repo
stringclasses 1
value | number
int64 1
25.3k
| state
stringclasses 2
values | title
stringlengths 1
487
| body
stringlengths 0
234k
⌀ | created_at
stringlengths 19
19
| closed_at
stringlengths 19
19
| comments
stringlengths 0
293k
|
---|---|---|---|---|---|---|---|
transformers | 1,087 | closed | Decode now calls private property instead of public method | Removes the warning raised when the decode method is called. | 08-22-2019 21:26:43 | 08-22-2019 21:26:43 | # [Codecov](https://codecov.io/gh/huggingface/pytorch-transformers/pull/1087?src=pr&el=h1) Report
> Merging [#1087](https://codecov.io/gh/huggingface/pytorch-transformers/pull/1087?src=pr&el=desc) into [master](https://codecov.io/gh/huggingface/pytorch-transformers/commit/e00b4ff1de0591d5093407b16e665e5c86028f04?src=pr&el=desc) will **decrease** coverage by `0.01%`.
> The diff coverage is `33.33%`.
[](https://codecov.io/gh/huggingface/pytorch-transformers/pull/1087?src=pr&el=tree)
```diff
@@ Coverage Diff @@
## master #1087 +/- ##
=========================================
- Coverage 79.61% 79.6% -0.02%
=========================================
Files 42 42
Lines 6898 6898
=========================================
- Hits 5492 5491 -1
- Misses 1406 1407 +1
```
| [Impacted Files](https://codecov.io/gh/huggingface/pytorch-transformers/pull/1087?src=pr&el=tree) | Coverage Δ | |
|---|---|---|
| [pytorch\_transformers/tokenization\_utils.py](https://codecov.io/gh/huggingface/pytorch-transformers/pull/1087/diff?src=pr&el=tree#diff-cHl0b3JjaF90cmFuc2Zvcm1lcnMvdG9rZW5pemF0aW9uX3V0aWxzLnB5) | `85.9% <33.33%> (-0.33%)` | :arrow_down: |
------
[Continue to review full report at Codecov](https://codecov.io/gh/huggingface/pytorch-transformers/pull/1087?src=pr&el=continue).
> **Legend** - [Click here to learn more](https://docs.codecov.io/docs/codecov-delta)
> `Δ = absolute <relative> (impact)`, `ø = not affected`, `? = missing data`
> Powered by [Codecov](https://codecov.io/gh/huggingface/pytorch-transformers/pull/1087?src=pr&el=footer). Last update [e00b4ff...2ba1a14](https://codecov.io/gh/huggingface/pytorch-transformers/pull/1087?src=pr&el=lastupdated). Read the [comment docs](https://docs.codecov.io/docs/pull-request-comments).
<|||||>Yes! Thanks @LysandreJik LGTM |
transformers | 1,086 | closed | ProjectedAdaptiveLogSoftmax log_prob computation dimensions error | ## 🐛 Bug
<!-- Important information -->
Model I am using (Bert, XLNet....): TransformerXL
The problem arise when using:
* [x] the official example scripts: (give details)
* [ ] my own modified scripts: (give details)
The tasks I am working on is:
* [ ] an official GLUE/SQUaD task: (give the name)
* [x] my own task or dataset: (give details)
## To Reproduce
Steps to reproduce the behavior:
```python
from pytorch_transformers.modeling_transfo_xl_utilities import ProjectedAdaptiveLogSoftmax
import torch
s = ProjectedAdaptiveLogSoftmax(10000, 8, 8, [1000, 2000, 8000])
outputs = torch.randn(5, 3, 8)
outputs = outputs.view(-1, outputs.size(-1))
log_prob = s.log_prob(outputs)
```
Error:
> Traceback (most recent call last):
File "<input>", line 5, in <module>
File "/usr/local/Cellar/python/3.7.3/Frameworks/Python.framework/Versions/3.7/lib/python3.7/site-packages/pytorch_transformers/modeling_transfo_xl_utilities.py", line 254, in log_prob
logprob_i = head_logprob[:, -i] + tail_logprob_i
RuntimeError: The size of tensor a (15) must match the size of tensor b (1000) at non-singleton dimension 1
I think the code should be:
```python
def log_prob(self, hidden):
r""" Computes log probabilities for all :math:`n\_classes`
From: https://github.com/pytorch/pytorch/blob/master/torch/nn/modules/adaptive.py
Args:
hidden (Tensor): a minibatch of examples
Returns:
log-probabilities of for each class :math:`c`
in range :math:`0 <= c <= n\_classes`, where :math:`n\_classes` is a
parameter passed to ``AdaptiveLogSoftmaxWithLoss`` constructor.
Shape:
- Input: :math:`(N, in\_features)`
- Output: :math:`(N, n\_classes)`
"""
if self.n_clusters == 0:
logit = self._compute_logit(hidden, self.out_layers[0].weight,
self.out_layers[0].bias, self.out_projs[0])
return F.log_softmax(logit, dim=-1)
else:
# construct weights and biases
weights, biases = [], []
for i in range(len(self.cutoffs)):
if self.div_val == 1:
l_idx, r_idx = self.cutoff_ends[i], self.cutoff_ends[i + 1]
weight_i = self.out_layers[0].weight[l_idx:r_idx]
bias_i = self.out_layers[0].bias[l_idx:r_idx]
else:
weight_i = self.out_layers[i].weight
bias_i = self.out_layers[i].bias
if i == 0:
weight_i = torch.cat(
[weight_i, self.cluster_weight], dim=0)
bias_i = torch.cat(
[bias_i, self.cluster_bias], dim=0)
weights.append(weight_i)
biases.append(bias_i)
head_weight, head_bias, head_proj = weights[0], biases[0], self.out_projs[0]
head_logit = self._compute_logit(hidden, head_weight, head_bias, head_proj)
out = hidden.new_empty((head_logit.size(0), self.n_token))
head_logprob = F.log_softmax(head_logit, dim=1)
cutoff_values = [0] + self.cutoffs
for i in range(len(cutoff_values) - 1):
start_idx, stop_idx = cutoff_values[i], cutoff_values[i + 1]
if i == 0:
out[:, :self.cutoffs[0]] = head_logprob[:, :self.cutoffs[0]]
else:
weight_i, bias_i, proj_i = weights[i], biases[i], self.out_projs[i]
tail_logit_i = self._compute_logit(hidden, weight_i, bias_i, proj_i)
tail_logprob_i = F.log_softmax(tail_logit_i, dim=1)
logprob_i = head_logprob[:, -1].unsqueeze(1) + tail_logprob_i
out[:, start_idx:stop_idx] = logprob_i
return out
```
The change here is on the third to last line, you guys did `logprob_i = head_logprob[:, -1] + tail_logprob_i`. This isn't fitting in dimensions, so I think unsqueezing it will fix the problem, the class [AdaptiveLogSoftmaxWithLoss](https://pytorch.org/docs/stable/_modules/torch/nn/modules/adaptive.html) had to unsqueeze the `head_logprob`.
Another problem that I ran into is that, with the original code, your second to last line is
`out[:, start_idx, stop_idx] = logprob_i`, but `out` only has 2 dimensions, so I think you meant `start_idx:stop_idx` instead. Let me know if I'm wrong.
## Environment
* OS: OSX Mojave
* Python version: 3.7
* PyTorch version: 1.1.0
* PyTorch Transformers version (or branch): Master
* Using GPU ? No
* Distributed of parallel setup ? None | 08-22-2019 15:39:22 | 08-22-2019 15:39:22 | This issue has been automatically marked as stale because it has not had recent activity. It will be closed if no further activity occurs. Thank you for your contributions.
|
transformers | 1,085 | closed | RuntimeError: Creating MTGP constants failed. at /opt/conda/conda-bld/pytorch_1533739672741/work/aten/src/THC/THCTensorRandom.cu:34 | Hi there, I am trying to fine tune the roberta model, but I meet the following errors below. Basically I use input_ids, token_type_ids, attention_ mask as inputs. Below are the command I use:
```
outputs_pos = model(input_ids=pos_data, token_type_ids=pos_segs, attention_mask=pos_mask)[0]
```
```
The data are as follow:
input_ids:
[[50262 354 10 410 26604 15983 148 6690 0 0 0 0
0 0 0 0 0 0 0 0]]
[[50263 170 218 3695 4056 7471 4056 27 90 216 10 319
59 5 3038 9 26604 148 6690 15 47 8 110 1928
4 407 24 3695 4056 7471 4056 27 29 275 7 3000
5 1280 47 120 349 183 4 318 47 3695 4056 7471
4056 27 241 5283 6 3000 26604 7 1878 7259 1023 27809
349 183 4 152 16 59 5 1280 11 112 2537 14989
290 12 15810 12988 9 3895 50 65 316 12 15810 4946
9 3895 4 0 0 0 0 0 0 0 0 0
0 0 0 0 0 0 0 0 0 0 0 0
0 0 0 0 0 0 0 0 0 0 0 0
0 0 0 0 0 0 0 0 0 0 0 0
0 0 0 0 0 0 0 0 0 0 0 0
0 0 0 0 0 0 0 0 0 0 0 0
0 0 0 0 0 0 0 0 0 0 0 0
0 0 0 0 0 0 0 0 0 0 0 0
0 0 0 0 0 0 0 0 0 0 0 0
0 0 0 0 0 0 0 0]]
[[50263 243 16 3489 1522 13 5283 390 7 3529 7548 142
3218 33 2343 7 3364 1402 1795 9 4441 7548 148 6690
4 635 6 5283 390 197 1306 49 26604 14797 16 874
1878 17844 228 183 4 0 0 0 0 0 0 0
0 0 0 0 0 0 0 0 0 0 0 0
0 0 0 0 0 0 0 0 0 0 0 0
0 0 0 0 0 0 0 0 0 0 0 0
0 0 0 0 0 0 0 0 0 0 0 0
0 0 0 0 0 0 0 0 0 0 0 0
0 0 0 0 0 0 0 0 0 0 0 0
0 0 0 0 0 0 0 0 0 0 0 0
0 0 0 0 0 0 0 0 0 0 0 0
0 0 0 0 0 0 0 0 0 0 0 0
0 0 0 0 0 0 0 0 0 0 0 0
0 0 0 0 0 0 0 0 0 0 0 0
0 0 0 0 0 0 0 0 0 0 0 0
0 0 0 0 0 0 0 0]]
token_type_ids:
tensor([[50262, 354, 10, 410, 26604, 15983, 148, 6690, 0, 0,
0, 0, 0, 0, 0, 0, 0, 0, 0, 0]])
tensor([[50263, 170, 218, 3695, 4056, 7471, 4056, 27, 90, 216,
10, 319, 59, 5, 3038, 9, 26604, 148, 6690, 15,
47, 8, 110, 1928, 4, 407, 24, 3695, 4056, 7471,
4056, 27, 29, 275, 7, 3000, 5, 1280, 47, 120,
349, 183, 4, 318, 47, 3695, 4056, 7471, 4056, 27,
241, 5283, 6, 3000, 26604, 7, 1878, 7259, 1023, 27809,
349, 183, 4, 152, 16, 59, 5, 1280, 11, 112,
2537, 14989, 290, 12, 15810, 12988, 9, 3895, 50, 65,
316, 12, 15810, 4946, 9, 3895, 4, 0, 0, 0,
0, 0, 0, 0, 0, 0, 0, 0, 0, 0,
0, 0, 0, 0, 0, 0, 0, 0, 0, 0,
0, 0, 0, 0, 0, 0, 0, 0, 0, 0,
0, 0, 0, 0, 0, 0, 0, 0, 0, 0,
0, 0, 0, 0, 0, 0, 0, 0, 0, 0,
0, 0, 0, 0, 0, 0, 0, 0, 0, 0,
0, 0, 0, 0, 0, 0, 0, 0, 0, 0,
0, 0, 0, 0, 0, 0, 0, 0, 0, 0,
0, 0, 0, 0, 0, 0, 0, 0, 0, 0,
0, 0, 0, 0, 0, 0, 0, 0, 0, 0,
0, 0, 0, 0, 0, 0, 0, 0, 0, 0]])
tensor([[50263, 243, 16, 3489, 1522, 13, 5283, 390, 7, 3529,
7548, 142, 3218, 33, 2343, 7, 3364, 1402, 1795, 9,
4441, 7548, 148, 6690, 4, 635, 6, 5283, 390, 197,
1306, 49, 26604, 14797, 16, 874, 1878, 17844, 228, 183,
4, 0, 0, 0, 0, 0, 0, 0, 0, 0,
0, 0, 0, 0, 0, 0, 0, 0, 0, 0,
0, 0, 0, 0, 0, 0, 0, 0, 0, 0,
0, 0, 0, 0, 0, 0, 0, 0, 0, 0,
0, 0, 0, 0, 0, 0, 0, 0, 0, 0,
0, 0, 0, 0, 0, 0, 0, 0, 0, 0,
0, 0, 0, 0, 0, 0, 0, 0, 0, 0,
0, 0, 0, 0, 0, 0, 0, 0, 0, 0,
0, 0, 0, 0, 0, 0, 0, 0, 0, 0,
0, 0, 0, 0, 0, 0, 0, 0, 0, 0,
0, 0, 0, 0, 0, 0, 0, 0, 0, 0,
0, 0, 0, 0, 0, 0, 0, 0, 0, 0,
0, 0, 0, 0, 0, 0, 0, 0, 0, 0,
0, 0, 0, 0, 0, 0, 0, 0, 0, 0,
0, 0, 0, 0, 0, 0, 0, 0, 0, 0,
0, 0, 0, 0, 0, 0, 0, 0, 0, 0]])
mask_attention:
tensor([[1., 1., 1., 1., 1., 1., 1., 1., 0., 0., 0., 0., 0., 0., 0., 0., 0., 0.,
0., 0.]])
tensor([[1., 1., 1., 1., 1., 1., 1., 1., 1., 1., 1., 1., 1., 1., 1., 1., 1., 1.,
1., 1., 1., 1., 1., 1., 1., 1., 1., 1., 1., 1., 1., 1., 1., 1., 1., 1.,
1., 1., 1., 1., 1., 1., 1., 1., 1., 1., 1., 1., 1., 1., 1., 1., 1., 1.,
1., 1., 1., 1., 1., 1., 1., 1., 1., 1., 1., 1., 1., 1., 1., 1., 1., 1.,
1., 1., 1., 1., 1., 1., 1., 1., 1., 1., 1., 1., 1., 1., 1., 0., 0., 0.,
0., 0., 0., 0., 0., 0., 0., 0., 0., 0., 0., 0., 0., 0., 0., 0., 0., 0.,
0., 0., 0., 0., 0., 0., 0., 0., 0., 0., 0., 0., 0., 0., 0., 0., 0., 0.,
0., 0., 0., 0., 0., 0., 0., 0., 0., 0., 0., 0., 0., 0., 0., 0., 0., 0.,
0., 0., 0., 0., 0., 0., 0., 0., 0., 0., 0., 0., 0., 0., 0., 0., 0., 0.,
0., 0., 0., 0., 0., 0., 0., 0., 0., 0., 0., 0., 0., 0., 0., 0., 0., 0.,
0., 0., 0., 0., 0., 0., 0., 0., 0., 0., 0., 0., 0., 0., 0., 0., 0., 0.,
0., 0.]])
tensor([[1., 1., 1., 1., 1., 1., 1., 1., 1., 1., 1., 1., 1., 1., 1., 1., 1., 1.,
1., 1., 1., 1., 1., 1., 1., 1., 1., 1., 1., 1., 1., 1., 1., 1., 1., 1.,
1., 1., 1., 1., 1., 0., 0., 0., 0., 0., 0., 0., 0., 0., 0., 0., 0., 0.,
0., 0., 0., 0., 0., 0., 0., 0., 0., 0., 0., 0., 0., 0., 0., 0., 0., 0.,
0., 0., 0., 0., 0., 0., 0., 0., 0., 0., 0., 0., 0., 0., 0., 0., 0., 0.,
0., 0., 0., 0., 0., 0., 0., 0., 0., 0., 0., 0., 0., 0., 0., 0., 0., 0.,
0., 0., 0., 0., 0., 0., 0., 0., 0., 0., 0., 0., 0., 0., 0., 0., 0., 0.,
0., 0., 0., 0., 0., 0., 0., 0., 0., 0., 0., 0., 0., 0., 0., 0., 0., 0.,
0., 0., 0., 0., 0., 0., 0., 0., 0., 0., 0., 0., 0., 0., 0., 0., 0., 0.,
0., 0., 0., 0., 0., 0., 0., 0., 0., 0., 0., 0., 0., 0., 0., 0., 0., 0.,
0., 0., 0., 0., 0., 0., 0., 0., 0., 0., 0., 0., 0., 0., 0., 0., 0., 0.,
0., 0.]])
I add special tokens '[CLS]', '[SEP]' in the tokenizer which id is 50262, 50263.
Then I get the following error, can anyone gives some hints, thanks:
A sequence with no special tokens has been passed to the RoBERTa model. This model requires special tokens in order to work. Please specify add_special_tokens=True in your encoding.
/opt/conda/conda-bld/pytorch_1533739672741/work/aten/src/THC/THCTensorIndex.cu:362: void indexSelectLargeIndex(TensorInfo<T, IndexType>, TensorInfo<T, IndexType>, TensorInfo<long, IndexType>, int, int, IndexType, IndexType, long) [with T = float, IndexType = unsigned int, DstDim = 2, SrcDim = 2, IdxDim = -2, IndexIsMajor = true]: block: [222,0,0], thread: [96,0,0] Assertion `srcIndex < srcSelectDimSize` failed.
/opt/conda/conda-bld/pytorch_1533739672741/work/aten/src/THC/THCTensorIndex.cu:362: void indexSelectLargeIndex(TensorInfo<T, IndexType>, TensorInfo<T, IndexType>, TensorInfo<long, IndexType>, int, int, IndexType, IndexType, long) [with T = float, IndexType = unsigned int, DstDim = 2, SrcDim = 2, IdxDim = -2, IndexIsMajor = true]: block: [222,0,0], thread: [97,0,0] Assertion `srcIndex < srcSelectDimSize` failed.
/opt/conda/conda-bld/pytorch_1533739672741/work/aten/src/THC/THCTensorIndex.cu:362: void indexSelectLargeIndex(TensorInfo<T, IndexType>, TensorInfo<T, IndexType>, TensorInfo<long, IndexType>, int, int, IndexType, IndexType, long) [with T = float, IndexType = unsigned int, DstDim = 2, SrcDim = 2, IdxDim = -2, IndexIsMajor = true]: block: [222,0,0], thread: [98,0,0] Assertion `srcIndex < srcSelectDimSize` failed.
/opt/conda/conda-bld/pytorch_1533739672741/work/aten/src/THC/THCTensorIndex.cu:362: void indexSelectLargeIndex(TensorInfo<T, IndexType>, TensorInfo<T, IndexType>, TensorInfo<long, IndexType>, int, int, IndexType, IndexType, long) [with T = float, IndexType = unsigned int, DstDim = 2, SrcDim = 2, IdxDim = -2, IndexIsMajor = true]: block: [222,0,0], thread: [99,0,0] Assertion `srcIndex < srcSelectDimSize` failed.
/opt/conda/conda-bld/pytorch_1533739672741/work/aten/src/THC/THCTensorIndex.cu:362: void indexSelectLargeIndex(TensorInfo<T, IndexType>, TensorInfo<T, IndexType>, TensorInfo<long, IndexType>, int, int, IndexType, IndexType, long) [with T = float, IndexType = unsigned int, DstDim = 2, SrcDim = 2, IdxDim = -2, IndexIsMajor = true]: block: [222,0,0], thread: [100,0,0] Assertion `srcIndex < srcSelectDimSize` failed.
/opt/conda/conda-bld/pytorch_1533739672741/work/aten/src/THC/THCTensorIndex.cu:362: void indexSelectLargeIndex(TensorInfo<T, IndexType>, TensorInfo<T, IndexType>, TensorInfo<long, IndexType>, int, int, IndexType, IndexType, long) [with T = float, IndexType = unsigned int, DstDim = 2, SrcDim = 2, IdxDim = -2, IndexIsMajor = true]: block: [222,0,0], thread: [101,0,0] Assertion `srcIndex < srcSelectDimSize` failed.
/opt/conda/conda-bld/pytorch_1533739672741/work/aten/src/THC/THCTensorIndex.cu:362: void indexSelectLargeIndex(TensorInfo<T, IndexType>, TensorInfo<T, IndexType>, TensorInfo<long, IndexType>, int, int, IndexType, IndexType, long) [with T = float, IndexType = unsigned int, DstDim = 2, SrcDim = 2, IdxDim = -2, IndexIsMajor = true]: block: [222,0,0], thread: [102,0,0] Assertion `srcIndex < srcSelectDimSize` failed.
/opt/conda/conda-bld/pytorch_1533739672741/work/aten/src/THC/THCTensorIndex.cu:362: void indexSelectLargeIndex(TensorInfo<T, IndexType>, TensorInfo<T, IndexType>, TensorInfo<long, IndexType>, int, int, IndexType, IndexType, long) [with T = float, IndexType = unsigned int, DstDim = 2, SrcDim = 2, IdxDim = -2, IndexIsMajor = true]: block: [222,0,0], thread: [103,0,0] Assertion `srcIndex < srcSelectDimSize` failed.
/opt/conda/conda-bld/pytorch_1533739672741/work/aten/src/THC/THCTensorIndex.cu:362: void indexSelectLargeIndex(TensorInfo<T, IndexType>, TensorInfo<T, IndexType>, TensorInfo<long, IndexType>, int, int, IndexType, IndexType, long) [with T = float, IndexType = unsigned int, DstDim = 2, SrcDim = 2, IdxDim = -2, IndexIsMajor = true]: block: [222,0,0], thread: [104,0,0] Assertion `srcIndex < srcSelectDimSize` failed.
/opt/conda/conda-bld/pytorch_1533739672741/work/aten/src/THC/THCTensorIndex.cu:362: void indexSelectLargeIndex(TensorInfo<T, IndexType>, TensorInfo<T, IndexType>, TensorInfo<long, IndexType>, int, int, IndexType, IndexType, long) [with T = float, IndexType = unsigned int, DstDim = 2, SrcDim = 2, IdxDim = -2, IndexIsMajor = true]: block: [222,0,0], thread: [105,0,0] Assertion `srcIndex < srcSelectDimSize` failed.
/opt/conda/conda-bld/pytorch_1533739672741/work/aten/src/THC/THCTensorIndex.cu:362: void indexSelectLargeIndex(TensorInfo<T, IndexType>, TensorInfo<T, IndexType>, TensorInfo<long, IndexType>, int, int, IndexType, IndexType, long) [with T = float, IndexType = unsigned int, DstDim = 2, SrcDim = 2, IdxDim = -2, IndexIsMajor = true]: block: [222,0,0], thread: [106,0,0] Assertion `srcIndex < srcSelectDimSize` failed.
/opt/conda/conda-bld/pytorch_1533739672741/work/aten/src/THC/THCTensorIndex.cu:362: void indexSelectLargeIndex(TensorInfo<T, IndexType>, TensorInfo<T, IndexType>, TensorInfo<long, IndexType>, int, int, IndexType, IndexType, long) [with T = float, IndexType = unsigned int, DstDim = 2, SrcDim = 2, IdxDim = -2, IndexIsMajor = true]: block: [222,0,0], thread: [107,0,0] Assertion `srcIndex < srcSelectDimSize` failed.
/opt/conda/conda-bld/pytorch_1533739672741/work/aten/src/THC/THCTensorIndex.cu:362: void indexSelectLargeIndex(TensorInfo<T, IndexType>, TensorInfo<T, IndexType>, TensorInfo<long, IndexType>, int, int, IndexType, IndexType, long) [with T = float, IndexType = unsigned int, DstDim = 2, SrcDim = 2, IdxDim = -2, IndexIsMajor = true]: block: [222,0,0], thread: [108,0,0] Assertion `srcIndex < srcSelectDimSize` failed.
/opt/conda/conda-bld/pytorch_1533739672741/work/aten/src/THC/THCTensorIndex.cu:362: void indexSelectLargeIndex(TensorInfo<T, IndexType>, TensorInfo<T, IndexType>, TensorInfo<long, IndexType>, int, int, IndexType, IndexType, long) [with T = float, IndexType = unsigned int, DstDim = 2, SrcDim = 2, IdxDim = -2, IndexIsMajor = true]: block: [222,0,0], thread: [109,0,0] Assertion `srcIndex < srcSelectDimSize` failed.
/opt/conda/conda-bld/pytorch_1533739672741/work/aten/src/THC/THCTensorIndex.cu:362: void indexSelectLargeIndex(TensorInfo<T, IndexType>, TensorInfo<T, IndexType>, TensorInfo<long, IndexType>, int, int, IndexType, IndexType, long) [with T = float, IndexType = unsigned int, DstDim = 2, SrcDim = 2, IdxDim = -2, IndexIsMajor = true]: block: [222,0,0], thread: [110,0,0] Assertion `srcIndex < srcSelectDimSize` failed.
/opt/conda/conda-bld/pytorch_1533739672741/work/aten/src/THC/THCTensorIndex.cu:362: void indexSelectLargeIndex(TensorInfo<T, IndexType>, TensorInfo<T, IndexType>, TensorInfo<long, IndexType>, int, int, IndexType, IndexType, long) [with T = float, IndexType = unsigned int, DstDim = 2, SrcDim = 2, IdxDim = -2, IndexIsMajor = true]: block: [222,0,0], thread: [111,0,0] Assertion `srcIndex < srcSelectDimSize` failed.
/opt/conda/conda-bld/pytorch_1533739672741/work/aten/src/THC/THCTensorIndex.cu:362: void indexSelectLargeIndex(TensorInfo<T, IndexType>, TensorInfo<T, IndexType>, TensorInfo<long, IndexType>, int, int, IndexType, IndexType, long) [with T = float, IndexType = unsigned int, DstDim = 2, SrcDim = 2, IdxDim = -2, IndexIsMajor = true]: block: [222,0,0], thread: [112,0,0] Assertion `srcIndex < srcSelectDimSize` failed.
/opt/conda/conda-bld/pytorch_1533739672741/work/aten/src/THC/THCTensorIndex.cu:362: void indexSelectLargeIndex(TensorInfo<T, IndexType>, TensorInfo<T, IndexType>, TensorInfo<long, IndexType>, int, int, IndexType, IndexType, long) [with T = float, IndexType = unsigned int, DstDim = 2, SrcDim = 2, IdxDim = -2, IndexIsMajor = true]: block: [222,0,0], thread: [113,0,0] Assertion `srcIndex < srcSelectDimSize` failed.
/opt/conda/conda-bld/pytorch_1533739672741/work/aten/src/THC/THCTensorIndex.cu:362: void indexSelectLargeIndex(TensorInfo<T, IndexType>, TensorInfo<T, IndexType>, TensorInfo<long, IndexType>, int, int, IndexType, IndexType, long) [with T = float, IndexType = unsigned int, DstDim = 2, SrcDim = 2, IdxDim = -2, IndexIsMajor = true]: block: [222,0,0], thread: [114,0,0] Assertion `srcIndex < srcSelectDimSize` failed.
/opt/conda/conda-bld/pytorch_1533739672741/work/aten/src/THC/THCTensorIndex.cu:362: void indexSelectLargeIndex(TensorInfo<T, IndexType>, TensorInfo<T, IndexType>, TensorInfo<long, IndexType>, int, int, IndexType, IndexType, long) [with T = float, IndexType = unsigned int, DstDim = 2, SrcDim = 2, IdxDim = -2, IndexIsMajor = true]: block: [222,0,0], thread: [115,0,0] Assertion `srcIndex < srcSelectDimSize` failed.
/opt/conda/conda-bld/pytorch_1533739672741/work/aten/src/THC/THCTensorIndex.cu:362: void indexSelectLargeIndex(TensorInfo<T, IndexType>, TensorInfo<T, IndexType>, TensorInfo<long, IndexType>, int, int, IndexType, IndexType, long) [with T = float, IndexType = unsigned int, DstDim = 2, SrcDim = 2, IdxDim = -2, IndexIsMajor = true]: block: [222,0,0], thread: [116,0,0] Assertion `srcIndex < srcSelectDimSize` failed.
/opt/conda/conda-bld/pytorch_1533739672741/work/aten/src/THC/THCTensorIndex.cu:362: void indexSelectLargeIndex(TensorInfo<T, IndexType>, TensorInfo<T, IndexType>, TensorInfo<long, IndexType>, int, int, IndexType, IndexType, long) [with T = float, IndexType = unsigned int, DstDim = 2, SrcDim = 2, IdxDim = -2, IndexIsMajor = true]: block: [222,0,0], thread: [117,0,0] Assertion `srcIndex < srcSelectDimSize` failed.
/opt/conda/conda-bld/pytorch_1533739672741/work/aten/src/THC/THCTensorIndex.cu:362: void indexSelectLargeIndex(TensorInfo<T, IndexType>, TensorInfo<T, IndexType>, TensorInfo<long, IndexType>, int, int, IndexType, IndexType, long) [with T = float, IndexType = unsigned int, DstDim = 2, SrcDim = 2, IdxDim = -2, IndexIsMajor = true]: block: [222,0,0], thread: [118,0,0] Assertion `srcIndex < srcSelectDimSize` failed.
/opt/conda/conda-bld/pytorch_1533739672741/work/aten/src/THC/THCTensorIndex.cu:362: void indexSelectLargeIndex(TensorInfo<T, IndexType>, TensorInfo<T, IndexType>, TensorInfo<long, IndexType>, int, int, IndexType, IndexType, long) [with T = float, IndexType = unsigned int, DstDim = 2, SrcDim = 2, IdxDim = -2, IndexIsMajor = true]: block: [222,0,0], thread: [119,0,0] Assertion `srcIndex < srcSelectDimSize` failed.
/opt/conda/conda-bld/pytorch_1533739672741/work/aten/src/THC/THCTensorIndex.cu:362: void indexSelectLargeIndex(TensorInfo<T, IndexType>, TensorInfo<T, IndexType>, TensorInfo<long, IndexType>, int, int, IndexType, IndexType, long) [with T = float, IndexType = unsigned int, DstDim = 2, SrcDim = 2, IdxDim = -2, IndexIsMajor = true]: block: [222,0,0], thread: [120,0,0] Assertion `srcIndex < srcSelectDimSize` failed.
/opt/conda/conda-bld/pytorch_1533739672741/work/aten/src/THC/THCTensorIndex.cu:362: void indexSelectLargeIndex(TensorInfo<T, IndexType>, TensorInfo<T, IndexType>, TensorInfo<long, IndexType>, int, int, IndexType, IndexType, long) [with T = float, IndexType = unsigned int, DstDim = 2, SrcDim = 2, IdxDim = -2, IndexIsMajor = true]: block: [222,0,0], thread: [121,0,0] Assertion `srcIndex < srcSelectDimSize` failed.
/opt/conda/conda-bld/pytorch_1533739672741/work/aten/src/THC/THCTensorIndex.cu:362: void indexSelectLargeIndex(TensorInfo<T, IndexType>, TensorInfo<T, IndexType>, TensorInfo<long, IndexType>, int, int, IndexType, IndexType, long) [with T = float, IndexType = unsigned int, DstDim = 2, SrcDim = 2, IdxDim = -2, IndexIsMajor = true]: block: [222,0,0], thread: [122,0,0] Assertion `srcIndex < srcSelectDimSize` failed.
/opt/conda/conda-bld/pytorch_1533739672741/work/aten/src/THC/THCTensorIndex.cu:362: void indexSelectLargeIndex(TensorInfo<T, IndexType>, TensorInfo<T, IndexType>, TensorInfo<long, IndexType>, int, int, IndexType, IndexType, long) [with T = float, IndexType = unsigned int, DstDim = 2, SrcDim = 2, IdxDim = -2, IndexIsMajor = true]: block: [222,0,0], thread: [123,0,0] Assertion `srcIndex < srcSelectDimSize` failed.
/opt/conda/conda-bld/pytorch_1533739672741/work/aten/src/THC/THCTensorIndex.cu:362: void indexSelectLargeIndex(TensorInfo<T, IndexType>, TensorInfo<T, IndexType>, TensorInfo<long, IndexType>, int, int, IndexType, IndexType, long) [with T = float, IndexType = unsigned int, DstDim = 2, SrcDim = 2, IdxDim = -2, IndexIsMajor = true]: block: [222,0,0], thread: [124,0,0] Assertion `srcIndex < srcSelectDimSize` failed.
/opt/conda/conda-bld/pytorch_1533739672741/work/aten/src/THC/THCTensorIndex.cu:362: void indexSelectLargeIndex(TensorInfo<T, IndexType>, TensorInfo<T, IndexType>, TensorInfo<long, IndexType>, int, int, IndexType, IndexType, long) [with T = float, IndexType = unsigned int, DstDim = 2, SrcDim = 2, IdxDim = -2, IndexIsMajor = true]: block: [222,0,0], thread: [125,0,0] Assertion `srcIndex < srcSelectDimSize` failed.
/opt/conda/conda-bld/pytorch_1533739672741/work/aten/src/THC/THCTensorIndex.cu:362: void indexSelectLargeIndex(TensorInfo<T, IndexType>, TensorInfo<T, IndexType>, TensorInfo<long, IndexType>, int, int, IndexType, IndexType, long) [with T = float, IndexType = unsigned int, DstDim = 2, SrcDim = 2, IdxDim = -2, IndexIsMajor = true]: block: [222,0,0], thread: [126,0,0] Assertion `srcIndex < srcSelectDimSize` failed.
/opt/conda/conda-bld/pytorch_1533739672741/work/aten/src/THC/THCTensorIndex.cu:362: void indexSelectLargeIndex(TensorInfo<T, IndexType>, TensorInfo<T, IndexType>, TensorInfo<long, IndexType>, int, int, IndexType, IndexType, long) [with T = float, IndexType = unsigned int, DstDim = 2, SrcDim = 2, IdxDim = -2, IndexIsMajor = true]: block: [222,0,0], thread: [127,0,0] Assertion `srcIndex < srcSelectDimSize` failed.
/opt/conda/conda-bld/pytorch_1533739672741/work/aten/src/THC/THCTensorIndex.cu:362: void indexSelectLargeIndex(TensorInfo<T, IndexType>, TensorInfo<T, IndexType>, TensorInfo<long, IndexType>, int, int, IndexType, IndexType, long) [with T = float, IndexType = unsigned int, DstDim = 2, SrcDim = 2, IdxDim = -2, IndexIsMajor = true]: block: [222,0,0], thread: [32,0,0] Assertion `srcIndex < srcSelectDimSize` failed.
/opt/conda/conda-bld/pytorch_1533739672741/work/aten/src/THC/THCTensorIndex.cu:362: void indexSelectLargeIndex(TensorInfo<T, IndexType>, TensorInfo<T, IndexType>, TensorInfo<long, IndexType>, int, int, IndexType, IndexType, long) [with T = float, IndexType = unsigned int, DstDim = 2, SrcDim = 2, IdxDim = -2, IndexIsMajor = true]: block: [222,0,0], thread: [33,0,0] Assertion `srcIndex < srcSelectDimSize` failed.
/opt/conda/conda-bld/pytorch_1533739672741/work/aten/src/THC/THCTensorIndex.cu:362: void indexSelectLargeIndex(TensorInfo<T, IndexType>, TensorInfo<T, IndexType>, TensorInfo<long, IndexType>, int, int, IndexType, IndexType, long) [with T = float, IndexType = unsigned int, DstDim = 2, SrcDim = 2, IdxDim = -2, IndexIsMajor = true]: block: [222,0,0], thread: [34,0,0] Assertion `srcIndex < srcSelectDimSize` failed.
/opt/conda/conda-bld/pytorch_1533739672741/work/aten/src/THC/THCTensorIndex.cu:362: void indexSelectLargeIndex(TensorInfo<T, IndexType>, TensorInfo<T, IndexType>, TensorInfo<long, IndexType>, int, int, IndexType, IndexType, long) [with T = float, IndexType = unsigned int, DstDim = 2, SrcDim = 2, IdxDim = -2, IndexIsMajor = true]: block: [222,0,0], thread: [35,0,0] Assertion `srcIndex < srcSelectDimSize` failed.
/opt/conda/conda-bld/pytorch_1533739672741/work/aten/src/THC/THCTensorIndex.cu:362: void indexSelectLargeIndex(TensorInfo<T, IndexType>, TensorInfo<T, IndexType>, TensorInfo<long, IndexType>, int, int, IndexType, IndexType, long) [with T = float, IndexType = unsigned int, DstDim = 2, SrcDim = 2, IdxDim = -2, IndexIsMajor = true]: block: [222,0,0], thread: [36,0,0] Assertion `srcIndex < srcSelectDimSize` failed.
/opt/conda/conda-bld/pytorch_1533739672741/work/aten/src/THC/THCTensorIndex.cu:362: void indexSelectLargeIndex(TensorInfo<T, IndexType>, TensorInfo<T, IndexType>, TensorInfo<long, IndexType>, int, int, IndexType, IndexType, long) [with T = float, IndexType = unsigned int, DstDim = 2, SrcDim = 2, IdxDim = -2, IndexIsMajor = true]: block: [222,0,0], thread: [37,0,0] Assertion `srcIndex < srcSelectDimSize` failed.
/opt/conda/conda-bld/pytorch_1533739672741/work/aten/src/THC/THCTensorIndex.cu:362: void indexSelectLargeIndex(TensorInfo<T, IndexType>, TensorInfo<T, IndexType>, TensorInfo<long, IndexType>, int, int, IndexType, IndexType, long) [with T = float, IndexType = unsigned int, DstDim = 2, SrcDim = 2, IdxDim = -2, IndexIsMajor = true]: block: [222,0,0], thread: [38,0,0] Assertion `srcIndex < srcSelectDimSize` failed.
/opt/conda/conda-bld/pytorch_1533739672741/work/aten/src/THC/THCTensorIndex.cu:362: void indexSelectLargeIndex(TensorInfo<T, IndexType>, TensorInfo<T, IndexType>, TensorInfo<long, IndexType>, int, int, IndexType, IndexType, long) [with T = float, IndexType = unsigned int, DstDim = 2, SrcDim = 2, IdxDim = -2, IndexIsMajor = true]: block: [222,0,0], thread: [39,0,0] Assertion `srcIndex < srcSelectDimSize` failed.
/opt/conda/conda-bld/pytorch_1533739672741/work/aten/src/THC/THCTensorIndex.cu:362: void indexSelectLargeIndex(TensorInfo<T, IndexType>, TensorInfo<T, IndexType>, TensorInfo<long, IndexType>, int, int, IndexType, IndexType, long) [with T = float, IndexType = unsigned int, DstDim = 2, SrcDim = 2, IdxDim = -2, IndexIsMajor = true]: block: [222,0,0], thread: [40,0,0] Assertion `srcIndex < srcSelectDimSize` failed.
/opt/conda/conda-bld/pytorch_1533739672741/work/aten/src/THC/THCTensorIndex.cu:362: void indexSelectLargeIndex(TensorInfo<T, IndexType>, TensorInfo<T, IndexType>, TensorInfo<long, IndexType>, int, int, IndexType, IndexType, long) [with T = float, IndexType = unsigned int, DstDim = 2, SrcDim = 2, IdxDim = -2, IndexIsMajor = true]: block: [222,0,0], thread: [41,0,0] Assertion `srcIndex < srcSelectDimSize` failed.
/opt/conda/conda-bld/pytorch_1533739672741/work/aten/src/THC/THCTensorIndex.cu:362: void indexSelectLargeIndex(TensorInfo<T, IndexType>, TensorInfo<T, IndexType>, TensorInfo<long, IndexType>, int, int, IndexType, IndexType, long) [with T = float, IndexType = unsigned int, DstDim = 2, SrcDim = 2, IdxDim = -2, IndexIsMajor = true]: block: [222,0,0], thread: [42,0,0] Assertion `srcIndex < srcSelectDimSize` failed.
/opt/conda/conda-bld/pytorch_1533739672741/work/aten/src/THC/THCTensorIndex.cu:362: void indexSelectLargeIndex(TensorInfo<T, IndexType>, TensorInfo<T, IndexType>, TensorInfo<long, IndexType>, int, int, IndexType, IndexType, long) [with T = float, IndexType = unsigned int, DstDim = 2, SrcDim = 2, IdxDim = -2, IndexIsMajor = true]: block: [222,0,0], thread: [43,0,0] Assertion `srcIndex < srcSelectDimSize` failed.
/opt/conda/conda-bld/pytorch_1533739672741/work/aten/src/THC/THCTensorIndex.cu:362: void indexSelectLargeIndex(TensorInfo<T, IndexType>, TensorInfo<T, IndexType>, TensorInfo<long, IndexType>, int, int, IndexType, IndexType, long) [with T = float, IndexType = unsigned int, DstDim = 2, SrcDim = 2, IdxDim = -2, IndexIsMajor = true]: block: [222,0,0], thread: [44,0,0] Assertion `srcIndex < srcSelectDimSize` failed.
/opt/conda/conda-bld/pytorch_1533739672741/work/aten/src/THC/THCTensorIndex.cu:362: void indexSelectLargeIndex(TensorInfo<T, IndexType>, TensorInfo<T, IndexType>, TensorInfo<long, IndexType>, int, int, IndexType, IndexType, long) [with T = float, IndexType = unsigned int, DstDim = 2, SrcDim = 2, IdxDim = -2, IndexIsMajor = true]: block: [222,0,0], thread: [45,0,0] Assertion `srcIndex < srcSelectDimSize` failed.
/opt/conda/conda-bld/pytorch_1533739672741/work/aten/src/THC/THCTensorIndex.cu:362: void indexSelectLargeIndex(TensorInfo<T, IndexType>, TensorInfo<T, IndexType>, TensorInfo<long, IndexType>, int, int, IndexType, IndexType, long) [with T = float, IndexType = unsigned int, DstDim = 2, SrcDim = 2, IdxDim = -2, IndexIsMajor = true]: block: [222,0,0], thread: [46,0,0] Assertion `srcIndex < srcSelectDimSize` failed.
/opt/conda/conda-bld/pytorch_1533739672741/work/aten/src/THC/THCTensorIndex.cu:362: void indexSelectLargeIndex(TensorInfo<T, IndexType>, TensorInfo<T, IndexType>, TensorInfo<long, IndexType>, int, int, IndexType, IndexType, long) [with T = float, IndexType = unsigned int, DstDim = 2, SrcDim = 2, IdxDim = -2, IndexIsMajor = true]: block: [222,0,0], thread: [47,0,0] Assertion `srcIndex < srcSelectDimSize` failed.
/opt/conda/conda-bld/pytorch_1533739672741/work/aten/src/THC/THCTensorIndex.cu:362: void indexSelectLargeIndex(TensorInfo<T, IndexType>, TensorInfo<T, IndexType>, TensorInfo<long, IndexType>, int, int, IndexType, IndexType, long) [with T = float, IndexType = unsigned int, DstDim = 2, SrcDim = 2, IdxDim = -2, IndexIsMajor = true]: block: [222,0,0], thread: [48,0,0] Assertion `srcIndex < srcSelectDimSize` failed.
/opt/conda/conda-bld/pytorch_1533739672741/work/aten/src/THC/THCTensorIndex.cu:362: void indexSelectLargeIndex(TensorInfo<T, IndexType>, TensorInfo<T, IndexType>, TensorInfo<long, IndexType>, int, int, IndexType, IndexType, long) [with T = float, IndexType = unsigned int, DstDim = 2, SrcDim = 2, IdxDim = -2, IndexIsMajor = true]: block: [222,0,0], thread: [49,0,0] Assertion `srcIndex < srcSelectDimSize` failed.
/opt/conda/conda-bld/pytorch_1533739672741/work/aten/src/THC/THCTensorIndex.cu:362: void indexSelectLargeIndex(TensorInfo<T, IndexType>, TensorInfo<T, IndexType>, TensorInfo<long, IndexType>, int, int, IndexType, IndexType, long) [with T = float, IndexType = unsigned int, DstDim = 2, SrcDim = 2, IdxDim = -2, IndexIsMajor = true]: block: [222,0,0], thread: [50,0,0] Assertion `srcIndex < srcSelectDimSize` failed.
/opt/conda/conda-bld/pytorch_1533739672741/work/aten/src/THC/THCTensorIndex.cu:362: void indexSelectLargeIndex(TensorInfo<T, IndexType>, TensorInfo<T, IndexType>, TensorInfo<long, IndexType>, int, int, IndexType, IndexType, long) [with T = float, IndexType = unsigned int, DstDim = 2, SrcDim = 2, IdxDim = -2, IndexIsMajor = true]: block: [222,0,0], thread: [51,0,0] Assertion `srcIndex < srcSelectDimSize` failed.
/opt/conda/conda-bld/pytorch_1533739672741/work/aten/src/THC/THCTensorIndex.cu:362: void indexSelectLargeIndex(TensorInfo<T, IndexType>, TensorInfo<T, IndexType>, TensorInfo<long, IndexType>, int, int, IndexType, IndexType, long) [with T = float, IndexType = unsigned int, DstDim = 2, SrcDim = 2, IdxDim = -2, IndexIsMajor = true]: block: [222,0,0], thread: [52,0,0] Assertion `srcIndex < srcSelectDimSize` failed.
/opt/conda/conda-bld/pytorch_1533739672741/work/aten/src/THC/THCTensorIndex.cu:362: void indexSelectLargeIndex(TensorInfo<T, IndexType>, TensorInfo<T, IndexType>, TensorInfo<long, IndexType>, int, int, IndexType, IndexType, long) [with T = float, IndexType = unsigned int, DstDim = 2, SrcDim = 2, IdxDim = -2, IndexIsMajor = true]: block: [222,0,0], thread: [53,0,0] Assertion `srcIndex < srcSelectDimSize` failed.
/opt/conda/conda-bld/pytorch_1533739672741/work/aten/src/THC/THCTensorIndex.cu:362: void indexSelectLargeIndex(TensorInfo<T, IndexType>, TensorInfo<T, IndexType>, TensorInfo<long, IndexType>, int, int, IndexType, IndexType, long) [with T = float, IndexType = unsigned int, DstDim = 2, SrcDim = 2, IdxDim = -2, IndexIsMajor = true]: block: [222,0,0], thread: [54,0,0] Assertion `srcIndex < srcSelectDimSize` failed.
/opt/conda/conda-bld/pytorch_1533739672741/work/aten/src/THC/THCTensorIndex.cu:362: void indexSelectLargeIndex(TensorInfo<T, IndexType>, TensorInfo<T, IndexType>, TensorInfo<long, IndexType>, int, int, IndexType, IndexType, long) [with T = float, IndexType = unsigned int, DstDim = 2, SrcDim = 2, IdxDim = -2, IndexIsMajor = true]: block: [222,0,0], thread: [55,0,0] Assertion `srcIndex < srcSelectDimSize` failed.
/opt/conda/conda-bld/pytorch_1533739672741/work/aten/src/THC/THCTensorIndex.cu:362: void indexSelectLargeIndex(TensorInfo<T, IndexType>, TensorInfo<T, IndexType>, TensorInfo<long, IndexType>, int, int, IndexType, IndexType, long) [with T = float, IndexType = unsigned int, DstDim = 2, SrcDim = 2, IdxDim = -2, IndexIsMajor = true]: block: [222,0,0], thread: [56,0,0] Assertion `srcIndex < srcSelectDimSize` failed.
/opt/conda/conda-bld/pytorch_1533739672741/work/aten/src/THC/THCTensorIndex.cu:362: void indexSelectLargeIndex(TensorInfo<T, IndexType>, TensorInfo<T, IndexType>, TensorInfo<long, IndexType>, int, int, IndexType, IndexType, long) [with T = float, IndexType = unsigned int, DstDim = 2, SrcDim = 2, IdxDim = -2, IndexIsMajor = true]: block: [222,0,0], thread: [57,0,0] Assertion `srcIndex < srcSelectDimSize` failed.
/opt/conda/conda-bld/pytorch_1533739672741/work/aten/src/THC/THCTensorIndex.cu:362: void indexSelectLargeIndex(TensorInfo<T, IndexType>, TensorInfo<T, IndexType>, TensorInfo<long, IndexType>, int, int, IndexType, IndexType, long) [with T = float, IndexType = unsigned int, DstDim = 2, SrcDim = 2, IdxDim = -2, IndexIsMajor = true]: block: [222,0,0], thread: [58,0,0] Assertion `srcIndex < srcSelectDimSize` failed.
/opt/conda/conda-bld/pytorch_1533739672741/work/aten/src/THC/THCTensorIndex.cu:362: void indexSelectLargeIndex(TensorInfo<T, IndexType>, TensorInfo<T, IndexType>, TensorInfo<long, IndexType>, int, int, IndexType, IndexType, long) [with T = float, IndexType = unsigned int, DstDim = 2, SrcDim = 2, IdxDim = -2, IndexIsMajor = true]: block: [222,0,0], thread: [59,0,0] Assertion `srcIndex < srcSelectDimSize` failed.
/opt/conda/conda-bld/pytorch_1533739672741/work/aten/src/THC/THCTensorIndex.cu:362: void indexSelectLargeIndex(TensorInfo<T, IndexType>, TensorInfo<T, IndexType>, TensorInfo<long, IndexType>, int, int, IndexType, IndexType, long) [with T = float, IndexType = unsigned int, DstDim = 2, SrcDim = 2, IdxDim = -2, IndexIsMajor = true]: block: [222,0,0], thread: [60,0,0] Assertion `srcIndex < srcSelectDimSize` failed.
/opt/conda/conda-bld/pytorch_1533739672741/work/aten/src/THC/THCTensorIndex.cu:362: void indexSelectLargeIndex(TensorInfo<T, IndexType>, TensorInfo<T, IndexType>, TensorInfo<long, IndexType>, int, int, IndexType, IndexType, long) [with T = float, IndexType = unsigned int, DstDim = 2, SrcDim = 2, IdxDim = -2, IndexIsMajor = true]: block: [222,0,0], thread: [61,0,0] Assertion `srcIndex < srcSelectDimSize` failed.
/opt/conda/conda-bld/pytorch_1533739672741/work/aten/src/THC/THCTensorIndex.cu:362: void indexSelectLargeIndex(TensorInfo<T, IndexType>, TensorInfo<T, IndexType>, TensorInfo<long, IndexType>, int, int, IndexType, IndexType, long) [with T = float, IndexType = unsigned int, DstDim = 2, SrcDim = 2, IdxDim = -2, IndexIsMajor = true]: block: [222,0,0], thread: [62,0,0] Assertion `srcIndex < srcSelectDimSize` failed.
/opt/conda/conda-bld/pytorch_1533739672741/work/aten/src/THC/THCTensorIndex.cu:362: void indexSelectLargeIndex(TensorInfo<T, IndexType>, TensorInfo<T, IndexType>, TensorInfo<long, IndexType>, int, int, IndexType, IndexType, long) [with T = float, IndexType = unsigned int, DstDim = 2, SrcDim = 2, IdxDim = -2, IndexIsMajor = true]: block: [222,0,0], thread: [63,0,0] Assertion `srcIndex < srcSelectDimSize` failed.
/opt/conda/conda-bld/pytorch_1533739672741/work/aten/src/THC/THCTensorIndex.cu:362: void indexSelectLargeIndex(TensorInfo<T, IndexType>, TensorInfo<T, IndexType>, TensorInfo<long, IndexType>, int, int, IndexType, IndexType, long) [with T = float, IndexType = unsigned int, DstDim = 2, SrcDim = 2, IdxDim = -2, IndexIsMajor = true]: block: [222,0,0], thread: [0,0,0] Assertion `srcIndex < srcSelectDimSize` failed.
/opt/conda/conda-bld/pytorch_1533739672741/work/aten/src/THC/THCTensorIndex.cu:362: void indexSelectLargeIndex(TensorInfo<T, IndexType>, TensorInfo<T, IndexType>, TensorInfo<long, IndexType>, int, int, IndexType, IndexType, long) [with T = float, IndexType = unsigned int, DstDim = 2, SrcDim = 2, IdxDim = -2, IndexIsMajor = true]: block: [222,0,0], thread: [1,0,0] Assertion `srcIndex < srcSelectDimSize` failed.
/opt/conda/conda-bld/pytorch_1533739672741/work/aten/src/THC/THCTensorIndex.cu:362: void indexSelectLargeIndex(TensorInfo<T, IndexType>, TensorInfo<T, IndexType>, TensorInfo<long, IndexType>, int, int, IndexType, IndexType, long) [with T = float, IndexType = unsigned int, DstDim = 2, SrcDim = 2, IdxDim = -2, IndexIsMajor = true]: block: [222,0,0], thread: [2,0,0] Assertion `srcIndex < srcSelectDimSize` failed.
/opt/conda/conda-bld/pytorch_1533739672741/work/aten/src/THC/THCTensorIndex.cu:362: void indexSelectLargeIndex(TensorInfo<T, IndexType>, TensorInfo<T, IndexType>, TensorInfo<long, IndexType>, int, int, IndexType, IndexType, long) [with T = float, IndexType = unsigned int, DstDim = 2, SrcDim = 2, IdxDim = -2, IndexIsMajor = true]: block: [222,0,0], thread: [3,0,0] Assertion `srcIndex < srcSelectDimSize` failed.
/opt/conda/conda-bld/pytorch_1533739672741/work/aten/src/THC/THCTensorIndex.cu:362: void indexSelectLargeIndex(TensorInfo<T, IndexType>, TensorInfo<T, IndexType>, TensorInfo<long, IndexType>, int, int, IndexType, IndexType, long) [with T = float, IndexType = unsigned int, DstDim = 2, SrcDim = 2, IdxDim = -2, IndexIsMajor = true]: block: [222,0,0], thread: [4,0,0] Assertion `srcIndex < srcSelectDimSize` failed.
/opt/conda/conda-bld/pytorch_1533739672741/work/aten/src/THC/THCTensorIndex.cu:362: void indexSelectLargeIndex(TensorInfo<T, IndexType>, TensorInfo<T, IndexType>, TensorInfo<long, IndexType>, int, int, IndexType, IndexType, long) [with T = float, IndexType = unsigned int, DstDim = 2, SrcDim = 2, IdxDim = -2, IndexIsMajor = true]: block: [222,0,0], thread: [5,0,0] Assertion `srcIndex < srcSelectDimSize` failed.
/opt/conda/conda-bld/pytorch_1533739672741/work/aten/src/THC/THCTensorIndex.cu:362: void indexSelectLargeIndex(TensorInfo<T, IndexType>, TensorInfo<T, IndexType>, TensorInfo<long, IndexType>, int, int, IndexType, IndexType, long) [with T = float, IndexType = unsigned int, DstDim = 2, SrcDim = 2, IdxDim = -2, IndexIsMajor = true]: block: [222,0,0], thread: [6,0,0] Assertion `srcIndex < srcSelectDimSize` failed.
/opt/conda/conda-bld/pytorch_1533739672741/work/aten/src/THC/THCTensorIndex.cu:362: void indexSelectLargeIndex(TensorInfo<T, IndexType>, TensorInfo<T, IndexType>, TensorInfo<long, IndexType>, int, int, IndexType, IndexType, long) [with T = float, IndexType = unsigned int, DstDim = 2, SrcDim = 2, IdxDim = -2, IndexIsMajor = true]: block: [222,0,0], thread: [7,0,0] Assertion `srcIndex < srcSelectDimSize` failed.
/opt/conda/conda-bld/pytorch_1533739672741/work/aten/src/THC/THCTensorIndex.cu:362: void indexSelectLargeIndex(TensorInfo<T, IndexType>, TensorInfo<T, IndexType>, TensorInfo<long, IndexType>, int, int, IndexType, IndexType, long) [with T = float, IndexType = unsigned int, DstDim = 2, SrcDim = 2, IdxDim = -2, IndexIsMajor = true]: block: [222,0,0], thread: [8,0,0] Assertion `srcIndex < srcSelectDimSize` failed.
/opt/conda/conda-bld/pytorch_1533739672741/work/aten/src/THC/THCTensorIndex.cu:362: void indexSelectLargeIndex(TensorInfo<T, IndexType>, TensorInfo<T, IndexType>, TensorInfo<long, IndexType>, int, int, IndexType, IndexType, long) [with T = float, IndexType = unsigned int, DstDim = 2, SrcDim = 2, IdxDim = -2, IndexIsMajor = true]: block: [222,0,0], thread: [9,0,0] Assertion `srcIndex < srcSelectDimSize` failed.
/opt/conda/conda-bld/pytorch_1533739672741/work/aten/src/THC/THCTensorIndex.cu:362: void indexSelectLargeIndex(TensorInfo<T, IndexType>, TensorInfo<T, IndexType>, TensorInfo<long, IndexType>, int, int, IndexType, IndexType, long) [with T = float, IndexType = unsigned int, DstDim = 2, SrcDim = 2, IdxDim = -2, IndexIsMajor = true]: block: [222,0,0], thread: [10,0,0] Assertion `srcIndex < srcSelectDimSize` failed.
/opt/conda/conda-bld/pytorch_1533739672741/work/aten/src/THC/THCTensorIndex.cu:362: void indexSelectLargeIndex(TensorInfo<T, IndexType>, TensorInfo<T, IndexType>, TensorInfo<long, IndexType>, int, int, IndexType, IndexType, long) [with T = float, IndexType = unsigned int, DstDim = 2, SrcDim = 2, IdxDim = -2, IndexIsMajor = true]: block: [222,0,0], thread: [11,0,0] Assertion `srcIndex < srcSelectDimSize` failed.
/opt/conda/conda-bld/pytorch_1533739672741/work/aten/src/THC/THCTensorIndex.cu:362: void indexSelectLargeIndex(TensorInfo<T, IndexType>, TensorInfo<T, IndexType>, TensorInfo<long, IndexType>, int, int, IndexType, IndexType, long) [with T = float, IndexType = unsigned int, DstDim = 2, SrcDim = 2, IdxDim = -2, IndexIsMajor = true]: block: [222,0,0], thread: [12,0,0] Assertion `srcIndex < srcSelectDimSize` failed.
/opt/conda/conda-bld/pytorch_1533739672741/work/aten/src/THC/THCTensorIndex.cu:362: void indexSelectLargeIndex(TensorInfo<T, IndexType>, TensorInfo<T, IndexType>, TensorInfo<long, IndexType>, int, int, IndexType, IndexType, long) [with T = float, IndexType = unsigned int, DstDim = 2, SrcDim = 2, IdxDim = -2, IndexIsMajor = true]: block: [222,0,0], thread: [13,0,0] Assertion `srcIndex < srcSelectDimSize` failed.
/opt/conda/conda-bld/pytorch_1533739672741/work/aten/src/THC/THCTensorIndex.cu:362: void indexSelectLargeIndex(TensorInfo<T, IndexType>, TensorInfo<T, IndexType>, TensorInfo<long, IndexType>, int, int, IndexType, IndexType, long) [with T = float, IndexType = unsigned int, DstDim = 2, SrcDim = 2, IdxDim = -2, IndexIsMajor = true]: block: [222,0,0], thread: [14,0,0] Assertion `srcIndex < srcSelectDimSize` failed.
/opt/conda/conda-bld/pytorch_1533739672741/work/aten/src/THC/THCTensorIndex.cu:362: void indexSelectLargeIndex(TensorInfo<T, IndexType>, TensorInfo<T, IndexType>, TensorInfo<long, IndexType>, int, int, IndexType, IndexType, long) [with T = float, IndexType = unsigned int, DstDim = 2, SrcDim = 2, IdxDim = -2, IndexIsMajor = true]: block: [222,0,0], thread: [15,0,0] Assertion `srcIndex < srcSelectDimSize` failed.
/opt/conda/conda-bld/pytorch_1533739672741/work/aten/src/THC/THCTensorIndex.cu:362: void indexSelectLargeIndex(TensorInfo<T, IndexType>, TensorInfo<T, IndexType>, TensorInfo<long, IndexType>, int, int, IndexType, IndexType, long) [with T = float, IndexType = unsigned int, DstDim = 2, SrcDim = 2, IdxDim = -2, IndexIsMajor = true]: block: [222,0,0], thread: [16,0,0] Assertion `srcIndex < srcSelectDimSize` failed.
/opt/conda/conda-bld/pytorch_1533739672741/work/aten/src/THC/THCTensorIndex.cu:362: void indexSelectLargeIndex(TensorInfo<T, IndexType>, TensorInfo<T, IndexType>, TensorInfo<long, IndexType>, int, int, IndexType, IndexType, long) [with T = float, IndexType = unsigned int, DstDim = 2, SrcDim = 2, IdxDim = -2, IndexIsMajor = true]: block: [222,0,0], thread: [17,0,0] Assertion `srcIndex < srcSelectDimSize` failed.
/opt/conda/conda-bld/pytorch_1533739672741/work/aten/src/THC/THCTensorIndex.cu:362: void indexSelectLargeIndex(TensorInfo<T, IndexType>, TensorInfo<T, IndexType>, TensorInfo<long, IndexType>, int, int, IndexType, IndexType, long) [with T = float, IndexType = unsigned int, DstDim = 2, SrcDim = 2, IdxDim = -2, IndexIsMajor = true]: block: [222,0,0], thread: [18,0,0] Assertion `srcIndex < srcSelectDimSize` failed.
/opt/conda/conda-bld/pytorch_1533739672741/work/aten/src/THC/THCTensorIndex.cu:362: void indexSelectLargeIndex(TensorInfo<T, IndexType>, TensorInfo<T, IndexType>, TensorInfo<long, IndexType>, int, int, IndexType, IndexType, long) [with T = float, IndexType = unsigned int, DstDim = 2, SrcDim = 2, IdxDim = -2, IndexIsMajor = true]: block: [222,0,0], thread: [19,0,0] Assertion `srcIndex < srcSelectDimSize` failed.
/opt/conda/conda-bld/pytorch_1533739672741/work/aten/src/THC/THCTensorIndex.cu:362: void indexSelectLargeIndex(TensorInfo<T, IndexType>, TensorInfo<T, IndexType>, TensorInfo<long, IndexType>, int, int, IndexType, IndexType, long) [with T = float, IndexType = unsigned int, DstDim = 2, SrcDim = 2, IdxDim = -2, IndexIsMajor = true]: block: [222,0,0], thread: [20,0,0] Assertion `srcIndex < srcSelectDimSize` failed.
/opt/conda/conda-bld/pytorch_1533739672741/work/aten/src/THC/THCTensorIndex.cu:362: void indexSelectLargeIndex(TensorInfo<T, IndexType>, TensorInfo<T, IndexType>, TensorInfo<long, IndexType>, int, int, IndexType, IndexType, long) [with T = float, IndexType = unsigned int, DstDim = 2, SrcDim = 2, IdxDim = -2, IndexIsMajor = true]: block: [222,0,0], thread: [21,0,0] Assertion `srcIndex < srcSelectDimSize` failed.
/opt/conda/conda-bld/pytorch_1533739672741/work/aten/src/THC/THCTensorIndex.cu:362: void indexSelectLargeIndex(TensorInfo<T, IndexType>, TensorInfo<T, IndexType>, TensorInfo<long, IndexType>, int, int, IndexType, IndexType, long) [with T = float, IndexType = unsigned int, DstDim = 2, SrcDim = 2, IdxDim = -2, IndexIsMajor = true]: block: [222,0,0], thread: [22,0,0] Assertion `srcIndex < srcSelectDimSize` failed.
/opt/conda/conda-bld/pytorch_1533739672741/work/aten/src/THC/THCTensorIndex.cu:362: void indexSelectLargeIndex(TensorInfo<T, IndexType>, TensorInfo<T, IndexType>, TensorInfo<long, IndexType>, int, int, IndexType, IndexType, long) [with T = float, IndexType = unsigned int, DstDim = 2, SrcDim = 2, IdxDim = -2, IndexIsMajor = true]: block: [222,0,0], thread: [23,0,0] Assertion `srcIndex < srcSelectDimSize` failed.
/opt/conda/conda-bld/pytorch_1533739672741/work/aten/src/THC/THCTensorIndex.cu:362: void indexSelectLargeIndex(TensorInfo<T, IndexType>, TensorInfo<T, IndexType>, TensorInfo<long, IndexType>, int, int, IndexType, IndexType, long) [with T = float, IndexType = unsigned int, DstDim = 2, SrcDim = 2, IdxDim = -2, IndexIsMajor = true]: block: [222,0,0], thread: [24,0,0] Assertion `srcIndex < srcSelectDimSize` failed.
/opt/conda/conda-bld/pytorch_1533739672741/work/aten/src/THC/THCTensorIndex.cu:362: void indexSelectLargeIndex(TensorInfo<T, IndexType>, TensorInfo<T, IndexType>, TensorInfo<long, IndexType>, int, int, IndexType, IndexType, long) [with T = float, IndexType = unsigned int, DstDim = 2, SrcDim = 2, IdxDim = -2, IndexIsMajor = true]: block: [222,0,0], thread: [25,0,0] Assertion `srcIndex < srcSelectDimSize` failed.
/opt/conda/conda-bld/pytorch_1533739672741/work/aten/src/THC/THCTensorIndex.cu:362: void indexSelectLargeIndex(TensorInfo<T, IndexType>, TensorInfo<T, IndexType>, TensorInfo<long, IndexType>, int, int, IndexType, IndexType, long) [with T = float, IndexType = unsigned int, DstDim = 2, SrcDim = 2, IdxDim = -2, IndexIsMajor = true]: block: [222,0,0], thread: [26,0,0] Assertion `srcIndex < srcSelectDimSize` failed.
/opt/conda/conda-bld/pytorch_1533739672741/work/aten/src/THC/THCTensorIndex.cu:362: void indexSelectLargeIndex(TensorInfo<T, IndexType>, TensorInfo<T, IndexType>, TensorInfo<long, IndexType>, int, int, IndexType, IndexType, long) [with T = float, IndexType = unsigned int, DstDim = 2, SrcDim = 2, IdxDim = -2, IndexIsMajor = true]: block: [222,0,0], thread: [27,0,0] Assertion `srcIndex < srcSelectDimSize` failed.
/opt/conda/conda-bld/pytorch_1533739672741/work/aten/src/THC/THCTensorIndex.cu:362: void indexSelectLargeIndex(TensorInfo<T, IndexType>, TensorInfo<T, IndexType>, TensorInfo<long, IndexType>, int, int, IndexType, IndexType, long) [with T = float, IndexType = unsigned int, DstDim = 2, SrcDim = 2, IdxDim = -2, IndexIsMajor = true]: block: [222,0,0], thread: [28,0,0] Assertion `srcIndex < srcSelectDimSize` failed.
/opt/conda/conda-bld/pytorch_1533739672741/work/aten/src/THC/THCTensorIndex.cu:362: void indexSelectLargeIndex(TensorInfo<T, IndexType>, TensorInfo<T, IndexType>, TensorInfo<long, IndexType>, int, int, IndexType, IndexType, long) [with T = float, IndexType = unsigned int, DstDim = 2, SrcDim = 2, IdxDim = -2, IndexIsMajor = true]: block: [222,0,0], thread: [29,0,0] Assertion `srcIndex < srcSelectDimSize` failed.
/opt/conda/conda-bld/pytorch_1533739672741/work/aten/src/THC/THCTensorIndex.cu:362: void indexSelectLargeIndex(TensorInfo<T, IndexType>, TensorInfo<T, IndexType>, TensorInfo<long, IndexType>, int, int, IndexType, IndexType, long) [with T = float, IndexType = unsigned int, DstDim = 2, SrcDim = 2, IdxDim = -2, IndexIsMajor = true]: block: [222,0,0], thread: [30,0,0] Assertion `srcIndex < srcSelectDimSize` failed.
/opt/conda/conda-bld/pytorch_1533739672741/work/aten/src/THC/THCTensorIndex.cu:362: void indexSelectLargeIndex(TensorInfo<T, IndexType>, TensorInfo<T, IndexType>, TensorInfo<long, IndexType>, int, int, IndexType, IndexType, long) [with T = float, IndexType = unsigned int, DstDim = 2, SrcDim = 2, IdxDim = -2, IndexIsMajor = true]: block: [222,0,0], thread: [31,0,0] Assertion `srcIndex < srcSelectDimSize` failed.
Traceback (most recent call last):
File "main_roberta.py", line 502, in <module>
main()
File "main_roberta.py", line 472, in main
train(model, opt, crit, optimizer, scheduler, training_data, validation_data)
File "main_roberta.py", line 220, in train
outputs_pos = model(input_ids=pos_data, token_type_ids=pos_segs, attention_mask=pos_mask)[0]#, pos_segs, pos_mask)
File "/home/1917/anaconda3/lib/python3.7/site-packages/torch/nn/modules/module.py", line 477, in __call__
result = self.forward(*input, **kwargs)
File "/home/1917/pytorch-transformers/pytorch_transformers/modeling_roberta.py", line 314, in forward
attention_mask=attention_mask, head_mask=head_mask)
File "/home/1917/anaconda3/lib/python3.7/site-packages/torch/nn/modules/module.py", line 477, in __call__
result = self.forward(*input, **kwargs)
File "/home/1917/pytorch-transformers/pytorch_transformers/modeling_roberta.py", line 173, in forward
return super(RobertaModel, self).forward(input_ids, token_type_ids, attention_mask, position_ids, head_mask)
File "/home/1917/pytorch-transformers/pytorch_transformers/modeling_bert.py", line 712, in forward
embedding_output = self.embeddings(input_ids, position_ids=position_ids, token_type_ids=token_type_ids)
File "/home/1917/anaconda3/lib/python3.7/site-packages/torch/nn/modules/module.py", line 477, in __call__
result = self.forward(*input, **kwargs)
File "/home/1917/pytorch-transformers/pytorch_transformers/modeling_roberta.py", line 64, in forward
return super(RobertaEmbeddings, self).forward(input_ids, token_type_ids=token_type_ids, position_ids=position_ids)
File "/home/1917/pytorch-transformers/pytorch_transformers/modeling_bert.py", line 270, in forward
embeddings = self.dropout(embeddings)
File "/home/1917/anaconda3/lib/python3.7/site-packages/torch/nn/modules/module.py", line 477, in __call__
result = self.forward(*input, **kwargs)
File "/home/1917/anaconda3/lib/python3.7/site-packages/torch/nn/modules/dropout.py", line 53, in forward
return F.dropout(input, self.p, self.training, self.inplace)
File "/home/1917/anaconda3/lib/python3.7/site-packages/torch/nn/functional.py", line 595, in dropout
return _functions.dropout.Dropout.apply(input, p, training, inplace)
File "/home/1917/anaconda3/lib/python3.7/site-packages/torch/nn/_functions/dropout.py", line 40, in forward
ctx.noise.bernoulli_(1 - ctx.p).div_(1 - ctx.p)
RuntimeError: Creating MTGP constants failed. at /opt/conda/conda-bld/pytorch_1533739672741/work/aten/src/THC/THCTensorRandom.cu:34
``` | 08-22-2019 13:06:15 | 08-22-2019 13:06:15 | Hi! Could you provide us with the script that you use to add the cls and sep tokens?
Please be aware that RoBERTa already has those tokens that you can access using `tokenizer.sep_token` as well as `tokenizer.cls_token`.
The error you're showing often happens when you're trying to access an index that is not in the embedding matrix. My guess is that even though you've added the tokens to the tokenizer, you have not resized the model's embedding matrix accordingly. You can see how it's done in the [tokenizer example](https://huggingface.co/pytorch-transformers/main_classes/tokenizer.html).<|||||>Hi @LysandreJik, thanks for the information, below is the script I use to create the id of the text. Basically I use a single script to create the text id, then I feed the id to the model in another script.
I check the main file and I did resize the embedding matrix with
```
model = RobertaForSequenceClassification.from_pretrained('roberta-base', num_labels=1)
model.resize_token_embeddings(50264)
```
```
The same error would occur with using '<s>' and '<\s>'. However if I just input the id number to the model without input the token type and mask, the model will work fine but the performance is almost zero. Below are the script to create the text id:
```
```
from pytorch_transformers import *
tokenizer = RobertaTokenizer.from_pretrained('roberta-base')
tokenizer.add_tokens(['[CLS]', '[SEP]'])
def trans(txt):
return tokenizer.encode(txt)
def make_data(line):
qury, docp, docn = trans('[CLS]' + ' ' + line[0]), trans('[SEP]' + ' ' + line[1]), trans('[SEP]' + ' ' + line[2])
return ','.join(str(x) for x in qury) + '\t' + ','.join(str(x) for x in docp) + '\t' + ','.join(str(x) for x in docn) + '\n'
if __name__ == '__main__':
with open("data_file.txt") as file:
data = file.readlines()
with open("output_file.txt", "w") as file:
for line in data:
line = line.strip('\n').split('\t')
if len(line) < 3:
continue
output = make_data(line)
file.write(output)
```
So I think one of the important information is that the model works fine when only input the text id whereas when input the inputs_id, token_type and attention_mask, there will be the error above.<|||||>I'm not sure I understand what you're trying to do. Are you trying to add the CLS and SEP tokens to your sequences before they are fed to the RoBERTa model? If that's the case you can use the native
```
roberta_tokenizer.encode(text, add_special_tokens=True)
```
for single sequences and
```
roberta_tokenizer.encode(text1, text2, add_special_tokens=True)
```
for sequence pairs.
This will put the correct CLS and SEP tokens that were used during RoBERTa's pre-training.
In your first message, it seems to me that you are padding your sequence with `0`, which is RoBERTa's token for CLS. If you're looking to pad your sequence you should probably use RoBERTa's pad tokens, which are 1:
```
roberta_tokenizer.pad_token # <pad>
roberta_tokenzer.encoder[roberta_tokenizer.pad_token] # 1
```<|||||>This issue has been automatically marked as stale because it has not had recent activity. It will be closed if no further activity occurs. Thank you for your contributions.
|
transformers | 1,084 | closed | Xlnet for multi-label classification | Can you provide me with the xlnet code to deal with the multi-label classification task, please | 08-22-2019 12:18:30 | 08-22-2019 12:18:30 | you can try fast-bert. https://github.com/kaushaltrivedi/fast-bert.
its built on top of pytorch-transformers and supports multi-label classification for both BERT and XLNet.<|||||>This issue has been automatically marked as stale because it has not had recent activity. It will be closed if no further activity occurs. Thank you for your contributions.
|
transformers | 1,083 | closed | hwo to get RoBERTaTokenizer vocab.json and also merge file | ## ❓ Questions & Help
<!-- A clear and concise description of the question. -->
hello, I trained the robert on my customized corpus following the fairseq instruction. I am confused how to generate the robert vocab.json and also merge.txt because I want to use the pytorch-transformer RoBERTaTokenizer. I only have a dict.txt in my data | 08-22-2019 11:16:10 | 08-22-2019 11:16:10 | @thomwolf @LysandreJik @julien-c <|||||>Hi! RoBERTa's tokenizer is based on the GPT-2 tokenizer.
**Please note that except if you have completely re-trained RoBERTa from scratch, there is usually no need to change the `vocab.json` and `merges.txt` file.**
Currently we do not have a built-in way of creating your vocab/merges files, neither for GPT-2 nor for RoBERTa. I'm describing the process we followed for RoBERTa, hoping that you will be able to solve your problem following a similar process.
Encoding a sentence is done according to the following process:
Say you start with this text:
```
What's up with the tokenizer?
```
The tokenizer first tokenizes according to the merges file:
```
['What', "'s", 'Ġup', 'Ġwith', 'Ġthe', 'Ġtoken', 'izer', '?']
```
And then, according to the values in the `vocab.json`, these tokens are then replaced by their indices:
```
[ 'What', "'s", 'Ġup', 'Ġwith', 'Ġthe', 'Ġtoken', 'izer', '?']
---- becomes ----
[ 2061, 338, 510, 351, 262, 11241, 7509, 30]
```
The dict.txt file generated from RoBERTa actually modifies the `vocab.json` from the original GPT-2 by shifting the indices.
If you open the dict.txt file you should see values such as (the values shown here are the first values of the native RoBERTa `dict.txt`):
```
13 850314647
262 800385005
11 800251374
284 432911125
```
which are token indices ordered by the highest occurence. For the first example, the token `13` in the GPT-2 tokenizer is the token `.`: `gpt2_tokenizer.encode('.')` returns `[13]`
In order to get the appropriate RoBERTa `vocab.json` we remapped the original GPT-2 `vocab.json` with this dict. The first four values are the special tokens:
```
{"<s>": 0, "<pad>": 1, "</s>": 2, "<unk>": 3}
```
Following those values, are the values from the `dict.txt` ordered by index. For example:
```
gpt2_tokenizer.decode(13) -> '.' # INDEX 0 (13 is on the 1st line of the dict.txt)
gpt2_tokenizer.decode(262) -> ' the' # INDEX 1 (262 is on the 2nd line of the dict.txt)
gpt2_tokenizer.decode(11) -> ',' # INDEX 2 (11 is on the third line of the dict.txt)
gpt2_tokenizer.decode(284) -> to' # INDEX 3 (284 is on the fourth line of the dict.txt)
```
The vocab then becomes:
```
{"<s>": 0, "<pad>": 1, "</s>": 2, "<unk>": 3, ".": 4, "Ġthe": 5, ",": 6, "Ġto": 7}
```
That's how you create the `vocab.json`. The `merges.txt` file is unchanged.<|||||>@julien-c Thanks for your reply!
Hi, I am pre-training RoBERTa in my own corpus, which consists of numbers
> 4758 7647 16712 6299 11255 6068 695 23 19536 7142 7009 9655 10524 4864 7379 17348 7501 17225 14123 13711 7133 11255 21097 3277 6068 695 4190 1269 4526 12266 2161 17597 15274
23 6484 17225 8217 16374 11122 5592 21224 7251 11188 533 9685 11487 4246 19311 19851 8038 15822 9435 15274
1027 1269 14461 4815 12617 14123 3268 3390 8197 19019 16908 20958 15033 16541 19421 19429 7664 17253 4246 11123 1884 15274
5863 17166 21224 13159 2289 11944 8205 17083 13426 21224 17225 17186 14499 6225 16201 400 5635 3219 16498 15274
each separated line represents a paragraph
So I skip the BPE encode, I just binarize my data into language format, using
> TEXT=examples/language_model/wikitext-103
fairseq-preprocess \
--only-source \
--trainpref $TEXT/wiki.train.tokens \
--validpref $TEXT/wiki.valid.tokens \
--testpref $TEXT/wiki.test.tokens \
--destdir data-bin/wikitext-103 \
--workers 20
The vocab.json I think I can construct by myself but the merges.txt I didn't use the BPE, So I wondering if I just use an empty file to mean no merging.<|||||>This issue has been automatically marked as stale because it has not had recent activity. It will be closed if no further activity occurs. Thank you for your contributions.
<|||||>> @julien-c Thanks for your reply!
>
> Hi, I am pre-training RoBERTa in my own corpus, which consists of numbers
>
> > 4758 7647 16712 6299 11255 6068 695 23 19536 7142 7009 9655 10524 4864 7379 17348 7501 17225 14123 13711 7133 11255 21097 3277 6068 695 4190 1269 4526 12266 2161 17597 15274
> > 23 6484 17225 8217 16374 11122 5592 21224 7251 11188 533 9685 11487 4246 19311 19851 8038 15822 9435 15274
> > 1027 1269 14461 4815 12617 14123 3268 3390 8197 19019 16908 20958 15033 16541 19421 19429 7664 17253 4246 11123 1884 15274
> > 5863 17166 21224 13159 2289 11944 8205 17083 13426 21224 17225 17186 14499 6225 16201 400 5635 3219 16498 15274
>
> each separated line represents a paragraph
>
> So I skip the BPE encode, I just binarize my data into language format, using
>
> > TEXT=examples/language_model/wikitext-103
> > fairseq-preprocess
> > --only-source
> > --trainpref $TEXT/wiki.train.tokens
> > --validpref $TEXT/wiki.valid.tokens
> > --testpref $TEXT/wiki.test.tokens \
> > --destdir data-bin/wikitext-103
> > --workers 20
>
> The vocab.json I think I can construct by myself but the merges.txt I didn't use the BPE, So I wondering if I just use an empty file to mean no merging.
I want to know this too<|||||>U guys can get vocab.txt and merges.txt from:
https://huggingface.co/transformers/v1.1.0/_modules/pytorch_transformers/tokenization_roberta.html
the works still come from huggingface.<|||||>@songtaoshi I have a similar problem. Did you get your issue resolved. <|||||>For another new language and a totally new dataset, preparing my own merges.txt and vocab.json is for sure necessary:
Check this:
https://towardsdatascience.com/transformers-from-scratch-creating-a-tokenizer-7d7418adb403
this is a step-by-step tutorial on how to use "oscar" dataset to train your own byte-level bpe tokenizer (which exactly outputs "merges.txt" and "vocab.json".
### 1. data prepare ###
>>> import datasets
>>> dataset = datasets.load_dataset('oscar', 'unshuffled_deduplicated_la')
>>> from tqdm.auto import tqdm
>>> text_data = []
>>> file_count = 0
>>> for sample in tqdm(dataset['train']):
... sample = sample['text'].replace('\n', '')
... text_data.append(sample)
... if len(text_data) == 5000:
... with open(f'./oscar_la/text_{file_count}.txt', 'w', encoding='utf-8') as fp:
... fp.write('\n'.join(text_data))
... text_data = []
... file_count += 1
...
>>> with open(f'./oscar_la/text_{file_count}.txt', 'w', encoding='utf-8') as fp:
... fp.write('\n'.join(text_data))
...
>>> from pathlib import Path
>>> paths = [str(x) for x in Path('./oscar_la').glob('*.txt')]
>>> paths
['oscar_la/text_1.txt', 'oscar_la/text_2.txt', 'oscar_la/text_3.txt', 'oscar_la/text_0.txt']
### 2. train ###
>>> from tokenizers import ByteLevelBPETokenizer
>>> tokenizer = ByteLevelBPETokenizer()
>>> tokenizer.train(files=paths, vocab_size=30522, min_frequency=2, special_tokens=['<s>', '<pad>', '</s>', '<unk>', '<mask>'])
### 3. save ###
>>> tokenizer.save_model('./oscar_la/blbpe')
['./oscar_la/blbpe/vocab.json', './oscar_la/blbpe/merges.txt']
<|||||>@Xianchao-Wu
Thanks, that helped me a lot!<|||||>您发给我的信件已经收到,我会尽快查收并回复您。Your e-mail has been received, I will reply as soon as possible.邢璐茜<|||||>>
Can you please give any reference to the code or explain how can we generate tokens for a given using the merges.txt file?<|||||>您发给我的信件已经收到,我会尽快查收并回复您。Your e-mail has been received, I will reply as soon as possible.邢璐茜 |
transformers | 1,082 | closed | Getting tokenization ERROR while running run_generation.py | ## 🐛 Bug
<!-- Important information -->
Model I am using (GPT-2....):
Language I am using the model on (English):
The problem arise when using:
* [ ] the official example scripts: (give details)
pytorch-transformers/examples/run_generation.py \
The tasks I am working on is:
* [ ] my own task or dataset: (give details)
-> just simple next sentence prediction. My actual text 'Saw her in the park yesterday'
## To Reproduce
Steps to reproduce the behavior:
1.
2.
3.
<!-- If you have a code sample, error messages, stack traces, please provide it here as well. -->
Error message I got is
ERROR - pytorch_transformers.tokenization_utils - Using sep_token, but it is not set yet.
And then the next sentence that it predicts has nothing to do with my given sentence.
## Expected behavior
<!-- A clear and concise description of what you expected to happen. -->
-> Not give the error. and work like it is supposed to,
## Environment
* OS: Google Colab
* Python version:
* PyTorch version:
* PyTorch Transformers version (or branch):
* Using GPU ? Yes
* Distributed of parallel setup ?
* Any other relevant information:
## Additional context
I am pretty sure that the problem is not so hard to solve. But I am a noob here. So please forgive me . | 08-22-2019 05:44:55 | 08-22-2019 05:44:55 | Hi! Yes currently there's a small issue with the tokenizer that outputs this warning during the decoding of the sentence. It will be fixed very shortly.
It won't affect your training however, as it is only a warning :)<|||||>This issue has been automatically marked as stale because it has not had recent activity. It will be closed if no further activity occurs. Thank you for your contributions.
|
transformers | 1,081 | closed | Fix distributed barrier hang | This is bug reported in issue #998 (and is also valid for `run_squad.py`).
What is happening?
When launching a distributed training on one of the task of the GLUE benchmark (for instance this suggested command in the README [here](https://github.com/huggingface/pytorch-transformers#fine-tuning-bert-model-on-the-mrpc-classification-task) for GLUE or [here](https://github.com/huggingface/pytorch-transformers#run_squadpy-fine-tuning-on-squad-for-question-answering) for SQUAD), the training is performed in a distributed setting (expected behavior). Evaluation can be tricky for certain metrics in a distributed setting so the evaluation is performed solely by the master process (cf L476: `if args.do_eval and args.local_rank in [-1, 0]:`).
During the evaluation, the process hangs/gets stucked at L290 (`torch.distributed.barrier()`). It turns out that all the processes except the master one already exit at L476 and thus never enter the symmetric `torch.distributed.barrier()` at L254-255. It means that the master process is waiting at L290 for his process friends who already left the party without telling him (printing a `torch.distributed.get_world_size()` at L290 during evaluation reveals torch is expecting `$NGPU` processes).
Adding a `and not evaluate` condition both at L254 and L289 is a solution to fix the bug (the master process is the only surviving process at evaluation, so no need to wait for others...)
| 08-22-2019 04:44:10 | 08-22-2019 04:44:10 | Ok great, thanks a lot @VictorSanh |
transformers | 1,080 | closed | 51 lm | 08-22-2019 04:28:15 | 08-22-2019 04:28:15 | ||
transformers | 1,079 | closed | Fix "No such file or directory" for SQuAD v1.1 | This solves the exception for SQuAD v1.1 evaluation without predicted null_odds file.
Traceback (most recent call last):
File "./examples/run_squad.py", line 521, in <module>
File "./examples/run_squad.py", line 510, in main
for checkpoint in checkpoints:
File "./examples/run_squad.py", line 257, in evaluate
na_prob_file=output_null_log_odds_file)
File "/home/zhangzs/pytorch-transformers-master/examples/utils_squad_evaluate.py", line 291, in main
with open(OPTS.na_prob_file) as f:
FileNotFoundError: [Errno 2] No such file or directory: 'squad/squad-debug/null_odds_.json' | 08-22-2019 03:19:22 | 08-22-2019 03:19:22 | # [Codecov](https://codecov.io/gh/huggingface/pytorch-transformers/pull/1079?src=pr&el=h1) Report
> Merging [#1079](https://codecov.io/gh/huggingface/pytorch-transformers/pull/1079?src=pr&el=desc) into [master](https://codecov.io/gh/huggingface/pytorch-transformers/commit/e00b4ff1de0591d5093407b16e665e5c86028f04?src=pr&el=desc) will **decrease** coverage by `0.02%`.
> The diff coverage is `n/a`.
[](https://codecov.io/gh/huggingface/pytorch-transformers/pull/1079?src=pr&el=tree)
```diff
@@ Coverage Diff @@
## master #1079 +/- ##
==========================================
- Coverage 79.61% 79.58% -0.03%
==========================================
Files 42 42
Lines 6898 6898
==========================================
- Hits 5492 5490 -2
- Misses 1406 1408 +2
```
| [Impacted Files](https://codecov.io/gh/huggingface/pytorch-transformers/pull/1079?src=pr&el=tree) | Coverage Δ | |
|---|---|---|
| [pytorch\_transformers/tokenization\_bert.py](https://codecov.io/gh/huggingface/pytorch-transformers/pull/1079/diff?src=pr&el=tree#diff-cHl0b3JjaF90cmFuc2Zvcm1lcnMvdG9rZW5pemF0aW9uX2JlcnQucHk=) | `93.89% <0%> (-0.94%)` | :arrow_down: |
------
[Continue to review full report at Codecov](https://codecov.io/gh/huggingface/pytorch-transformers/pull/1079?src=pr&el=continue).
> **Legend** - [Click here to learn more](https://docs.codecov.io/docs/codecov-delta)
> `Δ = absolute <relative> (impact)`, `ø = not affected`, `? = missing data`
> Powered by [Codecov](https://codecov.io/gh/huggingface/pytorch-transformers/pull/1079?src=pr&el=footer). Last update [e00b4ff...61f14c5](https://codecov.io/gh/huggingface/pytorch-transformers/pull/1079?src=pr&el=lastupdated). Read the [comment docs](https://docs.codecov.io/docs/pull-request-comments).
<|||||>I think I fixed the issue about month ago?
https://github.com/huggingface/pytorch-transformers/blob/e00b4ff1de0591d5093407b16e665e5c86028f04/examples/run_squad.py#L248-L251<|||||>Thx! It looks fine now. My version is out of date. I'll close the comment. |
transformers | 1,078 | closed | Index misplacement of Vocab.txt BUG BUG BUG | ## 🐛 Bug
<!-- Important information -->
Model I am using (BertTokenizer):
Language I am using the model on (Chinese):
The problem arise when using:
**pytorch tokenizer**
```
t = tokenizer.tokenize('[CLS]哦我[SEP]')
i = tokenizer.convert_tokens_to_ids(t)
print(i)
[101, 1522, 2770, 102]
```
**tensorflow tokenizer**
```
t = tokenizer.tokenize('[CLS]哦我[SEP]')
i = tokenizer.convert_tokens_to_ids(t)
print(i)
[101, 1521, 2769, 102]
```
> Due to index misalignment, When the last word (##😎)in the Vocab.txt appears in the training set ,out of range error. | 08-22-2019 03:14:15 | 08-22-2019 03:14:15 | |
transformers | 1,077 | closed | Pruning changes so that deleted heads are kept on save/load | The models saved with pruned heads will now be loaded correctly with a correct state dict and a correct configuration file. The changes in head structure are available in the config file via the property `config.pruned_heads`.
Pruned heads can be loaded from the config file:
```
config = GPT2Config(n_layer=4, n_head=4, pruned_heads={0: [1], 1: [2, 3]})
model = GPT2Model(config=config)
print([h.attn.n_head for h in model.h])
# [3, 2, 4, 4]
```
They are kept upon save:
```
model.save_pretrained("checkpoint")
model = GPT2Model.from_pretrained("checkpoint")
print([h.attn.n_head for h in model.h], model.config.pruned_heads)
# [3, 2, 4, 4] {0: [1], 1: [2, 3]}
```
And heads can be additionaly pruned, raising a warning if a head has already been pruned:
```
model.prune_heads({1: [1, 2], 3: [2]})
print([h.attn.n_head for h in model.h])
# Tried to remove head 2 of layer 1 but it was already removed. The current removed heads are {1: [1, 2], 3: [2]}
# [3, 1, 4, 3]
```
It is implemented for GPT, GPT-2, BERT, RoBERTa as well as XLM.
| 08-22-2019 01:39:48 | 08-22-2019 01:39:48 | # [Codecov](https://codecov.io/gh/huggingface/pytorch-transformers/pull/1077?src=pr&el=h1) Report
> Merging [#1077](https://codecov.io/gh/huggingface/pytorch-transformers/pull/1077?src=pr&el=desc) into [master](https://codecov.io/gh/huggingface/pytorch-transformers/commit/d7a4c3252ed5e630b7fb6e4b4616daddfe574fc5?src=pr&el=desc) will **increase** coverage by `0.46%`.
> The diff coverage is `100%`.
[](https://codecov.io/gh/huggingface/pytorch-transformers/pull/1077?src=pr&el=tree)
```diff
@@ Coverage Diff @@
## master #1077 +/- ##
==========================================
+ Coverage 80.38% 80.84% +0.46%
==========================================
Files 46 46
Lines 7749 7859 +110
==========================================
+ Hits 6229 6354 +125
+ Misses 1520 1505 -15
```
| [Impacted Files](https://codecov.io/gh/huggingface/pytorch-transformers/pull/1077?src=pr&el=tree) | Coverage Δ | |
|---|---|---|
| [pytorch\_transformers/tests/modeling\_common\_test.py](https://codecov.io/gh/huggingface/pytorch-transformers/pull/1077/diff?src=pr&el=tree#diff-cHl0b3JjaF90cmFuc2Zvcm1lcnMvdGVzdHMvbW9kZWxpbmdfY29tbW9uX3Rlc3QucHk=) | `78.02% <100%> (+4.94%)` | :arrow_up: |
| [pytorch\_transformers/modeling\_distilbert.py](https://codecov.io/gh/huggingface/pytorch-transformers/pull/1077/diff?src=pr&el=tree#diff-cHl0b3JjaF90cmFuc2Zvcm1lcnMvbW9kZWxpbmdfZGlzdGlsYmVydC5weQ==) | `96.77% <100%> (+0.03%)` | :arrow_up: |
| [pytorch\_transformers/modeling\_xlnet.py](https://codecov.io/gh/huggingface/pytorch-transformers/pull/1077/diff?src=pr&el=tree#diff-cHl0b3JjaF90cmFuc2Zvcm1lcnMvbW9kZWxpbmdfeGxuZXQucHk=) | `78.83% <100%> (-0.08%)` | :arrow_down: |
| [pytorch\_transformers/modeling\_utils.py](https://codecov.io/gh/huggingface/pytorch-transformers/pull/1077/diff?src=pr&el=tree#diff-cHl0b3JjaF90cmFuc2Zvcm1lcnMvbW9kZWxpbmdfdXRpbHMucHk=) | `90.02% <100%> (+3.98%)` | :arrow_up: |
| [pytorch\_transformers/modeling\_bert.py](https://codecov.io/gh/huggingface/pytorch-transformers/pull/1077/diff?src=pr&el=tree#diff-cHl0b3JjaF90cmFuc2Zvcm1lcnMvbW9kZWxpbmdfYmVydC5weQ==) | `88.03% <100%> (+0.04%)` | :arrow_up: |
| [pytorch\_transformers/modeling\_roberta.py](https://codecov.io/gh/huggingface/pytorch-transformers/pull/1077/diff?src=pr&el=tree#diff-cHl0b3JjaF90cmFuc2Zvcm1lcnMvbW9kZWxpbmdfcm9iZXJ0YS5weQ==) | `75.89% <100%> (ø)` | :arrow_up: |
| [pytorch\_transformers/modeling\_transfo\_xl.py](https://codecov.io/gh/huggingface/pytorch-transformers/pull/1077/diff?src=pr&el=tree#diff-cHl0b3JjaF90cmFuc2Zvcm1lcnMvbW9kZWxpbmdfdHJhbnNmb194bC5weQ==) | `57% <100%> (-0.12%)` | :arrow_down: |
| [pytorch\_transformers/modeling\_openai.py](https://codecov.io/gh/huggingface/pytorch-transformers/pull/1077/diff?src=pr&el=tree#diff-cHl0b3JjaF90cmFuc2Zvcm1lcnMvbW9kZWxpbmdfb3BlbmFpLnB5) | `81.95% <100%> (+0.11%)` | :arrow_up: |
| [pytorch\_transformers/modeling\_gpt2.py](https://codecov.io/gh/huggingface/pytorch-transformers/pull/1077/diff?src=pr&el=tree#diff-cHl0b3JjaF90cmFuc2Zvcm1lcnMvbW9kZWxpbmdfZ3B0Mi5weQ==) | `84.03% <100%> (+0.19%)` | :arrow_up: |
| [pytorch\_transformers/modeling\_xlm.py](https://codecov.io/gh/huggingface/pytorch-transformers/pull/1077/diff?src=pr&el=tree#diff-cHl0b3JjaF90cmFuc2Zvcm1lcnMvbW9kZWxpbmdfeGxtLnB5) | `87.08% <100%> (+0.34%)` | :arrow_up: |
| ... and [1 more](https://codecov.io/gh/huggingface/pytorch-transformers/pull/1077/diff?src=pr&el=tree-more) | |
------
[Continue to review full report at Codecov](https://codecov.io/gh/huggingface/pytorch-transformers/pull/1077?src=pr&el=continue).
> **Legend** - [Click here to learn more](https://docs.codecov.io/docs/codecov-delta)
> `Δ = absolute <relative> (impact)`, `ø = not affected`, `? = missing data`
> Powered by [Codecov](https://codecov.io/gh/huggingface/pytorch-transformers/pull/1077?src=pr&el=footer). Last update [d7a4c32...11600ed](https://codecov.io/gh/huggingface/pytorch-transformers/pull/1077?src=pr&el=lastupdated). Read the [comment docs](https://docs.codecov.io/docs/pull-request-comments).
<|||||>Let's have a talk about this one before merging (see my comment above)<|||||>Ok great, I think this is also ready to merge, now. Let's merge. |
transformers | 1,076 | closed | can this project select the specific version of BERT? | ## ❓ Questions & Help
I dont know if this project can select the version of BERT which I need. For example, i want use BERT-wwm not BERT-basic, what should i do? Can you help me, plz.
<!-- A clear and concise description of the question. --> | 08-22-2019 01:29:33 | 08-22-2019 01:29:33 | Hi. You can check the documentation about the different checkpoints available for each model [here](https://huggingface.co/pytorch-transformers/pretrained_models.html).
If you're looking for BERT whole word masking, there are the following pretrained models that might be of interest: `bert-large-uncased-whole-word-masking`, `bert-large-cased-whole-word-masking`, `bert-large-uncased-whole-word-masking-finetuned-squad` and `bert-large-cased-whole-word-masking-finetuned-squad`.<|||||>@LysandreJik Thanks for your advice. But, in my situation, I have to use my corpus to train a new BERT with whole word mask, so I cant use the pre-trained BERT model, what I should do in this situation?<|||||>Training an entire BERT model from scratch takes a lot of resources, and we don't have any scripts/examples that show how to do it with our library.
You could look at [Microsoft's repository](https://github.com/microsoft/AzureML-BERT) that uses our implementation to pre-train/fine-tune BERT.<|||||>@LysandreJik notice that the bert had been updated by adding whole word mask, do you updating your pytorch-transformer with this trick when you convert BERT from tf to pytorch |
transformers | 1,075 | closed | reraise EnvironmentError in modeling_utils.py | When an EnvironmentError occurs in modeling_utils.py, currently the code returns None. This causes a TypeError saying None is not iterable in the statement
config, model_kwargs = cls.config_class.from_pretrained(
pretrained_model_name_or_path, *model_args,
cache_dir=cache_dir, return_unused_kwargs=True,
force_download=force_download,
**kwargs
) | 08-21-2019 22:35:14 | 08-21-2019 22:35:14 | # [Codecov](https://codecov.io/gh/huggingface/pytorch-transformers/pull/1075?src=pr&el=h1) Report
> Merging [#1075](https://codecov.io/gh/huggingface/pytorch-transformers/pull/1075?src=pr&el=desc) into [master](https://codecov.io/gh/huggingface/pytorch-transformers/commit/e00b4ff1de0591d5093407b16e665e5c86028f04?src=pr&el=desc) will **decrease** coverage by `0.02%`.
> The diff coverage is `n/a`.
[](https://codecov.io/gh/huggingface/pytorch-transformers/pull/1075?src=pr&el=tree)
```diff
@@ Coverage Diff @@
## master #1075 +/- ##
==========================================
- Coverage 79.61% 79.58% -0.03%
==========================================
Files 42 42
Lines 6898 6898
==========================================
- Hits 5492 5490 -2
- Misses 1406 1408 +2
```
| [Impacted Files](https://codecov.io/gh/huggingface/pytorch-transformers/pull/1075?src=pr&el=tree) | Coverage Δ | |
|---|---|---|
| [pytorch\_transformers/modeling\_utils.py](https://codecov.io/gh/huggingface/pytorch-transformers/pull/1075/diff?src=pr&el=tree#diff-cHl0b3JjaF90cmFuc2Zvcm1lcnMvbW9kZWxpbmdfdXRpbHMucHk=) | `83.41% <ø> (ø)` | :arrow_up: |
| [pytorch\_transformers/tokenization\_bert.py](https://codecov.io/gh/huggingface/pytorch-transformers/pull/1075/diff?src=pr&el=tree#diff-cHl0b3JjaF90cmFuc2Zvcm1lcnMvdG9rZW5pemF0aW9uX2JlcnQucHk=) | `93.89% <0%> (-0.94%)` | :arrow_down: |
------
[Continue to review full report at Codecov](https://codecov.io/gh/huggingface/pytorch-transformers/pull/1075?src=pr&el=continue).
> **Legend** - [Click here to learn more](https://docs.codecov.io/docs/codecov-delta)
> `Δ = absolute <relative> (impact)`, `ø = not affected`, `? = missing data`
> Powered by [Codecov](https://codecov.io/gh/huggingface/pytorch-transformers/pull/1075?src=pr&el=footer). Last update [e00b4ff...14eef67](https://codecov.io/gh/huggingface/pytorch-transformers/pull/1075?src=pr&el=lastupdated). Read the [comment docs](https://docs.codecov.io/docs/pull-request-comments).
<|||||># [Codecov](https://codecov.io/gh/huggingface/pytorch-transformers/pull/1075?src=pr&el=h1) Report
> Merging [#1075](https://codecov.io/gh/huggingface/pytorch-transformers/pull/1075?src=pr&el=desc) into [master](https://codecov.io/gh/huggingface/pytorch-transformers/commit/e00b4ff1de0591d5093407b16e665e5c86028f04?src=pr&el=desc) will **decrease** coverage by `0.02%`.
> The diff coverage is `n/a`.
[](https://codecov.io/gh/huggingface/pytorch-transformers/pull/1075?src=pr&el=tree)
```diff
@@ Coverage Diff @@
## master #1075 +/- ##
==========================================
- Coverage 79.61% 79.58% -0.03%
==========================================
Files 42 42
Lines 6898 6898
==========================================
- Hits 5492 5490 -2
- Misses 1406 1408 +2
```
| [Impacted Files](https://codecov.io/gh/huggingface/pytorch-transformers/pull/1075?src=pr&el=tree) | Coverage Δ | |
|---|---|---|
| [pytorch\_transformers/modeling\_utils.py](https://codecov.io/gh/huggingface/pytorch-transformers/pull/1075/diff?src=pr&el=tree#diff-cHl0b3JjaF90cmFuc2Zvcm1lcnMvbW9kZWxpbmdfdXRpbHMucHk=) | `83.41% <ø> (ø)` | :arrow_up: |
| [pytorch\_transformers/tokenization\_bert.py](https://codecov.io/gh/huggingface/pytorch-transformers/pull/1075/diff?src=pr&el=tree#diff-cHl0b3JjaF90cmFuc2Zvcm1lcnMvdG9rZW5pemF0aW9uX2JlcnQucHk=) | `93.89% <0%> (-0.94%)` | :arrow_down: |
------
[Continue to review full report at Codecov](https://codecov.io/gh/huggingface/pytorch-transformers/pull/1075?src=pr&el=continue).
> **Legend** - [Click here to learn more](https://docs.codecov.io/docs/codecov-delta)
> `Δ = absolute <relative> (impact)`, `ø = not affected`, `? = missing data`
> Powered by [Codecov](https://codecov.io/gh/huggingface/pytorch-transformers/pull/1075?src=pr&el=footer). Last update [e00b4ff...14eef67](https://codecov.io/gh/huggingface/pytorch-transformers/pull/1075?src=pr&el=lastupdated). Read the [comment docs](https://docs.codecov.io/docs/pull-request-comments).
<|||||># [Codecov](https://codecov.io/gh/huggingface/pytorch-transformers/pull/1075?src=pr&el=h1) Report
> Merging [#1075](https://codecov.io/gh/huggingface/pytorch-transformers/pull/1075?src=pr&el=desc) into [master](https://codecov.io/gh/huggingface/pytorch-transformers/commit/e00b4ff1de0591d5093407b16e665e5c86028f04?src=pr&el=desc) will **decrease** coverage by `0.02%`.
> The diff coverage is `n/a`.
[](https://codecov.io/gh/huggingface/pytorch-transformers/pull/1075?src=pr&el=tree)
```diff
@@ Coverage Diff @@
## master #1075 +/- ##
==========================================
- Coverage 79.61% 79.58% -0.03%
==========================================
Files 42 42
Lines 6898 6898
==========================================
- Hits 5492 5490 -2
- Misses 1406 1408 +2
```
| [Impacted Files](https://codecov.io/gh/huggingface/pytorch-transformers/pull/1075?src=pr&el=tree) | Coverage Δ | |
|---|---|---|
| [pytorch\_transformers/modeling\_utils.py](https://codecov.io/gh/huggingface/pytorch-transformers/pull/1075/diff?src=pr&el=tree#diff-cHl0b3JjaF90cmFuc2Zvcm1lcnMvbW9kZWxpbmdfdXRpbHMucHk=) | `83.41% <ø> (ø)` | :arrow_up: |
| [pytorch\_transformers/tokenization\_bert.py](https://codecov.io/gh/huggingface/pytorch-transformers/pull/1075/diff?src=pr&el=tree#diff-cHl0b3JjaF90cmFuc2Zvcm1lcnMvdG9rZW5pemF0aW9uX2JlcnQucHk=) | `93.89% <0%> (-0.94%)` | :arrow_down: |
------
[Continue to review full report at Codecov](https://codecov.io/gh/huggingface/pytorch-transformers/pull/1075?src=pr&el=continue).
> **Legend** - [Click here to learn more](https://docs.codecov.io/docs/codecov-delta)
> `Δ = absolute <relative> (impact)`, `ø = not affected`, `? = missing data`
> Powered by [Codecov](https://codecov.io/gh/huggingface/pytorch-transformers/pull/1075?src=pr&el=footer). Last update [e00b4ff...14eef67](https://codecov.io/gh/huggingface/pytorch-transformers/pull/1075?src=pr&el=lastupdated). Read the [comment docs](https://docs.codecov.io/docs/pull-request-comments).
<|||||># [Codecov](https://codecov.io/gh/huggingface/pytorch-transformers/pull/1075?src=pr&el=h1) Report
> Merging [#1075](https://codecov.io/gh/huggingface/pytorch-transformers/pull/1075?src=pr&el=desc) into [master](https://codecov.io/gh/huggingface/pytorch-transformers/commit/e00b4ff1de0591d5093407b16e665e5c86028f04?src=pr&el=desc) will **decrease** coverage by `0.02%`.
> The diff coverage is `n/a`.
[](https://codecov.io/gh/huggingface/pytorch-transformers/pull/1075?src=pr&el=tree)
```diff
@@ Coverage Diff @@
## master #1075 +/- ##
==========================================
- Coverage 79.61% 79.58% -0.03%
==========================================
Files 42 42
Lines 6898 6898
==========================================
- Hits 5492 5490 -2
- Misses 1406 1408 +2
```
| [Impacted Files](https://codecov.io/gh/huggingface/pytorch-transformers/pull/1075?src=pr&el=tree) | Coverage Δ | |
|---|---|---|
| [pytorch\_transformers/modeling\_utils.py](https://codecov.io/gh/huggingface/pytorch-transformers/pull/1075/diff?src=pr&el=tree#diff-cHl0b3JjaF90cmFuc2Zvcm1lcnMvbW9kZWxpbmdfdXRpbHMucHk=) | `83.41% <ø> (ø)` | :arrow_up: |
| [pytorch\_transformers/tokenization\_bert.py](https://codecov.io/gh/huggingface/pytorch-transformers/pull/1075/diff?src=pr&el=tree#diff-cHl0b3JjaF90cmFuc2Zvcm1lcnMvdG9rZW5pemF0aW9uX2JlcnQucHk=) | `93.89% <0%> (-0.94%)` | :arrow_down: |
------
[Continue to review full report at Codecov](https://codecov.io/gh/huggingface/pytorch-transformers/pull/1075?src=pr&el=continue).
> **Legend** - [Click here to learn more](https://docs.codecov.io/docs/codecov-delta)
> `Δ = absolute <relative> (impact)`, `ø = not affected`, `? = missing data`
> Powered by [Codecov](https://codecov.io/gh/huggingface/pytorch-transformers/pull/1075?src=pr&el=footer). Last update [e00b4ff...14eef67](https://codecov.io/gh/huggingface/pytorch-transformers/pull/1075?src=pr&el=lastupdated). Read the [comment docs](https://docs.codecov.io/docs/pull-request-comments).
<|||||># [Codecov](https://codecov.io/gh/huggingface/pytorch-transformers/pull/1075?src=pr&el=h1) Report
> Merging [#1075](https://codecov.io/gh/huggingface/pytorch-transformers/pull/1075?src=pr&el=desc) into [master](https://codecov.io/gh/huggingface/pytorch-transformers/commit/e00b4ff1de0591d5093407b16e665e5c86028f04?src=pr&el=desc) will **not change** coverage.
> The diff coverage is `n/a`.
[](https://codecov.io/gh/huggingface/pytorch-transformers/pull/1075?src=pr&el=tree)
```diff
@@ Coverage Diff @@
## master #1075 +/- ##
=======================================
Coverage 79.61% 79.61%
=======================================
Files 42 42
Lines 6898 6898
=======================================
Hits 5492 5492
Misses 1406 1406
```
| [Impacted Files](https://codecov.io/gh/huggingface/pytorch-transformers/pull/1075?src=pr&el=tree) | Coverage Δ | |
|---|---|---|
| [pytorch\_transformers/modeling\_utils.py](https://codecov.io/gh/huggingface/pytorch-transformers/pull/1075/diff?src=pr&el=tree#diff-cHl0b3JjaF90cmFuc2Zvcm1lcnMvbW9kZWxpbmdfdXRpbHMucHk=) | `83.41% <ø> (ø)` | :arrow_up: |
| [pytorch\_transformers/tokenization\_utils.py](https://codecov.io/gh/huggingface/pytorch-transformers/pull/1075/diff?src=pr&el=tree#diff-cHl0b3JjaF90cmFuc2Zvcm1lcnMvdG9rZW5pemF0aW9uX3V0aWxzLnB5) | `86.22% <ø> (ø)` | :arrow_up: |
------
[Continue to review full report at Codecov](https://codecov.io/gh/huggingface/pytorch-transformers/pull/1075?src=pr&el=continue).
> **Legend** - [Click here to learn more](https://docs.codecov.io/docs/codecov-delta)
> `Δ = absolute <relative> (impact)`, `ø = not affected`, `? = missing data`
> Powered by [Codecov](https://codecov.io/gh/huggingface/pytorch-transformers/pull/1075?src=pr&el=footer). Last update [e00b4ff...c603d09](https://codecov.io/gh/huggingface/pytorch-transformers/pull/1075?src=pr&el=lastupdated). Read the [comment docs](https://docs.codecov.io/docs/pull-request-comments).
<|||||># [Codecov](https://codecov.io/gh/huggingface/pytorch-transformers/pull/1075?src=pr&el=h1) Report
> Merging [#1075](https://codecov.io/gh/huggingface/pytorch-transformers/pull/1075?src=pr&el=desc) into [master](https://codecov.io/gh/huggingface/pytorch-transformers/commit/e00b4ff1de0591d5093407b16e665e5c86028f04?src=pr&el=desc) will **decrease** coverage by `0.02%`.
> The diff coverage is `n/a`.
[](https://codecov.io/gh/huggingface/pytorch-transformers/pull/1075?src=pr&el=tree)
```diff
@@ Coverage Diff @@
## master #1075 +/- ##
==========================================
- Coverage 79.61% 79.58% -0.03%
==========================================
Files 42 42
Lines 6898 6898
==========================================
- Hits 5492 5490 -2
- Misses 1406 1408 +2
```
| [Impacted Files](https://codecov.io/gh/huggingface/pytorch-transformers/pull/1075?src=pr&el=tree) | Coverage Δ | |
|---|---|---|
| [pytorch\_transformers/modeling\_utils.py](https://codecov.io/gh/huggingface/pytorch-transformers/pull/1075/diff?src=pr&el=tree#diff-cHl0b3JjaF90cmFuc2Zvcm1lcnMvbW9kZWxpbmdfdXRpbHMucHk=) | `83.41% <ø> (ø)` | :arrow_up: |
| [pytorch\_transformers/tokenization\_bert.py](https://codecov.io/gh/huggingface/pytorch-transformers/pull/1075/diff?src=pr&el=tree#diff-cHl0b3JjaF90cmFuc2Zvcm1lcnMvdG9rZW5pemF0aW9uX2JlcnQucHk=) | `93.89% <0%> (-0.94%)` | :arrow_down: |
------
[Continue to review full report at Codecov](https://codecov.io/gh/huggingface/pytorch-transformers/pull/1075?src=pr&el=continue).
> **Legend** - [Click here to learn more](https://docs.codecov.io/docs/codecov-delta)
> `Δ = absolute <relative> (impact)`, `ø = not affected`, `? = missing data`
> Powered by [Codecov](https://codecov.io/gh/huggingface/pytorch-transformers/pull/1075?src=pr&el=footer). Last update [e00b4ff...14eef67](https://codecov.io/gh/huggingface/pytorch-transformers/pull/1075?src=pr&el=lastupdated). Read the [comment docs](https://docs.codecov.io/docs/pull-request-comments).
<|||||>Indeed, good practice.
Do you think you could update the `from_pretrained()` method of the `PretrainedConfig` and `PreTrainedTokenizer` classes as well?<|||||>done<|||||>Thanks a lot @abhishekraok! |
transformers | 1,074 | closed | Shortcut to special tokens' ids - fix GPT2 & RoBERTa tokenizers - improved testing for GPT/GPT-2 | This PR:
- Add shortcut to each special tokens with `_id` properties (e.g. `tokenizer.cls_token_id` for the id in the vocabulary of the `tokenizer.cls_token`)
- Fix GPT2 and RoBERTa tokenizer so that sentences to be tokenized always begins with at least one space (see note by fairseq authors: https://github.com/pytorch/fairseq/blob/master/fairseq/models/roberta/hub_interface.py#L38-L56)
- Fix and clean up byte-level BPE tests
- Update Roberta tokenizer to depend on GPT2
- Update GPT2DoubleHeadModel docstring so that the given example is clear and works well
- Update the test classes for OpenAI GPT and GPT-2 to now depend on `CommonTestCases.CommonModelTester` so that these models are tested against other common tests. | 08-21-2019 22:16:22 | 08-21-2019 22:16:22 | # [Codecov](https://codecov.io/gh/huggingface/pytorch-transformers/pull/1074?src=pr&el=h1) Report
> Merging [#1074](https://codecov.io/gh/huggingface/pytorch-transformers/pull/1074?src=pr&el=desc) into [master](https://codecov.io/gh/huggingface/pytorch-transformers/commit/f7978490b20ca3a8861bddb72689a464f0c59e84?src=pr&el=desc) will **decrease** coverage by `0.29%`.
> The diff coverage is `89.23%`.
[](https://codecov.io/gh/huggingface/pytorch-transformers/pull/1074?src=pr&el=tree)
```diff
@@ Coverage Diff @@
## master #1074 +/- ##
========================================
- Coverage 80.7% 80.4% -0.3%
========================================
Files 46 46
Lines 7411 7529 +118
========================================
+ Hits 5981 6054 +73
- Misses 1430 1475 +45
```
| [Impacted Files](https://codecov.io/gh/huggingface/pytorch-transformers/pull/1074?src=pr&el=tree) | Coverage Δ | |
|---|---|---|
| [pytorch\_transformers/modeling\_openai.py](https://codecov.io/gh/huggingface/pytorch-transformers/pull/1074/diff?src=pr&el=tree#diff-cHl0b3JjaF90cmFuc2Zvcm1lcnMvbW9kZWxpbmdfb3BlbmFpLnB5) | `81.84% <ø> (+7.07%)` | :arrow_up: |
| [pytorch\_transformers/tokenization\_xlnet.py](https://codecov.io/gh/huggingface/pytorch-transformers/pull/1074/diff?src=pr&el=tree#diff-cHl0b3JjaF90cmFuc2Zvcm1lcnMvdG9rZW5pemF0aW9uX3hsbmV0LnB5) | `89.18% <100%> (ø)` | :arrow_up: |
| [...h\_transformers/tests/tokenization\_tests\_commons.py](https://codecov.io/gh/huggingface/pytorch-transformers/pull/1074/diff?src=pr&el=tree#diff-cHl0b3JjaF90cmFuc2Zvcm1lcnMvdGVzdHMvdG9rZW5pemF0aW9uX3Rlc3RzX2NvbW1vbnMucHk=) | `100% <100%> (ø)` | :arrow_up: |
| [pytorch\_transformers/tokenization\_bert.py](https://codecov.io/gh/huggingface/pytorch-transformers/pull/1074/diff?src=pr&el=tree#diff-cHl0b3JjaF90cmFuc2Zvcm1lcnMvdG9rZW5pemF0aW9uX2JlcnQucHk=) | `94.88% <100%> (ø)` | :arrow_up: |
| [pytorch\_transformers/tests/modeling\_common\_test.py](https://codecov.io/gh/huggingface/pytorch-transformers/pull/1074/diff?src=pr&el=tree#diff-cHl0b3JjaF90cmFuc2Zvcm1lcnMvdGVzdHMvbW9kZWxpbmdfY29tbW9uX3Rlc3QucHk=) | `73% <100%> (-21.74%)` | :arrow_down: |
| [pytorch\_transformers/tokenization\_gpt2.py](https://codecov.io/gh/huggingface/pytorch-transformers/pull/1074/diff?src=pr&el=tree#diff-cHl0b3JjaF90cmFuc2Zvcm1lcnMvdG9rZW5pemF0aW9uX2dwdDIucHk=) | `96.69% <100%> (+0.02%)` | :arrow_up: |
| [pytorch\_transformers/tokenization\_xlm.py](https://codecov.io/gh/huggingface/pytorch-transformers/pull/1074/diff?src=pr&el=tree#diff-cHl0b3JjaF90cmFuc2Zvcm1lcnMvdG9rZW5pemF0aW9uX3hsbS5weQ==) | `83.33% <100%> (ø)` | :arrow_up: |
| [...torch\_transformers/tests/tokenization\_gpt2\_test.py](https://codecov.io/gh/huggingface/pytorch-transformers/pull/1074/diff?src=pr&el=tree#diff-cHl0b3JjaF90cmFuc2Zvcm1lcnMvdGVzdHMvdG9rZW5pemF0aW9uX2dwdDJfdGVzdC5weQ==) | `97.36% <100%> (+0.07%)` | :arrow_up: |
| [pytorch\_transformers/tokenization\_roberta.py](https://codecov.io/gh/huggingface/pytorch-transformers/pull/1074/diff?src=pr&el=tree#diff-cHl0b3JjaF90cmFuc2Zvcm1lcnMvdG9rZW5pemF0aW9uX3JvYmVydGEucHk=) | `100% <100%> (+3.7%)` | :arrow_up: |
| [pytorch\_transformers/modeling\_gpt2.py](https://codecov.io/gh/huggingface/pytorch-transformers/pull/1074/diff?src=pr&el=tree#diff-cHl0b3JjaF90cmFuc2Zvcm1lcnMvbW9kZWxpbmdfZ3B0Mi5weQ==) | `83.84% <100%> (+8%)` | :arrow_up: |
| ... and [9 more](https://codecov.io/gh/huggingface/pytorch-transformers/pull/1074/diff?src=pr&el=tree-more) | |
------
[Continue to review full report at Codecov](https://codecov.io/gh/huggingface/pytorch-transformers/pull/1074?src=pr&el=continue).
> **Legend** - [Click here to learn more](https://docs.codecov.io/docs/codecov-delta)
> `Δ = absolute <relative> (impact)`, `ø = not affected`, `? = missing data`
> Powered by [Codecov](https://codecov.io/gh/huggingface/pytorch-transformers/pull/1074?src=pr&el=footer). Last update [f797849...50e615f](https://codecov.io/gh/huggingface/pytorch-transformers/pull/1074?src=pr&el=lastupdated). Read the [comment docs](https://docs.codecov.io/docs/pull-request-comments).
<|||||>Ok this one is also good to go. Let's merge. |
transformers | 1,073 | closed | Unable to get hidden states and attentions BertForSequenceClassification | I am able to instantiate the model etc. without the `output_` named arguments, but it fails when I include them. This is the latest master of pytorch_transformers installed via pip+git.
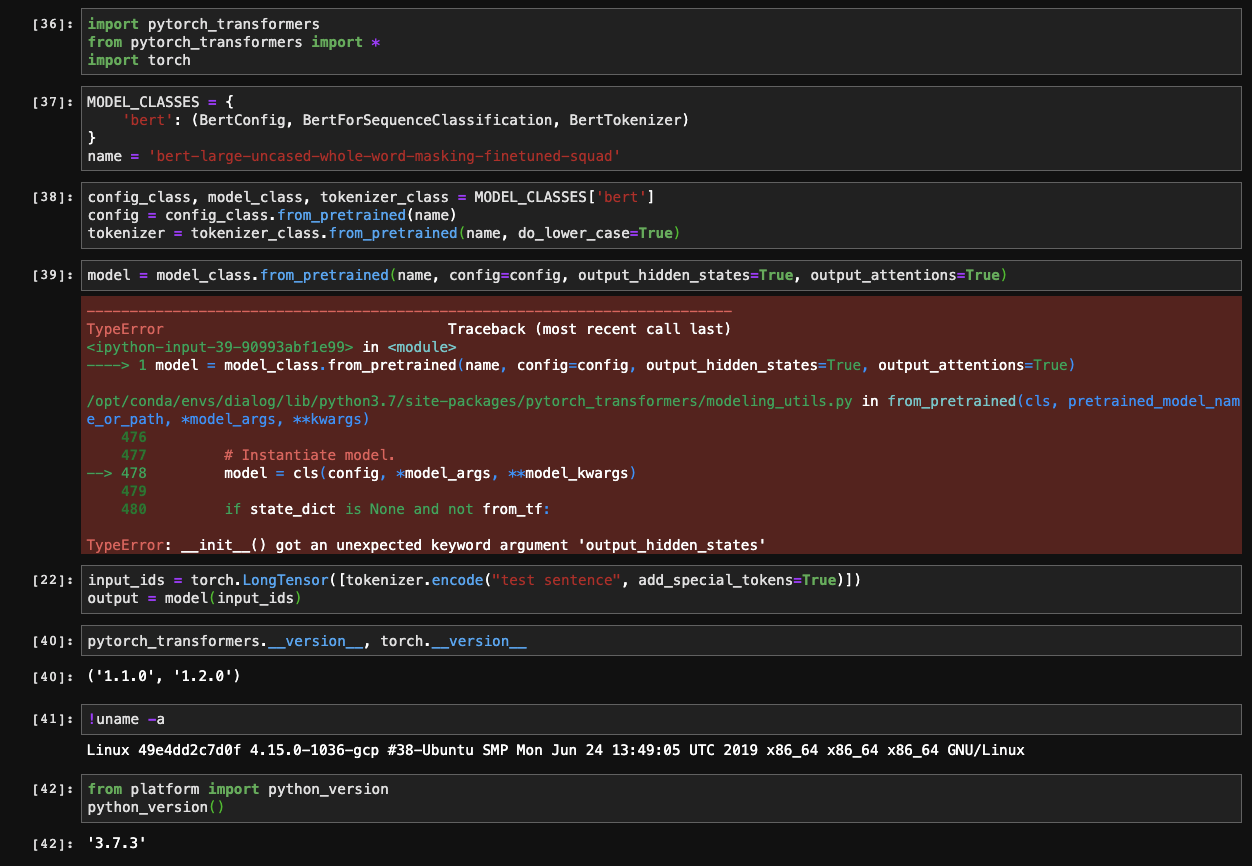
| 08-21-2019 18:09:22 | 08-21-2019 18:09:22 | Hi!
The two arguments `output_hidden_states` and `output_attentions` are arguments to be given to the configuration.
Here, you would do as follows:
```
config = config_class.from_pretrained(name, output_hidden_states=True, output_attentions=True)
tokenizer = tokenizer_class.from_pretrained(name, do_lower_case=True)
model = model.from_pretrained(name, config=config)
input_ids = torch.LongTensor([tok.encode("test sentence", add_special_tokens=True)])
output = model(input_ids)
# (logits, hidden_states, attentions)
```
You can have more information on the configuration object [here](https://huggingface.co/pytorch-transformers/main_classes/configuration.html).
Hope that helps!<|||||>Juste a few additional details:
The behavior of the added named arguments provided to `model_class.from_pretrained()` depends on whether you supply a configuration or not (see the [doc/docstrings](https://huggingface.co/pytorch-transformers/main_classes/model.html#pytorch_transformers.PreTrainedModel.from_pretrained)).
First, note that *you don't have to supply a configuration* to `model_class.from_pretrained()`. If you don't, the relevant configuration will be automatically downloaded. You can supply a configuration file if you want to control in details the parameters of the model.
As a consequence, if you supply a configuration, we assume you have already set up all the configuration parameters you need and then just forward the named arguments provided to `model_class.from_pretrained()` to the model `__init__`.
If you don't supply configuration, the relevant configuration will be automatically downloaded and the named arguments provided to `model_class.from_pretrained()` will be first passed to the configuration class initialization function (from_pretrained()). Each key of `kwargs` that corresponds to a configuration attribute will be used to override said attribute with the supplied `kwargs` value. Remaining keys that do not correspond to any configuration attribute will be passed to the underlying model’s __init__ function. This is a way to quickly set up a model with a personalized configuration.
TL;DR, you have a few ways to prepare a model like one you want:
```python
# First possibility: prepare a modified configuration yourself and use it when you
# load the model:
config = config_class.from_pretrained(name, output_hidden_states=True)
model = model.from_pretrained(name, config=config)
# Second possibility: small variant of the first possibility:
config = config_class.from_pretrained(name)
config.output_hidden_states = True
model = model.from_pretrained(name, config=config)
# Third possibility: the quickest to write, do all in one take:
model = model.from_pretrained(name, output_hidden_states=True)
# This last variant doesn't work because model.from_pretrained() will assume
# the configuration you provide is already fully prepared and doesn't know what
# to do with the provided output_hidden_states argument
config = config_class.from_pretrained(name)
model = model.from_pretrained(name, config=config, output_hidden_states=True)
```<|||||>@LysandreJik and @thomwolf, thanks for your detailed answers. This is the best documentation of the relationship between config and the model class. I think I picked up the pattern I used in my notebook from the README, particularly this one:
https://github.com/huggingface/pytorch-transformers/blob/master/README.md#quick-tour
```
model = model_class.from_pretrained(pretrained_weights,
output_hidden_states=True,
output_attentions=True)
```
I might have picked up the config class use from here:
https://github.com/huggingface/pytorch-transformers/blob/master/examples/run_squad.py#L467
My thinking was the named arguments in `model.from_pretrained` override the config. I actually like the "second possibility" style a lot for doing that. It's explicit and very clear.
```
# Second possibility: small variant of the first possibility:
config = config_class.from_pretrained(name)
config.output_hidden_states = True
model = model.from_pretrained(name, config=config)
```
Thanks again for the clarity.
|
transformers | 1,072 | closed | Missing tf variables in convert_pytorch_checkpoint_to_tf.py | ## 🐛 Bug
<!-- Important information -->
Model I am using (Bert, XLNet....): bert-base-uncased
Language I am using the model on (English, Chinese....): English
The problem arise when using:
* [x] the official example scripts: (give details)
* [ ] my own modified scripts: (give details)
The tasks I am working on is:
* [x] an official GLUE/SQUaD task: (give the name)
* [ ] my own task or dataset: (give details)
## To Reproduce
Steps to reproduce the behavior:
1. Fine tune BERT model using examples/run_glue.py
2. Use convert_pytorch_checkpoint_to_tf.py
3. Use run_classifier.py provided by BERT GitHub repo to do the prediction task.
Tensorflow will fail in loading the converted checkpoint, due to the missing variables 'global_step' and 'output_bias' (and maybe other variables) | 08-21-2019 17:23:19 | 08-21-2019 17:23:19 | Indeed.
But I don't think we will aim for two-sided compatibility with the original Bert repo anyway.
In your case, you will need to adjust the original Bert repo code to be able to load the converted pytorch model (remove the unused variables or, more simple, tweak the checkpoint loading method).<|||||>Great. Thanks for your help, @thomwolf . Closing the ticket. |
transformers | 1,071 | closed | Support for Tensorflow (& or Keras) | ## 🚀 Feature
pytorch-transformers the best NLP processing library based on the transformer model. However it has only extensive support for PyTorch, just like it's name suggests. It would be really helpful for the entire Machine Learning community to use it in their legacy project which might have been written in Tensorflow and transitioning to PyTorch is either not feasible or company policy.
> NOTE: I'm speaking on behalf of [pytorch-transfomers]() fans who have this same challenge.
<!-- A clear and concise description of the feature proposal. Please provide a link to the paper and code in case they exist. -->
## Motivation
I work in a company that has being using TensorFlow since its inception and has extensive codebase written in TensorFlow, however, it is not feasible to rewrite or utilize PyTorch in our system. And this isn't just peculiar to my company, I believe many other Machine Learning engineers face this issue as well.
That being said, It would be nice if your API could use some TensorFlow operations and pre-trained model that could utilize [TF-Hub](https://www.tensorflow.org/hub/) at the very least.
Adopting too many toolchain (e.g PyTorch, TensorFlow, Keras, MXNet, etc) isn't something that large codebase does (for easy maintainability amongst teams and whatnot).
<!-- Please outline the motivation for the proposal. Is your feature request related to a problem? e.g., I'm always frustrated when [...]. If this is related to another GitHub issue, please link here too. -->
## Additional context
Saving tensorflow checkpoints isn't just enough, it would be really helpful if you could either add-on the stable [Tensorflow r1.14](https://www.tensorflow.org/api_docs/python/tf) or [TensorFlow 2.0](https://www.tensorflow.org/beta/) beta version.
<blockquote class="twitter-tweet"><p lang="en" dir="ltr">I love pytorch-transformer 🤗 Great job <a href="https://twitter.com/huggingface?ref_src=twsrc%5Etfw">@huggingface</a> <br>Could you maybe support <a href="https://twitter.com/TensorFlow?ref_src=twsrc%5Etfw">@TensorFlow</a> too?</p>— Victor I. Afolabi (@victor_iyi) <a href="https://twitter.com/victor_iyi/status/1162456581381992452?ref_src=twsrc%5Etfw">August 16, 2019</a></blockquote> <script async src="https://platform.twitter.com/widgets.js" charset="utf-8"></script> | 08-21-2019 13:47:35 | 08-21-2019 13:47:35 | Probably not what you want to hear but you should probably look into rebuilding your infrastructure to also allow pytorch models. As someone who also uses tensorflow due to legacy systems, I wouldn't want the huggingface team to waste time struggling with tensorflow idiocracies and the currently in-flux API. <|||||>This was merely a suggestion for the TensorFlow communities as well. Not just the PyTorch community. Plus it's really hard work (next to impossible) to convert thousands of lines of TensorFlow code to PyTorch, in any case.<|||||>Sorry, I didn't mean what I said as an attack on you or anyone using tf. My intention was to present a counterpoint. I do think this is a valid suggestion, even though I disagree with it.<|||||>Gotcha! No hard feelings, so I guess it's not going to be accepted?<|||||>It might. According to this issue, it seems that 50% are for and 50% are against.<|||||>Hey guys,
We (mostly @thomwolf) have done some preliminary research into potential (partial) support for TF2, but so far we haven't committed to any specific implementation or timeframe.
Feel free to keep the discussion going in this issue, it's insightful. Thanks!<|||||>Yes, thanks for that. I think it'll go a long way. Not just with me, but other `tensorflow` & `keras` users. _Especially those that aren't really an expert in it._
On the other hand, maybe if it were to be possible _(**DISCLAIMER:** I'm not positive if it has already been implemented and shipped)_, to provide an intuitive API that saves any `pytorch-transformers` models into `tensorflow`'s checkpoints (`chkpt`) _or_ protocol buffers (`pb`) and _(or)_ `keras`'s HDF5 (`h5`) files. So it can be loaded by the `tf.estimator.Estimator` API or `keras.Model` easily.
I apologize if what I said doesn't make much sense to the developers with years of exporting & importing `tensorflow` & `keras` models. But I think the goal of `pytorch-transformers` is to make life easier for everyone! 😃
> Suggestion: The work flow could be something like implementing a simple script with the fantastic `pytorch-transformers` API, then either _exporting the trained model_ or _exporting the model architecture to be loaded as a `tf` or `keras` model_, which has been their entire codebase from inception.<|||||>> Just a suggestion
Also, it might be a lot of work switching between frameworks. So I suggest, it's best to either set a backend (`tensorflow`, or `keras`) while working with `pytorch-transformers`, without any change in `pytorch-transformers`'s API.
Although it might be difficult to add-on, but I think this will help individuals and companies that have used `tensorflow` and `keras` their entire "career" and aren't all that willing to integrate `pytorch` into their system. Not because it's not great, but because of their "design decisions" and "company rules" won't allow it.<|||||>This issue has been automatically marked as stale because it has not had recent activity. It will be closed if no further activity occurs. Thank you for your contributions.
|
transformers | 1,070 | closed | Fix the gpt2 quickstart example | You need to add the SEP (seperator) token to the tokenizer, otherwise the tokenizer.decode will fail with this error:
`ERROR:pytorch_transformers.tokenization_utils:Using sep_token, but it is not set yet.` | 08-21-2019 12:01:33 | 08-21-2019 12:01:33 | # [Codecov](https://codecov.io/gh/huggingface/pytorch-transformers/pull/1070?src=pr&el=h1) Report
> Merging [#1070](https://codecov.io/gh/huggingface/pytorch-transformers/pull/1070?src=pr&el=desc) into [master](https://codecov.io/gh/huggingface/pytorch-transformers/commit/6f877d9daf36788bad4fd228930939fed6ab12bd?src=pr&el=desc) will **not change** coverage.
> The diff coverage is `n/a`.
[](https://codecov.io/gh/huggingface/pytorch-transformers/pull/1070?src=pr&el=tree)
```diff
@@ Coverage Diff @@
## master #1070 +/- ##
=======================================
Coverage 79.61% 79.61%
=======================================
Files 42 42
Lines 6898 6898
=======================================
Hits 5492 5492
Misses 1406 1406
```
------
[Continue to review full report at Codecov](https://codecov.io/gh/huggingface/pytorch-transformers/pull/1070?src=pr&el=continue).
> **Legend** - [Click here to learn more](https://docs.codecov.io/docs/codecov-delta)
> `Δ = absolute <relative> (impact)`, `ø = not affected`, `? = missing data`
> Powered by [Codecov](https://codecov.io/gh/huggingface/pytorch-transformers/pull/1070?src=pr&el=footer). Last update [6f877d9...3248388](https://codecov.io/gh/huggingface/pytorch-transformers/pull/1070?src=pr&el=lastupdated). Read the [comment docs](https://docs.codecov.io/docs/pull-request-comments).
<|||||>This issue was fixed upstream with #1087
Thanks @oliverguhr |
transformers | 1,069 | closed | ru language | which pre-trained model can work for russian language? i want get only vectors | 08-21-2019 10:03:14 | 08-21-2019 10:03:14 | of course i can use bert aka multi-language but they work very bad in my mind for ru <|||||>I think XLM models is better than mBERT. There are XLM models for 17 and 100 languages including ru.<|||||>cool
can u help me where i can download pre-trained xlm from ru because [here](https://huggingface.co/pytorch-transformers/pretrained_models.html) i can't find models for RU?<|||||>i think [here](https://github.com/facebookresearch/XLM) i can get pre-trained<|||||>@vtrokhymenko Yes, it's here<|||||>While wanting to understand how to convert a BERT Tensorflow model to one that works in pytorch-transformers, I stumbled upon RuBERT from DeepPavlov.
https://github.com/fredriko/bert-tensorflow-pytorch-spacy-conversion<|||||>or this
```
import tensorflow as tf
from bert_dp.modeling import BertConfig, BertModel
from deeppavlov.models.preprocessors.bert_preprocessor import BertPreprocessor
bert_config = BertConfig.from_json_file('./rubert_cased_L-12_H-768_A-12_v1/bert_config.json')
input_ids = tf.placeholder(shape=(None, None), dtype=tf.int32)
input_mask = tf.placeholder(shape=(None, None), dtype=tf.int32)
token_type_ids = tf.placeholder(shape=(None, None), dtype=tf.int32)
bert = BertModel(config=bert_config,
is_training=False,
input_ids=input_ids,
input_mask=input_mask,
token_type_ids=token_type_ids,
use_one_hot_embeddings=False)
preprocessor = BertPreprocessor(vocab_file='./rubert_cased_L-12_H-768_A-12_v1/vocab.txt',
do_lower_case=False,
max_seq_length=512)
with tf.Session() as sess:
# Load model
tf.train.Saver().restore(sess, './rubert_cased_L-12_H-768_A-12_v1/bert_model.ckpt')
# Get predictions
features = preprocessor(["Bert z ulicy Sezamkowej"])[0]
print(sess.run(bert.sequence_output, feed_dict={input_ids: [features.input_ids],
input_mask: [features.input_mask],
token_type_ids: [features.input_type_ids]}))
features = preprocessor(["Это", "Берт", "с", "Улицы", "Сезам"])[0]
print(sess.run(bert.sequence_output, feed_dict={input_ids: [features.input_ids],
input_mask: [features.input_mask],
token_type_ids: [features.input_type_ids]}))
``` |
transformers | 1,068 | closed | LM fine-tuning for non-english dataset (hindi) | ## ❓ Questions & Help
Previously, I made this movie review sentiment classifier app using this wonderful library.
(Links:
https://deployment-247905.appspot.com/
https://towardsdatascience.com/battle-of-the-heavyweights-bert-vs-ulmfit-faceoff-91a582a7c42b)
Now I am looking to build a language model that will be fine-tuned on Hindi movie songs.
Out of the pretrained models I see "bert-base-multilingual-cased" and "xlm-mlm-xnli15-1024" as the ones that I can use (that support hindi language). From what I understand, GPT/GPT-2/Transformer-XL/XLNet are auto-regressive models that can be used for text generation whereas BERT or XLM are trained using masked language models (MLM) so they won't do a good job in text generation. Is that a fair statement?
Anyways, just to play around I modified run_generation.py script to also include XLM.
This gave below error:
```
File "run_generation_xlm.py", line 128, in sample_sequence
next_token_logits = outputs[0][0, -1, :] / temperature
IndexError: too many indices for tensor of dimension 2
```
So I simply removed the first index after which it could at least run.
`next_token_logits = outputs[0][-1, :] / temperature`
However the results are lousy:
```
Model prompt >>> i had lunch
just i-only day cousin from me the the the the me, the the,,,,,,,,,,,,,,,,,,,,,,,,,,,,,,,,,,,,,,,,,,, " ",,,,,,,,,,,,,,,,,,,,,,,,,,,,,,,,,,,,,,,,
Model prompt >>> i had lunch
) could me freaking these prone right so mostly so his f**king i word february our so as made gig february more " tina <special4>and dy f**k r man roll ride ride ride ride ride ride ride ride ride ride ride ride ride ride ride riding riding riding riding riding riding riding riding riding riding riding riding riding riding riding it it how how how i the all all know know and and and and and and and and and and and and and and and and and and and and and and and and and and and and
```
Questions:
1) Can I use BERT or XLM for automatic text generation? The reason to pick these is coz of availability of pretrained models.
2) Are there instructions available to fine-tune any of the model for non-english datasets?
Thanks.
PS: I'm looking for a buddy to work together with in solving such problems. If you are interested please get in touch with me. | 08-21-2019 09:32:06 | 08-21-2019 09:32:06 | Hello! Thanks for showcasing the library in your article!
You are totally correct about the auto-regressive models (XLNet, Transformer-XL, GPT-2 etc). Those models can efficiently predict the next work in a sequence as they attend to the left side of the sequence, usually trained with causal language modeling (CLM).
Using BERT or RoBERTa for text generation won't work as it was trained using a bi-directional context with masked language modeling (MLM). However, XLM has several checkpoints with different training schemes, you can see them [here](https://github.com/facebookresearch/XLM#ii-cross-lingual-language-model-pretraining-xlm).
Some of them were trained using CLM (see `xlm-clm-enfr-1024` and `xlm-clm-ende-1024`), so they should be able to generate coherent sequences of text.
Unfortunately, if you're reaching for Hindi, you probably won't be able to fine-tune any model to it. To the best of my knowledge, fine-tuning models that were trained on a specific language to other languages does not yield good results.
Some efforts have been done training models from scratch to other languages: see [deepset's German BERT](https://deepset.ai/german-bert) or [Morizeyao's chinese GPT-2](https://github.com/Morizeyao/GPT2-Chinese, maybe this could guide you.
Hope that helps.<|||||>Thank you Lysandre for the links. I'll check them out.
So if I understand correctly, I'd need a `xlm-clm-enhi-1024` model to use for hindi language. Is that right?
These checkpoints I suppose were created by HuggingFace team. Any plans to include other languages (in my case hindi) or share the steps so that we can do it ourselves?
That would be a big help. Thanks.
<|||||>Hi @nikhilno1, the checkpoints for XLM were created by the authors of XLM, Guillaume Lample and Alexis Conneau from FAIR.
You should ask on the [official XLM repository](https://github.com/facebookresearch/XLM).<|||||>Oh. When I searched for "xlm-clm-enfr-1024" I only got hits within pytorch-transformers, so I assumed it was created by HF. Thanks, I'll check with the XLM authors.<|||||>This issue has been automatically marked as stale because it has not had recent activity. It will be closed if no further activity occurs. Thank you for your contributions.
|
transformers | 1,067 | closed | Fix bug in run_openai_gpt.py file. | Add a example for add special tokens to OpenAIGPTTokenizer, and resize the embedding layer of OpenAIGPTModel. | 08-21-2019 07:12:39 | 08-21-2019 07:12:39 | run_gpt2.py file has been test on ROCStories corpus, it runs fine and return auccuracy of 76%, lower than GPT1.<|||||>This issue has been automatically marked as stale because it has not had recent activity. It will be closed if no further activity occurs. Thank you for your contributions.
|
transformers | 1,066 | closed | `run_squad.py` not using the dev cache | ## 🐛 Bug
<!-- Important information -->
Model I am using (Bert, XLNet....): Bert (but it's independent of the model)
Language I am using the model on (English, Chinese....): English (but it's independent of the language)
The problem arise when using:
* [ X] the official example scripts: `examples/run_squad.py`
The tasks I am working on is:
* [ X] an official GLUE/SQUaD task: (give the name)
It's not really a bug, more an unnecessary repetition of some operations.
It seems like the dev set is binarized (tokenized + tokens_to_id) for every single evaluation of a checkpoint even if the binarized data are already cached (from the previous evaluation for instance). It is particularly striking when adding the flag `--eval_all_checkpoints`.
It arises when calling `dataset, examples, features = load_and_cache_examples(args, tokenizer, evaluate=True, output_examples=True)` in the `evaluate` function. The cache is never used because of the argument `output_examples=True`:
```python
if os.path.exists(cached_features_file) and not args.overwrite_cache and not output_examples:
logger.info("Loading features from cached file %s", cached_features_file)
features = torch.load(cached_features_file)
```
From my understanding, except if the tokenizer changes between two checkpoints (which is not the case), the computed features are always the same.
The command I use:
```bash
python -m torch.distributed.launch --nproc_per_node=8 ./examples/run_squad.py \
--model_type bert \
--model_name_or_path bert-base-uncased \
--do_train \
--do_eval \
--do_lower_case \
--train_file $SQUAD_DIR/train-v1.1.json \
--predict_file $SQUAD_DIR/dev-v1.1.json \
--learning_rate 3e-5 \
--num_train_epochs 2 \
--max_seq_length 384 \
--doc_stride 128 \
--output_dir ../models/bert-base-uncased_finetuned_squad/ \
--per_gpu_eval_batch_size=3 \
--per_gpu_train_batch_size=3 \
--eval_all_checkpoints
``` | 08-21-2019 04:07:17 | 08-21-2019 04:07:17 | This issue has been automatically marked as stale because it has not had recent activity. It will be closed if no further activity occurs. Thank you for your contributions.
|
transformers | 1,065 | closed | Has anyone reproduced RoBERTa scores on Squad dataset? | I have been working on and made some modifications to run_squad.py in examples folder and is currently having problem reproduce the scores.
If we can have help (or even a PR) on RoBERTa in run_squad.py that would be great. | 08-21-2019 00:56:55 | 08-21-2019 00:56:55 | @Morizeyao Were you able to find any answers to this?<|||||>Can you give us more info on what you tried and which results you obtained?<|||||>Sorry I was no longer working with the RoBERTa solution and switched to XLNet. Sadly the RoBERTa tries are overwritten. :(<|||||>This issue has been automatically marked as stale because it has not had recent activity. It will be closed if no further activity occurs. Thank you for your contributions.
|
transformers | 1,064 | closed | Adding gpt-2 large (774M parameters) model | Per request #1061
Also, fix a small restriction in a few conversion scripts (easier loading from original JSON configuration files). | 08-21-2019 00:32:43 | 08-21-2019 00:32:43 | Oops @LysandreJik forgot to add it in the [list of pretrained models of the doc](https://huggingface.co/pytorch-transformers/pretrained_models.html)<|||||>@thomwolf Added it with 2f93971
<|||||>You're the best! |
transformers | 1,063 | closed | Can't load the RobertaTokenizer from AutoTokenizer.from_pretrained interface | ## 🐛 Bug
<!-- Important information -->
Model I am using (Bert, XLNet....): `AutoModel`
Language I am using the model on (English, Chinese....): English
The problem arise when using:
* [ ] the official example scripts: (give details)
* [x] my own modified scripts: (give details)
I tried to load a downloaded copy of `roberta-base` with `AutoTokenizer` and I get the following error:
```
Model name 'pretrained_models/roberta-base' was not found in model name list (bert-base-uncased, bert-large-uncased, bert-base-cased, bert-large-cased, bert-base-multilingual-uncased, bert-base-multilingual-cased, bert-base-chinese, bert-base-german-cased, bert-large-uncased-whole-word-masking, bert-large-cased-whole-word-masking, bert-large-uncased-whole-word-masking-finetuned-squad, bert-large-cased-whole-word-masking-finetuned-squad, bert-base-cased-finetuned-mrpc). We assumed 'pretrained_models/roberta-base' was a path or url but couldn't find tokenizer filesat this path or url.
```
The tasks I am working on is:
* [ ] an official GLUE/SQUaD task: (give the name)
* [x] my own task or dataset: (give details)
## To Reproduce
The compute nodes of the cluster I am working on are air-gapped, so I downloaded the `roberta-base` model weights, config and vocab files likes so
```bash
$ mkdir -p pretrained_models/roberta-base
$ wget https://s3.amazonaws.com/models.huggingface.co/bert/roberta-base-pytorch_model.bin -O pretrained_models/roberta-base/pytorch_model.bin
$ wget https://s3.amazonaws.com/models.huggingface.co/bert/roberta-base-config.json -O pretrained_models/roberta-base/config.json
$ wget https://s3.amazonaws.com/models.huggingface.co/bert/roberta-base-vocab.json -O pretrained_models/roberta-base/vocab.json
$ wget https://s3.amazonaws.com/models.huggingface.co/bert/roberta-base-merges.txt -O pretrained_models/roberta-base/merges.txt
$ ls pretrained_models/roberta-base
$ config.json merges.txt pytorch_model.bin vocab.json
```
Steps to reproduce the behavior:
```python
>>> from pytorch_transformers import AutoTokenizer
>>> AutoTokenizer.from_pretrained('pretrained_models/roberta-base')
Model name 'pretrained_models/roberta-base' was not found in model name list (bert-base-uncased, bert-large-uncased, bert-base-cased, bert-large-cased, bert-base-multilingual-uncased, bert-base-multilingual-cased, bert-base-chinese, bert-base-german-cased, bert-large-uncased-whole-word-masking, bert-large-cased-whole-word-masking, bert-large-uncased-whole-word-masking-finetuned-squad, bert-large-cased-whole-word-masking-finetuned-squad, bert-base-cased-finetuned-mrpc). We assumed 'pretrained_models/roberta-base' was a path or url but couldn't find tokenizer filesat this path or url.
>>>
```
## Expected behavior
I expect `AutoTokenizer` to return a `RobertaTokenizer` object initialized with the `vocab.json` and `merges.txt` file from `pretrained_models/roberta-base`.
## Environment
* OS: Ubuntu 18.04
* Python version: 3.7.0
* PyTorch version: 1.2.0
* PyTorch Transformers version (or branch): 1.1.0
* Using GPU ? Yes
* Distributed of parallel setup ? No
* Any other relevant information: Compute nodes are air-gapped so I must download the model on a login node.
## Additional context
If I try to simply provide `roberta-base` to `AutoTokenizer`, I get the same issue
```python
>>> from pytorch_transformers import AutoTokenizer
>>> AutoTokenizer.from_pretrained('roberta-base')
Model name 'roberta-base' was not found in model name list (bert-base-uncased, bert-large-uncased, bert-base-cased, bert-large-cased, bert-base-multilingual-uncased, bert-base-multilingual-cased, bert-base-chinese, bert-base-german-cased, bert-large-uncased-whole-word-masking, bert-large-cased-whole-word-masking, bert-large-uncased-whole-word-masking-finetuned-squad, bert-large-cased-whole-word-masking-finetuned-squad, bert-base-cased-finetuned-mrpc). We assumed 'roberta-base' was a path or url but couldn't find tokenizer filesat this path or url.
```
If I rename `pretrained_models/roberta-base/vocab.json` to `pretrained_models/roberta-base/vocab.txt`, then `AutoModel` returns a `BertTokenizer` object
```bash
$ mv pretrained_models/roberta-base/vocab.json pretrained_models/roberta-base/vocab.txt
```
```python
>>> from pytorch_transformers import AutoTokenizer
>>> AutoTokenizer.from_pretrained('pretrained_models/roberta-base')
<pytorch_transformers.tokenization_bert.BertTokenizer object at 0x7f0de6605588>
>>>
``` | 08-20-2019 22:30:47 | 08-20-2019 22:30:47 | This should be fixed on master, can you try to install from master?
(clone the repo and `pip install -e .`).<|||||>Ah, that solved it. Great, thanks a lot!
<|||||>@thomwolf, is this fixed in the latest pip release version?<|||||>Yes, it is available in the latest pip release. |
transformers | 1,062 | closed | Example in OpenAIGPTDoubleHeadsModel can't run | I tried to run the example from OpenAIGPTDoubleHeadsModel. But it went wrong.
Although the tokenizer added new index for special tokens, the embedding in OpenAIGPTDoubleHeadModel didn't add new embeddings for them, which leads to index out of range.
```
tokenizer = OpenAIGPTTokenizer.from_pretrained('openai-gpt')
model = OpenAIGPTDoubleHeadsModel.from_pretrained('openai-gpt')
tokenizer.add_special_tokens({'cls_token': '[CLS]'}) # Add a [CLS] to the vocabulary (we should train it also!)
choices = ["Hello, my dog is cute [CLS]", "Hello, my cat is cute [CLS]"]
input_ids = torch.tensor([tokenizer.encode(s) for s in choices]).unsqueeze(0) # Batch size 1, 2 choices
mc_token_ids = torch.tensor([input_ids.size(-1), input_ids.size(-1)]).unsqueeze(0) # Batch size 1
outputs = model(input_ids, mc_token_ids)
lm_prediction_scores, mc_prediction_scores = outputs[:2]
``` | 08-20-2019 21:10:51 | 08-20-2019 21:10:51 | Yes, you need to resize the embeddings as well.
There is [an example](https://huggingface.co/pytorch-transformers/main_classes/tokenizer.html#pytorch_transformers.PreTrainedTokenizer.add_special_tokens) in the doc of the `add_special_tokens`method, that I copy here:
```
# Let's see how to add a new classification token to GPT-2
tokenizer = GPT2Tokenizer.from_pretrained('gpt2')
model = GPT2Model.from_pretrained('gpt2')
special_tokens_dict = {'cls_token': '<CLS>'}
num_added_toks = tokenizer.add_special_tokens(special_tokens_dict)
print('We have added', num_added_toks, 'tokens')
model.resize_token_embeddings(len(tokenizer)) # Notice: resize_token_embeddings expect to receive the full size of the new vocabulary, i.e. the length of the tokenizer.
assert tokenizer.cls_token == '<CLS>'
```<|||||>Ah, I see. Now it works. Thanks a lot. |
transformers | 1,061 | closed | GPT2 774M weights released! | ## 🚀 Feature
Hi! OpenAI released the 774M weights in GPT2, is it possible to integrate this into pytorch-transformers?
https://twitter.com/OpenAI/status/1163843803884601344
Also, sorry for the obnoxiously quick ask! Thanks for all the great work you do for the community.
Thanks! | 08-20-2019 16:13:29 | 08-20-2019 16:13:29 | I did the following:
1. Run `download_model.py 774` from [here](https://github.com/openai/gpt-2)
2. Create a file named `config.json` with the following contents (Might be correct but I am not super sure):
```json
{
"vocab_size": 50257,
"n_ctx": 1024,
"n_embd": 1280,
"n_head": 20,
"n_layer": 36,
"n_positions": 1024,
"embd_pdrop":0.1,
"attn_pdrop": 0.1,
"resid_pdrop": 0.1,
"layer_norm_epsilon": 1e-5,
"initializer_range": 0.02
}
```
3. Clone this repo
4. Run ```python .\pytorch-transformers\pytorch_transformers\convert_gpt2_checkpoint_to_pytorch.py --gpt2_checkpoint_path models/774M --pytorch_dump_folder_path ./ --gpt2_config_file config.json```
5. Use it with
```
config = GPT2Config.from_pretrained("config.json")
model = GPT2LMHeadModel.from_pretrained("pytorch_model.bin", config=config)
```
6. Realize there's no way you can fine-tune this your PC's GPU you need to rent something with more memory.<|||||>We've added it on master.
You can install from source and use the shortcut name `gpt2-large` to use it (but beware, it's big!)<|||||>Question: Will the gpt2-large be added to Write With Transformer? I've been eagerly looking forward to that since the moment the 774M was released!<|||||>@zacharymacleod Glad you asked! We're definitely planning on adding it in the near future :)<|||||>Seems to me as if this has been addressed via #1064 . Closing the feature request now! |
transformers | 1,060 | closed | Fix typo. configuratoin -> configuration | 08-20-2019 12:43:20 | 08-20-2019 12:43:20 | Thanks! |
|
transformers | 1,059 | closed | Better use of spacy tokenizer in open ai and xlm tokenizers | When you do: `spacy.load('en', disable=['parser', 'tagger', 'ner', 'textcat'])` There is a high risk of throwing an exception if the user did not install the model before.
Te easiest way to use the spaCy tokenizer is the one I propose here. This way there is no need for the user to download any spaCy model.
More info here: https://spacy.io/api/tokenizer#init | 08-20-2019 12:18:24 | 08-20-2019 12:18:24 | # [Codecov](https://codecov.io/gh/huggingface/pytorch-transformers/pull/1059?src=pr&el=h1) Report
> Merging [#1059](https://codecov.io/gh/huggingface/pytorch-transformers/pull/1059?src=pr&el=desc) into [master](https://codecov.io/gh/huggingface/pytorch-transformers/commit/933841d903a032d93b5100220dc72db9d1283eca?src=pr&el=desc) will **decrease** coverage by `0.02%`.
> The diff coverage is `0%`.
[](https://codecov.io/gh/huggingface/pytorch-transformers/pull/1059?src=pr&el=tree)
```diff
@@ Coverage Diff @@
## master #1059 +/- ##
==========================================
- Coverage 79.6% 79.57% -0.03%
==========================================
Files 42 42
Lines 6863 6865 +2
==========================================
Hits 5463 5463
- Misses 1400 1402 +2
```
| [Impacted Files](https://codecov.io/gh/huggingface/pytorch-transformers/pull/1059?src=pr&el=tree) | Coverage Δ | |
|---|---|---|
| [pytorch\_transformers/tokenization\_openai.py](https://codecov.io/gh/huggingface/pytorch-transformers/pull/1059/diff?src=pr&el=tree#diff-cHl0b3JjaF90cmFuc2Zvcm1lcnMvdG9rZW5pemF0aW9uX29wZW5haS5weQ==) | `81.51% <0%> (-0.7%)` | :arrow_down: |
| [pytorch\_transformers/tokenization\_xlm.py](https://codecov.io/gh/huggingface/pytorch-transformers/pull/1059/diff?src=pr&el=tree#diff-cHl0b3JjaF90cmFuc2Zvcm1lcnMvdG9rZW5pemF0aW9uX3hsbS5weQ==) | `83.06% <0%> (-0.68%)` | :arrow_down: |
------
[Continue to review full report at Codecov](https://codecov.io/gh/huggingface/pytorch-transformers/pull/1059?src=pr&el=continue).
> **Legend** - [Click here to learn more](https://docs.codecov.io/docs/codecov-delta)
> `Δ = absolute <relative> (impact)`, `ø = not affected`, `? = missing data`
> Powered by [Codecov](https://codecov.io/gh/huggingface/pytorch-transformers/pull/1059?src=pr&el=footer). Last update [933841d...388e325](https://codecov.io/gh/huggingface/pytorch-transformers/pull/1059?src=pr&el=lastupdated). Read the [comment docs](https://docs.codecov.io/docs/pull-request-comments).
<|||||>Indeed! |
transformers | 1,058 | closed | Initialising XLMTokenizer | ## ❓ Questions & Help
To initialise the XLMTokenizer, both the vocab file and the merges.txt file are needed. If I am pre-training XLM, how do I obtain the merges.txt file?
| 08-20-2019 09:59:41 | 08-20-2019 09:59:41 | To pretrain XLM, you should use the original (PyTorch) codebase and training scripts which are [here](https://github.com/facebookresearch/XLM)<|||||>This issue has been automatically marked as stale because it has not had recent activity. It will be closed if no further activity occurs. Thank you for your contributions.
|
transformers | 1,057 | closed | Add a few of typos corrections, bugs fixes and small improvements | - Add a `force_download` option to `from_pretrained` methods to override a corrupted file.
- Add a `proxies` option to `from_pretrained` methods to be able to use proxies.
- Update models doc (superseded #984)
- Fix a small bug when using Bert's `save_vocabulary` method with the path to a file instead of a directory (#1014)
- Detailed doc strings following #808
- Detailed doc strings following #1034 | 08-20-2019 09:03:54 | 08-20-2019 09:03:54 | # [Codecov](https://codecov.io/gh/huggingface/pytorch-transformers/pull/1057?src=pr&el=h1) Report
> Merging [#1057](https://codecov.io/gh/huggingface/pytorch-transformers/pull/1057?src=pr&el=desc) into [master](https://codecov.io/gh/huggingface/pytorch-transformers/commit/c589862b783b94a8408b40c6dc9bf4a14b2ee391?src=pr&el=desc) will **decrease** coverage by `<.01%`.
> The diff coverage is `91.66%`.
[](https://codecov.io/gh/huggingface/pytorch-transformers/pull/1057?src=pr&el=tree)
```diff
@@ Coverage Diff @@
## master #1057 +/- ##
==========================================
- Coverage 79.6% 79.59% -0.01%
==========================================
Files 42 42
Lines 6863 6867 +4
==========================================
+ Hits 5463 5466 +3
- Misses 1400 1401 +1
```
| [Impacted Files](https://codecov.io/gh/huggingface/pytorch-transformers/pull/1057?src=pr&el=tree) | Coverage Δ | |
|---|---|---|
| [pytorch\_transformers/modeling\_roberta.py](https://codecov.io/gh/huggingface/pytorch-transformers/pull/1057/diff?src=pr&el=tree#diff-cHl0b3JjaF90cmFuc2Zvcm1lcnMvbW9kZWxpbmdfcm9iZXJ0YS5weQ==) | `75.89% <ø> (ø)` | :arrow_up: |
| [pytorch\_transformers/modeling\_xlnet.py](https://codecov.io/gh/huggingface/pytorch-transformers/pull/1057/diff?src=pr&el=tree#diff-cHl0b3JjaF90cmFuc2Zvcm1lcnMvbW9kZWxpbmdfeGxuZXQucHk=) | `79.01% <ø> (ø)` | :arrow_up: |
| [pytorch\_transformers/modeling\_transfo\_xl.py](https://codecov.io/gh/huggingface/pytorch-transformers/pull/1057/diff?src=pr&el=tree#diff-cHl0b3JjaF90cmFuc2Zvcm1lcnMvbW9kZWxpbmdfdHJhbnNmb194bC5weQ==) | `57.53% <ø> (ø)` | :arrow_up: |
| [pytorch\_transformers/modeling\_openai.py](https://codecov.io/gh/huggingface/pytorch-transformers/pull/1057/diff?src=pr&el=tree#diff-cHl0b3JjaF90cmFuc2Zvcm1lcnMvbW9kZWxpbmdfb3BlbmFpLnB5) | `74.76% <ø> (ø)` | :arrow_up: |
| [pytorch\_transformers/modeling\_xlm.py](https://codecov.io/gh/huggingface/pytorch-transformers/pull/1057/diff?src=pr&el=tree#diff-cHl0b3JjaF90cmFuc2Zvcm1lcnMvbW9kZWxpbmdfeGxtLnB5) | `86.66% <ø> (ø)` | :arrow_up: |
| [pytorch\_transformers/modeling\_bert.py](https://codecov.io/gh/huggingface/pytorch-transformers/pull/1057/diff?src=pr&el=tree#diff-cHl0b3JjaF90cmFuc2Zvcm1lcnMvbW9kZWxpbmdfYmVydC5weQ==) | `87.98% <ø> (ø)` | :arrow_up: |
| [pytorch\_transformers/modeling\_gpt2.py](https://codecov.io/gh/huggingface/pytorch-transformers/pull/1057/diff?src=pr&el=tree#diff-cHl0b3JjaF90cmFuc2Zvcm1lcnMvbW9kZWxpbmdfZ3B0Mi5weQ==) | `75.84% <ø> (ø)` | :arrow_up: |
| [pytorch\_transformers/tokenization\_bert.py](https://codecov.io/gh/huggingface/pytorch-transformers/pull/1057/diff?src=pr&el=tree#diff-cHl0b3JjaF90cmFuc2Zvcm1lcnMvdG9rZW5pemF0aW9uX2JlcnQucHk=) | `94.83% <0%> (-0.45%)` | :arrow_down: |
| [pytorch\_transformers/modeling\_utils.py](https://codecov.io/gh/huggingface/pytorch-transformers/pull/1057/diff?src=pr&el=tree#diff-cHl0b3JjaF90cmFuc2Zvcm1lcnMvbW9kZWxpbmdfdXRpbHMucHk=) | `83.33% <100%> (+0.08%)` | :arrow_up: |
| [pytorch\_transformers/file\_utils.py](https://codecov.io/gh/huggingface/pytorch-transformers/pull/1057/diff?src=pr&el=tree#diff-cHl0b3JjaF90cmFuc2Zvcm1lcnMvZmlsZV91dGlscy5weQ==) | `71.22% <100%> (ø)` | :arrow_up: |
| ... and [1 more](https://codecov.io/gh/huggingface/pytorch-transformers/pull/1057/diff?src=pr&el=tree-more) | |
------
[Continue to review full report at Codecov](https://codecov.io/gh/huggingface/pytorch-transformers/pull/1057?src=pr&el=continue).
> **Legend** - [Click here to learn more](https://docs.codecov.io/docs/codecov-delta)
> `Δ = absolute <relative> (impact)`, `ø = not affected`, `? = missing data`
> Powered by [Codecov](https://codecov.io/gh/huggingface/pytorch-transformers/pull/1057?src=pr&el=footer). Last update [c589862...6d0aa73](https://codecov.io/gh/huggingface/pytorch-transformers/pull/1057?src=pr&el=lastupdated). Read the [comment docs](https://docs.codecov.io/docs/pull-request-comments).
|
transformers | 1,056 | closed | Swap of optimizer.step and scheduler.step for lm finetuning examples | Prior to PyTorch 1.1.0, the learning rate scheduler was expected to be called before the optimizer’s update; 1.1.0 changed this behavior in a BC-breaking way. If you use the learning rate scheduler (calling scheduler.step()) before the optimizer’s update (calling optimizer.step()), this will skip the first value of the learning rate schedule. If you are unable to reproduce results after upgrading to PyTorch 1.1.0, please check if you are calling scheduler.step() at the wrong time.
This is my first time very simple PR, please correct me if there's anything done wrong xD.
[link](https://pytorch.org/docs/stable/optim.html#how-to-adjust-learning-rate) | 08-20-2019 08:01:50 | 08-20-2019 08:01:50 | # [Codecov](https://codecov.io/gh/huggingface/pytorch-transformers/pull/1056?src=pr&el=h1) Report
> Merging [#1056](https://codecov.io/gh/huggingface/pytorch-transformers/pull/1056?src=pr&el=desc) into [master](https://codecov.io/gh/huggingface/pytorch-transformers/commit/c589862b783b94a8408b40c6dc9bf4a14b2ee391?src=pr&el=desc) will **not change** coverage.
> The diff coverage is `n/a`.
[](https://codecov.io/gh/huggingface/pytorch-transformers/pull/1056?src=pr&el=tree)
```diff
@@ Coverage Diff @@
## master #1056 +/- ##
======================================
Coverage 79.6% 79.6%
======================================
Files 42 42
Lines 6863 6863
======================================
Hits 5463 5463
Misses 1400 1400
```
------
[Continue to review full report at Codecov](https://codecov.io/gh/huggingface/pytorch-transformers/pull/1056?src=pr&el=continue).
> **Legend** - [Click here to learn more](https://docs.codecov.io/docs/codecov-delta)
> `Δ = absolute <relative> (impact)`, `ø = not affected`, `? = missing data`
> Powered by [Codecov](https://codecov.io/gh/huggingface/pytorch-transformers/pull/1056?src=pr&el=footer). Last update [c589862...d86b49a](https://codecov.io/gh/huggingface/pytorch-transformers/pull/1056?src=pr&el=lastupdated). Read the [comment docs](https://docs.codecov.io/docs/pull-request-comments).
<|||||>Looks good to me, thanks @Morizeyao! |
transformers | 1,055 | closed | Fix #1015 (tokenizer defaults to use_lower_case=True when loading from trained models) | This PR fixes the issue where the tokenizer always defaults to `use_lower_case=True` when loading from trained models. It returns the control to the command-line arguments. | 08-19-2019 20:09:00 | 08-19-2019 20:09:00 | # [Codecov](https://codecov.io/gh/huggingface/pytorch-transformers/pull/1055?src=pr&el=h1) Report
> Merging [#1055](https://codecov.io/gh/huggingface/pytorch-transformers/pull/1055?src=pr&el=desc) into [master](https://codecov.io/gh/huggingface/pytorch-transformers/commit/c589862b783b94a8408b40c6dc9bf4a14b2ee391?src=pr&el=desc) will **not change** coverage.
> The diff coverage is `n/a`.
[](https://codecov.io/gh/huggingface/pytorch-transformers/pull/1055?src=pr&el=tree)
```diff
@@ Coverage Diff @@
## master #1055 +/- ##
======================================
Coverage 79.6% 79.6%
======================================
Files 42 42
Lines 6863 6863
======================================
Hits 5463 5463
Misses 1400 1400
```
------
[Continue to review full report at Codecov](https://codecov.io/gh/huggingface/pytorch-transformers/pull/1055?src=pr&el=continue).
> **Legend** - [Click here to learn more](https://docs.codecov.io/docs/codecov-delta)
> `Δ = absolute <relative> (impact)`, `ø = not affected`, `? = missing data`
> Powered by [Codecov](https://codecov.io/gh/huggingface/pytorch-transformers/pull/1055?src=pr&el=footer). Last update [c589862...3bffd2e](https://codecov.io/gh/huggingface/pytorch-transformers/pull/1055?src=pr&el=lastupdated). Read the [comment docs](https://docs.codecov.io/docs/pull-request-comments).
<|||||>That's great, thanks @qipeng.
Do you think you could do the same fix on the `run_glue` example?<|||||>Added tokenizer fix in `run_glue.py` and fixed `do_train` logic in `run_squad.py`<|||||>Great, thanks a lot @qipeng! |
transformers | 1,054 | closed | simple example of BERT input features : position_ids and head_mask | ## Background:
the documentation does a great job in explaining the particularities of BERT input features (input_ids, token_types_ids etc …) however for some (if not most) tasks other inputs features are required and I think it would help the users if they were explained with examples.
## Question:
could we add to the documentation examples of how to get **position_ids** and **head_mask** for a given text input ?
I have seen that they are requested in BertForClassification class (in (pytorch_transformers/modeling_bert) and that they are explained in the BERT_INPUTS_DOCSTRING but I have not seen an example of how to get them.
The documentation says
**position_ids**: Indices of positions of each input sequence tokens in the position embeddings. Selected in the range : [0, config.max_position_embeddings - 1]
**head_mask**: Mask to nullify selected heads of the self-attention modules.
0 for masked and 1 for not masked
but it is not clear to me how to get them from a given text input.
## example of other inputs features :
I experimented with creating input features from a dataframe and I came up with the function below which tries to make explicit each step in input features. I think it could be useful for a tutorial. I would like to add the position_ids and head_mask
q1 = {'text' :["Who was Jim Henson ?",
"Jim Henson was an American puppeteer",
"I love Mom's cooking",
"I love you too !",
"No way",
"This is the kid",
"Yes"
],
'label' : [1, 0, 1, 1, 0, 1, 0]}
import pandas as pd
xdf = pd.DataFrame(q1)
from pytorch_transformers import BertTokenizer
from torch.utils.data import TensorDataset
xtokenizer = BertTokenizer.from_pretrained('bert-base-uncased')
def text_to_bertfeatures(df,
col_text,
col_labels=None,
max_length=6,
cls_token='[CLS]',
sep_token='[SEP]'):
''' create a tensordataset with bert input features
input:
- data frame with a column for text and a column for labels
-maximum sequence length
-special tokens
output:
tensor dataset with
**input_ids**: Indices of input sequence tokens in the vocabulary.
**labels** (if specified)
**token_type_ids**: Segment token indices to indicate first and second portions of the inputs. 0 for sentence A and 1 for sentence B
in the glue example they are called *segment_ids*
**attention_mask**: Mask to avoid performing attention on padding token indices. 0 for masked and 1 for not masked
in the glue example they are called *input_mask*
TO DO:
This is for tasks requiring a single "sequence/sentence" input
like classification , it could be modified for two sentences tasks
eventually option to pad left
'''
xlst_text = df[col_text]
# input text with special tokens
x_input_txt_sptokens = [cls_token + ' ' + x + ' ' + sep_token for x in xlst_text]
# input tokens
x_input_tokens = [xtokenizer.tokenize(x_text) for x_text in x_input_txt_sptokens]
# input ids
x_input_ids_int = [xtokenizer.convert_tokens_to_ids(xtoks) for xtoks in x_input_tokens]
# inputs with maximal length
x_input_ids_maxlen = [xtoks[0:max_length] for xtoks in x_input_ids_int]
# Input paaded with zeros on the right
x_input_ids_padded = [xtoks + [0] * (max_length - len(xtoks)) for xtoks in x_input_ids_maxlen]
# token_type_ids
token_type_ids_int = [[1 for x in tok_ids] for tok_ids in x_input_ids_padded]
# attention mask
attention_mask_int = [[int(x > 0) for x in tok_ids] for tok_ids in x_input_ids_padded]
# inputs to tensors
input_ids = torch.tensor(x_input_ids_padded, dtype=torch.long)
token_type_ids = torch.tensor(token_type_ids_int, dtype=torch.long)
attention_mask = torch.tensor(attention_mask_int, dtype=torch.long)
# labels if any:
if col_labels:
labels_int = [int(x) for x in list(df[col_labels])]
labels = torch.tensor(labels_int, dtype=torch.long)
xdset = TensorDataset(input_ids, token_type_ids, attention_mask, labels)
else:
xdset = TensorDataset(input_ids, token_type_ids, attention_mask)
return xdset
text_to_bertfeatures(df = xdf,
col_text = 'text',
col_labels = 'label',
max_length = 6,
cls_token='[CLS]',
sep_token='[SEP]') | 08-19-2019 19:21:28 | 08-19-2019 19:21:28 | Hi,
If you read the documentation [here](https://huggingface.co/pytorch-transformers/model_doc/bert.html#bertfortokenclassification) you will see that `position_ids` and `head_mask` are not required inputs but are optional.
No need to give them if you don't want to (and you probably don't unless you are doing complex stuff like custom position or head masking).<|||||>thanks Thomas.
Very helpful comment about need for this only for custom positioning.
In my case I indeed do not need it.
I am closing the issue to avoid clogging the list of open issues.
P.S: I also take the occasion to thank you (and all other contributors) for this amazing work.
We do not take for granted the fact that the most advanced models are accessible in so short time after their publication. Thank you.
|
transformers | 1,053 | closed | reproducing bert results on snli and mnli | Hi
I have finetuned bert for snli and mnli for 6 epochs for none of them I could reproduce bert results on these datasets. I also encountered degenerate solution which get around 47 accuracy, could you assist me how I can avoid this issue? so when there are several checkpoints, I always evaluate the last one after 6 epochs, thanks. | 08-19-2019 12:29:22 | 08-19-2019 12:29:22 | This issue has been automatically marked as stale because it has not had recent activity. It will be closed if no further activity occurs. Thank you for your contributions.
|
transformers | 1,052 | closed | Fix RobertaEmbeddings | First of all, the original implementation can define `segment_embeddings` depending on `num_segments` argument. Actually, their model(Roberta) didn't use `segment_embeddings` because they found the effectiveness of `FULL/DOC SENTENCE` setting of inputs.
And `position_embeddings` should use `padding_idx` to ignore padded inputs. Also the embedding matrix's size should be `padding_idx + max_seq_length + 1`. (e.g If `padding_idx=1` and `max_seq_length=512`, `maxtrix size = (1 + 512 + 1) = 514`.
Last, `position_ids` should be made by considering the previous feature. Below is simple test to make `position_ids` to reflect `padding_idx` of `input_ids`
```
input_ids = torch.randint(0,1000,(3,10))
padding_idx = 0
### dummy padded input
input_ids[:,-2] = padding_idx
input_ids[:,-1] = padding_idx
input_ids[0][-3] = padding_idx
input_ids[-1][-3] = padding_idx
input_ids
>>> tensor([[946, 783, 399, 951, 496, 400, 350, 0, 0, 0],
[905, 445, 410, 406, 526, 1, 255, 811, 0, 0],
[815, 669, 813, 708, 475, 232, 190, 0, 0, 0]])
```
```
mask = input_ids.ne(padding_idx).int()
position_ids = (torch.cumsum(mask, dim=1).type_as(mask) * mask).long() + padding_idx
position_ids
>>> tensor([[1, 2, 3, 4, 5, 6, 7, 0, 0, 0],
[1, 2, 3, 4, 5, 6, 7, 8, 0, 0],
[1, 2, 3, 4, 5, 6, 7, 0, 0, 0]])
``` | 08-19-2019 04:52:08 | 08-19-2019 04:52:08 | # [Codecov](https://codecov.io/gh/huggingface/pytorch-transformers/pull/1052?src=pr&el=h1) Report
> Merging [#1052](https://codecov.io/gh/huggingface/pytorch-transformers/pull/1052?src=pr&el=desc) into [master](https://codecov.io/gh/huggingface/pytorch-transformers/commit/40acf6b52a5250608c2b90edd955835131971d5a?src=pr&el=desc) will **increase** coverage by `0.11%`.
> The diff coverage is `92%`.
[](https://codecov.io/gh/huggingface/pytorch-transformers/pull/1052?src=pr&el=tree)
```diff
@@ Coverage Diff @@
## master #1052 +/- ##
==========================================
+ Coverage 79.57% 79.68% +0.11%
==========================================
Files 42 42
Lines 6863 6881 +18
==========================================
+ Hits 5461 5483 +22
+ Misses 1402 1398 -4
```
| [Impacted Files](https://codecov.io/gh/huggingface/pytorch-transformers/pull/1052?src=pr&el=tree) | Coverage Δ | |
|---|---|---|
| [pytorch\_transformers/modeling\_bert.py](https://codecov.io/gh/huggingface/pytorch-transformers/pull/1052/diff?src=pr&el=tree#diff-cHl0b3JjaF90cmFuc2Zvcm1lcnMvbW9kZWxpbmdfYmVydC5weQ==) | `88% <100%> (+0.02%)` | :arrow_up: |
| [pytorch\_transformers/modeling\_roberta.py](https://codecov.io/gh/huggingface/pytorch-transformers/pull/1052/diff?src=pr&el=tree#diff-cHl0b3JjaF90cmFuc2Zvcm1lcnMvbW9kZWxpbmdfcm9iZXJ0YS5weQ==) | `77.51% <91.66%> (+1.62%)` | :arrow_up: |
| [pytorch\_transformers/tokenization\_bert.py](https://codecov.io/gh/huggingface/pytorch-transformers/pull/1052/diff?src=pr&el=tree#diff-cHl0b3JjaF90cmFuc2Zvcm1lcnMvdG9rZW5pemF0aW9uX2JlcnQucHk=) | `95.28% <0%> (+0.94%)` | :arrow_up: |
| [pytorch\_transformers/file\_utils.py](https://codecov.io/gh/huggingface/pytorch-transformers/pull/1052/diff?src=pr&el=tree#diff-cHl0b3JjaF90cmFuc2Zvcm1lcnMvZmlsZV91dGlscy5weQ==) | `74.1% <0%> (+2.87%)` | :arrow_up: |
------
[Continue to review full report at Codecov](https://codecov.io/gh/huggingface/pytorch-transformers/pull/1052?src=pr&el=continue).
> **Legend** - [Click here to learn more](https://docs.codecov.io/docs/codecov-delta)
> `Δ = absolute <relative> (impact)`, `ø = not affected`, `? = missing data`
> Powered by [Codecov](https://codecov.io/gh/huggingface/pytorch-transformers/pull/1052?src=pr&el=footer). Last update [40acf6b...e2a628a](https://codecov.io/gh/huggingface/pytorch-transformers/pull/1052?src=pr&el=lastupdated). Read the [comment docs](https://docs.codecov.io/docs/pull-request-comments).
<|||||>This looks reasonable to me but I'd need to take a better look at it.
Maybe @myleott do you have time to take a quick glance?<|||||>This issue has been automatically marked as stale because it has not had recent activity. It will be closed if no further activity occurs. Thank you for your contributions.
|
transformers | 1,051 | closed | BUG: run_openai_gpt.py load ROCStories data error | ## 🐛 Bug
Model I am using (Bert, XLNet....): GPT
Language I am using the model on (English, Chinese....): English
The problem arise when using:
* [ 1 ] the official example scripts: run_openai_gpt.py
The tasks I am working on is:
* [ 1 ] an official GLUE/SQUaD task: ROCStories
**The method of preprocess of ROCStories use ids 0 pad input, while 0 is actually the id of unk_tokens.**
The code of method is as fellowing:
```
def pre_process_datasets(encoded_datasets, input_len, cap_length, start_token, delimiter_token, clf_token):
tensor_datasets = []
for dataset in encoded_datasets:
n_batch = len(dataset)
input_ids = np.zeros((n_batch, 2, input_len), dtype=np.int64)
for i, (story, cont1, cont2, mc_label), in enumerate(dataset):
with_cont1 = [start_token] + story[:cap_length] + [delimiter_token] + cont1[:cap_length] + [clf_token]
with_cont2 = [start_token] + story[:cap_length] + [delimiter_token] + cont2[:cap_length] + [clf_token]
input_ids[i, 0, :len(with_cont1)] = with_cont1
input_ids[i, 1, :len(with_cont2)] = with_cont2
```
input_ids initialize with 0, which is the id of unk_token, rather than id of pad_token. | 08-19-2019 01:44:55 | 08-19-2019 01:44:55 | Hi @nine09, thanks for the report. Any way you could fix it cleanly and open a pull request?<|||||>> Hi @nine09, thanks for the report. Any way you could fix it cleanly and open a pull request?
Bien sur! But I want to have some clues about whether GPTTokenizer already have pad_token, otherwise add a new pad_token need resize embedding of GPTModel.<|||||>I have fix the bug at #1067, that change add a pad_token to GPTTokenizer so that solved this problem. |
transformers | 1,050 | closed | Error in converting tensorflow checkpoints to pytorch | @thomwolf
I downloaded tensorflow checkpoints for domain specific bert model and extracted the zip file into the folder **pretrained_bert** which contains the following the three files
model.ckpt.data-00000-of-00001
model.ckpt.index
model.ckpt.meta
I used the following code to convert tensorflow checkpoints to pytorch
```
import torch
from pytorch_transformers.modeling_bert import BertConfig, BertForPreTraining, load_tf_weights_in_bert
tf_checkpoint_path="pretrained_bert/model.ckpt"
bert_config_file = "bert-base-cased-config.json"
pytorch_dump_path="pytorch_bert"
config = BertConfig.from_json_file(bert_config_file)
print("Building PyTorch model from configuration: {}".format(str(config)))
model = BertForPreTraining(config)
# Load weights from tf checkpoint
load_tf_weights_in_bert(model, config, tf_checkpoint_path)
# Save pytorch-model
print("Save PyTorch model to {}".format(pytorch_dump_path))
torch.save(model.state_dict(), pytorch_dump_path)
```
I got this error when ran the above code
**NotFoundError: Unsuccessful TensorSliceReader constructor:** Failed to find any matching files for pretrained_bert/model.ckpt
Any help is really appreciated............ | 08-18-2019 13:27:47 | 08-18-2019 13:27:47 | For me it worked to convert checkpoints without specifying the exact checkpoint. So only pointing to the folder of the checkpoint:
`tf_checkpoint_path="pretrained_bert"`<|||||>This issue has been automatically marked as stale because it has not had recent activity. It will be closed if no further activity occurs. Thank you for your contributions.
|
transformers | 1,049 | closed | BUG: run_openai_gpt.py bug of GPTTokenizer and GPTDoubleHeadsModel | ## 🐛 Bug
<!-- Important information -->
Model I am using (Bert, XLNet....): GPT
Language I am using the model on (English, Chinese....): ENGLISH
The problem arise when using:
* [ 1 ] the official example scripts: run_openai_gpt.py
The tasks I am working on is:
* [ 1 ] an official GLUE/SQUaD task: ROCSroties
**Run run_openai_gpt.py file error, Traceback are as fellow:**
```
Traceback (most recent call last):
File "/opt/lyon.li/gpt-2/examples/single_model_scripts/run_openai_gpt.py", line 288, in <module>
main()
File "/opt/lyon.li/gpt-2/examples/single_model_scripts/run_openai_gpt.py", line 158, in main
model = OpenAIGPTDoubleHeadsModel.from_pretrained(args.model_name, num_special_tokens=len(special_tokens))
File "/opt/lyon.li/gpt-2/pytorch_transformers/modeling_utils.py", line 474, in from_pretrained
model = cls(config, *model_args, **model_kwargs)
TypeError: __init__() got an unexpected keyword argument 'num_special_tokens'
```
It seems like GPTTokenizer and GPTModel fail to add special tokens. Run fellowing demo give me result like this.
```
special_tokens = ['_start_', '_delimiter_', '_classify_']
tokenizer = OpenAIGPTTokenizer.from_pretrained(args.model_name, special_tokens=special_tokens)
special_tokens_ids = list(tokenizer.convert_tokens_to_ids(token) for token in special_tokens)
```
return
```
special_tokens_ids=[0, 0, 0]
```
That means every special tokens was mapped to unk_token when tokenize.
When init GPTModel it directly report error because `num_special_tokens`.
Anyone have some ideas about why it dose not works, thanks. | 08-18-2019 13:08:40 | 08-18-2019 13:08:40 | Yes, the `run_openai_gpt.py` example still needs to be updated to the new pytorch-transformers release. We haven't found time to do it yet.<|||||>I have pull request at #1067, this change fix the bug I mentioned above. |
transformers | 1,048 | closed | Very bad performances with BertModel on sentence classification | ## ❓ Questions & Help
I'm trying to use the raw BertModel for predictions over a dataset containing a set of dialogues. In the original work I had 3 different losses but I've noticed that the losses are very high and not going down epoch after epoch. So I started to take only one loss (intent classification) and I've tried to overfit a small portions of the training dataset with a set of 50 samples. Anyway the results have not changed.
I've tried 2 solutions for the intent classification:
- Linear layer on top of the [CLS] embedding -> loss after 100 epochs = 2.4
- 2layer-LSTM to encode the bert hiddens of the last layer + linear-> loss after 100 epochs = 1.1
The input shape is:
`[CLS] what's the weather like [SEP] [PAD] .... [PAD]`
I've thought also to use biLSTM but at this point I think that something goes wrong... the sentences are very simple "check the weather for tomorrow" (weather intent) and contains only 3 intents to classify.
- The BertModel is the raw one pretrained with "bert-base-cased".
- The batch size is 1 because I had memory issue with BERT. i'm working with dialogue granularity and so I have an 3D-input of shape DIALOGUE_NUM x SENTENCE_NUM x SENTENCE_LEN while BERT expects a 2D-input tensor. By putting batch size of 1 I've found a work around to the problem.
- The optimizer is Adam with a learning rate of 0.001, by increasing it at 0.01 performances got worse.
- The loss is the Cross-Entropy loss to which I pass the output logits.
The BERT paper said that the finetuning process can achieve great performances within few epochs... Anyone has an idea why I cannot achieve these? | 08-18-2019 10:00:15 | 08-18-2019 10:00:15 | I still trying but the system seems to be in underfitting, i do not understand why it performs so poor<|||||>I've never used BERT for sentence classification tasks, but in regard to the batch size and memory constraints, you could use gradient accumulation to have a bigger effective batch size (see [examples/run_squad.py]](https://github.com/huggingface/pytorch-transformers/blob/b33a385091de604afb566155ec03329b84c96926/examples/run_squad.py#L137-L153)).
I didn't fully understand your input's shape, but it seems like you should drop dialogue and sentence number dimensions and work with shape (batch_size, sentence_length) as BERT expects. What information do you have on the first two dimensions?<|||||>I need to work with dialogue granularity because I have different predictions to do: prediction of the action to perform on the KB (insert/fetch), prediction of the intent and prediction of the end-of-dialogue(eod). The first two prediction are done on the first sentence of the dialogue, while eod prediction is done by concatenating the current dialogue with the first sentence of the next one, in this way the model can notice a sort of discontinuity and so an eod. The system is end-to-end, I perform the joint prediction of this 3 labels.
The loss of the eod classifier is computed for each sentence in the dialogue, the other two loss only once per "batch".
BERT receives always a 2D tensor [NUM_SENTENCES x SEQ_LEN] so I don't think this could be a problem. My losses (CrossEntropyLoss) are quite high after 50 epochs:
- 0.54 for eod
- 0.45 for action
- 1.1 for intent
So I've tried to overfit with the intent prediction only a smaller dataset of 20 sample but the results are the same. I've tried with less samples but the situation doesn't change...
I perform the gradient accumulation as following:
```
_GRADIENT_RATE = 16
for e in enumerate(_N_EPOCHS):
train_losses = []
model.train()
for idx, (batch, intent) in training_generator:
logits = model(batch)
loss = criterion(logits, target)
loss.backward()
if idx % _GRADIENT_RATE == 0 or idx == dataset.__len__()-1:
optimizer.step()
optimizer.zero_grad()
```
I cannot understand why my model is underfitted, I also thought about some errors with the loading of the pretrained model but I have already checked it.
```
class BertClass(nn.Module):
def __init__():
.....
def build_nn(self):
self._bert = BertModel.from_pretrained('bert_base_cased')
self._intent_classifier = nn.Linear(768, 3)
def forward(self, input, ...):
.... computing attention and segment mask ...
bert_hiddens, bert_cls_out = self._bert(input, segment_mask, attention_mask)
logits = self._intent_classifier(bert_cls_out)
return logits
```
I also modify the learning rate multiplying it by 0.1 after epochs 10, 20, 40<|||||>Ok I solved. Of course was my mistake.. this is my first real deep learning project and I have to learn a lot of things. Anyway my error was with learning rate, it was 2 order of magnitude greater wrt the ones suggested in the paper. Thank you for the support |
transformers | 1,047 | closed | Issue using Roberta | ## 🐛 Bug
<!-- Important information -->
Model I am using (Bert, XLNet....): Roberta
Language I am using the model on (English, Chinese....): English
The problem arise when using:
* [x] the official example scripts: (run_glue.py)
* [ ] my own modified scripts: (give details)

The tasks I am working on is:
* [x] an official GLUE/SQUaD task: (MNLI/MRPC)
* [ ] my own task or dataset: (give details)
## To Reproduce
Steps to reproduce the behavior:
just tried running run_glue.py
see image
<!-- If you have a code sample, error messages, stack traces, please provide it here as well. -->
## Expected behavior
<!-- A clear and concise description of what you expected to happen. -->
## Environment
* OS: Ubuntu
* Python version: 3.7.3
* PyTorch version: 1.1.0
* PyTorch Transformers version (or branch): 1.1.0
* Using GPU ? yes v100
* Distributed of parallel setup ?
* Any other relevant information:
## Additional context
<!-- Add any other context about the problem here. --> | 08-18-2019 02:49:57 | 08-18-2019 02:49:57 | Hello!
It seems you're having trouble accessing the file on our S3 bucket. Could it be your firewall?
If you paste the URL in your browser: https://s3.amazonaws.com/models.huggingface.co/bert/roberta-base-pytorch_model.bin
Can you download it or are you blocked?<|||||>Hi
You can close it . I managed to change firewall settings |
transformers | 1,046 | closed | Update README after RoBERTa addition | 08-17-2019 16:48:30 | 08-17-2019 16:48:30 | # [Codecov](https://codecov.io/gh/huggingface/pytorch-transformers/pull/1046?src=pr&el=h1) Report
> Merging [#1046](https://codecov.io/gh/huggingface/pytorch-transformers/pull/1046?src=pr&el=desc) into [master](https://codecov.io/gh/huggingface/pytorch-transformers/commit/d8923270e6c497862f990a3c72e40cc1ddd01d4e?src=pr&el=desc) will **increase** coverage by `0.05%`.
> The diff coverage is `n/a`.
[](https://codecov.io/gh/huggingface/pytorch-transformers/pull/1046?src=pr&el=tree)
```diff
@@ Coverage Diff @@
## master #1046 +/- ##
==========================================
+ Coverage 79.6% 79.65% +0.05%
==========================================
Files 42 42
Lines 6863 6863
==========================================
+ Hits 5463 5467 +4
+ Misses 1400 1396 -4
```
| [Impacted Files](https://codecov.io/gh/huggingface/pytorch-transformers/pull/1046?src=pr&el=tree) | Coverage Δ | |
|---|---|---|
| [pytorch\_transformers/file\_utils.py](https://codecov.io/gh/huggingface/pytorch-transformers/pull/1046/diff?src=pr&el=tree#diff-cHl0b3JjaF90cmFuc2Zvcm1lcnMvZmlsZV91dGlscy5weQ==) | `74.1% <0%> (+2.87%)` | :arrow_up: |
------
[Continue to review full report at Codecov](https://codecov.io/gh/huggingface/pytorch-transformers/pull/1046?src=pr&el=continue).
> **Legend** - [Click here to learn more](https://docs.codecov.io/docs/codecov-delta)
> `Δ = absolute <relative> (impact)`, `ø = not affected`, `? = missing data`
> Powered by [Codecov](https://codecov.io/gh/huggingface/pytorch-transformers/pull/1046?src=pr&el=footer). Last update [d892327...b97b7d9](https://codecov.io/gh/huggingface/pytorch-transformers/pull/1046?src=pr&el=lastupdated). Read the [comment docs](https://docs.codecov.io/docs/pull-request-comments).
<|||||>Thanks! |
|
transformers | 1,045 | closed | mnli results for BERT | Hi
I cannot reproduce the MNLI results of BERT, for how many epochs I need to finetune bert?
thanks | 08-17-2019 13:02:20 | 08-17-2019 13:02:20 | This issue has been automatically marked as stale because it has not had recent activity. It will be closed if no further activity occurs. Thank you for your contributions.
|
transformers | 1,044 | closed | Correct truncation for RoBERTa in 2-input GLUE | Extend the truncation fix to the two-input case.
(Example: currently throws if running MRPC with `max_seq_length=32`.) | 08-16-2019 20:02:18 | 08-16-2019 20:02:18 | Looks good to me, thanks!<|||||>Actually, for single-sentence inputs, do we expect one or two terminating `</s>`s? Currently we will generate two, I think.<|||||>@LysandreJik we can now update the GLUE scripts to use the newly added option `add_special_tokens` (added to all the tokenizers), don't you think?<|||||>Indeed, we should use it. I'll add that soon. |
transformers | 1,043 | closed | Unable to load custom tokens | ## 🐛 Bug
<!-- Important information -->
Model I am using (Bert, XLNet....): GPT2
Language I am using the model on (English, Chinese....): English
I am unable to load the custom tokens that were added to GPT2 tokenizer while training.
Code used while training
```
SPECIAL_TOKENS = ["bos_token","eos_token","persona, "personb", "pad_token"]
tokenizer = tokenizer_class.from_pretrained("gpt2",unk_token="unk_token")
model = model_class.from_pretrained("gpt2")
tokenizer.add_tokens(SPECIAL_TOKENS)
model.resize_token_embeddings(len(tokenizer))
#To save the tokenizer
tokenizer.save_pretrained(directory)
```
While Loading
```
tokenizer = GPT2Tokenizer.from_pretrained('./experiment1_conversation/runs/new/')
```

I run into the issue of unable to convert the custom tokens and it produces *None *. Is there something wrong in the way I am loading the tokenizer or saving it?
I get
## Environment
* OS: Ubuntu
* Python version: 3.6.8
* PyTorch version:1.1
* PyTorch Transformers version (or branch):1.1
* Using GPU ? Yes
| 08-16-2019 17:53:55 | 08-16-2019 17:53:55 | Hello, thanks for the bug report.
Could you please show me what's inside the directory `experiment1_conversation/runs/new` and `experiment1_conversation/runs/old`?<|||||>@LysandreJik the previous issue I had posted was due to a mistake on my side. I updated the issue. <|||||>@LysandreJik This is the content inside the directory.
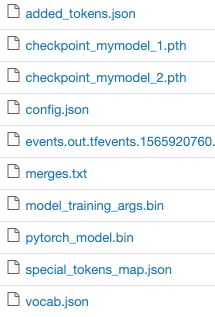
<|||||>No, the way you're saving your tokenizer is correct. If you study what's inside the `added_tokens.json`, you should have:
```
{"bos_token": 50257, "eos_token": 50258, "persona": 50259, "personb": 50260, "pad_token": 50261}
```
Following your procedure, when I print `tokenizer.convert_tokens_to_ids(["bos_token"])` after loading from the saved directory, I get `[50257]`, which is correct.
Could you show me what is inside of your `added_tokens.json`?<|||||>@LysandreJik The added_tokens.json is saved in the wrong way for me.
```{"50257": "bos_token", "50258": "eos_token", "50259": "persona", "50260": "personb", "50261": "pad_token"}```
Any reason for this?<|||||>What are `tokenizer_class` and `model_class` instances of? Are they instances of `GPT2Tokenizer` and `GPT2Model`?
Do you get the same result if you run this script?
```python
from pytorch_transformers import GPT2Tokenizer, GPT2Model
import os
os.makedirs("save_it_here")
SPECIAL_TOKENS = ["bos_token", "eos_token", "persona", "personb", "pad_token"]
tokenizer = GPT2Tokenizer.from_pretrained("gpt2")
model = GPT2Model.from_pretrained("gpt2")
tokenizer.add_tokens(SPECIAL_TOKENS)
model.resize_token_embeddings(len(tokenizer))
# To save the tokenizer
tokenizer.save_pretrained("save_it_here")
tokenizer = GPT2Tokenizer.from_pretrained("save_it_here")
print(tokenizer.convert_tokens_to_ids(["bos_token"]))
```<|||||>The above script produces the same results as what you got. I will investigate how mine went wrong when training. Thanks for the help. I will close this for now?<|||||>Alright, glad I could help. Don't hesitate to re-open if you see something weird :). |
transformers | 1,042 | closed | fix #1041 | 08-16-2019 15:46:35 | 08-16-2019 15:46:35 | ||
transformers | 1,041 | closed | Issue in running run_glue.py in Roberta, XLNet, XLM in latest release | ## 🐛 Bug
<!-- Important information -->
Model I am using (Bert, XLNet....): Roberta, XLM, XLNet
Language I am using the model on (English, Chinese....): English
The problem arise when using:
* [x] the official example scripts: run_glue.py
The tasks I am working on is:
* [x] my own task or dataset: binary classification on my own dataset
## To Reproduce
Steps to reproduce the behavior:
1. Upon running glue.py with the following : python ~/pytorch-transformers/examples/run_glue.py --task_name cola --do_train --do_eval --do_lower_case --data_dir ~/bert-data/ --model_type roberta --model_name_or_path roberta-base --max_seq_length 512 --learning_rate 2e-5 --num_train_epochs 1.0 --output_dir ~/data/roberta-1/
Getting the following error:
```
08/16/2019 14:18:21 - WARNING - pytorch_transformers.tokenization_utils - Token indices sequence length is longer than the specified maximum sequence length for this model (513 > 512). Running this sequence through the model will result in indexing errors
Traceback (most recent call last):
File "/home/pytorch-transformers/examples/run_glue.py", line 494, in <module>
main()
File "/home/pytorch-transformers/examples/run_glue.py", line 447, in main
train_dataset = load_and_cache_examples(args, args.task_name, tokenizer, evaluate=False)
File "/home/pytorch-transformers/examples/run_glue.py", line 283, in load_and_cache_examples
pad_token_segment_id=4 if args.model_type in ['xlnet'] else 0,
File "/home/pytorch-transformers/examples/utils_glue.py", line 485, in convert_examples_to_features
assert len(input_ids) == max_seq_length
AssertionError
```
2. Trying the same as above with XLNet and XLM, gives the following error:
```
08/16/2019 14:26:59 - INFO - __main__ - Creating features from dataset file at /home/new-bert-data/keyword_data/
Traceback (most recent call last):
File "/home/pytorch-transformers/examples/run_glue.py", line 494, in <module>
main()
File "/home/pytorch-transformers/examples/run_glue.py", line 447, in main
train_dataset = load_and_cache_examples(args, args.task_name, tokenizer, evaluate=False)
File "/home/pytorch-transformers/examples/run_glue.py", line 282, in load_and_cache_examples
pad_token=tokenizer.encoder[tokenizer.pad_token] if args.model_type in ['roberta'] else tokenizer.vocab[tokenizer.pad_token],
AttributeError: 'XLMTokenizer' object has no attribute 'vocab'
```
## Environment
* OS: Debian
* Python version: 3.6.9
* PyTorch version: 1.2.0
* PyTorch Transformers version (or branch): 1.1.0
* Using GPU: Yes
* Distributed of parallel setup: Multi-GPU
| 08-16-2019 14:28:22 | 08-16-2019 14:28:22 | Thanks for the report! I'm looking into it.<|||||>Could you try the changes in [this commit ](https://github.com/huggingface/pytorch-transformers/commit/a93966e608cac8e80b4ff355d7c61f712b6da7f4)on your own dataset and tell me if you still have errors?<|||||>@LysandreJik Ya this code works fine. Thanks for the quick fix<|||||>Great, glad I could help! |
transformers | 1,040 | closed | Fix bug of multi-gpu training in lm finetuning | Current code will raise error when running multi-gpu training (n_gpu > 1 & local_rank = -1).
| 08-16-2019 04:22:50 | 08-16-2019 04:22:50 | # [Codecov](https://codecov.io/gh/huggingface/pytorch-transformers/pull/1040?src=pr&el=h1) Report
> Merging [#1040](https://codecov.io/gh/huggingface/pytorch-transformers/pull/1040?src=pr&el=desc) into [master](https://codecov.io/gh/huggingface/pytorch-transformers/commit/9d0029e215f5ad0836d6be87458aab5142783af4?src=pr&el=desc) will **not change** coverage.
> The diff coverage is `n/a`.
[](https://codecov.io/gh/huggingface/pytorch-transformers/pull/1040?src=pr&el=tree)
```diff
@@ Coverage Diff @@
## master #1040 +/- ##
=======================================
Coverage 79.55% 79.55%
=======================================
Files 42 42
Lines 6863 6863
=======================================
Hits 5460 5460
Misses 1403 1403
```
------
[Continue to review full report at Codecov](https://codecov.io/gh/huggingface/pytorch-transformers/pull/1040?src=pr&el=continue).
> **Legend** - [Click here to learn more](https://docs.codecov.io/docs/codecov-delta)
> `Δ = absolute <relative> (impact)`, `ø = not affected`, `? = missing data`
> Powered by [Codecov](https://codecov.io/gh/huggingface/pytorch-transformers/pull/1040?src=pr&el=footer). Last update [9d0029e...856a63d](https://codecov.io/gh/huggingface/pytorch-transformers/pull/1040?src=pr&el=lastupdated). Read the [comment docs](https://docs.codecov.io/docs/pull-request-comments).
<|||||>Make sense, thanks @FeiWang96! |
transformers | 1,039 | closed | Minor bug in evaluation phase in example code for SQUAD | In run_squad.py, the model saving code is outside the loop which performs training (if args.do_train - line 477).
Due to this, after finetuning a model with --do_train, if we only run --do_eval, the existing trained model gets overwritten before being loaded for testing.
**Simple fix:** Pushing model saving code inside the training loop will exhibit desired behavior during testing | 08-15-2019 23:21:01 | 08-15-2019 23:21:01 | I fixed it in #923 weeks ago.
waiting merge<|||||>Thanks, I did not come across it earlier! I'll close this issue. |
transformers | 1,038 | closed | Adding new tokens to GPT tokenizer | ## 🐛 Bug
<!-- Important information -->
Model I am using (Bert, XLNet....): GPT2
Language I am using the model on (English, Chinese....): English
I am having saving GPT2Tokenizer when custom new tokens are added to it. Tried out two specific methods.
1. Using special_mapping dictionary and save_vocabulary and save_pretrained method
2. Using special_mapping list and save_vocabulary and save_pretrained method
## To Reproduce
```SPECIAL_TOKENS = ["<bos>", "<eos>", "PersonA", "PersonB", "<pad>"]
tokenizer_class = GPT2Tokenizer if "gpt2" in args.model_checkpoint else OpenAIGPTTokenizer
tokenizer = tokenizer_class.from_pretrained(args.model_checkpoint,unk_token="unk_token")
tokenizer.add_tokens(SPECIAL_TOKENS)
model.resize_token_embeddings(len(tokenizer))
tokenize.save_vocabulary(filedir)
```
the above method only save the current vocab json without any of the new tokens being added. When save_vocabulary is replaced with save_pretrained(filedir) a new file called special_mappings.json is created with only 3 special tokens `{"bos_token": "<|endoftext|>", "eos_token": "<|endoftext|>", "unk_token": "unk_token"}`
## Expected behavior
<!-- A clear and concise description of what you expected to happen. -->
## Environment
* OS: Ubunti
* Python version: 3.6.8
* PyTorch version:1.1
* PyTorch Transformers version (or branch):1
* Using GPU ?Yes
## Additional context
If there is anything wrong with the code, please do let me know. | 08-15-2019 21:05:05 | 08-15-2019 21:05:05 | |
transformers | 1,037 | closed | wrong generation of training sentence pairs. method: get_corpus_line, in finetune_on_pregenerated.py | ## 🐛 Bug
<!-- Important information -->
Model I am using:
BERT
Language I am using the model on (English, Chinese....):
The problem arise when using:
* [ ] the official example scripts: (give details)
The tasks I am working on is:
I am running on my own text corpus the official example to fine-tune BERT
Steps to reproduce the behavior:
1. create my_corpus.txt:
AAA
BBB
CCC
DDD
EEE
FFF
2. run python3 simple_lm_finetuning.py
--train_corpus my_corpus.txt
--bert_model bert-base-uncased
--do_lower_case
--output_dir finetuned_lm/
--do_train
<!-- If you have a code sample, error messages, stack traces, please provide it here as well. -->
## Expected behavior
I expected to see the following first 3 outputs of get_corpus_line method, ie, t1 t2:
1)
t1=AAA
t2=BBB
2)
t1=CCC
t2=DDD
3)
t1=EEE
t2=FFF
But received:
1)
t1=AAA
t2=BBB
2)
t1=CCC
t2=DDD
3) **!!!!!!! (HERE)
t1=DDD
t2=AAA
## Additional context
It seems we need to make self.line_buffer equal to None whenever we close the file.
Possible silution:
line 118:
if cur_id != 0 and (cur_id % len(self) == 0):
self.file.close()
self.file = open(self.corpus_path, "r", encoding=self.encoding)
***self.line_buffer = None***
<!-- Add any other context about the problem here. --> | 08-15-2019 18:17:38 | 08-15-2019 18:17:38 | Hi @Evgeneus, does your proposed solution solve the problem on your side?<|||||>> Hi @Evgeneus, does your proposed solution solve the problem on your side?
Hi @thomwolf, seems yes.<|||||>This issue has been automatically marked as stale because it has not had recent activity. It will be closed if no further activity occurs. Thank you for your contributions.
|
transformers | 1,036 | closed | Customize BertTokenizer and Feature Extraction from Bert Model | ## ❓ Questions & Help
Hello everybody, I tuned Bert follow [this example](https://github.com/huggingface/pytorch-transformers/tree/master/examples/lm_finetuning) with my corpus in my country language - Vietnamese.
So now I have 2 question that concerns:
1. With my corpus, in my country language Vietnamese, I don't want use Bert Tokenizer from `from_pretrained` BertTokenizer classmethod, so it get tokenizer from pretrained bert models.
Now I want use only BasicTokenize - whitespace split only, so i must customize this function with it's output are same with output of `from_pretrained` function. Anyone has better solution, can you help me ?
2. I want only get embeded vector so I can use with my problem, aren't Next Sentence Prediction task, so I thinked I will get last hidden layer from Bert Model used this follow code:
`model_state_dict = torch.load(output_model_file)
model = pytorch_transformers.BertModel.from_pretrained('bert-base-multilingual-cased', do_lower_case=False, state_dict=model_state_dict)
tokenizer = BertTokenizer.from_pretrained('bert-base-multilingual-cased', do_lower_case=False, state_dict=model_state_dict)
input_ids = torch.tensor(tokenizer.encode(sent)).unsqueeze(0) # Batch size 1
outputs = model(input_ids)`
Is that right, anyone has better solution, can you help me ?
Sorry for about my English, can anyone help me ? | 08-15-2019 12:32:55 | 08-15-2019 12:32:55 | 1. ) Not really sure what your meaning here, but use whatever tokenizer that you used to tokenise your corpus; a tokenizer just converts words into integers anyways.
2. ) You are pretty much right if all you want is the hidden states, `outputs = model(input_ids)` will create a tuple with the hidden layers. You can then use these vectors as inputs to different classifiers.
Only thing is that by doing it this way the BERT model ends up having frozen weights. Now it might just be that BERT has already found the best representation for your downstream predictions, but more than likely it has not. Instead, it's much better to allow BERT to be fine tuned.
(Just to let you know, BERT can be fine tuned on a binary classification problem straight out the box, more than likely will offer better performance than hand engineering a classifier).<|||||>@andrewpatterson2018 thank you for your help, my first question is from paragraph, BertTokenizer split its into words like:
'I am going to school' -> ['I', 'am', 'go', '##ing', 'to', 'school']
But I want its to be like: -> ['I', 'am', 'going', 'to', 'school']
Because in my language word structure is different from English. I want WhiteSpaceSplit only.
Do you have any solution ?
Thank you very much !<|||||>You shouldn't change the Tokenizer, because the Tokenizer produces the vocabulary that the Embedding layer expects. Considering the example you gave:
'I am going to school' -> ['I', 'am', 'go', '##ing', 'to', 'school']
Whitespace tokenization -> ['I', 'am', 'going', 'to', 'school']
The word "going" was split into "go ##ing" because BERT uses WordPiece embeddings and `bert-base-multilingual-cased` vocabulary does not contain the word `going`. You could write your own tokenizer that performs whitespace tokenization, but you would have to map all unknown tokens to the [UNK] token. The final tokenization would be:
['I', 'am', '[UNK]', 'to', 'school']
The performance will most certainly drop, because you would have embeddings for a really small percentage of your tokens.
What you probably want is to change the vocabulary BERT uses. This requires generating a new vocabulary for your corpus and pretraining BERT from scratch (you can initialize with the weights of `bert-base-multilingual-cased`) replacing the Embedding layer.<|||||>@fabiocapsouza thank you very much !
But now I want use BERT to fine tuned with my corpus, so I want use `bert-base-multilingual-cased` as initial weights.
I understand that don't change vocabulary by BERT, when I tuned, I go to folder, open vocab.txt, and this that file has been added vocabulary in my corpus but those words are tokenizer by using the BERT's BasicTokenizer, but what I want is that it gets tokenizer my way. I understand the output of the tokenizer to match the BERT encoder. Will I have to re-code all functions?
Because BERT tokenizer in addition to tokenize is masked, will I have to re-code to match my tokenize method ?
Thank you !<|||||>This issue has been automatically marked as stale because it has not had recent activity. It will be closed if no further activity occurs. Thank you for your contributions.
<|||||>> @fabiocapsouza thank you very much !
> But now I want use BERT to fine tuned with my corpus, so I want use `bert-base-multilingual-cased` as initial weights.
> I understand that don't change vocabulary by BERT, when I tuned, I go to folder, open vocab.txt, and this that file has been added vocabulary in my corpus but those words are tokenizer by using the BERT's BasicTokenizer, but what I want is that it gets tokenizer my way. I understand the output of the tokenizer to match the BERT encoder. Will I have to re-code all functions?
> Because BERT tokenizer in addition to tokenize is masked, will I have to re-code to match my tokenize method ?
> Thank you !
Did you make your own tokenizer that was not generating ## in the vocab file? |
transformers | 1,035 | closed | Merge pull request #1 from huggingface/master | update | 08-15-2019 05:56:53 | 08-15-2019 05:56:53 | # [Codecov](https://codecov.io/gh/huggingface/pytorch-transformers/pull/1035?src=pr&el=h1) Report
> Merging [#1035](https://codecov.io/gh/huggingface/pytorch-transformers/pull/1035?src=pr&el=desc) into [master](https://codecov.io/gh/huggingface/pytorch-transformers/commit/a7b4cfe9194bf93c7044a42c9f1281260ce6279e?src=pr&el=desc) will **decrease** coverage by `0.31%`.
> The diff coverage is `n/a`.
[](https://codecov.io/gh/huggingface/pytorch-transformers/pull/1035?src=pr&el=tree)
```diff
@@ Coverage Diff @@
## master #1035 +/- ##
=========================================
- Coverage 79.22% 78.9% -0.32%
=========================================
Files 38 34 -4
Lines 6406 6192 -214
=========================================
- Hits 5075 4886 -189
+ Misses 1331 1306 -25
```
| [Impacted Files](https://codecov.io/gh/huggingface/pytorch-transformers/pull/1035?src=pr&el=tree) | Coverage Δ | |
|---|---|---|
| [pytorch\_transformers/file\_utils.py](https://codecov.io/gh/huggingface/pytorch-transformers/pull/1035/diff?src=pr&el=tree#diff-cHl0b3JjaF90cmFuc2Zvcm1lcnMvZmlsZV91dGlscy5weQ==) | `71.01% <0%> (-3.09%)` | :arrow_down: |
| [pytorch\_transformers/tokenization\_bert.py](https://codecov.io/gh/huggingface/pytorch-transformers/pull/1035/diff?src=pr&el=tree#diff-cHl0b3JjaF90cmFuc2Zvcm1lcnMvdG9rZW5pemF0aW9uX2JlcnQucHk=) | `93.2% <0%> (-1.95%)` | :arrow_down: |
| [...transformers/tests/tokenization\_transfo\_xl\_test.py](https://codecov.io/gh/huggingface/pytorch-transformers/pull/1035/diff?src=pr&el=tree#diff-cHl0b3JjaF90cmFuc2Zvcm1lcnMvdGVzdHMvdG9rZW5pemF0aW9uX3RyYW5zZm9feGxfdGVzdC5weQ==) | `96.42% <0%> (-0.55%)` | :arrow_down: |
| [...rch\_transformers/tests/tokenization\_openai\_test.py](https://codecov.io/gh/huggingface/pytorch-transformers/pull/1035/diff?src=pr&el=tree#diff-cHl0b3JjaF90cmFuc2Zvcm1lcnMvdGVzdHMvdG9rZW5pemF0aW9uX29wZW5haV90ZXN0LnB5) | `96.77% <0%> (-0.45%)` | :arrow_down: |
| [...ytorch\_transformers/tests/tokenization\_xlm\_test.py](https://codecov.io/gh/huggingface/pytorch-transformers/pull/1035/diff?src=pr&el=tree#diff-cHl0b3JjaF90cmFuc2Zvcm1lcnMvdGVzdHMvdG9rZW5pemF0aW9uX3hsbV90ZXN0LnB5) | `96.77% <0%> (-0.45%)` | :arrow_down: |
| [...orch\_transformers/tests/tokenization\_xlnet\_test.py](https://codecov.io/gh/huggingface/pytorch-transformers/pull/1035/diff?src=pr&el=tree#diff-cHl0b3JjaF90cmFuc2Zvcm1lcnMvdGVzdHMvdG9rZW5pemF0aW9uX3hsbmV0X3Rlc3QucHk=) | `97.05% <0%> (-0.45%)` | :arrow_down: |
| [...torch\_transformers/tests/tokenization\_gpt2\_test.py](https://codecov.io/gh/huggingface/pytorch-transformers/pull/1035/diff?src=pr&el=tree#diff-cHl0b3JjaF90cmFuc2Zvcm1lcnMvdGVzdHMvdG9rZW5pemF0aW9uX2dwdDJfdGVzdC5weQ==) | `96.87% <0%> (-0.43%)` | :arrow_down: |
| [pytorch\_transformers/tests/optimization\_test.py](https://codecov.io/gh/huggingface/pytorch-transformers/pull/1035/diff?src=pr&el=tree#diff-cHl0b3JjaF90cmFuc2Zvcm1lcnMvdGVzdHMvb3B0aW1pemF0aW9uX3Rlc3QucHk=) | `98.57% <0%> (-0.41%)` | :arrow_down: |
| [pytorch\_transformers/optimization.py](https://codecov.io/gh/huggingface/pytorch-transformers/pull/1035/diff?src=pr&el=tree#diff-cHl0b3JjaF90cmFuc2Zvcm1lcnMvb3B0aW1pemF0aW9uLnB5) | `96.29% <0%> (-0.34%)` | :arrow_down: |
| [...torch\_transformers/tests/tokenization\_bert\_test.py](https://codecov.io/gh/huggingface/pytorch-transformers/pull/1035/diff?src=pr&el=tree#diff-cHl0b3JjaF90cmFuc2Zvcm1lcnMvdGVzdHMvdG9rZW5pemF0aW9uX2JlcnRfdGVzdC5weQ==) | `98.38% <0%> (-0.13%)` | :arrow_down: |
| ... and [14 more](https://codecov.io/gh/huggingface/pytorch-transformers/pull/1035/diff?src=pr&el=tree-more) | |
------
[Continue to review full report at Codecov](https://codecov.io/gh/huggingface/pytorch-transformers/pull/1035?src=pr&el=continue).
> **Legend** - [Click here to learn more](https://docs.codecov.io/docs/codecov-delta)
> `Δ = absolute <relative> (impact)`, `ø = not affected`, `? = missing data`
> Powered by [Codecov](https://codecov.io/gh/huggingface/pytorch-transformers/pull/1035?src=pr&el=footer). Last update [a7b4cfe...181f1e9](https://codecov.io/gh/huggingface/pytorch-transformers/pull/1035?src=pr&el=lastupdated). Read the [comment docs](https://docs.codecov.io/docs/pull-request-comments).
<|||||>,l |
transformers | 1,034 | closed | Getting embedding from XLM in differnet languages | ## ❓ Questions & Help
Hi,
I'm trying to get a cross-lingual embedding from the XLM model, but can't figure out how.
In the project original github, you need to give the tokenizer the language of each of the tokens, but it doesn't seem the case here.
Will appreciated any help on the matter :) | 08-14-2019 20:20:55 | 08-14-2019 20:20:55 | Hi, are you referring to the sentence embeddings that are generated in the [XLM notebook](https://github.com/facebookresearch/XLM/blob/master/generate-embeddings.ipynb)?<|||||>Yes. Although I'm using it to get the word embeddings (see [here](https://github.com/facebookresearch/XLM/issues/17)).
Maybe I'm missing something, but as far as I understand, the model uses a language embedding that is added to the token embedding, so it seem it will need that information. Am I missing something?<|||||>Well, neither the official XLM notebook @LysandreJik linked to nor the XLM repo issue @OfirArviv linked to are mentioning the need to give language ids so I'm not sure exactly why they would be needed.
Maybe this is a question for the original authors of XLM?<|||||>Hi @thomwolf!
I believe they already answered this question in [this](https://github.com/facebookresearch/XLM/issues/103#issuecomment-501682382) [issue](https://github.com/facebookresearch/XLM/issues/103#issuecomment-501682649):
So it will be useful if we can provide models with lang ids, preferably during training as well.
<|||||>Ok I see.
So you need to input a `torch.LongTensor` with the `language id` for each token in your input sequence in the model (see inputs [here](https://huggingface.co/pytorch-transformers/model_doc/xlm.html#pytorch_transformers.XLMModel)).
Right now the conversion mapping from language to ids (and vice-versa) can be found in the configuration of the model (see for ex [here](https://s3.amazonaws.com/models.huggingface.co/bert/xlm-mlm-xnli15-1024-config.json)).
Here is an example:
```python
from pytorch_transformers import XLMModel
model = XLMModel.from_pretrained('xlm-mlm-xnli15-1024')
lang2id_dict = model.config.lang2id
id2lang_dict = model.config.id2lang
```
If you only want the conversion dictionary and not the model, just load only the configuration:
```python
from pytorch_transformers import XLMConfig
config = XLMConfig.from_pretrained('xlm-mlm-xnli15-1024')
lang2id_dict =config.lang2id
id2lang_dict =config.id2lang
```
I'll add more details on that in the docstring. |
transformers | 1,033 | closed | GPT2 Tokenizer got an expected argument `skip_special_tokens` | ## 🐛 Bug
Model I am using -> GPT2
Language I am using the model on - >English
The problem arise when using:
I keep running into this error when trying to use the GPT2 model and GPT2 tokenizer while decoding.
Keep getting the following error when I run the piece of code below:
tokenizer.decode(response_ids, skip_special_tokens=True)
Error:
TypeError: decode() got an unexpected keyword argument 'skip_special_tokens'
* OS: Ubuntu
* Python version: 3.6.8
* PyTorch version: 1.1.0
* PyTorch Transformers version (or branch): 1.0.0
* Using GPU - Yes
| 08-14-2019 18:46:54 | 08-14-2019 18:46:54 | I'm having a hard time reproducing it on my side on a clean install. Could you provide a sample that throws the error?<|||||>@LysandreJik I will give you the code with sample input and output asap. |
transformers | 1,032 | closed | GPT2 Tokenizer got an expected argument `skip_special_tokens` | ## ❓ Questions & Help
I keep running into this error when trying to use the GPT2 model and GPT2 tokenizer while decoding.
Keep getting the following error when I run the piece of code below:
``tokenizer.decode(response_ids, skip_special_tokens=True)``
Error:
``TypeError: decode() got an unexpected keyword argument 'skip_special_tokens'``
| 08-14-2019 18:35:35 | 08-14-2019 18:35:35 | Could you please submit a bug report with the version of the library you're using?<|||||>will close this issue. Opened a bug report. |
transformers | 1,031 | closed | Efficient data loading functionality | ## 🚀 Feature
Efficient data loader for huge dataset with lazy loading!
## Motivation
<!-- Please outline the motivation for the proposal. Is your feature request related to a problem? e.g., I'm always frustrated when [...]. If this is related to another GitHub issue, please link here too. -->
I am working with a huge dataset consisting of 120m examples (~40G raw text) in a single csv file. I tried to follow the [run_glue](https://github.com/huggingface/pytorch-transformers/blob/master/examples/run_glue.py) distributed training example, however this is too slow as it first creates all the examples and cache it [here](https://github.com/huggingface/pytorch-transformers/blob/master/examples/run_glue.py#L251). Basically only first process in distributed training process the dataset and others just use the cache.
Is there any data loader (or a working example) that would be efficient for training the model on such a huge dataset?
## Additional context
<!-- Add any other context or screenshots about the feature request here. --> | 08-14-2019 16:50:02 | 08-14-2019 16:50:02 | This issue has been automatically marked as stale because it has not had recent activity. It will be closed if no further activity occurs. Thank you for your contributions.
<|||||>@thomwolf Is there an efficient implementation for this? Could you re-open the issue please. <|||||>CSV generally has slower load times. How about benchmarking with pickle, parquet, and feather? Pytorch's dataloader can handle multiple files and multiple lines per file
https://discuss.pytorch.org/t/dataloaders-multiple-files-and-multiple-rows-per-column-with-lazy-evaluation/11769 |
transformers | 1,030 | closed | Tokenizer not found after conversion from TF checkpoint to PyTorch | ## 🐛 Bug
<!-- Important information -->
Model I am using (Bert, XLNet....): GPT2
Language I am using the model on (English, Chinese....): English
The problem arise when using:
* [x] the official example scripts: run_generation.py, convert_tf_checkpoint_to_pytorch.py
* [ ] my own modified scripts: (give details)
The tasks I am working on is:
* [ ] an official GLUE/SQUaD task: (give the name)
* [x] my own task or dataset: Text generation. I finetuned a gpt2 model using Tensorflow and I converted the checkpoint using the `convert_tf_checkpoint_to_pytorch.py` script to PyTorch. Running `run_generation.py` from the examples folder results in an error. It seems like the tokenizer is not loaded from the converted model. (Maybe it is not saved?)
## To Reproduce
Steps to reproduce the behavior:
1. Have a tensorflow checkpoint.
2. Convert it with `python pytorch_transformers gpt2 path/to/checkpoint path/to/save/model`
3. Run `python run_generation.py --model_type gpt2 --model_name_or_path path/to/saved/model --top_p 0.9 --prompt "Hello Huggingface"`
This results in the following error:
<!-- If you have a code sample, error messages, stack traces, please provide it here as well. -->
`Traceback (most recent call last):
File "run_generation.py", line 195, in <module>
main()
File "run_generation.py", line 175, in main
context_tokens = tokenizer.encode(raw_text)
AttributeError: 'NoneType' object has no attribute 'encode'`
## Expected behavior
Text generation like using "gpt2" as `model_name_or_path`.
<!-- A clear and concise description of what you expected to happen. -->
## Environment
* OS: Windows 10
* Python version: 3.7
* PyTorch version: 1.1
* PyTorch Transformers version (or branch): 1.0
* Using GPU ? Yes, but doesn't work with CPU either
* Distributed of parallel setup ? No
* Any other relevant information:
## Additional context
I manged to get it working by substituting the loading of the tokenizer with "gpt2", that way the tokenizer is loaded not from my fine-tuned model, but from the cache of the 117M version. Is the tokenizer actually trained?
Right now I have 3 files in the models folder: `config.json`, `pytorch_model.bin` and `vocab.bpe`. Am I missing a file?
<!-- Add any other context about the problem here. --> | 08-14-2019 11:42:04 | 08-14-2019 11:42:04 | Hi, no the tokenizer is not trained. You can just load the original `gpt2` one.<|||||>Shouldn't the tokenizer then be loaded from `args.model_type` and not `args.model_name_or_path`? Or do they differ from `gpt2` to `gpt2-medium`?<|||||>This issue has been automatically marked as stale because it has not had recent activity. It will be closed if no further activity occurs. Thank you for your contributions.
|
transformers | 1,029 | closed | if cutoffs=[], convert_transfo_xl_checkpoint_to_pytorch.py has a bug | ## 🐛 Bug
<!-- Important information -->
Model I am using (Bert, XLNet....): transformer_xl
Language I am using the model on (English, Chinese....): I train xl model base on own dataset
<!-- If you have a code sample, error messages, stack traces, please provide it here as well. -->
<!-- A clear and concise description of what you expected to happen. -->
## Environment
* OS: centos 7.3
* Python version: python3.6
* PyTorch version: torch1.1
* PyTorch Transformers version (or branch): 1.0.0
* Using GPU: yes
* Distributed of parallel setup ? no
* Any other relevant information: no
## detail context
<!-- Add any other context about the problem here. -->
AttributeError: 'ProjectedAdaptiveLogSoftmax' object has no attribute 'cluster_weight'
I see 'ProjectedAdaptiveLogSoftmax' code, if len(cutoffs) - 1 > 0, will have attribute 'cluster_weight'
| 08-14-2019 09:27:38 | 08-14-2019 09:27:38 | Hi!
Yes, you should indeed specify `cutoffs` in your `TransfoXLConfig` or the adaptive softmax won't be able to create its clusters. We should probably put a more explicit error.<|||||>Hello, @LysandreJik
The checkpoint of https://github.com/kimiyoung/transformer-xl doesn't have cutoff_N when adaptive softmax is not used. Does PyTorch-Transformers support TransfoXLConfig.adaptive = False? If supported, should it read checkpoint without explicit error? The content of checkpoint is like this without adaptive softmax.
```
transformer/adaptive_embed/lookup_table (DT_FLOAT) [32768,512]
transformer/adaptive_embed/lookup_table/Adam (DT_FLOAT) [32768,512]
transformer/adaptive_embed/lookup_table/Adam_1 (DT_FLOAT) [32768,512]
transformer/adaptive_softmax/bias (DT_FLOAT) [32768]
transformer/adaptive_softmax/bias/Adam (DT_FLOAT) [32768]
transformer/adaptive_softmax/bias/Adam_1 (DT_FLOAT) [32768]
transformer/layer_0/ff/LayerNorm/beta (DT_FLOAT) [512]
```<|||||>This issue has been automatically marked as stale because it has not had recent activity. It will be closed if no further activity occurs. Thank you for your contributions.
|
transformers | 1,028 | closed | add data utils | 08-14-2019 09:17:51 | 08-14-2019 09:17:51 | ||
transformers | 1,027 | closed | Re-implemented tokenize() iteratively in PreTrainedTokenizer. | Firstly, Thanks a lot for this amazing library. Great work!
### Motivation
The `tokenize()` function in `PreTrainedTokenizer` uses the nested `split_on_tokens` recursive function which is called for all the added tokens (& special tokens). However, if the number of added tokens is large (e.g. > 1000), which is often the case with domain-specific texts, a `RuntimeError` is thrown due to reaching the maximum recursion depth.
### Changes
To address the issue, I re-implemented the `tokenize()` method in `PreTrainedTokenizer` iteratively.
My solution works faster than the original recursive code which features a large number of list copying because of slicing on line 482:
```python
return sum((split_on_tokens(tok_list[1:], sub_text.strip()) + [tok] \
for sub_text in split_text), [])[:-1]
```
### Results
I carefully tested the new function against the original recursive one. They produce exactly the same tokenization on all of my experiments. | 08-14-2019 09:17:39 | 08-14-2019 09:17:39 | The failed test log reads:
```
ERROR pytorch_transformers.modeling_utils:modeling_utils.py:160 Couldn't reach server at 'https://s3.amazonaws.com/models.huggingface.co/bert/bert-large-uncased-whole-word-masking-config.json' to download pretrained model configuration file.
```
This shouldn't be from my end.<|||||># [Codecov](https://codecov.io/gh/huggingface/pytorch-transformers/pull/1027?src=pr&el=h1) Report
> Merging [#1027](https://codecov.io/gh/huggingface/pytorch-transformers/pull/1027?src=pr&el=desc) into [master](https://codecov.io/gh/huggingface/pytorch-transformers/commit/9beaa85b071078f84037f6a036ea042f551a8623?src=pr&el=desc) will **increase** coverage by `0.02%`.
> The diff coverage is `96%`.
[](https://codecov.io/gh/huggingface/pytorch-transformers/pull/1027?src=pr&el=tree)
```diff
@@ Coverage Diff @@
## master #1027 +/- ##
==========================================
+ Coverage 79.6% 79.62% +0.02%
==========================================
Files 42 42
Lines 6864 6886 +22
==========================================
+ Hits 5464 5483 +19
- Misses 1400 1403 +3
```
| [Impacted Files](https://codecov.io/gh/huggingface/pytorch-transformers/pull/1027?src=pr&el=tree) | Coverage Δ | |
|---|---|---|
| [pytorch\_transformers/tokenization\_utils.py](https://codecov.io/gh/huggingface/pytorch-transformers/pull/1027/diff?src=pr&el=tree#diff-cHl0b3JjaF90cmFuc2Zvcm1lcnMvdG9rZW5pemF0aW9uX3V0aWxzLnB5) | `86.13% <96%> (+0.01%)` | :arrow_up: |
------
[Continue to review full report at Codecov](https://codecov.io/gh/huggingface/pytorch-transformers/pull/1027?src=pr&el=continue).
> **Legend** - [Click here to learn more](https://docs.codecov.io/docs/codecov-delta)
> `Δ = absolute <relative> (impact)`, `ø = not affected`, `? = missing data`
> Powered by [Codecov](https://codecov.io/gh/huggingface/pytorch-transformers/pull/1027?src=pr&el=footer). Last update [9beaa85...d30cbaf](https://codecov.io/gh/huggingface/pytorch-transformers/pull/1027?src=pr&el=lastupdated). Read the [comment docs](https://docs.codecov.io/docs/pull-request-comments).
<|||||>That's nice, thanks a lot Mikayel! |
transformers | 1,026 | closed | loads the tokenizer for each checkpoint, to solve the reproducability… | Hi
I observed that if you run "run_glue" code with the same parameters in the following ways:
1) run with both --do_train and --do_eval
2) run without --do_train but only --do_eval, but set the modelpath to use the trained models from step 1
The obtained evaluation results in these two cases are not the same, and to make the results reproducible it is needed to reload tokenizer from checkpoints.
Thanks. | 08-14-2019 09:02:57 | 08-14-2019 09:02:57 | # [Codecov](https://codecov.io/gh/huggingface/pytorch-transformers/pull/1026?src=pr&el=h1) Report
> Merging [#1026](https://codecov.io/gh/huggingface/pytorch-transformers/pull/1026?src=pr&el=desc) into [master](https://codecov.io/gh/huggingface/pytorch-transformers/commit/a7b4cfe9194bf93c7044a42c9f1281260ce6279e?src=pr&el=desc) will **decrease** coverage by `0.31%`.
> The diff coverage is `n/a`.
[](https://codecov.io/gh/huggingface/pytorch-transformers/pull/1026?src=pr&el=tree)
```diff
@@ Coverage Diff @@
## master #1026 +/- ##
=========================================
- Coverage 79.22% 78.9% -0.32%
=========================================
Files 38 34 -4
Lines 6406 6192 -214
=========================================
- Hits 5075 4886 -189
+ Misses 1331 1306 -25
```
| [Impacted Files](https://codecov.io/gh/huggingface/pytorch-transformers/pull/1026?src=pr&el=tree) | Coverage Δ | |
|---|---|---|
| [pytorch\_transformers/file\_utils.py](https://codecov.io/gh/huggingface/pytorch-transformers/pull/1026/diff?src=pr&el=tree#diff-cHl0b3JjaF90cmFuc2Zvcm1lcnMvZmlsZV91dGlscy5weQ==) | `71.01% <0%> (-3.09%)` | :arrow_down: |
| [pytorch\_transformers/tokenization\_bert.py](https://codecov.io/gh/huggingface/pytorch-transformers/pull/1026/diff?src=pr&el=tree#diff-cHl0b3JjaF90cmFuc2Zvcm1lcnMvdG9rZW5pemF0aW9uX2JlcnQucHk=) | `93.2% <0%> (-1.95%)` | :arrow_down: |
| [...transformers/tests/tokenization\_transfo\_xl\_test.py](https://codecov.io/gh/huggingface/pytorch-transformers/pull/1026/diff?src=pr&el=tree#diff-cHl0b3JjaF90cmFuc2Zvcm1lcnMvdGVzdHMvdG9rZW5pemF0aW9uX3RyYW5zZm9feGxfdGVzdC5weQ==) | `96.42% <0%> (-0.55%)` | :arrow_down: |
| [...rch\_transformers/tests/tokenization\_openai\_test.py](https://codecov.io/gh/huggingface/pytorch-transformers/pull/1026/diff?src=pr&el=tree#diff-cHl0b3JjaF90cmFuc2Zvcm1lcnMvdGVzdHMvdG9rZW5pemF0aW9uX29wZW5haV90ZXN0LnB5) | `96.77% <0%> (-0.45%)` | :arrow_down: |
| [...ytorch\_transformers/tests/tokenization\_xlm\_test.py](https://codecov.io/gh/huggingface/pytorch-transformers/pull/1026/diff?src=pr&el=tree#diff-cHl0b3JjaF90cmFuc2Zvcm1lcnMvdGVzdHMvdG9rZW5pemF0aW9uX3hsbV90ZXN0LnB5) | `96.77% <0%> (-0.45%)` | :arrow_down: |
| [...orch\_transformers/tests/tokenization\_xlnet\_test.py](https://codecov.io/gh/huggingface/pytorch-transformers/pull/1026/diff?src=pr&el=tree#diff-cHl0b3JjaF90cmFuc2Zvcm1lcnMvdGVzdHMvdG9rZW5pemF0aW9uX3hsbmV0X3Rlc3QucHk=) | `97.05% <0%> (-0.45%)` | :arrow_down: |
| [...torch\_transformers/tests/tokenization\_gpt2\_test.py](https://codecov.io/gh/huggingface/pytorch-transformers/pull/1026/diff?src=pr&el=tree#diff-cHl0b3JjaF90cmFuc2Zvcm1lcnMvdGVzdHMvdG9rZW5pemF0aW9uX2dwdDJfdGVzdC5weQ==) | `96.87% <0%> (-0.43%)` | :arrow_down: |
| [pytorch\_transformers/tests/optimization\_test.py](https://codecov.io/gh/huggingface/pytorch-transformers/pull/1026/diff?src=pr&el=tree#diff-cHl0b3JjaF90cmFuc2Zvcm1lcnMvdGVzdHMvb3B0aW1pemF0aW9uX3Rlc3QucHk=) | `98.57% <0%> (-0.41%)` | :arrow_down: |
| [pytorch\_transformers/optimization.py](https://codecov.io/gh/huggingface/pytorch-transformers/pull/1026/diff?src=pr&el=tree#diff-cHl0b3JjaF90cmFuc2Zvcm1lcnMvb3B0aW1pemF0aW9uLnB5) | `96.29% <0%> (-0.34%)` | :arrow_down: |
| [...torch\_transformers/tests/tokenization\_bert\_test.py](https://codecov.io/gh/huggingface/pytorch-transformers/pull/1026/diff?src=pr&el=tree#diff-cHl0b3JjaF90cmFuc2Zvcm1lcnMvdGVzdHMvdG9rZW5pemF0aW9uX2JlcnRfdGVzdC5weQ==) | `98.38% <0%> (-0.13%)` | :arrow_down: |
| ... and [14 more](https://codecov.io/gh/huggingface/pytorch-transformers/pull/1026/diff?src=pr&el=tree-more) | |
------
[Continue to review full report at Codecov](https://codecov.io/gh/huggingface/pytorch-transformers/pull/1026?src=pr&el=continue).
> **Legend** - [Click here to learn more](https://docs.codecov.io/docs/codecov-delta)
> `Δ = absolute <relative> (impact)`, `ø = not affected`, `? = missing data`
> Powered by [Codecov](https://codecov.io/gh/huggingface/pytorch-transformers/pull/1026?src=pr&el=footer). Last update [a7b4cfe...3d47a7f](https://codecov.io/gh/huggingface/pytorch-transformers/pull/1026?src=pr&el=lastupdated). Read the [comment docs](https://docs.codecov.io/docs/pull-request-comments).
<|||||>Ok, looks good to me, thanks @rabeehk <|||||>Hi Thomas
There is really some reproducibility issue in the codes, and this solves it
only
for the case when I think one does not evaluate on all the checkpoints,
please
undo this commit just to be sure not to break the codes, I will send you a
new
pull request soon when it is test for both cases.
thank you.
Best regards,
Rabeeh
On Fri, Aug 30, 2019 at 2:16 PM Thomas Wolf <[email protected]>
wrote:
> Merged #1026
> <https://github.com/huggingface/pytorch-transformers/pull/1026> into
> master.
>
> —
> You are receiving this because you were mentioned.
> Reply to this email directly, view it on GitHub
> <https://github.com/huggingface/pytorch-transformers/pull/1026?email_source=notifications&email_token=ABP4ZCFNY7Y4FNDVAV6CWK3QHEFQBA5CNFSM4ILSUUMKYY3PNVWWK3TUL52HS4DFWZEXG43VMVCXMZLOORHG65DJMZUWGYLUNFXW5KTDN5WW2ZLOORPWSZGOTLFNQXI#event-2596984925>,
> or mute the thread
> <https://github.com/notifications/unsubscribe-auth/ABP4ZCBELPM2QN44SHXIZMTQHEFQBANCNFSM4ILSUUMA>
> .
>
|
transformers | 1,025 | closed | puzzling issue regarding evaluation phase | Hi
I observe that if you run the run_glue code on WNLI, and activate both do_train and do_eval once you get one accuracy, if you run_glue with only eval with the path to the trained model,
you get another accuracy. This is very puzzling, thanks for your help | 08-14-2019 08:35:41 | 08-14-2019 08:35:41 | This issue has been automatically marked as stale because it has not had recent activity. It will be closed if no further activity occurs. Thank you for your contributions.
|
transformers | 1,024 | closed | fail to download vocabulary behind proxy server | ## ❓ Questions & Help
I work behind a proxy server. Following this [issue](https://github.com/huggingface/pytorch-transformers/issues/856), I manually download the `config.json` and `pytorch_model.bin` and the model can successfully load config and model weights.
However, in running `tokenizer = BertTokenizer.from_pretrained('bert-base-cased', do_lower_case=False)`,
I get:
INFO:pytorch_transformers.file_utils:https://s3.amazonaws.com/models.huggingface.co/bert/bert-base-cased-vocab.txt not found in cache, downloading to /tmp/tmpkat40bei
ERROR:pytorch_transformers.tokenization_utils:Couldn't reach server to download vocabulary.
If I download it manually, where should I put this vocab.txt?
Thanks!
| 08-14-2019 07:01:15 | 08-14-2019 07:01:15 | Put it in the same directory as your config.json file<|||||>Which directory is it by default?<|||||>> Which directory is it by default?
Have you found the solution? |
transformers | 1,023 | closed | fix issue #824 | fix issue #824 | 08-13-2019 15:26:36 | 08-13-2019 15:26:36 | Thanks for this @tuvuumass! |
transformers | 1,022 | closed | "mask_padding_with_zero" for xlnet | ## ❓ Questions & Help
<!-- A clear and concise description of the question. -->
From the source code in [xlnet repo](https://github.com/zihangdai/xlnet/blob/master/classifier_utils.py) line113-115
I see the comment
`
The mask has 0 for real tokens and 1 for padding tokens. Only real
tokens are attended to.
input_mask = [0] * len(input_ids)
`
But in this repo, I found the code for generate input_mask in examples/utils_glue.py
`
input_mask = [1 if mask_padding_with_zero else 0] * len(input_ids)
`
and `mask_padding_with_zero` for xlnet and bert is all set True.
I'm confused if this is a bug. | 08-13-2019 09:12:35 | 08-13-2019 09:12:35 | It is not. We've added an option to input a negative mask in XLNet so it can use the same input pattern as the other models.
If you take a look at the inputs of XLNetModel [here](https://huggingface.co/pytorch-transformers/model_doc/xlnet.html#pytorch_transformers.XLNetModel), you will see both possible masks: `attention_mask` (the original XLNet mask), `input_mask` the negative we use in the SQuAD example.<|||||>Oh, I see. Great work to maintain consistency with other models. |
transformers | 1,021 | closed | When I set fp16_opt_level == O2 or O3, I can not use multiple GPU | ## ❓ Questions & Help
<!-- A clear and concise description of the question. -->
| 08-13-2019 06:49:51 | 08-13-2019 06:49:51 | We need more information, like a full error log and the detailed command line you used for instance.<|||||>This issue has been automatically marked as stale because it has not had recent activity. It will be closed if no further activity occurs. Thank you for your contributions.
|
transformers | 1,020 | closed | Intended Behaviour for Impossible (out-of-span) SQuAD Features | ## ❓ Questions & Help
Hello! We have a quick question regarding the featurization for BERT/XLNet Question Answering.
We noticed a confusing contradiction in your current `utils_squad` implementation: regardless of how the `version_2_with_negative` flag is set, you do not discard “impossible” features (chunks of a context). Instead of discarding them, you train on them but with the span start and end indices pointing to the [CLS] token. However, this comment in your code indicates that you *do* intend to discard such features (at least for SQuAD 1.1 we would assume): https://github.com/huggingface/pytorch-transformers/blob/a7b4cfe9194bf93c7044a42c9f1281260ce6279e/examples/utils_squad.py#L332-L333.
We noticed that this behavior is the same with the Google TensorFlow BERT repository, though we see no reference in their paper to training SQuAD 1.1 with impossible contexts. Should we assume for SQuAD 1.1 the `max_sequence_length` was just always longer that all SQuAD contexts, and thus no "impossible" features were produced?
Ultimately, we are wondering if this behavior is intentional or not for purely extractive QA (like SQuAD 1.1, as opposed to 2.0)? Are you aware of anyone using “impossible" inputs to train a model for extractive QA without an abstention objective?
Thank you for your time and insights! | 08-13-2019 01:50:21 | 08-13-2019 01:50:21 | This issue has been automatically marked as stale because it has not had recent activity. It will be closed if no further activity occurs. Thank you for your contributions.
|
transformers | 1,019 | closed | Fine-tuning approach for Bert and GPT2 classifiers | ## ❓ Questions & Help
Hey folks, when we are fine-tuning BERT or GPT2 model for a classification task via classes like GPT2DoubleHeadsModel or BertForSequenceClassification, what is the recommended fine-tuning strategy? I assume all transformer layers of the base model are unfrozen for fine-tuning. Does this result in catastrophic forgetting in practice? Do people use gradual unfreezing (as in ULMFiT)? | 08-12-2019 22:26:46 | 08-12-2019 22:26:46 | AFAIK, BERT doesn't make use of gradual unfreezing. Instead, during fine-tuning all model parameters are trainable. It can result in catastrophic forgetting, if you train it for long enough/ large enough learning rate, which is why we usually fine tune for 1-2 epochs at a low learning rate.
When it comes to doing it yourself, you'll should be able to just tweak the number of epochs/train steps and then find which number gives you the best results. IMO anymore than a couple epochs will result in overfitting/forgetting.
Hope that helps.
https://arxiv.org/pdf/1905.05583.pdf<|||||>This issue can be closed.<|||||>Thanks @andrewpatterson2018. |
transformers | 1,018 | closed | Add LM-only finetuning script for GPT modules | A simple script adapted from `run_openai_gpt.py` to allow LM-only finetuning. Pre-processing is changed to accept arbitrary text files which are then chunked and a simple dataset caching scheme is added. | 08-12-2019 20:54:20 | 08-12-2019 20:54:20 | Closing because I noticed this is a special case of #987 |
transformers | 1,017 | closed | the execution order of `scheduler.step()` and `optimizer.step()` | ## ❓ Questions & Help
About current readme, related to the execution order of `scheduler.step()` and `optimizer.step()`
https://github.com/huggingface/pytorch-transformers#optimizers-bertadam--openaiadam-are-now-adamw-schedules-are-standard-pytorch-schedules
```python
### In PyTorch-Transformers, optimizer and schedules are splitted and instantiated like this:
optimizer = AdamW(model.parameters(), lr=lr, correct_bias=False) # To reproduce BertAdam specific behavior set correct_bias=False
scheduler = WarmupLinearSchedule(optimizer, warmup_steps=num_warmup_steps, t_total=num_total_steps) # PyTorch scheduler
### and used like this:
for batch in train_data:
loss = model(batch)
loss.backward()
torch.nn.utils.clip_grad_norm_(model.parameters(), max_grad_norm) # Gradient clipping is not in AdamW anymore (so you can use amp without issue)
**scheduler.step()**
**optimizer.step()**
optimizer.zero_grad()
```
While following the example code, i meet the warning which indicate the order is not expected according to the pytorch official document.
```bash
/lib/python3.6/site-packages/torch/optim/lr_scheduler.py:82: UserWarning: Detected call of `lr_scheduler.step()` before `optimizer.step()`. In PyTorch 1.1.0 and later, you should call them in the opposite order:
`optimizer.step()` before `lr_scheduler.step()`. Failure to do this will result in PyTorch skipping the first value of the learning rate schedule.See more details at https://pytorch.org/docs/stable/optim.html#how-to-adjust-learning-rate
```
I wonder if the readme need to be update to fit this announcement.
Thx | 08-12-2019 20:14:25 | 08-12-2019 20:14:25 | I believe the order doesn't actually matter as long as it reflects what you're trying to do with your learning rate.<|||||>Readme fixed, thanks! |
transformers | 1,016 | closed | inconsistent between class name (Pretrained vs PreTrained) | ## ❓ Questions & Help
https://github.com/huggingface/pytorch-transformers/blob/1b35d05d4b3c121a9740544aa6f884f1039780b1/pytorch_transformers/__init__.py#L37
I notice there are `Pre**t**rainedConfig`, `Pre**T**rainedModel` and `Pre**T**rainedTokenizer` have different naming case which is confusing.
Is this naming style expected? Or just typo?
thx | 08-12-2019 19:53:05 | 08-12-2019 19:53:05 | Hi, it isn't really expected and I agree that it can be a bit confusing, but now that it's like that we'll probably keep is so as to not make a breaking change.<|||||>This issue has been automatically marked as stale because it has not had recent activity. It will be closed if no further activity occurs. Thank you for your contributions.
|
transformers | 1,015 | closed | Logic issue with evaluating cased models in `run_squad.py` | ## 🐛 Bug
<!-- Important information -->
Model I am using (Bert, XLNet....): Bert
Language I am using the model on (English, Chinese....): English
The problem arise when using:
* [x] the official example scripts: (give details) `run_squad.py` with cased models
* [ ] my own modified scripts: (give details)
The tasks I am working on is:
* [x] an official GLUE/SQUaD task: (give the name) squad
* [ ] my own task or dataset: (give details)
## To Reproduce
Steps to reproduce the behavior:
1. Finetune a cased model with `--do_train` and `--do_eval` (the latter is optional)
2. Use `--do_eval` to make predictions.
## Expected behavior
<!-- A clear and concise description of what you expected to happen. -->
At evaluation time, the tokenizer should also be cased, but because it's loading from a path and not using a model name, the `from_pretrained` method in `BertTokenizer` fails to identify casing information, and the `BasicTokenizer` defaults to uncased (`do_lower_case=True`).
## Environment
(most of this is probably not relevant anyway)
* OS: Ubuntu 16.04
* Python version: 3.6.8
* PyTorch version: 1.2.0
* PyTorch Transformers version (or branch): master
* Using GPU ? Yes
* Distributed of parallel setup ? DataParallel
* Any other relevant information:
## Additional context
One solution is to add `do_lower_case=args.do_lower_case` in the kwargs here:
https://github.com/huggingface/pytorch-transformers/blob/master/examples/run_squad.py#L501 | 08-12-2019 19:02:47 | 08-12-2019 19:02:47 | Thanks for the bug report, will look into it.<|||||>Looks good to me, do you want to push a PR to fix this as you proposed @qipeng?<|||||>Done. See #1055!<|||||>Found another one: https://github.com/huggingface/pytorch-transformers/blob/master/examples/run_squad.py#L484
It seems like it should be
```python
if args.do_train and (args.local_rank == -1 or torch.distributed.get_rank() == 0):
```
instead of
```python
if args.do_train and args.local_rank == -1 or torch.distributed.get_rank() == 0:
```
?
I.e., this block shouldn't go through unless `args.do_train` is set explicitly IMO.<|||||>Yes, good catch @qipeng and thanks for the PR, do you want to add this fix to your PR as well?<|||||>Updated my PR! |
transformers | 1,014 | closed | BertTokenizer.save_vocabulary() doesn't work as docstring described | ## 🐛 Bug
https://github.com/huggingface/pytorch-transformers/blob/1b35d05d4b3c121a9740544aa6f884f1039780b1/pytorch_transformers/tokenization_bert.py#L169-L174
## Expected behavior
It's obvious that when `vocab_path` is not a directory, the `vocab_file` is not defined.
I believe replacing all `vocab_path` with `vocab_file` solves this issue, vice versa. | 08-12-2019 18:58:22 | 08-12-2019 18:58:22 | |
transformers | 1,013 | closed | XLNet / sentence padding | My samples have different lengths and I want to apply the padding to bring them to the same length, because my purpose is to create sentence embeddings batchwise. For that all sentences must have the same length, otherwise it is not possible to create a tensor.
How does padding work in use of XLNet model?
the snippet below shows my first try to do it with XLNet, I apply maxpooling on the model output.
```
class MaxPoolingChannel(torch.nn.AdaptiveMaxPool1d):
def forward(self, input):
input = input[0]
input = input.transpose(1,2)
result = torch.nn.AdaptiveMaxPool1d(1)(input)
return result.transpose(2,1)
tokenizer = XLNetTokenizer.from_pretrained('xlnet-base-cased')
model = XLNetModel.from_pretrained('xlnet-base-cased')
model.eval()
input_ids = torch.tensor(tokenizer.encode("Hello, my dog is cute")).unsqueeze(0) # Batch size 1
input_ids_2 = torch.tensor(tokenizer.encode("Hello, my dog is cute <pad>")).unsqueeze(0)
input_ids_3 = torch.tensor(tokenizer.encode("Hello, my dog is cute <pad> <pad>")).unsqueeze(0)
with torch.no_grad():
model = torch.nn.Sequential(model, MaxPoolingChannel(1))
res = model(input_ids)
res_2 = model(input_ids_2)
res_3 = model(input_ids_3)
print(cosine_similarity(res.detach().numpy()[0][0],res_2.detach().numpy()[0][0]))
print(cosine_similarity(res.detach().numpy()[0][0],res_3.detach().numpy()[0][0]))
```
There is a thread #790 (about document embeddings), however the point in regard to padding in XLNet has not been touched.
Thanks! | 08-12-2019 17:53:43 | 08-12-2019 17:53:43 | Hi!
By concatenating the `<pad>` value to the end of your sentences you are successfully padding them. It can be observed by identifying the encoded sentence, which shows that a `5` value (which is the padding index in the tokenizer dictionary) is appended to the end of your token sequences.
Once you have padded your sentences, you can tell the model to ignore the padded values by specifying an `attention_mask` or an `input_mask`, as described in [the documentation.](https://huggingface.co/pytorch-transformers/model_doc/xlnet.html#xlnetmodel)<|||||>I did a comparison in all dimensions between the outputs, they are different
```
input_ids = torch.tensor(tokenizer.encode("Hello, my dog is cute")).unsqueeze(0) # Batch size 1
input_ids_2 = torch.tensor(tokenizer.encode("Hello, my dog is cute <pad>")).unsqueeze(0)
mask_2 = torch.ones((1, input_ids_2.shape[1], input_ids_2.shape[1]), dtype=torch.float)
mask_2[:, :, -1] = 0.0
input_ids_3 = torch.tensor(tokenizer.encode("Hello, my dog is cute <pad> <pad>")).unsqueeze(0)
mask_3 = torch.zeros((1, input_ids_3.shape[1], input_ids_3.shape[1]), dtype=torch.float)
mask_3[:, :, 0:-2] = 1
for i in range(1):
with torch.no_grad():
outputs = model(input_ids)
res = MaxPoolingChannel(1)(outputs)
outputs_2 = model(input_ids_2, attention_mask=mask_2[:, 0])
res_2 = MaxPoolingChannel(1)(outputs_2)
outputs_3 = model(input_ids_3, attention_mask=mask_3[:, 0])
res_3 = MaxPoolingChannel(1)(outputs_3)
for i in range(outputs[0][0,:].shape[0]):
print("Hello, my dog is cute/Hello, my dog is cute <pad> dim#:", i,cosine_similarity(outputs[0][0,i].numpy(),outputs_2[0][0,i].numpy()))
print('-------------------')
for i in range(outputs[0][0,:].shape[0]):
print("Hello, my dog is cute/Hello, my dog is cute <pad> <pad> dim#:", i,cosine_similarity(outputs[0][0,i].numpy(),outputs_3[0][0,i].numpy()))
print('-------------------')
for i in range(outputs_2[0][0,:].shape[0]):
print("Hello, my dog is cute <pad>/Hello, my dog is cute <pad> <pad> dim#:", i,cosine_similarity(outputs_2[0][0,i].numpy(),outputs_3[0][0,i].numpy()))
```
here are outputs
```
Hello, my dog is cute/Hello, my dog is cute <pad> dim#: 0 0.9999999413703398
Hello, my dog is cute/Hello, my dog is cute <pad> dim#: 1 1.0000000465438699
Hello, my dog is cute/Hello, my dog is cute <pad> dim#: 2 1.000000000000007
Hello, my dog is cute/Hello, my dog is cute <pad> dim#: 3 0.9999999620304815
Hello, my dog is cute/Hello, my dog is cute <pad> dim#: 4 1.0000000000015001
Hello, my dog is cute/Hello, my dog is cute <pad> dim#: 5 0.9999999502016026
Hello, my dog is cute/Hello, my dog is cute <pad> dim#: 6 1.000000047706968
-------------------
Hello, my dog is cute/Hello, my dog is cute <pad> <pad> dim#: 0 1.0000000000000617
Hello, my dog is cute/Hello, my dog is cute <pad> <pad> dim#: 1 0.9999999534561627
Hello, my dog is cute/Hello, my dog is cute <pad> <pad> dim#: 2 1.0000000000001106
Hello, my dog is cute/Hello, my dog is cute <pad> <pad> dim#: 3 1.0000000000000115
Hello, my dog is cute/Hello, my dog is cute <pad> <pad> dim#: 4 0.9999999518847271
Hello, my dog is cute/Hello, my dog is cute <pad> <pad> dim#: 5 1.0000000000003175
Hello, my dog is cute/Hello, my dog is cute <pad> <pad> dim#: 6 1.0000000954140886
-------------------
Hello, my dog is cute <pad>/Hello, my dog is cute <pad> <pad> dim#: 0 0.999999941370278
Hello, my dog is cute <pad>/Hello, my dog is cute <pad> <pad> dim#: 1 0.999999906912401
Hello, my dog is cute <pad>/Hello, my dog is cute <pad> <pad> dim#: 2 1.000000000000062
Hello, my dog is cute <pad>/Hello, my dog is cute <pad> <pad> dim#: 3 1.000000037969543
Hello, my dog is cute <pad>/Hello, my dog is cute <pad> <pad> dim#: 4 1.00000004811622
Hello, my dog is cute <pad>/Hello, my dog is cute <pad> <pad> dim#: 5 0.9999999502025548
Hello, my dog is cute <pad>/Hello, my dog is cute <pad> <pad> dim#: 6 1.0000000477071729
Hello, my dog is cute <pad>/Hello, my dog is cute <pad> <pad> dim#: 7 1.00000000000002
```<|||||>the values are not the same, they are a slightly different (an exact consistency is not possible?)
```
-------------------
0 -0.7767028212547302
1 0.15364784002304077
2 -0.5269558429718018
3 -0.04860188066959381
4 0.14985302090644836
5 -0.6860541105270386
6 -1.598402738571167
-------------------
0 -0.7766993641853333
1 0.15364792943000793
2 -0.5269524455070496
3 -0.04859305918216705
4 0.1498618721961975
5 -0.6860424280166626
6 -1.5983952283859253
7 -0.921322226524353
8 -0.6499249935150146
```
would be the result of some picked dimension of an unpadded and a padded sentence.
```
for i in range(outputs[0][0,:].shape[0]):
print(i, outputs[0][0][i, 0].item())
print('-------------------')
for i in range(outputs_3[0][0,:].shape[0]):
print(i, outputs_3[0][0][i, 0].item())
```
dropout inplace is false on all layers in the model<|||||>This issue has been automatically marked as stale because it has not had recent activity. It will be closed if no further activity occurs. Thank you for your contributions.
|
transformers | 1,012 | closed | inconsistency of the model (XLNet) output / related to #475 #735 | Related to #475 #735
Unfortunately, I lost the overview regarding this issue.
what is the final solution for that problem?
```
tokenizer = XLNetTokenizer.from_pretrained('xlnet-base-cased')
config = XLNetConfig.from_pretrained('xlnet-base-cased')
config.output_hidden_states=True
xlnet_model = XLNetModel(config)
xlnet_model.from_pretrained('xlnet-base-cased')
xlnet_model.eval()
```
this configuration is still inconsistent.
best regards | 08-12-2019 13:05:40 | 08-12-2019 13:05:40 | if I don't load the config the results are consistent
```
tokenizer = XLNetTokenizer.from_pretrained("xlnet-base-cased")
model = XLNetLMHeadModel.from_pretrained("xlnet-base-cased")
model.eval()
```
<|||||>You should do this:
```
tokenizer = XLNetTokenizer.from_pretrained('xlnet-base-cased')
config = XLNetConfig.from_pretrained('xlnet-base-cased')
config.output_hidden_states=True
model = XLNetLMHeadModel.from_pretrained('xlnet-base-cased', config=config)
```<|||||>This issue has been automatically marked as stale because it has not had recent activity. It will be closed if no further activity occurs. Thank you for your contributions.
|
transformers | 1,011 | closed | run_classifier.py missing from examples dir | Hi, it seems that run_classifier is removed (or changed name?) from the examples dir. I am working on NER task with bert, can anyone suggest where I can find the sample/tutorial training/prediction code? | 08-12-2019 12:17:39 | 08-12-2019 12:17:39 | It is now replaced by run_glue.py in the /examples folder<|||||>@ningjize Got it, thanks.<|||||>This issue has been automatically marked as stale because it has not had recent activity. It will be closed if no further activity occurs. Thank you for your contributions.
|
transformers | 1,010 | closed | Order of inputs of forward function problematic for jit with Classification models | ## TL;DR
Due to order of args of `forward` in classification models, `device` gets hardcoded during jit tracing or causes unwanted overhead. Easy solution (but possibly breaking):
```
# change this
# classification BERT
class BertForSequenceClassification(BertPreTrainedModel):
...
def forward(self, input_ids, token_type_ids=None, attention_mask=None, labels=None,
position_ids=None, head_mask=None):
...
# to this
# classification BERT
class BertForSequenceClassification(BertPreTrainedModel):
...
def forward(self, input_ids, token_type_ids=None, attention_mask=None,
position_ids=None, head_mask=None, labels=None):
...
```
## Long Version
The order of the inputs of the models is problematic for jit tracing, because you separate the inputs of the base BERT models in the classifications models. Confusing in words, but easy to see in code:
```
# base BERT
class BertModel(BertPreTrainedModel):
...
def forward(self, input_ids, token_type_ids=None, attention_mask=None, position_ids=None, head_mask=None):
...
# classification BERT
# notice the order where labels comes in
class BertForSequenceClassification(BertPreTrainedModel):
...
def forward(self, input_ids, token_type_ids=None, attention_mask=None, labels=None,
position_ids=None, head_mask=None):
...
```
The problem arises because `torch.jit.trace` does not use the python logic when creating the embedding layer. [This line](https://github.com/huggingface/pytorch-transformers/blob/master/pytorch_transformers/modeling_bert.py#L259), `position_ids = torch.arange(seq_length, dtype=torch.long, device=input_ids.device)`, becomes `position_ids = torch.arange(seq_length, dtype=torch.long, device=torch.device("[device at time of jit]"))`. Importantly, `model.to(device)` will not change this hardcoded device in the embeddings. Thus the torch device gets hardcoded into the whole network and one can't use `model.to(device)` as expected. One could circumvent this problem by explicitly passing `position_ids` at the time of tracing, but the `torch.jit.trace` function only takes a tuple of inputs. Because `labels` comes before `position_ids`, you cannot jit trace the function without putting in dummy labels and doing the extra overhead of calculating the loss, which you don't want for a graph used solely for inference.
The simple solution is to change the order of your arguments to make the `labels` argument come after the arguments in the base bert model. Of course, this could break existing scripts that rely on this order, although the current examples use kwargs so it should be a problem.
```
# classification BERT
class BertForSequenceClassification(BertPreTrainedModel):
...
def forward(self, input_ids, token_type_ids=None, attention_mask=None,
position_ids=None, head_mask=None, labels=None):
...
```
If this were done then one could do:
```
# model = any of the classification models
msl = 15 # max sequence length, which gets hardcoded into the network
inputs = [
torch.ones(1, msl, dtype=torch.long()), # input_ids
torch.ones(1, msl, dtype=torch.long()), # segment_ids
torch.ones(1, msl, dtype=torch.long()), # attention_masks
torch.ones(1, msl, dtype=torch.long()), # position_ids
]
traced_model = torch.jit.trace(model, input)
```
Finally, and this is a judgement call, it's illogical to stick the labels parameter into the middle of the list of parameters, it probably should be at the end. But that is a minor, minor gripe in an otherwise fantastically built library. | 08-12-2019 10:48:10 | 08-12-2019 10:48:10 | Thanks for giving such an in-depth review of the issue, it is very helpful. I indeed see this can be problematic, I'll have a look into it.<|||||>Thanks a lot for the details @dhpollack!
As you probably guessed, the strange order of the arguments is the results of trying to minimize the number of breaking changes (for people who rely on the positions to feed keyword arguments) while adding additional functionalities to the library.
The resulting situation is not very satisfactory indeed.
Personally, I think it's probably time to reorder the keyword arguments.<|||||>#1195 seems to have solved this. |
transformers | 1,009 | closed | GPT2 Sentence Probability: Necessary to Prepend "<|endoftext|>"? | When computing sentence probability, do we need to prepend the sentence with a dummy start token (e.g. <|endoftext|>) to get the full sentence probability? I am currently using the following implemention (from https://github.com/huggingface/pytorch-transformers/issues/473):
```
model = GPT2LMHeadModel.from_pretrained("gpt2")
model.eval()
tokenizer = GPT2Tokenizer.from_pretrained("gpt2")
def score(sentence):
tokenize_input = tokenizer.tokenize(sentence)
tensor_input = torch.tensor([tokenizer.convert_tokens_to_ids(tokenize_input)])
loss=model(tensor_input, lm_labels=tensor_input)
return -loss[0] * len(tokenize_input)
a=['there is a book on the desk',
'there is a plane on the desk',
'there is a book in the desk']
print([score(i) for i in a])
```
With this implementation, say for the sentence "there is a book on the desk", is it taking into consideration all the words when computing the full sentence probability (i.e. it's computing P(there|<|endoftext|>) \* P(is|there,<|endoftext|>) \* ... * P(desk|the,...))? If not, what's the right way to prepend the dummy start token? | 08-12-2019 07:46:31 | 08-12-2019 07:46:31 | Dig into this a little, and it looks like the answer is yes:
```
text = "the book is on the desk."
tokenizer = GPT2Tokenizer.from_pretrained('gpt2')
model = GPT2LMHeadModel.from_pretrained('gpt2')
input_ids = torch.tensor(tokenizer.encode(text)).unsqueeze(0) # Batch size 1
tokenize_input = tokenizer.tokenize(text)
#50256 is the token_id for <|endoftext|>
tensor_input = torch.tensor([ [50256] + tokenizer.convert_tokens_to_ids(tokenize_input)])
with torch.no_grad():
outputs = model(tensor_input, labels=tensor_input)
loss, logits = outputs[:2]
print("a=", loss*len(tokenize_input))
lp = 0.0
for i in range(len(tokenize_input)):
masked_index = i
predicted_score = logits[0, masked_index]
predicted_prob = softmax(np.array(predicted_score))
lp += np.log(predicted_prob[tokenizer.convert_tokens_to_ids([tokenize_input[i]])[0]])
print("b=", lp)
```
produces:
a= tensor(32.5258)
b= -32.52579879760742
Without prepending [50256]:
a= tensor(30.4421)
b= -59.90513229370117
<|||||>@jhlau hello, out of curiosity, why are you multiplying the loss with length of tokenize_input? <|||||>The loss returned is the average loss (i.e. it is already divided by the length); since I am interested in getting the sentence probability, I need to revert that.<|||||>Instead of hard-coding `50256` better to use:
```
tokenizer.convert_tokens_to_ids(tokenizer.special_tokens_map['eos_token'])
```
<|||||>You can also use `tokenizer. eos_token_id` ([doc](https://huggingface.co/transformers/main_classes/tokenizer.html#transformers.PreTrainedTokenizer.eos_token_id))<|||||>Hope this question is simple to answer: How can I run the probability calculation entirely on gpu? When I start with numpy in the for loop I am supposed to put my data back on cpu right? I'd like to avoid that as long as possible.<|||||>@jhlau your code does not seem to be correct to me. Refer to [this](https://github.com/simonepri/lm-scorer/blob/master/lm_scorer/models/gpt2.py#L20-L60) or #2026 for a (hopefully) correct implementation.
You can also try [`lm-scorer`](https://github.com/simonepri/lm-scorer), a tiny wrapper around `transformers` I wrote that allows you to get sentences probabilities using models that support it (only GPT2 models are implemented at the time of writing).
> I included this here because this issue is still the first result when searching from GitHub/Google about using transformers' models to get sentences probabilities and I think it might be useful to many.
<|||||>I see. So I should be using self.tokenizer.bos_token and self.tokenizer.eos_token to start and end a sentence properly (instead of the hardcoded 50526 |endoftext| token). I'll give it a run and see if I find much difference.<|||||>> The loss returned is the average loss (i.e. it is already divided by the length); since I am interested in getting the sentence probability, I need to revert that.
I think this is incorrect. If you multiply by length, you will get higher probability for long sentences even if they make no sense. The average aims to normalize so that the probability is independent of the number of tokens. Does that make sense?<|||||>I understand that of course. I need the full sentence probability because I intend to do other types of normalisation myself (e.g. based unigram frequencies). I am not saying returning the average loss is wrong - I was just clarifying to another user why I multiplied the average loss with length (because I need the full sentence probability).<|||||>> I understand that of course. I need the full sentence probability because I intend to do other types of normalisation myself (e.g. based unigram frequencies). I am not saying returning the average loss is wrong - I was just clarifying to another user why I multiplied the average loss with length (because I need the full sentence probability).
AAAAh I see. Thanks<|||||>> When computing sentence probability, do we need to prepend the sentence with a dummy start token (e.g. <|endoftext|>) to get the full sentence probability? I am currently using the following implemention (from #473):
>
> ```
> model = GPT2LMHeadModel.from_pretrained("gpt2")
> model.eval()
> tokenizer = GPT2Tokenizer.from_pretrained("gpt2")
>
> def score(sentence):
> tokenize_input = tokenizer.tokenize(sentence)
> tensor_input = torch.tensor([tokenizer.convert_tokens_to_ids(tokenize_input)])
> loss=model(tensor_input, lm_labels=tensor_input)
> return -loss[0] * len(tokenize_input)
>
> a=['there is a book on the desk',
> 'there is a plane on the desk',
> 'there is a book in the desk']
> print([score(i) for i in a])
> ```
>
> With this implementation, say for the sentence "there is a book on the desk", is it taking into consideration all the words when computing the full sentence probability (i.e. it's computing P(there|<|endoftext|>) * P(is|there,<|endoftext|>) * ... * P(desk|the,...))? If not, what's the right way to prepend the dummy start token?
```sent_probability = math.exp(-1.0 * loss * (num_of_word_piece - 1))```
num_of_word_piece is the num of encoded ids by the tokenizer.
When calculating sent probability, it is appropriate to prepend "<|endoftext|>" in front of the sent text.
tokenizer will tokenize the "<|endoftext|>" into one token_id, which is tokenizer.eos_token_id.
The loss is calculated from the cross-entropy of `shift_logits` and `shift_labels`. By default, cross_entropy gives the mean reduction. And in this case, it is the mean reduction of `num_of_word_piece - 1` word_pieces.
<|||||>For anyone who's interested in **batching** the above process, here's the code:
```python
lines = [tokenizer.eos_token + line for line in lines]
tok_res = tokenizer.batch_encode_plus(lines, return_tensors='pt', pad_to_max_length=True)
input_ids = tok_res['input_ids']
attention_mask = tok_res['attention_mask']
lines_len = torch.sum(tok_res['attention_mask'], dim=1)
outputs = gpt2_model(input_ids=input_ids, attention_mask=attention_mask, labels=input_ids)
loss, logits = outputs[:2]
for line_ind in range(len(lines)):
line_log_prob = 0.0
for token_ind in range(lines_len[line_ind] - 1):
token_prob = F.softmax(logits[line_ind, token_ind], dim=0)
token_id = input_ids[line_ind, token_ind + 1]
line_log_prob += torch.log(token_prob[token_id])
print(f'line_log_prob:{line_log_prob}')
```
A caveat was that `token_type_ids` from `tokenizer.batch_encode_plus` should not be passed to the `gpt2_model` in order to obtain the same results as the line-by-line inference.<|||||>I think there's a mistake in the approach taken here.
It seems like the OP concluded that you can score the whole sentence including the first word, by appending a `bos_token` (`<|endoftext|>`) at the beginning of the string.
From what I understand, though, this is probably not a good idea, since it is __unlike training__, as mentioned by @thomwolf in another thread (https://github.com/huggingface/transformers/issues/473#issuecomment-482280934) (emphasis mine):
> Unfortunately, given __the way the model is trained (without using a token indicating the beginning of a sentence)__, I would say it does not make sense to try to get a score for a sentence with only one word.
So, the right way to get a sentence's probability would be
In [1]:
```python
import torch
import torch.nn.functional as F
import numpy as np
from tqdm import tqdm
from transformers import AutoTokenizer, AutoModelForCausalLM, AutoModelForMaskedLM
from transformers import logging
model_spec = 'gpt2'
model = AutoModelForCausalLM.from_pretrained(model_spec)
tokenizer = AutoTokenizer.from_pretrained(model_spec)
def score(sentence):
ids = tokenizer(sentence, return_tensors="pt").input_ids[0]
with torch.no_grad():
outs = model(input_ids=ids, labels=ids)
return -outs.loss * (len(ids) - 1) # the first word is not predicted
text = "the book is on the table."
print("sentence score = ", score(text).item())
```
Out [1]:
> sentence score = -23.651351928710938
We can verify where this score comes from.
In the spirit of the OP, I'll print each word's logprob and then sum
In [2]:
```python
ids = tokenizer(text, return_tensors="pt").input_ids[0]
with torch.no_grad():
outs = model(input_ids=ids, labels=ids)
logits = outs.logits
logprob = 0.0
print("", "id", "token", "logprob", sep='\t')
for i in range(len(ids)-1):
predicted_logprob = torch.log_softmax(logits[i], dim=-1)
logprob_i = predicted_logprob[ids[i+1]]
print(i, ids[i+1].item(), tokenizer.decode(ids[i+1]), logprob_i.item(), sep='\t')
logprob += logprob_i
print("total logprob = ", logprob.item(), sep = "\t")
```
Out [2]:
id token logprob
0 1492 book -7.818896770477295
1 318 is -1.9839171171188354
2 319 on -4.946821212768555
3 262 the -1.473121166229248
4 3084 table -4.56355619430542
5 13 . -2.865037441253662
total logprob = -23.651350021362305
Basically, I think we shouldn't prepend anything, if it wasn't like that in training, and so we shouldn't include the first word's score when we score a sentence from GPT2. Am I wrong? |
transformers | 1,008 | closed | How can I use only one layer transformer via this repository? | ## ❓ Questions & Help
I want to use only one layer transformer on the head of some backbone model. Can I use this repository in a simple way? | 08-12-2019 03:06:25 | 08-12-2019 03:06:25 | This repository is especially useful if you're looking to use a pre-trained transformer of the same architecture than that of BERT, GPT, GPT-2, XLM, XLNet or TransfoXL.
If you're looking at using a simple transformer of your own making, how about using the newly released [torch.nn.Transformer](https://pytorch.org/docs/stable/nn.html?highlight=transformer#torch.nn.Transformer)?<|||||>Thanks! But since I use pytorch v1.0.1 with cuda8.0, it's not convenient to upgrade to v1.2.0 and use torch.nn.Transformer.<|||||>If you're looking to use an existing architecture and modifying a few things (like the number of layers, or embedding size), you can always do so by specifying these values in a config file.
As you were saying you would like to use a one-layer transformer on top of some backbone model, you could create a config and specify `num_hidden_layers = 1` and `num_attention_heads = 1` to have a very simple one-layer single-headed transformer.
The documentation for the `BertConfig` file can be found [here](https://huggingface.co/pytorch-transformers/model_doc/bert.html#bertconfig). Each model has its own configuration file.
If you're looking to build a Transformer from scratch, something you could do is re-use some of our model's logic to create your own transformer. For example if you want to use our Attention for GPT-2, you could always import it like this:
```python
from pytorch_transformers.modeling_gpt2 import Attention
```
You can then re-use it as a part of your code, building your own Transformer architecture.
Hope that helps.<|||||>Thanks for your patience! I will try what you told me. It really helps me a lot!
Thanks again. |
transformers | 1,007 | closed | can somebody share an example of how to use GPT2 model for multiclass classification problem with fine tuning Language model ? | ## ❓ Questions & Help
I have huge text corpus without label and few data points with label.
can somebody guide on how to use GPT2 model for multi class classification problem with fine tuned Language model ? | 08-11-2019 19:17:18 | 08-11-2019 19:17:18 | This issue has been automatically marked as stale because it has not had recent activity. It will be closed if no further activity occurs. Thank you for your contributions.
|
transformers | 1,006 | closed | Update README.md | I assume that it should test the `re-load` functionality after testing the `save` functionality, however I'm also surprised that nobody points this out after such a long time, so maybe I've misunderstood the purpose. This PR is just in case :) | 08-11-2019 13:37:30 | 08-11-2019 13:37:30 | You're right, it should! Thanks for pointing it out! |
transformers | 1,005 | closed | Can't get attribute 'Corpus' on <module '__main__' from 'convert_transfo_xl_checkpoint_to_pytorch.py'> | I trained my data with the original transformer_xl repo, but I use convert_transfo_xl_checkpoint_to_pytorch.py to transfer tf to pytorch,
error occurs:
AttributeError: Can't get attribute 'Corpus' on <module '__main__' from 'convert_transfo_xl_checkpoint_to_pytorch.py'>
to use my data, What code do I want to change? | 08-11-2019 11:15:20 | 08-11-2019 11:15:20 | |
transformers | 1,004 | closed | Refactoring old run_swag.py | Pytorch-transformers! Nice work!
Refactoring old run_swag.py.
## Motivation:
I have seen the swag PR1 #951 and related issues #931
According to @thomwolf 's comments on PR1, I think it's necessary to adopt code styles of [run_squad.py](https://github.com/huggingface/pytorch-transformers/blob/master/examples/run_squad.py) in run_swag.py so that we can easily take advantage of the new powerful pytorch_transformers.
## Changes:
I refactored the old run_swag.py following [run_squad.py](https://github.com/huggingface/pytorch-transformers/blob/master/examples/run_squad.py) and tested it on bert_base_uncased pretrained model, on Tesla P100.
## Tests:
```shell
export SWAG_DIR=/path/to/SWAG
python -m torch.distributed.launch --nproc_per_node 1 run_swag.py \
--train_file SWAG_DIR/train.csv \
--predict_file SWAG_DIR/val.csv \
--model_type bert \
--model_name_or_path bert-base-uncased \
--max_seq_length 80 \
--do_train \
--do_eval \
--do_lower_case \
--output_dir ../models/swag_output \
--per_gpu_train_batch_size 32 \
--per_gpu_eval_batch_size 32 \
--learning_rate 2e-5 \
--gradient_accumulation_steps 2 \
--num_train_epochs 3.0 \
--logging_steps 200 \
--save_steps 200
```
Results:
```
eval_accuracy = 0.8016595021493552
eval_loss = 0.5581122178810473
```
I have also tested the ``--fp16`` and the acc is 0.801.
Other args have been tested: ``--evaluate_during_training``, ``--eval_all_checkpoints``, ``--overwrite_output_dir``, `--overwrite_cache``.
Things have not been tested: multi-gpu, distributed trianing. since I only have one gpu and one computer.
## Questions:
It seems the performance is worse than the pytorch-pretrain-bert results. Is this gap of result normal (0.82 and 0.86)?
## Future work:
I think it's good to add multiple choice model in XLnet since there are many multiple choice datasets such as RACE.
Thank you all!
| 08-11-2019 08:04:12 | 08-11-2019 08:04:12 | # [Codecov](https://codecov.io/gh/huggingface/pytorch-transformers/pull/1004?src=pr&el=h1) Report
> Merging [#1004](https://codecov.io/gh/huggingface/pytorch-transformers/pull/1004?src=pr&el=desc) into [master](https://codecov.io/gh/huggingface/pytorch-transformers/commit/e768f2322abd2a2f60a3a6d64a6a94c2d957fe89?src=pr&el=desc) will **decrease** coverage by `0.39%`.
> The diff coverage is `20.75%`.
[](https://codecov.io/gh/huggingface/pytorch-transformers/pull/1004?src=pr&el=tree)
```diff
@@ Coverage Diff @@
## master #1004 +/- ##
=========================================
- Coverage 81.16% 80.77% -0.4%
=========================================
Files 57 57
Lines 8039 8092 +53
=========================================
+ Hits 6525 6536 +11
- Misses 1514 1556 +42
```
| [Impacted Files](https://codecov.io/gh/huggingface/pytorch-transformers/pull/1004?src=pr&el=tree) | Coverage Δ | |
|---|---|---|
| [pytorch\_transformers/modeling\_xlnet.py](https://codecov.io/gh/huggingface/pytorch-transformers/pull/1004/diff?src=pr&el=tree#diff-cHl0b3JjaF90cmFuc2Zvcm1lcnMvbW9kZWxpbmdfeGxuZXQucHk=) | `74.52% <16%> (-2.9%)` | :arrow_down: |
| [pytorch\_transformers/modeling\_roberta.py](https://codecov.io/gh/huggingface/pytorch-transformers/pull/1004/diff?src=pr&el=tree#diff-cHl0b3JjaF90cmFuc2Zvcm1lcnMvbW9kZWxpbmdfcm9iZXJ0YS5weQ==) | `64.96% <25%> (-10.27%)` | :arrow_down: |
------
[Continue to review full report at Codecov](https://codecov.io/gh/huggingface/pytorch-transformers/pull/1004?src=pr&el=continue).
> **Legend** - [Click here to learn more](https://docs.codecov.io/docs/codecov-delta)
> `Δ = absolute <relative> (impact)`, `ø = not affected`, `? = missing data`
> Powered by [Codecov](https://codecov.io/gh/huggingface/pytorch-transformers/pull/1004?src=pr&el=footer). Last update [e768f23...8960988](https://codecov.io/gh/huggingface/pytorch-transformers/pull/1004?src=pr&el=lastupdated). Read the [comment docs](https://docs.codecov.io/docs/pull-request-comments).
<|||||>run_multiple_choice.py and utils_multiple_choice.py with roberta and xlnet have been tested on RACE, SWAG, ARC Challenge.
1. roebrta large: RACE dev 0.84, SWAG dev 0.88, ARC Challenge 0.65
2. xlnet large: RACE dev 0.81, ARC challenge 0.63<|||||>This looks really great. Thanks for updating and testing this script @erenup
A few questions and remarks:
- do we still need to keep `run_swag` now that there is a `run_multiple_choice`?
- there should be docstrings for the new classes, can you add them, taking inspiration from the other model's docstring?
- do you want to add an example on how to use the script in the doc, for instance you can add a section [here](https://github.com/huggingface/pytorch-transformers/blob/master/docs/source/examples.rst) with the commands you used to run the script and indicate the results you got with this commands for each models (good for later reference)<|||||>@thomwolf Thank you!
- SWAG dataset has been considered as one of the multiple-choice setting datasets and has a corresponding data processor in `utils_multiple_choice.py`. So I think `run_swag` will not be needed. It's also easy to add a new data processor for other multiple-choice datasets in `utils_multiple_choice.py`.
- Docstrings will be added soon.
- Sure, I'd like to add an example on how to use `run_multiple_choice`.<|||||>Hi @thomwolf, Docstrings of the multiple-choice models have been added. An example of run_multiple_choice.py has been added in the README of examples. Thank you.<|||||>Ok this looks clean and almost ready to merge, just added a quick comment to fix in the code (order of calls to step).
A few things for the merge as we have re-organized the examples folder, can you:
- move `run_swag` to `examples/contrib`
- move your `run_multiple_choice` scripts to the main `examples` folder? <|||||>Hi @thomwolf. I have moved run_multiple_choice.py and utils_multiple_choice.py to examples, run_swag.py to example/contrib and scheduler.step after optimizer.step. I have also done a test of the example/contrib/run_swag.py on current pytorch-transformers. run_swag.py can get a normal result of dev 0.809 of bert-base-uncased model. Thank you.<|||||>Awesome, thanks a lot for this contribution @erenup 🔥
Merging now<|||||>> run_multiple_choice.py and utils_multiple_choice.py with roberta and xlnet have been tested on RACE, SWAG, ARC Challenge.
>
> 1. roebrta large: RACE dev 0.84, SWAG dev 0.88, ARC Challenge 0.65
> 2. xlnet large: RACE dev 0.81, ARC challenge 0.63
Could you share your run -configuration on RACE and ARC dataset?
On SWAG, I could got 0.82 folllowing the suggested setting.
To the RACE,the best performance is 0.62. (maxLength 256, lr 1e-6, cal_gradient 8 etc). The loss is easy over-fittting.
But to the ARC. In the process of data. It show an error like this.
line 638, in _create_examples
contexts=[options[0]["para"].replace("_", ""), options[1]["para"].replace("_", ""),
KeyError: 'para'
(I have check the raw_data. the options item has no 'para' .
Could you give me a hit how to convert the dataset of ARC?
Thank you!<|||||>Hi, @PantherYan
For RACE, I checked my parameters. I run RACE with 4 P40 GPUs with roberta large:
``Namespace(adam_epsilon=1e-08, cache_dir='', config_name='', data_dir='data/RACE/', device=device(type='cuda'), do_eval=True, do_lower_case=True, do_test=False, do_train=True, eval_all_checkpoints=False, evaluate_during_training=False, fp16=False, fp16_opt_level='O1', gradient_accumulation_steps=3, learning_rate=1e-05, local_rank=-1, logging_steps=50, max_grad_norm=1.0, max_seq_length=384, max_steps=-1, model_name_or_path='roberta-large', model_type='roberta', n_gpu=4, no_cuda=False, num_train_epochs=5.0, output_dir='models_bert/race_large', overwrite_cache=False, overwrite_output_dir=False, per_gpu_eval_batch_size=2, per_gpu_train_batch_size=2, save_steps=2000, seed=42, server_ip='', server_port='', task_name='race', tokenizer_name='', train_batch_size=8, warmup_steps=0, weight_decay=0.0)``, you can have a try.
For ARC, you need to ask ai2 for the retrieved text named `para` for the corresponding task of ARC Challenge, ARC Easy, OpenBookqa. you can find more details in [this page](https://leaderboard.allenai.org/arc/submission/blcotvl7rrltlue6bsv0)<|||||>
> Hi, @PantherYan
> For RACE, I checked my parameters. I run RACE with 4 P40 GPUs with roberta large:
> `Namespace(adam_epsilon=1e-08, cache_dir='', config_name='', data_dir='data/RACE/', device=device(type='cuda'), do_eval=True, do_lower_case=True, do_test=False, do_train=True, eval_all_checkpoints=False, evaluate_during_training=False, fp16=False, fp16_opt_level='O1', gradient_accumulation_steps=3, learning_rate=1e-05, local_rank=-1, logging_steps=50, max_grad_norm=1.0, max_seq_length=384, max_steps=-1, model_name_or_path='roberta-large', model_type='roberta', n_gpu=4, no_cuda=False, num_train_epochs=5.0, output_dir='models_bert/race_large', overwrite_cache=False, overwrite_output_dir=False, per_gpu_eval_batch_size=2, per_gpu_train_batch_size=2, save_steps=2000, seed=42, server_ip='', server_port='', task_name='race', tokenizer_name='', train_batch_size=8, warmup_steps=0, weight_decay=0.0)`, you can have a try.
>
> For ARC, you need to ask ai2 for the retrieved text named `para` for the corresponding task of ARC Challenge, ARC Easy, OpenBookqa. you can find more details in [this page](https://leaderboard.allenai.org/arc/submission/blcotvl7rrltlue6bsv0)
Thanks a lot for your prompt reply! Appreciate!
It seems is a TensorFlow-version setting. I will try on the PyTorch. I only have 4 2080Ti (11GB), is the max-lenght batch-size or model size(like roberta-base) influence the performance significantly? I will run a comparison and post it out.
For the ARC. Thanks, I have write a email to AI2 for the help.
Thank you!<|||||>> Hi, @PantherYan
> For RACE, I checked my parameters. I run RACE with 4 P40 GPUs with roberta large:
> `Namespace(adam_epsilon=1e-08, cache_dir='', config_name='', data_dir='data/RACE/', device=device(type='cuda'), do_eval=True, do_lower_case=True, do_test=False, do_train=True, eval_all_checkpoints=False, evaluate_during_training=False, fp16=False, fp16_opt_level='O1', gradient_accumulation_steps=3, learning_rate=1e-05, local_rank=-1, logging_steps=50, max_grad_norm=1.0, max_seq_length=384, max_steps=-1, model_name_or_path='roberta-large', model_type='roberta', n_gpu=4, no_cuda=False, num_train_epochs=5.0, output_dir='models_bert/race_large', overwrite_cache=False, overwrite_output_dir=False, per_gpu_eval_batch_size=2, per_gpu_train_batch_size=2, save_steps=2000, seed=42, server_ip='', server_port='', task_name='race', tokenizer_name='', train_batch_size=8, warmup_steps=0, weight_decay=0.0)`, you can have a try.
>>>>>>>>>>>>>>>>>>>>>>>>>>>>>>>>>>>>>>>>>>>>>>>>>>>>>>>>>>>>>>>>>>>
Thank you for your sharing your training configuration to guid us.
I used the pytorch backend, and strictly following your configure setting, except roberta-base and the batch_size= 2(per_gpu_train_batch_size)*4(gpu_num) , which you set [ train_batch_size=8]. In other words, you setting batch_size = 8, and my setting batch_size =2.
>>>>>-------- Here is my acc on test dataset: 69.36, loss 0.8339.
>>>>> Is the batch_size inflenced my test perfermance? or the loss or convergence enough?
data/nlp/MCQA/RACE/cached_test_roberta-base_384_race
11/01/2019 01:49:55 - INFO - __main__ - ***** Running evaluation *****
11/01/2019 01:49:55 - INFO - __main__ - Num examples = 4934
11/01/2019 01:49:55 - INFO - __main__ - Batch size = 8
11/01/2019 01:53:38 - INFO - __main__ - ***** Eval results is test:True *****
11/01/2019 01:53:38 - INFO - __main__ - eval_acc = 0.6945683015808675
11/01/2019 01:53:38 - INFO - __main__ - eval_loss = 0.8386425418383782
11/01/2019 01:53:38 - INFO - __main__ - best steps of eval acc is the following checkpoints: 13000
>>>>>> I give up my training logs
11/01/2019 00:31:22 - INFO - transformers.configuration_utils - Configuration saved in models_race/roberta-base/checkpoint-12000/config.json
11/01/2019 00:31:23 - INFO - transformers.modeling_utils - Model weights saved in models_race/roberta-base/checkpoint-12000/pytorch_model.bin
11/01/2019 00:31:23 - INFO - __main__ - Saving model checkpoint to models_race/roberta-base/checkpoint-12000
11/01/2019 01:12:20 - INFO - __main__ - Loading features from cached file /workspace/data/nlp/MCQA/RACE/cached_dev_roberta-base_384_race
11/01/2019 01:12:22 - INFO - __main__ - ***** Running evaluation *****
11/01/2019 01:12:22 - INFO - __main__ - Num examples = 4887
11/01/2019 01:12:22 - INFO - __main__ - Batch size = 8
11/01/2019 01:16:00 - INFO - __main__ - ***** Eval results is test:False *****
11/01/2019 01:16:00 - INFO - __main__ - eval_acc = 0.7086146920401064
11/01/2019 01:16:00 - INFO - __main__ - eval_loss = 0.8062708838591306
11/01/2019 01:16:00 - INFO - __main__ - Loading features from cached file /workspace/data/nlp/MCQA/RACE/cached_test_roberta-base_384_race
11/01/2019 01:16:02 - INFO - __main__ - ***** Running evaluation *****
11/01/2019 01:16:02 - INFO - __main__ - Num examples = 4934
11/01/2019 01:16:02 - INFO - __main__ - Batch size = 8
11/01/2019 01:19:42 - INFO - __main__ - ***** Eval results is test:True *****
11/01/2019 01:19:42 - INFO - __main__ - eval_acc = 0.6935549250101337
11/01/2019 01:19:42 - INFO - __main__ - eval_loss = 0.8339384843925892
11/01/2019 01:19:42 - INFO - __main__ - test acc: 0.6935549250101337, loss: 0.8339384843925892, global steps: 13000
11/01/2019 01:19:42 - INFO - __main__ - Average loss: 0.6908835964873433 at global step: 13000
11/01/2019 01:19:42 - INFO - transformers.configuration_utils - Configuration saved in models_race/roberta-base/checkpoint-13000/config.json
11/01/2019 01:19:43 - INFO - transformers.modeling_utils - Model weights saved in models_race/roberta-base/checkpoint-13000/pytorch_model.bin
11/01/2019 01:19:43 - INFO - __main__ - Saving model checkpoint to models_race/roberta-base/checkpoint-13000
11/01/2019 01:49:44 - INFO - __main__ - global_step = 13730, average loss = 0.8482715931345925
>>>>>>
@erenup Could I learn your training loss and test loss after 5 epochs?
I have runed several times, the accuray still around 70%s. Is it influencd by the roberta-large model or batch_size ?
Looking forward your reply.
Thank you!
<|||||>Hi @PantherYan I did not run race dataset with roberta base. In my experience, I thought the results of RACE with roberta base make sense, Since Bert large can only reach about 71~72. You can check the [leaderboard ](http://www.qizhexie.com/data/RACE_leaderboard.html) for reference.<|||||>> Hi @PantherYan I did not run race dataset with roberta base. In my experience, I thought the results of RACE with roberta base make sense, Since Bert large can only reach about 71~72. You can check the [leaderboard ](http://www.qizhexie.com/data/RACE_leaderboard.html) for reference.
@erenup
I appreciate for your quick reply.
Thank you!
<|||||>@erenup
You are nice!<|||||>>
>
> > run_multiple_choice.py and utils_multiple_choice.py with roberta and xlnet have been tested on RACE, SWAG, ARC Challenge.
> >
> > 1. roebrta large: RACE dev 0.84, SWAG dev 0.88, ARC Challenge 0.65
> > 2. xlnet large: RACE dev 0.81, ARC challenge 0.63
>
> Could you share your run -configuration on RACE and ARC dataset?
> On SWAG, I could got 0.82 folllowing the suggested setting.
> To the RACE,the best performance is 0.62. (maxLength 256, lr 1e-6, cal_gradient 8 etc). The loss is easy over-fittting.
> But to the ARC. In the process of data. It show an error like this.
>
> line 638, in _create_examples contexts=[options[0]["para"].replace("_", ""), options[1]["para"].replace("_", ""),
>
> KeyError: 'para'
> (I have check the raw_data. the options item has no 'para' .
> Could you give me a hit how to convert the dataset of ARC?
> Thank you!
I also met the problem of missing item "para", have you got some methods for converting raw corpus?
Thank you! <|||||>Please see PatherYan's comments and [mine](https://github.com/huggingface/transformers/pull/1004#issuecomment-546900263) |
transformers | 1,003 | closed | Can't GPT-2 set special_tokens? (or unk tokens) | ## ❓ Questions & Help
<!-- A clear and concise description of the question. -->
In GPT, we can set special tokens. (I also did it branch 0.6.2)
https://github.com/huggingface/pytorch-transformers/blob/v1.0.0/pytorch_transformers/modeling_openai.py
But, in GPT-2, It seems like no way to add special tokens.
https://github.com/huggingface/pytorch-transformers/blob/b33a385091de604afb566155ec03329b84c96926/pytorch_transformers/modeling_gpt2.py#L619
I also saw #994. They said it's impossible.
It it true? and do you have any plan to add it? | 08-10-2019 14:12:38 | 08-10-2019 14:12:38 | I also saw #468. It will be probably added soon.
But, If someone informs new information about this, I'll thank for that.<|||||>Passing them like so works for me:
`GPT2Tokenizer.from_pretrained(args.model_name, unk_token="<|endoftext|>")`
You can all pass a list to `tokenizer.add_tokens`, then call `model.resize_token_embeddings(len(tokenizer))`.<|||||>@aburkard Thank you so much!!!! I'll try it.
<|||||>Hi, in GPT-2 there wasn't the option at first but we've added it down the line. It is available if you compile this repo from source from the master branch, or you can wait for the version 1.1 which should drop sometimes this week. In this version you're able to add special tokens to GPT-2.<|||||>Release 1.1.0 is here :-)<|||||>This issue has been automatically marked as stale because it has not had recent activity. It will be closed if no further activity occurs. Thank you for your contributions.
|
transformers | 1,002 | closed | How to make a new line when using gpt2 to generate lyrics? | ## ❓ Questions & Help
<!-- A clear and concise description of the question. -->
I use pre-trained gpt2 to generate lyrics (text generation). I can generate a long string of lyrics. While how to break the line, I try to add "\n" into it. But it seems that is not a good idea.
Thanks! | 08-10-2019 13:40:35 | 08-10-2019 13:40:35 | Did you pre-train your model while keeping all the line returns or did you remove them? You can keep them during the training so that the model learns to predict them.
If you remove them during training and wish to apply them later on, I guess you can always just create the long string of lyrics and split them with line returns.<|||||>Actually, I remove them during. Do you think keeping all the line returns during training is a better way? I mean input the whole song (use encode(text) method) instead of splitting each line into echo tokens -> ids .
By the way, Can I input POS of text into the model? Is it necessary?<|||||>I think keeping the line returns during your training is a good idea. The model is very likely to learn their position and frequency.
You can input the `position_ids` in your forward, but it is not necessary. If no position information is provided, the model will create it on its own.<|||||>This issue has been automatically marked as stale because it has not had recent activity. It will be closed if no further activity occurs. Thank you for your contributions.
|
transformers | 1,001 | closed | How do a put a different classifier on top of BertForSequenceClassification? | Hi,
Thanks for providing an efficient and easy-to-use implementation of BERT and other models.
I am working on a project that requires me to do binary classification of sentences. I am using `BertForSequenceClassification` for that but I am not getting good results i.e. my loss function doesn't converge. I noticed that by default there is only a single LinearClassifier on top of the BERT model. Is is possible to change that?
Thanks,
Shivin | 08-10-2019 07:19:49 | 08-10-2019 07:19:49 | Sure, one way you could go about it would be to create a new class similar to `BertForSequenceClassification` and implement your own custom final classifier.
The lib is pretty modular so you can usually subclass/extend what you need.<|||||>You can also replace `self.classifier` with your own model.
```
model = BertForSequenceClassification.from_pretrained("bert-base-multilingual-cased")
model.classifier = new_classifier
```
where `new_classifier` is any pytorch model that you want.<|||||>ok... Thanks a lot. I will try it.<|||||>@dhpollack Maybe its a little unrelated to this issue, but still I'll state the situation. I am using the BERT model to classify sentences on two different datasets. It is working fine on the first dataset but not on the second. Is it possible that it is because BERT has saved its weights according to the first dataset and is loading that for the second one also and thus not performing well. For example. the model configuration looks like this for BOTH the datasets. I suspect whether it should have the same vocabulary size.
```
INFO:pytorch_pretrained_bert.modeling:Model config {
"attention_probs_dropout_prob": 0.1,
"hidden_act": "gelu",
"hidden_dropout_prob": 0.1,
"hidden_size": 768,
"initializer_range": 0.02,
"intermediate_size": 3072,
"max_position_embeddings": 512,
"num_attention_heads": 12,
"num_hidden_layers": 12,
"type_vocab_size": 2,
"vocab_size": 28996
}
```
It shows the same message on both the datasets
```
INFO:pytorch_pretrained_bert.tokenization:loading vocabulary file https://s3.amazonaws.com/models.huggingface.co/bert/bert-base-cased-vocab.txt from cache at /home/pytorch/.pytorch_pretrained_bert/5e8a2b4893d13790ed4150ca1906be5f7a03d6c4ddf62296c383f6db42814db2.e13dbb970cb325137104fb2e5f36fe865f27746c6b526f6352861b1980eb80b1
INFO:pytorch_pretrained_bert.modeling:loading archive file https://s3.amazonaws.com/models.huggingface.co/bert/bert-base-cased.tar.gz from cache at cache/a803ce83ca27fecf74c355673c434e51c265fb8a3e0e57ac62a80e38ba98d384.681017f415dfb33ec8d0e04fe51a619f3f01532ecea04edbfd48c5d160550d9c
INFO:pytorch_pretrained_bert.modeling:extracting archive file cache/a803ce83ca27fecf74c355673c434e51c265fb8a3e0e57ac62a80e38ba98d384.681017f415dfb33ec8d0e04fe51a619f3f01532ecea04edbfd48c5d160550d9c to temp dir /tmp/tmpgummmons
```
How can effectively use BERT for two different datasets?<|||||>@shivin9 this is definitely not related to the classifier layer. Also, it's a little unclear what you what to do. Are you training on one dataset and then doing inference on another? If that's the case, then you do something like
```
# training
model = BertForSequenceClassification.from_pretrained("bert-base-cased")
...
model.save_pretrained("/tmp/trained_model_dir")
# inference
model = BertForSequenceClassification.from_pretrained("/tmp/trained_model_dir")
```
But as I said, it's unclear. If you are training on both datasets and getting good results on one but not the other than it probably has to do with your preprocessing. Good luck solving your problem.<|||||>Hi, I have a related question. I am experimenting with BERT for classification task. When I use `` `BertForSequenceClassification.from_pretrained ```, I can get 100% accuracy for a small data set. But if I have a customized classification head as shown below which is almost similar to ` `BertForSequenceClassification`` I get bad accuracy.
here is my customized classification head:
```
class Bertclfhead(nn.Module):
def __init__(self, config, adapt_args, bertmodel):
super().__init__()
self.num_labels = adapt_args.num_classes
self.config = config
self.bert = bertmodel
self.dropout = nn.Dropout(config['hidden_dropout_prob'])
self.classifier = nn.Linear(config['hidden_size'], adapt_args.num_classes)
def forward(self, input_ids, token_type_ids=None, attention_mask=None, labels=None, position_ids=None, head_mask=None):
outputs = self.bert(input_ids, position_ids=position_ids, token_type_ids=token_type_ids,
attention_mask=attention_mask, head_mask=head_mask)
pooled_output = outputs[1] # see note below
pooled_output = self.dropout(pooled_output)
logits = self.classifier(pooled_output)
outputs = (logits,) + outputs[2:] # add hidden states and attention if they are here
if labels is not None:
if self.num_labels == 1:
# We are doing regression
loss_fct = MSELoss()
loss = loss_fct(logits.view(-1), labels.view(-1))
else:
loss_fct = CrossEntropyLoss()
loss = loss_fct(logits.view(-1, self.num_labels), labels.view(-1))
outputs = (loss,) + outputs
return outputs # (loss), logits, (hidden_states), (attentions)
```
and I initialize my model like this:
```
model = Bertclfhead(bertconfig, adapt_args, BertModel.from_pretrained('bert-base-uncased'))
```
am I missing something?<|||||>@dhpollack I am first training on `x` and then inferring on `x`. Then I'm training on `y` and inferring on `y`.
I am also trying to put a BiLSTM on top of BERT but it seems that BERT doesn't output the vectors in the required format i.e. `(#batches, seq_len, input_dim)`. Do you have any idea how it can be solved? Right now BERT is just outputting a (BATCH_SIZE, 768) sized vector. 768 being the size of hidden layer.<|||||>@shivin9 you should read the docs. You want to output of the hidden layers but I think an lstm on top of Bert is overkill. What you are getting now is the output of the pooling layer.
Also you should close this issue since it's clear this is not an issue with the library. <|||||>Yeah sure. thanks for the help.<|||||>@mehdimashayekhi Do you solve it? Ihave the same question! By directly use `BertForSequenceClassification` and custom a classification similar to `BertForSequenceClassification` , the results totally different.<|||||>>
>
> @dhpollack I am first training on `x` and then inferring on `x`. Then I'm training on `y` and inferring on `y`.
>
> I am also trying to put a BiLSTM on top of BERT but it seems that BERT doesn't output the vectors in the required format i.e. `(#batches, seq_len, input_dim)`. Do you have any idea how it can be solved? Right now BERT is just outputting a (BATCH_SIZE, 768) sized vector. 768 being the size of hidden layer.
Were you able to resolve this?<|||||>Re dhpollack's August 12 comment. Maybe something got changed between then and now but I found you also have to set the model's number of labels to get that to work.
```
model.classifier = torch.nn.Linear(768, 8)
model.num_labels = 8
``` |
transformers | 1,000 | closed | Running on GPU? | ## ❓ Questions & Help
Hello,
I have a straightforward question I think, which I am curious about.
When I load a pretrained model and use it to tokenise and extract embeddings, is the model running on a GPU or CPU? The reason why I am asking is that using bert is very slow. In particular approximately 100 times slower than the https://pypi.org/project/bert-embedding/
Any ideas?
Cheers,
Dimitar
<!-- A clear and concise description of the question. --> | 08-09-2019 21:12:58 | 08-09-2019 21:12:58 | Hi!
When you're talking about extracting the embeddings using a pre-trained model, what are you talking about exactly?
Are you talking about using the tokenizer like :
```python
tokenizer.encode(text)
```
which returns the word ids?
Are you talking about using the embedding layer inside the model like :
```python
model.embeddings.word_embeddings(value)
```
Or are you talking about the encoded representation returned by the transformer after a forward pass like
```
model(value)
```
For the first one, using the tokenizer, you are simply using a python dictionary so it will run on CPU. For the next two, it depends on where you put your model. If you simply loaded it, it will be on CPU, but if you put in on a specific device using `model.to(device)`, then it will be on the specified device.<|||||>Yes, thank you. I messed up the model. <|||||> summarizer_cnn = pipeline('summarization')
summary_cnn = summarizer_cnn(sum_data)
#where sum_data is a textual data of 1000 length.
When I load a pretrained model and use it to extract summary, the model is running on a CPU instead of CPU and that is the reason bert is very slow. how to run the pretrained model and script on GPU. |
transformers | 999 | closed | Multi_Head Attention in BERT different from Transformer? | I have been digging through the code to understand the whole architecture of BERT (great job by the way, it's really easy to follow), and I noticed the way Multi-Headed Attention is implemented is different than from the original Transformer (unless I'm missing something). In particular, instead of using learnable weights to project the original keys, queries and values into different subspaces, they are just broken up into smaller vectors, each with different components of the originals. I am referring to the `self.transpose_for_scores` method of the `BertSelfAttention` class.
I was just wondering if there is any reason for this, as I have not seen it mentioned on the original paper. Maybe there would just be too many parameters if they included those weights? | 08-09-2019 20:20:13 | 08-09-2019 20:20:13 | Hi!
In the forward pass of the BertSelfAttention model you’re getting the hidden state of the previous layer which is of size `(batch_size, sequence_length, 768)` (768 being the embedding dimension).
The first step of the attention is to obtain the `mixed_query_layer`, `mixed_key_layer` as well as the `mixed_value_layer`, which are all of size `(batch_size, sequence_length, 768)`.
The 768 here isn’t actually directly related to the embedding size, but it is related to the number of heads (12) and the dimension of the query/key/value (64) vectors (12 * 64 = 768).
What we’re doing in the `transpose_for_scores` function is that we are reshaping our query/key/value layers so that they are of shape `(batch_size, number_of_heads, sequence_length, qkv_dimension)` -> `(batch_size, 12, sequence_length, 64)`.
It is then easy to compute the attention scores and apply the attention mask.
Is that helpful?<|||||>Thanks for the answer! I think I understand the code, but if you take a look at the [equation](https://imgur.com/a/WdqVG3J) from the [transformer paper](https://arxiv.org/pdf/1706.03762.pdf), here Q = `mixed_query_layer `, K = `mixed_key_layer ` and V = `mixed_value_layer`, and each of them are being multiplied by a different weight W_i^Q, W_i^K and W_i^V for each attention head i.
I don't see any equivalent to these weights on your code, instead as you say you just reshape Q, K and V, do the self-attention on each Q_i, K_i, V_i and then concat and multiply by W^0 (`BertSelfOutput`). I have yet to look at the transformer code, so maybe the notation in the paper is misleading and they actually did exactly what BERT is doing?<|||||>I believe that our implementation respects the formula in the paper. It is indeed Google's own implementation for BERT, you can check out their code and how they computed the attention scores here:
Resizing the [query layer](https://github.com/google-research/bert/blob/master/modeling.py#L690-L692)
Resizing the [key layer](https://github.com/google-research/bert/blob/master/modeling.py#L695-L696)
Resizing the [values layer](https://github.com/google-research/bert/blob/master/modeling.py#L727-L729)
Our BERT code is very similar to the original TF code to make the import/export of weights easy, so you would find the same ideas in both implementations.<|||||>Yes, I checked the code of the Transformer and you are right, the Multi-Head Attention is implemented in the exact same way as BERT (both original and this repo, of course). The Transformer paper explains a slightly different Multi-Head Attention, at least to my understanding, and it actually looks more powerful. Anyway, closing this issue as my doubt has been solved. Thanks again for your answers!<|||||>Glad I could help!<|||||>I had the same question, so I followed both steps in the implementation and paper. Since BertSelfAttention computes all heads in parallel, they look equivalent.
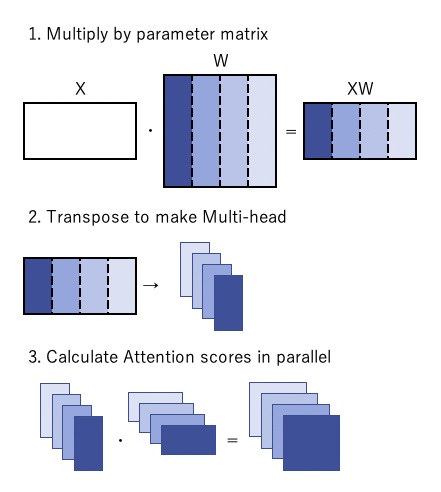
|
transformers | 998 | closed | Running the pytorch.distributed.launch example of Glue hangs at evaluation | ## 🐛 Bug
Model I am using (Bert, XLNet....): BERT base uncased
Language I am using the model on (English, Chinese....): English
The problem arise when using:
* [x] the official example scripts: (give details) The glue distributed example from Readme
## To Reproduce
Steps to reproduce the behavior:
1. Run the glue example from documentation on a multi-gpu machine with 4 GPUs (The only change I made was switch the base model to BERT uncased base) and number of GPUs to 4
2. Training completes fine
3. Script tries to evaluate - hangs at:
08/09/2019 18:02:56 - INFO - __main__ - Loading features from cached file /home/taavi/hackathon/glue_data/MRPC/cached_dev_bert-base-uncased_128_mrpc
## Expected behavior
Expected to get eval results and for the script to exit with 0.
## Environment
* OS: Centos 7
* Python version: 3.6
* PyTorch version: 1.1.0
* PyTorch Transformers version (or branch): Current master
* Using GPU: Yes, 4
* Distributed of parallel setup: distributed on 1 machine with 4 GPUs | 08-09-2019 18:03:56 | 08-09-2019 18:03:56 | More precisely it hangs on line 280:
if args.local_rank == 0:
HERE ---> torch.distributed.barrier() # Make sure only the first process in distributed training process the dataset, and the others will use the cache
# Convert to Tensors and build dataset
all_input_ids = torch.tensor([f.input_ids for f in features], dtype=torch.long)
all_input_mask = torch.tensor([f.input_mask for f in features], dtype=torch.long)
<|||||>What exact command are you using to run the script?<|||||>I also encountered similar problems when I run the example of squad. And my pytorch and Python environment are consistent with you.
My running script is:
```
python -m torch.distributed.launch --nproc_per_node=4 ./examples/run_squad.py \
--model_type bert \
--model_name_or_path bert-large-uncased-whole-word-masking \
--do_eval \
--do_lower_case \
--train_file $SQUAD_DIR/train-v1.1.json \
--predict_file $SQUAD_DIR/dev-v1.1.json \
--learning_rate 3e-5 \
--num_train_epochs 2 \
--max_seq_length 384 \
--doc_stride 128 \
--output_dir ../models/wwm_uncased_finetuned_squad/ \
--per_gpu_eval_batch_size=1 \
--per_gpu_train_batch_size=1 \
--save_steps 10000
```
Please Help!
What is more, training is OK!But the evaluation has the above problem<|||||>> I also encountered similar problems when I run the example of squad. And my pytorch and Python environment are consistent with you.
> My running script is:
>
> ```
> python -m torch.distributed.launch --nproc_per_node=4 ./examples/run_squad.py \
> --model_type bert \
> --model_name_or_path bert-large-uncased-whole-word-masking \
> --do_eval \
> --do_lower_case \
> --train_file $SQUAD_DIR/train-v1.1.json \
> --predict_file $SQUAD_DIR/dev-v1.1.json \
> --learning_rate 3e-5 \
> --num_train_epochs 2 \
> --max_seq_length 384 \
> --doc_stride 128 \
> --output_dir ../models/wwm_uncased_finetuned_squad/ \
> --per_gpu_eval_batch_size=1 \
> --per_gpu_train_batch_size=1 \
> --save_steps 10000
> ```
>
> Please Help!
> What exact command are you using to run the script?
I think I have encountered a similar problem, I have already reported my running script.<|||||>This is what I was running.
python -m torch.distributed.launch --nproc_per_node 4 ./examples/run_glue.py \
--model_type bert \
--model_name_or_path bert-base-uncased \
--task_name MRPC \
--do_train \
--do_eval \
--do_lower_case \
--data_dir $GLUE_DIR/MRPC/ \
--max_seq_length 128 \
--per_gpu_eval_batch_size=8 \
--per_gpu_train_batch_size=8 \
--learning_rate 2e-5 \
--num_train_epochs 3.0 \
--output_dir /tmp/mrpc_output/ \
--overwrite_output_dir \
--overwrite_cache \
The issue seems to be that the processes other than main never enter the evaluation section and the main process waits on a barrier for them to come join the party.
I managed to fix the issue with this change, I can push a PR if you're like. Squad seems to have the same problem.
```diff
# Evaluation
results = {}
- if args.do_eval and args.local_rank in [-1, 0]:
+ if args.do_eval:
+ if args.local_rank != -1:
+ torch.distributed.barrier()
```<|||||>We should not allow running the example script in distributed mode when only evaluation is done since the evaluation can only be done on a single GPU anyway (the reason is that the metrics cannot be computed in a distributed setting as some of the GLUE metrics are not additive with regards to the size of the evaluation dataset).
In your case, the answer is just to not run the script in distributed mode when you only do evaluation.<|||||>This issue has been automatically marked as stale because it has not had recent activity. It will be closed if no further activity occurs. Thank you for your contributions.
|
transformers | 997 | closed | Is XLA feature existed in current repo? | ## ❓ Questions & Help
I find https://news.developer.nvidia.com/nvidia-achieves-4x-speedup-on-bert-neural-network/ says tensorflow XLA has higher speed on bert, however, the pull request in this repo it mentioned https://github.com/huggingface/pytorch-pretrained-BERT/pull/116 didn't implement something like XLA. Is the XLA feature already exist? | 08-09-2019 15:19:29 | 08-09-2019 15:19:29 | Hi, there is no XLA/TPU support with the current library. Maybe in a future release! |
transformers | 996 | closed | Small typo fix in logger | I noticed two small typos when converting from Tensorflow checkpoints to PyTorch. | 08-09-2019 10:38:48 | 08-09-2019 10:38:48 | Great, thanks ! |
transformers | 995 | closed | BERT with sequence pairs & padding | ## ❓ Questions & Help
I am having trouble understanding how to setup BERT when doing a classification task like STS, for example, inputting two sentences and getting a classification of some sorts. I am using `BertForSequenceClassification` for this purpose. However, what boggles me is how to set up `attention_mask` and `token_type_ids` when using padding.
Let's assume two sentences: `I made a discovery.` and `I discovered something.`
Currently, I'll prepare the input as follows (assume padding).
1. Input IDs (encoded): `[CLS] I made a discovery. [SEP] I discovered something. [SEP] [PAD] [PAD] [PAD]`
2. `token_type_ids`: everything `0` by the first `[SEP]` (also included), **after** which everything will be marked as `1` (padding included).
3. `attention_mask`: `1` for everything but the padding.
And, of course, labels are trivial as they are not affected by padding. Anything wrong with my setup? Am I missing anything? | 08-09-2019 09:46:46 | 08-09-2019 09:46:46 | Hi! Yes, I think your understanding is correct. Your setup seems fine to me!<|||||>regarding the token_type_ids, shall we mark [PAD] token as 0 or 1? [PAD] by default does not belong to any of the two input sequences. Therefore it is ambiguous to determine whether it should be 0 or 1.<|||||>The default padding value for `token_type_ids` is 0 which is defined by `tokenizer._pad_token_type_id`. You can specify it to 1 by `tokenizer._pad_token_type_id=1`. |
transformers | 994 | closed | Pretrained GPT2 mdoels does not load unk special symbol | ## 🐛 Bug
<!-- Important information -->
Im using GPT2 (on pytorch-transformers 1.0.0) using the introductory tutorial but it seems that the tokenizer does not load the unk special symbol from the pretrained dictionary.
`
tokenizer = GPT2Tokenizer.from_pretrained('gpt2')
model = GPT2Model.from_pretrained('gpt2')
print(tokenizer.unk_token)
`
The output from the above code is as follows:
`Using unk_token, but it is not set yet.
None`
Is this the expected behavior?
Thank you!
## Environment
* OS: Linux
* Python version: 3.7.3
* PyTorch version:1.1.0
* PyTorch Transformers version (or branch):1.0.0
* Using GPU ? yes
* Distributed of parallel setup ? no
* Any other relevant information: -
| 08-08-2019 20:53:10 | 08-08-2019 20:53:10 | Hi! GPT-2 does not have an unknown token because of its byte-level BPE. This is a warning so it should not affect your code, but maybe we should do something about this warning for models that do not have unknown tokens. cc @thomwolf.<|||||>However, it seems that having a defined _unk_ symbol is necessary to run other methods, like
`def add_tokens(self, new_tokens)`
If the unk_token is set to None, add_special_tokens() breaks calling add_tokens() because the None type that is returned from convert_tokens_to_ids.<|||||>Yes, it's already on master if you compile from source and will be in the next (1.1) release (which will likely be released next week).<|||||>Thank you very much! |
transformers | 993 | closed | RuntimeError: Invalid index in gather at ../aten/src/TH/generic/THTensorEvenMoreMath.cpp:469 (GPT2DoubleHeadsModel) | ## 🐛 Bug
Model I am using (Bert, XLNet....): GPT2DoubleHeadsModel
Language I am using the model on (English, Chinese....): English
The problem arise when using:
* [x] the official example scripts: Trying out documentation
* [ ] my own modified scripts:
## To Reproduce
Steps to reproduce the behavior:
```python
tokenizer = GPT2Tokenizer.from_pretrained('gpt2')
model = GPT2DoubleHeadsModel.from_pretrained('gpt2')
choices = ["Hello, my dog is cute [CLS]", "Hello, my cat is cute [CLS]"] # Assume you've added [CLS] to the vocabulary
input_ids = torch.tensor([tokenizer.encode(s) for s in choices]).unsqueeze(0) # Batch size 1, 2 choices
mc_token_ids = torch.tensor([-1, -1]).unsqueeze(0) # Batch size 1
outputs = model(input_ids, mc_token_ids)
lm_prediction_scores, mc_prediction_scores = outputs[:2]
```
This is from the documentation of [GPT2DoubleHeadsModel](https://github.com/huggingface/pytorch-transformers/blob/f2b300df6bd46ad16580f0313bc4b30ddde8515d/pytorch_transformers/modeling_gpt2.py#L617)
The error:
> Traceback (most recent call last):
File "<input>", line 6, in <module>
File "/usr/local/Cellar/python/3.7.3/Frameworks/Python.framework/Versions/3.7/lib/python3.7/site-packages/torch/nn/modules/module.py", line 547, in __call__
result = self.forward(*input, **kwargs)
File "/usr/local/Cellar/python/3.7.3/Frameworks/Python.framework/Versions/3.7/lib/python3.7/site-packages/pytorch_transformers/modeling_gpt2.py", line 718, in forward
mc_logits = self.multiple_choice_head(hidden_states, mc_token_ids).squeeze(-1)
File "/usr/local/Cellar/python/3.7.3/Frameworks/Python.framework/Versions/3.7/lib/python3.7/site-packages/torch/nn/modules/module.py", line 547, in __call__
result = self.forward(*input, **kwargs)
File "/usr/local/Cellar/python/3.7.3/Frameworks/Python.framework/Versions/3.7/lib/python3.7/site-packages/pytorch_transformers/modeling_utils.py", line 774, in forward
output = hidden_states.gather(-2, token_ids).squeeze(-2) # shape (bsz, XX, hidden_size)
RuntimeError: Invalid index in gather at ../aten/src/TH/generic/THTensorEvenMoreMath.cpp:469
Using cls_token, but it is not set yet.
Using mask_token, but it is not set yet.
Using pad_token, but it is not set yet.
Using sep_token, but it is not set yet.
Using unk_token, but it is not set yet.
## Environment
* OS: MacOS Mojave 10.14.4
* Python version: 3.7
* PyTorch version: 1.2.0
* PyTorch Transformers version (or branch): latest
* Using GPU ? No
* Distributed of parallel setup ? No
* Any other relevant information:
| 08-08-2019 17:27:18 | 08-08-2019 17:27:18 | Indeed, there seems to be a problem here. I'll look into it.<|||||>Thanks for the report, the error was in the docstring, we cannot use `-1` as the index for the last token, it has to be the positive index of the CLS token (in the case of the example `9`.<|||||>The fix seems to have led to other issues. I'm getting the error:
----> 1 outputs = model(input_ids, mc_token_ids)
2 lm_prediction_scores, mc_prediction_scores = outputs[:2]
/opt/anaconda/anaconda3/envs/huggingface_env/lib/python3.7/site-packages/torch/nn/modules/module.py in __call__(self, *input, **kwargs)
545 result = self._slow_forward(*input, **kwargs)
546 else:
--> 547 result = self.forward(*input, **kwargs)
548 for hook in self._forward_hooks.values():
549 hook_result = hook(self, input, result)
/opt/anaconda/anaconda3/envs/huggingface_env/lib/python3.7/site-packages/pytorch_transformers/modeling_gpt2.py in forward(self, input_ids, mc_token_ids, lm_labels, mc_labels, token_type_ids, position_ids, past, head_mask)
710 position_ids=None, past=None, head_mask=None):
711 transformer_outputs = self.transformer(input_ids, position_ids=position_ids, token_type_ids=token_type_ids,
--> 712 past=past, head_mask=head_mask)
713 hidden_states = transformer_outputs[0]
714
/opt/anaconda/anaconda3/envs/huggingface_env/lib/python3.7/site-packages/torch/nn/modules/module.py in __call__(self, *input, **kwargs)
545 result = self._slow_forward(*input, **kwargs)
546 else:
--> 547 result = self.forward(*input, **kwargs)
548 for hook in self._forward_hooks.values():
549 hook_result = hook(self, input, result)
/opt/anaconda/anaconda3/envs/huggingface_env/lib/python3.7/site-packages/pytorch_transformers/modeling_gpt2.py in forward(self, input_ids, position_ids, token_type_ids, past, head_mask)
493 position_ids = position_ids.view(-1, position_ids.size(-1))
494
--> 495 inputs_embeds = self.wte(input_ids)
496 position_embeds = self.wpe(position_ids)
497 if token_type_ids is not None:
/opt/anaconda/anaconda3/envs/huggingface_env/lib/python3.7/site-packages/torch/nn/modules/module.py in __call__(self, *input, **kwargs)
545 result = self._slow_forward(*input, **kwargs)
546 else:
--> 547 result = self.forward(*input, **kwargs)
548 for hook in self._forward_hooks.values():
549 hook_result = hook(self, input, result)
/opt/anaconda/anaconda3/envs/huggingface_env/lib/python3.7/site-packages/torch/nn/modules/sparse.py in forward(self, input)
112 return F.embedding(
113 input, self.weight, self.padding_idx, self.max_norm,
--> 114 self.norm_type, self.scale_grad_by_freq, self.sparse)
115
116 def extra_repr(self):
/opt/anaconda/anaconda3/envs/huggingface_env/lib/python3.7/site-packages/torch/nn/functional.py in embedding(input, weight, padding_idx, max_norm, norm_type, scale_grad_by_freq, sparse)
1465 # remove once script supports set_grad_enabled
1466 _no_grad_embedding_renorm_(weight, input, max_norm, norm_type)
-> 1467 return torch.embedding(weight, input, padding_idx, scale_grad_by_freq, sparse)
1468
1469
RuntimeError: index out of range: Tried to access index 50257 out of table with 50256 rows. at /pytorch/aten/src/TH/generic/THTensorEvenMoreMath.cpp:237<|||||>What exact series of command did you use to get this error (maybe open a new issue with more details) |
transformers | 992 | closed | Any idea how to use pytorch-transformers for Entity Linking? | Thanks @huggingface for such a great library.
I am interested to use pytorch-transformers for entity linking. Any idea how to that?
Any help in this regard is highly appreciated.
| 08-08-2019 12:36:28 | 08-08-2019 12:36:28 | This issue has been automatically marked as stale because it has not had recent activity. It will be closed if no further activity occurs. Thank you for your contributions.
|
transformers | 991 | closed | Supress long sequence tokenization warning | ## ❓ Questions & Help
<!-- A clear and concise description of the question. -->
While tokenizing some sequences longer than 512 i get this error. I am aware that bert can't handle sequences longer than 512 so i split it later.
> Token indices sequence length is longer than the specified maximum sequence length for this model (619 > 512). Running this sequence through the model will result in indexing errors
Is there a way to supress this warning? | 08-08-2019 12:05:12 | 08-08-2019 12:05:12 | This is simply a warning, it won't change your results. I think it's important we keep it for people that are unaware that sequences have a max length of 512, so there's currently no option to suppress that warning.<|||||>Just a note: if you want to avoid displaying the warning, you can raise the level of the logger with `logging.getLogger("pytorch_pretrained_bert.tokenization").setLevel(logging.ERROR)`.
We did that in the Transfer Learning tutorial code (see [here](https://github.com/huggingface/naacl_transfer_learning_tutorial/blob/master/utils.py#L134))
This will avoid display all the logging message under the error level though, so use it with care ;-)<|||||>> Just a note: if you want to avoid displaying the warning, you can raise the level of the logger with `logging.getLogger("pytorch_pretrained_bert.tokenization").setLevel(logging.ERROR)`.
>
> We did that in the Transfer Learning tutorial code (see [here](https://github.com/huggingface/naacl_transfer_learning_tutorial/blob/master/utils.py#L134))
>
> This will avoid display all the logging message under the error level though, so use it with care ;-)
Just a small fix. Because the names have changed, use this instead:
`logging.getLogger("pytorch_transformers.tokenization_utils").setLevel(logging.ERROR)`<|||||>I encountered this problem too. As of Oct. 16, 2019, the correct way to suppress warning is:
`logging.getLogger("transformers.tokenization_utils").setLevel(logging.ERROR)`<|||||>I'm not sure from which version, but the above doesn't work anymore.
If any one stumbles on the same problem, try this:
`logging.getLogger("transformers.tokenization_utils_base").setLevel(logging.ERROR)`<|||||>See the global solution here: https://github.com/huggingface/transformers/issues/3050#issuecomment-682167272<|||||>from transformers.utils import logging
logging.set_verbosity(40)
This will set your logger to only display Errors (no warnings).
See more here -> https://huggingface.co/docs/transformers/main_classes/logging |
transformers | 990 | closed | bert-base-multilingual-uncased vocabulary not consecutive | ## 🐛 Bug
When I was checking out bert-base-multilingual-uncased vocabulary. I receive the warning "Saving vocabulary to ./vocab.txt: vocabulary indices are not consecutive. Please check that the vocabulary is not corrupted"
I ran the similar command on two different machine and got the same warning.
from pytorch_transformers import *
tokenizer = BertTokenizer.from_pretrained('bert-base-multilingual-uncased',do_lower_case=True)
tokenizer.save_vocabulary('./')
I ran it on
* OS:
* Python version: python3.5
* PyTorch version: pytorch1.0.1.post2
* PyTorch Transformers version (or branch): 1.0
* Using GPU ? Yes
* Distributed of parallel setup ?no | 08-08-2019 10:08:18 | 08-08-2019 10:08:18 | H! Could you please specify on which OS you have this error? I cannot reproduce this on MacOS 10.15, nor on Ubuntu 18.04 with both Python 3.5 and 3.6.<|||||>Hi, @LysandreJik
I tried these on Ubuntu 16.04.6 LTS.<|||||>@ntubertchen
Just in case helpful for you -- I had exactly the same issue with release 1.0 of pytorch-transformer, when I worked with multilingual BERT base models. (Ubuntu 19.04, training MRPC model with run_glue.py script.) Always at the end of training (e.g. MRPC), it gave me the above warning. And the eval results were quite strange. (e.g. often much lower than expected). Due to this, and also Chinese model broken issue; I installed master branch as of now (commits after Roberta models added), and the error went away. no more such warnings, and stable result.
Maybe you should try to install from current master (which will install locally built 1.1); it helped me. I think some code has been changed on handling multilingual vocab file. <|||||>Thanks @gilnoh. @ntubertchen can you let me know if you still have the same problem with the current (1.1.0) release ?<|||||>Yes, we had an issue in Bert tokenizer that made it lose a token in the Chinese vocabulary.
This is fixed now with the merge of #860.<|||||>This issue has been automatically marked as stale because it has not had recent activity. It will be closed if no further activity occurs. Thank you for your contributions.
|
transformers | 989 | closed | Using hidden states from BERT (Similar to using precomputed hidden states in GPT2 model "past" argument) | Hi,
In question and answer model using BERT, I will be querying context and around 50 questions on the same context. To reduce latency for obtaining results, I would like to cache hidden states of context before prediction.
For each question answer prediction on the same context, can the model use precomputed hidden states of context and get answer predictions?
Similar to GPT2, past can be used to reuse precomputed hidden state in a subsequent predictions.
Thank you,
| 08-08-2019 09:35:04 | 08-08-2019 09:35:04 | Hi, no, Bert doesn't have a cached hidden-states option.<|||||>This issue has been automatically marked as stale because it has not had recent activity. It will be closed if no further activity occurs. Thank you for your contributions.
|
transformers | 988 | closed | seq2seq model with transformer | Hi
I am urgently looking for a sequence to sequence model with transformer with script to
finetuning and training, I appreciate telling me which of the implementations in this repo could do a sequence to sequence model?
thanks
Best regards
Julia | 08-08-2019 09:20:43 | 08-08-2019 09:20:43 | Are you looking for LSTM/RNN-based seq2seq architectures or Transformer-based architectures? This repository does not host any LSTM/RNN architectures.
You can find information on all our (transformer) [models here](https://huggingface.co/pytorch-transformers/pretrained_models.html), and [examples using them here](https://huggingface.co/pytorch-transformers/examples.html).<|||||>I am looking for transformer based, pretrained model, I am not sure which of the implemented models in this repo I can use for seq2seq model? thanks for your help<|||||>The models hosted on this repo unfortunately probably cannot be used in a traditional sequence-to-sequence manner like translation (if that's what you have in mind).<|||||>yes, exactly, I am looking for such models, even gpt model cannot be used
for this purpose?
or gpt2 by conditioning?
Are you aware of clean implementation for seq2seq model with any of these
pretrained models hosted in your repo?
thanks.
On Thu, Aug 8, 2019 at 5:10 PM Lysandre Debut <[email protected]>
wrote:
> The models hosted on this repo unfortunately probably cannot be used in a
> traditional sequence-to-sequence manner like translation (if that's what
> you have in mind).
>
> —
> You are receiving this because you authored the thread.
> Reply to this email directly, view it on GitHub
> <https://github.com/huggingface/pytorch-transformers/issues/988?email_source=notifications&email_token=AM3GZM7GUYVJHJ6YKKQK3PDQDQZM5A5CNFSM4IKIFMC2YY3PNVWWK3TUL52HS4DFVREXG43VMVBW63LNMVXHJKTDN5WW2ZLOORPWSZGOD335YCY#issuecomment-519560203>,
> or mute the thread
> <https://github.com/notifications/unsubscribe-auth/AM3GZM2PJO6J63NC4DPTFW3QDQZM5ANCNFSM4IKIFMCQ>
> .
>
<|||||>Hi @juliahane, maybe take a look at `fairseq`<|||||>Hi
Thanks, Do you mind also suggest me a good implementation with lstm for
seq2seq model, I need some implementation with high quality of decoding,
thanks.
On Thu, Aug 8, 2019 at 6:52 PM Julien Chaumond <[email protected]>
wrote:
> Hi @juliahane <https://github.com/juliahane>, maybe take a look at fairseq
>
> —
> You are receiving this because you were mentioned.
> Reply to this email directly, view it on GitHub
> <https://github.com/huggingface/pytorch-transformers/issues/988?email_source=notifications&email_token=AM3GZMZG265MHPY53WI2HNTQDRFLDA5CNFSM4IKIFMC2YY3PNVWWK3TUL52HS4DFVREXG43VMVBW63LNMVXHJKTDN5WW2ZLOORPWSZGOD34HSYQ#issuecomment-519600482>,
> or mute the thread
> <https://github.com/notifications/unsubscribe-auth/AM3GZMYF5YYWWJMDVOXH3LTQDRFLDANCNFSM4IKIFMCQ>
> .
>
<|||||>Hi
I found FairSeq implementation not really clean and modular code. Are you
aware of more work which extend BERT, GPT, ... to a language model with
decoder?
thanks
Julia
On Thu, Aug 8, 2019 at 9:07 PM julia hane <[email protected]> wrote:
> Hi
> Thanks, Do you mind also suggest me a good implementation with lstm for
> seq2seq model, I need some implementation with high quality of decoding,
> thanks.
>
> On Thu, Aug 8, 2019 at 6:52 PM Julien Chaumond <[email protected]>
> wrote:
>
>> Hi @juliahane <https://github.com/juliahane>, maybe take a look at
>> fairseq
>>
>> —
>> You are receiving this because you were mentioned.
>> Reply to this email directly, view it on GitHub
>> <https://github.com/huggingface/pytorch-transformers/issues/988?email_source=notifications&email_token=AM3GZMZG265MHPY53WI2HNTQDRFLDA5CNFSM4IKIFMC2YY3PNVWWK3TUL52HS4DFVREXG43VMVBW63LNMVXHJKTDN5WW2ZLOORPWSZGOD34HSYQ#issuecomment-519600482>,
>> or mute the thread
>> <https://github.com/notifications/unsubscribe-auth/AM3GZMYF5YYWWJMDVOXH3LTQDRFLDANCNFSM4IKIFMCQ>
>> .
>>
>
<|||||>Then you should have a look at the "Cross-lingual Language Model Pretraining" from Lample and Conneau: https://arxiv.org/abs/1901.07291
Implementation of supervised and unsupervised NMT can be found here: https://github.com/facebookresearch/XLM#iii-applications-supervised--unsupervised-mt :)<|||||>Hi
thanks a lot. I was wondering if you could also suggest me a good
implementation for seq2seq with LSTMs in pytorch with good accuracy.
I have a deadline and I cannot find any, I really appreciate your help.
thanks
Julia
On Thu, Aug 8, 2019 at 11:41 PM Stefan Schweter <[email protected]>
wrote:
> Then you should have a look at the "Cross-lingual Language Model
> Pretraining" from Lample and Conneau: https://arxiv.org/abs/1901.07291
>
> Implementation of supervised and unsupervised NMT can be found here:
> https://github.com/facebookresearch/XLM#iii-applications-supervised--unsupervised-mt
> :)
>
> —
> You are receiving this because you were mentioned.
> Reply to this email directly, view it on GitHub
> <https://github.com/huggingface/pytorch-transformers/issues/988?email_source=notifications&email_token=AM3GZM3URUZ6GWYI7A4WPFTQDSHKBA5CNFSM4IKIFMC2YY3PNVWWK3TUL52HS4DFVREXG43VMVBW63LNMVXHJKTDN5WW2ZLOORPWSZGOD347NXQ#issuecomment-519698142>,
> or mute the thread
> <https://github.com/notifications/unsubscribe-auth/AM3GZM2PRHPLQBS7XBGCUBLQDSHKBANCNFSM4IKIFMCQ>
> .
>
<|||||>Hey Julia, without a specific task in mind I can't think of anything relevant, but browsing [paperswithcode.com with a seq2seq search](https://paperswithcode.com/search?q_meta=&q=seq2seq) yields quite a few interesting results.<|||||>Hi
My task is a autoencoding text. So encoding and decoding it in one
language. Thanks
On Wed, Aug 14, 2019, 5:18 PM Lysandre Debut <[email protected]>
wrote:
> Hey Julia, without a specific task in mind I can't think of anything
> relevant, but browsing paperswithcode.com with a seq2seq search
> <https://paperswithcode.com/search?q_meta=&q=seq2seq> yields quite a few
> interesting results.
>
> —
> You are receiving this because you were mentioned.
> Reply to this email directly, view it on GitHub
> <https://github.com/huggingface/pytorch-transformers/issues/988?email_source=notifications&email_token=AM3GZMZ3PDZUSW7QWNQV3CDQEQO2XA5CNFSM4IKIFMC2YY3PNVWWK3TUL52HS4DFVREXG43VMVBW63LNMVXHJKTDN5WW2ZLOORPWSZGOD4JEKTA#issuecomment-521291084>,
> or mute the thread
> <https://github.com/notifications/unsubscribe-auth/AM3GZM62USCZO4Z5UGUTOBTQEQO2XANCNFSM4IKIFMCQ>
> .
>
<|||||>I was wondering if you could tell me which of these are a fast sequence to
sequence implementation,
this is really hard for me to figure out which one to use. thanks
On Wed, Aug 14, 2019 at 5:19 PM julia hane <[email protected]> wrote:
> Hi
> My task is a autoencoding text. So encoding and decoding it in one
> language. Thanks
>
> On Wed, Aug 14, 2019, 5:18 PM Lysandre Debut <[email protected]>
> wrote:
>
>> Hey Julia, without a specific task in mind I can't think of anything
>> relevant, but browsing paperswithcode.com with a seq2seq search
>> <https://paperswithcode.com/search?q_meta=&q=seq2seq> yields quite a few
>> interesting results.
>>
>> —
>> You are receiving this because you were mentioned.
>> Reply to this email directly, view it on GitHub
>> <https://github.com/huggingface/pytorch-transformers/issues/988?email_source=notifications&email_token=AM3GZMZ3PDZUSW7QWNQV3CDQEQO2XA5CNFSM4IKIFMC2YY3PNVWWK3TUL52HS4DFVREXG43VMVBW63LNMVXHJKTDN5WW2ZLOORPWSZGOD4JEKTA#issuecomment-521291084>,
>> or mute the thread
>> <https://github.com/notifications/unsubscribe-auth/AM3GZM62USCZO4Z5UGUTOBTQEQO2XANCNFSM4IKIFMCQ>
>> .
>>
>
<|||||>I did checked this implementations you sent me, I honestly cannot find a
single good seq2seq one with lstm, and I really appreciate your help
On Wed, Aug 14, 2019 at 5:39 PM julia hane <[email protected]> wrote:
> I was wondering if you could tell me which of these are a fast sequence to
> sequence implementation,
> this is really hard for me to figure out which one to use. thanks
>
> On Wed, Aug 14, 2019 at 5:19 PM julia hane <[email protected]> wrote:
>
>> Hi
>> My task is a autoencoding text. So encoding and decoding it in one
>> language. Thanks
>>
>> On Wed, Aug 14, 2019, 5:18 PM Lysandre Debut <[email protected]>
>> wrote:
>>
>>> Hey Julia, without a specific task in mind I can't think of anything
>>> relevant, but browsing paperswithcode.com with a seq2seq search
>>> <https://paperswithcode.com/search?q_meta=&q=seq2seq> yields quite a
>>> few interesting results.
>>>
>>> —
>>> You are receiving this because you were mentioned.
>>> Reply to this email directly, view it on GitHub
>>> <https://github.com/huggingface/pytorch-transformers/issues/988?email_source=notifications&email_token=AM3GZMZ3PDZUSW7QWNQV3CDQEQO2XA5CNFSM4IKIFMC2YY3PNVWWK3TUL52HS4DFVREXG43VMVBW63LNMVXHJKTDN5WW2ZLOORPWSZGOD4JEKTA#issuecomment-521291084>,
>>> or mute the thread
>>> <https://github.com/notifications/unsubscribe-auth/AM3GZM62USCZO4Z5UGUTOBTQEQO2XANCNFSM4IKIFMCQ>
>>> .
>>>
>>
<|||||>@juliahane `fairseq` has an example of how to use a LSTM (encoder & decoder) for a seq2seq model:
https://fairseq.readthedocs.io/en/latest/tutorial_simple_lstm.html
Additionally, you could also check out Joey NMT, which has a very nice and clear codebase:
https://github.com/joeynmt/joeynmt<|||||>Hi
Thanks, Fairseq to me is not following a good coding practice although
Facebook has published it,
but the second one looks much better, thank you.
I was wondering if you could tell me if torchtext is faster than using
dataloader in pytorch for seq2seq applications?
I wonder how torchtext impact the speed and if this is really better than
dataloader
thanks
On Fri, Aug 16, 2019 at 1:42 PM Stefan Schweter <[email protected]>
wrote:
> @juliahane <https://github.com/juliahane> fairseq has an example of how
> to use a LSTM (encoder & decoder) for a seq2seq model:
>
> https://fairseq.readthedocs.io/en/latest/tutorial_simple_lstm.html
>
> Additionally, you could also check out Joey NMT, which has a very nice and
> clear codebase:
>
> https://github.com/joeynmt/joeynmt
>
> —
> You are receiving this because you were mentioned.
> Reply to this email directly, view it on GitHub
> <https://github.com/huggingface/pytorch-transformers/issues/988?email_source=notifications&email_token=AM3GZMZGVNRAT6FJLOEZAB3QE2HB3A5CNFSM4IKIFMC2YY3PNVWWK3TUL52HS4DFVREXG43VMVBW63LNMVXHJKTDN5WW2ZLOORPWSZGOD4OMY7A#issuecomment-521981052>,
> or mute the thread
> <https://github.com/notifications/unsubscribe-auth/AM3GZM3YNYMZ32LXZCCPSA3QE2HB3ANCNFSM4IKIFMCQ>
> .
>
<|||||>This issue has been automatically marked as stale because it has not had recent activity. It will be closed if no further activity occurs. Thank you for your contributions.
<|||||>Merging with #1506 |
Subsets and Splits
No community queries yet
The top public SQL queries from the community will appear here once available.