repo
stringclasses 1
value | number
int64 1
25.3k
| state
stringclasses 2
values | title
stringlengths 1
487
| body
stringlengths 0
234k
⌀ | created_at
stringlengths 19
19
| closed_at
stringlengths 19
19
| comments
stringlengths 0
293k
|
---|---|---|---|---|---|---|---|
transformers | 10,716 | closed | Language model for wav2vec2.0 decoding | Hello, I implemented [wav2vec2.0 code](https://huggingface.co/blog/fine-tune-xlsr-wav2vec2) and a language model is not used for decoding. How can I add a language model (let's say a language model which is trained with KenLM) for decoding?
thanks in advance. | 03-15-2021 12:51:47 | 03-15-2021 12:51:47 | Hello, thanks for opening an issue! We try to keep the github issues for bugs/feature requests.
Could you ask your question on the [forum](https://discusss.huggingface.co) instead?
You can ping @patrickvonplaten on the forum as he's the best suited to help you out.
Thanks!<|||||>I moved the question to the forum and @patrickvonplaten said that the feature is not supported for now, but will be soon.
https://discuss.huggingface.co/t/language-model-for-wav2vec2-0-decoding/4434<|||||>I'm now working on this topic full time.
We will most likely foster a closer collaboration between [pyctcdecode](https://github.com/kensho-technologies/pyctcdecode) and Transformers. [Here](https://github.com/patrickvonplaten/Wav2Vec2_PyCTCDecode) is a github repo that shows how to use `pyctcdecode` with Wav2Vec2 for LM supported decoding. It works quite well with KenLM. |
transformers | 10,715 | closed | Pegasus-Large Question | Is [Pegasus Large model checkpoint](https://huggingface.co/google/pegasus-large) trained on any downstream task? Or is it only trained on pre-training task of gap sentence? | 03-15-2021 09:48:33 | 03-15-2021 09:48:33 | `pegasus-large` is a pre-trained checkpoint. It's not fine-tuned on downstream task. The finetuned checkpoint name will have the name of the datsets in them.
Also please the [forum](https://discuss.huggingface.co/) to ask such questions.
Thanks.<|||||>This issue has been automatically marked as stale because it has not had recent activity. If you think this still needs to be addressed please comment on this thread.
Please note that issues that do not follow the [contributing guidelines](https://github.com/huggingface/transformers/blob/master/CONTRIBUTING.md) are likely to be ignored. |
transformers | 10,714 | closed | [Wav2Vec2] Make wav2vec2 test deterministic | # What does this PR do?
The Wav2Vec2 tests are not deterministic on some machines. This PR should force all tests to use the expected samples. | 03-15-2021 08:03:36 | 03-15-2021 08:03:36 | |
transformers | 10,713 | closed | Is any possible with pipeline for using local model? | Hallo
I have fine tuned a model in my local PC. I have read the Doc for Pipeline to search a way to use local model in Pipeline. But didn't find. Someone who knows how should I do? | 03-15-2021 07:23:13 | 03-15-2021 07:23:13 | As mentioned in the [docs](https://huggingface.co/transformers/main_classes/pipelines.html), you can either provide a model identifier from the hub or a model that inherits from `PreTrainedModel` or `TFPreTrainedModel`.
So suppose you have fine-tuned a model and stored it into a variable called `model`, then you can initialize a corresponding pipeline by providing this model variable at initialization. Make sure that the model you're providing is suitable for the pipeline.
So suppose that you want to use the question answering pipeline, and you have a local `xxxForQuestionAnswering` model, then you can provide it as follows:
```
from transformers import pipeline
model = ...
nlp = pipeline (task='question-answering', model=model)
```
<|||||>> docs
Thanks a lot. |
transformers | 10,712 | closed | Update modeling_tf_pytorch_utils.py | # What does this PR do?
Fix a bug in convert_tf_weight_name_to_pt_weight_name().
Similar to the kernel parameters, the recurrent_kernel parameters in the LSTM networks need to be transposed, too.
Fixes # (issue)
## Before submitting
- [x] This PR fixes a typo or improves the docs (you can dismiss the other checks if that's the case).
- [x] Did you read the [contributor guideline](https://github.com/huggingface/transformers/blob/master/CONTRIBUTING.md#start-contributing-pull-requests),
Pull Request section?
- [ ] Was this discussed/approved via a Github issue or the [forum](https://discuss.huggingface.co/)? Please add a link
to it if that's the case.
- [x] Did you make sure to update the documentation with your changes? Here are the
[documentation guidelines](https://github.com/huggingface/transformers/tree/master/docs), and
[here are tips on formatting docstrings](https://github.com/huggingface/transformers/tree/master/docs#writing-source-documentation).
- [ ] Did you write any new necessary tests?
## Who can review?
Anyone in the community is free to review the PR once the tests have passed. Feel free to tag
members/contributors which may be interested in your PR.
## Memo
If the recurrent_kernel parameters are not transposed, it will cause the model parameters not to be loaded correctly, resulting in model migration failure.
| 03-15-2021 07:12:52 | 03-15-2021 07:12:52 | This issue has been automatically marked as stale because it has not had recent activity. If you think this still needs to be addressed please comment on this thread.
Please note that issues that do not follow the [contributing guidelines](https://github.com/huggingface/transformers/blob/master/CONTRIBUTING.md) are likely to be ignored.<|||||>> This issue has been automatically marked as stale because it has not had recent activity. If you think this still needs to be addressed please comment on this thread.
>
> Please note that issues that do not follow the [contributing guidelines](https://github.com/huggingface/transformers/blob/master/CONTRIBUTING.md) are likely to be ignored.
This issue causes incorrect parameter transfer between PyTorch and TensorFlow when the LSTM neural networks are used in code.<|||||>This issue has been automatically marked as stale because it has not had recent activity. If you think this still needs to be addressed please comment on this thread.
Please note that issues that do not follow the [contributing guidelines](https://github.com/huggingface/transformers/blob/master/CONTRIBUTING.md) are likely to be ignored. |
transformers | 10,711 | closed | 'Trainer' object has no attribute 'log_metrics' | ## Environment info
<!-- You can run the command `transformers-cli env` and copy-and-paste its output below.
Don't forget to fill out the missing fields in that output! -->
- `transformers` version:4.3.3
- Platform:Pytorch
- Python version:3.7.0
- PyTorch version (GPU?):GPU
- Tensorflow version (GPU?):
- Using GPU in script?:
- Using distributed or parallel set-up in script?:NO
### Who can help
<!-- Your issue will be replied to more quickly if you can figure out the right person to tag with @
If you know how to use git blame, that is the easiest way, otherwise, here is a rough guide of **who to tag**.
Please tag fewer than 3 people.
Models:
- albert, bert, xlm: @LysandreJik
- blenderbot, bart, marian, pegasus, encoderdecoder, t5: @patrickvonplaten, @patil-suraj
- longformer, reformer, transfoxl, xlnet: @patrickvonplaten
- fsmt: @stas00
- funnel: @sgugger
- gpt2: @patrickvonplaten, @LysandreJik
- rag: @patrickvonplaten, @lhoestq
- tensorflow: @jplu
Library:
- benchmarks: @patrickvonplaten
- deepspeed: @stas00
- ray/raytune: @richardliaw, @amogkam
- text generation: @patrickvonplaten
- tokenizers: @LysandreJik
- trainer: @sgugger
- pipelines: @LysandreJik
Documentation: @sgugger
HF projects:
- nlp datasets: [different repo](https://github.com/huggingface/nlp)
- rust tokenizers: [different repo](https://github.com/huggingface/tokenizers)
Examples:
- maintained examples (not research project or legacy): @sgugger, @patil-suraj
- research_projects/bert-loses-patience: @JetRunner
- research_projects/distillation: @VictorSanh
-->
## Information
Model I am using (Bert, XLNet ...):
The problem arises when using:
* [x] the official example scripts: (give details below)
https://github.com/huggingface/transformers/blob/master/examples/language-modeling/run_mlm.py
* [ ] my own modified scripts: (give details below)
The tasks I am working on is:
* [x] an official GLUE/SQUaD task: (give the name)
* [ ] my own task or dataset: (give details below)
## To reproduce
Steps to reproduce the behavior:
1.python run_mlm.py --model_name_or_path release_model/ --dataset_name wikitext --dataset_config_name wikitext-2-raw-v1 --do_train --do_eval --output_dir test-mlm --max_seq_length 128
2.Got an error:
```
[INFO|trainer.py:1408] 2021-03-15 11:10:32,884 >> Saving model checkpoint to test-mlm
[INFO|configuration_utils.py:304] 2021-03-15 11:10:32,886 >> Configuration saved in test-mlm/config.json
[INFO|modeling_utils.py:817] 2021-03-15 11:10:33,863 >> Model weights saved in test-mlm/pytorch_model.bin
Traceback (most recent call last):
File "run_mlm.py", line 475, in <module>
main()
File "run_mlm.py", line 450, in main
trainer.log_metrics("train", metrics)
AttributeError: 'Trainer' object has no attribute 'log_metrics'
```
<!-- If you have code snippets, error messages, stack traces please provide them here as well.
Important! Use code tags to correctly format your code. See https://help.github.com/en/github/writing-on-github/creating-and-highlighting-code-blocks#syntax-highlighting
Do not use screenshots, as they are hard to read and (more importantly) don't allow others to copy-and-paste your code.-->
## Expected behavior
<!-- A clear and concise description of what you would expect to happen. -->
The script finish without error. | 03-15-2021 03:12:28 | 03-15-2021 03:12:28 | Btw ,these 3 APIs show similar error:
**API:**
```
trainer.log_metrics("train", metrics)
trainer.save_metrics("train", metrics)
trainer.save_state()
```
**error**
```
Traceback (most recent call last):
File "run_mlm.py", line 476, in <module>
main()
File "run_mlm.py", line 452, in main
trainer.save_state()
AttributeError: 'Trainer' object has no attribute 'save_state'
```<|||||>See #10446 <|||||>Indeed. Please search the issues before opening one that is an exact duplicate of an existing one (see the link above for a resolution of your problem). |
transformers | 10,710 | closed | independent training / eval with local files | # What does this PR do?
<!--
Congratulations! You've made it this far! You're not quite done yet though.
Once merged, your PR is going to appear in the release notes with the title you set, so make sure it's a great title that fully reflects the extent of your awesome contribution.
Then, please replace this with a description of the change and which issue is fixed (if applicable). Please also include relevant motivation and context. List any dependencies (if any) that are required for this change.
Once you're done, someone will review your PR shortly (see the section "Who can review?" below to tag some potential reviewers). They may suggest changes to make the code even better. If no one reviewed your PR after a week has passed, don't hesitate to post a new comment @-mentioning the same persons---sometimes notifications get lost.
-->
<!-- Remove if not applicable -->
Allows running evaluation on local files without specifying a train file.
## Before submitting
- [ ] This PR fixes a typo or improves the docs (you can dismiss the other checks if that's the case).
- [x] Did you read the [contributor guideline](https://github.com/huggingface/transformers/blob/master/CONTRIBUTING.md#start-contributing-pull-requests),
Pull Request section?
- [ ] Was this discussed/approved via a Github issue or the [forum](https://discuss.huggingface.co/)? Please add a link
to it if that's the case.
- [ ] Did you make sure to update the documentation with your changes? Here are the
[documentation guidelines](https://github.com/huggingface/transformers/tree/master/docs), and
[here are tips on formatting docstrings](https://github.com/huggingface/transformers/tree/master/docs#writing-source-documentation).
- [ ] Did you write any new necessary tests?
## Who can review?
Anyone in the community is free to review the PR once the tests have passed. Feel free to tag
members/contributors which may be interested in your PR.
<!-- Your PR will be replied to more quickly if you can figure out the right person to tag with @
If you know how to use git blame, that is the easiest way, otherwise, here is a rough guide of **who to tag**.
Please tag fewer than 3 people.
Models:
- albert, bert, xlm: @LysandreJik
- blenderbot, bart, marian, pegasus, encoderdecoder, t5: @patrickvonplaten, @patil-suraj
- longformer, reformer, transfoxl, xlnet: @patrickvonplaten
- fsmt: @stas00
- funnel: @sgugger
- gpt2: @patrickvonplaten, @LysandreJik
- rag: @patrickvonplaten, @lhoestq
- tensorflow: @jplu
Library:
- benchmarks: @patrickvonplaten
- deepspeed: @stas00
- ray/raytune: @richardliaw, @amogkam
- text generation: @patrickvonplaten
- tokenizers: @n1t0, @LysandreJik
- trainer: @sgugger
- pipelines: @LysandreJik
Documentation: @sgugger
HF projects:
- nlp datasets: [different repo](https://github.com/huggingface/nlp)
- rust tokenizers: [different repo](https://github.com/huggingface/tokenizers)
Examples:
- maintained examples (not research project or legacy): @sgugger, @patil-suraj
- research_projects/bert-loses-patience: @JetRunner
- research_projects/distillation: @VictorSanh
-->
- maintained examples (not research project or legacy): @sgugger | 03-14-2021 23:11:11 | 03-14-2021 23:11:11 | Thanks! |
transformers | 10,709 | closed | Wrong link to super class | # What does this PR do?
Documentation was referring to slow tokenizer class while it should be the fast tokenizer.
## Before submitting
- [X] This PR fixes a typo or improves the docs (you can dismiss the other checks if that's the case).
- [X] Did you read the [contributor guideline](https://github.com/huggingface/transformers/blob/master/CONTRIBUTING.md#start-contributing-pull-requests),
Pull Request section?
- [ ] Was this discussed/approved via a Github issue or the [forum](https://discuss.huggingface.co/)? Please add a link
to it if that's the case.
- [ ] Did you make sure to update the documentation with your changes? Here are the
[documentation guidelines](https://github.com/huggingface/transformers/tree/master/docs), and
[here are tips on formatting docstrings](https://github.com/huggingface/transformers/tree/master/docs#writing-source-documentation).
- [ ] Did you write any new necessary tests?
## Who can review?
Anyone in the community is free to review the PR once the tests have passed. Feel free to tag
members/contributors which may be interested in your PR.
Documentation: @sgugger | 03-14-2021 15:43:57 | 03-14-2021 15:43:57 | |
transformers | 10,708 | closed | ValueError: Unsupported value type BatchEncoding returned by IteratorSpec._serialize | I am trying to prediction on a data-point, but keep getting an error,
```
from transformers import TFDistilBertForSequenceClassification, DistilBertTokenizerFast
# initialize longformer tokenizer and model
tokenizer = DistilBertTokenizerFast.from_pretrained("distilbert-base-uncased", do_lower_case=True)
model = TFDistilBertForSequenceClassification.from_pretrained("MODEL")
data = tokenizer.encode_plus(
sentence,
padding="max_length",
add_special_tokens=True,
max_length=512,
truncation=True,
)
data['input_ids'] = tf.convert_to_tensor(np.reshape(data['input_ids'], (1, -1)))
data['attention_mask'] = tf.convert_to_tensor(np.reshape(data['attention_mask'], (1, -1)))
model.predict(data)
```
```
---------------------------------------------------------------------------
ValueError Traceback (most recent call last)
<ipython-input-95-c8644be457c1> in <module>
----> 1 model.predict(data)
~/Desktop/Work/apps/env/lib/python3.8/site-packages/tensorflow/python/keras/engine/training.py in predict(self, x, batch_size, verbose, steps, callbacks, max_queue_size, workers, use_multiprocessing)
1692 for step in data_handler.steps():
1693 callbacks.on_predict_batch_begin(step)
-> 1694 tmp_batch_outputs = self.predict_function(iterator)
1695 if data_handler.should_sync:
1696 context.async_wait()
~/Desktop/Work/apps/env/lib/python3.8/site-packages/tensorflow/python/eager/def_function.py in __call__(self, *args, **kwds)
865 tracing_count = self.experimental_get_tracing_count()
866 with trace.Trace(self._name) as tm:
--> 867 result = self._call(*args, **kwds)
868 compiler = "xla" if self._jit_compile else "nonXla"
869 new_tracing_count = self.experimental_get_tracing_count()
~/Desktop/Work/apps/env/lib/python3.8/site-packages/tensorflow/python/eager/def_function.py in _call(self, *args, **kwds)
900 # In this case we have not created variables on the first call. So we can
901 # run the first trace but we should fail if variables are created.
--> 902 results = self._stateful_fn(*args, **kwds)
903 if self._created_variables:
904 raise ValueError("Creating variables on a non-first call to a function"
~/Desktop/Work/apps/env/lib/python3.8/site-packages/tensorflow/python/eager/function.py in __call__(self, *args, **kwargs)
3015 with self._lock:
3016 (graph_function,
-> 3017 filtered_flat_args) = self._maybe_define_function(args, kwargs)
3018 return graph_function._call_flat(
3019 filtered_flat_args, captured_inputs=graph_function.captured_inputs) # pylint: disable=protected-access
~/Desktop/Work/apps/env/lib/python3.8/site-packages/tensorflow/python/eager/function.py in _maybe_define_function(self, args, kwargs)
3395
3396 cache_key_context = self._cache_key_context()
-> 3397 cache_key = self._cache_key(args, kwargs, cache_key_context)
3398
3399 try:
~/Desktop/Work/apps/env/lib/python3.8/site-packages/tensorflow/python/eager/function.py in _cache_key(self, args, kwargs, cache_key_context, include_tensor_ranks_only)
3176 input_signature = pywrap_tfe.TFE_Py_EncodeArg(inputs,
3177 include_tensor_ranks_only)
-> 3178 hashable_input_signature = _make_input_signature_hashable(input_signature)
3179 else:
3180 del args, kwargs
~/Desktop/Work/apps/env/lib/python3.8/site-packages/tensorflow/python/eager/function.py in _make_input_signature_hashable(elem)
112 """
113 try:
--> 114 hash(elem)
115 except TypeError:
116 # TODO(slebedev): consider using nest.
~/Desktop/Work/apps/env/lib/python3.8/site-packages/tensorflow/python/framework/type_spec.py in __hash__(self)
311
312 def __hash__(self):
--> 313 return hash(self.__get_cmp_key())
314
315 def __reduce__(self):
~/Desktop/Work/apps/env/lib/python3.8/site-packages/tensorflow/python/framework/type_spec.py in __get_cmp_key(self)
349 """Returns a hashable eq-comparable key for `self`."""
350 # TODO(b/133606651): Decide whether to cache this value.
--> 351 return (type(self), self.__make_cmp_key(self._serialize()))
352
353 def __make_cmp_key(self, value):
~/Desktop/Work/apps/env/lib/python3.8/site-packages/tensorflow/python/framework/type_spec.py in __make_cmp_key(self, value)
367 ])
368 if isinstance(value, tuple):
--> 369 return tuple([self.__make_cmp_key(v) for v in value])
370 if isinstance(value, list):
371 return (list, tuple([self.__make_cmp_key(v) for v in value]))
~/Desktop/Work/apps/env/lib/python3.8/site-packages/tensorflow/python/framework/type_spec.py in <listcomp>(.0)
367 ])
368 if isinstance(value, tuple):
--> 369 return tuple([self.__make_cmp_key(v) for v in value])
370 if isinstance(value, list):
371 return (list, tuple([self.__make_cmp_key(v) for v in value]))
~/Desktop/Work/apps/env/lib/python3.8/site-packages/tensorflow/python/framework/type_spec.py in __make_cmp_key(self, value)
367 ])
368 if isinstance(value, tuple):
--> 369 return tuple([self.__make_cmp_key(v) for v in value])
370 if isinstance(value, list):
371 return (list, tuple([self.__make_cmp_key(v) for v in value]))
~/Desktop/Work/apps/env/lib/python3.8/site-packages/tensorflow/python/framework/type_spec.py in <listcomp>(.0)
367 ])
368 if isinstance(value, tuple):
--> 369 return tuple([self.__make_cmp_key(v) for v in value])
370 if isinstance(value, list):
371 return (list, tuple([self.__make_cmp_key(v) for v in value]))
~/Desktop/Work/apps/env/lib/python3.8/site-packages/tensorflow/python/framework/type_spec.py in __make_cmp_key(self, value)
379 return (np.ndarray, value.shape,
380 TypeSpec.__nested_list_to_tuple(value.tolist()))
--> 381 raise ValueError("Unsupported value type %s returned by "
382 "%s._serialize" %
383 (type(value).__name__, type(self).__name__))
ValueError: Unsupported value type BatchEncoding returned by IteratorSpec._serialize
```
This is the input, I don't see any mistake in the input as well,
`Tensorflow :- 2.5.0-dev20210311`
```
{'input_ids': <tf.Tensor: shape=(1, 512), dtype=int64, numpy=
array([[ 101, 10373, 25933, 20974, 13006, 2980, 21397, 4012, 4813,
2086, 4341, 6823, 2086, 20519, 4852, 10198, 3325, 6605,
3375, 3934, 2312, 2235, 4094, 2565, 2622, 2968, 2203,
2203, 3001, 2458, 2166, 23490, 2968, 2974, 7300, 6959,
2974, 3136, 6032, 3325, 2551, 10317, 5338, 4044, 2832,
8048, 3003, 2844, 4813, 6581, 4105, 11647, 2583, 6464,
2958, 6578, 22859, 8754, 21466, 3893, 10266, 3798, 2136,
2372, 5198, 3237, 3247, 11153, 3237, 2968, 2195, 3454,
2764, 3266, 4800, 10521, 6895, 28296, 5649, 8185, 2098,
5500, 2780, 9605, 2136, 2372, 2164, 6327, 17088, 6605,
3674, 18402, 3934, 2780, 7453, 3144, 2650, 2501, 12771,
3934, 9531, 2051, 5166, 3737, 4118, 20792, 6605, 6502,
9871, 3325, 2086, 2551, 2976, 2231, 6401, 2780, 12746,
16134, 6310, 2544, 16134, 6605, 3934, 5994, 28585, 2974,
4526, 9084, 2449, 6194, 7142, 7620, 2306, 6605, 7781,
2279, 4245, 4684, 5097, 11924, 16380, 5281, 19905, 25870,
2500, 6605, 4736, 4114, 6581, 17826, 6970, 28823, 4807,
4813, 3001, 2933, 3266, 11103, 2892, 8360, 3934, 2434,
4145, 2345, 7375, 4208, 2968, 4073, 12725, 2536, 3450,
14206, 4187, 4130, 3450, 4719, 3934, 5147, 2949, 2051,
2306, 5166, 25276, 2152, 3737, 4781, 3113, 8013, 14206,
3450, 8518, 2426, 2622, 2136, 2372, 4722, 7640, 6327,
4411, 2734, 3113, 2622, 3289, 5676, 2622, 6503, 6134,
6413, 4807, 4722, 6327, 22859, 4953, 14670, 9531, 3570,
5166, 2622, 8503, 3450, 2164, 5337, 3550, 24162, 2622,
9920, 5935, 8220, 4342, 2949, 12653, 2192, 2622, 8116,
3085, 2015, 23946, 20271, 3530, 2588, 3314, 13248, 2967,
2949, 2695, 7375, 3319, 6709, 2752, 7620, 10779, 2136,
2372, 8013, 8048, 3921, 10908, 2836, 4806, 7411, 4781,
2836, 9312, 2873, 5704, 2443, 7748, 2780, 12706, 5300,
6481, 5326, 7142, 7620, 6078, 10471, 5906, 2109, 2622,
2968, 3120, 3642, 2544, 2491, 16473, 10813, 3451, 2689,
8678, 3488, 5461, 29494, 10787, 3029, 8651, 2366, 2195,
3934, 5704, 2443, 25505, 2724, 2164, 3772, 3040, 10912,
6605, 2051, 27554, 11376, 19795, 3145, 4391, 6567, 12725,
3463, 4041, 2680, 7396, 10908, 2635, 2599, 3788, 4722,
22859, 7846, 2622, 20283, 4187, 5246, 9990, 4415, 2396,
2594, 10924, 4751, 11157, 10035, 8635, 2147, 7528, 29445,
6194, 3266, 4684, 16380, 7375, 2081, 3154, 3266, 2048,
2590, 2951, 25095, 3934, 2951, 2697, 4600, 2109, 3934,
11506, 2367, 4127, 2951, 2066, 2865, 4684, 2951, 2449,
3563, 2592, 2136, 2565, 2622, 10489, 3375, 3795, 3454,
5884, 10843, 16316, 3454, 12139, 11100, 5225, 3144, 23259,
6503, 3454, 6162, 2449, 3289, 2164, 8720, 5813, 10831,
3314, 4254, 2565, 6959, 2195, 3454, 3934, 6401, 2976,
4034, 2306, 1044, 7898, 2565, 3208, 2877, 3947, 7396,
3674, 4411, 3266, 2832, 7620, 2967, 3271, 10938, 3151,
29003, 16134, 3024, 11433, 6143, 3257, 8182, 2449, 5918,
2864, 6578, 4106, 2203, 2203, 6653, 2346, 2863, 2361,
24977, 4208, 2968, 4073, 12725, 2536, 3450, 14206, 4187,
4130, 3450, 4719, 3934, 5147, 2949, 2051, 2306, 5166,
25276, 2152, 3737, 4781, 3113, 8013, 14206, 3450, 8518,
2426, 2622, 2136, 2372, 4722, 7640, 6327, 4411, 2734,
3113, 2622, 3289, 5676, 2622, 6503, 6134, 2780, 3454,
2458, 2190, 6078, 2109, 2622, 2968, 3120, 3642, 2544,
2491, 16473, 10813, 3266, 9123, 3433, 3934, 4083, 19875,
6364, 17953, 2361, 2109, 22969, 2376, 8013, 102]])>, 'attention_mask': <tf.Tensor: shape=(1, 512), dtype=int64, numpy=
array([[1, 1, 1, 1, 1, 1, 1, 1, 1, 1, 1, 1, 1, 1, 1, 1, 1, 1, 1, 1, 1, 1,
1, 1, 1, 1, 1, 1, 1, 1, 1, 1, 1, 1, 1, 1, 1, 1, 1, 1, 1, 1, 1, 1,
1, 1, 1, 1, 1, 1, 1, 1, 1, 1, 1, 1, 1, 1, 1, 1, 1, 1, 1, 1, 1, 1,
1, 1, 1, 1, 1, 1, 1, 1, 1, 1, 1, 1, 1, 1, 1, 1, 1, 1, 1, 1, 1, 1,
1, 1, 1, 1, 1, 1, 1, 1, 1, 1, 1, 1, 1, 1, 1, 1, 1, 1, 1, 1, 1, 1,
1, 1, 1, 1, 1, 1, 1, 1, 1, 1, 1, 1, 1, 1, 1, 1, 1, 1, 1, 1, 1, 1,
1, 1, 1, 1, 1, 1, 1, 1, 1, 1, 1, 1, 1, 1, 1, 1, 1, 1, 1, 1, 1, 1,
1, 1, 1, 1, 1, 1, 1, 1, 1, 1, 1, 1, 1, 1, 1, 1, 1, 1, 1, 1, 1, 1,
1, 1, 1, 1, 1, 1, 1, 1, 1, 1, 1, 1, 1, 1, 1, 1, 1, 1, 1, 1, 1, 1,
1, 1, 1, 1, 1, 1, 1, 1, 1, 1, 1, 1, 1, 1, 1, 1, 1, 1, 1, 1, 1, 1,
1, 1, 1, 1, 1, 1, 1, 1, 1, 1, 1, 1, 1, 1, 1, 1, 1, 1, 1, 1, 1, 1,
1, 1, 1, 1, 1, 1, 1, 1, 1, 1, 1, 1, 1, 1, 1, 1, 1, 1, 1, 1, 1, 1,
1, 1, 1, 1, 1, 1, 1, 1, 1, 1, 1, 1, 1, 1, 1, 1, 1, 1, 1, 1, 1, 1,
1, 1, 1, 1, 1, 1, 1, 1, 1, 1, 1, 1, 1, 1, 1, 1, 1, 1, 1, 1, 1, 1,
1, 1, 1, 1, 1, 1, 1, 1, 1, 1, 1, 1, 1, 1, 1, 1, 1, 1, 1, 1, 1, 1,
1, 1, 1, 1, 1, 1, 1, 1, 1, 1, 1, 1, 1, 1, 1, 1, 1, 1, 1, 1, 1, 1,
1, 1, 1, 1, 1, 1, 1, 1, 1, 1, 1, 1, 1, 1, 1, 1, 1, 1, 1, 1, 1, 1,
1, 1, 1, 1, 1, 1, 1, 1, 1, 1, 1, 1, 1, 1, 1, 1, 1, 1, 1, 1, 1, 1,
1, 1, 1, 1, 1, 1, 1, 1, 1, 1, 1, 1, 1, 1, 1, 1, 1, 1, 1, 1, 1, 1,
1, 1, 1, 1, 1, 1, 1, 1, 1, 1, 1, 1, 1, 1, 1, 1, 1, 1, 1, 1, 1, 1,
1, 1, 1, 1, 1, 1, 1, 1, 1, 1, 1, 1, 1, 1, 1, 1, 1, 1, 1, 1, 1, 1,
1, 1, 1, 1, 1, 1, 1, 1, 1, 1, 1, 1, 1, 1, 1, 1, 1, 1, 1, 1, 1, 1,
1, 1, 1, 1, 1, 1, 1, 1, 1, 1, 1, 1, 1, 1, 1, 1, 1, 1, 1, 1, 1, 1,
1, 1, 1, 1, 1, 1]])>}
``` | 03-14-2021 15:02:19 | 03-14-2021 15:02:19 | |
transformers | 10,707 | closed | Inheriting from BartForConditionalGeneration into a new class - weight not initializing | 03-14-2021 13:37:39 | 03-14-2021 13:37:39 | ||
transformers | 10,706 | closed | Trainer crashes when saving checkpoint | ## Environment info
- `transformers` version: 4.3.3
- Platform: Linux-4.4.0-161-generic-x86_64-with-glibc2.10
- Python version: 3.8.8
- PyTorch version (GPU?): 1.8.0+cu101 (True)
- Tensorflow version (GPU?): not installed (NA)
- Using GPU in script?: Yes, distributed
- Using distributed or parallel set-up in script?: Yes
```
+-----------------------------------------------------------------------------+
| NVIDIA-SMI 418.87.00 Driver Version: 418.87.00 CUDA Version: 10.1 |
|-------------------------------+----------------------+----------------------+
| GPU Name Persistence-M| Bus-Id Disp.A | Volatile Uncorr. ECC |
| Fan Temp Perf Pwr:Usage/Cap| Memory-Usage | GPU-Util Compute M. |
|===============================+======================+======================|
| 0 Tesla P100-PCIE... Off | 00000000:02:00.0 Off | 0 |
| N/A 47C P0 33W / 250W | 16181MiB / 16280MiB | 0% Default |
+-------------------------------+----------------------+----------------------+
| 1 Tesla P100-PCIE... Off | 00000000:81:00.0 Off | 0 |
| N/A 42C P0 34W / 250W | 16173MiB / 16280MiB | 0% Default |
+-------------------------------+----------------------+----------------------+
```
### Who can help
- trainer: @sgugger
## Information
Model I am using (Bert, XLNet ...): "bert-base-multilingual-cased"
The problem arises when using:
* [ ] the official example scripts: (give details below)
* [X] my own modified scripts: (give details below)
The tasks I am working on is:
* [ ] an official GLUE/SQUaD task: (give the name)
* [X] my own task or dataset: (give details below)
## To reproduce
Steps to reproduce the behavior:
```python
from transformers import AutoTokenizer
tokenizer = AutoTokenizer.from_pretrained(model_name, do_lower_case=False)
def preprocess_function(examples):
tokenized = tokenizer(examples["text"], truncation=True)
tokenized["labels"] = [1 if elem == "sexist" else 0 for elem in examples["task1"]]
return tokenized
encoded_dataset = dataset.map(preprocess_function, batched=True)
from transformers import AutoConfig, AutoModelForSequenceClassification, TrainingArguments, Trainer
import datasets
model_name = "bert-base-multilingual-cased"
config = AutoConfig.from_pretrained(
model_name,
num_labels=len(np.unique(encoded_dataset["train"]["labels"])),
)
model = AutoModelForSequenceClassification.from_pretrained(model_name, config=config)
training_arguments = TrainingArguments(
output_dir=f"{model_name}",
do_train=True,
do_eval=True,
evaluation_strategy="steps",
per_device_train_batch_size=16,
per_device_eval_batch_size=16,
learning_rate=2e-5,
num_train_epochs=5,
label_names=["labels"],
load_best_model_at_end=True,
metric_for_best_model=datasets.load_metric("accuracy"),
eval_steps=50,
)
def compute_metrics(eval_pred):
predictions, labels = eval_pred
predictions = np.argmax(predictions, axis=1)
return metric.compute(predictions=predictions, references=labels)
trainer = Trainer(
model,
training_arguments,
train_dataset=encoded_dataset["train"],
eval_dataset=encoded_dataset["validation"],
tokenizer=tokenizer,
compute_metrics=compute_metrics,
)
trainer.train()
```
The error:
```
---------------------------------------------------------------------------
TypeError Traceback (most recent call last)
<ipython-input-44-974384763956> in <module>
10 #callbacks=[EarlyStoppingCallback(early_stopping_patience=4)], # Por alguna razón, casca
11 )
---> 12 trainer.train()
~/.local/lib/python3.8/site-packages/transformers/trainer.py in train(self, resume_from_checkpoint, trial, **kwargs)
981 self.control = self.callback_handler.on_step_end(self.args, self.state, self.control)
982
--> 983 self._maybe_log_save_evaluate(tr_loss, model, trial, epoch)
984
985 if self.control.should_epoch_stop or self.control.should_training_stop:
~/.local/lib/python3.8/site-packages/transformers/trainer.py in _maybe_log_save_evaluate(self, tr_loss, model, trial, epoch)
1060
1061 if self.control.should_save:
-> 1062 self._save_checkpoint(model, trial, metrics=metrics)
1063 self.control = self.callback_handler.on_save(self.args, self.state, self.control)
1064
~/.local/lib/python3.8/site-packages/transformers/trainer.py in _save_checkpoint(self, model, trial, metrics)
1085 self.store_flos()
1086
-> 1087 self.save_model(output_dir)
1088 if self.deepspeed:
1089 self.deepspeed.save_checkpoint(output_dir)
~/.local/lib/python3.8/site-packages/transformers/trainer.py in save_model(self, output_dir)
1376 self._save_tpu(output_dir)
1377 elif self.is_world_process_zero():
-> 1378 self._save(output_dir)
1379
1380 # If on sagemaker and we are saving the main model (not a checkpoint so output_dir=None), save a copy to
~/.local/lib/python3.8/site-packages/transformers/trainer.py in _save(self, output_dir)
1419
1420 # Good practice: save your training arguments together with the trained model
-> 1421 torch.save(self.args, os.path.join(output_dir, "training_args.bin"))
1422
1423 def store_flos(self):
~/miniconda3/envs/transformers4/lib/python3.8/site-packages/torch/serialization.py in save(obj, f, pickle_module, pickle_protocol, _use_new_zipfile_serialization)
370 if _use_new_zipfile_serialization:
371 with _open_zipfile_writer(opened_file) as opened_zipfile:
--> 372 _save(obj, opened_zipfile, pickle_module, pickle_protocol)
373 return
374 _legacy_save(obj, opened_file, pickle_module, pickle_protocol)
~/miniconda3/envs/transformers4/lib/python3.8/site-packages/torch/serialization.py in _save(obj, zip_file, pickle_module, pickle_protocol)
474 pickler = pickle_module.Pickler(data_buf, protocol=pickle_protocol)
475 pickler.persistent_id = persistent_id
--> 476 pickler.dump(obj)
477 data_value = data_buf.getvalue()
478 zip_file.write_record('data.pkl', data_value, len(data_value))
TypeError: cannot pickle '_thread.lock' object
```
It doesn't matter the save steps, etc. When it tries to save the model, I get that error. I don't think that I can post the dataset here but it doesn't look like a dataset problem. I'm following the sequence classification notebook but I changed some things to use binary classification and load my own dataset.
I also get a different error when using the early stopping callback:
```python
from transformers import EarlyStoppingCallback
trainer = Trainer(
model,
training_arguments,
train_dataset=encoded_dataset["train"],
eval_dataset=encoded_dataset["validation"],
tokenizer=tokenizer,
compute_metrics=compute_metrics,
callbacks=[EarlyStoppingCallback(early_stopping_patience=4)],
)
trainer.train()
```
```
---------------------------------------------------------------------------
AttributeError Traceback (most recent call last)
<ipython-input-46-17322e623d42> in <module>
10 callbacks=[EarlyStoppingCallback(early_stopping_patience=4)], # Por alguna razón, casca
11 )
---> 12 trainer.train()
~/.local/lib/python3.8/site-packages/transformers/trainer.py in train(self, resume_from_checkpoint, trial, **kwargs)
981 self.control = self.callback_handler.on_step_end(self.args, self.state, self.control)
982
--> 983 self._maybe_log_save_evaluate(tr_loss, model, trial, epoch)
984
985 if self.control.should_epoch_stop or self.control.should_training_stop:
~/.local/lib/python3.8/site-packages/transformers/trainer.py in _maybe_log_save_evaluate(self, tr_loss, model, trial, epoch)
1056 metrics = None
1057 if self.control.should_evaluate:
-> 1058 metrics = self.evaluate()
1059 self._report_to_hp_search(trial, epoch, metrics)
1060
~/.local/lib/python3.8/site-packages/transformers/trainer.py in evaluate(self, eval_dataset, ignore_keys, metric_key_prefix)
1522 xm.master_print(met.metrics_report())
1523
-> 1524 self.control = self.callback_handler.on_evaluate(self.args, self.state, self.control, output.metrics)
1525 return output.metrics
1526
~/.local/lib/python3.8/site-packages/transformers/trainer_callback.py in on_evaluate(self, args, state, control, metrics)
360 def on_evaluate(self, args: TrainingArguments, state: TrainerState, control: TrainerControl, metrics):
361 control.should_evaluate = False
--> 362 return self.call_event("on_evaluate", args, state, control, metrics=metrics)
363
364 def on_save(self, args: TrainingArguments, state: TrainerState, control: TrainerControl):
~/.local/lib/python3.8/site-packages/transformers/trainer_callback.py in call_event(self, event, args, state, control, **kwargs)
375 def call_event(self, event, args, state, control, **kwargs):
376 for callback in self.callbacks:
--> 377 result = getattr(callback, event)(
378 args,
379 state,
~/.local/lib/python3.8/site-packages/transformers/trainer_callback.py in on_evaluate(self, args, state, control, metrics, **kwargs)
527 def on_evaluate(self, args, state, control, metrics, **kwargs):
528 metric_to_check = args.metric_for_best_model
--> 529 if not metric_to_check.startswith("eval_"):
530 metric_to_check = f"eval_{metric_to_check}"
531 metric_value = metrics.get(metric_to_check)
AttributeError: 'Accuracy' object has no attribute 'startswith'
```
| 03-14-2021 11:35:33 | 03-14-2021 11:35:33 | As indicated in the [documentation](https://huggingface.co/transformers/main_classes/trainer.html#trainingarguments) `metric_for_best_model` _Must be the name of a metric returned by the evaluation with or without the prefix "eval\_"_. You passed a Metric object to it instead of the name returned by your `compute_metric` function, which is what caused your error.<|||||>Thank you so much, I'm sorry for that |
transformers | 10,705 | closed | Please provide format of the dataset to finetuning wav2vec using run_asr.py script | Hi @patrickvonplaten, thanks for the great work, could you please provide some examples for the dataset format to train the wav2vec model using run_asr.py script. | 03-14-2021 09:12:11 | 03-14-2021 09:12:11 | We are organizing a "fine-tuning XLSR-53" event. Check this announcement: https://discuss.huggingface.co/t/open-to-the-community-xlsr-wav2vec2-fine-tuning-week-for-low-resource-languages/4467. Would be awesome if you want to participate :-)<|||||>This issue has been automatically marked as stale because it has not had recent activity. If you think this still needs to be addressed please comment on this thread.
Please note that issues that do not follow the [contributing guidelines](https://github.com/huggingface/transformers/blob/master/CONTRIBUTING.md) are likely to be ignored. |
transformers | 10,704 | closed | How to generate texts in huggingface in a batch way? | I am new to huggingface. My task is quite simple, where I want to generate contents based on the given titles. The below codes is of low efficiency, that the GPU Util is only about 15%. It seems that it makes generation one by one. How can I improve the code to process and generate the contents in a batch way?
```
df_test = pd.read_csv("./ag_news/test.csv").sample(frac=1)
from transformers import pipeline
text_generator = pipeline("text-generation")
rows = df_test.sample(1000)
titles = rows['title'].tolist()
contents = rows['content'].tolist()
generate_texts = text_generator(titles, max_length=40, do_sample=False)
``` | 03-14-2021 07:59:57 | 03-14-2021 07:59:57 | Looking at the [source code](https://github.com/huggingface/transformers/blob/4c32f9f26e6a84f0d9843fec8757e6ce640bb44e/src/transformers/pipelines/text_generation.py#L108) of the text-generation pipeline, it seems that the texts are indeed generated one by one, so it's not ideal for batch generation.
In order to genere contents in a batch, you'll have to use GPT-2 (or another generation model from the hub) directly, like so (this is based on PR #7552):
```
from transformers import GPT2Tokenizer, GPT2LMHeadModel
import torch
tokenizer = GPT2Tokenizer.from_pretrained('gpt2')
tokenizer.padding_side = "left"
tokenizer.pad_token = tokenizer.eos_token # to avoid an error
model = GPT2LMHeadModel.from_pretrained('gpt2')
device = 'cuda' if torch.cuda.is_available() else 'cpu'
texts = ["this is a first prompt", "this is a second prompt"]
encoding = tokenizer(texts, padding=True, return_tensors='pt').to(device)
with torch.no_grad():
generated_ids = model.generate(**encoding)
generated_texts = tokenizer.batch_decode(generated_ids, skip_special_tokens=True)
```
In my case, this prints out:
`['this is a first prompt for the user to enter the password.\n\nThe password is a string', "this is a second prompt, but it's not a full-screen one.\n\nThe first"]`<|||||>> Looking at the [source code](https://github.com/huggingface/transformers/blob/4c32f9f26e6a84f0d9843fec8757e6ce640bb44e/src/transformers/pipelines/text_generation.py#L108) of the text-generation pipeline, it seems that the texts are indeed generated one by one, so it's not ideal for batch generation.
>
> In order to genere contents in a batch, you'll have to use GPT-2 (or another generation model from the hub) directly, like so (this is based on PR #7552):
>
> ```
> from transformers import GPT2Tokenizer, GPT2LMHeadModel
> import torch
>
> tokenizer = GPT2Tokenizer.from_pretrained('gpt2')
> tokenizer.padding_side = "left"
> tokenizer.pad_token = tokenizer.eos_token # to avoid an error
> model = GPT2LMHeadModel.from_pretrained('gpt2')
>
> device = 'cuda' if torch.cuda.is_available() else 'cpu'
>
> texts = ["this is a first prompt", "this is a second prompt"]
> encoding = tokenizer(texts, return_tensors='pt').to(device)
> with torch.no_grad():
> generated_ids = model.generate(**encoding)
> generated_texts = tokenizer.batch_decode(generated_ids, skip_special_tokens=True)
> ```
>
> In my case, this prints out:
> `['this is a first prompt for the user to enter the password.\n\nThe password is a string', "this is a second prompt, but it's not a full-screen one.\n\nThe first"]`
thanks. It seems that the standard workflow is to organize the components of `tokenizer`, `generate` and `batch_decode`
in a cascade way. <|||||>This issue has been automatically marked as stale because it has not had recent activity. If you think this still needs to be addressed please comment on this thread.
Please note that issues that do not follow the [contributing guidelines](https://github.com/huggingface/transformers/blob/master/CONTRIBUTING.md) are likely to be ignored.<|||||>@NielsRogge Is padding on the left still the way to go for batched generation? It seems odd to require a workaround for such a common feature |
transformers | 10,703 | closed | DebertaTokenizer Rework closes #10258 | # What does this PR do?
Fixes #10258
@BigBird01 Please upload these [files](https://drive.google.com/drive/folders/1gH5EMABR94iHO7SCb_AdNGCOOIdSloxh?usp=sharing) to your deberta repositories.
@huggingface: Please don't merge before @BigBird01 has uploaded the files to his repository.
## Before submitting
- [ ] This PR fixes a typo or improves the docs (you can dismiss the other checks if that's the case).
- [X] Did you read the [contributor guideline](https://github.com/huggingface/transformers/blob/master/CONTRIBUTING.md#start-contributing-pull-requests),
Pull Request section?
- [X] Was this discussed/approved via a Github issue or the [forum](https://discuss.huggingface.co/)? Please add a link
to it if that's the case.
- [ ] Did you make sure to update the documentation with your changes? Here are the
[documentation guidelines](https://github.com/huggingface/transformers/tree/master/docs), and
[here are tips on formatting docstrings](https://github.com/huggingface/transformers/tree/master/docs#writing-source-documentation).
- [ ] Did you write any new necessary tests?
## Who can review?
- tokenizers: @LysandreJik, @BigBird01
| 03-14-2021 06:05:35 | 03-14-2021 06:05:35 | This is great @cronoik! I just tested it and reproduced identical results between the previous version and this one. Fantastic!
Could you add an integration tests for the DeBERTa tokenizer to ensure the implementations don't diverge? You can just copy paste the [test for ALBERT tokenizers](https://github.com/huggingface/transformers/blob/master/tests/test_tokenization_albert.py#L106) and change the values to DeBERTa.
If you don't have time, let us know and we'll take care of it.<|||||>@LysandreJik
I will give it a try.<|||||>Hi,
a new test is now added for the debertatokenizer. I stuck more to the robertatokenizer test since the debertatokenizer is basically a robertatokenizer.
I can not interpret the failed check. I assume it is because the model hub is missing the new files? @LysandreJik could you please have a look?<|||||>I am trying out this PR with the following:
```
tokenizer = DebertaTokenizer.from_pretrained("microsoft/deberta-base")
target_tokenized = tokenizer.tokenize("Some test text")
```
but I see the following error (`TypeError: expected str, bytes or os.PathLike object, not NoneType`):
```
def __init__(
self,
vocab_file,
merges_file,
errors="replace",
unk_token="<|endoftext|>",
bos_token="<|endoftext|>",
eos_token="<|endoftext|>",
add_prefix_space=False,
**kwargs
):
bos_token = AddedToken(bos_token, lstrip=False, rstrip=False) if isinstance(bos_token, str) else bos_token
eos_token = AddedToken(eos_token, lstrip=False, rstrip=False) if isinstance(eos_token, str) else eos_token
unk_token = AddedToken(unk_token, lstrip=False, rstrip=False) if isinstance(unk_token, str) else unk_token
super().__init__(
errors=errors,
unk_token=unk_token,
bos_token=bos_token,
eos_token=eos_token,
add_prefix_space=add_prefix_space,
**kwargs,
)
> with open(vocab_file, encoding="utf-8") as vocab_handle:
E TypeError: expected str, bytes or os.PathLike object, not NoneType
../../../../jeswan_transformers/src/transformers/models/gpt2/tokenization_gpt2.py:179: TypeError
```
@LysandreJik potentially related to @cronoik's error above <|||||>@jeswan that will not work since the required files are not uploaded to the model hub yet. You can download them from the [link](https://drive.google.com/drive/folders/1gH5EMABR94iHO7SCb_AdNGCOOIdSloxh?usp=sharing) and load the tokenizer from local.<|||||>@cronoik thanks for your effort. I just uploaded the files to the model repository and left one comment to the changes. <|||||>@BigBird01 Thank you for the review. I had only tested single sentences and completely ignored the sentence pairs before pushing.
@LysandreJik Can you please help me with the test error? <|||||>@sgugger Thanks for the review. I have pushed/accepted your change requests.<|||||>Thanks for your efforts @cronoik! |
transformers | 10,702 | closed | Performance Issue in doing inferencing hugging face models | ## Environment info
<!-- You can run the command `transformers-cli env` and copy-and-paste its output below.
Don't forget to fill out the missing fields in that output! -->
- `transformers` version:3.2.0
- Platform:windows azure with GPU
- Python version: 3.6
- PyTorch version (GPU?):CUDA 10.1
- Tensorflow version (GPU?):
- Using GPU in script?:
- Using distributed or parallel set-up in script?:
We have created a flask end point to cater to multiple ML models such as Longformer Question answeing,zero shot learning, paraphrasing.But when these functions are called from flask end point the CPU percentage utilisation increases to more than 97% even though the GPU is already in place. Any idea why CPU takes a hit as this is just used for inferencing purpose.
Also is there a way to check the memory size allocated to a variable when we load the torch pretrained model in the variable and pass this as an argument in the function. If there are concurrent calls does this memory requirement increases or is the same memory is being referred. | 03-14-2021 05:35:55 | 03-14-2021 05:35:55 | Hello, thanks for opening an issue! We try to keep the github issues for bugs/feature requests.
Could you ask your question on the [forum](https://discusss.huggingface.co) instead?
Thanks! |
transformers | 10,701 | closed | Seq2Seq Model with PreTrained BERT Model is Throwing Error During Training: ValueError("You cannot specify both input_ids and inputs_embeds at the same time") | Hi,
I tried creating a seq2seq model using pretrained BERT model following your tutorials:
https://github.com/bentrevett/pytorch-sentiment-analysis/blob/master/6%20-%20Transformers%20for%20Sentiment%20Analysis.ipynb
https://github.com/bentrevett/pytorch-seq2seq/blob/master/1%20-%20Sequence%20to%20Sequence%20Learning%20with%20Neural%20Networks.ipynb
However during training, I am getting the following error:
```
AttributeError Traceback (most recent call last)
<ipython-input-63-472071541d41> in <module>()
8 start_time = time.time()
9
---> 10 train_loss = train(model, train_iterator, optimizer, criterion, CLIP)
11 valid_loss = evaluate(model, valid_iterator, criterion)
12
6 frames
/usr/local/lib/python3.7/dist-packages/transformers/models/bert/modeling_bert.py in forward(self, input_ids, attention_mask, token_type_ids, position_ids, head_mask, inputs_embeds, encoder_hidden_states, encoder_attention_mask, past_key_values, use_cache, output_attentions, output_hidden_states, return_dict)
917 raise ValueError("You cannot specify both input_ids and inputs_embeds at the same time")
918 elif input_ids is not None:
--> 919 input_shape = input_ids.size()
920 batch_size, seq_length = input_shape
921 elif inputs_embeds is not None:
AttributeError: 'Field' object has no attribute 'size'
```
I am sharing my code for your review in the following github repo:
https://github.com/Ninja16180/BERT/blob/main/Training_Seq2Seq_Model_using_Pre-Trained_BERT_Model.ipynb
Also, request you to kindly review the Encoder and Decoder classes which have been modified to incorporate pretrained bert embedding.
Thanks in advance! | 03-14-2021 04:43:44 | 03-14-2021 04:43:44 | Hi @Ninja16180
Could you please post a short code snippet to reproduce the issue? Thanks.<|||||>Hi Suraj,
I was able to find out the issue; there was a variable name I wrongly passed into the decoder class and hence the error.
Correction made:
`embedded = self.bert(sent2)[0] should be embedded = self.bert(input)[0] `
Thus closing this issue. |
transformers | 10,700 | closed | Trying to implement "nielsr/luke-large" gives "KeyError: 'luke'" | ## Environment info
- `transformers` version: 4.1.1
- Platform: Windows-10-10.0.19041-SP0
- Python version: 3.8.3
- PyTorch version (GPU?): 1.7.1+cpu (False)
- Tensorflow version (GPU?): not installed (NA)
- Using GPU in script?: No
- Using distributed or parallel set-up in script?: No
### Who can help
@LysandreJik I guess, because it's an `AutoTokenizer`-related issue.
## Information
I'm trying to use an implementation of LUKE ([paper](https://arxiv.org/abs/2010.01057)) ([implementation](https://huggingface.co/nielsr/luke-large/tree/main)).
The problem arises when using:
* my own modified scripts
The task I am working on is:
I don't think this is relevant.
## To reproduce
Steps to reproduce the behavior:
1. `from transformers import AutoTokenizer, AutoModel`
2. `tokenizer = AutoTokenizer.from_pretrained("nielsr/luke-large")`
Running gives the following error:
```
---------------------------------------------------------------------------
KeyError Traceback (most recent call last)
<ipython-input-12-c1614eef2346> in <module>
4
----> 5 luke_tokenizer = AutoTokenizer.from_pretrained("nielsr/luke-large")
6
c:\...\venv\lib\site-packages\transformers\models\auto\tokenization_auto.py in from_pretrained(cls, pretrained_model_name_or_path, *inputs, **kwargs)
343 config = kwargs.pop("config", None)
344 if not isinstance(config, PretrainedConfig):
--> 345 config = AutoConfig.from_pretrained(pretrained_model_name_or_path, **kwargs)
346
347 use_fast = kwargs.pop("use_fast", True)
c:\...\venv\lib\site-packages\transformers\models\auto\configuration_auto.py in from_pretrained(cls, pretrained_model_name_or_path, **kwargs)
350
351 if "model_type" in config_dict:
--> 352 config_class = CONFIG_MAPPING[config_dict["model_type"]]
353 return config_class.from_dict(config_dict, **kwargs)
354 else:
KeyError: 'luke'
```
## Expected behavior
I'm expecting no error to be thrown.
| 03-13-2021 16:04:49 | 03-13-2021 16:04:49 | Thanks for your interest! LUKE is not part of the master branch yet.
Actually, the current implementation of LUKE is here (at my `adding_luke_v2` branch): https://github.com/NielsRogge/transformers/tree/adding_luke_v2/src/transformers/models/luke
Note that it is work-in-progress, but you can already use the base `EntityAwareAttentionModel` and the head models. It's mostly the tokenizer that needs some work.
cc'ing the original author for visibility: @ikuyamada <|||||>Thanks, Niels!
As far as I'm concerned, this can be closed. |
transformers | 10,699 | closed | TF BART models - Add `cross_attentions` to model output and fix cross-attention head masking | This PR fixes some missing and invalid things around `cross_attentions` for the TensorFlow implementation of BART models:
- `Bart`,
- `Blenderbot` / `Blenderbot_small`,
- `Marian`,
- `MBart`,
- `Pegasus`.
More specifically, this PR includes:
- Enable returning `cross_attentions`
- Add class `TFBaseModelOutputWithCrossAttentions` (according to the PyTorch counterpart) to support output containing `cross_attentions`
- Fix attention head masking for the cross-attention module (by the introduction of `cross_attn_head_mask` and `cross_attn_layer_head_mask`)
- Implement `test_head_masking` for `cross_attn_head_mask`
- Fix some little typos in docs
- Update model templates - implement `head_mask`, `decoder_head_mask`, `cross_attn_head_mask` and code around `cross_attentions` to the TF encoder-decoder models
<hr>
Partially fixes: #10698
<hr>
**Reviewers:** @jplu @patrickvonplaten @LysandreJik @sgugger | 03-13-2021 11:48:19 | 03-13-2021 11:48:19 | Currently, there are some Torch/TF equivalent tests failing but it should be settled down once #10605 be merged.<|||||>@jplu - I'm gonna run all slow tests today and will let you know if everything works or not.<|||||>@jplu - I ran all (slow) non-GPU tests and it seems to me everything is passing :) <|||||>Ok, if all the tests for the involved models, including the slow ones, are passing, it is fine to merge for me.<|||||>This issue has been automatically marked as stale because it has not had recent activity. If you think this still needs to be addressed please comment on this thread.
Please note that issues that do not follow the [contributing guidelines](https://github.com/huggingface/transformers/blob/master/CONTRIBUTING.md) are likely to be ignored.<|||||>still open <|||||>@jplu @patrickvonplaten After merging #10605 and rebasing the branch, all tests have passed now :)<|||||>Great, thanks @stancld!
This looks good to merge for me.<|||||>Pinging @Rocketknight1 for a final review here. Seq2Seq models like BART should also return cross attentions in TF (not just in PT)<|||||>This is a big PR but LGTM! I haven't exhaustively checked everything but the changes seem correct and innocuous, so if it passes tests I'm happy to merge it. |
transformers | 10,698 | closed | Add `cross_attentions` to the output of TensorFlow encoder-decoder models | # 🚀 Feature request
TensorFlow encoder-decoder models cannot return `cross_attentions` as do their PyTorch counterparts.
## Motivation
It would be nice to narrow the gap between PyTorch and Tensorflow implementations.
## Your contribution
I've been working on PR fixing this issue.
## Reviewers
@jplu and whoever else within the community | 03-13-2021 09:13:32 | 03-13-2021 09:13:32 | This issue has been automatically marked as stale because it has not had recent activity. If you think this still needs to be addressed please comment on this thread.
Please note that issues that do not follow the [contributing guidelines](https://github.com/huggingface/transformers/blob/master/CONTRIBUTING.md) are likely to be ignored. |
transformers | 10,697 | closed | Fix Wav2Vec2 classes imports | # What does this PR do?
Fixes imports for the following classes:
* Wav2Vec2CTCTokenizer
* Wav2Vec2FeatureExtractor
* Wav2Vec2Processor
In order to fine tune FB's Wav2Vec2 XLSR model, these classes need to be accessible. Importing using the instructions in the current [blog post](https://huggingface.co/blog/fine-tune-xlsr-wav2vec2) won't work, i.e. using `from transformers import Wav2Vec2CTCTokenizer` will fail. This PR fixes that.
## Before submitting
- [ ] This PR fixes a typo or improves the docs (you can dismiss the other checks if that's the case).
- [x] Did you read the [contributor guideline](https://github.com/huggingface/transformers/blob/master/CONTRIBUTING.md#start-contributing-pull-requests),
Pull Request section?
- [ ] Was this discussed/approved via a Github issue or the [forum](https://discuss.huggingface.co/)? Please add a link
to it if that's the case.
- [ ] Did you make sure to update the documentation with your changes? Here are the
[documentation guidelines](https://github.com/huggingface/transformers/tree/master/docs), and
[here are tips on formatting docstrings](https://github.com/huggingface/transformers/tree/master/docs#writing-source-documentation).
- [ ] Did you write any new necessary tests?
## Who can review?
@patrickvonplaten, I'd appreciate it if you could give this a look. Thanks!
| 03-13-2021 07:11:48 | 03-13-2021 07:11:48 | |
transformers | 10,696 | closed | OSerror, when loading 'wav2vec2-large-xlsr-53' Model of Wav2vec2 | ## Environment info
- `transformers` version: 4.3.3
- Platform: Linux-4.15.0-29-generic-x86_64-with-glibc2.10
- Python version: 3.8.8
- PyTorch version (GPU?): 1.8.0 (False)
- Tensorflow version (GPU?): not installed (NA)
@patrickvonplaten
## Information
Model I am using Wav2vec2.0:
The problem arises when using:
Scipts:
import soundfile as sf
import torch
from transformers import AutoTokenizer, AutoModel,Wav2Vec2ForCTC, Wav2Vec2Tokenizer
tokenizer4 = AutoTokenizer.from_pretrained("facebook/wav2vec2-large-xlsr-53")
model4 = AutoModel.from_pretrained("facebook/wav2vec2-large-xlsr-53")
OSError:
OSError: Can't load tokenizer for 'facebook/wav2vec2-large-xlsr-53'. Make sure that:
- 'facebook/wav2vec2-large-xlsr-53' is a correct model identifier listed on 'https://huggingface.co/models'
- or 'facebook/wav2vec2-large-xlsr-53' is the correct path to a directory containing relevant tokenizer files
The tasks I am working on is:
* an official wav2vec task: facebook/wav2vec2-large-xlsr-53
## To reproduce
Steps to reproduce the behavior:
Follow the instructions
https://huggingface.co/facebook/wav2vec2-large-xlsr-53
## Expected behavior
I try to use xlsr model as the pre-trained model to finetune my own ASR model, but the xlsr model, especially tokenizer, can't be loaded smoothly. Could you tell me how to modify it? Thank you very much!
| 03-13-2021 04:23:04 | 03-13-2021 04:23:04 | The model doesn't contains the tokenizer and preprocessing files.
Checkout this notebook:
https://huggingface.co/blog/fine-tune-xlsr-wav2vec2
To build your own vocab etc<|||||>@flozi00 Thank you!
> The model doesn't contains the tokenizer and preprocessing files.
> Checkout this notebook:
> https://huggingface.co/blog/fine-tune-xlsr-wav2vec2
>
> To build your own vocab etc
<|||||>Forget about `transformers.AutoProcessor`. This class is used to load a general `processor` model. By call the constructor of this class you have to submit `feature_extractor` and `tokenizer`, however `Wav2Vec2` just extract the features from raw speech data. Then, there is no `tokenizer` has been defined for it. To load the `processor` you can use `transformers.Wav2Vec2FeatureExtractor` as follow:
```
from transformers import Wav2Vec2FeatureExtractor
processor = Wav2Vec2FeatureExtractor.from_pretrained('facebook/wav2vec2-large-xlsr-53')
```<|||||>> Forget about `transformers.AutoProcessor`. This class is used to load a general `processor` model. By call the constructor of this class you have to submit `feature_extractor` and `tokenizer`, however `Wav2Vec2` just extract the features from raw speech data. Then, there is no `tokenizer` has been defined for it. To load the `processor` you can use `transformers.Wav2Vec2FeatureExtractor` as follow:
>
> ```
> from transformers import Wav2Vec2FeatureExtractor
>
> processor = Wav2Vec2FeatureExtractor.from_pretrained('facebook/wav2vec2-large-xlsr-53')
> ```
Using this approach, I got segmentation fault on the same wav2vec2-large-xlsr-53 model.
Output:
```bash
Some weights of the model checkpoint at facebook/wav2vec2-large-xlsr-53 were not used when initializing Wav2Vec2Model: ['quantizer.weight_proj.bias', 'project_q.weight', 'project_q.bias', 'quantizer.codevectors', 'quantizer.weight_proj.weight', 'project_hid.bias', 'project_hid.weight']
- This IS expected if you are initializing Wav2Vec2Model from the checkpoint of a model trained on another task or with another architecture (e.g. initializing a BertForSequenceClassification model from a BertForPreTraining model).
- This IS NOT expected if you are initializing Wav2Vec2Model from the checkpoint of a model that you expect to be exactly identical (initializing a BertForSequenceClassification model from a BertForSequenceClassification model).
Processing 24393.wav
Segmentation fault (core dumped)
``` |
transformers | 10,695 | closed | Merge from huggingface/transformer master | 03-13-2021 01:27:18 | 03-13-2021 01:27:18 | ||
transformers | 10,694 | closed | [Wav2Vec2] Fix documentation inaccuracy | # What does this PR do?
This PR resolves two Wav2Vec2 documentation statements that I believe are typos.
<!--
Congratulations! You've made it this far! You're not quite done yet though.
Once merged, your PR is going to appear in the release notes with the title you set, so make sure it's a great title that fully reflects the extent of your awesome contribution.
Then, please replace this with a description of the change and which issue is fixed (if applicable). Please also include relevant motivation and context. List any dependencies (if any) that are required for this change.
Once you're done, someone will review your PR shortly (see the section "Who can review?" below to tag some potential reviewers). They may suggest changes to make the code even better. If no one reviewed your PR after a week has passed, don't hesitate to post a new comment @-mentioning the same persons---sometimes notifications get lost.
-->
<!-- Remove if not applicable -->
Fixes # (issue)
## Before submitting
- [x] This PR fixes a typo or improves the docs (you can dismiss the other checks if that's the case).
- [x] Did you read the [contributor guideline](https://github.com/huggingface/transformers/blob/master/CONTRIBUTING.md#start-contributing-pull-requests),
Pull Request section?
- [ ] Was this discussed/approved via a Github issue or the [forum](https://discuss.huggingface.co/)? Please add a link
to it if that's the case.
- [ ] Did you make sure to update the documentation with your changes? Here are the
[documentation guidelines](https://github.com/huggingface/transformers/tree/master/docs), and
[here are tips on formatting docstrings](https://github.com/huggingface/transformers/tree/master/docs#writing-source-documentation).
- [ ] Did you write any new necessary tests?
## Who can review?
Anyone in the community is free to review the PR once the tests have passed. Feel free to tag
members/contributors which may be interested in your PR.
Based on revision history, I assume @patrickvonplaten is an appropriate reviewer.
<!-- Your PR will be replied to more quickly if you can figure out the right person to tag with @
If you know how to use git blame, that is the easiest way, otherwise, here is a rough guide of **who to tag**.
Please tag fewer than 3 people.
Models:
- albert, bert, xlm: @LysandreJik
- blenderbot, bart, marian, pegasus, encoderdecoder, t5: @patrickvonplaten, @patil-suraj
- longformer, reformer, transfoxl, xlnet: @patrickvonplaten
- fsmt: @stas00
- funnel: @sgugger
- gpt2: @patrickvonplaten, @LysandreJik
- rag: @patrickvonplaten, @lhoestq
- tensorflow: @jplu
Library:
- benchmarks: @patrickvonplaten
- deepspeed: @stas00
- ray/raytune: @richardliaw, @amogkam
- text generation: @patrickvonplaten
- tokenizers: @n1t0, @LysandreJik
- trainer: @sgugger
- pipelines: @LysandreJik
Documentation: @sgugger
HF projects:
- nlp datasets: [different repo](https://github.com/huggingface/nlp)
- rust tokenizers: [different repo](https://github.com/huggingface/tokenizers)
Examples:
- maintained examples (not research project or legacy): @sgugger, @patil-suraj
- research_projects/bert-loses-patience: @JetRunner
- research_projects/distillation: @VictorSanh
-->
| 03-13-2021 00:59:02 | 03-13-2021 00:59:02 | Great! Thanks a lot for correcting those :-)
<|||||>Hey @MikeG112, sorry could you run `make style` once to fix the code quality issue? Then we can merge :-)<|||||>Hey @patrickvonplaten, absolutely, I added the changes made by `make style`. Thanks for the review and the wav2vec2 implementation :) |
transformers | 10,693 | closed | mBART Large-50 MMT provides incorrect translation when the source and target language are the same | mBART Large-50 MMT provides incorrect translation when the source and target language are the same, e.g. when translating from "en_XX" to "en_XX"
## Environment info
<!-- You can run the command `transformers-cli env` and copy-and-paste its output below.
Don't forget to fill out the missing fields in that output! -->
- `transformers` version: 4.4.0.dev0
- Platform: Linux-4.19.112+-x86_64-with-Ubuntu-18.04-bionic
- Python version: 3.7.10
- PyTorch version (GPU?): 1.8.0+cu101 (False)
- Tensorflow version (GPU?): 2.4.1 (False)
- Using GPU in script?: No
- Using distributed or parallel set-up in script?: No
### Who can help
@patrickvonplaten, @patil-suraj
## Information
Model I am using (Bert, XLNet ...):
`facebook/mbart-large-50-one-to-many-mmt`
The problem arises when using:
* [ ] the official example scripts: (give details below)
* [ ] my own modified scripts: (give details below)
The tasks I am working on is:
* [ ] an official GLUE/SQUaD task: (give the name)
* [ ] my own task or dataset: (give details below)
## To reproduce
```python
from transformers import MBartForConditionalGeneration, MBart50TokenizerFast
article_en = "The head of the United Nations says there is no military solution in Syria"
model = MBartForConditionalGeneration.from_pretrained("facebook/mbart-large-50-one-to-many-mmt")
tokenizer = MBart50TokenizerFast.from_pretrained("facebook/mbart-large-50-one-to-many-mmt", src_lang="en_XX")
model_inputs = tokenizer(article_en, return_tensors="pt")
generated_tokens = model.generate(
**model_inputs,
forced_bos_token_id=tokenizer.lang_code_to_id["en_XX"]
)
decoded = tokenizer.batch_decode(generated_tokens, skip_special_tokens=True)
print(decoded)
```
Returns:
```
['Şeful Naţiunilor Unite declară că nu există o soluţie militară în Siria']
```
## Expected behavior
<!-- A clear and concise description of what you would expect to happen. -->
Should return something in english, preferably the same content as the original input | 03-13-2021 00:45:50 | 03-13-2021 00:45:50 | This issue has been automatically marked as stale because it has not had recent activity. If you think this still needs to be addressed please comment on this thread.
Please note that issues that do not follow the [contributing guidelines](https://github.com/huggingface/transformers/blob/master/CONTRIBUTING.md) are likely to be ignored.<|||||>@patil-suraj Was this resolved? If so I'll close this issue<|||||>HI @xhlulu
I don't think if this is an issue with the model implementation, also the model is not really expected to do well on paraphrasing (which English-English), I've seen few other issues where models output text in the wrong language but it's the same with original model in `fairseq` as well. From my experience, multilingual models tend to do this in few cases.<|||||>Thanks, that makes sense! Glad you clarified it 😊 |
transformers | 10,692 | closed | Add RemBERT model code to huggingface | Add RemBERT model to Huggingface ( https://arxiv.org/abs/2010.12821 ).
This adds code to support the RemBERT model in Huggingface.
In terms of implementation, this is roughly a scaled up version of mBERT with ALBERT-like factorized embeddings and tokenizer.
Still needs to be done:
- [x] Check results validity
- [x] Upload model to model hub
- [x] FastTokenizer version
- [x] More testing
- [x] TF code
Fixes #9711
## Before submitting
- [x] Did you read the [contributor guideline](https://github.com/huggingface/transformers/blob/master/CONTRIBUTING.md#start-contributing-pull-requests),
Pull Request section?
- [x] Did you make sure to update the documentation with your changes? Here are the
[documentation guidelines](https://github.com/huggingface/transformers/tree/master/docs), and
[here are tips on formatting docstrings](https://github.com/huggingface/transformers/tree/master/docs#writing-source-documentation).
- [x] Did you write any new necessary tests?
## Who can review?
@LysandreJik seems appropriate here. | 03-12-2021 23:50:29 | 03-12-2021 23:50:29 | @LysandreJik I've been mostly following: https://huggingface.co/transformers/add_new_model.html so far.
Trying to add the tokenizer. I think I am good for the slow one but not sure what to do for the fast one, in particular how to generate the tokenizer.json style files (e.g: https://huggingface.co/t5-small/resolve/main/tokenizer.json ). Do you have any pointers to that?
I also see that the doc mentions that there is no fast version for sentencepiece, which this model uses. Is that the case given T5 seems to have one?
Edit: Seem to have found a way to add a FastTokenizer version, doc still seems out of sync.<|||||>For the TF code, I'm struggling a bit to initialize an output embedding layer in `TFRemBertLMPredictionHead()` that interacts well with `_resize_token_embeddings`. Welcoming any suggestions as what the right approach there is.<|||||>@LysandreJik : They are still some things to iron out but I think this is ready for a first look.
What is missing:
- Model hub upload
- Minor discrepancy between model and original tf implementation
What I'd like some input on:
- I'm having some issue on the `TFRemBertLMPredictionHead` implementation. I'd like to initialize a new projection from hidden_size to vocab_size (embeddings are decoupled) but I'm struggling to find how to make my implementation compatible with all the `get_bias`, `set_bias` details so that it's `resize_embeddings` friendly. Any chance you could help here? This is the culprit for tests failing AFAICT.
- Model hub upload: should this be done on top level (how) or on the Google org model hub?
- I'm finding a discrepancy between this implementation and the original tf one. Results are exactly equal up to the first hidden layer (so embeddings and upprojection). On the first layer it differs but by small amounts (~0.002), difference eventually increases up to 0.007. Any idea what are common culprits here? This is just the standard BERT model and differences are small so maybe numerical stability?
<|||||>> Model hub upload: should this be done on top level (how) or on the Google org model hub?
Toplevel models were for legacy (historical) integrations and we now namespace all models. If this work was conducted at Google yes google is the right namespace! Do you want us to add you to the `google` org?<|||||>> A difference of *e-3 doesn't look too bad, but looking at the integration test you have provided, it seems that the difference is noticeable. Is it possible that a bias is missing, or something to do with attention masks?
Not impossible but given the transformer section is simply Bert I doubt it. Also does seem like the results would change more.
> If it proves impossible to get the two implementations closer to each other, then we'll rely on a fine-tuning tests: if we can obtain similar results on a same dataset with the two implementations, then we'll be good to go.
I've tried to do that for a bit, unfortunately hard to fine-tune this model on a colab on XNLI (training gets interrupted too early on). Will try to see if I can get a better finetuning setup.
<|||||>> > Model hub upload: should this be done on top level (how) or on the Google org model hub?
>
> Toplevel models were for legacy (historical) integrations and we now namespace all models. If this work was conducted at Google yes google is the right namespace! Do you want us to add you to the `google` org?
That would be helpful, though I'm no longer affiliated with Google so not sure what the usual policy is there. If it is ok that will be easier than having to send the checkpoints to @hwchung so he uploads them.<|||||>> That would be helpful, though I'm no longer affiliated with Google so not sure what the usual policy is there.
Ultimately the org admins should decide, but for now I think it's perfectly acceptable if you're a part of the org. I added you manually.<|||||>@Iwontbecreative I opened a PR on your branch that should fix all the failing tests here: https://github.com/Iwontbecreative/transformers/pull/1
I've separated each test suite (TF, style, docs) in three commits if you want to have a look at smaller portions at a time.<|||||>Thanks Lysandre. Have not forgotten about this issue, just need one more approval from Google to open source the checkpoint so waiting for this.<|||||>Sure @Iwontbecreative, let us know when all is good!<|||||>This issue has been automatically marked as stale because it has not had recent activity. If you think this still needs to be addressed please comment on this thread.
Please note that issues that do not follow the [contributing guidelines](https://github.com/huggingface/transformers/blob/master/CONTRIBUTING.md) are likely to be ignored.<|||||>Reopen: this is still in progress, just got the open sourcing done from google last Friday. Travelling for a few days and then can tackle this. See: https://github.com/google-research/google-research/tree/master/rembert<|||||>That's great news @Iwontbecreative!<|||||>(Finally) some updates on this now that the Google open sourcing is done.
* Updated the PR for the lates transformers
* Included @LysandreJik's change (manually instead of merging your PR since I messed up the order, sorry about that)
* Uploaded the model to the model hub under `iwontbecreative/rembert`
Main issue that still exists: the discrepancy between my implementation and the tf one. Welcoming ideas on this one. The code should now be easier for everyone to test now that the tf version is available and mine is uploaded to the model hub.
@LysandreJik think this is ready for another look. Thanks for the patience here!
<|||||>Welcome back! We'll take a look shortly. Do you have an example of code to run to quickly test this version with the TF one?<|||||>Sadly the tensorflow code to run the model is not available externally.
I tried to replicate for some time this morning but it is hard because it relies on https://github.com/google-research/bert which has dependencies that are not available on pip anymore...
The main change to the modelling code is here:
https://github.com/google-research/bert/blob/master/modeling.py#L813-L823
needing to be replaced with:
https://github.com/google-research/albert/blob/master/modeling.py#L1083-L1088
on the tokenization front, it is mainly replacing `BertTokenizer` with `AlbertTokenizer`
I do however have example inputs and outputs run by my coauthor:
### Model outputs
Example modelling outputs at several layers for different input_ids:
https://pastebin.com/t9bPFmeM
This is the `[batch, length, :3]` section of the `[batch, length, hidden]` outputs.
### Tokenization outputs
https://pastebin.com/j6D6YE1e<|||||>Fine-tuning was, here is what I was able to run, comnparing performance on XNLI:
https://docs.google.com/spreadsheets/d/1gWWSLo7XxEZkXpX272tQoZBXTgs96IFvh-fwqVqihM0/edit#gid=0
Performance matches in English but does seem to be lower on other languages. We used more hyperparam tuning at Google but I do not think that explains the whole difference for those languages. I think there might be a subtle difference that is both causing the results to differ slightly and the worse fine-tuning outcomes. The model is still much better than random so most of it should be there.<|||||>Performance does look pretty similar, and good enough for me to merge it.
There are a few `# Copied from` statements missing though as said in my previous message, in both the PyTorch and TensorFlow implementations. Do you mind adding them? If you're lacking time let me know and I'll do it myself.<|||||>Hi @LysandreJik
- Added copy statements
- Merged with last master
- Uploaded model to google org
Seems like it is mostly ready, though tests fail at the `utils/check_copies.py` stage of `make quality`.
I am actually not sure what the issue is in this instance, any chance you could help investigate/fix/merge after?<|||||>Actually, managed to find the issue
`utils/check_copies.py` crashes without a helpful error message if the "Copied from" statement is before the decorator. I was just overeager with my copied from statements.
Also renamed rembert-large to rembert since this is the only version we are open-sourcing at this time.
Edit: Not sure why the model templates check is failing, but think this should be ready for merge with one last review. <|||||>Fantastic, thanks a lot @Iwontbecreative! I'll take a final look, fix the model templates issue and ping another reviewer.<|||||>@patrickvonplaten Thanks for the helpful feedback. Incorporated most of it. See the comment on possible needed changes to the cookiecutter templates to address on of your comments in the future.
For the discrepancy in results, see my answer above.
@sgugger Regarding older template: Yes, this PR ended up being delayed due to slow open-sourcing process at Google, so the templates were a bit out of date. Thanks for catching most of the mistakes.
<|||||>Hi @LysandreJik, any last remaining steps before this can be merged? Would like to get this in to avoid further rebases if possible. <|||||>I think this can be merged - thanks for your effort @Iwontbecreative, fantastic addition!<|||||>I'm not entirely sure why there was 88 authors involved or 250 commits squashed into a single one - but I did verify only your changes were merged.
Could you let me know how you handled the merge/rebasing of this branch so that I may understand what happened w.r.t the number of commits included?<|||||>I think I just merged the master's changes into my branch to ensure it was up to date with upstream. Maybe I needed to rebase?<|||||>Hi @Iwontbecreative thanks for adding the RemBERT model! Do you have a list of the 110 languages used in the pretraining of the model?<|||||>Sure, here's the list:
['af', 'am', 'ar', 'az', 'be', 'bg', 'bg-Latn', 'bn', 'bs', 'ca', 'ceb', 'co', 'cs', 'cy', 'da', 'de', 'el', 'el-Latn', 'en', 'eo', 'es', 'et', 'eu', 'fa', 'fi', 'fil', 'fr', 'fy', 'ga', 'gd', 'gl', 'gu', 'ha', 'haw', 'hi', 'hi-Latn', 'hmn', 'hr', 'ht', 'hu', 'hy', 'id', 'ig', 'is', 'it', 'iw', 'ja', 'ja-Latn', 'jv', 'ka', 'kk', 'km', 'kn', 'ko', 'ku', 'ky', 'la', 'lb', 'lo', 'lt', 'lv', 'mg', 'mi', 'mk', 'ml', 'mn', 'mr', 'ms', 'mt', 'my', 'ne', 'nl', 'no', 'ny', 'pa', 'pl', 'ps', 'pt', 'ro', 'ru', 'ru-Latn', 'sd', 'si', 'sk', 'sl', 'sm', 'sn', 'so', 'sq', 'sr', 'st', 'su', 'sv', 'sw', 'ta', 'te', 'tg', 'th', 'tr', 'uk', 'ur', 'uz', 'vi', 'xh', 'yi', 'yo', 'zh', 'zh-Hans', 'zh-Hant', 'zh-Latn', 'zu']
cf https://github.com/google-research/google-research/tree/master/rembert
|
transformers | 10,691 | closed | Naming convention for (pytorch) checkpoints broken? | # 🚀 Feature request
In previous versions of the library and sample files, checkpoints were saved with some naming convention that had `checkpoint` in the name file. Subsequent jobs could look in the output directory and check if any checkpoint is available first; if found, it would load the checkpoint and the corresponding config and continue training from where it left off; if not found, it would check for the model_path_or_name.
I'm under the impression that this convention broke, from what I can tell. When using the utilities from the library, for pytorch models, the model is saved with the name `pytorch_model.bin` (WEIGHTS_NAME in file_utils.py) and when looking to load a checkpoint PREFIX_CHECKPOINT_DIR = "checkpoint" from trainer_utils.py is used. So it doesn't match and it starts training from scratch.
One (local) way to fix this is to rewrite searching for a checkpoint instead of using the one in the library.
Is there any other option that allows a pipeline of jobs without using different scripts (e.g., one script that loads the original pretrained bert model, for example, and all subsequent runs use a different script that point the model_path to the local path where the pytorch_model.bin is saved).
I guess the feature request is to bring this feature back. One way to do it is to use command line args for checkpoint names instead of using hardcoded naming in the files.
## Motivation
Cascading/pipelined training jobs: one job starts, takes a checkpoint, the next one picks up from the last checkpoint. The same script is used for either first or intermediate job in the pipeline.
| 03-12-2021 19:12:51 | 03-12-2021 19:12:51 | I think I might have come up with a bandaid solution for now. If the output dir exists and overwrite output dir flag is not set, load the configuration from the output dir and resume training from `pytorch_model.bin`. I'm going to give this a try, I think it's going to work. <|||||>Yes, as I expected, it worked. I modified the sample scripts as follows:
```
if os.path.isdir(training_args.output_dir) and training_args.do_train and not training_args.overwrite_output_dir:
# last_checkpoint = get_last_checkpoint(training_args.output_dir)
# check there is a WEIGHTS_NAME model in the output directory and use that as the last_checkpoint
if os.path.isfile(os.path.join(training_args.output_dir, WEIGHTS_NAME)):
# use it as checkpoint
last_checkpoint = training_args.output_dir
```
The ramification of this change is that the checkpoint is going to be replaced upon training completion (which so happens is what I needed).
I still think the checkpoint naming conventions should be reconciled (re: WEIGHTS_NAME and PREFIX_CHECKPOINT_DIR) so I'll leave this feature request open. <|||||>Nothing has changed in the way checkpoints are named since version 2 at least, e.g. the checkpoints are saved in `args.output_dir/checkpoin-xxx` where xxx is the number of training steps elapsed since the beginning of training.
The change you are suggesting would remove the ability for people to resume training from the last checkpoint saved which is not something we want. If you want to start your training from a specific saved model, you can pass along
`--model_name_or_path path_to_folder_with_saved_model`.<|||||>@sgugger Thanks for the reply. I think I wanted the behavior that you're talking about (not what I ended up doing, i.e., looking for a saved model directly in the output dir).
Based on what you just said, I looked again at the code and there are two ways to save a checkpoint: `save_model` and `_save_checkpoint`. It so happens that the sample text classification scripts use `save_model` directly which does not create the `checkpoint-xxx` directory. Underneath, `_save_checkpoint` calls `save_model` but with the dir `checkpoint-xxx` which was the behavior that I wanted.
BTW, what's the "right" way of saving models/checkpoints? the `_` in `_save_checkpoint` makes me believe it's supposed to be a utility function and there is another API function.
So I guess what's happening is the sample script calls `save_model` when the training ends (saving the `pytorch_model.bin` directly in the output dir) and that confused me. Bottom line: the current sample script for text classification can't resume training from the last checkpoint saved (because it doesn't "save a checkpoint" it "saves a model" https://github.com/huggingface/transformers/blob/master/examples/text-classification/run_glue.py#L466).
I completely agree with you that that is a behavior everybody wants, i.e., resuming training from last checkpoint. If you agree, I'll change this issue to read save checkpoint in sample text classification script.<|||||>I think you are confusing:
- saving checkpoints during training, done automatically by the `Trainer` every `save_steps` (unless you are using a different strategy)
and
- saving the final model, which is done at the end of training.<|||||>Got it, thanks for clarifying the terminology!
PS: It so happens that I needed a checkpoint to be saved at the end of training, now I understand how that's done. |
transformers | 10,690 | closed | enable loading Mbart50Tokenizer with AutoTokenizer | # What does this PR do?
Currently `MBart50Tokenizer`, `MBart50TokenizerFast` can't be loaded using `AutoTokenizer` because they use the `MBartConfig` which is associated with `MBartTokenizer`.
This PR enables loading `MBart50Tokenizer(Fast)` by adding them to the `NO_CONFIG_TOKENIZER` list. I've also added the `tokenizer_type` argument in the respective models' config file on the hub.
cc @Narsil | 03-12-2021 17:40:11 | 03-12-2021 17:40:11 | Very nice ! |
transformers | 10,689 | closed | Fix mixed precision for TFGPT2LMHeadModel | Fixed the loss of precision when using mixed_precision.
Not sure it's the right way to do this, correct me if it's wrong.
related issue: https://github.com/huggingface/transformers/issues/8559#issuecomment-797528526
- gpt2: @patrickvonplaten, @LysandreJik
- tensorflow: @jplu | 03-12-2021 14:53:10 | 03-12-2021 14:53:10 | > This is not the proper way to proceed. Here you force the dtype to float32 which is not correct, because you might encounter conflicts with other values that are in float16.
Yes, you're right, I should not force the dtype to float32, maybe I should force it to float32 when using `mixed_precision`.
> To get similar results, you have to increase the number of epochs. Usually, I get a similar model after multiplying the number of epochs by 2 or 2.5.
But in my sample:
I always get 0.98 accuracy after 18 epochs without using the `mixed_precision`,
but sometimes the accuracy will stop at 0.7333 when using the `mixed_precision` policy, even I train it after 100 epochs.
I think there must something wrong. I have updated my sample, have a look: https://colab.research.google.com/github/mymusise/gpt2-quickly/blob/main/examples/mixed_precision_test.ipynb<|||||>> Yes, you're right, I should not force the dtype to float32, maybe I should force it to float32 when using mixed_precision.
No! This would be even worse. Set the layer norm and the embeddings directly to `float32` is a better temporary fix.
> I always get 0.98 accuracy after 18 epochs without using the mixed_precision, but sometimes the accuracy will stop at 0.7333 when using the mixed_precision policy, even I train it after 100 epochs.
Having a lower accuracy in mixed precision is normal, but not that much. How much do you get by setting the layer norm and the embeddings directly to `float32` in mixed precision?
I cannot run any test for now as I don't have access to a computer so I cannot really use your Colab.<|||||>> No! This would be even worse. Set the layer norm and the embeddings directly to `float32` is a better temporary fix.
My apologies, I didn't get it, do you mean the first commit is better? :joy:
> Having a lower accuracy in mixed precision is normal, but not that much. How much do you get by setting the layer norm and the embeddings directly to `float32` in mixed precision?
>
> I cannot run any test for now as I don't have access to a computer so I cannot really use your Colab.
Sorry to disturb your rest time. I can get 0.99 accuracy after setting the layer norm and the embeddings directly to `float32` in mixed precision.
Here's a partial screenshot:
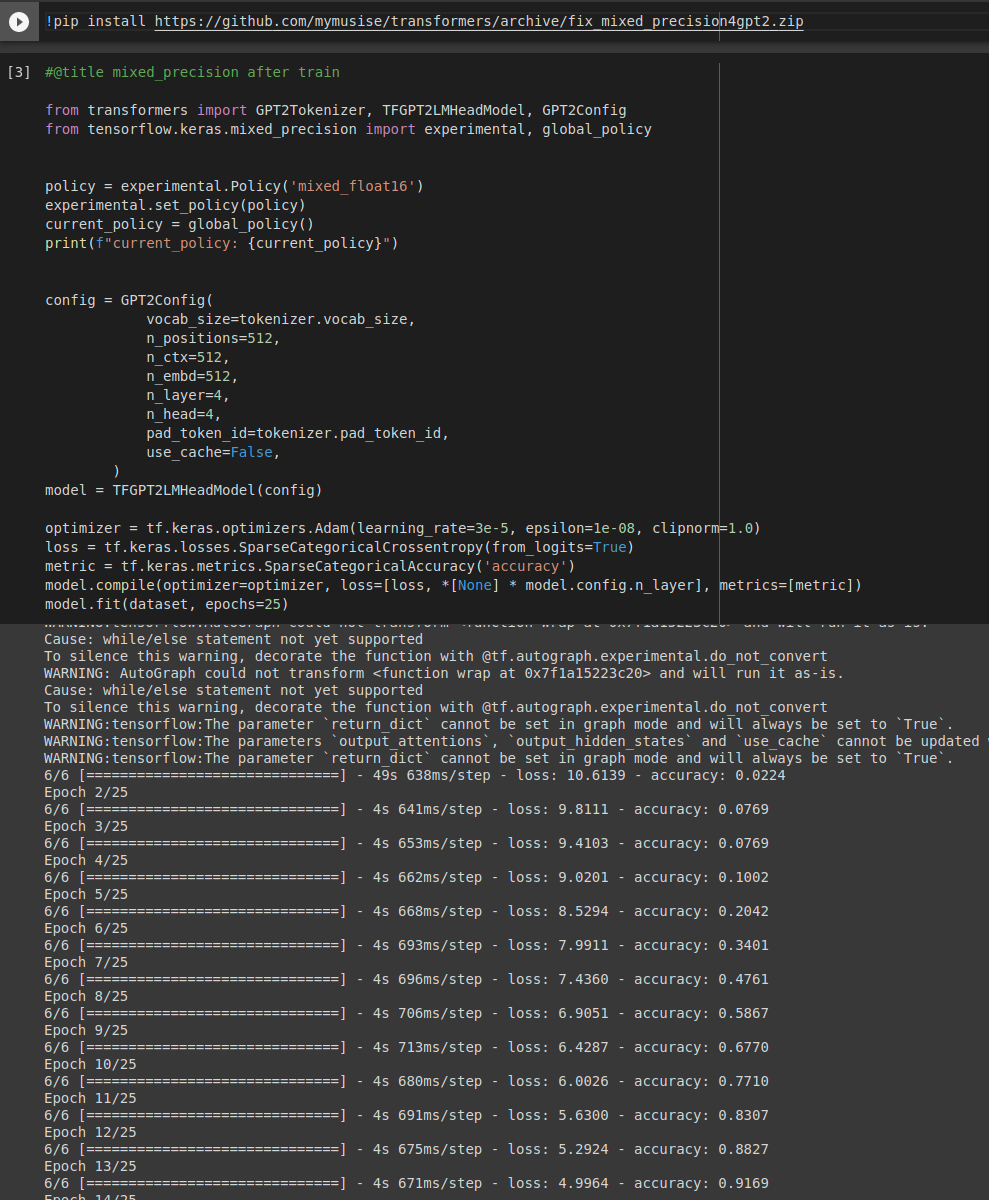
<|||||>> My apologies, I didn't get it, do you mean the first commit is better?
Yes.
> Sorry to disturb your rest time. I can get 0.99 accuracy after setting the layer norm and the embeddings directly to float32 in mixed precision.
Thanks for the screenshot. Can you please revert your last commit then.<|||||>> Thanks for the screenshot. Can you please revert your last commit then.
Sure!<|||||>This issue has been automatically marked as stale because it has not had recent activity. If you think this still needs to be addressed please comment on this thread.
Please note that issues that do not follow the [contributing guidelines](https://github.com/huggingface/transformers/blob/master/CONTRIBUTING.md) are likely to be ignored.<|||||>This issue has been automatically marked as stale because it has not had recent activity. If you think this still needs to be addressed please comment on this thread.
Please note that issues that do not follow the [contributing guidelines](https://github.com/huggingface/transformers/blob/master/CONTRIBUTING.md) are likely to be ignored.<|||||>@Rocketknight1
Hello, what should I do to help with this?<|||||>This issue has been automatically marked as stale because it has not had recent activity. If you think this still needs to be addressed please comment on this thread.
Please note that issues that do not follow the [contributing guidelines](https://github.com/huggingface/transformers/blob/master/CONTRIBUTING.md) are likely to be ignored. |
transformers | 10,688 | closed | Adding required flags to non-default arguments in hf_argparser | Signed-off-by: Adam Pocock <[email protected]>
# What does this PR do?
Fixes #10677. I didn't update the docs as I think this is the intended behaviour, but I can do if you think this change would be unexpected.
## Before submitting
- [ ] This PR fixes a typo or improves the docs (you can dismiss the other checks if that's the case).
- [x] Did you read the [contributor guideline](https://github.com/huggingface/transformers/blob/master/CONTRIBUTING.md#start-contributing-pull-requests),
Pull Request section?
- [x] Was this discussed/approved via a Github issue or the [forum](https://discuss.huggingface.co/)? Please add a link
to it if that's the case. Issue #10677.
- [ ] Did you make sure to update the documentation with your changes? Here are the
[documentation guidelines](https://github.com/huggingface/transformers/tree/master/docs), and
[here are tips on formatting docstrings](https://github.com/huggingface/transformers/tree/master/docs#writing-source-documentation).
- [x] Did you write any new necessary tests?
## Who can review?
@sgugger | 03-12-2021 14:43:00 | 03-12-2021 14:43:00 | No problem. I can't see what test failed in CircleCI as it wants to bind to my Github account and access private things in my org. Is there any way to see the failures without letting it into my account? |
transformers | 10,687 | closed | Multiple fixes in SageMakerTrainer | # What does this PR do?
This PR adds quite a few fixes to the `SageMakerTrainer` to make sure example scripts run fully. In particular it fixes:
- save made the training hanging forever
- predict didn't work
- evaluation required using `drop_last=True` which is not something anyone wants.
The goal is now to test a little bit more that functionality before merging the `SageMakerTrainer` into the main `Trainer` (otherwise one can't use model parallelism in seq2seq examples or QA example). The plan is to have them merged in the v4.5.0. | 03-12-2021 14:05:27 | 03-12-2021 14:05:27 | |
transformers | 10,686 | closed | fix backend tokenizer args override: key mismatch | # What does this PR do?
Related to #10390
Turns out it was a simple key mismatch - leaving as draft for now just to see the results of the full test suites, but hopeful this will fix the main problem for the related issue. | 03-12-2021 13:41:54 | 03-12-2021 13:41:54 | I'm talking with @n1t0 soon to get a better sense of where it might make sense as well yes! Will update then<|||||>went looking for similar case with this regex `(\[|\()"do_lower_case`, found only one more: 7e42461
I think this is it, will move onto #10121 once this is merged<|||||>There does seem to be an issue remaining though, as the tests in the suite currently fail with:
```
=========================== short test summary info ============================
FAILED tests/test_tokenization_auto.py::AutoTokenizerTest::test_do_lower_case
=== 1 failed, 5143 passed, 2103 skipped, 2207 warnings in 266.40s (0:04:26) ====
``` |
transformers | 10,685 | closed | Distributed barrier before loading model | # What does this PR do?
This PR adds a distributed barrier before the final load when the option `load_best_model_at_end` is selected. This is because a process might get to that point before process 0 has finished saving the checkpoint that is re-loaded, which would result in an error.
Fixes #10666 | 03-12-2021 13:35:55 | 03-12-2021 13:35:55 | |
transformers | 10,684 | closed | Question answering: a couple of things after fine-tuning a model | Hello everybody
First of all, I'm kinda new to HF and transformers library, so I apologize if my questions may be be trivial.
I followed the very well explained guide provided [here](https://github.com/huggingface/notebooks/blob/master/examples/question_answering.ipynbl) to fine-tune a pre-trained model (for the moment, I used the standard SQuaD dataset). The model has been fine-tuned correctly, saved and evaluated, giving as expected the following results: `{'exact_match': 76.80227057710502, 'f1': 84.96565168555021}`
That said, here are my questions:
1. Now that I have this model, how can I use it to answer to a "custom" question on a specific context? I am assuming that I will need to preprocess the question+context in a way similar to the preprocessing of training dataset, but how can I exactly do that? I will have to use this model in another script, so having the possibility to have a function that gets as input (model, context, question) and gives me as output the predicted answer (possibly with its probability) would be great, is there some piece of code that does this?
2. As final goal I will have to fine-tune an italian language model. I'm assuming that this depends on the value of `model_checkpoint`, so here I would have to select an italian pre-trained model (for example [dbmdz/bert-base-italian-cased](https://huggingface.co/dbmdz/bert-base-italian-cased)), is that correct? And if I want to use a multilanguage model, how do I specify the language I want to use?
3. (this may be a very dumb question) Can I fine-tune an already fine-tuned model (for example [this model](https://huggingface.co/mrm8488/bert-italian-finedtuned-squadv1-it-alfa))? Would it make sense? I'm asking this because in the future I will likely be able to expand my training set, so I want to know if I have to restart from a pre-trained model or if I can fine-tune an already tuned model several times.
Thanks a lot for your patience.
Claudio | 03-12-2021 12:42:43 | 03-12-2021 12:42:43 | Here's an answer to your questions:
> 1. Now that I have this model, how can I use it to answer to a "custom" question on a specific context? I am assuming that I will need to preprocess the question+context in a way similar to the preprocessing of training dataset, but how can I exactly do that?
Yes, I've created a small Colab notebook to illustrate inference for question answering models: https://colab.research.google.com/drive/1F-4rWIDythF4B8hS6SdNx9x3h3ffg2zw?usp=sharing
Btw, this is also explained in the [docs](https://huggingface.co/transformers/task_summary.html#extractive-question-answering).
> 2\. As final goal I will have to fine-tune an italian language model. I'm assuming that this depends on the value of `model_checkpoint`, so here I would have to select an italian pre-trained model (for example [dbmdz/bert-base-italian-cased](https://huggingface.co/dbmdz/bert-base-italian-cased)), is that correct? And if I want to use a multilanguage model, how do I specify the language I want to use?
If you want to fine-tune on an Italian dataset, then it's indeed advised to start from a pre-trained Italian model. If you want to use a multilanguage model, you don't need to specify any language you want to use, because it's a general-purpose model. Just make sure that Italian is one of the languages on which the multi-lingual model was pre-trained.
> 3\. (this may be a very dumb question) Can I fine-tune an already fine-tuned model (for example [this model](https://huggingface.co/mrm8488/bert-italian-finedtuned-squadv1-it-alfa))? Would it make sense?
Yes you can, and it's maybe the best thing to do, because as this model is already fine-tuned on Italian questions, then it will already have reasonable performance out-of-the-box. You can just improve it a little more by fine-tuning on your specific dataset.
Btw, it's advised to ask such questions on the [forum](https://discuss.huggingface.co/) rather than here, as the authors of HuggingFace like to keep Github issues for bugs and feature requests.
Cheers!<|||||>Many thanks @NielsRogge , I discovered the existence of the forum 20 minutes after posting this, my bad :(
I will use the forum now to ask some more things.
Thansk a lot! |
transformers | 10,683 | closed | Add util for deleting cached models programmatically | # What does this PR do?
<!--
Congratulations! You've made it this far! You're not quite done yet though.
Once merged, your PR is going to appear in the release notes with the title you set, so make sure it's a great title that fully reflects the extent of your awesome contribution.
Then, please replace this with a description of the change and which issue is fixed (if applicable). Please also include relevant motivation and context. List any dependencies (if any) that are required for this change.
Once you're done, someone will review your PR shortly (see the section "Who can review?" below to tag some potential reviewers). They may suggest changes to make the code even better. If no one reviewed your PR after a week has passed, don't hesitate to post a new comment @-mentioning the same persons---sometimes notifications get lost.
-->
<!-- Remove if not applicable -->
This was util was discussed in #8803 and had two parts to it. The first was addressed by #8836 which allows for cached models' names and information to be retrieved programmatically. This PR builds on that and allows a cached model and its associated .lock and .json files to be deleted by passing in the unique model url returned by `file_utils.get_cached_models()`.
This PR will only delete a model file and its associated metadata files, its worth noting that tokenizers and config metadata will be left behind as they have separate unique file identifiers to the model.
## Before submitting
- [ ] This PR fixes a typo or improves the docs (you can dismiss the other checks if that's the case).
- [X] Did you read the [contributor guideline](https://github.com/huggingface/transformers/blob/master/CONTRIBUTING.md#start-contributing-pull-requests),
Pull Request section?
- [X] Was this discussed/approved via a Github issue or the [forum](https://discuss.huggingface.co/)? Please add a link
to it if that's the case.
- [X] Did you make sure to update the documentation with your changes? Here are the
[documentation guidelines](https://github.com/huggingface/transformers/tree/master/docs), and
[here are tips on formatting docstrings](https://github.com/huggingface/transformers/tree/master/docs#writing-source-documentation).
- [X] Did you write any new necessary tests?
## Who can review?
Anyone in the community is free to review the PR once the tests have passed. Feel free to tag
members/contributors which may be interested in your PR.
@LysandreJik this is continuation of #8836 and #8803
<!-- Your PR will be replied to more quickly if you can figure out the right person to tag with @
If you know how to use git blame, that is the easiest way, otherwise, here is a rough guide of **who to tag**.
Please tag fewer than 3 people.
Models:
- albert, bert, xlm: @LysandreJik
- blenderbot, bart, marian, pegasus, encoderdecoder, t5: @patrickvonplaten, @patil-suraj
- longformer, reformer, transfoxl, xlnet: @patrickvonplaten
- fsmt: @stas00
- funnel: @sgugger
- gpt2: @patrickvonplaten, @LysandreJik
- rag: @patrickvonplaten, @lhoestq
- tensorflow: @jplu
Library:
- benchmarks: @patrickvonplaten
- deepspeed: @stas00
- ray/raytune: @richardliaw, @amogkam
- text generation: @patrickvonplaten
- tokenizers: @n1t0, @LysandreJik
- trainer: @sgugger
- pipelines: @LysandreJik
Documentation: @sgugger
HF projects:
- nlp datasets: [different repo](https://github.com/huggingface/nlp)
- rust tokenizers: [different repo](https://github.com/huggingface/tokenizers)
Examples:
- maintained examples (not research project or legacy): @sgugger, @patil-suraj
- research_projects/bert-loses-patience: @JetRunner
- research_projects/distillation: @VictorSanh
-->
| 03-12-2021 12:35:40 | 03-12-2021 12:35:40 | This issue has been automatically marked as stale because it has not had recent activity. If you think this still needs to be addressed please comment on this thread.
Please note that issues that do not follow the [contributing guidelines](https://github.com/huggingface/transformers/blob/master/CONTRIBUTING.md) are likely to be ignored. |
transformers | 10,682 | closed | Token Classification: How to tokenize and align labels with overflow and stride? | Hello Huggingface,
I try to solve a token classification task where the documents are longer than the model's max length.
I modified the `tokenize_and_align_labels` function from [example token classification notebook](https://github.com/huggingface/notebooks/blob/master/examples/token_classification.ipynb). I set the tokenizer option `return_overflowing_tokens=True` and rewrote the function to map labels for the overflowing tokens:
```python
tokenizer_settings = {'is_split_into_words':True,'return_offsets_mapping':True,
'padding':True, 'truncation':True, 'stride':0,
'max_length':tokenizer.model_max_length, 'return_overflowing_tokens':True}
def tokenize_and_align_labels(examples):
tokenized_inputs = tokenizer(examples["tokens"], **tokenizer_settings)
labels = []
for i,document in enumerate(tokenized_inputs.encodings):
doc_encoded_labels = []
last_word_id = None
for word_id in document.word_ids:
if word_id == None: #or last_word_id == word_id:
doc_encoded_labels.append(-100)
else:
document_id = tokenized_inputs.overflow_to_sample_mapping[i]
label = examples[task][document_id][word_id]
doc_encoded_labels.append(int(label))
last_word_id = word_id
labels.append(doc_encoded_labels)
tokenized_inputs["labels"] = labels
return tokenized_inputs
```
Executing this code will result in an error:
```
exception has occurred: ArrowInvalid
Column 5 named task1 expected length 820 but got length 30
```
It looks like the input 30 examples can't be mapped to the 820 examples after the slicing. How can I solve this issue?
## Environment info
Google Colab runing Code:
https://github.com/huggingface/notebooks/blob/master/examples/token_classification.ipynb
### Who can help
Library:
- tokenizers: @LysandreJik
## Information
Model I am using (Bert ):
The problem arises when using:
* [ ] the official example scripts: (give details below)
* [x] my own modified scripts: (give details below)
The tasks I am working on is:
* [x] an official conll2003 task:
* [x] my own task or dataset:
## To reproduce
Steps to reproduce the behaviour:
1. Replace the tokenize_and_align_labels function with the function given above.
2. Add examples longer than max_length
3. run `tokenized_datasets = datasets.map(tokenize_and_align_labels, batched=True)` cell.
| 03-12-2021 12:10:03 | 03-12-2021 12:10:03 | Hi,
would it be possible to ask this question on HuggingFace's [forum](https://discuss.huggingface.co/)? Some people like Sylvain (who created the tutorial you mention) are very active there, and are happy to help you. The authors like to keep Github issues for bugs caused by the Transformers library or feature requests.
Thanks!<|||||>I moved this question to: https://discuss.huggingface.co/t/token-classification-how-to-tokenize-and-align-labels-with-overflow-and-stride/4353 |
transformers | 10,681 | closed | Tests run on Docker | This PR updates the GPU-based tests to run on docker images. This:
- Simplifies maintenance
- Horizontal scaling is made much simpler
- Environment can be managed with a single line change (the docker image) hello pytorch 1.3-1.7 tests!
- Setups a notification service to get alerted when scheduled tests fail.
Co-authored-by: Morgan <[email protected]> | 03-12-2021 11:51:53 | 03-12-2021 11:51:53 | @stas00 I hear you regarding the installation of dependencies. We are installing every dependency except the main one (PT/TF) on the GPU machine, so I would say most errors are caught.
Furthermore, we are installing these exact dependencies on the CircleCI runs, so I believe we are testing that already on every commit.
If there is a scenario I am missing, please let me know and I will do my best to adjust.
All other comments have been adressed in the last three commits.<|||||>Ah, ok, for some reason I thought that if we are using a docker image then we can skip wasting time and resources on installing the same things million times a day and just run the tests right away, and in that case only an occasional test that installs from scratch will be needed. But perhaps this further speed up can be done in some future iteration.
Nothing more from my side.
Thank you, @LysandreJik |
transformers | 10,680 | closed | [TFMarian] Slow integration tests are failing | After having uploaded the TF weights of:
- https://huggingface.co/Helsinki-NLP/opus-mt-mt-en/commit/552db365bf294f7a2604fadcedfca0ed5b29bd66
- https://huggingface.co/Helsinki-NLP/opus-mt-en-zh/commit/137ef1a50f7a0eaf22a7d5685d07b66bb670ddd1
- https://huggingface.co/Helsinki-NLP/opus-mt-en-ROMANCE/commit/1854185e5a3183d8c73360b1cd53f63c2fb0ed46
and merged this PR:
https://github.com/huggingface/transformers/pull/10664
the TFMarian slow integration tests are falling. This doesn't seem to be an easy issue and needs further investigation.
cc @patrickvonplaten @patil-suraj | 03-12-2021 11:32:06 | 03-12-2021 11:32:06 | This is a very weird bug actually and can be reproduced the easiest ass follows:
```python
#!/usr/bin/env python3
from transformers import TFMarianMTModel, MarianMTModel, MarianTokenizer
tokenizer = MarianTokenizer.from_pretrained("Helsinki-NLP/opus-mt-mt-en")
model_pt = MarianMTModel.from_pretrained("Helsinki-NLP/opus-mt-mt-en")
model_tf = TFMarianMTModel.from_pretrained("Helsinki-NLP/opus-mt-mt-en", from_pt=True)
model_tf.save_pretrained("./")
model_tf = TFMarianMTModel.from_pretrained("./")
input_str = "My name is Wolfgang and I live in Berlin"
input_str = "Billi messu b'mod ġentili, Ġesù fejjaq raġel li kien milqut bil - marda kerha tal - ġdiem."
output_tokens_pt = model_pt.generate(tokenizer(input_str, return_tensors="pt").input_ids)
output_tokens_tf = model_tf.generate(tokenizer(input_str, return_tensors="tf").input_ids)
print("Pt:", tokenizer.batch_decode(output_tokens_pt))
print("Tf:", tokenizer.batch_decode(output_tokens_tf))
```
fails while commenting out the save and load lines works:
```python
#!/usr/bin/env python3
from transformers import TFMarianMTModel, MarianMTModel, MarianTokenizer
tokenizer = MarianTokenizer.from_pretrained("Helsinki-NLP/opus-mt-mt-en")
model_pt = MarianMTModel.from_pretrained("Helsinki-NLP/opus-mt-mt-en")
model_tf = TFMarianMTModel.from_pretrained("Helsinki-NLP/opus-mt-mt-en", from_pt=True)
input_str = "My name is Wolfgang and I live in Berlin"
input_str = "Billi messu b'mod ġentili, Ġesù fejjaq raġel li kien milqut bil - marda kerha tal - ġdiem."
output_tokens_pt = model_pt.generate(tokenizer(input_str, return_tensors="pt").input_ids)
output_tokens_tf = model_tf.generate(tokenizer(input_str, return_tensors="tf").input_ids)
print("Pt:", tokenizer.batch_decode(output_tokens_pt))
print("Tf:", tokenizer.batch_decode(output_tokens_tf))
```
Most other TFMarian models work correctly. This is pretty weird though and will need more time for investigation (cc @patil-suraj for info)<|||||>Thanks for your investigation!<|||||>This issue has been automatically marked as stale because it has not had recent activity. If you think this still needs to be addressed please comment on this thread.
Please note that issues that do not follow the [contributing guidelines](https://github.com/huggingface/transformers/blob/master/CONTRIBUTING.md) are likely to be ignored.<|||||>Unstale<|||||>This issue has been automatically marked as stale because it has not had recent activity. If you think this still needs to be addressed please comment on this thread.
Please note that issues that do not follow the [contributing guidelines](https://github.com/huggingface/transformers/blob/master/CONTRIBUTING.md) are likely to be ignored. |
transformers | 10,679 | closed | [Tests] RAG | This PR shortens the RAG tests by simply reducing the batch size to 8. It's not ideal because RAG is a fairly complex model and IMO, it's good that we have such "big" integration tests. Maybe we should move those tests to a different `@require_large_gpu` decorator? | 03-12-2021 11:23:09 | 03-12-2021 11:23:09 | |
transformers | 10,678 | closed | T5-base out of memory on one 2080 GPU with batchsize 4, sequence length 100 | I want to finetune T5 on totto dataset but failed.
This is werid that it is OOM suddenly when it is training about 10% of one epoch.
And before that it is normal. about 3500M.
Very grateful for help! thx!
here is my simple code:
```
import torch
from transformers import T5Tokenizer, T5ForConditionalGeneration,Adafactor
import pandas as pd
tokenizer = T5Tokenizer.from_pretrained('../t5-base')
model = T5ForConditionalGeneration.from_pretrained('../t5-base', return_dict=True)
if torch.cuda.is_available():
dev = torch.device("cuda:0")
print("Running on the GPU")
else:
dev = torch.device("cpu")
print("Running on the CPU")
model.to(dev)
train_df=pd.read_csv('../totto_data/tt.csv', index_col=[0])
train_df=train_df.iloc[:50000,:]
train_df=train_df.sample(frac = 1)
optimizer = Adafactor(model.parameters(),lr=1e-3,
eps=(1e-30, 1e-3),
clip_threshold=1.0,
decay_rate=-0.8,
beta1=None,
weight_decay=0.0,
relative_step=False,
scale_parameter=False,
warmup_init=False)
num_of_epochs = 6
batch_size=4
num_of_batches=len(train_df)//batch_size
model.train()
for epoch in range(1,num_of_epochs+1):
print('Running epoch: {}'.format(epoch))
running_loss=0
print(epoch)
for i in range(num_of_batches):
inputbatch=[]
labelbatch=[]
if i % 1000==0:
print(i/num_of_batches)
new_df=train_df[i*batch_size:i*batch_size+batch_size]
for indx,row in new_df.iterrows():
input = row['input_text']+'</s>'
labels = row['target_text']+'</s>'
inputbatch.append(input)
labelbatch.append(labels)
inputbatch=tokenizer.batch_encode_plus(inputbatch,padding=True,max_length=100,return_tensors='pt')["input_ids"]
labelbatch=tokenizer.batch_encode_plus(labelbatch,padding=True,max_length=100,return_tensors="pt") ["input_ids"]
inputbatch=inputbatch.to(dev)
labelbatch=labelbatch.to(dev)
# clear out the gradients of all Variables
optimizer.zero_grad()
# Forward propogation
outputs = model(input_ids=inputbatch, labels=labelbatch)
loss = outputs.loss
loss_num=loss.item()
logits = outputs.logits
running_loss+=loss_num
# calculating the gradients
loss.backward()
#updating the params
optimizer.step()
torch.cuda.empty_cache()
running_loss=running_loss/int(num_of_batches)
print('Epoch: {} , Running loss: {}'.format(epoch,running_loss))
torch.save(model.state_dict(),'./finetune/pytoch_model.bin'+str(epoch+1))
```
and this is my python libraries:
backcall 0.2.0
backports.functools-lru-cache 1.6.1
certifi 2020.12.5
chardet 4.0.0
click 7.1.2
decorator 4.4.2
filelock 3.0.12
idna 2.10
importlib-metadata 3.7.2
ipykernel 5.5.0
ipython 7.21.0
ipython-genutils 0.2.0
jedi 0.18.0
joblib 1.0.1
jsonlines 2.0.0
jupyter-client 6.1.11
jupyter-core 4.7.1
mkl-fft 1.3.0
mkl-random 1.2.0
mkl-service 2.3.0
numpy 1.19.2
olefile 0.46
packaging 20.9
pandas 1.2.3
parso 0.8.1
pexpect 4.8.0
pickleshare 0.7.5
Pillow 8.1.2
pip 21.0.1
prompt-toolkit 3.0.16
ptyprocess 0.7.0
Pygments 2.8.1
pyparsing 2.4.7
python-dateutil 2.8.1
pytz 2021.1
pyzmq 22.0.3
regex 2020.11.13
requests 2.25.1
sacremoses 0.0.43
sentencepiece 0.1.95
setuptools 49.6.0.post20210108
six 1.15.0
tokenizers 0.10.1
torch 1.8.0
torchaudio 0.8.0a0+a751e1d
torchvision 0.9.0
tornado 6.1
tqdm 4.59.0
traitlets 5.0.5
transformers 4.3.3
typing-extensions 3.7.4.3
urllib3 1.26.3
wcwidth 0.2.5
wheel 0.36.2
zipp 3.4.1
| 03-12-2021 06:45:12 | 03-12-2021 06:45:12 | I don't think you should use `torch.cuda.empty_cache()`, as explained on [PyTorch's forum](https://discuss.pytorch.org/t/about-torch-cuda-empty-cache/34232/2), "This function should not be used by the end-user except in very edge cases.".
Also, you can set `truncation=True`, because currently you're only padding examples, but not truncating examples that are too long.
Btw, it's better to ask training related questions which are not bugs caused by the Transformers library on the [forum](https://discuss.huggingface.co/) rather than here.<|||||>@NielsRogge
Thanks for your help! It works!
I use `torch.cuda.empty_cache()` because I have no idea but try it.
And I will go to the website you said then.
Thank you again! |
transformers | 10,677 | closed | hf_argparser doesn't set the required flag on non-defaulted enums | ## Environment info
- `transformers` version: 3.0.0-4.3.3
- Platform: macOS
- Python version: 3.9
- PyTorch version (GPU?): n/a
- Tensorflow version (GPU?): n/a
- Using GPU in script?: n/a
- Using distributed or parallel set-up in script?: n/a
### Who can help
I'm not sure who the owner is of hf_argparser.
## Information
We're using hf_argparser to parse our experiment config into dataclasses before training.
## To reproduce
Steps to reproduce the behavior:
1. Add an enum argument without a default to a dataclass
2. Parse the command line arguments without supplying the enum argument
3. Should have raised an exception and printed the usage, instead defaults the value to `None`.
## Expected behavior
It should raise an exception. The issue is on https://github.com/huggingface/transformers/blob/master/src/transformers/hf_argparser.py#L100, the if statement should have an else which sets `kwargs["required"]=True`, the same way line [134](https://github.com/huggingface/transformers/blob/master/src/transformers/hf_argparser.py#L134) does.
I can work up a patch if you agree this is an issue. I think it will also occur with anything that falls into [this branch](https://github.com/huggingface/transformers/blob/master/src/transformers/hf_argparser.py#L118) of the if too.
| 03-12-2021 02:47:41 | 03-12-2021 02:47:41 | I agree with your assessment and your proposed fix, so by all means, please suggest a PR! Thanks! |
transformers | 10,676 | closed | Improve the speed of adding tokens from added_tokens.json | # 🚀 Feature request
<!-- A clear and concise description of the feature proposal.
Please provide a link to the paper and code in case they exist. -->
~~Make `PreTrainedTokenizer.unique_no_split_tokens` a type `Set[str]`, or use a temporary `Set[str]` variable for adding tokens from `added_tokens.json`.~~
(**Update**) Found one old PR related to this: https://github.com/huggingface/transformers/pull/6461
So instead of changing its type to `Set[str]`, it would be great to slightly modify the way how tokens are added to `PreTrainedTokenizer.unique_no_split_tokens`. Assume `unique_no_split_tokens` is always ordered and deduped during the token adding process, we could do something like below:
```python
import bisect
# add this function to transformers/src/transformers/tokenization_utils.py
def _insert_one_token(token_list: List[str], new_token: str):
# search if new_token is already in the ordered token_list
insertion_idx = bisect.bisect_left(token_list, new_token)
if insertion_idx < len(token_list) and token_list[
insertion_idx] == new_token:
# new_token is in token_list, don't add
return
else:
token_list.insert(insertion_idx, new_token)
```
Then at https://github.com/huggingface/transformers/blob/26a33cfd8c2d6923f41ab98683f33172e8948ff3/src/transformers/tokenization_utils.py#L200-L205
Do something like this:
```python
if special_tokens:
if len(new_tokens) == 1:
_insert_one_token(self.unique_no_split_tokens, new_tokens[0])
else:
self.unique_no_split_tokens = sorted(set(self.unique_no_split_tokens).union(set(new_tokens)))
else:
# Or on the newly added tokens
if len(tokens_to_add) == 1:
_insert_one_token(self.unique_no_split_tokens, tokens_to_add[0])
else:
self.unique_no_split_tokens = sorted(set(self.unique_no_split_tokens).union(set(tokens_to_add)))
```
My local tests show that this can reduce the token adding time from 9 mins (see details below) down to about 1 seconds.
## Motivation
<!-- Please outline the motivation for the proposal. Is your feature request
related to a problem? e.g., I'm always frustrated when [...]. If this is related
to another GitHub issue, please link here too. -->
Currently the `unique_no_split_tokens` is of type `List[str]`: https://github.com/huggingface/transformers/blob/26a33cfd8c2d6923f41ab98683f33172e8948ff3/src/transformers/tokenization_utils.py#L123
This causes a performance issue if the number of added tokens from `added_tokens.json` is large, when running `tokenizer.from_pretrained()`. For example, it takes 9 minutes to add about 50000 new tokens on a MacBook Pro (2.6 GHz Intel Core i7). Specifically, the issue is mainly caused by:
https://github.com/huggingface/transformers/blob/26a33cfd8c2d6923f41ab98683f33172e8948ff3/src/transformers/tokenization_utils.py#L200-L205
Tokens in `added_tokens.json` are added **one by one,** https://github.com/huggingface/transformers/blob/26a33cfd8c2d6923f41ab98683f33172e8948ff3/src/transformers/tokenization_utils_base.py#L1827-L1832
so the `set` operation will be repeated 50000 times, with more and more number of elements. ~~By switching to `Set[str]` or using a temporary `Set[str]` variable for token adding purpose, it would significantly lower the overhead when adding tokens from `added_tokens.json`, and also helps a few `in` presence checks in a few places.~~ (**Update**: Like pointed out earlier, we don't need to change to `Set[str]`, just using a more efficient way to insert one token into the ordered list should be good enough.)
| 03-11-2021 22:47:40 | 03-11-2021 22:47:40 | @lhoestq If this looks good to you, I can help create a PR. Thanks!<|||||>Hi !
This looks like a great solution, feel free to open a PR ! :)<|||||>Great, thanks! Just made a PR here: https://github.com/huggingface/transformers/pull/10780 |
transformers | 10,675 | closed | Reformer _pad_to_mult_of_chunk_length seems incorrect | ## Environment info
- `transformers` version: 4.3.3
- Platform: Linux-4.15.0-136-generic-x86_64-with-glibc2.10
- Python version: 3.8.5
- PyTorch version (GPU?): 1.7.1 (True)
- Tensorflow version (GPU?): not installed (NA)
- Using GPU in script?: yes
- Using distributed or parallel set-up in script?: no
### Who can help
Original Author of the method: @patrickvonplaten
## Information
Model I am using: Reformer
The problem arises when using:
* [x] the official example scripts: (give details below)
* [ ] my own modified scripts: (give details below)
The tasks I am working on is:
* [ ] an official GLUE/SQUaD task: (give the name)
* [x] my own task or dataset: (give details below)
Mnist
## Bug Description
For input_ids,position_ids=None and inputs_embeds!=None _pad_to_mult_of_chunk_length produces unexpected results.
When position_ids are None, the input embeds are padded with overlapping position_ids. Line
[Link to method](https://github.com/huggingface/transformers/blame/90ecc29656ce37fdbe7279cf586511ed678c0cb7/src/transformers/models/reformer/modeling_reformer.py#L2176)
Would produced
```padded_inputs_embeds```
with positional encoding for `[0,padding_length] `
## Expected behavior
`padded_input_embeds` should have a positional encoding in the range of [max_seq_length-padding_length, max_seq_length]
[Link to method](https://github.com/huggingface/transformers/blame/90ecc29656ce37fdbe7279cf586511ed678c0cb7/src/transformers/models/reformer/modeling_reformer.py#L2176)
Should be changed to:
```
padded_inputs_embeds = self.embeddings(padded_input_ids, position_ids,start_idx_pos_encodings=inputs_embeds.shape[-1])
```
I am not sure at what extend, this affects the model or attention mechanism or whether the effect is cancelled out by the masking mechanism.
I can create a pull request if @patrickvonplaten approves?
| 03-11-2021 22:32:41 | 03-11-2021 22:32:41 | Hey @fostiropoulos,
Sorry to answer this late - could you provide a code example to reproduce the error? <|||||>@patrickvonplaten It would take me time to come up with a full working minimal example in colab.
However you can try a model that you supply only the input_embeds and leave input_ids,position_ids to `None` during test time and when the sequence requires padding.
The positional encoding will use a ids (`torch.arange`) from index 0 (`start_idx_pos_encodings=0`) to `padded_sequence_length` for the padded ids. It should have been the start position of the end of the input embedding e.g. (`start_idx_pos_encodings=seq_len`)
It shouldn't affect the final results because the padded tokens are discarded at the end, but it is not the expected behavior. It would cause errors if padding happens outside of test time or the function is used elsewhere. <|||||>@patrickvonplaten does padding affect anything during sampling? e.g. the pad token being 0 vs 100 or any other random int. The casual attention / mask should only make it so that each token depends only on previously seen tokens (beyond the pad). Am I correct? <|||||>This issue has been automatically marked as stale because it has not had recent activity. If you think this still needs to be addressed please comment on this thread.
Please note that issues that do not follow the [contributing guidelines](https://github.com/huggingface/transformers/blob/master/CONTRIBUTING.md) are likely to be ignored. |
transformers | 10,674 | closed | [trainer] loss = NaN with label_smoothing and full-fp16 eval | It looks like our `--label_smoothing_factor` Trainer's feature doesn't handle fp16 well. It's a problem with the deepspeed zero3 I'm integrating right now, since it evals in fp16, but also can be reproduced with the recently added `--fp16_full_eval` trainer option.
To reproduce:
```
export BS=16; rm -r output_dir; PYTHONPATH=src USE_TF=0 CUDA_VISIBLE_DEVICES=0 python examples/seq2seq/run_seq2seq.py --model_name_or_path t5-small --output_dir output_dir --adam_eps 1e-06 --do_eval --evaluation_strategy=steps --label_smoothing 0.1 --learning_rate 3e-5 --logging_first_step --logging_steps 1000 --max_source_length 128 --max_target_length 128 --num_train_epochs 1 --overwrite_output_dir --per_device_eval_batch_size $BS --predict_with_generate --eval_steps 25000 --sortish_sampler --task translation_en_to_ro --val_max_target_length 128 --warmup_steps 500 --max_val_samples 500 --dataset_name wmt16 --dataset_config "ro-en" --source_prefix "translate English to Romanian: " --fp16_full_eval
```
```
***** eval metrics *****
eval_bleu = 24.1257
eval_gen_len = 39.554
eval_loss = nan
eval_mem_cpu_alloc_delta = 56MB
eval_mem_cpu_peaked_delta = 0MB
eval_mem_gpu_alloc_delta = 116MB
eval_mem_gpu_peaked_delta = 374MB
eval_runtime = 25.3246
eval_samples = 500
eval_samples_per_second = 19.744
init_mem_cpu_alloc_delta = 2MB
init_mem_cpu_peaked_delta = 0MB
init_mem_gpu_alloc_delta = 0MB
init_mem_gpu_peaked_delta = 0MB
```
If someone in the community would like to have a look at solving this puzzle, please refer to the discussion of this Issue.
Basically, we would like to try to find a way to perform label smoothing under full fp16 while finding a way to handle NaNs so that the final loss is not a NaN.
And for the reference value running the same script w/o `--fp16_full_eval` should give you the "golden" `eval_loss` - i.e. ideally it should be about the same with `--fp16_full_eval` (if possible that is).
Thank you!
@sgugger | 03-11-2021 20:43:13 | 03-11-2021 20:43:13 | I am unsure what you want me to fix. Yes evaluation in FP16 is not as precise as evaluation in FP32 and results in NaNs more easily, in particular in the loss, that's precisely the reason I was reluctant to add the --fp16_full_eval option.<|||||>well, first of all I'm reporting this behavior.
Secondly, it looks like DeepSpeed Zero3 for now requires that we continue using the fp16 model for inference. We are discussing other possibilities but they aren't there yet. This is because of all the hooks that get installed into the model during training. But if we were to remove all those hooks suddenly the model won't fit into the gpu memory - if it was spread out over multiple gpus in the first place.
Which is why I added ` --fp16_full_eval` in first place. To enable fitting the model onto the gpu memory during inference if it fit during training (with fp32 master weights being offloaded to cpu). e.g. fitting t5-11b (45GB at fp32) into 40GB gpu. Can train with DeepSpeed zero2, so should be able to do inference too.
Finally, according to DeepSpeed devs a model trained in mixed precision shouldn't need to be put back into fp32 and should provide similar results only slightly imprecise.
we will need a way to get the fp32 model out of DeepSpeed training https://github.com/microsoft/DeepSpeed/issues/800<|||||>I understand all of that, and maybe a fine-tuned model in mixed precision will have better results that don't get any Nan losses on the evaluation dataset, but it only takes one sample of the whole evaluation dataset with a slightly bigger loss than usual to get one Nan that will then drive the whole evaluation loss to NaN. And with label smoothing enabled it only takes one log_probs over all the possibilities to get to that.
Not sure it really matters since you still have proper metrics (the predictions are not driven to Nan, just the loss). Maybe we could try a flag to deactivate label smoothing when running evaluation, but then the loss wouldn't be comparable to the training loss, so not sure if it would really be useful either.
<|||||>Thank you for elucidating the situation and suggesting workarounds, @sgugger
Would it be of a useful value if we approximated the nans with something that would lead to a non-nan loss? since NaN can come from a variety of combinations of inf/0 operations, do we know which one is it? and then perhaps pick a corresponding substitution that might lead to a sufficiently good estimate?
<|||||>Yes, we could maybe track when it arises and where it comes from exactly as a first step. Won't have time to dive into this in the near future, but if someone wants to tackle that issue to that effect and report here, that would be awesome!<|||||>I'm interested in taking a stab on this!<|||||>Awesome! Thank you, @vladdy
Just be aware that the following PR should be merged shortly: https://github.com/huggingface/transformers/pull/10611
and so the script in the reproduction line will most likely be renamed to `run_translation.py` and will slightly change the cl args.
<|||||>@vladdy, while you research this - it'd be great to understand the cause of NaNs - so if you discover which operation leads to it please do share. Thank you!<|||||>Before falling asleep this idea came to me, tried it this morning and it worked:
```
--- a/src/transformers/trainer_pt_utils.py
+++ b/src/transformers/trainer_pt_utils.py
@@ -390,7 +390,9 @@ class LabelSmoother:
def __call__(self, model_output, labels):
logits = model_output["logits"] if isinstance(model_output, dict) else model_output[0]
+ #logits = logits.to(dtype=torch.float32)
log_probs = -torch.nn.functional.log_softmax(logits, dim=-1)
+ log_probs = log_probs.to(dtype=torch.float32)
if labels.dim() == log_probs.dim() - 1:
labels = labels.unsqueeze(-1)
```
Basically, flip `logits` or `log_probs` back to fp32 and the problem goes away.
So the problem here has nothing to do with full fp16 inference, but with how label smoothing is calculated.
The issue comes from:
```
smoothed_loss = log_probs.sum(dim=-1, keepdim=True)
```
in fp32, the return values are huge:
```
[ 863637.3750],
[ 864242.0000],
[ 865449.0000],
[ 866092.9375],
[ 867702.4375],
```
and in fp16 these turn `inf`.
So either:
1. we do what I proposed on top of this comment, which will double the size of the `log_probs` tensor (4 times if we apply it to `logits`, rather than `log_probs`) . This will of course depend on the size of the dictionary and `target_max_len` - so say:
```
bs * max_len * dict_size * 2 more bytes
32 * 128 * 32000 * 2 = 262MB - huge!
```
2. or we change the calculation to scale down huge numbers back to a numerical range where `sum` over fp16 numbers doesn't overflow. Note that `smoothed_loss` does another `sum` towards the end which would definitely blow things up.
3. same as (1) but switch the calculations to `.cpu()` - a bit slower but no extra gpu memory will be required.
Of course number 2 is a better solution since it doesn't require much more memory to solve this problem and will require a change in algorithm to avoid going into huge numbers.
The other question is: do we deal with the label smoother separately or do we have other parts which may be affected in which case we should change the logits back to fp32 when prediction has completed. But as explained above this will come at a large gpu memory cost.<|||||>Yeah, I came to a similar conclusion regarding the cause and when I wanted to post an update on it, I saw @stas00's response above.
If I'm not wrong, Apex tried to solve [this compatibility issue between fp16 and losses](https://github.com/NVIDIA/apex/tree/a109f856840ebb3ff5578e0bddfc4cffd4b96ed0/apex/fp16_utils), but I'm not sure how much of that could be reused in addition to already stated options. @stas00, please let me know if you want to continue driving this and I'll try to find some other issue for my contribution.<|||||>@vladdy, by all means please continue, I was just sharing what I have discovered and calculated that this won't be an efficient solution memory requirement-wise. And I now have a better understanding of where NaN came from.
As you're suggesting the most efficient generic solution would be around loss scaling. We are doing it already during the training, so this is just some of the same for label smoothing.
But we definitely don't want to depend on apex for this.
I haven't looked closely but I think the idea is to scale the `log_probs` into a much smaller size, while ensuring that the scaled numbers and the sum of 30-60k elements remain within the dynamic range of fp16 (plus there is one more sum of sums at the end!). If we were to implement it in a non-vectorized way it'd be the simplest to create an fp32 variable and add the fp16 bits to it, so it won't overflow. It won't take any extra memory, but that won't be efficient speed wise.
And of course perhaps you can think of other solutions. Anything that doesn't require an extra GPU memory to perform label smoothing is goodness.
Perhaps pytorch has some ready-made solutions too...<|||||>Any progress on this, @vladdy? I have one idea that may work, but would love to hear what you have discovered in your research.<|||||>@stas00, I have not found anything better than switching to fp32 for that operation. The rest of the approaches appear to be more complicated or not as generic as I think we want them to be. What idea did you have in mind?<|||||>Thank you for sharing the results of your research, @vladdy. So the need is the same, it's all about how to do it efficiently and not defeat the purpose of keeping things at fp16.
My discovery was switching to fp32 only for the aggregate and have it done by pytorch on the hardware level:
```
diff --git a/src/transformers/trainer_pt_utils.py b/src/transformers/trainer_pt_utils.py
index ae8e24949..c2071733c 100644
--- a/src/transformers/trainer_pt_utils.py
+++ b/src/transformers/trainer_pt_utils.py
@@ -399,7 +399,8 @@ class LabelSmoother:
# will ignore them in any case.
labels.clamp_min_(0)
nll_loss = log_probs.gather(dim=-1, index=labels)
- smoothed_loss = log_probs.sum(dim=-1, keepdim=True)
+ # works for fp16 input tensor too, by internally upcasting it to fp32
+ smoothed_loss = log_probs.sum(dim=-1, keepdim=True, dtype=torch.float32)
nll_loss.masked_fill_(padding_mask, 0.0)
smoothed_loss.masked_fill_(padding_mask, 0.0)
```
I was shocked that it took ~0 extra memory over pure fp16 solution (that is not even extra peak memory!) - that is it was doing the conversion to fp32 on the hardware level. At least that was so on my hardware - it might be not so on an older gpu that doesn't support fp16 natively.
This is pretty amazing that it can do that!
I came to this idea while researching bfloat16 where its aggregate operations too require fp32 aggregates - so I thought why not try the same for our case and it seems to work. Similarly sometimes they use fp64 for fp32 inputs if they are too big, but I don't think we run into those things here.
What do you think?
<|||||>I think, this simple solution makes sense to be applied as it is also generic enough to cover all the cases. I doubt it is possible to find a better approach within short time and it does not appear it is necessary to spend more time on this (at least, for now). Feel free to do the PR as you offered it!<|||||>Dear @stas00
Thank you very much for filing this issue. I am training mt5-small model and with deepspeed without label smoothing, I am getting NaNs, so far could not managed to fix it. I greatly appreciate your suggestions on this. if you think this can be appropriate I will open up a separate issue for mt5 model getting NaNs with deepspeed, and if not I follow this issue. Thank you very much<|||||>@vladdy, thank you for doing your research and validating my suggestion.
@dorost1234, the PR is here: https://github.com/huggingface/transformers/pull/10815 or you can just change it manually for your test - it's just one line.
Please let me know if it fixes your problem, if it's about eval_loss. If it doesn't, or it's about something else - then yes please open a separate issue. Based on your comments elsewhere the issue about mt5 and NaNs already exists, but not with deepspeed so definitely open one. Perhaps the Deepspeed team has some insights about this situation.
<|||||>Thank you so much @stas00 for the great response, I applied your PR and with deepspeed now mt5-small for me is not getting nan anymore, this is an incredible job you are doing, thanks a lot, I still getting nans with mt5-small with fp16, even after your PR, for this I made a separate issue here https://github.com/huggingface/transformers/issues/10819 I did not tag you since with deepspeed with your applied magic PR it is not getting nans so far, while still If you have time to give me an advice I would be really grateful. <|||||>@stas00 I tested mt5-small with run_translation.py model without this PR this also works fine without nans, if one does not use smoothing, with this PR this becomes much slower for me with deepspeed. is there a way to keep the speed as great as before?<|||||>Please post the exact command lines that you're referring to.
As I wrote in https://github.com/huggingface/transformers/pull/10815 I definitely can see a 25% slowdown when enabling --fp16_full_eval and opened an issue about it https://github.com/huggingface/transformers/issues/10816
I don't see any speed difference with https://github.com/huggingface/transformers/pull/10815 w/o deepspeed, so once you show me what command line use then I can test.
edit: Oh, I see you posted them in https://github.com/huggingface/transformers/issues/10819 - all is good then - I can test now. |
transformers | 10,673 | closed | Add auto_wrap option in fairscale integration | # What does this PR do?
This PR adds support for the `auto_wrap` function added by fairscale to automatically wrap the model's modules in the `FSDP` container (necessary for ZeRO-DP3).
cc @stas00 So you are informed for when you want to experiment more with fairscale. | 03-11-2021 20:14:22 | 03-11-2021 20:14:22 | |
transformers | 10,672 | closed | fix typing error for HfArgumentParser for Optional[bool] | `TrainingArguments` uses the `Optional[bool]` type for [a couple arguments](https://github.com/huggingface/transformers/blob/master/src/transformers/training_args.py#L443). I ran into the following error when using transformers v4.3.3 with python 3.8:
`"TrainingArguments" TypeError: issubclass() arg 1 must be a class` | 03-11-2021 18:48:31 | 03-11-2021 18:48:31 | No, `Optional[bool]` are dealt with later on at [this line](https://github.com/huggingface/transformers/blob/master/src/transformers/hf_argparser.py#L101) and need to stay wrapped until then.
Without more information on your error it's hard to help find the right fix.<|||||>Hi @sugger, thank you for the quick response and for pointing that out. Sorry I did not catch it.
I also see that you put a [tentative fix](https://github.com/huggingface/transformers/commit/fa1a8d102f273ee8118546f7b84133ab58032ac5) for this issue, but also just wanted to check to make sure that `Optional[bool]` values are caught by the expected if statement.
Looking more into the issue, I'm actually seeing that `disable_tqdm` has its typing changed from `Optional[bool]` to `Union[bool, None]` during the `dataclasses.fields` parsing. So it is not getting caught by the line you reference and then the error occurs on the next if statement.
I'm running Python 3.8 on ubuntu 18.04, maybe dataclasses parses optionals differently in other versions.
Printout of `disable_tqdm` fields value:
```
>>> [field for field in dataclasses.fields(TrainingArguments) if "tqdm" in field.name][0]
Field(name='disable_tqdm',type=typing.Union[bool, NoneType],default=None,default_factory=<dataclasses._MISSING_TYPE object at 0x7f2e6e7ff1f0>,init=True,repr=True,hash=None,compare=True,metadata=mappingproxy({'help': 'Whether or not to disable the tqdm progress bars.'}),_field_type=_FIELD)
```
dataclasses parsing of generic optional bool type:
```
>>> @dataclass
... class OptionalBool:
... value: Optional[bool]
...
>>> dataclasses.fields(OptionalBool)
(Field(name='value',type=typing.Union[bool, NoneType],default=<dataclasses._MISSING_TYPE object at 0x7f2e6e7ff1f0>,default_factory=<dataclasses._MISSING_TYPE object at 0x7f2e6e7ff1f0>,init=True,repr=True,hash=None,compare=True,metadata=mappingproxy({}),_field_type=_FIELD),)
```
To address this, would it be fine to change the `is Optional[bool]` check [here](https://github.com/huggingface/transformers/blob/master/src/transformers/hf_argparser.py#L101) to `==`?
```
>>> Optional[bool] is Union[bool, None]
False
>>> Optional[bool] == Union[bool, None]
True
```
Thanks again for looking into this so quickly.<|||||>I think this change is acceptable, thanks! Trying to check what the failure in the test is and if it's spurious. |
transformers | 10,671 | closed | Fixes Pegasus tokenization tests | Non rectangular if padding is not set. | 03-11-2021 18:35:16 | 03-11-2021 18:35:16 | |
transformers | 10,670 | closed | Fix integration slow tests | # What does this PR do?
This PR fixes the following slow tests which are failing because of the change of beahvior in the `Embeddings` layer in PyTorh 1.8. This is done by adding an attention mask to ignore the padding token and checking a slice that does not contain the padding hidden states.
```
tests/test_modeling_albert.py::AlbertModelIntegrationTest::test_inference_no_head_absolute_embedding
tests/test_modeling_bert.py::BertModelIntegrationTest::test_inference_no_head_absolute_embedding
tests/test_modeling_bert.py::BertModelIntegrationTest::test_inference_no_head_relative_embedding_key
tests/test_modeling_bert.py::BertModelIntegrationTest::test_inference_no_head_relative_embedding_key_query
tests/test_modeling_convbert.py::ConvBertModelIntegrationTest::test_inference_masked_lm
tests/test_modeling_deberta.py::DebertaModelIntegrationTest::test_inference_no_head
tests/test_modeling_deberta_v2.py::DebertaV2ModelIntegrationTest::test_inference_no_head
tests/test_modeling_distilbert.py::DistilBertModelIntergrationTest::test_inference_no_head_absolute_embedding
tests/test_modeling_electra.py::ElectraModelIntegrationTest::test_inference_no_head_absolute_embedding
tests/test_modeling_squeezebert.py::SqueezeBertModelIntegrationTest::test_inference_classification_head
```
It also fixes
```
tests/test_modeling_mbart.py::MBartEnroIntegrationTest::test_enro_generate_batch
```
that was failing since the change in `prepare_seq2seq_batch`. For some reason a word is different but it was consistent in PyTorch 1.7/PyTorch 1.8 so I changed the desired target. @patil-suraj if you want to take a closer look, I'll leave it to you. | 03-11-2021 18:33:25 | 03-11-2021 18:33:25 | |
transformers | 10,669 | closed | MT5 integration test: adjust loss difference | @patrickvonplaten, this test didn't pass. If you can double check that it has the expected outputs, that would be great. The difference I'm seeing on my machine is of 1.1e-4, which is slightly higher than the value proposed here of 1e-4. | 03-11-2021 18:15:34 | 03-11-2021 18:15:34 | |
transformers | 10,668 | closed | Add DeBERTa to MODEL_FOR_PRETRAINING_MAPPING | # What does this PR do?
<!--
Congratulations! You've made it this far! You're not quite done yet though.
Once merged, your PR is going to appear in the release notes with the title you set, so make sure it's a great title that fully reflects the extent of your awesome contribution.
Then, please replace this with a description of the change and which issue is fixed (if applicable). Please also include relevant motivation and context. List any dependencies (if any) that are required for this change.
Once you're done, someone will review your PR shortly (see the section "Who can review?" below to tag some potential reviewers). They may suggest changes to make the code even better. If no one reviewed your PR after a week has passed, don't hesitate to post a new comment @-mentioning the same persons---sometimes notifications get lost.
-->
This PR adds DebertaForMaskedLM to MODEL_FOR_PRETRAINING_MAPPING since DeBERTa is currently missing from this dict.
## Before submitting
- [ ] This PR fixes a typo or improves the docs (you can dismiss the other checks if that's the case).
- [X] Did you read the [contributor guideline](https://github.com/huggingface/transformers/blob/master/CONTRIBUTING.md#start-contributing-pull-requests),
Pull Request section?
- [ ] Was this discussed/approved via a Github issue or the [forum](https://discuss.huggingface.co/)? Please add a link
to it if that's the case.
- [ ] Did you make sure to update the documentation with your changes? Here are the
[documentation guidelines](https://github.com/huggingface/transformers/tree/master/docs), and
[here are tips on formatting docstrings](https://github.com/huggingface/transformers/tree/master/docs#writing-source-documentation).
- [ ] Did you write any new necessary tests?
@patrickvonplaten
@sgugger
<!-- Your PR will be replied to more quickly if you can figure out the right person to tag with @
If you know how to use git blame, that is the easiest way, otherwise, here is a rough guide of **who to tag**.
Please tag fewer than 3 people.
Models:
- albert, bert, xlm: @LysandreJik
- blenderbot, bart, marian, pegasus, encoderdecoder, t5: @patrickvonplaten, @patil-suraj
- longformer, reformer, transfoxl, xlnet: @patrickvonplaten
- fsmt: @stas00
- funnel: @sgugger
- gpt2: @patrickvonplaten, @LysandreJik
- rag: @patrickvonplaten, @lhoestq
- tensorflow: @jplu
Library:
- benchmarks: @patrickvonplaten
- deepspeed: @stas00
- ray/raytune: @richardliaw, @amogkam
- text generation: @patrickvonplaten
- tokenizers: @n1t0, @LysandreJik
- trainer: @sgugger
- pipelines: @LysandreJik
Documentation: @sgugger
HF projects:
- nlp datasets: [different repo](https://github.com/huggingface/nlp)
- rust tokenizers: [different repo](https://github.com/huggingface/tokenizers)
Examples:
- maintained examples (not research project or legacy): @sgugger, @patil-suraj
- research_projects/bert-loses-patience: @JetRunner
- research_projects/distillation: @VictorSanh
-->
| 03-11-2021 17:33:52 | 03-11-2021 17:33:52 | @LysandreJik Added!<|||||>Very cool, thanks! Will merge once all the tests are green. |
transformers | 10,667 | closed | [S2T] fix example in docs | # What does this PR do?
`attention_mask` should always be passed for the `S2T` model. This PR fixes the examples in the doc. | 03-11-2021 16:50:46 | 03-11-2021 16:50:46 | |
transformers | 10,666 | closed | training LayouttLM 1 epoch in distributed more results in error | Not sure whether this issue should be posted here or rather in the pytorch repo, please let me know if it is not a transformer issue.
When training LayoutLM with the Trainer in distributed mode for only one epoch, with setting `load_best_model_at_end` to `True`, I get an error when the model is loaded at the end. According to the error message, the config.json file for the model cannot be found although it is there. This issue does **not** arise when not training in distributed mode or when training in distributed mode for more than one epoch.
```
import os
import torch
from transformers import EvaluationStrategy, Trainer
from transformers.training_args import TrainingArguments
from transformers import (
LayoutLMConfig,
LayoutLMForTokenClassification,
)
training_args = TrainingArguments(
output_dir="output_dir", # output directory
do_train=True,
do_eval=False,
do_predict=False,
evaluation_strategy=EvaluationStrategy.EPOCH,
num_train_epochs=1, # total # of training epochs
per_device_train_batch_size=8, # batch size per device during training
per_device_eval_batch_size=8, # batch size for evaluation
weight_decay=0.0005, # strength of weight decay
learning_rate=0.00000001,
logging_steps=0, # it logs when running evaluation so no need to log on step interval
save_steps=0,
seed=42,
overwrite_output_dir=True,
save_total_limit=10,
load_best_model_at_end=True,
metric_for_best_model="f1",
greater_is_better=True, # higher f1 score is better
fp16=True,
local_rank=-1,
gradient_accumulation_steps=2,
warmup_steps=300,
)
model_dir = "layoutlm_pretrained_model"
train_dataset = []
validation_dataset = []
config = LayoutLMConfig.from_pretrained(
os.path.join(model_dir, "config.json"), num_labels=64, cache_dir=None
)
model = LayoutLMForTokenClassification.from_pretrained(
model_dir,
from_tf=bool(".ckpt" in model_dir),
config=config,
cache_dir=None,
)
device = torch.device("cuda")
model.train().to(device)
trainer = Trainer(
model=model, # the instantiated 🤗 Transformers model to be trained
args=training_args, # training arguments, defined above
train_dataset=train_dataset, # training dataset
eval_dataset=validation_dataset, # validation dataset
)
trainer.train()
``` | 03-11-2021 16:32:28 | 03-11-2021 16:32:28 | Hi! Do you mind posting the error message with the stacktrace? Thank you!
Pinging @sgugger <|||||>Here you go:
```
Training completed. Do not forget to share your model on huggingface.co/models =)
Loading best model from /mnt/pipeline/636d29be-97f9-458a-8174-70ae425a2a6a/pytorch_model/checkpoint-8 (score: 0.00027643400138217).
Saving model checkpoint to /mnt/pipeline/636d29be-97f9-458a-8174-70ae425a2a6a/pytorch_model/checkpoint-8
Configuration saved in /mnt/pipeline/636d29be-97f9-458a-8174-70ae425a2a6a/pytorch_model/checkpoint-8/config.json
404 Client Error: Not Found for url:
https://huggingface.co//mnt/pipeline/636d29be-97f9-458a-8174-70ae425a2a6a/pytorch_model/checkpoint-8/resolve/main/config.json
2021-03-11 12:17:57 ERROR layoutlm_model_training_script Can't load config for '/mnt/pipeline/636d29be-97f9-458a-8174-70ae425a2a6a/pytorch_model/checkpoint-8'. Make sure that:
- '/mnt/pipeline/636d29be-97f9-458a-8174-70ae425a2a6a/pytorch_model/checkpoint-8' is a correct model identifier listed on '
https://huggingface.co/models
'
- or '/mnt/pipeline/636d29be-97f9-458a-8174-70ae425a2a6a/pytorch_model/checkpoint-8' is the correct path to a directory containing a config.json file
Traceback (most recent call last):
File "/usr/local/lib/python3.8/dist-packages/transformers/configuration_utils.py", line 399, in get_config_dict
resolved_config_file = cached_path(
File "/usr/local/lib/python3.8/dist-packages/transformers/file_utils.py", line 1077, in cached_path
output_path = get_from_cache(
File "/usr/local/lib/python3.8/dist-packages/transformers/file_utils.py", line 1215, in get_from_cache
r.raise_for_status()
File "/usr/local/lib/python3.8/dist-packages/requests/models.py", line 943, in raise_for_status
raise HTTPError(http_error_msg, response=self)
requests.exceptions.HTTPError: 404 Client Error: Not Found for url:
https://huggingface.co//mnt/pipeline/636d29be-97f9-458a-8174-70ae425a2a6a/pytorch_model/checkpoint-8/resolve/main/config.json
During handling of the above exception, another exception occurred:
Traceback (most recent call last):
File "nlp_ner_layoutlm/train_pipeline/training_step/training_script.py", line 50, in <module>
train_model(
File "/app/nlp_ner_layoutlm/layoutlm/utils_train.py", line 260, in train_model
trainer.train()
File "/usr/local/lib/python3.8/dist-packages/transformers/trainer.py", line 868, in train
self.model = self.model.from_pretrained(self.state.best_model_checkpoint)
File "/usr/local/lib/python3.8/dist-packages/transformers/modeling_utils.py", line 948, in from_pretrained
config, model_kwargs = cls.config_class.from_pretrained(
File "/usr/local/lib/python3.8/dist-packages/transformers/configuration_utils.py", line 360, in from_pretrained
config_dict, kwargs = cls.get_config_dict(pretrained_model_name_or_path, **kwargs)
File "/usr/local/lib/python3.8/dist-packages/transformers/configuration_utils.py", line 418, in get_config_dict
raise EnvironmentError(msg)
OSError: Can't load config for '/mnt/pipeline/636d29be-97f9-458a-8174-70ae425a2a6a/pytorch_model/checkpoint-8'. Make sure that:
- '/mnt/pipeline/636d29be-97f9-458a-8174-70ae425a2a6a/pytorch_model/checkpoint-8' is a correct model identifier listed on '
https://huggingface.co/models
'
- or '/mnt/pipeline/636d29be-97f9-458a-8174-70ae425a2a6a/pytorch_model/checkpoint-8' is the correct path to a directory containing a config.json file
Traceback (most recent call last):
File "/usr/local/lib/python3.8/dist-packages/transformers/configuration_utils.py", line 399, in get_config_dict
resolved_config_file = cached_path(
File "/usr/local/lib/python3.8/dist-packages/transformers/file_utils.py", line 1077, in cached_path
output_path = get_from_cache(
File "/usr/local/lib/python3.8/dist-packages/transformers/file_utils.py", line 1215, in get_from_cache
r.raise_for_status()
File "/usr/local/lib/python3.8/dist-packages/requests/models.py", line 943, in raise_for_status
raise HTTPError(http_error_msg, response=self)
requests.exceptions.HTTPError: 404 Client Error: Not Found for url:
https://huggingface.co//mnt/pipeline/636d29be-97f9-458a-8174-70ae425a2a6a/pytorch_model/checkpoint-8/resolve/main/config.json
During handling of the above exception, another exception occurred:
Traceback (most recent call last):
File "nlp_ner_layoutlm/train_pipeline/training_step/training_script.py", line 50, in <module>
train_model(
File "/app/nlp_ner_layoutlm/layoutlm/utils_train.py", line 260, in train_model
trainer.train()
File "/usr/local/lib/python3.8/dist-packages/transformers/trainer.py", line 868, in train
self.model = self.model.from_pretrained(self.state.best_model_checkpoint)
File "/usr/local/lib/python3.8/dist-packages/transformers/modeling_utils.py", line 948, in from_pretrained
config, model_kwargs = cls.config_class.from_pretrained(
File "/usr/local/lib/python3.8/dist-packages/transformers/configuration_utils.py", line 360, in from_pretrained
config_dict, kwargs = cls.get_config_dict(pretrained_model_name_or_path, **kwargs)
File "/usr/local/lib/python3.8/dist-packages/transformers/configuration_utils.py", line 418, in get_config_dict
raise EnvironmentError(msg)
OSError: Can't load config for '/mnt/pipeline/636d29be-97f9-458a-8174-70ae425a2a6a/pytorch_model/checkpoint-8'. Make sure that:
- '/mnt/pipeline/636d29be-97f9-458a-8174-70ae425a2a6a/pytorch_model/checkpoint-8' is a correct model identifier listed on '
https://huggingface.co/models
'
- or '/mnt/pipeline/636d29be-97f9-458a-8174-70ae425a2a6a/pytorch_model/checkpoint-8' is the correct path to a directory containing a config.json file
```
<|||||>And what is inside the folder `/mnt/pipeline/636d29be-97f9-458a-8174-70ae425a2a6a/pytorch_model/checkpoint-8`?<|||||>config.json
optimizer.pt
pytorch_model.bin
sheduler.pt
trainer_state.json
training_args.bin
Everything that is needed to load the model.
I checked the config file, it looks entirely normal.<|||||>Oh, I think I know why: it's possible the process 1 arrived at that line before the process 0 finished its save and since there is no barrier, it failed loading the model since it wasn't there yet. Will make a fix for that.<|||||>That makes sense, judging by our logs process 1 wasn't finished yet, as we had a log of saving a checkpoint after the error message from process 0. I cannot share the logs since the pod they were one is already gone...<|||||>If you can checkout the PR mentioned above and see if it solves your issue, that would be great!<|||||>I have to make some extra changes in my code to be able to use that commit ( was using 4.1.0 previously, and there are some breaking changes apparently).<|||||>It works now. I did get a weird error though (not related):
`ValueError: <EvaluationStrategy.EPOCH: 'epoch'> is not a valid IntervalStrategy, please select one of ['no', 'steps', 'epoch']`
Looks like it's not possible anymore to pass EvaluationStrategy.EPOCH as an evaluation_strategy to the Trainer anymore... With version 4.1.0 this was possible. <|||||>Oh it's a bug in the backward compatibility (will fix today). It should work if you pass "epoch" instead of `EvaluationStrategy.EPOCH`.<|||||>yes, that's what I did.
Any idea when these fixes will be released?<|||||>We'll be releasing v4.4.0 in the coming days, which will have the fix. The fix is available on `master` as of now!<|||||>ok, thanks for the fast response! |
transformers | 10,665 | closed | W2v2 test require torch | The object `WAV_2_VEC_2_PRETRAINED_MODEL_ARCHIVE_LIST` requires torch to be installed to not be `None`. This adds the required `@require_torch`. | 03-11-2021 16:31:18 | 03-11-2021 16:31:18 | |
transformers | 10,664 | closed | TensorFlow tests: having from_pt set to True requires torch to be installed. | Some tests were executed without having torch installed, while they require torch. Namely, all the tests that have a `from_pt=True` requirement require torch to be installed.
This is a draft PR as several of the requirements to merge this PR are not met:
- The Marian models do not have their tensorflow variant available on the hub
- Neither do the RAG models
The easy option is to only set `@requires_torch`, but since we have no slow test suite that runs both PT + TF that's not a good workaround.
How do you want to proceed @patrickvonplaten ?
| 03-11-2021 16:23:21 | 03-11-2021 16:23:21 | @LysandreJik - I'll upload the respective weights today and then check that all these slow tests here work without `from_pt`<|||||>Uploaded all the TF weights and checked that:
`RUN_SLOW=1 pytest tests/test_modeling_tf_rag.py`
`RUN_SLOW=1 pytest tests/test_modeling_tf_blenderbot.py`
pass.
For some reason `RUN_SLOW=1 pytest tests/test_modeling_tf_marian.py` now throws an error. I've opened a new issue for this here: https://github.com/huggingface/transformers/issues/10680 |
transformers | 10,663 | closed | Onnx fix test | GPT2 `past_keys_values` format seems to have changed since last time I checked, now exporting for each layer tuple with 2 elements.
PyTorch's ONNX exporter doesn't seem to handle this format, so it was crashing with an error.
The PR assumes we don't currently support exporting `past_keys_values` for GPT2 and then disable the return of such values when constructing the model.
In order to support this behavior, `pipeline()` now ha a `model_kwargs: Dict[str, Any]` parameter which forwards the dict of parameters to model's `from_pretrained(..., **model_kwargs)`. | 03-11-2021 16:21:57 | 03-11-2021 16:21:57 | Merging now to rebase the slow tests and re-run them. |
transformers | 10,662 | closed | Specify minimum version for sacrebleu | The `_tests_requirements.txt` require an install of sacrebleu without any version specified. However, some `sacrebleu` versions don't have the same API. I've had problems with version `1.2.10`, and @lhoestq confirmed the issue is not present in `1.4.12`.
The error was the following:
```
def _compute(
self,
predictions,
references,
smooth_method="exp",
smooth_value=None,
force=False,
lowercase=False,
tokenize=scb.DEFAULT_TOKENIZER,
use_effective_order=False,
):
references_per_prediction = len(references[0])
if any(len(refs) != references_per_prediction for refs in references):
raise ValueError("Sacrebleu requires the same number of references for each prediction")
transformed_references = [[refs[i] for refs in references] for i in range(references_per_prediction)]
> output = scb.corpus_bleu(
sys_stream=predictions,
ref_streams=transformed_references,
smooth_method=smooth_method,
smooth_value=smooth_value,
force=force,
lowercase=lowercase,
tokenize=tokenize,
use_effective_order=use_effective_order,
)
E TypeError: corpus_bleu() got an unexpected keyword argument 'smooth_method'
/mnt/cache/modules/datasets_modules/metrics/sacrebleu/b390045b3d1dd4abf6a95c4a2a11ee3bcc2b7620b076204d0ddc353fa649fd86/sacrebleu.py:114: TypeError
```
Full stack trace:
```
E File "/__w/transformers/transformers/src/transformers/trainer_seq2seq.py", line 74, in evaluate
E return super().evaluate(eval_dataset, ignore_keys=ignore_keys, metric_key_prefix=metric_key_prefix)
E File "/__w/transformers/transformers/src/transformers/trainer.py", line 1650, in evaluate
E output = self.prediction_loop(
E File "/__w/transformers/transformers/src/transformers/trainer.py", line 1823, in prediction_loop
E metrics = self.compute_metrics(EvalPrediction(predictions=preds, label_ids=label_ids))
E File "/__w/transformers/transformers/examples/seq2seq/run_seq2seq.py", line 563, in compute_metrics
E result = metric.compute(predictions=decoded_preds, references=decoded_labels)
E File "/opt/conda/lib/python3.8/site-packages/datasets/metric.py", line 403, in compute
E output = self._compute(predictions=predictions, references=references, **kwargs)
E File "/mnt/cache/modules/datasets_modules/metrics/sacrebleu/b390045b3d1dd4abf6a95c4a2a11ee3bcc2b7620b076204d0ddc353fa649fd86/sacrebleu.py", line 114, in _compute
E output = scb.corpus_bleu(
```
I'm unsure about the minimum version required here, I just know that 1.2.10 doesn't work. Please advise if you think a better minimum version would be better. | 03-11-2021 16:16:00 | 03-11-2021 16:16:00 | @patil-suraj, you must have meant `1.4.12`
|
transformers | 10,661 | closed | Fix Marian/TFMarian tokenization tests | Fixing a few tests that are failing in the slow tests suite.
cc @patrickvonplaten (Marian) and @sgugger (author of the recent changes) | 03-11-2021 15:29:24 | 03-11-2021 15:29:24 | |
transformers | 10,660 | closed | fix: #10628 expanduser path in TrainingArguments |
## Who can review?
- trainer: @sgugger
| 03-11-2021 15:21:33 | 03-11-2021 15:21:33 | Thanks a lot for fixing that issue! |
transformers | 10,659 | closed | How to use deepspeed finetune RAG? | Hi Stas!@stas00 Thanks for your great work! I have a question that is it possible to use deepspeed finetune RAG (finetune_rag.py)? Thanks! | 03-11-2021 15:14:08 | 03-11-2021 15:14:08 | Thank you for your kind words, @qixintechnology
I haven't tried it with RAG, but I don't see any reason why it shouldn't work - if you encounter any problems please open a specific issue with details so that we could reproduce it. |
transformers | 10,658 | closed | GPT2DoubleHeadsModel made parallelizable | # What does this PR do?
GPT2DoubleHeadsModel made parallelizable; it is also reflected in the test_modeling_gpt2.py suite
<!--
Congratulations! You've made it this far! You're not quite done yet though.
Once merged, your PR is going to appear in the release notes with the title you set, so make sure it's a great title that fully reflects the extent of your awesome contribution.
Then, please replace this with a description of the change and which issue is fixed (if applicable). Please also include relevant motivation and context. List any dependencies (if any) that are required for this change.
Once you're done, someone will review your PR shortly (see the section "Who can review?" below to tag some potential reviewers). They may suggest changes to make the code even better. If no one reviewed your PR after a week has passed, don't hesitate to post a new comment @-mentioning the same persons---sometimes notifications get lost.
-->
<!-- Remove if not applicable -->
Fixes # (issue)
## Before submitting
- [ ] This PR fixes a typo or improves the docs (you can dismiss the other checks if that's the case).
- [X] Did you read the [contributor guideline](https://github.com/huggingface/transformers/blob/master/CONTRIBUTING.md#start-contributing-pull-requests),
Pull Request section?
- [ ] Was this discussed/approved via a Github issue or the [forum](https://discuss.huggingface.co/)? Please add a link
to it if that's the case.
- [ ] Did you make sure to update the documentation with your changes? Here are the
[documentation guidelines](https://github.com/huggingface/transformers/tree/master/docs), and
[here are tips on formatting docstrings](https://github.com/huggingface/transformers/tree/master/docs#writing-source-documentation).
- [ ] Did you write any new necessary tests?
## Who can review?
Anyone in the community is free to review the PR once the tests have passed. Feel free to tag
members/contributors which may be interested in your PR.
<!-- Your PR will be replied to more quickly if you can figure out the right person to tag with @
If you know how to use git blame, that is the easiest way, otherwise, here is a rough guide of **who to tag**.
Please tag fewer than 3 people.
Models:
- albert, bert, xlm: @LysandreJik
- blenderbot, bart, marian, pegasus, encoderdecoder, t5: @patrickvonplaten, @patil-suraj
- longformer, reformer, transfoxl, xlnet: @patrickvonplaten
- fsmt: @stas00
- funnel: @sgugger
- gpt2: @patrickvonplaten, @LysandreJik
- rag: @patrickvonplaten, @lhoestq
- tensorflow: @jplu
Library:
- benchmarks: @patrickvonplaten
- deepspeed: @stas00
- ray/raytune: @richardliaw, @amogkam
- text generation: @patrickvonplaten
- tokenizers: @n1t0, @LysandreJik
- trainer: @sgugger
- pipelines: @LysandreJik
Documentation: @sgugger
HF projects:
- nlp datasets: [different repo](https://github.com/huggingface/nlp)
- rust tokenizers: [different repo](https://github.com/huggingface/tokenizers)
Examples:
- maintained examples (not research project or legacy): @sgugger, @patil-suraj
- research_projects/bert-loses-patience: @JetRunner
- research_projects/distillation: @VictorSanh
-->
| 03-11-2021 14:41:49 | 03-11-2021 14:41:49 | @patrickvonplaten , @LysandreJik<|||||>Also pinging @stas00 here - does it make sense to add parallelize ability to GPT2DoubleHeadsModel?<|||||>If it's being used then yes since `GPT2LMHeadModel` has it.<|||||>@alexorona, do you want to take a look at this?<|||||>@stas00 yeah, so I've been using the `GPT2DoubleHeadsModel` for my tasks since reading [this medium](https://medium.com/huggingface/how-to-build-a-state-of-the-art-conversational-ai-with-transfer-learning-2d818ac26313) (I guess a lot of people in the dialogue community also followed that tutorial).
And seeing `parallelize()` implemented with the `GPT2LMHeadModel` got me curious to have the double-headed one working like that as well :)<|||||>@LysandreJik, we parked any further activity on extending naive MP to other `transformers` models and their sub-classes because while it solved the immediate need it's not a good long term solution due to very inefficient gpu utilization.
We are working on integrating ZeRO-3 from DeepSpeed and fairscale which will automatically solve this scalability issue and will make the naive MP approach redundant and we can then decide whether it makes sense to keep it.
Until we sort it out and we can reliable know that ZeRO solves this problem and it's accessible to all users you can definitely merge this since @ishalyminov clearly has a good use for it.<|||||>@ishalyminov Great work here! You're bringing up an important point. I've had this some question myself. @thomwolf It might be useful to add a few sentences to [this Medium article](https://medium.com/huggingface/how-to-build-a-state-of-the-art-conversational-ai-with-transfer-learning-2d818ac26313) clarifying whether distractors are likely to improve final performance in tasks that are ultimately concerned with generative text. It's not entirely clear if your approach using distractors and a double-headed model is an artifact of the competition setup or whether it's an approach you would recommend for anyone trying to fine-tune a transformer for chatbot-style tasks. If someone only cares about language modeling, do you think a double-headed approach with distractors and a classification task would usually produce a better chatbot than simply focusing on LM? Does it matter if the chatbot is attempting to model several discrete personalities present in the dataset?<|||||>@alexorona thanks! Yeah it would be very interesting to hear more about @thomwolf's ConvAI experience:) As for me, I didn't conduct any evaluation of how the NSP task affects the resulting LM (and indeed, there are some works out there that don't use this secondary task at all).
But for what it's worth, we found the NSP head to be beneficial for hybrid [generative/retrieval](https://github.com/microsoft/GRTr) GPT-2 based dialogue architectures.
Also I guess the multi-task setup makes the model intuitively more versatile for a range of downstream tasks, as was originally proposed in the [BERT paper](https://arxiv.org/pdf/1810.04805.pdf) - would be really useful if there was an experimental evaluation proving or disproving this for the case of ConvAI GPT-2. |
transformers | 10,657 | closed | S2S + M2M100 should be available in tokenization_auto | cc @patil-suraj
Was it a choice not to add these to the tokenizer auto, or is it because that's not covered in the template? | 03-11-2021 14:35:25 | 03-11-2021 14:35:25 | |
transformers | 10,656 | closed | Fix broken link | # What does this PR do?
Fixes a broken link in model_doc/pegasus
Link was pointing to: https://github.com/huggingface/transformers/blob/master/examples/seq2seq/finetune_pegasus_xsum.sh
File had been moved to: https://github.com/huggingface/transformers/blob/master/examples/research_projects/seq2seq-distillation/finetune_pegasus_xsum.sh
Fixes # (issue)
[#9257](https://github.com/huggingface/transformers/issues/9257)
## Before submitting
- [x] This PR fixes a typo or improves the docs (you can dismiss the other checks if that's the case).
- [ ] Did you read the [contributor guideline](https://github.com/huggingface/transformers/blob/master/CONTRIBUTING.md#start-contributing-pull-requests),
Pull Request section?
- [ ] Was this discussed/approved via a Github issue or the [forum](https://discuss.huggingface.co/)? Please add a link
to it if that's the case.
- [ ] Did you make sure to update the documentation with your changes? Here are the
[documentation guidelines](https://github.com/huggingface/transformers/tree/master/docs), and
[here are tips on formatting docstrings](https://github.com/huggingface/transformers/tree/master/docs#writing-source-documentation).
- [ ] Did you write any new necessary tests?
## Who can review?
Anyone | 03-11-2021 14:18:48 | 03-11-2021 14:18:48 | |
transformers | 10,655 | closed | MarianMT - tokenizer.supported_language_codes -> 'NoneType' object has no attribute 'supported_language_codes' | ## Environment info
- colab.research.google.com
### Who can help
@patrickvonplaten
Models:
- MarianMT
Examples:
https://huggingface.co/transformers/model_doc/marian.html
## Information
I'm trying to run example code in colab but it fails
`from transformers import MarianMTModel, MarianTokenizer
src_text = [
'>>fra<< this is a sentence in english that we want to translate to french',
'>>por<< This should go to portuguese',
'>>esp<< And this to Spanish'
]
model_name = 'Helsinki-NLP/opus-mt-en-roa'
tokenizer = MarianTokenizer.from_pretrained(model_name)
print(tokenizer.supported_language_codes)
model = MarianMTModel.from_pretrained(model_name)
translated = model.generate(**tokenizer.prepare_seq2seq_batch(src_text, return_tensors="pt"))
tgt_text = [tokenizer.decode(t, skip_special_tokens=True) for t in translated]`
---> 10 print(tokenizer.supported_language_codes)
AttributeError: 'NoneType' object has no attribute 'supported_language_codes'
Could you please provide working translation sample.
| 03-11-2021 14:00:39 | 03-11-2021 14:00:39 | Hi @gagy3798
I couldn't reproduce the issue on master and with the latest pypi version as well. What is your transformers version?
(please always make sure to post the env info when opening an issue)<|||||>Hi @patil-suraj
please try it on this colab https://colab.research.google.com/drive/1z9UtSETxVrDhYnH1eN9lyMv2g-YTFNrz?usp=sharing
transformers 4.3.3
<|||||>probably `sentencepiece` is not installed. Please install `sentencepiece` and restart the colab. That should resolve the issue.<|||||>Ok, thank you. |
transformers | 10,654 | closed | Allow private model hosting and resolution | # 🚀 Feature request
It would be great to provide model hosting and automatic naming resolution on remote storage outside of HF hub/infra. Currently, it is possible to store a model on, say, S3 and resolve it via AutoModel and AutoConfig. However, in this case, a user has to explicitly specify the full path to the configuration file or the model's pytorch_model.bin file.
It would be great if private remote storage could be registered with the same resolution mechanism reserved for HF so that:
`model = AutoModel.from_pretrained('my_org/my_model')`
`config = AutoConfig.from_pretrained('my_org/my_model')`
could be resolved to an actual remote storage path just like HF default resolution mechanism resolves config, models and tokenizers on HF hub.
## Motivation
During the model development lifecycle, organizations often produce many models for internal testing and benchmarking before producing the final model for publishing. Storing all the models during the development phase on the HF hub is sometimes impractical, and some organizations might need stricter control of model storage.
## Your contribution
I've investigated a bit feature request's implementation scope and it doesn't seem to require a big rewrite. The name --> URL naming resolution is done in `file_utils.py`. One could follow how models are resolved for `HUGGINGFACE_CO_PREFIX`
| 03-11-2021 13:35:40 | 03-11-2021 13:35:40 | I think it's a brilliant idea.
So just to validate I understood your proposal correclty, in addition to checking the usual places, it'll first check the env var `HUGGINGFACE_CO_PREFIX` and join it with `model_path` and check if it's available - and if not proceed with the normal algorithm.
So in your example using bash, it'd check `$HUGGINGFACE_CO_PREFIX/my_org/my_model`, which might be `https://some.place.on.earth/data/my_org/my_model`, right?
@LysandreJik, @julien-c - what do you think?<|||||>Yes, pretty much that's it. I think the top flat namespace where `bert-base-uncased`, `t5-base` and all other LMs "live" should never be allowed to resolve to anything else except HF hub (not just for security reasons). However, for the other 2+ level namespaces, i.e. `my_org/my_model` if users can register resolver - that would be great. In the Java world (where I come from), there are these notions of resources and resource bundles that could be dropped in predefined file locations and picked up by the library/framework. Not sure how this is done in Python, but perhaps [pkg_resources](https://setuptools.readthedocs.io/en/latest/pkg_resources.html#resourcemanager-api) could be used. I believe this would be a better approach than registering these resolvers via some HF API. Although perhaps that should be left as an option.
I would love to hear the opinions of others!<|||||>Hi,
I think the simplest way (and I've seen users and organizations do that) would be to extend `AutoModel` to sync your model files from your remote storage before loading it locally
Something like:
```python
class MyAutoModel:
@classmethod
def from_pretrained(cls, id):
subprocess.run(f"aws s3 sync s3://mymodels/{id}/ {local_path}")
return AutoModel.from_pretrained(local_path)
```
ie. all methods are guaranteed to work to load from local paths so pretty trivial to use them to load from anywhere<|||||>This issue has been automatically marked as stale because it has not had recent activity. If you think this still needs to be addressed please comment on this thread.
Please note that issues that do not follow the [contributing guidelines](https://github.com/huggingface/transformers/blob/master/CONTRIBUTING.md) are likely to be ignored. |
transformers | 10,653 | closed | Fix Longformer tokenizer filename | Fixes https://github.com/huggingface/transformers/issues/10642
| 03-11-2021 13:33:23 | 03-11-2021 13:33:23 | |
transformers | 10,652 | closed | Infernal tokenizer loading trained | ## Environment info
<!-- You can run the command `transformers-cli env` and copy-and-paste its output below.
Don't forget to fill out the missing fields in that output! -->
- `transformers` version: 4.4.dev0
- Platform: Ubuntu 18
- Python version: 3.7
- PyTorch version (GPU?): 1.7.1 (YES)
- Tensorflow version (GPU?):
- Using GPU in script?: YES
- Using distributed or parallel set-up in script?: NO
### Who can help
@LysandreJik @patrickvonplaten @patil-suraj @sgugger @n1t0
## Information
Model I am using (Bert, XLNet ...): DeBerta
The problem arises when using:
* [ x] the official example scripts: (give details below)
* [ ] my own modified scripts: (give details below)
The tasks I am working on is:
* [ ] an official GLUE/SQUaD task: (give the name)
* [ x] my own task or dataset: (give details below)
## To reproduce
Steps to reproduce the behavior:
1. Use, for example, the OSCAR corpus in spanish, then use Tokenizers library to train your BPETokenizer (the one Deberta needs).
2. Try to load DebertaTokenizer from the .json generated by Tokenizers.
The code used for training the tokenizer was the following:
```{python}
import glob
import os
import random
from tokenizers import Tokenizer
from tokenizers.models import BPE
from tokenizers.trainers import BpeTrainer
if __name__ == "__main__":
tokenizer = Tokenizer(BPE())
# tokenizer = ByteLevelBPETokenizer(add_prefix_space=False)
trainer = BpeTrainer(
special_tokens=["[UNK]", "[CLS]", "[SEP]", "[PAD]", "[MASK]"],
vocab_size=50265,
continuing_subword_prefix="\u0120",
min_frequency=2,
)
# t = AutoTokenizer.from_pretrained("microsoft/deberta-base")
files = glob.glob("cleaned_train_data/*.csv")
files_sample = random.choices(files, k=250)
tokenizer.train(
files=files_sample,
trainer=trainer,
)
os.makedirs("bpe_tokenizer_0903", exist_ok=True)
tokenizer.save("bpe_tokenizer_0903")
```
The problem is that the DebertaTokenizer from transformers needs a different set of files to the ones Tokenizers generate. It's ironic that it's also a Huggingface library, because there doesn't seem to be much integration between the 2. Well, as this was the case, I tried many things. First, I tried adding added_tokens.json, special_tokens_map.json, vocab.json, vocab.txt, merges.txt... All these files are included in tokenizer.json (the file generated by huggingface/Tokenizers). However, none of those worked. Then, I tried looking at the files that are saved when you load a DebertaTokenizer from microsoft checkpoints, so that I could copy the structure of the saved folder. I tried to do so, but for the bpe_encoder.bin, there were some difficulties. I used my merges for bpe_encoder["vocab"], as the vocab in the Microsoft bpe_encoder.bin seemed to be merges, and in bpe_encoder["encoder"] I put the vocab dict. For the field bpe_encoder["dict_map"], I couldn't replicate it as token frequencies are not saved by Tokenizers, so I invented them with a random number. However, when I try to train with this tokenizer, it throws a KeyError on step 5, which is strange because when I try to tokenize that concrete token: 'Ŀ', it does indeed tokenize it (by doing DebertaTokenizer.from_pretrained(my_path)("Ŀ"))...
I think all those problems are caused mainly because there is a complete disconnection between Transformers and Tokenizers library, as the tokenizers trained with Tokenizers are not integrable with Transformers, which doesn't make much sense to me, because Tokenizers is supposed to be used to train Tokenizers that are later used in Transformers...
Could please anyone tell me how can I train a Deberta Tokenizer that is, from the beginning, saved with the files needed by Transformers DebertaTokenizer?? Is there any version of Tokenizers in which, when you train a BPETokenizer, it saves the files required by Transformers?
Thank you very much.
## Expected behavior
It is expected that if 2 libraries are from the same company and the mission of one of the two is to build tools that are later used by the other, the 2 libraries expect and produce the same objects for the same tasks, as it doesn't make sense that you can train a BPETokenizer that you cannot later use as a tokenizer in Transformers. So, what is expected is that if DebertaTokenizer uses BPETokenizer, and this tokenizer expects to receive bpe_encoder.bin, special_tokens_map.json and tokenizer_config.json, then when you train a BPETokenizer with Tokenizers library it should save those objects, not a tokenizer.json file that is useless for later use in Transformers library. | 03-11-2021 13:05:15 | 03-11-2021 13:05:15 | The tokenizers library powers the fast tokenizers, not the Python "slow" tokenizers. As there is no fast tokenizer for deberta, you can't use the tokenizers library for that model.
You can check which tokenizers have a version backed by the Tokenizers library in [this table](https://huggingface.co/transformers/index.html#bigtable).<|||||>Then, how could we convert the "fast" BPETokenizer to the "slow" BPETokenizer used by Deberta? @sgugger <|||||>Another important thing. I have checked the table and it says that Roberta is able to use fast tokenizer. Deberta, as stated in the paper, uses exactly the same tokenizer as Roberta, so the obvious question is: if Deberta uses Roberta tokenizer, and Roberta tokenizer can be used in "fast" mode, why cannot Deberta be used in "fast" mode??<|||||>Another issue is that when I try to use the BPE Tokenizer trained with huggingface/tokenizers with Roberta directly, it works:
```{python}
tok = RobertaTokenizer.from_pretrained("bpe_tokenizer_0903", use_fast=True)
```
However, when I try to use this same tokenizer for training a language model, it fails:
```{bash}
python -u transformers/examples/language-modeling/run_mlm_wwm.py \
--model_type deberta \
--config_name ./bpe_tokenizer_0903/config.json \
--tokenizer_name ./bpe_tokenizer_0903 \
--train_file ./prueba_tr.txt \
--validation_file ./final_valid.txt \
--output_dir ./roberta_1102 \
--overwrite_output_dir \
--do_train \
--do_eval \
--evaluation_strategy steps \
--per_device_train_batch_size 1 \
--per_device_eval_batch_size 2 \
--gradient_accumulation_steps 2 \
--learning_rate 6e-4 \
--save_steps 10 \
--logging_steps 10 \
--overwrite_cache \
--max_seq_length 128 \
--eval_accumulation_steps 10 \
--load_best_model_at_end \
--run_name deberta_0902 \
--save_total_limit 10 --warmup_steps 1750 \
--adam_beta2 0.98 --adam_epsilon 1e-6 --weight_decay 0.01 --num_train_epochs 1
```
The error message is the following:
```
Traceback (most recent call last):
File "transformers/examples/language-modeling/run_mlm_wwm.py", line 399, in <module>
main()
File "transformers/examples/language-modeling/run_mlm_wwm.py", line 286, in main
use_fast=model_args.use_fast_tokenizer,
File "/home/alejandro.vaca/data_rigoberta/transformers/src/transformers/models/auto/tokenization_auto.py", line 401, in from_pretrained
return tokenizer_class_fast.from_pretrained(pretrained_model_name_or_path, *inputs, **kwargs)
File "/home/alejandro.vaca/data_rigoberta/transformers/src/transformers/tokenization_utils_base.py", line 1719, in from_pretrained
resolved_vocab_files, pretrained_model_name_or_path, init_configuration, *init_inputs, **kwargs
File "/home/alejandro.vaca/data_rigoberta/transformers/src/transformers/tokenization_utils_base.py", line 1790, in _from_pretrained
tokenizer = cls(*init_inputs, **init_kwargs)
File "/home/alejandro.vaca/data_rigoberta/transformers/src/transformers/models/roberta/tokenization_roberta_fast.py", line 173, in __init__
**kwargs,
File "/home/alejandro.vaca/data_rigoberta/transformers/src/transformers/models/gpt2/tokenization_gpt2_fast.py", line 145, in __init__
**kwargs,
File "/home/alejandro.vaca/data_rigoberta/transformers/src/transformers/tokenization_utils_fast.py", line 87, in __init__
fast_tokenizer = TokenizerFast.from_file(fast_tokenizer_file)
Exception: data did not match any variant of untagged enum ModelWrapper at line 1 column 1138661
```
Why doesn't it fail when I try to load the tokenizer with RobertaTokenizer.from_pretrained() but it does fail when I try to run run_mlm_wwm.py ? @sgugger @patrickvonplaten @LysandreJik <|||||>This issue has been automatically marked as stale because it has not had recent activity. If you think this still needs to be addressed please comment on this thread.
Please note that issues that do not follow the [contributing guidelines](https://github.com/huggingface/transformers/blob/master/CONTRIBUTING.md) are likely to be ignored. |
transformers | 10,651 | closed | added support for exporting of T5 models to onnx with past_key_values. | # What does this PR do?
>by applying this fix I was able to create **[fastT5](https://pypi.org/project/fastt5/)** library. which increases the T5 model inference speed up to 5x. for more details check out my [GitHub](https://github.com/Ki6an/fastT5) repo.
addressing [this ](https://github.com/huggingface/transformers/issues/10645)issue and [this ](https://github.com/huggingface/transformers/pull/9733)PR
while exporting T5 decoder model to onnx with `past_key_values`
was getting this error.
```python
/usr/local/lib/python3.7/dist-packages/transformers/models/t5/modeling_t5.py in forward(self, hidden_states, mask, key_value_states, position_bias, past_key_value, layer_head_mask, query_length, use_cache, output_attentions)
497 position_bias = position_bias + mask # (batch_size, n_heads, seq_length, key_length)
498
--> 499 scores += position_bias
500 attn_weights = F.softmax(scores.float(), dim=-1).type_as(
501 scores
RuntimeError: output with shape [5, 8, 1, 2] doesn't match the broadcast shape [5, 8, 2, 2]
```
the reason is while `torch-jit-tracing` the `seq_lenth` is converted to type `<class 'torch.Tensor'>` in this line [424](https://github.com/huggingface/transformers/blob/26a33cfd8c2d6923f41ab98683f33172e8948ff3/src/transformers/models/t5/modeling_t5.py#L424) ` batch_size, seq_length = hidden_states.shape[:2]`
next, tracing throws the following warning at line [494](https://github.com/huggingface/transformers/blob/26a33cfd8c2d6923f41ab98683f33172e8948ff3/src/transformers/models/t5/modeling_t5.py#L494)
```python
/usr/local/lib/python3.7/dist-packages/transformers/models/t5/modeling_t5.py:494:
TracerWarning: Converting a tensor to a Python index might cause the trace to be incorrect. We can't record the data flow of Python values, so this value will be treated as a constant in the future. This means that the trace might not generalize to other inputs!
position_bias = position_bias[:, :, -seq_length:, :]
```
so it keeps `position_bias` as constant and we get the error at line [499](https://github.com/huggingface/transformers/blob/26a33cfd8c2d6923f41ab98683f33172e8948ff3/src/transformers/models/t5/modeling_t5.py#L499). because of the shape mismatch of `positon_bais` and `scores`.
to solve this issue, we can create a variable `int_seq_length` that will stay as `<class 'int'>` throughout the whole process. & we will use this variable in line `position_bias = position_bias[:, :, -int_seq_length:, :]`.
now, tracing no longer throws the warning of `position_bias` being constant and we won't get the error: shape mismatch of `positon_bais` and `scores`.
by following this simple fix I was able to export t5 to onnx as shown in this [notebook ](https://colab.research.google.com/drive/1Q5GSqOOrhO-7NQLpZPZ1C7YrkAot3TJg?usp=sharing). & also was able to create ['fastT5'](https://github.com/Ki6an/fastT5) repo :)
t5: @patrickvonplaten, @patil-suraj
| 03-11-2021 12:53:34 | 03-11-2021 12:53:34 | @patrickvonplaten, @patil-suraj any updates on this PR or [10645](https://github.com/huggingface/transformers/issues/10645) issue?<|||||>@mfuntowicz or @Narsil do you have 2min to give your feedback on this maybe? :-)<|||||>+1 for this<|||||>+1, this is needed for fastT5<|||||>Sorry, I failed to see the first mention.
Yes this is needed for T5. It's a relatively small change, so probably worth it.
@Ki6an thanks for the notebook !
Just a note for everyone reading this, dynamic sizes that are **too** general might affect performance (for instance at runtime, batch_size=1 can be enforced for `encoder_input_ids`. This can lead to some performance gains using `onnxruntime`.
Enforcing batch_size = num_beams *can* also lead to improvements. <|||||>hey, @patrickvonplaten, @patil-suraj, @mfuntowicz could you please have another look at this PR.<|||||>If this enables ONNX, I'm totally fine with the PR, but I'm no expert in ONNX at all...
I leave it no @Narsil to merge the PR if it looks good to him |
transformers | 10,650 | closed | DistilBertTokenizerFast ignores "do_lower_case=False" parameter | Hi, hope all is well :)
It looks like DistilBertTokenizerFast doesn't take do_lower_case into account.
```
from transformers import DistilBertTokenizerFast, DistilBertTokenizer
PRE_TRAINED_MODEL_NAME = "distilbert-base-uncased"
tokenizer_f = DistilBertTokenizerFast.from_pretrained(PRE_TRAINED_MODEL_NAME, do_lower_case=False)
tokenizer_s = DistilBertTokenizer.from_pretrained(PRE_TRAINED_MODEL_NAME, do_lower_case=False)
sample = "Hello, world. How are you?"
tokens_f = tokenizer_f.tokenize(sample)
tokens_s = tokenizer_s.tokenize(sample)
print(tokens_f)
print(tokens_s)
```
output:
```
['hello', ',', 'world', '.', 'how', 'are', 'you', '?']
['[UNK]', ',', 'world', '.', '[UNK]', 'are', 'you', '?']
```
expected:
```
['[UNK]', ',', 'world', '.', '[UNK]', 'are', 'you', '?']
['[UNK]', ',', 'world', '.', '[UNK]', 'are', 'you', '?']
```
packages:
```
argon2-cffi==20.1.0
async-generator==1.10
attrs==20.3.0
backcall==0.2.0
bleach==3.3.0
certifi==2020.12.5
cffi==1.14.5
chardet==4.0.0
click==7.1.2
decorator==4.4.2
defusedxml==0.7.1
entrypoints==0.3
filelock==3.0.12
idna==2.10
ipykernel==5.5.0
ipython==7.21.0
ipython-genutils==0.2.0
ipywidgets==7.6.3
jedi==0.18.0
Jinja2==2.11.3
joblib==1.0.1
jsonschema==3.2.0
jupyter-client==6.1.11
jupyter-core==4.7.1
jupyterlab-pygments==0.1.2
jupyterlab-widgets==1.0.0
MarkupSafe==1.1.1
mistune==0.8.4
nbclient==0.5.3
nbconvert==6.0.7
nbformat==5.1.2
nest-asyncio==1.5.1
notebook==6.2.0
numpy==1.20.1
packaging==20.9
pandocfilters==1.4.3
parso==0.8.1
pexpect==4.8.0
pickleshare==0.7.5
prometheus-client==0.9.0
prompt-toolkit==3.0.16
ptyprocess==0.7.0
pycparser==2.20
Pygments==2.8.1
pyparsing==2.4.7
pyrsistent==0.17.3
python-dateutil==2.8.1
pyzmq==22.0.3
regex==2020.11.13
requests==2.25.1
sacremoses==0.0.43
Send2Trash==1.5.0
six==1.15.0
terminado==0.9.2
testpath==0.4.4
tokenizers==0.10.1
torch==1.8.0+cu111
tornado==6.1
tqdm==4.59.0
traitlets==5.0.5
transformers==4.3.3
typing-extensions==3.7.4.3
urllib3==1.26.3
wcwidth==0.2.5
webencodings==0.5.1
widgetsnbextension==3.5.1
```
Python version:
`Python 3.8.6`
System:
PopOS 20, happy to provide more info on system specs such as hardware if needed | 03-11-2021 12:39:43 | 03-11-2021 12:39:43 | Hi @PierceEigirthon! Thanks for submitting, we're aware of this bug, it's related to #10390 and on my backlog<|||||>I'll close this one as duplicate, you'll be able to follow progress on #10390 ;) |
transformers | 10,649 | closed | [Question] How do I prevent a lack of VRAM halfway through training a (Pegasus) model? | I'm taking a pre-trained pegasus model (specifically, google/pegasus-cnn_dailymail, and I'm using Huggingface transformers through Pytorch) and I want to finetune it on my own data. This is however quite a large dataset and I've run into the problem of running out of VRAM halfway through training, which because of the size of the dataset can be a few days after training even started, which makes a trial-and-error approach very inefficient.
I'm wondering how I can make sure ahead of time that it doesn't run out of memory. I would think that the memory usage of the model is in some way proportional to the size of the input, so I've passed truncation=True, padding=True, max_length=1024 to my tokenizer, which if my understanding is correct should make all the outputs of the tokenizer of the same size per line. Considering that the batch size is also a constant, I would think that the amount of VRAM in use should be stable. So I should just be able to cut up the dataset into managable parts, just looking at the ram/vram use of the first run, and infer that it will run smoothly from start to finish.
However, the opposite seems to be true. I've been observing the amount of VRAM used at any time and it can vary wildly, from ~12GB at one time to suddenly requiring more than 24GB and crashing (because I don't have more than 24GB).
So, how do I make sure that the amount of vram in use will stay within reasonable bounds for the full duration of the training process, and avoid it crashing due to a lack of vram when I'm already days into the training process?
| 03-11-2021 12:32:52 | 03-11-2021 12:32:52 | I think I may have been an idiot; shortly after posting this I found that instead of `padding=True` I should set `padding='max_length'`. Woops. |
transformers | 10,648 | closed | [XLSR-Wav2Vec2] Add multi-lingual Wav2Vec2 models | The model is identical to Wav2Vec2, but comes with a new paper and new checkpoints.
So this PR only adds a new doc page and a tiny change in the conversion script.
Check out the new models here: https://huggingface.co/models?search=wav2vec2-large-xlsr | 03-11-2021 12:17:33 | 03-11-2021 12:17:33 | |
transformers | 10,647 | closed | Update README.md | correct spell error: 'nether'
| 03-11-2021 11:44:34 | 03-11-2021 11:44:34 | |
transformers | 10,646 | closed | seq2seq BertGeneration model failed "ValueError: You have to specify either input_ids or inputs_embeds" | ## Environment info
<!-- You can run the command `transformers-cli env` and copy-and-paste its output below.
Don't forget to fill out the missing fields in that output! -->
- `transformers` version: 4.4.0.dev0
- blenderbot, bart, marian, pegasus, encoderdecoder, t5: @patrickvonplaten, @patil-suraj
```
python examples/seq2seq/run_seq2seq.py \
--model_name_or_path google/roberta2roberta_L-24_discofuse \
--do_train \
--do_eval \
--task summarization \
--train_file path_to_csv_or_jsonlines_file \
--validation_file path_to_csv_or_jsonlines_file \
--output_dir /tmp/tst-summarization \
--per_device_train_batch_size=4 \
--per_device_eval_batch_size=4 \
--overwrite_output_dir \
--predict_with_generate \
--max_train_samples 500 \
--max_val_samples 500
```
path_to_csv_or_jsonlines_file:
```
text,summary
google map, gg map
google translate, gg translate
```
t5-small works perfectly. But BertGeneration model has the following error
error:
```
File "/Users/gyin/Documents/working/transformers/src/transformers/models/bert_generation/modeling_bert_generation.py", line 361, in forward
raise ValueError("You have to specify either input_ids or inputs_embeds")
ValueError: You have to specify either input_ids or inputs_embeds
```
| 03-11-2021 11:32:52 | 03-11-2021 11:32:52 | hi @gyin-ai
Thank you for reporting the issue. The `run_seq2seq.py` currently does not work for encoder-decoder models. This is because the encoder-decoder models expect both `decoder_input_ids` and `labels` whereas the script only passes the `labels`. Which is causing the above error.
You could refer to this [notebook](https://colab.research.google.com/github/patrickvonplaten/notebooks/blob/master/RoBERTaShared_for_BBC_XSum.ipynb) to see how to use `Trainer` for encoder-decoder models. Also, you easily adapt the `run_seq2seq.py` script for this, I think you'll only need to change the data collator [here](https://github.com/huggingface/transformers/blob/master/examples/seq2seq/run_seq2seq.py#L521) to return both the `labels` and `decoder_input_ids`<|||||>@patil-suraj can I ask whether `batch["decoder_input_ids"]` should be `inputs.input_ids` instead of `outputs.input_ids`?
```
def process_data_to_model_inputs(batch):
# Tokenizer will automatically set [BOS] <text> [EOS]
inputs = tokenizer(batch["document"], padding="max_length", truncation=True, max_length=encoder_max_length)
outputs = tokenizer(batch["summary"], padding="max_length", truncation=True, max_length=decoder_max_length)
batch["input_ids"] = inputs.input_ids
batch["attention_mask"] = inputs.attention_mask
batch["decoder_input_ids"] = outputs.input_ids
batch["labels"] = outputs.input_ids.copy()
# mask loss for padding
batch["labels"] = [
[-100 if token == tokenizer.pad_token_id else token for token in labels] for labels in batch["labels"]
]
batch["decoder_attention_mask"] = outputs.attention_mask
return batch
```
here is the example from EncoderDecoderModel
```
>>> from transformers import EncoderDecoderModel, BertTokenizer
>>> import torch
>>> tokenizer = BertTokenizer.from_pretrained('bert-base-uncased')
>>> model = EncoderDecoderModel.from_encoder_decoder_pretrained('bert-base-uncased', 'bert-base-uncased') # initialize Bert2Bert from pre-trained checkpoints
>>> # forward
>>> input_ids = torch.tensor(tokenizer.encode("Hello, my dog is cute", add_special_tokens=True)).unsqueeze(0) # Batch size 1
>>> outputs = model(input_ids=input_ids, decoder_input_ids=input_ids)
>>> # training
>>> outputs = model(input_ids=input_ids, decoder_input_ids=input_ids, labels=input_ids)
```<|||||>The `labels` and `decoder_input_ids` always correspond to output. so it should be `outputs.input_ids` |
transformers | 10,645 | closed | export T5 model to onnx with past_key_values | ## Environment info
- `transformers` version: 4.3.3
- torch version 1.7.0
- Platform: Linux-4.19.112+-x86_64-with-Ubuntu-18.04-bionic
- Python version: 3.7.10
- PyTorch version (GPU?): 1.7.0 (False)
- Tensorflow version (GPU?): 2.4.1 (False)
- Using GPU in script?: <fill in>
- Using distributed or parallel set-up in script?: <fill in>
Models:
- t5: @patrickvonplaten, @patil-suraj
while exporting `T5 decoder` with `past_key_values`, I'm getting the following error,
````python
/usr/local/lib/python3.7/dist-packages/torch/onnx/utils.py:1109: UserWarning: Provided key encoder_hidden_states for dynamic axes is not a valid input/output name
warnings.warn("Provided key {} for dynamic axes is not a valid input/output name".format(key))
/usr/local/lib/python3.7/dist-packages/transformers/models/t5/modeling_t5.py:646: TracerWarning: Converting a tensor to a Python boolean might cause the trace to be incorrect. We can't record the data flow of Python values, so this value will be treated as a constant in the future. This means that the trace might not generalize to other inputs!
if torch.isinf(hidden_states).any():
/usr/local/lib/python3.7/dist-packages/transformers/models/t5/modeling_t5.py:684: TracerWarning: Converting a tensor to a Python boolean might cause the trace to be incorrect. We can't record the data flow of Python values, so this value will be treated as a constant in the future. This means that the trace might not generalize to other inputs!
if torch.isinf(hidden_states).any():
/usr/local/lib/python3.7/dist-packages/transformers/modeling_utils.py:244: TracerWarning: Converting a tensor to a Python boolean might cause the trace to be incorrect. We can't record the data flow of Python values, so this value will be treated as a constant in the future. This means that the trace might not generalize to other inputs!
if causal_mask.shape[1] < attention_mask.shape[1]:
/usr/local/lib/python3.7/dist-packages/transformers/models/t5/modeling_t5.py:494: TracerWarning: Converting a tensor to a Python index might cause the trace to be incorrect. We can't record the data flow of Python values, so this value will be treated as a constant in the future. This means that the trace might not generalize to other inputs!
position_bias = position_bias[:, :, -seq_length:, :]
---------------------------------------------------------------------------
RuntimeError Traceback (most recent call last)
<ipython-input-7-baab8a36e37d> in <module>()
----> 1 generate_onnx_representation(model_to_be_converted, 't5')
25 frames
/usr/local/lib/python3.7/dist-packages/transformers/models/t5/modeling_t5.py in forward(self, hidden_states, mask, key_value_states, position_bias, past_key_value, layer_head_mask, query_length, use_cache, output_attentions)
497 position_bias = position_bias + mask # (batch_size, n_heads, seq_length, key_length)
498
--> 499 scores += position_bias
500 attn_weights = F.softmax(scores.float(), dim=-1).type_as(
501 scores
RuntimeError: output with shape [5, 8, 1, 2] doesn't match the broadcast shape [5, 8, 2, 2]
``` | 03-11-2021 10:24:44 | 03-11-2021 10:24:44 | @LysandreJik @mfuntowicz - how do we deal with ONNX issues currently? <|||||>This issue has been automatically marked as stale because it has not had recent activity. If you think this still needs to be addressed please comment on this thread.
Please note that issues that do not follow the [contributing guidelines](https://github.com/huggingface/transformers/blob/master/CONTRIBUTING.md) are likely to be ignored. |
transformers | 10,644 | closed | Numeracy | # What does this PR do?
<!--
Congratulations! You've made it this far! You're not quite done yet though.
Once merged, your PR is going to appear in the release notes with the title you set, so make sure it's a great title that fully reflects the extent of your awesome contribution.
Then, please replace this with a description of the change and which issue is fixed (if applicable). Please also include relevant motivation and context. List any dependencies (if any) that are required for this change.
Once you're done, someone will review your PR shortly (see the section "Who can review?" below to tag some potential reviewers). They may suggest changes to make the code even better. If no one reviewed your PR after a week has passed, don't hesitate to post a new comment @-mentioning the same persons---sometimes notifications get lost.
-->
<!-- Remove if not applicable -->
Fixes # (issue)
## Before submitting
- [ ] This PR fixes a typo or improves the docs (you can dismiss the other checks if that's the case).
- [ ] Did you read the [contributor guideline](https://github.com/huggingface/transformers/blob/master/CONTRIBUTING.md#start-contributing-pull-requests),
Pull Request section?
- [ ] Was this discussed/approved via a Github issue or the [forum](https://discuss.huggingface.co/)? Please add a link
to it if that's the case.
- [ ] Did you make sure to update the documentation with your changes? Here are the
[documentation guidelines](https://github.com/huggingface/transformers/tree/master/docs), and
[here are tips on formatting docstrings](https://github.com/huggingface/transformers/tree/master/docs#writing-source-documentation).
- [ ] Did you write any new necessary tests?
## Who can review?
Anyone in the community is free to review the PR once the tests have passed. Feel free to tag
members/contributors which may be interested in your PR.
<!-- Your PR will be replied to more quickly if you can figure out the right person to tag with @
If you know how to use git blame, that is the easiest way, otherwise, here is a rough guide of **who to tag**.
Please tag fewer than 3 people.
Models:
- albert, bert, xlm: @LysandreJik
- blenderbot, bart, marian, pegasus, encoderdecoder, t5: @patrickvonplaten, @patil-suraj
- longformer, reformer, transfoxl, xlnet: @patrickvonplaten
- fsmt: @stas00
- funnel: @sgugger
- gpt2: @patrickvonplaten, @LysandreJik
- rag: @patrickvonplaten, @lhoestq
- tensorflow: @jplu
Library:
- benchmarks: @patrickvonplaten
- deepspeed: @stas00
- ray/raytune: @richardliaw, @amogkam
- text generation: @patrickvonplaten
- tokenizers: @n1t0, @LysandreJik
- trainer: @sgugger
- pipelines: @LysandreJik
Documentation: @sgugger
HF projects:
- nlp datasets: [different repo](https://github.com/huggingface/nlp)
- rust tokenizers: [different repo](https://github.com/huggingface/tokenizers)
Examples:
- maintained examples (not research project or legacy): @sgugger, @patil-suraj
- research_projects/bert-loses-patience: @JetRunner
- research_projects/distillation: @VictorSanh
-->
| 03-11-2021 10:17:48 | 03-11-2021 10:17:48 | |
transformers | 10,643 | closed | Space token cannot be add when is_split_into_words = True | for example,
```python
>>> tokenizer = BertTokenizer.from_pretrained('bert-base-chinese')
>>> tokenizer.add_tokens(' ')
1
```
```python
>>> tokenizer.encode('你好 世界', add_special_tokens=False)
[872, 1962, 21128, 686, 4518]
>>> tokenizer.encode(['你','好',' ', '世', '界'], is_split_into_words=True, add_special_tokens=False)
[872, 1962, 686, 4518]
```
Obviously, the blank token is ignored. But if you change it to another token like ‘[balabala]’, it works.
So what is the proper way to do this?
| 03-11-2021 09:14:48 | 03-11-2021 09:14:48 | This issue has been automatically marked as stale because it has not had recent activity. If you think this still needs to be addressed please comment on this thread.
Please note that issues that do not follow the [contributing guidelines](https://github.com/huggingface/transformers/blob/master/CONTRIBUTING.md) are likely to be ignored. |
transformers | 10,642 | closed | Unable To Load Pretrained Longformer Models' Tokenizers | ## Environment info
- `transformers` version: 4.4.0.dev0
- Platform: Windows
- Python version : 3.7.10
- Using GPU in script?: Issue is with both
- Using distributed or parallel set-up in script?: Single device
@patrickvonplaten (because the issue is with longformers)
@LysandreJik (because the issue is with tokenizers)
## Information
Model I am using : Longformer.
The problem arises when loading tokenizer using from_pretrained() function.
The tasks I am working on is Question Answering but it does not matter since I am facing this issue while loading any kind of Longformer:
## To reproduce
Steps to reproduce the behavior:
1. Install Transformers
2. import Transformers
3. run tokenizer = transformers.AutoTokenizer.from_pretrained(MODEL_NAME)
## Reference Code:
```
!pip3 install git+https://github.com/huggingface/transformers
import transformers
DEEP_LEARNING_MODEL_NAME = "mrm8488/longformer-base-4096-finetuned-squadv2" # Not working for 4.4.0.dev0
# DEEP_LEARNING_MODEL_NAME = "a-ware/longformer-QA" # Not working for 4.4.0.dev0
# DEEP_LEARNING_MODEL_NAME = "valhalla/longformer-base-4096-finetuned-squadv1" # Not working for 4.4.0.dev0
# DEEP_LEARNING_MODEL_NAME = "allenai/longformer-base-4096" # Not working for 4.4.0.dev0
# DEEP_LEARNING_MODEL_NAME = "deepset/roberta-base-squad2" # Working for 4.4.0.dev0
# DEEP_LEARNING_MODEL_NAME = "mrm8488/bert-base-portuguese-cased-finetuned-squad-v1-pt" # Working for 4.4.0.dev0
tokenizer = transformers.AutoTokenizer.from_pretrained(DEEP_LEARNING_MODEL_NAME)
```
## Reference Colab notebook:
https://colab.research.google.com/drive/1v10E77og3-7B2_aFfYhrHvzBZzRlo7wo#scrollTo=2zHj2lMsFuv3
## Further Information:
- This issue started appearing today. It **was working fine till yesterday.**
- This **issue is only with 4.4.0** dev version. This issue **does not** occur for pip install transformers (which is currently on version **4.3.3**)
- The issue is only while loading tokenizers, not models
- The issue is only while loading longformers (any longformer model). Other models' tokenizers are loaded correctly (for example 'deepset/roberta-base-squad2' tokenizer can be loaded correctly) | 03-11-2021 07:42:06 | 03-11-2021 07:42:06 | Indeed, I can reproduce and traced it back to https://github.com/huggingface/transformers/issues/10624. Investigating!<|||||>Found the issue, opening a PR shortly.<|||||>It should now be fixed on `master`. Thanks a lot for using the `master` branch and letting us know of the issue! |
transformers | 10,641 | closed | Unable to reduce time in summarization! | ## Environment info
<!-- You can run the command `transformers-cli env` and copy-and-paste its output below.
Don't forget to fill out the missing fields in that output! -->
- `transformers` version: `t5-large`
- Platform: Amazon Sagemaker
- Python version: 3.7
- Tensorflow version (GPU?):2.3
- Using distributed or parallel set-up in script?: Unable to implement it properly.
### Who can help
@LysandreJik, @patil-suraj, @jplu
### Problem:
Unable to reduce the summarization time.
The tasks I am working on is:
I am using pretrained transformer of T5(`TFT5ForConditionalGeneration`) for text summarization.
Brief script:
```
inputs = tokenizer("summarize: " + text, return_tensors="tf").input_ids
outputs = model.generate(
inputs,
max_length=200,
min_length=5,
num_beams=5,)
```
I tried to use distributed strategy of tensorflow. But it doesn't made any improvement.
```
strategy = tf.distribute.MirroredStrategy()
strategy = tf.distribute.MirroredStrategy(devices=["/gpu:0", "/gpu:1"])
```
## Expected behavior
I am hoping that if we increase the number of **GPU**, time must be reduced. But It is not happening in this case.
| 03-11-2021 07:33:09 | 03-11-2021 07:33:09 | Hello!
The generation part of TensorFlow can only be run on eagermode, hence doesn't matter how you execute it, you will not be able to run it "fast". It is planned to bring a graph execution for the generation, but no ETA yet. Sorry for the inconvenience. |
transformers | 10,640 | closed | Nonetype when using deepspeed | ## Environment info
<!-- You can run the command `transformers-cli env` and copy-and-paste its output below.
Don't forget to fill out the missing fields in that output! -->
- `transformers` version: 4.3.3
- Platform: linux
- Python version: 3.7
- PyTorch version (GPU?): 1.8
- Tensorflow version (GPU?): -
- Using GPU in script?: -
- Using distributed or parallel set-up in script?: -
### Who can help
<!-- Your issue will be replied to more quickly if you can figure out the right person to tag with @
If you know how to use git blame, that is the easiest way, otherwise, here is a rough guide of **who to tag**.
Please tag fewer than 3 people.
Models:
- albert, bert, xlm: @LysandreJik
- blenderbot, bart, marian, pegasus, encoderdecoder, t5: @patrickvonplaten, @patil-suraj
- longformer, reformer, transfoxl, xlnet: @patrickvonplaten
- fsmt: @stas00
- funnel: @sgugger
- gpt2: @patrickvonplaten, @LysandreJik
- rag: @patrickvonplaten, @lhoestq
- tensorflow: @jplu
Library:
- benchmarks: @patrickvonplaten
- deepspeed: @stas00
- ray/raytune: @richardliaw, @amogkam
- text generation: @patrickvonplaten
- tokenizers: @LysandreJik
- trainer: @sgugger
- pipelines: @LysandreJik
Documentation: @sgugger
HF projects:
- nlp datasets: [different repo](https://github.com/huggingface/nlp)
- rust tokenizers: [different repo](https://github.com/huggingface/tokenizers)
Examples:
- maintained examples (not research project or legacy): @sgugger, @patil-suraj
- research_projects/bert-loses-patience: @JetRunner
- research_projects/distillation: @VictorSanh
-->
deepspeed: @stas00
## Information
Hi there,
I am training run_mlm.py with wikipedia datasets with deepspeed, and for some datasets, I am getting this error below:
Do you have an idea why this might happen with deepspeed? looks like not to be a memory issue rather a None bug occuring:
```
File "run_mlm.py", line 525, in <module>
main()
File "run_mlm.py", line 491, in main
train_result = trainer.train(resume_from_checkpoint=checkpoint)
File "/user/diba/libs/anaconda3/envs/transformer/lib/python3.7/site-packages/transformers/trainer.py", line 968, in train
self.deepspeed.step()
File "/user/diba/libs/anaconda3/envs/transformer/lib/python3.7/site-packages/deepspeed/runtime/engine.py", line 959, in step
self._take_model_step(lr_kwargs)
File "/user/diba/libs/anaconda3/envs/transformer/lib/python3.7/site-packages/deepspeed/runtime/engine.py", line 914, in _take_model_step
self.optimizer.step()
File "/user/diba/libs/anaconda3/envs/transformer/lib/python3.7/site-packages/deepspeed/runtime/zero/stage2.py", line 1425, in step
self.optimizer.step()
File "/user/diba/libs/anaconda3/envs/transformer/lib/python3.7/site-packages/torch/optim/optimizer.py", line 89, in wrapper
return func(*args, **kwargs)
File "/user/diba/libs/anaconda3/envs/transformer/lib/python3.7/site-packages/torch/autograd/grad_mode.py", line 27, in decorate_context
return func(*args, **kwargs)
File "/user/diba/libs/anaconda3/envs/transformer/lib/python3.7/site-packages/torch/optim/adamw.py", line 121, in step
group['eps'])
File "/user/diba/libs/anaconda3/envs/transformer/lib/python3.7/site-packages/torch/optim/_functional.py", line 136, in adamw
denom = (exp_avg_sq.sqrt() / math.sqrt(bias_correction2)).add_(eps)
RuntimeError: [enforce fail at CPUAllocator.cpp:67] . DefaultCPUAllocator: can't allocate memory: you tried to allocate 2329531392 bytes. Error code 12 (Cannot allocate memory)
Exception ignored in: <function tqdm.__del__ at 0x7f9b52ef4440>
Traceback (most recent call last):
File "/user/diba/libs/anaconda3/envs/transformer/lib/python3.7/site-packages/tqdm/std.py", line 1090, in __del__
File "/user/diba/libs/anaconda3/envs/transformer/lib/python3.7/site-packages/tqdm/std.py", line 1280, in close
File "/user/diba/libs/anaconda3/envs/transformer/lib/python3.7/site-packages/tqdm/std.py", line 574, in _decr_instances
File "/user/diba/libs/anaconda3/envs/transformer/lib/python3.7/site-packages/tqdm/_monitor.py", line 51, in exit
File "/user/diba/libs/anaconda3/envs/transformer/lib/python3.7/threading.py", line 522, in set
File "/user/diba/libs/anaconda3/envs/transformer/lib/python3.7/threading.py", line 365, in notify_all
File "/user/diba/libs/anaconda3/envs/transformer/lib/python3.7/threading.py", line 348, in notify
TypeError: 'NoneType' object is not callable
```
## To reproduce
Sorry a bit hard to reproduce, I have modified a bit data collator of language modeling to let it do the T5 pretraining, which is currently not added in huggingface repo. If you could give me some advice from the error, it is greatly appreciated
| 03-11-2021 06:49:59 | 03-11-2021 06:49:59 | Hi! I think the error you're looking for is actually a bit above to the error you're mentioning, namely:
```
RuntimeError: [enforce fail at CPUAllocator.cpp:67] . DefaultCPUAllocator: can't allocate memory: you tried to allocate 2329531392 bytes. Error code 12 (Cannot allocate memory)
```
which would indicate a memory issue!<|||||>What @LysandreJik said - check if you're close to using up all your gpu memory?
You can try to reduce `allgather_bucket_size` and `reduce_bucket_size` sizes,
https://huggingface.co/transformers/main_classes/trainer.html#zero
and see if it solves the problem.
In general if you see the traceback happening inside deepspeed most likely you will want to file an Issue at https://github.com/microsoft/DeepSpeed/ - Deepspeed is pretty much an independent engine.<|||||>This issue has been automatically marked as stale because it has not had recent activity. If you think this still needs to be addressed please comment on this thread.
Please note that issues that do not follow the [contributing guidelines](https://github.com/huggingface/transformers/blob/master/CONTRIBUTING.md) are likely to be ignored. |
transformers | 10,639 | closed | Support Quantization Aware Fine-tuning in all models (pytorch) | # 🚀 Feature request
Pytorch supports mimicking quantization errors while training the models.
Here is the [tutorial](https://pytorch.org/tutorials/recipes/quantization.html#quantization-aware-training) on this. For our NLP transformers, it requires a "fake quantization" operation to be done on the embeddings. I found this [repository](https://github.com/IntelLabs/nlp-architect/blob/0f00215dcaf81f8a9b296035834310d77015f085/nlp_architect/models/transformers/quantized_bert.py) converting BERT to support this.
## Motivation
I think quantization aware fine-tuning (if it works) will help a lot of use-cases where dynamic quantization alone doesn't suffice in maintaining the performance of the quantized model. Supporting it out of the box will remove the duplication of model code in end use cases.
## Your contribution
I can work on this ASAP. Would appreciate initial thoughts on what a the MVP for it would be, any thoughts on the API (should we take in a "qat" boolean in config?), any pitfalls that I should be aware of, etc. | 03-11-2021 06:03:47 | 03-11-2021 06:03:47 | Hello! Would [I-BERT](https://huggingface.co/transformers/master/model_doc/ibert.html), available on `master` and contributed by @kssteven418 be of interest?<|||||>@LysandreJik, Thanks for the useful reference. I guess the i-BERT model has manually implemented the architectural components (kernels, int8 layer norm etc) to make quantization work for BERT. If I am not wrong, their objective is to train BERT as much as possible in int8. The qat in torch takes the approach of training model in floating point fully but incorporating noise in gradients that mimic noise due to quantization. So it's basically throwing the "optimizing for quantization error" part to gradient descent, foregoing any need for altering architectures or fp32/16 training regime.
This approach would be broader and apply for all the architectures without re-implementation. Maybe we can have a "qat" flag in config, that can be used to perform fake quantization and dequantization (which introduces quantization noise to parts of the gradients).<|||||>Do you have an idea of the changes required for that? Could you do PoC and show us so that we can discuss over it?<|||||>@LysandreJik Can you take a look at this [implementation](https://github.com/IntelLabs/nlp-architect/blob/0f00215dcaf81f8a9b296035834310d77015f085/nlp_architect/models/transformers/quantized_bert.py). It's a functioning qat aware BERT fine-tuning implementation. The process is described in this paper, [Q8BERT: Quantized 8Bit BERT](https://arxiv.org/abs/1910.06188).<|||||>This issue has been automatically marked as stale because it has not had recent activity. If you think this still needs to be addressed please comment on this thread.
Please note that issues that do not follow the [contributing guidelines](https://github.com/huggingface/transformers/blob/master/CONTRIBUTING.md) are likely to be ignored.<|||||>This is a feature I'd like to see as well, as dynamic quantization leads to a huge accuracy drop in my use case. My understanding is that a possible implementation of QAT could also easily be expanded to support static quantization.<|||||>@sai-prasanna is it possible to load Bert-base (FP32 model) weights into Q8Bert ? |
transformers | 10,638 | closed | Fix decoding score comparison when using logits processors or warpers | When doing beam search or other decoding search strategies, the logit scores are normalized (with `log_softmax`) so the comparisons between the beams (hypotheses) are meaningful. However, the logit processors or warpers may change the scores, and thus may not be normalized anymore.
For example, say you have a beam size of 2. During beam search at some point, beam A is better than B (higher score). You use `prefix_allowed_tokens_fn`, which in turn through a logit processor narrows down the options of the next tokens to only one. Then masks out all tokens with `-inf` but one. The score vector may look like `[-inf, ..., -2.13, ..., -inf]`. This is output and now the scores are not normalized anymore. This filter is not applied to B. Now beam search selects B, which actually keeping the hypothesis A meant having the same probability since the normalized vector should have been `[-inf, ..., 0, ..., -inf]`. In that case, hypothesis A would have been kept (and that's what actually should happen). This erroneous behavior can happen with any logit processor that doesn't normalize its output, which I see it's often the case.
So that's why I moved the `log_softmax` to after the logit processor/warper application. I also checked if any logit processor needed the normalization for its input. It doesn't seem to be the case (though I'm not 100% sure). They can still individually apply a normalization if they need to. Maybe the documentation could be changed, by the way:
https://github.com/huggingface/transformers/blob/26a33cfd8c2d6923f41ab98683f33172e8948ff3/src/transformers/generation_logits_process.py#L37-L39
## Before submitting
- [ ] This PR fixes a typo or improves the docs (you can dismiss the other checks if that's the case).
- [x] Did you read the [contributor guideline](https://github.com/huggingface/transformers/blob/master/CONTRIBUTING.md#start-contributing-pull-requests),
Pull Request section?
- [ ] Was this discussed/approved via a Github issue or the [forum](https://discuss.huggingface.co/)? Please add a link
to it if that's the case.
- [x] Did you make sure to update the documentation with your changes? Here are the
[documentation guidelines](https://github.com/huggingface/transformers/tree/master/docs), and
[here are tips on formatting docstrings](https://github.com/huggingface/transformers/tree/master/docs#writing-source-documentation).
- [x] Did you write any new necessary tests?
I feel I should tag @patrickvonplaten, @patil-suraj
| 03-11-2021 03:11:23 | 03-11-2021 03:11:23 | The failing test is `test_90_generation_from_short_input`, which generates "have you ever heard of sam harris? he is an american singer and songwriter. have you heard of him?" instead of "have you ever been to a sam club? it's a great club in the south." or "have you ever heard of sam harris? he's an american singer, songwriter, and actor.".
I honestly don't know what's the expected behavior there, so not sure if it's flaky or not. The weird thing is that this test seems to be greedy search, not beam search.<|||||>Actually, I just looked more closely and the failing test does use beam search (the beam size is specified in the config). This is an example of something that changes since it uses a `NoRepeatNGramLogitsProcessor`, a `MinLengthLogitsProcessor`, and a `ForcedEOSTokenLogitsProcessor`.<|||||>This issue has been automatically marked as stale because it has not had recent activity. If you think this still needs to be addressed please comment on this thread.
Please note that issues that do not follow the [contributing guidelines](https://github.com/huggingface/transformers/blob/master/CONTRIBUTING.md) are likely to be ignored.<|||||>I'm gonna address it, it's been in my mind. Please don't mark it as stale!<|||||>I've added the `WIP` label so that the stale bot doesn't close it!<|||||>@patrickvonplaten sorry for the big delay.
I changed the normalization to be a logit warper now. What do you think of it, and its documentation?
Also, what if we set a deprecation for it? And take advantage of some breaking change in the future and make it the default?<|||||>The failing tests are flaky, right?<|||||>Could we add one tests for the new logits processor as well? :-)<|||||>@patrickvonplaten can you remove the WIP label? This should be done.
Also, the latest time a test failed, it seemed to be flaky. It should be good to go :rocket: <|||||>_The documentation is not available anymore as the PR was closed or merged._<|||||>@patrickvonplaten friendly reminder on this!<|||||>Also, should we add a flag in `generate` so this logit processor gets added to the list? Such as `renormalize_logits`.<|||||>PR looks good to go for me - thanks @bryant1410. Yes indeed could you maybe add a flag `renormalize_logits` to `generate()`?<|||||>> PR looks good to go for me - thanks @bryant1410. Yes indeed could you maybe add a flag `renormalize_logits` to `generate()`?
Okay, @patrickvonplaten I did this change.
What do you think about also making `renormalize_logits=True` in the future? So then adding some deprecation or warning that this value is gonna change? Or that it should be set to `False` to keep BC?<|||||>Oh, and btw, note I also applied it to the warpers (so it's applied to both the processors and warpers).<|||||>Should the attribute be added to the configs such that the following can be applied?
```python
renormalize_logits if renormalize_logits is not None else self.config.renormalize_logits
```<|||||>> Should the attribute be added to the configs such that the following can be applied?
>
> ```python
> renormalize_logits if renormalize_logits is not None else self.config.renormalize_logits
> ```
No need for this I think since it's quite a specific logit processor<|||||>@bryant1410, could you also update RAG's generate method to incorporate you changes? The test currently fails with
```TypeError: _get_logits_processor() missing 1 required positional argument: 'renormalize_logits'```
It should be easy to adapt here: https://github.com/huggingface/transformers/blob/febe42b5daf4b416f4613e9d7f68617ee983bb40/src/transformers/models/rag/modeling_rag.py#L1608<|||||>> @bryant1410, could you also update RAG's generate method to incorporate you changes? The test currently fails with `TypeError: _get_logits_processor() missing 1 required positional argument: 'renormalize_logits'`
>
> It should be easy to adapt here:
>
> https://github.com/huggingface/transformers/blob/febe42b5daf4b416f4613e9d7f68617ee983bb40/src/transformers/models/rag/modeling_rag.py#L1608
Done. What about this?
> What do you think about also making `renormalize_logits=True` in the future? So then adding some deprecation or warning that this value is gonna change? Or that it should be set to `False` to keep BC?<|||||>Good for merge for me! Let's see what @gante says <|||||>> Good for merge for me! Let's see what @gante says
Okay! What about the comment/idea on making it `renormalize_logits=True` in the future? So then adding some deprecation or warning that this value is gonna change?<|||||>> > Good for merge for me! Let's see what @gante says
>
> Okay! What about the comment/idea on making it `renormalize_logits=True` in the future? So then adding some deprecation or warning that this value is gonna change?
Don't really think that's possible due to backwards breaking changes tbh<|||||>> Don't really think that's possible due to backwards breaking changes tbh
I understand. However, eventually, the breaking change is gonna happen because of some accumulated "debt" that gets big enough, after many different fixes or wanted features. Like it happens in other libraries. It could happen after some major version change (e.g., v5), which it's a great opportunity to change a lot of desired changes that are breaking.
One approach to track this is to deprecate the value and say when it's gonna be changed (e.g., v5). It could be with a warning, some comment in the docstring, or maybe just a doc that tracks down which is gonna be changed. I guess what I'm saying is to add this change to that list (is it worth it, in your opinion?). BTW, do you have in this repo such a list of things that are eventually gonna be changed (maybe implicitly tracked in various comments)?
What are your thoughts? Maybe you think differently?<|||||>> To ensure this change stays future-proof, I'd like to discuss an additional change. The new logit processor, when it exists in the list of logit processors to be applied, must be the last one. Should we raise an exception when it isn't? (e.g. it has to be the last one in [this list](https://github.com/huggingface/transformers/blob/main/src/transformers/generation_logits_process.py#L82), when it exists) cc @patrickvonplaten
Makes sense to me. However, what if the user wants to do something custom, by manually adding this processor logit somewhere? If we add a check and an exception, then the user would face it in this custom scenario. Or maybe it's a bit far-fetched?<|||||>> Makes sense to me. However, what if the user wants to do something custom, by manually adding this processor logit somewhere? If we add a check and an exception, then the user would face it in this custom scenario. Or maybe it's a bit far-fetched?
Uhmm I see. We can go with the low effort, low cost, and low consequence alternative (see the following suggestion)<|||||>@bryant1410 regarding the `renormalize_logits` default value, I've added it to a v5 wishlist, to discuss internally when we decide to do the next major change :)
Since there are no other outstanding requests and CI is green, I'm merging the PR 💪 |
transformers | 10,637 | closed | Remove special treatment for custom vocab files | # What does this PR do?
This PR follows up from #10624 and removes the ability to specify a custom vocab file that doesn't lie in the model repo for a tokenizer (which prevents using the versioning system for those tokenizers).
It also cleans up a tiny bit the `from_pretrained` method, mainly:
- use f-strings instead of `.format()`
- remove double try since you can have several except in cascade
- use a FutureWarning for a deprecation warning that was just sent to the logs and set an end date | 03-10-2021 20:49:18 | 03-10-2021 20:49:18 | |
transformers | 10,636 | closed | Layout lm tf 2 | # What does this PR do?
<!--
Congratulations! You've made it this far! You're not quite done yet though.
Once merged, your PR is going to appear in the release notes with the title you set, so make sure it's a great title that fully reflects the extent of your awesome contribution.
Then, please replace this with a description of the change and which issue is fixed (if applicable). Please also include relevant motivation and context. List any dependencies (if any) that are required for this change.
Once you're done, someone will review your PR shortly (see the section "Who can review?" below to tag some potential reviewers). They may suggest changes to make the code even better. If no one reviewed your PR after a week has passed, don't hesitate to post a new comment @-mentioning the same persons---sometimes notifications get lost.
-->
<!-- Remove if not applicable -->
This PR adds TF version of LayoutLM for issue [(10312)](https://github.com/huggingface/transformers/issues/10312)
## Before submitting
- [ ] This PR fixes a typo or improves the docs (you can dismiss the other checks if that's the case).
- [x] Did you read the [contributor guideline](https://github.com/huggingface/transformers/blob/master/CONTRIBUTING.md#start-contributing-pull-requests),
Pull Request section?
- [x] Was this discussed/approved via a Github issue or the [forum](https://discuss.huggingface.co/)? Please add a link
to it if that's the case.
- [x] Did you make sure to update the documentation with your changes? Here are the
[documentation guidelines](https://github.com/huggingface/transformers/tree/master/docs), and
[here are tips on formatting docstrings](https://github.com/huggingface/transformers/tree/master/docs#writing-source-documentation).
- [x] Did you write any new necessary tests?
## Who can review?
Anyone in the community is free to review the PR once the tests have passed. Feel free to tag
members/contributors which may be interested in your PR.
<!-- Your PR will be replied to more quickly if you can figure out the right person to tag with @
If you know how to use git blame, that is the easiest way, otherwise, here is a rough guide of **who to tag**.
Please tag fewer than 3 people.
Models:
- albert, bert, xlm: @LysandreJik
- blenderbot, bart, marian, pegasus, encoderdecoder, t5: @patrickvonplaten, @patil-suraj
- longformer, reformer, transfoxl, xlnet: @patrickvonplaten
- fsmt: @stas00
- funnel: @sgugger
- gpt2: @patrickvonplaten, @LysandreJik
- rag: @patrickvonplaten, @lhoestq
- tensorflow: @jplu
Library:
- benchmarks: @patrickvonplaten
- deepspeed: @stas00
- ray/raytune: @richardliaw, @amogkam
- text generation: @patrickvonplaten
- tokenizers: @n1t0, @LysandreJik
- trainer: @sgugger
- pipelines: @LysandreJik
Documentation: @sgugger
HF projects:
- nlp datasets: [different repo](https://github.com/huggingface/nlp)
- rust tokenizers: [different repo](https://github.com/huggingface/tokenizers)
Examples:
- maintained examples (not research project or legacy): @sgugger, @patil-suraj
- research_projects/bert-loses-patience: @JetRunner
- research_projects/distillation: @VictorSanh
-->
| 03-10-2021 19:50:58 | 03-10-2021 19:50:58 | @LysandreJik Thanks to you all tests passed! It's ready for an in depth review.
I've already uploaded TF2 model files under:
- https://huggingface.co/atahmasb/tf-layoutlm-base-uncased
- https://huggingface.co/atahmasb/tf-layoutlm-large-uncased
would you please copy them to the main repos when the code is merged?
Also, it seems like I can't add you or others from the team as reviewers<|||||>> I think this is starting to look great! Fantastic that you've added all models.
>
> Could you add an additional integration test, to ensure that the current implementation doesn't diverge?
>
> Something like what is done in the PT version of LayoutLM:
>
> https://github.com/huggingface/transformers/blob/8715d20c97b3975c1d89cf0c0cca45af91badd1d/tests/test_modeling_layoutlm.py#L262-L283
>
> I'm asking others to review.
sure, will do<|||||>> Thanks a lot for adding this model! There is one last problem with the examples in the docstrings (we can't use the base ones since we need to provide bounding boxes), otherwise it's good to be merged!
Thanks, will fix it.<|||||>> I think this is starting to look great! Fantastic that you've added all models.
>
> Could you add an additional integration test, to ensure that the current implementation doesn't diverge?
>
> Something like what is done in the PT version of LayoutLM:
>
> https://github.com/huggingface/transformers/blob/8715d20c97b3975c1d89cf0c0cca45af91badd1d/tests/test_modeling_layoutlm.py#L262-L283
>
> I'm asking others to review.
for the tf layoutlm integration tests to pass on CI, the tf model files should live under `microsoft/tayoutlm-base-uncased` or I have to use their location under my account in the model registry which is `atahmasb/tf-layoutlm-base-uncased`. Do you want me to use the temp location under my account for now?<|||||>Yes sure let's use a temporary reference for now and update it right before we merge.<|||||>@LysandreJik I cleaned up the initialisation file, the conflicts are resolved now! Anything else before it can be merged?<|||||>Cool! I just moved the weights to the microsoft organization. Could you update the links/checkpoint identifiers in your PR and test that it has the expected behavior? Thanks!<|||||>> Cool! I just moved the weights to the microsoft organization. Could you update the links/checkpoint identifiers in your PR and test that it has the expected behavior? Thanks!
it's done! for some reasons one of the tests that has nothing to do with my code failed on CI this time. do you have any idea why? Also it seems like i can't re run the tests on CI without making a change in the code
```
FAILED tests/test_modeling_prophetnet.py::ProphetNetModelTest::test_attn_mask_model
=== 1 failed, 5133 passed, 2140 skipped, 2209 warnings in 282.78s (0:04:42) ====
```<|||||>> Yes, this is a flaky test that's under the process of being fixed so you needn't worry about it.
>
> This looks good to me, thanks for your work @atahmasb!!
Thanks for your help along the way!<|||||>> There are a few things left to fix, then this should be good to merge!
Thanks for catching those! They are fixed [here](https://github.com/huggingface/transformers/pull/10636/commits/3bee70daf2a71066335d360761ce5c7bb432500a) |
transformers | 10,635 | closed | Document Trainer limitation on custom models | # What does this PR do?
As discussed in #10629, documenting the limitations of the `Trainer` when working with custom models. | 03-10-2021 19:37:31 | 03-10-2021 19:37:31 | |
transformers | 10,634 | closed | Issues with Multi-GPU | - `transformers` version: 4.3.3
- Platform: Linux-4.15.0-132-generic-x86_64-with-Ubuntu-18.04-bionic
- Python version: 3.6.9
- PyTorch version (GPU?): 1.8.0 (True)
- Tensorflow version (GPU?): 2.3.0 (True)
- Using GPU in script?: Yes, multi GeForce RTX 2080 Ti GPUs
- Using distributed or parallel set-up in script?: DataParallel
- NVIDIA-SMI 440.33.01 Driver Version: 440.33.01 CUDA Version: 10.2
I have tried to run the IMDb review sequence classification from https://huggingface.co/transformers/custom_datasets.html on two GPUs, using `DataParallel`:
```
import os
os.environ["CUDA_VISIBLE_DEVICES"]="6,7"
import time
import torch
import torch.nn as nn
from pathlib import Path
from sklearn.model_selection import train_test_split
from transformers import DistilBertTokenizerFast
from transformers import DistilBertForSequenceClassification, Trainer, TrainingArguments
def read_imdb_split(split_dir):
split_dir = Path(split_dir)
texts = []
labels = []
for label_dir in ["pos", "neg"]:
for text_file in (split_dir/label_dir).iterdir():
texts.append(text_file.read_text())
labels.append(0 if label_dir is "neg" else 1)
return texts, labels
class IMDbDataset(torch.utils.data.Dataset):
def __init__(self, encodings, labels):
self.encodings = encodings
self.labels = labels
def __getitem__(self, idx):
item = {key: torch.tensor(val[idx]) for key, val in self.encodings.items()}
item['labels'] = torch.tensor(self.labels[idx])
return item
def __len__(self):
return len(self.labels)
device = torch.device("cuda" if torch.cuda.is_available() else "cpu")
n_gpus = torch.cuda.device_count()
train_texts, train_labels = read_imdb_split('aclImdb/train')
test_texts, test_labels = read_imdb_split('aclImdb/test')
train_texts, val_texts, train_labels, val_labels = train_test_split(train_texts, train_labels, test_size=.2)
tokenizer = DistilBertTokenizerFast.from_pretrained('distilbert-base-uncased')
train_encodings = tokenizer(train_texts, truncation=True, padding=True)
val_encodings = tokenizer(val_texts, truncation=True, padding=True)
test_encodings = tokenizer(test_texts, truncation=True, padding=True)
train_dataset = IMDbDataset(train_encodings, train_labels)
val_dataset = IMDbDataset(val_encodings, val_labels)
test_dataset = IMDbDataset(test_encodings, test_labels)
training_args = TrainingArguments(
output_dir='./results', # output directory
num_train_epochs=3, # total number of training epochs
per_device_train_batch_size=16, # batch size per device during training
per_device_eval_batch_size=64, # batch size for evaluation
warmup_steps=500, # number of warmup steps for learning rate scheduler
weight_decay=0.01, # strength of weight decay
logging_dir='./logs', # directory for storing logs
logging_steps=10,
)
model = DistilBertForSequenceClassification.from_pretrained("distilbert-base-uncased")
if n_gpus > 1:
model = nn.DataParallel(model)
model.to(device)
trainer = Trainer(
model=model, # the instantiated 🤗 Transformers model to be trained
args=training_args, # training arguments, defined above
train_dataset=train_dataset, # training dataset
eval_dataset=val_dataset # evaluation dataset
)
trainer.train()
```
For **torch==1.8.0**, no matter I use single GPU or multi GPU, I encounter the same CUDA error (shown below). For **torch==1.7.1**, I am able to run the code on single GPU with no issue. However, with multi-GPU, the `Input, output and indices must be on the current device` error occurs (also shown below).
With **torch==1.8.0**:
```
2021-03-10 19:16:07.624155: I tensorflow/stream_executor/platform/default/dso_loader.cc:48] Successfully opened dynamic library libcudart.so.10.1
Some weights of the model checkpoint at distilbert-base-uncased were not used when initializing DistilBertForSequenceClassification: ['vocab_transform.weight', 'vocab_transform.bias', 'vocab_layer_norm.weight', 'vocab_layer_norm.bias', 'vocab_projector.weight', 'vocab_projector.bias']
- This IS expected if you are initializing DistilBertForSequenceClassification from the checkpoint of a model trained on another task or with another architecture (e.g. initializing a BertForSequenceClassification model from a BertForPreTraining model).
- This IS NOT expected if you are initializing DistilBertForSequenceClassification from the checkpoint of a model that you expect to be exactly identical (initializing a BertForSequenceClassification model from a BertForSequenceClassification model).
Some weights of DistilBertForSequenceClassification were not initialized from the model checkpoint at distilbert-base-uncased and are newly initialized: ['pre_classifier.weight', 'pre_classifier.bias', 'classifier.weight', 'classifier.bias']
You should probably TRAIN this model on a down-stream task to be able to use it for predictions and inference.
0%| | 0/1875 [00:00<?, ?it/s]Traceback (most recent call last):
File "test.py", line 80, in <module>
trainer.train()
File "/mnt/sdb/env1/lib/python3.6/site-packages/transformers/trainer.py", line 940, in train
tr_loss += self.training_step(model, inputs)
File "/mnt/sdb/env1/lib/python3.6/site-packages/transformers/trainer.py", line 1304, in training_step
loss = self.compute_loss(model, inputs)
File "/mnt/sdb/env1/lib/python3.6/site-packages/transformers/trainer.py", line 1334, in compute_loss
outputs = model(**inputs)
File "/mnt/sdb/env1/lib/python3.6/site-packages/torch/nn/modules/module.py", line 889, in _call_impl
result = self.forward(*input, **kwargs)
File "/mnt/sdb/env1/lib/python3.6/site-packages/torch/nn/parallel/data_parallel.py", line 167, in forward
outputs = self.parallel_apply(replicas, inputs, kwargs)
File "/mnt/sdb/env1/lib/python3.6/site-packages/torch/nn/parallel/data_parallel.py", line 177, in parallel_apply
return parallel_apply(replicas, inputs, kwargs, self.device_ids[:len(replicas)])
File "/mnt/sdb/env1/lib/python3.6/site-packages/torch/nn/parallel/parallel_apply.py", line 86, in parallel_apply
output.reraise()
File "/mnt/sdb/env1/lib/python3.6/site-packages/torch/_utils.py", line 429, in reraise
raise self.exc_type(msg)
RuntimeError: Caught RuntimeError in replica 0 on device 0.
Original Traceback (most recent call last):
File "/mnt/sdb/env1/lib/python3.6/site-packages/torch/nn/parallel/parallel_apply.py", line 61, in _worker
output = module(*input, **kwargs)
File "/mnt/sdb/env1/lib/python3.6/site-packages/torch/nn/modules/module.py", line 889, in _call_impl
result = self.forward(*input, **kwargs)
File "/mnt/sdb/env1/lib/python3.6/site-packages/torch/nn/parallel/data_parallel.py", line 167, in forward
outputs = self.parallel_apply(replicas, inputs, kwargs)
File "/mnt/sdb/env1/lib/python3.6/site-packages/torch/nn/parallel/data_parallel.py", line 177, in parallel_apply
return parallel_apply(replicas, inputs, kwargs, self.device_ids[:len(replicas)])
File "/mnt/sdb/env1/lib/python3.6/site-packages/torch/nn/parallel/parallel_apply.py", line 86, in parallel_apply
output.reraise()
File "/mnt/sdb/env1/lib/python3.6/site-packages/torch/_utils.py", line 429, in reraise
raise self.exc_type(msg)
RuntimeError: Caught RuntimeError in replica 0 on device 0.
Original Traceback (most recent call last):
File "/mnt/sdb/env1/lib/python3.6/site-packages/torch/nn/parallel/parallel_apply.py", line 61, in _worker
output = module(*input, **kwargs)
File "/mnt/sdb/env1/lib/python3.6/site-packages/torch/nn/modules/module.py", line 889, in _call_impl
result = self.forward(*input, **kwargs)
File "/mnt/sdb/env1/lib/python3.6/site-packages/transformers/models/distilbert/modeling_distilbert.py", line 623, in forward
return_dict=return_dict,
File "/mnt/sdb/env1/lib/python3.6/site-packages/torch/nn/modules/module.py", line 889, in _call_impl
result = self.forward(*input, **kwargs)
File "/mnt/sdb/env1/lib/python3.6/site-packages/transformers/models/distilbert/modeling_distilbert.py", line 487, in forward
return_dict=return_dict,
File "/mnt/sdb/env1/lib/python3.6/site-packages/torch/nn/modules/module.py", line 889, in _call_impl
result = self.forward(*input, **kwargs)
File "/mnt/sdb/env1/lib/python3.6/site-packages/transformers/models/distilbert/modeling_distilbert.py", line 309, in forward
x=hidden_state, attn_mask=attn_mask, head_mask=head_mask[i], output_attentions=output_attentions
File "/mnt/sdb/env1/lib/python3.6/site-packages/torch/nn/modules/module.py", line 889, in _call_impl
result = self.forward(*input, **kwargs)
File "/mnt/sdb/env1/lib/python3.6/site-packages/transformers/models/distilbert/modeling_distilbert.py", line 256, in forward
output_attentions=output_attentions,
File "/mnt/sdb/env1/lib/python3.6/site-packages/torch/nn/modules/module.py", line 889, in _call_impl
result = self.forward(*input, **kwargs)
File "/mnt/sdb/env1/lib/python3.6/site-packages/transformers/models/distilbert/modeling_distilbert.py", line 177, in forward
q = shape(self.q_lin(query)) # (bs, n_heads, q_length, dim_per_head)
File "/mnt/sdb/env1/lib/python3.6/site-packages/torch/nn/modules/module.py", line 889, in _call_impl
result = self.forward(*input, **kwargs)
File "/mnt/sdb/env1/lib/python3.6/site-packages/torch/nn/modules/linear.py", line 94, in forward
return F.linear(input, self.weight, self.bias)
File "/mnt/sdb/env1/lib/python3.6/site-packages/torch/nn/functional.py", line 1753, in linear
return torch._C._nn.linear(input, weight, bias)
RuntimeError: CUDA error: CUBLAS_STATUS_INTERNAL_ERROR when calling `cublasCreate(handle)`
```
With **torch==1.7.1**:
```
2021-03-10 19:22:14.938302: I tensorflow/stream_executor/platform/default/dso_loader.cc:48] Successfully opened dynamic library libcudart.so.10.1
Some weights of the model checkpoint at distilbert-base-uncased were not used when initializing DistilBertForSequenceClassification: ['vocab_transform.weight', 'vocab_transform.bias', 'vocab_layer_norm.weight', 'vocab_layer_norm.bias', 'vocab_projector.weight', 'vocab_projector.bias']
- This IS expected if you are initializing DistilBertForSequenceClassification from the checkpoint of a model trained on another task or with another architecture (e.g. initializing a BertForSequenceClassification model from a BertForPreTraining model).
- This IS NOT expected if you are initializing DistilBertForSequenceClassification from the checkpoint of a model that you expect to be exactly identical (initializing a BertForSequenceClassification model from a BertForSequenceClassification model).
Some weights of DistilBertForSequenceClassification were not initialized from the model checkpoint at distilbert-base-uncased and are newly initialized: ['pre_classifier.weight', 'pre_classifier.bias', 'classifier.weight', 'classifier.bias']
You should probably TRAIN this model on a down-stream task to be able to use it for predictions and inference.
0%| | 0/1875 [00:00<?, ?it/s]Traceback (most recent call last):
File "test.py", line 80, in <module>
trainer.train()
File "/mnt/sdb/env1/lib/python3.6/site-packages/transformers/trainer.py", line 940, in train
tr_loss += self.training_step(model, inputs)
File "/mnt/sdb/env1/lib/python3.6/site-packages/transformers/trainer.py", line 1304, in training_step
loss = self.compute_loss(model, inputs)
File "/mnt/sdb/env1/lib/python3.6/site-packages/transformers/trainer.py", line 1334, in compute_loss
outputs = model(**inputs)
File "/mnt/sdb/env1/lib/python3.6/site-packages/torch/nn/modules/module.py", line 727, in _call_impl
result = self.forward(*input, **kwargs)
File "/mnt/sdb/env1/lib/python3.6/site-packages/torch/nn/parallel/data_parallel.py", line 161, in forward
outputs = self.parallel_apply(replicas, inputs, kwargs)
File "/mnt/sdb/env1/lib/python3.6/site-packages/torch/nn/parallel/data_parallel.py", line 171, in parallel_apply
return parallel_apply(replicas, inputs, kwargs, self.device_ids[:len(replicas)])
File "/mnt/sdb/env1/lib/python3.6/site-packages/torch/nn/parallel/parallel_apply.py", line 86, in parallel_apply
output.reraise()
File "/mnt/sdb/env1/lib/python3.6/site-packages/torch/_utils.py", line 428, in reraise
raise self.exc_type(msg)
RuntimeError: Caught RuntimeError in replica 0 on device 0.
Original Traceback (most recent call last):
File "/mnt/sdb/env1/lib/python3.6/site-packages/torch/nn/parallel/parallel_apply.py", line 61, in _worker
output = module(*input, **kwargs)
File "/mnt/sdb/env1/lib/python3.6/site-packages/torch/nn/modules/module.py", line 727, in _call_impl
result = self.forward(*input, **kwargs)
File "/mnt/sdb/env1/lib/python3.6/site-packages/torch/nn/parallel/data_parallel.py", line 161, in forward
outputs = self.parallel_apply(replicas, inputs, kwargs)
File "/mnt/sdb/env1/lib/python3.6/site-packages/torch/nn/parallel/data_parallel.py", line 171, in parallel_apply
return parallel_apply(replicas, inputs, kwargs, self.device_ids[:len(replicas)])
File "/mnt/sdb/env1/lib/python3.6/site-packages/torch/nn/parallel/parallel_apply.py", line 86, in parallel_apply
output.reraise()
File "/mnt/sdb/env1/lib/python3.6/site-packages/torch/_utils.py", line 428, in reraise
raise self.exc_type(msg)
RuntimeError: Caught RuntimeError in replica 1 on device 1.
Original Traceback (most recent call last):
File "/mnt/sdb/env1/lib/python3.6/site-packages/torch/nn/parallel/parallel_apply.py", line 61, in _worker
output = module(*input, **kwargs)
File "/mnt/sdb/env1/lib/python3.6/site-packages/torch/nn/modules/module.py", line 727, in _call_impl
result = self.forward(*input, **kwargs)
File "/mnt/sdb/env1/lib/python3.6/site-packages/transformers/models/distilbert/modeling_distilbert.py", line 623, in forward
return_dict=return_dict,
File "/mnt/sdb/env1/lib/python3.6/site-packages/torch/nn/modules/module.py", line 727, in _call_impl
result = self.forward(*input, **kwargs)
File "/mnt/sdb/env1/lib/python3.6/site-packages/transformers/models/distilbert/modeling_distilbert.py", line 480, in forward
inputs_embeds = self.embeddings(input_ids) # (bs, seq_length, dim)
File "/mnt/sdb/env1/lib/python3.6/site-packages/torch/nn/modules/module.py", line 727, in _call_impl
result = self.forward(*input, **kwargs)
File "/mnt/sdb/env1/lib/python3.6/site-packages/transformers/models/distilbert/modeling_distilbert.py", line 107, in forward
word_embeddings = self.word_embeddings(input_ids) # (bs, max_seq_length, dim)
File "/mnt/sdb/env1/lib/python3.6/site-packages/torch/nn/modules/module.py", line 727, in _call_impl
result = self.forward(*input, **kwargs)
File "/mnt/sdb/env1/lib/python3.6/site-packages/torch/nn/modules/sparse.py", line 126, in forward
self.norm_type, self.scale_grad_by_freq, self.sparse)
File "/mnt/sdb/env1/lib/python3.6/site-packages/torch/nn/functional.py", line 1852, in embedding
return torch.embedding(weight, input, padding_idx, scale_grad_by_freq, sparse)
RuntimeError: Input, output and indices must be on the current device
```
With **torch==1.5.0**:
```
2021-03-10 19:23:51.586005: I tensorflow/stream_executor/platform/default/dso_loader.cc:48] Successfully opened dynamic library libcudart.so.10.1
Some weights of the model checkpoint at distilbert-base-uncased were not used when initializing DistilBertForSequenceClassification: ['vocab_transform.weight', 'vocab_transform.bias', 'vocab_layer_norm.weight', 'vocab_layer_norm.bias', 'vocab_projector.weight', 'vocab_projector.bias']
- This IS expected if you are initializing DistilBertForSequenceClassification from the checkpoint of a model trained on another task or with another architecture (e.g. initializing a BertForSequenceClassification model from a BertForPreTraining model).
- This IS NOT expected if you are initializing DistilBertForSequenceClassification from the checkpoint of a model that you expect to be exactly identical (initializing a BertForSequenceClassification model from a BertForSequenceClassification model).
Some weights of DistilBertForSequenceClassification were not initialized from the model checkpoint at distilbert-base-uncased and are newly initialized: ['pre_classifier.weight', 'pre_classifier.bias', 'classifier.weight', 'classifier.bias']
You should probably TRAIN this model on a down-stream task to be able to use it for predictions and inference.
0%| | 0/1875 [00:00<?, ?it/s]Traceback (most recent call last):
File "test.py", line 80, in <module>
trainer.train()
File "/mnt/sdb/env1/lib/python3.6/site-packages/transformers/trainer.py", line 940, in train
tr_loss += self.training_step(model, inputs)
File "/mnt/sdb/env1/lib/python3.6/site-packages/transformers/trainer.py", line 1304, in training_step
loss = self.compute_loss(model, inputs)
File "/mnt/sdb/env1/lib/python3.6/site-packages/transformers/trainer.py", line 1334, in compute_loss
outputs = model(**inputs)
File "/mnt/sdb/env1/lib/python3.6/site-packages/torch/nn/modules/module.py", line 550, in __call__
result = self.forward(*input, **kwargs)
File "/mnt/sdb/env1/lib/python3.6/site-packages/torch/nn/parallel/data_parallel.py", line 155, in forward
outputs = self.parallel_apply(replicas, inputs, kwargs)
File "/mnt/sdb/env1/lib/python3.6/site-packages/torch/nn/parallel/data_parallel.py", line 165, in parallel_apply
return parallel_apply(replicas, inputs, kwargs, self.device_ids[:len(replicas)])
File "/mnt/sdb/env1/lib/python3.6/site-packages/torch/nn/parallel/parallel_apply.py", line 85, in parallel_apply
output.reraise()
File "/mnt/sdb/env1/lib/python3.6/site-packages/torch/_utils.py", line 395, in reraise
raise self.exc_type(msg)
RuntimeError: Caught RuntimeError in replica 0 on device 0.
Original Traceback (most recent call last):
File "/mnt/sdb/env1/lib/python3.6/site-packages/torch/nn/parallel/parallel_apply.py", line 60, in _worker
output = module(*input, **kwargs)
File "/mnt/sdb/env1/lib/python3.6/site-packages/torch/nn/modules/module.py", line 550, in __call__
result = self.forward(*input, **kwargs)
File "/mnt/sdb/env1/lib/python3.6/site-packages/torch/nn/parallel/data_parallel.py", line 155, in forward
outputs = self.parallel_apply(replicas, inputs, kwargs)
File "/mnt/sdb/env1/lib/python3.6/site-packages/torch/nn/parallel/data_parallel.py", line 165, in parallel_apply
return parallel_apply(replicas, inputs, kwargs, self.device_ids[:len(replicas)])
File "/mnt/sdb/env1/lib/python3.6/site-packages/torch/nn/parallel/parallel_apply.py", line 85, in parallel_apply
output.reraise()
File "/mnt/sdb/env1/lib/python3.6/site-packages/torch/_utils.py", line 395, in reraise
raise self.exc_type(msg)
RuntimeError: Caught RuntimeError in replica 1 on device 1.
Original Traceback (most recent call last):
File "/mnt/sdb/env1/lib/python3.6/site-packages/torch/nn/parallel/parallel_apply.py", line 60, in _worker
output = module(*input, **kwargs)
File "/mnt/sdb/env1/lib/python3.6/site-packages/torch/nn/modules/module.py", line 550, in __call__
result = self.forward(*input, **kwargs)
File "/mnt/sdb/env1/lib/python3.6/site-packages/transformers/models/distilbert/modeling_distilbert.py", line 623, in forward
return_dict=return_dict,
File "/mnt/sdb/env1/lib/python3.6/site-packages/torch/nn/modules/module.py", line 550, in __call__
result = self.forward(*input, **kwargs)
File "/mnt/sdb/env1/lib/python3.6/site-packages/transformers/models/distilbert/modeling_distilbert.py", line 480, in forward
inputs_embeds = self.embeddings(input_ids) # (bs, seq_length, dim)
File "/mnt/sdb/env1/lib/python3.6/site-packages/torch/nn/modules/module.py", line 550, in __call__
result = self.forward(*input, **kwargs)
File "/mnt/sdb/env1/lib/python3.6/site-packages/transformers/models/distilbert/modeling_distilbert.py", line 107, in forward
word_embeddings = self.word_embeddings(input_ids) # (bs, max_seq_length, dim)
File "/mnt/sdb/env1/lib/python3.6/site-packages/torch/nn/modules/module.py", line 550, in __call__
result = self.forward(*input, **kwargs)
File "/mnt/sdb/env1/lib/python3.6/site-packages/torch/nn/modules/sparse.py", line 114, in forward
self.norm_type, self.scale_grad_by_freq, self.sparse)
File "/mnt/sdb/env1/lib/python3.6/site-packages/torch/nn/functional.py", line 1724, in embedding
return torch.embedding(weight, input, padding_idx, scale_grad_by_freq, sparse)
RuntimeError: arguments are located on different GPUs at /pytorch/aten/src/THC/generic/THCTensorIndex.cu:403
```
| 03-10-2021 19:25:01 | 03-10-2021 19:25:01 | Had to remove the following:
```
device = torch.device("cuda" if torch.cuda.is_available() else "cpu")
n_gpus = torch.cuda.device_count()
if n_gpus > 1:
model = nn.DataParallel(model)
model.to(device)
```
Then everything is running for torch==1.7.1 for both GPUs. So `Trainer()` sorts everything by itself? |
transformers | 10,633 | closed | Extend trainer logging for sm | # What does this PR do?
adds a helper function to `logging.py` to add a native logging handler if needed (`add_handler`). Adds in the `Trainer` a logging `StreamHandler(sys.stdout)` with `sys.stdout` when training is run on sagemaker to forward logs. | 03-10-2021 18:31:49 | 03-10-2021 18:31:49 | |
transformers | 10,632 | closed | Ensure metric results are JSON-serializable | # What does this PR do?
Metrics returned from numpy (with an `np.mean()` for instance) are not real Python floats but `np.float32` (or other type) objects that are not serializable. This causes problems when the metrics are saved in JSON format in the `Trainer`, for instance when using `load_best_model_at_end`. This PR fixes that by recursively applying a `.item()` on the metrics dictionary.
Fixes #10299 | 03-10-2021 17:52:35 | 03-10-2021 17:52:35 | |
transformers | 10,631 | closed | Help using Speech2Text | Hey @patil-suraj (and anyone who can help),
Sorry, I'm still a beginner compared to the rest of the folks here so sorry if my question is a little basic.
But I'm trying to build a pipeline to manually transcribe Youtube videos (that aren't transcribed correctly by Google) and I was considering using your [model ](https://huggingface.co/facebook/s2t-small-librispeech-asr)for it.
Here's my unfinished code on [Google Colab](https://colab.research.google.com/drive/15SgSw1KmD-sxdf6Zd953fJIPSmisHd6M?usp=sharing); the last line throws an error:
```
!pip install git+https://github.com/huggingface/transformers
!pip install youtube-dl path.py soundfile librosa sentencepiece torchaudio
import youtube_dl
from path import Path as Path
import tempfile
import textwrap
import librosa
import soundfile as sf
import torch
from transformers import Speech2TextProcessor, Speech2TextForConditionalGeneration
model = Speech2TextForConditionalGeneration.from_pretrained("facebook/s2t-small-librispeech-asr")
processor = Speech2TextProcessor.from_pretrained("facebook/s2t-small-librispeech-asr")
wrapper = textwrap.TextWrapper(width=70)
mydir = tempfile.TemporaryDirectory()
dirname = mydir.name + "/tmp.wav"
!youtube-dl -o $dirname -ci -f 'bestvideo[ext=mp4]+bestaudio' -x --audio-format wav https://www.youtube.com/watch?v=d5yfUuHYWho
filename = dirname + ".wav"
speech, rate = sf.read(filename)
speech = librosa.resample(speech.T, rate, 16000)
features = processor(speech, sampling_rate=16000, padding=True, return_tensors="pt")
```
And here's the error produced:
```
---------------------------------------------------------------------------
AssertionError Traceback (most recent call last)
<ipython-input-8-8fc3e2d943e0> in <module>()
----> 1 features = processor(speech, sampling_rate=16000, padding=True, return_tensors="pt")
5 frames
/usr/local/lib/python3.7/dist-packages/torchaudio/compliance/kaldi.py in _get_waveform_and_window_properties(waveform, channel, sample_frequency, frame_shift, frame_length, round_to_power_of_two, preemphasis_coefficient)
147 assert 2 <= window_size <= len(
148 waveform), ('choose a window size {} that is [2, {}]'
--> 149 .format(window_size, len(waveform)))
150 assert 0 < window_shift, '`window_shift` must be greater than 0'
151 assert padded_window_size % 2 == 0, 'the padded `window_size` must be divisible by two.' \
AssertionError: choose a window size 400 that is [2, 2]
```
Can anyone point me in the right direction? Thanks. | 03-10-2021 17:05:30 | 03-10-2021 17:05:30 | Your speech loading code is incorrect; instead try the following:
```python
from IPython.display import Audio
speech, rate = librosa.load(filename, sr=16000)
Audio(speech, rate=rate)
```<|||||>When I run this line
`processor = Speech2TextProcessor.from_pretrained("facebook/s2t-small-librispeech-asr")`
I am getting the following error: "AttributeError: type object 'Speech2TextProcessor' has no attribute 'from_pretrained'". Did this part was recently changed in the repository?
EDIT: sorry, my mistake. The previous installation was causing trouble. After uninstalling everything and installing again it is working fine.<|||||>As @elgeish said, the speech loading code was causing the issue. Glad to know that you resolved it!<|||||>Success! Thanks
On Wed, Mar 10, 2021, 20:25 rodrigoheck ***@***.***> wrote:
> When I run this line
>
> processor =
> Speech2TextProcessor.from_pretrained("facebook/s2t-small-librispeech-asr")
>
> I am getting the following error: "AttributeError: type object
> 'Speech2TextProcessor' has no attribute 'from_pretrained'". Did this part
> was recently changed in the repository?
>
> —
> You are receiving this because you authored the thread.
> Reply to this email directly, view it on GitHub
> <https://github.com/huggingface/transformers/issues/10631#issuecomment-796383135>,
> or unsubscribe
> <https://github.com/notifications/unsubscribe-auth/AECXP3AO6IAKWJYWUGB42BTTDAS2PANCNFSM4Y6OTVBA>
> .
>
|
transformers | 10,630 | closed | I get different results everytime I run run_squad.py | Is it possible to have deterministic results using the run_squad.py script?
It has the set_seed() method but still, it gives different results every time I run it.
How can I get same results across all runs? | 03-10-2021 14:50:23 | 03-10-2021 14:50:23 | This issue has been automatically marked as stale because it has not had recent activity. If you think this still needs to be addressed please comment on this thread.
Please note that issues that do not follow the [contributing guidelines](https://github.com/huggingface/transformers/blob/master/CONTRIBUTING.md) are likely to be ignored. |
transformers | 10,629 | closed | Using `label` in Trainer leads to TypeError | ## Environment info
- `transformers` version: 4.3.3
- Platform: Linux-4.19.112+-x86_64-with-Ubuntu-18.04-bionic
- Python version: 3.7.10
- PyTorch version (GPU?): 1.8.0+cu101 (True)
- Tensorflow version (GPU?): 2.4.1 (True)
- Using GPU in script?: Not explicitly.
- Using distributed or parallel set-up in script?: No
### Who can help
@LysandreJik
## Information
My dataset is defined as follows:
```python
"""Implements MNIST Dataset"""
from torch.utils.data import Dataset
from torchvision import datasets, transforms
from torchvision.transforms import Grayscale, ToTensor, Normalize
class Mnist(Dataset):
def __init__(self, config):
self.config = config
transformations = [Grayscale(num_output_channels=1),ToTensor(),Normalize(mean=[0.0],std=[1.0])]
self.transform = (
transforms.Compose(transformations)
)
self.dataset = datasets.MNIST(
config.load_dataset_args.path,
download=True,
train=self.config.split == "train",
transform=self.transform,
)
def __len__(self):
return len(self.dataset)
def __getitem__(self, example_idx):
# essential to return as dict, hence the roundabout way of loading the dataset
img, label = self.dataset[example_idx]
return {"image": img, "label": label}
```
Model I am using - a custom CNN, defined as follows:
```python
"""Implementation of a custom CNN with random weights."""
from torch.nn import (
BatchNorm2d,
Conv2d,
Linear,
MaxPool2d,
Module,
ReLU,
Sequential,
CrossEntropyLoss,
)
class SimpleCnn(Module):
def __init__(self):
super(SimpleCnn, self).__init__()
self.cnn_layers = Sequential(
Conv2d(1, 32, kernel_size=3, stride=1, padding=1),
BatchNorm2d(32),
ReLU(),
MaxPool2d(kernel_size=2, stride=2),
Conv2d(32, 32, kernel_size=3, stride=1, padding=1),
BatchNorm2d(32),
ReLU(),
MaxPool2d(kernel_size=2, stride=2),
Conv2d(32, 32, kernel_size=3, stride=1, padding=1),
BatchNorm2d(32),
ReLU(),
MaxPool2d(kernel_size=2, stride=2),
)
self.linear_layers = Linear(32 * 3 * 3, 10)
self.loss_fn = CrossEntropyLoss()
def forward(self, image, label=None):
out = self.cnn_layers(image)
out = out.view(out.size(0), -1)
out = self.linear_layers(out)
if label is not None:
loss = self.loss_fn(out, label)
return loss, out
return out
```
The problem arises when using:
Trainer with a custom `label_names` as `['label']`. I provide `label_names` as `['label']` in `TrainingArguments`. The following error occurs on `trainer.train()`:
```python
Traceback (most recent call last):
File "hf_train.py", line 97, in <module>
trainer.train()
File "/usr/local/lib/python3.7/dist-packages/transformers/trainer.py", line 943, in train
tr_loss += self.training_step(model, inputs)
File "/usr/local/lib/python3.7/dist-packages/transformers/trainer.py", line 1307, in training_step
loss = self.compute_loss(model, inputs)
File "/usr/local/lib/python3.7/dist-packages/transformers/trainer.py", line 1337, in compute_loss
outputs = model(**inputs)
File "/usr/local/lib/python3.7/dist-packages/torch/nn/modules/module.py", line 889, in _call_impl
result = self.forward(*input, **kwargs)
TypeError: forward() got an unexpected keyword argument 'labels'
```
I tried printing batch keys, using `torch.utils.data.DataLoader` inside, and after the function call to `get_train_dataloader` in `trainer.py`:
```
dict_keys(['image', 'label']) #Inside
dict_keys(['labels', 'image']) #Immediately after call
```
I don't understand how it gets converted to `labels` on its own.
## To reproduce
Steps to reproduce the behavior:
1. Load any dataset with one output key as `['label']`.
2. Provide `['label']` as the label_names to `TrainingArguments`
3. Run `trainer.train()`.
One can also try using `load_dataset('mnist')` directly from the `datasets` library. This error will get thrown.
This is not expected. Strangely enough, changing every `'label'` to `'class_label'` or `'labels'` works perfectly. I don't know why this would happen.
| 03-10-2021 10:54:48 | 03-10-2021 10:54:48 | Pinging @sgugger <|||||>Yes, the default data collator always change `label` to `labels` because Hugging Face models expect that argument while Hugging Face datasets usually have `label`. You can work around this by using the default data collator of PyTorch and pass it to the `Trainer`, but you should make your model work like the one in Transformers to avoid any other issues we didn't think of (so use a `labels` argument and always return tuples).
I'll see what I can do to avoid this specific bug in the future.<|||||>Thanks a lot @sgugger, @LysandreJik. Should I close this issue?
EDIT:
I think that this could be mentioned in the docs where custom Trainer/TrainingArguments are discussed. What do you think?<|||||>Like I said, will try to solve the bug in itself. My recommendation was more in general to avoid any other bugs :-) <|||||>Thanks again @sgugger :) |
transformers | 10,628 | closed | expanduser path in Trainer | the `output_dir` passed to TrainingArguments is not expanded (the behaviour is probably the same for logging_dir)
### Who can help
Library:
- trainer: @sgugger
## To reproduce
Directly using os.makedirs but this is what happens in Trainer
```py
In [7]: !mkdir ~/foo
In [8]: !cd ~/foo
/mnt/beegfs/home/lerner/foo
In [10]: os.makedirs("~/bar")
In [14]: !realpath "~/bar"
/mnt/beegfs/home/lerner/foo/~/bar
```
## To fix
Call os.path.expanduser before making dir
```py
In [10]: os.makedirs(os.path.expanduser("~/bar"))
In [18]: cd
/mnt/beegfs/home/lerner
In [21]: !realpath bar
/mnt/beegfs/home/lerner/bar
```
| 03-10-2021 09:46:45 | 03-10-2021 09:46:45 | Sounds reasonable. Would you like to make a PR with this change?<|||||>Can do.
I should expand the path in TrainingArguments right? for logging_dir also?<|||||>Yes, `output_dir` and `logging_dir`, preferable in the postinit of `TrainingArguments` so it's done as early as possible. |
transformers | 10,627 | closed | considering `pad_to_multiple_of` for run_mlm.py | ## Environment info
<!-- You can run the command `transformers-cli env` and copy-and-paste its output below.
Don't forget to fill out the missing fields in that output! -->
- `transformers` version: 4.3.3
- Platform: linux
- Python version: 3.7
- PyTorch version (GPU?): 1.8
- Tensorflow version (GPU?): -
- Using GPU in script?: -
- Using distributed or parallel set-up in script?: -
### Who can help
@sgugger, @patil-suraj
## Information
I have seen in huggingface codes such as run_seq2seq.py that for the case of fp16, they pad to multiple of 8 like below, perhpas for efficiency purpose:
```
data_collator = DataCollatorForSeq2Seq(
tokenizer,
label_pad_token_id=label_pad_token_id,
pad_to_multiple_of=8 if training_args.fp16 else None,
)
```
For run_mlm.py codes, the padding condition in case of fp16 is not considered, could it be a bug and possibly performance could get better in run_mlm.py if this was set? kindly appreciate having a look.
thanks
| 03-10-2021 09:44:40 | 03-10-2021 09:44:40 | Indeed, we could use that when the line by line option is set (otherwise there is just no padding). Would you like to make a PR with this? |
transformers | 10,626 | closed | Average checkpoints | Is it possible to average weights of several checkpoints? Some thing like https://github.com/pytorch/fairseq/blob/master/scripts/average_checkpoints.py | 03-10-2021 08:52:01 | 03-10-2021 08:52:01 | Hello, thanks for opening an issue! We try to keep the github issues for bugs/feature requests.
Could you ask your question on the [forum](https://discusss.huggingface.co) instead?
Thanks! |
transformers | 10,625 | closed | Model "deberta-v2--xxlarge-mnli" doesn't work!!! | Whenever i try to load the tokenizer by
```python
from transformers import AutoTokenizer, AutoModel
tokenizer = AutoTokenizer.from_pretrained('microsoft/deberta-v2-xxlarge-mnli')
```
it returns this issue:
```
config_class = CONFIG_MAPPING[config_dict["model_type"]]
KeyError: 'deberta-v2'
```
Is this model not available in Transformers 4.3.3 (the latest release)? | 03-10-2021 08:09:37 | 03-10-2021 08:09:37 | No, DeBERTa-v2 is not available in v4.3.3, it's only available from source as of now. Version v4.4.0 should be released end of this week or early next week, and will have DeBERTa-v2.<|||||>Yes, exactly what i think! Thanks Lysandre for confirming this. |
transformers | 10,624 | closed | Copy tokenizer files in each of their repo | # What does this PR do?
This PR cleans the maps in the tokenizer files to make sure each checkpoint has the proper tokenization files. This will allow us to remove custom code that mapped some checkpoints to special files (like BART using RoBERTa vocab files) and take full advantage of the versioning systems for those checkpoints. All checkpoints changed have been properly copied in the corresponding model repos in parallel.
For instance, to accomodate the move on the fast BART tokenizers, the following commits have been on the model hub:
- in [facebook/bart-base](https://huggingface.co/facebook/bart-base/commit/c2469fb7e666a5c5629a161f17c9ef23c85217f7)
- in [facebook/bart-large](https://huggingface.co/facebook/bart-large/commit/22fa33834dccc11df99c4fc5fcc96c67f806dfdb)
- in [facebook/bart-large-mnli](https://huggingface.co/facebook/bart-large-mnli/commit/6a35c499ad1087bad8d9c348a05b1fa10c5ad47d)
- in [facebook/bart-large-cnn](https://huggingface.co/facebook/bart-large-cnn/commit/18614750f248f300641757e8e44e6afce801d664)
- in [facebook/bart-large-xsum](https://huggingface.co/facebook/bart-large-xsum/commit/96ea79a741cd376cdc8a740b225330773da151f0)
- in [yjernite/bart_eli5](https://huggingface.co/yjernite/bart_eli5/commit/38797dd2ef06f5542c6f7db853518703f6b3da21)
In the PR I've also uniformized the way the maps are structured across models, to make it easier to alter (and ultimately remove) them in the future via automatic scripts. | 03-10-2021 04:10:56 | 03-10-2021 04:10:56 | Love it!
Maybe a good practice to link to a sample of the related commits on hf.co: for instance here https://huggingface.co/facebook/bart-base/commit/c2469fb7e666a5c5629a161f17c9ef23c85217f7<|||||>I think I did around 50 of them in various repos to move all the tokenizers files, so a bit hard to keep track of all of them.<|||||>Yep just link one, or a small sample.
Makes it easier to see what this PR entails on hf-hub side |
transformers | 10,623 | closed | Invalid pytorch_model.bin for TAPAS-large | Hi,
I was downloading `google/tapas-large` binaries from [HF models](https://huggingface.co/google/tapas-large/tree/main) and for pytorch_model.bin, a zip file was getting downloaded which is not a `bin` file like other models.
folder structure is like - `archive/data/.*File`, `archive/data.pkl` ,` archive/version.File`. Same for tapas-base as well.
These cannot be used for loading a model (`TapasForQuestionAnswering.from_pretrained('path/to/binaries directory')`)
plz suggest how to proceed further.. | 03-10-2021 03:14:09 | 03-10-2021 03:14:09 | Hi, not sure why this happens, cc @julien-c.
A workaround is to load the model using `model = TapasModel.from_pretrained("google/tapas-base")` and then use `model.save_pretrained("./")` to save the `config.json` and `pytorch_model.bin` file to a local directory. <|||||>I don't remember how those models were uploaded so not sure why this is happening.
cc'ing @Pierrci for visibility
In the meantime you can just rename the file to .bin<|||||>@saichandrapandraju the file on tapas-large is a bin file, but since pytorch 1.6.0, bin files are now zip-based. You can check the documentation of [torch.save](https://pytorch.org/docs/stable/generated/torch.save.html) which states:
> The 1.6 release of PyTorch switched torch.save to use a new zipfile-based file format. torch.load still retains the ability to load files in the old format. If for any reason you want torch.save to use the old format, pass the kwarg _use_new_zipfile_serialization=False.
Are you using a torch version inferior to 1.6.0 to try to load the models?<|||||>As pointed out by @julien-c, it is actually downloaded as a zip file, which seems to be the case for several models (my guess is that it does that when the file is zip-based, like all models saved with torch >1.6).
Downloading through git doesn't have that issue.<|||||>Ok will close this as:
- we found the root cause but it's outside our control (`lfs` adds an auto content-type)
- there are several workarounds like `git clone`ing the repo or just renaming the file (`from_pretrained` also works as usual)
Thanks for investigating @Pierrci and @LysandreJik 🥇 |
transformers | 10,622 | closed | wav2vec2: adding single-char tokens to tokenizer causes tokenization mistakes | ## Environment info
- `transformers` version: 4.4.0.dev0
- Platform: Linux-5.8.0-44-generic-x86_64-with-glibc2.10
- Python version: 3.8.8
- PyTorch version (GPU?): 1.8.0 (True)
- Tensorflow version (GPU?): 2.4.1 (False)
- Using GPU in script?: N/A
- Using distributed or parallel set-up in script?: N/A
### Who can help
@patrickvonplaten and @LysandreJik
Issue is probably related to interactions of the following:
https://github.com/huggingface/transformers/blob/9a8c168f56fe3c0e21d554a577ac03beb004ef89/src/transformers/tokenization_utils.py#L213
https://github.com/huggingface/transformers/blob/11fdde02719dbd20651c9f43cc6f54959fc6ede6/src/transformers/tokenization_utils.py#L352
https://github.com/huggingface/transformers/blob/cb38ffcc5e0ae2fac653342ac36dc75c15ea178f/src/transformers/models/wav2vec2/tokenization_wav2vec2.py#L184
This is a corner case: `add_tokens` adds new tokens to `self.unique_no_split_tokens` -- causing `tokenize()` to skip calling `Wav2Vec2CTCTokenizer._tokenize()`
This is probably not the case with most tokenizers since their vocab includes most, if not all, commonly used single-characters tokens without including them in `self.unique_no_split_tokens`. I faced this while debugging my code for https://github.com/huggingface/transformers/pull/10581 to add support for Buckwalter Arabic transliteration. The issue is not limited to adding single-char tokens but rather when words (space-separated) start or end with a newly added token.
## Information
Model I am using (Bert, XLNet ...): wav2vec2
The problem arises when using:
* [ ] the official example scripts: (give details below)
* [x] my own modified scripts: adding tokens to ASR vocab
The tasks I am working on is:
* [ ] an official GLUE/SQUaD task: (give the name)
* [x] my own task or dataset: training an ASR with extended vocab
## To reproduce
Steps to reproduce the behavior:
```python
from transformers import Wav2Vec2Processor
tokenizer = Wav2Vec2Processor.from_pretrained('facebook/wav2vec2-base').tokenizer
tokenizer.add_tokens('x')
token_ids = tokenizer('C x A').input_ids
decoded = tokenizer.decode(token_ids)
print(decoded, token_ids)
# CxA [19, 32, 7]
```
## Expected behavior
Should have printed `C x A [19, 4, 32, 4, 7]`
| 03-10-2021 03:05:16 | 03-10-2021 03:05:16 | My workaround right now is to keep a reference to the original `tokenizer.unique_no_split_tokens` before adding tokens then restoring it afterwards:
```python
from transformers import Wav2Vec2Processor
tokenizer = Wav2Vec2Processor.from_pretrained('facebook/wav2vec2-base').tokenizer
unique_no_split_tokens = tokenizer.unique_no_split_tokens
tokenizer.add_tokens('x')
tokenizer.unique_no_split_tokens = unique_no_split_tokens
token_ids = tokenizer('C x A').input_ids
decoded = tokenizer.decode(token_ids)
print(decoded, token_ids)
# C x A [19, 4, 32, 4, 7]
```<|||||>Hey @elgeish,
Sorry for replying that late! Yes, you are absolutely right here :-)
I think we should overwrite the `add_tokens(self, ...)` function in
`src/transformers/models/wav2vec2/tokenization_wav2vec2.py` with the "hack" just as you did:
```python
def _add_tokens(self, new_tokens: Union[List[str], List[AddedToken]], special_tokens: bool = False) -> int:
# copy past the function from `src/transformers/tokenization_utils.py`
# + add the "hack":
unique_no_split_tokens = tokenizer.unique_no_split_tokens
tokenizer.unique_no_split_tokens = unique_no_split_tokens
```
If you want and have some time, it would be amazing if you could open a PR :-)
<|||||>This issue has been automatically marked as stale because it has not had recent activity. If you think this still needs to be addressed please comment on this thread.
Please note that issues that do not follow the [contributing guidelines](https://github.com/huggingface/transformers/blob/master/CONTRIBUTING.md) are likely to be ignored.<|||||>Hey @patrickvonplaten,
Shall I open a PR for this issue.<|||||>Hey @Muktan,
yes this would be great :-) |
transformers | 10,621 | closed | Fixes an issue in `text-classification` where MNLI eval/test datasets are not being preprocessed. | # What does this PR do?
In https://github.com/huggingface/transformers/commit/dfd16af8322788e6dd58e8396e0d6f2f5312bf99 for `run_glue.py`, `{train|eval|test}_dataset` was split out and preprocessed individually. However, this misses `datasets["{validation|test}_mismatched"]` which is appended to the `{eval|test}_dataset` only when MNLI is used.
When running evaluation on MNLI, that means we eventually hit an un-preprocessed dataset which leads to a stack trace like this:
```
Traceback (most recent call last):
File "/root/anaconda3/envs/pytorch/lib/python3.6/site-packages/torch_xla/distributed/xla_multiprocessing.py", line 329, in _mp_start_fn
_start_fn(index, pf_cfg, fn, args)
File "/root/anaconda3/envs/pytorch/lib/python3.6/site-packages/torch_xla/distributed/xla_multiprocessing.py", line 323, in _start_fn
fn(gindex, *args)
File "/transformers/examples/text-classification/run_glue.py", line 532, in _mp_fn
main()
File "/transformers/examples/text-classification/run_glue.py", line 493, in main
metrics = trainer.evaluate(eval_dataset=eval_dataset)
File "/root/anaconda3/envs/pytorch/lib/python3.6/site-packages/transformers/trainer.py", line 1657, in evaluate
metric_key_prefix=metric_key_prefix,
File "/root/anaconda3/envs/pytorch/lib/python3.6/site-packages/transformers/trainer.py", line 1788, in prediction_loop
loss, logits, labels = self.prediction_step(model, inputs, prediction_loss_only, ignore_keys=ignore_keys)
File "/root/anaconda3/envs/pytorch/lib/python3.6/site-packages/transformers/trainer.py", line 1899, in prediction_step
loss, outputs = self.compute_loss(model, inputs, return_outputs=True)
File "/root/anaconda3/envs/pytorch/lib/python3.6/site-packages/transformers/trainer.py", line 1458, in compute_loss
outputs = model(**inputs)
File "/root/anaconda3/envs/pytorch/lib/python3.6/site-packages/torch/nn/modules/module.py", line 1015, in _call_impl
return forward_call(*input, **kwargs)
File "/root/anaconda3/envs/pytorch/lib/python3.6/site-packages/transformers/models/distilbert/modeling_distilbert.py", line 625, in forward
return_dict=return_dict,
File "/root/anaconda3/envs/pytorch/lib/python3.6/site-packages/torch/nn/modules/module.py", line 1015, in _call_impl
return forward_call(*input, **kwargs)
File "/root/anaconda3/envs/pytorch/lib/python3.6/site-packages/transformers/models/distilbert/modeling_distilbert.py", line 471, in forward
raise ValueError("You have to specify either input_ids or inputs_embeds")
ValueError: You have to specify either input_ids or inputs_embeds
```
This commit resolves this by moving the `dataset.map(preprocess...)` to the beginning.
<!--
Congratulations! You've made it this far! You're not quite done yet though.
Once merged, your PR is going to appear in the release notes with the title you set, so make sure it's a great title that fully reflects the extent of your awesome contribution.
Then, please replace this with a description of the change and which issue is fixed (if applicable). Please also include relevant motivation and context. List any dependencies (if any) that are required for this change.
Once you're done, someone will review your PR shortly (see the section "Who can review?" below to tag some potential reviewers). They may suggest changes to make the code even better. If no one reviewed your PR after a week has passed, don't hesitate to post a new comment @-mentioning the same persons---sometimes notifications get lost.
-->
<!-- Remove if not applicable -->
Fixes # 10620
## Before submitting
- [ ] This PR fixes a typo or improves the docs (you can dismiss the other checks if that's the case).
- [ x] Did you read the [contributor guideline](https://github.com/huggingface/transformers/blob/master/CONTRIBUTING.md#start-contributing-pull-requests),
Pull Request section?
- [ ] Was this discussed/approved via a Github issue or the [forum](https://discuss.huggingface.co/)? Please add a link
to it if that's the case.
- [ ] Did you make sure to update the documentation with your changes? Here are the
[documentation guidelines](https://github.com/huggingface/transformers/tree/master/docs), and
[here are tips on formatting docstrings](https://github.com/huggingface/transformers/tree/master/docs#writing-source-documentation).
- [ ] Did you write any new necessary tests?
## Who can review?
Anyone in the community is free to review the PR once the tests have passed. Feel free to tag
members/contributors which may be interested in your PR.
<!-- Your PR will be replied to more quickly if you can figure out the right person to tag with @
If you know how to use git blame, that is the easiest way, otherwise, here is a rough guide of **who to tag**.
Please tag fewer than 3 people.
Models:
- albert, bert, xlm: @LysandreJik
- blenderbot, bart, marian, pegasus, encoderdecoder, t5: @patrickvonplaten, @patil-suraj
- longformer, reformer, transfoxl, xlnet: @patrickvonplaten
- fsmt: @stas00
- funnel: @sgugger
- gpt2: @patrickvonplaten, @LysandreJik
- rag: @patrickvonplaten, @lhoestq
- tensorflow: @jplu
Library:
- benchmarks: @patrickvonplaten
- deepspeed: @stas00
- ray/raytune: @richardliaw, @amogkam
- text generation: @patrickvonplaten
- tokenizers: @n1t0, @LysandreJik
- trainer: @sgugger
- pipelines: @LysandreJik
Documentation: @sgugger
HF projects:
- nlp datasets: [different repo](https://github.com/huggingface/nlp)
- rust tokenizers: [different repo](https://github.com/huggingface/tokenizers)
Examples:
- maintained examples (not research project or legacy): @sgugger, @patil-suraj
- research_projects/bert-loses-patience: @JetRunner
- research_projects/distillation: @VictorSanh
-->
| 03-10-2021 01:08:52 | 03-10-2021 01:08:52 | |
transformers | 10,620 | closed | MNLI eval/test dataset is not being preprocessed in `run_glue.py` | ## Environment info
<!-- You can run the command `transformers-cli env` and copy-and-paste its output below.
Don't forget to fill out the missing fields in that output! -->
- `transformers` version: 4.4.0.dev0
- Platform: Linux-4.9.0-14-amd64-x86_64-with-debian-9.13
- Python version: 3.6.10
- PyTorch version (GPU?): 1.8.0 (False)
- Tensorflow version (GPU?): not installed (NA)
- Using GPU in script?: no, using TPU
- Using distributed or parallel set-up in script?: distributed
### Who can help
<!-- Your issue will be replied to more quickly if you can figure out the right person to tag with @
If you know how to use git blame, that is the easiest way, otherwise, here is a rough guide of **who to tag**.
Please tag fewer than 3 people.
Models:
- albert, bert, xlm: @LysandreJik
- blenderbot, bart, marian, pegasus, encoderdecoder, t5: @patrickvonplaten, @patil-suraj
- longformer, reformer, transfoxl, xlnet: @patrickvonplaten
- fsmt: @stas00
- funnel: @sgugger
- gpt2: @patrickvonplaten, @LysandreJik
- rag: @patrickvonplaten, @lhoestq
- tensorflow: @jplu
Library:
- benchmarks: @patrickvonplaten
- deepspeed: @stas00
- ray/raytune: @richardliaw, @amogkam
- text generation: @patrickvonplaten
- tokenizers: @LysandreJik
- trainer: @sgugger
- pipelines: @LysandreJik
Documentation: @sgugger
HF projects:
- nlp datasets: [different repo](https://github.com/huggingface/nlp)
- rust tokenizers: [different repo](https://github.com/huggingface/tokenizers)
Examples:
- maintained examples (not research project or legacy): @sgugger, @patil-suraj
- research_projects/bert-loses-patience: @JetRunner
- research_projects/distillation: @VictorSanh
-->
N/A, I have a fix upcoming 👍
## Information
Model I am using (Bert, XLNet ...): Any model within `examples/text-classification/run_glue.py` that uses MNLI
The problem arises when using:
* [x] the official example scripts: (give details below)
* [ ] my own modified scripts: (give details below)
The tasks I am working on is:
* [x] an official GLUE/SQUaD task: (MNLI)
* [ ] my own task or dataset: (give details below)
Essentially, the issue is that in https://github.com/huggingface/transformers/commit/dfd16af8322788e6dd58e8396e0d6f2f5312bf99 for `run_glue.py`, `{train|eval|test}_dataset` was split out and preprocessed individually. However, this misses `datasets["{validation|test}_mismatched"]` which is appended to the `{eval|test}_dataset` only when MNLI is used.
## To reproduce
Steps to reproduce the behavior:
1. Run the `run_glue.py` example on an MNLI dataset and include eval. The full command I'm using on a v2-8 TPU is:
```
python examples/xla_spawn.py --num_cores 8 examples/text-classification/run_glue.py --logging_dir=./tensorboard-metrics --task_name MNLI --cache_dir ./cache_dir --do_eval --max_seq_length 128 --learning_rate 3e-5 --output_dir MNLI --logging_steps 30 --save_steps 3000 --tpu_metrics_debug --model_name_or_path bert-base-cased --per_device_eval_batch_size 64 --overwrite_output_dir
```
This results in:
```
Traceback (most recent call last):
File "/root/anaconda3/envs/pytorch/lib/python3.6/site-packages/torch_xla/distributed/xla_multiprocessing.py", line 329, in _mp_start_fn
_start_fn(index, pf_cfg, fn, args)
File "/root/anaconda3/envs/pytorch/lib/python3.6/site-packages/torch_xla/distributed/xla_multiprocessing.py", line 323, in _start_fn
fn(gindex, *args)
File "/transformers/examples/text-classification/run_glue.py", line 532, in _mp_fn
main()
File "/transformers/examples/text-classification/run_glue.py", line 493, in main
metrics = trainer.evaluate(eval_dataset=eval_dataset)
File "/root/anaconda3/envs/pytorch/lib/python3.6/site-packages/transformers/trainer.py", line 1657, in evaluate
metric_key_prefix=metric_key_prefix,
File "/root/anaconda3/envs/pytorch/lib/python3.6/site-packages/transformers/trainer.py", line 1788, in prediction_loop
loss, logits, labels = self.prediction_step(model, inputs, prediction_loss_only, ignore_keys=ignore_keys)
File "/root/anaconda3/envs/pytorch/lib/python3.6/site-packages/transformers/trainer.py", line 1899, in prediction_step
loss, outputs = self.compute_loss(model, inputs, return_outputs=True)
File "/root/anaconda3/envs/pytorch/lib/python3.6/site-packages/transformers/trainer.py", line 1458, in compute_loss
outputs = model(**inputs)
File "/root/anaconda3/envs/pytorch/lib/python3.6/site-packages/torch/nn/modules/module.py", line 1015, in _call_impl
return forward_call(*input, **kwargs)
File "/root/anaconda3/envs/pytorch/lib/python3.6/site-packages/transformers/models/distilbert/modeling_distilbert.py", line 625, in forward
return_dict=return_dict,
File "/root/anaconda3/envs/pytorch/lib/python3.6/site-packages/torch/nn/modules/module.py", line 1015, in _call_impl
return forward_call(*input, **kwargs)
File "/root/anaconda3/envs/pytorch/lib/python3.6/site-packages/transformers/models/distilbert/modeling_distilbert.py", line 471, in forward
raise ValueError("You have to specify either input_ids or inputs_embeds")
ValueError: You have to specify either input_ids or inputs_embeds
```
## Expected behavior
Dataset should be preprocessed for the entirety of the dataset.
Fix: https://github.com/huggingface/transformers/pull/10621 | 03-10-2021 01:08:12 | 03-10-2021 01:08:12 | Fixed by #10621
Thanks for flagging and fixing :-) |
transformers | 10,619 | closed | wav2vec2: `convert_tokens_to_string` contracts legitimately repeated characters | ## Environment info
- `transformers` version: 4.4.0.dev0
- Platform: Linux-5.8.0-44-generic-x86_64-with-glibc2.10
- Python version: 3.8.8
- PyTorch version (GPU?): 1.8.0 (True)
- Tensorflow version (GPU?): 2.4.1 (False)
- Using GPU in script?: N/A
- Using distributed or parallel set-up in script?: N/A
### Who can help
@patrickvonplaten - issue is most probably due to https://github.com/huggingface/transformers/blob/cb38ffcc5e0ae2fac653342ac36dc75c15ea178f/src/transformers/models/wav2vec2/tokenization_wav2vec2.py#L203
## Information
Model I am using (Bert, XLNet ...): wav2vec2
The problem arises when using:
* [x] the official example scripts: run_asr.py
* [ ] my own modified scripts: (give details below)
The tasks I am working on is:
* [x] an official GLUE/SQUaD task: wav2vec2
* [ ] my own task or dataset: (give details below)
## To reproduce
```python
from transformers import Wav2Vec2Processor
tokenizer = Wav2Vec2Processor.from_pretrained('facebook/wav2vec2-base').tokenizer
tokenizer.decode(tokenizer('CARRY').input_ids)
# CARY
```
Decoder should have returned `'CARRY'` instead. | 03-10-2021 01:05:25 | 03-10-2021 01:05:25 | Can you try this?
```python
from transformers import Wav2Vec2Processor
tokenizer = Wav2Vec2Processor.from_pretrained('facebook/wav2vec2-base').tokenizer
tokenizer.decode(tokenizer('CARRY').input_ids, group_tokens=False)
# CARRY
```
Because we need to decode the predicted tokens with CTC, `"RR"` is decoded to `"R"` by default. See this blog post for more information: https://distill.pub/2017/ctc/<|||||>Yeah looks good! Thanks!<|||||>By the way, I totally get why it's needed for CTC, I mistook it for the tokenizer used to decode the final results but noticed it wasn't the case. The final results work as expected. Sorry for the false alarm! |
transformers | 10,618 | closed | Run_qa crashes because of parser = HfArgumentParser((ModelArguments, DataTrainingArguments, TrainingArguments)) | ## Environment info
- `transformers` version: 4.3.3
- Platform: linux
- Python version:3.7, 3.8, 3.9 reproed across all three
- PyTorch version (GPU?): 1.7, tried 1.8 with same behavior
- Tensorflow version (GPU?):N/A
- Using GPU in script?: yes
- Using distributed or parallel set-up in script?: Yes 2 gpu
### Who can help
@sgugger, @patil-suraj
## Information
Model I am using (Bert, XLNet ...): bert-base-uncased
The problem arises when using:
* [ X] the official example scripts: (give details below)
* [ ] my own modified scripts: (give details below)
The tasks I am working on is:
* [ X] an official GLUE/SQUaD task: (give the name)
* [ ] my own task or dataset: (give details below)
SQUAD 1.0
## To reproduce
Steps to reproduce the behavior:
1. Install clean transformers environment
2. run the run_qa.py script with instructions as specified
3. crash
If you go ahead and create a new environment and install the most recent version of the transformer and try to run the run_qa.py script(SQUAD) it crashes because of a parser issue.
python run_qa.py --model_name_or_path bert-base-uncased --dataset_name squad --do_train --per_device_train_batch_size 8 --learning_rate 3e-5 --max_seq_length 384 --doc_stride 128 --output_dir output --overwrite_output_dir --cache_dir cache --preprocessing_num_workers 4 --seed 42 --num_train_epochs 1
Traceback (most recent call last):
File "run_qa.py", line 1095, in <module>
main()
File "run_qa.py", line 902, in main
parser = HfArgumentParser((ModelArguments, DataTrainingArguments, TrainingArguments))
File "/home/spacemanidol/miniconda3/envs/sparseml/lib/python3.7/site-packages/transformers/hf_argparser.py", line 52, in __init__
self._add_dataclass_arguments(dtype)
File "/home/spacemanidol/miniconda3/envs/sparseml/lib/python3.7/site-packages/transformers/hf_argparser.py", line 93, in _add_dataclass_arguments
elif hasattr(field.type, "__origin__") and issubclass(field.type.__origin__, List):
File "/home/spacemanidol/miniconda3/envs/sparseml/lib/python3.7/typing.py", line 721, in __subclasscheck__
return issubclass(cls, self.__origin__)
TypeError: issubclass() arg 1 must be a clas
## Expected behavior
<!-- A clear and concise description of what you would expect to happen. -->
Run and produce a BERT-QA model | 03-09-2021 22:59:26 | 03-09-2021 22:59:26 | This is weird and linked to your environment somehow.
@stas00 Was this the error you encountered when `dataclasses` is installed in Python 3.7 or was it a different one?<|||||>no, that was not that error. I tested `run_qa.py` w/ dataclasses on py38 and it didn't fail.
the datasets error was: `AttributeError: module 'typing' has no attribute '_ClassVar'`
https://github.com/huggingface/transformers/issues/8638<|||||>I just tried this on 2 new servers with a fresh conda environment and reproduced behavior.
Steps.
```bash
conda create -n test python=3.8
conda activate test
pip install transformers datasets torch
python run_qa.py --model_name_or_path bert-base-uncased --dataset_name squad --do_train --per_device_train_batch_size 8 --learning_rate 3e-5 --max_seq_length 384 --doc_stride 128 --output_dir bert-base-uncased-qa/ --overwrite_output_dir --cache_dir cache --preprocessing_num_workers 4 --seed 42 --num_train_epochs 1
```
<|||||>I have also reproed with venv and regular environment on multiple machines<|||||>The suggested commands work fine on my side, so can't reproduce the issue. <|||||>I have pushed a fix (on master by mistake but it's pretty harmless) a tentative fix to remove the line that caused you problem and replace it by a regex. Let me know if it fixes your issue or not (I can't confirm myself since I can't reproduce).<|||||>FWIW, I followed your new conda env steps and couldn't reproduce the problem.
@spacemanidol, fyi I edited your comment to fix the conda create line as it had the commands reversed.<|||||>Can confirm this works. |
transformers | 10,617 | closed | Request: Ignore Dataset transforms when iterating to the most recent checkpoint when resuming training | # 🚀 Feature request
It'd be great if, when resuming training from a checkpoint and using a Dataset with a format/transform function applied, the dataset's format/transform function could be ignored while iterating up to the last checkpoint step.
@lhoestq @sgugger
## Motivation
I doubt it's much of an issue most of the time, but I've started playing with `dataset.set_transform()` for doing some heavy preprocessing, and just iterating through samples to the current checkpoint step can take a ridiculously long time compared to a dataset without a transform applied. And I don't think there's any case where the transformed sample would be used, right?
See [this conversation in the forum](https://discuss.huggingface.co/t/understanding-set-transform/3740/6?u=jncasey) for more backstory and my rudimentary thoughts on how I'd accomplish it.
## Your contribution
I'm hesitant to try updating any of the trainer code myself since it's so complicated, and needs to cover so many edge cases I'm not familiar with.
| 03-09-2021 22:22:27 | 03-09-2021 22:22:27 | This is already there :-) Just pass along `--ignore_data_skip` in your script or `ignore_data_skip=True` in your `TrainingArguments`.<|||||>Wow, that was fast! :)
That loads the model from the checkpoint and advances the dataset to the next sample that would have been trained in the original run?
From my reading of the code I assumed that it reloaded the model and started the training over at the first sample of the dataset.
<|||||>Ah sorry I misunderstood your feature request. Indeed it starts from the first sample instead of iterating. What you ask is a bit more complicated and will require a minimum version of datasets. It's possible but will take some time.<|||||>Sorry for the confusion from my writing! And thanks, as always, for your work on this amazing project.<|||||>This issue has been automatically marked as stale because it has not had recent activity. If you think this still needs to be addressed please comment on this thread.
Please note that issues that do not follow the [contributing guidelines](https://github.com/huggingface/transformers/blob/master/CONTRIBUTING.md) are likely to be ignored. |
Subsets and Splits
No community queries yet
The top public SQL queries from the community will appear here once available.