ-quantized version of the model already exists.
</Tip>
Once your model is quantized, you can push the model and tokenizer to the Hub where it can be easily shared and accessed. Use the [`~PreTrainedModel.push_to_hub`] method to save the [`GPTQConfig`]:
```py
quantized_model.push_to_hub("opt-125m-gptq")
tokenizer.push_to_hub("opt-125m-gptq")
```
You could also save your quantized model locally with the [`~PreTrainedModel.save_pretrained`] method. If the model was quantized with the `device_map` parameter, make sure to move the entire model to a GPU or CPU before saving it. For example, to save the model on a CPU:
```py
quantized_model.save_pretrained("opt-125m-gptq")
tokenizer.save_pretrained("opt-125m-gptq")
# if quantized with device_map set
quantized_model.to("cpu")
quantized_model.save_pretrained("opt-125m-gptq")
```
Reload a quantized model with the [`~PreTrainedModel.from_pretrained`] method, and set `device_map="auto"` to automatically distribute the model on all available GPUs to load the model faster without using more memory than needed.
```py
from transformers import AutoModelForCausalLM
model = AutoModelForCausalLM.from_pretrained("{your_username}/opt-125m-gptq", device_map="auto")
```
### ExLlama
[ExLlama](https://github.com/turboderp/exllama) is a Python/C++/CUDA implementation of the [Llama](model_doc/llama) model that is designed for faster inference with 4-bit GPTQ weights (check out these [benchmarks](https://github.com/huggingface/optimum/tree/main/tests/benchmark#gptq-benchmark)). The ExLlama kernel is activated by default when you create a [`GPTQConfig`] object. To boost inference speed even further, use the [ExLlamaV2](https://github.com/turboderp/exllamav2) kernels by configuring the `exllama_config` parameter:
```py
import torch
from transformers import AutoModelForCausalLM, GPTQConfig
gptq_config = GPTQConfig(bits=4, exllama_config={"version":2})
model = AutoModelForCausalLM.from_pretrained("{your_username}/opt-125m-gptq", device_map="auto", quantization_config=gptq_config)
```
<Tip warning={true}>
Only 4-bit models are supported, and we recommend deactivating the ExLlama kernels if you're finetuning a quantized model with PEFT.
</Tip>
The ExLlama kernels are only supported when the entire model is on the GPU. If you're doing inference on a CPU with AutoGPTQ (version > 0.4.2), then you'll need to disable the ExLlama kernel. This overwrites the attributes related to the ExLlama kernels in the quantization config of the config.json file.
```py
import torch
from transformers import AutoModelForCausalLM, GPTQConfig
gptq_config = GPTQConfig(bits=4, use_exllama=False)
model = AutoModelForCausalLM.from_pretrained("{your_username}/opt-125m-gptq", device_map="cpu", quantization_config=gptq_config)
```
## bitsandbytes
[bitsandbytes](https://github.com/TimDettmers/bitsandbytes) is the easiest option for quantizing a model to 8 and 4-bit. 8-bit quantization multiplies outliers in fp16 with non-outliers in int8, converts the non-outlier values back to fp16, and then adds them together to return the weights in fp16. This reduces the degradative effect outlier values have on a model's performance. 4-bit quantization compresses a model even further, and it is commonly used with [QLoRA](https://hf.co/papers/2305.14314) to finetune quantized LLMs.
To use bitsandbytes, make sure you have the following libraries installed:
<hfoptions id="bnb">
<hfoption id="8-bit">
```bash
pip install transformers accelerate bitsandbytes>0.37.0
```
</hfoption>
<hfoption id="4-bit">
```bash
pip install bitsandbytes>=0.39.0
pip install --upgrade accelerate
pip install --upgrade transformers
```
</hfoption>
</hfoptions>
Now you can quantize a model with the `load_in_8bit` or `load_in_4bit` parameters in the [`~PreTrainedModel.from_pretrained`] method. This works for any model in any modality, as long as it supports loading with Accelerate and contains `torch.nn.Linear` layers.
<hfoptions id="bnb">
<hfoption id="8-bit">
Quantizing a model in 8-bit halves the memory-usage, and for large models, set `device_map="auto"` to efficiently use the GPUs available:
```py
from transformers import AutoModelForCausalLM
model_8bit = AutoModelForCausalLM.from_pretrained("bigscience/bloom-1b7", device_map="auto", load_in_8bit=True)
```
By default, all the other modules such as `torch.nn.LayerNorm` are converted to `torch.float16`. You can change the data type of these modules with the `torch_dtype` parameter if you want:
```py
import torch
from transformers import AutoModelForCausalLM
model_8bit = AutoModelForCausalLM.from_pretrained("facebook/opt-350m", load_in_8bit=True, torch_dtype=torch.float32)
model_8bit.model.decoder.layers[-1].final_layer_norm.weight.dtype
```
Once a model is quantized to 8-bit, you can't push the quantized weights to the Hub unless you're using the latest version of Transformers and bitsandbytes. If you have the latest versions, then you can push the 8-bit model to the Hub with the [`~PreTrainedModel.push_to_hub`] method. The quantization config.json file is pushed first, followed by the quantized model weights.
```py
from transformers import AutoModelForCausalLM, AutoTokenizer
model = AutoModelForCausalLM.from_pretrained("bigscience/bloom-560m", device_map="auto", load_in_8bit=True)
tokenizer = AutoTokenizer.from_pretrained("bigscience/bloom-560m")
model.push_to_hub("bloom-560m-8bit")
```
</hfoption>
<hfoption id="4-bit">
Quantizing a model in 4-bit reduces your memory-usage by 4x, and for large models, set `device_map="auto"` to efficiently use the GPUs available:
```py
from transformers import AutoModelForCausalLM
model_4bit = AutoModelForCausalLM.from_pretrained("bigscience/bloom-1b7", device_map="auto", load_in_4bit=True)
```
By default, all the other modules such as `torch.nn.LayerNorm` are converted to `torch.float16`. You can change the data type of these modules with the `torch_dtype` parameter if you want:
```py
import torch
from transformers import AutoModelForCausalLM
model_4bit = AutoModelForCausalLM.from_pretrained("facebook/opt-350m", load_in_4bit=True, torch_dtype=torch.float32)
model_4bit.model.decoder.layers[-1].final_layer_norm.weight.dtype
```
If you have `bitsandbytes>=0.41.3`, you can serialize 4-bit models and push them on Hugging Face Hub. Simply call `model.push_to_hub()` after loading it in 4-bit precision. You can also save the serialized 4-bit models locally with `model.save_pretrained()` command.
</hfoption>
</hfoptions>
<Tip warning={true}>
Training with 8-bit and 4-bit weights are only supported for training *extra* parameters.
</Tip>
You can check your memory footprint with the `get_memory_footprint` method:
```py
print(model.get_memory_footprint())
```
Quantized models can be loaded from the [`~PreTrainedModel.from_pretrained`] method without needing to specify the `load_in_8bit` or `load_in_4bit` parameters:
```py
from transformers import AutoModelForCausalLM, AutoTokenizer
model = AutoModelForCausalLM.from_pretrained("{your_username}/bloom-560m-8bit", device_map="auto")
```
### 8-bit
<Tip>
Learn more about the details of 8-bit quantization in this [blog post](https://huggingface.co/blog/hf-bitsandbytes-integration)!
</Tip>
This section explores some of the specific features of 8-bit models, such as offloading, outlier thresholds, skipping module conversion, and finetuning.
#### Offloading
8-bit models can offload weights between the CPU and GPU to support fitting very large models into memory. The weights dispatched to the CPU are actually stored in **float32**, and aren't converted to 8-bit. For example, to enable offloading for the [bigscience/bloom-1b7](https://huggingface.co/bigscience/bloom-1b7) model, start by creating a [`BitsAndBytesConfig`]:
```py
from transformers import AutoModelForCausalLM, BitsAndBytesConfig
quantization_config = BitsAndBytesConfig(llm_int8_enable_fp32_cpu_offload=True)
```
Design a custom device map to fit everything on your GPU except for the `lm_head`, which you'll dispatch to the CPU:
```py
device_map = {
"transformer.word_embeddings": 0,
"transformer.word_embeddings_layernorm": 0,
"lm_head": "cpu",
"transformer.h": 0,
"transformer.ln_f": 0,
}
```
Now load your model with the custom `device_map` and `quantization_config`:
```py
model_8bit = AutoModelForCausalLM.from_pretrained(
"bigscience/bloom-1b7",
device_map=device_map,
quantization_config=quantization_config,
)
```
#### Outlier threshold
An "outlier" is a hidden state value greater than a certain threshold, and these values are computed in fp16. While the values are usually normally distributed ([-3.5, 3.5]), this distribution can be very different for large models ([-60, 6] or [6, 60]). 8-bit quantization works well for values ~5, but beyond that, there is a significant performance penalty. A good default threshold value is 6, but a lower threshold may be needed for more unstable models (small models or finetuning).
To find the best threshold for your model, we recommend experimenting with the `llm_int8_threshold` parameter in [`BitsAndBytesConfig`]:
```py
from transformers import AutoModelForCausalLM, BitsAndBytesConfig
model_id = "bigscience/bloom-1b7"
quantization_config = BitsAndBytesConfig(
llm_int8_threshold=10,
)
model_8bit = AutoModelForCausalLM.from_pretrained(
model_id,
device_map=device_map,
quantization_config=quantization_config,
)
```
#### Skip module conversion
For some models, like [Jukebox](model_doc/jukebox), you don't need to quantize every module to 8-bit which can actually cause instability. With Jukebox, there are several `lm_head` modules that should be skipped using the `llm_int8_skip_modules` parameter in [`BitsAndBytesConfig`]:
```py
from transformers import AutoModelForCausalLM, AutoTokenizer, BitsAndBytesConfig
model_id = "bigscience/bloom-1b7"
quantization_config = BitsAndBytesConfig(
llm_int8_skip_modules=["lm_head"],
)
model_8bit = AutoModelForCausalLM.from_pretrained(
model_id,
device_map="auto",
quantization_config=quantization_config,
)
```
#### Finetuning
With the [PEFT](https://github.com/huggingface/peft) library, you can finetune large models like [flan-t5-large](https://huggingface.co/google/flan-t5-large) and [facebook/opt-6.7b](https://huggingface.co/facebook/opt-6.7b) with 8-bit quantization. You don't need to pass the `device_map` parameter for training because it'll automatically load your model on a GPU. However, you can still customize the device map with the `device_map` parameter if you want to (`device_map="auto"` should only be used for inference).
### 4-bit
<Tip>
Try 4-bit quantization in this [notebook](https://colab.research.google.com/drive/1ge2F1QSK8Q7h0hn3YKuBCOAS0bK8E0wf) and learn more about it's details in this [blog post](https://huggingface.co/blog/4bit-transformers-bitsandbytes).
</Tip>
This section explores some of the specific features of 4-bit models, such as changing the compute data type, using the Normal Float 4 (NF4) data type, and using nested quantization.
#### Compute data type
To speedup computation, you can change the data type from float32 (the default value) to bf16 using the `bnb_4bit_compute_dtype` parameter in [`BitsAndBytesConfig`]:
```py
import torch
from transformers import BitsAndBytesConfig
quantization_config = BitsAndBytesConfig(load_in_4bit=True, bnb_4bit_compute_dtype=torch.bfloat16)
```
#### Normal Float 4 (NF4)
NF4 is a 4-bit data type from the [QLoRA](https://hf.co/papers/2305.14314) paper, adapted for weights initialized from a normal distribution. You should use NF4 for training 4-bit base models. This can be configured with the `bnb_4bit_quant_type` parameter in the [`BitsAndBytesConfig`]:
```py
from transformers import BitsAndBytesConfig
nf4_config = BitsAndBytesConfig(
load_in_4bit=True,
bnb_4bit_quant_type="nf4",
)
model_nf4 = AutoModelForCausalLM.from_pretrained(model_id, quantization_config=nf4_config)
```
For inference, the `bnb_4bit_quant_type` does not have a huge impact on performance. However, to remain consistent with the model weights, you should use the `bnb_4bit_compute_dtype` and `torch_dtype` values.
#### Nested quantization
Nested quantization is a technique that can save additional memory at no additional performance cost. This feature performs a second quantization of the already quantized weights to save an addition 0.4 bits/parameter. For example, with nested quantization, you can finetune a [Llama-13b](https://huggingface.co/meta-llama/Llama-2-13b) model on a 16GB NVIDIA T4 GPU with a sequence length of 1024, a batch size of 1, and enabling gradient accumulation with 4 steps.
```py
from transformers import BitsAndBytesConfig
double_quant_config = BitsAndBytesConfig(
load_in_4bit=True,
bnb_4bit_use_double_quant=True,
)
model_double_quant = AutoModelForCausalLM.from_pretrained("meta-llama/Llama-2-13b", quantization_config=double_quant_config)
```
## Optimum
The [Optimum](https://huggingface.co/docs/optimum/index) library supports quantization for Intel, Furiosa, ONNX Runtime, GPTQ, and lower-level PyTorch quantization functions. Consider using Optimum for quantization if you're using specific and optimized hardware like Intel CPUs, Furiosa NPUs or a model accelerator like ONNX Runtime.
## Benchmarks
To compare the speed, throughput, and latency of each quantization scheme, check the following benchmarks obtained from the [optimum-benchmark](https://github.com/huggingface/optimum-benchmark) library. The benchmark was run on a NVIDIA A1000 for the [TheBloke/Mistral-7B-v0.1-AWQ](https://huggingface.co/TheBloke/Mistral-7B-v0.1-AWQ) and [TheBloke/Mistral-7B-v0.1-GPTQ](https://huggingface.co/TheBloke/Mistral-7B-v0.1-GPTQ) models. These were also tested against the bitsandbytes quantization methods as well as a native fp16 model.
<div class="flex gap-4">
<div>
<img class="rounded-xl" src="https://huggingface.co/datasets/huggingface/documentation-images/resolve/main/quantization/forward_memory_plot.png" alt="forward peak memory per batch size" />
<figcaption class="mt-2 text-center text-sm text-gray-500">forward peak memory/batch size</figcaption>
</div>
<div>
<img class="rounded-xl" src="https://huggingface.co/datasets/huggingface/documentation-images/resolve/main/quantization/generate_memory_plot.png" alt="generate peak memory per batch size" />
<figcaption class="mt-2 text-center text-sm text-gray-500">generate peak memory/batch size</figcaption>
</div>
</div>
<div class="flex gap-4">
<div>
<img class="rounded-xl" src="https://huggingface.co/datasets/huggingface/documentation-images/resolve/main/quantization/generate_throughput_plot.png" alt="generate throughput per batch size" />
<figcaption class="mt-2 text-center text-sm text-gray-500">generate throughput/batch size</figcaption>
</div>
<div>
<img class="rounded-xl" src="https://huggingface.co/datasets/huggingface/documentation-images/resolve/main/quantization/forward_latency_plot.png" alt="forward latency per batch size" />
<figcaption class="mt-2 text-center text-sm text-gray-500">forward latency/batch size</figcaption>
</div>
</div>
The benchmarks indicate AWQ quantization is the fastest for inference, text generation, and has the lowest peak memory for text generation. However, AWQ has the largest forward latency per batch size. For a more detailed discussion about the pros and cons of each quantization method, read the [Overview of natively supported quantization schemes in 🤗 Transformers](https://huggingface.co/blog/overview-quantization-transformers) blog post.
### Fused AWQ modules
The [TheBloke/Mistral-7B-OpenOrca-AWQ](https://huggingface.co/TheBloke/Mistral-7B-OpenOrca-AWQ) model was benchmarked with `batch_size=1` with and without fused modules.
<figcaption class="text-center text-gray-500 text-lg">Unfused module</figcaption>
| Batch Size | Prefill Length | Decode Length | Prefill tokens/s | Decode tokens/s | Memory (VRAM) |
|-------------:|-----------------:|----------------:|-------------------:|------------------:|:----------------|
| 1 | 32 | 32 | 60.0984 | 38.4537 | 4.50 GB (5.68%) |
| 1 | 64 | 64 | 1333.67 | 31.6604 | 4.50 GB (5.68%) |
| 1 | 128 | 128 | 2434.06 | 31.6272 | 4.50 GB (5.68%) |
| 1 | 256 | 256 | 3072.26 | 38.1731 | 4.50 GB (5.68%) |
| 1 | 512 | 512 | 3184.74 | 31.6819 | 4.59 GB (5.80%) |
| 1 | 1024 | 1024 | 3148.18 | 36.8031 | 4.81 GB (6.07%) |
| 1 | 2048 | 2048 | 2927.33 | 35.2676 | 5.73 GB (7.23%) |
<figcaption class="text-center text-gray-500 text-lg">Fused module</figcaption>
| Batch Size | Prefill Length | Decode Length | Prefill tokens/s | Decode tokens/s | Memory (VRAM) |
|-------------:|-----------------:|----------------:|-------------------:|------------------:|:----------------|
| 1 | 32 | 32 | 81.4899 | 80.2569 | 4.00 GB (5.05%) |
| 1 | 64 | 64 | 1756.1 | 106.26 | 4.00 GB (5.05%) |
| 1 | 128 | 128 | 2479.32 | 105.631 | 4.00 GB (5.06%) |
| 1 | 256 | 256 | 1813.6 | 85.7485 | 4.01 GB (5.06%) |
| 1 | 512 | 512 | 2848.9 | 97.701 | 4.11 GB (5.19%) |
| 1 | 1024 | 1024 | 3044.35 | 87.7323 | 4.41 GB (5.57%) |
| 1 | 2048 | 2048 | 2715.11 | 89.4709 | 5.57 GB (7.04%) |
The speed and throughput of fused and unfused modules were also tested with the [optimum-benchmark](https://github.com/huggingface/optimum-benchmark) library.
<div class="flex gap-4">
<div>
<img class="rounded-xl" src="https://huggingface.co/datasets/huggingface/documentation-images/resolve/main/quantization/fused_forward_memory_plot.png" alt="generate throughput per batch size" />
<figcaption class="mt-2 text-center text-sm text-gray-500">forward peak memory/batch size</figcaption>
</div>
<div>
<img class="rounded-xl" src="https://huggingface.co/datasets/huggingface/documentation-images/resolve/main/quantization/fused_generate_throughput_plot.png" alt="forward latency per batch size" />
<figcaption class="mt-2 text-center text-sm text-gray-500">generate throughput/batch size</figcaption>
</div>
</div>
| transformers/docs/source/en/quantization.md/0 | {
"file_path": "transformers/docs/source/en/quantization.md",
"repo_id": "transformers",
"token_count": 12286
} | 257 |
<!--Copyright 2024 The HuggingFace Team. All rights reserved.
Licensed under the Apache License, Version 2.0 (the "License"); you may not use this file except in compliance with
the License. You may obtain a copy of the License at
http://www.apache.org/licenses/LICENSE-2.0
Unless required by applicable law or agreed to in writing, software distributed under the License is distributed on
an "AS IS" BASIS, WITHOUT WARRANTIES OR CONDITIONS OF ANY KIND, either express or implied. See the License for the
specific language governing permissions and limitations under the License.
⚠️ Note that this file is in Markdown but contain specific syntax for our doc-builder (similar to MDX) that may not be
rendered properly in your Markdown viewer.
-->
# Mask Generation
Mask generation is the task of generating semantically meaningful masks for an image.
This task is very similar to [image segmentation](semantic_segmentation), but many differences exist. Image segmentation models are trained on labeled datasets and are limited to the classes they have seen during training; they return a set of masks and corresponding classes, given an image.
Mask generation models are trained on large amounts of data and operate in two modes.
- Prompting mode: In this mode, the model takes in an image and a prompt, where a prompt can be a 2D point location (XY coordinates) in the image within an object or a bounding box surrounding an object. In prompting mode, the model only returns the mask over the object
that the prompt is pointing out.
- Segment Everything mode: In segment everything, given an image, the model generates every mask in the image. To do so, a grid of points is generated and overlaid on the image for inference.
Mask generation task is supported by [Segment Anything Model (SAM)](model_doc/sam). It's a powerful model that consists of a Vision Transformer-based image encoder, a prompt encoder, and a two-way transformer mask decoder. Images and prompts are encoded, and the decoder takes these embeddings and generates valid masks.
<div class="flex justify-center">
<img src="https://huggingface.co/datasets/huggingface/documentation-images/resolve/main/transformers/tasks/sam.png" alt="SAM Architecture"/>
</div>
SAM serves as a powerful foundation model for segmentation as it has large data coverage. It is trained on
[SA-1B](https://ai.meta.com/datasets/segment-anything/), a dataset with 1 million images and 1.1 billion masks.
In this guide, you will learn how to:
- Infer in segment everything mode with batching,
- Infer in point prompting mode,
- Infer in box prompting mode.
First, let's install `transformers`:
```bash
pip install -q transformers
```
## Mask Generation Pipeline
The easiest way to infer mask generation models is to use the `mask-generation` pipeline.
```python
>>> from transformers import pipeline
>>> checkpoint = "facebook/sam-vit-base"
>>> mask_generator = pipeline(model=checkpoint, task="mask-generation")
```
Let's see the image.
```python
from PIL import Image
import requests
img_url = "https://huggingface.co/datasets/huggingface/documentation-images/resolve/main/bee.jpg"
image = Image.open(requests.get(img_url, stream=True).raw).convert("RGB")
```
<div class="flex justify-center">
<img src="https://huggingface.co/datasets/huggingface/documentation-images/resolve/main/bee.jpg" alt="Example Image"/>
</div>
Let's segment everything. `points-per-batch` enables parallel inference of points in segment everything mode. This enables faster inference, but consumes more memory. Moreover, SAM only enables batching over points and not the images. `pred_iou_thresh` is the IoU confidence threshold where only the masks above that certain threshold are returned.
```python
masks = mask_generator(image, points_per_batch=128, pred_iou_thresh=0.88)
```
The `masks` looks like the following:
```bash
{'masks': [array([[False, False, False, ..., True, True, True],
[False, False, False, ..., True, True, True],
[False, False, False, ..., True, True, True],
...,
[False, False, False, ..., False, False, False],
[False, False, False, ..., False, False, False],
[False, False, False, ..., False, False, False]]),
array([[False, False, False, ..., False, False, False],
[False, False, False, ..., False, False, False],
[False, False, False, ..., False, False, False],
...,
'scores': tensor([0.9972, 0.9917,
...,
}
```
We can visualize them like this:
```python
import matplotlib.pyplot as plt
plt.imshow(image, cmap='gray')
for i, mask in enumerate(masks["masks"]):
plt.imshow(mask, cmap='viridis', alpha=0.1, vmin=0, vmax=1)
plt.axis('off')
plt.show()
```
Below is the original image in grayscale with colorful maps overlaid. Very impressive.
<div class="flex justify-center">
<img src="https://huggingface.co/datasets/huggingface/documentation-images/resolve/main/bee_segmented.png" alt="Visualized"/>
</div>
## Model Inference
### Point Prompting
You can also use the model without the pipeline. To do so, initialize the model and
the processor.
```python
from transformers import SamModel, SamProcessor
device = torch.device('cuda' if torch.cuda.is_available() else 'cpu')
model = SamModel.from_pretrained("facebook/sam-vit-base").to(device)
processor = SamProcessor.from_pretrained("facebook/sam-vit-base")
```
To do point prompting, pass the input point to the processor, then take the processor output
and pass it to the model for inference. To post-process the model output, pass the outputs and
`original_sizes` and `reshaped_input_sizes` we take from the processor's initial output. We need to pass these
since the processor resizes the image, and the output needs to be extrapolated.
```python
input_points = [[[2592, 1728]]] # point location of the bee
inputs = processor(image, input_points=input_points, return_tensors="pt").to(device)
with torch.no_grad():
outputs = model(**inputs)
masks = processor.image_processor.post_process_masks(outputs.pred_masks.cpu(), inputs["original_sizes"].cpu(), inputs["reshaped_input_sizes"].cpu())
```
We can visualize the three masks in the `masks` output.
```python
import torch
import matplotlib.pyplot as plt
import numpy as np
fig, axes = plt.subplots(1, 4, figsize=(15, 5))
axes[0].imshow(image)
axes[0].set_title('Original Image')
mask_list = [masks[0][0][0].numpy(), masks[0][0][1].numpy(), masks[0][0][2].numpy()]
for i, mask in enumerate(mask_list, start=1):
overlayed_image = np.array(image).copy()
overlayed_image[:,:,0] = np.where(mask == 1, 255, overlayed_image[:,:,0])
overlayed_image[:,:,1] = np.where(mask == 1, 0, overlayed_image[:,:,1])
overlayed_image[:,:,2] = np.where(mask == 1, 0, overlayed_image[:,:,2])
axes[i].imshow(overlayed_image)
axes[i].set_title(f'Mask {i}')
for ax in axes:
ax.axis('off')
plt.show()
```
<div class="flex justify-center">
<img src="https://huggingface.co/datasets/huggingface/documentation-images/resolve/main/transformers/tasks/masks.png" alt="Visualized"/>
</div>
### Box Prompting
You can also do box prompting in a similar fashion to point prompting. You can simply pass the input box in the format of a list
`[x_min, y_min, x_max, y_max]` format along with the image to the `processor`. Take the processor output and directly pass it
to the model, then post-process the output again.
```python
# bounding box around the bee
box = [2350, 1600, 2850, 2100]
inputs = processor(
image,
input_boxes=[[[box]]],
return_tensors="pt"
).to("cuda")
with torch.no_grad():
outputs = model(**inputs)
mask = processor.image_processor.post_process_masks(
outputs.pred_masks.cpu(),
inputs["original_sizes"].cpu(),
inputs["reshaped_input_sizes"].cpu()
)[0][0][0].numpy()
```
You can visualize the bounding box around the bee as shown below.
```python
import matplotlib.patches as patches
fig, ax = plt.subplots()
ax.imshow(image)
rectangle = patches.Rectangle((2350, 1600, 500, 500, linewidth=2, edgecolor='r', facecolor='none')
ax.add_patch(rectangle)
ax.axis("off")
plt.show()
```
<div class="flex justify-center">
<img src="https://huggingface.co/datasets/huggingface/documentation-images/resolve/main/transformers/tasks/bbox.png" alt="Visualized Bbox"/>
</div>
You can see the inference output below.
```python
fig, ax = plt.subplots()
ax.imshow(image)
ax.imshow(mask, cmap='viridis', alpha=0.4)
ax.axis("off")
plt.show()
```
<div class="flex justify-center">
<img src="https://huggingface.co/datasets/huggingface/documentation-images/resolve/main/transformers/tasks/box_inference.png" alt="Visualized Inference"/>
</div>
| transformers/docs/source/en/tasks/mask_generation.md/0 | {
"file_path": "transformers/docs/source/en/tasks/mask_generation.md",
"repo_id": "transformers",
"token_count": 2851
} | 258 |
<!--Copyright 2023 The HuggingFace Team. All rights reserved.
Licensed under the Apache License, Version 2.0 (the "License"); you may not use this file except in compliance with
the License. You may obtain a copy of the License at
http://www.apache.org/licenses/LICENSE-2.0
Unless required by applicable law or agreed to in writing, software distributed under the License is distributed on
an "AS IS" BASIS, WITHOUT WARRANTIES OR CONDITIONS OF ANY KIND, either express or implied. See the License for the
specific language governing permissions and limitations under the License.
⚠️ Note that this file is in Markdown but contain specific syntax for our doc-builder (similar to MDX) that may not be
rendered properly in your Markdown viewer.
-->
# Zero-shot object detection
[[open-in-colab]]
Traditionally, models used for [object detection](object_detection) require labeled image datasets for training,
and are limited to detecting the set of classes from the training data.
Zero-shot object detection is supported by the [OWL-ViT](../model_doc/owlvit) model which uses a different approach. OWL-ViT
is an open-vocabulary object detector. It means that it can detect objects in images based on free-text queries without
the need to fine-tune the model on labeled datasets.
OWL-ViT leverages multi-modal representations to perform open-vocabulary detection. It combines [CLIP](../model_doc/clip) with
lightweight object classification and localization heads. Open-vocabulary detection is achieved by embedding free-text queries with the text encoder of CLIP and using them as input to the object classification and localization heads.
associate images and their corresponding textual descriptions, and ViT processes image patches as inputs. The authors
of OWL-ViT first trained CLIP from scratch and then fine-tuned OWL-ViT end to end on standard object detection datasets using
a bipartite matching loss.
With this approach, the model can detect objects based on textual descriptions without prior training on labeled datasets.
In this guide, you will learn how to use OWL-ViT:
- to detect objects based on text prompts
- for batch object detection
- for image-guided object detection
Before you begin, make sure you have all the necessary libraries installed:
```bash
pip install -q transformers
```
## Zero-shot object detection pipeline
The simplest way to try out inference with OWL-ViT is to use it in a [`pipeline`]. Instantiate a pipeline
for zero-shot object detection from a [checkpoint on the Hugging Face Hub](https://huggingface.co/models?other=owlvit):
```python
>>> from transformers import pipeline
>>> checkpoint = "google/owlv2-base-patch16-ensemble"
>>> detector = pipeline(model=checkpoint, task="zero-shot-object-detection")
```
Next, choose an image you'd like to detect objects in. Here we'll use the image of astronaut Eileen Collins that is
a part of the [NASA](https://www.nasa.gov/multimedia/imagegallery/index.html) Great Images dataset.
```py
>>> import skimage
>>> import numpy as np
>>> from PIL import Image
>>> image = skimage.data.astronaut()
>>> image = Image.fromarray(np.uint8(image)).convert("RGB")
>>> image
```
<div class="flex justify-center">
<img src="https://huggingface.co/datasets/huggingface/documentation-images/resolve/main/transformers/tasks/zero-sh-obj-detection_1.png" alt="Astronaut Eileen Collins"/>
</div>
Pass the image and the candidate object labels to look for to the pipeline.
Here we pass the image directly; other suitable options include a local path to an image or an image url. We also pass text descriptions for all items we want to query the image for.
```py
>>> predictions = detector(
... image,
... candidate_labels=["human face", "rocket", "nasa badge", "star-spangled banner"],
... )
>>> predictions
[{'score': 0.3571370542049408,
'label': 'human face',
'box': {'xmin': 180, 'ymin': 71, 'xmax': 271, 'ymax': 178}},
{'score': 0.28099656105041504,
'label': 'nasa badge',
'box': {'xmin': 129, 'ymin': 348, 'xmax': 206, 'ymax': 427}},
{'score': 0.2110239565372467,
'label': 'rocket',
'box': {'xmin': 350, 'ymin': -1, 'xmax': 468, 'ymax': 288}},
{'score': 0.13790413737297058,
'label': 'star-spangled banner',
'box': {'xmin': 1, 'ymin': 1, 'xmax': 105, 'ymax': 509}},
{'score': 0.11950037628412247,
'label': 'nasa badge',
'box': {'xmin': 277, 'ymin': 338, 'xmax': 327, 'ymax': 380}},
{'score': 0.10649408400058746,
'label': 'rocket',
'box': {'xmin': 358, 'ymin': 64, 'xmax': 424, 'ymax': 280}}]
```
Let's visualize the predictions:
```py
>>> from PIL import ImageDraw
>>> draw = ImageDraw.Draw(image)
>>> for prediction in predictions:
... box = prediction["box"]
... label = prediction["label"]
... score = prediction["score"]
... xmin, ymin, xmax, ymax = box.values()
... draw.rectangle((xmin, ymin, xmax, ymax), outline="red", width=1)
... draw.text((xmin, ymin), f"{label}: {round(score,2)}", fill="white")
>>> image
```
<div class="flex justify-center">
<img src="https://huggingface.co/datasets/huggingface/documentation-images/resolve/main/transformers/tasks/zero-sh-obj-detection_2.png" alt="Visualized predictions on NASA image"/>
</div>
## Text-prompted zero-shot object detection by hand
Now that you've seen how to use the zero-shot object detection pipeline, let's replicate the same
result manually.
Start by loading the model and associated processor from a [checkpoint on the Hugging Face Hub](https://huggingface.co/models?other=owlvit).
Here we'll use the same checkpoint as before:
```py
>>> from transformers import AutoProcessor, AutoModelForZeroShotObjectDetection
>>> model = AutoModelForZeroShotObjectDetection.from_pretrained(checkpoint)
>>> processor = AutoProcessor.from_pretrained(checkpoint)
```
Let's take a different image to switch things up.
```py
>>> import requests
>>> url = "https://unsplash.com/photos/oj0zeY2Ltk4/download?ixid=MnwxMjA3fDB8MXxzZWFyY2h8MTR8fHBpY25pY3xlbnwwfHx8fDE2Nzc0OTE1NDk&force=true&w=640"
>>> im = Image.open(requests.get(url, stream=True).raw)
>>> im
```
<div class="flex justify-center">
<img src="https://huggingface.co/datasets/huggingface/documentation-images/resolve/main/transformers/tasks/zero-sh-obj-detection_3.png" alt="Beach photo"/>
</div>
Use the processor to prepare the inputs for the model. The processor combines an image processor that prepares the
image for the model by resizing and normalizing it, and a [`CLIPTokenizer`] that takes care of the text inputs.
```py
>>> text_queries = ["hat", "book", "sunglasses", "camera"]
>>> inputs = processor(text=text_queries, images=im, return_tensors="pt")
```
Pass the inputs through the model, post-process, and visualize the results. Since the image processor resized images before
feeding them to the model, you need to use the [`~OwlViTImageProcessor.post_process_object_detection`] method to make sure the predicted bounding
boxes have the correct coordinates relative to the original image:
```py
>>> import torch
>>> with torch.no_grad():
... outputs = model(**inputs)
... target_sizes = torch.tensor([im.size[::-1]])
... results = processor.post_process_object_detection(outputs, threshold=0.1, target_sizes=target_sizes)[0]
>>> draw = ImageDraw.Draw(im)
>>> scores = results["scores"].tolist()
>>> labels = results["labels"].tolist()
>>> boxes = results["boxes"].tolist()
>>> for box, score, label in zip(boxes, scores, labels):
... xmin, ymin, xmax, ymax = box
... draw.rectangle((xmin, ymin, xmax, ymax), outline="red", width=1)
... draw.text((xmin, ymin), f"{text_queries[label]}: {round(score,2)}", fill="white")
>>> im
```
<div class="flex justify-center">
<img src="https://huggingface.co/datasets/huggingface/documentation-images/resolve/main/transformers/tasks/zero-sh-obj-detection_4.png" alt="Beach photo with detected objects"/>
</div>
## Batch processing
You can pass multiple sets of images and text queries to search for different (or same) objects in several images.
Let's use both an astronaut image and the beach image together.
For batch processing, you should pass text queries as a nested list to the processor and images as lists of PIL images,
PyTorch tensors, or NumPy arrays.
```py
>>> images = [image, im]
>>> text_queries = [
... ["human face", "rocket", "nasa badge", "star-spangled banner"],
... ["hat", "book", "sunglasses", "camera"],
... ]
>>> inputs = processor(text=text_queries, images=images, return_tensors="pt")
```
Previously for post-processing you passed the single image's size as a tensor, but you can also pass a tuple, or, in case
of several images, a list of tuples. Let's create predictions for the two examples, and visualize the second one (`image_idx = 1`).
```py
>>> with torch.no_grad():
... outputs = model(**inputs)
... target_sizes = [x.size[::-1] for x in images]
... results = processor.post_process_object_detection(outputs, threshold=0.1, target_sizes=target_sizes)
>>> image_idx = 1
>>> draw = ImageDraw.Draw(images[image_idx])
>>> scores = results[image_idx]["scores"].tolist()
>>> labels = results[image_idx]["labels"].tolist()
>>> boxes = results[image_idx]["boxes"].tolist()
>>> for box, score, label in zip(boxes, scores, labels):
... xmin, ymin, xmax, ymax = box
... draw.rectangle((xmin, ymin, xmax, ymax), outline="red", width=1)
... draw.text((xmin, ymin), f"{text_queries[image_idx][label]}: {round(score,2)}", fill="white")
>>> images[image_idx]
```
<div class="flex justify-center">
<img src="https://huggingface.co/datasets/huggingface/documentation-images/resolve/main/transformers/tasks/zero-sh-obj-detection_4.png" alt="Beach photo with detected objects"/>
</div>
## Image-guided object detection
In addition to zero-shot object detection with text queries, OWL-ViT offers image-guided object detection. This means
you can use an image query to find similar objects in the target image.
Unlike text queries, only a single example image is allowed.
Let's take an image with two cats on a couch as a target image, and an image of a single cat
as a query:
```py
>>> url = "http://images.cocodataset.org/val2017/000000039769.jpg"
>>> image_target = Image.open(requests.get(url, stream=True).raw)
>>> query_url = "http://images.cocodataset.org/val2017/000000524280.jpg"
>>> query_image = Image.open(requests.get(query_url, stream=True).raw)
```
Let's take a quick look at the images:
```py
>>> import matplotlib.pyplot as plt
>>> fig, ax = plt.subplots(1, 2)
>>> ax[0].imshow(image_target)
>>> ax[1].imshow(query_image)
```
<div class="flex justify-center">
<img src="https://huggingface.co/datasets/huggingface/documentation-images/resolve/main/transformers/tasks/zero-sh-obj-detection_5.png" alt="Cats"/>
</div>
In the preprocessing step, instead of text queries, you now need to use `query_images`:
```py
>>> inputs = processor(images=image_target, query_images=query_image, return_tensors="pt")
```
For predictions, instead of passing the inputs to the model, pass them to [`~OwlViTForObjectDetection.image_guided_detection`]. Draw the predictions
as before except now there are no labels.
```py
>>> with torch.no_grad():
... outputs = model.image_guided_detection(**inputs)
... target_sizes = torch.tensor([image_target.size[::-1]])
... results = processor.post_process_image_guided_detection(outputs=outputs, target_sizes=target_sizes)[0]
>>> draw = ImageDraw.Draw(image_target)
>>> scores = results["scores"].tolist()
>>> boxes = results["boxes"].tolist()
>>> for box, score, label in zip(boxes, scores, labels):
... xmin, ymin, xmax, ymax = box
... draw.rectangle((xmin, ymin, xmax, ymax), outline="white", width=4)
>>> image_target
```
<div class="flex justify-center">
<img src="https://huggingface.co/datasets/huggingface/documentation-images/resolve/main/transformers/tasks/zero-sh-obj-detection_6.png" alt="Cats with bounding boxes"/>
</div>
| transformers/docs/source/en/tasks/zero_shot_object_detection.md/0 | {
"file_path": "transformers/docs/source/en/tasks/zero_shot_object_detection.md",
"repo_id": "transformers",
"token_count": 3901
} | 259 |
<!--Copyright 2022 The HuggingFace Team. All rights reserved.
Licensed under the Apache License, Version 2.0 (the "License"); you may not use this file except in compliance with
the License. You may obtain a copy of the License at
http://www.apache.org/licenses/LICENSE-2.0
Unless required by applicable law or agreed to in writing, software distributed under the License is distributed on
an "AS IS" BASIS, WITHOUT WARRANTIES OR CONDITIONS OF ANY KIND, either express or implied. See the License for the
specific language governing permissions and limitations under the License.
⚠️ Note that this file is in Markdown but contain specific syntax for our doc-builder (similar to MDX) that may not be
rendered properly in your Markdown viewer.
-->
# Carga instancias preentrenadas con un AutoClass
Con tantas arquitecturas diferentes de Transformer puede ser retador crear una para tu checkpoint. Como parte de la filosofía central de 🤗 Transformers para hacer que la biblioteca sea fácil, simple y flexible de usar; una `AutoClass` automáticamente infiere y carga la arquitectura correcta desde un checkpoint dado. El método `from_pretrained` te permite cargar rápidamente un modelo preentrenado para cualquier arquitectura, por lo que no tendrás que dedicar tiempo y recursos para entrenar uno desde cero. Producir este tipo de código con checkpoint implica que si funciona con uno, funcionará también con otro (siempre que haya sido entrenado para una tarea similar) incluso si la arquitectura es distinta.
<Tip>
Recuerda, la arquitectura se refiere al esqueleto del modelo y los checkpoints son los pesos para una arquitectura dada. Por ejemplo, [BERT](https://huggingface.co/google-bert/bert-base-uncased) es una arquitectura, mientras que `google-bert/bert-base-uncased` es un checkpoint. Modelo es un término general que puede significar una arquitectura o un checkpoint.
</Tip>
En este tutorial, aprenderás a:
* Cargar un tokenizador pre-entrenado.
* Cargar un extractor de características (feature extractor en inglés) pre-entrenado.
* Cargar un procesador pre-entrenado.
* Cargar un modelo pre-entrenado.
## AutoTokenizer
Casi cualquier tarea de Procesamiento de Lenguaje Natural comienza con un tokenizador. Un tokenizador convierte tu input a un formato que puede ser procesado por el modelo.
Carga un tokenizador con [`AutoTokenizer.from_pretrained`]:
```py
>>> from transformers import AutoTokenizer
>>> tokenizer = AutoTokenizer.from_pretrained("google-bert/bert-base-uncased")
```
Luego tokeniza tu input como lo mostrado a continuación:
```py
>>> sequence = "In a hole in the ground there lived a hobbit."
>>> print(tokenizer(sequence))
{'input_ids': [101, 1999, 1037, 4920, 1999, 1996, 2598, 2045, 2973, 1037, 7570, 10322, 4183, 1012, 102],
'token_type_ids': [0, 0, 0, 0, 0, 0, 0, 0, 0, 0, 0, 0, 0, 0, 0],
'attention_mask': [1, 1, 1, 1, 1, 1, 1, 1, 1, 1, 1, 1, 1, 1, 1]}
```
## AutoFeatureExtractor
Para tareas de audio y visión, un extractor de características procesa la señal de audio o imagen al formato de input correcto.
Carga un extractor de características con [`AutoFeatureExtractor.from_pretrained`]:
```py
>>> from transformers import AutoFeatureExtractor
>>> feature_extractor = AutoFeatureExtractor.from_pretrained(
... "ehcalabres/wav2vec2-lg-xlsr-en-speech-emotion-recognition"
... )
```
## AutoProcessor
Las tareas multimodales requieren un procesador que combine dos tipos de herramientas de preprocesamiento. Por ejemplo, el modelo [LayoutLMV2](model_doc/layoutlmv2) requiere que un extractor de características maneje las imágenes y que un tokenizador maneje el texto; un procesador combina ambas.
Carga un procesador con [`AutoProcessor.from_pretrained`]:
```py
>>> from transformers import AutoProcessor
>>> processor = AutoProcessor.from_pretrained("microsoft/layoutlmv2-base-uncased")
```
## AutoModel
<frameworkcontent>
<pt>
Finalmente, las clases `AutoModelFor` te permiten cargar un modelo preentrenado para una tarea dada (revisa [aquí](model_doc/auto) para conocer la lista completa de tareas disponibles). Por ejemplo, cargue un modelo para clasificación de secuencias con [`AutoModelForSequenceClassification.from_pretrained`]:
```py
>>> from transformers import AutoModelForSequenceClassification
>>> model = AutoModelForSequenceClassification.from_pretrained("distilbert/distilbert-base-uncased")
```
Reutiliza fácilmente el mismo checkpoint para cargar una aquitectura para alguna tarea diferente:
```py
>>> from transformers import AutoModelForTokenClassification
>>> model = AutoModelForTokenClassification.from_pretrained("distilbert/distilbert-base-uncased")
```
Generalmente recomendamos utilizar las clases `AutoTokenizer` y `AutoModelFor` para cargar instancias pre-entrenadas de modelos. Ésto asegurará que cargues la arquitectura correcta en cada ocasión. En el siguiente [tutorial](preprocessing), aprende a usar tu tokenizador recién cargado, el extractor de características y el procesador para preprocesar un dataset para fine-tuning.
</pt>
<tf>
Finalmente, la clase `TFAutoModelFor` te permite cargar tu modelo pre-entrenado para una tarea dada (revisa [aquí](model_doc/auto) para conocer la lista completa de tareas disponibles). Por ejemplo, carga un modelo para clasificación de secuencias con [`TFAutoModelForSequenceClassification.from_pretrained`]:
```py
>>> from transformers import TFAutoModelForSequenceClassification
>>> model = TFAutoModelForSequenceClassification.from_pretrained("distilbert/distilbert-base-uncased")
```
Reutiliza fácilmente el mismo checkpoint para cargar una aquitectura para alguna tarea diferente:
```py
>>> from transformers import TFAutoModelForTokenClassification
>>> model = TFAutoModelForTokenClassification.from_pretrained("distilbert/distilbert-base-uncased")
```
Generalmente recomendamos utilizar las clases `AutoTokenizer` y `TFAutoModelFor` para cargar instancias de modelos pre-entrenados. Ésto asegurará que cargues la arquitectura correcta cada vez. En el siguiente [tutorial](preprocessing), aprende a usar tu tokenizador recién cargado, el extractor de características y el procesador para preprocesar un dataset para fine-tuning.
</tf>
</frameworkcontent>
| transformers/docs/source/es/autoclass_tutorial.md/0 | {
"file_path": "transformers/docs/source/es/autoclass_tutorial.md",
"repo_id": "transformers",
"token_count": 2066
} | 260 |
<!--Copyright 2020 The HuggingFace Team. All rights reserved.
Licensed under the Apache License, Version 2.0 (the "License"); you may not use this file except in compliance with
the License. You may obtain a copy of the License at
http://www.apache.org/licenses/LICENSE-2.0
Unless required by applicable law or agreed to in writing, software distributed under the License is distributed on
an "AS IS" BASIS, WITHOUT WARRANTIES OR CONDITIONS OF ANY KIND, either express or implied. See the License for the
specific language governing permissions and limitations under the License.
⚠️ Note that this file is in Markdown but contain specific syntax for our doc-builder (similar to MDX) that may not be
rendered properly in your Markdown viewer.
-->
# Perplejidad de los modelos de longitud fija
[[open-in-colab]]
La perplejidad, perplexity en inglés (PPL), es una de las métricas más comunes para evaluar modelos de lenguaje. Antes de sumergirnos, debemos tener en cuenta que esta métrica se aplica específicamente a modelos de lenguaje clásicos (a veces llamados modelos autorregresivos o causales) y no está bien definida para modelos de lenguaje enmascarados como BERT (ver [resumen del modelo](model_summary)).
La perplejidad se define como la media negativa exponenciada del log-likelihood de una secuencia. Si tenemos una secuencia tokenizada \\(X = (x_0, x_1, \dots, x_t)\\), entonces la perplejidad de \\(X\\) es,
$$\text{PPL}(X) = \exp \left\{ {-\frac{1}{t}\sum_i^t \log p_\theta (x_i|x_{<i}) } \right\}$$
donde \\(\log p_\theta (x_i|x_{<i})\\) es el log-likelihood del token i-ésimo condicionado a los tokens precedentes \\(x_{<i}\\) según nuestro modelo. De manera intuitiva, se puede pensar en esto como una evaluación de la capacidad del modelo para predecir de manera uniforme entre el conjunto de tokens especificados en un corpus. Es importante destacar que el procedimiento de tokenización tiene un impacto directo en la perplejidad de un modelo, lo cual siempre debe tenerse en cuenta al comparar diferentes modelos.
Esto también es equivalente a la exponenciación de la entropía cruzada entre los datos y las predicciones del modelo. Para obtener más intuición sobre la perplejidad y su relación con los Bits Por Carácter (BPC) y la compresión de datos, echa un vistazo a esta [fantástica publicación en el blog de "The Gradient"](https://thegradient.pub/understanding-evaluation-metrics-for-language-models/).
## Cálculo de PPL con modelos de longitud fija
Si no estuviéramos limitados por el tamaño del contexto de un modelo, evaluaríamos la perplejidad (PPL) del modelo auto regresivamente factorizando una secuencia y condicionándonos en toda la subsecuencia precedente en cada paso, como se muestra a continuación.
<img width="600" alt="Full decomposition of a sequence with unlimited context length" src="https://huggingface.co/datasets/huggingface/documentation-images/resolve/main/ppl_full.gif"/>
Sin embargo, al trabajar con modelos aproximados, generalmente tenemos una restricción en la cantidad de tokens que el modelo puede procesar. La versión más grande de [GPT-2](model_doc/gpt2), por ejemplo, tiene una longitud fija de 1024 tokens, por lo que no podemos calcular \\(p_\theta(x_t|x_{<t})\\) directamente cuando \\(t\\) es mayor que 1024.
En cambio, la secuencia se divide típicamente en subsecuencias iguales al tamaño máximo de entrada del modelo. Si el tamaño máximo de entrada, de un modelo es \\(k\\), entonces aproximamos la probabilidad de un token \\(x_t\\) condicionándonos solo en los \\(k-1\\) tokens que lo preceden en lugar de todo el contexto. Al evaluar la perplejidad del modelo en una secuencia, un enfoque tentador pero sub óptimo es dividir la secuencia en fragmentos independientes y sumar los log-likelihood descompuestos de cada segmento de manera independiente.
<img width="600" alt="Suboptimal PPL not taking advantage of full available context" src="https://huggingface.co/datasets/huggingface/documentation-images/resolve/main/ppl_chunked.gif"/>
Esto es rápido de calcular, ya que la perplejidad de cada segmento se puede calcular en un solo pase hacia adelante, pero sirve como una aproximación pobre de la perplejidad completamente factorizada y generalmente dará como resultado una PPL más alta (peor) porque el modelo tendrá menos contexto en la mayoría de los pasos de predicción.
En cambio, la PPL de modelos de longitud fija debería evaluarse con una estrategia de ventana deslizante. Esto implica deslizar repetidamente la ventana de contexto para que el modelo tenga más contexto al hacer cada predicción.
<img width="600" alt="Sliding window PPL taking advantage of all available context" src="https://huggingface.co/datasets/huggingface/documentation-images/resolve/main/ppl_sliding.gif"/>
Esta es una aproximación más cercana a la verdadera descomposición de la probabilidad de la secuencia y generalmente dará como resultado una puntuación más favorable. La desventaja es que requiere un pase hacia adelante separado para cada token en el corpus. Un buen compromiso práctico es emplear una ventana deslizante estratificada, moviendo el contexto con pasos más grandes en lugar de deslizarse de 1 token a la vez. Esto permite que la computación avance mucho más rápido, mientras le da al modelo un contexto amplio para hacer
predicciones en cada paso.
## Ejemplo: Cálculo de la perplejidad con GPT-2 en 🤗 Transformers
Demostremos este proceso con GPT-2.
```python
from transformers import GPT2LMHeadModel, GPT2TokenizerFast
device = "cuda"
model_id = "openai-community/gpt2-large"
model = GPT2LMHeadModel.from_pretrained(model_id).to(device)
tokenizer = GPT2TokenizerFast.from_pretrained(model_id)
```
Carguemos el conjunto de datos WikiText-2 y evaluemos la perplejidad utilizando algunas estrategias de ventana deslizante diferentes. Dado que este conjunto de datos es pequeño y solo estamos realizando un pase hacia adelante sobre el conjunto, podemos cargar y codificar todo el conjunto de datos en la memoria.
```python
from datasets import load_dataset
test = load_dataset("wikitext", "wikitext-2-raw-v1", split="test")
encodings = tokenizer("\n\n".join(test["text"]), return_tensors="pt")
```
Con 🤗 Transformers, simplemente podemos pasar los `input_ids` como las `labels` a nuestro modelo, y la media negativa del log-likelihood para cada token se devuelve como la pérdida. Sin embargo, con nuestro enfoque de ventana deslizante, hay superposición en los tokens que pasamos al modelo en cada iteración. No queremos que el log-likelihood de los tokens que estamos tratando solo como contexto se incluya en nuestra pérdida, por lo que podemos establecer estos objetivos en `-100` para que se ignoren. El siguiente es un ejemplo de cómo podríamos hacer esto con un paso de `512`. Esto significa que el modelo tendrá al menos `512` tokens como contexto al calcular el log-likelihood condicional de cualquier token (siempre que haya `512` tokens precedentes disponibles para condicionar).
```python
import torch
from tqdm import tqdm
max_length = model.config.n_positions
stride = 512
seq_len = encodings.input_ids.size(1)
nlls = []
prev_end_loc = 0
for begin_loc in tqdm(range(0, seq_len, stride)):
end_loc = min(begin_loc + max_length, seq_len)
trg_len = end_loc - prev_end_loc # puede ser diferente del paso en el último bucle
input_ids = encodings.input_ids[:, begin_loc:end_loc].to(device)
target_ids = input_ids.clone()
target_ids[:, :-trg_len] = -100
with torch.no_grad():
outputs = model(input_ids, labels=target_ids)
# la pérdida se calcula utilizando CrossEntropyLoss, que promedia las etiquetas válidas
# N.B. el modelo solo calcula la pérdida sobre trg_len - 1 etiquetas, porque desplaza las etiqueta internamente
# a la izquierda por 1.
neg_log_likelihood = outputs.loss
nlls.append(neg_log_likelihood)
prev_end_loc = end_loc
if end_loc == seq_len:
break
ppl = torch.exp(torch.stack(nlls).mean())
```
Ejecuta esto con la longitud de paso igual a la longitud máxima de entrada es equivalente a la estrategia sub óptima,
sin ventana deslizante, que discutimos anteriormente. Cuanto menor sea el paso, más contexto tendrá el modelo para
realizar cada predicción y, por lo general, mejor será la perplejidad informada.
Cuando ejecutamos lo anterior con `stride = 1024`, es decir, sin superposición, la PPL resultante es `19.44`, que es
aproximadamente la misma que la `19.93` informada en el artículo de GPT-2. Al utilizar `stride = 512` y, por lo tanto,
emplear nuestra estrategia de ventana deslizante, esto disminuye a `16.45`. Esto no solo es una puntuación más favorable, sino que se calcula de una manera más cercana a la verdadera descomposición autorregresiva de la probabilidad de una secuencia.
| transformers/docs/source/es/perplexity.md/0 | {
"file_path": "transformers/docs/source/es/perplexity.md",
"repo_id": "transformers",
"token_count": 3119
} | 261 |
<!--Copyright 2022 The HuggingFace Team. All rights reserved.
Licensed under the Apache License, Version 2.0 (the "License"); you may not use this file except in compliance with
the License. You may obtain a copy of the License at
http://www.apache.org/licenses/LICENSE-2.0
Unless required by applicable law or agreed to in writing, software distributed under the License is distributed on
an "AS IS" BASIS, WITHOUT WARRANTIES OR CONDITIONS OF ANY KIND, either express or implied. See the License for the
specific language governing permissions and limitations under the License.
⚠️ Note that this file is in Markdown but contain specific syntax for our doc-builder (similar to MDX) that may not be
rendered properly in your Markdown viewer.
-->
# Allenamento distribuito con 🤗 Accelerate
La parallelizzazione è emersa come strategia per allenare modelli sempre più grandi su hardware limitato e accelerarne la velocità di allenamento di diversi ordini di magnitudine. In Hugging Face, abbiamo creato la libreria [🤗 Accelerate](https://huggingface.co/docs/accelerate) per aiutarti ad allenare in modo semplice un modello 🤗 Transformers su qualsiasi tipo di configurazione distribuita, sia che si tratti di più GPU su una sola macchina o di più GPU su più macchine. In questo tutorial, imparerai come personalizzare il training loop nativo di PyTorch per consentire l'addestramento in un ambiente distribuito.
## Configurazione
Inizia installando 🤗 Accelerate:
```bash
pip install accelerate
```
Poi importa e crea un oggetto [`Accelerator`](https://huggingface.co/docs/accelerate/package_reference/accelerator#accelerate.Accelerator). `Accelerator` rileverà automaticamente il tuo setup distribuito e inizializzerà tutte le componenti necessarie per l'allenamento. Non dovrai allocare esplicitamente il tuo modello su un device.
```py
>>> from accelerate import Accelerator
>>> accelerator = Accelerator()
```
## Preparati ad accelerare
Il prossimo passo è quello di passare tutti gli oggetti rilevanti per l'allenamento al metodo [`prepare`](https://huggingface.co/docs/accelerate/package_reference/accelerator#accelerate.Accelerator.prepare). Questo include i tuoi DataLoaders per l'allenamento e per la valutazione, un modello e un ottimizzatore:
```py
>>> train_dataloader, eval_dataloader, model, optimizer = accelerator.prepare(
... train_dataloader, eval_dataloader, model, optimizer
... )
```
## Backward
Infine, sostituisci il tipico metodo `loss.backward()` nel tuo loop di allenamento con il metodo [`backward`](https://huggingface.co/docs/accelerate/package_reference/accelerator#accelerate.Accelerator.backward) di 🤗 Accelerate:
```py
>>> for epoch in range(num_epochs):
... for batch in train_dataloader:
... outputs = model(**batch)
... loss = outputs.loss
... accelerator.backward(loss)
... optimizer.step()
... lr_scheduler.step()
... optimizer.zero_grad()
... progress_bar.update(1)
```
Come puoi vedere nel seguente codice, hai solo bisogno di aggiungere quattro righe in più di codice al tuo training loop per abilitare l'allenamento distribuito!
```diff
+ from accelerate import Accelerator
from transformers import AdamW, AutoModelForSequenceClassification, get_scheduler
+ accelerator = Accelerator()
model = AutoModelForSequenceClassification.from_pretrained(checkpoint, num_labels=2)
optimizer = AdamW(model.parameters(), lr=3e-5)
- device = torch.device("cuda") if torch.cuda.is_available() else torch.device("cpu")
- model.to(device)
+ train_dataloader, eval_dataloader, model, optimizer = accelerator.prepare(
+ train_dataloader, eval_dataloader, model, optimizer
+ )
num_epochs = 3
num_training_steps = num_epochs * len(train_dataloader)
lr_scheduler = get_scheduler(
"linear",
optimizer=optimizer,
num_warmup_steps=0,
num_training_steps=num_training_steps
)
progress_bar = tqdm(range(num_training_steps))
model.train()
for epoch in range(num_epochs):
for batch in train_dataloader:
- batch = {k: v.to(device) for k, v in batch.items()}
outputs = model(**batch)
loss = outputs.loss
- loss.backward()
+ accelerator.backward(loss)
optimizer.step()
lr_scheduler.step()
optimizer.zero_grad()
progress_bar.update(1)
```
## Allenamento
Una volta che hai aggiunto le righe di codice rilevanti, lancia il tuo allenamento in uno script o in un notebook come Colaboratory.
### Allenamento con uno script
Se stai eseguendo il tuo allenamento da uno script, esegui il comando seguente per creare e salvare un file di configurazione:
```bash
accelerate config
```
Poi lancia il tuo allenamento con:
```bash
accelerate launch train.py
```
### Allenamento con un notebook
La libreria 🤗 Accelerate può anche essere utilizzata in un notebook se stai pianificando di utilizzare le TPU di Colaboratory. Inserisci tutto il codice legato all'allenamento in una funzione, e passala al `notebook_launcher`:
```py
>>> from accelerate import notebook_launcher
>>> notebook_launcher(training_function)
```
Per maggiori informazioni relative a 🤗 Accelerate e le sue numerose funzionalità, fai riferimento alla [documentazione](https://huggingface.co/docs/accelerate). | transformers/docs/source/it/accelerate.md/0 | {
"file_path": "transformers/docs/source/it/accelerate.md",
"repo_id": "transformers",
"token_count": 1891
} | 262 |
<!--Copyright 2022 The HuggingFace Team. All rights reserved.
Licensed under the Apache License, Version 2.0 (the "License"); you may not use this file except in compliance with
the License. You may obtain a copy of the License at
http://www.apache.org/licenses/LICENSE-2.0
Unless required by applicable law or agreed to in writing, software distributed under the License is distributed on
an "AS IS" BASIS, WITHOUT WARRANTIES OR CONDITIONS OF ANY KIND, either express or implied. See the License for the
⚠️ Note that this file is in Markdown but contain specific syntax for our doc-builder (similar to MDX) that may not be
rendered properly in your Markdown viewer.
-->
# Inferenza Efficiente su CPU
Questa guida si concentra sull'inferenza di modelli di grandi dimensioni in modo efficiente sulla CPU.
## `BetterTransformer` per inferenza più rapida
Abbiamo integrato di recente `BetterTransformer` per fare inferenza più rapidamente con modelli per testi, immagini e audio. Visualizza la documentazione sull'integrazione [qui](https://huggingface.co/docs/optimum/bettertransformer/overview) per maggiori dettagli.
## PyTorch JIT-mode (TorchScript)
TorchScript è un modo di creare modelli serializzabili e ottimizzabili da codice PyTorch. Ogni programmma TorchScript può esere salvato da un processo Python e caricato in un processo dove non ci sono dipendenze Python.
Comparandolo con l'eager mode di default, jit mode in PyTorch normalmente fornisce prestazioni migliori per l'inferenza del modello da parte di metodologie di ottimizzazione come la operator fusion.
Per una prima introduzione a TorchScript, vedi la Introduction to [PyTorch TorchScript tutorial](https://pytorch.org/tutorials/beginner/Intro_to_TorchScript_tutorial.html#tracing-modules).
### IPEX Graph Optimization con JIT-mode
Intel® Extension per PyTorch fornnisce ulteriori ottimizzazioni in jit mode per i modelli della serie Transformers. Consigliamo vivamente agli utenti di usufruire dei vantaggi di Intel® Extension per PyTorch con jit mode. Alcuni operator patterns usati fequentemente dai modelli Transformers models sono già supportati in Intel® Extension per PyTorch con jit mode fusions. Questi fusion patterns come Multi-head-attention fusion, Concat Linear, Linear+Add, Linear+Gelu, Add+LayerNorm fusion and etc. sono abilitati e hanno buone performance. I benefici della fusion è fornito agli utenti in modo trasparente. In base alle analisi, il ~70% dei problemi più popolari in NLP question-answering, text-classification, and token-classification possono avere benefici sulle performance grazie ai fusion patterns sia per Float32 precision che per BFloat16 Mixed precision.
Vedi maggiori informazioni per [IPEX Graph Optimization](https://intel.github.io/intel-extension-for-pytorch/cpu/latest/tutorials/features/graph_optimization.html).
#### Installazione di IPEX
I rilasci di IPEX seguono PyTorch, verifica i vari approcci per [IPEX installation](https://intel.github.io/intel-extension-for-pytorch/).
### Utilizzo del JIT-mode
Per abilitare JIT-mode in Trainer per evaluation e prediction, devi aggiungere `jit_mode_eval` negli argomenti di Trainer.
<Tip warning={true}>
per PyTorch >= 1.14.0. JIT-mode potrebe giovare a qualsiasi modello di prediction e evaluaion visto che il dict input è supportato in jit.trace
per PyTorch < 1.14.0. JIT-mode potrebbe giovare ai modelli il cui ordine dei parametri corrisponde all'ordine delle tuple in ingresso in jit.trace, come i modelli per question-answering.
Nel caso in cui l'ordine dei parametri seguenti non corrisponda all'ordine delle tuple in ingresso in jit.trace, come nei modelli di text-classification, jit.trace fallirà e lo cattureremo con una eccezione al fine di renderlo un fallback. Il logging è usato per notificare gli utenti.
</Tip>
Trovi un esempo con caso d'uso in [Transformers question-answering](https://github.com/huggingface/transformers/tree/main/examples/pytorch/question-answering)
- Inference using jit mode on CPU:
<pre>python run_qa.py \
--model_name_or_path csarron/bert-base-uncased-squad-v1 \
--dataset_name squad \
--do_eval \
--max_seq_length 384 \
--doc_stride 128 \
--output_dir /tmp/ \
--no_cuda \
<b>--jit_mode_eval </b></pre>
- Inference with IPEX using jit mode on CPU:
<pre>python run_qa.py \
--model_name_or_path csarron/bert-base-uncased-squad-v1 \
--dataset_name squad \
--do_eval \
--max_seq_length 384 \
--doc_stride 128 \
--output_dir /tmp/ \
--no_cuda \
<b>--use_ipex \</b>
<b>--jit_mode_eval</b></pre>
| transformers/docs/source/it/perf_infer_cpu.md/0 | {
"file_path": "transformers/docs/source/it/perf_infer_cpu.md",
"repo_id": "transformers",
"token_count": 1497
} | 263 |
<!--Copyright 2022 The HuggingFace Team. All rights reserved.
Licensed under the Apache License, Version 2.0 (the "License"); you may not use this file except in compliance with
the License. You may obtain a copy of the License at
http://www.apache.org/licenses/LICENSE-2.0
Unless required by applicable law or agreed to in writing, software distributed under the License is distributed on
an "AS IS" BASIS, WITHOUT WARRANTIES OR CONDITIONS OF ANY KIND, either express or implied. See the License for the
specific language governing permissions and limitations under the License.
⚠️ Note that this file is in Markdown but contain specific syntax for our doc-builder (similar to MDX) that may not be
rendered properly in your Markdown viewer.
-->
# 🤗 Accelerate を用いた分散学習
モデルが大きくなるにつれて、限られたハードウェアでより大きなモデルを訓練し、訓練速度を大幅に上昇させるための方法として並列処理が浮上してきました。1台のマシンに複数のGPUがあっても、複数のマシンにまたがる複数のGPUがあっても、あらゆるタイプの分散処理セットアップ上でユーザーが簡単に 🤗 Transformers モデルを訓練できるように、 Hugging Face では [🤗 Accelerate](https://huggingface.co/docs/accelerate) ライブラリを作成しました。このチュートリアルでは、PyTorch の訓練ループをカスタマイズして、分散処理環境での訓練を可能にする方法について学びます。
## セットアップ
はじめに 🤗 Accelerate をインストールしましょう:
```bash
pip install accelerate
```
そしたらインポートして [`~accelerate.Accelerator`] オブジェクトを作成しましょう。[`~accelerate.Accelerator`] は分散処理セットアップを自動的に検出し、訓練のために必要な全てのコンポーネントを初期化します。モデルをデバイスに明示的に配置する必要はありません。
```py
>>> from accelerate import Accelerator
>>> accelerator = Accelerator()
```
## Accelerate する準備をしましょう
次に、関連する全ての訓練オブジェクトを [`~accelerate.Accelerator.prepare`] メソッドに渡します。これには、訓練と評価それぞれのDataloader、モデル、optimizer が含まれます:
```py
>>> train_dataloader, eval_dataloader, model, optimizer = accelerator.prepare(
... train_dataloader, eval_dataloader, model, optimizer
... )
```
## Backward
最後に訓練ループ内の `loss.backward()` を 🤗 Accelerate の [`~accelerate.Accelerator.backward`] メソッドで置き換えます:
```py
>>> for epoch in range(num_epochs):
... for batch in train_dataloader:
... outputs = model(**batch)
... loss = outputs.loss
... accelerator.backward(loss)
... optimizer.step()
... lr_scheduler.step()
... optimizer.zero_grad()
... progress_bar.update(1)
```
以下のコードで確認できる通り、訓練ループに4行のコードを追加するだけで分散学習が可能です!
```diff
+ from accelerate import Accelerator
from transformers import AdamW, AutoModelForSequenceClassification, get_scheduler
+ accelerator = Accelerator()
model = AutoModelForSequenceClassification.from_pretrained(checkpoint, num_labels=2)
optimizer = AdamW(model.parameters(), lr=3e-5)
- device = torch.device("cuda") if torch.cuda.is_available() else torch.device("cpu")
- model.to(device)
+ train_dataloader, eval_dataloader, model, optimizer = accelerator.prepare(
+ train_dataloader, eval_dataloader, model, optimizer
+ )
num_epochs = 3
num_training_steps = num_epochs * len(train_dataloader)
lr_scheduler = get_scheduler(
"linear",
optimizer=optimizer,
num_warmup_steps=0,
num_training_steps=num_training_steps
)
progress_bar = tqdm(range(num_training_steps))
model.train()
for epoch in range(num_epochs):
for batch in train_dataloader:
- batch = {k: v.to(device) for k, v in batch.items()}
outputs = model(**batch)
loss = outputs.loss
- loss.backward()
+ accelerator.backward(loss)
optimizer.step()
lr_scheduler.step()
optimizer.zero_grad()
progress_bar.update(1)
```
## 訓練する
関連するコードを追加したら、スクリプトまたは Colaboratory などのノートブックで訓練を開始します。
### スクリプトで訓練する
スクリプトから訓練をしている場合は、設定ファイルを作成・保存するために以下のコマンドを実行してください:
```bash
accelerate config
```
そして次のようにして訓練を開始します:
```bash
accelerate launch train.py
```
### ノートブックで訓練する
Colaboratory の TPU の利用をお考えの場合、🤗 Accelerate はノートブック上で実行することもできます。訓練に必要な全てのコードを関数に含め、[`~accelerate.notebook_launcher`] に渡してください:
```py
>>> from accelerate import notebook_launcher
>>> notebook_launcher(training_function)
```
🤗 Accelerate と豊富な機能についてもっと知りたい方は[ドキュメント](https://huggingface.co/docs/accelerate)を参照してください。
| transformers/docs/source/ja/accelerate.md/0 | {
"file_path": "transformers/docs/source/ja/accelerate.md",
"repo_id": "transformers",
"token_count": 2185
} | 264 |
<!--Copyright 2023 The HuggingFace Team. All rights reserved.
Licensed under the Apache License, Version 2.0 (the "License"); you may not use this file except in compliance with
the License. You may obtain a copy of the License at
http://www.apache.org/licenses/LICENSE-2.0
Unless required by applicable law or agreed to in writing, software distributed under the License is distributed on
an "AS IS" BASIS, WITHOUT WARRANTIES OR CONDITIONS OF ANY KIND, either express or implied. See the License for the
⚠️ Note that this file is in Markdown but contain specific syntax for our doc-builder (similar to MDX) that may not be
rendered properly in your Markdown viewer.
-->
# Hyperparameter Search using Trainer API
🤗 Transformersは、🤗 Transformersモデルのトレーニングを最適化する[`Trainer`]クラスを提供し、独自のトレーニングループを手動で記述せずにトレーニングを開始するのが簡単になります。[`Trainer`]はハイパーパラメーター検索のAPIも提供しています。このドキュメントでは、それを例示します。
## Hyperparameter Search backend
[`Trainer`]は現在、4つのハイパーパラメーター検索バックエンドをサポートしています:
[optuna](https://optuna.org/)、[sigopt](https://sigopt.com/)、[raytune](https://docs.ray.io/en/latest/tune/index.html)、および[wandb](https://wandb.ai/site/sweeps)。
これらを使用する前に、ハイパーパラメーター検索バックエンドをインストールする必要があります。
```bash
pip install optuna/sigopt/wandb/ray[tune]
```
## How to enable Hyperparameter search in example
ハイパーパラメータの検索スペースを定義し、異なるバックエンドには異なるフォーマットが必要です。
Sigoptの場合、sigopt [object_parameter](https://docs.sigopt.com/ai-module-api-references/api_reference/objects/object_parameter) を参照してください。それは以下のようなものです:
```py
>>> def sigopt_hp_space(trial):
... return [
... {"bounds": {"min": 1e-6, "max": 1e-4}, "name": "learning_rate", "type": "double"},
... {
... "categorical_values": ["16", "32", "64", "128"],
... "name": "per_device_train_batch_size",
... "type": "categorical",
... },
... ]
```
Optunaに関しては、[object_parameter](https://optuna.readthedocs.io/en/stable/tutorial/10_key_features/002_configurations.html#sphx-glr-tutorial-10-key-features-002-configurations-py)をご覧ください。以下のようになります:
```py
>>> def optuna_hp_space(trial):
... return {
... "learning_rate": trial.suggest_float("learning_rate", 1e-6, 1e-4, log=True),
... "per_device_train_batch_size": trial.suggest_categorical("per_device_train_batch_size", [16, 32, 64, 128]),
... }
```
Optunaは、多目的のハイパーパラメータ最適化(HPO)を提供しています。 `hyperparameter_search` で `direction` を渡し、複数の目的関数値を返すための独自の `compute_objective` を定義することができます。 Pareto Front(`List[BestRun]`)は `hyperparameter_search` で返され、[test_trainer](https://github.com/huggingface/transformers/blob/main/tests/trainer/test_trainer.py) のテストケース `TrainerHyperParameterMultiObjectOptunaIntegrationTest` を参照する必要があります。これは以下のようになります。
```py
>>> best_trials = trainer.hyperparameter_search(
... direction=["minimize", "maximize"],
... backend="optuna",
... hp_space=optuna_hp_space,
... n_trials=20,
... compute_objective=compute_objective,
... )
```
Ray Tuneに関して、[object_parameter](https://docs.ray.io/en/latest/tune/api/search_space.html)を参照してください。以下のようになります:
```py
>>> def ray_hp_space(trial):
... return {
... "learning_rate": tune.loguniform(1e-6, 1e-4),
... "per_device_train_batch_size": tune.choice([16, 32, 64, 128]),
... }
```
Wandbについては、[object_parameter](https://docs.wandb.ai/guides/sweeps/configuration)をご覧ください。これは以下のようになります:
```py
>>> def wandb_hp_space(trial):
... return {
... "method": "random",
... "metric": {"name": "objective", "goal": "minimize"},
... "parameters": {
... "learning_rate": {"distribution": "uniform", "min": 1e-6, "max": 1e-4},
... "per_device_train_batch_size": {"values": [16, 32, 64, 128]},
... },
... }
```
`model_init` 関数を定義し、それを [`Trainer`] に渡す例を示します:
```py
>>> def model_init(trial):
... return AutoModelForSequenceClassification.from_pretrained(
... model_args.model_name_or_path,
... from_tf=bool(".ckpt" in model_args.model_name_or_path),
... config=config,
... cache_dir=model_args.cache_dir,
... revision=model_args.model_revision,
... token=True if model_args.use_auth_token else None,
... )
```
[`Trainer`] を `model_init` 関数、トレーニング引数、トレーニングデータセット、テストデータセット、および評価関数と共に作成してください:
```py
>>> trainer = Trainer(
... model=None,
... args=training_args,
... train_dataset=small_train_dataset,
... eval_dataset=small_eval_dataset,
... compute_metrics=compute_metrics,
... tokenizer=tokenizer,
... model_init=model_init,
... data_collator=data_collator,
... )
```
ハイパーパラメーターの探索を呼び出し、最良のトライアル パラメーターを取得します。バックエンドは `"optuna"` / `"sigopt"` / `"wandb"` / `"ray"` である可能性があります。方向は `"minimize"` または `"maximize"` であり、目標をより大きくするか小さくするかを示します。
`compute_objective` 関数を独自に定義することもできます。定義されていない場合、デフォルトの `compute_objective` が呼び出され、F1などの評価メトリックの合計が目標値として返されます。
```py
>>> best_trial = trainer.hyperparameter_search(
... direction="maximize",
... backend="optuna",
... hp_space=optuna_hp_space,
... n_trials=20,
... compute_objective=compute_objective,
... )
```
## Hyperparameter search For DDP finetune
現在、DDP(Distributed Data Parallel)のためのハイパーパラメーター検索は、Optuna と SigOpt に対して有効になっています。ランクゼロプロセスのみが検索トライアルを生成し、他のランクに引数を渡します。
| transformers/docs/source/ja/hpo_train.md/0 | {
"file_path": "transformers/docs/source/ja/hpo_train.md",
"repo_id": "transformers",
"token_count": 2841
} | 265 |
<!--Copyright 2020 The HuggingFace Team. All rights reserved.
Licensed under the Apache License, Version 2.0 (the "License"); you may not use this file except in compliance with
the License. You may obtain a copy of the License at
http://www.apache.org/licenses/LICENSE-2.0
Unless required by applicable law or agreed to in writing, software distributed under the License is distributed on
an "AS IS" BASIS, WITHOUT WARRANTIES OR CONDITIONS OF ANY KIND, either express or implied. See the License for the
specific language governing permissions and limitations under the License.
⚠️ Note that this file is in Markdown but contain specific syntax for our doc-builder (similar to MDX) that may not be
rendered properly in your Markdown viewer.
-->
# ALBERT
<div class="flex flex-wrap space-x-1">
<a href="https://huggingface.co/models?filter=albert">
<img alt="Models" src="https://img.shields.io/badge/All_model_pages-albert-blueviolet">
</a>
<a href="https://huggingface.co/spaces/docs-demos/albert-base-v2">
<img alt="Spaces" src="https://img.shields.io/badge/%F0%9F%A4%97%20Hugging%20Face-Spaces-blue">
</a>
</div>
## 概要
ALBERTモデルは、「[ALBERT: A Lite BERT for Self-supervised Learning of Language Representations](https://arxiv.org/abs/1909.11942)」という論文でZhenzhong Lan、Mingda Chen、Sebastian Goodman、Kevin Gimpel、Piyush Sharma、Radu Soricutによって提案されました。BERTのメモリ消費を減らしトレーニングを高速化するためのパラメータ削減技術を2つ示しています:
- 埋め込み行列を2つの小さな行列に分割する。
- グループ間で分割された繰り返し層を使用する。
論文の要旨は以下の通りです:
*自然言語表現の事前学習時にモデルのサイズを増やすと、下流タスクのパフォーマンスが向上することがしばしばあります。しかし、ある時点でさらなるモデルの増大は、GPU/TPUのメモリ制限、長い訓練時間、予期せぬモデルの劣化といった問題のために困難になります。これらの問題に対処するために、我々はBERTのメモリ消費を低減し、訓練速度を高めるための2つのパラメータ削減技術を提案します。包括的な実証的証拠は、我々の提案方法が元のBERTに比べてはるかによくスケールするモデルを生み出すことを示しています。また、文間の一貫性をモデリングに焦点を当てた自己教師あり損失を使用し、複数の文が含まれる下流タスクに一貫して助けとなることを示します。その結果、我々の最良のモデルは、BERT-largeに比べてパラメータが少ないにもかかわらず、GLUE、RACE、SQuADベンチマークで新たな最先端の結果を確立します。*
このモデルは[lysandre](https://huggingface.co/lysandre)により提供されました。このモデルのjaxバージョンは[kamalkraj](https://huggingface.co/kamalkraj)により提供されました。オリジナルのコードは[こちら](https://github.com/google-research/ALBERT)で見ることができます。
## 使用上のヒント
- ALBERTは絶対位置埋め込みを使用するモデルなので、通常、入力を左側ではなく右側にパディングすることが推奨されます。
- ALBERTは繰り返し層を使用するためメモリ使用量は小さくなりますが、同じ数の(繰り返し)層を反復しなければならないため、隠れ層の数が同じであればBERTのようなアーキテクチャと同様の計算コストがかかります。
- 埋め込みサイズEは隠れサイズHと異なりますが、これは埋め込みが文脈に依存しない(一つの埋め込みベクトルが一つのトークンを表す)のに対し、隠れ状態は文脈に依存する(1つの隠れ状態がトークン系列を表す)ため、H >> Eとすることがより論理的です。また、埋め込み行列のサイズはV x Eと大きいです(Vは語彙サイズ)。E < Hであれば、パラメータは少なくなります。
- 層はパラメータを共有するグループに分割されています(メモリ節約のため)。次文予測(NSP: Next Sentence Prediction)は文の順序予測に置き換えられます:入力では、2つの文AとB(それらは連続している)があり、Aに続いてBを与えるか、Bに続いてAを与えます。モデルはそれらが入れ替わっているかどうかを予測する必要があります。
## 参考資料
- [テキスト分類タスクガイド](../tasks/sequence_classification)
- [トークン分類タスクガイド](../tasks/token_classification)
- [質問応答タスクガイド](../tasks/question_answering)
- [マスクされた言語モデルタスクガイド](../tasks/masked_language_modeling)
- [多肢選択タスクガイド](../tasks/multiple_choice)
## AlbertConfig
[[autodoc]] AlbertConfig
## AlbertTokenizer
[[autodoc]] AlbertTokenizer
- build_inputs_with_special_tokens
- get_special_tokens_mask
- create_token_type_ids_from_sequences
- save_vocabulary
## AlbertTokenizerFast
[[autodoc]] AlbertTokenizerFast
## Albert specific outputs
[[autodoc]] models.albert.modeling_albert.AlbertForPreTrainingOutput
[[autodoc]] models.albert.modeling_tf_albert.TFAlbertForPreTrainingOutput
<frameworkcontent>
<pt>
## AlbertModel
[[autodoc]] AlbertModel
- forward
## AlbertForPreTraining
[[autodoc]] AlbertForPreTraining
- forward
## AlbertForMaskedLM
[[autodoc]] AlbertForMaskedLM
- forward
## AlbertForSequenceClassification
[[autodoc]] AlbertForSequenceClassification
- forward
## AlbertForMultipleChoice
[[autodoc]] AlbertForMultipleChoice
## AlbertForTokenClassification
[[autodoc]] AlbertForTokenClassification
- forward
## AlbertForQuestionAnswering
[[autodoc]] AlbertForQuestionAnswering
- forward
</pt>
<tf>
## TFAlbertModel
[[autodoc]] TFAlbertModel
- call
## TFAlbertForPreTraining
[[autodoc]] TFAlbertForPreTraining
- call
## TFAlbertForMaskedLM
[[autodoc]] TFAlbertForMaskedLM
- call
## TFAlbertForSequenceClassification
[[autodoc]] TFAlbertForSequenceClassification
- call
## TFAlbertForMultipleChoice
[[autodoc]] TFAlbertForMultipleChoice
- call
## TFAlbertForTokenClassification
[[autodoc]] TFAlbertForTokenClassification
- call
## TFAlbertForQuestionAnswering
[[autodoc]] TFAlbertForQuestionAnswering
- call
</tf>
<jax>
## FlaxAlbertModel
[[autodoc]] FlaxAlbertModel
- __call__
## FlaxAlbertForPreTraining
[[autodoc]] FlaxAlbertForPreTraining
- __call__
## FlaxAlbertForMaskedLM
[[autodoc]] FlaxAlbertForMaskedLM
- __call__
## FlaxAlbertForSequenceClassification
[[autodoc]] FlaxAlbertForSequenceClassification
- __call__
## FlaxAlbertForMultipleChoice
[[autodoc]] FlaxAlbertForMultipleChoice
- __call__
## FlaxAlbertForTokenClassification
[[autodoc]] FlaxAlbertForTokenClassification
- __call__
## FlaxAlbertForQuestionAnswering
[[autodoc]] FlaxAlbertForQuestionAnswering
- __call__
</jax>
</frameworkcontent>
| transformers/docs/source/ja/model_doc/albert.md/0 | {
"file_path": "transformers/docs/source/ja/model_doc/albert.md",
"repo_id": "transformers",
"token_count": 2960
} | 266 |
<!--Copyright 2021 The HuggingFace Team. All rights reserved.
Licensed under the Apache License, Version 2.0 (the "License"); you may not use this file except in compliance with
the License. You may obtain a copy of the License at
http://www.apache.org/licenses/LICENSE-2.0
Unless required by applicable law or agreed to in writing, software distributed under the License is distributed on
an "AS IS" BASIS, WITHOUT WARRANTIES OR CONDITIONS OF ANY KIND, either express or implied. See the License for the
specific language governing permissions and limitations under the License.
⚠️ Note that this file is in Markdown but contain specific syntax for our doc-builder (similar to MDX) that may not be
rendered properly in your Markdown viewer.
-->
# BigBirdPegasus
## Overview
BigBird モデルは、[Big Bird: Transformers for Longer Sequences](https://arxiv.org/abs/2007.14062) で提案されました。
ザヒール、マンジルとグルガネシュ、グルとダベイ、クマール・アヴィナヴァとエインズリー、ジョシュアとアルベルティ、クリスとオンタノン、
サンティアゴとファム、フィリップとラブラ、アニルードとワン、キーファンとヤン、リーなど。 BigBird は注目度が低い
BERT などの Transformer ベースのモデルをさらに長いシーケンスに拡張する、Transformer ベースのモデル。まばらに加えて
アテンションと同様に、BigBird は入力シーケンスにランダム アテンションだけでなくグローバル アテンションも適用します。理論的には、
まばらで全体的でランダムな注意を適用すると、完全な注意に近づくことが示されていますが、
長いシーケンスでは計算効率が大幅に向上します。より長いコンテキストを処理できる機能の結果として、
BigBird は、質問応答や
BERT または RoBERTa と比較した要約。
論文の要約は次のとおりです。
*BERT などのトランスフォーマーベースのモデルは、NLP で最も成功した深層学習モデルの 1 つです。
残念ながら、それらの中核的な制限の 1 つは、シーケンスに対する二次依存性 (主にメモリに関する) です。
完全な注意メカニズムによる長さです。これを解決するために、BigBird は、まばらな注意メカニズムを提案します。
この二次依存関係を線形に削減します。 BigBird がシーケンス関数の汎用近似器であることを示します。
チューリングは完全であるため、二次完全注意モデルのこれらの特性が保存されます。途中、私たちの
理論分析により、O(1) 個のグローバル トークン (CLS など) を持つ利点の一部が明らかになり、
スパース注意メカニズムの一部としてのシーケンス。提案されたスパース アテンションは、次の長さのシーケンスを処理できます。
同様のハードウェアを使用して以前に可能であったものの 8 倍。より長いコンテキストを処理できる機能の結果として、
BigBird は、質問応答や要約などのさまざまな NLP タスクのパフォーマンスを大幅に向上させます。私達も
ゲノミクスデータへの新しいアプリケーションを提案します。*
## Usage tips
- BigBird の注意がどのように機能するかについての詳細な説明については、[このブログ投稿](https://huggingface.co/blog/big-bird) を参照してください。
- BigBird には、**original_full** と **block_sparse** の 2 つの実装が付属しています。シーケンス長が 1024 未満の場合、次を使用します。
**block_sparse** を使用してもメリットがないため、**original_full** を使用することをお勧めします。
- コードは現在、3 ブロックと 2 グローバル ブロックのウィンドウ サイズを使用しています。
- シーケンスの長さはブロック サイズで割り切れる必要があります。
- 現在の実装では **ITC** のみがサポートされています。
- 現在の実装では **num_random_blocks = 0** はサポートされていません。
- BigBirdPegasus は [PegasusTokenizer](https://github.com/huggingface/transformers/blob/main/src/transformers/models/pegasus/tokenization_pegasus.py) を使用します。
- BigBird は絶対位置埋め込みを備えたモデルであるため、通常は入力を右側にパディングすることをお勧めします。
左。
元のコードは [こちら](https://github.com/google-research/bigbird) にあります。
## ドキュメント リソース
- [テキスト分類タスクガイド](../tasks/sequence_classification)
- [質問回答タスク ガイド](../tasks/question_answering)
- [因果言語モデリング タスク ガイド](../tasks/language_modeling)
- [翻訳タスクガイド](../tasks/translation)
- [要約タスクガイド](../tasks/summarization)
## BigBirdPegasusConfig
[[autodoc]] BigBirdPegasusConfig
- all
## BigBirdPegasusModel
[[autodoc]] BigBirdPegasusModel
- forward
## BigBirdPegasusForConditionalGeneration
[[autodoc]] BigBirdPegasusForConditionalGeneration
- forward
## BigBirdPegasusForSequenceClassification
[[autodoc]] BigBirdPegasusForSequenceClassification
- forward
## BigBirdPegasusForQuestionAnswering
[[autodoc]] BigBirdPegasusForQuestionAnswering
- forward
## BigBirdPegasusForCausalLM
[[autodoc]] BigBirdPegasusForCausalLM
- forward
| transformers/docs/source/ja/model_doc/bigbird_pegasus.md/0 | {
"file_path": "transformers/docs/source/ja/model_doc/bigbird_pegasus.md",
"repo_id": "transformers",
"token_count": 2264
} | 267 |
<!--Copyright 2021 The HuggingFace Team. All rights reserved.
Licensed under the Apache License, Version 2.0 (the "License"); you may not use this file except in compliance with
the License. You may obtain a copy of the License at
http://www.apache.org/licenses/LICENSE-2.0
Unless required by applicable law or agreed to in writing, software distributed under the License is distributed on
an "AS IS" BASIS, WITHOUT WARRANTIES OR CONDITIONS OF ANY KIND, either express or implied. See the License for the
specific language governing permissions and limitations under the License.
⚠️ Note that this file is in Markdown but contain specific syntax for our doc-builder (similar to MDX) that may not be
rendered properly in your Markdown viewer.
-->
# CLIP
## Overview
CLIP モデルは、Alec Radford、Jong Wook Kim、Chris Hallacy、Aditya Ramesh、Gabriel Goh Sandhini Agarwal, Girish Sastry, Amanda Askell, Pamela Mishkin, Jack Clark, Gretchen Krueger, Ilya Sutskever [Learning Transferable Visual Models From Natural Language Supervision](https://arxiv.org/abs/2103.00020) で提案されました。
サンディニ・アガルワル、ギリッシュ・サストリー、アマンダ・アスケル、パメラ・ミシュキン、ジャック・クラーク、グレッチェン・クルーガー、イリヤ・サツケヴァー。クリップ
(Contrastive Language-Image Pre-Training) は、さまざまな (画像、テキスト) ペアでトレーニングされたニューラル ネットワークです。かもね
直接最適化することなく、与えられた画像から最も関連性の高いテキスト スニペットを予測するように自然言語で指示されます。
GPT-2 および 3 のゼロショット機能と同様に、タスクに対して。
論文の要約は次のとおりです。
*最先端のコンピューター ビジョン システムは、あらかじめ定められたオブジェクト カテゴリの固定セットを予測するようにトレーニングされています。これ
制限された形式の監視では、指定するために追加のラベル付きデータが必要となるため、一般性と使いやすさが制限されます。
その他の視覚的なコンセプト。画像に関する生のテキストから直接学習することは、
より広範な監督源。どのキャプションが表示されるかを予測するという単純な事前トレーニング タスクが有効であることを示します。
400 のデータセットで SOTA 画像表現を最初から学習するための効率的かつスケーラブルな方法はどの画像ですか
インターネットから収集された数百万の(画像、テキスト)ペア。事前トレーニング後、自然言語を使用して参照します。
視覚的な概念を学習し(または新しい概念を説明し)、下流のタスクへのモデルのゼロショット転送を可能にします。私たちは勉強します
30 を超えるさまざまな既存のコンピューター ビジョン データセットでタスクをまたがってベンチマークを行うことにより、このアプローチのパフォーマンスを評価します。
OCR、ビデオ内のアクション認識、地理的位置特定、およびさまざまな種類のきめ細かいオブジェクト分類など。の
モデルはほとんどのタスクに簡単に移行でき、多くの場合、必要がなくても完全に監視されたベースラインと競合します。
データセット固有のトレーニングに適しています。たとえば、ImageNet ゼロショットではオリジナルの ResNet-50 の精度と一致します。
トレーニングに使用された 128 万のトレーニング サンプルを使用する必要はありません。コードをリリースし、事前トレーニング済み
モデルの重みはこの https URL で確認できます。*
このモデルは [valhalla](https://huggingface.co/valhalla) によって提供されました。元のコードは [ここ](https://github.com/openai/CLIP) にあります。
## Usage tips and example
CLIP は、マルチモーダルなビジョンおよび言語モデルです。画像とテキストの類似性やゼロショット画像に使用できます。
分類。 CLIP は、ViT のようなトランスフォーマーを使用して視覚的特徴を取得し、因果言語モデルを使用してテキストを取得します
特徴。次に、テキストと視覚の両方の特徴が、同じ次元の潜在空間に投影されます。ドット
投影された画像とテキストの特徴間の積が同様のスコアとして使用されます。
画像を Transformer エンコーダに供給するために、各画像は固定サイズの重複しないパッチのシーケンスに分割されます。
これらは線形に埋め込まれます。 [CLS] トークンは、イメージ全体の表現として機能するために追加されます。作家たち
また、絶対位置埋め込みを追加し、結果として得られるベクトルのシーケンスを標準の Transformer エンコーダに供給します。
[`CLIPImageProcessor`] を使用して、モデルの画像のサイズ変更 (または再スケール) および正規化を行うことができます。
[`CLIPTokenizer`] はテキストのエンコードに使用されます。 [`CLIPProcessor`] はラップします
[`CLIPImageProcessor`] と [`CLIPTokenizer`] を両方の単一インスタンスに統合
テキストをエンコードして画像を準備します。次の例は、次のメソッドを使用して画像とテキストの類似性スコアを取得する方法を示しています。
[`CLIPProcessor`] と [`CLIPModel`]。
```python
>>> from PIL import Image
>>> import requests
>>> from transformers import CLIPProcessor, CLIPModel
>>> model = CLIPModel.from_pretrained("openai/clip-vit-base-patch32")
>>> processor = CLIPProcessor.from_pretrained("openai/clip-vit-base-patch32")
>>> url = "http://images.cocodataset.org/val2017/000000039769.jpg"
>>> image = Image.open(requests.get(url, stream=True).raw)
>>> inputs = processor(text=["a photo of a cat", "a photo of a dog"], images=image, return_tensors="pt", padding=True)
>>> outputs = model(**inputs)
>>> logits_per_image = outputs.logits_per_image # this is the image-text similarity score
>>> probs = logits_per_image.softmax(dim=1) # we can take the softmax to get the label probabilities
```
## Resources
CLIP を使い始めるのに役立つ公式 Hugging Face およびコミュニティ (🌎 で示されている) リソースのリスト。
- [リモート センシング (衛星) 画像とキャプションを使用した CLIP の微調整](https://huggingface.co/blog/fine-tune-clip-rsicd)、[RSICD データセット] を使用して CLIP を微調整する方法に関するブログ投稿(https://github.com/201528014227051/RSICD_optimal) と、データ拡張によるパフォーマンスの変化の比較。
- この [サンプル スクリプト](https://github.com/huggingface/transformers/tree/main/examples/pytorch/contrastive-image-text) は、プレ- [COCO データセット](https://cocodataset.org/#home) を使用してトレーニングされたビジョンおよびテキスト エンコーダー。
<PipelineTag pipeline="image-to-text"/>
- 画像キャプションのビーム検索による推論に事前トレーニング済み CLIP を使用する方法に関する [ノートブック](https://colab.research.google.com/drive/1tuoAC5F4sC7qid56Z0ap-stR3rwdk0ZV?usp=sharing)。 🌎
**画像検索**
- 事前トレーニングされた CLIP を使用した画像検索と MRR (平均相互ランク) スコアの計算に関する [ノートブック](https://colab.research.google.com/drive/1bLVwVKpAndpEDHqjzxVPr_9nGrSbuOQd?usp=sharing)。 🌎
- 画像の取得と類似性スコアの表示に関する [ノートブック](https://colab.research.google.com/github/deep-diver/image_search_with_natural_language/blob/main/notebooks/Image_Search_CLIP.ipynb)。 🌎
- 多言語 CLIP を使用して画像とテキストを同じベクトル空間にマッピングする方法に関する [ノートブック](https://colab.research.google.com/drive/1xO-wC_m_GNzgjIBQ4a4znvQkvDoZJvH4?usp=sharing)。 🌎
- を使用してセマンティック イメージ検索で CLIP を実行する方法に関する [ノートブック](https://colab.research.google.com/github/vivien000/clip-demo/blob/master/clip.ipynb#scrollTo=uzdFhRGqiWkR) [Unsplash](https://unsplash.com) および [TMDB](https://www.themoviedb.org/) データセット。 🌎
**説明可能性**
- 入力トークンと画像セグメントの類似性を視覚化する方法に関する [ノートブック](https://colab.research.google.com/github/hila-chefer/Transformer-MM-Explainability/blob/main/CLIP_explainability.ipynb)。 🌎
ここに含めるリソースの送信に興味がある場合は、お気軽にプル リクエストを開いてください。審査させていただきます。
リソースは、既存のリソースを複製するのではなく、何か新しいものを示すことが理想的です。
## CLIPConfig
[[autodoc]] CLIPConfig
- from_text_vision_configs
## CLIPTextConfig
[[autodoc]] CLIPTextConfig
## CLIPVisionConfig
[[autodoc]] CLIPVisionConfig
## CLIPTokenizer
[[autodoc]] CLIPTokenizer
- build_inputs_with_special_tokens
- get_special_tokens_mask
- create_token_type_ids_from_sequences
- save_vocabulary
## CLIPTokenizerFast
[[autodoc]] CLIPTokenizerFast
## CLIPImageProcessor
[[autodoc]] CLIPImageProcessor
- preprocess
## CLIPFeatureExtractor
[[autodoc]] CLIPFeatureExtractor
## CLIPProcessor
[[autodoc]] CLIPProcessor
<frameworkcontent>
<pt>
## CLIPModel
[[autodoc]] CLIPModel
- forward
- get_text_features
- get_image_features
## CLIPTextModel
[[autodoc]] CLIPTextModel
- forward
## CLIPTextModelWithProjection
[[autodoc]] CLIPTextModelWithProjection
- forward
## CLIPVisionModelWithProjection
[[autodoc]] CLIPVisionModelWithProjection
- forward
## CLIPVisionModel
[[autodoc]] CLIPVisionModel
- forward
</pt>
<tf>
## TFCLIPModel
[[autodoc]] TFCLIPModel
- call
- get_text_features
- get_image_features
## TFCLIPTextModel
[[autodoc]] TFCLIPTextModel
- call
## TFCLIPVisionModel
[[autodoc]] TFCLIPVisionModel
- call
</tf>
<jax>
## FlaxCLIPModel
[[autodoc]] FlaxCLIPModel
- __call__
- get_text_features
- get_image_features
## FlaxCLIPTextModel
[[autodoc]] FlaxCLIPTextModel
- __call__
## FlaxCLIPTextModelWithProjection
[[autodoc]] FlaxCLIPTextModelWithProjection
- __call__
## FlaxCLIPVisionModel
[[autodoc]] FlaxCLIPVisionModel
- __call__
</jax>
</frameworkcontent>
| transformers/docs/source/ja/model_doc/clip.md/0 | {
"file_path": "transformers/docs/source/ja/model_doc/clip.md",
"repo_id": "transformers",
"token_count": 4545
} | 268 |
<!--Copyright 2022 The HuggingFace Team. All rights reserved.
Licensed under the Apache License, Version 2.0 (the "License"); you may not use this file except in compliance with
the License. You may obtain a copy of the License at
http://www.apache.org/licenses/LICENSE-2.0
Unless required by applicable law or agreed to in writing, software distributed under the License is distributed on
an "AS IS" BASIS, WITHOUT WARRANTIES OR CONDITIONS OF ANY KIND, either express or implied. See the License for the
specific language governing permissions and limitations under the License.
⚠️ Note that this file is in Markdown but contain specific syntax for our doc-builder (similar to MDX) that may not be
rendered properly in your Markdown viewer.
-->
# Decision Transformer
## Overview
Decision Transformer モデルは、[Decision Transformer: Reinforcement Learning via Sequence Modeling](https://arxiv.org/abs/2106.01345) で提案されました。
Lili Chen, Kevin Lu, Aravind Rajeswaran, Kimin Lee, Aditya Grover, Michael Laskin, Pieter Abbeel, Aravind Srinivas, Igor Mordatch.
論文の要約は次のとおりです。
*強化学習(RL)をシーケンスモデリング問題として抽象化するフレームワークを紹介します。
これにより、Transformer アーキテクチャのシンプルさとスケーラビリティ、および関連する進歩を活用できるようになります。
GPT-x や BERT などの言語モデリングで。特に、Decision Transformer というアーキテクチャを紹介します。
RL の問題を条件付きシーケンス モデリングとして投げかけます。値関数に適合する以前の RL アプローチとは異なり、
ポリシー勾配を計算すると、Decision Transformer は因果的にマスクされたアルゴリズムを利用して最適なアクションを出力するだけです。
変成器。望ましいリターン (報酬)、過去の状態、アクションに基づいて自己回帰モデルを条件付けすることにより、
Decision Transformer モデルは、望ましいリターンを達成する将来のアクションを生成できます。そのシンプルさにも関わらず、
Decision Transformer は、最先端のモデルフリーのオフライン RL ベースラインのパフォーマンスと同等、またはそれを超えています。
Atari、OpenAI Gym、Key-to-Door タスク*
このバージョンのモデルは、状態がベクトルであるタスク用です。
このモデルは、[edbeeching](https://huggingface.co/edbeeching) によって提供されました。元のコードは [ここ](https://github.com/kzl/decion-transformer) にあります。
## DecisionTransformerConfig
[[autodoc]] DecisionTransformerConfig
## DecisionTransformerGPT2Model
[[autodoc]] DecisionTransformerGPT2Model
- forward
## DecisionTransformerModel
[[autodoc]] DecisionTransformerModel
- forward
| transformers/docs/source/ja/model_doc/decision_transformer.md/0 | {
"file_path": "transformers/docs/source/ja/model_doc/decision_transformer.md",
"repo_id": "transformers",
"token_count": 1073
} | 269 |
<!--Copyright 2023 The HuggingFace Team. All rights reserved.
Licensed under the Apache License, Version 2.0 (the "License"); you may not use this file except in compliance with
the License. You may obtain a copy of the License at
http://www.apache.org/licenses/LICENSE-2.0
Unless required by applicable law or agreed to in writing, software distributed under the License is distributed on
an "AS IS" BASIS, WITHOUT WARRANTIES OR CONDITIONS OF ANY KIND, either express or implied. See the License for the
⚠️ Note that this file is in Markdown but contain specific syntax for our doc-builder (similar to MDX) that may not be
rendered properly in your Markdown viewer.
-->
# Efficient Inference on a Multiple GPUs
この文書には、複数のGPUで効率的に推論を行う方法に関する情報が含まれています。
<Tip>
注意: 複数のGPUセットアップは、[単一のGPUセクション](./perf_infer_gpu_one)で説明されているほとんどの戦略を使用できます。ただし、より良い使用法のために使用できる簡単なテクニックについても認識しておく必要があります。
</Tip>
## Flash Attention 2
Flash Attention 2の統合は、複数のGPUセットアップでも機能します。詳細については、[単一のGPUセクション](./perf_infer_gpu_one#Flash-Attention-2)の適切なセクションをご覧ください。
## BetterTransformer
[BetterTransformer](https://huggingface.co/docs/optimum/bettertransformer/overview)は、🤗 TransformersモデルをPyTorchネイティブの高速実行パスを使用するように変換し、その下でFlash Attentionなどの最適化されたカーネルを呼び出します。
BetterTransformerは、テキスト、画像、音声モデルの単一GPUおよび複数GPUでの高速推論もサポートしています。
<Tip>
Flash Attentionは、fp16またはbf16 dtypeを使用しているモデルにのみ使用できます。BetterTransformerを使用する前に、モデルを適切なdtypeにキャストしてください。
</Tip>
### Decoder models
テキストモデル、特にデコーダーベースのモデル(GPT、T5、Llamaなど)の場合、BetterTransformer APIはすべての注意操作を[`torch.nn.functional.scaled_dot_product_attention`オペレーター](https://pytorch.org/docs/master/generated/torch.nn.functional.scaled_dot_product_attention)(SDPA)を使用するように変換します。これはPyTorch 2.0以降でのみ使用可能です。
モデルをBetterTransformerに変換するには:
```python
from transformers import AutoModelForCausalLM
model = AutoModelForCausalLM.from_pretrained("facebook/opt-350m")
# convert the model to BetterTransformer
model.to_bettertransformer()
# Use it for training or inference
```
SDPAは、ハードウェアや問題のサイズなどの特定の設定で[Flash Attention](https://arxiv.org/abs/2205.14135)カーネルを呼び出すこともできます。Flash Attentionを有効にするか、特定の設定(ハードウェア、問題のサイズ)で利用可能かを確認するには、[`torch.backends.cuda.sdp_kernel`](https://pytorch.org/docs/master/backends.html#torch.backends.cuda.sdp_kernel)をコンテキストマネージャとして使用します。
```diff
import torch
from transformers import AutoModelForCausalLM, AutoTokenizer
tokenizer = AutoTokenizer.from_pretrained("facebook/opt-350m")
model = AutoModelForCausalLM.from_pretrained("facebook/opt-350m").to("cuda")
# convert the model to BetterTransformer
model.to_bettertransformer()
input_text = "Hello my dog is cute and"
inputs = tokenizer(input_text, return_tensors="pt").to("cuda")
+ with torch.backends.cuda.sdp_kernel(enable_flash=True, enable_math=False, enable_mem_efficient=False):
outputs = model.generate(**inputs)
print(tokenizer.decode(outputs[0], skip_special_tokens=True))
```
もしトレースバックで次のようなエラーメッセージが表示された場合:
```bash
RuntimeError: No available kernel. Aborting execution.
```
当日、Flash Attentionのカバレッジが広範囲である可能性があるPyTorch Nightlyバージョンを試すようにお勧めします。
```bash
pip3 install -U --pre torch torchvision torchaudio --index-url https://download.pytorch.org/whl/nightly/cu118
```
[このブログ投稿](https://pytorch.org/blog/out-of-the-box-acceleration/)をチェックして、BetterTransformer + SDPA APIで可能なことについて詳しく学びましょう。
### Encoder Models
推論中のエンコーダーモデルでは、BetterTransformerはエンコーダーレイヤーのforward呼び出しを、エンコーダーレイヤーの[`torch.nn.TransformerEncoderLayer`](https://pytorch.org/docs/stable/generated/torch.nn.TransformerEncoderLayer.html)の相当するものにディスパッチします。これにより、エンコーダーレイヤーの高速実装が実行されます。
`torch.nn.TransformerEncoderLayer`の高速実装はトレーニングをサポートしていないため、代わりに`torch.nn.functional.scaled_dot_product_attention`にディスパッチされます。これにより、ネストされたテンソルを活用しないFlash AttentionまたはMemory-Efficient Attentionの融合カーネルを使用できます。
BetterTransformerのパフォーマンスの詳細については、この[ブログ投稿](https://medium.com/pytorch/bettertransformer-out-of-the-box-performance-for-huggingface-transformers-3fbe27d50ab2)をご覧いただけます。また、エンコーダーモデル用のBetterTransformerについては、この[ブログ](https://pytorch.org/blog/a-better-transformer-for-fast-transformer-encoder-inference/)で詳しく学ぶことができます。
## Advanced usage: mixing FP4 (or Int8) and BetterTransformer
モデルの最良のパフォーマンスを得るために、上記で説明した異なる方法を組み合わせることができます。例えば、FP4ミックスプレシジョン推論+Flash Attentionを使用したBetterTransformerを組み合わせることができます。
```py
import torch
from transformers import AutoModelForCausalLM, AutoTokenizer, BitsAndBytesConfig
quantization_config = BitsAndBytesConfig(
load_in_4bit=True,
bnb_4bit_compute_dtype=torch.float16
)
tokenizer = AutoTokenizer.from_pretrained("facebook/opt-350m")
model = AutoModelForCausalLM.from_pretrained("facebook/opt-350m", quantization_config=quantization_config)
input_text = "Hello my dog is cute and"
inputs = tokenizer(input_text, return_tensors="pt").to("cuda")
with torch.backends.cuda.sdp_kernel(enable_flash=True, enable_math=False, enable_mem_efficient=False):
outputs = model.generate(**inputs)
print(tokenizer.decode(outputs[0], skip_special_tokens=True))
``` | transformers/docs/source/ja/perf_infer_gpu_many.md/0 | {
"file_path": "transformers/docs/source/ja/perf_infer_gpu_many.md",
"repo_id": "transformers",
"token_count": 2561
} | 270 |
<!---
Copyright 2023 The HuggingFace Team. All rights reserved.
Licensed under the Apache License, Version 2.0 (the "License");
you may not use this file except in compliance with the License.
You may obtain a copy of the License at
http://www.apache.org/licenses/LICENSE-2.0
Unless required by applicable law or agreed to in writing, software
distributed under the License is distributed on an "AS IS" BASIS,
WITHOUT WARRANTIES OR CONDITIONS OF ANY KIND, either express or implied.
See the License for the specific language governing permissions and
limitations under the License.
⚠️ Note that this file is in Markdown but contain specific syntax for our doc-builder (similar to MDX) that may not be
rendered properly in your Markdown viewer.
-->
# Checks on a Pull Request
🤗 Transformersリポジトリでプルリクエストを開くと、追加しているパッチが既存のものを壊していないことを確認するために、かなりの数のチェックが実行されます。これらのチェックには、次の4つのタイプがあります:
- 通常のテスト
- ドキュメンテーションのビルド
- コードとドキュメンテーションのスタイル
- リポジトリ全体の一貫性
このドキュメントでは、これらのさまざまなチェックとその背後にある理由、そしてそれらのいずれかがあなたのプルリクエストで失敗した場合のローカルでのデバッグ方法について説明します。
なお、理想的には、開発者用のインストールが必要です:
```bash
pip install transformers[dev]
```
または編集可能なインストールの場合:
```bash
pip install -e .[dev]
```
トランスフォーマーズのリポジトリ内で作業しています。トランスフォーマーズのオプションの依存関係の数が増えたため、すべてを取得できない可能性があります。開発用インストールが失敗した場合、作業しているディープラーニングフレームワーク(PyTorch、TensorFlow、および/またはFlax)をインストールし、次の手順を実行してください。
```bash
pip install transformers[quality]
```
または編集可能なインストールの場合:
```bash
pip install -e .[quality]
```
## Tests
`ci/circleci: run_tests_` で始まるすべてのジョブは、Transformersのテストスイートの一部を実行します。これらのジョブは、特定の環境でライブラリの一部に焦点を当てて実行されます。たとえば、`ci/circleci: run_tests_pipelines_tf` は、TensorFlowのみがインストールされた環境でパイプラインのテストを実行します。
テストスイートの一部のみが実行されるように注意してください。テストスイートは、変更前と変更後のPRのライブラリの違いを決定し、その違いに影響を受けるテストを選択するためのユーティリティが実行されます。このユーティリティは、ローカルで以下のコマンドを実行して実行できます:
```bash
python utils/tests_fetcher.py
```
1. リポジトリのルートからスクリプトを実行します。これは次のステップを実行します:
1. 差分内の各ファイルをチェックし、変更がコード内にあるか、コメントやドキュメンテーション文字列のみにあるかを確認します。実際のコード変更があるファイルのみを保持します。
2. 内部のマップを構築します。このマップは、ライブラリのソースコードの各ファイルが再帰的に影響を与えるすべてのファイルを提供します。モジュールAがモジュールBに影響を与えるとは、モジュールBがモジュールAをインポートする場合を指します。再帰的な影響を得るには、モジュールAからモジュールBへのモジュールのチェーンが必要で、各モジュールは前のモジュールをインポートする必要があります。
3. このマップをステップ1で収集したファイルに適用します。これにより、PRに影響を受けるモデルファイルのリストが得られます。
4. これらのファイルをそれに対応するテストファイルにマップし、実行するテストのリストを取得します。
2. スクリプトをローカルで実行する場合、ステップ1、3、および4の結果が表示され、実行するテストがわかります。スクリプトはまた、`test_list.txt` という名前のファイルを作成します。このファイルには実行するテストのリストが含まれており、次のコマンドでローカルで実行できます:
```bash
python -m pytest -n 8 --dist=loadfile -rA -s $(cat test_list.txt)
```
## Documentation build
`build_pr_documentation` ジョブは、ドキュメンテーションのビルドを行い、あなたのPRがマージされた後にすべてが正常に表示されることを確認します。ボットがプレビューのドキュメンテーションへのリンクをPRに追加します。PRに対する変更は、プレビューに自動的に反映されます。ドキュメンテーションのビルドに失敗した場合、失敗したジョブの隣にある「詳細」をクリックして、何が問題になっているかを確認できます。多くの場合、問題は`toctree`内のファイルが不足しているなど、単純なものです。
ドキュメンテーションをローカルでビルドまたはプレビューしたい場合は、[docsフォルダ内の`README.md`](https://github.com/huggingface/transformers/tree/main/docs)をご覧ください。
## Code and documentation style
すべてのソースファイル、例、テストには、`black`と`ruff`を使用してコードのフォーマットが適用されます。また、ドックストリングと`rst`ファイルのフォーマット、Transformersの`__init__.py`ファイルで実行される遅延インポートの順序についてもカスタムツールが存在します(`utils/style_doc.py`と`utils/custom_init_isort.py`)。これらすべては、以下を実行することで起動できます。
```bash
make style
```
CIは、`ci/circleci: check_code_quality` チェック内でこれらのチェックが適用されていることを確認します。また、`ruff` を実行し、未定義の変数や使用されていない変数がある場合にエラーを報告します。このチェックをローカルで実行するには、以下のコマンドを使用してください。
```bash
make quality
```
時間がかかることがあります。したがって、現在のブランチで変更したファイルのみで同じことを実行するには、次のコマンドを実行します。
```bash
make fixup
```
この最後のコマンドは、リポジトリの整合性のためのすべての追加のチェックも実行します。それらを詳しく見てみましょう。
## Repository consistency
これには、あなたのPRがリポジトリを適切な状態に保ったままであることを確認するためのすべてのテストが含まれており、ci/`circleci: check_repository_consistency` チェックによって実行されます。ローカルでこのチェックを実行するには、以下を実行します。
```bash
make repo-consistency
```
これを確認します:
- `utils/check_repo.py` によって実行される、init に追加されたすべてのオブジェクトが文書化されています。
- `utils/check_inits.py` によって実行される、すべての `__init__.py` ファイルがその2つのセクションで同じ内容を持っています。
- `utils/check_copies.py` によって実行される、他のモジュールからのコピーとして識別されたすべてのコードが元のコードと一致しています。
- `utils/check_config_docstrings.py` によって実行される、すべての設定クラスには少なくとも1つの有効なチェックポイントがドキュメント文字列に記載されています。
- `utils/check_config_attributes.py` によって実行される、すべての設定クラスには、対応するモデリングファイルで使用されている属性のみが含まれています。
- `utils/check_copies.py` によって実行される、README とドキュメントのインデックスの翻訳が、メインのREADME と同じモデルリストを持っています。
- `utils/check_table.py` によって実行される、ドキュメンテーションの自動生成テーブルが最新であることを確認します。
- `utils/check_dummies.py` によって実行される、すべてのオブジェクトが利用可能であり、オプションの依存関係がすべてインストールされていなくても問題ありません。
このチェックが失敗する場合、最初の2つの項目は手動で修正する必要があり、最後の4つはコマンドを実行して自動的に修正できます。
```bash
make fix-copies
```
追加のチェックポイントは、新しいモデルを追加するPull Request(PR)に関連しています。主に次の点を確認します:
- すべての追加されたモデルは、Auto-mapping(`utils/check_repo.py`で実行)に含まれています。
<!-- TODO Sylvain、共通のテストが実装されていることを確認するチェックを追加してください。-->
- すべてのモデルが適切にテストされています(`utils/check_repo.py`で実行)。
<!-- TODO Sylvain、以下を追加してください
- すべてのモデルがメインのREADMEおよびメインのドキュメント内に追加されています。
- 使用されているすべてのチェックポイントが実際にHubに存在しています
-->
### Check copies
Transformersライブラリは、モデルコードに関して非常に意見があるため、各モデルは他のモデルに依存せずに完全に1つのファイルに実装する必要があります。したがって、特定のモデルのコードのコピーが元のコードと一貫しているかどうかを確認する仕組みを追加しました。これにより、バグ修正がある場合、他の影響を受けるモデルをすべて確認し、変更を伝達するかコピーを破棄するかを選択できます。
<Tip>
ファイルが別のファイルの完全なコピーである場合、それを`utils/check_copies.py`の`FULL_COPIES`定数に登録する必要があります。
</Tip>
この仕組みは、`# Copied from xxx`という形式のコメントに依存しています。`xxx`は、コピーされているクラスまたは関数の完全なパスを含む必要があります。例えば、`RobertaSelfOutput`は`BertSelfOutput`クラスの直接のコピーですので、[こちら](https://github.com/huggingface/transformers/blob/2bd7a27a671fd1d98059124024f580f8f5c0f3b5/src/transformers/models/roberta/modeling_roberta.py#L289)にコメントがあります。
```py
# Copied from transformers.models.bert.modeling_bert.BertSelfOutput
```
注意点として、これをクラス全体に適用する代わりに、コピー元の関連メソッドに適用できます。たとえば、[こちら](https://github.com/huggingface/transformers/blob/2bd7a27a671fd1d98059124024f580f8f5c0f3b5/src/transformers/models/roberta/modeling_roberta.py#L598)では、`RobertaPreTrainedModel._init_weights` が `BertPreTrainedModel` からコピーされており、以下のコメントがあります:
```py
# Copied from transformers.models.bert.modeling_bert.BertAttention with Bert->Roberta
```
注:矢印の周りにはスペースが含まれていてはいけません(もちろん、そのスペースが置換パターンの一部である場合を除きます)。
カンマで区切られた複数のパターンを追加できます。例えば、ここでは `CamemberForMaskedLM` は `RobertaForMaskedLM` の直接のコピーで、2つの置換があります: `Roberta` から `Camembert` へ、そして `ROBERTA` から `CAMEMBERT` へと置換されます。[こちら](https://github.com/huggingface/transformers/blob/15082a9dc6950ecae63a0d3e5060b2fc7f15050a/src/transformers/models/camembert/modeling_camembert.py#L929)で、この作業はコメント付きで行われています。
```py
# Copied from transformers.models.roberta.modeling_roberta.RobertaForMaskedLM with Roberta->Camembert, ROBERTA->CAMEMBERT
```
もし順序が重要な場合(以前の置換と競合する可能性があるため)、置換は左から右に実行されます。
<Tip>
もし置換がフォーマットを変更する場合(たとえば、短い名前を非常に長い名前に置き換える場合など)、自動フォーマッタを適用した後にコピーが確認されます。
</Tip>
パターンが同じ置換の異なるケース(大文字と小文字のバリアントがある)の場合、オプションとして `all-casing` を追加するだけの別の方法もあります。[こちら](https://github.com/huggingface/transformers/blob/15082a9dc6950ecae63a0d3e5060b2fc7f15050a/src/transformers/models/mobilebert/modeling_mobilebert.py#L1237)は、`MobileBertForSequenceClassification` 内の例で、コメントがついています。
```py
# Copied from transformers.models.bert.modeling_bert.BertForSequenceClassification with Bert->MobileBert all-casing
```
この場合、コードは「BertForSequenceClassification」からコピーされ、次のように置換されます:
- `Bert` を `MobileBert` に置き換える(例:`init`で `MobileBertModel` を使用する場合)
- `bert` を `mobilebert` に置き換える(例:`self.mobilebert` を定義する場合)
- `BERT` を `MOBILEBERT` に置き換える(定数 `MOBILEBERT_INPUTS_DOCSTRING` 内で)
| transformers/docs/source/ja/pr_checks.md/0 | {
"file_path": "transformers/docs/source/ja/pr_checks.md",
"repo_id": "transformers",
"token_count": 5982
} | 271 |
<!--Copyright 2023 The HuggingFace Team. All rights reserved.
Licensed under the Apache License, Version 2.0 (the "License"); you may not use this file except in compliance with
the License. You may obtain a copy of the License at
http://www.apache.org/licenses/LICENSE-2.0
Unless required by applicable law or agreed to in writing, software distributed under the License is distributed on
an "AS IS" BASIS, WITHOUT WARRANTIES OR CONDITIONS OF ANY KIND, either express or implied. See the License for the
specific language governing permissions and limitations under the License.
⚠️ Note that this file is in Markdown but contain specific syntax for our doc-builder (similar to MDX) that may not be
rendered properly in your Markdown viewer.
-->
# Monocular depth estimation
単眼奥行き推定は、シーンの奥行き情報を画像から予測することを含むコンピューター ビジョン タスクです。
単一の画像。言い換えれば、シーン内のオブジェクトの距離を距離から推定するプロセスです。
単一カメラの視点。
単眼奥行き推定には、3D 再構築、拡張現実、自動運転、
そしてロボット工学。モデルがオブジェクト間の複雑な関係を理解する必要があるため、これは困難な作業です。
シーンとそれに対応する深度情報(照明条件などの要因の影響を受ける可能性があります)
オクルージョンとテクスチャ。
<Tip>
このチュートリアルで説明するタスクは、次のモデル アーキテクチャでサポートされています。
<!--This tip is automatically generated by `make fix-copies`, do not fill manually!-->
[DPT](../model_doc/dpt), [GLPN](../model_doc/glpn)
<!--End of the generated tip-->
</Tip>
このガイドでは、次の方法を学びます。
* 深度推定パイプラインを作成する
* 手動で深度推定推論を実行します
始める前に、必要なライブラリがすべてインストールされていることを確認してください。
```bash
pip install -q transformers
```
## Depth estimation pipeline
深度推定をサポートするモデルで推論を試す最も簡単な方法は、対応する [`pipeline`] を使用することです。
[Hugging Face Hub のチェックポイント](https://huggingface.co/models?pipeline_tag=Depth-estimation&sort=downloads) からパイプラインをインスタンス化します。
```py
>>> from transformers import pipeline
>>> checkpoint = "vinvino02/glpn-nyu"
>>> depth_estimator = pipeline("depth-estimation", model=checkpoint)
```
次に、分析する画像を選択します。
```py
>>> from PIL import Image
>>> import requests
>>> url = "https://unsplash.com/photos/HwBAsSbPBDU/download?ixid=MnwxMjA3fDB8MXxzZWFyY2h8MzR8fGNhciUyMGluJTIwdGhlJTIwc3RyZWV0fGVufDB8MHx8fDE2Nzg5MDEwODg&force=true&w=640"
>>> image = Image.open(requests.get(url, stream=True).raw)
>>> image
```
<div class="flex justify-center">
<img src="https://huggingface.co/datasets/huggingface/documentation-images/resolve/main/transformers/tasks/depth-estimation-example.jpg" alt="Photo of a busy street"/>
</div>
画像をパイプラインに渡します。
```py
>>> predictions = depth_estimator(image)
```
パイプラインは 2 つのエントリを含む辞書を返します。最初のものは`predicted_ Depth`と呼ばれ、次の値を持つテンソルです。
深さは各ピクセルのメートル単位で表されます。
2 番目の`depth`は、深度推定結果を視覚化する PIL 画像です。
視覚化された結果を見てみましょう。
```py
>>> predictions["depth"]
```
<div class="flex justify-center">
<img src="https://huggingface.co/datasets/huggingface/documentation-images/resolve/main/transformers/tasks/depth-visualization.png" alt="Depth estimation visualization"/>
</div>
## Depth estimation inference by hand
深度推定パイプラインの使用方法を理解したので、同じ結果を手動で複製する方法を見てみましょう。
まず、[Hugging Face Hub のチェックポイント](https://huggingface.co/models?pipeline_tag=Depth-estimation&sort=downloads) からモデルと関連プロセッサをロードします。
ここでは、前と同じチェックポイントを使用します。
```py
>>> from transformers import AutoImageProcessor, AutoModelForDepthEstimation
>>> checkpoint = "vinvino02/glpn-nyu"
>>> image_processor = AutoImageProcessor.from_pretrained(checkpoint)
>>> model = AutoModelForDepthEstimation.from_pretrained(checkpoint)
```
必要な画像変換を処理する`image_processor`を使用して、モデルの画像入力を準備します。
サイズ変更や正規化など:
```py
>>> pixel_values = image_processor(image, return_tensors="pt").pixel_values
```
準備された入力をモデルに渡します。
```py
>>> import torch
>>> with torch.no_grad():
... outputs = model(pixel_values)
... predicted_depth = outputs.predicted_depth
```
結果を視覚化します。
```py
>>> import numpy as np
>>> # interpolate to original size
>>> prediction = torch.nn.functional.interpolate(
... predicted_depth.unsqueeze(1),
... size=image.size[::-1],
... mode="bicubic",
... align_corners=False,
... ).squeeze()
>>> output = prediction.numpy()
>>> formatted = (output * 255 / np.max(output)).astype("uint8")
>>> depth = Image.fromarray(formatted)
>>> depth
```
<div class="flex justify-center">
<img src="https://huggingface.co/datasets/huggingface/documentation-images/resolve/main/transformers/tasks/depth-visualization.png" alt="Depth estimation visualization"/>
</div>
| transformers/docs/source/ja/tasks/monocular_depth_estimation.md/0 | {
"file_path": "transformers/docs/source/ja/tasks/monocular_depth_estimation.md",
"repo_id": "transformers",
"token_count": 2274
} | 272 |
<!--Copyright 2023 The HuggingFace Team. All rights reserved.
Licensed under the Apache License, Version 2.0 (the "License"); you may not use this file except in compliance with
the License. You may obtain a copy of the License at
http://www.apache.org/licenses/LICENSE-2.0
Unless required by applicable law or agreed to in writing, software distributed under the License is distributed on
an "AS IS" BASIS, WITHOUT WARRANTIES OR CONDITIONS OF ANY KIND, either express or implied. See the License for the
specific language governing permissions and limitations under the License.
⚠️ Note that this file is in Markdown but contain specific syntax for our doc-builder (similar to MDX) that may not be
rendered properly in your Markdown viewer.
-->
# Testing
🤗 Transformersモデルがどのようにテストされ、新しいテストを書いて既存のテストを改善できるかを見てみましょう。
このリポジトリには2つのテストスイートがあります:
1. `tests` -- 一般的なAPI用のテスト
2. `examples` -- APIの一部ではないさまざまなアプリケーション用のテスト
## How transformers are tested
1. PRが提出されると、9つのCircleCiジョブでテストされます。PRへの新しいコミットごとに再テストされます。これらのジョブは、[この設定ファイル](https://github.com/huggingface/transformers/tree/main/.circleci/config.yml)で定義されており、必要な場合は同じ環境を自分のマシンで再現できます。
これらのCIジョブは `@slow` テストを実行しません。
2. [GitHub Actions](https://github.com/huggingface/transformers/actions)によって実行される3つのジョブがあります:
- [torch hub integration](https://github.com/huggingface/transformers/tree/main/.github/workflows/github-torch-hub.yml): torch hubの統合が動作するかどうかを確認します。
- [self-hosted (push)](https://github.com/huggingface/transformers/tree/main/.github/workflows/self-push.yml): `main` にコミットが行われた場合に、GPUで高速テストを実行します。このジョブは、`main` でのコミットが以下のフォルダーのコードを更新した場合にのみ実行されます:`src`、`tests`、`.github`(追加されたモデルカード、ノートブックなどの実行を防ぐため)。
- [self-hosted runner](https://github.com/huggingface/transformers/tree/main/.github/workflows/self-scheduled.yml): GPUで `tests` と `examples` の通常のテストと遅いテストを実行します。
```bash
RUN_SLOW=1 pytest tests/
RUN_SLOW=1 pytest examples/
```
結果は[here](https://github.com/huggingface/transformers/actions)で観察できます。
## Running tests
### Choosing which tests to run
このドキュメントは、テストを実行する方法の多くの詳細について説明しています。すべてを読んだ後でも、さらに詳細が必要な場合は、[こちら](https://docs.pytest.org/en/latest/usage.html)で見つけることができます。
以下は、テストを実行するためのいくつかの最も便利な方法です。
すべて実行します:
```console
pytest
```
または:
```bash
make test
```
後者は次のように定義されることに注意してください。
```bash
python -m pytest -n auto --dist=loadfile -s -v ./tests/
```
以下は、pytestに渡す設定情報です。
- テストプロセスをCPUコアの数と同じだけ実行するように指示します。ただし、RAMが十分でない場合は注意が必要です。
- 同じファイルからのすべてのテストは、同じテストプロセスで実行されるようにします。
- 出力のキャプチャを行いません。
- 冗長モードで実行します。
### Getting the list of all tests
テストスイートのすべてのテスト:
```bash
pytest --collect-only -q
```
指定されたテスト ファイルのすべてのテスト:
```bash
pytest tests/test_optimization.py --collect-only -q
```
### Run a specific test module
個別のテスト モジュールを実行するには:
```bash
pytest tests/utils/test_logging.py
```
### Run specific tests
ほとんどのテストでunittestが使用されているため、特定のサブテストを実行するには、それらのテストを含むunittestクラスの名前を知っている必要があります。例えば、それは次のようになるかもしれません:
```bash
pytest tests/test_optimization.py::OptimizationTest::test_adam_w
```
テストの実行方法:
テストファイル: `tests/test_optimization.py`
クラス名: `OptimizationTest`
テスト関数の名前: `test_adam_w`
ファイルに複数のクラスが含まれている場合は、特定のクラスのテストのみを実行することを選択できます。例えば:
```bash
pytest tests/test_optimization.py::OptimizationTest
```
テストクラス内のすべてのテストを実行します。
前述の通り、`OptimizationTest` クラスに含まれるテストを実行するには、次のコマンドを実行できます:
```bash
pytest tests/test_optimization.py::OptimizationTest --collect-only -q
```
キーワード式を使用してテストを実行できます。
名前に `adam` が含まれるテストのみを実行するには:
```bash
pytest -k adam tests/test_optimization.py
```
`and`および`or`は、すべてのキーワードが一致するか、いずれかを示すために使用できます。`not`は否定するために使用できます。
`adam`という名前を含むテストを除いてすべてのテストを実行するには:
```bash
pytest -k "not adam" tests/test_optimization.py
```
以下は、提供されたテキストの日本語訳です。
```bash
pytest -k "ada and not adam" tests/test_optimization.py
```
たとえば、`test_adafactor`と`test_adam_w`の両方を実行するには、以下のコマンドを使用できます:
```bash
pytest -k "test_adam_w or test_adam_w" tests/test_optimization.py
```
注意: ここでは、`or` を使用しています。キーワードのいずれか一つが一致すれば、両方を含めるためです。
両方のパターンを含むテストのみを含めたい場合は、`and` を使用してください。
```bash
pytest -k "test and ada" tests/test_optimization.py
```
### Run `accelerate` tests
時々、モデルに対して `accelerate` テストを実行する必要があります。たとえば、`OPT` 実行に対してこれらのテストを実行したい場合、コマンドに `-m accelerate_tests` を追加するだけで済みます:
```bash
RUN_SLOW=1 pytest -m accelerate_tests tests/models/opt/test_modeling_opt.py
```
### Run documentation tests
ドキュメンテーションの例が正しいかどうかをテストするには、`doctests` が合格しているかを確認する必要があります。
例として、[`WhisperModel.forward` のドックストリング](https://github.com/huggingface/transformers/blob/main/src/transformers/models/whisper/modeling_whisper.py#L1017-L1035)を使用しましょう。
```python
r"""
Returns:
Example:
```python
>>> import torch
>>> from transformers import WhisperModel, WhisperFeatureExtractor
>>> from datasets import load_dataset
>>> model = WhisperModel.from_pretrained("openai/whisper-base")
>>> feature_extractor = WhisperFeatureExtractor.from_pretrained("openai/whisper-base")
>>> ds = load_dataset("hf-internal-testing/librispeech_asr_dummy", "clean", split="validation")
>>> inputs = feature_extractor(ds[0]["audio"]["array"], return_tensors="pt")
>>> input_features = inputs.input_features
>>> decoder_input_ids = torch.tensor([[1, 1]]) * model.config.decoder_start_token_id
>>> last_hidden_state = model(input_features, decoder_input_ids=decoder_input_ids).last_hidden_state
>>> list(last_hidden_state.shape)
[1, 2, 512]
```"""
```
指定したファイル内のすべてのドックストリング例を自動的にテストするために、以下の行を実行してください:
```bash
pytest --doctest-modules <path_to_file_or_dir>
```
ファイルにマークダウン拡張子がある場合は、`--doctest-glob="*.md"`引数を追加する必要があります。
### Run only modified tests
[pytest-picked](https://github.com/anapaulagomes/pytest-picked)を使用すると、未ステージングのファイルまたは現在のブランチ(Gitに従って)に関連するテストを実行できます。これは、変更内容に関連するテストのみ実行されるため、変更が何も壊れていないことを迅速に確認する素晴らしい方法です。変更されていないファイルに関連するテストは実行されません。
```bash
pip install pytest-picked
```
```bash
pytest --picked
```
すべてのテストは、変更されたがまだコミットされていないファイルとフォルダから実行されます。
### Automatically rerun failed tests on source modification
[pytest-xdist](https://github.com/pytest-dev/pytest-xdist)は、非常に便利な機能を提供しており、すべての失敗したテストを検出し、ファイルを修正する間にそれらの失敗したテストを連続して再実行することができます。そのため、修正を行った後にpytestを再起動する必要がありません。すべてのテストが合格するまで繰り返され、その後再度フルランが実行されます。
```bash
pip install pytest-xdist
```
モードに入るには: `pytest -f`または`pytest --looponfail`
ファイルの変更は、`looponfailroots`ルートディレクトリとその内容全体(再帰的に)を見て検出されます。この値のデフォルトが機能しない場合、`setup.cfg`で設定オプションを変更してプロジェクト内で変更できます。
```ini
[tool:pytest]
looponfailroots = transformers tests
```
または `pytest.ini`/`tox.ini` ファイル:
```ini
[pytest]
looponfailroots = transformers tests
```
ファイルの変更を探すことは、iniファイルのディレクトリを基準にして指定されたディレクトリ内でのみ行われます。
[pytest-watch](https://github.com/joeyespo/pytest-watch) は、この機能の代替実装です。
### Skip a test module
特定のテストモジュールを除外してすべてのテストモジュールを実行したい場合、実行するテストの明示的なリストを指定することができます。例えば、`test_modeling_*.py` テストを除外してすべてを実行するには次のようにします:
```bash
pytest *ls -1 tests/*py | grep -v test_modeling*
```
### Clearing state
CIビルドおよび速度に対する隔離が重要な場合(キャッシュに対して)、キャッシュをクリアする必要があります:
```bash
pytest --cache-clear tests
```
### Running tests in parallel
前述のように、`make test` は `pytest-xdist` プラグインを介してテストを並列実行します(`-n X` 引数、例: `-n 2` で2つの並列ジョブを実行)。
`pytest-xdist` の `--dist=` オプションを使用すると、テストがどのようにグループ化されるかを制御できます。`--dist=loadfile` は同じファイルにあるテストを同じプロセスに配置します。
テストの実行順序が異なり予測不可能であるため、`pytest-xdist` を使用してテストスイートを実行すると失敗が発生する場合(つまり、いくつかの未検出の連動テストがある場合)、[pytest-replay](https://github.com/ESSS/pytest-replay) を使用してテストを同じ順序で再生し、その後、失敗するシーケンスを最小限にするのに役立ちます。
### Test order and repetition
潜在的な相互依存性や状態に関連するバグ(ティアダウン)を検出するために、テストを複数回、連続して、ランダムに、またはセットで繰り返すことは有用です。そして、単純な複数回の繰り返しは、DLのランダム性によって明らかになるいくつかの問題を検出するのに役立ちます。
#### Repeat tests
- [pytest-flakefinder](https://github.com/dropbox/pytest-flakefinder):
```bash
pip install pytest-flakefinder
```
そして、すべてのテストを複数回実行します (デフォルトでは 50 回)。
```bash
pytest --flake-finder --flake-runs=5 tests/test_failing_test.py
```
<Tip>
このプラグインは、`pytest-xdist` の `-n` フラグでは動作しません。
</Tip>
<Tip>
別のプラグイン `pytest-repeat` もありますが、これは `unittest` では動作しません。
</Tip>
#### Run tests in a random order
```bash
pip install pytest-random-order
```
重要: `pytest-random-order` が存在すると、テストは自動的にランダム化されます。設定の変更や変更は必要ありません。
コマンドラインオプションは必須です。
前に説明したように、これにより、結合されたテスト (1 つのテストの状態が別のテストの状態に影響を与える) の検出が可能になります。いつ
`pytest-random-order` がインストールされていると、そのセッションに使用されたランダム シードが出力されます。例:
```bash
pytest tests
[...]
Using --random-order-bucket=module
Using --random-order-seed=573663
```
そのため、指定された特定のシーケンスが失敗した場合、その正確なシードを追加することでそれを再現できます。例:
```bash
pytest --random-order-seed=573663
[...]
Using --random-order-bucket=module
Using --random-order-seed=573663
```
特定のテストのリストを使用しない場合、またはまったくリストを使用しない場合、同じテストの正確な順序を再現します。テストのリストを手動で絞り込み始めると、シードに依存せず、テストが失敗した正確な順序で手動でリストを指定する必要があります。これには、`--random-order-bucket=none` を使用してランダム化を無効にするようpytestに指示する必要があります。例えば、次のようにします:
```bash
pytest --random-order-bucket=none tests/test_a.py tests/test_c.py tests/test_b.py
```
すべてのテストのシャッフルを無効にするには:
```bash
pytest --random-order-bucket=none
```
デフォルトでは、`--random-order-bucket=module` が暗黙的に適用され、モジュールレベルでファイルをシャッフルします。また、`class`、`package`、`global`、および`none` レベルでシャッフルすることもできます。詳細については、その[ドキュメンテーション](https://github.com/jbasko/pytest-random-order)を参照してください。
別のランダム化の代替手段は、[`pytest-randomly`](https://github.com/pytest-dev/pytest-randomly) です。このモジュールは非常に似た機能/インターフェースを持っていますが、`pytest-random-order` で利用可能なバケットモードを持っていません。インストール後に自動的に有効になるという同じ問題があります。
### Look and feel variations
#### pytest-sugar
[pytest-sugar](https://github.com/Frozenball/pytest-sugar) は、外観と操作性を向上させ、プログレスバーを追加し、即座に失敗したテストとアサーションを表示するプラグインです。インストール後に自動的にアクティブ化されます。
```bash
pip install pytest-sugar
```
これを使用せずにテストを実行するには、次を実行します。
```bash
pytest -p no:sugar
```
またはアンインストールします。
#### Report each sub-test name and its progress
`pytest` による単一またはグループのテストの場合 (`pip install pytest-pspec` の後):
```bash
pytest --pspec tests/test_optimization.py
```
#### Instantly shows failed tests
[pytest-instafail](https://github.com/pytest-dev/pytest-instafail) では、失敗とエラーが即座に表示されます。
テストセッションが終了するまで待機します。
```bash
pip install pytest-instafail
```
```bash
pytest --instafail
```
### To GPU or not to GPU
GPU が有効な設定で、CPU のみモードでテストするには、`CUDA_VISIBLE_DEVICES=""`を追加します。
```bash
CUDA_VISIBLE_DEVICES="" pytest tests/utils/test_logging.py
```
または、複数の GPU がある場合は、`pytest` でどれを使用するかを指定できます。たとえば、
2 番目の GPU GPU `0` と `1` がある場合は、次を実行できます。
```bash
CUDA_VISIBLE_DEVICES="1" pytest tests/utils/test_logging.py
```
これは、異なるGPUで異なるタスクを実行したい場合に便利です。
一部のテストはCPUのみで実行する必要があり、他のテストはCPU、GPU、またはTPUで実行する必要があり、また別のテストは複数のGPUで実行する必要があります。次のスキップデコレーターは、テストのCPU/GPU/TPUに関する要件を設定するために使用されます:
- `require_torch` - このテストはtorchの下でのみ実行されます。
- `require_torch_gpu` - `require_torch` に加えて、少なくとも1つのGPUが必要です。
- `require_torch_multi_gpu` - `require_torch` に加えて、少なくとも2つのGPUが必要です。
- `require_torch_non_multi_gpu` - `require_torch` に加えて、0または1つのGPUが必要です。
- `require_torch_up_to_2_gpus` - `require_torch` に加えて、0、1、または2つのGPUが必要です。
- `require_torch_xla` - `require_torch` に加えて、少なくとも1つのTPUが必要です。
以下の表にGPUの要件を示します:
| n gpus | decorator |
|--------+--------------------------------|
| `>= 0` | `@require_torch` |
| `>= 1` | `@require_torch_gpu` |
| `>= 2` | `@require_torch_multi_gpu` |
| `< 2` | `@require_torch_non_multi_gpu` |
| `< 3` | `@require_torch_up_to_2_gpus` |
たとえば、使用可能な GPU が 2 つ以上あり、pytorch がインストールされている場合にのみ実行する必要があるテストを次に示します。
```python no-style
@require_torch_multi_gpu
def test_example_with_multi_gpu():
```
テストに `tensorflow` が必要な場合は、`require_tf` デコレータを使用します。例えば:
```python no-style
@require_tf
def test_tf_thing_with_tensorflow():
```
これらのデコレータは積み重ねることができます。たとえば、テストが遅く、pytorch で少なくとも 1 つの GPU が必要な場合は、次のようになります。
設定方法:
```python no-style
@require_torch_gpu
@slow
def test_example_slow_on_gpu():
```
`@parametrized` のような一部のデコレータはテスト名を書き換えるため、`@require_*` スキップ デコレータをリストする必要があります。
最後にそれらが正しく動作するようにします。正しい使用例は次のとおりです
```python no-style
@parameterized.expand(...)
@require_torch_multi_gpu
def test_integration_foo():
```
この順序の問題は `@pytest.mark.parametrize` には存在しません。最初または最後に配置しても、それでも問題は解決されます。
仕事。ただし、それは非単体テストでのみ機能します。
内部テスト:
- 利用可能な GPU の数:
```python
from transformers.testing_utils import get_gpu_count
n_gpu = get_gpu_count() # works with torch and tf
```
### Testing with a specific PyTorch backend or device
特定のtorchデバイスでテストスイートを実行するには、`TRANSFORMERS_TEST_DEVICE="$device"` を追加します。ここで `$device` は対象のバックエンドです。例えば、CPUでテストするには以下のようにします:
```bash
TRANSFORMERS_TEST_DEVICE="cpu" pytest tests/utils/test_logging.py
```
この変数は、`mps`などのカスタムまたはあまり一般的ではない PyTorch バックエンドをテストするのに役立ちます。また、特定の GPU をターゲットにしたり、CPU 専用モードでテストしたりすることで、`CUDA_VISIBLE_DEVICES`と同じ効果を達成するために使用することもできます。
特定のデバイスでは、初めて「torch」をインポートした後、追加のインポートが必要になります。これは、環境変数 `TRANSFORMERS_TEST_BACKEND` を使用して指定できます。
```bash
TRANSFORMERS_TEST_BACKEND="torch_npu" pytest tests/utils/test_logging.py
```
### Distributed training
`pytest` は直接的に分散トレーニングを処理することはできません。試みると、サブプロセスは正しい処理を行わず、自分自身が `pytest` であると思い込んでテストスイートをループで実行し続けます。ただし、通常のプロセスを生成し、それから複数のワーカーを生成し、IOパイプを管理するプロセスを生成すれば機能します。
これを使用するいくつかのテストがあります:
- [test_trainer_distributed.py](https://github.com/huggingface/transformers/tree/main/tests/trainer/test_trainer_distributed.py)
- [test_deepspeed.py](https://github.com/huggingface/transformers/tree/main/tests/deepspeed/test_deepspeed.py)
実行ポイントにすぐに移動するには、これらのテスト内で `execute_subprocess_async` 呼び出しを検索してください。
これらのテストを実行するには、少なくとも2つのGPUが必要です:
```bash
CUDA_VISIBLE_DEVICES=0,1 RUN_SLOW=1 pytest -sv tests/test_trainer_distributed.py
```
### Output capture
テストの実行中に、`stdout` および `stderr` に送信された出力はキャプチャされます。テストまたはセットアップメソッドが失敗した場合、通常、それに対応するキャプチャされた出力が失敗のトレースバックと共に表示されます。
出力のキャプチャを無効にし、`stdout` と `stderr` を通常通りに取得するには、`-s` または `--capture=no` を使用してください:
これらのテストを実行するには少なくとも2つのGPUが必要です:
```bash
pytest -s tests/utils/test_logging.py
```
テスト結果を JUnit 形式の出力に送信するには:
```bash
py.test tests --junitxml=result.xml
```
### Color control
色を持たないようにする(例:黄色のテキストを白い背景に表示すると読みにくいです):
```bash
pytest --color=no tests/utils/test_logging.py
```
### Sending test report to online pastebin service
テスト失敗ごとに URL を作成します。
```bash
pytest --pastebin=failed tests/utils/test_logging.py
```
これにより、テスト実行情報がリモートのPasteサービスに送信され、各エラーに対してURLが提供されます。通常通りテストを選択するか、たとえば特定のエラーのみを送信したい場合は `-x` を追加で指定できます。
テストセッション全体のログに対するURLを作成する方法:
```bash
pytest --pastebin=all tests/utils/test_logging.py
```
## Writing tests
🤗 transformersのテストは `unittest` を基にしていますが、 `pytest` で実行されるため、ほとんどの場合、両方のシステムの機能を使用できます。
[こちら](https://docs.pytest.org/en/stable/unittest.html)でサポートされている機能を読むことができますが、重要なことは、ほとんどの `pytest` のフィクスチャが動作しないことです。パラメータ化も同様ですが、似たような方法で動作する `parameterized` モジュールを使用しています。
### Parametrization
同じテストを異なる引数で複数回実行する必要があることがよくあります。これはテスト内部から行うこともできますが、その場合、そのテストを単一の引数セットで実行する方法はありません。
```python
# test_this1.py
import unittest
from parameterized import parameterized
class TestMathUnitTest(unittest.TestCase):
@parameterized.expand(
[
("negative", -1.5, -2.0),
("integer", 1, 1.0),
("large fraction", 1.6, 1),
]
)
def test_floor(self, name, input, expected):
assert_equal(math.floor(input), expected)
```
デフォルトでは、このテストは3回実行され、それぞれの実行で `test_floor` の最後の3つの引数がパラメータリストの対応する引数に割り当てられます。
そして、`negative` と `integer` パラメータのセットのみを実行することもできます:
```bash
pytest -k "negative and integer" tests/test_mytest.py
```
または、`Negative`のサブテストを除くすべての場合、次のようになります。
```bash
pytest -k "not negative" tests/test_mytest.py
```
`-k` フィルターを使用することに加えて、各サブテストの正確な名前を調べ、その正確な名前を使用して任意のサブテストまたはすべてのサブテストを実行することができます。
```bash
pytest test_this1.py --collect-only -q
```
すると次のものがリストされます:
```bash
test_this1.py::TestMathUnitTest::test_floor_0_negative
test_this1.py::TestMathUnitTest::test_floor_1_integer
test_this1.py::TestMathUnitTest::test_floor_2_large_fraction
```
したがって、2 つの特定のサブテストのみを実行できるようになりました。
```bash
pytest test_this1.py::TestMathUnitTest::test_floor_0_negative test_this1.py::TestMathUnitTest::test_floor_1_integer
```
`transformers`の開発者依存関係にすでに含まれているモジュール[parameterized](https://pypi.org/project/parameterized/) は、`unittests` と `pytest` テストの両方で機能します。
ただし、テストが `unittest` でない場合、`pytest.mark.parametrize` を使用することができます(または既存のテストのいくつかで、主に `examples` の下で使用されているのを見ることができます)。
次に、同じ例を示しますが、今度は `pytest` の `parametrize` マーカーを使用しています:
```python
# test_this2.py
import pytest
@pytest.mark.parametrize(
"name, input, expected",
[
("negative", -1.5, -2.0),
("integer", 1, 1.0),
("large fraction", 1.6, 1),
],
)
def test_floor(name, input, expected):
assert_equal(math.floor(input), expected)
```
`parameterized` と同様に、`pytest.mark.parametrize` を使用すると、`-k` フィルタが役立たない場合でも、サブテストの実行を細かく制御できます。ただし、このパラメータ化関数はサブテストの名前をわずかに異なるものにします。以下にその例を示します:
```bash
pytest test_this2.py --collect-only -q
```
すると次のものがリストされます:
```bash
test_this2.py::test_floor[integer-1-1.0]
test_this2.py::test_floor[negative--1.5--2.0]
test_this2.py::test_floor[large fraction-1.6-1]
```
これで、特定のテストのみを実行できるようになりました。
```bash
pytest test_this2.py::test_floor[negative--1.5--2.0] test_this2.py::test_floor[integer-1-1.0]
```
前の例と同様に。
### Files and directories
テストの中で、現在のテストファイルからの相対位置を知る必要があることがよくあります。しかし、これは簡単なことではありません。なぜなら、テストは複数のディレクトリから呼び出されるか、異なる深さのサブディレクトリに存在することがあるからです。`transformers.test_utils.TestCasePlus` というヘルパークラスは、すべての基本パスを整理し、簡単にアクセスできるようにすることで、この問題を解決します。
- `pathlib` オブジェクト(すべて完全に解決されたもの):
- `test_file_path` - 現在のテストファイルのパス、つまり `__file__`
- `test_file_dir` - 現在のテストファイルを含むディレクトリ
- `tests_dir` - `tests` テストスイートのディレクトリ
- `examples_dir` - `examples` テストスイートのディレクトリ
- `repo_root_dir` - リポジトリのディレクトリ
- `src_dir` - `transformers` サブディレクトリが存在する場所
- パスの文字列表現――上記と同じですが、これらは `pathlib` オブジェクトではなく文字列としてパスを返します:
- `test_file_path_str`
- `test_file_dir_str`
- `tests_dir_str`
- `examples_dir_str`
- `repo_root_dir_str`
- `src_dir_str`
これらを使用し始めるには、テストが `transformers.test_utils.TestCasePlus` のサブクラスに存在することを確認するだけです。例:
```python
from transformers.testing_utils import TestCasePlus
class PathExampleTest(TestCasePlus):
def test_something_involving_local_locations(self):
data_dir = self.tests_dir / "fixtures/tests_samples/wmt_en_ro"
```
もし、`pathlib` を介してパスを操作する必要がない場合、または単に文字列としてパスが必要な場合は、`pathlib` オブジェクトに `str()` を呼び出すか、`_str` で終わるアクセサを使用できます。例:
```python
from transformers.testing_utils import TestCasePlus
class PathExampleTest(TestCasePlus):
def test_something_involving_stringified_locations(self):
examples_dir = self.examples_dir_str
```
### Temporary files and directories
一意の一時ファイルとディレクトリの使用は、並列テストの実行には欠かせません。これにより、テストがお互いのデータを上書きしないようにします。また、これらを作成した各テストの終了時に一時ファイルとディレクトリが削除されることを望みます。そのため、これらのニーズを満たすパッケージである `tempfile` のようなパッケージの使用は重要です。
しかし、テストのデバッグ時には、一時ファイルやディレクトリに何が格納されているかを確認できる必要があり、テストを再実行するたびにランダムに変更されないその正確なパスを知りたいと思います。
`transformers.test_utils.TestCasePlus` というヘルパークラスは、このような目的に最適です。これは `unittest.TestCase` のサブクラスであるため、テストモジュールで簡単に継承することができます。
以下はその使用例です:
```python
from transformers.testing_utils import TestCasePlus
class ExamplesTests(TestCasePlus):
def test_whatever(self):
tmp_dir = self.get_auto_remove_tmp_dir()
```
このコードはユニークな一時ディレクトリを作成し、`tmp_dir` をその場所に設定します。
- ユニークな一時ディレクトリを作成します:
```python
def test_whatever(self):
tmp_dir = self.get_auto_remove_tmp_dir()
```
`tmp_dir` には、作成された一時ディレクトリへのパスが含まれます。期間終了後は自動的に削除されます
テスト。
- 任意の一時ディレクトリを作成し、テストの開始前にそれが空であることを確認し、テスト後には空にしないでください。
```python
def test_whatever(self):
tmp_dir = self.get_auto_remove_tmp_dir("./xxx")
```
これは、特定のディレクトリを監視し、前のテストがそこにデータを残さないことを確認したい場合に、デバッグに役立ちます。
- `before` と `after` 引数を直接オーバーライドすることで、デフォルトの動作をオーバーライドできます。以下のいずれかの動作に導きます:
- `before=True`:テストの開始時に常に一時ディレクトリがクリアされます。
- `before=False`:一時ディレクトリが既に存在する場合、既存のファイルはそのままになります。
- `after=True`:テストの終了時に常に一時ディレクトリが削除されます。
- `after=False`:テストの終了時に常に一時ディレクトリはそのままになります。
<Tip>
`rm -r`の相当を安全に実行するために、明示的な `tmp_dir` が使用される場合、プロジェクトリポジトリのチェックアウトのサブディレクトリのみが許可されます。誤って `/tmp` などのファイルシステムの重要な部分が削除されないように、常に `./` から始まるパスを渡してください。
</Tip>
<Tip>
各テストは複数の一時ディレクトリを登録でき、要求がない限りすべて自動で削除されます。
</Tip>
### Temporary sys.path override
別のテストからインポートするために一時的に `sys.path` をオーバーライドする必要がある場合、`ExtendSysPath` コンテキストマネージャを使用できます。例:
```python
import os
from transformers.testing_utils import ExtendSysPath
bindir = os.path.abspath(os.path.dirname(__file__))
with ExtendSysPath(f"{bindir}/.."):
from test_trainer import TrainerIntegrationCommon # noqa
```
### Skipping tests
これは、バグが見つかり、新しいテストが作成された場合であっても、バグがまだ修正されていない場合に役立ちます。メインリポジトリにコミットできるようにするには、`make test` の実行中にそれをスキップする必要があります。
メソッド:
- **skip** は、テストが特定の条件が満たされた場合にのみパスすることを期待しており、それ以外の場合は pytest がテストの実行をスキップします。一般的な例は、Windows専用のテストを非Windowsプラットフォームでスキップする場合、または現在利用できない外部リソースに依存するテストをスキップする場合です(例: データベースが利用できない場合)。
- **xfail** は、何らかの理由でテストが失敗することを期待しています。一般的な例は、まだ実装されていない機能のテストや、まだ修正されていないバグのテストです。テストが予想される失敗にもかかわらずパスした場合(pytest.mark.xfailでマークされたテスト)、それはxpassとしてテストサマリーに報告されます。
これらの2つの間の重要な違いの1つは、`skip` はテストを実行しない点であり、`xfail` は実行します。したがって、バグのあるコードが他のテストに影響を与える場合は、`xfail` を使用しないでください。
#### Implementation
- テスト全体を無条件にスキップする方法は次のとおりです:
```python no-style
@unittest.skip("this bug needs to be fixed")
def test_feature_x():
```
または pytest 経由:
```python no-style
@pytest.mark.skip(reason="this bug needs to be fixed")
```
または `xfail` の方法:
```python no-style
@pytest.mark.xfail
def test_feature_x():
```
- テスト内の内部チェックに基づいてテストをスキップする方法は次のとおりです。
```python
def test_feature_x():
if not has_something():
pytest.skip("unsupported configuration")
```
またはモジュール全体:
```python
import pytest
if not pytest.config.getoption("--custom-flag"):
pytest.skip("--custom-flag is missing, skipping tests", allow_module_level=True)
```
または `xfail` の方法:
```python
def test_feature_x():
pytest.xfail("expected to fail until bug XYZ is fixed")
```
- 一部のインポートが欠落している場合にモジュール内のすべてのテストをスキップする方法は次のとおりです。
```python
docutils = pytest.importorskip("docutils", minversion="0.3")
```
- 条件に基づいてテストをスキップします。
```python no-style
@pytest.mark.skipif(sys.version_info < (3,6), reason="requires python3.6 or higher")
def test_feature_x():
```
または:
```python no-style
@unittest.skipIf(torch_device == "cpu", "Can't do half precision")
def test_feature_x():
```
またはモジュール全体をスキップします。
```python no-style
@pytest.mark.skipif(sys.platform == 'win32', reason="does not run on windows")
class TestClass():
def test_feature_x(self):
```
詳細、例、および方法についての詳細は[こちら](https://docs.pytest.org/en/latest/skipping.html)を参照してください。
### Slow tests
テストライブラリは着実に成長しており、テストの一部は数分かかります。そのため、CIでテストスイートの完了を待つのは1時間待つ余裕がないことがあります。したがって、いくつかの例外を除いて、遅いテストは以下の例のようにマークすべきです:
```python no-style
from transformers.testing_utils import slow
@slow
def test_integration_foo():
```
テストが`@slow`としてマークされたら、そのようなテストを実行するには、環境変数 `RUN_SLOW=1`を設定します。例:
```bash
RUN_SLOW=1 pytest tests
```
`@parameterized` のようなデコレータはテスト名を書き換えるため、`@slow` および他のスキップデコレータ `@require_*` は正しく動作するためには、最後にリストアップする必要があります。以下は正しい使用例の一例です:
```python no-style
@parameterized.expand(...)
@slow
def test_integration_foo():
```
このドキュメントの冒頭で説明したように、遅いテストは定期的なスケジュールに従って実行され、PRのCIチェックでは実行されません。そのため、一部の問題がPRの提出時に見落とされ、マージされる可能性があります。そのような問題は次回のスケジュールされたCIジョブで検出されます。しかし、それはまた、PRを提出する前に自分のマシンで遅いテストを実行する重要性を意味しています。
どのテストを遅いテストとしてマークすべきかを選択するための、おおまかな意思決定メカニズムが次に示されています:
- テストがライブラリの内部コンポーネントの1つに焦点を当てている場合(例: モデリングファイル、トークン化ファイル、パイプライン)、そのテストは遅いテストスイートで実行する必要があります。それがライブラリの他の側面、たとえばドキュメンテーションや例に焦点を当てている場合、それらのテストは遅いテストスイートで実行する必要があります。そして、このアプローチを洗練させるために例外を設ける必要があります。
- 重いウェイトセットや約50MB以上のデータセットをダウンロードする必要があるすべてのテスト(例: モデル統合テスト、トークナイザ統合テスト、パイプライン統合テスト)は遅いテストとして設定する必要があります。新しいモデルを追加する場合、統合テスト用にランダムなウェイトを持つ小さなバージョンを作成し、ハブにアップロードする必要があります。これについては以下の段落で詳しく説明します。
- 特に高速化されていないトレーニングを行う必要があるすべてのテストは遅いテストとして設定する必要があります。
- 一部の「遅い」であるべきでないテストが非常に遅い場合、およびそれらを `@slow` として設定する必要がある場合には例外を導入できます。大容量のファイルをディスクに保存および読み込みする自動モデリングテストは、`@slow` としてマークされたテストの良い例です。
- CIで1秒未満でテストが完了する場合(ダウンロードを含む)、それは通常のテストであるべきです。
すべての非遅いテストは、さまざまな内部要素を完全にカバーする必要がありますが、高速である必要があります。たとえば、特別に作成された小さなモデル(レイヤー数が最小限で、語彙サイズが小さいなど)を使用して、かなりのカバレッジを実現できます。その後、`@slow` テストでは大規模な遅いモデルを使用して質的なテストを実行できます。これらを使用するには、以下のように *tiny* モデルを探してください:
```bash
grep tiny tests examples
```
[スクリプトの例](https://github.com/huggingface/transformers/tree/main/scripts/fsmt/fsmt-make-tiny-model.py)があり、これにより tiny-wmt19-en-de のような小さなモデルが作成されます。特定のモデルのアーキテクチャに簡単に調整できます。
実行時間を誤って測定することが簡単です。たとえば、巨大なモデルのダウンロードに関するオーバーヘッドがある場合、ローカルでテストするとダウンロードされたファイルがキャッシュされ、ダウンロード時間が計測されなくなります。したがって、CIログの実行速度レポート(`pytest --durations=0 tests` の出力)を確認してください。
このレポートは、遅いテストとしてマークされていない遅い外れ値や、高速に書き直す必要があるテストを見つけるのにも役立ちます。テストスイートがCIで遅くなり始めた場合、このレポートのトップリストには最も遅いテストが表示されます。
### Testing the stdout/stderr output
`stdout` および/または `stderr` に書き込む関数をテストするために、テストは `pytest` の [capsys システム](https://docs.pytest.org/en/latest/capture.html) を使用してこれらのストリームにアクセスできます。以下はその方法です:
```python
import sys
def print_to_stdout(s):
print(s)
def print_to_stderr(s):
sys.stderr.write(s)
def test_result_and_stdout(capsys):
msg = "Hello"
print_to_stdout(msg)
print_to_stderr(msg)
out, err = capsys.readouterr() # consume the captured output streams
# optional: if you want to replay the consumed streams:
sys.stdout.write(out)
sys.stderr.write(err)
# test:
assert msg in out
assert msg in err
```
そしてもちろん、ほとんどの場合、`stderr`は例外の一部として提供されるため、そのような場合には try/excel を使用する必要があります。
ケース:
```python
def raise_exception(msg):
raise ValueError(msg)
def test_something_exception():
msg = "Not a good value"
error = ""
try:
raise_exception(msg)
except Exception as e:
error = str(e)
assert msg in error, f"{msg} is in the exception:\n{error}"
```
stdout をキャプチャするもう 1 つのアプローチは、`contextlib.redirect_stdout`を使用することです。
```python
from io import StringIO
from contextlib import redirect_stdout
def print_to_stdout(s):
print(s)
def test_result_and_stdout():
msg = "Hello"
buffer = StringIO()
with redirect_stdout(buffer):
print_to_stdout(msg)
out = buffer.getvalue()
# optional: if you want to replay the consumed streams:
sys.stdout.write(out)
# test:
assert msg in out
```
stdout をキャプチャする際の重要な潜在的な問題は、通常の `print` でこれまでに出力された内容をリセットする可能性がある `\r` 文字が含まれている可能性があることです。`pytest` 自体には問題はありませんが、`pytest -s` ではこれらの文字がバッファに含まれるため、`-s` ありとなしでテストを実行できるようにするには、`re.sub(r'~.*\r', '', buf, 0, re.M)` を使用してキャプチャされた出力に対して追加のクリーンアップを行う必要があります。
しかし、その後、`\r` が含まれているかどうかにかかわらず、すべての操作を自動的に処理するヘルパーコンテキストマネージャラッパーがあります。したがって、次のように簡単に行えます:
```python
from transformers.testing_utils import CaptureStdout
with CaptureStdout() as cs:
function_that_writes_to_stdout()
print(cs.out)
```
完全なテスト例は次のとおりです。
```python
from transformers.testing_utils import CaptureStdout
msg = "Secret message\r"
final = "Hello World"
with CaptureStdout() as cs:
print(msg + final)
assert cs.out == final + "\n", f"captured: {cs.out}, expecting {final}"
```
`stderr` をキャプチャしたい場合は、代わりに `CaptureStderr` クラスを使用してください。
```python
from transformers.testing_utils import CaptureStderr
with CaptureStderr() as cs:
function_that_writes_to_stderr()
print(cs.err)
```
両方のストリームを一度にキャプチャする必要がある場合は、親の `CaptureStd` クラスを使用します。
```python
from transformers.testing_utils import CaptureStd
with CaptureStd() as cs:
function_that_writes_to_stdout_and_stderr()
print(cs.err, cs.out)
```
また、テストの問題のデバッグを支援するために、デフォルトで、これらのコンテキスト マネージャーは終了時にキャプチャされたストリームを自動的に再生します。
文脈から。
### Capturing logger stream
ロガーの出力を検証する必要がある場合は、`CaptureLogger`を使用できます。
```python
from transformers import logging
from transformers.testing_utils import CaptureLogger
msg = "Testing 1, 2, 3"
logging.set_verbosity_info()
logger = logging.get_logger("transformers.models.bart.tokenization_bart")
with CaptureLogger(logger) as cl:
logger.info(msg)
assert cl.out, msg + "\n"
```
### Testing with environment variables
特定のテストで環境変数の影響をテストしたい場合は、ヘルパー デコレータを使用できます。
`transformers.testing_utils.mockenv`
```python
from transformers.testing_utils import mockenv
class HfArgumentParserTest(unittest.TestCase):
@mockenv(TRANSFORMERS_VERBOSITY="error")
def test_env_override(self):
env_level_str = os.getenv("TRANSFORMERS_VERBOSITY", None)
```
場合によっては、外部プログラムを呼び出す必要があるため、`os.environ` に`PYTHONPATH`を設定してインクルードする必要があります。
複数のローカル パス。ヘルパー クラス `transformers.test_utils.TestCasePlus` が役に立ちます。
```python
from transformers.testing_utils import TestCasePlus
class EnvExampleTest(TestCasePlus):
def test_external_prog(self):
env = self.get_env()
# now call the external program, passing `env` to it
```
テストファイルが `tests` テストスイートまたは `examples` のどちらにあるかに応じて
`env[PYTHONPATH]` を使用して、これら 2 つのディレクトリのいずれかを含めます。また、テストが確実に行われるようにするための `src` ディレクトリも含めます。
現在のリポジトリに対して実行され、最後に、テストが実行される前にすでに設定されていた `env[PYTHONPATH]` を使用して実行されます。
何かあれば呼ばれます。
このヘルパー メソッドは `os.environ` オブジェクトのコピーを作成するため、元のオブジェクトはそのまま残ります。
### Getting reproducible results
状況によっては、テストのランダム性を削除したい場合があります。同一の再現可能な結果セットを取得するには、
シードを修正する必要があります:
```python
seed = 42
# python RNG
import random
random.seed(seed)
# pytorch RNGs
import torch
torch.manual_seed(seed)
torch.backends.cudnn.deterministic = True
if torch.cuda.is_available():
torch.cuda.manual_seed_all(seed)
# numpy RNG
import numpy as np
np.random.seed(seed)
# tf RNG
tf.random.set_seed(seed)
```
### Debugging tests
警告が発生した時点でデバッガーを開始するには、次の手順を実行します。
```bash
pytest tests/utils/test_logging.py -W error::UserWarning --pdb
```
## Working with github actions workflows
セルフプッシュのワークフローCIジョブをトリガーするには、以下の手順を実行する必要があります:
1. `transformers` のリモートリポジトリで新しいブランチを作成します(フォークではなく、元のリポジトリで行います)。
2. ブランチの名前は `ci_` または `ci-` で始まる必要があります(`main` もトリガーしますが、`main` ではPRを作成できません)。また、特定のパスでのみトリガーされます - このドキュメントが書かれた後に変更された場合に備えて、最新の定義は[こちら](https://github.com/huggingface/transformers/blob/main/.github/workflows/self-push.yml)の *push:* にあります。
3. このブランチからPRを作成します。
4. その後、このジョブが[ここ](https://github.com/huggingface/transformers/actions/workflows/self-push.yml)に表示されます。ジョブはバックログがある場合、すぐに実行されないことがあります。
## Testing Experimental CI Features
CI機能のテストは通常のCIの正常な動作に干渉する可能性があるため、新しいCI機能を追加する場合、以下の手順に従う必要があります。
1. テストが必要なものをテストするための新しい専用のジョブを作成します。
2. 新しいジョブは常に成功する必要があるため、常にグリーン ✓(詳細は以下参照)を表示する必要があります。
3. さまざまな種類のPR(ユーザーフォークブランチ、非フォークブランチ、github.com UIから直接ファイルを編集するブランチ、さまざまな強制プッシュなど)が実行されるまでいくつかの日間実行し、実験的なジョブのログを監視します(意図的に常にグリーンになるようになっている全体のジョブの緑ではなく)。
4. すべてが安定していることが明確になったら、新しい変更を既存のジョブに統合します。
このように、CI機能自体の実験が通常のワークフローに干渉しないようにできます。
では、新しいCI機能が開発中である間、ジョブを常に成功させるにはどうすればいいでしょうか?
TravisCIのような一部のCIは `ignore-step-failure` をサポートし、全体のジョブを成功として報告しますが、この文書が作成された時点ではCircleCIとGithub Actionsはそれをサポートしていません。
したがって、以下のワークアラウンドを使用できます:
1. bashスクリプト内で潜在的な失敗を抑制するために実行コマンドの冒頭に `set +euo pipefail` を記述します。
2. 最後のコマンドは成功する必要があります。たとえば `echo "done"` または単に `true` を使用できます。
以下は例です:
```yaml
- run:
name: run CI experiment
command: |
set +euo pipefail
echo "setting run-all-despite-any-errors-mode"
this_command_will_fail
echo "but bash continues to run"
# emulate another failure
false
# but the last command must be a success
echo "during experiment do not remove: reporting success to CI, even if there were failures"
```
単純なコマンドの場合は、次のようにすることもできます。
```bash
cmd_that_may_fail || true
```
もちろん、結果に満足したら、実験的なステップやジョブを通常のジョブと統合し、`set +euo pipefail` などの追加した要素を削除して、実験的なジョブが通常のCIの動作に干渉しないようにします。
このプロセス全体は、実験的なステップに対して `allow-failure` のようなものを設定し、PRの全体のステータスに影響を与えずに失敗させることができれば、はるかに簡単になったでしょう。しかし、前述の通り、現在はCircleCIとGithub Actionsはこの機能をサポートしていません。
この機能に関しての投票や、CIに特有のスレッドでその進捗状況を確認できます:
- [Github Actions:](https://github.com/actions/toolkit/issues/399)
- [CircleCI:](https://ideas.circleci.com/ideas/CCI-I-344)
| transformers/docs/source/ja/testing.md/0 | {
"file_path": "transformers/docs/source/ja/testing.md",
"repo_id": "transformers",
"token_count": 22732
} | 273 |
<!--Copyright 2020 The HuggingFace Team. All rights reserved.
Licensed under the Apache License, Version 2.0 (the "License"); you may not use this file except in compliance with
the License. You may obtain a copy of the License at
http://www.apache.org/licenses/LICENSE-2.0
Unless required by applicable law or agreed to in writing, software distributed under the License is distributed on
an "AS IS" BASIS, WITHOUT WARRANTIES OR CONDITIONS OF ANY KIND, either express or implied. See the License for the
specific language governing permissions and limitations under the License.
⚠️ Note that this file is in Markdown but contain specific syntax for our doc-builder (similar to MDX) that may not be
rendered properly in your Markdown viewer.
-->
# BERTology
BERT와 같은 대규모 트랜스포머의 내부 동작을 조사하는 연구 분야가 점점 더 중요해지고 있습니다.
혹자는 "BERTology"라 칭하기도 합니다. 이 분야의 좋은 예시는 다음과 같습니다:
- BERT는 고전적인 NLP 파이프라인의 재발견 - Ian Tenney, Dipanjan Das, Ellie Pavlick:
https://arxiv.org/abs/1905.05950
- 16개의 헤드가 정말로 1개보다 나은가? - Paul Michel, Omer Levy, Graham Neubig:
https://arxiv.org/abs/1905.10650
- BERT는 무엇을 보는가? BERT의 어텐션 분석 - Kevin Clark, Urvashi Khandelwal, Omer Levy, Christopher D. Manning:
https://arxiv.org/abs/1906.04341
- CAT-probing: 프로그래밍 언어에 대해 사전훈련된 모델이 어떻게 코드 구조를 보는지 알아보기 위한 메트릭 기반 접근 방법:
https://arxiv.org/abs/2210.04633
우리는 이 새로운 연구 분야의 발전을 돕기 위해, BERT/GPT/GPT-2 모델에 내부 표현을 살펴볼 수 있는 몇 가지 기능을 추가했습니다.
이 기능들은 주로 Paul Michel의 훌륭한 작업을 참고하여 개발되었습니다
(https://arxiv.org/abs/1905.10650):
- BERT/GPT/GPT-2의 모든 은닉 상태에 접근하기,
- BERT/GPT/GPT-2의 각 헤드의 모든 어텐션 가중치에 접근하기,
- 헤드의 출력 값과 그래디언트를 검색하여 헤드 중요도 점수를 계산하고 https://arxiv.org/abs/1905.10650에서 설명된 대로 헤드를 제거하는 기능을 제공합니다.
이러한 기능들을 이해하고 직접 사용해볼 수 있도록 [bertology.py](https://github.com/huggingface/transformers/tree/main/examples/research_projects/bertology/run_bertology.py) 예제 스크립트를 추가했습니다. 이 예제 스크립트에서는 GLUE에 대해 사전훈련된 모델에서 정보를 추출하고 모델을 가지치기(prune)해봅니다.
| transformers/docs/source/ko/bertology.md/0 | {
"file_path": "transformers/docs/source/ko/bertology.md",
"repo_id": "transformers",
"token_count": 1557
} | 274 |
<!---
Copyright 2021 The HuggingFace Team. All rights reserved.
Licensed under the Apache License, Version 2.0 (the "License");
you may not use this file except in compliance with the License.
You may obtain a copy of the License at
http://www.apache.org/licenses/LICENSE-2.0
Unless required by applicable law or agreed to in writing, software
distributed under the License is distributed on an "AS IS" BASIS,
WITHOUT WARRANTIES OR CONDITIONS OF ANY KIND, either express or implied.
See the License for the specific language governing permissions and
limitations under the License.
⚠️ Note that this file is in Markdown but contain specific syntax for our doc-builder (similar to MDX) that may not be
rendered properly in your Markdown viewer.
-->
# 성능 및 확장성 [[performance-and-scalability]]
점점 더 큰 규모의 트랜스포머 모델을 훈련하고 프로덕션에 배포하는 데에는 다양한 어려움이 따릅니다. 훈련 중에는 모델이 사용 가능한 GPU 메모리보다 더 많은 메모리를 필요로 하거나 훈련 속도가 매우 느릴 수 있으며, 추론을 위해 배포할 때는 제품 환경에서 요구되는 처리량으로 인해 과부하가 발생할 수 있습니다. 이 문서는 이러한 문제를 극복하고 사용 사례에 가장 적합한 설정을 찾도록 도움을 주기 위해 설계되었습니다. 훈련과 추론으로 가이드를 분할했는데, 이는 각각 다른 문제와 해결 방법이 있기 때문입니다. 그리고 각 가이드에는 다양한 종류의 하드웨어 설정에 대한 별도의 가이드가 있습니다(예: 훈련을 위한 단일 GPU vs 다중 GPU 또는 추론을 위한 CPU vs GPU).
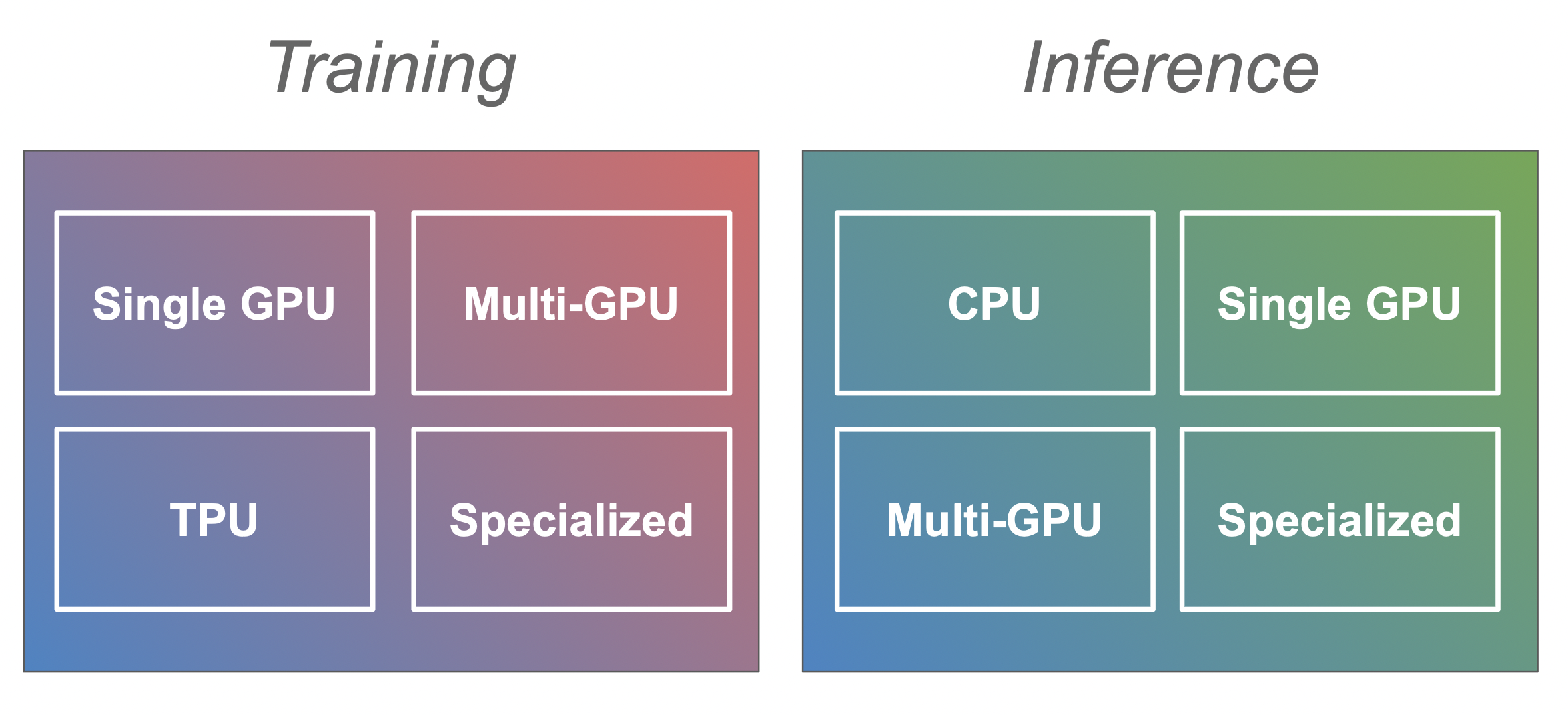
이 문서는 사용자의 상황에 유용할 수 있는 방법들에 대한 개요 및 시작점 역할을 합니다.
## 훈련 [[training]]
효율적인 트랜스포머 모델 훈련에는 GPU나 TPU와 같은 가속기가 필요합니다. 가장 일반적인 경우는 단일 GPU만 사용하는 경우지만, 다중 GPU 및 CPU 훈련에 대한 섹션도 있습니다(곧 더 많은 내용이 추가될 예정).
<Tip>
참고: 단일 GPU 섹션에서 소개된 대부분의 전략(예: 혼합 정밀도 훈련 또는 그라디언트 누적)은 일반적인 모델 훈련에도 적용되므로, 다중 GPU나 CPU 훈련과 같은 섹션을 살펴보기 전에 꼭 참고하시길 바랍니다.
</Tip>
### 단일 GPU [[single-gpu]]
단일 GPU에서 대규모 모델을 훈련하는 것은 어려울 수 있지만, 이를 가능하게 하는 여러 가지 도구와 방법이 있습니다. 이 섹션에서는 혼합 정밀도 훈련, 그라디언트 누적 및 체크포인팅, 효율적인 옵티마이저, 최적의 배치 크기를 결정하기 위한 전략 등에 대해 논의합니다.
[단일 GPU 훈련 섹션으로 이동](perf_train_gpu_one)
### 다중 GPU [[multigpu]]
단일 GPU에서 훈련하는 것이 너무 느리거나 대규모 모델에 적합하지 않은 경우도 있습니다. 다중 GPU 설정으로 전환하는 것은 논리적인 단계이지만, 여러 GPU에서 한 번에 훈련하려면 각 GPU마다 모델의 전체 사본을 둘지, 혹은 모델 자체도 여러 GPU에 분산하여 둘지 등 새로운 결정을 내려야 합니다. 이 섹션에서는 데이터, 텐서 및 파이프라인 병렬화에 대해 살펴봅니다.
[다중 GPU 훈련 섹션으로 이동](perf_train_gpu_many)
### CPU [[cpu]]
[CPU 훈련 섹션으로 이동](perf_train_cpu)
### TPU [[tpu]]
[_곧 제공될 예정_](perf_train_tpu)
### 특수한 하드웨어 [[specialized-hardware]]
[_곧 제공될 예정_](perf_train_special)
## 추론 [[inference]]
제품 및 서비스 환경에서 대규모 모델을 효율적으로 추론하는 것은 모델을 훈련하는 것만큼 어려울 수 있습니다. 이어지는 섹션에서는 CPU 및 단일/다중 GPU 설정에서 추론을 진행하는 단계를 살펴봅니다.
### CPU [[cpu]]
[CPU 추론 섹션으로 이동](perf_infer_cpu)
### 단일 GPU [[single-gpu]]
[단일 GPU 추론 섹션으로 이동](perf_infer_gpu_one)
### 다중 GPU [[multigpu]]
[다중 GPU 추론 섹션으로 이동](perf_infer_gpu_many)
### 특수한 하드웨어 [[specialized-hardware]]
[_곧 제공될 예정_](perf_infer_special)
## 하드웨어 [[hardware]]
하드웨어 섹션에서는 자신만의 딥러닝 장비를 구축할 때 유용한 팁과 요령을 살펴볼 수 있습니다.
[하드웨어 섹션으로 이동](perf_hardware)
## 기여하기 [[contribute]]
이 문서는 완성되지 않은 상태이며, 추가해야 할 내용이나 수정 사항이 많이 있습니다. 따라서 추가하거나 수정할 내용이 있으면 주저하지 말고 PR을 열어 주시거나, 자세한 내용을 논의하기 위해 Issue를 시작해 주시기 바랍니다.
A가 B보다 좋다고 하는 기여를 할 때는, 재현 가능한 벤치마크와/또는 해당 정보의 출처 링크를 포함해주세요(당신으로부터의 직접적인 정보가 아닌 경우). | transformers/docs/source/ko/performance.md/0 | {
"file_path": "transformers/docs/source/ko/performance.md",
"repo_id": "transformers",
"token_count": 3692
} | 275 |
<!--Copyright 2022 The HuggingFace Team. All rights reserved.
Licensed under the Apache License, Version 2.0 (the "License"); you may not use this file except in compliance with
the License. You may obtain a copy of the License at
http://www.apache.org/licenses/LICENSE-2.0
Unless required by applicable law or agreed to in writing, software distributed under the License is distributed on
an "AS IS" BASIS, WITHOUT WARRANTIES OR CONDITIONS OF ANY KIND, either express or implied. See the License for the
specific language governing permissions and limitations under the License.
⚠️ Note that this file is in Markdown but contain specific syntax for our doc-builder (similar to MDX) that may not be
rendered properly in your Markdown viewer.
-->
# 이미지 분류[[image-classification]]
[[open-in-colab]]
<Youtube id="tjAIM7BOYhw"/>
이미지 분류는 이미지에 레이블 또는 클래스를 할당합니다. 텍스트 또는 오디오 분류와 달리 입력은
이미지를 구성하는 픽셀 값입니다. 이미지 분류에는 자연재해 후 피해 감지, 농작물 건강 모니터링, 의료 이미지에서 질병의 징후 검사 지원 등
다양한 응용 사례가 있습니다.
이 가이드에서는 다음을 설명합니다:
1. [Food-101](https://huggingface.co/datasets/food101) 데이터 세트에서 [ViT](model_doc/vit)를 미세 조정하여 이미지에서 식품 항목을 분류합니다.
2. 추론을 위해 미세 조정 모델을 사용합니다.
<Tip>
이 튜토리얼에서 설명하는 작업은 다음 모델 아키텍처에 의해 지원됩니다:
<!--This tip is automatically generated by `make fix-copies`, do not fill manually!-->
[BEiT](../model_doc/beit), [BiT](../model_doc/bit), [ConvNeXT](../model_doc/convnext), [ConvNeXTV2](../model_doc/convnextv2), [CvT](../model_doc/cvt), [Data2VecVision](../model_doc/data2vec-vision), [DeiT](../model_doc/deit), [DiNAT](../model_doc/dinat), [EfficientFormer](../model_doc/efficientformer), [EfficientNet](../model_doc/efficientnet), [FocalNet](../model_doc/focalnet), [ImageGPT](../model_doc/imagegpt), [LeViT](../model_doc/levit), [MobileNetV1](../model_doc/mobilenet_v1), [MobileNetV2](../model_doc/mobilenet_v2), [MobileViT](../model_doc/mobilevit), [NAT](../model_doc/nat), [Perceiver](../model_doc/perceiver), [PoolFormer](../model_doc/poolformer), [RegNet](../model_doc/regnet), [ResNet](../model_doc/resnet), [SegFormer](../model_doc/segformer), [Swin Transformer](../model_doc/swin), [Swin Transformer V2](../model_doc/swinv2), [VAN](../model_doc/van), [ViT](../model_doc/vit), [ViT Hybrid](../model_doc/vit_hybrid), [ViTMSN](../model_doc/vit_msn)
<!--End of the generated tip-->
</Tip>
시작하기 전에, 필요한 모든 라이브러리가 설치되어 있는지 확인하세요:
```bash
pip install transformers datasets evaluate
```
Hugging Face 계정에 로그인하여 모델을 업로드하고 커뮤니티에 공유하는 것을 권장합니다. 메시지가 표시되면, 토큰을 입력하여 로그인하세요:
```py
>>> from huggingface_hub import notebook_login
>>> notebook_login()
```
## Food-101 데이터 세트 가져오기[[load-food101-dataset]]
🤗 Datasets 라이브러리에서 Food-101 데이터 세트의 더 작은 부분 집합을 가져오는 것으로 시작합니다. 이렇게 하면 전체 데이터 세트에 대한
훈련에 많은 시간을 할애하기 전에 실험을 통해 모든 것이 제대로 작동하는지 확인할 수 있습니다.
```py
>>> from datasets import load_dataset
>>> food = load_dataset("food101", split="train[:5000]")
```
데이터 세트의 `train`을 [`~datasets.Dataset.train_test_split`] 메소드를 사용하여 훈련 및 테스트 세트로 분할하세요:
```py
>>> food = food.train_test_split(test_size=0.2)
```
그리고 예시를 살펴보세요:
```py
>>> food["train"][0]
{'image': <PIL.JpegImagePlugin.JpegImageFile image mode=RGB size=512x512 at 0x7F52AFC8AC50>,
'label': 79}
```
데이터 세트의 각 예제에는 두 개의 필드가 있습니다:
- `image`: 식품 항목의 PIL 이미지
- `label`: 식품 항목의 레이블 클래스
모델이 레이블 ID에서 레이블 이름을 쉽게 가져올 수 있도록
레이블 이름을 정수로 매핑하고, 정수를 레이블 이름으로 매핑하는 사전을 만드세요:
```py
>>> labels = food["train"].features["label"].names
>>> label2id, id2label = dict(), dict()
>>> for i, label in enumerate(labels):
... label2id[label] = str(i)
... id2label[str(i)] = label
```
이제 레이블 ID를 레이블 이름으로 변환할 수 있습니다:
```py
>>> id2label[str(79)]
'prime_rib'
```
## 전처리[[preprocess]]
다음 단계는 이미지를 텐서로 처리하기 위해 ViT 이미지 프로세서를 가져오는 것입니다:
```py
>>> from transformers import AutoImageProcessor
>>> checkpoint = "google/vit-base-patch16-224-in21k"
>>> image_processor = AutoImageProcessor.from_pretrained(checkpoint)
```
<frameworkcontent>
<pt>
이미지에 몇 가지 이미지 변환을 적용하여 과적합에 대해 모델을 더 견고하게 만듭니다. 여기서 Torchvision의 [`transforms`](https://pytorch.org/vision/stable/transforms.html) 모듈을 사용하지만, 원하는 이미지 라이브러리를 사용할 수도 있습니다.
이미지의 임의 부분을 크롭하고 크기를 조정한 다음, 이미지 평균과 표준 편차로 정규화하세요:
```py
>>> from torchvision.transforms import RandomResizedCrop, Compose, Normalize, ToTensor
>>> normalize = Normalize(mean=image_processor.image_mean, std=image_processor.image_std)
>>> size = (
... image_processor.size["shortest_edge"]
... if "shortest_edge" in image_processor.size
... else (image_processor.size["height"], image_processor.size["width"])
... )
>>> _transforms = Compose([RandomResizedCrop(size), ToTensor(), normalize])
```
그런 다음 전처리 함수를 만들어 변환을 적용하고 이미지의 `pixel_values`(모델에 대한 입력)를 반환하세요:
```py
>>> def transforms(examples):
... examples["pixel_values"] = [_transforms(img.convert("RGB")) for img in examples["image"]]
... del examples["image"]
... return examples
```
전체 데이터 세트에 전처리 기능을 적용하려면 🤗 Datasets [`~datasets.Dataset.with_transform`]을 사용합니다. 데이터 세트의 요소를 가져올 때 변환이 즉시 적용됩니다:
```py
>>> food = food.with_transform(transforms)
```
이제 [`DefaultDataCollator`]를 사용하여 예제 배치를 만듭니다. 🤗 Transformers의 다른 데이터 콜레이터와 달리, `DefaultDataCollator`는 패딩과 같은 추가적인 전처리를 적용하지 않습니다.
```py
>>> from transformers import DefaultDataCollator
>>> data_collator = DefaultDataCollator()
```
</pt>
</frameworkcontent>
<frameworkcontent>
<tf>
과적합을 방지하고 모델을 보다 견고하게 만들기 위해 데이터 세트의 훈련 부분에 데이터 증강을 추가합니다.
여기서 Keras 전처리 레이어로 훈련 데이터에 대한 변환(데이터 증강 포함)과
검증 데이터에 대한 변환(중앙 크로핑, 크기 조정, 정규화만)을 정의합니다.
`tf.image` 또는 다른 원하는 라이브러리를 사용할 수 있습니다.
```py
>>> from tensorflow import keras
>>> from tensorflow.keras import layers
>>> size = (image_processor.size["height"], image_processor.size["width"])
>>> train_data_augmentation = keras.Sequential(
... [
... layers.RandomCrop(size[0], size[1]),
... layers.Rescaling(scale=1.0 / 127.5, offset=-1),
... layers.RandomFlip("horizontal"),
... layers.RandomRotation(factor=0.02),
... layers.RandomZoom(height_factor=0.2, width_factor=0.2),
... ],
... name="train_data_augmentation",
... )
>>> val_data_augmentation = keras.Sequential(
... [
... layers.CenterCrop(size[0], size[1]),
... layers.Rescaling(scale=1.0 / 127.5, offset=-1),
... ],
... name="val_data_augmentation",
... )
```
다음으로 한 번에 하나의 이미지가 아니라 이미지 배치에 적절한 변환을 적용하는 함수를 만듭니다.
```py
>>> import numpy as np
>>> import tensorflow as tf
>>> from PIL import Image
>>> def convert_to_tf_tensor(image: Image):
... np_image = np.array(image)
... tf_image = tf.convert_to_tensor(np_image)
... # `expand_dims()` is used to add a batch dimension since
... # the TF augmentation layers operates on batched inputs.
... return tf.expand_dims(tf_image, 0)
>>> def preprocess_train(example_batch):
... """Apply train_transforms across a batch."""
... images = [
... train_data_augmentation(convert_to_tf_tensor(image.convert("RGB"))) for image in example_batch["image"]
... ]
... example_batch["pixel_values"] = [tf.transpose(tf.squeeze(image)) for image in images]
... return example_batch
... def preprocess_val(example_batch):
... """Apply val_transforms across a batch."""
... images = [
... val_data_augmentation(convert_to_tf_tensor(image.convert("RGB"))) for image in example_batch["image"]
... ]
... example_batch["pixel_values"] = [tf.transpose(tf.squeeze(image)) for image in images]
... return example_batch
```
🤗 Datasets [`~datasets.Dataset.set_transform`]를 사용하여 즉시 변환을 적용하세요:
```py
food["train"].set_transform(preprocess_train)
food["test"].set_transform(preprocess_val)
```
최종 전처리 단계로 `DefaultDataCollator`를 사용하여 예제 배치를 만듭니다. 🤗 Transformers의 다른 데이터 콜레이터와 달리
`DefaultDataCollator`는 패딩과 같은 추가 전처리를 적용하지 않습니다.
```py
>>> from transformers import DefaultDataCollator
>>> data_collator = DefaultDataCollator(return_tensors="tf")
```
</tf>
</frameworkcontent>
## 평가[[evaluate]]
훈련 중에 평가 지표를 포함하면 모델의 성능을 평가하는 데 도움이 되는 경우가 많습니다.
🤗 [Evaluate](https://huggingface.co/docs/evaluate/index) 라이브러리로 평가 방법을 빠르게 가져올 수 있습니다. 이 작업에서는
[accuracy](https://huggingface.co/spaces/evaluate-metric/accuracy) 평가 지표를 가져옵니다. (🤗 Evaluate [빠른 둘러보기](https://huggingface.co/docs/evaluate/a_quick_tour)를 참조하여 평가 지표를 가져오고 계산하는 방법에 대해 자세히 알아보세요):
```py
>>> import evaluate
>>> accuracy = evaluate.load("accuracy")
```
그런 다음 예측과 레이블을 [`~evaluate.EvaluationModule.compute`]에 전달하여 정확도를 계산하는 함수를 만듭니다:
```py
>>> import numpy as np
>>> def compute_metrics(eval_pred):
... predictions, labels = eval_pred
... predictions = np.argmax(predictions, axis=1)
... return accuracy.compute(predictions=predictions, references=labels)
```
이제 `compute_metrics` 함수를 사용할 준비가 되었으며, 훈련을 설정하면 이 함수로 되돌아올 것입니다.
## 훈련[[train]]
<frameworkcontent>
<pt>
<Tip>
[`Trainer`]를 사용하여 모델을 미세 조정하는 방법에 익숙하지 않은 경우, [여기](../training#train-with-pytorch-trainer)에서 기본 튜토리얼을 확인하세요!
</Tip>
이제 모델을 훈련시킬 준비가 되었습니다! [`AutoModelForImageClassification`]로 ViT를 가져옵니다. 예상되는 레이블 수, 레이블 매핑 및 레이블 수를 지정하세요:
```py
>>> from transformers import AutoModelForImageClassification, TrainingArguments, Trainer
>>> model = AutoModelForImageClassification.from_pretrained(
... checkpoint,
... num_labels=len(labels),
... id2label=id2label,
... label2id=label2id,
... )
```
이제 세 단계만 거치면 끝입니다:
1. [`TrainingArguments`]에서 훈련 하이퍼파라미터를 정의하세요. `image` 열이 삭제되기 때문에 미사용 열을 제거하지 않는 것이 중요합니다. `image` 열이 없으면 `pixel_values`을 생성할 수 없습니다. 이 동작을 방지하려면 `remove_unused_columns=False`로 설정하세요! 다른 유일한 필수 매개변수는 모델 저장 위치를 지정하는 `output_dir`입니다. `push_to_hub=True`로 설정하면 이 모델을 허브에 푸시합니다(모델을 업로드하려면 Hugging Face에 로그인해야 합니다). 각 에폭이 끝날 때마다, [`Trainer`]가 정확도를 평가하고 훈련 체크포인트를 저장합니다.
2. [`Trainer`]에 모델, 데이터 세트, 토크나이저, 데이터 콜레이터 및 `compute_metrics` 함수와 함께 훈련 인수를 전달하세요.
3. [`~Trainer.train`]을 호출하여 모델을 미세 조정하세요.
```py
>>> training_args = TrainingArguments(
... output_dir="my_awesome_food_model",
... remove_unused_columns=False,
... evaluation_strategy="epoch",
... save_strategy="epoch",
... learning_rate=5e-5,
... per_device_train_batch_size=16,
... gradient_accumulation_steps=4,
... per_device_eval_batch_size=16,
... num_train_epochs=3,
... warmup_ratio=0.1,
... logging_steps=10,
... load_best_model_at_end=True,
... metric_for_best_model="accuracy",
... push_to_hub=True,
... )
>>> trainer = Trainer(
... model=model,
... args=training_args,
... data_collator=data_collator,
... train_dataset=food["train"],
... eval_dataset=food["test"],
... tokenizer=image_processor,
... compute_metrics=compute_metrics,
... )
>>> trainer.train()
```
훈련이 완료되면, 모든 사람이 모델을 사용할 수 있도록 [`~transformers.Trainer.push_to_hub`] 메소드로 모델을 허브에 공유하세요:
```py
>>> trainer.push_to_hub()
```
</pt>
</frameworkcontent>
<frameworkcontent>
<tf>
<Tip>
Keras를 사용하여 모델을 미세 조정하는 방법에 익숙하지 않은 경우, 먼저 [기본 튜토리얼](./training#train-a-tensorflow-model-with-keras)을 확인하세요!
</Tip>
TensorFlow에서 모델을 미세 조정하려면 다음 단계를 따르세요:
1. 훈련 하이퍼파라미터를 정의하고 옵티마이저와 학습률 스케쥴을 설정합니다.
2. 사전 훈련된 모델을 인스턴스화합니다.
3. 🤗 Dataset을 `tf.data.Dataset`으로 변환합니다.
4. 모델을 컴파일합니다.
5. 콜백을 추가하고 훈련을 수행하기 위해 `fit()` 메소드를 사용합니다.
6. 커뮤니티와 공유하기 위해 모델을 🤗 Hub에 업로드합니다.
하이퍼파라미터, 옵티마이저 및 학습률 스케쥴을 정의하는 것으로 시작합니다:
```py
>>> from transformers import create_optimizer
>>> batch_size = 16
>>> num_epochs = 5
>>> num_train_steps = len(food["train"]) * num_epochs
>>> learning_rate = 3e-5
>>> weight_decay_rate = 0.01
>>> optimizer, lr_schedule = create_optimizer(
... init_lr=learning_rate,
... num_train_steps=num_train_steps,
... weight_decay_rate=weight_decay_rate,
... num_warmup_steps=0,
... )
```
그런 다음 레이블 매핑과 함께 [`TFAuto ModelForImageClassification`]으로 ViT를 가져옵니다:
```py
>>> from transformers import TFAutoModelForImageClassification
>>> model = TFAutoModelForImageClassification.from_pretrained(
... checkpoint,
... id2label=id2label,
... label2id=label2id,
... )
```
데이터 세트를 [`~datasets.Dataset.to_tf_dataset`]와 `data_collator`를 사용하여 `tf.data.Dataset` 형식으로 변환하세요:
```py
>>> # converting our train dataset to tf.data.Dataset
>>> tf_train_dataset = food["train"].to_tf_dataset(
... columns="pixel_values", label_cols="label", shuffle=True, batch_size=batch_size, collate_fn=data_collator
... )
>>> # converting our test dataset to tf.data.Dataset
>>> tf_eval_dataset = food["test"].to_tf_dataset(
... columns="pixel_values", label_cols="label", shuffle=True, batch_size=batch_size, collate_fn=data_collator
... )
```
`compile()`를 사용하여 훈련 모델을 구성하세요:
```py
>>> from tensorflow.keras.losses import SparseCategoricalCrossentropy
>>> loss = tf.keras.losses.SparseCategoricalCrossentropy(from_logits=True)
>>> model.compile(optimizer=optimizer, loss=loss)
```
예측에서 정확도를 계산하고 모델을 🤗 Hub로 푸시하려면 [Keras callbacks](../main_classes/keras_callbacks)를 사용하세요.
`compute_metrics` 함수를 [KerasMetricCallback](../main_classes/keras_callbacks#transformers.KerasMetricCallback)에 전달하고,
[PushToHubCallback](../main_classes/keras_callbacks#transformers.PushToHubCallback)을 사용하여 모델을 업로드합니다:
```py
>>> from transformers.keras_callbacks import KerasMetricCallback, PushToHubCallback
>>> metric_callback = KerasMetricCallback(metric_fn=compute_metrics, eval_dataset=tf_eval_dataset)
>>> push_to_hub_callback = PushToHubCallback(
... output_dir="food_classifier",
... tokenizer=image_processor,
... save_strategy="no",
... )
>>> callbacks = [metric_callback, push_to_hub_callback]
```
이제 모델을 훈련할 준비가 되었습니다! 훈련 및 검증 데이터 세트, 에폭 수와 함께 `fit()`을 호출하고,
콜백을 사용하여 모델을 미세 조정합니다:
```py
>>> model.fit(tf_train_dataset, validation_data=tf_eval_dataset, epochs=num_epochs, callbacks=callbacks)
Epoch 1/5
250/250 [==============================] - 313s 1s/step - loss: 2.5623 - val_loss: 1.4161 - accuracy: 0.9290
Epoch 2/5
250/250 [==============================] - 265s 1s/step - loss: 0.9181 - val_loss: 0.6808 - accuracy: 0.9690
Epoch 3/5
250/250 [==============================] - 252s 1s/step - loss: 0.3910 - val_loss: 0.4303 - accuracy: 0.9820
Epoch 4/5
250/250 [==============================] - 251s 1s/step - loss: 0.2028 - val_loss: 0.3191 - accuracy: 0.9900
Epoch 5/5
250/250 [==============================] - 238s 949ms/step - loss: 0.1232 - val_loss: 0.3259 - accuracy: 0.9890
```
축하합니다! 모델을 미세 조정하고 🤗 Hub에 공유했습니다. 이제 추론에 사용할 수 있습니다!
</tf>
</frameworkcontent>
<Tip>
이미지 분류를 위한 모델을 미세 조정하는 자세한 예제는 다음 [PyTorch notebook](https://colab.research.google.com/github/huggingface/notebooks/blob/main/examples/image_classification.ipynb)을 참조하세요.
</Tip>
## 추론[[inference]]
좋아요, 이제 모델을 미세 조정했으니 추론에 사용할 수 있습니다!
추론을 수행하고자 하는 이미지를 가져와봅시다:
```py
>>> ds = load_dataset("food101", split="validation[:10]")
>>> image = ds["image"][0]
```
<div class="flex justify-center">
<img src="https://huggingface.co/datasets/huggingface/documentation-images/resolve/main/beignets-task-guide.png" alt="image of beignets"/>
</div>
미세 조정 모델로 추론을 시도하는 가장 간단한 방법은 [`pipeline`]을 사용하는 것입니다. 모델로 이미지 분류를 위한 `pipeline`을 인스턴스화하고 이미지를 전달합니다:
```py
>>> from transformers import pipeline
>>> classifier = pipeline("image-classification", model="my_awesome_food_model")
>>> classifier(image)
[{'score': 0.31856709718704224, 'label': 'beignets'},
{'score': 0.015232225880026817, 'label': 'bruschetta'},
{'score': 0.01519392803311348, 'label': 'chicken_wings'},
{'score': 0.013022331520915031, 'label': 'pork_chop'},
{'score': 0.012728818692266941, 'label': 'prime_rib'}]
```
원한다면, `pipeline`의 결과를 수동으로 복제할 수도 있습니다:
<frameworkcontent>
<pt>
이미지를 전처리하기 위해 이미지 프로세서를 가져오고 `input`을 PyTorch 텐서로 반환합니다:
```py
>>> from transformers import AutoImageProcessor
>>> import torch
>>> image_processor = AutoImageProcessor.from_pretrained("my_awesome_food_model")
>>> inputs = image_processor(image, return_tensors="pt")
```
입력을 모델에 전달하고 logits을 반환합니다:
```py
>>> from transformers import AutoModelForImageClassification
>>> model = AutoModelForImageClassification.from_pretrained("my_awesome_food_model")
>>> with torch.no_grad():
... logits = model(**inputs).logits
```
확률이 가장 높은 예측 레이블을 가져오고, 모델의 `id2label` 매핑을 사용하여 레이블로 변환합니다:
```py
>>> predicted_label = logits.argmax(-1).item()
>>> model.config.id2label[predicted_label]
'beignets'
```
</pt>
</frameworkcontent>
<frameworkcontent>
<tf>
이미지를 전처리하기 위해 이미지 프로세서를 가져오고 `input`을 TensorFlow 텐서로 반환합니다:
```py
>>> from transformers import AutoImageProcessor
>>> image_processor = AutoImageProcessor.from_pretrained("MariaK/food_classifier")
>>> inputs = image_processor(image, return_tensors="tf")
```
입력을 모델에 전달하고 logits을 반환합니다:
```py
>>> from transformers import TFAutoModelForImageClassification
>>> model = TFAutoModelForImageClassification.from_pretrained("MariaK/food_classifier")
>>> logits = model(**inputs).logits
```
확률이 가장 높은 예측 레이블을 가져오고, 모델의 `id2label` 매핑을 사용하여 레이블로 변환합니다:
```py
>>> predicted_class_id = int(tf.math.argmax(logits, axis=-1)[0])
>>> model.config.id2label[predicted_class_id]
'beignets'
```
</tf>
</frameworkcontent>
| transformers/docs/source/ko/tasks/image_classification.md/0 | {
"file_path": "transformers/docs/source/ko/tasks/image_classification.md",
"repo_id": "transformers",
"token_count": 11866
} | 276 |
<!--Copyright 2023 The HuggingFace Team. All rights reserved.
Licensed under the Apache License, Version 2.0 (the "License"); you may not use this file except in compliance with
the License. You may obtain a copy of the License at
http://www.apache.org/licenses/LICENSE-2.0
Unless required by applicable law or agreed to in writing, software distributed under the License is distributed on
an "AS IS" BASIS, WITHOUT WARRANTIES OR CONDITIONS OF ANY KIND, either express or implied. See the License for the
specific language governing permissions and limitations under the License.
⚠️ Note that this file is in Markdown but contain specific syntax for our doc-builder (similar to MDX) that may not be
rendered properly in your Markdown viewer.
-->
# 🤗 Transformers로 작업을 해결하는 방법[[how-transformers-solve-tasks]]
[🤗 Transformers로 할 수 있는 작업](task_summary)에서 자연어 처리(NLP), 음성 및 오디오, 컴퓨터 비전 작업 등의 중요한 응용을 배웠습니다. 이 페이지에서는 모델이 이러한 작업을 어떻게 해결하는지 자세히 살펴보고 내부에서 어떤 일이 일어나는지 설명합니다. 주어진 작업을 해결하는 많은 방법이 있으며, 일부 모델은 특정 기술을 구현하거나 심지어 새로운 방식으로 작업에 접근할 수도 있지만, Transformer 모델의 경우 일반적인 아이디어는 동일합니다. 유연한 아키텍처 덕분에 대부분의 모델은 인코더, 디코더 또는 인코더-디코더 구조의 변형입니다. Transformer 모델뿐만 아니라 우리의 라이브러리에는 오늘날 컴퓨터 비전 작업에 사용되는 몇 가지 합성곱 신경망(CNNs)도 있습니다. 또한, 우리는 현대 CNN의 작동 방식에 대해 설명할 것입니다.
작업이 어떻게 해결되는지 설명하기 위해, 유용한 예측을 출력하고자 모델 내부에서 어떤 일이 일어나는지 살펴봅니다.
- 오디오 분류 및 자동 음성 인식(ASR)을 위한 [Wav2Vec2](model_doc/wav2vec2)
- 이미지 분류를 위한 [Vision Transformer (ViT)](model_doc/vit) 및 [ConvNeXT](model_doc/convnext)
- 객체 탐지를 위한 [DETR](model_doc/detr)
- 이미지 분할을 위한 [Mask2Former](model_doc/mask2former)
- 깊이 추정을 위한 [GLPN](model_doc/glpn)
- 인코더를 사용하는 텍스트 분류, 토큰 분류 및 질의응답과 같은 NLP 작업을 위한 [BERT](model_doc/bert)
- 디코더를 사용하는 텍스트 생성과 같은 NLP 작업을 위한 [GPT2](model_doc/gpt2)
- 인코더-디코더를 사용하는 요약 및 번역과 같은 NLP 작업을 위한 [BART](model_doc/bart)
<Tip>
더 나아가기 전에, 기존 Transformer 아키텍처에 대한 기본적인 지식을 숙지하는 것이 좋습니다. 인코더, 디코더 및 어텐션의 작동 방식을 알면 다양한 Transformer 모델이 어떻게 작동하는지 이해하는 데 도움이 됩니다. 시작 단계거나 복습이 필요한 경우, 더 많은 정보를 위해 [코스](https://huggingface.co/course/chapter1/4?fw=pt)를 확인하세요!
</Tip>
## 음성 및 오디오[[speech-and-audio]]
[Wav2Vec2](model_doc/wav2vec2)는 레이블이 지정되지 않은 음성 데이터에 대해 사전훈련된 모델로, 오디오 분류 및 자동 음성 인식을 위해 레이블이 지정된 데이터로 미세 조정합니다.
<div class="flex justify-center">
<img src="https://huggingface.co/datasets/huggingface/documentation-images/resolve/main/wav2vec2_architecture.png"/>
</div>
이 모델에는 4가지 주요 구성 요소가 있습니다:
1. *특징 인코더(feature encoder)*는 원시 오디오 파형(raw audio waveform)을 가져와서 제로 평균 및 단위 분산으로 표준화하고, 각각 20ms 길이의 특징 벡터의 시퀀스로 변환합니다.
2. 오디오 파형은 본질적으로 연속적이기 때문에, 텍스트 시퀀스를 단어로 나누는 것과 같이 분할할 수 없습니다. 그래서 *양자화 모듈(quantization module)*로 전달되는 특징 벡터는 이산형 음성 단위를 학습하기 위한 것입니다. 음성 단위는 *코드북(codebook)*(어휘집이라고 생각할 수 있습니다)이라는 코드단어(codewords) 콜렉션에서 선택됩니다. 코드북에서 연속적인 오디오 입력을 가장 잘 나타내는 벡터 또는 음성 단위가 선택되어 모델을 통과합니다.
3. 특징 벡터의 절반은 무작위로 마스크가 적용되며, 마스크된 특징 벡터는 *상대적 위치 임베딩*을 추가하는 Transformer 인코더인 *문맥 네트워크(context network)*로 전달됩니다.
4. 문맥 네트워크의 사전훈련 목표는 *대조적 작업(contrastive task)*입니다. 모델은 잘못된 예측 시퀀스에서 마스크된 예측의 실제 양자화된 음성 표현을 예측하며, 모델이 가장 유사한 컨텍스트 벡터와 양자화된 음성 단위(타겟 레이블)를 찾도록 권장합니다.
이제 wav2vec2가 사전훈련되었으므로, 오디오 분류 또는 자동 음성 인식을 위해 데이터에 맞춰 미세 조정할 수 있습니다!
### 오디오 분류[[audio-classification]]
사전훈련된 모델을 오디오 분류에 사용하려면, 기본 Wav2Vec2 모델 상단에 시퀀스 분류 헤드를 추가하면 됩니다. 분류 헤드는 인코더의 은닉 상태(hidden states)를 받는 선형 레이어입니다. 은닉 상태는 각각 길이가 다른 오디오 프레임에서 학습된 특징을 나타냅니다. 고정 길이의 벡터 하나를 만들기 위해, 은닉 상태는 먼저 풀링되고, 클래스 레이블에 대한 로짓으로 변환됩니다. 가장 가능성이 높은 클래스를 찾기 위해 로짓과 타겟 사이의 교차 엔트로피 손실이 계산됩니다.
오디오 분류에 직접 도전할 준비가 되셨나요? 완전한 [오디오 분류 가이드](tasks/audio_classification)를 확인하여 Wav2Vec2를 미세 조정하고 추론에 사용하는 방법을 학습하세요!
### 자동 음성 인식[[automatic-speech-recognition]]
사전훈련된 모델을 자동 음성 인식에 사용하려면, [연결주의적 시간 분류(CTC, Connectionist Temporal Classification)](glossary#connectionist-temporal-classification-ctc)를 위해 기본 Wav2Vec2 모델 상단에 언어 모델링 헤드를 추가합니다. 언어 모델링 헤드는 인코더의 은닉 상태를 받아서 로짓으로 변환합니다. 각 로짓은 토큰 클래스(토큰 수는 작업의 어휘에서 나타납니다)를 나타냅니다. CTC 손실은 텍스트로 디코딩된 토큰에서 가장 가능성이 높은 토큰 시퀀스를 찾기 위해 로짓과 타겟 사이에서 계산됩니다.
자동 음성 인식에 직접 도전할 준비가 되셨나요? 완전한 [자동 음성 인식 가이드](tasks/asr)를 확인하여 Wav2Vec2를 미세 조정하고 추론에 사용하는 방법을 학습하세요!
## 컴퓨터 비전[[computer-vision]]
컴퓨터 비전 작업에 접근하는 2가지 방법이 있습니다:
1. 이미지를 패치 시퀀스로 분리하고 Transformer로 병렬 처리합니다.
2. [ConvNeXT](model_doc/convnext)와 같은 현대 CNN을 사용합니다. 이는 합성곱 레이어를 기반으로 하지만 현대 네트워크 설계를 적용합니다.
<Tip>
세 번째 방법은 Transformer와 합성곱(예를 들어, [Convolutional Vision Transformer](model_doc/cvt) 또는 [LeViT](model_doc/levit))을 결합하는 것입니다. 우리는 살펴볼 두 가지 방법만 결합하기 때문에 여기서 이 방법을 다루지 않습니다.
</Tip>
ViT와 ConvNeXT는 일반적으로 이미지 분류에서 사용되지만, 물체 감지, 분할, 깊이 추정과 같은 다른 비전 작업에는 각각 DETR, Mask2Former, GLPN이 더 적합하므로 이러한 모델을 살펴보겠습니다.
### 이미지 분류[[image-classification]]
ViT와 ConvNeXT 모두 이미지 분류에 사용될 수 있지만, ViT는 어텐션 메커니즘을, ConvNeXT는 합성곱을 사용하는 것이 주된 차이입니다.
#### Transformer[[transformer]]
[ViT](model_doc/vit)은 합성곱을 전적으로 순수 Transformer 아키텍처로 대체합니다. 기존 Transformer에 익숙하다면, ViT를 이해하는 방법의 대부분을 이미 파악했다고 볼 수 있습니다.
<div class="flex justify-center">
<img src="https://huggingface.co/datasets/huggingface/documentation-images/resolve/main/transformers/model_doc/vit_architecture.jpg"/>
</div>
ViT가 도입한 주요 변경 사항은 이미지가 Transformer로 어떻게 전달되는지에 있습니다:
1. 이미지는 서로 중첩되지 않는 정사각형 패치로 분할되고, 각 패치는 벡터 또는 *패치 임베딩(patch embedding)*으로 변환됩니다. 패치 임베딩은 적절한 입력 차원을 만드는 2D 합성곱 계층에서 생성됩니다(기본 Transformer의 경우 각 패치의 임베딩마다 768개의 값이 필요합니다). 224x224 픽셀 이미지가 있다면, 16x16 이미지 패치 196개로 분할할 수 있습니다. 텍스트가 단어로 토큰화되는 것처럼, 이미지도 패치 시퀀스로 "토큰화"됩니다.
2. *학습 가능한 임베딩(learnable embedding)*(특수한 `[CLS]` 토큰)이 BERT와 같이 패치 임베딩의 시작 부분에 추가됩니다. `[CLS]` 토큰의 마지막 은닉 상태는 부착된 분류 헤드의 입력으로 사용되고, 다른 출력은 무시됩니다. 이 토큰은 모델이 이미지의 표현을 인코딩하는 방법을 학습하는 데 도움이 됩니다.
3. 패치와 학습 가능한 임베딩에 마지막으로 추가할 것은 *위치 임베딩*입니다. 왜냐하면 모델은 이미지 패치의 순서를 모르기 때문입니다. 위치 임베딩도 학습 가능하며, 패치 임베딩과 동일한 크기를 가집니다. 최종적으로, 모든 임베딩이 Transformer 인코더에 전달됩니다.
4. `[CLS]` 토큰을 포함한 출력은 다층 퍼셉트론 헤드(MLP)에 전달됩니다. ViT의 사전훈련 목표는 단순히 분류입니다. 다른 분류 헤드와 같이, MLP 헤드는 출력을 클래스 레이블에 대해 로짓으로 변환하고 교차 엔트로피 손실을 계산하여 가장 가능성이 높은 클래스를 찾습니다.
이미지 분류에 직접 도전할 준비가 되셨나요? 완전한 [이미지 분류 가이드](tasks/image_classification)를 확인하여 ViT를 미세 조정하고 추론에 사용하는 방법을 학습하세요!
#### CNN[[cnn]]
<Tip>
이 섹션에서는 합성곱에 대해 간략하게 설명합니다. 그러나 이미지의 모양과 크기가 어떻게 변화하는지에 대한 사전 이해가 있다면 도움이 될 것입니다. 합성곱에 익숙하지 않은 경우, fastai book의 [합성곱 신경망 챕터](https://github.com/fastai/fastbook/blob/master/13_convolutions.ipynb)를 확인하세요!
</Tip>
[ConvNeXT](model_doc/convnext)는 성능을 높이기 위해 새로운 현대 네트워크 설계를 적용한 CNN 구조입니다. 그러나 합성곱은 여전히 모델의 핵심입니다. 높은 수준의 관점에서 볼 때, [합성곱](glossary#convolution)은 작은 행렬(*커널*)에 이미지 픽셀의 작은 윈도우를 곱하는 연산입니다. 이는 특정 텍스쳐(texture)이나 선의 곡률과 같은 일부 특징을 계산합니다. 그러고 다음 픽셀 윈도우로 넘어가는데, 여기서 합성곱이 이동하는 거리를 *보폭(stride)*이라고 합니다.
<div class="flex justify-center">
<img src="https://huggingface.co/datasets/huggingface/documentation-images/resolve/main/convolution.gif"/>
</div>
<small>패딩이나 보폭이 없는 기본 합성곱, <a href="https://arxiv.org/abs/1603.07285">딥러닝을 위한 합성곱 연산 가이드</a></small>
이 출력을 다른 합성곱 레이어에 전달할 수 있으며, 각 연속적인 레이어를 통해 네트워크는 핫도그나 로켓과 같이 더 복잡하고 추상적인 것을 학습합니다. 합성곱 레이어 사이에 풀링 레이어를 추가하여 차원을 줄이고 특징의 위치 변화에 대해 모델을 더 견고하게 만드는 것이 일반적입니다.
<div class="flex justify-center">
<img src="https://huggingface.co/datasets/huggingface/documentation-images/resolve/main/convnext_architecture.png"/>
</div>
ConvNeXT는 CNN을 5가지 방식으로 현대화합니다:
1. 각 단계의 블록 수를 변경하고 더 큰 보폭과 그에 대응하는 커널 크기로 이미지를 "패치화(patchify)"합니다. 겹치지 않는 슬라이딩 윈도우는 ViT가 이미지를 패치로 분할하는 방법과 유사하게 이 패치화 전략을 만듭니다.
2. *병목(bottleneck)* 레이어는 채널 수를 줄였다가 다시 복원합니다. 왜냐하면 1x1 합성곱을 수행하는 것이 더 빠르고, 깊이를 늘릴 수 있기 때문입니다. 역 병목(inverted bottlenect)은 채널 수를 확장하고 축소함으로써 그 반대로 수행하므로, 메모리 효율이 더 높습니다.
3. 병목 레이어의 일반적인 3x3 합성곱 레이어를 각 입력 채널에 개별적으로 합성곱을 적용한 다음 마지막에 쌓는 *깊이별 합성곱(depthwise convolution)*으로 대체합니다. 이는 네트워크 폭이 넓혀 성능이 향상됩니다.
4. ViT는 어텐션 메커니즘 덕분에 한 번에 더 많은 이미지를 볼 수 있는 전역 수신 필드를 가지고 있습니다. ConvNeXT는 커널 크기를 7x7로 늘려 이 효과를 재현하려고 시도합니다.
5. 또한 ConvNeXT는 Transformer 모델을 모방하는 몇 가지 레이어 설계를 변경합니다. 활성화 및 정규화 레이어가 더 적고, 활성화 함수가 ReLU 대신 GELU로 전환되고, BatchNorm 대신 LayerNorm을 사용합니다.
합성곱 블록의 출력은 분류 헤드로 전달되며, 분류 헤드는 출력을 로짓으로 변환하고 교차 엔트로피 손실을 계산하여 가장 가능성이 높은 레이블을 찾습니다.
### 객체 탐지[[object-detection]]
[DETR](model_doc/detr), *DEtection TRansformer*는 CNN과 Transformer 인코더-디코더를 결합한 종단간(end-to-end) 객체 탐지 모델입니다.
<div class="flex justify-center">
<img src="https://huggingface.co/datasets/huggingface/documentation-images/resolve/main/detr_architecture.png"/>
</div>
1. 사전훈련된 CNN *백본(backbone)*은 픽셀 값으로 나타낸 이미지를 가져와 저해상도 특징 맵을 만듭니다. 특징 맵에 대해 1x1 합성곱을 적용하여 차원을 줄이고, 고수준 이미지 표현을 가진 새로운 특징 맵을 생성합니다. Transformer는 시퀀스 모델이기 때문에 특징 맵을 위치 임베딩과 결합된 특징 벡터의 시퀀스로 평탄화합니다.
2. 특징 벡터는 어텐션 레이어를 사용하여 이미지 표현을 학습하는 인코더에 전달됩니다. 다음으로, 인코더의 은닉 상태는 디코더에서 *객체 쿼리*와 결합됩니다. 객체 쿼리는 이미지의 다른 영역에 초점을 맞춘 학습된 임베딩으로 학습되고, 각 어텐션 레이어를 진행하면서 갱신됩니다. 디코더의 은닉 상태는 각 객체 쿼리에 대한 바운딩 박스 좌표와 클래스 레이블을 예측하는 순방향 네트워크에 전달되며, 객체가 없는 경우 `no object`가 출력됩니다.
DETR은 각 객체 쿼리를 병렬로 디코딩하여 *N* 개의 최종 예측을 출력합니다. 여기서 *N*은 쿼리 수입니다. 한 번에 하나의 요소를 예측하는 일반적인 자기회귀 모델과 달리, 객체 탐지는 한 번에 *N* 개의 예측을 수행하는 집합 예측 작업(`바운딩 박스`, `클래스 레이블`)입니다.
3. DETR은 훈련 중 *이분 매칭 손실(bipartite matching loss)*을 사용하여 고정된 수의 예측과 고정된 실제 정답 레이블(ground truth labels) 세트를 비교합니다. *N*개의 레이블 세트에 실제 정답 레이블보다 적은 경우, `no object` 클래스로 패딩됩니다. 이 손실 함수는 DETR이 예측과 실제 정답 레이블 간 1:1 대응을 찾도록 권장합니다. 바운딩 박스 또는 클래스 레이블 중 하나라도 잘못된 경우, 손실이 발생합니다. 마찬가지로, 존재하지 않는 객체를 예측하는 경우, 패널티를 받습니다. 이로 인해 DETR은 이미지에서 눈에 잘 띄는 물체 하나에 집중하는 대신, 다른 객체를 찾도록 권장됩니다.
객체 탐지 헤드가 DETR 상단에 추가되어 클래스 레이블과 바운딩 박스의 좌표를 찾습니다. 객체 탐지 헤드에는 두 가지 구성 요소가 있습니다: 디코더 은닉 상태를 클래스 레이블의 로짓으로 변환하는 선형 레이어 및 바운딩 박스를 예측하는 MLP
객체 탐지에 직접 도전할 준비가 되셨나요? 완전한 [객체 탐지 가이드](tasks/object_detection)를 확인하여 DETR을 미세 조정하고 추론에 사용하는 방법을 학습하세요!
### 이미지 분할[[image-segmentation]]
[Mask2Former](model_doc/mask2former)는 모든 유형의 이미지 분할 작업을 해결하는 범용 아키텍처입니다. 전통적인 분할 모델은 일반적으로 시멘틱(semantic) 또는 파놉틱(panoptic) 분할과 같은 이미지 분할의 특정 하위 작업에 맞춰 조정됩니다. Mask2Former는 모든 작업을 *마스크 분류* 문제로 구성합니다. 마스크 분류는 픽셀을 *N*개 세그먼트로 그룹화하고, 주어진 이미지에 대해 *N*개의 마스크와 그에 대응하는 클래스 레이블을 예측합니다. 이 섹션에서 Mask2Former의 작동 방법을 설명한 다음, 마지막에 SegFormer를 미세 조정해볼 수 있습니다.
<div class="flex justify-center">
<img src="https://huggingface.co/datasets/huggingface/documentation-images/resolve/main/mask2former_architecture.png"/>
</div>
Mask2Former에는 3가지 주요 구성 요소가 있습니다:
1. [Swin](model_doc/swin) 백본이 이미지를 받아 3개의 연속된 3x3 합성곱에서 저해상도 이미지 특징 맵을 생성합니다.
2. 특징 맵은 *픽셀 디코더*에 전달됩니다. 이 디코더는 저해상도 특징을 고해상도 픽셀 임베딩으로 점진적으로 업샘플링합니다. 픽셀 디코더는 실제로 원본 이미지의 1/32, 1/16, 1/8 해상도의 다중 스케일 특징(저해상도 및 고해상도 특징 모두 포함)을 생성합니다.
3. 이러한 서로 다른 크기의 특징 맵은 고해상도 특징에서 작은 객체를 포착하기 위해 한 번에 하나의 Transformer 디코더 레이어에 연속적으로 공급됩니다. Mask2Former의 핵심은 디코더의 *마스크 어텐션* 메커니즘입니다. 전체 이미지를 참조할 수 있는 크로스 어텐션(cross-attention)과 달리, 마스크 어텐션은 이미지의 특정 영역에만 집중합니다. 이는 이미지의 지역적 특징만으로 모델이 충분히 학습할 수 있기 때문에 더 빠르고 성능이 우수합니다.
4. [DETR](tasks_explained#object-detection)과 같이, Mask2Former는 학습된 객체 쿼리를 사용하고 이를 픽셀 디코더에서의 이미지 특징과 결합하여 예측 집합(`클래스 레이블`, `마스크 예측`)을 생성합니다. 디코더의 은닉 상태는 선형 레이어로 전달되어 클래스 레이블에 대한 로짓으로 변환됩니다. 로짓과 클래스 레이블 사이의 교차 엔트로피 손실을 계산하여 가장 가능성이 높은 것을 찾습니다.
마스크 예측은 픽셀 임베딩과 최종 디코더 은닉 상태를 결합하여 생성됩니다. 시그모이드 교차 엔트로피 및 Dice 손실은 로짓과 실제 정답 마스크(ground truth mask) 사이에서 계산되어 가장 가능성이 높은 마스크를 찾습니다.
이미지 분할에 직접 도전할 준비가 되셨나요? 완전한 [이미지 분할 가이드](tasks/semantic_segmentation)를 확인하여 SegFormer를 미세 조정하고 추론에 사용하는 방법을 학습하세요!
### 깊이 추정[[depth-estimation]]
[GLPN](model_doc/glpn), *Global-Local Path Network*는 [SegFormer](model_doc/segformer) 인코더와 경량 디코더를 결합한 깊이 추정을 위한 Transformer입니다.
<div class="flex justify-center">
<img src="https://huggingface.co/datasets/huggingface/documentation-images/resolve/main/glpn_architecture.jpg"/>
</div>
1. ViT와 같이, 이미지는 패치 시퀀스로 분할되지만, 이미지 패치가 더 작다는 점이 다릅니다. 이는 세그멘테이션이나 깊이 추정과 같은 밀도 예측 작업에 더 적합합니다. 이미지 패치는 패치 임베딩으로 변환되어(패치 임베딩이 생성되는 방법은 [이미지 분류](#image-classification) 섹션을 참조하세요), 인코더로 전달됩니다.
2. 인코더는 패치 임베딩을 받아, 여러 인코더 블록에 전달합니다. 각 블록은 어텐션 및 Mix-FFN 레이어로 구성됩니다. 후자의 목적은 위치 정보를 제공하는 것입니다. 각 인코더 블록의 끝에는 계층적 표현을 생성하기 위한 *패치 병합(patch merging)* 레이어가 있습니다. 각 인접한 패치 그룹의 특징은 연결되고, 연결된 특징에 선형 레이어가 적용되어 패치 수를 1/4의 해상도로 줄입니다. 이는 다음 인코더 블록의 입력이 되며, 이러한 전체 프로세스는 1/8, 1/16, 1/32 해상도의 이미지 특징을 가질 때까지 반복됩니다.
3. 경량 디코더는 인코더에서 마지막 특징 맵(1/32 크기)을 가져와 1/16 크기로 업샘플링합니다. 여기서, 특징은 *선택적 특징 융합(SFF, Selective Feature Fusion)* 모듈로 전달됩니다. 이 모듈은 각 특징에 대해 어텐션 맵에서 로컬 및 전역 특징을 선택하고 결합한 다음, 1/8로 업샘플링합니다. 이 프로세스는 디코딩된 특성이 원본 이미지와 동일한 크기가 될 때까지 반복됩니다. 출력은 두 개의 합성곱 레이어를 거친 다음, 시그모이드 활성화가 적용되어 각 픽셀의 깊이를 예측합니다.
## 자연어처리[[natural-language-processing]]
Transformer는 초기에 기계 번역을 위해 설계되었고, 그 이후로는 사실상 모든 NLP 작업을 해결하기 위한 기본 아키텍처가 되었습니다. 어떤 작업은 Transformer의 인코더 구조에 적합하며, 다른 작업은 디코더에 더 적합합니다. 또 다른 작업은 Transformer의 인코더-디코더 구조를 모두 활용합니다.
### 텍스트 분류[[text-classification]]
[BERT](model_doc/bert)는 인코더 전용 모델이며, 텍스트의 풍부한 표현을 학습하기 위해 양방향의 단어에 주목함으로써 심층 양방향성(deep bidirectionality)을 효과적으로 구현한 최초의 모델입니다.
1. BERT는 [WordPiece](tokenizer_summary#wordpiece) 토큰화를 사용하여 문장의 토큰 임베딩을 생성합니다. 단일 문장과 한 쌍의 문장을 구분하기 위해 특수한 `[SEP]` 토큰이 추가됩니다. 모든 텍스트 시퀀스의 시작 부분에는 특수한 `[CLS]` 토큰이 추가됩니다. `[CLS]` 토큰이 있는 최종 출력은 분류 작업을 위한 분류 헤드로 입력에 사용됩니다. BERT는 또한 한 쌍의 문장에서 각 토큰이 첫 번째 문장인지 두 번째 문장에 속하는지 나타내는 세그먼트 임베딩(segment embedding)을 추가합니다.
2. BERT는 마스크드 언어 모델링과 다음 문장 예측, 두 가지 목적으로 사전훈련됩니다. 마스크드 언어 모델링에서는 입력 토큰의 일부가 무작위로 마스킹되고, 모델은 이를 예측해야 합니다. 이는 모델이 모든 단어를 보고 다음 단어를 "예측"할 수 있는 양방향성 문제를 해결합니다. 예측된 마스크 토큰의 최종 은닉 상태는 어휘에 대한 소프트맥스가 있는 순방향 네트워크로 전달되어 마스크된 단어를 예측합니다.
두 번째 사전훈련 대상은 다음 문장 예측입니다. 모델은 문장 B가 문장 A 다음에 오는지 예측해야 합니다. 문장 B가 다음 문장인 경우와 무작위 문장인 경우 각각 50%의 확률로 발생합니다. 다음 문장인지 아닌지에 대한 예측은 두 개의 클래스(`IsNext` 및 `NotNext`)에 대한 소프트맥스가 있는 순방향 네트워크로 전달됩니다.
3. 입력 임베딩은 여러 인코더 레이어를 거쳐서 최종 은닉 상태를 출력합니다.
사전훈련된 모델을 텍스트 분류에 사용하려면, 기본 BERT 모델 상단에 시퀀스 분류 헤드를 추가합니다. 시퀀스 분류 헤드는 최종 은닉 상태를 받는 선형 레이어이며, 로짓으로 변환하기 위해 선형 변환을 수행합니다. 교차 엔트로피 손실은 로짓과 타겟 간에 계산되어 가장 가능성이 높은 레이블을 찾습니다.
텍스트 분류에 직접 도전할 준비가 되셨나요? 완전한 [텍스트 분류 가이드](tasks/sequence_classification)를 확인하여 DistilBERT를 미세 조정하고 추론에 사용하는 방법을 학습하세요!
### 토큰 분류[[token-classification]]
개체명 인식(Named Entity Recognition, NER)과 같은 토큰 분류 작업에 BERT를 사용하려면, 기본 BERT 모델 상단에 토큰 분류 헤드를 추가합니다. 토큰 분류 헤드는 최종 은닉 상태를 받는 선형 레이어이며, 로짓으로 변환하기 위해 선형 변환을 수행합니다. 교차 엔트로피 손실은 로짓과 각 토큰 간에 계산되어 가장 가능성이 높은 레이블을 찾습니다.
토큰 분류에 직접 도전할 준비가 되셨나요? 완전한 [토큰 분류 가이드](tasks/token_classification)를 확인하여 DistilBERT를 미세 조정하고 추론에 사용하는 방법을 학습하세요!
### 질의응답[[question-answering]]
질의응답에 BERT를 사용하려면, 기본 BERT 모델 위에 스팬(span) 분류 헤드를 추가합니다. 이 선형 레이어는 최종 은닉 상태를 받고, 답변에 대응하는 `스팬`의 시작과 끝 로그를 계산하기 위해 선형 변환을 수행합니다. 교차 엔트로피 손실은 로짓과 각 레이블 위치 간에 계산되어 답변에 대응하는 가장 가능성이 높은 텍스트의 스팬을 찾습니다.
질의응답에 직접 도전할 준비가 되셨나요? 완전한 [질의응답 가이드](tasks/question_answering)를 확인하여 DistilBERT를 미세 조정하고 추론에 사용하는 방법을 학습하세요!
<Tip>
💡 사전훈련된 BERT를 다양한 작업에 사용하는 것이 얼마나 쉬운지 주목하세요. 사전훈련된 모델에 특정 헤드를 추가하기만 하면 은닉 상태를 원하는 출력으로 조작할 수 있습니다!
</Tip>
### 텍스트 생성[[text-generation]]
[GPT-2](model_doc/gpt2)는 대량의 텍스트에 대해 사전훈련된 디코딩 전용 모델입니다. 프롬프트를 주어지면 설득력 있는 (항상 사실은 아니지만!) 텍스트를 생성하고 명시적으로 훈련되지 않았음에도 불구하고 질의응답과 같은 다른 NLP 작업을 완수할 수 있습니다.
<div class="flex justify-center">
<img src="https://huggingface.co/datasets/huggingface/documentation-images/resolve/main/gpt2_architecture.png"/>
</div>
1. GPT-2는 단어를 토큰화하고 토큰 임베딩을 생성하기 위해 [바이트 페어 인코딩(BPE, byte pair encoding)](tokenizer_summary#bytepair-encoding-bpe)을 사용합니다. 위치 인코딩은 시퀀스에서 각 토큰의 위치를 나타내기 위해 토큰 임베딩에 추가됩니다. 입력 임베딩은 여러 디코더 블록을 거쳐 일부 최종 은닉 상태를 출력합니다. 각 디코더 블록 내에서 GPT-2는 *마스크드 셀프 어텐션(masked self-attention)* 레이어를 사용합니다. 이는 GPT-2가 이후 토큰(future tokens)에 주의를 기울일 수 없도록 합니다. 왼쪽에 있는 토큰에만 주의를 기울일 수 있습니다. 마스크드 셀프 어텐션에서는 어텐션 마스크를 사용하여 이후 토큰에 대한 점수(score)를 `0`으로 설정하기 때문에 BERT의 [`mask`] 토큰과 다릅니다.
2. 디코더의 출력은 언어 모델링 헤드에 전달되며, 언어 모델링 헤드는 은닉 상태를 로짓으로 선형 변환을 수행합니다. 레이블은 시퀀스의 다음 토큰으로, 로짓을 오른쪽으로 하나씩 이동하여 생성됩니다. 교차 엔트로피 손실은 이동된 로짓과 레이블 간에 계산되어 가장 가능성이 높은 다음 토큰을 출력합니다.
GPT-2의 사전훈련 목적은 전적으로 [인과적 언어 모델링](glossary#causal-language-modeling)에 기반하여, 시퀀스에서 다음 단어를 예측하는 것입니다. 이는 GPT-2가 텍스트 생성에 관련된 작업에 특히 우수하도록 합니다.
텍스트 생성에 직접 도전할 준비가 되셨나요? 완전한 [인과적 언어 모델링 가이드](tasks/language_modeling#causal-language-modeling)를 확인하여 DistilGPT-2를 미세 조정하고 추론에 사용하는 방법을 학습하세요!
<Tip>
텍스트 생성에 대한 자세한 내용은 [텍스트 생성 전략](generation_strategies) 가이드를 확인하세요!
</Tip>
### 요약[[summarization]]
[BART](model_doc/bart) 및 [T5](model_doc/t5)와 같은 인코더-디코더 모델은 요약 작업의 시퀀스-투-시퀀스 패턴을 위해 설계되었습니다. 이 섹션에서 BART의 작동 방법을 설명한 다음, 마지막에 T5를 미세 조정해볼 수 있습니다.
<div class="flex justify-center">
<img src="https://huggingface.co/datasets/huggingface/documentation-images/resolve/main/bart_architecture.png"/>
</div>
1. BART의 인코더 아키텍처는 BERT와 매우 유사하며 텍스트의 토큰 및 위치 임베딩을 받습니다. BART는 입력을 변형시키고 디코더로 재구성하여 사전훈련됩니다. 특정 변형 기법이 있는 다른 인코더와는 달리, BART는 모든 유형의 변형을 적용할 수 있습니다. 그러나 *text infilling* 변형 기법이 가장 잘 작동합니다. Text Infiling에서는 여러 텍스트 스팬을 **단일** [`mask`] 토큰으로 대체합니다. 이는 모델이 마스크된 토큰을 예측해야 하고, 모델에 누락된 토큰의 수를 예측하도록 가르치기 때문에 중요합니다. 입력 임베딩과 마스크된 스팬이 인코더를 거쳐 최종 은닉 상태를 출력하지만, BERT와 달리 BART는 마지막에 단어를 예측하는 순방향 네트워크를 추가하지 않습니다.
2. 인코더의 출력은 디코더로 전달되며, 디코더는 인코더의 출력에서 마스크 토큰과 변형되지 않은 토큰을 예측해야 합니다. 이는 디코더가 원본 텍스트를 복원하는 데 도움이 되는 추가적인 문맥을 얻도록 합니다. 디코더의 출력은 언어 모델링 헤드에 전달되며, 언어 모델링 헤드는 은닉 상태를 로짓으로 선형 변환을 수행합니다. 교차 엔트로피 손실은 로짓과 토큰이 오른쪽으로 이동된 레이블 간에 계산됩니다.
요약에 직접 도전할 준비가 되셨나요? 완전한 [요약 가이드](tasks/summarization)를 확인하여 T5를 미세 조정하고 추론에 사용하는 방법을 학습하세요!
<Tip>
텍스트 생성에 대한 자세한 내용은 [텍스트 생성 전략](generation_strategies) 가이드를 확인하세요!
</Tip>
### 번역[[translation]]
번역은 시퀀스-투-시퀀스 작업의 또 다른 예로, [BART](model_doc/bart) 또는 [T5](model_doc/t5)와 같은 인코더-디코더 모델을 사용할 수 있습니다. 이 섹션에서 BART의 작동 방법을 설명한 다음, 마지막에 T5를 미세 조정해볼 수 있습니다.
BART는 원천 언어를 타겟 언어로 디코딩할 수 있는 입력에 매핑하기 위해 무작위로 초기화된 별도의 인코더를 추가하여 번역에 적용합니다. 이 새로운 인코더의 임베딩은 원본 단어 임베딩 대신 사전훈련된 인코더로 전달됩니다. 원천 인코더는 모델 출력의 교차 엔트로피 손실로부터 원천 인코더, 위치 임베딩, 입력 임베딩을 갱신하여 훈련됩니다. 첫 번째 단계에서는 모델 파라미터가 고정되고, 두 번째 단계에서는 모든 모델 파라미터가 함께 훈련됩니다.
BART는 이후 번역을 위해 다양한 언어로 사전훈련된 다국어 버전의 mBART로 확장되었습니다.
번역에 직접 도전할 준비가 되셨나요? 완전한 [번역 가이드](tasks/summarization)를 확인하여 T5를 미세 조정하고 추론에 사용하는 방법을 학습하세요!
<Tip>
텍스트 생성에 대한 자세한 내용은 [텍스트 생성 전략](generation_strategies) 가이드를 확인하세요!
</Tip> | transformers/docs/source/ko/tasks_explained.md/0 | {
"file_path": "transformers/docs/source/ko/tasks_explained.md",
"repo_id": "transformers",
"token_count": 25797
} | 277 |
<!--Copyright 2020 The HuggingFace Team. All rights reserved.
Licensed under the Apache License, Version 2.0 (the "License"); you may not use this file except in compliance with
the License. You may obtain a copy of the License at
http://www.apache.org/licenses/LICENSE-2.0
Unless required by applicable law or agreed to in writing, software distributed under the License is distributed on
an "AS IS" BASIS, WITHOUT WARRANTIES OR CONDITIONS OF ANY KIND, either express or implied. See the License for the
specific language governing permissions and limitations under the License.
⚠️ Note that this file is in Markdown but contain specific syntax for our doc-builder (similar to MDX) that may not be
rendered properly in your Markdown viewer.
-->
# Compartilhando modelos customizados
A biblioteca 🤗 Transformers foi projetada para ser facilmente extensível. Cada modelo é totalmente codificado em uma determinada subpasta
do repositório sem abstração, para que você possa copiar facilmente um arquivo de modelagem e ajustá-lo às suas necessidades.
Se você estiver escrevendo um modelo totalmente novo, pode ser mais fácil começar do zero. Neste tutorial, mostraremos
como escrever um modelo customizado e sua configuração para que possa ser usado com Transformers, e como você pode compartilhá-lo
com a comunidade (com o código em que se baseia) para que qualquer pessoa possa usá-lo, mesmo se não estiver presente na biblioteca 🤗 Transformers.
Ilustraremos tudo isso em um modelo ResNet, envolvendo a classe ResNet do
[biblioteca timm](https://github.com/rwightman/pytorch-image-models) em um [`PreTrainedModel`].
## Escrevendo uma configuração customizada
Antes de mergulharmos no modelo, vamos primeiro escrever sua configuração. A configuração de um modelo é um objeto que
terá todas as informações necessárias para construir o modelo. Como veremos na próxima seção, o modelo só pode
ter um `config` para ser inicializado, então realmente precisamos que esse objeto seja o mais completo possível.
Em nosso exemplo, pegaremos alguns argumentos da classe ResNet que podemos querer ajustar. Diferentes
configurações nos dará os diferentes tipos de ResNets que são possíveis. Em seguida, apenas armazenamos esses argumentos,
após verificar a validade de alguns deles.
```python
from transformers import PretrainedConfig
from typing import List
class ResnetConfig(PretrainedConfig):
model_type = "resnet"
def __init__(
self,
block_type="bottleneck",
layers: List[int] = [3, 4, 6, 3],
num_classes: int = 1000,
input_channels: int = 3,
cardinality: int = 1,
base_width: int = 64,
stem_width: int = 64,
stem_type: str = "",
avg_down: bool = False,
**kwargs,
):
if block_type not in ["basic", "bottleneck"]:
raise ValueError(f"`block_type` must be 'basic' or bottleneck', got {block_type}.")
if stem_type not in ["", "deep", "deep-tiered"]:
raise ValueError(f"`stem_type` must be '', 'deep' or 'deep-tiered', got {stem_type}.")
self.block_type = block_type
self.layers = layers
self.num_classes = num_classes
self.input_channels = input_channels
self.cardinality = cardinality
self.base_width = base_width
self.stem_width = stem_width
self.stem_type = stem_type
self.avg_down = avg_down
super().__init__(**kwargs)
```
As três coisas importantes a serem lembradas ao escrever sua própria configuração são:
- você tem que herdar de `PretrainedConfig`,
- o `__init__` do seu `PretrainedConfig` deve aceitar quaisquer kwargs,
- esses `kwargs` precisam ser passados para a superclasse `__init__`.
A herança é para garantir que você obtenha todas as funcionalidades da biblioteca 🤗 Transformers, enquanto as outras duas
restrições vêm do fato de um `PretrainedConfig` ter mais campos do que os que você está configurando. Ao recarregar um
config com o método `from_pretrained`, esses campos precisam ser aceitos pelo seu config e então enviados para a
superclasse.
Definir um `model_type` para sua configuração (aqui `model_type="resnet"`) não é obrigatório, a menos que você queira
registrar seu modelo com as classes automáticas (veja a última seção).
Com isso feito, você pode facilmente criar e salvar sua configuração como faria com qualquer outra configuração de modelo da
biblioteca. Aqui está como podemos criar uma configuração resnet50d e salvá-la:
```py
resnet50d_config = ResnetConfig(block_type="bottleneck", stem_width=32, stem_type="deep", avg_down=True)
resnet50d_config.save_pretrained("custom-resnet")
```
Isso salvará um arquivo chamado `config.json` dentro da pasta `custom-resnet`. Você pode então recarregar sua configuração com o
método `from_pretrained`:
```py
resnet50d_config = ResnetConfig.from_pretrained("custom-resnet")
```
Você também pode usar qualquer outro método da classe [`PretrainedConfig`], como [`~PretrainedConfig.push_to_hub`] para
carregar diretamente sua configuração para o Hub.
## Escrevendo um modelo customizado
Agora que temos nossa configuração ResNet, podemos continuar escrevendo o modelo. Na verdade, escreveremos dois: um que
extrai os recursos ocultos de um lote de imagens (como [`BertModel`]) e um que é adequado para classificação de imagem
(como [`BertForSequenceClassification`]).
Como mencionamos antes, escreveremos apenas um wrapper solto do modelo para mantê-lo simples para este exemplo. A única
coisa que precisamos fazer antes de escrever esta classe é um mapa entre os tipos de bloco e as classes de bloco reais. Então o
modelo é definido a partir da configuração passando tudo para a classe `ResNet`:
```py
from transformers import PreTrainedModel
from timm.models.resnet import BasicBlock, Bottleneck, ResNet
from .configuration_resnet import ResnetConfig
BLOCK_MAPPING = {"basic": BasicBlock, "bottleneck": Bottleneck}
class ResnetModel(PreTrainedModel):
config_class = ResnetConfig
def __init__(self, config):
super().__init__(config)
block_layer = BLOCK_MAPPING[config.block_type]
self.model = ResNet(
block_layer,
config.layers,
num_classes=config.num_classes,
in_chans=config.input_channels,
cardinality=config.cardinality,
base_width=config.base_width,
stem_width=config.stem_width,
stem_type=config.stem_type,
avg_down=config.avg_down,
)
def forward(self, tensor):
return self.model.forward_features(tensor)
```
Para o modelo que irá classificar as imagens, vamos apenas alterar o método forward:
```py
import torch
class ResnetModelForImageClassification(PreTrainedModel):
config_class = ResnetConfig
def __init__(self, config):
super().__init__(config)
block_layer = BLOCK_MAPPING[config.block_type]
self.model = ResNet(
block_layer,
config.layers,
num_classes=config.num_classes,
in_chans=config.input_channels,
cardinality=config.cardinality,
base_width=config.base_width,
stem_width=config.stem_width,
stem_type=config.stem_type,
avg_down=config.avg_down,
)
def forward(self, tensor, labels=None):
logits = self.model(tensor)
if labels is not None:
loss = torch.nn.cross_entropy(logits, labels)
return {"loss": loss, "logits": logits}
return {"logits": logits}
```
Em ambos os casos, observe como herdamos de `PreTrainedModel` e chamamos a inicialização da superclasse com o `config`
(um pouco parecido quando você escreve um `torch.nn.Module`). A linha que define o `config_class` não é obrigatória, a menos que
você deseje registrar seu modelo com as classes automáticas (consulte a última seção).
<Tip>
Se o seu modelo for muito semelhante a um modelo dentro da biblioteca, você poderá reutilizar a mesma configuração desse modelo.
</Tip>
Você pode fazer com que seu modelo retorne o que você quiser,porém retornando um dicionário como fizemos para
`ResnetModelForImageClassification`, com a função de perda incluída quando os rótulos são passados, vai tornar seu modelo diretamente
utilizável dentro da classe [`Trainer`]. Você pode usar outro formato de saída, desde que esteja planejando usar seu próprio
laço de treinamento ou outra biblioteca para treinamento.
Agora que temos nossa classe do modelo, vamos criar uma:
```py
resnet50d = ResnetModelForImageClassification(resnet50d_config)
```
Novamente, você pode usar qualquer um dos métodos do [`PreTrainedModel`], como [`~PreTrainedModel.save_pretrained`] ou
[`~PreTrainedModel.push_to_hub`]. Usaremos o segundo na próxima seção e veremos como enviar os pesos e
o código do nosso modelo. Mas primeiro, vamos carregar alguns pesos pré-treinados dentro do nosso modelo.
Em seu próprio caso de uso, você provavelmente estará treinando seu modelo customizado em seus próprios dados. Para este tutorial ser rápido,
usaremos a versão pré-treinada do resnet50d. Como nosso modelo é apenas um wrapper em torno dele, será
fácil de transferir esses pesos:
```py
import timm
pretrained_model = timm.create_model("resnet50d", pretrained=True)
resnet50d.model.load_state_dict(pretrained_model.state_dict())
```
Agora vamos ver como ter certeza de que quando fazemos [`~PreTrainedModel.save_pretrained`] ou [`~PreTrainedModel.push_to_hub`], o
código do modelo é salvo.
## Enviando o código para o Hub
<Tip warning={true}>
Esta API é experimental e pode ter algumas pequenas alterações nas próximas versões.
</Tip>
Primeiro, certifique-se de que seu modelo esteja totalmente definido em um arquivo `.py`. Ele pode contar com importações relativas para alguns outros arquivos
desde que todos os arquivos estejam no mesmo diretório (ainda não suportamos submódulos para este recurso). Para o nosso exemplo,
vamos definir um arquivo `modeling_resnet.py` e um arquivo `configuration_resnet.py` em uma pasta no
diretório de trabalho atual chamado `resnet_model`. O arquivo de configuração contém o código para `ResnetConfig` e o arquivo de modelagem
contém o código do `ResnetModel` e `ResnetModelForImageClassification`.
```
.
└── resnet_model
├── __init__.py
├── configuration_resnet.py
└── modeling_resnet.py
```
O `__init__.py` pode estar vazio, apenas está lá para que o Python detecte que o `resnet_model` possa ser usado como um módulo.
<Tip warning={true}>
Se estiver copiando arquivos de modelagem da biblioteca, você precisará substituir todas as importações relativas na parte superior do arquivo
para importar do pacote `transformers`.
</Tip>
Observe que você pode reutilizar (ou subclasse) uma configuração/modelo existente.
Para compartilhar seu modelo com a comunidade, siga estas etapas: primeiro importe o modelo ResNet e a configuração do
arquivos criados:
```py
from resnet_model.configuration_resnet import ResnetConfig
from resnet_model.modeling_resnet import ResnetModel, ResnetModelForImageClassification
```
Então você tem que dizer à biblioteca que deseja copiar os arquivos de código desses objetos ao usar o `save_pretrained`
e registrá-los corretamente com uma determinada classe automáticas (especialmente para modelos), basta executar:
```py
ResnetConfig.register_for_auto_class()
ResnetModel.register_for_auto_class("AutoModel")
ResnetModelForImageClassification.register_for_auto_class("AutoModelForImageClassification")
```
Observe que não há necessidade de especificar uma classe automática para a configuração (há apenas uma classe automática,
[`AutoConfig`]), mas é diferente para os modelos. Seu modelo customizado pode ser adequado para muitas tarefas diferentes, então você
tem que especificar qual das classes automáticas é a correta para o seu modelo.
Em seguida, vamos criar a configuração e os modelos como fizemos antes:
```py
resnet50d_config = ResnetConfig(block_type="bottleneck", stem_width=32, stem_type="deep", avg_down=True)
resnet50d = ResnetModelForImageClassification(resnet50d_config)
pretrained_model = timm.create_model("resnet50d", pretrained=True)
resnet50d.model.load_state_dict(pretrained_model.state_dict())
```
Agora para enviar o modelo para o Hub, certifique-se de estar logado. Ou execute no seu terminal:
```bash
huggingface-cli login
```
ou a partir do notebook:
```py
from huggingface_hub import notebook_login
notebook_login()
```
Você pode então enviar para seu próprio namespace (ou uma organização da qual você é membro) assim:
```py
resnet50d.push_to_hub("custom-resnet50d")
```
Além dos pesos do modelo e da configuração no formato json, isso também copiou o modelo e
configuração `.py` na pasta `custom-resnet50d` e carregou o resultado para o Hub. Você pode conferir o resultado
neste [repositório de modelos](https://huggingface.co/sgugger/custom-resnet50d).
Consulte o [tutorial de compartilhamento](model_sharing) para obter mais informações sobre o método push_to_hub.
## Usando um modelo com código customizado
Você pode usar qualquer configuração, modelo ou tokenizador com arquivos de código customizados em seu repositório com as classes automáticas e
o método `from_pretrained`. Todos os arquivos e códigos carregados no Hub são verificados quanto a malware (consulte a documentação de [Segurança do Hub](https://huggingface.co/docs/hub/security#malware-scanning) para obter mais informações), mas você ainda deve
revisar o código do modelo e o autor para evitar a execução de código malicioso em sua máquina. Defina `trust_remote_code=True` para usar
um modelo com código customizado:
```py
from transformers import AutoModelForImageClassification
model = AutoModelForImageClassification.from_pretrained("sgugger/custom-resnet50d", trust_remote_code=True)
```
Também é fortemente recomendado passar um hash de confirmação como uma `revisão` para garantir que o autor dos modelos não
atualize o código com novas linhas maliciosas (a menos que você confie totalmente nos autores dos modelos).
```py
commit_hash = "ed94a7c6247d8aedce4647f00f20de6875b5b292"
model = AutoModelForImageClassification.from_pretrained(
"sgugger/custom-resnet50d", trust_remote_code=True, revision=commit_hash
)
```
Observe que ao navegar no histórico de commits do repositório do modelo no Hub, há um botão para copiar facilmente o commit
hash de qualquer commit.
## Registrando um modelo com código customizado para as classes automáticas
Se você estiver escrevendo uma biblioteca que estende 🤗 Transformers, talvez queira estender as classes automáticas para incluir seus próprios
modelos. Isso é diferente de enviar o código para o Hub no sentido de que os usuários precisarão importar sua biblioteca para
obter os modelos customizados (ao contrário de baixar automaticamente o código do modelo do Hub).
Desde que sua configuração tenha um atributo `model_type` diferente dos tipos de modelo existentes e que as classes do seu modelo
tenha os atributos `config_class` corretos, você pode simplesmente adicioná-los às classes automáticas assim:
```py
from transformers import AutoConfig, AutoModel, AutoModelForImageClassification
AutoConfig.register("resnet", ResnetConfig)
AutoModel.register(ResnetConfig, ResnetModel)
AutoModelForImageClassification.register(ResnetConfig, ResnetModelForImageClassification)
```
Observe que o primeiro argumento usado ao registrar sua configuração customizada para [`AutoConfig`] precisa corresponder ao `model_type`
de sua configuração customizada. E o primeiro argumento usado ao registrar seus modelos customizados, para qualquer necessidade de classe de modelo automático
deve corresponder ao `config_class` desses modelos.
| transformers/docs/source/pt/custom_models.md/0 | {
"file_path": "transformers/docs/source/pt/custom_models.md",
"repo_id": "transformers",
"token_count": 5915
} | 278 |
<!---
Copyright 2020 The HuggingFace Team. All rights reserved.
Licensed under the Apache License, Version 2.0 (the "License");
you may not use this file except in compliance with the License.
You may obtain a copy of the License at
http://www.apache.org/licenses/LICENSE-2.0
Unless required by applicable law or agreed to in writing, software
distributed under the License is distributed on an "AS IS" BASIS,
WITHOUT WARRANTIES OR CONDITIONS OF ANY KIND, either express or implied.
See the License for the specific language governing permissions and
limitations under the License.
-->
# Examples
We host a wide range of example scripts for multiple learning frameworks. Simply choose your favorite: [TensorFlow](https://github.com/huggingface/transformers/tree/main/examples/tensorflow), [PyTorch](https://github.com/huggingface/transformers/tree/main/examples/pytorch) or [JAX/Flax](https://github.com/huggingface/transformers/tree/main/examples/flax).
We also have some [research projects](https://github.com/huggingface/transformers/tree/main/examples/research_projects), as well as some [legacy examples](https://github.com/huggingface/transformers/tree/main/examples/legacy). Note that unlike the main examples these are not actively maintained, and may require specific older versions of dependencies in order to run.
While we strive to present as many use cases as possible, the example scripts are just that - examples. It is expected that they won't work out-of-the-box on your specific problem and that you will be required to change a few lines of code to adapt them to your needs. To help you with that, most of the examples fully expose the preprocessing of the data, allowing you to tweak and edit them as required.
Please discuss on the [forum](https://discuss.huggingface.co/) or in an [issue](https://github.com/huggingface/transformers/issues) a feature you would like to implement in an example before submitting a PR; we welcome bug fixes, but since we want to keep the examples as simple as possible it's unlikely that we will merge a pull request adding more functionality at the cost of readability.
## Important note
**Important**
To make sure you can successfully run the latest versions of the example scripts, you have to **install the library from source** and install some example-specific requirements. To do this, execute the following steps in a new virtual environment:
```bash
git clone https://github.com/huggingface/transformers
cd transformers
pip install .
```
Then cd in the example folder of your choice and run
```bash
pip install -r requirements.txt
```
To browse the examples corresponding to released versions of 🤗 Transformers, click on the line below and then on your desired version of the library:
<details>
<summary>Examples for older versions of 🤗 Transformers</summary>
<ul>
<li><a href="https://github.com/huggingface/transformers/tree/v4.21.0/examples">v4.21.0</a></li>
<li><a href="https://github.com/huggingface/transformers/tree/v4.20.1/examples">v4.20.1</a></li>
<li><a href="https://github.com/huggingface/transformers/tree/v4.19.4/examples">v4.19.4</a></li>
<li><a href="https://github.com/huggingface/transformers/tree/v4.18.0/examples">v4.18.0</a></li>
<li><a href="https://github.com/huggingface/transformers/tree/v4.17.0/examples">v4.17.0</a></li>
<li><a href="https://github.com/huggingface/transformers/tree/v4.16.2/examples">v4.16.2</a></li>
<li><a href="https://github.com/huggingface/transformers/tree/v4.15.0/examples">v4.15.0</a></li>
<li><a href="https://github.com/huggingface/transformers/tree/v4.14.1/examples">v4.14.1</a></li>
<li><a href="https://github.com/huggingface/transformers/tree/v4.13.0/examples">v4.13.0</a></li>
<li><a href="https://github.com/huggingface/transformers/tree/v4.12.5/examples">v4.12.5</a></li>
<li><a href="https://github.com/huggingface/transformers/tree/v4.11.3/examples">v4.11.3</a></li>
<li><a href="https://github.com/huggingface/transformers/tree/v4.10.3/examples">v4.10.3</a></li>
<li><a href="https://github.com/huggingface/transformers/tree/v4.9.2/examples">v4.9.2</a></li>
<li><a href="https://github.com/huggingface/transformers/tree/v4.8.2/examples">v4.8.2</a></li>
<li><a href="https://github.com/huggingface/transformers/tree/v4.7.0/examples">v4.7.0</a></li>
<li><a href="https://github.com/huggingface/transformers/tree/v4.6.1/examples">v4.6.1</a></li>
<li><a href="https://github.com/huggingface/transformers/tree/v4.5.1/examples">v4.5.1</a></li>
<li><a href="https://github.com/huggingface/transformers/tree/v4.4.2/examples">v4.4.2</a></li>
<li><a href="https://github.com/huggingface/transformers/tree/v4.3.3/examples">v4.3.3</a></li>
<li><a href="https://github.com/huggingface/transformers/tree/v4.2.2/examples">v4.2.2</a></li>
<li><a href="https://github.com/huggingface/transformers/tree/v4.1.1/examples">v4.1.1</a></li>
<li><a href="https://github.com/huggingface/transformers/tree/v4.0.1/examples">v4.0.1</a></li>
<li><a href="https://github.com/huggingface/transformers/tree/v3.5.1/examples">v3.5.1</a></li>
<li><a href="https://github.com/huggingface/transformers/tree/v3.4.0/examples">v3.4.0</a></li>
<li><a href="https://github.com/huggingface/transformers/tree/v3.3.1/examples">v3.3.1</a></li>
<li><a href="https://github.com/huggingface/transformers/tree/v3.2.0/examples">v3.2.0</a></li>
<li><a href="https://github.com/huggingface/transformers/tree/v3.1.0/examples">v3.1.0</a></li>
<li><a href="https://github.com/huggingface/transformers/tree/v3.0.2/examples">v3.0.2</a></li>
<li><a href="https://github.com/huggingface/transformers/tree/v2.11.0/examples">v2.11.0</a></li>
<li><a href="https://github.com/huggingface/transformers/tree/v2.10.0/examples">v2.10.0</a></li>
<li><a href="https://github.com/huggingface/transformers/tree/v2.9.1/examples">v2.9.1</a></li>
<li><a href="https://github.com/huggingface/transformers/tree/v2.8.0/examples">v2.8.0</a></li>
<li><a href="https://github.com/huggingface/transformers/tree/v2.7.0/examples">v2.7.0</a></li>
<li><a href="https://github.com/huggingface/transformers/tree/v2.6.0/examples">v2.6.0</a></li>
<li><a href="https://github.com/huggingface/transformers/tree/v2.5.1/examples">v2.5.1</a></li>
<li><a href="https://github.com/huggingface/transformers/tree/v2.4.0/examples">v2.4.0</a></li>
<li><a href="https://github.com/huggingface/transformers/tree/v2.3.0/examples">v2.3.0</a></li>
<li><a href="https://github.com/huggingface/transformers/tree/v2.2.0/examples">v2.2.0</a></li>
<li><a href="https://github.com/huggingface/transformers/tree/v2.1.0/examples">v2.1.1</a></li>
<li><a href="https://github.com/huggingface/transformers/tree/v2.0.0/examples">v2.0.0</a></li>
<li><a href="https://github.com/huggingface/transformers/tree/v1.2.0/examples">v1.2.0</a></li>
<li><a href="https://github.com/huggingface/transformers/tree/v1.1.0/examples">v1.1.0</a></li>
<li><a href="https://github.com/huggingface/transformers/tree/v1.0.0/examples">v1.0.0</a></li>
</ul>
</details>
Alternatively, you can switch your cloned 🤗 Transformers to a specific version (for instance with v3.5.1) with
```bash
git checkout tags/v3.5.1
```
and run the example command as usual afterward.
## Running the Examples on Remote Hardware with Auto-Setup
[run_on_remote.py](./run_on_remote.py) is a script that launches any example on remote self-hosted hardware,
with automatic hardware and environment setup. It uses [Runhouse](https://github.com/run-house/runhouse) to launch
on self-hosted hardware (e.g. in your own cloud account or on-premise cluster) but there are other options
for running remotely as well. You can easily customize the example used, command line arguments, dependencies,
and type of compute hardware, and then run the script to automatically launch the example.
You can refer to
[hardware setup](https://runhouse-docs.readthedocs-hosted.com/en/latest/api/python/cluster.html#hardware-setup)
for more information about hardware and dependency setup with Runhouse, or this
[Colab tutorial](https://colab.research.google.com/drive/1sh_aNQzJX5BKAdNeXthTNGxKz7sM9VPc) for a more in-depth
walkthrough.
You can run the script with the following commands:
```bash
# First install runhouse:
pip install runhouse
# For an on-demand V100 with whichever cloud provider you have configured:
python run_on_remote.py \
--example pytorch/text-generation/run_generation.py \
--model_type=openai-community/gpt2 \
--model_name_or_path=openai-community/gpt2 \
--prompt "I am a language model and"
# For byo (bring your own) cluster:
python run_on_remote.py --host <cluster_ip> --user <ssh_user> --key_path <ssh_key_path> \
--example <example> <args>
# For on-demand instances
python run_on_remote.py --instance <instance> --provider <provider> \
--example <example> <args>
```
You can also adapt the script to your own needs.
| transformers/examples/README.md/0 | {
"file_path": "transformers/examples/README.md",
"repo_id": "transformers",
"token_count": 3302
} | 279 |
#!/usr/bin/env python
# coding=utf-8
# Copyright 2021 The HuggingFace Team All rights reserved.
#
# Licensed under the Apache License, Version 2.0 (the "License");
# you may not use this file except in compliance with the License.
# You may obtain a copy of the License at
#
# http://www.apache.org/licenses/LICENSE-2.0
#
# Unless required by applicable law or agreed to in writing, software
# distributed under the License is distributed on an "AS IS" BASIS,
# WITHOUT WARRANTIES OR CONDITIONS OF ANY KIND, either express or implied.
# See the License for the specific language governing permissions and
# limitations under the License.
"""
Fine-tuning the library models for question answering.
"""
# You can also adapt this script on your own question answering task. Pointers for this are left as comments.
import json
import logging
import math
import os
import random
import sys
import time
import warnings
from dataclasses import asdict, dataclass, field
from enum import Enum
from pathlib import Path
from typing import Any, Callable, Dict, Optional, Tuple
import datasets
import evaluate
import jax
import jax.numpy as jnp
import numpy as np
import optax
from datasets import load_dataset
from flax import struct, traverse_util
from flax.jax_utils import pad_shard_unpad, replicate, unreplicate
from flax.training import train_state
from flax.training.common_utils import get_metrics, onehot, shard
from huggingface_hub import HfApi
from tqdm import tqdm
from utils_qa import postprocess_qa_predictions
import transformers
from transformers import (
AutoConfig,
AutoTokenizer,
EvalPrediction,
FlaxAutoModelForQuestionAnswering,
HfArgumentParser,
PreTrainedTokenizerFast,
is_tensorboard_available,
)
from transformers.utils import check_min_version, send_example_telemetry
logger = logging.getLogger(__name__)
# Will error if the minimal version of Transformers is not installed. Remove at your own risks.
check_min_version("4.40.0.dev0")
Array = Any
Dataset = datasets.arrow_dataset.Dataset
PRNGKey = Any
# region Arguments
@dataclass
class TrainingArguments:
output_dir: str = field(
metadata={"help": "The output directory where the model predictions and checkpoints will be written."},
)
overwrite_output_dir: bool = field(
default=False,
metadata={
"help": (
"Overwrite the content of the output directory. "
"Use this to continue training if output_dir points to a checkpoint directory."
)
},
)
do_train: bool = field(default=False, metadata={"help": "Whether to run training."})
do_eval: bool = field(default=False, metadata={"help": "Whether to run eval on the dev set."})
do_predict: bool = field(default=False, metadata={"help": "Whether to run predictions on the test set."})
per_device_train_batch_size: int = field(
default=8, metadata={"help": "Batch size per GPU/TPU core/CPU for training."}
)
per_device_eval_batch_size: int = field(
default=8, metadata={"help": "Batch size per GPU/TPU core/CPU for evaluation."}
)
learning_rate: float = field(default=5e-5, metadata={"help": "The initial learning rate for AdamW."})
weight_decay: float = field(default=0.0, metadata={"help": "Weight decay for AdamW if we apply some."})
adam_beta1: float = field(default=0.9, metadata={"help": "Beta1 for AdamW optimizer"})
adam_beta2: float = field(default=0.999, metadata={"help": "Beta2 for AdamW optimizer"})
adam_epsilon: float = field(default=1e-8, metadata={"help": "Epsilon for AdamW optimizer."})
adafactor: bool = field(default=False, metadata={"help": "Whether or not to replace AdamW by Adafactor."})
num_train_epochs: float = field(default=3.0, metadata={"help": "Total number of training epochs to perform."})
warmup_steps: int = field(default=0, metadata={"help": "Linear warmup over warmup_steps."})
logging_steps: int = field(default=500, metadata={"help": "Log every X updates steps."})
save_steps: int = field(default=500, metadata={"help": "Save checkpoint every X updates steps."})
eval_steps: int = field(default=None, metadata={"help": "Run an evaluation every X steps."})
seed: int = field(default=42, metadata={"help": "Random seed that will be set at the beginning of training."})
push_to_hub: bool = field(
default=False, metadata={"help": "Whether or not to upload the trained model to the model hub after training."}
)
hub_model_id: str = field(
default=None, metadata={"help": "The name of the repository to keep in sync with the local `output_dir`."}
)
hub_token: str = field(default=None, metadata={"help": "The token to use to push to the Model Hub."})
def __post_init__(self):
if self.output_dir is not None:
self.output_dir = os.path.expanduser(self.output_dir)
def to_dict(self):
"""
Serializes this instance while replace `Enum` by their values (for JSON serialization support). It obfuscates
the token values by removing their value.
"""
d = asdict(self)
for k, v in d.items():
if isinstance(v, Enum):
d[k] = v.value
if isinstance(v, list) and len(v) > 0 and isinstance(v[0], Enum):
d[k] = [x.value for x in v]
if k.endswith("_token"):
d[k] = f"<{k.upper()}>"
return d
@dataclass
class ModelArguments:
"""
Arguments pertaining to which model/config/tokenizer we are going to fine-tune from.
"""
model_name_or_path: str = field(
metadata={"help": "Path to pretrained model or model identifier from huggingface.co/models"}
)
config_name: Optional[str] = field(
default=None, metadata={"help": "Pretrained config name or path if not the same as model_name"}
)
tokenizer_name: Optional[str] = field(
default=None, metadata={"help": "Pretrained tokenizer name or path if not the same as model_name"}
)
cache_dir: Optional[str] = field(
default=None,
metadata={"help": "Path to directory to store the pretrained models downloaded from huggingface.co"},
)
model_revision: str = field(
default="main",
metadata={"help": "The specific model version to use (can be a branch name, tag name or commit id)."},
)
token: str = field(
default=None,
metadata={
"help": (
"The token to use as HTTP bearer authorization for remote files. If not specified, will use the token "
"generated when running `huggingface-cli login` (stored in `~/.huggingface`)."
)
},
)
use_auth_token: bool = field(
default=None,
metadata={
"help": "The `use_auth_token` argument is deprecated and will be removed in v4.34. Please use `token` instead."
},
)
trust_remote_code: bool = field(
default=False,
metadata={
"help": (
"Whether or not to allow for custom models defined on the Hub in their own modeling files. This option "
"should only be set to `True` for repositories you trust and in which you have read the code, as it will "
"execute code present on the Hub on your local machine."
)
},
)
dtype: Optional[str] = field(
default="float32",
metadata={
"help": (
"Floating-point format in which the model weights should be initialized and trained. Choose one of"
" `[float32, float16, bfloat16]`."
)
},
)
@dataclass
class DataTrainingArguments:
"""
Arguments pertaining to what data we are going to input our model for training and eval.
"""
dataset_name: Optional[str] = field(
default=None, metadata={"help": "The name of the dataset to use (via the datasets library)."}
)
dataset_config_name: Optional[str] = field(
default=None, metadata={"help": "The configuration name of the dataset to use (via the datasets library)."}
)
train_file: Optional[str] = field(default=None, metadata={"help": "The input training data file (a text file)."})
validation_file: Optional[str] = field(
default=None,
metadata={"help": "An optional input evaluation data file to evaluate the perplexity on (a text file)."},
)
test_file: Optional[str] = field(
default=None,
metadata={"help": "An optional input test data file to evaluate the perplexity on (a text file)."},
)
overwrite_cache: bool = field(
default=False, metadata={"help": "Overwrite the cached training and evaluation sets"}
)
preprocessing_num_workers: Optional[int] = field(
default=None,
metadata={"help": "The number of processes to use for the preprocessing."},
)
max_seq_length: int = field(
default=384,
metadata={
"help": (
"The maximum total input sequence length after tokenization. Sequences longer "
"than this will be truncated, sequences shorter will be padded."
)
},
)
pad_to_max_length: bool = field(
default=False,
metadata={
"help": (
"Whether to pad all samples to `max_seq_length`. If False, will pad the samples dynamically when"
" batching to the maximum length in the batch (which can be faster on GPU but will be slower on TPU)."
)
},
)
max_train_samples: Optional[int] = field(
default=None,
metadata={
"help": (
"For debugging purposes or quicker training, truncate the number of training examples to this "
"value if set."
)
},
)
max_eval_samples: Optional[int] = field(
default=None,
metadata={
"help": (
"For debugging purposes or quicker training, truncate the number of evaluation examples to this "
"value if set."
)
},
)
max_predict_samples: Optional[int] = field(
default=None,
metadata={
"help": (
"For debugging purposes or quicker training, truncate the number of prediction examples to this "
"value if set."
)
},
)
version_2_with_negative: bool = field(
default=False, metadata={"help": "If true, some of the examples do not have an answer."}
)
null_score_diff_threshold: float = field(
default=0.0,
metadata={
"help": (
"The threshold used to select the null answer: if the best answer has a score that is less than "
"the score of the null answer minus this threshold, the null answer is selected for this example. "
"Only useful when `version_2_with_negative=True`."
)
},
)
doc_stride: int = field(
default=128,
metadata={"help": "When splitting up a long document into chunks, how much stride to take between chunks."},
)
n_best_size: int = field(
default=20,
metadata={"help": "The total number of n-best predictions to generate when looking for an answer."},
)
max_answer_length: int = field(
default=30,
metadata={
"help": (
"The maximum length of an answer that can be generated. This is needed because the start "
"and end predictions are not conditioned on one another."
)
},
)
def __post_init__(self):
if (
self.dataset_name is None
and self.train_file is None
and self.validation_file is None
and self.test_file is None
):
raise ValueError("Need either a dataset name or a training/validation file/test_file.")
else:
if self.train_file is not None:
extension = self.train_file.split(".")[-1]
assert extension in ["csv", "json"], "`train_file` should be a csv or a json file."
if self.validation_file is not None:
extension = self.validation_file.split(".")[-1]
assert extension in ["csv", "json"], "`validation_file` should be a csv or a json file."
if self.test_file is not None:
extension = self.test_file.split(".")[-1]
assert extension in ["csv", "json"], "`test_file` should be a csv or a json file."
# endregion
# region Create a train state
def create_train_state(
model: FlaxAutoModelForQuestionAnswering,
learning_rate_fn: Callable[[int], float],
num_labels: int,
training_args: TrainingArguments,
) -> train_state.TrainState:
"""Create initial training state."""
class TrainState(train_state.TrainState):
"""Train state with an Optax optimizer.
The two functions below differ depending on whether the task is classification
or regression.
Args:
logits_fn: Applied to last layer to obtain the logits.
loss_fn: Function to compute the loss.
"""
logits_fn: Callable = struct.field(pytree_node=False)
loss_fn: Callable = struct.field(pytree_node=False)
# We use Optax's "masking" functionality to not apply weight decay
# to bias and LayerNorm scale parameters. decay_mask_fn returns a
# mask boolean with the same structure as the parameters.
# The mask is True for parameters that should be decayed.
def decay_mask_fn(params):
flat_params = traverse_util.flatten_dict(params)
# find out all LayerNorm parameters
layer_norm_candidates = ["layernorm", "layer_norm", "ln"]
layer_norm_named_params = {
layer[-2:]
for layer_norm_name in layer_norm_candidates
for layer in flat_params.keys()
if layer_norm_name in "".join(layer).lower()
}
flat_mask = {path: (path[-1] != "bias" and path[-2:] not in layer_norm_named_params) for path in flat_params}
return traverse_util.unflatten_dict(flat_mask)
tx = optax.adamw(
learning_rate=learning_rate_fn,
b1=training_args.adam_beta1,
b2=training_args.adam_beta2,
eps=training_args.adam_epsilon,
weight_decay=training_args.weight_decay,
mask=decay_mask_fn,
)
def cross_entropy_loss(logits, labels):
start_loss = optax.softmax_cross_entropy(logits[0], onehot(labels[0], num_classes=num_labels))
end_loss = optax.softmax_cross_entropy(logits[1], onehot(labels[1], num_classes=num_labels))
xentropy = (start_loss + end_loss) / 2.0
return jnp.mean(xentropy)
return TrainState.create(
apply_fn=model.__call__,
params=model.params,
tx=tx,
logits_fn=lambda logits: logits,
loss_fn=cross_entropy_loss,
)
# endregion
# region Create learning rate function
def create_learning_rate_fn(
train_ds_size: int, train_batch_size: int, num_train_epochs: int, num_warmup_steps: int, learning_rate: float
) -> Callable[[int], jnp.ndarray]:
"""Returns a linear warmup, linear_decay learning rate function."""
steps_per_epoch = train_ds_size // train_batch_size
num_train_steps = steps_per_epoch * num_train_epochs
warmup_fn = optax.linear_schedule(init_value=0.0, end_value=learning_rate, transition_steps=num_warmup_steps)
decay_fn = optax.linear_schedule(
init_value=learning_rate, end_value=0, transition_steps=num_train_steps - num_warmup_steps
)
schedule_fn = optax.join_schedules(schedules=[warmup_fn, decay_fn], boundaries=[num_warmup_steps])
return schedule_fn
# endregion
# region train data iterator
def train_data_collator(rng: PRNGKey, dataset: Dataset, batch_size: int):
"""Returns shuffled batches of size `batch_size` from truncated `train dataset`, sharded over all local devices."""
steps_per_epoch = len(dataset) // batch_size
perms = jax.random.permutation(rng, len(dataset))
perms = perms[: steps_per_epoch * batch_size] # Skip incomplete batch.
perms = perms.reshape((steps_per_epoch, batch_size))
for perm in perms:
batch = dataset[perm]
batch = {k: np.array(v) for k, v in batch.items()}
batch = shard(batch)
yield batch
# endregion
# region eval data iterator
def eval_data_collator(dataset: Dataset, batch_size: int):
"""Returns batches of size `batch_size` from `eval dataset`. Sharding handled by `pad_shard_unpad` in the eval loop."""
batch_idx = np.arange(len(dataset))
steps_per_epoch = math.ceil(len(dataset) / batch_size)
batch_idx = np.array_split(batch_idx, steps_per_epoch)
for idx in batch_idx:
batch = dataset[idx]
batch = {k: np.array(v) for k, v in batch.items()}
yield batch
# endregion
def main():
# region Argument parsing
# See all possible arguments in src/transformers/training_args.py
# or by passing the --help flag to this script.
# We now keep distinct sets of args, for a cleaner separation of concerns.
parser = HfArgumentParser((ModelArguments, DataTrainingArguments, TrainingArguments))
if len(sys.argv) == 2 and sys.argv[1].endswith(".json"):
# If we pass only one argument to the script and it's the path to a json file,
# let's parse it to get our arguments.
model_args, data_args, training_args = parser.parse_json_file(json_file=os.path.abspath(sys.argv[1]))
else:
model_args, data_args, training_args = parser.parse_args_into_dataclasses()
if model_args.use_auth_token is not None:
warnings.warn(
"The `use_auth_token` argument is deprecated and will be removed in v4.34. Please use `token` instead.",
FutureWarning,
)
if model_args.token is not None:
raise ValueError("`token` and `use_auth_token` are both specified. Please set only the argument `token`.")
model_args.token = model_args.use_auth_token
# Sending telemetry. Tracking the example usage helps us better allocate resources to maintain them. The
# information sent is the one passed as arguments along with your Python/PyTorch versions.
send_example_telemetry("run_qa", model_args, data_args, framework="flax")
# endregion
# region Logging
# Make one log on every process with the configuration for debugging.
logging.basicConfig(
format="%(asctime)s - %(levelname)s - %(name)s - %(message)s",
datefmt="%m/%d/%Y %H:%M:%S",
level=logging.INFO,
)
# Setup logging, we only want one process per machine to log things on the screen.
logger.setLevel(logging.INFO if jax.process_index() == 0 else logging.ERROR)
if jax.process_index() == 0:
datasets.utils.logging.set_verbosity_warning()
transformers.utils.logging.set_verbosity_info()
else:
datasets.utils.logging.set_verbosity_error()
transformers.utils.logging.set_verbosity_error()
# endregion
# Handle the repository creation
if training_args.push_to_hub:
# Retrieve of infer repo_name
repo_name = training_args.hub_model_id
if repo_name is None:
repo_name = Path(training_args.output_dir).absolute().name
# Create repo and retrieve repo_id
api = HfApi()
repo_id = api.create_repo(repo_name, exist_ok=True, token=training_args.hub_token).repo_id
# region Load Data
# Get the datasets: you can either provide your own CSV/JSON/TXT training and evaluation files (see below)
# or just provide the name of one of the public datasets available on the hub at https://huggingface.co/datasets/
# (the dataset will be downloaded automatically from the datasets Hub).
#
# For CSV/JSON files, this script will use the column called 'text' or the first column if no column called
# 'text' is found. You can easily tweak this behavior (see below).
#
# In distributed training, the load_dataset function guarantee that only one local process can concurrently
# download the dataset.
if data_args.dataset_name is not None:
# Downloading and loading a dataset from the hub.
raw_datasets = load_dataset(
data_args.dataset_name,
data_args.dataset_config_name,
cache_dir=model_args.cache_dir,
token=model_args.token,
)
else:
# Loading the dataset from local csv or json file.
data_files = {}
if data_args.train_file is not None:
data_files["train"] = data_args.train_file
extension = data_args.train_file.split(".")[-1]
if data_args.validation_file is not None:
data_files["validation"] = data_args.validation_file
extension = data_args.validation_file.split(".")[-1]
if data_args.test_file is not None:
data_files["test"] = data_args.test_file
extension = data_args.test_file.split(".")[-1]
raw_datasets = load_dataset(
extension,
data_files=data_files,
field="data",
cache_dir=model_args.cache_dir,
token=model_args.token,
)
# See more about loading any type of standard or custom dataset (from files, python dict, pandas DataFrame, etc) at
# https://huggingface.co/docs/datasets/loading_datasets.
# endregion
# region Load pretrained model and tokenizer
#
# Load pretrained model and tokenizer
config = AutoConfig.from_pretrained(
model_args.config_name if model_args.config_name else model_args.model_name_or_path,
cache_dir=model_args.cache_dir,
revision=model_args.model_revision,
token=model_args.token,
trust_remote_code=model_args.trust_remote_code,
)
tokenizer = AutoTokenizer.from_pretrained(
model_args.tokenizer_name if model_args.tokenizer_name else model_args.model_name_or_path,
cache_dir=model_args.cache_dir,
use_fast=True,
revision=model_args.model_revision,
token=model_args.token,
trust_remote_code=model_args.trust_remote_code,
)
# endregion
# region Tokenizer check: this script requires a fast tokenizer.
if not isinstance(tokenizer, PreTrainedTokenizerFast):
raise ValueError(
"This example script only works for models that have a fast tokenizer. Checkout the big table of models at"
" https://huggingface.co/transformers/index.html#supported-frameworks to find the model types that meet"
" this requirement"
)
# endregion
# region Preprocessing the datasets
# Preprocessing is slightly different for training and evaluation.
if training_args.do_train:
column_names = raw_datasets["train"].column_names
elif training_args.do_eval:
column_names = raw_datasets["validation"].column_names
else:
column_names = raw_datasets["test"].column_names
question_column_name = "question" if "question" in column_names else column_names[0]
context_column_name = "context" if "context" in column_names else column_names[1]
answer_column_name = "answers" if "answers" in column_names else column_names[2]
# Padding side determines if we do (question|context) or (context|question).
pad_on_right = tokenizer.padding_side == "right"
if data_args.max_seq_length > tokenizer.model_max_length:
logger.warning(
f"The max_seq_length passed ({data_args.max_seq_length}) is larger than the maximum length for the "
f"model ({tokenizer.model_max_length}). Using max_seq_length={tokenizer.model_max_length}."
)
max_seq_length = min(data_args.max_seq_length, tokenizer.model_max_length)
# Training preprocessing
def prepare_train_features(examples):
# Some of the questions have lots of whitespace on the left, which is not useful and will make the
# truncation of the context fail (the tokenized question will take a lots of space). So we remove that
# left whitespace
examples[question_column_name] = [q.lstrip() for q in examples[question_column_name]]
# Tokenize our examples with truncation and maybe padding, but keep the overflows using a stride. This results
# in one example possible giving several features when a context is long, each of those features having a
# context that overlaps a bit the context of the previous feature.
tokenized_examples = tokenizer(
examples[question_column_name if pad_on_right else context_column_name],
examples[context_column_name if pad_on_right else question_column_name],
truncation="only_second" if pad_on_right else "only_first",
max_length=max_seq_length,
stride=data_args.doc_stride,
return_overflowing_tokens=True,
return_offsets_mapping=True,
padding="max_length",
)
# Since one example might give us several features if it has a long context, we need a map from a feature to
# its corresponding example. This key gives us just that.
sample_mapping = tokenized_examples.pop("overflow_to_sample_mapping")
# The offset mappings will give us a map from token to character position in the original context. This will
# help us compute the start_positions and end_positions.
offset_mapping = tokenized_examples.pop("offset_mapping")
# Let's label those examples!
tokenized_examples["start_positions"] = []
tokenized_examples["end_positions"] = []
for i, offsets in enumerate(offset_mapping):
# We will label impossible answers with the index of the CLS token.
input_ids = tokenized_examples["input_ids"][i]
cls_index = input_ids.index(tokenizer.cls_token_id)
# Grab the sequence corresponding to that example (to know what is the context and what is the question).
sequence_ids = tokenized_examples.sequence_ids(i)
# One example can give several spans, this is the index of the example containing this span of text.
sample_index = sample_mapping[i]
answers = examples[answer_column_name][sample_index]
# If no answers are given, set the cls_index as answer.
if len(answers["answer_start"]) == 0:
tokenized_examples["start_positions"].append(cls_index)
tokenized_examples["end_positions"].append(cls_index)
else:
# Start/end character index of the answer in the text.
start_char = answers["answer_start"][0]
end_char = start_char + len(answers["text"][0])
# Start token index of the current span in the text.
token_start_index = 0
while sequence_ids[token_start_index] != (1 if pad_on_right else 0):
token_start_index += 1
# End token index of the current span in the text.
token_end_index = len(input_ids) - 1
while sequence_ids[token_end_index] != (1 if pad_on_right else 0):
token_end_index -= 1
# Detect if the answer is out of the span (in which case this feature is labeled with the CLS index).
if not (offsets[token_start_index][0] <= start_char and offsets[token_end_index][1] >= end_char):
tokenized_examples["start_positions"].append(cls_index)
tokenized_examples["end_positions"].append(cls_index)
else:
# Otherwise move the token_start_index and token_end_index to the two ends of the answer.
# Note: we could go after the last offset if the answer is the last word (edge case).
while token_start_index < len(offsets) and offsets[token_start_index][0] <= start_char:
token_start_index += 1
tokenized_examples["start_positions"].append(token_start_index - 1)
while offsets[token_end_index][1] >= end_char:
token_end_index -= 1
tokenized_examples["end_positions"].append(token_end_index + 1)
return tokenized_examples
processed_raw_datasets = {}
if training_args.do_train:
if "train" not in raw_datasets:
raise ValueError("--do_train requires a train dataset")
train_dataset = raw_datasets["train"]
if data_args.max_train_samples is not None:
# We will select sample from whole data if argument is specified
max_train_samples = min(len(train_dataset), data_args.max_train_samples)
train_dataset = train_dataset.select(range(max_train_samples))
# Create train feature from dataset
train_dataset = train_dataset.map(
prepare_train_features,
batched=True,
num_proc=data_args.preprocessing_num_workers,
remove_columns=column_names,
load_from_cache_file=not data_args.overwrite_cache,
)
if data_args.max_train_samples is not None:
# Number of samples might increase during Feature Creation, We select only specified max samples
max_train_samples = min(len(train_dataset), data_args.max_train_samples)
train_dataset = train_dataset.select(range(max_train_samples))
processed_raw_datasets["train"] = train_dataset
# Validation preprocessing
def prepare_validation_features(examples):
# Some of the questions have lots of whitespace on the left, which is not useful and will make the
# truncation of the context fail (the tokenized question will take a lots of space). So we remove that
# left whitespace
examples[question_column_name] = [q.lstrip() for q in examples[question_column_name]]
# Tokenize our examples with truncation and maybe padding, but keep the overflows using a stride. This results
# in one example possible giving several features when a context is long, each of those features having a
# context that overlaps a bit the context of the previous feature.
tokenized_examples = tokenizer(
examples[question_column_name if pad_on_right else context_column_name],
examples[context_column_name if pad_on_right else question_column_name],
truncation="only_second" if pad_on_right else "only_first",
max_length=max_seq_length,
stride=data_args.doc_stride,
return_overflowing_tokens=True,
return_offsets_mapping=True,
padding="max_length",
)
# Since one example might give us several features if it has a long context, we need a map from a feature to
# its corresponding example. This key gives us just that.
sample_mapping = tokenized_examples.pop("overflow_to_sample_mapping")
# For evaluation, we will need to convert our predictions to substrings of the context, so we keep the
# corresponding example_id and we will store the offset mappings.
tokenized_examples["example_id"] = []
for i in range(len(tokenized_examples["input_ids"])):
# Grab the sequence corresponding to that example (to know what is the context and what is the question).
sequence_ids = tokenized_examples.sequence_ids(i)
context_index = 1 if pad_on_right else 0
# One example can give several spans, this is the index of the example containing this span of text.
sample_index = sample_mapping[i]
tokenized_examples["example_id"].append(examples["id"][sample_index])
# Set to None the offset_mapping that are not part of the context so it's easy to determine if a token
# position is part of the context or not.
tokenized_examples["offset_mapping"][i] = [
(o if sequence_ids[k] == context_index else None)
for k, o in enumerate(tokenized_examples["offset_mapping"][i])
]
return tokenized_examples
if training_args.do_eval:
if "validation" not in raw_datasets:
raise ValueError("--do_eval requires a validation dataset")
eval_examples = raw_datasets["validation"]
if data_args.max_eval_samples is not None:
# We will select sample from whole data
max_eval_samples = min(len(eval_examples), data_args.max_eval_samples)
eval_examples = eval_examples.select(range(max_eval_samples))
# Validation Feature Creation
eval_dataset = eval_examples.map(
prepare_validation_features,
batched=True,
num_proc=data_args.preprocessing_num_workers,
remove_columns=column_names,
load_from_cache_file=not data_args.overwrite_cache,
)
if data_args.max_eval_samples is not None:
# During Feature creation dataset samples might increase, we will select required samples again
max_eval_samples = min(len(eval_dataset), data_args.max_eval_samples)
eval_dataset = eval_dataset.select(range(max_eval_samples))
processed_raw_datasets["validation"] = eval_dataset
if training_args.do_predict:
if "test" not in raw_datasets:
raise ValueError("--do_predict requires a test dataset")
predict_examples = raw_datasets["test"]
if data_args.max_predict_samples is not None:
# We will select sample from whole data
predict_examples = predict_examples.select(range(data_args.max_predict_samples))
# Predict Feature Creation
predict_dataset = predict_examples.map(
prepare_validation_features,
batched=True,
num_proc=data_args.preprocessing_num_workers,
remove_columns=column_names,
load_from_cache_file=not data_args.overwrite_cache,
)
if data_args.max_predict_samples is not None:
# During Feature creation dataset samples might increase, we will select required samples again
max_predict_samples = min(len(predict_dataset), data_args.max_predict_samples)
predict_dataset = predict_dataset.select(range(max_predict_samples))
processed_raw_datasets["test"] = predict_dataset
# endregion
# region Metrics and Post-processing:
def post_processing_function(examples, features, predictions, stage="eval"):
# Post-processing: we match the start logits and end logits to answers in the original context.
predictions = postprocess_qa_predictions(
examples=examples,
features=features,
predictions=predictions,
version_2_with_negative=data_args.version_2_with_negative,
n_best_size=data_args.n_best_size,
max_answer_length=data_args.max_answer_length,
null_score_diff_threshold=data_args.null_score_diff_threshold,
output_dir=training_args.output_dir,
prefix=stage,
)
# Format the result to the format the metric expects.
if data_args.version_2_with_negative:
formatted_predictions = [
{"id": k, "prediction_text": v, "no_answer_probability": 0.0} for k, v in predictions.items()
]
else:
formatted_predictions = [{"id": k, "prediction_text": v} for k, v in predictions.items()]
references = [{"id": ex["id"], "answers": ex[answer_column_name]} for ex in examples]
return EvalPrediction(predictions=formatted_predictions, label_ids=references)
metric = evaluate.load(
"squad_v2" if data_args.version_2_with_negative else "squad", cache_dir=model_args.cache_dir
)
def compute_metrics(p: EvalPrediction):
return metric.compute(predictions=p.predictions, references=p.label_ids)
# Create and fill numpy array of size len_of_validation_data * max_length_of_output_tensor
def create_and_fill_np_array(start_or_end_logits, dataset, max_len):
"""
Create and fill numpy array of size len_of_validation_data * max_length_of_output_tensor
Args:
start_or_end_logits(:obj:`tensor`):
This is the output predictions of the model. We can only enter either start or end logits.
eval_dataset: Evaluation dataset
max_len(:obj:`int`):
The maximum length of the output tensor. ( See the model.eval() part for more details )
"""
step = 0
# create a numpy array and fill it with -100.
logits_concat = np.full((len(dataset), max_len), -100, dtype=np.float64)
# Now since we have create an array now we will populate it with the outputs of the model.
for i, output_logit in enumerate(start_or_end_logits): # populate columns
# We have to fill it such that we have to take the whole tensor and replace it on the newly created array
# And after every iteration we have to change the step
batch_size = output_logit.shape[0]
cols = output_logit.shape[1]
if step + batch_size < len(dataset):
logits_concat[step : step + batch_size, :cols] = output_logit
else:
logits_concat[step:, :cols] = output_logit[: len(dataset) - step]
step += batch_size
return logits_concat
# endregion
# region Training steps and logging init
train_dataset = processed_raw_datasets["train"]
eval_dataset = processed_raw_datasets["validation"]
# Log a few random samples from the training set:
for index in random.sample(range(len(train_dataset)), 3):
logger.info(f"Sample {index} of the training set: {train_dataset[index]}.")
# Define a summary writer
has_tensorboard = is_tensorboard_available()
if has_tensorboard and jax.process_index() == 0:
try:
from flax.metrics.tensorboard import SummaryWriter
summary_writer = SummaryWriter(training_args.output_dir)
summary_writer.hparams({**training_args.to_dict(), **vars(model_args), **vars(data_args)})
except ImportError as ie:
has_tensorboard = False
logger.warning(
f"Unable to display metrics through TensorBoard because some package are not installed: {ie}"
)
else:
logger.warning(
"Unable to display metrics through TensorBoard because the package is not installed: "
"Please run pip install tensorboard to enable."
)
def write_train_metric(summary_writer, train_metrics, train_time, step):
summary_writer.scalar("train_time", train_time, step)
train_metrics = get_metrics(train_metrics)
for key, vals in train_metrics.items():
tag = f"train_{key}"
for i, val in enumerate(vals):
summary_writer.scalar(tag, val, step - len(vals) + i + 1)
def write_eval_metric(summary_writer, eval_metrics, step):
for metric_name, value in eval_metrics.items():
summary_writer.scalar(f"eval_{metric_name}", value, step)
num_epochs = int(training_args.num_train_epochs)
rng = jax.random.PRNGKey(training_args.seed)
dropout_rngs = jax.random.split(rng, jax.local_device_count())
train_batch_size = int(training_args.per_device_train_batch_size) * jax.local_device_count()
per_device_eval_batch_size = int(training_args.per_device_eval_batch_size)
eval_batch_size = per_device_eval_batch_size * jax.local_device_count()
# endregion
# region Load model
model = FlaxAutoModelForQuestionAnswering.from_pretrained(
model_args.model_name_or_path,
config=config,
cache_dir=model_args.cache_dir,
revision=model_args.model_revision,
token=model_args.token,
trust_remote_code=model_args.trust_remote_code,
seed=training_args.seed,
dtype=getattr(jnp, model_args.dtype),
)
learning_rate_fn = create_learning_rate_fn(
len(train_dataset),
train_batch_size,
training_args.num_train_epochs,
training_args.warmup_steps,
training_args.learning_rate,
)
state = create_train_state(model, learning_rate_fn, num_labels=max_seq_length, training_args=training_args)
# endregion
# region Define train step functions
def train_step(
state: train_state.TrainState, batch: Dict[str, Array], dropout_rng: PRNGKey
) -> Tuple[train_state.TrainState, float]:
"""Trains model with an optimizer (both in `state`) on `batch`, returning a pair `(new_state, loss)`."""
dropout_rng, new_dropout_rng = jax.random.split(dropout_rng)
start_positions = batch.pop("start_positions")
end_positions = batch.pop("end_positions")
targets = (start_positions, end_positions)
def loss_fn(params):
logits = state.apply_fn(**batch, params=params, dropout_rng=dropout_rng, train=True)
loss = state.loss_fn(logits, targets)
return loss
grad_fn = jax.value_and_grad(loss_fn)
loss, grad = grad_fn(state.params)
grad = jax.lax.pmean(grad, "batch")
new_state = state.apply_gradients(grads=grad)
metrics = jax.lax.pmean({"loss": loss, "learning_rate": learning_rate_fn(state.step)}, axis_name="batch")
return new_state, metrics, new_dropout_rng
p_train_step = jax.pmap(train_step, axis_name="batch", donate_argnums=(0,))
# endregion
# region Define eval step functions
def eval_step(state, batch):
logits = state.apply_fn(**batch, params=state.params, train=False)
return state.logits_fn(logits)
p_eval_step = jax.pmap(eval_step, axis_name="batch")
# endregion
# region Define train and eval loop
logger.info(f"===== Starting training ({num_epochs} epochs) =====")
train_time = 0
# make sure weights are replicated on each device
state = replicate(state)
train_time = 0
step_per_epoch = len(train_dataset) // train_batch_size
total_steps = step_per_epoch * num_epochs
epochs = tqdm(range(num_epochs), desc=f"Epoch ... (1/{num_epochs})", position=0)
for epoch in epochs:
train_start = time.time()
train_metrics = []
# Create sampling rng
rng, input_rng = jax.random.split(rng)
# train
for step, batch in enumerate(
tqdm(
train_data_collator(input_rng, train_dataset, train_batch_size),
total=step_per_epoch,
desc="Training...",
position=1,
),
1,
):
state, train_metric, dropout_rngs = p_train_step(state, batch, dropout_rngs)
train_metrics.append(train_metric)
cur_step = epoch * step_per_epoch + step
if cur_step % training_args.logging_steps == 0 and cur_step > 0:
# Save metrics
train_metric = unreplicate(train_metric)
train_time += time.time() - train_start
if has_tensorboard and jax.process_index() == 0:
write_train_metric(summary_writer, train_metrics, train_time, cur_step)
epochs.write(
f"Step... ({cur_step}/{total_steps} | Training Loss: {train_metric['loss']}, Learning Rate:"
f" {train_metric['learning_rate']})"
)
train_metrics = []
if (
training_args.do_eval
and (cur_step % training_args.eval_steps == 0 or cur_step % step_per_epoch == 0)
and cur_step > 0
):
eval_metrics = {}
all_start_logits = []
all_end_logits = []
# evaluate
for batch in tqdm(
eval_data_collator(eval_dataset, eval_batch_size),
total=math.ceil(len(eval_dataset) / eval_batch_size),
desc="Evaluating ...",
position=2,
):
_ = batch.pop("example_id")
_ = batch.pop("offset_mapping")
predictions = pad_shard_unpad(p_eval_step)(
state, batch, min_device_batch=per_device_eval_batch_size
)
start_logits = np.array(predictions[0])
end_logits = np.array(predictions[1])
all_start_logits.append(start_logits)
all_end_logits.append(end_logits)
max_len = max([x.shape[1] for x in all_start_logits]) # Get the max_length of the tensor
# concatenate the numpy array
start_logits_concat = create_and_fill_np_array(all_start_logits, eval_dataset, max_len)
end_logits_concat = create_and_fill_np_array(all_end_logits, eval_dataset, max_len)
# delete the list of numpy arrays
del all_start_logits
del all_end_logits
outputs_numpy = (start_logits_concat, end_logits_concat)
prediction = post_processing_function(eval_examples, eval_dataset, outputs_numpy)
eval_metrics = compute_metrics(prediction)
logger.info(f"Step... ({cur_step}/{total_steps} | Evaluation metrics: {eval_metrics})")
if has_tensorboard and jax.process_index() == 0:
write_eval_metric(summary_writer, eval_metrics, cur_step)
if (cur_step % training_args.save_steps == 0 and cur_step > 0) or (cur_step == total_steps):
# save checkpoint after each epoch and push checkpoint to the hub
if jax.process_index() == 0:
params = jax.device_get(unreplicate(state.params))
model.save_pretrained(training_args.output_dir, params=params)
tokenizer.save_pretrained(training_args.output_dir)
if training_args.push_to_hub:
api.upload_folder(
commit_message=f"Saving weights and logs of step {cur_step}",
folder_path=training_args.output_dir,
repo_id=repo_id,
repo_type="model",
token=training_args.hub_token,
)
epochs.desc = f"Epoch ... {epoch + 1}/{num_epochs}"
# endregion
# Eval after training
if training_args.do_eval:
eval_metrics = {}
all_start_logits = []
all_end_logits = []
eval_loader = eval_data_collator(eval_dataset, eval_batch_size)
for batch in tqdm(
eval_loader, total=math.ceil(len(eval_dataset) / eval_batch_size), desc="Evaluating ...", position=2
):
_ = batch.pop("example_id")
_ = batch.pop("offset_mapping")
predictions = pad_shard_unpad(p_eval_step)(state, batch, min_device_batch=per_device_eval_batch_size)
start_logits = np.array(predictions[0])
end_logits = np.array(predictions[1])
all_start_logits.append(start_logits)
all_end_logits.append(end_logits)
max_len = max([x.shape[1] for x in all_start_logits]) # Get the max_length of the tensor
# concatenate the numpy array
start_logits_concat = create_and_fill_np_array(all_start_logits, eval_dataset, max_len)
end_logits_concat = create_and_fill_np_array(all_end_logits, eval_dataset, max_len)
# delete the list of numpy arrays
del all_start_logits
del all_end_logits
outputs_numpy = (start_logits_concat, end_logits_concat)
prediction = post_processing_function(eval_examples, eval_dataset, outputs_numpy)
eval_metrics = compute_metrics(prediction)
if jax.process_index() == 0:
eval_metrics = {f"eval_{metric_name}": value for metric_name, value in eval_metrics.items()}
path = os.path.join(training_args.output_dir, "eval_results.json")
with open(path, "w") as f:
json.dump(eval_metrics, f, indent=4, sort_keys=True)
if __name__ == "__main__":
main()
| transformers/examples/flax/question-answering/run_qa.py/0 | {
"file_path": "transformers/examples/flax/question-answering/run_qa.py",
"repo_id": "transformers",
"token_count": 20250
} | 280 |
#### Fine-tuning BERT on SQuAD1.0 with relative position embeddings
The following examples show how to fine-tune BERT models with different relative position embeddings. The BERT model
`google-bert/bert-base-uncased` was pretrained with default absolute position embeddings. We provide the following pretrained
models which were pre-trained on the same training data (BooksCorpus and English Wikipedia) as in the BERT model
training, but with different relative position embeddings.
* `zhiheng-huang/bert-base-uncased-embedding-relative-key`, trained from scratch with relative embedding proposed by
Shaw et al., [Self-Attention with Relative Position Representations](https://arxiv.org/abs/1803.02155)
* `zhiheng-huang/bert-base-uncased-embedding-relative-key-query`, trained from scratch with relative embedding method 4
in Huang et al. [Improve Transformer Models with Better Relative Position Embeddings](https://arxiv.org/abs/2009.13658)
* `zhiheng-huang/bert-large-uncased-whole-word-masking-embedding-relative-key-query`, fine-tuned from model
`google-bert/bert-large-uncased-whole-word-masking` with 3 additional epochs with relative embedding method 4 in Huang et al.
[Improve Transformer Models with Better Relative Position Embeddings](https://arxiv.org/abs/2009.13658)
##### Base models fine-tuning
```bash
export CUDA_VISIBLE_DEVICES=0,1,2,3,4,5,6,7
torchrun --nproc_per_node=8 ./examples/question-answering/run_squad.py \
--model_name_or_path zhiheng-huang/bert-base-uncased-embedding-relative-key-query \
--dataset_name squad \
--do_train \
--do_eval \
--learning_rate 3e-5 \
--num_train_epochs 2 \
--max_seq_length 512 \
--doc_stride 128 \
--output_dir relative_squad \
--per_device_eval_batch_size=60 \
--per_device_train_batch_size=6
```
Training with the above command leads to the following results. It boosts the BERT default from f1 score of 88.52 to 90.54.
```bash
'exact': 83.6802270577105, 'f1': 90.54772098174814
```
The change of `max_seq_length` from 512 to 384 in the above command leads to the f1 score of 90.34. Replacing the above
model `zhiheng-huang/bert-base-uncased-embedding-relative-key-query` with
`zhiheng-huang/bert-base-uncased-embedding-relative-key` leads to the f1 score of 89.51. The changing of 8 gpus to one
gpu training leads to the f1 score of 90.71.
##### Large models fine-tuning
```bash
export CUDA_VISIBLE_DEVICES=0,1,2,3,4,5,6,7
torchrun --nproc_per_node=8 ./examples/question-answering/run_squad.py \
--model_name_or_path zhiheng-huang/bert-large-uncased-whole-word-masking-embedding-relative-key-query \
--dataset_name squad \
--do_train \
--do_eval \
--learning_rate 3e-5 \
--num_train_epochs 2 \
--max_seq_length 512 \
--doc_stride 128 \
--output_dir relative_squad \
--per_gpu_eval_batch_size=6 \
--per_gpu_train_batch_size=2 \
--gradient_accumulation_steps 3
```
Training with the above command leads to the f1 score of 93.52, which is slightly better than the f1 score of 93.15 for
`google-bert/bert-large-uncased-whole-word-masking`.
#### Distributed training
Here is an example using distributed training on 8 V100 GPUs and Bert Whole Word Masking uncased model to reach a F1 > 93 on SQuAD1.1:
```bash
torchrun --nproc_per_node=8 ./examples/question-answering/run_squad.py \
--model_name_or_path google-bert/bert-large-uncased-whole-word-masking \
--dataset_name squad \
--do_train \
--do_eval \
--learning_rate 3e-5 \
--num_train_epochs 2 \
--max_seq_length 384 \
--doc_stride 128 \
--output_dir ./examples/models/wwm_uncased_finetuned_squad/ \
--per_device_eval_batch_size=3 \
--per_device_train_batch_size=3 \
```
Training with the previously defined hyper-parameters yields the following results:
```bash
f1 = 93.15
exact_match = 86.91
```
This fine-tuned model is available as a checkpoint under the reference
[`google-bert/bert-large-uncased-whole-word-masking-finetuned-squad`](https://huggingface.co/google-bert/bert-large-uncased-whole-word-masking-finetuned-squad).
## Results
Larger batch size may improve the performance while costing more memory.
##### Results for SQuAD1.0 with the previously defined hyper-parameters:
```python
{
"exact": 85.45884578997162,
"f1": 92.5974600601065,
"total": 10570,
"HasAns_exact": 85.45884578997162,
"HasAns_f1": 92.59746006010651,
"HasAns_total": 10570
}
```
##### Results for SQuAD2.0 with the previously defined hyper-parameters:
```python
{
"exact": 80.4177545691906,
"f1": 84.07154997729623,
"total": 11873,
"HasAns_exact": 76.73751686909581,
"HasAns_f1": 84.05558584352873,
"HasAns_total": 5928,
"NoAns_exact": 84.0874684608915,
"NoAns_f1": 84.0874684608915,
"NoAns_total": 5945
}
``` | transformers/examples/legacy/question-answering/README.md/0 | {
"file_path": "transformers/examples/legacy/question-answering/README.md",
"repo_id": "transformers",
"token_count": 1768
} | 281 |
# Copyright 2020 The HuggingFace Team. All rights reserved.
#
# Licensed under the Apache License, Version 2.0 (the "License");
# you may not use this file except in compliance with the License.
# You may obtain a copy of the License at
#
# http://www.apache.org/licenses/LICENSE-2.0
#
# Unless required by applicable law or agreed to in writing, software
# distributed under the License is distributed on an "AS IS" BASIS,
# WITHOUT WARRANTIES OR CONDITIONS OF ANY KIND, either express or implied.
# See the License for the specific language governing permissions and
# limitations under the License.
import re
from filelock import FileLock
try:
import nltk
NLTK_AVAILABLE = True
except (ImportError, ModuleNotFoundError):
NLTK_AVAILABLE = False
if NLTK_AVAILABLE:
with FileLock(".lock") as lock:
nltk.download("punkt", quiet=True)
def add_newline_to_end_of_each_sentence(x: str) -> str:
"""This was added to get rougeLsum scores matching published rougeL scores for BART and PEGASUS."""
re.sub("<n>", "", x) # remove pegasus newline char
assert NLTK_AVAILABLE, "nltk must be installed to separate newlines between sentences. (pip install nltk)"
return "\n".join(nltk.sent_tokenize(x))
| transformers/examples/legacy/seq2seq/sentence_splitter.py/0 | {
"file_path": "transformers/examples/legacy/seq2seq/sentence_splitter.py",
"repo_id": "transformers",
"token_count": 403
} | 282 |
# Copyright 2020 The HuggingFace Team. All rights reserved.
#
# Licensed under the Apache License, Version 2.0 (the "License");
# you may not use this file except in compliance with the License.
# You may obtain a copy of the License at
#
# http://www.apache.org/licenses/LICENSE-2.0
#
# Unless required by applicable law or agreed to in writing, software
# distributed under the License is distributed on an "AS IS" BASIS,
# WITHOUT WARRANTIES OR CONDITIONS OF ANY KIND, either express or implied.
# See the License for the specific language governing permissions and
# limitations under the License.
python finetune_trainer.py \
--model_name_or_path=facebook/mbart-large-cc25 \
--data_dir $ENRO_DIR \
--output_dir mbart_cc25_enro --overwrite_output_dir \
--learning_rate=3e-5 \
--warmup_steps 500 \
--fp16 \
--label_smoothing 0.1 \
--adam_eps 1e-06 \
--src_lang en_XX --tgt_lang ro_RO \
--freeze_embeds \
--per_device_train_batch_size=4 --per_device_eval_batch_size=4 \
--max_source_length 128 --max_target_length 128 --val_max_target_length 128 --test_max_target_length 128\
--sortish_sampler \
--num_train_epochs 6 \
--save_steps 25000 --eval_steps 25000 --logging_steps 1000 \
--do_train --do_eval --do_predict \
--evaluation_strategy steps \
--predict_with_generate --logging_first_step \
--task translation \
"$@"
| transformers/examples/legacy/seq2seq/train_mbart_cc25_enro.sh/0 | {
"file_path": "transformers/examples/legacy/seq2seq/train_mbart_cc25_enro.sh",
"repo_id": "transformers",
"token_count": 501
} | 283 |
#!/usr/bin/env python
# coding=utf-8
# Copyright 2021 The HuggingFace Inc. team. All rights reserved.
#
# Licensed under the Apache License, Version 2.0 (the "License");
# you may not use this file except in compliance with the License.
# You may obtain a copy of the License at
#
# http://www.apache.org/licenses/LICENSE-2.0
#
# Unless required by applicable law or agreed to in writing, software
# distributed under the License is distributed on an "AS IS" BASIS,
# WITHOUT WARRANTIES OR CONDITIONS OF ANY KIND, either express or implied.
# See the License for the specific language governing permissions and
# limitations under the License.
"""
Fine-tuning XLNet for question answering with beam search using 🤗 Accelerate.
"""
# You can also adapt this script on your own question answering task. Pointers for this are left as comments.
import argparse
import json
import logging
import math
import os
import random
from pathlib import Path
import datasets
import evaluate
import numpy as np
import torch
from accelerate import Accelerator
from accelerate.logging import get_logger
from accelerate.utils import set_seed
from datasets import load_dataset
from huggingface_hub import HfApi
from torch.utils.data import DataLoader
from tqdm.auto import tqdm
from utils_qa import postprocess_qa_predictions_with_beam_search
import transformers
from transformers import (
AdamW,
DataCollatorWithPadding,
EvalPrediction,
SchedulerType,
XLNetConfig,
XLNetForQuestionAnswering,
XLNetTokenizerFast,
default_data_collator,
get_scheduler,
)
from transformers.utils import check_min_version, send_example_telemetry
from transformers.utils.versions import require_version
# Will error if the minimal version of Transformers is not installed. Remove at your own risks.
check_min_version("4.40.0.dev0")
require_version("datasets>=1.8.0", "To fix: pip install -r examples/pytorch/question-answering/requirements.txt")
logger = get_logger(__name__)
def save_prefixed_metrics(results, output_dir, file_name: str = "all_results.json", metric_key_prefix: str = "eval"):
"""
Save results while prefixing metric names.
Args:
results: (:obj:`dict`):
A dictionary of results.
output_dir: (:obj:`str`):
An output directory.
file_name: (:obj:`str`, `optional`, defaults to :obj:`all_results.json`):
An output file name.
metric_key_prefix: (:obj:`str`, `optional`, defaults to :obj:`eval`):
A metric name prefix.
"""
# Prefix all keys with metric_key_prefix + '_'
for key in list(results.keys()):
if not key.startswith(f"{metric_key_prefix}_"):
results[f"{metric_key_prefix}_{key}"] = results.pop(key)
with open(os.path.join(output_dir, file_name), "w") as f:
json.dump(results, f, indent=4)
def parse_args():
parser = argparse.ArgumentParser(description="Finetune a transformers model on a Question Answering task")
parser.add_argument(
"--dataset_name",
type=str,
default=None,
help="The name of the dataset to use (via the datasets library).",
)
parser.add_argument(
"--dataset_config_name",
type=str,
default=None,
help="The configuration name of the dataset to use (via the datasets library).",
)
parser.add_argument(
"--train_file", type=str, default=None, help="A csv or a json file containing the training data."
)
parser.add_argument(
"--preprocessing_num_workers", type=int, default=1, help="A csv or a json file containing the training data."
)
parser.add_argument("--do_predict", action="store_true", help="Eval the question answering model")
parser.add_argument(
"--validation_file", type=str, default=None, help="A csv or a json file containing the validation data."
)
parser.add_argument(
"--test_file", type=str, default=None, help="A csv or a json file containing the Prediction data."
)
parser.add_argument(
"--max_seq_length",
type=int,
default=384,
help=(
"The maximum total input sequence length after tokenization. Sequences longer than this will be truncated,"
" sequences shorter will be padded if `--pad_to_max_length` is passed."
),
)
parser.add_argument(
"--pad_to_max_length",
action="store_true",
help="If passed, pad all samples to `max_seq_length`. Otherwise, dynamic padding is used.",
)
parser.add_argument(
"--model_name_or_path",
type=str,
help="Path to pretrained model or model identifier from huggingface.co/models.",
required=True,
)
parser.add_argument(
"--per_device_train_batch_size",
type=int,
default=8,
help="Batch size (per device) for the training dataloader.",
)
parser.add_argument(
"--per_device_eval_batch_size",
type=int,
default=8,
help="Batch size (per device) for the evaluation dataloader.",
)
parser.add_argument(
"--learning_rate",
type=float,
default=5e-5,
help="Initial learning rate (after the potential warmup period) to use.",
)
parser.add_argument("--weight_decay", type=float, default=0.0, help="Weight decay to use.")
parser.add_argument("--num_train_epochs", type=int, default=3, help="Total number of training epochs to perform.")
parser.add_argument(
"--max_train_steps",
type=int,
default=None,
help="Total number of training steps to perform. If provided, overrides num_train_epochs.",
)
parser.add_argument(
"--gradient_accumulation_steps",
type=int,
default=1,
help="Number of updates steps to accumulate before performing a backward/update pass.",
)
parser.add_argument(
"--lr_scheduler_type",
type=SchedulerType,
default="linear",
help="The scheduler type to use.",
choices=["linear", "cosine", "cosine_with_restarts", "polynomial", "constant", "constant_with_warmup"],
)
parser.add_argument(
"--num_warmup_steps", type=int, default=0, help="Number of steps for the warmup in the lr scheduler."
)
parser.add_argument("--output_dir", type=str, default=None, help="Where to store the final model.")
parser.add_argument("--seed", type=int, default=None, help="A seed for reproducible training.")
parser.add_argument(
"--doc_stride",
type=int,
default=128,
help="When splitting up a long document into chunks how much stride to take between chunks.",
)
parser.add_argument(
"--n_best_size",
type=int,
default=20,
help="The total number of n-best predictions to generate when looking for an answer.",
)
parser.add_argument(
"--null_score_diff_threshold",
type=float,
default=0.0,
help=(
"The threshold used to select the null answer: if the best answer has a score that is less than "
"the score of the null answer minus this threshold, the null answer is selected for this example. "
"Only useful when `version_2_with_negative=True`."
),
)
parser.add_argument(
"--version_2_with_negative",
action="store_true",
help="If true, some of the examples do not have an answer.",
)
parser.add_argument(
"--max_answer_length",
type=int,
default=30,
help=(
"The maximum length of an answer that can be generated. This is needed because the start "
"and end predictions are not conditioned on one another."
),
)
parser.add_argument(
"--max_train_samples",
type=int,
default=None,
help=(
"For debugging purposes or quicker training, truncate the number of training examples to this "
"value if set."
),
)
parser.add_argument(
"--max_eval_samples",
type=int,
default=None,
help=(
"For debugging purposes or quicker training, truncate the number of evaluation examples to this "
"value if set."
),
)
parser.add_argument(
"--overwrite_cache", action="store_true", help="Overwrite the cached training and evaluation sets"
)
parser.add_argument(
"--max_predict_samples",
type=int,
default=None,
help="For debugging purposes or quicker training, truncate the number of prediction examples to this",
)
parser.add_argument("--push_to_hub", action="store_true", help="Whether or not to push the model to the Hub.")
parser.add_argument(
"--hub_model_id", type=str, help="The name of the repository to keep in sync with the local `output_dir`."
)
parser.add_argument("--hub_token", type=str, help="The token to use to push to the Model Hub.")
parser.add_argument(
"--checkpointing_steps",
type=str,
default=None,
help="Whether the various states should be saved at the end of every n steps, or 'epoch' for each epoch.",
)
parser.add_argument(
"--resume_from_checkpoint",
type=str,
default=None,
help="If the training should continue from a checkpoint folder.",
)
parser.add_argument(
"--with_tracking",
action="store_true",
help="Whether to load in all available experiment trackers from the environment and use them for logging.",
)
args = parser.parse_args()
# Sanity checks
if (
args.dataset_name is None
and args.train_file is None
and args.validation_file is None
and args.test_file is None
):
raise ValueError("Need either a dataset name or a training/validation/test file.")
else:
if args.train_file is not None:
extension = args.train_file.split(".")[-1]
assert extension in ["csv", "json"], "`train_file` should be a csv or a json file."
if args.validation_file is not None:
extension = args.validation_file.split(".")[-1]
assert extension in ["csv", "json"], "`validation_file` should be a csv or a json file."
if args.test_file is not None:
extension = args.test_file.split(".")[-1]
assert extension in ["csv", "json"], "`test_file` should be a csv or a json file."
if args.push_to_hub:
assert args.output_dir is not None, "Need an `output_dir` to create a repo when `--push_to_hub` is passed."
return args
def main():
args = parse_args()
# Sending telemetry. Tracking the example usage helps us better allocate resources to maintain them. The
# information sent is the one passed as arguments along with your Python/PyTorch versions.
send_example_telemetry("run_qa_beam_search_no_trainer", args)
# Initialize the accelerator. We will let the accelerator handle device placement for us in this example.
# If we're using tracking, we also need to initialize it here and it will pick up all supported trackers
# in the environment
accelerator_log_kwargs = {}
if args.with_tracking:
accelerator_log_kwargs["log_with"] = args.report_to
accelerator_log_kwargs["project_dir"] = args.output_dir
accelerator = Accelerator(gradient_accumulation_steps=args.gradient_accumulation_steps, **accelerator_log_kwargs)
# Make one log on every process with the configuration for debugging.
logging.basicConfig(
format="%(asctime)s - %(levelname)s - %(name)s - %(message)s",
datefmt="%m/%d/%Y %H:%M:%S",
level=logging.INFO,
)
logger.info(accelerator.state, main_process_only=False)
if accelerator.is_local_main_process:
datasets.utils.logging.set_verbosity_warning()
transformers.utils.logging.set_verbosity_info()
else:
datasets.utils.logging.set_verbosity_error()
transformers.utils.logging.set_verbosity_error()
# If passed along, set the training seed now.
if args.seed is not None:
set_seed(args.seed)
# Handle the repository creation
if accelerator.is_main_process:
if args.push_to_hub:
# Retrieve of infer repo_name
repo_name = args.hub_model_id
if repo_name is None:
repo_name = Path(args.output_dir).absolute().name
# Create repo and retrieve repo_id
api = HfApi()
repo_id = api.create_repo(repo_name, exist_ok=True, token=args.hub_token).repo_id
with open(os.path.join(args.output_dir, ".gitignore"), "w+") as gitignore:
if "step_*" not in gitignore:
gitignore.write("step_*\n")
if "epoch_*" not in gitignore:
gitignore.write("epoch_*\n")
elif args.output_dir is not None:
os.makedirs(args.output_dir, exist_ok=True)
accelerator.wait_for_everyone()
# Get the datasets: you can either provide your own CSV/JSON/TXT training and evaluation files (see below)
# or just provide the name of one of the public datasets available on the hub at https://huggingface.co/datasets/
# (the dataset will be downloaded automatically from the datasets Hub).
#
# For CSV/JSON files, this script will use the column called 'text' or the first column if no column called
# 'text' is found. You can easily tweak this behavior (see below).
#
# In distributed training, the load_dataset function guarantee that only one local process can concurrently
# download the dataset.
if args.dataset_name is not None:
# Downloading and loading a dataset from the hub.
raw_datasets = load_dataset(args.dataset_name, args.dataset_config_name)
else:
data_files = {}
if args.train_file is not None:
data_files["train"] = args.train_file
extension = args.train_file.split(".")[-1]
if args.validation_file is not None:
data_files["validation"] = args.validation_file
extension = args.validation_file.split(".")[-1]
if args.test_file is not None:
data_files["test"] = args.test_file
extension = args.test_file.split(".")[-1]
raw_datasets = load_dataset(extension, data_files=data_files, field="data")
# See more about loading any type of standard or custom dataset (from files, python dict, pandas DataFrame, etc) at
# https://huggingface.co/docs/datasets/loading_datasets.
# Load pretrained model and tokenizer
#
# In distributed training, the .from_pretrained methods guarantee that only one local process can concurrently
# download model & vocab.
config = XLNetConfig.from_pretrained(args.model_name_or_path)
tokenizer = XLNetTokenizerFast.from_pretrained(args.model_name_or_path)
model = XLNetForQuestionAnswering.from_pretrained(
args.model_name_or_path, from_tf=bool(".ckpt" in args.model_name_or_path), config=config
)
# Preprocessing the datasets.
# Preprocessing is slightly different for training and evaluation.
column_names = raw_datasets["train"].column_names
question_column_name = "question" if "question" in column_names else column_names[0]
context_column_name = "context" if "context" in column_names else column_names[1]
answer_column_name = "answers" if "answers" in column_names else column_names[2]
# Padding side determines if we do (question|context) or (context|question).
pad_on_right = tokenizer.padding_side == "right"
if args.max_seq_length > tokenizer.model_max_length:
logger.warning(
f"The max_seq_length passed ({args.max_seq_length}) is larger than the maximum length for the "
f"model ({tokenizer.model_max_length}). Using max_seq_length={tokenizer.model_max_length}."
)
max_seq_length = min(args.max_seq_length, tokenizer.model_max_length)
# Training preprocessing
def prepare_train_features(examples):
# Some of the questions have lots of whitespace on the left, which is not useful and will make the
# truncation of the context fail (the tokenized question will take a lots of space). So we remove that
# left whitespace
examples[question_column_name] = [q.lstrip() for q in examples[question_column_name]]
# Tokenize our examples with truncation and maybe padding, but keep the overflows using a stride. This results
# in one example possible giving several features when a context is long, each of those features having a
# context that overlaps a bit the context of the previous feature.
tokenized_examples = tokenizer(
examples[question_column_name if pad_on_right else context_column_name],
examples[context_column_name if pad_on_right else question_column_name],
truncation="only_second" if pad_on_right else "only_first",
max_length=max_seq_length,
stride=args.doc_stride,
return_overflowing_tokens=True,
return_offsets_mapping=True,
return_special_tokens_mask=True,
return_token_type_ids=True,
padding="max_length",
)
# Since one example might give us several features if it has a long context, we need a map from a feature to
# its corresponding example. This key gives us just that.
sample_mapping = tokenized_examples.pop("overflow_to_sample_mapping")
# The offset mappings will give us a map from token to character position in the original context. This will
# help us compute the start_positions and end_positions.
offset_mapping = tokenized_examples.pop("offset_mapping")
# The special tokens will help us build the p_mask (which indicates the tokens that can't be in answers).
special_tokens = tokenized_examples.pop("special_tokens_mask")
# Let's label those examples!
tokenized_examples["start_positions"] = []
tokenized_examples["end_positions"] = []
tokenized_examples["is_impossible"] = []
tokenized_examples["cls_index"] = []
tokenized_examples["p_mask"] = []
for i, offsets in enumerate(offset_mapping):
# We will label impossible answers with the index of the CLS token.
input_ids = tokenized_examples["input_ids"][i]
cls_index = input_ids.index(tokenizer.cls_token_id)
tokenized_examples["cls_index"].append(cls_index)
# Grab the sequence corresponding to that example (to know what is the context and what is the question).
sequence_ids = tokenized_examples["token_type_ids"][i]
for k, s in enumerate(special_tokens[i]):
if s:
sequence_ids[k] = 3
context_idx = 1 if pad_on_right else 0
# Build the p_mask: non special tokens and context gets 0.0, the others get 1.0.
# The cls token gets 1.0 too (for predictions of empty answers).
tokenized_examples["p_mask"].append(
[
0.0 if (not special_tokens[i][k] and s == context_idx) or k == cls_index else 1.0
for k, s in enumerate(sequence_ids)
]
)
# One example can give several spans, this is the index of the example containing this span of text.
sample_index = sample_mapping[i]
answers = examples[answer_column_name][sample_index]
# If no answers are given, set the cls_index as answer.
if len(answers["answer_start"]) == 0:
tokenized_examples["start_positions"].append(cls_index)
tokenized_examples["end_positions"].append(cls_index)
tokenized_examples["is_impossible"].append(1.0)
else:
# Start/end character index of the answer in the text.
start_char = answers["answer_start"][0]
end_char = start_char + len(answers["text"][0])
# Start token index of the current span in the text.
token_start_index = 0
while sequence_ids[token_start_index] != context_idx:
token_start_index += 1
# End token index of the current span in the text.
token_end_index = len(input_ids) - 1
while sequence_ids[token_end_index] != context_idx:
token_end_index -= 1
# Detect if the answer is out of the span (in which case this feature is labeled with the CLS index).
if not (offsets[token_start_index][0] <= start_char and offsets[token_end_index][1] >= end_char):
tokenized_examples["start_positions"].append(cls_index)
tokenized_examples["end_positions"].append(cls_index)
tokenized_examples["is_impossible"].append(1.0)
else:
# Otherwise move the token_start_index and token_end_index to the two ends of the answer.
# Note: we could go after the last offset if the answer is the last word (edge case).
while token_start_index < len(offsets) and offsets[token_start_index][0] <= start_char:
token_start_index += 1
tokenized_examples["start_positions"].append(token_start_index - 1)
while offsets[token_end_index][1] >= end_char:
token_end_index -= 1
tokenized_examples["end_positions"].append(token_end_index + 1)
tokenized_examples["is_impossible"].append(0.0)
return tokenized_examples
if "train" not in raw_datasets:
raise ValueError("--do_train requires a train dataset")
train_dataset = raw_datasets["train"]
if args.max_train_samples is not None:
# We will select sample from whole data if argument is specified
train_dataset = train_dataset.select(range(args.max_train_samples))
# Create train feature from dataset
with accelerator.main_process_first():
train_dataset = train_dataset.map(
prepare_train_features,
batched=True,
num_proc=args.preprocessing_num_workers,
remove_columns=column_names,
load_from_cache_file=not args.overwrite_cache,
desc="Running tokenizer on train dataset",
)
if args.max_train_samples is not None:
# Number of samples might increase during Feature Creation, We select only specified max samples
train_dataset = train_dataset.select(range(args.max_train_samples))
# Validation preprocessing
def prepare_validation_features(examples):
# Some of the questions have lots of whitespace on the left, which is not useful and will make the
# truncation of the context fail (the tokenized question will take a lots of space). So we remove that
# left whitespace
examples[question_column_name] = [q.lstrip() for q in examples[question_column_name]]
# Tokenize our examples with truncation and maybe padding, but keep the overflows using a stride. This results
# in one example possible giving several features when a context is long, each of those features having a
# context that overlaps a bit the context of the previous feature.
tokenized_examples = tokenizer(
examples[question_column_name if pad_on_right else context_column_name],
examples[context_column_name if pad_on_right else question_column_name],
truncation="only_second" if pad_on_right else "only_first",
max_length=max_seq_length,
stride=args.doc_stride,
return_overflowing_tokens=True,
return_offsets_mapping=True,
return_special_tokens_mask=True,
return_token_type_ids=True,
padding="max_length",
)
# Since one example might give us several features if it has a long context, we need a map from a feature to
# its corresponding example. This key gives us just that.
sample_mapping = tokenized_examples.pop("overflow_to_sample_mapping")
# The special tokens will help us build the p_mask (which indicates the tokens that can't be in answers).
special_tokens = tokenized_examples.pop("special_tokens_mask")
# For evaluation, we will need to convert our predictions to substrings of the context, so we keep the
# corresponding example_id and we will store the offset mappings.
tokenized_examples["example_id"] = []
# We still provide the index of the CLS token and the p_mask to the model, but not the is_impossible label.
tokenized_examples["cls_index"] = []
tokenized_examples["p_mask"] = []
for i, input_ids in enumerate(tokenized_examples["input_ids"]):
# Find the CLS token in the input ids.
cls_index = input_ids.index(tokenizer.cls_token_id)
tokenized_examples["cls_index"].append(cls_index)
# Grab the sequence corresponding to that example (to know what is the context and what is the question).
sequence_ids = tokenized_examples["token_type_ids"][i]
for k, s in enumerate(special_tokens[i]):
if s:
sequence_ids[k] = 3
context_idx = 1 if pad_on_right else 0
# Build the p_mask: non special tokens and context gets 0.0, the others 1.0.
tokenized_examples["p_mask"].append(
[
0.0 if (not special_tokens[i][k] and s == context_idx) or k == cls_index else 1.0
for k, s in enumerate(sequence_ids)
]
)
# One example can give several spans, this is the index of the example containing this span of text.
sample_index = sample_mapping[i]
tokenized_examples["example_id"].append(examples["id"][sample_index])
# Set to None the offset_mapping that are not part of the context so it's easy to determine if a token
# position is part of the context or not.
tokenized_examples["offset_mapping"][i] = [
(o if sequence_ids[k] == context_idx else None)
for k, o in enumerate(tokenized_examples["offset_mapping"][i])
]
return tokenized_examples
if "validation" not in raw_datasets:
raise ValueError("--do_eval requires a validation dataset")
eval_examples = raw_datasets["validation"]
if args.max_eval_samples is not None:
# We will select sample from whole data
eval_examples = eval_examples.select(range(args.max_eval_samples))
# Validation Feature Creation
with accelerator.main_process_first():
eval_dataset = eval_examples.map(
prepare_validation_features,
batched=True,
num_proc=args.preprocessing_num_workers,
remove_columns=column_names,
load_from_cache_file=not args.overwrite_cache,
desc="Running tokenizer on validation dataset",
)
if args.max_eval_samples is not None:
# During Feature creation dataset samples might increase, we will select required samples again
eval_dataset = eval_dataset.select(range(args.max_eval_samples))
if args.do_predict:
if "test" not in raw_datasets:
raise ValueError("--do_predict requires a test dataset")
predict_examples = raw_datasets["test"]
if args.max_predict_samples is not None:
# We will select sample from whole data
predict_examples = predict_examples.select(range(args.max_predict_samples))
# Predict Feature Creation
with accelerator.main_process_first():
predict_dataset = predict_examples.map(
prepare_validation_features,
batched=True,
num_proc=args.preprocessing_num_workers,
remove_columns=column_names,
load_from_cache_file=not args.overwrite_cache,
desc="Running tokenizer on prediction dataset",
)
if args.max_predict_samples is not None:
# During Feature creation dataset samples might increase, we will select required samples again
predict_dataset = predict_dataset.select(range(args.max_predict_samples))
# Log a few random samples from the training set:
for index in random.sample(range(len(train_dataset)), 3):
logger.info(f"Sample {index} of the training set: {train_dataset[index]}.")
# DataLoaders creation:
if args.pad_to_max_length:
# If padding was already done ot max length, we use the default data collator that will just convert everything
# to tensors.
data_collator = default_data_collator
else:
# Otherwise, `DataCollatorWithPadding` will apply dynamic padding for us (by padding to the maximum length of
# the samples passed). When using mixed precision, we add `pad_to_multiple_of=8` to pad all tensors to multiple
# of 8s, which will enable the use of Tensor Cores on NVIDIA hardware with compute capability >= 7.5 (Volta).
data_collator = DataCollatorWithPadding(tokenizer, pad_to_multiple_of=(8 if accelerator.use_fp16 else None))
train_dataloader = DataLoader(
train_dataset, shuffle=True, collate_fn=data_collator, batch_size=args.per_device_train_batch_size
)
eval_dataset_for_model = eval_dataset.remove_columns(["example_id", "offset_mapping"])
eval_dataloader = DataLoader(
eval_dataset_for_model, collate_fn=data_collator, batch_size=args.per_device_eval_batch_size
)
if args.do_predict:
predict_dataset_for_model = predict_dataset.remove_columns(["example_id", "offset_mapping"])
predict_dataloader = DataLoader(
predict_dataset_for_model, collate_fn=data_collator, batch_size=args.per_device_eval_batch_size
)
# Post-processing:
def post_processing_function(examples, features, predictions, stage="eval"):
# Post-processing: we match the start logits and end logits to answers in the original context.
predictions, scores_diff_json = postprocess_qa_predictions_with_beam_search(
examples=examples,
features=features,
predictions=predictions,
version_2_with_negative=args.version_2_with_negative,
n_best_size=args.n_best_size,
max_answer_length=args.max_answer_length,
start_n_top=model.config.start_n_top,
end_n_top=model.config.end_n_top,
output_dir=args.output_dir,
prefix=stage,
)
# Format the result to the format the metric expects.
if args.version_2_with_negative:
formatted_predictions = [
{"id": k, "prediction_text": v, "no_answer_probability": scores_diff_json[k]}
for k, v in predictions.items()
]
else:
formatted_predictions = [{"id": k, "prediction_text": v} for k, v in predictions.items()]
references = [{"id": ex["id"], "answers": ex[answer_column_name]} for ex in examples]
return EvalPrediction(predictions=formatted_predictions, label_ids=references)
metric = evaluate.load("squad_v2" if args.version_2_with_negative else "squad")
def create_and_fill_np_array(start_or_end_logits, dataset, max_len):
"""
Create and fill numpy array of size len_of_validation_data * max_length_of_output_tensor
Args:
start_or_end_logits(:obj:`tensor`):
This is the output predictions of the model. We can only enter either start or end logits.
eval_dataset: Evaluation dataset
max_len(:obj:`int`):
The maximum length of the output tensor. ( See the model.eval() part for more details )
"""
step = 0
# create a numpy array and fill it with -100.
logits_concat = np.full((len(dataset), max_len), -100, dtype=np.float32)
# Now since we have create an array now we will populate it with the outputs gathered using accelerator.gather_for_metrics
for i, output_logit in enumerate(start_or_end_logits): # populate columns
# We have to fill it such that we have to take the whole tensor and replace it on the newly created array
# And after every iteration we have to change the step
batch_size = output_logit.shape[0]
cols = output_logit.shape[1]
if step + batch_size < len(dataset):
logits_concat[step : step + batch_size, :cols] = output_logit
else:
logits_concat[step:, :cols] = output_logit[: len(dataset) - step]
step += batch_size
return logits_concat
# Optimizer
# Split weights in two groups, one with weight decay and the other not.
no_decay = ["bias", "LayerNorm.weight"]
optimizer_grouped_parameters = [
{
"params": [p for n, p in model.named_parameters() if not any(nd in n for nd in no_decay)],
"weight_decay": args.weight_decay,
},
{
"params": [p for n, p in model.named_parameters() if any(nd in n for nd in no_decay)],
"weight_decay": 0.0,
},
]
optimizer = AdamW(optimizer_grouped_parameters, lr=args.learning_rate)
# Scheduler and math around the number of training steps.
overrode_max_train_steps = False
num_update_steps_per_epoch = math.ceil(len(train_dataloader) / args.gradient_accumulation_steps)
if args.max_train_steps is None:
args.max_train_steps = args.num_train_epochs * num_update_steps_per_epoch
overrode_max_train_steps = True
lr_scheduler = get_scheduler(
name=args.lr_scheduler_type,
optimizer=optimizer,
num_warmup_steps=args.num_warmup_steps * accelerator.num_processes,
num_training_steps=args.max_train_steps
if overrode_max_train_steps
else args.max_train_steps * accelerator.num_processes,
)
# Prepare everything with our `accelerator`.
model, optimizer, train_dataloader, eval_dataloader, lr_scheduler = accelerator.prepare(
model, optimizer, train_dataloader, eval_dataloader, lr_scheduler
)
# We need to recalculate our total training steps as the size of the training dataloader may have changed.
num_update_steps_per_epoch = math.ceil(len(train_dataloader) / args.gradient_accumulation_steps)
if overrode_max_train_steps:
args.max_train_steps = args.num_train_epochs * num_update_steps_per_epoch
# Afterwards we recalculate our number of training epochs
args.num_train_epochs = math.ceil(args.max_train_steps / num_update_steps_per_epoch)
# Figure out how many steps we should save the Accelerator states
checkpointing_steps = args.checkpointing_steps
if checkpointing_steps is not None and checkpointing_steps.isdigit():
checkpointing_steps = int(checkpointing_steps)
# We need to initialize the trackers we use, and also store our configuration
if args.with_tracking:
experiment_config = vars(args)
# TensorBoard cannot log Enums, need the raw value
experiment_config["lr_scheduler_type"] = experiment_config["lr_scheduler_type"].value
accelerator.init_trackers("qa_beam_search_no_trainer", experiment_config)
# Train!
total_batch_size = args.per_device_train_batch_size * accelerator.num_processes * args.gradient_accumulation_steps
logger.info("***** Running training *****")
logger.info(f" Num examples = {len(train_dataset)}")
logger.info(f" Num Epochs = {args.num_train_epochs}")
logger.info(f" Instantaneous batch size per device = {args.per_device_train_batch_size}")
logger.info(f" Total train batch size (w. parallel, distributed & accumulation) = {total_batch_size}")
logger.info(f" Gradient Accumulation steps = {args.gradient_accumulation_steps}")
logger.info(f" Total optimization steps = {args.max_train_steps}")
# Only show the progress bar once on each machine.
progress_bar = tqdm(range(args.max_train_steps), disable=not accelerator.is_local_main_process)
completed_steps = 0
starting_epoch = 0
# Potentially load in the weights and states from a previous save
if args.resume_from_checkpoint:
if args.resume_from_checkpoint is not None or args.resume_from_checkpoint != "":
checkpoint_path = args.resume_from_checkpoint
path = os.path.basename(args.resume_from_checkpoint)
else:
# Get the most recent checkpoint
dirs = [f.name for f in os.scandir(os.getcwd()) if f.is_dir()]
dirs.sort(key=os.path.getctime)
path = dirs[-1] # Sorts folders by date modified, most recent checkpoint is the last
checkpoint_path = path
path = os.path.basename(checkpoint_path)
accelerator.print(f"Resumed from checkpoint: {checkpoint_path}")
accelerator.load_state(checkpoint_path)
# Extract `epoch_{i}` or `step_{i}`
training_difference = os.path.splitext(path)[0]
if "epoch" in training_difference:
starting_epoch = int(training_difference.replace("epoch_", "")) + 1
resume_step = None
completed_steps = starting_epoch * num_update_steps_per_epoch
else:
# need to multiply `gradient_accumulation_steps` to reflect real steps
resume_step = int(training_difference.replace("step_", "")) * args.gradient_accumulation_steps
starting_epoch = resume_step // len(train_dataloader)
completed_steps = resume_step // args.gradient_accumulation_steps
resume_step -= starting_epoch * len(train_dataloader)
# update the progress_bar if load from checkpoint
progress_bar.update(completed_steps)
for epoch in range(starting_epoch, args.num_train_epochs):
model.train()
if args.with_tracking:
total_loss = 0
if args.resume_from_checkpoint and epoch == starting_epoch and resume_step is not None:
# We skip the first `n` batches in the dataloader when resuming from a checkpoint
active_dataloader = accelerator.skip_first_batches(train_dataloader, resume_step)
else:
active_dataloader = train_dataloader
for step, batch in enumerate(active_dataloader):
with accelerator.accumulate(model):
outputs = model(**batch)
loss = outputs.loss
# We keep track of the loss at each epoch
if args.with_tracking:
total_loss += loss.detach().float()
accelerator.backward(loss)
optimizer.step()
lr_scheduler.step()
optimizer.zero_grad()
# Checks if the accelerator has performed an optimization step behind the scenes
if accelerator.sync_gradients:
progress_bar.update(1)
completed_steps += 1
if isinstance(checkpointing_steps, int):
if completed_steps % checkpointing_steps == 0:
accelerator.save_state(f"step_{completed_steps}")
if completed_steps >= args.max_train_steps:
break
if args.push_to_hub and epoch < args.num_train_epochs - 1:
accelerator.wait_for_everyone()
unwrapped_model = accelerator.unwrap_model(model)
unwrapped_model.save_pretrained(
args.output_dir, is_main_process=accelerator.is_main_process, save_function=accelerator.save
)
if accelerator.is_main_process:
tokenizer.save_pretrained(args.output_dir)
api.upload_folder(
commit_message=f"Training in progress epoch {epoch}",
folder_path=args.output_dir,
repo_id=repo_id,
repo_type="model",
token=args.hub_token,
)
# initialize all lists to collect the batches
all_start_top_log_probs = []
all_start_top_index = []
all_end_top_log_probs = []
all_end_top_index = []
all_cls_logits = []
model.eval()
for step, batch in enumerate(eval_dataloader):
with torch.no_grad():
outputs = model(**batch)
start_top_log_probs = outputs.start_top_log_probs
start_top_index = outputs.start_top_index
end_top_log_probs = outputs.end_top_log_probs
end_top_index = outputs.end_top_index
cls_logits = outputs.cls_logits
if not args.pad_to_max_length: # necessary to pad predictions and labels for being gathered
start_top_log_probs = accelerator.pad_across_processes(start_top_log_probs, dim=1, pad_index=-100)
start_top_index = accelerator.pad_across_processes(start_top_index, dim=1, pad_index=-100)
end_top_log_probs = accelerator.pad_across_processes(end_top_log_probs, dim=1, pad_index=-100)
end_top_index = accelerator.pad_across_processes(end_top_index, dim=1, pad_index=-100)
cls_logits = accelerator.pad_across_processes(cls_logits, dim=1, pad_index=-100)
all_start_top_log_probs.append(accelerator.gather_for_metrics(start_top_log_probs).cpu().numpy())
all_start_top_index.append(accelerator.gather_for_metrics(start_top_index).cpu().numpy())
all_end_top_log_probs.append(accelerator.gather_for_metrics(end_top_log_probs).cpu().numpy())
all_end_top_index.append(accelerator.gather_for_metrics(end_top_index).cpu().numpy())
all_cls_logits.append(accelerator.gather_for_metrics(cls_logits).cpu().numpy())
max_len = max([x.shape[1] for x in all_end_top_log_probs]) # Get the max_length of the tensor
# concatenate all numpy arrays collected above
start_top_log_probs_concat = create_and_fill_np_array(all_start_top_log_probs, eval_dataset, max_len)
start_top_index_concat = create_and_fill_np_array(all_start_top_index, eval_dataset, max_len)
end_top_log_probs_concat = create_and_fill_np_array(all_end_top_log_probs, eval_dataset, max_len)
end_top_index_concat = create_and_fill_np_array(all_end_top_index, eval_dataset, max_len)
cls_logits_concat = np.concatenate(all_cls_logits, axis=0)
# delete the list of numpy arrays
del start_top_log_probs
del start_top_index
del end_top_log_probs
del end_top_index
del cls_logits
outputs_numpy = (
start_top_log_probs_concat,
start_top_index_concat,
end_top_log_probs_concat,
end_top_index_concat,
cls_logits_concat,
)
prediction = post_processing_function(eval_examples, eval_dataset, outputs_numpy)
eval_metric = metric.compute(predictions=prediction.predictions, references=prediction.label_ids)
logger.info(f"Evaluation metrics: {eval_metric}")
if args.do_predict:
# initialize all lists to collect the batches
all_start_top_log_probs = []
all_start_top_index = []
all_end_top_log_probs = []
all_end_top_index = []
all_cls_logits = []
model.eval()
for step, batch in enumerate(predict_dataloader):
with torch.no_grad():
outputs = model(**batch)
start_top_log_probs = outputs.start_top_log_probs
start_top_index = outputs.start_top_index
end_top_log_probs = outputs.end_top_log_probs
end_top_index = outputs.end_top_index
cls_logits = outputs.cls_logits
if not args.pad_to_max_length: # necessary to pad predictions and labels for being gathered
start_top_log_probs = accelerator.pad_across_processes(start_top_log_probs, dim=1, pad_index=-100)
start_top_index = accelerator.pad_across_processes(start_top_index, dim=1, pad_index=-100)
end_top_log_probs = accelerator.pad_across_processes(end_top_log_probs, dim=1, pad_index=-100)
end_top_index = accelerator.pad_across_processes(end_top_index, dim=1, pad_index=-100)
cls_logits = accelerator.pad_across_processes(cls_logits, dim=1, pad_index=-100)
all_start_top_log_probs.append(accelerator.gather_for_metrics(start_top_log_probs).cpu().numpy())
all_start_top_index.append(accelerator.gather_for_metrics(start_top_index).cpu().numpy())
all_end_top_log_probs.append(accelerator.gather_for_metrics(end_top_log_probs).cpu().numpy())
all_end_top_index.append(accelerator.gather_for_metrics(end_top_index).cpu().numpy())
all_cls_logits.append(accelerator.gather_for_metrics(cls_logits).cpu().numpy())
max_len = max([x.shape[1] for x in all_end_top_log_probs]) # Get the max_length of the tensor
# concatenate all numpy arrays collected above
start_top_log_probs_concat = create_and_fill_np_array(all_start_top_log_probs, predict_dataset, max_len)
start_top_index_concat = create_and_fill_np_array(all_start_top_index, predict_dataset, max_len)
end_top_log_probs_concat = create_and_fill_np_array(all_end_top_log_probs, predict_dataset, max_len)
end_top_index_concat = create_and_fill_np_array(all_end_top_index, predict_dataset, max_len)
cls_logits_concat = np.concatenate(all_cls_logits, axis=0)
# delete the list of numpy arrays
del start_top_log_probs
del start_top_index
del end_top_log_probs
del end_top_index
del cls_logits
outputs_numpy = (
start_top_log_probs_concat,
start_top_index_concat,
end_top_log_probs_concat,
end_top_index_concat,
cls_logits_concat,
)
prediction = post_processing_function(predict_examples, predict_dataset, outputs_numpy)
predict_metric = metric.compute(predictions=prediction.predictions, references=prediction.label_ids)
logger.info(f"Predict metrics: {predict_metric}")
if args.with_tracking:
log = {
"squad_v2" if args.version_2_with_negative else "squad": eval_metric,
"train_loss": total_loss,
"epoch": epoch,
"step": completed_steps,
}
if args.do_predict:
log["squad_v2_predict" if args.version_2_with_negative else "squad_predict"] = predict_metric
accelerator.log(log)
if args.checkpointing_steps == "epoch":
accelerator.save_state(f"epoch_{epoch}")
if args.output_dir is not None:
accelerator.wait_for_everyone()
unwrapped_model = accelerator.unwrap_model(model)
unwrapped_model.save_pretrained(
args.output_dir, is_main_process=accelerator.is_main_process, save_function=accelerator.save
)
if accelerator.is_main_process:
tokenizer.save_pretrained(args.output_dir)
if args.push_to_hub:
api.upload_folder(
commit_message="End of training",
folder_path=args.output_dir,
repo_id=repo_id,
repo_type="model",
token=args.hub_token,
)
logger.info(json.dumps(eval_metric, indent=4))
save_prefixed_metrics(eval_metric, args.output_dir)
if __name__ == "__main__":
main()
| transformers/examples/pytorch/question-answering/run_qa_beam_search_no_trainer.py/0 | {
"file_path": "transformers/examples/pytorch/question-answering/run_qa_beam_search_no_trainer.py",
"repo_id": "transformers",
"token_count": 19980
} | 284 |
#!/usr/bin/env python
# coding=utf-8
# Copyright 2018 Google AI, Google Brain and Carnegie Mellon University Authors and the HuggingFace Inc. team.
# Copyright (c) 2018, NVIDIA CORPORATION. All rights reserved.
#
# Licensed under the Apache License, Version 2.0 (the "License");
# you may not use this file except in compliance with the License.
# You may obtain a copy of the License at
#
# http://www.apache.org/licenses/LICENSE-2.0
#
# Unless required by applicable law or agreed to in writing, software
# distributed under the License is distributed on an "AS IS" BASIS,
# WITHOUT WARRANTIES OR CONDITIONS OF ANY KIND, either express or implied.
# See the License for the specific language governing permissions and
# limitations under the License.
""" Conditional text generation with the auto-regressive models of the library (GPT/GPT-2/CTRL/Transformer-XL/XLNet)
"""
import argparse
import inspect
import logging
from typing import Tuple
import torch
from accelerate import PartialState
from accelerate.utils import set_seed
from transformers import (
AutoTokenizer,
BloomForCausalLM,
BloomTokenizerFast,
CTRLLMHeadModel,
CTRLTokenizer,
GenerationMixin,
GPT2LMHeadModel,
GPT2Tokenizer,
GPTJForCausalLM,
LlamaForCausalLM,
LlamaTokenizer,
OpenAIGPTLMHeadModel,
OpenAIGPTTokenizer,
OPTForCausalLM,
TransfoXLLMHeadModel,
TransfoXLTokenizer,
XLMTokenizer,
XLMWithLMHeadModel,
XLNetLMHeadModel,
XLNetTokenizer,
)
from transformers.modeling_outputs import CausalLMOutputWithPast
logging.basicConfig(
format="%(asctime)s - %(levelname)s - %(name)s - %(message)s",
datefmt="%m/%d/%Y %H:%M:%S",
level=logging.INFO,
)
logger = logging.getLogger(__name__)
MAX_LENGTH = int(10000) # Hardcoded max length to avoid infinite loop
MODEL_CLASSES = {
"gpt2": (GPT2LMHeadModel, GPT2Tokenizer),
"ctrl": (CTRLLMHeadModel, CTRLTokenizer),
"openai-gpt": (OpenAIGPTLMHeadModel, OpenAIGPTTokenizer),
"xlnet": (XLNetLMHeadModel, XLNetTokenizer),
"transfo-xl": (TransfoXLLMHeadModel, TransfoXLTokenizer),
"xlm": (XLMWithLMHeadModel, XLMTokenizer),
"gptj": (GPTJForCausalLM, AutoTokenizer),
"bloom": (BloomForCausalLM, BloomTokenizerFast),
"llama": (LlamaForCausalLM, LlamaTokenizer),
"opt": (OPTForCausalLM, GPT2Tokenizer),
}
# Padding text to help Transformer-XL and XLNet with short prompts as proposed by Aman Rusia
# in https://github.com/rusiaaman/XLNet-gen#methodology
# and https://medium.com/@amanrusia/xlnet-speaks-comparison-to-gpt-2-ea1a4e9ba39e
PREFIX = """In 1991, the remains of Russian Tsar Nicholas II and his family
(except for Alexei and Maria) are discovered.
The voice of Nicholas's young son, Tsarevich Alexei Nikolaevich, narrates the
remainder of the story. 1883 Western Siberia,
a young Grigori Rasputin is asked by his father and a group of men to perform magic.
Rasputin has a vision and denounces one of the men as a horse thief. Although his
father initially slaps him for making such an accusation, Rasputin watches as the
man is chased outside and beaten. Twenty years later, Rasputin sees a vision of
the Virgin Mary, prompting him to become a priest. Rasputin quickly becomes famous,
with people, even a bishop, begging for his blessing. <eod> </s> <eos>"""
#
# Functions to prepare models' input
#
def prepare_ctrl_input(args, _, tokenizer, prompt_text):
if args.temperature > 0.7:
logger.info("CTRL typically works better with lower temperatures (and lower top_k).")
encoded_prompt = tokenizer.encode(prompt_text, add_special_tokens=False)
if not any(encoded_prompt[0] == x for x in tokenizer.control_codes.values()):
logger.info("WARNING! You are not starting your generation from a control code so you won't get good results")
return prompt_text
def prepare_xlm_input(args, model, tokenizer, prompt_text):
# kwargs = {"language": None, "mask_token_id": None}
# Set the language
use_lang_emb = hasattr(model.config, "use_lang_emb") and model.config.use_lang_emb
if hasattr(model.config, "lang2id") and use_lang_emb:
available_languages = model.config.lang2id.keys()
if args.xlm_language in available_languages:
language = args.xlm_language
else:
language = None
while language not in available_languages:
language = input("Using XLM. Select language in " + str(list(available_languages)) + " >>> ")
model.config.lang_id = model.config.lang2id[language]
# kwargs["language"] = tokenizer.lang2id[language]
# TODO fix mask_token_id setup when configurations will be synchronized between models and tokenizers
# XLM masked-language modeling (MLM) models need masked token
# is_xlm_mlm = "mlm" in args.model_name_or_path
# if is_xlm_mlm:
# kwargs["mask_token_id"] = tokenizer.mask_token_id
return prompt_text
def prepare_xlnet_input(args, _, tokenizer, prompt_text):
prefix = args.prefix if args.prefix else args.padding_text if args.padding_text else PREFIX
prompt_text = prefix + prompt_text
return prompt_text
def prepare_transfoxl_input(args, _, tokenizer, prompt_text):
prefix = args.prefix if args.prefix else args.padding_text if args.padding_text else PREFIX
prompt_text = prefix + prompt_text
return prompt_text
PREPROCESSING_FUNCTIONS = {
"ctrl": prepare_ctrl_input,
"xlm": prepare_xlm_input,
"xlnet": prepare_xlnet_input,
"transfo-xl": prepare_transfoxl_input,
}
def adjust_length_to_model(length, max_sequence_length):
if length < 0 and max_sequence_length > 0:
length = max_sequence_length
elif 0 < max_sequence_length < length:
length = max_sequence_length # No generation bigger than model size
elif length < 0:
length = MAX_LENGTH # avoid infinite loop
return length
def sparse_model_config(model_config):
embedding_size = None
if hasattr(model_config, "hidden_size"):
embedding_size = model_config.hidden_size
elif hasattr(model_config, "n_embed"):
embedding_size = model_config.n_embed
elif hasattr(model_config, "n_embd"):
embedding_size = model_config.n_embd
num_head = None
if hasattr(model_config, "num_attention_heads"):
num_head = model_config.num_attention_heads
elif hasattr(model_config, "n_head"):
num_head = model_config.n_head
if embedding_size is None or num_head is None or num_head == 0:
raise ValueError("Check the model config")
num_embedding_size_per_head = int(embedding_size / num_head)
if hasattr(model_config, "n_layer"):
num_layer = model_config.n_layer
elif hasattr(model_config, "num_hidden_layers"):
num_layer = model_config.num_hidden_layers
else:
raise ValueError("Number of hidden layers couldn't be determined from the model config")
return num_layer, num_head, num_embedding_size_per_head
def generate_past_key_values(model, batch_size, seq_len):
num_block_layers, num_attention_heads, num_embedding_size_per_head = sparse_model_config(model.config)
if model.config.model_type == "bloom":
past_key_values = tuple(
(
torch.empty(int(num_attention_heads * batch_size), num_embedding_size_per_head, seq_len)
.to(model.dtype)
.to(model.device),
torch.empty(int(num_attention_heads * batch_size), seq_len, num_embedding_size_per_head)
.to(model.dtype)
.to(model.device),
)
for _ in range(num_block_layers)
)
else:
past_key_values = tuple(
(
torch.empty(batch_size, num_attention_heads, seq_len, num_embedding_size_per_head)
.to(model.dtype)
.to(model.device),
torch.empty(batch_size, num_attention_heads, seq_len, num_embedding_size_per_head)
.to(model.dtype)
.to(model.device),
)
for _ in range(num_block_layers)
)
return past_key_values
def prepare_jit_inputs(inputs, model, tokenizer):
batch_size = len(inputs)
dummy_input = tokenizer.batch_encode_plus(inputs, return_tensors="pt")
dummy_input = dummy_input.to(model.device)
if model.config.use_cache:
dummy_input["past_key_values"] = generate_past_key_values(model, batch_size, 1)
dummy_input["attention_mask"] = torch.cat(
[
torch.zeros(dummy_input["attention_mask"].shape[0], 1)
.to(dummy_input["attention_mask"].dtype)
.to(model.device),
dummy_input["attention_mask"],
],
-1,
)
return dummy_input
class _ModelFallbackWrapper(GenerationMixin):
__slots__ = ("_optimized", "_default")
def __init__(self, optimized, default):
self._optimized = optimized
self._default = default
def __call__(self, *args, **kwargs):
if kwargs["past_key_values"] is None and self._default.config.use_cache:
kwargs["past_key_values"] = generate_past_key_values(self._default, kwargs["input_ids"].shape[0], 0)
kwargs.pop("position_ids", None)
for k in list(kwargs.keys()):
if kwargs[k] is None or isinstance(kwargs[k], bool):
kwargs.pop(k)
outputs = self._optimized(**kwargs)
lm_logits = outputs[0]
past_key_values = outputs[1]
fixed_output = CausalLMOutputWithPast(
loss=None,
logits=lm_logits,
past_key_values=past_key_values,
hidden_states=None,
attentions=None,
)
return fixed_output
def __getattr__(self, item):
return getattr(self._default, item)
def prepare_inputs_for_generation(
self, input_ids, past_key_values=None, inputs_embeds=None, use_cache=None, **kwargs
):
return self._default.prepare_inputs_for_generation(
input_ids, past_key_values=past_key_values, inputs_embeds=inputs_embeds, use_cache=use_cache, **kwargs
)
def _reorder_cache(
self, past_key_values: Tuple[Tuple[torch.Tensor]], beam_idx: torch.Tensor
) -> Tuple[Tuple[torch.Tensor]]:
"""
This function is used to re-order the `past_key_values` cache if [`~PretrainedModel.beam_search`] or
[`~PretrainedModel.beam_sample`] is called. This is required to match `past_key_values` with the correct
beam_idx at every generation step.
"""
return self._default._reorder_cache(past_key_values, beam_idx)
def main():
parser = argparse.ArgumentParser()
parser.add_argument(
"--model_type",
default=None,
type=str,
required=True,
help="Model type selected in the list: " + ", ".join(MODEL_CLASSES.keys()),
)
parser.add_argument(
"--model_name_or_path",
default=None,
type=str,
required=True,
help="Path to pre-trained model or shortcut name selected in the list: " + ", ".join(MODEL_CLASSES.keys()),
)
parser.add_argument("--prompt", type=str, default="")
parser.add_argument("--length", type=int, default=20)
parser.add_argument("--stop_token", type=str, default=None, help="Token at which text generation is stopped")
parser.add_argument(
"--temperature",
type=float,
default=1.0,
help="temperature of 1.0 has no effect, lower tend toward greedy sampling",
)
parser.add_argument(
"--repetition_penalty", type=float, default=1.0, help="primarily useful for CTRL model; in that case, use 1.2"
)
parser.add_argument("--k", type=int, default=0)
parser.add_argument("--p", type=float, default=0.9)
parser.add_argument("--prefix", type=str, default="", help="Text added prior to input.")
parser.add_argument("--padding_text", type=str, default="", help="Deprecated, the use of `--prefix` is preferred.")
parser.add_argument("--xlm_language", type=str, default="", help="Optional language when used with the XLM model.")
parser.add_argument("--seed", type=int, default=42, help="random seed for initialization")
parser.add_argument(
"--use_cpu",
action="store_true",
help="Whether or not to use cpu. If set to False, " "we will use gpu/npu or mps device if available",
)
parser.add_argument("--num_return_sequences", type=int, default=1, help="The number of samples to generate.")
parser.add_argument(
"--fp16",
action="store_true",
help="Whether to use 16-bit (mixed) precision (through NVIDIA apex) instead of 32-bit",
)
parser.add_argument("--jit", action="store_true", help="Whether or not to use jit trace to accelerate inference")
args = parser.parse_args()
# Initialize the distributed state.
distributed_state = PartialState(cpu=args.use_cpu)
logger.warning(f"device: {distributed_state.device}, 16-bits inference: {args.fp16}")
if args.seed is not None:
set_seed(args.seed)
# Initialize the model and tokenizer
try:
args.model_type = args.model_type.lower()
model_class, tokenizer_class = MODEL_CLASSES[args.model_type]
except KeyError:
raise KeyError("the model {} you specified is not supported. You are welcome to add it and open a PR :)")
tokenizer = tokenizer_class.from_pretrained(args.model_name_or_path)
if tokenizer.pad_token is None:
tokenizer.pad_token = tokenizer.eos_token
model = model_class.from_pretrained(args.model_name_or_path)
# Set the model to the right device
model.to(distributed_state.device)
if args.fp16:
model.half()
max_seq_length = getattr(model.config, "max_position_embeddings", 0)
args.length = adjust_length_to_model(args.length, max_sequence_length=max_seq_length)
logger.info(args)
prompt_text = args.prompt if args.prompt else input("Model prompt >>> ")
# Different models need different input formatting and/or extra arguments
requires_preprocessing = args.model_type in PREPROCESSING_FUNCTIONS.keys()
if requires_preprocessing:
prepare_input = PREPROCESSING_FUNCTIONS.get(args.model_type)
preprocessed_prompt_text = prepare_input(args, model, tokenizer, prompt_text)
if model.__class__.__name__ in ["TransfoXLLMHeadModel"]:
tokenizer_kwargs = {"add_space_before_punct_symbol": True}
else:
tokenizer_kwargs = {}
encoded_prompt = tokenizer.encode(
preprocessed_prompt_text, add_special_tokens=False, return_tensors="pt", **tokenizer_kwargs
)
else:
prefix = args.prefix if args.prefix else args.padding_text
encoded_prompt = tokenizer.encode(prefix + prompt_text, add_special_tokens=False, return_tensors="pt")
encoded_prompt = encoded_prompt.to(distributed_state.device)
if encoded_prompt.size()[-1] == 0:
input_ids = None
else:
input_ids = encoded_prompt
if args.jit:
jit_input_texts = ["enable jit"]
jit_inputs = prepare_jit_inputs(jit_input_texts, model, tokenizer)
torch._C._jit_set_texpr_fuser_enabled(False)
model.config.return_dict = False
if hasattr(model, "forward"):
sig = inspect.signature(model.forward)
else:
sig = inspect.signature(model.__call__)
jit_inputs = tuple(jit_inputs[key] for key in sig.parameters if jit_inputs.get(key, None) is not None)
traced_model = torch.jit.trace(model, jit_inputs, strict=False)
traced_model = torch.jit.freeze(traced_model.eval())
traced_model(*jit_inputs)
traced_model(*jit_inputs)
model = _ModelFallbackWrapper(traced_model, model)
output_sequences = model.generate(
input_ids=input_ids,
max_length=args.length + len(encoded_prompt[0]),
temperature=args.temperature,
top_k=args.k,
top_p=args.p,
repetition_penalty=args.repetition_penalty,
do_sample=True,
num_return_sequences=args.num_return_sequences,
)
# Remove the batch dimension when returning multiple sequences
if len(output_sequences.shape) > 2:
output_sequences.squeeze_()
generated_sequences = []
for generated_sequence_idx, generated_sequence in enumerate(output_sequences):
print(f"=== GENERATED SEQUENCE {generated_sequence_idx + 1} ===")
generated_sequence = generated_sequence.tolist()
# Decode text
text = tokenizer.decode(generated_sequence, clean_up_tokenization_spaces=True)
# Remove all text after the stop token
text = text[: text.find(args.stop_token) if args.stop_token else None]
# Add the prompt at the beginning of the sequence. Remove the excess text that was used for pre-processing
total_sequence = (
prompt_text + text[len(tokenizer.decode(encoded_prompt[0], clean_up_tokenization_spaces=True)) :]
)
generated_sequences.append(total_sequence)
print(total_sequence)
return generated_sequences
if __name__ == "__main__":
main()
| transformers/examples/pytorch/text-generation/run_generation.py/0 | {
"file_path": "transformers/examples/pytorch/text-generation/run_generation.py",
"repo_id": "transformers",
"token_count": 6877
} | 285 |
# coding=utf-8
# Copyright 2018 The Google AI Language Team Authors and The HuggingFace Inc. team.
# Copyright (c) 2018, NVIDIA CORPORATION. All rights reserved.
#
# Licensed under the Apache License, Version 2.0 (the "License");
# you may not use this file except in compliance with the License.
# You may obtain a copy of the License at
#
# http://www.apache.org/licenses/LICENSE-2.0
#
# Unless required by applicable law or agreed to in writing, software
# distributed under the License is distributed on an "AS IS" BASIS,
# WITHOUT WARRANTIES OR CONDITIONS OF ANY KIND, either express or implied.
# See the License for the specific language governing permissions and
# limitations under the License.
""" Finetuning the library models for sequence classification on HANS."""
import logging
import os
from dataclasses import dataclass, field
from typing import Dict, List, Optional
import numpy as np
import torch
from utils_hans import HansDataset, InputFeatures, hans_processors, hans_tasks_num_labels
import transformers
from transformers import (
AutoConfig,
AutoModelForSequenceClassification,
AutoTokenizer,
HfArgumentParser,
Trainer,
TrainingArguments,
default_data_collator,
set_seed,
)
from transformers.trainer_utils import is_main_process
logger = logging.getLogger(__name__)
@dataclass
class ModelArguments:
"""
Arguments pertaining to which model/config/tokenizer we are going to fine-tune from.
"""
model_name_or_path: str = field(
metadata={"help": "Path to pretrained model or model identifier from huggingface.co/models"}
)
config_name: Optional[str] = field(
default=None, metadata={"help": "Pretrained config name or path if not the same as model_name"}
)
tokenizer_name: Optional[str] = field(
default=None, metadata={"help": "Pretrained tokenizer name or path if not the same as model_name"}
)
cache_dir: Optional[str] = field(
default=None,
metadata={"help": "Where do you want to store the pretrained models downloaded from huggingface.co"},
)
@dataclass
class DataTrainingArguments:
"""
Arguments pertaining to what data we are going to input our model for training and eval.
"""
task_name: str = field(
metadata={"help": "The name of the task to train selected in the list: " + ", ".join(hans_processors.keys())}
)
data_dir: str = field(
metadata={"help": "The input data dir. Should contain the .tsv files (or other data files) for the task."}
)
max_seq_length: int = field(
default=128,
metadata={
"help": (
"The maximum total input sequence length after tokenization. Sequences longer "
"than this will be truncated, sequences shorter will be padded."
)
},
)
overwrite_cache: bool = field(
default=False, metadata={"help": "Overwrite the cached training and evaluation sets"}
)
def hans_data_collator(features: List[InputFeatures]) -> Dict[str, torch.Tensor]:
"""
Data collator that removes the "pairID" key if present.
"""
batch = default_data_collator(features)
_ = batch.pop("pairID", None)
return batch
def main():
# See all possible arguments in src/transformers/training_args.py
# or by passing the --help flag to this script.
# We now keep distinct sets of args, for a cleaner separation of concerns.
parser = HfArgumentParser((ModelArguments, DataTrainingArguments, TrainingArguments))
model_args, data_args, training_args = parser.parse_args_into_dataclasses()
if (
os.path.exists(training_args.output_dir)
and os.listdir(training_args.output_dir)
and training_args.do_train
and not training_args.overwrite_output_dir
):
raise ValueError(
f"Output directory ({training_args.output_dir}) already exists and is not empty. Use"
" --overwrite_output_dir to overcome."
)
# Setup logging
logging.basicConfig(
format="%(asctime)s - %(levelname)s - %(name)s - %(message)s",
datefmt="%m/%d/%Y %H:%M:%S",
level=logging.INFO if training_args.local_rank in [-1, 0] else logging.WARN,
)
logger.warning(
"Process rank: %s, device: %s, n_gpu: %s, distributed training: %s, 16-bits training: %s",
training_args.local_rank,
training_args.device,
training_args.n_gpu,
bool(training_args.local_rank != -1),
training_args.fp16,
)
# Set the verbosity to info of the Transformers logger (on main process only):
if is_main_process(training_args.local_rank):
transformers.utils.logging.set_verbosity_info()
transformers.utils.logging.enable_default_handler()
transformers.utils.logging.enable_explicit_format()
logger.info("Training/evaluation parameters %s", training_args)
# Set seed
set_seed(training_args.seed)
try:
num_labels = hans_tasks_num_labels[data_args.task_name]
except KeyError:
raise ValueError("Task not found: %s" % (data_args.task_name))
# Load pretrained model and tokenizer
#
# Distributed training:
# The .from_pretrained methods guarantee that only one local process can concurrently
# download model & vocab.
config = AutoConfig.from_pretrained(
model_args.config_name if model_args.config_name else model_args.model_name_or_path,
num_labels=num_labels,
finetuning_task=data_args.task_name,
cache_dir=model_args.cache_dir,
)
tokenizer = AutoTokenizer.from_pretrained(
model_args.tokenizer_name if model_args.tokenizer_name else model_args.model_name_or_path,
cache_dir=model_args.cache_dir,
)
model = AutoModelForSequenceClassification.from_pretrained(
model_args.model_name_or_path,
from_tf=bool(".ckpt" in model_args.model_name_or_path),
config=config,
cache_dir=model_args.cache_dir,
)
# Get datasets
train_dataset = (
HansDataset(
data_dir=data_args.data_dir,
tokenizer=tokenizer,
task=data_args.task_name,
max_seq_length=data_args.max_seq_length,
overwrite_cache=data_args.overwrite_cache,
)
if training_args.do_train
else None
)
eval_dataset = (
HansDataset(
data_dir=data_args.data_dir,
tokenizer=tokenizer,
task=data_args.task_name,
max_seq_length=data_args.max_seq_length,
overwrite_cache=data_args.overwrite_cache,
evaluate=True,
)
if training_args.do_eval
else None
)
# Initialize our Trainer
trainer = Trainer(
model=model,
args=training_args,
train_dataset=train_dataset,
eval_dataset=eval_dataset,
data_collator=hans_data_collator,
)
# Training
if training_args.do_train:
trainer.train(
model_path=model_args.model_name_or_path if os.path.isdir(model_args.model_name_or_path) else None
)
trainer.save_model()
# For convenience, we also re-save the tokenizer to the same directory,
# so that you can share your model easily on huggingface.co/models =)
if trainer.is_world_master():
tokenizer.save_pretrained(training_args.output_dir)
# Evaluation
if training_args.do_eval:
logger.info("*** Evaluate ***")
output = trainer.predict(eval_dataset)
preds = output.predictions
preds = np.argmax(preds, axis=1)
pair_ids = [ex.pairID for ex in eval_dataset]
output_eval_file = os.path.join(training_args.output_dir, "hans_predictions.txt")
label_list = eval_dataset.get_labels()
if trainer.is_world_master():
with open(output_eval_file, "w") as writer:
writer.write("pairID,gold_label\n")
for pid, pred in zip(pair_ids, preds):
writer.write("ex" + str(pid) + "," + label_list[int(pred)] + "\n")
trainer._log(output.metrics)
def _mp_fn(index):
# For xla_spawn (TPUs)
main()
if __name__ == "__main__":
main()
| transformers/examples/research_projects/adversarial/run_hans.py/0 | {
"file_path": "transformers/examples/research_projects/adversarial/run_hans.py",
"repo_id": "transformers",
"token_count": 3302
} | 286 |
Subsets and Splits
No community queries yet
The top public SQL queries from the community will appear here once available.