repo
stringclasses 1
value | number
int64 1
25.3k
| state
stringclasses 2
values | title
stringlengths 1
487
| body
stringlengths 0
234k
⌀ | created_at
stringlengths 19
19
| closed_at
stringlengths 19
19
| comments
stringlengths 0
293k
|
---|---|---|---|---|---|---|---|
transformers | 10,616 | closed | changing ".view()" to ".reshape()" for pytorch | New Version of PyTorch uses ".reshape()" instead of ".view()".
There might be some issue if still using ".view()". | 03-09-2021 22:18:18 | 03-09-2021 22:18:18 | This issue has been automatically marked as stale because it has not had recent activity. If you think this still needs to be addressed please comment on this thread.
Please note that issues that do not follow the [contributing guidelines](https://github.com/huggingface/transformers/blob/master/CONTRIBUTING.md) are likely to be ignored. |
transformers | 10,615 | closed | Fix tests of TrainerCallback | # What does this PR do?
When introducing the `report_to` argument, I must have messed something up. Bottomline is that the tests of `TrainerCallback` can fail depending on what is installed in the env (TensorBoard for instance), this PR fixes that. | 03-09-2021 21:24:31 | 03-09-2021 21:24:31 | |
transformers | 10,614 | closed | Not able to convert T5 tf checkpoints | Hi,
I was trying to convert some tf checkpoints for T5 into PyTorch using ```transformers-cli convert```, and am getting the following error:
> Traceback (most recent call last):
File "/home/william18026/miniconda3/bin/transformers-cli", line 32, in <module>
service.run()
File "/home/william18026/miniconda3/lib/python3.7/site-packages/transformers/commands/convert.py", line 158, in run
raise ValueError("--model_type should be selected in the list [bert, gpt, gpt2, transfo_xl, xlnet, xlm]")
ValueError: --model_type should be selected in the list [bert, gpt, gpt2, transfo_xl, xlnet, xlm]
My initial attempt was with transformers==4.3.3, but then I also tried using the source version (4.4.0dev) and the editable clone, but got the same error with all. T5 seems to have been added to the pipeline in #9654, but for some reason, it's not working at the user (/my) end.
What could I be doing wrong? | 03-09-2021 19:50:45 | 03-09-2021 19:50:45 | It seems that T5 was added to the conversion scripts two months ago in https://github.com/huggingface/transformers/pull/9654 as you've mentioned.
However, in your error there is no mention of "t5":
```
ValueError: --model_type should be selected in the list [bert, gpt, gpt2, transfo_xl, xlnet, xlm]
```
But on master it clearly shows there should be one:
```
"--model_type should be selected in the list [bert, gpt, gpt2, t5, transfo_xl, xlnet, xlm, lxmert]"
```
Are you certain you're launching the command in the correct environment? Could you share the result of `transformers-cli env`?
---
Also, this conversion command is far from being complete, I think it could use some of your templating magic @sgugger if you ever feel like it :)
It isn't high priority as we already have the model-specific conversion scripts.<|||||>This issue has been automatically marked as stale because it has not had recent activity. If you think this still needs to be addressed please comment on this thread.
Please note that issues that do not follow the [contributing guidelines](https://github.com/huggingface/transformers/blob/master/CONTRIBUTING.md) are likely to be ignored. |
transformers | 10,613 | closed | OOM issues with save_pretrained models | Posted this issue to the HuggingFace forums without a response.
Having a weird issue with DialoGPT Large model deployment. From PyTorch 1.8.0 and Transformers 4.3.3 using model.save_pretrained and tokenizer.save_pretrained, the exported pytorch_model.bin is almost twice the size of the model card repo and results in OOM on a reasonably equipped machine that when using the standard transformers download process it works fine (I am building a CI pipeline to containerize the model hence the pre-populated model requirement):
```
Model card:
pytorch_model.bin 1.6GB
model.save_pretrained and tokenizer.save_pretrained:
-rw-r--r-- 1 jrandel jrandel 800 Mar 6 16:51 config.json
-rw-r--r-- 1 jrandel jrandel 446K Mar 6 16:51 merges.txt
-rw-r--r-- 1 jrandel jrandel 3.0G Mar 6 16:51 pytorch_model.bin
-rw-r--r-- 1 jrandel jrandel 357 Mar 6 16:51 special_tokens_map.json
-rw-r--r-- 1 jrandel jrandel 580 Mar 6 16:51 tokenizer_config.json
-rw-r--r-- 1 jrandel jrandel 780K Mar 6 16:51 vocab.json
```
When I download the model card files directly however, I’m getting the following errors:
```
curl -L https://huggingface.co/microsoft/DialoGPT-large/resolve/main/config.json -o ./model/config.json
curl -L https://huggingface.co/microsoft/DialoGPT-large/resolve/main/pytorch_model.bin -o ./model/pytorch_model.bin
curl https://huggingface.co/microsoft/DialoGPT-large/resolve/main/tokenizer_config.json -o ./model/tokenizer_config.json
curl https://huggingface.co/microsoft/DialoGPT-large/resolve/main/config.json -o ./model/config.json
curl https://huggingface.co/microsoft/DialoGPT-large/resolve/main/merges.txt -o ./model/merges.txt
curl https://huggingface.co/microsoft/DialoGPT-large/resolve/main/special_tokens_map.json -o ./model/special_tokens_map.json
curl https://huggingface.co/microsoft/DialoGPT-large/resolve/main/vocab.json -o ./model/vocab.json
<snip>
tokenizer = AutoTokenizer.from_pretrained("model/")
File "/var/lang/lib/python3.8/site-packages/transformers/models/auto/tokenization_auto.py", line 395, in from_pretrained
return tokenizer_class_fast.from_pretrained(pretrained_model_name_or_path, *inputs, **kwargs)
File "/var/lang/lib/python3.8/site-packages/transformers/tokenization_utils_base.py", line 1788, in from_pretrained
return cls._from_pretrained(
File "/var/lang/lib/python3.8/site-packages/transformers/tokenization_utils_base.py", line 1801, in _from_pretrained
slow_tokenizer = (cls.slow_tokenizer_class)._from_pretrained(
File "/var/lang/lib/python3.8/site-packages/transformers/tokenization_utils_base.py", line 1876, in _from_pretrained
special_tokens_map = json.load(special_tokens_map_handle)
File "/var/lang/lib/python3.8/json/__init__.py", line 293, in load
return loads(fp.read(),
File "/var/lang/lib/python3.8/json/__init__.py", line 357, in loads
return _default_decoder.decode(s)
File "/var/lang/lib/python3.8/json/decoder.py", line 337, in decode
obj, end = self.raw_decode(s, idx=_w(s, 0).end())
File "/var/lang/lib/python3.8/json/decoder.py", line 355, in raw_decode
raise JSONDecodeError("Expecting value", s, err.value) from None
json.decoder.JSONDecodeError: Expecting value: line 1 column 1 (char 0)
During handling of the above exception, another exception occurred:
Traceback (most recent call last):
File "/var/runtime/bootstrap.py", line 481, in <module>
main()
File "/var/runtime/bootstrap.py", line 458, in main
lambda_runtime_client.post_init_error(to_json(error_result))
File "/var/runtime/lambda_runtime_client.py", line 42, in post_init_error
response = runtime_connection.getresponse()
File "/var/lang/lib/python3.8/http/client.py", line 1347, in getresponse
response.begin()
File "/var/lang/lib/python3.8/http/client.py", line 307, in begin
version, status, reason = self._read_status()
File "/var/lang/lib/python3.8/http/client.py", line 276, in _read_status
raise RemoteDisconnected("Remote end closed connection without"
http.client.RemoteDisconnected: Remote end closed connection without response
time="2021-03-08T09:01:39.33" level=warning msg="First fatal error stored in appctx: Runtime.ExitError"
time="2021-03-08T09:01:39.33" level=warning msg="Process 14(bootstrap) exited: Runtime exited with error: exit status 1"
time="2021-03-08T09:01:39.33" level=error msg="Init failed" InvokeID= error="Runtime exited with error: exit status 1"
time="2021-03-08T09:01:39.33" level=warning msg="Failed to send default error response: ErrInvalidInvokeID"
time="2021-03-08T09:01:39.33" level=error msg="INIT DONE failed: Runtime.ExitError"
time="2021-03-08T09:01:39.33" level=warning msg="Reset initiated: ReserveFail"
```
So what would be causing the large file variance between save_pretrained models and the model card repo? And any ideas why the directly downloaded model card files aren’t working in this example?
Thanks in advance | 03-09-2021 17:14:06 | 03-09-2021 17:14:06 | This issue has been automatically marked as stale because it has not had recent activity. If you think this still needs to be addressed please comment on this thread.
Please note that issues that do not follow the [contributing guidelines](https://github.com/huggingface/transformers/blob/master/CONTRIBUTING.md) are likely to be ignored.<|||||>This is a pretty big deal one would think. An almost 100% bloat of the model checkpoint when exporting compared to the model card...?<|||||>This is impacting me as well, is it possible for us to reopen. I am happy to provide more relevant deatils<|||||>This seems to be a duplicate of https://github.com/huggingface/transformers/issues/11222 |
transformers | 10,612 | open | Implementing efficient self attention in T5 | # 🌟 New model addition
My teammates and I (including @ice-americano) would like to use efficient self attention methods such as Linformer, Performer and Nystromformer
## Model description
These new methods serve as approximations of regular attention, but reduce complexity from quadratic in the inputs to linear. We would like to add a parameter to T5 where users can specify an efficient attention method to use instead of regular attention. Ideally, this would be implemented across all models, but the models tend to have varying implementations of attention, rendering this generalization fairly tedious.
## Open source status
* [x] the model implementation is available: repos are https://github.com/mlpen and https://github.com/lucidrains/performer-pytorch
* [ ] the model weights are available: N/A
* [x] who are the authors: @mlpen and @lucidrains
| 03-09-2021 16:29:30 | 03-09-2021 16:29:30 | There are already some PRs regarding these models, I'm working on adding the Linformer (#10587), there's also a PR for the Performer (#9325, see further down the thread - people can already train T5 with Performer). |
transformers | 10,611 | closed | split seq2seq script into summarization & translation | keeping the original script for tests cc @stas00
fix #10164 | 03-09-2021 16:01:41 | 03-09-2021 16:01:41 | cc @stas00 `run_seq2seq` is left as is for now. At some point, if your tests migrate from that script to either the new ones or another one, we will remove it.<|||||>> cc @stas00 `run_seq2seq` is left as is for now. At some point, if your tests migrate from that script to either the new ones or another one, we will remove it.
I know we discussed to potentially leave the all-in-one script for performance testing, but it's very likely we will be using a different approach that Morgan created.
Therefore please don't leave this on me - please sync the tests with these changes and remove the do-it-all script. Thank you.
<|||||>May I suggest that the examples are inconsistent script naming-wise, some are very abbreviated `run_clm.py`, others are the extreme opposite `run_summarization.py` - that's a way too much to type - won't `run_sum.py` be sufficient?
For "to type" I mean when referring to them in documents, Issues, etc. There is no file-completion there.<|||||>> May I suggest that the examples are inconsistent with script naming-wise, some are very abbreviated `run_clm.py`, others are the extreme opposite `run_summarization.py` - that's a way too much to type - won't `run_sum.py` be sufficient?
my personal preference goes to clarity so `run_summarization.py` trumps `run_sum.py` - but consistency is also important - unsure about the best tradeoff here
edit: if we were to shorten the script names, what would be the matching acronym for `run_translation.py`?<|||||>`run_trans.py`
and just to clarify, I'm just flagging the inconsistency and my preference to type less, and in no way suggesting to interfere with this process - if most of you prefer the long names - go for it. <|||||>Also while we are at it or perhaps after the split - different PR, this further proposed improvement could be applied:
https://github.com/huggingface/transformers/issues/10164
<|||||>I'm not seeing the benefit of shortening to `run_sum` and `run_trans` when tab-complete will give you the full name. In the language-modeling folder, the acronym was used because `run_causal_language_modeling`/`run_masked_language_modeling` is really long, same for question-answering.
If summarization and translation took two words at least, we could use the acronym to shorten the name, but since they don't I would go for the full name.<|||||>> I'm not seeing the benefit of shortening to `run_sum` and `run_trans` when tab-complete will give you the full name. In the language-modeling folder, the acronym was used because `run_causal_language_modeling`/`run_masked_language_modeling` is really long, same for question-answering.
>
> If summarization and translation took two words at least, we could use the acronym to shorten the name, but since they don't I would go for the full name.
I can't see how one is much longer than the other:
```
run_causal_language_modeling
run_summarization
```
Would `run_causal_lm` and `run_masked_lm` be perhaps a good middle ground if you want example names to be of the explicit type?
<|||||>> I'm not seeing the benefit of shortening to `run_sum` and `run_trans` when tab-complete will give you the full name. In the language-modeling folder, the acronym was used because `run_causal_language_modeling`/`run_masked_language_modeling` is really long, same for question-answering.
>
> If summarization and translation took two words at least, we could use the acronym to shorten the name, but since they don't I would go for the full name.
My current understanding is that consistency primes for now, and the change for more explicit script names should be done in a separate PR, if there is indeed consensus that explicit names are better here.<|||||>Very nice PR overall!
I don't really agree though with the `sum`, `trans` and the "one-size-fits-it-all" design choices. Maybe we can settle on displaying a warning for T5 @stas00 ?<|||||>> I don't really agree though with the `sum`, `trans`
As long as other examples are consistently named then it works.
> and the "one-size-fits-it-all" design choices.
It doesn't sound like we are reaching a consensus here. I outlined that entering the same data more than once in the same input is error-prone. Perhaps there is another way to fix this w/o "one-size-fits-it-all"
> Maybe we can settle on displaying a warning for T5 @stas00 ?
Yes, please. At the very least.<|||||>@theo-m, @stas00 - if it's fine for you maybe we can change the script names to `run_summarization.py` and `run_translation.py` then and replace the automatic setting of `prefix` for T5 with a warning instead. Would that work? I'm more than happy to merge this PR then<|||||>If the group prefers it this way then it is fine as you propose.<|||||>> If the group prefers it this way then it is fine as you propose.
Okey, maybe @sgugger, @patil-suraj and @LysandreJik can give their final word then as well<|||||>I would prefer explicit names (`run_summarization.py` and `run_translation.py`) and not handling T5 prefixes automatically.<|||||>I have already given my opinion on the names. For the `source_prefix` I have no strong opinion as I don't see any "good" solution sadly. I'm fine with the warning.<|||||>Seems to me this could be merged, last call for maintainers @patrickvonplaten @sgugger @LysandreJik (thumbs up on this message will be interpreted as a go 😉 ) |
transformers | 10,610 | closed | Trigger add sm information | The PR adds functionality to identify more telemetry information when training is run. | 03-09-2021 15:38:34 | 03-09-2021 15:38:34 | |
transformers | 10,609 | closed | SortedDL for contiguous LM | Hi there,
I am currently implementing LM re-training of a RoBERTa model using the `Trainer` API. Since I have a huge training corpus, I was wondering if there is a functionality in the `Trainer` or the corresponding `DataCollatorForLanguageModeling` that allows for sorted batching as in `fastai`?
More precisely, I would like to feed in all my training data as a contiguous text stream and let the respective functions handle sorted batching irrespective of the sequence length of the individual sequences.
Best,
Simon | 03-09-2021 13:13:25 | 03-09-2021 13:13:25 | This issue is more suited for the forum, but maybe @sgugger has some hints to share!<|||||>I'm not sure what you would want to sort a single text stream. The `Trainer` supports `--group_by_length` but that's when you have multiple texts.
Note that `DataCollatorForLanguageModeling` only performs random masking on your prepared data, nothing more.<|||||>Sorry, maybe I was imprecise here (still trying to wrap my head around a lot of these new concepts). Essentially, what I was wondering if batches for language modeling are constructed in a way that is similar to the approach that you described in [Chapter 10:
NLP Deep Dive](https://github.com/fastai/fastbook/blob/master/10_nlp.ipynb) (section 'Putting Our Texts into Batches for a Language Model') of the book you co-authored. If I got it correctly, that would allow me to train the transformer over variable length input sequences without worrying about sequences being truncated due to the constraints imposed by `tokenizer.max_len_single_sentence` (overflowing parts would simply end up at the appropriate position in the nex mini-batch).<|||||>I think you may be referring to the LM DataLoader. This kind of preprocessing is done using the `datasets` library on our side. Take a look at the [run_clm](https://github.com/huggingface/transformers/blob/master/examples/language-modeling/run_clm.py) or [run_mlm](https://github.com/huggingface/transformers/blob/master/examples/language-modeling/run_mlm.py) examples (in run_mlm the part that is not in the block "line_by_line") or the [language modeling notebook](https://colab.research.google.com/github/huggingface/notebooks/blob/master/examples/language_modeling.ipynb) to see how.<|||||>Thanks a lot! Indeed, the `group_text()` function was exactly what I was looking for. <|||||>Hey @sgugger,
sorry for reopening this one. I am still not 100% sure if I conveyed my issue properly the first time. Hence, let me briefly restate it using an example:
Let's say I chunk my text stream of length 100 (let's say including 3 sentences) into blocks of `block_size=5`, resulting in 20 blocks. Now, I'd like to feed them into my model using a `batch_size=5`, resulting in 4 batches à 5 text blocks.
I am still not 100% sure how they are fed into the model using the `DataCollatorForLanguageModeling` and `Trainer` API:
```python
# Variant A
batch_1 = [block1, block2, block3, block4, block5]
# ...
batch_4 = [block16, block17, block18, block19, block20]
```
```python
# Variant B
batch_1 = [block1, block5, block9, block13, block17]
batch_2 = [block2, block6, block10, block14, block18]
# ...
batch_4 = [block4, block8, block12, block16, block20]
```
If I got it correctly, the method presented in the book referred to in the previous comment relates to *variant B*.<|||||>You will need to write your own data collator for that as this is not in the Transformers library: contrary to LSTMs, Transformers do not have a state so we don't care about the ordering across batches for those models.<|||||>This makes entirely sense, thanks for lifting this barrier in my head! |
transformers | 10,608 | closed | Image feature extractor design | # What does this PR do?
Here we can discuss how to design the `ImageFeatureExtractor` class, and the `ViTFeatureExtractor` subclass.
The hierarchy looks as follows:
`FeatureExtractorMixin` -> `ImageFeatureExtractor` -> `ViTFeatureExtractor`. The `FeatureExtractorMixin` defines common properties among `SequenceFeatureExtractors` (for speech recognition) and `ImageFeatureExtractors` (for vision related tasks), namely saving utilities and the general `BatchFeature` class.
Notes:
- `ImageFeatureExtractor` is based on `SequenceFeatureExtractor`, but with some changes: renamed `max_length` to `max_resolution`, renamed `PaddingStrategy.LONGEST` to `PaddingStrategy.LARGEST` (to pad to the resolution of the largest image in a batch), renamed `PaddingStrategy.MAX_LENGTH` to `PaddingStrategy.MAX_RESOLUTION`.
- Currently, this `ImageFeatureExtractor` class defines common properties among feature extractors for vision models, which are now `image_mean`, `image_std` and `padding_value`. Each concrete FeatureExtractor then provides values for these 3 attributes, and defines any additional attributes.
- Currently, the `ImageFeatureExtractor` class only defines `pad` and `_pad` methods (which should be updated to work for 2D images), but I guess we can add general image transformation methods (such as resize, normalize), and maybe also a `__call__` method. These are now all defined in `ViTFeatureExtractor`.
## Who can review?
@patrickvonplaten @patil-suraj @LysandreJik
| 03-09-2021 12:56:18 | 03-09-2021 12:56:18 | |
transformers | 10,607 | closed | Can't load config for hosted model, works when downloaded | I have recently (19 hours ago) uploaded a new model to huggingface: https://huggingface.co/EMBEDDIA/sloberta
When attempting to load it with `model = AutoModelForMaskedLM.from_pretrained("EMBEDDIA/sloberta")` I get the following error:
```
Traceback (most recent call last):
File "/home/mulcar/.conda/envs/transformerslatest/lib/python3.8/site-packages/transformers/configuration_utils.py", line 353, in get_config_dict
raise EnvironmentError
OSError
During handling of the above exception, another exception occurred:
Traceback (most recent call last):
File "<stdin>", line 1, in <module>
File "/home/mulcar/.conda/envs/transformerslatest/lib/python3.8/site-packages/transformers/modeling_auto.py", line 1105, in from_pretrained
config, kwargs = AutoConfig.from_pretrained(
File "/home/mulcar/.conda/envs/transformerslatest/lib/python3.8/site-packages/transformers/configuration_auto.py", line 272, in from_pretrained
config_dict, _ = PretrainedConfig.get_config_dict(pretrained_model_name_or_path, **kwargs)
File "/home/mulcar/.conda/envs/transformerslatest/lib/python3.8/site-packages/transformers/configuration_utils.py", line 362, in get_config_dict
raise EnvironmentError(msg)
OSError: Can't load config for 'EMBEDDIA/sloberta'. Make sure that:
- 'EMBEDDIA/sloberta' is a correct model identifier listed on 'https://huggingface.co/models'
- or 'EMBEDDIA/sloberta' is the correct path to a directory containing a config.json file
```
If I download the model from the huggingface.co, eg. cloning the model's repo, it loads perfectly fine. Is there a waiting time before a new model is completely added to the system? Or is there an other issue going on? | 03-09-2021 10:52:26 | 03-09-2021 10:52:26 | Hello! I can do `model = AutoModelForMaskedLM.from_pretrained("EMBEDDIA/sloberta")` without any issues on my end.
Could it be linked to a connection issue?
```py
>>> from transformers import AutoModelForMaskedLM
>>> model = AutoModelForMaskedLM.from_pretrained("EMBEDDIA/sloberta")
Downloading: 100%|██████████| 520/520 [00:00<00:00, 215kB/s]
Downloading: 100%|██████████| 443M/443M [00:38<00:00, 11.5MB/s]
```<|||||>Tested from two computers on two different networks, didn't work. Managed to load it on Google Colab, though.
Anyway, it does work now. I was foiled by conda loading an old version of transformers instead of a newer one. Thanks! |
transformers | 10,606 | closed | [M2M100] remove final_logits_bias | # What does this PR do?
M2M100 does not need `final_logits_bias`, this PR removes it from the `M2M100ForConditionalGeneration` | 03-09-2021 09:02:03 | 03-09-2021 09:02:03 | |
transformers | 10,605 | closed | Fix cross-attention head mask for Torch encoder-decoder models | 1. This PR fixes head masking for the cross-attention module in the following models:
- BART,
- Blenderbot,
- Blenderbot_small,
- FSMT,
- LED,
- M2M_100,
- Marian,
- MBart,
- Pegasus.
- T5
2. This PR also contains slight changes in docstrings so that it will be clear that `head_mask` is related to the config of an encoder and the shape of `decoder_head_mask` and `cross_head_mask` depends on the config of a decoder.
3. This PR enables `test_headmasking` for M2M_100 model.
<hr>
**Reviewers:** @patrickvonplaten @patil-suraj
<hr>
Fixes: #10540 | 03-09-2021 08:57:45 | 03-09-2021 08:57:45 | Hi @patrickvonplaten & @patil-suraj, my PR does not pass one test, however, I am not able to reproduce this error on my local (I can't even find the file at `src/transformers/models/new_enc_dec/modeling_new_enc_dec.py` in the repo, which is the one where should be a problem with a copy inconsistency)<|||||>Hi @stancld thank you for your work! The issue is because you have updated all model files (thank you!!), but you haven't updated the template. The template is used when adding a new model, it's available [here](https://github.com/huggingface/transformers/tree/master/templates/adding_a_new_model).
For example, these lines should probably be updated to include the `cross_head_mask`:
https://github.com/huggingface/transformers/blob/44f64132a5f50726f9de4467ed745421c3b11ab3/templates/adding_a_new_model/cookiecutter-template-%7B%7Bcookiecutter.modelname%7D%7D/modeling_%7B%7Bcookiecutter.lowercase_modelname%7D%7D.py#L2070-L2085<|||||>@LysandreJik - Thank you very much for the clarification :)<|||||>Wonderful, the tests pass! Thanks for handling the templates.<|||||>@patil-suraj Thank you for your review and the suggestions. I agree with the change in variable naming. The new one is more accurate. I will change it everywhere accordingly :) <|||||>Hi @patrickvonplaten, I've just rebased this branch to the current master to keep this PR up to date. Could you please review this one? Thanks a lot! :) <|||||>Hey @stancld, Patrick is off for a couple of weeks but will take a look at this as soon as he's back :)<|||||>The PR looks good to me :-)
Think we just need to fix the docstring. @stancld - let me know if you need help regarding the docstring<|||||>@patrickvonplaten The docstring should be fixed now. I forgot a line from a conflict there.. :) |
transformers | 10,604 | closed | fix flaky m2m100 test | # What does this PR do?
The `test_retain_grad_hidden_states_attentions` test is sometimes failing for `M2M100`, with the error
` AttributeError: 'NoneType' object has no attribute 'retain_grad'`
This is because of `layerdrop` sometimes a layer is skipped and the `encoder_attenion/decoder_attentions/cross_attentions` can be `None`.
This PR sets the `config.encoder_layerdrop` and `config.decoder_layerdrop` to 0 in tests to make the tests deterministic. | 03-09-2021 07:30:22 | 03-09-2021 07:30:22 | |
transformers | 10,603 | closed | AlbertForSequenceClassification random output | I use AlbertForSequenceClassification interface as follows:
`import torch
from transformers import BertTokenizer,AlbertConfig,AlbertForSequenceClassification
import numpy
pretrained = "./albert_chinese_base"
tokenizer = BertTokenizer.from_pretrained(pretrained)
config = AlbertConfig.from_json_file('./albert_chinese_base/config.json')
config.output_hidden_states = True
model = AlbertForSequenceClassification.from_pretrained(pretrained, config = config)
inputtext = "今天心情情很好啊,买了很多东西,我特别喜欢,终于有了自己喜欢的电子产品,这次总算可以好好学习了"
max_length = 128
tokenized_text=tokenizer.encode_plus(inputtext, add_special_tokens = True, # add [CLS], [SEP]
max_length = max_length, # max length of the text that can go to BERT
pad_to_max_length = True, # add [PAD] tokens
return_attention_mask = True, # add attention mask to not focus on pad tokens
return_tensors="pt")
outputs=model(input_ids=tokenized_text["input_ids"], token_type_ids=tokenized_text["token_type_ids"], attention_mask=tokenized_text["attention_mask"])
print(outputs.logits)`
but, when I run this code, an error occured as follows:
`Some weights of the model checkpoint at ./albert_chinese_base were not used when initializing AlbertForSequenceClassification: ['predictions.bias', 'predictions.LayerNorm.weight', 'predictions.LayerNorm.bias', 'predictions.dense.weight', 'predictions.dense.bias', 'predictions.decoder.weight', 'predictions.decoder.bias']
-This IS expected if you are initializing AlbertForSequenceClassification from the checkpoint of a model trained on another task or with another architecture (e.g. initializing a BertForSequenceClassification model from a BertForPreTraining model).
-This IS NOT expected if you are initializing AlbertForSequenceClassification from the checkpoint of a model that you expect to be exactly identical (initializing a BertForSequenceClassification model from a BertForSequenceClassification model).
Some weights of AlbertForSequenceClassification were not initialized from the model checkpoint at ./albert_chinese_base and are newly initialized: ['classifier.weight', 'classifier.bias']`
And I print outputs.logits value, found that the value is different every time you run,the logits value like this:
tensor([[0.3077, 0.1200]], grad_fn=)
tensor([[-0.3245, -0.3117]], grad_fn=)
so, I wonder AlbertForSequenceClassification model is not initialized correctly the value of ['classifier.weight', 'classifier.bias'] and
random value every time I run, and BertForSequenceClassification result output is right,so how can I slove the problem? | 03-09-2021 06:37:09 | 03-09-2021 06:37:09 | Hello! You're loading a model called `albert_chinese_base`; my guess is that this model only contains the base transformer model and not the sequence classification head that you need.
Does that make sense? You should use a model fine-tuned on sequence classification, and not a base model, if you want to do sequence classification. Of course, that model will be fine-tuned to a specific sequence classification task so it can't be used in any context.<|||||>This issue has been automatically marked as stale because it has not had recent activity. If you think this still needs to be addressed please comment on this thread.
Please note that issues that do not follow the [contributing guidelines](https://github.com/huggingface/transformers/blob/master/CONTRIBUTING.md) are likely to be ignored. |
transformers | 10,602 | closed | [examples template] added max_sample args and metrics changes | # What does this PR do?
This PR adds the same as https://github.com/huggingface/transformers/pull/10551 and https://github.com/huggingface/transformers/pull/10436 to the cookie-cutter template.
Fixes #10423
## Before submitting
- [ ] This PR fixes a typo or improves the docs (you can dismiss the other checks if that's the case).
- [x] Did you read the [contributor guideline](https://github.com/huggingface/transformers/blob/master/CONTRIBUTING.md#start-contributing-pull-requests),
Pull Request section?
- [x] Was this discussed/approved via a Github issue or the [forum](https://discuss.huggingface.co/)? Please add a link
to it if that's the case.
- [ ] Did you make sure to update the documentation with your changes? Here are the
[documentation guidelines](https://github.com/huggingface/transformers/tree/master/docs), and
[here are tips on formatting docstrings](https://github.com/huggingface/transformers/tree/master/docs#writing-source-documentation).
- [ ] Did you write any new necessary tests?
## review:
@stas00 | 03-09-2021 05:22:31 | 03-09-2021 05:22:31 | Ya @stas00,
Actually I figured that and tested visually with the example tamplate not the model template |
transformers | 10,601 | closed | Speedup tf tests | Fyi @sgugger @patrickvonplaten @stas00, I'm temporarily marking these tests as slow as they take more than 1h30 minutes and prevent the CI from completing, therefore preventing any relevant information from the TF tests.
I'm working on improving the CI times so this is temporary (will revert by Friday). | 03-09-2021 02:43:55 | 03-09-2021 02:43:55 | This single change made the tests on the TF CI pass from 6+ hours (non slow) to 29/31 minutes: https://github.com/huggingface/transformers/actions/runs/635711849
Will look for a way to reduce their time. |
transformers | 10,600 | closed | [docs] How to solve "Title level inconsistent" sphinx error | This PR documents an easy solution to the "Title level inconsistent" puzzle when adding a new sub-section to an `.rst` doc.
@sgugger | 03-09-2021 01:41:58 | 03-09-2021 01:41:58 | |
transformers | 10,599 | closed | Pass encoder outputs into GenerationMixin | # What does this PR do?
For encoder-decoder models such as T5, `GenerationMixin.generate()` currently runs both the encoder and the decoder. This PR allows one to pass already-computed `encoder_outputs` into this method, thus only the decoder will be run.
The flexibility to skip the encoder in the generation utilities is useful for several different scenarios, such as:
- The T5 encoder can encode `inputs_embeds` instead of `input_ids`. However, this is not possible within the generation utilities because only `input_ids` is accepted. With the changes in this PR, one can encode the `inputs_embeds` separately, and pass the encoder outputs to `generate()`. This is a partial solution to the issue https://github.com/huggingface/transformers/issues/6535.
- In some applications, the same encoder outputs are reused in different decoding processes. It would be computationally efficient not having to recompute the encoder outputs.
- This would also allow altering the encoder outputs for the purpose of incorporating additional information, etc. (In general, I think it is good practice to offer this option, where the encoding process is "decoupled" from the generation utilities.)
## Before submitting
- [ ] This PR fixes a typo or improves the docs (you can dismiss the other checks if that's the case).
- [x] Did you read the [contributor guideline](https://github.com/huggingface/transformers/blob/master/CONTRIBUTING.md#start-contributing-pull-requests),
Pull Request section?
- [x] Was this discussed/approved via a Github issue or the [forum](https://discuss.huggingface.co/)? Please add a link
to it if that's the case.
- [x] Did you make sure to update the documentation with your changes? Here are the
[documentation guidelines](https://github.com/huggingface/transformers/tree/master/docs), and
[here are tips on formatting docstrings](https://github.com/huggingface/transformers/tree/master/docs#writing-source-documentation).
- [ ] Did you write any new necessary tests?
## Who can review?
Anyone in the community is free to review the PR once the tests have passed. Feel free to tag
members/contributors which may be interested in your PR.
<!-- Your PR will be replied to more quickly if you can figure out the right person to tag with @
If you know how to use git blame, that is the easiest way, otherwise, here is a rough guide of **who to tag**.
Please tag fewer than 3 people.
Models:
- albert, bert, xlm: @LysandreJik
- blenderbot, bart, marian, pegasus, encoderdecoder, t5: @patrickvonplaten, @patil-suraj
- longformer, reformer, transfoxl, xlnet: @patrickvonplaten
- fsmt: @stas00
- funnel: @sgugger
- gpt2: @patrickvonplaten, @LysandreJik
- rag: @patrickvonplaten, @lhoestq
- tensorflow: @jplu
Library:
- benchmarks: @patrickvonplaten
- deepspeed: @stas00
- ray/raytune: @richardliaw, @amogkam
- text generation: @patrickvonplaten
- tokenizers: @n1t0, @LysandreJik
- trainer: @sgugger
- pipelines: @LysandreJik
Documentation: @sgugger
HF projects:
- nlp datasets: [different repo](https://github.com/huggingface/nlp)
- rust tokenizers: [different repo](https://github.com/huggingface/tokenizers)
Examples:
- maintained examples (not research project or legacy): @sgugger, @patil-suraj
- research_projects/bert-loses-patience: @JetRunner
- research_projects/distillation: @VictorSanh
-->
| 03-08-2021 23:40:18 | 03-08-2021 23:40:18 | Hi @ymfa , thanks a lot for the PR!
- The `generate` method does allow you to pass `input_embeds` as a keyword argument (`**model_kwargs` arg). If `input_embeds` is passed then T5 or any other model will use those instead of `input_ids`. If you look at the `generate` method signature, you can see that `input_ids` is optional.
- I think we can allow to pass `encoder_outputs` directly to `generate`. Pinging @patrickvonplaten <|||||>Hey @ymfa thanks for your PR and your thoughtful explanations in the description!
The design philosophy of `generate()` is that the 99% cases should be covered by `generate()` and for specific cases the sub-generated generate functions should be called directly as it is the case in the examples here:
https://github.com/huggingface/transformers/blob/0d909f6bd8ca0bc1ec8f42e089b64b4fffc4d230/src/transformers/generation_utils.py#L1592
As you can see, you only need to add a couple more lines when directly using `beam_search(...)` instead `generate(...)`. Could this solve your use case?
<|||||>Hi @patil-suraj , thanks for your comment.
I do realise that I can pass additional arguments via `**model_kwargs`. However, it doesn't work if I pass `inputs_embeds` instead of `input_ids`, because there are a number of places in `generate()` that depend on `input_ids`.
Specifically, this is the error I got (model is T5ForConditionalGeneration):
```
>>> model.generate(inputs_embeds=input_embeded)
Traceback (most recent call last):
...
ValueError: `bos_token_id` has to be defined when no `input_ids` are provided.
```
If I do pass `bos_token_id` (which shouldn't be necessary), this is the error (it's because the `input_ids` are created from `bos_token_id` and passed to the encoder):
```
>>> model.generate(inputs_embeds=input_embeded, bos_token_id=0)
Traceback (most recent call last):
...
ValueError: You cannot specify both inputs and inputs_embeds at the same time
```
So in the end, I am now using the method in this PR to achieve this purpose.<|||||>Thanks @patrickvonplaten .
To be honest I haven't found a way to make `beam_search()` work even for the simple use case of passing ids only. In this example (model is T5ForConditionalGeneration), `input_ids_batch` is the just 5 identical `input_ids` stacked together for the beam size.
```
>>> input_ids_batch.shape
torch.Size([5, 15])
>>> model.beam_search(input_ids_batch, beam_scorer)
Traceback (most recent call last):
...
File "...python3.7/site-packages/transformers/models/t5/modeling_t5.py", line 871, in forward
raise ValueError(f"You have to specify either {err_msg_prefix}inputs or {err_msg_prefix}inputs_embeds")
ValueError: You have to specify either inputs or inputs_embeds
```
When I pass the `encoder_outputs`:
```
>>> model.beam_search(decoder_input_ids_batch, beam_scorer, encoder_outputs=encoder_outputs)
Traceback (most recent call last):
...
File "...python3.7/site-packages/transformers/models/t5/modeling_t5.py", line 498, in forward
scores += position_bias
RuntimeError: The size of tensor a (3) must match the size of tensor b (15) at non-singleton dimension 3
```
I don't think it would be a simpler PR if I change `beam_search()` instead of `generate()`. It would also be less general as it doesn't apply to the other decoding methods, and it would require the user to prepare `beam_scorer` and `decoder_input_ids_batch`. <|||||>> scores += position_bias
I think you just need to broadcast your `decoder_input_ids_batch` to be of the same size as `encoder_outputs`, then it should work.
Overall, I'm not really in favor of adding this new special use case. To me it's not naturally to do `model.generate(None, decoder_input_ids=..., encoder_outputs=encoder_outputs)` lot of people won't understand that the first argument has in fact to be `None` since it corresponds to the encoder input ids. For such a case it should be relatively easy to make it work with `beam_search` to be honest. The philosophy here is that that both `decoder_input_ids` and `encoder_outputs.last_hidden_state` have to be of the sample batch dimensions, so `encoder_outputs.shape][0] == decoder_input_ids.shape[0]` . Then the command is as simple as:
```
model.beam_search(decoder_input_ids, beam_scorer, encoder_outputs)
```
The problem is that if we allow to many specific use cases for `generate()` the method becomes quite cluttered with if-statements again and I would like to avoid it. In this case, I think it's much cleaner to directly call the `beam_search` method tbh.<|||||>What do you think @patil-suraj ?<|||||>I've made a change so that the method signature of `generate()` remains the same as before. There is not "clutterness" added into this method now. This PR can be regarded as a fix, by properly handling the case when `encoder_outputs` is passed as one of the `model_kwargs`.
You're right that decoder_input_ids and encoder_outputs.last_hidden_state have to be of the sample batch dimensions. However, this means both of them need to be broadcast according to the beam size, which is not done automatically. A user would have to broadcast these tensors and objects manually in order to call `beam_search()`.
To be honest, I have been using the patch I submitted here for doing both beam search and sampling. The easy-to-use generation utility is one of my main reasons to choose the transformers package. I don't think it is a good idea to limit the potential based on "cleanness." On the contrary, I even suggest refactoring `generate()` more systematically, so that `input_ids` is no longer used like a central "currency" in this method.<|||||>Hey @ymfa,
It's actually a good point that changing between different generation methods while using `encoder_outputs` is not very user-friendly and "pre-computing" encoder_outputs is quite a common use case for all seq2seq models. Also since only the "helper" methods `_prepare...` are changed, I think I'm fine with the PR now! Thanks for being persistent here!
@patil-suraj @LysandreJik - it would be nice you could take a look as well.<|||||>I think `generate` now deserves its own doc page where we could explain this and maybe some more details like what the method supports, what are its limitations and what features won't be supported etc. It's changed significantly after the refactor and the design now follows a strict philosophy. It would be better to document that. What do you think @patrickvonplaten?
@ymfa The PR is good to merge, I'll merge it once you add helpful comments in `_prepare_input_ids_for_generation` as suggested by Patrick and Lysandre. |
transformers | 10,598 | closed | Check layer types for Optimizer construction | # What does this PR do?
As pointed out on the [forum](https://discuss.huggingface.co/t/parameter-groups-and-gpt2-layernorm/4239), `Trainer` currently excludes form weight decay layernorm layers by using a name pattern, which is not consistently followed by all models.
This PR actual checks the layer types and adds some tests. | 03-08-2021 21:11:35 | 03-08-2021 21:11:35 | |
transformers | 10,597 | closed | No model card for roberta-large-finetuned-wsc | # 🚀 Feature request
<!-- A clear and concise description of the feature proposal.
Please provide a link to the paper and code in case they exist. -->
## Motivation
I can not finetune this model `roberta-large-finetuned-wsc`, it doesn't have model card.
## Your contribution
Please fix this!
| 03-08-2021 21:03:20 | 03-08-2021 21:03:20 | Are you talking about this model? https://huggingface.co/mrm8488/roberta-large-finetuned-wsc<|||||>> Are you talking about this model? https://huggingface.co/mrm8488/roberta-large-finetuned-wsc
Yes, this model i can not call by transformers. So how can i use it?<|||||>You can't call it from transformers? Do you get an error?
It seems I can load it:
```py
>>> from transformers import AutoModelForMaskedLM
>>> model = AutoModelForMaskedLM.from_pretrained("mrm8488/roberta-large-finetuned-wsc")
```<|||||>> You can't call it from transformers? Do you get an error?
> It seems I can load it:
>
> ```python
> >>> from transformers import AutoModelForMaskedLM
> >>> model = AutoModelForMaskedLM.from_pretrained("mrm8488/roberta-large-finetuned-wsc")
> ```
I couldn't load it from "AutoModel". Thanks for your snippet! Anyways, should i finetune this for text classification task by removing Language Modeling head on top? Just for experiments!<|||||>You can load it in a text-classification auto model in order to fine-tune it to text-classification:
```py
>>> from transformers import AutoModelForSequenceClassification
... model = AutoModelForSequenceClassification.from_pretrained("mrm8488/roberta-large-finetuned-wsc")
```
It tells you that the LM head layers were discarded, and that it initialized randomly the layers for text-classification:
```
Some weights of the model checkpoint at mrm8488/roberta-large-finetuned-wsc were not used when initializing RobertaForSequenceClassification: ['lm_head.bias', 'lm_head.dense.weight', 'lm_head.dense.bias', 'lm_head.layer_norm.weight', 'lm_head.layer_norm.bias', 'lm_head.decoder.weight', 'lm_head.decoder.bias', 'roberta.pooler.dense.weight', 'roberta.pooler.dense.bias']
- This IS expected if you are initializing RobertaForSequenceClassification from the checkpoint of a model trained on another task or with another architecture (e.g. initializing a BertForSequenceClassification model from a BertForPreTraining model).
- This IS NOT expected if you are initializing RobertaForSequenceClassification from the checkpoint of a model that you expect to be exactly identical (initializing a BertForSequenceClassification model from a BertForSequenceClassification model).
Some weights of RobertaForSequenceClassification were not initialized from the model checkpoint at mrm8488/roberta-large-finetuned-wsc and are newly initialized: ['classifier.dense.weight', 'classifier.dense.bias', 'classifier.out_proj.weight', 'classifier.out_proj.bias']
You should probably TRAIN this model on a down-stream task to be able to use it for predictions and inference.
```
You should now fine-tune this on a text-classification dataset so that the randomly initialized layers may be trained!<|||||>Oh wow, i don't fine tune for classification by this way. I directly removed the LM head though. But still thank you Lysandre!<|||||>Happy to help! |
transformers | 10,596 | closed | Fairscale FSDP fix model save | # What does this PR do?
This PR fixes the fact training with fairscale fully-sharded wrapper was hanging: it looks like recent changes in fairscale make a synchronization during the model state dict call, which results in the training hanging if we don't call that state_dict method on all processes. This PR addresses that. | 03-08-2021 20:03:12 | 03-08-2021 20:03:12 | |
transformers | 10,595 | closed | Fix version control with anchors | # What does this PR do?
In urls containing an anchor (such as https://huggingface.co/transformers/master/installation.html#caching-models), the version controller was not finding the right version (basically because the page wasn't ending with .html). This PR fixes that.
Fixes #10559 | 03-08-2021 15:16:14 | 03-08-2021 15:16:14 | |
transformers | 10,594 | closed | [FeatureExtractorSavingUtils] Refactor PretrainedFeatureExtractor | # What does this PR do?
This PR refactors the class `PreTrainedFeatureExtractor`. The following changes are done to move functionality that is shared between sequence and image feature extractors into a separate file. This should unblock the PRs of [DETR](https://github.com/huggingface/transformers/pull/9998), [VIT](https://github.com/huggingface/transformers/pull/10513), and [CLIP](https://github.com/huggingface/transformers/pull/10426)
- `PreTrainedFeatureExtractor` is renamed to `PreTrainedSequenceFeatureExtractor` because it implicitly assumed that the it will treat only sequential inputs (a.k.a sequence of float values or a sequence of float vectors). `PreTrainedFeatureExtractor` was too general
- All functionality that is shared between Image and Speech feature extractors (which IMO all relates to "saving" utilities) is moved to a `FeatureExtractorSavingUtilsMixin`
- `BatchFeature` is moved from the `feature_extraction_sequence_utils.py` to `feature_extraction_common_utils.py` to be used by the `PreTrainedImageFeatureExtractor` class as well
- The tests are refactored accordingly
The following things were assumed before applying the changes.
- In the mid-term future there will only be three modalities in HF: text, sequential features (value sequence, vector sequence), image features (2D non-sequential array)
- Models, such as ViT, DETR & CLIP will call their "preprocessor" `VITFeatureExtractor`, .... IMO, feature extractor is also a fitting name for image recognition (see: https://en.wikipedia.org/wiki/Feature_extraction) so that it is assumed that for image-text or image-only models there will be a `PreTrainedImageFeatureExtractor`, a `VITFeatureExtractor`, (and maybe a VITTokenizer & VITProcessor as well, but not necessary). For vision-text models that do require both a tokenizer and a feature extractor such as CLIP it is assumed that the classes `CLIPFeatureExtractor` and `CLIPTokenizer` are wrapped into a `CLIPProcessor` class similar to `Wav2Vec2Processor`. I think this is the most important assumption that is taken here, so we should make sure we are on the same page here @LysandreJik @sgugger @patil-suraj @NielsRogge
- Image - Text or Image - only models won't require a `BatchImageFeature` or `BatchImage`, but can just use `BatchFeature`. From looking at the code in @NielsRogge's PR here: https://github.com/huggingface/transformers/pull/10513 this seems to be the case.
# Backwards compatibility:
The class `PreTrainedFeatureExtractor` was accessible via:
```python
from transformers import PreTrainedFeatureExtractor
```
but is now replaced by `PreTrainedSequenceFeatureExtractor`. However, since `PreTrainedFeatureExtractor` so far was only available on master, this change is OK IMO. | 03-08-2021 13:35:49 | 03-08-2021 13:35:49 | |
transformers | 10,593 | closed | Enable torch 1.8.0 on GPU CI | This enables torch 1.8.0 on the GPU CI, and disables the torch-scatter tests today as they're creating issues and blocking the CI pipeline. | 03-08-2021 12:11:28 | 03-08-2021 12:11:28 | |
transformers | 10,592 | closed | CUBLAS_STATUS_INTERNAL_ERROR at examples/question-answering/run_qa.py | ## Environment info
<!-- You can run the command `transformers-cli env` and copy-and-paste its output below.
Don't forget to fill out the missing fields in that output! -->
- `transformers` version: 4.4.0.dev0
- Platform: Linux-5.10.20-1-lts-x86_64-with-glibc2.2.5
- Python version: 3.8.3
- PyTorch version (GPU?): 1.8.0 (True)
- Tensorflow version (GPU?): not installed (NA)
- Using GPU in script?: Yes
- Using distributed or parallel set-up in script?: No
### Who can help
- @LysandreJik
- @sgugger
## Information
Model I am using (Bert, XLNet ...):
The problem arises when using:
* [x] the official example scripts: (give details below)
* [ ] my own modified scripts: (give details below)
The tasks I am working on is:
* [x] an official GLUE/SQUaD task: (give the name)
* [ ] my own task or dataset: (give details below)
## To reproduce
```
#!/bin/bash
python3 -m venv env
source env/bin/activate
pip install torch
pip install datasets
git clone https://github.com/huggingface/transformers.git
pip install -e transformers/
python transformers/examples/question-answering/run_qa.py \
--model_name_or_path bert-base-uncased \
--dataset_name squad \
--do_train \
--do_eval \
--per_device_train_batch_size 1 \
--learning_rate 3e-5 \
--num_train_epochs 4 \
--max_seq_length 384 \
--doc_stride 128 \
--output_dir ./models/
```
```
03/08/2021 09:54:08 - WARNING - __main__ - Process rank: -1, device: cuda:0, n_gpu: 2distributed training: False, 16-bits training: False
03/08/2021 09:54:08 - INFO - __main__ - Training/evaluation parameters TrainingArguments(output_dir=./models/, overwrite_output_dir=False, do_train=True, do_eval=True, do_predict=False, evaluation_strategy=IntervalStrategy.NO, prediction_loss_only=False, per_device_train_batch_size=1, per_device_eval_batch_size=8, gradient_accumulation_steps=1, eval_accumulation_steps=None, learning_rate=3e-05, weight_decay=0.0, adam_beta1=0.9, adam_beta2=0.999, adam_epsilon=1e-08, max_grad_norm=1.0, num_train_epochs=4.0, max_steps=-1, lr_scheduler_type=SchedulerType.LINEAR, warmup_ratio=0.0, warmup_steps=0, logging_dir=runs/Mar08_09-54-06_inf-105-gpu-1, logging_strategy=IntervalStrategy.STEPS, logging_first_step=False, logging_steps=500, save_strategy=IntervalStrategy.STEPS, save_steps=500, save_total_limit=None, no_cuda=False, seed=42, fp16=False, fp16_opt_level=O1, fp16_backend=auto, fp16_full_eval=False, local_rank=-1, tpu_num_cores=None, tpu_metrics_debug=False, debug=False, dataloader_drop_last=False, eval_steps=500, dataloader_num_workers=0, past_index=-1, run_name=./models/, disable_tqdm=False, remove_unused_columns=True, label_names=None, load_best_model_at_end=False, metric_for_best_model=None, greater_is_better=None, ignore_data_skip=False, sharded_ddp=[], deepspeed=None, label_smoothing_factor=0.0, adafactor=False, group_by_length=False, report_to=[], ddp_find_unused_parameters=None, dataloader_pin_memory=True, skip_memory_metrics=False, _n_gpu=2)
03/08/2021 09:54:08 - WARNING - datasets.builder - Reusing dataset squad (/home/blozano/.cache/huggingface/datasets/squad/plain_text/1.0.0/0fd9e01360d229a22adfe0ab7e2dd2adc6e2b3d6d3db03636a51235947d4c6e9)
[INFO|configuration_utils.py:463] 2021-03-08 09:54:09,206 >> loading configuration file https://huggingface.co/bert-base-uncased/resolve/main/config.json from cache at /home/blozano/.cache/huggingface/transformers/3c61d016573b14f7f008c02c4e51a366c67ab274726fe2910691e2a761acf43e.637c6035640bacb831febcc2b7f7bee0a96f9b30c2d7e9ef84082d9f252f3170
[INFO|configuration_utils.py:499] 2021-03-08 09:54:09,207 >> Model config BertConfig {
"architectures": [
"BertForMaskedLM"
],
"attention_probs_dropout_prob": 0.1,
"gradient_checkpointing": false,
"hidden_act": "gelu",
"hidden_dropout_prob": 0.1,
"hidden_size": 768,
"initializer_range": 0.02,
"intermediate_size": 3072,
"layer_norm_eps": 1e-12,
"max_position_embeddings": 512,
"model_type": "bert",
"num_attention_heads": 12,
"num_hidden_layers": 12,
"pad_token_id": 0,
"position_embedding_type": "absolute",
"transformers_version": "4.4.0.dev0",
"type_vocab_size": 2,
"use_cache": true,
"vocab_size": 30522
}
[INFO|configuration_utils.py:463] 2021-03-08 09:54:09,509 >> loading configuration file https://huggingface.co/bert-base-uncased/resolve/main/config.json from cache at /home/blozano/.cache/huggingface/transformers/3c61d016573b14f7f008c02c4e51a366c67ab274726fe2910691e2a761acf43e.637c6035640bacb831febcc2b7f7bee0a96f9b30c2d7e9ef84082d9f252f3170
[INFO|configuration_utils.py:499] 2021-03-08 09:54:09,510 >> Model config BertConfig {
"architectures": [
"BertForMaskedLM"
],
"attention_probs_dropout_prob": 0.1,
"gradient_checkpointing": false,
"hidden_act": "gelu",
"hidden_dropout_prob": 0.1,
"hidden_size": 768,
"initializer_range": 0.02,
"intermediate_size": 3072,
"layer_norm_eps": 1e-12,
"max_position_embeddings": 512,
"model_type": "bert",
"num_attention_heads": 12,
"num_hidden_layers": 12,
"pad_token_id": 0,
"position_embedding_type": "absolute",
"transformers_version": "4.4.0.dev0",
"type_vocab_size": 2,
"use_cache": true,
"vocab_size": 30522
}
[INFO|tokenization_utils_base.py:1721] 2021-03-08 09:54:10,138 >> loading file https://huggingface.co/bert-base-uncased/resolve/main/vocab.txt from cache at /home/blozano/.cache/huggingface/transformers/45c3f7a79a80e1cf0a489e5c62b43f173c15db47864303a55d623bb3c96f72a5.d789d64ebfe299b0e416afc4a169632f903f693095b4629a7ea271d5a0cf2c99
[INFO|tokenization_utils_base.py:1721] 2021-03-08 09:54:10,138 >> loading file https://huggingface.co/bert-base-uncased/resolve/main/tokenizer.json from cache at /home/blozano/.cache/huggingface/transformers/534479488c54aeaf9c3406f647aa2ec13648c06771ffe269edabebd4c412da1d.7f2721073f19841be16f41b0a70b600ca6b880c8f3df6f3535cbc704371bdfa4
[INFO|modeling_utils.py:1051] 2021-03-08 09:54:10,501 >> loading weights file https://huggingface.co/bert-base-uncased/resolve/main/pytorch_model.bin from cache at /home/blozano/.cache/huggingface/transformers/a8041bf617d7f94ea26d15e218abd04afc2004805632abc0ed2066aa16d50d04.faf6ea826ae9c5867d12b22257f9877e6b8367890837bd60f7c54a29633f7f2f
[WARNING|modeling_utils.py:1158] 2021-03-08 09:54:12,594 >> Some weights of the model checkpoint at bert-base-uncased were not used when initializing BertForQuestionAnswering: ['cls.predictions.bias', 'cls.predictions.transform.dense.weight', 'cls.predictions.transform.dense.bias', 'cls.predictions.decoder.weight', 'cls.seq_relationship.weight', 'cls.seq_relationship.bias', 'cls.predictions.transform.LayerNorm.weight', 'cls.predictions.transform.LayerNorm.bias']
- This IS expected if you are initializing BertForQuestionAnswering from the checkpoint of a model trained on another task or with another architecture (e.g. initializing a BertForSequenceClassification model from a BertForPreTraining model).
- This IS NOT expected if you are initializing BertForQuestionAnswering from the checkpoint of a model that you expect to be exactly identical (initializing a BertForSequenceClassification model from a BertForSequenceClassification model).
[WARNING|modeling_utils.py:1169] 2021-03-08 09:54:12,594 >> Some weights of BertForQuestionAnswering were not initialized from the model checkpoint at bert-base-uncased and are newly initialized: ['qa_outputs.weight', 'qa_outputs.bias']
You should probably TRAIN this model on a down-stream task to be able to use it for predictions and inference.
03/08/2021 09:54:12 - WARNING - datasets.arrow_dataset - Loading cached processed dataset at /home/blozano/.cache/huggingface/datasets/squad/plain_text/1.0.0/0fd9e01360d229a22adfe0ab7e2dd2adc6e2b3d6d3db03636a51235947d4c6e9/cache-a560de6b2f76743b.arrow
03/08/2021 09:54:12 - WARNING - datasets.arrow_dataset - Loading cached processed dataset at /home/blozano/.cache/huggingface/datasets/squad/plain_text/1.0.0/0fd9e01360d229a22adfe0ab7e2dd2adc6e2b3d6d3db03636a51235947d4c6e9/cache-15b011eed342eca6.arrow
[INFO|trainer.py:471] 2021-03-08 09:54:15,885 >> The following columns in the evaluation set don't have a corresponding argument in `BertForQuestionAnswering.forward` and have been ignored: example_id, offset_mapping.
[INFO|trainer.py:929] 2021-03-08 09:54:15,937 >> ***** Running training *****
[INFO|trainer.py:930] 2021-03-08 09:54:15,937 >> Num examples = 88524
[INFO|trainer.py:931] 2021-03-08 09:54:15,937 >> Num Epochs = 4
[INFO|trainer.py:932] 2021-03-08 09:54:15,937 >> Instantaneous batch size per device = 1
[INFO|trainer.py:933] 2021-03-08 09:54:15,937 >> Total train batch size (w. parallel, distributed & accumulation) = 2
[INFO|trainer.py:934] 2021-03-08 09:54:15,937 >> Gradient Accumulation steps = 1
[INFO|trainer.py:935] 2021-03-08 09:54:15,937 >> Total optimization steps = 177048
0%| | 0/177048 [00:00<?, ?it/s]Traceback (most recent call last):
File "transformers/examples/question-answering/run_qa.py", line 507, in <module>
main()
File "transformers/examples/question-answering/run_qa.py", line 481, in main
train_result = trainer.train(resume_from_checkpoint=checkpoint)
File "/home/blozano/finetune_qa/transformers/src/transformers/trainer.py", line 1036, in train
tr_loss += self.training_step(model, inputs)
File "/home/blozano/finetune_qa/transformers/src/transformers/trainer.py", line 1420, in training_step
loss = self.compute_loss(model, inputs)
File "/home/blozano/finetune_qa/transformers/src/transformers/trainer.py", line 1452, in compute_loss
outputs = model(**inputs)
File "/home/blozano/finetune_qa/env/lib/python3.8/site-packages/torch/nn/modules/module.py", line 889, in _call_impl
result = self.forward(*input, **kwargs)
File "/home/blozano/finetune_qa/env/lib/python3.8/site-packages/torch/nn/parallel/data_parallel.py", line 167, in forward
outputs = self.parallel_apply(replicas, inputs, kwargs)
File "/home/blozano/finetune_qa/env/lib/python3.8/site-packages/torch/nn/parallel/data_parallel.py", line 177, in parallel_apply
return parallel_apply(replicas, inputs, kwargs, self.device_ids[:len(replicas)])
File "/home/blozano/finetune_qa/env/lib/python3.8/site-packages/torch/nn/parallel/parallel_apply.py", line 86, in parallel_apply
output.reraise()
File "/home/blozano/finetune_qa/env/lib/python3.8/site-packages/torch/_utils.py", line 429, in reraise
raise self.exc_type(msg)
RuntimeError: Caught RuntimeError in replica 0 on device 0.
Original Traceback (most recent call last):
File "/home/blozano/finetune_qa/env/lib/python3.8/site-packages/torch/nn/parallel/parallel_apply.py", line 61, in _worker
output = module(*input, **kwargs)
File "/home/blozano/finetune_qa/env/lib/python3.8/site-packages/torch/nn/modules/module.py", line 889, in _call_impl
result = self.forward(*input, **kwargs)
File "/home/blozano/finetune_qa/transformers/src/transformers/models/bert/modeling_bert.py", line 1775, in forward
outputs = self.bert(
File "/home/blozano/finetune_qa/env/lib/python3.8/site-packages/torch/nn/modules/module.py", line 889, in _call_impl
result = self.forward(*input, **kwargs)
File "/home/blozano/finetune_qa/transformers/src/transformers/models/bert/modeling_bert.py", line 971, in forward
encoder_outputs = self.encoder(
File "/home/blozano/finetune_qa/env/lib/python3.8/site-packages/torch/nn/modules/module.py", line 889, in _call_impl
result = self.forward(*input, **kwargs)
File "/home/blozano/finetune_qa/transformers/src/transformers/models/bert/modeling_bert.py", line 568, in forward
layer_outputs = layer_module(
File "/home/blozano/finetune_qa/env/lib/python3.8/site-packages/torch/nn/modules/module.py", line 889, in _call_impl
result = self.forward(*input, **kwargs)
File "/home/blozano/finetune_qa/transformers/src/transformers/models/bert/modeling_bert.py", line 456, in forward
self_attention_outputs = self.attention(
File "/home/blozano/finetune_qa/env/lib/python3.8/site-packages/torch/nn/modules/module.py", line 889, in _call_impl
result = self.forward(*input, **kwargs)
File "/home/blozano/finetune_qa/transformers/src/transformers/models/bert/modeling_bert.py", line 387, in forward
self_outputs = self.self(
File "/home/blozano/finetune_qa/env/lib/python3.8/site-packages/torch/nn/modules/module.py", line 889, in _call_impl
result = self.forward(*input, **kwargs)
File "/home/blozano/finetune_qa/transformers/src/transformers/models/bert/modeling_bert.py", line 253, in forward
mixed_query_layer = self.query(hidden_states)
File "/home/blozano/finetune_qa/env/lib/python3.8/site-packages/torch/nn/modules/module.py", line 889, in _call_impl
result = self.forward(*input, **kwargs)
File "/home/blozano/finetune_qa/env/lib/python3.8/site-packages/torch/nn/modules/linear.py", line 94, in forward
return F.linear(input, self.weight, self.bias)
File "/home/blozano/finetune_qa/env/lib/python3.8/site-packages/torch/nn/functional.py", line 1753, in linear
return torch._C._nn.linear(input, weight, bias)
RuntimeError: CUDA error: CUBLAS_STATUS_INTERNAL_ERROR when calling `cublasCreate(handle)`
```
```
NVIDIA-SMI 460.56 Driver Version: 460.56 CUDA Version: 11.2
```
## Expected behavior
The expected default behavior as stated in transformers/examples/question-answering/README.md
| 03-08-2021 09:02:00 | 03-08-2021 09:02:00 | Hi! I don't think torch supports CUDA 11.2 yet. See https://github.com/pytorch/pytorch/issues/50232#issuecomment-777703998<|||||>I had a similar issue with torch 1.8 and solved it by downgrading to 1.7.1<|||||>> Hi! I don't think torch supports CUDA 11.2 yet. See [pytorch/pytorch#50232 (comment)](https://github.com/pytorch/pytorch/issues/50232#issuecomment-777703998)
Thanks for the quick response. I just tested the script with CUDA11.1 and it worked just fine.<|||||>This issue has been automatically marked as stale because it has not had recent activity. If you think this still needs to be addressed please comment on this thread.
Please note that issues that do not follow the [contributing guidelines](https://github.com/huggingface/transformers/blob/master/CONTRIBUTING.md) are likely to be ignored. |
transformers | 10,591 | closed | Fix typo in docstring for pipeline | # What does this PR do?
Fixed typo in docstring for pipeline ("conversation" -> "conversational")
<!--
Congratulations! You've made it this far! You're not quite done yet though.
Once merged, your PR is going to appear in the release notes with the title you set, so make sure it's a great title that fully reflects the extent of your awesome contribution.
Then, please replace this with a description of the change and which issue is fixed (if applicable). Please also include relevant motivation and context. List any dependencies (if any) that are required for this change.
Once you're done, someone will review your PR shortly (see the section "Who can review?" below to tag some potential reviewers). They may suggest changes to make the code even better. If no one reviewed your PR after a week has passed, don't hesitate to post a new comment @-mentioning the same persons---sometimes notifications get lost.
-->
<!-- Remove if not applicable -->
Fixes # (issue)
## Before submitting
- [x] This PR fixes a typo or improves the docs (you can dismiss the other checks if that's the case).
- [ ] Did you read the [contributor guideline](https://github.com/huggingface/transformers/blob/master/CONTRIBUTING.md#start-contributing-pull-requests),
Pull Request section?
- [ ] Was this discussed/approved via a Github issue or the [forum](https://discuss.huggingface.co/)? Please add a link
to it if that's the case.
- [ ] Did you make sure to update the documentation with your changes? Here are the
[documentation guidelines](https://github.com/huggingface/transformers/tree/master/docs), and
[here are tips on formatting docstrings](https://github.com/huggingface/transformers/tree/master/docs#writing-source-documentation).
- [ ] Did you write any new necessary tests?
## Who can review?
Anyone in the community is free to review the PR once the tests have passed. Feel free to tag
members/contributors which may be interested in your PR.
<!-- Your PR will be replied to more quickly if you can figure out the right person to tag with @
If you know how to use git blame, that is the easiest way, otherwise, here is a rough guide of **who to tag**.
Please tag fewer than 3 people.
Models:
- albert, bert, xlm: @LysandreJik
- blenderbot, bart, marian, pegasus, encoderdecoder, t5: @patrickvonplaten, @patil-suraj
- longformer, reformer, transfoxl, xlnet: @patrickvonplaten
- fsmt: @stas00
- funnel: @sgugger
- gpt2: @patrickvonplaten, @LysandreJik
- rag: @patrickvonplaten, @lhoestq
- tensorflow: @jplu
Library:
- benchmarks: @patrickvonplaten
- deepspeed: @stas00
- ray/raytune: @richardliaw, @amogkam
- text generation: @patrickvonplaten
- tokenizers: @n1t0, @LysandreJik
- trainer: @sgugger
- pipelines: @LysandreJik
Documentation: @sgugger
HF projects:
- nlp datasets: [different repo](https://github.com/huggingface/nlp)
- rust tokenizers: [different repo](https://github.com/huggingface/tokenizers)
Examples:
- maintained examples (not research project or legacy): @sgugger, @patil-suraj
- research_projects/bert-loses-patience: @JetRunner
- research_projects/distillation: @VictorSanh
-->
| 03-08-2021 09:01:58 | 03-08-2021 09:01:58 | |
transformers | 10,590 | closed | [M2M100] fix positional embeddings | # What does this PR do?
The torchscript tests for `M2M100` are failing on master. This is because the `weights` in `M2M100SinusoidalPositionalEmbedding` are initially not on the same device as the rest of the parameters.
The PR makes the `weights` as `nn.Parameter` so they'll be on the same device. | 03-08-2021 08:58:11 | 03-08-2021 08:58:11 | |
transformers | 10,589 | closed | Small question about BertForMaskedLM usage on TF model | Hi everyone,
I was using BertForMaskedLM to predict possible candidate words in the content.
For example:
**cat like to drink [MASK], so am I.**
on [tf1 bert-pretrained model](https://storage.googleapis.com/bert_models/2018_10_18/uncased_L-12_H-768_A-12.zip)
output would be
`[[{'sequence': '[CLS] cat like to drink it, so am i. [SEP]', 'score': 0.22914603352546692, 'token': 2009, 'token_str': 'i t'}, {'sequence': '[CLS] cat like to drink water, so am i. [SEP]', 'score': 0.1088637188076973, 'token': 2300, 'token_str': 'w a t e r'}, {'sequence': '[CLS] cat like to drink blood, so am i. [SEP]', 'score': 0.1075243279337883, 'token': 2668, 'token_str': 'b l o o d'}]]`
However, in [tf2 bert-pretrained model](https://storage.googleapis.com/cloud-tpu-checkpoints/bert/keras_bert/uncased_L-12_H-768_A-12.tar.gz)
output gives,
`[[{'sequence': '[CLS] cat like to drink∘, so am i. [SEP]', 'score': 0.0002078865800285712, 'token': 30126, 'token_str': '# # ∘'}, {'sequence': '[CLS] cat like to drink zinc, so am i. [SEP]', 'score': 0.00020266805950086564, 'token': 15813, 'token_str': 'z i n c'}, {'sequence': '[CLS] cat like to drink organic, so am i. [SEP]', 'score': 0.00019718357361853123, 'token': 7554, 'token_str': 'o r g a n i c'}]]
`
It seems tf2 will randomly give unreasonable words, but I have no idea why causing this.
I notice the implementation of BERT on TF1 and TF2 are different (I think so? since tf2 use keras as main core implementation), however, does the architecture vary from TF1 pretrained model to TF2 that cause BertForMaskedLM give different embedding? Does BertForMaskedLM support reading weight on TF2 model?
The code I am using are something like this
```
config = BertConfig.from_pretrained("./pretrained/tf2")
config.is_decoder=False
tokenizer = BertTokenizer.from_pretrained("./pretrained/tf2")
model = BertForMaskedLM.from_pretrained("./pretrained/tf2",config=config,from_tf=True)
```
tf1 checkpoint in FileZilla
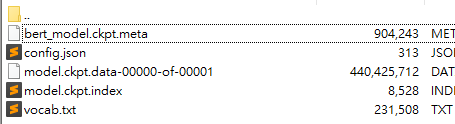
tf2
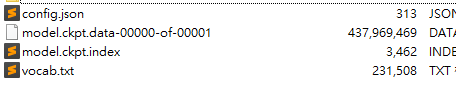
Am I using wrong API(like should I use other API instead other than BertForMaskedLM) or any place I have to change to make it work?
Any suggestion or reflection are sincerely grateful, thanks in advance! | 03-08-2021 08:41:02 | 03-08-2021 08:41:02 | Hi! In order to use either of those weights you'll have to convert them to a HuggingFace format.
For that you have two available scripts:
- From [TF1](https://github.com/huggingface/transformers/blob/master/src/transformers/models/bert/convert_bert_original_tf_checkpoint_to_pytorch.py)
- From [TF2](https://github.com/huggingface/transformers/blob/master/src/transformers/models/bert/convert_bert_original_tf2_checkpoint_to_pytorch.py)<|||||>Hi @LysandreJik! Thanks for always being kind and active to solve our questions, I have checked both TF1 and TF2 convert script which TF1 works perfectly to convert bert_model.ckpt to pytorch_model.bin, while the TF2 will always give error message as picture here:

To add on, in my task I keep training checkpoint model on my dataset by running [run_pretraining.py ](https://github.com/tensorflow/models/blob/master/official/nlp/bert/run_pretraining.py), so according to the script description on TF2 convert script, I believe the problem is mlm head which I have to add something above the convert script to convert model right? or keep running run_pretraining.py won't give such header on it?

If the header exist, how can I solve this problem? Even though I tried BERT for months but I am not that confident to say I understand how it works. In #9941 I have tried to add elif condition on m_name but seems it's a bad approach on this question.
Again, thanks for the reply! Really appreciated for giving me such direction to it!
<|||||>Hmmm, I see this is an issue indeed! Could you let me know how you obtained your TF2 checkpoint so that I may check it on my side?
You're welcome, happy to help :)<|||||>Sure! Due to the [repository](https://github.com/tensorflow/models/tree/master/official/nlp/bert#access-to-pretrained-checkpoints) haven't release chinese model yet, so the way I obtained this model is by these steps,
1. Download [bert-base-chinese](https://storage.googleapis.com/bert_models/2018_11_03/chinese_L-12_H-768_A-12.zip) from google-bert
2. Use this [script](https://github.com/tensorflow/models/blob/master/official/nlp/bert/tf2_encoder_checkpoint_converter.py) to convert tf1 checkpoint to tf2 with following args
`python tf2_encoder_checkpoint_converter.py --checkpoint_to_convert=$BASE_DIR/bert_model.ckpt --converted_checkpoint_path=tmp/ --bert_config_file=$BASE_DIR/bert_config.json`
note: $BASE_DIR is the path to the model directory (which is the model done by step 1 & 2)
3. Using [create_pretraining_data.py](https://github.com/tensorflow/models/blob/master/official/nlp/data/create_pretraining_data.py) to create the dataset that I want to keep training by its domain, the simple data I use can be found [here](https://drive.google.com/file/d/163IAWfgQZ1TIN6WYzWdoRwmFXDzGIRxj/view?usp=sharing).
The args I use are like this
`python create_pretraining_data.py --input_file=./sample.txt --output_file=ModelRecord --vocab_file=./$BASE_DIR/vocab.txt --max_seq_length=128 --max_predictions_per_seq=19 --masked_lm_prob=0.15 --random_seed=46 --dupe_factor=1`
4. Now we have ModelRecord, use tf2 version [run_pretraining.py](https://github.com/tensorflow/models/blob/master/official/nlp/bert/run_pretraining.py) to training model on these instances in ModelRecord. The args I use look like this
`python run_pretraining.py --input_file=ModelRecord --output_dir=tmp/Model_L128_B32 --bert_config_file=bert_config.json --max_seq_length=128 --max_predictions_per_seq=19 --do_train=True --train_batch_size=32 --num_train_steps=2000000 --num_warmup_steps=2000 --learning_rate=1e-5 --save_checkpoints_step=60000 --keep_checkpoint_max=4`
5. After training, the directory in Model_L128_B32(where model saved) containing these checkpoint,
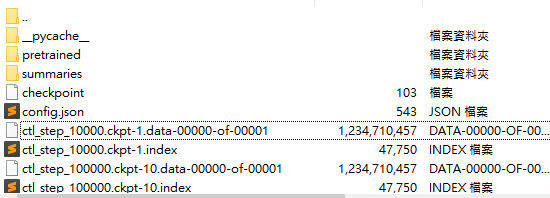
I load the checkpoint in directory "pretrained", and rename the checkpoint I want to evaluate to model.ckpt.data-00000-of-00001, ckpt_index to model.ckpt.index, with config and vocab in the directory.
### System Info
My environments are: (using pipreqs)
Python version: 3.7.5
CUDA used to build PyTorch: cuda_11.0_bu.TC445_37.28845127_0
OS: Ubuntu 18.04.5 LTS
GCC version: 7.5.0
### Versions of relevant libraries: (using pipreqs)
numpy==1.19.5
transformers==4.2.2
six==1.15.0
torch==1.7.1+cu110
tensorflow_gpu==2.2.0
gin_config==0.1.1
absl_py==0.11.0
tensorflow_hub==0.11.0
sentencepiece==0.1.94
absl==0.0
torchvision==0.8.2+cu110
bert4keras==0.9.9
gin==0.1.006
tensorflow==2.4.1
I think that's all of it, if I miss any step please inform me with no hesitate, I might get wrong :P
Best regards!
<|||||>I see, the conversion script should work for that use-case. Is there a way for you to share the checkpoints you have obtained so that I can take a look? You can share them through the hub under your username.<|||||>I test for a while and also follow what #8504 did, but now sure why not success
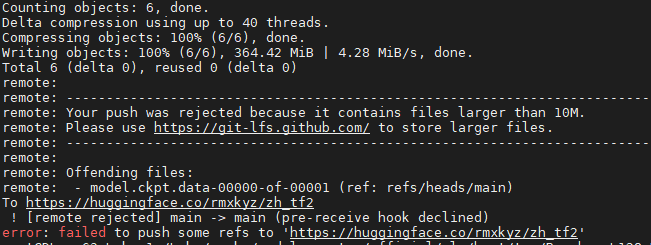
instead, I upload to [mydrive](https://drive.google.com/drive/folders/1e1xHXZQSEpHBI0YF6xLpUY2Ne8Zi7Cjl?usp=sharing), I will try it again tomorrow see if I miss something.<|||||>Ah, it's probably because you didn't install git-lfs/didn't track the files! Doing this in the repo should help:
```
git-lfs install
git-lfs track <name_of_your_large_file>
```
You can check it's being correctly tracked:
```
git-lfs track
```
check for the name of your large file and ensure it's being tracked by git-lfs:
```
Objects to be committed:
.gitattributes (Git: 31aaf10)
README.md (Git: 358442a)
config.json (Git: 57a54a8)
[...]
pytorch_model.bin (LFS: 6a9a9a5)
^^^
[...]
special_tokens_map.json (Git: e3ec7ab)
tokenizer_config.json (Git: ab033df)
vocab.txt (Git: 4d96f93)
```
Then you should be able to push without any issue<|||||>LGTM! The only issue I encounter is I don't actually know how these file being add.
```
pytorch_model.bin (LFS: 6a9a9a5)
^^^
```
My occasion for track and commit is
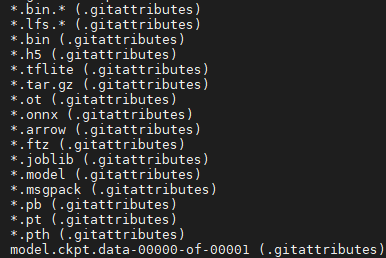
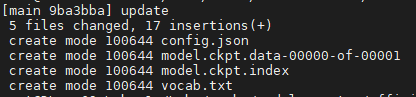
I assume this will be shown at the git commit -m "comment" phase? Anyway I finally upload my [model](https://huggingface.co/rmxkyz/zh_tf2/tree/main), weeee!
<|||||>Fantastic! I don't have time to look at it today, but I'll try and do that on Monday. Thanks!<|||||>Thanks for your kindly support! I am not rush with this experiment, so do it ease when you're free :D<|||||>This issue has been automatically marked as stale because it has not had recent activity. If you think this still needs to be addressed please comment on this thread.
Please note that issues that do not follow the [contributing guidelines](https://github.com/huggingface/transformers/blob/master/CONTRIBUTING.md) are likely to be ignored. |
transformers | 10,588 | closed | Can't reproduce xlm-roberta-large finetuned result on XNLI | # ❓ Questions & Help
I'm trying to finetune `xlm-roberta-large` on MNLI English training data and make zero-shot classification on XNLI dataset.
However, I found that `xlm-roberta-large` is super sensitive to hyper parameters. The reported average accuracy is 80.9, while my model can only achieve 79.74, which is 1% less than the reported accuracy.
I used Adam optimizer with 5e-6 learning rate and the batch size is 16. Any one can suggest better hyperparameters to reproduce the XNLI result of `xlm-roberta-large`?
| 03-08-2021 08:39:29 | 03-08-2021 08:39:29 | Hello, thanks for opening an issue! We try to keep the github issues for bugs/feature requests.
Could you ask your question on the [forum](https://discusss.huggingface.co) instead?
Thanks! |
transformers | 10,587 | open | [WIP] Add Linformer | # What does this PR do?
This PR adds [Linformer: Self-Attention with Linear Complexity](https://arxiv.org/abs/2006.04768) by Facebook AI. Contrary to the regular Transformer, it has linear complexity in both space and time w.r.t. the sequence length, allowing it to be trained on much larger sequence lengths.
I've created both a PT and TF version using the CookieCutter template (BERT-based, encoder-only). The only difference with BERT is that:
- `LinformerSelfAttention` looks a bit different compared to `BertSelfAttention`. The Linformer model adds 2 projection matrices to the keys and values, which project the sequence dimension (which is of size 512 by default) to a lower dimension k (such as `k=256`). As you'll see, I do not use the `extended_attention_mask` function which is used by other models. Instead, I cast the attention mask across the different attention heads by simply writing `attention_mask[:,None,:,None]`, which I then multiply by the keys and values, before projecting to the lower dimension.
- One can choose to use the same projection matrix for both keys and values. This is determined by the `share_projection` attribute of `LinformerConfig`.
- Linformer comes with 2 limitations: it cannot be used in the autoregressive case (see [this](https://github.com/lucidrains/linformer/issues/4#issuecomment-777781537)) - hence I removed the `is_decoder` logic, and it assumes a fixed sequence length. The latter is determined by the `seq_length` attribute of LinformerConfig, which is set to 512 by default. So if you provide `input_ids` and so on to the model, their length must be equal to the value of `seq_length`.
Fixes the following issues:
#4967
#5201
## Before submitting
- [ ] Did you write any new necessary tests? Yes I did, however I need some help to make sure all pass. Current status: for PyTorch, 30 are passing, 7 are failing. For Tensorflow, 26 passed, 9 failed. Note that there are currently no integration tests, as no weights were shared by the authors yet. However, they plan to release them as seen [here](https://github.com/pytorch/fairseq/issues/2795#issuecomment-720680448).
- [ ] Also, it seems that the original authors relied on RoBERTa rather than BERT, so we might have to let `LinformerTokenizer` inherit from `RobertaTokenizer` rather than `BertTokenizer`.
## Who can review?
@patil-suraj @LysandreJik
| 03-08-2021 08:15:22 | 03-08-2021 08:15:22 | This issue has been automatically marked as stale because it has not had recent activity. If you think this still needs to be addressed please comment on this thread.
Please note that issues that do not follow the [contributing guidelines](https://github.com/huggingface/transformers/blob/master/CONTRIBUTING.md) are likely to be ignored.<|||||>Unstale<|||||>This Linformer's ForSequenceClassification is not work. I finished mlm pretrain with 1.14loss(100epoch, wiki-cn). But in afqmf, acc only reach 0.6899<|||||>Hi @luoda888,
great to see you're already using Linformer! Did you pretrain Linformer on several GPUs using the `run_mlm.py` script? What is afqmf?
Note that in the [original paper](https://arxiv.org/abs/2006.04768), they state that "All models are pretrained with the masked-language-modeling (MLM) objective, and the training for all experiments are parallelized across 64 Tesla V100 GPUs with 250k updates."<|||||>@NielsRogge since the weights for `linformer` are not yet released, maybe we can add this model in the the `research_projects` dir, similar to [performer](https://github.com/huggingface/transformers/tree/master/examples/research_projects/performer) and add a training/fine-tuning script there.<|||||>> Hi @luoda888,
>
> great to see you're already using Linformer! Did you pretrain Linformer on several GPUs using the `run_mlm.py` script? What is afqmf?
>
> Note that in the [original paper](https://arxiv.org/abs/2006.04768), they state that "All models are pretrained with the masked-language-modeling (MLM) objective, and the training for all experiments are parallelized across 64 Tesla V100 GPUs with 250k updates."
Thanks reply.
1. I pre-trained the linformer model from scratch, using Wikipedia Chinese corpus. Set text maxlen=128, k=64, on 10 nodes (each with 8*32g V100) (which means I use 80*v100), 2.5 hours of pre-training. Using LinkerForMaskedLM, mlm loss reached 1.14 when training stopped.
2. I use the trained model for validation on downstream tasks, and afqmf is the semantic similarity (text matching) task in Chinese (https://github.com/CLUEbenchmark/CLUE). According to the report, the accuracy of bert-base-chinese is 74.16% when the parameter settings of bs=16, lr=2e-5, maxlen=128, epoch=3 are fine-tuned. I used the parameters of bs=256, lr=2e-5, maxlen=128, epoch=3 and the bert-base-chinese accuracy was 72.81%. However, when the LinformerForSequenceClassifier is used for classification, the AUC is only 0.5, and the accuracy is only 0.6899 (that is, all predictions are 0 categories).
3. For the specific training details, I use the Trainer interface, distributed pre-training. The config of the informer is seq_length=128, LinformerConfig(vocab_size=vocab_size, seq_length=seq_length, share_projection=True, k=seq_length//2) . I use BertWordPieceTokenizer for tokenizer, random mask (DataCollatorForLanguageModeling). with FP16 & train_batch_size=256, gradient_accumulation_steps=1, dataloader_num_workers=4, model.save_pretrained(output_files).
_**I analyze possible causes.**_
1. The fine-tuning script can run properly, and the bert-base-chinese open source weight is executed as expected.
2. Is it possible that the tokenizer is faulty? Inconsistent in pre-training and fine-tuning.
3. Press BertConfig again to perform pre-training. The fine-tuning effect is also AUC = 0.5. So I'm guessing that Bert Word Piece Tokenizer has some problems with Chinese.
If you don't mind, I'd like to share the code with you to locate the problem.<|||||>> @NielsRogge since the weights for `linformer` are not yet released, maybe we can add this model in the the `research_projects` dir, similar to [performer](https://github.com/huggingface/transformers/tree/master/examples/research_projects/performer) and add a training/fine-tuning script there.
I think it's a very good idea, and it would be nice to have a torch-only version available instead of jax, flax. Also, I've observed that transformers_plus_performers reproduce the performer paper, and I don't know if you've noticed.<|||||>I'd love you to come up with a pre-training script from the beginning on the Chinese Wikipedia and how to fine-tune the Chinese downstream tasks. In addition, it is possible to design more pre-training tasks (SpanMask + SBO, StuncBert(TGS), ASP, etc.) and design more fine-tuned multi-task learning (MT-DNN).
BTW, nezha is also can add in models. https://github.com/lonePatient/NeZha_Chinese_PyTorch. I test it in Chinese~
BTWWW, PGD & FGM & FreeAT & FreeLB & SMART also can add in modeling_language or fine-tune~~~~<|||||>That's really interesting to read! Wow, 80 V100's, that's a lot.
Some remarks:
* I still need someone to verify my implementation of `LinformerSelfAttention` in `modeling_linformer.py`, as I'm currently the only one that implemented it, I'd like to have a second opinion to know for sure my implementation is correct. I used [this implementation](https://github.com/mlpen/Nystromformer/blob/main/code/attention_linformer.py) (from the author of Nyströmformer, who benchmarked several efficient self-attention implementations) as a reference. I also checked @lucidrains' implementation, available [here](https://github.com/lucidrains/linformer). However, as you see the loss going down, it might indeed be a tokenizer issue (I'm fairly sure my implementation is correct).
* In both the original paper and the Nyströmformer implementation, it looks like they rely on a RoBERTa encoder rather than BERT, hence they also use `RobertaTokenizer` rather than `BertTokenizer`. So this is something that might have to be updated (as mentioned at the top of this PR).
* Did you use `BertTokenizer.from_pretrained("bert-base-chinese")`? Or did you train the tokenizer on Chinese Wikipedia before using it?
And indeed, it would be great if we can provide the same functionality for Chinese, and introduce pre-training/fine-tuning scripts for Chinese. <|||||>> That's really interesting to read! Wow, 80 V100's, that's a lot.
>
> Some remarks:
>
> * I still need someone to verify my implementation of `LinformerSelfAttention` in `modeling_linformer.py`, as I'm currently the only one that implemented it, I'd like to have a second opinion to know for sure my implementation is correct. I used [this implementation](https://github.com/mlpen/Nystromformer/blob/main/code/attention_linformer.py) (from the author of Nyströmformer, who benchmarked several efficient self-attention implementations) as a reference. I also checked @lucidrains' implementation, available [here](https://github.com/lucidrains/linformer). However, as you see the loss going down, it might indeed be a tokenizer issue (I'm fairly sure my implementation is correct).
> * In both the original paper and the Nyströmformer implementation, it looks like they rely on a RoBERTa encoder rather than BERT, hence they also use `RobertaTokenizer` rather than `BertTokenizer`. So this is something that might have to be updated (as mentioned at the top of this PR).
> * Did you use `BertTokenizer.from_pretrained("bert-base-chinese")`? Or did you train the tokenizer on Chinese Wikipedia before using it?
>
> And indeed, it would be great if we can provide the same functionality for Chinese, and introduce pre-training/fine-tuning scripts for Chinese.
```
limit_alphabat = 30000
special_tokens = ["[PAD]", "[UNK]", "[CLS]", "[SEP]", "[MASK]"]
tokenizer = tokenizers.BertWordPieceTokenizer(clean_text=True, handle_chinese_chars=True, strip_accents=True, lowercase=True)
tokenizer.train(
files,
vocab_size = args.vocab_size,
min_frequency = args.min_frequency,
show_progress = True,
special_tokens = special_tokens,
limit_alphabet = limit_alphabat,
wordpieces_prefix = "##",
)
try:
os.mkdir(output_files)
except:
pass
tokenizer.save_model(output_files)
```
MultiProcessing for tokenizer (1.3GB corpus is too large). Copy K times for dp mask (follow roberta)
```
def _convert_to_transformer_inputs(question, answer, tokenizer, max_sequence_length):
"""Converts tokenized input to ids, masks and segments for transformer (including bert)"""
def return_id(str1, str2, truncation_strategy, length):
inputs = tokenizer.encode_plus(str1, str2,
add_special_tokens=True,
max_length=length,
truncation_strategy=truncation_strategy,
truncation=True
)
input_ids = inputs["input_ids"]
input_masks = [1] * len(input_ids)
input_segments = inputs["token_type_ids"]
padding_length = length - len(input_ids)
padding_id = tokenizer.pad_token_id
input_ids = input_ids + ([padding_id] * padding_length)
input_masks = input_masks + ([0] * padding_length)
input_segments = input_segments + ([0] * padding_length)
return [input_ids, input_masks, input_segments]
input_ids_q, input_masks_q, input_segments_q = return_id(
question, answer, 'longest_first', max_sequence_length)
return input_ids_q
def compute_input_arrays(lines, tokenizer, max_sequence_length):
input_ids = Parallel(backend='multiprocessing', n_jobs=args.n_jobs, batch_size=512)\
(delayed(_convert_to_transformer_inputs)(line, None, tokenizer, max_sequence_length) for line in tqdm(lines))
return [i for i in np.asarray(input_ids, dtype=np.int32)]
tokenizer = tfs.BertTokenizer.from_pretrained(output_files + '/vocab.txt', maxlen=512)
with open(files, encoding="utf-8") as f:
lines = [line for line in tqdm(f.read().splitlines()) if (len(line) > 0 and not line.isspace())]
dataset = compute_input_arrays(lines, tokenizer, args.maxlen)
dp_mask = args.dp_mask
shuffle = deepcopy(dataset)
for i in tqdm(range(dp_mask)):
random.shuffle(shuffle)
dataset.extend(shuffle)
np.save(files + "-dpmask.npy", dataset)
logger.info("Sentence Cut Finish")
```
1. In many Chinese pre-training models, Roberta Tokenizer is often used as Bert Tokenizer, such as hfl:/roberta-chinese-wwm.
2. The same problem occurs when I use the BertTokenizer to train the Bert. After the same parameters are trained, the AUC is still 0.5.
```
class LineByLineTextDataset(Dataset):
"""
This will be superseded by a framework-agnostic approach soon.
"""
def __init__(self, tokenizer: PreTrainedTokenizer, file_path: str, block_size: int):
assert os.path.isfile(file_path), f"Input file path {file_path} not found"
# Here, we do not cache the features, operating under the assumption
# that we will soon use fast multithreaded tokenizers from the
# `tokenizers` repo everywhere =)
lines = np.load(files + '-dpmask.npy')
if args.debug:
lines = lines[:20000]
self.examples = [{"input_ids": torch.tensor(e, dtype=torch.long)} for e in lines]
def __len__(self):
return len(self.examples)
def __getitem__(self, i) -> Dict[str, torch.tensor]:
return self.examples[i]
vocab_size = 21128
seq_length = args.max_seq_length
config = LinformerConfig(vocab_size=vocab_size, seq_length=seq_length, share_projection=True, k=seq_length//2)
model = LinformerForMaskedLM(config=config)
tokenizer = tfs.BertTokenizer.from_pretrained(output_files + '/vocab.txt', maxlen=seq_length)
dataset = LineByLineTextDataset(tokenizer=tokenizer, file_path=files, block_size=seq_length)
datacol = tfs.DataCollatorForLanguageModeling(tokenizer=tokenizer, mlm=True, mlm_probability=0.15)
train_args = tfs.TrainingArguments(
output_dir = output_files,
overwrite_output_dir = True,
num_train_epochs = args.train_epochs,
per_device_train_batch_size = args.train_batch_size,
gradient_accumulation_steps = args.gradient_accumulation_steps,
save_steps = 10000,
logging_steps = 500,
save_total_limit = 10,
fp16 = args.fp16,
prediction_loss_only = True,
dataloader_num_workers = args.num_workers,
local_rank = args.local_rank,
disable_tqdm = False,
)
logger.info("TrainingArguments Init")
logger.info(train_args)
decay_parameters = get_parameter_names(model, [torch.nn.LayerNorm])
decay_parameters = [name for name in decay_parameters if "bias" not in name]
optimizer_grouped_parameters = [
{ "params": [p for n, p in model.named_parameters() if n in decay_parameters], "weight_decay": 0.0,},
{ "params": [p for n, p in model.named_parameters() if n not in decay_parameters], "weight_decay": 0.0,}
]
optimizer_cls = torch.optim.Adam(optimizer_grouped_parameters, lr=args.learning_rate)
lr_cls = torch.optim.lr_scheduler.CosineAnnealingLR(optimizer_cls, T_max=5, eta_min=0)
trainer = tfs.Trainer(
model = model,
args = train_args,
data_collator = datacol,
train_dataset = dataset,
optimizers = (optimizer_cls, lr_cls),
)
trainer.train()
model.save_pretrained(output_files)
```<|||||>> I still need someone to verify my implementation of LinformerSelfAttention in modeling_linformer.py, as I'm currently the only one that implemented it, I'd like to have a second opinion to know for sure my implementation is correct.
I would be happy to take a look now. Would you mind opening another PR and adding this in `research_projects/linformer` dir ? Just the modeling and config file should be enough.
Also, would it be possible to make the implem more similar to the official implem in `fairseq` ? That way we could compare apples to apples. We could then train a small model in `fairseq` and then port and compare it with this implem , that would make it a bit easier to verify the model.<|||||>> > I still need someone to verify my implementation of LinformerSelfAttention in modeling_linformer.py, as I'm currently the only one that implemented it, I'd like to have a second opinion to know for sure my implementation is correct.
>
> I would be happy to take a look now. Would you mind opening another PR and adding this in `research_projects/linformer` dir ? Just the modeling and config file should be enough.
>
> Also, would it be possible to make the implem more similar to the official implem in `fairseq` ? That way we could compare apples to apples. We could then train a small model in `fairseq` and then port and compare it with this implem , that would make it a bit easier to verify the model.
lol. I can provide GPUs for testing.<|||||>This issue has been automatically marked as stale because it has not had recent activity. If you think this still needs to be addressed please comment on this thread.
Please note that issues that do not follow the [contributing guidelines](https://github.com/huggingface/transformers/blob/master/CONTRIBUTING.md) are likely to be ignored.<|||||>unstale<|||||>What happened to this?
asking for a friend :D <|||||>Hi,
Well actually I wonder if we could investigate the issue with fine-tuning LinFormer.
@luoda888 would you be able to pre-train the model on English data (Books/Wikipedia)?<|||||>Hello,
Sounds preety cool tbh,
i'll try to tinker with. lets see if i go anywhere<|||||>> Hi,
>
> Well actually I wonder if we could investigate the issue with fine-tuning LinFormer.
>
> @luoda888 would you be able to pre-train the model on English data (Books/Wikipedia)?
I will try it |
transformers | 10,586 | closed | from_pretrained: check that the pretrained model is for the right model architecture | # What does this PR do?
Adding Checks to the from_pretrained workflow to check the model name passed belongs to the model being initiated.
Same checks need to be added for Tokenizer.
<!--
Congratulations! You've made it this far! You're not quite done yet though.
Once merged, your PR is going to appear in the release notes with the title you set, so make sure it's a great title that fully reflects the extent of your awesome contribution.
Then, please replace this with a description of the change and which issue is fixed (if applicable). Please also include relevant motivation and context. List any dependencies (if any) that are required for this change.
Once you're done, someone will review your PR shortly (see the section "Who can review?" below to tag some potential reviewers). They may suggest changes to make the code even better. If no one reviewed your PR after a week has passed, don't hesitate to post a new comment @-mentioning the same persons---sometimes notifications get lost.
-->
<!-- Remove if not applicable -->
Fixes https://github.com/huggingface/transformers/issues/10293
## Before submitting
- [ ] This PR fixes a typo or improves the docs (you can dismiss the other checks if that's the case).
- [ ] Did you read the [contributor guideline](https://github.com/huggingface/transformers/blob/master/CONTRIBUTING.md#start-contributing-pull-requests),
Pull Request section?
- [ ] Was this discussed/approved via a Github issue or the [forum](https://discuss.huggingface.co/)? Please add a link
to it if that's the case.
- [ ] Did you make sure to update the documentation with your changes? Here are the
[documentation guidelines](https://github.com/huggingface/transformers/tree/master/docs), and
[here are tips on formatting docstrings](https://github.com/huggingface/transformers/tree/master/docs#writing-source-documentation).
- [ ] Did you write any new necessary tests?
## Who can review?
Anyone in the community is free to review the PR once the tests have passed. Feel free to tag
members/contributors which may be interested in your PR.
<!-- Your PR will be replied to more quickly if you can figure out the right person to tag with @
If you know how to use git blame, that is the easiest way, otherwise, here is a rough guide of **who to tag**.
Please tag fewer than 3 people.
Models:
- albert, bert, xlm: @LysandreJik
- blenderbot, bart, marian, pegasus, encoderdecoder, t5: @patrickvonplaten, @patil-suraj
- longformer, reformer, transfoxl, xlnet: @patrickvonplaten
- fsmt: @stas00
- funnel: @sgugger
- gpt2: @patrickvonplaten, @LysandreJik
- rag: @patrickvonplaten, @lhoestq
- tensorflow: @jplu
Library:
- benchmarks: @patrickvonplaten
- deepspeed: @stas00
- ray/raytune: @richardliaw, @amogkam
- text generation: @patrickvonplaten
- tokenizers: @n1t0, @LysandreJik
- trainer: @sgugger
- pipelines: @LysandreJik
Documentation: @sgugger
HF projects:
- nlp datasets: [different repo](https://github.com/huggingface/nlp)
- rust tokenizers: [different repo](https://github.com/huggingface/tokenizers)
Examples:
- maintained examples (not research project or legacy): @sgugger, @patil-suraj
- research_projects/bert-loses-patience: @JetRunner
- research_projects/distillation: @VictorSanh
-->
| 03-08-2021 06:40:11 | 03-08-2021 06:40:11 | Hi @vimarshc, thank you for opening this PR! Could you:
- rebase your PR on the most recent master so that the failing tests don't fail anymore
- run `make fixup` at the root of your repository to fix your code quality issue (More information related to this on step 5 of [this document](https://github.com/huggingface/transformers/blob/master/CONTRIBUTING.md#start-contributing-pull-requests)<|||||>Awesome!
Would you like to attempt to add a test for this check?
We need to use tiny models so it's fast and I made the suggestions here:
https://github.com/huggingface/transformers/issues/10293#issuecomment-784630105
If you're not sure how to do it please let me know and I will add a test.
<|||||>Hi @stas00,
I'd like to add the tests myself if that's ok. I have to add the same checks for the `from_pretrained` for Tokenizer however it's not as straightforward. The Tokenizer's `from_pretrained` is written with some assumptions in mind and I'm not entirely sure where to add the check. Here's the `from_pretrained` method for Tokenizers.
Regardless, I shall try to add the test for this assertion I've already added and the changes mentioned by @LysandreJik in the next 24 hours.
<|||||>OK, so your change works for the model and the config:
```
PYTHONPATH=src python -c 'from transformers import PegasusForConditionalGeneration; PegasusForConditionalGeneration.from_pretrained("patrickvonplaten/t5-tiny-random")'
Traceback (most recent call last):
File "<string>", line 1, in <module>
File "/mnt/nvme1/code/huggingface/transformers-master/src/transformers/modeling_utils.py", line 975, in from_pretrained
config, model_kwargs = cls.config_class.from_pretrained(
File "/mnt/nvme1/code/huggingface/transformers-master/src/transformers/configuration_utils.py", line 387, in from_pretrained
assert (
AssertionError: You tried to initiate a model of type 'pegasus' with a pretrained model of type 't5'
```
same for:
```
PYTHONPATH=src python -c 'from transformers import PegasusConfig; PegasusConfig.from_pretrained("patrickvonplaten/t5-tiny-random")'
```
As you discovered - and I didn't know - the tokenizer doesn't seem to need the config file, so it doesn't look there is a way to check that the tokenizer being downloaded is of the right kind. I will ask.
And yes, it's great if you can add the test - thank you.
I restyled your PR to fit our style guide - we don't use `format` and you need to run the code through `make fixup` or `make style` (slower) before committing - otherwise CIs may fail. Which is what @LysandreJik was requesting.
https://github.com/huggingface/transformers/blob/master/CONTRIBUTING.md#start-contributing-pull-requests
So please `git pull` your branch to get my updates.<|||||>Hi @stas00,
Thanks for the update.
Will take a pull, add the test and go through the checklist before pushing the changes.
Will try to push in a few hours. <|||||>I'm puzzled. why did you undo my fix? If you want to restore it, it was:
```
--- a/src/transformers/configuration_utils.py
+++ b/src/transformers/configuration_utils.py
@@ -384,6 +384,9 @@ class PretrainedConfig(object):
"""
config_dict, kwargs = cls.get_config_dict(pretrained_model_name_or_path, **kwargs)
+ assert (
+ config_dict["model_type"] == cls.model_type
+ ), f"You tried to initiate a model of type '{cls.model_type}' with a pretrained model of type '{config_dict['model_type']}'"
return cls.from_dict(config_dict, **kwargs)
@classmethod
```<|||||>Hi,
Apologies.
I rebased my branch and assumed had to force push which deleted your changes. <|||||>Hi,
I have added the tests.
Everything seems to be working fine.
However, I pushed after taking a pull from the master, and yet it's showing a merge conflict. Not sure how that got there. <|||||>you messed up your PR branch - so this PR now contains dozens of unrelated changes.
You can do a soft reset to the last good sha, e.g.:
```
git reset --soft d70a770
git commit
git push -f
```
Just save somewhere your newly added test code first.
<|||||>I think you picked the wrong sha and ended up with an even worse situation. Try `d70a770` as I suggested.<|||||>OK, so looking at the errors - need to solve 2 issues:
### Issue 1.
```
assert (
> config_dict["model_type"] == cls.model_type
), f"You tried to initiate a model of type '{cls.model_type}' with a pretrained model of type '{config_dict['model_type']}'"
E KeyError: 'model_type'
```
so some models don't have the `model_type` key.
@vimarshc, I suppose you need to edit the code to skip this assert if we don't have the data.
You can verify that your change works with this test:
```
pytest -sv tests/test_trainer.py::TrainerIntegrationTest -k test_early_stopping_callback
```
I looked at the config.json generated by this test and it's:
```
{
"a": 0,
"architectures": [
"RegressionPreTrainedModel"
],
"b": 0,
"double_output": false,
"transformers_version": "4.4.0.dev0"
}
```
so far from being complete.
### Issue 2
This one looks trickier:
```
E AssertionError: You tried to initiate a model of type 'blenderbot-small' with a pretrained model of type 'blenderbot'
```
We will ask for help with this one.<|||||>@patrickvonplaten, @patil-suraj - your help is needed here.
BlenderbotSmall has an inconsistency. It declares its model type as "blenderbot-small":
```
src/transformers/models/auto/configuration_auto.py: ("blenderbot-small", BlenderbotSmallConfig),
src/transformers/models/auto/configuration_auto.py: ("blenderbot-small", "BlenderbotSmall"),
src/transformers/models/blenderbot_small/configuration_blenderbot_small.py: model_type = "blenderbot-small"
```
but the pretrained models all use `model_type: blenderbot`: https://huggingface.co/facebook/blenderbot-90M/blob/main/config.json
So this new sanity check this PR is trying to add fails.
```
config_dict, kwargs = cls.get_config_dict(pretrained_model_name_or_path, **kwargs)
> assert (
config_dict["model_type"] == cls.model_type
), f"You tried to initiate a model of type '{cls.model_type}' with a pretrained model of type '{config_dict['model_type']}'"
E AssertionError: You tried to initiate a model of type 'blenderbot-small' with a pretrained model of type 'blenderbot'
```
What shall we do?
It's possible that that part of the config object needs to be re-designed, so that there is a top architecture/type and then perhaps sub-types?
<|||||>Hi @stas00
Will add the check you mentioned today.
<|||||>Looks good, @vimarshc
So we are down to one failing test:
```
tests/test_modeling_blenderbot_small.py::Blenderbot90MIntegrationTests::test_90_generation_from_short_input
```<|||||>I wonder if we could sort of cheat and do:
```
if not cls.model_type in config_dict["model_type"]: assert ...
```
so this will check whether the main type matches as a substring of a sub-type. It's not a precise solution, but will probably catch the majority of mismatches.
Actually for t5/mt5 it's reversed. `model_type` are t5 and mt5, but both may have `T5ForConditionalGeneration` as `architecture`.
https://huggingface.co/google/mt5-base/blob/main/config.json#L16 since `MT5ForConditionalGeneration` is a copy of `T5ForConditionalGeneration` with the only difference of having `model_type = "mt5"`
So I think this check could fail in some situations. In which case we could perhaps check if one is a subset of another in either direction?
```
if not (cls.model_type in config_dict["model_type"] or config_dict["model_type"] in cls.model_type): assert ...
```
So this proposes a sort of fuzzy-match.
<|||||>>BlenderbotSmall has an inconsistency. It declares its model type as "blenderbot-small":
@stas00 You are right. Before the BART refactor all `blenderbot` models shared the same model class, but the config was not updated after the refactor. The `model_type` on the hub should be `blenderbot-small`. I will fix that.<|||||>I updated the config https://huggingface.co/facebook/blenderbot-90M/blob/main/config.json.
And actually, there's a new version of `blenderbot-90M` , https://huggingface.co/facebook/blenderbot_small-90M
It's actually the same model, but with the proper name. The blenderbot small test uses `blenderbot-90M` which should be changed to use this new model.<|||||>Hi @stas00,
The fuzzy match approach will not work for the case 'distilbert' vs 'bert'. <|||||>> Hi @stas00,
> The fuzzy match approach will not work for the case 'distilbert' vs 'bert'.
That's an excellent counter-example! As I proposed that it might mostly work ;)
But it looks like your original solution will now work after @patil-suraj fixing.
some unrelated test is failing - I rebased this branch - let's see if it will be green now.<|||||>> I updated the config https://huggingface.co/facebook/blenderbot-90M/blob/main/config.json.
>
> And actually, there's a new version of `blenderbot-90M` , https://huggingface.co/facebook/blenderbot_small-90M
>
> It's actually the same model, but with the proper name. The blenderbot small test uses `blenderbot-90M` which should be changed to use this new model.
Thank you, Suraj!
Since it's sort of related to this PR, do you want to push the change in here, or do it in another PR?<|||||>Oh bummer, we have 2 more in TF land:
```
FAILED tests/test_modeling_tf_flaubert.py::TFFlaubertModelTest::test_compile_tf_model
FAILED tests/test_modeling_tf_flaubert.py::TFFlaubertModelTest::test_save_load
```
same issue for both tests:
```
E AssertionError: You tried to initiate a model of type 'xlm' with a pretrained model of type 'flaubert'
```
@LysandreJik, who can help resolving this one? Thank you!
<|||||>Yes, I'll take a look as soon as possible!<|||||>I fixed the tests related to FlauBERT. Flax test is a flaky test that @patrickvonplaten is working on solving, and should not block this PR.<|||||>Thank you for taking care of this, @LysandreJik
I suppose we will take care of potentially doing the same for the Tokenizer validation in another PR.<|||||>With the tokenizer it'll likely be a bit more complex, as it is perfectly possible to have decoupled models/tokenizers, e.g., a BERT model and a different tokenizer like it is the case in [BERTweet (config.json)](https://huggingface.co/vinai/bertweet-base/blob/main/config.json).<|||||>Indeed, I think this will require a change where there is a required `tokenizer_config.json` which identifies itself which arch it belongs to, so while it should be possible to mix a model and tokenizer from different architectures, this shouldn't fail with random misleading errors like:
```
python -c 'from transformers import BartTokenizer; BartTokenizer.from_pretrained("prajjwal1/bert-tiny")'
Traceback (most recent call last):
File "<string>", line 1, in <module>
File "/mnt/nvme1/code/huggingface/transformers-master/src/transformers/tokenization_utils_base.py", line 1693, in from_pretrained
raise EnvironmentError(msg)
OSError: Can't load tokenizer for 'prajjwal1/bert-tiny'. Make sure that:
- 'prajjwal1/bert-tiny' is a correct model identifier listed on 'https://huggingface.co/models'
- or 'prajjwal1/bert-tiny' is the correct path to a directory containing relevant tokenizer files
```
but to indicate to the user that they got either the wrong tokenizer class or the the tokenizer identifier, since the above error is invalid - it's the correct identifier
As can be seen from:
```
python -c 'from transformers import BertTokenizer; BertTokenizer.from_pretrained("prajjwal1/bert-tiny")'
```
which works.
(and it erroneously says "model identifier" and there is no model here, but that's an unrelated minor issue).
And of course there are many other ways I have seen this mismatch to fail, usually a lot noisier when it's missing some file.
<|||||>@LysandreJik, I rebased this PR and it looks good. v4.4.0 is out so we can probably merge this one now.
Thank you.<|||||>Indeed, this is great! Thanks a lot @vimarshc and @stas00 for working on this.<|||||>So should I create a new issue for doing the same for the Tokenizers? I think it'd be much more complicated since we don't save any tokenizer data at the moment that puts the tokenizer in any category/architecture.<|||||>Hi,
Thanks, @stas00 for providing the guidance to close this issue. This is my first contribution to transformers so you can imagine my excitement. :D
I understand that a similar change for Tokenizer will be a bit more complicated. Would love to take a shot at fixing that as well. :) <|||||>I'm glad to hear it was a good experience for you, @vimarshc.
I'm not quite sure yet how to tackle the same for tokenizers. I will try to remember to tag you if we can think of an idea on how to approach this task. |
transformers | 10,585 | closed | [run_seq2seq] fix nltk lookup | Hmm, CI crashes every so often on
```
try:
nltk.data.find("tokenizers/punkt")
except LookupError:
```
introduced in this PR: https://github.com/huggingface/transformers/pull/10407
https://app.circleci.com/pipelines/github/huggingface/transformers/20635/workflows/989fde0b-e543-4620-9d9a-f213ad53dd9b/jobs/176742
```
__________________ ERROR collecting examples/test_examples.py __________________
examples/test_examples.py:51: in <module>
import run_seq2seq
examples/seq2seq/run_seq2seq.py:54: in <module>
nltk.data.find("tokenizers/punkt")
../.local/lib/python3.6/site-packages/nltk/data.py:539: in find
return FileSystemPathPointer(p)
../.local/lib/python3.6/site-packages/nltk/compat.py:41: in _decorator
return init_func(*args, **kwargs)
../.local/lib/python3.6/site-packages/nltk/data.py:315: in __init__
raise IOError("No such file or directory: %r" % _path)
E OSError: No such file or directory: '/home/circleci/nltk_data/tokenizers/punkt/PY3'
```
which is odd, re-running the job fixed the problem.
So trying to mend it with:
```
try:
nltk.data.find("tokenizers/punkt")
except (LookupError, OSError):
```
not sure why the Exception was different here.
@sgugger, @LysandreJik
| 03-08-2021 06:00:18 | 03-08-2021 06:00:18 | I'm going to merge this since the issue could be interfering with other PRs. |
transformers | 10,584 | closed | [examples tests] various fixes | This PR is fixing slow examples tests that currently fail on scheduled CI
2 more tests will be fixed by https://github.com/huggingface/transformers/pull/10551
This PR:
Sharded DDP issues:
* fixes fully sharded ddp enum - and the corresponding tests
* 2 sharded ddp tests currently hang with master fairscale - add skip until this is sorted out - didn't want to step on @sgugger's toes - so for now just skipping
Tests:
* changes a large group of tests to check loss is not nan
* fix `test_run_seq2seq_slow` test
- missed by my PR https://github.com/huggingface/transformers/pull/10428
- make it more resilient - was failing quality-wise on multi-gpu
* then we have an issue with apex - once run in a worker directly it breaks other tests running directly in the same pytest worker:
```
# XXX: apex breaks the trainer if it's run twice e.g. run_seq2seq.main() from the same
# program and it breaks other tests that run from the same pytest worker, therefore until this is
# sorted out it must be run only in an external program, that is distributed=True in this
# test and only under one or more gpus - if we want cpu will need to make a special test
#
# specifically to the problem traced it to self.optimizer.step() - if it's run 2nd time via
# 2nd main() call it botches the future eval.
```
I'm not quite sure what happens but I think no-one will run into this in a normal situation, here we basically end up running:
```
main()
main()
```
in the same process. We have some internal state that doesn't get reset.
So as I wrote above I used a workaround of running the apex integration test in a separate process so that it doesn't affect the rest of the test suite.
Of course if you have ideas on what the problem is and how to fix it I'm all ears. It's very simple to reproduce it:
```
def test_run_seq2seq_apex(self):
self.run_seq2seq_quick(distributed=True, extra_args_str="--fp16 --fp16_backend=apex")
# test 2nd time - was getting eval_loss': nan'
# to reproduce the problem set distributed=False
self.run_seq2seq_quick(distributed=True, extra_args_str="--fp16 --fp16_backend=apex")
```
I can move it out into a separate PR if it's too much for one.
@LysandreJik, @sgugger | 03-08-2021 05:53:57 | 03-08-2021 05:53:57 | It's still hanging waiting for something. If I'm not mistaken it's hanging in saving the model. but there is another thread that might be relevant:
```
Thread 0x00007f6dd984b740 (most recent call first):
File "/home/stas/anaconda3/envs/main-38/lib/python3.8/site-packages/torch/_utils.py", line 45 in _type
File "/home/stas/anaconda3/envs/main-38/lib/python3.8/site-packages/torch/cuda/__init__.py", line 496 in type
File "/home/stas/anaconda3/envs/main-38/lib/python3.8/site-packages/torch/storage.py", line 72 in cpu
File "/home/stas/anaconda3/envs/main-38/lib/python3.8/site-packages/torch/serialization.py", line 488 in _save
File "/home/stas/anaconda3/envs/main-38/lib/python3.8/site-packages/torch/serialization.py", line 372 in save
File "/mnt/nvme1/code/huggingface/transformers-test-fix/src/transformers/modeling_utils.py", line 835 in save_pretrained
File "/mnt/nvme1/code/huggingface/transformers-test-fix/src/transformers/trainer.py", line 1535 in _save
File "/mnt/nvme1/code/huggingface/transformers-test-fix/src/transformers/trainer.py", line 1496 in save_model
File "/mnt/nvme1/code/huggingface/transformers-test-fix/src/transformers/trainer.py", line 1204 in _save_checkpoint
File "/mnt/nvme1/code/huggingface/transformers-test-fix/src/transformers/trainer.py", line 1179 in _maybe_log_save_evaluate
File "/mnt/nvme1/code/huggingface/transformers-test-fix/src/transformers/trainer.py", line 1088 in train
File "/mnt/nvme1/code/huggingface/transformers-test-fix/examples/seq2seq/run_seq2seq.py", line 590 in main
File "/mnt/nvme1/code/huggingface/transformers-test-fix/examples/seq2seq/run_seq2seq.py", line 654 in <module>
Thread 0x00007f59389d2740 (most recent call first):
File "/mnt/nvme1/code/huggingface/transformers-test-fix/src/transformers/trainer.py", line 1552 in store_flos
File "/mnt/nvme1/code/huggingface/transformers-test-fix/src/transformers/trainer.py", line 1132 in train
File "/mnt/nvme1/code/huggingface/transformers-test-fix/examples/seq2seq/run_seq2seq.py", line 590 in main
File "/mnt/nvme1/code/huggingface/transformers-test-fix/examples/seq2seq/run_seq2seq.py", line 654 in <module>
```
Right after:
```
Saving model checkpoint to /tmp/tmp4rujlsox/checkpoint-1 'epoch': 1.0}
stderr: Configuration saved in /tmp/tmp4rujlsox/checkpoint-1/config.json
```
You can add:
```
--- a/examples/seq2seq/run_seq2seq.py
+++ b/examples/seq2seq/run_seq2seq.py
@@ -649,4 +649,6 @@ def _mp_fn(index):
if __name__ == "__main__":
+ import faulthandler
+ faulthandler.dump_traceback_later(20, repeat=True)
main()
```
To get the hanging bt.
And it's best debug outside of pytest, otherwise some bt gets messed up it seems.<|||||>Oh? It was running to completion on my side after the removal. So let's merge as is and I will look into the failure when I have time. |
transformers | 10,583 | closed | [trainer] fix double wrapping + test | We have an issue with
```
trainer.train()
trainer.train()
```
under any environment that requires model wrapping - we currently get the wrapping multiple times - and things may kind of work - but most of the time it breaks badly - thanks to apex for complaining noisily when it's being wrapped second time.
i.e. we get things like `DataParallel(DataParallel(model))`
This PR fixes this problem and adds a test.
@sgugger, @LysandreJik | 03-08-2021 05:31:10 | 03-08-2021 05:31:10 | |
transformers | 10,582 | closed | wrong model used for BART Summarization example | I'm pretty sure that `bart-large` was not trained for summarization, I replaced it with `bart-large-cnn` which is a model that was fine-tuned for summarization
# What does this PR do?
replace model used in Summarization example
| 03-08-2021 03:37:27 | 03-08-2021 03:37:27 | |
transformers | 10,581 | closed | wav2vec2: support datasets other than LibriSpeech | # What does this PR do?
Building on #10145, I'm adding support for the two other speech datasets (besides LibriSpeech) for ASR at the time of writing (`timit_asr` and `arabic_speech_corpus`), which require the following:
* Custom validation split name
* On-the-fly resampling support to target feature extractor's sampling rate (via `librosa` -- see `requirements.txt`)
* Max duration (in seconds) filter to remove outliers (which may crash GPU training when running OOM)
* Verbose logging to help debug custom datasets, including reverse transliteration (via `lang-trans` -- see `requirements.txt`)
* Pre-processing: tokenization and text normalization using orthography rules
* Casing (`do_lower_case`)
* Custom vocab for tokens used in orthography (e.g., Buckwalter transliteration for Arabic)
* Custom word delimiter token (when the default, `"|"`, is used in orthography)
* Transformations similar to those in `jiwer` to normalize transcripts for training
* Removing special words (e.g., "sil" which can be used to indicate silence)
* Translation table (e.g., "-" -> " " to break compounds like "quarter-century-old")
* Cleaning up characters not in vocab (after applying the rules above)
Arabic model: https://huggingface.co/elgeish/wav2vec2-large-xlsr-53-arabic
TIMIT models: https://huggingface.co/elgeish/wav2vec2-base-timit-asr and https://huggingface.co/elgeish/wav2vec2-large-lv60-timit-asr
## Who can review?
@patrickvonplaten @sgugger @LysandreJik @patil-suraj @SeanNaren
| 03-08-2021 01:01:33 | 03-08-2021 01:01:33 | Thanks to a fix for `timit_asr` that @patrickvonplaten made, now I have some good results using `wav2vec2-base`:
<img width="1050" alt="timit_asr_pr_ 10581" src="https://user-images.githubusercontent.com/6879673/110573935-0435e480-8111-11eb-8e0e-845af4e2eab7.png">
I'm running one for `arabic_speech_corpus` using `wav2vec2-base` as well. @patrickvonplaten let me know when you have the rest of configs for https://huggingface.co/facebook/wav2vec2-large-xlsr uploaded so I can try it as well (or a workaround). Thanks!<|||||>Thanks for adding this functionality! Your script worked succesfully when I fine-tuned `wav2vec2-base` , `xlsr` (https://dl.fbaipublicfiles.com/fairseq/wav2vec/xlsr_53_56k.pt) and `wa2vec2-large-100k` (multilingual Large Model from https://github.com/facebookresearch/voxpopuli#pre-trained-models pre-trained on VoxPopuli dataset) on TIMIT dataset. If fine-tuning on some another custom dataset, is it enough to set `--orthography` to `timit` in `run_asr.py` if the transcriptions are lowercased and `librispeech` if they are uppercased?<|||||>> Thanks for adding this functionality! Your script worked succesfully when I fine-tuned `wav2vec2-base` , `xlsr` (https://dl.fbaipublicfiles.com/fairseq/wav2vec/xlsr_53_56k.pt) and `wa2vec2-large-100k` (multilingual Large Model from https://github.com/facebookresearch/voxpopuli#pre-trained-models pre-trained on VoxPopuli dataset) on TIMIT dataset.
Thanks, @Getmany1 - you can do me a favor and run it with `arabic_speech_corpus` dataset and `--target_feature_extractor_sampling_rate --orthography buckwalter` on `xlsr` to verify it works with extended vocab. Unfortunately I can't fit `xlsr` on my machine.
> If fine-tuning on some another custom dataset, is it enough to set `--orthography` to `timit` in `run_asr.py` if the transcriptions are lowercased and `librispeech` if they are uppercased?
For the most part, yes! Run it with `--verbose_logging` to see how the orthography rules pre-processed the text. Keep us posted!<|||||>`XLSR `model I used didn't work with this setup: the training loss is _nan_ when I tried to fine-tune on Arabic corpus. If I got it correctly, with `--orthography buckwalter` you modify the tokenizer only. However, if you load e.g.
`model = Wav2Vec2ForCTC.from_pretrained("facebook/wav2vec2-large-xlsr-53")`
and check it structure
`print(model.state_dict)`
you'll see that the last layer of the network is the LM head with the default vocabulary size:
`(lm_head): Linear(in_features=1024, out_features=32, bias=True)`
If I understand correctly, you need to convert the model manually if you want to have a letter vocabulary different from english.
I converted the fairseq xlsr checkpoint using this script
`transformers/src/transformers/models/wav2vec2/convert_wav2vec2_original_pytorch_checkpoint_to_pytorch.py`
together with "custom", Swedish letter dictionary, and then succeeded to fine-tune it on a Swedish corpus.
I guess you need to do the same for Arabic in order to have proper LM head on top of the model.<|||||>Yes, thanks! I missed that step (which is similar to `PreTrainedModel.resize_token_embeddings()`). I'm adding a method for that: `Wav2Vec2ForCTC.resize_lm_head()` which simply calls `PreTrainedModel.model._get_resized_lm_head()` and updates model config. The LM head looks good when inspecting the return value. I'll add some unit tests as well.
Now I'm fine-tuning it on `wav2vec2-base` but I'm not expecting great results given the phonetic differences with Arabic. If you can try it again with `xlsr`, I'd appreciate it!<|||||>Thanks @elgeish, the method seems to work correctly. Now the loss is not `nan` any more during the fine-tuning of the `xlsr` model. Training loss:
```
{'loss': 584.0067, 'learning_rate': 8.333333333333333e-05, 'epoch': 0.28}
{'loss': 291.7098, 'learning_rate': 0.00016666666666666666, 'epoch': 0.55}
```
Validation loss:
```
{'eval_loss': 374.4364929199219, 'eval_wer': 1.0, 'eval_runtime': 22.3424, 'eval_samples_per_second': 4.476, 'epoch': 0.28}
{'eval_loss': 374.20855712890625, 'eval_wer': 1.0, 'eval_runtime': 22.8504, 'eval_samples_per_second': 4.376, 'epoch': 0.55}
```
Predictions after epoch 0.55:
```
03/12/2021 12:07:54 - DEBUG - __main__ - reference: "wayaquwlu lEulamA'u <in~ahu min gayri lmuraj~aHi >an tuTaw~ira lbaktiyryA lmuEdiyapu muqAwamapan Did~a lEilAji ljadiyd >al~a*iy >aSbaHa mutAHan biAlfiEl fiy $akli marhamin lil>amrADi ljildiy~api"
03/12/2021 12:07:54 - DEBUG - __main__ - prediction: ""
03/12/2021 12:07:54 - DEBUG - __main__ - reference: "wayumkinuka lHuSuwlu EalY taTbiyqAtin lilt~adriybAti l>asAsiy~api maj~Anan"
03/12/2021 12:07:54 - DEBUG - __main__ - prediction: ""
```
Unfortunately, after that I always run out of memory in Colab. The recordings in the Arabic corpus are probably very long. As a result even with `batch_size=1` 16Gb of GPU memory is not enough:
```
RuntimeError: CUDA out of memory. Tried to allocate 80.00 MiB (GPU 0; 15.90 GiB total capacity; 14.80 GiB already allocated; 87.75 MiB free; 14.94 GiB reserved in total by PyTorch)
2% 1050/54390 [07:16<6:09:57, 2.40it/s]
```
If you have more than 16Gb of GPU memory available, I can share the `xlsr` model to try it out on your machine.<|||||>I added a `--max_duration_in_seconds` filter. I'm seeing ok results now fine-tuning `wav2vec2-base` after 500 steps, for example:
```
reference: "wamin tilka ls~ilaE >al$~Ayu lS~iyniy~u wAlwaraqu wAlbAruwdu wAlbuwSilapu"
prediction: "wamin tiloka Als~ila>a$~aAyu AS~iyniy~u walowaraqu waAlobaAruwdu waAlobuwSilapu"
```
I've also started fine-tuning [wav2vec2-large-xlsr-53](https://huggingface.co/facebook/wav2vec2-large-xlsr-53) after adding missing files locally on my machine.
For future PRs, I'm thinking of:
* Supporting other languages and examples from [Patrick's awesome blog post](https://huggingface.co/blog/fine-tune-xlsr-wav2vec2), which I need to read
* CER and WER transformations for other languages (e.g., ignoring tashkil in Arabic)
* Supporting [Lhotse](https://lhotse.readthedocs.io/en/latest/corpus.html) datasets, which provide a ton of speech-specific functionality
Before adding UTs, I want to check with @patrickvonplaten the code here is on the right track.
@Getmany1 you've been super helpful, thank you!<|||||>> Hey @elgeish,
>
> Thanks a lot for your PR! Sorry that I only reviewed it now. In general I like the changes you have made to `run_wav2vec2.py` :-)
>
> A couple of things, I'd like to change:
>
> * Remove the change from `modeling_wav2vec2.py`. I don't really want a resize lm head method in the model there -> it's too much of an edge case IMO. You can simply first instantiate the processor then use the tokenizer length to load the model with `Wav2Vec2ForCTC.from_pretrained(model_path, vocab_size=len(tokenizer))`. This way we don't need a `resize_lm_head()` method.
> * Can you give me more context on the `buckwalter.json` file?
> * Can you also add more text to the README.md that explains a bit how to use the `run_wav2vec2.py` script? E.g. what does the orthography class do, what is the buckwalter vocab?
Thank you! I responded inline. I'll update `README.md` as well. I think you mean `run_asr.py`, no?<|||||>Great the PR looks good to me now! Thanks a lot for doing this :-) The failures seem unrelated, so I'll rerun the tests again to make the CI happy |
transformers | 10,580 | closed | Issue when customizing loss in Trainer | Hi everyone,
I am a student and therefore not yet very familiar with the way issues report work on git, so I aplogize in advance if this is not the proper place to post this message.
I'm trying to customize the loss to use a weighted CrossEntropyLoss, I browsed the issues reports and saw that this matter was already mentionned and a solution was brought by the developpers (I think it was @sgugger) of the Transformers library, I tried to follow their code snipets as much as possible but always ended up with the same error.
I'm working with the last version of Transformers and this is my code:
```
config = AutoConfig.from_pretrained("bert-base-cased", num_labels=2, finetuning_task="SST-2")
# Test with modified trainer for weighted CrossEntropyLoss
model = AutoModelForSequenceClassification.from_pretrained(
"dmis-lab/biobert-base-cased-v1.1",
from_tf=False,
config=config)
from torch import FloatTensor
classDistribution_raw = [97, 3]
classDistribution = [0.8, 0.2]
normedWeights = [1 - (x / sum(classDistribution)) for x in classDistribution]
normedWeights = FloatTensor(normedWeights).cuda()
from torch.nn import CrossEntropyLoss
class MyTrainer(Trainer):
def compute_loss(self, model, inputs, return_outputs=False):
if "labels" in inputs:
labels = inputs.pop("labels")
outputs = model(**inputs)
logits = outputs.logits
loss_function = CrossEntropyLoss(weight = normedWeights)
if self.args.past_index >= 0:
self._past = outputs[self.args.past_index]
if labels is not None:
loss = loss_function(logits, labels)
else:
# We don't use .loss here since the model may return tuples instead of ModelOutput.
loss = outputs["loss"] if isinstance(outputs, dict) else outputs[0]
return (loss, outputs) if return_outputs else loss
trainer = MyTrainer(
model=model,
args=training_args,
train_dataset=train_dataset,
eval_dataset=eval_dataset,
compute_metrics=compute_metrics_fn,
tokenizer=tokenizer,
)
```
And this is the error I keep getting:
`'NoneType' object has no attribute 'detach'`
I'm probably doing something wrong but I can't understand what.
Thanks in advance for your answers, I stay availble if u need any more details about my set up or my code,
Best regards,
Arthur Ledaguenel | 03-07-2021 21:59:42 | 03-07-2021 21:59:42 | I think you may be experiencing a bug that was fixed since then (I would need the whole error message to be sure) so before we dive further, could you see if an [install from source](https://huggingface.co/transformers/installation.html#installing-from-source) solves your problem?<|||||>Hi @sgugger,
Thank you very much for the quick answer, I tested with the installation from source and he it worked ! |
transformers | 10,579 | closed | request about deepspeed tutorial | Dear @stas00
You have created this great tutorial here that without you it was really very hard near impossible to be able to train these large models, thank you so much
https://github.com/huggingface/transformers/issues/8771
Do you mind updating your comment, including how numbers would change if you add distributed training and deepspeed and how the command should be in this case? so to further speedup deepspeed on multiple GPUs
I also get this message with deepspeed with transformer 4.3.3 running the tutorial:
[deepscale] OVERFLOW! Rank 0 Skipping step. Attempted loss scale: 131072.0, reducing to 65536.0
could you tell me if this shows any issue in training? sorry I am very unfamiliar with deepspeed.
One more question, in tutorial you did not use --fp16, could you add a few comments if we can use it with deepspeed?
this is such a great toturial and would be great if we could have all info in one place.
thank you so much for the hard work and a lot of help
| 03-07-2021 19:51:17 | 03-07-2021 19:51:17 | @dorost1234, thank you for the kind words. I'm glad to hear it was useful.
In general to answer the bulk of your questions - you will find the full documentation here:
https://huggingface.co/transformers/master/main_classes/trainer.html#deepspeed
Please let me know if you still have any question after reading it.
> [deepscale] OVERFLOW! Rank 0 Skipping step. Attempted loss scale: 131072.0, reducing to 65536.0
I shall document this. This is just a warning that DeepSpeed prints when it delays the optimizer stepping due to fp16 dynamic scaling. I definitely want to document that since it's alarming and hard to understand. and how to get the optimizer kick on the first step.
Also I started working on a notebook, which you can try - should work on jupyter or colab:
https://github.com/stas00/porting/blob/master/transformers/deepspeed/DeepSpeed_on_colab_CLI.ipynb
Please let me know what you'd like to be added to it.
<|||||>This issue has been automatically marked as stale because it has not had recent activity. If you think this still needs to be addressed please comment on this thread.
Please note that issues that do not follow the [contributing guidelines](https://github.com/huggingface/transformers/blob/master/CONTRIBUTING.md) are likely to be ignored. |
transformers | 10,578 | closed | Why HFArgumentParser.parse_dict(TrainerArguments) return tuple instead of dict? | I guess it is not certainly bug however I could not almost understand why` HfArgumentParser.parsedict() `return `(*outputs,)`. As can be seen in [docs](https://huggingface.co/transformers/_modules/transformers/hf_argparser.html#HfArgumentParser
)
I am trying to fine-tune BERT for Token Classification using Trainer class and my aim is to turn argparse object to TrainingArguments. I converted ArgumentParser object to dictionary then using HfArgumentParser.parsedict() turn it to TrainingArguments object. However I realized that ` HfArgumentParser.parsedict() ` returns `(TrainingArguments, )` tuple which cause following error in Trainer initializer.
```console
Traceback (most recent call last):
File "transformers_ner.py", line 344, in <module>
finetune_model(args)
File "transformers_ner.py", line 308, in finetune_model
args=train_args
File "/home/akali/.local/lib/python3.6/site-packages/transformers/trainer.py", line 237, in __init__
set_seed(self.args.seed)
AttributeError: 'tuple' object has no attribute 'seed'
```
I get train_args by:
```python
# ArgParser --> Training Arguments
HFParser = HfArgumentParser(TrainingArguments)
train_args = HFParser.parse_dict(args)
```
I know I can get TrainingArguments object by doing` train_args[0] `however, isn't it meaningless that `HfArgumentParser.parsedict()` return tuple instead of directly TrainingArguments.
Thanks.
| 03-07-2021 18:40:52 | 03-07-2021 18:40:52 | hi @akalieren
the `parse_dict` or `parse_args_into_dataclasses` methods always return a `tuple` of parsed arguments for each `dataclass` that was used to initialize `HfArgumentParser`. Here you're initializing it with just `TrainingArguments` so `parse_dict` returns a `tuple` of length 1.
Hope this helps.<|||||>Thanks for quick response @patil-suraj,
I am understanding it returns `tuple` of initializing given data classes; however, my question is that is there a reason why it returns `tuple` instead of just `*outputs`.
I hope this is not kind of misunderstanding, and grateful about your time.
Thanks.
<|||||>`return *outputs` is not a valid python statement. Also `outputs` here is a list and it's just a good python practice to return return `tuple` instead of `list` when returning multiple values.<|||||>I tried it and realized that you are right. Thanks a lot for your interest and kind responses.
I am closing this issue. Wish healthy days. |
transformers | 10,577 | closed | seq2seq example with T5 does not run due to issue with loading tokernizer | ## Environment info
<!-- You can run the command `transformers-cli env` and copy-and-paste its output below.
Don't forget to fill out the missing fields in that output! -->
- `transformers` version: 4.3.3
- Platform: linux
- Python version: 3.7
- PyTorch version (GPU?): 1.8
- Tensorflow version (GPU?): -
- Using GPU in script?: -
- Using distributed or parallel set-up in script?: -
### Who can help
<!-- Your issue will be replied to more quickly if you can figure out the right person to tag with @
If you know how to use git blame, that is the easiest way, otherwise, here is a rough guide of **who to tag**.
Please tag fewer than 3 people.
Models:
- albert, bert, xlm: @LysandreJik
- blenderbot, bart, marian, pegasus, encoderdecoder, t5: @patrickvonplaten, @patil-suraj
- longformer, reformer, transfoxl, xlnet: @patrickvonplaten
- fsmt: @stas00
- funnel: @sgugger
- gpt2: @patrickvonplaten, @LysandreJik
- rag: @patrickvonplaten, @lhoestq
- tensorflow: @jplu
Library:
- benchmarks: @patrickvonplaten
- deepspeed: @stas00
- ray/raytune: @richardliaw, @amogkam
- text generation: @patrickvonplaten
- tokenizers: @LysandreJik
- trainer: @sgugger
- pipelines: @LysandreJik
Documentation: @sgugger
HF projects:
- nlp datasets: [different repo](https://github.com/huggingface/nlp)
- rust tokenizers: [different repo](https://github.com/huggingface/tokenizers)
Examples:
- maintained examples (not research project or legacy): @sgugger, @patil-suraj
- research_projects/bert-loses-patience: @JetRunner
- research_projects/distillation: @VictorSanh
-->
@patrickvonplaten, @patil-suraj
## Information
Hi
I am trying to run run_seq2seq.py example on mt5 model
` python run_seq2seq.py --model_name_or_path google/mt5-small --dataset_name wmt16 --dataset_config_name ro-en --source_prefix "translate English to Romanian: " --task translation_en_to_ro --output_dir /test/test_large --do_train --do_eval --predict_with_generate --max_train_samples 500 --max_val_samples 500 --tokenizer_name google/mt5-small
`
getting this error:
```
Traceback (most recent call last):
File "run_seq2seq.py", line 539, in <module>
main()
File "run_seq2seq.py", line 309, in main
use_auth_token=True if model_args.use_auth_token else None,
File "/dara/libs/anaconda3/envs/fast/lib/python3.7/site-packages/transformers/models/auto/tokenization_auto.py", line 379, in from_pretrained
return tokenizer_class.from_pretrained(pretrained_model_name_or_path, *inputs, **kwargs)
File "/dara/libs/anaconda3/envs/fast/lib/python3.7/site-packages/transformers/tokenization_utils_base.py", line 1789, in from_pretrained
resolved_vocab_files, pretrained_model_name_or_path, init_configuration, *init_inputs, **kwargs
File "/dara/libs/anaconda3/envs/fast/lib/python3.7/site-packages/transformers/tokenization_utils_base.py", line 1860, in _from_pretrained
tokenizer = cls(*init_inputs, **init_kwargs)
File "/dara/libs/anaconda3/envs/fast/lib/python3.7/site-packages/transformers/models/t5/tokenization_t5_fast.py", line 147, in __init__
**kwargs,
File "/dara/libs/anaconda3/envs/fast/lib/python3.7/site-packages/transformers/tokenization_utils_fast.py", line 103, in __init__
"Couldn't instantiate the backend tokenizer from one of: "
ValueError: Couldn't instantiate the backend tokenizer from one of: (1) a `tokenizers` library serialization file, (2) a slow tokenizer instance to convert or (3) an equivalent slow tokenizer class to instantiate and convert. You need to have sentencepiece installed to convert a slow tokenizer to a fast one.
```
thank you for your help
| 03-07-2021 18:34:51 | 03-07-2021 18:34:51 | solved with installing sentencepiece, I appreciate adding a file mentioning requirements.txt thanks <|||||>Hi @dorost1234 ,
Glad you resolved the issue. Your `transformers` version is old, we have now added the `sentencepiece` dependency in `requirements.txt`.
https://github.com/huggingface/transformers/blob/master/examples/seq2seq/requirements.txt#L2 |
transformers | 10,576 | closed | Movement pruning for DistilGPT2 - pre_trained model, issue while using dynamic_quantization | ## Environment info
- `transformers` version: 4.3.3
- Platform: Ubuntu 20.04
- Python version: 3.8.8
- PyTorch version (GPU?): 1.4.0 (False)
- Tensorflow version (GPU?): 2.4.1 (False)
- Using GPU in script?: False
- Using distributed or parallel set-up in script?: False
### Who can help
[@VictorSanh](https://github.com/VictorSanh)
## Information
I am following through the Saving PruneBERT [notebook](https://github.com/huggingface/transformers/blob/b11386e158e86e62d4041eabd86d044cd1695737/examples/movement-pruning/Saving_PruneBERT.ipynb) from the *examples/movement-pruning/* directory, to have a pruned and quantized model for DistilGPT2.
In cell 4:
```
# Elementary representation: we decompose the quantized tensors into (scale, zero_point, int_repr).
# See https://pytorch.org/docs/stable/quantization.html
# We further leverage the fact that int_repr is sparse matrix to optimize the storage: we decompose int_repr into
# its CSR representation (data, indptr, indices).
elementary_qtz_st = {}
for name, param in qtz_st.items():
if "dtype" not in name and param.is_quantized:
print("Decompose quantization for", name)
# We need to extract the scale, the zero_point and the int_repr for the quantized tensor and modules
scale = param.q_scale() # torch.tensor(1,) - float32
zero_point = param.q_zero_point() # torch.tensor(1,) - int32
elementary_qtz_st[f"{name}.scale"] = scale
elementary_qtz_st[f"{name}.zero_point"] = zero_point
# We assume the int_repr is sparse and compute its CSR representation
# Only the FCs in the encoder are actually sparse
int_repr = param.int_repr() # torch.tensor(nb_rows, nb_columns) - int8
int_repr_cs = sparse.csr_matrix(int_repr) # scipy.sparse.csr.csr_matrix
elementary_qtz_st[f"{name}.int_repr.data"] = int_repr_cs.data # np.array int8
elementary_qtz_st[f"{name}.int_repr.indptr"] = int_repr_cs.indptr # np.array int32
assert max(int_repr_cs.indices) < 65535 # If not, we shall fall back to int32
elementary_qtz_st[f"{name}.int_repr.indices"] = np.uint16(int_repr_cs.indices) # np.array uint16
elementary_qtz_st[f"{name}.int_repr.shape"] = int_repr_cs.shape # tuple(int, int)
else:
elementary_qtz_st[name] = param
```
my model throws the below error:
```AttributeError: 'NoneType' object has no attribute 'is_quantized'```
which on digging into quantizing step in cell 2, shows a significant difference between the used BERT and DistilGPT2 (the one am using) quantized version:
1. The BERT quantized first few layers look like:
```
bert.embeddings.position_ids
bert.embeddings.word_embeddings.weight
bert.embeddings.position_embeddings.weight
bert.embeddings.token_type_embeddings.weight
bert.embeddings.LayerNorm.weight
bert.embeddings.LayerNorm.bias
bert.encoder.layer.0.attention.self.query.scale
bert.encoder.layer.0.attention.self.query.zero_point
bert.encoder.layer.0.attention.self.query._packed_params.weight
bert.encoder.layer.0.attention.self.query._packed_params.bias
bert.encoder.layer.0.attention.self.key.scale
bert.encoder.layer.0.attention.self.key.zero_point
bert.encoder.layer.0.attention.self.key._packed_params.weight
bert.encoder.layer.0.attention.self.key._packed_params.bias
bert.encoder.layer.0.attention.self.value.scale
bert.encoder.layer.0.attention.self.value.zero_point
bert.encoder.layer.0.attention.self.value._packed_params.weight
bert.encoder.layer.0.attention.self.value._packed_params.bias
```
2. The quantized DistilGPT2 first few layers look like:
```
transformer.wte.weight
transformer.wpe.weight
transformer.h.0.ln_1.weight
transformer.h.0.ln_1.bias
transformer.h.0.attn.bias
transformer.h.0.attn.masked_bias
transformer.h.0.attn.c_attn.weight
transformer.h.0.attn.c_attn.bias
transformer.h.0.attn.c_proj.weight
transformer.h.0.attn.c_proj.bias
transformer.h.0.ln_2.weight
transformer.h.0.ln_2.bias
```
3. As you would notice, there is a clear difference in the way layers are formed after quantization
a) BERT has ```.scale``` and ```.zero_point``` added to every layer after embeddings, whereas the DistilGPT2 layers do not get these 2 extras.
b) any ```.weight``` and ```.bias``` are converted to ```._packed_params.weight``` and ```._packed_params.bias``` respectively.
4. I beleive this is why when processing the cell 4:
a) It does not even go to all layers that are missing ```._packed_params``` and just tries to process the last layer which is
```
lm_head.scale
lm_head.zero_point
lm_head._packed_params.weight
lm_head._packed_params.bias
```
b) Where it fails with the error mentioned just before point 1.
## To reproduce
Steps to reproduce the behavior:
1. Clone `transformers` and follow the steps to install the `movement-pruning` example
2. Upgrade torch to v1.4
3. Try to run the `Saving_PruneBERT.ipynb` notebook with 1 change, in the cell 2 instantiate the model class with below line
```model = AutoModelForCausalLM.from_pretrained('distilgpt2')``` | 03-07-2021 18:29:29 | 03-07-2021 18:29:29 | This issue has been automatically marked as stale because it has not had recent activity. If you think this still needs to be addressed please comment on this thread.
Please note that issues that do not follow the [contributing guidelines](https://github.com/huggingface/transformers/blob/master/CONTRIBUTING.md) are likely to be ignored. |
transformers | 10,575 | closed | bug in run_finetune | ## Environment info
<!-- You can run the command `transformers-cli env` and copy-and-paste its output below.
Don't forget to fill out the missing fields in that output! -->
- `transformers` version: 4.3.3
- Platform: linux
- Python version: 3.7
- PyTorch version (GPU?): 1.8
- Tensorflow version (GPU?): -
- Using GPU in script?: -
- Using distributed or parallel set-up in script?: -
### Who can help
<!-- Your issue will be replied to more quickly if you can figure out the right person to tag with @
If you know how to use git blame, that is the easiest way, otherwise, here is a rough guide of **who to tag**.
Please tag fewer than 3 people.
Models:
- albert, bert, xlm: @LysandreJik
- blenderbot, bart, marian, pegasus, encoderdecoder, t5: @patrickvonplaten, @patil-suraj
- longformer, reformer, transfoxl, xlnet: @patrickvonplaten
- fsmt: @stas00
- funnel: @sgugger
- gpt2: @patrickvonplaten, @LysandreJik
- rag: @patrickvonplaten, @lhoestq
- tensorflow: @jplu
Library:
- benchmarks: @patrickvonplaten
- deepspeed: @stas00
- ray/raytune: @richardliaw, @amogkam
- text generation: @patrickvonplaten
- tokenizers: @LysandreJik
- trainer: @sgugger
- pipelines: @LysandreJik
Documentation: @sgugger
HF projects:
- nlp datasets: [different repo](https://github.com/huggingface/nlp)
- rust tokenizers: [different repo](https://github.com/huggingface/tokenizers)
Examples:
- maintained examples (not research project or legacy): @sgugger, @patil-suraj
- research_projects/bert-loses-patience: @JetRunner
- research_projects/distillation: @VictorSanh
-->
@patrickvonplaten, @patil-suraj
## Information
I am running run_seq2seq.py getting
ImportError: cannot import name 'is_offline_mode' from 'transformers.file_utils'
## To reproduce
Steps to reproduce the behavior:
python run_seq2seq.py
thnaks | 03-07-2021 18:05:39 | 03-07-2021 18:05:39 | sorry my mistake to use last version of examples <|||||>hi how you solved this. i also face this error. i can't find "is_offline_mode" and "get_full_repo_name" under transformers.utils. i use https://github.com/huggingface/transformers/tree/main/examples/pytorch/summarization<|||||>This should work if you are using `transformers` master/main |
transformers | 10,574 | closed | The dimension of Feature extraction |
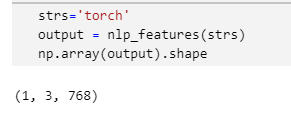
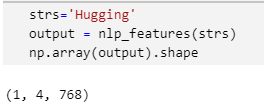
why this happened?
| 03-07-2021 14:42:24 | 03-07-2021 14:42:24 | How did you create the `nlp_features` function?
The sequence length is different due to different tokenization. <|||||>This issue has been automatically marked as stale because it has not had recent activity. If you think this still needs to be addressed please comment on this thread.
Please note that issues that do not follow the [contributing guidelines](https://github.com/huggingface/transformers/blob/master/CONTRIBUTING.md) are likely to be ignored. |
transformers | 10,573 | closed | Update data_collator.py | 加上中文註解
| 03-07-2021 08:33:52 | 03-07-2021 08:33:52 | |
transformers | 10,572 | closed | Import error for class Speech2TextProcessor, Speech2TextTransformerForConditionalGeneration | ## Environment info
- `transformers` version: 4.4.0.dev0
- Platform: Windows-10-10.0.19041-SP0
- Python version: 3.8.8
- PyTorch version (GPU?): 1.7.1+cpu (False)
- Tensorflow version (GPU?): not installed (NA)
- Using GPU in script?: <fill in>
- Using distributed or parallel set-up in script?: <fill in>
### Who can help
Models:
- Speech2TextProcessor, Speech2TextTransformerForConditionalGeneration @patil-suraj
## Information
Model I am using (Speech2TextProcessor, Speech2TextTransformerForConditionalGeneration):
The problem arises when using:
* trying to import the model
## To reproduce
Steps to reproduce the behavior:
1. first install master brach
2. then try to import the Speech2TextProcessor, Speech2TextTransformerForConditionalGeneration class then showing import error
```
---------------------------------------------------------------------------
ImportError Traceback (most recent call last)
<ipython-input-1-8149e8a8d76d> in <module>
1 import torch
----> 2 from transformers import Speech2TextProcessor, Speech2TextTransformerForConditionalGeneration
3 from datasets import load_dataset
4 import soundfile as sf
ImportError: cannot import name 'Speech2TextProcessor' from 'transformers' (unknown location)
```
## Expected behavior
able to run the code from _https://huggingface.co/facebook/s2t-large-librispeech-asr_ from docs
one suggestion, if any fine-tuning script for s2t-large-librispeech-asr model it would be a great help
thankyou | 03-07-2021 07:13:36 | 03-07-2021 07:13:36 | hi @amiyamandal-dev
Thank you for your interest in `S2T`. It's still a work in progress and not available on master yet. If you want to try it, you could checkout this PR #10175<|||||>Hey @amiyamandal-dev ,
The model is now available on [master](https://huggingface.co/transformers/master/model_doc/speech_to_text.html)! You could install transformers from the source if you want to try it. |
transformers | 10,571 | closed | Advice on creating/wrapping `PreTrainedModel` to be compatible with the codebase? | ## Environment info
<!-- You can run the command `transformers-cli env` and copy-and-paste its output below.
Don't forget to fill out the missing fields in that output! -->
- `transformers` version: NA
- Platform: NA
- Python version: NA
- PyTorch version (GPU?): NA
- Tensorflow version (GPU?): NA
- Using GPU in script?: NA
- Using distributed or parallel set-up in script?: NA
### Who can help
@patrickvonplaten
<!-- Your issue will be replied to more quickly if you can figure out the right person to tag with @
If you know how to use git blame, that is the easiest way, otherwise, here is a rough guide of **who to tag**.
Please tag fewer than 3 people.
Models:
- albert, bert, xlm: @LysandreJik
- blenderbot, bart, marian, pegasus, encoderdecoder, t5: @patrickvonplaten, @patil-suraj
- longformer, reformer, transfoxl, xlnet: @patrickvonplaten
- fsmt: @stas00
- funnel: @sgugger
- gpt2: @patrickvonplaten, @LysandreJik
- rag: @patrickvonplaten, @lhoestq
- tensorflow: @jplu
Library:
- benchmarks: @patrickvonplaten
- deepspeed: @stas00
- ray/raytune: @richardliaw, @amogkam
- text generation: @patrickvonplaten
- tokenizers: @LysandreJik
- trainer: @sgugger
- pipelines: @LysandreJik
Documentation: @sgugger
HF projects:
- nlp datasets: [different repo](https://github.com/huggingface/nlp)
- rust tokenizers: [different repo](https://github.com/huggingface/tokenizers)
Examples:
- maintained examples (not research project or legacy): @sgugger, @patil-suraj
- research_projects/bert-loses-patience: @JetRunner
- research_projects/distillation: @VictorSanh
-->
## Information
Model I am using (Bert, XLNet ...):
The problem arises when using:
* [ ] the official example scripts: (give details below)
* [X] my own modified scripts: (give details below)
The tasks I am working on is:
* [ ] an official GLUE/SQUaD task: (give the name)
* [ ] my own task or dataset: (give details below)
## To reproduce
Thanks for the amazing library!
I'm curious if there are instructions on creating a `PreTrainedModel` subclass or creating an `nn.Module` that behaves like a `PreTrainedModel`? Suppose I want to wrap the existing model with some simple additional capabilities inside an `nn.Module`, what are some of the methods that I need to implement/override -- so that they can work well with existing examples?
I'm aware of some tutorials on creating a new model, but that seems pretty complicated and involved -- whereas I'm interested in just adding a couple of simple features.
For example, in the Seq2Seq example, I have noticed that the function signature of `model.forward` determines what data will (not) be passed to the model (as in [`trainer._remove_unused_columns`](https://github.com/huggingface/transformers/blob/master/src/transformers/trainer.py#L458)), and the existence of `model.prepare_decoder_input_ids_from_labels` also influences the input data (as in [`DataCollatorForSeq2Seq .__call__`](https://github.com/huggingface/transformers/blob/master/src/transformers/data/data_collator.py#L292)).
It'd be great if someone could point me to some guidance on tweaking the model to be compatible with the rest of the codebase. Thanks in advance for your time!
Steps to reproduce the behavior:
1.
2.
3.
<!-- If you have code snippets, error messages, stack traces please provide them here as well.
Important! Use code tags to correctly format your code. See https://help.github.com/en/github/writing-on-github/creating-and-highlighting-code-blocks#syntax-highlighting
Do not use screenshots, as they are hard to read and (more importantly) don't allow others to copy-and-paste your code.-->
## Expected behavior
<!-- A clear and concise description of what you would expect to happen. -->
| 03-07-2021 03:45:34 | 03-07-2021 03:45:34 | Hey @HanGuo97,
We try to keep the GitHub issues for bug reports. Do you mind asking your question on the forum instead? Also there might already be similar questions on the forum, such as https://discuss.huggingface.co/t/create-a-custom-model-that-works-with-any-pretrained-transformer-body/4186. Thanks!<|||||>Got it, thanks for letting me know! |
transformers | 10,570 | closed | fix tf doc bug | I found there are a different between tfBertForPretraining and BertForPretraining.
I Have create a forum at `https://discuss.huggingface.co/t/different-doc-with-bertforpretraining-and-tfbertforpretraining/4167` and get a response.
| 03-07-2021 02:53:17 | 03-07-2021 02:53:17 | |
transformers | 10,569 | closed | offline mode for firewalled envs (part 2) | In https://github.com/huggingface/transformers/pull/10407 I noticed I missed a few places where `local_files_only` should be overridden for the offline mode, so this PR completes the process.
Also rewrote the test to be more readable. Could test TF/Flax too but I don't have tiny models to run quick tests on.
@LysandreJik, @sgugger | 03-07-2021 00:12:37 | 03-07-2021 00:12:37 | |
transformers | 10,568 | closed | Ner label re alignment | # What does this PR do?
<!--
Congratulations! You've made it this far! You're not quite done yet though.
Once merged, your PR is going to appear in the release notes with the title you set, so make sure it's a great title that fully reflects the extent of your awesome contribution.
Then, please replace this with a description of the change and which issue is fixed (if applicable). Please also include relevant motivation and context. List any dependencies (if any) that are required for this change.
Once you're done, someone will review your PR shortly (see the section "Who can review?" below to tag some potential reviewers). They may suggest changes to make the code even better. If no one reviewed your PR after a week has passed, don't hesitate to post a new comment @-mentioning the same persons---sometimes notifications get lost.
-->
<!-- Remove if not applicable -->
Fixes #10263
## Before submitting
- [ ] This PR fixes a typo or improves the docs (you can dismiss the other checks if that's the case).
- [x] Did you read the [contributor guideline](https://github.com/huggingface/transformers/blob/master/CONTRIBUTING.md#start-contributing-pull-requests),
Pull Request section?
- [x] Was this discussed/approved via a Github issue or the [forum](https://discuss.huggingface.co/)? Please add a link
to it if that's the case. [link](https://github.com/huggingface/transformers/issues/10263#issuecomment-781648059)
- [ ] Did you make sure to update the documentation with your changes? Here are the
[documentation guidelines](https://github.com/huggingface/transformers/tree/master/docs), and
[here are tips on formatting docstrings](https://github.com/huggingface/transformers/tree/master/docs#writing-source-documentation).
- [ ] Did you write any new necessary tests?
## Who can review?
- pipelines: @LysandreJik, @Narsil, @joshdevins
## What capabilities have been added ?
label realignment: token predictions for subwords can be realigned with 4 different strategies
- default: reset all subword token predictions except for first token
- first: the prediction for the first token in the word is assigned to all subword tokens
- max: the highest confidence prediction among the subword tokens is assigned to all subword tokens
- average: the average pool of the predictions for all subwords is assigned to all subword tokens
- ignore subwords: enable ignoring subwords by merging tokens
## What are the expected changes from the current behavior?
- New flag subword_label_re_alignment enables realignment.
- Already existing flag ignore_subwords actually enables merging subwords.
## Example use cases with code sample enabled by the PR
```
ner = transformers.pipeline(
'ner',
model='elastic/distilbert-base-cased-finetuned-conll03-english',
tokenizer='elastic/distilbert-base-cased-finetuned-conll03-english',
ignore_labels=[],
ignore_subwords=False,
subword_label_re_alignment='average'
)
ner('Mark Musterman')
[
{
'word': 'Mark',
'score': 0.999686598777771,
'index': 1,
'start': 0,
'end': 4,
'is_subword': False,
'entity': 'B-PER'
},
{
'word': 'Must',
'score': 0.9995412826538086,
'index': 2,
'start': 5,
'end': 9,
'is_subword': False,
'entity': 'I-PER'
},
{
'word': '##erman',
'score': 0.9996127486228943,
'index': 3,
'start': 9,
'end': 14,
'is_subword': True,
'entity': 'I-PER'
}
]
ner = transformers.pipeline(
'ner',
model='elastic/distilbert-base-cased-finetuned-conll03-english',
tokenizer='elastic/distilbert-base-cased-finetuned-conll03-english',
ignore_labels=[],
ignore_subwords=True,
subword_label_re_alignment='average'
)
ner('Mark Musterman')
[
{
'word': 'Mark',
'score': 0.999686598777771,
'index': 1,
'start': 0,
'end': 4,
'is_subword': False,
'entity': 'B-PER'
},
{
'word': 'Musterman',
'score': 0.9995412826538086,
'index': 2,
'start': 5,
'end': 9,
'is_subword': False,
'entity': 'I-PER'
}
]
```
## Previous use cases with code sample that see the behavior changes
```
ner = transformers.pipeline(
'ner',
model='elastic/distilbert-base-cased-finetuned-conll03-english',
tokenizer='elastic/distilbert-base-cased-finetuned-conll03-english',
ignore_labels=[],
ignore_subwords=True
)
ner('Mark Musterman')
[
{
'word': 'Mark',
'score': 0.999686598777771,
'entity': 'B-PER',
'index': 1,
'start': 0,
'end': 4
},
{
'word': 'Must',
'score': 0.9995412826538086,
'entity': 'I-PER',
'index': 2,
'start': 5,
'end': 9
},
{
'word': '##erman',
'score': 0.9996127486228943,
'entity': 'I-PER',
'index': 3,
'start': 9,
'end': 14
}
]
``` | 03-06-2021 21:50:05 | 03-06-2021 21:50:05 | Thank you for addressing this! I left some minor comments/questions.<|||||>@LysandreJik I think this is ready for review now.<|||||>Hey @elk-cloner, @francescorubbo! That's an amazing work you've done here. The added tests are a wonderful addition, and will ensure the pipeline is as robust as it can be.
To make reviews easier, could you please fill in the PR description or add a comment mentioning the changes? For example:
- What capabilities have been added
- What are the expected changes from the current behavior
And optionally, if you have the time to:
- Example use cases with code sample enabled by the PR
- Previous use cases with code sample that see the behavior changes
If you don't have time to do any of that, that's perfectly fine - just let me know and I'll take care of it as soon as I have a bit of availability.
Thanks again for the great work you've done here!<|||||>This looks good. I'm wondering if you can add some tests to verify the expected behaviour of two other scenarios from the bug report.
Specifically, the tests in the PR seem to ensure:
Accenture → A ##cc ##ent ##ure → B-ORG O O O → Accenture (ORG)
...but does not make assertions for mixed B/I/O labels in the same word:
Max Mustermann → Max Must ##erman ##n → B-PER I-PER I-PER O → Max Mustermann (PER)
...or inner entity labels surrounded by O labels:
Elasticsearch → El ##astic ##sea #rch → O O I-MISC O → Elasticsearch (MISC)
<|||||>@joshdevins Thank you for suggesting to test those additional scenarios. Testing for those helped me identify some bugs in the previous implementation. I believe the new test should cover all three scenarios now.<|||||>@LysandreJik I'll add the requested notes here, as I don't seem to have permissions to edit the PR description. Maybe @elk-cloner can transfer some of the info there.
> What capabilities have been added
## label realignment
Token predictions for subwords can be realigned with 4 different strategies
- default: reset all subword token predictions except for first token
- first: the prediction for the first token in the word is assigned to all subword tokens
- max: the highest confidence prediction among the subword tokens is assigned to all subword tokens
- average: the average pool of the predictions for all subwords is assigned to all subword tokens
- ignore subwords: enable ignoring subwords by merging tokens
> What are the expected changes from the current behavior
## New flag `subword_label_re_alignment` enables realignment.
Already existing flag `ignore_subwords` actually enables merging subwords.
> Example use cases with code sample enabled by the PR
```
ner = transformers.pipeline('ner',
model='elastic/distilbert-base-cased-finetuned-conll03-english',
tokenizer='elastic/distilbert-base-cased-finetuned-conll03-english',
ignore_labels = [],
ignore_subwords=False,
subword_label_re_alignment='average'
)
ner('Mark Musterman')
[{'word': 'Mark',
'score': 0.999686598777771,
'index': 1,
'start': 0,
'end': 4,
'is_subword': False,
'entity': 'B-PER'},
{'word': 'Must',
'score': 0.9995412826538086,
'index': 2,
'start': 5,
'end': 9,
'is_subword': False,
'entity': 'I-PER'},
{'word': '##erman',
'score': 0.9996127486228943,
'index': 3,
'start': 9,
'end': 14,
'is_subword': True,
'entity': 'I-PER'}]
ner = transformers.pipeline('ner',
model='elastic/distilbert-base-cased-finetuned-conll03-english',
tokenizer='elastic/distilbert-base-cased-finetuned-conll03-english',
ignore_labels = [],
ignore_subwords=True,
subword_label_re_alignment='average'
)
ner('Mark Musterman')
[{'word': 'Mark',
'score': 0.999686598777771,
'index': 1,
'start': 0,
'end': 4,
'is_subword': False,
'entity': 'B-PER'},
{'word': 'Musterman',
'score': 0.9995412826538086,
'index': 2,
'start': 5,
'end': 9,
'is_subword': False,
'entity': 'I-PER'}]
```
> Previous use cases with code sample that see the behavior changes
```
ner = transformers.pipeline('ner',
model='elastic/distilbert-base-cased-finetuned-conll03-english',
tokenizer='elastic/distilbert-base-cased-finetuned-conll03-english',
ignore_labels = [],
ignore_subwords=True
)
ner('Mark Musterman')
[{'word': 'Mark',
'score': 0.999686598777771,
'entity': 'B-PER',
'index': 1,
'start': 0,
'end': 4},
{'word': 'Must',
'score': 0.9995412826538086,
'entity': 'I-PER',
'index': 2,
'start': 5,
'end': 9},
{'word': '##erman',
'score': 0.9996127486228943,
'entity': 'I-PER',
'index': 3,
'start': 9,
'end': 14}]
```<|||||>Thank you, @francescorubbo, I added them to PR.<|||||>I haven't looked at the code changes yet, but looking at the proposed functionality changes.
Referring to [this comment](https://github.com/huggingface/transformers/issues/10263#issuecomment-782187601):
> As a general principle, I would argue that if `grouped_entities=True`, we should never be returning sub-words alone.
> Either they're part of a word that has a label, or they're not. I honestly still don't understand what the flag `ignore_subwords` is supposed to control 🤷
It used to be that `grouped_entities=True` wouldn't treat subwords differently, `ignore_subwords` was added as a way to provide the current default behaviour, while still allowing `ignore_subwords=False` to be set for backwards compatibility.
Indeed I had [similar thoughts](https://github.com/huggingface/transformers/pull/5970#issuecomment-693374424) about how the subwords should be treated, & if there was need for a custom strategy (average,max etc)
I like the below proposal as it can be seen as an expansion of the current logic:
> I would propose two flags:
>
> * `grouped_entities` (boolean) -- note that this implies subword grouping/label realignment (see below)
>
> * `True` will group all words into larger entities, e.g. Max Mustermann -> B-PER I-PER -> "Max Musterman" (PER)
> * `False` will leave words separated, , e.g. Max Mustermann -> B-PER I-PER -> "Max Musterman" (PER)
> * `subword_label_realignment` (boolean or strategy name)
>
> * `True` will use the default for the way the NER fine-tuning was performed, see default suggestions above
> * `False` will leave sub-words alone -- note that this implies that `grouped_entities` should be ignores
> * strategy name -- based on the above strategies
❗ Except that subword_label_realignment=False shouldn't ignore `grouped_entities`. `grouped_entities` flag refers to B-I grouping not subword grouping. We shouldn't enforce subword grouping with `grouped_entities` flag ! We don't know what user cases there might be that use that combination.
👉 So my proposed generalized version would be like this:
`grouped_entities` (Current behaviour is left as is):
* `True` will group all words into larger entities, e.g. Max Mustermann -> B-PER I-PER -> "Max Musterman" (PER)
* `False` will leave words separated, , e.g. Max Mustermann -> B-PER I-PER -> "Max Musterman" (B-PER I-PER)"
`subword_label_realignment` (strategy name) (Replaces `ignore_subwords`)
* none: Don't treat subwords differently (equal to old ignore_subwords=False)
* first: the prediction for the first token in the word is assigned to the word (equal to old ignore_subwords=True, the current default behaviour)
* max: the highest confidence prediction among the wordpiece tokens is assigned to the word (New feature)
* average: the average pool of the predictions among the wordpiece tokens is assigned to the word (New feature)
Here `subword_label_realignment` becomes actually an expansion of the `ignore_subwords` flag.
Also I don't understand what the below mode is supposed to mean @francescorubbo @elk-cloner
> default: reset all subword token predictions except for first token<|||||>Thank you for the feedback, @LysandreJik @sgugger @cceyda !
I've refactored things as follows:
- the new argument is named `aggregation_strategy` and only determines how score and label of the word are computed if the `ignore_subwords` argument is `True`
- the possible strategies are mapped to the `AggregationStrategy` enum
- expected results for the tests are moved into json fixtures
Note that I didn't push the refactor as far as @cceyda suggested because I wanted to preserve backward-compatibility, as also requested by @LysandreJik .
For some reason merging the latest master is causing the code quality check to fail on files unrelated to this PR...any thought on that?<|||||>There was a new release of the black library which touched a lot of files, so you will need to rebase your PR on master to have the quality tests pass again.<|||||>> There was a new release of the black library which touched a lot of files, so you will need to rebase your PR on master to have the quality tests pass again.
I did merge master (see 031f3ef39db9b7164bad783ca17086cdcf000389). Shouldn't it address that?<|||||>I think originally there was also mention of saving the `aggregation_strategy` to the model config?
since it makes the most sense to use the same strategy the model was trained on, ignoring subwords or else.<|||||>> I think originally there was also mention of saving the aggregation_strategy to the model config?
> since it makes the most sense to use the same strategy the model was trained on, ignoring subwords or else.
@cceyda Yes, this was my original proposal, but I think it might be too much for one PR. I would not close the original issue (https://github.com/huggingface/transformers/issues/10263) until the other items are addressed, but perhaps a new/smaller PR can address saving the strategy used at training/evaluation time to the model config file.<|||||>ugh...this ^ is why I hate rebasing on big project repos...
@sgugger from a cursory look the 215 (!) file diffs look legit, please let me know if this PR needs any more work before you can merge.<|||||>@LysandreJik @sgugger Is there more work needed for this PR? If the rebase is an issue, I can create a new PR with only the relevant changes, but we would loose the commit history.<|||||>We can't see the diff of the PR anymore after the rebase, so you should close this one and open a new one from the same branch please. (GitHub completely sucks at properly showing rebases, unless you force push after the rebase.) |
transformers | 10,567 | closed | XLSR-53 | # 🚀 Feature request
Is it possible to use XLSR-53 with transformers in the near future?
| 03-06-2021 21:15:31 | 03-06-2021 21:15:31 | Apparently, someone [just did it](https://huggingface.co/facebook/wav2vec2-large-xlsr). But there are some files missing and it currently unusable. Hopefully the author will soon update it :)<|||||>Pinging @patrickvonplaten for knowledge :)<|||||>Yeah, I just added the pretrained checkpoint. I'll release a notebook by the middle/end of next week on how to fine-tune the checkpoint. Please ping me here again if you can't find it :-)<|||||>@patrickvonplaten Thanks a lot! Cant wait to use it and see how it performs :) <|||||>@patrickvonplaten cool! is it possible to use with Transformers XLSR-53 finetuned with Fairseq?<|||||>will release a notebook either tomorrow or on Monday about it :-)<|||||>@patrickvonplaten can't wait :)<|||||>Notebook is available here: https://huggingface.co/blog/fine-tune-xlsr-wav2vec2 :-) <|||||>We are organizing a "fine-tuning XLSR-53" event. Check this announcement: https://discuss.huggingface.co/t/open-to-the-community-xlsr-wav2vec2-fine-tuning-week-for-low-resource-languages/4467. Would be awesome if you want to participate :-)<|||||>@patrickvonplaten
Hey buddy!
First and foremost I want to thank you again for all your effort! Really appreciate it!
Got another litte question:
Fine tuned a wav2vec-large-xlsr-53 model on Swiss German (bernese dialect) as laid out in one of your blogs.
Currently trying to add an already existing 6-Gram-KenLM on top.
Could you give me some hints on how to do it? Or is it yet not even possible?
Kind regards
Yves :wink:
<|||||>Hey Yves,
Here a forum post regarding this issue: https://discuss.huggingface.co/t/language-model-for-wav2vec2-0-decoding/4434<|||||>Hi all,
I am following up on this issue: I am trying to use the pre-trained Wav2Vec2-XLSR-53 (https://huggingface.co/facebook/wav2vec2-large-xlsr-53) and according to the documentation, it should be available as:
```
from transformers import AutoTokenizer, AutoModel
tokenizer = AutoTokenizer.from_pretrained("facebook/wav2vec2-large-xlsr-53")
model = AutoModel.from_pretrained("facebook/wav2vec2-large-xlsr-53")
```
The model is available, but the tokenizer is not found (error: OSError: Can't load tokenizer for 'facebook/wav2vec2-large-xlsr-53'. Make sure that: (...) ). I tried using Transformers 4.2.2 and 4.5.0 as well as cloning the repository -- no luck. I am able to successfully load e.g. the French version:
` tokenizer = AutoTokenizer.from_pretrained("facebook/wav2vec2-large-xlsr-53-french")
`
But not the base XLSR tokenizer?
Thanks so much for the brilliant work!<|||||>Hey @gretatuckute
Check out my HuggingFace Profile https://huggingface.co/Yves. There you'll find what you're after.
If you ask @patrickvonplaten he could also invite you the wav2vec xlsr slack channel :)
Cheers
Yves<|||||>Hi @yagan93, thank you for getting back! On your HF profile I only see the Swiss-German tokenizer? <|||||>@gretatuckute You just got to swop the models and make little adjustments. Check out this notebook for details information on how to do so. <|||||>Closed by https://github.com/huggingface/transformers/pull/10648 |
transformers | 10,566 | closed | from_pretrained() - some model weights not initialized message | ## Environment info
- `transformers` version: 4.0.1
- Platform: Linux-4.15.0-132-generic-x86_64-with-glibc2.10
- Python version: 3.8.5
- PyTorch version (GPU?): 1.7.1+cu110 (True)
- Tensorflow version (GPU?): not installed (NA)
- Using GPU in script?: <fill in>
- Using distributed or parallel set-up in script?: no
Note also: cookiecutter dependency is not included in pip install transformers so transformers-cli env initially fails
### Who can help
(T5) @patrickvonplaten, @patil-suraj, @sshleifer
When using `T5ForConditionalGeneration.from_pretrained('t5-base')`, I get the following warning at load:
```
Some weights of the model checkpoint at t5-large were not used when initializing T5ForConditionalGeneration: ['decoder.block.0.layer.1.EncDecAttention.relative_attention_bias.weight']
- This IS expected if you are initializing T5ForConditionalGeneration from the checkpoint of a model trained on another task or with another architecture (e.g. initializing a BertForSequenceClassification model from a BertForPreTraining m
odel).
- This IS NOT expected if you are initializing T5ForConditionalGeneration from the checkpoint of a model that you expect to be exactly identical (initializing a BertForSequenceClassification model from a BertForSequenceClassification mode
l).
```
If I load from a checkpoint that I create (i.e. local file), I get the same message.
But I think that all weights are, in fact, identical:
- evaluation code on the model I finetune before saving AND
- evaluation code on the model I finetune, save, and then reload
are identical.
This suggests that *all* weights are identical, since performance is identical. This contradicts the warning message.
Questions:
1) Are some weights actually not being loaded? If so, how could I observe identical behavior on metrics. Or is this warning wrong?
2) If this warning is correct, how can I force the model to fully load the model exactly as I saved it.
3) Is there any other difference (randomly initialized head, randomly initialized weights) between the t5 that is pretrained and the T5ForConditionalGeneration?
| 03-06-2021 19:46:11 | 03-06-2021 19:46:11 | Duplicate of https://github.com/huggingface/transformers/issues/8933 . This wrong waring should have been fixed in newer versions, see: https://github.com/huggingface/transformers/blob/63c295ac05962b03701bdda87a90595b5f864075/src/transformers/models/t5/modeling_t5.py#L1188<|||||>Great! So *all* weights are in fact loaded from pretrained, or when loading from a checkpoint, then, right? |
transformers | 10,565 | closed | Mismatch between input and target batch_sizes while training FSMT model | Code to reproduce
```python
tokenizer = get_fsmt_tokenizer()
tokenizer.model_max_length=100
model = get_fsmt_model()
freeze_embeds(model)
freeze_encoder(model)
train_dataset = YandexRuEnDataset("data", split="train")
val_dataset = YandexRuEnDataset("data", split="valid")
training_args = TrainingArguments(
output_dir='./results', # output directory
num_train_epochs=3, # total # of training epochs
per_device_train_batch_size=16, # batch size per device during training
per_device_eval_batch_size=64, # batch size for evaluation
warmup_steps=5000, # number of warmup steps for learning rate scheduler
weight_decay=0.01, # strength of weight decay
logging_dir='./logs', # directory for storing logs
fp16=True,
fp16_opt_level='O2',
save_steps=20000
)
trainer = Seq2SeqTrainer(
model=model, # the instantiated 🤗 Transformers model to be trained
args=training_args, # training arguments, defined above
train_dataset=train_dataset, # training dataset
eval_dataset=val_dataset, # evaluation dataset
tokenizer=tokenizer,
data_collator=collate_sentences(tokenizer)
)
trainer.train()
```
Dataset class
```python
class YandexRuEnDataset(Dataset):
def __init__(self, root_data, split):
src = open(f"{root_data}/corpus.en_ru.1m.ru", "r").readlines()
tgt = open(f"{root_data}/corpus.en_ru.1m.en", "r").readlines()
# src = src[:int(0.1*len(src))]
# tgt = tgt[:int(0.1 * len(tgt))]
X_train, X_test, y_train, y_test = train_test_split(src, tgt, test_size=0.33, random_state=228)
if split == "train":
self.src = X_train
self.trg = y_train
elif split == "valid":
self.src = X_test
self.trg = y_test
def __len__(self):
return len(self.src)
def __getitem__(self, idx):
src = self.src[idx]
trg = self.trg[idx]
return src, trg
def collate_sentences(tokenizer: Tokenizer):
def collate_fn(batch):
batch = list(zip(*batch))
X_batch = list(batch[0])
y_batch = list(batch[1])
batch = tokenizer.prepare_seq2seq_batch(
src_texts=X_batch, tgt_texts=y_batch,
padding=True, truncation=True,
return_tensors='pt'
)
return batch
return collate_fn
```
Exception
```
File "/home/farit/PycharmProjects/NMT/train.py", line 40, in <module>
trainer.train()
File "/home/farit/anaconda3/envs/NMT/lib/python3.8/site-packages/transformers/trainer.py", line 940, in train
tr_loss += self.training_step(model, inputs)
File "/home/farit/anaconda3/envs/NMT/lib/python3.8/site-packages/transformers/trainer.py", line 1302, in training_step
loss = self.compute_loss(model, inputs)
File "/home/farit/anaconda3/envs/NMT/lib/python3.8/site-packages/transformers/trainer.py", line 1334, in compute_loss
outputs = model(**inputs)
File "/home/farit/anaconda3/envs/NMT/lib/python3.8/site-packages/torch/nn/modules/module.py", line 727, in _call_impl
result = self.forward(*input, **kwargs)
File "/home/farit/anaconda3/envs/NMT/lib/python3.8/site-packages/transformers/models/fsmt/modeling_fsmt.py", line 1180, in forward
masked_lm_loss = loss_fct(lm_logits.view(-1, self.config.tgt_vocab_size), labels.view(-1))
File "/home/farit/anaconda3/envs/NMT/lib/python3.8/site-packages/torch/nn/modules/module.py", line 727, in _call_impl
result = self.forward(*input, **kwargs)
File "/home/farit/anaconda3/envs/NMT/lib/python3.8/site-packages/torch/nn/modules/loss.py", line 961, in forward
return F.cross_entropy(input, target, weight=self.weight,
File "/home/farit/anaconda3/envs/NMT/lib/python3.8/site-packages/torch/nn/functional.py", line 2468, in cross_entropy
return nll_loss(log_softmax(input, 1), target, weight, None, ignore_index, None, reduction)
File "/home/farit/anaconda3/envs/NMT/lib/python3.8/site-packages/torch/nn/functional.py", line 2261, in nll_loss
raise ValueError('Expected input batch_size ({}) to match target batch_size ({}).'
ValueError: Expected input batch_size (1440) to match target batch_size (1600).
```` | 03-06-2021 17:10:56 | 03-06-2021 17:10:56 | This issue has been automatically marked as stale because it has not had recent activity. If you think this still needs to be addressed please comment on this thread.
Please note that issues that do not follow the [contributing guidelines](https://github.com/huggingface/transformers/blob/master/CONTRIBUTING.md) are likely to be ignored. |
transformers | 10,564 | closed | [Causal Language Modeling] seems not as expected | # Problem
Causal Models is only attended to the left context. Therefore causal models should not depend on the right tokens.
For example, The word embedding of "I" will be unchanged no matter what is in the right In GPT2. Since Causal Language Model are uni-directional self-attention.
```
from transformers import AutoModel,AutoTokenizer, AutoConfig
import torch
# gpt
gpt_model = AutoModel.from_pretrained('gpt2')
gpt_tokenizer = AutoTokenizer.from_pretrained('gpt2')
embeddings = gpt_model.get_input_embeddings()
# create ids of encoded input vectors
decoder_input_ids = gpt_tokenizer("<pad> Ich will ein", return_tensors="pt", add_special_tokens=False).input_ids
# pass decoder input_ids and encoded input vectors to decoder
lm_logits = gpt_model(decoder_input_ids).last_hidden_state
# change the decoder input slightly
decoder_input_ids_perturbed = gpt_tokenizer("<pad> Ich will das", return_tensors="pt", add_special_tokens=False).input_ids
lm_logits_perturbed = gpt_model(decoder_input_ids_perturbed).last_hidden_state
# compare values of word embedding of "I" for input_ids and perturbed input_ids
print("Is encoding for `Ich` equal to its perturbed version?: ", torch.allclose(lm_logits[0, 0], lm_logits_perturbed[0, 0], atol=1e-3))
```
Result
```
Is encoding for `Ich` equal to its perturbed version?: True
```
However, when it comes to other models, the result is not following the assumption, the logits will be changed when changing the right side input? What is the reason? Is it a bug? I really want to know the answer, thank you!
BERT
```
Is encoding for `Ich` equal to its perturbed version?: False
```
BART
```
Is encoding for `Ich` equal to its perturbed version?: False
```
Roberta
```
Is encoding for `Ich` equal to its perturbed version?: False
```
Experiment notebook [colab](https://colab.research.google.com/drive/15V37RWAL40vhrk-uBIh9m99j1gZMLjUy?usp=sharing)
## Environment info
- `transformers` version: 4.3.3
- Platform: Linux-4.19.112+-x86_64-with-Ubuntu-18.04-bionic
- Python version: 3.7.10
- PyTorch version (GPU?): 1.7.1+cu101 (False)
- Tensorflow version (GPU?): 2.4.1 (False)
- Using GPU in script?: <fill in>
- Using distributed or parallel set-up in script?: <fill in>
### Who can help
- @patrickvonplaten
- @LysandreJik
- @patil-suraj
<!-- Your issue will be replied to more quickly if you can figure out the right person to tag with @
If you know how to use git blame, that is the easiest way, otherwise, here is a rough guide of **who to tag**.
Please tag fewer than 3 people.
Models:
- albert, bert, xlm: @LysandreJik
- blenderbot, bart, marian, pegasus, encoderdecoder, t5: @patrickvonplaten, @patil-suraj
- longformer, reformer, transfoxl, xlnet: @patrickvonplaten
- fsmt: @stas00
- funnel: @sgugger
- gpt2: @patrickvonplaten, @LysandreJik
- rag: @patrickvonplaten, @lhoestq
- tensorflow: @jplu
Library:
- benchmarks: @patrickvonplaten
- deepspeed: @stas00
- ray/raytune: @richardliaw, @amogkam
- text generation: @patrickvonplaten
- tokenizers: @LysandreJik
- trainer: @sgugger
- pipelines: @LysandreJik
Documentation: @sgugger
HF projects:
- nlp datasets: [different repo](https://github.com/huggingface/nlp)
- rust tokenizers: [different repo](https://github.com/huggingface/tokenizers)
Examples:
- maintained examples (not research project or legacy): @sgugger, @patil-suraj
- research_projects/bert-loses-patience: @JetRunner
- research_projects/distillation: @VictorSanh
-->
## Information
Model I am using (GPT, Bert, RoBerta, BART ForCausalLM):
The problem arises when using:
* [ x] the official example scripts: https://huggingface.co/blog/encoder-decoder#decoder
## To reproduce
Experiment notebook [colab](https://colab.research.google.com/drive/15V37RWAL40vhrk-uBIh9m99j1gZMLjUy?usp=sharing)
## Expected behavior
Causal Models should not be affected by the right context?
| 03-06-2021 15:36:34 | 03-06-2021 15:36:34 | This is not a problem.
When the model predicts the word next to "Ich" (given "Ich"), the word "Ich" cannot attend the words in the future positions (e.g., "will", "ein", etc).
However, when the model predicts the word next to "ein" (given "Ich will ein"), the word "Ich" can attend "will" and "ein", which is not cheating.
So, the word embeddings of "Ich" in the different right contexts are different.<|||||>> This is not a problem.
> When the model predicts the word next to "Ich" (given "Ich"), the word "Ich" cannot attend the words in the future positions (e.g., "will", "ein", etc).
> However, when the model predicts the word next to "ein" (given "Ich will ein"), the word "Ich" can attend "will" and "ein", which is not cheating.
> So, the word embeddings of "Ich" in the different right contexts are different.
I agree this is true for transformer encoder models, but for decode models, due to 'casual mask', the left context should not be affected by the right context. That‘s why GPT "Ich" hidden will not be changed.
Therefore, I am curious why CausalLM models can not apply this rule.
<|||||>> > This is not a problem.
> > When the model predicts the word next to "Ich" (given "Ich"), the word "Ich" cannot attend the words in the future positions (e.g., "will", "ein", etc).
> > However, when the model predicts the word next to "ein" (given "Ich will ein"), the word "Ich" can attend "will" and "ein", which is not cheating.
> > So, the word embeddings of "Ich" in the different right contexts are different.
>
> I agree this is true for transformer encoder models, but for decode models, due to 'casual mask', the left context should not be affected by the right context. That‘s why GPT "Ich" hidden will not be changed.
>
> Therefore, I am curious why CausalLM models can not apply this rule.
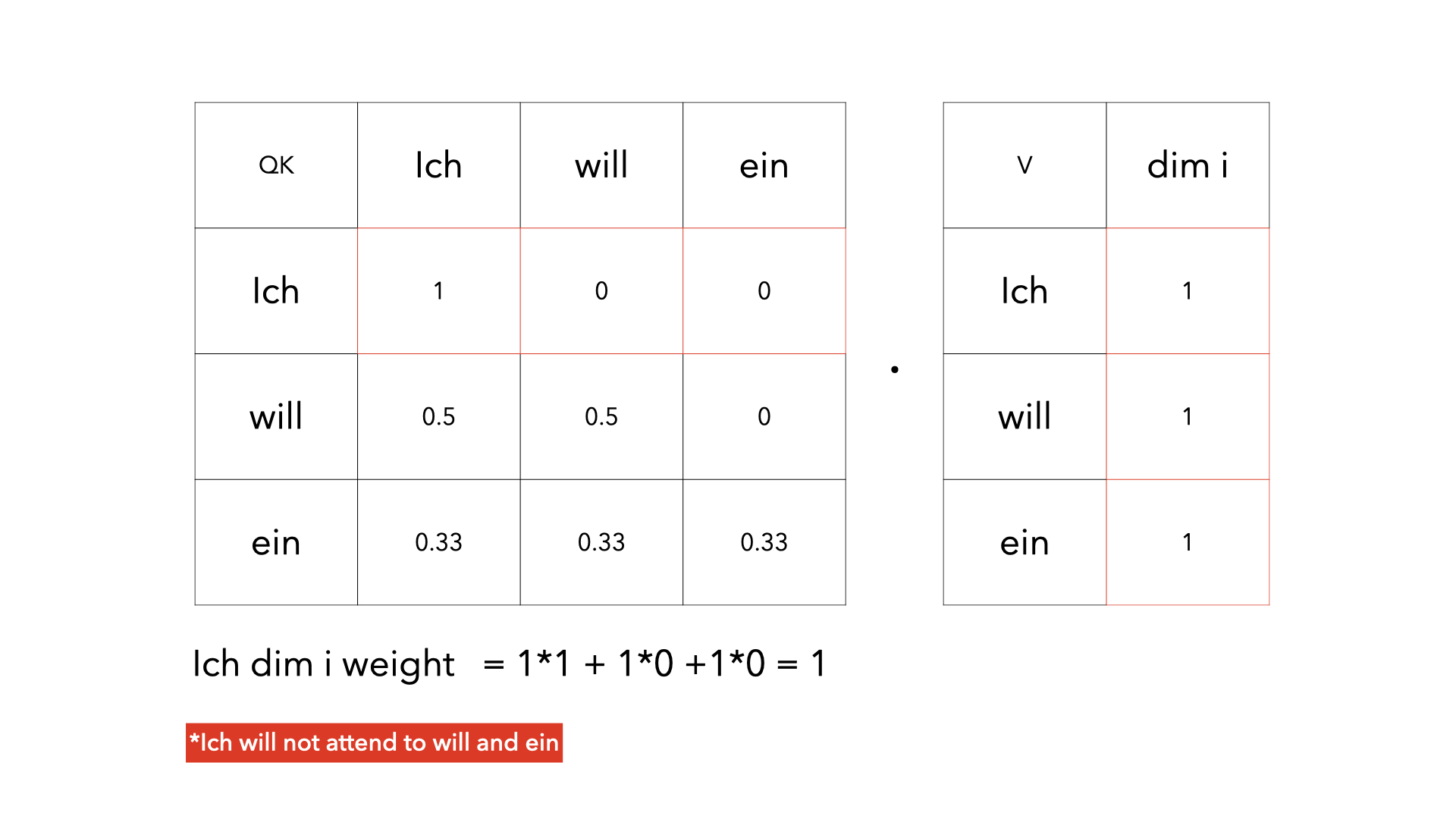
<|||||>> This is not a problem.
> When the model predicts the word next to "Ich" (given "Ich"), the word "Ich" cannot attend the words in the future positions (e.g., "will", "ein", etc).
> However, when the model predicts the word next to "ein" (given "Ich will ein"), the word "Ich" can attend "will" and "ein", which is not cheating.
> So, the word embeddings of "Ich" in the different right contexts are different.
I think that the previous hidden state of the token should not change, since the change of the previous hidden state, there is no way to compute the loss with tokens in once in CausalLM<|||||>I was talking about decoder, not encoder.
The attention masks vary according to a decoding step.
(In the following, "->" means "attends to")
When the model predicts the next word given "Ich":
- "Ich" -> None
When the model predicts the next word given "Ich will ein":
- "Ich" -> "will" and "ein"
- "will" -> "Ich" and "ein"
- "ein" -> "Ich" and "will"
Please see the "The Illustrated Masked Self-Attention" section in the following page.
https://jalammar.github.io/illustrated-gpt2/<|||||>> I was talking about decoder, not encoder.
> The attention masks vary according to a decoding step.
>
> (In the following, "->" means "attends to")
> When the model predicts the next word given "Ich":
>
> * "Ich" -> None
>
> When the model predicts the next word given "Ich will ein":
>
> * "Ich" -> "will" and "ein"
> * "will" -> "Ich" and "ein"
> * "ein" -> "Ich" and "will"
>
> Please see the "The Illustrated Masked Self-Attention" section in the following page.
> https://jalammar.github.io/illustrated-gpt2/
https://huggingface.co/blog/encoder-decoder#decoder
auto-regressive models, such as GPT2, have the same architecture as transformer-based decoder models if one removes the cross-attention layer
On a side-note, autoencoding models, such as Bert, have the same architecture as transformer-based encoder models.
So, without involving cross-attention, the main difference between transformer encoder and decoder is that encoder uses bi-directional self-attention, decoder uses uni-directional self-attention layer instead.
Ich weight will attend to "will", but it's for "will" token weight, not for Ich token.
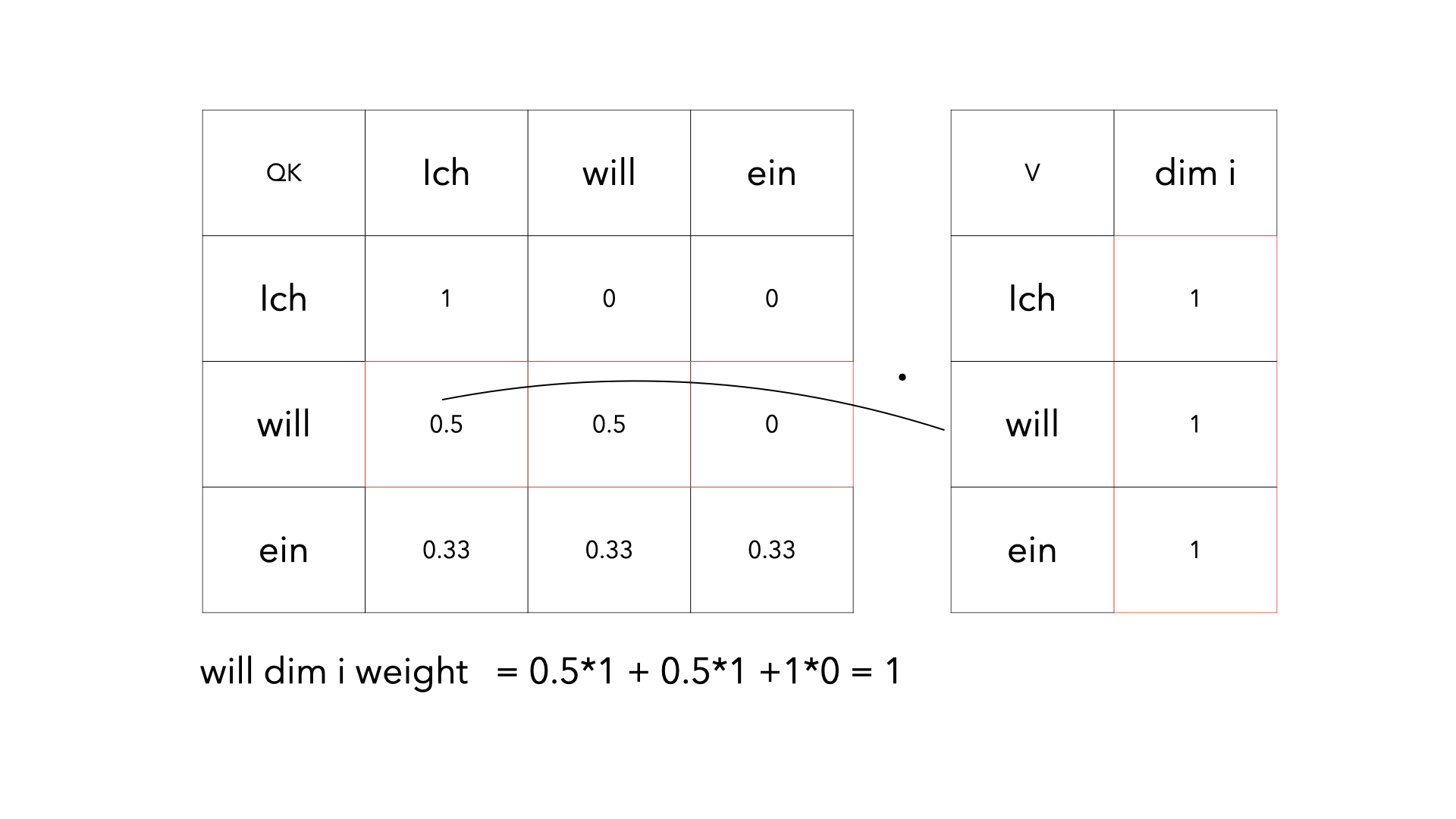
<|||||>All the theory is right.
I got the reason, it is because of the bias...
In `from_pretrained` function, it will call model.eval() by default which will disable all the bias in model.
https://github.com/huggingface/transformers/blob/88a951e3cc00f56b94d9b93dbc35a3812cd88747/src/transformers/modeling_utils.py#L1190
However in `from_config`, it won't call model.eval by default, so the result is affected by bias.
https://github.com/huggingface/transformers/blob/d26b37e744ea980977e266adf48736451b73c583/src/transformers/models/auto/modeling_auto.py#L750
Therefore, I suggest that we should call model.eval() in `from_config` as same as `from_pretrained `
- @patrickvonplaten
- @LysandreJik
- @patil-suraj<|||||>`model.eval()` does not disable the bias in the model as far as I know. `model.eval()` simply puts the model into "non training" mode meaning that dropout layers are not applied, etc.. . I don't think we need to add a `model.eval()` to the `from_config()` function.<|||||>> `model.eval()` does not disable the bias in the model as far as I know. `model.eval()` simply puts the model into "non training" mode meaning that dropout layers are not applied, etc.. . I don't think we need to add a `model.eval()` to the `from_config()` function.
I don't know why I said `bias` 😂, It should be dropout.
from_config() is more likely for training, so it should be fine not to add `model.eval()` by default.
Thanks for your reply~ |
transformers | 10,563 | closed | I have trained Bert on my own data which has been converted to IDs by using BertForMaskedLM, but when I use the model for the further fine-tuned, I found this error | @LysandreJik
## code info
<!-- You can run the command `transformers-cli env` and copy-and-paste its output below.
Don't forget to fill out the missing fields in that output! -->
here's my model:

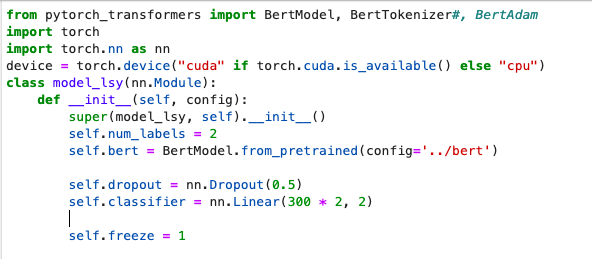
## Information
Model I am using my own pre-tained Bert model which is stored in the path "../bert":
The problem arises when using:

here are the files in the path "../bert":
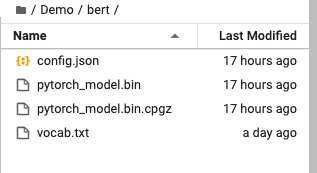
The tasks I am working on is: text matching:
* [ ] my own dataset is like:
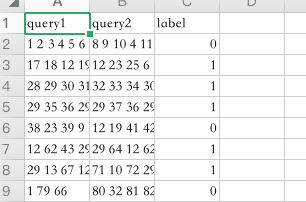
## Expected behavior
<!-- A clear and concise description of what you would expect to happen. -->
what exactly is the problem? | 03-06-2021 15:16:50 | 03-06-2021 15:16:50 | |
transformers | 10,562 | closed | Stale bot updated | This is an updated version of the stale bot.
**It is easier to review the file than the diff, you can find the file [here](https://github.com/huggingface/transformers/blob/d1e516ea0fe9e641a75a89e5a7522392f7dbd59d/scripts/stale.py).**
It sends a warning message after 23 days of inactivity, and closes the issue/PR if no activity is detected in the following 7 days.
It ignores the following labels (case insensitive):
- `Good First Issue`
- `Good Second Issue`
- `Feature Request`
- `New Model`
- `WIP`
If there are assignees on the issue/PR, then it puts the following comment: `f"This issue has been stale for a while, ping @{assignee.login}"`
I propose to leave the PR like it is, and I'll push an empty commit daily to check the result of the stale bot test (I'll remove other tests to ensure that we don't spend unnecessary CI credits). Once we verify that it works as expected for a few days, I propose to merge it.
You may check the results of the first run here: https://github.com/huggingface/transformers/runs/2045189559?check_suite_focus=true (Second commit was rate limited) | 03-06-2021 06:49:58 | 03-06-2021 06:49:58 | @stas00, @sgugger, please review this PR. Here is a visualization of what would be done by the bot, were it to be merged today: https://github.com/huggingface/transformers/pull/10562/checks?check_run_id=2113061781
I have verified that all 11 issues that would be closed have received a warning 10 days ago. Thank you. |
transformers | 10,561 | closed | [examples tests on multigpu] resolving require_torch_non_multi_gpu_but_fix_me | A while ago I added `@require_torch_non_multi_gpu_but_fix_me` to quickly allow us to start running example tests on multigpu, so this PR resolves that temporary band-aid.
This PR:
* fixes a few tests to make them run on multi-gpu
* removes the decorator where it's not needed after testing that it works
* leaves the dropped legacy tests untouched - since they don't run on CI
* eliminates `@require_torch_non_multi_gpu_but_fix_me` from existence since it's no longer needed
* the only test I couldn't figure out is https://github.com/huggingface/transformers/issues/10560 but it's not worse off than it was - added some refactoring to it and prepared it for multi-gpu if someone knows how to fix it
Note: 2 slow tests in `examples/test_examples.py` currently fail because of yet another missed thing in ported `run_seq2seq.py` - but these should be resolved by https://github.com/huggingface/transformers/pull/10551 once that one is merged, so we can merge this PR after it.
@LysandreJik
| 03-06-2021 04:16:55 | 03-06-2021 04:16:55 | |
transformers | 10,560 | closed | [examples] run_glue_deebert.py distrbuted fails | I'm working on making the tests work under multiple gpus and run into and this one that proved to be stubborn, for some reason it doesn't work under any DP scheme. I don't know anything about this script, To reproduce:
Note - you need at least 2 gpus:
Actually it fails with 1 gpu too (just change to --nproc_per_node=1)
```
python -m torch.distributed.launch --nproc_per_node=2 examples/research_projects/deebert/run_glue_deebert.py --model_type roberta --model_name_or_path roberta-base --task_name MRPC --do_train --do_eval --do_lower_case --data_dir ./tests/fixtures/tests_samples/MRPC/ --max_seq_length 128 --per_gpu_eval_batch_size=1 --per_gpu_train_batch_size=8 --learning_rate 2e-4 --num_train_epochs 3 --overwrite_output_dir --seed 42 --output_dir ./examples/deebert/saved_models/roberta-base/MRPC/two_stage --plot_data_dir ./examples/deebert/results/ --save_steps 0 --overwrite_cache --eval_after_first_stage
W reducer.cpp:1084] Warning: find_unused_parameters=True was specified in DDP constructor, but did not find any unused parameters in the forward pass. This flag results in an extra traversal of the autograd graph every iteration, which can adversely affect performance. If your model indeed never has any unused parameters in the forward pass, consider turning this flag off. Note that this warning may be a false positive if your model has flow control causing later iterations to have unused parameters. (function operator())
Traceback (most recent call last):
File "examples/research_projects/deebert/run_glue_deebert.py", line 730, in <module>
main()
File "examples/research_projects/deebert/run_glue_deebert.py", line 645, in main
global_step, tr_loss = train(args, train_dataset, model, tokenizer)
File "examples/research_projects/deebert/run_glue_deebert.py", line 176, in train
outputs = model(**inputs)
File "/home/stas/anaconda3/envs/main-38/lib/python3.8/site-packages/torch/nn/modules/module.py", line 872, in _call_impl
return forward_call(*input, **kwargs)
File "/home/stas/anaconda3/envs/main-38/lib/python3.8/site-packages/torch/nn/parallel/distributed.py", line 705, in forward
if self.reducer._rebuild_buckets():
RuntimeError: Expected to have finished reduction in the prior iteration before starting a new one. This error indicates that your module has parameters that were not used in producing loss. Since `find_unused_parameters=True` is enabled, this likely means that not all `forward` outputs participate in computing loss. You can fix this by making sure all `forward` function outputs participate in calculating loss.
If you already have done the above, then the distributed data parallel module wasn't able to locate the output tensors in the return value of your module's `forward` function. Please include the loss function and the structure of the return value of `forward` of your module when reporting this issue (e.g. list, dict, iterable).
[W reducer.cpp:1084] Warning: find_unused_parameters=True was specified in DDP constructor, but did not find any unused parameters in the forward pass. This flag results in an extra traversal of the autograd graph every iteration, which can adversely affect performance. If your model indeed never has any unused parameters in the forward pass, consider turning this flag off. Note that this warning may be a false positive if your model has flow control causing later iterations to have unused parameters. (function operator())
Iteration: 100%1/1 [00:00<00:00, 1.83it/s]
Iteration: 0%| | 0/1 [00:00<?, ?it/s]
Epoch: 33 | 1/3 [00:00<00:01, 1.82it/s]
Traceback (most recent call last):
File "examples/research_projects/deebert/run_glue_deebert.py", line 730, in <module>
main()
File "examples/research_projects/deebert/run_glue_deebert.py", line 645, in main
global_step, tr_loss = train(args, train_dataset, model, tokenizer)
File "examples/research_projects/deebert/run_glue_deebert.py", line 176, in train
outputs = model(**inputs)
File "/home/stas/anaconda3/envs/main-38/lib/python3.8/site-packages/torch/nn/modules/module.py", line 872, in _call_impl
return forward_call(*input, **kwargs)
File "/home/stas/anaconda3/envs/main-38/lib/python3.8/site-packages/torch/nn/parallel/distributed.py", line 705, in forward
if self.reducer._rebuild_buckets():
RuntimeError: Expected to have finished reduction in the prior iteration before starting a new one. This error indicates that your module has parameters that were not used in producing loss. Since `find_unused_parameters=True` is enabled, this likely means that not all `forward` outputs participate in computing loss. You can fix this by making sure all `forward` function outputs participate in calculating loss.
If you already have done the above, then the distributed data parallel module wasn't able to locate the output tensors in the return value of your module's `forward` function. Please include the loss function and the structure of the return value of `forward` of your module when reporting this issue (e.g. list, dict, iterable).
Killing subprocess 2242528
Killing subprocess 2242529
Traceback (most recent call last):
File "/home/stas/anaconda3/envs/main-38/lib/python3.8/runpy.py", line 194, in _run_module_as_main
return _run_code(code, main_globals, None,
File "/home/stas/anaconda3/envs/main-38/lib/python3.8/runpy.py", line 87, in _run_code
exec(code, run_globals)
File "/home/stas/anaconda3/envs/main-38/lib/python3.8/site-packages/torch/distributed/launch.py", line 340, in <module>
main()
File "/home/stas/anaconda3/envs/main-38/lib/python3.8/site-packages/torch/distributed/launch.py", line 326, in main
sigkill_handler(signal.SIGTERM, None) # not coming back
File "/home/stas/anaconda3/envs/main-38/lib/python3.8/site-packages/torch/distributed/launch.py", line 301, in sigkill_handler
raise subprocess.CalledProcessError(returncode=last_return_code, cmd=cmd)
subprocess.CalledProcessError: Command '['/home/stas/anaconda3/envs/main-38/bin/python', '-u', 'examples/research_projects/deebert/run_glue_deebert.py', '--local_rank=1', '--model_type', 'roberta', '--model_name_or_path', 'roberta-base', '--task_name', 'MRPC', '--do_train', '--do_eval', '--do_lower_case', '--data_dir', './tests/fixtures/tests_samples/MRPC/', '--max_seq_length', '128', '--per_gpu_eval_batch_size=1', '--per_gpu_train_batch_size=8', '--learning_rate', '2e-4', '--num_train_epochs', '3', '--overwrite_output_dir', '--seed', '42', '--output_dir', './examples/deebert/saved_models/roberta-base/MRPC/two_stage', '--plot_data_dir', './examples/deebert/results/', '--save_steps', '0', '--overwrite_cache', '--eval_after_first_stage']' returned non-zero exit status 1.
```
@LysandreJik | 03-06-2021 04:01:00 | 03-06-2021 04:01:00 | Pinging @JetRunner <|||||>@stas00 Well it is just not designed for DP or DDP. DeeBERT is for accelerating inference with bs=1 (especially on CPU). I don't believe it should support DP.<|||||>But yes theoretically it can support multi-GPU training but I'm not sure if it's necessary?<|||||>That's good enough for me, I will leave it at 0 or 1-gpu - no problem - thank you for elaborating about the needs of this example, @JetRunner! |
transformers | 10,559 | closed | [website] installation doc blues | Switching docs to a different branch seems to be broken from at least one doc, e.g. going from
https://huggingface.co/transformers/master/installation.html#caching-models
upper left corner - if you want to switch to a different branch it sends you to a 404 page on all of them:
it has an issue with the base url, note how it links to: https://huggingface.co/transformers/master/master
master twice, so now it prefixes all branches with master/branch
ok narrowed it down - it happens specifically on the installation page: https://huggingface.co/transformers/master/installation.html
I tried a bunch of other pages and it seems to be ok there.
oddly I don't see anything unusual about installation.md in toctree or its content.
if you look at the snapshot - instead of showing `master` for the currently selected version it shows a chunk of the url instead.
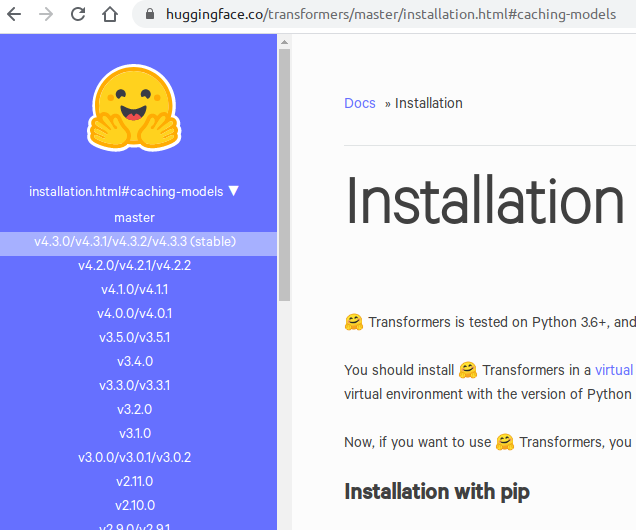
@sgugger | 03-06-2021 03:31:17 | 03-06-2021 03:31:17 | |
transformers | 10,558 | closed | Dear developer, does transformers have the support to translate Chinese text into English? | # 🚀 Feature request
<!-- A clear and concise description of the feature proposal.
Please provide a link to the paper and code in case they exist. -->
## Motivation
<!-- Please outline the motivation for the proposal. Is your feature request
related to a problem? e.g., I'm always frustrated when [...]. If this is related
to another GitHub issue, please link here too. -->
## Your contribution
<!-- Is there any way that you could help, e.g. by submitting a PR?
Make sure to read the CONTRIBUTING.MD readme:
https://github.com/huggingface/transformers/blob/master/CONTRIBUTING.md -->
| 03-06-2021 02:35:07 | 03-06-2021 02:35:07 | Hi @j2538318409,
I think Mbart model from facebook can do that for you [mbart](https://huggingface.co/facebook/mbart-large-50-many-to-many-mmt),
You need to specify `zh_CN` as the source language and `en_XX` as the target language.
This [colab notebook](https://github.com/bhadreshpsavani/UnderstandingNLP/blob/master/MultilingualMBart.ipynb) does translation from English to hindi. You can use the same for doing translation from Chinese to English by modifying `src_lang` and `trg_lang`.
There are other translation models also available in huggingface like [Helsinki-NLP/opus-mt-zh-en](https://huggingface.co/Helsinki-NLP/opus-mt-zh-en). You can find more details on [huggingface models section](https://huggingface.co/models?pipeline_tag=translation)
I hope this will be helpful to you!<|||||>https://huggingface.co/transformers/master/model_doc/m2m_100.html
M2M is in master since today, is this what you are looking for ?<|||||>This issue has been automatically marked as stale because it has not had recent activity. If you think this still needs to be addressed please comment on this thread.
Please note that issues that do not follow the [contributing guidelines](https://github.com/huggingface/transformers/blob/master/CONTRIBUTING.md) are likely to be ignored. |
transformers | 10,557 | closed | [RAG] Expected RAG output after fine tuning | Hi there.
Perhaps the following isn’t even a real issue, but I’m a bit confused with the current outputs I got.
I’m trying to fine tune RAG on a bunch of question-answer pairs I have (for while, not that much, < 1k ones). I have splitted them as suggested (train.source, train.target, val.source…). After running the ```finetune_rag.py```, the outputs generated were **only two files (~2 kB)**:
- git_log.json
- hparams.pkl
Is that right? Because I was expecting *a big binary file or something like that containing the weight matrices*, so I could use them afterwards in a new trial.
Could you please tell me what’s the point I’m missing here?
----------------------
I provide more details below. Btw, I have two NVIDIA RTX 3090, 24GB each, but they were barely used in the whole process (which took ~3 hours).
**Command:**
```
python finetune_rag.py \
--data_dir rag_manual_qa_finetuning \
--output_dir output_ft \
--model_name_or_path rag-sequence-base \
--model_type rag_sequence \
--gpus 2 \
--distributed_retriever pytorch
```
**Logs** (in fact, it’s strange but the logs even seem to be generated in duplicate - I don’t know why):
```
loading configuration file rag-sequence-base/config.json
Model config RagConfig {
"architectures": [
"RagSequenceForGeneration"
],
"dataset": "wiki_dpr",
"dataset_split": "train",
"do_deduplication": true,
"do_marginalize": false,
"doc_sep": " // ",
"exclude_bos_score": false,
"forced_eos_token_id": 2,
"generator": {
"_name_or_path": "",
"_num_labels": 3,
"activation_dropout": 0.0,
"activation_function": "gelu",
"add_bias_logits": false,
"add_cross_attention": false,
"add_final_layer_norm": false,
"architectures": [
"BartModel",
"BartForMaskedLM",
"BartForSequenceClassification"
],
"attention_dropout": 0.0,
"bad_words_ids": null,
"bos_token_id": 0,
"chunk_size_feed_forward": 0,
"classif_dropout": 0.0,
"classifier_dropout": 0.0,
"d_model": 1024,
"decoder_attention_heads": 16,
"decoder_ffn_dim": 4096,
"decoder_layerdrop": 0.0,
"decoder_layers": 12,
"decoder_start_token_id": 2,
"diversity_penalty": 0.0,
"do_sample": false,
"dropout": 0.1,
"early_stopping": false,
"encoder_attention_heads": 16,
"encoder_ffn_dim": 4096,
"encoder_layerdrop": 0.0,
"encoder_layers": 12,
"encoder_no_repeat_ngram_size": 0,
"eos_token_id": 2,
"extra_pos_embeddings": 2,
"finetuning_task": null,
"force_bos_token_to_be_generated": false,
"forced_bos_token_id": null,
"forced_eos_token_id": 2,
"gradient_checkpointing": false,
"id2label": {
"0": "LABEL_0",
"1": "LABEL_1",
"2": "LABEL_2"
},
"init_std": 0.02,
"is_decoder": false,
"is_encoder_decoder": true,
"label2id": {
"LABEL_0": 0,
"LABEL_1": 1,
"LABEL_2": 2
},
"length_penalty": 1.0,
"max_length": 20,
"max_position_embeddings": 1024,
"min_length": 0,
"model_type": "bart",
"no_repeat_ngram_size": 0,
"normalize_before": false,
"normalize_embedding": true,
"num_beam_groups": 1,
"num_beams": 1,
"num_hidden_layers": 12,
"num_return_sequences": 1,
"output_attentions": false,
"output_hidden_states": false,
"output_past": false,
"output_scores": false,
"pad_token_id": 1,
"prefix": " ",
"pruned_heads": {},
"repetition_penalty": 1.0,
"return_dict": false,
"return_dict_in_generate": false,
"scale_embedding": false,
"sep_token_id": null,
"static_position_embeddings": false,
"task_specific_params": {
"summarization": {
"early_stopping": true,
"length_penalty": 2.0,
"max_length": 142,
"min_length": 56,
"no_repeat_ngram_size": 3,
"num_beams": 4
}
},
"temperature": 1.0,
"tie_encoder_decoder": false,
"tie_word_embeddings": true,
"tokenizer_class": null,
"top_k": 50,
"top_p": 1.0,
"torchscript": false,
"transformers_version": "4.4.0.dev0",
"use_bfloat16": false,
"use_cache": true,
"vocab_size": 50265
},
"index_name": "exact",
"index_path": null,
"is_encoder_decoder": true,
"label_smoothing": 0.0,
"max_combined_length": 300,
"model_type": "rag",
"n_docs": 5,
"output_retrieved": false,
"passages_path": null,
"question_encoder": {
"_name_or_path": "",
"add_cross_attention": false,
"architectures": [
"DPRQuestionEncoder"
],
"attention_probs_dropout_prob": 0.1,
"bad_words_ids": null,
"bos_token_id": null,
"chunk_size_feed_forward": 0,
"decoder_start_token_id": null,
"diversity_penalty": 0.0,
"do_sample": false,
"early_stopping": false,
"encoder_no_repeat_ngram_size": 0,
"eos_token_id": null,
"finetuning_task": null,
"forced_bos_token_id": null,
"forced_eos_token_id": null,
"gradient_checkpointing": false,
"hidden_act": "gelu",
"hidden_dropout_prob": 0.1,
"hidden_size": 768,
"id2label": {
"0": "LABEL_0",
"1": "LABEL_1"
},
"initializer_range": 0.02,
"intermediate_size": 3072,
"is_decoder": false,
"is_encoder_decoder": false,
"label2id": {
"LABEL_0": 0,
"LABEL_1": 1
},
"layer_norm_eps": 1e-12,
"length_penalty": 1.0,
"max_length": 20,
"max_position_embeddings": 512,
"min_length": 0,
"model_type": "dpr",
"no_repeat_ngram_size": 0,
"num_attention_heads": 12,
"num_beam_groups": 1,
"num_beams": 1,
"num_hidden_layers": 12,
"num_return_sequences": 1,
"output_attentions": false,
"output_hidden_states": false,
"output_scores": false,
"pad_token_id": 0,
"position_embedding_type": "absolute",
"prefix": null,
"projection_dim": 0,
"pruned_heads": {},
"repetition_penalty": 1.0,
"return_dict": false,
"return_dict_in_generate": false,
"sep_token_id": null,
"task_specific_params": null,
"temperature": 1.0,
"tie_encoder_decoder": false,
"tie_word_embeddings": true,
"tokenizer_class": null,
"top_k": 50,
"top_p": 1.0,
"torchscript": false,
"transformers_version": "4.4.0.dev0",
"type_vocab_size": 2,
"use_bfloat16": false,
"use_cache": true,
"vocab_size": 30522
},
"reduce_loss": false,
"retrieval_batch_size": 8,
"retrieval_vector_size": 768,
"title_sep": " / ",
"use_cache": true,
"use_dummy_dataset": false,
"vocab_size": null
}
Model name 'rag-sequence-base' not found in model shortcut name list (facebook/dpr-question_encoder-single-nq-base, facebook/dpr-question_encoder-multiset-base). Assuming 'rag-sequence-base' is a path, a model identifier, or url to a directory containing tokenizer files.
Didn't find file rag-sequence-base/question_encoder_tokenizer/tokenizer.json. We won't load it.
Didn't find file rag-sequence-base/question_encoder_tokenizer/added_tokens.json. We won't load it.
loading file rag-sequence-base/question_encoder_tokenizer/vocab.txt
loading file None
loading file None
loading file rag-sequence-base/question_encoder_tokenizer/special_tokens_map.json
loading file rag-sequence-base/question_encoder_tokenizer/tokenizer_config.json
Model name 'rag-sequence-base' not found in model shortcut name list (facebook/bart-base, facebook/bart-large, facebook/bart-large-mnli, facebook/bart-large-cnn, facebook/bart-large-xsum, yjernite/bart_eli5). Assuming 'rag-sequence-base' is a path, a model identifier, or url to a directory containing tokenizer files.
Didn't find file rag-sequence-base/generator_tokenizer/tokenizer.json. We won't load it.
Didn't find file rag-sequence-base/generator_tokenizer/added_tokens.json. We won't load it.
loading file rag-sequence-base/generator_tokenizer/vocab.json
loading file rag-sequence-base/generator_tokenizer/merges.txt
loading file None
loading file None
loading file rag-sequence-base/generator_tokenizer/special_tokens_map.json
loading file rag-sequence-base/generator_tokenizer/tokenizer_config.json
Loading passages from wiki_dpr
Downloading: 9.64kB [00:00, 10.8MB/s]
Downloading: 67.5kB [00:00, 59.5MB/s]
WARNING:datasets.builder:Using custom data configuration psgs_w100.nq.no_index-dummy=False,with_index=False
Downloading and preparing dataset wiki_dpr/psgs_w100.nq.no_index (download: 66.09 GiB, generated: 73.03 GiB, post-processed: Unknown size, total: 139.13 GiB) to /home/usp/.cache/huggingface/datasets/wiki_dpr/psgs_w100.nq.no_index-dummy=False,with_index=False/0.0.0/91b145e64f5bc8b55a7b3e9f730786ad6eb19cd5bc020e2e02cdf7d0cb9db9c1...
Downloading: 100%|█████████████████████████| 4.69G/4.69G [07:11<00:00, 10.9MB/s]
Downloading: 100%|█████████████████████████| 1.33G/1.33G [02:27<00:00, 9.00MB/s]
Downloading: 100%|█████████████████████████| 1.33G/1.33G [02:36<00:00, 8.47MB/s]
Downloading: 100%|█████████████████████████| 1.33G/1.33G [02:37<00:00, 8.41MB/s]
Downloading: 100%|█████████████████████████| 1.33G/1.33G [02:38<00:00, 8.36MB/s]
Downloading: 100%|█████████████████████████| 1.33G/1.33G [02:40<00:00, 8.25MB/s]
Downloading: 100%|█████████████████████████| 1.33G/1.33G [02:58<00:00, 7.45MB/s]
Downloading: 100%|█████████████████████████| 1.33G/1.33G [02:58<00:00, 7.43MB/s]
Downloading: 100%|█████████████████████████| 1.33G/1.33G [03:00<00:00, 7.34MB/s]
Downloading: 100%|█████████████████████████| 1.33G/1.33G [03:04<00:00, 7.17MB/s]
Downloading: 100%|█████████████████████████| 1.33G/1.33G [03:05<00:00, 7.13MB/s]
Downloading: 100%|█████████████████████████| 1.33G/1.33G [03:07<00:00, 7.06MB/s]
Downloading: 100%|█████████████████████████| 1.33G/1.33G [03:10<00:00, 6.94MB/s]
Downloading: 100%|█████████████████████████| 1.33G/1.33G [03:24<00:00, 6.48MB/s]
Downloading: 100%|█████████████████████████| 1.32G/1.32G [03:27<00:00, 6.38MB/s]
Downloading: 100%|█████████████████████████| 1.33G/1.33G [03:33<00:00, 6.21MB/s]
Downloading: 100%|█████████████████████████| 1.33G/1.33G [04:57<00:00, 4.45MB/s]
Downloading: 100%|█████████████████████████| 1.33G/1.33G [02:36<00:00, 8.47MB/s]
Downloading: 100%|█████████████████████████| 1.33G/1.33G [02:28<00:00, 8.94MB/s]
Downloading: 100%|█████████████████████████| 1.33G/1.33G [02:44<00:00, 8.03MB/s]
Downloading: 100%|█████████████████████████| 1.33G/1.33G [02:55<00:00, 7.54MB/s]
Downloading: 100%|█████████████████████████| 1.33G/1.33G [02:28<00:00, 8.92MB/s]
Downloading: 100%|█████████████████████████| 1.33G/1.33G [02:28<00:00, 8.90MB/s]
Downloading: 100%|█████████████████████████| 1.33G/1.33G [02:56<00:00, 7.49MB/s]
Downloading: 100%|█████████████████████████| 1.33G/1.33G [03:19<00:00, 6.63MB/s]
Downloading: 100%|█████████████████████████| 1.33G/1.33G [02:53<00:00, 7.63MB/s]
Downloading: 100%|█████████████████████████| 1.33G/1.33G [03:00<00:00, 7.33MB/s]
Downloading: 100%|█████████████████████████| 1.33G/1.33G [03:11<00:00, 6.92MB/s]
Downloading: 100%|█████████████████████████| 1.33G/1.33G [03:14<00:00, 6.80MB/s]
Downloading: 100%|█████████████████████████| 1.32G/1.32G [03:06<00:00, 7.10MB/s]
Downloading: 100%|█████████████████████████| 1.33G/1.33G [03:35<00:00, 6.16MB/s]
Downloading: 100%|█████████████████████████| 1.33G/1.33G [03:50<00:00, 5.76MB/s]
Downloading: 100%|█████████████████████████| 1.33G/1.33G [02:28<00:00, 8.93MB/s]
Downloading: 100%|█████████████████████████| 1.33G/1.33G [02:32<00:00, 8.67MB/s]
Downloading: 100%|█████████████████████████| 1.33G/1.33G [03:07<00:00, 7.05MB/s]
Downloading: 100%|█████████████████████████| 1.33G/1.33G [02:53<00:00, 7.62MB/s]
Downloading: 100%|█████████████████████████| 1.33G/1.33G [03:22<00:00, 6.56MB/s]
Downloading: 100%|█████████████████████████| 1.33G/1.33G [02:47<00:00, 7.93MB/s]
Downloading: 100%|█████████████████████████| 1.33G/1.33G [02:26<00:00, 9.06MB/s]
Downloading: 100%|█████████████████████████| 1.33G/1.33G [02:40<00:00, 8.25MB/s]
Downloading: 100%|█████████████████████████| 1.33G/1.33G [02:42<00:00, 8.17MB/s]
Downloading: 100%|█████████████████████████| 1.33G/1.33G [02:54<00:00, 7.59MB/s]
Downloading: 100%|█████████████████████████| 1.33G/1.33G [02:41<00:00, 8.22MB/s]
Downloading: 100%|█████████████████████████| 1.33G/1.33G [03:18<00:00, 6.69MB/s]
Downloading: 100%|█████████████████████████| 1.33G/1.33G [02:30<00:00, 8.83MB/s]
Downloading: 100%|█████████████████████████| 1.33G/1.33G [03:00<00:00, 7.34MB/s]
Downloading: 100%|█████████████████████████| 1.33G/1.33G [02:20<00:00, 9.44MB/s]
Downloading: 100%|█████████████████████████| 1.33G/1.33G [02:24<00:00, 9.19MB/s]
Downloading: 100%|█████████████████████████| 1.33G/1.33G [02:21<00:00, 9.38MB/s]
Downloading: 100%|█████████████████████████| 1.33G/1.33G [02:18<00:00, 9.59MB/s]
Downloading: 100%|█████████████████████████| 1.33G/1.33G [02:19<00:00, 9.53MB/s]
0 examples [00:00, ? examples/s]2021-03-05 12:11:39.666323: I tensorflow/stream_executor/platform/default/dso_loader.cc:49] Successfully opened dynamic library libcudart.so.11.0
Dataset wiki_dpr downloaded and prepared to /home/usp/.cache/huggingface/datasets/wiki_dpr/psgs_w100.nq.no_index-dummy=False,with_index=False/0.0.0/91b145e64f5bc8b55a7b3e9f730786ad6eb19cd5bc020e2e02cdf7d0cb9db9c1. Subsequent calls will reuse this data.
loading weights file rag-sequence-base/pytorch_model.bin
All model checkpoint weights were used when initializing RagSequenceForGeneration.
Some weights of RagSequenceForGeneration were not initialized from the model checkpoint at rag-sequence-base and are newly initialized: ['rag.generator.lm_head.weight']
You should probably TRAIN this model on a down-stream task to be able to use it for predictions and inference.
loading configuration file rag-sequence-base/config.json
Model config RagConfig {
"architectures": [
"RagSequenceForGeneration"
],
"dataset": "wiki_dpr",
"dataset_split": "train",
"do_deduplication": true,
"do_marginalize": false,
"doc_sep": " // ",
"exclude_bos_score": false,
"forced_eos_token_id": 2,
"generator": {
"_name_or_path": "",
"_num_labels": 3,
"activation_dropout": 0.0,
"activation_function": "gelu",
"add_bias_logits": false,
"add_cross_attention": false,
"add_final_layer_norm": false,
"architectures": [
"BartModel",
"BartForMaskedLM",
"BartForSequenceClassification"
],
"attention_dropout": 0.0,
"bad_words_ids": null,
"bos_token_id": 0,
"chunk_size_feed_forward": 0,
"classif_dropout": 0.0,
"classifier_dropout": 0.0,
"d_model": 1024,
"decoder_attention_heads": 16,
"decoder_ffn_dim": 4096,
"decoder_layerdrop": 0.0,
"decoder_layers": 12,
"decoder_start_token_id": 2,
"diversity_penalty": 0.0,
"do_sample": false,
"dropout": 0.1,
"early_stopping": false,
"encoder_attention_heads": 16,
"encoder_ffn_dim": 4096,
"encoder_layerdrop": 0.0,
"encoder_layers": 12,
"encoder_no_repeat_ngram_size": 0,
"eos_token_id": 2,
"extra_pos_embeddings": 2,
"finetuning_task": null,
"force_bos_token_to_be_generated": false,
"forced_bos_token_id": null,
"forced_eos_token_id": 2,
"gradient_checkpointing": false,
"id2label": {
"0": "LABEL_0",
"1": "LABEL_1",
"2": "LABEL_2"
},
"init_std": 0.02,
"is_decoder": false,
"is_encoder_decoder": true,
"label2id": {
"LABEL_0": 0,
"LABEL_1": 1,
"LABEL_2": 2
},
"length_penalty": 1.0,
"max_length": 20,
"max_position_embeddings": 1024,
"min_length": 0,
"model_type": "bart",
"no_repeat_ngram_size": 0,
"normalize_before": false,
"normalize_embedding": true,
"num_beam_groups": 1,
"num_beams": 1,
"num_hidden_layers": 12,
"num_return_sequences": 1,
"output_attentions": false,
"output_hidden_states": false,
"output_past": false,
"output_scores": false,
"pad_token_id": 1,
"prefix": " ",
"pruned_heads": {},
"repetition_penalty": 1.0,
"return_dict": false,
"return_dict_in_generate": false,
"scale_embedding": false,
"sep_token_id": null,
"static_position_embeddings": false,
"task_specific_params": {
"summarization": {
"early_stopping": true,
"length_penalty": 2.0,
"max_length": 142,
"min_length": 56,
"no_repeat_ngram_size": 3,
"num_beams": 4
}
},
"temperature": 1.0,
"tie_encoder_decoder": false,
"tie_word_embeddings": true,
"tokenizer_class": null,
"top_k": 50,
"top_p": 1.0,
"torchscript": false,
"transformers_version": "4.4.0.dev0",
"use_bfloat16": false,
"use_cache": true,
"vocab_size": 50265
},
"index_name": "exact",
"index_path": null,
"is_encoder_decoder": true,
"label_smoothing": 0.0,
"max_combined_length": 300,
"model_type": "rag",
"n_docs": 5,
"output_retrieved": false,
"passages_path": null,
"question_encoder": {
"_name_or_path": "",
"add_cross_attention": false,
"architectures": [
"DPRQuestionEncoder"
],
"attention_probs_dropout_prob": 0.1,
"bad_words_ids": null,
"bos_token_id": null,
"chunk_size_feed_forward": 0,
"decoder_start_token_id": null,
"diversity_penalty": 0.0,
"do_sample": false,
"early_stopping": false,
"encoder_no_repeat_ngram_size": 0,
"eos_token_id": null,
"finetuning_task": null,
"forced_bos_token_id": null,
"forced_eos_token_id": null,
"gradient_checkpointing": false,
"hidden_act": "gelu",
"hidden_dropout_prob": 0.1,
"hidden_size": 768,
"id2label": {
"0": "LABEL_0",
"1": "LABEL_1"
},
"initializer_range": 0.02,
"intermediate_size": 3072,
"is_decoder": false,
"is_encoder_decoder": false,
"label2id": {
"LABEL_0": 0,
"LABEL_1": 1
},
"layer_norm_eps": 1e-12,
"length_penalty": 1.0,
"max_length": 20,
"max_position_embeddings": 512,
"min_length": 0,
"model_type": "dpr",
"no_repeat_ngram_size": 0,
"num_attention_heads": 12,
"num_beam_groups": 1,
"num_beams": 1,
"num_hidden_layers": 12,
"num_return_sequences": 1,
"output_attentions": false,
"output_hidden_states": false,
"output_scores": false,
"pad_token_id": 0,
"position_embedding_type": "absolute",
"prefix": null,
"projection_dim": 0,
"pruned_heads": {},
"repetition_penalty": 1.0,
"return_dict": false,
"return_dict_in_generate": false,
"sep_token_id": null,
"task_specific_params": null,
"temperature": 1.0,
"tie_encoder_decoder": false,
"tie_word_embeddings": true,
"tokenizer_class": null,
"top_k": 50,
"top_p": 1.0,
"torchscript": false,
"transformers_version": "4.4.0.dev0",
"type_vocab_size": 2,
"use_bfloat16": false,
"use_cache": true,
"vocab_size": 30522
},
"reduce_loss": false,
"retrieval_batch_size": 8,
"retrieval_vector_size": 768,
"title_sep": " / ",
"use_cache": true,
"use_dummy_dataset": false,
"vocab_size": null
}
Model name 'rag-sequence-base' not found in model shortcut name list (facebook/dpr-question_encoder-single-nq-base, facebook/dpr-question_encoder-multiset-base). Assuming 'rag-sequence-base' is a path, a model identifier, or url to a directory containing tokenizer files.
Didn't find file rag-sequence-base/question_encoder_tokenizer/tokenizer.json. We won't load it.
Didn't find file rag-sequence-base/question_encoder_tokenizer/added_tokens.json. We won't load it.
loading file rag-sequence-base/question_encoder_tokenizer/vocab.txt
loading file None
loading file None
loading file rag-sequence-base/question_encoder_tokenizer/special_tokens_map.json
loading file rag-sequence-base/question_encoder_tokenizer/tokenizer_config.json
Model name 'rag-sequence-base' not found in model shortcut name list (facebook/bart-base, facebook/bart-large, facebook/bart-large-mnli, facebook/bart-large-cnn, facebook/bart-large-xsum, yjernite/bart_eli5). Assuming 'rag-sequence-base' is a path, a model identifier, or url to a directory containing tokenizer files.
Didn't find file rag-sequence-base/generator_tokenizer/tokenizer.json. We won't load it.
Didn't find file rag-sequence-base/generator_tokenizer/added_tokens.json. We won't load it.
loading file rag-sequence-base/generator_tokenizer/vocab.json
loading file rag-sequence-base/generator_tokenizer/merges.txt
loading file None
loading file None
loading file rag-sequence-base/generator_tokenizer/special_tokens_map.json
loading file rag-sequence-base/generator_tokenizer/tokenizer_config.json
GPU available: True, used: True
INFO:lightning:GPU available: True, used: True
TPU available: False, using: 0 TPU cores
INFO:lightning:TPU available: False, using: 0 TPU cores
LOCAL_RANK: 0 - CUDA_VISIBLE_DEVICES: [0,1]
INFO:lightning:LOCAL_RANK: 0 - CUDA_VISIBLE_DEVICES: [0,1]
```
| 03-06-2021 00:07:39 | 03-06-2021 00:07:39 | Pinging @lhoestq and @patrickvonplaten <|||||>Hello there,
I am having the exact same issue when trying to finetune rag. I used the masters version of transformers.
I tried a couple of different things like:
- My own dataset and wikipedia default one
- In a physical machine and in colab
- With ray and pytorch
- With the rag-sequence-base and rag-sequence-nq
They all returned the same documents:
git_log.json
hparams.pkl
Also, I realized that if the folder with the trained data is empty, the results are the same.
I am not sure if I am doing something wrong with the implementation or I am not just using the hparams correctly.
Thanks in advance
Marcos Menon
<|||||>Hi ! If I recall correctly the model is saved using pytorch lightning [on_save_checkpoint](https://pytorch-lightning.readthedocs.io/en/0.4.9/LightningModule/RequiredTrainerInterface/#on_save_checkpoint).
So the issue might come from the checkpointing config at
https://github.com/huggingface/transformers/blob/2295d783d5787bcd4c99ea0ddb2a9403697fc126/examples/research_projects/rag/callbacks_rag.py#L36-L43<|||||>Hi, @lhoestq. Thanks for your quick response.
From the log output, I believe the system **is not even starting the network training**. Hence, I guess this issue is even a step **before the saving step** - also because I did not change any code provided by the main transformers library.
Another reason for it: the **output logs don't change**, even when I run the ```!python finetune_rag.py ...``` keeping my ```data_dir``` totally **empty**. So, I think the system is not training at all **or** maybe there is a mistake in my input, so the code skips the training.
Anyway, bellow, there's a sample of the training data I'm using. They all have one question per line in the source and the respective expected answer in the target (fine-tune for a QA task).
**train.source**
```
How big is the Brazilian coastline?
Why was the Port of Santos known as the port of death in the past?
Which Brazilian state has the largest number of coastal cities?
```
**train.target**
```
7,491 km.
The Yellow Fever.
Bahia state.
```<|||||>Oh ok. Maybe this is because you need the `do_train` flag ? See here:
https://github.com/huggingface/transformers/blob/fcf10214e00ede3a3a4d8507022bc8c679c9aff4/examples/research_projects/rag/finetune_rag.sh#L15-L16<|||||>@lhoestq, that's it; it has solved the problem - actually, quite a simple thing.
Since the central ideia of the fine-tune itself is to provide a way to _train_ the model, I guess it'd be nice to have these params shown in the [README](https://github.com/huggingface/transformers/tree/master/examples/research_projects/rag) too - despite of their immediate need, there's no mention of them there.
Anyway, thank you again, @lhoestq.<|||||>You're totally right they must be in the README. Feel free to open a PR to add it, if you want to contribute :)<|||||>So, that's right. Meanwhile, I'm going to close this issue :)<|||||>@nakasato @MMenonJ I am also fine-tuning the RAG for my custom dataset. I am using rag-token model. Although I use an already trained rag, the loss starts around 70. Can you let me know how your loss changes? At what value it starts?<|||||>Hi, @shamanez. Sure: in my last training round, with a dataset of ~30MB (for DPR) and 2400 question-answer pairs in the training data for fine-tune, the loss started off at 118.2, and ended at 30.2, after 100 epochs. I'm using a rag-sequence-base model. In different settings I've tried so far, however, it's common to see the same pattern: it starts around ~130 and ends around ~30.
Nevertheless, maybe because of the extreme specificity of my data (abstracts data), or because of the quality of the question-answer pairs I have (which were generated automatically with a T5 model), the final results were a lot nonsense, in this case.
Btw, since you're also working with RAG, perhaps we can exchange our working experience. Feel free to send me an email ;)<|||||>Thanks a lot. I did some modifications to RAG .. like end to end training of the retrival. Now the code is allmost finish. I will share it very soon with documentation. <|||||>Cool. Good job! ;) |
transformers | 10,556 | closed | Layoutlm tf | # What does this PR do?
<!--
Congratulations! You've made it this far! You're not quite done yet though.
Once merged, your PR is going to appear in the release notes with the title you set, so make sure it's a great title that fully reflects the extent of your awesome contribution.
Then, please replace this with a description of the change and which issue is fixed (if applicable). Please also include relevant motivation and context. List any dependencies (if any) that are required for this change.
Once you're done, someone will review your PR shortly (see the section "Who can review?" below to tag some potential reviewers). They may suggest changes to make the code even better. If no one reviewed your PR after a week has passed, don't hesitate to post a new comment @-mentioning the same persons---sometimes notifications get lost.
-->
<!-- Remove if not applicable -->
This PR adds TF version of LayoutLM for issue [(10312)](https://github.com/huggingface/transformers/issues/10312)
## Before submitting
- [ ] This PR fixes a typo or improves the docs (you can dismiss the other checks if that's the case).
- [ ] Did you read the [contributor guideline](https://github.com/huggingface/transformers/blob/master/CONTRIBUTING.md#start-contributing-pull-requests),
Pull Request section?
- [X] Was this discussed/approved via a Github issue or the [forum](https://discuss.huggingface.co/)? Please add a link
to it if that's the case.
- [X] Did you make sure to update the documentation with your changes? Here are the
[documentation guidelines](https://github.com/huggingface/transformers/tree/master/docs), and
[here are tips on formatting docstrings](https://github.com/huggingface/transformers/tree/master/docs#writing-source-documentation).
- [X] Did you write any new necessary tests?
## Who can review?
Anyone in the community is free to review the PR once the tests have passed. Feel free to tag
members/contributors which may be interested in your PR.
<!-- Your PR will be replied to more quickly if you can figure out the right person to tag with @
If you know how to use git blame, that is the easiest way, otherwise, here is a rough guide of **who to tag**.
Please tag fewer than 3 people.
Models:
- albert, bert, xlm: @LysandreJik
- blenderbot, bart, marian, pegasus, encoderdecoder, t5: @patrickvonplaten, @patil-suraj
- longformer, reformer, transfoxl, xlnet: @patrickvonplaten
- fsmt: @stas00
- funnel: @sgugger
- gpt2: @patrickvonplaten, @LysandreJik
- rag: @patrickvonplaten, @lhoestq
- tensorflow: @jplu
Library:
- benchmarks: @patrickvonplaten
- deepspeed: @stas00
- ray/raytune: @richardliaw, @amogkam
- text generation: @patrickvonplaten
- tokenizers: @n1t0, @LysandreJik
- trainer: @sgugger
- pipelines: @LysandreJik
Documentation: @sgugger
HF projects:
- nlp datasets: [different repo](https://github.com/huggingface/nlp)
- rust tokenizers: [different repo](https://github.com/huggingface/tokenizers)
Examples:
- maintained examples (not research project or legacy): @sgugger, @patil-suraj
- research_projects/bert-loses-patience: @JetRunner
- research_projects/distillation: @VictorSanh
-->
| 03-05-2021 23:59:57 | 03-05-2021 23:59:57 | Oh, no! Did you have some issues with a rebase? Can we help in any way?<|||||>> Oh, no! Did you have some issues with a rebase? Can we help in any way?
I do! For some reason when I rebased I was not able to push my changes. It was rejected because my branch was diverged too much form the remote. Then my option was to pull all changes which results in many file changes that are not mine. <|||||>Ah! Would you like me to try and retrieve your commits and push them on a new branch of your repository? I can take care of the rebasing as well.<|||||>> Ah! Would you like me to try and retrieve your commits and push them on a new branch of your repository? I can take care of the rebasing as well.
that would be great, ty.<|||||>I'm getting permission denied on your fork, can you invite me to it so I can push the new branch? Thanks!<|||||>> I'm getting permission denied on your fork, can you invite me to it so I can push the new branch? Thanks!
done. Let me know if there was any access issues and Thanks again for helping me with this<|||||>You can find the branch [here](https://github.com/atahmasb/transformers/tree/layout-lm-tf-2)! I've rebased it for you, and fixed the code quality issues. The `TFLayoutLMForSequenceClassification` class was in double so I removed one of them. Let me know if this shouldn't have been removed!<|||||>> You can find the branch [here](https://github.com/atahmasb/transformers/tree/layout-lm-tf-2)! I've rebased it for you, and fixed the code quality issues. The `TFLayoutLMForSequenceClassification` class was in double so I removed one of them. Let me know if this shouldn't have been removed!
Thanks! You're awesome! |
transformers | 10,555 | closed | Add new GLUE example with no Trainer. | # What does this PR do?
This PR adds a new GLUE example that does not use the `Trainer`, leveraging [accelerate](https://github.com/huggingface/accelerate) for the distributed training. The necessary instructions are added in the text-classification README.
cc @JetRunner as it should be of interest to you. | 03-05-2021 22:19:11 | 03-05-2021 22:19:11 | This kind of logging is very useful for researchers. Let's add them back?
https://github.com/google-research/bert/blob/master/run_classifier.py#L871<|||||>In a nutshell, I'll burst into tears if we can just have Google's `run_classifier.py` back but with `accelerate` :)<|||||>Maybe we should tag other researchers (even external) to give some feedback. cc @VictorSanh @TevenLeScao <|||||>Addressed most of your comments except the logging/saving steps. I do not have time to add this right now, so I suggest we merge the current version and someone from the community can finish it. |
transformers | 10,554 | closed | Fixed dead link in Trainer documentation | # What does this PR do?
<!--
Congratulations! You've made it this far! You're not quite done yet though.
Once merged, your PR is going to appear in the release notes with the title you set, so make sure it's a great title that fully reflects the extent of your awesome contribution.
Then, please replace this with a description of the change and which issue is fixed (if applicable). Please also include relevant motivation and context. List any dependencies (if any) that are required for this change.
Once you're done, someone will review your PR shortly (see the section "Who can review?" below to tag some potential reviewers). They may suggest changes to make the code even better. If no one reviewed your PR after a week has passed, don't hesitate to post a new comment @-mentioning the same persons---sometimes notifications get lost.
-->
<!-- Remove if not applicable -->
Fixes #10548
## Before submitting
- [x] This PR fixes a typo or improves the docs (you can dismiss the other checks if that's the case).
- [ ] Did you read the [contributor guideline](https://github.com/huggingface/transformers/blob/master/CONTRIBUTING.md#start-contributing-pull-requests),
Pull Request section?
- [x] Was this discussed/approved via a Github issue or the [forum](https://discuss.huggingface.co/)? Please add a link
to it if that's the case.
- [ ] Did you make sure to update the documentation with your changes? Here are the
[documentation guidelines](https://github.com/huggingface/transformers/tree/master/docs), and
[here are tips on formatting docstrings](https://github.com/huggingface/transformers/tree/master/docs#writing-source-documentation).
- [ ] Did you write any new necessary tests?
## Who can review?
Anyone in the community is free to review the PR once the tests have passed. Feel free to tag
members/contributors which may be interested in your PR.
<!-- Your PR will be replied to more quickly if you can figure out the right person to tag with @
If you know how to use git blame, that is the easiest way, otherwise, here is a rough guide of **who to tag**.
Please tag fewer than 3 people.
Models:
- albert, bert, xlm: @LysandreJik
- blenderbot, bart, marian, pegasus, encoderdecoder, t5: @patrickvonplaten, @patil-suraj
- longformer, reformer, transfoxl, xlnet: @patrickvonplaten
- fsmt: @stas00
- funnel: @sgugger
- gpt2: @patrickvonplaten, @LysandreJik
- rag: @patrickvonplaten, @lhoestq
- tensorflow: @jplu
Library:
- benchmarks: @patrickvonplaten
- deepspeed: @stas00
- ray/raytune: @richardliaw, @amogkam
- text generation: @patrickvonplaten
- tokenizers: @n1t0, @LysandreJik
- trainer: @sgugger
- pipelines: @LysandreJik
Documentation: @sgugger
HF projects:
- nlp datasets: [different repo](https://github.com/huggingface/nlp)
- rust tokenizers: [different repo](https://github.com/huggingface/tokenizers)
Examples:
- maintained examples (not research project or legacy): @sgugger, @patil-suraj
- research_projects/bert-loses-patience: @JetRunner
- research_projects/distillation: @VictorSanh
-->
| 03-05-2021 19:44:35 | 03-05-2021 19:44:35 | |
transformers | 10,553 | closed | Transformers upgrade | Transformers upgrade - redoing all ec-ml related changes | 03-05-2021 17:37:49 | 03-05-2021 17:37:49 | |
transformers | 10,552 | closed | Handle padding in decoder_inputs_id when using generate | # What does this PR do?
<!--
Congratulations! You've made it this far! You're not quite done yet though.
Once merged, your PR is going to appear in the release notes with the title you set, so make sure it's a great title that fully reflects the extent of your awesome contribution.
Then, please replace this with a description of the change and which issue is fixed (if applicable). Please also include relevant motivation and context. List any dependencies (if any) that are required for this change.
Once you're done, someone will review your PR shortly (see the section "Who can review?" below to tag some potential reviewers). They may suggest changes to make the code even better. If no one reviewed your PR after a week has passed, don't hesitate to post a new comment @-mentioning the same persons---sometimes notifications get lost.
-->
<!-- Remove if not applicable -->
Fixes #10478 for pytorch version of `generate()` in generate_utils.py
As described in the issue, when `decoder_input_ids` have different lengths and require padding, generation continues after the padding tokens. This PR modifies that behavior so that tokens are generated before the padding for each element in the batch.
## Before submitting
- [ ] This PR fixes a typo or improves the docs (you can dismiss the other checks if that's the case).
- [x] Did you read the [contributor guideline](https://github.com/huggingface/transformers/blob/master/CONTRIBUTING.md#start-contributing-pull-requests),
Pull Request section?
- [x] Was this discussed/approved via a Github issue or the [forum](https://discuss.huggingface.co/)? Please add a link
to it if that's the case.
- [ ] Did you make sure to update the documentation with your changes? Here are the
[documentation guidelines](https://github.com/huggingface/transformers/tree/master/docs), and
[here are tips on formatting docstrings](https://github.com/huggingface/transformers/tree/master/docs#writing-source-documentation).
- [ ] Did you write any new necessary tests?
## Who can review?
I am adding those who I see more often in the git blame of the file:
@patrickvonplaten, @patil-suraj, @yjernite
<!-- Your PR will be replied to more quickly if you can figure out the right person to tag with @
If you know how to use git blame, that is the easiest way, otherwise, here is a rough guide of **who to tag**.
Please tag fewer than 3 people.
Models:
- albert, bert, xlm: @LysandreJik
- blenderbot, bart, marian, pegasus, encoderdecoder, t5: @patrickvonplaten, @patil-suraj
- longformer, reformer, transfoxl, xlnet: @patrickvonplaten
- fsmt: @stas00
- funnel: @sgugger
- gpt2: @patrickvonplaten, @LysandreJik
- rag: @patrickvonplaten, @lhoestq
- tensorflow: @jplu
Library:
- benchmarks: @patrickvonplaten
- deepspeed: @stas00
- ray/raytune: @richardliaw, @amogkam
- text generation: @patrickvonplaten
- tokenizers: @n1t0, @LysandreJik
- trainer: @sgugger
- pipelines: @LysandreJik
Documentation: @sgugger
HF projects:
- nlp datasets: [different repo](https://github.com/huggingface/nlp)
- rust tokenizers: [different repo](https://github.com/huggingface/tokenizers)
Examples:
- maintained examples (not research project or legacy): @sgugger, @patil-suraj
- research_projects/bert-loses-patience: @JetRunner
- research_projects/distillation: @VictorSanh
-->
This is my first PR, so let me use it to thank everyone involved in this library for all the cool work :) | 03-05-2021 17:10:55 | 03-05-2021 17:10:55 | Some tests failed due to pad_token_id being None. Is this really a possibility for any Transformer model?<|||||>Added a check for `token_pad_id` equal to `None` and tests pass, but it is not very elegant, any feedback will be appreciated.<|||||>I just noticed that one test, `run_tests_flax`, failed, however no changes are made that should affect that. @patrickvonplaten or @patil-suraj, when you have the time let me know if there's anything else I should be aware of regarding that test.<|||||>Hi @LittlePea13
Thanks a lot for the PR.
I understand the problem but it seems like an edge case and overall I'm not really in favor of supporting this. The philosophy of `generate` is to keep it simple and extensible and not try to cover all use-cases.
We generally try to keep such if/else statements to a minimum. This change will introduce a lot of complexity in the code.
Also with this change, we won't be able to use `use_cache`, which will slow down generation significantly.
One could always just call generate multiple times if the `decoder_input_ids` are of different length. I would rater batch the sentences together which require the `decoder_input_ids` of the same length and then pass those to `generate` instead of passing `decoder_input_ids` of different lengths. Which would cover this use-case.
But thanks a lot for your work! It's a good practice to first propose and discuss the solution in the issues before opening a PR.
What do you think @patrickvonplaten?<|||||>Hi @patil-suraj thanks for the feedback, I agree that this introduces something specific, and I am not too happy on how it is dealt with for the reasons you point out. However, it seemed useful to have a way to deal with it since it is what one would expect if one inputs `decoder_input_ids` to the `generate` function with some padding.
I opened an issue but was too impatient and opened a PR (sorry about that), basically because I needed this for my own work and coded it anyways.
Perhaps this doesn't belong here, but in any case I feel like including extra documentation about `decoder_input_ids` [here](https://huggingface.co/transformers/main_classes/model.html?highlight=beam%20search#transformers.generation_utils.GenerationMixin.generate) would be beneficial, maybe explaining this behavior (ie. they have to be of the same length).<|||||>Hey @LittlePea13,
Thanks for raising awareness for your problem and thanks for opening a PR! I agree with @patil-suraj here and would prefer to not include such specific code in `generate()`.
In general the philosophy for more specific use cases of `generate()` is to directly use the "sub"-generate methods, such as `sample()`, `greedy_search()`, and `beam_search()` as explained here: https://discuss.huggingface.co/t/big-generate-refactor/1857
I think in your use case, we could do a similar trick that what was done for GPT2 for batched inference:
https://discuss.huggingface.co/t/batch-generation-with-gpt2/1517
This means that instead of passing `["This is <PAD> <PAD>", "This is a sentence"]` as `decoder_input_ids` you could pass `["<PAD> <PAD> This is", "This is a sentence"]` to make `generate()` work. Could you try this out? Also, I'd recommend to directly use `beam_search()` instead of `generate()` in your example. If it doesn't work feel free to post on the forum: https://discuss.huggingface.co/ and tag me - I'll try to help you make it work then :-) <|||||>I think a well-written forum post would also be a great way of documenting this behavior. However I do think, it's a bit too specific for the general docs of `generate()` since they don't even include `decoder_input_ids` as an input argument to the function.<|||||>@patil-suraj and I will keep an eye out if more people run into this problem! Thanks a lot for bringing it up in any case :-)<|||||>Thanks both for the review. Indeed, I didn't think of just moving the padding to the left, much more elegant. I tried it out and it works but it produces different outputs than without padding.
The issue here is that by using directly the different "sub"-generate methods is not possible to apply the same changes, so if one wants to have the same results as if there was no difference in the sentences lengths they would still need to do a similar tweak as the one here on each method.
But this is very narrow, I don't even know if it affects performance in my case when compared to moving padding to the left. I am closing this and in case someone has a similar issue you can just refer to the changes here.
Cheers! |
transformers | 10,551 | closed | Added max_sample_ arguments | # What does this PR do?
<!--
Congratulations! You've made it this far! You're not quite done yet though.
Once merged, your PR is going to appear in the release notes with the title you set, so make sure it's a great title that fully reflects the extent of your awesome contribution.
Then, please replace this with a description of the change and which issue is fixed (if applicable). Please also include relevant motivation and context. List any dependencies (if any) that are required for this change.
Once you're done, someone will review your PR shortly (see the section "Who can review?" below to tag some potential reviewers). They may suggest changes to make the code even better. If no one reviewed your PR after a week has passed, don't hesitate to post a new comment @-mentioning the same persons---sometimes notifications get lost.
-->
<!-- Remove if not applicable -->
Fixes #10437 #10423
## Before submitting
- [ ] This PR fixes a typo or improves the docs (you can dismiss the other checks if that's the case).
- [x] Did you read the [contributor guideline](https://github.com/huggingface/transformers/blob/master/CONTRIBUTING.md#start-contributing-pull-requests),
Pull Request section?
- [x] Was this discussed/approved via a Github issue or the [forum](https://discuss.huggingface.co/)? #10437
- [ ] Did you make sure to update the documentation with your changes? Here are the
[documentation guidelines](https://github.com/huggingface/transformers/tree/master/docs), and
[here are tips on formatting docstrings](https://github.com/huggingface/transformers/tree/master/docs#writing-source-documentation).
- [ ] Did you write any new necessary tests?
## Notes:
All the PyTorch-based examples except the below two files will have support for the arguments by adding these changes.
1. The same changes can be implemented for `run_mlm_flax.py` but since I couldn't test the changes I didn't make changes to that file.
2. `run_generation.py`
* I have reverted the code changes for three TF-based examples since it was giving an error and we want to keep it as it is.
* Test/Predict code addition is still pending. I will do it next.
## review:
@stas00 @sgugger
<!-- Your PR will be replied to more quickly if you can figure out the right person to tag with @
If you know how to use git blame, that is the easiest way, otherwise, here is a rough guide of **who to tag**.
Please tag fewer than 3 people.
Models:
- albert, bert, xlm: @LysandreJik
- blenderbot, bart, marian, pegasus, encoderdecoder, t5: @patrickvonplaten, @patil-suraj
- longformer, reformer, transfoxl, xlnet: @patrickvonplaten
- fsmt: @stas00
- funnel: @sgugger
- gpt2: @patrickvonplaten, @LysandreJik
- rag: @patrickvonplaten, @lhoestq
- tensorflow: @jplu
Library:
- benchmarks: @patrickvonplaten
- deepspeed: @stas00
- ray/raytune: @richardliaw, @amogkam
- text generation: @patrickvonplaten
- tokenizers: @n1t0, @LysandreJik
- trainer: @sgugger
- pipelines: @LysandreJik
Documentation: @sgugger
HF projects:
- nlp datasets: [different repo](https://github.com/huggingface/nlp)
- rust tokenizers: [different repo](https://github.com/huggingface/tokenizers)
Examples:
- maintained examples (not research project or legacy): @sgugger, @patil-suraj
- research_projects/bert-loses-patience: @JetRunner
- research_projects/distillation: @VictorSanh
-->
| 03-05-2021 16:38:57 | 03-05-2021 16:38:57 | Thank you for having a closer look that I did, @sgugger.
Ideally we should have tests that would have caught this<|||||>Hi @stas00,
How can we add test cases for this testing? If we check `max_train_samples` and `max_valid_samples` from metrics and add assert statement that might be possible.
<|||||>> How can we add test cases for this testing? If we check `max_train_samples` and `max_valid_samples` from metrics and add assert statement that might be possible.
Yes, that's exactly the idea<|||||>Hi @stas00,
What should I do if I got this error while using git,
```
$ git push origin argument-addition
To https://github.com/bhadreshpsavani/transformers.git
! [rejected] argument-addition -> argument-addition (non-fast-forward)
error: failed to push some refs to 'https://github.com/bhadreshpsavani/transformers.git'
hint: Updates were rejected because the tip of your current branch is behind
hint: its remote counterpart. Integrate the remote changes (e.g.
hint: 'git pull ...') before pushing again.
hint: See the 'Note about fast-forwards' in 'git push --help' for details.
```<|||||>I found that I need to use this command `git push -f origin argument-addition` with fast-forward flag.
Thanks, @stas00 I used your rebase script. It's cool! I did it the first time!<|||||>Some unrelated to your work CI tests were failing so I rebased your PR branch to master, and then they passed. You may have not noticed that.
So you needed to do `git pull` before continuing pushing. and if you already made some changes and `git pull` doesn't work because an update was made in files that you locally modify, you normally do:
```
git stash
git pull
git stash pop
```
and deal with merge conflicts if any emerge.
In general force-pushing should only be reserved for when a bad mistake was made and you need to undo some damage.
So your force-pushing undid the changes I pushed. But since you then rebased it's the same as what I did. No damage done in this situation.
But please be careful in the future and first understand why you think of doing force pushing.<|||||>Okay @stas00,
I will be careful while using force push I will use `stash`.
Now I understood<|||||>Hello @stas00 and @sgugger,
I have made the suggested changes
Please let me know if any other changes are required
Thanks<|||||>@LysandreJik I think this is ready for final review and merge if you're happy with it. |
transformers | 10,550 | closed | How to get best model from hyperparameter search easily | Hi,
after doing a hyperparameter search(by calling `hyperparameter_search`on the trainer object) , I asked myself how to easily get the best model out of it. Currently, I'm using Ray Tune as a backend.
Given [the code in integrations.py](https://github.com/huggingface/transformers/blob/54e55b52d4886d4c63e592310b4253e01c606285/src/transformers/integrations.py#L238) the trial id, objective and chosen hyperparameters are stored in class [BestRun](https://github.com/huggingface/transformers/blob/54e55b52d4886d4c63e592310b4253e01c606285/src/transformers/trainer_utils.py#L116) which is then returned by the hyperparameter_search function. But here the model is somehow missing or am I wrong? One option would be to retrained from the given hyperparameters but this is not possible in PBT because the perturbation is applied during hyperparameter search (and cannot be repeated).
The only thing I currently see is to load the model based on the run_id and compose the corresponding file path.
But maybe there is an easier way to do it (or is this the expected way?). I also tried out the parameter `load_best_model_at_end=True` in [TrainingArguments](https://huggingface.co/transformers/main_classes/trainer.html#trainingarguments) which is used in [this example](https://docs.ray.io/en/master/tune/examples/pbt_transformers.html) but it does not help.
One proposal would be to add a parameter in BestRun which would contain the model or even better load it directly into the trainer (such that the `predict` and `save_model` functions also work).
Is this a reasonable feature request? In case yes, I'd be happy to create a pull request.
In other documentations they show how they extract the checkpoint directly:
- [Ray Docs](https://docs.ray.io/en/master/tune/tutorials/tune-serve-integration-mnist.html#configuring-the-search-space-and-starting-ray-tune)
```
best_trial = analysis.get_best_trial("mean_accuracy", "max", "last")
best_accuracy = best_trial.metric_analysis["mean_accuracy"]["last"]
best_trial_config = best_trial.config
best_checkpoint = best_trial.checkpoint.value
```
- [Colab example for PBT](https://colab.research.google.com/drive/1tQgAKgcKQzheoh503OzhS4N9NtfFgmjF?usp=sharing#scrollTo=TxKyvQ6WNlvG)
```
best_config = analysis.get_best_config(metric="eval_acc", mode="max")
print(best_config)
best_checkpoint = recover_checkpoint(
analysis.get_best_trial(metric="eval_acc",
mode="max").checkpoint.value)
```
Best regards
Sven | 03-05-2021 16:36:06 | 03-05-2021 16:36:06 | cc @sgugger <|||||>Yes there is nothing available for that right now. I believe the to `run_hp_search` functions should save the checkpoints of the non-aborted training and at least return the location of the best checkpoint in the BestRun namedtuple, as well as load the best model fine-tuned at the end if `load_best_model_at_end=True`. If you want to tackle this, we'd love to get a PR!<|||||>This issue has been automatically marked as stale because it has not had recent activity. If you think this still needs to be addressed please comment on this thread.
Please note that issues that do not follow the [contributing guidelines](https://github.com/huggingface/transformers/blob/master/CONTRIBUTING.md) are likely to be ignored. |
transformers | 10,549 | closed | Fix embeddings for PyTorch 1.8 | # What does this PR do?
This PR fixes several embeddings layer with the recent breaking change introduced in PyTorch 1.8. Up until PyTorch 1.7, the `padding_idx` passed to an embedding layer was used to initialize the corresponding row in the weights to 0 but ignored afterwards.
Now, this `padding_idx` is used at every forward pass and ignores the potential weights of the padding index (spoiler alert, all pretrained models I checked have a nonzero one).
To solve this, this PR removes all `padding_idx` passed to embeddings layer. As we were in any case re-initializing them in the `_init_weights` function, the zero weight for that index was ignored in any case. This PR thus introduces no breaking change on our side while dealing with the breaking change in PyTorch.
| 03-05-2021 16:34:47 | 03-05-2021 16:34:47 | |
transformers | 10,548 | closed | Dead link to optuna.create_study under hyperparamter_search in Trainer | I noticed the hyperlink to the documentation of optuna's create_study under ```kwargs``` in the ```hyperparameter_search``` method of Trainer is outdated.
https://huggingface.co/transformers/main_classes/trainer.html
### Who can help
Documentation: @sgugger
New URL (I'm guessing): https://optuna.readthedocs.io/en/stable/reference/generated/optuna.study.create_study.html
Old (currently used): https://optuna.readthedocs.io/en/stable/reference/alias_generated/optuna.create_study.html#optuna.create_study
| 03-05-2021 16:01:56 | 03-05-2021 16:01:56 | Thanks for flagging! Do you want to make a PR to fix it?<|||||>> Thanks for flagging! Do you want to make a PR to fix it?
I tried (#10554). Did I do it correctly?<|||||>Looks okay to me! Let's just wait to check the tests pass. Thanks! :-) |
transformers | 10,547 | closed | [Wav2Vec2 Example Script] Typo | # What does this PR do?
<!--
Congratulations! You've made it this far! You're not quite done yet though.
Once merged, your PR is going to appear in the release notes with the title you set, so make sure it's a great title that fully reflects the extent of your awesome contribution.
Then, please replace this with a description of the change and which issue is fixed (if applicable). Please also include relevant motivation and context. List any dependencies (if any) that are required for this change.
Once you're done, someone will review your PR shortly (see the section "Who can review?" below to tag some potential reviewers). They may suggest changes to make the code even better. If no one reviewed your PR after a week has passed, don't hesitate to post a new comment @-mentioning the same persons---sometimes notifications get lost.
-->
<!-- Remove if not applicable -->
Fix a typo. Script should be as generic as possible
## Before submitting
- [ ] This PR fixes a typo or improves the docs (you can dismiss the other checks if that's the case).
- [ ] Did you read the [contributor guideline](https://github.com/huggingface/transformers/blob/master/CONTRIBUTING.md#start-contributing-pull-requests),
Pull Request section?
- [ ] Was this discussed/approved via a Github issue or the [forum](https://discuss.huggingface.co/)? Please add a link
to it if that's the case.
- [ ] Did you make sure to update the documentation with your changes? Here are the
[documentation guidelines](https://github.com/huggingface/transformers/tree/master/docs), and
[here are tips on formatting docstrings](https://github.com/huggingface/transformers/tree/master/docs#writing-source-documentation).
- [ ] Did you write any new necessary tests?
## Who can review?
Anyone in the community is free to review the PR once the tests have passed. Feel free to tag
members/contributors which may be interested in your PR.
<!-- Your PR will be replied to more quickly if you can figure out the right person to tag with @
If you know how to use git blame, that is the easiest way, otherwise, here is a rough guide of **who to tag**.
Please tag fewer than 3 people.
Models:
- albert, bert, xlm: @LysandreJik
- blenderbot, bart, marian, pegasus, encoderdecoder, t5: @patrickvonplaten, @patil-suraj
- longformer, reformer, transfoxl, xlnet: @patrickvonplaten
- fsmt: @stas00
- funnel: @sgugger
- gpt2: @patrickvonplaten, @LysandreJik
- rag: @patrickvonplaten, @lhoestq
- tensorflow: @jplu
Library:
- benchmarks: @patrickvonplaten
- deepspeed: @stas00
- ray/raytune: @richardliaw, @amogkam
- text generation: @patrickvonplaten
- tokenizers: @n1t0, @LysandreJik
- trainer: @sgugger
- pipelines: @LysandreJik
Documentation: @sgugger
HF projects:
- nlp datasets: [different repo](https://github.com/huggingface/nlp)
- rust tokenizers: [different repo](https://github.com/huggingface/tokenizers)
Examples:
- maintained examples (not research project or legacy): @sgugger, @patil-suraj
- research_projects/bert-loses-patience: @JetRunner
- research_projects/distillation: @VictorSanh
-->
| 03-05-2021 15:16:42 | 03-05-2021 15:16:42 | |
transformers | 10,546 | closed | Fix torch 1.8.0 segmentation fault | The ONNX test fails on PyTorch 1.8.0 due to a segmentation fault. This is a draft PR to try different things out. | 03-05-2021 14:46:16 | 03-05-2021 14:46:16 | |
transformers | 10,545 | closed | Fixing conversation test for torch 1.8 | # What does this PR do?
<!--
Congratulations! You've made it this far! You're not quite done yet though.
Once merged, your PR is going to appear in the release notes with the title you set, so make sure it's a great title that fully reflects the extent of your awesome contribution.
Then, please replace this with a description of the change and which issue is fixed (if applicable). Please also include relevant motivation and context. List any dependencies (if any) that are required for this change.
Once you're done, someone will review your PR shortly (see the section "Who can review?" below to tag some potential reviewers). They may suggest changes to make the code even better. If no one reviewed your PR after a week has passed, don't hesitate to post a new comment @-mentioning the same persons---sometimes notifications get lost.
-->
<!-- Remove if not applicable -->
Fixes # (issue)
## Before submitting
- [ ] This PR fixes a typo or improves the docs (you can dismiss the other checks if that's the case).
- [ ] Did you read the [contributor guideline](https://github.com/huggingface/transformers/blob/master/CONTRIBUTING.md#start-contributing-pull-requests),
Pull Request section?
- [ ] Was this discussed/approved via a Github issue or the [forum](https://discuss.huggingface.co/)? Please add a link
to it if that's the case.
- [ ] Did you make sure to update the documentation with your changes? Here are the
[documentation guidelines](https://github.com/huggingface/transformers/tree/master/docs), and
[here are tips on formatting docstrings](https://github.com/huggingface/transformers/tree/master/docs#writing-source-documentation).
- [ ] Did you write any new necessary tests?
## Who can review?
Anyone in the community is free to review the PR once the tests have passed. Feel free to tag
members/contributors which may be interested in your PR.
<!-- Your PR will be replied to more quickly if you can figure out the right person to tag with @
If you know how to use git blame, that is the easiest way, otherwise, here is a rough guide of **who to tag**.
Please tag fewer than 3 people.
Models:
- albert, bert, xlm: @LysandreJik
- blenderbot, bart, marian, pegasus, encoderdecoder, t5: @patrickvonplaten, @patil-suraj
- longformer, reformer, transfoxl, xlnet: @patrickvonplaten
- fsmt: @stas00
- funnel: @sgugger
- gpt2: @patrickvonplaten, @LysandreJik
- rag: @patrickvonplaten, @lhoestq
- tensorflow: @jplu
Library:
- benchmarks: @patrickvonplaten
- deepspeed: @stas00
- ray/raytune: @richardliaw, @amogkam
- text generation: @patrickvonplaten
- tokenizers: @n1t0, @LysandreJik
- trainer: @sgugger
- pipelines: @LysandreJik
Documentation: @sgugger
HF projects:
- nlp datasets: [different repo](https://github.com/huggingface/nlp)
- rust tokenizers: [different repo](https://github.com/huggingface/tokenizers)
Examples:
- maintained examples (not research project or legacy): @sgugger, @patil-suraj
- research_projects/bert-loses-patience: @JetRunner
- research_projects/distillation: @VictorSanh
--> | 03-05-2021 13:36:50 | 03-05-2021 13:36:50 | |
transformers | 10,544 | closed | Handle padding in decoder_inputs_id when using generate | # What does this PR do?
<!--
Congratulations! You've made it this far! You're not quite done yet though.
Once merged, your PR is going to appear in the release notes with the title you set, so make sure it's a great title that fully reflects the extent of your awesome contribution.
Then, please replace this with a description of the change and which issue is fixed (if applicable). Please also include relevant motivation and context. List any dependencies (if any) that are required for this change.
Once you're done, someone will review your PR shortly (see the section "Who can review?" below to tag some potential reviewers). They may suggest changes to make the code even better. If no one reviewed your PR after a week has passed, don't hesitate to post a new comment @-mentioning the same persons---sometimes notifications get lost.
-->
<!-- Remove if not applicable -->
Fixes #10478 for pytorch version of `generate()` in generate_utils.py
As described in the issue, when `decoder_input_ids` have different lengths and require padding, generation continues after the padding tokens. This PR modifies that behavior so that tokens are generated before the padding for each element in the batch.
## Before submitting
- [ ] This PR fixes a typo or improves the docs (you can dismiss the other checks if that's the case).
- [x] Did you read the [contributor guideline](https://github.com/huggingface/transformers/blob/master/CONTRIBUTING.md#start-contributing-pull-requests),
Pull Request section?
- [x] Was this discussed/approved via a Github issue or the [forum](https://discuss.huggingface.co/)? Please add a link
to it if that's the case.
- [ ] Did you make sure to update the documentation with your changes? Here are the
[documentation guidelines](https://github.com/huggingface/transformers/tree/master/docs), and
[here are tips on formatting docstrings](https://github.com/huggingface/transformers/tree/master/docs#writing-source-documentation).
- [ ] Did you write any new necessary tests?
## Who can review?
I am adding those who I see more often in the git blame of the file:
@patrickvonplaten, @patil-suraj, @yjernite
<!-- Your PR will be replied to more quickly if you can figure out the right person to tag with @
If you know how to use git blame, that is the easiest way, otherwise, here is a rough guide of **who to tag**.
Please tag fewer than 3 people.
Models:
- albert, bert, xlm: @LysandreJik
- blenderbot, bart, marian, pegasus, encoderdecoder, t5: @patrickvonplaten, @patil-suraj
- longformer, reformer, transfoxl, xlnet: @patrickvonplaten
- fsmt: @stas00
- funnel: @sgugger
- gpt2: @patrickvonplaten, @LysandreJik
- rag: @patrickvonplaten, @lhoestq
- tensorflow: @jplu
Library:
- benchmarks: @patrickvonplaten
- deepspeed: @stas00
- ray/raytune: @richardliaw, @amogkam
- text generation: @patrickvonplaten
- tokenizers: @n1t0, @LysandreJik
- trainer: @sgugger
- pipelines: @LysandreJik
Documentation: @sgugger
HF projects:
- nlp datasets: [different repo](https://github.com/huggingface/nlp)
- rust tokenizers: [different repo](https://github.com/huggingface/tokenizers)
Examples:
- maintained examples (not research project or legacy): @sgugger, @patil-suraj
- research_projects/bert-loses-patience: @JetRunner
- research_projects/distillation: @VictorSanh
-->
This is my first PR, so let me use it to thank everyone involved in this library for all the cool work :) | 03-05-2021 12:50:05 | 03-05-2021 12:50:05 | |
transformers | 10,543 | closed | Similar issue like #1091 in Blenderbot | Tokenizer and model are not in sync. I am using "facebook/blenderbot-400M-distill"
Tokenizer has 8009 base tokens where as model has 8008.
Could you please help me with this?
`from transformers import BlenderbotTokenizer, BlenderbotForConditionalGeneration
mname = "facebook/blenderbot-400M-distill"
model = BlenderbotForConditionalGeneration.from_pretrained(mname)
tokenizer = BlenderbotTokenizer.from_pretrained(mname, local_files_only=True)
print(len(tokenizer))
print(model.config.to_dict()['vocab_size'])`
Here is the output that I get.
8009
8008
## Environment info
- `transformers` version: 4.3.2
- Platform: Windows-10-10.0.18362-SP0
- Python version: 3.8.6
- PyTorch version (GPU?): 1.7.1+cpu (False)
- Tensorflow version (GPU?): 2.3.1 (False)
- Using GPU in script?: <fill in>
- Using distributed or parallel set-up in script?: <fill in>
- blenderbot, bart, marian, pegasus, encoderdecoder, t5: @patrickvonplaten, @patil-suraj
| 03-05-2021 12:04:45 | 03-05-2021 12:04:45 | Do you encounter any errors because of the mismatch in length?<|||||>This issue has been automatically marked as stale because it has not had recent activity. If you think this still needs to be addressed please comment on this thread.
Please note that issues that do not follow the [contributing guidelines](https://github.com/huggingface/transformers/blob/master/CONTRIBUTING.md) are likely to be ignored. |
transformers | 10,542 | closed | OSError: Can't load weights for 'facebook/mbart-large-cc25' when using TFMBartModel | ## Environment info
- `transformers` version: 4.3.3
- Platform: Windows 10
- Python version: 3.8
- PyTorch version (GPU?): - No
- Tensorflow version (GPU?): 2.4.1, Yes
- Using GPU in script?: Yes (I think it is indifferent for this issue)
- Using distributed or parallel set-up in script?: No
## Issue Description
Firstly, I would to thank you this extraordinary contribution to NLP. We are starting to apply transformers to our NLP problem and we want to test the pretrained mBART model. I have tried to load the TF version of this model following your documentation: https://huggingface.co/transformers/master/model_doc/mbart.html#tfmbartmodel
Unfortunately, we are experiencing an error in _"model = TFMBartModel.from_pretrained('facebook/mbart-large-cc25')"_.
**Error Traceback:**
404 Client Error: Not Found for url: https://huggingface.co/facebook/mbart-large-cc25/resolve/main/tf_model.h5
Traceback (most recent call last):
File "c:\users\vicen\anaconda3\envs\signon_2\lib\site-packages\transformers\modeling_tf_utils.py", line 1203, in from_pretrained
resolved_archive_file = cached_path(
File "c:\users\vicen\anaconda3\envs\signon_2\lib\site-packages\transformers\file_utils.py", line 1078, in cached_path
output_path = get_from_cache(
File "c:\users\vicen\anaconda3\envs\signon_2\lib\site-packages\transformers\file_utils.py", line 1216, in get_from_cache
r.raise_for_status()
File "c:\users\vicen\anaconda3\envs\signon_2\lib\site-packages\requests\models.py", line 943, in raise_for_status
raise HTTPError(http_error_msg, response=self)
HTTPError: 404 Client Error: Not Found for url: https://huggingface.co/facebook/mbart-large-cc25/resolve/main/tf_model.h5
During handling of the above exception, another exception occurred:
Traceback (most recent call last):
File "G:\Mi unidad\D4.2\Proof of Concept simCLR for MT\test_tokenizer.py", line 46, in <module>
bart_model = TFMBartModel.from_pretrained('facebook/mbart-large-cc25')
File "c:\users\vicen\anaconda3\envs\signon_2\lib\site-packages\transformers\modeling_tf_utils.py", line 1219, in from_pretrained
raise EnvironmentError(msg)
OSError: Can't load weights for 'facebook/mbart-large-cc25'. Make sure that:
- 'facebook/mbart-large-cc25' is a correct model identifier listed on 'https://huggingface.co/models'
- or 'facebook/mbart-large-cc25' is the correct path to a directory containing a file named one of tf_model.h5, pytorch_model.bin.
It seems that .h5 file with pretrained weights are not available in your repository. If it is not possible to update it, do you any way to transform pytorch .bin to TF .h5?
Thank you in advance!!!
| 03-05-2021 11:50:04 | 03-05-2021 11:50:04 | Hi! Yes, you can load the PyTorch weights into a Transformer model by adding `from_pt=True` in the `from_pretrained` method.<|||||>Thank you very much @LysandreJik!
I have tried two ways:
**Option 1 Using from_pt = True**
_bart_model = TFMBartModel.from_pretrained("facebook/mbart-large-cc25", from_pt=True)_
This worked well, but the following message appeared:
Some weights of the PyTorch model were not used when initializing the TF 2.0 model TFMBartModel: ['final_logits_bias', 'model.encoder.embed_tokens.weight', 'model.decoder.embed_tokens.weight']
- This IS expected if you are initializing TFMBartModel from a PyTorch model trained on another task or with another architecture (e.g. initializing a TFBertForSequenceClassification model from a BertForPreTraining model).
- This IS NOT expected if you are initializing TFMBartModel from a PyTorch model that you expect to be exactly identical (e.g. initializing a TFBertForSequenceClassification model from a BertForSequenceClassification model).
All the weights of TFMBartModel were initialized from the PyTorch model.
If your task is similar to the task the model of the checkpoint was trained on, you can already use TFMBartModel for predictions without further training.
Furthermore, I had memory problems when trying this way in GPU.
**Option 2 Using MBartConfig**
_configuration = MBartConfig(name_or_path = "facebook/mbart-large-cc25")
bart_model = TFMBartModel(configuration)_
I have checked the outputs for the same input, and they were different. So I think that Option 1 did not properly loaded all the weights. So, I recommend using Option 2! Hope this helps!
<|||||>Hi! The option 2 you mention isn't loading any weights on the model itself. You're instantiating a configuration that is similar to `facebook/mbart-large-cc25`, and initializing a model with random weights following that configuration.<|||||>If you run inference twice through the model loaded with option 1, do you get different inputs?<|||||>Hi @LysandreJik,
The outputs were different between option 1 & 2, but it is obvious if they do not load the same weights. But other fact is that when I load the model with option 1 several times, the outputs are different. Meanwhile, if I load once the model and predict twice, the outputs are the same. Could be it due to dropout?
To avoid memory problems with option 1, I am going to load the model in CPU and export the TF weights to h5 file. Then load them with GPU settings.<|||||>**[CPU] Saving pretrained model**
I have tried loading the pretrained mBART model in CPU settings and save it in TF formar with the following code:
_mbart_cpu = TFMBartModel.from_pretrained("facebook/mbart-large-cc25", from_pt=True)
mbart_cpu.save_pretrained('saved_models/')_
No errors appeared
**[GPU] Loading pretrained weights**
After exporting pretrained mBART, I tried loading it with GPU settings as follows:
_mbart_in_gpu = TFMBartModel.from_pretrained("saved_models")_
However, the following error appeared:
**Traceback (most recent call last):**
File "G:\Mi unidad\D4.2\Proof of Concept simCLR for MT\load_in_GPU.py", line 23, in <module>
mbart_model_2 = TFMBartModel.from_pretrained("saved_models")
File "c:\users\vicen\anaconda3\envs\signon_2\lib\site-packages\transformers\modeling_tf_utils.py", line 1244, in from_pretrained
missing_keys, unexpected_keys = load_tf_weights(model, resolved_archive_file)
File "c:\users\vicen\anaconda3\envs\signon_2\lib\site-packages\transformers\modeling_tf_utils.py", line 532, in load_tf_weights
K.batch_set_value(weight_value_tuples)
File "c:\users\vicen\anaconda3\envs\signon_2\lib\site-packages\tensorflow\python\util\dispatch.py", line 201, in wrapper
return target(*args, **kwargs)
File "c:\users\vicen\anaconda3\envs\signon_2\lib\site-packages\tensorflow\python\keras\backend.py", line 3706, in batch_set_value
x.assign(np.asarray(value, dtype=dtype(x)))
File "c:\users\vicen\anaconda3\envs\signon_2\lib\site-packages\tensorflow\python\ops\resource_variable_ops.py", line 892, in assign
assign_op = gen_resource_variable_ops.assign_variable_op(
File "c:\users\vicen\anaconda3\envs\signon_2\lib\site-packages\tensorflow\python\ops\gen_resource_variable_ops.py", line 144, in assign_variable_op
_ops.raise_from_not_ok_status(e, name)
File "c:\users\vicen\anaconda3\envs\signon_2\lib\site-packages\tensorflow\python\framework\ops.py", line 6862, in raise_from_not_ok_status
six.raise_from(core._status_to_exception(e.code, message), None)
File "<string>", line 3, in raise_from
**InternalError: Failed copying input tensor from /job:localhost/replica:0/task:0/device:CPU:0 to /job:localhost/replica:0/task:0/device:GPU:0 in order to run AssignVariableOp: Dst tensor is not initialized. [Op:AssignVariableOp]**
Thank you in advance for your help!
<|||||>Pinging @patrickvonplaten and @patil-suraj <|||||>Thank you very much @LysandreJik!<|||||>Hey @SantiagoEG
the reason for the issue is that `TFMBartModel` is not TF counterpart of `MBartModel`, the counterpart is `TFMBartMainLayer`
as you can see here
pt `MBartModel` : https://github.com/huggingface/transformers/blob/master/src/transformers/models/mbart/modeling_mbart.py#L1096
tf `TFMBartModel`: https://github.com/huggingface/transformers/blob/master/src/transformers/models/mbart/modeling_tf_mbart.py#L1178
the structure is different, `TFMBartModel` does not contain the shared token embeddings layer, but instead, it wraps `TFMBartMainLayer `, which is why we can’t do `TFMBartModel.from_pretrained(..., from_pt=True)`
instead, we need to load weights using `TFMBartForConditionalGeneration` and then we can load `TFMBartModel` using the saved `TFMBartForConditionalGeneration`
```python
tf_model = TFMBartForConditionalGeneration.from_pretrained("facebook/mbart-large-cc25", from_pt=True)
tf_model.save_pretrained("tf_model")
tf_mbart_model = TFMBartModel.from_pretrained("tf_model")<|||||>This issue has been automatically marked as stale because it has not had recent activity. If you think this still needs to be addressed please comment on this thread.
Please note that issues that do not follow the [contributing guidelines](https://github.com/huggingface/transformers/blob/master/CONTRIBUTING.md) are likely to be ignored. |
transformers | 10,541 | closed | Facing Issue while running `run_tf_multiple_choice.py` from examples | ## Environment info
<!-- You can run the command `transformers-cli env` and copy-and-paste its output below.
Don't forget to fill out the missing fields in that output! -->
- `transformers` version: 4.4.0.dev0
- Platform: Colab
- Python version: NA
- PyTorch version (GPU?): NA
- Tensorflow version (GPU?):
- Using GPU in script?: No
- Using distributed or parallel set-up in script?: No
### Who can help
<!-- Your issue will be replied to more quickly if you can figure out the right person to tag with @
If you know how to use git blame, that is the easiest way, otherwise, here is a rough guide of **who to tag**.
Please tag fewer than 3 people.
Models:
- albert, bert, xlm: @LysandreJik
- blenderbot, bart, marian, pegasus, encoderdecoder, t5: @patrickvonplaten, @patil-suraj
- longformer, reformer, transfoxl, xlnet: @patrickvonplaten
- fsmt: @stas00
- funnel: @sgugger
- gpt2: @patrickvonplaten, @LysandreJik
- rag: @patrickvonplaten, @lhoestq
- tensorflow: @jplu
Library:
- benchmarks: @patrickvonplaten
- deepspeed: @stas00
- ray/raytune: @richardliaw, @amogkam
- text generation: @patrickvonplaten
- tokenizers: @LysandreJik
- trainer: @sgugger
- pipelines: @LysandreJik
Documentation: @sgugger
HF projects:
- nlp datasets: [different repo](https://github.com/huggingface/nlp)
- rust tokenizers: [different repo](https://github.com/huggingface/tokenizers)
Examples:
- maintained examples (not research project or legacy): @sgugger, @patil-suraj
- research_projects/bert-loses-patience: @JetRunner
- research_projects/distillation: @VictorSanh
-->
## Information
I was trying to train `bert-base-cased` on Multiple Choice task with below script provided on Readme of the task
```
export SWAG_DIR=/path/to/swag_data_dir
python ./examples/multiple-choice/run_tf_multiple_choice.py \
--task_name swag \
--model_name_or_path bert-base-cased \
--do_train \
--do_eval \
--data_dir $SWAG_DIR \
--learning_rate 5e-5 \
--num_train_epochs 3 \
--max_seq_length 80 \
--output_dir models_bert/swag_base \
--per_gpu_eval_batch_size=16 \
--per_device_train_batch_size=16 \
--gradient_accumulation_steps 2 \
--overwrite_output
```
I got below error
```
Invalid argument: ValueError: `generator` yielded an element of shape (4, 1, 80) where an element of shape (None, None) was expected.
Traceback (most recent call last):
File "/usr/local/lib/python3.7/dist-packages/tensorflow/python/ops/script_ops.py", line 249, in __call__
ret = func(*args)
File "/usr/local/lib/python3.7/dist-packages/tensorflow/python/autograph/impl/api.py", line 620, in wrapper
return func(*args, **kwargs)
File "/usr/local/lib/python3.7/dist-packages/tensorflow/python/data/ops/dataset_ops.py", line 938, in generator_py_func
(ret_array.shape, expected_shape))
ValueError: `generator` yielded an element of shape (4, 1, 80) where an element of shape (None, None) was expected.
```
The problem arises when using:
* [x] the official example scripts: (give details below)
* [ ] my own modified scripts: (give details below)
The tasks I am working on is:
* [x] an official GLUE/SQUaD task: (give the name) swag
* [ ] my own task or dataset: (give details below)
## To reproduce
Steps to reproduce the behavior:
Follow this colab [Notebook ](https://github.com/bhadreshpsavani/UnderstandingNLP/blob/master/CheckingTFScripts.ipynb) to run the script and reproduce the issue.
<!-- If you have code snippets, error messages, stack traces please provide them here as well.
Important! Use code tags to correctly format your code. See https://help.github.com/en/github/writing-on-github/creating-and-highlighting-code-blocks#syntax-highlighting
Do not use screenshots, as they are hard to read and (more importantly) don't allow others to copy-and-paste your code.-->
## Expected behavior
It should execute the script and train the model without the given error.
Note: The colab notebook given in the readme is not working, It's outdated maybe!
**I removed `--logging-dir logs \` from the script because it was giving me another error**
Tagging SMEs:
@LysandreJik @jplu | 03-05-2021 10:25:29 | 03-05-2021 10:25:29 | Even for the `run_tf_squad.py` script, I am facing the issue.
Here is the [colab notebook](https://github.com/bhadreshpsavani/UnderstandingNLP/blob/master/Check_run_tf_squad.ipynb) with issue and Traceback logs
Is there anything else I need to use while running the script?<|||||>Hello!
The multiple choice example needs to be reworked. A PR to fix the squad example is available https://github.com/huggingface/transformers/pull/10275. Be aware that some arguments are not implemented on the TF side.
The TF examples are under rework and should become more reliable in a near future.<|||||>I am closing this issue since it is already WIP |
transformers | 10,540 | closed | 🐛 Bug in attention head mask for cross-attention module in encoder-decoder models | Currently, encoder-decoder models use either `head_mask` or `decoder_head_mask` for masking attention heads in cross-attention modules. Both cases are not perfectly correct. Furthermore, MHA in cross-attention modules shares the parameters with the decoder, i.e. `shape = (decoder.num_layers, decoder.num_attention_heads)`, therefore, the usage of encoder `head_mask` in the cross-attention module may lead to errors due to the shape mismatch.
<hr>
**My contribution:** I will take care of this issue this weekend.
<hr>
**Reviewers:** @patil-suraj @patrickvonplaten
| 03-05-2021 10:00:33 | 03-05-2021 10:00:33 | This issue has been automatically marked as stale because it has not had recent activity. If you think this still needs to be addressed please comment on this thread.
Please note that issues that do not follow the [contributing guidelines](https://github.com/huggingface/transformers/blob/master/CONTRIBUTING.md) are likely to be ignored.<|||||>Unstale<|||||>Hey @stancld,
Sorry for being so unresponsive here - I'm happy to change the behavior and provide 3 masks |
transformers | 10,539 | closed | Wave2vec custom training tokenizer bug | ## Environment info
<!-- You can run the command `transformers-cli env` and copy-and-paste its output below.
Don't forget to fill out the missing fields in that output! -->
- `transformers` version: master
- Platform: win 10
- Python version: 3.8
- PyTorch version (GPU?): GPU
- Tensorflow version (GPU?):
- Using GPU in script?:
- Using distributed or parallel set-up in script?:
### Who can help
<!-- Your issue will be replied to more quickly if you can figure out the right person to tag with @
If you know how to use git blame, that is the easiest way, otherwise, here is a rough guide of **who to tag**.
Please tag fewer than 3 people.
HF projects:
- nlp datasets: [different repo](https://github.com/huggingface/nlp)
- rust tokenizers: [different repo](https://github.com/huggingface/tokenizers)
-->
@patrickvonplaten
## Information
Model I am using (Bert, XLNet ...):
The problem arises when using:
* [X] the official example scripts: (give details below)
wave2vec Training example
* [ ] my own modified scripts: (give details below)
The tasks I am working on is:
* [ ] an official GLUE/SQUaD task: (give the name)
* [ ] my own task or dataset: (give details below)
## To reproduce
Steps to reproduce the behavior:
I was just running the wave2vec Training on pretrained base modell with the german common voice dataset. I modified the dataset that it fits into the format of librispeech so there are no changes in the example script.
<!-- If you have code snippets, error messages, stack traces please provide them here as well.
Important! Use code tags to correctly format your code. See https://help.github.com/en/github/writing-on-github/creating-and-highlighting-code-blocks#syntax-highlighting
Do not use screenshots, as they are hard to read and (more importantly) don't allow others to copy-and-paste your code.-->
## Expected behavior
<!-- A clear and concise description of what you would expect to happen. -->
While Training the wer on eval dataset is exactly 1.
After Training, while evaluation I recognized that the transcribed predictions are unk tokens only and the tokenizer is missing. I am using the pretrained tokenizer that's why.
The words and sentences seems to be correct (counting the same on both sides), only instead of words only the unk tokens are returned after detokenizing. | 03-05-2021 09:30:02 | 03-05-2021 09:30:02 | I will add a notebook on how to fine-tune Wav2Vec2 on languages other than English next week (think I'll also go for the German Common Voice dataset). We only today added the multi-lingual checkpoint, so you probably used the English checkpoint which cannot handle German. If you didn't see the notebook within ~1,2 weeks, please ping me here again<|||||>Thanks for your answer
For understanding, why does the english checkpoint does not support german ?
I didn't see any reason for that, where is the point I was blind at ?<|||||>@flozi00 did you use a default English tokenizer/processor? You need to load a custom tokenizer, e.g. by `tokenizer = Wav2Vec2CTCTokenizer(vocab_file='path/to/custom/vocab.json')`, where vocab_file is the path to vocabulary of German characters. After that you can create a custom Wav2Vec2Processor:
`processor = Wav2Vec2Processor(feature_extractor=Wav2Vec2FeatureExtractor.from_pretrained("facebook/wav2vec2-base"), tokenizer=tokenizer)`
Regarding the model, I'm not completely sure whether you can load the pretrained base model directly or instead convert the fairseq pytorch checkpoint manually. If I understand correctly, the script for converting Wav2Vec2 checkpoint requires letter dictionary (e.g. https://dl.fbaipublicfiles.com/fairseq/wav2vec/dict.ltr.txt). So if the Huggingface Transformers Wav2Vec2 model stores this letter dictionary, you probably need to convert the model manually with your own dict.ltr.txt with German letters included, as well as set the vocabulary size during conversion. |
transformers | 10,538 | closed | Transfomer-xl padding token | When dealing with a batch consisting of sequences of different lengths, how do I choose parameters so that padding_token is not involved in the computation. | 03-05-2021 09:20:03 | 03-05-2021 09:20:03 | Hello, thanks for opening an issue! We try to keep the github issues for bugs/feature requests.
Could you ask your question on the [forum](https://discusss.huggingface.co) instead?
Thanks! |
transformers | 10,537 | closed | Fix example of custom Trainer to reflect signature of compute_loss | # What does this PR do?
<!--
Congratulations! You've made it this far! You're not quite done yet though.
Once merged, your PR is going to appear in the release notes with the title you set, so make sure it's a great title that fully reflects the extent of your awesome contribution.
Then, please replace this with a description of the change and which issue is fixed (if applicable). Please also include relevant motivation and context. List any dependencies (if any) that are required for this change.
Once you're done, someone will review your PR shortly (see the section "Who can review?" below to tag some potential reviewers). They may suggest changes to make the code even better. If no one reviewed your PR after a week has passed, don't hesitate to post a new comment @-mentioning the same persons---sometimes notifications get lost.
-->
<!-- Remove if not applicable -->
Fixes out-of-date example of custom `Trainer` in docs.
Since several people have asked about multi-label classification in the forum and in #10232 I thought it might be useful to use this as the example.
I also took the liberty of tightening the grammar a bit in the preceding text 😃
## Before submitting
- [x] This PR fixes a typo or improves the docs (you can dismiss the other checks if that's the case).
- [ ] Did you read the [contributor guideline](https://github.com/huggingface/transformers/blob/master/CONTRIBUTING.md#start-contributing-pull-requests),
Pull Request section?
- [x] Was this discussed/approved via a Github issue or the [forum](https://discuss.huggingface.co/)? Please add a link
to it if that's the case.
- [ ] Did you make sure to update the documentation with your changes? Here are the
[documentation guidelines](https://github.com/huggingface/transformers/tree/master/docs), and
[here are tips on formatting docstrings](https://github.com/huggingface/transformers/tree/master/docs#writing-source-documentation).
- [ ] Did you write any new necessary tests?
Forum link: https://discuss.huggingface.co/t/custom-loss-compute-loss-got-an-unexpected-keyword-argument-return-outputs/4148?u=lewtun
## Who can review?
Anyone in the community is free to review the PR once the tests have passed. Feel free to tag
members/contributors which may be interested in your PR.
<!-- Your PR will be replied to more quickly if you can figure out the right person to tag with @
If you know how to use git blame, that is the easiest way, otherwise, here is a rough guide of **who to tag**.
Please tag fewer than 3 people.
Models:
- albert, bert, xlm: @LysandreJik
- blenderbot, bart, marian, pegasus, encoderdecoder, t5: @patrickvonplaten, @patil-suraj
- longformer, reformer, transfoxl, xlnet: @patrickvonplaten
- fsmt: @stas00
- funnel: @sgugger
- gpt2: @patrickvonplaten, @LysandreJik
- rag: @patrickvonplaten, @lhoestq
- tensorflow: @jplu
Library:
- benchmarks: @patrickvonplaten
- deepspeed: @stas00
- ray/raytune: @richardliaw, @amogkam
- text generation: @patrickvonplaten
- tokenizers: @n1t0, @LysandreJik
- trainer: @sgugger
- pipelines: @LysandreJik
Documentation: @sgugger
HF projects:
- nlp datasets: [different repo](https://github.com/huggingface/nlp)
- rust tokenizers: [different repo](https://github.com/huggingface/tokenizers)
Examples:
- maintained examples (not research project or legacy): @sgugger, @patil-suraj
- research_projects/bert-loses-patience: @JetRunner
- research_projects/distillation: @VictorSanh
-->
@sgugger | 03-05-2021 09:19:27 | 03-05-2021 09:19:27 | Not sure why the tests are failing since I only tweaked the docs - perhaps it's a problem with the CI on your end? |
transformers | 10,536 | closed | Enabling multilingual models for translation pipelines. | # What does this PR do?
Enables mutlilingual translation for pipelines.
Some models can target multiple languages/ language pairs. Before this PR, there was no simple way to exploit that within the Translation pipeline.
## Move away from `translation_XX_to_YY`.
Because src_lang, tgt_lang pairs can be used for a single model, we need to move away from this task naming scheme.
Currently it was done, as being able to use `src_lang` and `tgt_lang` both within `pipeline(.... src_lang=XX, tgt_lang=yy)` and at call time `translator = pipeline(...); translation("input string", src_lang=XX, tgt_lang=YY)`
## Rely on the model's tokenizer to build the inputs.
We now have at least 3 (m2m. mbart50, T5) different models that prepare input ids in a different manner. In order to avoid switches within a pipeline that would depend on a model, instead `tokenizer` can optionally implement `_build_translation_input_ids`. That enables to put model specific logic within their model files and use all their custom methods over there.
This is in line with https://github.com/huggingface/transformers/pull/10002.
## Misc
- `ensure_tensor_on_device` now supports non tensor members
- Added a `deep_round` test utility to enable testing nested structures that contain tensors, floats and so on.
<!--
Congratulations! You've made it this far! You're not quite done yet though.
Once merged, your PR is going to appear in the release notes with the title you set, so make sure it's a great title that fully reflects the extent of your awesome contribution.
Then, please replace this with a description of the change and which issue is fixed (if applicable). Please also include relevant motivation and context. List any dependencies (if any) that are required for this change.
Once you're done, someone will review your PR shortly (see the section "Who can review?" below to tag some potential reviewers). They may suggest changes to make the code even better. If no one reviewed your PR after a week has passed, don't hesitate to post a new comment @-mentioning the same persons---sometimes notifications get lost.
-->
<!-- Remove if not applicable -->
Fixes # (issue)
## Before submitting
- [ ] This PR fixes a typo or improves the docs (you can dismiss the other checks if that's the case).
- [ ] Did you read the [contributor guideline](https://github.com/huggingface/transformers/blob/master/CONTRIBUTING.md#start-contributing-pull-requests),
Pull Request section?
- [ ] Was this discussed/approved via a Github issue or the [forum](https://discuss.huggingface.co/)? Please add a link
to it if that's the case.
- [ ] Did you make sure to update the documentation with your changes? Here are the
[documentation guidelines](https://github.com/huggingface/transformers/tree/master/docs), and
[here are tips on formatting docstrings](https://github.com/huggingface/transformers/tree/master/docs#writing-source-documentation).
- [ ] Did you write any new necessary tests?
## Who can review?
Anyone in the community is free to review the PR once the tests have passed. Feel free to tag
members/contributors which may be interested in your PR.
<!-- Your PR will be replied to more quickly if you can figure out the right person to tag with @
If you know how to use git blame, that is the easiest way, otherwise, here is a rough guide of **who to tag**.
Please tag fewer than 3 people.
Models:
- albert, bert, xlm: @LysandreJik
- blenderbot, bart, marian, pegasus, encoderdecoder, t5: @patrickvonplaten, @patil-suraj
- longformer, reformer, transfoxl, xlnet: @patrickvonplaten
- fsmt: @stas00
- funnel: @sgugger
- gpt2: @patrickvonplaten, @LysandreJik
- rag: @patrickvonplaten, @lhoestq
- tensorflow: @jplu
Library:
- benchmarks: @patrickvonplaten
- deepspeed: @stas00
- ray/raytune: @richardliaw, @amogkam
- text generation: @patrickvonplaten
- tokenizers: @n1t0, @LysandreJik
- trainer: @sgugger
- pipelines: @LysandreJik
Documentation: @sgugger
HF projects:
- nlp datasets: [different repo](https://github.com/huggingface/nlp)
- rust tokenizers: [different repo](https://github.com/huggingface/tokenizers)
Examples:
- maintained examples (not research project or legacy): @sgugger, @patil-suraj
- research_projects/bert-loses-patience: @JetRunner
- research_projects/distillation: @VictorSanh
--> | 03-05-2021 08:39:20 | 03-05-2021 08:39:20 | @LysandreJik
I added a method here `deep_round` to try and make test equality a bit sane.
torchTensor(...) == torch.Tensor(..) does not work (understandably).
Any sort of float comparison is also flaky.
`deep_round` simply tries to make `assertEqual` work in a sane way for any sort of nested structure to make test comparisons simpler to read and write.
Here the tests just need to make sure that the actual output of `_build_translation_input_ids` is actually correct, writing it in that way make its much more readable IMO (than having to extract each element and call `allclose` on them). It actually allowed me to see that mbart has a different encoding scheme.
How do you feel about such a function to improve small sanity checks ?
Another route would be to create something like `assertAlmostEqual` that behaves similarly but I think it's a bit less simple to reason about.
Finally we could stick to not using any such helper functions.<|||||>When this PR is ready, could you complete the description of the PR? It would help to understand what we're reviewing, and would be better for the release notes and posterity. Thanks!<|||||>Good catch ! Updated and completed !
I think this is ready to merge if you're ok with it.<|||||>I'm asking @patrickvonplaten and @sgugger for review as they're more acquainted with mBART-like tokenizers and their review would be helpful. |
transformers | 10,535 | closed | tensorflow model convert onnx | I use this code to transfer TFBertModel to onnx:
”convert(framework="tf", model=model, tokenizer=tokenizer, output=Path("onnx_tf/segment.onnx"), opset=12)”
and the output log as follows:
`Using framework TensorFlow: 2.2.0, keras2onnx: 1.7.0
Found input input_ids with shape: {0: 'batch', 1: 'sequence'}
Found input token_type_ids with shape: {0: 'batch', 1: 'sequence'}
Found input attention_mask with shape: {0: 'batch', 1: 'sequence'}
Found output output_0 with shape: {0: 'batch'}
Found output output_1 with shape: {0: 'batch', 1: 'sequence'}
Found output output_2 with shape: {0: 'batch', 1: 'sequence'}
Found output output_3 with shape: {0: 'batch', 1: 'sequence'}
Found output output_4 with shape: {0: 'batch', 1: 'sequence'}
Found output output_5 with shape: {0: 'batch', 1: 'sequence'}
Found output output_6 with shape: {0: 'batch', 1: 'sequence'}
Found output output_7 with shape: {0: 'batch', 1: 'sequence'}
Found output output_8 with shape: {0: 'batch', 1: 'sequence'}
Found output output_9 with shape: {0: 'batch', 1: 'sequence'}
Found output output_10 with shape: {0: 'batch', 1: 'sequence'}
Found output output_11 with shape: {0: 'batch', 1: 'sequence'}
Found output output_12 with shape: {0: 'batch', 1: 'sequence'}
Found output output_13 with shape: {0: 'batch', 1: 'sequence'}`
but,I run the output onnx,an error is occured as follows:
logits = session.run(None, inputs_onnx)
File "/usr/lib64/python3.6/site-packages/onnxruntime/capi/onnxruntime_inference_collection.py", line 124, in run
return self._sess.run(output_names, input_feed, run_options)
onnxruntime.capi.onnxruntime_pybind11_state.InvalidArgument: [ONNXRuntimeError] : 2 : INVALID_ARGUMENT : Invalid Feed Input Name:token_type_ids:0
transformers 3.0.2 | 03-05-2021 07:27:32 | 03-05-2021 07:27:32 | and when I upgrade transformers version to 4.3.3, onnx version is 1.8.1,another error occurred:
Traceback (most recent call last):
File "test_segment.py", line 104, in <module>
session = onnxruntime.InferenceSession(output_model_path, sess_options, providers=['CPUExecutionProvider'])
File "/usr/lib64/python3.6/site-packages/onnxruntime/capi/onnxruntime_inference_collection.py", line 206, in __init__
self._create_inference_session(providers, provider_options)
File "/usr/lib64/python3.6/site-packages/onnxruntime/capi/onnxruntime_inference_collection.py", line 226, in _create_inference_session
sess = C.InferenceSession(session_options, self._model_path, True, self._read_config_from_model)
onnxruntime.capi.onnxruntime_pybind11_state.Fail: [ONNXRuntimeError] : 1 : FAIL : Load model from onnx_tf/segment.onnx failed:Fatal error: BroadcastTo is not a registered function/op<|||||>This issue has been automatically marked as stale because it has not had recent activity. If you think this still needs to be addressed please comment on this thread.
Please note that issues that do not follow the [contributing guidelines](https://github.com/huggingface/transformers/blob/master/CONTRIBUTING.md) are likely to be ignored. |
transformers | 10,534 | closed | VisualBERT | This PR adds VisualBERT (See Closed Issue #5095). | 03-05-2021 04:42:25 | 03-05-2021 04:42:25 | Hi @gchhablani
This is great! You can ping me if you need any help with this model.
Also, we have now added a step-by-step doc for how to add a model, you can find it here
https://huggingface.co/transformers/add_new_model.html
Also have a look at the `cookiecutter` tool, which will help you generate lots of boilerplate code.
https://github.com/huggingface/transformers/tree/master/templates/adding_a_new_model<|||||>Hi @patil-suraj
Thanks a lot.
I wanted to add this model in 2020, but I faced a lot of issues and got busy with some other work. Seems like the code structure has changed quite a bit after that. I'll check the shared links and get back.<|||||>Hi @patil-suraj,
I have started adding some code. For comparison, you can see: https://github.com/uclanlp/visualbert/blob/master/pytorch_pretrained_bert/modeling.py.
Please look at the `VisualBertEmbeddings` and `VisualBertModel`. I have checked these using dummy tensors. I am adding/fixing other kinds of down-stream models. Please tell me if you think this is going in the right direction, and if there are any things that to need to be kept in mind.
I skipped testing the original repository for now, they don't have an entry point, and require a bunch of installs for loading /handling the dataset(s) which are huge.
There are several different checkpoints, even for the pre-trained models (with different embedding dimensions, etc.). For each we'll have to make a separate checkpoint, I guess.
In addition, I was wondering if we want to provide encoder-decoder features (`cross-attention`, `past_key_value`, etc.). I don't think it has been used in the original code, but it will certainly be a nice feature to have in case we have some task in the future which involves generation of text given an image and a text (probably there is something already).
Thanks :)<|||||>Hi @patil-suraj
I would appreciate some feedback :) It'll help me know if I am going in the right direction,
Thanks,
Gunjan<|||||>Hey @gchhablani
I'm thinking about this and will get back to you tomorrow :)<|||||>This issue has been automatically marked as stale because it has not had recent activity. If you think this still needs to be addressed please comment on this thread.
Please note that issues that do not follow the [contributing guidelines](https://github.com/huggingface/transformers/blob/master/CONTRIBUTING.md) are likely to be ignored.<|||||>Unstale<|||||>Hi @LysandreJik
Thanks for reviewing.
I'll make the suggested changes in a few (4-5) hours.
The `position_embeddings_type` is not exactly being used by the authors. They use absolute embeddings. They do have an `embedding_strategy_type` argument but it is unused and kept as `'plain'`.
Yes, almost all of it is copied from BERT. The new additions are the embeddings and the model classes.
Initially, I did have plans to add actual examples and notebooks initially in the same PR :stuck_out_tongue: I guess I'll work on it now.<|||||>@LysandreJik Do you wanna take a final look?<|||||>:') Thanks a lot for all your help @patil-suraj @LysandreJik @sgugger @patrickvonplaten
Edit:
I will be working on another PR soon to add more/better examples, and to use the `SimpleDetector` as used by the original authors. Probably will also attempt to create `TF`/`Flax` models.<|||||>The PR looks awesome! I still think however that we should add `get_visual_embeddings` with a Processor in this PR to have it complete. @gchhablani @patil-suraj - do you think it would be too time-consuming to add a `VisualBERTFeatureExtractor` here? Just don't really think people will be able to run an example after we merge it at the moment<|||||>@patrickvonplaten here `visual_embeddings` comes from a cnn backbone (ResNet) or object detection model. So I don't think
we can add `VisualBERTFeatureExtractor`. The plan is to add the cnn model and a demo notebook in `research_projects` dir in a follow-up a PR.<|||||>> @patrickvonplaten here `visual_embeddings` comes from a cnn backbone (ResNet) or object detection model. So I don't think
> we can add `VisualBERTFeatureExtractor`. The plan is to add the cnn model and a demo notebook in `research_projects` dir in a follow-up a PR.
Ok! Do we have another model that could give us those embeddings? ViT maybe?<|||||>@patrickvonplaten, I am not sure about ViT as I haven't used or read about it yet. The VisualBERT authors used Detectron-based (MaskRCNN with FPN-ResNet-101 backbone) features for 3 out of 4 tasks. Each "token" is actually an object detected and the token features/embeddings from the detectron classifier layer. In case of VCR task, they use a ResNet on given box coordinates.
Unless ViT has/is an extractor similar to this, if we could use ViT, it'd be very different from the original and might not work with the provided pre-trained weights. :/
<|||||>Adding a common Fast/Faster/MaskRCNN feature extractor, however, will help with LXMERT/VisualBERT and other models I'm planning to contribute in the future - ViLBERT (#11986), VL-BERT, (and possibly MCAN).
**Edit**: There's already an example for LXMERT: https://github.com/huggingface/transformers/blob/master/examples/research_projects/lxmert/modeling_frcnn.py which I'll build upon.<|||||>Ok! Good to merge for me then<|||||>maybe not promote it yet<|||||>@patrickvonplaten Yes, won't be promoted before adding an example.
The plan forward is to add a detector and example notebook in `research_projects` dir in a follow-up PR.
Verified that all slow tests are passing :)
Merging!<|||||>Great model addition @gchhablani. Small note: can you fix the code examples of the HTML page?
Currently they look like this:
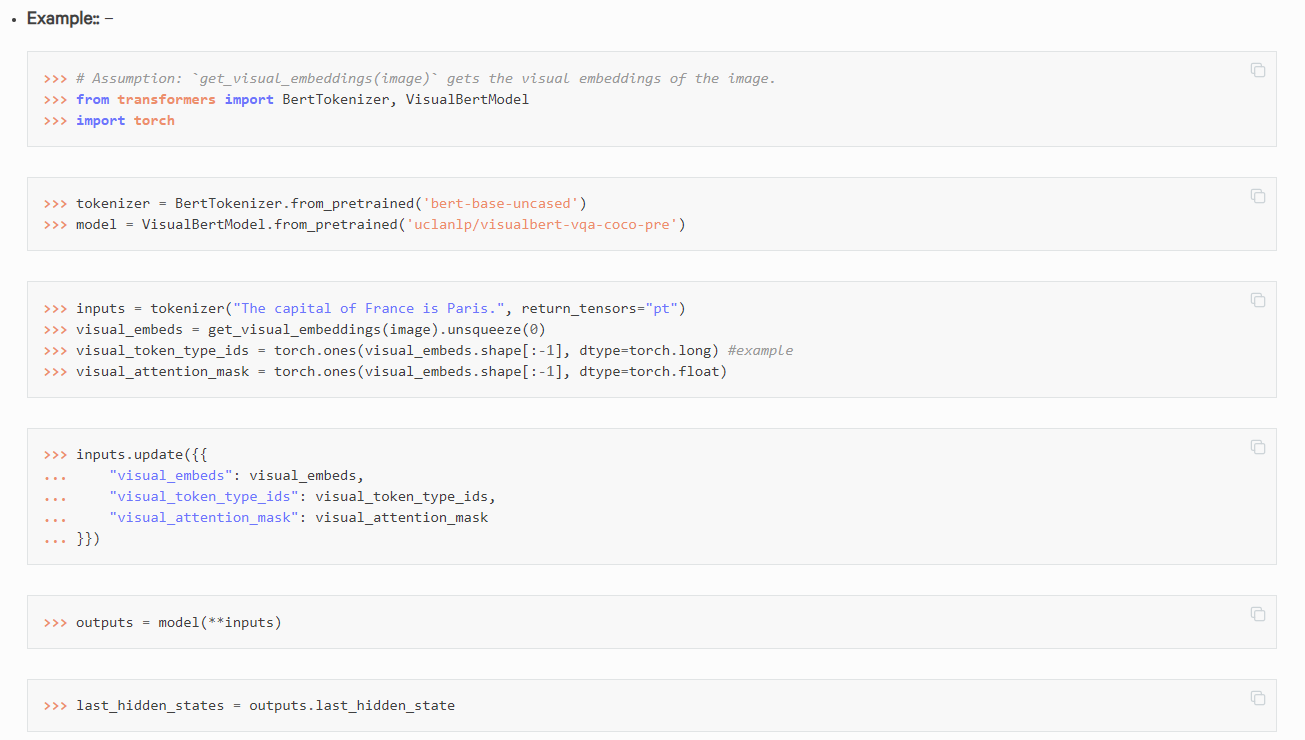
The Returns: statement should be below the Example: statement in `modeling_visual_bert.py`. Sorry for nitpicking ;) |
transformers | 10,533 | closed | RAG with RAY workers keep repetitive copies of knowledge base as .nfs files until the process is done. |
As mentioned in [this PR](https://github.com/huggingface/transformers/pull/10410), I update the **my_knowledge_dataset** object all the time. I save the new my_knowledge_dataset in the same place by removing previously saved stuff. But still, I see there are always some hidden files left. Please check the screenshot below.


I did some checks and found that these .nfs files being used by RAY. But my local KB is 30GB, so I do not want to add a .nfs file in every iteration. Is there a way to get over this?
@amogkam
@lhoestq
| 03-05-2021 04:33:01 | 03-05-2021 04:33:01 | I don't know what those .nfs are used for in Ray, is it safe to remove them @amogkam ? |
transformers | 10,532 | closed | Calling Inference API returns input text | - `transformers` version: 4.4.0dev0
- Platform: MACosx
- Python version: 3.7
- PyTorch version (GPU?): N/A
- Tensorflow version (GPU?): N/A
- Using GPU in script?: No
- Using distributed or parallel set-up in script?: No
### Who can help
Library:
@patrickvonplaten
@LysandreJik
@sgugger
## Information
Model I am using (Bert, XLNet ...): TransformerXL
The problem arises when using:
* [ ] the official example scripts: (give details below)
* [x] my own modified scripts: (give details below)
The tasks I am working on is:
* [ ] an official GLUE/SQUaD task: (give the name)
* [x] my own task or dataset: (give details below)
## To reproduce
Steps to reproduce the behavior:
1. Upload private model to hub
2. Follow the tutorial around calling the Inference API (pasted below)
I've trained a `TransformerXLLMHeadModel` and am using the equivalent tokenizer class `TransfoXLTokenizer` on a custom dataset. I've saved both of these classes and have verified that loading the directory using the Auto classes succeeds and that the model and tokenizer are usable. When attempting to call the Inference API, I only get back my input text.
### Specific code from tutorial
```python
import json
import requests
API_TOKEN = "api_1234"
API_URL = "https://api-inference.huggingface.co/models/private/model"
headers = {"Authorization": f"Bearer {API_TOKEN}"}
def query(payload):
data = json.dumps(payload)
response = requests.request("POST", API_URL, headers=headers, data=data)
return json.loads(response.content.decode("utf-8"))
data = query({"inputs": "Begin 8Bars ", "temperature": .85, "num_return_sequences": 5})
# data = [{'generated_text': 'Begin First '}]
```
## Expected behavior
When calling the Inference API on my private model I would expect it to return additional output rather than just my input text.
| 03-05-2021 04:17:48 | 03-05-2021 04:17:48 | Could you try to add `"max_length": 200` to your payload ? (also cc @Narsil )<|||||>Hi @gstranger ,
Can you reproduce the problem locally ? It could be that your model simply produces EOS token with high probability (leading to having the exact same prompt as output)
If not, do you mind telling us your username by DM `[email protected]` so we can investigate this issue ?<|||||>Hello @Narsil ,
When I run the same model locally, without either `max_length` or `min_length` I receive additional output, typically it will generate about 20-40 tokens. Also @patrickvonplaten when I add either that parameter or the `min_length` parameter the model still returns in less than a second with the same input text. I've sent an email with additional information. <|||||>Hi @gstranger,
It does seem like a `max_length`. Your config defines it as `20` which is not long enough for the prompt that gets automatically added (because it's a transfo-xl model): https://github.com/huggingface/transformers/blob/master/src/transformers/pipelines/text_generation.py#L23
You can override both `max_length` and `prefix` within the config to override the default `transfo-xl` behavior (depending on how it was trained it might lead to significant perf boost, or loss).
By default the API will read the config first. Replying also with more information by email with more information. Just sharing here so that the community can get help too.
<|||||>This issue has been automatically marked as stale because it has not had recent activity. If you think this still needs to be addressed please comment on this thread.
Please note that issues that do not follow the [contributing guidelines](https://github.com/huggingface/transformers/blob/master/CONTRIBUTING.md) are likely to be ignored. |
transformers | 10,531 | closed | Typo correction. | # What does this PR do?
Fix a typo: DEBERTA_PRETRAINED_MODEL_ARCHIVE_LIST => DEBERTA_V2_PRETRAINED_MODEL_ARCHIVE_LIST in line 31.
<!--
Congratulations! You've made it this far! You're not quite done yet though.
Once merged, your PR is going to appear in the release notes with the title you set, so make sure it's a great title that fully reflects the extent of your awesome contribution.
Then, please replace this with a description of the change and which issue is fixed (if applicable). Please also include relevant motivation and context. List any dependencies (if any) that are required for this change.
Once you're done, someone will review your PR shortly (see the section "Who can review?" below to tag some potential reviewers). They may suggest changes to make the code even better. If no one reviewed your PR after a week has passed, don't hesitate to post a new comment @-mentioning the same persons---sometimes notifications get lost.
-->
<!-- Remove if not applicable -->
Fixes #10529
## Before submitting
- [x] This PR fixes a typo or improves the docs (you can dismiss the other checks if that's the case).
- [] Did you read the [contributor guideline](https://github.com/huggingface/transformers/blob/master/CONTRIBUTING.md#start-contributing-pull-requests),
Pull Request section?
- [] Was this discussed/approved via a Github issue or the [forum](https://discuss.huggingface.co/)? Please add a link
to it if that's the case.
- [] Did you make sure to update the documentation with your changes? Here are the
[documentation guidelines](https://github.com/huggingface/transformers/tree/master/docs), and
[here are tips on formatting docstrings](https://github.com/huggingface/transformers/tree/master/docs#writing-source-documentation).
- [] Did you write any new necessary tests?
## Who can review?
Anyone in the community is free to review the PR once the tests have passed. Feel free to tag
members/contributors which may be interested in your PR.
<!-- Your PR will be replied to more quickly if you can figure out the right person to tag with @
If you know how to use git blame, that is the easiest way, otherwise, here is a rough guide of **who to tag**.
Please tag fewer than 3 people.
Models:
- albert, bert, xlm: @LysandreJik
- blenderbot, bart, marian, pegasus, encoderdecoder, t5: @patrickvonplaten, @patil-suraj
- longformer, reformer, transfoxl, xlnet: @patrickvonplaten
- fsmt: @stas00
- funnel: @sgugger
- gpt2: @patrickvonplaten, @LysandreJik
- rag: @patrickvonplaten, @lhoestq
- tensorflow: @jplu
Library:
- benchmarks: @patrickvonplaten
- deepspeed: @stas00
- ray/raytune: @richardliaw, @amogkam
- text generation: @patrickvonplaten
- tokenizers: @n1t0, @LysandreJik
- trainer: @sgugger
- pipelines: @LysandreJik
Documentation: @sgugger
HF projects:
- nlp datasets: [different repo](https://github.com/huggingface/nlp)
- rust tokenizers: [different repo](https://github.com/huggingface/tokenizers)
Examples:
- maintained examples (not research project or legacy): @sgugger, @patil-suraj
- research_projects/bert-loses-patience: @JetRunner
- research_projects/distillation: @VictorSanh
-->
| 03-05-2021 03:58:52 | 03-05-2021 03:58:52 | |
transformers | 10,530 | closed | Test/Predict on summarization task | ## Environment info
<!-- You can run the command `transformers-cli env` and copy-and-paste its output below.
Don't forget to fill out the missing fields in that output! -->
- `transformers` version: 4.4.0.dev0
- Platform: Linux-5.3.0-53-generic-x86_64-with-glibc2.10
- Python version: 3.8.3
- PyTorch version (GPU?): 1.7.1 (True)
- Tensorflow version (GPU?): not installed (NA)
- Using GPU in script?: <fill in>
- Using distributed or parallel set-up in script?: <fill in>
### Who can help
<!-- Your issue will be replied to more quickly if you can figure out the right person to tag with @
If you know how to use git blame, that is the easiest way, otherwise, here is a rough guide of **who to tag**.
Please tag fewer than 3 people.
@patrickvonplaten, @patil-suraj
Models:
- albert, bert, xlm: @LysandreJik
- blenderbot, bart, marian, pegasus, encoderdecoder, t5: @patrickvonplaten, @patil-suraj
- longformer, reformer, transfoxl, xlnet: @patrickvonplaten
- fsmt: @stas00
- funnel: @sgugger
- gpt2: @patrickvonplaten, @LysandreJik
- rag: @patrickvonplaten, @lhoestq
- tensorflow: @jplu
Library:
- benchmarks: @patrickvonplaten
- deepspeed: @stas00
- ray/raytune: @richardliaw, @amogkam
- text generation: @patrickvonplaten
- tokenizers: @LysandreJik
- trainer: @sgugger
- pipelines: @LysandreJik
Documentation: @sgugger
HF projects:
- nlp datasets: [different repo](https://github.com/huggingface/nlp)
- rust tokenizers: [different repo](https://github.com/huggingface/tokenizers)
Examples:
- maintained examples (not research project or legacy): @sgugger, @patil-suraj
- research_projects/bert-loses-patience: @JetRunner
- research_projects/distillation: @VictorSanh
-->
- maintained examples using bart: @patrickvonplaten, @patil-suraj
## Information
Model I am using (Bart):
The problem arises when using:
* [ ] only have train and eval example, do not have test/predict example script
* [ ] my own modified scripts: (give details below)
* [ ] CUDA_VISIBLE_DEVICES=2,3 python examples/seq2seq/run_seq2seq.py \
--model_name_or_path /home/yxzhou/experiment/ASBG/output/xsum_bart_large/ \
--do_predict \
--task summarization \
--dataset_name xsum \
--output_dir /home/yxzhou/experiment/ASBG/output/xsum_bart_large/test/ \
--num_beams 1 \
The tasks I am working on is:
* [ ] CNNDAILYMAIL , XSUM
## Expected behavior
<!-- A clear and concise description of what you would expect to happen. -->
Use the above script to test on CNNDAILYMAIL and XSUM dataset, the program seems will be always stuck at training_step (e.g., 26/709)
Could you please kindly provide a test/predict example script of the summarization task (e.g., XSUM). Thank you so much! | 03-05-2021 03:41:56 | 03-05-2021 03:41:56 | Hi,
could you please ask questions related to training of models on the [forum](https://discuss.huggingface.co/)?
All questions related to fine-tuning a model for summarization on CNN can be found [here](https://discuss.huggingface.co/search?q=summarization%20cnn) for example.
<|||||>> Hi,
>
> could you please ask questions related to training of models on the [forum](https://discuss.huggingface.co/)?
>
> All questions related to fine-tuning a model for summarization on CNN can be found [here](https://discuss.huggingface.co/search?q=summarization%20cnn) for example.
@NielsRogge Oh, thank you for your reminder, that really helped!<|||||>This issue has been automatically marked as stale because it has not had recent activity. If you think this still needs to be addressed please comment on this thread.
Please note that issues that do not follow the [contributing guidelines](https://github.com/huggingface/transformers/blob/master/CONTRIBUTING.md) are likely to be ignored. |
transformers | 10,529 | closed | Typo in deberta_v2/__init__.py | https://github.com/huggingface/transformers/blob/c503a1c15ec1b11e69a3eaaf06edfa87c05a2849/src/transformers/models/deberta_v2/__init__.py#L31
Should be '' DEBERTA_V2_PRETRAINED_MODEL_ARCHIVE_LIST ''. | 03-05-2021 02:36:33 | 03-05-2021 02:36:33 | That's correct! Do you want to open a PR to fix it? |
transformers | 10,528 | closed | Different vocab_size between model and tokenizer of mT5 | ## Environment info
<!-- You can run the command `transformers-cli env` and copy-and-paste its output below.
Don't forget to fill out the missing fields in that output! -->
- `transformers` version: 4.1.1
- Platform: ubuntu 18.04
- Python version: 3.8.5
- PyTorch version (GPU?): 1.7.1
### Who can help
@patrickvonplaten
<!-- Your issue will be replied to more quickly if you can figure out the right person to tag with @
If you know how to use git blame, that is the easiest way, otherwise, here is a rough guide of **who to tag**.
Please tag fewer than 3 people.
Models:
- albert, bert, xlm: @LysandreJik
- blenderbot, bart, marian, pegasus, encoderdecoder, t5: @patrickvonplaten, @patil-suraj
- longformer, reformer, transfoxl, xlnet: @patrickvonplaten
- fsmt: @stas00
- funnel: @sgugger
- gpt2: @patrickvonplaten, @LysandreJik
- rag: @patrickvonplaten, @lhoestq
- tensorflow: @jplu
Library:
- benchmarks: @patrickvonplaten
- deepspeed: @stas00
- ray/raytune: @richardliaw, @amogkam
- text generation: @patrickvonplaten
- tokenizers: @LysandreJik
- trainer: @sgugger
- pipelines: @LysandreJik
Documentation: @sgugger
HF projects:
- nlp datasets: [different repo](https://github.com/huggingface/nlp)
- rust tokenizers: [different repo](https://github.com/huggingface/tokenizers)
Examples:
- maintained examples (not research project or legacy): @sgugger, @patil-suraj
- research_projects/bert-loses-patience: @JetRunner
- research_projects/distillation: @VictorSanh
-->
## To reproduce
Steps to reproduce the behavior:
```python
from transformers import AutoModelForSeq2SeqLM
from transformers import AutoTokenizer
mt5s = ['google/mt5-base', 'google/mt5-small', 'google/mt5-large', 'google/mt5-xl', 'google/mt5-xxl']
for mt5 in mt5s:
model = AutoModelForSeq2SeqLM.from_pretrained(mt5)
tokenizer = AutoTokenizer.from_pretrained(mt5)
print()
print(mt5)
print(f"tokenizer vocab: {tokenizer.vocab_size}, model vocab: {model.config.vocab_size}")
```
This is problematic in case when one addes some (special) tokens to tokenizer and resizes the token embedding of the model with `model.resize_token_embedding(len(tokenizer))`
<!-- If you have code snippets, error messages, stack traces please provide them here as well.
Important! Use code tags to correctly format your code. See https://help.github.com/en/github/writing-on-github/creating-and-highlighting-code-blocks#syntax-highlighting
Do not use screenshots, as they are hard to read and (more importantly) don't allow others to copy-and-paste your code.-->
## Expected behavior
vocab_size for model and tokenizer should be the same?
<!-- A clear and concise description of what you would expect to happen. -->
| 03-05-2021 02:24:38 | 03-05-2021 02:24:38 | Hello! This is a duplicate of https://github.com/huggingface/transformers/issues/4875, https://github.com/huggingface/transformers/issues/10144 and https://github.com/huggingface/transformers/issues/9247
@patrickvonplaten, maybe we could do something about this in the docs? In the docs we recommend doing this:
```py
model.resize_token_embedding(len(tokenizer))
```
but this is unfortunately false for T5!<|||||>@LysandreJik @cih9088 , actually I think doing:
```python
model.resize_token_embedding(len(tokenizer))
```
is fine -> it shouldn't throw an error & logically it should also be correct...Can you try it out?
<|||||>```python
model.resize_token_embedding(len(tokenizer))
```
This works perfectly fine but here is the thing.
One might add the `model.resize_token_embedding(len(tokenizer))` in their code and use other configuration packages such as `hydra` from Facebook to train models with additional tokens or without them dynamically at runtime.
He would naturally think that `vocab_size` of tokenizer (no tokens added) and `vocab_size` of model are the same because other models are.
Eventually, he fine-tunes the `google/mt5-base` model without added tokens but because of `model.resize_token_embedding(len(tokenizer))`, model he will fine-tune is not the same with `google/mt5-base`.
After training, he wants to load the trained model to test but the model complains about inconsistent embedding size between a loaded model which is `google/mt5-base`, and the trained model which has a smaller size of token embedding.
Of course, we could resize token embedding before loading model, but what matters is inconsistency with other models I think.
I reckon that people would not very much care about how the dictionary is composed in tokenizer. Maybe we add some dummy tokens to the tokenizer to keep consistency with other huggingface models or add documentation about it (I could not find any).
What do you think?<|||||>This issue has been automatically marked as stale because it has not had recent activity. If you think this still needs to be addressed please comment on this thread.
Please note that issues that do not follow the [contributing guidelines](https://github.com/huggingface/transformers/blob/master/CONTRIBUTING.md) are likely to be ignored.<|||||>
> Hello! This is a duplicate of #4875, #10144 and #9247
>
> @patrickvonplaten, maybe we could do something about this in the docs? In the docs we recommend doing this:
>
> ```python
> model.resize_token_embedding(len(tokenizer))
> ```
>
> but this is unfortunately false for T5!
What is the correct way to resize_token_embedding for T5/mT5? |
transformers | 10,527 | closed | Refactoring checkpoint names for multiple models | Hi, @sgugger reupload without datasets dir and added tf_modeling files, removed extra decorator in distilbert.
Linked to #10193, this PR refactors the checkpoint names in one private constant.
one note: longformer_tf has two checkpoints "allenai/longformer-base-4096" & "allenai/longformer-large-4096-finetuned-triviaqa". I set the checkpoint constant to "allenai/longformer-base-4096" and left the one decorator with "allenai/longformer-large-4096-finetuned-triviaqa".
Fixes #10193
## Before submitting
- [ ] This PR fixes a typo or improves the docs (you can dismiss the other checks if that's the case).
- [x] Did you read the [contributor guideline](https://github.com/huggingface/transformers/blob/master/CONTRIBUTING.md#start-contributing-pull-requests),
Pull Request section?
- [x] Was this discussed/approved via a Github issue or the [forum](https://discuss.huggingface.co/)? Please add a link
to it if that's the case.
- [ ] Did you make sure to update the documentation with your changes? Here are the
[documentation guidelines](https://github.com/huggingface/transformers/tree/master/docs), and
[here are tips on formatting docstrings](https://github.com/huggingface/transformers/tree/master/docs#writing-source-documentation).
- [ ] Did you write any new necessary tests?
| 03-05-2021 01:40:49 | 03-05-2021 01:40:49 | ...The test pasts on my local machine, I ran make test, style, quality, fixup. I dont know why this failed..<|||||>I just rebased the PR to ensure that the tests pass. We'll merge if all is green!<|||||>Thanks guys |
transformers | 10,526 | closed | Fix Adafactor documentation (recommend correct settings) | This PR fixes documentation to reflect optimal settings for Adafactor:
- fix an impossible arg combination erroneously proposed in the example
- use the correct link to the adafactor paper where `clip_treshold` is discussed
- document the recommended `scale_parameter=False`
- add other recommended settings combinations, which are quite different from the original
- re-org notes
- make the errors less ambiguous
Fixes #7789
@sgugger
(edited by @stas00 to reflect it's pre-merge state as the PR evolved since it's original submission) | 03-04-2021 23:03:39 | 03-04-2021 23:03:39 | Is this part correct?
> Recommended T5 finetuning settings:
> - Scheduled LR warm-up to fixed LR
> - disable relative updates
> - use clip threshold: https://arxiv.org/abs/2004.14546
In particular:
- are we supposed to do scheduled LR? adafactor handles this no?
- we *should not* disable relative updates
- i don't know what clip threshold means in this context<|||||>@sshleifer can you accept this documentation change?<|||||>No but @stas00 can!<|||||>@jsrozner, thank you for the PR.
Reading https://github.com/huggingface/transformers/issues/7789 it appears that the `Recommended T5 finetuning settings` are invalid.
So if we are fixing this, in addition to changing the example the prose above it should be synced as well.
I don't know where the original recommendation came from - do you by chance have a source we could point to for the corrected recommendation? If you know that is, if not, please don't worry.
Thank you.
<|||||>I receive the following error when using this the "recommended way":
```{python}
Traceback (most recent call last):
File "./folder_aws/transformers/examples/seq2seq/run_seq2seq.py", line 759, in <module>
main()
File "./folder_aws/transformers/examples/seq2seq/run_seq2seq.py", line 651, in main
train_result = trainer.train(resume_from_checkpoint=checkpoint)
File "/home/alejandro.vaca/SpainAI_Hackaton_2020/folder_aws/transformers/src/transformers/trainer.py", line 909, in train
self.create_optimizer_and_scheduler(num_training_steps=max_steps)
File "/home/alejandro.vaca/SpainAI_Hackaton_2020/folder_aws/transformers/src/transformers/trainer.py", line 660, in create_optimizer_and_scheduler
self.optimizer = optimizer_cls(optimizer_grouped_parameters, **optimizer_kwargs)
File "/home/alejandro.vaca/SpainAI_Hackaton_2020/folder_aws/transformers/src/transformers/optimization.py", line 452, in __init__
raise ValueError("warmup_init requires relative_step=True")
ValueError: warmup_init requires relative_step=True
```
Following this example in documentation:
```{python}
Adafactor(model.parameters(), lr=1e-3, relative_step=False, warmup_init=True)
```
@sshleifer @stas00 @jsrozner <|||||>@alexvaca0, what was the command line you run when you received this error?
HF Trainer doesn't set `warmup_init=True`. Unless you modified the script?
https://github.com/huggingface/transformers/blob/21e86f99e6b91af2e4df3790ba6c781e85fa0eb5/src/transformers/trainer.py#L649-L651
It is possible that the whole conflict comes from misunderstanding how this optimizer has to be used?
> To use a manual (external) learning rate schedule you should set `scale_parameter=False` and `relative_step=False`.
which is what the Trainer does at the moment.
and:
> relative_step (:obj:`bool`, `optional`, defaults to :obj:`True`):
> If True, time-dependent learning rate is computed instead of external learning rate
Is it possible that you are trying to use both an external and the internal scheduler at the same time?
It'd help a lot of you could show us the code that breaks, (perhaps on colab?) and how you invoke it. Basically, help us reproduce it.
Thank you.<|||||>Hi, @stas00 , first thank you very much for looking into it so fast. I forgot to say it, but yes, I changed the code in Trainer because I was trying to use the recommended settings for training T5 (I mean, setting an external learning rate with warmup_init = True as in the documentation. `Training without LR warmup or clip threshold is not recommended. Additional optimizer operations like gradient clipping should not be used alongside Adafactor.` From your answer, I understand that Trainer was designed for using Adam, as it uses the external learning rate scheduler and doesn't let you pass None as learning rate. Is there a workaround to be able to use the Trainer class with Adafactor following Adafactor settings recommended in the documentation? I'd also like to try using Adafactor without specifying the learning rate, would that be possible? I think maybe this documentation causes a little bit of confusion, because when you set the parameters specified in it `Adafactor(model.parameters(), lr=1e-3, relative_step=False, warmup_init=True)` it breaks.
<|||||>OK, so first, as @jsrozner PR goes and others commented, the current recommendation appears to be invalid.
So we want to gather all the different combinations that work and identify which of them provides the best outcome. I originally replied to this PR asking if someone knows of an authoritative paper we could copy the recommendation from - i.e. finding someone who already did the quality studies, so that we won't have it. So I'm all ears if any of you knows of such source.
Now to your commentary, @alexvaca0, while HF trainer has Adam as the default it has `--adafactor` which enables it in the mode with using the external scheduler. Surely, we could change the Trainer to skip the external scheduler (or perhaps simpler feeding it some no-op scheduler) and instead use this recommendation if @sgugger agrees with that. But we first need to see that it provides a better outcome in the general case. Or alternatively to make `--adafactor` configurable so it could support more than just one way.
For me personally I want to understand first the different combinations, what are the impacts and how many of those combinations should we expose through the Trainer. e.g. like apex optimization level, we could have named combos `--adafactor setup1`, `--adafactor setup2` and would activate the corresponding configuration. But first let's compile the list of desirable combos.
Would any of the current participants be interested in taking a lead on that? I'm asking you since you are already trying to get the best outcome with your data and so are best positioned to judge which combinations work the best for what situation.
Once we compiled the data it'd be trivial to update the documented recommendation and potentially extend HF Trainer to support more than one setting for Adafactor.
<|||||>I only ported the `--adafactor` option s it was implemented for the `Seq2SeqTrainer` to keep the commands using it working as they were. The `Trainer` does not have vocation to support all the optimizers and all their possible setups, just one sensible default that works well, that is the reason you can:
- pass an `optimizer` at init
- subclass and override `create_optimizer`.
In retrospect, I shouldn't have ported the Adafactor option and it should have stayed just in the script using it.<|||||>Thank you for your feedback, @sgugger.
So let's leave the trainer as it is and let's then solve this for Adafactor as just an optimizer and then document the best combinations. <|||||>Per my comment on #7789, I observed that
> I can confirm that `Adafactor(lr=1e-3, relative_step=False, warmup_init=False)` seems to break training (i.e. I observe no learning over 4 epochs, whereas `Adafactor(model.parameters(), relative_step=True, warmup_init=True, lr=None)` works well (much better than adam)
Given that relative_step and warmup_init must take on the same value, it seems like there is only one configuration that is working?
But, this is also confusing (see my comment above): https://github.com/huggingface/transformers/pull/10526#issuecomment-791012771
> > Recommended T5 finetuning settings:
> > - Scheduled LR warm-up to fixed LR
> > - disable relative updates
> > - use clip threshold: https://arxiv.org/abs/2004.14546
>
> In particular:
> - are we supposed to do scheduled LR? adafactor handles this no?
> - we *should not* disable relative updates
> - i don't know what clip threshold means in this context
<|||||>I first validated that HF `Adafactor` is 100% identical to the latest [fairseq version](https://github.com/pytorch/fairseq/blob/5273bbb7c18a9b147e3f0cfc97121cc945a962bd/fairseq/optim/adafactor.py).
I then tried to find out the source of these recommendations and found:
1. https://discuss.huggingface.co/t/t5-finetuning-tips/684/3
```
lr=0.001, scale_parameter=False, relative_step=False
```
2. https://discuss.huggingface.co/t/t5-finetuning-tips/684/22 which is your comment @jsrozner where you propose that the opposite combination works well:
```
lr=None, relative_step=True, warmup_init=True
```
If both found to be working, I propose we solve this conundrum by documenting this as following:
```
Recommended T5 finetuning settings (https://discuss.huggingface.co/t/t5-finetuning-tips/684/3):
- Scheduled LR warm-up to fixed LR
- disable relative updates
- scale_parameter=False
- use clip threshold: https://arxiv.org/abs/2004.14546
Example::
Adafactor(model.parameters(), scale_parameter=False, relative_step=False, warmup_init=False, lr=1e-3)
Others reported the following combination to work well::
Adafactor(model.parameters(), scale_parameter=False, relative_step=True, warmup_init=True, lr=None)
- Training without LR warmup or clip threshold is not recommended. Additional optimizer operations like
gradient clipping should not be used alongside Adafactor.
```
https://discuss.huggingface.co/t/t5-finetuning-tips/684/22 Also highly recommends to turn `scale_parameter=False` - so I added that to the documentation and the example above in both cases. Please correct me if I'm wrong.
And @jsrozner's correction in this PR is absolutely right to the point.
Please let me know if my proposal makes sense, in particular I'd like your validation, @jsrozner, since I added your alternative proposal. And don't have any other voices to agree or disagree with it.
Thank you!
<|||||>> Is this part correct?
>
> > ```
> > Recommended T5 finetuning settings:
> > - Scheduled LR warm-up to fixed LR
> > - disable relative updates
> > - use clip threshold: https://arxiv.org/abs/2004.14546
> > ```
>
> In particular:
>
> * are we supposed to do scheduled LR? adafactor handles this no?
see my last comment - it depends on whether we use the external LR scheduler or not.
> * we _should not_ disable relative updates
see my last comment - it depends on `warmup_init`'s value
> * i don't know what clip threshold means in this context
this?
```
def __init__(
[....]
clip_threshold=1.0,
```<|||||>I'm running some experiments, playing around with Adafactor parameters. I'll post here which configuration has best results. From T5 paper, they used the following parameters for fine-tuning: Adafactor with *constant* lr 1e-3, with batch size 128, if I understood the paper well. Therefore, I find it appropriate the documentation changes mentioned above, leaving the recommendations from the paper while mentioning other configs that have worked well for other users. In my case, for example, the configuration from the paper doesn't work very well and I quickly overfit. <|||||>Finally, I'm trying to understand the confusing:
```
- use clip threshold: https://arxiv.org/abs/2004.14546
[...]
gradient clipping should not be used alongside Adafactor.
```
As the paper explains these are 2 different types of clipping.
Since the code is:
```
update.div_((self._rms(update) / group["clip_threshold"]).clamp_(min=1.0))
```
this probably means that the default `clip_threshold=1.0` is in effect disables clip threshold.
I can't find any mentioning of clip threshold in https://arxiv.org/abs/2004.14546 - is this a wrong paper? Perhaps it needed to link to the original paper https://arxiv.org/abs/1804.04235 where clipping is actually discussed? I think it's the param `d` in the latter paper and it proposes to get the best results with `d=1.0` without learning rate warmup:
page 5 from https://arxiv.org/pdf/1804.04235:
> We added update clipping to the previously described fast-
> decay experiments. For the experiment without learning rate
> warmup, update clipping with d = 1 significantly amelio-
> rated the instability problem – see Table 2 (A) vs. (H). With
> d = 2, the instability was not improved. Update clipping
> did not significantly affect the experiments with warmup
> (with no instability problems).
So I will change the doc to a non-ambiguous:
`use clip_threshold=1.0 `<|||||>OK, so here is the latest proposal. I re-organized the notes:
```
Recommended T5 finetuning settings (https://discuss.huggingface.co/t/t5-finetuning-tips/684/3):
- Training without LR warmup or clip_threshold is not recommended.
* use scheduled LR warm-up to fixed LR
* use clip_threshold=1.0 (https://arxiv.org/abs/1804.04235)
- Disable relative updates
- Use scale_parameter=False
- Additional optimizer operations like gradient clipping should not be used alongside Adafactor
Example::
Adafactor(model.parameters(), scale_parameter=False, relative_step=False, warmup_init=False, lr=1e-3)
Others reported the following combination to work well::
Adafactor(model.parameters(), scale_parameter=False, relative_step=True, warmup_init=True, lr=None)
```
I added these into this PR, please have a look.
<|||||>Let's just wait to hear from both @jsrozner and @alexvaca0 to ensure that my edits are valid before merging.<|||||>I observed that `Adafactor(lr=1e-3, relative_step=False, warmup_init=False)` failed to lead to any learning. I guess this is because I didn't change `scale_parameter` to False? I can try rerunning with scale_param false.
And when I ran with `Adafactor(model.parameters(), relative_step=True, warmup_init=True, lr=None)`, I *did not* set `scale_parameter=False`. Before adding the "others seem to have success with ..." bit, we should check on the effect of scale_parameter.
Regarding clip_threshold - just confirming that the comment is correct that when using adafactor we should *not* have any other gradient clipping (e.g. `nn.utils.clip_grad_norm_()`)?
Semi-related per @alexvaca0, regarding T5 paper's recommended batch_size: is the 128 recommendation agnostic to the length of input sequences? Or is there a target number of tokens per batch that would be optimal? (E.g. input sequences of max length 10 tokens vs input sequences of max length 100 tokens -- should we expect 128 to work optimally for both?)
But most importantly, shouldn't we change the defaults so that a call to `Adafactor(model.paramaters())` == `Adafactor(model.parameters(), scale_parameter=False, relative_step=False, warmup_init=False, lr=1e-3)`
i.e, we default to what we suggest?<|||||>> I observed that Adafactor(lr=1e-3, relative_step=False, warmup_init=False) failed to lead to any learning. I guess this is because I didn't change scale_parameter to False? I can try rerunning with scale_param false.
Yes, please and thank you!
> Regarding clip_threshold - just confirming that the comment is correct that when using adafactor we should not have any other gradient clipping (e.g. nn.utils.clip_grad_norm_())?
Thank you for validating this, @jsrozner
Is the current incarnation of the doc clear wrt this subject matter or should we add an explicit example?
One thing I'm concerned about is that the Trainer doesn't validate this and will happily run `clip_grad_norm` with Adafactor
Might we need to add to:
https://github.com/huggingface/transformers/blob/9f8fa4e9730b8e658bcd5625610cc70f3a019818/src/transformers/trainer.py#L649-L651
```
if self.args.max_grad_norm:
raise ValueError("don't use max_grad_norm with adafactor")
```
> But most importantly, shouldn't we change the defaults so that a call to Adafactor(model.paramaters()) == `Adafactor(model.parameters(), scale_parameter=False, relative_step=False, warmup_init=False, lr=1e-3)`
Since we copied the code verbatim from fairseq, it might be a good idea to keep the defaults the same? I'm not attached to either way. @sgugger what do you think?
edit: I don't think we can/should since it may break people's code that relies on the current defaults.<|||||>> > Regarding clip_threshold - just confirming that the comment is correct that when using adafactor we should not have any other gradient clipping (e.g. nn.utils.clip_grad_norm_())?
>
> Thank you for validating this, @jsrozner
Sorry, I didn't validate this. I wanted to confirm with you all that this is correct.
> > But most importantly, shouldn't we change the defaults so that a call to Adafactor(model.paramaters()) == `Adafactor(model.parameters(), scale_parameter=False, relative_step=False, warmup_init=False, lr=1e-3)`
>
> Since we copied the code verbatim from fairseq, it might be a good idea to keep the defaults the same? I'm not attached to either way. @sgugger what do you think?
Alternative is to not provide defaults for these values and force the user to read documentation and decide what he/she wants. Can provide the default implementation as well as Adafactor's recommended settings
<|||||>> I'm running some experiments, playing around with Adafactor parameters. I'll post here which configuration has best results. From T5 paper, they used the following parameters for fine-tuning: Adafactor with _constant_ lr 1e-3, with batch size 128, if I understood the paper well. Therefore, I find it appropriate the documentation changes mentioned above, leaving the recommendations from the paper while mentioning other configs that have worked well for other users. In my case, for example, the configuration from the paper doesn't work very well and I quickly overfit.
@alexvaca0
What set of adafactor params did you find work well when you were finetuning t5?<|||||>> Sorry, I didn't validate this. I wanted to confirm with you all that this is correct.
I meant validating as in reading over and checking that it makes sense. So all is good. Thank you for extra clarification so we were on the same page, @jsrozner
> Alternative is to not provide defaults for these values and force the user to read documentation and decide what he/she wants. Can provide the default implementation as well as Adafactor's recommended settings
as I appended to my initial comment, this would be a breaking change. So if it's crucial that we do that, this would need to happen in the next major release.
<|||||>Or maybe add a warning message that indicates that default params may not be optimal? It will be logged only a single time at optimizer init so not too annoying.
`log.warning('Initializing Adafactor. If you are using default settings, it is recommended that you read the documentation to ensure that these are optimal for your use case.)`<|||||>But we now changed it propose two different ways - which one is the recommended one? The one used by the Trainer?
Since it's pretty clear that there is more than one way, surely the user will find their way to the doc if they aren't happy with the results.
<|||||>I ran my model under three different adafactor setups:
```python
optimizer = Adafactor(self.model.parameters(),
relative_step=True,
warmup_init=True)
```
```python
optimizer = Adafactor(self.model.parameters(),
relative_step=True,
warmup_init=True,
scale_parameter=False)
```
```python
optimizer = Adafactor(self.model.parameters(),
lr=1e-3,
relative_step=False,
warmup_init=False,
scale_parameter=False)
```
I track exact match and NLL on the dev set. Epochs are tracked at the bottom. They start at 11 because of how I'm doing things. (i.e. x=11 => epoch=1)
Note that I'm training a t5-small model on 80,000 examples, so maybe there's some variability with the sort of model we're training?
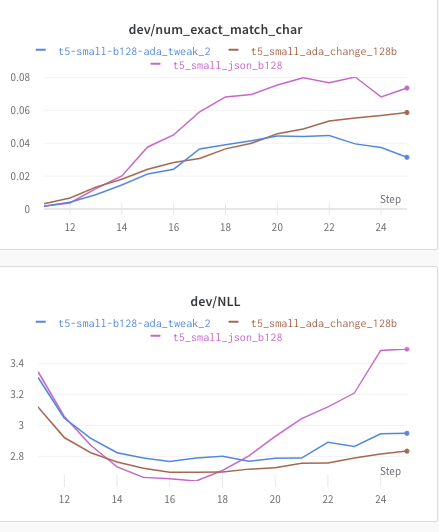
(Image works if you navigate to the link, but seems not to appear?)
purple is (1)
blue is (2) and by far the worst (i.e. shows that scale_param should be set to True if we are using relative_step)
brown is (3)
In particular, it looks like scale_param should be True for the setting under " Others reported the following combination to work well::"
On the other hand, it looks like for a t5-large model, (3) does better than (1) (although I also had substantially different batch sizes). <|||||>Thank you, @jsrozner, for running these experiments and the analysis.
So basically we should adjust " Others reported the following combination to work well::" to `scale_param=True`, correct?
Interestingly we end up with 2 almost total opposites.<|||||>@jsrozner Batch size and learning rate configuration go hand in hand, therefore it's difficult to know about your last reflexion, as having different different batch sizes lead to different gradient estimations (in particular, the lower the batch size, the worse your gradient estimation is), the larger your batch size, the larger your learning rate can be without negatively affecting performance.
For the rest of the experiments, thanks a lot, the comparison is very clear and this will be very helpful for those of us who want to have some "default" parameters to start from. I see a clear advantage to leave the learning rate as None, as when setting an external learning rate we typically have to make experiments to find the optimal one for that concrete problem, so if it provides results similar or better to the ones provided by the paper's recommended parameters, I'd go with that as default.
I hope I can have my experiments done soon, which will be with t5-large probably, to see if they coincide with your findings.<|||||>OK, let's merge this and if we need to make updates for any new findings we will do it then. <|||||>Although I didn't really run an experiment, I have found that my settings for adafactor (relative step, warmup, scale all true) do well when training t5-large, also.
@alexvaca0 please post your results when you have them! |
transformers | 10,525 | closed | fine-tune Pegasus with xsum using Colab but generation results have no difference | Hi. I tried to fine-tune pegasus large with xsum dataset using Colab (Pro). I was able to finish the fine-tuning with batch size 1, and 2000 epochs in about 40 minutes (larger batch size crashed colab). The working Colab notebook I used is shared at https://colab.research.google.com/drive/1RyUsYDAo6bA1RZICMb-FxYLszBcDY81X?usp=sharing
However, the generated summary seems to be the same for the pegasus large model (https://huggingface.co/google/pegasus-large) and the fine-tuned model. But the generated result using pegasus xsum model (https://huggingface.co/google/pegasus-xsum) is different and much better.
The training loss is already 0 and I am not sure what I have done wrong. Any help and pointers are highly appreciated.
@sshleifer
| 03-04-2021 20:43:02 | 03-04-2021 20:43:02 | Hi,
could you please ask this question on the [forum](https://discuss.huggingface.co/)? We're happy to help you there!
Questions regarding training of models are a perfect use case for the forum :) for example, [here](https://discuss.huggingface.co/search?q=pegasus) you can find all questions related to fine-tuning PEGASUS.<|||||>@NielsRogge thanks a lot! I will post the question in forum 😄🤝<|||||>I think this issue can be closed - I used another input text and the generated text is different - I guess the fine-tuned model is different but for some input text, the generated result is exactly the same as the large model - interesting to know why though :). |
transformers | 10,524 | closed | Change/remove default maximum length in run_glue.py | I propose one of the following:
1) A vast majority of models have maximum sequence length as 512. 128 as a maximum length is very misleading because of this reason and hence I suggest we revise it to 512.
2) Totally remove this variable and set maximum length only based on model’s maximum length. The tokenizers library used in this example already has code to take the minimum of (max_seq_length, max_model_seq_length). Why not just remove this redundancy and directly set it based on the model’s largest acceptable sequence length
# What does this PR do?
<!--
Congratulations! You've made it this far! You're not quite done yet though.
Once merged, your PR is going to appear in the release notes with the title you set, so make sure it's a great title that fully reflects the extent of your awesome contribution.
Then, please replace this with a description of the change and which issue is fixed (if applicable). Please also include relevant motivation and context. List any dependencies (if any) that are required for this change.
Once you're done, someone will review your PR shortly (see the section "Who can review?" below to tag some potential reviewers). They may suggest changes to make the code even better. If no one reviewed your PR after a week has passed, don't hesitate to post a new comment @-mentioning the same persons---sometimes notifications get lost.
-->
<!-- Remove if not applicable -->
Fixes # (issue)
## Before submitting
- [ ] This PR fixes a typo or improves the docs (you can dismiss the other checks if that's the case).
- [ ] Did you read the [contributor guideline](https://github.com/huggingface/transformers/blob/master/CONTRIBUTING.md#start-contributing-pull-requests),
Pull Request section?
- [ ] Was this discussed/approved via a Github issue or the [forum](https://discuss.huggingface.co/)? Please add a link
to it if that's the case.
- [ ] Did you make sure to update the documentation with your changes? Here are the
[documentation guidelines](https://github.com/huggingface/transformers/tree/master/docs), and
[here are tips on formatting docstrings](https://github.com/huggingface/transformers/tree/master/docs#writing-source-documentation).
- [ ] Did you write any new necessary tests?
## Who can review?
Anyone in the community is free to review the PR once the tests have passed. Feel free to tag
members/contributors which may be interested in your PR.
<!-- Your PR will be replied to more quickly if you can figure out the right person to tag with @
If you know how to use git blame, that is the easiest way, otherwise, here is a rough guide of **who to tag**.
Please tag fewer than 3 people.
Models:
- albert, bert, xlm: @LysandreJik
- blenderbot, bart, marian, pegasus, encoderdecoder, t5: @patrickvonplaten, @patil-suraj
- longformer, reformer, transfoxl, xlnet: @patrickvonplaten
- fsmt: @stas00
- funnel: @sgugger
- gpt2: @patrickvonplaten, @LysandreJik
- rag: @patrickvonplaten, @lhoestq
- tensorflow: @jplu
Library:
- benchmarks: @patrickvonplaten
- deepspeed: @stas00
- ray/raytune: @richardliaw, @amogkam
- text generation: @patrickvonplaten
- tokenizers: @n1t0, @LysandreJik
- trainer: @sgugger
- pipelines: @LysandreJik
Documentation: @sgugger
HF projects:
- nlp datasets: [different repo](https://github.com/huggingface/nlp)
- rust tokenizers: [different repo](https://github.com/huggingface/tokenizers)
Examples:
- maintained examples (not research project or legacy): @sgugger, @patil-suraj
- research_projects/bert-loses-patience: @JetRunner
- research_projects/distillation: @VictorSanh
-->
| 03-04-2021 19:18:52 | 03-04-2021 19:18:52 | @sgugger Can you review this extremely simple PR<|||||>Hi there. The default is actually the same as the legacy script and I don't see any reason to change it. It provides the results given in the README which are consistent with the paper.
Also a default of 512 won't work with models that have a smaller max_length like distilbert.<|||||>This issue has been automatically marked as stale because it has not had recent activity. If you think this still needs to be addressed please comment on this thread.
Please note that issues that do not follow the [contributing guidelines](https://github.com/huggingface/transformers/blob/master/CONTRIBUTING.md) are likely to be ignored.<|||||>Oh. Yeah, I didn’t know distilbert had a smaller max_len. I’d seen max_len at least greater than or equal to 512 until now. |
transformers | 10,523 | closed | BERT as encoder - position ids | Hello,
I have an EncoderDecoderModel.from_encoder_decoder_pretrained which is using BERT as both the decoder and encoder.
I would like to adjust position_ids for the encoder input, however, looking at [this documentation](https://huggingface.co/transformers/model_doc/encoderdecoder.html#transformers.EncoderDecoderModel.forward) it seems like there is no such argument.
How can I do this?
Sorry if this is an obvious question, I'm new to this stuff.
Thanks! | 03-04-2021 18:56:21 | 03-04-2021 18:56:21 | Hello! In the documentation you pointed to, you'll see that there is the `kwargs` argument, which accepts any keyword argument. The doc says:
```
(optional) Remaining dictionary of keyword arguments. Keyword arguments come in two flavors:
Without a prefix which will be input as **encoder_kwargs for the encoder forward function.
With a decoder_ prefix which will be input as **decoder_kwargs for the decoder forward function.
```
Have you tried passing `position_ids` to the `__call__` method of your model instantiated with the `EncoderDecoderModel.from_encoder_decoder_pretrained`? It should work!<|||||>Thanks so much! |
transformers | 10,522 | closed | Inconsistent API output for Q&A models between eager mode and torchscripted | ## Environment info
- `transformers` version: 4.3.3
- Platform: Linux-5.4.0-1037-aws-x86_64-with-glibc2.10
- Python version:3.8.6
- PyTorch version (GPU?): 1.7.1 (True)
- Tensorflow version (GPU?):
- Using GPU in script?: Yes
- Using distributed or parallel set-up in script?: No
## Information
@sgugger I am using question-answering models from auto class, have tried, Bert-base/Roberta-base/distilbert-base-cased-distilled-squad.
The problem arises when using:
Accessing the start and end logits through the output of the model is inconsistent between eager mode and Torchscripted(traced) model. For the eager mode, it is possible to use "outputs.start_logits, outputs.end_logits" however for
Torchscripted model, it returns a tuple, as it was in older version (i.e. 2.10).
## To reproduce
```
import transformers
import os
import torch
from transformers import (AutoModelForSequenceClassification, AutoTokenizer, AutoModelForQuestionAnswering,
AutoModelForTokenClassification, AutoConfig)
device = torch.device('cuda' if torch.cuda.is_available() else 'cpu')
pretrained_model_name = 'distilbert-base-cased-distilled-squad'
max_length = 30
config_torchscript = AutoConfig.from_pretrained(pretrained_model_name,torchscript=True)
model_torchscript = AutoModelForQuestionAnswering.from_pretrained(pretrained_model_name,config=config_torchscript)
tokenizer = AutoTokenizer.from_pretrained(pretrained_model_name,do_lower_case=True)
config = AutoConfig.from_pretrained(pretrained_model_name)
model = AutoModelForQuestionAnswering.from_pretrained(pretrained_model_name,config=config)
dummy_input = "This is a dummy input for torch jit trace"
inputs = tokenizer.encode_plus(dummy_input,max_length = int(max_length),pad_to_max_length = True, add_special_tokens = True, return_tensors = 'pt')
input_ids = inputs["input_ids"].to(device)
model.to(device).eval()
model_torchscript.to(device).eval()
traced_model = torch.jit.trace(model_torchscript, [input_ids])
outputs = model(input_ids)
print(outputs.start_logits, outputs.end_logits)
print("******************************* eager mode passed **************")
traced_outputs = traced_model(input_ids)
print(traced_outputs.start_logits, traced_outputs.end_logits)
print("******************************* traced mode passed **************")
```
Steps to reproduce the behavior:
1. install transformers.
2. Run the above code snippet
## Expected behavior
Being able to access the start and end logits consistently between eager and torchscript mode.
| 03-04-2021 18:28:43 | 03-04-2021 18:28:43 | `torchscript` does not support anything else than tuple outputs, so you can't rely on the attributes when using it and transformers automatically sets `return_dict=False` in this case.
You need to access to the output fields with indices.<|||||>@sgugger Thanks for the explanations.<|||||>This issue has been automatically marked as stale because it has not had recent activity. If you think this still needs to be addressed please comment on this thread.
Please note that issues that do not follow the [contributing guidelines](https://github.com/huggingface/transformers/blob/master/CONTRIBUTING.md) are likely to be ignored. |
transformers | 10,521 | closed | Removes overwrites for output_dir | # What does this PR do?
This PR removes the overwrites of the `output_dir` when running training on `SageMaker` and it removes the automatic save if `output_dir` is `None`.
The overwrites have been removed since it prevents saving checkpoints to a different dir like `opt/ml/checkpoints` impossible and it is not the "transformers" way. We can keep it the same as running training somewhere else and provide documentation on what you need to do. | 03-04-2021 15:39:02 | 03-04-2021 15:39:02 | |
transformers | 10,520 | closed | Unable to translate Arabic to many other languages in MBart-50 | ## Environment info
<!-- You can run the command `transformers-cli env` and copy-and-paste its output below.
Don't forget to fill out the missing fields in that output! -->
- `transformers` version: 4.4.0.dev0
- Platform: Linux-4.19.112+-x86_64-with-Ubuntu-18.04-bionic
- Python version: 3.7.10
- PyTorch version (GPU?): 1.7.1+cu101 (True)
- Tensorflow version (GPU?): 2.4.1 (True)
- Using GPU in script?: <fill in>
- Using distributed or parallel set-up in script?: <fill in>
### Who can help
<!-- Your issue will be replied to more quickly if you can figure out the right person to tag with @
If you know how to use git blame, that is the easiest way, otherwise, here is a rough guide of **who to tag**.
Please tag fewer than 3 people.
Models:
- albert, bert, xlm: @LysandreJik
- blenderbot, bart, marian, pegasus, encoderdecoder, t5: @patrickvonplaten, @patil-suraj
- longformer, reformer, transfoxl, xlnet: @patrickvonplaten
- fsmt: @stas00
- funnel: @sgugger
- gpt2: @patrickvonplaten, @LysandreJik
- rag: @patrickvonplaten, @lhoestq
- tensorflow: @jplu
Library:
- benchmarks: @patrickvonplaten
- deepspeed: @stas00
- ray/raytune: @richardliaw, @amogkam
- text generation: @patrickvonplaten
- tokenizers: @LysandreJik
- trainer: @sgugger
- pipelines: @LysandreJik
Documentation: @sgugger
HF projects:
- nlp datasets: [different repo](https://github.com/huggingface/nlp)
- rust tokenizers: [different repo](https://github.com/huggingface/tokenizers)
Examples:
- maintained examples (not research project or legacy): @sgugger, @patil-suraj
- research_projects/bert-loses-patience: @JetRunner
- research_projects/distillation: @VictorSanh
-->
@patrickvonplaten
## Information
Model I am using (Bert, XLNet ...): Mbart-50
The problem arises when using:
* [ X] the official example scripts: (give details below)
* [ ] my own modified scripts: (give details below)
The tasks I am working on is:
* [ X] an official GLUE/SQUaD task: (give the name)
* [ ] my own task or dataset: (give details below)
## To reproduce
```
# translate Arabic to Hindi
article_ar = "الأمين العام للأمم المتحدة يقول إنه لا يوجد حل عسكري في سوريا."
tokenizer.src_lang = "ar_AR"
encoded_ar = tokenizer(article_ar, return_tensors="pt")
generated_tokens = model.generate(
**encoded_ar,
forced_bos_token_id=tokenizer.lang_code_to_id["hi_IN"]
)
tokenizer.batch_decode(generated_tokens, skip_special_tokens=True)
```
**Result: ['Secretary of the United Nations says there is no military solution to Syria.']**
<!-- If you have code snippets, error messages, stack traces please provide them here as well.
Important! Use code tags to correctly format your code. See https://help.github.com/en/github/writing-on-github/creating-and-highlighting-code-blocks#syntax-highlighting
Do not use screenshots, as they are hard to read and (more importantly) don't allow others to copy-and-paste your code.-->
## Expected behavior
The result should be in Hindi, but it weirdly it is in English. Arabic --> Hindi is an example. But if you test Arabic -->xx_XX you will obtain the same behavior except for a few ones (such as `fr_XX` or `de_DE`)
<!-- A clear and concise description of what you would expect to happen. -->
| 03-04-2021 15:34:22 | 03-04-2021 15:34:22 | Hi @lecidhugo
Thank you for reporting this. Is this for one particular example or is it happening for all examples?<|||||>Hi @patil-suraj,
Thank you for your reply.
Indeed, it is for all examples and for many languages<|||||>Thanks. I'll look into it next week.<|||||>This issue has been automatically marked as stale because it has not had recent activity. If you think this still needs to be addressed please comment on this thread.
Please note that issues that do not follow the [contributing guidelines](https://github.com/huggingface/transformers/blob/master/CONTRIBUTING.md) are likely to be ignored.<|||||>Unstale
<|||||>This issue has been automatically marked as stale because it has not had recent activity. If you think this still needs to be addressed please comment on this thread.
Please note that issues that do not follow the [contributing guidelines](https://github.com/huggingface/transformers/blob/master/CONTRIBUTING.md) are likely to be ignored. |
transformers | 10,519 | open | Adding option to truncation from beginning instead of end, for both longest_first and longest_second | # 🚀 Feature request
<!-- A clear and concise description of the feature proposal.
Please provide a link to the paper and code in case they exist. -->
The current [truncation strategies](https://huggingface.co/transformers/preprocessing.html#everything-you-always-wanted-to-know-about-padding-and-truncation) only provide truncation from end of the input sentence. This may be enough for most cases, but is not applicable for dialog tasks.
Say, you are concatenating an input dialog context to BERT. If the input length > max_length (256/ 512), then the tokens are truncated from the ending i.e. the most recent utterances in the dialog.
In some cases, you want the most recent utterances to be included in the input and if truncation needs to be done, then the oldest utterances be truncated i.e. truncation from the beginning.
This can be done manually in own code base outside of Transformers library, but it's not ideal.
Truncation outside of model will be done most likely on full words to truncate from beginning and fit them to model max length (say you truncate from beginning and reduce input to 254 words). But, these words will be converted to subwords when fed to BertTokenizer and the final input will be > 256, thus resulting in words being dropped from the last utterance again.
To do this properly outside of the Transformers library, one would need to instantiate the same Tokenizer object, tokenize each input and then truncate from beginning, then convert ids back to tokens and then either reconstruct the input from the truncated tokenized version or skip the tokenizer call inside the Transformers library.
## Motivation
<!-- Please outline the motivation for the proposal. Is your feature request
related to a problem? e.g., I'm always frustrated when [...]. If this is related
to another GitHub issue, please link here too. -->
## Your contribution
<!-- Is there any way that you could help, e.g. by submitting a PR?
Make sure to read the CONTRIBUTING.MD readme:
https://github.com/huggingface/transformers/blob/master/CONTRIBUTING.md -->
| 03-04-2021 15:27:07 | 03-04-2021 15:27:07 | Previous mention of this idea
https://github.com/huggingface/transformers/issues/4476#issuecomment-677823688<|||||>Here's my workaround (from the previous issue): https://github.com/huggingface/transformers/issues/4476#issuecomment-951445067<|||||>May be of interest to @SaulLu @NielsRogge <|||||>This is indeed a feature that is requested and would make sense to have!
To my knowledge @NielsRogge has started a [PR to add this feature](https://github.com/huggingface/transformers/pull/12913). Unfortunately, the feature requires development also in the Tokenizers library and nobody has yet got the bandwidth to support [the dedicated issue](https://github.com/huggingface/tokenizers/issues/779) for this feature.<|||||>> the feature requires development
Links provided show that only fast tokenizers are stopping this ("requested and would make sense to have" feature) to be implemented ("since july.... July, Karl!"). May this be implemented for ordinary tokenizers faster, and for faster tokenizers later, when they solve dedicated issues?
In my opinion, having left truncation for "slow" tokenizers now, is better than not having one at all (or in distant future releases). It is awaited (https://github.com/huggingface/transformers/issues/4476#event-3364051747) for more than a year now at least.<|||||>Seems this was added in #14947 about a month ago, so hopefully it will be in a near-future release! |
transformers | 10,518 | closed | Converting models for tensoflowjs (node) | ## Environment info
- `transformers` version: 4.3.3
- Platform: Linux-5.11.2-zen1-1-zen-x86_64-with-glibc2.2.5
- Python version: 3.8.8 (also 3.9.2 but tensorflowjs is not available in 3.9.2)
- PyTorch version (GPU?): 1.7.1 (True)
- Tensorflow version (GPU?): 2.4.1 (False)
- Using GPU in script?: No
- Using distributed or parallel set-up in script?: No
### Who can help
- tensorflow: @jplu
## Information
Model I am using (Bert, XLNet ...): Any tensorflow ones
The problem arises when using:
* [X] the official example scripts: (give details below)
* [X] my own modified scripts: (give details below)
The tasks I am working on is:
* [X] my own task or dataset: (give details below)
## To reproduce
Steps to reproduce the behavior:
1. Load a tensforflow model (e.g : `model = TFAutoModel.from_pretrained("distilbert-base-uncased")`)
2. Convert it to H5 with `model.save_pretrained(path)`
3. Try to load it in tensorflowjs and get `UnhandledPromiseRejectionWarning: TypeError: Cannot read property 'model_config' of null`
## Expected behavior
Be able to load a model in the LayerModel format as it's the only one which allows finetuning.
## More informations.
I know the question was already posted in https://github.com/huggingface/transformers/issues/4073
I need to do finetuning so onnx, graphModels & cie should be avoided.
Seems that the H5 model just need the config file which seems saved on the side with the custom HF script.
I went to read some issues on tensorflowjs (exemple : [this](https://github.com/tensorflow/tfjs/issues/2455) or [that](https://github.com/tensorflow/tfjs/issues/931)) and the problem is that the HF model contains only the weights and not the architecture. The goal would be to adapt the `save_pretrained` function to save the architecture as well. I guess it's complex because of the `Saving the model to HDF5 format requires the model to be a Functional model or a Sequential model.` error in described bellow.
Seems also that only H5 model can be converted to a trainable LayerModel.
I'm willing to work on a PR or to help as i'm working on a web stack (nodejs) and I need this.
I made a drawing of all models (that I'm aware of) to summarize loading converting :
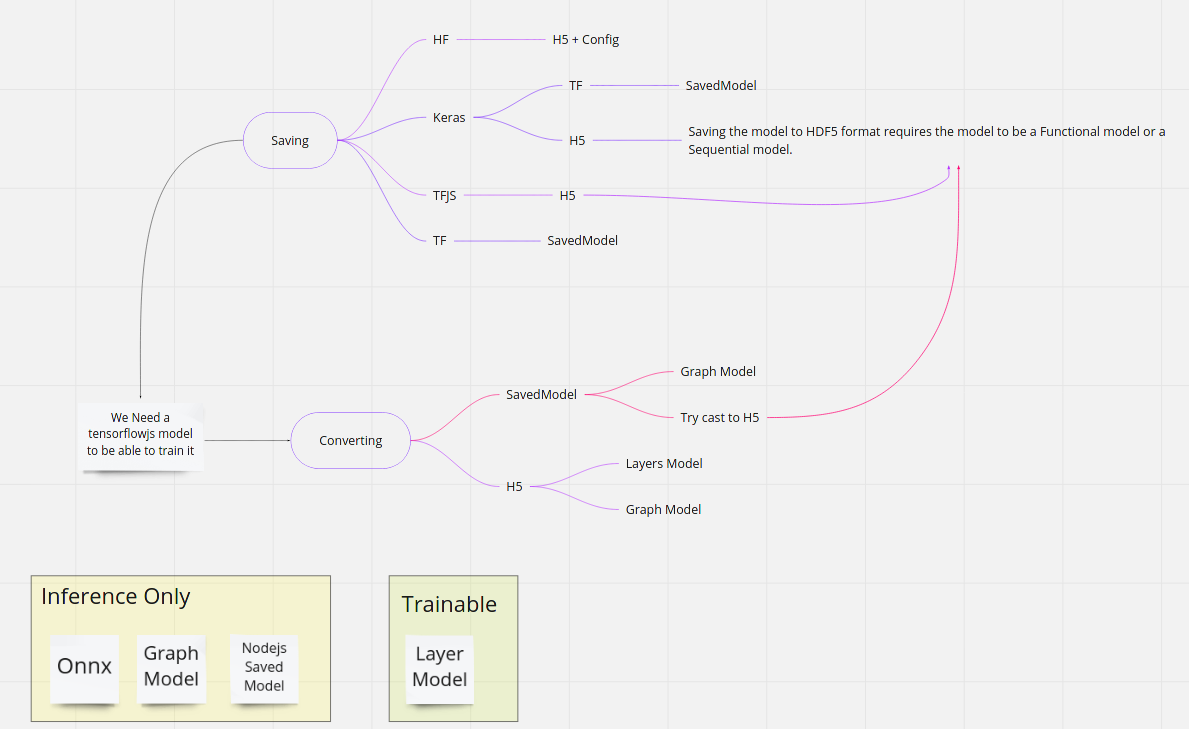
## Also tried :
Use the nodejs `tf.node.loadSavedModel` which return only a saved model which I cannot use as the base structure with something like :
```
const bert = await tf.loadLayersModel(`file://${this._bert_model_path}/model.json`)
this._clf = tf.sequential();
this._clf.add(bert); // Raise Error
this._clf.add(tf.layers.dense({ units: 768, useBias: true }));
etc...
this._clf.compile(....)
this._clf.train(...)
```
Look for other libraries to train models (libtorch : incomplete, onnx training: only in python etc..)
Should I also write an issue on tensorflowjs ?
Thanks in advance for you time and have a great day. | 03-04-2021 15:13:07 | 03-04-2021 15:13:07 | Hello!
Thanks for reporting this issue! Did you try to convert your H5 file to be able to use it with `tensorflowjs_converter --input_format keras path/to/my_model.h5 path/to/tfjs_target_dir`? You can also have a SavedModel version with:
1. `model = TFAutoModel.from_pretrained("distilbert-base-uncased")`
2. `model.save_pretrained(path, saved_model=True)`
H5 and SavedModel conversion process are nicely explained in https://www.tensorflow.org/js/tutorials/conversion/import_keras and https://www.tensorflow.org/js/tutorials/conversion/import_saved_model<|||||>Hi @jplu thanks for the fast reply !
Yes I used the `tensorflowjs_converter` to convert the H5 huggingFace model to a TFJS layerModel (.json).
If not using a h5 but a savedModel I don't know how to then use it in tensorflowJS.... all my researches seems to indicate that a savedModel can only do inference but i would like to finetune it.
My goal is just to finetune Bert in nodejs (could be with other framework than tfjs if you know some (like pytorch node bindings or some fancy framework but I found nothing that allow training))
## Attempts :
### HuggingFace :
1. Load model with huggingFace : `model = TFAutoModel.from_pretrained(model_name)`
2. Save it (H5 format) : `model.save_pretrained(path_saved)`
3. convert it to tfjs.json Layer format :
```python
dispatch_keras_h5_to_tfjs_layers_model_conversion(
h5_path='path_saved/tf_model.h5',
output_dir=my_tfjs_output_dir
)
```
(`dispatch_keras_h5_to_tfjs_layers_model_conversion` is the function called when using `tensorflowjs_convert`, i'm calling it from python code)
4. try to load it in nodejs : `const bert = await tf.loadLayersModel('file://${my_tfjs_output_dir}/model.json')`
5. Get the error `UnhandledPromiseRejectionWarning: TypeError: Cannot read property 'model_config' of null`
### Keras or tfjs saving (to have the `model_config`)
1. Load model with huggingFace : `model = TFAutoModel.from_pretrained(model_name)`
2. Save it (H5 format) :
```python
tf.keras.models.save_model(
model=model,
filepath=path_saved,
signatures=tf.function(model.call).get_concrete_function([
tf.TensorSpec([1, 512], tf.int32, name="input_ids"),
tf.TensorSpec([1, 512], tf.int32, name="attention_mask")
]),
save_format='h5'
)
```
or
`tfjs.converters.save_keras_model( model, path_saved)`
3. Get the error `Saving the model to HDF5 format requires the model to be a Functional model or a Sequential model.`
### Other formats :
1. Load model with huggingFace : `model = TFAutoModel.from_pretrained(model_name)`
2. Save it (SavedModel format) : `model.save_pretrained(path_saved)`
4. try to load it in nodejs : `const bert = await tf.node.loadSavedModel(path_saved, ['serve'], "serving_default")`
5. Cannot train it or use it in another model with `this._clf = tf.sequential(); this._clf.add(bert);`
#### GraphModel or onnx model are for inference only
Sorry for this (too) long reply.
Thanks for your help (I guess many people try to attempt the same),
Have a great day <|||||>Indeed you cannot train or fine tune a SavedModel in other envs than Python.
Currently to load a model you are forced to have the config file because it is required as an argument to init a model. Then the H5 as well. Furthermore, the TF implementations are using subclass models which are not compliant with most of the internal TF process (such as what you are trying to do in your described process when saving a model).
Then, I suggest you to fine tune your model in Python, create a SavedModel, and then use it in JS as described in the link I shared. Sorry for the inconvenience.<|||||>Okay so SOA is training custom models in tfjs but finetunning is almost impossible. It's what I thought but I'm glad you confirmed it.
Do you know any other solutions that would allow me to finetune a model in a webstack (nodejs / typescript) ?
I'm still hoping that one day pytorch will have node bindings...
Thanks again for your time.
I guess we can close the issue.<|||||>> Do you know any other solutions that would allow me to finetune a model in a webstack (nodejs / typescript) ?
No, sorry this is not really my domain. And sorry again for the inconvenience on this. |
transformers | 10,517 | closed | Not always consider a local model a checkpoint in run_glue | # What does this PR do?
In the `run_glue` script, a local model is automatically considered a checkpoint (which is there to enable users to do --model_path_or_name path_to_specific_checkpoint`) but when using a local model, it can crash if the number of labels is different (cf #10502). This PR fixes that by checking the number of labels inside the potential checkpoint before passing it to the `Trainer`. Another possible fix is to check for the presence of files like `trainer_state.json` (but this only works if the checkpoint was created with a recent version of Transformers).
Fixes #10502
| 03-04-2021 14:49:36 | 03-04-2021 14:49:36 |
Subsets and Splits
No community queries yet
The top public SQL queries from the community will appear here once available.