repo
stringclasses 1
value | number
int64 1
25.3k
| state
stringclasses 2
values | title
stringlengths 1
487
| body
stringlengths 0
234k
⌀ | created_at
stringlengths 19
19
| closed_at
stringlengths 19
19
| comments
stringlengths 0
293k
|
---|---|---|---|---|---|---|---|
transformers | 1,289 | closed | Adding Adapters | From: https://arxiv.org/pdf/1902.00751.pdf
Open to feedback!
* Implementing adapters requires a couple more hyperparameters that need to go into the BertConfig. Do let me know if there is an alternative to modifying the core Config object (maybe a subclass would work better?)
* If `use_adapter` is False, the adapter modules are not created, so there should be no issue with changes in `state_dicts`/weights if they're not enabled.
* Added a utility function for extracting the adapter parameters from the model, to facilitate tuning only the adapter layers. In practice, a user should tune the adapter layers (+layer norm) and the final classifier layers, the latter of which varies depending on the model.
* I believe this should work seamlessly with RoBERTa. | 09-18-2019 20:45:15 | 09-18-2019 20:45:15 | # [Codecov](https://codecov.io/gh/huggingface/pytorch-transformers/pull/1289?src=pr&el=h1) Report
> Merging [#1289](https://codecov.io/gh/huggingface/pytorch-transformers/pull/1289?src=pr&el=desc) into [master](https://codecov.io/gh/huggingface/pytorch-transformers/commit/0d1dad6d5323cf627cb8d7ddd428856ab8475f6b?src=pr&el=desc) will **increase** coverage by `0.22%`.
> The diff coverage is `39.39%`.
[](https://codecov.io/gh/huggingface/pytorch-transformers/pull/1289?src=pr&el=tree)
```diff
@@ Coverage Diff @@
## master #1289 +/- ##
==========================================
+ Coverage 80.77% 80.99% +0.22%
==========================================
Files 57 57
Lines 8092 8072 -20
==========================================
+ Hits 6536 6538 +2
+ Misses 1556 1534 -22
```
| [Impacted Files](https://codecov.io/gh/huggingface/pytorch-transformers/pull/1289?src=pr&el=tree) | Coverage Δ | |
|---|---|---|
| [pytorch\_transformers/configuration\_bert.py](https://codecov.io/gh/huggingface/pytorch-transformers/pull/1289/diff?src=pr&el=tree#diff-cHl0b3JjaF90cmFuc2Zvcm1lcnMvY29uZmlndXJhdGlvbl9iZXJ0LnB5) | `88.57% <100%> (+1.47%)` | :arrow_up: |
| [pytorch\_transformers/modeling\_bert.py](https://codecov.io/gh/huggingface/pytorch-transformers/pull/1289/diff?src=pr&el=tree#diff-cHl0b3JjaF90cmFuc2Zvcm1lcnMvbW9kZWxpbmdfYmVydC5weQ==) | `85.17% <31.03%> (-3.16%)` | :arrow_down: |
| [pytorch\_transformers/modeling\_xlnet.py](https://codecov.io/gh/huggingface/pytorch-transformers/pull/1289/diff?src=pr&el=tree#diff-cHl0b3JjaF90cmFuc2Zvcm1lcnMvbW9kZWxpbmdfeGxuZXQucHk=) | `77.42% <0%> (+2.89%)` | :arrow_up: |
| [pytorch\_transformers/modeling\_roberta.py](https://codecov.io/gh/huggingface/pytorch-transformers/pull/1289/diff?src=pr&el=tree#diff-cHl0b3JjaF90cmFuc2Zvcm1lcnMvbW9kZWxpbmdfcm9iZXJ0YS5weQ==) | `75.22% <0%> (+10.26%)` | :arrow_up: |
------
[Continue to review full report at Codecov](https://codecov.io/gh/huggingface/pytorch-transformers/pull/1289?src=pr&el=continue).
> **Legend** - [Click here to learn more](https://docs.codecov.io/docs/codecov-delta)
> `Δ = absolute <relative> (impact)`, `ø = not affected`, `? = missing data`
> Powered by [Codecov](https://codecov.io/gh/huggingface/pytorch-transformers/pull/1289?src=pr&el=footer). Last update [0d1dad6...cd97cad](https://codecov.io/gh/huggingface/pytorch-transformers/pull/1289?src=pr&el=lastupdated). Read the [comment docs](https://docs.codecov.io/docs/pull-request-comments).
<|||||>I think our goal here should be that you'd be able to extend the relevant classes without having modify to modify the lib's code.
Thoughts @LysandreJik @thomwolf?<|||||>Yes, I agree with @julien-c.
Having adapters is a nice addition but we should have a mechanism that lets us extend the base code (of each model) instead of modifying it for each type of adapting mechanism.
One way we could do that is to have standardized pointers to a selection of relevant portions of the models (also asked by Matt Newman for some AllenNLP extension of the models).<|||||>Would that be on the roadmap soon? I can resubmit my PR after there's a more generalized approach for extending model functionality.<|||||>Thinking about it again, I think the approach you proposed is the right one if we want to integrate adapters in the library as a permanent option.
But I have two questions:
- do we want to integrate adapters as a permanent option? My quick tests with adapters for the NAACL tutorial on transfer learning were giving mixed results. Do you have clear indications and benchmarks that they are useful in practice, @zphang?
- if we do integrate them in the library we would want to have them for all the models.
If we don't want to integrate them as a permanent option then we would have to find a general way to extend the models easily so people can add stuff like this in a simple way. This is open for comments.<|||||>In the meantime, I've actual moved to using an implementation that doesn't involve modifying the Transformers library. Here's an example (pardon the hacks):
https://github.com/zphang/nlprunners/blob/9caaf94ea99102a9980012b934a4373dc4996108/nlpr/proj/adapters/modeling.py#L55-L135
It involves swapping out relevant portions of the model with modified/similar layers. Given the differences between the major transformer models, I think this would be the more sustainable and less intrusive (as long as the underlying transformer code doesn't change too often).
Performance-wise, my experience has been that adapters work roughly as advertised: consistently slightly less well than fine-tuning the whole model, but only the adapter layers + classifier head need to be tuned.<|||||>I think the repository you are linking to is private Jason<|||||>Oops! Here's a gist of the code: https://gist.github.com/zphang/8eb4717b6f74c82a8ca4637ae9236e21<|||||>This issue has been automatically marked as stale because it has not had recent activity. It will be closed if no further activity occurs. Thank you for your contributions.
<|||||>Closing for now, we'll continue the work on this topic in the more general #2165 |
transformers | 1,288 | closed | Typo with LM Fine tuning script | Typo with LM Fine tuning script | 09-18-2019 20:42:47 | 09-18-2019 20:42:47 | # [Codecov](https://codecov.io/gh/huggingface/transformers/pull/1288?src=pr&el=h1) Report
> Merging [#1288](https://codecov.io/gh/huggingface/transformers/pull/1288?src=pr&el=desc) into [master](https://codecov.io/gh/huggingface/transformers/commit/2dc8cb87341223e86220516951bb4ad84f880b4a?src=pr&el=desc) will **decrease** coverage by `3.92%`.
> The diff coverage is `n/a`.
[](https://codecov.io/gh/huggingface/transformers/pull/1288?src=pr&el=tree)
```diff
@@ Coverage Diff @@
## master #1288 +/- ##
==========================================
- Coverage 84.69% 80.77% -3.93%
==========================================
Files 84 57 -27
Lines 12596 8092 -4504
==========================================
- Hits 10668 6536 -4132
+ Misses 1928 1556 -372
```
| [Impacted Files](https://codecov.io/gh/huggingface/transformers/pull/1288?src=pr&el=tree) | Coverage Δ | |
|---|---|---|
| [transformers/tests/conftest.py](https://codecov.io/gh/huggingface/transformers/pull/1288/diff?src=pr&el=tree#diff-dHJhbnNmb3JtZXJzL3Rlc3RzL2NvbmZ0ZXN0LnB5) | | |
| [transformers/modeling\_tf\_transfo\_xl\_utilities.py](https://codecov.io/gh/huggingface/transformers/pull/1288/diff?src=pr&el=tree#diff-dHJhbnNmb3JtZXJzL21vZGVsaW5nX3RmX3RyYW5zZm9feGxfdXRpbGl0aWVzLnB5) | | |
| [transformers/data/processors/utils.py](https://codecov.io/gh/huggingface/transformers/pull/1288/diff?src=pr&el=tree#diff-dHJhbnNmb3JtZXJzL2RhdGEvcHJvY2Vzc29ycy91dGlscy5weQ==) | | |
| [transformers/modeling\_gpt2.py](https://codecov.io/gh/huggingface/transformers/pull/1288/diff?src=pr&el=tree#diff-dHJhbnNmb3JtZXJzL21vZGVsaW5nX2dwdDIucHk=) | | |
| [transformers/tests/modeling\_tf\_openai\_gpt\_test.py](https://codecov.io/gh/huggingface/transformers/pull/1288/diff?src=pr&el=tree#diff-dHJhbnNmb3JtZXJzL3Rlc3RzL21vZGVsaW5nX3RmX29wZW5haV9ncHRfdGVzdC5weQ==) | | |
| [transformers/modeling\_tf\_xlm.py](https://codecov.io/gh/huggingface/transformers/pull/1288/diff?src=pr&el=tree#diff-dHJhbnNmb3JtZXJzL21vZGVsaW5nX3RmX3hsbS5weQ==) | | |
| [transformers/optimization.py](https://codecov.io/gh/huggingface/transformers/pull/1288/diff?src=pr&el=tree#diff-dHJhbnNmb3JtZXJzL29wdGltaXphdGlvbi5weQ==) | | |
| [transformers/file\_utils.py](https://codecov.io/gh/huggingface/transformers/pull/1288/diff?src=pr&el=tree#diff-dHJhbnNmb3JtZXJzL2ZpbGVfdXRpbHMucHk=) | | |
| [transformers/tokenization\_bert.py](https://codecov.io/gh/huggingface/transformers/pull/1288/diff?src=pr&el=tree#diff-dHJhbnNmb3JtZXJzL3Rva2VuaXphdGlvbl9iZXJ0LnB5) | | |
| [transformers/tests/tokenization\_distilbert\_test.py](https://codecov.io/gh/huggingface/transformers/pull/1288/diff?src=pr&el=tree#diff-dHJhbnNmb3JtZXJzL3Rlc3RzL3Rva2VuaXphdGlvbl9kaXN0aWxiZXJ0X3Rlc3QucHk=) | | |
| ... and [131 more](https://codecov.io/gh/huggingface/transformers/pull/1288/diff?src=pr&el=tree-more) | |
------
[Continue to review full report at Codecov](https://codecov.io/gh/huggingface/transformers/pull/1288?src=pr&el=continue).
> **Legend** - [Click here to learn more](https://docs.codecov.io/docs/codecov-delta)
> `Δ = absolute <relative> (impact)`, `ø = not affected`, `? = missing data`
> Powered by [Codecov](https://codecov.io/gh/huggingface/transformers/pull/1288?src=pr&el=footer). Last update [2dc8cb8...f7978f7](https://codecov.io/gh/huggingface/transformers/pull/1288?src=pr&el=lastupdated). Read the [comment docs](https://docs.codecov.io/docs/pull-request-comments).
<|||||>Thanks, updated a bit to use `format` |
transformers | 1,287 | closed | TransfoXLLMHeadModel compatibility with pytorch 1.1.0 | TransfoXLLMHeadModel._forward uses torch.Tensor.bool, which is not present in pytorch 1.1.0 | 09-18-2019 20:20:54 | 09-18-2019 20:20:54 | Should be fixed on master and the new release (2.0) |
transformers | 1,286 | closed | Evaluation result.txt path suggestion | ## 🚀 Feature
At pytorch-transformers/examples/**run_lm_finetuning**.py and **run_glue**.py
There is a line ```output_eval_file = os.path.join(eval_output_dir, "eval_results.txt")```
When setting evaluate_during_training **True**, `output_eval_file` will keep being overwritten.
I think `output_eval_file` can be assigned like
`output_eval_file = os.path.join(eval_output_dir, prefix, "eval_results.txt")`
Meanwhile, in the main() function
```result = evaluate(args, model, tokenizer, prefix=global_step)```
change into
```
result = evaluate(args, model, tokenizer, prefix=checkpoint.split('/')[-1] if checkpoint.find('checkpoint') != -1 else "")
```
Just a little suggestion
| 09-18-2019 16:41:42 | 09-18-2019 16:41:42 | Yes, why not, do you want to submit a PR for that?<|||||>> Yes, why not, do you want to submit a PR for that?
Thanks~
By the way, is there any code formatting requirement or a contribution docs for developers?
<|||||>This issue has been automatically marked as stale because it has not had recent activity. It will be closed if no further activity occurs. Thank you for your contributions.
<|||||>seems like the first checkpoint's eval results does not have a prefix? |
transformers | 1,285 | closed | GPT2 Tokenizer Decoding Adding Space | ## 🐛 Bug
The GPT-2 tokenizer's decoder now adds a space at the beginning of the string upon decoding.
(Potentially causing #1254)
Model I am using (Bert, XLNet....): GPT2
Language I am using the model on (English, Chinese....): English
The problem arise when using:
* [ ] the official example scripts: (give details)
* [x] my own modified scripts: (give details)
The tasks I am working on is:
* [ ] an official GLUE/SQUaD task: (give the name)
* [x] my own task or dataset: (give details)
## To Reproduce
Steps to reproduce the behavior:
1. Run the following code:
```bash
from pytorch_transformers.tokenization_gpt2 import GPT2Tokenizer
tokenizer = GPT2Tokenizer.from_pretrained('gpt2')
tokenizer.decode(tokenizer.encode("test phrase"))
```
<!-- If you have a code sample, error messages, stack traces, please provide it here as well. -->
## Expected behavior
The expected decoded string is "test phrase". However, currently it produces " test phrase".
## Environment
* OS: OSX
* Python version: 3.7.3
* PyTorch version: 1.1.0
* PyTorch Transformers version (or branch): master (#e768f2322abd2a2f60a3a6d64a6a94c2d957fe89)
* Using GPU ? No
* Distributed of parallel setup ? No
* Any other relevant information:
| 09-18-2019 15:42:20 | 09-18-2019 15:42:20 | Also getting this effect when using the reproduction code on my system.<|||||>It's not a bug. This is an artefact produced by BPE as explained here https://github.com/huggingface/pytorch-transformers/blob/d483cd8e469126bed081c59473bdf64ce74c8b36/pytorch_transformers/tokenization_gpt2.py#L106
I think the solution is to process whitespaces after the tokeniser. |
transformers | 1,284 | closed | Fix fp16 masking in PoolerEndLogits | Necessary to run xlnet squad fine-tuning with `--fp16 --fp16_opt_level="O2"`, otherwise loss is immediately `NaN` and fine-tuning cannot proceed.
Similar to #1249 | 09-18-2019 13:33:52 | 09-18-2019 13:33:52 | # [Codecov](https://codecov.io/gh/huggingface/transformers/pull/1284?src=pr&el=h1) Report
> Merging [#1284](https://codecov.io/gh/huggingface/transformers/pull/1284?src=pr&el=desc) into [master](https://codecov.io/gh/huggingface/transformers/commit/2dc8cb87341223e86220516951bb4ad84f880b4a?src=pr&el=desc) will **decrease** coverage by `<.01%`.
> The diff coverage is `66.66%`.
[](https://codecov.io/gh/huggingface/transformers/pull/1284?src=pr&el=tree)
```diff
@@ Coverage Diff @@
## master #1284 +/- ##
==========================================
- Coverage 84.69% 84.68% -0.01%
==========================================
Files 84 84
Lines 12596 12598 +2
==========================================
+ Hits 10668 10669 +1
- Misses 1928 1929 +1
```
| [Impacted Files](https://codecov.io/gh/huggingface/transformers/pull/1284?src=pr&el=tree) | Coverage Δ | |
|---|---|---|
| [transformers/modeling\_utils.py](https://codecov.io/gh/huggingface/transformers/pull/1284/diff?src=pr&el=tree#diff-dHJhbnNmb3JtZXJzL21vZGVsaW5nX3V0aWxzLnB5) | `92.44% <66.66%> (-0.25%)` | :arrow_down: |
------
[Continue to review full report at Codecov](https://codecov.io/gh/huggingface/transformers/pull/1284?src=pr&el=continue).
> **Legend** - [Click here to learn more](https://docs.codecov.io/docs/codecov-delta)
> `Δ = absolute <relative> (impact)`, `ø = not affected`, `? = missing data`
> Powered by [Codecov](https://codecov.io/gh/huggingface/transformers/pull/1284?src=pr&el=footer). Last update [2dc8cb8...c50783e](https://codecov.io/gh/huggingface/transformers/pull/1284?src=pr&el=lastupdated). Read the [comment docs](https://docs.codecov.io/docs/pull-request-comments).
<|||||>LGTM @slayton58 thanks |
transformers | 1,283 | closed | Is training from scratch possible now? | Do the models support training from scratch, together with original (paper) parameters? | 09-18-2019 09:04:55 | 09-18-2019 09:04:55 | You can just instanciate the models without the `.from_pretraining()` like so:
```python
config = BertConfig(**optionally your favorite parameters**)
model = BertForPretraining(config)
```
I added a flag to `run_lm_finetuning.py` that gets checked in the `main()`. Maybe this snipped helps (note, I am only using this with Bert w/o next sentence prediction).
```python
# check if instead initialize freshly
if args.do_fresh_init:
config = config_class()
tokenizer = tokenizer_class()
if args.block_size <= 0:
args.block_size = tokenizer.max_len # Our input block size will be the max possible for the model
args.block_size = min(args.block_size, tokenizer.max_len)
model = model_class(config=config)
else:
config = config_class.from_pretrained(args.config_name if args.config_name else args.model_name_or_path)
tokenizer = tokenizer_class.from_pretrained(args.tokenizer_name if args.tokenizer_name else args.model_name_or_path)
if args.block_size <= 0:
args.block_size = tokenizer.max_len # Our input block size will be the max possible for the model
args.block_size = min(args.block_size, tokenizer.max_len)
model = model_class.from_pretrained(args.model_name_or_path, from_tf=bool('.ckpt' in args.model_name_or_path), config=config)
model.to(args.device)
```<|||||>Hi,
thanks for the quick response.
I am more interested in the XLNet and TransformerXL models. Would they have the same interface?
<|||||>I don’t know firsthand, but suppose so and it is fundamentally an easy problem to reinitialize weights randomly before any kind of training in pytorch :)
Good luck,
Zacharias
Am 18. Sep. 2019, 1:56 PM +0200 schrieb Stamenov <[email protected]>:
> Hi,
> thanks for the quick response.
> I am more interested in the XLNet and TransformerXL models. Would they have the same interface?
> —
> You are receiving this because you commented.
> Reply to this email directly, view it on GitHub, or mute the thread.
<|||||>I think XLNet requires a very specific training procedure, see #943 :+1:
"For XLNet, the implementation in this repo is missing some key functionality (the permutation generation function and an analogue of the dataset record generator) which you'd have to implement yourself."
<|||||>https://github.com/huggingface/pytorch-transformers/issues/1283#issuecomment-532598578
Hmm, tokenizers' constructors require a `vocab_file` parameter...<|||||>This issue has been automatically marked as stale because it has not had recent activity. It will be closed if no further activity occurs. Thank you for your contributions.
<|||||>@Stamenov Did you figure out how to pretrain XLNet? I'm interested in that as well.<|||||>No, I haven't. According to some recent tweet, huggingface could prioritize putting more effort into providing interfaces for self pre-training.<|||||>You can now leave `--model_name_or_path` to None in `run_language_modeling.py` to train a model from scratch.
See also https://huggingface.co/blog/how-to-train |
transformers | 1,282 | closed | start_position=0 in utils_squad.py when span is impossible | Hi,
https://github.com/huggingface/pytorch-transformers/blob/e768f2322abd2a2f60a3a6d64a6a94c2d957fe89/examples/utils_squad.py#L340-L351
when answer is out of span, start_position should be cls_index rather than 0 as L350
And in
https://github.com/huggingface/pytorch-transformers/blob/e768f2322abd2a2f60a3a6d64a6a94c2d957fe89/examples/run_squad.py#L253-L259
when using multi gpu and set evaluate_during_training=True, we may add
`model_tmp = model.module if hasattr(model, 'module') else model` in order to get `model_tmp.config. start_n_top` rather than `model.config.start_n_top` | 09-18-2019 08:53:09 | 09-18-2019 08:53:09 | |
transformers | 1,280 | closed | FineTuning using single sentence document | Hello
roBERTa is not using the next sentence prediction objective. I want to fine-tune the pre-trained model on an unlabelled corpus of domain-specific text (ULMFIT style intermediate pretraining).
The bottleneck is my examples are single short sentences, instead of a document with multiple sentences
The INPUT format here says it requires a file with one sentence per line and one blank line between documents. For me, each document has a single sentence
https://github.com/huggingface/pytorch-transformers/tree/b62abe87c94f8df4d5fdc2e9202da651be9c331d/examples/lm_finetuning
The last time I raised an issue this was an expected behavior I know
Re : https://github.com/huggingface/pytorch-transformers/issues/272
How to do it now :) ? Any help is appreciated . | 09-18-2019 04:40:48 | 09-18-2019 04:40:48 | Hi Tuhin, you can use `examples/run_lm_finetuning.py` now. The scripts in `examples/lm_finetuning` are deprecated (removed on master now).<|||||>Thomas i checked the wiki text 2 format and its confusing to me . Do we have to seperate documents by new lines ? My input file is a set of single sentence documents one per line . Do i need a new line after each sentence ?
My format is
sent1
sent2
sent3
Do i need to have
sent1
sent2
sent3 ?
I am currently running without a new line after each sentence and getting 09/19/2019 03:37:22 - WARNING - pytorch_transformers.tokenization_utils - Token indices sequence length is longer than the specified maximum sequence length for this model (3989110 >
512). Running this sequence through the model will result in indexing errors
<|||||>Also while running
def mask_tokens(inputs, tokenizer, args):
- [ ] labels = inputs.clone()
- [ ] # We sample a few tokens in each sequence for masked-LM training (with probability args.mlm_probability defaults to 0.15 in Bert/RoBERTa)
- [ ] **masked_indices = torch.bernoulli(torch.full(labels.shape, args.mlm_probability)).bool()**
- [ ]
Getting error
AttributeError: 'Tensor' object has no attribute 'bool'
Line 110
<|||||>you need to update your pytorch version. I believe bool() function was intrcoduced in torch 1.1.0 or 1.2.0<|||||>> I am currently running without a new line after each sentence and getting 09/19/2019 03:37:22 - WARNING - pytorch_transformers.tokenization_utils - Token indices sequence length is longer than the specified maximum sequence length for this model (3989110 >
> 512). Running this sequence through the model will result in indexing errors
I'm also getting this error. Is there more detail on the expected input format for the LM pretraining?<|||||>This issue has been automatically marked as stale because it has not had recent activity. It will be closed if no further activity occurs. Thank you for your contributions.
|
transformers | 1,279 | closed | connection limit of pregenerate_training_data.py | ## ❓ Questions & Help
Inside pregenerate_training_data.py, one can use multiprocessing to process each epoch in parallel.
However, since the communication between process is limited by pickle limit size. We can only transfer arguments less than 1GB and argument: docs are very likely to exceed this limit.
I save the docs as pickle file and ask every process to read on their own. | 09-18-2019 03:16:47 | 09-18-2019 03:16:47 | This issue has been automatically marked as stale because it has not had recent activity. It will be closed if no further activity occurs. Thank you for your contributions.
|
transformers | 1,278 | closed | 'Default process group is not initialized' Error | I get this error when finetuning bert using code in `lm_finetuning/` folder, when I try to run it several GPUs.
```
Traceback (most recent call last):
File "finetune_on_pregenerated.py", line 330, in <module>
main()
File "finetune_on_pregenerated.py", line 323, in main
if n_gpu > 1 and torch.distributed.get_rank() == 0 or n_gpu <=1 :
File "/opt/anaconda/lib/python3.6/site-packages/torch/distributed/distributed_c10d.py", line 562, in get_rank
_check_default_pg()
File "/opt/anaconda/lib/python3.6/site-packages/torch/distributed/distributed_c10d.py", line 191, in _check_default_pg
"Default process group is not initialized"
AssertionError: Default process group is not initialized
```
sample training data(*epoch_0.json*):
```
{"tokens": ["[CLS]", "i", "'", "ve", "got", "the", "[MASK]", "scenario", "in", "mind", "do", "you", "[MASK]", "[MASK]", "##k", "[SEP]", "i", "prefer", "that", "over", "red", "##dit", "[SEP]"], "segment_ids": [0, 0, 0, 0, 0, 0, 0, 0, 0, 0, 0, 0, 0, 0, 0, 0, 1, 1, 1, 1, 1, 1, 1], "is_random_next": false, "masked_lm_positions": [6, 12, 13], "masked_lm_labels": ["perfect", "have", "ki"]}
{"tokens": ["[CLS]", "she", "message", "##d", "me", "suggesting", "i", "was", "ignorant", "because", "i", "[MASK]", "##t", "know", "the", "feeling", "and", "restriction", "that", "panties", "[MASK]", "on", "women", "[MASK]", ".", ".", ".", "seriously", ".", ".", "[MASK]", "panties", "she", "[MASK]", "[MASK]", "men", "don", "##t", "know", "how", "bad", "it", "is", "to", "wear", "panties", "because", "society", "[MASK]", "##t", "let", "women", "speak", "up", "about", "it", "[SEP]", "[MASK]", "yourself", "lucky", ".", ".", "[MASK]", "bullet", "dodged", "[SEP]"], "segment_ids": [0, 0, 0, 0, 0, 0, 0, 0, 0, 0, 0, 0, 0, 0, 0, 0, 0, 0, 0, 0, 0, 0, 0, 0, 0, 0, 0, 0, 0, 0, 0, 0, 0, 0, 0, 0, 0, 0, 0, 0, 0, 0, 0, 0, 0, 0, 0, 0, 0, 0, 0, 0, 0, 0, 0, 0, 0, 1, 1, 1, 1, 1, 1, 1, 1, 1], "is_random_next": false, "masked_lm_positions": [11, 20, 22, 23, 30, 33, 34, 48, 57, 62], "masked_lm_labels": ["didn", "put", "women", ".", ".", "said", "that", "won", "consider", "."]}
{"tokens": ["[CLS]", "[MASK]", "enough", "my", "first", "name", "[MASK]", "actually", "lisa", "i", "[MASK]", "##t", "ha", "[MASK]", "minded", "[MASK]", "b", "##ds", "##m", "vi", "##ds", "at", "12", "13", "not", "made", "to", "do", "actual", "sex", "like", "u", "said", "but", "the", "[MASK]", "displayed", "play", "whipped", "on", "[MASK]", "vi", "##ds", "i", "think", "the", "pe", "##dos", "[MASK]", "'", "ve", "enjoyed", "watching", "[MASK]", "[SEP]", "this", "probably", "[MASK]", "[MASK]", "'", "t", "what", "[MASK]", "had", "in", "mind", "though", "sorry", "but", "i", "thought", "it", "funny", "when", "the", "first", "word", "was", "lisa", "an", "that", "'", "s", "my", "emil", "[SEP]"], "segment_ids": [0, 0, 0, 0, 0, 0, 0, 0, 0, 0, 0, 0, 0, 0, 0, 0, 0, 0, 0, 0, 0, 0, 0, 0, 0, 0, 0, 0, 0, 0, 0, 0, 0, 0, 0, 0, 0, 0, 0, 0, 0, 0, 0, 0, 0, 0, 0, 0, 0, 0, 0, 0, 0, 0, 0, 1, 1, 1, 1, 1, 1, 1, 1, 1, 1, 1, 1, 1, 1, 1, 1, 1, 1, 1, 1, 1, 1, 1, 1, 1, 1, 1, 1, 1, 1, 1], "is_random_next": false, "masked_lm_positions": [1, 6, 10, 13, 15, 35, 40, 48, 53, 57, 58, 62, 84], "masked_lm_labels": ["funny", "is", "would", "##v", "doing", "being", "the", "would", "them", "is", "n", "u", "name"]}
```
*epoch_0_metrics.json*
```
{"num_training_examples": 3, "max_seq_len": 256}
```
Reproducing:
```
export CUDA_VISIBLE_DEVICES=6,7
python3 finetune_on_pregenerated.py --pregenerated_data training/ --bert_model bert-base-uncased --do_lower_case --output_dir finetuned_lm/ --epochs 1 --train_batch_size 16
```
The code works fine when training on one single GPU.
Thanks. | 09-18-2019 01:50:39 | 09-18-2019 01:50:39 | You can use `example/run_lm_finetuning` now, the scripts in the `example/lm_finetuning/` folder are deprecated (removed on master).<|||||>What kind input format is good for `example/run_lm_finetuning.py`?<|||||>Hi, there's an example using WikiText-2 in the [documentation](https://huggingface.co/pytorch-transformers/examples.html#language-model-fine-tuning).
A file containing text is really all that's needed! You can change the way the file is used in `TextDataset` to better reflect the text you're fine-tuning the model to.<|||||>Thanks, Lysandre.<|||||>This issue has been automatically marked as stale because it has not had recent activity. It will be closed if no further activity occurs. Thank you for your contributions.
|
transformers | 1,277 | closed | No language embedding weights in pre-trained xlm models. | I'm trying to train a one-shot classification model using the given XLM pre-trained weights.
However, I noticed that for both `xlm-mlm-17-1280` and `xlm-mlm-100-1280`, That I kept receiving the warning `weights of XLMForSequenceClassification not initialized from pre-trained model: ['lang_embeddings.weight']`. I then looked into the state_dict of those two checkpoints and saw that indeed there were no weights that matched that key. Should that weight exist in the state_dict somewhere? | 09-17-2019 22:40:23 | 09-17-2019 22:40:23 | Indeed, I've checked and the 100 and 17 language models don't use language indices.
Just supply `langs=None`.
You can see that in the official notebook from Facebook: https://github.com/facebookresearch/XLM/blob/master/generate-embeddings.ipynb<|||||>I see. I missed this issue here which explains it pretty well. https://github.com/huggingface/pytorch-transformers/issues/1034
Thanks for the help! |
transformers | 1,276 | closed | Write with Transformer: Please, add an autosave to browser cache! | ## 🚀 Feature
In Write with Transformer, the writing should be periodically saved to the browser cache, so that if the user accidentally refreshes the page, their work that they may have spent hours on won't be lost.
## Motivation
I just lost several hours worth of writing because I accidentally refreshed the page.
## Additional context
:( | 09-17-2019 20:56:43 | 09-17-2019 20:56:43 | Hi @varkarrus, thank you for your feature request. There is a "save & publish" button on the top right-hand side, which saves your document on a specific URL. Does this fit your needs?<|||||>Does it begin autosaving after you do that? If so, then probably!<|||||>Nope, it does not currently auto-save 🙁
One quick fix I've thought about would be to pop a window alert if closing a tab that's got non-saved changes.<|||||>Oh, even that alone would be a lifesaver!<|||||>This issue has been automatically marked as stale because it has not had recent activity. It will be closed if no further activity occurs. Thank you for your contributions.
|
transformers | 1,275 | closed | Implement fine-tuning BERT on CoNLL-2003 named entity recognition task | I added a script for fine-tuning BERT on the CoNLL-2003 named entity recognition task, as an example for token classification. This was requested in #1216.
I followed the structure of the run_glue example, and implemented the data processing in a way suitable for all transformer models (although currently token classification is only implemented for BERT).
The training procedure is as described in the original BERT paper: Only the first sub-token of each CoNLL-tokenized word is classified and contributes to the loss, the remaining sub-tokens are ignored. | 09-17-2019 14:13:16 | 09-17-2019 14:13:16 | Thanks for adding this :+1:
I've one suggestion for some improvement (rfc): can we make the `get_labels()` function a bit more configurable? E.g. reading the labels from a file `labels.txt` would be great, so I could use other datasets (e.g. GermEval, which has more labels) 🤔 What do you think 🤗<|||||>That would be useful for fine-tuning on other datasets, I agree. I tried to keep it very close to the run_glue example for the beginning (where the labels are also fixed), and wait for feedback from the maintainers to know if these kind of extensions are wanted or not ;-)
How about adding a new CLI argument where the user can specify a path to a labels file, and we use the default CoNLL labels when no path was specified?<|||||>@stecklin Would be great to have a cli argument for that (+ like your proposed suggestion) :heart: <|||||>This looks awesome, thanks a lot @stecklin and @stefan-it!
Happy to review this when you folks think it's ready.
And ping me if I can help otherwise.<|||||>Results are pretty good. I wrote an additional prediction script that outputs a CoNLL compatible format, so that I could verify the results with the official CoNLL evaluation script. Here I fine-tuned a `bert-base-cased` model (5 epochs):
Development set:
```bash
processed 51362 tokens with 5942 phrases; found: 5997 phrases; correct: 5661.
accuracy: 99.10%; precision: 94.40%; recall: 95.27%; FB1: 94.83
LOC: precision: 96.74%; recall: 96.90%; FB1: 96.82 1840
MISC: precision: 89.54%; recall: 91.00%; FB1: 90.26 937
ORG: precision: 92.24%; recall: 92.24%; FB1: 92.24 1341
PER: precision: 96.06%; recall: 97.99%; FB1: 97.02 1879
```
Test set:
```bash
processed 46435 tokens with 5648 phrases; found: 5712 phrases; correct: 5185.
accuracy: 98.26%; precision: 90.77%; recall: 91.80%; FB1: 91.29
LOC: precision: 92.12%; recall: 93.23%; FB1: 92.67 1688
MISC: precision: 79.75%; recall: 82.48%; FB1: 81.09 726
ORG: precision: 89.23%; recall: 90.31%; FB1: 89.77 1681
PER: precision: 95.92%; recall: 95.92%; FB1: 95.92 1617
```<|||||># [Codecov](https://codecov.io/gh/huggingface/transformers/pull/1275?src=pr&el=h1) Report
> Merging [#1275](https://codecov.io/gh/huggingface/transformers/pull/1275?src=pr&el=desc) into [master](https://codecov.io/gh/huggingface/transformers/commit/80889a0226b8f8022fd9ff65ed6bce71b60ba800?src=pr&el=desc) will **not change** coverage.
> The diff coverage is `n/a`.
[](https://codecov.io/gh/huggingface/transformers/pull/1275?src=pr&el=tree)
```diff
@@ Coverage Diff @@
## master #1275 +/- ##
=======================================
Coverage 85.98% 85.98%
=======================================
Files 91 91
Lines 13579 13579
=======================================
Hits 11676 11676
Misses 1903 1903
```
------
[Continue to review full report at Codecov](https://codecov.io/gh/huggingface/transformers/pull/1275?src=pr&el=continue).
> **Legend** - [Click here to learn more](https://docs.codecov.io/docs/codecov-delta)
> `Δ = absolute <relative> (impact)`, `ø = not affected`, `? = missing data`
> Powered by [Codecov](https://codecov.io/gh/huggingface/transformers/pull/1275?src=pr&el=footer). Last update [80889a0...c55badc](https://codecov.io/gh/huggingface/transformers/pull/1275?src=pr&el=lastupdated). Read the [comment docs](https://docs.codecov.io/docs/pull-request-comments).
<|||||>The labels can be configured now :heavy_check_mark:
@stefan-it Do we want to add your prediction script as well? I think that would be very useful, after all NER prediction is not as straightforward as e.g. sequence classification prediction.<|||||>@stecklin Thanks 😊 I'm currently using this script:
https://gist.github.com/stefan-it/c39b63eb0043182010f2f61138751e0f
It mainly copies parts from the `evaluate` function.
But I think a more elegant way would be to fully re-use the evaluate function. The function currently returns the evaluation result, but maybe it could return a tuple of results and predicted tags?<|||||>@stefan-it I followed your suggestion, the evaluate function now returns the results and the predictions. I added the argument `--do_predict` to predict on a test set.
@thomwolf I think now would be a good moment for you to have a look. Let me know your feedback!<|||||>The mentioned script compatible with new "Transformers" source code ? <|||||>This looks awesome, thank you for the script. I did something similar that worked but this code is totally better. Thanks @stecklin !!<|||||>Ok I've reviewed the PR and it looks great, thanks a lot @stecklin and @stefan-it.
I've rebased, switched from `pytorch-transformers` to `transformers` and added `seqeval` in the requirements.
The only missing element is to add a simple usage explanation in the examples readme file at `examples/README.md` which explain:
- how to download the training/testing data,
- an example of command-line to run the script, and
- an example of results with this command line.
@stefan-it do you want to share the command line you use for the above results?<|||||> @thomwolf No problem, here's an example for GermEval 2014 (German NER):
# Data (Download and pre-processing steps)
Data can be obtained from the [GermEval 2014](https://sites.google.com/site/germeval2014ner/data) shared task page.
Here are the commands for downloading and pre-processing train, dev and test datasets. The original data format has four (tab-separated) columns, in a pre-processing step only the two relevant columns (token and outer span NER annotation) are extracted:
```bash
curl -L 'https://sites.google.com/site/germeval2014ner/data/NER-de-train.tsv?attredirects=0&d=1' \
| grep -v "^#" | cut -f 2,3 | tr '\t' ' ' > train.txt.tmp
curl -L 'https://sites.google.com/site/germeval2014ner/data/NER-de-dev.tsv?attredirects=0&d=1' \
| grep -v "^#" | cut -f 2,3 | tr '\t' ' ' > dev.txt.tmp
curl -L 'https://sites.google.com/site/germeval2014ner/data/NER-de-test.tsv?attredirects=0&d=1' \
| grep -v "^#" | cut -f 2,3 | tr '\t' ' ' > test.txt.tmp
```
The GermEval 2014 dataset contains some strange "control character" tokens like `'\x96', '\u200e', '\x95', '\xad' or '\x80'`. One problem with these tokens is, that `BertTokenizer` returns an empty token for them, resulting in misaligned `InputExample`s. I wrote a script that a) filters these tokens and b) splits longer sentences into smaller ones (once the max. subtoken length is reached).
```bash
wget "https://raw.githubusercontent.com/stefan-it/fine-tuned-berts-seq/master/scripts/preprocess.py"
```
Let's define some variables that we need for further pre-processing steps and training the model:
```bash
export MAX_LENGTH=128
export BERT_MODEL=bert-base-multilingual-cased
```
Run the pre-processing script on training, dev and test datasets:
```bash
python3 preprocess.py train.txt.tmp $BERT_MODEL $MAX_LENGTH > train.txt
python3 preprocess.py dev.txt.tmp $BERT_MODEL $MAX_LENGTH > dev.txt
python3 preprocess.py test.txt.tmp $BERT_MODEL $MAX_LENGTH > test.txt
```
The GermEval 2014 dataset has much more labels than CoNLL-2002/2003 datasets, so an own set of labels must be used:
```bash
cat train.txt dev.txt test.txt | cut -d " " -f 2 | grep -v "^$"| sort | uniq > labels.txt
```
# Training
Additional environment variables must be set:
```bash
export OUTPUT_DIR=germeval-model
export BATCH_SIZE=32
export NUM_EPOCHS=3
export SAVE_STEPS=750
export SEED=1
```
To start training, just run:
```bash
python3 run_ner.py --data_dir ./ \
--model_type bert \
--labels ./labels.txt \
--model_name_or_path $BERT_MODEL \
--output_dir $OUTPUT_DIR \
--max_seq_length $MAX_LENGTH \
--num_train_epochs $NUM_EPOCHS \
--per_gpu_train_batch_size $BATCH_SIZE \
--save_steps $SAVE_STEPS \
--seed $SEED \
--do_train \
--do_eval \
--do_predict
```
If your GPU supports half-precision training, just add the `--fp16` flag. After training, the model will be both evaluated on development and test datasets.
# Evaluation
Evaluation on development dataset outputs the following for our example:
```bash
10/04/2019 00:42:06 - INFO - __main__ - ***** Eval results *****
10/04/2019 00:42:06 - INFO - __main__ - f1 = 0.8623348017621146
10/04/2019 00:42:06 - INFO - __main__ - loss = 0.07183869666975543
10/04/2019 00:42:06 - INFO - __main__ - precision = 0.8467916366258111
10/04/2019 00:42:06 - INFO - __main__ - recall = 0.8784592370979806
```
On the test dataset the following results could be achieved:
```bash
10/04/2019 00:42:42 - INFO - __main__ - ***** Eval results *****
10/04/2019 00:42:42 - INFO - __main__ - f1 = 0.8614389652384803
10/04/2019 00:42:42 - INFO - __main__ - loss = 0.07064602487454782
10/04/2019 00:42:42 - INFO - __main__ - precision = 0.8604651162790697
10/04/2019 00:42:42 - INFO - __main__ - recall = 0.8624150210424085
```
Please let me know if you have more questions 🤗<|||||>Hi, great work, thanks for sharing!
I think the argument `overwrite_cache` is not used in the code. I suspect there is a missing if check in the `load_and_cache_examples()` function.<|||||>There was something strange with git on this branch (32 files changed...) so I had to do a rebase and force push on your PR @stecklin. Please do a `git reset --hard` to be up-to-date with the new clean state on the remote repo.
Now it looks in order for merging with master.<|||||>@stefan-it Not able to reproduce the above results.
The best I can for dev dataset get is this :
11/27/2019 18:16:38 - INFO - __main__ - ***** Eval results *****
11/27/2019 18:16:38 - INFO - __main__ - f1 = 0.12500000000000003
11/27/2019 18:16:38 - INFO - __main__ - loss = 1.6597001552581787
11/27/2019 18:16:38 - INFO - __main__ - precision = 0.2
11/27/2019 18:16:38 - INFO - __main__ - recall = 0.09090909090909091
Any pointers on what I am missing ?
<|||||>@oneraghavan What version/commit of `transformers` are you using? Do you use the GermEval dataset or another one? I'll check the example :)<|||||>@stefan-it Thanks for quick response :) . I am using commit from oct 24. Has anything changed since then ? I am following the same steps said in examples/readme.md . Let me know if you want me to check with latest commit .<|||||>Could you try to use the latest `master` version? I re-do the experiment on GermEval, here are the results:
Evaluation on dev set:
```bash
f1 = 0.8702821546353977
loss = 0.07410008722260086
precision = 0.8530890804597702
recall = 0.8881824981301422
```
Evaluation on test set:
```bash
f1 = 0.860249697946033
loss = 0.07239935705435063
precision = 0.8561808561808562
recall = 0.8643573972159275
```<|||||>@stefan-it I tried with the latest master. Not able to reproduce. I am exactly following the instructions given in readme.md. The following are the results I am getting .
11/28/2019 09:34:50 - INFO - __main__ - ***** Eval results *****
11/28/2019 09:34:50 - INFO - __main__ - f1 = 0.12500000000000003
11/28/2019 09:34:50 - INFO - __main__ - loss = 1.1732935905456543
11/28/2019 09:34:50 - INFO - __main__ - precision = 0.2
11/28/2019 09:34:50 - INFO - __main__ - recall = 0.09090909090909091
Can you check if there are any other parameters that is being given in run_ner.py parameters . How much epoch are you training ? <|||||>In order to reproduce the conll score reported in BERT paper (92.4 bert-base and 92.8 bert-large) one trick is to apply a truecaser on article titles (all upper case sentences) as preprocessing step for conll train/dev/test. This can be simply done with the following method.
```
#https://github.com/daltonfury42/truecase
#pip install truecase
import truecase
import re
# original tokens
#['FULL', 'FEES', '1.875', 'REOFFER', '99.32', 'SPREAD', '+20', 'BP']
def truecase_sentence(tokens):
word_lst = [(w, idx) for idx, w in enumerate(tokens) if all(c.isalpha() for c in w)]
lst = [w for w, _ in word_lst if re.match(r'\b[A-Z\.\-]+\b', w)]
if len(lst) and len(lst) == len(word_lst):
parts = truecase.get_true_case(' '.join(lst)).split()
# the trucaser have its own tokenization ...
# skip if the number of word dosen't match
if len(parts) != len(word_lst): return tokens
for (w, idx), nw in zip(word_lst, parts):
tokens[idx] = nw
# truecased tokens
#['Full', 'fees', '1.875', 'Reoffer', '99.32', 'spread', '+20', 'BP']
```
Also, i found useful to use : very small learning rate (5e-6) \ large batch size (128) \ high epoch num (>40).
With these configurations and preprocessing, I was able to reach 92.8 with bert-large. |
transformers | 1,274 | closed | Fixes #1263, add tokenization_with_offsets, gets tokens with offsets in the original text | This is similar to the utils_squad approach to getting offsets for the tokens but can also be used in other places where the tokens should have a correspondence to the original text to fix #1263.
| 09-17-2019 14:13:00 | 09-17-2019 14:13:00 | I think this is an interesting addition and I like the way the PR is structured in general.
Before I dive in, could you lay down the status of the PR in terms of supported models, python version (we will still keep python 2 support for now), know issues and TO-DOs?<|||||>The fully supported models are BERT, GPT2, XLNet, and RoBERTa. For these models tokenize_with_offsets always produces the same tokens as tokenize and the subword tokens are well-aligned to the original text, typically as well as possible.
XLM has a known issue with patterns like '... inc. reported ...'
tokxlm.tokenize_with_offsets('inc. reported')
['inc</w>', '.</w>', 'reported</w>']
tokxlm.tokenize('inc. reported')
['inc.</w>', 'reported</w>']
Because tokenize_with_offsets passes whitespace separated 'chunks' (utils_squad style) the xlm tokenizer doesn't get to look ahead to see that a lowercase word follows the period.
TransfoXL just needs the latest commit (not in this PR), while OpenAIGPT has an issue with '\n</w>' tokens, which tokenize_with_offsets never produces.
Everything works in Python 2 except GPT2 (and therefore RoBERTa) as well as XLNet. I think this is the case even without this PR. I'm not experienced with Python 2 though, so maybe I am just missing something.
<|||||># [Codecov](https://codecov.io/gh/huggingface/pytorch-transformers/pull/1274?src=pr&el=h1) Report
> Merging [#1274](https://codecov.io/gh/huggingface/pytorch-transformers/pull/1274?src=pr&el=desc) into [master](https://codecov.io/gh/huggingface/pytorch-transformers/commit/45de034bf899af678d844351ff21ea0444815ddb?src=pr&el=desc) will **decrease** coverage by `0.97%`.
> The diff coverage is `59.04%`.
[](https://codecov.io/gh/huggingface/pytorch-transformers/pull/1274?src=pr&el=tree)
```diff
@@ Coverage Diff @@
## master #1274 +/- ##
==========================================
- Coverage 81.16% 80.19% -0.98%
==========================================
Files 57 60 +3
Lines 8039 8405 +366
==========================================
+ Hits 6525 6740 +215
- Misses 1514 1665 +151
```
| [Impacted Files](https://codecov.io/gh/huggingface/pytorch-transformers/pull/1274?src=pr&el=tree) | Coverage Δ | |
|---|---|---|
| [...tests/regression\_test\_tokenization\_with\_offsets.py](https://codecov.io/gh/huggingface/pytorch-transformers/pull/1274/diff?src=pr&el=tree#diff-cHl0b3JjaF90cmFuc2Zvcm1lcnMvdGVzdHMvcmVncmVzc2lvbl90ZXN0X3Rva2VuaXphdGlvbl93aXRoX29mZnNldHMucHk=) | `0% <0%> (ø)` | |
| [pytorch\_transformers/tokenization\_bert.py](https://codecov.io/gh/huggingface/pytorch-transformers/pull/1274/diff?src=pr&el=tree#diff-cHl0b3JjaF90cmFuc2Zvcm1lcnMvdG9rZW5pemF0aW9uX2JlcnQucHk=) | `95.79% <100%> (+0.12%)` | :arrow_up: |
| [pytorch\_transformers/tokenization\_gpt2.py](https://codecov.io/gh/huggingface/pytorch-transformers/pull/1274/diff?src=pr&el=tree#diff-cHl0b3JjaF90cmFuc2Zvcm1lcnMvdG9rZW5pemF0aW9uX2dwdDIucHk=) | `96.87% <100%> (+0.18%)` | :arrow_up: |
| [...ytorch\_transformers/tests/tokenization\_xlm\_test.py](https://codecov.io/gh/huggingface/pytorch-transformers/pull/1274/diff?src=pr&el=tree#diff-cHl0b3JjaF90cmFuc2Zvcm1lcnMvdGVzdHMvdG9rZW5pemF0aW9uX3hsbV90ZXN0LnB5) | `97.87% <100%> (+0.14%)` | :arrow_up: |
| [...ch\_transformers/tests/tokenization\_offsets\_test.py](https://codecov.io/gh/huggingface/pytorch-transformers/pull/1274/diff?src=pr&el=tree#diff-cHl0b3JjaF90cmFuc2Zvcm1lcnMvdGVzdHMvdG9rZW5pemF0aW9uX29mZnNldHNfdGVzdC5weQ==) | `100% <100%> (ø)` | |
| [...transformers/tests/tokenization\_transfo\_xl\_test.py](https://codecov.io/gh/huggingface/pytorch-transformers/pull/1274/diff?src=pr&el=tree#diff-cHl0b3JjaF90cmFuc2Zvcm1lcnMvdGVzdHMvdG9rZW5pemF0aW9uX3RyYW5zZm9feGxfdGVzdC5weQ==) | `97.36% <100%> (+0.3%)` | :arrow_up: |
| [...ch\_transformers/tests/tokenization\_roberta\_test.py](https://codecov.io/gh/huggingface/pytorch-transformers/pull/1274/diff?src=pr&el=tree#diff-cHl0b3JjaF90cmFuc2Zvcm1lcnMvdGVzdHMvdG9rZW5pemF0aW9uX3JvYmVydGFfdGVzdC5weQ==) | `92.85% <100%> (+0.4%)` | :arrow_up: |
| [pytorch\_transformers/tokenization\_transfo\_xl.py](https://codecov.io/gh/huggingface/pytorch-transformers/pull/1274/diff?src=pr&el=tree#diff-cHl0b3JjaF90cmFuc2Zvcm1lcnMvdG9rZW5pemF0aW9uX3RyYW5zZm9feGwucHk=) | `34.54% <100%> (+0.36%)` | :arrow_up: |
| [pytorch\_transformers/tokenization\_xlm.py](https://codecov.io/gh/huggingface/pytorch-transformers/pull/1274/diff?src=pr&el=tree#diff-cHl0b3JjaF90cmFuc2Zvcm1lcnMvdG9rZW5pemF0aW9uX3hsbS5weQ==) | `82.98% <100%> (+0.28%)` | :arrow_up: |
| [pytorch\_transformers/tokenization\_openai.py](https://codecov.io/gh/huggingface/pytorch-transformers/pull/1274/diff?src=pr&el=tree#diff-cHl0b3JjaF90cmFuc2Zvcm1lcnMvdG9rZW5pemF0aW9uX29wZW5haS5weQ==) | `82.4% <100%> (+0.58%)` | :arrow_up: |
| ... and [11 more](https://codecov.io/gh/huggingface/pytorch-transformers/pull/1274/diff?src=pr&el=tree-more) | |
------
[Continue to review full report at Codecov](https://codecov.io/gh/huggingface/pytorch-transformers/pull/1274?src=pr&el=continue).
> **Legend** - [Click here to learn more](https://docs.codecov.io/docs/codecov-delta)
> `Δ = absolute <relative> (impact)`, `ø = not affected`, `? = missing data`
> Powered by [Codecov](https://codecov.io/gh/huggingface/pytorch-transformers/pull/1274?src=pr&el=footer). Last update [45de034...328e698](https://codecov.io/gh/huggingface/pytorch-transformers/pull/1274?src=pr&el=lastupdated). Read the [comment docs](https://docs.codecov.io/docs/pull-request-comments).
<|||||>Would love to see this feature available - what can I do to help get it merged in?<|||||>We are currently working on a larger project around this and should come to this PR pretty soon (next week I hope).<|||||>@michaelrglass How does this handle the destructive normalisation that occurs in eg BertTokenizer? Specifically, logic like [this](https://github.com/huggingface/transformers/blob/master/transformers/tokenization_bert.py#L330) means that the normalisation isn't length preserving, and it may not be possible to find the (normalised) token in the original input text.<|||||>> @michaelrglass How does this handle the destructive normalisation that occurs in eg BertTokenizer? Specifically, logic like [this](https://github.com/huggingface/transformers/blob/master/transformers/tokenization_bert.py#L330) means that the normalisation isn't length preserving, and it may not be possible to find the (normalised) token in the original input text.
IMO it's too error-prone to run a destructive tokenizer and then try to align the sequences after the fact. I wrote https://github.com/microsoft/bistring for this exact kind of problem. Otherwise it's very tricky to align substrings of the modified text with the original text, as both NFD and filtering out nonspacing marks can shift the positions of chars significantly.<|||||>This issue has been automatically marked as stale because it has not had recent activity. It will be closed if no further activity occurs. Thank you for your contributions.
|
transformers | 1,273 | closed | ModuleNotFoundError: No module named 'pytorch_transformers.modeling' using convert_pytorch_checkpoint_to_tf.py | ## 🐛 Bug
<!-- Important information -->
Model I am using (Bert, XLNet....):
BERT
Language I am using the model on (English, Chinese....):
Chinese
The problem arise when using:
* [✔️ ] the official example scripts: (give details)
run convert_pytorch_checkpoint_to_tf.py to generate the tf check point
## To Reproduce
Steps to reproduce the behavior:
```
python3 /Users/xxx/py3ml/lib/python3.6/site-packages/pytorch_transformers/convert_pytorch_checkpoint_to_tf.py
Traceback (most recent call last):
File "/Users/xxx/py3ml/lib/python3.6/site-packages/pytorch_transformers/convert_pytorch_checkpoint_to_tf.py", line 23, in <module>
from pytorch_transformers.modeling import BertModel
ModuleNotFoundError: No module named 'pytorch_transformers.modeling'
```
## Expected behavior
convert it
<!-- A clear and concise description of what you expected to happen. -->
## Environment
* OS: macOS 10.14
* Python version: 3.6
* PyTorch version: 1.2
* PyTorch Transformers version (or branch):1.2.0
* Using GPU ? no
* Distributed of parallel setup ? no
* Any other relevant information:
I change the code to
`from pytorch_transformer import BertModel`
It works fine
## Additional context
<!-- Add any other context about the problem here. --> | 09-17-2019 05:32:40 | 09-17-2019 05:32:40 | This issue has been automatically marked as stale because it has not had recent activity. It will be closed if no further activity occurs. Thank you for your contributions.
|
transformers | 1,272 | closed | How long does it take? (BERT Model Finetuning using Masked ML objective) | I am about to finetune a multilingual BERT model using English and Chinese text from the legal domain.
My corpus is around 27GB, how long should I expect to train 3 epochs (default parameters) using a Google TPU? | 09-16-2019 21:10:39 | 09-16-2019 21:10:39 | This issue has been automatically marked as stale because it has not had recent activity. It will be closed if no further activity occurs. Thank you for your contributions.
|
transformers | 1,271 | closed | get NaN loss when I run the example code run_squad.py | ## ❓ Questions & Help
<!-- A clear and concise description of the question. -->
I use the example run_squad.py code, and use the Readme's hyper-parameters, but I got nan loss when I trained a few batches. And I use the `autograd.detect_anomaly()` want to catch that.
The more information is below:
> File "/users4/zkwang/miniconda3/envs/MRC/lib/python3.6/site-packages/torch/nn/parallel/parallel_apply.py", line 59, in _worker
output = module(*input, **kwargs)
self._target(*self._args, **self._kwargs)
File "/users4/zkwang/miniconda3/envs/MRC/lib/python3.6/site-packages/torch/nn/parallel/parallel_apply.py", line 59, in _worker
output = module(*input, **kwargs)
File "/users4/zkwang/miniconda3/envs/MRC/lib/python3.6/site-packages/torch/nn/modules/module.py", line 493, in __call__
result = self.forward(*input, **kwargs)
File "/users4/zkwang/miniconda3/envs/MRC/lib/python3.6/site-packages/pytorch_transformers/modeling_bert.py", line 1211, in forward
attention_mask=attention_mask, head_mask=head_mask)
File "/users4/zkwang/miniconda3/envs/MRC/lib/python3.6/site-packages/torch/nn/modules/module.py", line 493, in __call__
result = self.forward(*input, **kwargs)
File "/users4/zkwang/miniconda3/envs/MRC/lib/python3.6/site-packages/pytorch_transformers/modeling_bert.py", line 713, in forward
head_mask=head_mask)
File "/users4/zkwang/miniconda3/envs/MRC/lib/python3.6/site-packages/torch/nn/modules/module.py", line 493, in __call__
result = self.forward(*input, **kwargs)
File "/users4/zkwang/miniconda3/envs/MRC/lib/python3.6/site-packages/pytorch_transformers/modeling_bert.py", line 434, in forward
layer_outputs = layer_module(hidden_states, attention_mask, head_mask[i])
File "/users4/zkwang/miniconda3/envs/MRC/lib/python3.6/site-packages/torch/nn/modules/module.py", line 493, in __call__
result = self.forward(*input, **kwargs)
File "/users4/zkwang/miniconda3/envs/MRC/lib/python3.6/site-packages/pytorch_transformers/modeling_bert.py", line 414, in forward
intermediate_output = self.intermediate(attention_output)
File "/users4/zkwang/miniconda3/envs/MRC/lib/python3.6/site-packages/torch/nn/modules/module.py", line 493, in __call__
result = self.forward(*input, **kwargs)
File "/users4/zkwang/miniconda3/envs/MRC/lib/python3.6/site-packages/pytorch_transformers/modeling_bert.py", line 386, in forward
hidden_states = self.intermediate_act_fn(hidden_states)
File "/users4/zkwang/miniconda3/envs/MRC/lib/python3.6/site-packages/pytorch_transformers/modeling_bert.py", line 145, in gelu
return x * 0.5 * (1.0 + torch.erf(x / math.sqrt(2.0)))
Traceback (most recent call last):
File "run_squad.py", line 544, in <module>
main()
File "run_squad.py", line 490, in main
global_step, tr_loss = train(args, train_dataset, model, tokenizer)
File "run_squad.py", line 165, in train
loss.backward()
File "/users4/zkwang/miniconda3/envs/MRC/lib/python3.6/site-packages/torch/tensor.py", line 107, in backward
torch.autograd.backward(self, gradient, retain_graph, create_graph)
File "/users4/zkwang/miniconda3/envs/MRC/lib/python3.6/site-packages/torch/autograd/__init__.py", line 93, in backward
allow_unreachable=True) # allow_unreachable flag
RuntimeError: Function 'MulBackward0' returned nan values in its 0th output.
My environment PyTorch version: torch 1.1.0, pytorch-transformers 1.2.0, and use 4 Titan X gpus for train. I don't know why the official code cause this result, could someone help me about that... | 09-16-2019 18:00:39 | 09-16-2019 18:00:39 | Maybe the learning rate is too high?<|||||>I change a GPU node and this situation doesn't appear. I will change the learning rate and see results. Thanks a lot. |
transformers | 1,270 | closed | BERT returns different embedding for same sentence | I am using pre-trained BERT for creating features, for same sentence it produces different result in two different runs. Do we have to set some random state to produce consistent result? I am using pytorch-transformers for reading pre-trained model.
| 09-16-2019 11:31:00 | 09-16-2019 11:31:00 | Are you initializing from a pretrained model? If no, than this is normal behaviour: your weights are randomly initialized. If yes, make sure your model is in evaluation mode (```model.eval()```), this disables dropout and other random modules.<|||||>Thank you for quick response @srslynow . how to initialize weights and biases fora pre-trained model ? I thought weights and biases freezes after training.
you were right , I missed the model.eval() that's the reason I was getting slightly different embedding on each run becz of dropout layer.
|
transformers | 1,269 | closed | could you add an option to transfer variables from float32 to float16 in GPT2 model to reduce model size and accelerate the inference speed | ## 🚀 Feature
could you add an option to transfer variables from float32 to float16 in GPT2 model
## Motivation
reduce model size and accelerate the inference speed
## Additional context
<!-- Add any other context or screenshots about the feature request here. --> | 09-16-2019 01:56:39 | 09-16-2019 01:56:39 | You should give a look at NVIDIA's apex library and PyTorch `model.half()` method.<|||||>This issue has been automatically marked as stale because it has not had recent activity. It will be closed if no further activity occurs. Thank you for your contributions.
<|||||>@thomwolf Do you know if the gpt2 model needs to be pre-trained with apex support in order to use NVIDIA's apex library (e.g. O1 mode) at inference time? Was mixed precision used during the training of the gpt2 model? Is there a way I can verify that?<|||||>Do we have models trained with mixed precision enabled for gpt2? I can't find them in huggingface's repo. |
transformers | 1,268 | closed | How to use pytorch-transformers for transfer learning? | ## ❓ Questions & Help
```python
pretrained_weights='bert-base-uncased'
tokenizer = tokenizer_class.from_pretrained(pretrained_weights)
model = model_class.from_pretrained(pretrained_weights)
features = []
input_ids = torch.tensor([self.tokenizer.encode(phrase, add_special_tokens=True)])
with torch.no_grad():
# Models outputs are tuples
outputs = self.model(input_ids)
last_hidden_states = outputs[0]
avg_pool_hidden_states = np.average(last_hidden_states[0], axis=0))
return avg_pool_hidden_states
```
I am working on Sentence Similarity problem, given sentence S, find similar sentences W. So I want to encode sentence S and find the closest top-K sentences from W.
I have read the documentations, but I want to confirm few things how do I do. the following:
- How do I get the second to last layer?
- Am I average pooling the last hidden states correctly? | 09-15-2019 17:42:59 | 09-15-2019 17:42:59 | Hey, so not exactly a direct answer to your question, but bert outright doesn't do amazing on sentence similarity. This repo here should help with your question and their paper does a great job at explaining how their method works https://github.com/UKPLab/sentence-transformers. I think you will find better results with that. Hope it helps.<|||||>This issue has been automatically marked as stale because it has not had recent activity. It will be closed if no further activity occurs. Thank you for your contributions.
|
transformers | 1,267 | closed | Accuracy not increasing with BERT Large model | I experimented with `BERT_base_cased` and `BERT_large_cased` model for multi class text classification. With BERT_base_cased, I got satisfactory results. When I tried with BERT_large_cased model, the accuracy is same for all the epochs
```
Epoch: 01 | Epoch Time: 0m 57s
*******train_loss,train_acc,valid_loss,valid_ac**********
5.200893470219204 2.790178544819355 4.977107011354887 3.6057692021131516
Epoch: 02 | Epoch Time: 0m 57s
*******train_loss,train_acc,valid_loss,valid_ac**********
5.085730476038797 2.287946455180645 4.954357807452862 3.6057692021131516
Epoch: 03 | Epoch Time: 0m 58s
*******train_loss,train_acc,valid_loss,valid_ac**********
5.019492668764932 2.901785634458065 4.961122549497164 3.6057692021131516
Epoch: 04 | Epoch Time: 0m 58s
*******train_loss,train_acc,valid_loss,valid_ac**********
5.0052995937211175 3.57142873108387 4.9535566843473 3.6057692021131516
Epoch: 05 | Epoch Time: 0m 58s
*******train_loss,train_acc,valid_loss,valid_ac**********
5.003523528575897 3.23660708963871 4.9652618261484 3.6057692021131516
Epoch: 06 | Epoch Time: 0m 58s
*******train_loss,train_acc,valid_loss,valid_ac**********
5.010107040405273 3.29241082072258 4.96296108686007 3.6057692021131516
Epoch: 07 | Epoch Time: 0m 58s
*******train_loss,train_acc,valid_loss,valid_ac**********
5.028377030576978 2.678571455180645 4.94510478239793 3.6057692021131516
Epoch: 08 | Epoch Time: 0m 58s
*******train_loss,train_acc,valid_loss,valid_ac**********
5.04387321642467 2.901785634458065 4.9411917466383715 3.6057692021131516
Epoch: 09 | Epoch Time: 0m 58s
*******train_loss,train_acc,valid_loss,valid_ac**********
5.027528064591544 3.18080373108387 4.940045246711144 3.6057692021131516
Epoch: 10 | Epoch Time: 0m 58s
*******train_loss,train_acc,valid_loss,valid_ac**********
5.023407867976597 3.29241082072258 4.940378886002761 3.6057692021131516
Epoch: 11 | Epoch Time: 0m 58s
*******train_loss,train_acc,valid_loss,valid_ac**********
5.015415557793209 3.125 4.939220135028545 3.6057692021131516
Epoch: 12 | Epoch Time: 0m 58s
*******train_loss,train_acc,valid_loss,valid_ac**********
5.018008896282741 3.29241082072258 4.9386150653545675 3.6057692021131516
Epoch: 13 | Epoch Time: 0m 58s
*******train_loss,train_acc,valid_loss,valid_ac**********
5.003824523517063 2.957589365541935 4.938107490539551 3.6057692021131516
Epoch: 14 | Epoch Time: 0m 58s
*******train_loss,train_acc,valid_loss,valid_ac**********
5.003440124647958 3.069196455180645 4.93824944129357 3.6057692021131516
Epoch: 15 | Epoch Time: 0m 58s
*******train_loss,train_acc,valid_loss,valid_ac**********
5.012082431997571 3.069196455180645 4.9383643590486965 3.6057692021131516
Epoch: 16 | Epoch Time: 0m 58s
*******train_loss,train_acc,valid_loss,valid_ac**********
5.009286454745701 3.01339291036129 4.93832148038424 3.6057692021131516
Epoch: 17 | Epoch Time: 0m 58s
*******train_loss,train_acc,valid_loss,valid_ac**********
5.006769972188132 2.901785634458065 4.937925778902494 3.6057692021131516
Epoch: 18 | Epoch Time: 0m 58s
*******train_loss,train_acc,valid_loss,valid_ac**********
5.006464583533151 3.125 4.937762847313514 3.6057692021131516
Epoch: 19 | Epoch Time: 0m 58s
*******train_loss,train_acc,valid_loss,valid_ac**********
5.004164610590253 2.957589365541935 4.937491783728967 3.6057692021131516
Epoch: 20 | Epoch Time: 0m 57s
*******train_loss,train_acc,valid_loss,valid_ac**********
5.013612789767129 2.957589365541935 4.937890896430383 3.6057692021131516
Epoch: 21 | Epoch Time: 0m 58s
*******train_loss,train_acc,valid_loss,valid_ac**********
4.997398240225656 2.511160634458065 4.937900726611797 3.6057692021131516
```
With `BERT_base_cased`, there is no such problem. But with `BERT_large_cased`, why accuracy is same in all the epochs? Any help is really appreciated............. @thomwolf @nreimers | 09-15-2019 14:17:07 | 09-15-2019 14:17:07 | Hi, I experienced this also in several experiments. BERT large is extremely sensitive to the random seed. Try some other seeds and you will likely get a performance at least on oar with the base model.
I haven't it studied further why the large model is so sensitive to the random seed, but it appears that the gradient for some step destroys the model, from which on you only get bad scores. Might be some exploding gradient or some Nan issues.
Best,
Nils Reimers <|||||>I find this issue as well -- no convergence with the large model.
Potentially related:
https://github.com/huggingface/transformers/issues/753
https://github.com/huggingface/transformers/issues/92<|||||>This issue has been automatically marked as stale because it has not had recent activity. It will be closed if no further activity occurs. Thank you for your contributions.
|
transformers | 1,266 | closed | Fine-tune distilbert-base-uncased under run_glue | ## ❓ Questions & Help
I am a bit confused on how to fine-tune distilbert. I see three options
1. Fine-tune bert for the task and then use distillation.distiller
2. Fine-tune bert for the task and then use distillation.train
3. Fine-tune distilbert-base-uncased directly for the task using run_glue.py
I tried the 3rd option under run_glue.py as follows
add distilbert to MODEL_CLASSES
```MODEL_CLASSES = {
'bert': (BertConfig, BertForSequenceClassification, BertTokenizer),
'distilbert': (DistilBertConfig, DistilBertForSequenceClassification, DistilBertTokenizer),
'xlnet': (XLNetConfig, XLNetForSequenceClassification, XLNetTokenizer),
'xlm': (XLMConfig, XLMForSequenceClassification, XLMTokenizer),
'roberta': (RobertaConfig, RobertaForSequenceClassification, RobertaTokenizer),
}
```
and add a flag `--model_type=distilbert`
Which of the three methods above should be used? | 09-15-2019 14:04:06 | 09-15-2019 14:04:06 | Hello @YosiMass,
The simplest/more direct way to do transfer learning is indeed the 3rd solution.
If you use `run_glue.py`, the modification you made is correct. You also have to be careful since DistilBERT doesn't take `token_type_embeddings` as input --> [here](https://github.com/huggingface/pytorch-transformers/blob/master/examples/run_glue.py#L131) and [here](https://github.com/huggingface/pytorch-transformers/blob/master/examples/run_glue.py#L221).
I'll add these modifications in a few days directly to these scripts so that it's seamless to use DistilBERT with run_squad or run_glue.<|||||>Thanks @VictorSanh.
Yes, I handled the missing token_types as follows. In run_glue.train and run_glue.evaluate I changed From
```
inputs = {'input_ids': batch[0],
'attention_mask': batch[1],
'token_type_ids': batch[2] if args.model_type in ['bert', 'xlnet'] else None, # XLM and RoBERTa don't use segment_ids
'labels': batch[3]}
```
To
```
if args.model_type not in ['distilbert']:
inputs = {'input_ids': batch[0],
'attention_mask': batch[1],
'token_type_ids': batch[2] if args.model_type in ['bert', 'xlnet'] else None, # XLM and RoBERTa don't use segment_ids
'labels': batch[3]}
else:
inputs = {'input_ids': batch[0],
'attention_mask': batch[1],
'labels': batch[3]}
```<|||||>> handled the missing token_types as follows. In run_glue.train and run_glue.evaluate I changed From
It looks good to me! |
transformers | 1,265 | closed | different results shown each time when I run the example code for BertForMultipleChoice | When I ran the following example provided for BertForMultipleChoice in the documentation, I've got different results each time when I run it. Does it mean that BertForMultipleChoice is only provided to fine-tune the BERT model with RocStories/SWAG like datasets, and no pretrained models (after the fine-tuning) are provided?
________________________
import torch
from pytorch_transformers import BertTokenizer, BertModel, BertConfig
from pytorch_transformers import BertForNextSentencePrediction, BertForMultipleChoice
import numpy as np
tokenizer = BertTokenizer.from_pretrained('bert-base-uncased')
model = BertForMultipleChoice.from_pretrained('bert-base-uncased')
model.eval()
choices = ["Hello, my dog is cute", "Hello, my cat is amazing"]
input_ids = torch.tensor([tokenizer.encode(s) for s in choices]).unsqueeze(0) # Batch size 1, 2 choices
labels = torch.tensor(1).unsqueeze(0) # Batch size 1
outputs = model(input_ids, labels=labels)
loss, classification_scores = outputs[:2]
print(classification_scores) | 09-15-2019 07:20:06 | 09-15-2019 07:20:06 | Yes!
You have to fine-tune BertForMultipleChoice to be able to use it.
<|||||>This issue has been automatically marked as stale because it has not had recent activity. It will be closed if no further activity occurs. Thank you for your contributions.
<|||||>Does Bert add extra hidden layers that are randomly initialized on top of the pre trained network when using BertForMultipleChoice? Would these added hidden layers be the only hidden layers that are adjusted during the learning process? |
transformers | 1,264 | closed | Error running openai-gpt on ROCstories | ## 🐛 Bug
<!-- Important information -->
The model I am using: OpenAIGPT
The language I am using the model on: English
The problem arises when using:
* [ ] the official example scripts: When I try to run examples/single_model_script/run_openai_gpt.py I get this error:
```
Traceback (most recent call last):
File "/home/rohola/Codes/Python/pytorch-transformers/examples/single_model_scripts/run_openai_gpt.py", line 288, in <module>
main()
File "/home/rohola/Codes/Python/pytorch-transformers/examples/single_model_scripts/run_openai_gpt.py", line 158, in main
model = OpenAIGPTDoubleHeadsModel.from_pretrained(args.model_name, num_special_tokens=len(special_tokens))
File "/home/rohola/Codes/Python/pytorch-transformers/pytorch_transformers/modeling_utils.py", line 330, in from_pretrained
model = cls(config, *model_args, **model_kwargs)
TypeError: __init__() got an unexpected keyword argument 'num_special_tokens'
```
The tasks I am working on is:
* ROCstories
## To Reproduce
Steps to reproduce the behavior:
1. Just run the "run_openai_gpt.py "
## Environment
* OS: Ubuntu 16.04
* Python version: 3.6
* PyTorch version: 1.1.0
* PyTorch Transformers version (or branch): The last commit
* Using GPU: True
* Distributed of parallel setup: No
* Any other relevant information:
## Additional context
Even when I remove that argument I get another error:
```
Traceback (most recent call last):
File "/home/rohola/Codes/Python/pytorch-transformers/examples/single_model_scripts/run_openai_gpt.py", line 288, in <module>
main()
File "/home/rohola/Codes/Python/pytorch-transformers/examples/single_model_scripts/run_openai_gpt.py", line 224, in main
losses = model(input_ids, mc_token_ids, lm_labels, mc_labels)
File "/home/rohola/Codes/Python/pytorch-transformers/env/lib/python3.6/site-packages/torch/nn/modules/module.py", line 493, in __call__
result = self.forward(*input, **kwargs)
File "/home/rohola/Codes/Python/pytorch-transformers/pytorch_transformers/modeling_openai.py", line 601, in forward
head_mask=head_mask)
File "/home/rohola/Codes/Python/pytorch-transformers/env/lib/python3.6/site-packages/torch/nn/modules/module.py", line 493, in __call__
result = self.forward(*input, **kwargs)
File "/home/rohola/Codes/Python/pytorch-transformers/pytorch_transformers/modeling_openai.py", line 425, in forward
hidden_states = inputs_embeds + position_embeds + token_type_embeds
RuntimeError: The size of tensor a (78) must match the size of tensor b (16) at non-singleton dimension 1
``` | 09-14-2019 15:51:23 | 09-14-2019 15:51:23 | Ok should be fixed now on master with e768f23 |
transformers | 1,263 | closed | Offsets in original text from tokenizers | ## 🚀 Feature
A new method for tokenizers: tokenize_with_offsets. In addition to returning the tokens, it returns the spans in the original text that the tokens correspond to.
After
tokens, offsets = tokenizer.tokenize_with_offsets(text)
then
tokens[i] maps to text[offsets[i, 0]:offsets[i, 1]]
## Motivation
I find it useful to be able to get the spans in the original text where the tokens come from. This is useful for example in extractive question answering, where the model predicts a sequence of tokens, but the user would like to see a highlighted passage.
## Additional context
I have a version of this in a fork: https://github.com/michaelrglass/pytorch-transformers
There is a test (regression_test_tokenization_with_offsets) that verifies the tokenization with offsets gives the same tokenization for many models - still working on XLM.
The test data I used is available from https://ibm.box.com/s/228183fe95ptn8eb9n0zq4i2y7picq4r
Since this touches several files, and works for the majority (but not all) of the tokenizers, I thought creating an issue for discussion would be better than an immediate pull request. | 09-13-2019 23:29:36 | 09-13-2019 23:29:36 | I am glad to see someone is working on this and really appreciate your work.
Currently I'm using LCS the original xlnet also uses to align the token and raw input to extract the answer highlighting.
This is really painful as it's slow and may fail at some corner case.
Can't wait to see your pulled feature merged!<|||||>Commenting in #1274 thread<|||||>This issue has been automatically marked as stale because it has not had recent activity. It will be closed if no further activity occurs. Thank you for your contributions.
|
transformers | 1,262 | closed | run_generation.py 'encode' error for gpt2 and xlnet | Hello:
Been using nshepperd's tf repo and various excellent forks for fine-tuning and inference without issue.
Wanted to check out py-torch transformers and compare. First test is simple conditional sampling from the pytorch models:
python3 run_generation.py --model_type=xlnet --length=20 --model_name_or_path=models/xlnet-large-cased
Loads config.json and model weights then Model prompt >>>
Enter anything: Hello there huggingface. What's up?
then ...
Traceback (most recent call last):
File "run_generation.py", line 195, in <module>
main()
File "run_generation.py", line 175, in main
context_tokens = tokenizer.encode(raw_text)
AttributeError: 'NoneType' object has no attribute 'encode'
Same error when switching to gpt2, in this case gpt-large.
Is there a modification to the script required? Is the terminal syntax incorrect?
Can't move on to fine-tuning until basic inference sorted out.
Tested both pytorch 1.2.0 and 1.1.0. Same error.
OS: ubuntu 18.04
Python version: python 3.6.8
PyTorch version: torch 1.2.0 tested and changed to 1.1.0 to match transformer
PyTorch Transformers version (or branch): pytorch-transformers 1.2.0 tested and changed to 1.1.0 to match above
Using GPU ? yes
Distributed of parallel setup ? no
All help appreciated. Would like to test distributed fine-tuning but need the basic repo working first on local machine.
Cheers
| 09-13-2019 15:24:33 | 09-13-2019 15:24:33 | Closing own non-issue. Needed to download missing files listed in the four applicable tokenizer scripts. Working 100%. On to fine-tuning. |
transformers | 1,261 | closed | SequenceSummary / quenstion regarding summary types | in the class SequenceSummary(nn.Module) which is a part of {BERT, XLNet}ForSequenceClassification:
https://github.com/huggingface/pytorch-transformers/blob/32e1332acf6fd1ad372b81c296d43be441d3b0b1/pytorch_transformers/modeling_utils.py#L643-L644
in case of XLNet we can see that the last token will be taken, for BERT it is otherwise the first one.
https://github.com/huggingface/pytorch-transformers/blob/32e1332acf6fd1ad372b81c296d43be441d3b0b1/pytorch_transformers/modeling_utils.py#L692
Why only the first one or the last one? Why not to apply the max or average pooling over all token from the output?
Thanks a lot! | 09-13-2019 13:41:31 | 09-13-2019 13:41:31 | That's how it's done in the respective original implementations.<|||||>it is very interesting. All other tokens will not be considered.
Do you know whether other architectures has been tried out? Initially, when I had taken the transformer-model I created another output architecture. It is very interesting, whether this "end architecture" affects the performance significantly
Thanks a lot!<|||||>I found this project doc [1], there are four variants of the end architecture, their performance is almost equal.
I will be glad if you can provide other papers regarding this issue.
[1] - http://web.stanford.edu/class/cs224n/reports/custom/15785631.pdf<|||||>This issue has been automatically marked as stale because it has not had recent activity. It will be closed if no further activity occurs. Thank you for your contributions.
|
transformers | 1,260 | closed | XLNet tokenizer returns empty list instead of string for some indexes | ## 🐛 Bug
<!-- Important information -->
Model I am using (Bert, XLNet....):
XLNet
Language I am using the model on (English, Chinese....):
English
The problem arise when using:
* [ ] my own modified scripts: (give details)
The tasks I am working on is:
* [ ] my own task or dataset: (give details)
## To Reproduce
Steps to reproduce the behavior:
1. Create tokenizer: tokenizer = XLNetTokenizer.from_pretrained(path)
2. Try to decode index 4: tokenizer.decode(4)
3. You get empty list [] although expected is a string
<!-- If you have a code sample, error messages, stack traces, please provide it here as well. -->
## Expected behavior
Decode method of the tokenizer should return a string and not a list.
## Environment
* OS:
* Python version: 3.7
* PyTorch version: 1.1.0
* PyTorch Transformers version (or branch): 1.2.0
| 09-13-2019 13:41:29 | 09-13-2019 13:41:29 | Hi,
`tokenizer.decode()` expect a sequence of ids as indicated in the doc/docstring: https://huggingface.co/pytorch-transformers/main_classes/tokenizer.html#pytorch_transformers.PreTrainedTokenizer.decode<|||||>well, as far as i see, the tokenizer can accept both - sequence or single index. For example:
`tokenizer = XLNetTokenizer.from_pretrained('xlnet-base-cased')`
`tokenizer.decode(3)`
produce `'<cls>'` as well as `tokenizer.decode([3])`.
And the problem happens in both cases:
`tokenizer.decode([4])` produces empty string. And, what is even more strange, if index 4 is in the sequence, the result is a list instead of a string. For example:
`tokenizer.decode([35,109])` gives `'I new'`, but `tokenizer.decode([35,109,4])` generates list instead of a string `['I new']`<|||||>ok, it looks like 4 is an index of a separation token and it turns one sequence into sequence of sequences each of which will be decoded into string. So if index 4 presents in the sequence, the result will be list of strings. This behavior is quite unintuitive. If it was created purposely, should be properly documented. I've got very strange bug in my code that was hard to track, since index 4 was predicted very rarely and out of 500 predictions 1 was buggy. <|||||>Thanks for the bug report. Indeed this was an unwanted behavior. Fixed on master now. |
transformers | 1,259 | closed | Cannot install the library | ## ❓ Questions & Help
<!-- A clear and concise description of the question. -->
Traceback (most recent call last):
File "/usr/lib/python2.7/dist-packages/pip/basecommand.py", line 215, in main
status = self.run(options, args)
File "/usr/lib/python2.7/dist-packages/pip/commands/install.py", line 353, in run
wb.build(autobuilding=True)
File "/usr/lib/python2.7/dist-packages/pip/wheel.py", line 749, in build
self.requirement_set.prepare_files(self.finder)
File "/usr/lib/python2.7/dist-packages/pip/req/req_set.py", line 380, in prepare_files
ignore_dependencies=self.ignore_dependencies))
File "/usr/lib/python2.7/dist-packages/pip/req/req_set.py", line 620, in _prepare_file
session=self.session, hashes=hashes)
File "/usr/lib/python2.7/dist-packages/pip/download.py", line 821, in unpack_url
hashes=hashes
File "/usr/lib/python2.7/dist-packages/pip/download.py", line 659, in unpack_http_url
hashes)
File "/usr/lib/python2.7/dist-packages/pip/download.py", line 882, in _download_http_url
_download_url(resp, link, content_file, hashes)
File "/usr/lib/python2.7/dist-packages/pip/download.py", line 603, in _download_url
hashes.check_against_chunks(downloaded_chunks)
File "/usr/lib/python2.7/dist-packages/pip/utils/hashes.py", line 46, in check_against_chunks
for chunk in chunks:
File "/usr/lib/python2.7/dist-packages/pip/download.py", line 571, in written_chunks
for chunk in chunks:
File "/usr/lib/python2.7/dist-packages/pip/utils/ui.py", line 139, in iter
for x in it:
File "/usr/lib/python2.7/dist-packages/pip/download.py", line 560, in resp_read
decode_content=False):
File "/usr/share/python-wheels/urllib3-1.22-py2.py3-none-any.whl/urllib3/response.py", line 436, in stream
data = self.read(amt=amt, decode_content=decode_content)
File "/usr/share/python-wheels/urllib3-1.22-py2.py3-none-any.whl/urllib3/response.py", line 384, in read
data = self._fp.read(amt)
File "/usr/share/python-wheels/CacheControl-0.11.7-py2.py3-none-any.whl/cachecontrol/filewrapper.py", line 63, in read
self._close()
File "/usr/share/python-wheels/CacheControl-0.11.7-py2.py3-none-any.whl/cachecontrol/filewrapper.py", line 50, in _close
self.__callback(self.__buf.getvalue())
File "/usr/share/python-wheels/CacheControl-0.11.7-py2.py3-none-any.whl/cachecontrol/controller.py", line 275, in cache_response
self.serializer.dumps(request, response, body=body),
File "/usr/share/python-wheels/CacheControl-0.11.7-py2.py3-none-any.whl/cachecontrol/serialize.py", line 87, in dumps
).encode("utf8"),
MemoryError
| 09-12-2019 22:32:17 | 09-12-2019 22:32:17 | This issue has been automatically marked as stale because it has not had recent activity. It will be closed if no further activity occurs. Thank you for your contributions.
|
transformers | 1,258 | closed | fix padding_idx of RoBERTa model | The padding index of the pretrained RoBERTa model is 1, and 0 is assigned to `<s>` token. The padding index of the current RoBERTa model is set to be 0, therefore `<s>` is treated as padding.
This PR aims to fix this problem. | 09-12-2019 22:20:31 | 09-12-2019 22:20:31 | LGTM but let's have @julien-c or @LysandreJik confirm<|||||>lgtm too<|||||>It would be appreciated if you review this PR! @LysandreJik <|||||>@ikuyamada Out of curiosity, in which cases did you need to specify this `padding_idx`? It shouldn't have any impact on the inference so are you training a model from scratch? (with @LysandreJik)<|||||>Merged in https://github.com/huggingface/transformers/commit/a6a6d9e6382961dc92a1a08d1bab05a52dc815f9<|||||>big drawback is that we initialize the embeddings multiple times.
@ikuyamada do you have an idea to improve this?<|||||>Thank you for merging this PR!
> Out of curiosity, in which cases did you need to specify this padding_idx? It shouldn't have any impact on the inference so are you training a model from scratch?
In my understanding, the embedding corresponding to `padding_idx` is not updated while training (pre-training or fine-tuning). Because the embedding of the token `<s>` may play some roles for computing contextualized embeddings for other tokens, and the output embedding of the `<s>` token is used for computing a feature vector for some fine-tuning tasks, I think the embedding should be updated while training.
> big drawback is that we initialize the embeddings multiple times.
> @ikuyamada do you have an idea to improve this?
We can avoid this by removing [the constructor call of the `BertEmbeddings`](https://github.com/huggingface/transformers/blob/master/transformers/modeling_roberta.py#L44) and simply initialize the `token_type_embeddings`, `LayerNorm`, and `dropout` in the constructor of the `RobertaEmbeddings`.
If you prefer this implementation, I will create a PR again! :)
@julien-c <|||||>> In my understanding, the embedding corresponding to padding_idx is not updated while training (pre-training or fine-tuning). Because the embedding of the token `<s>` may play some roles for computing contextualized embeddings for other tokens, and the output embedding of the `<s>` token is used for computing a feature vector for some fine-tuning tasks, I think the embedding should be updated while training.
Yes you are correct.
> If you prefer this implementation, I will create a PR again! :)
That would be great, thank you. |
transformers | 1,257 | closed | Training time increased from 45 min per epoch to 6 hours per epoch in colab | ## 📚 Migration
<!-- Important information -->
Model I am using (Bert): PyTorch-pretrained-Bert and BERT in pytorch-transformers
Language I am using the model on : English
The problem arise when using:
* the official example scripts: run_squad.py in pytorch-transformers
The tasks I am working on is:
* an official GLUE/SQUaD task: Fine Tuning SQuAD
Details of the issue:
Hi finetuned model over SQuAD with the following code in colab:
%run run_squad.py --bert_model bert-base-uncased \
--do_train \
--do_predict \
--do_lower_case \
--fp16 \
--train_file SQUAD_DIR/train-v1.1.json \
--predict_file SQUAD_DIR/dev-v1.1.json \
--train_batch_size 12 \
--learning_rate 3e-5 \
--num_train_epochs 2.0 \
--max_seq_length 384 \
--doc_stride 128 \
--output_dir debug_squad9
The above code took 45 min for one epoch with "exact_match": 81.94891201513718, "f1": 89.02481046726041. I ran this code(PyTorch-pretrained-Bert) somewhere between July 20th - 27th 2019.
And now the same file with the above code(PyTorch-pretrained-Bert) is taking the 6 hours for one epoch. Why is that?
I have tested with pytorch-transformers as well, It is also taking 6 hours for one epoch.
I am unable to understand. Is there any change with apex or implementation ??
## Environment
* OS: google colab
* Python version: Python 3.6.8
* PyTorch version: 1.2
* PyTorch Transformers version (or branch): the existing version pytorch-transformers
* Using GPU ? - Yes I guess K80 single GPU
* Distributed of parallel setup ? No
* Any other relevant information:
- The above environment details on based on the exiting run which took 6 hours for one epoch.
- I haven't collected the environment information when I tested it for the first time over PyTorch-pretrained-Bert.
## Checklist
- [yes ] I have read the migration guide in the readme.
- [yes ] I checked if a related official extension example runs on my machine.
## Additional context
Is there anything new that I am missing from pytorch-Transformers or implementation change in PyTorch-pretrained-Bert ?? | 09-12-2019 16:14:34 | 09-12-2019 16:14:34 | Hi,
Found a solution to my problem to some extent. I had cloned the latest apex repo and testing out with PyTorch-pretrained-Bert which is causing the problem to take more time for execution. I took the older apex repo and tested the code and working as earlier (45 min per epoch)
But when I was using the pytorch-transformers with latest apex repo it is taking 6 hours for one epoch.
Is that usual to take 6 hours for one epoch in colab ??
<|||||>This issue has been automatically marked as stale because it has not had recent activity. It will be closed if no further activity occurs. Thank you for your contributions.
|
transformers | 1,256 | closed | Could you please implement a Adafactor optimizer? :) | ## 🚀 Feature
Could you please implement a Adafactor optimizer? :)
( https://arxiv.org/abs/1804.04235 )
## Motivation
In contrast to Adam it requires much less GPU memory.
I tried to use the FairSeq implementation for the pytorch-transformers, but I'm no expert and I couldn't get it done.
Could you please do that? :)
## Additional context
<!-- Add any other context or screenshots about the feature request here. --> | 09-12-2019 14:41:24 | 09-12-2019 14:41:24 | What didn't work for you with the fairseq implementation?
It seems pretty self-contained: https://github.com/pytorch/fairseq/blob/master/fairseq/optim/adafactor.py#L65-L213<|||||>This issue has been automatically marked as stale because it has not had recent activity. It will be closed if no further activity occurs. Thank you for your contributions.
<|||||>FYI @sshleifer -- I was wrong -- able to train T5-large even batch==1 with FP32, no gradient check-pointing and ADAM. Given that T5 team strongly recommends AdaFactor -- giving it a try, other pieces perhaps being more difficult... |
transformers | 1,255 | closed | examples/lm_finetuning/simple_lm_finetuning.py crashes with cublas runtime error | ## Possible bug: simple_lm_finetuning.py crashes with cublas runtime error🐛 Bug
<!-- Important information -->
### TL;DR
I'm trying to finetune the existing English **bert-base-uncased** model according to the examples in `examples/lm_finetuning/README.md` on IMDB data, but fail. The `simple_lm_finetuning.py` script crashes with a cublas runtime error.
### VERBOSE
I have tried the following on a local machine, as well as on GCP, with two different datasets.
I have formatted the input data according to the specification in `examples/lm_finetuning/README.md` and stored it in a file called `imdb_corpus_1.txt` (essentially using the information kindly provided in https://medium.com/dsnet/running-pytorch-transformers-on-custom-datasets-717fd9e10fe2 wrt to the data and preprocessing)
To reproduce the issue, run the following on a suitable dataset.
The command:
```
~/pytorch-transformers/examples/lm_finetuning$ python3 simple_lm_finetuning.py --train_corpus ~/imdb_corpus_1_small.txt --bert_model bert-base-uncased --do_lo
wer_case --output_dir finetuned_lm --do_train
```
results in output ending with the following stacktrace:
```
raceback (most recent call last):
File "simple_lm_finetuning.py", line 641, in <module>
main()
File "simple_lm_finetuning.py", line 591, in main
outputs = model(input_ids, segment_ids, input_mask, lm_label_ids, is_next)
File "/home/fredrik_olsson/venv/pytorch-transformers/lib/python3.7/site-packages/torch/nn/modules/module.py", line 547, in __call__
result = self.forward(*input, **kwargs)
File "/home/fredrik_olsson/venv/pytorch-transformers/lib/python3.7/site-packages/pytorch_transformers/modeling_bert.py", line 694, in forward
head_mask=head_mask)
File "/home/fredrik_olsson/venv/pytorch-transformers/lib/python3.7/site-packages/torch/nn/modules/module.py", line 547, in __call__
result = self.forward(*input, **kwargs)
File "/home/fredrik_olsson/venv/pytorch-transformers/lib/python3.7/site-packages/pytorch_transformers/modeling_bert.py", line 623, in forward
head_mask=head_mask)
File "/home/fredrik_olsson/venv/pytorch-transformers/lib/python3.7/site-packages/torch/nn/modules/module.py", line 547, in __call__
result = self.forward(*input, **kwargs)
File "/home/fredrik_olsson/venv/pytorch-transformers/lib/python3.7/site-packages/pytorch_transformers/modeling_bert.py", line 344, in forward
layer_outputs = layer_module(hidden_states, attention_mask, head_mask[i])
File "/home/fredrik_olsson/venv/pytorch-transformers/lib/python3.7/site-packages/torch/nn/modules/module.py", line 547, in __call__
result = self.forward(*input, **kwargs)
File "/home/fredrik_olsson/venv/pytorch-transformers/lib/python3.7/site-packages/pytorch_transformers/modeling_bert.py", line 322, in forward
attention_outputs = self.attention(hidden_states, attention_mask, head_mask)
File "/home/fredrik_olsson/venv/pytorch-transformers/lib/python3.7/site-packages/torch/nn/modules/module.py", line 547, in __call__
result = self.forward(*input, **kwargs)
File "/home/fredrik_olsson/venv/pytorch-transformers/lib/python3.7/site-packages/pytorch_transformers/modeling_bert.py", line 279, in forward
self_outputs = self.self(input_tensor, attention_mask, head_mask)
File "/home/fredrik_olsson/venv/pytorch-transformers/lib/python3.7/site-packages/torch/nn/modules/module.py", line 547, in __call__
result = self.forward(*input, **kwargs)
File "/home/fredrik_olsson/venv/pytorch-transformers/lib/python3.7/site-packages/pytorch_transformers/modeling_bert.py", line 199, in forward
mixed_query_layer = self.query(hidden_states)
File "/home/fredrik_olsson/venv/pytorch-transformers/lib/python3.7/site-packages/torch/nn/modules/module.py", line 547, in __call__
result = self.forward(*input, **kwargs)
File "/home/fredrik_olsson/venv/pytorch-transformers/lib/python3.7/site-packages/torch/nn/modules/linear.py", line 87, in forward
return F.linear(input, self.weight, self.bias)
File "/home/fredrik_olsson/venv/pytorch-transformers/lib/python3.7/site-packages/torch/nn/functional.py", line 1371, in linear
output = input.matmul(weight.t())
RuntimeError: cublas runtime error : resource allocation failed at /pytorch/aten/src/THC/THCGeneral.cpp:216
```
*However*, the following command, also pertaining to finetuning, successfully executes:
```
~/pytorch-transformers/examples$ python3 run_lm_finetuning.py --train_data_file ~/imdb_corpus.txt --output_dir fredriko --model_name_or_path bert-base
-uncased --mlm --do_train --do_lower_case --evaluate_during_training --overwrite_output_dir
```
## Environment
* OS: ubuntu
* Python version: python 3.7.3
* PyTorch version: torch 1.2.0
* PyTorch Transformers version (or branch): pytorch-transformers 1.2.0
* Using GPU ? yes
* Distributed of parallel setup ? no
* Any other relevant information: | 09-12-2019 13:22:43 | 09-12-2019 13:22:43 | Additional info: simple_lm_finetuning.py works with pytorch-transformers version 1.1.0, but not with version 1.2.0.<|||||>Maybe related to the change in order of parameters to BertModel's forward method ?
See #1246<|||||>Hi, the lm finetuning examples are now replaced by `examples/run_lm_finetuning` |
transformers | 1,254 | closed | Write With Transformer adding spaces? | This issue didn't happen before, but now whenever you use the autocomplete it always adds a space to the beginning, even when a space is not needed. I.E: when adding a comma / period to the end of a sentence, when starting a new line, or (most egregiously) when finishing a word that was only partially written.
As an aside, I wouldn't mind waiting a few extra seconds to get auto-fill suggestions that are longer than two words. | 09-12-2019 13:19:04 | 09-12-2019 13:19:04 | Commenting to say I have also noticed this. Also I would assume the small amount of tokens being generated per autocomplete lately is because of compute concerns, not time concerns. It is a bit limiting.<|||||>Also having this problem!<|||||>This issue has been automatically marked as stale because it has not had recent activity. It will be closed if no further activity occurs. Thank you for your contributions.
|
transformers | 1,253 | closed | Running XLNet on Squad | ## ❓ Questions & Help
<!-- A clear and concise description of the question. -->
This is the padding problem. In GLUE codes in the examples, the padding for XLNet is on the left of the input, but in Squad codes, the padding is on right. I was wondering which one is correct.
Also, the inputs of `convert_examples_to_features` are different in Glue and Squad, where Squad uses most of default values like `pad_token, sep_token, pad_token_segment_id and cis_token_segment_id`, but Glue use the value of `tokenizer`. which one is correct?
Or the example codes are out-of-date? Thanks | 09-12-2019 03:01:41 | 09-12-2019 03:01:41 | Seems like the run_squad script is in bad shape now. It just doesn't work.<|||||>same question.. also running this script with XLNet on Squad, is ~10 F1 points below BERT-Large-WWM. The difference in preprocessing as pointed out above could be one of the reasons.<|||||>This issue has been automatically marked as stale because it has not had recent activity. It will be closed if no further activity occurs. Thank you for your contributions.
|
transformers | 1,252 | closed | Max encoding length + corresponding tests | The encoding function eases the encoding of sequences across tokenizers. The addition of the `head_mask` return further removes the pressure on the user to manually check the added special tokens.
There is currently no easy method to truncate the encoded sequences while keeping the special tokens intact. This PR aims to change this by providing a `max_length` flag to be passed to the encoding function. This flag works even when no special tokens are involved (e.g. for GPT-2).
The second sequence is truncated while the first stays intact. If the first sequence is longer than the specified maximum length, a warning is sent and no sequences are truncated. | 09-11-2019 16:24:33 | 09-11-2019 16:24:33 | # [Codecov](https://codecov.io/gh/huggingface/pytorch-transformers/pull/1252?src=pr&el=h1) Report
> Merging [#1252](https://codecov.io/gh/huggingface/pytorch-transformers/pull/1252?src=pr&el=desc) into [glue-example](https://codecov.io/gh/huggingface/pytorch-transformers/commit/5583711822f79d8b3b7e7ba2560748cc0cf5654f?src=pr&el=desc) will **increase** coverage by `0.08%`.
> The diff coverage is `90.9%`.
[](https://codecov.io/gh/huggingface/pytorch-transformers/pull/1252?src=pr&el=tree)
```diff
@@ Coverage Diff @@
## glue-example #1252 +/- ##
===============================================
+ Coverage 81.32% 81.4% +0.08%
===============================================
Files 57 57
Lines 8074 8104 +30
===============================================
+ Hits 6566 6597 +31
+ Misses 1508 1507 -1
```
| [Impacted Files](https://codecov.io/gh/huggingface/pytorch-transformers/pull/1252?src=pr&el=tree) | Coverage Δ | |
|---|---|---|
| [...h\_transformers/tests/tokenization\_tests\_commons.py](https://codecov.io/gh/huggingface/pytorch-transformers/pull/1252/diff?src=pr&el=tree#diff-cHl0b3JjaF90cmFuc2Zvcm1lcnMvdGVzdHMvdG9rZW5pemF0aW9uX3Rlc3RzX2NvbW1vbnMucHk=) | `100% <100%> (ø)` | :arrow_up: |
| [pytorch\_transformers/tokenization\_utils.py](https://codecov.io/gh/huggingface/pytorch-transformers/pull/1252/diff?src=pr&el=tree#diff-cHl0b3JjaF90cmFuc2Zvcm1lcnMvdG9rZW5pemF0aW9uX3V0aWxzLnB5) | `90.02% <80%> (+0.57%)` | :arrow_up: |
------
[Continue to review full report at Codecov](https://codecov.io/gh/huggingface/pytorch-transformers/pull/1252?src=pr&el=continue).
> **Legend** - [Click here to learn more](https://docs.codecov.io/docs/codecov-delta)
> `Δ = absolute <relative> (impact)`, `ø = not affected`, `? = missing data`
> Powered by [Codecov](https://codecov.io/gh/huggingface/pytorch-transformers/pull/1252?src=pr&el=footer). Last update [5583711...a804892](https://codecov.io/gh/huggingface/pytorch-transformers/pull/1252?src=pr&el=lastupdated). Read the [comment docs](https://docs.codecov.io/docs/pull-request-comments).
|
transformers | 1,251 | closed | Why you need DistilBertModel class? | ## ❓ Questions & Help
<!-- A clear and concise description of the question. -->
You have `DistilBertModel`, `DistilBertForSequenceClassification`, etc. in `modeling_distilbert.py`. Why you need these classes? How about using `BertModel`, `BertForSequenceClassification`, etc.?
I found the weight names are different (e.g., `transformer.layer.0.attention.q_lin.weight`and `bert.encoder.layer.0.attention.self.query.weight`), but I think it's better to use the same weight names.
(A conversion script from distilbert weights to normal bert weights is useful to use `BertModel`.)
Thanks. | 09-11-2019 14:35:28 | 09-11-2019 14:35:28 | This is a smaller model compared to the original, and is thus better suitable for usage on embedded devices / devices without large gpu's. Their blog posts explains this: https://medium.com/huggingface/distilbert-8cf3380435b5.<|||||>Hello @tomohideshibata,
True, we could use the same code base for BERT and DistilBERT.
For now, I prefer keeping them separate mainly for clarity since the architectures are slightly different:
- No token_type_embeddings in DistilBERT
- No Sequence Pooler in DistilBERT
Handling these two in BertModel would unnecessarily (slightly) complexify the code and I'd like to keep it clean.
Another caveat: I use torch's `nn.LayerNorm` in DistilBERT while BERT uses a custom `BertLayerNorm`. There might be slightly different edge cases (I have to check).
But overall, you can totally implement a single class for BERT and DistilBERT on your side. I would suggest to have a look at [this script](https://github.com/huggingface/pytorch-transformers/blob/master/examples/distillation/scripts/extract_for_distil.py) to have the mapping between the names.<|||||>@VictorSanh Thanks for your comments. I will try to make a conversion script from DistilBERT to BERT weights. |
transformers | 1,250 | closed | R-BERT implementation | ## 🚀 Feature
An implementation of the R-BERT architecture for relationship classification
## Motivation
Hi @Huggingface. A recent paper describes an architecture for relationship classification called [R-BERT](https://arxiv.org/pdf/1905.08284.pdf), which claims SOTA performance on the Semeval 2010 Task 8 challenge. However, no code was provided with the paper. [I’ve written an implementation of this](https://github.com/azdatascience/pytorch-transformers/blob/rbert/examples/run_semeval.py) and can confirm it produces very good results (F1=89.07 using the official Semeval scoring script). Is this suitable for merging into the pytorch-transformers repo, or should it exist as a separate package? | 09-11-2019 08:09:31 | 09-11-2019 08:09:31 | This is mostly a new head for Bert, right?
If so, yes I think it could be a nice addition.
Is the tokenizer different as well?<|||||>That's correct. The new head is [here](https://github.com/azdatascience/pytorch-transformers/blob/rbert/pytorch_transformers/modeling_bert.py#L832) (Think that's in the right place). A new [tokenizer](https://github.com/azdatascience/pytorch-transformers/blob/rbert/pytorch_transformers/tokenization_rbert.py) is required as well, as it needs to insert some special characters surrounding the entities of interest. <|||||>Ok, I think we can accept a PR for that if you want to submit one.
Two notes on that:
- the tokenizer can inherit from `BertTokenizer` and you can probably mostly override the methods called `add_special_tokens_single_sentence` and `add_special_tokens_sentences_pair` to insert the special characters.
- the model and tokenizer should have tests (check how we test the other models, it's pretty simple) and docstring.
- adding an example similar to `run_glue` would be nice also I think or just a usage example in the docstring.<|||||>This issue has been automatically marked as stale because it has not had recent activity. It will be closed if no further activity occurs. Thank you for your contributions.
|
transformers | 1,249 | closed | fixed: hard coding for max and min number will out of range in fp16, which will cause nan. | 09-11-2019 07:49:49 | 09-11-2019 07:49:49 | # [Codecov](https://codecov.io/gh/huggingface/pytorch-transformers/pull/1249?src=pr&el=h1) Report
> Merging [#1249](https://codecov.io/gh/huggingface/pytorch-transformers/pull/1249?src=pr&el=desc) into [master](https://codecov.io/gh/huggingface/pytorch-transformers/commit/364920e216c16d73c782a61a4cf6652e541fbe18?src=pr&el=desc) will **decrease** coverage by `0.21%`.
> The diff coverage is `61.11%`.
[](https://codecov.io/gh/huggingface/pytorch-transformers/pull/1249?src=pr&el=tree)
```diff
@@ Coverage Diff @@
## master #1249 +/- ##
==========================================
- Coverage 81.23% 81.02% -0.21%
==========================================
Files 57 57
Lines 8029 8035 +6
==========================================
- Hits 6522 6510 -12
- Misses 1507 1525 +18
```
| [Impacted Files](https://codecov.io/gh/huggingface/pytorch-transformers/pull/1249?src=pr&el=tree) | Coverage Δ | |
|---|---|---|
| [pytorch\_transformers/modeling\_transfo\_xl.py](https://codecov.io/gh/huggingface/pytorch-transformers/pull/1249/diff?src=pr&el=tree#diff-cHl0b3JjaF90cmFuc2Zvcm1lcnMvbW9kZWxpbmdfdHJhbnNmb194bC5weQ==) | `54.43% <60%> (-0.18%)` | :arrow_down: |
| [pytorch\_transformers/modeling\_utils.py](https://codecov.io/gh/huggingface/pytorch-transformers/pull/1249/diff?src=pr&el=tree#diff-cHl0b3JjaF90cmFuc2Zvcm1lcnMvbW9kZWxpbmdfdXRpbHMucHk=) | `90.03% <66.66%> (-0.25%)` | :arrow_down: |
| [pytorch\_transformers/file\_utils.py](https://codecov.io/gh/huggingface/pytorch-transformers/pull/1249/diff?src=pr&el=tree#diff-cHl0b3JjaF90cmFuc2Zvcm1lcnMvZmlsZV91dGlscy5weQ==) | `66.66% <0%> (-4.85%)` | :arrow_down: |
| [...orch\_transformers/tests/tokenization\_utils\_test.py](https://codecov.io/gh/huggingface/pytorch-transformers/pull/1249/diff?src=pr&el=tree#diff-cHl0b3JjaF90cmFuc2Zvcm1lcnMvdGVzdHMvdG9rZW5pemF0aW9uX3V0aWxzX3Rlc3QucHk=) | `92% <0%> (-4%)` | :arrow_down: |
| [...h\_transformers/tests/tokenization\_tests\_commons.py](https://codecov.io/gh/huggingface/pytorch-transformers/pull/1249/diff?src=pr&el=tree#diff-cHl0b3JjaF90cmFuc2Zvcm1lcnMvdGVzdHMvdG9rZW5pemF0aW9uX3Rlc3RzX2NvbW1vbnMucHk=) | `97.43% <0%> (-2.57%)` | :arrow_down: |
| [pytorch\_transformers/tokenization\_gpt2.py](https://codecov.io/gh/huggingface/pytorch-transformers/pull/1249/diff?src=pr&el=tree#diff-cHl0b3JjaF90cmFuc2Zvcm1lcnMvdG9rZW5pemF0aW9uX2dwdDIucHk=) | `95.86% <0%> (-0.83%)` | :arrow_down: |
| [pytorch\_transformers/tokenization\_transfo\_xl.py](https://codecov.io/gh/huggingface/pytorch-transformers/pull/1249/diff?src=pr&el=tree#diff-cHl0b3JjaF90cmFuc2Zvcm1lcnMvdG9rZW5pemF0aW9uX3RyYW5zZm9feGwucHk=) | `33.89% <0%> (-0.29%)` | :arrow_down: |
------
[Continue to review full report at Codecov](https://codecov.io/gh/huggingface/pytorch-transformers/pull/1249?src=pr&el=continue).
> **Legend** - [Click here to learn more](https://docs.codecov.io/docs/codecov-delta)
> `Δ = absolute <relative> (impact)`, `ø = not affected`, `? = missing data`
> Powered by [Codecov](https://codecov.io/gh/huggingface/pytorch-transformers/pull/1249?src=pr&el=footer). Last update [364920e...8bdee1c](https://codecov.io/gh/huggingface/pytorch-transformers/pull/1249?src=pr&el=lastupdated). Read the [comment docs](https://docs.codecov.io/docs/pull-request-comments).
<|||||>LGTM, thanks @ziliwang |
|
transformers | 1,247 | closed | KnowBert | As has amazingly and remarkably become a standard response to a new model announcement, will this new transformer model be implemented:
https://arxiv.org/pdf/1909.04164.pdf - KnowBert at EMNLP19. | 09-11-2019 01:23:01 | 09-11-2019 01:23:01 | Hi,
We only add models when there are pretrained weights released.
This doesn't seem to be the case for KnowBert, or maybe I missed them?<|||||>Yes, looking into if they are releasing pretrained weights, I incorrectly assumed they were. <|||||>Wow! Really looking forward to this.
I really feel that models that combine text and unstructured data are not getting enough attention.
This is so relevant for creating great AI products because I dare to say that in most real life applications you do not deal with text only data. Metadata is crucial!<|||||>The authors released the model. Any update on integrating it into huggingface?<|||||>We'd welcome a community or author-contributed implementation!
(Also might look into integrating it ourselves at some point, but bandwidth is low)
[Update: [link to the implem + weights](https://github.com/allenai/kb)]<|||||>This issue has been automatically marked as stale because it has not had recent activity. It will be closed if no further activity occurs. Thank you for your contributions.
|
transformers | 1,246 | closed | breaking change | Great job, just in case it went unnoticed:
from revision 995e38b7af1aa325b994246e1bfcc7bf7c9b6b4f
to revision 2c177a87eb5faab8a0abee907ff75898b4886689
examples are broken due to changed orders of parameters in
pytorch_transformers/modeling_bert.py
```
< def forward(self, input_ids, token_type_ids=None, attention_mask=None, labels=None,
< position_ids=None, head_mask=None):
< outputs = self.bert(input_ids, position_ids=position_ids, token_type_ids=token_type_ids,
< attention_mask=attention_mask, head_mask=head_mask)
---
> def forward(self, input_ids, attention_mask=None, token_type_ids=None,
> position_ids=None, head_mask=None, labels=None):
>
``` | 09-10-2019 18:44:41 | 09-10-2019 18:44:41 | Indeed. What do you mean by "examples"? The docstrings examples?<|||||>Most of examples folder.
In particular run_swag.py and lm finetuning scripts.<|||||>just came here to say the same. <|||||>Indeed, I've fixed and cleaned up the examples in 8334993 (the lm finetuning examples are now replaced by `run_lm_finetuning`). Also indicated more clearly which examples are not actively maintained and tested.<|||||>This issue has been automatically marked as stale because it has not had recent activity. It will be closed if no further activity occurs. Thank you for your contributions.
|
transformers | 1,245 | closed | Different performance between pip install vs. download zip code | ## ❓ Questions & Help
<!-- A clear and concise description of the question. -->
Hi guys,
I meet a weird problem. Basically I am using BERT for sentence pair classification, such as MNLI, RTE tasks.
I installed the pytorch_transformers by "pip install pytorch-transformers" and
> from pytorch_transformers.modeling_bert import BertForSequenceClassification
everything works fine, the performance on RTE can reach 70%.
Instead, I downloaded the zip code to local since I want to do some modification about the BertForSequenceClassification in my future projects. (let's say I renamed the unzipped folder into "my_pytorch_transformers"), and now import as:
> from my_pytorch_transformers.modeling_bert import BertForSequenceClassification
Now, everything was not the same; the performance was just 53%, and it showed difference from the beginning of iterations.
So, what's wrong here? I thought maybe some initializations are different between the default installation and my local code? But I always used the pretrained BERT "bert-large-uncased", ....I can not figure out where the difference comes from.
Thanks for any hints
| 09-10-2019 18:21:52 | 09-10-2019 18:21:52 | sorry I found the problem: the pip source code and the zip code downloaded are different, especially for the "BertForSequenceClassification" class |
transformers | 1,244 | closed | unconditional generation with run_generation.py | ## ❓ Questions & Help
Is it possible to have unconditional generation with ``` run_generation.py``` ? I realized the previous script ```run_gpt2.py``` had this option.
Can we use the same ```start_token``` trick? | 09-10-2019 17:55:56 | 09-10-2019 17:55:56 | Hi, by unconditional generation do you mean generating a sequence from no context? If so, if using GPT-2, you can set your initial context to be: `<|endoftext|>`. This will generate sequences with no other initial context.
You could do so like this:
```
from pytorch_transformers import GPT2Tokenizer
tokenizer = GPT2Tokenizer.from_pretrained("gpt2")
context = [tokenizer.encoder["<|endoftext|>"]]
```<|||||>@LysandreJik Yeah I did that but It is not performing good, it generated the start like this:
```
"<|endoftext|>ingly anticipation passations, out he didn't realize that any or products in order to stand on. As Eric's disappointment, he threw into those o-bag's fanware vugeless chainsas. Finally, Chris went on a hob, and grabbed the crurne cartocos juice!"
```
I wonder if I can add a starting special token (sth like [CLS]) to my inputs and finetune gpt2 with this added vocabulary?
I have asked this [here](https://github.com/huggingface/pytorch-transformers/issues/1145) though<|||||>This issue has been automatically marked as stale because it has not had recent activity. It will be closed if no further activity occurs. Thank you for your contributions.
<|||||>> @LysandreJik Yeah I did that but It is not performing good, it generated the start like this:
>
> ```
> "<|endoftext|>ingly anticipation passations, out he didn't realize that any or products in order to stand on. As Eric's disappointment, he threw into those o-bag's fanware vugeless chainsas. Finally, Chris went on a hob, and grabbed the crurne cartocos juice!"
> ```
>
> I wonder if I can add a starting special token (sth like [CLS]) to my inputs and finetune gpt2 with this added vocabulary?
> I have asked this [here](https://github.com/huggingface/pytorch-transformers/issues/1145) though
Hi Have you figured out this issue? |
transformers | 1,243 | closed | Can pytorch-transformers be used to get XLM sentence embeddings for multiple languages? | ## ❓ Questions & Help
I tried to create a class to get the XLM sentence embeddings in multiple languages.
```
class XLMSentenceEmbeddings(pt.XLMPreTrainedModel):
def __init__(self, config):
super(XLMSentenceEmbeddings, self).__init__(config)
self.transformer = pt.XLMModel(config)
def forward(self, input_ids, lengths=None, position_ids=None, langs=None, token_type_ids=None,
attention_mask=None, cache=None, labels=None, head_mask=None):
transformer_outputs = self.transformer(input_ids, lengths=lengths, position_ids=position_ids, token_type_ids=token_type_ids, langs=langs, attention_mask=attention_mask, cache=cache, head_mask=head_mask)
return transformer_outputs[0][:, 0, :]
```
But if I try this code I get the same result if I set the language ids to Dutch or if I don't.
```
tokenizer = pt.XLMTokenizer.from_pretrained('xlm-mlm-100-1280')
xlm_model = XLMSentenceEmbeddings.from_pretrained('xlm-mlm-100-1280')
sentence = 'een nederlandstalige zin'
lang = 'nl'
input_ids = torch.tensor(tokenizer.encode(sentence, lang=lang)).unsqueeze(0)
with torch.no_grad():
xlm_model.eval()
output_without_lang = xlm_model(input_ids).numpy()
output_with_lang = xlm_model(input_ids, langs=xlm_model.transformer.config.lang2id[lang]*torch.ones(input_ids.size(), dtype=int)).numpy()
np.sum(output_without_lang - output_with_lang)
```
The output of this sum at the end always returns zero.
If I change the config to use the language embeddings like so: `config.use_lang_emb = True` my results are random each time which seems to suggest the embeddings are not included in the model. Should I change a different config? Or is the only way to get the sentence embeddings to train the model again? | 09-10-2019 17:36:29 | 09-10-2019 17:36:29 | This issue has been automatically marked as stale because it has not had recent activity. It will be closed if no further activity occurs. Thank you for your contributions.
|
transformers | 1,242 | closed | Special tokens / XLNet | it is necessary to add [CLS], [SEP] tokens in case of XLNet transformers?
Thanks!
*I used only the tokenizer.encode() function even if the sample had several sentences and I didn't set any special tokens. I think it was not the right way, isn't? It was done for a classification task. | 09-10-2019 16:41:32 | 09-10-2019 16:41:32 | Hi, in the case of sequence classification, XLNet does indeed use special tokens. For sentence pairs, it looks like this:
```
A [SEP] B [SEP][CLS]
```
You can either create those yourself or use the flag `add_special_tokens` from the `encode` function as follows:
```
tokenizer.encode(a, b, add_special_tokens=True)
```
which will return the correct list of tokens according to the tokenizer you used (which should be the `XLNetTokenizer` in your case)<|||||>@LysandreJik how to deal with more than two sentences? In the same way?<|||||>I'm not a dev of this lib but just stumbling upon this whilst searching from something else so I'll reply ;)
I think for more than 2 sentences you can use A [SEP] B [SEP] C [SEP] [CLS] for the encoding, and then specify token_type_ids as explained [there](https://github.com/huggingface/pytorch-transformers/blob/32e1332acf6fd1ad372b81c296d43be441d3b0b1/pytorch_transformers/modeling_xlnet.py#L505) to tell the model which token belongs to which segment. <|||||>regarding token_type_ids:
@LysandreJik wrote here about two sentences,
https://github.com/huggingface/pytorch-transformers/issues/1208#issuecomment-528515647
> If I recall correctly the XLNet model has 0 for the first sequence token_type_ids, 1 for the second sequence, and 2 for the last (cls) token.
what is to do for the third, fourth, fifth ... sentences ? 0 and 1 alternating?<|||||>I think you can put 0 for first sentence, 1 for second, 2 for third etc..
but the actual indices do not matter because the encoding is relative (see
XLNet paper section 2.5), the only important thing is that tokens from a
same sentence have the same token_type_ids. XLnet was made this way in
order to handle an arbitrary number of sentences at finetuning. At least
that is the way I understand it.
Le ven. 13 sept. 2019 à 15:55, cherepanovic <[email protected]> a
écrit :
> regarding token_type_ids:
>
> @LysandreJik <https://github.com/LysandreJik> wrote here about two
> sentences,
>
> #1208 (comment)
> <https://github.com/huggingface/pytorch-transformers/issues/1208#issuecomment-528515647>
>
> If I recall correctly the XLNet model has 0 for the first sequence
> token_type_ids, 1 for the second sequence, and 2 for the last (cls) token.
>
> what is to do for the third, fourth, fifth ... sentences ?
>
> —
> You are receiving this because you commented.
> Reply to this email directly, view it on GitHub
> <https://github.com/huggingface/pytorch-transformers/issues/1242?email_source=notifications&email_token=AD6A5ZI5KDS2BLIQLBJB5QLQJOLVJA5CNFSM4IVKBXC2YY3PNVWWK3TUL52HS4DFVREXG43VMVBW63LNMVXHJKTDN5WW2ZLOORPWSZGOD6VCZMA#issuecomment-531246256>,
> or mute the thread
> <https://github.com/notifications/unsubscribe-auth/AD6A5ZNAFKI6SQPLVVSKYLLQJOLVJANCNFSM4IVKBXCQ>
> .
>
<|||||>> Hi, in the case of sequence classification, XLNet does indeed use special tokens. For sentence pairs, it looks like this:
@LysandreJik
you are speaking about sentence pairs, what is to do with several sentences, could you please give an advice
thanks a lot<|||||>This issue has been automatically marked as stale because it has not had recent activity. It will be closed if no further activity occurs. Thank you for your contributions.
<|||||>> > Hi, in the case of sequence classification, XLNet does indeed use special tokens. For sentence pairs, it looks like this:
>
> @LysandreJik
> you are speaking about sentence pairs, what is to do with several sentences, could you please give an advice
>
> thanks a lot
so have you got the answer about how to deal with more than two sentences?<|||||>@sloth2012
>>so have you got the answer about how to deal with more than two sentences?
no, but I did it in this way [SEP] A.B.C [CLS], in this way |
transformers | 1,241 | closed | Fixing typo in gpt2 for doc site's class link | 09-10-2019 16:13:17 | 09-10-2019 16:13:17 | 👍 |
|
transformers | 1,240 | closed | ModuleNotFoundError in distillation/scripts/binarized_data.py | Hello,
importing logger (still? I've seen previous issues, but this is the first time I'm running the code myself) throws the ModuleNotFoundError.
```
Traceback (most recent call last):
File "examples/distillation/scripts/binarized_data.py", line 25, in <module>
from examples.distillation.utils import logger
ModuleNotFoundError: No module named 'examples.distillation'
``` | 09-10-2019 15:45:49 | 09-10-2019 15:45:49 | Hello @MatejUlcar
Thanks for pointing that out. I fixed it once and for all by having a local logger (and not importing the global one) in commit 32e1332acf6fd1ad372b81c296d43be441d3b0b1. |
transformers | 1,239 | closed | how to finetuning with roberta-large | ## 🐛 Bug
<!-- Important information -->
Model I am using (Roberta-large):
Language I am using the model on (English, ):
The problem arise when using:
* [ ] my own modified scripts: (give details)
export CUDA_VISIBLE_DEVICES=2
export GLUE_DIR=/home/zhaoguangxiang/bert/glue_data
DATA=MNLI
NUM_CLASSES=3
LR=1e-5
MAX_SENTENCES=32
TOTAL_NUM_UPDATES=123873
WARMUP_UPDATES=7432
for seed in 42
do
python3 examples/run_glue.py \
--model_type bert \
--model_name_or_path https://s3.amazonaws.com/models.huggingface.co/bert/roberta-large-pytorch_model.bin \
--task_name ${DATA} \
--do_train \
--do_eval \
--do_lower_case \
--data_dir $GLUE_DIR/${DATA} \
--save_steps 10000 \
--logging_steps 1000 \
--max_seq_length 512 \
--max_steps ${TOTAL_NUM_UPDATES} --warmup_steps ${WARMUP_UPDATES} --learning_rate ${LR} \
--per_gpu_eval_batch_size 32 \
--per_gpu_train_batch_size 32 \
--seed ${seed} \
--output_dir checkpoint/roberta_${DATA}_output_seed${seed}/
done
The tasks I am working on is:
* [ ] an official GLUE/SQUaD task: (give the name)
## To Reproduce
Steps to reproduce the behavior:
1. run scripts
2. i will see

<!-- If you have a code sample, error messages, stack traces, please provide it here as well. -->
## Expected behavior
load or download roeberta and s tart training
## Environment
* PyTorch Transformers version (or branch): latest
* Using GPU ? yes
* Distributed of parallel setup ? no
| 09-10-2019 12:10:08 | 09-10-2019 12:10:08 | What do you mean by "latest" version of PyTorch tranformers.
Are you using a release or installing from source from master?<|||||>> What do you mean by "latest" version of PyTorch tranformers.
> Are you using a release or installing from source from master?
Thanks for your reply.
pytorch transformers version 1.1.0;
downloading the code from master;
examples of finetuning roberta were not given in the docs.
<|||||>this is not a bug, since i write the wrong model_type, that should be 'roberta' |
transformers | 1,238 | closed | BLUE | In this PR:
- I add BertForMultiLabelClassification, RobertaForTokenClassification, RobertaForMultiLabelClassification.
- I add examples for Finetuning the BERT, RoBERTa models for tasks on BLUE (https://github.com/ncbi-nlp/BLUE_Benchmark). BLUE (Biomedical Language Understanding Evaluation) is similar to GLUE, but for Biomedical data. The "run_blue", "utils_blue" are customized from "run_glue", "utils_glue", but more sufficient, because it contains not only sequence classification, but also token classification, multi-label classification. People may also have more options for examples of fine-tuning BERT/RoBERTa.
- I also add test function to test_examples as well as test data (HOC) | 09-10-2019 11:37:11 | 09-10-2019 11:37:11 | @LysandreJik @julien-c one of you want to give a look?<|||||># [Codecov](https://codecov.io/gh/huggingface/pytorch-transformers/pull/1238?src=pr&el=h1) Report
> Merging [#1238](https://codecov.io/gh/huggingface/pytorch-transformers/pull/1238?src=pr&el=desc) into [master](https://codecov.io/gh/huggingface/pytorch-transformers/commit/2c177a87eb5faab8a0abee907ff75898b4886689?src=pr&el=desc) will **decrease** coverage by `0.43%`.
> The diff coverage is `30.88%`.
[](https://codecov.io/gh/huggingface/pytorch-transformers/pull/1238?src=pr&el=tree)
```diff
@@ Coverage Diff @@
## master #1238 +/- ##
==========================================
- Coverage 81.23% 80.79% -0.44%
==========================================
Files 57 57
Lines 8029 8092 +63
==========================================
+ Hits 6522 6538 +16
- Misses 1507 1554 +47
```
| [Impacted Files](https://codecov.io/gh/huggingface/pytorch-transformers/pull/1238?src=pr&el=tree) | Coverage Δ | |
|---|---|---|
| [pytorch\_transformers/modeling\_bert.py](https://codecov.io/gh/huggingface/pytorch-transformers/pull/1238/diff?src=pr&el=tree#diff-cHl0b3JjaF90cmFuc2Zvcm1lcnMvbW9kZWxpbmdfYmVydC5weQ==) | `85.68% <27.27%> (-2.65%)` | :arrow_down: |
| [pytorch\_transformers/modeling\_roberta.py](https://codecov.io/gh/huggingface/pytorch-transformers/pull/1238/diff?src=pr&el=tree#diff-cHl0b3JjaF90cmFuc2Zvcm1lcnMvbW9kZWxpbmdfcm9iZXJ0YS5weQ==) | `62.33% <32.6%> (-12.9%)` | :arrow_down: |
| [pytorch\_transformers/tests/modeling\_common\_test.py](https://codecov.io/gh/huggingface/pytorch-transformers/pull/1238/diff?src=pr&el=tree#diff-cHl0b3JjaF90cmFuc2Zvcm1lcnMvdGVzdHMvbW9kZWxpbmdfY29tbW9uX3Rlc3QucHk=) | `73.19% <0%> (-0.13%)` | :arrow_down: |
------
[Continue to review full report at Codecov](https://codecov.io/gh/huggingface/pytorch-transformers/pull/1238?src=pr&el=continue).
> **Legend** - [Click here to learn more](https://docs.codecov.io/docs/codecov-delta)
> `Δ = absolute <relative> (impact)`, `ø = not affected`, `? = missing data`
> Powered by [Codecov](https://codecov.io/gh/huggingface/pytorch-transformers/pull/1238?src=pr&el=footer). Last update [2c177a8...3cbe79a](https://codecov.io/gh/huggingface/pytorch-transformers/pull/1238?src=pr&el=lastupdated). Read the [comment docs](https://docs.codecov.io/docs/pull-request-comments).
<|||||>@thomwolf : Hi, all checks have passed. Please review it again ;). <|||||>This PR is out of date.
I updated a new one here (https://github.com/huggingface/transformers/pull/1440). |
transformers | 1,237 | closed | Issue in fine-tuning distilbert on Squad 1.0 | When I tried to finetune the distillbert (using run_squad.py in examples folder), the model reaches the F1 score of 17.43 on dev set but you have mentioned that the F1 score is 86.2. Can you help me with what I am doing wrong at the time of fine-tuning?
Below is the command that I am using
python ./examples/run_squad.py \
--model_type bert \
--model_name_or_path /root/distilbert_training \
--do_train \
--do_eval \
--do_lower_case \
--train_file train-v1.1.json \
--predict_file dev-v1.1.json \
--learning_rate 3e-5 \
--num_train_epochs 2 \
--max_seq_length 384 \
--doc_stride 128 \
--output_dir /root/output/ \
--per_gpu_eval_batch_size=3 \
--per_gpu_train_batch_size=3 | 09-10-2019 10:16:31 | 09-10-2019 10:16:31 | Hello @pragnakalpdev,
Did you change the `run_squad.py` file to include distilbert ([here](https://github.com/huggingface/pytorch-transformers/blob/master/examples/run_squad.py#L58) for instance)?
Can you check the warnings ``Weights from XXX not used in YYY``?<|||||>Hello @VictorSanh,
Thank You for your response. I got the F1 score 86.4.
But now when I am facing issue in the evaluation process.
I had performed the evaluation on my custom file that has a single paragraph and 5 questions, and its taking approx 10 seconds to generate the prediction.json file.
I want the inference time about 1-2seconds, what can I do for that?<|||||>Hello @pragnakalpdev7,
Good!
How long is your paragraph? Is the inference time during the training/fine-tuning the same as during test? (a forward pass during training should be slightly slower).
If you're already using `run_squad.py`, there is no easy/direct way to accelerate the inference.<|||||>Hello @VictorSanh ,
My paragraph is less than 1000 characters, and yes i am already using the run_squad.py for inference.
and i didn't understand this - "Is the inference time during the training/fine-tuning the same as during test? (a forward pass during training should be slightly slower)."
For fine-tuning it took 4 hours on 1 GPU, and now i am using the fined-tuned model for inference which is taking 8 - 9 seconds.<|||||>I mean that if a forward pass already takes ~2 sec during training on your machine, it is not likely to go down to 0.1 sec during test. The reason why a forward pass is on average slightly faster during test is that the flag `torch.no_grad` deactivate the autograd engine.<|||||>Hi @pragnakalpdev , can I ask you how you solved the first problem to achieve good performance? I'm in a similar situation and any hints would help.<|||||>Hi @pragnakalpdev could you indeed please comment how you solved the first problem (bad F1) to achieve good performance?<|||||>This issue has been automatically marked as stale because it has not had recent activity. It will be closed if no further activity occurs. Thank you for your contributions.
<|||||>Hi, can anyone tell me where(exactly in which directory or in colab exactly where) to run that code to fine-tune the bert model? I can't able to fine-tune my bert model for QnA purposes. Please reply. |
transformers | 1,236 | closed | Roberta for squad | Hi,
Please add Roberta for squad.
Thanks
Mahesh | 09-10-2019 06:35:53 | 09-10-2019 06:35:53 | This issue has been automatically marked as stale because it has not had recent activity. It will be closed if no further activity occurs. Thank you for your contributions.
|
transformers | 1,235 | closed | Quick questions about details | ## ❓ Questions & Help
<!-- A clear and concise description of the question. -->
Can someone explain the difference between `mem_len`, `mlen`, and `ext_len` in `TransfoXLModel`? While the documentation has stated below
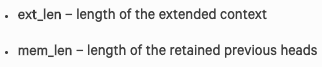
Unfortunately, I'm still confused especially `mem_len` and `mlen`. Thank you so much. | 09-10-2019 04:21:50 | 09-10-2019 04:21:50 | This issue has been automatically marked as stale because it has not had recent activity. It will be closed if no further activity occurs. Thank you for your contributions.
|
transformers | 1,234 | closed | ❓ How to finetune `token_type_ids` of RoBERTa ? | ## ❓ Questions & Help
RoBERTa model does not use `token_type_ids`.
However it is mentioned in the documentation :
> you will have to train it during finetuning
Indeed, I would like to train it during finetuning. I tried to load the model with :
`model = RobertaModel.from_pretrained('roberta-base', type_vocab_size=2)`
But I received the error :
> RuntimeError: Error(s) in loading state_dict for RobertaModel:
size mismatch for roberta.embeddings.token_type_embeddings.weight: copying a param with shape torch.Size([1, 768]) from checkpoint, the shape in current model is torch.Size([2, 768]).
---
So **how can I create my RoBERTa model from the pretrained checkpoint, in order to finetune the use of `token ids` ?** | 09-10-2019 02:05:18 | 09-10-2019 02:05:18 | What I have done is :
```python
model = RobertaModel.from_pretrained('roberta-base')
model.config.type_vocab_size = 2
single_emb = model.embeddings.token_type_embeddings
model.embeddings.token_type_embeddings = torch.nn.Embedding(2, single_emb.embedding_dim)
model.embeddings.token_type_embeddings.weight = torch.nn.Parameter(single_emb.weight.repeat([2, 1]))
```
But it seems quite clumsy...
**What is the 'official' way to go ?**<|||||>Just using it without doing anything special doesn't work?
```
model = RobertaModel.from_pretrained('roberta-base')
model(inputs_ids, token_type_ids=token_type_ids)
```<|||||>Roberta does not use segment IDs in pre-training.
As you mentioned in #1114, we can use it as BERT, but we should pass only 0 (if token_type_ids contain 1, it will throw an error).
I would like to fine-tune RoBERTa using a vocabulary of 2 for the token_type_ids (so the token_type_ids can contain 0 or 1).
Hopefully by doing this, RoBERTa can learn the difference between `token_type_id = 0` and `token_type_id = 1` after fine-tuning.
Did I misunderstand issue #1114 ?<|||||>Yes, just feed `token_type_ids` during finetuning.
The embeddings for 2 token type ids are there, they are just not trained.
Nothing special to do to activate them.<|||||>@thomwolf
I'm sorry, I still don't get it, and I still think we need to modify the model after loading the pretrained checkpoint..
---
Can you try this code and see if we have the same output ?
```python
from pytorch_transformers import XLNetModel, XLNetTokenizer, RobertaTokenizer, RobertaModel
import torch
model = RobertaModel.from_pretrained('roberta-base')
tokenizer = RobertaTokenizer.from_pretrained('roberta-base')
print("Config show size of {}\n".format(model.config.type_vocab_size))
src = torch.tensor([tokenizer.encode("<s> My name is Roberta. </s>")])
segs = torch.zeros_like(src)
print("Using segment ids : {}".format(segs))
outputs = model(src, token_type_ids=segs)
print("Output = {}\n".format(outputs[0].size()))
segs[:, 4:] = torch.tensor([1, 1, 1, 1])
print("Using segment ids : {}".format(segs))
outputs = model(src, token_type_ids=segs)
print("Output = {}".format(outputs[0].size()))
```
My output show :
> Config show size of 1
Using segment ids : tensor([[0, 0, 0, 0, 0, 0, 0, 0]])
Output = torch.Size([1, 8, 768])
Using segment ids : tensor([[0, 0, 0, 0, 1, 1, 1, 1]])
>RuntimeError Traceback (most recent call last)
<ipython-input-15-85c5c590aed9> in <module>()
14 segs[:, 4:] = torch.tensor([1, 1, 1, 1])
15 print("Using segment ids : {}".format(segs))
---> 16 outputs = model(src, token_type_ids=segs)
17 print("Output = {}".format(outputs[0].size()))
8 frames
/usr/local/lib/python3.6/dist-packages/torch/nn/functional.py in embedding(input, weight, padding_idx, max_norm, norm_type, scale_grad_by_freq, sparse)
1504 # remove once script supports set_grad_enabled
1505 _no_grad_embedding_renorm_(weight, input, max_norm, norm_type)
-> 1506 return torch.embedding(weight, input, padding_idx, scale_grad_by_freq, sparse)
1507
1508
RuntimeError: index out of range at /pytorch/aten/src/TH/generic/THTensorEvenMoreMath.cpp:193
---
Which in my opinion makes sense because :
```python
print(model.embeddings.token_type_embeddings.weight.size())
```
show :
> torch.Size([1, 768])
(And we need [2, 768] if we want to use 2 types of segment IDs)<|||||>Yes. The problem is in the config file of Roberta model the type_vocab_size = 1 while for bert it's 2. This cause the problem. I'm trying to set it manually to 2 to see what happens.<|||||>You are right, we've dived deeply into this issue with @LysandreJik and unfortunately there no solution that would, at the same time, keep full backward compatibility for people who have been using RoBERTa up to now and allow to train and fine-tune token type embeddings for RoBERTa.
So, unfortunately, it won't be possible to fine-tune token type embeddings with RoBERTa.
We'll remove the pointers to this possibility in the doc and docstring.<|||||>I just simply set all token_type_ids to 0 and I can finetune on SQuAD 2.0. I can achive 86.8 F1 score, which looks reasonable though still worse than the reported 89.4 F1 score.<|||||>Thanks for the investigation @thomwolf !
It makes sense to **not** allow finetuning token type embeddings with RoBERTa (because of pretraining).
**However, it's still possible to load the pretrained model and manually modify it to allow finetuning, right ?**
If so, maybe we can add an example of how to do such a thing. My code for this is :
```python
# Load pretrained model
model = RobertaModel.from_pretrained('roberta-base')
# Update config to finetune token type embeddings
model.config.type_vocab_size = 2
# Create a new Embeddings layer, with 2 possible segments IDs instead of 1
model.embeddings.token_type_embeddings = nn.Embedding(2, model.config.hidden_size)
# Initialize it
model.embeddings.token_type_embeddings.weight.data.normal_(mean=0.0, std=model.config.initializer_range)
```
_It seems to work, but I would like some feedback, if I missed something :)_
<|||||>@tbright17
By setting all token types IDs to 0, you're not actually using it. It's fine, because anyway RoBERTa does not use it, but people might need to use it for some downstream tasks. This issue is about this case :)<|||||>@Colanim
I think Thomas's fix is okay. If you need token_type_ids for some tasks, you can always add new arguments to the forward method. There is no need to use token_type_ids as an argument for the RobertaModel class.<|||||>I think there is some confusion here ^^
As I understood, Thomas didn't fix anything. The current API of RoBERTa already handle `token_type_ids` in the forward method, but to use it you need to set all `token_type_ids` to 0 (as you mentioned).
It makes sense (see pretraining of RoBERTa) and should not be changed, as Thomas mentioned. Only documentation may need to be updated.
---
But I opened this issue because for my task I need to use 2 types of `token_type_ids` (`0` and `1`). I was asking how to do this with the current API, what do I need to modify, etc...<|||||>Okay I see. Sorry for the confusion. <|||||>@Colanim
Thanks for raising this issue. I was experiencing it too recently where I tried to use the token type ids created by `RobertaTokenizer.create_token_type_ids_from_sequences()` but when I used it as the model's input, I will get an index out of range error.
I like the way you manually fixed the token type embedding layer. Do you by any chance have a comparison of performance with and without the adjustment you made? And if so, what was the downstream task that you were using Roberta for? I am curious as I would like to do relationship classification for two sequence inputs. <|||||>@wise-east
Sorry I didn't compare with and without. I used RoBERTa for text summarization, and I think it has only little impact on performance (for my task). <|||||>This issue has been automatically marked as stale because it has not had recent activity. It will be closed if no further activity occurs. Thank you for your contributions.
<|||||>same probleam, it seems that roberta have no nsp tasks , so segments id have no means for roberta<|||||>@astariul As you mentioned, you use the token_type_ids in text summarization task. So have you do some comparison on the performance between using token_type_ids or not.<|||||>>
@thomwolf @astariul Why not just add a `resize_token_type_embeddings` method, just like there is a `resize_token_embeddings` method?<|||||>is there any convenient way to construct `token_type_ids` from RobertaTokenizer ? I tried the following way:

|
transformers | 1,233 | closed | Fix to prevent crashing on assert len(tokens_b)>=1 | Thank you for the awesome library! One little issue that I have sometimes with somewhat "noisy" text is that Bert tokenizer fails to process some weird stuff. In such a case, one unexpectedly gets no tokens and the converter fails on assert with a message like this one:
```
Epoch: 0%| | 0/1 [00:00<?, ?it/s] Traceback (most recent call last):
File "../pytorch-transformers/examples/lm_finetuning/pregenerate_training_data.py", line 354, in <module>
main()
File "../pytorch-transformers/examples/lm_finetuning/pregenerate_training_data.py", line 350, in main
create_training_file(docs, vocab_list, args, epoch)
File "../pytorch-transformers/examples/lm_finetuning/pregenerate_training_data.py", line 276, in create_training_file
whole_word_mask=args.do_whole_word_mask, vocab_list=vocab_list)
File "../pytorch-transformers/examples/lm_finetuning/pregenerate_training_data.py", line 244, in create_instances_from_document
assert len(tokens_b) >= 1
AssertionError
``` | 09-10-2019 00:01:36 | 09-10-2019 00:01:36 | Ok, merging.
Note that we are in the process of deprecating these finetuning scripts and replacing them with the common `run_lm_finetuning.py` which handles several models.<|||||>Thank you @thomwolf sorry didn't realize this was deprecated. |
transformers | 1,232 | closed | Can't reproduce XNLI zero-shot results from MBERT in Chinese | ## ❓ Questions & Help
Hi guys,
I am trying to reproduce the XNLI zero-shot transfer results from MBERT.
With the same code and same checkpoint but different language for the test set, I am not able to reproduce the results for Chinese, Arabic, and Urdu. Does anyone encounter the same problem? Thanks!
Model |English | Chinese | Arabic | German | Spanish | Urdu
-- |-- | -- | -- | -- | -- | --
From MBERT github page | 81.4 | 63.8 | 62.1 | 70.5 | 74.3 | 58.3
My results | 82.076 | 36.088 | 35.327 | 68.782 | 70.419 | 35.170
| 09-09-2019 21:13:54 | 09-09-2019 21:13:54 | Hey @edchengg, I'm running into the same problem. Were you able to figure this out? thanks
<|||||>Anyone else who stumbles here.
**Fix**: Just use bert-based-multilingual-cased as shown here https://huggingface.co/transformers/v2.3.0/examples.html.
When I used Google's mBERT and made it pyTorch compatible using the convert_tf_original..... script from src/transformers, somehow it doesn't learn properly. Couldn't figure out why, hence opening a new issue here : https://github.com/huggingface/transformers/issues/5019<|||||>@bsinghpratap Did you manage to run the script in only evaluation mode? When I try to evaluate an mBERT model trained on MNLI, it just freezes at 99%.<|||||>Yeah, I ran it on eval mode as well. Works fine for me. |
transformers | 1,231 | closed | Unable to load DistilBertModel after training | ## ❓ Questions & Help
I'm following the example to train a DistilBert model from scratch from: examples/distillation/README.md
I perform the training step:
```
python examples/distillation/train.py --dump_path ser_dir/sm_training_1 --data_file data/sm_bin_text.bert-base-uncased.pickle --token_counts data/sm_token_counts.bert-base-uncased.pickle --force --fp16
```
Which completes successfully, however the `dump_path` doesn't contain a `pytorch_model.bin` file so I cannot load in the model:
```py
from pytorch_transformers import DistilBertModel
DistilBertModel.from_pretrained("ser_dir/sm_training_1")
Model name 'ser_dir/sm_training_1' was not found in model name list (distilbert-base-uncased, distilbert-base-uncased-distilled-squad). We assumed 'ser_dir/sm_training_1/pytorch_model.bin' was a path or url but couldn't find any file associated to this path or url.
Traceback (most recent call last):
...
OSError: file ser_dir/sm_training_1/pytorch_model.bin not found
```
Content of serialization directory:
```sh
ls ser_dir/sm_training_1/
checkpoint.pth config.json git_log.json log model_epoch_0.pth model_epoch_1.pth model_epoch_2.pth parameters.json
```
I also tried to load it as a regular `BertModel`. Is there something else I need to do to load in a basic .bin file then apply the checkpoint weights on top? I cannot find any tutorials or examples specifying the next steps on this process.
Thanks! | 09-09-2019 18:51:26 | 09-09-2019 18:51:26 | Hi @dalefwillis,
You simply have to rename your last checkpoint (I guess in your case it's _"model_epoch_2.pth"_) to _"pytorch_model.bin"_ --> `mv model_epoch_2.pth pytorch_model.bin`.
I updated the training code so that the very last _"model_epoch_*.pth"_ checkpoint is also saved as _"pytorch_model.bin"_ so that you don't have to do this manip manually.<|||||>Thanks for the fast response! I just tried it and it works. |
transformers | 1,230 | closed | How to deal with oov tokens with pretrained models | ## ❓ Questions & Help
Can you, please, give an advice on how to handle out of vocabulary word? Just to use `[UNK]` token or there is a way to add this token to vocabulary and thus to train embedding for it?
Also, I noticed that oov words by default return multiple tokens. In my task (sequence tagging) I would like to save token-label correspondence. | 09-09-2019 16:03:44 | 09-09-2019 16:03:44 | Hello! We have a method called `add_tokens` in our tokenizers that does just that. [Here's the relevant information](https://huggingface.co/pytorch-transformers/main_classes/tokenizer.html#pytorch_transformers.PreTrainedTokenizer.add_tokens) in the documentation.<|||||>Thanks a lot for your answer. That's exactly what I was looking for. |
transformers | 1,229 | closed | changes in evaluate function in run_lm_finetuning.py | changed the return value of `evaluate` function from `results` to `result` and also removed unused empty dict `results` | 09-09-2019 14:38:06 | 09-09-2019 14:38:06 | # [Codecov](https://codecov.io/gh/huggingface/pytorch-transformers/pull/1229?src=pr&el=h1) Report
> Merging [#1229](https://codecov.io/gh/huggingface/pytorch-transformers/pull/1229?src=pr&el=desc) into [master](https://codecov.io/gh/huggingface/pytorch-transformers/commit/84d346b68707f3c43903b122baae76ae022ef420?src=pr&el=desc) will **not change** coverage.
> The diff coverage is `n/a`.
[](https://codecov.io/gh/huggingface/pytorch-transformers/pull/1229?src=pr&el=tree)
```diff
@@ Coverage Diff @@
## master #1229 +/- ##
=======================================
Coverage 81.22% 81.22%
=======================================
Files 57 57
Lines 8027 8027
=======================================
Hits 6520 6520
Misses 1507 1507
```
------
[Continue to review full report at Codecov](https://codecov.io/gh/huggingface/pytorch-transformers/pull/1229?src=pr&el=continue).
> **Legend** - [Click here to learn more](https://docs.codecov.io/docs/codecov-delta)
> `Δ = absolute <relative> (impact)`, `ø = not affected`, `? = missing data`
> Powered by [Codecov](https://codecov.io/gh/huggingface/pytorch-transformers/pull/1229?src=pr&el=footer). Last update [84d346b...4b082bd](https://codecov.io/gh/huggingface/pytorch-transformers/pull/1229?src=pr&el=lastupdated). Read the [comment docs](https://docs.codecov.io/docs/pull-request-comments).
<|||||>Thanks! |
transformers | 1,228 | closed | Trying to fix the head masking test | Reviving this PR from @LysandreJik which tried to fix the head masking failing test by making random seed accessible anywhere within the common tests. | 09-09-2019 13:04:23 | 09-09-2019 13:04:23 | @LysandreJik was this still WIP or finished?<|||||>It solved the problems with head masking -> finished! |
transformers | 1,227 | closed | class DistilBertForMultiLabelSequenceClassification() | ## 🚀 Feature
Distil BERT For Multi-Label Sequence Classification
## Motivation
To do multi-label text classification using DistilBERT
## Additional context
None | 09-09-2019 11:04:40 | 09-09-2019 11:04:40 | This issue has been automatically marked as stale because it has not had recent activity. It will be closed if no further activity occurs. Thank you for your contributions.
|
transformers | 1,226 | closed | Question on the position embedding of DistilBERT | ## ❓ Questions & Help
To the best of my knowledge, sinusoidal position embedding is used in the training procedure of DistilBERT, which is computed by [create_sinusoidal_embeddings](https://github.com/huggingface/pytorch-transformers/blob/master/pytorch_transformers/modeling_distilbert.py#L52).
When I compute the position embedding by using `create_sinusoidal_embeddings` with `n_pos=512` and `dim=768`, I got the following position embedding tensor:
```python
Parameter containing:
tensor([[ 0.0000e+00, 1.0000e+00, 0.0000e+00, ..., 1.0000e+00,
0.0000e+00, 1.0000e+00],
[ 8.4147e-01, 5.4030e-01, 8.2843e-01, ..., 1.0000e+00,
1.0243e-04, 1.0000e+00],
[ 9.0930e-01, -4.1615e-01, 9.2799e-01, ..., 1.0000e+00,
2.0486e-04, 1.0000e+00],
...,
[ 6.1950e-02, 9.9808e-01, 5.3551e-01, ..., 9.9857e-01,
5.2112e-02, 9.9864e-01],
[ 8.7333e-01, 4.8714e-01, 9.9957e-01, ..., 9.9857e-01,
5.2214e-02, 9.9864e-01],
[ 8.8177e-01, -4.7168e-01, 5.8419e-01, ..., 9.9856e-01,
5.2317e-02, 9.9863e-01]])
```
However, when I looked into the position embedding from the pre-trained DistilBERT checkpoint files (`distilbert-base-uncased` and `distilbert-base-uncased-distilled-squad`,), I got the position embedding tensor as follows:
```python
tensor([[ 1.7505e-02, -2.5631e-02, -3.6642e-02, ..., 3.3437e-05,
6.8312e-04, 1.5441e-02],
[ 7.7580e-03, 2.2613e-03, -1.9444e-02, ..., 2.8910e-02,
2.9753e-02, -5.3247e-03],
[-1.1287e-02, -1.9644e-03, -1.1573e-02, ..., 1.4908e-02,
1.8741e-02, -7.3140e-03],
...,
[ 1.7418e-02, 3.4903e-03, -9.5621e-03, ..., 2.9599e-03,
4.3435e-04, -2.6949e-02],
[ 2.1687e-02, -6.0216e-03, 1.4736e-02, ..., -5.6118e-03,
-1.2590e-02, -2.8085e-02],
[ 2.6413e-03, -2.3298e-02, 5.4922e-03, ..., 1.7537e-02,
2.7550e-02, -7.7656e-02]])
```
I am wondering if I missed or misunderstood something in details. Why is there a difference between these two position embedding tensors? I think the sinusoidal position embedding should be unchanged during training.
Thanks a lot!
| 09-09-2019 01:24:12 | 09-09-2019 01:24:12 | Hello @gpengzhi
You're right, the name is quite confusing: the second matrix of embeddings that you're showing is actually initialized from `bert-base-uncased` (compare with `bert = BertModel.from_pretrained('bert-base-uncased'); print(bert.embeddings.position_embeddings.weight)`). Once initialized, these position embeddings are frozen (both distillation or fine-tuning)
Victor |
transformers | 1,225 | closed | Bert output last hidden state | ## ❓ Questions & Help
Hi,
Suppose we have an utterance of length 24 (considering special tokens) and we right-pad it with 0 to max length of 64.
If we use Bert pertained model to get the last hidden states, the output would be of size [1, 64, 768].
Can we use just the first 24 as the hidden states of the utterance? I mean is it right to say that the output[0, :24, :] has all the required information?
I realized that from index 24:64, the outputs has float values as well. | 09-08-2019 21:51:29 | 09-08-2019 21:51:29 | Hello! I believe that you are currently computing values for your padding indices, resulting in your confusion. There is a parameter `attention_mask` to be passed to the `forward`/`__call__` method which will prevent the values to be computed for the padded indices!<|||||>@LysandreJik thanks for replying.
Consider the example given in the modeling_bert.py script:
```
tokenizer = BertTokenizer.from_pretrained('bert-base-uncased')
model = BertModel.from_pretrained('bert-base-uncased')
input_ids = torch.tensor(tokenizer.encode("Hello, my dog is cute")).unsqueeze(0) # Batch size 1
padding = [0] * ( 128 - len(input_ids))
input_ids += padding
attn_mask = input_ids.ne(0) # I added this to create a mask for padded indices
outputs = model(input_ids, attention_mask=attn_mask)
last_hidden_states = outputs[0] # The last hidden-state is the first element of the output tuple
```
even with passing attention_mask parameter, it still compute values for the padded indices.
Am I doing something wrong?<|||||>> Can we use just the first 24 as the hidden states of the utterance? I mean is it right to say that the output[0, :24, :] has all the required information?
> I realized that from index 24:64, the outputs has float values as well.
yes, the remaining indices are values of padding embeddings, you can try/prove it out by different length of padding
take a look at that post #1013 (XLNet) and #278 (Bert)<|||||>@cherepanovic Thanks for your reply.
Oh See, I tried padding w/wo passing attention mask and I realized the output would be completely different for all indices.
So I understand that when we use padding we must pass the attention mask for sure, this way the output (on non padded indices) would be equal (not exactly, but almost) to when we don't use padding at all, right?
<|||||>> would be equal (not exactly, but almost)
right<|||||>@cherepanovic Just my very main question is are the output values in the padded indices, create noise or in other word misleading? or can we just make use of the whole output without being worried that for example the last 20 indices in the output is for padded tokens.<|||||>@ehsan-soe can you describe your intent more precisely <|||||>This issue has been automatically marked as stale because it has not had recent activity. It will be closed if no further activity occurs. Thank you for your contributions.
<|||||>**would be equal (not exactly, but almost)**
Why are they not exactly the same? (assuming all random seeds are set the same) |
transformers | 1,224 | closed | Remove duplicate hidden_states of the last layer in BertEncoder in modeling_bert.py | ## 🚀 Feature
<!-- A clear and concise description of the feature proposal. Please provide a link to the paper and code in case they exist. -->
For "class BertEncoder" in modeling_bert.py, remove duplicate hidden_states of the last layer
## Motivation
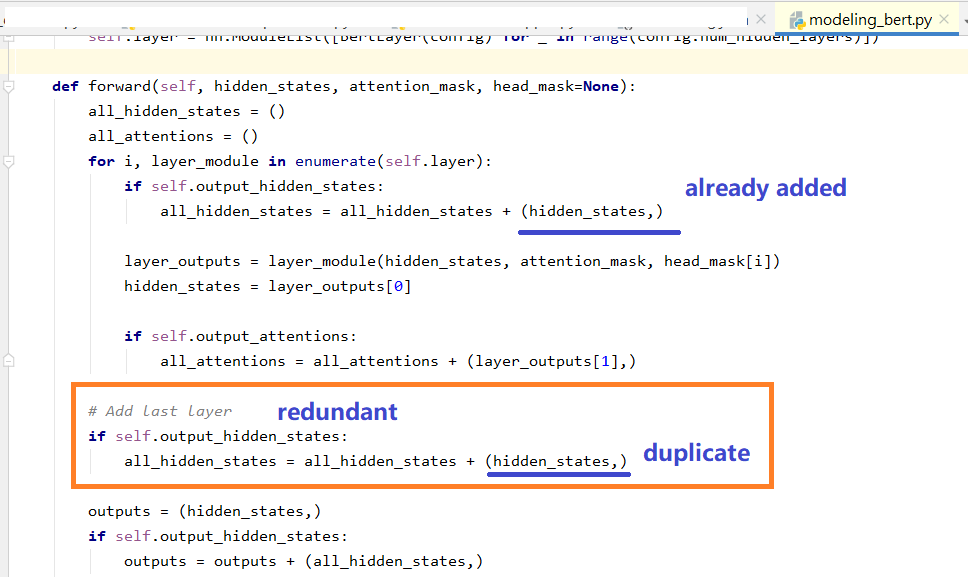
Bert-Base models have 12 layers instead of 13 layers. But when config.output_hidden_states is true, "len(all_hidden_states)" is printed 13 instead of 12. It seems that the two lines under "# Add last layer" is improper, since the last layer's hidden_states are already added.
| 09-08-2019 17:32:34 | 09-08-2019 17:32:34 | Hi! Indeed, the BERT-base only has 12 layers. The `all_hidden_states` is 13-dimensional however because it keeps track of the inputs as well.
In the code you have shown, the `hidden_states` variable is computed between the two underlined variables you mentioned. None of it is redundant :)! <|||||>> Hi! Indeed, the BERT-base only has 12 layers. The `all_hidden_states` is 13-dimensional however because it keeps track of the inputs as well.
>
> In the code you have shown, the `hidden_states` variable is computed between the two underlined variables you mentioned. None of it is redundant :)!
Thanks, I see :)<|||||>This issue has been automatically marked as stale because it has not had recent activity. It will be closed if no further activity occurs. Thank you for your contributions.
|
transformers | 1,223 | closed | [RuntimeError: sizes must be non-negative] : XLnet, Large and Base | ## 🐛 Bug
<!-- Important information -->
Model I am using (Bert, XLNet....): XLNet
Language I am using the model on (English, Chinese....): English
The problem arise when using:
* [ ] the official example scripts: (give details)
* [x] my own modified scripts: (give details)
The tasks I am working on is:
* [ ] an official GLUE/SQUaD task: (give the name)
* [x] my own task or dataset: (give details)
## To Reproduce
Steps to reproduce the behavior:
1.
2.
3.
<!-- If you have a code sample, error messages, stack traces, please provide it here as well. -->
## Expected behavior
<!-- A clear and concise description of what you expected to happen. -->
## Environment
* OS: Ubuntu
* Python version: 3.5.2
* PyTorch version: 1.20, torch 0.4.1
* PyTorch Transformers version (or branch): latest
* Using GPU ? YES
* Distributed of parallel setup ? No
* Any other relevant information:
## Additional context
The issue reads almost exactly like this - https://github.com/huggingface/pytorch-transformers/issues/924
Except - the problem still appears to persist as I have pytorch 1.2 installed./
To be sure I have to use CODA 9.0 - not sure if that is causing the issue. Note - I can run - XLM, Bert and Roberta in the exact same data and code -jut swapping out the modelname .
<!-- Add any other context about the problem here. --> | 09-08-2019 06:16:46 | 09-08-2019 06:16:46 | Can you post a simple example showing the behavior and a detailed error message?<|||||>-----------------------------------------------------------------------
RuntimeError Traceback (most recent call last)
<ipython-input-1-e5cfbf5c4eca> in <module>
127 train_df.to_csv('data/train.tsv', sep='\t', index=False, header=False)
128 dev_df.to_csv('data/dev.tsv', sep='\t', index=False, header=False)
--> 129 results= run_model(args,device)
130 cv_results.append(results[0])
131 r = pa.DataFrame(cv_results)
~/xlm/run_model.py in run_model(args, device)
65 train_dataset = load_and_cache_examples(task, tokenizer,args,processor,logger,False, undersample_scale_factor=1)
66 #stop
---> 67 global_step, tr_loss = train(train_dataset, model, tokenizer,args,logger,device)
68 logger.info(" global_step = %s, average loss = %s", global_step, tr_loss)
69
~/xlm/train.py in train(train_dataset, model, tokenizer, args, logger, device)
64 'token_type_ids': batch[2] if args['model_type'] in ['bert', 'xlnet'] else None, # XLM don't use segment_ids
65 'labels': batch[3]}
---> 66 outputs = model(**inputs)
67 loss = outputs[0] # model outputs are always tuple in pytorch-transformers (see doc)
68 print("\r%f" % loss, end='')
~/.local/lib/python3.5/site-packages/torch/nn/modules/module.py in __call__(self, *input, **kwargs)
475 result = self._slow_forward(*input, **kwargs)
476 else:
--> 477 result = self.forward(*input, **kwargs)
478 for hook in self._forward_hooks.values():
479 hook_result = hook(self, input, result)
~/.local/lib/python3.5/site-packages/pytorch_transformers/modeling_xlnet.py in forward(self, input_ids, token_type_ids, input_mask, attention_mask, mems, perm_mask, target_mapping, labels, head_mask)
1120 input_mask=input_mask, attention_mask=attention_mask,
1121 mems=mems, perm_mask=perm_mask, target_mapping=target_mapping,
-> 1122 head_mask=head_mask)
1123 output = transformer_outputs[0]
1124
~/.local/lib/python3.5/site-packages/torch/nn/modules/module.py in __call__(self, *input, **kwargs)
475 result = self._slow_forward(*input, **kwargs)
476 else:
--> 477 result = self.forward(*input, **kwargs)
478 for hook in self._forward_hooks.values():
479 hook_result = hook(self, input, result)
~/.local/lib/python3.5/site-packages/pytorch_transformers/modeling_xlnet.py in forward(self, input_ids, token_type_ids, input_mask, attention_mask, mems, perm_mask, target_mapping, head_mask)
883 if data_mask is not None:
884 # all mems can be attended to
--> 885 mems_mask = torch.zeros([data_mask.shape[0], mlen, bsz]).to(data_mask)
886 data_mask = torch.cat([mems_mask, data_mask], dim=1)
887 if attn_mask is None:
RuntimeError: sizes must be non-negative<|||||>Works perfectly with xlm, bert and roberta<|||||>Ok, this should be fixed on master with 45de034.
You can test it either by installing from source or using `torch.hub` and tell us if it doesn't work. |
transformers | 1,222 | closed | Citing DistilBERT | Currently, my understanding is citing the repo/codebase should be done by via a link (i.e. in the paper as a footnote) as there is no citation (i.e. in BibTeX style) yet.
For citing DistilBERT (the released model and distillation approach), how should this be done? | 09-08-2019 05:07:49 | 09-08-2019 05:07:49 | Hello @rishibommasani
Thank you for your question.
For citing DistilBERT, you're right, there is no formal write-up like an arXiv paper yet (it's definitely in our TODO stack). For the moment, I would recommend citing the blogpost as an URL.
Victor |
transformers | 1,221 | closed | Hi there, is bert-large-uncased-whole-word-masking-finetuned-squad trained for Squad 1.0 or 2.0? | I mean, whether the training data contains examples with no answer? | 09-07-2019 23:47:24 | 09-07-2019 23:47:24 | Hi! I believe this checkpoint originates from the training specified [there](https://huggingface.co/pytorch-transformers/examples.html#squad).
The SQuAD version would then be 1.1!<|||||>This issue has been automatically marked as stale because it has not had recent activity. It will be closed if no further activity occurs. Thank you for your contributions.
|
transformers | 1,220 | closed | RuntimeError: Gather got an input of invalid size: got [2, 3, 12, 256, 64], but expected [2, 4, 12, 256, 64] (gather at /opt/conda/conda-bld/pytorch_1544199946412/work/torch/csrc/cuda/comm.cpp:227) | ## ❓ Questions & Help
Hi,
I am running a modified version of ```run_lm_finetuning.py```, it was working fine and model checkpoints have been saved, until the last step of the first epoch (9677/9678), where I got this error:
```
Traceback (most recent call last):████████████████████████████████████████████████████████████████████████████████████████████████████████████████████████████████████████████████████████████████████████████████████████████████████████▉| 9677/9678 [2:01:24<00:00, 1.36it/s]
File "my_run_lm_finetuning.py", line 588, in <module>
main()
File "my_run_lm_finetuning.py", line 542, in main
global_step, tr_loss = train(args, train_dataset, model, bert_model_fintuned, tokenizer, bert_tokenizer)
File "my_run_lm_finetuning.py", line 260, in train
outputs = model(inputs, masked_lm_labels=labels) if args.mlm else model(inputs, enc_output, labels=labels)
File "/home/anaconda3/envs/py36/lib/python3.6/site-packages/torch/nn/modules/module.py", line 489, in __call__
result = self.forward(*input, **kwargs)
File "/home/anaconda3/envs/py36/lib/python3.6/site-packages/torch/nn/parallel/data_parallel.py", line 144, in forward
return self.gather(outputs, self.output_device)
File "/home/anaconda3/envs/py36/lib/python3.6/site-packages/torch/nn/parallel/data_parallel.py", line 156, in gather
return gather(outputs, output_device, dim=self.dim)
File "/home/anaconda3/envs/py36/lib/python3.6/site-packages/torch/nn/parallel/scatter_gather.py", line 67, in gather
return gather_map(outputs)
File "/home/anaconda3/envs/py36/lib/python3.6/site-packages/torch/nn/parallel/scatter_gather.py", line 62, in gather_map
return type(out)(map(gather_map, zip(*outputs)))
File "/home/anaconda3/envs/py36/lib/python3.6/site-packages/torch/nn/parallel/scatter_gather.py", line 62, in gather_map
return type(out)(map(gather_map, zip(*outputs)))
File "/home/anaconda3/envs/py36/lib/python3.6/site-packages/torch/nn/parallel/scatter_gather.py", line 54, in gather_map
return Gather.apply(target_device, dim, *outputs)
File "/home/anaconda3/envs/py36/lib/python3.6/site-packages/torch/nn/parallel/_functions.py", line 68, in forward
return comm.gather(inputs, ctx.dim, ctx.target_device)
File "/home/anaconda3/envs/py36/lib/python3.6/site-packages/torch/cuda/comm.py", line 166, in gather
return torch._C._gather(tensors, dim, destination)
RuntimeError: Gather got an input of invalid size: got [2, 3, 12, 256, 64], but expected [2, 4, 12, 256, 64] (gather at /opt/conda/conda-bld/pytorch_1544199946412/work/torch/csrc/cuda/comm.cpp:227)
frame #0: c10::Error::Error(c10::SourceLocation, std::string const&) + 0x45 (0x7f3c52b7fcc5 in /home/anaconda3/envs/py36/lib/python3.6/site-packages/torch/lib/libc10.so)
frame #1: torch::cuda::gather(c10::ArrayRef<at::Tensor>, long, c10::optional<int>) + 0x4d8 (0x7f3c936eaba8 in /home/anaconda3/envs/py36/lib/python3.6/site-packages/torch/lib/libtorch_python.so)
frame #2: <unknown function> + 0x4f99de (0x7f3c936ed9de in /home/anaconda3/envs/py36/lib/python3.6/site-packages/torch/lib/libtorch_python.so)
frame #3: <unknown function> + 0x111e36 (0x7f3c93305e36 in /home/anaconda3/envs/py36/lib/python3.6/site-packages/torch/lib/libtorch_python.so)
<omitting python frames>
frame #14: THPFunction_apply(_object*, _object*) + 0x5dd (0x7f3c9350140d in /home/anaconda3/envs/py36/lib/python3.6/site-packages/torch/lib/libtorch_python.so)
```
Note that in this experiment I used a fine-tuned version of Bert (I fine-tuned it using your previous script in lm_finetune folder) and there I have the ```max_seq_length =256```, however when running this (```run_lm_finetuning.py```) , I have ```block_size=128```.
Any idea of what is the error for? | 09-07-2019 18:48:26 | 09-07-2019 18:48:26 | This is a wild guess since I don't have access to your modified version, but I feel like this has to do with a mismatch in the batch size (expecting a batch size of 4 but receiving a batch size of 3).
Could you check your input tensor and label tensor sizes and get back to me so I can try and reproduce it on my end?<|||||>@LysandreJik I saved them inputs and reload it. It is of size [7, 256].
The thing is I don't know why the error is having a size which is 5 dimensional rather than 3 or even in the attention split, the size should be of dimension 4 [batchsize, sequence_length, head, head_feature]
Also, how should I know where the error exactly come from? like which line of code in the modeling scripts cause this.<|||||>I tried to save the specific batch of inputs before the program gives this error and terminate. Out of the program, I used load the inputs and pass it to the line of code that cause the error, and this doesn't give me any error. However, when trying to train the model inside the script this throws error.
I guess it might have to do sth with parallel/distributed training<|||||>Was a solution to this issue found? I'm receiving the same error. It works with batch size = 1 but if I can use a larger batch size I'd like to. <|||||>@isabelcachola for some dataset it works and for some it gives this error. I am getting the same error again now for the last step of the first batch. yours' the same?
The problem is due to parallel and distributed/ multi gpu training I guess.
I have two gpus but when I run, only one of my gpus get occupied.
Any thought on that?<|||||>@isabelcachola one thing that I tried which seems to be working and didn't throw error is to set args.n_gpu= 1, then it would do distributed training.
but not sure if this is a right way of getting around the issue.<|||||>@isabelcachola this script doesn't save the best model,it saves the last one, right? <|||||>@ehsan-soe I fixed the problem by truncating incomplete batches. So if there are 2001 examples and my batch size = 2, then I truncate the last example and train on the first 2000. This has fixed it for me both with and without distributed. My load_and_cache function now looks like this
```
def load_and_cache_examples(args, tokenizer, evaluate=False, fpath=None):
if fpath:
dataset = TextDataset(tokenizer, args, fpath)
else:
dataset = TextDataset(tokenizer, args, args.eval_data_path if evaluate else args.train_data_path)
# Ignore incomplete batches
# If you don't do this, you'll get an error at the end of training
n = len(dataset) % args.per_gpu_train_batch_size
if n != 0:
dataset.examples = dataset.examples[:-n]
return dataset
```<|||||>This issue has been automatically marked as stale because it has not had recent activity. It will be closed if no further activity occurs. Thank you for your contributions.
<|||||>I am having this same issue trying to train a GPT2LmHead model on 4 Tesla V100s<|||||>@zbloss Look at my [answer above](https://github.com/huggingface/transformers/issues/1220#issuecomment-557237248) and see if that solves your issue <|||||>This issue has been automatically marked as stale because it has not had recent activity. It will be closed if no further activity occurs. Thank you for your contributions.
<|||||>"dataloader_drop_last = True " may help?
You can refer to this [pr](https://github.com/huggingface/transformers/pull/4757#issuecomment-638970242)<|||||>I think this can solve it.
Duplicate of #https://github.com/huggingface/transformers/issues/1220#issuecomment-557237248
Also, you can set the parameter `drop_last` in your DataLoader like this:
`tain_text = DataLoader(train_dataset, batch_size=args.batch_size, shuffle=True, drop_last=True)`
<|||||>I am facing the same issue while using gpt2-medium.
The train text dataset constructed like below,
from transformers import TextDataset
train_dataset = TextDataset(
tokenizer=gpt2_tokenizer,
file_path=train_path,
block_size=128)
@ChaooMa Can you please tel how to use 'drop_last' parameter here?
<|||||>Has this problem been solved? I have the same problem.
> I am facing the same issue while using gpt2-medium.
>
> The train text dataset constructed like below,
>
> from transformers import TextDataset
>
> train_dataset = TextDataset( tokenizer=gpt2_tokenizer, file_path=train_path, block_size=128)
>
> @ChaooMa Can you please tel how to use 'drop_last' parameter here?
<|||||>Same
> Has this problem been solved? I have the same problem.
>
> > I am facing the same issue while using gpt2-medium.
> > The train text dataset constructed like below,
> > from transformers import TextDataset
> > train_dataset = TextDataset( tokenizer=gpt2_tokenizer, file_path=train_path, block_size=128)
> > @ChaooMa Can you please tel how to use 'drop_last' parameter here?
|
transformers | 1,219 | closed | fix tokenize(): potential bug of splitting pretrained tokens with newly added tokens | In the tokenizer base class, `split_on_token()` attempts to split input text by each of the added tokens. Because it uses `text.split(tok)`, it may accidentally split a token in the pretrained vocabulary at the middle.
For example a new token "ht" is added to the vocabulary. Then "light" will be split into `["lig", ""]`. But as "light" is a token in the pretrained vocabulary, it probably should be left intact to be processed by `self._tokenize()`.
Hence in this pull request, `text.split()` is replaced with `re.split()`, which will split only at word boundaries (`[^A-Za-z0-9_]` in regular expression). This behavior can be enabled by specifying a new `tokenize()` argument: `additional_tokens_as_full_words_only=True` (default: False). If it's specified in `tokenizer.encode(text, ...)`, it will still take effect, as this argument will be passed down to `tokenize()`.
On languages that have no or different word boundaries as above (such as Chinese or Japanese), this behavior may produce undesirable results, and the user can revert to the old `text.split()` by not specifying `additional_tokens_as_full_words_only` (it will take the default value `False`).
An explanation of the argument `additional_tokens_as_full_words_only` has been added to the docstring of `tokenize()`. A test function `test_add_partial_tokens_tokenizer()` has been added to `tokenization_bert_test.py`.
| 09-07-2019 08:29:28 | 09-07-2019 08:29:28 | # [Codecov](https://codecov.io/gh/huggingface/transformers/pull/1219?src=pr&el=h1) Report
> Merging [#1219](https://codecov.io/gh/huggingface/transformers/pull/1219?src=pr&el=desc) into [master](https://codecov.io/gh/huggingface/transformers/commit/80faf22b4ac194061a08fde09ad8b202118c151e?src=pr&el=desc) will **increase** coverage by `7.67%`.
> The diff coverage is `100%`.
[](https://codecov.io/gh/huggingface/transformers/pull/1219?src=pr&el=tree)
```diff
@@ Coverage Diff @@
## master #1219 +/- ##
==========================================
+ Coverage 73.24% 80.91% +7.67%
==========================================
Files 87 46 -41
Lines 14989 7903 -7086
==========================================
- Hits 10979 6395 -4584
+ Misses 4010 1508 -2502
```
| [Impacted Files](https://codecov.io/gh/huggingface/transformers/pull/1219?src=pr&el=tree) | Coverage Δ | |
|---|---|---|
| [...torch\_transformers/tests/tokenization\_bert\_test.py](https://codecov.io/gh/huggingface/transformers/pull/1219/diff?src=pr&el=tree#diff-cHl0b3JjaF90cmFuc2Zvcm1lcnMvdGVzdHMvdG9rZW5pemF0aW9uX2JlcnRfdGVzdC5weQ==) | `98.98% <100%> (ø)` | |
| [pytorch\_transformers/tokenization\_utils.py](https://codecov.io/gh/huggingface/transformers/pull/1219/diff?src=pr&el=tree#diff-cHl0b3JjaF90cmFuc2Zvcm1lcnMvdG9rZW5pemF0aW9uX3V0aWxzLnB5) | `80.62% <100%> (ø)` | |
| [src/transformers/tokenization\_t5.py](https://codecov.io/gh/huggingface/transformers/pull/1219/diff?src=pr&el=tree#diff-c3JjL3RyYW5zZm9ybWVycy90b2tlbml6YXRpb25fdDUucHk=) | | |
| [src/transformers/tokenization\_ctrl.py](https://codecov.io/gh/huggingface/transformers/pull/1219/diff?src=pr&el=tree#diff-c3JjL3RyYW5zZm9ybWVycy90b2tlbml6YXRpb25fY3RybC5weQ==) | | |
| [src/transformers/configuration\_roberta.py](https://codecov.io/gh/huggingface/transformers/pull/1219/diff?src=pr&el=tree#diff-c3JjL3RyYW5zZm9ybWVycy9jb25maWd1cmF0aW9uX3JvYmVydGEucHk=) | | |
| [src/transformers/tokenization\_distilbert.py](https://codecov.io/gh/huggingface/transformers/pull/1219/diff?src=pr&el=tree#diff-c3JjL3RyYW5zZm9ybWVycy90b2tlbml6YXRpb25fZGlzdGlsYmVydC5weQ==) | | |
| [src/transformers/modeling\_tf\_auto.py](https://codecov.io/gh/huggingface/transformers/pull/1219/diff?src=pr&el=tree#diff-c3JjL3RyYW5zZm9ybWVycy9tb2RlbGluZ190Zl9hdXRvLnB5) | | |
| [src/transformers/configuration\_distilbert.py](https://codecov.io/gh/huggingface/transformers/pull/1219/diff?src=pr&el=tree#diff-c3JjL3RyYW5zZm9ybWVycy9jb25maWd1cmF0aW9uX2Rpc3RpbGJlcnQucHk=) | | |
| [src/transformers/configuration\_ctrl.py](https://codecov.io/gh/huggingface/transformers/pull/1219/diff?src=pr&el=tree#diff-c3JjL3RyYW5zZm9ybWVycy9jb25maWd1cmF0aW9uX2N0cmwucHk=) | | |
| [src/transformers/modeling\_roberta.py](https://codecov.io/gh/huggingface/transformers/pull/1219/diff?src=pr&el=tree#diff-c3JjL3RyYW5zZm9ybWVycy9tb2RlbGluZ19yb2JlcnRhLnB5) | | |
| ... and [125 more](https://codecov.io/gh/huggingface/transformers/pull/1219/diff?src=pr&el=tree-more) | |
------
[Continue to review full report at Codecov](https://codecov.io/gh/huggingface/transformers/pull/1219?src=pr&el=continue).
> **Legend** - [Click here to learn more](https://docs.codecov.io/docs/codecov-delta)
> `Δ = absolute <relative> (impact)`, `ø = not affected`, `? = missing data`
> Powered by [Codecov](https://codecov.io/gh/huggingface/transformers/pull/1219?src=pr&el=footer). Last update [80faf22...d97a223](https://codecov.io/gh/huggingface/transformers/pull/1219?src=pr&el=lastupdated). Read the [comment docs](https://docs.codecov.io/docs/pull-request-comments).
<|||||>Great, thanks @askerlee <|||||>This issue has been automatically marked as stale because it has not had recent activity. It will be closed if no further activity occurs. Thank you for your contributions.
<|||||>Oh so it won't be merged? Anyway it's just a small issue @thomwolf<|||||>Thanks for the heads up. I forgot to follow up on this one.
It's good to merge indeed (rebased on mater).
cc @LysandreJik <|||||>Hum actually this seems to break a number of tokenization tests.
Do you want to give it a look @askerlee?<|||||>@thomwolf sure. will fix it ASAP.
<|||||>cc @LysandreJik who is working on fixing #2096 and following #2101 which are both related to this PR.<|||||>Hey @askerlee, thanks for your pull request. I'm currently working on it and adapting it to all models, I've updated the tests so that they fit the current master so don't worry about it.<|||||>@LysandreJik thank you so much! Sorry have been through very busy days. Do I need to do anything now? <|||||>This issue has been automatically marked as stale because it has not had recent activity. It will be closed if no further activity occurs. Thank you for your contributions.
|
transformers | 1,218 | closed | How to set the weight decay in other layers after BERT output? | ## ❓ Questions & Help
<!-- A clear and concise description of the question. -->
I notice that we should set weight decay of bias and LayerNorm.weight to zero and set weight decay of other parameter in BERT to 0.01. But how to set the weight decay of other layer such as the classifier after BERT? Thanks | 09-07-2019 00:04:41 | 09-07-2019 00:04:41 | @RoderickGu. maybe try:
```python
bert_param_optimizer = list(model.bert.named_parameters())
lstm_param_optimizer = list(model.bilstm.named_parameters())
crf_param_optimizer = list(model.crf.named_parameters())
linear_param_optimizer = list(model.classifier.named_parameters())
no_decay = ['bias', 'LayerNorm.weight']
optimizer_grouped_parameters = [
{'params': [p for n, p in bert_param_optimizer if not any(nd in n for nd in no_decay)], 'weight_decay': 0.01,
'lr': args.learning_rate},
{'params': [p for n, p in bert_param_optimizer if any(nd in n for nd in no_decay)], 'weight_decay': 0.0,
'lr': args.learning_rate},
{'params': [p for n, p in lstm_param_optimizer if not any(nd in n for nd in no_decay)], 'weight_decay': 0.01,
'lr': 0.001},
{'params': [p for n, p in lstm_param_optimizer if any(nd in n for nd in no_decay)], 'weight_decay': 0.0,
'lr': 0.001},
{'params': [p for n, p in crf_param_optimizer if not any(nd in n for nd in no_decay)], 'weight_decay': 0.01,
'lr': 0.001},
{'params': [p for n, p in crf_param_optimizer if any(nd in n for nd in no_decay)], 'weight_decay': 0.0,
'lr': 0.001},
{'params': [p for n, p in linear_param_optimizer if not any(nd in n for nd in no_decay)], 'weight_decay': 0.01,
'lr': 0.001},
{'params': [p for n, p in linear_param_optimizer if any(nd in n for nd in no_decay)], 'weight_decay': 0.0,
'lr': 0.001}
]
```<|||||>@lonePatient Thanks for your answer! Do you mean that if there is a linear layer after the BERT, the weights of the linear layer will get a weight decay, but the bias of the linear layer will not? Besides, I wonder since your code covers each part in the model, if your answer is equivalent to:
`param_optimizer = list(model.named_parameters())
optimizer_grouped_parameters = [
{'params': [p for n, p in param_optimizer if not any(nd in n for nd in no_decay)], 'weight_decay': 0.01, 'lr': args.learning_rate},
{'params': [p for n, p in param_optimizer if any(nd in n for nd in no_decay)], 'weight_decay': 0.0, 'lr': args.learning_rate}
]`<|||||>This issue has been automatically marked as stale because it has not had recent activity. It will be closed if no further activity occurs. Thank you for your contributions.
|
transformers | 1,217 | closed | Fixing head masking test | Try to fix the Nan in head masking tests by removing them. | 09-06-2019 21:28:21 | 09-06-2019 21:28:21 | # [Codecov](https://codecov.io/gh/huggingface/pytorch-transformers/pull/1217?src=pr&el=h1) Report
> Merging [#1217](https://codecov.io/gh/huggingface/pytorch-transformers/pull/1217?src=pr&el=desc) into [master](https://codecov.io/gh/huggingface/pytorch-transformers/commit/ee027c89f2b8fd0338df39b7e0b48345ea132e99?src=pr&el=desc) will **increase** coverage by `0.38%`.
> The diff coverage is `100%`.
[](https://codecov.io/gh/huggingface/pytorch-transformers/pull/1217?src=pr&el=tree)
```diff
@@ Coverage Diff @@
## master #1217 +/- ##
=========================================
+ Coverage 80.92% 81.3% +0.38%
=========================================
Files 57 57
Lines 8014 8018 +4
=========================================
+ Hits 6485 6519 +34
+ Misses 1529 1499 -30
```
| [Impacted Files](https://codecov.io/gh/huggingface/pytorch-transformers/pull/1217?src=pr&el=tree) | Coverage Δ | |
|---|---|---|
| [pytorch\_transformers/tests/modeling\_common\_test.py](https://codecov.io/gh/huggingface/pytorch-transformers/pull/1217/diff?src=pr&el=tree#diff-cHl0b3JjaF90cmFuc2Zvcm1lcnMvdGVzdHMvbW9kZWxpbmdfY29tbW9uX3Rlc3QucHk=) | `73.37% <100%> (+0.17%)` | :arrow_up: |
| [pytorch\_transformers/tokenization\_transfo\_xl.py](https://codecov.io/gh/huggingface/pytorch-transformers/pull/1217/diff?src=pr&el=tree#diff-cHl0b3JjaF90cmFuc2Zvcm1lcnMvdG9rZW5pemF0aW9uX3RyYW5zZm9feGwucHk=) | `34.17% <0%> (+0.18%)` | :arrow_up: |
| [pytorch\_transformers/tokenization\_gpt2.py](https://codecov.io/gh/huggingface/pytorch-transformers/pull/1217/diff?src=pr&el=tree#diff-cHl0b3JjaF90cmFuc2Zvcm1lcnMvdG9rZW5pemF0aW9uX2dwdDIucHk=) | `96.69% <0%> (+0.82%)` | :arrow_up: |
| [pytorch\_transformers/modeling\_utils.py](https://codecov.io/gh/huggingface/pytorch-transformers/pull/1217/diff?src=pr&el=tree#diff-cHl0b3JjaF90cmFuc2Zvcm1lcnMvbW9kZWxpbmdfdXRpbHMucHk=) | `90.27% <0%> (+1.51%)` | :arrow_up: |
| [...orch\_transformers/tests/tokenization\_utils\_test.py](https://codecov.io/gh/huggingface/pytorch-transformers/pull/1217/diff?src=pr&el=tree#diff-cHl0b3JjaF90cmFuc2Zvcm1lcnMvdGVzdHMvdG9rZW5pemF0aW9uX3V0aWxzX3Rlc3QucHk=) | `96% <0%> (+4%)` | :arrow_up: |
| [pytorch\_transformers/file\_utils.py](https://codecov.io/gh/huggingface/pytorch-transformers/pull/1217/diff?src=pr&el=tree#diff-cHl0b3JjaF90cmFuc2Zvcm1lcnMvZmlsZV91dGlscy5weQ==) | `71.51% <0%> (+4.84%)` | :arrow_up: |
| [...h\_transformers/tests/tokenization\_tests\_commons.py](https://codecov.io/gh/huggingface/pytorch-transformers/pull/1217/diff?src=pr&el=tree#diff-cHl0b3JjaF90cmFuc2Zvcm1lcnMvdGVzdHMvdG9rZW5pemF0aW9uX3Rlc3RzX2NvbW1vbnMucHk=) | `100% <0%> (+5.98%)` | :arrow_up: |
| [pytorch\_transformers/tokenization\_xlnet.py](https://codecov.io/gh/huggingface/pytorch-transformers/pull/1217/diff?src=pr&el=tree#diff-cHl0b3JjaF90cmFuc2Zvcm1lcnMvdG9rZW5pemF0aW9uX3hsbmV0LnB5) | `89.18% <0%> (+7.2%)` | :arrow_up: |
------
[Continue to review full report at Codecov](https://codecov.io/gh/huggingface/pytorch-transformers/pull/1217?src=pr&el=continue).
> **Legend** - [Click here to learn more](https://docs.codecov.io/docs/codecov-delta)
> `Δ = absolute <relative> (impact)`, `ø = not affected`, `? = missing data`
> Powered by [Codecov](https://codecov.io/gh/huggingface/pytorch-transformers/pull/1217?src=pr&el=footer). Last update [ee027c8...01b9255](https://codecov.io/gh/huggingface/pytorch-transformers/pull/1217?src=pr&el=lastupdated). Read the [comment docs](https://docs.codecov.io/docs/pull-request-comments).
<|||||>Superseded by #1203 |
transformers | 1,216 | closed | Is there any sample code for fine-tuning BERT on sequence labeling tasks, e.g., NER on CoNLL-2003? | ## ❓ Questions & Help
Is there any sample code for fine-tuning BERT on sequence labeling tasks, e.g., NER on CoNLL-2003, using BertForTokenClassification?
<!-- A clear and concise description of the question. --> | 09-06-2019 18:38:07 | 09-06-2019 18:38:07 | Hi @tuvuumass,
Issue https://github.com/huggingface/pytorch-transformers/issues/64 is a good start for sequence labeling tasks. It also points to some repositories that show how to fine-tune BERT with PyTorch-Transformers (with focus on NER).
Nevertheless, it would be awesome to get some kind of fine-tuning examples (*reference implementation*) integrated into this outstanding PyTorch-Transformers library 🤗 Maybe `run_glue.py` could be a good start 🤔<|||||>Thanks, @stefan-it. I found #64 too. But it seems like none of the repositories in #64 could replicate BERT's results (i.e., 96.6 dev F1 and 92.8 test F1 for BERT large, 96.4 dev F1 and 92.4 test F1 for BERT base). Yes, I agree that it would be great if there is a fine-tuning example for sequence labeling tasks.<|||||>Yes I think it would be nice to have a clean example showing how the model can be trained and used on a token classification task like NER.
We won’t have the bandwidth/use-case to do that internally but if someone in the community has a (preferably self contained) script he can share, happy to welcome a PR and include it in the repo.
Maybe you have something Stefan?<|||||>Update on that:
I used the data preprocessing functions and `forward` implementation from @kamalkraj's [BERT-NER](https://github.com/kamalkraj/BERT-NER) ported it from `pytorch-pretrained-bert` to `pytorch-transformers`, and integrated it into a `run_glue` copy 😅
Fine-tuning is working - evaluation on dev set (using a BERT base and cased model):
```bash
precision recall f1-score support
PER 0.9713 0.9745 0.9729 1842
MISC 0.8993 0.9197 0.9094 922
LOC 0.9769 0.9679 0.9724 1837
ORG 0.9218 0.9403 0.9310 1341
micro avg 0.9503 0.9562 0.9533 5942
macro avg 0.9507 0.9562 0.9534 5942
```
Evaluation on test set:
```bash
09/09/2019 23:20:02 - INFO - __main__ -
precision recall f1-score support
LOC 0.9309 0.9287 0.9298 1668
MISC 0.7937 0.8276 0.8103 702
PER 0.9614 0.9549 0.9581 1617
ORG 0.8806 0.9145 0.8972 1661
micro avg 0.9066 0.9194 0.9130 5648
macro avg 0.9078 0.9194 0.9135 5648
```
Trained for 5 epochs using the default parameters from `run_glue`. Each epoch took ~5 minutes on a RTX 2080 TI.
However, it's an early implementation and maybe (with a little help from @kamalkraj) we can integrate it here 🤗<|||||>@stefan-it could you pls share your fork? thanks :)<|||||>@olix20 Here's the first draft of an implementation:
https://gist.github.com/stefan-it/feb6c35bde049b2c19d8dda06fa0a465
(Just a gist at the moment) :)<|||||>After working with [BERT-NER](https://github.com/kamalkraj/BERT-NER) for a few days now, I tried to come up with a script that could be integrated here.
Compared to that repo and @stefan-it's gist, I tried to do the following:
* Use the default BertForTokenClassification class instead modifying the forward pass in a subclass. For that to work, I changed the way label ids are stored: I use the real label ids for the first sub-token of each word and padding ids for the remaining sub-tokens. Padding ids get ignored in the cross entropy loss function, instead of picking only the desired tokens in a for loop before feeding them to the loss computation.
* Log metrics to tensorboard.
* Remove unnecessary parts copied over from glue (e.g. DataProcessor class).<|||||>BERT-NER using tensorflow 2.0
https://github.com/kamalkraj/BERT-NER-TF<|||||>This issue has been automatically marked as stale because it has not had recent activity. It will be closed if no further activity occurs. Thank you for your contributions.
<|||||>Similar, can we use conll type/format data to fine tune BERT for relation extraction..!!? |
transformers | 1,215 | closed | Cut off sequences of length greater than max_length= 512 for roberta | ## 🚀 Feature
<!-- A clear and concise description of the feature proposal. Please provide a link to the paper and code in case they exist. -->
Roberta uses max_length of 512 but text to tokenize is variable length. Is there an option to cut off source text to maximum length during tokenization process?
## Motivation
Most text are not fixed sizes but are still required for end goal of using roberta on new datasets. The roberta model will throw an error when it encounters a text size > 512. Any help is appreciated to allow tokenization and limit the size to maximum length for pretrained encoders. Alternative is to let the pretrained encoder adapt by increasing positional embedding size so an error is not thrown or use average pooling. I m not sure how this would be implemented and allow fine tuning.
<!-- Please outline the motivation for the proposal. Is your feature request related to a problem? e.g., I'm always frustrated when [...]. If this is related to another GitHub issue, please link here too. -->
I can't seem to figure out how to circumvent the text length problem as new data text length can go as large as 2500 or more but roberta is only 512 max.
## Additional context
<!-- Add any other context or screenshots about the feature request here. --> | 09-06-2019 16:41:04 | 09-06-2019 16:41:04 | Hi! Indeed RoBERTa has a max length of 512. Why don't you slice your text?<|||||>I was hoping the tokenizer could take care of it as a functionality? The actual hope is not to throw an error but allow training with it by increasing the positional encoding as a way to allow training on the whole text length. Is this possible?<|||||>This issue has been automatically marked as stale because it has not had recent activity. It will be closed if no further activity occurs. Thank you for your contributions.
|
transformers | 1,214 | closed | Better examples | Refactored the examples section: removed old and not up-to-date examples and added new examples for fine-tuning and generation.
The `examples` file is not in the `/doc/source` folder anymore but in the `/examples` folder. It is therefore visible when users open the folder on GitHub.
Note: In order to generate the current documentation, a symlink has to be done between the `examples/README.md` file to a `docs/source/examples.md`. The corresponding documentation has been added to the documentation README, alongside the command necessary to create the symlink.
**The new examples are visible on: http://lysand.re/examples.html** | 09-06-2019 16:15:20 | 09-06-2019 16:15:20 | There were indeed quite a few artifacts. I fixed them in the two latest commits.<|||||># [Codecov](https://codecov.io/gh/huggingface/pytorch-transformers/pull/1214?src=pr&el=h1) Report
> Merging [#1214](https://codecov.io/gh/huggingface/pytorch-transformers/pull/1214?src=pr&el=desc) into [master](https://codecov.io/gh/huggingface/pytorch-transformers/commit/5ac8b62265efac24f0dbfab271d2bce534179993?src=pr&el=desc) will **not change** coverage.
> The diff coverage is `n/a`.
[](https://codecov.io/gh/huggingface/pytorch-transformers/pull/1214?src=pr&el=tree)
```diff
@@ Coverage Diff @@
## master #1214 +/- ##
=======================================
Coverage 81.29% 81.29%
=======================================
Files 57 57
Lines 8015 8015
=======================================
Hits 6516 6516
Misses 1499 1499
```
------
[Continue to review full report at Codecov](https://codecov.io/gh/huggingface/pytorch-transformers/pull/1214?src=pr&el=continue).
> **Legend** - [Click here to learn more](https://docs.codecov.io/docs/codecov-delta)
> `Δ = absolute <relative> (impact)`, `ø = not affected`, `? = missing data`
> Powered by [Codecov](https://codecov.io/gh/huggingface/pytorch-transformers/pull/1214?src=pr&el=footer). Last update [5ac8b62...3f91338](https://codecov.io/gh/huggingface/pytorch-transformers/pull/1214?src=pr&el=lastupdated). Read the [comment docs](https://docs.codecov.io/docs/pull-request-comments).
<|||||>Awesome! Merging |
transformers | 1,213 | closed | Fine-tuned RoBERTa models on CPU | ## ❓ Questions & Help
Because of using FusedLayerNorm as BertLayerNorm from apex library after finetuning a saved model isn't possible to use on CPU with apex and Cuda installed. What will be the easiest way to run finetuned models on a server without a GPU? I see that the easiest way to just use the python version of BertLayerNorm during training but maybe there is another way. | 09-06-2019 11:00:35 | 09-06-2019 11:00:35 | This issue has been automatically marked as stale because it has not had recent activity. It will be closed if no further activity occurs. Thank you for your contributions.
|
transformers | 1,212 | closed | LSTM returns nan after using the pretrained BERT embedding as input | Hello ,
i'm using the pretrained Bert model (from pytorch-transformers) to get the contextual embedding of a written text , i summed the last 4 hidden layers outputs (i red that the concatenation of the last four layers usually produce the best results )
than i use a LSTM layer with attention to get the paragraph level embedding from the word embedding produced by the Bert model
the output should be a score range [-1,1]
I tried the rmsprop , adam ... optimizers with MSEloss and always after just few batches iterations the lstm layer produces nan values .
any suggestions will be greatly appreciated | 09-06-2019 09:41:04 | 09-06-2019 09:41:04 | i found that the problem is related to the data
i will close this issue , or if anyone has the permission he could delete this :) |
transformers | 1,211 | closed | How to fine tune small dataset? | ## ❓ Questions & Help
<!-- A clear and concise description of the question. -->
Most people test bert on large dataset, but when it comes to small dataset, I assume the fine tune process and batch size maybe different. Besides, the dataset domain is twitter domain, which is kind of different from BERT pretrained corpus.
Could anyone gives some suggestions on finetuning BERT on small twitter dataset? Thanks in advance for any help. | 09-06-2019 08:12:17 | 09-06-2019 08:12:17 | Usually, you should train more epochs. Optimal batch size from 8 to 16. I'm not sure but maybe learning rate should be lower in this case. You can try<|||||>Thanks for your suggestion. I will try that. Besides, do you think I should modify the warmup step or just set it as 10% of total step just like in the original paper? <|||||>Influence of warmup steps is not clear for me. it looks like it's not so important for final quality but can speed up training a bit<|||||>@avostryakov Thanks for your help!<|||||>@avostryakov I wonder if I could ask another question. I know in BERT, weight decay of some layer is set to be 0 while others are set to be 0.01. So how to set the weight decay for other layer like the linear layer after bert output in finetuning? <|||||>First of all, I'm not sure that weight decay is really important. I tried 0.1 and 0.01 for RoBERTa without a difference in quality. But ok, you can look in run_glue.py for this code:
```
no_decay = ['bias', 'LayerNorm.weight']
optimizer_grouped_parameters = [
{'params': [p for n, p in model.named_parameters() if not any(nd in n for nd in no_decay)], 'weight_decay': args.weight_decay},
{'params': [p for n, p in model.named_parameters() if any(nd in n for nd in no_decay)], 'weight_decay': 0.0}
]
optimizer = AdamW(optimizer_grouped_parameters, lr=args.learning_rate, eps=args.adam_epsilon)```
As you can see you can set any decay weight for any parameter or layer, you need to know it's name.<|||||>@avostryakov Thanks very much, maybe the learning rate is more important than weight decay <|||||>This issue has been automatically marked as stale because it has not had recent activity. It will be closed if no further activity occurs. Thank you for your contributions.
<|||||>Hey @RoderickGu, Do you have any intuition now? If so could you share them with public? <|||||>@ereday First I think you should follow the most methods in BERT fine-tune process, such as use adamw. Besides, you could use a small batch size. Since dataset is small, I also suggested you to run it several times for the best learning rate. Hope this helps. |
transformers | 1,210 | closed | Finetuning distilbert-base-uncased | ## ❓ Questions & Help
When trying to finetune distilbert-base-uncased on my own dataset
I receive the following error message:
ERROR - pytorch_transformers.tokenization_utils - Model name 'distilbert-base-uncased' was not found in model name list (bert-base-uncased,bert-large-uncased, bert-base-cased, bert-large-cased, bert-base-multilingual-uncased, bert-base-multilingual-cased, bert-base-chinese, bert-base-german-cased, bert-large-uncased-whole-word-masking, bert-large-cased-whole-word-masking, bert-large-uncased-whole-word-masking-finetuned-squad, bert-large-cased-whole-word-masking-finetuned-squad, bert-base-cased-finetuned-mrpc).
How do I add distilbert-base-uncased to the model name list (I assume I will somehow have to modify PYTORCH-TRANSFORMERS_CACHE)
code used:
python simple_lm_finetuning.py \
--train_corpus dataset.txt \
--bert_model distilbert-base-uncased \
--do_lower_case \
--output_dir finetuned_lm/ \
--do_train | 09-06-2019 06:31:40 | 09-06-2019 06:31:40 | Hi! Yes we're in the process of adding DistilBERT to the examples. Until then, you can simply edit the script to add it.
Please note that the `simple_lm_finetuning` script is now deprecated in favor of `run_lm_finetuning`.<|||||>Hi there @aah39 , I came across the same issue in run_glue.py when I tried to fine tune distilbert_base_uncased. Later I found the fix was easy: just change the model_type to be distilbert when running the script (I saw run_lm_finetuning has this input parameter as well)
After this change, when running the script, it will load the cache from DISTILBERT_PRETRAINED_CONFIG_ARCHIVE_MAP instead of BERT_PRETRAINED_CONFIG_ARCHIVE_MAP<|||||>I think this is not an issue anymore since it's been fixed in the https://github.com/huggingface/transformers/commit/88368c2a16d26bc2d00dc28f79196c81373d3a71
|
transformers | 1,209 | closed | run_squad.py predictions | ## ❓ Can anyone explain how start_logit and end_logit value is been determined ? And how it can used to measure the reliability of the answer span
In some issues I saw that if start_logit and end_logit = -1 then it is concluded to be unanswerable question.
There are some cases where I get the
> "1": [
{
"text": "hover your mouse pointer",
"probability": 1.0,
"start_logit": -12.987695693969727,
"end_logit": -12.40383529663086
}
],
In this place, what these values of start_logit and end_logit actually mean ? Since logit is natural log of odds, what is considered here as odds ?
In some cases, if the number of nbest predictions is 1, then [here in utils_squad.py](https://github.com/huggingface/pytorch-transformers/blob/master/examples/utils_squad.py#L613-L614) the answer span is made `empty`, does it means that it is the right answer ?
if len(nbest)==1:
nbest.insert(0,
_NbestPrediction(text="empty", start_logit=0.0, end_logit=0.0))
But in the [same file](https://github.com/huggingface/pytorch-transformers/blob/master/examples/utils_squad.py#L618-L620), when there is no nbest predictions, again the answer span is made `empty`
if not nbest:
nbest.append(
_NbestPrediction(text="empty", start_logit=0.0, end_logit=0.0))
For two different situations why the same answer is been set ? | 09-06-2019 02:16:05 | 09-06-2019 02:16:05 | This issue has been automatically marked as stale because it has not had recent activity. It will be closed if no further activity occurs. Thank you for your contributions.
<|||||>The comments say this situation is when the answer is a single null , but I don't see any conditionals to filter for this. hmmm did you figure it out? |
transformers | 1,208 | closed | How to set the token_type_ids in XLNet correctly? | Hi, I am fine-tuning an XLNet, and I want to use type embedding to indicate different parts of a sequence. I am facing a difficulty that `“indices are selected in the vocabulary (unlike BERT which has a specific vocabulary for segment indices)”` which is the description of `token_type_ids` in the official document. Does that mean the type embedding and token embedding share the same vocabulary? In that case, how can I select the right indices for the types? If I use `0` and `1` for types, is there a collision between types and the special tokens (like `UNK`)?
Thanks in advance!
| 09-05-2019 14:52:03 | 09-05-2019 14:52:03 | Hello! We have an example using `token_type_ids` in our `run_glue` script. You can look at how we build the features in the [`utils_glue`, especially concerning the `segment_ids`](https://github.com/huggingface/pytorch-transformers/blob/master/examples/utils_glue.py#L456-L484) which are the `token_type_ids` that will be fed to the model.
If I recall correctly the XLNet model has `0` for the first sequence `token_type_ids`, `1` for the second sequence, and `2` for the last (cls) token.
<|||||>> Hello! We have an example using `token_type_ids` in our `run_glue` script. You can look at how we build the features in the [`utils_glue`, especially concerning the `segment_ids`](https://github.com/huggingface/pytorch-transformers/blob/master/examples/utils_glue.py#L456-L484) which are the `token_type_ids` that will be fed to the model.
>
> If I recall correctly the XLNet model has `0` for the first sequence `token_type_ids`, `1` for the second sequence, and `2` for the last (cls) token.
Thank you for your explanation!
I still have a question, the code in `utils_glue` is for BERT. As far as I know, the token embeddings and type embeddings are selected from two embedding matrices in BERT, therefore, the type index `0` won't give you a `PAD` token embedding. In XLNet, the type indices are selected in the vocabulary, in which `0` index represents `UNK` token and `1` index represents `BOS` token.
Do I misunderstand the meaning of `"indices are selected in the vocabulary"`, or we can freely use the `BOS` `EOP` `EOD` tokens for our type embeddings?
<|||||>Oh, that's a typo in XLNet docstring that I thought we had corrected already.
Thanks for reminding us of that.
The type indices in XLNet are not selected in the vocabulary, they can be arbitrary.
In XLNet segment ids (what we call `token_type_ids in the repo) don't correspond to embeddings, they are just numbers and the only important thing is that they have to be different for tokens which belong to different segments, hence the flexibility in the exact values (XLNet is using relative segment difference with just two segment embeddings: 0 if the segment id of two tokens are the same, 1 if not). See [here](https://github.com/huggingface/pytorch-transformers/blob/master/pytorch_transformers/modeling_xlnet.py#L926-L928). |
transformers | 1,207 | closed | convert_roberta_checkpoint_to_pytorch.py 514 max position? | ## ❓ Questions & Help
<!-- A clear and concise description of the question. -->
I m not sure if this is intentional but why is max position 514. I m assuming the origingal roberta model is 512 like bert or is this incorrect? This is the only place i find this reference as well. | 09-05-2019 13:13:03 | 09-05-2019 13:13:03 | This issue has been automatically marked as stale because it has not had recent activity. It will be closed if no further activity occurs. Thank you for your contributions.
|
transformers | 1,206 | closed | the best way to cut the upper layers | Salut,
what would be the best way to cut the upper layers of transformers (from 12 cutting 5 upper layer results 7 layers model for the use)?
Best regards | 09-05-2019 10:40:45 | 09-05-2019 10:40:45 | Hi, don't know which model you are using so I can't answer precisely but here is the general workflow:
1. load the relevant pretrained configuration with `config = config_class.from_pretrained('your-model-of-interest')`
2. Reduce the number of layers in the configuration with for example: `config.num_hidden_layers = 5` (here you have to check the correct attribute for your model).
3. Use the modified config to build and instantiate your model: `model = model_class.from_pretrained('your-model-of-interest', config=config)`.
Pretty easy, isn't it?<|||||>>>Pretty easy, isn't it?
indeed!
<|||||>```
config = XLNetConfig.from_pretrained('xlnet-base-cased')
config.num_hidden_layers = 3
```
raised this error
`AttributeError: can't set attribute`<|||||>config.n_layer = 3 does it work<|||||>> Hi, don't know which model you are using so I can't answer precisely but here is the general workflow:
>
> 1. load the relevant pretrained configuration with `config = config_class.from_pretrained('your-model-of-interest')`
>
> 2. Reduce the number of layers in the configuration with for example: `config.num_hidden_layers = 5` (here you have to check the correct attribute for your model).
>
> 3. Use the modified config to build and instantiate your model: `model = model_class.from_pretrained('your-model-of-interest', config=config)`.
>
>
> Pretty easy, isn't it?
I assume this gives the upper 5 layers. Is there a way to get the lower 5 layers ? <|||||>it's kind of annoying and non-intuitive, but @cherepanovic, the reason why you're seeing this message is that there are several parameters in many of the configs that are not parameters, but properties. I suppose the authors did this to emphasize that they should not be changed after the model is initialized? Not sure. But here they are:
```
@property
def max_position_embeddings(self):
return self.n_positions
@property
def hidden_size(self):
return self.n_embd
@property
def num_attention_heads(self):
return self.n_head
@property
def num_hidden_layers(self):
return self.n_layer
```
You can change these, after initialization, by referring to the actual parameters that the property returns.
IMO it certainly isn't "pretty easy", as doubly-named parameters/properties is kinda poor practice, and it would've been easy from a coding perspective to put getters and setters in there as well. |
transformers | 1,205 | closed | Fix typo | 09-05-2019 10:25:23 | 09-05-2019 10:25:23 | # [Codecov](https://codecov.io/gh/huggingface/pytorch-transformers/pull/1205?src=pr&el=h1) Report
> Merging [#1205](https://codecov.io/gh/huggingface/pytorch-transformers/pull/1205?src=pr&el=desc) into [master](https://codecov.io/gh/huggingface/pytorch-transformers/commit/0b52642d379bed155e8aa4f4088588bfd8ceaa88?src=pr&el=desc) will **increase** coverage by `0.44%`.
> The diff coverage is `95.65%`.
[](https://codecov.io/gh/huggingface/pytorch-transformers/pull/1205?src=pr&el=tree)
```diff
@@ Coverage Diff @@
## master #1205 +/- ##
==========================================
+ Coverage 80.83% 81.27% +0.44%
==========================================
Files 46 46
Lines 7878 7877 -1
==========================================
+ Hits 6368 6402 +34
+ Misses 1510 1475 -35
```
| [Impacted Files](https://codecov.io/gh/huggingface/pytorch-transformers/pull/1205?src=pr&el=tree) | Coverage Δ | |
|---|---|---|
| [...h\_transformers/tests/tokenization\_tests\_commons.py](https://codecov.io/gh/huggingface/pytorch-transformers/pull/1205/diff?src=pr&el=tree#diff-cHl0b3JjaF90cmFuc2Zvcm1lcnMvdGVzdHMvdG9rZW5pemF0aW9uX3Rlc3RzX2NvbW1vbnMucHk=) | `100% <100%> (ø)` | :arrow_up: |
| [pytorch\_transformers/tokenization\_utils.py](https://codecov.io/gh/huggingface/pytorch-transformers/pull/1205/diff?src=pr&el=tree#diff-cHl0b3JjaF90cmFuc2Zvcm1lcnMvdG9rZW5pemF0aW9uX3V0aWxzLnB5) | `89.37% <91.66%> (+8.89%)` | :arrow_up: |
------
[Continue to review full report at Codecov](https://codecov.io/gh/huggingface/pytorch-transformers/pull/1205?src=pr&el=continue).
> **Legend** - [Click here to learn more](https://docs.codecov.io/docs/codecov-delta)
> `Δ = absolute <relative> (impact)`, `ø = not affected`, `? = missing data`
> Powered by [Codecov](https://codecov.io/gh/huggingface/pytorch-transformers/pull/1205?src=pr&el=footer). Last update [0b52642...5c6cac1](https://codecov.io/gh/huggingface/pytorch-transformers/pull/1205?src=pr&el=lastupdated). Read the [comment docs](https://docs.codecov.io/docs/pull-request-comments).
<|||||>Thanks a lot for that!
Took the occasion to add regression tests and clean up a bit the base class. |
|
transformers | 1,204 | closed | Can't trace any model with pytorch-transformers 1.2 | ## 🐛 Bug
I get an error whenever I try to trace any model from pytorch-transformers 1.2.0.
When I roll back to 1.1 everything is fine.
## To Reproduce
```python
from pytorch_transformers import BertModel
import torch
model = BertModel.from_pretrained("bert-base-uncased", torchscript=True)
model.to('cuda')
model.eval()
ids = torch.LongTensor([[1, 2, 3]]).cuda()
tok = torch.zeros_like(ids)
att = torch.ones_like(ids)
torch.jit.trace(model, (ids, tok, att, ids))
```
This script produces the following error:
```
Traceback (most recent call last):
File "/home/louisj/.local/lib/python3.5/site-packages/torch/jit/__init__.py", line 545, in run_mod_and_filter_tensor_outputs
outs = wrap_retval(mod(*_clone_inputs(inputs)))
File "/home/louisj/.local/lib/python3.5/site-packages/torch/nn/modules/module.py", line 493, in __call__
result = self.forward(*input, **kwargs)
RuntimeError: r ASSERT FAILED at /pytorch/aten/src/ATen/core/jit_type.h:142, please report a bug to PyTorch. (expect at /pytorch/aten/src/ATen/core/jit_type.h:142)
frame #0: std::function<std::string ()>::operator()() const + 0x11 (0x7f273aa91441 in /home/louisj/.local/lib/python3.5/site-packages/torch/lib/libc10.so)
frame #1: c10::Error::Error(c10::SourceLocation, std::string const&) + 0x2a (0x7f273aa90d7a in /home/louisj/.local/lib/python3.5/site-packages/torch/lib/libc10.so)
frame #2: std::shared_ptr<c10::DimensionedTensorType const> c10::Type::expect<c10::DimensionedTensorType const>() + 0x140 (0x7f27397ff810 in /home/louisj/.local/lib/python3.5/site-packages/torch/lib/libtorch.so.1)
frame #3: torch::jit::fuser::compileKernel(torch::jit::fuser::KernelSpec const&, torch::jit::fuser::ArgSpec const&, std::vector<long, std::allocator<long> > const&, c10::Device) + 0xa5a (0x7f27397fbdca in /home/louisj/.local/lib/python3.5/site-packages/torch/lib/libtorch.so.1)
frame #4: torch::jit::fuser::runFusion(long, std::vector<c10::IValue, std::allocator<c10::IValue> >&, std::string*) + 0x5b0 (0x7f2739803c20 in /home/louisj/.local/lib/python3.5/site-packages/torch/lib/libtorch.so.1)
frame #5: torch::jit::runFusion(long, std::vector<c10::IValue, std::allocator<c10::IValue> >&) + 0x13 (0x7f2739733bc3 in /home/louisj/.local/lib/python3.5/site-packages/torch/lib/libtorch.so.1)
frame #6: <unknown function> + 0xb2b066 (0x7f273973d066 in /home/louisj/.local/lib/python3.5/site-packages/torch/lib/libtorch.so.1)
frame #7: <unknown function> + 0xa8ebe6 (0x7f27396a0be6 in /home/louisj/.local/lib/python3.5/site-packages/torch/lib/libtorch.so.1)
frame #8: torch::jit::InterpreterState::run(std::vector<c10::IValue, std::allocator<c10::IValue> >&) + 0x22 (0x7f273969c202 in /home/louisj/.local/lib/python3.5/site-packages/torch/lib/libtorch.so.1)
frame #9: <unknown function> + 0xa7685d (0x7f273968885d in /home/louisj/.local/lib/python3.5/site-packages/torch/lib/libtorch.so.1)
frame #10: <unknown function> + 0x457617 (0x7f277a2cd617 in /home/louisj/.local/lib/python3.5/site-packages/torch/lib/libtorch_python.so)
frame #11: <unknown function> + 0x130d0c (0x7f2779fa6d0c in /home/louisj/.local/lib/python3.5/site-packages/torch/lib/libtorch_python.so)
<omitting python frames>
frame #14: python3() [0x4fbfce]
frame #16: python3() [0x574db6]
frame #20: python3() [0x4ec2e3]
frame #22: python3() [0x4fbfce]
frame #24: python3() [0x574db6]
frame #27: python3() [0x5401ef]
frame #30: python3() [0x4ec3f7]
frame #33: python3() [0x5401ef]
frame #35: python3() [0x53fc97]
frame #37: python3() [0x53fc97]
frame #39: python3() [0x60cb42]
frame #44: __libc_start_main + 0xf0 (0x7f279df7d830 in /lib/x86_64-linux-gnu/libc.so.6)
During handling of the above exception, another exception occurred:
Traceback (most recent call last):
File "session.py", line 10, in <module>
torch.jit.trace(model, (ids, tok, att, ids))
File "/home/louisj/.local/lib/python3.5/site-packages/torch/jit/__init__.py", line 702, in trace
_check_trace([example_inputs], func, executor_options, traced, check_tolerance, _force_outplace)
File "/home/louisj/.local/lib/python3.5/site-packages/torch/autograd/grad_mode.py", line 43, in decorate_no_grad
return func(*args, **kwargs)
File "/home/louisj/.local/lib/python3.5/site-packages/torch/jit/__init__.py", line 583, in _check_trace
traced_outs = run_mod_and_filter_tensor_outputs(module, inputs, 'trace')
File "/home/louisj/.local/lib/python3.5/site-packages/torch/jit/__init__.py", line 551, in run_mod_and_filter_tensor_outputs
' with test inputs.\nException:\n' + indent(str(e)))
torch.jit.TracingCheckError: Tracing failed sanity checks!
Encountered an exception while running the trace with test inputs.
Exception:
r ASSERT FAILED at /pytorch/aten/src/ATen/core/jit_type.h:142, please report a bug to PyTorch. (expect at /pytorch/aten/src/ATen/core/jit_type.h:142)
frame #0: std::function<std::string ()>::operator()() const + 0x11 (0x7f273aa91441 in /home/louisj/.local/lib/python3.5/site-packages/torch/lib/libc10.so)
frame #1: c10::Error::Error(c10::SourceLocation, std::string const&) + 0x2a (0x7f273aa90d7a in /home/louisj/.local/lib/python3.5/site-packages/torch/lib/libc10.so)
frame #2: std::shared_ptr<c10::DimensionedTensorType const> c10::Type::expect<c10::DimensionedTensorType const>() + 0x140 (0x7f27397ff810 in /home/louisj/.local/lib/python3.5/site-packages/torch/lib/libtorch.so.1)
frame #3: torch::jit::fuser::compileKernel(torch::jit::fuser::KernelSpec const&, torch::jit::fuser::ArgSpec const&, std::vector<long, std::allocator<long> > const&, c10::Device) + 0xa5a (0x7f27397fbdca in /home/louisj/.local/lib/python3.5/site-packages/torch/lib/libtorch.so.1)
frame #4: torch::jit::fuser::runFusion(long, std::vector<c10::IValue, std::allocator<c10::IValue> >&, std::string*) + 0x5b0 (0x7f2739803c20 in /home/louisj/.local/lib/python3.5/site-packages/torch/lib/libtorch.so.1)
frame #5: torch::jit::runFusion(long, std::vector<c10::IValue, std::allocator<c10::IValue> >&) + 0x13 (0x7f2739733bc3 in /home/louisj/.local/lib/python3.5/site-packages/torch/lib/libtorch.so.1)
frame #6: <unknown function> + 0xb2b066 (0x7f273973d066 in /home/louisj/.local/lib/python3.5/site-packages/torch/lib/libtorch.so.1)
frame #7: <unknown function> + 0xa8ebe6 (0x7f27396a0be6 in /home/louisj/.local/lib/python3.5/site-packages/torch/lib/libtorch.so.1)
frame #8: torch::jit::InterpreterState::run(std::vector<c10::IValue, std::allocator<c10::IValue> >&) + 0x22 (0x7f273969c202 in /home/louisj/.local/lib/python3.5/site-packages/torch/lib/libtorch.so.1)
frame #9: <unknown function> + 0xa7685d (0x7f273968885d in /home/louisj/.local/lib/python3.5/site-packages/torch/lib/libtorch.so.1)
frame #10: <unknown function> + 0x457617 (0x7f277a2cd617 in /home/louisj/.local/lib/python3.5/site-packages/torch/lib/libtorch_python.so)
frame #11: <unknown function> + 0x130d0c (0x7f2779fa6d0c in /home/louisj/.local/lib/python3.5/site-packages/torch/lib/libtorch_python.so)
<omitting python frames>
frame #14: python3() [0x4fbfce]
frame #16: python3() [0x574db6]
frame #20: python3() [0x4ec2e3]
frame #22: python3() [0x4fbfce]
frame #24: python3() [0x574db6]
frame #27: python3() [0x5401ef]
frame #30: python3() [0x4ec3f7]
frame #33: python3() [0x5401ef]
frame #35: python3() [0x53fc97]
frame #37: python3() [0x53fc97]
frame #39: python3() [0x60cb42]
frame #44: __libc_start_main + 0xf0 (0x7f279df7d830 in /lib/x86_64-linux-gnu/libc.so.6)
```
## Environment
* OS: Ubuntu 16.04.6 LTS
* Python version: Python 3.5.2
* PyTorch version: '1.1.0'
* PyTorch Transformers version (or branch): '1.2.0'
* Using GPU ? yes
* Distributed of parallel setup ? no
* Any other relevant information:
I installed pytorch-transformers with pip:
```
pip3 install --user pytorch-transformers==1.2
``` | 09-05-2019 09:21:19 | 09-05-2019 09:21:19 | Hi, thank you for reporting this. I can reproduce it on my side. It seems to be a problem relative to the model being on `cuda`, as it doesn't fail if you don't put the model/ids on `cuda`.
This doesn't fail:
```py
from pytorch_transformers import BertModel
import torch
model = BertModel.from_pretrained("bert-base-uncased", torchscript=True)
model.eval()
ids = torch.LongTensor([[1, 2, 3]])
tok = torch.zeros_like(ids)
att = torch.ones_like(ids)
torch.jit.trace(model, (ids, tok, att, ids))
```<|||||>Traced models on cpu may not be convertible to cuda due to hard coded tensor creation in torchscript. I tried a while ago and it wasn't working, and I found an issue (#1010) referencing a similar problem.<|||||>Yes, with the merge of #1195, the jit tracing issue of #1010 should now be fixed on master.
You test installing from source and see if it solves your issue. <|||||>I ran BERT on GPU after tracing it on CPU, and it works fine! Any information about when this fix will be available from the official distribution?<|||||>This issue has been automatically marked as stale because it has not had recent activity. It will be closed if no further activity occurs. Thank you for your contributions.
<|||||>Hi, while the model outputs has multi outputs like ``sequence_output`` and ``pooled_output``,how to get one of them in C++? |
transformers | 1,203 | closed | [2.0] TF 2.0 support | Currently converted models:
- [x] BERT
- [x] GPT-2
- [x] XLNet
- [x] XLM
- [x] Transformer-XL
- [x] GPT
- [x] RoBERTa
- [x] DistilBert
With TF 2.0 Keras imperative interface and Eager, the workflow and models are suprisingly similar:
```python
import numpy
import torch
import tensorflow as tf
from pytorch_transformers import BertModel, TFBertModel, BertTokenizer
tokenizer = BertTokenizer.from_pretrained('bert-base-uncased')
pytorch_model = BertModel.from_pretrained('bert-base-uncased')
tf_model = TFBertModel.from_pretrained('bert-base-uncased')
text = "[CLS] Who was Jim Henson ? Jim [MASK] was a puppeteer [SEP]"
tokens = tokenizer.encode(text)
pytorch_inputs = torch.tensor([tokens])
tf_inputs = tf.constant([tokens])
with torch.no_grad():
pytorch_outputs = pytorch_model(pytorch_inputs)
tf_output = tf_model(tf_inputs, training=False)
numpy.amax(numpy.abs(pytorch_outputs[0].numpy() - tf_output[0].numpy()))
# >>> 2.861023e-06 => we are good, a few 1e-6 is the expected difference
# between TF and PT arising from internals computation ops
```
The convention is to use the same name for classes as the original PyTorch classes but prefixed with `TF`.
If you want to install and use this development branch, you should install from the `tf2` branch like this:
- install TF 2.0: `pip install tensorflow==2.0.0-rc0`
- install pytorch-transformers from the `tf2` branch: `pip install https://github.com/huggingface/pytorch-transformers/archive/tf2.zip`
TO-DO / not forget:
- [ ] check weights initialization
- [x] add weights tying
- [x] add example with losses using `model.compile` /`model.fit`
- [ ] take care of having the two possible gelu implementations for Bert
- [ ] untangle Transfo-XL tokenizer from `torch.load` and `torch.save`
- [x] test that all dropout modules are desactivated when training=False (check determinism)
- [ ] clean up our FP16 support (for PyTorch as well) with (i) an adjustment of masking values and (ii) an adjustment of LayerNorm epsilon (add an attribute in configuration files). | 09-05-2019 08:23:57 | 09-05-2019 08:23:57 | # [Codecov](https://codecov.io/gh/huggingface/transformers/pull/1203?src=pr&el=h1) Report
> Merging [#1203](https://codecov.io/gh/huggingface/transformers/pull/1203?src=pr&el=desc) into [master](https://codecov.io/gh/huggingface/transformers/commit/4a233e5b2c18f0cf508f6b917cd1e02954764699?src=pr&el=desc) will **increase** coverage by `4.27%`.
> The diff coverage is `89.06%`.
[](https://codecov.io/gh/huggingface/transformers/pull/1203?src=pr&el=tree)
```diff
@@ Coverage Diff @@
## master #1203 +/- ##
==========================================
+ Coverage 80.45% 84.73% +4.27%
==========================================
Files 57 84 +27
Lines 8090 12573 +4483
==========================================
+ Hits 6509 10654 +4145
- Misses 1581 1919 +338
```
| [Impacted Files](https://codecov.io/gh/huggingface/transformers/pull/1203?src=pr&el=tree) | Coverage Δ | |
|---|---|---|
| [transformers/tokenization\_auto.py](https://codecov.io/gh/huggingface/transformers/pull/1203/diff?src=pr&el=tree#diff-dHJhbnNmb3JtZXJzL3Rva2VuaXphdGlvbl9hdXRvLnB5) | `70.96% <ø> (ø)` | |
| [transformers/modeling\_roberta.py](https://codecov.io/gh/huggingface/transformers/pull/1203/diff?src=pr&el=tree#diff-dHJhbnNmb3JtZXJzL21vZGVsaW5nX3JvYmVydGEucHk=) | `70.8% <ø> (ø)` | |
| [transformers/modeling\_auto.py](https://codecov.io/gh/huggingface/transformers/pull/1203/diff?src=pr&el=tree#diff-dHJhbnNmb3JtZXJzL21vZGVsaW5nX2F1dG8ucHk=) | `53.94% <ø> (ø)` | |
| [transformers/configuration\_distilbert.py](https://codecov.io/gh/huggingface/transformers/pull/1203/diff?src=pr&el=tree#diff-dHJhbnNmb3JtZXJzL2NvbmZpZ3VyYXRpb25fZGlzdGlsYmVydC5weQ==) | `89.74% <ø> (ø)` | |
| [transformers/configuration\_openai.py](https://codecov.io/gh/huggingface/transformers/pull/1203/diff?src=pr&el=tree#diff-dHJhbnNmb3JtZXJzL2NvbmZpZ3VyYXRpb25fb3BlbmFpLnB5) | `89.13% <ø> (ø)` | |
| [transformers/modeling\_transfo\_xl\_utilities.py](https://codecov.io/gh/huggingface/transformers/pull/1203/diff?src=pr&el=tree#diff-dHJhbnNmb3JtZXJzL21vZGVsaW5nX3RyYW5zZm9feGxfdXRpbGl0aWVzLnB5) | `53.89% <ø> (ø)` | |
| [transformers/modeling\_openai.py](https://codecov.io/gh/huggingface/transformers/pull/1203/diff?src=pr&el=tree#diff-dHJhbnNmb3JtZXJzL21vZGVsaW5nX29wZW5haS5weQ==) | `80.4% <ø> (ø)` | |
| [transformers/optimization.py](https://codecov.io/gh/huggingface/transformers/pull/1203/diff?src=pr&el=tree#diff-dHJhbnNmb3JtZXJzL29wdGltaXphdGlvbi5weQ==) | `96.62% <ø> (ø)` | |
| [transformers/configuration\_xlm.py](https://codecov.io/gh/huggingface/transformers/pull/1203/diff?src=pr&el=tree#diff-dHJhbnNmb3JtZXJzL2NvbmZpZ3VyYXRpb25feGxtLnB5) | `93.33% <ø> (ø)` | |
| [transformers/tests/conftest.py](https://codecov.io/gh/huggingface/transformers/pull/1203/diff?src=pr&el=tree#diff-dHJhbnNmb3JtZXJzL3Rlc3RzL2NvbmZ0ZXN0LnB5) | `90% <ø> (ø)` | |
| ... and [113 more](https://codecov.io/gh/huggingface/transformers/pull/1203/diff?src=pr&el=tree-more) | |
------
[Continue to review full report at Codecov](https://codecov.io/gh/huggingface/transformers/pull/1203?src=pr&el=continue).
> **Legend** - [Click here to learn more](https://docs.codecov.io/docs/codecov-delta)
> `Δ = absolute <relative> (impact)`, `ø = not affected`, `? = missing data`
> Powered by [Codecov](https://codecov.io/gh/huggingface/transformers/pull/1203?src=pr&el=footer). Last update [4a233e5...80bf868](https://codecov.io/gh/huggingface/transformers/pull/1203?src=pr&el=lastupdated). Read the [comment docs](https://docs.codecov.io/docs/pull-request-comments).
<|||||>Ok big merge |
transformers | 1,202 | closed | Learning word-pieces garble the predictions | ## 🐛 Bug
<!-- Important information -->
Model I am using (Bert, XLNet....): Bert
Language I am using the model on (English, Chinese....): English
The tasks I am working on is:
* [ x] my own task or dataset: Sentiment Analysis on chatbot conversations
Word-pieces change the prediction completely, I fail to understand why.
If i do a token to token similarity also, by considering the token vector to be the average of wordpieces or the first token vector to see if they carry the same context, they don't.
If I had lemmatized the training set, these pieces would have not learnt anything really.
But that is not intuitive.
<img width="571" alt="Screenshot 2019-09-05 at 12 01 26 PM" src="https://user-images.githubusercontent.com/25073753/64317678-07a95680-cfd6-11e9-8849-f9c11f8531ae.png">
<img width="574" alt="Screenshot 2019-09-05 at 11 59 48 AM" src="https://user-images.githubusercontent.com/25073753/64317679-07a95680-cfd6-11e9-900a-96ec5d318b12.png">
<img width="594" alt="Screenshot 2019-09-05 at 11 59 53 AM" src="https://user-images.githubusercontent.com/25073753/64317680-07a95680-cfd6-11e9-9950-2957c284e6e8.png">
[CLS] token vector is fed to the classifier. Changes need to be done internally.
How can I handle such cases?
Any leads would be helpful.
Thanks in advance. | 09-05-2019 06:40:10 | 09-05-2019 06:40:10 | This issue has been automatically marked as stale because it has not had recent activity. It will be closed if no further activity occurs. Thank you for your contributions.
|
transformers | 1,201 | closed | [2.0] - Split configuration and modeling files | Refactor to split configuration and modeling files so we can share configuration easily between various frameworks.
This PR is quite annoying to rebase so we should probably merge it pretty soon. | 09-04-2019 22:29:39 | 09-04-2019 22:29:39 | # [Codecov](https://codecov.io/gh/huggingface/pytorch-transformers/pull/1201?src=pr&el=h1) Report
> Merging [#1201](https://codecov.io/gh/huggingface/pytorch-transformers/pull/1201?src=pr&el=desc) into [master](https://codecov.io/gh/huggingface/pytorch-transformers/commit/0b52642d379bed155e8aa4f4088588bfd8ceaa88?src=pr&el=desc) will **increase** coverage by `0.03%`.
> The diff coverage is `89.57%`.
[](https://codecov.io/gh/huggingface/pytorch-transformers/pull/1201?src=pr&el=tree)
```diff
@@ Coverage Diff @@
## master #1201 +/- ##
==========================================
+ Coverage 80.83% 80.86% +0.03%
==========================================
Files 46 57 +11
Lines 7878 8016 +138
==========================================
+ Hits 6368 6482 +114
- Misses 1510 1534 +24
```
| [Impacted Files](https://codecov.io/gh/huggingface/pytorch-transformers/pull/1201?src=pr&el=tree) | Coverage Δ | |
|---|---|---|
| [pytorch\_transformers/modeling\_auto.py](https://codecov.io/gh/huggingface/pytorch-transformers/pull/1201/diff?src=pr&el=tree#diff-cHl0b3JjaF90cmFuc2Zvcm1lcnMvbW9kZWxpbmdfYXV0by5weQ==) | `53.94% <100%> (+3.94%)` | :arrow_up: |
| [pytorch\_transformers/tests/modeling\_xlnet\_test.py](https://codecov.io/gh/huggingface/pytorch-transformers/pull/1201/diff?src=pr&el=tree#diff-cHl0b3JjaF90cmFuc2Zvcm1lcnMvdGVzdHMvbW9kZWxpbmdfeGxuZXRfdGVzdC5weQ==) | `95.91% <100%> (+0.02%)` | :arrow_up: |
| [pytorch\_transformers/tests/modeling\_common\_test.py](https://codecov.io/gh/huggingface/pytorch-transformers/pull/1201/diff?src=pr&el=tree#diff-cHl0b3JjaF90cmFuc2Zvcm1lcnMvdGVzdHMvbW9kZWxpbmdfY29tbW9uX3Rlc3QucHk=) | `73.19% <100%> (-4.83%)` | :arrow_down: |
| [pytorch\_transformers/tests/modeling\_gpt2\_test.py](https://codecov.io/gh/huggingface/pytorch-transformers/pull/1201/diff?src=pr&el=tree#diff-cHl0b3JjaF90cmFuc2Zvcm1lcnMvdGVzdHMvbW9kZWxpbmdfZ3B0Ml90ZXN0LnB5) | `93.06% <100%> (+0.06%)` | :arrow_up: |
| [...rch\_transformers/tests/modeling\_distilbert\_test.py](https://codecov.io/gh/huggingface/pytorch-transformers/pull/1201/diff?src=pr&el=tree#diff-cHl0b3JjaF90cmFuc2Zvcm1lcnMvdGVzdHMvbW9kZWxpbmdfZGlzdGlsYmVydF90ZXN0LnB5) | `99.06% <100%> (-0.02%)` | :arrow_down: |
| [pytorch\_transformers/modeling\_gpt2.py](https://codecov.io/gh/huggingface/pytorch-transformers/pull/1201/diff?src=pr&el=tree#diff-cHl0b3JjaF90cmFuc2Zvcm1lcnMvbW9kZWxpbmdfZ3B0Mi5weQ==) | `83.83% <100%> (-0.2%)` | :arrow_down: |
| [pytorch\_transformers/modeling\_roberta.py](https://codecov.io/gh/huggingface/pytorch-transformers/pull/1201/diff?src=pr&el=tree#diff-cHl0b3JjaF90cmFuc2Zvcm1lcnMvbW9kZWxpbmdfcm9iZXJ0YS5weQ==) | `75.22% <100%> (-0.67%)` | :arrow_down: |
| [pytorch\_transformers/modeling\_xlnet.py](https://codecov.io/gh/huggingface/pytorch-transformers/pull/1201/diff?src=pr&el=tree#diff-cHl0b3JjaF90cmFuc2Zvcm1lcnMvbW9kZWxpbmdfeGxuZXQucHk=) | `77.84% <100%> (-0.99%)` | :arrow_down: |
| [pytorch\_transformers/tests/modeling\_openai\_test.py](https://codecov.io/gh/huggingface/pytorch-transformers/pull/1201/diff?src=pr&el=tree#diff-cHl0b3JjaF90cmFuc2Zvcm1lcnMvdGVzdHMvbW9kZWxpbmdfb3BlbmFpX3Rlc3QucHk=) | `93% <100%> (+0.07%)` | :arrow_up: |
| [pytorch\_transformers/modeling\_utils.py](https://codecov.io/gh/huggingface/pytorch-transformers/pull/1201/diff?src=pr&el=tree#diff-cHl0b3JjaF90cmFuc2Zvcm1lcnMvbW9kZWxpbmdfdXRpbHMucHk=) | `90.27% <100%> (+0.2%)` | :arrow_up: |
| ... and [34 more](https://codecov.io/gh/huggingface/pytorch-transformers/pull/1201/diff?src=pr&el=tree-more) | |
------
[Continue to review full report at Codecov](https://codecov.io/gh/huggingface/pytorch-transformers/pull/1201?src=pr&el=continue).
> **Legend** - [Click here to learn more](https://docs.codecov.io/docs/codecov-delta)
> `Δ = absolute <relative> (impact)`, `ø = not affected`, `? = missing data`
> Powered by [Codecov](https://codecov.io/gh/huggingface/pytorch-transformers/pull/1201?src=pr&el=footer). Last update [0b52642...85df4f7](https://codecov.io/gh/huggingface/pytorch-transformers/pull/1201?src=pr&el=lastupdated). Read the [comment docs](https://docs.codecov.io/docs/pull-request-comments).
|
transformers | 1,200 | closed | Distributed device ordinal question | ## ❓ Questions & Help
In the following line, we set the device ordinal to be local rank. However, suppose we have four independent nodes each with only one GPU. Then the 4th node (rank 3) will execute this line with the device ordinal 3, but it really only has 1 GPU, so it'll be invalid to ask for the GPU 3. So won't that break things? Shouldn't it be `torch.device("cuda", 0)`?
https://github.com/huggingface/pytorch-transformers/blob/0287d264e913e10018a95a2723115dc9121e5fc6/examples/run_glue.py#L403 | 09-04-2019 22:21:59 | 09-04-2019 22:21:59 | No, `local_rank` is only the local rank on each node.<|||||>Ah I see, thanks! A tangential question, considering the distributed setting only, would it be the same if we simply call `.cuda()` for the model and tensors instead of passing around the device?<|||||>This issue has been automatically marked as stale because it has not had recent activity. It will be closed if no further activity occurs. Thank you for your contributions.
|
transformers | 1,199 | closed | Fixing TransformerXL bool issue #1169 | Fixing #1169 regarding using uint or bool masks in Transformer-XL and PyTorch 1.1.0 and 1.2.0.
Hopefully, this solution will be compatible upward with the future PyTorch releases. | 09-04-2019 20:38:44 | 09-04-2019 20:38:44 | # [Codecov](https://codecov.io/gh/huggingface/pytorch-transformers/pull/1199?src=pr&el=h1) Report
> Merging [#1199](https://codecov.io/gh/huggingface/pytorch-transformers/pull/1199?src=pr&el=desc) into [master](https://codecov.io/gh/huggingface/pytorch-transformers/commit/0b52642d379bed155e8aa4f4088588bfd8ceaa88?src=pr&el=desc) will **decrease** coverage by `0.01%`.
> The diff coverage is `33.33%`.
[](https://codecov.io/gh/huggingface/pytorch-transformers/pull/1199?src=pr&el=tree)
```diff
@@ Coverage Diff @@
## master #1199 +/- ##
==========================================
- Coverage 80.83% 80.81% -0.02%
==========================================
Files 46 46
Lines 7878 7881 +3
==========================================
+ Hits 6368 6369 +1
- Misses 1510 1512 +2
```
| [Impacted Files](https://codecov.io/gh/huggingface/pytorch-transformers/pull/1199?src=pr&el=tree) | Coverage Δ | |
|---|---|---|
| [pytorch\_transformers/modeling\_transfo\_xl.py](https://codecov.io/gh/huggingface/pytorch-transformers/pull/1199/diff?src=pr&el=tree#diff-cHl0b3JjaF90cmFuc2Zvcm1lcnMvbW9kZWxpbmdfdHJhbnNmb194bC5weQ==) | `56.9% <33.33%> (-0.1%)` | :arrow_down: |
------
[Continue to review full report at Codecov](https://codecov.io/gh/huggingface/pytorch-transformers/pull/1199?src=pr&el=continue).
> **Legend** - [Click here to learn more](https://docs.codecov.io/docs/codecov-delta)
> `Δ = absolute <relative> (impact)`, `ø = not affected`, `? = missing data`
> Powered by [Codecov](https://codecov.io/gh/huggingface/pytorch-transformers/pull/1199?src=pr&el=footer). Last update [0b52642...38b79b5](https://codecov.io/gh/huggingface/pytorch-transformers/pull/1199?src=pr&el=lastupdated). Read the [comment docs](https://docs.codecov.io/docs/pull-request-comments).
<|||||># [Codecov](https://codecov.io/gh/huggingface/pytorch-transformers/pull/1199?src=pr&el=h1) Report
> Merging [#1199](https://codecov.io/gh/huggingface/pytorch-transformers/pull/1199?src=pr&el=desc) into [master](https://codecov.io/gh/huggingface/pytorch-transformers/commit/0b52642d379bed155e8aa4f4088588bfd8ceaa88?src=pr&el=desc) will **decrease** coverage by `0.01%`.
> The diff coverage is `42.85%`.
[](https://codecov.io/gh/huggingface/pytorch-transformers/pull/1199?src=pr&el=tree)
```diff
@@ Coverage Diff @@
## master #1199 +/- ##
==========================================
- Coverage 80.83% 80.81% -0.02%
==========================================
Files 46 46
Lines 7878 7881 +3
==========================================
+ Hits 6368 6369 +1
- Misses 1510 1512 +2
```
| [Impacted Files](https://codecov.io/gh/huggingface/pytorch-transformers/pull/1199?src=pr&el=tree) | Coverage Δ | |
|---|---|---|
| [pytorch\_transformers/modeling\_transfo\_xl.py](https://codecov.io/gh/huggingface/pytorch-transformers/pull/1199/diff?src=pr&el=tree#diff-cHl0b3JjaF90cmFuc2Zvcm1lcnMvbW9kZWxpbmdfdHJhbnNmb194bC5weQ==) | `56.9% <42.85%> (-0.1%)` | :arrow_down: |
------
[Continue to review full report at Codecov](https://codecov.io/gh/huggingface/pytorch-transformers/pull/1199?src=pr&el=continue).
> **Legend** - [Click here to learn more](https://docs.codecov.io/docs/codecov-delta)
> `Δ = absolute <relative> (impact)`, `ø = not affected`, `? = missing data`
> Powered by [Codecov](https://codecov.io/gh/huggingface/pytorch-transformers/pull/1199?src=pr&el=footer). Last update [0b52642...0be6a2a](https://codecov.io/gh/huggingface/pytorch-transformers/pull/1199?src=pr&el=lastupdated). Read the [comment docs](https://docs.codecov.io/docs/pull-request-comments).
<|||||>Looks great to me! |
transformers | 1,198 | closed | How to fine-tune xlnet on SQuAD with the parameter setting provided in the paper? | From [here](https://arxiv.org/pdf/1906.08237.pdf) on page 16, it seems we should set Layer-wise lr decay to 0.75. However, I didn't find a way to do so in `run_squad.py`. Could someone provide a sample command line that could run this fine-tune task with the given parameters?
Thanks! | 09-04-2019 19:38:25 | 09-04-2019 19:38:25 | Here is my attempt to do layer-wise lr decay. It didn't help with the model performance though. Fixing the preprocessing code helped a lot, but still a few points lower than what they reported in the paper and lower than BERT large WWM model. See my comment in #947
```
lr_layer_decay = 0.75
n_layers = 24
no_lr_layer_decay_group = []
lr_layer_decay_groups = {k:[] for k in range(n_layers)}
for n, p in model.named_parameters():
name_split = n.split(".")
if name_split[1] == "layer":
lr_layer_decay_groups[int(name_split[2])].append(p)
else:
no_lr_layer_decay_group.append(p)
optimizer_grouped_parameters = [{"params": no_lr_layer_decay_group, "lr": learning_rate}]
for i in range(n_layers):
parameters_group = {"params": lr_layer_decay_groups[i], "lr": learning_rate * (lr_layer_decay ** (n_layers - i - 1))}
optimizer_grouped_parameters.append(parameters_group)
optimizer = AdamW(optimizer_grouped_parameters, lr=learning_rate, eps=1e-6)
```<|||||>This issue has been automatically marked as stale because it has not had recent activity. It will be closed if no further activity occurs. Thank you for your contributions.
|
transformers | 1,197 | closed | Fix loading of question answering bert from tf weights. | I've got an attribute error when loading pretrained tf weights for question answering (bert) in `load_tf_weights_in_bert` at:
```
elif l[0] == 'squad':
pointer = getattr(pointer, 'classifier')
```
since `BertForQuestionAnswering` does not have a 'classifier' attribute but `qa_outputs`. I've added a try except, which resolves the error. | 09-04-2019 13:24:07 | 09-04-2019 13:24:07 | # [Codecov](https://codecov.io/gh/huggingface/pytorch-transformers/pull/1197?src=pr&el=h1) Report
> Merging [#1197](https://codecov.io/gh/huggingface/pytorch-transformers/pull/1197?src=pr&el=desc) into [master](https://codecov.io/gh/huggingface/pytorch-transformers/commit/89fd3450a61b5efd76d2524df2454e0a0e4ca070?src=pr&el=desc) will **not change** coverage.
> The diff coverage is `100%`.
[](https://codecov.io/gh/huggingface/pytorch-transformers/pull/1197?src=pr&el=tree)
```diff
@@ Coverage Diff @@
## master #1197 +/- ##
=======================================
Coverage 80.83% 80.83%
=======================================
Files 46 46
Lines 7878 7878
=======================================
Hits 6368 6368
Misses 1510 1510
```
| [Impacted Files](https://codecov.io/gh/huggingface/pytorch-transformers/pull/1197?src=pr&el=tree) | Coverage Δ | |
|---|---|---|
| [pytorch\_transformers/modeling\_bert.py](https://codecov.io/gh/huggingface/pytorch-transformers/pull/1197/diff?src=pr&el=tree#diff-cHl0b3JjaF90cmFuc2Zvcm1lcnMvbW9kZWxpbmdfYmVydC5weQ==) | `88.03% <100%> (ø)` | :arrow_up: |
------
[Continue to review full report at Codecov](https://codecov.io/gh/huggingface/pytorch-transformers/pull/1197?src=pr&el=continue).
> **Legend** - [Click here to learn more](https://docs.codecov.io/docs/codecov-delta)
> `Δ = absolute <relative> (impact)`, `ø = not affected`, `? = missing data`
> Powered by [Codecov](https://codecov.io/gh/huggingface/pytorch-transformers/pull/1197?src=pr&el=footer). Last update [89fd345...d6fb182](https://codecov.io/gh/huggingface/pytorch-transformers/pull/1197?src=pr&el=lastupdated). Read the [comment docs](https://docs.codecov.io/docs/pull-request-comments).
<|||||>This issue has been automatically marked as stale because it has not had recent activity. It will be closed if no further activity occurs. Thank you for your contributions.
|
transformers | 1,196 | closed | RoBERTa/GPT2 tokenization | Hi,
I've one question regarding to the tokenization logic.
I'm using the RoBERTa tokenizer from `fairseq`:
```python
In [15]: tokens = roberta.encode("Berlin and Munich have a lot of puppeteer to see .")
In [16]: tokens
Out[16]:
tensor([ 0, 26795, 2614, 8, 10489, 33, 10, 319, 9, 32986,
9306, 254, 7, 192, 479, 2])
```
Interestingly, Berlin will be splitted into two subwords (with ids 26795 and 2614).
When I use the `pytorch-transformer` implementation:
```
In [21]: tokens = tokenizer.tokenize("<s>Berlin and Munich have a lot of puppeteer to see .</s>")
In [22]: indexed_tokens = tokenizer.convert_tokens_to_ids(tokens)
In [23]: indexed_tokens
Out[23]: [0, 5459, 8, 10489, 33, 10, 319, 9, 32986, 9306, 254, 7, 192, 479, 2]
```
Berlin is not splitted 😅
The `roberta.encode` method will return one subword for Berlin, when I start the sentence with a space - which tokenizer is correct here 🤔 | 09-04-2019 12:44:52 | 09-04-2019 12:44:52 | This is a more complex question than it may seem but in general, I think both will be pretty similar in practice.
This is related to the fact that the GPT-2 tokenizer (also used by RoBERTa) requires a space before all the words (see [this wise note](https://github.com/pytorch/fairseq/blob/master/fairseq/models/roberta/hub_interface.py#L38-L56) in fairseq about it).
Now at the beginning of a string you don't have a space which can result in strange behaviors.
Here is an example of the resulting behavior on RoBERTa. You would expect that the strings `Berlin and Munich` and `Munich and Berlin` are tokenized similarly with only the order of the tokens modified but they are not:
```
>>> roberta.encode("Berlin and Munich")
tensor([ 0, 26795, 2614, 8, 10489, 2])
>>> roberta.encode("Munich and Berlin")
tensor([ 0, 448, 879, 1725, 8, 5459, 2])
```
In this example, the first word is split and not the second.
In our tokenizer, to avoid this behavior we decided to always add a space at the beginning of a string (multiple spaces doesn't have an effect so it's ok to always add one) so that the tokenization can be consistent.
A side effect of this (indicated in the doc/docstring) is that the encoding/decoding process doesn't preserve the absence of a space at the beginning of a string but on the other hand the resulting behavior is more consistent.
```
>>> tokenizer.encode("Berlin and Munich", add_special_tokens=True)
[0, 5459, 8, 10489, 2]
>>> tokenizer.encode("Munich and Berlin", add_special_tokens=True)
[0, 10489, 8, 5459, 2]
```
Here is a short discussion from my point of view but it would but nice, I think, to have @myleott inputs on this as well.<|||||>Thanks for your explanation :+1:
I just ran an experiment for a downstream task (English NER) and F1-score decreased around 0.5% 😟
I'll repeat that experiment with one commit before 0517e7a1cb4a70bdf32f8d11b56df8d3911d1792 (that introduced the whitespace rule) to find out where this performance drop comes from.<|||||>Update on that: I used 3bcbebd440c220adbaab657f2d13dac7c89f6453 and re-do my experiment on NER. Now the final F1-score is 92.26 (consistent with a prior result that was 92.31) - in contrast to 91.81 for the latest 1.2.0 version 🤔
Would it possible to add a flag that uses the "original" tokenization 🤔<|||||>We'll see what we can do (cc @LysandreJik @julien-c).
Is this difference significantly different with regards to seed run variability?<|||||>I made a few more experiments with the same dataset and different runs:
| Version | Run 1 | Run 2 | Run 3 | Avg.
| ------- | ----- | ----- | ----- | ----
| 1.2.0 | 91.81 | 91.82 | 91.78 | 91.80
| 3bcbebd | 92.31 | 92.26 | 92.38 | 92.32
On average, the difference is 0.52%.<|||||>Thanks a lot for the detailed experiments Stefan.
The comparison is pretty consistently in favor of the original tokenization so I guess we will switch back to the fairseq tokenization as default and add an option to use the "consistent-tokenization".
cc @LysandreJik @julien-c |
transformers | 1,195 | closed | [2.0] Reodering arguments for torch jit #1010 and future TF2.0 compatibility | Torch jit (cf #1010) and TF 2.0 (cf #1104) are more strict than PyTorch on having a specific order of arguments for easy use.
This PR refactor the order of the keyword arguments to make them as natural as possible.
This will be a breaking change for people using positional order to input keyword arguments in the forward pass of the models, hence is delayed to the 2.0 release. | 09-04-2019 10:50:17 | 09-04-2019 10:50:17 | # [Codecov](https://codecov.io/gh/huggingface/pytorch-transformers/pull/1195?src=pr&el=h1) Report
> Merging [#1195](https://codecov.io/gh/huggingface/pytorch-transformers/pull/1195?src=pr&el=desc) into [master](https://codecov.io/gh/huggingface/pytorch-transformers/commit/0b52642d379bed155e8aa4f4088588bfd8ceaa88?src=pr&el=desc) will **decrease** coverage by `0.4%`.
> The diff coverage is `86.59%`.
[](https://codecov.io/gh/huggingface/pytorch-transformers/pull/1195?src=pr&el=tree)
```diff
@@ Coverage Diff @@
## master #1195 +/- ##
==========================================
- Coverage 80.83% 80.42% -0.41%
==========================================
Files 46 46
Lines 7878 7892 +14
==========================================
- Hits 6368 6347 -21
- Misses 1510 1545 +35
```
| [Impacted Files](https://codecov.io/gh/huggingface/pytorch-transformers/pull/1195?src=pr&el=tree) | Coverage Δ | |
|---|---|---|
| [pytorch\_transformers/modeling\_xlnet.py](https://codecov.io/gh/huggingface/pytorch-transformers/pull/1195/diff?src=pr&el=tree#diff-cHl0b3JjaF90cmFuc2Zvcm1lcnMvbW9kZWxpbmdfeGxuZXQucHk=) | `78.83% <100%> (ø)` | :arrow_up: |
| [pytorch\_transformers/tests/modeling\_bert\_test.py](https://codecov.io/gh/huggingface/pytorch-transformers/pull/1195/diff?src=pr&el=tree#diff-cHl0b3JjaF90cmFuc2Zvcm1lcnMvdGVzdHMvbW9kZWxpbmdfYmVydF90ZXN0LnB5) | `96.29% <100%> (ø)` | :arrow_up: |
| [...rch\_transformers/tests/modeling\_distilbert\_test.py](https://codecov.io/gh/huggingface/pytorch-transformers/pull/1195/diff?src=pr&el=tree#diff-cHl0b3JjaF90cmFuc2Zvcm1lcnMvdGVzdHMvbW9kZWxpbmdfZGlzdGlsYmVydF90ZXN0LnB5) | `99.08% <100%> (ø)` | :arrow_up: |
| [pytorch\_transformers/modeling\_distilbert.py](https://codecov.io/gh/huggingface/pytorch-transformers/pull/1195/diff?src=pr&el=tree#diff-cHl0b3JjaF90cmFuc2Zvcm1lcnMvbW9kZWxpbmdfZGlzdGlsYmVydC5weQ==) | `96.77% <100%> (ø)` | :arrow_up: |
| [pytorch\_transformers/modeling\_xlm.py](https://codecov.io/gh/huggingface/pytorch-transformers/pull/1195/diff?src=pr&el=tree#diff-cHl0b3JjaF90cmFuc2Zvcm1lcnMvbW9kZWxpbmdfeGxtLnB5) | `87.08% <100%> (ø)` | :arrow_up: |
| [pytorch\_transformers/modeling\_bert.py](https://codecov.io/gh/huggingface/pytorch-transformers/pull/1195/diff?src=pr&el=tree#diff-cHl0b3JjaF90cmFuc2Zvcm1lcnMvbW9kZWxpbmdfYmVydC5weQ==) | `88.03% <100%> (ø)` | :arrow_up: |
| [...ytorch\_transformers/tests/modeling\_roberta\_test.py](https://codecov.io/gh/huggingface/pytorch-transformers/pull/1195/diff?src=pr&el=tree#diff-cHl0b3JjaF90cmFuc2Zvcm1lcnMvdGVzdHMvbW9kZWxpbmdfcm9iZXJ0YV90ZXN0LnB5) | `78.81% <100%> (ø)` | :arrow_up: |
| [pytorch\_transformers/modeling\_transfo\_xl.py](https://codecov.io/gh/huggingface/pytorch-transformers/pull/1195/diff?src=pr&el=tree#diff-cHl0b3JjaF90cmFuc2Zvcm1lcnMvbW9kZWxpbmdfdHJhbnNmb194bC5weQ==) | `56.9% <42.85%> (-0.1%)` | :arrow_down: |
| [pytorch\_transformers/modeling\_openai.py](https://codecov.io/gh/huggingface/pytorch-transformers/pull/1195/diff?src=pr&el=tree#diff-cHl0b3JjaF90cmFuc2Zvcm1lcnMvbW9kZWxpbmdfb3BlbmFpLnB5) | `81.08% <76.47%> (-0.88%)` | :arrow_down: |
| [pytorch\_transformers/modeling\_gpt2.py](https://codecov.io/gh/huggingface/pytorch-transformers/pull/1195/diff?src=pr&el=tree#diff-cHl0b3JjaF90cmFuc2Zvcm1lcnMvbW9kZWxpbmdfZ3B0Mi5weQ==) | `83.13% <76.47%> (-0.91%)` | :arrow_down: |
| ... and [8 more](https://codecov.io/gh/huggingface/pytorch-transformers/pull/1195/diff?src=pr&el=tree-more) | |
------
[Continue to review full report at Codecov](https://codecov.io/gh/huggingface/pytorch-transformers/pull/1195?src=pr&el=continue).
> **Legend** - [Click here to learn more](https://docs.codecov.io/docs/codecov-delta)
> `Δ = absolute <relative> (impact)`, `ø = not affected`, `? = missing data`
> Powered by [Codecov](https://codecov.io/gh/huggingface/pytorch-transformers/pull/1195?src=pr&el=footer). Last update [0b52642...7fba47b](https://codecov.io/gh/huggingface/pytorch-transformers/pull/1195?src=pr&el=lastupdated). Read the [comment docs](https://docs.codecov.io/docs/pull-request-comments).
<|||||>Ok merging |
transformers | 1,194 | closed | How to finetune DistilBERT on custom data? | ## ❓ Questions & Help
I want to build classifier by using DistillBERT. I would like to know how to finetune it on the custom dataset and build classifier on it.
Thansk! | 09-04-2019 10:43:04 | 09-04-2019 10:43:04 | Hello @008karan,
There is the class `DistilBertForSequenceClassification` for classification tasks. Its used is really similar to `BertForSequenceClassification`: the main difference is that `DistilBertForSequenceClassification` does not need `token_type_ids` as inputs.<|||||>This issue has been automatically marked as stale because it has not had recent activity. It will be closed if no further activity occurs. Thank you for your contributions.
|
transformers | 1,193 | closed | how to get distilbert-base-uncased-distilled-squad? | ## ❓ Questions & Help
<!-- A clear and concise description of the question. -->
I think it is initialize a six layer bert and distill with a 12 layer bert, then save the checkpoint file, is it right? | 09-04-2019 09:45:42 | 09-04-2019 09:45:42 | Hello @RyanHuangNLP,
I am not sure to get your question.
Do you mean that you want to play with the model (do inferences)? If so, did you try `qa_model = DistilBertForQuestionAnswering.from_pretrained('distilbert-base-uncased-distilled-squad')`?<|||||>@VictorSanh I wonder how to get the 'distilbert-base-uncased-distilled-squad' pretrain model, just use the first six layer of the base one or initialize a six layer bert?<|||||>If you do:
```
qa_model = DistilBertForQuestionAnswering.from_pretrained('distilbert-base-uncased-distilled-squad')
```
You will get the 'distilbert-base-uncased-distilled-squad' pretrain model.
Nothing more to do.<|||||>I am sorry not clearly expressed, my question is how to get the 'distilbert-base-uncased-distilled-squad' pretrain model, I know that use that code can get the six layer layer, but how to train the pretrain model is what I concern, there is no six layer bert release<|||||>Ok, I understand your question now @RyanHuangNLP.
It is finetuned from `distilbert-base-uncased`. More precisely, the model we release is finetuned AND distilled at the same time: the loss is computed from the classic qa loss (see `run_squad.py` and `DistilBertForQuestionAnswering`) plus the distillation supervision from a BERT SQuAD model (second loss).
We haven't released the script for doing that (and we plan to do it in the near future) but it is a simple adaptation of `run_squad.py` (mostly adding a second loss i.e. distillation).<|||||>do we have any release date of that run_squad_adapted.py @VictorSanh ? :D<|||||>This issue has been automatically marked as stale because it has not had recent activity. It will be closed if no further activity occurs. Thank you for your contributions.
|
transformers | 1,192 | closed | Finetuning BertModel to extract textual features for VQA shows bad results | ## ❓ Questions & Help
I am trying to use Bert as a textual feature extractor for VQA.
This is the code for tokenizing question text in VQA.
```
self.tokenizer = pytorch_transformers.BertTokenizer.from_pretrained(
'bert-base-uncased')
q = self.tokenizer.encode(q_text, add_special_tokens=True)
```
This code is for extracting features from Bert.
```
self.bert = pytorch_transformers.BertModel.from_pretrained('bert-base-uncased',
output_attentions=True)
question_mask_cls = torch.arange(lengths[0]).to(self.device)
lengths_cls = lengths
q_mask_cls = question_mask_cls[None, :] < lengths_cls[:, None]
q_mask_cls = q_mask_cls.to(torch.float32)
question_embed_t, _, src_attn_list = self.bert(question_padded,
attention_mask=q_mask_cls)
output, tgt_attn_list, tgt_src_attn_list = self.q_decoder(
tgt=obj_feature_bbox_cls.permute(1, 0, 2),
memory=question_embed_t.permute(1, 0, 2),
memory_key_padding_mask=memory_key_padding_mask,
tgt_key_padding_mask=tgt_key_padding_mask)
```
If I free the parameters of Bert, it gives better results. But when I fine tune the whole model, the model does not seem to learn. I've tried the Adam optimizer of pytorch and AdamW provided by this repository. Both of them does not work.
```
optimizer = pytorch_transformers.optimization.AdamW(model.parameters(), lr=3e-5)
```
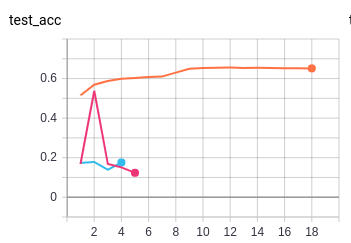
The orange curve shows the model with Bert parameters freezed, while the pink and skyblue curve shows the model that trains Bert parameters.
Are there any potential issues I am missing?
<!-- A clear and concise description of the question. --> | 09-04-2019 09:30:37 | 09-04-2019 09:30:37 | It turned out the learning rate was too high (1e-4) using 3e-5 showed good results. |
transformers | 1,191 | closed | how to use 'spiece.model' to create the xlnet_tokenizer | ## ❓ Questions & Help
For some reason, my computer can not connect to Internet, which means I can not use the code "tokenizer = tokenizer_class.from_pretrained('xlnet-base-cased', do_lower_case = True)" to create the tokenizer.
The Piece model (spiece.model) is used for (de)tokenization, how can I use it to create the tokenizer?
I have tried :
`
from pytorch_transformers import XLNetTokenizer
config = {
'vocab_path' : path.sep.join([BASIC_DIR,'pretrained/pytorch_xlnet_pretrained/spiece.model'])
}
tokenizer = XLNetTokenizer.from_pretrained(config['vocab_path'], do_lower_case = True)
`
it doesn't work. | 09-04-2019 07:15:54 | 09-04-2019 07:15:54 | First, note that you can give proxies to the `from_pretrained` method if your connection problem comes from a proxy (see the doc/docstring for an example).
You can also download the sentence piece model from our S3 bucket (see the top of the `tokenization_xlnet.py` file for the url) and save it in a folder with the name `spiece.model`, then just give this folder path to the `from_pretrained` method.<|||||>This issue has been automatically marked as stale because it has not had recent activity. It will be closed if no further activity occurs. Thank you for your contributions.
|
transformers | 1,190 | closed | Fix reference of import in XLM tokenization | 09-04-2019 01:52:36 | 09-04-2019 01:52:36 | # [Codecov](https://codecov.io/gh/huggingface/pytorch-transformers/pull/1190?src=pr&el=h1) Report
> Merging [#1190](https://codecov.io/gh/huggingface/pytorch-transformers/pull/1190?src=pr&el=desc) into [master](https://codecov.io/gh/huggingface/pytorch-transformers/commit/0287d264e913e10018a95a2723115dc9121e5fc6?src=pr&el=desc) will **decrease** coverage by `0.19%`.
> The diff coverage is `0%`.
[](https://codecov.io/gh/huggingface/pytorch-transformers/pull/1190?src=pr&el=tree)
```diff
@@ Coverage Diff @@
## master #1190 +/- ##
=========================================
- Coverage 80.85% 80.65% -0.2%
=========================================
Files 46 46
Lines 7876 7878 +2
=========================================
- Hits 6368 6354 -14
- Misses 1508 1524 +16
```
| [Impacted Files](https://codecov.io/gh/huggingface/pytorch-transformers/pull/1190?src=pr&el=tree) | Coverage Δ | |
|---|---|---|
| [pytorch\_transformers/tokenization\_xlm.py](https://codecov.io/gh/huggingface/pytorch-transformers/pull/1190/diff?src=pr&el=tree#diff-cHl0b3JjaF90cmFuc2Zvcm1lcnMvdG9rZW5pemF0aW9uX3hsbS5weQ==) | `82.7% <0%> (-0.71%)` | :arrow_down: |
| [...orch\_transformers/tests/tokenization\_utils\_test.py](https://codecov.io/gh/huggingface/pytorch-transformers/pull/1190/diff?src=pr&el=tree#diff-cHl0b3JjaF90cmFuc2Zvcm1lcnMvdGVzdHMvdG9rZW5pemF0aW9uX3V0aWxzX3Rlc3QucHk=) | `92% <0%> (-4%)` | :arrow_down: |
| [...h\_transformers/tests/tokenization\_tests\_commons.py](https://codecov.io/gh/huggingface/pytorch-transformers/pull/1190/diff?src=pr&el=tree#diff-cHl0b3JjaF90cmFuc2Zvcm1lcnMvdGVzdHMvdG9rZW5pemF0aW9uX3Rlc3RzX2NvbW1vbnMucHk=) | `97.16% <0%> (-2.84%)` | :arrow_down: |
| [pytorch\_transformers/modeling\_utils.py](https://codecov.io/gh/huggingface/pytorch-transformers/pull/1190/diff?src=pr&el=tree#diff-cHl0b3JjaF90cmFuc2Zvcm1lcnMvbW9kZWxpbmdfdXRpbHMucHk=) | `88.61% <0%> (-1.46%)` | :arrow_down: |
| [pytorch\_transformers/file\_utils.py](https://codecov.io/gh/huggingface/pytorch-transformers/pull/1190/diff?src=pr&el=tree#diff-cHl0b3JjaF90cmFuc2Zvcm1lcnMvZmlsZV91dGlscy5weQ==) | `70.42% <0%> (-1.41%)` | :arrow_down: |
| [pytorch\_transformers/tokenization\_gpt2.py](https://codecov.io/gh/huggingface/pytorch-transformers/pull/1190/diff?src=pr&el=tree#diff-cHl0b3JjaF90cmFuc2Zvcm1lcnMvdG9rZW5pemF0aW9uX2dwdDIucHk=) | `95.86% <0%> (-0.83%)` | :arrow_down: |
| [pytorch\_transformers/tokenization\_transfo\_xl.py](https://codecov.io/gh/huggingface/pytorch-transformers/pull/1190/diff?src=pr&el=tree#diff-cHl0b3JjaF90cmFuc2Zvcm1lcnMvdG9rZW5pemF0aW9uX3RyYW5zZm9feGwucHk=) | `33.89% <0%> (-0.29%)` | :arrow_down: |
------
[Continue to review full report at Codecov](https://codecov.io/gh/huggingface/pytorch-transformers/pull/1190?src=pr&el=continue).
> **Legend** - [Click here to learn more](https://docs.codecov.io/docs/codecov-delta)
> `Δ = absolute <relative> (impact)`, `ø = not affected`, `? = missing data`
> Powered by [Codecov](https://codecov.io/gh/huggingface/pytorch-transformers/pull/1190?src=pr&el=footer). Last update [0287d26...a15562e](https://codecov.io/gh/huggingface/pytorch-transformers/pull/1190?src=pr&el=lastupdated). Read the [comment docs](https://docs.codecov.io/docs/pull-request-comments).
<|||||>Yes, thanks a lot @shijie-wu! |
|
transformers | 1,189 | closed | Roberta tokenizer fails on certain unicode characters | ## 🐛 Bug
<!-- Important information -->
Model I am using: `Roberta`:
Language I am using the model on (English, Chinese....): English
The problem arise when using:
- The `roberta-base` tokenizer and tokenizing unicode accents
```
from pytorch_transformers import *
tokenizer = RobertaTokenizer.from_pretrained('roberta-base')
phrase = "I visited the Côte d'Azur"
for word in phrase.split():
print(tokenizer.tokenize(word))
```
this outputs:
```
['I']
['vis', 'ited']
['the']
['C', 'ô', 'te']
['d', "'", 'Az', 'ur']
```
## Expected behavior
```
['I']
['vis', 'ited']
['the']
['C', 'ô', 'te']
['d', "'", 'Az', 'ur']
```
## Environment
* OS: MacOS 10.14.6
* Python version: 3.6
* PyTorch version: 1.1.0.post2
* PyTorch Transformers version (or branch): 1.1.0
* Using GPU ? no
* Distributed of parallel setup ? no
* Any other relevant information: xlnet and bert do not face this same tokenization issue. It appears that the issue comes from the gpt2-tokenizer
## Additional context
<!-- Add any other context about the problem here. --> | 09-04-2019 00:53:45 | 09-04-2019 00:53:45 | Does `fairseq` exhibit the same behavior?
If it does, I would ask upstream. But in any case, I'm not sure it's a bug (it's just the internal encoding used by the neural net)<|||||>I think you need to tokenize by XLM-Roberta-Tokenizer.
I tokenized for Korean. |
transformers | 1,188 | closed | BertEncoder head_mask not subscript-able error when not passed | ## 🐛 Bug
BertEncoder takes in head_mask parameters with default value of None but on https://github.com/huggingface/pytorch-transformers/blob/master/pytorch_transformers/modeling_bert.py#L431, ith index is checked without checking if head_mask is None. If by default nothing is passed this results in error.
## Fix
Check head_mask is not None in https://github.com/huggingface/pytorch-transformers/blob/master/pytorch_transformers/modeling_bert.py#L431 | 09-03-2019 23:56:41 | 09-03-2019 23:56:41 | Got same issue. If no `head_mask` is given to `.forward` method of `BertEncoder`, then the following code will cause `TypeError: 'NoneType' object is not subscriptable`.
https://github.com/huggingface/transformers/blob/a701c9b32126f1e6974d9fcb3a5c3700527d8559/transformers/modeling_bert.py#L348
I wonder if there will be a case of using `BertEncoder` without any `head_mask`. Though, this issue should be addressed by checking whether `head_mask` is None, and expand them as size `[config.num_hidden_layers]`.<|||||>This issue has been automatically marked as stale because it has not had recent activity. It will be closed if no further activity occurs. Thank you for your contributions.
<|||||>As bot closed the issue and it is still existing, I provided a fix it in the PR linked above. |
Subsets and Splits
No community queries yet
The top public SQL queries from the community will appear here once available.