repo
stringclasses 1
value | number
int64 1
25.3k
| state
stringclasses 2
values | title
stringlengths 1
487
| body
stringlengths 0
234k
⌀ | created_at
stringlengths 19
19
| closed_at
stringlengths 19
19
| comments
stringlengths 0
293k
|
---|---|---|---|---|---|---|---|
transformers | 11,217 | closed | Question about validation_set | I want to ask a simple question. The parameters of the model have been set before model training. What is the purpose of the validation set in model training? Thank you! | 04-13-2021 07:24:36 | 04-13-2021 07:24:36 | Hi,
You can use Stackoverflow for that: https://stats.stackexchange.com/questions/19048/what-is-the-difference-between-test-set-and-validation-set
We like to keep Github issues for feature requests/bug reports. There's also the [forum](https://discuss.huggingface.co/) where you can ask training-related questions.
Thanks!<|||||>This issue has been automatically marked as stale because it has not had recent activity. If you think this still needs to be addressed please comment on this thread.
Please note that issues that do not follow the [contributing guidelines](https://github.com/huggingface/transformers/blob/master/CONTRIBUTING.md) are likely to be ignored. |
transformers | 11,216 | closed | Load BART-base error | ## Environment info
<!-- You can run the command `transformers-cli env` and copy-and-paste its output below.
Don't forget to fill out the missing fields in that output! -->
- `transformers` version: 4.5.0
- simpletransformers: 0.61.4
- Platform: CentOS
- Python version: Python 3.8.2
- PyTorch version (GPU?): torch-1.8.1 (yes)
- Tensorflow version (GPU?):
- Using GPU in script?:
- Using distributed or parallel set-up in script?:
Models:
- bart: @patrickvonplaten, @patil-suraj
## Information
Model I am using (Bert, XLNet ...): Bart
The problem arises when using:
* [ ] the official example scripts: (give details below)
* [X] my own modified scripts: (give details below)
The tasks I am working on is:
* [ ] an official GLUE/SQUaD task: (give the name)
* [X] my own task or dataset: (give details below)
## To reproduce
Steps to reproduce the behavior:
I downloaded the bart-base and un zip it. I have the following code:
`
from sklearn.model_selection import train_test_split
from simpletransformers.seq2seq import Seq2SeqModel, Seq2SeqArgs
model_args = Seq2SeqArgs()
model_args.do_sample = True
model_args.eval_batch_size = 64
model_args.evaluate_during_training = True
model_args.evaluate_during_training_steps = 2500
model_args.evaluate_during_training_verbose = True
model_args.fp16 = False
model_args.learning_rate = 5e-5
model_args.max_length = 128
model_args.max_seq_length = 128
model_args.num_beams = None
model_args.num_return_sequences = 3
model_args.num_train_epochs = 2
model_args.overwrite_output_dir = True
model_args.reprocess_input_data = True
model_args.save_eval_checkpoints = False
model_args.save_steps = -1
model_args.top_k = 50
model_args.top_p = 0.95
model_args.train_batch_size = 8
model_args.use_multiprocessing = False
model_args.wandb_project = "Paraphrasing with BART"
model = Seq2SeqModel(
encoder_decoder_type="bart",
encoder_decoder_name="/home/ahmad2/.cache/huggingface/transformers/bart-base",
args=model_args,
use_cuda = False,
from_tf=True,
}`
However, the above code throws the following error:
`Traceback (most recent call last):
File "original_BART.py", line 109, in <module>
model = Seq2SeqModel(
File "/home/ahmad2/.local/lib/python3.8/site-packages/simpletransformers/seq2seq/seq2seq_model.py", line 275, in __init__
self.model = model_class.from_pretrained(encoder_decoder_name)
File "/home/ahmad2/.local/lib/python3.8/site-packages/transformers/modeling_utils.py", line 1065, in from_pretrained
raise OSError(
OSError: Unable to load weights from pytorch checkpoint file for '/home/ahmad2/.cache/huggingface/transformers/bart-base' at '/home/ahmad2/.cache/huggingface/transformers/bart-base/pytorch_model.bin'If you tried to load a PyTorch model from a TF 2.0 checkpoint, please set from_tf=True.`
I am not sure if there is an issue with the above code. Thanks in advance!
| 04-12-2021 23:43:29 | 04-12-2021 23:43:29 | This seems to be an issue with `simpletransformers` so please post it there since we won't get time to look into other code bases to fix such issues.<|||||>This issue has been automatically marked as stale because it has not had recent activity. If you think this still needs to be addressed please comment on this thread.
Please note that issues that do not follow the [contributing guidelines](https://github.com/huggingface/transformers/blob/master/CONTRIBUTING.md) are likely to be ignored. |
transformers | 11,215 | closed | It don't find simple logic sequences | 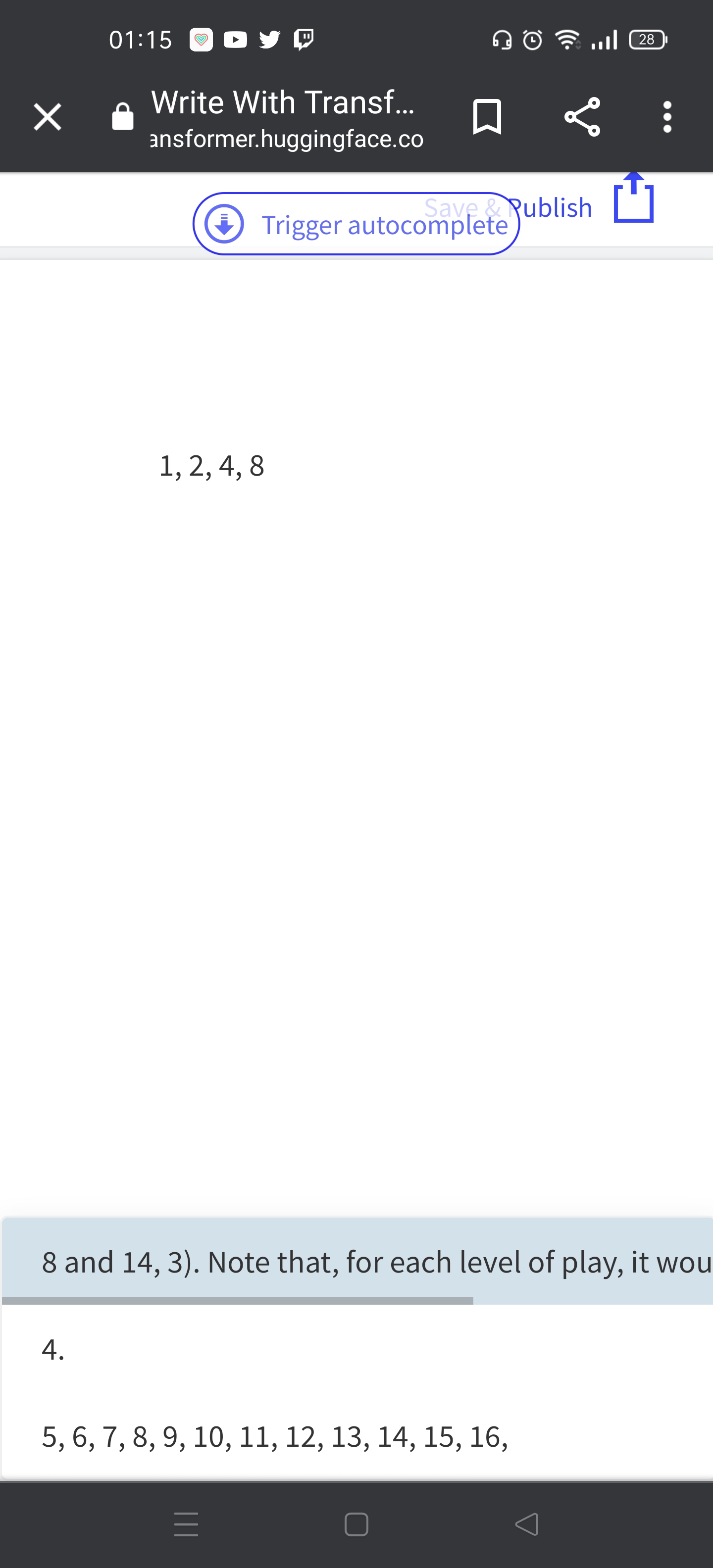
| 04-12-2021 23:17:57 | 04-12-2021 23:17:57 | This issue has been automatically marked as stale because it has not had recent activity. If you think this still needs to be addressed please comment on this thread.
Please note that issues that do not follow the [contributing guidelines](https://github.com/huggingface/transformers/blob/master/CONTRIBUTING.md) are likely to be ignored. |
transformers | 11,214 | closed | Import torch.utils.checkpoint in ProphetNet | Fix https://github.com/huggingface/transformers/issues/11193 | 04-12-2021 22:47:10 | 04-12-2021 22:47:10 | |
transformers | 11,213 | closed | Fix GPT-2 warnings | There was a forgotten code path when identifying missing weights.
When loading from a pytorch checkpoint to a tensorflow checkpoint, there was no issue, but doing so the other way around wouldn't check the `_keys_to_ignore_on_load_missing` and `_keys_to_ignore_on_load_unexpected` variables before printing a warning.
closes https://github.com/huggingface/transformers/issues/11192 | 04-12-2021 22:01:54 | 04-12-2021 22:01:54 | |
transformers | 11,212 | closed | Add Matt as the TensorFlow reference | 04-12-2021 20:59:42 | 04-12-2021 20:59:42 | ||
transformers | 11,211 | closed | Beam search on BART seq2seq | ## Environment info
<!-- You can run the command `transformers-cli env` and copy-and-paste its output below.
Don't forget to fill out the missing fields in that output! -->
- `transformers` version: 4.5.0.dev0
- Platform: Linux-3.10.0-1160.15.2.el7.x86_64-x86_64-with-glibc2.10
- Python version: 3.8.5
- PyTorch version (GPU?): 1.8.1 (False)
- Tensorflow version (GPU?): not installed (NA)
- Using GPU in script?: yes
- Using distributed or parallel set-up in script?: ?
### Who can help
@patrickvonplaten, @patil-suraj
## Information
Model I am using (Bert, XLNet ...): BART seq2seq
The problem arises when using:
* [x] the official example scripts: (give details below)
* [ ] my own modified scripts: (give details below)
The tasks I am working on is:
* [ ] an official GLUE/SQUaD task: (give the name)
* [x] my own task or dataset: (give details below)
## To reproduce
Steps to reproduce the behavior:
1. I'm attempting to use beam search and have the model output the 10 best possible predictions for each test item.
2. I found the parameter `num_beams`, which I am using though it does not appear to work by itself. No error occurs, but only 1 output per test item is produced.
3. I thought I should use the parameter `num_return_sequences` as well, but it does not appear to be a possible argument for this model and I have not been able to find anything comparable.
Here is my command:
```
python transformers/examples/seq2seq/run_translation.py \
--model_name_or_path facebook/bart-base \
--do_train \
--do_predict \
--source_lang en \
--target_lang lf \
--source_prefix "translate English to Logical Forms: " \
--train_file folds/0_train.json \
--test_file folds/0_val.json \
--num_train_epochs=5 \
--num_beams=10 \
--output_dir ./test_results_beam \
--per_device_train_batch_size=4 \
--per_device_eval_batch_size=4 \
--overwrite_output_dir \
--predict_with_generate
```
## Expected behavior
Outputting the 10 best predictions per test item.
| 04-12-2021 20:08:53 | 04-12-2021 20:08:53 | Hi @ashleylew
The `run_translation.py` uses the `Seq2SeqTrainer` which does not pass the `num_return_sequences` argument to `generate`, this is because if multiple sequences are returned then its not clear what sequence should be used to compute the metrics.
you could generate test set predictions by using the `generate` method and passing the `num_return_sequences` argument. But if you want to do this using `Seq2SeqTrainer` then you would need to modify it.<|||||>This issue has been automatically marked as stale because it has not had recent activity. If you think this still needs to be addressed please comment on this thread.
Please note that issues that do not follow the [contributing guidelines](https://github.com/huggingface/transformers/blob/master/CONTRIBUTING.md) are likely to be ignored. |
transformers | 11,210 | closed | Documentation enhancement - model_type | # 🚀 Feature request
Please provide a clear explanation of what the valid values would be for "model_type". I think that the answer is any model name you would use in from_pretrained() but I am not sure.
## Motivation
Clarity of the parameter and saving time on trial and error and guesswork.
## Your contribution
If the assumption above is correct, I am willing to write up the answer if it will help. If you have a HTML page with the list of valid values (maybe on a model page) we can just add a link to that.
| 04-12-2021 19:33:54 | 04-12-2021 19:33:54 | This issue has been automatically marked as stale because it has not had recent activity. If you think this still needs to be addressed please comment on this thread.
Please note that issues that do not follow the [contributing guidelines](https://github.com/huggingface/transformers/blob/master/CONTRIBUTING.md) are likely to be ignored. |
transformers | 11,209 | open | [RFC] introduce `config.trained_precision` | # 🚀 Feature request
As we are discovering that `bf16`-pretrained models don't do well on `fp16` "regime" (and surely vice-versa), and some models are pre-trained in `fp32` and surely won't do well on either `bf16` or `fp16`, and the problem is going to grow as more `bf16`-supporting hardware comes out, I propose we start requiring that the model tells the user which mode it was pretrained under.
So I suggest we add `config.trained_precision` which currently would be one of `fp16`, `bf16`, `fp32`, `unknown`.
I haven't thoughts it through on how to derive this automatically during `save_pretrained`, but when porting checkpoints the porter can figure that out and manually set this in the conversion script.
For example, from what I understood gtp-neo if `bf16` for all but `2.7B` version, which is `fp32`.
@sgugger, @LysandreJik | 04-12-2021 16:54:09 | 04-12-2021 16:54:09 | Would that information not be better on the respective model cards? I think that's more on that side that it should go with the rest of the training setup.<|||||>1. It'd be difficult to enforce that it's being filled out
2. It'd be difficult to tell the user at run time that model A pre-trained in fp32, is attempted to be run in fp16 or bf16
But if we can't do it on config level at the very least it could be a required card entry (but I don't think anything is required in the cards).<|||||>How would you enforce it being filled out via the config? You would get the default for pretty much all models too: if a user is too lazy to fill the model card they will also be too lazy to fill the config.
I don't understand what you mean in 2, could you elaborate? Why would it be bad to fine-tune a model trained in FP32 in FP16 or bfloat16?<|||||>> How would you enforce it being filled out via the config? You would get the default for pretty much all models too: if a user is too lazy to fill the model card they will also be too lazy to fill the config.
Hmm, right. I was thinking about the tests, which can enforce the field existing in the config object but have no way to enforce the values.
> I don't understand what you mean in 2, could you elaborate? Why would it be bad to fine-tune a model trained in FP32 in FP16 or bfloat16?
It won't work out of the box and will require finetuning, which may not succeed if running into infs/nans. I suppose I was thinking more about inference, which won't work w/o finetuning first. If the model was pre-trained in mixed precision it can be used in fp16 inference, but this won't be the case if it was pretrained in fp32.<|||||>I think this feature would be welcome indeed and would save us a lot of trouble as we've seen in the past. Regarding whether we want to have this in the model card or in the configuration, I guess it really depends on whether we want to enforce that with errors or warnings.
I think the main point of having that field is to actually warn the user when they're doing inference with a model trained with a different precision, and to that extent, having it in the configuration makes more sense.
I also think the configuration is here to detail how a checkpoint is configured: how the architecture fits the weights (hidden size, layers) and how it should be used (model type, architecture). I think it would make sense to have this as a configuration field, as not knowing that can result in an unusable checkpoint in other environments. I think that's different from other training-related arguments, such as `gradient_checkpointing`, which don't really make sense once ported to a different environment.<|||||>Excellent! So that makes the 2 of us who think it would be most strategically placed in the model config.
So let's look at specifics. I can think of the following:
1. at conversion point - it'd be a responsibility of the porter to fill it out - but could also look at the imported state_dict first - perhaps the weights are already in non-fp32 (some models are saved in `.half()` so in this situation it could be derived automatically)
2. at `save_pretrained` - this is the difficult one. what do we set here? As `save_pretrained` has no way to determine how the model was trained. So we will need to require the precision to be passed explicitly then? The Trainer can be adapted since it knows the precision, but for non-Trainer users will have to specify it explicitly.
3. rewriting history. what do we do about the thousands of models already on the hub? do a massive script that will push `config.trained_precision = unknown` and then over time start correcting this? at least for the main/popular models and problematic ones - m?t5/pegasus/gpt-neo
any others cases that I missed?
what would be a good not too long keyword for this one? would `config.trained_precision` be not too long and clear enough?<|||||>I think the name is good. I would leave it to a default of `"unknown"` for all existing models, so that we don't have to add it anywhere (especially when we don't have the info). I would personally not try to guess it too much and only set that information when we have it from the people who trained the model.
For 2, I don't think we should try to guess it either when people are not using the `Trainer` and just focus on the trainer. We just need to add a `model.config.trained_precision = xxx` from the args and the env at the beginning of training, then the `save_pretrained` method, which also saves the config, will properly handle that.
For 3, I would only populate the popular models, for which we have the info.<|||||>> I think the name is good. I would leave it to a default of `"unknown"` for all existing models, so that we don't have to add it anywhere (especially when we don't have the info). I would personally not try to guess it too much and only set that information when we have it from the people who trained the model.
But we could require this new key for when new models are added. That's why I thought that if we were to massively rewrite the hub's config with `trained_precision = unknown` then we could start enforcing this new field.
> For 2, I don't think we should try to guess it either when people are not using the `Trainer` and just focus on the trainer. We just need to add a `model.config.trained_precision = xxx` from the args and the env at the beginning of training, then the `save_pretrained` method, which also saves the config, will properly handle that.
Yes!
The only trouble here is that someone taking a model in fp32, training it for 10 steps in mixed precision doesn't quite qualify it for fp16.
----------------
I think the problem is that we can't make `save_pretrained` require this new field (for outside Trainer) since it'd be a breaking change.
And also the main event where this field needs to be set is when the model is ported from another system (since that's where the current problems all originate from). So how could we at the very least enforce this in conversion scripts?
<|||||>Made a [wiki post](https://discuss.huggingface.co/t/compiling-data-on-how-models-were-trained-fp16-fp32-bf16/5671) - hoping to gather more info via the community input, so that we can have enough data to do some initial seeding of this new field.
<|||||>This PR is related - adding `config.torch_dtype` field: https://github.com/huggingface/transformers/pull/12316
I guess I can tackle this one next in line.
<|||||>New development:
8-bit quantized models have arrived: https://github.com/huggingface/transformers/issues/14839 - need to make sure we don't load those in fp32!
|
transformers | 11,208 | closed | Issue: Trainer error on `evaluate()` in multithreaded/distributed context (shape mismatch) | ## Environment info
<!-- You can run the command `transformers-cli env` and copy-and-paste its output below.
Don't forget to fill out the missing fields in that output! -->
- `transformers` version: 4.3.3
- Platform: Linux-5.4.83.1.fi-x86_64-with-centos-7.8.2003-Core
- Python version: 3.7.3
- PyTorch version (GPU?): 1.8.0 (True)
- Tensorflow version (GPU?): not installed (NA)
- Using GPU in script?: yes
- Using distributed or parallel set-up in script?: yes - multinode/multigpu and multigpu settings.
### Who can help
@LysandreJik @sgugger
## Information
Model I am using (GPT2):
The problem arises when using:
* [ ] the official example scripts: (give details below)
* [X] my own modified scripts: (give details below)
The tasks I am working on is:
* [ ] an official GLUE/SQUaD task: (give the name)
* [X] my own task or dataset: (give details below)
## To reproduce
Steps to reproduce the behavior: I have witnessed this error in two contexts
Using a custom `torch.utils.data.IterableDataset`.
First:
1. specify `dataloader_num_workers` > 1 in `TrainingArguments` and run `trainer.train()` with an eval dataset
Second:
1. In distributed setting, fire up multiple training instances on separate nodes using the `torch.distributed.launch` command, run `trainer.train()` with an eval dataset
Error message:
```
File "/mnt/home/dberenberg/projects/metagenomics/huggingface_meta/lib/python3.7/site-packages/transformers/trainer.py", line 1655, in prediction_loop
eval_losses_gatherer.add_arrays(self._gather_and_numpify(losses_host, "eval_losses"))
File "/mnt/home/dberenberg/projects/metagenomics/huggingface_meta/lib/python3.7/site-packages/transformers/trainer_pt_utils.py", line 338, in add_arrays
slice_len = self._nested_set_tensors(self._storage, arrays)
File "/mnt/home/dberenberg/projects/metagenomics/huggingface_meta/lib/python3.7/site-packages/transformers/trainer_pt_utils.py", line 354, in _nested_set_tensors
storage[self._offsets[i] : self._offsets[i] + slice_len] = arrays[i * slice_len : (i + 1) * slice_len]
ValueError: could not broadcast input array from shape (104,) into shape (96,)
```
The broadcast input array shape varies. In the first case, the broadcast shape will be `dataloader_num_workers` * `expected_shape` (in this case (96,)). Above exhibits the second case error message.
## Expected behavior
The `evaluate` loop should run without error.
## Dataset information
The dataset object is an `IterableDataset` that is `abc.Sized`.
## Script information
The script is fairly generic, involving training and evaluating GPT2 via the `Trainer` object for next-token prediction.
| 04-12-2021 16:34:38 | 04-12-2021 16:34:38 | I am unsure on what you want us to do: all the example scripts have been tested with evaluation and work in a distributed setup. Unless you share your script, there is little we can do to fix an issue we have not encountered.
Also make sure you have the latest version of Transformers installed as this bug might have been fixed already.<|||||>@sgugger Hello and thank you for the reply! Understood it's not clear how to help here. Unfortunately the error persists in version `4.5.0`. For a minimal example, will you need data or is code good enough? It's a very nonstandard dataset, composed of DNA strings ( and only 4 coding tokens plus a pad), but the only nonstandard way I am interacting with `transformers` is by feeding the custom datasets to a `Trainer`.
training code:
```python
#!/usr/bin/env python
# -*- coding: utf-8 -*-
import os
import sys
import logging
from typing import Optional
import shutil
import argparse
import itertools
from pathlib import Path
from functools import partial
import torch
from tokenizers import ByteLevelBPETokenizer
from tokenizers.pre_tokenizers import Whitespace
import transformers
from min_data import DNADataset, read_list, chunk_fasta
def arguments():
parser = argparse.ArgumentParser(description="Train GPT-2 model on DNA data.")
parser.add_argument("--partitions", type=Path, nargs=2,
help="Train, validation partition files")
parser.add_argument("--session", type=Path,
help="Training session directory; models are saved here along with other important metadata")
parser.add_argument("--log-to", type=Path,
help="Tensorboard logging root directory; logs will write to log_dir/session_name",
default="tensorboard_logs",
dest='log_dir')
parser.add_argument("--tokenizer",
type=Path,
help="Specify pre-trained tokenizer if it exists",
required=True)
# architecture specs
parser.add_argument("--n-layer", type=int, default=4, help="# of layers")
parser.add_argument("--n-embed", type=int, default=16, help="embedding dim.")
parser.add_argument("--n-inner", type=int, default=1024, help="hidden dim.")
parser.add_argument("--chunk-size", type=int, default=2000, help="max base pair width", dest="chunksize")
parser.add_argument("--lr", default=1e-4, type=float)
# training/logging specs
parser.add_argument("--train-epochs", type=int, default=1)
parser.add_argument("--save-steps", type=int, default=250)
parser.add_argument("--save-up-to", type=int, default=5)
parser.add_argument("--batch-size", type=int, default=8)
parser.add_argument("--lens", nargs=2, type=int, default=(10_000, 2000))
parser.add_argument("--progress-bar", action='store_true', default=False, dest='tqdm')
parser.add_argument("--local_rank", type=int, default=-1)
return parser
def get_training_args(args: argparse.Namespace):
output_dir = args.session / "outputs"
save_steps = args.save_steps
save_limit = args.save_up_to
batch_size = args.batch_size
log_dir = args.log_dir / args.session.name
lr = args.lr
max_steps = args.train_epochs * args.lens[0]
return transformers.TrainingArguments(
output_dir=str(output_dir),
overwrite_output_dir=True,
ddp_find_unused_parameters=False,
per_device_train_batch_size=batch_size,
per_device_eval_batch_size=batch_size,
evaluation_strategy="steps",
learning_rate=lr,
local_rank=args.local_rank,
disable_tqdm=not args.tqdm,
max_steps=max_steps,
eval_steps=save_steps,
save_steps=save_steps,
prediction_loss_only=True,
#logging_dir=str(log_dir),
save_total_limit=save_limit
)
def construct_dataset_from(fasta_list: Path, tokenizer, **kwargs):
dataset = DNADataset( read_list(fasta_list), tokenizer, **kwargs )
return dataset
class DNATrainer(transformers.Trainer): # overwritten because error occur wrt sampler for IterableDataset, still seems necessary in 4.5.0
def _get_eval_sampler(self, eval_dataset: Dataset) -> Optional[torch.utils.data.sampler.Sampler]:
if isinstance(eval_dataset, torch.utils.data.IterableDataset):
return None
if __name__ == '__main__':
args: argparse.Namespace = arguments()
# get tokenizer, gpt2 config, training arguments
tokenizer: tokenizers.ByteLevelBPETokenizer = LOAD_TOKENIZER(args) # standard loading of tokenizer
config: transformers.GPT2Config = GET_GPT2_CONFIG(args) # generates a GPT2Config
training_args: transformers.TrainingArguments = get_training_args(args)
model = transformers.GPT2LMHeadModel(config=config)
model.resize_token_embeddings(len(tokenizer))
model.train()
kwargs = {'pad_token': '<pad>', 'chunksize': args.chunksize}
train_part, val_part = args.partitions # list of files for each dataset to stream from
train_data = construct_dataset_from(train_part, tokenizer, asserted_len=args.lens[0], **kwargs)
val_data = construct_dataset_from(val_part, tokenizer, asserted_len=args.lens[1], **kwargs)
collator = transformers.DataCollatorForLanguageModeling(tokenizer=tokenizer, mlm=False)
trainer = DNATrainer(model=model,
args=training_args,
data_collator=collator,
train_dataset=train_data,
eval_dataset=val_data,
)
trainer.train()
```<|||||>Oh, but you're overriding the sampler part of the `Trainer` code. There is no way distributed evaluation can work then, as it relies on this.<|||||>Aha! Thank you! I'm sure this is the right track, but now I am back to an error in how the `Trainer` chooses a sampler and constructs the `DataLoader`: (this is `transformers` version `4.5.0`)
```
ValueError: DataLoader with IterableDataset: expected unspecified sampler option, but got sampler=<transformers.trainer_pt_utils.SequentialDistributedSampler object at 0x1554e8f1e2b0>
self._maybe_log_save_evaluate(tr_loss, model, trial, epoch)
File "/mnt/home/dberenberg/projects/metagenomics/huggingface_meta/lib/python3.7/site-packages/transformers/trainer.py", line 1265, in _maybe_log_save_evaluate
eval_dataloader = self.get_eval_dataloader(eval_dataset)
File "/mnt/home/dberenberg/projects/metagenomics/huggingface_meta/lib/python3.7/site-packages/transformers/trainer.py", line 612, in get_eval_dataloader
pin_memory=self.args.dataloader_pin_memory,
File "/mnt/home/dberenberg/projects/metagenomics/huggingface_meta/lib/python3.7/site-packages/torch/utils/data/dataloader.py", line 231, in __init__
metrics = self.evaluate()
File "/mnt/home/dberenberg/projects/metagenomics/huggingface_meta/lib/python3.7/site-packages/transformers/trainer.py", line 1754, in evaluate
"sampler option, but got sampler={}".format(sampler))
ValueError: DataLoader with IterableDataset: expected unspecified sampler option, but got sampler=<transformers.trainer_pt_utils.SequentialDistributedSampler object at 0x1554e8039dd8>
eval_dataloader = self.get_eval_dataloader(eval_dataset)
File "/mnt/home/dberenberg/projects/metagenomics/huggingface_meta/lib/python3.7/site-packages/transformers/trainer.py", line 612, in get_eval_dataloader
pin_memory=self.args.dataloader_pin_memory,
File "/mnt/home/dberenberg/projects/metagenomics/huggingface_meta/lib/python3.7/site-packages/torch/utils/data/dataloader.py", line 231, in __init__
"sampler option, but got sampler={}".format(sampler))
ValueError: DataLoader with IterableDataset: expected unspecified sampler option, but got sampler=<transformers.trainer_pt_utils.SequentialDistributedSampler object at 0x1554f2898c88>
```<|||||>Yes you need to forego the inheritance to `IterableDataset` as PyTorch does not let you take a sampler for those, so you will nee to implement a `__getitem__` instead of the `__iter__` for the evaluation.<|||||>Ok, that makes sense. So just to conclude, `transformers.Trainer` won't work in distributed setting with an `torch.utils.data.IterableDataset`, in principal due to the fact that `IterableDataset`s are not amenable to that use case, since it isn't clear how describe a distributed sampling procedure for them. Is that correct? Thanks in advance<|||||>That's correct, especially for distributed evaluation. |
transformers | 11,207 | closed | Replace error by warning when loading an architecture in another | # What does this PR do?
#10586 introduced a breaking change by mistake by removing the possibility to do something like:
```
from transformers import BertGenerationEncoder
model = BertGenerationEncoder.from_pretrained("bert-large-uncased", bos_token_id=101, eos_token_id=102)
```
which is perfectly acceptable and [documented](https://huggingface.co/transformers/model_doc/bertgeneration.html?highlight=bertgeneration)
This PR reverts the hard error and replaces it with a warning.
Fixes #11184 | 04-12-2021 15:01:25 | 04-12-2021 15:01:25 | Would you consider this solution instead?
```
--- a/src/transformers/models/bert_generation/configuration_bert_generation.py
+++ b/src/transformers/models/bert_generation/configuration_bert_generation.py
@@ -78,7 +78,7 @@ class BertGenerationConfig(PretrainedConfig):
>>> # Accessing the model configuration
>>> configuration = model.config
"""
- model_type = "bert-generation"
+ model_type = "bert"
def __init__(
self,
```
So it's adjusted to say that it's actually a `bert`-type of model that it works with. And if some model can work with 5 types or any, it could list those 5 types or have `any`.
perhaps we need to split this field - one of them should say the type of model it works with and keep this one that is just a unique identifier of the sub-type of the model.
So e.g. `model.config.works_with = "(type1|...|typeN|any)"`
<|||||>Then the all the calls to `from_pretrained` with models that have a `model_type` of `bert-generation` will fail (and there are several checkpoints on the hub with that model type). I also don't think this is the only instance where it's possible to load some model's weights in another model, it's just the first one that is reported.<|||||>see my edits - perhaps we need a specific config entry that lists all the model types the model can work with?
So that field doesn't control too many functions?<|||||>But why would we actively prevent a user to load some weights in another model if that doesn't cause any error? Of course they would not work as is, but perhaps it could be a strategy of transfer learning.<|||||>Load the model weights with the model class it was pre-trained with, then do whatever you want with those weights - copy them into the new model, etc. Nothing stops the user here from using those weights. i.e. unless I'm missing something here we aren't preventing anything.
I just fail to see how loading weights in a model class that is totally different can be of any direct use, even for transfer learning. If you can see such ways do you have an example?
That's said if you strongly feel that the enforcement of the match is not logical, then I'm totally fine with the proposed change.<|||||>I don't have strong opinions but the change made was breaking for existing use-cases. I have no way to know which other use cases have been broken by it too, so leaving the warning makes the most sense to me to avoid having to do a new patch release in ten days if a user comes with another case of `XxxModel.from_pretrained(yyy_model)` not working anymore.
Let's see what @LysandreJik thinks!
<|||||>I agree wrt breaking changes. How far are we from v5.0? We could postpone the enforcement until then and use your proposed change until then.
But functionality-wise do you agree that the model type match enforcement would be useful and that it doesn't prevent the user from using the weights from a mismatched model?<|||||>The issue with raising an error is that I'm nearly 100% sure we're forgetting some use-cases. Bert Generation is one example, but I really wouldn't be surprised that there exist other niche use-cases and that we're involuntarily blocking those. Printing a warning instead seems safer, and while it's not as visible as an error, a user that doesn't obtain whatever performance they're looking for will look at the warnings and should still understand where the issue is coming from.
> How far are we from v5.0?
It isn't on the horizon yet. The breaking changes we've wanted to make until now are mostly cosmetic, so there's nothing pushing us to release a breaking release as of now.
LGTM, thanks for taking care of this @sgugger.<|||||>That's all said - we ideally should start stirring users towards loading models with the exact classes that created them, and once loaded do whatever is wanted (copy weights, etc.). What is happening now in the edge cases is a misuse of not having a strict verification - it kind of works, so "why not" seems to be the way. If this is done, e.g. by changing the documentation, this issue will just disappear and we can reinstate the assert-check.
I was just thinking about this whole issue of warnings and how they don't quite work. A warning sign on the road is not surrounded by 20 other signs - it stands alone and acts as a warning - loud and clear. A warning in the logs is like a vendor in the bazaar shouting how good his wares are - nobody can hear unless you're right in front of that vendor.
Just 2 days ago my [PR](https://github.com/huggingface/transformers/pull/11168) trying to help with invalid warning, ended up introducing a bug which I didn't see because it got covered up by yet another warning. The first warning was from incomplete design. And the second warning was covering a real bug.
Warnings should be a last resort and usually indicate that some aspect of the software design isn't fully thought out. IMHO, of course. |
transformers | 11,206 | closed | Sagemaker test docs update for framework upgrade | # What does this PR do?
This PR resolves the last todo in the sagemaker test `Readme.md` and increase a test metric to stable the test for `model_parallelism`. | 04-12-2021 14:59:09 | 04-12-2021 14:59:09 | |
transformers | 11,205 | closed | Rework examples/ to overwrite cache_dir for datasets too. | # 🚀 Feature request
Currently, you can pass [cache_dir](https://github.com/huggingface/transformers/blob/ef102c48865d70ff354b8ba1488d3fa8bfc116d8/examples/seq2seq/run_summarization.py#L79) into the `examples/` script to overwrite the `cache_dir` of [model config and tokenizers](https://github.com/huggingface/transformers/blob/ef102c48865d70ff354b8ba1488d3fa8bfc116d8/examples/seq2seq/run_summarization.py#L336).
What would be the best way to adjust this to be able to use the `cache_dir` parameter for `datasets` [load_dataset](https://github.com/huggingface/transformers/blob/ef102c48865d70ff354b8ba1488d3fa8bfc116d8/examples/seq2seq/run_summarization.py#L313) method and `transformers`
## Motivation
When running training on Amazon SageMaker the cache_dir (`~/.cache/`) is not mounted to an EBS and cannot be increased. Therefore we need an option to point the `cache_dir` to an EBS backed directory for using the `examples/` scripts with large datasets.
| 04-12-2021 13:34:24 | 04-12-2021 13:34:24 | Well someone would need to go other all the examples and add it as an argument in those calls to `load_dataset`.<|||||>Would you still keep it in
```python
@dataclass
class ModelArguments:
```
I can adjust the examples in the near future. I just wanted to align on we can adjust this. <|||||>We can switch it but to be honest it's more an internal class to the script than something of real significance so we don't really care. |
transformers | 11,204 | closed | ModuleNotFoundError: No module named 'transformers.modeling_camembert' | ## Environment info
<!-- You can run the command `transformers-cli env` and copy-and-paste its output below.
Don't forget to fill out the missing fields in that output! -->
`transformers` version: 4.2.2
-Python version: 3.7.9
- PyTorch version (GPU?):1.7.1
- Tensorflow version (GPU?) : 2.4.1
- Using GPU in script?:
- Using distributed or parallel set-up in script?:
### Who can help
<!-- Your issue will be replied to more quickly if you can figure out the right person to tag with @
If you know how to use git blame, that is the easiest way, otherwise, here is a rough guide of **who to tag**.
Please tag fewer than 3 people.
Models:
- albert, bert, xlm: @LysandreJik
- blenderbot, bart, marian, pegasus, encoderdecoder, t5: @patrickvonplaten, @patil-suraj
- longformer, reformer, transfoxl, xlnet: @patrickvonplaten
- fsmt: @stas00
- funnel: @sgugger
- gpt2: @patrickvonplaten, @LysandreJik
- rag: @patrickvonplaten, @lhoestq
- tensorflow: @LysandreJik
Library:
- benchmarks: @patrickvonplaten
- deepspeed: @stas00
- ray/raytune: @richardliaw, @amogkam
- text generation: @patrickvonplaten
- tokenizers: @LysandreJik
- trainer: @sgugger
- pipelines: @LysandreJik
Documentation: @sgugger
Model hub:
- for issues with a model report at https://discuss.huggingface.co/ and tag the model's creator.
HF projects:
- datasets: [different repo](https://github.com/huggingface/datasets)
- rust tokenizers: [different repo](https://github.com/huggingface/tokenizers)
Examples:
- maintained examples (not research project or legacy): @sgugger, @patil-suraj
- research_projects/bert-loses-patience: @JetRunner
- research_projects/distillation: @VictorSanh
-->
## Information
Model I am using (Bert, XLNet ...):
The problem arises when using:
* [ ] the official example scripts: (give details below)
* [ ] my own modified scripts: (give details below)
The tasks I am working on is:
* [ ] an official GLUE/SQUaD task: (give the name)
* [ ] my own task or dataset: (give details below)
## To reproduce
Steps to reproduce the behavior:
1.
2.
3.
<!-- If you have code snippets, error messages, stack traces please provide them here as well.
Important! Use code tags to correctly format your code. See https://help.github.com/en/github/writing-on-github/creating-and-highlighting-code-blocks#syntax-highlighting
Do not use screenshots, as they are hard to read and (more importantly) don't allow others to copy-and-paste your code.-->
## Expected behavior
<!-- A clear and concise description of what you would expect to happen. -->
| 04-12-2021 12:20:03 | 04-12-2021 12:20:03 | Which version of Transformers are you using?
If you're using one of the latest versions of Transformers, `modeling_camembert.py` will be located at `transformers.models.camembert.modeling_camembert.py`. <|||||>This issue has been automatically marked as stale because it has not had recent activity. If you think this still needs to be addressed please comment on this thread.
Please note that issues that do not follow the [contributing guidelines](https://github.com/huggingface/transformers/blob/master/CONTRIBUTING.md) are likely to be ignored. |
transformers | 11,203 | closed | How to extract the specific output using the method "encoder_output[0]" | Dear Transformers Team
Thank you very much for Transformers which provides me solutions for relation extraction problems. I have a question. My input is like " [E1]Jack[/E2] was born in [E2]London[/E2]". I want to only extract the sequence output of "[E1] and "[E2]" with the method of "encoder_output[0]". And then concat the [CLS] output and sequence output of "[E1] and "[E2]". Could you help me solve the problem? This is a question that I took more than a month to thank aout the solution but I am not able to solve it. Thank you very much for your help. Thank you! | 04-12-2021 12:17:40 | 04-12-2021 12:17:40 | Hello, thanks for opening an issue! We try to keep the github issues for bugs/feature requests.
Could you ask your question on the [forum](https://discusss.huggingface.co) instead?
Thanks! |
transformers | 11,202 | closed | Fix TFBert embedding tf variables with the same name - Fixes problems with checkpoints under tf.distribute.Strategy | # What does this PR do?
Removes usage of tf.name_scope() in BERT like models and replaces it with layers.
Ideally all erroneous use of tf.name_scope() should be fixed across all models, but this PR will at least make the TFBert like models work.
<!-- Remove if not applicable -->
Fixes # (issue)
#11169
## Models:
- bert: @LysandreJik
| 04-12-2021 12:03:42 | 04-12-2021 12:03:42 | Hi! I'm the new Tensorflow maintainer at Hugging Face. Your PR looks good, and you're right that unique weight names is a better strategy than relying on `name_scope`. Now that you've raised the issue, doing a check for that across the whole codebase is definitely on my to-do list.
The main issue for us is backward compatibility and ensuring that cross-loading weights from PyTorch checkpoints still works as expected after the change. Can you leave this with me for a few days until I get a chance to review that properly? Hopefully there are no issues, but I don't want to be the guy who breaks the whole codebase in his first week, lol.<|||||>> Hi! I'm the new Tensorflow maintainer at Hugging Face. Your PR looks good, and you're right that unique weight names is a better strategy than relying on `name_scope`. Now that you've raised the issue, doing a check for that across the whole codebase is definitely on my to-do list.
>
> The main issue for us is backward compatibility and ensuring that cross-loading weights from PyTorch checkpoints still works as expected after the change. Can you leave this with me for a few days until I get a chance to review that properly? Hopefully there are no issues, but I don't want to be the guy who breaks the whole codebase in his first week, lol.
Hi @Rocketknight1 that sounds great! Thx for taking a look at this :) Just to clarify a bit: It is my current understanding that tf.name_scope() makes absolutely no difference when it comes to variables in tf 2.x and is not comparable to the old tf.compat.v1.variable_scope. There is, to the best of my knowledge, no point in adding these name scopes for variables in tf 2.x.
They might still be useful for grouping certain ops in the graph under logical names, but TensorFlow 2.x generally relies on the object hierarchy of tf.Module subclass objects rather than global variable names / name spaces. See: https://www.tensorflow.org/guide/migrate#2_use_python_objects_to_track_variables_and_losses<|||||>I checked the test logs and we have several failing tests involving loading model weights, so it seems like there might be backward compatibility issues with this change, even though you're totally right about the `name_scope()` issues. So unfortunately, I probably can't merge this PR as is.
I'd like to resolve the underlying problem, though - if you want to try to figure out the compatibility issues yourself you can, or if not (which would be completely understandable, lol) I'll try to take a look when I get a chance.<|||||>@Rocketknight1 Hey, I think I have pretty much fixed the issues. But all of this arcane template usage is giving me a headache, any ideas where/how I might be make the final test pass?
(Model templates runner / run_tests_templates)
The error message form the test suggests running "make fix-copies" but that does not seem to do anything in the current state.<|||||>Hey! Don't worry too much about the template issues, we can fix those up for you before we merge it.
This is something that will affect a few teams, though - we're currently in the process of making sure everyone knows about it and they don't think it'll catastrophically break anything, but we might have to make some changes, which will probably be Monday because it's 7pm at the French office on a Friday right now!
Thanks again for the work you put into this and for identifying the problem, though - I'll try to keep you updated as we figure out if we can use your solution, or which tweaks we'll have to make to make it fit in with our other projects.<|||||>> Hey! Don't worry too much about the template issues, we can fix those up for you before we merge it.
>
> This is something that will affect a few teams, though - we're currently in the process of making sure everyone knows about it and they don't think it'll catastrophically break anything, but we might have to make some changes, which will probably be Monday because it's 7pm at the French office on a Friday right now!
>
> Thanks again for the work you put into this and for identifying the problem, though - I'll try to keep you updated as we figure out if we can use your solution, or which tweaks we'll have to make to make it fit in with our other projects.
Have a great weekend :) <|||||>So this seems to be taking quite a while. Is there anything I can do to help and/or expedite this process? Thx in advance. <|||||>I'm sorry about the delay! I've checked with everyone and we think it's okay, but there's an issue with ensuring this code stays in sync with the other BERT-based models. It's going slowly because I only started a couple of weeks ago, so I'm very paranoid about breaking things, and I'm double-checking things as I go.<|||||>> I'm sorry about the delay! I've checked with everyone and we think it's okay, but there's an issue with ensuring this code stays in sync with the other BERT-based models. It's going slowly because I only started a couple of weeks ago, so I'm very paranoid about breaking things, and I'm double-checking things as I go.
No worries :) You should be moving fast and breaking things. That's what you have tests for ;)
Also why on earth are you guys doing all of this templating in the first place? It seems like a total maintenance nightmare and a textbook example of what not to do? You could cleanup and remove like 80% of your code for your MLMs with some standard object oriented programming? Or is there something I'm not seeing here?<|||||>It's a good question! The underlying idea is that we want code to be self-contained and easy to separate from the rest of the library, so that users can work on the model they care about in isolation without needing to understand our whole hierarchy of abstractions and imports.
It's also helpful because we care a lot about supporting a variety of models that were trained outside of Hugging Face, which often involves reproducing their particular quirks rather than just importing the same single function in every case.<|||||>Any updates? I would like to contribute in any way I can :) Other people in my organisation are starting to use HugginFace transformers at the same scale as me and will likely face the same issues as I did.
Is there a branch I can follow?<|||||>This issue has been automatically marked as stale because it has not had recent activity. If you think this still needs to be addressed please comment on this thread.
Please note that issues that do not follow the [contributing guidelines](https://github.com/huggingface/transformers/blob/master/CONTRIBUTING.md) are likely to be ignored.<|||||>Was this ever fixed?<|||||>No, but we're seeing other issues being caused by the same underlying problem, such as #12245 . I'm very aware of it, but finding a way to fix it without breaking changes to backward compatibility is difficult! It might be something that'll have to wait until a major release when we can break a lot of things at once. |
transformers | 11,201 | closed | Issue: List index out of range when using Seq2SeqTrainer | ## Environment info
- `transformers` version: v4.5.0
- Platform: Google Colab
- Python version: Python 3.7
- Using GPU in script? Yes
## Who can help
- tokenizers: @LysandreJik
- trainer: @sgugger
## Information
I am using a pre-trained Bert in order to train an abstractive summarization model. The problem arises when using my own colab notebook. The error arises during validation, sometimes sooner or later. The code is very similar to: https://colab.research.google.com/drive/1WIk2bxglElfZewOHboPFNj8H44_VAyKE?usp=sharing#scrollTo=Gw3IZYrfKl4Z
## To reproduce
Here are a few code snippiets to reproduce this behavior:
```ruby
import transformers as ft
training_args = ft.Seq2SeqTrainingArguments(
predict_with_generate=True,
evaluation_strategy="steps",
per_device_train_batch_size=batch_size,
per_device_eval_batch_size=batch_size,
output_dir=path_output,
warmup_steps=1000,
save_steps=2000,
logging_steps=100,
eval_steps=2000,
save_total_limit=1,
fp16=True
)
trainer = ft.Seq2SeqTrainer(
model=tf2tf,
args=training_args,
compute_metrics=compute_metrics,
train_dataset=train_data,
eval_dataset=val_data,
tokenizer=tokenizer
)
trainer.train()
```
Error message:
```ruby
---------------------------------------------------------------------------
IndexError Traceback (most recent call last)
<ipython-input-23-38bced663988> in <module>()
9 )
10
---> 11 trainer.train()
12 frames
/usr/local/lib/python3.7/dist-packages/datasets/arrow_dataset.py in <genexpr>(.0)
878 @staticmethod
879 def _unnest(py_dict):
--> 880 return dict((key, array[0]) for key, array in py_dict.items())
881
882 @staticmethod
IndexError: list index out of range
```
## Expected behavior
The training should go through without errors, as in previous versions. I would be happy if someone knows what I need to adjust in the code to make it run. Thanks :) | 04-12-2021 11:29:47 | 04-12-2021 11:29:47 | The error seems to come from your dataset, and you did not share the code you used to create and process it, so there is little we can do to help.<|||||>Thanks for the quick reply. Here is the code I used to prepare the data:
```
train_data = datasets.load_dataset("cnn_dailymail", "3.0.0", split="train")
val_data = datasets.load_dataset("cnn_dailymail", "3.0.0", split="validation[:10%]")
test_data = datasets.load_dataset("cnn_dailymail", "3.0.0", split="test[:5%]")
encoder_max_length = 512
decoder_max_length = 128
batch_size = 4 # 16
def process_data_to_model_inputs(batch):
inputs = tokenizer(batch["article"], padding="max_length", truncation=True, max_length=encoder_max_length)
outputs = tokenizer(batch["highlights"], padding="max_length", truncation=True, max_length=decoder_max_length)
batch["input_ids"] = inputs.input_ids
batch["attention_mask"] = inputs.attention_mask
batch["decoder_input_ids"] = outputs.input_ids
batch["decoder_attention_mask"] = outputs.attention_mask
batch["labels"] = outputs.input_ids.copy()
batch["labels"] = [[-100 if token == tokenizer.pad_token_id else token for token in labels] for labels in batch["labels"]]
return batch
train_data = train_data.shuffle()
train_data = train_data.map(
process_data_to_model_inputs,
batched=True,
batch_size=batch_size,
remove_columns=["article", "highlights"] # "id"
)
train_data.set_format(
type="torch",
columns=["input_ids",
"attention_mask",
"decoder_input_ids",
"decoder_attention_mask",
"labels"]
)
val_data = val_data.shuffle()
val_data = val_data.map(
process_data_to_model_inputs,
batched=True,
remove_columns=["article", "highlights"] # id
)
val_data.set_format(
type="torch",
columns=["input_ids",
"attention_mask",
"decoder_input_ids",
"decoder_attention_mask",
"labels"]
)
```
Then I loaded the pre-trained models and set parameters as in the original notebook. If more information is needed, please let me know.<|||||>The evaluation runs without any problem on my side, with the code you provided and the rest from the notebook you mentioned. Are you sure you have the latest version of the `Datasets` library installed?
Otherwise, could you share a colab or a full script reproducing the error?<|||||>Thank you! An update to the latest version of `Datasets` solved my problem. |
transformers | 11,200 | closed | Issue: Adding new tokens to bert tokenizer in QA | **WARNING**: This issue is a replica of this other [issue](https://github.com/huggingface/notebooks/issues/21) open by me, I ask you sorry if I have open it in the wrong place.
Hello Huggingface's team (@sgugger , @joeddav, @LysandreJik)
I have a problem with this code base
notebooks/examples/question_answering.ipynb - [link](https://github.com/huggingface/notebooks/blob/master/examples/question_answering.ipynb)
`
ENV: Google Colab -
transformers Version: 4.5.0;
datasets Version: 1.5.0;
torch Version: 1.8.1+cu101;
`
I am trying to add some domain tokens in the bert-base-cased tokenizer
```python3
model_checkpoint = 'bert-base-cased'
tokenizer = AutoTokenizer.from_pretrained(model_checkpoint)
list_of_domain_tokens = ["token1", "token2", "token3"]
tokenizer.add_tokens(list_of_domain_tokens)
...
...
model = AutoModelForQuestionAnswering.from_pretrained(model_checkpoint)
print(model.device) # cpu
model.resize_token_embeddings(len(tokenizer))
trainer = Trainer(...)
```
Then during the trainer.fit() call it report the attached error.
Can you please tell me where I'm wrong?
The tokenizer output is the usual bert inputs expressed in the form of List[List[int]] eg inputs_ids and attention_mask.
So I can't figure out where the problem is with the device
`Input, output and indices must be on the current device`
Kind Regards,
Andrea | 04-12-2021 10:23:12 | 04-12-2021 10:23:12 | I am unable to reproduce: the notebook with your added code works smoothly on my side.<|||||>Thank you @sgugger.
I want ask you sorry, I can't figure out on what's going on my side.
Now I have cloned again the notebook and the example works.
In the next days I want test it again and I will tell you more about it.
Thank you again for your help
Kind Regards,
Andrea<|||||>Hi @sgugger,
I worked on the notebook and I found the problem.
I have not yet had the opportunity to test it with the original squad dataset but this happens to me both on colab and on my machine.
I warn you it seems an absurd and paradoxical situation, moreover I in no way manage the device.
I can provide you with a video while running the notebook.
As you can see from the screenshot I am forced to keep two versions of the training args, one original from the notebook and one customized by me.
If I perform these operations I get the error
1) I instantiate my training args
2) I instantiate the Trainer
3) I run trainer.fit
I get the error `Input, output and indices must be on the current device`
To solve I have to:
Instantiate the original training args of the notebook, instantiate the trainer, perform the fit to check that it has started and then do it all over again with the training args I customized.
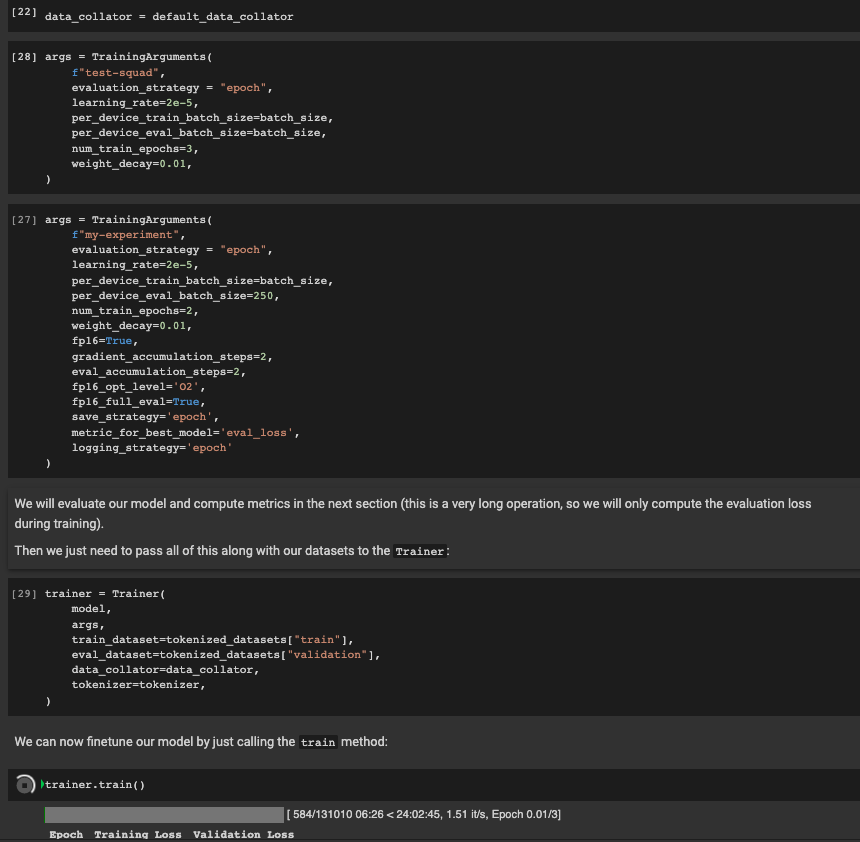
Kind regards,
Andrea<|||||>Hi @sgugger,
I can confirm, the same bug happens in the original notebook with this TrainingArguments (I have tested with squad v2), the temporary fix is to start the train with the original one, stop it and then run with the customized args. <|||||>It looks like a bug in colab (from the screenshots I assume that is what you are using for training?) since I didn't get any error on my side by executing this as a notebook.<|||||>Hi @sgugger
Do you have tested the notebook replacing the trainer args with the following?
```python3
args = TrainingArguments(
f"my-experiment",
evaluation_strategy = "epoch",
learning_rate=2e-5,
per_device_train_batch_size=batch_size,
per_device_eval_batch_size=250,
num_train_epochs=2,
weight_decay=0.01,
fp16=True,
gradient_accumulation_steps=2,
eval_accumulation_steps=2,
fp16_opt_level='O2',
fp16_full_eval=True,
save_strategy='epoch',
metric_for_best_model='eval_loss',
logging_strategy='epoch'
)
```
Because I encountered the same issue on my machine.
Can you kindly test with it? Please
To test it: remove the old trainer args use the attached one and run the trainer.fit
Kind regards,
Andrea<|||||>Ah you're right, I must have made a mistake. This comes from the option `fp16_full_eval=True`.
@stas00 I'm not sure what the best place is for fixing this but if someone uses `fp16_full_eval=True` with training, the model is never sent to the proper device and training fails.<|||||>But there is no `do_train` in the args at https://github.com/huggingface/transformers/issues/11200#issuecomment-822566973
The logic is very explicit to not place on the device only for non-train when`fp16_full_eval=True` is used:
```
if (
self.is_model_parallel
or (args.deepspeed and args.do_train)
or (args.fp16_full_eval and not args.do_train)
or (self.sharded_ddp in [ShardedDDPOption.ZERO_DP_2, ShardedDDPOption.ZERO_DP_3])
):
self.place_model_on_device = False
```
You need to add `do_train=True` to your `TrainingArguments`, otherwise it defaults to eval only because you have `evaluation_strategy` set.
<|||||>Hi @stas00 & @sgugger,
> You need to add `do_train=True` to your `TrainingArguments`, otherwise it defaults to eval only because you have `evaluation_strategy` set.
Ok so `do_train=True` is also compatible with `fp16_full_eval=True`?
My objective is to train the model and pick the best one at the lowest point of eval loss.
Regarding the notebook, can I use the same Trainer object for fit and predict? Because these Booleans are never set in the notebook. I mean when I am doing trainer.predict() is obvious for the trainer to set model.eval() and torch.no_grad()?
Thank you both,
Andrea<|||||>> Ok so do_train=True is also compatible with fp16_full_eval=True?
Why did you think it shouldn't be compatible?
The only reason there is a special case for non-training is to avoid placing the full model on device before it was `half()`'ed - as it might not fit in its full size, but might fit in `half()`.
> Regarding the notebook, can I use the same Trainer object for fit and predict? Because these Booleans are never set in the notebook. I mean when I am doing trainer.predict() is obvious for the trainer to set model.eval() and torch.no_grad()?
Of course. It was designed for you to pass all the init args at once and then you can call all its functions.
<|||||>@stas00 Ok clear, I have just checked and the trainer works perfectly.
What do you think to place a warning to alert the user when call trainer.fit having the trainer.do_train = False?
Because it's clear in the point of view of performance as you said but the documentation don't bring out this things for this reason I have open then issue.
Kind regards,
Andrea<|||||>Oh, I see. Until recently `do_train` was sort of optional when using user's custom code and what you're saying we need to then require `do_train=True` if `trainer.train()` is called. But we started relying on `do_train` for more than just knowing to call `train()` from scripts. This makes sense to me.
@sgugger, do you agree if we add this?
```
def train(...):
[...]
if not self.args.do_train:
raise ValueError("To use `train` please make sure you set `do_train=True` when instantiating the Trainer object")
```
<|||||>I would rather avoid adding this, as users have been used to not have to set that argument to True when not using example scripts. Can we just add the proper line in `train` to put the model on the device if it was not done already?
(Sorry I didn't catch you were using `do_train` in the PR you added that test, I should have caught it and commented there.)<|||||>We will probably have to rethink the design then, since it's not a simple "put on device if it wasn't already" - there are multiple cases when it shouldn't happen. For now added a hardcoded workaround: https://github.com/huggingface/transformers/pull/11322
|
transformers | 11,199 | closed | Add examples/bert-loses-patience who can help | # What does this PR do?
hello
<!--
Congratulations! You've made it this far! You're not quite done yet though.
Once merged, your PR is going to appear in the release notes with the title you set, so make sure it's a great title that fully reflects the extent of your awesome contribution.
Then, please replace this with a description of the change and which issue is fixed (if applicable). Please also include relevant motivation and context. List any dependencies (if any) that are required for this change.
Once you're done, someone will review your PR shortly (see the section "Who can review?" below to tag some potential reviewers). They may suggest changes to make the code even better. If no one reviewed your PR after a week has passed, don't hesitate to post a new comment @-mentioning the same persons---sometimes notifications get lost.
-->
<!-- Remove if not applicable -->
Fixes # (issue)
## Before submitting
- [ ] This PR fixes a typo or improves the docs (you can dismiss the other checks if that's the case).
- [ ] Did you read the [contributor guideline](https://github.com/huggingface/transformers/blob/master/CONTRIBUTING.md#start-contributing-pull-requests),
Pull Request section?
- [ ] Was this discussed/approved via a Github issue or the [forum](https://discuss.huggingface.co/)? Please add a link
to it if that's the case.
- [ ] Did you make sure to update the documentation with your changes? Here are the
[documentation guidelines](https://github.com/huggingface/transformers/tree/master/docs), and
[here are tips on formatting docstrings](https://github.com/huggingface/transformers/tree/master/docs#writing-source-documentation).
- [ ] Did you write any new necessary tests?
## Who can review?
Anyone in the community is free to review the PR once the tests have passed. Feel free to tag
members/contributors which may be interested in your PR.
<!-- Your PR will be replied to more quickly if you can figure out the right person to tag with @
If you know how to use git blame, that is the easiest way, otherwise, here is a rough guide of **who to tag**.
Please tag fewer than 3 people.
Models:
- albert, bert, xlm: @LysandreJik
- blenderbot, bart, marian, pegasus, encoderdecoder, t5: @patrickvonplaten, @patil-suraj
- longformer, reformer, transfoxl, xlnet: @patrickvonplaten
- fsmt: @stas00
- funnel: @sgugger
- gpt2: @patrickvonplaten, @LysandreJik
- rag: @patrickvonplaten, @lhoestq
- tensorflow: @LysandreJik
Library:
- benchmarks: @patrickvonplaten
- deepspeed: @stas00
- ray/raytune: @richardliaw, @amogkam
- text generation: @patrickvonplaten
- tokenizers: @n1t0, @LysandreJik
- trainer: @sgugger
- pipelines: @LysandreJik
Documentation: @sgugger
HF projects:
- datasets: [different repo](https://github.com/huggingface/datasets)
- rust tokenizers: [different repo](https://github.com/huggingface/tokenizers)
Examples:
- maintained examples (not research project or legacy): @sgugger, @patil-suraj
- research_projects/bert-loses-patience: @JetRunner
- research_projects/distillation: @VictorSanh
-->
| 04-12-2021 10:02:00 | 04-12-2021 10:02:00 | This issue has been automatically marked as stale because it has not had recent activity. If you think this still needs to be addressed please comment on this thread.
Please note that issues that do not follow the [contributing guidelines](https://github.com/huggingface/transformers/blob/master/CONTRIBUTING.md) are likely to be ignored. |
transformers | 11,198 | closed | trainer.evaluate() expects batch_size to match target batch_size | @LysandreJik
@sgugger
## Environment info
<!-- You can run the command `transformers-cli env` and copy-and-paste its output below.
Don't forget to fill out the missing fields in that output! -->
- `transformers` version: 4.0.1
- Platform: Windows/Ubuntu 18.04.3
- Python version: 3.7.6
- PyTorch version (GPU?): 1.7.1 CPU
- Using distributed or parallel set-up in script?: Nope
## Information
Model I am using ('deepset/gbert-base'):
The problem arises when using:
* [ ] the official example scripts: (give details below)
* [x] my own modified scripts: (give details below)
The problem I get is the following when I call the trainer.evaluate() function:
```Bash
Traceback (most recent call last):
File "fine_tune_bert.py", line 174, in <module>
trainer.evaluate()
File "/home/rouven/anaconda3/lib/python3.7/site-packages/transformers/trainer.py", line 1259, in evaluate
ignore_keys=ignore_keys,
File "/home/rouven/anaconda3/lib/python3.7/site-packages/transformers/trainer.py", line 1363, in prediction_loop
loss, logits, labels = self.prediction_step(model, inputs, prediction_loss_only, ignore_keys=ignore_keys)
File "/home/rouven/anaconda3/lib/python3.7/site-packages/transformers/trainer.py", line 1469, in prediction_step
outputs = model(**inputs)
File "/home/rouven/anaconda3/lib/python3.7/site-packages/torch/nn/modules/module.py", line 727, in _call_impl
result = self.forward(*input, **kwargs)
File "/home/rouven/anaconda3/lib/python3.7/site-packages/transformers/models/bert/modeling_bert.py", line 1363, in forward
loss = loss_fct(logits.view(-1, self.num_labels), labels.view(-1))
File "/home/rouven/anaconda3/lib/python3.7/site-packages/torch/nn/modules/module.py", line 727, in _call_impl
result = self.forward(*input, **kwargs)
File "/home/rouven/anaconda3/lib/python3.7/site-packages/torch/nn/modules/loss.py", line 962, in forward
ignore_index=self.ignore_index, reduction=self.reduction)
File "/home/rouven/anaconda3/lib/python3.7/site-packages/torch/nn/functional.py", line 2468, in cross_entropy
return nll_loss(log_softmax(input, 1), target, weight, None, ignore_index, None, reduction)
File "/home/rouven/anaconda3/lib/python3.7/site-packages/torch/nn/functional.py", line 2262, in nll_loss
.format(input.size(0), target.size(0)))
ValueError: Expected input batch_size (18) to match target batch_size (6).
```
I'm doing a multiclass classification problem. With six classes, that is why i'm replacing the classifyer here.
```Python
model = BertForSequenceClassification.from_pretrained('deepset/gbert-base', proxies=charite_proxy)
model.classifier = torch.nn.Linear(768, 6)
```
I had the same problem with the trainer.train() call before overwriting the compute_loss function. Which looks like this now:
```Python
class MultilabelTrainer(Trainer):
def compute_loss(self, model, inputs, return_outputs=False):
labels = inputs.pop("labels")
outputs = model(**inputs)
logits = outputs[0]
global weigths
global lambda_reg
reg_lambda = lambda_reg
weight = weights
criterior = CrossEntropyLoss(weight=weight.to(device))
loss = criterior(logits, labels)
loss += calculate_l2_reg(model, reg_lambda)
return (loss, outputs) if return_outputs else loss
```
Further my training setup looks like this:
```Python
EPOCHS = 3
LEARNING_RATE = 2e-5
BATCH_SIZE = 32
training_args = TrainingArguments(
output_dir='./results', # output directory
num_train_epochs=EPOCHS, # total # of training epochs
per_device_train_batch_size=BATCH_SIZE, # batch size per device during training
per_device_eval_batch_size=BATCH_SIZE, # batch size for evaluation
warmup_steps=500, # number of warmup steps for learning rate scheduler
weight_decay=0.01, # strength of weight decay
logging_dir='./logs', # directory for storing logs
no_cuda = True,
seed = seed,
learning_rate = LEARNING_RATE
)
model.train()
trainer = MultilabelTrainer(
model=model, # the instantiated 🤗 Transformers model to be trained
args=training_args, # training arguments, defined above
train_dataset=dataset, # training dataset
eval_dataset=test_dataset # evaluation dataset
)
trainer.train()
trainer.evaluate()
```
The tasks I am working on is:
* [ ] an official GLUE/SQUaD task: (give the name)
* [x] my own task or dataset: (give details below)
I'm not quite sure what you would need to know, but it is a dataset consisting of ~60k examples with 1 of 6 possible labels.
## Expected behavior
The expected behavior would be to get the evaluation metrics from the trainer.evaluate() call.
Hope you can help me.
Cheers
Rouven
| 04-12-2021 09:50:06 | 04-12-2021 09:50:06 | You will need to update to the last version of Transformers (I'm seeing 4.0.1 in your report), we fixed this issue so the evaluation loop uses the `compute_loss` function too.<|||||>> You will need to update to the last version of Transformers (I'm seeing 4.0.1 in your report), we fixed this issue so the evaluation loop uses the `compute_loss` function too.
Thanks sgugger!
I also found this out after taking a dive into your code base. I overwrote the prediction_step function in my case, since i dont know if the rest of my code supports transformers 4.5.0.
You can close the issue now! :) |
transformers | 11,197 | closed | [T5] Add 3D attention mask to T5 model (2) (#9643) | # What does this PR do?
It allows for 3D attention mask in T5 model (modeling_t5.py) with an accompanying test.
Fixes #9643
This is a clean version for an earlier PR #10903.
This is a solution for allowing the 3D attention mask in the T5 model by making it broadcastable. It is based on what is used in BERT.
<!--
Congratulations! You've made it this far! You're not quite done yet though.
Once merged, your PR is going to appear in the release notes with the title you set, so make sure it's a great title that fully reflects the extent of your awesome contribution.
Then, please replace this with a description of the change and which issue is fixed (if applicable). Please also include relevant motivation and context. List any dependencies (if any) that are required for this change.
Once you're done, someone will review your PR shortly (see the section "Who can review?" below to tag some potential reviewers). They may suggest changes to make the code even better. If no one reviewed your PR after a week has passed, don't hesitate to post a new comment @-mentioning the same persons---sometimes notifications get lost.
-->
<!-- Remove if not applicable -->
## Who can review?
@patrickvonplaten
Anyone in the community is free to review the PR once the tests have passed. Feel free to tag
members/contributors which may be interested in your PR.
<!-- Your PR will be replied to more quickly if you can figure out the right person to tag with @
If you know how to use git blame, that is the easiest way, otherwise, here is a rough guide of **who to tag**.
Please tag fewer than 3 people.
Models:
- albert, bert, xlm: @LysandreJik
- blenderbot, bart, marian, pegasus, encoderdecoder, t5: @patrickvonplaten, @patil-suraj
- longformer, reformer, transfoxl, xlnet: @patrickvonplaten
- fsmt: @stas00
- funnel: @sgugger
- gpt2: @patrickvonplaten, @LysandreJik
- rag: @patrickvonplaten, @lhoestq
- tensorflow: @LysandreJik
Library:
- benchmarks: @patrickvonplaten
- deepspeed: @stas00
- ray/raytune: @richardliaw, @amogkam
- text generation: @patrickvonplaten
- tokenizers: @n1t0, @LysandreJik
- trainer: @sgugger
- pipelines: @LysandreJik
Documentation: @sgugger
HF projects:
- datasets: [different repo](https://github.com/huggingface/datasets)
- rust tokenizers: [different repo](https://github.com/huggingface/tokenizers)
Examples:
- maintained examples (not research project or legacy): @sgugger, @patil-suraj
- research_projects/bert-loses-patience: @JetRunner
- research_projects/distillation: @VictorSanh
-->
| 04-12-2021 07:45:59 | 04-12-2021 07:45:59 | This issue has been automatically marked as stale because it has not had recent activity. If you think this still needs to be addressed please comment on this thread.
Please note that issues that do not follow the [contributing guidelines](https://github.com/huggingface/transformers/blob/master/CONTRIBUTING.md) are likely to be ignored.<|||||>Great job @lexhuismans !
Error is unrelated -> merging |
transformers | 11,196 | closed | Added translation example script | This PR adds the translation example script using the Accelerate library.
@sgugger
| 04-12-2021 05:13:03 | 04-12-2021 05:13:03 | Hey @rajvi-k, I believe there is just the styling issue left to fix before we can merge this. Just run `make style` on your branch! |
transformers | 11,195 | closed | Getting no attribute 'output_attentions' error when upgrading to latest huggingface transformers | # 📚 Migration
## Information
I am getting `torch.nn.modules.module.ModuleAttributeError: 'CaptionBertSelfAttention' object has no attribute 'output_attentions'` error when upgrading my code from pytorch-transformers to latest version of huggingface transformers.
Model I am using (Bert, XLNet ...): Bert
Language I am using the model on (English, Chinese ...): English
The problem arises when using:
* [ ] the official example scripts: (give details below): not sure
* [ ] my own modified scripts: (give details below): yes
The tasks I am working on is:
* [ ] an official GLUE/SQUaD task: (give the name): no
* [ ] my own task or dataset: (give details below): no
## Details
<!-- A clear and concise description of the migration issue.
If you have code snippets, please provide it here as well.
Important! Use code tags to correctly format your code. See https://help.github.com/en/github/writing-on-github/creating-and-highlighting-code-blocks#syntax-highlighting
Do not use screenshots, as they are hard to read and (more importantly) don't allow others to copy-and-paste your code.
-->
I am trying to upgrade my code which uses pytorch-transformers to use latest version of Huggingface transformers. However when I try to use the latest version of huggingface transformers, I get below error:
```
Traceback (most recent call last):
File "oscar/run_captioning.py", line 1014, in <module>
main()
File "oscar/run_captioning.py", line 989, in main
last_checkpoint = train(args, train_dataloader, val_dataloader, model, tokenizer)
File "oscar/run_captioning.py", line 479, in train
outputs = model(**inputs)
File "/usr/local/lib64/python3.6/site-packages/torch/nn/modules/module.py", line 727, in _call_impl
result = self.forward(*input, **kwargs)
File "/home/default/ephemeral_drive/work/image_captioning/Oscar_latest/oscar/modeling/modeling_bert.py", line 450, in forward
return self.encode_forward(*args, **kwargs)
File "/home/default/ephemeral_drive/work/image_captioning/Oscar_latest/oscar/modeling/modeling_bert.py", line 458, in encode_forward
encoder_history_states=encoder_history_states)
File "/usr/local/lib64/python3.6/site-packages/torch/nn/modules/module.py", line 727, in _call_impl
result = self.forward(*input, **kwargs)
File "/home/default/ephemeral_drive/work/image_captioning/Oscar_latest/oscar/modeling/modeling_bert.py", line 281, in forward
encoder_history_states=encoder_history_states)
File "/usr/local/lib64/python3.6/site-packages/torch/nn/modules/module.py", line 727, in _call_impl
result = self.forward(*input, **kwargs)
File "/home/default/ephemeral_drive/work/image_captioning/Oscar_latest/oscar/modeling/modeling_bert.py", line 115, in forward
history_state)
File "/usr/local/lib64/python3.6/site-packages/torch/nn/modules/module.py", line 727, in _call_impl
result = self.forward(*input, **kwargs)
File "/home/default/ephemeral_drive/work/image_captioning/Oscar_latest/oscar/modeling/modeling_bert.py", line 146, in forward
head_mask, history_state)
File "/usr/local/lib64/python3.6/site-packages/torch/nn/modules/module.py", line 727, in _call_impl
result = self.forward(*input, **kwargs)
File "/home/default/ephemeral_drive/work/image_captioning/Oscar_latest/oscar/modeling/modeling_bert.py", line 88, in forward
self_outputs = self.self(input_tensor, attention_mask, head_mask, history_state)
File "/usr/local/lib64/python3.6/site-packages/torch/nn/modules/module.py", line 727, in _call_impl
result = self.forward(*input, **kwargs)
File "/home/default/ephemeral_drive/work/image_captioning/Oscar_latest/oscar/modeling/modeling_bert.py", line 73, in forward
outputs = (context_layer, attention_probs) if self.output_attentions else (context_layer,)
File "/usr/local/lib64/python3.6/site-packages/torch/nn/modules/module.py", line 779, in __getattr__
type(self).__name__, name))
torch.nn.modules.module.ModuleAttributeError: 'CaptionBertSelfAttention' object has no attribute 'output_attentions'
```
This branch of my code is using the latest version of transformers which gives above error - https://github.com/gsrivas4/Oscar_latest/tree/latest_transformer. This another branch of my code is using older version of transformers (https://github.com/huggingface/transformers/tree/067923d3267325f525f4e46f357360c191ba562e) which runs without any error - https://github.com/gsrivas4/Oscar_latest/tree/old_transformers. I have added README.md files to run both the branches.
So far, based on my debugging the issue I understand that `self.output_attentions` is defined in the older version of transformers here - https://github.com/huggingface/transformers/blob/067923d3267325f525f4e46f357360c191ba562e/pytorch_transformers/modeling_bert.py#L281. However, in the latest version of transformers `self.output_attentions` is not defined in the class `BertSelfAttention` - https://github.com/huggingface/transformers/blob/master/src/transformers/models/bert/modeling_bert.py#L213-L236. As `self.output_attentions` is not defined the latest version of transformers, which causes the error.
I have checked the migration document and did not the find steps needed or guidelines about how to resolve the issue caused by upgrading huggingface transformers - https://huggingface.co/transformers/migration.html#migrating-from-transformers-v3-x-to-v4-x.
It would be really helpful to know how to resolve the error.
## Environment info
<!-- You can run the command `python transformers-cli env` and copy-and-paste its output below.
Don't forget to fill out the missing fields in that output! -->
- `transformers` version: https://github.com/huggingface/transformers
- Platform: x86_64 GNU/Linux
- Python version: 3.6.8
- PyTorch version (GPU?): 1.7.0+cu101 (GPU)
- Tensorflow version (GPU?): 2.3.0 (GPU)
- Using GPU in script?: yes
- Using distributed or parallel set-up in script?: no
<!-- IMPORTANT: which version of the former library do you use? -->
* `pytorch-transformers` or `pytorch-pretrained-bert` version (or branch): https://github.com/huggingface/transformers/tree/067923d3267325f525f4e46f357360c191ba562e
## Checklist
- [ yes] I have read the migration guide in the readme.
([pytorch-transformers](https://github.com/huggingface/transformers#migrating-from-pytorch-transformers-to-transformers);
[pytorch-pretrained-bert](https://github.com/huggingface/transformers#migrating-from-pytorch-pretrained-bert-to-transformers))
- [yes ] I checked if a related official extension example runs on my machine.
| 04-12-2021 03:33:34 | 04-12-2021 03:33:34 | #### Update:
I changed the following line of code from
`outputs = (context_layer, attention_probs) if self.output_attentions else (context_layer,)` to
`outputs = (context_layer,)`
and my code seems to run fine with the latest transformers - https://github.com/gsrivas4/Oscar_latest/blob/latest_transformer/oscar/modeling/modeling_bert.py#L74-L75. However, I am still not sure if this change can break something in code logically.<|||||>This issue has been automatically marked as stale because it has not had recent activity. If you think this still needs to be addressed please comment on this thread.
Please note that issues that do not follow the [contributing guidelines](https://github.com/huggingface/transformers/blob/master/CONTRIBUTING.md) are likely to be ignored. |
transformers | 11,194 | closed | Transfer learning on bert | We take pretrained bert model and passing our dataset we save model on .bin file and we take predictions.
But how can we retrain a model with new data set on top of generated bin file
Please help me on this issue | 04-12-2021 02:12:36 | 04-12-2021 02:12:36 | Hello, thanks for opening an issue! We try to keep the github issues for bugs/feature requests.
Could you ask your question on the [forum](https://discusss.huggingface.co) instead?
Thanks! |
transformers | 11,193 | closed | ProphetNet with AttributeError: module 'torch.utils' has no attribute 'checkpoint' | ## Environment info
- `transformers` version: 4.5.0
- Platform: Linux-5.4.0-70-generic-x86_64-with-debian-buster-sid
- Python version: 3.7.6
- PyTorch version (GPU?): 1.7.1 (True)
- Tensorflow version (GPU?): not installed (NA)
- Using GPU in script?: Yes
- Using distributed or parallel set-up in script?: No
### Who can help
@patrickvonplaten
## Information
Model I am using ProphetNet:
The problem arises when using:
my own modified scripts (simplified):
```python
self.model = ProphetNetForConditionalGeneration.from_pretrained(self.pretrained_model_path, config=self.config)
outputs = self.model(
input_ids,
attention_mask=input_att,
decoder_input_ids=decoder_input_ids,
decoder_attention_mask=decoder_input_att,
use_cache=False
)
```
And rised:
```
File "/home/ruc/tty/TextBox/textbox/model/Seq2Seq/prophetnet.py", line 89, in forward
use_cache=False
File "/home/ruc/anaconda3/lib/python3.7/site-packages/torch/nn/modules/module.py", line 727, in _call_impl
result = self.forward(*input, **kwargs)
File "/home/ruc/anaconda3/lib/python3.7/site-packages/transformers/models/prophetnet/modeling_prophetnet.py", line 1841, in forward
return_dict=return_dict,
File "/home/ruc/anaconda3/lib/python3.7/site-packages/torch/nn/modules/module.py", line 727, in _call_impl
result = self.forward(*input, **kwargs)
File "/home/ruc/anaconda3/lib/python3.7/site-packages/transformers/models/prophetnet/modeling_prophetnet.py", line 1725, in forward
return_dict=return_dict,
File "/home/ruc/anaconda3/lib/python3.7/site-packages/torch/nn/modules/module.py", line 727, in _call_impl
result = self.forward(*input, **kwargs)
File "/home/ruc/anaconda3/lib/python3.7/site-packages/transformers/models/prophetnet/modeling_prophetnet.py", line 1272, in forward
layer_outputs = torch.utils.checkpoint.checkpoint(
AttributeError: module 'torch.utils' has no attribute 'checkpoint'
```
I think it is the same problem as [#9617](https://github.com/huggingface/transformers/issues/9617) and [#9919](https://github.com/huggingface/transformers/issues/9919). | 04-12-2021 02:05:28 | 04-12-2021 02:05:28 | |
transformers | 11,192 | closed | Loading a model saved with `TFGPT2LMHeadModel.save_pretrained` with `GPT2LMHeadModel.from_pretrained(..., from_tf=True)` | ## Environment info
- `transformers` version: 4.5.0
- Platform: Linux-4.19.0-16-cloud-amd64-x86_64-with-glibc2.10
- Python version: 3.8.8
- PyTorch version (GPU?): 1.8.1+cu102 (False)
- Tensorflow version (GPU?): 2.4.1 (False)
- Using GPU in script?: No
- Using distributed or parallel set-up in script?: No
### Who can help
@patrickvonplaten, @LysandreJik
## Information
Hello,
(My problem seems related to https://github.com/huggingface/transformers/issues/5588)
I fine-tuned a `TFGPT2LMHeadModel` and saved it with `.save_pretrained`, giving me a `tf_model.h5` and a `config.json` files.
I try loading it with
```
model = transformers.GPT2LMHeadModel.from_pretrained(
".", from_tf=True, config="./config.json"
)
```.
The path is fine.
I get the following messages:
```
All TF 2.0 model weights were used when initializing GPT2LMHeadModel.
Some weights of GPT2LMHeadModel were not initialized from the TF 2.0 model and are newly initialized: ['transformer.h.0.attn.bias', 'transformer.h.0.attn.masked_bias', 'transformer.h.1.attn.bias', 'transformer.h.1.attn.masked_bias', 'transformer.h.2.attn.bias', 'transformer.h.2.attn.masked_bias', 'transformer.h.3.attn.bias', 'transformer.h.3.attn.masked_bias', 'transformer.h.4.attn.bias', 'transformer.h.4.attn.masked_bias', 'transformer.h.5.attn.bias', 'transformer.h.5.attn.masked_bias', 'transformer.h.6.attn.bias', 'transformer.h.6.attn.masked_bias', 'transformer.h.7.attn.bias', 'transformer.h.7.attn.masked_bias', 'transformer.h.8.attn.bias', 'transformer.h.8.attn.masked_bias', 'transformer.h.9.attn.bias', 'transformer.h.9.attn.masked_bias', 'transformer.h.10.attn.bias', 'transformer.h.10.attn.masked_bias', 'transformer.h.11.attn.bias', 'transformer.h.11.attn.masked_bias', 'transformer.h.12.attn.bias', 'transformer.h.12.attn.masked_bias', 'transformer.h.13.attn.bias', 'transformer.h.13.attn.masked_bias', 'transformer.h.14.attn.bias', 'transformer.h.14.attn.masked_bias', 'transformer.h.15.attn.bias', 'transformer.h.15.attn.masked_bias', 'transformer.h.16.attn.bias', 'transformer.h.16.attn.masked_bias', 'transformer.h.17.attn.bias', 'transformer.h.17.attn.masked_bias', 'transformer.h.18.attn.bias', 'transformer.h.18.attn.masked_bias', 'transformer.h.19.attn.bias', 'transformer.h.19.attn.masked_bias', 'transformer.h.20.attn.bias', 'transformer.h.20.attn.masked_bias', 'transformer.h.21.attn.bias', 'transformer.h.21.attn.masked_bias', 'transformer.h.22.attn.bias', 'transformer.h.22.attn.masked_bias', 'transformer.h.23.attn.bias', 'transformer.h.23.attn.masked_bias', 'transformer.h.24.attn.bias', 'transformer.h.24.attn.masked_bias', 'transformer.h.25.attn.bias', 'transformer.h.25.attn.masked_bias', 'transformer.h.26.attn.bias', 'transformer.h.26.attn.masked_bias', 'transformer.h.27.attn.bias', 'transformer.h.27.attn.masked_bias', 'transformer.h.28.attn.bias', 'transformer.h.28.attn.masked_bias', 'transformer.h.29.attn.bias', 'transformer.h.29.attn.masked_bias', 'transformer.h.30.attn.bias', 'transformer.h.30.attn.masked_bias', 'transformer.h.31.attn.bias', 'transformer.h.31.attn.masked_bias', 'transformer.h.32.attn.bias', 'transformer.h.32.attn.masked_bias', 'transformer.h.33.attn.bias', 'transformer.h.33.attn.masked_bias', 'transformer.h.34.attn.bias', 'transformer.h.34.attn.masked_bias', 'transformer.h.35.attn.bias', 'transformer.h.35.attn.masked_bias', 'transformer.h.36.attn.bias', 'transformer.h.36.attn.masked_bias', 'transformer.h.37.attn.bias', 'transformer.h.37.attn.masked_bias', 'transformer.h.38.attn.bias', 'transformer.h.38.attn.masked_bias', 'transformer.h.39.attn.bias', 'transformer.h.39.attn.masked_bias', 'transformer.h.40.attn.bias', 'transformer.h.40.attn.masked_bias', 'transformer.h.41.attn.bias', 'transformer.h.41.attn.masked_bias', 'transformer.h.42.attn.bias', 'transformer.h.42.attn.masked_bias', 'transformer.h.43.attn.bias', 'transformer.h.43.attn.masked_bias', 'transformer.h.44.attn.bias', 'transformer.h.44.attn.masked_bias', 'transformer.h.45.attn.bias', 'transformer.h.45.attn.masked_bias', 'transformer.h.46.attn.bias', 'transformer.h.46.attn.masked_bias', 'transformer.h.47.attn.bias', 'transformer.h.47.attn.masked_bias', 'lm_head.weight']
You should probably TRAIN this model on a down-stream task to be able to use it for predictions and inference.
```
It means that the conversion hasn't worked, right?
Can I just use the model for generation? Should I change the way the model is saved ?
| 04-12-2021 01:38:15 | 04-12-2021 01:38:15 | Actually that's not an issue, this warning shouldn't be here. I'll open a PR to remove it shortly.<|||||>If you try generating text with it, you should get sensible results!<|||||>Great to hear, thanks. |
transformers | 11,191 | closed | Decoding throws Segmentation Fault | ## Environment info
<!-- You can run the command `transformers-cli env` and copy-and-paste its output below.
Don't forget to fill out the missing fields in that output! -->
- `transformers` version: 4.4.2
- Platform: Linux-5.8.0-48-generic-x86_64-with-glibc2.10
- Python version: 3.8.5
- PyTorch version (GPU?): 1.8.1 (True)
- Tensorflow version (GPU?): 2.4.1 (False)
- Using GPU in script?: <fill in>
- Using distributed or parallel set-up in script?: <fill in>
Models:
- bert
## Information
A very simple decoding step throws `24272 segmentation fault (core dumped)` .
## To reproduce
Steps to reproduce the behavior:
```
from transformers import AutoTokenizer
tokenizer = AutoTokenizer.from_pretrained("bert-base-uncased")
print(tokenizer.decode(token_ids=torch.tensor([3446])))
```
<!-- If you have code snippets, error messages, stack traces please provide them here as well.
Important! Use code tags to correctly format your code. See https://help.github.com/en/github/writing-on-github/creating-and-highlighting-code-blocks#syntax-highlighting
Do not use screenshots, as they are hard to read and (more importantly) don't allow others to copy-and-paste your code.-->
## Expected behavior
It should print `'rate'`
| 04-11-2021 20:42:49 | 04-11-2021 20:42:49 | I get `rate` printed out on my setup! Do you mind sharing your `pip list`?<|||||>This was mentioned in #4857 and #5359 ....fiixed it after reinstalling. <|||||>For me, i didn't have sentencepiece installed. I needed to import torch before transformers to fix this |
transformers | 11,190 | closed | wav2vec 2.0 doesn't appear to do vector quantization | In the [paper](https://arxiv.org/abs/2006.11477) from FAIR, they describe wav2vec 2.0 as using a vector quantization module to learn discrete vectors of speech units (section 2.) As far as I know, this should be happening between `Wav2Vec2FeatureExtractor` and `Wav2Vec2FeatureProjection`.
The HuggingFace implementation doesn't seem to do any vector quantization. Is this a correct implementation? | 04-11-2021 20:13:16 | 04-11-2021 20:13:16 | Hi, I think VQ only works for Pretraining, it doesn't look like Transformers currently support Pretrain<|||||>Vector quantization is only required for pretraining which is currently not supported. It should be added soon: https://github.com/huggingface/transformers/issues/10873.<|||||>Thanks! Just to clarify, was the [base model](https://huggingface.co/facebook/wav2vec2-base) pretrained with quantization, and it's just that the port to HF doesn't include the quantization module?<|||||>The port didn't include the quantization module - we should re-port the model :-) <|||||>It was trained with quantization if I remember correctly<|||||>This issue has been automatically marked as stale because it has not had recent activity. If you think this still needs to be addressed please comment on this thread.
Please note that issues that do not follow the [contributing guidelines](https://github.com/huggingface/transformers/blob/master/CONTRIBUTING.md) are likely to be ignored. |
transformers | 11,189 | closed | correct the input_ids value and batch_sentences value. | I found two places with minor typo in transformers/docs/source/preprocessing.rst.
First, the example output does not match the input in Base use section. "Hello, I'm a single sentence!" should be mapped to [101, 8667, 117, 146, 112, 182, 170, 1423, 5650, 106, 102] rather than [101, 138, 18696, 155, 1942, 3190, 1144, 1572, 13745, 1104, 159, 9664, 2107, 102] which is the original edition. (A quick check is that they do not match in length)
Second, the example input does not match the output in the same section. For consistency, I changed the sentence to be identical to the example given above it by adding a comma. | 04-11-2021 19:08:34 | 04-11-2021 19:08:34 | This issue has been automatically marked as stale because it has not had recent activity. If you think this still needs to be addressed please comment on this thread.
Please note that issues that do not follow the [contributing guidelines](https://github.com/huggingface/transformers/blob/master/CONTRIBUTING.md) are likely to be ignored. |
transformers | 11,188 | closed | Fix typo | # What does this PR do?
<!--
Congratulations! You've made it this far! You're not quite done yet though.
Once merged, your PR is going to appear in the release notes with the title you set, so make sure it's a great title that fully reflects the extent of your awesome contribution.
Then, please replace this with a description of the change and which issue is fixed (if applicable). Please also include relevant motivation and context. List any dependencies (if any) that are required for this change.
Once you're done, someone will review your PR shortly (see the section "Who can review?" below to tag some potential reviewers). They may suggest changes to make the code even better. If no one reviewed your PR after a week has passed, don't hesitate to post a new comment @-mentioning the same persons---sometimes notifications get lost.
-->
<!-- Remove if not applicable -->
## Before submitting
- [x] This PR fixes a typo or improves the docs (you can dismiss the other checks if that's the case).
- [x] Did you read the [contributor guideline](https://github.com/huggingface/transformers/blob/master/CONTRIBUTING.md#start-contributing-pull-requests),
Pull Request section?
- [ ] Was this discussed/approved via a Github issue or the [forum](https://discuss.huggingface.co/)? Please add a link
to it if that's the case.
- [ ] Did you make sure to update the documentation with your changes? Here are the
[documentation guidelines](https://github.com/huggingface/transformers/tree/master/docs), and
[here are tips on formatting docstrings](https://github.com/huggingface/transformers/tree/master/docs#writing-source-documentation).
- [ ] Did you write any new necessary tests?
## Who can review?
Anyone in the community is free to review the PR once the tests have passed. Feel free to tag
members/contributors which may be interested in your PR.
<!-- Your PR will be replied to more quickly if you can figure out the right person to tag with @
If you know how to use git blame, that is the easiest way, otherwise, here is a rough guide of **who to tag**.
Please tag fewer than 3 people.
Models:
- albert, bert, xlm: @LysandreJik
- blenderbot, bart, marian, pegasus, encoderdecoder, t5: @patrickvonplaten, @patil-suraj
- longformer, reformer, transfoxl, xlnet: @patrickvonplaten
- fsmt: @stas00
- funnel: @sgugger
- gpt2: @patrickvonplaten, @LysandreJik
- rag: @patrickvonplaten, @lhoestq
- tensorflow: @LysandreJik
Library:
- benchmarks: @patrickvonplaten
- deepspeed: @stas00
- ray/raytune: @richardliaw, @amogkam
- text generation: @patrickvonplaten
- tokenizers: @n1t0, @LysandreJik
- trainer: @sgugger
- pipelines: @LysandreJik
Documentation: @sgugger
HF projects:
- datasets: [different repo](https://github.com/huggingface/datasets)
- rust tokenizers: [different repo](https://github.com/huggingface/tokenizers)
Examples:
- maintained examples (not research project or legacy): @sgugger, @patil-suraj
- research_projects/bert-loses-patience: @JetRunner
- research_projects/distillation: @VictorSanh
-->
| 04-11-2021 13:21:09 | 04-11-2021 13:21:09 | |
transformers | 11,187 | closed | ELECTRA-large-discriminator results are not stable | I'm fine tuning ELECTRA-large-discriminator (https://huggingface.co/google/electra-large-discriminator) for my classification task.
The problem is that the results are not stable. First time I fine tuned it with validation accuracy around 97%. The second try, i got 7x% accuracy. The third is 5x% accuracy.
I just did the same job with BERT, RoBERTa,...
Anyone has any thoughts on this problem?
| 04-11-2021 12:27:28 | 04-11-2021 12:27:28 | This issue has been automatically marked as stale because it has not had recent activity. If you think this still needs to be addressed please comment on this thread.
Please note that issues that do not follow the [contributing guidelines](https://github.com/huggingface/transformers/blob/master/CONTRIBUTING.md) are likely to be ignored. |
transformers | 11,186 | closed | strange memory usage for t5 models | ## Environment info
<!-- You can run the command `transformers-cli env` and copy-and-paste its output below.
Don't forget to fill out the missing fields in that output! -->
- `transformers` version: 4.4.3
- Platform: linux
- Python version: 3.7
- PyTorch version (GPU?): yes
- Tensorflow version (GPU?): -
- Using GPU in script?: no
- Using distributed or parallel set-up in script?: no
### Who can help
<!-- Your issue will be replied to more quickly if you can figure out the right person to tag with @
If you know how to use git blame, that is the easiest way, otherwise, here is a rough guide of **who to tag**.
Please tag fewer than 3 people.
Models:
- albert, bert, xlm: @LysandreJik
- blenderbot, bart, marian, pegasus, encoderdecoder, t5: @patrickvonplaten, @patil-suraj
- longformer, reformer, transfoxl, xlnet: @patrickvonplaten
- fsmt: @stas00
- funnel: @sgugger
- gpt2: @patrickvonplaten, @LysandreJik
- rag: @patrickvonplaten, @lhoestq
- tensorflow: @LysandreJik
Library:
- benchmarks: @patrickvonplaten
- deepspeed: @stas00
- ray/raytune: @richardliaw, @amogkam
- text generation: @patrickvonplaten
- tokenizers: @LysandreJik
- trainer: @sgugger
- pipelines: @LysandreJik
Documentation: @sgugger
Model hub:
- for issues with a model report at https://discuss.huggingface.co/ and tag the model's creator.
HF projects:
- datasets: [different repo](https://github.com/huggingface/datasets)
- rust tokenizers: [different repo](https://github.com/huggingface/tokenizers)
Examples:
- maintained examples (not research project or legacy): @sgugger, @patil-suraj
- research_projects/bert-loses-patience: @JetRunner
- research_projects/distillation: @VictorSanh
-->
t5: @patrickvonplaten, @patil-suraj
## Information
Hi
I am having a hard time with training t5 models for classification using seq2seq examples on paws-x dataset, I am often getting out of memory error for even small batch sizes, and there must be a bug in seq2seq model with t5 causing large usage of memory, thanks for having a look
```
Traceback (most recent call last):
File "run_seq2seq.py", line 593, in <module>
main()
File "run_seq2seq.py", line 551, in main
train_result = trainer.train(resume_from_checkpoint=checkpoint)
File "/users/dorood/seq2seq/third_party/trainers/trainer.py", line 321, in train
tr_loss += self.training_step(model, inputs)
File "/users/dorood/libs/anaconda3/envs/test2/lib/python3.7/site-packages/transformers/trainer.py", line 1485, in training_step
loss = self.compute_loss(model, inputs)
File "/users/dorood/libs/anaconda3/envs/test2/lib/python3.7/site-packages/transformers/trainer.py", line 1517, in compute_loss
outputs = model(**inputs)
File "/users/dorood/libs/anaconda3/envs/test2/lib/python3.7/site-packages/torch/nn/modules/module.py", line 889, in _call_impl
result = self.forward(*input, **kwargs)
File "/users/dorood/seq2seq/third_party/models/t5/modeling_t5.py", line 1751, in forward
lang=lang
File "/users/dorood/test2/lib/python3.7/site-packages/torch/nn/modules/module.py", line 889, in _call_impl
result = self.forward(*input, **kwargs)
File "/users/dorood/seq2seq/third_party/models/t5/modeling_t5.py", line 1115, in forward
task=task
File "/users/dorood/test2/lib/python3.7/site-packages/torch/nn/modules/module.py", line 889, in _call_impl
result = self.forward(*input, **kwargs)
File "/users/dorood/seq2seq/third_party/models/t5/modeling_t5.py", line 752, in forward
output_attentions=output_attentions,
File "/users/dorood/libs/anaconda3/envs/test2/lib/python3.7/site-packages/torch/nn/modules/module.py", line 889, in _call_impl
result = self.forward(*input, **kwargs)
File "/users/dorood/seq2seq/third_party/models/t5/modeling_t5.py", line 653, in forward
output_attentions=output_attentions,
File "/users/dorood/libs/anaconda3/envs/test2/lib/python3.7/site-packages/torch/nn/modules/module.py", line 889, in _call_impl
result = self.forward(*input, **kwargs)
File "/users/dorood/seq2seq/third_party/models/t5/modeling_t5.py", line 557, in forward
attn_output = unshape(torch.matmul(attn_weights, value_states)) # (batch_size, seq_length, dim)
RuntimeError: CUDA out of memory. Tried to allocate 42.00 MiB (GPU 0; 23.70 GiB total capacity; 21.14 GiB already allocated; 1.69 MiB free; 22.36 GiB reserved in total by PyTorch)
``` | 04-11-2021 11:34:34 | 04-11-2021 11:34:34 | Which model are you using? What's the command you're using to launch the training?<|||||>Hi
I will close this issue and open up a proper reporting as I see the issue is arising from loading a checkpointin trainer class |
transformers | 11,185 | closed | Loading pretrained mBART model always generate the same output | Hi,
I trained mBART with pytorch lightning with the model MBartForConditionalGeneration.from_pretrained('facebook/mbart-large-cc25') to do summaries. As output I got a checkpoint.ckpt. Moreover, I used model.model.save_pretrained to have a config.json and pytorch_model.bin.
During the training and testing, I saw what the model was generating as summaries and I got satisfying results.
However, when I load it back into transformers, the output it generates is always the same no matter the input. I can see that this output comes from my training data but during the training and testing the model was not doing this. The model was in fact generating output with a relation to the input which is not the case here as it always outputs the same thing.
I suppose there must be a mistake on how I load and use the pretrained model but I don't know what.
This is how I do it:
```
configuration = MBartConfig.from_json_file("config.json")
model = MBartForConditionalGeneration.from_pretrained("pytorch_model.bin",
config="configuration")
tokenizer = MBartTokenizer.from_pretrained('facebook/mbart-large-cc25')
inputs = tokenizer([ARTICLE_TO_SUMMARIZE], max_length=1024, return_tensors='pt')
summary_ids = model.generate(inputs['input_ids'], num_beams=4, max_length=150, early_stopping=True)
print([tokenizer.decode(g, skip_special_tokens=True, clean_up_tokenization_spaces=False) for g in summary_ids])
```
Thanks in advance for the help | 04-11-2021 11:06:24 | 04-11-2021 11:06:24 | |
transformers | 11,184 | closed | Can not instantiate BertGenerationEncoder or BertGenerationDecoder from bert model | ## Environment info
<!-- You can run the command `transformers-cli env` and copy-and-paste its output below.
Don't forget to fill out the missing fields in that output! -->
- `transformers` version: 4.5.0
- Platform: Ubuntu 18.04.5 LTS
- Python version: 3.6.9
- PyTorch version (GPU?): 1.8.1 (Quadro GV100 )
- Tensorflow version (GPU?):
- Using GPU in script?:
- Using distributed or parallel set-up in script?:
### Who can help
<!-- Your issue will be replied to more quickly if you can figure out the right person to tag with @
If you know how to use git blame, that is the easiest way, otherwise, here is a rough guide of **who to tag**.
Please tag fewer than 3 people.
Models:
- albert, bert, xlm: @LysandreJik
- blenderbot, bart, marian, pegasus, encoderdecoder, t5: @patrickvonplaten, @patil-suraj
- longformer, reformer, transfoxl, xlnet: @patrickvonplaten
- fsmt: @stas00
- funnel: @sgugger
- gpt2: @patrickvonplaten, @LysandreJik
- rag: @patrickvonplaten, @lhoestq
- tensorflow: @LysandreJik
Library:
- benchmarks: @patrickvonplaten
- deepspeed: @stas00
- ray/raytune: @richardliaw, @amogkam
- text generation: @patrickvonplaten
- tokenizers: @LysandreJik
- trainer: @sgugger
- pipelines: @LysandreJik
Documentation: @sgugger
Model hub:
- for issues with a model report at https://discuss.huggingface.co/ and tag the model's creator.
HF projects:
- datasets: [different repo](https://github.com/huggingface/datasets)
- rust tokenizers: [different repo](https://github.com/huggingface/tokenizers)
Examples:
- maintained examples (not research project or legacy): @sgugger, @patil-suraj
- research_projects/bert-loses-patience: @JetRunner
- research_projects/distillation: @VictorSanh
-->
@LysandreJik
@sgugger, @patil-suraj
## Information
Model I am using BertGeneration:
The problem arises when using:
* [ ] the official example scripts: (give details below)
## To reproduce
Steps to reproduce the behavior:
1. encoder = BertGenerationEncoder.from_pretrained("bert-large-uncased", bos_token_id=101, eos_token_id=102)
https://huggingface.co/transformers/model_doc/bertgeneration.html?highlight=bertgeneration
2. I have got following error
File "python3.6/site-packages/transformers/modeling_utils.py", line 988, in from_pretrained
**kwargs,
File "python3.6/site-packages/transformers/configuration_utils.py", line 405, in from_pretrained
), f"You tried to initiate a model of type '{cls.model_type}' with a pretrained model of type '{config_dict['model_type']}'"
AssertionError: You tried to initiate a model of type 'bert-generation' with a pretrained model of type 'bert'
3.
<!-- If you have code snippets, error messages, stack traces please provide them here as well.
Important! Use code tags to correctly format your code. See https://help.github.com/en/github/writing-on-github/creating-and-highlighting-code-blocks#syntax-highlighting
Do not use screenshots, as they are hard to read and (more importantly) don't allow others to copy-and-paste your code.-->
## Expected behavior
The same script works when using the previous version 4.4.2
<!-- A clear and concise description of what you would expect to happen. -->
| 04-11-2021 01:43:29 | 04-11-2021 01:43:29 | I met the same issues.<|||||>This was fixed by #11207 and was released in patch 4.5.1. Please install the latest version and let us know if it works for you!<|||||>It works in patch 4.5.1, thanks.
I still wonder what is the difference between the following two methods to instantiate the BERT encoder-decoder model.
Probably I should ask in another thread.
model_name='bert-base-multilingual-cased'
encoder = BertGenerationEncoder.from_pretrained(model_name, bos_token_id=bos_token_id, eos_token_id=eos_token_id)
decoder = BertGenerationDecoder.from_pretrained(model_name, add_cross_attention=True, is_decoder=True, bos_token_id=bos_token_id, eos_token_id=eos_token_id)
bert2bert = EncoderDecoderModel(encoder=encoder, decoder=decoder)
v.s.
model_name='bert-base-multilingual-cased'
bert2bert = EncoderDecoderModel.from_encoder_decoder_pretrained(model_name, model_name)
bert2bert.config.decoder.decoder_start_token_id = bos_token_id
bert2bert.config.encoder.bos_token_id = bos_token_id
bert2bert.config.encoder.eos_token_id = eos_token_id
bert2bert.config.encoder.pad_token_id = pad_token_id
<|||||>Hi @ken-arf
Both methods are doing the same thing.
The difference is that in the second method you don't need to initialize the encoder and decoder, you could just pass the name of the model two the `from_encoder_decoder_pretrained` method and takes care of initializing the encoder, decoder and adding cross_attention in the decoder etc.<|||||>Hi Suraj
Thank you for your answer, I understand. I used both methods interchangeably, so that sounds good to me. |
transformers | 11,183 | closed | Replaced `which` with `who` | # What does this PR do?
<!--
Congratulations! You've made it this far! You're not quite done yet though.
Once merged, your PR is going to appear in the release notes with the title you set, so make sure it's a great title that fully reflects the extent of your awesome contribution.
Then, please replace this with a description of the change and which issue is fixed (if applicable). Please also include relevant motivation and context. List any dependencies (if any) that are required for this change.
Once you're done, someone will review your PR shortly (see the section "Who can review?" below to tag some potential reviewers). They may suggest changes to make the code even better. If no one reviewed your PR after a week has passed, don't hesitate to post a new comment @-mentioning the same persons---sometimes notifications get lost.
-->
<!-- Remove if not applicable -->
Fixes # (issue)
## Before submitting
- [ ] This PR fixes a typo or improves the docs (you can dismiss the other checks if that's the case).
- [ ] Did you read the [contributor guideline](https://github.com/huggingface/transformers/blob/master/CONTRIBUTING.md#start-contributing-pull-requests),
Pull Request section?
- [ ] Was this discussed/approved via a Github issue or the [forum](https://discuss.huggingface.co/)? Please add a link
to it if that's the case.
- [ ] Did you make sure to update the documentation with your changes? Here are the
[documentation guidelines](https://github.com/huggingface/transformers/tree/master/docs), and
[here are tips on formatting docstrings](https://github.com/huggingface/transformers/tree/master/docs#writing-source-documentation).
- [ ] Did you write any new necessary tests?
## Who can review?
Anyone in the community is free to review the PR once the tests have passed. Feel free to tag
members/contributors which may be interested in your PR.
<!-- Your PR will be replied to more quickly if you can figure out the right person to tag with @
If you know how to use git blame, that is the easiest way, otherwise, here is a rough guide of **who to tag**.
Please tag fewer than 3 people.
Models:
- albert, bert, xlm: @LysandreJik
- blenderbot, bart, marian, pegasus, encoderdecoder, t5: @patrickvonplaten, @patil-suraj
- longformer, reformer, transfoxl, xlnet: @patrickvonplaten
- fsmt: @stas00
- funnel: @sgugger
- gpt2: @patrickvonplaten, @LysandreJik
- rag: @patrickvonplaten, @lhoestq
- tensorflow: @LysandreJik
Library:
- benchmarks: @patrickvonplaten
- deepspeed: @stas00
- ray/raytune: @richardliaw, @amogkam
- text generation: @patrickvonplaten
- tokenizers: @n1t0, @LysandreJik
- trainer: @sgugger
- pipelines: @LysandreJik
Documentation: @sgugger
HF projects:
- datasets: [different repo](https://github.com/huggingface/datasets)
- rust tokenizers: [different repo](https://github.com/huggingface/tokenizers)
Examples:
- maintained examples (not research project or legacy): @sgugger, @patil-suraj
- research_projects/bert-loses-patience: @JetRunner
- research_projects/distillation: @VictorSanh
-->
| 04-10-2021 23:50:53 | 04-10-2021 23:50:53 | |
transformers | 11,182 | closed | Minor typos fixed | # What does this PR do?
Fixes minor typos.
## Before submitting
- [X] This PR fixes a typo or improves the docs (you can dismiss the other checks if that's the case).
- [X] Did you read the [contributor guideline](https://github.com/huggingface/transformers/blob/master/CONTRIBUTING.md#start-contributing-pull-requests),
Pull Request section?
- [ ] Was this discussed/approved via a Github issue or the [forum](https://discuss.huggingface.co/)? Please add a link
to it if that's the case.
- [ ] Did you make sure to update the documentation with your changes? Here are the
[documentation guidelines](https://github.com/huggingface/transformers/tree/master/docs), and
[here are tips on formatting docstrings](https://github.com/huggingface/transformers/tree/master/docs#writing-source-documentation).
- [ ] Did you write any new necessary tests?
## Who can review?
Anyone in the community is free to review the PR once the tests have passed. Feel free to tag
members/contributors which may be interested in your PR.
Documentation: @sgugger
| 04-10-2021 23:47:57 | 04-10-2021 23:47:57 | |
transformers | 11,181 | closed | How to kill bad starts when pre-training from scratch | ### Environment info
transformers version: 4.4.3
Platform: linux
Python version: 3.8.5
PyTorch version (GPU?): -
Tensorflow version (GPU?): 2.4.1
Using GPU in script?: yes
Using distributed or parallel set-up in script?: parallel
### Information
Hi!
I am pre-training a RoBERTa model from scratch and was wondering about the possibility of killing bad starts. Because the model will be initiated with random weights when pre-training from scratch, and these initial weights might influence the performance of the final model, I want to do my best to at least not get the worst weight initialization. I have heard that it might be a possibility to calculate perplexity and let that score be decisive of whether to kill the training process or not. Does anyone have experience with how to do this, or does someone have a better idea to review weight initialization and kill bad starts?
| 04-10-2021 15:00:47 | 04-10-2021 15:00:47 | This issue has been automatically marked as stale because it has not had recent activity. If you think this still needs to be addressed please comment on this thread.
Please note that issues that do not follow the [contributing guidelines](https://github.com/huggingface/transformers/blob/master/CONTRIBUTING.md) are likely to be ignored. |
transformers | 11,180 | open | Sequential constraints? | fairseq has an implementation of unordered and ordered multi-token constraints: see their [PR](https://github.com/pytorch/fairseq/pull/2402) and [example](https://github.com/pytorch/fairseq/tree/1bba712622b8ae4efb3eb793a8a40da386fe11d0/examples/constrained_decoding).
This is more advanced than the single-token constraints that have been occasionally [requested here](https://github.com/huggingface/transformers/issues/10485) mainly due to the bookkeeping involved; see the papers referenced in the fairseq PR.
Has anyone looked into porting this feature? fairseq's [constraint tracking logic](https://github.com/pytorch/fairseq/blob/master/fairseq/token_generation_constraints.py) looks to be well-factored and could probably be adopted verbatim, license permitting. The beam search modifications ([fairseq implementation](https://github.com/pytorch/fairseq/blob/ee0d5a0f65a25e5f5372776402aac5cb9c4adbf1/fairseq/search.py#L210)) may be able to be implemented as a `LogitsProcessor`, or maybe even just a `prefix_allowed_tokens_fn`, but the papers propose some additional logic around making sure that a partial constraint stays in the beam; I'm not sure whether those hooks are sufficient to implement that logic (or how essential it is to the functionality).
(I've found one [related issue](https://github.com/huggingface/transformers/issues/1163).) | 04-10-2021 14:46:16 | 04-10-2021 14:46:16 | Has anyone working on this yet?<|||||>This issue has been automatically marked as stale because it has not had recent activity. If you think this still needs to be addressed please comment on this thread.
Please note that issues that do not follow the [contributing guidelines](https://github.com/huggingface/transformers/blob/master/CONTRIBUTING.md) are likely to be ignored.<|||||>If no one else beats me to it, I may tackle this mid-summer. No promises. <|||||>@kcarnold any update?
There is another recent paper on constrained decoding with complex constraints (fairseq only has positive constraints): [NeuroLogic Decoding: (Un)supervised Neural Text Generation with Predicate Logic Constraints
](https://arxiv.org/abs/2010.12884) |
transformers | 11,179 | closed | Why couldn't I use encoder_hidden_states when position_ids is not None? GPT2Model.foward() | `device` is required in GPT2Model.foward() if I'd like to use encoder_hidden_states.
```
if self.config.add_cross_attention and encoder_hidden_states is not None:
encoder_batch_size, encoder_sequence_length, _ = encoder_hidden_states.size()
encoder_hidden_shape = (encoder_batch_size, encoder_sequence_length)
if encoder_attention_mask is None:
encoder_attention_mask = torch.ones(encoder_hidden_shape, device=device)
encoder_attention_mask = self.invert_attention_mask(encoder_attention_mask)
else:
encoder_attention_mask = None
```
https://github.com/huggingface/transformers/blob/26212c14e5570aff40b90c11495d97dada4272fb/src/transformers/models/gpt2/modeling_gpt2.py#L682
But the only place that sets `device` is in another *if statement*.
```python
if position_ids is None:
device = input_ids.device if input_ids is not None else inputs_embeds.device
position_ids = torch.arange(past_length, input_shape[-1] + past_length, dtype=torch.long, device=device)
position_ids = position_ids.unsqueeze(0).view(-1, input_shape[-1])
```
https://github.com/huggingface/transformers/blob/26212c14e5570aff40b90c11495d97dada4272fb/src/transformers/models/gpt2/modeling_gpt2.py#L653
And I was wondering why is it required to have 'position_ids==None' when I just want to use `encoder_hidden_states` .
Am I missing something?
I ran into this problem when trying to use GPT2LMHeadModel for image captioning tasks. | 04-10-2021 12:44:13 | 04-10-2021 12:44:13 | Ah, I think this is an issue indeed, the device statement shouldn't be inside that `if` statement. Do you want to open a PR to fix this?<|||||>Sure. <|||||>Fixed by #11292 |
transformers | 11,178 | closed | Use MSELoss with single class label in (M)BartForSequenceClassification | # What does this PR do?
Similar to `BertForSequenceClassification`, `(M)BartForSequenceClassification` now uses a regression loss in case `num_labels` equals 1 (as already documented for both model classes). E.g. required when running the GLUE script for STS-B with these model classes.
## Before submitting
- [x] Did you read the [contributor guideline](https://github.com/huggingface/transformers/blob/master/CONTRIBUTING.md#start-contributing-pull-requests),
Pull Request section?
## Who can review?
@patrickvonplaten @patil-suraj
<!-- Your PR will be replied to more quickly if you can figure out the right person to tag with @
If you know how to use git blame, that is the easiest way, otherwise, here is a rough guide of **who to tag**.
Please tag fewer than 3 people.
Models:
- albert, bert, xlm: @LysandreJik
- blenderbot, bart, marian, pegasus, encoderdecoder, t5: @patrickvonplaten, @patil-suraj
- longformer, reformer, transfoxl, xlnet: @patrickvonplaten
- fsmt: @stas00
- funnel: @sgugger
- gpt2: @patrickvonplaten, @LysandreJik
- rag: @patrickvonplaten, @lhoestq
- tensorflow: @LysandreJik
Library:
- benchmarks: @patrickvonplaten
- deepspeed: @stas00
- ray/raytune: @richardliaw, @amogkam
- text generation: @patrickvonplaten
- tokenizers: @n1t0, @LysandreJik
- trainer: @sgugger
- pipelines: @LysandreJik
Documentation: @sgugger
HF projects:
- datasets: [different repo](https://github.com/huggingface/datasets)
- rust tokenizers: [different repo](https://github.com/huggingface/tokenizers)
Examples:
- maintained examples (not research project or legacy): @sgugger, @patil-suraj
- research_projects/bert-loses-patience: @JetRunner
- research_projects/distillation: @VictorSanh
-->
| 04-10-2021 12:05:21 | 04-10-2021 12:05:21 | |
transformers | 11,177 | closed | TypeError: expected str, bytes or os.PathLike object, not NoneType | 04-10-2021 07:00:08 | 04-10-2021 07:00:08 | from transformers import LongformerModel, LongformerTokenizer, RobertaTokenizer, AutoTokenizer
pretrain_model_path = 'schen/longformer-chinese-base-4096'
tokenizer = LongformerTokenizer.from_pretrained(pretrain_model_path)
<|||||>This issue has been automatically marked as stale because it has not had recent activity. If you think this still needs to be addressed please comment on this thread.
Please note that issues that do not follow the [contributing guidelines](https://github.com/huggingface/transformers/blob/master/CONTRIBUTING.md) are likely to be ignored. |
|
transformers | 11,176 | closed | bug fix | # What does this PR do?
<!--
Congratulations! You've made it this far! You're not quite done yet though.
Once merged, your PR is going to appear in the release notes with the title you set, so make sure it's a great title that fully reflects the extent of your awesome contribution.
Then, please replace this with a description of the change and which issue is fixed (if applicable). Please also include relevant motivation and context. List any dependencies (if any) that are required for this change.
Once you're done, someone will review your PR shortly (see the section "Who can review?" below to tag some potential reviewers). They may suggest changes to make the code even better. If no one reviewed your PR after a week has passed, don't hesitate to post a new comment @-mentioning the same persons---sometimes notifications get lost.
-->
<!-- Remove if not applicable -->
Fixes # (issue)
## Before submitting
- [ ] This PR fixes a typo or improves the docs (you can dismiss the other checks if that's the case).
- [ ] Did you read the [contributor guideline](https://github.com/huggingface/transformers/blob/master/CONTRIBUTING.md#start-contributing-pull-requests),
Pull Request section?
- [ ] Was this discussed/approved via a Github issue or the [forum](https://discuss.huggingface.co/)? Please add a link
to it if that's the case.
- [ ] Did you make sure to update the documentation with your changes? Here are the
[documentation guidelines](https://github.com/huggingface/transformers/tree/master/docs), and
[here are tips on formatting docstrings](https://github.com/huggingface/transformers/tree/master/docs#writing-source-documentation).
- [ ] Did you write any new necessary tests?
## Who can review?
Anyone in the community is free to review the PR once the tests have passed. Feel free to tag
members/contributors which may be interested in your PR.
<!-- Your PR will be replied to more quickly if you can figure out the right person to tag with @
If you know how to use git blame, that is the easiest way, otherwise, here is a rough guide of **who to tag**.
Please tag fewer than 3 people.
Models:
- albert, bert, xlm: @LysandreJik
- blenderbot, bart, marian, pegasus, encoderdecoder, t5: @patrickvonplaten, @patil-suraj
- longformer, reformer, transfoxl, xlnet: @patrickvonplaten
- fsmt: @stas00
- funnel: @sgugger
- gpt2: @patrickvonplaten, @LysandreJik
- rag: @patrickvonplaten, @lhoestq
- tensorflow: @LysandreJik
Library:
- benchmarks: @patrickvonplaten
- deepspeed: @stas00
- ray/raytune: @richardliaw, @amogkam
- text generation: @patrickvonplaten
- tokenizers: @n1t0, @LysandreJik
- trainer: @sgugger
- pipelines: @LysandreJik
Documentation: @sgugger
HF projects:
- datasets: [different repo](https://github.com/huggingface/datasets)
- rust tokenizers: [different repo](https://github.com/huggingface/tokenizers)
Examples:
- maintained examples (not research project or legacy): @sgugger, @patil-suraj
- research_projects/bert-loses-patience: @JetRunner
- research_projects/distillation: @VictorSanh
-->
| 04-10-2021 06:12:59 | 04-10-2021 06:12:59 | This issue has been automatically marked as stale because it has not had recent activity. If you think this still needs to be addressed please comment on this thread.
Please note that issues that do not follow the [contributing guidelines](https://github.com/huggingface/transformers/blob/master/CONTRIBUTING.md) are likely to be ignored. |
transformers | 11,175 | closed | MemoryError: when we run_language_model.py to train an English Adapter | run run_language_model.py
https://github.com/Adapter-Hub/adapter-transformers/blob/master/examples/contrib/legacy/run_language_modeling.py
in such codes:
python3 run_language_modeling.py \
--output_dir=/mnt/localdata/cao/output_language_adapter_en/ \
--model_type=bert \
--model_name_or_path=bert-base-multilingual-cased \
--do_train \
--train_data_file=/mnt/localdata/cao/data_for_model/EN_train_updated.txt \
--do_eval \
--eval_data_file=/mnt/localdata/cao/data_for_model/EN_valid.txt \
--mlm \
--language en \
--train_adapter \
--adapter_config pfeiffer \
--per_gpu_train_batch_size 4 \
--per_gpu_eval_batch_size 4 \
--learning_rate 5e-5 \
--dataloader_num_workers 32 \
--cache_dir /mnt/localdata/cao/en_cache_dir/
get error:
with open(file_path, encoding="utf-8") as f:
text = f.read()
MemoryError
the train_data_file is around 6GB, I think it is the loading problem.
So how can we load large files? | 04-10-2021 04:25:08 | 04-10-2021 04:25:08 | You could try the another one [run_language_modeling.py](https://github.com/Adapter-Hub/adapter-transformers/blob/master/examples/contrib/legacy/run_language_modeling.py) with `line-by-line` and add a `batch_size` along with `batched=True` to eliminate the memory error:
```
tokenized_datasets = datasets.map(
...
batched=True,
batch_size=200,
...
)
```<|||||>This issue has been automatically marked as stale because it has not had recent activity. If you think this still needs to be addressed please comment on this thread.
Please note that issues that do not follow the [contributing guidelines](https://github.com/huggingface/transformers/blob/master/CONTRIBUTING.md) are likely to be ignored. |
transformers | 11,174 | closed | Using BART for Mask Infilling makes all the first tokens missing | I'm fine-tuning BART ```"facebook/bart-large"``` model for mask infilling. My dataset looks like below.
The original sentence BART should predict is `taste the rainbow.`, and the input data it gets is `<mask> taste <mask> rainbow <mask>`, or it should predict `global asset management`, given `<mask> global <mask> asset <mask>`.
Generally it works well but only the first tokens are missing. BART's prediction for the first data was `aste the rainbow.` and the prediction for the second was `asset management.`. I don't know why this is happening. `taste` and `global` was given in input, why BART is missing those? Even the first token in original sentence is not given in the input BART predictions always drop the first token. Given `<mask> happiest <mask> place <mask>`, it should predict `the happiest place on earth.`, but it gives me `happiest place on earth.`
I'm not sure this is related, but I gave `force_bos_token_to_be_generated` options to be `True`, and still not working
```
config = BartConfig(force_bos_token_to_be_generated=True)
model = BartForConditionalGeneration.from_pretrained("facebook/bart-large", config = config)
```
I would appreciate any help. Thanks
| 04-10-2021 02:36:31 | 04-10-2021 02:36:31 | This issue has been automatically marked as stale because it has not had recent activity. If you think this still needs to be addressed please comment on this thread.
Please note that issues that do not follow the [contributing guidelines](https://github.com/huggingface/transformers/blob/master/CONTRIBUTING.md) are likely to be ignored.<|||||>Works fine when using the model.generate function |
transformers | 11,173 | closed | Encoder-Decoder Models Can't Generate using Apex | ## Environment info
<!-- You can run the command `transformers-cli env` and copy-and-paste its output below.
Don't forget to fill out the missing fields in that output! -->
- `transformers` version: 4.4.2
- Platform: Linux-5.4.0-1041-aws-x86_64-with-debian-buster-sid
- Python version: 3.7.10
- PyTorch version (GPU?): 1.7.1+cu101 (True)
- Tensorflow version (GPU?): not installed (NA)
- Using GPU in script?: yes
- Using distributed or parallel set-up in script?: no
### Who can help
@patrickvonplaten , @patil-suraj
## Information
Model I am using (Bert, XLNet ...): T5, ProphetNet
The problem arises when using:
* [ ] the official example scripts: (give details below)
* [X] my own modified scripts: (give details below)
The tasks I am working on is:
* [ ] an official GLUE/SQUaD task: (give the name)
* [X] my own task or dataset: (give details below)
## To reproduce
``` Python
>>> from apex import amp
>>> from transformers import ProphetNetForConditionalGeneration, ProphetNetTokenizer
>>> tokenizer = ProphetNetTokenizer.from_pretrained("microsoft/prophetnet-large-uncased-squad-qg")
>>> model = ProphetNetForConditionalGeneration.from_pretrained("microsoft/prophetnet-large-uncased-squad-qg")
>>> model = model.to("cuda")
>>> model = amp.initialize(model, opt_level="O2") # comment out this line and it works fine
>>> encoder_inputs = tokenizer( ["Hello, I am"], return_tensors="pt", truncation=True, padding=True)["input_ids"].to("cuda")
>>> model.generate(encoder_inputs, num_beams=5, do_sample=True, max_length=32)
Traceback (most recent call last):
File "ex.py", line 8, in <module>
model.generate(encoder_inputs, num_beams=5, do_sample=True, max_length=32)
File "/home/ubuntu/RLDiverseQG/env/lib/python3.7/site-packages/torch/autograd/grad_mode.py", line 26, in decorate_context
return func(*args, **kwargs)
File "/home/ubuntu/RLDiverseQG/env/lib/python3.7/site-packages/transformers/generation_utils.py", line 1093, in generate
**model_kwargs,
File "/home/ubuntu/RLDiverseQG/env/lib/python3.7/site-packages/transformers/generation_utils.py", line 1990, in beam_sample
output_hidden_states=output_hidden_states,
File "/home/ubuntu/RLDiverseQG/env/lib/python3.7/site-packages/torch/nn/modules/module.py", line 727, in _call_impl
result = self.forward(*input, **kwargs)
File "/home/ubuntu/RLDiverseQG/env/lib/python3.7/site-packages/apex/amp/_initialize.py", line 197, in new_fwd
**applier(kwargs, input_caster))
File "/home/ubuntu/RLDiverseQG/env/lib/python3.7/site-packages/transformers/models/prophetnet/modeling_prophetnet.py", line 1841, in forward
return_dict=return_dict,
File "/home/ubuntu/RLDiverseQG/env/lib/python3.7/site-packages/torch/nn/modules/module.py", line 727, in _call_impl
result = self.forward(*input, **kwargs)
File "/home/ubuntu/RLDiverseQG/env/lib/python3.7/site-packages/transformers/models/prophetnet/modeling_prophetnet.py", line 1732, in forward
encoder_hidden_states=encoder_outputs[0],
KeyError: 0
```
You can switch out ProphetNet for T5ForConditionalGeneration and get the same error
## Expected behavior
I expect using apex shouldn't affect the code's functionality.
I figured out the main cause of the error: apex converts `BaseModelOutput` objects into dictionaries, but a lot of the code functionality relies on receiving the former. I don't know if there is a way to avoid this.
It is a pretty tedious fix to go over all of the places where this assumption is made and change direct indexing or attribute accesses to use `.get` but I believe that would be the solution to this problem. Hopefully, this is some helpful direction. I am also happy to help with this! | 04-09-2021 23:20:45 | 04-09-2021 23:20:45 | I was able to get a fix working for ProphetNetForConditionalGeneration<|||||>Hi @ManavR123
> I figured out the main cause of the error: apex converts BaseModelOutput objects into dictionaries, but a lot of the code functionality relies on receiving the former. I don't know if there is a way to avoid this.
You could pass `return_dict=False` to `forward` if you don't want the mode to return the output as model output classes, when `return_dict` is `False`, `tuple` is returned<|||||>This issue has been automatically marked as stale because it has not had recent activity. If you think this still needs to be addressed please comment on this thread.
Please note that issues that do not follow the [contributing guidelines](https://github.com/huggingface/transformers/blob/master/CONTRIBUTING.md) are likely to be ignored. |
transformers | 11,172 | closed | Run CI on deepspeed and fairscale | Adds additional workflows for DeepSpeed and Fairscale | 04-09-2021 20:48:42 | 04-09-2021 20:48:42 | |
transformers | 11,171 | closed | Error in running run_tf_text_classification.py | ## Environment info
<!-- You can run the command `transformers-cli env` and copy-and-paste its output below.
Don't forget to fill out the missing fields in that output! -->
- `transformers` version: 4.4.2
- Platform: Windows-10-10.0.18362-SP0
- Python version: 3.8.0
- PyTorch version (GPU?): 1.7.1+cpu (False)
- Tensorflow version (GPU?): 2.4.1 (False)
- Using GPU in script?: No
- Using distributed or parallel set-up in script?: No
### Who can help
<!-- Your issue will be replied to more quickly if you can figure out the right person to tag with @
If you know how to use git blame, that is the easiest way, otherwise, here is a rough guide of **who to tag**.
Please tag fewer than 3 people.
Models:
- albert, bert, xlm: @LysandreJik
- blenderbot, bart, marian, pegasus, encoderdecoder, t5: @patrickvonplaten, @patil-suraj
- longformer, reformer, transfoxl, xlnet: @patrickvonplaten
- fsmt: @stas00
- funnel: @sgugger
- gpt2: @patrickvonplaten, @LysandreJik
- rag: @patrickvonplaten, @lhoestq
- tensorflow: @LysandreJik
Library:
- benchmarks: @patrickvonplaten
- deepspeed: @stas00
- ray/raytune: @richardliaw, @amogkam
- text generation: @patrickvonplaten
- tokenizers: @LysandreJik
- trainer: @sgugger
- pipelines: @LysandreJik
Documentation: @sgugger
Model hub:
- for issues with a model report at https://discuss.huggingface.co/ and tag the model's creator.
HF projects:
- datasets: [different repo](https://github.com/huggingface/datasets)
- rust tokenizers: [different repo](https://github.com/huggingface/tokenizers)
Examples:
- maintained examples (not research project or legacy): @sgugger, @patil-suraj
- research_projects/bert-loses-patience: @JetRunner
- research_projects/distillation: @VictorSanh
-->
## Information
Model I am using (Bert, XLNet ...): microsoft/deberta-base
The problem arises when using: run_tf_text_classification.py
## To reproduce
Steps to reproduce the behavior:
just run
python run_tf_text_classification.py --model_name_or_path microsoft/deberta-base --output_dir classificationoutput --train_file PreparedData.csv --label_column_id 1 --do_train
[PreparedData.zip](https://github.com/huggingface/transformers/files/6288064/PreparedData.zip)
## Stack trace
[INFO|training_args.py:631] 2021-04-09 13:21:17,622 >> PyTorch: setting up devices
[INFO|training_args.py:554] 2021-04-09 13:21:17,629 >> The default value for the training argument `--report_to` will change in v5 (from all installed integrations to none). In v5, you will need to use `--report_to all` to get the same behavior as now. You should start updating your code and make this info disappear :-).
[INFO|training_args_tf.py:192] 2021-04-09 13:21:17,635 >> Tensorflow: setting up strategy
04/09/2021 13:21:18 - INFO - __main__ - n_replicas: 1, distributed training: False, 16-bits training: False
04/09/2021 13:21:18 - INFO - __main__ - Training/evaluation parameters TFTrainingArguments(output_dir='classificationoutput', overwrite_output_dir=False, do_train=True, do_eval=None, do_predict=False, evaluation_strategy=<IntervalStrategy.NO: 'no'>, prediction_loss_only=False, per_device_train_batch_size=8, per_device_eval_batch_size=8, per_gpu_train_batch_size=None, per_gpu_eval_batch_size=None, gradient_accumulation_steps=1, eval_accumulation_steps=None, learning_rate=5e-05, weight_decay=0.0, adam_beta1=0.9, adam_beta2=0.999, adam_epsilon=1e-08, max_grad_norm=1.0, num_train_epochs=3.0, max_steps=-1, lr_scheduler_type=<SchedulerType.LINEAR: 'linear'>, warmup_ratio=0.0, warmup_steps=0, logging_dir='runs\\Apr09_13-21-17_GC8SQLQ2E', logging_strategy=<IntervalStrategy.STEPS: 'steps'>, logging_first_step=False, logging_steps=500, save_strategy=<IntervalStrategy.STEPS: 'steps'>, save_steps=500, save_total_limit=None, no_cuda=False, seed=42, fp16=False, fp16_opt_level='O1', fp16_backend='auto', fp16_full_eval=False, local_rank=-1, tpu_num_cores=None, tpu_metrics_debug=False, debug=False, dataloader_drop_last=False, eval_steps=500, dataloader_num_workers=0, past_index=-1, run_name='classificationoutput', disable_tqdm=False, remove_unused_columns=True, label_names=None, load_best_model_at_end=False, metric_for_best_model=None, greater_is_better=None, ignore_data_skip=False, sharded_ddp=[], deepspeed=None, label_smoothing_factor=0.0, adafactor=False, group_by_length=False, report_to=['tensorboard'], ddp_find_unused_parameters=None, dataloader_pin_memory=True, skip_memory_metrics=False, tpu_name=None, tpu_zone=None, gcp_project=None, poly_power=1.0, xla=False)
[INFO|configuration_utils.py:463] 2021-04-09 13:21:19,339 >> loading configuration file https://huggingface.co/microsoft/deberta-base/resolve/main/config.json from cache at C:\Users\ XXXXXXXX/.cache\huggingface\transformers\e313266bff73867debdfa78c78a9a4966d5e78281ac4ed7048c178b16a37eba7.fb501413b9cef9cef6babdc543bb4153cbec58d52bce077647efba3e3f14ccf3
[INFO|configuration_utils.py:499] 2021-04-09 13:21:19,340 >> Model config DebertaConfig {
"attention_probs_dropout_prob": 0.1,
"hidden_act": "gelu",
"hidden_dropout_prob": 0.1,
"hidden_size": 768,
"initializer_range": 0.02,
"intermediate_size": 3072,
"layer_norm_eps": 1e-07,
"max_position_embeddings": 512,
"max_relative_positions": -1,
"model_type": "deberta",
"num_attention_heads": 12,
"num_hidden_layers": 12,
"pad_token_id": 0,
"pooler_dropout": 0,
"pooler_hidden_act": "gelu",
"pooler_hidden_size": 768,
"pos_att_type": [
"c2p",
"p2c"
],
"position_biased_input": false,
"relative_attention": true,
"transformers_version": "4.4.2",
"type_vocab_size": 0,
"vocab_size": 50265
}
[INFO|tokenization_utils_base.py:1702] 2021-04-09 13:21:20,647 >> loading file https://huggingface.co/microsoft/deberta-base/resolve/main/bpe_encoder.bin from cache at C:\Users\ XXXXXXXX/.cache\huggingface\transformers\b5857926db0a74705bc948686137f046f6ecbc4342162fa03c873a7407eb90ef.d9f36b1bee7c5e05c6b209f4839d4f94d59c2e71c73b1ad67935d66c41c24ff7
[INFO|tokenization_utils_base.py:1702] 2021-04-09 13:21:20,648 >> loading file https://huggingface.co/microsoft/deberta-base/resolve/main/added_tokens.json from cache at None
[INFO|tokenization_utils_base.py:1702] 2021-04-09 13:21:20,648 >> loading file https://huggingface.co/microsoft/deberta-base/resolve/main/special_tokens_map.json from cache at None
[INFO|tokenization_utils_base.py:1702] 2021-04-09 13:21:20,649 >> loading file https://huggingface.co/microsoft/deberta-base/resolve/main/tokenizer_config.json from cache at C:\Users\ XXXXXXXX/.cache\huggingface\transformers\c2bc27a1c7529c177696ff76b1e74cba8667be14e202359f20f9114e407f43e2.a39abb1c6179fb264c2db685f9a056b7cb8d4bc48d729888d292a2280debf8e2
[INFO|tokenization_utils_base.py:1702] 2021-04-09 13:21:20,650 >> loading file https://huggingface.co/microsoft/deberta-base/resolve/main/tokenizer.json from cache at None
04/09/2021 13:21:21 - WARNING - datasets.builder - Using custom data configuration default-337be17b0e590a88
Downloading and preparing dataset csv/default (download: Unknown size, generated: Unknown size, post-processed: Unknown size, total: Unknown size) to C:\Users\ XXXXXXXX\.cache\huggingface\datasets\csv\default-337be17b0e590a88\0.0.0\2dc6629a9ff6b5697d82c25b73731dd440507a69cbce8b425db50b751e8fcfd0...
Dataset csv downloaded and prepared to C:\Users\ XXXXXXXX\.cache\huggingface\datasets\csv\default-337be17b0e590a88\0.0.0\2dc6629a9ff6b5697d82c25b73731dd440507a69cbce8b425db50b751e8fcfd0. Subsequent calls will reuse this data.
---------------------------------------------------------------------------
ValueError Traceback (most recent call last)
~\run_tf_text_classification.py in <module>
350
351 if __name__ == "__main__":
--> 352 main()
~\run_tf_text_classification.py in main()
284 )
285
--> 286 train_dataset, eval_dataset, test_ds, label2id = get_tfds(
287 train_file=data_args.train_file,
288 eval_file=data_args.dev_file,
~\run_tf_text_classification.py in get_tfds(train_file, eval_file, test_file, tokenizer, label_column_id, max_seq_length)
121 print(ds[k])
122 '''
--> 123 transformed_ds[k] = ds[k].map(
124 lambda example: tokenizer.batch_encode_plus(
125 (example[features_name[0]], example[features_name[1]]),
c:\python38\lib\site-packages\datasets\arrow_dataset.py in map(self, function, with_indices, input_columns, batched, batch_size, drop_last_batch, remove_columns, keep_in_memory, load_from_cache_file, cache_file_name, writer_batch_size, features, disable_nullable, fn_kwargs, num_proc, suffix_template, new_fingerprint)
1405 test_inputs = self[:2] if batched else self[0]
1406 test_indices = [0, 1] if batched else 0
-> 1407 update_data = does_function_return_dict(test_inputs, test_indices)
1408 logger.info("Testing finished, running the mapping function on the dataset")
1409
c:\python38\lib\site-packages\datasets\arrow_dataset.py in does_function_return_dict(inputs, indices)
1376 fn_args = [inputs] if input_columns is None else [inputs[col] for col in input_columns]
1377 processed_inputs = (
-> 1378 function(*fn_args, indices, **fn_kwargs) if with_indices else function(*fn_args, **fn_kwargs)
1379 )
1380 does_return_dict = isinstance(processed_inputs, Mapping)
~\run_tf_text_classification.py in <lambda>(example)
122 '''
123 transformed_ds[k] = ds[k].map(
--> 124 lambda example: tokenizer.batch_encode_plus(
125 (example[features_name[0]], example[features_name[1]]),
126 truncation=True,
c:\python38\lib\site-packages\transformers\tokenization_utils_base.py in batch_encode_plus(self, batch_text_or_text_pairs, add_special_tokens, padding, truncation, max_length, stride, is_split_into_words, pad_to_multiple_of, return_tensors, return_token_type_ids, return_attention_mask, return_overflowing_tokens, return_special_tokens_mask, return_offsets_mapping, return_length, verbose, **kwargs)
2432 )
2433
-> 2434 return self._batch_encode_plus(
2435 batch_text_or_text_pairs=batch_text_or_text_pairs,
2436 add_special_tokens=add_special_tokens,
c:\python38\lib\site-packages\transformers\tokenization_utils.py in _batch_encode_plus(self, batch_text_or_text_pairs, add_special_tokens, padding_strategy, truncation_strategy, max_length, stride, is_split_into_words, pad_to_multiple_of, return_tensors, return_token_type_ids, return_attention_mask, return_overflowing_tokens, return_special_tokens_mask, return_offsets_mapping, return_length, verbose, **kwargs)
529 ids, pair_ids = ids_or_pair_ids
530
--> 531 first_ids = get_input_ids(ids)
532 second_ids = get_input_ids(pair_ids) if pair_ids is not None else None
533 input_ids.append((first_ids, second_ids))
c:\python38\lib\site-packages\transformers\tokenization_utils.py in get_input_ids(text)
509 return text
510 else:
--> 511 raise ValueError(
512 "Input is not valid. Should be a string, a list/tuple of strings or a list/tuple of integers."
513 )
ValueError: Input is not valid. Should be a string, a list/tuple of strings or a list/tuple of integers.
| 04-09-2021 19:35:45 | 04-09-2021 19:35:45 | @Rocketknight1 can you help in this<|||||>@sgugger can you help in this<|||||>Hi, we have a new, simpler and more robust text classification in TensorFlow script contributed by @Rocketknight1 [here](https://github.com/huggingface/transformers/blob/master/examples/tensorflow/text-classification/run_text_classification.py), could you check it out?<|||||>Hi @LysandreJik and @Rocketknight1
I used it run_text_classification.py in jupyter
%run run_text_classification.py \
--model_name_or_path roberta-base \
--output_dir classificationoutput \
--train_file PreparedData.csv \
--validation_file PreparedData.csv \
--do_train
PreparedData.csv looks like below
sentence,label
sent1,l1
sent2,l1
sent3,l2
sent3,l2
I got following error
---------------------------------------------------------------------------
TypeError Traceback (most recent call last)
~\run_text_classification.py in <module>
532
533 if __name__ == "__main__":
--> 534 main()
~\run_text_classification.py in main()
492
493 callbacks = [SavePretrainedCallback(output_dir=training_args.output_dir)]
--> 494 model.fit(
495 training_dataset, validation_data=eval_dataset, epochs=training_args.num_train_epochs, callbacks=callbacks
496 )
c:\python38\lib\site-packages\tensorflow\python\keras\engine\training.py in fit(self, x, y, batch_size, epochs, verbose, callbacks, validation_split, validation_data, shuffle, class_weight, sample_weight, initial_epoch, steps_per_epoch, validation_steps, validation_batch_size, validation_freq, max_queue_size, workers, use_multiprocessing)
1086 self._maybe_load_initial_epoch_from_ckpt(initial_epoch))
1087 logs = None
-> 1088 for epoch, iterator in data_handler.enumerate_epochs():
1089 self.reset_metrics()
1090 callbacks.on_epoch_begin(epoch)
c:\python38\lib\site-packages\tensorflow\python\keras\engine\data_adapter.py in enumerate_epochs(self)
1132 with self._truncate_execution_to_epoch():
1133 data_iterator = iter(self._dataset)
-> 1134 for epoch in range(self._initial_epoch, self._epochs):
1135 if self._insufficient_data: # Set by `catch_stop_iteration`.
1136 break
TypeError: 'float' object cannot be interpreted as an integer
<|||||>Good catch, thank you! This was totally my fault, and has now been fixed in #11379 . If you pull the latest version of the library, training should work.<|||||>This issue has been automatically marked as stale because it has not had recent activity. If you think this still needs to be addressed please comment on this thread.
Please note that issues that do not follow the [contributing guidelines](https://github.com/huggingface/transformers/blob/master/CONTRIBUTING.md) are likely to be ignored. |
transformers | 11,170 | closed | [examples/translation] support mBART-50 and M2M100 fine-tuning | # What does this PR do?
the `run_translation.py` does not support fine-tuning mBART-50 and M2M100 as we need to set `src_lang` and `tgt_lang` attributes, but the script only checks for `MBartTokenizer`, this PR
- adds the `MULTILINGUAL_TOKENIZERS` list where we can add all tokenizers which require setting `src_lang` and `target_lang` attributes, this avoids having multiple if/else statements (Thanks Sylvain!)
- adds the `--forced_bos_token` argument which is used to set the `config.forced_bos_token_id` attribute which is required for mBART-50 and M2M100 during generation to be able to force the target language token as the first generated token. We could use the `--target_language` argument to set this, but this attribute shouldn't be set auto-magically as generations change completely depending upon the forced id, so IMO it's better to ask the user to explicitly provide it.
| 04-09-2021 18:14:21 | 04-09-2021 18:14:21 | @patil-suraj , can you please help in suggesting how to finetune m2m100 on more than one-pair.I am able to finetune for one lang pair using below script:
CUDA_VISIBLE_DEVICES=0,1,2,3,6 python -m torch.distributed.run --nproc_per_node=5 run_translation.py --model_name_or_path=m2m100_418M_new_token --do_train --do_eval --source_lang ja --target_lang en --fp16=True --evaluation_strategy epoch --output_dir bigfrall --per_device_train_batch_size=48 --per_device_eval_batch_size=48 --overwrite_output_dir --forced_bos_token "en" --train_file orig_manga/orig/train_exp_frame_50k.json --validation_file orig_manga/orig/valid_exp_frame_50k.json --tokenizer_name tokenizer_new_token --num_train_epochs 50 --save_total_limit=5 --save_strategy=epoch --load_best_model_at_end=True --predict_with_generate
But, now I want to finetune it on ja-en and ja-zh pairs. How to pass these both languages?<|||||>Hi @nikhiljaiswal !
It would be nice if you ask this question on the [forum ](https://discuss.huggingface.co/). PR comments won't be a good place to discuss this. Thanks! |
transformers | 11,169 | closed | Unable to resume checkpoints with TFBertModel using tf.distribute.Strategy and a custom LM head that shares the underlying TFBertEmbeddings layer | ## Environment info
- `transformers` version: 4.5.0
- Platform: Linux (Ubuntu 18.04, 20.04 + CentOs)
- Python version: 3.7.4
- PyTorch version (GPU?): N/A
- Tensorflow version (GPU?): 2.4.1 GPU
- Using GPU in script?: Yes
- Using distributed or parallel set-up in script?: Yes, using MirroredStrategy
Models:
- BERT: @LysandreJik
## Information
Model I am using (Bert, XLNet ...):
The problem arises when using:
* TFBertModel
* tf.distribute.MirroredStrategy
* A custom LM head that shares a TFBertEmbeddings layer with TFBertModel
The tasks I am working on is:
* [x] my own task and dataset: (not relevant)
## To reproduce
Steps to reproduce the behavior:
1. Train a BERT model, with MirroredStrategy, based on the TFBertModel class using using a custom head with a shared TFBertEmbeddings layer
2. Stop training
3. Attempt to resume using checkpoints
<!-- If you have code snippets, error messages, stack traces please provide them here as well.
Important! Use code tags to correctly format your code. See https://help.github.com/en/github/writing-on-github/creating-and-highlighting-code-blocks#syntax-highlighting
Do not use screenshots, as they are hard to read and (more importantly) don't allow others to copy-and-paste your code.-->
## Expected behavior
I should be able to re-load checkpoints trained with shared layers under a MirroredStrategy
## Problem
Someone has used tf.name_scope() and assumed that would change anything about the variable names in tf 2.x (It does not, it only modifies the names of ops)
See the build method of TFBertEmbeddings: https://github.com/huggingface/transformers/blob/cd56f3fe7eae4a53a9880e3f5e8f91877a78271c/src/transformers/models/bert/modeling_tf_bert.py#L155
In the above referenced code the variable name "embeddings" is used for both of the variables created with the property names "token_type_embeddings" and "position_embeddings". This does not matter in most cases as TensorFlow 2.x will use the property names of the variables, i.e. the object hierarchy path, and not the variable name given to the add_weight member function of a given Keras layer.
But it does matter in this case, it would seem, as the Distribution Strategy has issues resolving where to assign the saved variable from a checkpoint given that they have the same name.
## Proposed solution
Stop the meaningless use of tf.name_scope() (See: https://www.tensorflow.org/api_docs/python/tf/name_scope)
Give variables different names with the add_weight member function
## Stack trace
`ValueError: in user code: /opt/ml/code/unsilo_ml/python/supervisors/TF2custom_keras_loop_supervisor.py:395 train_epoch * loss, local_global_step = distributed_train_step(x) /opt/ml/code/unsilo_ml/python/supervisors/TF2custom_keras_loop_supervisor.py:359 distributed_train_step * per_replica_losses, per_replica_global_step = self.dist_strategy.run( /opt/ml/code/unsilo_ml/python/supervisors/TF2custom_keras_loop_supervisor.py:313 train_step * predictions = keras_model(features, training=True) /opt/ml/code/unsilo_ml/python/models/base_models/base_model.py:72 call * return self.build_forward_pass(training=training, inputs=inputs) /opt/ml/code/unsilo_ml/python/models/multitask_model.py:103 build_forward_pass * inputs_with_encoder_output = self.prepare_inputs_with_encoder_output( /opt/ml/code/unsilo_ml/python/models/multitask_model.py:147 prepare_inputs_with_encoder_output * encoder_outputs = self.encoder(inputs, training=training) /opt/ml/code/unsilo_ml/python/modules/encoders/util_encoders/pipe_encoder.py:18 call * encoder_output = self.resolve_tensor_dict( /opt/ml/code/unsilo_ml/python/modules/encoders/bert_encoder.py:83 call * hidden_states = self.bert_model( /opt/conda/lib/python3.7/site-packages/transformers/models/bert/modeling_tf_bert.py:887 call * outputs = self.bert( /opt/conda/lib/python3.7/site-packages/transformers/models/bert/modeling_tf_bert.py:645 call * embedding_output = self.embeddings( /opt/conda/lib/python3.7/site-packages/tensorflow/python/keras/engine/base_layer.py:1008 __call__ ** self._maybe_build(inputs) /opt/conda/lib/python3.7/site-packages/tensorflow/python/keras/engine/base_layer.py:2710 _maybe_build self.build(input_shapes) # pylint:disable=not-callable /opt/conda/lib/python3.7/site-packages/transformers/models/bert/modeling_tf_bert.py:159 build initializer=get_initializer(self.initializer_range), /opt/conda/lib/python3.7/site-packages/tensorflow/python/keras/engine/base_layer.py:639 add_weight caching_device=caching_device) /opt/conda/lib/python3.7/site-packages/tensorflow/python/training/tracking/base.py:810 _add_variable_with_custom_getter **kwargs_for_getter) /opt/conda/lib/python3.7/site-packages/tensorflow/python/keras/engine/base_layer_utils.py:142 make_variable shape=variable_shape if variable_shape else None) /opt/conda/lib/python3.7/site-packages/tensorflow/python/ops/variables.py:260 __call__ return cls._variable_v1_call(*args, **kwargs) /opt/conda/lib/python3.7/site-packages/tensorflow/python/ops/variables.py:221 _variable_v1_call shape=shape) /opt/conda/lib/python3.7/site-packages/tensorflow/python/ops/variables.py:67 getter return captured_getter(captured_previous, **kwargs) /opt/conda/lib/python3.7/site-packages/tensorflow/python/distribute/shared_variable_creator.py:69 create_new_variable v = next_creator(**kwargs) /opt/conda/lib/python3.7/site-packages/tensorflow/python/ops/variables.py:67 getter return captured_getter(captured_previous, **kwargs) /opt/conda/lib/python3.7/site-packages/tensorflow/python/distribute/distribute_lib.py:2083 creator_with_resource_vars created = self._create_variable(next_creator, **kwargs) /opt/conda/lib/python3.7/site-packages/tensorflow/python/distribute/mirrored_strategy.py:489 _create_variable distribute_utils.VARIABLE_POLICY_MAPPING, **kwargs) /opt/conda/lib/python3.7/site-packages/tensorflow/python/distribute/distribute_utils.py:311 create_mirrored_variable value_list = real_mirrored_creator(**kwargs) /opt/conda/lib/python3.7/site-packages/tensorflow/python/distribute/mirrored_strategy.py:481 _real_mirrored_creator v = next_creator(**kwargs) /opt/conda/lib/python3.7/site-packages/tensorflow/python/ops/variables.py:67 getter return captured_getter(captured_previous, **kwargs) /opt/conda/lib/python3.7/site-packages/tensorflow/python/eager/def_function.py:714 variable_capturing_scope lifted_initializer_graph=lifted_initializer_graph, **kwds) /opt/conda/lib/python3.7/site-packages/tensorflow/python/ops/variables.py:264 __call__ return super(VariableMetaclass, cls).__call__(*args, **kwargs) /opt/conda/lib/python3.7/site-packages/tensorflow/python/eager/def_function.py:227 __init__ initial_value = initial_value() /opt/conda/lib/python3.7/site-packages/tensorflow/python/training/tracking/base.py:82 __call__ self._checkpoint_position, shape, shard_info=shard_info) /opt/conda/lib/python3.7/site-packages/tensorflow/python/training/tracking/base.py:117 __init__ self.wrapped_value.set_shape(shape) /opt/conda/lib/python3.7/site-packages/tensorflow/python/framework/ops.py:1217 set_shape (self.shape, shape)) ValueError: Tensor's shape (512, 768) is not compatible with supplied shape [2, 768] | ValueError: in user code: /opt/ml/code/unsilo_ml/python/supervisors/TF2custom_keras_loop_supervisor.py:395 train_epoch * loss, local_global_step = distributed_train_step(x) /opt/ml/code/unsilo_ml/python/supervisors/TF2custom_keras_loop_supervisor.py:359 distributed_train_step * per_replica_losses, per_replica_global_step = self.dist_strategy.run( /opt/ml/code/unsilo_ml/python/supervisors/TF2custom_keras_loop_supervisor.py:313 train_step * predictions = keras_model(features, training=True) /opt/ml/code/unsilo_ml/python/models/base_models/base_model.py:72 call * return self.build_forward_pass(training=training, inputs=inputs) /opt/ml/code/unsilo_ml/python/models/multitask_model.py:103 build_forward_pass * inputs_with_encoder_output = self.prepare_inputs_with_encoder_output( /opt/ml/code/unsilo_ml/python/models/multitask_model.py:147 prepare_inputs_with_encoder_output * encoder_outputs = self.encoder(inputs, training=training) /opt/ml/code/unsilo_ml/python/modules/encoders/util_encoders/pipe_encoder.py:18 call * encoder_output = self.resolve_tensor_dict( /opt/ml/code/unsilo_ml/python/modules/encoders/bert_encoder.py:83 call * hidden_states = self.bert_model( /opt/conda/lib/python3.7/site-packages/transformers/models/bert/modeling_tf_bert.py:887 call * outputs = self.bert( /opt/conda/lib/python3.7/site-packages/transformers/models/bert/modeling_tf_bert.py:645 call * embedding_output = self.embeddings( /opt/conda/lib/python3.7/site-packages/tensorflow/python/keras/engine/base_layer.py:1008 __call__ ** self._maybe_build(inputs) /opt/conda/lib/python3.7/site-packages/tensorflow/python/keras/engine/base_layer.py:2710 _maybe_build self.build(input_shapes) # pylint:disable=not-callable /opt/conda/lib/python3.7/site-packages/transformers/models/bert/modeling_tf_bert.py:159 build initializer=get_initializer(self.initializer_range), /opt/conda/lib/python3.7/site-packages/tensorflow/python/keras/engine/base_layer.py:639 add_weight caching_device=caching_device) /opt/conda/lib/python3.7/site-packages/tensorflow/python/training/tracking/base.py:810 _add_variable_with_custom_getter **kwargs_for_getter) /opt/conda/lib/python3.7/site-packages/tensorflow/python/keras/engine/base_layer_utils.py:142 make_variable shape=variable_shape if variable_shape else None) /opt/conda/lib/python3.7/site-packages/tensorflow/python/ops/variables.py:260 __call__ return cls._variable_v1_call(*args, **kwargs) /opt/conda/lib/python3.7/site-packages/tensorflow/python/ops/variables.py:221 _variable_v1_call shape=shape) /opt/conda/lib/python3.7/site-packages/tensorflow/python/ops/variables.py:67 getter return captured_getter(captured_previous, **kwargs) /opt/conda/lib/python3.7/site-packages/tensorflow/python/distribute/shared_variable_creator.py:69 create_new_variable v = next_creator(**kwargs) /opt/conda/lib/python3.7/site-packages/tensorflow/python/ops/variables.py:67 getter return captured_getter(captured_previous, **kwargs) /opt/conda/lib/python3.7/site-packages/tensorflow/python/distribute/distribute_lib.py:2083 creator_with_resource_vars created = self._create_variable(next_creator, **kwargs) /opt/conda/lib/python3.7/site-packages/tensorflow/python/distribute/mirrored_strategy.py:489 _create_variable distribute_utils.VARIABLE_POLICY_MAPPING, **kwargs) /opt/conda/lib/python3.7/site-packages/tensorflow/python/distribute/distribute_utils.py:311 create_mirrored_variable value_list = real_mirrored_creator(**kwargs) /opt/conda/lib/python3.7/site-packages/tensorflow/python/distribute/mirrored_strategy.py:481 _real_mirrored_creator v = next_creator(**kwargs) /opt/conda/lib/python3.7/site-packages/tensorflow/python/ops/variables.py:67 getter return captured_getter(captured_previous, **kwargs) /opt/conda/lib/python3.7/site-packages/tensorflow/python/eager/def_function.py:714 variable_capturing_scope lifted_initializer_graph=lifted_initializer_graph, **kwds) /opt/conda/lib/python3.7/site-packages/tensorflow/python/ops/variables.py:264 __call__ return super(VariableMetaclass, cls).__call__(*args, **kwargs) /opt/conda/lib/python3.7/site-packages/tensorflow/python/eager/def_function.py:227 __init__ initial_value = initial_value() /opt/conda/lib/python3.7/site-packages/tensorflow/python/training/tracking/base.py:82 __call__ self._checkpoint_position, shape, shard_info=shard_info) /opt/conda/lib/python3.7/site-packages/tensorflow/python/training/tracking/base.py:117 __init__ self.wrapped_value.set_shape(shape) /opt/conda/lib/python3.7/site-packages/tensorflow/python/framework/ops.py:1217 set_shape (self.shape, shape)) ValueError: Tensor's shape (512, 768) is not compatible with supplied shape [2, 768]` | 04-09-2021 17:35:30 | 04-09-2021 17:35:30 | I have tested it locally under tf.distribute.OneDeviceStrategy and the problem seems to be the same.
```
Traceback (most recent call last):
File "/home/marhlder/unsilo/unsilo-ml/unsilo_ml/python/run.py", line 73, in <module>
cli()
File "/home/marhlder/anaconda3/envs/unsilo-ml/lib/python3.7/site-packages/click/core.py", line 829, in __call__
return self.main(*args, **kwargs)
File "/home/marhlder/anaconda3/envs/unsilo-ml/lib/python3.7/site-packages/click/core.py", line 782, in main
rv = self.invoke(ctx)
File "/home/marhlder/anaconda3/envs/unsilo-ml/lib/python3.7/site-packages/click/core.py", line 1259, in invoke
return _process_result(sub_ctx.command.invoke(sub_ctx))
File "/home/marhlder/anaconda3/envs/unsilo-ml/lib/python3.7/site-packages/click/core.py", line 1066, in invoke
return ctx.invoke(self.callback, **ctx.params)
File "/home/marhlder/anaconda3/envs/unsilo-ml/lib/python3.7/site-packages/click/core.py", line 610, in invoke
return callback(*args, **kwargs)
File "/home/marhlder/unsilo/unsilo-ml/unsilo_ml/python/run.py", line 31, in train
provisioner.train()
File "/home/marhlder/unsilo/unsilo-ml/mlops/provisioning/local/local.py", line 72, in train
**self.entry_point_parameters,
File "/home/marhlder/unsilo/unsilo-ml//unsilo_ml/python/provisioner_entry_point.py", line 78, in run
tc.train(tracker=tracker)
File "/home/marhlder/unsilo/unsilo-ml/unsilo_ml/python/module_composition/train_composer.py", line 83, in train
return self.model_supervisor.train(**kwargs)
File "/home/marhlder/unsilo/unsilo-ml/unsilo_ml/python/supervisors/TF2custom_keras_loop_supervisor.py", line 463, in train
train_epoch()
File "/home/marhlder/anaconda3/envs/unsilo-ml/lib/python3.7/site-packages/tensorflow/python/eager/def_function.py", line 828, in __call__
result = self._call(*args, **kwds)
File "/home/marhlder/anaconda3/envs/unsilo-ml/lib/python3.7/site-packages/tensorflow/python/eager/def_function.py", line 871, in _call
self._initialize(args, kwds, add_initializers_to=initializers)
File "/home/marhlder/anaconda3/envs/unsilo-ml/lib/python3.7/site-packages/tensorflow/python/eager/def_function.py", line 726, in _initialize
*args, **kwds))
File "/home/marhlder/anaconda3/envs/unsilo-ml/lib/python3.7/site-packages/tensorflow/python/eager/function.py", line 2969, in _get_concrete_function_internal_garbage_collected
graph_function, _ = self._maybe_define_function(args, kwargs)
File "/home/marhlder/anaconda3/envs/unsilo-ml/lib/python3.7/site-packages/tensorflow/python/eager/function.py", line 3361, in _maybe_define_function
graph_function = self._create_graph_function(args, kwargs)
File "/home/marhlder/anaconda3/envs/unsilo-ml/lib/python3.7/site-packages/tensorflow/python/eager/function.py", line 3206, in _create_graph_function
capture_by_value=self._capture_by_value),
File "/home/marhlder/anaconda3/envs/unsilo-ml/lib/python3.7/site-packages/tensorflow/python/framework/func_graph.py", line 990, in func_graph_from_py_func
func_outputs = python_func(*func_args, **func_kwargs)
File "/home/marhlder/anaconda3/envs/unsilo-ml/lib/python3.7/site-packages/tensorflow/python/eager/def_function.py", line 634, in wrapped_fn
out = weak_wrapped_fn().__wrapped__(*args, **kwds)
File "/home/marhlder/anaconda3/envs/unsilo-ml/lib/python3.7/site-packages/tensorflow/python/framework/func_graph.py", line 977, in wrapper
raise e.ag_error_metadata.to_exception(e)
ValueError: in user code:
/home/marhlder/unsilo/unsilo-ml/unsilo_ml/python/supervisors/TF2custom_keras_loop_supervisor.py:395 train_epoch *
loss, local_global_step = distributed_train_step(x)
/home/marhlder/unsilo/unsilo-ml/unsilo_ml/python/supervisors/TF2custom_keras_loop_supervisor.py:359 distributed_train_step *
per_replica_losses, per_replica_global_step = self.dist_strategy.run(
/home/marhlder/unsilo/unsilo-ml/unsilo_ml/python/supervisors/TF2custom_keras_loop_supervisor.py:313 train_step *
predictions = keras_model(features, training=True)
/home/marhlder/unsilo/unsilo-ml/unsilo_ml/python/models/base_models/base_model.py:72 call *
return self.build_forward_pass(training=training, inputs=inputs)
/home/marhlder/unsilo/unsilo-ml/unsilo_ml/python/models/multitask_model.py:103 build_forward_pass *
inputs_with_encoder_output = self.prepare_inputs_with_encoder_output(
/home/marhlder/unsilo/unsilo-ml/unsilo_ml/python/models/multitask_model.py:147 prepare_inputs_with_encoder_output *
encoder_outputs = self.encoder(inputs, training=training)
/home/marhlder/unsilo/unsilo-ml/unsilo_ml/python/modules/encoders/util_encoders/pipe_encoder.py:18 call *
encoder_output = self.resolve_tensor_dict(
/home/marhlder/unsilo/unsilo-ml/unsilo_ml/python/modules/encoders/bert_encoder.py:83 call *
hidden_states = self.bert_model(
/home/marhlder/anaconda3/envs/unsilo-ml/lib/python3.7/site-packages/transformers/models/bert/modeling_tf_bert.py:887 call *
outputs = self.bert(
/home/marhlder/anaconda3/envs/unsilo-ml/lib/python3.7/site-packages/transformers/models/bert/modeling_tf_bert.py:645 call *
embedding_output = self.embeddings(
/home/marhlder/anaconda3/envs/unsilo-ml/lib/python3.7/site-packages/tensorflow/python/keras/engine/base_layer.py:1008 __call__ **
self._maybe_build(inputs)
/home/marhlder/anaconda3/envs/unsilo-ml/lib/python3.7/site-packages/tensorflow/python/keras/engine/base_layer.py:2710 _maybe_build
self.build(input_shapes) # pylint:disable=not-callable
/home/marhlder/anaconda3/envs/unsilo-ml/lib/python3.7/site-packages/transformers/models/bert/modeling_tf_bert.py:159 build
initializer=get_initializer(self.initializer_range),
/home/marhlder/anaconda3/envs/unsilo-ml/lib/python3.7/site-packages/tensorflow/python/keras/engine/base_layer.py:639 add_weight
caching_device=caching_device)
/home/marhlder/anaconda3/envs/unsilo-ml/lib/python3.7/site-packages/tensorflow/python/training/tracking/base.py:810 _add_variable_with_custom_getter
**kwargs_for_getter)
/home/marhlder/anaconda3/envs/unsilo-ml/lib/python3.7/site-packages/tensorflow/python/keras/engine/base_layer_utils.py:142 make_variable
shape=variable_shape if variable_shape else None)
/home/marhlder/anaconda3/envs/unsilo-ml/lib/python3.7/site-packages/tensorflow/python/ops/variables.py:260 __call__
return cls._variable_v1_call(*args, **kwargs)
/home/marhlder/anaconda3/envs/unsilo-ml/lib/python3.7/site-packages/tensorflow/python/ops/variables.py:221 _variable_v1_call
shape=shape)
/home/marhlder/anaconda3/envs/unsilo-ml/lib/python3.7/site-packages/tensorflow/python/ops/variables.py:67 getter
return captured_getter(captured_previous, **kwargs)
/home/marhlder/anaconda3/envs/unsilo-ml/lib/python3.7/site-packages/tensorflow/python/distribute/distribute_lib.py:2083 creator_with_resource_vars
created = self._create_variable(next_creator, **kwargs)
/home/marhlder/anaconda3/envs/unsilo-ml/lib/python3.7/site-packages/tensorflow/python/distribute/one_device_strategy.py:278 _create_variable
return next_creator(**kwargs)
/home/marhlder/anaconda3/envs/unsilo-ml/lib/python3.7/site-packages/tensorflow/python/ops/variables.py:67 getter
return captured_getter(captured_previous, **kwargs)
/home/marhlder/anaconda3/envs/unsilo-ml/lib/python3.7/site-packages/tensorflow/python/eager/def_function.py:714 variable_capturing_scope
lifted_initializer_graph=lifted_initializer_graph, **kwds)
/home/marhlder/anaconda3/envs/unsilo-ml/lib/python3.7/site-packages/tensorflow/python/ops/variables.py:264 __call__
return super(VariableMetaclass, cls).__call__(*args, **kwargs)
/home/marhlder/anaconda3/envs/unsilo-ml/lib/python3.7/site-packages/tensorflow/python/eager/def_function.py:227 __init__
initial_value = initial_value()
/home/marhlder/anaconda3/envs/unsilo-ml/lib/python3.7/site-packages/tensorflow/python/training/tracking/base.py:82 __call__
self._checkpoint_position, shape, shard_info=shard_info)
/home/marhlder/anaconda3/envs/unsilo-ml/lib/python3.7/site-packages/tensorflow/python/training/tracking/base.py:117 __init__
self.wrapped_value.set_shape(shape)
/home/marhlder/anaconda3/envs/unsilo-ml/lib/python3.7/site-packages/tensorflow/python/framework/ops.py:1217 set_shape
(self.shape, shape))
ValueError: Tensor's shape (512, 768) is not compatible with supplied shape [2, 768]
Process finished with exit code 1
```
<|||||>#11202 <|||||>This issue has been automatically marked as stale because it has not had recent activity. If you think this still needs to be addressed please comment on this thread.
Please note that issues that do not follow the [contributing guidelines](https://github.com/huggingface/transformers/blob/master/CONTRIBUTING.md) are likely to be ignored. |
transformers | 11,168 | closed | [examples run_clm] fix _LazyModule hasher error | This PR fixes a problem I introduced in https://github.com/huggingface/transformers/pull/11145 and reported in https://github.com/huggingface/transformers/issues/11166
`datasets.fingerprint.Hasher` fails to run
```
hasher = Hasher()
hasher.update(tokenize_function)
```
getting:
```
TypeError: cannot pickle '_LazyModule' object
```
Because the logger object contains a lazy import.
The error was subtle as the exception was caught and not propagated but instead a warning was logged, which I didn't notice in the first place. Warnings aren't a great way to communicate problems. So we were getting now:
> [WARNING|tokenization_utils_base.py:3144] 2021-04-09 09:46:31,368 >> Token indices sequence length is longer than the specified maximum sequence length for this model (1462828 > 1024). Running this sequence through the model will result in indexing errors
> [WARNING|run_clm.py:326] 2021-04-09 09:46:31,368 >> ^^^^^^^^^^^^^^^^ Please ignore the warning above - this long input will be chunked into smaller bits before being passed to the model.
> 04/09/2021 09:46:31 - WARNING - datasets.fingerprint - Parameter 'function'=<function main.<locals>.tokenize_function at 0x7f434d90da60> of the transform datasets.arrow_dataset.Dataset._map_single couldn't be hashed properly, a random hash was used instead. Make sure your transforms and parameters are serializable with pickle or dill for the dataset fingerprinting and caching to work. If you reuse this transform, the caching mechanism will consider it to be different from the previous calls and recompute everything. This warning is only showed once. Subsequent hashing failures won't be showed.
So I fixed this by moving the logger object fetching to outside of the function to be hashed and then it all works.
Fixes: https://github.com/huggingface/transformers/issues/11166
@sgugger
| 04-09-2021 16:53:33 | 04-09-2021 16:53:33 | |
transformers | 11,167 | closed | added json dump and extraction of train run time | # What does this PR do?
This PR adjusts to the latest metric exposing changes that `train_runtime` is now logged as `hh:mm:ss.ms`. So instead of extracting the `train_runtime` from the logs it is using the `sagemaker-sdk` to get the full train time. Additionally, I added a JSON dump for all tests to share the result easier, when opening a new PR to upgrade the HF DLC. | 04-09-2021 16:52:02 | 04-09-2021 16:52:02 | |
transformers | 11,166 | closed | [run_clm] tokenize_function clarification makes it non-hashable => no-reusing cache | ## Environment info
<!-- You can run the command `transformers-cli env` and copy-and-paste its output below.
Don't forget to fill out the missing fields in that output! -->
- `transformers` version: master at commit acc851e1ff92835d2a3ee9774d9d0abfda6e3f36 (from yesterday)
- Platform:
- Python version:
- PyTorch version (GPU?):
- Tensorflow version (GPU?):
- Using GPU in script?:
- Using distributed or parallel set-up in script?:
### Who can help
@stas00 since you opened the PR #11145
## Information
Model I am using (Bert, XLNet ...):
The problem arises when using:
* [x ] the official example scripts: (give details below)
* [ ] my own modified scripts: (give details below)
## To reproduce
I am running the minimal command:
```bash
CUDA_VISIBLE_DEVICES=0 python examples/language-modeling/run_clm.py \
--model_name_or_path gpt2 \
--dataset_name ./data/bk --block_size 1024 \
--do_train \
--output_dir debug --overwrite_output_dir \
--preprocessing_num_workers 5
```
When it gets to line [331](https://github.com/huggingface/transformers/blob/60607465708814fe22aaa18b26a3aab3df110c1c/examples/language-modeling/run_clm.py#L331), datasets.map gives this warning:
> [WARNING|tokenization_utils_base.py:3143] 2021-04-09 15:48:53,408 >> Token indices sequence length is longer than the specified maximum sequence length for this model (191443 > 1024). Running this sequence through the model will result in indexing errors
> [WARNING|run_clm.py:333] 2021-04-09 15:48:53,408 >> ^^^^^^^^^^^^^^^^ Please ignore the warning above - this long input will be chunked into smaller bits before being passed to the model.
> 04/09/2021 15:48:53 - WARNING - 17900 - datasets.fingerprint - Parameter 'function'=<function tokenize_function at 0x7f747662c268> of the transform datasets.arrow_dataset._map_single couldn't be hashed properly, a random hash was used instead. Make sure your transforms and parameters are serializable with pickle or dill for the dataset fingerprinting and caching to work. If you reuse this transform, the caching mechanism will consider it to be different from the previous calls and recompute everything. This warning is only showed once. Subsequent hashing failures won't be showed.
Basically, something went wrong when trying to hash the `tokenize_function` (to produce the cache file name) => it doesn't use the pre-processed cache for the next launch.
The `tokenize_function` was originally
```python
def tokenize_function(examples):
output = tokenizer(examples[text_column_name])
return output
```
and became:
```python
def tokenize_function(examples):
tok_logger = transformers.utils.logging.get_logger("transformers.tokenization_utils_base")
with CaptureLogger(tok_logger) as cl:
output = tokenizer(examples[text_column_name])
# clm input could be much much longer than block_size
if "Token indices sequence length is longer than the" in cl.out:
tok_logger.warning(
"^^^^^^^^^^^^^^^^ Please ignore the warning above - this long input will be chunked into smaller bits before being passed to the model."
)
return output
```
| 04-09-2021 16:01:37 | 04-09-2021 16:01:37 | Thank you for the report, @VictorSanh!
I can reproduce the problem separately:
```
import transformers
from transformers import AutoTokenizer
from transformers.testing_utils import CaptureLogger
tokenizer = AutoTokenizer.from_pretrained("t5-small")
def tokenize_function(examples):
tok_logger = transformers.utils.logging.get_logger("transformers.tokenization_utils_base")
with CaptureLogger(tok_logger) as cl:
output = tokenizer(examples[text_column_name])
# clm input could be much much longer than block_size
if "Token indices sequence length is longer than the" in cl.out:
tok_logger.warning(
"^^^^^^^^^^^^^^^^ Please ignore the warning above - this long input will be chunked into smaller bits before being passed to the model."
)
return output
def tokenize_function2(examples):
return tokenizer(examples[text_column_name])
```
This works (original function)
```
from datasets.fingerprint import Hasher
hasher = Hasher()
hasher.update(tokenize_function2)
```
This crashes:
```
from datasets.fingerprint import Hasher
hasher = Hasher()
hasher.update(tokenize_function)
```
```
TypeError: cannot pickle '_LazyModule' object
```
I thought I made a mistake on my side, but I saw this problem yesterday in a totally different situation:
https://github.com/huggingface/datasets/issues/2194
Let me investigate some more and will get back to you.
Until then to enable your work please just put back:
```
def tokenize_function(examples):
return tokenizer(examples[text_column_name])
```<|||||>This should fix the problem: https://github.com/huggingface/transformers/pull/11168
<|||||>you rock!<|||||>> This should fix the problem: #11168
I modified my code according to your way, but still didn't solve the problem.
I run the official example scripts run_clm.py with multiprocessing
```
Traceback (most recent call last):
File "/usr/local/anaconda3/lib/python3.6/multiprocessing/process.py", line 258, in _bootstrap
self.run()
File "/usr/local/anaconda3/lib/python3.6/multiprocessing/process.py", line 93, in run
self._target(*self._args, **self._kwargs)
File "/media/cfs/gonglixing/9Nctl/gpt_v2/run_clm_v2.py", line 484, in init_process
fn(rank, size)
File "/media/cfs/gonglixing/9Nctl/gpt_v2/run_clm_v2.py", line 350, in main
load_from_cache_file=not data_args.overwrite_cache,
File "/media/cfs/gonglixing/.pylib/lib/python3.6/site-packages/datasets/dataset_dict.py", line 489, in map
for k, dataset in self.items()
File "/media/cfs/gonglixing/.pylib/lib/python3.6/site-packages/datasets/dataset_dict.py", line 489, in <dictcomp>
for k, dataset in self.items()
File "/media/cfs/gonglixing/.pylib/lib/python3.6/site-packages/datasets/arrow_dataset.py", line 1693, in map
transformed_shards = [r.get() for r in results]
File "/media/cfs/gonglixing/.pylib/lib/python3.6/site-packages/datasets/arrow_dataset.py", line 1693, in <listcomp>
transformed_shards = [r.get() for r in results]
File "/media/cfs/gonglixing/.pylib/lib/python3.6/site-packages/multiprocess/pool.py", line 644, in get
raise self._value
File "/media/cfs/gonglixing/.pylib/lib/python3.6/site-packages/multiprocess/pool.py", line 424, in _handle_tasks
put(task)
File "/media/cfs/gonglixing/.pylib/lib/python3.6/site-packages/multiprocess/connection.py", line 209, in send
self._send_bytes(_ForkingPickler.dumps(obj))
File "/media/cfs/gonglixing/.pylib/lib/python3.6/site-packages/multiprocess/reduction.py", line 54, in dumps
cls(buf, protocol, *args, **kwds).dump(obj)
File "/media/cfs/gonglixing/.pylib/lib/python3.6/site-packages/dill/_dill.py", line 498, in dump
StockPickler.dump(self, obj)
File "/usr/local/anaconda3/lib/python3.6/pickle.py", line 409, in dump
self.save(obj)
File "/usr/local/anaconda3/lib/python3.6/pickle.py", line 476, in save
f(self, obj) # Call unbound method with explicit self
File "/usr/local/anaconda3/lib/python3.6/pickle.py", line 751, in save_tuple
save(element)
File "/usr/local/anaconda3/lib/python3.6/pickle.py", line 476, in save
f(self, obj) # Call unbound method with explicit self
File "/media/cfs/gonglixing/.pylib/lib/python3.6/site-packages/dill/_dill.py", line 990, in save_module_dict
StockPickler.save_dict(pickler, obj)
File "/usr/local/anaconda3/lib/python3.6/pickle.py", line 821, in save_dict
self._batch_setitems(obj.items())
File "/usr/local/anaconda3/lib/python3.6/pickle.py", line 847, in _batch_setitems
save(v)
File "/usr/local/anaconda3/lib/python3.6/pickle.py", line 476, in save
f(self, obj) # Call unbound method with explicit self
File "/media/cfs/gonglixing/.pylib/lib/python3.6/site-packages/dill/_dill.py", line 1496, in save_function
obj.__dict__, fkwdefaults), obj=obj)
File "/usr/local/anaconda3/lib/python3.6/pickle.py", line 610, in save_reduce
save(args)
File "/usr/local/anaconda3/lib/python3.6/pickle.py", line 476, in save
f(self, obj) # Call unbound method with explicit self
File "/usr/local/anaconda3/lib/python3.6/pickle.py", line 751, in save_tuple
save(element)
File "/usr/local/anaconda3/lib/python3.6/pickle.py", line 476, in save
f(self, obj) # Call unbound method with explicit self
File "/media/cfs/gonglixing/.pylib/lib/python3.6/site-packages/dill/_dill.py", line 990, in save_module_dict
StockPickler.save_dict(pickler, obj)
File "/usr/local/anaconda3/lib/python3.6/pickle.py", line 821, in save_dict
self._batch_setitems(obj.items())
File "/usr/local/anaconda3/lib/python3.6/pickle.py", line 847, in _batch_setitems
save(v)
File "/usr/local/anaconda3/lib/python3.6/pickle.py", line 496, in save
rv = reduce(self.proto)
TypeError: can't pickle _LazyModule objects
```<|||||>Since we can't see your custom code, it's hard to tell why you have a problem. At least checking your traceback it doesn't match the current `run_clm.py` version in master. Perhaps you are running an unmodified code that still has the original problem?
Perhaps give a try to `run_clm.py` in master?
If it doesn't work, please open a new Issue and give us all the required details to be able to reproduce the problem. And tag me to it. Thank you.<|||||>> Since we can't see your custom code, it's hard to tell why you have a problem. At least checking your traceback it doesn't match the current `run_clm.py` version in master. Perhaps you are running an unmodified code that still has the original problem?
>
> Perhaps give a try to `run_clm.py` in master?
>
> If it doesn't work, please open a new Issue and give us all the required details to be able to reproduce the problem. And tag me to it. Thank you.
I tried the `run_clm.py` in master, but it still doesn't work. I will create a new issue.
Thanks for your reply!
|
transformers | 11,165 | closed | tokenizer.encode_plus returns torch.tensors loaded on the desired device | # 🚀 Feature request
add device attribute to tokenizer.encode_plus so when it returns a torch.tensors it loads it on the desired device
## Motivation
- to pass the output of tokenizer to the model, one only can unpack the returned output using ** without bothering about the content of tokenizer, that only true when with cpu, but for gpu u need to unpack the output and load each input to device, and then pass them to the model.
this process will be also frustrating if you don't know the keys from the output or when you want to switch from a model to another -eg from bert to roberta, roberta doesnt need token_type_ids-.
| 04-09-2021 14:39:33 | 04-09-2021 14:39:33 | Hi! You can cast the `BatchEncoding` output by `encode_plus` to your device:
```py
model_input = tokenizer.encode_plus(xxx, return_tensors="pt")
model_input.to("cuda")
```<|||||>niiice, thank you!!
|
transformers | 11,164 | closed | error while training wave2vec on arabic text | Traceback (most recent call last):
File "/content/transformers/examples/research_projects/wav2vec2/run_asr.py", line 480, in <module>
main()
File "/content/transformers/examples/research_projects/wav2vec2/run_asr.py", line 430, in main
num_proc=2,
File "/usr/local/lib/python3.7/dist-packages/datasets/dataset_dict.py", line 448, in map
for k, dataset in self.items()
File "/usr/local/lib/python3.7/dist-packages/datasets/dataset_dict.py", line 448, in <dictcomp>
for k, dataset in self.items()
File "/usr/local/lib/python3.7/dist-packages/datasets/arrow_dataset.py", line 1289, in map
update_data = does_function_return_dict(test_inputs, test_indices)
File "/usr/local/lib/python3.7/dist-packages/datasets/arrow_dataset.py", line 1260, in does_function_return_dict
function(*fn_args, indices, **fn_kwargs) if with_indices else function(*fn_args, **fn_kwargs)
File "/content/transformers/examples/research_projects/wav2vec2/run_asr.py", line 423, in prepare_dataset
batch["labels"] = processor(batch[data_args.target_text_column]).input_ids
File "/usr/local/lib/python3.7/dist-packages/transformers/models/wav2vec2/processing_wav2vec2.py", line 117, in __call__
return self.current_processor(*args, **kwargs)
File "/usr/local/lib/python3.7/dist-packages/transformers/tokenization_utils_base.py", line 2266, in __call__
**kwargs,
File "/usr/local/lib/python3.7/dist-packages/transformers/tokenization_utils_base.py", line 2451, in batch_encode_plus
**kwargs,
File "/usr/local/lib/python3.7/dist-packages/transformers/tokenization_utils.py", line 543, in _batch_encode_plus
verbose=verbose,
File "/usr/local/lib/python3.7/dist-packages/transformers/tokenization_utils.py", line 606, in _batch_prepare_for_model
return_attention_mask=return_attention_mask,
File "/usr/local/lib/python3.7/dist-packages/transformers/tokenization_utils_base.py", line 2579, in pad
while len(required_input[index]) == 0:
IndexError: list index out of range
[ ]
| 04-09-2021 14:05:44 | 04-09-2021 14:05:44 | Hello, Can you provide more information about the dataset you are using. The error message alone seems very vague, but pointing towards input.<|||||>Its my custom dataset. Arabic audio and arabic transcription<|||||>This issue has been automatically marked as stale because it has not had recent activity. If you think this still needs to be addressed please comment on this thread.
Please note that issues that do not follow the [contributing guidelines](https://github.com/huggingface/transformers/blob/master/CONTRIBUTING.md) are likely to be ignored. |
transformers | 11,163 | closed | Make `get_special_tokens_mask` consider all tokens | # What does this PR do?
As discovered via #11155, some tokenizers do not return the proper special tokens mask in the `get_special_tokens_mask` when `already_has_special_tokens=True` because they only check for the CLS and SEP tokens. This PR fixes that by delegating to the superclass the call when `already_has_special_tokens=True` (the generic method checks for all special tokens).
It also seems from the error message [here](https://github.com/huggingface/transformers/blob/b9b60c1630f63b54b10380ef8bf30ec323985553/src/transformers/tokenization_utils_base.py#L3091) that the `get_special_tokens_mask` method is not supposed to be implemented for fast tokenziers when `already_has_special_tokens=True`, so this PR removes this method from the fast tokenizers where it exists, except for a select few that have a different implementation.
Fixes #11155 | 04-09-2021 14:01:10 | 04-09-2021 14:01:10 | Thanks a lot for your super-fast feedback! Your projects are big inspiration to me. Thank you. |
transformers | 11,162 | closed | ZeroDivisionError: float division by zero after some epochs while training using run_mmimdb.py | I am trying to train image+test model using the run_mmiddb.py(https://github.com/huggingface/transformers/tree/master/examples/research_projects/mm-imdb). I have two classes in this task.
Initially i ran it for 1 epoch with below input parameters and it went well::
--model_name_or_path bert-base-cased \
--max_seq_length 512 \
--stride_len 112 \
--num_image_embeds 3 \
--per_gpu_train_batch_size 8 \
--per_gpu_eval_batch_size 16 \
--gradient_accumulation_steps 20 \
--patience 5 \
--fp16 \
and from log i have "Num examples = 5703" while training and in evaluation "Num examples = 1176".
Now, when i am running it for 20 epochs and reduced gradient_accumulation_steps to 6. It gave error as
"ZeroDivisionError: float division by zero"
maybe after 13 epochs i guess(I lost log some of it).
Also the loss from startig onwards is "nan". I dont know how. I see the loss fucntion as
"criterion = nn.BCEWithLogitsLoss(pos_weight=label_weights)"
Even if loss is "nan" from starting, I see 'macro_f1' going up from 55 to 58 and again back to 55 before this above mentioned final error.
Any suggestion/solution is appreciated. Thanks | 04-09-2021 13:53:14 | 04-09-2021 13:53:14 | This issue has been automatically marked as stale because it has not had recent activity. If you think this still needs to be addressed please comment on this thread.
Please note that issues that do not follow the [contributing guidelines](https://github.com/huggingface/transformers/blob/master/CONTRIBUTING.md) are likely to be ignored. |
transformers | 11,161 | closed | Correct typographical error in README.md | Corrected a typo ('Downlowd' to 'Download')
# What does this PR do?
<!--
Congratulations! You've made it this far! You're not quite done yet though.
Once merged, your PR is going to appear in the release notes with the title you set, so make sure it's a great title that fully reflects the extent of your awesome contribution.
Then, please replace this with a description of the change and which issue is fixed (if applicable). Please also include relevant motivation and context. List any dependencies (if any) that are required for this change.
Once you're done, someone will review your PR shortly (see the section "Who can review?" below to tag some potential reviewers). They may suggest changes to make the code even better. If no one reviewed your PR after a week has passed, don't hesitate to post a new comment @-mentioning the same persons---sometimes notifications get lost.
-->
<!-- Remove if not applicable -->
Fixes # (issue)
## Before submitting
- [x] This PR fixes a typo or improves the docs (you can dismiss the other checks if that's the case).
- [ ] Did you read the [contributor guideline](https://github.com/huggingface/transformers/blob/master/CONTRIBUTING.md#start-contributing-pull-requests),
Pull Request section?
- [ ] Was this discussed/approved via a Github issue or the [forum](https://discuss.huggingface.co/)? Please add a link
to it if that's the case.
- [ ] Did you make sure to update the documentation with your changes? Here are the
[documentation guidelines](https://github.com/huggingface/transformers/tree/master/docs), and
[here are tips on formatting docstrings](https://github.com/huggingface/transformers/tree/master/docs#writing-source-documentation).
- [ ] Did you write any new necessary tests?
## Who can review?
Anyone in the community is free to review the PR once the tests have passed. Feel free to tag
members/contributors which may be interested in your PR.
<!-- Your PR will be replied to more quickly if you can figure out the right person to tag with @
If you know how to use git blame, that is the easiest way, otherwise, here is a rough guide of **who to tag**.
Please tag fewer than 3 people.
Models:
- albert, bert, xlm: @LysandreJik
- blenderbot, bart, marian, pegasus, encoderdecoder, t5: @patrickvonplaten, @patil-suraj
- longformer, reformer, transfoxl, xlnet: @patrickvonplaten
- fsmt: @stas00
- funnel: @sgugger
- gpt2: @patrickvonplaten, @LysandreJik
- rag: @patrickvonplaten, @lhoestq
- tensorflow: @LysandreJik
Library:
- benchmarks: @patrickvonplaten
- deepspeed: @stas00
- ray/raytune: @richardliaw, @amogkam
- text generation: @patrickvonplaten
- tokenizers: @n1t0, @LysandreJik
- trainer: @sgugger
- pipelines: @LysandreJik
Documentation: @sgugger
HF projects:
- datasets: [different repo](https://github.com/huggingface/datasets)
- rust tokenizers: [different repo](https://github.com/huggingface/tokenizers)
Examples:
- maintained examples (not research project or legacy): @sgugger, @patil-suraj
- research_projects/bert-loses-patience: @JetRunner
- research_projects/distillation: @VictorSanh
-->
| 04-09-2021 12:20:41 | 04-09-2021 12:20:41 | |
transformers | 11,160 | closed | Why optimizer need split parameter group? | I want to ask some question about follow codes:
```
no_decay = ['bias', 'LayerNorm.weight']
optimizer_grouped_parameters = [
{'params': [p for n, p in model.named_parameters() if not any(nd in n for nd in no_decay)], 'weight_decay': 0.01},
{'params': [p for n, p in model.named_parameters() if any(nd in n for nd in no_decay)], 'weight_decay': 0.0}
]
optimizer = AdamW(optimizer_grouped_parameters, lr=1e-5)
```
if i hide `{'params': [p for n, p in model.named_parameters() if any(nd in n for nd in no_decay)], 'weight_decay': 0.0}`.
Would it cause other problems? and why need split parameters group.
reference: https://huggingface.co/transformers/training.html#fine-tuning-in-native-pytorch | 04-09-2021 10:16:24 | 04-09-2021 10:16:24 | Difficult to tell what happens when you apply weight_decay to those layers as well. I think you should just give it a try and tells us what has happened.
The shown code that applies different weight_decay to different parameters is in line with the original bert implementation ([link](https://github.com/google-research/bert/blob/eedf5716ce1268e56f0a50264a88cafad334ac61/optimization.py#L65)).<|||||>This issue has been automatically marked as stale because it has not had recent activity. If you think this still needs to be addressed please comment on this thread.
Please note that issues that do not follow the [contributing guidelines](https://github.com/huggingface/transformers/blob/master/CONTRIBUTING.md) are likely to be ignored. |
transformers | 11,159 | closed | LM finetuning on domain specific unlabelled data | Hello Team,
Thanks a lot for the awesome work!
Can you please tell me how to finetune a(any) MLM model on domain specific corpus ? I am following this [link](https://colab.research.google.com/github/huggingface/notebooks/blob/master/examples/language_modeling.ipynb) obtained from the huggingface documentation. Is this the procedure I should be following ? if this is how it is done, how will this update the vocabulary to adapt to new tokens of my domain specific corpus ?
Thanks in advance. | 04-09-2021 10:14:24 | 04-09-2021 10:14:24 | Hello, thanks for opening an issue! We try to keep the github issues for bugs/feature requests.
Could you ask your question on the [forum](https://discusss.huggingface.co) instead?
Thanks! |
transformers | 11,158 | closed | Why padding tokens can be masked in albert model? Is it bug or right? | I tried to run run_mlm.py for bert model and albert model.
"pad" token is not masked when I run bert-base-uncased model , but "pad" token can be masked when I run albert-base-v2
[bert command]
```
% python run_mlm.py --model_name_or_path bert-base-uncased --dataset_name wikitext --dataset_config_name wikitext-2-raw-v1 --do_train --do_eval --output_dir ./tmp/test-mlm --line_by_line
```
[albert command]
```
% python run_mlm.py --model_name_or_path albert-base-v2 --dataset_name wikitext --dataset_config_name wikitext-2-raw-v1 --do_train --do_eval --output_dir ./tmp/test-mlm --line_by_line
```
In examples/language-modeliing/run_mlm.py, I try to call tokenizer.get_special_tokens_mask.
```
tokenizer = AutoTokenizer.from_pretrained(model_args.model_name_or_path, **tokenizer_kwargs)
print(tokenizer.get_special_tokens_mask([0, 100, 101, 102, 2, 3, 4], already_has_special_tokens=True))
```
"get_special_tokens_mask" function is called from "class PreTrainedTokenizerBase" when I run bert-base-uncased, but "get_special_tokens_mask" function is called from "class AlbertTokenizerFast" whenn I run albert-base-v2.
In PretrainedToknizerBase class,
```
def get_special_tokens_mask(
self, token_ids_0: List[int], token_ids_1: Optional[List[int]] = None, already_has_special_tokens: bool = False
) -> List[int]:
all_special_ids = self.all_special_ids # cache the property
special_tokens_mask = [1 if token in all_special_ids else 0 for token in token_ids_0]
return special_tokens_mask
```
However in AlbertTokenizerFast class,
```
def get_special_tokens_mask(
self, token_ids_0: List[int], token_ids_1: Optional[List[int]] = None, already_has_special_tokens: bool = False
) -> List[int]:
if already_has_special_tokens:
if token_ids_1 is not None:
raise ValueError(
"You should not supply a second sequence if the provided sequence of "
"ids is already formatted with special tokens for the model."
)
return list(map(lambda x: 1 if x in [self.sep_token_id, self.cls_token_id] else 0, token_ids_0))
if token_ids_1 is not None:
return [1] + ([0] * len(token_ids_0)) + [1] + ([0] * len(token_ids_1)) + [1]
return [1] + ([0] * len(token_ids_0)) + [1]
```
=> These two functions are different. Thus when I use bert, all_special_ids( it contains cls, sep, pad id) are ids which cannot be masked. But when i use albert, only cls, sep ids cannot be masked. Thus pad token can be masked when i use albert.
I don't know why the functions are called from different class when I run bert-base-uncased or albert.
Do you know why??
And is it correct that pad token will be masked in albert model??
| 04-09-2021 08:09:00 | 04-09-2021 08:09:00 | Related to #11163 by @sgugger <|||||>This is solved by #11163 |
transformers | 11,157 | closed | model_path should be ignored as the checkpoint path | # What does this PR do?
When the directory which holds the transformer model is given the command line argument, the script `run_xnli.py` arises the following error. This PR fixes this problem.
```sh
$ python3 run_xnli.py \
--model_name_or_path ./NICT_BERT-base_JapaneseWikipedia_32K_BPE \
--do_train \
--do_eval \
--max_seq_length 128 \
--per_device_train_batch_size 32 \
--learning_rate 2e-5 \
--num_train_epochs 3 \
--language en \
--fp16 \
--fp16_opt_level O2 \
--output_dir /tmp/xnli/
(snip)
[INFO|trainer.py:1013] 2021-04-09 15:27:01,518 >> ***** Running training *****
[INFO|trainer.py:1014] 2021-04-09 15:27:01,518 >> Num examples = 392702
[INFO|trainer.py:1015] 2021-04-09 15:27:01,518 >> Num Epochs = 3
[INFO|trainer.py:1016] 2021-04-09 15:27:01,518 >> Instantaneous batch size per device = 32
[INFO|trainer.py:1017] 2021-04-09 15:27:01,518 >> Total train batch size (w. parallel, distributed & accumulation) = 256
[INFO|trainer.py:1018] 2021-04-09 15:27:01,518 >> Gradient Accumulation steps = 1
[INFO|trainer.py:1019] 2021-04-09 15:27:01,518 >> Total optimization steps = 4602
0%| | 0/4602 [00:00<?, ?it/s]/pytorch/aten/src/THCUNN/ClassNLLCriterion.cu:108: cunn_ClassNLLCriterion_updateOutput_kernel: block: [0,0,0], thread: [0,0,0] Assertion `t >= 0 && t < n_classes` failed.
/pytorch/aten/src/THCUNN/ClassNLLCriterion.cu:108: cunn_ClassNLLCriterion_updateOutput_kernel: block: [0,0,0], thread: [2,0,0] Assertion `t >= 0 && t < n_classes` failed.
/pytorch/aten/src/THCUNN/ClassNLLCriterion.cu:108: cunn_ClassNLLCriterion_updateOutput_kernel: block: [0,0,0], thread: [3,0,0] Assertion `t >= 0 && t < n_classes` failed.
/pytorch/aten/src/THCUNN/ClassNLLCriterion.cu:108: cunn_ClassNLLCriterion_updateOutput_kernel: block: [0,0,0], thread: [6,0,0] Assertion `t >= 0 && t < n_classes` failed.
/pytorch/aten/src/THCUNN/ClassNLLCriterion.cu:108: cunn_ClassNLLCriterion_updateOutput_kernel: block: [0,0,0], thread: [9,0,0] Assertion `t >= 0 && t < n_classes` failed.
/pytorch/aten/src/THCUNN/ClassNLLCriterion.cu:108: cunn_ClassNLLCriterion_updateOutput_kernel: block: [0,0,0], thread: [10,0,0] Assertion `t >= 0 && t < n_classes` failed.
/pytorch/aten/src/THCUNN/ClassNLLCriterion.cu:108: cunn_ClassNLLCriterion_updateOutput_kernel: block: [0,0,0], thread: [12,0,0] Assertion `t >= 0 && t < n_classes` failed.
/pytorch/aten/src/THCUNN/ClassNLLCriterion.cu:108: cunn_ClassNLLCriterion_updateOutput_kernel: block: [0,0,0], thread: [23,0,0] Assertion `t >= 0 && t < n_classes` failed.
/pytorch/aten/src/THCUNN/ClassNLLCriterion.cu:108: cunn_ClassNLLCriterion_updateOutput_kernel: block: [0,0,0], thread: [24,0,0] Assertion `t >= 0 && t < n_classes` failed.
/pytorch/aten/src/THCUNN/ClassNLLCriterion.cu:108: cunn_ClassNLLCriterion_updateOutput_kernel: block: [0,0,0], thread: [29,0,0] Assertion `t >= 0 && t < n_classes` failed.
Traceback (most recent call last):
File "run_xnli.py", line 351, in <module>
main()
File "run_xnli.py", line 325, in main
train_result = trainer.train(model_path=model_path)
File "/home/foo/.local/lib/python3.6/site-packages/transformers/trainer.py", line 1120, in train
tr_loss += self.training_step(model, inputs)
File "/home/foo/.local/lib/python3.6/site-packages/transformers/trainer.py", line 1522, in training_step
loss = self.compute_loss(model, inputs)
File "/home/foo/.local/lib/python3.6/site-packages/transformers/trainer.py", line 1556, in compute_loss
outputs = model(**inputs)
File "/home/foo/.local/lib/python3.6/site-packages/torch/nn/modules/module.py", line 889, in _call_impl
result = self.forward(*input, **kwargs)
File "/home/foo/.local/lib/python3.6/site-packages/torch/nn/parallel/data_parallel.py", line 167, in forward
outputs = self.parallel_apply(replicas, inputs, kwargs)
File "/home/foo/.local/lib/python3.6/site-packages/torch/nn/parallel/data_parallel.py", line 177, in parallel_apply
return parallel_apply(replicas, inputs, kwargs, self.device_ids[:len(replicas)])
File "/home/foo/.local/lib/python3.6/site-packages/torch/nn/parallel/parallel_apply.py", line 86, in parallel_apply
output.reraise()
File "/home/foo/.local/lib/python3.6/site-packages/torch/_utils.py", line 429, in reraise
raise self.exc_type(msg)
RuntimeError: Caught RuntimeError in replica 1 on device 1.
Original Traceback (most recent call last):
File "/home/foo/.local/lib/python3.6/site-packages/torch/nn/parallel/parallel_apply.py", line 61, in _worker
output = module(*input, **kwargs)
File "/home/foo/.local/lib/python3.6/site-packages/torch/nn/modules/module.py", line 889, in _call_impl
result = self.forward(*input, **kwargs)
File "/home/foo/.local/lib/python3.6/site-packages/transformers/models/bert/modeling_bert.py", line 1510, in forward
return_dict=return_dict,
File "/home/foo/.local/lib/python3.6/site-packages/torch/nn/modules/module.py", line 889, in _call_impl
result = self.forward(*input, **kwargs)
File "/home/foo/.local/lib/python3.6/site-packages/transformers/models/bert/modeling_bert.py", line 981, in forward
return_dict=return_dict,
File "/home/foo/.local/lib/python3.6/site-packages/torch/nn/modules/module.py", line 889, in _call_impl
result = self.forward(*input, **kwargs)
File "/home/foo/.local/lib/python3.6/site-packages/transformers/models/bert/modeling_bert.py", line 575, in forward
output_attentions,
File "/home/foo/.local/lib/python3.6/site-packages/torch/nn/modules/module.py", line 889, in _call_impl
result = self.forward(*input, **kwargs)
File "/home/foo/.local/lib/python3.6/site-packages/transformers/models/bert/modeling_bert.py", line 461, in forward
past_key_value=self_attn_past_key_value,
File "/home/foo/.local/lib/python3.6/site-packages/torch/nn/modules/module.py", line 889, in _call_impl
result = self.forward(*input, **kwargs)
File "/home/foo/.local/lib/python3.6/site-packages/transformers/models/bert/modeling_bert.py", line 394, in forward
output_attentions,
File "/home/foo/.local/lib/python3.6/site-packages/torch/nn/modules/module.py", line 889, in _call_impl
result = self.forward(*input, **kwargs)
File "/home/foo/.local/lib/python3.6/site-packages/transformers/models/bert/modeling_bert.py", line 312, in forward
attention_scores = attention_scores + attention_mask
RuntimeError: CUDA error: device-side assert triggered
0%| | 0/4602 [00:30<?, ?it/s]
```
I think that the directory specified by the command line argument has already been used as the model path of the trainer, and think that it should be ignored as the checkpoint path.
<!--
Congratulations! You've made it this far! You're not quite done yet though.
Once merged, your PR is going to appear in the release notes with the title you set, so make sure it's a great title that fully reflects the extent of your awesome contribution.
Then, please replace this with a description of the change and which issue is fixed (if applicable). Please also include relevant motivation and context. List any dependencies (if any) that are required for this change.
Once you're done, someone will review your PR shortly (see the section "Who can review?" below to tag some potential reviewers). They may suggest changes to make the code even better. If no one reviewed your PR after a week has passed, don't hesitate to post a new comment @-mentioning the same persons---sometimes notifications get lost.
-->
<!-- Remove if not applicable -->
## Before submitting
- [ ] This PR fixes a typo or improves the docs (you can dismiss the other checks if that's the case).
- [x] Did you read the [contributor guideline](https://github.com/huggingface/transformers/blob/master/CONTRIBUTING.md#start-contributing-pull-requests),
Pull Request section?
- [ ] Was this discussed/approved via a Github issue or the [forum](https://discuss.huggingface.co/)? Please add a link
to it if that's the case.
- [x] Did you make sure to update the documentation with your changes? Here are the
[documentation guidelines](https://github.com/huggingface/transformers/tree/master/docs), and
[here are tips on formatting docstrings](https://github.com/huggingface/transformers/tree/master/docs#writing-source-documentation).
- [ ] Did you write any new necessary tests?
## Who can review?
Anyone in the community is free to review the PR once the tests have passed. Feel free to tag
members/contributors which may be interested in your PR.
<!-- Your PR will be replied to more quickly if you can figure out the right person to tag with @
If you know how to use git blame, that is the easiest way, otherwise, here is a rough guide of **who to tag**.
Please tag fewer than 3 people.
Models:
- albert, bert, xlm: @LysandreJik
- blenderbot, bart, marian, pegasus, encoderdecoder, t5: @patrickvonplaten, @patil-suraj
- longformer, reformer, transfoxl, xlnet: @patrickvonplaten
- fsmt: @stas00
- funnel: @sgugger
- gpt2: @patrickvonplaten, @LysandreJik
- rag: @patrickvonplaten, @lhoestq
- tensorflow: @LysandreJik
Library:
- benchmarks: @patrickvonplaten
- deepspeed: @stas00
- ray/raytune: @richardliaw, @amogkam
- text generation: @patrickvonplaten
- tokenizers: @n1t0, @LysandreJik
- trainer: @sgugger
- pipelines: @LysandreJik
Documentation: @sgugger
HF projects:
- datasets: [different repo](https://github.com/huggingface/datasets)
- rust tokenizers: [different repo](https://github.com/huggingface/tokenizers)
Examples:
- maintained examples (not research project or legacy): @sgugger, @patil-suraj
- research_projects/bert-loses-patience: @JetRunner
- research_projects/distillation: @VictorSanh
-->
| 04-09-2021 06:36:12 | 04-09-2021 06:36:12 | No this will then make it impossible to resume from a checkpoint if you pass `--output_dir path_to_specific_checkpoint`. This should only be ignored if the checkpoints are the wrong number of labels like [here](https://github.com/huggingface/transformers/blob/45fc8c7951f978c0f8f13c8bab52c744cd5c4784/examples/text-classification/run_glue.py#L454) in run_glue.<|||||>Thanks for your comment. I have just improved the patch according to your comment. Could you review it again? |
transformers | 11,156 | closed | Multi-`train_dataset` in Huggingface Trainer | # 🚀 Feature request
The current Huggingface Trainer Supports, a single `train_dataset` (torch.utils.data.dataset.Dataset). While it makes sense for most of the training setups, there are still some cases where it is convenient to have a list of `train_dataset`. The trainer can randomly select or follow a specific sampling strategy to select the samples from each of the `train_dataset`. Usually the papers mentioned in the `motivation` sections use a multinomial distribution with a penalty hyperparameter (\alpha). An example is attached below with code.
## Motivation
1. Easy Multi-task learning setup.
2. Multi-lingual pre-training mentioned in [XLM](https://github.com/facebookresearch/XLM), [mT5](https://arxiv.org/abs/2010.11934)
3. Even for LM fine-tuning [MultiMix](https://arxiv.org/abs/2004.13240) requires this feature.
## Your contribution
The sampling strategy for each of the `train_dataset` (torch.utils.data.dataset.Dataset) can be varied by a penalty variable (\alpha). The sample code for multinomial distribution based sampling strategy is below,
```
def multinomial_prob(dataset_len, alpha=.5):
tot_number_of_sent_in_all_lang = 0
prob = OrderedDict()
for k, v in dataset_len.items():
tot_number_of_sent_in_all_lang += v
for k, v in dataset_len.items():
neu = v
den = tot_number_of_sent_in_all_lang
p = neu/den
prob[k] = p
q = OrderedDict()
q_den = 0.0
for k, v in prob.items():
q_den += (v**alpha)
sum_ = 0.0
for k, v in prob.items():
q[k] = (v**alpha)/q_den
sum_ += q[k]
assert math.fabs(1-sum_) < 1e-5
return q
```
```
def iterator_selection_prob(alpha, train_datasets, logger=None):
dataset_len = OrderedDict()
for k, v in train_datasets.items():
dataset_len[k] = len(v)
for k, v in dataset_len.items():
logger.info("Total Number of samples in {} : {}".format(k, v))
prob = multinomial_prob(dataset_len, alpha=alpha)
logger.info("Language iterator selection probability.")
ret_prob_index, ret_prob_list = [], []
for k,v in prob.items():
ret_prob_index.append(k)
ret_prob_list.append(v)
for k, v in zip(ret_prob_index, ret_prob_list):
logger.info("{} : {}".format(k, v))
return dataset_len, ret_prob_index, ret_prob_list
```
Inside the training loop, we could integrate like the following (the sample code may not match with the `Trainer` code). This is just an example.
```
for step in range(args.max_steps*args.gradient_accumulation_steps):
model.train()
iterator_id = np.random.choice(range(tot_num_of_iterator), p=lang_prob)
try:
batch = train_iterators[iterator_id].__next__()
except StopIteration:
train_iterators[iterator_id] = iter(train_data_loader[iterator_id][1])
batch = train_iterators[iterator_id].__next__()
num_of_batch_trained[ iterator_id ] += 1
``` | 04-09-2021 05:36:52 | 04-09-2021 05:36:52 | This can all be done in one `Dataset` that randomly picks elements from subdatasets, so there is no need to add anything to the `Trainer` to support this.<|||||>Hi! Sorry to bother you again. I could not find any example code, that's why I opened the issue.
Later after your comment, I search through the [repo](https://huggingface.co/docs/datasets/) but could not find any class name SubDataset [here](https://huggingface.co/docs/datasets/search.html?q=SubDataset&check_keywords=yes&area=default#).
After Searching in the repository, I found some related [examples](https://github.com/huggingface/datasets/blob/67574a8d74796bc065a8b9b49ec02f7b1200c172/datasets/wmt16/wmt_utils.py) with the same `SubDataset` keyword. Is that what you mean?
@sgugger <|||||>All the links point to the Datasets library, so you shuold maybe open an issue there or ask on the Datasets category of the [forums](https://discuss.huggingface.co/)?<|||||>Sure, thank you for the reply and closing the issue. |
transformers | 11,155 | closed | [BUG] padding tokens are also masked in DataCollatorForLanguageModeling | ## Environment info
<!-- You can run the command `transformers-cli env` and copy-and-paste its output below.
Don't forget to fill out the missing fields in that output! -->
- `transformers` version: 4.3.2
- Platform: Linux
- Python version: 3.6
- PyTorch version (GPU?): 1.7.1 GPU
- Tensorflow version (GPU?): N/A
- Using GPU in script?: Yes
- Using distributed or parallel set-up in script?: Sagemaker distributed data parallel
### Who can help
@sgugger
<!-- Your issue will be replied to more quickly if you can figure out the right person to tag with @
If you know how to use git blame, that is the easiest way, otherwise, here is a rough guide of **who to tag**.
Please tag fewer than 3 people.
Models:
- albert, bert, xlm: @LysandreJik
- blenderbot, bart, marian, pegasus, encoderdecoder, t5: @patrickvonplaten, @patil-suraj
- longformer, reformer, transfoxl, xlnet: @patrickvonplaten
- fsmt: @stas00
- funnel: @sgugger
- gpt2: @patrickvonplaten, @LysandreJik
- rag: @patrickvonplaten, @lhoestq
- tensorflow: @LysandreJik
Library:
- benchmarks: @patrickvonplaten
- deepspeed: @stas00
- ray/raytune: @richardliaw, @amogkam
- text generation: @patrickvonplaten
- tokenizers: @LysandreJik
- trainer: @sgugger
- pipelines: @LysandreJik
Documentation: @sgugger
Model hub:
- for issues with a model report at https://discuss.huggingface.co/ and tag the model's creator.
HF projects:
- datasets: [different repo](https://github.com/huggingface/datasets)
- rust tokenizers: [different repo](https://github.com/huggingface/tokenizers)
Examples:
- maintained examples (not research project or legacy): @sgugger, @patil-suraj
- research_projects/bert-loses-patience: @JetRunner
- research_projects/distillation: @VictorSanh
-->
## Information
Model I am using (Bert, XLNet ...): All models that use DataCollatorForLanguageModeling.
The bug is introduced in this [PR](https://github.com/huggingface/transformers/pull/8308).
3 lines (241-243) are removed by mistake from this [line](https://github.com/huggingface/transformers/pull/8308/commits/74b3d7abce96c79bf8c35517857b4032b3d85a21#diff-046566f2b40a246c7d533457cd7f6f07830516da845b904086f36b3cfe0d5965L241).
Now padding tokens are also masked in MLM.
The problem arises when using:
* [x] the official example scripts: (give details below)
* [ ] my own modified scripts: (give details below)
The tasks I am working on is:
* [ ] an official GLUE/SQUaD task: (give the name)
* [ ] my own task or dataset: (give details below)
## To reproduce
Steps to reproduce the behavior:
```
from transformers import DataCollatorForLanguageModeling
from transformers import AutoTokenizer
tokenizer = AutoTokenizer.from_pretrained('albert-base-v2')
data_collator = DataCollatorForLanguageModeling(
tokenizer=tokenizer, mlm=True, mlm_probability=0.15
)
tok = tokenizer('hello how are you!', add_special_tokens=True, truncation=True, max_length=256, padding='max_length')
data_collator([tok['input_ids']])
```
From the output you can easily see that the padding tokens are masked. Add back the three removed lines fix this bug.
## Expected behavior
padding token is not supposed to be mask-able in MLM.
| 04-09-2021 03:27:36 | 04-09-2021 03:27:36 | I have similar issues.
"pad" token is not masked when I run bert-base-uncased model , but "pad" token can be masked when I run albert-base-v2
In examples/language-modeliing/run_mlm.py, I try to call tokenizer.get_special_tokens_mask.
```
print(tokenizer.get_special_tokens_mask([0, 100, 101, 102, 2, 3, 4], already_has_special_tokens=True))
```
Interestingly, "get_special_tokens_mask" function is called from "class PreTrainedTokenizerBase" when I run bert-base-uncased, but "get_special_tokens_mask" function is called from "class AlbertTokenizerFast" whenn I run albert-base-v2.
In PretrainedToknizerBase class,
```
def get_special_tokens_mask(
self, token_ids_0: List[int], token_ids_1: Optional[List[int]] = None, already_has_special_tokens: bool = False
) -> List[int]:
all_special_ids = self.all_special_ids # cache the property
special_tokens_mask = [1 if token in all_special_ids else 0 for token in token_ids_0]
return special_tokens_mask
```
However in AlbertTokenizerFast class,
```
def get_special_tokens_mask(
self, token_ids_0: List[int], token_ids_1: Optional[List[int]] = None, already_has_special_tokens: bool = False
) -> List[int]:
if already_has_special_tokens:
if token_ids_1 is not None:
raise ValueError(
"You should not supply a second sequence if the provided sequence of "
"ids is already formatted with special tokens for the model."
)
return list(map(lambda x: 1 if x in [self.sep_token_id, self.cls_token_id] else 0, token_ids_0))
if token_ids_1 is not None:
return [1] + ([0] * len(token_ids_0)) + [1] + ([0] * len(token_ids_1)) + [1]
return [1] + ([0] * len(token_ids_0)) + [1]
```
=> These two functions are different. Thus when I use bert, all_special_ids( it contains cls, sep, pad id) are ids which cannot be masked. But when i use albert, only cls, sep ids cannot be masked. Thus pad token can be masked when i use albert.
I don't know why the functions are called from different class when I run bert-base-uncased or albert.
Do you know why??
And is it correct that pad token will be masked in albert model??
[bert command]
```
% python run_mlm.py --model_name_or_path bert-base-uncased --dataset_name wikitext --dataset_config_name wikitext-2-raw-v1 --do_train --do_eval --output_dir ./tmp/test-mlm --line_by_line
```
[albert command]
```
% python run_mlm.py --model_name_or_path albert-base-v2 --dataset_name wikitext --dataset_config_name wikitext-2-raw-v1 --do_train --do_eval --output_dir ./tmp/test-mlm --line_by_line
```
<|||||>Thanks for reporting! This is actually a bug in the `get_special_tokens_mask` method of most tokenizers. I will push a fix soon. In the meantime, you can workaround the problem by passing the `special_token_mask` the tokenizer returns to the data collator (which will actually be faster since it will avoid being recomputed):
```
tokenizer = AutoTokenizer.from_pretrained('albert-base-v2')
data_collator = DataCollatorForLanguageModeling(
tokenizer=tokenizer, mlm=True, mlm_probability=0.15
)
tok = tokenizer('hello how are you!',return_special_tokens_mask=True, truncation=True, max_length=256, padding='max_length')
data_collator([tok])
``` |
transformers | 11,154 | closed | Using run_language_modeling.py to train an English adapter | # 📚 Migration
## Information
<!-- Important information -->
Model I am using bert-base-multilingual-cased:
Language I am using the model on English:
The problem arises when using:
When I entered the codes in the command line and run, the process just stuck here for a long time and did nothing, I tried many times and it always couldn’t start to train?
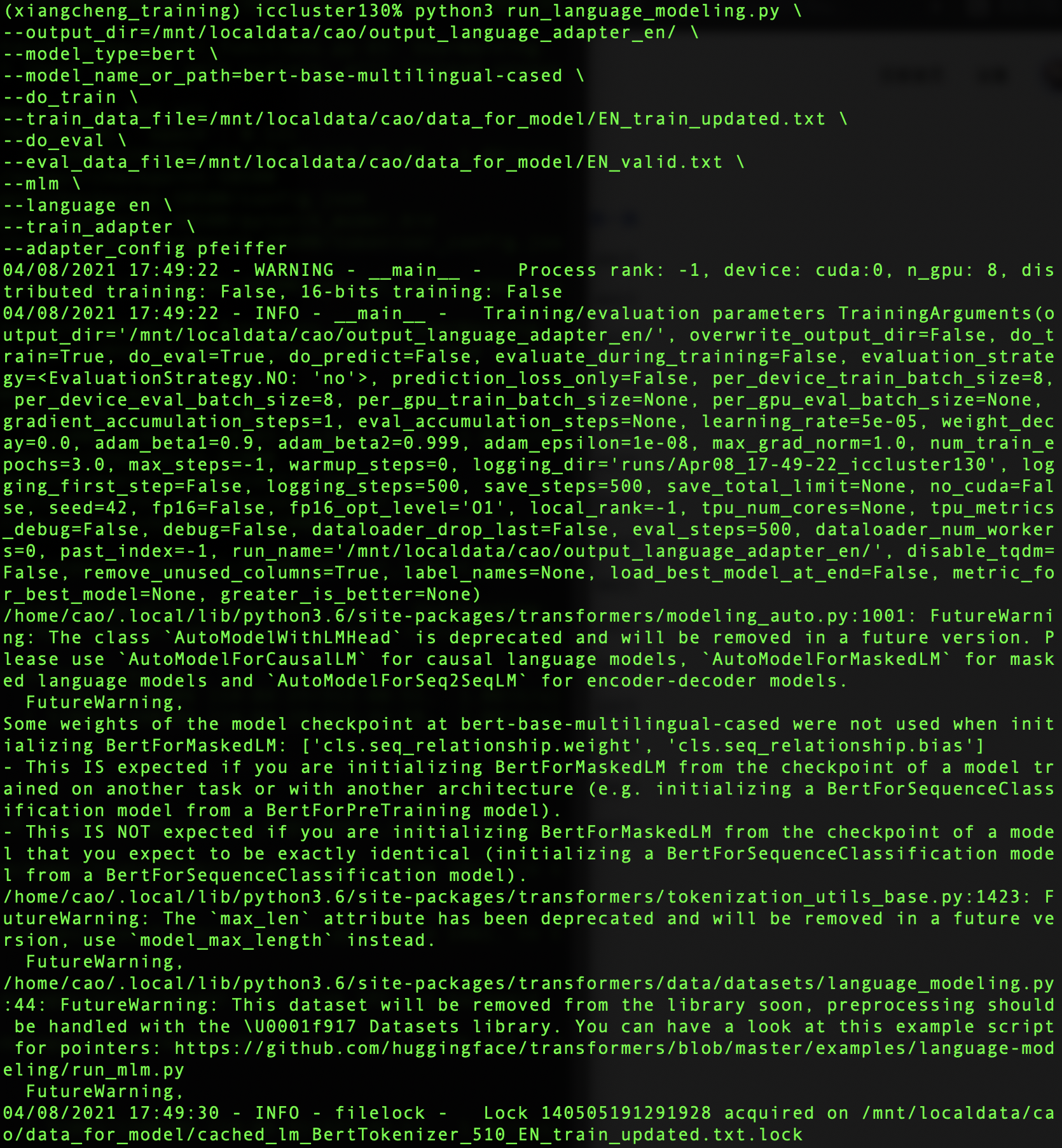
The tasks I am working on is:
* Train an English adapter using this script: https://github.com/Adapter-Hub/adapter-transformers/blob/master/examples/contrib/legacy/run_language_modeling.py
* I wrote this in command line:
* python3 run_language_modeling.py \
--output_dir=xxx \
--model_type=bert \
--model_name_or_path=bert-base-multilingual-cased \
--do_train \
--train_data_file=xxx/a.txt \
--do_eval \
--eval_data_file=xxx/b.txt \
--mlm \
--language en \
--train_adapter \
--adapter_config pfeiffer \
--per_gpu_train_batch_size 4 \
--per_gpu_eval_batch_size 4 \
--learning_rate 5e-5
## Details
<!-- A clear and concise description of the migration issue.
If you have code snippets, please provide it here as well.
Important! Use code tags to correctly format your code. See https://help.github.com/en/github/writing-on-github/creating-and-highlighting-code-blocks#syntax-highlighting
Do not use screenshots, as they are hard to read and (more importantly) don't allow others to copy-and-paste your code.
-->
## Environment info
<!-- You can run the command `python transformers-cli env` and copy-and-paste its output below.
Don't forget to fill out the missing fields in that output! -->
- `transformers` version:
- Platform:
- Python version:
- PyTorch version (GPU?):
- Tensorflow version (GPU?):
- Using GPU in script?:
- Using distributed or parallel set-up in script?:
<!-- IMPORTANT: which version of the former library do you use? -->
* `pytorch-transformers` or `pytorch-pretrained-bert` version (or branch):
## Checklist
- [ ] I have read the migration guide in the readme.
([pytorch-transformers](https://github.com/huggingface/transformers#migrating-from-pytorch-transformers-to-transformers);
[pytorch-pretrained-bert](https://github.com/huggingface/transformers#migrating-from-pytorch-pretrained-bert-to-transformers))
- [ ] I checked if a related official extension example runs on my machine.
| 04-09-2021 03:23:18 | 04-09-2021 03:23:18 | This issue has been automatically marked as stale because it has not had recent activity. If you think this still needs to be addressed please comment on this thread.
Please note that issues that do not follow the [contributing guidelines](https://github.com/huggingface/transformers/blob/master/CONTRIBUTING.md) are likely to be ignored. |
transformers | 11,153 | closed | cannot import name 'BigBirdModel' from 'transformers' | when I write the “from transformers import BigBirdModel”, the error is “cannot import name 'BigBirdConfig' from 'transformers'”,how to solve the problem? thank you. | 04-09-2021 03:02:04 | 04-09-2021 03:02:04 | Hello! Please respect the issue template so that we can help you. Big Bird is only available in the latest transformers version, do you have this version in your setup?<|||||>This issue has been automatically marked as stale because it has not had recent activity. If you think this still needs to be addressed please comment on this thread.
Please note that issues that do not follow the [contributing guidelines](https://github.com/huggingface/transformers/blob/master/CONTRIBUTING.md) are likely to be ignored. |
transformers | 11,152 | closed | typo | doc typo | 04-09-2021 02:23:55 | 04-09-2021 02:23:55 | |
transformers | 11,151 | closed | [setup] make fairscale and deepspeed setup extras | Based on a request adding support for:
```
pip install transformers[deepspeed]
pip install transformers[fairscale]
```
so moving the version minimums into `setup.py`, and also had to add a helper function `dep_version_check`
@LysandreJik, @sgugger | 04-08-2021 21:44:27 | 04-08-2021 21:44:27 | |
transformers | 11,150 | closed | Add support for multiple models for one config in auto classes | # What does this PR do?
This PR adds support for having multiple models with the same config be in the same auto class. For instance `FunnelBaseModel` and `FunnelModel` are both valid models for the class `AutoModel` but since they both rely on `FunnelConfig`, only `FunnelModel` was in the model mapping for `AutoModel`.
The mechanism when loading changes slightly: if the mapping finds a tuple for the config at hand, it will look into the `architectures` field and return the model in the tuple corresponding to the architecture found there, or the first model of the tuple as a default.
While diving into this, I realized that TF and Flax pretrained models do not populate the field architectures of their configs, so I added support for this.
The rest of the changes are need to adapt to the fact some model mappings now can have tuple values. | 04-08-2021 19:51:11 | 04-08-2021 19:51:11 | |
transformers | 11,149 | closed | Enable option for subword regularization in `XLMRobertaTokenizer` | # What does this PR do?
I would like to use [subword regularization](https://github.com/google/sentencepiece#subword-regularization-and-bpe-dropout) from [google/sentencepiece](https://github.com/google/sentencepiece). The reason is that it might be used to improve downstream task performance.
Since `XLMRobertaTokenizer` already uses `SentencePieceProcessor` from `google/sentencepiece` there are only some minor modifications needed.
3 additional parameters are added to the constructor of `XLMRobertaTokenizer`. This are:
```python
enable_sampling=False,
nbest_size=-1,
alpha=0.1,
```
The default values are selected so that this is no breaking change.
In the `_tokenize(self, text)` function there was a call to `self.sp_model.EncodeAsPieces(text)`. This call does ignore the parameters for subword regularization. That is why it had to be replaced by a call to `self.sp_model.encode(text, out_type=str)`.
Since `XLMRobertaTokenizerFast` is an independent implentation which does not use `google/sentencepiece` it is not in the scope of the PR to add subword regularization to the fast tokenizer.
## To-do
- [x] check if tests pass
- [x] check if tests can / should be added
- [x] add a link to a page where we can see all kwargs
## Who can review?
@LysandreJik @stefan-it | 04-08-2021 19:21:49 | 04-08-2021 19:21:49 | It would be awesome if you ( @LysandreJik and @stefan-it ) could give some feedback on this - although some tests still fail.
Is it a good idea? Would you merge it if everything is cleaned up?<|||||>I added a test, everything is green and IMO ready for review. @LysandreJik @stefan-it<|||||>@LysandreJik and @n1t0 I think that would be a good idea but IMO it should not be done in the scope of this PR.
Because the slow tokenizer just delegates the work to [google/sentencepiece](https://github.com/google/sentencepiece) this PR is very easy but adding that to the Rust Tokenizer would be way more work afaik.<|||||>Hey @LysandreJik and @n1t0
I think this PR is somehow stuck...
AFAIK my change is ok for you.
What about merging it and moving the part for the fast tokenizer to a seperate issue?<|||||>@LysandreJik
- Requested changes are made and marked as resolved.
- Inline questions are answered and marked as resolved.
- CI is green.
IMO ready for merge.<|||||>@sgugger all green again :-)<|||||>> Perfect! If you feel up to the task, I think all (slow) sentencepiece-based tokenizers could benefit from this addition.
see #11417 |
transformers | 11,148 | closed | [setup] extras[docs] must include 'all' | Currently `pip install -e .[docs]` doesn't necessarily lead to a successful `make docs`, so this PR makes `extras["docs"]` fully self-contained.
@sgugger, @LysandreJik | 04-08-2021 19:12:04 | 04-08-2021 19:12:04 | |
transformers | 11,147 | closed | Add fairscale and deepspeed back to the CI | Add fairscale and deepspeed back to the CI, they were erroneously removed in https://github.com/huggingface/transformers/pull/10681.
| 04-08-2021 17:52:41 | 04-08-2021 17:52:41 | |
transformers | 11,146 | closed | [tests] relocate core integration tests | This PR
* moves `deepspeed`/`fairscale`/extended trainer tests from `examples` to `tests`
* updates docs to point to the new sample config files
* adds a new `testing_utils.py`'s context manager `ExtendSysPath` that allows temporary `sys.path` changing to import something locally in the tests and uses it (otherwise sagemaker tests were breaking because they contain `__init__.py`) + doc
Hopefully, it'll be the new home for integration tests for awhile, - specifically for deepspeed tests as the DeepSpeed team would like to run our tests as part of their CIs.
We still need to split off the `fairscale` tests once we start working on this integration again, so for now just moving as is.
@sgugger | 04-08-2021 17:39:26 | 04-08-2021 17:39:26 | @sgugger, @LysandreJik - so after this move we have a problem of dependencies now - the extended integration tests need be able to check score metrics, but the main tests don't have `sacrebleu` and other dependencies installed. How do we resolve this conundrum?
Lysandre replied on slack to add them to `extras["testing"]` - so doing that.<|||||>I think we can add
```
sacrebleu >= 1.4.12
rouge-score
nltk
```
to the testing extra. It should be all you need. |
transformers | 11,145 | closed | [run_clm] clarify why we get the tokenizer warning on long input | Solving https://github.com/huggingface/transformers/issues/11108 this PR adds a clarification of why the warning is printed by the tokenizer when `run_clm.py` sends a huge input to tokenize against a short `block_size`. It's not great, but at least now the user will know that the warning is not warranted to be a warning in this particular situation.
> [WARNING|tokenization_utils_base.py:3138] 2021-04-06 21:29:29,790 >> Token indices sequence length is longer than the specified maximum sequence length for this model (1462828 > 1024). Running this sequence through the model will result in indexing errors
So after this PR the we end up an extra warning:
```
[WARNING|tokenization_utils_base.py:3143] 2021-04-07 21:09:22,144 >> Token indices sequence length is longer than the specified maximum sequence length for this model (1462828 > 1024). Running this sequence through the model will result in indexing errors
[WARNING|run_clm.py:326] 2021-04-07 21:13:14,300 >> ^^^^^^^^^^^^^^^^ Please ignore the warning above - it's just a long input
```
The correct solution would be to redesign the API to notify the tokenizer that in some cases the input doesn't have to be less than `block_size`.
Fixes: https://github.com/huggingface/transformers/issues/11108
@sgugger | 04-08-2021 15:59:56 | 04-08-2021 15:59:56 | |
transformers | 11,144 | closed | [trainer] solve "scheduler before optimizer step" warning | As discussed in https://github.com/huggingface/transformers/issues/11106 fp16 scaler leads to a warning:
> torch/optim/lr_scheduler.py:132: UserWarning: Detected call of lr_scheduler.step() before optimizer.step(). In PyTorch 1.1.0 and later, you should call them in the opposite order: optimizer.step() before lr_scheduler.step(). Failure to do this will result in PyTorch skipping the first value of the learning rate schedule. See more details at https://pytorch.org/docs/stable/optim.html#how-to-adjust-learning-rate
> warnings.warn("Detected call of lr_scheduler.step() before optimizer.step().
because the optimizer may get skipped until the right scale is found, so we shouldn't run `lr_scheduler.step()` when that happens.
@ptrblck provided a workaround here:
https://discuss.pytorch.org/t/model-weights-not-getting-updated-when-using-autocast/117286/10?u=ptrblck
This is also reported at pytoch: https://github.com/pytorch/pytorch/issues/55585
So the solution is we check the scale before and after and if it changed, then the optimizer wasn't run and we skip the scheduler step then.
Fixes: https://github.com/huggingface/transformers/issues/11106
@sgugger | 04-08-2021 15:53:00 | 04-08-2021 15:53:00 | I'm a bit torn about this solution: it solves the problem exposed by the warning but it creates another problem with no warning, which I had flagged in #11106 as
> If we somehow manage to catch those skipped optimizer steps and delay the scheduler steps, then we won't respect the number of steps in the scheduler, leading to some wrong end learning rates.
I have no idea if it's better to skip the beginning or end values for the learning rate though.
Also the test is wrong I think it should be
```
optimizer_was_run = scale_before <= scale_after
```
as the scale factor can be multiplied by the `growth_factor` (after a long period without decrease) without skipping a step. It's when it decreases that we know the step was skipped.<|||||>> I'm a bit torn about this solution: it solves the problem exposed by the warning but it creates another problem with no warning, which I had flagged in #11106 as
>
> > If we somehow manage to catch those skipped optimizer steps and delay the scheduler steps, then we won't respect the number of steps in the scheduler, leading to some wrong end learning rates.
If the scheduler and the optimizer are now synchronized why would this happen?
I think it's the external step counter that is out of sync, so we are off at the total number of steps the Trainer does and the optimizer/scheduler see - so the end may be cut off as some "promised steps" won't be seen by the scheduler.
deepspeed already runs `scheduler.step()` only if `optimizer.step()` was run so it's in the same boat.
> I have no idea if it's better to skip the beginning or end values for the learning rate though.
I'd say that potentially cutting of the end is safer.
Also the optimizer stepping could be skipped in the middle of the run as well.
> ```
> optimizer_was_run = scale_before <= scale_after
> ```
Fixed, thank you for catching this!<|||||>> If the scheduler and the optimizer are now synchronized why would this happen?
The scheduler was built with a certain number of total steps (for instance go linearly from 1e-4 to 0 in 500 steps). So by skipping those initial steps, we won't be seeing the last learning rates.<|||||>> The scheduler was built with a certain number of total steps (for instance go linearly from 1e-4 to 0 in 500 steps). So by skipping those initial steps, we won't be seeing the last learning rates.
Yes! My apologies I understood this from your earlier comment. I just meant that until this PR the scheduler was not synced with optimizer.
So best to truncate the last learning rates where it's usually fixed, or doesn't quite matter if it's a huge run and if it is cyclical it doesn't matter for sure if I understand the situation correctly. Let's perhaps ask it differently - in what situations do you think this mismatch/missing few last steps would practically matter? <|||||>Thinking more and I think it's actually better to skip at the end, since the "short" part of a scheduler is often the warmup (for instance if we set `warum_steps=50`). So I revert my previous objection and I'm okay with the PR :-)<|||||>That's a very good point!
And this will also synchronize with the behavior one gets under deepspeed.
Thank you for this brainstorming, @sgugger! |
transformers | 11,143 | closed | Training loss is not logged correctly when doing evaluation with Trainer | ## Environment info
<!-- You can run the command `transformers-cli env` and copy-and-paste its output below.
Don't forget to fill out the missing fields in that output! -->
- `transformers` version: 4.4.2
- Platform: Linux-5.4.0-62-generic-x86_64-with-glibc2.10
- Python version: 3.8.8
- PyTorch version (GPU?): 1.8.1+cu102 (True)
- Tensorflow version (GPU?): 2.4.1 (False)
- Using GPU in script?: Yes
- Using distributed or parallel set-up in script?: No
### Who can help
@sgugger
## Information
Model I am using (Bert, XLNet ...): Reformer
The problem arises when using:
* [ ] the official example scripts: (give details below)
* [x] my own modified scripts: (give details below)
The tasks I am working on is:
* [ ] an official GLUE/SQUaD task: (give the name)
* [x] my own task or dataset: (give details below)
I'm doing a text generation with Reformer using my own dataset.
## To reproduce
Steps to reproduce the behavior:
1. Set `logging_steps=10` and `evaluation_strategy="steps`, `eval_steps=20` in `TrainingArguments`
<!-- If you have code snippets, error messages, stack traces please provide them here as well.
Important! Use code tags to correctly format your code. See https://help.github.com/en/github/writing-on-github/creating-and-highlighting-code-blocks#syntax-highlighting
Do not use screenshots, as they are hard to read and (more importantly) don't allow others to copy-and-paste your code.-->
I'm doing training in Jupyter notebook. With the settings above, the logging output shows training loss and validation loss every 20 steps (which is the `eval_steps`). However, I want the training loss to be logged at higher frequency than the validation loss, for example at 10 steps like above. This is because running evaluation will take some time for large validation set, while I still want to monitor mini-batch training loss.
When inspecting logs with Tensor Board, no training loss is logged at all (even the values every 20 steps).
If I disable evaluation (`evaluation_strategy="no"`), the training loss is logged every 10 steps as expected.
## Expected behavior
When enabling evaluation in Trainer, training loss should be logged every `logging_steps`, while validation loss is logged every `eval_steps`
| 04-08-2021 15:24:21 | 04-08-2021 15:24:21 | I am not able to reproduce, on my side I do see logs every logging_steps for the training loss and learning rate, and every eval_steps for the validation loss and metrics, both in the console and TensorBoard. Could you try again with a source install?<|||||>After I restarted TensorBoard, training loss showed up correctly again. Maybe something went wrong with TensorBoard. Thank you for your prompt response! FYI it worked correctly with my current version `transformers=4.4.2`. It was a silly mistake from my side. |
transformers | 11,142 | closed | [Community notebooks] Add Wav2Vec notebook for creating captions for YT Clips | # What does this PR do?
<!--
Congratulations! You've made it this far! You're not quite done yet though.
Once merged, your PR is going to appear in the release notes with the title you set, so make sure it's a great title that fully reflects the extent of your awesome contribution.
Then, please replace this with a description of the change and which issue is fixed (if applicable). Please also include relevant motivation and context. List any dependencies (if any) that are required for this change.
Once you're done, someone will review your PR shortly (see the section "Who can review?" below to tag some potential reviewers). They may suggest changes to make the code even better. If no one reviewed your PR after a week has passed, don't hesitate to post a new comment @-mentioning the same persons---sometimes notifications get lost.
-->
<!-- Remove if not applicable -->
Adds a notebook for performing inference with wav2vec. The notebook aims to serve as a reference for people wanting to use wav2cec to build useful audio applications.
Includes:
- Extracting audio from movies
- Preparing audio for tokenization
- Wav2Vec inference
## Before submitting
- [X] This PR fixes a typo or improves the docs (you can dismiss the other checks if that's the case).
- [ ] Did you read the [contributor guideline](https://github.com/huggingface/transformers/blob/master/CONTRIBUTING.md#start-contributing-pull-requests),
Pull Request section?
- [ ] Was this discussed/approved via a Github issue or the [forum](https://discuss.huggingface.co/)? Please add a link
to it if that's the case.
- [ ] Did you make sure to update the documentation with your changes? Here are the
[documentation guidelines](https://github.com/huggingface/transformers/tree/master/docs), and
[here are tips on formatting docstrings](https://github.com/huggingface/transformers/tree/master/docs#writing-source-documentation).
- [ ] Did you write any new necessary tests?
## Who can review?
Anyone in the community is free to review the PR once the tests have passed. Feel free to tag
members/contributors which may be interested in your PR.
<!-- Your PR will be replied to more quickly if you can figure out the right person to tag with @
If you know how to use git blame, that is the easiest way, otherwise, here is a rough guide of **who to tag**.
Please tag fewer than 3 people.
Models:
- albert, bert, xlm: @LysandreJik
- blenderbot, bart, marian, pegasus, encoderdecoder, t5: @patrickvonplaten, @patil-suraj
- longformer, reformer, transfoxl, xlnet: @patrickvonplaten
- fsmt: @stas00
- funnel: @sgugger
- gpt2: @patrickvonplaten, @LysandreJik
- rag: @patrickvonplaten, @lhoestq
- tensorflow: @LysandreJik
Library:
- benchmarks: @patrickvonplaten
- deepspeed: @stas00
- ray/raytune: @richardliaw, @amogkam
- text generation: @patrickvonplaten
- tokenizers: @n1t0, @LysandreJik
- trainer: @sgugger
- pipelines: @LysandreJik
Documentation: @sgugger
HF projects:
- datasets: [different repo](https://github.com/huggingface/datasets)
- rust tokenizers: [different repo](https://github.com/huggingface/tokenizers)
Examples:
- maintained examples (not research project or legacy): @sgugger, @patil-suraj
- research_projects/bert-loses-patience: @JetRunner
- research_projects/distillation: @VictorSanh
-->
| 04-08-2021 15:05:13 | 04-08-2021 15:05:13 | Very nice! @patil-suraj do you want to give this a look and merge if it looks good to you too?<|||||>Very cool! LGTM, thanks a lot for adding this! |
transformers | 11,141 | closed | Don't duplicate logs in TensorBoard and handle --use_env | # What does this PR do?
This PR fixes a few bugs in the `Trainer` and `TrainingArguments`. First it cleans up the `TensorBoardCallback` to make sure the logs are not duplicated (I think they were not for some convoluted logic with the `tb_writer` never set but now I'm sure).
The second part is more important and handles support for when a user launches a training script using `Trainer` with the `--use_env` option (for instance when using `accelerate launch`). In this case the argument `local_rank` is not passed directly, it's just set in the environment and we did not detect it. | 04-08-2021 13:36:54 | 04-08-2021 13:36:54 | |
transformers | 11,140 | closed | Updates SageMaker docs for updating DLCs | # What does this PR do?
Adds a Link to an example PR for what content someone needs to put into the PR comment. | 04-08-2021 12:32:01 | 04-08-2021 12:32:01 | |
transformers | 11,139 | closed | OOM issue with prediction | Hi! I fine-tuned the bart model on XSum (both training and validation are fine). However, the OOM appeared during the prediction using the same machine. @patrickvonplaten @patil-suraj Here is my code:
```
python3 run_summarization.py \
--output_dir ./tmp/xsum-test/ \
--overwrite_output_dir \
--text_column text \
--summary_column summary \
--per_device_eval_batch_size 1 \
--do_predict \
--model_name_or_path ./tmp/xsum-summarization/checkpoint-15000 \
--max_source_length=512 \
--max_target_length=128 \
--val_max_target_length=60 \
--test_path data/multi \
--num_beams 6 \
```
The error is:
```
***** Running Prediction *****
Num examples = 11334
Batch size = 1
4%|▍ | 465/11334 [00:54<42:38, 4.25it/s]Traceback (most recent call last):
File "run_summarization.py", line 587, in <module>
main()
File "run_summarization.py", line 559, in main
num_beams=data_args.num_beams,
File "/lustre/home/ec156/xx6/transformers/src/transformers/trainer_seq2seq.py", line 121, in predict
return super().predict(test_dataset, ignore_keys=ignore_keys, metric_key_prefix=metric_key_prefix)
File "/lustre/home/ec156/xx6/transformers/src/transformers/trainer.py", line 1824, in predict
test_dataloader, description="Prediction", ignore_keys=ignore_keys, metric_key_prefix=metric_key_prefix
File "/lustre/home/ec156/xx6/transformers/src/transformers/trainer.py", line 1900, in prediction_loop
preds_host = logits if preds_host is None else nested_concat(preds_host, logits, padding_index=-100)
File "/lustre/home/ec156/xx6/transformers/src/transformers/trainer_pt_utils.py", line 96, in nested_concat
return type(tensors)(nested_concat(t, n, padding_index=padding_index) for t, n in zip(tensors, new_tensors))
File "/lustre/home/ec156/xx6/transformers/src/transformers/trainer_pt_utils.py", line 96, in <genexpr>
return type(tensors)(nested_concat(t, n, padding_index=padding_index) for t, n in zip(tensors, new_tensors))
File "/lustre/home/ec156/xx6/transformers/src/transformers/trainer_pt_utils.py", line 98, in nested_concat
return torch_pad_and_concatenate(tensors, new_tensors, padding_index=padding_index)
File "/lustre/home/ec156/xx6/transformers/src/transformers/trainer_pt_utils.py", line 66, in torch_pad_and_concatenate
result = tensor1.new_full(new_shape, padding_index)
RuntimeError: CUDA out of memory. Tried to allocate 932.00 MiB (GPU 0; 15.78 GiB total capacity; 12.89 GiB already allocated; 913.69 MiB free; 13.79 GiB reserved in total by PyTorch)
4%|▍ | 465/11334 [00:55<21:27, 8.44it/s]srun: error: r2i4n0: task 0: Exited with exit code 1
``` | 04-08-2021 09:44:47 | 04-08-2021 09:44:47 | Hi @XinnuoXu
you should pass the `--predict_with_generate` arg for summarization evaluation, this will use the `generate` method to generate the summaries.
I think one possible reason for this issue is that when `predict_with_generate` is not passed the final hidden_states from the model are used as predictions which are of shape `[bs, seq_len, vocab_size]`, which is quite large, hence OOM.<|||||>This issue has been automatically marked as stale because it has not had recent activity. If you think this still needs to be addressed please comment on this thread.
Please note that issues that do not follow the [contributing guidelines](https://github.com/huggingface/transformers/blob/master/CONTRIBUTING.md) are likely to be ignored. |
transformers | 11,138 | closed | Fix typing error in Trainer class (prediction_step) | # What does this PR do?
<!--
Congratulations! You've made it this far! You're not quite done yet though.
Once merged, your PR is going to appear in the release notes with the title you set, so make sure it's a great title that fully reflects the extent of your awesome contribution.
Then, please replace this with a description of the change and which issue is fixed (if applicable). Please also include relevant motivation and context. List any dependencies (if any) that are required for this change.
Once you're done, someone will review your PR shortly (see the section "Who can review?" below to tag some potential reviewers). They may suggest changes to make the code even better. If no one reviewed your PR after a week has passed, don't hesitate to post a new comment @-mentioning the same persons---sometimes notifications get lost.
-->
<!-- Remove if not applicable -->
This is a minor fix. The argument types and docstring of `transformers.trainer.prediction_step` are incorrect. This error was introduced in transformers==3.4.0, specifically in #7767 where documentation was not updated properly.
The current docs indicate that `prediction_step` returns a 3-Tuple of Optionals (loss, logits and labels) and that the type of _loss_ is `float`. Indeed, if returned, `loss` is always a `torch.Tensor` as the only performed operations in this function are `.mean()`, `.detach()` and `.cpu()`, but **not** `.item()`. In transformers<3.4.0, there was indeed a `.item()` operation, but in #7767 this behavior was changed but the docstring and types were not updated.
## Before submitting
- [x] This PR fixes a typo or improves the docs (you can dismiss the other checks if that's the case).
- [ ] Did you read the [contributor guideline](https://github.com/huggingface/transformers/blob/master/CONTRIBUTING.md#start-contributing-pull-requests),
Pull Request section?
- [ ] Was this discussed/approved via a Github issue or the [forum](https://discuss.huggingface.co/)? Please add a link
to it if that's the case.
- [x] Did you make sure to update the documentation with your changes? Here are the
[documentation guidelines](https://github.com/huggingface/transformers/tree/master/docs), and
[here are tips on formatting docstrings](https://github.com/huggingface/transformers/tree/master/docs#writing-source-documentation).
- [ ] Did you write any new necessary tests?
## Who can review?
Anyone in the community is free to review the PR once the tests have passed. Feel free to tag
members/contributors which may be interested in your PR.
@sgugger
<!-- Your PR will be replied to more quickly if you can figure out the right person to tag with @
If you know how to use git blame, that is the easiest way, otherwise, here is a rough guide of **who to tag**.
Please tag fewer than 3 people.
Models:
- albert, bert, xlm: @LysandreJik
- blenderbot, bart, marian, pegasus, encoderdecoder, t5: @patrickvonplaten, @patil-suraj
- longformer, reformer, transfoxl, xlnet: @patrickvonplaten
- fsmt: @stas00
- funnel: @sgugger
- gpt2: @patrickvonplaten, @LysandreJik
- rag: @patrickvonplaten, @lhoestq
- tensorflow: @LysandreJik
Library:
- benchmarks: @patrickvonplaten
- deepspeed: @stas00
- ray/raytune: @richardliaw, @amogkam
- text generation: @patrickvonplaten
- tokenizers: @n1t0, @LysandreJik
- trainer: @sgugger
- pipelines: @LysandreJik
Documentation: @sgugger
HF projects:
- datasets: [different repo](https://github.com/huggingface/datasets)
- rust tokenizers: [different repo](https://github.com/huggingface/tokenizers)
Examples:
- maintained examples (not research project or legacy): @sgugger, @patil-suraj
- research_projects/bert-loses-patience: @JetRunner
- research_projects/distillation: @VictorSanh
-->
| 04-08-2021 09:40:35 | 04-08-2021 09:40:35 | |
transformers | 11,137 | closed | Inference time got very high, very low CUDA activity | ## Environment info
<!-- You can run the command `transformers-cli env` and copy-and-paste its output below.
Don't forget to fill out the missing fields in that output! -->
- `transformers` version: 4.6.0.dev0
- Platform: Windows-10-10.0.19041-SP0
- Python version: 3.8.8
- PyTorch version (GPU?): 1.8.1 (True)
- Tensorflow version (GPU?): 2.4.1 (True)
- Using GPU in script?: Yes
- Using distributed or parallel set-up in script?: no
## Information
I am using Trainer() for some BERT/DistilBERT experiments.
After I upgraded to the latest git master version, the inference time got very high.
Noted that I have very low CUDA activity (CPU is also very low).
Seems to be something messed up around training.
The problem arises when using:
* [ ] the official example scripts: (give details below)
* [x] my own modified scripts: (give details below)
The tasks I am working on is:
* [ ] an official GLUE/SQUaD task: (give the name)
* [x] my own task or dataset: (give details below)
## To reproduce
Steps to reproduce the behavior:
1. pip install transformers==4.4.2
2. Run script below, and inspect inference time, CUDA activity.
3. pip install git+https://github.com/huggingface/transformers
4. Run script below, and inspect inference time, CUDA activity (note that there is no/or very low CUDA activity, and inference time got very high).
`from transformers import Trainer, TrainingArguments
from transformers import DistilBertForSequenceClassification, DistilBertTokenizerFast
from datasets import load_dataset
from sklearn.metrics import accuracy_score, precision_recall_fscore_support
select_model = 'distilbert-base-uncased'
model = DistilBertForSequenceClassification.from_pretrained(select_model, num_labels=2, force_download=False)
tokenizer = DistilBertTokenizerFast.from_pretrained(select_model, force_download=False)
#model.config
train_dataset, val_dataset = load_dataset('imdb', split=['train[:20%]', 'test[:20%]'])
train_dataset = train_dataset.rename_column('text','sentence')
val_dataset = val_dataset.rename_column('text','sentence')
sentence_lenght = 5
def tokenize(batch):
return tokenizer(batch['sentence'], padding=True, truncation=True, max_length=sentence_lenght) # batch['text']
train_dataset = train_dataset.map(tokenize, batched=True, batch_size=len(train_dataset))
val_dataset = val_dataset.map(tokenize, batched=True, batch_size=len(val_dataset))
train_dataset.set_format('torch', columns=['input_ids', 'attention_mask', 'label'])
val_dataset.set_format('torch', columns=['input_ids', 'attention_mask', 'label'])
def compute_metrics(pred):
labels = pred.label_ids
preds = pred.predictions.argmax(-1)
precision, recall, f1, _ = precision_recall_fscore_support(labels, preds, average='binary')
acc = accuracy_score(labels, preds)
return {
'accuracy': acc,
'f1': f1,
'precision': precision,
'recall': recall
}
training_args = TrainingArguments(
run_name='experiments_distilBert_01',
output_dir='./results',
num_train_epochs=4,
per_device_train_batch_size=16,
per_device_eval_batch_size=16,
warmup_steps=500,
learning_rate=2e-5,
weight_decay=0.01,
evaluation_strategy="epoch",
logging_dir='./logs',
logging_steps=250,
save_strategy='no',
report_to=['tensorboard'],
# deepspeed='./ds_config.json'
fp16=False,
fp16_backend='auto',
disable_tqdm=False,
load_best_model_at_end=True
)
trainer = Trainer(
model=model,
args=training_args,
tokenizer=tokenizer,
train_dataset=train_dataset,
eval_dataset=val_dataset,
compute_metrics=compute_metrics
)
trainer.train()
trainer.evaluate()`
## Expected behavior
Scripts execute at least with the same inference time.
| 04-08-2021 08:49:58 | 04-08-2021 08:49:58 | I'm having a similar issue as well but occurring at both test and training time - <3% GPU utilization. When executing the `run_qa.py` script using the command line arguments in the first example of the question answering example, it takes much longer than when I was running `transformers` v4.3.3.
However, the script seems to run fine on our cluster (also running v4.5.0) using 2080Ti's.
- transformers version: 4.5.0
- Platform: Windows 10 Version 2004 (OS Build 19041.264)
- Python version: 3.9,2
- PyTorch version (GPU?): 1.8.1 (RTX 3090)
- Tensorflow version (GPU?): None
- Using GPU in script?: Yes
- Using distributed or parallel set-up in script?: no
<|||||>This issue has been automatically marked as stale because it has not had recent activity. If you think this still needs to be addressed please comment on this thread.
Please note that issues that do not follow the [contributing guidelines](https://github.com/huggingface/transformers/blob/master/CONTRIBUTING.md) are likely to be ignored.<|||||>Installing the latest 4.6.0.dev0 dev release from git is still very slow on GoogleColab, compared to the latest official when running text classification on BERT, distillBert or Xlm-r.<|||||>I have the same issue.<|||||>Hello! You mention Google Colab, do you have a notebook to share so that we can take a look? Thank you.<|||||>This issue has been automatically marked as stale because it has not had recent activity. If you think this still needs to be addressed please comment on this thread.
Please note that issues that do not follow the [contributing guidelines](https://github.com/huggingface/transformers/blob/master/CONTRIBUTING.md) are likely to be ignored. |
transformers | 11,136 | closed | Trainer callbacks such as on_epoch_end do not pass in the documented eval dataloader | ## Environment info
<!-- You can run the command `transformers-cli env` and copy-and-paste its output below.
Don't forget to fill out the missing fields in that output! -->
- `transformers` version: 4.4.2
- Platform: ubuntu 20
- Python version: 3.8.5
- PyTorch version (GPU?): Happens on GPU and no GPU
- Tensorflow version (GPU?): not used
- Using GPU in script?: I check if it is available and use it if it is
- Using distributed or parallel set-up in script?: no
### Who can help
@sgugger
## Information
Model I am using (Bert, XLNet ...): Bert
The problem arises when using:
* [ ] the official example scripts: (give details below)
* [X] my own modified scripts: (give details below)
The tasks I am working on is:
* [ ] an official GLUE/SQUaD task: (give the name)
* [X ] my own task or dataset: (give details below)
## To reproduce
Create an on_epoch_end callback and attempt to use the documented eval_dataloader. You will find it is not passed in.
Even printing out the kwargs shows the training dataloader but not the eval dataloader. If you look at trainer.py at line 1048 you will see that all the correct arguments are attached to the callback except the eval_dataloader.
Additionally the documentation on the website is wrong as it describes the eval dataloader as the dataloader used for training just like the train dataloader.
Steps to reproduce the behavior:
1. Create on_epoch_end callback
2.attempt to use the documented eval_dataloader
<!-- If you have code snippets, error messages, stack traces please provide them here as well.
Important! Use code tags to correctly format your code. See https://help.github.com/en/github/writing-on-github/creating-and-highlighting-code-blocks#syntax-highlighting
Do not use screenshots, as they are hard to read and (more importantly) don't allow others to copy-and-paste your code.-->
## Expected behavior
I would expect thee able to access the eval dataloader in the callback
I am happy to help with this if it is as simple as it seems.
| 04-08-2021 08:25:57 | 04-08-2021 08:25:57 | It seems like the solution would be to just add
```
self.callback_handler.eval_dataloader = eval_dataloader
```
below line 1051 in trainer.py and then fix the documentation.
I see the eval_dataloader can be attached in the prediction_loop function but that doesn't seem to take effect when my callback is called during training. I want to see how my metrics change at the end of every epoch so I need to use the eval dataloader.<|||||>The evaluation dataloader does not exist at this step, it is only accessible in the evaluation loop, which is why it's attached [here only](https://github.com/huggingface/transformers/blob/5bf5d50c8dae2e54327a754aa476f13a0308f844/src/transformers/trainer.py#L1892).
It will exist and be passed to the `on_epoch_end` event but only if one evaluation loop has run before. The problem you might be encountering is that if you have set your `evaluation_strategy` to `epochs`, the evaluation dataloader will not be present at the first `on_epoch_end`: that's because this is the event that triggers the evaluation after each epoch in the main `DefaultFlowCallback`. <|||||>This issue has been automatically marked as stale because it has not had recent activity. If you think this still needs to be addressed please comment on this thread.
Please note that issues that do not follow the [contributing guidelines](https://github.com/huggingface/transformers/blob/master/CONTRIBUTING.md) are likely to be ignored. |
transformers | 11,135 | closed | Adding FastSpeech2 | # What does this PR do?
This is a draft PR for Fastspeech2 which includes melgan and a custom g2p pythorch module. See https://huggingface.co/ontocord/fastspeech2-en
## Who can review?
Anyone in the community is free to review the PR once the tests have passed. Feel free to tag
members/contributors which may be interested in your PR.
@patil-suraj
Models:
- Fastspeech2
| 04-08-2021 07:33:38 | 04-08-2021 07:33:38 | Hey @ontocord,
Thanks a lot for opening this pull request :-)
We are very much open to adding FastSpeech2 to `transformers`! One thing that is quite important to us is that we stick as much as possible to the original implementation of the model.
IMO, the easiest way to approach this would be to translate [this](TF code) to PyTorch: https://github.com/TensorSpeech/TensorFlowTTS/blob/master/tensorflow_tts/models/fastspeech2.py and add it to our lib.
Let me know if it would also be ok/interesting for you to stay closer to the official code -> I'm very happy to help you get this PR merged then :-)<|||||>Happy for you to close this PR in favor of an official implementation. Just as an aside, the G2P (GRU->GRU) code is actually based on the original impementation from the Fastspeech2 paper. But it uses https://github.com/Kyubyong/g2p which is slower than pytorh and based on Numpy. I re-wrote the G2P in pytorch based on the G2P author's notes, and retrained it so it's faster.
From the paper:
"To alleviate the mispronunciation problem, we convert the
text sequence into the phoneme sequence (Arik et al., 2017; Wang et al., 2017; Shen et al., 2018;
Sun et al., 2019) with an open-source grapheme-to-phoneme tool5
...
5https://github.com/Kyubyong/g2p"
I think this module is really one of the things that keeps the Fastspeech2 model (and tacotron 2 and similar models) from generalizing to more languages. In theory you could just train on character level, but it's harder. DM if you want to discuss work arounds...<|||||>Hey @ontocord @patrickvonplaten, I was wondering if there has been a followup to this PR. I'd love to see transformer TTS models like FastSpeech2 in this library and would be more than happy to help contribute if possible!<|||||>I also think we should eventually add models like FastSpeech2 to the library. Gently ping to @anton-l here who was interested in this addition as well.<|||||>@patrickvonplaten @anton-l Do we only add models with official weights from the paper authors? AFAIK FastSpeech2 has plenty of unofficial implementations with weights, but there is no official repository ([PwC](https://paperswithcode.com/paper/fastspeech-2-fast-and-high-quality-end-to-end)). I think we should reach out to the author (Yi Ren is on GitHub), and if that doesn't work out, consider which implementation/weights we want to port. What do you think?
Also if you'd prefer, I'll open a new issue dedicated to this discussion instead of hijacking this PR.<|||||>I think we should definitely reach out to the original authors! Feel free to contact them :-)<|||||>Just emailed the first author and cc'd both you and Anton! I'll keep you posted. <|||||>I would be interested in working on something more generic than fastspeech2 which needs a g2p module. It’s not truly end to end.
> On Jan 3, 2022, at 7:55 AM, Jake Tae ***@***.***> wrote:
>
>
> Just emailed the first author and cc'd both you and Anton! I'll keep you posted.
>
> —
> Reply to this email directly, view it on GitHub, or unsubscribe.
> Triage notifications on the go with GitHub Mobile for iOS or Android.
> You are receiving this because you were mentioned.
|
transformers | 11,134 | closed | Problem with data download | Hello can I ask which is the directory that the downloaded stuff is stored? I am trying to bundle these data into a docker image and every time the image is built transformers is downloading the 440M data from the beggining | 04-08-2021 06:59:24 | 04-08-2021 06:59:24 | @chatzich your question seems similar to this - #2323. <|||||>This issue has been automatically marked as stale because it has not had recent activity. If you think this still needs to be addressed please comment on this thread.
Please note that issues that do not follow the [contributing guidelines](https://github.com/huggingface/transformers/blob/master/CONTRIBUTING.md) are likely to be ignored. |
transformers | 11,133 | closed | Typo fix of the name of BertLMHeadModel in BERT doc | # What does this PR do?
Typo fix in BERT doc.
I was confused that I couldn't find the implementation and discussion log of `BertModelLMHeadModel,` and found that `BertLMHeadModel` is the correct name.
It was titled `BertModelLMHeadModel` in the BERT doc, and it seems `BertLMHeadModel` is the intended name.
## Before submitting
- [x] This PR fixes a typo or improves the docs (you can dismiss the other checks if that's the case).
- [x] Did you read the [contributor guideline](https://github.com/huggingface/transformers/blob/master/CONTRIBUTING.md#start-contributing-pull-requests),
Pull Request section?
- [ ] Was this discussed/approved via a Github issue or the [forum](https://discuss.huggingface.co/)? Please add a link
to it if that's the case.
- [ ] Did you make sure to update the documentation with your changes? Here are the
[documentation guidelines](https://github.com/huggingface/transformers/tree/master/docs), and
[here are tips on formatting docstrings](https://github.com/huggingface/transformers/tree/master/docs#writing-source-documentation).
- [ ] Did you write any new necessary tests?
## Who can review?
Documentation: @sgugger
| 04-08-2021 05:21:04 | 04-08-2021 05:21:04 | |
transformers | 11,132 | closed | Clear add labels to token classification example | I have spent a lot of time looking for a clear example on how to add labels to an existing model. For example, I would like to train Bert to recognize addresses in addition to the B-PER, B-ORG, etc. labels. So I think I would do the following
1. Add B-ADDRESS, B-CITY, B-STATE, etc. to a portion of a data set (like take a small subset of conll2003 or custom data.)
2. Add the labels to the id2label and label2id - BUT, where do I do this? In the config object? Is that all since the model does not expect the new labels?
3. Set the label count variable (in config again?)
4. Train on the new datasets (conll2003 & the new data) using the config file?
So in addition to the questions above, I would think that I could remove the head and do some transfer learning - meaning that I don't have to re-train with the conll2003 data. I should be able to just add training with the new data so that I have Bert+conll2003+my new data but I am only training on my new data. However, I don't see an example of this with HF either.
Sorry if I am just missing it. Here are some of the links I have looked at:
https://huggingface.co/transformers/custom_datasets.html#token-classification-with-w-nut-emerging-entities
https://huggingface.co/transformers/custom_datasets.html#fine-tuning-with-trainer
https://discuss.huggingface.co/t/retrain-reuse-fine-tuned-models-on-different-set-of-labels/346/4 ** GOOD INFO but not complete
https://github.com/huggingface/transformers/tree/master/examples/token-classification
@sgugger was in the thread above so he may be able to help?
| 04-08-2021 02:01:09 | 04-08-2021 02:01:09 | Have you looked at the [run_ner](https://github.com/huggingface/transformers/blob/master/examples/token-classification/run_ner.py) example and its corresponding [notebook](https://github.com/huggingface/notebooks/blob/master/examples/token_classification.ipynb)?<|||||>Thanks for getting back to me so quickly @sgugger.
I am using what looks like the same exact version of run_ner with a different notebook. However, I don't see anything in the notebook you provided or run_ner that adds new labels. For example, if you look at the cell 8 of the notebook you linked, you see that it is only using the labels loaded with the model. What if I wanted to find addresses or some other entity type?
Thanks for your help!
Gregg
<|||||>I am confused, the labels are loaded from the dataset, not the model. If you have another dataset with other labels, the rest of the notebook will work the same way.<|||||>Sorry,
What I want to do is load the Bert model for NER trained from Conll2003 and use transfer learning to add addition training with new, additional data tagged with additional labels.
In the end, I want to take advantage of the existing training and add my own; teaching it to recognize more entity types.
I have seen that some people seem to have done this but I haven't found the complete list of steps. <|||||>This issue has been automatically marked as stale because it has not had recent activity. If you think this still needs to be addressed please comment on this thread.
Please note that issues that do not follow the [contributing guidelines](https://github.com/huggingface/transformers/blob/master/CONTRIBUTING.md) are likely to be ignored. |
transformers | 11,131 | closed | Update training.rst | # What does this PR do?
fix a typo in tutorial
| 04-08-2021 01:41:57 | 04-08-2021 01:41:57 | This issue has been automatically marked as stale because it has not had recent activity. If you think this still needs to be addressed please comment on this thread.
Please note that issues that do not follow the [contributing guidelines](https://github.com/huggingface/transformers/blob/master/CONTRIBUTING.md) are likely to be ignored. |
transformers | 11,130 | closed | Fix LogitsProcessor documentation | # What does this PR do?
<!--
Congratulations! You've made it this far! You're not quite done yet though.
Once merged, your PR is going to appear in the release notes with the title you set, so make sure it's a great title that fully reflects the extent of your awesome contribution.
Then, please replace this with a description of the change and which issue is fixed (if applicable). Please also include relevant motivation and context. List any dependencies (if any) that are required for this change.
Once you're done, someone will review your PR shortly (see the section "Who can review?" below to tag some potential reviewers). They may suggest changes to make the code even better. If no one reviewed your PR after a week has passed, don't hesitate to post a new comment @-mentioning the same persons---sometimes notifications get lost.
-->
<!-- Remove if not applicable -->
Fixes document related to LogitsProcessor
## Before submitting
- [x] This PR fixes a typo or improves the docs (you can dismiss the other checks if that's the case).
- [x] Did you read the [contributor guideline](https://github.com/huggingface/transformers/blob/master/CONTRIBUTING.md#start-contributing-pull-requests),
Pull Request section?
- [ ] Was this discussed/approved via a Github issue or the [forum](https://discuss.huggingface.co/)? Please add a link
to it if that's the case.
- [x] Did you make sure to update the documentation with your changes? Here are the
[documentation guidelines](https://github.com/huggingface/transformers/tree/master/docs), and
[here are tips on formatting docstrings](https://github.com/huggingface/transformers/tree/master/docs#writing-source-documentation).
- [ ] Did you write any new necessary tests?
## Who can review?
Anyone in the community is free to review the PR once the tests have passed. Feel free to tag
members/contributors which may be interested in your PR.
<!-- Your PR will be replied to more quickly if you can figure out the right person to tag with @
If you know how to use git blame, that is the easiest way, otherwise, here is a rough guide of **who to tag**.
Please tag fewer than 3 people.
Models:
- albert, bert, xlm: @LysandreJik
- blenderbot, bart, marian, pegasus, encoderdecoder, t5: @patrickvonplaten, @patil-suraj
- longformer, reformer, transfoxl, xlnet: @patrickvonplaten
- fsmt: @stas00
- funnel: @sgugger
- gpt2: @patrickvonplaten, @LysandreJik
- rag: @patrickvonplaten, @lhoestq
- tensorflow: @LysandreJik
Library:
- benchmarks: @patrickvonplaten
- deepspeed: @stas00
- ray/raytune: @richardliaw, @amogkam
- text generation: @patrickvonplaten
- tokenizers: @n1t0, @LysandreJik
- trainer: @sgugger
- pipelines: @LysandreJik
Documentation: @sgugger
HF projects:
- datasets: [different repo](https://github.com/huggingface/datasets)
- rust tokenizers: [different repo](https://github.com/huggingface/tokenizers)
Examples:
- maintained examples (not research project or legacy): @sgugger, @patil-suraj
- research_projects/bert-loses-patience: @JetRunner
- research_projects/distillation: @VictorSanh
-->
| 04-08-2021 01:21:39 | 04-08-2021 01:21:39 | |
transformers | 11,129 | open | denoising with sentence permutation, and language sampling | # 🚀 Feature request
<!-- A clear and concise description of the feature proposal.
Please provide a link to the paper and code in case they exist. -->
## Motivation
When training or fine tuning models, data collator provided in huggingface isn't enough.
For example, if we want to further pretrain `mBART` or `XLM-R`, where language sampling or sentence permutation are needed, which is hard to do with huggingface datasets API since it loads all language datasets at first.
Thanks!
| 04-07-2021 22:08:57 | 04-07-2021 22:08:57 | |
transformers | 11,128 | closed | Run mlm pad to multiple for fp16 | # What does this PR do?
This PR uses padding to a multiple of 8 in the run_mlm.py language modeling example, when fp16 is used. Since the DataCollatorForLanguageModeling did not initially accept the pad_to_multiple_of option, that functionality was added.
Fixes #10627
## Before submitting
- [X] Did you write any new necessary tests?
## Who can review?
@sgugger | 04-07-2021 21:19:53 | 04-07-2021 21:19:53 | |
transformers | 11,127 | closed | Fix and refactor check_repo | # What does this PR do?
This PR fixes issues that a user may have with `make quality`/`make fixup` when all backends are not installed: in that case `requires_backends` is imported in the main init on top of the dummy objects and the script complains it's not documented.
The PR also refactors the white-list for the model that are not in an Auto-class, which was mostly containing Encoder and Decoder pieces of seq2seq models. | 04-07-2021 19:35:28 | 04-07-2021 19:35:28 | |
transformers | 11,126 | closed | Create embeddings vectors for the context parameter of QuestionAnsweringPipeline for reusability. | Create embeddings vectors for the context parameter of QuestionAnsweringPipeline for reusability.
**Scinario**
For each time we pass question and context to QuestionAnsweringPipeline, the context vector is created. Is there a way to create this context for once and just pass the question to save time and make inference quicker. | 04-07-2021 18:45:21 | 04-07-2021 18:45:21 | This issue has been automatically marked as stale because it has not had recent activity. If you think this still needs to be addressed please comment on this thread.
Please note that issues that do not follow the [contributing guidelines](https://github.com/huggingface/transformers/blob/master/CONTRIBUTING.md) are likely to be ignored. |
transformers | 11,125 | closed | Not very good answers | When I try to feed in this context from a long txt file lets say below:
[New_Spark_Questions.txt](https://github.com/huggingface/transformers/files/6273496/New_Spark_Questions.txt)
and I feed in a question from that txt file: Would police or the FBI ever be able to access DNA or other information collected?
it is not giving me a very good answer: I get Answer: 'help speed up the progress of autism research' with score 0.4306815266609192. How do we see all the cores available, how does the model decide which answer is the best?
| 04-07-2021 17:34:12 | 04-07-2021 17:34:12 | The text you are providing is probably too long for the model. Most Transformer models accept a sequence length of 512 tokens.
Which model did you use?<|||||>I just used the general q-a pipeline:
from google.colab import files
uploaded = files.upload()
filename = "New_Spark_Questions.txt"
new_file = uploaded[filename].decode("utf-8")
!pip3 install sentencepiece
!pip3 install git+https://github.com/huggingface/transformers
question = "Why should I take part in SPARK?"
from transformers import pipeline
qa = pipeline("question-answering")
answer = qa(question=question, context=new_file)
print(f"Question: {question}")
print(f"Answer: '{answer['answer']}' with score {answer['score']}")
How does the q-a pipeline decide the score?
Also how do we use a model like Bert, XLNet, etc. on a q-a pipeline
Does the input in the q-a pipeline have to be a dictionary?<|||||>This issue has been automatically marked as stale because it has not had recent activity. If you think this still needs to be addressed please comment on this thread.
Please note that issues that do not follow the [contributing guidelines](https://github.com/huggingface/transformers/blob/master/CONTRIBUTING.md) are likely to be ignored. |
transformers | 11,124 | closed | ALBERT pretrained tokenizer loading failed on Google Colab | I tried the example code for ALBERT model on Google Colab:
```
from transformers import AlbertTokenizer, AlbertModel
import torch
tokenizer = AlbertTokenizer.from_pretrained('albert-base-v2')
model = AlbertModel.from_pretrained('albert-base-v2')
inputs = tokenizer("Hello, my dog is cute", return_tensors="pt")
outputs = model(**inputs)
last_hidden_states = outputs.last_hidden_state
```
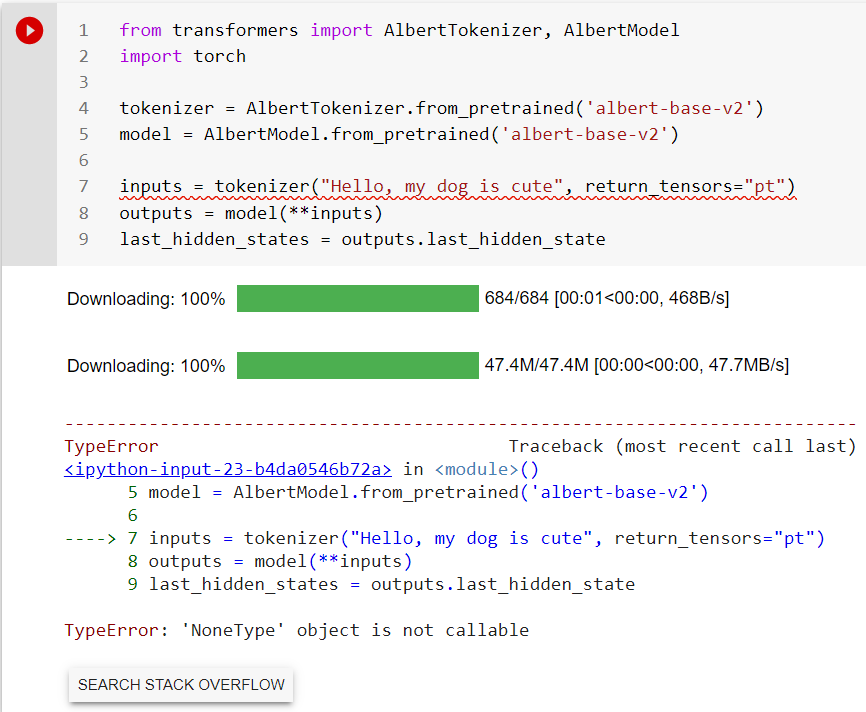
Error Message:
```
---------------------------------------------------------------------------
TypeError Traceback (most recent call last)
<ipython-input-23-b4da0546b72a> in <module>()
5 model = AlbertModel.from_pretrained('albert-base-v2')
6
----> 7 inputs = tokenizer("Hello, my dog is cute", return_tensors="pt")
8 outputs = model(**inputs)
9 last_hidden_states = outputs.last_hidden_state
TypeError: 'NoneType' object is not callable
```
It seems that the ALBERT tokenizer failed to load correctly. And I tried BERT's pretrained tokenizer and it could be loaded correctly instead.
BERT tokenizer:
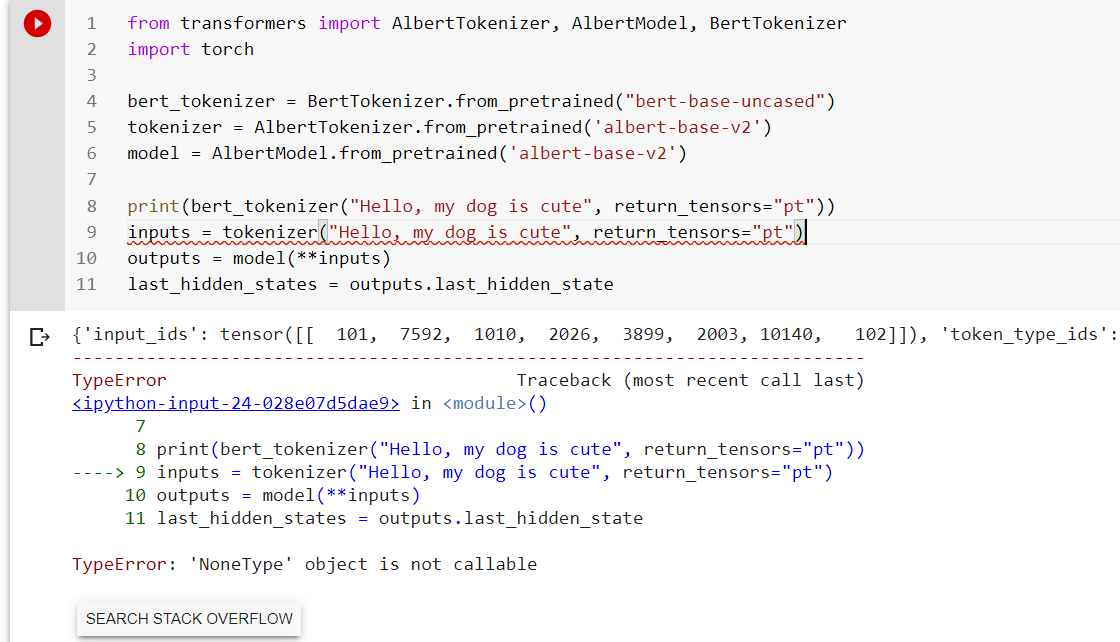
| 04-07-2021 17:32:31 | 04-07-2021 17:32:31 | Have you installed the [sentencepiece](https://github.com/google/sentencepiece) library?<|||||>> Have you installed the [sentencepiece](https://github.com/google/sentencepiece) library?
Yes.
```
!pip install transformers
!pip install sentencepiece
```
```
Requirement already satisfied: transformers in /usr/local/lib/python3.7/dist-packages (4.5.0)
Requirement already satisfied: numpy>=1.17 in /usr/local/lib/python3.7/dist-packages (from transformers) (1.19.5)
Requirement already satisfied: tqdm>=4.27 in /usr/local/lib/python3.7/dist-packages (from transformers) (4.41.1)
Requirement already satisfied: requests in /usr/local/lib/python3.7/dist-packages (from transformers) (2.23.0)
Requirement already satisfied: importlib-metadata; python_version < "3.8" in /usr/local/lib/python3.7/dist-packages (from transformers) (3.8.1)
Requirement already satisfied: filelock in /usr/local/lib/python3.7/dist-packages (from transformers) (3.0.12)
Requirement already satisfied: sacremoses in /usr/local/lib/python3.7/dist-packages (from transformers) (0.0.44)
Requirement already satisfied: tokenizers<0.11,>=0.10.1 in /usr/local/lib/python3.7/dist-packages (from transformers) (0.10.2)
Requirement already satisfied: regex!=2019.12.17 in /usr/local/lib/python3.7/dist-packages (from transformers) (2019.12.20)
Requirement already satisfied: packaging in /usr/local/lib/python3.7/dist-packages (from transformers) (20.9)
Requirement already satisfied: chardet<4,>=3.0.2 in /usr/local/lib/python3.7/dist-packages (from requests->transformers) (3.0.4)
Requirement already satisfied: certifi>=2017.4.17 in /usr/local/lib/python3.7/dist-packages (from requests->transformers) (2020.12.5)
Requirement already satisfied: urllib3!=1.25.0,!=1.25.1,<1.26,>=1.21.1 in /usr/local/lib/python3.7/dist-packages (from requests->transformers) (1.24.3)
Requirement already satisfied: idna<3,>=2.5 in /usr/local/lib/python3.7/dist-packages (from requests->transformers) (2.10)
Requirement already satisfied: zipp>=0.5 in /usr/local/lib/python3.7/dist-packages (from importlib-metadata; python_version < "3.8"->transformers) (3.4.1)
Requirement already satisfied: typing-extensions>=3.6.4; python_version < "3.8" in /usr/local/lib/python3.7/dist-packages (from importlib-metadata; python_version < "3.8"->transformers) (3.7.4.3)
Requirement already satisfied: six in /usr/local/lib/python3.7/dist-packages (from sacremoses->transformers) (1.15.0)
Requirement already satisfied: joblib in /usr/local/lib/python3.7/dist-packages (from sacremoses->transformers) (1.0.1)
Requirement already satisfied: click in /usr/local/lib/python3.7/dist-packages (from sacremoses->transformers) (7.1.2)
Requirement already satisfied: pyparsing>=2.0.2 in /usr/local/lib/python3.7/dist-packages (from packaging->transformers) (2.4.7)
Requirement already satisfied: sentencepiece in /usr/local/lib/python3.7/dist-packages (0.1.95)
```<|||||>I restart the runtime and it is fixed now. Seems like some strange compatibility issues with colab. |
transformers | 11,123 | closed | Adds use_auth_token with pipelines | # What does this PR do?
This PR adds `use_auth_token` as a named parameter to the `pipeline`. Also fixed `AutoConfig.from_pretrained` adding the `model_kwargs` as `**kwargs` to load private model with `use_auth_token`.
**Possible Usage for `pipeline` with `use_auth_token`:**
with model_kwargs
```python
hf_pipeline = pipeline('sentiment-analysis',
model='philschmid/sagemaker-getting-started',
tokenizer='philschmid/sagemaker-getting-started',
model_kwargs={"use_auth_token": "xxx"})
```
as named paramter
```python
hf_pipeline = pipeline('sentiment-analysis',
model='philschmid/sagemaker-getting-started',
tokenizer='philschmid/sagemaker-getting-started',
use_auth_token = "xxx")
```
cc @Narsil | 04-07-2021 16:11:46 | 04-07-2021 16:11:46 | |
transformers | 11,122 | closed | fixed max_length in beam_search() and group_beam_search() to use beam… | …_scorer.max_length
# What does this PR do?
Fixes the issue #11040
`beam_search()` and `group_beam_search()` uses `beam_scorer.max_length` if `max_length` is not explicitly passed.
<!--
Congratulations! You've made it this far! You're not quite done yet though.
Once merged, your PR is going to appear in the release notes with the title you set, so make sure it's a great title that fully reflects the extent of your awesome contribution.
Then, please replace this with a description of the change and which issue is fixed (if applicable). Please also include relevant motivation and context. List any dependencies (if any) that are required for this change.
Once you're done, someone will review your PR shortly (see the section "Who can review?" below to tag some potential reviewers). They may suggest changes to make the code even better. If no one reviewed your PR after a week has passed, don't hesitate to post a new comment @-mentioning the same persons---sometimes notifications get lost.
-->
<!-- Remove if not applicable -->
Fixes #11040
## Before submitting
- [ ] This PR fixes a typo or improves the docs (you can dismiss the other checks if that's the case).
- [x] Did you read the [contributor guideline](https://github.com/huggingface/transformers/blob/master/CONTRIBUTING.md#start-contributing-pull-requests),
Pull Request section?
- [ ] Was this discussed/approved via a Github issue or the [forum](https://discuss.huggingface.co/)? Please add a link
to it if that's the case.
- [ ] Did you make sure to update the documentation with your changes? Here are the
[documentation guidelines](https://github.com/huggingface/transformers/tree/master/docs), and
[here are tips on formatting docstrings](https://github.com/huggingface/transformers/tree/master/docs#writing-source-documentation).
- [] Did you write any new necessary tests?
## Who can review?
Anyone in the community is free to review the PR once the tests have passed. Feel free to tag
members/contributors which may be interested in your PR.
<!-- Your PR will be replied to more quickly if you can figure out the right person to tag with @
If you know how to use git blame, that is the easiest way, otherwise, here is a rough guide of **who to tag**.
Please tag fewer than 3 people.
Models:
- albert, bert, xlm: @LysandreJik
- blenderbot, bart, marian, pegasus, encoderdecoder, t5: @patrickvonplaten, @patil-suraj
- longformer, reformer, transfoxl, xlnet: @patrickvonplaten
- fsmt: @stas00
- funnel: @sgugger
- gpt2: @patrickvonplaten, @LysandreJik
- rag: @patrickvonplaten, @lhoestq
- tensorflow: @LysandreJik
Library:
- benchmarks: @patrickvonplaten
- deepspeed: @stas00
- ray/raytune: @richardliaw, @amogkam
- text generation: @patrickvonplaten
- tokenizers: @n1t0, @LysandreJik
- trainer: @sgugger
- pipelines: @LysandreJik
Documentation: @sgugger
HF projects:
- datasets: [different repo](https://github.com/huggingface/datasets)
- rust tokenizers: [different repo](https://github.com/huggingface/tokenizers)
Examples:
- maintained examples (not research project or legacy): @sgugger, @patil-suraj
- research_projects/bert-loses-patience: @JetRunner
- research_projects/distillation: @VictorSanh
-->
| 04-07-2021 14:41:32 | 04-07-2021 14:41:32 | hi @GeetDsa
Thanks a lot for the PR. I understand the issue and IMO what should be done here is to make sure to pass the same `max_length` to the `BeamScorer` and `beam_search` instead of changing the method.
This is because the overall philosophy of `generate` is that whenever some argument is `None` its value should explicitly default to the value specified in `config`. This how all generation methods work.<|||||>Thanks for the issue & PR @GeetDsa! I agree with @patil-suraj that we should not change the way `max_length` is set in `beam_search`.
Overall, the problem IMO is actually that `BeamScorer` has a `max_length` attribute... => this shouldn't be the case IMO:
- `BeamHypotheses` has a `max_length` attribute that is unused and can be removed
- `BeamSearchScorer` has a `max_length` attribute that is only used for the function `finalize` => the better approach here would be too pass `max_length` as an argument to `finalize(...)` IMO
This solution will then ensure that only one `max_length` is being used and should also help to refactor out `max_length` cc @Narsil longterm.
Do you want to give it a try @GeetDsa ? :-)<|||||>> Thanks for the issue & PR @GeetDsa! I agree with @patil-suraj that we should not change the way `max_length` is set in `beam_search`.
>
> Overall, the problem IMO is actually that `BeamScorer` has a `max_length` attribute... => this shouldn't be the case IMO:
>
> * `BeamHypotheses` has a `max_length` attribute that is unused and can be removed
> * `BeamSearchScorer` has a `max_length` attribute that is only used for the function `finalize` => the better approach here would be too pass `max_length` as an argument to `finalize(...)` IMO
>
> This solution will then ensure that only one `max_length` is being used and should also help to refactor out `max_length` cc @Narsil longterm.
>
> Do you want to give it a try @GeetDsa ? :-)
I can give a try :)
<|||||>> BeamHypotheses has a max_length attribute that is unused and can be removed
Nice !
> BeamSearchScorer has a max_length attribute that is only used for the function finalize => the better approach here would be too pass max_length as an argument to finalize(...) IMO
Seems easier.
@GeetDsa Do you think you could also add a test that reproduces your issue without your fix and that passes with the fix ? That will make backward compatibility easier to test (we're heading towards a direction to remove `max_length` as much as possible while maintaining backward compatbility)<|||||>I have created a new pull request #11378 ; @Narsil, I think it will be little hard and time consuming for me to implement a test as I am not well-versed with it.<|||||>This issue has been automatically marked as stale because it has not had recent activity. If you think this still needs to be addressed please comment on this thread.
Please note that issues that do not follow the [contributing guidelines](https://github.com/huggingface/transformers/blob/master/CONTRIBUTING.md) are likely to be ignored. |
transformers | 11,121 | closed | Errors in inference API | I understand that the inference API returns a json with "error" field if an error occurs. Where can I find the list of such possible errors? | 04-07-2021 14:18:32 | 04-07-2021 14:18:32 | Maybe of interest to @Narsil <|||||>@DeveloperInProgress .
Sorry, there is no exhaustive list of those as of yet (as a number of them are actual exceptions raised by transformers itself)
What I can say, is that Environment and ValueError are simply displayed as is and treated as user error (usually problem in the model configuration or inputs of the model).
Any other exception is raised as a server error (and looked at regularly).
Any "unknown error" is an error for which we can't find a good message, we try to accompany it (when it's possible) with any warnings that might have been raised earlier by transformers (for instance too long sequences make certain models crash, deep cuda errors are unusable as is, the warning is better).
Does that answer your question ?<|||||>@Narsil gotcha |
transformers | 11,120 | closed | Adds a note to resize the token embedding matrix when adding special … | …tokens
This was added to the `add_tokens` method, but was forgotten on the `add_special_tokens` method.
See the updated docs: https://191874-155220641-gh.circle-artifacts.com/0/docs/_build/html/internal/tokenization_utils.html?highlight=add_special_tokens#transformers.tokenization_utils_base.SpecialTokensMixin.add_special_tokens
closes https://github.com/huggingface/transformers/issues/11102 | 04-07-2021 13:31:39 | 04-07-2021 13:31:39 | |
transformers | 11,119 | closed | updated user permissions based on umask | # What does this PR do?
Fixes [#2065](https://github.com/huggingface/datasets/issues/2065) where cached model's permissions change depending on running user's umask.
## Who can review?
@thomwolf @stas00 please let me know if any other changes are required in this.
| 04-07-2021 13:14:19 | 04-07-2021 13:14:19 | Thank you, @bhavitvyamalik. This is excellent
Let's first review what we are trying to correct.
Looking under `~/.cache/huggingface/transformers/` I see:
```
-rw------- 1 stas stas 1.1K Oct 16 13:27 00209bab0f0b1af5ef50d4d8a2f8fb0589ec747d29d975f496d377312fc50ea7.688a102406298bdd2190bac9e0c6da7c3ac2bfa26aa40e9e07904fa
e563aeec3
-rw-rw-r-- 1 stas stas 158 Oct 16 13:27 00209bab0f0b1af5ef50d4d8a2f8fb0589ec747d29d975f496d377312fc50ea7.688a102406298bdd2190bac9e0c6da7c3ac2bfa26aa40e9e07904fa
e563aeec3.json
-rwxrwxr-x 1 stas stas 0 Oct 16 13:27 00209bab0f0b1af5ef50d4d8a2f8fb0589ec747d29d975f496d377312fc50ea7.688a102406298bdd2190bac9e0c6da7c3ac2bfa26aa40
e9e07904fae563aeec3.lock*
-rw------- 1 stas stas 4.9M Oct 14 11:56 002911b8e4cea0a107864f5b17f20c10f613d256e92e3c1247d6d174fbf56fe5.bf6ebaf6162cfbfbad2ce1909278a9ea1fbfe9284d318bff8bccddf
daa104205
-rw-rw-r-- 1 stas stas 130 Oct 14 11:56 002911b8e4cea0a107864f5b17f20c10f613d256e92e3c1247d6d174fbf56fe5.bf6ebaf6162cfbfbad2ce1909278a9ea1fbfe9284d318bff8bccddf
daa104205.json
```
So some files already have the correct perms `-rw-rw-r--`, but the others don't (`-rw-------` missing group/other perms)
If I try to get a new cached file:
```
PYTHONPATH="src" python -c "from transformers import AutoModel; AutoModel.from_pretrained('sshleifer/student_pegasus_xsum_16_8')"
```
we can see how the tempfile uses user-only perms while downloading it:
```
-rw------- 1 stas stas 246M Apr 7 10:06 tmplse9bwr1
```
and then your fix, adjusts the perms:
```
-rw-rw-r-- 1 stas stas 1.7G Apr 7 10:08 6636af980d08a3205d570f287ec5867d09d09c71d8d192861bf72e639a8c42fc.c7a07b57c0fbcb714c5b77aa08bea4f26ee23043f3c28e7c1af1153
a4bdfeea5
-rw-rw-r-- 1 stas stas 180 Apr 7 10:08 6636af980d08a3205d570f287ec5867d09d09c71d8d192861bf72e639a8c42fc.c7a07b57c0fbcb714c5b77aa08bea4f26ee23043f3c28e7c1af1153
a4bdfeea5.json
-rwxrwxr-x 1 stas stas 0 Apr 7 10:06 6636af980d08a3205d570f287ec5867d09d09c71d8d192861bf72e639a8c42fc.c7a07b57c0fbcb714c5b77aa08bea4f26ee23043f3c28
e7c1af1153a4bdfeea5.lock*
```
So this is goodness.<|||||>There is also a recipe subclassing `NamedTemporaryFile` https://stackoverflow.com/a/44130605/9201239 so it's even more atomic. But I'm not sure how that would work with resumes. I think your way is just fine for now and if we start doing more of that we will use a subclass that fixes perms internally.
<|||||>That makes sense, moving it from `cached_path` to `get_from_cache`. Let me push your suggested changes. Yeah, even I came across this subclassing `NamedTemporaryFile` when I had to fix this for Datasets but I felt adding more such tempfiles and then using subclassing would be more beneficial.<|||||>Any plans for asking user what file permission they want for this model?<|||||>> Any plans for asking user what file permission they want for this model?
Could you elaborate why would a user need to do that?
For shared environment this is a domain of `umask` and may be "sticky bit".
<|||||>When we started working on this feature for Datasets someone suggested this to us:
> For example, I might start a training run, downloading a dataset. Then, a couple of days later, a collaborator using the same repository might want to use the same dataset on the same shared filesystem, but won't be able to under the default permissions.
>
> Being able to specify directly in the top-level load_dataset() call seems important, but an equally valid option would be to just inherit from the running user's umask (this should probably be the default anyway).
>
> So basically, argument that takes a custom set of permissions, and by default, use the running user's umask!
Say if someone doesn't want the default running user's umask then they can specify what file permissions they want for that model. Incase they opt for this, we can avoid the umask part and directly `chmod` those permissions for the newly downloaded model. I'm not sure how useful would this be from the context from Transformers library.<|||||>Thank you for sharing the use case, that was helpful.
But this can be solved on the unix side of things. If you want a shared directory you can set it up as such. If you need to share files with other members of the group you put them into the same unix group.
IMHO, in general programs shouldn't mess with permissions directly. Other than the fix you just did which compensates for the temp facility restrictions.
<|||||>@LysandreJik, could you please have a look so that we could merge this? Thank you!<|||||>@stas00 @bhavitvyamalik I must say that I am not familiar with the umask command, but it seems = as @LysandreJik rightfully points out in my feature request https://github.com/huggingface/transformers/issues/12169#issuecomment-861467551 - that this may solve the issue that we were having.
In brief (but please read the whole issue if you have the time): we are trying to use a single shared cache directory for all our users to prevent duplicate models. This did not work as we were running into permission errors (due to `-rw-------` as @stas00 shows). Does this PR change the behaviour of created/downloaded files so that they adhere to the permission level of the current directory? Or at least that those files are accessible by all users?
Thanks!<|||||>I think, yes, this was the point of this PR. The problem is that `tempfile` forces user-only perms, so this PR restored them back to `umask`'s setting.
One other thing that helps is setting set group id bit `g+s`, which makes sub-dirs and files create under such dirs inherit the perms of the parent dir.
So your setup can be something like:
```
sudo find /shared/path -type d -execdir chmod g+rwxs {} \;
sudo find /shared/path -type f -execdir chmod g+rw {} \;
sudo chgrp -R shared_group_name /shared/path
```
where `/shared/path` is obvious and `shared_group_name` is the group name that all users that should have access belong to.
Finally, each user having `umask 0002` or `umask 0007` in their `~/.bashrc` will make sure that the files will be read/write-able by the group on creation. `0007` is if you don't want files to be readable by others.
Note that some unix programs don't respect set gid, e.g. `scp` ignores any sub-folders copied with `scp -r` and will set them to user's `umask` perms and drop setgid. But I don't think you'll be affected by it.<|||||>Thanks, this looks promising! We currently have a "hack" implemented that simply watches for new file changes and on the creation of a new file, changes the permissions. Not ideal, but seeing that some colleagues use older versions of transformers in their experiments, we will have to make do for now. |
transformers | 11,118 | closed | Some styling of the training table in Notebooks | # What does this PR do?
This PR removes the custom styling of the progress bar, because the default one is actually prettier and it can cause some lag on some browsers (like Safari) to recompute the style at each update of the progress bar.
It also removes the timing metrics which do not make much sense form the table (there are still in the log history). | 04-07-2021 13:10:34 | 04-07-2021 13:10:34 |
Subsets and Splits
No community queries yet
The top public SQL queries from the community will appear here once available.