repo
stringclasses 1
value | number
int64 1
25.3k
| state
stringclasses 2
values | title
stringlengths 1
487
| body
stringlengths 0
234k
⌀ | created_at
stringlengths 19
19
| closed_at
stringlengths 19
19
| comments
stringlengths 0
293k
|
---|---|---|---|---|---|---|---|
transformers | 8,302 | closed | Fix path to old run_language_modeling.py script | # What does this PR do?
<!--
Congratulations! You've made it this far! You're not quite done yet though.
Once merged, your PR is going to appear in the release notes with the title you set, so make sure it's a great title that fully reflects the extent of your awesome contribution.
Then, please replace this with a description of the change and which issue is fixed (if applicable). Please also include relevant motivation and context. List any dependencies (if any) that are required for this change.
Once you're done, someone will review your PR shortly (see the section "Who can review?" below to tag some potential reviewers). They may suggest changes to make the code even better. If no one reviewed your PR after a week has passed, don't hesitate to post a new comment @-mentioning the same persons---sometimes notifications get lost.
-->
<!-- Remove if not applicable -->
Fixes # (issue)
## Before submitting
- [ ] This PR fixes a typo or improves the docs (you can dismiss the other checks if that's the case).
- [ ] Did you read the [contributor guideline](https://github.com/huggingface/transformers/blob/master/CONTRIBUTING.md#start-contributing-pull-requests),
Pull Request section?
- [ ] Was this discussed/approved via a Github issue or the [forum](https://discuss.huggingface.co/)? Please add a link
to the it if that's the case.
- [ ] Did you make sure to update the documentation with your changes? Here are the
[documentation guidelines](https://github.com/huggingface/transformers/tree/master/docs), and
[here are tips on formatting docstrings](https://github.com/huggingface/transformers/tree/master/docs#writing-source-documentation).
- [ ] Did you write any new necessary tests?
## Who can review?
Anyone in the community is free to review the PR once the tests have passed. Feel free to tag
members/contributors which may be interested in your PR.
<!-- Your PR will be replied to more quickly if you can figure out the right person to tag with @
If you know how to use git blame, that is the easiest way, otherwise, here is a rough guide of **who to tag**.
Please tag fewer than 3 people.
albert, bert, XLM: @LysandreJik
GPT2: @LysandreJik, @patrickvonplaten
tokenizers: @mfuntowicz
Trainer: @sgugger
Benchmarks: @patrickvonplaten
Model Cards: @julien-c
Translation: @sshleifer
Summarization: @sshleifer
examples/distillation: @VictorSanh
nlp datasets: [different repo](https://github.com/huggingface/nlp)
rust tokenizers: [different repo](https://github.com/huggingface/tokenizers)
Text Generation: @patrickvonplaten, @TevenLeScao
Blenderbot, Bart, Marian, Pegasus: @sshleifer
T5: @patrickvonplaten
Rag: @patrickvonplaten, @lhoestq
EncoderDecoder: @patrickvonplaten
Longformer, Reformer: @patrickvonplaten
TransfoXL, XLNet: @TevenLeScao, @patrickvonplaten
examples/seq2seq: @sshleifer
examples/bert-loses-patience: @JetRunner
tensorflow: @jplu
examples/token-classification: @stefan-it
documentation: @sgugger
-->
| 11-04-2020 17:18:06 | 11-04-2020 17:18:06 | |
transformers | 8,301 | closed | Speedup doc build | # What does this PR do?
This PR speeds the doc build by pinning the version of sphinx to 3.2.1 | 11-04-2020 16:40:07 | 11-04-2020 16:40:07 | |
transformers | 8,300 | closed | adding model cards for distilled models | # Model cards for distilled models
As discussed on Slack, a bunch of model cards for distilled models (at least the ones I contributed to).
cc @julien-c
I never know which tags are auto-generated, so please correct me if I did something useless! | 11-04-2020 16:23:09 | 11-04-2020 16:23:09 | > I never know which tags are auto-generated, so please correct me if I did something useless!
looks good to me in terms of the tags 👍 |
transformers | 8,299 | closed | Model card: T5-base fine-tuned on QASC | # What does this PR do?
<!--
Congratulations! You've made it this far! You're not quite done yet though.
Once merged, your PR is going to appear in the release notes with the title you set, so make sure it's a great title that fully reflects the extent of your awesome contribution.
Then, please replace this with a description of the change and which issue is fixed (if applicable). Please also include relevant motivation and context. List any dependencies (if any) that are required for this change.
Once you're done, someone will review your PR shortly (see the section "Who can review?" below to tag some potential reviewers). They may suggest changes to make the code even better. If no one reviewed your PR after a week has passed, don't hesitate to post a new comment @-mentioning the same persons---sometimes notifications get lost.
-->
<!-- Remove if not applicable -->
Fixes # (issue)
## Before submitting
- [ ] This PR fixes a typo or improves the docs (you can dismiss the other checks if that's the case).
- [ ] Did you read the [contributor guideline](https://github.com/huggingface/transformers/blob/master/CONTRIBUTING.md#start-contributing-pull-requests),
Pull Request section?
- [ ] Was this discussed/approved via a Github issue or the [forum](https://discuss.huggingface.co/)? Please add a link
to the it if that's the case.
- [ ] Did you make sure to update the documentation with your changes? Here are the
[documentation guidelines](https://github.com/huggingface/transformers/tree/master/docs), and
[here are tips on formatting docstrings](https://github.com/huggingface/transformers/tree/master/docs#writing-source-documentation).
- [ ] Did you write any new necessary tests?
## Who can review?
Anyone in the community is free to review the PR once the tests have passed. Feel free to tag
members/contributors which may be interested in your PR.
<!-- Your PR will be replied to more quickly if you can figure out the right person to tag with @
If you know how to use git blame, that is the easiest way, otherwise, here is a rough guide of **who to tag**.
Please tag fewer than 3 people.
albert, bert, XLM: @LysandreJik
GPT2: @LysandreJik, @patrickvonplaten
tokenizers: @mfuntowicz
Trainer: @sgugger
Benchmarks: @patrickvonplaten
Model Cards: @julien-c
Translation: @sshleifer
Summarization: @sshleifer
examples/distillation: @VictorSanh
nlp datasets: [different repo](https://github.com/huggingface/nlp)
rust tokenizers: [different repo](https://github.com/huggingface/tokenizers)
Text Generation: @patrickvonplaten, @TevenLeScao
Blenderbot, Bart, Marian, Pegasus: @sshleifer
T5: @patrickvonplaten
Rag: @patrickvonplaten, @lhoestq
EncoderDecoder: @patrickvonplaten
Longformer, Reformer: @patrickvonplaten
TransfoXL, XLNet: @TevenLeScao, @patrickvonplaten
examples/seq2seq: @sshleifer
examples/bert-loses-patience: @JetRunner
tensorflow: @jplu
examples/token-classification: @stefan-it
documentation: @sgugger
-->
| 11-04-2020 16:03:58 | 11-04-2020 16:03:58 | Pretty cool!<|||||>Thank you so much, @julien-c :) More models are coming ;) |
transformers | 8,298 | closed | Fix validation file loading in scripts | # What does this PR do?
As pointed out in #8295, the validation file was not properly loaded in all the examples scripts (one typo copy-pasted several times). This PR fixes that.
<!-- Remove if not applicable -->
Fixes #8295
| 11-04-2020 15:34:39 | 11-04-2020 15:34:39 | |
transformers | 8,297 | closed | [s2s] 1 GPU test for run_distributed_eval | Add test coverage for run_distributed_eval.py that can run on 1 GPU.
The command:
```bash
python -m torch.distributed.launch --nproc_per_node=1 run_distributed_eval.py --model_name Helsinki-NLP/opus-mt-en-ro --save_dir opus_wmt_en_ro_gens --data_dir wmt_en_ro
```
works on 1 GPU.
After adding test coverage, we could try to improve API consistency between run_distributed_eval.py and run_eval.py . | 11-04-2020 15:17:15 | 11-04-2020 15:17:15 | wdyt @stas00 <|||||>I will work on that, thank you.<|||||>A minor correction to the command (corrected `data_dir`):
```
python -m torch.distributed.launch --nproc_per_node=2 run_distributed_eval.py --model_name Helsinki-NLP/opus-mt-en-ro --save_dir test_data/opus_wmt_en_ro_gens --data_dir test_data/wmt_en_ro
```
Question: why only 1 gpu? we currently don't have it tested at all.
<|||||>I thought 1 GPU test coverage would be runnable in current CI/by more users.
But if much easier to test 2 gpu/easy to add test for 2 GPU that is great!<|||||>Bottom line - run with as many GPUs as available.
Thank you for clarifying.
|
transformers | 8,296 | closed | Update README.md | fix website address
# What does this PR do?
<!--
Congratulations! You've made it this far! You're not quite done yet though.
Once merged, your PR is going to appear in the release notes with the title you set, so make sure it's a great title that fully reflects the extent of your awesome contribution.
Then, please replace this with a description of the change and which issue is fixed (if applicable). Please also include relevant motivation and context. List any dependencies (if any) that are required for this change.
Once you're done, someone will review your PR shortly (see the section "Who can review?" below to tag some potential reviewers). They may suggest changes to make the code even better. If no one reviewed your PR after a week has passed, don't hesitate to post a new comment @-mentioning the same persons---sometimes notifications get lost.
-->
<!-- Remove if not applicable -->
Fixes # (issue)
## Before submitting
- [ ] This PR fixes a typo or improves the docs (you can dismiss the other checks if that's the case).
- [ ] Did you read the [contributor guideline](https://github.com/huggingface/transformers/blob/master/CONTRIBUTING.md#start-contributing-pull-requests),
Pull Request section?
- [ ] Was this discussed/approved via a Github issue or the [forum](https://discuss.huggingface.co/)? Please add a link
to the it if that's the case.
- [ ] Did you make sure to update the documentation with your changes? Here are the
[documentation guidelines](https://github.com/huggingface/transformers/tree/master/docs), and
[here are tips on formatting docstrings](https://github.com/huggingface/transformers/tree/master/docs#writing-source-documentation).
- [ ] Did you write any new necessary tests?
## Who can review?
Anyone in the community is free to review the PR once the tests have passed. Feel free to tag
members/contributors which may be interested in your PR.
<!-- Your PR will be replied to more quickly if you can figure out the right person to tag with @
If you know how to use git blame, that is the easiest way, otherwise, here is a rough guide of **who to tag**.
Please tag fewer than 3 people.
albert, bert, XLM: @LysandreJik
GPT2: @LysandreJik, @patrickvonplaten
tokenizers: @mfuntowicz
Trainer: @sgugger
Benchmarks: @patrickvonplaten
Model Cards: @julien-c
Translation: @sshleifer
Summarization: @sshleifer
examples/distillation: @VictorSanh
nlp datasets: [different repo](https://github.com/huggingface/nlp)
rust tokenizers: [different repo](https://github.com/huggingface/tokenizers)
Text Generation: @patrickvonplaten, @TevenLeScao
Blenderbot, Bart, Marian, Pegasus: @sshleifer
T5: @patrickvonplaten
Rag: @patrickvonplaten, @lhoestq
EncoderDecoder: @patrickvonplaten
Longformer, Reformer: @patrickvonplaten
TransfoXL, XLNet: @TevenLeScao, @patrickvonplaten
examples/seq2seq: @sshleifer
examples/bert-loses-patience: @JetRunner
tensorflow: @jplu
examples/token-classification: @stefan-it
documentation: @sgugger
-->
| 11-04-2020 15:08:07 | 11-04-2020 15:08:07 | I have a newer pull req<|||||>I have a newer pull req |
transformers | 8,295 | closed | Validation data in `run_mlm.py` is the same as train data | Inspecting the script I found the following:
https://github.com/huggingface/transformers/blob/cb966e640b8b9d0f6e9c06c1655d078a917e5196/examples/language-modeling/run_mlm.py#L204
Am I missing something? Otherwise, I could send a PR. | 11-04-2020 14:56:27 | 11-04-2020 14:56:27 | Very good catch! Thanks for pointing it out, the PR mentioned above should fix this. |
transformers | 8,294 | closed | pipelines: Tentative fix for AutoModel for PegasusConfig. | # What does this PR do?
Original error lies in `pipeline(task='summarization',
model='google/pegasus-xsum')`.
- Code fails while trying to infer framework from model_name (str).
- It attempts to determine framework by running `AutoModel.from_pretrained(..)` then `TFAutoModel.from_pretrained(...)` and decides by seeing whichever works first.
Proposed fix by:
- implementing `AutoModel.from_pretrained('google/pegasus-xsum')` that is a
`PegasusConfig`. and returning a `PegasusForConditionalGeneration`.
Not sure if that's desirable as we are loading a
`ForConditionalGeneration` model by default (but it's the only available
anyway).
Other options that are available:
- load `BartModel` (Pegasus inherits from BartForConditionalGeneration)
from `PegasusConfig`, but unsure about side effects and odd to load
`Bart` from `Pegasus`.
- Change `get_framework` function from pipeline. That was my initial
choice but it seems understanding if a config is for a TF or Pytorch
model would require replicating some of `AutoModel` logic anyway
so doing that would lead to a discrepancy between the 2 code paths just
for Pegasus (and maybe BartConfig which also suffers some issues, but
that will be in a follow-up PR).
<!--
Congratulations! You've made it this far! You're not quite done yet though.
Once merged, your PR is going to appear in the release notes with the title you set, so make sure it's a great title that fully reflects the extent of your awesome contribution.
Then, please replace this with a description of the change and which issue is fixed (if applicable). Please also include relevant motivation and context. List any dependencies (if any) that are required for this change.
Once you're done, someone will review your PR shortly (see the section "Who can review?" below to tag some potential reviewers). They may suggest changes to make the code even better. If no one reviewed your PR after a week has passed, don't hesitate to post a new comment @-mentioning the same persons---sometimes notifications get lost.
-->
<!-- Remove if not applicable -->
## Before submitting
- [ ] This PR fixes a typo or improves the docs (you can dismiss the other checks if that's the case).
- [x] Did you read the [contributor guideline](https://github.com/huggingface/transformers/blob/master/CONTRIBUTING.md#start-contributing-pull-requests),
Pull Request section?
- [ ] Was this discussed/approved via a Github issue or the [forum](https://discuss.huggingface.co/)? Please add a link
to the it if that's the case.
- [ ] Did you make sure to update the documentation with your changes? Here are the
[documentation guidelines](https://github.com/huggingface/transformers/tree/master/docs), and
[here are tips on formatting docstrings](https://github.com/huggingface/transformers/tree/master/docs#writing-source-documentation).
- [ ] Did you write any new necessary tests?
## Who can review?
@LysandreJik
@sshleifer
Anyone in the community is free to review the PR once the tests have passed. Feel free to tag
members/contributors which may be interested in your PR.
<!-- Your PR will be replied to more quickly if you can figure out the right person to tag with @
If you know how to use git blame, that is the easiest way, otherwise, here is a rough guide of **who to tag**.
Please tag fewer than 3 people.
albert, bert, XLM: @LysandreJik
GPT2: @LysandreJik, @patrickvonplaten
tokenizers: @mfuntowicz
Trainer: @sgugger
Benchmarks: @patrickvonplaten
Model Cards: @julien-c
Translation: @sshleifer
Summarization: @sshleifer
examples/distillation: @VictorSanh
nlp datasets: [different repo](https://github.com/huggingface/nlp)
rust tokenizers: [different repo](https://github.com/huggingface/tokenizers)
Text Generation: @patrickvonplaten, @TevenLeScao
Blenderbot, Bart, Marian, Pegasus: @sshleifer
T5: @patrickvonplaten
Rag: @patrickvonplaten, @lhoestq
EncoderDecoder: @patrickvonplaten
Longformer, Reformer: @patrickvonplaten
TransfoXL, XLNet: @TevenLeScao, @patrickvonplaten
examples/seq2seq: @sshleifer
examples/bert-loses-patience: @JetRunner
tensorflow: @jplu
examples/token-classification: @stefan-it
documentation: @sgugger
--> | 11-04-2020 14:36:24 | 11-04-2020 14:36:24 | After thinking about it a bit, I don't think the `PegasusForConditionalGeneration` should be going there. The `MODEL_MAPPING` is a mapping to all the headless models, i.e., the models that output hidden states without processing them in a head.
Introducing `PegasusForConditionalGeneration` would result in a mismatch between every single other models defined in that mapping and this newly added model.
Adding `BartModel` would fail because of the configuration, as you've said, so imo the best thing to do here is to create a `PegasusModel` that inherits from `BartModel`, and use this in the `AutoModel`.<|||||>Why is summarization pipeline using AutoModel? Shouldn't it require a model with a head?<|||||>The pipeline is using `AutoModel` to load the weights to see if they load. It follows the current installed platform (PT or TF), but if both are installed, it first tries to load the checkpoint in `AutoModel`, and if it fails (wrongly formatted weights), it tries to load it in `TFAutoModel`.
This does mean that the model is loaded twice (once in `AutoModel` and another time in the appropriate auto model), which may not be the best performance-wise.
The easy fix here is to add a base model for Pegasus (and all models should have base models imo), the somewhat more robust fix is to load the checkpoint directly in the appropriate auto model.<|||||>> This does mean that the model is loaded twice (once in AutoModel and another time in the appropriate auto model), which may not be the best performance-wise.
Yes this is not ideal. If there was simpler way do determine appropriate framework from config that would be much better. Or attempt the AutoModel way but without going the full way (stopping at checking filenames).
> The easy fix here is to add a base model for Pegasus (and all models should have base models imo), the somewhat more robust fix is to load the checkpoint directly in the appropriate auto model.
That seems probably like the best solution. (at least in the short term)<|||||>> and all models should have base models imo
MarianMT, Pegasus, Blenderbot are all only published/trained/used for one task, why should they have base models?
What ever happened to `config.architectures`? Would that help?<|||||>Some configs (old ones maybe) don't have `architectures` defined.<|||||>> The pipeline is using `AutoModel` to load the weights to see if they load. It follows the current installed platform (PT or TF), but if both are installed, it first tries to load the checkpoint in `AutoModel`, and if it fails (wrongly formatted weights), it tries to load it in `TFAutoModel`.
Just a note that I'm not 100% sure that our design goal with Pipelines is to be able to load a model automatically in PT/TF without any user input (e.g. in case the model is only TF)
Besides, in most cases you would have access to the huggingface.co model list API so you would know if model has PT/TF files.<|||||>> Some configs (old ones maybe) don't have `architectures` defined.
Just a note that we can always backport architectures into the hosted config files (will be easier with the new model versioning system)<|||||>Obsolete. |
transformers | 8,293 | closed | [Generate Test] fix greedy generate test | # What does this PR do?
<!--
Congratulations! You've made it this far! You're not quite done yet though.
Once merged, your PR is going to appear in the release notes with the title you set, so make sure it's a great title that fully reflects the extent of your awesome contribution.
Then, please replace this with a description of the change and which issue is fixed (if applicable). Please also include relevant motivation and context. List any dependencies (if any) that are required for this change.
Once you're done, someone will review your PR shortly (see the section "Who can review?" below to tag some potential reviewers). They may suggest changes to make the code even better. If no one reviewed your PR after a week has passed, don't hesitate to post a new comment @-mentioning the same persons---sometimes notifications get lost.
-->
<!-- Remove if not applicable -->
`greedy_search` test was flaky. This PR should fix it.
## Before submitting
- [ ] This PR fixes a typo or improves the docs (you can dismiss the other checks if that's the case).
- [ ] Did you read the [contributor guideline](https://github.com/huggingface/transformers/blob/master/CONTRIBUTING.md#start-contributing-pull-requests),
Pull Request section?
- [ ] Was this discussed/approved via a Github issue or the [forum](https://discuss.huggingface.co/)? Please add a link
to the it if that's the case.
- [ ] Did you make sure to update the documentation with your changes? Here are the
[documentation guidelines](https://github.com/huggingface/transformers/tree/master/docs), and
[here are tips on formatting docstrings](https://github.com/huggingface/transformers/tree/master/docs#writing-source-documentation).
- [ ] Did you write any new necessary tests?
## Who can review?
Anyone in the community is free to review the PR once the tests have passed. Feel free to tag
members/contributors which may be interested in your PR.
<!-- Your PR will be replied to more quickly if you can figure out the right person to tag with @
If you know how to use git blame, that is the easiest way, otherwise, here is a rough guide of **who to tag**.
Please tag fewer than 3 people.
albert, bert, XLM: @LysandreJik
GPT2: @LysandreJik, @patrickvonplaten
tokenizers: @mfuntowicz
Trainer: @sgugger
Benchmarks: @patrickvonplaten
Model Cards: @julien-c
Translation: @sshleifer
Summarization: @sshleifer
examples/distillation: @VictorSanh
nlp datasets: [different repo](https://github.com/huggingface/nlp)
rust tokenizers: [different repo](https://github.com/huggingface/tokenizers)
Text Generation: @patrickvonplaten, @TevenLeScao
Blenderbot, Bart, Marian, Pegasus: @sshleifer
T5: @patrickvonplaten
Rag: @patrickvonplaten, @lhoestq
EncoderDecoder: @patrickvonplaten
Longformer, Reformer: @patrickvonplaten
TransfoXL, XLNet: @TevenLeScao, @patrickvonplaten
examples/seq2seq: @sshleifer
examples/bert-loses-patience: @JetRunner
tensorflow: @jplu
examples/token-classification: @stefan-it
documentation: @sgugger
-->
| 11-04-2020 14:29:35 | 11-04-2020 14:29:35 | Ping @LysandreJik |
transformers | 8,292 | closed | Fine Tune Bert Ner using TFBertForTokenClassification.from_pretrained | Hey,
I am new to the transformers Bert Training world and am trying to fine tune Bert model for NER on a coNll like dataset. But its not training the model and giving the below error
ValueError: No gradients provided for any variable: ['tf_bert_for_token_classification_8/classifier/kernel:0', 'tf_bert_for_token_classification_8/classifier/bias:0'].
Below is my code
```py
tr_inputs = tf.convert_to_tensor(tr_inputs)
val_inputs = tf.convert_to_tensor(val_inputs)
tr_tags = tf.convert_to_tensor(tr_tags)
val_tags = tf.convert_to_tensor(val_tags)
tr_masks = tf.convert_to_tensor(tr_masks)
val_masks = tf.convert_to_tensor(val_masks)
tr_segs = tf.convert_to_tensor(tr_segs)
val_segs = tf.convert_to_tensor(val_segs)
input_features_dict = {"input_ids":tr_inputs, "attention_mask":tr_masks, "token_type_ids":tr_segs, 'labels':tr_tags}
val_features_dict = {"input_ids":val_inputs, "attention_mask":val_masks, "token_type_ids":val_segs, 'labels':tr_tags}
train_data = tf.data.Dataset.from_tensor_slices(input_features_dict)
batch_train_data = train_data.batch(batch_num)
valid_data = tf.data.Dataset.from_tensor_slices(val_features_dict)
batch_valid_data = valid_data.batch(batch_num)
modell = TFBertForTokenClassification.from_pretrained('bert-base-uncased',num_labels=len(tag2idx))
modell.layers[2].activation = tf.keras.activations.softmax
modell.layers[0].trainable = False
modell.compile(optimizer=optimizer, loss=loss, metrics=[metrics])
modell.fit(batch_train_data, epochs=epochs, validation_data=batch_val_data)
```
Not sure what needs to be done. Any advice/pointers on this would be highly helpful for me. | 11-04-2020 14:01:07 | 11-04-2020 14:01:07 | What is your `metrics`?<|||||>```py
optimizer= AdamWeightDecay(
learning_rate=5e-5,
beta_1=0.9,
beta_2=0.999,
weight_decay_rate=0.01,
epsilon=1e-6,
exclude_from_weight_decay=['layer_norm', 'bias'])
optimizer._HAS_AGGREGATE_GRAD = False
loss=tf.keras.losses.SparseCategoricalCrossentropy(from_logits=True)
metrics=[tf.keras.metrics.SparseCategoricalAccuracy(name="acc")]
```<|||||>@jplu might know what's going on<|||||>Hello @aks2193!
Sorry for this, but for now you cannot use `.compile()` + `.fit()` to train a Token Classification model. To make it short, this is because a layer is not used and then the gradients will be None, something that `.fit()` cannot handle.
If you want to train a NER I suggest you to use the [example](https://github.com/huggingface/transformers/blob/master/examples/token-classification/run_tf_ner.py).<|||||>Hey @jplu
Thanks for the reply. I tried to follow the link and below is how I changed my code
modell = TFBertForTokenClassification.from_pretrained('bert-base-uncased',num_labels=len(tag2idx))
modell.layers[2].activation = tf.keras.activations.softmax
modell.layers[0].trainable = False
modell.compile(optimizer=optimizer, loss=loss, metrics=[metrics])
modell.fit(batch_train_data, epochs=epochs, validation_data=batch_val_data)
def compute_metrics(pred):
labels = pred.label_ids
preds = pred.predictions.argmax(-1)
precision, recall, f1, _ = precision_recall_fscore_support(labels, preds, average='binary')
acc = accuracy_score(labels, preds)
return {
'accuracy': acc,
'f1': f1,
'precision': precision,
'recall': recall
}
training_args = TFTrainingArguments(
output_dir='./bert_test', # output directory
num_train_epochs=5, # total # of training epochs
per_device_train_batch_size=32, # batch size per device during training
per_device_eval_batch_size=32, # batch size for evaluation
warmup_steps=500, # number of warmup steps for learning rate scheduler
weight_decay=0.01, # strength of weight decay
logging_dir='./logs', # directory for storing logs
learning_rate=3e-5,
)
trainer = TFTrainer(
model = modell,
args=training_args,
train_dataset=train_dataset,
eval_dataset=val_dataset,
compute_metrics=compute_metrics
)
trainer.train()
But I am still getting the below error
ValueError: Trying to create optimizer slot variable under the scope for tf.distribute.Strategy (<tensorflow.python.distribute.one_device_strategy.OneDeviceStrategy object at 0x7fb46c2dec50>), which is different from the scope used for the original variable (<tf.Variable 'tf_bert_for_token_classification_14/classifier/kernel:0' shape=(768, 10) dtype=float32
Make sure the slot variables are created under the same strategy scope. This may happen if you're restoring from a checkpoint outside the scope
Then I tried defining a strategy scope and include all the above code inside that
strategy = tf.distribute.OneDeviceStrategy(device="/cpu:0")
with strategy.scope():
The ABOVE CODE
On doing this getting the below error
Mixing different tf.distribute.Strategy objects: <tensorflow.python.distribute.one_device_strategy.OneDeviceStrategy object at 0x7fb446d98eb8> is not <tensorflow.python.distribute.one_device_strategy.OneDeviceStrategy object at 0x7fb45fe2d7b8>
How does this exactly work?
How do I define the strategy scope for all this calculations?<|||||>Hey @aks2193
I'm facing the same problem. Please let me know if you find a solution.<|||||>@aks2193
Try replacing `strategy = tf.distribute.OneDeviceStrategy(device="/cpu:0")` to `training_args.strategy.scope()`. Worked for me.<|||||>@alibi123 has right, you are not properly instanciate your model. Please use the example as it is. You example won't work as well with the `TFTrainer` if you are setting the activation to `softmax` because we don't compute the loss from the logits.<|||||>@jplu I have a question about restoring weights from the checkpoint. How to do it correctly?
This is how I try to load weights:
```
>>> model = TFBertForTokenClassification.from_pretrained(settings.BERT_NAME)
>>> model.load_weights('/models/exp1/checkpoint/ckpt-55')
```
I get very long exception message starting with:
```
Nothing except the root object matched a checkpointed value. Typically this means that the checkpoint does not match the Python program. The following objects have no matching checkpointed value: [<tf.Variable 't
```
Here's my training code:
```
dataset = get_dataset(in_fn, debug)
args = TFTrainingArguments(
os.path.join(os.path.join(settings.MODELS_DIR, save_name)),
overwrite_output_dir=True,
do_train=True,
logging_dir=os.path.join(settings.DATA_DIR, 'exp1_logs'),
save_total_limit=2,
)
with args.strategy.scope():
model = TFBertForTokenClassification.from_pretrained(settings.BERT_NAME)
trainer = TFTrainer(
model=model,
args=args,
train_dataset=dataset,
)
trainer.train()
```
`BERT_NAME = 'bert-base-multilingual-cased'`
I was also trying to use ckpt path in `.from_pretrained()` but also got errors regarding format.
<|||||>You cannot use Keras `load_weights` on a TF checkpoint. If you want to load your model you just have to use the path where you saved your model `model = TFBertForTokenClassification.from_pretrained("my_output_dir")`<|||||>@jplu Thank you for the quick response.
I've tried that in the first place, but get an error as well.
This is my output dir:
```
checkpoint$ ls
checkpoint ckpt-55.data-00000-of-00001 ckpt-55.index ckpt-56.data-00000-of-00001 ckpt-56.index
```
Here are my attempts with error messages:
1:
```
config = BertConfig.from_pretrained(settings.BERT_NAME)
>>> model = TFBertForTokenClassification.from_pretrained('/models/exp1/checkpoint', config=config)
Traceback (most recent call last):
File "<stdin>", line 1, in <module>
File "/usr/local/lib/python3.6/dist-packages/transformers/modeling_tf_utils.py", line 653, in from_pretrained
[WEIGHTS_NAME, TF2_WEIGHTS_NAME], pretrained_model_name_or_path
OSError: Error no file named ['pytorch_model.bin', 'tf_model.h5'] found in directory /models/exp1/checkpoint or `from_pt` set to False
```
2:
```
>>> model = TFBertForTokenClassification.from_pretrained('/models/exp1/checkpoint/ckpt-55', config=config)
Traceback (most recent call last):
File "/usr/local/lib/python3.6/dist-packages/transformers/modeling_tf_utils.py", line 711, in from_pretrained
load_tf_weights(model, resolved_archive_file)
File "/usr/local/lib/python3.6/dist-packages/transformers/modeling_tf_utils.py", line 268, in load_tf_weights
with h5py.File(resolved_archive_file, "r") as f:
File "/usr/local/lib/python3.6/dist-packages/h5py/_hl/files.py", line 408, in __init__
swmr=swmr)
File "/usr/local/lib/python3.6/dist-packages/h5py/_hl/files.py", line 173, in make_fid
fid = h5f.open(name, flags, fapl=fapl)
File "h5py/_objects.pyx", line 54, in h5py._objects.with_phil.wrapper
File "h5py/_objects.pyx", line 55, in h5py._objects.with_phil.wrapper
File "h5py/h5f.pyx", line 88, in h5py.h5f.open
OSError: Unable to open file (file signature not found)
During handling of the above exception, another exception occurred:
Traceback (most recent call last):
File "<stdin>", line 1, in <module>
File "/usr/local/lib/python3.6/dist-packages/transformers/modeling_tf_utils.py", line 714, in from_pretrained
"Unable to load weights from h5 file. "
OSError: Unable to load weights from h5 file. If you tried to load a TF 2.0 model from a PyTorch checkpoint, please set from_pt=True.
```
3: I've even tried `from_pt=True` even though I used TFTrainer and TFBert
```
>>> model = TFBertForTokenClassification.from_pretrained('/models/exp1/checkpoint/ckpt-55', config=config, from_pt=True)
Traceback (most recent call last):
File "<stdin>", line 1, in <module>
File "/usr/local/lib/python3.6/dist-packages/transformers/modeling_tf_utils.py", line 703, in from_pretrained
return load_pytorch_checkpoint_in_tf2_model(model, resolved_archive_file, allow_missing_keys=True)
File "/usr/local/lib/python3.6/dist-packages/transformers/modeling_tf_pytorch_utils.py", line 89, in load_pytorch_checkpoint_in_tf2_model
pt_state_dict = torch.load(pt_path, map_location="cpu")
File "/usr/local/lib/python3.6/dist-packages/torch/serialization.py", line 595, in load
return _legacy_load(opened_file, map_location, pickle_module, **pickle_load_args)
File "/usr/local/lib/python3.6/dist-packages/torch/serialization.py", line 764, in _legacy_load
magic_number = pickle_module.load(f, **pickle_load_args)
_pickle.UnpicklingError: invalid load key, '\x00'.
```
4: I've also tried to add config.json into the output dir
```
>>> model = TFBertForTokenClassification.from_pretrained('/models/exp1/checkpoint')
Traceback (most recent call last):
File "<stdin>", line 1, in <module>
File "/usr/local/lib/python3.6/dist-packages/transformers/modeling_tf_utils.py", line 653, in from_pretrained
[WEIGHTS_NAME, TF2_WEIGHTS_NAME], pretrained_model_name_or_path
OSError: Error no file named ['pytorch_model.bin', 'tf_model.h5'] found in directory /models/exp1/checkpoint or `from_pt` set to False
```<|||||>As I said, these are normal errors because: **You cannot use Keras load_weights on a TF checkpoint.** You have to use your output dir not the file or the checkpoint dir: `model = TFBertForTokenClassification.from_pretrained('/models/exp1')`.<|||||>@jplu Sorry for bothering. But still doesn't work. It expects `'pytorch_model.bin', 'tf_model.h5'`.
```
>>> model = TFBertForTokenClassification.from_pretrained('/models/exp1')
Traceback (most recent call last):
File "<stdin>", line 1, in <module>
File "/usr/local/lib/python3.6/dist-packages/transformers/modeling_tf_utils.py", line 653, in from_pretrained
[WEIGHTS_NAME, TF2_WEIGHTS_NAME], pretrained_model_name_or_path
OSError: Error no file named ['pytorch_model.bin', 'tf_model.h5'] found in directory /models/exp1 or `from_pt` set to False
>>> model = TFBertForTokenClassification.from_pretrained('/models/exp1', from_pt=True)
Traceback (most recent call last):
File "<stdin>", line 1, in <module>
File "/usr/local/lib/python3.6/dist-packages/transformers/modeling_tf_utils.py", line 653, in from_pretrained
[WEIGHTS_NAME, TF2_WEIGHTS_NAME], pretrained_model_name_or_path
OSError: Error no file named ['pytorch_model.bin', 'tf_model.h5'] found in directory /models/exp1 or `from_pt` set to False
```
But my output_dir only contains `checkpoint` dir<|||||>This is because you are trying to load a PyTorch model into a TensorFlow one with `from_pt=True`, remove this parameter. If not working it means that your models have not been properly saved.
Did you call the `save` method of the trainer?<|||||>No, I haven't. Sorry, my bad. I thought that I can use checkpoints.
Thanks for your help!<|||||>This issue has been automatically marked as stale because it has not had recent activity. It will be closed if no further activity occurs. Thank you for your contributions.
|
transformers | 8,291 | closed | could you please give me a torch example of xlm-roberta-(base/large) for multilingual-text question? | # ❓ Questions & Help
<!-- The GitHub issue tracker is primarly intended for bugs, feature requests,
new models and benchmarks, and migration questions. For all other questions,
we direct you to the Hugging Face forum: https://discuss.huggingface.co/ .
You can also try Stack Overflow (SO) where a whole community of PyTorch and
Tensorflow enthusiast can help you out. In this case, make sure to tag your
question with the right deep learning framework as well as the
huggingface-transformers tag:
https://stackoverflow.com/questions/tagged/huggingface-transformers
-->
## Details
I read the document of [XLM-RoBERTa](https://huggingface.co/transformers/model_doc/xlmroberta.html#overview), but i also have a lots of question. For example, `should be able to determine the correct language from the input ids.` how to determine?
If you have a example for how to use xlm-roberta about multilingual text question, please show me, Thank you very much!
| 11-04-2020 13:38:09 | 11-04-2020 13:38:09 | |
transformers | 8,290 | closed | finetuning T5 on translation on TPU, questions about clarifying the setup | Hi,
I'd like to run finetune.py with wmt dataset in TPU to train from scratch not finetune. I appreciate the response to some questions:
1) Why there are two versions of fine-tuning using Seq2SeqTrainer and finetune.py and which one is suitable for my usecase?
2) Seq2SeqTrainer does not support predict on TPU, is this the case for finetune.py as well?
3) when running on TPU, it is written to use xla_spawn.py, since the codes of finetune.py are with pytorch lightening, is this needed to launch them with xmp.spawn(mod._mp_fn, args=(), nprocs=args.num_cores)?
4) in the finetune.py dataloader, I see this is distributed based on number of gpus, but I cannot see this is distributed also when one uses tpus, Is this taking care of making dataloader distributed in case of using TPU automatically?
5) If using finetune.py with TPU, is there any specific setup I need to add for finetuning/training T5 on WMT?
6) I assume one need to use maybe something like this as a sampler for TPU distributed dataloaders, I see this is not the case in the codes of finetune.py, does data parallelism works in finetune.py?
7) when to use sortish_sampler/dynamic_sampler, do they work on TPUs?
```
sampler = torch.utils.data.distributed.DistributedSampler(
dataset,
num_replicas=xm.xrt_world_size(),
rank=xm.get_ordinal(),
shuffle=True)
```
8. fp16 does it work with TPUs as well?
9. with iterable datasets seems dataloader in finetune_trainer is not working with distributed detup on TPU, do you know how to implement it? am I missing something? thanks
thank you very much. | 11-04-2020 13:11:56 | 11-04-2020 13:11:56 | in the main page of examples you mention one can pass any script to run it on tpu, but inside the seq2seq it seems one needs to use finetune_trainer and not finetune.py for tpus, I am confused which one to use, thanks for your help<|||||>@sshleifer @patil-suraj Maybe we could improve the documentation here<|||||>Hi, thank you @LysandreJik, do you know which version of finetune.py to finetune_trainer.py are working with tpus? in the documentation it is written any example can be run with multiple tpus by using xla_spawn.py but I am not sure if this is true for finetune.py too. thanks <|||||>`finetune_trainer.py` works with TPU, here is [the wmt script](https://github.com/huggingface/transformers/blob/master/examples/seq2seq/builtin_trainer/finetune_tpu.sh)
1) They were developed at different times. We are trying to get them both working well.
2) finetune.py should not be used with TPU at all.
3) yes, see script.
4) see script
5) see script
6) see script
7) I would guess that those samplers don't work on TPU.
8) No it does not.
9) No idea, maybe @patil-suraj knows.
<|||||>thanks Sam for this, so finetune.py does not work? In the documentation,
this is written one can run all examples with xla_spawn on TPU, I
appreciate updating the README mentioning it.
thank you.
On Wed, Nov 4, 2020 at 8:06 PM Sam Shleifer <[email protected]>
wrote:
> finetune_trainer.py works with TPU, cc @patil-suraj
> <https://github.com/patil-suraj> .
>
> —
> You are receiving this because you authored the thread.
> Reply to this email directly, view it on GitHub
> <https://github.com/huggingface/transformers/issues/8290#issuecomment-721917741>,
> or unsubscribe
> <https://github.com/notifications/unsubscribe-auth/ARPXHH345RVTXO3EA7ZUPUTSOGQ3XANCNFSM4TKBDLQQ>
> .
>
<|||||>Correct, updated docs.<|||||>thank you Sam
On Wed, Nov 4, 2020 at 9:46 PM Sam Shleifer <[email protected]>
wrote:
> Correct, updated docs.
>
> —
> You are receiving this because you authored the thread.
> Reply to this email directly, view it on GitHub
> <https://github.com/huggingface/transformers/issues/8290#issuecomment-721966350>,
> or unsubscribe
> <https://github.com/notifications/unsubscribe-auth/ARPXHH4QZWN6KEL2BKSF7BTSOG4TVANCNFSM4TKBDLQQ>
> .
>
|
transformers | 8,289 | closed | Why do I use XLMRobertaTokenizer and return an error on token_type_ids? | # ❓ Questions & Help
<!-- The GitHub issue tracker is primarly intended for bugs, feature requests,
new models and benchmarks, and migration questions. For all other questions,
we direct you to the Hugging Face forum: https://discuss.huggingface.co/ .
You can also try Stack Overflow (SO) where a whole community of PyTorch and
Tensorflow enthusiast can help you out. In this case, make sure to tag your
question with the right deep learning framework as well as the
huggingface-transformers tag:
https://stackoverflow.com/questions/tagged/huggingface-transformers
-->
## Details
```python
encoded_pair = self.tokenizer(sent_ko, sent_cn,
padding='max_length', # Pad to max_length
truncation=True, # Truncate to max_length
max_length=self.maxlen,
return_tensors='pt') # Return torch.Tensor objects
token_ids = encoded_pair['input_ids'].squeeze(0)
attn_masks = encoded_pair['attention_mask'].squeeze(0)
token_type_ids = encoded_pair['token_type_ids'].squeeze(0)
```
```
File "/usr/local/lib/python3.6/dist-packages/transformers/tokenization_utils_base.py", line 234, in __getitem__
return self.data[item]
KeyError: 'token_type_ids'
``` | 11-04-2020 12:23:43 | 11-04-2020 12:23:43 | I also have this question |
transformers | 8,288 | closed | Training T5-large model for Question Answering | Are there are any specific documents that I can follow, to do the training of the t5 model for Question answering?
I found this (https://huggingface.co/transformers/custom_datasets.html#qa-squad) on your website and it is not allowing me to use t5 model instead of DistilBert. | 11-04-2020 12:00:07 | 11-04-2020 12:00:07 | This notebook should help: https://github.com/patil-suraj/exploring-T5/blob/master/T5_on_TPU.ipynb<|||||>This issue has been automatically marked as stale because it has not had recent activity. It will be closed if no further activity occurs. Thank you for your contributions.
|
transformers | 8,287 | closed | Fix typo in language-modeling README.md | # What does this PR do?
Fix the typo in `README.md` in the `language-modeling` folder.
<!-- Remove if not applicable -->
Fixes # (issue)
## Before submitting
- [x] This PR fixes a typo or improves the docs (you can dismiss the other checks if that's the case).
- [ ] Did you read the [contributor guideline](https://github.com/huggingface/transformers/blob/master/CONTRIBUTING.md#start-contributing-pull-requests),
Pull Request section?
- [ ] Was this discussed/approved via a Github issue or the [forum](https://discuss.huggingface.co/)? Please add a link
to the it if that's the case.
- [ ] Did you make sure to update the documentation with your changes? Here are the
[documentation guidelines](https://github.com/huggingface/transformers/tree/master/docs), and
[here are tips on formatting docstrings](https://github.com/huggingface/transformers/tree/master/docs#writing-source-documentation).
- [ ] Did you write any new necessary tests?
## Who can review?
Anyone in the community is free to review the PR once the tests have passed. Feel free to tag
members/contributors which may be interested in your PR.
<!-- Your PR will be replied to more quickly if you can figure out the right person to tag with @
If you know how to use git blame, that is the easiest way, otherwise, here is a rough guide of **who to tag**.
Please tag fewer than 3 people.
albert, bert, XLM: @LysandreJik
GPT2: @LysandreJik, @patrickvonplaten
tokenizers: @mfuntowicz
Trainer: @sgugger
Benchmarks: @patrickvonplaten
Model Cards: @julien-c
Translation: @sshleifer
Summarization: @sshleifer
examples/distillation: @VictorSanh
nlp datasets: [different repo](https://github.com/huggingface/nlp)
rust tokenizers: [different repo](https://github.com/huggingface/tokenizers)
Text Generation: @patrickvonplaten, @TevenLeScao
Blenderbot, Bart, Marian, Pegasus: @sshleifer
T5: @patrickvonplaten
Rag: @patrickvonplaten, @lhoestq
EncoderDecoder: @patrickvonplaten
Longformer, Reformer: @patrickvonplaten
TransfoXL, XLNet: @TevenLeScao, @patrickvonplaten
examples/seq2seq: @sshleifer
examples/bert-loses-patience: @JetRunner
tensorflow: @jplu
examples/token-classification: @stefan-it
documentation: @sgugger
-->
| 11-04-2020 11:14:27 | 11-04-2020 11:14:27 | |
transformers | 8,286 | closed | Improve QA pipeline error handling | # What does this PR do?
- The issue is that with previous code we would have the following:
```python
qa_pipeline = (...)
qa_pipeline(question="Where was he born ?", context="")
-> IndexError: Dimension out of range (expected to be in range of [-1, 0], but got 1)
```
The goal here is to improve this to actually return a ValueError
wherever possible.
While at it, I tried to simplify QuestionArgumentHandler's code to
make it smaller and more compat while keeping backward compat.
Quick note: For the tests, I feel they would be more readable if it was possible to write
```python
self.assertEqual(qa(.....), [SquadExample(None, Q, C, None, None,...)])
```
as it would cover both types, and length and deep equality.
However, it's not possible because SquadExample does not implement `__eq__`. It felt out of scope, but
if reviewers think it would be a nice addition, I'd be happy to implement it and change the test.
<!--
Congratulations! You've made it this far! You're not quite done yet though.
Once merged, your PR is going to appear in the release notes with the title you set, so make sure it's a great title that fully reflects the extent of your awesome contribution.
Then, please replace this with a description of the change and which issue is fixed (if applicable). Please also include relevant motivation and context. List any dependencies (if any) that are required for this change.
Once you're done, someone will review your PR shortly (see the section "Who can review?" below to tag some potential reviewers). They may suggest changes to make the code even better. If no one reviewed your PR after a week has passed, don't hesitate to post a new comment @-mentioning the same persons---sometimes notifications get lost.
-->
<!-- Remove if not applicable -->
## Before submitting
- [ ] This PR fixes a typo or improves the docs (you can dismiss the other checks if that's the case).
- [x] Did you read the [contributor guideline](https://github.com/huggingface/transformers/blob/master/CONTRIBUTING.md#start-contributing-pull-requests),
Pull Request section?
- [ ] Was this discussed/approved via a Github issue or the [forum](https://discuss.huggingface.co/)? Please add a link
to the it if that's the case.
- [ ] Did you make sure to update the documentation with your changes? Here are the
[documentation guidelines](https://github.com/huggingface/transformers/tree/master/docs), and
[here are tips on formatting docstrings](https://github.com/huggingface/transformers/tree/master/docs#writing-source-documentation).
- [ ] Did you write any new necessary tests?
## Who can review?
Anyone in the community is free to review the PR once the tests have passed. Feel free to tag
members/contributors which may be interested in your PR.
@mfuntowicz
@LysandreJik
<!-- Your PR will be replied to more quickly if you can figure out the right person to tag with @
If you know how to use git blame, that is the easiest way, otherwise, here is a rough guide of **who to tag**.
Please tag fewer than 3 people.
albert, bert, XLM: @LysandreJik
GPT2: @LysandreJik, @patrickvonplaten
tokenizers: @mfuntowicz
Trainer: @sgugger
Benchmarks: @patrickvonplaten
Model Cards: @julien-c
Translation: @sshleifer
Summarization: @sshleifer
examples/distillation: @VictorSanh
nlp datasets: [different repo](https://github.com/huggingface/nlp)
rust tokenizers: [different repo](https://github.com/huggingface/tokenizers)
Text Generation: @patrickvonplaten, @TevenLeScao
Blenderbot, Bart, Marian, Pegasus: @sshleifer
T5: @patrickvonplaten
Rag: @patrickvonplaten, @lhoestq
EncoderDecoder: @patrickvonplaten
Longformer, Reformer: @patrickvonplaten
TransfoXL, XLNet: @TevenLeScao, @patrickvonplaten
examples/seq2seq: @sshleifer
examples/bert-loses-patience: @JetRunner
tensorflow: @jplu
examples/token-classification: @stefan-it
documentation: @sgugger
--> | 11-04-2020 10:41:23 | 11-04-2020 10:41:23 | |
transformers | 8,285 | closed | RAG performance on Open-NQ dataset much lower than expected | # ❓ Questions & Help
<!-- The GitHub issue tracker is primarly intended for bugs, feature requests,
new models and benchmarks, and migration questions. For all other questions,
we direct you to the Hugging Face forum: https://discuss.huggingface.co/ .
You can also try Stack Overflow (SO) where a whole community of PyTorch and
Tensorflow enthusiast can help you out. In this case, make sure to tag your
question with the right deep learning framework as well as the
huggingface-transformers tag:
https://stackoverflow.com/questions/tagged/huggingface-transformers
-->
## Details
<!-- Description of your issue -->
One peculiar finding is that when we ran the rag-sequence-nq model along with the provided wiki_dpr index, all models and index files were used as is, on the open-NQ test split (3610 questions, https://github.com/google-research-datasets/natural-questions/tree/master/nq_open), we observed EM=27.2 performance, which was rather different from that in the paper, namely EM=44.5.
We are baffled. Has anyone seen lower performance using the transformers RAG models?
<!-- You should first ask your question on the forum or SO, and only if
you didn't get an answer ask it here on GitHub. -->
**A link to original question on the forum/Stack Overflow**: | 11-04-2020 09:52:24 | 11-04-2020 09:52:24 | Maybe @lhoestq @patrickvonplaten have an idea<|||||>Hey @gaobo1987,
We checked that the models match the performance as reported in the paper.
Did you run the model as stated in https://github.com/huggingface/transformers/blob/master/examples/rag/README.md ? <|||||>Which index did you use exactly with wiki_dpr ? This EM value is expected if you used the `compressed` one. For the `exact` one you might need to increase the efSearch parameter of the index. I ran some indexing experiments recently and I'll update the default parameters of the wiki_dpr index with the optimized ones that reproduce RAG's paper results.
EDIT: they've been updated a few weeks ago<|||||>> Hey @gaobo1987,
>
> We checked that the models match the performance as reported in the paper.
>
> Did you run the model as stated in https://github.com/huggingface/transformers/blob/master/examples/rag/README.md ?
Thanks for your reply @patrickvonplaten ,
we did not use the example run script there, but followed the code snippets provided in the huggingface documentation:
```python
from transformers import RagTokenizer, RagRetriever, RagSequenceForGeneration
import torch
tokenizer = RagTokenizer.from_pretrained("facebook/rag-sequence-nq")
retriever = RagRetriever.from_pretrained("facebook/rag-sequence-nq", index_name="exact", use_dummy_dataset=True)
# initialize with RagRetriever to do everything in one forward call
model = RagSequenceForGeneration.from_pretrained("facebook/rag-token-nq", retriever=retriever)
input_dict = tokenizer.prepare_seq2seq_batch("How many people live in Paris?", "In Paris, there are 10 million people.", return_tensors="pt")
input_ids = input_dict["input_ids"]
outputs = model(input_ids=input_ids, labels=input_dict["labels"])
# or use retriever seperately
model = RagSequenceForGeneration.from_pretrained("facebook/rag-sequence-nq", use_dummy_dataset=True)
# 1. Encode
question_hidden_states = model.question_encoder(input_ids)[0]
# 2. Retrieve
docs_dict = retriever(input_ids.numpy(), question_hidden_states.detach().numpy(), return_tensors="pt")
doc_scores = torch.bmm(question_hidden_states.unsqueeze(1), docs_dict["retrieved_doc_embeds"].float().transpose(1, 2)).squeeze(1)
# 3. Forward to generator
outputs = model(context_input_ids=docs_dict["context_input_ids"], context_attention_mask=docs_dict["context_attention_mask"], doc_scores=doc_scores, decoder_input_ids=input_dict["labels"])
```
see here: https://huggingface.co/transformers/model_doc/rag.html#ragsequenceforgeneration
We did use our own evaluation script for computing EM scores.
In general, we tried to follow the prescribed steps from official source as exactly as possible, as for the customized EM calculation, difference may arise there, but I believe the main source of performance difference lies somewhere else.<|||||>> Which index did you use exactly with wiki_dpr ? This EM value is expected if you used the `compressed` one. For the `exact` one you might need to increase the efSearch parameter of the index. I ran some indexing experiments recently and I'll update the default parameters of the wiki_dpr index with the optimised ones that reproduce RAG's paper results.
thanks for the reply @lhoestq , we used the "exact" mode of the wiki_dpr index, indeed, we haven't tried the "compressed" mode, nor did we tune the "exact" index. Thanks for the update, we will check the "compressed" alternative, and the parameter tuning of the "exact" index. Also great to know that you will update the default parameters!<|||||>This issue has been automatically marked as stale because it has not had recent activity. It will be closed if no further activity occurs. Thank you for your contributions.
<|||||>Hi, to provide an update on this issue. Recently I refactored my own RAG code based transformers-4.1.1, and obtained EM=40.7 performance on the open NQ dataset with rag-sequence-nq model (n_beams=4) and FAISS HNSW index with n_docs=5, efSearch=256 and efConstruction=200. Unfortunately it still didn't reach the expected 44.5 score. Are these sound parameters? Am I missing any? What is the best parameter combination used at Huggingface? Any advice is much appreciated, thanks! (Note that I couldn't use the original rag code as there is firewall restrictions on my server that prevented downloading the wiki_dpr.py script as well the arrow files for exact indexing, so I have to download these files on a much less powerful laptop and upload them to my server. Consequently, I am using a modified version of RagSequenceForGeneration along with a modified RagRetriever) @lhoestq <|||||>@gaobo1987
Can you please share how exactly you played around with the efSearch and efConstruction parameters?
As in where in the code did you make the changes??<|||||>hello @krishanudb , thanks for your reply. What I did is merely manually downloading the wiki_dpr-train.arrow file, then use it to construct a faiss hnsw index with efSearch=256, efConstruction=200, then save this index to disk. I wrote a wrapper around RagRetriever and RagSequenceForGeneration respectively so that rag can run directly on the aforementioned faiss index, instead of relying on huggingFace.Datasets utilities and other caching sub-routines. I did not change the models in any way. Could you provide an answer to my question regarding the best combination of parameters from huggingFace to reach the performance as reported in the original paper? Thanks for your time<|||||>@gaobo1987
There are several versions of the DPR model (single-nq vs multiset) as well as the precomputed passages wiki_dpr
I am not sure which one the authors used to get 44% EM but I think they have used the single-nq models for the tasks.
Make sure that you are using the 'right; model. Maybe the authors can shed more light on this..
Even I am facing the same issue... Not getting more than 40% EM no matter if I use the multiset or the nq-single models..<|||||>This issue has been automatically marked as stale because it has not had recent activity. If you think this still needs to be addressed please comment on this thread.
Please note that issues that do not follow the [contributing guidelines](https://github.com/huggingface/transformers/blob/master/CONTRIBUTING.md) are likely to be ignored. |
transformers | 8,284 | closed | [rag] missing a working End-to-end evaluation example | I'm going to try to write tests for `examples/rag` (https://github.com/huggingface/transformers/issues/7715), but first I'm trying to figure out how it works.
Would it be possible to add a full `End-to-end evaluation` invocation example in https://github.com/huggingface/transformers/blob/master/examples/rag/README.md#end-to-end-evaluation? i.e. with the correct data.
I tested https://github.com/huggingface/transformers/blob/master/examples/rag/README.md#retrieval-evaluation and it worked, but if I try to adapt the same params for e2e it crashes with:
```
$ python eval_rag.py --model_name_or_path facebook/rag-sequence-nq --model_type rag_sequence \
--evaluation_set output/biencoder-nq-dev.questions --gold_data_path output/biencoder-nq-dev.pages \
--predictions_path output/retrieval_preds.tsv --eval_mode e2e --gold_data_mode qa --n_docs 5 \
--print_predictions
2020-11-03 22:07:33.124277: I tensorflow/stream_executor/platform/default/dso_loader.cc:49] Successfully opened dynamic library libcudart.so.11.0
INFO:__main__:Evaluate the following checkpoints: ['facebook/rag-sequence-nq']
INFO:__main__:Calculating metrics based on an existing predictions file: output/retrieval_preds.tsv
Traceback (most recent call last):
File "eval_rag.py", line 314, in <module>
main(args)
File "eval_rag.py", line 280, in main
score_fn(args, args.predictions_path, args.gold_data_path)
File "eval_rag.py", line 46, in get_scores
data = pd.read_csv(gold_data_path, sep="\t", header=None)
File "/home/stas/anaconda3/envs/main-38/lib/python3.8/site-packages/pandas/io/parsers.py", line 686, in read_csv
return _read(filepath_or_buffer, kwds)
File "/home/stas/anaconda3/envs/main-38/lib/python3.8/site-packages/pandas/io/parsers.py", line 458, in _read
data = parser.read(nrows)
File "/home/stas/anaconda3/envs/main-38/lib/python3.8/site-packages/pandas/io/parsers.py", line 1196, in read
ret = self._engine.read(nrows)
File "/home/stas/anaconda3/envs/main-38/lib/python3.8/site-packages/pandas/io/parsers.py", line 2155, in read
data = self._reader.read(nrows)
File "pandas/_libs/parsers.pyx", line 847, in pandas._libs.parsers.TextReader.read
File "pandas/_libs/parsers.pyx", line 862, in pandas._libs.parsers.TextReader._read_low_memory
File "pandas/_libs/parsers.pyx", line 918, in pandas._libs.parsers.TextReader._read_rows
File "pandas/_libs/parsers.pyx", line 905, in pandas._libs.parsers.TextReader._tokenize_rows
File "pandas/_libs/parsers.pyx", line 2042, in pandas._libs.parsers.raise_parser_error
pandas.errors.ParserError: Error tokenizing data. C error: Expected 5 fields in line 2, saw 6
```
I think it needs a different input data.
And we need 2 functional examples: for `qa` and `ans` each.
I can handle adding this to the doc if you tell me what to add.
Thanks.
@patrickvonplaten, @lhoestq
| 11-04-2020 06:15:03 | 11-04-2020 06:15:03 | @stas00
Can you please write a test code for **finetune.sh**. <|||||>As you can see I'm waiting for this ticket to be addressed before I'm able to write the tests.
Perhaps you can address that, and then I will have all the info needed to write the tests.<|||||>Until then please file a normal issue about it. I haven't done any rag work yet, so that's why I'm asking for support.<|||||>@lhoestq is working on this at the moment :-) <|||||>Actually I'm working on the finetuning script example, not eval ;)
But maybe this can help with adding a test for the eval script example.<|||||>This issue has been automatically marked as stale because it has not had recent activity. It will be closed if no further activity occurs. Thank you for your contributions.
<|||||>stale |
transformers | 8,283 | closed | [tokenizers] convert_to_tensors: don't reconvert when the type is already right | I was trying to fix this warning:
```
src/transformers/tokenization_utils_base.py:608: UserWarning: To copy construct from a tensor,
it is recommended to use sourceTensor.clone().detach() or sourceTensor.clone().detach().requires_grad_(True),
rather than torch.tensor(sourceTensor).
tensor = as_tensor(value)
```
which appeared when running:
```
python eval_rag.py --model_name_or_path facebook/rag-sequence-nq --model_type rag_sequence --evaluation_set output/biencoder-nq-dev.questions --gold_data_path output/biencoder-nq-dev.pages --predictions_path output/retrieval_preds.tsv --eval_mode retrieval --k 1
```
This appears to have happened since `convert_to_tensors` was called with data which was already a tensor of the right type.
* [x] and ended up fixing it for pt and also adding the same fix for tf/jax/np. basically skip the conversion if the value is already of the required type and avoid the pytorch warning.
* [x] added tests for converting the already converted
* [x] while at it added a missing test for `test_batch_encoding_with_labels_jax`
I understand `lambda` isn't welcome, so I had to define a few helper functions for numpy/jax. `partial` would have done the trick, but `isinstance` doesn't accept keyword args.
@LysandreJik, @mfuntowicz | 11-04-2020 05:31:27 | 11-04-2020 05:31:27 | ping<|||||>Looks good to me. Thanks for handling this one @stas00 and sorry for the delay. |
transformers | 8,282 | closed | [blenderbot] regex fix | This PR fixes:
```
src/transformers/tokenization_blenderbot.py:163: DeprecationWarning: invalid escape sequence \s
token = re.sub("\s{2,}", " ", token)
```
@LysandreJik | 11-04-2020 02:37:31 | 11-04-2020 02:37:31 | |
transformers | 8,281 | closed | Create README.md | # What does this PR do?
<!--
Congratulations! You've made it this far! You're not quite done yet though.
Once merged, your PR is going to appear in the release notes with the title you set, so make sure it's a great title that fully reflects the extent of your awesome contribution.
Then, please replace this with a description of the change and which issue is fixed (if applicable). Please also include relevant motivation and context. List any dependencies (if any) that are required for this change.
Once you're done, someone will review your PR shortly (see the section "Who can review?" below to tag some potential reviewers). They may suggest changes to make the code even better. If no one reviewed your PR after a week has passed, don't hesitate to post a new comment @-mentioning the same persons---sometimes notifications get lost.
-->
<!-- Remove if not applicable -->
Fixes # (issue)
## Before submitting
- [x] This PR fixes a typo or improves the docs (you can dismiss the other checks if that's the case).
- [x] Did you read the [contributor guideline](https://github.com/huggingface/transformers/blob/master/CONTRIBUTING.md#start-contributing-pull-requests),
Pull Request section?
- [ ] Was this discussed/approved via a Github issue or the [forum](https://discuss.huggingface.co/)? Please add a link
to the it if that's the case.
- [ ] Did you make sure to update the documentation with your changes? Here are the
[documentation guidelines](https://github.com/huggingface/transformers/tree/master/docs), and
[here are tips on formatting docstrings](https://github.com/huggingface/transformers/tree/master/docs#writing-source-documentation).
- [ ] Did you write any new necessary tests?
## Who can review?
Anyone in the community is free to review the PR once the tests have passed. Feel free to tag
members/contributors which may be interested in your PR.
<!-- Your PR will be replied to more quickly if you can figure out the right person to tag with @
If you know how to use git blame, that is the easiest way, otherwise, here is a rough guide of **who to tag**.
Please tag fewer than 3 people.
albert, bert, XLM: @LysandreJik
GPT2: @LysandreJik, @patrickvonplaten
tokenizers: @mfuntowicz
Trainer: @sgugger
Benchmarks: @patrickvonplaten
Model Cards: @julien-c
Translation: @sshleifer
Summarization: @sshleifer
examples/distillation: @VictorSanh
nlp datasets: [different repo](https://github.com/huggingface/nlp)
rust tokenizers: [different repo](https://github.com/huggingface/tokenizers)
Text Generation: @patrickvonplaten, @TevenLeScao
Blenderbot, Bart, Marian, Pegasus: @sshleifer
T5: @patrickvonplaten
Rag: @patrickvonplaten, @lhoestq
EncoderDecoder: @patrickvonplaten
Longformer, Reformer: @patrickvonplaten
TransfoXL, XLNet: @TevenLeScao, @patrickvonplaten
examples/seq2seq: @sshleifer
examples/bert-loses-patience: @JetRunner
tensorflow: @jplu
examples/token-classification: @stefan-it
documentation: @sgugger
-->
| 11-04-2020 02:18:16 | 11-04-2020 02:18:16 | Very cool. Is possible, can you add metadata as described in https://huggingface.co/docs#what-metadata-can-i-add-to-my-model-card? |
transformers | 8,280 | closed | Translation finetuning error : TypeError: '>' not supported between instances of 'function' and 'int' | Dear huggingface team,
I'd like to train from scratch T5 on wmt19 (de-en), and I see these instructions in your page:
- you provided the script for finetune mbart_cc25, could I just change the model path and it works out of the box for training T5 on a translation task? any changes needed?
- when you use sortish sampler (line 256 finetune.py) you check the number of gpus, in case using tpus, shall I check the number of cores of tpus for distributed version of dataloader in line 256?
- does distributed tpu training works for seq2seq model? I wonder why the dataloader is not modified for tpu cores, is this by purpose and works fine for tpus too?
- I also gets these errors running the provided script, thank you for your help.
Best
Rabeeh
```
(test) rabeeh@brain1:~/ruse/hf/transformers/examples/seq2seq$ ./train_mbart_cc25_enro.sh --output_dir enro_finetune_baseline --label_smoothing 0.1 --fp16_opt_level=O1 --logger_name wandb --sortish_sampler
2020-11-04 01:41:53.720772: W tensorflow/stream_executor/platform/default/dso_loader.cc:59] Could not load dynamic library 'libcudart.so.10.1'; dlerror: libcudart.so.10.1: cannot open shared object file: No such file or directory; LD_LIBRARY_PATH: /usr/local/cuda/lib64:/usr/local/nccl2/lib:/usr/local/cuda/extras/CUPTI/lib64
2020-11-04 01:41:53.720823: I tensorflow/stream_executor/cuda/cudart_stub.cc:29] Ignore above cudart dlerror if you do not have a GPU set up on your machine.
Traceback (most recent call last):
File "finetune.py", line 442, in <module>
main(args)
File "finetune.py", line 383, in main
model: SummarizationModule = TranslationModule(args)
File "finetune.py", line 367, in __init__
super().__init__(hparams, **kwargs)
File "finetune.py", line 57, in __init__
if hparams.sortish_sampler and hparams.gpus > 1:
TypeError: '>' not supported between instances of 'function' and 'int'
``` | 11-04-2020 01:44:34 | 11-04-2020 01:44:34 | The issue solved with setting --gpus 1 explicitly. thanks. |
transformers | 8,279 | closed | Finetuning T5 on translation wmt19(de-en) | Dear huggingface team,
I'd like to train from scratch T5 on wmt19 (de-en), and I see these instructions in your page:
you provided the script for finetune mbart_cc25, could I just change the model path and it works out of the box for training T5 on a translation task?
I also gets these errors running the provided script, thank you for your help.
Best
Rabeeh
```
(test) rabeeh@brain1:~/ruse/hf/transformers/examples/seq2seq$ ./train_mbart_cc25_enro.sh --output_dir enro_finetune_baseline --label_smoothing 0.1 --fp16_opt_level=O1 --logger_name wandb --sortish_sampler
2020-11-04 01:41:53.720772: W tensorflow/stream_executor/platform/default/dso_loader.cc:59] Could not load dynamic library 'libcudart.so.10.1'; dlerror: libcudart.so.10.1: cannot open shared object file: No such file or directory; LD_LIBRARY_PATH: /usr/local/cuda/lib64:/usr/local/nccl2/lib:/usr/local/cuda/extras/CUPTI/lib64
2020-11-04 01:41:53.720823: I tensorflow/stream_executor/cuda/cudart_stub.cc:29] Ignore above cudart dlerror if you do not have a GPU set up on your machine.
Traceback (most recent call last):
File "finetune.py", line 442, in <module>
main(args)
File "finetune.py", line 383, in main
model: SummarizationModule = TranslationModule(args)
File "finetune.py", line 367, in __init__
super().__init__(hparams, **kwargs)
File "finetune.py", line 57, in __init__
if hparams.sortish_sampler and hparams.gpus > 1:
TypeError: '>' not supported between instances of 'function' and 'int'
``` | 11-04-2020 01:28:05 | 11-04-2020 01:28:05 | |
transformers | 8,278 | closed | [commit #29b536a]AttributeError: module 'numpy.random' has no attribute 'Generator' | ## Environment info
<!-- You can run the command `transformers-cli env` and copy-and-paste its output below.
Don't forget to fill out the missing fields in that output! -->
- `transformers` version: commit #29b536a
- Platform: Linux
- Python version: 3.7.4
- PyTorch version (GPU?): 1.4.0
- Tensorflow version (GPU?): N/A
- Using GPU in script?: yes
- Using distributed or parallel set-up in script?: yes
### Who can help
I don't know, anyone?
## Information
The problem arises when using:
```
import transformers
```
The tasks I am working on is:
(ANY)
## To reproduce
Steps to reproduce the behavior:
```
import transformers
```
## Error message
```
File "/home01/a1204a01/.local/bin/transformers-cli", line 6, in <module>
from transformers.commands.transformers_cli import main
File "/home01/a1204a01/.local/lib/python3.7/site-packages/transformers/__init__.py", line 22, in <module>
from .integrations import ( # isort:skip
File "/home01/a1204a01/.local/lib/python3.7/site-packages/transformers/integrations.py", line 81, in <module>
from .file_utils import is_torch_tpu_available # noqa: E402
File "/home01/a1204a01/.local/lib/python3.7/site-packages/transformers/file_utils.py", line 87, in <module>
import datasets # noqa: F401
File "/home01/a1204a01/.local/lib/python3.7/site-packages/datasets/__init__.py", line 27, in <module>
from .arrow_dataset import Dataset, concatenate_datasets
File "/home01/a1204a01/.local/lib/python3.7/site-packages/datasets/arrow_dataset.py", line 175, in <module>
class Dataset(DatasetInfoMixin, IndexableMixin):
File "/home01/a1204a01/.local/lib/python3.7/site-packages/datasets/arrow_dataset.py", line 1889, in Dataset
new_fingerprint: Optional[str] = None,
AttributeError: module 'numpy.random' has no attribute 'Generator'
```
## Expected behavior
Import
| 11-04-2020 01:04:24 | 11-04-2020 01:04:24 | Another error:
```
---------------------------------------------------------------------------
ImportError Traceback (most recent call last)
<ipython-input-7-279c49635b32> in <module>
----> 1 import transformers
/scratch/a1204a01/.conda/envs/notebook/lib/python3.7/site-packages/transformers/__init__.py in <module>
133
134 # Pipelines
--> 135 from .pipelines import (
136 Conversation,
137 ConversationalPipeline,
/scratch/a1204a01/.conda/envs/notebook/lib/python3.7/site-packages/transformers/pipelines.py in <module>
35 from .file_utils import add_end_docstrings, is_tf_available, is_torch_available
36 from .modelcard import ModelCard
---> 37 from .tokenization_auto import AutoTokenizer
38 from .tokenization_bert import BasicTokenizer
39 from .tokenization_utils import PreTrainedTokenizer
/scratch/a1204a01/.conda/envs/notebook/lib/python3.7/site-packages/transformers/tokenization_auto.py in <module>
117
118 if is_tokenizers_available():
--> 119 from .tokenization_albert_fast import AlbertTokenizerFast
120 from .tokenization_bart_fast import BartTokenizerFast
121 from .tokenization_bert_fast import BertTokenizerFast
/scratch/a1204a01/.conda/envs/notebook/lib/python3.7/site-packages/transformers/tokenization_albert_fast.py in <module>
21
22 from .file_utils import is_sentencepiece_available
---> 23 from .tokenization_utils_fast import PreTrainedTokenizerFast
24 from .utils import logging
25
/scratch/a1204a01/.conda/envs/notebook/lib/python3.7/site-packages/transformers/tokenization_utils_fast.py in <module>
28 from tokenizers.decoders import Decoder as DecoderFast
29
---> 30 from .convert_slow_tokenizer import convert_slow_tokenizer
31 from .file_utils import add_end_docstrings
32 from .tokenization_utils import PreTrainedTokenizer
/scratch/a1204a01/.conda/envs/notebook/lib/python3.7/site-packages/transformers/convert_slow_tokenizer.py in <module>
26
27 # from transformers.tokenization_openai import OpenAIGPTTokenizer
---> 28 from transformers.utils import sentencepiece_model_pb2 as model
29
30 from .file_utils import requires_sentencepiece
ImportError: cannot import name 'sentencepiece_model_pb2' from 'transformers.utils' (/home01/a1204a01/.local/lib/python3.7/site-packages/transformers/utils/__init__.py)
```<|||||>Hi! could you let us know how you installed `transformers`?<|||||>I built from source, by ```git clone``` and ```pip install .```
EDIT: Huh, it's now giving error ```ImportError: cannot import name 'is_main_process' from 'transformers.trainer_utils'```<|||||>Fixed by reinstalling python3 and reinstalling transformer with latest commit |
transformers | 8,277 | closed | SqueezeBert does not appear to properly generate text | ## Environment info
Google Colab
Using CPU with High Ram
### Who can help
@sgugger @forresti @LysandreJik
## Information
Model I am using: Squeezebert-uncased, squeezebert-mnli, etc.
The problem arises when using:
Trying to generate the likely output of the input sequence and predicting masked tokens.
## To reproduce
```
from torch import nn
from transformers import AutoModelForMaskedLM, AutoTokenizer
model = AutoModelForMaskedLM.from_pretrained('squeezebert/squeezebert-mnli')
tokenizer = AutoTokenizer.from_pretrained('squeezebert/squeezebert-mnli')
#model.tie_weights()
input_txt = ["[MASK] was an American [MASK] and lawyer who served as the 16th president of the United States from 1861 to 1865. [MASK] led the nation through the American Civil War, the country's greatest [MASK], [MASK], and [MASK] crisis. ", \
"George [MASK], who served as the first president of the United States from [MASK] to 1797, was an American political leader, [MASK] [MASK], statesman, and Founding Father. Previously, he led Patriot forces to [MASK] in the nation's War for Independence. ", \
"[MASK], the first African-American [MASK] of the [MASK] [MASK], is an American politician and attorney who served as the 44th [MASK] of the United States from [MASK] to 2017. [MASK] was a member of the [MASK] [MASK]. "]
#input_txt =
input_txt= [i.replace("[MASK]", tokenizer.mask_token) for i in input_txt] #
inputs = tokenizer(input_txt, return_tensors='pt', add_special_tokens=True, padding=True)
inputs['output_attentions'] = True
inputs['output_hidden_states'] = True
inputs['return_dict'] = True
outputs = model(**inputs)
if True:
predictions = outputs.logits
for pred in predictions:
print ("**")
sorted_preds, sorted_idx = pred.sort(dim=-1, descending=True)
for k in range(2):
predicted_index = [sorted_idx[i, k].item() for i in range(0,len(predictions[0]))]
predicted_token = ' '.join([tokenizer.convert_ids_to_tokens([predicted_index[x]])[0] for x in range(1,len(predictions[0]))]).replace('Ġ', ' ').replace(' ', ' ').replace('##', '')
print(predicted_token)
```
## Expected behavior
I expected at least the input to be echoed out, with the slots filling with Lincoln, Washington and Obama. This works for bert, distlbert, roberta, etc.
## Actual output
Some weights of the model checkpoint at squeezebert/squeezebert-mnli were not used when initializing SqueezeBertForMaskedLM: ['classifier.weight', 'classifier.bias']
- This IS expected if you are initializing SqueezeBertForMaskedLM from the checkpoint of a model trained on another task or with another architecture (e.g. initializing a BertForSequenceClassification model from a BertForPretraining model).
- This IS NOT expected if you are initializing SqueezeBertForMaskedLM from the checkpoint of a model that you expect to be exactly identical (initializing a BertForSequenceClassification model from a BertForSequenceClassification model).
Some weights of SqueezeBertForMaskedLM were not initialized from the model checkpoint at squeezebert/squeezebert-mnli and are newly initialized: ['lm_head.weight', 'lm_head.bias']
You should probably TRAIN this model on a down-stream task to be able to use it for predictions and inference.
odict_keys(['logits', 'hidden_states', 'attentions'])
**
tone lani rce soto olar rce ux wer lani bal bal vus novice rce rce rce lani owe frey owe gent naire tres che lani lani nae ui territories accusing oaks accusing ois lor resulting resulting rce lor rce rendering rce rce tres assist ois accusing rendering warns accusing gent
culture bowls hectares awan rce bal ade wd an rce mole hoe yde lani lani lani rce tres resulted bal resulted resulting tone consequently bowls fellow wo ois crafts oaks withdrew nations wu resulting fellow rce resulting verses motivated lori motivated motivated gent vus naire dealt warns gent warns tres
**
culture sas hari lani rce gaa lani novice rce rce rce rce tres nae jan thal rce rce rce awan olar v8 rce olar example rce select rce rce hore rden resulting lori resulting drive led bon peoples jal gau nae hoe lies lies lies lies lins lies resulting tone
continuum tone repeat gaa lani wo rce coven lani lani lani lani gle aw aw awan sco lani yde rce yde olar ux rce rce trait xie xie cao particular elder lani lani naturally blend lie aman commando folding rendering helps ois lete wi lins lins hoe independence sons tones
**
tone acts attribute trait pour pour trait % sities ub azi % acts lani rce awan act cao yde wd hoe hoe hoe hoe % vos vos rce hort hoe sept jan vers naire hum candle therefore lists chen hoe lie side mut hen mor lungs zoo lie side side
hum fever acts pour shropshire cz % sities isson penalties lie sities act acts bble pour yde ave shropshire yde lto ango ango pour lden rce hoe gil hoe tres aw nae dha therefore bisexual therefore lb mates rden too zoo forum naire dealt lag mole mess pore forum ior
| 11-03-2020 22:48:11 | 11-03-2020 22:48:11 | Hello! First of all, you're using the `squeezebert-mnli` checkpoint, which is a checkpoint that was fine-tuned on the MNLI dataset. It cannot be used to do masked language modeling.
I believe you should be using the `squeezebert-uncased` checkpoint instead.
However, even when using that checkpoint with the MLM pipeline I cannot obtain sensible results. Maybe @forresti can chime in and let us know if something's up!
<|||||>Thanks @LysandreJik . I used both squeezebert-mnli and squeezebert-uncased (not shown). Same type of results. Thanks for checking. @forresti any thoughts? Is there something wrong with the squeezbert tokenizer? <|||||>@ontocord Sorry for the slow reply. I will dig into this on Thursday this week.<|||||>@ontocord Thanks so much for bringing this to my attention! I was able to reproduce the issue. And, I think I was able to fix the issue in PR #8479.
Now, let's try running your example code with...
* PR #8479
* the `squeezebert-uncased` checkpoint
... this produces the following output:
```
Some weights of the model checkpoint at squeezebert/squeezebert-uncased were not used when initializing SqueezeBertForMaskedLM: ['cls.seq_relationship.weight', 'cls.seq_relationship.bias']
- This IS expected if you are initializing SqueezeBertForMaskedLM from the checkpoint of a model trained on another task or with another architecture (e.g. initializing a BertForSequenceClassification model from a BertForPreTraining model).
- This IS NOT expected if you are initializing SqueezeBertForMaskedLM from the checkpoint of a model that you expect to be exactly identical (initializing a BertForSequenceClassification model from a BertForSequenceClassification model).
Some weights of SqueezeBertForMaskedLM were not initialized from the model checkpoint at squeezebert/squeezebert-uncased and are newly initialized: ['transformer.embeddings.position_ids']
You should probably TRAIN this model on a down-stream task to be able to use it for predictions and inference.
**
he was an american politician and lawyer who served as the 16th president of the united states from 1861 to 1865 . he led the nation through the american civil war , the country ' s greatest war , war , and economic crisis . , war , economic economic war
johnson is a americans statesman & attorney and serve the interim 17th presidency in of confederate state in 1860 until 1866 " white lead a country throughout a america black wars and a nation ’ largest largest economic and famine and , political crises " and famine war and political crisis
**
george washington , who served as the first president of the united states from 1796 to 1797 , was an american political leader , patriot patriot , statesman , and founding father . previously , he led patriot forces to victory in the nation ' s war for independence . ,
james harrison s jr serve in inaugural inaugural presidency in s u united in 1789 until 1799 ) is a americans politician figure and military statesman and politician and , adoptive fathers " historically was his lead revolutionary troops in fight during a country ’ the fight of freedom " and
**
johnson , the first african - american president of the united states , is an american politician and attorney who served as the 44th president of the united states from 2016 to 2017 . he was a member of the republican party . , john the republican republican party . the
williams is , second black – americans governor in this colored senate islander was a americans political , lawyer , serves the a 43rd governor for of union state in 2015 until 2016 , she is an part the house democratic assembly " . james senate democratic democratic assembly party and
```
Alas, the model seems to think Obama's name is "Johnson," but it does get George Washington correct.
Anyway, does this output look a bit more like what you expected? :)<|||||>Thsnks a lot @forresti! This works as well with the fill-mask pipeline:
```py
>>> from transformers import AutoModelForMaskedLM, AutoTokenizer
>>> model = AutoModelForMaskedLM.from_pretrained('squeezebert/squeezebert-uncased')
>>> tokenizer = AutoTokenizer.from_pretrained('squeezebert/squeezebert-uncased')
>>> input_txt = [
... "George Washington, who served as the first [MASK] of the United States from 1789 to 1797, was an American political leader."
... ]
>>> from transformers import pipeline
>>> nlp = pipeline("fill-mask", model=model, tokenizer=tokenizer)
>>> print(nlp(input_txt))
[{'sequence': '[CLS] george washington, who served as the first president of the united states from 1789 to 1797, was an american political leader. [SEP]', 'score': 0.9644643664360046, 'token': 2343, 'token_str': 'president'}, {'sequence': '[CLS] george washington, who served as the first governor of the united states from 1789 to 1797, was an american political leader. [SEP]', 'score': 0.026940250769257545, 'token': 3099, 'token_str': 'governor'}, {'sequence': '[CLS] george washington, who served as the first king of the united states from 1789 to 1797, was an american political leader. [SEP]', 'score': 0.0013772461097687483, 'token': 2332, 'token_str': 'king'}, {'sequence': '[CLS] george washington, who served as the first lieutenant of the united states from 1789 to 1797, was an american political leader. [SEP]', 'score': 0.0012003666488453746, 'token': 3812, 'token_str': 'lieutenant'}, {'sequence': '[CLS] george washington, who served as the first secretary of the united states from 1789 to 1797, was an american political leader. [SEP]', 'score': 0.0008091009221971035, 'token': 3187, 'token_str': 'secretary'}]
```<|||||>Thank @forresti! Yes this fixes the problem! Thank you @LysandreJik as well! I noticed that different models have different capacities to store facts. Roughly based on the number of parameters, but not always. As a question, do you know of any models that are trained to identify a relationship and not a word in the mask:, leader($X, president,united_states,1789,1797) served as the first president of the united states from 1789 to 1797 ... in theory this should reduce the number of facts the model needs to learn as the relationships are already being learned by the attention mechanism, I belive.
|
transformers | 8,276 | closed | Support various BERT relative position embeddings (2nd) | # What does this PR do?
Creating a new PR for https://github.com/huggingface/transformers/pull/8108 to keep cleaner git history/commits.
The default BERT model `bert-base-uncased` was pre-trained with absolute position embeddings. We provide three pre-trained models which were pre-trained on the same training data (BooksCorpus and English Wikipedia) as in the BERT model training, but with different relative position embeddings (Shaw et al., Self-Attention with Relative Position Representations, https://arxiv.org/abs/1803.02155 and Huang et al., Improve Transformer Models with Better Relative Position Embeddings, https://arxiv.org/abs/2009.13658, accepted in findings of EMNLP 2020). We show how to fine-tune these pre-trained models on SQuAD1.1 data set. Our proposed relative position embedding method can boost the BERT base model (with default absolute position embedding) from f1 score of 88.52 to 90.54 with similar training/inference speed. It also boosts the `bert-large-uncased-whole-word-masking` model from 93.15 to 93.52 with 3 additional fine-tune epochs. See examples/question-answering/README.md for more details.
Fixes # (issue)
#8108
## Before submitting
- [ ] This PR fixes a typo or improves the docs (you can dismiss the other checks if that's the case).
- [X] Did you read the [contributor guideline](https://github.com/huggingface/transformers/blob/master/CONTRIBUTING.md#start-contributing-pull-requests),
Pull Request section?
- [ ] Was this discussed/approved via a Github issue or the [forum](https://discuss.huggingface.co/)? Please add a link
to the it if that's the case.
- [ ] Did you make sure to update the documentation with your changes? Here are the
[documentation guidelines](https://github.com/huggingface/transformers/tree/master/docs), and
[here are tips on formatting docstrings](https://github.com/huggingface/transformers/tree/master/docs#writing-source-documentation).
- [x] Did you write any new necessary tests?
## Who can review?
@patrickvonplaten @LysandreJik @julien-c
| 11-03-2020 22:28:26 | 11-03-2020 22:28:26 | Hey @zhiheng-huang,
it would be great if you could take a look at the failing tests :-) <|||||>Hey @patrickvonplaten, I fixed all failed tests except check_code_quality. Currently the relative embedding is implemented for BERT only. In check_code_quality, `utils/check_copies.py` tries to copy the relative embedding implementation from BERT model to other models including `albert`, `electra`, `roberta` etc. I understand this may make the relative embedding methods ready to be used in those models. However, we haven't pre-trained those type of models with relative embedding and thus cannot assess their effectiveness. Please advise if I should fix this failing test (by ensuring relative embedding implementation copied to those BERT variants) or leave it as is. <|||||>Hey @zhiheng-huang,
Sadly there is still a problem with the git commit history. As you can see 54 files are changed in this PR. Could you make sure to keep the commit tree clean. It is not really possible to review the PR otherwise :-/
Try to make use of `git rebase` to avoid appending the master's commit history to your branch maybe<|||||>In the worst case, you can just make the changes to the files you intend to change without `rebasing` or `merging` and then I can review and merge/rebase for you. <|||||>Rebased and removed the unintended merge commit. @patrickvonplaten, can you comment on the `utils/check_copies.py` question so we can move forward?<|||||>Hi @patrickvonplaten @LysandreJik, I see one approval already, is it ready to merge? If not, can you point to the embedding (for example absolute position embedding) unit tests so I can try to come up with similar tests?<|||||>Regarding tests, I think adding integration tests in the `test_modeling_bert.py` would be nice. What do you think @patrickvonplaten?
The BERT model doesn't have any such tests right now, but you can take inspiration from the `RobertaModelIntegrationTest` class in `test_modeling_roberta.py`, which you can find [here](https://github.com/huggingface/transformers/blob/master/tests/test_modeling_roberta.py#L402).
You could add a couple of tests, each testing that you get the expected results: this will ensure the implementation will not diverge in the future. If you need a checkpoint, you can use `lysandre/tiny-bert-random`, which is a very small model (with random values), so it will be very light on the CI.
Let me know if you need anything.<|||||>@patrickvonplaten @LysandreJik
1. Added forward test to ensure forward runs okay for `LayoutLM`, `Roberta`, `ELECTRA`, and `BERT` for three position embeddings: "absolute", "relative_key", "relative_key_query".
2. Added integration test for `BERT` check points `bert-base-uncased`, `zhiheng-huang/bert-base-uncased-embedding-relative-key`, and `zhiheng-huang/bert-base-uncased-embedding-relative-key-query` to ensure that models predictions match expected outputs.<|||||>@zhiheng-huang - Let me fix the CI later, don't worry about it :-) <|||||>> This looks good to me. Thanks a lot for your PR!
> Any reason ALBERT and Longformer don't get this new functionality? (But RoBERTa and ELECTRA do?)
Great question! I ALBERT should get this functionality (I just added it - great catch!). Longformer has weird attention_scores which does not work with those embeddings.<|||||>Good to merge! Thanks a mille @zhiheng-huang! <|||||>> Good to merge! Thanks a mille @zhiheng-huang!
Thanks! @patrickvonplaten @sgugger @LysandreJik |
transformers | 8,275 | closed | [CIs] Better reports everywhere | Continuing the work in https://github.com/huggingface/transformers/pull/8110 and https://github.com/huggingface/transformers/pull/8163 this PR does the following:
* [x] rename `pytest --make_reports` to `pytest --make-reports` for consistency with the rest of `pytest` opts that don't use `_`
* [x] move the `--make_reports` opt adding to a shared location and load it only once to avoid `pytest` failure
- some pytest plugins like `pytest-instafail` load `tests/conftest.py` even when running `examples`
- now we can run tests from both test suites at once
* [x] rename `reports/report_foo` to `reports/foo` - avoid repetition
* [x] install `--make_reports` in all CIs: circleci and github actions
* [x] make the reports available via artifacts
* [x] always cat short failure reports for github actions in its own "tab" since getting to artifacts there is a cumbersome process. I'm not sure this is needed in circleci jobs since each report in artifacts is available in 2 clicks, so I left the `cat *failures_short.txt` out on CircleCI jobs.
* [x] fixed a few issues in the github actions job configuration
@sgugger, @LysandreJik, @sshleifer
| 11-03-2020 21:17:03 | 11-03-2020 21:17:03 | And and update on proposing this multiple report files feature to be a core feature in pytest https://github.com/pytest-dev/pytest/issues/7972 - one of the developers vetoed it, so I guess it will remain just here for now. It makes no sense for this not to be a core feature of pytest, as we are just splitting the huge mess of everything being dumped to the terminal to just one file per report, but there was no invitation to discuss that - just NO. If someone wants to make it into a pytest plugin it'd surely be useful to others.<|||||>> I saw the discussion (or lack thereof) on pytest. Their loss! We don't mind having the post-processing in transformers.
Eventually we will either have to port it to pytest hooks or keep up with the pytest API changes, since currently the code uses `pytest` internals and could break should they change those. It's just so much simpler doing that than reinventing the wheel. |
transformers | 8,274 | closed | Data collator for token classification | # What does this PR do?
This PR adds a `DataCollatorForTokenClassification`, very similar to `DataCollatorWithPadding` but whose job is to pad the labels to the same size as the inputs.
In passing, it adds tests of `DataCollatorWithPadding` and cleans all the tests of various data collators that were marked as slow because they required a pretrained tokenizer. For the unit testing, no real tokenizer is needed since we just need the pas/mask token. | 11-03-2020 21:10:07 | 11-03-2020 21:10:07 | I just tried it, and noticed that it doesn't work if `features` is `List[Dict[str, torch.Tensor]]`,
because `tokenizer.pad()` will set `return_tensors` to `pt` if `input_ids` is `torch.Tensor` and `return_tensors` is `None`.
For example my dataset looked like this.
```python
def __getitem__(self, i):
return {k: torch.tensor(v, dtype=torch.long) for k,v in self.examples[i].items()}
```
Changing to this solves the problem.
```python
def __getitem__(self, i):
return self.examples[i]
```
Maybe I should have always used this and leave it to collator to tensorize the features.<|||||>Will have look. In general, yes it's better to have your examples be the results of the tokenization (so `Dict[str, List[int]]`) and let the data collator handles the conversion to tensors. |
transformers | 8,273 | closed | add evaluate doc - trainer.evaluate returns 'epoch' from training | Improved documentation - see #8184 | 11-03-2020 20:01:04 | 11-03-2020 20:01:04 | @sgugger changes are made...<|||||>Thanks! |
transformers | 8,272 | closed | Saving and reloading DistilBertForTokenClassification fine-tuned model | I am trying to reload a fine-tuned DistilBertForTokenClassification model. I am using transformers 3.4.0 and pytorch version 1.6.0+cu101. After using the Trainer to train the downloaded model, I save the model with trainer.save_model() and during my trouble shooting I saved the model in a **different** directory via model.save_pretrained(). I am using Google Colab and saving the model to my Google drive. Before closing out my session, I evaluated the model and got good test results, however, when I return to the notebook (or Factory restart the colab notebook) and try to reload the model, the predictions are terrible. Upon checking the both directories, the config.json file is there as is the pytorch_mode.bin. It seems the trained model is not getting saved in this directories, but rather just the original model is? The model will work just fine if I don't close out my notebook session, but upon returning (or factory resetting) the reloading of the model yields a model that does not give good predictions. Is the trained model getting saved in a cache temporarily? But the save_model() function saves the original model?
```
from transformers import DistilBertForTokenClassification
# load the pretrained model from huggingface
model = DistilBertForTokenClassification.from_pretrained('distilbert-base-uncased', num_labels=len(uniq_labels))
model.to('cuda');
from transformers import Trainer, TrainingArguments
training_args = TrainingArguments(
output_dir = model_dir + 'mitmovie_pt_distilbert_uncased/results', # output directory
#overwrite_output_dir = True,
evaluation_strategy='epoch',
num_train_epochs=3, # total number of training epochs
per_device_train_batch_size=16, # batch size per device during training
per_device_eval_batch_size=64, # batch size for evaluation
warmup_steps=500, # number of warmup steps for learning rate scheduler
weight_decay=0.01, # strength of weight decay
logging_dir = model_dir + 'mitmovie_pt_distilbert_uncased/logs', # directory for storing logs
logging_steps=10,
load_best_model_at_end = True
)
trainer = Trainer(
model = model, # the instantiated 🤗 Transformers model to be trained
args = training_args, # training arguments, defined above
train_dataset = train_dataset, # training dataset
eval_dataset = test_dataset # evaluation dataset
)
trainer.train()
trainer.evaluate()
model_dir = '/content/drive/My Drive/Colab Notebooks/models/'
trainer.save_model(model_dir + 'mitmovie_pt_distilbert_uncased/model')
# alternative saving method and folder
model.save_pretrained(model_dir + 'distilbert_testing')
```
Coming back to the notebook after restarting...
```from transformers import DistilBertForTokenClassification, DistilBertConfig, AutoModelForTokenClassification
# retreive the saved model
model = DistilBertForTokenClassification.from_pretrained(model_dir + 'mitmovie_pt_distilbert_uncased/model',
local_files_only=True)
model.to('cuda')
```
Model predictions are now terrible loading the model from either of the directories.
| 11-03-2020 19:41:50 | 11-03-2020 19:41:50 | I'm encountering the same problem. Were you able to solve it?<|||||>Could you add the following lines:
```py
from transformers import logging as hf_logging
hf_logging.set_verbosity_info()
```
before reloading the model, and paste the results here?
cc @sgugger <|||||>loading configuration file trained_models/checkpoint-8000/config.json
Model config DistilBertConfig {
"_name_or_path": "distilbert-base-uncased",
"activation": "gelu",
"architectures": [
"DistilBertForSequenceClassification"
],
"attention_dropout": 0.1,
"dim": 768,
"dropout": 0.1,
"hidden_dim": 3072,
"id2label": { ... },
"initializer_range": 0.02,
"label2id": { ... },
"max_position_embeddings": 512,
"model_type": "distilbert",
"n_heads": 12,
"n_layers": 6,
"pad_token_id": 0,
"qa_dropout": 0.1,
"seq_classif_dropout": 0.2,
"sinusoidal_pos_embds": false,
"tie_weights_": true,
"vocab_size": 30522
}
loading weights file trained_models/checkpoint-8000/pytorch_model.bin
All model checkpoint weights were used when initializing DistilBertForSequenceClassification.
All the weights of DistilBertForSequenceClassification were initialized from the model checkpoint at trained_models/checkpoint-8000.
If your task is similar to the task the model of the checkpoint was trained on, you can already use DistilBertForSequenceClassification for predictions without further training.<|||||>When I go and evaluate the model from this point (either manually or by making a Trainer and using trainer.evaluate()) I get terrible scores.
If I make a Trainer and try to continue training, I get terrible loss scores _except_ if I provide the checkpoint directory as part of the input to trainer.train(). If I supply the checkpoint directory there, the training appears to continue from the checkpoint, and if I train for ~300 more iterations, trainer.evaluate() gives decent performance but still not what I was seeing during the initial run. <|||||>Okay, that's interesting. Do you mind sharing with us your environment? You can run `!transformers-cli env` and put the result here, we'll look into it.<|||||>Thanks @LysandreJik.
- `transformers` version: 3.5.0
- Platform: Linux-5.4.0-1030-aws-x86_64-with-Ubuntu-18.04-bionic
- Python version: 3.6.9
- PyTorch version (GPU?): 1.6.0 (True)
- Tensorflow version (GPU?): 2.3.1 (False)
- Using GPU in script?: Yes, single K80 on AWS
- Using distributed or parallel set-up in script?: No<|||||>There is little we can do to debug without a reproducer, which we don't have as the initial code contains a `train_dataset` and an `eval_dataset` we don't have access to. I just tried the notebook on {GLUE](https://github.com/huggingface/notebooks/blob/master/examples/text_classification.ipynb) and ran until the end of training (before hyperparameter-search), saved the model with `trainer.save_model(some_path)`, the restarted the notebook, ran all the cells up until the training then a new one with
```
model = AutoModelForSequenceClassification.from_pretrained(some_path, local_files_only=True)
trainer.model = model.cuda()
trainer.evaluate()
```
and it gave the exact same results as the end of training, so the `from_pretrained` method works well with the distilbert models.<|||||>As an update, I find that it's not just Distilbert models which will not save/reload for me, but also an Albert model gives the same behavior. Evaluation at the end of training gives 68% accuracy on my problem, whereas save/reload/reevaluate gives <1% accuracy. Currently trying transformers 4.0 rather than 3.5.<|||||>Thanks for the update. As mentioned above, it does not help us fix this problem. We need a reliable reproducer for that.<|||||>@sgugger Turns out the problem was my fault. I was not keeping a consistent mapping of label names to integers across my runs. Once I corrected this my models performed identically after reload. Perhaps the OPs problem was similar. In any case, thanks for the help (the benchmark you linked helped me to debug) and sorry for the wild goose chase.<|||||>Glad you found the reason to your issue!<|||||>@mkreisel How are you "keeping a consistent mapping of label names to integers" across your runs now? Do you use a huggingface dataset ClassLabel ? I noticed in this original problem that it might be that the label mapping is somehow different after reloading the model when using num_labels in from_pretrained().<|||||>> There is little we can do to debug without a reproducer, which we don't have as the initial code contains a `train_dataset` and an `eval_dataset` we don't have access to. I just tried the notebook on {GLUE](https://github.com/huggingface/notebooks/blob/master/examples/text_classification.ipynb) and ran until the end of training (before hyperparameter-search), saved the model with `trainer.save_model(some_path)`, the restarted the notebook, ran all the cells up until the training then a new one with
>
> ```
> model = AutoModelForSequenceClassification.from_pretrained(some_path, local_files_only=True)
> trainer.model = model.cuda()
> trainer.evaluate()
> ```
>
> and it gave the exact same results as the end of training, so the `from_pretrained` method works well with the distilbert models.
@sgugger The issue is strictly with tokenclassification class. The index of the labels gets misaligned somehow when reloading a tokenclassification model. The problem happens across many model types: bert, distilbert, roberta, etc. If just giving num_labels = x when loading the model. I believe the issue has to do with the tokenizers and the fact that setting subwords equal to -100 creates another class when training the model, but that class is no longer available when you reload a pretrained tokenclassification model using from_pretrained(local_path). <|||||>@nhsmith85 I was doing index -> class mapping using my own dictionary, not using anything internal to HuggingFace. I created a dataset class as an extension of torch.utils.data.Dataset:
```
class RecordsDataset(torch.utils.data.Dataset):
def __init__(self, encodings, labels):
self.encodings = encodings
self.labels = labels
def __getitem__(self, idx):
item = {key: torch.tensor(val[idx]) for key, val in self.encodings.items()}
item['labels'] = torch.tensor(self.labels[idx])
return item
def __len__(self):
return len(self.labels)
```
At this point the text labels had already been mapped to integers. <|||||>This issue has been automatically marked as stale because it has not had recent activity. If you think this still needs to be addressed please comment on this thread.
Please note that issues that do not follow the [contributing guidelines](https://github.com/huggingface/transformers/blob/master/CONTRIBUTING.md) are likely to be ignored.<|||||>Hello, Did anyone manage to get a solution for this? I am facing a very similar issue on `ViTForImageClassification` on using "google/vit-base-patch16-224". Upon training, I am getting an accuracy of 0.75 and a very low loss. However, once I save and reload it, say after a day, the loss is back to ~10 and accuracy is 0.
Please find the necessary parts of my code here: https://gist.github.com/thevishnupradeep/d5efc0b0510d8a30d997cadd836d2c61<|||||>Also encountering the exact same problem with DistilBERT for QA.<|||||>As of July 2023 facing same issue with Bert model. Some one suggest a fix<|||||>Saved model performance is very bad compared to online model. Why???? |
transformers | 8,271 | closed | Low accuracy after load custom pretrained model in a text binary classification problem | ## Environment info
<!-- You can run the command `transformers-cli env` and copy-and-paste its output below.
Don't forget to fill out the missing fields in that output! -->
- `transformers` version: 3.4.0
- Platform: Linux-4.15.0-122-generic-x86_64-with-Ubuntu-18.04-bionic
- Python version: 3.6.9
- PyTorch version (GPU?): 1.5.1+cpu (False)
- Tensorflow version (GPU?): 2.3.0 (False)
- Using GPU in script?: No
- Using distributed or parallel set-up in script?: Distributed (not really sure)
### Who can help
@LysandreJik
## Information
Posted in StackOverflow. Received a comment with two similar issues regarding save and load custom models. The original question can be found at: https://stackoverflow.com/questions/64666510/huggingface-transformers-low-accuracy-after-load-custom-pretrained-model-in-a-t?noredirect=1#comment114344159_64666510
In a nutshell I am using BertForSequenceClassification (PyTorch) with ```dccuchile/bert-base-spanish-wwm-cased``` for solving a binary classification problem. I have trained the network and evaluate the model with a testing dataset (different from the training dataset). I have achieved an ```acc``` and ```val_acc``` between 0.85 and 0.9. However, after I save the model and retrieve it again in another script, the accuracy is similar to a random classifier (0.41).
The problem arises when using:
* [ ] the official example scripts: (give details below)
* [X] my own modified scripts: (give details below)
The tasks I am working on is:
* [ ] an official GLUE/SQUaD task: (give the name)
* [X] my own task or dataset: (give details below)
## To reproduce
This is the code I am using for training and evaluating (during training):
```
criterion = torch.nn.CrossEntropyLoss ()
criterion = criterion.to (device)
optimizer = AdamW (model.parameters(), lr=5e-5)
for epoch in range (4):
i = 0
# Train this epoch
model.train ()
for batch in train_loader:
optimizer.zero_grad ()
input_ids = batch['input_ids'].to (device)
attention_mask = batch['attention_mask'].to (device)
labels = batch['label'].to (device)
loss, _ = model (input_ids, attention_mask=attention_mask, labels=labels)
_, preds = torch.max (_, dim=1)
correct_predictions += torch.sum (preds == labels)
i += 1
acc = correct_predictions.item () / (batch_size * i)
loss.backward ()
optimizer.step ()
# Eval this epoch with the testing dataset
model = model.eval ()
correct_predictions = 0
with torch.no_grad ():
for batch in test_loader:
input_ids = batch['input_ids'].to (device)
attention_mask = batch['attention_mask'].to (device)
labels = batch['label'].to (device)
loss, _ = model (input_ids, attention_mask=attention_mask, labels=labels)
_, preds = torch.max (_, dim=1)
correct_predictions += torch.sum (preds == labels)
model.bert.save_pretrained ("my-model")
tokenizer.save_pretrained ("my-model")
```
After this step, I got good accuracy after the first epoch
Then, I load the model again in another script
```
model = BertForSequenceClassification.from_pretrained ("my-model")
# Eval this epoch with the testing dataset
model = model.eval ()
correct_predictions = 0
with torch.no_grad ():
for batch in test_loader:
input_ids = batch['input_ids'].to (device)
attention_mask = batch['attention_mask'].to (device)
labels = batch['label'].to (device)
loss, _ = model (input_ids, attention_mask=attention_mask, labels=labels)
_, preds = torch.max (_, dim=1)
correct_predictions += torch.sum (preds == labels)
print (correct_predictions.item () / len (test_df))
```
but the accuracy is similar as If I retrieved a non-trained model.
## Expected behavior
After load a model saved with ```save_pretrained```, the model should provide similar accuracy and loss for the same data.
| 11-03-2020 19:34:32 | 11-03-2020 19:34:32 | The problem is mine. As other user suggest me in Stackoverflow, I have to save the model this way
```
model.save_pretrained ("my-model")`
``` |
transformers | 8,270 | closed | improve documentation of training_args.py | Documentation for the following fields has been improved:
- do_train
- do_eval
- do_predict
Also see #8179 | 11-03-2020 18:56:16 | 11-03-2020 18:56:16 | Thanks a lot! |
transformers | 8,269 | closed | [wip/s2s/pl] attempt to sync metrics in DDP | This is broken.
Attempted to add
`AverageMetric` where you just dump python floats and they get averaged and the end, but not working on DDP.
### Failing command
(fails quickly at val sanity check)
```bash
cd examples/seq2seq
wget https://cdn-datasets.huggingface.co/translation/wmt_en_ro.tar.gz
tar -xzvf wmt_en_ro.tar.gz
export WANDB_PROJECT=dmar
export BS=64
export m=sshleifer/mar_enro_6_3_student
export MAX_LEN=128
python finetune.py \
--learning_rate=3e-4 \
--do_train \
--do_predict \
--fp16 \
--val_check_interval 0.25 \
--data_dir wmt_en_ro \
--max_source_length $MAX_LEN --max_target_length $MAX_LEN --val_max_target_length $MAX_LEN --test_max_target_length $MAX_LEN \
--freeze_encoder --freeze_embeds \
--train_batch_size=$BS --eval_batch_size=$BS \
--tokenizer_name Helsinki-NLP/opus-mt-en-ro --model_name_or_path $m \
--warmup_steps 500 --sortish_sampler --logger_name wandb \
--gpus 2 --fp16_opt_level=O1 --task translation --num_sanity_val_steps=1 --output_dir dmar_met_test_2gpu \
--num_train_epochs=2 --overwrite_output_dir
```
### Traceback
```bash
File "/home/shleifer/transformers_fork/examples/seq2seq/finetune.py", line 206, in <dictcomp>
pl_metrics = {f"pl_{prefix}_avg_{k}": v.compute().item() for k, v in self.metric_stores.items()}
File "/home/shleifer/miniconda/lib/python3.8/site-packages/pytorch_lightning/metrics/metric.py", line 214, in wrapped_func
self._sync_dist()
File "/home/shleifer/miniconda/lib/python3.8/site-packages/pytorch_lightning/metrics/metric.py", line 177, in _sync_dist
output_dict = apply_to_collection(
File "/home/shleifer/miniconda/lib/python3.8/site-packages/pytorch_lightning/utilities/apply_func.py", line 53, in apply_to_collection
return elem_type({k: apply_to_collection(v, dtype, function, *args, **kwargs)
File "/home/shleifer/miniconda/lib/python3.8/site-packages/pytorch_lightning/utilities/apply_func.py", line 53, in <dictcomp>
return elem_type({k: apply_to_collection(v, dtype, function, *args, **kwargs)
File "/home/shleifer/miniconda/lib/python3.8/site-packages/pytorch_lightning/utilities/apply_func.py", line 49, in apply_to_collection
return function(data, *args, **kwargs)
File "/home/shleifer/miniconda/lib/python3.8/site-packages/pytorch_lightning/utilities/distributed.py", line 100, in gather_all_tensors_if_available
torch.distributed.all_gather(gathered_result, result, group)
File "/home/shleifer/miniconda/lib/python3.8/site-packages/torch/distributed/distributed_c10d.py", line 1185, in all_gather
work = _default_pg.allgather([tensor_list], [tensor])
RuntimeError: Tensors must be CUDA and dense
``` | 11-03-2020 18:40:25 | 11-03-2020 18:40:25 | This issue has been automatically marked as stale because it has not had recent activity. If you think this still needs to be addressed please comment on this thread.
Please note that issues that do not follow the [contributing guidelines](https://github.com/huggingface/transformers/blob/master/CONTRIBUTING.md) are likely to be ignored. |
transformers | 8,268 | closed | Clean Trainer tests and datasets dep | # What does this PR do?
This PR removes the installation of datasets from master and uses the dependency already in `testing` instead. It also cleans up a bit the tests in Trainer by:
- using the decorator `requires_datasets` when needed
- using a temp dir for the output of one test, to avoid some files to be created when the user has optuna installed | 11-03-2020 17:28:36 | 11-03-2020 17:28:36 | |
transformers | 8,267 | closed | [Seq2Seq] Make Seq2SeqArguments an independent file | # What does this PR do?
By putting all `Seq2SeqTrainingArguments` logic in a separate file, the `Seq2SeqTrainer` and `Seq2SeqTrainingArguments` can be used as standalone files without having to download any additional files because of other dependencies.
| 11-03-2020 17:27:11 | 11-03-2020 17:27:11 | |
transformers | 8,266 | closed | german medbert model details | # What does this PR do?
- added details for German MedBERT
## Before submitting
- [x] This PR fixes a typo or improves the docs (you can dismiss the other checks if that's the case).
- [x] Did you read the [contributor guideline](https://github.com/huggingface/transformers/blob/master/CONTRIBUTING.md#start-contributing-pull-requests),
Pull Request section?
- [ ] Was this discussed/approved via a Github issue or the [forum](https://discuss.huggingface.co/)? Please add a link
to the it if that's the case.
- [x] Did you make sure to update the documentation with your changes? Here are the
[documentation guidelines](https://github.com/huggingface/transformers/tree/master/docs), and
[here are tips on formatting docstrings](https://github.com/huggingface/transformers/tree/master/docs#writing-source-documentation).
- [ ] Did you write any new necessary tests?
## Who can review?
Anyone in the community is free to review the PR once the tests have passed. Feel free to tag
members/contributors which may be interested in your PR.
<!-- Your PR will be replied to more quickly if you can figure out the right person to tag with @
If you know how to use git blame, that is the easiest way, otherwise, here is a rough guide of **who to tag**.
Please tag fewer than 3 people.
albert, bert, XLM: @LysandreJik
GPT2: @LysandreJik, @patrickvonplaten
tokenizers: @mfuntowicz
Trainer: @sgugger
Benchmarks: @patrickvonplaten
Model Cards: @julien-c
Translation: @sshleifer
Summarization: @sshleifer
examples/distillation: @VictorSanh
nlp datasets: [different repo](https://github.com/huggingface/nlp)
rust tokenizers: [different repo](https://github.com/huggingface/tokenizers)
Text Generation: @patrickvonplaten, @TevenLeScao
Blenderbot, Bart, Marian, Pegasus: @sshleifer
T5: @patrickvonplaten
Rag: @patrickvonplaten, @lhoestq
EncoderDecoder: @patrickvonplaten
Longformer, Reformer: @patrickvonplaten
TransfoXL, XLNet: @TevenLeScao, @patrickvonplaten
examples/seq2seq: @sshleifer
examples/bert-loses-patience: @JetRunner
tensorflow: @jplu
examples/token-classification: @stefan-it
documentation: @sgugger
-->
| 11-03-2020 16:56:58 | 11-03-2020 16:56:58 | |
transformers | 8,265 | closed | Is there a pre-trained BERT model with the sequence length of 2048? | Hello,
I want to use the pre-trained BERT model because I do not want to train the entire BERT model to analyze my data. Is there a pre-trained BERT model with sequence length 2048?
or are all pre-trained BERT model only have the sequence length of 512?
Thank you. | 11-03-2020 16:52:34 | 11-03-2020 16:52:34 | This issue has been automatically marked as stale because it has not had recent activity. It will be closed if no further activity occurs. Thank you for your contributions.
|
transformers | 8,264 | closed | New TensorFlow trainer version | Hello,
This PR is a proposal for an updated version of the current TensorFlow trainer. This new trainer brings the following improvements:
- Uses the Keras methods `.compile()` + `.fit()` instead of the custom training loop. This change brings a better integration with TensorFlow and the different strategies that can be used for training a model. It takes also advantages of all the optimizations done by the Google team for a proper training.
- Uses the Keras methods `.evaluate()` and `predict()` instead of the custom evaluation loop. Same advantages than for the training part.
- Uses the Keras callbacks and metrics. We can takes advantages of the callback and metric features proposed by default when training/evaluate a model with Keras. Also one can create its own callbacks and metrics and use them for the training/evaluation.
- Big reduction in terms of line of codes which makes it easier to maintain.
- Create a new optimizer for gradient accumulation to move the logic inside this new optimizer than inside the trainer instead.
Of course this is still far to be finished and there is still work to do but you can easily see the direction I'm thinking of.
@LysandreJik @sgugger I will be happy to ear your comments.
@thomwolf @lhoestq Here I have created a file where I put all the Keras metrics, but we should definitely think a way to integrate such metrics directly inside `datasets` where they will be better suited. | 11-03-2020 16:43:13 | 11-03-2020 16:43:13 | # 1. Input data
> The PyTorch side of the library (and all the PyTorch scripts) have datasets that eventually yield dictionaries containing all inputs of the model as well as the labels. In an ideal world, it would be great if we could leverage that format easily for TF as well (so that the same script can be used for PT and TF by just changing a few lines, especially when using the datasets library). I don't know if that's possible or not but one thing to explore more I believe.
Can you elaborate a bit more please? Do you mean that the input data given to the `.fit()` method should be a dictionary? If it is what you mean, it is already the case.
# 2. Optimizer and scheduler
> I like the new optimizer for gradient accumulation a lot. This feels like a very good design. Should we deprecate GradientAccumulator?
Yes, this should be deprecated because we won't use it anymore.
> But where are the schedulers? Is this something you intend to control via callbacks? If that's the case I didn't see one with a sensible default (the linear + warmup used in PT for instance).
The schedulers are directly inside the optimizer, if you look at the `create_optimizer` method you can see that the scheduler is first created and then given to the Adam optimizer as input for the `learning_rate` parameter. In the previous Trainer the scheduler was returned only for being used in the logging, the scheduling is done automatically internally in the `tf.keras.optimizers.Optimizer` class.
# 3. Callbacks
> Leveraging Keras callbacks is definitely a good idea. My only remark here is that is should be more customizable. On the PT side we have some default callbacks and the init takes a list of additional callbacks the user can add.
No worries, this will be added in the next push :)
# 4. Metrics
> I'm not in favor of adding a new file of metrics we will have to maintain forever. We should provide an adapter for datasets Metric object and rely on the datasets library (or existing Keras metrics if users prefer them) but they shouldn't be in the transformers library (on the PT we will soon deprecate the ones in the metric folder).
I fully agree, and this is why I asked @thomwolf and @lhoestq opinion's on this on the best way to integrate Keras metrics inside datasets :) For this will require, I think, a non negligeable amont of work in `datasets` that I would prefer to do not do alone.<|||||>> Can you elaborate a bit more please? Do you mean that the input data given to the `.fit()` method should be a dictionary? If it is what you mean, it is already the case.
If you look at the new example scripts (like [run_glue](https://github.com/huggingface/transformers/blob/master/examples/text-classification/run_glue.py)) the datasets are immediately sent to `Trainer` with no platform-specific processing needed. It would be really cool if we could just replace `Trainer` by `TFTrainer` in that script and have it work the same way. I'm not sure if the easiest for that is to change the input of `training_step` or do some internal processing of the dataset inside `TFTrainer`.<|||||>> If you look at the new example scripts (like run_glue) the datasets are immediately sent to Trainer with no platform-specific processing needed. It would be really cool if we could just replace Trainer by TFTrainer in that script and have it work the same way. I'm not sure if the easiest for that is to change the input of training_step or do some internal processing of the dataset inside TFTrainer.
Hum I see. As a first glance, I would say it will requires much more changes than just replace Trainer by TFTrainer, at least all the metrics part won't be compliant, and the way we create the model is different (in TF we have to do that in a strategy).<|||||>This issue has been automatically marked as stale because it has not had recent activity. If you think this still needs to be addressed please comment on this thread.
Please note that issues that do not follow the [contributing guidelines](https://github.com/huggingface/transformers/blob/master/CONTRIBUTING.md) are likely to be ignored. |
transformers | 8,263 | closed | GPT2 is not jit-traceable | ## Information
I would like to use Pytorch tracing on a pretrained GPT2 model, but I run into these warnings for the attention layers:
```
python3.8/site-packages/transformers/modeling_gpt2.py:164: TracerWarning: Converting a tensor to a Python float might cause the trace to be incorrect. We can't record the data flow of Python values, so this value will be treated as a constant in the future. This means that the trace might not generalize to other inputs!
w = w / (float(v.size(-1)) ** 0.5)
python3.8/site-packages/transformers/modeling_gpt2.py:169: TracerWarning: Converting a tensor to a Python index might cause the trace to be incorrect. We can't record the data flow of Python values, so this value will be treated as a constant in the future. This means that the trace might not generalize to other inputs!
mask = self.bias[:, :, ns - nd : ns, :ns]
```
The first warning concern the same line as the one reported in #3954 (and fixed by #3955).
## To reproduce
You can run the following:
```
import torch
from transformers import GPT2Tokenizer, GPT2LMHeadModel
tokenizer = GPT2Tokenizer.from_pretrained('gpt2')
model = GPT2LMHeadModel.from_pretrained('gpt2')
model.eval()
tokens=tokenizer('The cat is on the table.', return_tensors='pt')['input_ids']
with torch.jit.optimized_execution(True):
traced_model = torch.jit.trace(model, tokens)
```
## Environment info
- `transformers` version: 3.4.0
- Platform: Linux-5.4.0-52-generic-x86_64-with-glibc2.29
- Python version: 3.8.5
- PyTorch version (GPU?): 1.7.0+cpu (False)
- Using GPU in script?: NO
- Using distributed or parallel set-up in script?: NO
| 11-03-2020 16:29:36 | 11-03-2020 16:29:36 | Hi! I believe those are warnings and not errors? Does it change the expected results when tracing the model?<|||||>You are right, despite the warnings (and my - limited - understanding of what should work inside tracing), the output of the compiled model with different inputs are comparable to the base ones.
Thanks a lot!<|||||>Glad it works!<|||||>@gcompagnoni @LysandreJik
Hi, there
Why do I try the example below occurs error ? issue reported in #15598
```
import torch
from transformers import GPT2Tokenizer, GPT2LMHeadModel
tokenizer = GPT2Tokenizer.from_pretrained('gpt2')
model = GPT2LMHeadModel.from_pretrained('gpt2')
model.eval()
tokens=tokenizer('The cat is on the table.', return_tensors='pt')['input_ids']
with torch.jit.optimized_execution(True):
traced_model = torch.jit.trace(model, tokens)
```
|
transformers | 8,262 | closed | [distributed testing] forward the worker stderr to the parent process | As discussed on slack, this PR:
* on distributed failure reproduces the combined `stderr` of the worker processes in the exception of the test invoking the distributed process
This is so that the CI's new optimized reports will include the full error message.
@sgugger | 11-03-2020 16:24:18 | 11-03-2020 16:24:18 | |
transformers | 8,261 | closed | Encoder Decoder Model | Hi,
I am following the instructions written on the HuggingFace website to use an encoder-decoder model:
from transformers import EncoderDecoderModel, BertTokenizer
import torch
```
tokenizer = BertTokenizer.from_pretrained('bert-base-uncased')
model = EncoderDecoderModel.from_encoder_decoder_pretrained('bert-base-uncased', 'bert-base-uncased') # initialize Bert2Bert from pre-trained checkpoints
#model.save_pretrained('/content/drive/My Drive/NLP/'+'model_1')
# forward
input_ids = torch.tensor(tokenizer.encode("Hello, my dog is cute", add_special_tokens=True)).unsqueeze(0) # Batch size 1
outputs = model(input_ids=input_ids, decoder_input_ids=input_ids)
# training
outputs = model(input_ids=input_ids, decoder_input_ids=input_ids, labels=input_ids, return_dict=True)
#print(type(outputs)) #Seq2SeqLMOutput
loss, logits = outputs.loss, outputs.logits
# save and load from pretrained
#model.save_pretrained("bert2bert")
#model = EncoderDecoderModel.from_pretrained("bert2bert")
# generation
generated = model.generate(input_ids, decoder_start_token_id=model.config.decoder.pad_token_id)
generated
tensor([[ 0, 1012, 1010, 1010, 1010, 1010, 1010, 1010, 1010, 1010, 1010, 1010,
1010, 1010, 1010, 1010, 1010, 1010, 1010, 1010]])
```
However, I have no idea how to decode the generated output, can anybody pls help?
Thank you | 11-03-2020 15:36:27 | 11-03-2020 15:36:27 | Maybe I found out, is it:
```
for i, sample_output in enumerate(generated):
print("{}: {}".format(i, tokenizer.decode(sample_output, skip_special_tokens=True)))
```
?<|||||>You can also make use of `tokenizer.batch_decode(...)` |
transformers | 8,260 | closed | [fix] Skip tatoeba tests if Tatoeba-Challenge not cloned | 11-03-2020 14:48:26 | 11-03-2020 14:48:26 | ||
transformers | 8,259 | closed | Disable default sigmoid function for single label classification Inference API | # 🚀 Feature request
Allow people to disable the default sigmoid function in TextClassificationPipeline (maybe via model cards?).
## Motivation
When we use the sequence classification model (e.g. RobertaForSequenceClassification) for regression tasks, the output may have different ranges other than [0,1], it would be better to allow configurations for the sigmoid function in TextClassificationPipeline.
| 11-03-2020 13:31:55 | 11-03-2020 13:31:55 | Hi! Right now the sigmoid function is applied when the pipeline detects that there is a single label. You would like the option to disable the sigmoid function in that case?<|||||>@LysandreJik
Sorry for my late reply.
Yes, cuz when people are doing regression tasks using the single-label SequenceClassification model, the output range depends on the specific task. For example, when predicting age from the text, [0,1] output after a sigmoid function is not a good fit here. <|||||>Indeed, I understand! I'm adding an option to return the raw outputs in #8328 <|||||>Thank you! I'm also wondering if this will be reflected by the Inference API? The inference API is using the sequence classification pipeline, therefore the API output on my model page is different from the original model output, which might confuse potential users. <|||||>@Jiaxin-Pei see discussion in #8328<|||||>This issue has been automatically marked as stale because it has not had recent activity. It will be closed if no further activity occurs. Thank you for your contributions.
|
transformers | 8,258 | closed | Create README.md | # What does this PR do?
<!--
Congratulations! You've made it this far! You're not quite done yet though.
Once merged, your PR is going to appear in the release notes with the title you set, so make sure it's a great title that fully reflects the extent of your awesome contribution.
Then, please replace this with a description of the change and which issue is fixed (if applicable). Please also include relevant motivation and context. List any dependencies (if any) that are required for this change.
Once you're done, someone will review your PR shortly (see the section "Who can review?" below to tag some potential reviewers). They may suggest changes to make the code even better. If no one reviewed your PR after a week has passed, don't hesitate to post a new comment @-mentioning the same persons---sometimes notifications get lost.
-->
<!-- Remove if not applicable -->
Fixes # (issue)
## Before submitting
- [ ] This PR fixes a typo or improves the docs (you can dismiss the other checks if that's the case).
- [ ] Did you read the [contributor guideline](https://github.com/huggingface/transformers/blob/master/CONTRIBUTING.md#start-contributing-pull-requests),
Pull Request section?
- [ ] Was this discussed/approved via a Github issue or the [forum](https://discuss.huggingface.co/)? Please add a link
to the it if that's the case.
- [ ] Did you make sure to update the documentation with your changes? Here are the
[documentation guidelines](https://github.com/huggingface/transformers/tree/master/docs), and
[here are tips on formatting docstrings](https://github.com/huggingface/transformers/tree/master/docs#writing-source-documentation).
- [ ] Did you write any new necessary tests?
## Who can review?
Anyone in the community is free to review the PR once the tests have passed. Feel free to tag
members/contributors which may be interested in your PR.
<!-- Your PR will be replied to more quickly if you can figure out the right person to tag with @
If you know how to use git blame, that is the easiest way, otherwise, here is a rough guide of **who to tag**.
Please tag fewer than 3 people.
albert, bert, XLM: @LysandreJik
GPT2: @LysandreJik, @patrickvonplaten
tokenizers: @mfuntowicz
Trainer: @sgugger
Benchmarks: @patrickvonplaten
Model Cards: @julien-c
Translation: @sshleifer
Summarization: @sshleifer
examples/distillation: @VictorSanh
nlp datasets: [different repo](https://github.com/huggingface/nlp)
rust tokenizers: [different repo](https://github.com/huggingface/tokenizers)
Text Generation: @patrickvonplaten, @TevenLeScao
Blenderbot, Bart, Marian, Pegasus: @sshleifer
T5: @patrickvonplaten
Rag: @patrickvonplaten, @lhoestq
EncoderDecoder: @patrickvonplaten
Longformer, Reformer: @patrickvonplaten
TransfoXL, XLNet: @TevenLeScao, @patrickvonplaten
examples/seq2seq: @sshleifer
examples/bert-loses-patience: @JetRunner
tensorflow: @jplu
examples/token-classification: @stefan-it
documentation: @sgugger
-->
| 11-03-2020 13:16:49 | 11-03-2020 13:16:49 | Add model card for pedropei/question-intimacy |
transformers | 8,257 | closed | Create README.md | # What does this PR do?
<!--
Congratulations! You've made it this far! You're not quite done yet though.
Once merged, your PR is going to appear in the release notes with the title you set, so make sure it's a great title that fully reflects the extent of your awesome contribution.
Then, please replace this with a description of the change and which issue is fixed (if applicable). Please also include relevant motivation and context. List any dependencies (if any) that are required for this change.
Once you're done, someone will review your PR shortly (see the section "Who can review?" below to tag some potential reviewers). They may suggest changes to make the code even better. If no one reviewed your PR after a week has passed, don't hesitate to post a new comment @-mentioning the same persons---sometimes notifications get lost.
-->
<!-- Remove if not applicable -->
Fixes # (issue)
## Before submitting
- [ ] This PR fixes a typo or improves the docs (you can dismiss the other checks if that's the case).
- [ ] Did you read the [contributor guideline](https://github.com/huggingface/transformers/blob/master/CONTRIBUTING.md#start-contributing-pull-requests),
Pull Request section?
- [ ] Was this discussed/approved via a Github issue or the [forum](https://discuss.huggingface.co/)? Please add a link
to the it if that's the case.
- [ ] Did you make sure to update the documentation with your changes? Here are the
[documentation guidelines](https://github.com/huggingface/transformers/tree/master/docs), and
[here are tips on formatting docstrings](https://github.com/huggingface/transformers/tree/master/docs#writing-source-documentation).
- [ ] Did you write any new necessary tests?
## Who can review?
Anyone in the community is free to review the PR once the tests have passed. Feel free to tag
members/contributors which may be interested in your PR.
<!-- Your PR will be replied to more quickly if you can figure out the right person to tag with @
If you know how to use git blame, that is the easiest way, otherwise, here is a rough guide of **who to tag**.
Please tag fewer than 3 people.
albert, bert, XLM: @LysandreJik
GPT2: @LysandreJik, @patrickvonplaten
tokenizers: @mfuntowicz
Trainer: @sgugger
Benchmarks: @patrickvonplaten
Model Cards: @julien-c
Translation: @sshleifer
Summarization: @sshleifer
examples/distillation: @VictorSanh
nlp datasets: [different repo](https://github.com/huggingface/nlp)
rust tokenizers: [different repo](https://github.com/huggingface/tokenizers)
Text Generation: @patrickvonplaten, @TevenLeScao
Blenderbot, Bart, Marian, Pegasus: @sshleifer
T5: @patrickvonplaten
Rag: @patrickvonplaten, @lhoestq
EncoderDecoder: @patrickvonplaten
Longformer, Reformer: @patrickvonplaten
TransfoXL, XLNet: @TevenLeScao, @patrickvonplaten
examples/seq2seq: @sshleifer
examples/bert-loses-patience: @JetRunner
tensorflow: @jplu
examples/token-classification: @stefan-it
documentation: @sgugger
-->
| 11-03-2020 13:13:23 | 11-03-2020 13:13:23 | |
transformers | 8,256 | closed | [FIX] TextGenerationPipeline is currently broken. | # What does this PR do?
It's most likely due to #8180. What's missing is a multi vs single string
handler at the beginning of the pipe. And also there was no testing of this
pipeline.
This also changes Conversational pipeline tests which seemed to have also test failures
This was linked to having state within the input that get consumed and the tests did not recreate
them so we had a stale `Conversation` object for the new test.
<!--
Congratulations! You've made it this far! You're not quite done yet though.
Once merged, your PR is going to appear in the release notes with the title you set, so make sure it's a great title that fully reflects the extent of your awesome contribution.
Then, please replace this with a description of the change and which issue is fixed (if applicable). Please also include relevant motivation and context. List any dependencies (if any) that are required for this change.
Once you're done, someone will review your PR shortly (see the section "Who can review?" below to tag some potential reviewers). They may suggest changes to make the code even better. If no one reviewed your PR after a week has passed, don't hesitate to post a new comment @-mentioning the same persons---sometimes notifications get lost.
-->
<!-- Remove if not applicable -->
## Before submitting
- [ ] This PR fixes a typo or improves the docs (you can dismiss the other checks if that's the case).
- [x] Did you read the [contributor guideline](https://github.com/huggingface/transformers/blob/master/CONTRIBUTING.md#start-contributing-pull-requests),
Pull Request section?
- [ ] Was this discussed/approved via a Github issue or the [forum](https://discuss.huggingface.co/)? Please add a link
to the it if that's the case.
- [ ] Did you make sure to update the documentation with your changes? Here are the
[documentation guidelines](https://github.com/huggingface/transformers/tree/master/docs), and
[here are tips on formatting docstrings](https://github.com/huggingface/transformers/tree/master/docs#writing-source-documentation).
- [x] Did you write any new necessary tests?
## Who can review?
Anyone in the community is free to review the PR once the tests have passed. Feel free to tag
members/contributors which may be interested in your PR.
@thomwolf
<!-- Your PR will be replied to more quickly if you can figure out the right person to tag with @
If you know how to use git blame, that is the easiest way, otherwise, here is a rough guide of **who to tag**.
Please tag fewer than 3 people.
albert, bert, XLM: @LysandreJik
GPT2: @LysandreJik, @patrickvonplaten
tokenizers: @mfuntowicz
Trainer: @sgugger
Benchmarks: @patrickvonplaten
Model Cards: @julien-c
Translation: @sshleifer
Summarization: @sshleifer
examples/distillation: @VictorSanh
nlp datasets: [different repo](https://github.com/huggingface/nlp)
rust tokenizers: [different repo](https://github.com/huggingface/tokenizers)
Text Generation: @patrickvonplaten, @TevenLeScao
Blenderbot, Bart, Marian, Pegasus: @sshleifer
T5: @patrickvonplaten
Rag: @patrickvonplaten, @lhoestq
EncoderDecoder: @patrickvonplaten
Longformer, Reformer: @patrickvonplaten
TransfoXL, XLNet: @TevenLeScao, @patrickvonplaten
examples/seq2seq: @sshleifer
examples/bert-loses-patience: @JetRunner
tensorflow: @jplu
examples/token-classification: @stefan-it
documentation: @sgugger
--> | 11-03-2020 12:17:42 | 11-03-2020 12:17:42 | Ran all pipeline tests with @slow too to make sure:
```
==================================================================== warnings summary ====================================================================
.venv/lib/python3.8/site-packages/tensorflow/python/autograph/utils/testing.py:21
/home/nicolas/src/transformers/.venv/lib/python3.8/site-packages/tensorflow/python/autograph/utils/testing.py:21: DeprecationWarning: the imp module is
deprecated in favour of importlib; see the module's documentation for alternative uses
import imp
tests/test_pipelines_fill_mask.py::FillMaskPipelineTests::test_tf_fill_mask_results
/home/nicolas/src/transformers/src/transformers/pipelines.py:1200: FutureWarning: The `topk` argument is deprecated and will be removed in a future vers
ion, use `top_k` instead.
warnings.warn(
-- Docs: https://docs.pytest.org/en/stable/warnings.html
====================================================== 93 passed, 2 warnings in 2234.37s (0:37:14) =======================================================
``` |
transformers | 8,255 | closed | Create README.md | Initial commit
# What does this PR do?
<!--
Congratulations! You've made it this far! You're not quite done yet though.
Once merged, your PR is going to appear in the release notes with the title you set, so make sure it's a great title that fully reflects the extent of your awesome contribution.
Then, please replace this with a description of the change and which issue is fixed (if applicable). Please also include relevant motivation and context. List any dependencies (if any) that are required for this change.
Once you're done, someone will review your PR shortly (see the section "Who can review?" below to tag some potential reviewers). They may suggest changes to make the code even better. If no one reviewed your PR after a week has passed, don't hesitate to post a new comment @-mentioning the same persons---sometimes notifications get lost.
-->
<!-- Remove if not applicable -->
Fixes # (issue)
## Before submitting
- [ ] This PR fixes a typo or improves the docs (you can dismiss the other checks if that's the case).
- [ ] Did you read the [contributor guideline](https://github.com/huggingface/transformers/blob/master/CONTRIBUTING.md#start-contributing-pull-requests),
Pull Request section?
- [ ] Was this discussed/approved via a Github issue or the [forum](https://discuss.huggingface.co/)? Please add a link
to the it if that's the case.
- [ ] Did you make sure to update the documentation with your changes? Here are the
[documentation guidelines](https://github.com/huggingface/transformers/tree/master/docs), and
[here are tips on formatting docstrings](https://github.com/huggingface/transformers/tree/master/docs#writing-source-documentation).
- [ ] Did you write any new necessary tests?
## Who can review?
Anyone in the community is free to review the PR once the tests have passed. Feel free to tag
members/contributors which may be interested in your PR.
<!-- Your PR will be replied to more quickly if you can figure out the right person to tag with @
If you know how to use git blame, that is the easiest way, otherwise, here is a rough guide of **who to tag**.
Please tag fewer than 3 people.
albert, bert, XLM: @LysandreJik
GPT2: @LysandreJik, @patrickvonplaten
tokenizers: @mfuntowicz
Trainer: @sgugger
Benchmarks: @patrickvonplaten
Model Cards: @julien-c
Translation: @sshleifer
Summarization: @sshleifer
examples/distillation: @VictorSanh
nlp datasets: [different repo](https://github.com/huggingface/nlp)
rust tokenizers: [different repo](https://github.com/huggingface/tokenizers)
Text Generation: @patrickvonplaten, @TevenLeScao
Blenderbot, Bart, Marian, Pegasus: @sshleifer
T5: @patrickvonplaten
Rag: @patrickvonplaten, @lhoestq
EncoderDecoder: @patrickvonplaten
Longformer, Reformer: @patrickvonplaten
TransfoXL, XLNet: @TevenLeScao, @patrickvonplaten
examples/seq2seq: @sshleifer
examples/bert-loses-patience: @JetRunner
tensorflow: @jplu
examples/token-classification: @stefan-it
documentation: @sgugger
-->
| 11-03-2020 12:11:01 | 11-03-2020 12:11:01 | why is the model card not visible under transformer/model_cards/tartuNLP/EstBERT/README.md link?
I am quite new to git thingy :S |
transformers | 8,254 | closed | [Seq2Seq] Correct import in Seq2Seq Trainer | # What does this PR do?
Correct import as mentioned by @stas00 here: https://github.com/huggingface/transformers/pull/8194#discussion_r515690821
Pinging @stas00 for review as well here.
<!--
Congratulations! You've made it this far! You're not quite done yet though.
Once merged, your PR is going to appear in the release notes with the title you set, so make sure it's a great title that fully reflects the extent of your awesome contribution.
Then, please replace this with a description of the change and which issue is fixed (if applicable). Please also include relevant motivation and context. List any dependencies (if any) that are required for this change.
Once you're done, someone will review your PR shortly (see the section "Who can review?" below to tag some potential reviewers). They may suggest changes to make the code even better. If no one reviewed your PR after a week has passed, don't hesitate to post a new comment @-mentioning the same persons---sometimes notifications get lost.
-->
<!-- Remove if not applicable -->
## Before submitting
- [ ] This PR fixes a typo or improves the docs (you can dismiss the other checks if that's the case).
- [ ] Did you read the [contributor guideline](https://github.com/huggingface/transformers/blob/master/CONTRIBUTING.md#start-contributing-pull-requests),
Pull Request section?
- [ ] Was this discussed/approved via a Github issue or the [forum](https://discuss.huggingface.co/)? Please add a link
to the it if that's the case.
- [ ] Did you make sure to update the documentation with your changes? Here are the
[documentation guidelines](https://github.com/huggingface/transformers/tree/master/docs), and
[here are tips on formatting docstrings](https://github.com/huggingface/transformers/tree/master/docs#writing-source-documentation).
- [ ] Did you write any new necessary tests?
## Who can review?
Anyone in the community is free to review the PR once the tests have passed. Feel free to tag
members/contributors which may be interested in your PR.
<!-- Your PR will be replied to more quickly if you can figure out the right person to tag with @
If you know how to use git blame, that is the easiest way, otherwise, here is a rough guide of **who to tag**.
Please tag fewer than 3 people.
albert, bert, XLM: @LysandreJik
GPT2: @LysandreJik, @patrickvonplaten
tokenizers: @mfuntowicz
Trainer: @sgugger
Benchmarks: @patrickvonplaten
Model Cards: @julien-c
Translation: @sshleifer
Summarization: @sshleifer
examples/distillation: @VictorSanh
nlp datasets: [different repo](https://github.com/huggingface/nlp)
rust tokenizers: [different repo](https://github.com/huggingface/tokenizers)
Text Generation: @patrickvonplaten, @TevenLeScao
Blenderbot, Bart, Marian, Pegasus: @sshleifer
T5: @patrickvonplaten
Rag: @patrickvonplaten, @lhoestq
EncoderDecoder: @patrickvonplaten
Longformer, Reformer: @patrickvonplaten
TransfoXL, XLNet: @TevenLeScao, @patrickvonplaten
examples/seq2seq: @sshleifer
examples/bert-loses-patience: @JetRunner
tensorflow: @jplu
examples/token-classification: @stefan-it
documentation: @sgugger
-->
| 11-03-2020 10:59:23 | 11-03-2020 10:59:23 | |
transformers | 8,253 | closed | when the txt file has 5GB, a Killed prompt appears. | I am running run_language_modeling.py,
python run_language_modeling.py \
--output_dir ${model_dir} \
--tokenizer_name $data_dir/wordpiece-custom.json \
--config_name $data_dir/config.json \
--train_data_file "$data_dir/train.txt" \
--eval_data_file $data_dir/valid.txt \
--block_size=128 \
--do_train \
--per_device_train_batch_size 1 \
--gradient_accumulation_steps 64 \
--learning_rate 6e-4 \
--weight_decay 0.01 \
--adam_epsilon 1e-6 \
--adam_beta1 0.9 \
--adam_beta2 0.98 \
--max_steps 500_000 \
--warmup_steps 24_000 \
--fp16 \
--logging_dir ${model_dir}/tensorboard \
--save_steps 1000 \
--save_total_limit 20 \
--seed 108 \
--max_steps -1 \
--num_train_epochs 20 \
--overwrite_output_dir
when the txt file has 5GB, a Killed prompt appears.
| 11-03-2020 09:53:03 | 11-03-2020 09:53:03 | What is your machine's specs? It's probably an out of memory error.<|||||>This issue has been automatically marked as stale because it has not had recent activity. It will be closed if no further activity occurs. Thank you for your contributions.
|
transformers | 8,252 | closed | Updated Reformer to use caching during generation | # What does this PR do?
The current reformer implementation supports caching of buckets and states, but this is not used during generation. Running a generation example in debugging mode, such as
```python
from transformers import ReformerModelWithLMHead, ReformerTokenizer
model = ReformerModelWithLMHead.from_pretrained("google/reformer-crime-and-punishment").cuda()
tok = ReformerTokenizer.from_pretrained("google/reformer-crime-and-punishment")
output = tok.decode(
model.generate(tok.encode("Notwithstanding", return_tensors="pt").cuda(),
do_sample=True,
temperature=0.7,
max_length=100,
use_cache=True)[0])
```
One can see that the `past_buckets_states` passed to the attention are always `None` (at https://github.com/huggingface/transformers/blob/504ff7bb1234991eb07595c123b264a8a1064bd3/src/transformers/modeling_reformer.py#L365)
This is because the name of the past states for the reformer are neither `past_key_values` or `mems`.
This PR adds the name of the past states to the generation `past` allocation.
Generally, it may make sense to harmonize the `past` value for all models, so that the `generate` function generalizes better
## Who can review?
Text Generation: @patrickvonplaten, @TevenLeScao
Reformer: @patrickvonplaten
| 11-03-2020 09:30:00 | 11-03-2020 09:30:00 | Great catch!<|||||>Let's merge that quickly so that I can integrate it into https://github.com/huggingface/transformers/pull/6949/files#diff-b7601d397d5d60326ce61a9c91beaa2afa026014141052b32b07e1d044fbbe17<|||||>Actually, we would have to add in two spots of this `generate` version. Considering that we will merge the big generate refactor today, I just added your fix quickly here: https://github.com/huggingface/transformers/pull/6949/commits/12b54eceeb57229ffd940cadf47e6e159b101d8e
Mentioned your PR at the fix - hope it's ok for you to close this PR to avoid any more merge conflicts.
Thanks a lot! |
transformers | 8,251 | closed | Train BERT with CLI commands | I have downloaded the HuggingFace BERT model from the transformer repository found [here][1] and would like to train the model on custom NER labels by using the run_ner.py script as it is referenced [here][2] in the section "Named Entity Recognition".
I define model ("bert-base-german-cased"), data_dir ("Data/sentence_data.txt") and labels ("Data/labels.txt)" as defaults in the code.
Now I'm using this input for the command line:
```
python run_ner.py --output_dir="Models" --num_train_epochs=3 --logging_steps=100 --do_train --do_eval --do_predict
```
But all it does is telling me:
```
Some weights of the model checkpoint at bert-base-german-cased were not used when initializing BertForTokenClassification: ['cls.predictions.bias', 'cls.predictions.transform.dense.weight', 'cls.predictions.transform.dense.bias', 'cls.predictions.transform.LayerNorm.w
eight', 'cls.predictions.transform.LayerNorm.bias', 'cls.predictions.decoder.weight', 'cls.seq_relationship.weight', 'cls.seq_relationship.bias']
- This IS expected if you are initializing BertForTokenClassification from the checkpoint of a model trained on another task or with another architecture (e.g. initializing a BertForSequenceClassification model from a BertForPretraining model).
- This IS NOT expected if you are initializing BertForTokenClassification from the checkpoint of a model that you expect to be exactly identical (initializing a BertForSequenceClassification model from a BertForSequenceClassification model).
Some weights of BertForTokenClassification were not initialized from the model checkpoint at bert-base-german-cased and are newly initialized: ['classifier.weight', 'classifier.bias']
You should probably TRAIN this model on a down-stream task to be able to use it for predictions and inference.
```
After that it just stops, not ending the script, but simply waiting.
Does anyone know what could be the problem here? Am I missing a parameter?
My sentence_data.txt in CoNLL format looks like this (small snippet):
```
Strafverfahren O
gegen O
; O
wegen O
Diebstahls O
hat O
das O
Amtsgericht Ort
Leipzig Ort
- O
Strafrichter O
```
And that's how I defined my labels in labels.txt:
```
"Date", "Delikt", "Strafe_Tatbestand", "Schadensbetrag", "Geständnis_ja", "Vorstrafe_ja", "Vorstrafe_nein", "Ort",
"Strafe_Gesamtfreiheitsstrafe_Dauer", "Strafe_Gesamtsatz_Dauer", "Strafe_Gesamtsatz_Betrag"
```
[1]: https://github.com/huggingface/transformers
[2]: https://huggingface.co/transformers/task_summary.html | 11-03-2020 09:03:48 | 11-03-2020 09:03:48 | It's probably tokenizing. How big is your dataset?<|||||>This issue has been automatically marked as stale because it has not had recent activity. It will be closed if no further activity occurs. Thank you for your contributions.
|
transformers | 8,250 | closed | tokenizer.vocab key and values is change begin line 261? | transformers version 3.0.0
```python
tokenizer = BertTokenizer.from_pretrained('bert-base-chinese')
for v, i in tokenizer.vocab.items():
print(v, i)
```
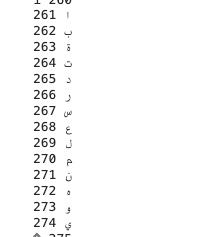
i find key and values maybe wrong position. | 11-03-2020 07:32:06 | 11-03-2020 07:32:06 | This issue has been automatically marked as stale because it has not had recent activity. It will be closed if no further activity occurs. Thank you for your contributions.
|
transformers | 8,249 | closed | [ray] Support `n_jobs` for Ray hyperparameter search on CPUs | # What does this PR do?
<!--
Congratulations! You've made it this far! You're not quite done yet though.
Once merged, your PR is going to appear in the release notes with the title you set, so make sure it's a great title that fully reflects the extent of your awesome contribution.
Then, please replace this with a description of the change and which issue is fixed (if applicable). Please also include relevant motivation and context. List any dependencies (if any) that are required for this change.
Once you're done, someone will review your PR shortly (see the section "Who can review?" below to tag some potential reviewers). They may suggest changes to make the code even better. If no one reviewed your PR after a week has passed, don't hesitate to post a new comment @-mentioning the same persons---sometimes notifications get lost.
-->
<!-- Remove if not applicable -->
Fixes # (issue)
## Before submitting
- [ ] This PR fixes a typo or improves the docs (you can dismiss the other checks if that's the case).
- [ ] Did you read the [contributor guideline](https://github.com/huggingface/transformers/blob/master/CONTRIBUTING.md#start-contributing-pull-requests),
Pull Request section?
- [ ] Was this discussed/approved via a Github issue or the [forum](https://discuss.huggingface.co/)? Please add a link
to the it if that's the case.
- [ ] Did you make sure to update the documentation with your changes? Here are the
[documentation guidelines](https://github.com/huggingface/transformers/tree/master/docs), and
[here are tips on formatting docstrings](https://github.com/huggingface/transformers/tree/master/docs#writing-source-documentation).
- [ ] Did you write any new necessary tests?
## Who can review?
Anyone in the community is free to review the PR once the tests have passed. Feel free to tag
members/contributors which may be interested in your PR.
<!-- Your PR will be replied to more quickly if you can figure out the right person to tag with @
If you know how to use git blame, that is the easiest way, otherwise, here is a rough guide of **who to tag**.
Please tag fewer than 3 people.
albert, bert, XLM: @LysandreJik
GPT2: @LysandreJik, @patrickvonplaten
tokenizers: @mfuntowicz
Trainer: @sgugger
Benchmarks: @patrickvonplaten
Model Cards: @julien-c
Translation: @sshleifer
Summarization: @sshleifer
examples/distillation: @VictorSanh
nlp datasets: [different repo](https://github.com/huggingface/nlp)
rust tokenizers: [different repo](https://github.com/huggingface/tokenizers)
Text Generation: @patrickvonplaten, @TevenLeScao
Blenderbot, Bart, Marian, Pegasus: @sshleifer
T5: @patrickvonplaten
Rag: @patrickvonplaten, @lhoestq
EncoderDecoder: @patrickvonplaten
Longformer, Reformer: @patrickvonplaten
TransfoXL, XLNet: @TevenLeScao, @patrickvonplaten
examples/seq2seq: @sshleifer
examples/bert-loses-patience: @JetRunner
tensorflow: @jplu
examples/token-classification: @stefan-it
documentation: @sgugger
--> | 11-02-2020 21:07:00 | 11-02-2020 21:07:00 | This issue has been automatically marked as stale because it has not had recent activity. If you think this still needs to be addressed please comment on this thread.
Please note that issues that do not follow the [contributing guidelines](https://github.com/huggingface/transformers/blob/master/CONTRIBUTING.md) are likely to be ignored. |
transformers | 8,248 | closed | Model card: GPT-2 fine-tuned on CommonGen | # What does this PR do?
<!--
Congratulations! You've made it this far! You're not quite done yet though.
Once merged, your PR is going to appear in the release notes with the title you set, so make sure it's a great title that fully reflects the extent of your awesome contribution.
Then, please replace this with a description of the change and which issue is fixed (if applicable). Please also include relevant motivation and context. List any dependencies (if any) that are required for this change.
Once you're done, someone will review your PR shortly (see the section "Who can review?" below to tag some potential reviewers). They may suggest changes to make the code even better. If no one reviewed your PR after a week has passed, don't hesitate to post a new comment @-mentioning the same persons---sometimes notifications get lost.
-->
<!-- Remove if not applicable -->
Fixes # (issue)
## Before submitting
- [ ] This PR fixes a typo or improves the docs (you can dismiss the other checks if that's the case).
- [ ] Did you read the [contributor guideline](https://github.com/huggingface/transformers/blob/master/CONTRIBUTING.md#start-contributing-pull-requests),
Pull Request section?
- [ ] Was this discussed/approved via a Github issue or the [forum](https://discuss.huggingface.co/)? Please add a link
to the it if that's the case.
- [ ] Did you make sure to update the documentation with your changes? Here are the
[documentation guidelines](https://github.com/huggingface/transformers/tree/master/docs), and
[here are tips on formatting docstrings](https://github.com/huggingface/transformers/tree/master/docs#writing-source-documentation).
- [ ] Did you write any new necessary tests?
## Who can review?
Anyone in the community is free to review the PR once the tests have passed. Feel free to tag
members/contributors which may be interested in your PR.
<!-- Your PR will be replied to more quickly if you can figure out the right person to tag with @
If you know how to use git blame, that is the easiest way, otherwise, here is a rough guide of **who to tag**.
Please tag fewer than 3 people.
albert, bert, XLM: @LysandreJik
GPT2: @LysandreJik, @patrickvonplaten
tokenizers: @mfuntowicz
Trainer: @sgugger
Benchmarks: @patrickvonplaten
Model Cards: @julien-c
Translation: @sshleifer
Summarization: @sshleifer
examples/distillation: @VictorSanh
nlp datasets: [different repo](https://github.com/huggingface/nlp)
rust tokenizers: [different repo](https://github.com/huggingface/tokenizers)
Text Generation: @patrickvonplaten, @TevenLeScao
Blenderbot, Bart, Marian, Pegasus: @sshleifer
T5: @patrickvonplaten
Rag: @patrickvonplaten, @lhoestq
EncoderDecoder: @patrickvonplaten
Longformer, Reformer: @patrickvonplaten
TransfoXL, XLNet: @TevenLeScao, @patrickvonplaten
examples/seq2seq: @sshleifer
examples/bert-loses-patience: @JetRunner
tensorflow: @jplu
examples/token-classification: @stefan-it
documentation: @sgugger
-->
| 11-02-2020 19:56:54 | 11-02-2020 19:56:54 | |
transformers | 8,247 | closed | Model card: CodeBERT fine-tuned for Insecure Code Detection | # What does this PR do?
<!--
Congratulations! You've made it this far! You're not quite done yet though.
Once merged, your PR is going to appear in the release notes with the title you set, so make sure it's a great title that fully reflects the extent of your awesome contribution.
Then, please replace this with a description of the change and which issue is fixed (if applicable). Please also include relevant motivation and context. List any dependencies (if any) that are required for this change.
Once you're done, someone will review your PR shortly (see the section "Who can review?" below to tag some potential reviewers). They may suggest changes to make the code even better. If no one reviewed your PR after a week has passed, don't hesitate to post a new comment @-mentioning the same persons---sometimes notifications get lost.
-->
<!-- Remove if not applicable -->
Fixes # (issue)
## Before submitting
- [ ] This PR fixes a typo or improves the docs (you can dismiss the other checks if that's the case).
- [ ] Did you read the [contributor guideline](https://github.com/huggingface/transformers/blob/master/CONTRIBUTING.md#start-contributing-pull-requests),
Pull Request section?
- [ ] Was this discussed/approved via a Github issue or the [forum](https://discuss.huggingface.co/)? Please add a link
to the it if that's the case.
- [ ] Did you make sure to update the documentation with your changes? Here are the
[documentation guidelines](https://github.com/huggingface/transformers/tree/master/docs), and
[here are tips on formatting docstrings](https://github.com/huggingface/transformers/tree/master/docs#writing-source-documentation).
- [ ] Did you write any new necessary tests?
## Who can review?
Anyone in the community is free to review the PR once the tests have passed. Feel free to tag
members/contributors which may be interested in your PR.
<!-- Your PR will be replied to more quickly if you can figure out the right person to tag with @
If you know how to use git blame, that is the easiest way, otherwise, here is a rough guide of **who to tag**.
Please tag fewer than 3 people.
albert, bert, XLM: @LysandreJik
GPT2: @LysandreJik, @patrickvonplaten
tokenizers: @mfuntowicz
Trainer: @sgugger
Benchmarks: @patrickvonplaten
Model Cards: @julien-c
Translation: @sshleifer
Summarization: @sshleifer
examples/distillation: @VictorSanh
nlp datasets: [different repo](https://github.com/huggingface/nlp)
rust tokenizers: [different repo](https://github.com/huggingface/tokenizers)
Text Generation: @patrickvonplaten, @TevenLeScao
Blenderbot, Bart, Marian, Pegasus: @sshleifer
T5: @patrickvonplaten
Rag: @patrickvonplaten, @lhoestq
EncoderDecoder: @patrickvonplaten
Longformer, Reformer: @patrickvonplaten
TransfoXL, XLNet: @TevenLeScao, @patrickvonplaten
examples/seq2seq: @sshleifer
examples/bert-loses-patience: @JetRunner
tensorflow: @jplu
examples/token-classification: @stefan-it
documentation: @sgugger
-->
| 11-02-2020 19:31:52 | 11-02-2020 19:31:52 | You can add the dataset(s) id(s) even for datasets not currently implemented in the `datasets` lib. That way, it will prompt us, or someone from the community, to add it at some point :)
Actually, did you take a look at how to implement a new `dataset`, @mrm8488? We can help, cc @lhoestq @thomwolf <|||||>I didn't know I could add the dataset `id` if it was not available at HF/Datasets. I will do it next times. Thanks for letting me know @julien-c. And yes, I was talking with @thomwolf and I will try to add this dataset to HF/Datasets ASAP (this weekend). |
transformers | 8,246 | closed | [Notebooks] Add new encoder-decoder notebooks | # What does this PR do?
<!--
Congratulations! You've made it this far! You're not quite done yet though.
Once merged, your PR is going to appear in the release notes with the title you set, so make sure it's a great title that fully reflects the extent of your awesome contribution.
Then, please replace this with a description of the change and which issue is fixed (if applicable). Please also include relevant motivation and context. List any dependencies (if any) that are required for this change.
Once you're done, someone will review your PR shortly (see the section "Who can review?" below to tag some potential reviewers). They may suggest changes to make the code even better. If no one reviewed your PR after a week has passed, don't hesitate to post a new comment @-mentioning the same persons---sometimes notifications get lost.
-->
<!-- Remove if not applicable -->
Adds 2 community notebooks
## Before submitting
- [x] This PR fixes a typo or improves the docs (you can dismiss the other checks if that's the case).
- [ ] Did you read the [contributor guideline](https://github.com/huggingface/transformers/blob/master/CONTRIBUTING.md#start-contributing-pull-requests),
Pull Request section?
- [ ] Was this discussed/approved via a Github issue or the [forum](https://discuss.huggingface.co/)? Please add a link
to the it if that's the case.
- [ ] Did you make sure to update the documentation with your changes? Here are the
[documentation guidelines](https://github.com/huggingface/transformers/tree/master/docs), and
[here are tips on formatting docstrings](https://github.com/huggingface/transformers/tree/master/docs#writing-source-documentation).
- [ ] Did you write any new necessary tests?
## Who can review?
Anyone in the community is free to review the PR once the tests have passed. Feel free to tag
members/contributors which may be interested in your PR.
<!-- Your PR will be replied to more quickly if you can figure out the right person to tag with @
If you know how to use git blame, that is the easiest way, otherwise, here is a rough guide of **who to tag**.
Please tag fewer than 3 people.
albert, bert, XLM: @LysandreJik
GPT2: @LysandreJik, @patrickvonplaten
tokenizers: @mfuntowicz
Trainer: @sgugger
Benchmarks: @patrickvonplaten
Model Cards: @julien-c
Translation: @sshleifer
Summarization: @sshleifer
examples/distillation: @VictorSanh
nlp datasets: [different repo](https://github.com/huggingface/nlp)
rust tokenizers: [different repo](https://github.com/huggingface/tokenizers)
Text Generation: @patrickvonplaten, @TevenLeScao
Blenderbot, Bart, Marian, Pegasus: @sshleifer
T5: @patrickvonplaten
Rag: @patrickvonplaten, @lhoestq
EncoderDecoder: @patrickvonplaten
Longformer, Reformer: @patrickvonplaten
TransfoXL, XLNet: @TevenLeScao, @patrickvonplaten
examples/seq2seq: @sshleifer
examples/bert-loses-patience: @JetRunner
tensorflow: @jplu
examples/token-classification: @stefan-it
documentation: @sgugger
-->
| 11-02-2020 19:20:33 | 11-02-2020 19:20:33 | |
transformers | 8,245 | closed | Add XLMProphetNetTokenizer to tokenization auto | Closes #8196 | 11-02-2020 18:57:05 | 11-02-2020 18:57:05 | |
transformers | 8,244 | closed | _shift_right when to use | Hi
In modeling_t5 there is a function called shift_right I wonder when it needs to be used, for which tasks? I sometimes see T5 finetuning without using it, not sure when this is suitable to use it thanks | 11-02-2020 18:53:22 | 11-02-2020 18:53:22 | Hey @rabeehkarimimahabadi, it's a convenience function that is used if `input_ids` and `labels` are provided but no `decoder_input_ids`. In this case this function automatically creates the correct `decoder_input_ids` as described here: https://huggingface.co/transformers/model_doc/t5.html?highlight=t5#training<|||||>This issue has been automatically marked as stale because it has not had recent activity. It will be closed if no further activity occurs. Thank you for your contributions.
|
transformers | 8,243 | closed | [EncoderDecoder] fix encoder decoder config model type bug | # What does this PR do?
<!--
Congratulations! You've made it this far! You're not quite done yet though.
Once merged, your PR is going to appear in the release notes with the title you set, so make sure it's a great title that fully reflects the extent of your awesome contribution.
Then, please replace this with a description of the change and which issue is fixed (if applicable). Please also include relevant motivation and context. List any dependencies (if any) that are required for this change.
Once you're done, someone will review your PR shortly (see the section "Who can review?" below to tag some potential reviewers). They may suggest changes to make the code even better. If no one reviewed your PR after a week has passed, don't hesitate to post a new comment @-mentioning the same persons---sometimes notifications get lost.
-->
<!-- Remove if not applicable -->
Small typo in config encoder-decoder class which leads to false config model type name
## Before submitting
- [ ] This PR fixes a typo or improves the docs (you can dismiss the other checks if that's the case).
- [ ] Did you read the [contributor guideline](https://github.com/huggingface/transformers/blob/master/CONTRIBUTING.md#start-contributing-pull-requests),
Pull Request section?
- [ ] Was this discussed/approved via a Github issue or the [forum](https://discuss.huggingface.co/)? Please add a link
to the it if that's the case.
- [ ] Did you make sure to update the documentation with your changes? Here are the
[documentation guidelines](https://github.com/huggingface/transformers/tree/master/docs), and
[here are tips on formatting docstrings](https://github.com/huggingface/transformers/tree/master/docs#writing-source-documentation).
- [ ] Did you write any new necessary tests?
## Who can review?
Anyone in the community is free to review the PR once the tests have passed. Feel free to tag
members/contributors which may be interested in your PR.
<!-- Your PR will be replied to more quickly if you can figure out the right person to tag with @
If you know how to use git blame, that is the easiest way, otherwise, here is a rough guide of **who to tag**.
Please tag fewer than 3 people.
albert, bert, XLM: @LysandreJik
GPT2: @LysandreJik, @patrickvonplaten
tokenizers: @mfuntowicz
Trainer: @sgugger
Benchmarks: @patrickvonplaten
Model Cards: @julien-c
Translation: @sshleifer
Summarization: @sshleifer
examples/distillation: @VictorSanh
nlp datasets: [different repo](https://github.com/huggingface/nlp)
rust tokenizers: [different repo](https://github.com/huggingface/tokenizers)
Text Generation: @patrickvonplaten, @TevenLeScao
Blenderbot, Bart, Marian, Pegasus: @sshleifer
T5: @patrickvonplaten
Rag: @patrickvonplaten, @lhoestq
EncoderDecoder: @patrickvonplaten
Longformer, Reformer: @patrickvonplaten
TransfoXL, XLNet: @TevenLeScao, @patrickvonplaten
examples/seq2seq: @sshleifer
examples/bert-loses-patience: @JetRunner
tensorflow: @jplu
examples/token-classification: @stefan-it
documentation: @sgugger
-->
| 11-02-2020 18:36:26 | 11-02-2020 18:36:26 | |
transformers | 8,242 | closed | Error converting tensorflow checkpoints | # ❓ Questions & Help
I'm trying to convert a BERT Tensorflow checkpoint to hugging face model
## Details
```
!transformers-cli convert \
--model_type bert \
--tf_checkpoint C:\Users\sacl\Panasonic-AI\POC\pretraining\content\PatentBERT\model.ckpt-181172 \
--config C:\Users\sacl\Panasonic-AI\POC\pretraining\content\PatentBERT\bert_config.json \
--pytorch_dump_output C:\Users\sacl\Panasonic-AI\POC\pretraining\content\PatentBERT\pytorch_model.bin
```
Full traceback:
> c:\programdata\anaconda3\envs\py37cuda10\lib\site-packages\tensorflow\python\framework\dtypes.py:516: FutureWarning: Passing (type, 1) or '1type' as a synonym of type is deprecated; in a future version of numpy, it will be understood as (type, (1,)) / '(1,)type'.
_np_qint8 = np.dtype([("qint8", np.int8, 1)])
c:\programdata\anaconda3\envs\py37cuda10\lib\site-packages\tensorflow\python\framework\dtypes.py:517: FutureWarning: Passing (type, 1) or '1type' as a synonym of type is deprecated; in a future version of numpy, it will be understood as (type, (1,)) / '(1,)type'.
_np_quint8 = np.dtype([("quint8", np.uint8, 1)])
c:\programdata\anaconda3\envs\py37cuda10\lib\site-packages\tensorflow\python\framework\dtypes.py:518: FutureWarning: Passing (type, 1) or '1type' as a synonym of type is deprecated; in a future version of numpy, it will be understood as (type, (1,)) / '(1,)type'.
_np_qint16 = np.dtype([("qint16", np.int16, 1)])
c:\programdata\anaconda3\envs\py37cuda10\lib\site-packages\tensorflow\python\framework\dtypes.py:519: FutureWarning: Passing (type, 1) or '1type' as a synonym of type is deprecated; in a future version of numpy, it will be understood as (type, (1,)) / '(1,)type'.
_np_quint16 = np.dtype([("quint16", np.uint16, 1)])
c:\programdata\anaconda3\envs\py37cuda10\lib\site-packages\tensorflow\python\framework\dtypes.py:520: FutureWarning: Passing (type, 1) or '1type' as a synonym of type is deprecated; in a future version of numpy, it will be understood as (type, (1,)) / '(1,)type'.
_np_qint32 = np.dtype([("qint32", np.int32, 1)])
c:\programdata\anaconda3\envs\py37cuda10\lib\site-packages\tensorflow\python\framework\dtypes.py:525: FutureWarning: Passing (type, 1) or '1type' as a synonym of type is deprecated; in a future version of numpy, it will be understood as (type, (1,)) / '(1,)type'.
np_resource = np.dtype([("resource", np.ubyte, 1)])
c:\programdata\anaconda3\envs\py37cuda10\lib\site-packages\tensorboard\compat\tensorflow_stub\dtypes.py:541: FutureWarning: Passing (type, 1) or '1type' as a synonym of type is deprecated; in a future version of numpy, it will be understood as (type, (1,)) / '(1,)type'.
_np_qint8 = np.dtype([("qint8", np.int8, 1)])
c:\programdata\anaconda3\envs\py37cuda10\lib\site-packages\tensorboard\compat\tensorflow_stub\dtypes.py:542: FutureWarning: Passing (type, 1) or '1type' as a synonym of type is deprecated; in a future version of numpy, it will be understood as (type, (1,)) / '(1,)type'.
_np_quint8 = np.dtype([("quint8", np.uint8, 1)])
c:\programdata\anaconda3\envs\py37cuda10\lib\site-packages\tensorboard\compat\tensorflow_stub\dtypes.py:543: FutureWarning: Passing (type, 1) or '1type' as a synonym of type is deprecated; in a future version of numpy, it will be understood as (type, (1,)) / '(1,)type'.
_np_qint16 = np.dtype([("qint16", np.int16, 1)])
c:\programdata\anaconda3\envs\py37cuda10\lib\site-packages\tensorboard\compat\tensorflow_stub\dtypes.py:544: FutureWarning: Passing (type, 1) or '1type' as a synonym of type is deprecated; in a future version of numpy, it will be understood as (type, (1,)) / '(1,)type'.
_np_quint16 = np.dtype([("quint16", np.uint16, 1)])
c:\programdata\anaconda3\envs\py37cuda10\lib\site-packages\tensorboard\compat\tensorflow_stub\dtypes.py:545: FutureWarning: Passing (type, 1) or '1type' as a synonym of type is deprecated; in a future version of numpy, it will be understood as (type, (1,)) / '(1,)type'.
_np_qint32 = np.dtype([("qint32", np.int32, 1)])
c:\programdata\anaconda3\envs\py37cuda10\lib\site-packages\tensorboard\compat\tensorflow_stub\dtypes.py:550: FutureWarning: Passing (type, 1) or '1type' as a synonym of type is deprecated; in a future version of numpy, it will be understood as (type, (1,)) / '(1,)type'.
np_resource = np.dtype([("resource", np.ubyte, 1)])
Converting TensorFlow checkpoint from C:\Users\sacl\Panasonic-AI\POC\pretraining\content\PatentBERT\model.ckpt-181172
Loading TF weight bert/embeddings/LayerNorm/beta with shape [768]
Loading TF weight bert/embeddings/LayerNorm/beta/adam_m with shape [768]
Loading TF weight bert/embeddings/LayerNorm/beta/adam_v with shape [768]
Loading TF weight bert/embeddings/LayerNorm/gamma with shape [768]
Loading TF weight bert/embeddings/LayerNorm/gamma/adam_m with shape [768]
Loading TF weight bert/embeddings/LayerNorm/gamma/adam_v with shape [768]
Loading TF weight bert/embeddings/position_embeddings with shape [512, 768]
Loading TF weight bert/embeddings/position_embeddings/adam_m with shape [512, 768]
Loading TF weight bert/embeddings/position_embeddings/adam_v with shape [512, 768]
Loading TF weight bert/embeddings/token_type_embeddings with shape [2, 768]
Loading TF weight bert/embeddings/token_type_embeddings/adam_m with shape [2, 768]
Loading TF weight bert/embeddings/token_type_embeddings/adam_v with shape [2, 768]
Loading TF weight bert/embeddings/word_embeddings with shape [30522, 768]
Loading TF weight bert/embeddings/word_embeddings/adam_m with shape [30522, 768]
Loading TF weight bert/embeddings/word_embeddings/adam_v with shape [30522, 768]
Loading TF weight bert/encoder/layer_0/attention/output/LayerNorm/beta with shape [768]
Loading TF weight bert/encoder/layer_0/attention/output/LayerNorm/beta/adam_m with shape [768]
Loading TF weight bert/encoder/layer_0/attention/output/LayerNorm/beta/adam_v with shape [768]
Loading TF weight bert/encoder/layer_0/attention/output/LayerNorm/gamma with shape [768]
Loading TF weight bert/encoder/layer_0/attention/output/LayerNorm/gamma/adam_m with shape [768]
Loading TF weight bert/encoder/layer_0/attention/output/LayerNorm/gamma/adam_v with shape [768]
Loading TF weight bert/encoder/layer_0/attention/output/dense/bias with shape [768]
Loading TF weight bert/encoder/layer_0/attention/output/dense/bias/adam_m with shape [768]
Loading TF weight bert/encoder/layer_0/attention/output/dense/bias/adam_v with shape [768]
Loading TF weight bert/encoder/layer_0/attention/output/dense/kernel with shape [768, 768]
Loading TF weight bert/encoder/layer_0/attention/output/dense/kernel/adam_m with shape [768, 768]
"vocab_size": 30522
}
Loading TF weight bert/encoder/layer_0/attention/output/dense/kernel/adam_v with shape [768, 768]
Loading TF weight bert/encoder/layer_0/attention/self/key/bias with shape [768]
Loading TF weight bert/encoder/layer_0/attention/self/key/bias/adam_m with shape [768]
Loading TF weight bert/encoder/layer_0/attention/self/key/bias/adam_v with shape [768]
Loading TF weight bert/encoder/layer_0/attention/self/key/kernel with shape [768, 768]
Loading TF weight bert/encoder/layer_0/attention/self/key/kernel/adam_m with shape [768, 768]
Loading TF weight bert/encoder/layer_0/attention/self/key/kernel/adam_v with shape [768, 768]
Loading TF weight bert/encoder/layer_0/attention/self/query/bias with shape [768]
Loading TF weight bert/encoder/layer_0/attention/self/query/bias/adam_m with shape [768]
Loading TF weight bert/encoder/layer_0/attention/self/query/bias/adam_v with shape [768]
Loading TF weight bert/encoder/layer_0/attention/self/query/kernel with shape [768, 768]
Loading TF weight bert/encoder/layer_0/attention/self/query/kernel/adam_m with shape [768, 768]
Loading TF weight bert/encoder/layer_0/attention/self/query/kernel/adam_v with shape [768, 768]
Loading TF weight bert/encoder/layer_0/attention/self/value/bias with shape [768]
Loading TF weight bert/encoder/layer_0/attention/self/value/bias/adam_m with shape [768]
Loading TF weight bert/encoder/layer_0/attention/self/value/bias/adam_v with shape [768]
Loading TF weight bert/encoder/layer_0/attention/self/value/kernel with shape [768, 768]
Loading TF weight bert/encoder/layer_0/attention/self/value/kernel/adam_m with shape [768, 768]
Loading TF weight bert/encoder/layer_0/attention/self/value/kernel/adam_v with shape [768, 768]
Loading TF weight bert/encoder/layer_0/intermediate/dense/bias with shape [3072]
Loading TF weight bert/encoder/layer_0/intermediate/dense/bias/adam_m with shape [3072]
Loading TF weight bert/encoder/layer_0/intermediate/dense/bias/adam_v with shape [3072]
Loading TF weight bert/encoder/layer_0/intermediate/dense/kernel with shape [768, 3072]
Loading TF weight bert/encoder/layer_0/intermediate/dense/kernel/adam_m with shape [768, 3072]
Loading TF weight bert/encoder/layer_0/intermediate/dense/kernel/adam_v with shape [768, 3072]
Loading TF weight bert/encoder/layer_0/output/LayerNorm/beta with shape [768]
Loading TF weight bert/encoder/layer_0/output/LayerNorm/beta/adam_m with shape [768]
Loading TF weight bert/encoder/layer_0/output/LayerNorm/beta/adam_v with shape [768]
Loading TF weight bert/encoder/layer_0/output/LayerNorm/gamma with shape [768]
Loading TF weight bert/encoder/layer_0/output/LayerNorm/gamma/adam_m with shape [768]
Loading TF weight bert/encoder/layer_0/output/LayerNorm/gamma/adam_v with shape [768]
Loading TF weight bert/encoder/layer_0/output/dense/bias with shape [768]
Loading TF weight bert/encoder/layer_0/output/dense/bias/adam_m with shape [768]
Loading TF weight bert/encoder/layer_0/output/dense/bias/adam_v with shape [768]
Loading TF weight bert/encoder/layer_0/output/dense/kernel with shape [3072, 768]
Loading TF weight bert/encoder/layer_0/output/dense/kernel/adam_m with shape [3072, 768]
Loading TF weight bert/encoder/layer_0/output/dense/kernel/adam_v with shape [3072, 768]
Loading TF weight bert/encoder/layer_1/attention/output/LayerNorm/beta with shape [768]
Loading TF weight bert/encoder/layer_1/attention/output/LayerNorm/beta/adam_m with shape [768]
Loading TF weight bert/encoder/layer_1/attention/output/LayerNorm/beta/adam_v with shape [768]
Loading TF weight bert/encoder/layer_1/attention/output/LayerNorm/gamma with shape [768]
Loading TF weight bert/encoder/layer_1/attention/output/LayerNorm/gamma/adam_m with shape [768]
Loading TF weight bert/encoder/layer_1/attention/output/LayerNorm/gamma/adam_v with shape [768]
Loading TF weight bert/encoder/layer_1/attention/output/dense/bias with shape [768]
Loading TF weight bert/encoder/layer_1/attention/output/dense/bias/adam_m with shape [768]
Loading TF weight bert/encoder/layer_1/attention/output/dense/bias/adam_v with shape [768]
Loading TF weight bert/encoder/layer_1/attention/output/dense/kernel with shape [768, 768]
Loading TF weight bert/encoder/layer_1/attention/output/dense/kernel/adam_m with shape [768, 768]
Loading TF weight bert/encoder/layer_1/attention/output/dense/kernel/adam_v with shape [768, 768]
Loading TF weight bert/encoder/layer_1/attention/self/key/bias with shape [768]
Loading TF weight bert/encoder/layer_1/attention/self/key/bias/adam_m with shape [768]
Loading TF weight bert/encoder/layer_1/attention/self/key/bias/adam_v with shape [768]
Loading TF weight bert/encoder/layer_1/attention/self/key/kernel with shape [768, 768]
Loading TF weight bert/encoder/layer_1/attention/self/key/kernel/adam_m with shape [768, 768]
Loading TF weight bert/encoder/layer_1/attention/self/key/kernel/adam_v with shape [768, 768]
Loading TF weight bert/encoder/layer_1/attention/self/query/bias with shape [768]
Loading TF weight bert/encoder/layer_1/attention/self/query/bias/adam_m with shape [768]
Loading TF weight bert/encoder/layer_1/attention/self/query/bias/adam_v with shape [768]
Loading TF weight bert/encoder/layer_1/attention/self/query/kernel with shape [768, 768]
Loading TF weight bert/encoder/layer_1/attention/self/query/kernel/adam_m with shape [768, 768]
Loading TF weight bert/encoder/layer_1/attention/self/query/kernel/adam_v with shape [768, 768]
Loading TF weight bert/encoder/layer_1/attention/self/value/bias with shape [768]
Loading TF weight bert/encoder/layer_1/attention/self/value/bias/adam_m with shape [768]
Loading TF weight bert/encoder/layer_1/attention/self/value/bias/adam_v with shape [768]
Loading TF weight bert/encoder/layer_1/attention/self/value/kernel with shape [768, 768]
Loading TF weight bert/encoder/layer_1/attention/self/value/kernel/adam_m with shape [768, 768]
Loading TF weight bert/encoder/layer_1/attention/self/value/kernel/adam_v with shape [768, 768]
Loading TF weight bert/encoder/layer_1/intermediate/dense/bias with shape [3072]
Loading TF weight bert/encoder/layer_1/intermediate/dense/bias/adam_m with shape [3072]
Loading TF weight bert/encoder/layer_1/intermediate/dense/bias/adam_v with shape [3072]
Loading TF weight bert/encoder/layer_1/intermediate/dense/kernel with shape [768, 3072]
Loading TF weight bert/encoder/layer_1/intermediate/dense/kernel/adam_m with shape [768, 3072]
Loading TF weight bert/encoder/layer_1/intermediate/dense/kernel/adam_v with shape [768, 3072]
Loading TF weight bert/encoder/layer_1/output/LayerNorm/beta with shape [768]
Loading TF weight bert/encoder/layer_1/output/LayerNorm/beta/adam_m with shape [768]
Loading TF weight bert/encoder/layer_1/output/LayerNorm/beta/adam_v with shape [768]
Loading TF weight bert/encoder/layer_1/output/LayerNorm/gamma with shape [768]
Loading TF weight bert/encoder/layer_1/output/LayerNorm/gamma/adam_m with shape [768]
Loading TF weight bert/encoder/layer_1/output/LayerNorm/gamma/adam_v with shape [768]
Loading TF weight bert/encoder/layer_1/output/dense/bias with shape [768]
Loading TF weight bert/encoder/layer_1/output/dense/bias/adam_m with shape [768]
Loading TF weight bert/encoder/layer_1/output/dense/bias/adam_v with shape [768]
Loading TF weight bert/encoder/layer_1/output/dense/kernel with shape [3072, 768]
Loading TF weight bert/encoder/layer_1/output/dense/kernel/adam_m with shape [3072, 768]
Loading TF weight bert/encoder/layer_1/output/dense/kernel/adam_v with shape [3072, 768]
Loading TF weight bert/encoder/layer_10/attention/output/LayerNorm/beta with shape [768]
Loading TF weight bert/encoder/layer_10/attention/output/LayerNorm/beta/adam_m with shape [768]
Loading TF weight bert/encoder/layer_10/attention/output/LayerNorm/beta/adam_v with shape [768]
Loading TF weight bert/encoder/layer_10/attention/output/LayerNorm/gamma with shape [768]
Loading TF weight bert/encoder/layer_10/attention/output/LayerNorm/gamma/adam_m with shape [768]
Loading TF weight bert/encoder/layer_10/attention/output/LayerNorm/gamma/adam_v with shape [768]
Loading TF weight bert/encoder/layer_10/attention/output/dense/bias with shape [768]
Loading TF weight bert/encoder/layer_10/attention/output/dense/bias/adam_m with shape [768]
Loading TF weight bert/encoder/layer_10/attention/output/dense/bias/adam_v with shape [768]
Loading TF weight bert/encoder/layer_10/attention/output/dense/kernel with shape [768, 768]
Loading TF weight bert/encoder/layer_10/attention/output/dense/kernel/adam_m with shape [768, 768]
Loading TF weight bert/encoder/layer_10/attention/output/dense/kernel/adam_v with shape [768, 768]
Loading TF weight bert/encoder/layer_10/attention/self/key/bias with shape [768]
Loading TF weight bert/encoder/layer_10/attention/self/key/bias/adam_m with shape [768]
Loading TF weight bert/encoder/layer_10/attention/self/key/bias/adam_v with shape [768]
Loading TF weight bert/encoder/layer_10/attention/self/key/kernel with shape [768, 768]
Loading TF weight bert/encoder/layer_10/attention/self/key/kernel/adam_m with shape [768, 768]
Loading TF weight bert/encoder/layer_10/attention/self/key/kernel/adam_v with shape [768, 768]
Loading TF weight bert/encoder/layer_10/attention/self/query/bias with shape [768]
Loading TF weight bert/encoder/layer_10/attention/self/query/bias/adam_m with shape [768]
Loading TF weight bert/encoder/layer_10/attention/self/query/bias/adam_v with shape [768]
Loading TF weight bert/encoder/layer_10/attention/self/query/kernel with shape [768, 768]
Loading TF weight bert/encoder/layer_10/attention/self/query/kernel/adam_m with shape [768, 768]
Loading TF weight bert/encoder/layer_10/attention/self/query/kernel/adam_v with shape [768, 768]
Loading TF weight bert/encoder/layer_10/attention/self/value/bias with shape [768]
Loading TF weight bert/encoder/layer_10/attention/self/value/bias/adam_m with shape [768]
Loading TF weight bert/encoder/layer_10/attention/self/value/bias/adam_v with shape [768]
Loading TF weight bert/encoder/layer_10/attention/self/value/kernel with shape [768, 768]
Loading TF weight bert/encoder/layer_10/attention/self/value/kernel/adam_m with shape [768, 768]
Loading TF weight bert/encoder/layer_10/attention/self/value/kernel/adam_v with shape [768, 768]
Loading TF weight bert/encoder/layer_10/intermediate/dense/bias with shape [3072]
Loading TF weight bert/encoder/layer_10/intermediate/dense/bias/adam_m with shape [3072]
Loading TF weight bert/encoder/layer_10/intermediate/dense/bias/adam_v with shape [3072]
Loading TF weight bert/encoder/layer_10/intermediate/dense/kernel with shape [768, 3072]
Loading TF weight bert/encoder/layer_10/intermediate/dense/kernel/adam_m with shape [768, 3072]
Loading TF weight bert/encoder/layer_10/intermediate/dense/kernel/adam_v with shape [768, 3072]
Loading TF weight bert/encoder/layer_10/output/LayerNorm/beta with shape [768]
Loading TF weight bert/encoder/layer_10/output/LayerNorm/beta/adam_m with shape [768]
Loading TF weight bert/encoder/layer_10/output/LayerNorm/beta/adam_v with shape [768]
Loading TF weight bert/encoder/layer_10/output/LayerNorm/gamma with shape [768]
Loading TF weight bert/encoder/layer_10/output/LayerNorm/gamma/adam_m with shape [768]
Loading TF weight bert/encoder/layer_10/output/LayerNorm/gamma/adam_v with shape [768]
Loading TF weight bert/encoder/layer_10/output/dense/bias with shape [768]
Loading TF weight bert/encoder/layer_10/output/dense/bias/adam_m with shape [768]
Loading TF weight bert/encoder/layer_10/output/dense/bias/adam_v with shape [768]
Loading TF weight bert/encoder/layer_10/output/dense/kernel with shape [3072, 768]
Loading TF weight bert/encoder/layer_10/output/dense/kernel/adam_m with shape [3072, 768]
Loading TF weight bert/encoder/layer_10/output/dense/kernel/adam_v with shape [3072, 768]
Loading TF weight bert/encoder/layer_11/attention/output/LayerNorm/beta with shape [768]
Loading TF weight bert/encoder/layer_11/attention/output/LayerNorm/beta/adam_m with shape [768]
Loading TF weight bert/encoder/layer_11/attention/output/LayerNorm/beta/adam_v with shape [768]
Loading TF weight bert/encoder/layer_11/attention/output/LayerNorm/gamma with shape [768]
Loading TF weight bert/encoder/layer_11/attention/output/LayerNorm/gamma/adam_m with shape [768]
Loading TF weight bert/encoder/layer_11/attention/output/LayerNorm/gamma/adam_v with shape [768]
Loading TF weight bert/encoder/layer_11/attention/output/dense/bias with shape [768]
Loading TF weight bert/encoder/layer_11/attention/output/dense/bias/adam_m with shape [768]
Loading TF weight bert/encoder/layer_11/attention/output/dense/bias/adam_v with shape [768]
Loading TF weight bert/encoder/layer_11/attention/output/dense/kernel with shape [768, 768]
Loading TF weight bert/encoder/layer_11/attention/output/dense/kernel/adam_m with shape [768, 768]
Loading TF weight bert/encoder/layer_11/attention/output/dense/kernel/adam_v with shape [768, 768]
Loading TF weight bert/encoder/layer_11/attention/self/key/bias with shape [768]
Loading TF weight bert/encoder/layer_11/attention/self/key/bias/adam_m with shape [768]
Loading TF weight bert/encoder/layer_11/attention/self/key/bias/adam_v with shape [768]
Loading TF weight bert/encoder/layer_11/attention/self/key/kernel with shape [768, 768]
Loading TF weight bert/encoder/layer_11/attention/self/key/kernel/adam_m with shape [768, 768]
Loading TF weight bert/encoder/layer_11/attention/self/key/kernel/adam_v with shape [768, 768]
Loading TF weight bert/encoder/layer_11/attention/self/query/bias with shape [768]
Loading TF weight bert/encoder/layer_11/attention/self/query/bias/adam_m with shape [768]
Loading TF weight bert/encoder/layer_11/attention/self/query/bias/adam_v with shape [768]
Loading TF weight bert/encoder/layer_11/attention/self/query/kernel with shape [768, 768]
Loading TF weight bert/encoder/layer_11/attention/self/query/kernel/adam_m with shape [768, 768]
Loading TF weight bert/encoder/layer_11/attention/self/query/kernel/adam_v with shape [768, 768]
Loading TF weight bert/encoder/layer_11/attention/self/value/bias with shape [768]
Loading TF weight bert/encoder/layer_11/attention/self/value/bias/adam_m with shape [768]
Loading TF weight bert/encoder/layer_11/attention/self/value/bias/adam_v with shape [768]
Loading TF weight bert/encoder/layer_11/attention/self/value/kernel with shape [768, 768]
Loading TF weight bert/encoder/layer_11/attention/self/value/kernel/adam_m with shape [768, 768]
Loading TF weight bert/encoder/layer_11/attention/self/value/kernel/adam_v with shape [768, 768]
Loading TF weight bert/encoder/layer_11/intermediate/dense/bias with shape [3072]
Loading TF weight bert/encoder/layer_11/intermediate/dense/bias/adam_m with shape [3072]
Loading TF weight bert/encoder/layer_11/intermediate/dense/bias/adam_v with shape [3072]
Loading TF weight bert/encoder/layer_11/intermediate/dense/kernel with shape [768, 3072]
Loading TF weight bert/encoder/layer_11/intermediate/dense/kernel/adam_m with shape [768, 3072]
Loading TF weight bert/encoder/layer_11/intermediate/dense/kernel/adam_v with shape [768, 3072]
Loading TF weight bert/encoder/layer_11/output/LayerNorm/beta with shape [768]
Loading TF weight bert/encoder/layer_11/output/LayerNorm/beta/adam_m with shape [768]
Loading TF weight bert/encoder/layer_11/output/LayerNorm/beta/adam_v with shape [768]
Loading TF weight bert/encoder/layer_11/output/LayerNorm/gamma with shape [768]
Loading TF weight bert/encoder/layer_11/output/LayerNorm/gamma/adam_m with shape [768]
Loading TF weight bert/encoder/layer_11/output/LayerNorm/gamma/adam_v with shape [768]
Loading TF weight bert/encoder/layer_11/output/dense/bias with shape [768]
Loading TF weight bert/encoder/layer_11/output/dense/bias/adam_m with shape [768]
Loading TF weight bert/encoder/layer_11/output/dense/bias/adam_v with shape [768]
Loading TF weight bert/encoder/layer_11/output/dense/kernel with shape [3072, 768]
Loading TF weight bert/encoder/layer_11/output/dense/kernel/adam_m with shape [3072, 768]
Loading TF weight bert/encoder/layer_11/output/dense/kernel/adam_v with shape [3072, 768]
Loading TF weight bert/encoder/layer_2/attention/output/LayerNorm/beta with shape [768]
Loading TF weight bert/encoder/layer_2/attention/output/LayerNorm/beta/adam_m with shape [768]
Loading TF weight bert/encoder/layer_2/attention/output/LayerNorm/beta/adam_v with shape [768]
Loading TF weight bert/encoder/layer_2/attention/output/LayerNorm/gamma with shape [768]
Loading TF weight bert/encoder/layer_2/attention/output/LayerNorm/gamma/adam_m with shape [768]
Loading TF weight bert/encoder/layer_2/attention/output/LayerNorm/gamma/adam_v with shape [768]
Loading TF weight bert/encoder/layer_2/attention/output/dense/bias with shape [768]
Loading TF weight bert/encoder/layer_2/attention/output/dense/bias/adam_m with shape [768]
Loading TF weight bert/encoder/layer_2/attention/output/dense/bias/adam_v with shape [768]
Loading TF weight bert/encoder/layer_2/attention/output/dense/kernel with shape [768, 768]
Loading TF weight bert/encoder/layer_2/attention/output/dense/kernel/adam_m with shape [768, 768]
Loading TF weight bert/encoder/layer_2/attention/output/dense/kernel/adam_v with shape [768, 768]
Loading TF weight bert/encoder/layer_2/attention/self/key/bias with shape [768]
Loading TF weight bert/encoder/layer_2/attention/self/key/bias/adam_m with shape [768]
Loading TF weight bert/encoder/layer_2/attention/self/key/bias/adam_v with shape [768]
Loading TF weight bert/encoder/layer_2/attention/self/key/kernel with shape [768, 768]
Loading TF weight bert/encoder/layer_2/attention/self/key/kernel/adam_m with shape [768, 768]
Loading TF weight bert/encoder/layer_2/attention/self/key/kernel/adam_v with shape [768, 768]
Loading TF weight bert/encoder/layer_2/attention/self/query/bias with shape [768]
Loading TF weight bert/encoder/layer_2/attention/self/query/bias/adam_m with shape [768]
Loading TF weight bert/encoder/layer_2/attention/self/query/bias/adam_v with shape [768]
Loading TF weight bert/encoder/layer_2/attention/self/query/kernel with shape [768, 768]
Loading TF weight bert/encoder/layer_2/attention/self/query/kernel/adam_m with shape [768, 768]
Loading TF weight bert/encoder/layer_2/attention/self/query/kernel/adam_v with shape [768, 768]
Loading TF weight bert/encoder/layer_2/attention/self/value/bias with shape [768]
Loading TF weight bert/encoder/layer_2/attention/self/value/bias/adam_m with shape [768]
Loading TF weight bert/encoder/layer_2/attention/self/value/bias/adam_v with shape [768]
Loading TF weight bert/encoder/layer_2/attention/self/value/kernel with shape [768, 768]
Loading TF weight bert/encoder/layer_2/attention/self/value/kernel/adam_m with shape [768, 768]
Loading TF weight bert/encoder/layer_2/attention/self/value/kernel/adam_v with shape [768, 768]
Loading TF weight bert/encoder/layer_2/intermediate/dense/bias with shape [3072]
Loading TF weight bert/encoder/layer_2/intermediate/dense/bias/adam_m with shape [3072]
Loading TF weight bert/encoder/layer_2/intermediate/dense/bias/adam_v with shape [3072]
Loading TF weight bert/encoder/layer_2/intermediate/dense/kernel with shape [768, 3072]
Loading TF weight bert/encoder/layer_2/intermediate/dense/kernel/adam_m with shape [768, 3072]
Loading TF weight bert/encoder/layer_2/intermediate/dense/kernel/adam_v with shape [768, 3072]
Loading TF weight bert/encoder/layer_2/output/LayerNorm/beta with shape [768]
Loading TF weight bert/encoder/layer_2/output/LayerNorm/beta/adam_m with shape [768]
Loading TF weight bert/encoder/layer_2/output/LayerNorm/beta/adam_v with shape [768]
Loading TF weight bert/encoder/layer_2/output/LayerNorm/gamma with shape [768]
Loading TF weight bert/encoder/layer_2/output/LayerNorm/gamma/adam_m with shape [768]
Loading TF weight bert/encoder/layer_2/output/LayerNorm/gamma/adam_v with shape [768]
Loading TF weight bert/encoder/layer_2/output/dense/bias with shape [768]
Loading TF weight bert/encoder/layer_2/output/dense/bias/adam_m with shape [768]
Loading TF weight bert/encoder/layer_2/output/dense/bias/adam_v with shape [768]
Loading TF weight bert/encoder/layer_2/output/dense/kernel with shape [3072, 768]
Loading TF weight bert/encoder/layer_2/output/dense/kernel/adam_m with shape [3072, 768]
Loading TF weight bert/encoder/layer_2/output/dense/kernel/adam_v with shape [3072, 768]
Loading TF weight bert/encoder/layer_3/attention/output/LayerNorm/beta with shape [768]
Loading TF weight bert/encoder/layer_3/attention/output/LayerNorm/beta/adam_m with shape [768]
Loading TF weight bert/encoder/layer_3/attention/output/LayerNorm/beta/adam_v with shape [768]
Loading TF weight bert/encoder/layer_3/attention/output/LayerNorm/gamma with shape [768]
Loading TF weight bert/encoder/layer_3/attention/output/LayerNorm/gamma/adam_m with shape [768]
Loading TF weight bert/encoder/layer_3/attention/output/LayerNorm/gamma/adam_v with shape [768]
Loading TF weight bert/encoder/layer_3/attention/output/dense/bias with shape [768]
Loading TF weight bert/encoder/layer_3/attention/output/dense/bias/adam_m with shape [768]
Loading TF weight bert/encoder/layer_3/attention/output/dense/bias/adam_v with shape [768]
Loading TF weight bert/encoder/layer_3/attention/output/dense/kernel with shape [768, 768]
Loading TF weight bert/encoder/layer_3/attention/output/dense/kernel/adam_m with shape [768, 768]
Loading TF weight bert/encoder/layer_3/attention/output/dense/kernel/adam_v with shape [768, 768]
Loading TF weight bert/encoder/layer_3/attention/self/key/bias with shape [768]
Loading TF weight bert/encoder/layer_3/attention/self/key/bias/adam_m with shape [768]
Loading TF weight bert/encoder/layer_3/attention/self/key/bias/adam_v with shape [768]
Loading TF weight bert/encoder/layer_3/attention/self/key/kernel with shape [768, 768]
Loading TF weight bert/encoder/layer_3/attention/self/key/kernel/adam_m with shape [768, 768]
Loading TF weight bert/encoder/layer_3/attention/self/key/kernel/adam_v with shape [768, 768]
Loading TF weight bert/encoder/layer_3/attention/self/query/bias with shape [768]
Loading TF weight bert/encoder/layer_3/attention/self/query/bias/adam_m with shape [768]
Loading TF weight bert/encoder/layer_3/attention/self/query/bias/adam_v with shape [768]
Loading TF weight bert/encoder/layer_3/attention/self/query/kernel with shape [768, 768]
Loading TF weight bert/encoder/layer_3/attention/self/query/kernel/adam_m with shape [768, 768]
Loading TF weight bert/encoder/layer_3/attention/self/query/kernel/adam_v with shape [768, 768]
Loading TF weight bert/encoder/layer_3/attention/self/value/bias with shape [768]
Loading TF weight bert/encoder/layer_3/attention/self/value/bias/adam_m with shape [768]
Loading TF weight bert/encoder/layer_3/attention/self/value/bias/adam_v with shape [768]
Loading TF weight bert/encoder/layer_3/attention/self/value/kernel with shape [768, 768]
Loading TF weight bert/encoder/layer_3/attention/self/value/kernel/adam_m with shape [768, 768]
Loading TF weight bert/encoder/layer_3/attention/self/value/kernel/adam_v with shape [768, 768]
Loading TF weight bert/encoder/layer_3/intermediate/dense/bias with shape [3072]
Loading TF weight bert/encoder/layer_3/intermediate/dense/bias/adam_m with shape [3072]
Loading TF weight bert/encoder/layer_3/intermediate/dense/bias/adam_v with shape [3072]
Loading TF weight bert/encoder/layer_3/intermediate/dense/kernel with shape [768, 3072]
Loading TF weight bert/encoder/layer_3/intermediate/dense/kernel/adam_m with shape [768, 3072]
Loading TF weight bert/encoder/layer_3/intermediate/dense/kernel/adam_v with shape [768, 3072]
Loading TF weight bert/encoder/layer_3/output/LayerNorm/beta with shape [768]
Loading TF weight bert/encoder/layer_3/output/LayerNorm/beta/adam_m with shape [768]
Loading TF weight bert/encoder/layer_3/output/LayerNorm/beta/adam_v with shape [768]
Loading TF weight bert/encoder/layer_3/output/LayerNorm/gamma with shape [768]
Loading TF weight bert/encoder/layer_3/output/LayerNorm/gamma/adam_m with shape [768]
Loading TF weight bert/encoder/layer_3/output/LayerNorm/gamma/adam_v with shape [768]
Loading TF weight bert/encoder/layer_3/output/dense/bias with shape [768]
Loading TF weight bert/encoder/layer_3/output/dense/bias/adam_m with shape [768]
Loading TF weight bert/encoder/layer_3/output/dense/bias/adam_v with shape [768]
Loading TF weight bert/encoder/layer_3/output/dense/kernel with shape [3072, 768]
Loading TF weight bert/encoder/layer_3/output/dense/kernel/adam_m with shape [3072, 768]
Loading TF weight bert/encoder/layer_3/output/dense/kernel/adam_v with shape [3072, 768]
Loading TF weight bert/encoder/layer_4/attention/output/LayerNorm/beta with shape [768]
Loading TF weight bert/encoder/layer_4/attention/output/LayerNorm/beta/adam_m with shape [768]
Loading TF weight bert/encoder/layer_4/attention/output/LayerNorm/beta/adam_v with shape [768]
Loading TF weight bert/encoder/layer_4/attention/output/LayerNorm/gamma with shape [768]
Loading TF weight bert/encoder/layer_4/attention/output/LayerNorm/gamma/adam_m with shape [768]
Loading TF weight bert/encoder/layer_4/attention/output/LayerNorm/gamma/adam_v with shape [768]
Loading TF weight bert/encoder/layer_4/attention/output/dense/bias with shape [768]
Loading TF weight bert/encoder/layer_4/attention/output/dense/bias/adam_m with shape [768]
Loading TF weight bert/encoder/layer_4/attention/output/dense/bias/adam_v with shape [768]
Loading TF weight bert/encoder/layer_4/attention/output/dense/kernel with shape [768, 768]
Loading TF weight bert/encoder/layer_4/attention/output/dense/kernel/adam_m with shape [768, 768]
Loading TF weight bert/encoder/layer_4/attention/output/dense/kernel/adam_v with shape [768, 768]
Loading TF weight bert/encoder/layer_4/attention/self/key/bias with shape [768]
Loading TF weight bert/encoder/layer_4/attention/self/key/bias/adam_m with shape [768]
Loading TF weight bert/encoder/layer_4/attention/self/key/bias/adam_v with shape [768]
Loading TF weight bert/encoder/layer_4/attention/self/key/kernel with shape [768, 768]
Loading TF weight bert/encoder/layer_4/attention/self/key/kernel/adam_m with shape [768, 768]
Loading TF weight bert/encoder/layer_4/attention/self/key/kernel/adam_v with shape [768, 768]
Loading TF weight bert/encoder/layer_4/attention/self/query/bias with shape [768]
Loading TF weight bert/encoder/layer_4/attention/self/query/bias/adam_m with shape [768]
Loading TF weight bert/encoder/layer_4/attention/self/query/bias/adam_v with shape [768]
Loading TF weight bert/encoder/layer_4/attention/self/query/kernel with shape [768, 768]
Loading TF weight bert/encoder/layer_4/attention/self/query/kernel/adam_m with shape [768, 768]
Loading TF weight bert/encoder/layer_4/attention/self/query/kernel/adam_v with shape [768, 768]
Loading TF weight bert/encoder/layer_4/attention/self/value/bias with shape [768]
Loading TF weight bert/encoder/layer_4/attention/self/value/bias/adam_m with shape [768]
Loading TF weight bert/encoder/layer_4/attention/self/value/bias/adam_v with shape [768]
Loading TF weight bert/encoder/layer_4/attention/self/value/kernel with shape [768, 768]
Loading TF weight bert/encoder/layer_4/attention/self/value/kernel/adam_m with shape [768, 768]
Loading TF weight bert/encoder/layer_4/attention/self/value/kernel/adam_v with shape [768, 768]
Loading TF weight bert/encoder/layer_4/intermediate/dense/bias with shape [3072]
Loading TF weight bert/encoder/layer_4/intermediate/dense/bias/adam_m with shape [3072]
Loading TF weight bert/encoder/layer_4/intermediate/dense/bias/adam_v with shape [3072]
Loading TF weight bert/encoder/layer_4/intermediate/dense/kernel with shape [768, 3072]
Loading TF weight bert/encoder/layer_4/intermediate/dense/kernel/adam_m with shape [768, 3072]
Loading TF weight bert/encoder/layer_4/intermediate/dense/kernel/adam_v with shape [768, 3072]
Loading TF weight bert/encoder/layer_4/output/LayerNorm/beta with shape [768]
Loading TF weight bert/encoder/layer_4/output/LayerNorm/beta/adam_m with shape [768]
Loading TF weight bert/encoder/layer_4/output/LayerNorm/beta/adam_v with shape [768]
Loading TF weight bert/encoder/layer_4/output/LayerNorm/gamma with shape [768]
Loading TF weight bert/encoder/layer_4/output/LayerNorm/gamma/adam_m with shape [768]
Loading TF weight bert/encoder/layer_4/output/LayerNorm/gamma/adam_v with shape [768]
Loading TF weight bert/encoder/layer_4/output/dense/bias with shape [768]
Loading TF weight bert/encoder/layer_4/output/dense/bias/adam_m with shape [768]
Loading TF weight bert/encoder/layer_4/output/dense/bias/adam_v with shape [768]
Loading TF weight bert/encoder/layer_4/output/dense/kernel with shape [3072, 768]
Loading TF weight bert/encoder/layer_4/output/dense/kernel/adam_m with shape [3072, 768]
Loading TF weight bert/encoder/layer_4/output/dense/kernel/adam_v with shape [3072, 768]
Loading TF weight bert/encoder/layer_5/attention/output/LayerNorm/beta with shape [768]
Loading TF weight bert/encoder/layer_5/attention/output/LayerNorm/beta/adam_m with shape [768]
Loading TF weight bert/encoder/layer_5/attention/output/LayerNorm/beta/adam_v with shape [768]
Loading TF weight bert/encoder/layer_5/attention/output/LayerNorm/gamma with shape [768]
Loading TF weight bert/encoder/layer_5/attention/output/LayerNorm/gamma/adam_m with shape [768]
Loading TF weight bert/encoder/layer_5/attention/output/LayerNorm/gamma/adam_v with shape [768]
Loading TF weight bert/encoder/layer_5/attention/output/dense/bias with shape [768]
Loading TF weight bert/encoder/layer_5/attention/output/dense/bias/adam_m with shape [768]
Loading TF weight bert/encoder/layer_5/attention/output/dense/bias/adam_v with shape [768]
Loading TF weight bert/encoder/layer_5/attention/output/dense/kernel with shape [768, 768]
Loading TF weight bert/encoder/layer_5/attention/output/dense/kernel/adam_m with shape [768, 768]
Loading TF weight bert/encoder/layer_5/attention/output/dense/kernel/adam_v with shape [768, 768]
Loading TF weight bert/encoder/layer_5/attention/self/key/bias with shape [768]
Loading TF weight bert/encoder/layer_5/attention/self/key/bias/adam_m with shape [768]
Loading TF weight bert/encoder/layer_5/attention/self/key/bias/adam_v with shape [768]
Loading TF weight bert/encoder/layer_5/attention/self/key/kernel with shape [768, 768]
Loading TF weight bert/encoder/layer_5/attention/self/key/kernel/adam_m with shape [768, 768]
Loading TF weight bert/encoder/layer_5/attention/self/key/kernel/adam_v with shape [768, 768]
Loading TF weight bert/encoder/layer_5/attention/self/query/bias with shape [768]
Loading TF weight bert/encoder/layer_5/attention/self/query/bias/adam_m with shape [768]
Loading TF weight bert/encoder/layer_5/attention/self/query/bias/adam_v with shape [768]
Loading TF weight bert/encoder/layer_5/attention/self/query/kernel with shape [768, 768]
Loading TF weight bert/encoder/layer_5/attention/self/query/kernel/adam_m with shape [768, 768]
Loading TF weight bert/encoder/layer_5/attention/self/query/kernel/adam_v with shape [768, 768]
Loading TF weight bert/encoder/layer_5/attention/self/value/bias with shape [768]
Loading TF weight bert/encoder/layer_5/attention/self/value/bias/adam_m with shape [768]
Loading TF weight bert/encoder/layer_5/attention/self/value/bias/adam_v with shape [768]
Loading TF weight bert/encoder/layer_5/attention/self/value/kernel with shape [768, 768]
Loading TF weight bert/encoder/layer_5/attention/self/value/kernel/adam_m with shape [768, 768]
Loading TF weight bert/encoder/layer_5/attention/self/value/kernel/adam_v with shape [768, 768]
Loading TF weight bert/encoder/layer_5/intermediate/dense/bias with shape [3072]
Loading TF weight bert/encoder/layer_5/intermediate/dense/bias/adam_m with shape [3072]
Loading TF weight bert/encoder/layer_5/intermediate/dense/bias/adam_v with shape [3072]
Loading TF weight bert/encoder/layer_5/intermediate/dense/kernel with shape [768, 3072]
Loading TF weight bert/encoder/layer_5/intermediate/dense/kernel/adam_m with shape [768, 3072]
Loading TF weight bert/encoder/layer_5/intermediate/dense/kernel/adam_v with shape [768, 3072]
Loading TF weight bert/encoder/layer_5/output/LayerNorm/beta with shape [768]
Loading TF weight bert/encoder/layer_5/output/LayerNorm/beta/adam_m with shape [768]
Loading TF weight bert/encoder/layer_5/output/LayerNorm/beta/adam_v with shape [768]
Loading TF weight bert/encoder/layer_5/output/LayerNorm/gamma with shape [768]
Loading TF weight bert/encoder/layer_5/output/LayerNorm/gamma/adam_m with shape [768]
Loading TF weight bert/encoder/layer_5/output/LayerNorm/gamma/adam_v with shape [768]
Loading TF weight bert/encoder/layer_5/output/dense/bias with shape [768]
Loading TF weight bert/encoder/layer_5/output/dense/bias/adam_m with shape [768]
Loading TF weight bert/encoder/layer_5/output/dense/bias/adam_v with shape [768]
Loading TF weight bert/encoder/layer_5/output/dense/kernel with shape [3072, 768]
Loading TF weight bert/encoder/layer_5/output/dense/kernel/adam_m with shape [3072, 768]
Loading TF weight bert/encoder/layer_5/output/dense/kernel/adam_v with shape [3072, 768]
Loading TF weight bert/encoder/layer_6/attention/output/LayerNorm/beta with shape [768]
Loading TF weight bert/encoder/layer_6/attention/output/LayerNorm/beta/adam_m with shape [768]
Loading TF weight bert/encoder/layer_6/attention/output/LayerNorm/beta/adam_v with shape [768]
Loading TF weight bert/encoder/layer_6/attention/output/LayerNorm/gamma with shape [768]
Loading TF weight bert/encoder/layer_6/attention/output/LayerNorm/gamma/adam_m with shape [768]
Loading TF weight bert/encoder/layer_6/attention/output/LayerNorm/gamma/adam_v with shape [768]
Loading TF weight bert/encoder/layer_6/attention/output/dense/bias with shape [768]
Loading TF weight bert/encoder/layer_6/attention/output/dense/bias/adam_m with shape [768]
Loading TF weight bert/encoder/layer_6/attention/output/dense/bias/adam_v with shape [768]
Loading TF weight bert/encoder/layer_6/attention/output/dense/kernel with shape [768, 768]
Loading TF weight bert/encoder/layer_6/attention/output/dense/kernel/adam_m with shape [768, 768]
Loading TF weight bert/encoder/layer_6/attention/output/dense/kernel/adam_v with shape [768, 768]
Loading TF weight bert/encoder/layer_6/attention/self/key/bias with shape [768]
Loading TF weight bert/encoder/layer_6/attention/self/key/bias/adam_m with shape [768]
Loading TF weight bert/encoder/layer_6/attention/self/key/bias/adam_v with shape [768]
Loading TF weight bert/encoder/layer_6/attention/self/key/kernel with shape [768, 768]
Loading TF weight bert/encoder/layer_6/attention/self/key/kernel/adam_m with shape [768, 768]
Loading TF weight bert/encoder/layer_6/attention/self/key/kernel/adam_v with shape [768, 768]
Loading TF weight bert/encoder/layer_6/attention/self/query/bias with shape [768]
Loading TF weight bert/encoder/layer_6/attention/self/query/bias/adam_m with shape [768]
Loading TF weight bert/encoder/layer_6/attention/self/query/bias/adam_v with shape [768]
Loading TF weight bert/encoder/layer_6/attention/self/query/kernel with shape [768, 768]
Loading TF weight bert/encoder/layer_6/attention/self/query/kernel/adam_m with shape [768, 768]
Loading TF weight bert/encoder/layer_6/attention/self/query/kernel/adam_v with shape [768, 768]
Loading TF weight bert/encoder/layer_6/attention/self/value/bias with shape [768]
Loading TF weight bert/encoder/layer_6/attention/self/value/bias/adam_m with shape [768]
Loading TF weight bert/encoder/layer_6/attention/self/value/bias/adam_v with shape [768]
Loading TF weight bert/encoder/layer_6/attention/self/value/kernel with shape [768, 768]
Loading TF weight bert/encoder/layer_6/attention/self/value/kernel/adam_m with shape [768, 768]
Loading TF weight bert/encoder/layer_6/attention/self/value/kernel/adam_v with shape [768, 768]
Loading TF weight bert/encoder/layer_6/intermediate/dense/bias with shape [3072]
Loading TF weight bert/encoder/layer_6/intermediate/dense/bias/adam_m with shape [3072]
Loading TF weight bert/encoder/layer_6/intermediate/dense/bias/adam_v with shape [3072]
Loading TF weight bert/encoder/layer_6/intermediate/dense/kernel with shape [768, 3072]
Loading TF weight bert/encoder/layer_6/intermediate/dense/kernel/adam_m with shape [768, 3072]
Loading TF weight bert/encoder/layer_6/intermediate/dense/kernel/adam_v with shape [768, 3072]
Loading TF weight bert/encoder/layer_6/output/LayerNorm/beta with shape [768]
Loading TF weight bert/encoder/layer_6/output/LayerNorm/beta/adam_m with shape [768]
Loading TF weight bert/encoder/layer_6/output/LayerNorm/beta/adam_v with shape [768]
Loading TF weight bert/encoder/layer_6/output/LayerNorm/gamma with shape [768]
Loading TF weight bert/encoder/layer_6/output/LayerNorm/gamma/adam_m with shape [768]
Loading TF weight bert/encoder/layer_6/output/LayerNorm/gamma/adam_v with shape [768]
Loading TF weight bert/encoder/layer_6/output/dense/bias with shape [768]
Loading TF weight bert/encoder/layer_6/output/dense/bias/adam_m with shape [768]
Loading TF weight bert/encoder/layer_6/output/dense/bias/adam_v with shape [768]
Loading TF weight bert/encoder/layer_6/output/dense/kernel with shape [3072, 768]
Loading TF weight bert/encoder/layer_6/output/dense/kernel/adam_m with shape [3072, 768]
Loading TF weight bert/encoder/layer_6/output/dense/kernel/adam_v with shape [3072, 768]
Loading TF weight bert/encoder/layer_7/attention/output/LayerNorm/beta with shape [768]
Loading TF weight bert/encoder/layer_7/attention/output/LayerNorm/beta/adam_m with shape [768]
Loading TF weight bert/encoder/layer_7/attention/output/LayerNorm/beta/adam_v with shape [768]
Loading TF weight bert/encoder/layer_7/attention/output/LayerNorm/gamma with shape [768]
Loading TF weight bert/encoder/layer_7/attention/output/LayerNorm/gamma/adam_m with shape [768]
Loading TF weight bert/encoder/layer_7/attention/output/LayerNorm/gamma/adam_v with shape [768]
Loading TF weight bert/encoder/layer_7/attention/output/dense/bias with shape [768]
Loading TF weight bert/encoder/layer_7/attention/output/dense/bias/adam_m with shape [768]
Loading TF weight bert/encoder/layer_7/attention/output/dense/bias/adam_v with shape [768]
Loading TF weight bert/encoder/layer_7/attention/output/dense/kernel with shape [768, 768]
Loading TF weight bert/encoder/layer_7/attention/output/dense/kernel/adam_m with shape [768, 768]
Loading TF weight bert/encoder/layer_7/attention/output/dense/kernel/adam_v with shape [768, 768]
Loading TF weight bert/encoder/layer_7/attention/self/key/bias with shape [768]
Loading TF weight bert/encoder/layer_7/attention/self/key/bias/adam_m with shape [768]
Loading TF weight bert/encoder/layer_7/attention/self/key/bias/adam_v with shape [768]
Loading TF weight bert/encoder/layer_7/attention/self/key/kernel with shape [768, 768]
Loading TF weight bert/encoder/layer_7/attention/self/key/kernel/adam_m with shape [768, 768]
Loading TF weight bert/encoder/layer_7/attention/self/key/kernel/adam_v with shape [768, 768]
Loading TF weight bert/encoder/layer_7/attention/self/query/bias with shape [768]
Loading TF weight bert/encoder/layer_7/attention/self/query/bias/adam_m with shape [768]
Loading TF weight bert/encoder/layer_7/attention/self/query/bias/adam_v with shape [768]
Loading TF weight bert/encoder/layer_7/attention/self/query/kernel with shape [768, 768]
Loading TF weight bert/encoder/layer_7/attention/self/query/kernel/adam_m with shape [768, 768]
Loading TF weight bert/encoder/layer_7/attention/self/query/kernel/adam_v with shape [768, 768]
Loading TF weight bert/encoder/layer_7/attention/self/value/bias with shape [768]
Loading TF weight bert/encoder/layer_7/attention/self/value/bias/adam_m with shape [768]
Loading TF weight bert/encoder/layer_7/attention/self/value/bias/adam_v with shape [768]
Loading TF weight bert/encoder/layer_7/attention/self/value/kernel with shape [768, 768]
Loading TF weight bert/encoder/layer_7/attention/self/value/kernel/adam_m with shape [768, 768]
Loading TF weight bert/encoder/layer_7/attention/self/value/kernel/adam_v with shape [768, 768]
Loading TF weight bert/encoder/layer_7/intermediate/dense/bias with shape [3072]
Loading TF weight bert/encoder/layer_7/intermediate/dense/bias/adam_m with shape [3072]
Loading TF weight bert/encoder/layer_7/intermediate/dense/bias/adam_v with shape [3072]
Loading TF weight bert/encoder/layer_7/intermediate/dense/kernel with shape [768, 3072]
Loading TF weight bert/encoder/layer_7/intermediate/dense/kernel/adam_m with shape [768, 3072]
Loading TF weight bert/encoder/layer_7/intermediate/dense/kernel/adam_v with shape [768, 3072]
Loading TF weight bert/encoder/layer_7/output/LayerNorm/beta with shape [768]
Loading TF weight bert/encoder/layer_7/output/LayerNorm/beta/adam_m with shape [768]
Loading TF weight bert/encoder/layer_7/output/LayerNorm/beta/adam_v with shape [768]
Loading TF weight bert/encoder/layer_7/output/LayerNorm/gamma with shape [768]
Loading TF weight bert/encoder/layer_7/output/LayerNorm/gamma/adam_m with shape [768]
Loading TF weight bert/encoder/layer_7/output/LayerNorm/gamma/adam_v with shape [768]
Loading TF weight bert/encoder/layer_7/output/dense/bias with shape [768]
Loading TF weight bert/encoder/layer_7/output/dense/bias/adam_m with shape [768]
Loading TF weight bert/encoder/layer_7/output/dense/bias/adam_v with shape [768]
Loading TF weight bert/encoder/layer_7/output/dense/kernel with shape [3072, 768]
Loading TF weight bert/encoder/layer_7/output/dense/kernel/adam_m with shape [3072, 768]
Loading TF weight bert/encoder/layer_7/output/dense/kernel/adam_v with shape [3072, 768]
Loading TF weight bert/encoder/layer_8/attention/output/LayerNorm/beta with shape [768]
Loading TF weight bert/encoder/layer_8/attention/output/LayerNorm/beta/adam_m with shape [768]
Loading TF weight bert/encoder/layer_8/attention/output/LayerNorm/beta/adam_v with shape [768]
Loading TF weight bert/encoder/layer_8/attention/output/LayerNorm/gamma with shape [768]
Loading TF weight bert/encoder/layer_8/attention/output/LayerNorm/gamma/adam_m with shape [768]
Loading TF weight bert/encoder/layer_8/attention/output/LayerNorm/gamma/adam_v with shape [768]
Loading TF weight bert/encoder/layer_8/attention/output/dense/bias with shape [768]
Loading TF weight bert/encoder/layer_8/attention/output/dense/bias/adam_m with shape [768]
Loading TF weight bert/encoder/layer_8/attention/output/dense/bias/adam_v with shape [768]
Loading TF weight bert/encoder/layer_8/attention/output/dense/kernel with shape [768, 768]
Loading TF weight bert/encoder/layer_8/attention/output/dense/kernel/adam_m with shape [768, 768]
Loading TF weight bert/encoder/layer_8/attention/output/dense/kernel/adam_v with shape [768, 768]
Loading TF weight bert/encoder/layer_8/attention/self/key/bias with shape [768]
Loading TF weight bert/encoder/layer_8/attention/self/key/bias/adam_m with shape [768]
Loading TF weight bert/encoder/layer_8/attention/self/key/bias/adam_v with shape [768]
Loading TF weight bert/encoder/layer_8/attention/self/key/kernel with shape [768, 768]
Loading TF weight bert/encoder/layer_8/attention/self/key/kernel/adam_m with shape [768, 768]
Loading TF weight bert/encoder/layer_8/attention/self/key/kernel/adam_v with shape [768, 768]
Loading TF weight bert/encoder/layer_8/attention/self/query/bias with shape [768]
Loading TF weight bert/encoder/layer_8/attention/self/query/bias/adam_m with shape [768]
Loading TF weight bert/encoder/layer_8/attention/self/query/bias/adam_v with shape [768]
Loading TF weight bert/encoder/layer_8/attention/self/query/kernel with shape [768, 768]
Loading TF weight bert/encoder/layer_8/attention/self/query/kernel/adam_m with shape [768, 768]
Loading TF weight bert/encoder/layer_8/attention/self/query/kernel/adam_v with shape [768, 768]
Loading TF weight bert/encoder/layer_8/attention/self/value/bias with shape [768]
Loading TF weight bert/encoder/layer_8/attention/self/value/bias/adam_m with shape [768]
Loading TF weight bert/encoder/layer_8/attention/self/value/bias/adam_v with shape [768]
Loading TF weight bert/encoder/layer_8/attention/self/value/kernel with shape [768, 768]
Loading TF weight bert/encoder/layer_8/attention/self/value/kernel/adam_m with shape [768, 768]
Loading TF weight bert/encoder/layer_8/attention/self/value/kernel/adam_v with shape [768, 768]
Loading TF weight bert/encoder/layer_8/intermediate/dense/bias with shape [3072]
Loading TF weight bert/encoder/layer_8/intermediate/dense/bias/adam_m with shape [3072]
Loading TF weight bert/encoder/layer_8/intermediate/dense/bias/adam_v with shape [3072]
Loading TF weight bert/encoder/layer_8/intermediate/dense/kernel with shape [768, 3072]
Loading TF weight bert/encoder/layer_8/intermediate/dense/kernel/adam_m with shape [768, 3072]
Loading TF weight bert/encoder/layer_8/intermediate/dense/kernel/adam_v with shape [768, 3072]
Loading TF weight bert/encoder/layer_8/output/LayerNorm/beta with shape [768]
Loading TF weight bert/encoder/layer_8/output/LayerNorm/beta/adam_m with shape [768]
Loading TF weight bert/encoder/layer_8/output/LayerNorm/beta/adam_v with shape [768]
Loading TF weight bert/encoder/layer_8/output/LayerNorm/gamma with shape [768]
Loading TF weight bert/encoder/layer_8/output/LayerNorm/gamma/adam_m with shape [768]
Loading TF weight bert/encoder/layer_8/output/LayerNorm/gamma/adam_v with shape [768]
Loading TF weight bert/encoder/layer_8/output/dense/bias with shape [768]
Loading TF weight bert/encoder/layer_8/output/dense/bias/adam_m with shape [768]
Loading TF weight bert/encoder/layer_8/output/dense/bias/adam_v with shape [768]
Loading TF weight bert/encoder/layer_8/output/dense/kernel with shape [3072, 768]
Loading TF weight bert/encoder/layer_8/output/dense/kernel/adam_m with shape [3072, 768]
Loading TF weight bert/encoder/layer_8/output/dense/kernel/adam_v with shape [3072, 768]
Loading TF weight bert/encoder/layer_9/attention/output/LayerNorm/beta with shape [768]
Loading TF weight bert/encoder/layer_9/attention/output/LayerNorm/beta/adam_m with shape [768]
Loading TF weight bert/encoder/layer_9/attention/output/LayerNorm/beta/adam_v with shape [768]
Loading TF weight bert/encoder/layer_9/attention/output/LayerNorm/gamma with shape [768]
Loading TF weight bert/encoder/layer_9/attention/output/LayerNorm/gamma/adam_m with shape [768]
Loading TF weight bert/encoder/layer_9/attention/output/LayerNorm/gamma/adam_v with shape [768]
Loading TF weight bert/encoder/layer_9/attention/output/dense/bias with shape [768]
Loading TF weight bert/encoder/layer_9/attention/output/dense/bias/adam_m with shape [768]
Loading TF weight bert/encoder/layer_9/attention/output/dense/bias/adam_v with shape [768]
Loading TF weight bert/encoder/layer_9/attention/output/dense/kernel with shape [768, 768]
Loading TF weight bert/encoder/layer_9/attention/output/dense/kernel/adam_m with shape [768, 768]
Loading TF weight bert/encoder/layer_9/attention/output/dense/kernel/adam_v with shape [768, 768]
Loading TF weight bert/encoder/layer_9/attention/self/key/bias with shape [768]
Loading TF weight bert/encoder/layer_9/attention/self/key/bias/adam_m with shape [768]
Loading TF weight bert/encoder/layer_9/attention/self/key/bias/adam_v with shape [768]
Loading TF weight bert/encoder/layer_9/attention/self/key/kernel with shape [768, 768]
Loading TF weight bert/encoder/layer_9/attention/self/key/kernel/adam_m with shape [768, 768]
Loading TF weight bert/encoder/layer_9/attention/self/key/kernel/adam_v with shape [768, 768]
Loading TF weight bert/encoder/layer_9/attention/self/query/bias with shape [768]
Loading TF weight bert/encoder/layer_9/attention/self/query/bias/adam_m with shape [768]
Loading TF weight bert/encoder/layer_9/attention/self/query/bias/adam_v with shape [768]
Loading TF weight bert/encoder/layer_9/attention/self/query/kernel with shape [768, 768]
Loading TF weight bert/encoder/layer_9/attention/self/query/kernel/adam_m with shape [768, 768]
Loading TF weight bert/encoder/layer_9/attention/self/query/kernel/adam_v with shape [768, 768]
Loading TF weight bert/encoder/layer_9/attention/self/value/bias with shape [768]
Loading TF weight bert/encoder/layer_9/attention/self/value/bias/adam_m with shape [768]
Loading TF weight bert/encoder/layer_9/attention/self/value/bias/adam_v with shape [768]
Loading TF weight bert/encoder/layer_9/attention/self/value/kernel with shape [768, 768]
Loading TF weight bert/encoder/layer_9/attention/self/value/kernel/adam_m with shape [768, 768]
Loading TF weight bert/encoder/layer_9/attention/self/value/kernel/adam_v with shape [768, 768]
Loading TF weight bert/encoder/layer_9/intermediate/dense/bias with shape [3072]
Loading TF weight bert/encoder/layer_9/intermediate/dense/bias/adam_m with shape [3072]
Loading TF weight bert/encoder/layer_9/intermediate/dense/bias/adam_v with shape [3072]
Loading TF weight bert/encoder/layer_9/intermediate/dense/kernel with shape [768, 3072]
Loading TF weight bert/encoder/layer_9/intermediate/dense/kernel/adam_m with shape [768, 3072]
Loading TF weight bert/encoder/layer_9/intermediate/dense/kernel/adam_v with shape [768, 3072]
Loading TF weight bert/encoder/layer_9/output/LayerNorm/beta with shape [768]
Loading TF weight bert/encoder/layer_9/output/LayerNorm/beta/adam_m with shape [768]
Loading TF weight bert/encoder/layer_9/output/LayerNorm/beta/adam_v with shape [768]
Loading TF weight bert/encoder/layer_9/output/LayerNorm/gamma with shape [768]
Loading TF weight bert/encoder/layer_9/output/LayerNorm/gamma/adam_m with shape [768]
Loading TF weight bert/encoder/layer_9/output/LayerNorm/gamma/adam_v with shape [768]
Loading TF weight bert/encoder/layer_9/output/dense/bias with shape [768]
Loading TF weight bert/encoder/layer_9/output/dense/bias/adam_m with shape [768]
Loading TF weight bert/encoder/layer_9/output/dense/bias/adam_v with shape [768]
Loading TF weight bert/encoder/layer_9/output/dense/kernel with shape [3072, 768]
Loading TF weight bert/encoder/layer_9/output/dense/kernel/adam_m with shape [3072, 768]
Loading TF weight bert/encoder/layer_9/output/dense/kernel/adam_v with shape [3072, 768]
Loading TF weight bert/pooler/dense/bias with shape [768]
Loading TF weight bert/pooler/dense/bias/adam_m with shape [768]
Loading TF weight bert/pooler/dense/bias/adam_v with shape [768]
Loading TF weight bert/pooler/dense/kernel with shape [768, 768]
Loading TF weight bert/pooler/dense/kernel/adam_m with shape [768, 768]
Loading TF weight bert/pooler/dense/kernel/adam_v with shape [768, 768]
Loading TF weight global_step with shape []
Loading TF weight output_bias with shape [656]
Loading TF weight output_bias/adam_m with shape [656]
Loading TF weight output_bias/adam_v with shape [656]
Loading TF weight output_weights with shape [656, 768]
Loading TF weight output_weights/adam_m with shape [656, 768]
Loading TF weight output_weights/adam_v with shape [656, 768]
Initialize PyTorch weight ['bert', 'embeddings', 'LayerNorm', 'beta']
Skipping bert/embeddings/LayerNorm/beta/adam_m
Skipping bert/embeddings/LayerNorm/beta/adam_v
Initialize PyTorch weight ['bert', 'embeddings', 'LayerNorm', 'gamma']
Skipping bert/embeddings/LayerNorm/gamma/adam_m
Skipping bert/embeddings/LayerNorm/gamma/adam_v
Initialize PyTorch weight ['bert', 'embeddings', 'position_embeddings']
Skipping bert/embeddings/position_embeddings/adam_m
Skipping bert/embeddings/position_embeddings/adam_v
Initialize PyTorch weight ['bert', 'embeddings', 'token_type_embeddings']
Skipping bert/embeddings/token_type_embeddings/adam_m
Skipping bert/embeddings/token_type_embeddings/adam_v
Initialize PyTorch weight ['bert', 'embeddings', 'word_embeddings']
Skipping bert/embeddings/word_embeddings/adam_m
Skipping bert/embeddings/word_embeddings/adam_v
Initialize PyTorch weight ['bert', 'encoder', 'layer_0', 'attention', 'output', 'LayerNorm', 'beta']
Skipping bert/encoder/layer_0/attention/output/LayerNorm/beta/adam_m
Skipping bert/encoder/layer_0/attention/output/LayerNorm/beta/adam_v
Initialize PyTorch weight ['bert', 'encoder', 'layer_0', 'attention', 'output', 'LayerNorm', 'gamma']
Skipping bert/encoder/layer_0/attention/output/LayerNorm/gamma/adam_m
Skipping bert/encoder/layer_0/attention/output/LayerNorm/gamma/adam_v
Initialize PyTorch weight ['bert', 'encoder', 'layer_0', 'attention', 'output', 'dense', 'bias']
Skipping bert/encoder/layer_0/attention/output/dense/bias/adam_m
Skipping bert/encoder/layer_0/attention/output/dense/bias/adam_v
Initialize PyTorch weight ['bert', 'encoder', 'layer_0', 'attention', 'output', 'dense', 'kernel']
Skipping bert/encoder/layer_0/attention/output/dense/kernel/adam_m
Skipping bert/encoder/layer_0/attention/output/dense/kernel/adam_v
Initialize PyTorch weight ['bert', 'encoder', 'layer_0', 'attention', 'self', 'key', 'bias']
Skipping bert/encoder/layer_0/attention/self/key/bias/adam_m
Skipping bert/encoder/layer_0/attention/self/key/bias/adam_v
Initialize PyTorch weight ['bert', 'encoder', 'layer_0', 'attention', 'self', 'key', 'kernel']
Skipping bert/encoder/layer_0/attention/self/key/kernel/adam_m
Skipping bert/encoder/layer_0/attention/self/key/kernel/adam_v
Initialize PyTorch weight ['bert', 'encoder', 'layer_0', 'attention', 'self', 'query', 'bias']
Skipping bert/encoder/layer_0/attention/self/query/bias/adam_m
Skipping bert/encoder/layer_0/attention/self/query/bias/adam_v
Initialize PyTorch weight ['bert', 'encoder', 'layer_0', 'attention', 'self', 'query', 'kernel']
Skipping bert/encoder/layer_0/attention/self/query/kernel/adam_m
Skipping bert/encoder/layer_0/attention/self/query/kernel/adam_v
Initialize PyTorch weight ['bert', 'encoder', 'layer_0', 'attention', 'self', 'value', 'bias']
Skipping bert/encoder/layer_0/attention/self/value/bias/adam_m
Skipping bert/encoder/layer_0/attention/self/value/bias/adam_v
Initialize PyTorch weight ['bert', 'encoder', 'layer_0', 'attention', 'self', 'value', 'kernel']
Skipping bert/encoder/layer_0/attention/self/value/kernel/adam_m
Skipping bert/encoder/layer_0/attention/self/value/kernel/adam_v
Initialize PyTorch weight ['bert', 'encoder', 'layer_0', 'intermediate', 'dense', 'bias']
Skipping bert/encoder/layer_0/intermediate/dense/bias/adam_m
Skipping bert/encoder/layer_0/intermediate/dense/bias/adam_v
Initialize PyTorch weight ['bert', 'encoder', 'layer_0', 'intermediate', 'dense', 'kernel']
Skipping bert/encoder/layer_0/intermediate/dense/kernel/adam_m
Skipping bert/encoder/layer_0/intermediate/dense/kernel/adam_v
Initialize PyTorch weight ['bert', 'encoder', 'layer_0', 'output', 'LayerNorm', 'beta']
Skipping bert/encoder/layer_0/output/LayerNorm/beta/adam_m
Skipping bert/encoder/layer_0/output/LayerNorm/beta/adam_v
Initialize PyTorch weight ['bert', 'encoder', 'layer_0', 'output', 'LayerNorm', 'gamma']
Skipping bert/encoder/layer_0/output/LayerNorm/gamma/adam_m
Skipping bert/encoder/layer_0/output/LayerNorm/gamma/adam_v
Initialize PyTorch weight ['bert', 'encoder', 'layer_0', 'output', 'dense', 'bias']
Skipping bert/encoder/layer_0/output/dense/bias/adam_m
Skipping bert/encoder/layer_0/output/dense/bias/adam_v
Initialize PyTorch weight ['bert', 'encoder', 'layer_0', 'output', 'dense', 'kernel']
Skipping bert/encoder/layer_0/output/dense/kernel/adam_m
Skipping bert/encoder/layer_0/output/dense/kernel/adam_v
Initialize PyTorch weight ['bert', 'encoder', 'layer_1', 'attention', 'output', 'LayerNorm', 'beta']
Skipping bert/encoder/layer_1/attention/output/LayerNorm/beta/adam_m
Skipping bert/encoder/layer_1/attention/output/LayerNorm/beta/adam_v
Initialize PyTorch weight ['bert', 'encoder', 'layer_1', 'attention', 'output', 'LayerNorm', 'gamma']
Skipping bert/encoder/layer_1/attention/output/LayerNorm/gamma/adam_m
Skipping bert/encoder/layer_1/attention/output/LayerNorm/gamma/adam_v
Initialize PyTorch weight ['bert', 'encoder', 'layer_1', 'attention', 'output', 'dense', 'bias']
Skipping bert/encoder/layer_1/attention/output/dense/bias/adam_m
Skipping bert/encoder/layer_1/attention/output/dense/bias/adam_v
Initialize PyTorch weight ['bert', 'encoder', 'layer_1', 'attention', 'output', 'dense', 'kernel']
Skipping bert/encoder/layer_1/attention/output/dense/kernel/adam_m
Skipping bert/encoder/layer_1/attention/output/dense/kernel/adam_v
Initialize PyTorch weight ['bert', 'encoder', 'layer_1', 'attention', 'self', 'key', 'bias']
Skipping bert/encoder/layer_1/attention/self/key/bias/adam_m
Skipping bert/encoder/layer_1/attention/self/key/bias/adam_v
Initialize PyTorch weight ['bert', 'encoder', 'layer_1', 'attention', 'self', 'key', 'kernel']
Skipping bert/encoder/layer_1/attention/self/key/kernel/adam_m
Skipping bert/encoder/layer_1/attention/self/key/kernel/adam_v
Initialize PyTorch weight ['bert', 'encoder', 'layer_1', 'attention', 'self', 'query', 'bias']
Skipping bert/encoder/layer_1/attention/self/query/bias/adam_m
Skipping bert/encoder/layer_1/attention/self/query/bias/adam_v
Initialize PyTorch weight ['bert', 'encoder', 'layer_1', 'attention', 'self', 'query', 'kernel']
Skipping bert/encoder/layer_1/attention/self/query/kernel/adam_m
Skipping bert/encoder/layer_1/attention/self/query/kernel/adam_v
Initialize PyTorch weight ['bert', 'encoder', 'layer_1', 'attention', 'self', 'value', 'bias']
Skipping bert/encoder/layer_1/attention/self/value/bias/adam_m
Skipping bert/encoder/layer_1/attention/self/value/bias/adam_v
Initialize PyTorch weight ['bert', 'encoder', 'layer_1', 'attention', 'self', 'value', 'kernel']
Skipping bert/encoder/layer_1/attention/self/value/kernel/adam_m
Skipping bert/encoder/layer_1/attention/self/value/kernel/adam_v
Initialize PyTorch weight ['bert', 'encoder', 'layer_1', 'intermediate', 'dense', 'bias']
Skipping bert/encoder/layer_1/intermediate/dense/bias/adam_m
Skipping bert/encoder/layer_1/intermediate/dense/bias/adam_v
Initialize PyTorch weight ['bert', 'encoder', 'layer_1', 'intermediate', 'dense', 'kernel']
Skipping bert/encoder/layer_1/intermediate/dense/kernel/adam_m
Skipping bert/encoder/layer_1/intermediate/dense/kernel/adam_v
Initialize PyTorch weight ['bert', 'encoder', 'layer_1', 'output', 'LayerNorm', 'beta']
Skipping bert/encoder/layer_1/output/LayerNorm/beta/adam_m
Skipping bert/encoder/layer_1/output/LayerNorm/beta/adam_v
Initialize PyTorch weight ['bert', 'encoder', 'layer_1', 'output', 'LayerNorm', 'gamma']
Skipping bert/encoder/layer_1/output/LayerNorm/gamma/adam_m
Skipping bert/encoder/layer_1/output/LayerNorm/gamma/adam_v
Initialize PyTorch weight ['bert', 'encoder', 'layer_1', 'output', 'dense', 'bias']
Skipping bert/encoder/layer_1/output/dense/bias/adam_m
Skipping bert/encoder/layer_1/output/dense/bias/adam_v
Initialize PyTorch weight ['bert', 'encoder', 'layer_1', 'output', 'dense', 'kernel']
Skipping bert/encoder/layer_1/output/dense/kernel/adam_m
Skipping bert/encoder/layer_1/output/dense/kernel/adam_v
Initialize PyTorch weight ['bert', 'encoder', 'layer_10', 'attention', 'output', 'LayerNorm', 'beta']
Skipping bert/encoder/layer_10/attention/output/LayerNorm/beta/adam_m
Skipping bert/encoder/layer_10/attention/output/LayerNorm/beta/adam_v
Initialize PyTorch weight ['bert', 'encoder', 'layer_10', 'attention', 'output', 'LayerNorm', 'gamma']
Skipping bert/encoder/layer_10/attention/output/LayerNorm/gamma/adam_m
Skipping bert/encoder/layer_10/attention/output/LayerNorm/gamma/adam_v
Initialize PyTorch weight ['bert', 'encoder', 'layer_10', 'attention', 'output', 'dense', 'bias']
Skipping bert/encoder/layer_10/attention/output/dense/bias/adam_m
Skipping bert/encoder/layer_10/attention/output/dense/bias/adam_v
Initialize PyTorch weight ['bert', 'encoder', 'layer_10', 'attention', 'output', 'dense', 'kernel']
Skipping bert/encoder/layer_10/attention/output/dense/kernel/adam_m
Skipping bert/encoder/layer_10/attention/output/dense/kernel/adam_v
Initialize PyTorch weight ['bert', 'encoder', 'layer_10', 'attention', 'self', 'key', 'bias']
Skipping bert/encoder/layer_10/attention/self/key/bias/adam_m
Skipping bert/encoder/layer_10/attention/self/key/bias/adam_v
Initialize PyTorch weight ['bert', 'encoder', 'layer_10', 'attention', 'self', 'key', 'kernel']
Skipping bert/encoder/layer_10/attention/self/key/kernel/adam_m
Skipping bert/encoder/layer_10/attention/self/key/kernel/adam_v
Initialize PyTorch weight ['bert', 'encoder', 'layer_10', 'attention', 'self', 'query', 'bias']
Skipping bert/encoder/layer_10/attention/self/query/bias/adam_m
Skipping bert/encoder/layer_10/attention/self/query/bias/adam_v
Initialize PyTorch weight ['bert', 'encoder', 'layer_10', 'attention', 'self', 'query', 'kernel']
Skipping bert/encoder/layer_10/attention/self/query/kernel/adam_m
Skipping bert/encoder/layer_10/attention/self/query/kernel/adam_v
Initialize PyTorch weight ['bert', 'encoder', 'layer_10', 'attention', 'self', 'value', 'bias']
Skipping bert/encoder/layer_10/attention/self/value/bias/adam_m
Skipping bert/encoder/layer_10/attention/self/value/bias/adam_v
Initialize PyTorch weight ['bert', 'encoder', 'layer_10', 'attention', 'self', 'value', 'kernel']
Skipping bert/encoder/layer_10/attention/self/value/kernel/adam_m
Skipping bert/encoder/layer_10/attention/self/value/kernel/adam_v
Initialize PyTorch weight ['bert', 'encoder', 'layer_10', 'intermediate', 'dense', 'bias']
Skipping bert/encoder/layer_10/intermediate/dense/bias/adam_m
Skipping bert/encoder/layer_10/intermediate/dense/bias/adam_v
Initialize PyTorch weight ['bert', 'encoder', 'layer_10', 'intermediate', 'dense', 'kernel']
Skipping bert/encoder/layer_10/intermediate/dense/kernel/adam_m
Skipping bert/encoder/layer_10/intermediate/dense/kernel/adam_v
Initialize PyTorch weight ['bert', 'encoder', 'layer_10', 'output', 'LayerNorm', 'beta']
Skipping bert/encoder/layer_10/output/LayerNorm/beta/adam_m
Skipping bert/encoder/layer_10/output/LayerNorm/beta/adam_v
Initialize PyTorch weight ['bert', 'encoder', 'layer_10', 'output', 'LayerNorm', 'gamma']
Skipping bert/encoder/layer_10/output/LayerNorm/gamma/adam_m
Skipping bert/encoder/layer_10/output/LayerNorm/gamma/adam_v
Initialize PyTorch weight ['bert', 'encoder', 'layer_10', 'output', 'dense', 'bias']
Skipping bert/encoder/layer_10/output/dense/bias/adam_m
Skipping bert/encoder/layer_10/output/dense/bias/adam_v
Initialize PyTorch weight ['bert', 'encoder', 'layer_10', 'output', 'dense', 'kernel']
Skipping bert/encoder/layer_10/output/dense/kernel/adam_m
Skipping bert/encoder/layer_10/output/dense/kernel/adam_v
Initialize PyTorch weight ['bert', 'encoder', 'layer_11', 'attention', 'output', 'LayerNorm', 'beta']
Skipping bert/encoder/layer_11/attention/output/LayerNorm/beta/adam_m
Skipping bert/encoder/layer_11/attention/output/LayerNorm/beta/adam_v
Initialize PyTorch weight ['bert', 'encoder', 'layer_11', 'attention', 'output', 'LayerNorm', 'gamma']
Skipping bert/encoder/layer_11/attention/output/LayerNorm/gamma/adam_m
Skipping bert/encoder/layer_11/attention/output/LayerNorm/gamma/adam_v
Initialize PyTorch weight ['bert', 'encoder', 'layer_11', 'attention', 'output', 'dense', 'bias']
Skipping bert/encoder/layer_11/attention/output/dense/bias/adam_m
Skipping bert/encoder/layer_11/attention/output/dense/bias/adam_v
Initialize PyTorch weight ['bert', 'encoder', 'layer_11', 'attention', 'output', 'dense', 'kernel']
Skipping bert/encoder/layer_11/attention/output/dense/kernel/adam_m
Skipping bert/encoder/layer_11/attention/output/dense/kernel/adam_v
Initialize PyTorch weight ['bert', 'encoder', 'layer_11', 'attention', 'self', 'key', 'bias']
Skipping bert/encoder/layer_11/attention/self/key/bias/adam_m
Skipping bert/encoder/layer_11/attention/self/key/bias/adam_v
Initialize PyTorch weight ['bert', 'encoder', 'layer_11', 'attention', 'self', 'key', 'kernel']
Skipping bert/encoder/layer_11/attention/self/key/kernel/adam_m
Skipping bert/encoder/layer_11/attention/self/key/kernel/adam_v
Initialize PyTorch weight ['bert', 'encoder', 'layer_11', 'attention', 'self', 'query', 'bias']
Skipping bert/encoder/layer_11/attention/self/query/bias/adam_m
Skipping bert/encoder/layer_11/attention/self/query/bias/adam_v
Initialize PyTorch weight ['bert', 'encoder', 'layer_11', 'attention', 'self', 'query', 'kernel']
Skipping bert/encoder/layer_11/attention/self/query/kernel/adam_m
Skipping bert/encoder/layer_11/attention/self/query/kernel/adam_v
Initialize PyTorch weight ['bert', 'encoder', 'layer_11', 'attention', 'self', 'value', 'bias']
Skipping bert/encoder/layer_11/attention/self/value/bias/adam_m
Skipping bert/encoder/layer_11/attention/self/value/bias/adam_v
Initialize PyTorch weight ['bert', 'encoder', 'layer_11', 'attention', 'self', 'value', 'kernel']
Skipping bert/encoder/layer_11/attention/self/value/kernel/adam_m
Skipping bert/encoder/layer_11/attention/self/value/kernel/adam_v
Initialize PyTorch weight ['bert', 'encoder', 'layer_11', 'intermediate', 'dense', 'bias']
Skipping bert/encoder/layer_11/intermediate/dense/bias/adam_m
Skipping bert/encoder/layer_11/intermediate/dense/bias/adam_v
Initialize PyTorch weight ['bert', 'encoder', 'layer_11', 'intermediate', 'dense', 'kernel']
Skipping bert/encoder/layer_11/intermediate/dense/kernel/adam_m
Skipping bert/encoder/layer_11/intermediate/dense/kernel/adam_v
Initialize PyTorch weight ['bert', 'encoder', 'layer_11', 'output', 'LayerNorm', 'beta']
Skipping bert/encoder/layer_11/output/LayerNorm/beta/adam_m
Skipping bert/encoder/layer_11/output/LayerNorm/beta/adam_v
Initialize PyTorch weight ['bert', 'encoder', 'layer_11', 'output', 'LayerNorm', 'gamma']
Skipping bert/encoder/layer_11/output/LayerNorm/gamma/adam_m
Skipping bert/encoder/layer_11/output/LayerNorm/gamma/adam_v
Initialize PyTorch weight ['bert', 'encoder', 'layer_11', 'output', 'dense', 'bias']
Skipping bert/encoder/layer_11/output/dense/bias/adam_m
Skipping bert/encoder/layer_11/output/dense/bias/adam_v
Initialize PyTorch weight ['bert', 'encoder', 'layer_11', 'output', 'dense', 'kernel']
Skipping bert/encoder/layer_11/output/dense/kernel/adam_m
Skipping bert/encoder/layer_11/output/dense/kernel/adam_v
Initialize PyTorch weight ['bert', 'encoder', 'layer_2', 'attention', 'output', 'LayerNorm', 'beta']
Skipping bert/encoder/layer_2/attention/output/LayerNorm/beta/adam_m
Skipping bert/encoder/layer_2/attention/output/LayerNorm/beta/adam_v
Initialize PyTorch weight ['bert', 'encoder', 'layer_2', 'attention', 'output', 'LayerNorm', 'gamma']
Skipping bert/encoder/layer_2/attention/output/LayerNorm/gamma/adam_m
Skipping bert/encoder/layer_2/attention/output/LayerNorm/gamma/adam_v
Initialize PyTorch weight ['bert', 'encoder', 'layer_2', 'attention', 'output', 'dense', 'bias']
Skipping bert/encoder/layer_2/attention/output/dense/bias/adam_m
Skipping bert/encoder/layer_2/attention/output/dense/bias/adam_v
Initialize PyTorch weight ['bert', 'encoder', 'layer_2', 'attention', 'output', 'dense', 'kernel']
Skipping bert/encoder/layer_2/attention/output/dense/kernel/adam_m
Skipping bert/encoder/layer_2/attention/output/dense/kernel/adam_v
Initialize PyTorch weight ['bert', 'encoder', 'layer_2', 'attention', 'self', 'key', 'bias']
Skipping bert/encoder/layer_2/attention/self/key/bias/adam_m
Skipping bert/encoder/layer_2/attention/self/key/bias/adam_v
Initialize PyTorch weight ['bert', 'encoder', 'layer_2', 'attention', 'self', 'key', 'kernel']
Skipping bert/encoder/layer_2/attention/self/key/kernel/adam_m
Skipping bert/encoder/layer_2/attention/self/key/kernel/adam_v
Initialize PyTorch weight ['bert', 'encoder', 'layer_2', 'attention', 'self', 'query', 'bias']
Skipping bert/encoder/layer_2/attention/self/query/bias/adam_m
Skipping bert/encoder/layer_2/attention/self/query/bias/adam_v
Initialize PyTorch weight ['bert', 'encoder', 'layer_2', 'attention', 'self', 'query', 'kernel']
Skipping bert/encoder/layer_2/attention/self/query/kernel/adam_m
Skipping bert/encoder/layer_2/attention/self/query/kernel/adam_v
Initialize PyTorch weight ['bert', 'encoder', 'layer_2', 'attention', 'self', 'value', 'bias']
Skipping bert/encoder/layer_2/attention/self/value/bias/adam_m
Skipping bert/encoder/layer_2/attention/self/value/bias/adam_v
Initialize PyTorch weight ['bert', 'encoder', 'layer_2', 'attention', 'self', 'value', 'kernel']
Skipping bert/encoder/layer_2/attention/self/value/kernel/adam_m
Skipping bert/encoder/layer_2/attention/self/value/kernel/adam_v
Initialize PyTorch weight ['bert', 'encoder', 'layer_2', 'intermediate', 'dense', 'bias']
Skipping bert/encoder/layer_2/intermediate/dense/bias/adam_m
Skipping bert/encoder/layer_2/intermediate/dense/bias/adam_v
Initialize PyTorch weight ['bert', 'encoder', 'layer_2', 'intermediate', 'dense', 'kernel']
Skipping bert/encoder/layer_2/intermediate/dense/kernel/adam_m
Skipping bert/encoder/layer_2/intermediate/dense/kernel/adam_v
Initialize PyTorch weight ['bert', 'encoder', 'layer_2', 'output', 'LayerNorm', 'beta']
Skipping bert/encoder/layer_2/output/LayerNorm/beta/adam_m
Skipping bert/encoder/layer_2/output/LayerNorm/beta/adam_v
Initialize PyTorch weight ['bert', 'encoder', 'layer_2', 'output', 'LayerNorm', 'gamma']
Skipping bert/encoder/layer_2/output/LayerNorm/gamma/adam_m
Skipping bert/encoder/layer_2/output/LayerNorm/gamma/adam_v
Initialize PyTorch weight ['bert', 'encoder', 'layer_2', 'output', 'dense', 'bias']
Skipping bert/encoder/layer_2/output/dense/bias/adam_m
Skipping bert/encoder/layer_2/output/dense/bias/adam_v
Initialize PyTorch weight ['bert', 'encoder', 'layer_2', 'output', 'dense', 'kernel']
Skipping bert/encoder/layer_2/output/dense/kernel/adam_m
Skipping bert/encoder/layer_2/output/dense/kernel/adam_v
Initialize PyTorch weight ['bert', 'encoder', 'layer_3', 'attention', 'output', 'LayerNorm', 'beta']
Skipping bert/encoder/layer_3/attention/output/LayerNorm/beta/adam_m
Skipping bert/encoder/layer_3/attention/output/LayerNorm/beta/adam_v
Initialize PyTorch weight ['bert', 'encoder', 'layer_3', 'attention', 'output', 'LayerNorm', 'gamma']
Skipping bert/encoder/layer_3/attention/output/LayerNorm/gamma/adam_m
Skipping bert/encoder/layer_3/attention/output/LayerNorm/gamma/adam_v
Initialize PyTorch weight ['bert', 'encoder', 'layer_3', 'attention', 'output', 'dense', 'bias']
Skipping bert/encoder/layer_3/attention/output/dense/bias/adam_m
Skipping bert/encoder/layer_3/attention/output/dense/bias/adam_v
Initialize PyTorch weight ['bert', 'encoder', 'layer_3', 'attention', 'output', 'dense', 'kernel']
Skipping bert/encoder/layer_3/attention/output/dense/kernel/adam_m
Skipping bert/encoder/layer_3/attention/output/dense/kernel/adam_v
Initialize PyTorch weight ['bert', 'encoder', 'layer_3', 'attention', 'self', 'key', 'bias']
Skipping bert/encoder/layer_3/attention/self/key/bias/adam_m
Skipping bert/encoder/layer_3/attention/self/key/bias/adam_v
Initialize PyTorch weight ['bert', 'encoder', 'layer_3', 'attention', 'self', 'key', 'kernel']
Skipping bert/encoder/layer_3/attention/self/key/kernel/adam_m
Skipping bert/encoder/layer_3/attention/self/key/kernel/adam_v
Initialize PyTorch weight ['bert', 'encoder', 'layer_3', 'attention', 'self', 'query', 'bias']
Skipping bert/encoder/layer_3/attention/self/query/bias/adam_m
Skipping bert/encoder/layer_3/attention/self/query/bias/adam_v
Initialize PyTorch weight ['bert', 'encoder', 'layer_3', 'attention', 'self', 'query', 'kernel']
Skipping bert/encoder/layer_3/attention/self/query/kernel/adam_m
Skipping bert/encoder/layer_3/attention/self/query/kernel/adam_v
Initialize PyTorch weight ['bert', 'encoder', 'layer_3', 'attention', 'self', 'value', 'bias']
Skipping bert/encoder/layer_3/attention/self/value/bias/adam_m
Skipping bert/encoder/layer_3/attention/self/value/bias/adam_v
Initialize PyTorch weight ['bert', 'encoder', 'layer_3', 'attention', 'self', 'value', 'kernel']
Skipping bert/encoder/layer_3/attention/self/value/kernel/adam_m
Skipping bert/encoder/layer_3/attention/self/value/kernel/adam_v
Initialize PyTorch weight ['bert', 'encoder', 'layer_3', 'intermediate', 'dense', 'bias']
Skipping bert/encoder/layer_3/intermediate/dense/bias/adam_m
Skipping bert/encoder/layer_3/intermediate/dense/bias/adam_v
Initialize PyTorch weight ['bert', 'encoder', 'layer_3', 'intermediate', 'dense', 'kernel']
Skipping bert/encoder/layer_3/intermediate/dense/kernel/adam_m
Skipping bert/encoder/layer_3/intermediate/dense/kernel/adam_v
Initialize PyTorch weight ['bert', 'encoder', 'layer_3', 'output', 'LayerNorm', 'beta']
Skipping bert/encoder/layer_3/output/LayerNorm/beta/adam_m
Skipping bert/encoder/layer_3/output/LayerNorm/beta/adam_v
Initialize PyTorch weight ['bert', 'encoder', 'layer_3', 'output', 'LayerNorm', 'gamma']
Skipping bert/encoder/layer_3/output/LayerNorm/gamma/adam_m
Skipping bert/encoder/layer_3/output/LayerNorm/gamma/adam_v
Initialize PyTorch weight ['bert', 'encoder', 'layer_3', 'output', 'dense', 'bias']
Skipping bert/encoder/layer_3/output/dense/bias/adam_m
Skipping bert/encoder/layer_3/output/dense/bias/adam_v
Initialize PyTorch weight ['bert', 'encoder', 'layer_3', 'output', 'dense', 'kernel']
Skipping bert/encoder/layer_3/output/dense/kernel/adam_m
Skipping bert/encoder/layer_3/output/dense/kernel/adam_v
Initialize PyTorch weight ['bert', 'encoder', 'layer_4', 'attention', 'output', 'LayerNorm', 'beta']
Skipping bert/encoder/layer_4/attention/output/LayerNorm/beta/adam_m
Skipping bert/encoder/layer_4/attention/output/LayerNorm/beta/adam_v
Initialize PyTorch weight ['bert', 'encoder', 'layer_4', 'attention', 'output', 'LayerNorm', 'gamma']
Skipping bert/encoder/layer_4/attention/output/LayerNorm/gamma/adam_m
Skipping bert/encoder/layer_4/attention/output/LayerNorm/gamma/adam_v
Initialize PyTorch weight ['bert', 'encoder', 'layer_4', 'attention', 'output', 'dense', 'bias']
Skipping bert/encoder/layer_4/attention/output/dense/bias/adam_m
Skipping bert/encoder/layer_4/attention/output/dense/bias/adam_v
Initialize PyTorch weight ['bert', 'encoder', 'layer_4', 'attention', 'output', 'dense', 'kernel']
Skipping bert/encoder/layer_4/attention/output/dense/kernel/adam_m
Skipping bert/encoder/layer_4/attention/output/dense/kernel/adam_v
Initialize PyTorch weight ['bert', 'encoder', 'layer_4', 'attention', 'self', 'key', 'bias']
Skipping bert/encoder/layer_4/attention/self/key/bias/adam_m
Skipping bert/encoder/layer_4/attention/self/key/bias/adam_v
Initialize PyTorch weight ['bert', 'encoder', 'layer_4', 'attention', 'self', 'key', 'kernel']
Skipping bert/encoder/layer_4/attention/self/key/kernel/adam_m
Skipping bert/encoder/layer_4/attention/self/key/kernel/adam_v
Initialize PyTorch weight ['bert', 'encoder', 'layer_4', 'attention', 'self', 'query', 'bias']
Skipping bert/encoder/layer_4/attention/self/query/bias/adam_m
Skipping bert/encoder/layer_4/attention/self/query/bias/adam_v
Initialize PyTorch weight ['bert', 'encoder', 'layer_4', 'attention', 'self', 'query', 'kernel']
Skipping bert/encoder/layer_4/attention/self/query/kernel/adam_m
Skipping bert/encoder/layer_4/attention/self/query/kernel/adam_v
Initialize PyTorch weight ['bert', 'encoder', 'layer_4', 'attention', 'self', 'value', 'bias']
Skipping bert/encoder/layer_4/attention/self/value/bias/adam_m
Skipping bert/encoder/layer_4/attention/self/value/bias/adam_v
Initialize PyTorch weight ['bert', 'encoder', 'layer_4', 'attention', 'self', 'value', 'kernel']
Skipping bert/encoder/layer_4/attention/self/value/kernel/adam_m
Skipping bert/encoder/layer_4/attention/self/value/kernel/adam_v
Initialize PyTorch weight ['bert', 'encoder', 'layer_4', 'intermediate', 'dense', 'bias']
Skipping bert/encoder/layer_4/intermediate/dense/bias/adam_m
Skipping bert/encoder/layer_4/intermediate/dense/bias/adam_v
Initialize PyTorch weight ['bert', 'encoder', 'layer_4', 'intermediate', 'dense', 'kernel']
Skipping bert/encoder/layer_4/intermediate/dense/kernel/adam_m
Skipping bert/encoder/layer_4/intermediate/dense/kernel/adam_v
Initialize PyTorch weight ['bert', 'encoder', 'layer_4', 'output', 'LayerNorm', 'beta']
Skipping bert/encoder/layer_4/output/LayerNorm/beta/adam_m
Skipping bert/encoder/layer_4/output/LayerNorm/beta/adam_v
Initialize PyTorch weight ['bert', 'encoder', 'layer_4', 'output', 'LayerNorm', 'gamma']
Skipping bert/encoder/layer_4/output/LayerNorm/gamma/adam_m
Skipping bert/encoder/layer_4/output/LayerNorm/gamma/adam_v
Initialize PyTorch weight ['bert', 'encoder', 'layer_4', 'output', 'dense', 'bias']
Skipping bert/encoder/layer_4/output/dense/bias/adam_m
Skipping bert/encoder/layer_4/output/dense/bias/adam_v
Initialize PyTorch weight ['bert', 'encoder', 'layer_4', 'output', 'dense', 'kernel']
Skipping bert/encoder/layer_4/output/dense/kernel/adam_m
Skipping bert/encoder/layer_4/output/dense/kernel/adam_v
Initialize PyTorch weight ['bert', 'encoder', 'layer_5', 'attention', 'output', 'LayerNorm', 'beta']
Skipping bert/encoder/layer_5/attention/output/LayerNorm/beta/adam_m
Skipping bert/encoder/layer_5/attention/output/LayerNorm/beta/adam_v
Initialize PyTorch weight ['bert', 'encoder', 'layer_5', 'attention', 'output', 'LayerNorm', 'gamma']
Skipping bert/encoder/layer_5/attention/output/LayerNorm/gamma/adam_m
Skipping bert/encoder/layer_5/attention/output/LayerNorm/gamma/adam_v
Initialize PyTorch weight ['bert', 'encoder', 'layer_5', 'attention', 'output', 'dense', 'bias']
Skipping bert/encoder/layer_5/attention/output/dense/bias/adam_m
Skipping bert/encoder/layer_5/attention/output/dense/bias/adam_v
Initialize PyTorch weight ['bert', 'encoder', 'layer_5', 'attention', 'output', 'dense', 'kernel']
Skipping bert/encoder/layer_5/attention/output/dense/kernel/adam_m
Skipping bert/encoder/layer_5/attention/output/dense/kernel/adam_v
Initialize PyTorch weight ['bert', 'encoder', 'layer_5', 'attention', 'self', 'key', 'bias']
Skipping bert/encoder/layer_5/attention/self/key/bias/adam_m
Skipping bert/encoder/layer_5/attention/self/key/bias/adam_v
Initialize PyTorch weight ['bert', 'encoder', 'layer_5', 'attention', 'self', 'key', 'kernel']
Skipping bert/encoder/layer_5/attention/self/key/kernel/adam_m
Skipping bert/encoder/layer_5/attention/self/key/kernel/adam_v
Initialize PyTorch weight ['bert', 'encoder', 'layer_5', 'attention', 'self', 'query', 'bias']
Skipping bert/encoder/layer_5/attention/self/query/bias/adam_m
Skipping bert/encoder/layer_5/attention/self/query/bias/adam_v
Initialize PyTorch weight ['bert', 'encoder', 'layer_5', 'attention', 'self', 'query', 'kernel']
Skipping bert/encoder/layer_5/attention/self/query/kernel/adam_m
Skipping bert/encoder/layer_5/attention/self/query/kernel/adam_v
Initialize PyTorch weight ['bert', 'encoder', 'layer_5', 'attention', 'self', 'value', 'bias']
Skipping bert/encoder/layer_5/attention/self/value/bias/adam_m
Skipping bert/encoder/layer_5/attention/self/value/bias/adam_v
Initialize PyTorch weight ['bert', 'encoder', 'layer_5', 'attention', 'self', 'value', 'kernel']
Skipping bert/encoder/layer_5/attention/self/value/kernel/adam_m
Skipping bert/encoder/layer_5/attention/self/value/kernel/adam_v
Initialize PyTorch weight ['bert', 'encoder', 'layer_5', 'intermediate', 'dense', 'bias']
Skipping bert/encoder/layer_5/intermediate/dense/bias/adam_m
Skipping bert/encoder/layer_5/intermediate/dense/bias/adam_v
Initialize PyTorch weight ['bert', 'encoder', 'layer_5', 'intermediate', 'dense', 'kernel']
Skipping bert/encoder/layer_5/intermediate/dense/kernel/adam_m
Skipping bert/encoder/layer_5/intermediate/dense/kernel/adam_v
Initialize PyTorch weight ['bert', 'encoder', 'layer_5', 'output', 'LayerNorm', 'beta']
Skipping bert/encoder/layer_5/output/LayerNorm/beta/adam_m
Skipping bert/encoder/layer_5/output/LayerNorm/beta/adam_v
Initialize PyTorch weight ['bert', 'encoder', 'layer_5', 'output', 'LayerNorm', 'gamma']
Skipping bert/encoder/layer_5/output/LayerNorm/gamma/adam_m
Skipping bert/encoder/layer_5/output/LayerNorm/gamma/adam_v
Initialize PyTorch weight ['bert', 'encoder', 'layer_5', 'output', 'dense', 'bias']
Skipping bert/encoder/layer_5/output/dense/bias/adam_m
Skipping bert/encoder/layer_5/output/dense/bias/adam_v
Initialize PyTorch weight ['bert', 'encoder', 'layer_5', 'output', 'dense', 'kernel']
Skipping bert/encoder/layer_5/output/dense/kernel/adam_m
Skipping bert/encoder/layer_5/output/dense/kernel/adam_v
Initialize PyTorch weight ['bert', 'encoder', 'layer_6', 'attention', 'output', 'LayerNorm', 'beta']
Skipping bert/encoder/layer_6/attention/output/LayerNorm/beta/adam_m
Skipping bert/encoder/layer_6/attention/output/LayerNorm/beta/adam_v
Initialize PyTorch weight ['bert', 'encoder', 'layer_6', 'attention', 'output', 'LayerNorm', 'gamma']
Skipping bert/encoder/layer_6/attention/output/LayerNorm/gamma/adam_m
Skipping bert/encoder/layer_6/attention/output/LayerNorm/gamma/adam_v
Initialize PyTorch weight ['bert', 'encoder', 'layer_6', 'attention', 'output', 'dense', 'bias']
Skipping bert/encoder/layer_6/attention/output/dense/bias/adam_m
Skipping bert/encoder/layer_6/attention/output/dense/bias/adam_v
Initialize PyTorch weight ['bert', 'encoder', 'layer_6', 'attention', 'output', 'dense', 'kernel']
Skipping bert/encoder/layer_6/attention/output/dense/kernel/adam_m
Skipping bert/encoder/layer_6/attention/output/dense/kernel/adam_v
Initialize PyTorch weight ['bert', 'encoder', 'layer_6', 'attention', 'self', 'key', 'bias']
Skipping bert/encoder/layer_6/attention/self/key/bias/adam_m
Skipping bert/encoder/layer_6/attention/self/key/bias/adam_v
Initialize PyTorch weight ['bert', 'encoder', 'layer_6', 'attention', 'self', 'key', 'kernel']
Skipping bert/encoder/layer_6/attention/self/key/kernel/adam_m
Skipping bert/encoder/layer_6/attention/self/key/kernel/adam_v
Initialize PyTorch weight ['bert', 'encoder', 'layer_6', 'attention', 'self', 'query', 'bias']
Skipping bert/encoder/layer_6/attention/self/query/bias/adam_m
Skipping bert/encoder/layer_6/attention/self/query/bias/adam_v
Initialize PyTorch weight ['bert', 'encoder', 'layer_6', 'attention', 'self', 'query', 'kernel']
Skipping bert/encoder/layer_6/attention/self/query/kernel/adam_m
Skipping bert/encoder/layer_6/attention/self/query/kernel/adam_v
Initialize PyTorch weight ['bert', 'encoder', 'layer_6', 'attention', 'self', 'value', 'bias']
Skipping bert/encoder/layer_6/attention/self/value/bias/adam_m
Skipping bert/encoder/layer_6/attention/self/value/bias/adam_v
Initialize PyTorch weight ['bert', 'encoder', 'layer_6', 'attention', 'self', 'value', 'kernel']
Skipping bert/encoder/layer_6/attention/self/value/kernel/adam_m
Skipping bert/encoder/layer_6/attention/self/value/kernel/adam_v
Initialize PyTorch weight ['bert', 'encoder', 'layer_6', 'intermediate', 'dense', 'bias']
Skipping bert/encoder/layer_6/intermediate/dense/bias/adam_m
Skipping bert/encoder/layer_6/intermediate/dense/bias/adam_v
Initialize PyTorch weight ['bert', 'encoder', 'layer_6', 'intermediate', 'dense', 'kernel']
Skipping bert/encoder/layer_6/intermediate/dense/kernel/adam_m
Skipping bert/encoder/layer_6/intermediate/dense/kernel/adam_v
Initialize PyTorch weight ['bert', 'encoder', 'layer_6', 'output', 'LayerNorm', 'beta']
Skipping bert/encoder/layer_6/output/LayerNorm/beta/adam_m
Skipping bert/encoder/layer_6/output/LayerNorm/beta/adam_v
Initialize PyTorch weight ['bert', 'encoder', 'layer_6', 'output', 'LayerNorm', 'gamma']
Skipping bert/encoder/layer_6/output/LayerNorm/gamma/adam_m
Skipping bert/encoder/layer_6/output/LayerNorm/gamma/adam_v
Initialize PyTorch weight ['bert', 'encoder', 'layer_6', 'output', 'dense', 'bias']
Skipping bert/encoder/layer_6/output/dense/bias/adam_m
Skipping bert/encoder/layer_6/output/dense/bias/adam_v
Initialize PyTorch weight ['bert', 'encoder', 'layer_6', 'output', 'dense', 'kernel']
Skipping bert/encoder/layer_6/output/dense/kernel/adam_m
Skipping bert/encoder/layer_6/output/dense/kernel/adam_v
Initialize PyTorch weight ['bert', 'encoder', 'layer_7', 'attention', 'output', 'LayerNorm', 'beta']
Skipping bert/encoder/layer_7/attention/output/LayerNorm/beta/adam_m
Skipping bert/encoder/layer_7/attention/output/LayerNorm/beta/adam_v
Initialize PyTorch weight ['bert', 'encoder', 'layer_7', 'attention', 'output', 'LayerNorm', 'gamma']
Skipping bert/encoder/layer_7/attention/output/LayerNorm/gamma/adam_m
Skipping bert/encoder/layer_7/attention/output/LayerNorm/gamma/adam_v
Initialize PyTorch weight ['bert', 'encoder', 'layer_7', 'attention', 'output', 'dense', 'bias']
Skipping bert/encoder/layer_7/attention/output/dense/bias/adam_m
Skipping bert/encoder/layer_7/attention/output/dense/bias/adam_v
Initialize PyTorch weight ['bert', 'encoder', 'layer_7', 'attention', 'output', 'dense', 'kernel']
Skipping bert/encoder/layer_7/attention/output/dense/kernel/adam_m
Skipping bert/encoder/layer_7/attention/output/dense/kernel/adam_v
Initialize PyTorch weight ['bert', 'encoder', 'layer_7', 'attention', 'self', 'key', 'bias']
Skipping bert/encoder/layer_7/attention/self/key/bias/adam_m
Skipping bert/encoder/layer_7/attention/self/key/bias/adam_v
Initialize PyTorch weight ['bert', 'encoder', 'layer_7', 'attention', 'self', 'key', 'kernel']
Skipping bert/encoder/layer_7/attention/self/key/kernel/adam_m
Skipping bert/encoder/layer_7/attention/self/key/kernel/adam_v
Initialize PyTorch weight ['bert', 'encoder', 'layer_7', 'attention', 'self', 'query', 'bias']
Skipping bert/encoder/layer_7/attention/self/query/bias/adam_m
Skipping bert/encoder/layer_7/attention/self/query/bias/adam_v
Initialize PyTorch weight ['bert', 'encoder', 'layer_7', 'attention', 'self', 'query', 'kernel']
Skipping bert/encoder/layer_7/attention/self/query/kernel/adam_m
Skipping bert/encoder/layer_7/attention/self/query/kernel/adam_v
Initialize PyTorch weight ['bert', 'encoder', 'layer_7', 'attention', 'self', 'value', 'bias']
Skipping bert/encoder/layer_7/attention/self/value/bias/adam_m
Skipping bert/encoder/layer_7/attention/self/value/bias/adam_v
Initialize PyTorch weight ['bert', 'encoder', 'layer_7', 'attention', 'self', 'value', 'kernel']
Skipping bert/encoder/layer_7/attention/self/value/kernel/adam_m
Skipping bert/encoder/layer_7/attention/self/value/kernel/adam_v
Initialize PyTorch weight ['bert', 'encoder', 'layer_7', 'intermediate', 'dense', 'bias']
Skipping bert/encoder/layer_7/intermediate/dense/bias/adam_m
Skipping bert/encoder/layer_7/intermediate/dense/bias/adam_v
Initialize PyTorch weight ['bert', 'encoder', 'layer_7', 'intermediate', 'dense', 'kernel']
Skipping bert/encoder/layer_7/intermediate/dense/kernel/adam_m
Skipping bert/encoder/layer_7/intermediate/dense/kernel/adam_v
Initialize PyTorch weight ['bert', 'encoder', 'layer_7', 'output', 'LayerNorm', 'beta']
Skipping bert/encoder/layer_7/output/LayerNorm/beta/adam_m
Skipping bert/encoder/layer_7/output/LayerNorm/beta/adam_v
Initialize PyTorch weight ['bert', 'encoder', 'layer_7', 'output', 'LayerNorm', 'gamma']
Skipping bert/encoder/layer_7/output/LayerNorm/gamma/adam_m
Skipping bert/encoder/layer_7/output/LayerNorm/gamma/adam_v
Initialize PyTorch weight ['bert', 'encoder', 'layer_7', 'output', 'dense', 'bias']
Skipping bert/encoder/layer_7/output/dense/bias/adam_m
Skipping bert/encoder/layer_7/output/dense/bias/adam_v
Initialize PyTorch weight ['bert', 'encoder', 'layer_7', 'output', 'dense', 'kernel']
Skipping bert/encoder/layer_7/output/dense/kernel/adam_m
Skipping bert/encoder/layer_7/output/dense/kernel/adam_v
Initialize PyTorch weight ['bert', 'encoder', 'layer_8', 'attention', 'output', 'LayerNorm', 'beta']
Skipping bert/encoder/layer_8/attention/output/LayerNorm/beta/adam_m
Skipping bert/encoder/layer_8/attention/output/LayerNorm/beta/adam_v
Initialize PyTorch weight ['bert', 'encoder', 'layer_8', 'attention', 'output', 'LayerNorm', 'gamma']
Skipping bert/encoder/layer_8/attention/output/LayerNorm/gamma/adam_m
Skipping bert/encoder/layer_8/attention/output/LayerNorm/gamma/adam_v
Initialize PyTorch weight ['bert', 'encoder', 'layer_8', 'attention', 'output', 'dense', 'bias']
Skipping bert/encoder/layer_8/attention/output/dense/bias/adam_m
Skipping bert/encoder/layer_8/attention/output/dense/bias/adam_v
Initialize PyTorch weight ['bert', 'encoder', 'layer_8', 'attention', 'output', 'dense', 'kernel']
Skipping bert/encoder/layer_8/attention/output/dense/kernel/adam_m
Skipping bert/encoder/layer_8/attention/output/dense/kernel/adam_v
Initialize PyTorch weight ['bert', 'encoder', 'layer_8', 'attention', 'self', 'key', 'bias']
Skipping bert/encoder/layer_8/attention/self/key/bias/adam_m
Skipping bert/encoder/layer_8/attention/self/key/bias/adam_v
Initialize PyTorch weight ['bert', 'encoder', 'layer_8', 'attention', 'self', 'key', 'kernel']
Skipping bert/encoder/layer_8/attention/self/key/kernel/adam_m
Skipping bert/encoder/layer_8/attention/self/key/kernel/adam_v
Initialize PyTorch weight ['bert', 'encoder', 'layer_8', 'attention', 'self', 'query', 'bias']
Skipping bert/encoder/layer_8/attention/self/query/bias/adam_m
Skipping bert/encoder/layer_8/attention/self/query/bias/adam_v
Initialize PyTorch weight ['bert', 'encoder', 'layer_8', 'attention', 'self', 'query', 'kernel']
Skipping bert/encoder/layer_8/attention/self/query/kernel/adam_m
Skipping bert/encoder/layer_8/attention/self/query/kernel/adam_v
Initialize PyTorch weight ['bert', 'encoder', 'layer_8', 'attention', 'self', 'value', 'bias']
Skipping bert/encoder/layer_8/attention/self/value/bias/adam_m
Skipping bert/encoder/layer_8/attention/self/value/bias/adam_v
Initialize PyTorch weight ['bert', 'encoder', 'layer_8', 'attention', 'self', 'value', 'kernel']
Skipping bert/encoder/layer_8/attention/self/value/kernel/adam_m
Skipping bert/encoder/layer_8/attention/self/value/kernel/adam_v
Initialize PyTorch weight ['bert', 'encoder', 'layer_8', 'intermediate', 'dense', 'bias']
Skipping bert/encoder/layer_8/intermediate/dense/bias/adam_m
Skipping bert/encoder/layer_8/intermediate/dense/bias/adam_v
Initialize PyTorch weight ['bert', 'encoder', 'layer_8', 'intermediate', 'dense', 'kernel']
Skipping bert/encoder/layer_8/intermediate/dense/kernel/adam_m
Skipping bert/encoder/layer_8/intermediate/dense/kernel/adam_v
Initialize PyTorch weight ['bert', 'encoder', 'layer_8', 'output', 'LayerNorm', 'beta']
Skipping bert/encoder/layer_8/output/LayerNorm/beta/adam_m
Skipping bert/encoder/layer_8/output/LayerNorm/beta/adam_v
Initialize PyTorch weight ['bert', 'encoder', 'layer_8', 'output', 'LayerNorm', 'gamma']
Skipping bert/encoder/layer_8/output/LayerNorm/gamma/adam_m
Skipping bert/encoder/layer_8/output/LayerNorm/gamma/adam_v
Initialize PyTorch weight ['bert', 'encoder', 'layer_8', 'output', 'dense', 'bias']
Skipping bert/encoder/layer_8/output/dense/bias/adam_m
Skipping bert/encoder/layer_8/output/dense/bias/adam_v
Initialize PyTorch weight ['bert', 'encoder', 'layer_8', 'output', 'dense', 'kernel']
Skipping bert/encoder/layer_8/output/dense/kernel/adam_m
Skipping bert/encoder/layer_8/output/dense/kernel/adam_v
Initialize PyTorch weight ['bert', 'encoder', 'layer_9', 'attention', 'output', 'LayerNorm', 'beta']
Skipping bert/encoder/layer_9/attention/output/LayerNorm/beta/adam_m
Skipping bert/encoder/layer_9/attention/output/LayerNorm/beta/adam_v
Initialize PyTorch weight ['bert', 'encoder', 'layer_9', 'attention', 'output', 'LayerNorm', 'gamma']
Skipping bert/encoder/layer_9/attention/output/LayerNorm/gamma/adam_m
Skipping bert/encoder/layer_9/attention/output/LayerNorm/gamma/adam_v
Initialize PyTorch weight ['bert', 'encoder', 'layer_9', 'attention', 'output', 'dense', 'bias']
Skipping bert/encoder/layer_9/attention/output/dense/bias/adam_m
Skipping bert/encoder/layer_9/attention/output/dense/bias/adam_v
Initialize PyTorch weight ['bert', 'encoder', 'layer_9', 'attention', 'output', 'dense', 'kernel']
Skipping bert/encoder/layer_9/attention/output/dense/kernel/adam_m
Skipping bert/encoder/layer_9/attention/output/dense/kernel/adam_v
Initialize PyTorch weight ['bert', 'encoder', 'layer_9', 'attention', 'self', 'key', 'bias']
Skipping bert/encoder/layer_9/attention/self/key/bias/adam_m
Skipping bert/encoder/layer_9/attention/self/key/bias/adam_v
Initialize PyTorch weight ['bert', 'encoder', 'layer_9', 'attention', 'self', 'key', 'kernel']
Skipping bert/encoder/layer_9/attention/self/key/kernel/adam_m
Skipping bert/encoder/layer_9/attention/self/key/kernel/adam_v
Initialize PyTorch weight ['bert', 'encoder', 'layer_9', 'attention', 'self', 'query', 'bias']
Skipping bert/encoder/layer_9/attention/self/query/bias/adam_m
Skipping bert/encoder/layer_9/attention/self/query/bias/adam_v
Initialize PyTorch weight ['bert', 'encoder', 'layer_9', 'attention', 'self', 'query', 'kernel']
Skipping bert/encoder/layer_9/attention/self/query/kernel/adam_m
Skipping bert/encoder/layer_9/attention/self/query/kernel/adam_v
Initialize PyTorch weight ['bert', 'encoder', 'layer_9', 'attention', 'self', 'value', 'bias']
Skipping bert/encoder/layer_9/attention/self/value/bias/adam_m
Skipping bert/encoder/layer_9/attention/self/value/bias/adam_v
Initialize PyTorch weight ['bert', 'encoder', 'layer_9', 'attention', 'self', 'value', 'kernel']
Skipping bert/encoder/layer_9/attention/self/value/kernel/adam_m
Skipping bert/encoder/layer_9/attention/self/value/kernel/adam_v
Initialize PyTorch weight ['bert', 'encoder', 'layer_9', 'intermediate', 'dense', 'bias']
Skipping bert/encoder/layer_9/intermediate/dense/bias/adam_m
Skipping bert/encoder/layer_9/intermediate/dense/bias/adam_v
Initialize PyTorch weight ['bert', 'encoder', 'layer_9', 'intermediate', 'dense', 'kernel']
Skipping bert/encoder/layer_9/intermediate/dense/kernel/adam_m
Skipping bert/encoder/layer_9/intermediate/dense/kernel/adam_v
Initialize PyTorch weight ['bert', 'encoder', 'layer_9', 'output', 'LayerNorm', 'beta']
Skipping bert/encoder/layer_9/output/LayerNorm/beta/adam_m
Skipping bert/encoder/layer_9/output/LayerNorm/beta/adam_v
Initialize PyTorch weight ['bert', 'encoder', 'layer_9', 'output', 'LayerNorm', 'gamma']
Skipping bert/encoder/layer_9/output/LayerNorm/gamma/adam_m
Skipping bert/encoder/layer_9/output/LayerNorm/gamma/adam_v
Initialize PyTorch weight ['bert', 'encoder', 'layer_9', 'output', 'dense', 'bias']
Skipping bert/encoder/layer_9/output/dense/bias/adam_m
Skipping bert/encoder/layer_9/output/dense/bias/adam_v
Initialize PyTorch weight ['bert', 'encoder', 'layer_9', 'output', 'dense', 'kernel']
Skipping bert/encoder/layer_9/output/dense/kernel/adam_m
Skipping bert/encoder/layer_9/output/dense/kernel/adam_v
Initialize PyTorch weight ['bert', 'pooler', 'dense', 'bias']
Skipping bert/pooler/dense/bias/adam_m
Skipping bert/pooler/dense/bias/adam_v
Initialize PyTorch weight ['bert', 'pooler', 'dense', 'kernel']
Skipping bert/pooler/dense/kernel/adam_m
Skipping bert/pooler/dense/kernel/adam_v
Skipping global_step
Traceback (most recent call last):
File "c:\programdata\anaconda3\envs\py37cuda10\lib\runpy.py", line 193, in _run_module_as_main
"__main__", mod_spec)
File "c:\programdata\anaconda3\envs\py37cuda10\lib\runpy.py", line 85, in _run_code
exec(code, run_globals)
File "C:\ProgramData\Anaconda3\envs\py37cuda10\Scripts\transformers-cli.exe\__main__.py", line 7, in <module>
File "c:\programdata\anaconda3\envs\py37cuda10\lib\site-packages\transformers\commands\transformers_cli.py", line 33, in main
service.run()
File "c:\programdata\anaconda3\envs\py37cuda10\lib\site-packages\transformers\commands\convert.py", line 91, in run
convert_tf_checkpoint_to_pytorch(self._tf_checkpoint, self._config, self._pytorch_dump_output)
File "c:\programdata\anaconda3\envs\py37cuda10\lib\site-packages\transformers\convert_bert_original_tf_checkpoint_to_pytorch.py", line 36, in convert_tf_checkpoint_to_pytorch
load_tf_weights_in_bert(model, config, tf_checkpoint_path)
File "c:\programdata\anaconda3\envs\py37cuda10\lib\site-packages\transformers\modeling_bert.py", line 135, in load_tf_weights_in_bert
pointer = getattr(pointer, "bias")
File "c:\programdata\anaconda3\envs\py37cuda10\lib\site-packages\torch\nn\modules\module.py", line 779, in __getattr__
type(self).__name__, name))
torch.nn.modules.module.ModuleAttributeError: 'BertForPreTraining' object has no attribute 'bias' | 11-02-2020 17:46:42 | 11-02-2020 17:46:42 | I ran into the same problem<|||||>> I ran into the same problem
but I didn't get the error<|||||>Hi @nikhilbyte @chainesanbuenaventura
Any updates? I also have the same problem while converting TensorFlow model to PyTorch model?
Thanks
<|||||>This issue has been automatically marked as stale because it has not had recent activity. It will be closed if no further activity occurs. Thank you for your contributions.
<|||||>This issue has been automatically marked as stale because it has not had recent activity. If you think this still needs to be addressed please comment on this thread.
Please note that issues that do not follow the [contributing guidelines](https://github.com/huggingface/transformers/blob/master/CONTRIBUTING.md) are likely to be ignored. |
transformers | 8,241 | closed | Update model cards of deepset/roberta-base-squad2 v1 and v2 | Update model cards since deepset/roberta-base-squad2 is now being superced by deepset/robert-base-squad2-v2 | 11-02-2020 17:39:56 | 11-02-2020 17:39:56 | |
transformers | 8,240 | closed | Add line by line option to mlm/plm scripts | # What does this PR do?
The old `run_language_modeling` script was supporting the option to choose `line_by_line` or not for the datasets in MLM/PLM. This PR adds that option to `run_mlm` and `run_plm`. It also updates the README to present those options and adds a flag to disable dynamic batching on TPU: TPUs need all batches to always have the same size to avoid recompiling the code at each training/evaluation step.
All scripts are tested on ditributed GPU/TPU env with or without the new flags and train to expected ppl on wikitext-2
| 11-02-2020 16:53:43 | 11-02-2020 16:53:43 | Hi, I am currently executing the **run_mlm.py** file, because I do not know the entire code structure very well, and I am a little confused about the 262 lines of code in the **run_mlm.py** file. It is line 262 in the figure below.
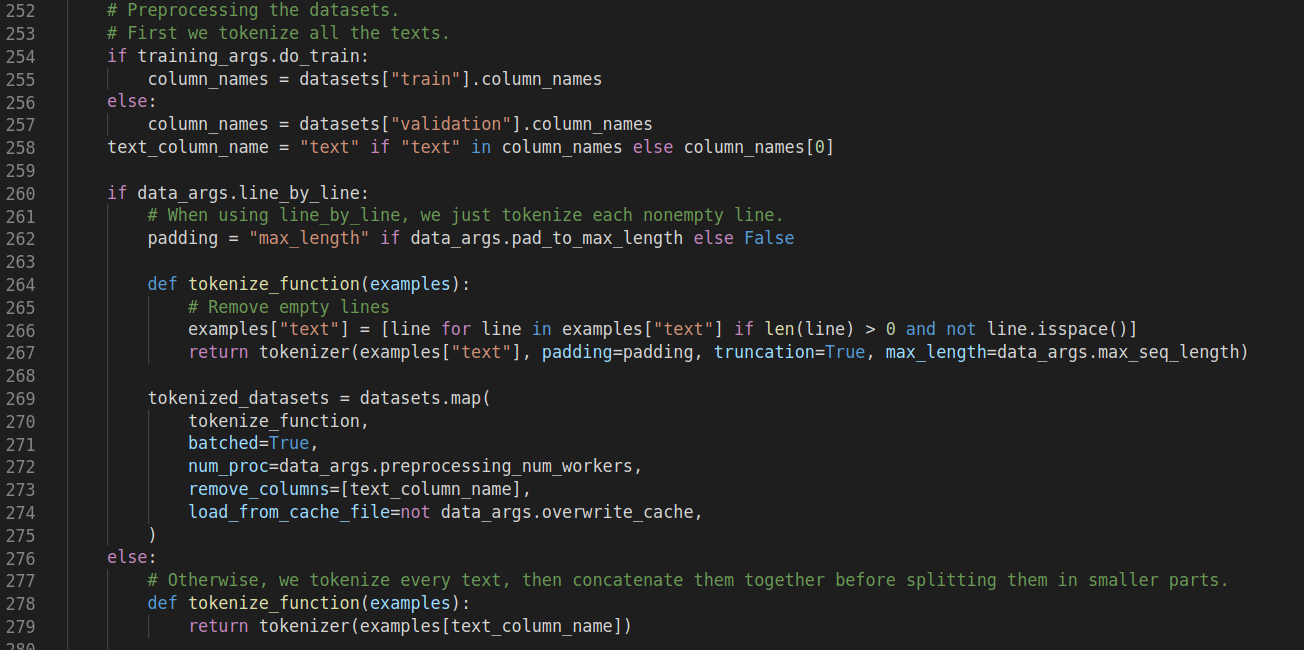
I think whether False in **padding = "max_length" if data_args.pad_to_max_length else False** should be True. Still say that True or False has no effect on the result. Thank you. Please ignore if I understand it wrong. :)
In addition, the following figure shows the usage of **tokenizer** in the Transformers documentation.
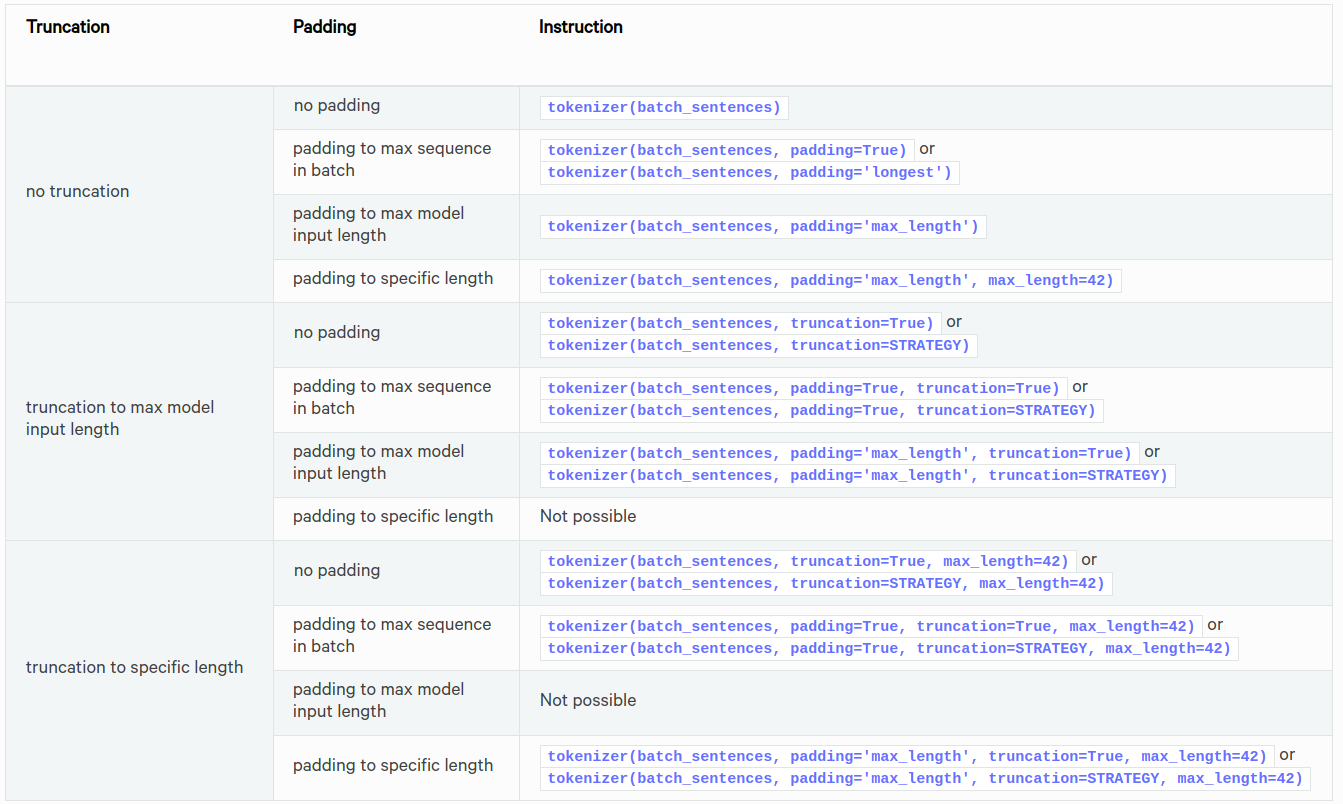
<|||||>Hi there, the test is as intended: the behavior is the following:
- if `data_args.pad_to_max_length` is True, then we will pad to the maximum length of the model.
- otherwise we don't pas (yet). Padding will done by the data collator so that we pad to the maximum length in the batch (dynamic padding).<|||||>I got it, thanks!<|||||>Hello, I still have a question to consult you. I want to train the **Translation Language Modeling (TLM)** in **XLM** (Paper: Cross-lingual Language Model Pretraining). The translation language modeling (**TLM**) is very similar to the **Masked Language Modeling (MLM)**, which only shows the difference in the form of input data. If I want to use the **run_mlm.py** file to achieve the effect of training the translation language modeling (**TLM**), can I just modify the composition of training data without modifying the source code of the **run_mlm.py** file? Is this feasible?
For example, for the masked language modeling (**MLM**), one row of my training data is a language, as shown below:
( **Row 1** ) polonium 's isotopes tend to decay with alpha or beta decay ( **en** ) .
( **Row 2** ) 231 and penetrated the armour of the Panzer IV behind it ( **en** ) .
( **Row 3** ) die Isotope von Polonium neigen dazu , mit dem Alpha- oder Beta-Zerfall zu zerfallen ( **de** ) .
( **Row 4** ) 231 und durchbrach die Rüstung des Panzers IV hinter ihm ( **de** ) .
**...**
For the translation language modeling (**TLM**), my training data is a combination of two parallel corpora (It is to splice the above data in pairs. The separator is **[/s]**.), as shown below:
( **Row 1** ) polonium 's isotopes tend to decay with alpha or beta decay ( **en** ) . **[/s]** die Isotope von Polonium neigen dazu , mit dem Alpha- oder Beta-Zerfall zu zerfallen ( **de** ) .
( **Row 2** ) 231 and penetrated the armour of the Panzer IV behind it ( **en** ) . **[/s]** 231 und durchbrach die Rüstung des Panzers IV hinter ihm ( **de** ) .
**...**
If I only modify the training data into a combination of two parallel corpora before executing the **run_mlm.py** file, can I achieve the effect of training the translation language modeling (**TLM**)?
Looking forward to your answer, thank you very much!<|||||>Hi @i-wanna-to this last question is something you should post on the forum for discussion at https://discuss.huggingface.co |
transformers | 8,239 | closed | Fix TensorBoardCallback for older versions of PyTorch | # What does this PR do?
It looks like the olad `SummaryWriter` class from `tensorboardX` does not have all the methods of the more recent class in PyTorch, this PR just checks the method is there before using it.
Fixes #8202 | 11-02-2020 15:36:15 | 11-02-2020 15:36:15 | |
transformers | 8,238 | closed | Patch reports | Patches the reports failures introduced by #8007.
Removes the examples tests from the multi-gpu tests for now.
Tests the pipelines in the TF suite. | 11-02-2020 15:26:17 | 11-02-2020 15:26:17 | |
transformers | 8,237 | closed | Fix bad import with PyTorch <= 1.4.1 | # What does this PR do?
`trainer_pt_utils` imports `SAVE_STATE_WARNING` from PyTorch, which only exists in 1.5.0 or later. This fixes that problem.
Fixes #8232 | 11-02-2020 15:13:21 | 11-02-2020 15:13:21 | FYI `SAVE_STATE_WARNING` has been removed 3 days ago: pytorch/pytorch#46813
So `transformers` needs to be recoded not to use that constant.
Looking at its use, this probably would suffice:
```
--- a/src/transformers/trainer_pt_utils.py
+++ b/src/transformers/trainer_pt_utils.py
@@ -34,7 +34,7 @@ from .utils import logging
if is_torch_tpu_available():
import torch_xla.core.xla_model as xm
-if version.parse(torch.__version__) <= version.parse("1.4.1"):
+if version.parse(torch.__version__) <= version.parse("1.4.1") or version.parse(torch.__version__) > version.parse("1.7.0"):
SAVE_STATE_WARNING = ""
else:
from torch.optim.lr_scheduler import SAVE_STATE_WARNING
```
and perhaps adding a note why this was needed in first place. |
transformers | 8,236 | closed | Weird Behavior in Finetuning Pegasus on a Custom Dataset/Longer Summaries Generated | ## Environment info
- `transformers` version: 3.4.0
- Platform: Linux-4.4.0-186-generic-x86_64-with-debian-stretch-sid
- Python version: 3.7.7
- PyTorch version (GPU?): 1.6.0 (True)
- Tensorflow version (GPU?): NA
- Using GPU in script?: Yes
- Using distributed or parallel set-up in script?:No
### Who can help
@sshleifer
## Information
I am using Pegasus
The problem arises when using my own modified scripts
The tasks I am working on summarization on my own dataset:
I am finetuning `google/pegasus-cnn_dailymail` on my own dataset
## Problem
I ran finetuning w/ `google/pegasus-cnn_dailymail` on my dataset about two weeks ago w/ similar code and got much better results. I have saved these checkpoints and will refer to them as the "old checkpoints"
Now I am running roughly the same script finetuning `google/pegasus-cnn_dailymail` on my dataset and for some reason Pegasus seems to produce a lot of irrelevant tokens (maybe doesn't know when to stop properly). I also saved these checkpoints and will refer to them as the "new checkpoints".
**Example**
```
Source:
"Yes, please confirm the medication above.
What do you think could be causing constipation? I eat well, exercise, drink a lot of water, etc."
Predicted Target (old checkpoint): "Thinks could be causing constipation. Eats well, drinks a lot of water, etc."
Predicted Target (new checkpoint): "Eats well. Exercised a lot of water above water. Constipation. Medications causing constipation. Is situated in the right-sided abdomen."
```
Both of the predicted targets were generated with the same decoding code so I do not think it is a problem there. Since the new checkpoint does not do as well as old I suspect I am doing something wrong in my training script.
Here is how I am doing my training step:
```
def _train_step(self, batch):
outputs = self._step(batch)
lm_logits = outputs.logits
labels = batch["target_input_ids"].to(self.device)
loss = F.cross_entropy(lm_logits.view(-1, lm_logits.shape[-1]),
labels.view(-1), ignore_index=0)
return loss
def _step(self, batch):
pad_token_id = self.tokenizer.pad_token_id
decoder_input_ids = shift_tokens_right(
batch["target_input_ids"], pad_token_id).to(self.device)
decoder_input_ids[:, 0] = self.tokenizer.pad_token_id
return self.model(
input_ids=batch["source_input_ids"].to(self.device),
attention_mask=batch["source_attention_mask"].to(self.device),
decoder_input_ids=decoder_input_ids,
decoder_attention_mask=batch["target_attention_mask"].to(
self.device),
use_cache=False,
return_dict=True,
)
```
I double checked this with code in `examples/seq2seq` and `modeling_bart` and it seems to be reasonable. Only difference is when I do the shift_tokens_right I make sure to use Pegasus's decoder_start_token_id of 0 = pad_token_id rather than eos. I tried w and w/o this and the results seem to be similar.
Also I trained both checkpoints with batch size of 4 accumulating 64 batches so effective batch size is 256 as suggested in the paper.
Any idea where I am going wrong with this? | 11-02-2020 14:53:31 | 11-02-2020 14:53:31 | Great Q!
we added `min_length=32` to many pegasus configs. Set `min_length=0` to fallback to the old behavior.
You shouldn't need to re-train. |
transformers | 8,235 | closed | doc: fix typo | # What does this PR do?
<!--
Congratulations! You've made it this far! You're not quite done yet though.
Once merged, your PR is going to appear in the release notes with the title you set, so make sure it's a great title that fully reflects the extent of your awesome contribution.
Then, please replace this with a description of the change and which issue is fixed (if applicable). Please also include relevant motivation and context. List any dependencies (if any) that are required for this change.
Once you're done, someone will review your PR shortly (see the section "Who can review?" below to tag some potential reviewers). They may suggest changes to make the code even better. If no one reviewed your PR after a week has passed, don't hesitate to post a new comment @-mentioning the same persons---sometimes notifications get lost.
-->
<!-- Remove if not applicable -->
Fixes a typo
## Before submitting
- [x] This PR fixes a typo or improves the docs (you can dismiss the other checks if that's the case).
- [ ] Did you read the [contributor guideline](https://github.com/huggingface/transformers/blob/master/CONTRIBUTING.md#start-contributing-pull-requests),
Pull Request section?
- [ ] Was this discussed/approved via a Github issue or the [forum](https://discuss.huggingface.co/)? Please add a link
to the it if that's the case.
- [ ] Did you make sure to update the documentation with your changes? Here are the
[documentation guidelines](https://github.com/huggingface/transformers/tree/master/docs), and
[here are tips on formatting docstrings](https://github.com/huggingface/transformers/tree/master/docs#writing-source-documentation).
- [ ] Did you write any new necessary tests?
## Who can review?
Anyone in the community is free to review the PR once the tests have passed. Feel free to tag
members/contributors which may be interested in your PR.
<!-- Your PR will be replied to more quickly if you can figure out the right person to tag with @
If you know how to use git blame, that is the easiest way, otherwise, here is a rough guide of **who to tag**.
Please tag fewer than 3 people.
albert, bert, XLM: @LysandreJik
GPT2: @LysandreJik, @patrickvonplaten
tokenizers: @mfuntowicz
Trainer: @sgugger
Benchmarks: @patrickvonplaten
Model Cards: @julien-c
Translation: @sshleifer
Summarization: @sshleifer
examples/distillation: @VictorSanh
nlp datasets: [different repo](https://github.com/huggingface/nlp)
rust tokenizers: [different repo](https://github.com/huggingface/tokenizers)
Text Generation: @patrickvonplaten, @TevenLeScao
Blenderbot, Bart, Marian, Pegasus: @sshleifer
T5: @patrickvonplaten
Rag: @patrickvonplaten, @lhoestq
EncoderDecoder: @patrickvonplaten
Longformer, Reformer: @patrickvonplaten
TransfoXL, XLNet: @TevenLeScao, @patrickvonplaten
examples/seq2seq: @sshleifer
examples/bert-loses-patience: @JetRunner
tensorflow: @jplu
examples/token-classification: @stefan-it
documentation: @sgugger
-->
| 11-02-2020 13:16:09 | 11-02-2020 13:16:09 | |
transformers | 8,234 | closed | filelock hangs for example script "run_language_modeling.py" | ## Environment info
- `transformers` version: 3.4.0
- Platform: Linux-3.10.0-957.27.2.el7.x86_64-x86_64-with-centos-7.8.2003-Core
- Python version: 3.6.9
- PyTorch version (GPU?): 1.7.0 (True)
- Tensorflow version (GPU?): not installed (NA)
- Using GPU in script?: yes
- Using distributed or parallel set-up in script?: no
### Who can help
@julien-c (most frequent commiter on `git log examples/language-modeling/run_language_modeling.py`)
## Information
Model I am using (Bert, XLNet ...): CamemBERT
The problem arises when using:
* [x] the official example scripts: (give details below)
* [ ] my own modified scripts: (give details below)
The tasks I am working on is:
* [x] an official GLUE/SQUaD task: (give the name) lm
* [ ] my own task or dataset: (give details below)
## To reproduce
Context: my training dir is ~200 files of ~30MB, as per documentation instructions to keep train files small for the tokenizer (however since I'm finetuning from CamemBERT I wouldn't expect a tokenizer "train" to be run?)
I'm unable to figure out why this freezes, looking for pointers
getting the same behaviour with a single 30MB training file
```
python run_language_modeling.py \
--output_dir=output \
--model_name_or_path=camembert-base \
--do_train \
--train_data_files='/home/theo_nabla_com/data/mydata-corpus/chunk*' \
--do_eval \
--eval_data_file=/home/theo_nabla_com/data/mydata-corpus/valid \
--mlm \
--whole_word_mask
10/29/2020 08:09:13 - WARNING - __main__ - Process rank: -1, device: cuda:0, n_gpu: 1, distributed training: False, 16-bits training: False
10/29/2020 08:09:13 - INFO - __main__ - Training/evaluation parameters TrainingArguments(output_dir='output', overwrite_output_dir=False, do_train=True, do_eval=True, do_predict=False, evaluate_during_training=False, evaluation_strategy=<EvaluationStrategy.NO: 'no'>, prediction_loss_only=False, per_device_train_batch_size=8, per_device_eval_batch_size=8, per_gpu_train_batch_size=None, per_gpu_eval_batch_size=None, gradient_accumulation_steps=1, eval_accumulation_steps=None, learning_rate=5e-05, weight_decay=0.0, adam_beta1=0.9, adam_beta2=0.999, adam_epsilon=1e-08, max_grad_norm=1.0, num_train_epochs=3.0, max_steps=-1, warmup_steps=0, logging_dir='runs/Oct29_08-09-13_google3-theo', logging_first_step=False, logging_steps=500, save_steps=500, save_total_limit=None, no_cuda=False, seed=42, fp16=False, fp16_opt_level='O1', local_rank=-1, tpu_num_cores=None, tpu_metrics_debug=False, debug=False, dataloader_drop_last=False, eval_steps=500, dataloader_num_workers=0, past_index=-1, run_name='output', disable_tqdm=False, remove_unused_columns=True, label_names=None, load_best_model_at_end=False, metric_for_best_model=None, greater_is_better=None)
10/29/2020 08:09:13 - DEBUG - urllib3.connectionpool - Starting new HTTPS connection (1): s3.amazonaws.com:443
10/29/2020 08:09:14 - DEBUG - urllib3.connectionpool - https://s3.amazonaws.com:443 "HEAD /models.huggingface.co/bert/camembert-base-config.json HTTP/1.1" 200 0
10/29/2020 08:09:14 - DEBUG - urllib3.connectionpool - Starting new HTTPS connection (1): s3.amazonaws.com:443
10/29/2020 08:09:14 - DEBUG - urllib3.connectionpool - https://s3.amazonaws.com:443 "HEAD /models.huggingface.co/bert/camembert-base-config.json HTTP/1.1" 200 0
10/29/2020 08:09:14 - DEBUG - urllib3.connectionpool - Starting new HTTPS connection (1): s3.amazonaws.com:443
10/29/2020 08:09:14 - DEBUG - urllib3.connectionpool - https://s3.amazonaws.com:443 "HEAD /models.huggingface.co/bert/camembert-base-sentencepiece.bpe.model HTTP/1.1" 200 0
/home/theo_nabla_com/code/transformers/src/transformers/modeling_auto.py:822: FutureWarning: The class `AutoModelWithLMHead` is deprecated and will be removed in a future version. Please use `AutoModelForCausalLM` for causal language models, `AutoModelForMaskedLM` for masked language models and `AutoModelForSeq2SeqLM` for encoder-decoder models.
FutureWarning,
10/29/2020 08:09:14 - DEBUG - urllib3.connectionpool - Starting new HTTPS connection (1): cdn.huggingface.co:443
10/29/2020 08:09:14 - DEBUG - urllib3.connectionpool - https://cdn.huggingface.co:443 "HEAD /camembert-base-pytorch_model.bin HTTP/1.1" 200 0
Some weights of CamembertForMaskedLM were not initialized from the model checkpoint at camembert-base and are newly initialized: ['lm_head.decoder.bias']
You should probably TRAIN this model on a down-stream task to be able to use it for predictions and inference.
/home/theo_nabla_com/code/transformers/src/transformers/tokenization_utils_base.py:1421: FutureWarning: The `max_len` attribute has been deprecated and will be removed in a future version, use `model_max_length` instead.
FutureWarning,
10/29/2020 08:09:19 - DEBUG - filelock - Attempting to acquire lock 140320072690936 on /home/theo_nabla_com/data/mydata-corpus/cached_lm_CamembertTokenizer_510_chunkaj.lock
10/29/2020 08:09:19 - INFO - filelock - Lock 140320072690936 acquired on /home/theo_nabla_com/data/mydata-corpus/cached_lm_CamembertTokenizer_510_chunkaj.lock
```
(posted this on the discuss but it didn't get attention, [here](https://discuss.huggingface.co/t/hang-in-language-modelling-script/1792)) | 11-02-2020 12:57:40 | 11-02-2020 12:57:40 | Pinging @sgugger<|||||>How long did you let the script hang for? It's probably tokenizing your dataset, which might take a while. Did you try with smaller files to see if it still hanged?<|||||>Hi @LysandreJik, I did try with a single 30MB file as reported, still hanging. It's hanging for hours.
Lately I've thought that it was because of a download, as per your source code where filelock is used, but I've used the model in a notebook before so it should be cached?
EDIT: I'm very sorry, it is actually running now on the small file, I'm baffled - could've sworn it was stuck this week-end.
Culprit could the tokenizer then indeed, but I'm unclear why the filelock would be the breaking point.
I had modified the script file to force the logging level to be debug, and it does get stuck for multiple hours on one of the files when using the globbing pattern with `--train_data_files`<|||||>The issue with the `run_language_modeling.py` script is that it does not leverage the fast tokenizers, so it can take a while to tokenize every file.
This script has been deprecated for a couple of days now, and we have introduced several different scripts in the [`examples/language-modeling` directory](https://github.com/huggingface/transformers/tree/master/examples/language-modeling).
These updated scripts now leverage the fast tokenizers by default, which should make it way faster to tokenize your files *and* you won't need to split your files into multiple small files anymore.
Let me know if you get to use that script, and if it fits your needs.<|||||>Yup I've actually tried right after my last comment to actually debug it and saw you had pushed a new script. Using it right now, seems to go smoothly for now (tokenizing the 7GB file, entering a third progress bar, first two lasted 40min each, i'm assuming it's the tokenizer).
Thanks, closing this!<|||||>Glad it works! |
transformers | 8,233 | closed | Contributing trained Greek<->English NMT models implemented with fairseq | Hi there, quick question that I couldn't answer by searching the docs:
I trained an EL-EN (Greek to English) and an EN-EL machine translation model using the fairseq implementation of the `transformer_iwslt_de_en `architecture on ~6GB of parallel corpora. Given that the models report a better BLEU score compared to the existing SotA, I would like to share them somehow. I thought that fairseq might offer a huggingface-like way to upload trained models but I couldn't find any, so I would appreciate any guidance.
If there's a straightforward way to convert and upload these as huggingface models it would be great!
Many thanks! | 11-02-2020 11:41:55 | 11-02-2020 11:41:55 | We'd love to help you share your models! Both @sshleifer and @stas00 worked on MT models and used Fairseq recently so might be able to help.<|||||>If I'm not mistaken the only difference between [wmt19](https://github.com/pytorch/fairseq/blob/master/examples/wmt19/README.md) and iwslt is the configuration of the layers. In which case it should be trivial to port it to `transformers` via [FSMT](https://huggingface.co/transformers/model_doc/fsmt.html). FSMT = FairSeqMachineTranslation.
You can try it yourself using the [conversion script](https://github.com/huggingface/transformers/blob/master/src/transformers/convert_fsmt_original_pytorch_checkpoint_to_pytorch.py) and if you get stuck please ask for help, pasting the code of what you have tried. You can see how it is used [here](https://github.com/huggingface/transformers/blob/master/scripts/fsmt/convert-facebook-wmt19.sh) and 2 more [here](https://github.com/huggingface/transformers/tree/master/scripts/fsmt).
The only thing the script can't automate at the moment is hyper param presetting, since they are not part of the model dump, we probably need to add clargs to optionally set those. Until now I embedded them in the script itself but that's not the best way to move forward. But let's handle that when everything else is working for you, the converted model will just use the default hparam settings.<|||||>Many thanks for the prompt response. I will try the script and update on the progress.
Apart from the model weights themselves, I assume I will need to take care of the preprocessing (Moses tokenization and fastBPE) as well, in order to load the model and perform inference without issues. <|||||>FSMT already does moses+bpe. No pre- or post-processing is required.<|||||>That's great, thx! I also just read it on the FSMT doc. ^_^<|||||>Edit: Updated with proper code block formatting.
Sorry for the delay @stas00! After updating to the latest transformers and fairseq versions, I had some progress.
OK so I followed the steps and it seems that the conversion starts succesfully using this command:
```
PYTHONPATH="src" python src/transformers/convert_fsmt_original_pytorch_checkpoint_to_pytorch.py --fsmt_checkpoint_path data/trans_elen/checkpoint_best.pt --pytorch_dump_folder_path data/wmt16-el-en-dist
```
But after a few seconds, it returns an error:
```
(base) earendil@C3PO$~/Desktop/conversion/transformers PYTHONPATH="src" python src/transformers/convert_fsmt_original_pytorch_checkpoint_to_pytorch.py --fsmt_checkpoint_path data/trans_elen/checkpoint_best.pt --pytorch_dump_folder_path data/wmt16-el-en-dist
/home/earendil/anaconda3/lib/python3.6/site-packages/tensorboard/compat/tensorflow_stub/dtypes.py:541: FutureWarning: Passing (type, 1) or '1type' as a synonym of type is deprecated; in a future version of numpy, it will be understood as (type, (1,)) / '(1,)type'.
_np_qint8 = np.dtype([("qint8", np.int8, 1)])
/home/earendil/anaconda3/lib/python3.6/site-packages/tensorboard/compat/tensorflow_stub/dtypes.py:542: FutureWarning: Passing (type, 1) or '1type' as a synonym of type is deprecated; in a future version of numpy, it will be understood as (type, (1,)) / '(1,)type'.
_np_quint8 = np.dtype([("quint8", np.uint8, 1)])
/home/earendil/anaconda3/lib/python3.6/site-packages/tensorboard/compat/tensorflow_stub/dtypes.py:543: FutureWarning: Passing (type, 1) or '1type' as a synonym of type is deprecated; in a future version of numpy, it will be understood as (type, (1,)) / '(1,)type'.
_np_qint16 = np.dtype([("qint16", np.int16, 1)])
/home/earendil/anaconda3/lib/python3.6/site-packages/tensorboard/compat/tensorflow_stub/dtypes.py:544: FutureWarning: Passing (type, 1) or '1type' as a synonym of type is deprecated; in a future version of numpy, it will be understood as (type, (1,)) / '(1,)type'.
_np_quint16 = np.dtype([("quint16", np.uint16, 1)])
/home/earendil/anaconda3/lib/python3.6/site-packages/tensorboard/compat/tensorflow_stub/dtypes.py:545: FutureWarning: Passing (type, 1) or '1type' as a synonym of type is deprecated; in a future version of numpy, it will be understood as (type, (1,)) / '(1,)type'.
_np_qint32 = np.dtype([("qint32", np.int32, 1)])
/home/earendil/anaconda3/lib/python3.6/site-packages/tensorboard/compat/tensorflow_stub/dtypes.py:550: FutureWarning: Passing (type, 1) or '1type' as a synonym of type is deprecated; in a future version of numpy, it will be understood as (type, (1,)) / '(1,)type'.
np_resource = np.dtype([("resource", np.ubyte, 1)])
/home/earendil/anaconda3/lib/python3.6/site-packages/tensorflow/python/framework/dtypes.py:516: FutureWarning: Passing (type, 1) or '1type' as a synonym of type is deprecated; in a future version of numpy, it will be understood as (type, (1,)) / '(1,)type'.
_np_qint8 = np.dtype([("qint8", np.int8, 1)])
/home/earendil/anaconda3/lib/python3.6/site-packages/tensorflow/python/framework/dtypes.py:517: FutureWarning: Passing (type, 1) or '1type' as a synonym of type is deprecated; in a future version of numpy, it will be understood as (type, (1,)) / '(1,)type'.
_np_quint8 = np.dtype([("quint8", np.uint8, 1)])
/home/earendil/anaconda3/lib/python3.6/site-packages/tensorflow/python/framework/dtypes.py:518: FutureWarning: Passing (type, 1) or '1type' as a synonym of type is deprecated; in a future version of numpy, it will be understood as (type, (1,)) / '(1,)type'.
_np_qint16 = np.dtype([("qint16", np.int16, 1)])
/home/earendil/anaconda3/lib/python3.6/site-packages/tensorflow/python/framework/dtypes.py:519: FutureWarning: Passing (type, 1) or '1type' as a synonym of type is deprecated; in a future version of numpy, it will be understood as (type, (1,)) / '(1,)type'.
_np_quint16 = np.dtype([("quint16", np.uint16, 1)])
/home/earendil/anaconda3/lib/python3.6/site-packages/tensorflow/python/framework/dtypes.py:520: FutureWarning: Passing (type, 1) or '1type' as a synonym of type is deprecated; in a future version of numpy, it will be understood as (type, (1,)) / '(1,)type'.
_np_qint32 = np.dtype([("qint32", np.int32, 1)])
/home/earendil/anaconda3/lib/python3.6/site-packages/tensorflow/python/framework/dtypes.py:525: FutureWarning: Passing (type, 1) or '1type' as a synonym of type is deprecated; in a future version of numpy, it will be understood as (type, (1,)) / '(1,)type'.
np_resource = np.dtype([("resource", np.ubyte, 1)])
Writing results to data/wmt16-el-en-dist
using checkpoint checkpoint_best.pt
/home/earendil/anaconda3/lib/python3.6/site-packages/hydra/_internal/hydra.py:71: UserWarning:
@hydra.main(strict) flag is deprecated and will removed in the next version.
See https://hydra.cc/docs/next/upgrades/0.11_to_1.0/strict_mode_flag_deprecated
warnings.warn(message=msg, category=UserWarning)
Traceback (most recent call last):
File "src/transformers/convert_fsmt_original_pytorch_checkpoint_to_pytorch.py", line 271, in <module>
convert_fsmt_checkpoint_to_pytorch(args.fsmt_checkpoint_path, args.pytorch_dump_folder_path)
File "src/transformers/convert_fsmt_original_pytorch_checkpoint_to_pytorch.py", line 118, in convert_fsmt_checkpoint_to_pytorch
src_lang = args["source_lang"]
KeyError: 'source_lang'
```
Which I cannot debug since I don't recall inputting any argument regarding src and tgt languages. Aren't these arguments acquired from the model checkpoint?<|||||>Could you please re-edit you comment and use proper code block formatting? it's impossible to figure out what it says since there are warnings mixed in - the new lines are needed to be able to parse it.
Please use the menu bar (`<>` button) or start/end with three backticks if you do it manually.
It should appear like so (I pasted a totally random error just as a demo):
```
"""
tens_ops = (input, weight)
if not torch.jit.is_scripting():
if any([type(t) is not Tensor for t in tens_ops]) and has_torch_function(tens_ops):
return handle_torch_function(linear, tens_ops, input, weight, bias=bias)
if input.dim() == 2 and bias is not None:
# fused op is marginally faster
ret = torch.addmm(bias, input, weight.t())
else:
> output = input.matmul(weight.t())
E RuntimeError: CUDA out of memory. Tried to allocate 100.00 MiB (GPU 0; 23.70 GiB total capacity; 21.83 GiB already allocated; 19.69 MiB free; 22.08 GiB reserved in total by PyTorch)
```
<|||||>Sorry, just fixed it.<|||||>Ah, much better - thank you!
So your model is different from wmt19's series, it fails here:
```
src_lang = args["source_lang"]
tgt_lang = args["target_lang"]
```
which comes from the checkpoint we are trying to convert.
Before it fails do:
```
print(args.keys())
```
and see what you have in there.
Most likely you're converting a different architecture, in which case this script won't work as is.
If you can't figure it out please send me the info at how to get the checkpoint and all the vocab/config files it comes with and I will have a look.
<|||||>The output of `print(args.keys())` is :
```
dict_keys(['_metadata', '_parent', '_content'])
```<|||||>OK, so this is a totally different arch then. In wmt19 the args contain a large set of model configuration, see: a few paragraphs into this section https://huggingface.co/blog/porting-fsmt#porting-weights-and-configuration
So where does it store the model configuration? Or does it not and there is just a fixed config - in which case what is it? how does one derive this from the checkpoint? Is it possible that you forgot to save it in the checkpoint? OR the code you were using for some reason wasn't saving it?
In addition to answering the above, please send me the download info (checkpoint file, and dict, config files) and I will see whether the FSMT arch can somehow be re-used.<|||||>I am not sure where the model configuration is saved, tbh. In my implementation I was just following the steps from this guide:
https://github.com/pytorch/fairseq/tree/master/examples/translation#training-a-new-model
but using my own data of course. If you check the following script:
```
CUDA_VISIBLE_DEVICES=0 fairseq-train \
data-bin/iwslt14.tokenized.de-en \
--arch transformer_iwslt_de_en --share-decoder-input-output-embed \
--optimizer adam --adam-betas '(0.9, 0.98)' --clip-norm 0.0 \
--lr 5e-4 --lr-scheduler inverse_sqrt --warmup-updates 4000 \
--dropout 0.3 --weight-decay 0.0001 \
--criterion label_smoothed_cross_entropy --label-smoothing 0.1 \
--max-tokens 4096 \
--eval-bleu \
--eval-bleu-args '{"beam": 5, "max_len_a": 1.2, "max_len_b": 10}' \
--eval-bleu-detok moses \
--eval-bleu-remove-bpe \
--eval-bleu-print-samples \
--best-checkpoint-metric bleu --maximize-best-checkpoint-metric
```
it seems that the `--arch transformer_iwslt_de_en` is enough for the trainer to understand the architecture (according to this [post](https://github.com/pytorch/fairseq/issues/1301), the key difference is in the ffn hidden dim ,iwslt_de_en is 1024 and transformer is 2048) .
I am uploading the files to a GDrive folder (it will take a while for the checkpoint) and will email you with the link if that's ok (mail found on your website).<|||||>Thank you for that info, @lighteternal.
I will have a look at the data you sent to me (thank you) and will get back to you.
<|||||>Let's continue over https://github.com/huggingface/transformers/pull/8374<|||||>Closing this, as it's solved by by @stas00 in #8374 |
transformers | 8,232 | closed | ImportError: cannot import name 'SAVE_STATE_WARNING' from 'torch.optim.lr_scheduler' | ## Environment info
<!-- You can run the command `transformers-cli env` and copy-and-paste its output below.
Don't forget to fill out the missing fields in that output! -->
- `transformers` version:
- Platform: ubuntu 18.04
- Python version: 3.7
- PyTorch version (GPU?): 1.4.0
- Tensorflow version (GPU?):
- Using GPU in script?: no
- Using distributed or parallel set-up in script?:no
### Who can help
Trainer: @sgugger
## Information
This import is not compatible with PyTorch 1.4.0
The problem arises when using:
* [ *] the official example scripts: (give details below)
The tasks I am working on is:
* [ *] an official GLUE/SQUaD task: (give the name)
## To reproduce
Steps to reproduce the behavior:
```python
>>> from transformers import PreTrainedTokenizer, is_tf_available, is_torch_available
Traceback (most recent call last):
File "<stdin>", line 1, in <module>
File "/home/yuchenlin/anaconda3/envs/mcqa/lib/python3.7/site-packages/transformers/__init__.py", line 611, in <module>
from .trainer import Trainer
File "/home/yuchenlin/anaconda3/envs/mcqa/lib/python3.7/site-packages/transformers/trainer.py", line 69, in <module>
from .trainer_pt_utils import (
File "/home/yuchenlin/anaconda3/envs/mcqa/lib/python3.7/site-packages/transformers/trainer_pt_utils.py", line 26, in <module>
from torch.optim.lr_scheduler import SAVE_STATE_WARNING
ImportError: cannot import name 'SAVE_STATE_WARNING' from 'torch.optim.lr_scheduler' (/home/yuchenlin/anaconda3/envs/mcqa/lib/python3.7/site-packages/torch/optim/lr_scheduler.py)
```
<!-- If you have code snippets, error messages, stack traces please provide them here as well.
Important! Use code tags to correctly format your code. See https://help.github.com/en/github/writing-on-github/creating-and-highlighting-code-blocks#syntax-highlighting
Do not use screenshots, as they are hard to read and (more importantly) don't allow others to copy-and-paste your code.-->
## Expected behavior
<!-- A clear and concise description of what you would expect to happen. -->
| 11-02-2020 11:20:56 | 11-02-2020 11:20:56 | Oh I didn't check when they added this. Do you know if PyTorch 1.4.0 is the last version without it? Will add a fix this morning.<|||||>thank you for the quick fix.<|||||>`SAVE_STATE_WARNING` has been removed 3 days ago: https://github.com/pytorch/pytorch/pull/46813
Need to update https://github.com/huggingface/transformers/pull/8237 to reflect this change. |
transformers | 8,231 | closed | Tf longformer for sequence classification | # What does this PR do?
<!--
Congratulations! You've made it this far! You're not quite done yet though.
Once merged, your PR is going to appear in the release notes with the title you set, so make sure it's a great title that fully reflects the extent of your awesome contribution.
Then, please replace this with a description of the change and which issue is fixed (if applicable). Please also include relevant motivation and context. List any dependencies (if any) that are required for this change.
Once you're done, someone will review your PR shortly (see the section "Who can review?" below to tag some potential reviewers). They may suggest changes to make the code even better. If no one reviewed your PR after a week has passed, don't hesitate to post a new comment @-mentioning the same persons---sometimes notifications get lost.
-->
<!-- Remove if not applicable -->
implement SequenceClassification, MultipleChoice and TokenClassification classes for TFLongformer.
Resolves #6401
## Before submitting
- [ ] This PR fixes a typo or improves the docs (you can dismiss the other checks if that's the case).
- [x] Did you read the [contributor guideline](https://github.com/huggingface/transformers/blob/master/CONTRIBUTING.md#start-contributing-pull-requests),
Pull Request section?
- [ ] Was this discussed/approved via a Github issue or the [forum](https://discuss.huggingface.co/)? Please add a link
to the it if that's the case.
- [ ] Did you make sure to update the documentation with your changes? Here are the
[documentation guidelines](https://github.com/huggingface/transformers/tree/master/docs), and
[here are tips on formatting docstrings](https://github.com/huggingface/transformers/tree/master/docs#writing-source-documentation).
- [x] Did you write any new necessary tests?
## Who can review?
Anyone in the community is free to review the PR once the tests have passed. Feel free to tag
members/contributors which may be interested in your PR.
<!-- Your PR will be replied to more quickly if you can figure out the right person to tag with @
If you know how to use git blame, that is the easiest way, otherwise, here is a rough guide of **who to tag**.
Please tag fewer than 3 people.
Longformer, Reformer: @patrickvonplaten
-->
| 11-02-2020 10:10:51 | 11-02-2020 10:10:51 | @elk-cloner - thanks a lot for taking a look into this!
Would be awesome to fix the TFLongformer related tests. There seem to be some obvious bug: `UnboundLocalError: local variable 'input_ids' referenced before assignment` .
I'll do a longer review once these tests are fixed :-) Lemme know if you need help at some point.<|||||>@patrickvonplaten i have passed all the tests but got stuck in `test_inputs_embeds` when it's checking `TFLongformerForMultipleChoice` model, i debugged my code and found out that `inputs_embeds` shape is not same when `TFLongformerEmbeddings` get call from [here](https://github.com/elk-cloner/transformers/blob/28ab848279d31970c9f3390a480041eca2beee82/src/transformers/modeling_tf_longformer.py#L2232)(test_inputs_embeds) and [here](https://github.com/elk-cloner/transformers/blob/28ab848279d31970c9f3390a480041eca2beee82/tests/test_modeling_tf_common.py#L702)(TFLongformerForMultipleChoice), but don't know how to fix it, can you help me ?<|||||>Hey @elk-cloner,
yeah this problem was not at all obvious! Thanks for letting me know :-) For Multiple Choice, we have to make sure that the position_ids stay 2-dimensional, which is only relevant for TFLongformer, but not for other TF models -> so we need this `if` fix here.
Feel free to ping me again, when you're ready with the PR or need help :-) <|||||>@patrickvonplaten all tests have passed, can you take a look ?<|||||>Hey @elk-cloner - the signature of the function calls should be done analogs to the one in other `modeling_tf_....py` files. Woud be great if you can fix that before we merge. <|||||>Good to merge IMO! <|||||>Checked the slow tests and everything passes. Great job @elk-cloner! Longformer is definitely not the easiest model<|||||>Would be awesome if @LysandreJik and @sgugger can take a final look, then we're good to merge. |
transformers | 8,230 | closed | Fixed emmental example. | Added information about loading of squad data in README.
Fixed BertLayerNorm which disappeared some time ago, replace with torch.nn.LayerNorm (which was buggy long time ago it seems).
| 11-02-2020 10:00:14 | 11-02-2020 10:00:14 | looks good to me!<|||||>looks good to me too!<|||||>This issue has been automatically marked as stale because it has not had recent activity. If you think this still needs to be addressed please comment on this thread.
Please note that issues that do not follow the [contributing guidelines](https://github.com/huggingface/transformers/blob/master/CONTRIBUTING.md) are likely to be ignored. |
transformers | 8,229 | closed | is it possible to extract the attention weights on test inputs when the pretrained model is fine-tuned on custom data? | # ❓ Questions & Help
I am wondering if it's possible to look into the attention weights on test data when the fine-tuned model is running. I tried to look for some docs for help but could not find useful guidance. Any pointers will be appreciated. Thanks a lot in advance.
<!-- The GitHub issue tracker is primarly intended for bugs, feature requests,
new models and benchmarks, and migration questions. For all other questions,
we direct you to the Hugging Face forum: https://discuss.huggingface.co/ .
You can also try Stack Overflow (SO) where a whole community of PyTorch and
Tensorflow enthusiast can help you out. In this case, make sure to tag your
question with the right deep learning framework as well as the
huggingface-transformers tag:
https://stackoverflow.com/questions/tagged/huggingface-transformers
-->
## Details
<!-- Description of your issue -->
<!-- You should first ask your question on the forum or SO, and only if
you didn't get an answer ask it here on GitHub. -->
**A link to original question on the forum/Stack Overflow**: | 11-02-2020 06:52:50 | 11-02-2020 06:52:50 | This issue has been automatically marked as stale because it has not had recent activity. It will be closed if no further activity occurs. Thank you for your contributions.
|
transformers | 8,228 | closed | segmentation fault (core dumped) proxychains4 python xxx.py | ## Environment info
<!-- You can run the command `transformers-cli env` and copy-and-paste its output below.
Don't forget to fill out the missing fields in that output! -->
- `transformers` version: 3.4.0
- Platform: ubuntu 18.04
- Python version: 3.6
- PyTorch version (GPU?): 1.4.0 GPU
- Tensorflow version (GPU?): 2.2.0 GPU
- Using GPU in script?: yes
- Using distributed or parallel set-up in script?: no
- Network: need to use http proxy to download files and I use the tool ProxyChains4
## To reproduce
Steps to reproduce the behavior:
1. Save the following code to file test.py
```python
from transformers import pipeline
classifier = pipeline('sentiment-analysis')
classifier('We are very happy to include pipeline into the transformers repository.')
```
2. Exec `proxychains4 python test.py`
3. The following error was raised
```shell
(test) ➜ test-transformer proxychains4 python test.py
[proxychains] config file found: /etc/proxychains4.conf
[proxychains] preloading /usr/lib/x86_64-linux-gnu/libproxychains.so.4
[proxychains] DLL init: proxychains-ng 4.12
[proxychains] DLL init: proxychains-ng 4.12
[proxychains] Strict chain ... 10.74.193.90:80 ... s3.amazonaws.com:443 ... OK
[proxychains] Strict chain ... 10.74.193.90:80 ... s3.amazonaws.com:443 ... OK
[proxychains] Strict chain ... 10.74.193.90:80 ... s3.amazonaws.com:443 ... OK
[proxychains] Strict chain ... 10.74.193.90:80 ... s3.amazonaws.com:443 ... OK
[proxychains] Strict chain ... 10.74.193.90:80 ... s3.amazonaws.com:443 ... OK
[proxychains] Strict chain ... 10.74.193.90:80 ... s3.amazonaws.com:443 ... OK
[proxychains] Strict chain ... 10.74.193.90:80 ... s3.amazonaws.com:443 ... OK
[proxychains] Strict chain ... 10.74.193.90:80 ... s3.amazonaws.com:443 ... OK
[proxychains] Strict chain ... 10.74.193.90:80 ... cdn.huggingface.co:443 ... OK
[1] 9790 segmentation fault (core dumped) proxychains4 python test.py
```
<!-- If you have code snippets, error messages, stack traces please provide them here as well.
Important! Use code tags to correctly format your code. See https://help.github.com/en/github/writing-on-github/creating-and-highlighting-code-blocks#syntax-highlighting
Do not use screenshots, as they are hard to read and (more importantly) don't allow others to copy-and-paste your code.-->
## Expected behavior
<!-- A clear and concise description of what you would expect to happen. -->
The model files can be downloaded without error
| 11-02-2020 04:06:21 | 11-02-2020 04:06:21 | This issue has been automatically marked as stale because it has not had recent activity. It will be closed if no further activity occurs. Thank you for your contributions.
|
transformers | 8,227 | closed | convert_graph_to_onnx.py and associated example notebook are broken for TensorFlow | ## Information
The `convert_graph_to_onnx.py` file and the associated [example notebook](https://github.com/huggingface/transformers/blob/master/notebooks/04-onnx-export.ipynb) appear to be broken for TensorFlow.
For ONNX-exported TensorFlow models, **only input tokens of length 5 are accepted**. Other inputs (e.g., `len(tokens)>5`) result in an error:
```
InvalidArgument: [ONNXRuntimeError] : 2 : INVALID_ARGUMENT : Got invalid dimensions for input: input_ids for the following indices
index: 1 Got: 6 Expected: 5
```
Also, If you run `session.get_inputs()` on ONNX-exported TensorFlow model, only `input_ids` key is listed as inputs (i.e., no `attention_mask`) while ONNX PyTorch behaves differently:
```python
# ONNX TensorFlow inputs for BERT model
print([input.name for input in cpu_model.get_inputs()])
# only prints 'input_ids' - no 'attention_mask'
# ONNX PyTorch inputs for BERT model
print([input.name for input in cpu_model.get_inputs()])
# prints ['input_ids', 'attention_mask', 'token_type_ids']
```
## How to Reproduce
In the [example notebook](https://github.com/huggingface/transformers/blob/master/notebooks/04-onnx-export.ipynb), uncomment this TensorFlow `convert` line:
```
convert(framework="tf", model="bert-base-cased", output="onnx-test-tf/bert-base-cased.onnx", opset=11)
```
I have also posted [this Google Colab notebook](https://colab.research.google.com/drive/1A2frWgfRlL5Ysf7xVVifx58NmEoxeYmu?usp=sharing) that more concisely reproduces this issue.
| 11-02-2020 02:05:24 | 11-02-2020 02:05:24 | ## Environment Info
- `transformers` version: 3.4.0
- Platform: Linux-4.15.0-108-generic-x86_64-with-Ubuntu-18.04-bionic
- Python version: 3.6.9
- PyTorch version (GPU?): 1.6.0+cpu (False)
- Tensorflow version (GPU?): 2.3.1 (False)
Also, reproduced on Google Colab, as indicated above.
## Who can help
TensorFlow: @jplu
ONNX: @mfuntowicz @sgugger @LysandreJik
<|||||>You have to create your own model with the size you need and then use the script to convert it. All the TF models are by default initialized with input sequence of 5 tokens.<|||||>@jplu Thanks. Would you mind clarifying what you mean by "create your own model with the size you need"? I'm creating and fine-tuning a model with `TFBertForSequenceClassification.from_pretrained` and was trying to use the example notebook to convert it. <|||||>What I mean is that you have to update the input shape of your model. When you do:
```
TFBertForSequenceClassification.from_pretrained("name")
```
You model is initialized with a [dummy input](https://github.com/huggingface/transformers/blob/master/src/transformers/modeling_tf_utils.py#L330) of [5 tokens](https://github.com/huggingface/transformers/blob/master/src/transformers/file_utils.py#L215). Then when you use your model the max length allowed is 5 by default.
If you want to use a model with a larger max length you have to update your input shape with:
```
from transformers import TFBertForSequenceClassification, BertTokenizerFast
import tensorflow as tf
my_model_name = "bert-base-cased" # replace here by the name of your model
tokenizer = BertTokenizerFast.from_pretrained(my_model_name )
model = TFBertForSequenceClassification.from_pretrained(my_model_name )
size = 510 # the max length you expect for your model. Don't forget the two extra tokens of start and end, here 510 + 2 to make 512 which is the max length allowed for all the models (except longformer).
inputs_dict = tokenizer("hello" * [size], return_tensors="tf")
model._saved_model_inputs_spec = None
model._set_save_spec(inputs_dict)
tf.saved_model.save(model, "path")
```
And then you can create your ONNX model afterward from your saved model that will take as input your proper input length.<|||||>@jplu Thank you!<|||||>This issue has been automatically marked as stale because it has not had recent activity. It will be closed if no further activity occurs. Thank you for your contributions.
<|||||>>
NotEncodableError: No encoder for object {'input_ids': TensorSpec(shape=(None, 128), dtype=tf.int32, name='input_ids/input_ids'), 'token_type_ids': TensorSpec(shape=(None, 128), dtype=tf.int32, name='token_type_ids'), 'attention_mask': TensorSpec(shape=(None, 128), dtype=tf.int32, name='attention_mask')} of type <class 'transformers.tokenization_utils_base.BatchEncoding'>.
I am using the mobileBert model. But when I follow this procedure to save model in Saved Model fromat, it gives the error above. Any suggestions? Thanks!
|
transformers | 8,226 | closed | [bart] 2 SinusoidalPositionalEmbedding fixes | This PR:
* `embedding_dim` param for `SinusoidalPositionalEmbedding` can now be odd.
* fixes a bug "RuntimeError: a view of a leaf Variable that requires grad is being used in an in-place operation" appearing in pytorch-1.8+ (this var requires no grad, so make it so before we do anything grad-related with it).
Fixes: #8021
@sshleifer, @LysandreJik | 11-02-2020 01:50:59 | 11-02-2020 01:50:59 | |
transformers | 8,225 | closed | When would pegasus be able to be exported in ONNX format? | It seems like it's not available now, I got this error:
`Error while converting the model: Unrecognized configuration class <class 'transformers.configuration_pegasus.PegasusConfig'> for this kind of AutoModel: AutoModel.
Model type should be one of RetriBertConfig, T5Config, DistilBertConfig, AlbertConfig, CamembertConfig, XLMRobertaConfig, BartConfig, LongformerConfig, RobertaConfig, LayoutLMConfig, SqueezeBertConfig, BertConfig, OpenAIGPTConfig, GPT2Config, MobileBertConfig, TransfoXLConfig, XLNetConfig, FlaubertConfig, FSMTConfig, XLMConfig, CTRLConfig, ElectraConfig, ReformerConfig, FunnelConfig, LxmertConfig, BertGenerationConfig, DebertaConfig, DPRConfig, XLMProphetNetConfig, ProphetNetConfig.`
Which is fair since pegasus is a new addition. Is it something the team plans to do soon?
Or can someone point me some resources on if there are other ways to export a pre-trained model from huggingface? I'm pretty new to the machine learning thing :p
Thanks all! | 11-01-2020 22:37:34 | 11-01-2020 22:37:34 | @patil-suraj has a partial solution that he just posted to the forums. he might be able to extend that to Pegasus/BART <|||||>I'm on it! Will ping here once I get it working.
@phosfuldev, you can refer to this post to see how T5 is exported to onnx
https://discuss.huggingface.co/t/speeding-up-t5-inference/1841<|||||>@sshleifer @patil-suraj Thank you!!<|||||>Thank you so much @patil-suraj for taking the initiative to export `Pegasus` to onnx. Eagerly waiting for it :) <|||||>Hi @patil-suraj
Please let us know if you have any update on exporting Pegasus to Onnx format.
Apologies for bothering you.
Thanks,
Karthik<|||||>I was about to open a new issue and then discovered this one. For reference, this is where I got stopped when trying to export a Pegasus model in ONNX format:
I am using a recent clone of the `transformers` repository, cloned on `feb 18 2021`
Unless I am doing something wrong, I think that the `convert_graph_to_onnx.py` script does not currently work with Pegasus models.
I tried it with both `pegasus_large`, and a model that I have fined-tuned, that is based, on `pegasus_large`, with a command like this....
command: `python3 -m transformers.convert_graph_to_onnx --framework pt --model ../models_foreign/pegasus_large ./onnx/onnx_model.onnx`
and in both cases, I got this console output....
console output
```
====== Converting model to ONNX ======
ONNX opset version set to: 11
Loading pipeline (model: ../models_foreign/pegasus_large, tokenizer: ../models_foreign/pegasus_large)
Some weights of PegasusModel were not initialized from the model checkpoint at ../models_foreign/pegasus_large and are newly initialized: ['model.encoder.embed_positions.weight', 'model.decoder.embed_positions.weight']
You should probably TRAIN this model on a down-stream task to be able to use it for predictions and inference.
Using framework PyTorch: 1.8.0a0+1606899
Error while converting the model: You have to specify either decoder_input_ids or decoder_inputs_embeds
```
<|||||>This issue has been automatically marked as stale because it has not had recent activity. If you think this still needs to be addressed please comment on this thread.
Please note that issues that do not follow the [contributing guidelines](https://github.com/huggingface/transformers/blob/master/CONTRIBUTING.md) are likely to be ignored. |
transformers | 8,224 | closed | Add encoder-decoder word embeddings tying by default | As discussed in #8158 config has `tie_encoder_decoder_word_embeds =True` parameter. `_tie_encoder_decoder_word_embeddings ` is called when Encoder Decoder model is initialized, if sizes are the same, the encoder word embedding matrix is assigned to decoder one, this may cause unexpected behavior if e.g. user chooses to init model with bert and gpt and their vocabs have same sizes but different words.
@patrickvonplaten | 11-01-2020 20:25:21 | 11-01-2020 20:25:21 | @alexyalunin - this is a great PR, thanks a lot! In general the function does exactly what I had in mind :-) I added some changes that I'd suggest we apply.
Also it would be great if we could add a test analogues to this one: https://github.com/huggingface/transformers/blob/93354bc7790ecf768690745db2407b7542264304/tests/test_modeling_encoder_decoder.py#L306 .
If you have any questions or need help, let me know!
Looking forward to merge this soon <|||||>> Thanks for the PR! Though I think the name of this option is kinda confusing, I can't think of a better one :)
I'm fine with the name<|||||>@patrickvonplaten finally I have found some time to finish this PR. I couldn't finish tests, you see I tried to initialize EncoderDecoder with a model and its copy and tie word embeddings, it seems like they are tied (I check by looking at `model.named_parameters()`, i.e these parameters do not have `decoder.word_embeddings`), but when I save the model and load it `decoder.word_embeddings` now appear in `model.named_parameters()`. I trained such a model for my project and after few epochs word embs for encoder and decoder are the same but they both appear in `model.named_parameters()`. Pls take a look. <|||||>This issue has been automatically marked as stale because it has not had recent activity. If you think this still needs to be addressed please comment on this thread.
Please note that issues that do not follow the [contributing guidelines](https://github.com/huggingface/transformers/blob/master/CONTRIBUTING.md) are likely to be ignored. |
transformers | 8,223 | closed | Create README.md | # What does this PR do?
<!--
Congratulations! You've made it this far! You're not quite done yet though.
Once merged, your PR is going to appear in the release notes with the title you set, so make sure it's a great title that fully reflects the extent of your awesome contribution.
Then, please replace this with a description of the change and which issue is fixed (if applicable). Please also include relevant motivation and context. List any dependencies (if any) that are required for this change.
Once you're done, someone will review your PR shortly (see the section "Who can review?" below to tag some potential reviewers). They may suggest changes to make the code even better. If no one reviewed your PR after a week has passed, don't hesitate to post a new comment @-mentioning the same persons---sometimes notifications get lost.
-->
<!-- Remove if not applicable -->
Fixes # (issue)
## Before submitting
- [ ] This PR fixes a typo or improves the docs (you can dismiss the other checks if that's the case).
- [x] Did you read the [contributor guideline](https://github.com/huggingface/transformers/blob/master/CONTRIBUTING.md#start-contributing-pull-requests),
Pull Request section?
- [ ] Was this discussed/approved via a Github issue or the [forum](https://discuss.huggingface.co/)? Please add a link
to the it if that's the case.
- [x] Did you make sure to update the documentation with your changes? Here are the
[documentation guidelines](https://github.com/huggingface/transformers/tree/master/docs), and
[here are tips on formatting docstrings](https://github.com/huggingface/transformers/tree/master/docs#writing-source-documentation).
- [ ] Did you write any new necessary tests?
## Who can review?
Anyone in the community is free to review the PR once the tests have passed. Feel free to tag
members/contributors which may be interested in your PR.
<!-- Your PR will be replied to more quickly if you can figure out the right person to tag with @
If you know how to use git blame, that is the easiest way, otherwise, here is a rough guide of **who to tag**.
Please tag fewer than 3 people.
albert, bert, XLM: @LysandreJik
GPT2: @LysandreJik, @patrickvonplaten
tokenizers: @mfuntowicz
Trainer: @sgugger
Benchmarks: @patrickvonplaten
Model Cards: @julien-c
Translation: @sshleifer
Summarization: @sshleifer
examples/distillation: @VictorSanh
nlp datasets: [different repo](https://github.com/huggingface/nlp)
rust tokenizers: [different repo](https://github.com/huggingface/tokenizers)
Text Generation: @patrickvonplaten, @TevenLeScao
Blenderbot, Bart, Marian, Pegasus: @sshleifer
T5: @patrickvonplaten
Rag: @patrickvonplaten, @lhoestq
EncoderDecoder: @patrickvonplaten
Longformer, Reformer: @patrickvonplaten
TransfoXL, XLNet: @TevenLeScao, @patrickvonplaten
examples/seq2seq: @sshleifer
examples/bert-loses-patience: @JetRunner
tensorflow: @jplu
examples/token-classification: @stefan-it
documentation: @sgugger
-->
| 11-01-2020 17:52:48 | 11-01-2020 17:52:48 | |
transformers | 8,222 | closed | Why is the accuracy rate of pre-trained GPT-2 model only ~26%? | Hello,
I have been trying to analyze HellaSwag dataset with the pre-trained GPT2DoubleHeadsModel. I fine-tuned the model by disabling any change fin weights of the main body (12 layers + embedding layer) while training weights of the multiple-choice head with moderate learning rate.
My understanding is that, since the main body of the model is already pre-trained, I should get a reasonably high accuracy rate for the HellaSwag task as long as I do a good job in training the weights from the multiple-choice head. However, the accuracy rate of the pre-trained GPT2DoubleHeadsModel on the HellaSwag task is only ~26% (although my training loss is only ~1.40).
Why is my accuracy rate so low? is this because I am not fine-tuning the weights of the main body of the model during the training? Any advice would be highly appreciated.
Thank you, | 11-01-2020 16:19:12 | 11-01-2020 16:19:12 | Hi, we try to keep the github issues for bugs only. Could you open a thread on the [forum](https://discuss.huggingface.co) instead? Thank you! |
transformers | 8,221 | closed | [GPT2] Loss NaN after some time with FP16 | ## Environment info
<!-- You can run the command `transformers-cli env` and copy-and-paste its output below.
Don't forget to fill out the missing fields in that output! -->
- `transformers` version: 3.4.0
- Platform: Linux-4.4.0-176-generic-x86_64-with-glibc2.17
- Python version: 3.8.6
- PyTorch version (GPU?): 1.7.0+cu101 (True)
- Tensorflow version (GPU?): not installed (NA)
- Using GPU in script?: yes
- Using distributed or parallel set-up in script?: yes, HF datasets
### Who can help
@LysandreJik
@sgugger
## Information
Model I am using (Bert, XLNet ...): GPT2
The problem arises when using:
* [ ] the official example scripts:
* [x] my own modified scripts: examples/language_modeling/run_language_modeling.py
The tasks I am working on is:
* [ ] an official GLUE/SQUaD task: (give the name)
* [x] my own task or dataset
## To reproduce
Steps to reproduce the behavior:
1. Run with ```--fp16 --n_ctx 2048```
2.
```
warnings.warn('Was asked to gather along dimension 0, but all '
[W python_anomaly_mode.cpp:104] Warning: Error detected in SoftmaxBackward. Traceback of forward call that caused the error:
File "/usr/lib/python3.8/threading.py", line 890, in _bootstrap
self._bootstrap_inner()
File "/usr/lib/python3.8/threading.py", line 932, in _bootstrap_inner
self.run()
File "/usr/lib/python3.8/threading.py", line 870, in run
self._target(*self._args, **self._kwargs)
File "/home/ubuntu/.local/lib/python3.8/site-packages/torch/nn/parallel/parallel_apply.py", line 61, in _worker
output = module(*input, **kwargs)
File "/home/ubuntu/.local/lib/python3.8/site-packages/torch/nn/modules/module.py", line 727, in _call_impl
result = self.forward(*input, **kwargs)
File "/home/ubuntu/.local/lib/python3.8/site-packages/transformers/modeling_gpt2.py", line 765, in forward
transformer_outputs = self.transformer(
File "/home/ubuntu/.local/lib/python3.8/site-packages/torch/nn/modules/module.py", line 727, in _call_impl
result = self.forward(*input, **kwargs)
File "/home/ubuntu/.local/lib/python3.8/site-packages/transformers/modeling_gpt2.py", line 645, in forward
outputs = block(
File "/home/ubuntu/.local/lib/python3.8/site-packages/torch/nn/modules/module.py", line 727, in _call_impl
result = self.forward(*input, **kwargs)
File "/home/ubuntu/.local/lib/python3.8/site-packages/transformers/modeling_gpt2.py", line 285, in forward
attn_outputs = self.attn(
File "/home/ubuntu/.local/lib/python3.8/site-packages/torch/nn/modules/module.py", line 727, in _call_impl
result = self.forward(*input, **kwargs)
File "/home/ubuntu/.local/lib/python3.8/site-packages/transformers/modeling_gpt2.py", line 235, in forward
attn_outputs = self._attn(query, key, value, attention_mask, head_mask, output_attentions)
File "/home/ubuntu/.local/lib/python3.8/site-packages/transformers/modeling_gpt2.py", line 176, in _attn
w = nn.Softmax(dim=-1)(w)
File "/home/ubuntu/.local/lib/python3.8/site-packages/torch/nn/modules/module.py", line 727, in _call_impl
result = self.forward(*input, **kwargs)
File "/home/ubuntu/.local/lib/python3.8/site-packages/torch/nn/modules/activation.py", line 1198, in forward
return F.softmax(input, self.dim, _stacklevel=5)
File "/home/ubuntu/.local/lib/python3.8/site-packages/torch/nn/functional.py", line 1512, in softmax
ret = input.softmax(dim)
(function _print_stack)
Traceback (most recent call last):
File "examples/language-modeling/run_language_modeling.py", line 349, in <module>
main()
File "examples/language-modeling/run_language_modeling.py", line 313, in main
trainer.train(model_path=model_path)
File "/home/ubuntu/.local/lib/python3.8/site-packages/transformers/trainer.py", line 756, in train
tr_loss += self.training_step(model, inputs)
File "/home/ubuntu/.local/lib/python3.8/site-packages/transformers/trainer.py", line 1065, in training_step
self.scaler.scale(loss).backward()
File "/home/ubuntu/.local/lib/python3.8/site-packages/torch/tensor.py", line 221, in backward
torch.autograd.backward(self, gradient, retain_graph, create_graph)
File "/home/ubuntu/.local/lib/python3.8/site-packages/torch/autograd/__init__.py", line 130, in backward
Variable._execution_engine.run_backward(
RuntimeError: Function 'SoftmaxBackward' returned nan values in its 0th output.
0%| | 0/19506024 [00:25<?, ?it/s]
```
## Expected behavior
Not print Nan
| 11-01-2020 14:54:58 | 11-01-2020 14:54:58 | We can't really help without seeing the code you are running. Some of the models do not support FP16 for instance, and we have no idea which model you are using.<|||||>Oh, sorry. It's the example script ```examples/language_modeling/run_language_modeling.py``` but with modified data loader.
full code below:
```
import logging
import math
import os
import glob
import datasets
from dataclasses import dataclass, field
from typing import Optional
from datasets import list_datasets, load_dataset
from transformers import (
CONFIG_MAPPING,
MODEL_WITH_LM_HEAD_MAPPING,
AutoConfig,
AutoModelWithLMHead,
AutoTokenizer,
DataCollatorForLanguageModeling,
DataCollatorForPermutationLanguageModeling,
HfArgumentParser,
LineByLineTextDataset,
PreTrainedTokenizer,
TextDataset,
Trainer,
TrainingArguments,
set_seed,
)
logger = logging.getLogger(__name__)
MODEL_CONFIG_CLASSES = list(MODEL_WITH_LM_HEAD_MAPPING.keys())
MODEL_TYPES = tuple(conf.model_type for conf in MODEL_CONFIG_CLASSES)
@dataclass
class ModelArguments:
"""
Arguments pertaining to which model/config/tokenizer we are going to fine-tune, or train from scratch.
"""
model_name_or_path: Optional[str] = field(
default=None,
metadata={
"help": "The model checkpoint for weights initialization. Leave None if you want to train a model from scratch."
},
)
model_type: Optional[str] = field(
default=None,
metadata={"help": "If training from scratch, pass a model type from the list: " + ", ".join(MODEL_TYPES)},
)
config_name: Optional[str] = field(
default=None, metadata={"help": "Pretrained config name or path if not the same as model_name"}
)
tokenizer_name: Optional[str] = field(
default=None, metadata={"help": "Pretrained tokenizer name or path if not the same as model_name"}
)
cache_dir: Optional[str] = field(
default=None, metadata={"help": "Where do you want to store the pretrained models downloaded from s3"}
)
@dataclass
class DataTrainingArguments:
"""
Arguments pertaining to what data we are going to input our model for training and eval.
"""
train_data_file: Optional[str] = field(
default=None, metadata={"help": "The input training data file (a text file)."}
)
eval_data_file: Optional[str] = field(
default=None,
metadata={"help": "An optional input evaluation data file to evaluate the perplexity on (a text file)."},
)
line_by_line: bool = field(
default=False,
metadata={"help": "Whether distinct lines of text in the dataset are to be handled as distinct sequences."},
)
mlm: bool = field(
default=False, metadata={"help": "Train with masked-language modeling loss instead of language modeling."}
)
mlm_probability: float = field(
default=0.15, metadata={"help": "Ratio of tokens to mask for masked language modeling loss"}
)
plm_probability: float = field(
default=1 / 6,
metadata={
"help": "Ratio of length of a span of masked tokens to surrounding context length for permutation language modeling."
},
)
max_span_length: int = field(
default=5, metadata={"help": "Maximum length of a span of masked tokens for permutation language modeling."}
)
block_size: int = field(
default=-1,
metadata={
"help": "Optional input sequence length after tokenization."
"The training dataset will be truncated in block of this size for training."
"Default to the model max input length for single sentence inputs (take into account special tokens)."
},
)
overwrite_cache: bool = field(
default=False, metadata={"help": "Overwrite the cached training and evaluation sets"}
)
arrow: bool = field(
default=True,
metadata={
"help": "Use Arrow-based HF NLP for optimization."
},
)
def get_dataset(
args: DataTrainingArguments,
tokenizer: PreTrainedTokenizer,
evaluate: bool = False,
cache_dir: Optional[str] = "./cache",
):
tokenizer.pad_token = "<|endoftext|>"
tokenizer._pad_token = "<|endoftext|>"
#tokenizer.pad_token_id = 50256
file_path = args.eval_data_file if evaluate else args.train_data_file
if True:
dataset = datasets.load_from_disk(file_path)
dataset.set_format(type='torch', columns=['input_ids'])
return dataset
if False:
dataset = load_dataset("text", data_files=[file_path], split='train')
dataset = dataset.map(lambda ex: tokenizer(ex["text"], add_special_tokens=True,
truncation=True, max_length=args.block_size), batched=True)
dataset.set_format(type='torch', columns=['input_ids'])
dataset.save_to_disk(file_path+'.arrow')
return dataset
if args.line_by_line:
return LineByLineTextDataset(tokenizer=tokenizer, file_path=file_path, block_size=args.block_size)
else:
return TextDataset(
tokenizer=tokenizer,
file_path=file_path,
block_size=args.block_size,
overwrite_cache=args.overwrite_cache,
cache_dir=cache_dir,
)
"""
dataset = load_dataset("text", data_files=file_path, split="train")
dataset = dataset.map(lambda ex: tokenizer(ex["text"], add_special_tokens=True,
truncation=True, max_length=args.block_size), batched=True)
dataset.set_format(type='torch', columns=['input_ids'])
return dataset
"""
def main():
# See all possible arguments in src/transformers/training_args.py
# or by passing the --help flag to this script.
# We now keep distinct sets of args, for a cleaner separation of concerns.
parser = HfArgumentParser((ModelArguments, DataTrainingArguments, TrainingArguments))
model_args, data_args, training_args = parser.parse_args_into_dataclasses()
if data_args.eval_data_file is None and training_args.do_eval:
raise ValueError(
"Cannot do evaluation without an evaluation data file. Either supply a file to --eval_data_file "
"or remove the --do_eval argument."
)
if (
os.path.exists(training_args.output_dir)
and os.listdir(training_args.output_dir)
and training_args.do_train
and not training_args.overwrite_output_dir
):
raise ValueError(
f"Output directory ({training_args.output_dir}) already exists and is not empty. Use --overwrite_output_dir to overcome."
)
# Setup logging
logging.basicConfig(
format="%(asctime)s - %(levelname)s - %(name)s - %(message)s",
datefmt="%m/%d/%Y %H:%M:%S",
level=logging.INFO if training_args.local_rank in [-1, 0] else logging.WARN,
)
logger.warning(
"Process rank: %s, device: %s, n_gpu: %s, distributed training: %s, 16-bits training: %s",
training_args.local_rank,
training_args.device,
training_args.n_gpu,
bool(training_args.local_rank != -1),
training_args.fp16,
)
logger.info("Training/evaluation parameters %s", training_args)
# Set seed
set_seed(training_args.seed)
# Load pretrained model and tokenizer
#
# Distributed training:
# The .from_pretrained methods guarantee that only one local process can concurrently
# download model & vocab.
if model_args.config_name:
config = AutoConfig.from_pretrained(model_args.config_name, cache_dir=model_args.cache_dir)
elif model_args.model_name_or_path:
config = AutoConfig.from_pretrained(model_args.model_name_or_path, cache_dir=model_args.cache_dir)
else:
config = CONFIG_MAPPING[model_args.model_type]()
logger.warning("You are instantiating a new config instance from scratch.")
if model_args.tokenizer_name:
tokenizer = AutoTokenizer.from_pretrained(model_args.tokenizer_name, cache_dir=model_args.cache_dir)
elif model_args.model_name_or_path:
tokenizer = AutoTokenizer.from_pretrained(model_args.model_name_or_path, cache_dir=model_args.cache_dir)
else:
raise ValueError(
"You are instantiating a new tokenizer from scratch. This is not supported, but you can do it from another script, save it,"
"and load it from here, using --tokenizer_name"
)
tokenizer.pad_token = "<|endoftext|>"
tokenizer._pad_token = "<|endoftext|>"
if model_args.model_name_or_path:
model = AutoModelWithLMHead.from_pretrained(
model_args.model_name_or_path,
from_tf=bool(".ckpt" in model_args.model_name_or_path),
config=config,
cache_dir=model_args.cache_dir,
)
else:
logger.info("Training new model from scratch")
model = AutoModelWithLMHead.from_config(config)
model.resize_token_embeddings(len(tokenizer))
if config.model_type in ["bert", "roberta", "distilbert", "camembert"] and not data_args.mlm:
raise ValueError(
"BERT and RoBERTa-like models do not have LM heads but masked LM heads. They must be run using the"
"--mlm flag (masked language modeling)."
)
if data_args.block_size <= 0:
data_args.block_size = tokenizer.max_len
# Our input block size will be the max possible for the model
else:
data_args.block_size = min(data_args.block_size, tokenizer.max_len)
# Get datasets
train_dataset = (
get_dataset(data_args, tokenizer=tokenizer, cache_dir=model_args.cache_dir) if training_args.do_train else None
)
eval_dataset = (
get_dataset(data_args, tokenizer=tokenizer, evaluate=True, cache_dir=model_args.cache_dir)
if training_args.do_eval
else None
)
if config.model_type == "xlnet":
data_collator = DataCollatorForPermutationLanguageModeling(
tokenizer=tokenizer,
plm_probability=data_args.plm_probability,
max_span_length=data_args.max_span_length,
)
else:
data_collator = DataCollatorForLanguageModeling(
tokenizer=tokenizer, mlm=data_args.mlm, mlm_probability=data_args.mlm_probability
)
# Initialize our Trainer
trainer = Trainer(
model=model,
args=training_args,
data_collator=data_collator,
train_dataset=train_dataset,
eval_dataset=eval_dataset,
prediction_loss_only=True,
)
# Training
if training_args.do_train:
model_path = (
model_args.model_name_or_path
if model_args.model_name_or_path is not None and os.path.isdir(model_args.model_name_or_path)
else None
)
trainer.train(model_path=model_path)
trainer.save_model()
# For convenience, we also re-save the tokenizer to the same directory,
# so that you can share your model easily on huggingface.co/models =)
if trainer.is_world_master():
tokenizer.save_pretrained(training_args.output_dir)
# Evaluation
results = {}
if training_args.do_eval:
logger.info("*** Evaluate ***")
eval_output = trainer.evaluate()
perplexity = math.exp(eval_output["eval_loss"])
result = {"perplexity": perplexity}
output_eval_file = os.path.join(training_args.output_dir, "eval_results_lm.txt")
if trainer.is_world_master():
with open(output_eval_file, "w") as writer:
logger.info("***** Eval results *****")
for key in sorted(result.keys()):
logger.info(" %s = %s", key, str(result[key]))
writer.write("%s = %s\n" % (key, str(result[key])))
results.update(result)
return results
def _mp_fn(index):
# For xla_spawn (TPUs)
main()
if __name__ == "__main__":
main()
```<|||||>Thanks, and which type of model do you use (e.g., what's the full command you launch)?<|||||>```
python3.7 examples/language-modeling/run_language_modeling.py --output_dir=kogpt1 --model_type=gpt2 --do_train --train_data_file=/home/ksjae/kogpt-2/data/NEWS_ARROW --overwrite_output_dir --per_device_train_batch_size=12 --per_device_eval_batch_size=8 --save_steps 10000 --num_train_epochs=1 --block_size 2048 --eval_steps 25000 --logging_steps=1000 --tokenizer_name kotok --model_name_or_path gpt2-medium --fp16
```<|||||>This issue has been automatically marked as stale because it has not had recent activity. It will be closed if no further activity occurs. Thank you for your contributions.
|
transformers | 8,220 | closed | Example for running T5 for translation | Hi,
I am having hard time finetuning T5-small on WMT-14 de/en and bleu score does go high. I followed the notebooks of question answering with T5, is there any specific point to consider for translation, so any specific parameter which needs to be given to model.generate ?
could you assist me with showing me some example codes you have made it work for translation task?
thanks | 11-01-2020 12:23:28 | 11-01-2020 12:23:28 | This issue has been automatically marked as stale because it has not had recent activity. It will be closed if no further activity occurs. Thank you for your contributions.
|
transformers | 8,219 | closed | Roberta weights are not initialized loading the bare Roberta | ## Environment info
<!-- You can run the command `transformers-cli env` and copy-and-paste its output below.
Don't forget to fill out the missing fields in that output! -->
- `transformers` version: '3.4.0'
- Platform: Colab
- Python version:
- PyTorch version (GPU?):
- Tensorflow version (GPU?): 2.3.0
- Using GPU in script?: yes
- Using distributed or parallel set-up in script?: no
### Who can help
<!-- Your issue will be replied to more quickly if you can figure out the right person to tag with @
If you know how to use git blame, that is the easiest way, otherwise, here is a rough guide of **who to tag**.
Please tag fewer than 3 people.
albert, bert, GPT2, XLM: @LysandreJik
tokenizers: @mfuntowicz
Trainer: @sgugger
Speed and Memory Benchmarks: @patrickvonplaten
Model Cards: @julien-c
Translation: @sshleifer
Summarization: @sshleifer
TextGeneration: @TevenLeScao
examples/distillation: @VictorSanh
nlp datasets: [different repo](https://github.com/huggingface/nlp)
rust tokenizers: [different repo](https://github.com/huggingface/tokenizers)
Text Generation: @TevenLeScao
blenderbot: @mariamabarham
Bart: @sshleifer
Marian: @sshleifer
T5: @patrickvonplaten
Longformer/Reformer: @patrickvonplaten
TransfoXL/XLNet: @TevenLeScao
examples/seq2seq: @sshleifer
examples/bert-loses-patience: @JetRunner
tensorflow: @jplu
examples/token-classification: @stefan-it
documentation: @sgugger
-->
## Information
Model I am using (Bert, XLNet ...):
The problem arises when using:
* [x] the official example scripts: (give details below)
* [*] my own modified scripts: (give details below)
class ROBERTA(transformers.TFRobertaModel):
def __init__(self, config, *inputs, **kwargs):
super(ROBERTA, self).__init__(config, *inputs, **kwargs)
self.roberta.call = tf.function(self.roberta.call)
def build_model():
# Define inputs (token_ids, mask_ids, seg_ids)
input_size = 2 * Each_seq_length + 4
token_inputs = tf.keras.layers.Input(shape=(input_size,), name='word_inputs', dtype='int32')
# Load model and collect encodings
roberta = ROBERTA.from_pretrained('roberta-base')
print(roberta.config)
roberta_encodings = roberta(token_inputs, training=True)[0]
# Keep [CLS] token encoding
doc_encoding = tf.squeeze(roberta_encodings[:, 0:1, :], axis=1)
# Apply dropout
doc_encoding = tf.keras.layers.Dropout(0.1)(doc_encoding)
# Final output (projection) layer
# predicted_labels, log_probs = CF_model(0.5, 8)(doc_encoding)
# In the case of one layer for prediction
# outputs = tf.keras.layers.Dense(1, activation='sigmoid', name='outputs')(doc_encoding)
# Wrap-up model
model = tf.keras.models.Model(inputs=[token_inputs], outputs=[outputs])
model.compile(optimizer=tf.keras.optimizers.Adam(lr=4e-6, epsilon=1e-8), loss=tf.keras.losses.BinaryCrossentropy())
return model
The tasks I am working on is:
* [ ] an official GLUE/SQUaD task: (give the name)
* [*] my own task or dataset: (give details below)
## To reproduce
sentence-pair classification
Steps to reproduce the behavior:
1.
2.
3.
<!-- If you have code snippets, error messages, stack traces please provide them here as well.
Important! Use code tags to correctly format your code. See https://help.github.com/en/github/writing-on-github/creating-and-highlighting-code-blocks#syntax-highlighting
Do not use screenshots, as they are hard to read and (more importantly) don't allow others to copy-and-paste your code.-->
## Expected behavior
previously, just the 'lm_head' layers were not initialized but now, using the same script more weights are not initialized including the encoder layers. Following error:
Some layers from the model checkpoint at roberta-base were not used when initializing ROBERTA: ['lm_head', 'encoder/layer_._3/attention/self/value/bias:0', 'encoder/layer_._10/attention/self/value/bias:0', 'encoder/layer_._10/attention/self/key/kernel:0', 'pooler/dense/bias:0', 'encoder/layer_._9/attention/self/query/kernel:0', 'encoder/layer_._10/attention/self/query/kernel:0', 'encoder/layer_._7/attention/output/dense/bias:0', 'embeddings/position_embeddings/embeddings:0', 'encoder/layer_._6/intermediate/dense/kernel:0', 'encoder/layer_._11/intermediate/dense/kernel:0', 'encoder/layer_._8/intermediate/dense/bias:0', 'encoder/layer_._10/attention/self/value/kernel:0', 'encoder/layer_._7/output/dense/bias:0', 'encoder/layer_._6/attention/self/value/bias:0', 'encoder/layer_._8/attention/output/dense/kernel:0', 'encoder/layer_._10/intermediate/dense/kernel:0', 'encoder/layer_._5/attention/self/value/kernel:0', 'encoder/layer_._6/attention/output/LayerNorm/gamma:0', 'encoder/layer_._7/attention/self/query/kernel:0', 'encoder/layer_._6/attention/self/query/kernel:0', 'encoder/layer_._6/attention/self/key/bias:0', 'encoder/layer_._8/attention/output/LayerNorm/gamma:0', 'encoder/layer_._2/output/dense/kernel:0', 'encoder/layer_._11/intermediate/dense/bias:0', 'encoder/layer_._6/output/dense/kernel:0', 'encoder/layer_._2/intermediate/dense/kernel:0', 'encoder/layer_._3/intermediate/dense/kernel:0', 'encoder/layer_._10/output/LayerNorm/beta:0', 'encoder/layer_._6/attention/self/query/bias:0', 'encoder/layer_._6/attention/output/LayerNorm/beta:0', 'encoder/layer_._9/attention/self/value/bias:0', 'encoder/layer_._8/attention/self/query/kernel:0', 'encoder/layer_._0/output/LayerNorm/gamma:0', 'encoder/layer_._11/attention/output/dense/bias:0', 'encoder/layer_._7/attention/self/value/bias:0', 'encoder/layer_._0/attention/output/dense/kernel:0', 'encoder/layer_._9/intermediate/dense/bias:0', 'encoder/layer_._2/attention/self/query/kernel:0', 'encoder/layer_._0/attention/self/key/bias:0', 'encoder/layer_._8/attention/output/LayerNorm/beta:0', 'encoder/layer_._1/attention/self/value/kernel:0', 'encoder/layer_._6/output/LayerNorm/gamma:0', 'encoder/layer_._1/attention/output/dense/bias:0', 'encoder/layer_._3/attention/self/query/bias:0', 'encoder/layer_._3/output/dense/bias:0', 'encoder/layer_._1/attention/self/key/kernel:0', 'encoder/layer_._8/attention/self/key/kernel:0', 'encoder/layer_._9/intermediate/dense/kernel:0', 'encoder/layer_._3/output/dense/kernel:0', 'encoder/layer_._2/output/LayerNorm/beta:0', 'encoder/layer_._7/attention/self/key/bias:0', 'encoder/layer_._5/attention/self/key/kernel:0', 'encoder/layer_._5/attention/self/query/bias:0', 'encoder/layer_._2/attention/output/dense/bias:0', 'encoder/layer_._4/intermediate/dense/kernel:0', 'encoder/layer_._1/intermediate/dense/bias:0', 'encoder/layer_._4/attention/self/value/kernel:0', 'encoder/layer_._11/attention/self/key/bias:0', 'encoder/layer_._5/output/dense/kernel:0', 'encoder/layer_._1/output/dense/bias:0', 'encoder/layer_._0/attention/self/value/bias:0', 'encoder/layer_._6/attention/self/key/kernel:0', 'encoder/layer_._9/attention/self/key/bias:0', 'encoder/layer_._7/output/LayerNorm/gamma:0', 'encoder/layer_._8/attention/output/dense/bias:0', 'encoder/layer_._10/attention/output/dense/bias:0', 'encoder/layer_._0/intermediate/dense/kernel:0', 'encoder/layer_._5/intermediate/dense/kernel:0', 'encoder/layer_._11/attention/self/value/kernel:0', 'encoder/layer_._8/attention/self/key/bias:0', 'encoder/layer_._8/output/dense/bias:0', 'encoder/layer_._8/intermediate/dense/kernel:0', 'encoder/layer_._7/attention/output/LayerNorm/beta:0', 'encoder/layer_._2/output/dense/bias:0', 'encoder/layer_._3/attention/output/dense/bias:0', 'encoder/layer_._0/output/dense/bias:0', 'encoder/layer_._9/attention/self/key/kernel:0', 'encoder/layer_._11/output/dense/bias:0', 'encoder/layer_._7/attention/self/query/bias:0', 'encoder/layer_._10/attention/self/key/bias:0', 'encoder/layer_._2/attention/output/dense/kernel:0', 'encoder/layer_._2/attention/self/query/bias:0', 'encoder/layer_._9/attention/output/dense/kernel:0', 'encoder/layer_._9/attention/output/LayerNorm/gamma:0', 'encoder/layer_._9/output/LayerNorm/gamma:0', 'encoder/layer_._0/attention/output/LayerNorm/beta:0', 'encoder/layer_._1/intermediate/dense/kernel:0', 'encoder/layer_._1/output/dense/kernel:0', 'encoder/layer_._1/attention/self/key/bias:0', 'encoder/layer_._2/attention/self/value/kernel:0', 'encoder/layer_._9/attention/self/value/kernel:0', 'encoder/layer_._10/intermediate/dense/bias:0', 'encoder/layer_._4/intermediate/dense/bias:0', 'encoder/layer_._6/output/LayerNorm/beta:0', 'encoder/layer_._7/output/LayerNorm/beta:0', 'encoder/layer_._11/attention/self/query/bias:0', 'encoder/layer_._0/intermediate/dense/bias:0', 'encoder/layer_._11/attention/output/dense/kernel:0', 'encoder/layer_._5/attention/self/query/kernel:0', 'encoder/layer_._8/attention/self/value/kernel:0', 'encoder/layer_._11/output/LayerNorm/beta:0', 'encoder/layer_._9/output/dense/bias:0', 'encoder/layer_._4/output/dense/bias:0', 'encoder/layer_._2/attention/self/key/bias:0', 'encoder/layer_._3/attention/self/query/kernel:0', 'encoder/layer_._4/attention/output/LayerNorm/gamma:0', 'encoder/layer_._1/attention/output/LayerNorm/beta:0', 'encoder/layer_._1/output/LayerNorm/beta:0', 'encoder/layer_._10/attention/output/LayerNorm/beta:0', 'encoder/layer_._3/attention/self/value/kernel:0', 'encoder/layer_._10/attention/self/query/bias:0', 'encoder/layer_._3/attention/self/key/bias:0', 'pooler/dense/kernel:0', 'encoder/layer_._1/attention/self/value/bias:0', 'encoder/layer_._7/attention/self/key/kernel:0', 'encoder/layer_._1/attention/output/dense/kernel:0', 'encoder/layer_._4/attention/self/key/kernel:0', 'encoder/layer_._8/output/dense/kernel:0', 'encoder/layer_._3/attention/output/LayerNorm/gamma:0', 'encoder/layer_._0/attention/self/value/kernel:0', 'encoder/layer_._3/attention/self/key/kernel:0', 'encoder/layer_._0/attention/self/query/kernel:0', 'encoder/layer_._3/intermediate/dense/bias:0', 'encoder/layer_._7/output/dense/kernel:0', 'encoder/layer_._10/output/dense/kernel:0', 'encoder/layer_._7/intermediate/dense/bias:0', 'embeddings/word_embeddings/weight:0', 'encoder/layer_._3/attention/output/LayerNorm/beta:0', 'encoder/layer_._0/attention/self/key/kernel:0', 'encoder/layer_._4/output/dense/kernel:0', 'encoder/layer_._5/output/LayerNorm/gamma:0', 'encoder/layer_._9/attention/output/dense/bias:0', 'encoder/layer_._0/attention/output/dense/bias:0', 'encoder/layer_._5/attention/output/LayerNorm/gamma:0', 'encoder/layer_._9/attention/output/LayerNorm/beta:0', 'encoder/layer_._11/output/LayerNorm/gamma:0', 'encoder/layer_._11/attention/output/LayerNorm/gamma:0', 'encoder/layer_._6/intermediate/dense/bias:0', 'encoder/layer_._2/attention/output/LayerNorm/gamma:0', 'encoder/layer_._5/output/dense/bias:0', 'encoder/layer_._0/output/dense/kernel:0', 'encoder/layer_._6/attention/output/dense/kernel:0', 'encoder/layer_._6/attention/output/dense/bias:0', 'encoder/layer_._1/attention/self/query/kernel:0', 'encoder/layer_._0/attention/self/query/bias:0', 'encoder/layer_._11/attention/self/value/bias:0', 'encoder/layer_._2/intermediate/dense/bias:0', 'embeddings/LayerNorm/beta:0', 'encoder/layer_._4/attention/output/dense/kernel:0', 'encoder/layer_._3/output/LayerNorm/beta:0', 'encoder/layer_._8/output/LayerNorm/gamma:0', 'encoder/layer_._10/attention/output/dense/kernel:0', 'encoder/layer_._11/output/dense/kernel:0', 'encoder/layer_._2/attention/output/LayerNorm/beta:0', 'encoder/layer_._7/attention/output/dense/kernel:0', 'encoder/layer_._9/attention/self/query/bias:0', 'encoder/layer_._4/attention/self/key/bias:0', 'encoder/layer_._2/output/LayerNorm/gamma:0', 'encoder/layer_._0/attention/output/LayerNorm/gamma:0', 'encoder/layer_._1/attention/output/LayerNorm/gamma:0', 'encoder/layer_._1/attention/self/query/bias:0', 'encoder/layer_._5/attention/output/LayerNorm/beta:0', 'encoder/layer_._10/output/dense/bias:0', 'encoder/layer_._8/output/LayerNorm/beta:0', 'encoder/layer_._5/output/LayerNorm/beta:0', 'embeddings/token_type_embeddings/embeddings:0', 'encoder/layer_._5/attention/output/dense/bias:0', 'encoder/layer_._4/output/LayerNorm/beta:0', 'encoder/layer_._4/attention/self/query/kernel:0', 'encoder/layer_._5/attention/output/dense/kernel:0', 'encoder/layer_._7/attention/self/value/kernel:0', 'encoder/layer_._7/intermediate/dense/kernel:0', 'encoder/layer_._11/attention/self/key/kernel:0', 'encoder/layer_._3/output/LayerNorm/gamma:0', 'encoder/layer_._10/output/LayerNorm/gamma:0', 'encoder/layer_._8/attention/self/query/bias:0', 'encoder/layer_._3/attention/output/dense/kernel:0', 'encoder/layer_._4/output/LayerNorm/gamma:0', 'encoder/layer_._10/attention/output/LayerNorm/gamma:0', 'encoder/layer_._4/attention/self/value/bias:0', 'encoder/layer_._11/attention/self/query/kernel:0', 'encoder/layer_._4/attention/output/dense/bias:0', 'encoder/layer_._4/attention/output/LayerNorm/beta:0', 'encoder/layer_._5/attention/self/key/bias:0', 'encoder/layer_._6/attention/self/value/kernel:0', 'encoder/layer_._5/attention/self/value/bias:0', 'encoder/layer_._11/attention/output/LayerNorm/beta:0', 'encoder/layer_._1/output/LayerNorm/gamma:0', 'encoder/layer_._2/attention/self/value/bias:0', 'encoder/layer_._9/output/dense/kernel:0', 'encoder/layer_._2/attention/self/key/kernel:0', 'encoder/layer_._9/output/LayerNorm/beta:0', 'encoder/layer_._7/attention/output/LayerNorm/gamma:0', 'encoder/layer_._5/intermediate/dense/bias:0', 'embeddings/LayerNorm/gamma:0', 'encoder/layer_._0/output/LayerNorm/beta:0', 'encoder/layer_._6/output/dense/bias:0', 'encoder/layer_._8/attention/self/value/bias:0', 'encoder/layer_._4/attention/self/query/bias:0']
<!-- A clear and concise description of what you would expect to happen. --> | 11-01-2020 11:16:37 | 11-01-2020 11:16:37 | This issue has been automatically marked as stale because it has not had recent activity. It will be closed if no further activity occurs. Thank you for your contributions.
|
transformers | 8,218 | closed | ValueError: decoder_start_token_id or bos_token_id has to be defined for encoder-decoder generation | Hi
I followed the tutorial for generation with T5, and I made my input as "Question: Question sequence ", and target as "Target sequence", then I use tokenizer.batch_encode_plus to encode the sentence and then I call generate as:
model.generate(batch[input_ids], attention_mask=..., max_length=..., early_stopping=True)
I got the following error:
File "/opt/conda/envs/pl/lib/python3.7/site-packages/transformers/generation_utils.py", line 398, in generate
"decoder_start_token_id or bos_token_id has to be defined for encoder-decoder generation"
ValueError: decoder_start_token_id or bos_token_id has to be defined for encoder-decoder generation
but the input format seems to be matching the tutorial. thanks for your help. | 11-01-2020 10:27:46 | 11-01-2020 10:27:46 | Hi,
could you assist in adding an example in tutorial for this, I followed the tutorial sample for summarization, Is the way I made my inputs ("Question: Question sequence ") correct? thanks
here is the tutorial sample:
```
>>> input_ids = tokenizer("summarize: studies have shown that owning a dog is good for you ", return_tensors="pt").input_ids # Batch size 1
>>> outputs = model.generate(input_ids)
```<|||||>solved when I load the config from a pretrained model, maybe this helps to add the extra info needed as the default setting :) |
transformers | 8,216 | closed | tokenizer's is_split_into_words seems not work | I input tokenized list of tokens, but it return different result(not count pad token). It seems tokenize pretokenized tokens, ignoring `is_split_into_words`. Please refer to the code below:
```
sent = "the latest investigation was authorized after the supreme court in 2007 found dcc and its founder , jim flavin , guilty of selling dcc 's ( euro ) 106 million ( then $ 130 million ) stake in fyffes after flavin -- also a fyffes director at the time -- received inside information about bad fyffes news in the pipeline ."
encoded_dict = tokenizer.encode_plus(
sent, # Sentence to encode.
add_special_tokens = False, # Add '[CLS]' and '[SEP]'
max_length = 314, # Pad & truncate all sentences.
padding = 'max_length',
return_attention_mask = True, # Construct attn. masks.
return_tensors = 'pt',
return_token_type_ids=False,# Return pytorch tensors.
truncation=False,
is_split_into_words=False)
input_ids = encoded_dict['input_ids']
tokenized = tokenizer.convert_ids_to_tokens([i.item() for i in input_ids.squeeze() if i > 1])
len(tokenized)
>> 79
print(tokenized)
>> ['the', 'latest', 'investigation', 'was', 'authorized', 'after', 'the', 'supreme', 'court', 'in', '2007', 'found', 'dc', '##c', 'and', 'its', 'founder', ',', 'jim', 'fl', '##avi', '##n', ',', 'guilty', 'of', 'selling', 'dc', '##c', "'", 's', '(', 'euro', ')', '106', 'million', '(', 'then', '$', '130', 'million', ')', 'stake', 'in', 'f', '##y', '##ffe', '##s', 'after', 'fl', '##avi', '##n', '-', '-', 'also', 'a', 'f', '##y', '##ffe', '##s', 'director', 'at', 'the', 'time', '-', '-', 'received', 'inside', 'information', 'about', 'bad', 'f', '##y', '##ffe', '##s', 'news', 'in', 'the', 'pipeline', '.']
###### tokenizing pretokenized tokens as list
encoded_dict = tokenizer.encode_plus(
tokenized, # Sentence to encode.
add_special_tokens = False, # Add '[CLS]' and '[SEP]'
max_length = 314, # Pad & truncate all sentences.
padding = 'max_length',
return_attention_mask = True, # Construct attn. masks.
return_tensors = 'pt',
return_token_type_ids=False,# Return pytorch tensors.
truncation=False,
is_split_into_words=True)
input_ids = encoded_dict['input_ids']
tokenized = tokenizer.convert_ids_to_tokens([i.item() for i in input_ids.squeeze() if i > 1])
len(tokenized)
>> 114 # it should be 79
print(tokenized)
>> ['the', 'latest', 'investigation', 'was', 'authorized', 'after', 'the', 'supreme', 'court', 'in', '2007', 'found', 'dc', '#', '#', 'c', 'and', 'its', 'founder', ',', 'jim', 'fl', '#', '#', 'av', '##i', '#', '#', 'n', ',', 'guilty', 'of', 'selling', 'dc', '#', '#', 'c', "'", 's', '(', 'euro', ')', '106', 'million', '(', 'then', '$', '130', 'million', ')', 'stake', 'in', 'f', '#', '#', 'y', '#', '#', 'ff', '##e', '#', '#', 's', 'after', 'fl', '#', '#', 'av', '##i', '#', '#', 'n', '-', '-', 'also', 'a', 'f', '#', '#', 'y', '#', '#', 'ff', '##e', '#', '#', 's', 'director', 'at', 'the', 'time', '-', '-', 'received', 'inside', 'information', 'about', 'bad', 'f', '#', '#', 'y', '#', '#', 'ff', '##e', '#', '#', 's', 'news', 'in', 'the', 'pipeline', '.']
``` | 11-01-2020 09:52:46 | 11-01-2020 09:52:46 | wrongly posted. I delete it. |
transformers | 8,215 | closed | Setting os.environ['CUDA_VISIBLE_DEVICES'] = ‘1’, but always training on GPU0, how to set it(GPT2)? | # ❓ Questions & Help
<!-- The GitHub issue tracker is primarly intended for bugs, feature requests,
new models and benchmarks, and migration questions. For all other questions,
we direct you to the Hugging Face forum: https://discuss.huggingface.co/ .
You can also try Stack Overflow (SO) where a whole community of PyTorch and
Tensorflow enthusiast can help you out. In this case, make sure to tag your
question with the right deep learning framework as well as the
huggingface-transformers tag:
https://stackoverflow.com/questions/tagged/huggingface-transformers
-->
## Details
<!-- Description of your issue -->
<!-- You should first ask your question on the forum or SO, and only if
you didn't get an answer ask it here on GitHub. -->
**A link to original question on the forum/Stack Overflow**: | 11-01-2020 09:40:11 | 11-01-2020 09:40:11 | Hi @TheoRenLi, this question should go on https://discuss.huggingface.co
We keep the issues of the repo for bug and features request (with clear descriptions).<|||||>This issue has been automatically marked as stale because it has not had recent activity. It will be closed if no further activity occurs. Thank you for your contributions.
|
transformers | 8,217 | closed | tokenizer "is_split_into_words" seems not work | I input tokenized list of tokens, but it return different result(not count pad token). It seems tokenize pretokenized tokens, ignoring `is_split_into_words`. Please refer to the code below:
```
sent = "the latest investigation was authorized after the supreme court in 2007 found dcc and its founder , jim flavin , guilty of selling dcc 's ( euro ) 106 million ( then $ 130 million ) stake in fyffes after flavin -- also a fyffes director at the time -- received inside information about bad fyffes news in the pipeline ."
encoded_dict = tokenizer.encode_plus(
sent, # Sentence to encode.
add_special_tokens = False, # Add '[CLS]' and '[SEP]'
max_length = 314, # Pad & truncate all sentences.
padding = 'max_length',
return_attention_mask = True, # Construct attn. masks.
return_tensors = 'pt',
return_token_type_ids=False,# Return pytorch tensors.
truncation=False,
is_split_into_words=False)
input_ids = encoded_dict['input_ids']
tokenized = tokenizer.convert_ids_to_tokens([i.item() for i in input_ids.squeeze() if i > 1])
len(tokenized)
>> 79
print(tokenized)
>> ['the', 'latest', 'investigation', 'was', 'authorized', 'after', 'the', 'supreme', 'court', 'in', '2007', 'found', 'dc', '##c', 'and', 'its', 'founder', ',', 'jim', 'fl', '##avi', '##n', ',', 'guilty', 'of', 'selling', 'dc', '##c', "'", 's', '(', 'euro', ')', '106', 'million', '(', 'then', '$', '130', 'million', ')', 'stake', 'in', 'f', '##y', '##ffe', '##s', 'after', 'fl', '##avi', '##n', '-', '-', 'also', 'a', 'f', '##y', '##ffe', '##s', 'director', 'at', 'the', 'time', '-', '-', 'received', 'inside', 'information', 'about', 'bad', 'f', '##y', '##ffe', '##s', 'news', 'in', 'the', 'pipeline', '.']
###### tokenizing pretokenized tokens as list
encoded_dict = tokenizer.encode_plus(
tokenized, # Sentence to encode.
add_special_tokens = False, # Add '[CLS]' and '[SEP]'
max_length = 314, # Pad & truncate all sentences.
padding = 'max_length',
return_attention_mask = True, # Construct attn. masks.
return_tensors = 'pt',
return_token_type_ids=False,# Return pytorch tensors.
truncation=False,
is_split_into_words=True)
input_ids = encoded_dict['input_ids']
tokenized = tokenizer.convert_ids_to_tokens([i.item() for i in input_ids.squeeze() if i > 1])
len(tokenized)
>> 114 # it should be 79
print(tokenized)
>> ['the', 'latest', 'investigation', 'was', 'authorized', 'after', 'the', 'supreme', 'court', 'in', '2007', 'found', 'dc', '#', '#', 'c', 'and', 'its', 'founder', ',', 'jim', 'fl', '#', '#', 'av', '##i', '#', '#', 'n', ',', 'guilty', 'of', 'selling', 'dc', '#', '#', 'c', "'", 's', '(', 'euro', ')', '106', 'million', '(', 'then', '$', '130', 'million', ')', 'stake', 'in', 'f', '#', '#', 'y', '#', '#', 'ff', '##e', '#', '#', 's', 'after', 'fl', '#', '#', 'av', '##i', '#', '#', 'n', '-', '-', 'also', 'a', 'f', '#', '#', 'y', '#', '#', 'ff', '##e', '#', '#', 's', 'director', 'at', 'the', 'time', '-', '-', 'received', 'inside', 'information', 'about', 'bad', 'f', '#', '#', 'y', '#', '#', 'ff', '##e', '#', '#', 's', 'news', 'in', 'the', 'pipeline', '.']
``` | 11-01-2020 09:19:09 | 11-01-2020 09:19:09 | the same issue, is there any workaround?<|||||>same issue, I think there is a bug in PreTrainedTokenizer class
```
def get_input_ids(text):
print(text)
if isinstance(text, str):
tokens = self.tokenize(text, **kwargs)
return self.convert_tokens_to_ids(tokens)
elif isinstance(text, (list, tuple)) and len(text) > 0 and isinstance(text[0], str):
if is_split_into_words:
tokens = list(
itertools.chain(*(self.tokenize(t, is_split_into_words=True, **kwargs) for t in text))
)
return self.convert_tokens_to_ids(tokens)
else:
return self.convert_tokens_to_ids(text)
```
in `if is_split_into_words` case (where the input is pretokenized words), the tokenizer should directly return ids.<|||||>Hello! I think all of the confusion here may be because you're expecting `is_split_into_words` to understand that the text was already pre-tokenized. This is not the case, it means that the string was split into words (not tokens), i.e., split on spaces.
@HenryPaik1, in your example, your list of words is the following:
```py
['the', 'latest', 'investigation', 'was', 'authorized', 'after', 'the', 'supreme', 'court', 'in', '2007', 'found', 'dc', '##c', 'and', 'its', 'founder', ',', 'jim', 'fl', '##avi', '##n', ',', 'guilty', 'of', 'selling', 'dc', '##c', "'", 's', '(', 'euro', ')', '106', 'million', '(', 'then', '$', '130', 'million', ')', 'stake', 'in', 'f', '##y', '##ffe', '##s', 'after', 'fl', '##avi', '##n', '-', '-', 'also', 'a', 'f', '##y', '##ffe', '##s', 'director', 'at', 'the', 'time', '-', '-', 'received', 'inside', 'information', 'about', 'bad', 'f', '##y', '##ffe', '##s', 'news', 'in', 'the', 'pipeline', '.']
```
Some of these strings are tokens, but not words. Running the encoding method on it once again means that you're re-tokenizing some of these tokens.
You can see it is the case, as the following token:
```py
[..., '##c', ...]
```
became:
```py
[..., '#', '#', 'c', ...]
```
I think in your case you're looking for the method `convert_tokens_to_ids`: your sequence is already tokenized, you only need the IDs. If you're looking to use `encode_plus` because you need padding/trunc/conversion to tensors, etc., then you can simply use it without specifying that the sequence is separated into words. Please be aware that the following code only works on python tokenizers, i.e., slow tokenizers.
```py
from transformers import BertTokenizer
tokenizer = BertTokenizer.from_pretrained("bert-base-cased")
sent = "the latest investigation was authorized after the supreme court in 2007 found dcc and its founder , jim flavin , guilty of selling dcc 's ( euro ) 106 million ( then $ 130 million ) stake in fyffes after flavin -- also a fyffes director at the time -- received inside information about bad fyffes news in the pipeline ."
encoded_dict = tokenizer.encode_plus(
sent, # Sentence to encode.
add_special_tokens = False, # Add '[CLS]' and '[SEP]'
max_length = 314, # Pad & truncate all sentences.
padding = 'max_length',
return_attention_mask = True, # Construct attn. masks.
return_tensors = 'pt',
truncation=False,
is_split_into_words=False)
input_ids = encoded_dict['input_ids']
tokenized = tokenizer.convert_ids_to_tokens([i.item() for i in input_ids.squeeze() if i > 1])
print(len(tokenized))
#80
###### tokenizing pretokenized tokens as list
encoded_dict = tokenizer.encode_plus(
tokenized, # Sentence to encode.
add_special_tokens = False, # Add '[CLS]' and '[SEP]'
max_length = 314, # Pad & truncate all sentences.
padding = 'max_length',
return_attention_mask = True, # Construct attn. masks.
return_tensors = 'pt',
truncation=False,
)
input_ids = encoded_dict['input_ids']
tokenized = tokenizer.convert_ids_to_tokens([i.item() for i in input_ids.squeeze() if i > 1])
print(len(tokenized))
# 80
```<|||||>@LysandreJik Thanks for your explanation. Yes, I want to use `encode_plus` for padding/trunc. It looks I thought the argument, `is_split_into_words`, the other way around. `is_split_into_words=True` seems for the "not tokenized sentence."
And if I understand correctly, you mean the part below is executed by python:
```
def get_input_ids(text):
if isinstance(text, str):
tokens = self.tokenize(text, **kwargs)
return self.convert_tokens_to_ids(tokens)
elif isinstance(text, (list, tuple)) and len(text) > 0 and isinstance(text[0], str):
if is_split_into_words:
####### this part ########
tokens = list(
itertools.chain(*(self.tokenize(t, is_split_into_words=True, **kwargs) for t in text))
)
####### this part ########
return self.convert_tokens_to_ids(tokens)
else:
return self.convert_tokens_to_ids(text)
```<|||||>The part you've highlighted is performing tokenization on each individual word (not token!). You can see here that if it was already tokenized, then applying a second tokenization would be incorrect.<|||||>@LysandreJik Understood, Thanks. I close the issue.<|||||>I think the tokenizer should support a new kwarg such as:
` is_already_tokens=False/True` |
transformers | 8,214 | closed | [Benchmark] | # 🖥 Benchmarking `transformers`
## Benchmark
Which part of `transformers` did you benchmark?
## Set-up
What did you run your benchmarks on? Please include details, such as: CPU, GPU? If using multiple GPUs, which parallelization did you use?
## Results
Put your results here!
| 11-01-2020 05:06:56 | 11-01-2020 05:06:56 | |
transformers | 8,213 | closed | Fix ignore files behavior in doctests | In the doc tests (which btw I'm aware they're disabled), the `ignore_files` uses a mutable default value, so it's modified (e.g., when `__init__.py` is appended), it modifies the value for the next function calls that also use the default value (that don't set the arg).
I also fixed typing issues in the file and other minor issues. | 11-01-2020 00:29:21 | 11-01-2020 00:29:21 | |
transformers | 8,212 | closed | Pickle error | While fine-tuning BERT with the new [script](https://github.com/huggingface/transformers/blob/master/examples/language-modeling/run_mlm.py) I am facing the issue as follows:
```
Traceback (most recent call last):
File "run_mlm.py", line 310, in <module>
main()
File "run_mlm.py", line 259, in main
load_from_cache_file=not data_args.overwrite_cache,
File "/home/ai-students/anaconda3/envs/env_nesara/lib/python3.6/site-packages/datasets/dataset_dict.py", line 300, in map
for k, dataset in self.items()
File "/home/ai-students/anaconda3/envs/env_nesara/lib/python3.6/site-packages/datasets/dataset_dict.py", line 300, in <dictcomp>
for k, dataset in self.items()
File "/home/ai-students/anaconda3/envs/env_nesara/lib/python3.6/site-packages/datasets/arrow_dataset.py", line 1256, in map
update_data=update_data,
File "/home/ai-students/anaconda3/envs/env_nesara/lib/python3.6/site-packages/datasets/arrow_dataset.py", line 156, in wrapper
out: Union["Dataset", "DatasetDict"] = func(self, *args, **kwargs)
File "/home/ai-students/anaconda3/envs/env_nesara/lib/python3.6/site-packages/datasets/fingerprint.py", line 158, in wrapper
self._fingerprint, transform, kwargs_for_fingerprint
File "/home/ai-students/anaconda3/envs/env_nesara/lib/python3.6/site-packages/datasets/fingerprint.py", line 105, in update_fingerprint
hasher.update(transform_args[key])
File "/home/ai-students/anaconda3/envs/env_nesara/lib/python3.6/site-packages/datasets/fingerprint.py", line 57, in update
self.m.update(self.hash(value).encode("utf-8"))
File "/home/ai-students/anaconda3/envs/env_nesara/lib/python3.6/site-packages/datasets/fingerprint.py", line 53, in hash
return cls.hash_default(value)
File "/home/ai-students/anaconda3/envs/env_nesara/lib/python3.6/site-packages/datasets/fingerprint.py", line 46, in hash_default
return cls.hash_bytes(dumps(value))
File "/home/ai-students/anaconda3/envs/env_nesara/lib/python3.6/site-packages/datasets/utils/py_utils.py", line 367, in dumps
dump(obj, file)
File "/home/ai-students/anaconda3/envs/env_nesara/lib/python3.6/site-packages/datasets/utils/py_utils.py", line 339, in dump
Pickler(file, recurse=True).dump(obj)
File "/home/ai-students/anaconda3/envs/env_nesara/lib/python3.6/site-packages/dill/_dill.py", line 446, in dump
StockPickler.dump(self, obj)
File "/home/ai-students/anaconda3/envs/env_nesara/lib/python3.6/pickle.py", line 409, in dump
self.save(obj)
File "/home/ai-students/anaconda3/envs/env_nesara/lib/python3.6/pickle.py", line 476, in save
f(self, obj) # Call unbound method with explicit self
File "/home/ai-students/anaconda3/envs/env_nesara/lib/python3.6/site-packages/dill/_dill.py", line 1438, in save_function
obj.__dict__, fkwdefaults), obj=obj)
File "/home/ai-students/anaconda3/envs/env_nesara/lib/python3.6/pickle.py", line 610, in save_reduce
save(args)
File "/home/ai-students/anaconda3/envs/env_nesara/lib/python3.6/pickle.py", line 476, in save
f(self, obj) # Call unbound method with explicit self
File "/home/ai-students/anaconda3/envs/env_nesara/lib/python3.6/pickle.py", line 751, in save_tuple
save(element)
File "/home/ai-students/anaconda3/envs/env_nesara/lib/python3.6/pickle.py", line 476, in save
f(self, obj) # Call unbound method with explicit self
File "/home/ai-students/anaconda3/envs/env_nesara/lib/python3.6/pickle.py", line 736, in save_tuple
save(element)
File "/home/ai-students/anaconda3/envs/env_nesara/lib/python3.6/pickle.py", line 476, in save
f(self, obj) # Call unbound method with explicit self
File "/home/ai-students/anaconda3/envs/env_nesara/lib/python3.6/site-packages/dill/_dill.py", line 1170, in save_cell
pickler.save_reduce(_create_cell, (f,), obj=obj)
File "/home/ai-students/anaconda3/envs/env_nesara/lib/python3.6/pickle.py", line 610, in save_reduce
save(args)
File "/home/ai-students/anaconda3/envs/env_nesara/lib/python3.6/pickle.py", line 476, in save
f(self, obj) # Call unbound method with explicit self
File "/home/ai-students/anaconda3/envs/env_nesara/lib/python3.6/pickle.py", line 736, in save_tuple
save(element)
File "/home/ai-students/anaconda3/envs/env_nesara/lib/python3.6/pickle.py", line 521, in save
self.save_reduce(obj=obj, *rv)
File "/home/ai-students/anaconda3/envs/env_nesara/lib/python3.6/pickle.py", line 605, in save_reduce
save(cls)
File "/home/ai-students/anaconda3/envs/env_nesara/lib/python3.6/pickle.py", line 476, in save
f(self, obj) # Call unbound method with explicit self
File "/home/ai-students/anaconda3/envs/env_nesara/lib/python3.6/site-packages/dill/_dill.py", line 1365, in save_type
obj.__bases__, _dict), obj=obj)
File "/home/ai-students/anaconda3/envs/env_nesara/lib/python3.6/pickle.py", line 610, in save_reduce
save(args)
File "/home/ai-students/anaconda3/envs/env_nesara/lib/python3.6/pickle.py", line 476, in save
f(self, obj) # Call unbound method with explicit self
File "/home/ai-students/anaconda3/envs/env_nesara/lib/python3.6/pickle.py", line 751, in save_tuple
save(element)
File "/home/ai-students/anaconda3/envs/env_nesara/lib/python3.6/pickle.py", line 476, in save
f(self, obj) # Call unbound method with explicit self
File "/home/ai-students/anaconda3/envs/env_nesara/lib/python3.6/site-packages/dill/_dill.py", line 933, in save_module_dict
StockPickler.save_dict(pickler, obj)
File "/home/ai-students/anaconda3/envs/env_nesara/lib/python3.6/pickle.py", line 821, in save_dict
self._batch_setitems(obj.items())
File "/home/ai-students/anaconda3/envs/env_nesara/lib/python3.6/pickle.py", line 847, in _batch_setitems
save(v)
File "/home/ai-students/anaconda3/envs/env_nesara/lib/python3.6/pickle.py", line 476, in save
f(self, obj) # Call unbound method with explicit self
File "/home/ai-students/anaconda3/envs/env_nesara/lib/python3.6/site-packages/dill/_dill.py", line 933, in save_module_dict
StockPickler.save_dict(pickler, obj)
File "/home/ai-students/anaconda3/envs/env_nesara/lib/python3.6/pickle.py", line 821, in save_dict
self._batch_setitems(obj.items())
File "/home/ai-students/anaconda3/envs/env_nesara/lib/python3.6/pickle.py", line 847, in _batch_setitems
save(v)
File "/home/ai-students/anaconda3/envs/env_nesara/lib/python3.6/pickle.py", line 507, in save
self.save_global(obj, rv)
File "/home/ai-students/anaconda3/envs/env_nesara/lib/python3.6/pickle.py", line 927, in save_global
(obj, module_name, name))
_pickle.PicklingError: Can't pickle typing.Union[str, NoneType]: it's not the same object as typing.Union
```
I am trying to run the same script with the already mentioned wikitext dataset. However, I am not able to run it successfully due to the above mentioned error.
@sgugger Could you please help me resolve this error?
| 10-31-2020 20:46:51 | 10-31-2020 20:46:51 | Can you give all the information you can about your environment and pip list and I’m pinging @lhoestq.
If you can manage to reproduce the error in a google colab or shareable environment that would be the top for debugging.<|||||>@VictorSanh got a similar issue once. Did you install transformers using `pip install -e .` ?<|||||>> Can you give all the information you can about your environment and pip list and I’m pinging @lhoestq.
>
> If you can manage to reproduce the error in a google colab or shareable environment that would be the top for debugging.
@thomwolf Please have a look at the [colab](https://colab.research.google.com/drive/1BlQF0-JYBVNsZXuIQsyVSGRQzsQzp0pl?usp=sharing). It is also reproducing the same error as before.<|||||>> @VictorSanh got a similar issue once. Did you install transformers using `pip install -e .` ?
@lhoestq Yes, I have installed it from source using:
```
git clone https://github.com/huggingface/transformers.git
cd transformers
pip install -e .
```
I also tried installing as suggested here in [examples](https://github.com/huggingface/transformers/tree/master/examples) as:
```
git clone https://github.com/huggingface/transformers
cd transformers
pip install .
pip install -r ./examples/requirements.txt
```<|||||>I am trying to train roberta model from scratch using run_mlm.py file. But, facing the same issue.
> Didn't find file ./model_output/tokenizer.json. We won't load it.
> Didn't find file ./model_output/added_tokens.json. We won't load it.
> Didn't find file ./model_output/special_tokens_map.json. We won't load it.
> Didn't find file ./model_output/tokenizer_config.json. We won't load it.
> loading file ./model_output/vocab.json
> loading file ./model_output/merges.txt
> loading file None
> loading file None
> loading file None
> loading file None
> Asking to truncate to max_length but no maximum length is provided and the model has no predefined maximum length. Defaultto no truncation.
> Traceback (most recent call last):
> File "transformers/examples/language-modeling/run_mlm.py", line 310, in <module>
> main()
> File "transformers/examples/language-modeling/run_mlm.py", line 259, in main
> load_from_cache_file=not data_args.overwrite_cache,
> File "/anaconda/envs/azureml_py36/lib/python3.6/site-packages/datasets/dataset_dict.py", line 300, in map
> for k, dataset in self.items()
> File "/anaconda/envs/azureml_py36/lib/python3.6/site-packages/datasets/dataset_dict.py", line 300, in <dictcomp>
> for k, dataset in self.items()
> File "/anaconda/envs/azureml_py36/lib/python3.6/site-packages/datasets/arrow_dataset.py", line 1256, in map
> update_data=update_data,
> File "/anaconda/envs/azureml_py36/lib/python3.6/site-packages/datasets/arrow_dataset.py", line 156, in wrapper
> out: Union["Dataset", "DatasetDict"] = func(self, *args, **kwargs)
> File "/anaconda/envs/azureml_py36/lib/python3.6/site-packages/datasets/fingerprint.py", line 158, in wrapper
> self._fingerprint, transform, kwargs_for_fingerprint
> File "/anaconda/envs/azureml_py36/lib/python3.6/site-packages/datasets/fingerprint.py", line 105, in update_fingerprint
> hasher.update(transform_args[key])
> File "/anaconda/envs/azureml_py36/lib/python3.6/site-packages/datasets/fingerprint.py", line 57, in update
> self.m.update(self.hash(value).encode("utf-8"))
> File "/anaconda/envs/azureml_py36/lib/python3.6/site-packages/datasets/fingerprint.py", line 53, in hash
> return cls.hash_default(value)
> File "/anaconda/envs/azureml_py36/lib/python3.6/site-packages/datasets/fingerprint.py", line 46, in hash_default
> return cls.hash_bytes(dumps(value))
> File "/anaconda/envs/azureml_py36/lib/python3.6/site-packages/datasets/utils/py_utils.py", line 367, in dumps
> dump(obj, file)
> File "/anaconda/envs/azureml_py36/lib/python3.6/site-packages/datasets/utils/py_utils.py", line 339, in dump
> Pickler(file, recurse=True).dump(obj)
> File "/anaconda/envs/azureml_py36/lib/python3.6/site-packages/dill/_dill.py", line 446, in dump
> StockPickler.dump(self, obj)
> File "/anaconda/envs/azureml_py36/lib/python3.6/pickle.py", line 409, in dump
> self.save(obj)
> File "/anaconda/envs/azureml_py36/lib/python3.6/pickle.py", line 476, in save
> f(self, obj) # Call unbound method with explicit self
> File "/anaconda/envs/azureml_py36/lib/python3.6/site-packages/dill/_dill.py", line 1438, in save_function
> obj.__dict__, fkwdefaults), obj=obj)
> File "/anaconda/envs/azureml_py36/lib/python3.6/pickle.py", line 610, in save_reduce
> save(args)
> File "/anaconda/envs/azureml_py36/lib/python3.6/pickle.py", line 476, in save
> f(self, obj) # Call unbound method with explicit self
> File "/anaconda/envs/azureml_py36/lib/python3.6/pickle.py", line 751, in save_tuple
> save(element)
> File "/anaconda/envs/azureml_py36/lib/python3.6/pickle.py", line 476, in save
> f(self, obj) # Call unbound method with explicit self
> File "/anaconda/envs/azureml_py36/lib/python3.6/pickle.py", line 736, in save_tuple
> save(element)
> File "/anaconda/envs/azureml_py36/lib/python3.6/pickle.py", line 476, in save
> f(self, obj) # Call unbound method with explicit self
> File "/anaconda/envs/azureml_py36/lib/python3.6/site-packages/dill/_dill.py", line 1170, in save_cell
> pickler.save_reduce(_create_cell, (f,), obj=obj)
> File "/anaconda/envs/azureml_py36/lib/python3.6/pickle.py", line 610, in save_reduce
> save(args)
> File "/anaconda/envs/azureml_py36/lib/python3.6/pickle.py", line 476, in save
> f(self, obj) # Call unbound method with explicit self
> File "/anaconda/envs/azureml_py36/lib/python3.6/pickle.py", line 736, in save_tuple
> save(element)
> File "/anaconda/envs/azureml_py36/lib/python3.6/pickle.py", line 521, in save
> self.save_reduce(obj=obj, *rv)
> File "/anaconda/envs/azureml_py36/lib/python3.6/pickle.py", line 605, in save_reduce
> save(cls)
> File "/anaconda/envs/azureml_py36/lib/python3.6/pickle.py", line 476, in save
> f(self, obj) # Call unbound method with explicit self
> File "/anaconda/envs/azureml_py36/lib/python3.6/site-packages/dill/_dill.py", line 1365, in save_type
> obj.__bases__, _dict), obj=obj)
> File "/anaconda/envs/azureml_py36/lib/python3.6/pickle.py", line 610, in save_reduce
> save(args)
> File "/anaconda/envs/azureml_py36/lib/python3.6/pickle.py", line 476, in save
> f(self, obj) # Call unbound method with explicit self
> File "/anaconda/envs/azureml_py36/lib/python3.6/pickle.py", line 751, in save_tuple
> save(element)
> File "/anaconda/envs/azureml_py36/lib/python3.6/pickle.py", line 476, in save
> f(self, obj) # Call unbound method with explicit self
> File "/anaconda/envs/azureml_py36/lib/python3.6/site-packages/dill/_dill.py", line 933, in save_module_dict
> StockPickler.save_dict(pickler, obj)
> File "/anaconda/envs/azureml_py36/lib/python3.6/pickle.py", line 821, in save_dict
> self._batch_setitems(obj.items())
> File "/anaconda/envs/azureml_py36/lib/python3.6/pickle.py", line 847, in _batch_setitems
> save(v)
> File "/anaconda/envs/azureml_py36/lib/python3.6/pickle.py", line 476, in save
> f(self, obj) # Call unbound method with explicit self
> File "/anaconda/envs/azureml_py36/lib/python3.6/site-packages/dill/_dill.py", line 933, in save_module_dict
> StockPickler.save_dict(pickler, obj)
> File "/anaconda/envs/azureml_py36/lib/python3.6/pickle.py", line 821, in save_dict
> self._batch_setitems(obj.items())
> File "/anaconda/envs/azureml_py36/lib/python3.6/pickle.py", line 847, in _batch_setitems
> save(v)
> File "/anaconda/envs/azureml_py36/lib/python3.6/pickle.py", line 507, in save
> self.save_global(obj, rv)
> File "/anaconda/envs/azureml_py36/lib/python3.6/pickle.py", line 927, in save_global
> (obj, module_name, name))
> _pickle.PicklingError: Can't pickle typing.Union[str, NoneType]: it's not the same object as typing.Union<|||||>I have the same problem, how to fix it?<|||||>
> > @VictorSanh got a similar issue once. Did you install transformers using `pip install -e .` ?
>
> @lhoestq Yes, I have installed it from source using:
>
> ```
> git clone https://github.com/huggingface/transformers.git
> cd transformers
> pip install -e .
> ```
>
> I also tried installing as suggested here in [examples](https://github.com/huggingface/transformers/tree/master/examples) as:
>
> ```
> git clone https://github.com/huggingface/transformers
> cd transformers
> pip install .
> pip install -r ./examples/requirements.txt
> ```
Yes @naturecreator, I had the same error last week. I managed to circumvent that by removing the editable mode when pip installing (from `pip install -e .` to a standard `pip install .`).
It worked for me both for python 3.6 and 3.7.<|||||>After the recent commit made to the [script](https://github.com/huggingface/transformers/blob/master/examples/language-modeling/run_mlm.py), it is running as expected without any errors.<|||||>Hey, I forked it and followed the solution given by @VictorSanh, but I am still getting this error. I am loading a custom dataset (text file) not a predefined one, and for Roberta-Base. Also using the --line_by_line parameter.Any ideas why this may be happening?<|||||>Tried by removing --line_by_line parameter. It works, but it is not taking line by line input anymore since we removed the parameter. I processed the file as a JSON for now. Is there a fix using the --line_by_line?<|||||>This an error that none of us on the team managed to fully reproduce, so if you could give us your full environment, that would be super helpful.<|||||>I would love to help. I am a bit new to this, do let me know if any more specifics are required. The versions of the required lib/lang are -
Python - 3.6.7
transformers - 3.4.0
pickle - 4.0
The command I ran was -
python3 run_mlm.py \
--model_name_or_path roberta-base \
--train_file train.txt \
--validation_file test.txt \
--do_train \
--do_eval \
--output_dir results/ \
--line_by_line \
<|||||>Ahah! Can reproduce! This will make investigation easier.<|||||>For future reference, here is how I create an env reproducing the bug, and the command that shows it (self-contained to the repo):
```
pyenv install 3.6.7
pyenv virtualenv 3.6.7 picklebug
pyenv activate picklebug
pip install --upgrade pip
pip install transformers[torch]
pip install datasets
cd git/transformers # Adapt to your local path to the cloned repo
pip install -e .
python examples/language-modeling/run_mlm.py \
--model_name_or_path roberta-base \
--train_file ./tests/fixtures/sample_text.txt \
--validation_file ./tests/fixtures/sample_text.txt \
--do_train \
--do_eval \
--output_dir /tmp/test=clm \
--line_by_line
```<|||||>The bug disappears for me with python 3.7.9 so if you can upgrade your python version, you should be good to go.<|||||>Further reduced, the bug appears in all python versions <= 3.6.12 but disappears in python 3.7.0.<|||||>Thanks, this was really helpful !!! |
transformers | 8,211 | closed | Appropriate dataset format for language modeling example | # What is the most memory efficient way/best way to format your dataset file for language modeling?
## Details
I am running run_clm.py and can only get my dataset to work with the smallest GPT2 model. I would like to experiment with gpt2-xl ideally but would settle for large and xlnet. I am using distributed training on TPU assuming that this improves memory. I have saved my file in a .txt. It is roughly 20mb with 82,000 samples of mean length 256 std 250 and is line delimited (where each line signifies a sample).
Is this the correct approach? I notice the .raw files used in training, are these smaller? Is there a way to pretokenize?
I hesitate to ask this on stack as it is not a bug.
@sgugger sorry to bother if this is a common issue I'd love to hear more no worries if it will require much work I can focus on that. Just lost for resources.
Thank you all! | 10-31-2020 19:15:40 | 10-31-2020 19:15:40 | General questions should be asked on the [forum](https://discuss.huggingface.co/) as we keep the issues for bugs.
`run_clm` doesn't use the approach of taking the different lines of a dataset (that's in `run_mlm` as it's usually done for masked language modeling) so you will have to tweak the example script to do this.
There is no way to pretokenzie, but the result of the tokenization will be cached, so it will only be run once on a given machine.<|||||>Thank you for the help! I won't make that mistake again my apologies. Editing the clm file now. New to open source software and excited to dig in and help please bear with me while I learn the ropes!<|||||>No worries, I'm just telling you for next time :-)
Good luck with your scripting, closing this issue for now. |
transformers | 8,210 | closed | Simple import issue for run_clm.py | ## Environment info
- `transformers` version: 3.4.0
- Platform: Linux-4.19.112+-x86_64-with-Ubuntu-18.04-bionic
- Python version: 3.6.9
- PyTorch version (GPU?): 1.6.0+cu101 (True)
- Tensorflow version (GPU?): 2.3.0 (True)
- Using GPU in script?: yes
- Using distributed or parallel set-up in script?: no
other details na on colab not sure how to find them sorry
### Who can help
@sgugger @TevenLeScao @LysandreJik
## Information
Model I am using (Bert, XLNet ...): GPT2
The problem arises when using:
* [ ] the official example scripts: (give details below)
run_clm.py
* [ ] my own modified scripts: (give details below)
na
The tasks I am working on is:
* [ ] an official GLUE/SQUaD task: (give the name)
language modeling for generation with custom data file
* [ ] my own task or dataset: (give details below)
my own dataset in a file train-4.txt which is line delimited
## To reproduce
Steps to reproduce the behavior:
1.run this script:
2. !python /content/transformers/examples/language-modeling/run_clm.py \
--model_name_or_path gpt2-medium \
--train_file /content/train-4.txt \
--do_train \
--output_dir /tmp/test-clm
This is the script:
!python /content/transformers/examples/language-modeling/run_clm.py \
--model_name_or_path gpt2-medium \
--train_file /content/train-4.txt \
--do_train \
--output_dir /tmp/test-clm
this is the error:
ImportError: cannot import name 'is_main_process'
## Expected behavior
working language modeling script
'is_main_process' should be importable from transformers.trainer.utils
Thank you!
Will be using this model down stream for some more advanced tasks trying to blow through finetuning so I can get a jump on the fun stuff. Any help would be appreciated, working on an academic art project and fast help would be deeply appreciated (no rush obviously) so creative iteration may commence. Thank you once again!
| 10-31-2020 18:35:54 | 10-31-2020 18:35:54 | Hi there, as mentioned in the README of the examples (in bold), you need to install transformers [from source](https://huggingface.co/transformers/installation.html#installing-from-source) to use that script.<|||||>I did thank, you for the rapid reply! Comfortable programmer, files not so much so again thank you for bearing with me. Installed via:
!pip install git+https://github.com/huggingface/transformers.git<|||||>Yep thats the issue sorry for being a moron and this can be closed. Perhaps someone of multicellular intelligence could explain why my !pip git+ solution is insufficient?
Thank you @sgugger<|||||>Mmmm, maybe you had the repo cached somewhere and it didn't update to the latest version? Glad your issue is fixed :-) <|||||>Maybe because you already had `transformers` installed in your environment, in which case you would have to supply the `-U` option to update to the specific version you're targeting <|||||>I have a issue of fine-tuning the T5 model(t5-base).
!python /content/transformers/examples/language-modeling/run_clm.py
--model_name_or_path t5-base
--train_file /content/train.txt
--do_train
--output_dir /tmp/test-clm
This code is not working. How can I fine-tune the t5-base model? |
transformers | 8,209 | closed | XLMRobertaTokenizer potential bug | ## Environment info
- `transformers` version: 3.4.0
- Platform: Linux-5.4.60-1-pve-x86_64-with-debian-buster-sid
- Python version: 3.6.3
- PyTorch version (GPU?): 1.7.0 (True)
- Tensorflow version (GPU?): not installed (NA)
- Using GPU in script?: no
- Using distributed or parallel set-up in script?: no
### Who can help
@LysandreJik
@mfuntowicz
## Information
I need to align tokens obtained with XLMRobertaTokenizer to original text. Normally, I use fast tokenizer API and token_to_chars method to get the mapping. However, when I use it with XLMRobertaTokenizer, there seems to be bug in the output (the returned indices of tokens tend to skip some tokens and sometimes do not correspond).
## To reproduce
```
from transformers import AutoTokenizer
input='walnut , 17.6.2007 22:20:59 , ip : *** . ***.108.25 , # 10305dobry den , nedavno me znicehonic zacal bolet nart prave nohy-spatne se s nim pohybuje , boli me pri chuzi .'
tokenizer = AutoTokenizer.from_pretrained('xlm-roberta-base', use_fast = True)
res = tokenizer(input_line)
print(res.tokens()[:5]
```
```
['<s>', '▁wal', 'nut', '▁', ',']
```
```
for i in range(15):
cur_char_word_ind = res.char_to_token(i)
print(input_line[i], cur_char_word_ind)
```
```
w 1
a 1
l 1
n 2
u 2
t 2
None
, 3
None
1 5
7 5
. 6
6 6
. 6
2 7
```
The line ```, 3 ``` is wrong as ```,``` should be aligned to fourth token ```,```. The fourth token is not used and is skipped.
## Expected behavior
Possibly
```
w 1
a 1
l 1
n 2
u 2
t 2
3
, 4
None
1 5
7 5
. 6
6 6
. 6
2 7
```
| 10-31-2020 13:42:10 | 10-31-2020 13:42:10 | Indeed this seems a bit strange.
Pining @n1t0 and @Narsil here (actually this should probably rather be an issue in the https://github.com/huggingface/tokenizers repo)<|||||>This issue has been automatically marked as stale because it has not had recent activity. It will be closed if no further activity occurs. Thank you for your contributions.
<|||||>@n1t0 Any update on this?<|||||>This issue has been automatically marked as stale because it has not had recent activity. If you think this still needs to be addressed please comment on this thread.
Please note that issues that do not follow the [contributing guidelines](https://github.com/huggingface/transformers/blob/master/CONTRIBUTING.md) are likely to be ignored. |
transformers | 8,208 | closed | Poor f1 score when validating existing models | I have been attempting to validate the results on several models, but have been unable to match them with the results posted on the model cards.
For example, I took an Albert model: https://huggingface.co/ahotrod/albert_xxlargev1_squad2_512
and I run:
```
python run_squad.py
--model_type albert
--model_name_or_path ahotrod/albert_xxlargev1_squad2_512
--do_eval
--predict_file ../squd/dev-v2.0.json
--per_gpu_eval_batch_size 8
--max_seq_length 512
--doc_stride 128
--output_dir ../squd/output/albert
--overwrite_output_dir
--threads 16
--verbose
--version_2_with_negative
```
I got:
```
exact: 77.39408742525058
f1: 81.6576936707378
total: 11873
HasAns_exact: 71.60931174089069
HasAns_f1': 80.14875117285251
HasAns_total': 5928
NoAns_exact': 83.16232127838519
NoAns_f1': 83.16232127838519
NoAns_total': 5945
best_exact': 77.38566495409754
best_exact_thresh': 0.0
best_f1': 81.64927119958456
best_f1_thresh': 0.0
```
While on the model card stated:
```
exact: 86.11134506864315
f1: 89.35371214945009
total': 11873
HasAns_exact': 83.56950067476383
HasAns_f1': 90.06353312254078
HasAns_total': 5928
NoAns_exact': 88.64592094196804
NoAns_f1': 88.64592094196804
NoAns_total': 5945
best_exact': 86.11134506864315
best_exact_thresh': 0.0
best_f1': 89.35371214944985
best_f1_thresh': 0.0
```
I did not alter any code, I was simply trying to validate the results. What am I overlooking here?
(the same diff also found using roberta models, bert, and others) | 10-31-2020 11:46:29 | 10-31-2020 11:46:29 | This issue has been automatically marked as stale because it has not had recent activity. It will be closed if no further activity occurs. Thank you for your contributions.
|
transformers | 8,207 | closed | Updated ConversationalPipeline to work with encoder-decoder models | # What does this PR do?
This PR extends the capabilities of the existing ConversationalPipeline to work with Encoder Decoder models (such as BlenderBot).
The pipeline has been modified as follows:
- history generated from concatenation of the inputs to the generated tokens for encoder-decoder (decoders use directly the generated tokens that contain the initial prompt)
- updated the cut-off position for generated tokens (1 for encoder-decoders, `input_length` for decoders)
- updated the clean-up script to remove all `pad_tokens` if `pad_token` != `eos_token`. Otherwise, remove pad_tokens from the second found if `pad_token` and `eos_token` are identical (previous behaviour of the pipeline). This is needed otherwise the models with a specific `eos_token` will keep an unnecessary `pad_token` that affects generation quality for subsequent rounds.
This has been tested with the BlenderBot 90M model (requires https://github.com/huggingface/transformers/pull/8205), producing the following output: https://gist.github.com/guillaume-be/380b98ec1ef91d0f6e3add5914dd92ce
## Who can review?
Text Generation: @patrickvonplaten, @TevenLeScao
Blenderbot, Bart, Marian, Pegasus: @sshleifer
| 10-31-2020 10:22:04 | 10-31-2020 10:22:04 | > Looks great! Can we maybe add one test with the small `BlenderbotModel` to `/home/patrick/hugging_face/transformers/tests/test_pipelines_conversational.py` ?
Thank you @patrickvonplaten , I added an integration test using BlenderBot 90M.<|||||>Merging, unrelated failure. |
transformers | 8,206 | closed | Sentence transformer Segmentation Fault - Pytorch 1.4.0, 2.80 | ## Environment info
<!-- You can run the command `transformers-cli env` and copy-and-paste its output below.
Don't forget to fill out the missing fields in that output! -->
- `transformers` version:
- Platform: Linux
- Python version: 3.7
- PyTorch version (GPU?): 1.4 (Both)
- Tensorflow version (GPU?):
- Using GPU in script?: Yes
- Using distributed or parallel set-up in script?: Doesn't Matter
### Who can help
@LysandreJik
<!-- Your issue will be replied to more quickly if you can figure out the right person to tag with @
If you know how to use git blame, that is the easiest way, otherwise, here is a rough guide of **who to tag**.
Please tag fewer than 3 people.
albert, bert, GPT2, XLM: @LysandreJik
tokenizers: @mfuntowicz
Trainer: @sgugger
Speed and Memory Benchmarks: @patrickvonplaten
Model Cards: @julien-c
Translation: @sshleifer
Summarization: @sshleifer
TextGeneration: @TevenLeScao
examples/distillation: @VictorSanh
nlp datasets: [different repo](https://github.com/huggingface/nlp)
rust tokenizers: [different repo](https://github.com/huggingface/tokenizers)
Text Generation: @TevenLeScao
blenderbot: @mariamabarham
Bart: @sshleifer
Marian: @sshleifer
T5: @patrickvonplaten
Longformer/Reformer: @patrickvonplaten
TransfoXL/XLNet: @TevenLeScao
examples/seq2seq: @sshleifer
examples/bert-loses-patience: @JetRunner
tensorflow: @jplu
examples/token-classification: @stefan-it
documentation: @sgugger
-->
## Information
Model I am using (Bert):
The problem arises when using:
* [ ] the official example scripts: (give details below)
## To reproduce
Steps to reproduce the behavior:
1. Open python console, with torch 1.4 installed
```python
import transformers
```
This crashes with error
```text
segementation fault
```
## Expected behavior
Works as normal without error
<!-- A clear and concise description of what you would expect to happen. -->
The only way to fix this is to force install sentencepiece==0.1.91 . The root cause is that https://github.com/huggingface/transformers/blob/v2.8.0/setup.py doesn't fix the version of sentencepiece.
| 10-31-2020 10:17:31 | 10-31-2020 10:17:31 | This will also be fixed by #8073 <|||||>This issue has been automatically marked as stale because it has not had recent activity. It will be closed if no further activity occurs. Thank you for your contributions.
|
transformers | 8,205 | closed | [Bug fix] Fixed value for BlenderBot pad token | # What does this PR do?
The current `BlenderbotSmallTokenizer` has an incorrect (probably a typo) value for the `pad_token`. This causes the BlenderBot model to crash on padded sequences (currently pads with a value that exceeds the embedding matrix size).
This PR fixes the behaviour and the tokenizer now pads correctly with `0`.
## Who can review?
Blenderbot, Bart, Marian, Pegasus: @sshleifer
| 10-31-2020 09:27:43 | 10-31-2020 09:27:43 | |
transformers | 8,204 | closed | [Benchmark] | # 🖥 Benchmarking `transformers`
## Benchmark
Which part of `transformers` did you benchmark?
## Set-up
What did you run your benchmarks on? Please include details, such as: CPU, GPU? If using multiple GPUs, which parallelization did you use?
## Results
Put your results here!
| 10-31-2020 04:04:40 | 10-31-2020 04:04:40 | |
transformers | 8,203 | closed | Add TFDPR | # What does this PR do?
Add `TFDPRContextEncoder, TFDPRQuestionEncoder` and `TFDPRReader` in `modeling_tf_dpr.py`, as well as other relevant files in the [checklist](https://github.com/huggingface/transformers/tree/master/templates/adding_a_new_model) .
Now the TF model works properly and can load Pytorch's weights successfully the same output as Pytorch's counterparts **except** small random noise (1e-5) which I suspect of some dtypes different , but I could not find the cause.
We can try playing TFDPR models and compare to Pytorch's ones [here in Colab](https://colab.research.google.com/drive/1czS_m9zy5k-iSJbzA_DP1k1xAAC_sdkf?usp=sharing)
<!--
Congratulations! You've made it this far! You're not quite done yet though.
Once merged, your PR is going to appear in the release notes with the title you set, so make sure it's a great title that fully reflects the extent of your awesome contribution.
Then, please replace this with a description of the change and which issue is fixed (if applicable). Please also include relevant motivation and context. List any dependencies (if any) that are required for this change.
Once you're done, someone will review your PR shortly (see the section "Who can review?" below to tag some potential reviewers). They may suggest changes to make the code even better. If no one reviewed your PR after a week has passed, don't hesitate to post a new comment @-mentioning the same persons---sometimes notifications get lost.
-->
## Before submitting
- [X] Did you read the [contributor guideline](https://github.com/huggingface/transformers/blob/master/CONTRIBUTING.md#start-contributing-pull-requests), Pull Request section?
- [X] Was this discussed/approved via a Github issue or the [forum](https://discuss.huggingface.co/)? Please add a link to the it if that's the case.
Here: https://github.com/huggingface/transformers/issues/8171
- [X] Did you make sure to update the documentation with your changes? Here are the [documentation guidelines](https://github.com/huggingface/transformers/tree/master/docs), and [here are tips on formatting docstrings](https://github.com/huggingface/transformers/tree/master/docs#writing-source-documentation).
- [X] Did you write any new necessary tests?
Yes, I write simple test whether TF and Pytorch models with pretrained weights give the same output (except very small random noise). The test is in Colab (shared above).
Also the model is passed all 27 tests in test_modeling_tf_dpr.py file (Please see the last cell in Colab above)
## Who can review?
@LysandreJik
# Details what were done according to Checklist
## Adding model/configuration/tokenization classes
Mostly done due to pre-existing of Pytorch's DPR.
- [X] Copy the python files from the present folder to the main folder and rename them, replacing `xxx` with your model
name.
- [X] Edit the files to replace `XXX` (with various casing) with your model name.
- [X] Copy-paste or create a simple configuration class for your model in the `configuration_...` file.
- [X] Copy-paste or create the code for your model in the `modeling_...` files (PyTorch and TF 2.0).
- [X] Copy-paste or create a tokenizer class for your model in the `tokenization_...` file.
## Adding conversion scripts
- [ ] Copy the conversion script (`convert_...`) from the present folder to the main folder.
- [ ] Edit this script to convert your original checkpoint weights to the current pytorch ones.
Not sure what to do since there already exists DPR pretrained weights.
## Adding tests:
- [X] Copy the python files from the `tests` sub-folder of the present folder to the `tests` subfolder of the main
folder and rename them, replacing `xxx` with your model name.
- [X] Edit the tests files to replace `XXX` (with various casing) with your model name.
- [X] Edit the tests code as needed.
The model is passed all 27 tests in test_modeling_tf_dpr.py file (Please see the last cell in Colab above) -- This is my updated 4 days after made a 1st PR.
## Documenting your model:
- [X] Make sure all your arguments are properly documented in your configuration and tokenizer.
- [X] Most of the documentation of the models is automatically generated, you just have to make sure that
`XXX_START_DOCSTRING` contains an introduction to the model you're adding and a link to the original
article and that `XXX_INPUTS_DOCSTRING` contains all the inputs of your model.
- [X] Create a new page `xxx.rst` in the folder `docs/source/model_doc` and add this file in `docs/source/index.rst`.
## Final steps
(Note the Pytorch DPR was already existed, so I assume I should check as "Done" )
- [X] Add import for all the relevant classes in `__init__.py`.
- [X] Add your configuration in `configuration_auto.py`.
- [X] Add your PyTorch and TF 2.0 model respectively in `modeling_auto.py` and `modeling_tf_auto.py`.
- [X] Add your tokenizer in `tokenization_auto.py`.
- [ ] Add a link to your conversion script in the main conversion utility (in `commands/convert.py`)
- [X] Edit the PyTorch to TF 2.0 conversion script to add your model in the `convert_pytorch_checkpoint_to_tf2.py`
file.
- [ ] Add a mention of your model in the doc: `README.md` and the documentation itself
in `docs/source/pretrained_models.rst`. Rune `make fix-copies` to update `docs/source/index.rst` with your changes.
- [X] Upload the pretrained weights, configurations and vocabulary files.
- [ ] Create model card(s) for your models on huggingface.co. For those last two steps, check the
[model sharing documentation](https://huggingface.co/transformers/model_sharing.html).
| 10-31-2020 03:09:32 | 10-31-2020 03:09:32 | Hi @LysandreJik , thanks for the great review!
I will fix and test the code as suggested.
However, I have a very newbie question about style and document fixing. I admit that I can access only Colab (I have only windows PC) so I am really not sure how to do `make fixup` and `make docs` (in my understanding, should run in linux environment) . Could you please make some suggestions on this issue ?<|||||>Hmmm I think you could do the following in your colab environment:
```py
# Clone the repo or your fork, I assume this is your working directory
!git clone https://github.com/huggingface/transformers
!cd transformers
# Install all the dev dependencies (you've probably done that already)
!pip install -e .[dev]
# Then you should be able to run `make fixup`
!make fixup
# Same for the docs!
!make docs
```
Let me know if that works!<|||||>@LysandreJik , thanks again and I could run the two make commands.
1) `make docs` , produced the error message which I have no clue, so I still need suggestion on this issue, sorry:
```
cd docs && make html SPHINXOPTS="-W"
make[1]: Entering directory '/content/transformers/docs'
Running Sphinx v1.8.5
Extension error:
Could not import extension recommonmark (exception: No module named recommonmark)
Makefile:19: recipe for target 'html' failed
make[1]: *** [html] Error 2
make[1]: Leaving directory '/content/transformers/docs'
Makefile:68: recipe for target 'docs' failed
make: *** [docs] Error 2
```
2) `make fixup` , required `make fix-copies` and then `make fixup` suggest me to add more tests on `test_modeling_tf_dpr.py` which I will investigate this issue and come back :D<|||||>Hey @ratthachat - great work! I helped you a bit with the docs and did some cleaning. I think we can merge the PR soon. It would be great if you could take a look at the comments above (a lot of them should already be resolved now) and also it would be awesome if you could add one integration test to both TF and PT (we forgot to do this originally for PyTorch).
An integration tests should be a slow test, where you can just statically type some input_ids vector and run it through one of the PyTorch pretrained models and test against its expected output. You should use the same input / expected output array then for Tensorflow, similar to how it's done here:
https://github.com/huggingface/transformers/blob/4185b115d4b3fd408265ffd91581698325652c47/tests/test_modeling_roberta.py#L423
Let me know if you have any questions!<|||||>Thanks very much for your great help, Patrick @patrickvonplaten !! I will get back to you guys as soon as possible.<|||||>Hi guys, with the great helps of Patrick, most comments of @LysandreJik were already dealt with. So I further addressed the rest as replied above. BTW, I did only very minimal and necessary changes, but many tests are now failed again , sorry.. I have no idea about this :( .
@patrickvonplaten I added one slow model integration test to `test_modeling_tf_dpr.py` . However, at the moment I still could not find a way to run original DPR repo to produce original output yet. So at the moment, the integration test is just a chek that TF and Pytorch `DPRQuestionEncoder` models produce the same output (with acceptable margin of error) -- i.e. the `tf.constant` expected slice comes from Pytorch's model.
(test can be played around here : https://colab.research.google.com/drive/1czS_m9zy5k-iSJbzA_DP1k1xAAC_sdkf?usp=sharing )
I will come back to add more model integration tests if I succeed to run the [original DPR](https://github.com/facebookresearch/DPR/blob/master/generate_dense_embeddings.py).<|||||>> Hi Patrick, I have a question. At the moment, we do not have native TF weights, so removing this is OK ?
I uploaded them a minute ago ;-) <|||||>Thanks so much everyone. Very happy :D 👯
See you guys again soon on TFRag (WIP)<|||||>@patrickvonplaten I think you didn't upload all of the weights on the model hub. I'm uploading the remaining weights now:
- `facebook/dpr-ctx_encoder-single-nq-base`
- `facebook/dpr-ctx_encoder-multiset-base`
- `facebook/dpr-question_encoder-multiset-base`
- `facebook/dpr-reader-single-nq-base`
- `facebook/dpr-reader-multiset-base`
<|||||>(Current slow CI is failing because it's trying to load some of them)<|||||>They're all uploaded. |
Subsets and Splits
No community queries yet
The top public SQL queries from the community will appear here once available.