repo
stringclasses 1
value | number
int64 1
25.3k
| state
stringclasses 2
values | title
stringlengths 1
487
| body
stringlengths 0
234k
⌀ | created_at
stringlengths 19
19
| closed_at
stringlengths 19
19
| comments
stringlengths 0
293k
|
---|---|---|---|---|---|---|---|
transformers | 887 | closed | No gradient clipping in AdamW | Hi!
After moving from pretrained-bert to transformers I've noticed that the new AdamW optimizer does not perform gradient clipping, even though both BertAdam and OpenAIAdam used to do it.
Also, in finetune_on_pregenerated example bias correction is turned off only for FusedAdam, but not for AdamW. | 07-24-2019 16:03:40 | 07-24-2019 16:03:40 | Yes, the LM fine-tuning example will be refactored.
Adding the removal of gradient clipping to the list of breaking changes, thanks.<|||||>This issue has been automatically marked as stale because it has not had recent activity. It will be closed if no further activity occurs. Thank you for your contributions.
|
transformers | 886 | closed | BERT uncased model outputs a tuple instead of a normal pytorch tensor | While finetuning the BERT uncased model for sequence classification as follows:
```
config = BertConfig.from_pretrained('bert-base-uncased')
tokenizer = BertTokenizer.from_pretrained('bert-base-uncased')
model = BertForSequenceClassification(config)
for layer, child in model.named_children():
if layer not in ['classifier']:
for param in child.parameters():
param.requires_grad = False
optimizer = optim.SGD(model.parameters(), lr=1e-3)
criterion = nn.CrossEntropyLoss()
for (data, target) in (train_loader):
data, target = data.to(device), target.to(device)
optimizer.zero_grad()
output = model(data)
target = target.float()
loss = criterion(output, target)
loss.backward()
optimizer.step()
```
The following error comes up :
```
1348 dim = _get_softmax_dim('log_softmax', input.dim(), _stacklevel)
1349 if dtype is None:
-> 1350 ret = input.log_softmax(dim)
1351 else:
1352 ret = input.log_softmax(dim, dtype=dtype)
AttributeError: 'tuple' object has no attribute 'log_softmax'
```
Here are the model output and target tensors :
```
--> output
(tensor([[-0.2530, 0.0788],
[-0.1457, -0.0624],
[-0.3478, -0.2125],
[-0.1337, 0.2051],
[ 0.0963, 0.3762],
[-0.0910, -0.0527],
[-0.1743, 0.2566],
[-0.2223, 0.4083],
[-0.1602, -0.0012],
[-0.0059, 0.2334],
[-0.3407, -0.1703],
[-0.1359, 0.0776],
[-0.2117, 0.1641],
[-0.3365, -0.1266],
[-0.1682, 0.0504],
[-0.2346, 0.2380]], device='cuda:0', grad_fn=<AddmmBackward>),)
--> target
tensor([0., 0., 1., 1., 1., 0., 0., 0., 1., 1., 1., 0., 0., 0., 1., 0.],
device='cuda:0')
``` | 07-24-2019 14:46:30 | 07-24-2019 14:46:30 | Hi, I was wondering how you managed to resolve this issue? I'm running into a similar problem. :) <|||||>Hi, the model outputs are well documented, they're *always* tuples, even if there's a single return value. You can check the documentation [here](https://huggingface.co/transformers/main_classes/output.html).<|||||>@jacqueline-he did you resolve the issue?<|||||>@WeeHyongTok Yes I have! I only needed to access what's inside the returned tuple. @LysandreJik's recommendation was very helpful. |
transformers | 885 | closed | Can lm_finetuning be used with non-english data ? | Hi,
My target domain is in German. Can I still use the scripts &codes under `lm_finetuning` folder to finetune pre-trained Bert models or are those only for English target domains? | 07-24-2019 14:11:50 | 07-24-2019 14:11:50 | @ereday Take the `simple_lm_finetuning.py` script for example. It has a `--bert_model` argument. When your target domain is German, then you should use the recently introduced [BERT model for german](https://github.com/huggingface/pytorch-transformers/pull/688) via passing `bert-base-german-cased`.
This should fine-tune the German BERT model :)<|||||>@stefan-it thanks alot. I wasn't aware of german specific bert model. Awesome! |
transformers | 884 | closed | Customized BertForTokenClassification Model | I try to customize BertForTokenClassification model by myself to perform sequence tagging and strictly follow the [original implementation](https://huggingface.co/pytorch-transformers/_modules/pytorch_transformers/modeling_bert.html#BertForTokenClassification). However, I cannot obtain the same results (lower scores) with those produced by BertForTokenClassification when I simply set the top-most tagging component/model as an Linear layer (i.e., the current model is identical to BertForTokenClassification). My code is below:
```python
class BertTagger(BertPreTrainedModel):
def __init__(self, bert_config):
super(BertTagger, self).__init__(bert_config)
self.num_labels = bert_config.num_labels
#self.tagger_config = TaggerConfig()
self.bert = BertModel(bert_config)
self.bert_dropout = nn.Dropout(bert_config.hidden_dropout_prob)
self.classifier = nn.Linear(bert_config.hidden_size, bert_config.num_labels)
self.apply(self.init_weights)
def forward(self, input_ids, token_type_ids=None, attention_mask=None, labels=None,
position_ids=None, head_mask=None):
outputs = self.bert(input_ids, position_ids=position_ids, token_type_ids=token_type_ids,
attention_mask=attention_mask, head_mask=head_mask)
# the hidden states of the last Bert Layer, shape: (bsz, seq_len, hsz)
tagger_input = outputs[0]
tagger_input = self.bert_dropout(tagger_input)
logits = self.classifier(tagger_input)
outputs = (logits,) + outputs[2:]
if labels is not None:
loss_fct = CrossEntropyLoss()
# Only keep active parts of the loss
if attention_mask is not None:
active_loss = attention_mask.view(-1) == 1
active_logits = logits.view(-1, self.num_labels)[active_loss]
active_labels = labels.view(-1)[active_loss]
loss = loss_fct(active_logits, active_labels)
else:
loss = loss_fct(logits.view(-1, self.num_labels), labels.view(-1))
outputs = (loss,) + outputs
return outputs
```
Has anyone encountered the same issue? | 07-24-2019 11:16:09 | 07-24-2019 11:16:09 | @lixin4ever I have the same question. Do you solve it?<|||||>I have solve it. Thank you!<|||||>@searchlink How do you solve this problem?<|||||>For reference, the updated resource link mentioned in the original post can be now found [here](https://huggingface.co/transformers/_modules/transformers/modeling_bert.html#BertForTokenClassification); It was affected by the renaming into `transformers`, too.<|||||>But I can't see any difference between "pytorch-transformers" and "transformers" except the line initializing BERT parameters. Have you met the same problem? @dennlinger <|||||>I was just pointing to the up-to-date reference. I'm currently looking into token classification using BERT (or in my case, I would prefer RoBERTa or other iterations of BERT, but unfortunately they seem not available yet).<|||||>So Token classification using BERT does not work?
<|||||>As you can see below, `BertForTokenClassification` works as expected with **PyTorch 1.3.1** and **Transformers 2.2.2** installed with `pip install transformers`.
```
Python 3.6.9 |Anaconda, Inc.| (default, Jul 30 2019, 19:07:31)
[GCC 7.3.0] on linux
Type "help", "copyright", "credits" or "license" for more information.
>>> import transformers
>>> from transformers import BertForTokenClassification
>>> from transformers import BertTokenizer
>>> tokenizer = BertTokenizer.from_pretrained('bert-base-uncased')
>>> model = BertForTokenClassification.from_pretrained('bert-base-uncased')
>>> text='Hello, my dog is cute'
>>> import torch
>>> input_ids = torch.tensor(tokenizer.encode(text, add_special_tokens=True)).unsqueeze(0) # Batch size 1
>>> input_ids
tensor([[ 101, 7592, 1010, 2026, 3899, 2003, 10140, 102]])
>>> labels = torch.tensor([1] * input_ids.size(1)).unsqueeze(0) # Batch size 1
>>> labels
tensor([[1, 1, 1, 1, 1, 1, 1, 1]])
>>> outputs = model(input_ids, labels=labels)
>>> outputs
(tensor(0.7529, grad_fn=<NllLossBackward>), tensor([[[ 0.5078, 0.1628],
[-0.0593, 0.0163],
[ 0.0308, -0.2312],
[ 0.0863, -0.1000],
[-0.2833, -0.2656],
[-0.2014, -0.5225],
[-0.2912, -0.1220],
[-0.2781, -0.2919]]], grad_fn=<AddBackward0>))
>>> len(outputs)
2
>>> loss=outputs[0]
>>> scores=outputs[1]
>>> loss
tensor(0.7529, grad_fn=<NllLossBackward>)
>>> scores
tensor([[[ 0.5078, 0.1628],
[-0.0593, 0.0163],
[ 0.0308, -0.2312],
[ 0.0863, -0.1000],
[-0.2833, -0.2656],
[-0.2014, -0.5225],
[-0.2912, -0.1220],
[-0.2781, -0.2919]]], grad_fn=<AddBackward0>)
>>>
```
It's working with **TensorFlow 2.0.0** and **Transformers 2.2.2** too!
```
Python 3.6.9 |Anaconda, Inc.| (default, Jul 30 2019, 19:07:31)
[GCC 7.3.0] on linux
Type "help", "copyright", "credits" or "license" for more information.
>>> import tensorflow as tf
/home/vidiemme/anaconda3/envs/huggingface/lib/python3.6/site-packages/tensorboard/compat/tensorflow_stub/dtypes.py:541: FutureWarning: Passing (type, 1) or '1type' as a synonym of type is deprecated; in a future version of numpy, it will be understood as (type, (1,)) / '(1,)type'.
_np_qint8 = np.dtype([("qint8", np.int8, 1)])
/home/vidiemme/anaconda3/envs/huggingface/lib/python3.6/site-packages/tensorboard/compat/tensorflow_stub/dtypes.py:542: FutureWarning: Passing (type, 1) or '1type' as a synonym of type is deprecated; in a future version of numpy, it will be understood as (type, (1,)) / '(1,)type'.
_np_quint8 = np.dtype([("quint8", np.uint8, 1)])
/home/vidiemme/anaconda3/envs/huggingface/lib/python3.6/site-packages/tensorboard/compat/tensorflow_stub/dtypes.py:543: FutureWarning: Passing (type, 1) or '1type' as a synonym of type is deprecated; in a future version of numpy, it will be understood as (type, (1,)) / '(1,)type'.
_np_qint16 = np.dtype([("qint16", np.int16, 1)])
/home/vidiemme/anaconda3/envs/huggingface/lib/python3.6/site-packages/tensorboard/compat/tensorflow_stub/dtypes.py:544: FutureWarning: Passing (type, 1) or '1type' as a synonym of type is deprecated; in a future version of numpy, it will be understood as (type, (1,)) / '(1,)type'.
_np_quint16 = np.dtype([("quint16", np.uint16, 1)])
/home/vidiemme/anaconda3/envs/huggingface/lib/python3.6/site-packages/tensorboard/compat/tensorflow_stub/dtypes.py:545: FutureWarning: Passing (type, 1) or '1type' as a synonym of type is deprecated; in a future version of numpy, it will be understood as (type, (1,)) / '(1,)type'.
_np_qint32 = np.dtype([("qint32", np.int32, 1)])
/home/vidiemme/anaconda3/envs/huggingface/lib/python3.6/site-packages/tensorboard/compat/tensorflow_stub/dtypes.py:550: FutureWarning: Passing (type, 1) or '1type' as a synonym of type is deprecated; in a future version of numpy, it will be understood as (type, (1,)) / '(1,)type'.
np_resource = np.dtype([("resource", np.ubyte, 1)])
>>> import transformers
>>> from transformers import BertTokenizer
>>> from transformers import TFBertForTokenClassification
>>> tokenizer = BertTokenizer.from_pretrained('bert-base-uncased')
>>> model = TFBertForTokenClassification.from_pretrained('bert-base-uncased')
2019-12-17 12:54:37.120123: I tensorflow/core/platform/cpu_feature_guard.cc:142] Your CPU supports instructions that this TensorFlow binary was not compiled to use: AVX2 FMA
2019-12-17 12:54:37.320081: I tensorflow/core/platform/profile_utils/cpu_utils.cc:94] CPU Frequency: 3408000000 Hz
2019-12-17 12:54:37.320815: I tensorflow/compiler/xla/service/service.cc:168] XLA service 0x55a42296edd0 executing computations on platform Host. Devices:
2019-12-17 12:54:37.320841: I tensorflow/compiler/xla/service/service.cc:175] StreamExecutor device (0): Host, Default Version
>>> text='Hello, my dog is cute'
>>> input_ids = tf.constant(tokenizer.encode(text))[None, :] # Batch size 1
>>> input_ids
<tf.Tensor: id=6056, shape=(1, 8), dtype=int32, numpy=
array([[ 101, 7592, 1010, 2026, 3899, 2003, 10140, 102]],
dtype=int32)>
>>> outputs = model(input_ids)
>>> len(outputs)
1
>>> outputs
(<tf.Tensor: id=7961, shape=(1, 8, 2), dtype=float32, numpy=
array([[[ 0.09657212, -0.51087016],
[ 0.28020248, -0.25160134],
[-0.09995201, 0.0843759 ],
[-0.12110823, 0.20886022],
[-0.03617962, 0.00401567],
[-0.03330922, 0.01042 ],
[-0.21674895, -0.1601235 ],
[ 0.1076538 , 0.19144017]]], dtype=float32)>,)
>>> scores = outputs[0]
>>> scores
<tf.Tensor: id=7961, shape=(1, 8, 2), dtype=float32, numpy=
array([[[ 0.09657212, -0.51087016],
[ 0.28020248, -0.25160134],
[-0.09995201, 0.0843759 ],
[-0.12110823, 0.20886022],
[-0.03617962, 0.00401567],
[-0.03330922, 0.01042 ],
[-0.21674895, -0.1601235 ],
[ 0.1076538 , 0.19144017]]], dtype=float32)>
```
> So Token classification using BERT does not work?<|||||>This issue has been automatically marked as stale because it has not had recent activity. It will be closed if no further activity occurs. Thank you for your contributions.
<|||||>This issue has been automatically marked as stale because it has not had recent activity. It will be closed if no further activity occurs. Thank you for your contributions.
|
transformers | 883 | closed | Upgrade to new FP16 | The original FP16_Optimizer and the old “Amp” API are deprecated and subject to removal at any time. Should we consider moving to the new one?
https://nvidia.github.io/apex/amp.html#for-users-of-the-old-fp16-optimizer | 07-24-2019 10:13:11 | 07-24-2019 10:13:11 | Just saw run_glue has the new one. |
transformers | 882 | closed | fix squad v1 error (na_prob_file should be None) | When running squad v1, na_prob_file should be None.
Or there will be an error when evaluate on testing data. | 07-24-2019 08:12:44 | 07-24-2019 08:12:44 | # [Codecov](https://codecov.io/gh/huggingface/pytorch-transformers/pull/882?src=pr&el=h1) Report
> Merging [#882](https://codecov.io/gh/huggingface/pytorch-transformers/pull/882?src=pr&el=desc) into [master](https://codecov.io/gh/huggingface/pytorch-transformers/commit/067923d3267325f525f4e46f357360c191ba562e?src=pr&el=desc) will **not change** coverage.
> The diff coverage is `n/a`.
[](https://codecov.io/gh/huggingface/pytorch-transformers/pull/882?src=pr&el=tree)
```diff
@@ Coverage Diff @@
## master #882 +/- ##
=======================================
Coverage 79.03% 79.03%
=======================================
Files 34 34
Lines 6234 6234
=======================================
Hits 4927 4927
Misses 1307 1307
```
------
[Continue to review full report at Codecov](https://codecov.io/gh/huggingface/pytorch-transformers/pull/882?src=pr&el=continue).
> **Legend** - [Click here to learn more](https://docs.codecov.io/docs/codecov-delta)
> `Δ = absolute <relative> (impact)`, `ø = not affected`, `? = missing data`
> Powered by [Codecov](https://codecov.io/gh/huggingface/pytorch-transformers/pull/882?src=pr&el=footer). Last update [067923d...a7fce6d](https://codecov.io/gh/huggingface/pytorch-transformers/pull/882?src=pr&el=lastupdated). Read the [comment docs](https://docs.codecov.io/docs/pull-request-comments).
<|||||>Thanks indeed, this should fix #891 |
transformers | 881 | closed | can not convert_tf_checkpoint_to_pytorch | ```
.
├── convert_tf_checkpoint_to_pytorch.py
├── uncased_L-12_H-768_A-12
│ ├── bert_config.json
│ ├── bert_model.ckpt.data-00000-of-00001
│ ├── bert_model.ckpt.index
│ ├── bert_model.ckpt.meta
│ └── vocab.txt
├── uncased_L-12_H-768_A-12.zip
└── Untitled.ipynb
```
```
(base) ➜ ckpt_to_bin git:(master) ✗ python convert.py --tf_checkpoint_path=./uncased_L-12_H-768_A-12 --bert_config_file=./uncased_L-12_H-768_A-12/bert_config.json --pytorch_dump_path=./uncased_L-12_H-768_A-12
Building PyTorch model from configuration: {
"attention_probs_dropout_prob": 0.1,
"finetuning_task": null,
"hidden_act": "gelu",
"hidden_dropout_prob": 0.1,
"hidden_size": 768,
"initializer_range": 0.02,
"intermediate_size": 3072,
"layer_norm_eps": 1e-12,
"max_position_embeddings": 512,
"num_attention_heads": 12,
"num_hidden_layers": 12,
"num_labels": 2,
"output_attentions": false,
"output_hidden_states": false,
"torchscript": false,
"type_vocab_size": 2,
"vocab_size": 30522
}
INFO:pytorch_transformers.modeling_bert:Converting TensorFlow checkpoint from /home/zxr/summary/bertsum/src/ckpt_to_bin/uncased_L-12_H-768_A-12
Traceback (most recent call last):
File "convert.py", line 65, in <module>
args.pytorch_dump_path)
File "convert.py", line 36, in convert_tf_checkpoint_to_pytorch
load_tf_weights_in_bert(model, config, tf_checkpoint_path)
File "/home/zxr/anaconda3/lib/python3.7/site-packages/pytorch_transformers/modeling_bert.py", line 83, in load_tf_weights_in_bert
init_vars = tf.train.list_variables(tf_path)
File "/home/zxr/anaconda3/lib/python3.7/site-packages/tensorflow/python/training/checkpoint_utils.py", line 95, in list_variables
reader = load_checkpoint(ckpt_dir_or_file)
File "/home/zxr/anaconda3/lib/python3.7/site-packages/tensorflow/python/training/checkpoint_utils.py", line 63, in load_checkpoint
"given directory %s" % ckpt_dir_or_file)
ValueError: Couldn't find 'checkpoint' file or checkpoints in given directory /home/zxr/summary/bertsum/src/ckpt_to_bin/uncased_L-12_H-768_A-12
``` | 07-24-2019 07:35:32 | 07-24-2019 07:35:32 | ```
python convert.py --tf_checkpoint_path=./uncased_L-12_H-768_A-12/bert_model.ckpt --bert_config_file=./uncased_L-12_H-768_A-12/bert_config.json --pytorch_dump_path=./uncased_L-12_H-768_A-12/bert_model.bin
``` |
transformers | 880 | closed | Printing Iteration every example problem | ```
Iteration: 0%| | 1/250 [00:00<03:28, 1.19it/s]
Iteration: 1%| | 2/250 [00:01<03:21, 1.23it/s]
Iteration: 1%| | 3/250 [00:02<03:17, 1.25it/s]
Iteration: 2%|▏ | 4/250 [00:03<03:14, 1.27it/s]
Iteration: 2%|▏ | 5/250 [00:03<03:11, 1.28it/s]
Iteration: 2%|▏ | 6/250 [00:04<03:09, 1.29it/s]
Iteration: 3%|▎ | 7/250 [00:05<03:05, 1.31it/s]
```
I dont know what is the error that is causing this error , can somebody help ?
and this is the code
```
train_iterator = trange(int(num_train_epochs), desc="Epoch", disable= local_rank not in [-1, 0])
set_seed(42)
Epochs = 0
for _ in train_iterator:
epoch_iterator = tqdm(train_dataloader, desc="Iteration", disable= local_rank not in [-1, 0])
#print('Check')
Epochs = Epochs + 1
for step, batch in enumerate(epoch_iterator):
model.train()
```
| 07-24-2019 07:07:29 | 07-24-2019 07:07:29 | Are you running in jupyter? This might be an artifact of how `tqdm` is interacting with whatever shell you're running it in. If you don't want to print anything, you could simply drop the `tdqm` wrapper and just iterate over `train_dataloader`. <|||||>This issue has been automatically marked as stale because it has not had recent activity. It will be closed if no further activity occurs. Thank you for your contributions.
|
transformers | 879 | closed | fix #878 | 07-24-2019 07:04:57 | 07-24-2019 07:04:57 | # [Codecov](https://codecov.io/gh/huggingface/pytorch-transformers/pull/879?src=pr&el=h1) Report
> Merging [#879](https://codecov.io/gh/huggingface/pytorch-transformers/pull/879?src=pr&el=desc) into [master](https://codecov.io/gh/huggingface/pytorch-transformers/commit/067923d3267325f525f4e46f357360c191ba562e?src=pr&el=desc) will **decrease** coverage by `0.01%`.
> The diff coverage is `0%`.
[](https://codecov.io/gh/huggingface/pytorch-transformers/pull/879?src=pr&el=tree)
```diff
@@ Coverage Diff @@
## master #879 +/- ##
==========================================
- Coverage 79.03% 79.02% -0.02%
==========================================
Files 34 34
Lines 6234 6235 +1
==========================================
Hits 4927 4927
- Misses 1307 1308 +1
```
| [Impacted Files](https://codecov.io/gh/huggingface/pytorch-transformers/pull/879?src=pr&el=tree) | Coverage Δ | |
|---|---|---|
| [pytorch\_transformers/tokenization\_utils.py](https://codecov.io/gh/huggingface/pytorch-transformers/pull/879/diff?src=pr&el=tree#diff-cHl0b3JjaF90cmFuc2Zvcm1lcnMvdG9rZW5pemF0aW9uX3V0aWxzLnB5) | `86.17% <0%> (-0.36%)` | :arrow_down: |
------
[Continue to review full report at Codecov](https://codecov.io/gh/huggingface/pytorch-transformers/pull/879?src=pr&el=continue).
> **Legend** - [Click here to learn more](https://docs.codecov.io/docs/codecov-delta)
> `Δ = absolute <relative> (impact)`, `ø = not affected`, `? = missing data`
> Powered by [Codecov](https://codecov.io/gh/huggingface/pytorch-transformers/pull/879?src=pr&el=footer). Last update [067923d...31bc1dd](https://codecov.io/gh/huggingface/pytorch-transformers/pull/879?src=pr&el=lastupdated). Read the [comment docs](https://docs.codecov.io/docs/pull-request-comments).
<|||||>Closing this for now. Feel free to re-open if you want to continue with this PR. |
|
transformers | 878 | closed | Fail to load pre-trained tokens. | The PreTrainedTokenizer fails to load tokenizer files when I load tokenizer files from local tokenizer files.
The error is caused by code line 174 - 182 in [tokenization_utils.py](https://github.com/huggingface/pytorch-transformers/blob/master/pytorch_transformers/tokenization_utils.py). The code assumes that there are three tokenizer files: added_tokens.json, special_tokens_map.json, vocab.txt. However, these path of tokenizer files will be the same path if the given parameter "pretrained_model_name_or_path" is full path of "vocab.txt". | 07-24-2019 07:02:30 | 07-24-2019 07:02:30 | Hi, how do you solve this problem? If we set `pretrained_model_name_or_path` as a path to vocab.txt, it still need the two files: added_tokens.json, special_tokens_map.json. Where can we get these files? <|||||>> Hi, how do you solve this problem? If we set `pretrained_model_name_or_path` as a path to vocab.txt, it still need the two files: added_tokens.json, special_tokens_map.json. Where can we get these files?
You can ignore these files: added_tokens.json, special_tokens_map.json. All you need to do is to modify some code lines in the file: tokenization_utils.py. I have modified it in my forked repository as you can see [here](https://github.com/xijiz/pytorch-transformers/commit/31bc1ddf4f68ad790da9874a3623cf22d62dc186). <|||||>This issue has been automatically marked as stale because it has not had recent activity. It will be closed if no further activity occurs. Thank you for your contributions.
|
transformers | 877 | closed | error when tried to migrate from pretrained-bert to transformers. | The code used to be:
`logits = model(input_ids, segment_ids, input_mask, labels=None)
if OUTPUT_MODE == "classification":
loss_fct = CrossEntropyLoss()
loss = loss_fct(logits.view(-1, num_labels), label_ids.view(-1))
elif OUTPUT_MODE == "regression":
loss_fct = MSELoss()
loss = loss_fct(logits.view(-1), label_ids.view(-1))
if GRADIENT_ACCUMULATION_STEPS > 1:
loss = loss / GRADIENT_ACCUMULATION_STEPS
loss.backward()
print("\r%f" % loss, end='')
tr_loss += loss.item()
nb_tr_examples += input_ids.size(0)
nb_tr_steps += 1
if (step + 1) % GRADIENT_ACCUMULATION_STEPS == 0:
optimizer.step()
optimizer.zero_grad()
global_step += 1`
According to readme, I changed it into:
`output = model(input_ids,labels=num_labels)
loss, logits = output[:2]
if GRADIENT_ACCUMULATION_STEPS > 1:
loss = loss / GRADIENT_ACCUMULATION_STEPS
loss.backward()
print("\r%f" % loss, end='')
tr_loss += loss.item()
nb_tr_examples += input_ids.size(0)
nb_tr_steps += 1
if (step + 1) % GRADIENT_ACCUMULATION_STEPS == 0:
optimizer.step()
optimizer.zero_grad()
global_step += 1`
And the issue I had before was 'tuple' object has no attribute 'view'.
After the change, I'm having a similar issue that says:
`Traceback (most recent call last):
File "C:/Users/Youchen Miao/PycharmProjects/BERT_sent3/to_feature.py", line 150, in <module>
output = model(input_ids,labels=num_labels)
File "C:\Users\Youchen Miao\PycharmProjects\BERT_sent2\BERT_sent3\lib\site-packages\torch\nn\modules\module.py", line 493, in __call__
result = self.forward(*input, **kwargs)
File "C:\Users\Youchen Miao\PycharmProjects\BERT_sent2\BERT_sent3\lib\site-packages\pytorch_transformers\modeling_bert.py", line 985, in forward
loss = loss_fct(logits.view(-1, self.num_labels), labels.view(-1))
AttributeError: 'int' object has no attribute 'view'` | 07-23-2019 22:18:56 | 07-23-2019 22:18:56 | The `labels` input for the model is not the number of labels but the tensor of labels (see the docstrings and doc).<|||||>> The `labels` input for the model is not the number of labels but the tensor of labels (see the docstrings and doc).
Thank you for the answer. I'm trying to train the model to do polarity classification for google reviews, this is how the code that computes "logits" looked like before in pytorch-pretrained-bert:
`logits = model(input_ids, segment_ids, input_mask, labels=None)`
Do I do after the migration now:
`output = model(input_ids,labels=None)`
`loss, logits = output[:2]`
in order to match the similar behaviors? Thanks.
p.s. I'm new to the field, picked up the code from the street and trying to figure out how to make it work, I'm sorry if the question is dumb.<|||||>How was this solved? I have the same problem and for me :
output = model(input_ids,labels=None)
loss, logits = output[:2]
does not solve it |
transformers | 876 | closed | How to use BERT for finding similar sentences or similar news? | I have used BERT NextSentencePredictor to find similar sentences or similar news, However, It's super slow. Even on Tesla V100 which is the fastest GPU till now. It takes around 10secs for a query title with around 3,000 articles. Is there a way to use BERT better for finding similar sentences or similar news given a corpus of news articles? | 07-23-2019 22:16:01 | 07-23-2019 22:16:01 | Hi,
BERT out-of-the-box is not the best option for this task, as the run-time in your setup scales with the number of sentences in your corpus. I.e., if you have 10,000 sentences/articles in your corpus, you need to classify 10k pairs with BERT, which is rather slow.
A better option is to generate sentence embeddings: Every sentence / article is mapped to a fixed sized vector. You need to map your 3k articles only once to a vector.
A new query is then also mapped to a vector. In this setup, you only need to run BERT for one sentence (at inference), independent how large your corpus is.
Then, you can use cosine-similiarity, or manhatten / euclidean distance to find sentence embeddings that are closest = that are the most similar.
I released today a framework which uses pytorch-transformers for exactly that purpose:
https://github.com/UKPLab/sentence-transformers
I also uploaded an example for semantic search, where each sentence in a corpus is mapped to a vector and than cosine-similarity is used to find the most similar sentences / vectors:
https://github.com/UKPLab/sentence-transformers/blob/master/examples/application_semantic_search.py
Let me know if you have further questions.<|||||>I think you can use [faiss](https://github.com/facebookresearch/faiss) for storing and finding similar embeddings. <|||||>@nreimers Amazing!! Thank you so much. What you created is a real-life savior! Can this be used for finding similar news(given title and abstract)? I ran the code and I have the following doubts.
Which model should I use?
bert-large-nli-stsb-mean-tokens vs bert-base-nli-mean-tokens vs bert-large-nli-mean-tokens (what are the datasets on which all these models are trained on?)
Can I use [faiss](https://github.com/facebookresearch/faiss) to compute the search/distance of the vectors instead of L2/Manhattan/Cosine distances?
Many thanks to @stefan-it for introducing me to [faiss](https://github.com/facebookresearch/faiss).
<|||||>@nreimers I don't think scipy.spatial.distance.cdist is good enough, it takes a lot of time to compute the results, almost 10 minutes on a corpus of 3.9k news articles. I think I should try using [faiss](https://github.com/facebookresearch/faiss). I don't know anything about [faiss](https://github.com/facebookresearch/faiss) but I will try.<|||||>Hi @Raghavendra15,
regarding the model I sadly cannot be helpful, you would need to test them. In general, sentence embeddings methods (like Inference, Universal Sentence Encoder or my git) work well for short text, i.e., for sentences. For longer text with multiple sentences their performance often decrease and average word embeddings or tf-idf is in many case a much better choice. For longer texts, all these sentence embeddings methods are not really needed.
It would be great if you have some training data. Then, it would be quite easy to fine-tune a model specifically for your task. It should achieve much better performances than the pre-trained models.
I think the issue is not scipy.spatial.distance.cdist. On a corpus with 100k embeddings and 1024 embedding size, it requires about 0.2 seconds per query (if you can batch queries, even less time is needed).
I think the issue might be the generation of the 4k sentence embeddings? Transformer networks like BERT are extremely slow on CPUs. However, on a GPU, the implementation can process about 2000 sentences per seconds. On a GPU, only about 40 sentences.
But the corpus must only be processed once and can then be stored & loaded from disk. At inference, you just need to generate one embedding for the respective query.
You can of course combine this with faiss. Faiss generates index structures that allow a quick search in vector space and is especially suitable if you have a high number (millions) of vectors. For 4k vectors, scipy takes about 0.008 seconds per queries to find the most similar vectors.
So either something is really strange with scipy on your computer, or the long run-time comes from the generation of the embeddings.<|||||>@nreimers Thank you very much for your response. You're absolutely right, most of the time taken is for generating the embedding for 4k sentences. I'm now confused between choosing this model over [XLNet](https://github.com/zihangdai/xlnet), XLNet has achieved the state of the art results.
By your comments on faiss, As long as I have a smaller dataset, results from faiss and scipy won't make any difference? However, If I had millions or billions of news articles then using faiss makes sense right? For smaller datasets, there is no difference in terms of quality of matches between faiss and scipy(the results are the same for computing the distances)?
I have one important question, If I want to train the model as you suggested which would yield better results, In that case, I should have labeled dataset right? However, for news, I only have titles and abstract about that news. Is there a way to train them without the labels? <|||||>Hi,
XLNet achieved state-of-the-art performance for supervised tasks like classification. But it is unclear if it generates also good embeddings for unsupervised tasks.
In the framework you can choose XLNet, but I was only able to produce results that are slightly below those of BERT.
Others also have problems getting a good performance with Xlnet for supervised tasks, as it appears that it is extremely sensitive to the hyper parameters.
If you have millions of docs, faiss makes sense. With scipy, you get exact scores. With faiss, the scores are fuzzy and the returned most similar vectors must not necessarily be the actual most similar vectors. There can be small variations. But I think the difference will be small.
Often you have in your data some structure, like categories or links between news articles. This structure can be used to fine-tune a model. Let's say you have links linking to similar events. Than you train the network with triplet loss with the two linked articles and one random other article as negative example.
This will give you a vector space where (possibly) linked articles are close. <|||||>@nreimers Thank you very much for your quick response.
Are the existing model "bert-large-nli-stsb-mean-tokens" better than the google news word2vec [google_news_300](https://drive.google.com/file/d/0B7XkCwpI5KDYNlNUTTlSS21pQmM/edit?usp=sharing), they claim that-" We are publishing pre-trained vectors trained on part of Google News dataset (about 100 billion words). The model contains 300-dimensional vectors for 3 million words and phrases."
Is the pretrained "bert-large-nli-stsb-mean-tokens" better than google's pre-trained news vectors?
For training the existing model to improve results for news similarity, the problem I have is I can't create a dataset to compute triplet loss. For triplet loss to work in the case of news similarity for query news ['**a**'], I need to find a news article ['**b**'] which is similar as a positive example and a dissimilar news article ['**c**'] as a negative example. Like <a,b> positive example and <a,c> negative example.
However, If I run the news every day then, new entities/topics are going to pop up every single day? I need to update my embeddings right? I don't know how to handle this situation.
<|||||>Google News vectors are just word vectors, you still need a strategy to derive sentence embeddings from these. But as mentioned earlier, average word embeddings is a promising idea for your task. Note, average word embeddings models will be added soon to the repository.
Constantly updating of the model is not needed. News are changing, but the used words remain the same. So training once should give you a model that can be used for a long time. <|||||>@nreimers Thank you very much! Any tentative date by when the average word embeddings will be added to the repository?
I want to know how to evaluate the results of similar sentences numerically, for example when I use your model to evaluate for a given news, finding similar news in the corpus.
Is there a way to measure numerically how good the similar sentences are in the below example? I used BLEU score, but the problem is, it's not an accurate measure of similarity. BLEU score doesn't consider the context of the sentence, it just blindly counts whether a word in the query sentence is present in the similar sentence regardless of where the word is placed.
For an item, I get related items.
In the below example, the first title in relatedItems is similar, however, the second item in "relatedItems" is not at all similar which talks about Stephen Colbert and Joe Biden.
Suppose I use word2vec model for the above task it might give me two totally different sentences as relatedItems, In that case, how can I evaluate both the models and claim numerically which one is better?
Example:
{"title": "Google Is Rolling Out A New Version Of Android Auto - Here's What You Can Expect",
"abstract": "The new Android Auto. Google If you use Android Auto, you're about to receive to a nice upgrade.",
}
"relatedItems":
[{
"title": "New Android ransomware is spreading through text messages",
"abstract": "There\u2019s a new type of Android ransomware making the rounds that leverages SMS to spread, according to a new report from cyberappsecurity com",
},
{
"title": "Stephen Colbert Brings Curtain Down On Democratic Debates With Joe Biden Tweaks",
"abstract": "Stephen Colbert closed his second of two live Late Show monologues with a spree of zingers directed at Joe Biden, mixing in plenty for the o",
}
]}
<|||||>Bleu wouldn't be a good measure, because the best similarity metric to find similar news would be: Bleu (of course).
What you would need is an annotated Corpus. For a given article, get for example the 20 articles with the highest tf idf similarity. Then annotate every pair as similar or not.
With this data you can compare different methods with Ndcg about how well they rank the 20 candidate articles.
Avg. Word embeddings should be included within the next two weeks to the repo. <|||||>@nreimers When you say -"Bleu wouldn't be a good measure, because the best similarity metric to find similar news would be: Bleu (of course)."
Do you mean when I get similar news like in the above example, BLEU is the best metric to measure how similar the two news articles are? Please correct me if I understood this wrong.
In the STS benchmark, I saw a pair in the training dataset with gold-standard human evaluated scores. The following paid had a score of 5, however, when I use BLEU scores for 1gram they don't get a score of 1. Instead, they get the following scores. BLEU looks for the exact word to be present in the reference sentence that's the problem I feel. There's no notion of similarity.
s=word_tokenize("The polar bear is sliding on the snow")
reference = [s]
candidate =word_tokenize("The polar bear is sliding across the snow")
print('Individual 1-gram: %f' % sentence_bleu(reference, candidate, weights=(1, 0, 0, 0)))
print('Individual 2-gram: %f' % sentence_bleu(reference, candidate, weights=(0, 1, 0, 0)))
print('Individual 3-gram: %f' % sentence_bleu(reference, candidate, weights=(0, 0, 1, 0)))
print('Individual 4-gram: %f' % sentence_bleu(reference, candidate, weights=(0, 0, 0, 1)))
Individual 1-gram: 0.875000
Individual 2-gram: 0.714286
Individual 3-gram: 0.500000
Individual 4-gram: 0.400000
reference sentence has 8 words out of which the candidate matches exactly 7 words, so 7/8 score for 1-gram matches.
I'm not sure how the STS benchmarks are evaluated, I'm currently looking into them. If you have any leads or a document I would be more than happy to read them.
Thank you very much for your help :)
<|||||>No, BLEU is a terrible idea for evaluation.
STS is usually evaluated using Pearson correlation between gold and predicted labels. But Pearson correlation is also a bad idea:
https://aclweb.org/anthology/C16-1009
I strongly recommend to use Spearman correlation for comparison. <|||||>@nreimers Kudos on the COLING paper! It's very well written. In the paper, you have mentioned How Pearson correlation can be misleading or ill-suited for the semantic text-similarity task. However, you did not suggest to use Spearman correlation instead of Pearson correlation. But for me, you suggested me to use Spearman correlation why? (That's my current understanding of the paper)
Can I use the Spearman rank correlation from scipy?
Basically, I want to compare the BERT output sentences from your model and output from word2vec to see which one gives better output.
So there is a reference sentence and I get a bunch of similar sentences as I mentioned in the previous example [ please refer to the JSON output in the previous comments].
Will the below code is the right way to do the comparison?
In your sentence transformer, you have used the same below package in SentenceEvaluator class. I couldn't figure out how to use that class for my comparison.
Will you please give me some idea in this regard?
Example code:
from scipy.stats import spearmanr
x = [1, 2, 3] ---> I will use BERT and word2vec embeddings here.
x_corr = [2, 4, 6]
corr, p_value = spearmanr(x, x_corr)
print (corr)
<|||||>Hi @Raghavendra15
The issue with pearson correlation is, that it assumes a linear correlation between the system output and gold labels. Adding a montone function to the system output can change the scores (make them better or worse), which does not really make sense in applications.
Assume you have a systems that predicts the perfect gold scores, however, the output is output=sqrt(gold_label).
This system would get a really low Pearson correlation. However, for every application, this system would be perfect, as it predicts the gold labels. With Spearman correlation, you don't have this issue. There, just the ranking of the scores are important.
In general I think the STS tasks (or the STS benchmark) are not really well suited to evaluated approaches. The STS tasks with Pearson/Spearman correlation weights every score similar, but in applications, we are often only interested in certain examples.
For example, if we search for pairs with the highest similarity, then we don't care how the scores are for low similarity pairs. A system that gives a perfect score for high similarity pairs and a random score for low similarity pairs would be great for this application. However, this system would get a low Pearson/Spearman correlation, as it fails to correctly order the somewhat-similar and unsimilar pairs.
If you want so estimate the similarity of two vectors, you should use cosine-similarity or Manhatten/Euclidean distance.
Spearman correlation is only used for the comparison to gold scores.
Assume you have the pairs:
x_1, y_1
x_2, y_2
...
for every (x_i, y_i) you have a score s_i from 0 ... 1 indicating a gold label score for their similarity.
You can check how good the embeddings are by computing the cosine similarity between the embeddings for (x_i, y_i) and then you compute the Spearman correlation between these computes cosine similarity scores and the gold score s_i.
Note: Currently I add methods to compute average word embeddings and similar methods to the repository. So a comparison will become easier.<|||||>@nreimers Last week you added the methods to compute average word embeddings should I use that method when I get a sentence embedding or will there be a pre-trained average word embedding weights?
In the below code I will get the embeddings once I pass the input strings. Should I use the compute avg word embedding method on top of this?
corpus = ['A man is eating a food.',
'A man is eating a piece of bread.' ]
corpus_embeddings = embedder.encode(corpus)
or
By any chance, pre-trained avg-word embedding weights will be uploaded to the repository by any time this week. <|||||>Hi @Raghavendra15
I just uploaded v0.2.0 to github and PyPi:
https://github.com/UKPLab/sentence-transformers
You can update with pip install -U sentence-transformers
I added an example for average word embeddings (+a DAN layer that is trainable):
https://github.com/UKPLab/sentence-transformers/blob/master/examples/training_stsbenchmark_avg_word_embeddings.py
You can also use it without the DAN layer. There is also a tokenizer implemented that allows the usage of the word2vec Google News vectors. These vectors contain phrases like 'New_York'. These phrases are detected by the tokenizer and mapped to the correct embedding for New_York. But there is currently no example for this in the repo. If you need help, let me know.
To get avg. word embeddings only (without DAN), the code must look like this:
```
# Map tokens to traditional word embeddings like GloVe
word_embedding_model = models.WordEmbeddings.from_text_file('glove.6B.300d.txt.gz')
# Apply mean pooling to get one fixed sized sentence vector
pooling_model = models.Pooling(word_embedding_model.get_word_embedding_dimension(),
pooling_mode_mean_tokens=True,
pooling_mode_cls_token=False,
pooling_mode_max_tokens=False)
model = SentenceTransformer(modules=[word_embedding_model, pooling_model])
corpus_embeddings = model.encode(corpus)
```
Next release will update include support for RoBERTa and add other sentence embeddings methods (like USE, LASER), which will be trainable.<|||||>@nreimers Thank you very much! You spoke my mind with RoBERTa, I was about to ask you about it. But with the avg-embedding approach, I won't be using BERT at all right?
In addition to that, I won't be training the model. I don't think I fully understand this. Earlier I would pass a pretrained weight model into SentenceTransformer, however now I won't pass anything related to BERT, does that mean I won't be using BERT?<|||||>@Raghavendra15
The framework offers you a lot of flexibility. You can choose between the following embedding approaches:
- BERT or XLNet (RoBERTa and other will follow)
- Traditional word embeddings like GloVe, word2vec etc.
Then, you can choose between different pooling modes: Mean pooling, max pooling, usage of the CLS token for BERT / XLNet.
Finally, if you like, you can add feed-forward networks to create a deep-averaging network.
If you have training data, I can recommend this combination:
BERT + mean-pooling
This gave the best performance for many cases.
If you have training data, you need a low computation time and performance is not that important, choose this combination:
GloVe embeddings (or something similar) + mean-pooling + 1 or 2 dense layers
If you don't have training data, choose:
GloVe embeddings (or something similar) + mean-pooling
As you can see, there are various options you can choose from, depending if you have training data and how important is a high speed vs. a good performance.
Once I have RoBERTa integrated, how suitable it is for the generation of sentence embeddings. My experiences with XLNet was that the performance is slightly below the performance of BERT for sentence embeddings. Maybe RoBERTa is better for sentence embeddings, maybe not.
Averaging BERT without fine-tuning on data gave really poor results. However, what you can of course try, is to use one of the existent pretrained BERT models like 'bert-base-nli-mean-tokens', which is BERT+mean-pooling, fine-tuned on NLI data to generate meaningful sentence embeddings.
<|||||>@nreimers Thank you very much! Why didn't you choose (word2vec) Google news vectors? Is there any particular reason for choosing Glove embedding over word2vec? I'm curious to know how RoBERTa will perform! 😃<|||||>@Raghavendra15
There are two reasons:
1) Google news word2vec is quite large, it requires about 12 GB of RAM to read it in. Not that ideal for an example script. GloVe embeddings are about 10 times smaller.
2) In most of my experiments, the Google news word2vec vectors did not yield good performances. GloVe embeddings were often a bit better. I especially like the embeddings by Levy et al (trained on dependencies) and by Komninos. I also conducted a larger comparison between word embeddings (https://arxiv.org/abs/1707.06799, Table 5).
But note, using the Google news word2vec vectors is quite easy. In training_stsbenchmark_avg_word_embeddings.py replace
```
word_embedding_model = models.WordEmbeddings.from_text_file('glove.6B.300d.txt.gz')
```
with
```
word_embedding_model = models.WordEmbeddings.from_text_file('GoogleNews-vectors-negative300.txt.gz')
```
First experiments with RoBERTa are done: On STSbenchmark, it increases the Spearman correlation by about 1 - 2 percentage points. I will see how it will perform on other datasets.
Best, Nils Reimers<|||||>This issue is very interesting, thanks for sharing your experiments and framework @nreimers!<|||||>@nreimers I read your paper on word embedding comparison, however, when I saw the GLEU scoreboard for STS benchmark Glove scored very less compared to word2vec, Isn't it contradictory to your paper? Also in your paper, the comparisons are on a certain set of tasks like Entity Recognition, NER but not on Semantic Textual Similarity. I don't know much about it, I'm trying to learn. Do my questions make sense?
Is there any significant difference between using glove.840B.300d.zip (contains 840 billion words vectors trained on the common crawl ) vs glove.6B.300d.txt.gz (contains 6 billion words vectors wikipedia+Gigaword), Is it like more words the better? also, they're trained on different datasets, will that make a huge difference when applied to news similarity?<|||||>See the GloVe website / paper for the differences. 6B was trained on 6 billion words from Wikipedia, 840B was trained on 840 Billion words from common crawl.
It depends on the task and data which one is more suitable. If you have a lot of rare words, and those play an important role for your task, 840B is often better. If you have clean data / only common words are important for your task, 6B often works better.
However, the differences are often only minor between the two versions.
In my paper I only compare embeddings for supervised task, only for sequence tagging.
In unsupervised tasks, you can get completely different results. Further, how word embeddings are averaged has a big impact. Some authors don't ignore stop words, instead they propose some complicated weighting scheme. If stop words are ignored, performances can be improved up tp 10 percentage points, sometimes outperforming complex weighting approaches.
Best,
Nils Reimers <|||||>Thank you for your work, Nils, it is brillant!
I would like to design a sentence level semantic search engine using email data (Enron dataset).
I am still a little bit confused about how I should be fine-tuning models on such dataset (maybe I am missing something obvious).
Thanks.
Gogan
<|||||>@ggndtes In general BM25 will be really hard to beat on this type of task. See this paper where they compare sentence embeddings with BM25 on an end-to-end retrieval task (given: question, find similar / duplicate questions in a large corpus):
https://arxiv.org/pdf/1811.08008.pdf
A complex sentence embedding method only achieves 1 - 2 percentage points improvement against BM25 (Table 2, Dual Encoder Paralex vs. Okapi BM 25).
Especially if you have more than just a sentence, carefully constructed BM25 for example with Elasticsearch is really really hard to beat. If you are interested in a production system, I would highly recommend to first try Elasticsearch (or similar), beating it will be difficult.
Back to your question how you can tune it:
The big question narrows down to: What are your queries, what are your documents. Are your documents complete emails? Or only email subjects? Or only sentences within emails?
Are your queries inputs from the user, email subjects or complete emails?
In general you would need to construct same sort of similarity. Currently I can only think of imperfect method to create similarity labels. One option would be: Triplet loss with 2 emails from the same inbox vs. one random other subject. But this would I think create rather bad embeddings.
Currently I can't think of a good method to create similarity labels for that dataset. And as mention, even with perfect labels, it will be really hard to beat BM25.
Best,
-Nils Reimers
<|||||>@nreimers The sentence encoder actually takes quite a lot of time to load the Glove embeddings, Is there a way where I can make it load from the disk or make it faster?<|||||>@Raghavendra15 When you run the code the first time, the embeddings are downloaded and stored in the path of the script. In follow-up executions, the embeddings file is loaded from disk.
GloVe embeddings are quite large, so loading it can take some time.
There are two ways to speed it up:
1) Limit the vocab size, i.e., don't load all the ~400k embeddings. Pass the parameter 'max_vocab_size' to the method 'from_text_file' when called.
2) Save the WordEmbeddings model to disc. In follow-up executions, you can load the (binary) model directly from disc and you don't have to read in and parse in the text file.
Should work something like this:
```
word_model = WordEmbeddings.from_text_file('my-glove-file.txt')
word_model.save('my/output/folder/GloveWordModel')
# In follow-up calls, should be faster
word_model = WordEmbeddings.load('my/output/folder/GloveWordModel')
```<|||||>@nreimers Wow!! It works blazingly fast!
I was trying to play with the below code. Thank you very much for the help :)
Code in In WordEmbeddings.py file:
```
with gzip.open(embeddings_file_path, "rt", encoding="utf8") if embeddings_file_path.endswith('.gz') else open(embeddings_file_path, encoding="utf8") as fIn:
iterator = tqdm(fIn, desc="Load Word Embeddings", unit="Embeddings")
for line in iterator:
```
Also, can I load the model similar to that for BERT pre-trained weights? such as the below code?
`embedder = SentenceTransformer('bert-large-nli-stsb-mean-tokens')`
Can I load the above pre-trained weights somehow just like you have `load` method for glove weights?
Is the avg embedding with Glove better than "bert-large-nli-stsb-mean-tokens" the BERT pre-trained model you have loaded in the repository? How's RoBERTa doing? Your work is amazing! Thank you so much again!
<|||||>@Raghavendra15 Sure you can:
```
word_embedding_model = models.WordEmbeddings.from_text_file('glove.6B.300d.txt.gz')
# Apply mean pooling to get one fixed sized sentence vector
pooling_model = models.Pooling(word_embedding_model.get_word_embedding_dimension(),
pooling_mode_mean_tokens=True,
pooling_mode_cls_token=False,
pooling_mode_max_tokens=False)
model = SentenceTransformer(modules=[word_embedding_model, pooling_model])
model.save('my/output/folder/avg-glove-embeddings')
# Load the Model:
model = SentenceTransformer('my/output/folder/avg-glove-embeddings')
```
Which model is better depends extremely on your data and on your task. The BERT models work good if you have clean data, which is not too domain specific and rather descriptive. This is due to the nature on which data it was fine-tuned (on NLI dataset).
Average GloVe embeddings works I think better if you have noisy data, really domain specific data or very short sentences or very large paragraphs.
Experiments with RoBERTa are finished. Paper will be uploaded next week to arxiv. In my experiments, I could not observe a major difference between BERT and RoBERTa for sentence embeddings: Sometimes BERT is a little bit better, sometimes RoBERTa. But nothing that is significant. XLNet was so far in general worse than BERT.
Best
-Nils Reimers<|||||>@nreimers Thanks! but my question is how can make the pretrained BERT model faster like loading the below model.
`embedder = SentenceTransformer('bert-large-nli-stsb-mean-tokens')
`
When I run the encoder for BERT it takes a lot of time like 10-15 minutes for 4k sentences.
`embedder.encode(corpus)` ---> This takes around 10minutes for "bert-large-nli-stsb-mean-tokens"
However, Glove model does the job in 30 secs. bert-large-nli-stsb-mean-tokens is similar to glove pretrained word vectors right? Then is there a way to convert speed up the BERT sentence encoder?<|||||>@Raghavendra15 No, the BERT model and average GloVe embeddings are completely different.
GloVe embeddings have one vector for each word in a language, for example, the word 'apple' is mapped to the vector 0.31 0.42 0.15 ....
To compute avg. GloVe embeddings, you just perform some memory lookup: Every word is mapped to the vector and then you compute the mean values.
BERT (https://arxiv.org/abs/1810.04805) is much more complex: Words in a sentence are first broken down to subwords, which are than mapped to vectors (which is the fast part).
But after that, a transformer network is run over the complete sentence: For BERT-base it has 12 layers, for BERT-large, it has 24 layers. This produces vectors for each word which depend on the context of the complete sentence.
If you have the two sentences:
- Apple is a healthy fruit
- Apple presented their new iPhone
With GloVe, Apple are mapped in both cases to the same vector. With BERT, the two Apple words are mapped to different embeddings. In the first case, it is mapped closer to words like Banana, Mango etc., in the second sentence, it is mapped closer to words like Microsoft, Google etc.
But this comes with a cost: Transformer networks are rather slow. This is especially true if you have only a CPU or an older GPU.
On a CPU, you can process with BERT about 80 sentences / second (with GloVe, more than 5k). On a Nividia V100 GPU, the speed is a bit better: About 2000 sentences / second (BERT-base).
The runtime for transformer networks is quadratic with the sentence length. If your sentence is twice as long, the runtime increases 4x.
So the only ways to speed-up the BERT model:
- Try to figure out what the optimal batch size is for your system (you can pass the batch size as a parameter to encode())
- Use the base, not the large model. The large model is multiple times slower than the base model.
- Be careful with your sentences lengths. Maybe truncate your sentences
- Get a better / faster GPU (or multiple GPUs). Running BERT on CPU is horrible.
I hope this of some help for you.
Best regards
-Nils Reimers
<|||||>This is an outstanding explanation Nils – you should blog or tweet, I'm sure lots of people would be interested in reading more from you!<|||||>@nreimers Brilliant explanation! :D You're a life saviour :-)
I need your help with this issue. Can I use sentence transformer for this case?
https://github.com/huggingface/pytorch-transformers/issues/1170<|||||>@nreimers Very patient brilliant explanation. Wish u a happy life.<|||||>@nreimers
Let's say you have a sufficient training set for information retrieval, such as that from fever.ai.
We used black-box Bayesian Optimization to train BM25 on Elasticsearch... producing close to the results described in the SOTA evidence retrieval from the UKP-Athene team, but were still a few % off SOTA, without entity extraction or any other ML preprocessing.
Shouldn't it be the case that a well trained encoder transformer with cosine-loss, with specific weights for a query and document / sentence in the result set, should be able to beat an arbitrary algorithm like BM25?
And that it could be deployed at scale using faiss or hsnw?<|||||>Hi @pertschuk
If the recall of BM25 is quite good, I would aim for re-ranking instead of a full semantic search.
In re-ranking, you retrieve e.g. 100 documents with your BM25 algorithm. Then, you run BERT to compare each document with your query to get one score (0...1).
Next, you sort these scores.
Your original ranking from BM25 is then replaced with these BERT-based scores ranking.
Sentence embeddings often have challenges in information retrieval as the false positive probability is higher than BM25. I.e., if you compare two dissimilar sentences with sentence embeddings, the probability of getting a high similarity score is higher for approaches like Sentence-BERT / InferSent / USE, than it is for BM25.
In Information Retrieval, you usually have a large set of unrelated docs, i.e., this higher false positive rate leads to really bad consequences that you find many unrelated documents, leading to a performance usually lower than BM25.
The re-ranking approach prevents this to happen: BM25 gives you a rather clean candidate set, and your neural re-ranking approach can then do the hard work and determine which of the n documents matches the query the best.
Best regards
Nils Reimers<|||||>Great thank you, this makes sense.
We are currently using reranking on top 9 documents, but maybe could increase this number since our reranking recall is quite high ~.95 based on a RoBERTA regression model.
link: https://github.com/koursaros-ai/koursaros/blob/master/examples/pipelines/factchecking/services/scorer/__main__.py
I guess then the challenge becomes the scale of re-ranking, because there would be ~700 sentences to rerank with this larger set, and we can maybe run 100/s on SoTA transformer.
I wrote a FEVER dataset loader and am currently training a sentence reranking model based on your cosine loss, I am hoping to achieve the greater performance afforded by precomputing embeddings and running KNN to rerank, I will publish results here when I have them. <|||||>Yes, larger candidate sets can actually be quite interesting.
What you can also try is the faster, destilled BERT from hugging face. It achieves similar results like BERT, but is faster.
Sometimes, a larger set with worse (but cheaper) models achieves better overall results than a small set with a better (but expensive) model.
Best
-Nils Reimers<|||||>> Hi,
> BERT out-of-the-box is not the best option for this task, as the run-time in your setup scales with the number of sentences in your corpus. I.e., if you have 10,000 sentences/articles in your corpus, you need to classify 10k pairs with BERT, which is rather slow.
>
> A better option is to generate sentence embeddings: Every sentence / article is mapped to a fixed sized vector. You need to map your 3k articles only once to a vector.
>
> A new query is then also mapped to a vector. In this setup, you only need to run BERT for one sentence (at inference), independent how large your corpus is.
>
> Then, you can use cosine-similiarity, or manhatten / euclidean distance to find sentence embeddings that are closest = that are the most similar.
>
> I released today a framework which uses pytorch-transformers for exactly that purpose:
> https://github.com/UKPLab/sentence-transformers
>
> I also uploaded an example for semantic search, where each sentence in a corpus is mapped to a vector and than cosine-similarity is used to find the most similar sentences / vectors:
> https://github.com/UKPLab/sentence-transformers/blob/master/examples/application_semantic_search.py
>
> Let me know if you have further questions.
Hi, Can t
> Hi,
> BERT out-of-the-box is not the best option for this task, as the run-time in your setup scales with the number of sentences in your corpus. I.e., if you have 10,000 sentences/articles in your corpus, you need to classify 10k pairs with BERT, which is rather slow.
>
> A better option is to generate sentence embeddings: Every sentence / article is mapped to a fixed sized vector. You need to map your 3k articles only once to a vector.
>
> A new query is then also mapped to a vector. In this setup, you only need to run BERT for one sentence (at inference), independent how large your corpus is.
>
> Then, you can use cosine-similiarity, or manhatten / euclidean distance to find sentence embeddings that are closest = that are the most similar.
>
> I released today a framework which uses pytorch-transformers for exactly that purpose:
> https://github.com/UKPLab/sentence-transformers
>
> I also uploaded an example for semantic search, where each sentence in a corpus is mapped to a vector and than cosine-similarity is used to find the most similar sentences / vectors:
> https://github.com/UKPLab/sentence-transformers/blob/master/examples/application_semantic_search.py
>
> Let me know if you have further questions.
Can this use GPUs, if so how ?<|||||>Hi @duttsh,
Yes, GPU is supported out of the box. You just need the necessary Cuda drivers and then you can train / perform inference on the GPU without any changes
Best regards
Nils Reimers <|||||>Thanks Nils
Sent from my iPhone
> On Oct 6, 2019, at 1:43 AM, Nils Reimers <[email protected]> wrote:
>
> Hi @duttsh,
> Yes, GPU is supported out of the box. You just need the necessary Cuda drivers and then you can train / perform inference on the GPU without any changes
>
> Best regards
> Nils Reimers
>
> —
> You are receiving this because you were mentioned.
> Reply to this email directly, view it on GitHub, or mute the thread.
<|||||>@nreimers (Nils) one more question, when will you have pre-trained models of RoBERTa available ? or if they are please send me the name.<|||||>@duttsh I can try to upload it, but in my experimemts I didn't see any improvements from Roberta for sentence embeddings.
Best regards
Nils Reimers <|||||>Thanks, can you please upload. Also I believe Roberta will increase the accuracy of inference. Right ?
Sent from my iPhone
> On Oct 6, 2019, at 7:18 PM, Nils Reimers <[email protected]> wrote:
>
> @duttsh I can try to upload it, but in my experimemts I didn't see any improvements from Roberta for sentence embeddings.
>
> Best regards
> Nils Reimers
>
> —
> You are receiving this because you were mentioned.
> Reply to this email directly, view it on GitHub, or mute the thread.
<|||||>@duttsh In my experiment, I didn't observe any differences between BERT and RoBERTa when used for different sentence embeddings tasks. <|||||>@nreimers thanks. If you could share he name if your RoBERTa model, would be great.<|||||>After a couple months of research, the best approach I've found for building semantic search is to integrate with an existing BM25 search platform such as Elasticsearch, and then **rerank** the top n results using a neural network regression trained to score a <query> <passage> combination, on a dataset such as [MS MARCO.](http://www.msmarco.org/)
Per @nreimers comment, something like BM25 produces a cleaner result set, and training a model to look at query passage pairs at the same time rather than training a cosine loss and comparing precomputed vectors enables it to use attention to more accurately rank passages.
Check out this project that implements such a system: https://github.com/koursaros-ai/nboost<|||||>Took me a long time to reply but thanks so much @nreimers for your incredibly clear explanations and responses.
Thanks also to @pertschuk for sharing the results of your research, this is very helpful.<|||||>Hi,
I'm looking into the question of finding prior art for patents.
This means for one patent application (around 20pages) we would like to find the closest 100 patents in a corpus of 100 million patents. The search results of patent offices could be used as training material.
We thought about tf-idf, word2vec, GloVe etc. So far transformers like BERT seemed to be too slow for such a task.
Now with SBERT and SRoBERTa and powerful AI accelerators, we ask ourselves, if we shouldn't be so quick to exclude transformers.
Any advice? Has anyone applied SBERT to such an amount of data? Anyone using AI accelerators such as Jetson?
<|||||>@wolf-tag
The two biggest issues with my research into building a transformer cosine loss solution based on SBert at scale (I was working with ~6million wikipedia articles, much smaller than all of the patents).
1. Evaluating the solution. To rebuild all of the 6 million vectors and put them into a FAISS index takes like (200/s to encode) takes like 6-8 hours, and then more time to actually query your test set and calculate something like MRR. Building a good model often requires **dozens** of evals, tweaking, etc.
2. Memory usage. There are various compression methods, but currently vector indexes are pretty memory-hungry. https://github.com/facebookresearch/faiss/wiki/Indexing-1G-vectors. This has possible solutions. It seems like probably to make this scalable, you would need much smaller embeddings than like 1024 for BERT large.
If you are well funded and have lots of GPU/ TPU and memory, it's feasible, and I would look at Patent-BERT, and incorporate that into sentence transformers.
One final thought to keep in mind - I have found that almost everything out there, patents included, have summaries (abstracts). At an even more micro scale, humans often tend to summarize a paragraph with the first sentence. You can leverage this to optimize your solutions, by choosing to look at the summary text instead of **all** of it.<|||||>Thank you for your quick reply.
Did I get it right: you used one vector for each wikipedia article which is the result of SBERT's pooling operation?
<|||||>Hi @wolf-tag
Personally I think tf-idf / BM25 is the best strategy for your task, due to various reasons.
**First**, it is important to differentiate between false positive and false negative rates:
False positive: A non-similar pair of docs is judged as similar, even they are not similar.
False negative: A similar pair of docs is judged as dissimilar.
TF-IDF/BM25 has a low false positive rate and a high false negative rate, i.e., if a pair is judged as similar, there is a high chance that they are actually similar.
Sentence embeddings methods (avg. GloVe embeddings, InferSent, USE, SBERT etc.) have a reverse characteristic: high false positive rates, low false negative rates. It seldom misses a similar pair, but a pair judged as similar must not necessarily be similar.
For Information Retrieval, you have an extrem imbalance. You have 1 search query and 100 Mio. documents, i.e., you perform 100 Mio pairwise comparisons.
Sentence embeddings with a high false positive rate will return many pairs where the embeddings think they are similar, but they are not. Your result set of 10 documents will be often completely garbage.
TF-IDF / BM25 might miss some relevant documents, but the 10 document you will find will be of high quality.
**Second**, in my experience, sentence embeddings methods work best for sentences. For (longer) documents, the results are often not that great. Here, word overlap (with tf-idf / BM25) is really hard to beat.
**Third**: In our experiments in Question Answering (given a question, find in Millions of answers on StackOverflow the correct one), TF-IDF / BM25 is extremely hard to beat. It often performs much better than sentence embeddings methods + it is much quicker.
So far our experiments with end-to-end representation learning for information retrieval rather failed.
What works quite good is a re-ranking approach: You use BM25 to retrieve the top 100 documents. Than, you take a neural approach like BERT to re-rank these 100 results and you present the top-10 results (the 10 results with the highest score according to the neural re-ranker) to the user. This often gives a nice boost to pure BM25 ranking, and the runtime is not too-bad, as you must only re-rank 100 documents.
Best regards
Nils Reimers <|||||>Dear Nils,
thank you for your detailed explanation.
Indeed, recent publications for the prior art task do hardly show any improvements when using word2vec, GloVe or doc2vec compared to tf-idf.
I was just curious as Google now uses BERT for the search engine, and I suppose they are more interested in high precision than in high recall, so somehow they seem to master the high false positive rates. Maybe they do so as recommended by you (re-ranking).
I hoped that one of the newer methods would somehow have a positive impact on this task. Just wishful thinking, I fear.<|||||>Hi @wolf-tag
an interesting paper could be this:
https://arxiv.org/abs/1811.08008
In Table 2 you see, that BM25 outperforms untrained sentence embeddings methods like avg. word2vec. If you have a lot of training data, you can tune the dual encoder so that it performs better than BM25 for the tested task (finding similar questions).
However, the task of finding similar questions involves rather short documents (often only a sentence). For longer documents, I would guess that BM25 still outperforms sentence embeddings methods.
In the paper it would have been interesting to compare the methods also against neural re-ranking, to see if the trained end-to-end retrieval is better or worse than the BM25 + re-ranking approach.
Best regards
Nils Reimers
<|||||>Thank you for the hint.
If TF-IDF / BM25 is still the best option for long documents, there seems to be a lot of room for improvement for future research, as this method does not use context, does not follow any semantic approach such as WSD, WordNet or synsets, does neither use trained models nor exploit available training data and does not use any language specific resources (e.g. stemming or noun phrase identification). Maybe some kind of challenge is needed to encourage research in this field.
Best regards,
Wolfgang
<|||||>Hey @nreimers deep thanks for all the info!
(Hopefully) quick question: what would be the optimal setup to find similarities (and build a search engine) between objects defined by a combination of senses?
For instance, consider a DB:
Object 1: "pizza", "street food", "Italian cuisine"
Object 2: "khachapuri", "street food", "Georgian cuisine", "cheese", "bread"
And then, a query "cheesy street food".
I'm using USE + hnswlib now, works pretty good, but only if the query string is more than 1 word. The more words, the better.<|||||>Hi @realsergii
Not sure if USE is the best match for that task. From the given example, I would again think that you would get quite far with BM25 and for example Elasticsearch. Elasticsearch is quite great for indexing complex objects and search over them.
Of course you would need to tune the search a bit, e.g. that longer n-grams give higher scores, maybe combined with stemming / lemmatization of words.
Otherwise, for individual words, I think word embeddings (like Word2vec / GloVe) are quite great. Sentence embeddings often have difficulties to give a good representation for words or short phrases, as these systems were not trained for it.
Also this repo could be interesting, which combines Elasticsearch BM25 with BERT re-ranking:
https://towardsdatascience.com/elasticsearch-meets-bert-building-search-engine-with-elasticsearch-and-bert-9e74bf5b4cf2
This could potentially also be combined with a simple average word embedding re-ranking approach.
I hope that helps.
Best
Nils Reimers
<|||||>@nreimers thanks Nils!
Just one more clarification - what would change if in my DB I replace each word/phrase with the 1st sentence of Wikipedia entry which is the closest to the respective word/phrase ?
So in that case, would USE or SBERT be a good choice?<|||||>Hi @realsergii
Sounds a bit complicated and you would have several other issues (how to find the correct article, what about small spelling variances).
Word embeddings are quite strong on finding similar words. As the context is rather small, I don't see too much benefit from using a sentence embedding methods to disambiguate words. 'Cheese' in your context will most often refer to the food, and not to e.g. a company or a strategy in a computer game.
Best
Nils Reimers
<|||||>Thanks @nreimers
My idea is not just to find similar words/phrases, but to find similar senses.
E.g. "welding" is similar to "joining", "building", in my understanding.
In order to comprehend this, a machine needs to know what are all of those concepts, described in more basic words, right?
One way to teach a machine is to create a vector from a sentence where the sense is described by Wikipedia (and thus, in more basic concepts).
Other way is to just get a sense (as a vector) (by word/phrase) from a model trained on Wikipedia and other sources.
This is my understanding.
Please suggest what sounds better.<|||||>Hi @realsergii
That is exactly what words embeddings are great for: to find similar words, e.g. welding is similar to joining / building.
Mapping words to Wikipedia definitions sounds unnecessary complicated and I doubt you get good results with this (compared to simple word embeddings). At the end, as you have a fixed word to Wikipedia article mapping, you will get a fixed word -> vector mapping. But it is much more complicated and the quality will be much lower.
I would train word2vec / GloVe on large amount of text from your domain and then you can use these word embeddings for comparing word similarities.
<|||||>That’s why using word embedding. Because tf-did just find lexicon
overlapping but not the similar semantic meaning
On Mon, Dec 23, 2019 at 03:58 Nils Reimers <[email protected]> wrote:
> Hi @realsergii <https://github.com/realsergii>
> That is exactly what words embeddings are great for: to find similar
> words, e.g. welding is similar to joining / building.
>
> Mapping words to Wikipedia definitions sounds unnecessary complicated and
> I doubt you get good results with this (compared to simple word
> embeddings). At the end, as you have a fixed word to Wikipedia article
> mapping, you will get a fixed word -> vector mapping. But it is much more
> complicated and the quality will be much lower.
>
> I would train word2vec / GloVe on large amount of text from your domain
> and then you can use these word embeddings for comparing word similarities.
>
> —
> You are receiving this because you are subscribed to this thread.
> Reply to this email directly, view it on GitHub
> <https://github.com/huggingface/transformers/issues/876?email_source=notifications&email_token=AIEAE4GQI2RXKMS4HDVY2Y3QZ7BIBA5CNFSM4IGKGJT2YY3PNVWWK3TUL52HS4DFVREXG43VMVBW63LNMVXHJKTDN5WW2ZLOORPWSZGOEHPYXRA#issuecomment-568298436>,
> or unsubscribe
> <https://github.com/notifications/unsubscribe-auth/AIEAE4EKRBKQQRZYQTNZXKDQZ7BIBANCNFSM4IGKGJTQ>
> .
>
<|||||>Thanks! So it feels like Elasticsearch 7.3+ with a bunch of `dense_vector`s from GloVe for all components of my objects (e.g. "khachapuri", "street food", "Georgian cuisine", "cheese", "bread") is the most proper data structure for my system (semantic search engine).
I don't even need MySQL (for text representation storage) + separate index based on e.g. Faiss (for vectors and index) and don't need to sync them. Everything can be inside Elasticsearch (however the query speed will be slower than Faiss but I can live with that for now).
@nreimers just want to clarify that I don't need to even look into BERT/USE etc direction, right?<|||||>@realsergii
If your queries and documents are only words or short phrases, I think there is no benefit from using BERT / USE. Sentence embeddings can be helpful when you have more text (at least a sentence) and when words can be used ambiguously (like the word apple).<|||||>@realsergii I mentioned it prior in this thread, but if you're using elastic search as your backend, check out [NBoost](https://github.com/koursaros-ai/nboost), which acts as a proxy on top of ES and uses BERT to rerank the n top results.
We recently released TinyBERT distilled version of the base models which are about 10x faster (critical when it comes to search). See https://arxiv.org/abs/1909.10351 for distilling custom models by the same method. <|||||>@Raghavendra15 @nreimers Did you end up trialing it out with Faiss ? What were the results. ?
I have a similar use case where I have a domain dataset (about 100k english sentences) related to fires, I want to find synthetic multilingual sentences in different languages (arabic, italian, chinese etc.). My thought was to download the Wikipedia corpus (source) for each language and embed both wikipedia and my fire data and find synthetic sentences.
By following this example [Semantic Similarity](https://github.com/UKPLab/sentence-transformers/blob/master/examples/application_semantic_search.py)
- I was able to download the multilingual trained models `distiluse-base-multilingual-cased`
- Embed about 4.2 Million Arabic sentences (took about 7hrs on p2.xlarge instance, 85% GPU utilization) and 100k Fire Sentences (took couple of minutes)
This is where it hangs/very slow -
- Running the similarity using cdist seems to run forever, had to cancel after running it for a day. I did not expect for it to take this long. Even though it was very straightforward. Figuring there should be a more optimized way of doing this.
Is there something wrong with the steps I have taken, appreciate any help.
Cheers !
Ayub<|||||>Update: I ran it with faiss library using FlatIndex (as it give the most accurate results). On a p2.xlarge instance it was amazingly fast- building and searching took only 30 mins. I could not compare the results to scipy's cdist but for a sample of 10,000. I saw than >90% of the results lie in the top 5 matches found by faiss distance.<|||||>@Raghavendra15 @mohammedayub44 FYI, two relevant papers that recently came out from Google and Microsoft:
- Pre-training embeddings for large-scale retrieval https://arxiv.org/abs/2002.03932
- TwinBERT: distillation for efficient retrieval https://arxiv.org/abs/2002.06275<|||||>How to train BERT on LinkedIn pages? <|||||>> Hi @wolf-tag
> Personally I think tf-idf / BM25 is the best strategy for your task, due to various reasons.
>
> **First**, it is important to differentiate between false positive and false negative rates:
> False positive: A non-similar pair of docs is judged as similar, even they are not similar.
> False negative: A similar pair of docs is judged as dissimilar.
>
> TF-IDF/BM25 has a low false positive rate and a high false negative rate, i.e., if a pair is judged as similar, there is a high chance that they are actually similar.
>
> Sentence embeddings methods (avg. GloVe embeddings, InferSent, USE, SBERT etc.) have a reverse characteristic: high false positive rates, low false negative rates. It seldom misses a similar pair, but a pair judged as similar must not necessarily be similar.
>
> For Information Retrieval, you have an extrem imbalance. You have 1 search query and 100 Mio. documents, i.e., you perform 100 Mio pairwise comparisons.
>
> Sentence embeddings with a high false positive rate will return many pairs where the embeddings think they are similar, but they are not. Your result set of 10 documents will be often completely garbage.
>
> TF-IDF / BM25 might miss some relevant documents, but the 10 document you will find will be of high quality.
>
> **Second**, in my experience, sentence embeddings methods work best for sentences. For (longer) documents, the results are often not that great. Here, word overlap (with tf-idf / BM25) is really hard to beat.
>
> **Third**: In our experiments in Question Answering (given a question, find in Millions of answers on StackOverflow the correct one), TF-IDF / BM25 is extremely hard to beat. It often performs much better than sentence embeddings methods + it is much quicker.
>
> So far our experiments with end-to-end representation learning for information retrieval rather failed.
>
> What works quite good is a re-ranking approach: You use BM25 to retrieve the top 100 documents. Than, you take a neural approach like BERT to re-rank these 100 results and you present the top-10 results (the 10 results with the highest score according to the neural re-ranker) to the user. This often gives a nice boost to pure BM25 ranking, and the runtime is not too-bad, as you must only re-rank 100 documents.
>
> Best regards
> Nils Reimers
I am working on a use case where I need to get similar documents (2-3 pages average), when I upload a 1 page document. For me reducing false negatives is a priority, at the same time I don't want too many false positives. Can I first implement an embedding model to get let's say 200 similar documents and then apply TFIDF/BM25 to filter out irrelevant documents <|||||>I just recently started on NLP and "AI" and have been following this thread. Having a similar use case (less than 10k documents --> find similar documents and also do a multi-label classification) I am very interested in your opinion on BERT-AL:
https://openreview.net/pdf?id=SklnVAEFDB<|||||>> @Raghavendra15 @nreimers Did you end up trialing it out with Faiss ? What were the results. ?
> I have a similar use case where I have a domain dataset (about 100k english sentences) related to fires, I want to find synthetic multilingual sentences in different languages (arabic, italian, chinese etc.). My thought was to download the Wikipedia corpus (source) for each language and embed both wikipedia and my fire data and find synthetic sentences.
>
> By following this example [Semantic Similarity](https://github.com/UKPLab/sentence-transformers/blob/master/examples/application_semantic_search.py)
>
> * I was able to download the multilingual trained models `distiluse-base-multilingual-cased`
> * Embed about 4.2 Million Arabic sentences (took about 7hrs on p2.xlarge instance, 85% GPU utilization) and 100k Fire Sentences (took couple of minutes)
>
> This is where it hangs/very slow -
>
> * Running the similarity using cdist seems to run forever, had to cancel after running it for a day. I did not expect for it to take this long. Even though it was very straightforward. Figuring there should be a more optimized way of doing this.
>
> Is there something wrong with the steps I have taken, appreciate any help.
>
> Cheers !
> Ayub
Did you consider using XLM-R for your multilingual approach? (Generates language independent embeddings for semantic similarity)<|||||>@timpal0l
I tested XLM-R for multilingual sentence embeddings.
If used out-of-the-box (without further fine-tuning), the results are really bad, far worse than mBERT (mBERT is also really bad without fine-tuning).
The vector spaces for XLM-R are not aligned across languages, i.e. the same sentence in two different languages are mapped to completely different points in vector space.
However, when fine-tuned, you can get quite nice results with XLM-R for cross-lingual tasks. Currently I prepare some code + paper + models, which will be release soon in the sentence-transformers repository.
Best
Nils Reimers
<|||||>@nreimers Thanks for you reply!
I see. I have a unlabelled corpus consisting of several languages that I wish to fine tune XLM-R (just update the language models weights to get more domain specific embeddings). Not a down stream task like classification.
I cant seem to find any example code of doing this, have you managed to do this with XLM-R using HuggingFace? Could you give me any pointers?
Cheers<|||||>Hi @timpal0l
I think this is the file you need
https://github.com/huggingface/transformers/blob/master/examples/run_language_modeling.py
I haven't tested it by myself.
Best
Nils Reimers<|||||>This issue has been automatically marked as stale because it has not had recent activity. It will be closed if no further activity occurs. Thank you for your contributions.
<|||||>Hi @nreimers, I am using sentence transformers for finding stack overflow duplicate questions. I want to train the model from scratch but I am facing some issues. My training set contains questions and its duplicates only. Is it possible to train the model from this type of training data set.<|||||>Hi @sajit9285
Just positive examples won't work. You somehow need to teach the network what is similar and what not.
But usually it is not an issue, as getting negative pairs is quite easy. The most simple strategy is just to sample two questions randomly. In 99,9999% of the cases they are non-duplicate and get the negative label.
A better strategy is to use hard negatives, as with the random strategy, your negatives are too easy to spot. One better way would to sample another random question with the same stackoverflow tag and treat it as negative. Or to find a similar question with ElasticSearch BM25 and to assume that it is a negative example<|||||>@nreimers Thanks for your reply. I will try your stated methods.
I used word2vec averaging method and sentence transformers with pretrained model('bert-base-nli-mean-tokens') for ranking similar questions, and I found word2vec averaging method (for sentence embeddings) performed better. May be the data has lots of tech terms!
That's why I am thinking of training the model from scratch. <|||||>@sajit9285 Yes, the NLI data sadly does not contain any computer science / programming specific examples, so it does not learn these terms. word2vec is trained on a much wider range of topics, so it has an understanding of programming terms.<|||||>@nreimers So will it work if I trained it from scratch as stated in the that github repo?<|||||>@sajit9285 As always, it depends on the quality of your training data. But I saw quite some good improvements for domain specific terms / sentences if you train it on appropriate training data<|||||>@sajit9285 Is it not better to use the existing weights as a base, rather than train something from scratch? <|||||>@nreimers I will give a try. Thanks :)<|||||>@timpal0l Yeah ofcourse, anytime they are better than random weights. <|||||>@nreimers You are a beast! A lot of questions I had were addressed on here! <|||||>@nreimers I have tried a lot replacing AllNLI files with my own dataset files in the same format. I have also changed labels (inside nliReader class' member function named get_labels) from 3 labels(contradiction,neutral,entailment) to two labels (true,false) for my task. But it is still printing those three labels and unable to detect my dataset. I tried a lot but now need your help now. I task that I trying to perform is fine tuning on bert which takes paired para/sentences as input.<|||||>Hello @nreimers. I run all models available in https://public.ukp.informatik.tu-darmstadt.de/reimers/sentence-transformers/v0.2/
only using
`model = SentenceTransformer(model_name)`
The model that gave the best results was: distiluse-base-multilingual-cased
The results are very similar than USE (Universal Sentence Enconder).
My questions are:
1. How I can improve the results of distiluse-base-multilingual-cased without clean all weird text cases that I have in dataset?
2. Should I need explore fine-tune parameters? How I can do that?
3. Should I need add more layers after the end layer? What are your suggestions?
4. There are any way to use GloVe pre-trained model with SBERT? If yes, how I can do that?
I want to understand how I can navigate in your beautiful SBERT to do a little/easy modifications that can bring me better results.
Thank you for your help.
<|||||>> Hi,
> BERT out-of-the-box is not the best option for this task, as the run-time in your setup scales with the number of sentences in your corpus. I.e., if you have 10,000 sentences/articles in your corpus, you need to classify 10k pairs with BERT, which is rather slow.
>
> A better option is to generate sentence embeddings: Every sentence / article is mapped to a fixed sized vector. You need to map your 3k articles only once to a vector.
>
> A new query is then also mapped to a vector. In this setup, you only need to run BERT for one sentence (at inference), independent how large your corpus is.
>
> Then, you can use cosine-similiarity, or manhatten / euclidean distance to find sentence embeddings that are closest = that are the most similar.
>
> I released today a framework which uses pytorch-transformers for exactly that purpose:
> https://github.com/UKPLab/sentence-transformers
>
> I also uploaded an example for semantic search, where each sentence in a corpus is mapped to a vector and than cosine-similarity is used to find the most similar sentences / vectors:
> https://github.com/UKPLab/sentence-transformers/blob/master/examples/application_semantic_search.py
>
> Let me know if you have further questions.
Hi there! Phenomenal work! I just had one question, how do transformer encodings (say BERT) compare against encodings from models like Google's Universal Sentence Encoder on a textual semantic similarity task? <|||||>Hi @algoromeo
Universal Sentence Encoder (USE) spans several different architectures. The USE large is based on transformer networks like BERT, i.e., the architectures are quite comparable. A big advantage of BERT is the language model pre-training, which induces a lot of information about language in the model. This pre-training is missing in USE.
USE also has CNN networks, which are faster and runtime scales better with the input length. But their performance is usually worse than the transformer based architectures. So you trade speed for lower accurarcy.<|||||>> Hi @algoromeo
> Universal Sentence Encoder (USE) spans several different architectures. The USE large is based on transformer networks like BERT, i.e., the architectures are quite comparable. A big advantage of BERT is the language model pre-training, which induces a lot of information about language in the model. This pre-training is missing in USE.
> USE also has CNN networks, which are faster and runtime scales better with the input length. But their performance is usually worse than the transformer based architectures. So you trade speed for lower accurarcy.
Thank you for your timely and apt reply! Gave me the much needed clarity! Cheers!<|||||>Hi @nreimers , thank you for your detailed explanation on many issues around sentence-bert and semantic textual similarity search. I am currently working on a social science project in which I am trying to measure the "cultural distinctiveness" (basically whether people are different from each other when they comment) of Reddit users based on their comments in certain posts.
I am thinking of treating all comments of each user as a document. Hopefully, I could obtain document embeddings using sentence transformers. Alternatively, I could use GloVe or Latent Semantic Analysis as embeddings of the document. After that, I am also hoping to compare each individual with the collectives he/she belongs to. So comparing text generated one user against text generated by a group of pre-defined people (and do that iteratively for every user in the dataset). Do you think sentence BERT is a suitable method to embed documents? Could you recommend any work related to the thing I am trying to do, please? Thank you!<|||||>Hi @SamALIENWARE
I am afraid that Sentence-BERT is not suitable for that.
BERT (&Co.) have a quadratic runtime and quadratic memory requirement with the text length. I.e., for long documents you would need extremely large memory and have an extremely long runtime. This is why BERT & Co. limit the length for the input document to 512 word pieces, which are about 300 words.
For your purpose I would use avg. GloVe embeddings (which are already implemented in the sentence-transformers project) or LSA/LDA (e.g. from Gensim).
Best
Nils Reimers<|||||>> Hi @SamALIENWARE
> I am afraid that Sentence-BERT is not suitable for that.
>
> BERT (&Co.) have a quadratic runtime and quadratic memory requirement with the text length. I.e., for long documents you would need extremely large memory and have an extremely long runtime. This is why BERT & Co. limit the length for the input document to 512 word pieces, which are about 300 words.
>
> For your purpose I would use avg. GloVe embeddings (which are already implemented in the sentence-transformers project) or LSA/LDA (e.g. from Gensim).
>
> Best
> Nils Reimers
Thanks a million, @nreimers ! I will definitely try your suggestions out.
I have tried distilled sentence BERT out yesterday. Perhaps its because there aren't that many data (19000+ users in my dataset), the "sentence" embeddings were acquired in a relatively short time period. Then I used k-means clustering on the embeddings and calculated the sum of the distance of each vector to the centroids of the clusters. I am thinking that the larger the sum, the more "distinct" the user's content is since it's semantically far from everyone else's.
So after I got embeddings using GloVe or LSA/LDA, do you think the euclidean distance to k-means centroids is a good representation of semantic textual similarity in a non-pairwise situation (1 vs. many)? Or is it better to stick to cosine similarity (calculate pairwise cosine similarity and then average), as the embedding models are trained using this metric?
Thank you again for your valuable time. I do appreciate it. Have a nice day!<|||||>> @nreimers , I have tried a lot replacing AllNLI files with my own dataset files in the same format. I have also changed labels (inside nliReader class' member function named get_labels) from 3 labels(contradiction,neutral,entailment) to two labels (true,false) for my task. But it is still printing those three labels and unable to detect my dataset. I tried a lot but now need your help now. I task that I trying to perform is fine tuning on bert which takes paired para/sentences as input.
<|||||>@nreimers Brillant Work!!! I just wanted to understand when we are doing evaluation we are using STS Bench Mark but when we have domain-specific data do we still need to STS or we can split our data into test and train and evaluate. <|||||>Hi @saurabhsaxena86
No, in that case you don't need STS. If your domain specific data is suitable, you can of course train on that.<|||||>Hey @nreimers, I am bit confused on how to go about training the model from scratch on my dataset. Is there some resource which I can refer to. I am having hard time figuring out how to create the dataloader and train the model on specific data.
<|||||>Hi @saurabhsaxena86 , can you please share the code on how you have trained the model on your domain specific data?
That would be of great help!<|||||>Hi @Shubhamsaboo
Currently only the these scripts with training examples exists:
https://github.com/UKPLab/sentence-transformers/tree/master/examples/training_transformers
More examples for training will be pushed soon. Further, I currently work on a more extensive documentation.<|||||>HI @nreimers , how can i find average feature vector from the embeddings given by sentence bert for large sentence similarity comparison ?<|||||>Hi @ankitkr3
Not sure what you mean. But you can use:
https://github.com/UKPLab/sentence-transformers/blob/master/sentence_transformers/util.py#L12
With quite large sets of sentences to compute the cosine sim between all of them.
This example might also be relevant:
https://github.com/UKPLab/sentence-transformers/blob/master/examples/training_quora_duplicate_questions/application_duplicate_questions_mining.py<|||||>hi @nreimers I want to calculate the similarity between two large/medium paragraphs. How can i achieve that with the current available models?<|||||>Hi @ankitkr3
Currently the models are trained and optimized for sentence length inputs. You can also input longer inputs, up to 510 word pieces (which is the limit for BERT).
One way to compare paragraphs and to get a similarity score would be to use Sentence Mover Distance:
https://www.aclweb.org/anthology/P19-1264v2.pdf
Code available here:
https://github.com/eaclark07/sms
I did not use this code / approach, but I heard that it can produce quite good results when you compare paragraphs with each other.<|||||>@nreimers are you planning to make it available for large sentences or paragraphs ?<|||||>This thread help-me a lot, thanks guys! A question: I have more or less 7000 documents with 300 words each in average and some text from users. My idea is use the text from users as queries to "retrieval" or "ranking" these documents,but I dont know which is the best strategy, STS task or Learn to Rank? Any ideas are welcome. <|||||>Hi @finardi
Do you have training data in the form (user-query, relevant_doc)? If yes, you can use the MultipleNegativesRankingLoss, which is a learn to rank loss function: https://www.sbert.net/docs/package_reference/losses.html#multiplenegativesrankingloss
<|||||>Hi @nreimers,
For a project I need a good document embeddings for news articles example from cnn.com, what if we use Bert for each paragraph of the article and then average all the embedding vectors, do you think that naive approach could lead to good performance? I am trying to search for similar news covering the same event from different news outlets. Example scenario could be that the company X goes bankrupt, cnn.com will cover the event, CNBC will cover the event, fox.com, and so on, so if the user selects one article the system will show other articles covering the same event, I will pre-filter the news from too far in the future and too far in the past because probably those news are not covering the same event. I have all the news in a database.
Do you think with a simple average word embedding offer good performance in this application? and I forget using Bert? for my application I want the best accuracy I can use GPUs for inference if that is required.
<|||||>Hi @bushjavier
I am not sure if BERT / SBERT will work that well for your task.
For documents, the best approach is usually to use TF-IDF / BM25. Often, these documents on the same events have so many word overlaps, that it is quite easy to identify similar documents.
Embedding approaches are more suitable if you want to compare sentences. There, the word overlap can be quite small where TD-IDF / BM25 fails. However, when you average them for larger text, it is quite unclear what the averaged embedding will look like.
Further, you have issues with docs with different lengths.
Assume you have doc A, reporting about event X.
Then you have doc B, reporting about event X but then also providing background information or reporting about other events. With BM25, this is no issue, it detects that the information of A is include in doc B. With averaged embeddings, the embeddings for doc A and B can be quite different.<|||||>@nreimers thanks for the crystal clear explanation! I will proceed with TF-IDF / BM25.<|||||>HI @nreimers, i've used bert embeddings with success to perform a smart search on a huge software manual. It works pretty well.
I use `'SentenceTransformer('distiluse-base-multilingual-cased')`'. In the corpus i set all the paragraph of the manual and i compare the user query with cosine similarity.
I get great results when the query is a sentence of some words: i.e. "what are supperted servers", "how to upgrade the program", etc. But when the query is a single word, or a single word mispelled or composed by random char (i.e. kjashdjkah) i get false positive often on the first corpus item.
I've made another project in which this issue is more evident: i made a document classificator using an OCR to scan the image and then comparse the results with some sentences. In this case some words read by the OCR are non sense, becouse not all the words are correctly found, and my results a set of words with some strange char. Like i said before in these cases i get false positive, often on the the first corpus item.
Is ther a way to avoid that?
kind regards
Gianluca<|||||>> HI @nreimers, i've used bert embeddings with success to perform a smart search on a huge software manual. It works pretty well.
> I use `'SentenceTransformer('distiluse-base-multilingual-cased')`'. In the corpus i set all the paragraph of the manual and i compare the user query with cosine similarity.
> I get great results when the query is a sentence of some words: i.e. "what are supperted servers", "how to upgrade the program", etc. But when the query is a **single word**, or a single word **mispelled** or composed by random char (i.e. kjashdjkah) i get false positive often on the first corpus item.
> I've made another project in which this issue is more evident: i made a document classificator using an OCR to scan the image and then comparse the results with some sentences. In this case some words read by the OCR are non sense, becouse not all the words are correctly found, and my results a set of words with some strange char. Like i said before in these cases i get false positive, often on the the first corpus item.
> Is ther a way to avoid that?
>
> kind regards
> Gianluca
Perhaps use word embeddings for single word documents instead of contextualized embeddings such as Bert. And for misspelled words - can't you perform a dictionary checkup to see if the word exists in a vocabulary or not?
<|||||>
> can't you perform a dictionary checkup to see if the word exists in a vocabulary or not?
I can't becouse some queries may contains acronyms or other technician abbreviation (i.e. F24) that aren't present in a vocabulary.
Now i'm trying the way suggested from @nreimers some post above: using bm24 and then sentence embeddings to update the score.
<|||||>> > can't you perform a dictionary checkup to see if the word exists in a vocabulary or not?
>
> I can't becouse some queries may contains acronyms or other technician abbreviation (i.e. F24) that aren't present in a vocabulary.
Cant you build your own word vector model on your domain specific data to learn acronyms and common terms that are not present in a vocabulary. And then allow for the x nearest neighbours?
<|||||>Man! this Github far more insightful than some of those NLP blogs out there!!! So thanks for the QNA's.
Here articles and sentences are used for semantic search but what about other datasets like well any DB(SQL or JSON)?
Well for an intuitive example suppose I have an object `{Product: Cool Refrigerator, Price: 5000}` and if i type a query like "refrigerators under 5000" the result should be `Result: Cool Refrigerators` there are models out there with similar solutions... but was curios if anyone had good references and solutions.
THanks<|||||>This Discussion is GOLD!!!
@nreimers - I salute your patience and knowledge-graphs embedded in your brains :). You answered almost every question with details. I accidentally bumped on this trying to read through issues.
I usually use Jina, Nboost and Sentence-Transformer depending on the problem statement along with transformers. I learnt a lot from this discussion. Thanks again to everyone who contributed. This discussion should be part of the Huggingface newsletter.<|||||>Hi, I m trying to merge the output of LDA with BERT.
@nreimers Could you please route me with right steps on how to merge the output of LDA vectors with BERT for topic modelling task. Which BERT pre-trained model will be best suited for this task. Sentence-transformer or any ?
<|||||>@sarojadevi Never worked with LDA and BERT. Can't help here, sorry.<|||||>@nreimers Hi, I have used sentence-transformer to finetune on my dateset which has Anchor,Positive,Negative.
I use tripletloss as loss function, but after training, the word embeddings are very similar, so they cosine-similar are all close to 1.
Could tell me how to solve this problem?
By the way, my goal is to find the sentences closest to the input sentence, and all the sentence is about 400 words. Do you have any better suggestions? <|||||>Hi @zitaozz
You can try this loss, where you only need anchor and positives (if suitable):
https://www.sbert.net/docs/package_reference/losses.html#multiplenegativesrankingloss
Do you mean word embeddings or sentence embeddings?
<|||||>@nreimers Thanks for you replying! But my dataset seems not suitable for this loss because some positive sentences are similar.
Yes, I mean sentence embeddings. I don' t know why they are very similar after training.<|||||>Hi @nreimers
Sorry to barge in on this issue, but i had a query.
I used the scibert model from the hugging face repository and finetuned it on the SNLI and MNLI datasets using the sentence transformer repository. So I was trying to perform a similarity match between a query sentence and a larger text (title and abstract from arxiv papers). However, I wasn't able to get really good performance (as in the matched samples didn't look too good).
I understand that bert is trained on single sentences and not on multiple. But do you have any recommendations as to how I can improve. Thanks :)<|||||>Hi @MukundVarmaT
As often, it depends on the training data. SNLI and MNLI are not really good training sets for this.
Have a look at this paper:
https://arxiv.org/abs/2004.07180
<|||||>Hi! I am going through the exactly same kind of problem as I have around 20M text data. Please help me with the idea as I have some code already for it. My idea is to extract the `CLS` token for all the text in the DB and save it in CSV or somewhere else. So when a new text comes in, instead of using the `Cosine Similarity/JAccard/MAnhattan/Euclidean` or other distances, I have to use some approximation like `LSH, ANN (ANNOY, sklearn.neighbor)` or the one given here `faiss` . How can that be done using your library? I have my code as:
```
tokenizer = BertTokenizer.from_pretrained('bert-base-uncased')
model = BertModel.from_pretrained('bert-base-uncased')
input_ids = torch.tensor(tokenizer.encode("Hello, my dog is cute")).unsqueeze(0) # Batch size 1
outputs = model(input_ids)
last_hidden_states = outputs[0] # The last hidden-state is the first element of the output tuple
Using Tensorflow:
import tensorflow as tf
from transformers import BertTokenizer, TFBertModel
tokenizer = BertTokenizer.from_pretrained('bert-base-uncased')
model = TFBertModel.from_pretrained('bert-base-uncased')
input_ids = tf.constant(tokenizer.encode("Hello, my dog is cute"))[None, :] # Batch size 1
outputs = model(input_ids)
last_hidden_states = outputs[0] # The last hidden-state is the first element of the output tuple
```
and I can get the `CLS` token as:
```
last_hidden_states = outputs[0]
cls_embedding = last_hidden_states[0][0]
```
Please tell me if it's the right way to use and how can I use any of the `LSH, ANNOT, faiss` or something like that?<|||||>@deshwalmahesh
As outlined in our paper, produces BERT out of the box rather bad sentence embeddings, especially if you want to use them to find similar news articles:
https://arxiv.org/abs/1908.10084
For finding similar news articles, I can recommend these 3 articles:
https://www.sbert.net/examples/applications/paraphrase-mining/README.html
https://www.sbert.net/examples/applications/parallel-sentence-mining/README.html
https://www.sbert.net/examples/applications/semantic-search/README.html
There, you also find examples how to use approximate nearest neighbor methods, for example, based on faiss and similar<|||||>@nreimers
> @ggndtes In general BM25 will be really hard to beat on this type of task. See this paper where they compare sentence embeddings with BM25 on an end-to-end retrieval task (given: question, find similar / duplicate questions in a large corpus):
> https://arxiv.org/pdf/1811.08008.pdf
In our experience (building a search engine for a specific domain), sentence-transformers and BM-25 produce different results and answer different use-cases. For short queries, sentence transformers seem to have a hard time formalizing the meaning and resort to finding lexically similar sentences. In this case, BM-25 is probably better, especially if enhanced with synonyms etc.
However. for longer queries (>=6 words) expressing a compound concept, sentence transformers (with appropriate training data) shine and are able to capture semantically similar yet lexically different sentences. Elasticsearch, on the other hand, failed miserably and managed to capture only part of the meaning.
Overall, it depends also on the goal. In many cases, the typical information needs are contained within a sentence/short paragraph. There is little meaning to a document besides a collection of such paragraphs (with some dependencies between them). The text is better represented as sentences rather than a long document, and that's where transformer-based semantic encoder can encode meaning well.
As a side note, there are still substantial challenges with negation and directionality.
<|||||>@tkorach Thanks for sharing.
I agree, we saw good improvements with dense retriever over the last years. But there are still issues:
- Domain shift: We see good results when there is sufficient training data. However, when you go to a specialized domain (for example publications on COVID 19, as done in TREC COVID), existent dense models perform often rather poorly and are out-performed by BM25. I.e., you need quite some training data to get good results with dense approaches
- In some cases users search for key words, for example, a specific error code they want further information: There, dense approach perform often rather poorly. So an interesting area is on how to combine sparse lexical approaches with dense approaches. Especially, when is the user searching for keywords and when not?
<|||||>> Especially, when is the user searching for keywords and when not?
These could actually represent different information needs. Searching for named entities (individuals, locations, medications etc.) could focus on topical similarity: the entity is atomic, and either exists in the document or not (edge cases like negation can be handled by rule-based approach). For this ask topical occurrence-based methods like BM-25 work well enough and have the advantage of generalizability.
Compound concepts (e.g. "the patient's nausea improved in response to the treatment") may represent another task. The information need can be described not as finding the most relevant documents, but rather as finding a passage of text that contain the same meaning. In essence, each search query is a binary classification tasks of each and every text in the corpus. In my experience, many customers ask for "search" but are actually looking for information extraction. Semantic similarity serve as a "poor-man's" zero-shot text classifier.
The use-cases may also differ. In the search projects we did, topical similarity was used to expand their information (e.g. a person travelling to Italy would like to read about Rome), while in semantic search the "search" is used to classify items in a population. This happens frequently when the documents are components of another unit (a medical record, an insurance claim, a legal lawsuit). In professional business settings the second use-case is far more common and the main task revolves, almost always, around classification of cases. This is in contrast to web-search engine, where the document is main unit (we don't search for the web sites themselves but for content within them).
To make a long story short, we found out that explaining these differences to our users help them understand what can they expect from the algorithm. Experience with Google might push users to downgrade the query to fewer keywords to improve results. With semantic-search (e.g. STS-based encoders), it's the opposite and a more elaborate query will facilitate capturing the meaning and generalizing to similarly-meaning yet lexically-different ones.
<|||||>@nreimers You had an answer before regarding semantic search, where you suggested doing BM25 to retrieve a first set of documents, and then to re-rank them with sentence embeddings.
1) I cant find that comment anymore.
2) With getting the first subset of the BM25 results, you will only get documents with lexical overlap / direct matches of words. And sure you can probably re-rank them a bit with the semantical information from sentence representations. But this subset throws away alot of documents where there is a high semantic match.. Would you agree?<|||||>@timpal0l There was quite an improvement over the years for semantic search.
But BM25 is still a really strong system in cases where you have a specialized domain or task and you don't have any training data. Also, BM25 is really strong if you retrieve longer texts (documents). Pre-trained dense retrieval models, for example on MS MARCO or NR, often have issues when you go to specialized domains or when your retrieval task is different than how it is models in MS MARCO / NQ.
Semantic search works well when you have training data or your task&domain is similar to something where you have training data. Also semantic search is quite beneficial when you retrieve short text like sentences.
First retrieving with BM25 and then re-ranking with sentence embeddings is not that sensible. What can make sense is to retrieve with BM25 and to re-rank with a BERT cross-encoder:
https://www.sbert.net/examples/applications/information-retrieval/README.html
Re-ranking with a cross-encoder can substantially boost the performances, but has sadly the downside of being slower and more compute intensive then retrieval with a bi-encoder embedding approach.<|||||>> @timpal0l There was quite an improvement over the years for semantic search.
>
Agree on that!
>
> But BM25 is still a really strong system in cases where you have a specialized domain or task and you don't have any training data.
>
Ok, by training data you mean like an annotated corpus in the style of SNLI, STS-B, or similar? But you mention in your sentence-bert paper that you can get around with the self-supervised approach, when you create triplets from wikipedia documents. Doesnt BM25 also require a corpus to calculate the tf-idfs weights from? You think this still is stronger then the self supervised sentence bert with triplet loss (on short sentences)?
>
>
> Also, BM25 is really strong if you retrieve longer texts (documents). Pre-trained dense retrieval models, for example on MS MARCO or NR, often have issues when you go to specialized domains or when your retrieval task is different than how it is models in MS MARCO / NQ.
>
Agree, I have seen similar results where simpler methods (tf-idf) works better on looong documents, do you think its cause its hard to create a good representation of a long document since the context can change a lot over document?
>
> Semantic search works well when you have training data or your task&domain is similar to something where you have training data. Also semantic search is quite beneficial when you retrieve short text like sentences.
>
> First retrieving with BM25 and then re-ranking with sentence embeddings is not that sensible. What can make sense is to retrieve with BM25 and to re-rank with a BERT cross-encoder:
> https://www.sbert.net/examples/applications/information-retrieval/README.html
>
Ok, with "sentence embeddings" I meant the BERT bi-encoder (siemiese bert / your sentence bert paper). Right, so you say that by using the cross-encoder, you re-rank all pairs with each other in the form of "sentence_n [SEP] sentence_n+1" style?
> Re-ranking with a cross-encoder can substantially boost the performances, but has sadly the downside of being slower and more compute intensive then retrieval with a bi-encoder embedding approach.
Makes sense its slower than using precalculated embeddings from the bi-encoder.
I might have missed something, but my first questions remains. Dont you possibly throw away matches when getting the first subset based only on the BM25 (lexical / word overlap) match. Sentences with non lexical overlap but with semantic similarity will not be captured to even be re-ranked..?
<|||||>
> > But BM25 is still a really strong system in cases where you have a specialized domain or task and you don't have any training data.
>
> Ok, by training data you mean like an annotated corpus in the style of SNLI, STS-B, or similar? But you mention in your sentence-bert paper that you can get around with the self-supervised approach, when you create triplets from wikipedia documents. Doesnt BM25 also require a corpus to calculate the tf-idfs weights from? You think this still is stronger then the self supervised sentence bert with triplet loss (on short sentences)?
It depends on your task, so you should have training data similar to your task. BM25 is primarily be used for search (information retrieval), so in the following I will focus on search. If you have a different task, like clustering, paraphrase mining, bitext mining etc., you need a different approach.
For search, you need training data similar to MS MARCO / Natural Questions: Given a query and a matching (relevant) passage answering the query.
The triplets created from Wikipedia perform extremely badly on search.
We have currently a paper on the way that presents new methods for training embeddings for semantic search if you don't have labeled training data, just a large set of unlabeled text. BM25 is a strong contestant, sometimes much better than dense approach, sometimes worse.
BM25 just need unlabeled data to compute idf values.
>
> > Also, BM25 is really strong if you retrieve longer texts (documents). Pre-trained dense retrieval models, for example on MS MARCO or NR, often have issues when you go to specialized domains or when your retrieval task is different than how it is models in MS MARCO / NQ.
>
> Agree, I have seen similar results where simpler methods (tf-idf) works better on looong documents, do you think its cause its hard to create a good representation of a long document since the context can change a lot over document?
I think mapping longer documents to a single, dense vector does not make sense.
If I combine two Wikipedia articles, e.g. Microsoft and Chlorine, then this is not that big of a problem for BM25 because the relevant word overlap between the two articles is rather low. But how should a single dense vector look like? Is it (Vector(Microsoft) + Vector(Chlorine)) / 2
Likely not.
> > Semantic search works well when you have training data or your task&domain is similar to something where you have training data. Also semantic search is quite beneficial when you retrieve short text like sentences.
> > First retrieving with BM25 and then re-ranking with sentence embeddings is not that sensible. What can make sense is to retrieve with BM25 and to re-rank with a BERT cross-encoder:
> > https://www.sbert.net/examples/applications/information-retrieval/README.html
>
> Ok, with "sentence embeddings" I meant the BERT bi-encoder (siemiese bert / your sentence bert paper). Right, so you say that by using the cross-encoder, you re-rank all pairs with each other in the form of "sentence_n [SEP] sentence_n+1" style?
Training with sentence_n [SEP] sentence_n+1 does not work (neither for bi- nor for cross-encoder).
You train the cross-encoder for:
query [SEP] paragraph
To output a score 0...1 if the paragraph is relevant for query. For this, you need labeled training data which paragraphs are relevant for which queries.
Then you compute the score for all paragraphs: query [SEP] paragraph1, query [SEP] paragraph2, query [SEP] paragraph3...
and re-rank them.
> > Re-ranking with a cross-encoder can substantially boost the performances, but has sadly the downside of being slower and more compute intensive then retrieval with a bi-encoder embedding approach.
>
> Makes sense its slower than using precalculated embeddings from the bi-encoder.
>
> I might have missed something, but my first questions remains. Dont you possibly throw away matches when getting the first subset based only on the BM25 (lexical / word overlap) match. Sentences with non lexical overlap but with semantic similarity will not be captured to even be re-ranked..?
Yes, of course you only get matches with word overlap. How bad this is, depends on your task. For many tasks, BM25 can get quite good recall values (e.g. recall@100), but the ranking of BM25 is bad => then re-ranking helps.
If BM25 does not find the relevant hits within the first 100 or 1000 hits, then re-ranking will not bring a benefit. As before and as always: It depends on your specific task
<|||||>> > > But BM25 is still a really strong system in cases where you have a specialized domain or task and you don't have any training data.
> >
> >
> > Ok, by training data you mean like an annotated corpus in the style of SNLI, STS-B, or similar? But you mention in your sentence-bert paper that you can get around with the self-supervised approach, when you create triplets from wikipedia documents. Doesnt BM25 also require a corpus to calculate the tf-idfs weights from? You think this still is stronger then the self supervised sentence bert with triplet loss (on short sentences)?
>
> It depends on your task, so you should have training data similar to your task. BM25 is primarily be used for search (information retrieval), so in the following I will focus on search. If you have a different task, like clustering, paraphrase mining, bitext mining etc., you need a different approach.
>
> For search, you need training data similar to MS MARCO / Natural Questions: Given a query and a matching (relevant) passage answering the query.
>
> The triplets created from Wikipedia perform extremely badly on search.
>
> We have currently a paper on the way that presents new methods for training embeddings for semantic search if you don't have labeled training data, just a large set of unlabeled text. BM25 is a strong contestant, sometimes much better than dense approach, sometimes worse.
>
> BM25 just need unlabeled data to compute idf values.
>
> > > Also, BM25 is really strong if you retrieve longer texts (documents). Pre-trained dense retrieval models, for example on MS MARCO or NR, often have issues when you go to specialized domains or when your retrieval task is different than how it is models in MS MARCO / NQ.
> >
> >
> > Agree, I have seen similar results where simpler methods (tf-idf) works better on looong documents, do you think its cause its hard to create a good representation of a long document since the context can change a lot over document?
>
> I think mapping longer documents to a single, dense vector does not make sense.
>
> If I combine two Wikipedia articles, e.g. Microsoft and Chlorine, then this is not that big of a problem for BM25 because the relevant word overlap between the two articles is rather low. But how should a single dense vector look like? Is it (Vector(Microsoft) + Vector(Chlorine)) / 2
> Likely not.
>
> > > Semantic search works well when you have training data or your task&domain is similar to something where you have training data. Also semantic search is quite beneficial when you retrieve short text like sentences.
> > > First retrieving with BM25 and then re-ranking with sentence embeddings is not that sensible. What can make sense is to retrieve with BM25 and to re-rank with a BERT cross-encoder:
> > > https://www.sbert.net/examples/applications/information-retrieval/README.html
> >
> >
> > Ok, with "sentence embeddings" I meant the BERT bi-encoder (siemiese bert / your sentence bert paper). Right, so you say that by using the cross-encoder, you re-rank all pairs with each other in the form of "sentence_n [SEP] sentence_n+1" style?
>
> Training with sentence_n [SEP] sentence_n+1 does not work (neither for bi- nor for cross-encoder).
>
> You train the cross-encoder for:
> query [SEP] paragraph
>
> To output a score 0...1 if the paragraph is relevant for query. For this, you need labeled training data which paragraphs are relevant for which queries.
>
> Then you compute the score for all paragraphs: query [SEP] paragraph1, query [SEP] paragraph2, query [SEP] paragraph3...
>
> and re-rank them.
>
> > > Re-ranking with a cross-encoder can substantially boost the performances, but has sadly the downside of being slower and more compute intensive then retrieval with a bi-encoder embedding approach.
> >
> >
> > Makes sense its slower than using precalculated embeddings from the bi-encoder.
> > I might have missed something, but my first questions remains. Dont you possibly throw away matches when getting the first subset based only on the BM25 (lexical / word overlap) match. Sentences with non lexical overlap but with semantic similarity will not be captured to even be re-ranked..?
>
> Yes, of course you only get matches with word overlap. How bad this is, depends on your task. For many tasks, BM25 can get quite good recall values (e.g. recall@100), but the ranking of BM25 is bad => then re-ranking helps.
>
> If BM25 does not find the relevant hits within the first 100 or 1000 hits, then re-ranking will not bring a benefit. As before and as always: It depends on your specific task
Thanks alot! Your answers are as always, very appreciated! Do you have any paper / results, where I can see how much the re-ranking with the cross-encoder increases the search results compared to only using a bi-encoder? :) <|||||>@timpal0l
I don't have perfect number.
For the TREC 19 Deep Learning dataset, which is based on the MS MARCO dataset, I get the following results (NDCG@10):
BM25 45.5
BM25 retrieve 1k, re-rank with bi-encoder: 68.4 [*]
BM25 retrieve 1k, re-rank with cross-encoder (electra-base): 72
[*] Note, this is re-ranking with a bi-encoder, not retrieval. Retrieval with a bi-encoder yields roughly the same performances for this dataset.
So I would assume that re-ranking with a cross-encoder is about 3 points better than retrieval with a bi-encoder.
For the bi-encoder, I noticed that it can be quite sensitive to noise. For example, for the query:
query: How many people live in London?
It can retrieve a passage like:
passage: It has 2,000 inhabitants.
Here, the cross-encoder easily identifies that the passage is not relevant for the query as there is not information about London in it. The cross-encoder has here a much harder time.
<|||||>What a fantastically informative read this thread has been, thanks to all for contributing to the discussion.
@nreimers I'm working on a semantic search project using the CORD-19 (covid) corpus of academic papers. Pass in a search phrase to surface a list of most relevant documents, showing an answer excerpt of similar sentence from surfaced doc.
Ideally I'd like to encode all articles so as not to miss what could be a vital article to the clinical researcher (PubMed prints ~100k articles, preprints ~100K), thats a lot of encoding! Would you advise just to use the abstract, possibly first n and last n sentences from body text, rather than the full article? I amusing the allenai-specter model as sentence transformer for this objective, any comments?
Would you still support BM25 as first pass then re-rank with cross-encoder for this semantic search task?
Regarding fine-tuning BERT-based model (first time fine tuning here) - would I take a split of the cord 19 articles and pass sentence pairs in, as per the [sentence-transformers NLI example](https://github.com/UKPLab/sentence-transformers/blob/master/examples/training/nli/training_nli.py). One thing Im not sure of is that the NLI example uses labeled data I believe, where the cord19 articles are unlabelled , so I just want to fine-tune the model for the domain semantics.
`train_samples.append(InputExample(texts=[row['sentence1'], row['sentence2']], label=label_id))`
Thank-you very much for any guidance.<|||||>Hi @corticalstack
I think the allenai SPECTER model is not that great for this. It was trained for the task of paper recommendation, i.e. you input a title and an abstract of an paper and the model can return similar papers.
For semantic search, where you input a query or question, I don't expect the model to work that well.
For the encoding: I would split a paper into paragraphs and then encode them individually.
We are currently working on code that will allow to train semantic search models in an unsupervised fashion. Code & documentation will be released this month. It also works quite well for the CORD-19 dataset (as evaluated on the TREC COVID shared task).
But for CORD-19 we saw that BM25 can be hard to beat. The dataset has many specific terminology, like specific names for virus types. Here, lexical search excels as it gives you exactly the papers that talk about this specific type of virus. So I would at least combine semantic search with lexical search.
The training examples currently available are only when you have labeled data. As mentioned, we will release this month code & docs for training models without labeled data using two different strategies.<|||||>@nreimers Really appreciate the swift feedback and sharing your expertise, thanks! I will take a look at splitting by paragraph, and will most certainly jump on the unsupervised feature as soon as it becomes available. What's the best channel to watch out for it becoming available?
Will focus on BM25, at least in combination with semantic.
Thanks again for all your shared knowledge in this thread, extremely educational. Oh, and the hard work on the repo!<|||||>@corticalstack I will likely post it on twitter once the tutorial for unsupervised semantic search training becomes available:
https://twitter.com/Nils_Reimers
Otherwise, it will appear on www.SBERT.net in a new section on the left side: Unsupervised Training.
Combing semantic search and BM25 results proved quite effective for me. I combined these two via:
final_score = bm25_score_i / max(bm25_score) + lambda*cos_score_i / max(cos_score)
With:
max(bm25_score) = The BM25 score of your best BM25 hit
max(cos_score) = The cosine similarity score of your best semantic search hit.
Lambda is a factor to balance between BM25 and semantic search, so that you can control which type of search should have the higher impact.
<|||||>Any suggestion on dataset for benchmarking semantic search please - I was thinking MS Marco but [this article says no](https://www.kdnuggets.com/2020/04/ms-marco-evaluate-semantic-search.html)<|||||>The situation is not as bad as described in the blog post. Every dataset for semantic search / information retrieval has a selection bias. Is is impossible to create a dataset without a selection bias, because otherwise you would need to label for every query 8 Million documents (for the MS MARCO case). This is of course not possible.
So as the author writes at the end:
> But despite all those remarks, the most important point here is that if we want to investigate the power and limitations of semantic vectors (pre-trained or not), we should ideally prioritize datasets that are less biased towards term-matching signals. This might be an obvious conclusion, but what is not obvious to us at this moment is where to find those datasets since the bias reported here are likely present in many other datasets due to similar data collection designs.
The described biases are well known and a long recognized problem. But there are no good solutions for it, especially not at scale.
So in conclusion:
- Is the MS MARCO dataset perfect: No
- Is the MS MARCO dataset useful for training and evaluation: Yes, I definitely think so. It is far better than many other datasets that could be used (STSbenchmark, SQuAD)
- Are there better datasets: The Natural Questions (NQ) dataset from Google is interesting, but more targeted on answering questions using Wikipedia. So for question-answer retrieval with Wikipedia, I think NQ is better. For broader retrieval, I cannot say yet which is better (MS MARCO / NQ). MS MARCO has also many keyword style queries and non-wikipedia-answerable queries like "weather san diego", which matches broader what people are searching for.
- Will a better score on MS MARCO mean better performance on my task: No. At some point, models will be too specialized on MS MARCO and its selection bias, i.e., they don't get better, they are just better overfitting on MS MARCO selection bias. I sadly don't know at which point (i.e. score range) this will happen.
- Is a perfect dataset for semantic search possible? Sadly not, creating one even for evaluation is rather expensive. And sadly you always have some selection bias in it<|||||>Grateful for any guidance. Consider the following question asked when performing a semantic search:
_"Which of the current covid19 vaccines in the clinic have reported the highest levels of neutralizing Abs after a single vaccination?_"
The intent behind the question is to get information back about covid19 vaccines generating high levels of antibodies as tested in the clinic (therefore not phase 1/2 trials with mice). With BERT variants, how may emphasis be placed on certain words. Having tried various search online search tools (e.g. PubMed) and my own draft search tool using off-the-shelf _distilbert-base-nli-stsb-mean-tokens_ and BM25, it seems quite a challenging questions with results not so good. Thanks for any thoughts.<|||||>@corticalstack
First note the difference between symmetric and asymmetric semantic search:
https://www.sbert.net/examples/applications/semantic-search/README.html#symmetric-vs-asymmetric-semantic-search
You have an asymmetric use case (answer and query are of different type), but you are using a model that is only suitable for a symmetric case (query and answer have the same amount of content).
Second: Queries like "what is the biggest/largest/highest..." are quite difficult. Sure, if a text mentions it (X is the largest...), then it can be retrieved. But if you only have sentences like (A is 3), (B is 5), (C is 1), then the model has no way to compare these results to find what is the largest. It has no sense for numbers and does not know what is large / the largest or small / the smallest.
<|||||>@nreimers Can you just do a brain dump into a book please? :)
When Googling for "_Symmetric vs asymmetric semantic search_", oddly enough your SBERT site is first hit! With seemingly few very few other relevant hits. Is this distinction so little discussed in the public domain? The "msmarco-distilbert-base-v2" sentence-transformer states training on a **passage retrieval** dataset. Is this the more common phrase under which such an asymetric task is discussed, would really like to read and understand more behind it.
<|||||>@corticalstack
It is not a common distinction, so no wonder that SBERT is on the first position.
For semantic search you have basically two cases:
**Paraphrase search (or symmetric semantic search)**
E.g., I have a query like "How can I learn Python via the internet" and you want to find a paraphrase like "How to learn Python using the web". Here, query & document are interchangeable, i.e., the relation is symmetric.
**QA Retrieval / Information Retrieval (or asymmetric semantic search)**
You have a short query (like "learning Python") and you want to find a long document providing you with the answer.
If you use a symmetric model for your QA retrieval (asym. search task), you usually end up with bad results.
Passage retrieval mainly describes just that you search for a query and want to retrieve a text passage.
You could also have doc retrieval, you search for a short query and want to retrieve a long document.
I think there is no clear terminological distinction yet between the two outlined cases.
<|||||>@corticalstack The code for Synthetic Query Generation is available:
https://github.com/UKPLab/sentence-transformers/tree/master/examples/unsupervised_learning/query_generation
It can be used when you want to train a semantic search model without having training data. For the given paragraphs in your corpus, it generates queries what people could ask about this paragraph.
It then uses this generated queries and the paragraph to train a model on it.
We saw really good results with this on various datasets:
https://github.com/UKPLab/sentence-transformers/tree/master/examples/unsupervised_learning/query_generation
Currently we prepare an extensive publication that explains it in more detail, provides more insights and also shows ways for optimization.<|||||>@nreimers Thanks so much for the update and great work here, shall try it in next week and report back. Look forward to reading more, any approximate expectation for release of publication?<|||||>@corticalstack As we run quite a lot of experiment for various domains and tasks, I expect that it will still take some time until we publish the paper. I think it will be available in about 2 months.<|||||>This issue has been automatically marked as stale because it has not had recent activity. If you think this still needs to be addressed please comment on this thread.
Please note that issues that do not follow the [contributing guidelines](https://github.com/huggingface/transformers/blob/master/CONTRIBUTING.md) are likely to be ignored.<|||||>@nreimers finally got around to testing the synthetic code generation, fascinating query generation and working to understand it better. Has the related paper been published?<|||||>Hi @corticalstack
We did some experiments in: https://arxiv.org/abs/2104.08663
But the longer (and more detailed) paper is sadly not ready yet.<|||||>@nreimers thanks very much for link to paper, will cite in my work<|||||>@nreimers Thanks so much for both the synthetic query generation and BEIR benchmarking utilities, they are fantastic. I've done the following for task of passage (paragraph) retrieval on CORD-19 covid-19 corpus:
- Used synthetic query generation over 10% sample of cord-19 collection, with model/tokenizer _BeIR/query-gen-msmarco-t5-large-v1 for ~600K query/text combinations_ (2 queries generated per paragraph)
- Generated queries to train _distilbert-base-uncased_ by encoding cord-19 article paragraphs, BEIR testing gives **trec_covid ndcg@10 score 0.468**
- Generated queries to train _sentence-transformers/msmarco-distilbert-base-v3_, BEIR testing gives **trec_covid ndcg@10 score 0.466**
- Base _msmarco-distilbert-base-v3_ without training in BEIR testing gives **trec_covid ndcg@10 score 0.477**
- BM25 achieves **trec_covid ndcg@10 score 0.615**
Training done pretty much as per your synthetic generation example:
[query generation train encoder](https://github.com/UKPLab/sentence-transformers/blob/master/examples/unsupervised_learning/query_generation/2_programming_train_bi-encoder.py)
For query generation seq2seq tokenizer Ive set max_length at 300, output query max_length at 64, and epochs for training at 3.
As per your paper [https://arxiv.org/abs/2104.08663](https://arxiv.org/abs/2104.08663), BM25 is hard to beat. But such a gap between BM25 and the neural models. Also identify the trained model performs slightly poorer than trained model.
Would welcome any pointers to try and up trec-covid score for neural models, as this benchmark seems the most comparable to the cord-19 corpus?
**Edit:** mean length of cord-19 article paragraph is 730, and title 100, after running query gen with max_length = 850, **trec_covid ndcg@10 score** up to **0.473** (from 0.466).
**Edit 2** With same cord-19 generated queries training (input max_length 850, query output max_length 180), and model msmarco-roberta-base-ance-fristp, **trec_covid ndcg@10 score 0.547**. Progress :)
**Edit 3** sampling just 0.5% cord-19 articles (525 articles), now with 4 queries generated per passage (prev=2), with model _castorini/ance-msmarco-passage_, **trec_covid ndcg@10 score 0.553**<|||||>Hi @corticalstack (cc @NThakur20)
We currently also perform some experiments with TREC-COVID-19. Here are some insights:
- When you train with cosine similarity, the model prefers to retrieve publications that have just a title. However, there is a strong annotation bias that such publications were seldom annotated and hence, are automatically marked as not relevant even though they might be relevant. I think a better setup for TREC-COVID-19 is to just focus on publications that have a title and a non-empty abstract
- When you train with dot-product, the model prefers longer documents and hence retrieves more docs with title & abstract, which results in a higher score. However, it is not clear if such a system is really better as annotators did not really annotate publications where the abstract is missing.
- We also observe that dense models retrieve a lot of docs that have not been seen by an annotator and are marked as not relevant. This is problematic, as we don't know if these publications are really irrelevant or not. The fraction of publications that BM25 retrieves and have not been seen by an annotator is a lot smaller, as annotators used BM25 to retrieve the candidates for annotations. So the difference between BM25 and dense models could be primarily due to this difference, that dense model retrieve good hit, but which have not been annotated. We don't know and more extensive annotations would be needed.
<|||||>@nreimers grateful if you can consider the following question: "_Which of the current vaccines in the clinic have reported the highest levels of neutralizing Abs after a single vaccination?_". Appreciate this question could be considered rather ambiguous. However, testing with BM25 and various base/trained dense models (synthetic query generation), top results focus on words like _neutralizing_ , missing the caveat in the question "after a single vaccination" . I'm finding results from models miss opening few words in questions which set the context, such as "Which of the current vaccines".
Another example is "_Which vaccines have been approved_" with documents scoring highly that contain "_approved_" but not related to vaccines.
A third example is "_What T-cell epitopes been identified in the Receptor Binding Motif (RBM) region of the S-glycoprotein Receptor Binding Domain (RBD) of the SARS-CoV-2 virus?_" which picks up documents containing _glycoprotein_ but misses the constraint "What t-cell epitopes". Other ranked documents discuss epitopes but not the T-cell types.
In that last example we are only interested in articles that specifically discuss t-cell epitopes and so the question is how we can enforce such constraints with dense models.
It seems that unless there is a Eureka moment hitting a golden paper that directly answers the question, then answers are very much somewhat relevant and can be useful but don't begin to answer the question fully. Typically , a human researcher, an expert in their field such as biomedical, will search multiple knowledge sources, collating snippets from papers in a spreadsheet, aggregating for a high-quality answer. I'm interested in how I can refine the dense model to better capture constraints like "which of the <constraint> have been ......", "what <constraint>.....reports/have been identified ........". Then, aggregating their relevant texts together to more fully answer the question, but that Im sure is another topic and challenge.<|||||>Hi @corticalstack
In that case I think a cross-encoder is better, that re-ranks your results. See: https://www.sbert.net/examples/applications/retrieve_rerank/README.html
Dense models have limitations that it is really hard to encode multiple constrains from a query into a vector space. Cross-Encoders, on the other hand, are a lot better on these constraint and fine-grade checking.
<|||||>@nreimers @NThakur20
On reading the original [T5](https://arxiv.org/pdf/1910.10683.pdf) paper, as a multi-task model I'm curious to know which task prefix you added to the input sequence and how "interrogatives" were added/coerced to the text output such as "what is", "which of the", "how to"? Is this something you can share? Thanks!
Edit: I assume its the T5 sequence-2-sequence (or text to text) task. Just checking the [docTTTTT](https://cs.uwaterloo.ca/~jimmylin/publications/Nogueira_Lin_2019_docTTTTTquery-v2.pdf) and original [doc2query](https://arxiv.org/pdf/1904.08375.pdf) papers for possible answers, but fascinating that training on collection of query datasets can consistently pose interrogatives.<|||||>@corticalstack
Hi there, yes, you are right. We use the T5 seq-2-seq model and directly train on the passage-query pairs without adding any prefix. This is also why we don't have any control over what interrogative words (like what, why, etc) the model outputs in the synthetic text.
The distribution of these words more or less resembles the one present in the training set (therefore, ours resembles the distribution in MSMARCO). One way to control these globally would be to alter the distribution in your train set. I can also think how adding prefixes like, "what: <what query>" and "when: <when query>" in the training set may allow you to do this for individual instances. I haven't experimented with this but similar past experiments suggest this could work.<|||||>Very interesting discussion!
I have a related and hopefully simpler task:
> finding multiword expressions (MWE) that are most similar to a reference MWE.
MWE can range from bigram short to 5-gram long phrases. Can I please ask if anyone has suggestions on the best approach for this? Would it be simply retraining a word embedding model by adding preprocessed phrases? Or is there a more recent approach that achieves this?
Much appreciated! <|||||>You could simply use the library NLTK and their phrases module, then train
your w2v model on your corpus that can contain MWE/phrases ! :)
lör 24 juli 2021 kl. 06:13 skrev Bo Wang ***@***.***>:
> Very interesting discussion!
>
> I have a related and hopefully simpler task: finding multiword expressions
> (MWE) that are most similar to a reference MWE. MWE can range from bigram
> short to 5-gram long phrases. Can I please ask if anyone has suggestions on
> the best approach for this? Would it be simply retraining a word embedding
> model by adding preprocessed phrases? Or is there a more recent approach
> that achieves this?
>
> Much appreciated!
>
> —
> You are receiving this because you were mentioned.
> Reply to this email directly, view it on GitHub
> <https://github.com/huggingface/transformers/issues/876#issuecomment-885996543>,
> or unsubscribe
> <https://github.com/notifications/unsubscribe-auth/ABSAYJXK33JELKT7XVOVOJ3TZI4UZANCNFSM4IGKGJTQ>
> .
>
<|||||>@timpal0l Thanks! I am actually wondering if there is a more recent approach of embedding MWEs and find the most similar MWEs? I imagine the search space for character-level embeddings is perhaps infinite. Also would it be possible to ask these large LMs such as GPT-3 to complete for example "I feel ___" providing a reference sentence for example "I feel lack of family support"?<|||||>I am using sentence-bert for creating the embeddings and the faisslibrary for the query search. Does anyone know how I can evaluate the retrieved outputs for a given query on my own dataset? |
transformers | 875 | closed | XLNet bidirectional input pipeline requires batch size at least 2 | This may not be a true bug since it's mentioned in the paper that
> each of the forward and backward directions takes half of the batch size
but when using the bidirectional input pipeline, any call to `XLNetModel.forward()` will raise an error of the form
```
RuntimeError: shape '[x, y, z]' is invalid for input of size 0
```
if the **batch size of the `input_ids` passed is less than 2**. This is because it halves (integer div) `bsz` in accordance with the above quote in the following block:
```
if self.bi_data:
fwd_pos_seq = torch.arange(beg, end, -1.0, dtype=torch.float)
bwd_pos_seq = torch.arange(-beg, -end, 1.0, dtype=torch.float)
if self.clamp_len > 0:
fwd_pos_seq = fwd_pos_seq.clamp(-self.clamp_len, self.clamp_len)
bwd_pos_seq = bwd_pos_seq.clamp(-self.clamp_len, self.clamp_len)
if bsz is not None:
fwd_pos_emb = self.positional_embedding(fwd_pos_seq, inv_freq, bsz//2)
bwd_pos_emb = self.positional_embedding(bwd_pos_seq, inv_freq, bsz//2)
```
The result is a batch size of `0`, which obviously wreaks havoc later on. It's only relevant if people are trying to run a MWE with really small input as I was for testing, but maybe an assert statement somewhere near the top of the `XLNetModel.forward()` function is a good idea, conditional on `bi_data` being `True`.
More generally, a shape mismatch is caused for the same reason if `bi_data` is `True` and `bsz` is any positive odd integer. | 07-23-2019 18:18:01 | 07-23-2019 18:18:01 | This issue has been automatically marked as stale because it has not had recent activity. It will be closed if no further activity occurs. Thank you for your contributions.
|
transformers | 874 | closed | Fine-tuning model and Generation | Hello!
I am beginner and I just wanted to run some experiments, but I've hit a road block. I am trying to generate text using `run_generator.py` after I fine-tune a model on my data using `simple_lm_finetuning.py`. I've looked around a bit, and I'm not sure how to go about this, or if this is possible at all. I don't see an option for `run_generator` to use BERT models, and I'm not sure how to bridge the two scripts.
Basically what I want to do is to fine-tune a model on my data and then generate text. Can this be done with `run_generator` and `simple_lm_finetuning`?
Thank you!
---
EDIT:
Forgot to add my code:
```
python pytorch-transformers/examples/lm_finetuning/simple_lm_finetuning.py \
--train_corpus data.txt \
--bert_model bert-base-uncased \
--do_lower_case \
--output_dir finetuned_lm/ \
--do_train
python pytorch-transformers/examples/run_generation.py \
--model_type=transfo-xl \
--length=20 \
--model_name_or_path='finetuned_lm'
``` | 07-23-2019 17:43:02 | 07-23-2019 17:43:02 | Having the same question - how to use bert for generation? <|||||>the same problem, how to train my own data for text generation?<|||||>We'll add an example for fine-tuning this month.<|||||>@thomwolf as I read in other issues, BERT model cannot be used to generate text directly (your reply https://github.com/huggingface/pytorch-transformers/issues/401#issuecomment-477111518).
What exact examples are you planning to add? Thanks.<|||||>@Bruno-bai did you figure out how to train own data?<|||||>Not really. Would appreciate a tutorial:)
On Mon, Aug 19, 2019 at 5:22 AM Vedang Mandhana <[email protected]>
wrote:
> @Bruno-bai <https://github.com/Bruno-bai> did you figure out how to train
> own data?
>
> —
> You are receiving this because you commented.
> Reply to this email directly, view it on GitHub
> <https://github.com/huggingface/pytorch-transformers/issues/874?email_source=notifications&email_token=AHZ2KL5Q2RIKUTGARMX6EY3QFINYTA5CNFSM4IGHELH2YY3PNVWWK3TUL52HS4DFVREXG43VMVBW63LNMVXHJKTDN5WW2ZLOORPWSZGOD4RUR4I#issuecomment-522406129>,
> or mute the thread
> <https://github.com/notifications/unsubscribe-auth/AHZ2KL3FS6CDZQN75KNXVPDQFINYTANCNFSM4IGHELHQ>
> .
>
<|||||>this would be very useful example to have. to finetune gpt2, xlnet, ... and run generation from the finetuned model. Don't know whether bert supports generation or not, but the ones that do..<|||||>I am too struggling with similar problem. I want to train a non-english (hindi) language model on my custom dataset and use it for text generation. From what I understood, BERT sucks at text generation as it uses MLM for training. The ones that do well (gpt,trans-xl,xlnet) don't have a pretrained multilingual model available.
@Bruno-bai @sakalouski are you looking for training own data for language generation? Coz I have done it for classification and can help with that.<|||||>Hi @thomwolf
> We'll add an example for fine-tuning this month.
Has this example been added yet?
Thanks<|||||>Hi @amin-nejad, the example has been added and is available [here](https://github.com/huggingface/pytorch-transformers/blob/master/examples/run_lm_finetuning.py).<|||||>Thanks @LysandreJik. Will this also work with Transformer-XL if we just modify the source code to include the Transformer-XL Config, LMHeadModel and Tokenizer as a model class? Or will it require more substantial changes?<|||||>Using `run_lm_finetuning.py` seemingly works for Transformer-XL if we additionally import the Transformer-XL Config, LMHeadModel and Tokenizer and modify the `MODEL_CLASSES` to include them. We also need to provide the `block_size` as a command line parameter. Training curves look reasonable and decoding also happens without errors using `run_generation.py` but the model output is pretty much always just a bunch of equals signs e.g. `= = = = = = = = =` etc. for me at least anyway. Clearly more substantial changes are required to `run_lm_finetuning.py` to make it work. If anyone knows what/why, please let me know<|||||>One thing we should do (maybe when we have some bandwidth for that with @LysandreJik) is to push back a PR to PyTorch repo to add an option to have biases on all clusters of PyTorch's Adaptive Softmax so we can rely on the official Adaptive Softmax implementation instead of having our own.
That would make the job of maintaining and upgrading Transformer-XL a lot easier as it's currently the most cumbersome code base to maintain.<|||||>This issue has been automatically marked as stale because it has not had recent activity. It will be closed if no further activity occurs. Thank you for your contributions.
|
transformers | 873 | closed | Add nn.Identity replacement for old PyTorch | Fix #869 to keep at least PyTorch 1.0.0 compatiblity. | 07-23-2019 15:53:06 | 07-23-2019 15:53:06 | |
transformers | 872 | closed | Updating schedules for state_dict saving/loading | This PR updates the schedules so that they can be saved/reloaded using the standard `state_dict()` and `load_state_dict()` methods of PyTorch [`LambdaLR` model](https://pytorch.org/docs/stable/optim.html#torch.optim.lr_scheduler.LambdaLR.load_state_dict).
Useful for continuing stopped training as mentioned in #839 | 07-23-2019 13:59:43 | 07-23-2019 13:59:43 | # [Codecov](https://codecov.io/gh/huggingface/pytorch-transformers/pull/872?src=pr&el=h1) Report
> Merging [#872](https://codecov.io/gh/huggingface/pytorch-transformers/pull/872?src=pr&el=desc) into [master](https://codecov.io/gh/huggingface/pytorch-transformers/commit/268c6cc160ba046d6a91747c5f281f82bd88a4d8?src=pr&el=desc) will **increase** coverage by `0.12%`.
> The diff coverage is `100%`.
[](https://codecov.io/gh/huggingface/pytorch-transformers/pull/872?src=pr&el=tree)
```diff
@@ Coverage Diff @@
## master #872 +/- ##
==========================================
+ Coverage 78.9% 79.03% +0.12%
==========================================
Files 34 34
Lines 6192 6228 +36
==========================================
+ Hits 4886 4922 +36
Misses 1306 1306
```
| [Impacted Files](https://codecov.io/gh/huggingface/pytorch-transformers/pull/872?src=pr&el=tree) | Coverage Δ | |
|---|---|---|
| [pytorch\_transformers/tests/optimization\_test.py](https://codecov.io/gh/huggingface/pytorch-transformers/pull/872/diff?src=pr&el=tree#diff-cHl0b3JjaF90cmFuc2Zvcm1lcnMvdGVzdHMvb3B0aW1pemF0aW9uX3Rlc3QucHk=) | `98.97% <100%> (+0.4%)` | :arrow_up: |
| [pytorch\_transformers/optimization.py](https://codecov.io/gh/huggingface/pytorch-transformers/pull/872/diff?src=pr&el=tree#diff-cHl0b3JjaF90cmFuc2Zvcm1lcnMvb3B0aW1pemF0aW9uLnB5) | `96.62% <100%> (+0.33%)` | :arrow_up: |
------
[Continue to review full report at Codecov](https://codecov.io/gh/huggingface/pytorch-transformers/pull/872?src=pr&el=continue).
> **Legend** - [Click here to learn more](https://docs.codecov.io/docs/codecov-delta)
> `Δ = absolute <relative> (impact)`, `ø = not affected`, `? = missing data`
> Powered by [Codecov](https://codecov.io/gh/huggingface/pytorch-transformers/pull/872?src=pr&el=footer). Last update [268c6cc...0740e63](https://codecov.io/gh/huggingface/pytorch-transformers/pull/872?src=pr&el=lastupdated). Read the [comment docs](https://docs.codecov.io/docs/pull-request-comments).
|
transformers | 871 | closed | fp16 is not work | GPU:v100
run run_glue.py with the command in the README:
```
python ./examples/run_glue.py \
--model_type bert \
--model_name_or_path bert-base-uncased \
--task_name $TASK_NAME \
--do_train \
--do_eval \
--do_lower_case \
--data_dir $GLUE_DIR/$TASK_NAME \
--max_seq_length 128 \
--per_gpu_eval_batch_size=8 \
--per_gpu_train_batch_size=8 \
--learning_rate 2e-5 \
--num_train_epochs 3.0 \
--output_dir /tmp/$TASK_NAME/
```
and i also run with --fp16, but spend the same time and gpu memory, why fp16 does not work | 07-23-2019 11:52:50 | 07-23-2019 11:52:50 | Duplicate of #868 |
transformers | 870 | closed | How to load a fine-tuned model pytorch_model.bin produced by run_bert_swag.py | Hi, guys! I have a little question about how to load a fine-tuned model 'pytorch_model.bin' produced by run_bert_swag.py.
When I load a fine-tuned model pytorch_model.bin with .from_pretrained methods, runtime error occurs as follow:
RuntimeError: storage has wrong size: expected 4357671300540823961 got 589824.
I fine-tuned a bert-base-uncased model.
| 07-23-2019 09:04:06 | 07-23-2019 09:04:06 | That's a strange error, what are the exact process you are using and full error log?<|||||>> That's a strange error, what are the exact process you are using and full error log?
Hi, thanks for the reply.
I used distributed training in one node with 2GPUs and my command is:
export SWAG_DIR=SWAG; export export CUDA_VISIBLE_DEVICES=2,3; python -m torch.distributed.launch --nproc_per_node=2 run_bert_swag.py --bert_model bert-base-uncased --do_train --do_lower_case --do_eval --data_dir $SWAG_DIR/data --train_batch_size 16 --learning_rate 2e-5 --num_train_epochs 1.0 --max_seq_length 80 --output_dir /home/disk1/chengqinyuan/pt_transformer_examples/swag_output --gradient_accumulation_steps 1
I modified run_swag.py with follow lines:
if args.do_train:
# Save a trained model, configuration and tokenizer
model_to_save = model.module if hasattr(model, 'module') else model # Only save the model it-self
# If we save using the predefined names, we can load using `from_pretrained`
output_model_file = os.path.join(args.output_dir, WEIGHTS_NAME)
output_config_file = os.path.join(args.output_dir, CONFIG_NAME)
# torch.save(model.state_dict(), output_model_file)
model_to_save.save_pretrained(args.output_dir)
model_to_save.config.to_json_file(output_config_file)
tokenizer.save_vocabulary(args.output_dir)
# Load a trained model and vocabulary that you have fine-tuned
model = BertForMultipleChoice.from_pretrained(args.output_dir, num_choices=4)
tokenizer = BertTokenizer.from_pretrained(args.output_dir, do_lower_case=args.do_lower_case)
else:
model = BertForMultipleChoice.from_pretrained(args.output_dir, num_choices=4)
tokenizer = BertTokenizer.from_pretrained(args.output_dir, do_lower_case=args.do_lower_case)
model.to(device)
And the error log is:
Traceback (most recent call last):
File "run_bert_swag.py", line 571, in <module>
main()
File "run_bert_swag.py", line 505, in main
model = BertForMultipleChoice.from_pretrained(args.output_dir)
File "/home/chengqinyuan/anaconda3/envs/py3/lib/python3.6/site-packages/pytorch_transformers-1.0.0-py3.6.egg/pytorch_transformers/modeling_utils.py", line 406, in from_pretrained
File "/home/chengqinyuan/anaconda3/envs/py3/lib/python3.6/site-packages/torch/serialization.py", line 387, in load
return _load(f, map_location, pickle_module, **pickle_load_args)
File "/home/chengqinyuan/anaconda3/envs/py3/lib/python3.6/site-packages/torch/serialization.py", line 581, in _load
deserialized_objects[key]._set_from_file(f, offset, f_should_read_directly)
RuntimeError: storage has wrong size: expected -4807048246308659860 got 589824
By the way, when I used the script to save a pre_trained model without fine-tune(I skipped the training process and then saved the model), it can normally load the saved model and do evaluation. But the error would occur when I loaded a fine-tuned model.
Or could you please give me a brief guideline to execute run_swag.py with pytorch_transformers. I followed the guideline at https://huggingface.co/pytorch-transformers/examples.html and encounter many bugs. Thank you very much!<|||||>I solved this error by use multi-GPU training instead of distributed training, it seems like something wrong in distributed training setting, thanks for your reply : )<|||||>> I solved this error by use multi-GPU training instead of distributed training, it seems like something wrong in distributed training setting, thanks for your reply : )
我在用单机多卡训练后保存模型也遇到了这个问题,请问你是怎么解决的,用DataParallel还是DistributedDataParallel?
|
transformers | 869 | closed | module 'torch.nn' has no attribute 'Identity' | Traceback (most recent call last):
File "trainer.py", line 17, in <module>
model = XLMForSequenceClassification(config)
File "/home/ankit/anaconda3/lib/python3.6/site-packages/pytorch_transformers/modeling_xlm.py", line 823, in __init__
self.sequence_summary = SequenceSummary(config)
File "/home/ankit/anaconda3/lib/python3.6/site-packages/pytorch_transformers/modeling_utils.py", line 734, in __init__
self.summary = nn.Identity()
AttributeError: module 'torch.nn' has no attribute 'Identity'
https://github.com/huggingface/pytorch-transformers/blob/2f869dc6651f9cf9253f4c5a43279027a0eccfc5/pytorch_transformers/modeling_utils.py#L734 | 07-23-2019 08:20:57 | 07-23-2019 08:20:57 | This was added in PyTorch 1.1.0 (see [changelog here](https://github.com/pytorch/pytorch/tree/v1.1.0) :)
So I guess you just have to update your PyTorch version!<|||||>Oh yes, I guess we can add a replacement to keep older PyTorch compatibility.
Would be sad to lose backward compatibility just for this.<|||||>Is this replacement added in any of the newer versions? |
transformers | 868 | closed | fp16 is broken | run run_glue.py with the parameter of --fp16, and return error:
```
RuntimeError: Incoming model is an instance of torch.nn.parallel.DistributedDataParallel. Parallel wrappers should only be applied to the model(s) AFTER
the model(s) have been returned from amp.initialize.
```
i find the reason is the wrong order of `amp.initialize` and `model = torch.nn.DataParallel`, [iIf DDP wrapping occurs before amp.initialize, amp.initialize will raise an error](https://github.com/NVIDIA/apex/blob/master/examples/imagenet/README.md), and it worked after i change the order | 07-23-2019 08:18:21 | 07-23-2019 08:18:21 | Indeed, thanks! Fixed in master |
transformers | 867 | closed | XLnet sentence vector | how can i get the XLnet sentence vector by pytorch-transformers. I use the sample but I only get the word vector. it drives me crazy | 07-23-2019 02:06:26 | 07-23-2019 02:06:26 | You can train the model on a downstream task to get a sentence vector related to your task or you can get a sentence vector by averaging or max-pooling the output sequence of token hidden-states.<|||||>Try doing:
```Python
model = model_class.from_pretrained(pretrained_weights,
output_hidden_states=True,
output_attentions=True)
sequence_summary = SequenceSummary(model.config)
es = torch.tensor([tokenizer.encode(s)])
# sentence embedding
t = sequence_summary(model(es)[0])<|||||>A simple strategy is to take the concatentation of the last hidden state, the mean-pooling, and the max-pooling (tends to be a reasonably good baseline pooling strategy, e.g. [ULMFit](https://arxiv.org/pdf/1801.06146.pdf))<|||||>@cpcdoy hey, and I have a question why the sentence is not stationary.<|||||>So, we should pool over all hidden states, and not just use the hidden state corresponding to `[CLS]`?<|||||>I would go with @rishibommasani's solution for a general "semantic" sentence embeddings or fine-tuning and using the `[CLS]` for a task-specific sentence embedding.<|||||>This issue has been automatically marked as stale because it has not had recent activity. It will be closed if no further activity occurs. Thank you for your contributions.
|
transformers | 866 | closed | Rework how PreTrainedModel.from_pretrained handles its arguments | Unification of the `from_pretrained` functions belonging to various modules (GPT2PreTrainedModel, OpenAIGPTPreTrainedModel, BertPreTrainedModel) brought changes to the function's argument handling which don't cause any issues within the repository itself (afaik), but have the potential to break a variety of downstream code (eg. my own).
In the last release of pytorch_transformers ([v0.6.2](https://github.com/huggingface/pytorch-transformers/tree/v0.6.2)), the `from_pretrained` functions took in `*args` and `**kwargs` and passed them directly to the relevant model's constructor (perhaps with some processing along the way). For a typical example, see `from_pretrained`'s signature in `modeling.py` here https://github.com/huggingface/pytorch-transformers/blob/b832d5bb8a6dfc5965015b828e577677eace601e/pytorch_pretrained_bert/modeling.py#L526
and the relevant usage of said arguments (after [some small modifications](https://github.com/huggingface/pytorch-transformers/blob/b832d5bb8a6dfc5965015b828e577677eace601e/pytorch_pretrained_bert/modeling.py#L553-L558)) https://github.com/huggingface/pytorch-transformers/blob/b832d5bb8a6dfc5965015b828e577677eace601e/pytorch_pretrained_bert/modeling.py#L600
In the [latest release](https://github.com/huggingface/pytorch-transformers/tree/v1.0.0), the function's signature remains unchanged but the `*args` and most of the `**kwargs` parameters, in particular pretty much anything not explicitly accessed in [[1]](https://github.com/huggingface/pytorch-transformers/blob/b33a385091de604afb566155ec03329b84c96926/pytorch_transformers/modeling_utils.py#L354-L358)
https://github.com/huggingface/pytorch-transformers/blob/b33a385091de604afb566155ec03329b84c96926/pytorch_transformers/modeling_utils.py#L354-L358
is ignored. If a key of `kwargs` is shared with the relevant model's configuration file then its value is still used to override said key (see the relevant logic [here](https://github.com/huggingface/pytorch-transformers/blob/b33a385091de604afb566155ec03329b84c96926/pytorch_transformers/modeling_utils.py#L138-L148)), but the current architecture breaks, for example, the following pattern which was previously possible.
```
class UsefulSubclass(BertForSequenceClassification)
def __init__(self, *args, useful_argument, **kwargs):
super().__init__(*args, **kwargs)
*logic*
...
bert = UsefulSubclass.from_pretrained(model_name, useful_argument=42).
```
What's more, if these arguments have default values declared in `__init__` then the entire pattern is broken **silently**: because these default values will **never** be overwritten via pretrained instantiation. Thus end users might continue running experiments passing different values of `useful_argument` to `from_pretrained`, unaware that **nothing is actually being changed**
As evidenced by issue #833, I'm not the only one whose code was broken. This commit implements behavior which is a compromise between the old and new behaviors. From [my docstring](https://github.com/xanlsh/pytorch-transformers/blob/764b2d3d2310458b77dc563913313ba0c6d826dd/pytorch_transformers/modeling_utils.py#L347-L351):
```
If config is None, then **kwargs will be passed to the model.
If config is *not* None, then kwargs will be used to
override any keys shared with the default configuration for the
given pretrained_model_name_or_path, and only the unshared
key/value pairs will be passed to the model.
```
It would actually be ideal to avoid mixing configuration and model parameters entirely (via some sort of `model_args` parameter for example): however this fix has the advantages of
1. Not breaking code written during the `pytorch-pretrained-bert` era
2. Preserving (to the extent possible) the usage of the `from_pretrained.**kwargs` parameter introduced with `pytorch-transformers`
--------------------------------------------------------------------------
I have also included various other (smaller) changes in this pull request:
* ~~Making `PreTrainedModel.__init__` not accept `*args` and `**kwargs` parameters which it has no use for and currently ignores~~ Apparently necessary for the tests to pass :(
* ~~Stop using the the "popping from kwargs" antipattern (see [[1]](https://github.com/huggingface/pytorch-transformers/blob/b33a385091de604afb566155ec03329b84c96926/pytorch_transformers/modeling_utils.py#L354-L358)). Keyword arguments with default values achieve the same thing more quickly, and are strictly more informative since they linters/autodoc modules can actually make use of them. I've replaced all instances that I could find, if this pattern exists elsewhere it should be removed.~~ Oops: turns out this is a Python 2 compatibility thing. With that said, is there really a need to continue supporting Python 2? Especially with its EOL coming up in just a few months, and especially when it necessitates such ugly code...
* Subsume the fix included in #864 , which would conflict (admittedly in a very minor fashion) with this PR.
* Remove some trailing whitespace which seems to have infiltrated the file | 07-22-2019 20:42:34 | 07-22-2019 20:42:34 | Hmm, well that's embarrassing. I'll inspect the failing tests some more to see what's up<|||||>Regarding python 2, yes we want to keep supporting it and thanks for taking care of it.
Google (which is still using python 2) is a major supplier of pretrained model and architectures and having python 2 support in the library make the job of re-implementing the models a lot easier (I can load TF and PT models side-by-side) :)<|||||>I have updated the readme breaking change section on this (ba52fe6)<|||||>Thanks for the feedback: In my latest commits I've updated the documentation as requested and renamed the `return_unused_args` parameter to `return_unused_kwargs` to remove any ambiguity.
I also removed the unused `*args` parameter from `PreTrainedConfig.from_pretrained`, which is the only actual interface/logic change<|||||>Looks good to me, thanks a lot @xanlsh! |
transformers | 865 | closed | Using Fp16 half precision makes Bert prediction slower. | When I use:
model = BertForMaskedLM.from_pretrained('bert-large-cased')
model = model.half()
model.eval()
model.to('cuda')
by adding Fp16:
model = model.half()
It runs around 50% slower. Why is that?
I run it on ubuntu 18.04, cuda 9, pytorch 1.1 and python 3.6.8
| 07-22-2019 20:01:12 | 07-22-2019 20:01:12 | gtx 1080. Is there any other way to make the predictions faster?<|||||>Hi, you need at least a Volta GPU to get benefits from fp16 unfortunately.<|||||>@thomwolf Does P100 applicable?<|||||>I don't think so<|||||>This issue has been automatically marked as stale because it has not had recent activity. It will be closed if no further activity occurs. Thank you for your contributions.
|
transformers | 864 | closed | Fixed PreTrainedModel.from_pretrained(...) not passing cache_dir to PretrainedConfig.from_pretrained(...) | See #863
It's not a beautiful solution, but neither is the practice of modifying incoming parameters via pop. 🤷♂ | 07-22-2019 19:17:26 | 07-22-2019 19:17:26 | Indeed thanks. We'll subsume this PR with #866 which add a few other stuff.
I agree with you on the `pop` pattern. We'll move away from this when the first one of these two events happens: (i) google stop open-sourcing interesting new models or (ii) google stop using python 2 internally ;)<|||||>Okay! :+1: |
transformers | 863 | closed | PreTrainedModel.from_pretrained(...) doesn't pass cache_dir to PretrainedConfig.from_pretrained(...) | The cache_dir key-value parameter does not work as intended in `PreTrainedModel.from_pretrained(...)`. It is popped from the kwargs, then `PretrainedConfig.from_pretrained(...)` is called which expects this parameter in the kwargs, but it's obviously not there anymore. A default location is used as a fallback, but this leads to strange behaviour if this default location doesn't exist or isn't writable (as it was in my case). | 07-22-2019 19:15:00 | 07-22-2019 19:15:00 | Fix with #866 |
transformers | 862 | closed | Bert encodings | Hi,
Really interesting work!
I want to use BERT embeddings for a downstream task. I have been following the [steps](https://github.com/huggingface/pytorch-transformers#quick-tour) here as:
```
import torch
from pytorch_transformers import BertModel, BertTokenizer
pretrained_weights = 'bert-base-uncased'
tokenizer = BertTokenizer.from_pretrained(pretrained_weights)
model = BertModel.from_pretrained(pretrained_weights)
raw_text = ["[CLS] This is first element [SEP] continuing statement",
"[CLS] second element of the list."]
encoding = tokenizer.encode(raw_text)
input_ids = torch.tensor(encoding)
last_hidden_states = model(input_ids)[0] # Models outputs are now tuples
print(last_hidden_states.size())
```
getting the error as:
```
File "/home/shubham/anaconda3/envs/test/lib/python3.6/site-packages/pytorch_transformers/tokenization_utils.py", line 356, in split_on_tokens
split_text = text.split(tok)
AttributeError: 'list' object has no attribute 'split'
```
@thomwolf Is there an easy way to pass the list instead of strings or should I use lambda functions (which might be slow)? Can we pass the maximum sequence length as well?
Also I need some advice related to the code structure. If I have pre-exisiting code with data loader, should I convert these embedding there (can't do finetuning then) or pass the raw strings and convert them before passing them to model (model run gets too slow)?
Is there any way where we can pass the vocabulary (and tensors) directly instead of passing raw strings? | 07-22-2019 17:43:30 | 07-22-2019 17:43:30 | I have borrowed most of the ideas from [utils](https://github.com/huggingface/pytorch-transformers/blob/master/examples/utils_glue.py#L391) in one of the examples to create a [script to extract embeddings](https://gist.github.com/shubhamagarwal92/37ccb747f7130a35a8e76aa66d60e014).
However, I am still curious if there is any way where we can pass the vocabulary (and tensors) directly instead of passing raw strings?
<|||||>Hi, I think your example is nice.
I'm not sure to understand what you are referring to when you want to "pass the vocabulary (and tensors) directly instead of passing raw strings". <|||||>Currently, I am trying to get the Bert embeddings in my encoder before I use `nn.Embedding` instead of pre-computing it.
Thus, I have to convert the tensors to raw strings using `vocab` before passing it through the bert model and hence the gist. <|||||>Sorry,I want to know why we pass the sentence(Hello, my dog is cute) directly instead of adding some tokens([CLS] Hello, my dog is cute [SEP]) in the @thomwolf .
```
>>> config = BertConfig.from_pretrained('bert-base-uncased')
>>> tokenizer = BertTokenizer.from_pretrained('bert-base-uncased')
>>> model = BertModel(config)
>>> input_ids = torch.tensor(tokenizer.encode("Hello, my dog is cute")).unsqueeze(0) # Batch size 1
>>> outputs = model(input_ids)
>>> last_hidden_states = outputs[0] # The last hidden-state is the first element of the output tuple
```<|||||>If you use the function such as [this](https://github.com/huggingface/pytorch-transformers/blob/master/examples/utils_glue.py#L391), it appends the special tokens automatically. I guess the example you mentioned needs to append these tokens.
@thomwolf could verify that for you! <|||||>Yeah we'll add the option to automatically add control tokens. It can be useful.<|||||>This issue has been automatically marked as stale because it has not had recent activity. It will be closed if no further activity occurs. Thank you for your contributions.
<|||||>> If you use the function such as [this](https://github.com/huggingface/pytorch-transformers/blob/master/examples/utils_glue.py#L391), it appends the special tokens automatically. I guess the example you mentioned needs to append these tokens.
>
> @thomwolf could verify that for you!
Yes, I understand one can set "add_special_tokens=True" for the same when encoding the document. |
transformers | 861 | closed | Deleting models | I would like to delete the 'bert-base-uncased' and 'bert-large-uncased' models and the tokenizer from my hardrive (working under Ubuntu 18.04). I assumed that uninstalling pytorch-pretrained-bert would do it, but it did not. Where are these models located at?
Thanks!
| 07-22-2019 16:32:13 | 07-22-2019 16:32:13 | Models are usually located under `~/.cache/torch/pytorch_pretrained_bert` (older version of this library) or `~/.cache/torch/pytorch_transformers` (now) :)<|||||>Thank you! I found it elsewhere, actually. What worked for me (quaintly) was:
find . -type f -size +1G -print 2>/dev/null
<|||||>hey, do you guys know where it is stored on windows? thanks<|||||>In my case, it gets stored in " /tmp/torch"<|||||>In my case I found a variable containing the default cache path.
Run the following in python:
```
from transformers import file_utils
print(file_utils.default_cache_path)
```
If it is not there, check your environmental variables.
In my current transformers version `PYTORCH_PRETRAINED_BERT_CACHE`, `PYTORCH_TRANSFORMERS_CACHE` and `TRANSFORMERS_CACHE` can overwrite the default cache path. |
transformers | 860 | closed | read().splitlines() -> readlines() | splitlines() does not work as what we expect here for bert-base-chinese because there is a '\u2028' (unicode line seperator) token in vocab file. Value of '\u2028'.splitlines() is ['', ''].
Perhaps we should use readlines() instead. | 07-22-2019 12:49:29 | 07-22-2019 12:49:29 | # [Codecov](https://codecov.io/gh/huggingface/pytorch-transformers/pull/860?src=pr&el=h1) Report
> Merging [#860](https://codecov.io/gh/huggingface/pytorch-transformers/pull/860?src=pr&el=desc) into [master](https://codecov.io/gh/huggingface/pytorch-transformers/commit/2f869dc6651f9cf9253f4c5a43279027a0eccfc5?src=pr&el=desc) will **not change** coverage.
> The diff coverage is `100%`.
[](https://codecov.io/gh/huggingface/pytorch-transformers/pull/860?src=pr&el=tree)
```diff
@@ Coverage Diff @@
## master #860 +/- ##
======================================
Coverage 78.9% 78.9%
======================================
Files 34 34
Lines 6192 6192
======================================
Hits 4886 4886
Misses 1306 1306
```
| [Impacted Files](https://codecov.io/gh/huggingface/pytorch-transformers/pull/860?src=pr&el=tree) | Coverage Δ | |
|---|---|---|
| [pytorch\_transformers/tokenization\_bert.py](https://codecov.io/gh/huggingface/pytorch-transformers/pull/860/diff?src=pr&el=tree#diff-cHl0b3JjaF90cmFuc2Zvcm1lcnMvdG9rZW5pemF0aW9uX2JlcnQucHk=) | `93.2% <100%> (ø)` | :arrow_up: |
------
[Continue to review full report at Codecov](https://codecov.io/gh/huggingface/pytorch-transformers/pull/860?src=pr&el=continue).
> **Legend** - [Click here to learn more](https://docs.codecov.io/docs/codecov-delta)
> `Δ = absolute <relative> (impact)`, `ø = not affected`, `? = missing data`
> Powered by [Codecov](https://codecov.io/gh/huggingface/pytorch-transformers/pull/860?src=pr&el=footer). Last update [2f869dc...bef0c62](https://codecov.io/gh/huggingface/pytorch-transformers/pull/860?src=pr&el=lastupdated). Read the [comment docs](https://docs.codecov.io/docs/pull-request-comments).
<|||||>Great, thanks! |
transformers | 859 | closed | Bug of BertTokenizer | when load a tokenizer from pretrain
```python
tokenizer = BertTokenizer.from_pretrained(vocab_path)
```
the vocab length is:
```python
len(tokenizer.vocab)
21128
```
but the last token of vocab is:
```python
next(reversed(tokenizer.vocab.items()))
('##😎', 21129)
```
| 07-22-2019 12:25:06 | 07-22-2019 12:25:06 | If you are loading the chinese model, this is probably related to #860 and #825.
Should be fixed now.<|||||>thanks! |
transformers | 858 | closed | CLS segment_id for BERT | Hello,
In your example for GLUE you set the CLS segment id token to 1 for BERT: https://github.com/huggingface/pytorch-transformers/blob/2f869dc6651f9cf9253f4c5a43279027a0eccfc5/examples/run_glue.py#L259
Reading the original reference implementation it seems that CLS should have a segment_id=0. This is also aligned with several comments & docstrings you have around the code. Is this a design choice? What is the impact on general performance? | 07-22-2019 12:23:08 | 07-22-2019 12:23:08 | Yes, this one was also mentioned in https://github.com/huggingface/pytorch-transformers/issues/810#issuecomment-512991164.
It is fixed now. |
transformers | 857 | closed | XLMForMaskedLM | Hi, I am currently training a BERT model using facebook XLM framework. I use the script in this repo to convert XLM format to PyTorch format. Is it possible to implement an `XLMForMaskedLM` which is just like `BertForMaskedLM` but use XLM trained BERT instead? | 07-22-2019 09:57:54 | 07-22-2019 09:57:54 | You can do that using `XLNetLMHeadModel` and custom masks as shown in the [`run_generation` example](https://github.com/huggingface/pytorch-transformers/blob/master/examples/run_generation.py#L115-L121).
But note that XLNet is rather bad on short text input completions as I discussed in https://github.com/huggingface/pytorch-transformers/issues/846#issuecomment-514228565<|||||>> #846 (comment)
Thanks for your reply! However, I mean the `XLM` version of BERT, instead of XLNet. Is it also convenient to do that for XLM?
Thanks!<|||||>Oh, right! You can just use `XLMWithLMHeadModel` with an input sequence containing XLM masked token `tokenizer.mask_token` (which is `<special1>` for XLM).<|||||>This issue has been automatically marked as stale because it has not had recent activity. It will be closed if no further activity occurs. Thank you for your contributions.
|
transformers | 856 | closed | manually download models | ERROR:pytorch_transformers.modeling_utils:Couldn't reach server at 'https://s3.amazonaws.com/models.huggingface.co/bert/bert-base-uncased-config.json' to download pretrained model configuration file.
ERROR:pytorch_transformers.modeling_utils:Couldn't reach server at 'https://s3.amazonaws.com/models.huggingface.co/bert/bert-base-uncased-pytorch_model.bin' to download pretrained weights.
ERROR:pytorch_transformers.tokenization_utils:Couldn't reach server to download vocabulary.
how can I point to these 2 files if I manually download these two to some path? | 07-22-2019 05:31:09 | 07-22-2019 05:31:09 | If you don't want/cannot to use the built-in download/caching method, you can download both files manually, save them in a directory and rename them respectively `config.json` and `pytorch_model.bin`
Then you can load the model using `model = BertModel.from_pretrained('path/to/your/directory')`<|||||>What if I try to run a GPT-2 example from docs Quickstart:
```
tokenizer = GPT2Tokenizer.from_pretrained('gpt2')
...
model = GPT2LMHeadModel.from_pretrained('gpt2')
```
and get this
```
INFO:pytorch_transformers.file_utils:https://s3.amazonaws.com/models.huggingface.co/bert/gpt2-vocab.json not found in cache, downloading to C:\Users\KHOVRI~1\AppData\Local\Temp\tmprm150emm
ERROR:pytorch_transformers.tokenization_utils:Couldn't reach server to download vocabulary.
```
Where should I put vocab file and get other files for GPT-2? I work under corporate proxy, maybe there is a way to write this proxy into the sort of config?<|||||>This issue has been automatically marked as stale because it has not had recent activity. It will be closed if no further activity occurs. Thank you for your contributions.
<|||||>> 错误:pytorch_transformers.modeling_utils:无法到达位于https://s3.amazonaws.com/models.huggingface.co/bert/bert-base-uncased-config.json的服务器,无法下载经过预先训练的模型配置文件。
> 错误:pytorch_transformers.modeling_utils:无法到达位于“ https://s3.amazonaws.com/models.huggingface.co/bert/bert-base-uncased-pytorch_model.bin ”的服务器以下载预先训练的权重。
> 错误:pytorch_transformers.tokenization_utils:无法访问服务器以下载词汇。
>
> 如果手动将这两个文件下载到某个路径,如何指向这两个文件?
I also encountered such a problem, the network speed is very slow, can not get off.However, I ran several times, about 10 times, and finally successfully ran without any error
<|||||>Same question. Thank you.<|||||>> If you don't want/cannot to use the built-in download/caching method, you can download both files manually, save them in a directory and rename them respectively `config.json` and `pytorch_model.bin`
>
> Then you can load the model using `model = BertModel.from_pretrained('path/to/your/directory')`
For posterity, those who get errors because of missing vocab.txt despite doing above, you can get it at `https://s3.amazonaws.com/models.huggingface.co/bert/bert-base-uncased-vocab.txt` and also rename it to `vocab.txt` in desired folder. Resolved my errors.<|||||>Hi swayson,
model = BertModel.from_pretrained('path/to/your/directory')
where we need to add above line of code for loading model?<|||||>You can find all the models here [https://stackoverflow.com/a/64280935/251674](https://stackoverflow.com/a/64280935/251674)<|||||>> If you don't want/cannot to use the built-in download/caching method, you can download both files manually, save them in a directory and rename them respectively `config.json` and `pytorch_model.bin`
>
> Then you can load the model using `model = BertModel.from_pretrained('path/to/your/directory')`
so great!<|||||>I tried downloading these models and then upload it in Jupyter lab to use in `Styleformer ` package. But the result seems to be broken. It works fine in Google Colab but fails when I try to manually upload and run.
Models: https://huggingface.co/prithivida/informal_to_formal_styletransfer
https://huggingface.co/prithivida/parrot_adequacy_on_BART<|||||>In my case, I want to load gpt2 pretrained model locally.
- First I download config.json and pytorch_model.bin from [hugginface model zoo](https://huggingface.co/gpt2/tree/main)
When I execute code below:
`gpt2_tok = GPT2Tokenizer.from_pretrained(myfolderpath, do_lower_case=False)`
Some error occurs like:
`TypeError: expected str bytes or os.pathlike object not nonetype`
- Later I follow the instruction @swayson provides to download vocab.json.
Same error occurs again but it indicates that I may need some kind "merges" file:
`with open(merges_file, encoding="utf-8") as merges_handle
...
TypeError: expected str bytes or os.pathlike object not nonetype`
- So I go back to [hugginface model zoo](https://huggingface.co/gpt2/tree/main) and download merges.txt.
Finally the code is executed successfully and the encoding process also works.
Hope this helps someone who also suffers from the internet connection problem. |
transformers | 855 | closed | modeling_xlnet.py lines 798 torch.eisum('i,d->id', pos_seq, inv_freq) | hi I run position embedding in modeling_xlnet.py , but it not work , why not torch.eisum('i,d->id', [pos_seq, inv_freq]) ?i use pytorch 0.4.1 | 07-22-2019 04:55:53 | 07-22-2019 04:55:53 | I don't understand your question.
Can you give more details and point to the exact code lines you are referring to?
You cannot provide specific position indices to XLNet if that's what you are trying to do. You have to use the built-in relative embeddings.<|||||>This issue has been automatically marked as stale because it has not had recent activity. It will be closed if no further activity occurs. Thank you for your contributions.
<|||||>For new PyTorch 1.0, the syntax should be `torch.eisum('i,d->id', pos_seq, inv_freq) `<|||||>This issue has been automatically marked as stale because it has not had recent activity. It will be closed if no further activity occurs. Thank you for your contributions.
|
transformers | 854 | closed | Get the different result at BertModel | At old version pytorch-pretrained-bert :
I used the BertModel to fine-tuned, loss will decrease.
But I used the New version BertModel to use the same data to finetune, but loss won't decrease.
> optimzer
>I have tried different optimzer AdamW ,BertAdam.
> learning rate
>0.1 0.01 0.001 ... 0.0000000001
> Batch Size
>1
> input I put **input_ids** and **token_type_ids**
> At old version return _,CLS,I take CLS
> At new version return [seq_len,1,768],[seq_len,768],I take [0,1,:] or [0,:] loss won't decrease.
I don't know what the detail I miss in the new version?
| 07-22-2019 04:31:33 | 07-22-2019 04:31:33 | Have you read in detail the [migration guide](https://github.com/huggingface/pytorch-transformers#migrating-from-pytorch-pretrained-bert-to-pytorch-transformers) of the readme?
There is also a new `run_glue` example which is an updated version of the previous `run_classifier` and that you can use as a starting point for designing your own fine-tuning scripts.<|||||>This issue has been automatically marked as stale because it has not had recent activity. It will be closed if no further activity occurs. Thank you for your contributions.
|
transformers | 853 | closed | Error loading converted pytorch checkpoint | I am using BioBert. After converting the tensorflow checkpoint to pytorch checkpoint, I want to load it to Bert model. I found that the old pytorch-pretrained-bert works perfect but the new pytorch-transformer fails.
Here is the successful run using pytorch-pretrained-bert:
> from pytorch_pretrained_bert import BertForSequenceClassification
> model = BertForSequenceClassification.from_pretrained("biobert_v1.1_pubmed/", num_labels=1)
> exit()
Here's the failure in pytorch_transformers:
> from pytorch_transformers import BertForSequenceClassification
> model = BertForSequenceClassification.from_pretrained("biobert_v1.1_pubmed/", num_labels=1)
>
> Model name 'biobert_v1.1_pubmed/' was not found in model name list (bert-base-uncased, bert-large-uncased, bert-base-cased, bert-large-cased, bert-base-multilingual-uncased, bert-base-multilingual-cased, bert-base-chinese, bert-base-german-cased, bert-large-uncased-whole-word-masking, bert-large-cased-whole-word-masking, bert-large-uncased-whole-word-masking-finetuned-squad, bert-large-cased-whole-word-masking-finetuned-squad, bert-base-cased-finetuned-mrpc). We assumed 'biobert_v1.1_pubmed/config.json' was a path or url but couldn't find any file associated to this path or url.
> Traceback (most recent call last):
> File "<stdin>", line 1, in <module>
> File "/home/michael/.local/lib/python3.7/site-packages/pytorch_transformers/modeling_utils.py", line 403, in from_pretrained
> model = cls(config)
> File "/home/michael/.local/lib/python3.7/site-packages/pytorch_transformers/modeling_bert.py", line 958, in __init__
> super(BertForSequenceClassification, self).__init__(config)
> File "/home/michael/.local/lib/python3.7/site-packages/pytorch_transformers/modeling_bert.py", line 548, in __init__
> super(BertPreTrainedModel, self).__init__(*inputs, **kwargs)
> File "/home/michael/.local/lib/python3.7/site-packages/pytorch_transformers/modeling_utils.py", line 206, in __init__
> self.__class__.__name__, self.__class__.__name__
> ValueError: Parameter config in `BertForSequenceClassification(config)` should be an instance of class `PretrainedConfig`. To create a model from a pretrained model use `model = BertForSequenceClassification.from_pretrained(PRETRAINED_MODEL_NAME)`
| 07-22-2019 04:01:29 | 07-22-2019 04:01:29 | Do you have a file named `biobert_v1.1_pubmed/config.json` as mentioned in the error?<|||||>Oh thanks. There is a config file name "bert_config.json" in the directory "biobert_v1.1_pubmed/". I changed the file name to "config.json" and it works!
I am wondering why the pytorch-pretrained-bert can load the checkpoint. Maybe it reads "bert_config.json" and pytorch-transformer reads "config.json"? |
transformers | 852 | closed | UserWarning: Was asked to gather along dimension 0, but all input tensors were scalars; will instead unsqueeze and return a vector. | When I finetune Bert with simple_lm_finetuning.py, there seems an error:
"UserWarning: Was asked to gather along dimension 0, but all input tensors were scalars; will instead unsqueeze and return a vector."
Will it influence the performance of the finetuning process ? Thanks in advance for any suggestion. | 07-22-2019 02:55:22 | 07-22-2019 02:55:22 | It should be fine. There are probably your output losses.<|||||>@thomwolf Thanks for your reply. Could you explain more about why this happens ? I am still confused though<|||||>Maybe it is caused by calculating the loss in the model's forward function.
<|||||>This issue has been automatically marked as stale because it has not had recent activity. It will be closed if no further activity occurs. Thank you for your contributions.
<|||||>I have the same problem in version 2.2.1, what can it be?<|||||>In my case, it doesn’t influence the results
On Mon, Jan 6, 2020 at 5:19 AM calusbr <[email protected]> wrote:
> I have the same problem in version 2.2.1, what can it be?
>
> —
> You are receiving this because you authored the thread.
> Reply to this email directly, view it on GitHub
> <https://github.com/huggingface/transformers/issues/852?email_source=notifications&email_token=AKRMVV4J4UT6HCXEEMOYBGDQ4MVV3A5CNFSM4IFUKP3KYY3PNVWWK3TUL52HS4DFVREXG43VMVBW63LNMVXHJKTDN5WW2ZLOORPWSZGOEIFNJAI#issuecomment-571135105>,
> or unsubscribe
> <https://github.com/notifications/unsubscribe-auth/AKRMVV37AGO5AXJNPSGUVLTQ4MVV3ANCNFSM4IFUKP3A>
> .
>
<|||||>In my case, the speed of training with 4 GPU is the same as 1 GPU. How solve the speed issues?<|||||>👀 |
transformers | 851 | closed | problem when calling resize_token_embeddings | When calling resize_token_embeddings, the model actually only modifies its embedding and decoder weight, whlie the decoder bias is unchanged. So whenever the forward function is called, the following error will be raised.
```python
RuntimeError: The size of tensor a (21215) must match the size of tensor b (21128) at non-singleton dimension 2
``` | 07-22-2019 02:08:29 | 07-22-2019 02:08:29 | Which model were you resizing?<|||||>I'm working on chinese BertForPreTraining model<|||||>This is strange because Bert's LM head has no bias...
Would need to have a more complete error message to be able to understand.<|||||>```python
class BertLMPredictionHead(nn.Module):
def __init__(self, config):
super(BertLMPredictionHead, self).__init__()
self.transform = BertPredictionHeadTransform(config)
# The output weights are the same as the input embeddings, but there is
# an output-only bias for each token.
self.decoder = nn.Linear(config.hidden_size,
config.vocab_size,
bias=False)
self.bias = nn.Parameter(torch.zeros(config.vocab_size))
def forward(self, hidden_states):
hidden_states = self.transform(hidden_states)
hidden_states = self.decoder(hidden_states) + self.bias
return hidden_states
```
```python
self.bias = nn.Parameter(torch.zeros(config.vocab_size))
```
For example, we load the pretrained model whose vocab size is 23189, and i add 1000 tokens and call resize_token_embeddings. Since the decoder weight is actually embedding weight, they are reshaped to (24189, hidden_size), but the bias is still 23189.<|||||>This issue has been automatically marked as stale because it has not had recent activity. It will be closed if no further activity occurs. Thank you for your contributions.
|
transformers | 850 | closed | Confused about the prune heads operation. | In codes there are a 'prune_heads' method for the 'BertAttention' class, which refers to the 'prune_linear_layer' operation. Not understanding the meaning of such operation. The codes of 'prune_linear_layer' is listed below. Thanks for any help!
def prune_linear_layer(layer, index, dim=0):
""" Prune a linear layer (a model parameters) to keep only entries in index.
Return the pruned layer as a new layer with requires_grad=True.
Used to remove heads.
"""
index = index.to(layer.weight.device)
W = layer.weight.index_select(dim, index).clone().detach()
if layer.bias is not None:
if dim == 1:
b = layer.bias.clone().detach()
else:
b = layer.bias[index].clone().detach()
new_size = list(layer.weight.size())
new_size[dim] = len(index)
new_layer = nn.Linear(new_size[1], new_size[0], bias=layer.bias is not None).to(layer.weight.device)
new_layer.weight.requires_grad = False
new_layer.weight.copy_(W.contiguous())
new_layer.weight.requires_grad = True
if layer.bias is not None:
new_layer.bias.requires_grad = False
new_layer.bias.copy_(b.contiguous())
new_layer.bias.requires_grad = True
return new_layer | 07-21-2019 14:21:07 | 07-21-2019 14:21:07 | Yes, I'll add a detailed example for this method in the coming weeks (update of the bertology script).
This can be used to remove heads in the model following the work of [Michel et al. (Are Sixteen Heads Really Better than One?)](http://arxiv.org/abs/1905.10650) among others.<|||||>Thanks a lot!<|||||>Hi @thomwolf, would it be possible to provide an example on how to prune or select some heads for a layer? when i just change the config file by setting
config.pruned_heads = {11:[1,2,3]} and use it in initializing the model, it throws an error.
```
size mismatch for bert.encoder.layer.11.attention.self.query.weight: copying a param with shape torch.Size([768
urrent model is torch.Size([576, 768]). and more.
```
so, the default query,key and vaule are set with 768 dim.
I assume we can not just prune heads and still load the pre-trained model because the word embedding and layer norm was setup up with 768 dim. <|||||>meanwhile i came across bertology.py script and realize that we can save a model after pruning. that works fine for me. now, i'm trying to load the saved model, and I get the opposite error.
```
size mismatch for bert.encoder.layer.11.attention.self.query.weight: copying a param with shape torch.Size([576, 768]) from checkpoint, the sh
ape in current model is torch.Size([768, 768]).
```
the error wouldn't go away after even changing the config file. |
transformers | 849 | closed | can't find utils_glue | import fails | 07-21-2019 13:38:55 | 07-21-2019 13:38:55 | It's hard to tell what could be the source of the error without describing with some minimum details what you tried to do/run and which version of the code you are running...
_If_ you are running `run_glue.py` in the examples, make sure that [`utils_glue.py`](https://github.com/huggingface/pytorch-transformers/blob/master/examples/utils_glue.py) is present in the same working directory in which you are executing the script.<|||||>Thanks David. Turns out that the import in e.g., `run_glue.py` should be
`from examples.utils_glue import ...`
instead of
`from utils_glue import ...`
Maybe it's a problem of PyCharm IDE<|||||>This issue has been automatically marked as stale because it has not had recent activity. It will be closed if no further activity occurs. Thank you for your contributions.
|
transformers | 848 | closed | adaptive softmax in transformer-xl | I guess there is some incomplete part for adaptive softmax in [modeling_transfo_xl.py](https://github.com/huggingface/pytorch-transformers/blob/master/pytorch_transformers/modeling_transfo_xl.py)
Actually, it is impossible to build model not using adaptive softmax even though `TransfoXLConfig` has `adaptive` parameter.
I can see that if `sample_softmax` is larger than -1, model uses sample softmax other than adaptive softmax, which seems to be the case of not using adaptive softmax.
However, in case of `sample_softmax` > -1 and `tie_weight`=True, there is a problem on [this line](https://github.com/huggingface/pytorch-transformers/blob/master/pytorch_transformers/modeling_transfo_xl.py#L1317)<code><pre>self.out_layer.weight = self.transformer.word_emb.weight</pre></code> because the model always use `AdaptiveEmbedding` as `word_emb`, which has no `weight` property.
Presumably we need some code for not using adaptive softmax and but using standard softmax and usual `nn.Embedding` as a word embedding.
Then we can tie weights between standard `nn.Embedding` and `nn.Linear` when we doesn't need adaptive softmax.
Can you consider about this problem? Thanks. | 07-21-2019 13:22:04 | 07-21-2019 13:22:04 | Yes. At the moment, this library is designed for loading pretrained models mostly and no one has open-sourced a Transformer-XL pretrained model using something else than adaptive softmax so I have not spent time adding these options.
Happy to welcome PR though.
The main thing of interest here, if you want to give it a try would be to add bias to all clusters in PyTorch official [`AdaptiveLogSoftmaxWithLoss`](https://pytorch.org/docs/stable/nn.html?highlight=adaptivelogsoftmaxwithloss#torch.nn.AdaptiveLogSoftmaxWithLoss) module so we could just use the official implementation without maintaining ours.<|||||>This issue has been automatically marked as stale because it has not had recent activity. It will be closed if no further activity occurs. Thank you for your contributions.
|
transformers | 847 | closed | typos | "ouputs" -> "outputs" | 07-21-2019 12:40:09 | 07-21-2019 12:40:09 | # [Codecov](https://codecov.io/gh/huggingface/pytorch-transformers/pull/847?src=pr&el=h1) Report
> Merging [#847](https://codecov.io/gh/huggingface/pytorch-transformers/pull/847?src=pr&el=desc) into [master](https://codecov.io/gh/huggingface/pytorch-transformers/commit/a615499076a67dceb8907ecdf8eadaff04bb8d6a?src=pr&el=desc) will **not change** coverage.
> The diff coverage is `n/a`.
[](https://codecov.io/gh/huggingface/pytorch-transformers/pull/847?src=pr&el=tree)
```diff
@@ Coverage Diff @@
## master #847 +/- ##
======================================
Coverage 78.9% 78.9%
======================================
Files 34 34
Lines 6192 6192
======================================
Hits 4886 4886
Misses 1306 1306
```
------
[Continue to review full report at Codecov](https://codecov.io/gh/huggingface/pytorch-transformers/pull/847?src=pr&el=continue).
> **Legend** - [Click here to learn more](https://docs.codecov.io/docs/codecov-delta)
> `Δ = absolute <relative> (impact)`, `ø = not affected`, `? = missing data`
> Powered by [Codecov](https://codecov.io/gh/huggingface/pytorch-transformers/pull/847?src=pr&el=footer). Last update [a615499...76be189](https://codecov.io/gh/huggingface/pytorch-transformers/pull/847?src=pr&el=lastupdated). Read the [comment docs](https://docs.codecov.io/docs/pull-request-comments).
<|||||>Indeed! |
transformers | 846 | closed | XLNET completely wrong and random output | I followed the example here: https://huggingface.co/pytorch-transformers/model_doc/xlnet.html#pytorch_transformers.XLNetModel
I found that I get completelly wrong output, I mean predicted word for the masked sentences are completelly irelevant and they change each run. I guess there is some bug, culd you please take a look at this:
**code:**
##############################
config = XLNetConfig.from_pretrained('xlnet-large-cased')
tokenizer = XLNetTokenizer.from_pretrained('xlnet-large-cased')'
model = XLNetLMHeadModel(config)
input_ids = torch.tensor(tokenizer.encode("Hello, my dog is very <..mask..> ")).unsqueeze(0) # We will
predict the masked token
print("input_ids",input_ids)
perm_mask = torch.zeros((1, input_ids.shape[1], input_ids.shape[1]), dtype=torch.float)
perm_mask[:, :, -1] = 1.0 # Previous tokens don't see last token
target_mapping = torch.zeros((1, 1, input_ids.shape[1]), dtype=torch.float) # Shape [1, 1, seq_length] => let's predict one token
target_mapping[0, 0, -1] = 1.0
predictions = model(input_ids, perm_mask=perm_mask, target_mapping=target_mapping)
predicted_k_indexes = torch.topk(predictions[0],k=10)
predicted_logits_list = predicted_k_indexes[0]
predicted_indexes_list = predicted_k_indexes[1]
print ("predicted <masked> words:")
for i,item in enumerate(predicted_indexes_list[0][0]):
the_index = predicted_indexes_list[0][0][i].item()
print("word and logits",tokenizer.decode(the_index),predicted_logits_list[0][0][i].item())
###########################
output (one example - it changes each run):
#################################
input_ids tensor([[ 17, 11368, 19, 94, 2288, 27, 172, 6]])
predicted <masked> words:
word and logits **emptiness** 2.7753820419311523
word and logits **Oklahoma** 2.61531400680542
word and logits **stars** 2.56619930267334
word and logits **bite** 2.5252184867858887
word and logits **Conte** 2.4745044708251953
word and logits **enforced** 2.4537196159362793
word and logits **antibody** 2.4416041374206543
word and logits **Got** 2.332545280456543
word and logits **Chev** 2.31380033493042
word and logits **MAG** 2.3047127723693848
####################################
| 07-20-2019 23:25:57 | 07-20-2019 23:25:57 | I solved one of the problems, using another way to load the model described bellow, but still it works way worse than BERT.
tokenizer = XLNetTokenizer.from_pretrained("xlnet-large-cased")
model = XLNetLMHeadModel.from_pretrained("xlnet-large-cased")
model.eval()
if torch.cuda.is_available(): model.to('cuda') #if we have a GPU
target_id = 5
input_ids = torch.tensor(tokenizer.encode("I believe my sister is <mask> because she eats a lot of vegetables .")).unsqueeze(0) # We will predict the masked token
perm_mask = torch.zeros((1, input_ids.shape[1], input_ids.shape[1]), dtype=torch.float)
perm_mask[:, :, target_id] = 1.0 # Previous tokens don't see last token
target_mapping = torch.zeros((1, 1, input_ids.shape[1]), dtype=torch.float) # Shape [1, 1, seq_length] => let's predict one token
target_mapping[0, 0, target_id] = 1.0 # Our first (and only) prediction will be the last token of the sequence (the masked token)
input_ids_tensor = input_ids.to("cuda")
target_mapping_tensor = target_mapping.to("cuda")
perm_mask_tensor = perm_mask.to("cuda")
with torch.no_grad():
predictions = model(input_ids_tensor, perm_mask=perm_mask_tensor, target_mapping=target_mapping_tensor)
predicted_k_indexes = torch.topk(predictions[0][0][0],k=10)
predicted_logits_list = predicted_k_indexes[0]
predicted_indexes_list = predicted_k_indexes[1]
print ("predicted word:",tokenizer.decode(input_ids[0][target_id].item()))
for i,item in enumerate(predicted_indexes_list):
the_index = predicted_indexes_list[i].item()
print("word and logits",tokenizer.decode(the_index),predicted_logits_list[i].item())
But the output is not so good, i believe Bert is better. I hope this is correct code to get masked word inside a sentence.
I am not sure if this line should be any different:
perm_mask[:, :, target_id] = 1.0 # Previous tokens don't see last token
output:
sentence: "I believe my sister is <mask> because she is a blonde ."
predicted word: <mask>
word and logits is -30.468482971191406
word and logits the -33.0710334777832
word and logits was -34.586158752441406
word and logits because -34.74900436401367
word and logits in -34.762718200683594
word and logits that -34.86489486694336
word and logits but -34.97043991088867
word and logits and -35.04599380493164
word and logits if -35.07524108886719
word and logits not -35.1640510559082
when i do not use perm_mask and call only:
predictions = model(input_ids_tensor, target_mapping=target_mapping_tensor)
I get a better, but still quite bad results, but it is at least interesting.
sentence: "I believe my sister is <mask> because she is a blonde ."
predicted word: <mask>
word and logits Colombian 25.14841651916504
word and logits a 25.1247615814209
word and logits the 25.11375617980957
word and logits Venezuelan 25.041296005249023
word and logits I 24.912843704223633
word and logits Beyonce 24.855722427368164
word and logits Jessica 24.557470321655273
word and logits in 24.518535614013672
word and logits paranoid 24.407917022705078
word and logits not 24.374282836914062
With bert base you get much better output, that makes much more sense [mainly adjectives]:
[('beautiful', 7.622010231018066), ('attractive', 6.6926116943359375), ('special', 6.309513568878174), ('crazy', 6.045520782470703), ('pretty', 5.968326091766357), ('lucky', 5.951317310333252), ('famous', 5.942074775695801), ('different', 5.920231819152832), ('gorgeous', 5.897611141204834), ('blonde', 5.834926605224609)]
<|||||>I also did comparasion with Bert, so far just one example, but I found that Bert is much much better. I am not sure why is that ... but there must be a reason.
<|||||>Agreed! There is a chance we are not using the permutation mask and target mapping correctly, but I am suspicious as the documentation's example is not working very well either.<|||||>The main reason you get bad performance is that XLNet is not good on short inputs (comes from the way it is pretrained, always having a long memory and only guessing a few words in the sequence).
The `run_generation` example [here](https://github.com/huggingface/pytorch-transformers/blob/master/examples/run_generation.py) will show you how to get better performances by adding a random text as initiator.
Aman Rusia also wrote a blog post about that [here](https://medium.com/@amanrusia/xlnet-speaks-comparison-to-gpt-2-ea1a4e9ba39e). We are using his solution in the `run_generation` example.
<|||||>Thanks, I am going to try the generation method and post the results here. Hope prediction is going to improve, but i guess that ading a lot of padding is make to slow the execution down a lot.<|||||>@Oxi84 Any luck with your results ? I still get pretty random results even while using this trick<|||||>Thank you suggestions.
After adding padding text, result is much more reasonable for predicting both middle masked token and text generation.
Some texting sample:
Input
`text = 'The quick brown fox jumps <mask> the lazy dog.'`
Output
```
The quick brown fox jumps above the lazy dog.
The quick brown fox jumps across the lazy dog.
```
Input
`text = 'The <mask> brown fox jumps over the lazy dog.'`
Output
```
The rapid brown fox jumps over the lazy dog.
The slow brown fox jumps over the lazy dog.
```
<|||||>hey guys,
Q1)
can someone give some more insight what @thomwolf explaining about?
'''
https://github.com/huggingface/transformers/issues/846
The main reason you get bad performance is that XLNet is not good on short inputs (comes from the way it is pretrained, always having a long memory and only guessing a few words in the sequence).
The run_generation example here will show you how to get better performances by adding a random text as initiator.
Aman Rusia also wrote a blog post about that here. We are using his solution in the run_generation example.
'''
I can't understand the difference the way both Bert and XLnetLM works for LMhead task.
Aren't both model having disadvantages if they have short sentence?
It seems he said **XLnet has huge disadvantage** on short input sentence
while Bert does not(or has less disadvantage). Any detail explanation could be useful !
Q2)
Also, I can't get the point of adding extra padding or adding random padding things to improve XLnetLMHead model. Any snippet or explanation could be appreciated too...(saw the link but could not fully understood). I experimented by just adding extra strings of line:'I believe my sister is <mask> because she is a blonde ' + '<eod> </s> <eos>' and it gives much better result than not having <eod> </s> <eos> at the end....
Q3)
https://github.com/huggingface/transformers/issues/846#issuecomment-513514039
Lastly, why do we have better result when we don't use perm_mask ? above link response shows that
not having perm_mask option does give at least better result...But isn't perm_mask supposed to help to get better prediction and what author of paper used for SOTA ?
isn't perm_mask allow model to not seeing the next <mask> tokens in the given input while can see the previous <mask> tokens? According to the paper and the original code, I could see that if permute order is 3->4->1->2, mask=1,3, then model cannot see masked<1> when it tried to predict masked<3> but the reverse is possible.
Many thanks in advance ! <|||||>I think these questions are not directly related to this repo. Maybe you should check out the [paper](https://arxiv.org/abs/1906.08237) or ask on [quora](https://www.quora.com/) or on [researchgate](https://explore.researchgate.net/display/support/Asking+questions)<|||||>This issue has been automatically marked as stale because it has not had recent activity. It will be closed if no further activity occurs. Thank you for your contributions.
|
transformers | 845 | closed | fixed version issues in run_openai_gpt | 07-20-2019 10:43:37 | 07-20-2019 10:43:37 | # [Codecov](https://codecov.io/gh/huggingface/pytorch-transformers/pull/845?src=pr&el=h1) Report
> Merging [#845](https://codecov.io/gh/huggingface/pytorch-transformers/pull/845?src=pr&el=desc) into [master](https://codecov.io/gh/huggingface/pytorch-transformers/commit/a615499076a67dceb8907ecdf8eadaff04bb8d6a?src=pr&el=desc) will **not change** coverage.
> The diff coverage is `n/a`.
[](https://codecov.io/gh/huggingface/pytorch-transformers/pull/845?src=pr&el=tree)
```diff
@@ Coverage Diff @@
## master #845 +/- ##
======================================
Coverage 78.9% 78.9%
======================================
Files 34 34
Lines 6192 6192
======================================
Hits 4886 4886
Misses 1306 1306
```
------
[Continue to review full report at Codecov](https://codecov.io/gh/huggingface/pytorch-transformers/pull/845?src=pr&el=continue).
> **Legend** - [Click here to learn more](https://docs.codecov.io/docs/codecov-delta)
> `Δ = absolute <relative> (impact)`, `ø = not affected`, `? = missing data`
> Powered by [Codecov](https://codecov.io/gh/huggingface/pytorch-transformers/pull/845?src=pr&el=footer). Last update [a615499...f63ff53](https://codecov.io/gh/huggingface/pytorch-transformers/pull/845?src=pr&el=lastupdated). Read the [comment docs](https://docs.codecov.io/docs/pull-request-comments).
<|||||>LGTM, thanks @rabeehk |
|
transformers | 844 | closed | Fixed typo | Fixed typo in README.md | 07-20-2019 08:50:11 | 07-20-2019 08:50:11 | # [Codecov](https://codecov.io/gh/huggingface/pytorch-transformers/pull/844?src=pr&el=h1) Report
> Merging [#844](https://codecov.io/gh/huggingface/pytorch-transformers/pull/844?src=pr&el=desc) into [master](https://codecov.io/gh/huggingface/pytorch-transformers/commit/a615499076a67dceb8907ecdf8eadaff04bb8d6a?src=pr&el=desc) will **not change** coverage.
> The diff coverage is `n/a`.
[](https://codecov.io/gh/huggingface/pytorch-transformers/pull/844?src=pr&el=tree)
```diff
@@ Coverage Diff @@
## master #844 +/- ##
======================================
Coverage 78.9% 78.9%
======================================
Files 34 34
Lines 6192 6192
======================================
Hits 4886 4886
Misses 1306 1306
```
------
[Continue to review full report at Codecov](https://codecov.io/gh/huggingface/pytorch-transformers/pull/844?src=pr&el=continue).
> **Legend** - [Click here to learn more](https://docs.codecov.io/docs/codecov-delta)
> `Δ = absolute <relative> (impact)`, `ø = not affected`, `? = missing data`
> Powered by [Codecov](https://codecov.io/gh/huggingface/pytorch-transformers/pull/844?src=pr&el=footer). Last update [a615499...6b3d9ad](https://codecov.io/gh/huggingface/pytorch-transformers/pull/844?src=pr&el=lastupdated). Read the [comment docs](https://docs.codecov.io/docs/pull-request-comments).
|
transformers | 843 | closed | Issue | 07-20-2019 03:51:49 | 07-20-2019 03:51:49 | @bmanishreddy Your issue contains no text, should it be closed?<|||||>Yeah .. my bad it can be closed <|||||>No worries, please close it so it doesn't create clutter. Thanks!<|||||>This issue has been automatically marked as stale because it has not had recent activity. It will be closed if no further activity occurs. Thank you for your contributions.
|
|
transformers | 842 | closed | 16 GB dataset for finetuning fail on reduce_memory | Hi, I am using 16GB dataset to finetune bert Model. When I do not use reduce_memory, which is loading dataset into memory first, it will use all of my 120GB memory and then crush because of out of memory. Now I am using reduce_memory model, with the increase of loading lines, the memory use is still increasing. But it is much slower in reduce_memory setting. So, I am thinking whether it would crush at the end. Could anyone have an answer for that ?
Sorry for the bothering, but it is too slow to run with reduce_memory setting, I have no idea weather it would crush or go well. I am afraid that it would keep loading for days and crushed finally. Thanks in advance for any suggestions | 07-20-2019 01:28:11 | 07-20-2019 01:28:11 | This issue has been automatically marked as stale because it has not had recent activity. It will be closed if no further activity occurs. Thank you for your contributions.
|
transformers | 841 | closed | Detaching Variables | Something I noticed in transitioning from Pretrained-BERT to Transformers is that for the purposes of using BERT as a feature extractor/probing the pretrained representations, I need to detach variables whereas I previously didn't. I am not sure if this is noted somewhere (I didn't see it in the section in the docs about transitioning) but found it be highly relevant to prevent unnecessary memory usage. | 07-20-2019 00:24:35 | 07-20-2019 00:24:35 | Maybe you are not using `with torch.grad()` when calling the model for inference?
I've added that in the readme example (it used to be mentioned there indeed).<|||||>This issue has been automatically marked as stale because it has not had recent activity. It will be closed if no further activity occurs. Thank you for your contributions.
|
transformers | 840 | closed | AttributeError: 'BertModel' object has no attribute '_load_from_state_dict' | Hi,
I am getting these error, even the i tried the model straight from the repository examples for the test cases.? anyone help me to understand this issues
Thanks for help in advance
| 07-19-2019 21:08:44 | 07-19-2019 21:08:44 | You are probably not using the new release of PyTorch-transformers.
Try `pip install pytorch-transformers --upgrade`.
And read the full [readme](https://github.com/huggingface/pytorch-transformers), there are several breaking changes.<|||||>@thomwolf Thanks for your update, it works for me !! |
transformers | 839 | closed | How to restore a training? | For example, I use "run_glue.py" to train a model and stop at Epoch 30, and how to restore the training process from Epoch 30? | 07-19-2019 19:58:37 | 07-19-2019 19:58:37 | You will have to modify the provided example to save/reload the model, optimizer and scheduler states.
I've updated the scheduler classes in #872 so that we can save/reload the schedulers with the standard PyTorch serialization practice:
```
torch.save(schedule.state_dict(), FILE_NAME) # save
schedule.load_state_dict(torch.load(FILE_NAME)) # reload
```<|||||>Thanks for the response~<|||||>@thomwolf - I happen to need save/resume training for `run_glue.py`; I'm willing to implement this and make a PR if I can get feedback about the overall approach.
It looks like I would want to save:
- `global_step`
- `step` (from this one could presumably skip to the right place in the `epoch_iterator` of `train`)
- optimizer, model, scheduler (these look like they should be trivially amenable to `torch.save`)
- random seed, torch's random seed, numpy's random seed
Based on the current set up, I'm not actually sure what the right way to preserve the `train_sampler` and `train_dataloader` states are (without also serializing them too, which seems like a waste, but is by far the easiest way to handle it if they are so amenable), since their states can't be recreated with the above information. In my case, punting on these is an acceptable option, as well as being wasteful and saving to disk.
Thoughts?<|||||>This issue has been automatically marked as stale because it has not had recent activity. It will be closed if no further activity occurs. Thank you for your contributions.
|
transformers | 838 | closed | Standardized head for Question Answering | Hi,
With some colleagues, we developed a QA system that uses a whole QA pipeline (Retriever, Reader, Ranker). We use your older version of `BertForQuestionAnswering` as Reader and now we wish to update it to be compatible with your new release and to add others models as well (XLNet, XLM).
Our system uses the logits outputted by the model in order to rank the answers between different paragraphs (using probabilities outputted by the softmax layer is an incorrect approach for such systems).
However, we understand that in your new API, the `forward` method of QA models now can only return probabilities. We suggest you add the option to output the raw logits as well.
We could of course overcome this by using the functions `self.start_logits` and `self.end_logits`, but we think that this feature can be useful for other users as well | 07-19-2019 15:03:36 | 07-19-2019 15:03:36 | Well, the output of the `BertForQuestionAnswering` model hasn't changed, the returned `start_score` and `end_score` are still scores before the softmax.
Can you point more specifically to the changes you are referring to?<|||||>I'm sorry. Indeed the implementation of `BertForQuestionAnswering` remains the same.
Actually, I was referring to the implementation of `XLNetForQuestionAnswering`, which is pretty different from `BertForQuestionAnswering` (I thought you had standardised the implementation for all QA models and I hadn't checked the `BERT` implementation before posting the issue here)
Please correct me if I am wrong, but I do not see the `forward()` method outputting the Start and End's logits here (only the softmax probas - `start_log_probs`and `end_log_probs`):
https://github.com/huggingface/pytorch-transformers/blob/268c6cc160ba046d6a91747c5f281f82bd88a4d8/pytorch_transformers/modeling_xlnet.py#L1226-L1290
Same with XLM:
https://github.com/huggingface/pytorch-transformers/blob/fec76a481d1ecfbf068d87735dd44ffc26158f6e/pytorch_transformers/modeling_xlm.py#L899-L921
https://github.com/huggingface/pytorch-transformers/blob/fec76a481d1ecfbf068d87735dd44ffc26158f6e/pytorch_transformers/modeling_utils.py#L649<|||||>Oh yes the official XLNet implementation uses a beam search for Question Answering so the output is more complex.
I'll see if I can come up with a standardized way to use both.<|||||>Thanks, I get it. Maybe caching the `start_logits` during the search and returning the logits of chosen End and Start positions would be a solution.
By the way, FYI the examples in the documentation for `XLNetForQuestionAnswering` and `XLMForQuestionAnswering` are incorrect, both show `start_scores` and `end_scores` in the outputs and the example in `XLNetForQuestionAnswering` uses `XLM` instead of `XLNet`
[`XLNetForQuestionAnswering`](https://huggingface.co/pytorch-transformers/model_doc/xlnet.html#xlnetforquestionanswering):
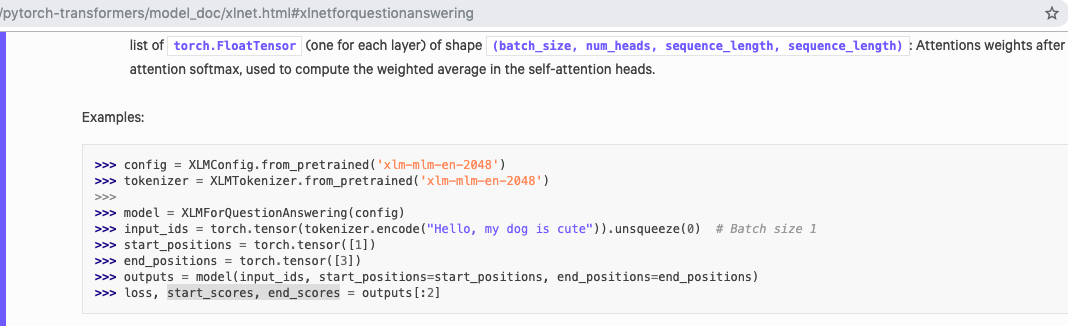
[`XLMForQuestionAnswering`](https://huggingface.co/pytorch-transformers/model_doc/xlm.html#xlmforquestionanswering):
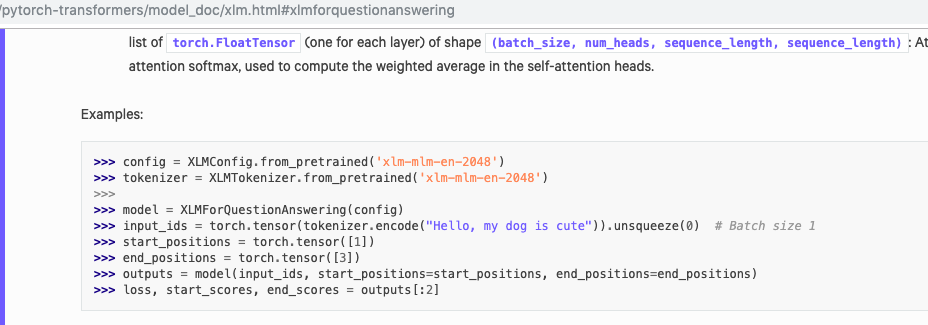
<|||||>This is silly/nitpicky - but can we change the title of this issue? Got very worried I had been using the BERT heads incorrectly until I read into the weeds of the comments..<|||||>Hello, any news on this standardized head? @thomwolf?
What do you think about my proposition about caching the `start_logits` during the Beam search and outputting both `start_logits` and `end_logits` as it is done with `BertForQuestionAnswering`?
Or do you have any other ideas?
I can try to work on that and do a PR if you need<|||||>This issue has been automatically marked as stale because it has not had recent activity. It will be closed if no further activity occurs. Thank you for your contributions.
|
transformers | 837 | closed | run_openai_gpt.py issues with Adamw | Hi
Adamw in this script has parameters not existing anymore, ...
Thanks for updates in advance. | 07-19-2019 14:13:50 | 07-19-2019 14:13:50 | Yes, so this should be fixed by (your own :) PR #845 Thanks again!<|||||>thanks :)
Best regards,
Rabeeh
On Tue, Jul 23, 2019 at 3:30 PM Thomas Wolf <[email protected]>
wrote:
> Yes, so this should be fixed by (your own :) PR #845
> <https://github.com/huggingface/pytorch-transformers/pull/845> Thanks
> again!
>
> —
> You are receiving this because you authored the thread.
> Reply to this email directly, view it on GitHub
> <https://github.com/huggingface/pytorch-transformers/issues/837?email_source=notifications&email_token=ABP4ZCAGTX6DNAYMBCHNORTQA4BYFA5CNFSM4IFGMGK2YY3PNVWWK3TUL52HS4DFVREXG43VMVBW63LNMVXHJKTDN5WW2ZLOORPWSZGOD2TDV6Y#issuecomment-514210555>,
> or mute the thread
> <https://github.com/notifications/unsubscribe-auth/ABP4ZCGDZR3TG2SS2LXIBTDQA4BYFANCNFSM4IFGMGKQ>
> .
>
<|||||>Thanks @rabeehk! #845 with flat learning rate gives a good result of 87.2% on ROCStories. Here are the args (the defaults in the file work well).
```
python run_openai_gpt.py \
--model_name openai-gpt \
--do_train \
--do_eval \
--train_dataset "./ROCStories/cloze_test_val__spring2016 - cloze_test_ALL_val.csv" \
--eval_dataset "./ROCStories/cloze_test_test__spring2016 - cloze_test_ALL_test.csv" \
--train_batch_size 8 \
--eval_batch_size 16 \
--num_train_epochs 3
```<|||||>@prrao87 great :) <|||||>This issue has been automatically marked as stale because it has not had recent activity. It will be closed if no further activity occurs. Thank you for your contributions.
|
transformers | 836 | closed | BertForNextSentencePrediction labels | Hi everyone!
I was reading trough the documentation, and, according to https://huggingface.co/pytorch-transformers/model_doc/bert.html#bertforsequenceclassification, it expects that `next_sentence_label` is `1` if B is **not** a next sequence for A, and `0` if B **is** a sequence for B.
That's somewhat counterintuitive, since most of the datasets (I believe) will assume this problem to be a binary classification.
Is my assumption correct? Should I flip my dataset before fine-tuning the model? | 07-19-2019 14:06:03 | 07-19-2019 14:06:03 | You should supply your own labels when using the `BertForSequenceClassification` class (`labels` input to the forward method). You can choose the labels you like.
The `BertForSequenceClassification` class is **not** related to the Next Sentence Classification task used during Bert pretraining. You can use the [BertForNextSentencePrediction](https://huggingface.co/pytorch-transformers/model_doc/bert.html#bertfornextsentenceprediction) class if you want to do next sentence prediction (classification).<|||||>Yes, I'm sorry I meant the [BertForNextSentencePrediction](https://huggingface.co/pytorch-transformers/model_doc/bert.html#bertfornextsentenceprediction) class.<|||||>Yes, in this case, you should follow the docstring: `0 indicates sequence B is a continuation of sequence A, 1 indicates sequence B is a random sequence.` |
transformers | 835 | closed | How to use the pretrain script with only token classification task ? | Hi, I need to train on my own twitter corpus, but most of the twitter contains only one sentence. Therefore I can not use the sentence prediction task to train the model. Will the script automatically use only token classification task when there is no next sentence ? Thanks in advance. | 07-19-2019 07:20:16 | 07-19-2019 07:20:16 | No, you probably need to adapt the example script to your exact task. |
transformers | 834 | closed | git pull pytorch-transformers?? | Hello,
I have git cloned 'pytorch-pretrained-bert' before there is a new release, pytorch-transformers
and I added many of the comments and new example files in the cloned project.
However, when I found there has been a new version released, git pulling didn't work
for conflicting files issues.
Is it because of the new release of 'pytorch-transformers' conflicts to the older version which
is totally different in name?
| 07-19-2019 06:07:16 | 07-19-2019 06:07:16 | I suspect this is more of a general `git` question. We'll close this unless there is something specific to the lib.
Good luck!<|||||>@julien-c ,
yeah, I had some of code mismatch issues and that falls into general github matters
and I got it done :)
Thank you :) |
transformers | 833 | closed | missing 1 required positional argument: 'num_classes' in 'from_pretrained' | I am running a [multiclass BERT classification](https://github.com/desireevl/Bert-Multi-Label-Text-Classification/blob/master/train_bert_multi_label.py) model and am receiving the following error:
`
Traceback (most recent call last):
File "train_bert_multi_label.py", line 144, in <module>
main()
File "train_bert_multi_label.py", line 78, in main
num_classes = len(id2label))
File "/opt/miniconda3/envs/tempenv/lib/python3.6/site-packages/pytorch_transformers/modeling_utils.py", line 403, in from_pretrained
model = cls(config)
TypeError: __init__() missing 1 required positional argument: 'num_classes'
`
The script worked fine when using `pytorch-pretrained-bert` and am guessing there is an issue from the new release.
Thanks for the great tools :) | 07-19-2019 06:00:59 | 07-19-2019 06:00:59 | Yes, @xanlsh is working on an update to reduce the effect of this breaking change in #866.
You should be able to keep your script unchanged.<|||||>@desireevl Since #866 has been merged, your code should work now<|||||>Thanks! |
transformers | 832 | closed | Training with wrong GPU count | Hi,
Thank you for your repo :)
I'm fine-tuning with 4 GPU (run_squad, bert model)
And I found that gpu count is wrong when to do distributed training.
I've got 1 GPU count and that's caused by source code below
Is there any reason to set n_gpu = 1 when to do distributed training?
if args.local_rank == -1 or args.no_cuda:
device = torch.device("cuda" if torch.cuda.is_available() and not args.no_cuda else "cpu")
n_gpu = torch.cuda.device_count()
else:
torch.cuda.set_device(args.local_rank)
device = torch.device("cuda", args.local_rank)
n_gpu = 1 <= this!!!
# Initializes the distributed backend which will take care of sychronizing nodes/GPUs
torch.distributed.init_process_group(backend='nccl')
| 07-19-2019 02:27:13 | 07-19-2019 02:27:13 | Yes, this is expected behavior. Each script in distributed training has ownership over one GPU only.
You can read this blog post for details on parallel and distributed training: https://medium.com/huggingface/training-larger-batches-practical-tips-on-1-gpu-multi-gpu-distributed-setups-ec88c3e51255 |
transformers | 831 | closed | finetune_on_pregenerate Loss.backwards() throw an error | In finetune_on_pregenerated.py, loss are tuples and thus loss.backward() is not going to work.
Original:
loss = model(input_ids, segment_ids, input_mask, lm_label_ids, is_next)
Update:
loss, _ , _ = model(input_ids, segment_ids, input_mask, lm_label_ids, is_next)
Is this fix correct? | 07-19-2019 00:42:46 | 07-19-2019 00:42:46 | Yes this example should have been updated now with #797. |
transformers | 830 | closed | AdamW does not have args warmup and t_total | In finetune_on_pregenerated.py, below code throw error cause AdamW does not have those two arguments. This can be fixed by comment out those two columns but not sure if that means warmup will be not effective after that?
optimizer = AdamW(optimizer_grouped_parameters,
lr=args.learning_rate)
#warmup=args.warmup_proportion,
# t_total=num_train_optimization_steps)
| 07-19-2019 00:39:41 | 07-19-2019 00:39:41 | Yes this example should have been updated now by #797.
Regarding `AdamW` and the schedule, details, and examples for the conversion are indicated in the migration section of the readme: https://github.com/huggingface/pytorch-transformers#Migrating-from-pytorch-pretrained-bert-to-pytorch-transformers<|||||>This issue has been automatically marked as stale because it has not had recent activity. It will be closed if no further activity occurs. Thank you for your contributions.
|
transformers | 829 | closed | RoBERTa support | https://twitter.com/sleepinyourhat/status/1151940994688016384
The code/parameters aren't out yet, but I figure it couldn't hurt to put in an obnoxious feature request now! | 07-18-2019 22:05:10 | 07-18-2019 22:05:10 | Working on the code/paper release as we speak :) It largely follows the existing masked_lm implementation in fairseq. Happy to help get this integrated here.<|||||>Hi @myleott great news :) I'm really excited about the release 🤗 I've some questions: do you plan to perform any comparisons between RoBERTa and BERT on NER (CoNLL-2003)?
I've read the [Cloze-driven Pretraining of Self-attention Networks](https://arxiv.org/abs/1903.07785) paper, and if I recall correctly, the implementation is currently done in the `bi_trans_lm` branch in `fairseq`, but do you have any updates on that? It would be awesome if a pre-trained CNN model from that paper could also be integrated into `pytorch-transformers` 😍<|||||>Sounds great @myleott. Keep us updated about the release!<|||||>Models and README are uploaded: https://github.com/pytorch/fairseq/tree/master/examples/roberta. We submitted the paper to arXiv today so it should be out Sunday evening.
> I've some questions: do you plan to perform any comparisons between RoBERTa and BERT on NER (CoNLL-2003)?
We haven't yet, but it would be interesting to explore. RoBERTa was trained on considerably more data than BERT, so I expect it would do well on NER tasks.<|||||>Paper is [out](https://arxiv.org/abs/1907.11692). Thanks @myleott!<|||||>Work in progress in #964 feel free to chime in :)<|||||>Example of how RoBERTa can be used to predict a masked token.
import torch
from pytorch_transformers import RobertaTokenizer, RobertaForMaskedLM
tokenizer = RobertaTokenizer.from_pretrained('roberta-large')
model = RobertaForMaskedLM.from_pretrained('roberta-large')
model.eval()
if torch.cuda.is_available(): model.to('cuda') #if we have a GPU
text = 'I believe my sister is <mask<m>> because she eats a lot of vegetables .'
tokenized_text = tokenizer.tokenize(text)
masked_index = tokenized_text.index(<mask<m>>)+1
#add_special_tokens adds a <s<s>> to the beginning and </s</s>> to the end of the text
input_ids = torch.tensor(tokenizer.encode(text,add_special_tokens=True)).unsqueeze(0)
input_ids_tensor = input_ids.to("cuda")
#with torch.no_grad():
outputs = model(input_ids_tensor, masked_lm_labels=input_ids_tensor)
loss, prediction_scores = outputs[:2]
#predicted_index = torch.argmax(prediction_scores[0, masked_index]).item()
#predicted_token = tokenizer.convert_ids_to_tokens([predicted_index])[0]
predicted_k_indexes = torch.topk(prediction_scores[0, masked_index],k=20)
predicted_logits_list = predicted_k_indexes[0]
predicted_indexes_list = predicted_k_indexes[1]
for i, item in enumerate(predicted_indexes_list):
the_index = predicted_indexes_list[i].item()
print("word and logits",tokenizer.decode(the_index),predicted_logits_list[i].item())
<|||||>Hi @pwolff, at first glance it looks ok to me. You don't need to send the `masked_lm_labels` if you don't use the loss though.<|||||>@thomwolf hello, I trained the robert on my customized corpus following the fairseq instruction. I am confused how to generate the robert vocab.json and also merge.txt because I want to use the pytorch-transformer RoBERTaTokenizer.<|||||>@stefan-it hello, I trained the robert on my customized corpus following the fairseq instruction. I am confused how to generate the robert vocab.json and also merge.txt because I want to use the pytorch-transformer RoBERTaTokenizer.<|||||>This issue has been automatically marked as stale because it has not had recent activity. It will be closed if no further activity occurs. Thank you for your contributions.
<|||||>@songtaoshi I think this can be done via `subword-nmt`, see this note:
https://github.com/pytorch/fairseq/issues/1163#issuecomment-534098220
<|||||>is this still an issue?<|||||>Nope, RoBERTa support was shipped in [v1.1.0](https://github.com/huggingface/transformers/releases/tag/1.1.0)
Thanks all! |
transformers | 828 | closed | CUDA error: invalid configuration argument when not using DataParallel | Good Evening,
We have a DGX2 system running the latest Nvidia pytorch docker container - 19.06. When attempting to use the gpt2 or gpt2-medium models to extract out embeddings we are getting the following error, but only when not using dataparallel: (note we are using apex here but an optimization level of 0, this issue occurs without apex)
```
[...]
File "[removed]", line 167, in forward
x1_emb, past = self.embedding(x1)
File "/opt/conda/lib/python3.6/site-packages/torch/nn/modules/module.py", line 494, in __call__
result = self.forward(*input, **kwargs)
File "/opt/conda/lib/python3.6/site-packages/pytorch_transformers/modeling_gpt2.py", line 515, in forward
outputs = block(hidden_states, layer_past, head_mask[i])
File "/opt/conda/lib/python3.6/site-packages/torch/nn/modules/module.py", line 494, in __call__
result = self.forward(*input, **kwargs)
File "/opt/conda/lib/python3.6/site-packages/pytorch_transformers/modeling_gpt2.py", line 332, in forward
output_attn = self.attn(self.ln_1(x), layer_past=layer_past, head_mask=head_mask)
File "/opt/conda/lib/python3.6/site-packages/torch/nn/modules/module.py", line 494, in __call__
result = self.forward(*input, **kwargs)
File "/opt/conda/lib/python3.6/site-packages/pytorch_transformers/modeling_gpt2.py", line 285, in forward
x = self.c_attn(x)
File "/opt/conda/lib/python3.6/site-packages/torch/nn/modules/module.py", line 494, in __call__
result = self.forward(*input, **kwargs)
File "/opt/conda/lib/python3.6/site-packages/pytorch_transformers/modeling_utils.py", line 490, in forward
x = torch.addmm(self.bias, x.view(-1, x.size(-1)), self.weight)
RuntimeError: CUDA error: invalid configuration argument
```
I found reference to this issue from Pytorch:
https://github.com/pytorch/pytorch/issues/2080
but it is reported as fixed so I'm not sure if this issue belongs to this repo or pytorch. | 07-18-2019 20:38:46 | 07-18-2019 20:38:46 | Further testing showed this was caused by the batch size being too high and the card running out of memory, and providing a misleading error. |
transformers | 827 | closed | xlnet input_mask and attention_mask type error | when I use:
```input_mask = (input_ids == 0)```
```perm_mask = perm_mask = torch.zeros((1, input_ids.shape[1], input_ids.shape[1]), dtype=torch.float, device=device)```
```perm_mask[:, :, -1] = 1.0 # Previous tokens don't see last token```
File "pytorch-transformers/pytorch_transformers/modeling_xlnet.py", line 881, in forward
data_mask = input_mask[None] + perm_mask
RuntimeError: expected backend CUDA and dtype Float but got backend CUDA and dtype Byte
input_mask is a Tensor but perm_mask is FloatTensor | 07-18-2019 19:25:01 | 07-18-2019 19:25:01 | Humm you are right, the docstrings are off, it would be more clear if they were all indicated as `torch.FloatTensor` (even though officially torch.Tensor is an alias for the default tensor type (torch.FloatTensor)). |
transformers | 826 | closed | Providing older documentation | Hey, would it be possible to release the previous documentation ? I'm working on previous version and can't find proper doc right now.
Thanks if you can help | 07-18-2019 17:09:06 | 07-18-2019 17:09:06 | Hi, you may go to https://github.com/huggingface/pytorch-transformers/releases, select the release you are working with and in its "Assets" download the repo and navigate the code, together with documentation<|||||>Hi, here is the older documentation: https://github.com/huggingface/pytorch-transformers/tree/v0.6.2 |
transformers | 825 | closed | Chinese BERT broken probably after `pytorch-transformer` release | I suspect that there is some recent code change that breaks the Chinese BERT.
I used the following PyTorch hub code to load the Chinese BERT tokenizer and print out some tokens in the vocab perhaps just a few days ago and everything was fine:
```python
import torch
GITHUB_REPO = "huggingface/pytorch-pretrained-BERT"
tokenizer = torch.hub.load(GITHUB_REPO, 'bertTokenizer', "bert-base-chinese")
# print some pre-determined tokens with their corresponding indices
indices = list(range(647, 657))
some_pairs = [(t, idx) for t, idx in vocab.items() if idx in indices]
for pair in some_pairs:
print(pair)
```
It used to produce the following result:
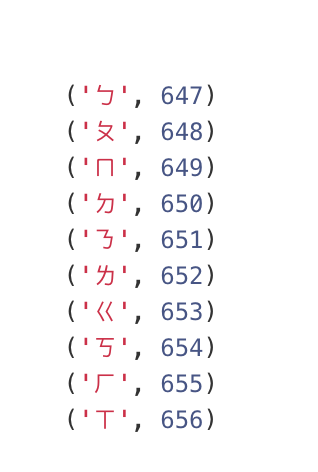
But after some recent commits or may the latest release, the voacab result slightly changed to even with the same code:
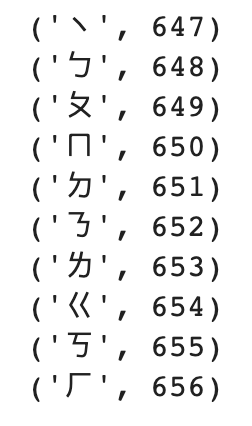
This difference should not happen since we're using the exact same model and code. And the following maskedLM task failed to predict the masked token accordingly and produced a broken result (which used to predict the correct result just a few days ago).
I already tried replacing `pytorch-pretrained-BERT` to `pytorch-transformers` but it still don't work.
I also tried to use the tokenizer directly from repo and it didn't work either.
```python
from pytorch_transformers import BertTokenizer, BertModel, BertForMaskedLM
tokenizer = BertTokenizer.from_pretrained("bert-base-chinese")
```
Please kindly provide some guide or suggestion about how to fix this problem. Chinese BERT may not be functioning as expected now. Thanks in advance.
| 07-18-2019 16:49:13 | 07-18-2019 16:49:13 | We did slightly change the way the tokenizer strip the spaces at the end of the words when loading the tokenizer, as discussed in issue #328, in particular here https://github.com/huggingface/pytorch-transformers/issues/328#issuecomment-503630929.
Now, I'm not exactly sure what is the right solution for both cases. I'll give a look, but I won't have time right now. If you want to investigate the source of the issue and compare with #328, it can help.<|||||>@thomwolf Thanks for the suggestion. After some twists, I can reproduce the desired result (though it's very hacky and we should come up with a better solution)
I used the previous version of `load_vocb` function to regenerate vocabulary, and it did reproduce the desired vocab:
https://github.com/huggingface/pytorch-transformers/blob/3763f8944dc3fef8afb0c525a2ced8a04889c14f/pytorch_pretrained_bert/tokenization.py#L56
```python
import collections
# previous version of `load_voacb`
def load_vocab(vocab_file):
"""Loads a vocabulary file into a dictionary."""
vocab = collections.OrderedDict()
index = 0
with open(vocab_file, "r", encoding="utf-8") as reader:
while True:
token = reader.readline()
if not token:
break
token = token.strip()
vocab[token] = index
index += 1
return vocab
# get the vocab file to regenerate vocb
!wget https://s3.amazonaws.com/models.huggingface.co/bert/bert-base-chinese-vocab.txt
# first load the latest version tokenizer and overwrite the vocab by previous version of `load_vocab`
tokenizer = torch.hub.load(GITHUB_REPO, 'bertTokenizer', "bert-base-chinese")
tokenizer.vocab = load_vocab("bert-base-chinese-vocab.txt")
# get the desired result as previously
indices = list(range(647, 657))
some_pairs = [(t, idx) for t, idx in vocab.items() if idx in indices]
for pair in some_pairs:
print(pair)
```
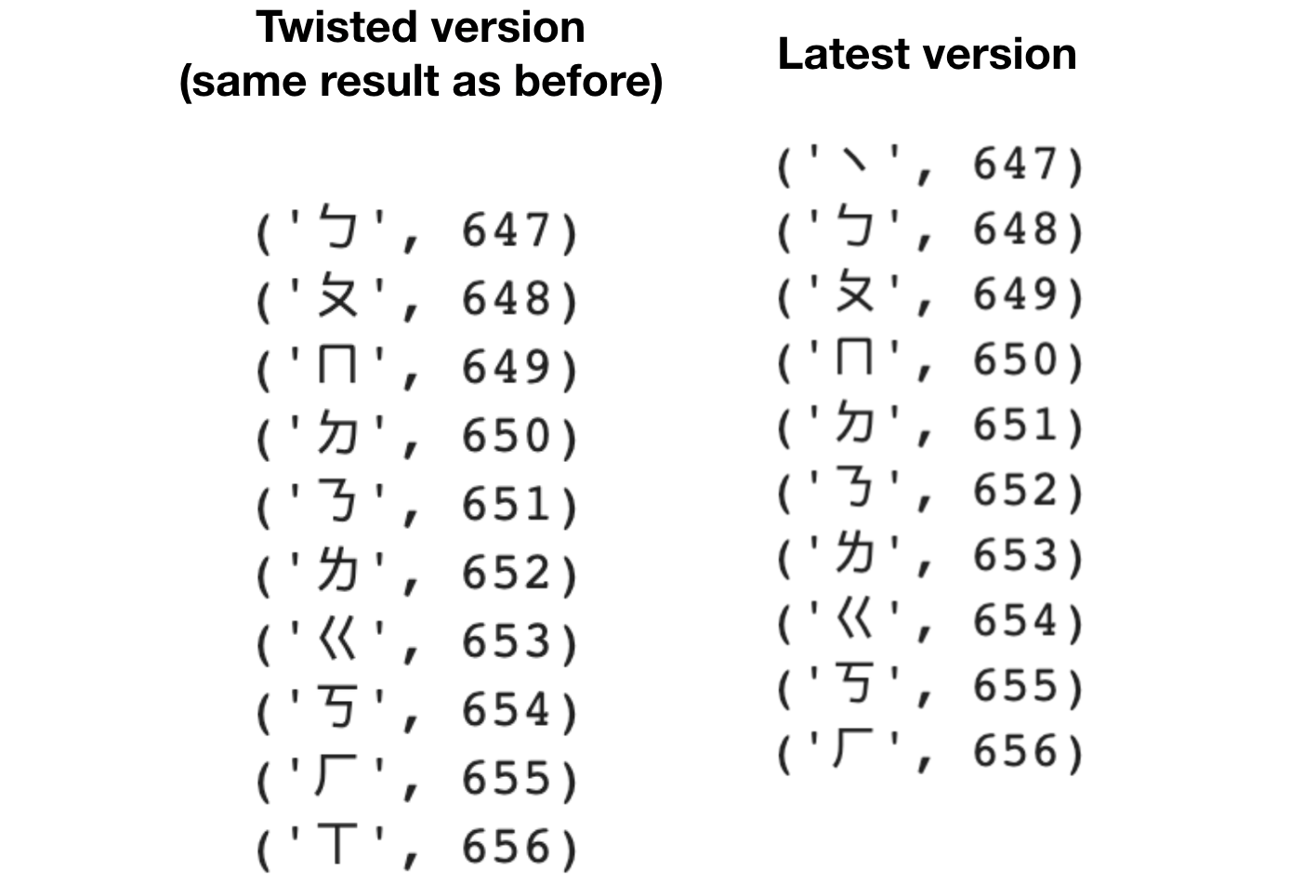
(left is the desired result acquired by using old `load_vab` function)
The vocab size is the same, but it seems that current / previous vocab index is kind of **offset by 1** so after getting the prediction (using twisted `tokenizer`) from the model, I have to **first add 1** to all predicted tokens and then convert them back to tokens:
```python
# predict masked token
maskedLM_model = torch.hub.load(GITHUB_REPO,
'bertForMaskedLM',
"bert-base-chinese")
maskedLM_model.eval()
with torch.no_grad():
outputs = maskedLM_model(tokens_tensor, segments_tensors)
predictions = outputs[0]
probs, indices = torch.topk(torch.softmax(predictions[0, masked_index], -1), k)
# HACKY HOTFIX HERE
indices += 1
# correct result
predicted_tokens = tokenizer.convert_ids_to_tokens(indices.tolist())
```
In sum, by:
- use previous `load_vocab` function
- add 1 to model output
I can reproduce the same correct result as before in this maskLM scenario. But of course, this is very hacky. We need a better solution.
<|||||>At the line 344 of bert-base-chinese vocab file the token is '\u2028', which is an unicode line separator.
I think using 'token = reader.readlines()' instead of 'token = reader.read().splitlines()' might solve the problem.<|||||>Have submitted a PR for this: https://github.com/huggingface/pytorch-transformers/pull/860<|||||>Great, thanks for investigating deeper @Yiqing-Zhou and @leemengtaiwan!<|||||>Thank you guys @Yiqing-Zhou and @thomwolf!
I have used the latest version of Chinese BERT and it seems that the vocab and accuracy of my downstream task work perfectly now. :)<|||||>Hi, @leemengtaiwan What kind of dataset are you running on?
I get the same issue even when I update to the latest version.
The same issue is also mention in #903
I'm running on Chinese-Style SQuAD dataset (DRCD).
I can train Chinese-Bert successfully about half year ago.
However, I could not train the model successfully but I can train Multi-Bert successfully.
@thomwolf Do you update Chinese-Bert recently? or there are still some bugs in preprocess step?<|||||>> Hi, @leemengtaiwan What kind of dataset are you running on?
@Liangtaiwan I'm using custom dataset (to be more specific, [WSDM Fake News Classification](https://www.kaggle.com/c/fake-news-pair-classification-challenge/) on Kaggle).
The updated version seems to work fine for me, but if you still encounter some issues, maybe you can create a separate issue and reference this issue if needed.
|
transformers | 824 | closed | Bertology example is probably broken | Hello!
I tried to run `run_bertology.py` in the example dir calling it with
```
export TASK_NAME=CoLA
python ./run_bertology.py --data_dir $GLUE_DIR/$TASK_NAME
--model_name bert-base-uncased
--task_name $TASK_NAME
--max_seq_length 128
--output_dir ./tmp/$TASK_NAME/
--try_masking
--metric_name mcc
```
But it fails with
> Traceback (most recent call last):
> File "./run_bertology.py", line 346, in <module>
> main()
> File "./run_bertology.py", line 327, in main
> eval_data = load_and_cache_examples(args, args.task_name, tokenizer, evaluate=True)
> File "/data/home/dfiocco/BERT/run_glue.py", line 245, in load_and_cache_examples
> list(filter(None, args.model_name_or_path.split('/'))).pop(),
> AttributeError: 'Namespace' object has no attribute 'model_name_or_path'
One fix to that problem should be (?) replacing all occurrences of `model_name` with `model_name_or_path` in `run_bertology.py`. Still, even with that "patch" running the code gives
> Traceback (most recent call last):
> File "./run_bertology.py", line 346, in <module>
> main()
> File "./run_bertology.py", line 341, in main
> head_mask = mask_heads(args, model, eval_dataloader)
> File "./run_bertology.py", line 175, in mask_heads
> print_2d_tensor(head_mask)
> UnboundLocalError: local variable 'head_mask' referenced before assignment
Trying another task (MRPC) I get instead
>
> Traceback (most recent call last):
> File "./run_bertology.py", line 346, in <module>
> main()
> File "./run_bertology.py", line 341, in main
> head_mask = mask_heads(args, model, eval_dataloader)
> File "./run_bertology.py", line 169, in mask_heads
> _, head_importance, preds, labels = compute_heads_importance(args, model, eval_dataloader, compute_entropy=False, head_mask=new_head_mask)
> File "./run_bertology.py", line 97, in compute_heads_importance
> head_importance += head_mask.grad.abs().detach()
> AttributeError: 'NoneType' object has no attribute 'abs'
Did anybody manage to run the Bertology example without hiccups?
| 07-18-2019 16:15:13 | 07-18-2019 16:15:13 | Yes, this example is still work in progress. Hopefully, I can finish it before ACL (but not sure).<|||||>This issue has been automatically marked as stale because it has not had recent activity. It will be closed if no further activity occurs. Thank you for your contributions.
|
transformers | 823 | closed | Updating simple_lm_finetuning.py for FP16 training | in simple_lm_finetuning the recent updated code doesn't work with the old optimizer specifications.
When not running with --fp16
`
optimizer = BertAdam(optimizer_grouped_parameters,
lr=args.learning_rate,
warmup=args.warmup_proportion,
t_total=num_train_optimization_steps)
// In PyTorch-Transformers, optimizer and schedules are splitted and instantiated like this:
optimizer = AdamW(model.parameters(), lr=args.learning_rate, correct_bias=False) # To reproduce BertAdam specific behavior set correct_bias=False
scheduler = WarmupLi
`
fixes the problem as suggested.
But when running --fp16
`scheduler = WarmupLinearSchedule(optimizer, warmup_steps=num_warmup_steps, t_total=num_train_optimization_steps) # PyTorch scheduler`
will error out with FP16_Optimizer saying that
> TypeError: FP16_Optimizer is not an Optimizer
Does the FuseAdam object need to be passed into WarmupLinearSchedule instead? | 07-18-2019 15:19:54 | 07-18-2019 15:19:54 | Hi, has this been fixed? I've tried updating my language modeling script to match but still getting errors.<|||||>Having the same problem at the moment ...<|||||>I guess the preferred way is to use `apex.amp` like in this example?
https://github.com/huggingface/pytorch-transformers/blob/master/examples/run_glue.py<|||||>This issue has been automatically marked as stale because it has not had recent activity. It will be closed if no further activity occurs. Thank you for your contributions.
|
transformers | 822 | closed | XLNet-large-cased on Squad 2.0: can't replicate results | I've been trying to replicate the numbers in the Squad 2.0 dev set (F1=86) with this script and the XLnet embeddings. So far the results are really off..{Opening a new issue as the previous one seems dedicated to SST-2}
`python run_squad.py --do_lower_case --do_train --do_eval --train_file $SQUAD_DIR/train-v2.0.json --predict_file $SQUAD_DIR/dev-v2.0.json --output_dir $SQUAD_DIR/output --version_2_with_negative --model_name xlnet-large-cased --save_steps 5000 --num_train_epochs 3 --overwrite_output_dir --model_type xlnet --per_gpu_train_batch_size 4 --gradient_accumulation_steps 1 --learning_rate 3e-5`
gives:
`07/18/2019 08:43:36 - INFO - __main__ - Results: {'exact': 3.217383980459867, 'f1': 7.001376535240158, 'total': 11873, 'HasAns_exact': 6.359649122807017, 'HasAns_f1': 13.938485762973412, 'HasAns_total': 5928, 'NoAns_exact': 0.08410428931875526, 'NoAns_f1': 0.08410428931875526, 'NoAns_total': 5945, 'best_exact': 50.07159100480081, 'best_exact_thresh': 0.0, 'best_f1': 50.07159100480081, 'best_f1_thresh': 0.0}`
| 07-18-2019 14:09:31 | 07-18-2019 14:09:31 | This is similar to what the authors ran in the paper (except I could fit only this on 3 v100 GPUs):
`python run_squad.py --do_lower_case --do_train --do_eval --train_file $SQUAD_DIR/train-v2.0.json --predict_file $SQUAD_DIR/dev-v2.0.json --output_dir $SQUAD_DIR/output --version_2_with_negative --model_name xlnet-large-cased --save_steps 5000 --num_train_epochs 3 --overwrite_output_dir --model_type xlnet --per_gpu_train_batch_size 2 --gradient_accumulation_steps 1 --max_seq_length 512 --max_answer_length 64 --adam_epsilon 1e-6 --learning_rate 3e-5 --num_train_epochs 2`
gives:
`07/18/2019 06:20:54 - INFO - __main__ - Results: {'exact': 2.0382380190347846, 'f1': 6.232918462554391, 'total': 11873, 'HasAns_exact': 3.9979757085020244, 'HasAns_f1': 12.399365874815837, 'HasAns_total': 5928, 'NoAns_exact': 0.08410428931875526, 'NoAns_f1': 0.08410428931875526, 'NoAns_total': 5945, 'best_exact': 50.07159100480081, 'best_exact_thresh': 0.0, 'best_f1': 50.07159100480081, 'best_f1_thresh': 0.0}`
<|||||>@thomwolf are you already working on this? I can work with you to try to solve it :) <|||||>with the same question... Also got weird results on other QA datasets like BoolQ, MultiRC.<|||||>@avisil not yet, I won't have time to work on this before ACL but you can start to have a look if you want. Such discrepancies pretty much always come, not from the model it-self but, from different settings for pre/post-processing the dataset or for the optimizer/optimization process.
If you want to start giving it a look, the way I usually check exact reproducibility on downstream tasks like GLUE/SQuAD is to directly import the pytorch-transformer's model in the tensorflow code (that's the main reason the library is python 2 compatible), load the pytorch model with the initialized tf model and run the models side by side on the same inputs (on separate GPUs) to check-in details the inputs/outputs/hidden-states and so-on. It's better to do it on a GPU version of the TF code so you can setup the optimizer your-self. I think somebody did a GPU version of the official SQuAD example, but you can also take inspiration from the multi-GPU adaptation I did of the TensorFlow code for GLUE, which is here: https://github.com/thomwolf/xlnet/blob/master/run_classifier_gpu.py.
In this fork, you can see how I import and run the PyTorch model along the TensorFlow one side by side.
In the case of SQuAD, I already know that they are a few differences which should be fixed:
- the pre-processing of the dataset is not exactly the same (parsing and tokenization logic is a lot more complex in the XLNet repo),
- XLNet was trained using discriminative learning (progressively decreasing learning rate along with the depth of the model).<|||||>I found similar problem on GLEU dataset.
With the command:
python run_glue.py --data_dir=./glue_data/SST-2 --model_type=xlnet --task_name=sst-2 --output_dir=./xlnet_glue --model_name_or_path=xlnet-base-cased --do_train --evaluate_during_training
The final result of SST-2 is only 0.836, which is way lower than the current SoTA.
Does anyone have a clue how to solve it?<|||||>@ntubertchen good parameters for SST-2 are in the (adequately titled) issue #795 <|||||>I encountered similar problem with bert-large models. No luck yet.<|||||>This issue has been automatically marked as stale because it has not had recent activity. It will be closed if no further activity occurs. Thank you for your contributions.
<|||||>looks like xlnet for squad 2.0 is broken:
```
python run_squad.py --version_2_with_negative --cache_dir ${CACHE_DIR} \
--model_type xlnet --model_name_or_path xlnet-large-cased \
--do_train --train_file $SQUAD_DIR/train-v2.0.json \
--do_eval --predict_file $SQUAD_DIR/dev-v2.0.json \
--gradient_accumulation_steps 4 --overwrite_output_dir \
--learning_rate "3e-5" --num_train_epochs 2 --max_seq_length 512 --doc_stride 128 \
--output_dir $SQUAD_DIR/output/" \
--fp16 --fp16_opt_level "O2" --per_gpu_train_batch_size 8 \
--per_gpu_eval_batch_size 8 --weight_decay=0.00 --save_steps 20000 --adam_epsilon 1e-6
```
gives:
```
Epoch: 0%| | 0/2 [00:00<?, ?it/s]
Iteration: 0%| | 0/16343 [00:00<?, ?it/s][ATraceback (most recent call last):
File "examples/run_squad.py", line 830, in <module>
main()
File "examples/run_squad.py", line 769, in main
global_step, tr_loss = train(args, train_dataset, model, tokenizer)
File "examples/run_squad.py", line 221, in train
inputs.update({"is_impossible": batch[7]})
IndexError: tuple index out of range
```
I added `is_impossible` to the features and dataloader, but the result was very low:
```
{'exact': 44.5717173418681, 'f1': 44.82239308319654, 'total': 11873, 'HasAns_exact': 0.0, 'HasAns_f1': 0.5020703570837503, 'HasAns_total': 5928, 'NoAns_exact': 89.01597981497056, 'NoAns_f1': 89.01597981497056, 'NoAns_total': 5945, 'best_exact': 50.07159100480081, 'best_exact_thresh': 0.0, 'best_f1': 50.07159100480081, 'best_f1_thresh': 0.0}
```<|||||>Thanks for reporting the bug @panl2015, should have been fixed with 073219b.<|||||>Thanks @LysandreJik ! I think that's how I fixed it locally to make it run but got the low result. Maybe I should try with your version to make sure I don't have other changes. |
transformers | 821 | closed | Couldn't reach server | Hi I am running the very first example in readme. I got these errors, thanks for your help
Couldn't reach server to download vocabulary.
Couldn't reach server at 'https://s3.amazonaws.com/models.huggingface.co/bert/bert-base-uncased-config.json' to download pretrained model configuration file.
Couldn't reach server at 'https://s3.amazonaws.com/models.huggingface.co/bert/bert-base-uncased-pytorch_model.bin' to download pretrained weights.
Traceback (most recent call last):
| 07-18-2019 12:03:44 | 07-18-2019 12:03:44 | If I click on the links you provided above, they are currently reachable for me...
It may be a silly suggestions, but could it be that your internet connection was momentarily down when the code tried download those files or somehow you are not allowed to reach data on s3?<|||||>I have an idea about it. We can download the file from local computer, and send the file to the location of pytorch_transformers, for example:
/root/anaconda3/lib/python3.6/site-packages/pytorch_transformers
After that, we need to modify the modeling_bert.py in this folder:
https://github.com/huggingface/pytorch-transformers/blob/master/pytorch_transformers/modeling_bert.py
<|||||>Are you behind a proxy maybe?
You can now give proxies parameters to the `from_pretrained` methods e.g.:
```python
proxies = {
"http": "http://10.10.1.10:3128",
"https": "https://10.10.1.10:1080",
}
model = BertModel.from_pretrained('bert-base-uncased', proxies=proxies)
```<|||||>This issue has been automatically marked as stale because it has not had recent activity. It will be closed if no further activity occurs. Thank you for your contributions.
<|||||>Can you try installing `pyopenssl` using this command.
`pip install pyopenssl`
This worked for me. I guess the requests library is unable to establish an SSL connection, due to which the downloads are failing. Installing `pyopenssl` should solve the problem.
<|||||>Hello, I am also facing this same error when running on AWS Lambda:
`module initialization error: Couldn't reach server at '{}' to download vocabulary files.`
I've added proxies and installed pyopenssl as suggested by @thomwolf and @saradhix. It doesn't solve this issue.
Do you have any further ideas please?
I am calling the "fill-mask" pipeline with "camembert-base".
Thank you!<|||||>> Can you try installing `pyopenssl` using this command.
> `pip install pyopenssl`
> This worked for me. I guess the requests library is unable to establish an SSL connection, due to which the downloads are failing. Installing `pyopenssl` should solve the problem.
@saradhix what are the changes you've made on the source code to use `pyopenssl` instead of `requests`?
Thanks!<|||||>@ZiedHY I made no changes other than installing the `pyopenssl` package. I guess the `requests` module might internally use the `pyopenssl` for making secure connections.<|||||>> Hello, I am also facing this same error when running on AWS Lambda:
> `module initialization error: Couldn't reach server at '{}' to download vocabulary files.`
>
> I've added proxies and installed pyopenssl as suggested by @thomwolf and @saradhix. It doesn't solve this issue.
>
> Do you have any further ideas please?
>
> I am calling the "fill-mask" pipeline with "camembert-base".
>
> Thank you!
This seems to be a different problem. Why is the server url not getting printed in the error message?
`Couldn't reach server at '{}'`
See the error message posted by @rabeehk at the top, which contains the full url. Your issue seems to be different.<|||||>Thank you @saradhix. You're right. It comes closer to this [issue](https://github.com/huggingface/transformers/issues/2116). |
transformers | 820 | closed | RuntimeError: Creating MTGP constants failed | Hi,
I successfully fine tuned a BertForTokenClassification model based on bert-base-cased in the past. However, I now encounter with an following error: (see full stack below)
```
RuntimeError: **Creating MTGP constants failed.** at /opt/conda/conda-bld/pytorch_1556653099582/work/aten/src/THC/THCTensorRandom.cu:33
terminate called after throwing an instance of 'c10::Error'
what(): CUDA error: device-side assert triggered (insert_events at /opt/conda/conda-bld/pytorch_1556653099582/work/c10/cuda/CUDACachingAllocator.cpp:564)
```
I cannot seem to track the source of the problem...
I made sure the sequence length is << 512 as required and defined in the bert config.
Please advise
**CUDA= 10
Torch = 1.0.0**
```
/opt/conda/conda-bld/pytorch_1556653099582/work/aten/src/THC/THCTensorIndex.cu:362: void indexSelectLargeIndex(TensorInfo<T, IndexType>, TensorInfo<T, IndexType>, TensorInfo<long, IndexType>, int, int, IndexType, IndexType, long) [with T = float, IndexType = unsigned int, DstDim = 2, SrcDim = 2, IdxDim = -2, IndexIsMajor = true]: block: [47,0,0], thread: [0,0,0] Assertion `srcIndex < srcSelectDimSize` failed.
/opt/conda/conda-bld/pytorch_1556653099582/work/aten/src/THC/THCTensorIndex.cu:362: void indexSelectLargeIndex(TensorInfo<T, IndexType>, TensorInfo<T, IndexType>, TensorInfo<long, IndexType>, int, int, IndexType, IndexType, long) [with T = float, IndexType = unsigned int, DstDim = 2, SrcDim = 2, IdxDim = -2, IndexIsMajor = true]: block: [47,0,0], thread: [1,0,0] Assertion `srcIndex < srcSelectDimSize` failed.
/opt/conda/conda-bld/pytorch_1556653099582/work/aten/src/THC/THCTensorIndex.cu:362: void indexSelectLargeIndex(TensorInfo<T, IndexType>, TensorInfo<T, IndexType>, TensorInfo<long, IndexType>, int, int, IndexType, IndexType, long) [with T = float, IndexType = unsigned int, DstDim = 2, SrcDim = 2, IdxDim = -2, IndexIsMajor = true]: block: [47,0,0], thread: [2,0,0] Assertion `srcIndex < srcSelectDimSize` failed.
/opt/conda/conda-bld/pytorch_1556653099582/work/aten/src/THC/THCTensorIndex.cu:362: void indexSelectLargeIndex(TensorInfo<T, IndexType>, TensorInfo<T, IndexType>, TensorInfo<long, IndexType>, int, int, IndexType, IndexType, long) [with T = float, IndexType = unsigned int, DstDim = 2, SrcDim = 2, IdxDim = -2, IndexIsMajor = true]: block: [47,0,0], thread: [3,0,0] Assertion `srcIndex < srcSelectDimSize` failed.
/opt/conda/conda-bld/pytorch_1556653099582/work/aten/src/THC/THCTensorIndex.cu:362: void indexSelectLargeIndex(TensorInfo<T, IndexType>, TensorInfo<T, IndexType>, TensorInfo<long, IndexType>, int, int, IndexType, IndexType, long) [with T = float, IndexType = unsigned int, DstDim = 2, SrcDim = 2, IdxDim = -2, IndexIsMajor = true]: block: [47,0,0], thread: [4,0,0] Assertion `srcIndex < srcSelectDimSize` failed.
/opt/conda/conda-bld/pytorch_1556653099582/work/aten/src/THC/THCTensorIndex.cu:362: void indexSelectLargeIndex(TensorInfo<T, IndexType>, TensorInfo<T, IndexType>, TensorInfo<long, IndexType>, int, int, IndexType, IndexType, long) [with T = float, IndexType = unsigned int, DstDim = 2, SrcDim = 2, IdxDim = -2, IndexIsMajor = true]: block: [47,0,0], thread: [5,0,0] Assertion `srcIndex < srcSelectDimSize` failed.
/opt/conda/conda-bld/pytorch_1556653099582/work/aten/src/THC/THCTensorIndex.cu:362: void indexSelectLargeIndex(TensorInfo<T, IndexType>, TensorInfo<T, IndexType>, TensorInfo<long, IndexType>, int, int, IndexType, IndexType, long) [with T = float, IndexType = unsigned int, DstDim = 2, SrcDim = 2, IdxDim = -2, IndexIsMajor = true]: block: [47,0,0], thread: [6,0,0] Assertion `srcIndex < srcSelectDimSize` failed.
/opt/conda/conda-bld/pytorch_1556653099582/work/aten/src/THC/THCTensorIndex.cu:362: void indexSelectLargeIndex(TensorInfo<T, IndexType>, TensorInfo<T, IndexType>, TensorInfo<long, IndexType>, int, int, IndexType, IndexType, long) [with T = float, IndexType = unsigned int, DstDim = 2, SrcDim = 2, IdxDim = -2, IndexIsMajor = true]: block: [47,0,0], thread: [7,0,0] Assertion `srcIndex < srcSelectDimSize` failed.
/opt/conda/conda-bld/pytorch_1556653099582/work/aten/src/THC/THCTensorIndex.cu:362: void indexSelectLargeIndex(TensorInfo<T, IndexType>, TensorInfo<T, IndexType>, TensorInfo<long, IndexType>, int, int, IndexType, IndexType, long) [with T = float, IndexType = unsigned int, DstDim = 2, SrcDim = 2, IdxDim = -2, IndexIsMajor = true]: block: [47,0,0], thread: [8,0,0] Assertion `srcIndex < srcSelectDimSize` failed.
/opt/conda/conda-bld/pytorch_1556653099582/work/aten/src/THC/THCTensorIndex.cu:362: void indexSelectLargeIndex(TensorInfo<T, IndexType>, TensorInfo<T, IndexType>, TensorInfo<long, IndexType>, int, int, IndexType, IndexType, long) [with T = float, IndexType = unsigned int, DstDim = 2, SrcDim = 2, IdxDim = -2, IndexIsMajor = true]: block: [47,0,0], thread: [9,0,0] Assertion `srcIndex < srcSelectDimSize` failed.
/opt/conda/conda-bld/pytorch_1556653099582/work/aten/src/THC/THCTensorIndex.cu:362: void indexSelectLargeIndex(TensorInfo<T, IndexType>, TensorInfo<T, IndexType>, TensorInfo<long, IndexType>, int, int, IndexType, IndexType, long) [with T = float, IndexType = unsigned int, DstDim = 2, SrcDim = 2, IdxDim = -2, IndexIsMajor = true]: block: [47,0,0], thread: [10,0,0] Assertion `srcIndex < srcSelectDimSize` failed.
/opt/conda/conda-bld/pytorch_1556653099582/work/aten/src/THC/THCTensorIndex.cu:362: void indexSelectLargeIndex(TensorInfo<T, IndexType>, TensorInfo<T, IndexType>, TensorInfo<long, IndexType>, int, int, IndexType, IndexType, long) [with T = float, IndexType = unsigned int, DstDim = 2, SrcDim = 2, IdxDim = -2, IndexIsMajor = true]: block: [47,0,0], thread: [11,0,0] Assertion `srcIndex < srcSelectDimSize` failed.
/opt/conda/conda-bld/pytorch_1556653099582/work/aten/src/THC/THCTensorIndex.cu:362: void indexSelectLargeIndex(TensorInfo<T, IndexType>, TensorInfo<T, IndexType>, TensorInfo<long, IndexType>, int, int, IndexType, IndexType, long) [with T = float, IndexType = unsigned int, DstDim = 2, SrcDim = 2, IdxDim = -2, IndexIsMajor = true]: block: [47,0,0], thread: [12,0,0] Assertion `srcIndex < srcSelectDimSize` failed.
/opt/conda/conda-bld/pytorch_1556653099582/work/aten/src/THC/THCTensorIndex.cu:362: void indexSelectLargeIndex(TensorInfo<T, IndexType>, TensorInfo<T, IndexType>, TensorInfo<long, IndexType>, int, int, IndexType, IndexType, long) [with T = float, IndexType = unsigned int, DstDim = 2, SrcDim = 2, IdxDim = -2, IndexIsMajor = true]: block: [47,0,0], thread: [13,0,0] Assertion `srcIndex < srcSelectDimSize` failed.
/opt/conda/conda-bld/pytorch_1556653099582/work/aten/src/THC/THCTensorIndex.cu:362: void indexSelectLargeIndex(TensorInfo<T, IndexType>, TensorInfo<T, IndexType>, TensorInfo<long, IndexType>, int, int, IndexType, IndexType, long) [with T = float, IndexType = unsigned int, DstDim = 2, SrcDim = 2, IdxDim = -2, IndexIsMajor = true]: block: [47,0,0], thread: [14,0,0] Assertion `srcIndex < srcSelectDimSize` failed.
/opt/conda/conda-bld/pytorch_1556653099582/work/aten/src/THC/THCTensorIndex.cu:362: void indexSelectLargeIndex(TensorInfo<T, IndexType>, TensorInfo<T, IndexType>, TensorInfo<long, IndexType>, int, int, IndexType, IndexType, long) [with T = float, IndexType = unsigned int, DstDim = 2, SrcDim = 2, IdxDim = -2, IndexIsMajor = true]: block: [47,0,0], thread: [15,0,0] Assertion `srcIndex < srcSelectDimSize` failed.
/opt/conda/conda-bld/pytorch_1556653099582/work/aten/src/THC/THCTensorIndex.cu:362: void indexSelectLargeIndex(TensorInfo<T, IndexType>, TensorInfo<T, IndexType>, TensorInfo<long, IndexType>, int, int, IndexType, IndexType, long) [with T = float, IndexType = unsigned int, DstDim = 2, SrcDim = 2, IdxDim = -2, IndexIsMajor = true]: block: [47,0,0], thread: [16,0,0] Assertion `srcIndex < srcSelectDimSize` failed.
/opt/conda/conda-bld/pytorch_1556653099582/work/aten/src/THC/THCTensorIndex.cu:362: void indexSelectLargeIndex(TensorInfo<T, IndexType>, TensorInfo<T, IndexType>, TensorInfo<long, IndexType>, int, int, IndexType, IndexType, long) [with T = float, IndexType = unsigned int, DstDim = 2, SrcDim = 2, IdxDim = -2, IndexIsMajor = true]: block: [47,0,0], thread: [17,0,0] Assertion `srcIndex < srcSelectDimSize` failed.
/opt/conda/conda-bld/pytorch_1556653099582/work/aten/src/THC/THCTensorIndex.cu:362: void indexSelectLargeIndex(TensorInfo<T, IndexType>, TensorInfo<T, IndexType>, TensorInfo<long, IndexType>, int, int, IndexType, IndexType, long) [with T = float, IndexType = unsigned int, DstDim = 2, SrcDim = 2, IdxDim = -2, IndexIsMajor = true]: block: [47,0,0], thread: [18,0,0] Assertion `srcIndex < srcSelectDimSize` failed.
/opt/conda/conda-bld/pytorch_1556653099582/work/aten/src/THC/THCTensorIndex.cu:362: void indexSelectLargeIndex(TensorInfo<T, IndexType>, TensorInfo<T, IndexType>, TensorInfo<long, IndexType>, int, int, IndexType, IndexType, long) [with T = float, IndexType = unsigned int, DstDim = 2, SrcDim = 2, IdxDim = -2, IndexIsMajor = true]: block: [47,0,0], thread: [19,0,0] Assertion `srcIndex < srcSelectDimSize` failed.
/opt/conda/conda-bld/pytorch_1556653099582/work/aten/src/THC/THCTensorIndex.cu:362: void indexSelectLargeIndex(TensorInfo<T, IndexType>, TensorInfo<T, IndexType>, TensorInfo<long, IndexType>, int, int, IndexType, IndexType, long) [with T = float, IndexType = unsigned int, DstDim = 2, SrcDim = 2, IdxDim = -2, IndexIsMajor = true]: block: [47,0,0], thread: [20,0,0] Assertion `srcIndex < srcSelectDimSize` failed.
/opt/conda/conda-bld/pytorch_1556653099582/work/aten/src/THC/THCTensorIndex.cu:362: void indexSelectLargeIndex(TensorInfo<T, IndexType>, TensorInfo<T, IndexType>, TensorInfo<long, IndexType>, int, int, IndexType, IndexType, long) [with T = float, IndexType = unsigned int, DstDim = 2, SrcDim = 2, IdxDim = -2, IndexIsMajor = true]: block: [47,0,0], thread: [21,0,0] Assertion `srcIndex < srcSelectDimSize` failed.
/opt/conda/conda-bld/pytorch_1556653099582/work/aten/src/THC/THCTensorIndex.cu:362: void indexSelectLargeIndex(TensorInfo<T, IndexType>, TensorInfo<T, IndexType>, TensorInfo<long, IndexType>, int, int, IndexType, IndexType, long) [with T = float, IndexType = unsigned int, DstDim = 2, SrcDim = 2, IdxDim = -2, IndexIsMajor = true]: block: [47,0,0], thread: [22,0,0] Assertion `srcIndex < srcSelectDimSize` failed.
/opt/conda/conda-bld/pytorch_1556653099582/work/aten/src/THC/THCTensorIndex.cu:362: void indexSelectLargeIndex(TensorInfo<T, IndexType>, TensorInfo<T, IndexType>, TensorInfo<long, IndexType>, int, int, IndexType, IndexType, long) [with T = float, IndexType = unsigned int, DstDim = 2, SrcDim = 2, IdxDim = -2, IndexIsMajor = true]: block: [47,0,0], thread: [23,0,0] Assertion `srcIndex < srcSelectDimSize` failed.
/opt/conda/conda-bld/pytorch_1556653099582/work/aten/src/THC/THCTensorIndex.cu:362: void indexSelectLargeIndex(TensorInfo<T, IndexType>, TensorInfo<T, IndexType>, TensorInfo<long, IndexType>, int, int, IndexType, IndexType, long) [with T = float, IndexType = unsigned int, DstDim = 2, SrcDim = 2, IdxDim = -2, IndexIsMajor = true]: block: [47,0,0], thread: [24,0,0] Assertion `srcIndex < srcSelectDimSize` failed.
/opt/conda/conda-bld/pytorch_1556653099582/work/aten/src/THC/THCTensorIndex.cu:362: void indexSelectLargeIndex(TensorInfo<T, IndexType>, TensorInfo<T, IndexType>, TensorInfo<long, IndexType>, int, int, IndexType, IndexType, long) [with T = float, IndexType = unsigned int, DstDim = 2, SrcDim = 2, IdxDim = -2, IndexIsMajor = true]: block: [47,0,0], thread: [25,0,0] Assertion `srcIndex < srcSelectDimSize` failed.
/opt/conda/conda-bld/pytorch_1556653099582/work/aten/src/THC/THCTensorIndex.cu:362: void indexSelectLargeIndex(TensorInfo<T, IndexType>, TensorInfo<T, IndexType>, TensorInfo<long, IndexType>, int, int, IndexType, IndexType, long) [with T = float, IndexType = unsigned int, DstDim = 2, SrcDim = 2, IdxDim = -2, IndexIsMajor = true]: block: [47,0,0], thread: [26,0,0] Assertion `srcIndex < srcSelectDimSize` failed.
/opt/conda/conda-bld/pytorch_1556653099582/work/aten/src/THC/THCTensorIndex.cu:362: void indexSelectLargeIndex(TensorInfo<T, IndexType>, TensorInfo<T, IndexType>, TensorInfo<long, IndexType>, int, int, IndexType, IndexType, long) [with T = float, IndexType = unsigned int, DstDim = 2, SrcDim = 2, IdxDim = -2, IndexIsMajor = true]: block: [47,0,0], thread: [27,0,0] Assertion `srcIndex < srcSelectDimSize` failed.
/opt/conda/conda-bld/pytorch_1556653099582/work/aten/src/THC/THCTensorIndex.cu:362: void indexSelectLargeIndex(TensorInfo<T, IndexType>, TensorInfo<T, IndexType>, TensorInfo<long, IndexType>, int, int, IndexType, IndexType, long) [with T = float, IndexType = unsigned int, DstDim = 2, SrcDim = 2, IdxDim = -2, IndexIsMajor = true]: block: [47,0,0], thread: [28,0,0] Assertion `srcIndex < srcSelectDimSize` failed.
/opt/conda/conda-bld/pytorch_1556653099582/work/aten/src/THC/THCTensorIndex.cu:362: void indexSelectLargeIndex(TensorInfo<T, IndexType>, TensorInfo<T, IndexType>, TensorInfo<long, IndexType>, int, int, IndexType, IndexType, long) [with T = float, IndexType = unsigned int, DstDim = 2, SrcDim = 2, IdxDim = -2, IndexIsMajor = true]: block: [47,0,0], thread: [29,0,0] Assertion `srcIndex < srcSelectDimSize` failed.
/opt/conda/conda-bld/pytorch_1556653099582/work/aten/src/THC/THCTensorIndex.cu:362: void indexSelectLargeIndex(TensorInfo<T, IndexType>, TensorInfo<T, IndexType>, TensorInfo<long, IndexType>, int, int, IndexType, IndexType, long) [with T = float, IndexType = unsigned int, DstDim = 2, SrcDim = 2, IdxDim = -2, IndexIsMajor = true]: block: [47,0,0], thread: [30,0,0] Assertion `srcIndex < srcSelectDimSize` failed.
/opt/conda/conda-bld/pytorch_1556653099582/work/aten/src/THC/THCTensorIndex.cu:362: void indexSelectLargeIndex(TensorInfo<T, IndexType>, TensorInfo<T, IndexType>, TensorInfo<long, IndexType>, int, int, IndexType, IndexType, long) [with T = float, IndexType = unsigned int, DstDim = 2, SrcDim = 2, IdxDim = -2, IndexIsMajor = true]: block: [47,0,0], thread: [31,0,0] Assertion `srcIndex < srcSelectDimSize` failed.
/opt/conda/conda-bld/pytorch_1556653099582/work/aten/src/THC/THCTensorIndex.cu:362: void indexSelectLargeIndex(TensorInfo<T, IndexType>, TensorInfo<T, IndexType>, TensorInfo<long, IndexType>, int, int, IndexType, IndexType, long) [with T = float, IndexType = unsigned int, DstDim = 2, SrcDim = 2, IdxDim = -2, IndexIsMajor = true]: block: [47,0,0], thread: [32,0,0] Assertion `srcIndex < srcSelectDimSize` failed.
/opt/conda/conda-bld/pytorch_1556653099582/work/aten/src/THC/THCTensorIndex.cu:362: void indexSelectLargeIndex(TensorInfo<T, IndexType>, TensorInfo<T, IndexType>, TensorInfo<long, IndexType>, int, int, IndexType, IndexType, long) [with T = float, IndexType = unsigned int, DstDim = 2, SrcDim = 2, IdxDim = -2, IndexIsMajor = true]: block: [47,0,0], thread: [33,0,0] Assertion `srcIndex < srcSelectDimSize` failed.
/opt/conda/conda-bld/pytorch_1556653099582/work/aten/src/THC/THCTensorIndex.cu:362: void indexSelectLargeIndex(TensorInfo<T, IndexType>, TensorInfo<T, IndexType>, TensorInfo<long, IndexType>, int, int, IndexType, IndexType, long) [with T = float, IndexType = unsigned int, DstDim = 2, SrcDim = 2, IdxDim = -2, IndexIsMajor = true]: block: [47,0,0], thread: [34,0,0] Assertion `srcIndex < srcSelectDimSize` failed.
/opt/conda/conda-bld/pytorch_1556653099582/work/aten/src/THC/THCTensorIndex.cu:362: void indexSelectLargeIndex(TensorInfo<T, IndexType>, TensorInfo<T, IndexType>, TensorInfo<long, IndexType>, int, int, IndexType, IndexType, long) [with T = float, IndexType = unsigned int, DstDim = 2, SrcDim = 2, IdxDim = -2, IndexIsMajor = true]: block: [47,0,0], thread: [35,0,0] Assertion `srcIndex < srcSelectDimSize` failed.
/opt/conda/conda-bld/pytorch_1556653099582/work/aten/src/THC/THCTensorIndex.cu:362: void indexSelectLargeIndex(TensorInfo<T, IndexType>, TensorInfo<T, IndexType>, TensorInfo<long, IndexType>, int, int, IndexType, IndexType, long) [with T = float, IndexType = unsigned int, DstDim = 2, SrcDim = 2, IdxDim = -2, IndexIsMajor = true]: block: [47,0,0], thread: [36,0,0] Assertion `srcIndex < srcSelectDimSize` failed.
/opt/conda/conda-bld/pytorch_1556653099582/work/aten/src/THC/THCTensorIndex.cu:362: void indexSelectLargeIndex(TensorInfo<T, IndexType>, TensorInfo<T, IndexType>, TensorInfo<long, IndexType>, int, int, IndexType, IndexType, long) [with T = float, IndexType = unsigned int, DstDim = 2, SrcDim = 2, IdxDim = -2, IndexIsMajor = true]: block: [47,0,0], thread: [37,0,0] Assertion `srcIndex < srcSelectDimSize` failed.
/opt/conda/conda-bld/pytorch_1556653099582/work/aten/src/THC/THCTensorIndex.cu:362: void indexSelectLargeIndex(TensorInfo<T, IndexType>, TensorInfo<T, IndexType>, TensorInfo<long, IndexType>, int, int, IndexType, IndexType, long) [with T = float, IndexType = unsigned int, DstDim = 2, SrcDim = 2, IdxDim = -2, IndexIsMajor = true]: block: [47,0,0], thread: [38,0,0] Assertion `srcIndex < srcSelectDimSize` failed.
/opt/conda/conda-bld/pytorch_1556653099582/work/aten/src/THC/THCTensorIndex.cu:362: void indexSelectLargeIndex(TensorInfo<T, IndexType>, TensorInfo<T, IndexType>, TensorInfo<long, IndexType>, int, int, IndexType, IndexType, long) [with T = float, IndexType = unsigned int, DstDim = 2, SrcDim = 2, IdxDim = -2, IndexIsMajor = true]: block: [47,0,0], thread: [39,0,0] Assertion `srcIndex < srcSelectDimSize` failed.
/opt/conda/conda-bld/pytorch_1556653099582/work/aten/src/THC/THCTensorIndex.cu:362: void indexSelectLargeIndex(TensorInfo<T, IndexType>, TensorInfo<T, IndexType>, TensorInfo<long, IndexType>, int, int, IndexType, IndexType, long) [with T = float, IndexType = unsigned int, DstDim = 2, SrcDim = 2, IdxDim = -2, IndexIsMajor = true]: block: [47,0,0], thread: [40,0,0] Assertion `srcIndex < srcSelectDimSize` failed.
/opt/conda/conda-bld/pytorch_1556653099582/work/aten/src/THC/THCTensorIndex.cu:362: void indexSelectLargeIndex(TensorInfo<T, IndexType>, TensorInfo<T, IndexType>, TensorInfo<long, IndexType>, int, int, IndexType, IndexType, long) [with T = float, IndexType = unsigned int, DstDim = 2, SrcDim = 2, IdxDim = -2, IndexIsMajor = true]: block: [47,0,0], thread: [41,0,0] Assertion `srcIndex < srcSelectDimSize` failed.
/opt/conda/conda-bld/pytorch_1556653099582/work/aten/src/THC/THCTensorIndex.cu:362: void indexSelectLargeIndex(TensorInfo<T, IndexType>, TensorInfo<T, IndexType>, TensorInfo<long, IndexType>, int, int, IndexType, IndexType, long) [with T = float, IndexType = unsigned int, DstDim = 2, SrcDim = 2, IdxDim = -2, IndexIsMajor = true]: block: [47,0,0], thread: [42,0,0] Assertion `srcIndex < srcSelectDimSize` failed.
/opt/conda/conda-bld/pytorch_1556653099582/work/aten/src/THC/THCTensorIndex.cu:362: void indexSelectLargeIndex(TensorInfo<T, IndexType>, TensorInfo<T, IndexType>, TensorInfo<long, IndexType>, int, int, IndexType, IndexType, long) [with T = float, IndexType = unsigned int, DstDim = 2, SrcDim = 2, IdxDim = -2, IndexIsMajor = true]: block: [47,0,0], thread: [43,0,0] Assertion `srcIndex < srcSelectDimSize` failed.
/opt/conda/conda-bld/pytorch_1556653099582/work/aten/src/THC/THCTensorIndex.cu:362: void indexSelectLargeIndex(TensorInfo<T, IndexType>, TensorInfo<T, IndexType>, TensorInfo<long, IndexType>, int, int, IndexType, IndexType, long) [with T = float, IndexType = unsigned int, DstDim = 2, SrcDim = 2, IdxDim = -2, IndexIsMajor = true]: block: [47,0,0], thread: [44,0,0] Assertion `srcIndex < srcSelectDimSize` failed.
/opt/conda/conda-bld/pytorch_1556653099582/work/aten/src/THC/THCTensorIndex.cu:362: void indexSelectLargeIndex(TensorInfo<T, IndexType>, TensorInfo<T, IndexType>, TensorInfo<long, IndexType>, int, int, IndexType, IndexType, long) [with T = float, IndexType = unsigned int, DstDim = 2, SrcDim = 2, IdxDim = -2, IndexIsMajor = true]: block: [47,0,0], thread: [45,0,0] Assertion `srcIndex < srcSelectDimSize` failed.
/opt/conda/conda-bld/pytorch_1556653099582/work/aten/src/THC/THCTensorIndex.cu:362: void indexSelectLargeIndex(TensorInfo<T, IndexType>, TensorInfo<T, IndexType>, TensorInfo<long, IndexType>, int, int, IndexType, IndexType, long) [with T = float, IndexType = unsigned int, DstDim = 2, SrcDim = 2, IdxDim = -2, IndexIsMajor = true]: block: [47,0,0], thread: [46,0,0] Assertion `srcIndex < srcSelectDimSize` failed.
/opt/conda/conda-bld/pytorch_1556653099582/work/aten/src/THC/THCTensorIndex.cu:362: void indexSelectLargeIndex(TensorInfo<T, IndexType>, TensorInfo<T, IndexType>, TensorInfo<long, IndexType>, int, int, IndexType, IndexType, long) [with T = float, IndexType = unsigned int, DstDim = 2, SrcDim = 2, IdxDim = -2, IndexIsMajor = true]: block: [47,0,0], thread: [47,0,0] Assertion `srcIndex < srcSelectDimSize` failed.
/opt/conda/conda-bld/pytorch_1556653099582/work/aten/src/THC/THCTensorIndex.cu:362: void indexSelectLargeIndex(TensorInfo<T, IndexType>, TensorInfo<T, IndexType>, TensorInfo<long, IndexType>, int, int, IndexType, IndexType, long) [with T = float, IndexType = unsigned int, DstDim = 2, SrcDim = 2, IdxDim = -2, IndexIsMajor = true]: block: [47,0,0], thread: [48,0,0] Assertion `srcIndex < srcSelectDimSize` failed.
/opt/conda/conda-bld/pytorch_1556653099582/work/aten/src/THC/THCTensorIndex.cu:362: void indexSelectLargeIndex(TensorInfo<T, IndexType>, TensorInfo<T, IndexType>, TensorInfo<long, IndexType>, int, int, IndexType, IndexType, long) [with T = float, IndexType = unsigned int, DstDim = 2, SrcDim = 2, IdxDim = -2, IndexIsMajor = true]: block: [47,0,0], thread: [49,0,0] Assertion `srcIndex < srcSelectDimSize` failed.
/opt/conda/conda-bld/pytorch_1556653099582/work/aten/src/THC/THCTensorIndex.cu:362: void indexSelectLargeIndex(TensorInfo<T, IndexType>, TensorInfo<T, IndexType>, TensorInfo<long, IndexType>, int, int, IndexType, IndexType, long) [with T = float, IndexType = unsigned int, DstDim = 2, SrcDim = 2, IdxDim = -2, IndexIsMajor = true]: block: [47,0,0], thread: [50,0,0] Assertion `srcIndex < srcSelectDimSize` failed.
/opt/conda/conda-bld/pytorch_1556653099582/work/aten/src/THC/THCTensorIndex.cu:362: void indexSelectLargeIndex(TensorInfo<T, IndexType>, TensorInfo<T, IndexType>, TensorInfo<long, IndexType>, int, int, IndexType, IndexType, long) [with T = float, IndexType = unsigned int, DstDim = 2, SrcDim = 2, IdxDim = -2, IndexIsMajor = true]: block: [47,0,0], thread: [51,0,0] Assertion `srcIndex < srcSelectDimSize` failed.
/opt/conda/conda-bld/pytorch_1556653099582/work/aten/src/THC/THCTensorIndex.cu:362: void indexSelectLargeIndex(TensorInfo<T, IndexType>, TensorInfo<T, IndexType>, TensorInfo<long, IndexType>, int, int, IndexType, IndexType, long) [with T = float, IndexType = unsigned int, DstDim = 2, SrcDim = 2, IdxDim = -2, IndexIsMajor = true]: block: [47,0,0], thread: [52,0,0] Assertion `srcIndex < srcSelectDimSize` failed.
/opt/conda/conda-bld/pytorch_1556653099582/work/aten/src/THC/THCTensorIndex.cu:362: void indexSelectLargeIndex(TensorInfo<T, IndexType>, TensorInfo<T, IndexType>, TensorInfo<long, IndexType>, int, int, IndexType, IndexType, long) [with T = float, IndexType = unsigned int, DstDim = 2, SrcDim = 2, IdxDim = -2, IndexIsMajor = true]: block: [47,0,0], thread: [53,0,0] Assertion `srcIndex < srcSelectDimSize` failed.
/opt/conda/conda-bld/pytorch_1556653099582/work/aten/src/THC/THCTensorIndex.cu:362: void indexSelectLargeIndex(TensorInfo<T, IndexType>, TensorInfo<T, IndexType>, TensorInfo<long, IndexType>, int, int, IndexType, IndexType, long) [with T = float, IndexType = unsigned int, DstDim = 2, SrcDim = 2, IdxDim = -2, IndexIsMajor = true]: block: [47,0,0], thread: [54,0,0] Assertion `srcIndex < srcSelectDimSize` failed.
/opt/conda/conda-bld/pytorch_1556653099582/work/aten/src/THC/THCTensorIndex.cu:362: void indexSelectLargeIndex(TensorInfo<T, IndexType>, TensorInfo<T, IndexType>, TensorInfo<long, IndexType>, int, int, IndexType, IndexType, long) [with T = float, IndexType = unsigned int, DstDim = 2, SrcDim = 2, IdxDim = -2, IndexIsMajor = true]: block: [47,0,0], thread: [55,0,0] Assertion `srcIndex < srcSelectDimSize` failed.
/opt/conda/conda-bld/pytorch_1556653099582/work/aten/src/THC/THCTensorIndex.cu:362: void indexSelectLargeIndex(TensorInfo<T, IndexType>, TensorInfo<T, IndexType>, TensorInfo<long, IndexType>, int, int, IndexType, IndexType, long) [with T = float, IndexType = unsigned int, DstDim = 2, SrcDim = 2, IdxDim = -2, IndexIsMajor = true]: block: [47,0,0], thread: [56,0,0] Assertion `srcIndex < srcSelectDimSize` failed.
/opt/conda/conda-bld/pytorch_1556653099582/work/aten/src/THC/THCTensorIndex.cu:362: void indexSelectLargeIndex(TensorInfo<T, IndexType>, TensorInfo<T, IndexType>, TensorInfo<long, IndexType>, int, int, IndexType, IndexType, long) [with T = float, IndexType = unsigned int, DstDim = 2, SrcDim = 2, IdxDim = -2, IndexIsMajor = true]: block: [47,0,0], thread: [57,0,0] Assertion `srcIndex < srcSelectDimSize` failed.
/opt/conda/conda-bld/pytorch_1556653099582/work/aten/src/THC/THCTensorIndex.cu:362: void indexSelectLargeIndex(TensorInfo<T, IndexType>, TensorInfo<T, IndexType>, TensorInfo<long, IndexType>, int, int, IndexType, IndexType, long) [with T = float, IndexType = unsigned int, DstDim = 2, SrcDim = 2, IdxDim = -2, IndexIsMajor = true]: block: [47,0,0], thread: [58,0,0] Assertion `srcIndex < srcSelectDimSize` failed.
/opt/conda/conda-bld/pytorch_1556653099582/work/aten/src/THC/THCTensorIndex.cu:362: void indexSelectLargeIndex(TensorInfo<T, IndexType>, TensorInfo<T, IndexType>, TensorInfo<long, IndexType>, int, int, IndexType, IndexType, long) [with T = float, IndexType = unsigned int, DstDim = 2, SrcDim = 2, IdxDim = -2, IndexIsMajor = true]: block: [47,0,0], thread: [59,0,0] Assertion `srcIndex < srcSelectDimSize` failed.
/opt/conda/conda-bld/pytorch_1556653099582/work/aten/src/THC/THCTensorIndex.cu:362: void indexSelectLargeIndex(TensorInfo<T, IndexType>, TensorInfo<T, IndexType>, TensorInfo<long, IndexType>, int, int, IndexType, IndexType, long) [with T = float, IndexType = unsigned int, DstDim = 2, SrcDim = 2, IdxDim = -2, IndexIsMajor = true]: block: [47,0,0], thread: [60,0,0] Assertion `srcIndex < srcSelectDimSize` failed.
/opt/conda/conda-bld/pytorch_1556653099582/work/aten/src/THC/THCTensorIndex.cu:362: void indexSelectLargeIndex(TensorInfo<T, IndexType>, TensorInfo<T, IndexType>, TensorInfo<long, IndexType>, int, int, IndexType, IndexType, long) [with T = float, IndexType = unsigned int, DstDim = 2, SrcDim = 2, IdxDim = -2, IndexIsMajor = true]: block: [47,0,0], thread: [61,0,0] Assertion `srcIndex < srcSelectDimSize` failed.
/opt/conda/conda-bld/pytorch_1556653099582/work/aten/src/THC/THCTensorIndex.cu:362: void indexSelectLargeIndex(TensorInfo<T, IndexType>, TensorInfo<T, IndexType>, TensorInfo<long, IndexType>, int, int, IndexType, IndexType, long) [with T = float, IndexType = unsigned int, DstDim = 2, SrcDim = 2, IdxDim = -2, IndexIsMajor = true]: block: [47,0,0], thread: [62,0,0] Assertion `srcIndex < srcSelectDimSize` failed.
/opt/conda/conda-bld/pytorch_1556653099582/work/aten/src/THC/THCTensorIndex.cu:362: void indexSelectLargeIndex(TensorInfo<T, IndexType>, TensorInfo<T, IndexType>, TensorInfo<long, IndexType>, int, int, IndexType, IndexType, long) [with T = float, IndexType = unsigned int, DstDim = 2, SrcDim = 2, IdxDim = -2, IndexIsMajor = true]: block: [47,0,0], thread: [63,0,0] Assertion `srcIndex < srcSelectDimSize` failed.
Traceback (most recent call last):
File "/tmp/train/named_entity_recognition/bert/train.py", line 288, in <module>
train(model, train_iter, optimizer, criterion, scheduler)
File "/tmp/train/named_entity_recognition/bert/train.py", line 69, in train
attention_mask=input_mask, labels=y)
File "/data/anaconda/envs/py36/lib/python3.6/site-packages/torch/nn/modules/module.py", line 493, in __call__
result = self.forward(*input, **kwargs)
File "/data/anaconda/envs/py36/lib/python3.6/site-packages/torch/nn/parallel/data_parallel.py", line 152, in forward
outputs = self.parallel_apply(replicas, inputs, kwargs)
File "/data/anaconda/envs/py36/lib/python3.6/site-packages/torch/nn/parallel/data_parallel.py", line 162, in parallel_apply
return parallel_apply(replicas, inputs, kwargs, self.device_ids[:len(replicas)])
File "/data/anaconda/envs/py36/lib/python3.6/site-packages/torch/nn/parallel/parallel_apply.py", line 83, in parallel_apply
raise output
File "/data/anaconda/envs/py36/lib/python3.6/site-packages/torch/nn/parallel/parallel_apply.py", line 59, in _worker
output = module(*input, **kwargs)
File "/data/anaconda/envs/py36/lib/python3.6/site-packages/torch/nn/modules/module.py", line 493, in __call__
result = self.forward(*input, **kwargs)
File "/data/anaconda/envs/py36/lib/python3.6/site-packages/pytorch_transformers/modeling_bert.py", line 1146, in forward
attention_mask=attention_mask, head_mask=head_mask)
File "/data/anaconda/envs/py36/lib/python3.6/site-packages/torch/nn/modules/module.py", line 493, in __call__
result = self.forward(*input, **kwargs)
File "/data/anaconda/envs/py36/lib/python3.6/site-packages/pytorch_transformers/modeling_bert.py", line 706, in forward
embedding_output = self.embeddings(input_ids, position_ids=position_ids, token_type_ids=token_type_ids)
File "/data/anaconda/envs/py36/lib/python3.6/site-packages/torch/nn/modules/module.py", line 493, in __call__
result = self.forward(*input, **kwargs)
File "/data/anaconda/envs/py36/lib/python3.6/site-packages/pytorch_transformers/modeling_bert.py", line 270, in forward
embeddings = self.dropout(embeddings)
File "/data/anaconda/envs/py36/lib/python3.6/site-packages/torch/nn/modules/module.py", line 493, in __call__
result = self.forward(*input, **kwargs)
File "/data/anaconda/envs/py36/lib/python3.6/site-packages/torch/nn/modules/dropout.py", line 58, in forward
return F.dropout(input, self.p, self.training, self.inplace)
File "/data/anaconda/envs/py36/lib/python3.6/site-packages/torch/nn/functional.py", line 830, in dropout
else _VF.dropout(input, p, training))
RuntimeError: Creating MTGP constants failed. at /opt/conda/conda-bld/pytorch_1556653099582/work/aten/src/THC/THCTensorRandom.cu:33
terminate called after throwing an instance of 'c10::Error'
what(): CUDA error: device-side assert triggered (insert_events at /opt/conda/conda-bld/pytorch_1556653099582/work/c10/cuda/CUDACachingAllocator.cpp:564)
frame #0: c10::Error::Error(c10::SourceLocation, std::string const&) + 0x45 (0x7f16db6a1dc5 in /data/anaconda/envs/py36/lib/python3.6/site-packages/torch/lib/libc10.so)
frame #1: <unknown function> + 0x14792 (0x7f16d9213792 in /data/anaconda/envs/py36/lib/python3.6/site-packages/torch/lib/libc10_cuda.so)
frame #2: c10::TensorImpl::release_resources() + 0x50 (0x7f16db691640 in /data/anaconda/envs/py36/lib/python3.6/site-packages/torch/lib/libc10.so)
frame #3: <unknown function> + 0x3067fb (0x7f168947f7fb in /data/anaconda/envs/py36/lib/python3.6/site-packages/torch/lib/libtorch.so.1)
frame #4: <unknown function> + 0x13ff1b (0x7f16db9faf1b in /data/anaconda/envs/py36/lib/python3.6/site-packages/torch/lib/libtorch_python.so)
frame #5: <unknown function> + 0x3bf384 (0x7f16dbc7a384 in /data/anaconda/envs/py36/lib/python3.6/site-packages/torch/lib/libtorch_python.so)
frame #6: <unknown function> + 0x3bf3d1 (0x7f16dbc7a3d1 in /data/anaconda/envs/py36/lib/python3.6/site-packages/torch/lib/libtorch_python.so)
frame #7: <unknown function> + 0x1993cf (0x5590367ed3cf in /data/anaconda/envs/py36/bin/python)
frame #8: <unknown function> + 0xf1a07 (0x559036745a07 in /data/anaconda/envs/py36/bin/python)
frame #9: <unknown function> + 0xf1a07 (0x559036745a07 in /data/anaconda/envs/py36/bin/python)
frame #10: <unknown function> + 0xf12b7 (0x5590367452b7 in /data/anaconda/envs/py36/bin/python)
frame #11: <unknown function> + 0xf1147 (0x559036745147 in /data/anaconda/envs/py36/bin/python)
frame #12: <unknown function> + 0xf115d (0x55903674515d in /data/anaconda/envs/py36/bin/python)
frame #13: <unknown function> + 0xf115d (0x55903674515d in /data/anaconda/envs/py36/bin/python)
frame #14: <unknown function> + 0xf115d (0x55903674515d in /data/anaconda/envs/py36/bin/python)
frame #15: <unknown function> + 0xe3ba7 (0x559036737ba7 in /data/anaconda/envs/py36/bin/python)
frame #16: <unknown function> + 0x168ea2 (0x5590367bcea2 in /data/anaconda/envs/py36/bin/python)
frame #17: _PyGC_CollectNoFail + 0x2a (0x559036844cfa in /data/anaconda/envs/py36/bin/python)
frame #18: PyImport_Cleanup + 0x278 (0x5590367f78e8 in /data/anaconda/envs/py36/bin/python)
frame #19: Py_FinalizeEx + 0x61 (0x5590368635f1 in /data/anaconda/envs/py36/bin/python)
frame #20: Py_Main + 0x35e (0x55903686e1fe in /data/anaconda/envs/py36/bin/python)
frame #21: main + 0xee (0x55903673702e in /data/anaconda/envs/py36/bin/python)
frame #22: __libc_start_main + 0xf0 (0x7f16e0060830 in /lib/x86_64-linux-gnu/libc.so.6)
frame #23: <unknown function> + 0x1c3e0e (0x559036817e0e in /data/anaconda/envs/py36/bin/python)
```
| 07-18-2019 10:29:49 | 07-18-2019 10:29:49 | Not sure this comes from pytorch-transformers or CUDA, see: https://github.com/pytorch/pytorch/issues/20489<|||||>This issue has been automatically marked as stale because it has not had recent activity. It will be closed if no further activity occurs. Thank you for your contributions.
|
transformers | 819 | closed | Output of BertModel does not match the last hidden layer from fixed feature vectors | Based on BERT documentation (https://github.com/google-research/bert#using-bert-to-extract-fixed-feature-vectors-like-elmo) we can extract the contextualized token embeddings of each hidden layer separately. However, when I extract the last hidden layer (layer -1), it does not match the `outputs[0]` from `pytorch_transformers.BertModel()` as described here: https://huggingface.co/pytorch-transformers/model_doc/bert.html#bertmodel
Just to remind that I am using the same pre-trained model (e.g. `bert-base-uncased`) and the same input (e.g. 'here is an example .') for both. | 07-18-2019 07:16:38 | 07-18-2019 07:16:38 | What is your exact command to _extract the last hidden layer (layer -1)_?
And what is your exact command to get _the outputs[0] from pytorch_transformers.BertModel()_ ?<|||||>To extract the last hidden layer (layer -1) from BERT, I run the `extract_features.py` as follows:
`python extract_features.py --input_file=tmp/input.txt --output_file=tmp/output.json --vocab_file=cased_L-12_H-768_A-12/vocab.txt --bert_config_file=cased_L-12_H-768_A-12/bert_config.json --init_checkpoint=cased_L-12_H-768_A-12/bert_model.ckpt --layers=-1 --max_seq_length=128 --batch_size=1`
where the `input_file` contains only one line e.g. 'here is an example .'
The output gives me the -1 hidden layer of each token separately.
To get the embeddings from the `outputs[0]`:
```
config = BertConfig.from_pretrained('bert-base-cased')
tokenizer = BertTokenizer.from_pretrained('bert-base-cased')
model = BertModel(config)
input_ids = torch.tensor(tokenizer.encode("here is an example .")).unsqueeze(0) # Batch size 1
outputs = model(input_ids)
last_hidden_states = outputs[0]
```
where `last_hidden_states` gives me a list of embeddings. I presume one for each token in the sentence in the same order they appear in the sentence.
Thanks
<|||||>Help me, i having same problem, how to extract feature from tuned .bin file, in bert's original doc, only init ckpt checkpoint used <|||||>@sasaadi, you should load the pretrained model with `model = BertModel.from_pretrained('bert-base-cased')`. In your example only the config (a dict of hyper-parameters) is loaded from the pretrained model, not the weights. <|||||>@thomwolf pytorch_transformers.BertModel.from_pretrained('bert-base-multilingual-cased', state_dict=model_state_dict)
Is this solution when you load from tuned model ?<|||||>@hungph-dev-ict to load from a fine-tuned checkpoint you reference it directly: `BertModel.from_pretrained('/path/to/finetuned/model')`.<|||||>The doc for the method referenced by @LysandreJik is [here](https://huggingface.co/pytorch-transformers/main_classes/model.html#pytorch_transformers.PreTrainedModel.from_pretrained)<|||||>@LysandreJik @thomwolf thank you very much.
Now this library has just added RoBERTa, I want tune it with my corpus, do you have any solution ?<|||||>This issue has been automatically marked as stale because it has not had recent activity. It will be closed if no further activity occurs. Thank you for your contributions.
|
transformers | 818 | closed | GPT sentence log loss: average or summed loss? | >>> config = GPT2Config.from_pretrained('gpt2')
>>> tokenizer = GPT2Tokenizer.from_pretrained('gpt2')
>>> model = GPT2LMHeadModel(config)
>>> input_ids = torch.tensor(tokenizer.encode("Hello, my dog is cute")).unsqueeze(0) # Batch size 1
>>> outputs = model(input_ids, labels=input_ids)
>>> loss, logits = outputs[:2]
For the loss value computed for the sentence, is it an average log loss or summed log loss? I had a look at CrossEntropyLoss in torch.nn and it seems to be an average loss, but thought I'd double check.
If there are multiple sentences in the input instead (so batch size > 1), what does it return? The average logloss over all tokens in the two sentences? | 07-18-2019 07:09:05 | 07-18-2019 07:09:05 | Yes, it's the average<|||||>Thanks for the prompt reply. Much appreciated. |
transformers | 817 | closed | from pytorch-pretrained-bert to pytorch-transformers,some problem | TypeError: forward() got an unexpected keyword argument 'output_all_encoded_layers'
| 07-18-2019 06:32:50 | 07-18-2019 06:32:50 | now you should use:
```
model = BertModel.from_pretrained('bert-base-cased', output_hidden_states=True)
outputs = model(input_ids)
all_hidden_states = outputs[-1]
```
Note that the first element in `all_hidden_states` (`all_hidden_states[0]`) is the output of the embedding layers (hence the fact that there is `num_layers + 1` elements in `all_hidden_states`).<|||||>This issue has been automatically marked as stale because it has not had recent activity. It will be closed if no further activity occurs. Thank you for your contributions.
|
transformers | 816 | closed | typos | README.md: "formely known as" -> "formerly known as" | 07-18-2019 06:17:43 | 07-18-2019 06:17:43 | # [Codecov](https://codecov.io/gh/huggingface/pytorch-transformers/pull/816?src=pr&el=h1) Report
> Merging [#816](https://codecov.io/gh/huggingface/pytorch-transformers/pull/816?src=pr&el=desc) into [master](https://codecov.io/gh/huggingface/pytorch-transformers/commit/71d597dad0a28ccc397308146844486e0031d701?src=pr&el=desc) will **not change** coverage.
> The diff coverage is `n/a`.
[](https://codecov.io/gh/huggingface/pytorch-transformers/pull/816?src=pr&el=tree)
```diff
@@ Coverage Diff @@
## master #816 +/- ##
======================================
Coverage 78.9% 78.9%
======================================
Files 34 34
Lines 6192 6192
======================================
Hits 4886 4886
Misses 1306 1306
```
------
[Continue to review full report at Codecov](https://codecov.io/gh/huggingface/pytorch-transformers/pull/816?src=pr&el=continue).
> **Legend** - [Click here to learn more](https://docs.codecov.io/docs/codecov-delta)
> `Δ = absolute <relative> (impact)`, `ø = not affected`, `? = missing data`
> Powered by [Codecov](https://codecov.io/gh/huggingface/pytorch-transformers/pull/816?src=pr&el=footer). Last update [71d597d...e5a18b3](https://codecov.io/gh/huggingface/pytorch-transformers/pull/816?src=pr&el=lastupdated). Read the [comment docs](https://docs.codecov.io/docs/pull-request-comments).
<|||||>Thanks! |
transformers | 815 | closed | Update Readme link for Fine Tune/Usage section | Incorrect link for `Quick tour: Fine-tuning/usage scripts` | 07-18-2019 05:51:44 | 07-18-2019 05:51:44 | # [Codecov](https://codecov.io/gh/huggingface/pytorch-transformers/pull/815?src=pr&el=h1) Report
> Merging [#815](https://codecov.io/gh/huggingface/pytorch-transformers/pull/815?src=pr&el=desc) into [master](https://codecov.io/gh/huggingface/pytorch-transformers/commit/71d597dad0a28ccc397308146844486e0031d701?src=pr&el=desc) will **not change** coverage.
> The diff coverage is `n/a`.
[](https://codecov.io/gh/huggingface/pytorch-transformers/pull/815?src=pr&el=tree)
```diff
@@ Coverage Diff @@
## master #815 +/- ##
======================================
Coverage 78.9% 78.9%
======================================
Files 34 34
Lines 6192 6192
======================================
Hits 4886 4886
Misses 1306 1306
```
------
[Continue to review full report at Codecov](https://codecov.io/gh/huggingface/pytorch-transformers/pull/815?src=pr&el=continue).
> **Legend** - [Click here to learn more](https://docs.codecov.io/docs/codecov-delta)
> `Δ = absolute <relative> (impact)`, `ø = not affected`, `? = missing data`
> Powered by [Codecov](https://codecov.io/gh/huggingface/pytorch-transformers/pull/815?src=pr&el=footer). Last update [71d597d...0d46b17](https://codecov.io/gh/huggingface/pytorch-transformers/pull/815?src=pr&el=lastupdated). Read the [comment docs](https://docs.codecov.io/docs/pull-request-comments).
|
transformers | 814 | closed | Is there any plan of developing softmax-weight function for using 12 hidden BERT layer? | Thanks for developing very nice/useful library.
My question is about using 12/(or in large model, more) hidden layer.
First, Does how to use hidden layers depend on the downstream task?
(Say, concat, average, only final layer, only mean of top 4 layer, etc...)
For using all layer, I think it's good to use softmax weight. During training , hidden layer's feature is fix but weight is learned for the task. So second question is, Is there any plan of developing softmax-weight function for using 12 hidden BERT layer?
Thanks | 07-18-2019 02:03:24 | 07-18-2019 02:03:24 | Yes we might add a module for scalar mixture of layers like the one of AllenNLP, for instance (https://github.com/allenai/allennlp/blob/master/allennlp/modules/scalar_mix.py).<|||||>I'm looking forward to see that in also pytorch-transformer.
Again, thanks! I'll keep track on this repository.<|||||>This issue has been automatically marked as stale because it has not had recent activity. It will be closed if no further activity occurs. Thank you for your contributions.
|
transformers | 813 | closed | How to use BertModel ? | I want to use bert-crf in my NER task. But this github only provide softmax as the classifier, I decided to write my own crf. But I am not sure how to use it. Here is an example. Please correct me if I am wrong.
sentence: Here is some text to encode
input: torch.tensor([tokenizer.encode("[CLS]" + "Here is some text to encode" + "[SEP]")]), which is
tensor([[ 101, 3446, 1110, 1199, 3087, 1106, 4035, 13775, 102]])
output shape: [1, 9, 768],
Becasue "encode" is devided into two pieceword.
Then I select output[1, 2, 3, 4, 5, 6] from output[0,1,2,3,4,5,6,7,8]to get the crf_input that is of shape [1, 6, 768].
That is how I think it should work. Any suggestion will be appreciated. | 07-18-2019 00:41:46 | 07-18-2019 00:41:46 | This issue has been discussed at [#64](https://github.com/huggingface/pytorch-transformers/issues/64#issuecomment-443703063).<|||||>This issue has been automatically marked as stale because it has not had recent activity. It will be closed if no further activity occurs. Thank you for your contributions.
<|||||>Hi - I tried to implement it, you can have a look at my implementation here: https://github.com/chnsh/BERT-NER-CoNLL, hope that helps<|||||>@chnsh I don't see any crf layer on your github repo.<|||||>@RoderickGu Were you able to implement bert-crf? |
transformers | 812 | closed | do I need to add sep and cls token in each sequence ? | It might be a stupid question, but I just notice the authors did not add "[cls]" and "[sep]" token in the example. I think whether those tokens are added automatically inside the module ? Thanks | 07-18-2019 00:22:57 | 07-18-2019 00:22:57 | They are not added automatically.<|||||>@thomwolf Thanks ! |
transformers | 811 | closed | Fix openai-gpt ROCStories example's issues with AdamW optimizer | Fixes the `AdamW` optimizer instance in the `openai-gpt` ROCStories example as per the new API. The default arguments for it are now set as per the [documentation](https://huggingface.co/pytorch-transformers/model_doc/bert.html?highlight=adamw#pytorch_transformers.AdamW). | 07-17-2019 21:54:47 | 07-17-2019 21:54:47 | # [Codecov](https://codecov.io/gh/huggingface/pytorch-transformers/pull/811?src=pr&el=h1) Report
> Merging [#811](https://codecov.io/gh/huggingface/pytorch-transformers/pull/811?src=pr&el=desc) into [master](https://codecov.io/gh/huggingface/pytorch-transformers/commit/71d597dad0a28ccc397308146844486e0031d701?src=pr&el=desc) will **not change** coverage.
> The diff coverage is `n/a`.
[](https://codecov.io/gh/huggingface/pytorch-transformers/pull/811?src=pr&el=tree)
```diff
@@ Coverage Diff @@
## master #811 +/- ##
======================================
Coverage 78.9% 78.9%
======================================
Files 34 34
Lines 6192 6192
======================================
Hits 4886 4886
Misses 1306 1306
```
------
[Continue to review full report at Codecov](https://codecov.io/gh/huggingface/pytorch-transformers/pull/811?src=pr&el=continue).
> **Legend** - [Click here to learn more](https://docs.codecov.io/docs/codecov-delta)
> `Δ = absolute <relative> (impact)`, `ø = not affected`, `? = missing data`
> Powered by [Codecov](https://codecov.io/gh/huggingface/pytorch-transformers/pull/811?src=pr&el=footer). Last update [71d597d...51d66f1](https://codecov.io/gh/huggingface/pytorch-transformers/pull/811?src=pr&el=lastupdated). Read the [comment docs](https://docs.codecov.io/docs/pull-request-comments).
<|||||>We should also probably add a linearly decreasing schedule (not in the optimizer anymore).
Did you try this version with flat learning rate? Does it have good performances?<|||||>I didn't run the training loop to completion but I looked at it now and it seems there's an issue with the evaluation routine (it crashes with an error). Will look into this as soon as I have time. <|||||>@thomwolf I used the code from #845 and the flat learning rate does the trick - eval accuracy of 87.2% after 3 training epochs. The defaults args in `run_openai_gpt.py` are good, just confirming some of them used for this result below.
```
python run_openai_gpt.py \
--model_name openai-gpt \
--do_train \
--do_eval \
--train_dataset "./ROCStories/cloze_test_val__spring2016 - cloze_test_ALL_val.csv" \
--eval_dataset "./ROCStories/cloze_test_test__spring2016 - cloze_test_ALL_test.csv" \
--train_batch_size 8 \
--eval_batch_size 16 \
--num_train_epochs 3
```
It makes sense to go ahead and close this. Thanks! |
transformers | 810 | closed | SEG_ID constants for XLNet misleading/off | https://github.com/huggingface/pytorch-transformers/blob/master/pytorch_transformers/tokenization_xlnet.py#L47 shows:
```
# Segments (not really needed)
SEG_ID_A = 0
SEG_ID_B = 1
SEG_ID_CLS = 2
SEG_ID_SEP = 3
SEG_ID_PAD = 4
```
These don't seem to be used anywhere in the repo, but I tried using them as a shortcut myself, and I'm not sure they're right. In contrast, for xlnet-base-cased, I get:
```
self._sep_id = tokenizer.convert_tokens_to_ids("<sep>")
self._cls_id = tokenizer.convert_tokens_to_ids("<cls>")
self._pad_id = tokenizer.convert_tokens_to_ids("<pad>")
print(self._cls_id, self._sep_id, self._pad_id)
```
```
3 4 5
``` | 07-17-2019 20:45:40 | 07-17-2019 20:45:40 | Yes I will remove them. They are used in the `run_glue.py` example (like in the original TF repo) but they don't have any reason to be in the library it-self.
In XLNet segment ids (what we call `token_type_ids in the repo) don't correspond to embeddings, they are just numbers and the only important thing is that they have to be different for tokens which belong to different segments, hence the flexibility in the exact values (XLNet is using relative segment difference with just two segment embeddings: 0 if the segment id of two tokens are the same, 1 if not). See [here](https://github.com/huggingface/pytorch-transformers/blob/master/pytorch_transformers/modeling_xlnet.py#L926-L928).
It's in the XLNet paper but I should probably add a word or two in the docstring as well.<|||||>Ah, got it. Thanks! I had assumed that these were part of the token vocabulary. I didn't realize that there were more than two segment types.<|||||>By the way, the default [here](https://github.com/huggingface/pytorch-transformers/blob/master/examples/run_glue.py#L259) seems off—the [CLS] token for BERT is marked as part of segment 1/B, while the paper shows it as part of 0/A with the rest of the first input.<|||||>But that's another issue, and I'm not fully certain. Closing. |
transformers | 809 | closed | Problem loading finetuned XLNet model | After fine-tuning an XLNet classification model and obtaining TF checkpoints I converted the checkpoint to pytorch_model.bin and config.json. I need to make prediction on input text, but I have problems loading the models correctly. Any help? | 07-17-2019 20:30:57 | 07-17-2019 20:30:57 | What task did you fine-tuned it on?
You can convert it by running the `convert_xlnet_checkpoint_to_pytorch.py` script with a `--finetuning_task` argument (see [here](https://github.com/huggingface/pytorch-transformers/blob/master/pytorch_transformers/convert_xlnet_checkpoint_to_pytorch.py#L94-L97))
<|||||>binary classification (sentiment) on my dataset
I tried the following
`import torch
from pytorch_transformers import XLNetConfig
from pytorch_transformers import XLNetTokenizer
from pytorch_transformers import XLNetModel
config = XLNetConfig.from_pretrained('./')
tokenizer = XLNetTokenizer.from_pretrained('./')
model = XLNetModel(config)
input_ids = torch.tensor(tokenizer.encode("Apple stocks increase")).unsqueeze(0) # Batch size 1
outputs = model(input_ids)
last_hidden_states = outputs[0]`
however, the output is not binary, the output tensor is :
tensor([[[-1.0645, -1.1170, -0.5549, ..., 0.7089, 0.2862, -0.2995],
[ 0.0198, -1.6598, -0.1760, ..., 0.1167, -0.1545, 0.1777],
[ 0.6514, -0.5614, -1.2180, ..., 1.0796, 1.1217, 1.0362]]],
grad_fn=<PermuteBackward>)
<|||||>the converting was done using:
`pytorch_transformers xlnet $TRANSFO_XL_CHECKPOINT_PATH $TRANSFO_XL_CONFIG_PAH $PYTORCH_DUMP_OUTPUT . `
I did not specified the task, because I though that it is not important.<|||||>It is important to match the last (classification) layer otherwise the conversion will fail.
If you give me more information on your TF training (like which script you usde for instance) I may be able to help.<|||||>I was using the:
`train_command = "python xlnet/run_classifier.py \
--do_train=True \
--do_eval=True \
--eval_all_ckpt=True \
--task_name=spam \
--data_dir="+DATA_DIR+" \
--output_dir="+OUTPUT_DIR+" \
--model_dir="+CHECKPOINT_DIR+" \
--uncased=False \
--spiece_model_file="+PRETRAINED_MODEL_DIR+"/spiece.model \
--model_config_path="+PRETRAINED_MODEL_DIR+"/xlnet_config.json \
--init_checkpoint="+PRETRAINED_MODEL_DIR+"/xlnet_model.ckpt \
--max_seq_length=128 \
--train_batch_size=8 \
--eval_batch_size=8 \
--num_hosts=1 \
--num_core_per_host=1 \
--learning_rate=2e-5 \
--train_steps=4000 \
--warmup_steps=500 \
--save_steps=500 \
--iterations=500"
! {train_command}`
It is similar to the imdb sentiment task
The colab is here
https://colab.research.google.com/drive/1nfWEEDxPOE8myb-hwGdoXs9nVXM3AVcz<|||||>I have modified the:
class ImdbProcessor(DataProcessor):<|||||>Here is the model after model.eval():
`XLNetModel(
(word_embedding): Embedding(32000, 1024)
(layer): ModuleList(
(0): XLNetLayer(
(rel_attn): XLNetRelativeAttention(
(layer_norm): XLNetLayerNorm()
(dropout): Dropout(p=0.1)
)
(ff): XLNetFeedForward(
(layer_norm): XLNetLayerNorm()
(layer_1): Linear(in_features=1024, out_features=4096, bias=True)
(layer_2): Linear(in_features=4096, out_features=1024, bias=True)
(dropout): Dropout(p=0.1)
)
(dropout): Dropout(p=0.1)
)
(1): XLNetLayer(
(rel_attn): XLNetRelativeAttention(
(layer_norm): XLNetLayerNorm()
(dropout): Dropout(p=0.1)
)
(ff): XLNetFeedForward(
(layer_norm): XLNetLayerNorm()
(layer_1): Linear(in_features=1024, out_features=4096, bias=True)
(layer_2): Linear(in_features=4096, out_features=1024, bias=True)
(dropout): Dropout(p=0.1)
)
(dropout): Dropout(p=0.1)
)
(2): XLNetLayer(
(rel_attn): XLNetRelativeAttention(
(layer_norm): XLNetLayerNorm()
(dropout): Dropout(p=0.1)
)
(ff): XLNetFeedForward(
(layer_norm): XLNetLayerNorm()
(layer_1): Linear(in_features=1024, out_features=4096, bias=True)
(layer_2): Linear(in_features=4096, out_features=1024, bias=True)
(dropout): Dropout(p=0.1)
)
(dropout): Dropout(p=0.1)
)
(3): XLNetLayer(
(rel_attn): XLNetRelativeAttention(
(layer_norm): XLNetLayerNorm()
(dropout): Dropout(p=0.1)
)
(ff): XLNetFeedForward(
(layer_norm): XLNetLayerNorm()
(layer_1): Linear(in_features=1024, out_features=4096, bias=True)
(layer_2): Linear(in_features=4096, out_features=1024, bias=True)
(dropout): Dropout(p=0.1)
)
(dropout): Dropout(p=0.1)
)
(4): XLNetLayer(
(rel_attn): XLNetRelativeAttention(
(layer_norm): XLNetLayerNorm()
(dropout): Dropout(p=0.1)
)
(ff): XLNetFeedForward(
(layer_norm): XLNetLayerNorm()
(layer_1): Linear(in_features=1024, out_features=4096, bias=True)
(layer_2): Linear(in_features=4096, out_features=1024, bias=True)
(dropout): Dropout(p=0.1)
)
(dropout): Dropout(p=0.1)
)
(5): XLNetLayer(
(rel_attn): XLNetRelativeAttention(
(layer_norm): XLNetLayerNorm()
(dropout): Dropout(p=0.1)
)
(ff): XLNetFeedForward(
(layer_norm): XLNetLayerNorm()
(layer_1): Linear(in_features=1024, out_features=4096, bias=True)
(layer_2): Linear(in_features=4096, out_features=1024, bias=True)
(dropout): Dropout(p=0.1)
)
(dropout): Dropout(p=0.1)
)
(6): XLNetLayer(
(rel_attn): XLNetRelativeAttention(
(layer_norm): XLNetLayerNorm()
(dropout): Dropout(p=0.1)
)
(ff): XLNetFeedForward(
(layer_norm): XLNetLayerNorm()
(layer_1): Linear(in_features=1024, out_features=4096, bias=True)
(layer_2): Linear(in_features=4096, out_features=1024, bias=True)
(dropout): Dropout(p=0.1)
)
(dropout): Dropout(p=0.1)
)
(7): XLNetLayer(
(rel_attn): XLNetRelativeAttention(
(layer_norm): XLNetLayerNorm()
(dropout): Dropout(p=0.1)
)
(ff): XLNetFeedForward(
(layer_norm): XLNetLayerNorm()
(layer_1): Linear(in_features=1024, out_features=4096, bias=True)
(layer_2): Linear(in_features=4096, out_features=1024, bias=True)
(dropout): Dropout(p=0.1)
)
(dropout): Dropout(p=0.1)
)
(8): XLNetLayer(
(rel_attn): XLNetRelativeAttention(
(layer_norm): XLNetLayerNorm()
(dropout): Dropout(p=0.1)
)
(ff): XLNetFeedForward(
(layer_norm): XLNetLayerNorm()
(layer_1): Linear(in_features=1024, out_features=4096, bias=True)
(layer_2): Linear(in_features=4096, out_features=1024, bias=True)
(dropout): Dropout(p=0.1)
)
(dropout): Dropout(p=0.1)
)
(9): XLNetLayer(
(rel_attn): XLNetRelativeAttention(
(layer_norm): XLNetLayerNorm()
(dropout): Dropout(p=0.1)
)
(ff): XLNetFeedForward(
(layer_norm): XLNetLayerNorm()
(layer_1): Linear(in_features=1024, out_features=4096, bias=True)
(layer_2): Linear(in_features=4096, out_features=1024, bias=True)
(dropout): Dropout(p=0.1)
)
(dropout): Dropout(p=0.1)
)
(10): XLNetLayer(
(rel_attn): XLNetRelativeAttention(
(layer_norm): XLNetLayerNorm()
(dropout): Dropout(p=0.1)
)
(ff): XLNetFeedForward(
(layer_norm): XLNetLayerNorm()
(layer_1): Linear(in_features=1024, out_features=4096, bias=True)
(layer_2): Linear(in_features=4096, out_features=1024, bias=True)
(dropout): Dropout(p=0.1)
)
(dropout): Dropout(p=0.1)
)
(11): XLNetLayer(
(rel_attn): XLNetRelativeAttention(
(layer_norm): XLNetLayerNorm()
(dropout): Dropout(p=0.1)
)
(ff): XLNetFeedForward(
(layer_norm): XLNetLayerNorm()
(layer_1): Linear(in_features=1024, out_features=4096, bias=True)
(layer_2): Linear(in_features=4096, out_features=1024, bias=True)
(dropout): Dropout(p=0.1)
)
(dropout): Dropout(p=0.1)
)
(12): XLNetLayer(
(rel_attn): XLNetRelativeAttention(
(layer_norm): XLNetLayerNorm()
(dropout): Dropout(p=0.1)
)
(ff): XLNetFeedForward(
(layer_norm): XLNetLayerNorm()
(layer_1): Linear(in_features=1024, out_features=4096, bias=True)
(layer_2): Linear(in_features=4096, out_features=1024, bias=True)
(dropout): Dropout(p=0.1)
)
(dropout): Dropout(p=0.1)
)
(13): XLNetLayer(
(rel_attn): XLNetRelativeAttention(
(layer_norm): XLNetLayerNorm()
(dropout): Dropout(p=0.1)
)
(ff): XLNetFeedForward(
(layer_norm): XLNetLayerNorm()
(layer_1): Linear(in_features=1024, out_features=4096, bias=True)
(layer_2): Linear(in_features=4096, out_features=1024, bias=True)
(dropout): Dropout(p=0.1)
)
(dropout): Dropout(p=0.1)
)
(14): XLNetLayer(
(rel_attn): XLNetRelativeAttention(
(layer_norm): XLNetLayerNorm()
(dropout): Dropout(p=0.1)
)
(ff): XLNetFeedForward(
(layer_norm): XLNetLayerNorm()
(layer_1): Linear(in_features=1024, out_features=4096, bias=True)
(layer_2): Linear(in_features=4096, out_features=1024, bias=True)
(dropout): Dropout(p=0.1)
)
(dropout): Dropout(p=0.1)
)
(15): XLNetLayer(
(rel_attn): XLNetRelativeAttention(
(layer_norm): XLNetLayerNorm()
(dropout): Dropout(p=0.1)
)
(ff): XLNetFeedForward(
(layer_norm): XLNetLayerNorm()
(layer_1): Linear(in_features=1024, out_features=4096, bias=True)
(layer_2): Linear(in_features=4096, out_features=1024, bias=True)
(dropout): Dropout(p=0.1)
)
(dropout): Dropout(p=0.1)
)
(16): XLNetLayer(
(rel_attn): XLNetRelativeAttention(
(layer_norm): XLNetLayerNorm()
(dropout): Dropout(p=0.1)
)
(ff): XLNetFeedForward(
(layer_norm): XLNetLayerNorm()
(layer_1): Linear(in_features=1024, out_features=4096, bias=True)
(layer_2): Linear(in_features=4096, out_features=1024, bias=True)
(dropout): Dropout(p=0.1)
)
(dropout): Dropout(p=0.1)
)
(17): XLNetLayer(
(rel_attn): XLNetRelativeAttention(
(layer_norm): XLNetLayerNorm()
(dropout): Dropout(p=0.1)
)
(ff): XLNetFeedForward(
(layer_norm): XLNetLayerNorm()
(layer_1): Linear(in_features=1024, out_features=4096, bias=True)
(layer_2): Linear(in_features=4096, out_features=1024, bias=True)
(dropout): Dropout(p=0.1)
)
(dropout): Dropout(p=0.1)
)
(18): XLNetLayer(
(rel_attn): XLNetRelativeAttention(
(layer_norm): XLNetLayerNorm()
(dropout): Dropout(p=0.1)
)
(ff): XLNetFeedForward(
(layer_norm): XLNetLayerNorm()
(layer_1): Linear(in_features=1024, out_features=4096, bias=True)
(layer_2): Linear(in_features=4096, out_features=1024, bias=True)
(dropout): Dropout(p=0.1)
)
(dropout): Dropout(p=0.1)
)
(19): XLNetLayer(
(rel_attn): XLNetRelativeAttention(
(layer_norm): XLNetLayerNorm()
(dropout): Dropout(p=0.1)
)
(ff): XLNetFeedForward(
(layer_norm): XLNetLayerNorm()
(layer_1): Linear(in_features=1024, out_features=4096, bias=True)
(layer_2): Linear(in_features=4096, out_features=1024, bias=True)
(dropout): Dropout(p=0.1)
)
(dropout): Dropout(p=0.1)
)
(20): XLNetLayer(
(rel_attn): XLNetRelativeAttention(
(layer_norm): XLNetLayerNorm()
(dropout): Dropout(p=0.1)
)
(ff): XLNetFeedForward(
(layer_norm): XLNetLayerNorm()
(layer_1): Linear(in_features=1024, out_features=4096, bias=True)
(layer_2): Linear(in_features=4096, out_features=1024, bias=True)
(dropout): Dropout(p=0.1)
)
(dropout): Dropout(p=0.1)
)
(21): XLNetLayer(
(rel_attn): XLNetRelativeAttention(
(layer_norm): XLNetLayerNorm()
(dropout): Dropout(p=0.1)
)
(ff): XLNetFeedForward(
(layer_norm): XLNetLayerNorm()
(layer_1): Linear(in_features=1024, out_features=4096, bias=True)
(layer_2): Linear(in_features=4096, out_features=1024, bias=True)
(dropout): Dropout(p=0.1)
)
(dropout): Dropout(p=0.1)
)
(22): XLNetLayer(
(rel_attn): XLNetRelativeAttention(
(layer_norm): XLNetLayerNorm()
(dropout): Dropout(p=0.1)
)
(ff): XLNetFeedForward(
(layer_norm): XLNetLayerNorm()
(layer_1): Linear(in_features=1024, out_features=4096, bias=True)
(layer_2): Linear(in_features=4096, out_features=1024, bias=True)
(dropout): Dropout(p=0.1)
)
(dropout): Dropout(p=0.1)
)
(23): XLNetLayer(
(rel_attn): XLNetRelativeAttention(
(layer_norm): XLNetLayerNorm()
(dropout): Dropout(p=0.1)
)
(ff): XLNetFeedForward(
(layer_norm): XLNetLayerNorm()
(layer_1): Linear(in_features=1024, out_features=4096, bias=True)
(layer_2): Linear(in_features=4096, out_features=1024, bias=True)
(dropout): Dropout(p=0.1)
)
(dropout): Dropout(p=0.1)
)
)
(dropout): Dropout(p=0.1)
)
`<|||||>You'll have to modify the conversion script because this is not a standard task and the number of labels won't be found in the list (see top of the conversion script) you should add `'spam': 2` in the list (if you have two labels indeed).
The other option is to directly load the TF model in PyTorch and save the pytorch model afterwards with something like this:
```
config = XLNetConfig.from_pretrained('xlnet-large-cased', num_labels=2, finetuning_task='spam')
model = XLNetForSequenceClassification.from_pretrained('path/to/your/tf/model.ckpt.index', config=config, from_tf=True)
model.save_pretrained('pytorch_model_saving_directory')
```<|||||>Perfect, tnx.
Just a minor question if I have classification model with 4 labels, I do the same changes spam:4?<|||||>Yes, change my `2` to `4`<|||||>Hi Thom,
I did:
`import torch
from pytorch_transformers import XLNetConfig
from pytorch_transformers import XLNetForSequenceClassification
config = XLNetConfig.from_pretrained('xlnet-large-cased', num_labels=2, finetuning_task='sentiment')
model = XLNetForSequenceClassification.from_pretrained('../model.ckpt-2500', config=config, from_tf=True)
model.save_pretrained('./test')`
Afterwards I get in the test folder the config.json and pytorch_model.bin.
I tried to run:
'import torch
from pytorch_transformers import XLNetConfig
from pytorch_transformers import XLNetTokenizer
from pytorch_transformers import XLNetModel
config = XLNetConfig.from_pretrained('./')
tokenizer = XLNetTokenizer.from_pretrained('./')
model = XLNetModel(config)
input_ids = torch.tensor(tokenizer.encode("Apple stocks increase")).unsqueeze(0) # Batch size 1
outputs = model(input_ids)
last_hidden_states = outputs[0]
print(last_hidden_states)
There was an error:
Model name './' was not found in model name list (xlnet-base-cased, xlnet-large-cased). We assumed './' was a path or url but couldn't find tokenizer filesat this path or url.
So I included the spiece.model into the same test folder, but still the results is:
tensor([[[ 0.0156, -0.9046, 0.9789, ..., -0.7764, 1.1309, 0.2862],
[ 0.4416, 0.0665, 2.0020, ..., 0.4117, 0.9779, -1.0588],
[ 0.6908, -0.0000, 0.1479, ..., 0.0032, 0.9871, -0.9482]]],
grad_fn=<PermuteBackward>)
Do u know what I am doing wrong? <|||||>While converting this is some of the warnings:
Weights not copied to PyTorch model: beta1_power, beta2_power, global_step, model/classification_finsent/logit/bias, model/classification_finsent/logit/bias/Adam, model/classification_finsent/logit/bias/Adam_1, model/classification_finsent/logit/kernel, model/classification_finsent/logit/kernel/Adam, model/classification_finsent/logit/kernel/Adam_1<|||||>In addition, I have tried the other approach, to modify the script, but the results are the same. I think that the problem is that the weights from the classification_finsent are not copied to pytorch model. Any suggestions on this<|||||>I have tried also in this way:
`import torch
from pytorch_transformers import XLNetConfig
from pytorch_transformers import XLNetTokenizer
from pytorch_transformers import XLNetForSequenceClassification
config = XLNetConfig.from_pretrained('/home/igor/Falcon/XLNet/sentiment_model/PyTorch/pytorch_transformer_script/test')
tokenizer = XLNetTokenizer.from_pretrained('/home/igor/Falcon/XLNet/sentiment_model/PyTorch/pytorch_transformer_script/test')
config.output_hidden_states=True
model = XLNetForSequenceClassification(config)
input_ids = torch.tensor(tokenizer.encode("Apple stocks increase rapidly")).unsqueeze(0) # Batch size 1
labels = torch.tensor([1]).unsqueeze(0) # Batch size 1
outputs = model(input_ids, labels=labels)
print(outputs[0])`
and the output now is:
tensor(0.3944, grad_fn=<NllLossBackward>)
<|||||>In the last attempt I did:
```python
import torch
from pytorch_transformers import XLNetConfig
from pytorch_transformers import XLNetTokenizer
from pytorch_transformers import XLNetForSequenceClassification
config = XLNetConfig.from_pretrained('xlnet-large-cased', num_labels=2, finetuning_task='finsent')
tokenizer = XLNetTokenizer.from_pretrained('xlnet-large-cased') #I am using the default tokenizer
model = XLNetForSequenceClassification.from_pretrained('/home/igor/Falcon/XLNet/sentiment_model/model.ckpt-2500',config=config, from_tf=True) #this is my finetuned model
model.eval() #in evaluation mode
input_ids = torch.tensor(tokenizer.encode("Apple stock increase and they are overwhelmed with the success")).unsqueeze(0) # Batch size 1
labels = torch.tensor([1]).unsqueeze(0) # Batch size 1
outputs = model(input_ids, labels=labels)
loss, logits = outputs[:2]
print(torch.nn.functional.softmax(logits.data))
```
However I am getting different prediction for the same input data, I did model.eval() to stop the dropout, but still the inference looks random.
In addition here is my config file printed:
```json
{
"attn_type": "bi",
"bi_data": false,
"clamp_len": -1,
"d_head": 64,
"d_inner": 4096,
"d_model": 1024,
"dropatt": 0.1,
"dropout": 0.1,
"end_n_top": 5,
"ff_activation": "gelu",
"finetuning_task": "finsent",
"init": "normal",
"init_range": 0.1,
"init_std": 0.02,
"initializer_range": 0.02,
"layer_norm_eps": 1e-12,
"max_position_embeddings": 512,
"mem_len": null,
"n_head": 16,
"n_layer": 24,
"n_token": 32000,
"num_labels": 2,
"output_attentions": false,
"output_hidden_states": false,
"reuse_len": null,
"same_length": false,
"start_n_top": 5,
"summary_activation": "tanh",
"summary_last_dropout": 0.1,
"summary_type": "last",
"summary_use_proj": true,
"torchscript": false,
"untie_r": true
}
```
It looks that I getting closer, but still the inference is strange<|||||>@thomwolf I finally succeed to import the checkpoint model and infer. Still I am not sure if this a valid approach:
```python
import torch
from pytorch_transformers import XLNetConfig
from pytorch_transformers import XLNetTokenizer
from pytorch_transformers import XLNetForSequenceClassification
seed = 0
torch.manual_seed(seed)
if torch.cuda.is_available():
torch.cuda.manual_seed_all(seed)
#load the initial config file from the XLNet model
config = XLNetConfig.from_pretrained('xlnet_config.json', num_labels=2, finetuning_task='finsent')
#the tokenizer I am using is the initial one (spiece.model)
tokenizer = XLNetTokenizer.from_pretrained('xlnet-large-cased')
model = XLNetForSequenceClassification.from_pretrained('/sentiment_over_imdb/model.ckpt-4000',config=config, from_tf=True)
model.eval()
def sentiment(data):
input_ids = torch.tensor(tokenizer.encode(data)).unsqueeze(0) # Batch size 1
labels = torch.tensor([1]).unsqueeze(0) # Batch size 1
outputs = model(input_ids, labels=labels)
loss, logits = outputs[:2]
output = torch.nn.functional.softmax(logits.data, dim=1)
sent = output.tolist()[0][1]
return sent
``` |
transformers | 808 | closed | GPT2 model does not have attention mask | Hello, in the doc string of GPT2 model, it says there is an optional input called [attention_mask](https://github.com/huggingface/pytorch-transformers/blob/f289e6cfe46885f260e4f2b3c8a164aa1a567e4c/pytorch_transformers/modeling_gpt2.py#L405) to avoid computing attention on paddings. But actually I cannot find the implementation and there is no such arguments either. | 07-17-2019 18:36:10 | 07-17-2019 18:36:10 | Indeed, I will remove this doctring, there is no attention_mask on GPT-2.<|||||>> Indeed, I will remove this doctring, there is no attention_mask on GPT-2.
But what to do if I do want to avoid computing attention on the paddings in the input sequences.<|||||>@Saner3 @thomwolf I have same question? don't we need that for paddings?<|||||>GPT-2 is a model with absolute position embeddings (like Bert) so you should always pad on the right to get best performances for this model (will add this information to the doc_string).
As it's a causal model (only attend to the left context), also means that the model will not attend to the padding tokens (which are on the right) for any real token anyway.
So in conclusion, no need to take special care of avoiding attention on padding.
Just don't use the output of the padded tokens for anything as they don't contain any reliable information (which is obvious I hope).<|||||>@thomwolf thanks much, and great job! |
transformers | 807 | closed | AttributeError: 'tuple' object has no attribute 'softmax' | I get the following error when I use the pytorch transformers, It used to work just fine in the previous pretrained-bert,
Original code: https://github.com/ceshine/pytorch-pretrained-BERT/blob/master/notebooks/Next%20Sentence%20Prediction.ipynb
Code which has error:
model.eval()
res = []
mb = progress_bar(eval_dataloader)
for input_ids, input_mask, segment_ids in mb:
input_ids = input_ids.to(device)
input_mask = input_mask.to(device)
segment_ids = segment_ids.to(device)
with torch.no_grad():
res.append(nn.functional.softmax(
model(input_ids, segment_ids, input_mask), dim=1
)[:, 0].detach().cpu().numpy())
res = np.concatenate(res)
Error stacktrace:
---------------------------------------------------------------------------
AttributeError Traceback (most recent call last)
<ipython-input-37-a816e24060d8> in <module>
24 with torch.no_grad():
25 res.append(nn.functional.softmax(
---> 26 model(input_ids, segment_ids, input_mask), dim=1
27 )[:, 0].detach().cpu().numpy())
28
/common/users/rs1693/my_venv/venv_bert/lib64/python3.6/site-packages/torch/nn/functional.py in softmax(input, dim, _stacklevel, dtype)
1261 dim = _get_softmax_dim('softmax', input.dim(), _stacklevel)
1262 if dtype is None:
-> 1263 ret = input.softmax(dim)
1264 else:
1265 ret = input.softmax(dim, dtype=dtype)
AttributeError: 'tuple' object has no attribute 'softmax'
I read many posts where they say to do the following:(But not sure where in the code I have to make these changes)
1. disable aux_logits when the model is created here by also passing aux_logits=False to the inception_v3 function.
2. Edit your train function to accept and unpack the returned tuple here to be something like:
output, aux = model(input_var)
But where in the above function I have to do this?
| 07-17-2019 17:53:07 | 07-17-2019 17:53:07 | HI,
I have the same problem!
What was the solution here?<|||||>Me too.<|||||>Need more information like version of python/pytorch/transformers (all the information requested in the issue templates actually)<|||||>> Need more information like version of python/pytorch/transformers (all the information requested in the issue templates actually)
I am experiencing this issue as well with the BertForNextSentencePrediction model and not having much luck with a solution. I'm using macOS Mojave 10.14.6, python 3.7, pytorch 1.3.1 and transformers 2.2.1.
Please let me know if there is any more details I can provide. Thanks!<|||||>You should open a new issue with a clean code example we can test and the associate full error message. |
transformers | 806 | closed | Fix a path so that a test can run on Windows | The path for a temporal file is hard coded, so the test fails on Windows. This PR changes that line to a more platform-natural path. | 07-17-2019 16:10:39 | 07-17-2019 16:10:39 | Ok for that, thanks @wschin |
transformers | 805 | closed | Where is "run_bert_classifier.py"? | Thanks for this great repo.
Is there any equivalent to [the previous run_bert_classifier.py](https://github.com/huggingface/pytorch-pretrained-BERT/tree/master/examples/run_bert_classifier.py)?
| 07-17-2019 14:57:53 | 07-17-2019 14:57:53 | It's now `run_glue.py`<|||||>Hi @thomwolf
Doc needs changes from run_bert_classifier to run_glue
https://huggingface.co/pytorch-transformers/examples.html<|||||>Hey, just another headsup @thomwolf
This Doc also needs changing for the run_bert_classifier.py:
https://huggingface.co/transformers/v1.1.0/examples.html#introduction<|||||>That's an old version of the doc @mtwright (notice the `v1.1.0`), you should check out the up to date one:
https://huggingface.co/transformers/examples.html#introduction<|||||>documentation is still broken, by the way<|||||>like, here, points to a bunch of files that do not exist. also not quite sure if these instructions work anymore:
https://huggingface.co/transformers/converting_tensorflow_models.html |
transformers | 804 | closed | Answers to Bullet/List Items by bert | Hi,
There are lists in document (Bullet items), and I am running BERT (non trained as well as squad trained). But seems BERT does not understands Bullets/lines starting with number or star.
Will any text preprocessing help? | 07-17-2019 13:31:49 | 07-17-2019 13:31:49 | This issue has been automatically marked as stale because it has not had recent activity. It will be closed if no further activity occurs. Thank you for your contributions.
|
transformers | 803 | closed | AssertionError in BERT-Quickstart example | Hey
I tried running the Quickstart example with my own little text. Everything works fine until I get to the ```assert tokenized_text ==... ``` part. When I try to enter my text instead of the Jim Henson text, I get the following error message: ```Traceback (most recent call last):
File "<stdin>", line 1, in <module>
AssertionError ```
I'm not sure if the error is an operation-problem or if it is an issue... | 07-17-2019 11:21:44 | 07-17-2019 11:21:44 | What is the full line of the assertion you are testing?
And what is your input text?<|||||>I think the mistake was with me, sorry |
transformers | 802 | closed | fp16+xlnet did not gain any speed increase | Hi,
I tried fp16 + xlnet, it did not work.
when I set opt_level='O2', the memory was half, but it was much slower than fp32.
when I set opt_level='O1', the memory was original, and it has similar speed with fp32.
Environment: v100, cuda, 10.1, torch 1.1
The environment is ok, because I tried bert + fp16 and it was much faster than fp32.
I thought it is the problem of torch.einsum, but I am not that sure.
I used the code here to test: https://github.com/huggingface/pytorch-transformers/blob/master/examples/run_squad.py | 07-17-2019 09:30:23 | 07-17-2019 09:30:23 | XLNet makes heavy use of `torch.einsum()` but I'm not sure this method is fp16 compatible.
It's also quite slow currently so maybe in the mid/long-term it would be good to change these einsum to standard matmul. I won't have time to do that very soon though.<|||||>As as a suggestion, you can add ```apex.amp.register_half_function(torch, 'einsum')``` somewhere near the top of your driver script (examples/run_squad.py for instance).
This forces `amp` to cast the inputs to einsum to `torch.half` before executing, allowing you to get the perf benefits of fp16 + TensorCores when appropriate.<|||||>This issue has been automatically marked as stale because it has not had recent activity. It will be closed if no further activity occurs. Thank you for your contributions.
<|||||>Hi, @slayton58, could `torch.einsum` be automatically processed by `apex.amp` now?
Your response will be appreciated! |
transformers | 801 | closed | import sys twice | 07-17-2019 09:12:19 | 07-17-2019 09:12:19 | Thanks! |
|
transformers | 800 | closed | attention_mask at run_squad.py | I think there's minor mistake in [run_squad.py](https://github.com/huggingface/pytorch-transformers/blob/5fe0b378d8/examples/run_squad.py#L298) at line 298
```
inputs = {'input_ids': batch[0],
'token_type_ids': None if args.model_type == 'xlm' else batch[1],
'attention_mask': batch[2],
'start_positions': batch[3],
'end_positions': batch[4]}
```
but i think batch[1] is attention_mask and batch[2] is segment_ids, so it should be like this
```
inputs = {'input_ids': batch[0],
'attention_mask': batch[1],
'token_type_ids': None if args.model_type == 'xlm' else batch[2],
'start_positions': batch[3],
'end_positions': batch[4]}
```
because the data is
```
dataset = TensorDataset(all_input_ids, all_input_mask, all_segment_ids,
all_start_positions, all_end_positions,
all_cls_index, all_p_mask)
```
| 07-17-2019 06:30:54 | 07-17-2019 06:30:54 | I have same issue. <|||||>Thanks @seanie12! |
transformers | 799 | closed | Error while adding new tokens to GPT2 tokenizer | A **NoneType Error** is encountered when I call `add_tokens()` to add new tokens to **GPT2 tokenizer** and the error is as following:
~~~~
File ".../pytorch_transformers/tokenization_utils.py", line 311, in add_tokens
if self.convert_tokens_to_ids(token) == self.convert_tokens_to_ids(self.unk_token):
File ".../pytorch_transformers/tokenization_utils.py", line 381, in convert_tokens_to_ids
for token in tokens:
TypeError: 'NoneType' object is not iterable
~~~~
The error comes from that it checks if the word id of a new `token` equals to that of the `unk_token` (as in tokenization_utils.py, line 311) but a **GPT2 tokenizer**'s `unk_token` is `None`. Therefore, the error happens when it tries iterating over `unk_token` in `convert_tokens_to_ids()` (as in tokenization_utils.py, line 381).
I think we can solve it by either
1) changing the if-condition in line 311 and not checking the new token's equality to `unk_token` (I also don't quite understand the logic behind checking the equality here)
or
2) dealing with `None` input in `convert_tokens_to_ids(self, tokens)`so it returns `[]` if `tokens is None`. | 07-17-2019 06:22:02 | 07-17-2019 06:22:02 | Added:
I also found that `_convert_token_to_id()` in tokenization_gpt2.py (line 182) uses `unk_token`, which is initially `None` in GPT2 tokenizer. This line of code can also lead to bugs.
~~~~
def _convert_token_to_id(self, token):
""" Converts a token (str/unicode) in an id using the vocab. """
if token in self.encoder:
return self.encoder.get(token)
return self.encoder.get(self.unk_token)
~~~~<|||||>Indeed, GPT-2 doesn't have a `unk_token` since it's supposed to be able to encode any string but this does have some unintended consequences since we also use the fact that a tokenizer returns the `unk_token` to check whether a token is in the current vocabulary or not.
I'll see how we can update this in the most coherent way. Probably mapping the special `<|endoftext|>` token as `unk_token` and using the same logic as the other models (returning the `unk_token` when the token is not in the vocabulary) is the simplest way to fix it.<|||||>What will be the quick fix for this? When running `run_generation.py` in examples, I could resolve the `None' error by adding special tokens to the tokenizer like below:
` special_tokens = {"cls_token":"[CLS]", "unk_token":"[UNK]"} `
` tokenier = tokenizer_class.from_pretrained("gpt2", cls_token="[CLS]", unk_token="[UNK]")`
` tokenizer.add_special_tokens(special_tokens) `
But then, I got a CUDA error (probably) due to the different embedding size of the model and tokenizer. So, I resized the model's token embedding size from 50257 to 50259 like below:
` model.resize_token_embeddings(len(tokenizer)) `
Then, it tokenizes the tokens correctly with the additional token encoders that have `tokenizer.added_tokens_encoder.keys()` with [CLS] and [UNK]. But, regardless of input, the gpt2 output seems to be wrong: a sequence [CLS] [CLS] ...


<|||||>@dykang
A quick fix would be cleaning the generated sentence by replacing unwanted tokens as "".
However, it is reasonable that the GPT2 outputs weird sentences given inputs including "[CLS]" as in your example because the new word embeddings are not trained. <|||||>Thanks, @ZHAOTING. However, GH-910 seems to only add unk token though. @thomwolf, would the PR be generalizable to add any special tokens as my earlier comment above? When special tokens are added, how do existing pre-trained gpt2 models work properly? <|||||>Adding some context. Is it possible to add [CLS] and [SEP] tokens to gpt2-medium in a non destructive way. After finetuning a bit following the structure indicated here:
```
# (Default, BERT/XLM pattern): [CLS] + A + [SEP] + B + [SEP]
# (XLNet/GPT pattern): A + [SEP] + B + [SEP] + [CLS]
```
it is clear that GPT2 will attempt to predict the BPE tokens for [CLS] as ` [C LS]`, but adding the [SEP] and [CLS] tokens produce only an output of [CLS] / [SEP] tokens despite any top_k, top_p, or temperature settings.
<|||||>I had a similar problem.
I tried to finetune a gpt2 model using the simpletransformers library.
The error seems to originate from
"/usr/local/lib/python3.6/site-packages/transformers/optimization.py"
This is the file where the optimizer AdamW is implemented.
In my case, I made a backup of the
"scheduler.pt" and "optimizer.pt"
files in my saved checkpoint.
In file
"/usr/local/lib/python3.6/site-packages/simpletransformers/language_modeling/language_modeling_model.py"
the optimizer and scheduler were also loaded from the checkpoint but this broke the code.
If these two files cannot be found in the path then your code will proceed with the rest of it.
This however remains a bug as your new optimizer and scheduler will start from scratch ignoring any "knowledge" they already contained about your previous optimization.
|
transformers | 798 | closed | [bug]BertAdam change to AdamW in example | https://github.com/huggingface/pytorch-transformers/blob/master/examples/lm_finetuning/simple_lm_finetuning.py#L35 BertAdam change to AdamW | 07-17-2019 05:59:02 | 07-17-2019 05:59:02 | Changing that line still causes an error in line 568, BertAdam has to be changed to AdamW as well and the warmup kwarg has to be removed.<|||||>#797 (specifically d6522e28732fd14a926440ef5f315e6a8e13792c) <|||||>have fix the error! I tested it on toy dataset.<|||||>@shibing624 this bug can be closed |
transformers | 797 | closed | fix some errors for distributed lm_finetuning | 1. makedirs
2. save models | 07-17-2019 01:18:50 | 07-17-2019 01:18:50 | # [Codecov](https://codecov.io/gh/huggingface/pytorch-transformers/pull/797?src=pr&el=h1) Report
> Merging [#797](https://codecov.io/gh/huggingface/pytorch-transformers/pull/797?src=pr&el=desc) into [master](https://codecov.io/gh/huggingface/pytorch-transformers/commit/5fe0b378d899f81eb0a7f2db0c4eb0234748e915?src=pr&el=desc) will **not change** coverage.
> The diff coverage is `n/a`.
[](https://codecov.io/gh/huggingface/pytorch-transformers/pull/797?src=pr&el=tree)
```diff
@@ Coverage Diff @@
## master #797 +/- ##
=======================================
Coverage 78.91% 78.91%
=======================================
Files 34 34
Lines 6193 6193
=======================================
Hits 4887 4887
Misses 1306 1306
```
------
[Continue to review full report at Codecov](https://codecov.io/gh/huggingface/pytorch-transformers/pull/797?src=pr&el=continue).
> **Legend** - [Click here to learn more](https://docs.codecov.io/docs/codecov-delta)
> `Δ = absolute <relative> (impact)`, `ø = not affected`, `? = missing data`
> Powered by [Codecov](https://codecov.io/gh/huggingface/pytorch-transformers/pull/797?src=pr&el=footer). Last update [5fe0b37...a7ba27b](https://codecov.io/gh/huggingface/pytorch-transformers/pull/797?src=pr&el=lastupdated). Read the [comment docs](https://docs.codecov.io/docs/pull-request-comments).
<|||||>Hi, do you want to update these examples to the new `pytorch-transformers` API at the same time?
Models now return `tuple` so we should take the first element of the model output as the loss and we should also update `BertAdam` to `AdamW`.<|||||>ok, I have a try, I‘m stilll use the old API. <|||||>Hi, I have updated the opt to the new API , please check. <|||||>Thanks @yzy5630! |
transformers | 796 | closed | Minor documentation updates | Hi,
this PR just updates some urls in the documentation :)
---
Thanks for your great work on PyTorch-Transformers 🤗 | 07-16-2019 21:45:23 | 07-16-2019 21:45:23 | Thanks Stefan! |
transformers | 795 | closed | XLNet-large-cased: hyper-parameters for fine-tuning on SST-2 | I tried to finetune XLNet on one of the classification tasks from GLUE (Ubuntu, GPU Titan RTX, CUDA 10.0, pytorch 1.1):
export GLUE_DIR=/path/to/glue
python ./examples/run_glue.py \
--model_type xlnet \
--model_name_or_path xlnet-large-cased \
--do_train \
--do_eval \
--task_name=sst-2 \
--data_dir=${GLUE_DIR}/SST-2 \
--output_dir=./proc_data/sst-2 \
--max_seq_length=128 \
--per_gpu_eval_batch_size=8 \
--per_gpu_train_batch_size=8 \
--gradient_accumulation_steps=1 \
--max_steps=1200 \
--model_name=xlnet-large-cased \
--overwrite_output_dir \
--overwrite_cache \
--warmup_steps=120
Training and evaluation work without errors but it looks like accuracy doesn't increase during training, I evaluated every 500 steps:
07/16/2019 22:29:30 - INFO - __main__ - ***** Eval results *****
07/16/2019 22:29:30 - INFO - __main__ - acc = 0.5091743119266054
07/16/2019 22:32:16 - INFO - __main__ - Loading features from cached file glue_data/SST-2/cached_dev_xlnet-large-cased_128_sst-2 | 999/8419 [05:37<41:47, 2.96it/s]
07/16/2019 22:32:17 - INFO - __main__ - ***** Running evaluation *****
07/16/2019 22:32:17 - INFO - __main__ - Num examples = 872
07/16/2019 22:32:17 - INFO - __main__ - Batch size = 8
07/16/2019 22:32:25 - INFO - __main__ - ***** Eval results *****
07/16/2019 22:32:25 - INFO - __main__ - acc = 0.5091743119266054
Finally the same acc:
07/16/2019 22:33:59 - INFO - __main__ - ***** Eval results *****
07/16/2019 22:33:59 - INFO - __main__ - acc = 0.5091743119266054
The same situation is with my own classification dataset. Accuracy wasn't changed during training. Something is wrong with finetuning of XLNet | 07-16-2019 21:42:09 | 07-16-2019 21:42:09 | I also tried to finetune xlnet base on squad 2.0 but the numbers on dev are pretty bad
`Results: {'exact': 3.0405120862461046, 'f1': 6.947601433150003, 'total': 11873, 'HasAns_exact': 6.056005398110662, 'HasAns_f1': 13.881388632893048, 'HasAns_total': 5928, 'NoAns_exact': 0.0336417157275021, 'NoAns_f1': 0.0336417157275021, 'NoAns_total': 5945, 'best_exact': 50.07159100480081, 'best_exact_thresh': 0.0, 'best_f1': 50.07159100480081, 'best_f1_thresh': 0.0}`<|||||>I suspect something is wrong with the evaluation code. Looking into it now.<|||||>@tbright17 Nothing wrong with evaluation. Accuracy and evaluation loss aren't changed during training. I used my own evaluation script, I used old BertAdam or OpenAIAdam optimizers without success.
@thomwolf Can you help?<|||||>I'll give a look, I've only tested XLNet on STS-B for the moment. You should check the hyper-parameters as well, they probably won't be the same as the ones of STS-B (some are mentioned in the XLNet paper).<|||||>First thing that comes to mind is that SST-2 is ~10 times bigger than STS-B (see the [GLUE paper](https://arxiv.org/abs/1804.07461)) so you need to increase the number of training step a lot if you want to do at least one full epoch on SST-2 training dataset (here you use the value for STS-B). And you should probably do several epochs, e.g. we do 6-7 epochs on STS-B). Check some examples of recommended hyper-parameters table 8 of the [xlnet paper](http://arxiv.org/abs/1906.08237).
You can also directly specify the number of epochs instead of the maximum number of steps in the script. You can see all the hyper-parameters of the script with `python ./run_glue.py --help`.<|||||>> First thing that comes to mind is that SST-2 is ~10 times bigger than STS-B (see the [GLUE paper](https://arxiv.org/abs/1804.07461)) so you need to increase the number of training step a lot if you want to do at least one full epoch on SST-2 training dataset (here you use the value for STS-B). And you should probably do several epochs, e.g. we do 6-7 epochs on STS-B). Check some examples of recommended hyper-parameters table 8 of the [xlnet paper](http://arxiv.org/abs/1906.08237).
>
> You can also directly specify the number of epochs instead of the maximum number of steps in the script. You can see all the hyper-parameters of the script with `python ./run_glue.py --help`.
I trained STS-B task with the same problem. You can see the following output with evaluation of every 100 steps (I added train and evaluation loss in output):
```
07/17/2019 13:09:55 - INFO - __main__ - ***** Running evaluation *****
07/17/2019 13:09:55 - INFO - __main__ - Num examples = 1500
07/17/2019 13:09:55 - INFO - __main__ - Batch size = 8
07/17/2019 13:10:09 - INFO - __main__ - ***** Eval results *****
07/17/2019 13:10:09 - INFO - __main__ - corr = -0.05367882385720809
07/17/2019 13:10:09 - INFO - __main__ - eval_loss = 2.8412214481133096##################################################################################################################| 188/188 [00:14<00:00, 13.41it/s]
07/17/2019 13:10:09 - INFO - __main__ - pearson = -0.041275192
07/17/2019 13:10:09 - INFO - __main__ - spearmanr = -0.06608245566229025
07/17/2019 13:10:09 - INFO - __main__ - Training loss: 307.258519500494
07/17/2019 13:10:41 - INFO - __main__ - Loading features from cached file ...glue_data/STS-B/cached_dev_xlnet-large-cased_128_sts-b | 199/719 [01:18<03:25, 2.53it/s]
07/17/2019 13:10:41 - INFO - __main__ - ***** Running evaluation *****
07/17/2019 13:10:41 - INFO - __main__ - Num examples = 1500
07/17/2019 13:10:41 - INFO - __main__ - Batch size = 8
07/17/2019 13:10:56 - INFO - __main__ - ***** Eval results *****
07/17/2019 13:10:56 - INFO - __main__ - corr = 0.13943037650184956
07/17/2019 13:10:56 - INFO - __main__ - eval_loss = 2.3762524007482733##################################################################################################################| 188/188 [00:14<00:00, 13.29it/s]
07/17/2019 13:10:56 - INFO - __main__ - pearson = 0.13502572
07/17/2019 13:10:56 - INFO - __main__ - spearmanr = 0.1438350282350605
07/17/2019 13:10:56 - INFO - __main__ - Training loss: 533.9101385176182
07/17/2019 13:11:28 - INFO - __main__ - Loading features from cached file .../glue_data/STS-B/cached_dev_xlnet-large-cased_128_sts-b | 299/719 [02:05<02:56, 2.39it/s]
07/17/2019 13:11:28 - INFO - __main__ - ***** Running evaluation *****
07/17/2019 13:11:28 - INFO - __main__ - Num examples = 1500
07/17/2019 13:11:28 - INFO - __main__ - Batch size = 8
07/17/2019 13:11:42 - INFO - __main__ - ***** Eval results *****
07/17/2019 13:11:42 - INFO - __main__ - corr = -0.0830871973267994
07/17/2019 13:11:42 - INFO - __main__ - eval_loss = 2.5565993221516305##################################################################################################################| 188/188 [00:14<00:00, 13.20it/s]
07/17/2019 13:11:42 - INFO - __main__ - pearson = -0.08915693
07/17/2019 13:11:42 - INFO - __main__ - spearmanr = -0.077017461524765
07/17/2019 13:11:42 - INFO - __main__ - Training loss: 761.6802722513676
07/17/2019 13:12:15 - INFO - __main__ - Loading features from cached file .../glue_data/STS-B/cached_dev_xlnet-large-cased_128_sts-b | 399/719 [02:52<02:18, 2.32it/s]
07/17/2019 13:12:15 - INFO - __main__ - ***** Running evaluation *****
07/17/2019 13:12:15 - INFO - __main__ - Num examples = 1500
07/17/2019 13:12:15 - INFO - __main__ - Batch size = 8
07/17/2019 13:12:29 - INFO - __main__ - ***** Eval results *****
07/17/2019 13:12:29 - INFO - __main__ - corr = -0.08715267932681456
07/17/2019 13:12:29 - INFO - __main__ - eval_loss = 2.398741365113157###################################################################################################################| 188/188 [00:14<00:00, 13.12it/s]
07/17/2019 13:12:29 - INFO - __main__ - pearson = -0.08428703
07/17/2019 13:12:29 - INFO - __main__ - spearmanr = -0.09001832616862088
07/17/2019 13:12:29 - INFO - __main__ - Training loss: 974.8287971913815
```
How you can see training loss is increasing, eval loss is almost the same, other metrics fluctuate around 0.<|||||>@thomwolf So, it looks like training is happening but in opposite direction for some reason<|||||>Maybe you haven't fully read the [explanation](https://github.com/huggingface/pytorch-transformers#fine-tuning-xlnet-model-on-the-sts-b-regression-task) accompanying the STS-B example in the readme?
It says "On this machine we thus have a batch size of 32, please increase `gradient_accumulation_steps` to reach the same batch size if you have a smaller machine."<|||||>@avostryakov Did you try to reduce the learning rate? I had a similar issue training with the TensorFlow version XLNet on only one GPU. I tried reducing the learning rate from 5e-5 to 1e-5, and it worked. Wish this can help you.<|||||>@thomwolf @tbright17 I got similar numbers like you Squad 2.0. Seems that the model probably isn't learning much. I'll print out the losses to explore. Also should we change the LR as well?
: the best I got with fine-tuning on Squad 2.0 with a `train_batch_size=8` and `gas=1` all others are default on a single v100 gpu was:
`07/16/2019 16:21:43 - INFO - __main__ - Results: {'exact': 26.438136949380947, 'f1': 28.470459931964722, 'total': 11873, 'HasAns_exact': 0.08434547908232119, 'HasAns_f1': 4.154819630940996, 'HasAns_total': 5928, 'NoAns_exact': 52.716568544995795, 'NoAns_f1': 52.716568544995795, 'NoAns_total': 5945, 'best_exact': 50.07159100480081, 'best_exact_thresh': 0.0, 'best_f1': 50.07159100480081, 'best_f1_thresh': 0.0}`<|||||>May also be a problem of batch size, the authors use a batch size between 32 and 128 in the paper.
What effective batch size do you have (printed during training)?
While we reproduce the official XLNet number on STS-B, I still have to work a bit on the SQuAD example for XLNet, the XLNet authors used a complex pre- and post-processing of the data (smarter than Bert's) that I haven't fully integrated into our `run_squad` example yet.<|||||>> Maybe you haven't fully read the [explanation accompanying the STS-B example in the readme](https://github.com/huggingface/pytorch-transformers#fine-tuning-xlnet-model-on-the-sts-b-regression-task)?
>
> It says "On this machine we thus have a batch size of 32, please increase `gradient_accumulation_steps` to reach the same batch size if you have a smaller machine."
@thomwolf You are right, STS-B started to train with batch size 32 and gradient_accumulation_steps = 2. Now I'm wondering why it so heavily depends on batch size. But it doesn't help for STS-2, I set max_steps=5000 (it's 5 epochs) and training and evaluation loss didn't change at all during training. I'm trying to train with learning rate 1e-5 how it was recommended by @alexpython1988 <|||||>@thomwolf maybe. Also my sequence length is `384`: the authors did mention they prolly did 512. Here's my batch size related printout: I think the number of examples seem a lil low. No? I think Squad has about 150K examples (ha and na questions) and with the `doc_stride` I think it should be more than 150k examples (I think).
`07/15/2019 13:23:32 - INFO - __main__ - ***** Running training *****`
`07/15/2019 13:23:32 - INFO - __main__ - Num examples = 133947`
`07/15/2019 13:23:32 - INFO - __main__ - Num Epochs = 3`
`07/15/2019 13:23:32 - INFO - __main__ - Instantaneous batch size per GPU = 4`
`07/15/2019 13:23:32 - INFO - __main__ - Total train batch size (w. parallel, distributed & accumulation) = 4`
`07/15/2019 13:23:32 - INFO - __main__ - Gradient Accumulation steps = 1`
`07/15/2019 13:23:32 - INFO - __main__ - Total optimization steps = 100461`
I saw in the [renatoviolin's repo](https://github.com/renatoviolin/xlnet/blob/master/run_squad_GPU.py) that they have the following which gives them `86F1` on a RTX2080:
`flags.DEFINE_integer("max_seq_length",
default=512, help="Max sequence length")
flags.DEFINE_integer("max_query_length",
default=64, help="Max query length")
flags.DEFINE_integer("doc_stride",
default=128, help="Doc stride")
flags.DEFINE_integer("max_answer_length",
default=64, help="Max answer length")`
Also, lr is different than ours (`5e-5` in this repo):
`flags.DEFINE_float("learning_rate", default=3e-5, help="initial learning rate")`
<|||||>Learning rate = 1e-5 helps to train STS-2 together with batch size 32 and accumulation steps = 2. I need more experiments but it works. Thanks, @thomwolf, and @alexpython1988!<|||||>Great to hear, good job and good luck @avostryakov! Feel free to share good hyper-parameters if you find a nice set and I can add them to the documentation (with credits).<|||||>> May also be a problem of batch size, the authors use a batch size between 32 and 128 in the paper.
>
> What effective batch size do you have (printed during training)?
>
> While we reproduce the official XLNet number on STS-B, I still have to work a bit on the SQuAD example for XLNet, the XLNet authors used a complex pre- and post-processing of the data (smarter than Bert's) that I haven't fully integrated into our `run_squad` example yet.
I was using per_gpu_train_batch 8 for squad 2.0. Powerful model is hard to tune maybe<|||||>> Great to hear, good job and good luck @avostryakov! Feel free to share good hyper-parameters if you find a nice set and I can add them to the documentation (with credits).
@thomwolf My the best result for SST-2 so far is 94.15 of accuracy (in xlnet's article 95.6). It's better than BERT-large. I trained with the following parameters:
```
python ./examples/run_glue.py \
--model_type xlnet \
--model_name_or_path xlnet-large-cased \
--do_train \
--evaluate_during_training \
--do_eval \
--logging_steps 500 \
--save_steps 3000 \
--task_name=sst-2 \
--data_dir=${GLUE_DIR}/SST-2 \
--output_dir=./proc_data/sst-2 \
--max_seq_length=128 \
--learning_rate 1e-5 \
--per_gpu_eval_batch_size=8 \
--per_gpu_train_batch_size=8 \
--gradient_accumulation_steps=1 \
--max_steps=16000 \
--model_name=xlnet-large-cased \
--overwrite_output_dir \
--overwrite_cache \
--warmup_steps=120 \
--fp16
```<|||||>@thomwolf Ok, the last result for SST-2 almost matched with XLNet article: Accuracy 95.4:
```
python ./examples/run_glue.py \
--model_type xlnet \
--model_name_or_path xlnet-large-cased \
--do_train \
--evaluate_during_training \
--do_eval \
--logging_steps 400 \
--save_steps 3000 \
--task_name=sst-2 \
--data_dir=${GLUE_DIR}/SST-2 \
--output_dir=./proc_data/sst-2 \
--max_seq_length=128 \
--learning_rate 1e-5 \
--per_gpu_eval_batch_size=16 \
--per_gpu_train_batch_size=16 \
--gradient_accumulation_steps=1 \
--max_steps=8000 \
--model_name=xlnet-large-cased \
--overwrite_output_dir \
--overwrite_cache \
--warmup_steps=120 \
--fp16
```
Thank you for your work!<|||||>This is great @avostryakov! Thanks for sharing the results!
I'm editing the issue title until I've time to add the hyperparameters to the doc.<|||||>Hi, how could I finetune the model for text generation? Is it possible just having raw text for the finetuning?<|||||>This issue has been automatically marked as stale because it has not had recent activity. It will be closed if no further activity occurs. Thank you for your contributions.
|
transformers | 794 | closed | Adding additional model loading functionality | (Porting over some functionality from my old fork)
This PR adds additional methods to `PreTrainedModel` for loading models for `state_dict`s. Currently, `from_pretrained()` does a lot of the heavy lifting, but is primarily designed to load from file/folders. This adds additional options for users with different model-loading workflows. | 07-16-2019 18:40:23 | 07-16-2019 18:40:23 | Hi Jason,
Can you give me a little more information on the model-loading workflow you are using so I can understand the whys and wherefores of these proposed modifications?<|||||>Hey Thomas,
Sorry for the delay. My thinking is this: the `from_pretrained` method current does two things: resolve the path/archive for loading a pretrained model, and the specialized model loading logic (e.g. handing the fact that the current model may have different heads from those in the loaded weights). My proposed change is to separate the two and allow the user to just do the second.
This would be useful in cases where the user already has access to the `state_dict` in memory (e.g. if they have a different model saving workflow/format).<|||||>Hi Jason,
There is a `state_dict` option in `from_pretrained` that, I think, let you do just that!
See here for instance: https://huggingface.co/pytorch-transformers/main_classes/model.html#pytorch_transformers.PreTrainedModel.from_pretrained<|||||>Closing this for now. Feel free to re-open if the provided solution doesn't solve your problem, Jason. |
transformers | 793 | closed | BertModel docstring missing pooled_output | The BERT docstring describes three outputs here:
https://github.com/huggingface/pytorch-transformers/blob/master/pytorch_transformers/modeling_bert.py#L626
But none of these correspond to the pooled_output output that's added here:
https://github.com/huggingface/pytorch-transformers/blob/master/pytorch_transformers/modeling_bert.py#L713
I may be missing something, but this looks like a dated docstring. | 07-16-2019 18:08:51 | 07-16-2019 18:08:51 | Damned, missed that one, you are right.
Adding the missing doc-string:
```
**pooler_output**: ``torch.FloatTensor`` of shape ``(batch_size, hidden_size)``
Last layer hidden-state of the first token of the sequence (classification token)
further processed by a Linear layer and a Tanh activation function. The Linear
layer weights are trained from the next sentence prediction (classification)
objective during Bert pretraining. This output is usually *not* a good summary
of the semantic content of the input, you're often better with averaging or pooling
the sequence of hidden-states for the whole input sequence.
```
We'll probably do a small release in a few days once we have gathered all the feedbacks from the main release. In the meantime, I'll set up PyTorch-Hub so people can get the models from master.<|||||>A minor edit with the final two optional outputs `hidden_states` and `attentions` are tuples, not lists.<|||||>cc @LysandreJik :)<|||||>The documentation is outdated regarding that issue. It should probably be re-compiled :-) |
transformers | 792 | closed | Issue running run_transfo_xl.py | Run code:
```
python run_transfo_xl.py --work_dir ../log
```
Output
```
07/16/2019 18:01:46 - INFO - __main__ - device: cuda
07/16/2019 18:01:46 - INFO - pytorch_transformers.tokenization_utils - loading file https://s3.amazonaws.com/models.huggingface.co/bert/transfo-xl-wt103-vocab.bin from cache at /home/korymathewson/.cache/torch/pytorch_transformers/b24cb708726fd43cbf1a382da9ed3908263e4fb8a156f9e0a4f45b7540c69caa.a6a9c41b856e5c31c9f125dd6a7ed4b833fbcefda148b627871d4171b25cffd1
07/16/2019 18:01:46 - INFO - pytorch_transformers.tokenization_utils - loading file https://s3.amazonaws.com/models.huggingface.co/bert/transfo-xl-wt103-vocab.bin from cache at /home/korymathewson/.cache/torch/pytorch_transformers/b24cb708726fd43cbf1a382da9ed3908263e4fb8a156f9e0a4f45b7540c69caa.a6a9c41b856e5c31c9f125dd6a7ed4b833fbcefda148b627871d4171b25cffd1
07/16/2019 18:01:47 - INFO - pytorch_transformers.tokenization_transfo_xl - loading corpus file https://s3.amazonaws.com/models.huggingface.co/bert/transfo-xl-wt103-corpus.bin from cache at /home/korymathewson/.cache/torch/pytorch_transformers/b927918d674805742f3febcd807b375d5819f40410b83d09e3c0fb8344394216.a7d11b2fa856afe836727fbd95638053f056c4a3ac571d7800faed25ce81a4e1
07/16/2019 18:01:53 - INFO - pytorch_transformers.modeling_utils - loading configuration file https://s3.amazonaws.com/models.huggingface.co/bert/transfo-xl-wt103-config.json from cache at /home/korymathewson/.cache/torch/pytorch_transformers/a6dfd6a3896b3ae4c1a3c5f26ff1f1827c26c15b679de9212a04060eaf1237df.aef76fb1064c932cd6a2a2be3f23ebbfa5f9b6e29e8e87b571c45b4a5d5d1b90
07/16/2019 18:01:53 - INFO - pytorch_transformers.modeling_utils - Model config {
"adaptive": true,
"attn_type": 0,
"clamp_len": 1000,
"cutoffs": [
20000,
40000,
200000
],
"d_embed": 1024,
"d_head": 64,
"d_inner": 4096,
"d_model": 1024,
"div_val": 4,
"dropatt": 0.0,
"dropout": 0.1,
"ext_len": 0,
"finetuning_task": null,
"init": "normal",
"init_range": 0.01,
"init_std": 0.02,
"mem_len": 1600,
"n_head": 16,
"n_layer": 18,
"n_token": 267735,
"num_labels": 2,
"output_attentions": false,
"output_hidden_states": false,
"pre_lnorm": false,
"proj_init_std": 0.01,
"same_length": true,
"sample_softmax": -1,
"tgt_len": 128,
"tie_projs": [
false,
true,
true,
true
],
"tie_weight": true,
"torchscript": false,
"untie_r": true
}
07/16/2019 18:01:53 - INFO - pytorch_transformers.modeling_utils - loading weights file https://s3.amazonaws.com/models.huggingface.co/bert/transfo-xl-wt103-pytorch_model.bin from cache at /home/korymathewson/.cache/torch/pytorch_transformers/12642ff7d0279757d8356bfd86a729d9697018a0c93ad042de1d0d2cc17fd57b.e9704971f27275ec067a00a67e6a5f0b05b4306b3f714a96e9f763d8fb612671
07/16/2019 18:02:06 - INFO - __main__ - Evaluating with bsz 10 tgt_len 128 ext_len 0 mem_len 1600 clamp_len 1000
Traceback (most recent call last):
File "run_transfo_xl.py", line 153, in <module>
main()
File "run_transfo_xl.py", line 134, in main
test_loss = evaluate(te_iter)
File "run_transfo_xl.py", line 117, in evaluate
loss, mems = ret
ValueError: too many values to unpack (expected 2)
``` | 07-16-2019 18:03:38 | 07-16-2019 18:03:38 | |
transformers | 791 | closed | RestructuredText table for pretrained models. | 07-16-2019 16:00:17 | 07-16-2019 16:00:17 | # [Codecov](https://codecov.io/gh/huggingface/pytorch-transformers/pull/791?src=pr&el=h1) Report
> Merging [#791](https://codecov.io/gh/huggingface/pytorch-transformers/pull/791?src=pr&el=desc) into [master](https://codecov.io/gh/huggingface/pytorch-transformers/commit/b33a385091de604afb566155ec03329b84c96926?src=pr&el=desc) will **not change** coverage.
> The diff coverage is `n/a`.
[](https://codecov.io/gh/huggingface/pytorch-transformers/pull/791?src=pr&el=tree)
```diff
@@ Coverage Diff @@
## master #791 +/- ##
=======================================
Coverage 78.91% 78.91%
=======================================
Files 34 34
Lines 6193 6193
=======================================
Hits 4887 4887
Misses 1306 1306
```
------
[Continue to review full report at Codecov](https://codecov.io/gh/huggingface/pytorch-transformers/pull/791?src=pr&el=continue).
> **Legend** - [Click here to learn more](https://docs.codecov.io/docs/codecov-delta)
> `Δ = absolute <relative> (impact)`, `ø = not affected`, `? = missing data`
> Powered by [Codecov](https://codecov.io/gh/huggingface/pytorch-transformers/pull/791?src=pr&el=footer). Last update [b33a385...9d381e7](https://codecov.io/gh/huggingface/pytorch-transformers/pull/791?src=pr&el=lastupdated). Read the [comment docs](https://docs.codecov.io/docs/pull-request-comments).
|
|
transformers | 790 | closed | XLNet Embeddings | How can I retrieve contextual word vectors for my dataset using XLNet ?
The usage and examples in the documentation do not include any guide to use XLNet.
Thanks. | 07-16-2019 10:27:47 | 07-16-2019 10:27:47 | I'm currently finishing to add the documentation but just use `XLNetModel` instead of `BertModel` in the usage example with `BertModel`<|||||>Thanks a lot, @thomwolf for the quick reply. I'll try it out.<|||||>Here is an example now: https://huggingface.co/pytorch-transformers/model_doc/xlnet.html#pytorch_transformers.XLNetModel<|||||>@thomwolf, I tried the following snippet. The similarity score changes every time I run the cell. That is, the embeddings or the weights are changing every time. Is this related to dropout?
```
config = XLNetConfig.from_pretrained('xlnet-large-cased')
tokenizer = XLNetTokenizer.from_pretrained('xlnet-large-cased')
model = XLNetModel(config)
input_ids = torch.tensor(tokenizer.encode("The apple juice is sour.")).unsqueeze(0)
input_ids_2 = torch.tensor(tokenizer.encode("The orange juice is sweet.")).unsqueeze(0)
outputs = model(input_ids)
outputs_2 = model(input_ids_2)
last_hidden_states = outputs[0]
last_hidden_states_2 = outputs_2[0]
apple = last_hidden_states[0][1]
orange = last_hidden_states_2[0][1]
x = apple
y = orange
cos_sim = dot(x.detach().numpy(),y.detach().numpy())/(norm(x.detach().numpy())*norm(y.detach().numpy()))
print(cos_sim)
```
<|||||>For me logits values changes as well ... using exactly the same settings as mentioned in the example.
Have you found a way to fix that?<|||||>@Oxi84 put `model.eval()` before you make the predictions. This fixed the problem of changing weights for me.<|||||>Thanks. For me it works when call like that:
tokenizer = XLNetTokenizer.from_pretrained("xlnet-large-cased")
model = XLNetLMHeadModel.from_pretrained("xlnet-large-cased")
model.eval()
However accuracy seems to be much lower that for Bert - with the code i wrote here: https://github.com/huggingface/pytorch-transformers/issues/846
Did you find that the accuracy is good or bad? I compared with Bert on few examples for masked word prediction and most of XLNet predicted word with the highest probability do not fit at all.
<|||||>@kushalj001 hi, how can I get the sentence vector<|||||>Hi, so it seems that creating a model with a configuration is primarily the problem here:
`model = XLNetLMHeadModel.from_pretrained("xlnet-large-cased")`
yields consistent outputs, but
`config = XLNetConfig.from_pretrained("xlnet-large-uncased")`
`model = XLNetModel(config)`
does not at all.
My question is, how is it possible to set configuration states (like getting hidden states of the model). I have run the glue STS-B fine tuning code to customize the model which is stored at `./proc_data/sts-b-100`, but when I load the model using code like this to get hidden states:
`config = XLNetConfig.from_pretrained('./proc_data/sts-b-110/')`
`config.output_hidden_states=True`
`tokenizer = XLNetTokenizer.from_pretrained('././proc_data/sts-b-110/')`
`model = XLNetForSequenceClassification(config)`
I get results that vary wildly across runs.
Specifically, I would like to get the hidden states of each layer from the fine tuned model and correlate it to the actual text similarity. I was thinking I'd load the model with XLNetForSequenceClassification, get all the hidden states setting the configuration to output hidden states and do such a correlation. Is my approach incorrect?<|||||>Looking at run_glue, it seems that actually outputs[1] is used for prediction? This is confusing because all the examples use [0] and the documentation is not very clear.
`outputs = model(**inputs)`
`tmp_eval_loss, logits = outputs[:2]`
From run_glue.py
<|||||>Ok, I figured the logits and loss issue out - the issue is that for XLNetForSequenceClassification, the second index does in fact have logits while the first has loss.<|||||>@thomwolf @Oxi84 while calculating word-embeddings of a document, i.e multiple sentences, is it necessary to pass the document sentence-wise? For my dataset, I removed punctuation as a part of the pre-processing step. So now, my whole document goes into the model. Does this hurt the model's performance? Does it make a lot of difference in terms of capturing the context of words?
Thanks<|||||>It should improve acuracy if the text is longer, but still for me Bert is way better ... on 20-40 words long text.<|||||>> It should improve acuracy if the text is longer, but still for me Bert is way better ... on 20-40 words long text.
Yeah, even for my experiments, BERT simply outperfoms XLNet. Still don't know why though.
When you say "it should improve accuracy", you mean that feeding sentences to calculate word-vec would be better, right?<|||||>Did you managed to try tensorflow version of XLNet, there is a chance it might be different from the pytorch version?<|||||>Maybe there is some bug, but its unlikely since the bechmark results with the XLnet pytorch are the same. But I gues this would the first thing to try to recheck.<|||||>> Did you managed to try tensorflow version of XLNet, there is a chance it might be different from the pytorch version?
Any simple way of doing this?<|||||>any updates regarding this issue? <|||||>@kushalj001 why remove the punctuation ? Is it domain specific or to improve accuracy?<|||||>> @kushalj001 why remove the punctuation ? Is it domain specific or to improve accuracy?
My dataset had a lot of random punctuation, ie misplaced single and double-quotes.
But also, do punctuations add any valuable information to the text? Apart from the period (which can be used to break a large para into sentences), does keeping other punctuation symbols make sense? <|||||>I will close this issue which dates back before we had the clean documentation up here: https://huggingface.co/pytorch-transformers/
Please open a new issue with a clear explanation of your specific problem if you have related issues. |
transformers | 789 | closed | XLNet text generation ability : inference is slow | I compared the inference time for generating text with the given [example script](https://github.com/huggingface/pytorch-pretrained-BERT/blob/xlnet/examples/run_generation.py) between XLNet & GPT-2, on CPU.
To generate 100 tokens, XLNet takes **3m22s** while GPT-2 takes **14s**. And it grows exponentially : for 500 tokens, XLNet takes **51m46s** while GPT-2 takes **2m52s**.
Due to bidirectionality of the model, each tokens' attention should be computed again to relate to the newly generated token.
To reduce the time needed, we should allow the model to use unidirectional attention over generated tokens (even if it means that some older tokens will not see some newly generated tokens, i.e. reducing bidirectionality).
---
According to the [original post of Aman Rusia](https://medium.com/@amanrusia/xlnet-speaks-comparison-to-gpt-2-ea1a4e9ba39e), doing so greatly decrease the quality of text.
However the post was updated as it was a mistake in the code. It seems fine to generate tokens with unidirectional attention. Please refer to [this issue](https://github.com/rusiaaman/XLnet-gen/issues/1#issuecomment-511508957) | 07-16-2019 00:23:34 | 07-16-2019 00:23:34 | I tried it, but text quality is lowered a lot and inference time does not change at all.
I simply changed `perm_mask` to be 0 over initial context and 1 over generated tokens.
---
Input :
> In Seoul, you can do a lot of things ! For example you can
Generated text with full bidirectionality :
> buy grocery stores and restaurants, or even buy liquor, tobacco, etc. Then you can go to the mall. Then you can visit shopping mall. Then you can go to the university, then you can visit an outdoor pool. You can visit the cinema. You can visit art galleries. Then you can visit a garden.<eop> Etc. etc. etc. After all, if you can buy items and enjoy them, then yes, you can enjoy them in Seoul. It is that simple.
Generated text with bidirectionality over context tokens, and unidirectionality over generated tokens :
> buy tons Free do hotel on you whichT Seoul, list and do you coffee non can many of you sit- shopping People you river boatou. and Koreans in long you into graduate train/ by teacher college c people there ho sister formst to in city plain daughtera kayak cat.: years World home. still home later N will plan yearses street his looks a marriage different by tell it too stunning out to what ice by person a, people a bag.
**Why is it that bad ?**<|||||>This issue has been automatically marked as stale because it has not had recent activity. It will be closed if no further activity occurs. Thank you for your contributions.
<|||||>Hi @Colanim,
Thanks for the issue - sorry that we overlooked it!
I will take a closer look into this. GPT2 uses key value state caching whet doing generation. Not sure whether XLNet does something similar. Will see if it'd be easy to add or not!<|||||>Sorry to answer that late. `XLNet` is known to be rather slow for text generation due to the needed padding to get it started.
`XLNet` uses `mems` which is similar to `past` to have a longer memory span.
Since the quality seems to degrade much when applying your suggestion, I don't think trying to add a `XLNet` enhancement for generation is of high priority at the moment...Sorry! But feel free to open a PR if you have a good solution :-) |
transformers | 788 | closed | bert-large config file | Here is the config file I download from path in modelling for bert large,
{
"attention_probs_dropout_prob": 0.1,
"directionality": "bidi",
"hidden_act": "gelu",
"hidden_dropout_prob": 0.1,
"hidden_size": 1024,
"initializer_range": 0.02,
"intermediate_size": 4096,
"max_position_embeddings": 512,
"num_attention_heads": 16,
"num_hidden_layers": 24,
"pooler_fc_size": 768,
"pooler_num_attention_heads": 12,
"pooler_num_fc_layers": 3,
"pooler_size_per_head": 128,
"pooler_type": "first_token_transform",
"type_vocab_size": 2,
"vocab_size": 28996
}
I am wondering what are the following params for? I can't find them in the modelling file and the checkpoint I download.
"pooler_fc_size": 768,
"pooler_num_attention_heads": 12,
"pooler_num_fc_layers": 3,
"pooler_size_per_head": 128,
"pooler_type": "first_token_transform", | 07-15-2019 13:52:49 | 07-15-2019 13:52:49 | This issue has been automatically marked as stale because it has not had recent activity. It will be closed if no further activity occurs. Thank you for your contributions.
|
Subsets and Splits
No saved queries yet
Save your SQL queries to embed, download, and access them later. Queries will appear here once saved.