repo
stringclasses 1
value | number
int64 1
25.3k
| state
stringclasses 2
values | title
stringlengths 1
487
| body
stringlengths 0
234k
⌀ | created_at
stringlengths 19
19
| closed_at
stringlengths 19
19
| comments
stringlengths 0
293k
|
---|---|---|---|---|---|---|---|
transformers | 1,489 | closed | Excessively Long text_b Raises Unnecessary Warnings in `encode_plus` | In `encode_plus`, `convert_ids_to_tokens` is called before truncating to `max_len`. However, if either text_a or text_b are longer than `max_len`, `convert_ids_to_tokens` will raise a warning. Since sequences are truncated to the right length afterwards in `encode_plus`, this warning is unnecessary. | 10-11-2019 00:56:24 | 10-11-2019 00:56:24 | This issue has been automatically marked as stale because it has not had recent activity. It will be closed if no further activity occurs. Thank you for your contributions.
|
transformers | 1,488 | closed | GLUE on TPU | This takes advantage of the pytorch 1.3 XLA implementation to fine-tune GLUE on a TPU.
MRPC fine-tuning in 3 epochs + evaluation takes a total of 6 minutes and 30 seconds. | 10-10-2019 23:35:02 | 10-10-2019 23:35:02 | # [Codecov](https://codecov.io/gh/huggingface/transformers/pull/1488?src=pr&el=h1) Report
> Merging [#1488](https://codecov.io/gh/huggingface/transformers/pull/1488?src=pr&el=desc) into [master](https://codecov.io/gh/huggingface/transformers/commit/f382a8decda82062bb6911f05b646f404eacfdd4?src=pr&el=desc) will **not change** coverage.
> The diff coverage is `n/a`.
[](https://codecov.io/gh/huggingface/transformers/pull/1488?src=pr&el=tree)
```diff
@@ Coverage Diff @@
## master #1488 +/- ##
=======================================
Coverage 85.59% 85.59%
=======================================
Files 91 91
Lines 13526 13526
=======================================
Hits 11578 11578
Misses 1948 1948
```
------
[Continue to review full report at Codecov](https://codecov.io/gh/huggingface/transformers/pull/1488?src=pr&el=continue).
> **Legend** - [Click here to learn more](https://docs.codecov.io/docs/codecov-delta)
> `Δ = absolute <relative> (impact)`, `ø = not affected`, `? = missing data`
> Powered by [Codecov](https://codecov.io/gh/huggingface/transformers/pull/1488?src=pr&el=footer). Last update [f382a8d...639f4b7](https://codecov.io/gh/huggingface/transformers/pull/1488?src=pr&el=lastupdated). Read the [comment docs](https://docs.codecov.io/docs/pull-request-comments).
<|||||>LGTM!<|||||>It is possible to save/load when on TPU, just move them to CPU and save them:
https://github.com/pytorch/xla/blob/master/API_GUIDE.md#saving-and-loading-xla-tensors |
transformers | 1,487 | closed | convert int to str before adding to a str | 10-10-2019 20:28:01 | 10-10-2019 20:28:01 | # [Codecov](https://codecov.io/gh/huggingface/transformers/pull/1487?src=pr&el=h1) Report
> Merging [#1487](https://codecov.io/gh/huggingface/transformers/pull/1487?src=pr&el=desc) into [master](https://codecov.io/gh/huggingface/transformers/commit/6596e3d56626c921b3920e313866b7412633b91a?src=pr&el=desc) will **not change** coverage.
> The diff coverage is `n/a`.
[](https://codecov.io/gh/huggingface/transformers/pull/1487?src=pr&el=tree)
```diff
@@ Coverage Diff @@
## master #1487 +/- ##
=======================================
Coverage 85.59% 85.59%
=======================================
Files 91 91
Lines 13526 13526
=======================================
Hits 11578 11578
Misses 1948 1948
```
------
[Continue to review full report at Codecov](https://codecov.io/gh/huggingface/transformers/pull/1487?src=pr&el=continue).
> **Legend** - [Click here to learn more](https://docs.codecov.io/docs/codecov-delta)
> `Δ = absolute <relative> (impact)`, `ø = not affected`, `? = missing data`
> Powered by [Codecov](https://codecov.io/gh/huggingface/transformers/pull/1487?src=pr&el=footer). Last update [6596e3d...dd904e2](https://codecov.io/gh/huggingface/transformers/pull/1487?src=pr&el=lastupdated). Read the [comment docs](https://docs.codecov.io/docs/pull-request-comments).
<|||||>Great, thanks! |
|
transformers | 1,486 | closed | Can you please share the pre-processed text dump of the bookcorpus and wikipediacorpus? | ## ❓ Questions & Help
I am trying to train distilbert with different architecture. If you can share the text dump for the pre-training, it would be great. Thanks!
<!-- A clear and concise description of the question. -->
| 10-10-2019 17:55:17 | 10-10-2019 17:55:17 | Hello @kamalravi
For the English Wikipedia data, I followed the scripts in XLM [here](https://github.com/facebookresearch/XLM#train-your-own-monolingual-bert-model). It downloads the latest dump and does the necessary pre-processing.
For BookCorpus, as you probably know, TBC is not distributed anymore and it's not clear to me whether I can distribute it here (I prefer not to). However, there is open-source options to collect a similar dataset (like [this one](https://github.com/soskek/bookcorpus)).
If you are ever interested in Reddit-based dataset, I used [OpenWebTextCorpus](https://skylion007.github.io/OpenWebTextCorpus/) following RoBERTa to distill DistilGPT2.
Having the raw text dumps, I simply use `scripts/binarized_data.py` to pre-process the data.
Victor |
transformers | 1,485 | closed | improve final answer extraction in utils_squad.py | Shouldn't ` get_final_text` use the specific optionally pre-trained tokenizer instead of generically using `BasicTokenizer` ?
[examples/utils_squad.py L911](https://github.com/huggingface/transformers/blob/6596e3d56626c921b3920e313866b7412633b91a/examples/utils_squad.py#L911) | 10-10-2019 15:13:19 | 10-10-2019 15:13:19 | Any update?<|||||>This issue has been automatically marked as stale because it has not had recent activity. It will be closed if no further activity occurs. Thank you for your contributions.
|
transformers | 1,484 | closed | Error while fine-tuning model for GPT2 | ## 🐛 Bug
<!-- Important information -->
Model I am using GPT2:
Language I am using the model on English:
The problem arise when using:
* [ ] the official example scripts: I run the run_lm_finetuning.py script from the examples folder
The tasks I am working on is:
* [ ] my own task or dataset: The WritingPrompts dataset.
## To Reproduce
Steps to reproduce the behavior:
1. Running the run_lm_generation.py on the WP dataset.
python run_lm_finetuning.py --output_dir=output_ft_gpt2 --model_type=gpt2 --model_name_or_path=gpt2 --do_train --train_data_file=../stories_data/writingPrompts/train.wp_target --do_eval --eval_data_file=../stories_data/writingPrompts/test.wp_target --block_size=128 --save_total_limit=100
Error: Traceback (most recent call last):
File "run_lm_finetuning.py", line 538, in <module>
main()
File "run_lm_finetuning.py", line 485, in main
train_dataset = load_and_cache_examples(args, tokenizer, evaluate=False)
File "run_lm_finetuning.py", line 97, in load_and_cache_examples
dataset = TextDataset(tokenizer, file_path=args.eval_data_file if evaluate else args.train_data_file, block_size=args.block_size)
File "run_lm_finetuning.py", line 80, in __init__
self.examples.append(tokenizer.build_inputs_with_special_tokens(tokenized_text[i:i+block_size]))
AttributeError: 'GPT2Tokenizer' object has no attribute 'build_inputs_with_special_tokens'
| 10-10-2019 14:45:57 | 10-10-2019 14:45:57 | Hi! It seems like you have taken the example from the latest version but that your library is not up to date. Could you tell me the version of your `transformers` library ?<|||||>Hi!
I am using the version 2.0.0.<|||||>If you´re using the version 2.0.0 you should use the [script that was used in this version](https://github.com/huggingface/transformers/blob/v2.0.0/examples/run_lm_finetuning.py). The current script works on version 2.1!<|||||>Thanks! |
transformers | 1,483 | closed | Create new | 10-10-2019 12:19:40 | 10-10-2019 12:19:40 | Hi @saksham7778. In order to keep the repository clean we would prefer that people open pull requests once a substantial amount of work has been done. Closing for now. |
|
transformers | 1,482 | closed | Integration of TF 2.0 models with other Keras modules | Add tests that TF 2.0 models can be integrated with other Keras modules.
Add more serialization tests for TF 2.0 and PyTorch models.
Fix TFSequenceSummary head and RoBERTa. | 10-10-2019 11:18:29 | 10-10-2019 11:18:29 | # [Codecov](https://codecov.io/gh/huggingface/transformers/pull/1482?src=pr&el=h1) Report
> Merging [#1482](https://codecov.io/gh/huggingface/transformers/pull/1482?src=pr&el=desc) into [master](https://codecov.io/gh/huggingface/transformers/commit/6596e3d56626c921b3920e313866b7412633b91a?src=pr&el=desc) will **increase** coverage by `0.44%`.
> The diff coverage is `97.67%`.
[](https://codecov.io/gh/huggingface/transformers/pull/1482?src=pr&el=tree)
```diff
@@ Coverage Diff @@
## master #1482 +/- ##
==========================================
+ Coverage 85.59% 86.04% +0.44%
==========================================
Files 91 91
Lines 13526 13566 +40
==========================================
+ Hits 11578 11673 +95
+ Misses 1948 1893 -55
```
| [Impacted Files](https://codecov.io/gh/huggingface/transformers/pull/1482?src=pr&el=tree) | Coverage Δ | |
|---|---|---|
| [transformers/modeling\_tf\_distilbert.py](https://codecov.io/gh/huggingface/transformers/pull/1482/diff?src=pr&el=tree#diff-dHJhbnNmb3JtZXJzL21vZGVsaW5nX3RmX2Rpc3RpbGJlcnQucHk=) | `98.59% <ø> (+2%)` | :arrow_up: |
| [transformers/modeling\_tf\_gpt2.py](https://codecov.io/gh/huggingface/transformers/pull/1482/diff?src=pr&el=tree#diff-dHJhbnNmb3JtZXJzL21vZGVsaW5nX3RmX2dwdDIucHk=) | `94.79% <ø> (+1.31%)` | :arrow_up: |
| [transformers/modeling\_tf\_openai.py](https://codecov.io/gh/huggingface/transformers/pull/1482/diff?src=pr&el=tree#diff-dHJhbnNmb3JtZXJzL21vZGVsaW5nX3RmX29wZW5haS5weQ==) | `96.04% <ø> (+1.43%)` | :arrow_up: |
| [transformers/modeling\_tf\_transfo\_xl.py](https://codecov.io/gh/huggingface/transformers/pull/1482/diff?src=pr&el=tree#diff-dHJhbnNmb3JtZXJzL21vZGVsaW5nX3RmX3RyYW5zZm9feGwucHk=) | `92.21% <ø> (+0.97%)` | :arrow_up: |
| [transformers/modeling\_tf\_bert.py](https://codecov.io/gh/huggingface/transformers/pull/1482/diff?src=pr&el=tree#diff-dHJhbnNmb3JtZXJzL21vZGVsaW5nX3RmX2JlcnQucHk=) | `96.6% <ø> (+0.89%)` | :arrow_up: |
| [transformers/modeling\_tf\_pytorch\_utils.py](https://codecov.io/gh/huggingface/transformers/pull/1482/diff?src=pr&el=tree#diff-dHJhbnNmb3JtZXJzL21vZGVsaW5nX3RmX3B5dG9yY2hfdXRpbHMucHk=) | `76.76% <0%> (-0.17%)` | :arrow_down: |
| [transformers/modeling\_gpt2.py](https://codecov.io/gh/huggingface/transformers/pull/1482/diff?src=pr&el=tree#diff-dHJhbnNmb3JtZXJzL21vZGVsaW5nX2dwdDIucHk=) | `84.19% <100%> (+0.2%)` | :arrow_up: |
| [transformers/modeling\_tf\_ctrl.py](https://codecov.io/gh/huggingface/transformers/pull/1482/diff?src=pr&el=tree#diff-dHJhbnNmb3JtZXJzL21vZGVsaW5nX3RmX2N0cmwucHk=) | `97.75% <100%> (+1.74%)` | :arrow_up: |
| [transformers/tests/modeling\_tf\_xlnet\_test.py](https://codecov.io/gh/huggingface/transformers/pull/1482/diff?src=pr&el=tree#diff-dHJhbnNmb3JtZXJzL3Rlc3RzL21vZGVsaW5nX3RmX3hsbmV0X3Rlc3QucHk=) | `95.74% <100%> (+0.12%)` | :arrow_up: |
| [transformers/tests/modeling\_tf\_common\_test.py](https://codecov.io/gh/huggingface/transformers/pull/1482/diff?src=pr&el=tree#diff-dHJhbnNmb3JtZXJzL3Rlc3RzL21vZGVsaW5nX3RmX2NvbW1vbl90ZXN0LnB5) | `96.53% <100%> (+1.14%)` | :arrow_up: |
| ... and [12 more](https://codecov.io/gh/huggingface/transformers/pull/1482/diff?src=pr&el=tree-more) | |
------
[Continue to review full report at Codecov](https://codecov.io/gh/huggingface/transformers/pull/1482?src=pr&el=continue).
> **Legend** - [Click here to learn more](https://docs.codecov.io/docs/codecov-delta)
> `Δ = absolute <relative> (impact)`, `ø = not affected`, `? = missing data`
> Powered by [Codecov](https://codecov.io/gh/huggingface/transformers/pull/1482?src=pr&el=footer). Last update [6596e3d...4b8f3e8](https://codecov.io/gh/huggingface/transformers/pull/1482?src=pr&el=lastupdated). Read the [comment docs](https://docs.codecov.io/docs/pull-request-comments).
|
transformers | 1,481 | closed | Does run_lm_finetuning.py finetune the entire BERT / Xlnet architecture | ## ❓ Questions & Help
<!-- A clear and concise description of the question. -->
1. When finetuning on data without a task, i.e. **unsupervised finetuning** by running the **run_lm_finetuning.py** script, does the code finetune all the weight layers of the model or just finetunes by adding an extra layer over the top of the architecture?
2. The correct way to do this is to first finetune the top n layers for some epochs and then finetune all the layers. Is this how the finetuning takes place through run_lm_finetuning.py ?
I think answer is no.
I went through the run_lm_finetuning.py code and it seems that the entire model is getting finetuned from the start.
Still having doubt on this as I have little experience with pytorch. | 10-10-2019 09:54:44 | 10-10-2019 09:54:44 | 1) Yes, the entire model is fine-tuned.
2) We follow the fine-tuning that takes place in the BERT paper:
> All of the parameters of BERT and W are fine-tuned jointly to maximize the log-probability of the correct label.<|||||>Thanks.
<|||||>Why would _The correct way to do this is to first finetune the top n layers for some epochs and then finetune all the layers._ be the "one and only correct way to do this"? I've seen this being done to finetune VGG19 (IR), for different tasks, but I haven't see papers showing that one technique will result in better performance than others. Do you have a paper reference? As @LysandreJik indicates, the BERT paper indicates what the authors thing is the most efficient approach.<|||||>Follow up on this thread: in practice, if we have a small training set, would it make sense to only finetune the top n layers?
In that case, do we want to consider adding an option that controls the number of trainable layers?<|||||>You can also consider replacing the classification head first if you find a pre-trained model suiting your task..<|||||>This issue has been automatically marked as stale because it has not had recent activity. It will be closed if no further activity occurs. Thank you for your contributions.
|
transformers | 1,480 | closed | Fixing CTRL tokenizer - Update error messages - XLM-MLM in run_generation | # CTRL tokenizer
We are trying to find a good full-python replacement for the fastBPE tokenizer originally used for CTRL.
We don't really want to depend on fastBPE, even though it's fast, because it's a cython package which means we may then have installation issues on specific platforms like Windows.
Current options are:
- test our own Bert whitespace tokenizer
- uses Moses which is already included (as sacredmoses) for XLM
- using a regex like GPT-2.
Currently favored option is sacredmoses.
cc @LysandreJik @keskarnitish @stefan-it
[UPDATE]: Updating this, upon deeper inspection, fastBPE tokenizer just basically [split on spaces only](https://github.com/glample/fastBPE/blob/master/fastBPE/fastBPE.hpp?fbclid=IwAR1Vp2WMLxDjpmBIpU6mkddeyxzi2vpvHOcm8fL4iaWL1m3tVbSfz-yZAcE#L652).
This tokenizer was used in CTRL which is confirmed by the fact that many vocabulary tokens in CTRL vocabulary contains end or start punctuation (see CTRL vocabulary [here](https://raw.githubusercontent.com/salesforce/ctrl/master/ctrl-vocab.json)).
So the most logical solution is thus just to split on spaces which is also the easiest solution :-)
# Error messages
Improved error messages of `from_pretrained` when files are not found
# XLM MLM in run_generation
Add support for XLM MLM models in run_generation (though these models are not really intended for that anyway). | 10-10-2019 08:15:21 | 10-10-2019 08:15:21 | # [Codecov](https://codecov.io/gh/huggingface/transformers/pull/1480?src=pr&el=h1) Report
> Merging [#1480](https://codecov.io/gh/huggingface/transformers/pull/1480?src=pr&el=desc) into [master](https://codecov.io/gh/huggingface/transformers/commit/036483fae538faff62f78448b38787f3adb94f97?src=pr&el=desc) will **increase** coverage by `0.06%`.
> The diff coverage is `n/a`.
[](https://codecov.io/gh/huggingface/transformers/pull/1480?src=pr&el=tree)
```diff
@@ Coverage Diff @@
## master #1480 +/- ##
==========================================
+ Coverage 85.53% 85.59% +0.06%
==========================================
Files 91 91
Lines 13539 13526 -13
==========================================
- Hits 11580 11578 -2
+ Misses 1959 1948 -11
```
| [Impacted Files](https://codecov.io/gh/huggingface/transformers/pull/1480?src=pr&el=tree) | Coverage Δ | |
|---|---|---|
| [transformers/tokenization\_ctrl.py](https://codecov.io/gh/huggingface/transformers/pull/1480/diff?src=pr&el=tree#diff-dHJhbnNmb3JtZXJzL3Rva2VuaXphdGlvbl9jdHJsLnB5) | `96.03% <ø> (+7.64%)` | :arrow_up: |
| [transformers/tokenization\_utils.py](https://codecov.io/gh/huggingface/transformers/pull/1480/diff?src=pr&el=tree#diff-dHJhbnNmb3JtZXJzL3Rva2VuaXphdGlvbl91dGlscy5weQ==) | `91.43% <ø> (+0.42%)` | :arrow_up: |
| [transformers/configuration\_utils.py](https://codecov.io/gh/huggingface/transformers/pull/1480/diff?src=pr&el=tree#diff-dHJhbnNmb3JtZXJzL2NvbmZpZ3VyYXRpb25fdXRpbHMucHk=) | `97.29% <ø> (ø)` | :arrow_up: |
| [transformers/modeling\_utils.py](https://codecov.io/gh/huggingface/transformers/pull/1480/diff?src=pr&el=tree#diff-dHJhbnNmb3JtZXJzL21vZGVsaW5nX3V0aWxzLnB5) | `92.44% <ø> (ø)` | :arrow_up: |
------
[Continue to review full report at Codecov](https://codecov.io/gh/huggingface/transformers/pull/1480?src=pr&el=continue).
> **Legend** - [Click here to learn more](https://docs.codecov.io/docs/codecov-delta)
> `Δ = absolute <relative> (impact)`, `ø = not affected`, `? = missing data`
> Powered by [Codecov](https://codecov.io/gh/huggingface/transformers/pull/1480?src=pr&el=footer). Last update [036483f...177a721](https://codecov.io/gh/huggingface/transformers/pull/1480?src=pr&el=lastupdated). Read the [comment docs](https://docs.codecov.io/docs/pull-request-comments).
<|||||>Updating this, upon deeper inspection, `fastBPE` tokenizer just basically [split on spaces only](https://github.com/glample/fastBPE/blob/master/fastBPE/fastBPE.hpp?fbclid=IwAR1Vp2WMLxDjpmBIpU6mkddeyxzi2vpvHOcm8fL4iaWL1m3tVbSfz-yZAcE#L652).
This tokenizer was used in CTRL which is confirmed by the fact that many vocabulary tokens in CTRL vocabulary contains end or start punctuation (see CTRL vocabulary [here](https://raw.githubusercontent.com/salesforce/ctrl/master/ctrl-vocab.json)).
So the most logical solution is thus just to split on spaces which is also the easiest solution :-)<|||||>Ok, merging. |
transformers | 1,479 | closed | How can I get the transformers' parameters? | ## ❓ Questions & Help
Hi, I am new to transformers. Does this library offer an interface to compute the total number of different model's parameters?
| 10-10-2019 07:42:15 | 10-10-2019 07:42:15 | The models we use inherit directly from `torch.nn.Module` for our pytorch models and `tf.keras.layers.Layer` for tensorflow modules. You can therefore get the total number of parameters as you would do with any other pytorch/tensorflow modules:
`sum(p.numel() for p in model.parameters() if p.requires_grad)` for pytorch and
`np.sum([np.prod(v.shape) for v in tf.trainable_variables])` for tensorflow, for example.<|||||>Got it! |
transformers | 1,478 | closed | bert-large-uncased-whole-word-masking-finetuned-squad or BertForQuestionAnswering? | ## ❓ Questions & Help
<!-- A clear and concise description of the question. -->
I'm trying to use the pre-trained model bert-large-uncased-whole-word-masking-finetuned-squad to get answer to a question from a text, and I'm able to run:
```
model = BertModel.from_pretrained('bert-large-uncased-whole-word-masking-finetuned-squad')
model.eval()
```
but what should I do next? There's some example code using `BertForQuestionAnswering `:
```
tokenizer = BertTokenizer.from_pretrained('bert-base-uncased')
model = BertForQuestionAnswering.from_pretrained('bert-base-uncased')
input_ids = torch.tensor(tokenizer.encode("Hello, my dog is cute")).unsqueeze(0) # Batch size 1
start_positions = torch.tensor([1])
end_positions = torch.tensor([3])
outputs = model(input_ids, start_positions=start_positions, end_positions=end_positions)
loss, start_scores, end_scores = outputs[:2]
```
But when I try the code above, I get the following error:
```
I1009 23:26:51.743415 4495961408 modeling_utils.py:337] loading weights file https://s3.amazonaws.com/models.huggingface.co/bert/bert-base-uncased-pytorch_model.bin from cache at /Users/ailabby/.cache/torch/transformers/aa1ef1aede4482d0dbcd4d52baad8ae300e60902e88fcb0bebdec09afd232066.36ca03ab34a1a5d5fa7bc3d03d55c4fa650fed07220e2eeebc06ce58d0e9a157
I1009 23:26:54.848274 4495961408 modeling_utils.py:405] Weights of BertForQuestionAnswering not initialized from pretrained model: ['qa_outputs.weight', 'qa_outputs.bias']
I1009 23:26:54.848431 4495961408 modeling_utils.py:408] Weights from pretrained model not used in BertForQuestionAnswering: ['cls.predictions.bias', 'cls.predictions.transform.dense.weight', 'cls.predictions.transform.dense.bias', 'cls.predictions.decoder.weight', 'cls.seq_relationship.weight', 'cls.seq_relationship.bias', 'cls.predictions.transform.LayerNorm.weight', 'cls.predictions.transform.LayerNorm.bias']
---------------------------------------------------------------------------
ValueError Traceback (most recent call last)
<ipython-input-48-0738102265a4> in <module>
5 end_positions = torch.tensor([3])
6 outputs = model(input_ids, start_positions=start_positions, end_positions=end_positions)
----> 7 loss, start_scores, end_scores = outputs[:2]
ValueError: not enough values to unpack (expected 3, got 2)
```
Should I use the pre-trained model bert-large-uncased-whole-word-masking-finetuned-squad or the BertForQuestionAnswering class, or both, to input a text and question and get an answer? Thanks for the help!
| 10-10-2019 06:30:43 | 10-10-2019 06:30:43 | Hey @jeffxtang in your last line you are asking for 3 outputs, but only index from [:2]. You need to change it to
```
loss, start_scores, end_scores = outputs[:3]
```
The documentation is off in that example. As for your last question, I don't entirely understand it; however, BertForQuestionAnswering is the architecture you are using and bert-large-uncased-whole-word-masking-finetuned-squad is the weights (fine tuned on Squad 1.1) you are using in that architecture.
Hope that helps!<|||||>Thanks @cformosa ! My bad, I should've checked the value of outputs instead of just asking for help :)
So my last question is how I can use the Bert model fine tuned on Squad in Python the same way as it's used in [iOS](https://developer.apple.com/machine-learning/models/#text), [which](https://developer.apple.com/documentation/coreml/finding_answers_to_questions_in_a_text_document) expects a text and a question as input then outputs a possible answer from the text. From your answer, BertForQuestionAnswering uses the pre-trained finetuned-on-squad weights so I should be able to just use the BertForQuestionAnswering class? <|||||>I think I'm getting closer to the solution - the code below returns `predictions` with shape [1, 14, 1024]:
```
model = BertModel.from_pretrained('bert-large-uncased-whole-word-masking-finetuned-squad')
model.eval()
tokenizer = BertTokenizer.from_pretrained('bert-base-uncased')
text = "[CLS] Who was Jim Henson ? [SEP] Jim Henson was a nice puppet [SEP]"
tokenized_text = tokenizer.tokenize(text)
indexed_tokens = tokenizer.convert_tokens_to_ids(tokenized_text)
segments_ids = [0, 0, 0, 0, 0, 0, 0, 1, 1, 1, 1, 1, 1, 1]
tokens_tensor = torch.tensor([indexed_tokens])
segments_tensors = torch.tensor([segments_ids])
with torch.no_grad():
outputs = model(tokens_tensor, token_type_ids=segments_tensors)
predictions = outputs[0]
```
So the model with the pre-trained weights `bert-large-uncased-whole-word-masking-finetuned-squad` gets an input with the question "Who was Jim Henson ?" and the text "Jim Henson was a nice puppet" and outputs info that can be used to get the "a nice puppet" answer's indexes (10 and 12) from the `text` value in the code. But why 1024 in the predictions's shape? (14 is the length of the text) I think I'd use argmax on predictions to find out the begin and end indexes of the answer, but how exactly? Thanks!<|||||>OK after a lot of reading and testing, I got my final complete little working program that ends up using `bert-large-uncased-whole-word-masking-finetuned-squad` with `BertForQuestionAnswering`:
```
import torch
from transformers import BertTokenizer, BertForQuestionAnswering
tokenizer = BertTokenizer.from_pretrained('bert-base-uncased')
model = BertForQuestionAnswering.from_pretrained('bert-large-uncased-whole-word-masking-finetuned-squad')
question, text = "Who was Jim Henson?", "Jim Henson was a nice puppet"
input_text = "[CLS] " + question + " [SEP] " + text + " [SEP]"
input_ids = tokenizer.encode(input_text)
token_type_ids = [0 if i <= input_ids.index(102) else 1 for i in range(len(input_ids))]
start_scores, end_scores = model(torch.tensor([input_ids]), token_type_ids=torch.tensor([token_type_ids]))
all_tokens = tokenizer.convert_ids_to_tokens(input_ids)
print(' '.join(all_tokens[torch.argmax(start_scores) : torch.argmax(end_scores)+1]))
# a nice puppet
```
Thanks huggingface for the cool stuff, although your documentation could be cooler :)<|||||>Yes we are always a bit behind on documentation, just too many projects at the same time.
If you want to submit a PR fixing this part of the documentation that you noticed was wrong, that would be the most awesome thing!<|||||>Totally understandable :) and would love to do a PR, but first, I'd like to understand whether what I did is THE right way or one of the right ways to use the `bert-large-uncased-whole-word-masking-finetuned-squad` model.
To be more specific: Can I use also `model = BertModel.from_pretrained('bert-large-uncased-whole-word-masking-finetuned-squad')` to get the right `start_score` and `end_score`? Or dp I have to use `model = BertForQuestionAnswering.from_pretrained('bert-large-uncased-whole-word-masking-finetuned-squad')`?
<|||||>Use `BertForQuestionAnswering`, otherwise your model will not initialize its final span classification layer. <|||||>Thanks for the info. PR created https://github.com/huggingface/transformers/pull/1502<|||||>> OK after a lot of reading and testing, I got my final complete little working program that ends up using `bert-large-uncased-whole-word-masking-finetuned-squad` with `BertForQuestionAnswering`:
>
> ```
> import torch
> from transformers import BertTokenizer, BertForQuestionAnswering
>
> tokenizer = BertTokenizer.from_pretrained('bert-base-uncased')
> model = BertForQuestionAnswering.from_pretrained('bert-large-uncased-whole-word-masking-finetuned-squad')
>
> question, text = "Who was Jim Henson?", "Jim Henson was a nice puppet"
> input_text = "[CLS] " + question + " [SEP] " + text + " [SEP]"
> input_ids = tokenizer.encode(input_text)
> token_type_ids = [0 if i <= input_ids.index(102) else 1 for i in range(len(input_ids))]
>
> start_scores, end_scores = model(torch.tensor([input_ids]), token_type_ids=torch.tensor([token_type_ids]))
> all_tokens = tokenizer.convert_ids_to_tokens(input_ids)
> print(' '.join(all_tokens[torch.argmax(start_scores) : torch.argmax(end_scores)+1]))
> # a nice puppet
> ```
>
> Thanks huggingface for the cool stuff, although your documentation could be cooler :)
@jeffxtang , thanks for sharing this.
There may be an issue with your output. For instance, question, text = "Was Jim Henson a nice puppet?", "Jim Henson was a nice puppet". You answer text could be part of question, because you are using the start_scores/end_scores of all_tokens. It is possible that highest score is within the question.
Thanks.
Luke
<|||||>Thanks @luke4u but I think that's what the Squad-fine-tuned Bert model is supposed to do - its iOS version also returns "Jim Henson was a nice puppet" for the question "Was Jim Henson a nice puppet?", although ideally the answer should be simply "yes". My understanding is that answers returned by the model always have the highest start and end scores located in the text (not the question) - maybe @thomwolf or @julien-c can please verify this?<|||||>This issue has been automatically marked as stale because it has not had recent activity. It will be closed if no further activity occurs. Thank you for your contributions.
<|||||>How I can further fine tune bert-large-uncased-whole-word-masking-finetuned-squad with our domain specific data set?<|||||>@sanigam, please take a look at the [fine-tuning/training](https://huggingface.co/transformers/training.html) documentation. If you're having trouble, please open a new thread with your specific issue on the [forum](https://discuss.huggingface.co). Thanks!<|||||>I tried using the suggested code for using BertForQuestionAnswering but got an error at the end
<img width="980" alt="Screenshot 2021-05-25 at 11 37 56 AM" src="https://user-images.githubusercontent.com/27727185/119447528-a8611c00-bd4d-11eb-943e-fc7b64f70e30.png">
|
transformers | 1,477 | closed | Much slower for inference, even when traced? | ## ❓ Questions & Help
When running inference using BERT-large on a T4 GPU using bert-as-a-service, I could get well over 100/s on sentence pair classification. (I am aware that this utilized TF's graph freezing and pruning)
When running inference with Roberta-large on a T4 GPU using native pytorch and fairseq, I was able to get 70-80/s for inference on sentence pairs.
Even with using the torchscript JIT tracing, **I still am only able to get 17/s on a T4** using the transformers implementation of Bert-large, using a batch size of 8 (which fills most of the memory).
The training performance is similarly worse (about 40% - 100% longer even with apex vs no apex before).
One of the primary differences I can think of is that now I am padding all up to max-seq length, and it does increase performance a lot to decrease this. Is there a way to not pad in transformers? And just pass a list of pytorch tensors in that can be dynamically sized?
Should I try the tensorflow implementations?
Thank you! | 10-10-2019 04:51:45 | 10-10-2019 04:51:45 | Can you fix this sentence? It seems some error slipped in there
> One of the primary differences I can think of is that now I am padding all up to max-seq length, and it does increase performance a lot decrease this.
As far as I know, you don't have to pad up to the max sequence length manually, and you can just pad up to the max sequence length _per batch_. That might save you some time.<|||||>Yeah sorry I meant it increases performance a lot **to** decrease the max-seq-len.
Good point.. I should definitely padding up to max length per batch, although I am not sure this will make huge difference as most of my inputs are of similar length and close to the max.
I guess before I dive deeper I'm looking for a starting place into an investigation of why, say, the implementation of roberta here https://github.com/pytorch/fairseq/tree/master/examples/roberta would be 2x faster on the same GPU than the implementation in transformers.
Does transformers make a conscious performance sacrifice in the name of modularity and extensibility? Or are there specific optimizations in fairseq (for example) that I am observing that have not been ported.
Would updating the new pytorch modules from 1.12 discussed in #1451 make a difference (it seems like there can be performance improvements by fusing kernels so pytorch requires fewer to run the same model, although I do not fully understand this https://pytorch.org/blog/optimizing-cuda-rnn-with-torchscript/)<|||||>I am not sure about any of this, but I do remember that the PyTorch developers do an effort to implement as much parity between CPU and CUDA with specific optimisations for both. As an example, their C++ implementation for activation functions does specific things when MKL is available and otherwise. I'm not sure whether `nn.Transformer` and `nn.MultiheadAttention` got optimised intensively as well.<|||||>@pertschuk these benchmarks are usually mostly dependant on stuff like data-processing, selected float precision, specific inference code (are you in a `torch.no_grad` context for instance) and basically all these things that are outside of the models themselves (which computational graphs are pretty much identical across frameworks).
If you have a (not too big) codebase for benchmarking and clear numbers, we can have a look.<|||||>Yeah, thank you - I am not directly creating a torch.no_grad context, I (perhaps wrongly) assumed this would be handled with a call to .eval(). Also it seems that in the new release pretrained models are by default not loaded in a trainable state? Aka no grad? But perhaps I don't understand correctly.
**Load the model** (takes ~10) and then trace **~2 seconds**
```python
self.model_config = config.from_pretrained(self.checkpoint, cache_dir=self.dir)
self.model_config.num_labels = len(self.config.labels)
self.model_config.torchscript = True
self.model = model.from_pretrained(self.checkpoint, config=self.model_config,
cache_dir=self.dir, **kwargs)
self.tokenizer = tokenizer.from_pretrained(self.checkpoint, cache_dir=self.dir)
self.model.eval()
self.trace_model()
```
Trace function:
```python
def trace_model(self):
examples = [
InputExample(
guid=1,
text_a="Once upon a time there was a boy",
text_b="He liked to write code all day long"
)
]
features = [self.example_to_feature(example) for example in examples]
all_inputs = self.features_to_inputs(features, True)
inputs = self.inputs_from_batch(all_inputs)
self.model = torch.jit.trace(self.model, self.tuple_inputs(inputs))
```
**Run inference** Runs ~18/samples per second or ~2.25 batches (each call to run) with batch size = 8 (helper functions are below). Max_seq_len = 256:
```python
def run(self, *args):
examples = [
InputExample(
guid=str(i),
text_a=arg[0],
text_b=None if len(arg) < 2 else arg[1]
) for i, arg in enumerate(zip(*args))
]
features = [self.example_to_feature(example) for example in examples]
all_inputs = self.features_to_inputs(features, True)
inputs = self.inputs_from_batch(all_inputs)
outputs = self.model(*self.tuple_inputs(inputs))
return self.pred_from_output(outputs)
```
Convert examples to features:
``` python
def example_to_feature(self, example):
inputs = self.tokenizer.encode_plus(
example.text_a,
example.text_b,
add_special_tokens=True,
max_length=self.max_length,
truncate_first_sequence=True # We're truncating the first sequence in priority
)
input_ids, token_type_ids = inputs["input_ids"][:self.max_length], \
inputs["token_type_ids"][:self.max_length]
attention_mask = [1] * len(input_ids)
# Zero-pad up to the sequence length.
if self.pad:
padding_length = self.max_length - len(input_ids)
if self.pad_on_left:
input_ids = ([self.pad_token] * padding_length) + input_ids
attention_mask = ([0] * padding_length) + attention_mask
token_type_ids = ([self.pad_token_segment_id] * padding_length) + token_type_ids
else:
input_ids = input_ids + ([self.pad_token] * padding_length)
attention_mask = attention_mask + ([0] * padding_length)
token_type_ids = token_type_ids + ([self.pad_token_segment_id] * padding_length)
if example.label is not None:
if self.config.task == "classification":
if example.label in self.label_map:
label = self.label_map[example.label]
else:
logger.warning("UNKNOWN LABEL %s, ignoring" % example.label)
return
elif self.config.task == "regression":
label = float(example.label)
else:
logger.error("Only supported tasks are classification and regression")
raise NotImplementedError()
else:
label = None
return InputFeatures(input_ids=input_ids,
attention_mask=attention_mask,
token_type_ids=token_type_ids,
label=label)
```
Convert features to inputs:
```python
def features_to_inputs(self, features, inference):
all_input_ids = torch.tensor([f.input_ids for f in features], dtype=torch.long).to(self.device)
all_attention_mask = torch.tensor([f.attention_mask for f in features], dtype=torch.long).to(self.device)
all_token_type_ids = torch.tensor([f.token_type_ids for f in features], dtype=torch.long).to(self.device)
if not inference:
if self.config.task == "classification":
all_labels = torch.tensor([f.label for f in features], dtype=torch.long).to(self.device)
elif self.config.task == "regression":
all_labels = torch.tensor([f.label for f in features], dtype=torch.float).to(self.device)
else:
raise NotImplementedError()
return all_input_ids, all_attention_mask, all_token_type_ids, all_labels
else:
return all_input_ids, all_attention_mask, all_token_type_ids
```
Return inputs from batch:
```python
def inputs_from_batch(self, batch):
inputs = {'input_ids': batch[0],
'attention_mask': batch[1]}
if self.config.arch != 'distilbert':
inputs['token_type_ids'] = batch[2] if self.config.arch in ['bert',
'xlnet'] else None
if len(batch) > 3:
inputs['labels'] = batch[3]
return inputs
```
source: https://github.com/koursaros-ai/koursaros/blob/master/koursaros/modeling/models/transformer_model.py<|||||>I cleaned and consolidated my code with dynamic padding to current batch size and torch.no_grad() context. Output is below. It seems like the native fairseq/ torchub implementation is a little less than 2x as fast as transformers.
```python
import transformers
from fairseq.data.data_utils import collate_tokens
import time
import torch.nn.functional as F
import torch.hub
MAX_LENGTH = 512
PAD = True
def benchmark_mnli(samples):
torch_hub_model = time_fn(torch.hub.load, 'pytorch/fairseq','roberta.large.mnli')
torch_hub_model.eval()
torch_hub_model.cuda()
try:
transformers_model = time_fn(transformers.RobertaModel.from_pretrained,
'roberta-large-mnli')
except:
transformers_model = time_fn(transformers.RobertaModel.from_pretrained,
'roberta-large-mnli', force_download=True)
transformers_tokenizer = time_fn(transformers.RobertaTokenizer.from_pretrained, 'roberta-large-mnli')
pred_functions = {
'transformers' : predict_transformers(transformers_model, transformers_tokenizer),
'torch_hub' : predict_roberta(torch_hub_model)
}
for framework, pred_fn in pred_functions.items():
print(f'Benchmarking {framework} with {samples} samples')
time_fn(benchmark, pred_fn, samples)
def predict_transformers(model, tokenizer):
device = torch.device("cuda" if torch.cuda.is_available() else "cpu")
model = model.to(device)
def predict_fn(*args):
inputs = time_fn(transformers_encode_batch, tokenizer, *args)
inputs_dict = {
'input_ids': torch.tensor(inputs[0], dtype=torch.long).to(device),
'attention_mask': torch.tensor(inputs[1], dtype=torch.long).to(device),
# 'token_type_ids': torch.tensor(inputs[2], dtype=torch.long)
}
outputs = model(**inputs_dict)
logits = outputs[0]
preds = F.log_softmax(logits, dim=-1)
return preds.tolist()
return predict_fn
def predict_roberta(model):
def pred_fn(*args):
batch = time_fn(collate_tokens, [model.encode(*arg)[:MAX_LENGTH] for arg in zip(*args)], pad_idx=1)
labels = model.predict('mnli', batch).tolist()
return labels
return pred_fn
def benchmark(pred_fn, n):
args = ['All work and no play.'] * 8, ['Make jack a very dull boy.'] * 8
for i in range(0, n):
assert(type(pred_fn(*args)) == list)
### HELPERS
def time_fn(fn, *args, **kwargs):
start = time.time()
res = fn(*args, **kwargs)
print(f'Took {time.time() - start} seconds to run {fn.__name__}')
return res
def transformer_to_features(tokenizer, *args):
inputs = tokenizer.encode_plus(
*args,
add_special_tokens=True,
max_length=MAX_LENGTH,
truncate_first_sequence=True
)
input_ids = inputs["input_ids"][:MAX_LENGTH]
return input_ids
def pad_up(input_ids, max_length):
padding_length = max_length - len(input_ids)
input_ids = ([0] * padding_length) + input_ids
attention_mask = ([0] * padding_length) + [1] * len(input_ids)
return (input_ids, attention_mask)
def transformers_encode_batch(tokenizer, *args):
assert(type(args[0]) == list)
all_input_ids = []
max_batch_len = 0
for sample in zip(*args):
input_ids = transformer_to_features(tokenizer, *sample)
all_input_ids.append(input_ids)
max_batch_len = max(max_batch_len, len(input_ids))
all_input_ids, all_attention_masks = zip(*[
pad_up(input_ids, max_batch_len) for input_ids in all_input_ids
])
return all_input_ids, all_attention_masks
if __name__ == '__main__':
with torch.no_grad():
benchmark_mnli(10)
```
Here is the output:
```
Took 11.221294641494751 seconds to run load
Took 10.316125392913818 seconds to run from_pretrained
Took 0.3631258010864258 seconds to run from_pretrained
Benchmarking transformers with 10 samples
Took 0.00434112548828125 seconds to run transformers_encode_batch
Took 0.0039653778076171875 seconds to run transformers_encode_batch
Took 0.003747701644897461 seconds to run transformers_encode_batch
Took 0.0035974979400634766 seconds to run transformers_encode_batch
Took 0.0037157535552978516 seconds to run transformers_encode_batch
Took 0.003725767135620117 seconds to run transformers_encode_batch
Took 0.0038688182830810547 seconds to run transformers_encode_batch
Took 0.004169464111328125 seconds to run transformers_encode_batch
Took 0.003767728805541992 seconds to run transformers_encode_batch
Took 0.003550291061401367 seconds to run transformers_encode_batch
Took 0.7687280178070068 seconds to run benchmark
Benchmarking torch_hub with 10 samples
Took 0.0001957416534423828 seconds to run collate_tokens
Took 8.797645568847656e-05 seconds to run collate_tokens
Took 6.890296936035156e-05 seconds to run collate_tokens
Took 6.961822509765625e-05 seconds to run collate_tokens
Took 6.914138793945312e-05 seconds to run collate_tokens
Took 6.961822509765625e-05 seconds to run collate_tokens
Took 7.05718994140625e-05 seconds to run collate_tokens
Took 9.202957153320312e-05 seconds to run collate_tokens
Took 6.961822509765625e-05 seconds to run collate_tokens
Took 7.700920104980469e-05 seconds to run collate_tokens
Took 0.4018120765686035 seconds to run benchmark
```
Or with a longer sample input:
```
Took 10.34562063217163 seconds to run load
Took 10.523965835571289 seconds to run from_pretrained
Took 0.4653303623199463 seconds to run from_pretrained
Benchmarking transformers with 10 samples
Took 0.007193565368652344 seconds to run transformers_encode_batch
Took 0.005567789077758789 seconds to run transformers_encode_batch
Took 0.005621671676635742 seconds to run transformers_encode_batch
Took 0.006003141403198242 seconds to run transformers_encode_batch
Took 0.0061550140380859375 seconds to run transformers_encode_batch
Took 0.005508899688720703 seconds to run transformers_encode_batch
Took 0.005594730377197266 seconds to run transformers_encode_batch
Took 0.005545854568481445 seconds to run transformers_encode_batch
Took 0.005563259124755859 seconds to run transformers_encode_batch
Took 0.0059223175048828125 seconds to run transformers_encode_batch
Took 1.5394785404205322 seconds to run benchmark
Benchmarking torch_hub with 10 samples
Took 0.0001571178436279297 seconds to run collate_tokens
Took 9.131431579589844e-05 seconds to run collate_tokens
Took 9.322166442871094e-05 seconds to run collate_tokens
Took 8.7738037109375e-05 seconds to run collate_tokens
Took 8.726119995117188e-05 seconds to run collate_tokens
Took 8.726119995117188e-05 seconds to run collate_tokens
Took 8.869171142578125e-05 seconds to run collate_tokens
Took 8.96453857421875e-05 seconds to run collate_tokens
Took 8.58306884765625e-05 seconds to run collate_tokens
Took 8.869171142578125e-05 seconds to run collate_tokens
Took 0.9851493835449219 seconds to run benchmark
```
I benchmarked the traced transformer model and it's about the same.<|||||>You closed this, but I'm curious to hear about your result and thoughts. So fairseq/HUB implementation is twice as fast as the transformers implementation? Do you have any intuition about why? |
transformers | 1,476 | closed | RuntimeError: Error(s) in loading state_dict for BertModel: | ## ❓ Questions & Help
<!-- A clear and concise description of the question. -->
Hello, I need to use "py torch_model.bin" in a model. I used your "convert_bert_original_tf_checkpoint_to_pytorch.py" to generate bin file, but I used "model_bert.load_state_dict (torch.load (init_checkpoint, map_location='cpu') to load it in my code, except for the following mistakes:
Traceback (most recent call last):
File "train.py", line 579, in <module>
Model, model_bert, tokenizer, bert_config = get_models (args, BERT_PT_PATH)
File "train.py", line 157, in get_models
Args.no_pretraining)
File "train.py", line 125, in get_bert
Model_bert. load_state_dict (torch. load (init_checkpoint, map_location='cpu'))
File "/ home/ubuntu/anaconda3/lib/python 3.7/site-packages/torch/nn/modules/module.py", line 777, in load_state_dict
Self. class. Name, "n T. join (error_msgs)"
Runtime Error: Error (s) in loading state_dict for BertModel:
Missing key (s) in state_dict: "embeddings. word_embeddings. weight", "embeddings. position_embeddings. weight".
thanks | 10-10-2019 01:53:09 | 10-10-2019 01:53:09 | This issue has been automatically marked as stale because it has not had recent activity. It will be closed if no further activity occurs. Thank you for your contributions.
<|||||>try:
device = torch.device("cuda" if torch.cuda.is_available() else "cpu")
model_path = None
model = my_model.load_state_dict(torch.load(model_path, map_location=device)) |
transformers | 1,475 | closed | data loader for varying length input | ## ❓ Questions & Help
<!-- A clear and concise description of the question. -->
| 10-09-2019 21:06:22 | 10-09-2019 21:06:22 | |
transformers | 1,474 | closed | 'LayerNorm' object has no attribute 'cls' | ## ❓ Questions & Help
<!-- A clear and concise description of the question. -->
I try to use `load_tf_weights_in_bert` to convert my fine-tuned tf classification model in Pytorch. I original trained the model by tensorflow BERT.
I used this code:
```import torch
from transformers.modeling_bert import BertConfig, BertForPreTraining, load_tf_weights_in_bert, BertForSequenceClassification
tf_checkpoint_path="./model.ckpt-98400"
bert_config_file = "./bert_config.json"
pytorch_dump_path="pytorch_bert"
config = BertConfig.from_json_file(bert_config_file)
config.num_labels = 21
print("Building PyTorch model from configuration: {}".format(str(config)))
model = BertForSequenceClassification(config)
# Load weights from tf checkpoint
load_tf_weights_in_bert(model, config, tf_checkpoint_path)
# Save pytorch-model
print("Save PyTorch model to {}".format(pytorch_dump_path))
torch.save(model.state_dict(), pytorch_dump_path)
```
I noticed this method to solve my problem: https://github.com/huggingface/transformers/issues/580#issuecomment-489519231
I add to line in `modeling_bert.py`.
``` elif l[0] == 'output_bias' or l[0] == 'beta':
pointer = getattr(pointer, 'cls')
pointer = getattr(pointer, 'bias')
elif l[0] == 'output_weights':
pointer = getattr(pointer, 'cls')
pointer = getattr(pointer, 'weight')
```
But I still get error `AttributeError: 'LayerNorm' object has no attribute 'cls'`
```
Building PyTorch model from configuration: {
"attention_probs_dropout_prob": 0.1,
"directionality": "bidi",
"finetuning_task": null,
"hidden_act": "gelu",
"hidden_dropout_prob": 0.1,
"hidden_size": 768,
"initializer_range": 0.02,
"intermediate_size": 3072,
"layer_norm_eps": 1e-12,
"max_position_embeddings": 512,
"num_attention_heads": 12,
"num_hidden_layers": 12,
"num_labels": 21,
"output_attentions": false,
"output_hidden_states": false,
"pooler_fc_size": 768,
"pooler_num_attention_heads": 12,
"pooler_num_fc_layers": 3,
"pooler_size_per_head": 128,
"pooler_type": "first_token_transform",
"pruned_heads": {},
"torchscript": false,
"type_vocab_size": 2,
"use_bfloat16": false,
"vocab_size": 119547
}
I1009 15:47:03.337315 47631408520768 modeling_bert.py:65] Converting TensorFlow checkpoint from ./model.ckpt-98400
I1009 15:47:03.344796 47631408520768 modeling_bert.py:71] Loading TF weight bert/embeddings/LayerNorm/beta with shape [768]
I1009 15:47:03.350771 47631408520768 modeling_bert.py:71] Loading TF weight bert/embeddings/LayerNorm/beta/adam_m with shape [768]
I1009 15:47:03.356130 47631408520768 modeling_bert.py:71] Loading TF weight bert/embeddings/LayerNorm/beta/adam_v with shape [768]
I1009 15:47:03.361214 47631408520768 modeling_bert.py:71] Loading TF weight bert/embeddings/LayerNorm/gamma with shape [768]
I1009 15:47:03.366278 47631408520768 modeling_bert.py:71] Loading TF weight bert/embeddings/LayerNorm/gamma/adam_m with shape [768]
I1009 15:47:03.371291 47631408520768 modeling_bert.py:71] Loading TF weight bert/embeddings/LayerNorm/gamma/adam_v with shape [768]
I1009 15:47:03.376359 47631408520768 modeling_bert.py:71] Loading TF weight bert/embeddings/position_embeddings with shape [512, 768]
I1009 15:47:03.383015 47631408520768 modeling_bert.py:71] Loading TF weight bert/embeddings/position_embeddings/adam_m with shape [512, 768]
I1009 15:47:03.388718 47631408520768 modeling_bert.py:71] Loading TF weight bert/embeddings/position_embeddings/adam_v with shape [512, 768]
I1009 15:47:03.394378 47631408520768 modeling_bert.py:71] Loading TF weight bert/embeddings/token_type_embeddings with shape [2, 768]
I1009 15:47:03.400012 47631408520768 modeling_bert.py:71] Loading TF weight bert/embeddings/token_type_embeddings/adam_m with shape [2, 768]
I1009 15:47:03.405249 47631408520768 modeling_bert.py:71] Loading TF weight bert/embeddings/token_type_embeddings/adam_v with shape [2, 768]
I1009 15:47:03.410350 47631408520768 modeling_bert.py:71] Loading TF weight bert/embeddings/word_embeddings with shape [119547, 768]
I1009 15:47:03.575059 47631408520768 modeling_bert.py:71] Loading TF weight bert/embeddings/word_embeddings/adam_m with shape [119547, 768]
I1009 15:47:03.743357 47631408520768 modeling_bert.py:71] Loading TF weight bert/embeddings/word_embeddings/adam_v with shape [119547, 768]
I1009 15:47:03.908991 47631408520768 modeling_bert.py:71] Loading TF weight bert/encoder/layer_0/attention/output/LayerNorm/beta with shape [768]
I1009 15:47:03.915453 47631408520768 modeling_bert.py:71] Loading TF weight bert/encoder/layer_0/attention/output/LayerNorm/beta/adam_m with shape [768]
I1009 15:47:03.921177 47631408520768 modeling_bert.py:71] Loading TF weight bert/encoder/layer_0/attention/output/LayerNorm/beta/adam_v with shape [768]
I1009 15:47:03.926633 47631408520768 modeling_bert.py:71] Loading TF weight bert/encoder/layer_0/attention/output/LayerNorm/gamma with shape [768]
I1009 15:47:03.932333 47631408520768 modeling_bert.py:71] Loading TF weight bert/encoder/layer_0/attention/output/LayerNorm/gamma/adam_m with shape [768]
I1009 15:47:03.937757 47631408520768 modeling_bert.py:71] Loading TF weight bert/encoder/layer_0/attention/output/LayerNorm/gamma/adam_v with shape [768]
I1009 15:47:03.942878 47631408520768 modeling_bert.py:71] Loading TF weight bert/encoder/layer_0/attention/output/dense/bias with shape [768]
I1009 15:47:03.947972 47631408520768 modeling_bert.py:71] Loading TF weight bert/encoder/layer_0/attention/output/dense/bias/adam_m with shape [768]
I1009 15:47:03.953052 47631408520768 modeling_bert.py:71] Loading TF weight bert/encoder/layer_0/attention/output/dense/bias/adam_v with shape [768]
I1009 15:47:03.958150 47631408520768 modeling_bert.py:71] Loading TF weight bert/encoder/layer_0/attention/output/dense/kernel with shape [768, 768]
I1009 15:47:03.964268 47631408520768 modeling_bert.py:71] Loading TF weight bert/encoder/layer_0/attention/output/dense/kernel/adam_m with shape [768, 768]
I1009 15:47:03.970259 47631408520768 modeling_bert.py:71] Loading TF weight bert/encoder/layer_0/attention/output/dense/kernel/adam_v with shape [768, 768]
I1009 15:47:03.976348 47631408520768 modeling_bert.py:71] Loading TF weight bert/encoder/layer_0/attention/self/key/bias with shape [768]
I1009 15:47:03.981755 47631408520768 modeling_bert.py:71] Loading TF weight bert/encoder/layer_0/attention/self/key/bias/adam_m with shape [768]
I1009 15:47:03.986970 47631408520768 modeling_bert.py:71] Loading TF weight bert/encoder/layer_0/attention/self/key/bias/adam_v with shape [768]
I1009 15:47:03.992337 47631408520768 modeling_bert.py:71] Loading TF weight bert/encoder/layer_0/attention/self/key/kernel with shape [768, 768]
I1009 15:47:03.998313 47631408520768 modeling_bert.py:71] Loading TF weight bert/encoder/layer_0/attention/self/key/kernel/adam_m with shape [768, 768]
I1009 15:47:04.004308 47631408520768 modeling_bert.py:71] Loading TF weight bert/encoder/layer_0/attention/self/key/kernel/adam_v with shape [768, 768]
I1009 15:47:04.010322 47631408520768 modeling_bert.py:71] Loading TF weight bert/encoder/layer_0/attention/self/query/bias with shape [768]
I1009 15:47:04.015577 47631408520768 modeling_bert.py:71] Loading TF weight bert/encoder/layer_0/attention/self/query/bias/adam_m with shape [768]
I1009 15:47:04.020884 47631408520768 modeling_bert.py:71] Loading TF weight bert/encoder/layer_0/attention/self/query/bias/adam_v with shape [768]
I1009 15:47:04.026228 47631408520768 modeling_bert.py:71] Loading TF weight bert/encoder/layer_0/attention/self/query/kernel with shape [768, 768]
I1009 15:47:04.032151 47631408520768 modeling_bert.py:71] Loading TF weight bert/encoder/layer_0/attention/self/query/kernel/adam_m with shape [768, 768]
I1009 15:47:04.038157 47631408520768 modeling_bert.py:71] Loading TF weight bert/encoder/layer_0/attention/self/query/kernel/adam_v with shape [768, 768]
I1009 15:47:04.044193 47631408520768 modeling_bert.py:71] Loading TF weight bert/encoder/layer_0/attention/self/value/bias with shape [768]
I1009 15:47:04.049786 47631408520768 modeling_bert.py:71] Loading TF weight bert/encoder/layer_0/attention/self/value/bias/adam_m with shape [768]
I1009 15:47:04.055586 47631408520768 modeling_bert.py:71] Loading TF weight bert/encoder/layer_0/attention/self/value/bias/adam_v with shape [768]
I1009 15:47:04.060960 47631408520768 modeling_bert.py:71] Loading TF weight bert/encoder/layer_0/attention/self/value/kernel with shape [768, 768]
I1009 15:47:04.067193 47631408520768 modeling_bert.py:71] Loading TF weight bert/encoder/layer_0/attention/self/value/kernel/adam_m with shape [768, 768]
I1009 15:47:04.073462 47631408520768 modeling_bert.py:71] Loading TF weight bert/encoder/layer_0/attention/self/value/kernel/adam_v with shape [768, 768]
I1009 15:47:04.079773 47631408520768 modeling_bert.py:71] Loading TF weight bert/encoder/layer_0/intermediate/dense/bias with shape [3072]
I1009 15:47:04.084890 47631408520768 modeling_bert.py:71] Loading TF weight bert/encoder/layer_0/intermediate/dense/bias/adam_m with shape [3072]
I1009 15:47:04.090381 47631408520768 modeling_bert.py:71] Loading TF weight bert/encoder/layer_0/intermediate/dense/bias/adam_v with shape [3072]
I1009 15:47:04.096321 47631408520768 modeling_bert.py:71] Loading TF weight bert/encoder/layer_0/intermediate/dense/kernel with shape [768, 3072]
I1009 15:47:04.105394 47631408520768 modeling_bert.py:71] Loading TF weight bert/encoder/layer_0/intermediate/dense/kernel/adam_m with shape [768, 3072]
I1009 15:47:04.114429 47631408520768 modeling_bert.py:71] Loading TF weight bert/encoder/layer_0/intermediate/dense/kernel/adam_v with shape [768, 3072]
I1009 15:47:04.123474 47631408520768 modeling_bert.py:71] Loading TF weight bert/encoder/layer_0/output/LayerNorm/beta with shape [768]
I1009 15:47:04.128776 47631408520768 modeling_bert.py:71] Loading TF weight bert/encoder/layer_0/output/LayerNorm/beta/adam_m with shape [768]
I1009 15:47:04.133818 47631408520768 modeling_bert.py:71] Loading TF weight bert/encoder/layer_0/output/LayerNorm/beta/adam_v with shape [768]
I1009 15:47:04.138939 47631408520768 modeling_bert.py:71] Loading TF weight bert/encoder/layer_0/output/LayerNorm/gamma with shape [768]
I1009 15:47:04.144054 47631408520768 modeling_bert.py:71] Loading TF weight bert/encoder/layer_0/output/LayerNorm/gamma/adam_m with shape [768]
I1009 15:47:04.149456 47631408520768 modeling_bert.py:71] Loading TF weight bert/encoder/layer_0/output/LayerNorm/gamma/adam_v with shape [768]
I1009 15:47:04.154736 47631408520768 modeling_bert.py:71] Loading TF weight bert/encoder/layer_0/output/dense/bias with shape [768]
I1009 15:47:04.159813 47631408520768 modeling_bert.py:71] Loading TF weight bert/encoder/layer_0/output/dense/bias/adam_m with shape [768]
I1009 15:47:04.165272 47631408520768 modeling_bert.py:71] Loading TF weight bert/encoder/layer_0/output/dense/bias/adam_v with shape [768]
I1009 15:47:04.170530 47631408520768 modeling_bert.py:71] Loading TF weight bert/encoder/layer_0/output/dense/kernel with shape [3072, 768]
I1009 15:47:04.179566 47631408520768 modeling_bert.py:71] Loading TF weight bert/encoder/layer_0/output/dense/kernel/adam_m with shape [3072, 768]
I1009 15:47:04.188571 47631408520768 modeling_bert.py:71] Loading TF weight bert/encoder/layer_0/output/dense/kernel/adam_v with shape [3072, 768]
I1009 15:47:04.197654 47631408520768 modeling_bert.py:71] Loading TF weight bert/encoder/layer_1/attention/output/LayerNorm/beta with shape [768]
I1009 15:47:04.203165 47631408520768 modeling_bert.py:71] Loading TF weight bert/encoder/layer_1/attention/output/LayerNorm/beta/adam_m with shape [768]
I1009 15:47:04.208374 47631408520768 modeling_bert.py:71] Loading TF weight bert/encoder/layer_1/attention/output/LayerNorm/beta/adam_v with shape [768]
I1009 15:47:04.213510 47631408520768 modeling_bert.py:71] Loading TF weight bert/encoder/layer_1/attention/output/LayerNorm/gamma with shape [768]
I1009 15:47:04.218636 47631408520768 modeling_bert.py:71] Loading TF weight bert/encoder/layer_1/attention/output/LayerNorm/gamma/adam_m with shape [768]
I1009 15:47:04.223767 47631408520768 modeling_bert.py:71] Loading TF weight bert/encoder/layer_1/attention/output/LayerNorm/gamma/adam_v with shape [768]
I1009 15:47:04.229122 47631408520768 modeling_bert.py:71] Loading TF weight bert/encoder/layer_1/attention/output/dense/bias with shape [768]
I1009 15:47:04.234088 47631408520768 modeling_bert.py:71] Loading TF weight bert/encoder/layer_1/attention/output/dense/bias/adam_m with shape [768]
I1009 15:47:04.239236 47631408520768 modeling_bert.py:71] Loading TF weight bert/encoder/layer_1/attention/output/dense/bias/adam_v with shape [768]
I1009 15:47:04.244547 47631408520768 modeling_bert.py:71] Loading TF weight bert/encoder/layer_1/attention/output/dense/kernel with shape [768, 768]
I1009 15:47:04.250508 47631408520768 modeling_bert.py:71] Loading TF weight bert/encoder/layer_1/attention/output/dense/kernel/adam_m with shape [768, 768]
I1009 15:47:04.256635 47631408520768 modeling_bert.py:71] Loading TF weight bert/encoder/layer_1/attention/output/dense/kernel/adam_v with shape [768, 768]
I1009 15:47:04.262910 47631408520768 modeling_bert.py:71] Loading TF weight bert/encoder/layer_1/attention/self/key/bias with shape [768]
I1009 15:47:04.268387 47631408520768 modeling_bert.py:71] Loading TF weight bert/encoder/layer_1/attention/self/key/bias/adam_m with shape [768]
I1009 15:47:04.273391 47631408520768 modeling_bert.py:71] Loading TF weight bert/encoder/layer_1/attention/self/key/bias/adam_v with shape [768]
I1009 15:47:04.278625 47631408520768 modeling_bert.py:71] Loading TF weight bert/encoder/layer_1/attention/self/key/kernel with shape [768, 768]
I1009 15:47:04.284610 47631408520768 modeling_bert.py:71] Loading TF weight bert/encoder/layer_1/attention/self/key/kernel/adam_m with shape [768, 768]
I1009 15:47:04.290718 47631408520768 modeling_bert.py:71] Loading TF weight bert/encoder/layer_1/attention/self/key/kernel/adam_v with shape [768, 768]
I1009 15:47:04.296881 47631408520768 modeling_bert.py:71] Loading TF weight bert/encoder/layer_1/attention/self/query/bias with shape [768]
I1009 15:47:04.302387 47631408520768 modeling_bert.py:71] Loading TF weight bert/encoder/layer_1/attention/self/query/bias/adam_m with shape [768]
I1009 15:47:04.307479 47631408520768 modeling_bert.py:71] Loading TF weight bert/encoder/layer_1/attention/self/query/bias/adam_v with shape [768]
I1009 15:47:04.312391 47631408520768 modeling_bert.py:71] Loading TF weight bert/encoder/layer_1/attention/self/query/kernel with shape [768, 768]
I1009 15:47:04.318128 47631408520768 modeling_bert.py:71] Loading TF weight bert/encoder/layer_1/attention/self/query/kernel/adam_m with shape [768, 768]
I1009 15:47:04.324467 47631408520768 modeling_bert.py:71] Loading TF weight bert/encoder/layer_1/attention/self/query/kernel/adam_v with shape [768, 768]
I1009 15:47:04.330887 47631408520768 modeling_bert.py:71] Loading TF weight bert/encoder/layer_1/attention/self/value/bias with shape [768]
I1009 15:47:04.336219 47631408520768 modeling_bert.py:71] Loading TF weight bert/encoder/layer_1/attention/self/value/bias/adam_m with shape [768]
I1009 15:47:04.341366 47631408520768 modeling_bert.py:71] Loading TF weight bert/encoder/layer_1/attention/self/value/bias/adam_v with shape [768]
I1009 15:47:04.346387 47631408520768 modeling_bert.py:71] Loading TF weight bert/encoder/layer_1/attention/self/value/kernel with shape [768, 768]
I1009 15:47:04.352337 47631408520768 modeling_bert.py:71] Loading TF weight bert/encoder/layer_1/attention/self/value/kernel/adam_m with shape [768, 768]
I1009 15:47:04.358580 47631408520768 modeling_bert.py:71] Loading TF weight bert/encoder/layer_1/attention/self/value/kernel/adam_v with shape [768, 768]
I1009 15:47:04.365348 47631408520768 modeling_bert.py:71] Loading TF weight bert/encoder/layer_1/intermediate/dense/bias with shape [3072]
I1009 15:47:04.371170 47631408520768 modeling_bert.py:71] Loading TF weight bert/encoder/layer_1/intermediate/dense/bias/adam_m with shape [3072]
I1009 15:47:04.376696 47631408520768 modeling_bert.py:71] Loading TF weight bert/encoder/layer_1/intermediate/dense/bias/adam_v with shape [3072]
I1009 15:47:04.381763 47631408520768 modeling_bert.py:71] Loading TF weight bert/encoder/layer_1/intermediate/dense/kernel with shape [768, 3072]
I1009 15:47:04.390794 47631408520768 modeling_bert.py:71] Loading TF weight bert/encoder/layer_1/intermediate/dense/kernel/adam_m with shape [768, 3072]
I1009 15:47:04.400085 47631408520768 modeling_bert.py:71] Loading TF weight bert/encoder/layer_1/intermediate/dense/kernel/adam_v with shape [768, 3072]
I1009 15:47:04.409393 47631408520768 modeling_bert.py:71] Loading TF weight bert/encoder/layer_1/output/LayerNorm/beta with shape [768]
I1009 15:47:04.414902 47631408520768 modeling_bert.py:71] Loading TF weight bert/encoder/layer_1/output/LayerNorm/beta/adam_m with shape [768]
I1009 15:47:04.420410 47631408520768 modeling_bert.py:71] Loading TF weight bert/encoder/layer_1/output/LayerNorm/beta/adam_v with shape [768]
I1009 15:47:04.425521 47631408520768 modeling_bert.py:71] Loading TF weight bert/encoder/layer_1/output/LayerNorm/gamma with shape [768]
I1009 15:47:04.430895 47631408520768 modeling_bert.py:71] Loading TF weight bert/encoder/layer_1/output/LayerNorm/gamma/adam_m with shape [768]
I1009 15:47:04.436064 47631408520768 modeling_bert.py:71] Loading TF weight bert/encoder/layer_1/output/LayerNorm/gamma/adam_v with shape [768]
I1009 15:47:04.441035 47631408520768 modeling_bert.py:71] Loading TF weight bert/encoder/layer_1/output/dense/bias with shape [768]
I1009 15:47:04.446047 47631408520768 modeling_bert.py:71] Loading TF weight bert/encoder/layer_1/output/dense/bias/adam_m with shape [768]
I1009 15:47:04.451446 47631408520768 modeling_bert.py:71] Loading TF weight bert/encoder/layer_1/output/dense/bias/adam_v with shape [768]
I1009 15:47:04.456856 47631408520768 modeling_bert.py:71] Loading TF weight bert/encoder/layer_1/output/dense/kernel with shape [3072, 768]
I1009 15:47:04.465820 47631408520768 modeling_bert.py:71] Loading TF weight bert/encoder/layer_1/output/dense/kernel/adam_m with shape [3072, 768]
I1009 15:47:04.474748 47631408520768 modeling_bert.py:71] Loading TF weight bert/encoder/layer_1/output/dense/kernel/adam_v with shape [3072, 768]
I1009 15:47:04.484099 47631408520768 modeling_bert.py:71] Loading TF weight bert/encoder/layer_10/attention/output/LayerNorm/beta with shape [768]
I1009 15:47:04.489739 47631408520768 modeling_bert.py:71] Loading TF weight bert/encoder/layer_10/attention/output/LayerNorm/beta/adam_m with shape [768]
I1009 15:47:04.495579 47631408520768 modeling_bert.py:71] Loading TF weight bert/encoder/layer_10/attention/output/LayerNorm/beta/adam_v with shape [768]
I1009 15:47:04.500854 47631408520768 modeling_bert.py:71] Loading TF weight bert/encoder/layer_10/attention/output/LayerNorm/gamma with shape [768]
I1009 15:47:04.506203 47631408520768 modeling_bert.py:71] Loading TF weight bert/encoder/layer_10/attention/output/LayerNorm/gamma/adam_m with shape [768]
I1009 15:47:04.511337 47631408520768 modeling_bert.py:71] Loading TF weight bert/encoder/layer_10/attention/output/LayerNorm/gamma/adam_v with shape [768]
I1009 15:47:04.516572 47631408520768 modeling_bert.py:71] Loading TF weight bert/encoder/layer_10/attention/output/dense/bias with shape [768]
I1009 15:47:04.521539 47631408520768 modeling_bert.py:71] Loading TF weight bert/encoder/layer_10/attention/output/dense/bias/adam_m with shape [768]
I1009 15:47:04.526908 47631408520768 modeling_bert.py:71] Loading TF weight bert/encoder/layer_10/attention/output/dense/bias/adam_v with shape [768]
I1009 15:47:04.532043 47631408520768 modeling_bert.py:71] Loading TF weight bert/encoder/layer_10/attention/output/dense/kernel with shape [768, 768]
I1009 15:47:04.538394 47631408520768 modeling_bert.py:71] Loading TF weight bert/encoder/layer_10/attention/output/dense/kernel/adam_m with shape [768, 768]
I1009 15:47:04.544577 47631408520768 modeling_bert.py:71] Loading TF weight bert/encoder/layer_10/attention/output/dense/kernel/adam_v with shape [768, 768]
I1009 15:47:04.550608 47631408520768 modeling_bert.py:71] Loading TF weight bert/encoder/layer_10/attention/self/key/bias with shape [768]
I1009 15:47:04.555929 47631408520768 modeling_bert.py:71] Loading TF weight bert/encoder/layer_10/attention/self/key/bias/adam_m with shape [768]
I1009 15:47:04.561278 47631408520768 modeling_bert.py:71] Loading TF weight bert/encoder/layer_10/attention/self/key/bias/adam_v with shape [768]
I1009 15:47:04.566563 47631408520768 modeling_bert.py:71] Loading TF weight bert/encoder/layer_10/attention/self/key/kernel with shape [768, 768]
I1009 15:47:04.573011 47631408520768 modeling_bert.py:71] Loading TF weight bert/encoder/layer_10/attention/self/key/kernel/adam_m with shape [768, 768]
I1009 15:47:04.579270 47631408520768 modeling_bert.py:71] Loading TF weight bert/encoder/layer_10/attention/self/key/kernel/adam_v with shape [768, 768]
I1009 15:47:04.585390 47631408520768 modeling_bert.py:71] Loading TF weight bert/encoder/layer_10/attention/self/query/bias with shape [768]
I1009 15:47:04.590508 47631408520768 modeling_bert.py:71] Loading TF weight bert/encoder/layer_10/attention/self/query/bias/adam_m with shape [768]
I1009 15:47:04.595518 47631408520768 modeling_bert.py:71] Loading TF weight bert/encoder/layer_10/attention/self/query/bias/adam_v with shape [768]
I1009 15:47:04.601018 47631408520768 modeling_bert.py:71] Loading TF weight bert/encoder/layer_10/attention/self/query/kernel with shape [768, 768]
I1009 15:47:04.607660 47631408520768 modeling_bert.py:71] Loading TF weight bert/encoder/layer_10/attention/self/query/kernel/adam_m with shape [768, 768]
I1009 15:47:04.614161 47631408520768 modeling_bert.py:71] Loading TF weight bert/encoder/layer_10/attention/self/query/kernel/adam_v with shape [768, 768]
I1009 15:47:04.620493 47631408520768 modeling_bert.py:71] Loading TF weight bert/encoder/layer_10/attention/self/value/bias with shape [768]
I1009 15:47:04.625949 47631408520768 modeling_bert.py:71] Loading TF weight bert/encoder/layer_10/attention/self/value/bias/adam_m with shape [768]
I1009 15:47:04.631571 47631408520768 modeling_bert.py:71] Loading TF weight bert/encoder/layer_10/attention/self/value/bias/adam_v with shape [768]
I1009 15:47:04.637210 47631408520768 modeling_bert.py:71] Loading TF weight bert/encoder/layer_10/attention/self/value/kernel with shape [768, 768]
I1009 15:47:04.643343 47631408520768 modeling_bert.py:71] Loading TF weight bert/encoder/layer_10/attention/self/value/kernel/adam_m with shape [768, 768]
I1009 15:47:04.649336 47631408520768 modeling_bert.py:71] Loading TF weight bert/encoder/layer_10/attention/self/value/kernel/adam_v with shape [768, 768]
I1009 15:47:04.655313 47631408520768 modeling_bert.py:71] Loading TF weight bert/encoder/layer_10/intermediate/dense/bias with shape [3072]
I1009 15:47:04.660685 47631408520768 modeling_bert.py:71] Loading TF weight bert/encoder/layer_10/intermediate/dense/bias/adam_m with shape [3072]
I1009 15:47:04.666079 47631408520768 modeling_bert.py:71] Loading TF weight bert/encoder/layer_10/intermediate/dense/bias/adam_v with shape [3072]
I1009 15:47:04.671396 47631408520768 modeling_bert.py:71] Loading TF weight bert/encoder/layer_10/intermediate/dense/kernel with shape [768, 3072]
I1009 15:47:04.680429 47631408520768 modeling_bert.py:71] Loading TF weight bert/encoder/layer_10/intermediate/dense/kernel/adam_m with shape [768, 3072]
I1009 15:47:04.689695 47631408520768 modeling_bert.py:71] Loading TF weight bert/encoder/layer_10/intermediate/dense/kernel/adam_v with shape [768, 3072]
I1009 15:47:04.698902 47631408520768 modeling_bert.py:71] Loading TF weight bert/encoder/layer_10/output/LayerNorm/beta with shape [768]
I1009 15:47:04.704335 47631408520768 modeling_bert.py:71] Loading TF weight bert/encoder/layer_10/output/LayerNorm/beta/adam_m with shape [768]
I1009 15:47:04.710071 47631408520768 modeling_bert.py:71] Loading TF weight bert/encoder/layer_10/output/LayerNorm/beta/adam_v with shape [768]
I1009 15:47:04.715506 47631408520768 modeling_bert.py:71] Loading TF weight bert/encoder/layer_10/output/LayerNorm/gamma with shape [768]
I1009 15:47:04.720938 47631408520768 modeling_bert.py:71] Loading TF weight bert/encoder/layer_10/output/LayerNorm/gamma/adam_m with shape [768]
I1009 15:47:04.726950 47631408520768 modeling_bert.py:71] Loading TF weight bert/encoder/layer_10/output/LayerNorm/gamma/adam_v with shape [768]
I1009 15:47:04.732291 47631408520768 modeling_bert.py:71] Loading TF weight bert/encoder/layer_10/output/dense/bias with shape [768]
I1009 15:47:04.737487 47631408520768 modeling_bert.py:71] Loading TF weight bert/encoder/layer_10/output/dense/bias/adam_m with shape [768]
I1009 15:47:04.742859 47631408520768 modeling_bert.py:71] Loading TF weight bert/encoder/layer_10/output/dense/bias/adam_v with shape [768]
I1009 15:47:04.748318 47631408520768 modeling_bert.py:71] Loading TF weight bert/encoder/layer_10/output/dense/kernel with shape [3072, 768]
I1009 15:47:04.757482 47631408520768 modeling_bert.py:71] Loading TF weight bert/encoder/layer_10/output/dense/kernel/adam_m with shape [3072, 768]
I1009 15:47:04.766298 47631408520768 modeling_bert.py:71] Loading TF weight bert/encoder/layer_10/output/dense/kernel/adam_v with shape [3072, 768]
I1009 15:47:04.775268 47631408520768 modeling_bert.py:71] Loading TF weight bert/encoder/layer_11/attention/output/LayerNorm/beta with shape [768]
I1009 15:47:04.780487 47631408520768 modeling_bert.py:71] Loading TF weight bert/encoder/layer_11/attention/output/LayerNorm/beta/adam_m with shape [768]
I1009 15:47:04.785785 47631408520768 modeling_bert.py:71] Loading TF weight bert/encoder/layer_11/attention/output/LayerNorm/beta/adam_v with shape [768]
I1009 15:47:04.791079 47631408520768 modeling_bert.py:71] Loading TF weight bert/encoder/layer_11/attention/output/LayerNorm/gamma with shape [768]
I1009 15:47:04.796396 47631408520768 modeling_bert.py:71] Loading TF weight bert/encoder/layer_11/attention/output/LayerNorm/gamma/adam_m with shape [768]
I1009 15:47:04.801618 47631408520768 modeling_bert.py:71] Loading TF weight bert/encoder/layer_11/attention/output/LayerNorm/gamma/adam_v with shape [768]
I1009 15:47:04.806764 47631408520768 modeling_bert.py:71] Loading TF weight bert/encoder/layer_11/attention/output/dense/bias with shape [768]
I1009 15:47:04.811792 47631408520768 modeling_bert.py:71] Loading TF weight bert/encoder/layer_11/attention/output/dense/bias/adam_m with shape [768]
I1009 15:47:04.817137 47631408520768 modeling_bert.py:71] Loading TF weight bert/encoder/layer_11/attention/output/dense/bias/adam_v with shape [768]
I1009 15:47:04.822446 47631408520768 modeling_bert.py:71] Loading TF weight bert/encoder/layer_11/attention/output/dense/kernel with shape [768, 768]
I1009 15:47:04.828487 47631408520768 modeling_bert.py:71] Loading TF weight bert/encoder/layer_11/attention/output/dense/kernel/adam_m with shape [768, 768]
I1009 15:47:04.834693 47631408520768 modeling_bert.py:71] Loading TF weight bert/encoder/layer_11/attention/output/dense/kernel/adam_v with shape [768, 768]
I1009 15:47:04.840850 47631408520768 modeling_bert.py:71] Loading TF weight bert/encoder/layer_11/attention/self/key/bias with shape [768]
I1009 15:47:04.846439 47631408520768 modeling_bert.py:71] Loading TF weight bert/encoder/layer_11/attention/self/key/bias/adam_m with shape [768]
I1009 15:47:04.851520 47631408520768 modeling_bert.py:71] Loading TF weight bert/encoder/layer_11/attention/self/key/bias/adam_v with shape [768]
I1009 15:47:04.856792 47631408520768 modeling_bert.py:71] Loading TF weight bert/encoder/layer_11/attention/self/key/kernel with shape [768, 768]
I1009 15:47:04.862816 47631408520768 modeling_bert.py:71] Loading TF weight bert/encoder/layer_11/attention/self/key/kernel/adam_m with shape [768, 768]
I1009 15:47:04.869044 47631408520768 modeling_bert.py:71] Loading TF weight bert/encoder/layer_11/attention/self/key/kernel/adam_v with shape [768, 768]
I1009 15:47:04.875606 47631408520768 modeling_bert.py:71] Loading TF weight bert/encoder/layer_11/attention/self/query/bias with shape [768]
I1009 15:47:04.880790 47631408520768 modeling_bert.py:71] Loading TF weight bert/encoder/layer_11/attention/self/query/bias/adam_m with shape [768]
I1009 15:47:04.886349 47631408520768 modeling_bert.py:71] Loading TF weight bert/encoder/layer_11/attention/self/query/bias/adam_v with shape [768]
I1009 15:47:04.892000 47631408520768 modeling_bert.py:71] Loading TF weight bert/encoder/layer_11/attention/self/query/kernel with shape [768, 768]
I1009 15:47:04.898570 47631408520768 modeling_bert.py:71] Loading TF weight bert/encoder/layer_11/attention/self/query/kernel/adam_m with shape [768, 768]
I1009 15:47:04.904909 47631408520768 modeling_bert.py:71] Loading TF weight bert/encoder/layer_11/attention/self/query/kernel/adam_v with shape [768, 768]
I1009 15:47:04.911062 47631408520768 modeling_bert.py:71] Loading TF weight bert/encoder/layer_11/attention/self/value/bias with shape [768]
I1009 15:47:04.916296 47631408520768 modeling_bert.py:71] Loading TF weight bert/encoder/layer_11/attention/self/value/bias/adam_m with shape [768]
I1009 15:47:04.921895 47631408520768 modeling_bert.py:71] Loading TF weight bert/encoder/layer_11/attention/self/value/bias/adam_v with shape [768]
I1009 15:47:04.927209 47631408520768 modeling_bert.py:71] Loading TF weight bert/encoder/layer_11/attention/self/value/kernel with shape [768, 768]
I1009 15:47:04.933332 47631408520768 modeling_bert.py:71] Loading TF weight bert/encoder/layer_11/attention/self/value/kernel/adam_m with shape [768, 768]
I1009 15:47:04.939111 47631408520768 modeling_bert.py:71] Loading TF weight bert/encoder/layer_11/attention/self/value/kernel/adam_v with shape [768, 768]
I1009 15:47:04.945370 47631408520768 modeling_bert.py:71] Loading TF weight bert/encoder/layer_11/intermediate/dense/bias with shape [3072]
I1009 15:47:04.950793 47631408520768 modeling_bert.py:71] Loading TF weight bert/encoder/layer_11/intermediate/dense/bias/adam_m with shape [3072]
I1009 15:47:04.955806 47631408520768 modeling_bert.py:71] Loading TF weight bert/encoder/layer_11/intermediate/dense/bias/adam_v with shape [3072]
I1009 15:47:04.961072 47631408520768 modeling_bert.py:71] Loading TF weight bert/encoder/layer_11/intermediate/dense/kernel with shape [768, 3072]
I1009 15:47:04.970463 47631408520768 modeling_bert.py:71] Loading TF weight bert/encoder/layer_11/intermediate/dense/kernel/adam_m with shape [768, 3072]
I1009 15:47:04.979833 47631408520768 modeling_bert.py:71] Loading TF weight bert/encoder/layer_11/intermediate/dense/kernel/adam_v with shape [768, 3072]
I1009 15:47:04.989530 47631408520768 modeling_bert.py:71] Loading TF weight bert/encoder/layer_11/output/LayerNorm/beta with shape [768]
I1009 15:47:04.995094 47631408520768 modeling_bert.py:71] Loading TF weight bert/encoder/layer_11/output/LayerNorm/beta/adam_m with shape [768]
I1009 15:47:05.000446 47631408520768 modeling_bert.py:71] Loading TF weight bert/encoder/layer_11/output/LayerNorm/beta/adam_v with shape [768]
I1009 15:47:05.005793 47631408520768 modeling_bert.py:71] Loading TF weight bert/encoder/layer_11/output/LayerNorm/gamma with shape [768]
I1009 15:47:05.011140 47631408520768 modeling_bert.py:71] Loading TF weight bert/encoder/layer_11/output/LayerNorm/gamma/adam_m with shape [768]
I1009 15:47:05.016781 47631408520768 modeling_bert.py:71] Loading TF weight bert/encoder/layer_11/output/LayerNorm/gamma/adam_v with shape [768]
I1009 15:47:05.022187 47631408520768 modeling_bert.py:71] Loading TF weight bert/encoder/layer_11/output/dense/bias with shape [768]
I1009 15:47:05.027360 47631408520768 modeling_bert.py:71] Loading TF weight bert/encoder/layer_11/output/dense/bias/adam_m with shape [768]
I1009 15:47:05.032415 47631408520768 modeling_bert.py:71] Loading TF weight bert/encoder/layer_11/output/dense/bias/adam_v with shape [768]
I1009 15:47:05.037586 47631408520768 modeling_bert.py:71] Loading TF weight bert/encoder/layer_11/output/dense/kernel with shape [3072, 768]
I1009 15:47:05.046856 47631408520768 modeling_bert.py:71] Loading TF weight bert/encoder/layer_11/output/dense/kernel/adam_m with shape [3072, 768]
I1009 15:47:05.055976 47631408520768 modeling_bert.py:71] Loading TF weight bert/encoder/layer_11/output/dense/kernel/adam_v with shape [3072, 768]
I1009 15:47:05.065228 47631408520768 modeling_bert.py:71] Loading TF weight bert/encoder/layer_2/attention/output/LayerNorm/beta with shape [768]
I1009 15:47:05.070782 47631408520768 modeling_bert.py:71] Loading TF weight bert/encoder/layer_2/attention/output/LayerNorm/beta/adam_m with shape [768]
I1009 15:47:05.075919 47631408520768 modeling_bert.py:71] Loading TF weight bert/encoder/layer_2/attention/output/LayerNorm/beta/adam_v with shape [768]
I1009 15:47:05.081003 47631408520768 modeling_bert.py:71] Loading TF weight bert/encoder/layer_2/attention/output/LayerNorm/gamma with shape [768]
I1009 15:47:05.086196 47631408520768 modeling_bert.py:71] Loading TF weight bert/encoder/layer_2/attention/output/LayerNorm/gamma/adam_m with shape [768]
I1009 15:47:05.091385 47631408520768 modeling_bert.py:71] Loading TF weight bert/encoder/layer_2/attention/output/LayerNorm/gamma/adam_v with shape [768]
I1009 15:47:05.096379 47631408520768 modeling_bert.py:71] Loading TF weight bert/encoder/layer_2/attention/output/dense/bias with shape [768]
I1009 15:47:05.101588 47631408520768 modeling_bert.py:71] Loading TF weight bert/encoder/layer_2/attention/output/dense/bias/adam_m with shape [768]
I1009 15:47:05.106899 47631408520768 modeling_bert.py:71] Loading TF weight bert/encoder/layer_2/attention/output/dense/bias/adam_v with shape [768]
I1009 15:47:05.112151 47631408520768 modeling_bert.py:71] Loading TF weight bert/encoder/layer_2/attention/output/dense/kernel with shape [768, 768]
I1009 15:47:05.117895 47631408520768 modeling_bert.py:71] Loading TF weight bert/encoder/layer_2/attention/output/dense/kernel/adam_m with shape [768, 768]
I1009 15:47:05.123686 47631408520768 modeling_bert.py:71] Loading TF weight bert/encoder/layer_2/attention/output/dense/kernel/adam_v with shape [768, 768]
I1009 15:47:05.129775 47631408520768 modeling_bert.py:71] Loading TF weight bert/encoder/layer_2/attention/self/key/bias with shape [768]
I1009 15:47:05.135063 47631408520768 modeling_bert.py:71] Loading TF weight bert/encoder/layer_2/attention/self/key/bias/adam_m with shape [768]
I1009 15:47:05.140272 47631408520768 modeling_bert.py:71] Loading TF weight bert/encoder/layer_2/attention/self/key/bias/adam_v with shape [768]
I1009 15:47:05.145637 47631408520768 modeling_bert.py:71] Loading TF weight bert/encoder/layer_2/attention/self/key/kernel with shape [768, 768]
I1009 15:47:05.151918 47631408520768 modeling_bert.py:71] Loading TF weight bert/encoder/layer_2/attention/self/key/kernel/adam_m with shape [768, 768]
I1009 15:47:05.158202 47631408520768 modeling_bert.py:71] Loading TF weight bert/encoder/layer_2/attention/self/key/kernel/adam_v with shape [768, 768]
I1009 15:47:05.164404 47631408520768 modeling_bert.py:71] Loading TF weight bert/encoder/layer_2/attention/self/query/bias with shape [768]
I1009 15:47:05.170278 47631408520768 modeling_bert.py:71] Loading TF weight bert/encoder/layer_2/attention/self/query/bias/adam_m with shape [768]
I1009 15:47:05.175527 47631408520768 modeling_bert.py:71] Loading TF weight bert/encoder/layer_2/attention/self/query/bias/adam_v with shape [768]
I1009 15:47:05.180814 47631408520768 modeling_bert.py:71] Loading TF weight bert/encoder/layer_2/attention/self/query/kernel with shape [768, 768]
I1009 15:47:05.187093 47631408520768 modeling_bert.py:71] Loading TF weight bert/encoder/layer_2/attention/self/query/kernel/adam_m with shape [768, 768]
I1009 15:47:05.193276 47631408520768 modeling_bert.py:71] Loading TF weight bert/encoder/layer_2/attention/self/query/kernel/adam_v with shape [768, 768]
I1009 15:47:05.199446 47631408520768 modeling_bert.py:71] Loading TF weight bert/encoder/layer_2/attention/self/value/bias with shape [768]
I1009 15:47:05.204873 47631408520768 modeling_bert.py:71] Loading TF weight bert/encoder/layer_2/attention/self/value/bias/adam_m with shape [768]
I1009 15:47:05.210621 47631408520768 modeling_bert.py:71] Loading TF weight bert/encoder/layer_2/attention/self/value/bias/adam_v with shape [768]
I1009 15:47:05.215833 47631408520768 modeling_bert.py:71] Loading TF weight bert/encoder/layer_2/attention/self/value/kernel with shape [768, 768]
I1009 15:47:05.221964 47631408520768 modeling_bert.py:71] Loading TF weight bert/encoder/layer_2/attention/self/value/kernel/adam_m with shape [768, 768]
I1009 15:47:05.228211 47631408520768 modeling_bert.py:71] Loading TF weight bert/encoder/layer_2/attention/self/value/kernel/adam_v with shape [768, 768]
I1009 15:47:05.234716 47631408520768 modeling_bert.py:71] Loading TF weight bert/encoder/layer_2/intermediate/dense/bias with shape [3072]
I1009 15:47:05.240120 47631408520768 modeling_bert.py:71] Loading TF weight bert/encoder/layer_2/intermediate/dense/bias/adam_m with shape [3072]
I1009 15:47:05.245725 47631408520768 modeling_bert.py:71] Loading TF weight bert/encoder/layer_2/intermediate/dense/bias/adam_v with shape [3072]
I1009 15:47:05.250889 47631408520768 modeling_bert.py:71] Loading TF weight bert/encoder/layer_2/intermediate/dense/kernel with shape [768, 3072]
I1009 15:47:05.260031 47631408520768 modeling_bert.py:71] Loading TF weight bert/encoder/layer_2/intermediate/dense/kernel/adam_m with shape [768, 3072]
I1009 15:47:05.269109 47631408520768 modeling_bert.py:71] Loading TF weight bert/encoder/layer_2/intermediate/dense/kernel/adam_v with shape [768, 3072]
I1009 15:47:05.278731 47631408520768 modeling_bert.py:71] Loading TF weight bert/encoder/layer_2/output/LayerNorm/beta with shape [768]
I1009 15:47:05.283982 47631408520768 modeling_bert.py:71] Loading TF weight bert/encoder/layer_2/output/LayerNorm/beta/adam_m with shape [768]
I1009 15:47:05.289493 47631408520768 modeling_bert.py:71] Loading TF weight bert/encoder/layer_2/output/LayerNorm/beta/adam_v with shape [768]
I1009 15:47:05.294659 47631408520768 modeling_bert.py:71] Loading TF weight bert/encoder/layer_2/output/LayerNorm/gamma with shape [768]
I1009 15:47:05.299949 47631408520768 modeling_bert.py:71] Loading TF weight bert/encoder/layer_2/output/LayerNorm/gamma/adam_m with shape [768]
I1009 15:47:05.305051 47631408520768 modeling_bert.py:71] Loading TF weight bert/encoder/layer_2/output/LayerNorm/gamma/adam_v with shape [768]
I1009 15:47:05.310529 47631408520768 modeling_bert.py:71] Loading TF weight bert/encoder/layer_2/output/dense/bias with shape [768]
I1009 15:47:05.315993 47631408520768 modeling_bert.py:71] Loading TF weight bert/encoder/layer_2/output/dense/bias/adam_m with shape [768]
I1009 15:47:05.321487 47631408520768 modeling_bert.py:71] Loading TF weight bert/encoder/layer_2/output/dense/bias/adam_v with shape [768]
I1009 15:47:05.326727 47631408520768 modeling_bert.py:71] Loading TF weight bert/encoder/layer_2/output/dense/kernel with shape [3072, 768]
I1009 15:47:05.335873 47631408520768 modeling_bert.py:71] Loading TF weight bert/encoder/layer_2/output/dense/kernel/adam_m with shape [3072, 768]
I1009 15:47:05.345036 47631408520768 modeling_bert.py:71] Loading TF weight bert/encoder/layer_2/output/dense/kernel/adam_v with shape [3072, 768]
I1009 15:47:05.354362 47631408520768 modeling_bert.py:71] Loading TF weight bert/encoder/layer_3/attention/output/LayerNorm/beta with shape [768]
I1009 15:47:05.359932 47631408520768 modeling_bert.py:71] Loading TF weight bert/encoder/layer_3/attention/output/LayerNorm/beta/adam_m with shape [768]
I1009 15:47:05.365148 47631408520768 modeling_bert.py:71] Loading TF weight bert/encoder/layer_3/attention/output/LayerNorm/beta/adam_v with shape [768]
I1009 15:47:05.370391 47631408520768 modeling_bert.py:71] Loading TF weight bert/encoder/layer_3/attention/output/LayerNorm/gamma with shape [768]
I1009 15:47:05.375550 47631408520768 modeling_bert.py:71] Loading TF weight bert/encoder/layer_3/attention/output/LayerNorm/gamma/adam_m with shape [768]
I1009 15:47:05.380681 47631408520768 modeling_bert.py:71] Loading TF weight bert/encoder/layer_3/attention/output/LayerNorm/gamma/adam_v with shape [768]
I1009 15:47:05.385793 47631408520768 modeling_bert.py:71] Loading TF weight bert/encoder/layer_3/attention/output/dense/bias with shape [768]
I1009 15:47:05.390934 47631408520768 modeling_bert.py:71] Loading TF weight bert/encoder/layer_3/attention/output/dense/bias/adam_m with shape [768]
I1009 15:47:05.396157 47631408520768 modeling_bert.py:71] Loading TF weight bert/encoder/layer_3/attention/output/dense/bias/adam_v with shape [768]
I1009 15:47:05.401309 47631408520768 modeling_bert.py:71] Loading TF weight bert/encoder/layer_3/attention/output/dense/kernel with shape [768, 768]
I1009 15:47:05.407191 47631408520768 modeling_bert.py:71] Loading TF weight bert/encoder/layer_3/attention/output/dense/kernel/adam_m with shape [768, 768]
I1009 15:47:05.413287 47631408520768 modeling_bert.py:71] Loading TF weight bert/encoder/layer_3/attention/output/dense/kernel/adam_v with shape [768, 768]
I1009 15:47:05.419596 47631408520768 modeling_bert.py:71] Loading TF weight bert/encoder/layer_3/attention/self/key/bias with shape [768]
I1009 15:47:05.424950 47631408520768 modeling_bert.py:71] Loading TF weight bert/encoder/layer_3/attention/self/key/bias/adam_m with shape [768]
I1009 15:47:05.430454 47631408520768 modeling_bert.py:71] Loading TF weight bert/encoder/layer_3/attention/self/key/bias/adam_v with shape [768]
I1009 15:47:05.435939 47631408520768 modeling_bert.py:71] Loading TF weight bert/encoder/layer_3/attention/self/key/kernel with shape [768, 768]
I1009 15:47:05.441898 47631408520768 modeling_bert.py:71] Loading TF weight bert/encoder/layer_3/attention/self/key/kernel/adam_m with shape [768, 768]
I1009 15:47:05.448148 47631408520768 modeling_bert.py:71] Loading TF weight bert/encoder/layer_3/attention/self/key/kernel/adam_v with shape [768, 768]
I1009 15:47:05.454164 47631408520768 modeling_bert.py:71] Loading TF weight bert/encoder/layer_3/attention/self/query/bias with shape [768]
I1009 15:47:05.459583 47631408520768 modeling_bert.py:71] Loading TF weight bert/encoder/layer_3/attention/self/query/bias/adam_m with shape [768]
I1009 15:47:05.465055 47631408520768 modeling_bert.py:71] Loading TF weight bert/encoder/layer_3/attention/self/query/bias/adam_v with shape [768]
I1009 15:47:05.470114 47631408520768 modeling_bert.py:71] Loading TF weight bert/encoder/layer_3/attention/self/query/kernel with shape [768, 768]
I1009 15:47:05.476166 47631408520768 modeling_bert.py:71] Loading TF weight bert/encoder/layer_3/attention/self/query/kernel/adam_m with shape [768, 768]
I1009 15:47:05.482553 47631408520768 modeling_bert.py:71] Loading TF weight bert/encoder/layer_3/attention/self/query/kernel/adam_v with shape [768, 768]
I1009 15:47:05.489023 47631408520768 modeling_bert.py:71] Loading TF weight bert/encoder/layer_3/attention/self/value/bias with shape [768]
I1009 15:47:05.494502 47631408520768 modeling_bert.py:71] Loading TF weight bert/encoder/layer_3/attention/self/value/bias/adam_m with shape [768]
I1009 15:47:05.500063 47631408520768 modeling_bert.py:71] Loading TF weight bert/encoder/layer_3/attention/self/value/bias/adam_v with shape [768]
I1009 15:47:05.505194 47631408520768 modeling_bert.py:71] Loading TF weight bert/encoder/layer_3/attention/self/value/kernel with shape [768, 768]
I1009 15:47:05.511651 47631408520768 modeling_bert.py:71] Loading TF weight bert/encoder/layer_3/attention/self/value/kernel/adam_m with shape [768, 768]
I1009 15:47:05.517767 47631408520768 modeling_bert.py:71] Loading TF weight bert/encoder/layer_3/attention/self/value/kernel/adam_v with shape [768, 768]
I1009 15:47:05.524090 47631408520768 modeling_bert.py:71] Loading TF weight bert/encoder/layer_3/intermediate/dense/bias with shape [3072]
I1009 15:47:05.529507 47631408520768 modeling_bert.py:71] Loading TF weight bert/encoder/layer_3/intermediate/dense/bias/adam_m with shape [3072]
I1009 15:47:05.534897 47631408520768 modeling_bert.py:71] Loading TF weight bert/encoder/layer_3/intermediate/dense/bias/adam_v with shape [3072]
I1009 15:47:05.540130 47631408520768 modeling_bert.py:71] Loading TF weight bert/encoder/layer_3/intermediate/dense/kernel with shape [768, 3072]
I1009 15:47:05.549204 47631408520768 modeling_bert.py:71] Loading TF weight bert/encoder/layer_3/intermediate/dense/kernel/adam_m with shape [768, 3072]
I1009 15:47:05.558589 47631408520768 modeling_bert.py:71] Loading TF weight bert/encoder/layer_3/intermediate/dense/kernel/adam_v with shape [768, 3072]
I1009 15:47:05.568243 47631408520768 modeling_bert.py:71] Loading TF weight bert/encoder/layer_3/output/LayerNorm/beta with shape [768]
I1009 15:47:05.573777 47631408520768 modeling_bert.py:71] Loading TF weight bert/encoder/layer_3/output/LayerNorm/beta/adam_m with shape [768]
I1009 15:47:05.578975 47631408520768 modeling_bert.py:71] Loading TF weight bert/encoder/layer_3/output/LayerNorm/beta/adam_v with shape [768]
I1009 15:47:05.584223 47631408520768 modeling_bert.py:71] Loading TF weight bert/encoder/layer_3/output/LayerNorm/gamma with shape [768]
I1009 15:47:05.589388 47631408520768 modeling_bert.py:71] Loading TF weight bert/encoder/layer_3/output/LayerNorm/gamma/adam_m with shape [768]
I1009 15:47:05.594596 47631408520768 modeling_bert.py:71] Loading TF weight bert/encoder/layer_3/output/LayerNorm/gamma/adam_v with shape [768]
I1009 15:47:05.600200 47631408520768 modeling_bert.py:71] Loading TF weight bert/encoder/layer_3/output/dense/bias with shape [768]
I1009 15:47:05.605475 47631408520768 modeling_bert.py:71] Loading TF weight bert/encoder/layer_3/output/dense/bias/adam_m with shape [768]
I1009 15:47:05.610627 47631408520768 modeling_bert.py:71] Loading TF weight bert/encoder/layer_3/output/dense/bias/adam_v with shape [768]
I1009 15:47:05.616336 47631408520768 modeling_bert.py:71] Loading TF weight bert/encoder/layer_3/output/dense/kernel with shape [3072, 768]
I1009 15:47:05.625247 47631408520768 modeling_bert.py:71] Loading TF weight bert/encoder/layer_3/output/dense/kernel/adam_m with shape [3072, 768]
I1009 15:47:05.634833 47631408520768 modeling_bert.py:71] Loading TF weight bert/encoder/layer_3/output/dense/kernel/adam_v with shape [3072, 768]
I1009 15:47:05.644434 47631408520768 modeling_bert.py:71] Loading TF weight bert/encoder/layer_4/attention/output/LayerNorm/beta with shape [768]
I1009 15:47:05.649833 47631408520768 modeling_bert.py:71] Loading TF weight bert/encoder/layer_4/attention/output/LayerNorm/beta/adam_m with shape [768]
I1009 15:47:05.655650 47631408520768 modeling_bert.py:71] Loading TF weight bert/encoder/layer_4/attention/output/LayerNorm/beta/adam_v with shape [768]
I1009 15:47:05.660920 47631408520768 modeling_bert.py:71] Loading TF weight bert/encoder/layer_4/attention/output/LayerNorm/gamma with shape [768]
I1009 15:47:05.666323 47631408520768 modeling_bert.py:71] Loading TF weight bert/encoder/layer_4/attention/output/LayerNorm/gamma/adam_m with shape [768]
I1009 15:47:05.671495 47631408520768 modeling_bert.py:71] Loading TF weight bert/encoder/layer_4/attention/output/LayerNorm/gamma/adam_v with shape [768]
I1009 15:47:05.676601 47631408520768 modeling_bert.py:71] Loading TF weight bert/encoder/layer_4/attention/output/dense/bias with shape [768]
I1009 15:47:05.681863 47631408520768 modeling_bert.py:71] Loading TF weight bert/encoder/layer_4/attention/output/dense/bias/adam_m with shape [768]
I1009 15:47:05.686859 47631408520768 modeling_bert.py:71] Loading TF weight bert/encoder/layer_4/attention/output/dense/bias/adam_v with shape [768]
I1009 15:47:05.692172 47631408520768 modeling_bert.py:71] Loading TF weight bert/encoder/layer_4/attention/output/dense/kernel with shape [768, 768]
I1009 15:47:05.698084 47631408520768 modeling_bert.py:71] Loading TF weight bert/encoder/layer_4/attention/output/dense/kernel/adam_m with shape [768, 768]
I1009 15:47:05.703886 47631408520768 modeling_bert.py:71] Loading TF weight bert/encoder/layer_4/attention/output/dense/kernel/adam_v with shape [768, 768]
I1009 15:47:05.709926 47631408520768 modeling_bert.py:71] Loading TF weight bert/encoder/layer_4/attention/self/key/bias with shape [768]
I1009 15:47:05.715487 47631408520768 modeling_bert.py:71] Loading TF weight bert/encoder/layer_4/attention/self/key/bias/adam_m with shape [768]
I1009 15:47:05.720532 47631408520768 modeling_bert.py:71] Loading TF weight bert/encoder/layer_4/attention/self/key/bias/adam_v with shape [768]
I1009 15:47:05.725626 47631408520768 modeling_bert.py:71] Loading TF weight bert/encoder/layer_4/attention/self/key/kernel with shape [768, 768]
I1009 15:47:05.731551 47631408520768 modeling_bert.py:71] Loading TF weight bert/encoder/layer_4/attention/self/key/kernel/adam_m with shape [768, 768]
I1009 15:47:05.737704 47631408520768 modeling_bert.py:71] Loading TF weight bert/encoder/layer_4/attention/self/key/kernel/adam_v with shape [768, 768]
I1009 15:47:05.743711 47631408520768 modeling_bert.py:71] Loading TF weight bert/encoder/layer_4/attention/self/query/bias with shape [768]
I1009 15:47:05.748904 47631408520768 modeling_bert.py:71] Loading TF weight bert/encoder/layer_4/attention/self/query/bias/adam_m with shape [768]
I1009 15:47:05.754198 47631408520768 modeling_bert.py:71] Loading TF weight bert/encoder/layer_4/attention/self/query/bias/adam_v with shape [768]
I1009 15:47:05.759485 47631408520768 modeling_bert.py:71] Loading TF weight bert/encoder/layer_4/attention/self/query/kernel with shape [768, 768]
I1009 15:47:05.765789 47631408520768 modeling_bert.py:71] Loading TF weight bert/encoder/layer_4/attention/self/query/kernel/adam_m with shape [768, 768]
I1009 15:47:05.772267 47631408520768 modeling_bert.py:71] Loading TF weight bert/encoder/layer_4/attention/self/query/kernel/adam_v with shape [768, 768]
I1009 15:47:05.778261 47631408520768 modeling_bert.py:71] Loading TF weight bert/encoder/layer_4/attention/self/value/bias with shape [768]
I1009 15:47:05.783635 47631408520768 modeling_bert.py:71] Loading TF weight bert/encoder/layer_4/attention/self/value/bias/adam_m with shape [768]
I1009 15:47:05.788740 47631408520768 modeling_bert.py:71] Loading TF weight bert/encoder/layer_4/attention/self/value/bias/adam_v with shape [768]
I1009 15:47:05.794108 47631408520768 modeling_bert.py:71] Loading TF weight bert/encoder/layer_4/attention/self/value/kernel with shape [768, 768]
I1009 15:47:05.800311 47631408520768 modeling_bert.py:71] Loading TF weight bert/encoder/layer_4/attention/self/value/kernel/adam_m with shape [768, 768]
I1009 15:47:05.806456 47631408520768 modeling_bert.py:71] Loading TF weight bert/encoder/layer_4/attention/self/value/kernel/adam_v with shape [768, 768]
I1009 15:47:05.812658 47631408520768 modeling_bert.py:71] Loading TF weight bert/encoder/layer_4/intermediate/dense/bias with shape [3072]
I1009 15:47:05.818162 47631408520768 modeling_bert.py:71] Loading TF weight bert/encoder/layer_4/intermediate/dense/bias/adam_m with shape [3072]
I1009 15:47:05.823700 47631408520768 modeling_bert.py:71] Loading TF weight bert/encoder/layer_4/intermediate/dense/bias/adam_v with shape [3072]
I1009 15:47:05.828876 47631408520768 modeling_bert.py:71] Loading TF weight bert/encoder/layer_4/intermediate/dense/kernel with shape [768, 3072]
I1009 15:47:05.837978 47631408520768 modeling_bert.py:71] Loading TF weight bert/encoder/layer_4/intermediate/dense/kernel/adam_m with shape [768, 3072]
I1009 15:47:05.847428 47631408520768 modeling_bert.py:71] Loading TF weight bert/encoder/layer_4/intermediate/dense/kernel/adam_v with shape [768, 3072]
I1009 15:47:05.856863 47631408520768 modeling_bert.py:71] Loading TF weight bert/encoder/layer_4/output/LayerNorm/beta with shape [768]
I1009 15:47:05.862351 47631408520768 modeling_bert.py:71] Loading TF weight bert/encoder/layer_4/output/LayerNorm/beta/adam_m with shape [768]
I1009 15:47:05.867667 47631408520768 modeling_bert.py:71] Loading TF weight bert/encoder/layer_4/output/LayerNorm/beta/adam_v with shape [768]
I1009 15:47:05.873092 47631408520768 modeling_bert.py:71] Loading TF weight bert/encoder/layer_4/output/LayerNorm/gamma with shape [768]
I1009 15:47:05.878434 47631408520768 modeling_bert.py:71] Loading TF weight bert/encoder/layer_4/output/LayerNorm/gamma/adam_m with shape [768]
I1009 15:47:05.883496 47631408520768 modeling_bert.py:71] Loading TF weight bert/encoder/layer_4/output/LayerNorm/gamma/adam_v with shape [768]
I1009 15:47:05.888758 47631408520768 modeling_bert.py:71] Loading TF weight bert/encoder/layer_4/output/dense/bias with shape [768]
I1009 15:47:05.894265 47631408520768 modeling_bert.py:71] Loading TF weight bert/encoder/layer_4/output/dense/bias/adam_m with shape [768]
I1009 15:47:05.899498 47631408520768 modeling_bert.py:71] Loading TF weight bert/encoder/layer_4/output/dense/bias/adam_v with shape [768]
I1009 15:47:05.904521 47631408520768 modeling_bert.py:71] Loading TF weight bert/encoder/layer_4/output/dense/kernel with shape [3072, 768]
I1009 15:47:05.913688 47631408520768 modeling_bert.py:71] Loading TF weight bert/encoder/layer_4/output/dense/kernel/adam_m with shape [3072, 768]
I1009 15:47:05.922709 47631408520768 modeling_bert.py:71] Loading TF weight bert/encoder/layer_4/output/dense/kernel/adam_v with shape [3072, 768]
I1009 15:47:05.931876 47631408520768 modeling_bert.py:71] Loading TF weight bert/encoder/layer_5/attention/output/LayerNorm/beta with shape [768]
I1009 15:47:05.937582 47631408520768 modeling_bert.py:71] Loading TF weight bert/encoder/layer_5/attention/output/LayerNorm/beta/adam_m with shape [768]
I1009 15:47:05.942691 47631408520768 modeling_bert.py:71] Loading TF weight bert/encoder/layer_5/attention/output/LayerNorm/beta/adam_v with shape [768]
I1009 15:47:05.947911 47631408520768 modeling_bert.py:71] Loading TF weight bert/encoder/layer_5/attention/output/LayerNorm/gamma with shape [768]
I1009 15:47:05.953302 47631408520768 modeling_bert.py:71] Loading TF weight bert/encoder/layer_5/attention/output/LayerNorm/gamma/adam_m with shape [768]
I1009 15:47:05.958757 47631408520768 modeling_bert.py:71] Loading TF weight bert/encoder/layer_5/attention/output/LayerNorm/gamma/adam_v with shape [768]
I1009 15:47:05.963871 47631408520768 modeling_bert.py:71] Loading TF weight bert/encoder/layer_5/attention/output/dense/bias with shape [768]
I1009 15:47:05.969243 47631408520768 modeling_bert.py:71] Loading TF weight bert/encoder/layer_5/attention/output/dense/bias/adam_m with shape [768]
I1009 15:47:05.974361 47631408520768 modeling_bert.py:71] Loading TF weight bert/encoder/layer_5/attention/output/dense/bias/adam_v with shape [768]
I1009 15:47:05.979577 47631408520768 modeling_bert.py:71] Loading TF weight bert/encoder/layer_5/attention/output/dense/kernel with shape [768, 768]
I1009 15:47:05.985836 47631408520768 modeling_bert.py:71] Loading TF weight bert/encoder/layer_5/attention/output/dense/kernel/adam_m with shape [768, 768]
I1009 15:47:05.991879 47631408520768 modeling_bert.py:71] Loading TF weight bert/encoder/layer_5/attention/output/dense/kernel/adam_v with shape [768, 768]
I1009 15:47:05.998053 47631408520768 modeling_bert.py:71] Loading TF weight bert/encoder/layer_5/attention/self/key/bias with shape [768]
I1009 15:47:06.003427 47631408520768 modeling_bert.py:71] Loading TF weight bert/encoder/layer_5/attention/self/key/bias/adam_m with shape [768]
I1009 15:47:06.008820 47631408520768 modeling_bert.py:71] Loading TF weight bert/encoder/layer_5/attention/self/key/bias/adam_v with shape [768]
I1009 15:47:06.014085 47631408520768 modeling_bert.py:71] Loading TF weight bert/encoder/layer_5/attention/self/key/kernel with shape [768, 768]
I1009 15:47:06.020233 47631408520768 modeling_bert.py:71] Loading TF weight bert/encoder/layer_5/attention/self/key/kernel/adam_m with shape [768, 768]
I1009 15:47:06.026285 47631408520768 modeling_bert.py:71] Loading TF weight bert/encoder/layer_5/attention/self/key/kernel/adam_v with shape [768, 768]
I1009 15:47:06.032388 47631408520768 modeling_bert.py:71] Loading TF weight bert/encoder/layer_5/attention/self/query/bias with shape [768]
I1009 15:47:06.038056 47631408520768 modeling_bert.py:71] Loading TF weight bert/encoder/layer_5/attention/self/query/bias/adam_m with shape [768]
I1009 15:47:06.043298 47631408520768 modeling_bert.py:71] Loading TF weight bert/encoder/layer_5/attention/self/query/bias/adam_v with shape [768]
I1009 15:47:06.048563 47631408520768 modeling_bert.py:71] Loading TF weight bert/encoder/layer_5/attention/self/query/kernel with shape [768, 768]
I1009 15:47:06.054869 47631408520768 modeling_bert.py:71] Loading TF weight bert/encoder/layer_5/attention/self/query/kernel/adam_m with shape [768, 768]
I1009 15:47:06.061104 47631408520768 modeling_bert.py:71] Loading TF weight bert/encoder/layer_5/attention/self/query/kernel/adam_v with shape [768, 768]
I1009 15:47:06.067418 47631408520768 modeling_bert.py:71] Loading TF weight bert/encoder/layer_5/attention/self/value/bias with shape [768]
I1009 15:47:06.072948 47631408520768 modeling_bert.py:71] Loading TF weight bert/encoder/layer_5/attention/self/value/bias/adam_m with shape [768]
I1009 15:47:06.078219 47631408520768 modeling_bert.py:71] Loading TF weight bert/encoder/layer_5/attention/self/value/bias/adam_v with shape [768]
I1009 15:47:06.083603 47631408520768 modeling_bert.py:71] Loading TF weight bert/encoder/layer_5/attention/self/value/kernel with shape [768, 768]
I1009 15:47:06.090325 47631408520768 modeling_bert.py:71] Loading TF weight bert/encoder/layer_5/attention/self/value/kernel/adam_m with shape [768, 768]
I1009 15:47:06.096712 47631408520768 modeling_bert.py:71] Loading TF weight bert/encoder/layer_5/attention/self/value/kernel/adam_v with shape [768, 768]
I1009 15:47:06.102761 47631408520768 modeling_bert.py:71] Loading TF weight bert/encoder/layer_5/intermediate/dense/bias with shape [3072]
I1009 15:47:06.108000 47631408520768 modeling_bert.py:71] Loading TF weight bert/encoder/layer_5/intermediate/dense/bias/adam_m with shape [3072]
I1009 15:47:06.113360 47631408520768 modeling_bert.py:71] Loading TF weight bert/encoder/layer_5/intermediate/dense/bias/adam_v with shape [3072]
I1009 15:47:06.118700 47631408520768 modeling_bert.py:71] Loading TF weight bert/encoder/layer_5/intermediate/dense/kernel with shape [768, 3072]
I1009 15:47:06.128049 47631408520768 modeling_bert.py:71] Loading TF weight bert/encoder/layer_5/intermediate/dense/kernel/adam_m with shape [768, 3072]
I1009 15:47:06.137369 47631408520768 modeling_bert.py:71] Loading TF weight bert/encoder/layer_5/intermediate/dense/kernel/adam_v with shape [768, 3072]
I1009 15:47:06.146910 47631408520768 modeling_bert.py:71] Loading TF weight bert/encoder/layer_5/output/LayerNorm/beta with shape [768]
I1009 15:47:06.152923 47631408520768 modeling_bert.py:71] Loading TF weight bert/encoder/layer_5/output/LayerNorm/beta/adam_m with shape [768]
I1009 15:47:06.158300 47631408520768 modeling_bert.py:71] Loading TF weight bert/encoder/layer_5/output/LayerNorm/beta/adam_v with shape [768]
I1009 15:47:06.163956 47631408520768 modeling_bert.py:71] Loading TF weight bert/encoder/layer_5/output/LayerNorm/gamma with shape [768]
I1009 15:47:06.169229 47631408520768 modeling_bert.py:71] Loading TF weight bert/encoder/layer_5/output/LayerNorm/gamma/adam_m with shape [768]
I1009 15:47:06.174710 47631408520768 modeling_bert.py:71] Loading TF weight bert/encoder/layer_5/output/LayerNorm/gamma/adam_v with shape [768]
I1009 15:47:06.179936 47631408520768 modeling_bert.py:71] Loading TF weight bert/encoder/layer_5/output/dense/bias with shape [768]
I1009 15:47:06.185377 47631408520768 modeling_bert.py:71] Loading TF weight bert/encoder/layer_5/output/dense/bias/adam_m with shape [768]
I1009 15:47:06.190515 47631408520768 modeling_bert.py:71] Loading TF weight bert/encoder/layer_5/output/dense/bias/adam_v with shape [768]
I1009 15:47:06.196292 47631408520768 modeling_bert.py:71] Loading TF weight bert/encoder/layer_5/output/dense/kernel with shape [3072, 768]
I1009 15:47:06.205745 47631408520768 modeling_bert.py:71] Loading TF weight bert/encoder/layer_5/output/dense/kernel/adam_m with shape [3072, 768]
I1009 15:47:06.215335 47631408520768 modeling_bert.py:71] Loading TF weight bert/encoder/layer_5/output/dense/kernel/adam_v with shape [3072, 768]
I1009 15:47:06.224854 47631408520768 modeling_bert.py:71] Loading TF weight bert/encoder/layer_6/attention/output/LayerNorm/beta with shape [768]
I1009 15:47:06.230671 47631408520768 modeling_bert.py:71] Loading TF weight bert/encoder/layer_6/attention/output/LayerNorm/beta/adam_m with shape [768]
I1009 15:47:06.235839 47631408520768 modeling_bert.py:71] Loading TF weight bert/encoder/layer_6/attention/output/LayerNorm/beta/adam_v with shape [768]
I1009 15:47:06.241382 47631408520768 modeling_bert.py:71] Loading TF weight bert/encoder/layer_6/attention/output/LayerNorm/gamma with shape [768]
I1009 15:47:06.246639 47631408520768 modeling_bert.py:71] Loading TF weight bert/encoder/layer_6/attention/output/LayerNorm/gamma/adam_m with shape [768]
I1009 15:47:06.251890 47631408520768 modeling_bert.py:71] Loading TF weight bert/encoder/layer_6/attention/output/LayerNorm/gamma/adam_v with shape [768]
I1009 15:47:06.257052 47631408520768 modeling_bert.py:71] Loading TF weight bert/encoder/layer_6/attention/output/dense/bias with shape [768]
I1009 15:47:06.262314 47631408520768 modeling_bert.py:71] Loading TF weight bert/encoder/layer_6/attention/output/dense/bias/adam_m with shape [768]
I1009 15:47:06.267627 47631408520768 modeling_bert.py:71] Loading TF weight bert/encoder/layer_6/attention/output/dense/bias/adam_v with shape [768]
I1009 15:47:06.272980 47631408520768 modeling_bert.py:71] Loading TF weight bert/encoder/layer_6/attention/output/dense/kernel with shape [768, 768]
I1009 15:47:06.279123 47631408520768 modeling_bert.py:71] Loading TF weight bert/encoder/layer_6/attention/output/dense/kernel/adam_m with shape [768, 768]
I1009 15:47:06.285258 47631408520768 modeling_bert.py:71] Loading TF weight bert/encoder/layer_6/attention/output/dense/kernel/adam_v with shape [768, 768]
I1009 15:47:06.291564 47631408520768 modeling_bert.py:71] Loading TF weight bert/encoder/layer_6/attention/self/key/bias with shape [768]
I1009 15:47:06.296821 47631408520768 modeling_bert.py:71] Loading TF weight bert/encoder/layer_6/attention/self/key/bias/adam_m with shape [768]
I1009 15:47:06.302075 47631408520768 modeling_bert.py:71] Loading TF weight bert/encoder/layer_6/attention/self/key/bias/adam_v with shape [768]
I1009 15:47:06.307455 47631408520768 modeling_bert.py:71] Loading TF weight bert/encoder/layer_6/attention/self/key/kernel with shape [768, 768]
I1009 15:47:06.313520 47631408520768 modeling_bert.py:71] Loading TF weight bert/encoder/layer_6/attention/self/key/kernel/adam_m with shape [768, 768]
I1009 15:47:06.319566 47631408520768 modeling_bert.py:71] Loading TF weight bert/encoder/layer_6/attention/self/key/kernel/adam_v with shape [768, 768]
I1009 15:47:06.325647 47631408520768 modeling_bert.py:71] Loading TF weight bert/encoder/layer_6/attention/self/query/bias with shape [768]
I1009 15:47:06.331008 47631408520768 modeling_bert.py:71] Loading TF weight bert/encoder/layer_6/attention/self/query/bias/adam_m with shape [768]
I1009 15:47:06.336525 47631408520768 modeling_bert.py:71] Loading TF weight bert/encoder/layer_6/attention/self/query/bias/adam_v with shape [768]
I1009 15:47:06.342104 47631408520768 modeling_bert.py:71] Loading TF weight bert/encoder/layer_6/attention/self/query/kernel with shape [768, 768]
I1009 15:47:06.348503 47631408520768 modeling_bert.py:71] Loading TF weight bert/encoder/layer_6/attention/self/query/kernel/adam_m with shape [768, 768]
I1009 15:47:06.354544 47631408520768 modeling_bert.py:71] Loading TF weight bert/encoder/layer_6/attention/self/query/kernel/adam_v with shape [768, 768]
I1009 15:47:06.361086 47631408520768 modeling_bert.py:71] Loading TF weight bert/encoder/layer_6/attention/self/value/bias with shape [768]
I1009 15:47:06.366425 47631408520768 modeling_bert.py:71] Loading TF weight bert/encoder/layer_6/attention/self/value/bias/adam_m with shape [768]
I1009 15:47:06.371979 47631408520768 modeling_bert.py:71] Loading TF weight bert/encoder/layer_6/attention/self/value/bias/adam_v with shape [768]
I1009 15:47:06.377128 47631408520768 modeling_bert.py:71] Loading TF weight bert/encoder/layer_6/attention/self/value/kernel with shape [768, 768]
I1009 15:47:06.383063 47631408520768 modeling_bert.py:71] Loading TF weight bert/encoder/layer_6/attention/self/value/kernel/adam_m with shape [768, 768]
I1009 15:47:06.389229 47631408520768 modeling_bert.py:71] Loading TF weight bert/encoder/layer_6/attention/self/value/kernel/adam_v with shape [768, 768]
I1009 15:47:06.395555 47631408520768 modeling_bert.py:71] Loading TF weight bert/encoder/layer_6/intermediate/dense/bias with shape [3072]
I1009 15:47:06.401404 47631408520768 modeling_bert.py:71] Loading TF weight bert/encoder/layer_6/intermediate/dense/bias/adam_m with shape [3072]
I1009 15:47:06.406746 47631408520768 modeling_bert.py:71] Loading TF weight bert/encoder/layer_6/intermediate/dense/bias/adam_v with shape [3072]
I1009 15:47:06.411887 47631408520768 modeling_bert.py:71] Loading TF weight bert/encoder/layer_6/intermediate/dense/kernel with shape [768, 3072]
I1009 15:47:06.420855 47631408520768 modeling_bert.py:71] Loading TF weight bert/encoder/layer_6/intermediate/dense/kernel/adam_m with shape [768, 3072]
I1009 15:47:06.430287 47631408520768 modeling_bert.py:71] Loading TF weight bert/encoder/layer_6/intermediate/dense/kernel/adam_v with shape [768, 3072]
I1009 15:47:06.439881 47631408520768 modeling_bert.py:71] Loading TF weight bert/encoder/layer_6/output/LayerNorm/beta with shape [768]
I1009 15:47:06.445644 47631408520768 modeling_bert.py:71] Loading TF weight bert/encoder/layer_6/output/LayerNorm/beta/adam_m with shape [768]
I1009 15:47:06.450891 47631408520768 modeling_bert.py:71] Loading TF weight bert/encoder/layer_6/output/LayerNorm/beta/adam_v with shape [768]
I1009 15:47:06.456028 47631408520768 modeling_bert.py:71] Loading TF weight bert/encoder/layer_6/output/LayerNorm/gamma with shape [768]
I1009 15:47:06.461563 47631408520768 modeling_bert.py:71] Loading TF weight bert/encoder/layer_6/output/LayerNorm/gamma/adam_m with shape [768]
I1009 15:47:06.467115 47631408520768 modeling_bert.py:71] Loading TF weight bert/encoder/layer_6/output/LayerNorm/gamma/adam_v with shape [768]
I1009 15:47:06.472524 47631408520768 modeling_bert.py:71] Loading TF weight bert/encoder/layer_6/output/dense/bias with shape [768]
I1009 15:47:06.478100 47631408520768 modeling_bert.py:71] Loading TF weight bert/encoder/layer_6/output/dense/bias/adam_m with shape [768]
I1009 15:47:06.483274 47631408520768 modeling_bert.py:71] Loading TF weight bert/encoder/layer_6/output/dense/bias/adam_v with shape [768]
I1009 15:47:06.488587 47631408520768 modeling_bert.py:71] Loading TF weight bert/encoder/layer_6/output/dense/kernel with shape [3072, 768]
I1009 15:47:06.497782 47631408520768 modeling_bert.py:71] Loading TF weight bert/encoder/layer_6/output/dense/kernel/adam_m with shape [3072, 768]
I1009 15:47:06.506931 47631408520768 modeling_bert.py:71] Loading TF weight bert/encoder/layer_6/output/dense/kernel/adam_v with shape [3072, 768]
I1009 15:47:06.516527 47631408520768 modeling_bert.py:71] Loading TF weight bert/encoder/layer_7/attention/output/LayerNorm/beta with shape [768]
I1009 15:47:06.522197 47631408520768 modeling_bert.py:71] Loading TF weight bert/encoder/layer_7/attention/output/LayerNorm/beta/adam_m with shape [768]
I1009 15:47:06.527507 47631408520768 modeling_bert.py:71] Loading TF weight bert/encoder/layer_7/attention/output/LayerNorm/beta/adam_v with shape [768]
I1009 15:47:06.532787 47631408520768 modeling_bert.py:71] Loading TF weight bert/encoder/layer_7/attention/output/LayerNorm/gamma with shape [768]
I1009 15:47:06.538027 47631408520768 modeling_bert.py:71] Loading TF weight bert/encoder/layer_7/attention/output/LayerNorm/gamma/adam_m with shape [768]
I1009 15:47:06.543310 47631408520768 modeling_bert.py:71] Loading TF weight bert/encoder/layer_7/attention/output/LayerNorm/gamma/adam_v with shape [768]
I1009 15:47:06.548686 47631408520768 modeling_bert.py:71] Loading TF weight bert/encoder/layer_7/attention/output/dense/bias with shape [768]
I1009 15:47:06.553850 47631408520768 modeling_bert.py:71] Loading TF weight bert/encoder/layer_7/attention/output/dense/bias/adam_m with shape [768]
I1009 15:47:06.559104 47631408520768 modeling_bert.py:71] Loading TF weight bert/encoder/layer_7/attention/output/dense/bias/adam_v with shape [768]
I1009 15:47:06.564384 47631408520768 modeling_bert.py:71] Loading TF weight bert/encoder/layer_7/attention/output/dense/kernel with shape [768, 768]
I1009 15:47:06.570770 47631408520768 modeling_bert.py:71] Loading TF weight bert/encoder/layer_7/attention/output/dense/kernel/adam_m with shape [768, 768]
I1009 15:47:06.576959 47631408520768 modeling_bert.py:71] Loading TF weight bert/encoder/layer_7/attention/output/dense/kernel/adam_v with shape [768, 768]
I1009 15:47:06.583284 47631408520768 modeling_bert.py:71] Loading TF weight bert/encoder/layer_7/attention/self/key/bias with shape [768]
I1009 15:47:06.588658 47631408520768 modeling_bert.py:71] Loading TF weight bert/encoder/layer_7/attention/self/key/bias/adam_m with shape [768]
I1009 15:47:06.593865 47631408520768 modeling_bert.py:71] Loading TF weight bert/encoder/layer_7/attention/self/key/bias/adam_v with shape [768]
I1009 15:47:06.598938 47631408520768 modeling_bert.py:71] Loading TF weight bert/encoder/layer_7/attention/self/key/kernel with shape [768, 768]
I1009 15:47:06.604933 47631408520768 modeling_bert.py:71] Loading TF weight bert/encoder/layer_7/attention/self/key/kernel/adam_m with shape [768, 768]
I1009 15:47:06.611174 47631408520768 modeling_bert.py:71] Loading TF weight bert/encoder/layer_7/attention/self/key/kernel/adam_v with shape [768, 768]
I1009 15:47:06.617308 47631408520768 modeling_bert.py:71] Loading TF weight bert/encoder/layer_7/attention/self/query/bias with shape [768]
I1009 15:47:06.622585 47631408520768 modeling_bert.py:71] Loading TF weight bert/encoder/layer_7/attention/self/query/bias/adam_m with shape [768]
I1009 15:47:06.627687 47631408520768 modeling_bert.py:71] Loading TF weight bert/encoder/layer_7/attention/self/query/bias/adam_v with shape [768]
I1009 15:47:06.633019 47631408520768 modeling_bert.py:71] Loading TF weight bert/encoder/layer_7/attention/self/query/kernel with shape [768, 768]
I1009 15:47:06.639252 47631408520768 modeling_bert.py:71] Loading TF weight bert/encoder/layer_7/attention/self/query/kernel/adam_m with shape [768, 768]
I1009 15:47:06.645524 47631408520768 modeling_bert.py:71] Loading TF weight bert/encoder/layer_7/attention/self/query/kernel/adam_v with shape [768, 768]
I1009 15:47:06.651858 47631408520768 modeling_bert.py:71] Loading TF weight bert/encoder/layer_7/attention/self/value/bias with shape [768]
I1009 15:47:06.657348 47631408520768 modeling_bert.py:71] Loading TF weight bert/encoder/layer_7/attention/self/value/bias/adam_m with shape [768]
I1009 15:47:06.662594 47631408520768 modeling_bert.py:71] Loading TF weight bert/encoder/layer_7/attention/self/value/bias/adam_v with shape [768]
I1009 15:47:06.667656 47631408520768 modeling_bert.py:71] Loading TF weight bert/encoder/layer_7/attention/self/value/kernel with shape [768, 768]
I1009 15:47:06.673909 47631408520768 modeling_bert.py:71] Loading TF weight bert/encoder/layer_7/attention/self/value/kernel/adam_m with shape [768, 768]
I1009 15:47:06.680046 47631408520768 modeling_bert.py:71] Loading TF weight bert/encoder/layer_7/attention/self/value/kernel/adam_v with shape [768, 768]
I1009 15:47:06.686088 47631408520768 modeling_bert.py:71] Loading TF weight bert/encoder/layer_7/intermediate/dense/bias with shape [3072]
I1009 15:47:06.691427 47631408520768 modeling_bert.py:71] Loading TF weight bert/encoder/layer_7/intermediate/dense/bias/adam_m with shape [3072]
I1009 15:47:06.696527 47631408520768 modeling_bert.py:71] Loading TF weight bert/encoder/layer_7/intermediate/dense/bias/adam_v with shape [3072]
I1009 15:47:06.701802 47631408520768 modeling_bert.py:71] Loading TF weight bert/encoder/layer_7/intermediate/dense/kernel with shape [768, 3072]
I1009 15:47:06.710916 47631408520768 modeling_bert.py:71] Loading TF weight bert/encoder/layer_7/intermediate/dense/kernel/adam_m with shape [768, 3072]
I1009 15:47:06.719931 47631408520768 modeling_bert.py:71] Loading TF weight bert/encoder/layer_7/intermediate/dense/kernel/adam_v with shape [768, 3072]
I1009 15:47:06.729498 47631408520768 modeling_bert.py:71] Loading TF weight bert/encoder/layer_7/output/LayerNorm/beta with shape [768]
I1009 15:47:06.734817 47631408520768 modeling_bert.py:71] Loading TF weight bert/encoder/layer_7/output/LayerNorm/beta/adam_m with shape [768]
I1009 15:47:06.739971 47631408520768 modeling_bert.py:71] Loading TF weight bert/encoder/layer_7/output/LayerNorm/beta/adam_v with shape [768]
I1009 15:47:06.745015 47631408520768 modeling_bert.py:71] Loading TF weight bert/encoder/layer_7/output/LayerNorm/gamma with shape [768]
I1009 15:47:06.750005 47631408520768 modeling_bert.py:71] Loading TF weight bert/encoder/layer_7/output/LayerNorm/gamma/adam_m with shape [768]
I1009 15:47:06.755349 47631408520768 modeling_bert.py:71] Loading TF weight bert/encoder/layer_7/output/LayerNorm/gamma/adam_v with shape [768]
I1009 15:47:06.760453 47631408520768 modeling_bert.py:71] Loading TF weight bert/encoder/layer_7/output/dense/bias with shape [768]
I1009 15:47:06.765552 47631408520768 modeling_bert.py:71] Loading TF weight bert/encoder/layer_7/output/dense/bias/adam_m with shape [768]
I1009 15:47:06.770809 47631408520768 modeling_bert.py:71] Loading TF weight bert/encoder/layer_7/output/dense/bias/adam_v with shape [768]
I1009 15:47:06.775943 47631408520768 modeling_bert.py:71] Loading TF weight bert/encoder/layer_7/output/dense/kernel with shape [3072, 768]
I1009 15:47:06.785551 47631408520768 modeling_bert.py:71] Loading TF weight bert/encoder/layer_7/output/dense/kernel/adam_m with shape [3072, 768]
I1009 15:47:06.795017 47631408520768 modeling_bert.py:71] Loading TF weight bert/encoder/layer_7/output/dense/kernel/adam_v with shape [3072, 768]
I1009 15:47:06.804197 47631408520768 modeling_bert.py:71] Loading TF weight bert/encoder/layer_8/attention/output/LayerNorm/beta with shape [768]
I1009 15:47:06.809925 47631408520768 modeling_bert.py:71] Loading TF weight bert/encoder/layer_8/attention/output/LayerNorm/beta/adam_m with shape [768]
I1009 15:47:06.815610 47631408520768 modeling_bert.py:71] Loading TF weight bert/encoder/layer_8/attention/output/LayerNorm/beta/adam_v with shape [768]
I1009 15:47:06.820969 47631408520768 modeling_bert.py:71] Loading TF weight bert/encoder/layer_8/attention/output/LayerNorm/gamma with shape [768]
I1009 15:47:06.825976 47631408520768 modeling_bert.py:71] Loading TF weight bert/encoder/layer_8/attention/output/LayerNorm/gamma/adam_m with shape [768]
I1009 15:47:06.831141 47631408520768 modeling_bert.py:71] Loading TF weight bert/encoder/layer_8/attention/output/LayerNorm/gamma/adam_v with shape [768]
I1009 15:47:06.836268 47631408520768 modeling_bert.py:71] Loading TF weight bert/encoder/layer_8/attention/output/dense/bias with shape [768]
I1009 15:47:06.841736 47631408520768 modeling_bert.py:71] Loading TF weight bert/encoder/layer_8/attention/output/dense/bias/adam_m with shape [768]
I1009 15:47:06.847084 47631408520768 modeling_bert.py:71] Loading TF weight bert/encoder/layer_8/attention/output/dense/bias/adam_v with shape [768]
I1009 15:47:06.852262 47631408520768 modeling_bert.py:71] Loading TF weight bert/encoder/layer_8/attention/output/dense/kernel with shape [768, 768]
I1009 15:47:06.858251 47631408520768 modeling_bert.py:71] Loading TF weight bert/encoder/layer_8/attention/output/dense/kernel/adam_m with shape [768, 768]
I1009 15:47:06.864098 47631408520768 modeling_bert.py:71] Loading TF weight bert/encoder/layer_8/attention/output/dense/kernel/adam_v with shape [768, 768]
I1009 15:47:06.870030 47631408520768 modeling_bert.py:71] Loading TF weight bert/encoder/layer_8/attention/self/key/bias with shape [768]
I1009 15:47:06.875577 47631408520768 modeling_bert.py:71] Loading TF weight bert/encoder/layer_8/attention/self/key/bias/adam_m with shape [768]
I1009 15:47:06.881125 47631408520768 modeling_bert.py:71] Loading TF weight bert/encoder/layer_8/attention/self/key/bias/adam_v with shape [768]
I1009 15:47:06.886339 47631408520768 modeling_bert.py:71] Loading TF weight bert/encoder/layer_8/attention/self/key/kernel with shape [768, 768]
I1009 15:47:06.892580 47631408520768 modeling_bert.py:71] Loading TF weight bert/encoder/layer_8/attention/self/key/kernel/adam_m with shape [768, 768]
I1009 15:47:06.898903 47631408520768 modeling_bert.py:71] Loading TF weight bert/encoder/layer_8/attention/self/key/kernel/adam_v with shape [768, 768]
I1009 15:47:06.905303 47631408520768 modeling_bert.py:71] Loading TF weight bert/encoder/layer_8/attention/self/query/bias with shape [768]
I1009 15:47:06.910978 47631408520768 modeling_bert.py:71] Loading TF weight bert/encoder/layer_8/attention/self/query/bias/adam_m with shape [768]
I1009 15:47:06.916463 47631408520768 modeling_bert.py:71] Loading TF weight bert/encoder/layer_8/attention/self/query/bias/adam_v with shape [768]
I1009 15:47:06.921678 47631408520768 modeling_bert.py:71] Loading TF weight bert/encoder/layer_8/attention/self/query/kernel with shape [768, 768]
I1009 15:47:06.927710 47631408520768 modeling_bert.py:71] Loading TF weight bert/encoder/layer_8/attention/self/query/kernel/adam_m with shape [768, 768]
I1009 15:47:06.933935 47631408520768 modeling_bert.py:71] Loading TF weight bert/encoder/layer_8/attention/self/query/kernel/adam_v with shape [768, 768]
I1009 15:47:06.940702 47631408520768 modeling_bert.py:71] Loading TF weight bert/encoder/layer_8/attention/self/value/bias with shape [768]
I1009 15:47:06.946018 47631408520768 modeling_bert.py:71] Loading TF weight bert/encoder/layer_8/attention/self/value/bias/adam_m with shape [768]
I1009 15:47:06.951382 47631408520768 modeling_bert.py:71] Loading TF weight bert/encoder/layer_8/attention/self/value/bias/adam_v with shape [768]
I1009 15:47:06.957077 47631408520768 modeling_bert.py:71] Loading TF weight bert/encoder/layer_8/attention/self/value/kernel with shape [768, 768]
I1009 15:47:06.963493 47631408520768 modeling_bert.py:71] Loading TF weight bert/encoder/layer_8/attention/self/value/kernel/adam_m with shape [768, 768]
I1009 15:47:06.969939 47631408520768 modeling_bert.py:71] Loading TF weight bert/encoder/layer_8/attention/self/value/kernel/adam_v with shape [768, 768]
I1009 15:47:06.976311 47631408520768 modeling_bert.py:71] Loading TF weight bert/encoder/layer_8/intermediate/dense/bias with shape [3072]
I1009 15:47:06.982092 47631408520768 modeling_bert.py:71] Loading TF weight bert/encoder/layer_8/intermediate/dense/bias/adam_m with shape [3072]
I1009 15:47:06.987428 47631408520768 modeling_bert.py:71] Loading TF weight bert/encoder/layer_8/intermediate/dense/bias/adam_v with shape [3072]
I1009 15:47:06.992400 47631408520768 modeling_bert.py:71] Loading TF weight bert/encoder/layer_8/intermediate/dense/kernel with shape [768, 3072]
I1009 15:47:07.001886 47631408520768 modeling_bert.py:71] Loading TF weight bert/encoder/layer_8/intermediate/dense/kernel/adam_m with shape [768, 3072]
I1009 15:47:07.011502 47631408520768 modeling_bert.py:71] Loading TF weight bert/encoder/layer_8/intermediate/dense/kernel/adam_v with shape [768, 3072]
I1009 15:47:07.020718 47631408520768 modeling_bert.py:71] Loading TF weight bert/encoder/layer_8/output/LayerNorm/beta with shape [768]
I1009 15:47:07.026179 47631408520768 modeling_bert.py:71] Loading TF weight bert/encoder/layer_8/output/LayerNorm/beta/adam_m with shape [768]
I1009 15:47:07.031653 47631408520768 modeling_bert.py:71] Loading TF weight bert/encoder/layer_8/output/LayerNorm/beta/adam_v with shape [768]
I1009 15:47:07.037088 47631408520768 modeling_bert.py:71] Loading TF weight bert/encoder/layer_8/output/LayerNorm/gamma with shape [768]
I1009 15:47:07.042681 47631408520768 modeling_bert.py:71] Loading TF weight bert/encoder/layer_8/output/LayerNorm/gamma/adam_m with shape [768]
I1009 15:47:07.047634 47631408520768 modeling_bert.py:71] Loading TF weight bert/encoder/layer_8/output/LayerNorm/gamma/adam_v with shape [768]
I1009 15:47:07.052863 47631408520768 modeling_bert.py:71] Loading TF weight bert/encoder/layer_8/output/dense/bias with shape [768]
I1009 15:47:07.058085 47631408520768 modeling_bert.py:71] Loading TF weight bert/encoder/layer_8/output/dense/bias/adam_m with shape [768]
I1009 15:47:07.063718 47631408520768 modeling_bert.py:71] Loading TF weight bert/encoder/layer_8/output/dense/bias/adam_v with shape [768]
I1009 15:47:07.069069 47631408520768 modeling_bert.py:71] Loading TF weight bert/encoder/layer_8/output/dense/kernel with shape [3072, 768]
I1009 15:47:07.078701 47631408520768 modeling_bert.py:71] Loading TF weight bert/encoder/layer_8/output/dense/kernel/adam_m with shape [3072, 768]
I1009 15:47:07.088213 47631408520768 modeling_bert.py:71] Loading TF weight bert/encoder/layer_8/output/dense/kernel/adam_v with shape [3072, 768]
I1009 15:47:07.097591 47631408520768 modeling_bert.py:71] Loading TF weight bert/encoder/layer_9/attention/output/LayerNorm/beta with shape [768]
I1009 15:47:07.103389 47631408520768 modeling_bert.py:71] Loading TF weight bert/encoder/layer_9/attention/output/LayerNorm/beta/adam_m with shape [768]
I1009 15:47:07.109018 47631408520768 modeling_bert.py:71] Loading TF weight bert/encoder/layer_9/attention/output/LayerNorm/beta/adam_v with shape [768]
I1009 15:47:07.114469 47631408520768 modeling_bert.py:71] Loading TF weight bert/encoder/layer_9/attention/output/LayerNorm/gamma with shape [768]
I1009 15:47:07.119768 47631408520768 modeling_bert.py:71] Loading TF weight bert/encoder/layer_9/attention/output/LayerNorm/gamma/adam_m with shape [768]
I1009 15:47:07.125015 47631408520768 modeling_bert.py:71] Loading TF weight bert/encoder/layer_9/attention/output/LayerNorm/gamma/adam_v with shape [768]
I1009 15:47:07.130143 47631408520768 modeling_bert.py:71] Loading TF weight bert/encoder/layer_9/attention/output/dense/bias with shape [768]
I1009 15:47:07.135096 47631408520768 modeling_bert.py:71] Loading TF weight bert/encoder/layer_9/attention/output/dense/bias/adam_m with shape [768]
I1009 15:47:07.140460 47631408520768 modeling_bert.py:71] Loading TF weight bert/encoder/layer_9/attention/output/dense/bias/adam_v with shape [768]
I1009 15:47:07.145726 47631408520768 modeling_bert.py:71] Loading TF weight bert/encoder/layer_9/attention/output/dense/kernel with shape [768, 768]
I1009 15:47:07.151650 47631408520768 modeling_bert.py:71] Loading TF weight bert/encoder/layer_9/attention/output/dense/kernel/adam_m with shape [768, 768]
I1009 15:47:07.157860 47631408520768 modeling_bert.py:71] Loading TF weight bert/encoder/layer_9/attention/output/dense/kernel/adam_v with shape [768, 768]
I1009 15:47:07.163643 47631408520768 modeling_bert.py:71] Loading TF weight bert/encoder/layer_9/attention/self/key/bias with shape [768]
I1009 15:47:07.168712 47631408520768 modeling_bert.py:71] Loading TF weight bert/encoder/layer_9/attention/self/key/bias/adam_m with shape [768]
I1009 15:47:07.174322 47631408520768 modeling_bert.py:71] Loading TF weight bert/encoder/layer_9/attention/self/key/bias/adam_v with shape [768]
I1009 15:47:07.179837 47631408520768 modeling_bert.py:71] Loading TF weight bert/encoder/layer_9/attention/self/key/kernel with shape [768, 768]
I1009 15:47:07.185978 47631408520768 modeling_bert.py:71] Loading TF weight bert/encoder/layer_9/attention/self/key/kernel/adam_m with shape [768, 768]
I1009 15:47:07.192014 47631408520768 modeling_bert.py:71] Loading TF weight bert/encoder/layer_9/attention/self/key/kernel/adam_v with shape [768, 768]
I1009 15:47:07.198294 47631408520768 modeling_bert.py:71] Loading TF weight bert/encoder/layer_9/attention/self/query/bias with shape [768]
I1009 15:47:07.203473 47631408520768 modeling_bert.py:71] Loading TF weight bert/encoder/layer_9/attention/self/query/bias/adam_m with shape [768]
I1009 15:47:07.209064 47631408520768 modeling_bert.py:71] Loading TF weight bert/encoder/layer_9/attention/self/query/bias/adam_v with shape [768]
I1009 15:47:07.214386 47631408520768 modeling_bert.py:71] Loading TF weight bert/encoder/layer_9/attention/self/query/kernel with shape [768, 768]
I1009 15:47:07.220609 47631408520768 modeling_bert.py:71] Loading TF weight bert/encoder/layer_9/attention/self/query/kernel/adam_m with shape [768, 768]
I1009 15:47:07.226944 47631408520768 modeling_bert.py:71] Loading TF weight bert/encoder/layer_9/attention/self/query/kernel/adam_v with shape [768, 768]
I1009 15:47:07.233198 47631408520768 modeling_bert.py:71] Loading TF weight bert/encoder/layer_9/attention/self/value/bias with shape [768]
I1009 15:47:07.238353 47631408520768 modeling_bert.py:71] Loading TF weight bert/encoder/layer_9/attention/self/value/bias/adam_m with shape [768]
I1009 15:47:07.243831 47631408520768 modeling_bert.py:71] Loading TF weight bert/encoder/layer_9/attention/self/value/bias/adam_v with shape [768]
I1009 15:47:07.249445 47631408520768 modeling_bert.py:71] Loading TF weight bert/encoder/layer_9/attention/self/value/kernel with shape [768, 768]
I1009 15:47:07.255527 47631408520768 modeling_bert.py:71] Loading TF weight bert/encoder/layer_9/attention/self/value/kernel/adam_m with shape [768, 768]
I1009 15:47:07.261869 47631408520768 modeling_bert.py:71] Loading TF weight bert/encoder/layer_9/attention/self/value/kernel/adam_v with shape [768, 768]
I1009 15:47:07.268050 47631408520768 modeling_bert.py:71] Loading TF weight bert/encoder/layer_9/intermediate/dense/bias with shape [3072]
I1009 15:47:07.273308 47631408520768 modeling_bert.py:71] Loading TF weight bert/encoder/layer_9/intermediate/dense/bias/adam_m with shape [3072]
I1009 15:47:07.278274 47631408520768 modeling_bert.py:71] Loading TF weight bert/encoder/layer_9/intermediate/dense/bias/adam_v with shape [3072]
I1009 15:47:07.283823 47631408520768 modeling_bert.py:71] Loading TF weight bert/encoder/layer_9/intermediate/dense/kernel with shape [768, 3072]
I1009 15:47:07.293444 47631408520768 modeling_bert.py:71] Loading TF weight bert/encoder/layer_9/intermediate/dense/kernel/adam_m with shape [768, 3072]
I1009 15:47:07.302655 47631408520768 modeling_bert.py:71] Loading TF weight bert/encoder/layer_9/intermediate/dense/kernel/adam_v with shape [768, 3072]
I1009 15:47:07.312628 47631408520768 modeling_bert.py:71] Loading TF weight bert/encoder/layer_9/output/LayerNorm/beta with shape [768]
I1009 15:47:07.318495 47631408520768 modeling_bert.py:71] Loading TF weight bert/encoder/layer_9/output/LayerNorm/beta/adam_m with shape [768]
I1009 15:47:07.323935 47631408520768 modeling_bert.py:71] Loading TF weight bert/encoder/layer_9/output/LayerNorm/beta/adam_v with shape [768]
I1009 15:47:07.329243 47631408520768 modeling_bert.py:71] Loading TF weight bert/encoder/layer_9/output/LayerNorm/gamma with shape [768]
I1009 15:47:07.334521 47631408520768 modeling_bert.py:71] Loading TF weight bert/encoder/layer_9/output/LayerNorm/gamma/adam_m with shape [768]
I1009 15:47:07.339932 47631408520768 modeling_bert.py:71] Loading TF weight bert/encoder/layer_9/output/LayerNorm/gamma/adam_v with shape [768]
I1009 15:47:07.345828 47631408520768 modeling_bert.py:71] Loading TF weight bert/encoder/layer_9/output/dense/bias with shape [768]
I1009 15:47:07.351069 47631408520768 modeling_bert.py:71] Loading TF weight bert/encoder/layer_9/output/dense/bias/adam_m with shape [768]
I1009 15:47:07.356699 47631408520768 modeling_bert.py:71] Loading TF weight bert/encoder/layer_9/output/dense/bias/adam_v with shape [768]
I1009 15:47:07.362353 47631408520768 modeling_bert.py:71] Loading TF weight bert/encoder/layer_9/output/dense/kernel with shape [3072, 768]
I1009 15:47:07.371929 47631408520768 modeling_bert.py:71] Loading TF weight bert/encoder/layer_9/output/dense/kernel/adam_m with shape [3072, 768]
I1009 15:47:07.381705 47631408520768 modeling_bert.py:71] Loading TF weight bert/encoder/layer_9/output/dense/kernel/adam_v with shape [3072, 768]
I1009 15:47:07.390963 47631408520768 modeling_bert.py:71] Loading TF weight bert/pooler/dense/bias with shape [768]
I1009 15:47:07.396645 47631408520768 modeling_bert.py:71] Loading TF weight bert/pooler/dense/bias/adam_m with shape [768]
I1009 15:47:07.401854 47631408520768 modeling_bert.py:71] Loading TF weight bert/pooler/dense/bias/adam_v with shape [768]
I1009 15:47:07.406987 47631408520768 modeling_bert.py:71] Loading TF weight bert/pooler/dense/kernel with shape [768, 768]
I1009 15:47:07.412913 47631408520768 modeling_bert.py:71] Loading TF weight bert/pooler/dense/kernel/adam_m with shape [768, 768]
I1009 15:47:07.419250 47631408520768 modeling_bert.py:71] Loading TF weight bert/pooler/dense/kernel/adam_v with shape [768, 768]
I1009 15:47:07.425417 47631408520768 modeling_bert.py:71] Loading TF weight global_step with shape []
I1009 15:47:07.430413 47631408520768 modeling_bert.py:71] Loading TF weight output_bias with shape [21]
I1009 15:47:07.435464 47631408520768 modeling_bert.py:71] Loading TF weight output_bias/adam_m with shape [21]
I1009 15:47:07.440396 47631408520768 modeling_bert.py:71] Loading TF weight output_bias/adam_v with shape [21]
I1009 15:47:07.445353 47631408520768 modeling_bert.py:71] Loading TF weight output_weights with shape [21, 768]
I1009 15:47:07.450436 47631408520768 modeling_bert.py:71] Loading TF weight output_weights/adam_m with shape [21, 768]
I1009 15:47:07.455591 47631408520768 modeling_bert.py:71] Loading TF weight output_weights/adam_v with shape [21, 768]
---------------------------------------------------------------------------
AttributeError Traceback (most recent call last)
<ipython-input-34-7cd6093dcd13> in <module>
15
16 # Load weights from tf checkpoint
---> 17 load_tf_weights_in_bert(model, config, tf_checkpoint_path)
18
19 # Save pytorch-model
~/py3.6/lib/python3.6/site-packages/transformers/modeling_bert.py in load_tf_weights_in_bert(model, config, tf_checkpoint_path)
~/py3.6/lib/python3.6/site-packages/torch/nn/modules/module.py in __getattr__(self, name)
589 return modules[name]
590 raise AttributeError("'{}' object has no attribute '{}'".format(
--> 591 type(self).__name__, name))
592
593 def __setattr__(self, name, value):
AttributeError: 'LayerNorm' object has no attribute 'cls'
``` | 10-09-2019 19:57:59 | 10-09-2019 19:57:59 | Hi @chiyuzhang94 . What do you get if you remove everything related to `cls` in
```
pointer = getattr(pointer, 'cls')
pointer = getattr(pointer, 'bias')
elif l[0] == 'output_weights':
pointer = getattr(pointer, 'cls')
pointer = getattr(pointer, 'weight')
```
?<|||||>> Hi @chiyuzhang94 . What do you get if you remove everything related to `cls` in
>
> ```
> pointer = getattr(pointer, 'cls')
> pointer = getattr(pointer, 'bias')
> elif l[0] == 'output_weights':
> pointer = getattr(pointer, 'cls')
> pointer = getattr(pointer, 'weight')
> ```
>
> ?
<|||||>Hi @rlouf
When I removed `cls` parts, I got the following error:
```
I1009 17:18:05.159223 47655893610048 modeling_bert.py:81] Skipping bert/encoder/layer_9/output/dense/bias/adam_m
I1009 17:18:05.159870 47655893610048 modeling_bert.py:81] Skipping bert/encoder/layer_9/output/dense/bias/adam_v
I1009 17:18:05.160566 47655893610048 modeling_bert.py:115] Initialize PyTorch weight ['bert', 'encoder', 'layer_9', 'output', 'dense', 'kernel']
I1009 17:18:05.161365 47655893610048 modeling_bert.py:81] Skipping bert/encoder/layer_9/output/dense/kernel/adam_m
I1009 17:18:05.162042 47655893610048 modeling_bert.py:81] Skipping bert/encoder/layer_9/output/dense/kernel/adam_v
I1009 17:18:05.162718 47655893610048 modeling_bert.py:115] Initialize PyTorch weight ['bert', 'pooler', 'dense', 'bias']
I1009 17:18:05.163373 47655893610048 modeling_bert.py:81] Skipping bert/pooler/dense/bias/adam_m
I1009 17:18:05.164020 47655893610048 modeling_bert.py:81] Skipping bert/pooler/dense/bias/adam_v
I1009 17:18:05.164690 47655893610048 modeling_bert.py:115] Initialize PyTorch weight ['bert', 'pooler', 'dense', 'kernel']
I1009 17:18:05.165374 47655893610048 modeling_bert.py:81] Skipping bert/pooler/dense/kernel/adam_m
I1009 17:18:05.166048 47655893610048 modeling_bert.py:81] Skipping bert/pooler/dense/kernel/adam_v
I1009 17:18:05.166695 47655893610048 modeling_bert.py:81] Skipping global_step
---------------------------------------------------------------------------
AttributeError Traceback (most recent call last)
<ipython-input-1-a2cbceaf2173> in <module>
15
16 # Load weights from tf checkpoint
---> 17 load_tf_weights_in_bert(model, config, tf_checkpoint_path)
18
19 # Save pytorch-model
~/py3.6/lib/python3.6/site-packages/transformers-2.1.0-py3.6.egg/transformers/modeling_bert.py in load_tf_weights_in_bert(model, config, tf_checkpoint_path)
90 pointer = getattr(pointer, 'weight')
91 elif l[0] == 'output_bias' or l[0] == 'beta':
---> 92 pointer = getattr(pointer, 'bias')
93 elif l[0] == 'output_weights':
94 pointer = getattr(pointer, 'weight')
~/py3.6/lib/python3.6/site-packages/torch/nn/modules/module.py in __getattr__(self, name)
589 return modules[name]
590 raise AttributeError("'{}' object has no attribute '{}'".format(
--> 591 type(self).__name__, name))
592
593 def __setattr__(self, name, value):
```<|||||>Hi @chiyuzhang94, as a side question which TensorFlow version did you use to train your bert model ?
Do you observe the same behavior by loading the .index file directly using:
```python
config = BertConfig.from_json_file('your/tf_model/config.json')
model = BertForSequenceClassification.from_pretrained('your/tf_model/xxxx.ckpt.index', from_tf=True, config=config)
```<|||||>Hi @mfuntowicz ,
I trained the model with tensorflow 1.12.0.
My am currently using tensorflow 1.13.1 and torch 1.2.0 for this converting task.
If I use your suggestion code, it is also same issue:
```
---------------------------------------------------------------------------
AttributeError Traceback (most recent call last)
<ipython-input-2-35a5c9b35e78> in <module>
12
13 print("Building PyTorch model from configuration: {}".format(str(config)))
---> 14 model = BertForSequenceClassification.from_pretrained(tf_checkpoint_path, from_tf=True, config=config)
15 # Load weights from tf checkpoint
16 # load_tf_weights_in_bert(model, config, tf_checkpoint_path)
~/py3.6/lib/python3.6/site-packages/transformers-2.1.0-py3.6.egg/transformers/modeling_utils.py in from_pretrained(cls, pretrained_model_name_or_path, *model_args, **kwargs)
352 if resolved_archive_file.endswith('.index'):
353 # Load from a TensorFlow 1.X checkpoint - provided by original authors
--> 354 model = cls.load_tf_weights(model, config, resolved_archive_file[:-6]) # Remove the '.index'
355 else:
356 # Load from our TensorFlow 2.0 checkpoints
~/py3.6/lib/python3.6/site-packages/transformers-2.1.0-py3.6.egg/transformers/modeling_bert.py in load_tf_weights_in_bert(model, config, tf_checkpoint_path)
90 pointer = getattr(pointer, 'weight')
91 elif l[0] == 'output_bias' or l[0] == 'beta':
---> 92 pointer = getattr(pointer, 'bias')
93 elif l[0] == 'output_weights':
94 pointer = getattr(pointer, 'weight')
~/py3.6/lib/python3.6/site-packages/torch/nn/modules/module.py in __getattr__(self, name)
589 return modules[name]
590 raise AttributeError("'{}' object has no attribute '{}'".format(
--> 591 type(self).__name__, name))
592
593 def __setattr__(self, name, value):
AttributeError: 'BertForSequenceClassification' object has no attribute 'bias'
```<|||||>This happens because you are trying to load weights the functions wasn't designed for. Unfortunately we cannot support every possible file. You will have to modify `modeling_bert.py` manually to support your file. The part you need to modify is:
```
for name, array in zip(names, arrays):
name = name.split('/')
# adam_v and adam_m are variables used in AdamWeightDecayOptimizer to calculated m and v
# which are not required for using pretrained model
if any(n in ["adam_v", "adam_m", "global_step"] for n in name):
logger.info("Skipping {}".format("/".join(name)))
continue
pointer = model
for m_name in name:
if re.fullmatch(r'[A-Za-z]+_\d+', m_name):
l = re.split(r'_(\d+)', m_name)
else:
l = [m_name]
if l[0] == 'kernel' or l[0] == 'gamma':
pointer = getattr(pointer, 'weight')
elif l[0] == 'output_bias' or l[0] == 'beta':
pointer = getattr(pointer, 'bias')
elif l[0] == 'output_weights':
pointer = getattr(pointer, 'weight')
elif l[0] == 'squad':
pointer = getattr(pointer, 'classifier')
else:
try:
pointer = getattr(pointer, l[0])
except AttributeError:
logger.info("Skipping {}".format("/".join(name)))
continue
if len(l) >= 2:
num = int(l[1])
pointer = pointer[num]
if m_name[-11:] == '_embeddings':
pointer = getattr(pointer, 'weight')
elif m_name == 'kernel':
array = np.transpose(array)
try:
assert pointer.shape == array.shape
except AssertionError as e:
e.args += (pointer.shape, array.shape)
raise
logger.info("Initialize PyTorch weight {}".format(name))
pointer.data = torch.from_numpy(array)
```<|||||>Hi @rlouf ,
Thanks for your answer. I think adding `pointer = getattr(pointer, 'cls')` to the two if-so section make sense. But I am wondering how I can deal with the question of 'LayerNorm' object has no attribute 'cls'. Could you please provide me any hint?<|||||>Separating out the conditional statement:
`elif l[0] == 'output_bias' or l[0] == 'beta':`
into two, while maintaining the original functionality in the beta conditional should work?<|||||>This issue has been automatically marked as stale because it has not had recent activity. It will be closed if no further activity occurs. Thank you for your contributions.
|
transformers | 1,473 | closed | Bug in CTRL generation | ## 🐛 Bug
Model: CTRL
Language: English
The problem arises when using:
* [x] Official example script [`run_generation.py`](https://github.com/huggingface/transformers/blob/master/examples/run_generation.py)
The tasks I am working on is:
* [x] Generating text with the CTRL model.
## To Reproduce
Steps to reproduce the behavior:
1. Run `python run_generation.py` with `model_type=ctrl`, `model_name_or_path=ctrl`, `temperature=0`, a decent length like `length=50`, and `repetition_penalty=1.2`.
2. Input a `Links`-based control code, such as `Links https://www.cnn.com/2018/09/20/us-president-meets-british-pm`, from the original paper.
Rather than generating relevant, English text, it will often generate assorted, garbled French. For example, for the above example it generated: `m m e et au neuen auge de la part des
\* * * * * * * * * * * * * * * * * * * * * * * * * * * * * * * * * * * *`.
## Expected behavior
It should generate relatively-coherent English text, relevant to the link URL. This is the behavior in the paper, and in the [`lower_memory` branch](https://github.com/salesforce/ctrl/tree/lower_memory) Colab notebook.
## Environment
* OS: MacOS
* Python version: 3.5.6, Anaconda.
* PyTorch version: 1.2.0
* PyTorch Transformers version (or branch): 2.1.0
* Using GPU ? No
* Any other relevant information: | 10-09-2019 18:43:13 | 10-09-2019 18:43:13 | Yes we have observed there was a difference in tokenization. We've temporarily fixed in 036483f, could you install from source and tell us if you manage to have good generations?
By following the recommended specs (temperature=0.2, top_k=5 and repetition_penalty=1.2), with the following input sentence: `Reviews Rating 4.0`, I obtained the following completion:
Reviews Rating 4.0 < GENERATION > "out of 5 stars. I received a copy from the author in exchange for an honest review.
Rating: 4.0
This is one book that you will not want to put down. It was very well written and kept me on my toes. The characters were so real it made them seem like people you know. You could feel their pain as they struggled with what happened to them. There are some twists and turns along the way but nothing too surprising. All in all this story had everything needed to make it a great book. If"
<|||||>Looks like that fixed it. Getting a similar completion to what you got for `Reviews Rating 4.0`:
```
Reviews Rating 4.0 out of 5 starsI received a copy from the author in exchange for an honest review.
Rating: 4.0
This was a great book that kept me interested and wanting to read more. It is about two people who have been together forever but are not
```
And for `Links https://www.cnn.com/2018/09/20/us-president-meets-british-pm`, I get:
```
Links https://www.cnn.com/2018/09/20/us-president-meets-british-pm (CNN)President Donald Trump said Friday he would meet with British Prime Minister Theresa May in Washington next week, a move that could help ease tensions between the two countries after months of escalating trade tensions.
The White House announced Trump's decision to hold talks
```
Should I leave this issue open, since you mentioned this is a temporary fix?<|||||>Following deeper investigations, this temporary solution is actually the correct one for CTRL.
See details in #1480. |
transformers | 1,472 | closed | Bug when finetuning model on Squad | ## 🐛 Bug
Model: Bert (bert-large-uncased-whole-word-masking)
The problem arises when using:
The official example script for finetuning on squad data:
```
python -m torch.distributed.launch --nproc_per_node=8 run_squad.py \
--model_type bert \
--model_name_or_path bert-large-uncased-whole-word-masking \
--do_train \
--do_eval \
--do_lower_case \
--train_file $SQUAD_DIR/train-v1.1.json \
--predict_file $SQUAD_DIR/dev-v1.1.json \
--learning_rate 3e-5 \
--num_train_epochs 2 \
--max_seq_length 384 \
--doc_stride 128 \
--output_dir ./models/wwm_uncased_finetuned_squad/ \
--per_gpu_eval_batch_size 3 \
--per_gpu_train_batch_size 3 \
--save_steps 1500 \
--logging_steps 250 \
--fp16
```
The tasks I am working on is:
* [x ] an official GLUE/SQUaD task: SQUaD
Here is the error log:
```
...
10/09/2019 17:03:29 - INFO - utils_squad - Writing predictions to: ./models/wwm_uncased_finetuned_squad/predictions_.json
10/09/2019 17:03:29 - INFO - utils_squad - Writing nbest to: ./models/wwm_uncased_finetuned_squad/nbest_predictions_.json
Traceback (most recent call last):
File "run_squad.py", line 537, in <module>
main()
File "run_squad.py", line 526, in main
result = evaluate(args, model, tokenizer, prefix=global_step)
File "run_squad.py", line 268, in evaluate
args.version_2_with_negative, args.null_score_diff_threshold)
File "/dl/huggingface-bert/transformers/examples/SQuAD_runs/rundir/utils_squad.py", line 511, in write_predictions
result = unique_id_to_result[feature.unique_id]
KeyError: 1000000000
```
## Additional context
When running on multiple gpus the above problem shows up.
| 10-09-2019 17:25:55 | 10-09-2019 17:25:55 | https://github.com/huggingface/transformers/issues/940<|||||>@ahotrod you have any fix for this bug?<|||||>> @ahotrod you have any fix for this bug?
@a-maci no unfortunately not, still searching. I'm considering rolling back to Transformers 2.0.0 or even pytorch-transformers 1.2.0, one or both of which didn't spawn this error in my earlier SQuAD replications.<|||||>> @ahotrod you have any fix for this bug?
@a-maci I needed XLNet fine-tuned on SQuAD 2.0 with 512 max_seq_length. I found "**A**" solution: went back to the original XLNet paper's github for the "native" code. I could fit 1 batch on each of (2) 1080Ti GPUs, 85,000 steps, ~14.5 hr of fine-tuning with results EM / F1: 84.5 / 87.1.
`INFO:tensorflow:Result | best_exact 84.52792049187232 | best_exact_thresh -2.716632127761841 | best_f1 87.12844471348052 | best_f1_thresh -2.447098970413208 | has_ans_exact 0.8733130904183536 | has_ans_f1 0.9327569452896122 | `
Possibly try the BERT paper's "native" code?<|||||>I've described the bug here: https://github.com/huggingface/transformers/issues/940#issuecomment-547686206
Workaround is either to use DataParallel (remove `-m torch.distributed.launch --nproc_per_node=8`) or don't eval in the same run (remove `--do_eval`). You can evaluate the model after training with:
```
python examples/run_squad.py \
--model_type bert \
--model_name_or_path bert-base-cased \
--do_eval \
--do_lower_case \
--predict_file $SQUAD_DIR/dev-v1.1.json \
--train_file $SQUAD_DIR/train-v1.1.json \
--output_dir ./models/wwm_uncased_finetuned_squad/
```<|||||>As mentioned in #940, happy to welcome a PR to fix this case if someone from the community wants to contribute (I don't have the bandwidth for this issue at the moment).<|||||>Maybe try changing `args.local_rank == -1` to `args.local_rank in [-1, 0]` at this line? https://github.com/huggingface/transformers/blob/079bfb32fba4f2b39d344ca7af88d79a3ff27c7c/examples/run_squad.py#L216
I think evaluate is only used in the main process (local_rank==0) if you're using multiple gpus
(reference: https://github.com/huggingface/transformers/blob/079bfb32fba4f2b39d344ca7af88d79a3ff27c7c/examples/run_squad.py#L543)<|||||>It makes more sense to just remove the `DistributedSampler` case entirely. The problem is that `all_results` doesn't get gathered from all GPUs. Unless you also implement a gather you shouldn't use `DistributedSampler` at all.<|||||>This issue has been automatically marked as stale because it has not had recent activity. It will be closed if no further activity occurs. Thank you for your contributions.
<|||||>Is there a fix for this ? Im seeing the same issue for running only evaluation on CPU too. <|||||>Are you trying to do multiprocess evaluation? A single CPU process should work, my WAR above is to run eval seperately as a single process. |
transformers | 1,471 | closed | Write with Transformer: Changing settings on Mobile? | ## ❓ Questions & Help
It's great to see new features and options, in particular the Max Time option to generate longer outputs. However, none of the Model Settings are available on mobile...?
In order to change the model settings on mobile, I had to download Firefox, mess with the CSS settings in about://config to make everything really tiny, zoom in on the extremely small settings box, slide things around, then set everything back, and I'd have to do most of those all over again if I end up closing / reloading the tab.
| 10-09-2019 13:30:43 | 10-09-2019 13:30:43 | You're right, the interface isn't well suited to mess with settings on mobile. It's on our roadmap!<|||||>awesome! I found a dumb workaround; saved a copy of the page, changed the default values, then put it in my dropbox!<|||||>Haha that’s a great hack!
Closing this for now, thanks |
transformers | 1,470 | closed | Plan for Albert? | ## 🚀 Feature
<!-- A clear and concise description of the feature proposal. Please provide a link to the paper and code in case they exist. -->
I think Albert is popular enough to not say anything more. The link to the paper is below.
https://arxiv.org/pdf/1909.11942v1.pdf
## Motivation
<!-- Please outline the motivation for the proposal. Is your feature request related to a problem? e.g., I'm always frustrated when [...]. If this is related to another GitHub issue, please link here too. -->
## Additional context
<!-- Add any other context or screenshots about the feature request here. -->
| 10-09-2019 13:30:10 | 10-09-2019 13:30:10 | Duplicate of #1370 |
transformers | 1,469 | closed | How much GPU memory is needed to run run_squad.py | ## ❓ Questions & Help
How much GPU memory is needed to run `run_squad.py`, I tried on `GTX 1050ti (4gb)` with the following setting and I am getting out of memory error
```
$ python3 examples/run_squad.py \
--model_type bert \
--model_name_or_path bert-base-cased \
--do_train \
--do_eval \
--do_lower_case \
--train_file $SQUAD_DIR/train-v1.1.json \
--predict_file $SQUAD_DIR/dev-v1.1.json \
--per_gpu_train_batch_size 12 \
--learning_rate 3e-5 \
--num_train_epochs 2.0 \
--max_seq_length 384 \
--doc_stride 128 \
--output_dir /tmp/debug_squad/ \
--save_steps 1000
``` | 10-09-2019 11:38:08 | 10-09-2019 11:38:08 | With 4 GB you're bound to issues with a batch size of 12. You could figure out the total memory usage of the model + calculate the memory footprints of tensors to determine the biggest batch size that would fit on your GPU.
Specifying a smaller batch size (like 1 or 2) would let you run the script, though.<|||||>> With 4 GB you're bound to issues with a batch size of 12. You could figure out the total memory usage of the model + calculate the memory footprints of tensors to determine the biggest batch size that would fit on your GPU.
>
> Specifying a smaller batch size (like 1 or 2) would let you run the script, though.
Hello @LysandreJik I am able to run the code with `batch size = 1`
|
transformers | 1,468 | closed | Scores using BertForNextSentencePrediction are not Interpretable. | ## ❓ Questions & Help
<!-- A clear and concise description of the question. -->
The output of BertForNextSentencePrediction are not Interpretable.
What is seq_relationship_score?
Input example and their respective output is defined below.
1:
text = "[CLS] How old are you? [SEP] I am 193 years old [SEP]"
output=(tensor([[ 3.5181, -2.2946]], grad_fn=<AddmmBackward>)
2:
text = "[CLS] How old are you? [SEP] I am from Paris. [SEP]"
output=tensor([[ 3.9515, -2.5397]], grad_fn=<AddmmBackward>)
Following is my code:
```
import torch
from transformers import *
import torch.nn.functional as F
tokenizer = BertTokenizer.from_pretrained('bert-base-uncased')
text = "[CLS] How old are you? [SEP] I am from Paris. [SEP]"
tokenized_text = tokenizer.tokenize(text)
segments_idss=[]
flag=0
for index,token in enumerate(tokenized_text):
if flag==0:
segments_idss.append(0)
else:
segments_idss.append(1)
if tokenized_text[index]=='[SEP]':
flag=1
indexed_tokens = tokenizer.convert_tokens_to_ids(tokenized_text)
tokens_tensor = torch.tensor([indexed_tokens])
segments_tensors = torch.tensor([segments_idss])
model = BertForNextSentencePrediction.from_pretrained('bert-base-uncased')
model.eval()
predictions = model(tokens_tensor, segments_tensors)
print("predictions->",predictions[0])
```
Can someone tell me, Why scores are similar for different sentences and how to use BertForNextSentencePrediction to find next sentence score?
| 10-09-2019 09:21:14 | 10-09-2019 09:21:14 | This issue has been automatically marked as stale because it has not had recent activity. It will be closed if no further activity occurs. Thank you for your contributions.
|
transformers | 1,467 | closed | Hf master | 10-09-2019 07:57:38 | 10-09-2019 07:57:38 | ||
transformers | 1,466 | closed | RuntimeError: storage has wrong size: expected -1451456236095606723 got 1024 | ## 🐛 Bug
## RuntimeError: storage has wrong size: expected -1451456236095606723 got 1024
<!-- Important information -->
Model I am using GPT-2:
Language I am using the model on English:
The problem arise when using:
* [ ] i was trained and build GPT-2 model with my own corpus.
* [ ] when i `*tested in different CPU systems this issue arised`
The tasks I am working on is:
* [ ] **Language Model fine-tuning task**
* [ ] my own task or dataset: (with my own corpus (1000 lines))
## To Reproduce
Steps to reproduce the behavior:
1. i checked transformers pip version
2. i checked torch version mismatching or not
3. and then **tested in different CPU systems. it is throwing this issue**.
> python run.py
> To use data.metrics please install scikit-learn. See https://scikit-learn.org/stable/index.html
> INFO:transformers.tokenization_utils:Model name '/home/dell/ashok/Masking_technique/gpt-2_modelfiles' not found in model shortcut name list (gpt2, gpt2-medium, gpt2-large). Assuming '/home/dell/ashok/Masking_technique/gpt-2_modelfiles' is a path or url to a directory containing tokenizer files.
> INFO:transformers.tokenization_utils:loading file /home/dell/ashok/Masking_technique/gpt-2_modelfiles/vocab.json
> INFO:transformers.tokenization_utils:loading file /home/dell/ashok/Masking_technique/gpt-2_modelfiles/merges.txt
> INFO:transformers.tokenization_utils:loading file /home/dell/ashok/Masking_technique/gpt-2_modelfiles/added_tokens.json
> INFO:transformers.tokenization_utils:loading file /home/dell/ashok/Masking_technique/gpt-2_modelfiles/special_tokens_map.json
> INFO:transformers.tokenization_utils:loading file /home/dell/ashok/Masking_technique/gpt-2_modelfiles/tokenizer_config.json
> INFO:transformers.configuration_utils:loading configuration file /home/dell/ashok/Masking_technique/gpt-2_modelfiles/config.json
> INFO:transformers.configuration_utils:Model config {
> "attn_pdrop": 0.1,
> "embd_pdrop": 0.1,
> "finetuning_task": null,
> "initializer_range": 0.02,
> "layer_norm_epsilon": 1e-05,
> "n_ctx": 1024,
> "n_embd": 768,
> "n_head": 12,
> "n_layer": 12,
> "n_positions": 1024,
> "num_labels": 1,
> "output_attentions": false,
> "output_hidden_states": false,
> "pruned_heads": {},
> "resid_pdrop": 0.1,
> "summary_activation": null,
> "summary_first_dropout": 0.1,
> "summary_proj_to_labels": true,
> "summary_type": "cls_index",
> "summary_use_proj": true,
> "torchscript": false,
> "use_bfloat16": false,
> "vocab_size": 50257
> }
>
> INFO:transformers.modeling_utils:loading weights file /home/dell/ashok/Masking_technique/gpt-2_modelfiles/pytorch_model.bin
> Traceback (most recent call last):
> File "run.py", line 19, in <module>
> model = GPT2LMHeadModel.from_pretrained('/home/dell/ashok/Masking_technique/gpt-2_modelfiles')
> File "/home/dell/ashok/Masking_technique/env_inference/lib/python3.6/site-packages/transformers/modeling_utils.py", line 345, in from_pretrained
> state_dict = torch.load(resolved_archive_file, map_location='cpu')
> File "/home/dell/ashok/Masking_technique/env_inference/lib/python3.6/site-packages/torch/serialization.py", line 386, in load
> return _load(f, map_location, pickle_module, **pickle_load_args)
> File "/home/dell/ashok/Masking_technique/env_inference/lib/python3.6/site-packages/torch/serialization.py", line 580, in _load
> deserialized_objects[key]._set_from_file(f, offset, f_should_read_directly)
> RuntimeError: storage has wrong size: expected -1451456236095606723 got 1024
## Expected behavior
<!-- A clear and concise description of what you expected to happen. -->
## Environment For inference
* OS: Ubuntu 16.04, 8 GB RAM
* Python version: Python 3.6.8
* PyTorch version: Version: 1.2.0+cpu
* PyTorch Transformers version (or branch): Version: 2.0.0
* Using GPU : No. CPU only
* Distributed of parallel setup : No
* Any other relevant information:
## Additional context
1. **when i tested model on trained environement, It is working fine. but different CPU systems it is throwing this issue.** | 10-09-2019 06:26:22 | 10-09-2019 06:26:22 | Hi, what do you mean by different CPU systems? Do you mean that you tried it with different CPU architectures like ARM/x86? On which CPU did it fail?<|||||>Thank you so much for your reply sir.
** Different CPUs means** - normal CPU system it is not working. I tested two more CPU system for inferencing own model. It was arising this issue.
CPU architecture is normal Ubuntu 16.04 & 18.04 64 bit 8 GB RAM.
I don't know much about CPU architecture sir. :relaxed:<|||||>This issue has been automatically marked as stale because it has not had recent activity. It will be closed if no further activity occurs. Thank you for your contributions.
|
transformers | 1,465 | closed | Multilabel Classification with TFBertForSequenceClassification | I'm currently trying to train a multi label classifier, but in my trained model I'm get the same output no matter the input that I put in.
I've modified the TFBertForSequenceClassification class to include a sigmoid activation output layer as shown below:
```
class TFBertForMultilabelClassification(TFBertPreTrainedModel):
def __init__(self, config, *inputs, **kwargs):
super(TFBertForMultilabelClassification, self).__init__(config, *inputs, **kwargs)
self.num_labels = config.num_labels
self.bert = TFBertMainLayer(config, name='bert')
self.dropout = tf.keras.layers.Dropout(config.hidden_dropout_prob)
self.classifier = tf.keras.layers.Dense(config.num_labels,
kernel_initializer=get_initializer(config.initializer_range),
name='classifier',
activation='sigmoid')
def call(self, inputs, **kwargs):
outputs = self.bert(inputs, **kwargs)
pooled_output = outputs[1]
pooled_output = self.dropout(pooled_output, training=kwargs.get('training', False))
logits = self.classifier(pooled_output)
outputs = (logits,) + outputs[2:] # add hidden states and attention if they are here
return outputs # logits, (hidden_states), (attentions)
```
Here is a method which converts my InputExamples to InputFeatures for BERT:
```
def convert_examples_to_features(examples, tokenizer, label_list, max_seq_length):
"""Converts examples to features using specified tokenizer
Args:
examples (list): Examples to convert.
tokenizer (obj): The tokenzier object.
label_list (list): A list of all the labels.
max_sequence_length (int): Maximum length of a sequence
Returns:
tf.Dataset: A tensorflow dataset.
"""
features = []
for ex_index, example in enumerate(examples):
# Encode inputs using tokenizer
inputs = tokenizer.encode_plus(
example.text_a[:max_seq_length],
add_special_tokens=True,
max_length=max_seq_length,
truncate_first_sequence=True
)
input_ids, token_type_ids = inputs["input_ids"], inputs["token_type_ids"]
# The mask has 1 for real tokens and 0 for padding tokens. Only real tokens are attended to.
attention_mask = [1] * len(input_ids)
# Zero-pad up to the sequence length.
padding_length = max_seq_length - len(input_ids)
input_ids = input_ids + ([0] * padding_length)
attention_mask = attention_mask + ([0] * padding_length)
token_type_ids = token_type_ids + ([0] * padding_length)
# Create features and add to feature list
features.append(
InputFeatures(input_ids=input_ids,
attention_mask=attention_mask,
token_type_ids=token_type_ids,
label=example.label))
# Generator for creating tensorflow dataset
def gen():
for ex in features:
yield ({'input_ids': ex.input_ids,
'attention_mask': ex.attention_mask,
'token_type_ids': ex.token_type_ids},
ex.label)
return tf.data.Dataset.from_generator(gen,
({'input_ids': tf.int32,
'attention_mask': tf.int32,
'token_type_ids': tf.int32},
tf.int64),
({'input_ids': tf.TensorShape([max_seq_length]),
'attention_mask': tf.TensorShape([max_seq_length]),
'token_type_ids': tf.TensorShape([max_seq_length])},
tf.TensorShape([len(label_list)])))
```
Then I used the following code to train my model:
```
# Get pretrained weights and model
tokenizer = BertTokenizer.from_pretrained('bert-base-uncased')
model = TFBertForMultilabelClassification.from_pretrained('bert-base-uncased', num_labels=len(label_list))
# Convert examples to features
train_dataset = convert_examples_to_features(train_examples, tokenizer, label_list, max_seq_length)
valid_dataset = convert_examples_to_features(valid_examples, tokenizer, label_list, max_seq_length)
test_dataset = convert_examples_to_features(test_examples, tokenizer, label_list, max_seq_length)
# Shuffle train data and put into batches
train_dataset = train_dataset.shuffle(100).batch(batch_size)
valid_dataset = valid_dataset.batch(batch_size)
test_dataset = test_dataset.batch(batch_size)
# Prepare training: instantiate optimizer, loss and learning rate schedule
optimizer = tf.keras.optimizers.Adam(learning_rate=learning_rate)
loss = tf.keras.losses.BinaryCrossentropy()
metric = tf.keras.metrics.CategoricalAccuracy()
# Compile the model
model.compile(optimizer=optimizer, loss=loss, metrics=[metric])
# Train and evaluate model
history = model.fit(train_dataset, epochs=num_epochs, validation_data=valid_dataset)
# Save the trained model
if not os.path.exists(export_dir):
os.makedirs(export_dir)
model.save_pretrained(export_dir)
```
I've also tried the model with a linear and relu activation function in addition to other optimizers and the result is still the same output no matter what input I put into the model. Does anyone have any insight to where my problem could be? | 10-08-2019 23:02:21 | 10-08-2019 23:02:21 | Have you figured out what the problem is? I'm facing the same thing....<|||||>Have you figured out what the problem is? I'm facing the same thing....<|||||>Hello, did you figure out the solution here?<|||||>Hey guys, sorry for the late update. Here's my solution: I set a lower learning rate and the problem is fixed. It seems that when we do transfer learning, we cannot set a high learning rate because the model is not well connected to the softmax layer you add.(Just some intuition) In addition, it's also possible that you forget to call model.eval() to invalidate the Dropout layer or something. But this is not the case for me.<|||||>> Hey guys, sorry for the late update. Here's my solution: I set a lower learning rate and the problem is fixed. It seems that when we do transfer learning, we cannot set a high learning rate because the model is not well connected to the softmax layer you add.(Just some intuition) In addition, it's also possible that you forget to call model.eval() to invalidate the Dropout layer or something. But this is not the case for me.
Do you also use TFBertForSequenceClassification for multi-label classification?Multi-label classification requires sigmoid function.<|||||>> Hey guys, sorry for the late update. Here's my solution: I set a lower learning rate and the problem is fixed. It seems that when we do transfer learning, we cannot set a high learning rate because the model is not well connected to the softmax layer you add.(Just some intuition) In addition, it's also possible that you forget to call model.eval() to invalidate the Dropout layer or something. But this is not the case for me.
I used your method but it was unsuccessful<|||||>> > Hey guys, sorry for the late update. Here's my solution: I set a lower learning rate and the problem is fixed. It seems that when we do transfer learning, we cannot set a high learning rate because the model is not well connected to the softmax layer you add.(Just some intuition) In addition, it's also possible that you forget to call model.eval() to invalidate the Dropout layer or something. But this is not the case for me.
>
> Do you also use TFBertForSequenceClassification for multi-label classification?Multi-label classification requires sigmoid function.
Typically, **sigmoid** function is used in binary classification problems, instead **softmax** function is used in multi-class classification problems<|||||>> > > Hey guys, sorry for the late update. Here's my solution: I set a lower learning rate and the problem is fixed. It seems that when we do transfer learning, we cannot set a high learning rate because the model is not well connected to the softmax layer you add.(Just some intuition) In addition, it's also possible that you forget to call model.eval() to invalidate the Dropout layer or something. But this is not the case for me.
> >
> >
> > Do you also use TFBertForSequenceClassification for multi-label classification?Multi-label classification requires sigmoid function.
>
> Typically, **sigmoid** function is used in binary classification problems, instead **softmax** function is used in multi-class classification problems
I use the sigmoid function, but the output of different words is the same
<|||||>@thomwolf Is this problem solved<|||||>I have facing the same issue<|||||>This issue has been automatically marked as stale because it has not had recent activity. It will be closed if no further activity occurs. Thank you for your contributions.
<|||||>Try adjusting learning rate, the dropout probability(`config.hidden_dropout_prob`) and batch size.<|||||>@venkatasg Can you share the hyperparameters that worked for your experiments? Trying to get a sense of order-of-magnitude for each of those.<|||||>The problem is probably that the model is overfitting to the data. For BERT, the following hyperparameters worked for me:
- batch size: 16/32
- learning rate: 1e-5, 2e-5
- dropouts: 0.1
- weight decay: 0
However, what works for me might not work for you. Keep experimenting with the hyperparameters(and random seeds)<|||||>I just finished a multi label classifier training and got the exact same result:
> same output no matter the input that I put in
<|||||>I've been facing a similar issue as described here - I got a good accuracy but the predictions just would not make any sense.
Luckily, I've received help from the [HuggingFace.co community](https://discuss.huggingface.co/t/fine-tune-for-multiclass-or-multilabel-multiclass/4035) and it turned out that one has to initialize the model with the correct labels because the model is otherwise learning something but its just not clear what numeric label represents what string...
`bert = TFAutoModel.from_pretrained(tranformersPreTrainedModelName, label2id=label2Index, id2label=index2label)
`
The full code for the solution that works for me with public data can [be found here](https://github.com/Dirkster99/PyNotes/blob/master/Transformers/LocalModelUsage_Finetuning/66_Transformer_4_Language_Classification_MultiClass.ipynb).
Hope this helps... |
transformers | 1,464 | closed | How is it possible to furthur tune gpt-2(or gpt) in a seq2seq manner? | Hi,
Can we futhur funetue gpt-2 pretrained model in a sequence 2 sequence manner, where we want to minimize the loss of log p(y|x).
In other words, our dataset has both source and target and we want to generate target given source.
But I want to start from using gpt-2 weights and then tune it.
| 10-08-2019 21:46:07 | 10-08-2019 21:46:07 | Hi, this is on our mid-term roadmap (seq2seq models).<|||||>@Hannabrahman In the original GPT2 paper (section 3.7 Translation) the authors used the format "english sentence = french sentence" to produce translations. You can definitely fine tune the model using this format to produce translations using the existing scripts if you structure your seq2seq data this way.<|||||>@dvaltchanov and @thomwolf thanks for pointing out to me.
Do you think for that, I need to pass another input to the forward method of GPTLMHead method which is a list containing the length of source sequence, so that I will be able to zero out the loss calculated for the tokens in source?
I mean did I have to zero out the lm_logits associated with source sequence tokens so that I do not count them in loss calculation?
Or it doesn't matter if we include the source tokens loss in our total loss?<|||||>@Hannabrahman Based on my tests, it doesn't matter if you include them. Your total loss will be higher but you're mainly interested in the validation loss on the translations anyway. As long as you use the "start of text" and "end of text" tokens to wrap your "sequence = sequence" text the model seems to be able to figure it out after a little bit of fine tuning.<|||||>@dvaltchanov Thanks.
Just one question since you had experimented this.
I want to finetune gpt on a new dataset using the format you said and [this script.](https://github.com/huggingface/transformers/blob/master/examples/run_lm_finetuning.py) which is for finetuning pretained model on new dataset.
1- should I add special tokens ( [SOS], some separator token for source and target, [EOS]) and train it like this:
```
# Add a [SOS], [SEP] and [EOS] to the vocabulary (we should train it also!)
tokenizer.add_special_tokens({'start_token': '[CLS]', 'sep_token': '[SEP]', 'end_token': '[EOS]'})
model.resize_token_embeddings(len(tokenizer)) # Update the model embeddings with the new vocabulary size
```
2- The instances in my dataset have different length ( 60-85 tokens). I have to either trim them to be the same size (it is not really good for my usecase), or use padding to pad them to same size. However, I read somewhere in this repo that gpt and gpt-2 doesnt handle right padding, how did you solve this issue while finetuning gpt on your own usecase and dataset?
Many thanks in advance.<|||||>@Hannabrahman Great questions:
1. This is up to you. The model can learn the sequence of known tokens (e.g. "[", "E", "OS", "]") and use that as a prompt. I used a sequence and found that it worked well enough so I did not try adding extra tokens. There is already an "<|endoftext|>" token in the vocabulary which you can leverage.
2. I created a custom data loader which concatenated the desired sample with randomly selected sequences from the data up to the desired length. E.g., A training sample may be a concat of sample translation #1 and #32 which would look like this: "[SOS] something in English_#1 = something in French_#1 [EOS] [SOS] something in English_#32 = something in French_#32 [EOS] [SOS] .. etc"
This then gets tokenized and truncated to the max length. This will allow the model to learn variable length sequences.
You can accomplish the same effect by concatenating all of your text into a single string and sampling sections of it. However, if you do this the model will learn associations between neighbouring samples over multiple epochs, so I recommend having something that shuffles the order of concatenated samples each epoch.
During generation you prompt with "[SOS] something in English = " and stop generating when it produces an [EOS] token.
<|||||>@dvaltchanov
regarding 2 - I didn't get it completely.
Where is the padding in your given batch example? Also, did you mean you concat all the instances back to back to create a single instance when you have #32 after #1 or #32 is probably another instance in the same batch? that being said the input is [bs, max_seq_len]? (bs = 2 in this example)
Also did you add a [pad] token to the vocabulary? because gpt and gpt2 doesnt have padding token. Or you follow the same strategy as in question 1
Do you have your custom data loader code somewhere so that I can take a look?<|||||>@Hannabrahman See my edited response above. I hope my clarification helps. <|||||>@dvaltchanov Thankss. Basically you followed the same approach as in [here](https://github.com/huggingface/transformers/blob/master/examples/run_lm_finetuning.py) . They read all the input into one long string and then truncate it in max_len. However it doesn't have any sampling or shuffling.
My data is stories and each story is around 60-80 tokens. I read all the stories in one long string and truncate each section to 128 tokens. The problem is sometimes the beginning of an story may goes into previous sample section. and the rest goes in to next section.<|||||>This issue has been automatically marked as stale because it has not had recent activity. It will be closed if no further activity occurs. Thank you for your contributions.
<|||||>Hi, is there a seq2seq example of GPT2 now?<|||||>Hi, any updates?<|||||>Hi everyone,
Given that [Alpaca](https://github.com/tatsu-lab/stanford_alpaca) (decoder-only model like GPT) was trained in a seq2seq manner, I realised we can learn from their code (cheers to OS!).
## Approach
The naive solution is to concatenate the source and target strings. However, the main issue here is that the loss is incurred in the next-word-prediction of the source strings.
To circumvent this, [Alpaca](https://github.com/tatsu-lab/stanford_alpaca/blob/main/train.py) simply ignored the loss in the source strings. Concretely:
```
def preprocess(
sources: Sequence[str],
targets: Sequence[str],
tokenizer: transformers.PreTrainedTokenizer,
) -> Dict:
"""Preprocess the data by tokenizing."""
examples = [s + t for s, t in zip(sources, targets)] # concatenate source and target strings
examples_tokenized, sources_tokenized = [_tokenize_fn(strings, tokenizer) for strings in (examples, sources)]
input_ids = examples_tokenized["input_ids"]
labels = copy.deepcopy(input_ids)
for label, source_len in zip(labels, sources_tokenized["input_ids_lens"]):
label[:source_len] = IGNORE_INDEX # the source string's loss is ignored with IGNORE_INDEX
return dict(input_ids=input_ids, labels=labels)
```
Note how the source string's loss is ignored with `IGNORE_INDEX`
## Implications
**Seq2Seq prompting.**
In concatenating the source and target strings, it may not be obvious to the model how to differentiate the source from target strings. I suspect that Alpaca/self-instruct circumvented this by making the differentiation clear via prompts:
```
PROMPT_DICT = {
"prompt_input": (
"Below is an instruction that describes a task, paired with an input that provides further context. "
"Write a response that appropriately completes the request.\n\n"
"### Instruction:\n{instruction}\n\n### Input:\n{input}\n\n### Response:"
),
"prompt_no_input": (
"Below is an instruction that describes a task. "
"Write a response that appropriately completes the request.\n\n"
"### Instruction:\n{instruction}\n\n### Response:"
),
}
```
Notice how `### Instruction:` tells the model where the source string is while `### Response:` tells the model where the target string is.
**Increased GPU Memory usage**. To my understanding, the `input` and `labels` will now both be the concatenated source and target strings. In contrast for seq2seq models, the `input` will only be the source strings while the `labels` will only be the target strings. Thus this neat trick incurs additional GPU memory.
**Packing is more intuitive with causal LM.** Packing is the act of packing training examples together to avoid padding. In causal LM, we can pack via
```
(source->target)[IGNORE_INDEX](source->target)[IGNORE_INDEX]...(source->target)[IGNORE_INDEX])
```
Notice how the target string immediately comes after the source. In contrast, packing for seq2seq LM will look like
```
Input: (source)[IGNORE_INDEX](source)[IGNORE_INDEX]...(source)[IGNORE_INDEX]
Target: (target)[IGNORE_INDEX](target)[IGNORE_INDEX]...(target)[IGNORE_INDEX]
```
To me, it's not intuitive that the model can match the ith target to the ith source string.
## Credits
Cheers to Alpaca, LlaMMA, and OS for finally solving this engineering puzzle for me! Do LMK if any parts don't make sense to you - I'm still learning myself.<|||||>Created training examples by concatenating inputs and targets like this: 'Document:{document}\nSummary:{Summary}'
and created text summary model with this. But the problem here is the model starts generating from Document not from Summary. Would be there anyway to handle this problem? |
transformers | 1,463 | closed | bert ids | ## ❓ Questions & Help
after I Use the BERT tokenizer to convert the tokens to their index numbers in the BERT vocabulary
input_ids = [tokenizer.convert_tokens_to_ids(x) for x in tokenized_texts]
How I can convert them back to the original sentence???
Thank you in advance | 10-08-2019 21:31:21 | 10-08-2019 21:31:21 | Hello, you should take a look at the `encode` and `decode` methods in the [documentation](https://huggingface.co/transformers/main_classes/tokenizer.html#transformers.PreTrainedTokenizer.decode).<|||||>Thank you so much that was very helpful<|||||>Glad I could help!<|||||>Hi
I am trying to use the code in this link (https://colab.research.google.com/drive/1pS-eegmUz9EqXJw22VbVIHlHoXjNaYuc#scrollTo=JggjeDC9m2MH) to plot my trained model
But, I am getting an error. any idea please??
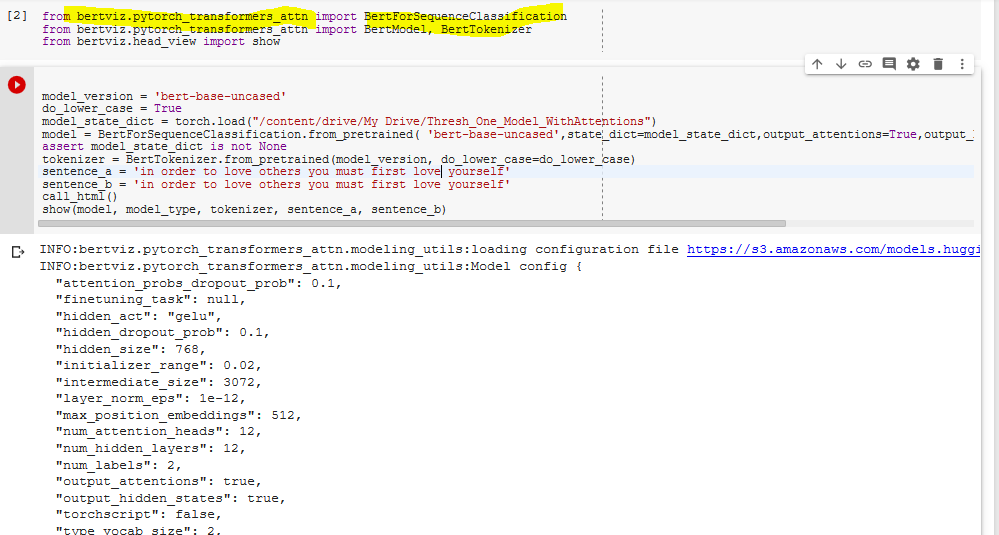
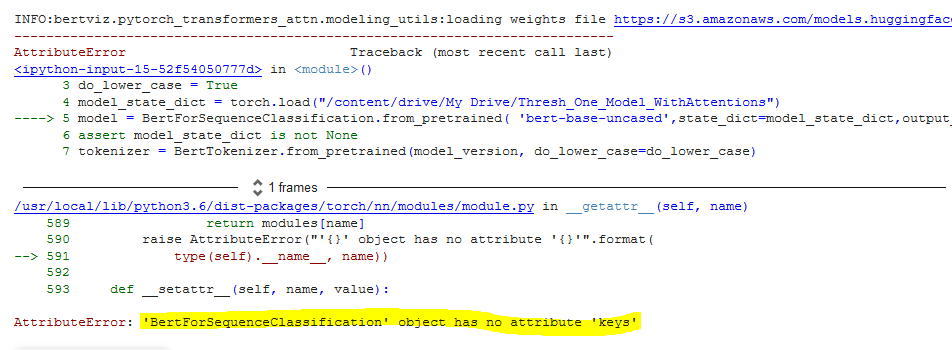
|
transformers | 1,462 | closed | Visualizing the Inner Workings of Attention | ## ❓ Questions & Help
what should I DO to plot my model by BertViz tool??
i am useing config = BertConfig.from_pretrained(“bert-base-uncased”,output_attentions=True,output_hidden_states=True, num_labels=2)
model = BertForSequenceClassification.from_pretrained(“bert-base-uncased”, config= config)
Thank you in advance
<!-- A clear and concise description of the question. -->
| 10-08-2019 21:23:59 | 10-08-2019 21:23:59 | This issue has been automatically marked as stale because it has not had recent activity. It will be closed if no further activity occurs. Thank you for your contributions.
|
transformers | 1,461 | closed | How can I use a TensorFlow 2.0 model for Named-Entity-Recognition (NER)? (using TFBertForTokenClassification ) | ## ❓ Questions & Help
<!-- A clear and concise description of the question. -->
How can I use a TensorFlow 2.0 model for Named-Entity-Recognition (NER)? (using TFBertForTokenClassification ) | 10-08-2019 20:40:04 | 10-08-2019 20:40:04 | Exactly the same question here! Can someone please provide us with a small tutorial or even some general guidelines?<|||||>Any response here? I was looking for something similar<|||||>This issue has been automatically marked as stale because it has not had recent activity. It will be closed if no further activity occurs. Thank you for your contributions.
|
transformers | 1,460 | closed | `decoder` without bias in BertLMPredictionHead | ## ❓ Questions & Help
What does this comment mean?
https://github.com/huggingface/transformers/blob/80bf868a268fa445926bc93f7fe15960853e828e/transformers/modeling_bert.py#L394-L407 | 10-08-2019 19:44:09 | 10-08-2019 19:44:09 | Hi, I believe that means that the decoder is a linear layer that has the same weights as the word embedding matrix. However, that embedding matrix does not have a bias, whereas the decoder does have a bias.
It is initialized to a vector of zeros here, but it can update its weights during training and has actual values in pre-trained models. For example:
```py
from transformers import BertForMaskedLM
bert = BertForMaskedLM.from_pretrained("bert-base-cased")
print(bert.cls.predictions.bias)
# tensor([-0.1788, -0.1758, -0.1752, ..., -0.3448, -0.3574, -0.3483], requires_grad=True)
```<|||||>Oh, I see. That makes sense. So it should share parameters with the embedding weights? Where is that enforced in the code?<|||||>Yes, exactly. You can see it in the [bert_modeling.py file, inside the BertForMaskedLM class](https://github.com/huggingface/transformers/blob/master/transformers/modeling_bert.py#L754-L759).<|||||>Thanks for the clarification!<|||||>hi,
I know it's an old issue but I had the same questions:
* it's not clear to me in the code where this parameter sharing is enforced
* is there any intuition why it is done ?
thanks in advance <|||||>@thibault-formal Hey, I had similar questions and asked them in the huggingface forum, I think my [post there](https://discuss.huggingface.co/t/understanding-bertlmpredictionhead/3618) could be helpful for you (if its still relevant). Your first point should be adressed by the explanation of my understanding and the second one is adressed by both replies.
Cheers! |
transformers | 1,459 | closed | Imports for Roberta conversion appear to be outdated | ## 🐛 Bug
<!-- Important information -->
I'm trying to convert a custom Roberta model (from fairseq checkpoints) to a Tensorflow model.
The problem arise when using:
* [x] the official example scripts: (give details)
* [ ] my own modified scripts: (give details)
The tasks I am working on is:
* [ ] an official GLUE/SQUaD task: (give the name)
* [x] my own task or dataset: (give details)
## To Reproduce
Steps to reproduce the behavior:
It is possible to load checkpoints saved from Roberta directly? From the documentation, it looks like it should be possible, but when I run the following code
```python
from transformers import TFRobertaModel
model = TFRobertaModel.from_pretrained('checkpoint_best.pt', from_pt=True)
```
I get
```
UnicodeDecodeError Traceback (most recent call last)
<ipython-input-2-47fa7f7cf639> in <module>()
----> 1 model = TFRobertaModel.from_pretrained('checkpoint_best.pt', from_pt=True)
~/venvs/transformers-tf/lib/python3.6/site-packages/transformers/modeling_tf_utils.py in from_pretrained(cls, pretrained_model_name_or_path, *model_args, **kwargs)
208 cache_dir=cache_dir, return_unused_kwargs=True,
209 force_download=force_download,
--> 210 **kwargs
211 )
212 else:
~/venvs/transformers-tf/lib/python3.6/site-packages/transformers/configuration_utils.py in from_pretrained(cls, pretrained_model_name_or_path, **kwargs)
152
153 # Load config
--> 154 config = cls.from_json_file(resolved_config_file)
155
156 if hasattr(config, 'pruned_heads'):
~/venvs/transformers-tf/lib/python3.6/site-packages/transformers/configuration_utils.py in from_json_file(cls, json_file)
184 """Constructs a `BertConfig` from a json file of parameters."""
185 with open(json_file, "r", encoding='utf-8') as reader:
--> 186 text = reader.read()
187 return cls.from_dict(json.loads(text))
188
/mnt/xfs1/sw/pkg/devel/python3/3.6.2/lib/python3.6/codecs.py in decode(self, input, final)
319 # decode input (taking the buffer into account)
320 data = self.buffer + input
--> 321 (result, consumed) = self._buffer_decode(data, self.errors, final)
322 # keep undecoded input until the next call
323 self.buffer = data[consumed:]
UnicodeDecodeError: 'utf-8' codec can't decode byte 0x80 in position 0: invalid start byte
```
I'm guessing since the checkpoint is in a binary, I need to first convert this to a json format.
It looks like it should be done [here](https://github.com/huggingface/transformers/blob/master/transformers/convert_roberta_original_pytorch_checkpoint_to_pytorch.py) . However, when I try to run that script, I get an error
```
(transformers-tf) [jmorton@pcn-7-01 checkpoints]$ python convert_roberta_original_pytorch_checkpoint_to_pytorch.py --help
To use data.metrics please install scikit-learn. See https://scikit-learn.org/stable/index.html
Traceback (most recent call last):
File "convert_roberta_original_pytorch_checkpoint_to_pytorch.py", line 26, in <module>
from transformers import (BertConfig, BertEncoder,
ImportError: cannot import name 'BertEncoder'
```
From what I can tell, those imports are dated and will need to be fixed anyways.
## Environment
* OS: Centos 7
* Python version: PY3.6
* PyTorch version: 1.2.0
* PyTorch Transformers version (or branch):2.0.0
* Using GPU ? Not yet
* Distributed of parallel setup ? Nope
* Any other relevant information:
| 10-08-2019 18:42:53 | 10-08-2019 18:42:53 | Getting the same error here<|||||>Will investigate, thanks for reporting. (And Hi, @louismartin :)<|||||>I had a similar issue trying to load BioBERT and I figured out what was going on in my case, sharing just in case that's what's going on in your case. In my case I converted TF BioBERT checkpoint to pytorch model. In my case the (first) problem was that I didn't provide a path to the config file.
My local scripts are adapted from the python code that runs Glue. I have a `--config_name` parameter that specifies the json file from which the configuration is loaded. If you don't provide that one, it tries to infer it by using the model_name_or_path - and that's what caused my problem.
Once I specified the config file, I had another problem that had to do with the following: `model_name_or_path` is supposed to be the path where you store the other info for the models and the model files are expected to follow a certain naming convention (e.g., in my case, it was looking for a `pytorch_model.bin` file; there is a similar file name for TF).
Hope this helps!
<|||||>This issue has been automatically marked as stale because it has not had recent activity. It will be closed if no further activity occurs. Thank you for your contributions.
<|||||>This was superseded, and closed, by #1512 |
transformers | 1,458 | closed | how to get word embedding vector in GPT-2 | ## ❓ Questions & Help
<!-- A clear and concise description of the question. -->
How can we get the word embedding vector in gpt-2? I follow the guidance in bert(model.embeddings.word_embeddings.weight). But it shows that ''GPT2LMHeadModel' object has no attribute 'embeddings''.
Please help me with that. Thank you in advance. | 10-08-2019 15:55:00 | 10-08-2019 15:55:00 | Hi, indeed GPT-2 has a slightly different implementation than BERT. In order to have access to the embeddings, you would have to do the following:
```py
from transformers import GPT2LMHeadModel
model = GPT2LMHeadModel.from_pretrained('gpt2') # or any other checkpoint
word_embeddings = model.transformer.wte.weight # Word Token Embeddings
position_embeddings = model.transformer.wpe.weight # Word Position Embeddings
```<|||||>> Hi, indeed GPT-2 has a slightly different implementation than BERT. In order to have access to the embeddings, you would have to do the following:
>
> ```python
> from transformers import GPT2LMHeadModel
>
> model = GPT2LMHeadModel.from_pretrained('gpt2') # or any other checkpoint
> word_embeddings = model.transformer.wte.weight # Word Token Embeddings
> position_embeddings = model.transformer.wpe.weight # Word Position Embeddings
> ```
Hi,
Thank you for your reply! So if I want to get the vector for 'man', it would be like this:
>tokenizer = GPT2Tokenizer.from_pretrained('gpt2')
>text_index = tokenizer.encode('man',add_prefix_space=True)
>vector = model.transformer.wte.weight[text_index,:]
Is it correct?
<|||||>Just wondering, how to transform word_vector to word? Imagine a word vector and change a few elements, how can I find closest word from gpt2 model?<|||||>> Just wondering, how to transform word_vector to word? Imagine a word vector and change a few elements, how can I find closest word from gpt2 model?
So for each token in dictionary there is a static embedding(on layer 0). You can use cosine similarity to find the closet static embedding to the transformed vector. That should help you find the word.<|||||>> > Just wondering, how to transform word_vector to word? Imagine a word vector and change a few elements, how can I find closest word from gpt2 model?
>
> So for each token in dictionary there is a static embedding(on layer 0). You can use cosine similarity to find the closet static embedding to the transformed vector. That should help you find the word.
Thanks. It means that for every word_vector I have to calculate vocab_size (~50K) cosine_sim manipulation. Is that right? <|||||>> > > Just wondering, how to transform word_vector to word? Imagine a word vector and change a few elements, how can I find closest word from gpt2 model?
> >
> >
> > So for each token in dictionary there is a static embedding(on layer 0). You can use cosine similarity to find the closet static embedding to the transformed vector. That should help you find the word.
>
> Thanks. It means that for every word_vector I have to calculate vocab_size (~50K) cosine_sim manipulation. Is that right?
I guess so. Unless you can use some property to first tighten the range.<|||||>> > > > Just wondering, how to transform word_vector to word? Imagine a word vector and change a few elements, how can I find closest word from gpt2 model?
> > >
> > >
> > > So for each token in dictionary there is a static embedding(on layer 0). You can use cosine similarity to find the closet static embedding to the transformed vector. That should help you find the word.
> >
> >
> > Thanks. It means that for every word_vector I have to calculate vocab_size (~50K) cosine_sim manipulation. Is that right?
>
> I guess so. Unless you can use some property to first tighten the range.
Ok. Three more questions, 1) is there any resource on how to generate fixed length sentence (a sentence with N words that ends with "." or "!" )? 2) what is the most effective underlying parameter for hyper-parameter tuning (eg. Temperature)? 3) Is there any slack channel to discuss these types of questions? <|||||>> > > > > Just wondering, how to transform word_vector to word? Imagine a word vector and change a few elements, how can I find closest word from gpt2 model?
> > > >
> > > >
> > > > So for each token in dictionary there is a static embedding(on layer 0). You can use cosine similarity to find the closet static embedding to the transformed vector. That should help you find the word.
> > >
> > >
> > > Thanks. It means that for every word_vector I have to calculate vocab_size (~50K) cosine_sim manipulation. Is that right?
> >
> >
> > I guess so. Unless you can use some property to first tighten the range.
>
> Ok. Three more questions, 1) is there any resource on how to generate fixed length sentence (a sentence with N words that ends with "." or "!" )? 2) what is the most effective underlying parameter for hyper-parameter tuning (eg. Temperature)? 3) Is there any slack channel to discuss these types of questions?
about 1) I don't think that there is any. You can use Web Scraping for such specified sentences. Also, you can download a corpus and use Regex to extract desired sentences.
2) I don't really know
3) If you find any, please share it with me too. Thanks! 😄 <|||||>> > Hi, indeed GPT-2 has a slightly different implementation than BERT. In order to have access to the embeddings, you would have to do the following:
> > ```python
> > from transformers import GPT2LMHeadModel
> >
> > model = GPT2LMHeadModel.from_pretrained('gpt2') # or any other checkpoint
> > word_embeddings = model.transformer.wte.weight # Word Token Embeddings
> > position_embeddings = model.transformer.wpe.weight # Word Position Embeddings
> > ```
>
> Hi,
>
> Thank you for your reply! So if I want to get the vector for 'man', it would be like this:
>
> > tokenizer = GPT2Tokenizer.from_pretrained('gpt2')
> > text_index = tokenizer.encode('man',add_prefix_space=True)
> > vector = model.transformer.wte.weight[text_index,:]
>
> Is it correct?
Did you succeed? I'm pursuing the same goal and I don't know how to validate my findings. I have tested some king - man + woman stuff, but it didn't work.<|||||>> > > Hi, indeed GPT-2 has a slightly different implementation than BERT. In order to have access to the embeddings, you would have to do the following:
> > > ```python
> > > from transformers import GPT2LMHeadModel
> > >
> > > model = GPT2LMHeadModel.from_pretrained('gpt2') # or any other checkpoint
> > > word_embeddings = model.transformer.wte.weight # Word Token Embeddings
> > > position_embeddings = model.transformer.wpe.weight # Word Position Embeddings
> > > ```
> >
> >
> > Hi,
> > Thank you for your reply! So if I want to get the vector for 'man', it would be like this:
> > > tokenizer = GPT2Tokenizer.from_pretrained('gpt2')
> > > text_index = tokenizer.encode('man',add_prefix_space=True)
> > > vector = model.transformer.wte.weight[text_index,:]
> >
> >
> > Is it correct?
>
> Did you succeed? I'm pursuing the same goal and I don't know how to validate my findings. I have tested some king - man + woman stuff, but it didn't work.
How did it go? I am stuck here too.<|||||>> > Hi, indeed GPT-2 has a slightly different implementation than BERT. In order to have access to the embeddings, you would have to do the following:
> > ```python
> > from transformers import GPT2LMHeadModel
> >
> > model = GPT2LMHeadModel.from_pretrained('gpt2') # or any other checkpoint
> > word_embeddings = model.transformer.wte.weight # Word Token Embeddings
> > position_embeddings = model.transformer.wpe.weight # Word Position Embeddings
> > ```
>
> Hi,
>
> Thank you for your reply! So if I want to get the vector for 'man', it would be like this:
>
> > tokenizer = GPT2Tokenizer.from_pretrained('gpt2')
> > text_index = tokenizer.encode('man',add_prefix_space=True)
> > vector = model.transformer.wte.weight[text_index,:]
>
> Is it correct?
How did it go?<|||||>> > > Hi, indeed GPT-2 has a slightly different implementation than BERT. In order to have access to the embeddings, you would have to do the following:
> > > ```python
> > > from transformers import GPT2LMHeadModel
> > >
> > > model = GPT2LMHeadModel.from_pretrained('gpt2') # or any other checkpoint
> > > word_embeddings = model.transformer.wte.weight # Word Token Embeddings
> > > position_embeddings = model.transformer.wpe.weight # Word Position Embeddings
> > > ```
> >
> >
> > Hi,
> > Thank you for your reply! So if I want to get the vector for 'man', it would be like this:
> > > tokenizer = GPT2Tokenizer.from_pretrained('gpt2')
> > > text_index = tokenizer.encode('man',add_prefix_space=True)
> > > vector = model.transformer.wte.weight[text_index,:]
> >
> >
> > Is it correct?
>
> How did it go?
Well, it is working. However, these weights/embeddings are "context-dependent" so one should not expect "king-queen+woman" lead to anything. <|||||>The code already posted here is correct:
```
model.transformer.wte.weight[input_ids,:]
```
where `input_ids` is a tensor of shape `(batch_size, sequence_length)`. This will give you a tensor of shape `(batch_size, sequence_length, embedding_dimension)`. For example, you can do this with the output of the tokenizer:
```
inputs = tokenizer(["Hello, my name"], return_tensors="pt")
embeds = model.transformer.wte.weight[input_ids, :]
```
You can validate that this is correct by passing the embeds into the model and checking that you get the same thing as when passing in the inputs:
```
outputs1 = model(input_ids=inputs.input_ids)
outputs2 = model(inputs_embeds=embeds)
assert torch.allclose(outputs1.logits, outputs2.logits)
```
or even
```
for layer1, layer2 in zip(outputs1.hidden_states, outputs2.hidden_states):
assert torch.allclose(layer1, layer2)
``` |
transformers | 1,457 | closed | when running run_squad.py it is showing no progress . stuck after feature building | ## ❓ Questions & Help
<!-- A clear and concise description of the question. -->
| 10-08-2019 15:38:53 | 10-08-2019 15:38:53 | when i use
export SQUAD_DIR=/path/to/SQUAD
python run_squad.py \ --model_type bert \ --model_name_or_path bert-base-cased \ --do_train \ --do_eval \ --do_lower_case \ --train_file $SQUAD_DIR/train-v1.1.json \ --predict_file $SQUAD_DIR/dev-v1.1.json \ --per_gpu_train_batch_size 12 \ --learning_rate 3e-5 \ --num_train_epochs 2.0 \ --max_seq_length 384 \ --doc_stride 128 \ --output_dir /tmp/debug_squad/
it is showing no progress. no gpu utilization.
<|||||>like this
0 0 0 0 0 0 0 0 0 0 0 0 0 0 0 0 0 0 0 0 0 0 0 0 0 0 0 0 0 0
10/08/2019 15:32:45 - INFO - utils_squad - input_mask: 1 1 1 1 1 1 1 1 1 1 1 1 1 1 1 1 1 1 1 1 1 1 1 1 1 1 1 1 1 1 1 1 1 1 1 1 1 1 1 1 1 1 1 1 1 1 1 1 1 1 1 1 1 1 1 1 1 1 1 1 1 1 1 1 1 1 1 1 1 1 1 1 1 1 1 1 1 1 1 1 1 1 1 1 1 1 1 1 1 1 1 1 1 1 1 1 1 1 1 1 1 1 1 1 1 1 1 1 1 1 1 1 1 1 1 1 1 1 1 1 1 1 1 1 1 1 1 1 1 1 1 1 1 1 1 1 1 1 1 1 1 1 1 1 1 1 1 1 1 1 1 1 1 1 1 1 1 1 1 1 1 1 0 0 0 0 0 0 0 0 0 0 0 0 0 0 0 0 0 0 0 0 0 0 0 0 0 0 0 0 0 0 0 0 0 0 0 0 0 0 0 0 0 0 0 0 0 0 0 0 0 0 0 0 0 0 0 0 0 0 0 0 0 0 0 0 0 0 0 0 0 0 0 0 0 0 0 0 0 0 0 0 0 0 0 0 0 0 0 0 0 0 0 0 0 0 0 0 0 0 0 0 0 0 0 0 0 0 0 0 0 0 0 0 0 0 0 0 0 0 0 0 0 0 0 0 0 0 0 0 0 0 0 0 0 0 0 0 0 0 0 0 0 0 0 0 0 0 0 0 0 0 0 0 0 0 0 0 0 0 0 0 0 0 0 0 0 0 0 0 0 0 0 0 0 0 0 0 0 0 0 0 0 0 0 0 0 0 0 0 0 0 0 0 0 0 0 0 0 0 0 0 0 0 0 0 0 0 0 0 0 0 0 0 0 0 0 0 0 0 0 0 0 0
10/08/2019 15:32:45 - INFO - utils_squad - segment_ids: 0 0 0 0 0 0 0 0 0 0 0 0 0 0 0 0 0 0 0 0 0 0 1 1 1 1 1 1 1 1 1 1 1 1 1 1 1 1 1 1 1 1 1 1 1 1 1 1 1 1 1 1 1 1 1 1 1 1 1 1 1 1 1 1 1 1 1 1 1 1 1 1 1 1 1 1 1 1 1 1 1 1 1 1 1 1 1 1 1 1 1 1 1 1 1 1 1 1 1 1 1 1 1 1 1 1 1 1 1 1 1 1 1 1 1 1 1 1 1 1 1 1 1 1 1 1 1 1 1 1 1 1 1 1 1 1 1 1 1 1 1 1 1 1 1 1 1 1 1 1 1 1 1 1 1 1 1 1 1 1 1 1 0 0 0 0 0 0 0 0 0 0 0 0 0 0 0 0 0 0 0 0 0 0 0 0 0 0 0 0 0 0 0 0 0 0 0 0 0 0 0 0 0 0 0 0 0 0 0 0 0 0 0 0 0 0 0 0 0 0 0 0 0 0 0 0 0 0 0 0 0 0 0 0 0 0 0 0 0 0 0 0 0 0 0 0 0 0 0 0 0 0 0 0 0 0 0 0 0 0 0 0 0 0 0 0 0 0 0 0 0 0 0 0 0 0 0 0 0 0 0 0 0 0 0 0 0 0 0 0 0 0 0 0 0 0 0 0 0 0 0 0 0 0 0 0 0 0 0 0 0 0 0 0 0 0 0 0 0 0 0 0 0 0 0 0 0 0 0 0 0 0 0 0 0 0 0 0 0 0 0 0 0 0 0 0 0 0 0 0 0 0 0 0 0 0 0 0 0 0 0 0 0 0 0 0 0 0 0 0 0 0 0 0 0 0 0 0 0 0 0 0 0 0
10/08/2019 15:32:45 - INFO - utils_squad - start_position: 49
10/08/2019 15:32:45 - INFO - utils_squad - end_position: 50
10/08/2019 15:32:45 - INFO - utils_squad - answer: the 1870s
<|||||>what is the meaning of this???? stuck somewhere in tokenization_bert.py<|||||>wait for 10-15 min ...it will work.<|||||>Hi, the program probably didn't hang but was still converting the examples to features, which can be a timely process.
If you want to have more information, you could always add a print statement notifying you of the current index it is converting to feature.
Please note that once you have done this conversion to features, these will be cached on your disk to be used the next time. This conversion is only done once.<|||||>I observed exactly the same, it took 18 minutes to log the next line in a p3.2xlarge host. Would be great to parallelize this portion(I notice only one cpu is running in this period.), and show a progress bar for converting the examples to features.
```
10/27/2019 02:45:46 - INFO - utils_squad - start_position: 47
10/27/2019 02:45:46 - INFO - utils_squad - end_position: 48
10/27/2019 02:45:46 - INFO - utils_squad - answer: the 1870s
10/27/2019 03:03:05 - INFO - __main__ - Saving features into cached file /home/ubuntu/SQuAD-explorer/dataset/cached_train_bert-base-uncased_384
10/27/2019 03:05:09 - INFO - __main__ - ***** Running training *****
```
<|||||>@cockroachzl @vikrant094
If you're running on a Linux variant OS you might try adding **`export OMP_NUM_THREADS=x`** at the top of your script file, where x is the number of cores, not threads, of your CPU. With this script file addition on my Ubuntu 18.04 machine, examples-to-features uses 2 of my 6 CPUs @ 100%, instead of just a single CPU. |
transformers | 1,456 | closed | questions on checkpoint and 'training_args.bin' in run_lm_finetuning.py | ## ❓ Questions & Help
<!-- A clear and concise description of the question. -->
2 questions:
1. there is a **checkpoint** save logical, but don't see any logical to load this checkpoint. nothe load method in code
1. there is '**training_args.bin**' has been store with checkpoint together. no load code to support it.
could you please tell how to use these checkpoint and 'training_args.bin' to **continue** training?
thanks.
| 10-08-2019 15:04:21 | 10-08-2019 15:04:21 | The model saved can be loaded by using the `model.from_pretrained(directory)` method. The training arguments are saved so that they can be re-used later. You can load them using the `torch.load(directory/training_args.bin)` method.<|||||>thanks for reply. <|||||>This issue has been automatically marked as stale because it has not had recent activity. It will be closed if no further activity occurs. Thank you for your contributions.
|
transformers | 1,455 | closed | [WIP] Add PretrainedEncoderDecoder class | In this PR we add the possibility to define encoder-decoder architectures. We:
- Added a `PreTrainedEncoderDecoder` class that can be initialized from pre-trained models;
- Modified the BERT model so it can behave as a decoder;
- Added a `Model2Model`class that simplifies the definition of an encoder-decoder when both encoder and decoder are based on the same model;
- Added relevant tests and updated the documentation;
- We also include a script to fine-tune an encoder-decoder model on the CNN/DailyMail dataset;
- We added a draft for a beam search.
Only the BERT model is available as a decoder right now. | 10-08-2019 14:24:14 | 10-08-2019 14:24:14 | # [Codecov](https://codecov.io/gh/huggingface/transformers/pull/1455?src=pr&el=h1) Report
> Merging [#1455](https://codecov.io/gh/huggingface/transformers/pull/1455?src=pr&el=desc) into [master](https://codecov.io/gh/huggingface/transformers/commit/ae1d03fc51bb22ed59517ee6f92c560417fdb049?src=pr&el=desc) will **decrease** coverage by `1.92%`.
> The diff coverage is `53.35%`.
[](https://codecov.io/gh/huggingface/transformers/pull/1455?src=pr&el=tree)
```diff
@@ Coverage Diff @@
## master #1455 +/- ##
==========================================
- Coverage 85.9% 83.97% -1.93%
==========================================
Files 91 87 -4
Lines 13653 12866 -787
==========================================
- Hits 11728 10804 -924
- Misses 1925 2062 +137
```
| [Impacted Files](https://codecov.io/gh/huggingface/transformers/pull/1455?src=pr&el=tree) | Coverage Δ | |
|---|---|---|
| [transformers/modeling\_utils.py](https://codecov.io/gh/huggingface/transformers/pull/1455/diff?src=pr&el=tree#diff-dHJhbnNmb3JtZXJzL21vZGVsaW5nX3V0aWxzLnB5) | `92.44% <ø> (ø)` | :arrow_up: |
| [transformers/modeling\_beam\_search.py](https://codecov.io/gh/huggingface/transformers/pull/1455/diff?src=pr&el=tree#diff-dHJhbnNmb3JtZXJzL21vZGVsaW5nX2JlYW1fc2VhcmNoLnB5) | `0% <0%> (ø)` | |
| [transformers/modeling\_xlm.py](https://codecov.io/gh/huggingface/transformers/pull/1455/diff?src=pr&el=tree#diff-dHJhbnNmb3JtZXJzL21vZGVsaW5nX3hsbS5weQ==) | `88.42% <100%> (ø)` | :arrow_up: |
| [transformers/configuration\_utils.py](https://codecov.io/gh/huggingface/transformers/pull/1455/diff?src=pr&el=tree#diff-dHJhbnNmb3JtZXJzL2NvbmZpZ3VyYXRpb25fdXRpbHMucHk=) | `97.33% <100%> (-1.34%)` | :arrow_down: |
| [transformers/tests/modeling\_common\_test.py](https://codecov.io/gh/huggingface/transformers/pull/1455/diff?src=pr&el=tree#diff-dHJhbnNmb3JtZXJzL3Rlc3RzL21vZGVsaW5nX2NvbW1vbl90ZXN0LnB5) | `74.68% <100%> (-1.34%)` | :arrow_down: |
| [transformers/tests/modeling\_bert\_test.py](https://codecov.io/gh/huggingface/transformers/pull/1455/diff?src=pr&el=tree#diff-dHJhbnNmb3JtZXJzL3Rlc3RzL21vZGVsaW5nX2JlcnRfdGVzdC5weQ==) | `96.92% <100%> (+0.53%)` | :arrow_up: |
| [transformers/modeling\_encoder\_decoder.py](https://codecov.io/gh/huggingface/transformers/pull/1455/diff?src=pr&el=tree#diff-dHJhbnNmb3JtZXJzL21vZGVsaW5nX2VuY29kZXJfZGVjb2Rlci5weQ==) | `67.69% <67.69%> (ø)` | |
| [transformers/modeling\_bert.py](https://codecov.io/gh/huggingface/transformers/pull/1455/diff?src=pr&el=tree#diff-dHJhbnNmb3JtZXJzL21vZGVsaW5nX2JlcnQucHk=) | `87.59% <86.79%> (-0.59%)` | :arrow_down: |
| [...ransformers/tests/modeling\_encoder\_decoder\_test.py](https://codecov.io/gh/huggingface/transformers/pull/1455/diff?src=pr&el=tree#diff-dHJhbnNmb3JtZXJzL3Rlc3RzL21vZGVsaW5nX2VuY29kZXJfZGVjb2Rlcl90ZXN0LnB5) | `96.29% <96.29%> (ø)` | |
| [transformers/modeling\_tf\_pytorch\_utils.py](https://codecov.io/gh/huggingface/transformers/pull/1455/diff?src=pr&el=tree#diff-dHJhbnNmb3JtZXJzL21vZGVsaW5nX3RmX3B5dG9yY2hfdXRpbHMucHk=) | `76.92% <0%> (-16.04%)` | :arrow_down: |
| ... and [45 more](https://codecov.io/gh/huggingface/transformers/pull/1455/diff?src=pr&el=tree-more) | |
------
[Continue to review full report at Codecov](https://codecov.io/gh/huggingface/transformers/pull/1455?src=pr&el=continue).
> **Legend** - [Click here to learn more](https://docs.codecov.io/docs/codecov-delta)
> `Δ = absolute <relative> (impact)`, `ø = not affected`, `? = missing data`
> Powered by [Codecov](https://codecov.io/gh/huggingface/transformers/pull/1455?src=pr&el=footer). Last update [ae1d03f...a88a0e4](https://codecov.io/gh/huggingface/transformers/pull/1455?src=pr&el=lastupdated). Read the [comment docs](https://docs.codecov.io/docs/pull-request-comments).
<|||||>Regarding the initialization of `Bert2Rnd` with pretrained-weights for the encoder and random initialization for the decoder (as per the name), I see two potential solutions:
1. Patch `from_pretrained` in `modeling_utils.py` by not attempting to load weights if `Decoder` is in the name. I don't like this solution at all: the burden of initialization should be borne by the instantiating class, and adding model-specific logic in this function will bite us in the 🍑 with almost 100% certainty at some point in the future.
2. Override `from_pretrained` in `Bert2Rnd`. This would be fairly simple if there was a way to fetch the config created by `from_pretrained` in the Base class. I imagined the following:
```python
@classmethod
def from_pretrained(cls, pretrained_model_or_path, *args, **kwargs):
pretrained_encoder, config = BertEncoder.from_pretrained(pretrained_model_or_path, *args, **kwargs)
model = cls(config)
model.encoder = pretrained_encoder
return model
```
Changing `PretrainedModel`'s `from_pretrained` to output the config as well as the model is a non-breaking change, thanks to python's magic ✨. The following code runs without problem:
```python
def magic_function(number):
return number, number+1
a = magic_function(10)
```
I'd appreciate your opinion since this is a central part of the library. In the meantime I'll dive into the way parameter loading works and see if I can find another solution.<|||||>Not sure I get the point of your `magic_function` stuff but yes solution 2 is the way to go.
You'll have to write a specific `from_pretrained` function for the seq2seq models.<|||||>Now I realize there was strictly zero point :smile:
I hope to have a functioning version by noon :crossed_fingers: <|||||>Here is something that "works" in the sense that:
1. All tests pass (with a new one that tests the initialization)
2. I can add an LM head at the top of the decoder and have a working `text -> Bert2Rand -> text` pipeline; the output is rubbish since the decoder is initialized randomly.
*Edit:* I just re-read the paper and it turns out they initialized the decoder with pretrained embeddings and not random embeddings. I’ll make the change.
To be able to generate meaningful text we would need to fine-tune the model. From here I can either:
- fine-tune the model for text generation (create `run_seq2seq_finetuning.py` and use `run_generation.py`)
- fine-tune for abstractive summarization (and create `run_abstractive_summarization.py`);
I’d vote for text generation for now as it is narrower in scope and won’t add yet another concept in the PR. Then we can finalize the API of the model and ship it + examples.
Tentative plan:
1. `Bert2Rnd` finetuning + text generation;
2. `UniLM`+ finetuning + text generation in a separate PR;
3. Abstractive summarization using `Bert2Rnd`and `UniLM`.
I am also strangely fascinated by the `BertShare`architecture (decoder sharing weights with encoder, asymmetry between them due to encoder-decoder attention only & outperforming everything else), but we can keep this one for later.<|||||>I implemented all elements necessary to reproduce the results from Lapata & Liu:
* Separate sentences in the document by `[SEP] [CLS]`. I currently did this in the `run_seq2seq_finetuning.py` file, but I could instead add a `add_special_tokens_sentence_representation` function in `tokenizer_bert.py` if you think it is cleaner.
* Add alternating `token_type_ids` for each sentence. Same remark as the previous point.
* Add a custom Optimizer class: they use separate optimizers for encoder & decoder + different learning schedules.
* Add the beloved beam-search in `modeling_beam_search.py.` It is a bit awkward, and I would like to have it well tested.
Things I need input on:
- [ ] Any mistake
- [ ] First and second point: is it worth adding two functions in `bert_tokenizer`?
- [ ] What do we do about beam search?<|||||>Ok LGTM, let's merge this and continue the work on summarization and T5 on separate PRs.<|||||>@rlouf This is a really great addition! Any plan to complete the run_summarization_finetuning.py end-to-end soon? Or any psuedo code to point me to the right direction would be great too. <|||||>> @rlouf This is a really great addition! Any plan to complete the run_summarization_finetuning.py end-to-end soon? Or any psuedo code to point me to the right direction would be great too.
Would something like this work?
1. Initialize a `TransformerBeamSearch` from a `PreTrainedEncoderDecoder`
2. Call the `forward` method of `TransformerBeamSearch` with encoder_input_ids and other necessary arguments
3. Use the tokenizer to convert results from step 2 back to text.
You mentioned `TransformerBeamSearch` is a draft version. Not sure how much more work is needed on it. Looks OK to me, but I'm new to seq2seq models. :)<|||||>@hlums Thanks! You can follow the `example-summarization` branch where we are currently completing the example (and solidifying the Beam Search).
The answers to your questions are in the `evaluate` function of the `run_summarization.py` example, and you are essentially right :) We will soon release the example with a short example of how to you use BeamSearch.<|||||>@rlouf , great addition. Is that possible to initialize Model2Model class with both the encoder/ decoder are going to be XLMRoberta model and pre-train it with my own data? |
transformers | 1,454 | closed | Change tensorboard imports to use built-in tensorboard if available | Related issue: #1427 | 10-08-2019 13:32:50 | 10-08-2019 13:32:50 | # [Codecov](https://codecov.io/gh/huggingface/transformers/pull/1454?src=pr&el=h1) Report
> Merging [#1454](https://codecov.io/gh/huggingface/transformers/pull/1454?src=pr&el=desc) into [master](https://codecov.io/gh/huggingface/transformers/commit/d688af19e5ce92c1395820a89e3f3b635eacc2ba?src=pr&el=desc) will **not change** coverage.
> The diff coverage is `n/a`.
[](https://codecov.io/gh/huggingface/transformers/pull/1454?src=pr&el=tree)
```diff
@@ Coverage Diff @@
## master #1454 +/- ##
=======================================
Coverage 84.72% 84.72%
=======================================
Files 84 84
Lines 12591 12591
=======================================
Hits 10668 10668
Misses 1923 1923
```
------
[Continue to review full report at Codecov](https://codecov.io/gh/huggingface/transformers/pull/1454?src=pr&el=continue).
> **Legend** - [Click here to learn more](https://docs.codecov.io/docs/codecov-delta)
> `Δ = absolute <relative> (impact)`, `ø = not affected`, `? = missing data`
> Powered by [Codecov](https://codecov.io/gh/huggingface/transformers/pull/1454?src=pr&el=footer). Last update [d688af1...5ce8d29](https://codecov.io/gh/huggingface/transformers/pull/1454?src=pr&el=lastupdated). Read the [comment docs](https://docs.codecov.io/docs/pull-request-comments).
<|||||>Fine with me, thanks!<|||||>My critique would be that just writing a general 'except' is not PEP-y. The correct error test should be checked. Then again, large parts of the whole package are not PEP-y so it might not be important for the developers. |
transformers | 1,453 | closed | DistilBert for Tensorflow doesn't work | Model: TFDistilBertForSequenceClassification
Language: English
Task: multi-label classification
Environment: google colab
When trying to use TF Distil Bert I get the below error after I have loaded the model and try to run model.fit() :
> TypeError: in converted code:
> relative to /usr/local/lib/python3.6/dist-packages:
>
> transformers/modeling_tf_distilbert.py:680 call *
> distilbert_output = self.distilbert(inputs, **kwargs)
> tensorflow_core/python/keras/engine/base_layer.py:842 __call__
> outputs = call_fn(cast_inputs, *args, **kwargs)
> transformers/modeling_tf_distilbert.py:447 call *
> tfmr_output = self.transformer([embedding_output, attention_mask, head_mask], training=training)
> tensorflow_core/python/keras/engine/base_layer.py:891 __call__
> outputs = self.call(cast_inputs, *args, **kwargs)
> transformers/modeling_tf_distilbert.py:382 call
> layer_outputs = layer_module([hidden_state, attn_mask, head_mask[i]], training=training)
> tensorflow_core/python/keras/engine/base_layer.py:891 __call__
> outputs = self.call(cast_inputs, *args, **kwargs)
> transformers/modeling_tf_distilbert.py:324 call
> sa_output = self.attention([x, x, x, attn_mask, head_mask], training=training)
> tensorflow_core/python/keras/engine/base_layer.py:891 __call__
> outputs = self.call(cast_inputs, *args, **kwargs)
> transformers/modeling_tf_distilbert.py:229 call
> assert 2 <= len(tf.shape(mask)) <= 3
> tensorflow_core/python/framework/ops.py:741 __len__
> "shape information.".format(self.name))
>
> TypeError: len is not well defined for symbolic Tensors. (tf_distil_bert_for_sequence_classification_1/distilbert/transformer/layer_._0/attention/Shape_2:0) Please call `x.shape` rather than `len(x)` for shape information.
The exact same procedure works if I use TF Bert but not Distil Bert. Does anyone know how to get around this problem? | 10-08-2019 11:21:08 | 10-08-2019 11:21:08 | I have been experiencing the same issue #1378.<|||||>Fixed on master with 23b7138, thanks.
Will be in this week's new release 2.1<|||||>thanks a lot |
transformers | 1,452 | closed | xlm-mlm-100-1280 model is not available for download | xlm-mlm-100-1280 model is not available for download, see:
https://s3.amazonaws.com/models.huggingface.co/bert/xlm-mlm-100-1280-tf_model.h5
The model for pytorch is available
| 10-08-2019 08:43:37 | 10-08-2019 08:43:37 | This issue has been automatically marked as stale because it has not had recent activity. It will be closed if no further activity occurs. Thank you for your contributions.
|
transformers | 1,451 | closed | nn.Transformer | ## 🚀
Use Pytorch's own attention and transformer modules.
## Motivation
Pytorch now offers modules like [nn.MultiheadAttention](https://pytorch.org/docs/stable/nn.html?highlight=attention#torch.nn.MultiheadAttention) and [nn.Transformer](https://pytorch.org/docs/stable/nn.html#transformer-layers). It would be nice to use the official Pytorch implementations in `transformers` now that they are available.
## Additional context
There is an offical Pytorch [tutorial](https://pytorch.org/tutorials/beginner/transformer_tutorial.html) that shows how nn.Transformer can be used and customized.
These modules are only available in Pytorch 1.1 (`nn.MultiHeadAttention`) and 1.2 (`nn.Transformer`). Using them would mean that anyone with Pytorch 1.0 would have to update their own version.
| 10-08-2019 00:13:35 | 10-08-2019 00:13:35 | Even though I am in favour of using as many built-ins as possible, I wonder whether it is not too early to do this. You will end up with a lot of pseudo-duplicate code: for those who are on 1.0 (no transformer-like support), 1.1 (only nn.*Attention), and 1.2 (full transformer). I don't know any statistics about people using `transformers` but I can imagine that many are still on PyTorch 1.0. <|||||>We have a small codebase on the side where we use `nn.Transformer` to build both a BERT-style and a GPT2-style model that are compatible with our pretrained weights, but we still think it's a bit too early to refactor/freeze the lib's internals. A lot of research is still going to focus on the models' internals so we don't want to overfit to the current architecture.<|||||>This issue has been automatically marked as stale because it has not had recent activity. It will be closed if no further activity occurs. Thank you for your contributions.
<|||||>@julien-c @BramVanroy just curious, do you guys still think it’s too early to use nn.Transformer?<|||||>A friendly ping to the maintainers to implement the in-built modules.<|||||>That's not something we can do because it will break all existing checkpoints. |
transformers | 1,450 | closed | Installation example #2 fails: cannot import name 'glue_compute_metrics' | ## 🐛 Bug
<!-- Important information -->
I am having issues with the official installation procedure, where running `python -m pytest -sv ./examples` fails with an opaque error message (below).
## To Reproduce
Steps to reproduce the behavior:
1. Create virtualenv
2. Install Pytorch (`pip install torch==1.2.0+cpu torchvision==0.4.0+cpu -f https://download.pytorch.org/whl/torch_stable.html`)
3. Install transformers (`pip install transformers`)
4. Install pytest (`pip install pytest`)
5. Run `python -m pytest -sv ./transformers/tests/`; no tests fail
6. Run `python -m pytest -sv ./examples/`; fails requiring tensorboardX
7. Install tensorboardX (`pip install tensorboardX`)
8. Run `python -m pytest -sv ./examples/`; fails with message:
```
==================================== ERRORS ====================================
__________________ ERROR collecting examples/test_examples.py __________________
ImportError while importing test module '/home/evancw/Projects/transformers/examples/test_examples.py'.
Hint: make sure your test modules/packages have valid Python names.
Traceback:
examples/test_examples.py:30: in <module>
import run_glue
examples/run_glue.py:49: in <module>
from transformers import glue_compute_metrics as compute_metrics
E ImportError: cannot import name 'glue_compute_metrics'
!!!!!!!!!!!!!!!!!!! Interrupted: 1 errors during collection !!!!!!!!!!!!!!!!!!!!
=============================== 1 error in 0.64s ===============================
```
<!-- If you have a code sample, error messages, stack traces, please provide it here as well. -->
## Expected behavior
<!-- A clear and concise description of what you expected to happen. -->
I would expect the tests to pass.
## Environment
* OS: Ubuntu 18.04.3 LTS
* Python version: 3.6.8
* PyTorch version: 1.2.0 + CPU
* PyTorch Transformers version (or branch): 2.0.0
* Using GPU ? No
* Distributed of parallel setup ? No
* Any other relevant information: System is completely clean before pip installs
## Additional context
Please let me know if there is any more information I can provide.
| 10-07-2019 23:44:59 | 10-07-2019 23:44:59 | @evanweissburg Hi, i got the same error..have you found any solution???
<|||||>Hello! I believe you must have sklearn installed in order to pass these tests. Please let me know if it doesn't work while having sklearn installed.<|||||>yeah got it .... i guess we need to run pip install -r ./examples/requirements.txt<|||||>Indeed!<|||||>Could we add this to the getting started documentation?
pip install -r ./examples/requirements.txt<|||||>Had the same issue. Probably a problem with sklearn. Installed with conda and it was fixed. |
transformers | 1,449 | closed | Can't replicate Language Model finetuning | I cannot replicate BioBERT results by using finetune_on_pregenerated.py with data generated using pregenerate_training_data.py.
I've noticed that the LM code has been removed from the repo in that last couple versions. Does this mean there were known issues with this process?
| 10-07-2019 22:15:46 | 10-07-2019 22:15:46 | Hello, this language model fine-tuning was community-maintained and is now deprecated. The example script to fine-tune on language modeling is now `run_lm_finetuning.py`. |
transformers | 1,448 | closed | Contribution guidelines | Here is a first draft to serve as a basis for discussion around contribution guidelines. Please mention anything that seems relevant to you / that you care about. | 10-07-2019 21:19:25 | 10-07-2019 21:19:25 | # [Codecov](https://codecov.io/gh/huggingface/transformers/pull/1448?src=pr&el=h1) Report
> Merging [#1448](https://codecov.io/gh/huggingface/transformers/pull/1448?src=pr&el=desc) into [master](https://codecov.io/gh/huggingface/transformers/commit/8fcc6507ce9d0922ddb60f4a31d4b9a839de1270?src=pr&el=desc) will **not change** coverage.
> The diff coverage is `n/a`.
[](https://codecov.io/gh/huggingface/transformers/pull/1448?src=pr&el=tree)
```diff
@@ Coverage Diff @@
## master #1448 +/- ##
=======================================
Coverage 84.72% 84.72%
=======================================
Files 84 84
Lines 12591 12591
=======================================
Hits 10668 10668
Misses 1923 1923
```
------
[Continue to review full report at Codecov](https://codecov.io/gh/huggingface/transformers/pull/1448?src=pr&el=continue).
> **Legend** - [Click here to learn more](https://docs.codecov.io/docs/codecov-delta)
> `Δ = absolute <relative> (impact)`, `ø = not affected`, `? = missing data`
> Powered by [Codecov](https://codecov.io/gh/huggingface/transformers/pull/1448?src=pr&el=footer). Last update [8fcc650...45de313](https://codecov.io/gh/huggingface/transformers/pull/1448?src=pr&el=lastupdated). Read the [comment docs](https://docs.codecov.io/docs/pull-request-comments).
|
transformers | 1,447 | closed | Provide requirements.txt for development dependencies | This PR adds the list of requirements needed to run the tests to the repo. Makes it easier for newcomers to contribute. | 10-07-2019 15:55:38 | 10-07-2019 15:55:38 | # [Codecov](https://codecov.io/gh/huggingface/transformers/pull/1447?src=pr&el=h1) Report
> Merging [#1447](https://codecov.io/gh/huggingface/transformers/pull/1447?src=pr&el=desc) into [master](https://codecov.io/gh/huggingface/transformers/commit/1615360c71f75da7b8aefd14c5d8a461486f865b?src=pr&el=desc) will **not change** coverage.
> The diff coverage is `n/a`.
[](https://codecov.io/gh/huggingface/transformers/pull/1447?src=pr&el=tree)
```diff
@@ Coverage Diff @@
## master #1447 +/- ##
=======================================
Coverage 84.72% 84.72%
=======================================
Files 84 84
Lines 12591 12591
=======================================
Hits 10668 10668
Misses 1923 1923
```
------
[Continue to review full report at Codecov](https://codecov.io/gh/huggingface/transformers/pull/1447?src=pr&el=continue).
> **Legend** - [Click here to learn more](https://docs.codecov.io/docs/codecov-delta)
> `Δ = absolute <relative> (impact)`, `ø = not affected`, `? = missing data`
> Powered by [Codecov](https://codecov.io/gh/huggingface/transformers/pull/1447?src=pr&el=footer). Last update [1615360...7afd00a](https://codecov.io/gh/huggingface/transformers/pull/1447?src=pr&el=lastupdated). Read the [comment docs](https://docs.codecov.io/docs/pull-request-comments).
|
transformers | 1,446 | closed | integer representation ambuiguty in tokenizer | ## ❓ Questions & Help
<!-- A clear and concise description of the question. -->
I use GPT-2 transformers model. Tokenizer.encode(' man') = 805, tokenizer.encode('man') = 805. But when in the sentence(e.g. Tokenizer.encode(' the man is a teacher') = [1169, 582, 318, 257, 4701], here the integer representing 'man' is 582. I think the problem is BPE used in transformer, where 850 is the integer representing the subtoken '-man' but not the word 'man'.
I wonder how I can set the tokenizer so I can get Tokenizer.encode(' man') = 582? | 10-07-2019 15:48:46 | 10-07-2019 15:48:46 | Hello! You should specify `add_prefix_space=True` in your encode method to obtain that behavior.<|||||>Thank you! That works! |
transformers | 1,445 | closed | Performance degradation with new version of this library (inference) | ## 🐛 Bug
<!-- Important information -->
Model I am using (Bert, XLNet....): GPT-2
Language I am using the model on (English, Chinese....): Russian
The problem arise when using:
* [ ] the official example scripts: (give details)
* [ x] my own modified scripts: (give details)
I do inference with a bit modified run_generation.py
The tasks I am working on is:
* [ ] an official GLUE/SQUaD task: (give the name)
* [ x] my own task or dataset: (give details)
I'm training Russian GPT-2
## To Reproduce
Steps to reproduce the behavior:
1. Use pytorch-tranformers library (1.2)
2. Use sample_sequence from run_generation.py on GPU
3. Use tranformers library (2.0)
4. Use sample_sequence from run_generation.py on GPU
5. Step 4 is running 5 times slower than step 2.
<!-- If you have a code sample, error messages, stack traces, please provide it here as well. -->
## Expected behavior
<!-- A clear and concise description of what you expected to happen. -->
The speed probably should stay the same.
## Environment
* OS: Ubuntu 18.04
* Python version: 3.7.3
* PyTorch version:
* PyTorch Transformers version (or branch): 1.2 vs 2.0
* Using GPU - yes
* Distributed of parallel setup - no
* Any other relevant information:
## Additional context
https://github.com/mgrankin/ru_transformers
<!-- Add any other context about the problem here. -->
| 10-07-2019 15:36:35 | 10-07-2019 15:36:35 | I'm so sorry, I haven't replaced all occurrences of `pytorch-tranformers` to `tranformers`. That was the source of the problem.<|||||>Glad to hear that! |
transformers | 1,444 | closed | XLNet - Finetuning - Layer-wise LR decay | ## ❓ Questions & Help
I'm trying to finetuning a XLNet using run_glue.py, but i haven't seen any references about **Layer-wise lr decay**, that were commented by the authors in the paper.
- Where can I set this parameter on finetuning optimizer?
- The *linear learning rate decay* commented in the paper is related to Warmup Scheduler ?(considering that after warmup_steps is reached, the lr rate begins to decay)
References:
(https://arxiv.org/pdf/1906.08237.pdf - page 16)
| 10-07-2019 15:29:35 | 10-07-2019 15:29:35 | No this means the layer rate is smaller deeper in the network, what is called "discriminative learning" in ULMFiT.
Check our NAACL Tutorial on Transfer Learning for more details, in particular, Hands-on n°5 slide 163 here: https://docs.google.com/presentation/d/1fIhGikFPnb7G5kr58OvYC3GN4io7MznnM0aAgadvJfc/edit?ts=5c8d09e7#slide=id.g5888218f39_54_89 <|||||>Thanks @thomwolf for the answer. |
transformers | 1,443 | closed | RuntimeError: cublas runtime error : resource allocation failed | ## 🐛 Bug
<!-- Important information -->
Model I am using Bert:
Language I am using the model on English:
The tasks I am working on is:
* [ ] Finetuned bert model with my own dataset.
* [ ] run_lm_finetuning.py
## To Reproduce
Steps to reproduce the behavior:
1. I was followesd this issue https://github.com/huggingface/transfer-learning-conv-ai/issues/10
2. i tried to reduced batch_size = 1
3. i tried `CUDA_LAUNCH_BLOCKING=1`
it is throwing,
`RuntimeError: CUDA error: out of memory`
<!-- If you have a code sample, error messages, stack traces, please provide it here as well. -->
> CUDA_VISIBLE_DEVICES=2 python run_lm_finetuning.py --output_dir=output --model_type=roberta --model_name_or_path=roberta-base --do_train --train_data_file=$TRAIN_FILE --do_eval --eval_data_file=$TEST_FILE --mlm --per_gpu_train_batch_size 1 --per_gpu_eval_batch_size 1
## Expected behavior
<!-- A clear and concise description of what you expected to happen. -->
```
Traceback (most recent call last):
File "run_lm_finetuning.py", line 497, in <module>
main()
File "run_lm_finetuning.py", line 451, in main
global_step, tr_loss = train(args, train_dataset, model, tokenizer)
File "run_lm_finetuning.py", line 189, in train
outputs = model(inputs, masked_lm_labels=labels) if args.mlm else model(inputs, labels=labels)
File "/media/user1/storage-1/Ashok_AI/mask_env/lib/python3.6/site-packages/torch/nn/modules/module.py", line 547, in __call__
result = self.forward(*input, **kwargs)
File "/media/user1/storage-1/Ashok_AI/mask_env/lib/python3.6/site-packages/transformers/modeling_roberta.py", line 237, in forward
head_mask=head_mask)
File "/media/user1/storage-1/Ashok_AI/mask_env/lib/python3.6/site-packages/torch/nn/modules/module.py", line 547, in __call__
result = self.forward(*input, **kwargs)
File "/media/user1/storage-1/Ashok_AI/mask_env/lib/python3.6/site-packages/transformers/modeling_roberta.py", line 177, in forward
head_mask=head_mask)
File "/media/user1/storage-1/Ashok_AI/mask_env/lib/python3.6/site-packages/transformers/modeling_bert.py", line 625, in forward
head_mask=head_mask)
File "/media/user1/storage-1/Ashok_AI/mask_env/lib/python3.6/site-packages/torch/nn/modules/module.py", line 547, in __call__
result = self.forward(*input, **kwargs)
File "/media/user1/storage-1/Ashok_AI/mask_env/lib/python3.6/site-packages/transformers/modeling_bert.py", line 346, in forward
layer_outputs = layer_module(hidden_states, attention_mask, head_mask[i])
File "/media/user1/storage-1/Ashok_AI/mask_env/lib/python3.6/site-packages/torch/nn/modules/module.py", line 547, in __call__
result = self.forward(*input, **kwargs)
File "/media/user1/storage-1/Ashok_AI/mask_env/lib/python3.6/site-packages/transformers/modeling_bert.py", line 324, in forward
attention_outputs = self.attention(hidden_states, attention_mask, head_mask)
File "/media/user1/storage-1/Ashok_AI/mask_env/lib/python3.6/site-packages/torch/nn/modules/module.py", line 547, in __call__
result = self.forward(*input, **kwargs)
File "/media/user1/storage-1/Ashok_AI/mask_env/lib/python3.6/site-packages/transformers/modeling_bert.py", line 281, in forward
self_outputs = self.self(input_tensor, attention_mask, head_mask)
File "/media/user1/storage-1/Ashok_AI/mask_env/lib/python3.6/site-packages/torch/nn/modules/module.py", line 547, in __call__
result = self.forward(*input, **kwargs)
File "/media/user1/storage-1/Ashok_AI/mask_env/lib/python3.6/site-packages/transformers/modeling_bert.py", line 200, in forward
mixed_query_layer = self.query(hidden_states)
File "/media/user1/storage-1/Ashok_AI/mask_env/lib/python3.6/site-packages/torch/nn/modules/module.py", line 547, in __call__
result = self.forward(*input, **kwargs)
File "/media/user1/storage-1/Ashok_AI/mask_env/lib/python3.6/site-packages/torch/nn/modules/linear.py", line 87, in forward
return F.linear(input, self.weight, self.bias)
File "/media/user1/storage-1/Ashok_AI/mask_env/lib/python3.6/site-packages/torch/nn/functional.py", line 1371, in linear
output = input.matmul(weight.t())
RuntimeError: cublas runtime error : resource allocation failed at /pytorch/aten/src/THC/THCGeneral.cpp:216
Epoch: 0%| | 0/1 [00:00<?, ?it/s]
Iteration: 0%|
```
## Environment
* OS: Linux
* Python version: 3.6
* PyTorch version: 1.2.0
* PyTorch Transformers version: latest
* Using GPU : yes, CUDA 10
* Distributed of parallel setup : yes
| 10-07-2019 11:57:34 | 10-07-2019 11:57:34 | What GPU do you have?<|||||>Thanks for your reply and support sir:)
NVIDIA TITAN RTX: 4 × 24 GB GPUs<|||||>Looks like your batch size may be too big?<|||||>Thank you so much for your support sir.
I given batch size = 1. May be the latest branch any issues will be present. I will check out previous master and then i will try sir. <|||||>Hi, I have the same error. Did you get this problem resolved?
<|||||>I have the same error too<|||||>It may be because of this [nn.embedding issue in pytorch](https://github.com/pytorch/pytorch/issues/24838) . I had the same error. See if you have padded correctly.. or have included some invalid token<|||||>Very similar issue with roberta-base (but not bert-base-cased/uncased):
RuntimeError: cublas runtime error : library not initialized at /opt/conda/conda-bld/pytorch_1573049306803/work/aten/src/THC/THCGeneral.cpp:216
I have checked and it isn't a problem with nn.embedding, nor a memory issue.<|||||>> Very similar issue with roberta-base (but not bert-base-cased/uncased):
>
> RuntimeError: cublas runtime error : library not initialized at /opt/conda/conda-bld/pytorch_1573049306803/work/aten/src/THC/THCGeneral.cpp:216
>
> I have checked and it isn't a problem with nn.embedding, nor a memory issue.
Very similar issue, when using camembert model which is based on roberta,
could you solve the issue ? any thoughts about it plz <|||||>>
>
> Very similar issue with roberta-base (but not bert-base-cased/uncased):
>
> RuntimeError: cublas runtime error : library not initialized at /opt/conda/conda-bld/pytorch_1573049306803/work/aten/src/THC/THCGeneral.cpp:216
>
> I have checked and it isn't a problem with nn.embedding, nor a memory issue.
@YDYordanov Same with you when using roberta-base, have you resolved it?<|||||>@YDYordanov @Hadjer13 I found the the solution. In my case , my input example has two sentences, so I use `token_type_ids` like I use in Bert, but it turns out that I pass the wrong `token_type_ids` to the `RobertaModel`. According to [the transformers doc](https://huggingface.co/transformers/model_doc/roberta.html#transformers.RobertaTokenizer.create_token_type_ids_from_sequences), **RoBERTa does not make use of token type ids**. So using `[0,0,..0,1,1..1,0,0,..]` as `token_type_ids` for Roberta is wrong, after I change it to all zeros, i.e. `[0,0,...,0,0]`, the error is fixed. Hope it can help someone!<|||||>> @YDYordanov @Hadjer13 I found the the solution. In my case , my input example has two sentences, so I use `token_type_ids` like I use in Bert, but it turns out that I pass the wrong `token_type_ids` to the `RobertaModel`. According to [the transformers doc](https://huggingface.co/transformers/model_doc/roberta.html#transformers.RobertaTokenizer.create_token_type_ids_from_sequences), **RoBERTa does not make use of token type ids**. So using `[0,0,..0,1,1..1,0,0,..]` as `token_type_ids` for Roberta is wrong, after I change it to all zeros, i.e. `[0,0,...,0,0]`, the error is fixed. Hope it can help someone!
thank you,<|||||>This issue has been automatically marked as stale because it has not had recent activity. It will be closed if no further activity occurs. Thank you for your contributions.
<|||||>> @YDYordanov @Hadjer13 I found the the solution. In my case , my input example has two sentences, so I use `token_type_ids` like I use in Bert, but it turns out that I pass the wrong `token_type_ids` to the `RobertaModel`. According to [the transformers doc](https://huggingface.co/transformers/model_doc/roberta.html#transformers.RobertaTokenizer.create_token_type_ids_from_sequences), **RoBERTa does not make use of token type ids**. So using `[0,0,..0,1,1..1,0,0,..]` as `token_type_ids` for Roberta is wrong, after I change it to all zeros, i.e. `[0,0,...,0,0]`, the error is fixed. Hope it can help someone!
I have already had this line in my code:
`transformer_params = {
'input_ids': input_ids,
'token_type_ids': (
segment_ids if args.model == 'bert-base-uncased' else None
),
'attention_mask': attention_mask,
}`
Still I am getting the error: `RuntimeError: cublas runtime error : resource allocation failed at /pytorch/aten/src/THC/THCGeneral.cpp:216`
Do you have any idea why? my teacher model is `bert-base-uncased` and when I set my student model as `roberta-base`, I am getting this error. |
transformers | 1,442 | closed | TFBertForSequenceClassification - Feeding List of InputExamples | ## ❓ Questions & Help
I used the "glue_convert_examples_to_features" function on my own InputExamples to get a List of InputFeatures. I want to do a Multi-Label Classification but I can not figure out how i need to feed the List of InputFeatures to the TFBertForSequenceClassification model.
train_dataset = glue_convert_examples_to_features(train_examples, tokenizer, max_length=512, task='metis_ton')
valid_dataset = glue_convert_examples_to_features(validation_examples, tokenizer, max_length=512, task='metis_ton')
optimizer = tf.keras.optimizers.Adam(learning_rate=3e-5, epsilon=1e-08, clipnorm=1.0)
loss = tf.keras.losses.SparseCategoricalCrossentropy(from_logits=True)
metric = tf.keras.metrics.SparseCategoricalAccuracy('accuracy')
model.compile(optimizer=optimizer, loss=loss, metrics=[metric])
history = model.fit(train_dataset, epochs=2, batch_size=16,
validation_data=valid_dataset, validation_steps=7)
In this case "metis_ton" is my own Procsesor with labels corresponding to my data.
When i try to feed the list directly to model.fit() i get the following error:
WARNING:tensorflow:Falling back from v2 loop because of error: Failed to find data adapter
that can handle input: (<class 'list'> containing values of types {"<class '
transformers.data.processors.utils.InputFeatures'>"}), <class 'NoneType'>
Please provide as model inputs either a single array or a list of arrays. You passed: inputs=[{
"attention_mask": [
1,
1,
...
I then tried to split the data in X and y:
input_ids = []
attention_mask = []
token_type_ids = []
train_y = []
for feature in train_dataset:
input_ids.append(feature.input_ids)
attention_mask.append(feature.attention_mask)
token_type_ids.append(feature.token_type_ids)
train_y.append(feature.label)
train_X = [input_ids, attention_mask, token_type_ids]
history = model.fit(train_X, train_Y, epochs=2, batch_size=16,
validation_data=valid_dataset, validation_steps=7)
In this case i get the error
Data cardinality is ambiguous:
x sizes: 3
y sizes: 362
Please provide data which shares the same first dimension.
Then i tried to reshape the train_X data:
train_X = list(map(list, zip(*train_X)))
train_X = np.asarray(train_X)
train_y = np.asarray(train_y)
train_X.shape
: (362, 3, 512)
Which results in the following error when calling model.fit():
ValueError: Cannot reshape a tensor with 768 elements to shape [1,1,512,1] (512 elements) for
'tf_bert_for_sequence_classification/bert/embeddings/LayerNorm/Reshape' (op: 'Reshape') with
input shapes: [768], [4] and with input tensors computed as partial shapes: input[1] = [1,1,512,1].
Right now im out of ideas what i could try, can someone help me out? | 10-07-2019 11:33:40 | 10-07-2019 11:33:40 | This issue has been automatically marked as stale because it has not had recent activity. It will be closed if no further activity occurs. Thank you for your contributions.
<|||||>Same problem here. My workaround:
```
def my_workaround(data):
'''Takes list of InputFeatures, returns arrays.'''
# List of dicts
data = [ feature.to_dict() for feature in data ]
# Make one list for each entry in the dicts
input_ids, attention_mask, token_type_ids, label = [], [], [], []
for data_dict in data:
input_ids.append(data_dict['input_ids'])
attention_mask.append(data_dict['attention_mask'])
token_type_ids.append(data_dict['token_type_ids'])
label.append(data_dict['label'])
# Stack in one array each
input_ids = np.vstack(input_ids)
attention_mask = np.vstack(attention_mask)
token_type_ids = np.vstack(token_type_ids)
label = np.vstack(label)
# Return
return label, input_ids, attention_mask, token_type_ids
y_train, *X_train = my_workaround(data_train)
```
It is not ideal, but I hope it helps :) <|||||>same problem,i havent got a solution |
transformers | 1,441 | closed | TF2 Mixed Precision, XLA, Distribution | ## 🚀 Feature
Hi there, I have benchmarked TF2 with the Transformers library. There are very positive results to be gained from the various TensorFlow 2.0 features:
- Automatic Mixed Precision (AMP)
- XLA compiler
- Distribution strategies (multi-GPU)
Here are the benefits (tested on CoLA, MRPC, SST-2):
- AMP: Between 1.4x to 1.6x decrease in overall time without change in batch size
- AMP+XLA: Up to 2.5x decrease in overall time on SST-2 (larger dataset)
- Distribution: Between 1.4x to 3.4x decrease in overall time on 4xV100
- Combined: Up to 5.7x decrease in overall training time, or 9.1x training throughput
Model quality (measured by validation accuracy) fluctuates slightly. Taking an average of 4 training runs for the single GPU results:
* CoLA: AMP results in slighter lower acc (0.820 vs 0.824)
* MRPC: AMP results in lower acc (0.823 vs 0.835)
* SST-2: AMP results in slighter lower acc (0.918 vs 0.922)
However, with 4xV100 (4x batch size), interesting AMP can result in better results:
* CoLA: AMP results in higher acc (0.828 vs 0.812)
* MRPC: AMP results in lower acc (0.817 vs 0.827)
* SST-2: AMP results in slightly lower acc (0.926 vs 0.929)
The benchmark script demonstrating the use of this features, and also allowing you to test on your own system, is available [here](https://github.com/NVAITC/benchmarking/blob/master/tf2/bert_dist.py).
Note: on some tasks (e.g. MRPC), dataset is too small. Hence overhead of compiling model with XLA and doing distribution strategy does not speed things up. XLA compile time is also the reason why although throughput can increase a lot (e.g. 2.7x for single GPU), overall (end-to-end) training speed-up is not as fast (as low as 1.4x)
The benefits as seen on SST-2 (larger dataset) is much clear.
All results can be seen at this [Google Sheet](https://docs.google.com/spreadsheets/d/1538MN224EzjbRL239sqSiUy6YY-rAjHyXhTzz_Zptls/).
## Motivation
I believe documentation and examples for this usage will be very useful to allow the community to train these models much faster on the hardware they might already have (V100/T4 on cloud, RTX GPUs in desktop etc.)
If possible maybe I could be guided to contribute some examples or documentation!
## Additional context
External material:
* Benchmark Script: https://github.com/NVAITC/benchmarking/blob/master/tf2/bert_dist.py
* Benchmark Results: https://docs.google.com/spreadsheets/d/1538MN224EzjbRL239sqSiUy6YY-rAjHyXhTzz_Zptls/
Testing was performed on an NVIDIA DGX Station with 4x V100 (16GB) with NVLink.
This might also answer part of #1426 (Benchmark Script) | 10-07-2019 10:10:14 | 10-07-2019 10:10:14 | Hi @tlkh, thank you for your work on the benchmarks!
We're planning to release some in-depths benchmarks by the end of the week/early next week. We'll add your work to it and we'll notify you once we have set-up an easier way to contribute benchmarks/examples!<|||||>This is really great @tlkh.
Do you think you could contribute an improved version of the `run_tf_glue` example with these best practices?
We could include your benchmarks and results in the examples readme.
Also, did you notice the same memory limitation mentioned in #1426?<|||||>@thomwolf
Delighted to contribute!
I haven't noticed the memory issues in #1426 on V100 (16GB) but I could see if I can replicate them on a Titan V (12GB).<|||||>Hey @tlkh as you've probably seen by now, we mentioned your work in the recent [Benchmarking blog post ](https://medium.com/huggingface/benchmarking-transformers-pytorch-and-tensorflow-e2917fb891c2) and added it to our [Benchmark section in our documentation](https://huggingface.co/transformers/benchmarks.html#tf2-with-mixed-precision-xla-distribution-tlkh). Thank you again for your work. |
transformers | 1,440 | closed | BLUE 2 | this PR seemed to be out of date due to being late considered (https://github.com/huggingface/transformers/pull/1238).
So I updated the code to be able to merge with the latest version.
In this PR:
- I add BertForMultiLabelClassification, RobertaForTokenClassification, RobertaForMultiLabelClassification.
- I add examples for Finetuning the BERT, RoBERTa models for tasks on BLUE (https://github.com/ncbi-nlp/BLUE_Benchmark). BLUE (Biomedical Language Understanding Evaluation) is similar to GLUE, but for Biomedical data. The "run_blue", "utils_blue" are customized from "run_glue", "utils_glue", but more sufficient, because it contains not only sequence classification, but also token classification, multi-label classification. People may also have more options for examples of fine-tuning BERT/RoBERTa.
- I also add test function to test_examples as well as test data | 10-07-2019 10:05:46 | 10-07-2019 10:05:46 | # [Codecov](https://codecov.io/gh/huggingface/transformers/pull/1440?src=pr&el=h1) Report
> Merging [#1440](https://codecov.io/gh/huggingface/transformers/pull/1440?src=pr&el=desc) into [master](https://codecov.io/gh/huggingface/transformers/commit/1615360c71f75da7b8aefd14c5d8a461486f865b?src=pr&el=desc) will **decrease** coverage by `1.21%`.
> The diff coverage is `30.88%`.
[](https://codecov.io/gh/huggingface/transformers/pull/1440?src=pr&el=tree)
```diff
@@ Coverage Diff @@
## master #1440 +/- ##
=========================================
- Coverage 84.72% 83.5% -1.22%
=========================================
Files 84 84
Lines 12591 12656 +65
=========================================
- Hits 10668 10569 -99
- Misses 1923 2087 +164
```
| [Impacted Files](https://codecov.io/gh/huggingface/transformers/pull/1440?src=pr&el=tree) | Coverage Δ | |
|---|---|---|
| [transformers/modeling\_bert.py](https://codecov.io/gh/huggingface/transformers/pull/1440/diff?src=pr&el=tree#diff-dHJhbnNmb3JtZXJzL21vZGVsaW5nX2JlcnQucHk=) | `85.54% <27.27%> (-2.63%)` | :arrow_down: |
| [transformers/modeling\_roberta.py](https://codecov.io/gh/huggingface/transformers/pull/1440/diff?src=pr&el=tree#diff-dHJhbnNmb3JtZXJzL21vZGVsaW5nX3JvYmVydGEucHk=) | `57.06% <32.6%> (-14.16%)` | :arrow_down: |
| [transformers/modeling\_tf\_pytorch\_utils.py](https://codecov.io/gh/huggingface/transformers/pull/1440/diff?src=pr&el=tree#diff-dHJhbnNmb3JtZXJzL21vZGVsaW5nX3RmX3B5dG9yY2hfdXRpbHMucHk=) | `10.48% <0%> (-66.44%)` | :arrow_down: |
| [transformers/tests/modeling\_tf\_common\_test.py](https://codecov.io/gh/huggingface/transformers/pull/1440/diff?src=pr&el=tree#diff-dHJhbnNmb3JtZXJzL3Rlc3RzL21vZGVsaW5nX3RmX2NvbW1vbl90ZXN0LnB5) | `87.5% <0%> (-7.5%)` | :arrow_down: |
| [transformers/modeling\_xlnet.py](https://codecov.io/gh/huggingface/transformers/pull/1440/diff?src=pr&el=tree#diff-dHJhbnNmb3JtZXJzL21vZGVsaW5nX3hsbmV0LnB5) | `71.25% <0%> (-0.9%)` | :arrow_down: |
------
[Continue to review full report at Codecov](https://codecov.io/gh/huggingface/transformers/pull/1440?src=pr&el=continue).
> **Legend** - [Click here to learn more](https://docs.codecov.io/docs/codecov-delta)
> `Δ = absolute <relative> (impact)`, `ø = not affected`, `? = missing data`
> Powered by [Codecov](https://codecov.io/gh/huggingface/transformers/pull/1440?src=pr&el=footer). Last update [1615360...e7ffd9a](https://codecov.io/gh/huggingface/transformers/pull/1440?src=pr&el=lastupdated). Read the [comment docs](https://docs.codecov.io/docs/pull-request-comments).
<|||||>Ok, I think this is great, sorry for the delay in reviewing the PR.<|||||>Do you want to just add the new RoBERTa models in the tests, at least [this line](https://github.com/huggingface/transformers/blob/master/transformers/tests/modeling_roberta_test.py#L38).
Also, optional but could be nice if you feel like it: add TF 2.0 counterparts to your new PyTorch heads (you can just copy-past-adapt) the relevant Bert heads.<|||||>This issue has been automatically marked as stale because it has not had recent activity. It will be closed if no further activity occurs. Thank you for your contributions.
|
transformers | 1,439 | closed | Input length is not equal to output length? | ## ❓ Questions & Help
<!-- A clear and concise description of the question. -->
| 10-07-2019 09:35:56 | 10-07-2019 09:35:56 | Hi @RichardHWD, I'm afraid we'll need a bit more information than what you have given.<|||||>@LysandreJik Sorry. In your example:
```
import torch
from transformers import *
model_class = BertModel
tokenizer_class = BertTokenizer
pretrained_weights = 'bert-base-uncased'
tokenizer = tokenizer_class.from_pretrained(pretrained_weights)
model = model_class.from_pretrained(pretrained_weights)
# Encode text
input_ids = torch.tensor([tokenizer.encode("Here is some text to encode", add_special_tokens=False)])
with torch.no_grad():
last_hidden_states = model(input_ids)[0] # Models outputs are now tuples
print(last_hidden_states.size())
print(last_hidden_states)
```
I set add_special_tokens=False, and sentence "Here is some text to encode" has 6 words. But the output size is [1, 7, 768]. I want an equal length embedding, how to fix it?<|||||>What’s the shape of input_ids?<|||||>(I suspect it's gonna be 7, you should look into what BertTokenizer does. Thanks!). |
transformers | 1,438 | closed | fix pytorch-transformers migration description in README | 10-07-2019 09:01:20 | 10-07-2019 09:01:20 | Yes! Thanks!<|||||># [Codecov](https://codecov.io/gh/huggingface/transformers/pull/1438?src=pr&el=h1) Report
> Merging [#1438](https://codecov.io/gh/huggingface/transformers/pull/1438?src=pr&el=desc) into [master](https://codecov.io/gh/huggingface/transformers/commit/904158ac4dbce046dd02be8382fdb8e52f0e691c?src=pr&el=desc) will **not change** coverage.
> The diff coverage is `n/a`.
[](https://codecov.io/gh/huggingface/transformers/pull/1438?src=pr&el=tree)
```diff
@@ Coverage Diff @@
## master #1438 +/- ##
=======================================
Coverage 84.72% 84.72%
=======================================
Files 84 84
Lines 12591 12591
=======================================
Hits 10668 10668
Misses 1923 1923
```
------
[Continue to review full report at Codecov](https://codecov.io/gh/huggingface/transformers/pull/1438?src=pr&el=continue).
> **Legend** - [Click here to learn more](https://docs.codecov.io/docs/codecov-delta)
> `Δ = absolute <relative> (impact)`, `ø = not affected`, `? = missing data`
> Powered by [Codecov](https://codecov.io/gh/huggingface/transformers/pull/1438?src=pr&el=footer). Last update [904158a...6dc6c71](https://codecov.io/gh/huggingface/transformers/pull/1438?src=pr&el=lastupdated). Read the [comment docs](https://docs.codecov.io/docs/pull-request-comments).
|
|
transformers | 1,437 | closed | how to do next word prediction in xlnet? | ## how to do next word prediction in xlnet?
First of all thanks for **huggingface - transformers**
community.
I am actually beginner for XLnet. I want to do Next word prediction by using XLnet. How can i do this?
and I have my own domain-specific datasets(1000 lines), finetune this dataset in xlnet.
is this dataset enoughfor us to get good results?
| 10-07-2019 04:46:21 | 10-07-2019 04:46:21 | Take a look at the example code [here](https://github.com/huggingface/transformers/blob/master/examples/run_generation.py). 1000 lines of text for fine-tuning shouldn't be an issue I think, since you're just fine-tuning. As always, try it out and you'll see.<|||||>Thank you so much for your reply<|||||>@BramVanroy But how to fine-tune xlnet for next-word-prediction ?
Is it correct to use perm_mask and target_mapping to simulate left-to-rigth ?<|||||>Can you direct me to a code on how to predict next word in tf 2.0?<|||||>You can see the [causal language modeling example in usage](https://huggingface.co/transformers/usage.html#causal-language-modeling). There's a TensorFlow toggle, and it showcases gpt-2. |
transformers | 1,436 | closed | Which model should I use for machine translation? | ## ❓ Questions & Help
I’m interested in training a model for translating articles from Spanish to English. There is too little information (Tutorials) about MT, should I use BERT, XLM or any other one? Also could you explain how to train the proposed model feeding the data, and output the predicted translation.
And is there a way to use XLNet so when translating chapters of a book it can remember the context of the previous ones and better translate?
There is even a model by Microsoft ([MASS](https://github.com/microsoft/MASS)) that looks simple to use, would you recommend it?
| 10-06-2019 23:37:04 | 10-06-2019 23:37:04 | Hi I recommend using XLM from Facebook for MT currently: https://github.com/facebookresearch/XLM
We may add some models for MT in the mid-term though.<|||||>[MASS](https://arxiv.org/pdf/1905.02450.pdf) reports higher BLEU-scores than [XLM](https://arxiv.org/abs/1901.07291) which is good in pretraining an encoder, but lacks in the training description of the decoder. So we could try to extend the XML-R #1769 encoder with MASS.<|||||>In which languages and domains are you interested?<|||||>I'm looking for translation mainly of Spanish and Chinese to English, mainly books and articles so maintaining an overall consistency of the terms and words is crucial.
Just a single way translation is enough and also it should be possible to further train the model on the already translated works.<|||||>Please help me on how to use xmlr for summarization? Also if there is any example based on xmlr.<|||||>This issue has been automatically marked as stale because it has not had recent activity. It will be closed if no further activity occurs. Thank you for your contributions.
|
transformers | 1,435 | closed | GPT2 Tokenizer | I want to know the pad token value for the gpt2 tokenizer. I have checked the [vocab](https://s3.amazonaws.com/models.huggingface.co/bert/gpt2-vocab.json) but couldn't find any.
Thanks,
Suchith | 10-06-2019 19:21:58 | 10-06-2019 19:21:58 | Hi! GPT-2 doesn't use padding tokens in its tokenizer. In order to manage padding, you should use the `attention_mask` detailed in the [documentation](https://huggingface.co/transformers/model_doc/gpt2.html#transformers.GPT2Model).<|||||>Closing as of now, feel free to reopen if @LysandreJik did not answer your question completely. |
transformers | 1,434 | closed | Remove unnecessary use of FusedLayerNorm in XLNet | Fix #1172 for XLNet | 10-06-2019 17:35:20 | 10-06-2019 17:35:20 | # [Codecov](https://codecov.io/gh/huggingface/transformers/pull/1434?src=pr&el=h1) Report
> Merging [#1434](https://codecov.io/gh/huggingface/transformers/pull/1434?src=pr&el=desc) into [master](https://codecov.io/gh/huggingface/transformers/commit/f3e0218fbb6bcc40b40f10089dae8876654edb23?src=pr&el=desc) will **decrease** coverage by `<.01%`.
> The diff coverage is `100%`.
[](https://codecov.io/gh/huggingface/transformers/pull/1434?src=pr&el=tree)
```diff
@@ Coverage Diff @@
## master #1434 +/- ##
==========================================
- Coverage 84.72% 84.72% -0.01%
==========================================
Files 84 84
Lines 12591 12590 -1
==========================================
- Hits 10668 10667 -1
Misses 1923 1923
```
| [Impacted Files](https://codecov.io/gh/huggingface/transformers/pull/1434?src=pr&el=tree) | Coverage Δ | |
|---|---|---|
| [transformers/modeling\_xlnet.py](https://codecov.io/gh/huggingface/transformers/pull/1434/diff?src=pr&el=tree#diff-dHJhbnNmb3JtZXJzL21vZGVsaW5nX3hsbmV0LnB5) | `72.09% <100%> (-0.05%)` | :arrow_down: |
------
[Continue to review full report at Codecov](https://codecov.io/gh/huggingface/transformers/pull/1434?src=pr&el=continue).
> **Legend** - [Click here to learn more](https://docs.codecov.io/docs/codecov-delta)
> `Δ = absolute <relative> (impact)`, `ø = not affected`, `? = missing data`
> Powered by [Codecov](https://codecov.io/gh/huggingface/transformers/pull/1434?src=pr&el=footer). Last update [f3e0218...1dea291](https://codecov.io/gh/huggingface/transformers/pull/1434?src=pr&el=lastupdated). Read the [comment docs](https://docs.codecov.io/docs/pull-request-comments).
<|||||>Ok, thanks! |
transformers | 1,433 | closed | Fix some typos in README | This PR fixes some typos in README.md and overall makes it slightly more readable.
No code changes. | 10-06-2019 17:17:14 | 10-06-2019 17:17:14 | # [Codecov](https://codecov.io/gh/huggingface/transformers/pull/1433?src=pr&el=h1) Report
> Merging [#1433](https://codecov.io/gh/huggingface/transformers/pull/1433?src=pr&el=desc) into [master](https://codecov.io/gh/huggingface/transformers/commit/f3e0218fbb6bcc40b40f10089dae8876654edb23?src=pr&el=desc) will **not change** coverage.
> The diff coverage is `n/a`.
[](https://codecov.io/gh/huggingface/transformers/pull/1433?src=pr&el=tree)
```diff
@@ Coverage Diff @@
## master #1433 +/- ##
=======================================
Coverage 84.72% 84.72%
=======================================
Files 84 84
Lines 12591 12591
=======================================
Hits 10668 10668
Misses 1923 1923
```
------
[Continue to review full report at Codecov](https://codecov.io/gh/huggingface/transformers/pull/1433?src=pr&el=continue).
> **Legend** - [Click here to learn more](https://docs.codecov.io/docs/codecov-delta)
> `Δ = absolute <relative> (impact)`, `ø = not affected`, `? = missing data`
> Powered by [Codecov](https://codecov.io/gh/huggingface/transformers/pull/1433?src=pr&el=footer). Last update [f3e0218...85d7c84](https://codecov.io/gh/huggingface/transformers/pull/1433?src=pr&el=lastupdated). Read the [comment docs](https://docs.codecov.io/docs/pull-request-comments).
<|||||>Great, thanks for the update! |
transformers | 1,432 | closed | How to return bert self attention, so that i can do visualization?? | ## ❓ Questions & Help
model = BertForSequenceClassification.from_pretrained("bert-base-uncased", num_labels= 2, output_attentions=True)
model.cuda()
I am useing the above code to return the attention weights, for visualizing the attention by BertViz.
But it gave me this error ( __init__( ) got an unexpected keyword argument 'output_attentions').
Also, please if you recommend any tutorial for beginner, which explain how to return the attention
<!-- A clear and concise description of the question. -->
| 10-06-2019 14:52:51 | 10-06-2019 14:52:51 | Hi! Could you specify which version of our library you are using? Thank you.<|||||>Hi
I am useing "pip install pytorch-pretrained-bert pytorch-nlp"<|||||>I believe the way to output attentions in `pytorch-pretrained-BERT` v0.6.2 was to specify the `output_all_encoded_layers` to `True` in the model forward call.
Please be aware that this version has been deprecated for some time now. The new version is called `transformers` and should be installed with `pip install transformers`.<|||||>
Thank for the quick reply
how about if i want to use transformers how to output the attention ??<|||||>If you want to use transformers to output the attention you can specify it in the config:
```py
config = BertConfig.from_pretrained("bert-base-cased", output_attentions=True, num_labels=2)
model = BertForSequenceClassification.from_pretrained("bert-base-cased", config=config)
```<|||||>> I believe the way to output attentions in pytorch-pretrained-BERT v0.6.2 was to specify the output_all_encoded_layers to True in the model forward call.
> Please be aware that this version has been deprecated for some time now. The new version is called transformers and should be installed with pip install transformers.
Do you mean some things like this
# Forward pass
loss = model(b_input_ids, token_type_ids=None, attention_mask=b_input_mask, labels=b_labels, output_all_encoded_layers = True)<|||||>Yes, that’s what I meant!<|||||>> Yes, that’s what I meant!
I am getting this error
TypeError: forward() got an unexpected keyword argument 'output_all_encoded_layers'<|||||>> Yes, that’s what I meant!
I am getting this error. any idea please
TypeError: forward() got an unexpected keyword argument 'output_all_encoded_layers'
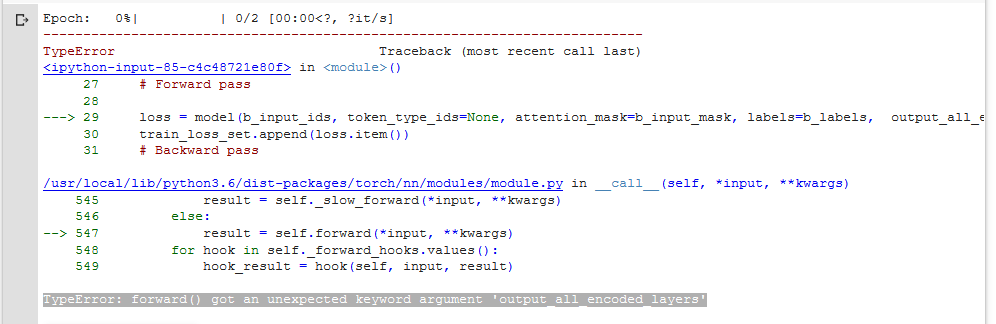
<|||||>Which version of the lib are you using in that example?<|||||>> Which version of the lib are you using in that example?
old one
pytorch-pretrained-bert pytorch-nlp<|||||>Is there any way you could update to `transformers`? That would make life easier.<|||||>This issue has been automatically marked as stale because it has not had recent activity. It will be closed if no further activity occurs. Thank you for your contributions.
|
transformers | 1,431 | closed | Fine-tune specific layers | Is there any easy way to fine-tune specific layers of the model instead of fine-tuning the complete model?
| 10-06-2019 10:48:13 | 10-06-2019 10:48:13 | In Pytorch or Tensorflow? If Pytorch, [this issue](https://github.com/huggingface/transformers/issues/400) might be of help.<|||||>In my scripts, I use the following code. Passing down a parameter 'freeze' (list) to the config that I use. All layers that start with any of the given strings will be frozen.
```python
# Freeze parts of pretrained model
# config['freeze'] can be "all" to freeze all layers,
# or any number of prefixes, e.g. ['embeddings', 'encoder']
if 'freeze' in config and config['freeze']:
for name, param in self.base_model.named_parameters():
if config['freeze'] == 'all' or 'all' in config['freeze'] or name.startswith(tuple(config['freeze'])):
param.requires_grad = False
logging.info(f"Froze layer {name}...")
```<|||||>Thanks. Your code works fine. I did the following:
```
if freeze_embeddings:
for param in list(model.bert.embeddings.parameters()):
param.requires_grad = False
print ("Froze Embedding Layer")
# freeze_layers is a string "1,2,3" representing layer number
if freeze_layers is not "":
layer_indexes = [int(x) for x in freeze_layers.split(",")]
for layer_idx in layer_indexes:
for param in list(model.bert.encoder.layer[layer_idx].parameters()):
param.requires_grad = False
print ("Froze Layer: ", layer_idx)
``` |
transformers | 1,430 | closed | AttributeError: 'BertOnlyMLMHead' object has no attribute 'bias' | ## ❓ Questions & Help
<!-- A clear and concise description of the question. -->
I was trying to load a RuBERT model from [DeepPavlov](http://docs.deeppavlov.ai/en/master/features/models/bert.html) but ran into this error. The model is in TensorFlow and the code I used to load it is:
```
config = BertConfig.from_json_file('rubert_cased_L-12_H-768_A-12_v2/bert_config.json')
model = BertForMaskedLM.from_pretrained('rubert_cased_L-12_H-768_A-12_v2/bert_model.ckpt.index', from_tf=True, config=config)
model.eval()
```
The error message is the following:
```
AttributeError Traceback (most recent call last)
<ipython-input-150-74d68b4b5d71> in <module>
1 config = BertConfig.from_json_file('rubert_cased_L-12_H-768_A-12_v2/bert_config.json')
----> 2 model = BertForMaskedLM.from_pretrained('rubert_cased_L-12_H-768_A-12_v2/bert_model.ckpt.index', from_tf=True, config=config)
3 model.eval()
c:\users\milin\appdata\local\programs\python\python36\lib\site-packages\transformers\modeling_utils.py in from_pretrained(cls, pretrained_model_name_or_path, *model_args, **kwargs)
352 if resolved_archive_file.endswith('.index'):
353 # Load from a TensorFlow 1.X checkpoint - provided by original authors
--> 354 model = cls.load_tf_weights(model, config, resolved_archive_file[:-6]) # Remove the '.index'
355 else:
356 # Load from our TensorFlow 2.0 checkpoints
c:\users\milin\appdata\local\programs\python\python36\lib\site-packages\transformers\modeling_bert.py in load_tf_weights_in_bert(model, config, tf_checkpoint_path)
90 pointer = getattr(pointer, 'weight')
91 elif l[0] == 'output_bias' or l[0] == 'beta':
---> 92 pointer = getattr(pointer, 'bias')
93 elif l[0] == 'output_weights':
94 pointer = getattr(pointer, 'weight')
c:\users\milin\appdata\local\programs\python\python36\lib\site-packages\torch\nn\modules\module.py in __getattr__(self, name)
533 return modules[name]
534 raise AttributeError("'{}' object has no attribute '{}'".format(
--> 535 type(self).__name__, name))
536
537 def __setattr__(self, name, value):
AttributeError: 'BertOnlyMLMHead' object has no attribute 'bias'
```
I've also tried to load the [official BERT models from Google](https://github.com/google-research/bert/blob/master/multilingual.md) and got the same result. | 10-06-2019 10:01:44 | 10-06-2019 10:01:44 | > ## ❓ Questions & Help
> I was trying to load a RuBERT model from [DeepPavlov](http://docs.deeppavlov.ai/en/master/features/models/bert.html) but ran into this error. The model is in TensorFlow and the code I used to load it is:
>
> ```
> config = BertConfig.from_json_file('rubert_cased_L-12_H-768_A-12_v2/bert_config.json')
> model = BertForMaskedLM.from_pretrained('rubert_cased_L-12_H-768_A-12_v2/bert_model.ckpt.index', from_tf=True, config=config)
> model.eval()
> ```
>
> The error message is the following:
>
> ```
> AttributeError Traceback (most recent call last)
> <ipython-input-150-74d68b4b5d71> in <module>
> 1 config = BertConfig.from_json_file('rubert_cased_L-12_H-768_A-12_v2/bert_config.json')
> ----> 2 model = BertForMaskedLM.from_pretrained('rubert_cased_L-12_H-768_A-12_v2/bert_model.ckpt.index', from_tf=True, config=config)
> 3 model.eval()
>
> c:\users\milin\appdata\local\programs\python\python36\lib\site-packages\transformers\modeling_utils.py in from_pretrained(cls, pretrained_model_name_or_path, *model_args, **kwargs)
> 352 if resolved_archive_file.endswith('.index'):
> 353 # Load from a TensorFlow 1.X checkpoint - provided by original authors
> --> 354 model = cls.load_tf_weights(model, config, resolved_archive_file[:-6]) # Remove the '.index'
> 355 else:
> 356 # Load from our TensorFlow 2.0 checkpoints
>
> c:\users\milin\appdata\local\programs\python\python36\lib\site-packages\transformers\modeling_bert.py in load_tf_weights_in_bert(model, config, tf_checkpoint_path)
> 90 pointer = getattr(pointer, 'weight')
> 91 elif l[0] == 'output_bias' or l[0] == 'beta':
> ---> 92 pointer = getattr(pointer, 'bias')
> 93 elif l[0] == 'output_weights':
> 94 pointer = getattr(pointer, 'weight')
>
> c:\users\milin\appdata\local\programs\python\python36\lib\site-packages\torch\nn\modules\module.py in __getattr__(self, name)
> 533 return modules[name]
> 534 raise AttributeError("'{}' object has no attribute '{}'".format(
> --> 535 type(self).__name__, name))
> 536
> 537 def __setattr__(self, name, value):
>
> AttributeError: 'BertOnlyMLMHead' object has no attribute 'bias'
> ```
>
> I've also tried to load the [official BERT models from Google](https://github.com/google-research/bert/blob/master/multilingual.md) and got the same result.
hey!
Have you solved this problem? I have the same problem!ROLAND JUNO-STAGE!<|||||> @lichunnan
After studying the manual more thoroughly, I found that you should [first convert the TensorFlow models to PyTorch](https://huggingface.co/transformers/converting_tensorflow_models.html) with [this script](https://github.com/huggingface/transformers/blob/master/transformers/convert_bert_original_tf_checkpoint_to_pytorch.py). |
transformers | 1,429 | closed | Checkpoint rotation | By default, no change in existing behavior. However, if you pass in an argument with `save_total_limit` flag and a natural number as value, then, your machine might not run out of space when fine-tuning. Because, it will only keep the latest `save_total_limit` number of checkpoints and delete the older checkpoints. | 10-06-2019 06:57:52 | 10-06-2019 06:57:52 | # [Codecov](https://codecov.io/gh/huggingface/transformers/pull/1429?src=pr&el=h1) Report
> Merging [#1429](https://codecov.io/gh/huggingface/transformers/pull/1429?src=pr&el=desc) into [master](https://codecov.io/gh/huggingface/transformers/commit/8fcc6507ce9d0922ddb60f4a31d4b9a839de1270?src=pr&el=desc) will **not change** coverage.
> The diff coverage is `n/a`.
[](https://codecov.io/gh/huggingface/transformers/pull/1429?src=pr&el=tree)
```diff
@@ Coverage Diff @@
## master #1429 +/- ##
=======================================
Coverage 84.72% 84.72%
=======================================
Files 84 84
Lines 12591 12591
=======================================
Hits 10668 10668
Misses 1923 1923
```
------
[Continue to review full report at Codecov](https://codecov.io/gh/huggingface/transformers/pull/1429?src=pr&el=continue).
> **Legend** - [Click here to learn more](https://docs.codecov.io/docs/codecov-delta)
> `Δ = absolute <relative> (impact)`, `ø = not affected`, `? = missing data`
> Powered by [Codecov](https://codecov.io/gh/huggingface/transformers/pull/1429?src=pr&el=footer). Last update [8fcc650...18c51b7](https://codecov.io/gh/huggingface/transformers/pull/1429?src=pr&el=lastupdated). Read the [comment docs](https://docs.codecov.io/docs/pull-request-comments).
<|||||>That's a nice addition, thanks! |
transformers | 1,428 | closed | Problem with word prediction with GPT2 | ## ❓ Questions & Help
I'm trying to understand how to obtain the probability of specific word predictions, but I am getting bad results. For example, according to the code below, the sequence "It seems that" is more likely followed by "ago" than by "we", which surely is not correct. What am I doing wrong?
```import sys
import torch
import numpy
from scipy.special import softmax
from pytorch_transformers import GPT2Config, GPT2Tokenizer, GPT2LMHeadModel
config = GPT2Config.from_pretrained('gpt2-medium')
tokenizer = GPT2Tokenizer.from_pretrained('gpt2-medium')
model = GPT2LMHeadModel(config)
item = "It seems that"
indexed_tokens = tokenizer.encode(item)
tokens_tensor = torch.tensor([indexed_tokens])
with torch.no_grad():
predictions = model(tokens_tensor)
results = predictions[0]
temp = results[0,-1,:]
temp = temp.numpy()
result = softmax(temp)
word_1 = tokenizer.encode('we')[0]
word_2 = tokenizer.encode('ago')[0]
print(result[word_1])
print(result[word_2])
```
This outputs:
1.0500242e-05
5.1639265e-05
But it should be the other way around (see [here](https://books.google.com/ngrams/graph?content=seems+that+ago%2C+seems+that+we&year_start=1800&year_end=2000&corpus=15&smoothing=3&share=&direct_url=t1%3B%2Cseems%20that%20we%3B%2Cc0)).
Thanks in advance. | 10-05-2019 23:34:10 | 10-05-2019 23:34:10 | Hi, indeed it should be the other way around! I believe it's due to a misconception, you're initializing your model as follows:
```py
config = GPT2Config.from_pretrained('gpt2-medium')
model = GPT2LMHeadModel(config)
```
However, as noted in the [documentation](https://huggingface.co/transformers/main_classes/configuration.html#transformers.PretrainedConfig): _A configuration file can be loaded and saved to disk. Loading the configuration file and using this file to initialize a model does not load the model weights. It only affects the model’s configuration._
In order to initialize the model weights as well, you should do:
```py
config = GPT2Config.from_pretrained('gpt2-medium')
model = GPT2LMHeadModel.from_pretrained("gpt2-medium", config=config)
```
or since you're loading the pre-trained configuration of the same pre-trained model, you could simply do:
```py
model = GPT2LMHeadModel.from_pretrained("gpt2-medium")
```
which already loads this configuration file.
Once you have done this change you should get as output:
```
1.248501e-06
3.727657e-09
```
which is more accurate :)<|||||>Yes! Thank you! I was looking for an error on the completely wrong place (must have re-written the latter part of the code about 5 different ways). |
transformers | 1,427 | closed | Replace TensorboardX with Pytorch's built in SummaryWriter | ## 🚀 Feature
Import `SummaryWriter` from `from torch.utils.tensorboard` instead of from `tensorboardX`
If you're interested, I can make a pull request to merge the changes that I made in my [fork](https://github.com/bkkaggle/transformers) into the main repository.
## Motivation
TensorboardX isn't needed anymore now that Pytorch 1.2 has been released. The relevant Pytorch docs are available [here](https://pytorch.org/docs/stable/tensorboard.html). | 10-05-2019 18:41:30 | 10-05-2019 18:41:30 | You cannot assume that suddenly _everyone_ is on 1.2. You'll need a fallback for people who are 1.x. Something like
```python
try:
from torch.utils.tensorboard import SummaryWriter
except AttributeError
from tensorboardX import SummaryWriter
```
That's a good way to 'ease into' a definite change.<|||||>Fixed.
I undid the commits removing tensorboardX from the requirements and added a try-except block around the imports to check if the user's pytorch version comes with tensorboard (It's 'experimental' in 1.1.0 and is now stable in 1.2.0).<|||||>I updated my fork to be in line with the recommendations for contributing in #1448
I created the pull request:<|||||>closing now that #1454 has been merged in |
transformers | 1,426 | closed | GPU Benchmarking + Accumulated Optimizer for TF2 | ## 🚀 Feature
- Create a GPU benchmarking section in Documentation (Wiki).
- Build and Include TF2 Optimizer with gradient accumulation.
```python
optimizer=AccumulatedOptimizer(Adam(lr=2e-5, clipnorm=1.0), accumulate_steps=4)
```
## Motivation
I experiment with transformers library on Tensorflow 2 for a week and what you offer to us the users seems really useful. It saves a ton of time and keeping us up-to-date with the latest advances on pre-trained models accelerate our research.
Although, this come with a great cost in computational resources (e.g., GPUs). For example the the use of BERT through Tensorflow Hub is much lighter than the one you offer in this great library. For example in a 12GB GPU (e.g., 1080Ti, RTX 2080Ti), someone can go up to batches of 8 of 512 tokens if he makes use of the Tensorflow Hub BERT-BASE module , while using transformers library will lead to 1/2 of the batch size (=4).
Based on the above facts I think we need three crucial things:
- An explanation on why this happens? It's seem weird to me that for a model with same parameters, we cannot go up to the same batching based on different implementations. I think understanding this mismatch is very interesting from a theoretical and practical point of view.
- A table with benchmarks given different GPUs, different batches, different max sequence
lengths. This will helps us find possible limitations and also find bugs in our code, if we do not meet the benchmarking.
- Given this limitation, I propose the release of a new TF2 optimizer that used gradient accumulation, thus we can go up to our batch size limit and use accumulation in order to avoid one back-propagation step per forward step.
| 10-05-2019 13:54:18 | 10-05-2019 13:54:18 | I would also like to see benchmarks, however this is a computationally heavy task. It might be useful to provide a benchmark script and a benchmark table. Contributors can then run the script on their available hardware, and add their results to the table - highlighting the used parameters and hardware.<|||||>Here's my findings from testing the Transformers library on a Titan V (12GB), which I'm also using to run my dual displays (about 400MB VRAM there).
The VRAM usage below is the VRAM allocated by TensorFlow, not necessarily the exact VRAM needed to run the model. TF tends to allocate VRAM in chunks once a smaller chunk is not sufficient. All experiments below use a token length of 512 tokens, and the same batch size for train and eval, and Adam optimizer. The script used is a minimally modified `run_tf_glue.py`.
| Batch Size | VRAM | Mixed Precision |
| ----------- | ---------- | ---------------- |
| 4 | 8723MB | No |
| 4 | 8723MB | Yes |
| 8 | 11265MB | No |
| 8 | 11265MB | Yes |
| 9 | 11265MB | No |
| 9 | 11265MB | Yes |
| 10 | OOM | No |
| 10 | OOM | Yes |
On 1080 Ti (11GB), I managed to run it at batch size 8, with VRAM usage of 10753MB.
From the results I got, one should be able to run at batch size 8 on a 2080 Ti (11GB), but I don't have a 2080 Ti to test. Worth nothing that you might not be able to run a display AND the training at the same time, as every last bit of VRAM seems to be required.
The script can be found [here](https://gist.github.com/tlkh/d252abcb3a5b59a7b8c47660997fd390#file-tf_run_glue-py).
I will test with the TF Hub BERT module at a later date if I have time, but from memory the VRAM usage seems to be similar.
cc @thomwolf who asked about it on #1441<|||||>Hi @tlkh, I was able to rerun your script `tf_run_glue.py` successfully in a 1080Ti. Then I tried to pass the core elements that affect GPU acceleration and optimization in my own code:
```python
gpus = tf.config.experimental.list_physical_devices('GPU')
if gpus:
for gpu in gpus:
tf.config.experimental.set_memory_growth(gpu, True)
USE_XLA = False
USE_AMP = False
tf.config.optimizer.set_jit(USE_XLA)
tf.config.optimizer.set_experimental_options({"auto_mixed_precision": USE_AMP})
# Compile t TFBertForSequenceClassification with Adam
# Load my data using a custom Keras Generator
# that yields a list of numpy ndarrays of shape: [(8,512), (8,512), (8,512)] to pass token_ids, mask_ids, segment_ids
# Call model.fit_generator(train_data=generator) on keras Model
```
This always lead me to an OOM error on specific steps of the network:
> tensorflow.python.framework.errors_impl.ResourceExhaustedError: OOM when allocating tensor with shape[8,12,512,512]
OR
> tensorflow.python.framework.errors_impl.ResourceExhaustedError: OOM when allocating tensor with shape[6,512,768]
OR
> tensorflow.python.framework.errors_impl.ResourceExhaustedError: OOM when allocating tensor with shape[6,512,3072]
All of them are internal functions/computations in transformers...
- Is there any reason to suspect that tf.Dataset objects are optimized compared to a keras Generator?
- I tried to activate XLA, but this leads to the following error:
> tensorflow.python.framework.errors_impl.NotFoundError: ./bin/ptxas not found
> [[{{node cluster_0_1/xla_compile}}]] [Op:__inference_call_6748]
and I can't find ptxas path on server....<|||||>> Is there any reason to suspect that tf.Dataset objects are optimized compared to a keras Generator?
I don't think the VRAM usage will defer, but tf.Dataset objects *should* be more optimized. I believe Keras Generators are supposed to be deprecated eventually.
> I tried to activate XLA
Your TensorFlow build needs to support XLA, although I believe it should be already built in by default.
If @iliaschalkidis don't mind sharing your code I could try running and see if I can replicate the problem.<|||||>@tlkh I just made this demo script that replicates the way I handle data and fit the model. In my server this script leads to OOM as well, as the actual project. I thought it's much easier than sharing the whole project, cover dependencies and load real datasets.
```python
import numpy as np
import tensorflow as tf
from transformers import TFBertForSequenceClassification
gpus = tf.config.experimental.list_physical_devices('GPU')
if gpus:
for gpu in gpus:
tf.config.experimental.set_memory_growth(gpu, True)
class SampleGenerator(tf.keras.utils.Sequence):
"""Generates data for Keras"""
def __len__(self):
# 10 batches of samples each
return 10
def __getitem__(self, index):
# Yield mock data batch
token_ids = np.zeros((8, 512), dtype=np.int32)
mask_ids = np.zeros((8, 512), dtype=np.int32)
segment_ids = np.zeros((8, 512), dtype=np.int32)
targets = np.zeros((8, 1000), dtype=np.int32)
return [token_ids, mask_ids, segment_ids], targets
# script parameters
BATCH_SIZE = 8
EVAL_BATCH_SIZE = BATCH_SIZE
USE_XLA = False
USE_AMP = False
tf.config.optimizer.set_jit(USE_XLA)
tf.config.optimizer.set_experimental_options({"auto_mixed_precision": USE_AMP})
# Load model from pretrained model/vocabulary
model = TFBertForSequenceClassification.from_pretrained('bert-base-uncased', num_labels=1000)
# Prepare datasets as Keras generators
train_generator = SampleGenerator()
val_generator = SampleGenerator()
# Prepare training: Compile tf.keras model with optimizer, loss and learning rate schedule
opt = tf.keras.optimizers.Adam(learning_rate=3e-5)
if USE_AMP:
# loss scaling is currently required when using mixed precision
opt = tf.keras.mixed_precision.experimental.LossScaleOptimizer(opt, 'dynamic')
model.compile(optimizer=opt, loss='categorical_crossentropy', metrics=['accuracy'])
model.fit_generator(generator=train_generator, epochs=2, validation_data=val_generator)
```<|||||>I replaced Keras generator with `tf.data.Dataset`:
```python
import numpy as np
import tensorflow as tf
from transformers import TFBertForSequenceClassification
gpus = tf.config.experimental.list_physical_devices('GPU')
if gpus:
for gpu in gpus:
tf.config.experimental.set_memory_growth(gpu, True)
# script parameters
BATCH_SIZE = 8
EVAL_BATCH_SIZE = BATCH_SIZE
USE_XLA = False
USE_AMP = False
tf.config.optimizer.set_jit(USE_XLA)
tf.config.optimizer.set_experimental_options({"auto_mixed_precision": USE_AMP})
# Load model from pretrained model/vocabulary
model = TFBertForSequenceClassification.from_pretrained('bert-base-uncased', num_labels=1000)
def gen():
for x1, x2, x3, y in zip(np.zeros((80, 512), dtype=np.int32),
np.zeros((80, 512), dtype=np.int32),
np.zeros((80, 512), dtype=np.int32),
np.zeros((80, 1000), dtype=np.int32)):
yield ({'input_ids': x1,
'attention_mask': x2,
'token_type_ids': x3}, y)
# Prepare dataset as tf.Dataset from generator
dataset = tf.data.Dataset.from_generator(gen,
({'input_ids': tf.int32,
'attention_mask': tf.int32,
'token_type_ids': tf.int32},
tf.int32),
({'input_ids': tf.TensorShape([None]),
'attention_mask': tf.TensorShape([None]),
'token_type_ids': tf.TensorShape([None])},
tf.TensorShape([None])))
train_dataset = dataset.shuffle(128).batch(BATCH_SIZE).repeat(-1)
# Prepare training: Compile tf.keras model with optimizer, loss and learning rate schedule
opt = tf.keras.optimizers.Adam(learning_rate=3e-5)
if USE_AMP:
# loss scaling is currently required when using mixed precision
opt = tf.keras.mixed_precision.experimental.LossScaleOptimizer(opt, 'dynamic')
model.compile(optimizer=opt, loss='categorical_crossentropy', metrics=['accuracy'])
# Train and evaluate using tf.keras.Model.fit()
model.fit(train_dataset, epochs=2, steps_per_epoch= 80//BATCH_SIZE)
```
The model know fits in the GPU... So it seems `tf.data.Dataset.from_generator()` is more memory efficient from Keras generators...<|||||>Interesting to know that the issue is Keras generators. Glad you have a way of running the model now!<|||||>> Interesting to know that the issue is Keras generators. Glad you have a way of running the model now!
Unfortunately, I still haven't. Because I need to refactor a lot aspects of my personal codebase in order to load the datasets in the same fashion as in this example... Let's hope this will not take more than a single day 😄 <|||||>This issue has been automatically marked as stale because it has not had recent activity. It will be closed if no further activity occurs. Thank you for your contributions.
|
transformers | 1,425 | closed | ELECTRA Model | ## 🚀 Feature
New Transformer based model: ELECTRA
## Motivation
Hi guys, did you see the following paper: https://openreview.net/forum?id=r1xMH1BtvB ? There is a new Transformer based model called ELECTRA that seems very interesting and promising. It would be very useful to have a implementation of the model in PyTorch.
## Additional context
Paper: https://openreview.net/forum?id=r1xMH1BtvB
| 10-04-2019 20:22:05 | 10-04-2019 20:22:05 | Hi @josecannete
Thanks for the tip! We are busy building other awesome things at the moment, but feel free to start a PR with a first draft and we will be happy to have a look at it 😄 <|||||>And note that it's probably better to wait for the author's original code and pretrained weights.<|||||>This issue has been automatically marked as stale because it has not had recent activity. It will be closed if no further activity occurs. Thank you for your contributions.
<|||||>Any news? Do you know if the code was released?<|||||>waiting...<|||||>The original code is available from here: https://github.com/google-research/electra<|||||>Anyone wants to give it a go? We can help!<|||||>We're on it! :hugs: <|||||>@LysandreJik I can help you with evaluating the model on downstream tasks to compare it with the original implementation - I'm currently training an ELECTRA model on GPU, so I'm highly interested in using it with Transformers 😅<|||||>@LysandreJik If it helps, I believe ELECTRA weights are drop-in replacements into the BERT codebase except we do not use a pooler layer and just take the final [CLS] hidden state for sentence representations.<|||||>waiting...+10086<|||||>Since v2.8.0 ELECTRA is in the library :)<|||||>@LysandreJik Is pretraining of Electra from scratch support available now?<|||||>Using default scripts `run_language_modeling.py`?<|||||>Hi, I'm trying to fine tune ELECTRA large discriminator for a downstream classification task. I took the [CLS] at the last hidden state as the sentence representation like some Autoencoding pretrained LM (BERT, RoBERTa,...). Is that right?
Just because my results are not stable. |
transformers | 1,424 | closed | Training on GLUE using TPUs | **_Disclaimer: This pull request is under active development and is being improved daily._**
This pull request aims to train a BERT model on GLUE, using a TPU. Several approaches are tested: keras' fit method (doesn't work yet), and a custom training loop using TPUStrategy.
The custom training loop currently worked until the 2nd of October on the `tf-nightly-2.0-preview`. The TPU should be `nightly` too. | 10-04-2019 18:24:55 | 10-04-2019 18:24:55 | # [Codecov](https://codecov.io/gh/huggingface/transformers/pull/1424?src=pr&el=h1) Report
> Merging [#1424](https://codecov.io/gh/huggingface/transformers/pull/1424?src=pr&el=desc) into [master](https://codecov.io/gh/huggingface/transformers/commit/b3cfd979460d6ff828741eddffc72c34417b5046?src=pr&el=desc) will **decrease** coverage by `0.05%`.
> The diff coverage is `50%`.
[](https://codecov.io/gh/huggingface/transformers/pull/1424?src=pr&el=tree)
```diff
@@ Coverage Diff @@
## master #1424 +/- ##
==========================================
- Coverage 84.72% 84.67% -0.06%
==========================================
Files 84 84
Lines 12591 12600 +9
==========================================
+ Hits 10668 10669 +1
- Misses 1923 1931 +8
```
| [Impacted Files](https://codecov.io/gh/huggingface/transformers/pull/1424?src=pr&el=tree) | Coverage Δ | |
|---|---|---|
| [transformers/modeling\_tf\_bert.py](https://codecov.io/gh/huggingface/transformers/pull/1424/diff?src=pr&el=tree#diff-dHJhbnNmb3JtZXJzL21vZGVsaW5nX3RmX2JlcnQucHk=) | `95.03% <50%> (-0.67%)` | :arrow_down: |
| [transformers/tokenization\_utils.py](https://codecov.io/gh/huggingface/transformers/pull/1424/diff?src=pr&el=tree#diff-dHJhbnNmb3JtZXJzL3Rva2VuaXphdGlvbl91dGlscy5weQ==) | `90.19% <0%> (-1.3%)` | :arrow_down: |
| [transformers/modeling\_gpt2.py](https://codecov.io/gh/huggingface/transformers/pull/1424/diff?src=pr&el=tree#diff-dHJhbnNmb3JtZXJzL21vZGVsaW5nX2dwdDIucHk=) | `83.98% <0%> (ø)` | :arrow_up: |
| [transformers/configuration\_gpt2.py](https://codecov.io/gh/huggingface/transformers/pull/1424/diff?src=pr&el=tree#diff-dHJhbnNmb3JtZXJzL2NvbmZpZ3VyYXRpb25fZ3B0Mi5weQ==) | `88.63% <0%> (ø)` | :arrow_up: |
| [transformers/modeling\_tf\_distilbert.py](https://codecov.io/gh/huggingface/transformers/pull/1424/diff?src=pr&el=tree#diff-dHJhbnNmb3JtZXJzL21vZGVsaW5nX3RmX2Rpc3RpbGJlcnQucHk=) | `96.61% <0%> (ø)` | :arrow_up: |
| [transformers/modeling\_tf\_gpt2.py](https://codecov.io/gh/huggingface/transformers/pull/1424/diff?src=pr&el=tree#diff-dHJhbnNmb3JtZXJzL21vZGVsaW5nX3RmX2dwdDIucHk=) | `93.47% <0%> (ø)` | :arrow_up: |
| [transformers/tokenization\_gpt2.py](https://codecov.io/gh/huggingface/transformers/pull/1424/diff?src=pr&el=tree#diff-dHJhbnNmb3JtZXJzL3Rva2VuaXphdGlvbl9ncHQyLnB5) | `96.72% <0%> (ø)` | :arrow_up: |
| [transformers/modeling\_utils.py](https://codecov.io/gh/huggingface/transformers/pull/1424/diff?src=pr&el=tree#diff-dHJhbnNmb3JtZXJzL21vZGVsaW5nX3V0aWxzLnB5) | `92.69% <0%> (+0.24%)` | :arrow_up: |
------
[Continue to review full report at Codecov](https://codecov.io/gh/huggingface/transformers/pull/1424?src=pr&el=continue).
> **Legend** - [Click here to learn more](https://docs.codecov.io/docs/codecov-delta)
> `Δ = absolute <relative> (impact)`, `ø = not affected`, `? = missing data`
> Powered by [Codecov](https://codecov.io/gh/huggingface/transformers/pull/1424?src=pr&el=footer). Last update [b3cfd97...111bf7c](https://codecov.io/gh/huggingface/transformers/pull/1424?src=pr&el=lastupdated). Read the [comment docs](https://docs.codecov.io/docs/pull-request-comments).
<|||||>Tensorflow official team have implemented this, I have tested it in TPU with tf-nightly vesion, it works well now, you can refer to https://github.com/tensorflow/models/tree/master/official/nlp |
transformers | 1,423 | closed | Problem loading trained keras model | I'm running the following line of code:
```
model = TFBertForSequenceClassification.from_pretrained(model_dir, num_labels=len(labels))
```
where model_dir is a directory containing a tf_model.h5 and a config.json file that was exported using the .save_pretrained() method.
However I get the following error shown below:
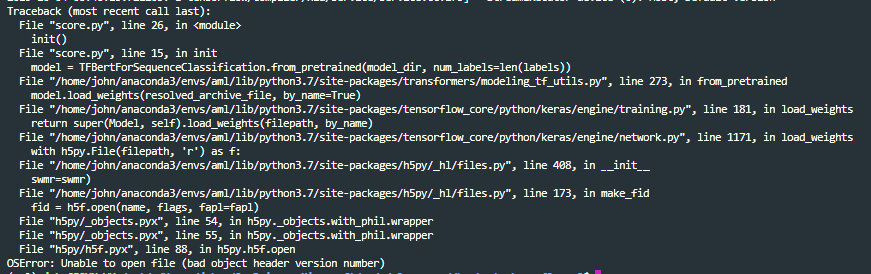
Could someone help here?
| 10-04-2019 16:05:17 | 10-04-2019 16:05:17 | It seems to me like your file is corrupted 😕<|||||>You can refresh the file in the cache with the `force_download` option (`model.from_pretrained(shortcut_name, force_download=True)`)<|||||>I think this worked @thomwolf, thanks!<|||||>Bringing this back up because it seems like the corrupted file actually happens even when it isn't in the cache. It seems like every 2/3 runs using .save_pretrained() results in a corrupted file for some reason.<|||||>Not sure we can do much about this here, we are just calling `tf.keras.Model.save_weights()` for that. Maybe ask upstream in the TensorFlow issues?<|||||>This issue has been automatically marked as stale because it has not had recent activity. It will be closed if no further activity occurs. Thank you for your contributions.
|
transformers | 1,422 | closed | Option to upload a trained model from gpt-2-simple to use with Write With Transformer | ## 🚀 Feature
I would like to be able to upload model checkpoints created in GPT-2-simple to use with Write with Transformer.
## Motivation
It would be really fun and allow people to use their own checkpoints without having to get them approved or anything or make them public.
## Additional context
none | 10-03-2019 23:15:01 | 10-03-2019 23:15:01 | That's on the long term horizon, but that'd be a cool feature, indeed. We are working on a way to let users of `🤗/transformers` upload their weights to share them with the community super easily.
Once we ship this, it would be doable to also host some of those on Write With Transformer. (with some *interesting* challenges on how to scale our infra to host lots of concurrent models, cc @LysandreJik :)<|||||>I'm training GPT-2 on all the Harry Potter books and I'd really love to play with it in Write With Transformer if you guys wanted to put it up, lol. (I know it's a really really small dataset but it's just for fun)<|||||>@torakoneko No ETA yet, but things are progressing on the aforementioned roadmap.<|||||>@julien-c Would it be possible to run it locally or on something like RunwayML with one's own checkpoint?<|||||>This issue has been automatically marked as stale because it has not had recent activity. It will be closed if no further activity occurs. Thank you for your contributions.
|
transformers | 1,421 | closed | Rbert - follow-up to #1301 - more robust configuration class loading | This PR update #1301 as discussed in the thread of #1308.
The configuration classes are updated to be more robust to the addition of new parameters (load defaults value first and then update with pretrained configuration if needed).
This incorporates the entity token ids directly in `BertConfig`.
cc @RichJackson | 10-03-2019 22:23:34 | 10-03-2019 22:23:34 | # [Codecov](https://codecov.io/gh/huggingface/transformers/pull/1421?src=pr&el=h1) Report
> :exclamation: No coverage uploaded for pull request base (`master@ecc4f1b`). [Click here to learn what that means](https://docs.codecov.io/docs/error-reference#section-missing-base-commit).
> The diff coverage is `97.95%`.
[](https://codecov.io/gh/huggingface/transformers/pull/1421?src=pr&el=tree)
```diff
@@ Coverage Diff @@
## master #1421 +/- ##
========================================
Coverage ? 84.8%
========================================
Files ? 84
Lines ? 12711
Branches ? 0
========================================
Hits ? 10779
Misses ? 1932
Partials ? 0
```
| [Impacted Files](https://codecov.io/gh/huggingface/transformers/pull/1421?src=pr&el=tree) | Coverage Δ | |
|---|---|---|
| [transformers/configuration\_distilbert.py](https://codecov.io/gh/huggingface/transformers/pull/1421/diff?src=pr&el=tree#diff-dHJhbnNmb3JtZXJzL2NvbmZpZ3VyYXRpb25fZGlzdGlsYmVydC5weQ==) | `89.74% <100%> (ø)` | |
| [transformers/configuration\_bert.py](https://codecov.io/gh/huggingface/transformers/pull/1421/diff?src=pr&el=tree#diff-dHJhbnNmb3JtZXJzL2NvbmZpZ3VyYXRpb25fYmVydC5weQ==) | `87.87% <100%> (ø)` | |
| [transformers/configuration\_gpt2.py](https://codecov.io/gh/huggingface/transformers/pull/1421/diff?src=pr&el=tree#diff-dHJhbnNmb3JtZXJzL2NvbmZpZ3VyYXRpb25fZ3B0Mi5weQ==) | `88.63% <100%> (ø)` | |
| [transformers/tests/modeling\_roberta\_test.py](https://codecov.io/gh/huggingface/transformers/pull/1421/diff?src=pr&el=tree#diff-dHJhbnNmb3JtZXJzL3Rlc3RzL21vZGVsaW5nX3JvYmVydGFfdGVzdC5weQ==) | `82.14% <100%> (ø)` | |
| [transformers/tests/modeling\_bert\_test.py](https://codecov.io/gh/huggingface/transformers/pull/1421/diff?src=pr&el=tree#diff-dHJhbnNmb3JtZXJzL3Rlc3RzL21vZGVsaW5nX2JlcnRfdGVzdC5weQ==) | `96.73% <100%> (ø)` | |
| [transformers/configuration\_openai.py](https://codecov.io/gh/huggingface/transformers/pull/1421/diff?src=pr&el=tree#diff-dHJhbnNmb3JtZXJzL2NvbmZpZ3VyYXRpb25fb3BlbmFpLnB5) | `89.13% <100%> (ø)` | |
| [transformers/configuration\_xlm.py](https://codecov.io/gh/huggingface/transformers/pull/1421/diff?src=pr&el=tree#diff-dHJhbnNmb3JtZXJzL2NvbmZpZ3VyYXRpb25feGxtLnB5) | `93.33% <100%> (ø)` | |
| [transformers/modeling\_roberta.py](https://codecov.io/gh/huggingface/transformers/pull/1421/diff?src=pr&el=tree#diff-dHJhbnNmb3JtZXJzL21vZGVsaW5nX3JvYmVydGEucHk=) | `74.39% <92.3%> (ø)` | |
| [transformers/modeling\_bert.py](https://codecov.io/gh/huggingface/transformers/pull/1421/diff?src=pr&el=tree#diff-dHJhbnNmb3JtZXJzL21vZGVsaW5nX2JlcnQucHk=) | `88.92% <96.15%> (ø)` | |
| [transformers/configuration\_xlnet.py](https://codecov.io/gh/huggingface/transformers/pull/1421/diff?src=pr&el=tree#diff-dHJhbnNmb3JtZXJzL2NvbmZpZ3VyYXRpb25feGxuZXQucHk=) | `91.22% <96.42%> (ø)` | |
------
[Continue to review full report at Codecov](https://codecov.io/gh/huggingface/transformers/pull/1421?src=pr&el=continue).
> **Legend** - [Click here to learn more](https://docs.codecov.io/docs/codecov-delta)
> `Δ = absolute <relative> (impact)`, `ø = not affected`, `? = missing data`
> Powered by [Codecov](https://codecov.io/gh/huggingface/transformers/pull/1421?src=pr&el=footer). Last update [ecc4f1b...ee0a99d](https://codecov.io/gh/huggingface/transformers/pull/1421?src=pr&el=lastupdated). Read the [comment docs](https://docs.codecov.io/docs/pull-request-comments).
<|||||>@thomwolf Is it possible to apply the changes on the TFxx classes as well?<|||||>hi @thomwolf anything I can do to help?<|||||>Thanks for the heads up. This one slipped out of my mind.
It's ready to merge I think (won't have time to do the TF conversion of the head).
Ok to merge @LysandreJik?<|||||>This actually needs deeper investigations to work with the new `input_embeds` inputs on master (skipping `input_ids`).<|||||>This issue has been automatically marked as stale because it has not had recent activity. It will be closed if no further activity occurs. Thank you for your contributions.
|
transformers | 1,420 | closed | ALBERT Model Incoming? | ALBERT: https://arxiv.org/abs/1909.11942v1 was just released. Are there plans to implement this in Transformers? | 10-03-2019 18:57:34 | 10-03-2019 18:57:34 | Duplicate of #1370 |
transformers | 1,419 | closed | question for one parameter matrix in transformers/GPT2 | ## ❓ Questions & Help
<!-- A clear and concise description of the question. -->
In transformers/gpt-2 model, there's a weight matrix called "transformer.h.0.attn.bias" whose size is torch.Size([1, 1, 1024, 1024]). I checked the original paper but still get confused by what it is for?
This parameter matrix is between the Layernomalization layer and the attention layer. Can anyone explain that? Thank you in advance.
| 10-03-2019 18:02:43 | 10-03-2019 18:02:43 | I dont think something like that is there, please have a look. Or paste everything from h.0 as it is here. Then it will be easy.<|||||>> I dont think something like that is there, please have a look. Or paste everything from h.0 as it is here. Then it will be easy.
Hi, thank you for your reply. Here's the parameter list from embedding layer to the first decoder layer.
['transformer.wte.weight', 'transformer.wpe.weight', 'transformer.h.0.ln_1.weight', 'transformer.h.0.ln_1.bias', 'transformer.h.0.attn.bias', 'transformer.h.0.attn.c_attn.weight', 'transformer.h.0.attn.c_attn.bias', 'transformer.h.0.attn.c_proj.weight', 'transformer.h.0.attn.c_proj.bias', 'transformer.h.0.ln_2.weight', 'transformer.h.0.ln_2.bias', 'transformer.h.0.mlp.c_fc.weight', 'transformer.h.0.mlp.c_fc.bias', 'transformer.h.0.mlp.c_proj.weight', 'transformer.h.0.mlp.c_proj.bias']
The fifth item is 'transformer.h.0.attn.bias'. I guess it may be some random noise but I can't find any reference for that.<|||||>Hi! Indeed there is a `bias` item in the attention layer. The name is probably not as accurate as it could be, as it does not represent a bias but a triangular matrix that is used when computing the attention score.
As a causal language model, GPT-2 should only look at its left context. This triangular matrix makes sure that the values on the right of the focused token are set to zero so that they do not affect the resulting attention score.
You don't need to worry about this matrix as the model initializes it on its own :).<|||||>> Hi! Indeed there is a `bias` item in the attention layer. The name is probably not as accurate as it could be, as it does not represent a bias but a triangular matrix that is used when computing the attention score.
>
> As a causal language model, GPT-2 should only look at its left context. This triangular matrix makes sure that the values on the right of the focused token are set to zero so that they do not affect the resulting attention score.
>
> You don't need to worry about this matrix as the model initializes it on its own :).
Hi, thank you for your reply. So the 'attn.bias' is actually used as the masking matrix for attention here. That's why its size is 1024, which is the length of the context in gpt2. Hope this can help others! |
transformers | 1,418 | closed | DistillBert Documentation Code Example fixes | Following code examples in the documentation are throwing errors:-
1. [DistilBertForQuestionAnswering](https://huggingface.co/transformers/model_doc/distilbert.html#transformers.DistilBertForQuestionAnswering)
```
tokenizer = DistilBertTokenizer.from_pretrained('distilbert-base-uncased')
model = DistilBertForQuestionAnswering.from_pretrained('distilbert-base-uncased')
input_ids = torch.tensor(tokenizer.encode("Hello, my dog is cute")).unsqueeze(0) # Batch size 1
start_positions = torch.tensor([1])
end_positions = torch.tensor([3])
outputs = model(input_ids, start_positions=start_positions, end_positions=end_positions)
loss, start_scores, end_scores = outputs[:2]
```
> ValueError: not enough values to unpack (expected 3, got 2)
2. [TFDistilBertForMaskedLM](https://huggingface.co/transformers/model_doc/distilbert.html#transformers.TFDistilBertForMaskedLM)
```
import tensorflow as tf
from transformers import DistilBertTokenizer, TFDistilBertForMaskedLM
tokenizer = DistilBertTokenizer.from_pretrained('distilbert-base-uncased')
model = TFDistilBertForMaskedLM.from_pretrained('distilbert-base-uncased')
input_ids = tf.constant(tokenizer.encode("Hello, my dog is cute"))[None, :] # Batch size 1
outputs = model(input_ids, masked_lm_labels=input_ids)
prediction_scores = outputs[0]
```
> TypeError: call() got an unexpected keyword argument 'masked_lm_labels'
3. [TFDistilBertForQuestionAnswering](https://huggingface.co/transformers/model_doc/distilbert.html#transformers.TFDistilBertForQuestionAnswering)
```
import tensorflow as tf
from transformers import BertTokenizer, TFDistilBertForQuestionAnswering
tokenizer = DistilBertTokenizer.from_pretrained('distilbert-base-uncased')
model = TFDistilBertForQuestionAnswering.from_pretrained('distilbert-base-uncased')
input_ids = tf.constant(tokenizer.encode("Hello, my dog is cute"))[None, :] # Batch size 1
start_positions = tf.constant([1])
end_positions = tf.constant([3])
outputs = model(input_ids, start_positions=start_positions, end_positions=end_positions)
start_scores, end_scores = outputs[:2]
```
> TypeError: call() got an unexpected keyword argument 'start_positions'
The first issue is just list indexing issue. Second and Third are due to implementation difference between Tensorflow and Pytorch DistillBERT. Tensorflow implementation doesn't have loss calculation inside `call`, but We do in `forward` for Pytorch. I have updated code examples in the docstring.
Let me know if you will be interested in a pull request for making same function API structure for Tensorflow implementation via adding loss calculation in `call function` similar to Pytorch.
This is my first issue. Let me know if you require any changes on pull request.
Regards 😃
Dharmendra | 10-03-2019 16:30:20 | 10-03-2019 16:30:20 | Indeed, thanks for the PR @drc10723 ! |
transformers | 1,417 | closed | How to replicate Arxiv-NLP but for different subject? | ## ❓ Questions & Help
<!-- A clear and concise description of the question. -->
Hi, I'm fairly new to NLP so apologies for my ignorance on some things.
If I wanted to fine tune text generation on a subject matter ( like Harry Potter), how would I do that?
Im looking to use XLNET and it seems like there isn't any support for fine tuning for that model. | 10-03-2019 15:27:36 | 10-03-2019 15:27:36 | This issue has been automatically marked as stale because it has not had recent activity. It will be closed if no further activity occurs. Thank you for your contributions.
|
transformers | 1,416 | closed | How to install transformers with pytorch only? | ## ❓ Questions & Help
Hi! Pytorch1.0 is installed and I'm installing the transformers with pip, everything is fine. But when I try:
```
import torch
from transformers import BertModel
```
then, an error occurred:
```
Traceback (most recent call last):
File "<stdin>", line 1, in <module>
File "/home/pc/miniconda3/lib/python3.7/site-packages/transformers/__init__.py", line 20, in <module>
from .file_utils import (TRANSFORMERS_CACHE, PYTORCH_TRANSFORMERS_CACHE, PYTORCH_PRETRAINED_BERT_CACHE,
File "/home/pc/miniconda3/lib/python3.7/site-packages/transformers/file_utils.py", line 30, in <module>
assert int(tf.__version__[0]) >= 2
AttributeError: module 'tensorflow' has no attribute '__version__'
```
It seems like it cannot work unless both the tensorflow and pytorch have been installed, is that right? And is there a way to run transformers with pytorch only? (I don't want to install tensorflow)
Thanks in advance! | 10-03-2019 15:19:44 | 10-03-2019 15:19:44 | Hi! No, you should be able to import every torch-related model without having TensorFlow installed.
As I understand it, our method for identifying if you had TensorFlow 2.0 installed broke because the TensorFlow version you have in your environment does not have the attribute `__version__`.
Could you provide the TensorFlow version you have installed so that we may patch this bug? In the meantime, uninstalling TensorFlow from this environment or creating a new environment without this TensorFlow version should work fine. Thanks.<|||||>Thanks a lot! Now I got it, I think there is something wrong with my miniconda, because there is a build-in incomplete tensorflow, which has no version, no functions,...nothing but a box. My conda version is 4.7.11. As I cannot uninstall the incomplete version of tensorflow, I just install the latest tensorflow and leave it there, not it works fine with pytorch. Cheers! |
transformers | 1,415 | closed | run_glue.py - Import Error | ## 🐛 Bug
<!-- Important information -->
Model I am using (Bert, XLNet....): XLNET (from example/run_glue.py)
The problem arise when using:
* [ ] the official example scripts: run_glue.py
- Stacktrace:
Traceback (most recent call last):
File "run_glue.py", line 49, in <module>
from transformers import glue_compute_metrics as compute_metrics
ImportError: cannot import name 'glue_compute_metrics'
The tasks I am working on is:
* [ ] an official GLUE/SQUaD task: MNLI
## To Reproduce
Steps to reproduce the behavior:
1. Execute run_glue.py after install requirements.
## Environment
* OS: Linux - Ubuntu
* Python version: 3.6
* PyTorch version: 1.2.0
* PyTorch Transformers version (or branch): tag 2.0.0
## Additional context
I found that some scripts related to glue tasks were not in *transformer* directory, which causes the import problem.But I really don't know if it may be a project setup issue or the files that contains glue utility code should be in */transformer* dir instead of */transformer/data/metrics*. | 10-03-2019 15:06:57 | 10-03-2019 15:06:57 | Hi! You may have seen this warning when importing from our library: `To use data.metrics please install scikit-learn. See https://scikit-learn.org/stable/index.html`.
Do you have this issue even with scikit-learn installed?<|||||>Hi @LysandreJik ,
Thanks for the answer. I already have scikit-learn but actually I'm working using a conda environment with *transformer* package installed using conda pip.
However, I solved this issue exporting the *PYTHONNOUSERSITE=1*, which enabled the scikit-learn installed in my conda environment. I discovered this problem because some stacktraces were pointing to files contained in local packages instead of my conda environment packages.
I'll close this issue. Thanks for the explanation.
*OBS*: Do you guys intend to publish a conda package of transformers?
<|||||>I face the exact same issue, even though `scikit-learn` is installed. The steps to reproduce are exactly identical, and I built from source.
I tried the `PYTHONNOUSERSITE=1` solution, but that does not change things because I can already import `sklearn` from the shell, and all required packages are in the conda environment.
Repeating the stack trace
```
Traceback (most recent call last):
File "<stdin>", line 1, in <module>
ImportError: cannot import name 'glue_compute_metrics' from 'transformers' (path/to/anaconda3/envs/nlp/lib/python3.7/site-packages/transformers/__init__.py)
```
# Environment
- OS: `Springdale Linux 7.7 (Verona)`
- Python version: `3.7.6`
- PyTorch version: `1.3.1`
- PyTorch Transformers version (or branch): `2.4.1`
> Hi! You may have seen this warning when importing from our library: `To use data.metrics please install scikit-learn. See https://scikit-learn.org/stable/index.html`.
>
> Do you have this issue even with scikit-learn installed?
> Yes :(<|||||>I fixed it by downgrading the python from 3.7.7 to 3.7.0:
```
conda install python=3.7.0
```<|||||>fixed for me by adding "import sklearn" to run_glue.py before the imports from transformers. |
transformers | 1,414 | closed | Instruction for Using XLM Text Generations | ## ❓ Questions & Help
<!-- A clear and concise description of the question. -->
Hi, I've reviewed every file in the document. I couldn't find an instruction to use **XLM** for text generation. What I really want to do is use a pre-trained **XLM** model for **English** text generation and examine the results. Then I would like to train a model or use pre-trained model for text generation in my language , which is **Turkish** , to examine the results .
How do I perform these operations step-by-step?
| 10-03-2019 13:23:10 | 10-03-2019 13:23:10 | I am working on similar situation. If anyone solves this problem please help me<|||||>It is really hard issue in my project. I can’t find anything as helpful about it and I really need this. I spent on this problem hours and hours. I found several resources but they didn’t have enough information. Please help us that title.<|||||>Hello! Thanks for opening this issue. As XLM indeed works slightly differently than other models, I have added it to the `run_generation.py` script.
Here's the difference from the other models: as a multilingual model, you can specify which language should be used when generating text. In order to do so, you should specify a language embedding during generation. Here's the way to do that:
Let's say we're using the pre-trained checkpoint `xlm-clm-1024-enfr`, which has two languages: English and French.
```py
import torch
from transformers import XLMTokenizer, XLMWithLMHeadModel
tokenizer = XLMTokenizer.from_pretrained("xlm-clm-1024-enfr")
```
You can see the different languages this tokenizer handles, as well as the ids of these languages using the `lang2id` attribute:
```py
print(tokenizer.lang2id) # {'en': 0, 'fr': 1}
```
These ids should be used when passing a language parameter during a model pass. Let's define our inputs:
```py
input_ids = torch.tensor([tokenizer.encode("Wikipedia was used to")]) # batch size of 1
```
We should now define the language embedding by using the previously defined language id. We want to create a tensor filled with the appropriate language ids, of the same size as `input_ids`. For english, the id is `0`:
```py
language_id = tokenizer.lang2id['en'] # 0
langs = torch.tensor([language_id] * input_ids.shape[1]) # torch.tensor([0, 0, 0, ..., 0])
# We reshape it to be of size (batch_size, sequence_length)
langs = langs.view(1, -1) # is now of shape [1, sequence_length] (we have a batch size of 1)
```
You can then feed it all as input to your model:
```py
outputs = model(input_ids, langs=langs)
```
You can see all of this implemented in the [`run_generation.py`](https://github.com/huggingface/transformers/blob/master/examples/run_generation.py) script, and how to decode the results. I hope this clears things up<|||||>Please also note that for accurate generation, only the `clm` models should be used. I believe the `mlm` could also be used, but would output worse text generation.
Furthermore, the `langs` value I explained works for the models that have `use_lang_emb` set to `True`. This is not the case for the 17 languages and 100 languages models.<|||||>I can't tell you how grateful I am for your answer and for updating the `run_generations` file. But I have one little problem.
I ran the model in the Colab environment with the following entry:
```
!python run_generation.py \
--model_type=xlm \
--model_name_or_path=xlm-clm-enfr-1024
```
Code gave me this error:
```
10/05/2019 20:17:55 - INFO - transformers.tokenization_utils - loading file https://s3.amazonaws.com/models.huggingface.co/bert/xlm-clm-enfr-1024-vocab.json from cache at /root/.cache/torch/transformers/e6f5fa1cd0da83c700ab5b38483774463b599ee8f73d995e6779dcd5f2777e84.892e5b45d85e254d5a121ca6986484acd0cf78f26b2d377b89be3771422779b6
10/05/2019 20:17:55 - INFO - transformers.tokenization_utils - loading file https://s3.amazonaws.com/models.huggingface.co/bert/xlm-mlm-enfr-1024-merges.txt from cache at /root/.cache/torch/transformers/6fcd506cac607ea4adeb88dddc38fef209ebeb4b2355132d43dc63b76863b81e.9da5d5f88a7619d42b4a6cc26c9bfd7c2186d3f0c3a1563b9d8176c58b44a745
10/05/2019 20:17:56 - INFO - transformers.configuration_utils - loading configuration file https://s3.amazonaws.com/models.huggingface.co/bert/xlm-clm-enfr-1024-config.json from cache at /root/.cache/torch/transformers/fbf61a111106c863e3566853bb101241339254ea07d761d4ba9d19642bcf471f.ba7ab938fe4de8fa5f7d97ad12ed8d5dfe6dc702abc18c55e9bd29db21fc7b8c
10/05/2019 20:17:56 - INFO - transformers.configuration_utils - Model config {
"asm": false,
"attention_dropout": 0.1,
"bos_index": 0,
"causal": false,
"dropout": 0.1,
"emb_dim": 1024,
"embed_init_std": 0.02209708691207961,
"end_n_top": 5,
"eos_index": 1,
"finetuning_task": null,
"gelu_activation": true,
"id2lang": {
"0": "en",
"1": "fr"
},
"init_std": 0.02,
"is_encoder": true,
"lang2id": {
"en": 0,
"fr": 1
},
"layer_norm_eps": 1e-12,
"mask_index": 5,
"max_position_embeddings": 512,
"max_vocab": -1,
"min_count": 0,
"n_heads": 8,
"n_langs": 2,
"n_layers": 6,
"n_words": 64139,
"num_labels": 2,
"output_attentions": false,
"output_hidden_states": false,
"pad_index": 2,
"pruned_heads": {},
"same_enc_dec": true,
"share_inout_emb": true,
"sinusoidal_embeddings": false,
"start_n_top": 5,
"summary_activation": null,
"summary_first_dropout": 0.1,
"summary_proj_to_labels": true,
"summary_type": "first",
"summary_use_proj": true,
"torchscript": false,
"unk_index": 3,
"use_bfloat16": false,
"use_lang_emb": true
}
10/05/2019 20:17:56 - INFO - transformers.modeling_utils - loading weights file https://s3.amazonaws.com/models.huggingface.co/bert/xlm-clm-enfr-1024-pytorch_model.bin from cache at /root/.cache/torch/transformers/bb34c23dd1c8c4a03862aa4347291a7bd0a405511ab9e6ac05c53ede177c2d09.ddfff42a040dae9a73f7b93c30f1b0a72bad65fa82637f63ab38ac9ed1bc425c
Namespace(device=device(type='cuda'), length=20, model_name_or_path='xlm-clm-enfr-1024', model_type='xlm', n_gpu=1, no_cuda=False, padding_text='', prompt='', seed=42, stop_token=None, temperature=1.0, top_k=0, top_p=0.9, xlm_lang='')
Using XLM. Select language in ['en', 'fr'] >>> en
Model prompt >>> Today is a nice day
0% 0/20 [00:00<?, ?it/s]Printing Inputs {'input_ids': tensor([[ 497, 29, 17, 3370, 206]], device='cuda:0'), 'langs': tensor([[0, 0, 0, 0, 0]])}
Traceback (most recent call last):
File "run_generation.py", line 220, in <module>
main()
File "run_generation.py", line 206, in main
device=args.device,
File "run_generation.py", line 130, in sample_sequence
outputs = model(**inputs) # Note: we could also use 'past' with GPT-2/Transfo-XL/XLNet (cached hidden-states)
File "/usr/local/lib/python3.6/dist-packages/torch/nn/modules/module.py", line 547, in __call__
result = self.forward(*input, **kwargs)
File "/usr/local/lib/python3.6/dist-packages/transformers/modeling_xlm.py", line 637, in forward
head_mask=head_mask)
File "/usr/local/lib/python3.6/dist-packages/torch/nn/modules/module.py", line 547, in __call__
result = self.forward(*input, **kwargs)
File "/usr/local/lib/python3.6/dist-packages/transformers/modeling_xlm.py", line 485, in forward
tensor = tensor + self.lang_embeddings(langs)
File "/usr/local/lib/python3.6/dist-packages/torch/nn/modules/module.py", line 547, in __call__
result = self.forward(*input, **kwargs)
File "/usr/local/lib/python3.6/dist-packages/torch/nn/modules/sparse.py", line 114, in forward
self.norm_type, self.scale_grad_by_freq, self.sparse)
File "/usr/local/lib/python3.6/dist-packages/torch/nn/functional.py", line 1467, in embedding
return torch.embedding(weight, input, padding_idx, scale_grad_by_freq, sparse)
RuntimeError: Expected object of backend CUDA but got backend CPU for argument #3 'index'
```
Where could i make mistake when runnig the code ? <|||||>Indeed, sorry about that I didn't assign the correct device to the new tensor. It should be fixed now.<|||||>Thank you for quick fix. I ran [run_generations](https://github.com/huggingface/transformers/blob/master/examples/run_generation.py) file in [all pre-trained XLM models](https://huggingface.co/transformers/pretrained_models.html) except for `xlm-mlm-en-2048` model ( I think the problem is that there is only one language in the model. So the model does have some parameters ) .
As you said, although it is not very successful in CLM models, I can get some results (I will continue to try) but I can't get meaningful results in MLM models.For example, for `xlm-mlm-ende-1024` model I wrote the outputs I received for 10 different inputs :
Outputs:
- ] ] ] ] ] ] ] ] ] " " " " " " " " " " "
- ] ] ] ] ] ] ] ] ] ] ] ] ] ] ] ] ] ] ] ]
- ] ] ] ] ] ) ) ) ) ) ) ) ) " ) ) ) ) ) )
- ] ] ] ] ] ] ] ] ] ] ] ) ) ) ) " " " " "
- ] ] ] ] ] • • • • • • • • • • • • • • •
- ] ] ] ] ] ] " " " " " " " " " " " " " "
- ] ] ] ] " " " " " " " " " " " " " " " "
- ] ] ] ] ] " " " " " " " " " " " " " " "
- stlike like like like like like like like like like like like like like like like like like like
- est blast stab at....docdocdocdocdocdocdoctooo"..
How can i generate meaningful outputs in mlm models ?<|||||>Unfortunately, MLM models won´t be of use useful for text generation. By nature, MLM models require left and right context to predict masked tokens, and in the case of text generation they only have access to the left context.
XLM is the only model in our library which was trained with both MLM and CLM; all other models in the `run_generation` script are CLM-only.<|||||>This issue has been automatically marked as stale because it has not had recent activity. It will be closed if no further activity occurs. Thank you for your contributions.
<|||||>@LysandreJik
> Indeed, sorry about that I didn't assign the correct device to the new tensor. It should be fixed now.
This is still a problem, even with updated torch. Please see #2360 |
transformers | 1,413 | closed | Adding New Vocabulary Tokens to the Models | ## ❓ Questions & Help
<!-- A clear and concise description of the question. -->
Hi,
How could I extend the vocabulary of the pre-trained models, e.g. by adding new tokens to the lookup table?
Any examples demonstrating this? | 10-03-2019 12:56:28 | 10-03-2019 12:56:28 | Hi, I believe this method does exactly what you're looking for: [add_tokens](https://huggingface.co/transformers/main_classes/tokenizer.html#transformers.PreTrainedTokenizer.add_tokens). There's an example right below it.<|||||>thanks @LysandreJik ! yes, that's exactly what I was looking for. A follow-up question: How could I initialize the embeddings of these "new tokens" to something I already have pre-computed? I assume currently, embedding for these new tokens will be randomly initialized.<|||||>You are right, these tokens will be randomly initialized. What I would do if I wanted to assign new values to this embedding (as an initialization), is to directly change the Embeddings `weight`. Here's an example with the `BertModel`.
```py
import torch
from transformers import BertTokenizer, BertModel
tokenizer = BertTokenizer.from_pretrained("bert-base-cased")
model = BertModel.from_pretrained("bert-base-cased")
print(len(tokenizer)) # 28996
tokenizer.add_tokens(["NEW_TOKEN"])
print(len(tokenizer)) # 28997
model.resize_token_embeddings(len(tokenizer))
# The new vector is added at the end of the embedding matrix
print(model.embeddings.word_embeddings.weight[-1, :])
# Randomly generated matrix
model.embeddings.word_embeddings.weight[-1, :] = torch.zeros([model.config.hidden_size])
print(model.embeddings.word_embeddings.weight[-1, :])
# outputs a vector of zeros of shape [768]
```<|||||>thanks @LysandreJik ! That should solve it quite neatly. I will reopen the issue in case I run into any issues. <|||||>Hello @LysandreJik ,
What is the difference between the following approaches?
1. to train a tokenizer from scratch such as pointed in [hugginface blog](https://huggingface.co/blog/how-to-train#2-train-a-tokenizer); or
2. to use [add_tokens](https://huggingface.co/transformers/main_classes/tokenizer.html#transformers.PreTrainedTokenizer.add_tokens) method?
Thank you in advance.
<|||||>Training a tokenizer from scratch would imply training a model from scratch as well - depending on the corpus used for the tokenizer, the tokens may be entirely different from another model's tokens trained on a similar corpus (except if you train the tokenizer using the exact same method and the exact same data).
Adding tokens adds tokens at the end of the tokenizer's vocabulary, essentially extending the vocabulary. The model's embedding matrix would need to be resized as well to take into account the new tokens, but all the other tokens would keep their representation as-is. Seeing as the new rows in the embedding matrix are randomly initialized, you would still need to fine-tune the model to a dataset containing such tokens.<|||||>@LysandreJik
I have a dutch medical dataset (for Namen Entity Recognition) which contains a lot of domain-specific words. The dutch BERT tokenizer therefor outputs a lot of [UNK] tokens when it tokenizes.
Given that I dispose over a corpus of 60k labelled tokens, and right now I have also a relatively small annotated corpus of 185k tokens, would it be best to:
- just add the most frequent out of vocab words to the vocab of the tokenizer
- start from a BERT checkpoint and do further pretraining on the unlabeled dataset (which is now of size 185k which is pretty small I assume..). There might be a possibility for me to obtain a much larger unannotated dataset of potentially millions of (unlabelled) tokens, but I was wondering if even millions of tokens is enough to do some meaningful further pretraining?
Thanks!<|||||>> Training a tokenizer from scratch would imply training a model from scratch as well - depending on the corpus used for the tokenizer, the tokens may be entirely different from another model's tokens trained on a similar corpus (except if you train the tokenizer using the exact same method and the exact same data).
>
> Adding tokens adds tokens at the end of the tokenizer's vocabulary, essentially extending the vocabulary. The model's embedding matrix would need to be resized as well to take into account the new tokens, but all the other tokens would keep their representation as-is. Seeing as the new rows in the embedding matrix are randomly initialized, you would still need to fine-tune the model to a dataset containing such tokens.
Hey I would like to fine-tune the model as you suggested at the end to the dataset containing such tokens. Can you help me out on how I can do that?<|||||>If I add unknown tokens to the tokenizer and train the model on, say sentence pair similarity, while I suppose the new tokens embeddings will not have the correct relationship with other tokens, will the model output still be able to find similarity correctly given sufficient training on the model?<|||||>@LysandreJik Thank you for your suggestion. However, I run into trouble because altering the embedding turns the embedding tensor into a non-leaf tensor and hence cannot be optimized i.e.
``` python
model.embeddings.word_embeddings.weight.is_leaf # False
```
I cannot figure out how to fix this (I am torch beginner; sorry). Do you have any suggestions? <|||||>facing same issue; getting false for is_leaf<|||||>`BertTokenizer.from_pretrained('bert-base-uncased', do_lower_case=True).get_vocab()` not return added token. How can I check if the new token is properly added to vocab dictionary? <|||||>> You are right, these tokens will be randomly initialized. What I would do if I wanted to assign new values to this embedding (as an initialization), is to directly change the Embeddings `weight`. Here's an example with the `BertModel`.
>
> ```python
> import torch
> from transformers import BertTokenizer, BertModel
>
> tokenizer = BertTokenizer.from_pretrained("bert-base-cased")
> model = BertModel.from_pretrained("bert-base-cased")
>
> print(len(tokenizer)) # 28996
> tokenizer.add_tokens(["NEW_TOKEN"])
> print(len(tokenizer)) # 28997
>
> model.resize_token_embeddings(len(tokenizer))
> # The new vector is added at the end of the embedding matrix
>
> print(model.embeddings.word_embeddings.weight[-1, :])
> # Randomly generated matrix
>
> model.embeddings.word_embeddings.weight[-1, :] = torch.zeros([model.config.hidden_size])
>
> print(model.embeddings.word_embeddings.weight[-1, :])
> # outputs a vector of zeros of shape [768]
> ```
Hi,
I tried this, but my code still stop in tokenizing the sentences section and doesn't pass it.
it may have lag or problem...
what should I do?<|||||>>
>
> > You are right, these tokens will be randomly initialized. What I would do if I wanted to assign new values to this embedding (as an initialization), is to directly change the Embeddings `weight`. Here's an example with the `BertModel`.
> > ```python
> > import torch
> > from transformers import BertTokenizer, BertModel
> >
> > tokenizer = BertTokenizer.from_pretrained("bert-base-cased")
> > model = BertModel.from_pretrained("bert-base-cased")
> >
> > print(len(tokenizer)) # 28996
> > tokenizer.add_tokens(["NEW_TOKEN"])
> > print(len(tokenizer)) # 28997
> >
> > model.resize_token_embeddings(len(tokenizer))
> > # The new vector is added at the end of the embedding matrix
> >
> > print(model.embeddings.word_embeddings.weight[-1, :])
> > # Randomly generated matrix
> >
> > model.embeddings.word_embeddings.weight[-1, :] = torch.zeros([model.config.hidden_size])
> >
> > print(model.embeddings.word_embeddings.weight[-1, :])
> > # outputs a vector of zeros of shape [768]
> > ```
>
> Hi,
> I tried this, but my code still stop in tokenizing the sentences section and doesn't pass it.
> it may have lag or problem...
> what should I do?
Have you solved the problem? If so, can you share it with us?<|||||>> > > You are right, these tokens will be randomly initialized. What I would do if I wanted to assign new values to this embedding (as an initialization), is to directly change the Embeddings `weight`. Here's an example with the `BertModel`.
> > > ```python
> > > import torch
> > > from transformers import BertTokenizer, BertModel
> > >
> > > tokenizer = BertTokenizer.from_pretrained("bert-base-cased")
> > > model = BertModel.from_pretrained("bert-base-cased")
> > >
> > > print(len(tokenizer)) # 28996
> > > tokenizer.add_tokens(["NEW_TOKEN"])
> > > print(len(tokenizer)) # 28997
> > >
> > > model.resize_token_embeddings(len(tokenizer))
> > > # The new vector is added at the end of the embedding matrix
> > >
> > > print(model.embeddings.word_embeddings.weight[-1, :])
> > > # Randomly generated matrix
> > >
> > > model.embeddings.word_embeddings.weight[-1, :] = torch.zeros([model.config.hidden_size])
> > >
> > > print(model.embeddings.word_embeddings.weight[-1, :])
> > > # outputs a vector of zeros of shape [768]
> > > ```
> >
> >
> > Hi,
> > I tried this, but my code still stop in tokenizing the sentences section and doesn't pass it.
> > it may have lag or problem...
> > what should I do?
>
> Have you solved the problem? If so, can you share it with us?
yes, it was because it takes a very long time to add all tokens. and I installed transformers from source:
pip install -U git+https://github.com/huggingface/transformers ,due to recently it was merged a PR that should speed this up dramatically and my problem solved.<|||||>thank you!
------------------ 原始邮件 ------------------
发件人: ***@***.***>;
发送时间: 2021年5月10日(星期一) 下午2:11
收件人: ***@***.***>;
抄送: "Patrick ***@***.***>; ***@***.***>;
主题: Re: [huggingface/transformers] Adding New Vocabulary Tokens to the Models (#1413)
You are right, these tokens will be randomly initialized. What I would do if I wanted to assign new values to this embedding (as an initialization), is to directly change the Embeddings weight. Here's an example with the BertModel.
import torch from transformers import BertTokenizer, BertModel tokenizer = BertTokenizer.from_pretrained("bert-base-cased") model = BertModel.from_pretrained("bert-base-cased") print(len(tokenizer)) # 28996 tokenizer.add_tokens(["NEW_TOKEN"]) print(len(tokenizer)) # 28997 model.resize_token_embeddings(len(tokenizer)) # The new vector is added at the end of the embedding matrix print(model.embeddings.word_embeddings.weight[-1, :]) # Randomly generated matrix model.embeddings.word_embeddings.weight[-1, :] = torch.zeros([model.config.hidden_size]) print(model.embeddings.word_embeddings.weight[-1, :]) # outputs a vector of zeros of shape [768]
Hi,
I tried this, but my code still stop in tokenizing the sentences section and doesn't pass it.
it may have lag or problem...
what should I do?
Have you solved the problem? If so, can you share it with us?
yes, it was because it takes a very long time to add all tokens. and I installed transformers from source:
pip install -U git+https://github.com/huggingface/transformers ,due to recently it was merged a PR that should speed this up dramatically and my problem solved.
—
You are receiving this because you commented.
Reply to this email directly, view it on GitHub, or unsubscribe.<|||||>> Training a tokenizer from scratch would imply training a model from scratch as well - depending on the corpus used for the tokenizer, the tokens may be entirely different from another model's tokens trained on a similar corpus (except if you train the tokenizer using the exact same method and the exact same data).
>
> Adding tokens adds tokens at the end of the tokenizer's vocabulary, essentially extending the vocabulary. The model's embedding matrix would need to be resized as well to take into account the new tokens, but all the other tokens would keep their representation as-is. Seeing as the new rows in the embedding matrix are randomly initialized, you would still need to fine-tune the model to a dataset containing such tokens.
Why can't we repurpose the existing 999 unused tokens [UNK] instead of extending the vocab size?
https://github.com/google-research/bert/issues/9#issuecomment-434796704<|||||>> You are right, these tokens will be randomly initialized. What I would do if I wanted to assign new values to this embedding (as an initialization), is to directly change the Embeddings `weight`. Here's an example with the `BertModel`.
>
> ```python
> import torch
> from transformers import BertTokenizer, BertModel
>
> tokenizer = BertTokenizer.from_pretrained("bert-base-cased")
> model = BertModel.from_pretrained("bert-base-cased")
>
> print(len(tokenizer)) # 28996
> tokenizer.add_tokens(["NEW_TOKEN"])
> print(len(tokenizer)) # 28997
>
> model.resize_token_embeddings(len(tokenizer))
> # The new vector is added at the end of the embedding matrix
>
> print(model.embeddings.word_embeddings.weight[-1, :])
> # Randomly generated matrix
>
> model.embeddings.word_embeddings.weight[-1, :] = torch.zeros([model.config.hidden_size])
>
> print(model.embeddings.word_embeddings.weight[-1, :])
> # outputs a vector of zeros of shape [768]
> ```
@LysandreJik when I ran your code the following error popped up. please help
**RuntimeError: a view of a leaf Variable that requires grad is being used in an in-place operation.**<|||||>> RuntimeError: a view of a leaf Variable that requires grad is being used in an in-place operation.
You can fix that error by temporarily disabling gradient calculation. (Because initializing the weights is not an operation that needs to be accounted for in backpropagation.)
```python
with torch.no_grad():
model.embeddings.word_embeddings.weight[-1, :] = torch.zeros([model.config.hidden_size])
```<|||||>why hidden_size? Is that specific to just Bert model? For Albert it should be different right?<|||||>How do we initialise the pre-existing embeddings for new tokens from old partitioned tokens?<|||||>> why hidden_size? Is that specific to just Bert model? For Albert it should be different right?
Hi, yes, I do believe the name can vary from model to model. For T5 model it seems to be `d_model`<|||||>> How do we initialise the pre-existing embeddings for new tokens from old partitioned tokens?
If I understand you correctly, we can initialise new tokens from already pre-trained ones with taking a mean of them:
```
with torch.no_grad():
for i, token in enumerate(reversed(added_tokens), start=1):
tokenized = tokenizer.tokenize(token)
tokenized_ids = tokenizer.convert_tokens_to_ids(tokenized)
model.embeddings.word_embeddings.weight[-i, :] = model.embeddings.word_embeddings.weight[tokenized_ids].mean(axis=0)
```<|||||>> > How do we initialise the pre-existing embeddings for new tokens from old partitioned tokens?
>
> If I understand you correctly, we can initialise new tokens from already pre-trained ones with taking a mean of them:
>
> ```
> with torch.no_grad():
> for i, token in enumerate(reversed(added_tokens), start=1):
> tokenized = tokenizer.tokenize(token)
> tokenized_ids = tokenizer.convert_tokens_to_ids(tokenized)
> model.embeddings.word_embeddings.weight[-i, :] = model.embeddings.word_embeddings.weight[tokenized_ids].mean(axis=0)
> ```
Ok. Thank you. Is this also correct?
```
model.resize_token_embeddings(len(tokenizer))
weights = model.roberta.embeddings.word_embeddings.weight
# initialize new embedding weights as mean of original tokens
with torch.no_grad():
emb = []
for i in range(len(joined_keywords)):
word = joined_keywords[i]
# first & last tokens are just string start/end; don't keep
tok_ids = tokenizer_org(word)["input_ids"][1:-1]
tok_weights = weights[tok_ids]
# average over tokens in original tokenization
weight_mean = torch.mean(tok_weights, axis=0)
emb.append(weight_mean)
weights[-len(joined_keywords):,:] = torch.vstack(emb).requires_grad_()
```<|||||>How should I save new tokenizer to use it in downstream model?
```
tokenizer_org = tr.BertTokenizer.from_pretrained("/home/pc/bert_base_multilingual_uncased")
tokenizer.add_tokens(joined_keywords)
model = tr.BertForMaskedLM.from_pretrained("/home/pc/bert_base_multilingual_uncased", return_dict=True)
# prepare input
text = ["Replace me by any text you'd like"]
encoded_input = tokenizer(text, truncation=True, padding=True, max_length=512, return_tensors="pt")
print(encoded_input)
# add embedding params for new vocab words
model.resize_token_embeddings(len(tokenizer))
weights = model.bert.embeddings.word_embeddings.weight
# initialize new embedding weights as mean of original tokens
with torch.no_grad():
emb = []
for i in range(len(joined_keywords)):
word = joined_keywords[i]
# first & last tokens are just string start/end; don't keep
tok_ids = tokenizer_org(word)["input_ids"][1:-1]
tok_weights = weights[tok_ids]
# average over tokens in original tokenization
weight_mean = torch.mean(tok_weights, axis=0)
emb.append(weight_mean)
weights[-len(joined_keywords):,:] = torch.vstack(emb).requires_grad_()
model.to(device)
```
`trainer.save_model("/home/pc/Bert_multilingual_exp_TCM/model_mlm_exp1")`
**It saves model, config, training_args. How to save the new tokenizer as well??**<|||||>I am not sure if anyone can help to answer this here but I cannot seems to be able to find an answer from anywhere:
what exactly is the difference between "token" and a "special token"?
I understand the following:
* what is a typical token
* what is a typical special token: MASK, UNK, SEP, etc
* when do you add a token (when you want to expand your vocab)
What I don't understand is, under what kind of capacity will you want to create a new special token, any examples what we need it for and when we want to create a special token other than those default special tokens? If an example uses a special token, why can't a normal token achieve the same objective?
```
tokenizer.add_tokens(['[EOT]'], special_tokens=True)
```
And I also dont quite understand the following description in the source documentation.
what difference does it do to our model if we set add_special_tokens to False?
```
add_special_tokens (bool, optional, defaults to True) — Whether or not to encode the sequences with the special tokens relative to their model.
```<|||||>> I am not sure if anyone can help to answer this here but I cannot seems to be able to find an answer from anywhere: what exactly is the difference between "token" and a "special token"?
>
> I understand the following:
>
> * what is a typical token
> * what is a typical special token: MASK, UNK, SEP, etc
> * when do you add a token (when you want to expand your vocab)
>
> What I don't understand is, under what kind of capacity will you want to create a new special token, any examples what we need it for and when we want to create a special token other than those default special tokens? If an example uses a special token, why can't a normal token achieve the same objective?
>
> ```
> tokenizer.add_tokens(['[EOT]'], special_tokens=True)
> ```
>
> And I also dont quite understand the following description in the source documentation. what difference does it do to our model if we set add_special_tokens to False?
>
> ```
> add_special_tokens (bool, optional, defaults to True) — Whether or not to encode the sequences with the special tokens relative to their model.
> ```
When you add a "special token" it will not be replaced by the "[MASK]" or replaced by a random word in the pre-training procedure. |
transformers | 1,412 | closed | How to use model.fit in GPT2 TF Model | ## ❓ Questions & Help
```import tensorflow as tf
from transformers import *
import numpy as np
# Load dataset, tokenizer, model from pretrained model/vocabulary
tokenizer = GPT2Tokenizer.from_pretrained('gpt2')
model = TFGPT2LMHeadModel.from_pretrainedmodel = TFGPT2LMHeadModel.from_pretrained('gpt2')
np.random.seed(0)
batch = 5
max_len = 750
inp = tar = np.random.randint(0, 50267, (batch, max_len))
dataset = tf.data.Dataset.from_tensor_slices((inp, tar))
dataset = dataset.batch(batch)
optimizer = tf.keras.optimizers.Adam(learning_rate=3e-5, epsilon=1e-08, clipnorm=1.0)
loss_function = tf.keras.losses.SparseCategoricalCrossentropy(from_logits=True)
metric = tf.keras.metrics.SparseCategoricalAccuracy('accuracy')
model.compile(optimizer=optimizer, loss=loss_function, metrics=[metric])
history = model.fit(dataset)
```
I would like to use ```model.fit``` on the dataset. Can anyone suggest me. Now getting following error
```ValueError: Error when checking model target: the list of Numpy arrays that you are passing to your model is not the size the model expected. Expected to see 13 array(s), but instead got the following list of 1 arrays: [<tf.Tensor 'IteratorGetNext:1' shape=(None, 750) dtype=int64>]...```
| 10-03-2019 09:32:54 | 10-03-2019 09:32:54 | Hello! Are you sure this is the script with which you get your error? The `model.fit` argument `epoch` doesn't exist (it should be `epochs`) and your model has not been compiled beforehand. Could you provide an example script which throws the error you're mentioning?<|||||>Hi , I tried to minimize the code as much as possible. I did add compile
and epoch was a typo. Will update new code.
On Fri, Oct 4, 2019, 12:10 AM Lysandre Debut <[email protected]>
wrote:
> Hello! Are you sure this is the script with which you get your error? The
> model.fit argument epoch doesn't exist (it should be epochs) and your
> model has not been compiled beforehand. Could you provide an example script
> which throws the error you're mentioning?
>
> —
> You are receiving this because you authored the thread.
> Reply to this email directly, view it on GitHub
> <https://github.com/huggingface/transformers/issues/1412?email_source=notifications&email_token=ACRE6KA765X6QGUG2K3FMQ3QMY4AXA5CNFSM4I5AVBUKYY3PNVWWK3TUL52HS4DFVREXG43VMVBW63LNMVXHJKTDN5WW2ZLOORPWSZGOEAJFPBA#issuecomment-538072964>,
> or mute the thread
> <https://github.com/notifications/unsubscribe-auth/ACRE6KA3PO2ED7PK4QPKUOLQMY4AXANCNFSM4I5AVBUA>
> .
>
<|||||>Hi @LysandreJik - I have updated the code. The issue remains the same.<|||||>Hi, thanks for updating your code. You should be careful with the model's output. The `TFGPT2LMHeadModel` outputs a list of 13 tensors: the first one is the one you're interested in, which is a tensor of logits across the vocabulary.
This tensor shape is `(batch_size, sequence_length, config.vocab_size)`, while you seem to be giving your models targets that have the same shape as your inputs.
The 12 following tensors are the "pre-computed hidden-states (key and values in the attention blocks)". You won't be using these for keras' fit method, so you should adapt your model compile method to only calculate the loss on the first output. [This Stack Overflow question](https://stackoverflow.com/questions/40446488/training-only-one-output-of-a-network-in-keras) talks about computing a loss for a single output in a multi-output model.
You can read the relevant documentation [here](https://huggingface.co/transformers/model_doc/gpt2.html#tfgpt2lmheadmodel).<|||||>This issue has been automatically marked as stale because it has not had recent activity. It will be closed if no further activity occurs. Thank you for your contributions.
<|||||>@s4sarath were you able to figure this out by chance? I'm having the same issue.<|||||>I'm having the same problem and I'm not sure @LysandreJik is correct. The output of `TFGPT2LMHeadModel` is a pair where the first item is the logits tensor and the second item is the twelve layer caches. So either
model.compile(..., loss = [SparseCategoricalCrossentropy(from_logits = True), None], ...)
or
model.compile(..., loss = [SparseCategoricalCrossentropy(from_logits = True), *[None]*12], ...)
ought to be the correct invocation. But neither of them works.<|||||>You're correct, they're the past, not the attentions. |
transformers | 1,411 | closed | Update run_glue.py | add DistilBert model shortcut name into ALL_MODELS | 10-03-2019 08:31:17 | 10-03-2019 08:31:17 | Great thanks! |
transformers | 1,410 | closed | migrate BertForQuestionAnswering from pytorch-pretrained-bert not produce the same result | ## 🐛 Bug
<!-- Important information -->
Model I am using (Bert, XLNet....): retrained Bert
Language I am using the model on (English, Chinese....): multilingual - vietnamese
The tasks I am working on is:
* an official GLUE/SQUaD task: SQUaD
* my own task or dataset: same format as SQUaD
## To Reproduce
Steps to reproduce the behavior:
I have trained a bert model on older pytorch-pretrained-bert and it works just fine. recently, I switch the code to the latest version of transformer. I use the following config:
> bert_model = './my_model'
> max_seq_length = 160
> doc_stride = 160
> predict_batch_size = 20
> n_best_size=20
> max_answer_length=30
> verbose_logging = False
> no_cuda = True
> seed= 42
> do_lower_case= True
> version_2_with_negative = True
> null_score_diff_threshold=0.0
> max_query_length = 64
> THRESH_HOLD = 0.95
I import 2 class:
`from transformers import BertForQuestionAnswering as bqa1`
`from pytorch_pretrained_bert.modeling import BertForQuestionAnswering as bqa2`
and load 2 model as following :
`model1 = bqa1.from_pretrained(args.bert_model)`
`model2 = bqa2.from_pretrained(args.bert_model)`
and input to models with the same tensors:
`input_ids = torch.ones((1,160),dtype = torch.int64)`
`segment_ids = torch.ones((1,160),dtype = torch.int64)`
`input_mask = torch.ones((1,160),dtype = torch.int64) `
`model(input_ids, segment_ids, input_mask)`
I also check if 2 model has same weights or not by using following guide [https://discuss.pytorch.org/t/check-if-models-have-same-weights/4351/3](guide).
I seed the randomness of torch before inference 2 model by using:
`seed = 0`
`torch.manual_seed(seed)`
`if torch.cuda.is_available():`
` torch.cuda.manual_seed_all(seed)`
but 2 models still produce difference results. | 10-03-2019 08:11:07 | 10-03-2019 08:11:07 | Hello! Have you put these models in `eval()` mode so as to deactivate the dropout modules?<|||||>For completeness sake: did you train both models with the same random seed? Or are you just trying to evaluate models that you trained?
My go-to method is:
```python
def set_seed(seed):
""" Set all seeds to make results reproducible (deterministic mode).
When seed is a false-y value or not supplied, disables deterministic mode. """
if seed:
logging.info(f"Running in deterministic mode with seed {seed}")
torch.manual_seed(seed)
torch.cuda.manual_seed_all(seed)
torch.backends.cudnn.deterministic = True
torch.backends.cudnn.benchmark = False
np.random.seed(seed)
random.seed(seed)
os.environ['PYTHONHASHSEED'] = str(seed)
else:
logging.info(f"Running in non-deterministic mode")
```<|||||>It does work when I put generated tensors into 2 models but doesn't when I put tensors I save before. Maybe I will rewrite the inference code with new code base and retrain the model.<|||||>Are you sure your model is in evaluation mode? <|||||>yes, I already put it in evaluation mode<|||||>This issue has been automatically marked as stale because it has not had recent activity. It will be closed if no further activity occurs. Thank you for your contributions.
|
transformers | 1,409 | closed | Evaluation result.txt path changing #1286 | Here is the suggestion that I mention in issues #1286
| 10-03-2019 04:53:09 | 10-03-2019 04:53:09 | Great, that looks good to me!<|||||>Ok, merging, thanks @brian41005 |
transformers | 1,408 | closed | Batched BertForNextSentencePrediction with variable length sentences | ## ❓ Questions & Help
What's the proper way to pad a batch of variable length sentences for the BertForNextSentencePrediction model?
I want to batch a list of sentences, and each sentence can have any length < max_seq_len. To fit them into a token tensor I assume I will need some form of padding?
Here's an example with 2 candidate sentences where the first sentence has no padding, and the second has 2 padded 0s.
```python
import torch
from pytorch_transformers import BertTokenizer, BertModel, BertForMaskedLM,BertForNextSentencePrediction
# Load pre-trained model tokenizer (vocabulary)
tokenizer = BertTokenizer.from_pretrained('bert-base-uncased')
# Tokenized inputs
text1 = "[CLS] Who was Jim ? [SEP] Jim Henson was a puppeteer [SEP]"
text2 = "[CLS] Who was Jim ? [SEP] Jim Henson was a puppeteer [SEP] [PAD] [PAD]"
tokenized_text1 = tokenizer.tokenize(text1)
tokenized_text2 = tokenizer.tokenize(text2)
# Convert token to vocabulary indices
indexed_tokens1 = tokenizer.convert_tokens_to_ids(tokenized_text1)
indexed_tokens2 = tokenizer.convert_tokens_to_ids(tokenized_text2)
# Define sentence A and B indices associated to 1st and 2nd sentences
segments_ids1 = [0, 0, 0, 0, 0, 0, 1, 1, 1, 1, 1, 1, 1]
segments_ids2 = [0, 0, 0, 0, 0, 0, 1, 1, 1, 1, 1, 1, 1, 0, 0]
# Convert inputs to PyTorch tensors
tokens_tensor1 = torch.tensor([indexed_tokens1])
tokens_tensor2 = torch.tensor([indexed_tokens2])
segments_tensors1 = torch.tensor([segments_ids1])
segments_tensors2 = torch.tensor([segments_ids2])
# Load pre-trained model (weights)
model = BertForNextSentencePrediction.from_pretrained('bert-base-uncased')
model.eval()
# Predict is Next Sentence ?
predictions1 = model(tokens_tensor1, segments_tensors1 )
predictions2 = model(tokens_tensor2, segments_tensors2 )
print(predictions1) #(tensor([[ 5.6165, -5.2786]], grad_fn=<AddmmBackward>),)
print(predictions2) #(tensor([[ 5.0919, -4.4939]], grad_fn=<AddmmBackward>),)
```
As the number padding 0s increases the the confidence of the model continues to decline. We haven't been able to find any documentation for setting up the padding sequences, especially for the segment ids. Any idea how we can set this up?
Thanks! | 10-03-2019 00:03:15 | 10-03-2019 00:03:15 | Hello!
1 - Indeed, if you want to have several sequences of variable length in a single batch, you should pad the shorter sequences.
2 - In the [`BertForNextSentencePrediction ` documentation](https://huggingface.co/transformers/model_doc/bert.html#bertfornextsentenceprediction) is written the following:
`attention_mask`: Mask to avoid performing attention on padding token indices. Mask values selected in `[0, 1]`: `1` for tokens that are NOT MASKED, `0` for MASKED tokens.
If using the `attention_mask`, there should be no difference between a model's predictions of a sequence and its padded counterpart.
3 - The segment ids padding indices can change according to the model. I believe it is `0` for most models, but `4` in the case of XLNet. You seem to be padding with `0` in your example, which is the way to go!<|||||>Thank you!!! This is exactly what I was missing.
```python3
import torch
from pytorch_transformers import BertTokenizer, BertModel, BertForMaskedLM,BertForNextSentencePrediction
# Load pre-trained model tokenizer (vocabulary)
tokenizer = BertTokenizer.from_pretrained('bert-base-uncased')
# Tokenized inputs
text1 = "[CLS] Who was Jim ? [SEP] Jim Henson was a puppeteer [SEP]"
text2 = "[CLS] Who was Jim ? [SEP] Jim Henson was a puppeteer [SEP] [PAD] [PAD]"
tokenized_text1 = tokenizer.tokenize(text1)
tokenized_text2 = tokenizer.tokenize(text2)
# Convert token to vocabulary indices
indexed_tokens1 = tokenizer.convert_tokens_to_ids(tokenized_text1)
indexed_tokens2 = tokenizer.convert_tokens_to_ids(tokenized_text2)
# Define sentence A and B indices associated to 1st and 2nd sentences
segments_ids1 = [0, 0, 0, 0, 0, 0, 1, 1, 1, 1, 1, 1, 1]
segments_ids2 = [0, 0, 0, 0, 0, 0, 1, 1, 1, 1, 1, 1, 1, 0, 0]
#Attention Mask [1] over tokens, [0] over padding
attention_mask = torch.FloatTensor([[1, 1, 1, 1, 1, 1, 1, 1, 1, 1, 1, 1, 1, 0, 0]])
# Convert inputs to PyTorch tensors
tokens_tensor1 = torch.tensor([indexed_tokens1])
tokens_tensor2 = torch.tensor([indexed_tokens2])
segments_tensors1 = torch.tensor([segments_ids1])
segments_tensors2 = torch.tensor([segments_ids2])
# Load pre-trained model (weights)
model = BertForNextSentencePrediction.from_pretrained('bert-base-uncased')
model.eval()
# Predict is Next Sentence ?
predictions1 = model(tokens_tensor1, segments_tensors1 )
predictions2 = model(tokens_tensor2, segments_tensors2, attention_mask=attention_mask )
print(predictions1) #(tensor([[ 5.6165, -5.2786]], grad_fn=<AddmmBackward>),)
print(predictions2) #(tensor([[ 5.6165, -5.2786]], grad_fn=<AddmmBackward>),)
```
With the attention mask now over tokens I get the same output without degradation.
:)
|
transformers | 1,407 | closed | GPT-2 Training on non-english text | ## ❓ Questions & Help
I wish to train a GPT-2 in different languages, like Portuguese and maybe some programming languages like C++ (and play with token predictions).
But I could not find any examples of how to take an X dataset (like c++ source files), create the tokens from it and train a GPT-2 to predict new tokens from the knowledge of this X dataset.
Is this even possible? (if yes, how could one do this?)
Thanks! | 10-02-2019 23:32:58 | 10-02-2019 23:32:58 | Hi! By "GPT-2 training" two different methods can be understood: training from scratch, and fine-tuning.
If you're looking at training GPT-2 on a different language such as Portuguese, then training from scratch seems necessary. You could use the [language modeling finetuning example](https://github.com/huggingface/transformers/blob/master/examples/run_lm_finetuning.py) as a start, but please be aware that training such a language model from scratch takes a humongous amount of power and data, which would cost a lot. I can point you to [this issue](https://github.com/huggingface/transformers/issues/1356) which discusses training such a model on French.
If you're looking at training your model on programming languages that have a lot of overlapping vocabulary with English (say Python with a lot of documentation), maybe you could fine-tune the original GPT-2 to your dataset (still using the [lm finetuning example](https://github.com/huggingface/transformers/blob/master/examples/run_lm_finetuning.py)), but I'm not sure of the results. <|||||>I'm training Russian GPT-2 at the moment. [I've tried to make Readme useful.](https://github.com/mgrankin/ru_transformers)<|||||>> If you're looking at training GPT-2 on a different language such as Portuguese, then training from scratch seems necessary.
It is definitely not necessary to start from scratch. I'd argue the opposite, it'd be useful to start with pre-trained GPT-2 even if you replacing the whole vocabulary (English -> Portuguese).<|||||>Alright @mgrankin, that's good to know, thanks!<|||||>> > If you're looking at training GPT-2 on a different language such as Portuguese, then training from scratch seems necessary.
>
> It is definitely not necessary to start from scratch. I'd argue the opposite, it'd be useful to start with pre-trained GPT-2 even if you replacing the whole vocabulary (English -> Portuguese).
But in $$$ terms it would still be closer to training from scratch than fine-tuning, right?<|||||>> I'm training Russian GPT-2 at the moment. [I've tried to make Readme useful.](https://github.com/mgrankin/ru_transformers)
Thank you @mgrankin for sharing your steps. I plan to do the same for Hindi language.
How much is it costing you to train?<|||||>> How much is it costing you to train?
It’s hard to tell overall cost because the training is in the process. I’ve got a workstation with 4 Titan RTX and I don’t use cloud GPUs at the moment. I use one GPU per model. The training already lasted about two weeks now and gpt2-medium gives me perplexity = 21 on my validation set.
Since PyTorch 1.3 was released recently with TPU support I’m thinking of trying to use TPU to speed up the training. I will update the repo in the next few days in case of success.
<|||||>> But in $$$ terms it would still be closer to training from scratch than fine-tuning, right?
Actually, in terms of quality it would be great if somebody try to train GPT2 on Portuguese from scratch vs fine-tune from pretrained English model. My guess that fine-tuning is better is based on intuition that non-random weights could be reused. Also, English is probably the most resourceful language and WebText is a great dataset. If you can build dataset with same or better quality you can give it a shot and train GPT-2 from scratch.
In terms of money it should be way cheaper to fine-tune. But I will say that with confidence then I'll finish the Russian GPT-2.
<|||||>Thanks for the answer @mgrankin i'm anxious to see your results!<|||||>> I'm training Russian GPT-2 at the moment. [I've tried to make Readme useful.](https://github.com/mgrankin/ru_transformers)
@mgrankin Could you explain to me how you trained your model from scratch with BERT?
I would like to train BERT from scratch for a textual base in PT-BR (8GB data). Is it possible to use the run_lm_finetuning.py code to perform this process without using the multi-language bert model?
I already have a vocab.txt for the PT-BR base and I don't want to load initial weights.
Is there any script or tutorial to perform this process step by step?<|||||>Hi, I also have a repo which allows to train gpt-2 language model on non-english text with a custom BPE tokenizer. But it uses a different gpt-2 implementation so currently it's unable to use pre-trained GPT-2 (although a conversion script should be possible, because it's a port of original TF implementation). Here is the link https://github.com/lopuhin/transformer-lm<|||||>Hello, this thread is what I'm looking (with the one about GPT-2 and BERT into French) for but I'm not sure I found the answer to my questions:
- how long does it take to go through GPT-2 on non-english text?
- what configuration of GPUs?
- what size of corpus?
Many thanks in advance for your answers!<|||||>Why don't you use [CamemBERT](https://camembert-model.fr/) model, which is dedicated to French language? **It's available in HuggingFace's Transformers** too (since few days ago, so try out :D)! If you want absolutely to use GPT2 model, I can answer to you too!
> Hello, this thread is what I'm looking (with the one about GPT-2 and BERT into French) for but I'm not sure I found the answer to my questions:
>
> * how long does it take to go through GPT-2 on non-english text?
> * what configuration of GPUs?
> * what size of corpus?
>
> Many thanks in advance for your answers!<|||||>Hi @piegu, please do not post the same message in two issues (that are linked with one another)<|||||>> Hi @piegu, please do not post the same message in two issues (that are linked with one another)
Hello @julien-c. Ok but then I have to update in this thread my question to French and Portuguese (same 3 questions about fine-tuning GPT-2 and BERT). Thank you.
<|||||>> Why don't you use [CamemBERT](https://camembert-model.fr/) model, which is dedicated to French language? **It's available in HuggingFace's Transformers** too (since few days ago, so try out :D)! If you want absolutely to use GPT2 model, I can answer to you too!
Thanks @TheEdoardo93. For sure I will test CamemBERT but it does not answer my 3 questions :-) Great if you can answer about GPT-2 at least. Thank you.<|||||>Hi @nikhilno1 , Did you manage to train it on Hindi?<|||||>Hi @GladiatorX, No I didn't. Life got in the way. :)
Would you like to work on it together?<|||||>@mgrankin Out of curiosity, how did you collect your 230 GB Russian dataset?
I would love to do something similar for another language, and I'm looking for tips<|||||>@BoxxiDev you can use something like a scraper/crawler like [Scrapy](https://scrapy.org/) (or something like it) on a russian site, and then you can use something like AWS Comprehend to get the language (or make a language detector yourself) and filter only Russian results.
to get tons of data use some distributed scraper on a cloud service like AWS.<|||||>@BoxxiDev Library projects have been working in Russia for a very long time, and they publish a torrent file with all the contents in fb2. [example](https://booktracker.org/viewtopic.php?t=1198)<|||||>Hi @nikhilno1 , +1,
Did you manage to train it on Hindi?
<|||||>> > If you're looking at training GPT-2 on a different language such as Portuguese, then training from scratch seems necessary.
>
> It is definitely not necessary to start from scratch. I'd argue the opposite, it'd be useful to start with pre-trained GPT-2 even if you replacing the whole vocabulary (English -> Portuguese).
@mgrankin you say that it is not necessary to train from scratch, but assumed the vocabulary will not overlap (let's say English and Russian), how you do it?
Also someone else is talking about BERT based models (like the French model CamemBERT), but those models are [MASK] token based models, so it would need a different approach for text generation à la GPT-2<|||||>@loretoparisi
By using progressive unfreezing. This's a technique from Transfer Learning. First, you freeze all layers and unfreeze only those layers that you expect to change the most - the embeddings and adjacent to the embeddings, you train them, you unfreeze a bit more layers, repeat. I’d advise taking a [course.fast.ai](https://course.fast.ai) course to be very comfortable with the concept.
You can look at the code [here](https://github.com/mgrankin/ru_transformers/blob/64d7a68e067737c35c7bf3986cb1845aaf54a163/tpu_lm_finetuning.py#L677).
<|||||>@mgrankin thank you, in the meanwhile I'm following this approach [BERT has a Mouth, and It Must Speak: BERT as a Markov Random Field Language Model](https://arxiv.org/abs/1902.04094)<|||||>>
> By using progressive unfreezing. This's a technique from Transfer Learning. First, you freeze all layers and unfreeze only those layers that you expect to change the most - the embeddings and adjacent to the embeddings, you train them, you unfreeze a bit more layers, repeat. I’d advise taking a [course.fast.ai](https://course.fast.ai) course to be very comfortable with the concept.
>
> You can look at the code [here](https://github.com/mgrankin/ru_transformers/blob/64d7a68e067737c35c7bf3986cb1845aaf54a163/tpu_lm_finetuning.py#L677).
Hi Mikhail. In your (great) code, you unfreeze groups of 3 layers (see [code](https://github.com/mgrankin/ru_transformers/blob/64d7a68e067737c35c7bf3986cb1845aaf54a163/tpu_lm_finetuning.py#L684) and below). There is a specific reason or it is the result of your tests? Thanks.
`need_grads = set(flat[:i_start+args.unfreeze_level*3]) | set(flat[-(i_end+args.unfreeze_level*3):])`<|||||>@piegu that's a heuristic, feel free to experiment with the number.<|||||>> Hi @nikhilno1 , +1,
> Did you manage to train it on Hindi?
Starting it now. Let me know if you want to work together.<|||||>> > Hi @nikhilno1 , +1,
> > Did you manage to train it on Hindi?
>
> Starting it now. Let me know if you want to work together.
@nikhilno1 Im interested to do this for tamil, were you able to figure our hindi ?<|||||>This issue has been automatically marked as stale because it has not had recent activity. It will be closed if no further activity occurs. Thank you for your contributions.
<|||||>Any official tutorial already available?
we really would like to see a way to train this on my language, using the
official framework.
On Thu, 4 Jun 2020 at 21:05, stale[bot] <[email protected]> wrote:
> This issue has been automatically marked as stale because it has not had
> recent activity. It will be closed if no further activity occurs. Thank you
> for your contributions.
>
> —
> You are receiving this because you authored the thread.
> Reply to this email directly, view it on GitHub
> <https://github.com/huggingface/transformers/issues/1407#issuecomment-639182158>,
> or unsubscribe
> <https://github.com/notifications/unsubscribe-auth/ACXKCCPVIM5QT3YDAT45D6DRVAZEXANCNFSM4I44TIKA>
> .
>
<|||||>This issue has been automatically marked as stale because it has not had recent activity. It will be closed if no further activity occurs. Thank you for your contributions.
<|||||>> > Hi @nikhilno1 , +1,
> > Did you manage to train it on Hindi?
>
> Starting it now. Let me know if you want to work together.
Were you able to train GPT-2 on Hindi?
<|||||>> > > Hi @nikhilno1 , +1,
> > > Did you manage to train it on Hindi?
> >
> >
> > Starting it now. Let me know if you want to work together.
>
> @nikhilno1 Im interested to do this for tamil, were you able to figure our hindi ?
Did you try Tamil?<|||||>Has anyone tried using GPT on multiscript text like Tamil + Devanagari + roman script text? The language of Whatsapps or Twitter msgs of Indian people.<|||||>> > I'm training Russian GPT-2 at the moment. [I've tried to make Readme useful.](https://github.com/mgrankin/ru_transformers)
>
> Thank you @mgrankin for sharing your steps. I plan to do the same for Hindi language.
> How much is it costing you to train?
Did you finish training for Hindi? |
transformers | 1,406 | closed | Distil update | Update Distil*
- update on distilbert weights
- add distilgpt2 weights
- link to the paper
- big update on code | 10-02-2019 20:32:53 | 10-02-2019 20:32:53 | # [Codecov](https://codecov.io/gh/huggingface/transformers/pull/1406?src=pr&el=h1) Report
> Merging [#1406](https://codecov.io/gh/huggingface/transformers/pull/1406?src=pr&el=desc) into [master](https://codecov.io/gh/huggingface/transformers/commit/63ed224b7c550ead5f9599187e665ded57ce80d4?src=pr&el=desc) will **not change** coverage.
> The diff coverage is `n/a`.
[](https://codecov.io/gh/huggingface/transformers/pull/1406?src=pr&el=tree)
```diff
@@ Coverage Diff @@
## master #1406 +/- ##
=======================================
Coverage 84.72% 84.72%
=======================================
Files 84 84
Lines 12591 12591
=======================================
Hits 10668 10668
Misses 1923 1923
```
------
[Continue to review full report at Codecov](https://codecov.io/gh/huggingface/transformers/pull/1406?src=pr&el=continue).
> **Legend** - [Click here to learn more](https://docs.codecov.io/docs/codecov-delta)
> `Δ = absolute <relative> (impact)`, `ø = not affected`, `? = missing data`
> Powered by [Codecov](https://codecov.io/gh/huggingface/transformers/pull/1406?src=pr&el=footer). Last update [63ed224...193bbda](https://codecov.io/gh/huggingface/transformers/pull/1406?src=pr&el=lastupdated). Read the [comment docs](https://docs.codecov.io/docs/pull-request-comments).
|
transformers | 1,405 | closed | Re-order XLNet attention head outputs for better perf | Significant performance boost over the original orderings. On an already somewhat optimised branch this gave me > 2x end-to-end throughput on a squad xlnet fine-tuning task (batch 8, seq-length 512, fp16, amp opt level = O2)
Justifying this is the contraction
```
attn_vec = torch.einsum('bnij,jbnd->ibnd', attn_prob, v_head_h)
```
Given how `torch.einsum` and tensor contractions work, this is a batched gemm with batch dimension `bn` and gemm dimension `(i x j) * (j x d)`. Moving `bn` to be the first dimensions for the first input eliminates a sizable transpose that would otherwise need to be done. | 10-02-2019 18:41:42 | 10-02-2019 18:41:42 | Remaining CI failures are valid, they look to assume a `ijbn` ordering for all attention-based things, which no longer holds.
I'm happy to add additional functionality to get these tests passing, but I'd like input on how you'd like that done (I'd lean to passing an optional `expected_attention_size` which is `[key_len, batch_size, num_heads]` by default, and checking that instead of assembling the expected sizes on-the-fly in the test(s))<|||||>Please ignore above :) test errors were due to a missed transpose on my part (if attention outputs are returned, they need to be transposed from `bnij` to `ijbn` ordering to keep the interface from attention <-> the rest of the code the same as before.)<|||||>This is a great work, thanks a lot @slayton58.
Can you confirm this has no noticeable impact on downstream performances (in terms of evaluation metrics), for instance on your SQuAD tests?<|||||>@thomwolf I have been testing against the config from https://github.com/huggingface/transformers/issues/947#issue-476001056 with seq-length=512, and obtained a consistent f1 score of 83 across both `ijbn` and `bnij` attention head orderings (also across fp32/fp16 O1/fp16 O2)
When there's a PR issued for the changes in https://github.com/huggingface/transformers/issues/947#issuecomment-535989890 I'd be happy to go ahead and repro those numbers with this change if you'd like the additional security.<|||||>Awesome, ok let's merge this then. |
transformers | 1,404 | closed | How to speedup BERT eval | ## ❓ Questions & Help
Is there a simple way to speedup `.eval()` when using the BERT model
Specifically I am using `BertForSequenceClassification`. I have finetuned a the model separately on my own data and I am trying to get hidden representations after doing `model.eval()` as follows:
`last_hidden_layer, all_hidden_states = model(input_ids)`
However for each input it is taking about 2.3 seconds on `cpu` and 2.6 seconds on `gpu`. Is there a way where I can do faster than this? | 10-02-2019 18:01:45 | 10-02-2019 18:01:45 | Did you try using DistilBERT? Inference should be ~ 60% faster<|||||>Turns out I wasn't using the using the gpu correctly. I moved the model and the inputs to gpu by doing `.to(device)` and it became 100x faster. Thanks for the suggestion. |
transformers | 1,403 | closed | Is it possible to modify the parameters in GPT-2? | ## ❓ Questions & Help
<!-- A clear and concise description of the question. -->
I wonder whether it's possible to modify the parameters in GPT-2? Since we can not train GPT-2, modifying the parameters and observing the changes in results will be helpful. Thank you in advance! | 10-02-2019 17:32:42 | 10-02-2019 17:32:42 | Hi! GPT-2, like all models in this library, directly inherit from pytorch's `nn.Module`, so you're free to finetune them or modify their parameters as you wish.<|||||>> Hi! GPT-2, like all models in this library, directly inherit from pytorch's `nn.Module`, so you're free to finetune them or modify their parameters as you wish.
Thank you for your help! |
transformers | 1,402 | closed | Defining Models in TF 2.0 and Extending Them | ## ❓ Questions & Help
Hi,
Thanks for the awesome library 😊
I saw the examples on fine-tuning the models. My question is, how could we get model definitions i.e the layered architectures (model.summary) in Keras.
Any example notebooks demonstrating how we could get the model definitions and extend the architectures (by subclassing or manually tweaking the layers)?
Cheers,
Vikas | 10-02-2019 17:13:51 | 10-02-2019 17:13:51 | This issue has been automatically marked as stale because it has not had recent activity. It will be closed if no further activity occurs. Thank you for your contributions.
|
transformers | 1,401 | closed | XLM add new models | hi
can u add to your libs new pretrained models by XLM, like `mlm_17_1280.pth` & `mlm_100_1280.pth`? | 10-02-2019 15:15:45 | 10-02-2019 15:15:45 | Hi! Those models actually are available, we just forgot to add them to the documentation :). Thanks for letting us know! |
transformers | 1,400 | closed | Fix typo: initialy -> initially | 10-02-2019 15:02:36 | 10-02-2019 15:02:36 | Great thanks!<|||||># [Codecov](https://codecov.io/gh/huggingface/transformers/pull/1400?src=pr&el=h1) Report
> Merging [#1400](https://codecov.io/gh/huggingface/transformers/pull/1400?src=pr&el=desc) into [master](https://codecov.io/gh/huggingface/transformers/commit/391db836ab7ed2ca61c51a7cf1b135b6ab92be58?src=pr&el=desc) will **not change** coverage.
> The diff coverage is `n/a`.
[](https://codecov.io/gh/huggingface/transformers/pull/1400?src=pr&el=tree)
```diff
@@ Coverage Diff @@
## master #1400 +/- ##
=======================================
Coverage 84.72% 84.72%
=======================================
Files 84 84
Lines 12591 12591
=======================================
Hits 10668 10668
Misses 1923 1923
```
| [Impacted Files](https://codecov.io/gh/huggingface/transformers/pull/1400?src=pr&el=tree) | Coverage Δ | |
|---|---|---|
| [transformers/modeling\_tf\_xlm.py](https://codecov.io/gh/huggingface/transformers/pull/1400/diff?src=pr&el=tree#diff-dHJhbnNmb3JtZXJzL21vZGVsaW5nX3RmX3hsbS5weQ==) | `88.23% <ø> (ø)` | :arrow_up: |
| [transformers/modeling\_tf\_bert.py](https://codecov.io/gh/huggingface/transformers/pull/1400/diff?src=pr&el=tree#diff-dHJhbnNmb3JtZXJzL21vZGVsaW5nX3RmX2JlcnQucHk=) | `95.7% <ø> (ø)` | :arrow_up: |
| [transformers/modeling\_bert.py](https://codecov.io/gh/huggingface/transformers/pull/1400/diff?src=pr&el=tree#diff-dHJhbnNmb3JtZXJzL21vZGVsaW5nX2JlcnQucHk=) | `88.17% <ø> (ø)` | :arrow_up: |
| [transformers/modeling\_tf\_distilbert.py](https://codecov.io/gh/huggingface/transformers/pull/1400/diff?src=pr&el=tree#diff-dHJhbnNmb3JtZXJzL21vZGVsaW5nX3RmX2Rpc3RpbGJlcnQucHk=) | `96.61% <ø> (ø)` | :arrow_up: |
------
[Continue to review full report at Codecov](https://codecov.io/gh/huggingface/transformers/pull/1400?src=pr&el=continue).
> **Legend** - [Click here to learn more](https://docs.codecov.io/docs/codecov-delta)
> `Δ = absolute <relative> (impact)`, `ø = not affected`, `? = missing data`
> Powered by [Codecov](https://codecov.io/gh/huggingface/transformers/pull/1400?src=pr&el=footer). Last update [391db83...0c39053](https://codecov.io/gh/huggingface/transformers/pull/1400?src=pr&el=lastupdated). Read the [comment docs](https://docs.codecov.io/docs/pull-request-comments).
|
|
transformers | 1,399 | closed | Generate Variable Length Text With GPT2 | This might be obviously explained in the documentation, but I've been browsing through the code for a while and can't seem to find a resolution, so thank you in advance for your help.
As demoed with Write with Transformers, it seems to generate variable length text suggestions. I was wondering how this would be possible with the transformers library given, and how it would be possible to interface the largest version of GPT2 to do so.
Thank you! | 10-02-2019 00:25:26 | 10-02-2019 00:25:26 | Hi! In Write With Transformer, we use the context to predict the following token. We then add that token to the initial context, to generate the following one. This way we can generate long sequences according to a given token.
In that app we stop generating tokens once we have reached a given time, or once we have seen an end of sentence token. We, therefore, don't generate variable length text suggestions, we just adjust the batch according to end tokens identified in our results.<|||||>Thanks for the explanation! |
transformers | 1,398 | closed | Fixed typo in docs README | 10-02-2019 00:22:06 | 10-02-2019 00:22:06 | # [Codecov](https://codecov.io/gh/huggingface/transformers/pull/1398?src=pr&el=h1) Report
> Merging [#1398](https://codecov.io/gh/huggingface/transformers/pull/1398?src=pr&el=desc) into [master](https://codecov.io/gh/huggingface/transformers/commit/391db836ab7ed2ca61c51a7cf1b135b6ab92be58?src=pr&el=desc) will **not change** coverage.
> The diff coverage is `n/a`.
[](https://codecov.io/gh/huggingface/transformers/pull/1398?src=pr&el=tree)
```diff
@@ Coverage Diff @@
## master #1398 +/- ##
=======================================
Coverage 84.72% 84.72%
=======================================
Files 84 84
Lines 12591 12591
=======================================
Hits 10668 10668
Misses 1923 1923
```
------
[Continue to review full report at Codecov](https://codecov.io/gh/huggingface/transformers/pull/1398?src=pr&el=continue).
> **Legend** - [Click here to learn more](https://docs.codecov.io/docs/codecov-delta)
> `Δ = absolute <relative> (impact)`, `ø = not affected`, `? = missing data`
> Powered by [Codecov](https://codecov.io/gh/huggingface/transformers/pull/1398?src=pr&el=footer). Last update [391db83...cd69bc9](https://codecov.io/gh/huggingface/transformers/pull/1398?src=pr&el=lastupdated). Read the [comment docs](https://docs.codecov.io/docs/pull-request-comments).
<|||||>👍 |
|
transformers | 1,397 | closed | remove token type inputs from roberta - fix #1234 | 10-01-2019 23:57:13 | 10-01-2019 23:57:13 | ||
transformers | 1,396 | closed | Fix syntax typo in README.md | 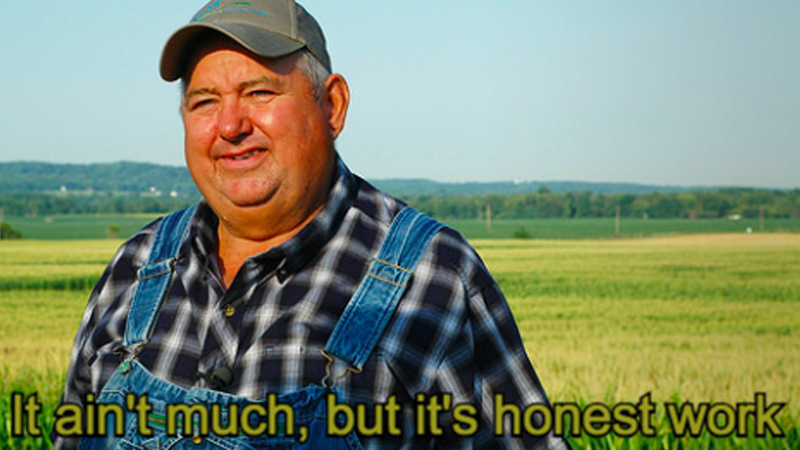
| 10-01-2019 18:58:13 | 10-01-2019 18:58:13 | Thanks :)<|||||>Your welcome, you are doing a great job!<|||||># [Codecov](https://codecov.io/gh/huggingface/transformers/pull/1396?src=pr&el=h1) Report
> Merging [#1396](https://codecov.io/gh/huggingface/transformers/pull/1396?src=pr&el=desc) into [master](https://codecov.io/gh/huggingface/transformers/commit/5c3b32d44d0164aaa9b91405f48e53cf53a82b35?src=pr&el=desc) will **not change** coverage.
> The diff coverage is `n/a`.
[](https://codecov.io/gh/huggingface/transformers/pull/1396?src=pr&el=tree)
```diff
@@ Coverage Diff @@
## master #1396 +/- ##
=======================================
Coverage 84.69% 84.69%
=======================================
Files 84 84
Lines 12596 12596
=======================================
Hits 10668 10668
Misses 1928 1928
```
------
[Continue to review full report at Codecov](https://codecov.io/gh/huggingface/transformers/pull/1396?src=pr&el=continue).
> **Legend** - [Click here to learn more](https://docs.codecov.io/docs/codecov-delta)
> `Δ = absolute <relative> (impact)`, `ø = not affected`, `? = missing data`
> Powered by [Codecov](https://codecov.io/gh/huggingface/transformers/pull/1396?src=pr&el=footer). Last update [5c3b32d...6b92911](https://codecov.io/gh/huggingface/transformers/pull/1396?src=pr&el=lastupdated). Read the [comment docs](https://docs.codecov.io/docs/pull-request-comments).
|
transformers | 1,395 | closed | Masking of special tokens in masked LM finetuning. | ## 🐛 Bug
roBERTa throws repeated warnings about the absence of special tokens in masked LM fine-tuning with `run_lm_finetuning.py`:
```
WARNING - transformers.modeling_roberta - A sequence with no special tokens has been passed to the RoBERTa model. This model requires special tokens in order to work. Please specify add_special_tokens=True in your encoding.
```
This made it look like there was a preprocessing problem, but it appears as though the random masking of tokens applies to the special tokens as well, both for BERT and roBERTa training with that script. It's not clear from the original papers if that's meant to happen, but I assumed it's not. The wording in section 3.3.1 of the BERT paper suggests they might not: They "mask 15% of all _wordpiece_ tokens at random". Their implementation would probably shed light but I just wanted to check with you, since masking of special tokens will affect the representation of [CLS]. Is this masking meant to happen?
Note that BERT does not throw such a warning, but the masking of special tokens also applies to that model.
Model I am using (Bert, XLNet....): BERT and roBERTa
Language I am using the model on (English, Chinese....): English WikiText 2 data.
The problem arise when using:
* [x] the official example scripts: run_lm_finetuning.py
* [ ] my own modified scripts: (give details)
The tasks I am working on is:
* [x] an official GLUE/SQUaD task: language model fine-tuning with run_lm_finetuning.py
* [] my own task or dataset: (give details)
## To Reproduce
Steps to reproduce the behavior:
Download the wikitext-2 data, and then run:
```
python run_lm_finetuning.py --output_dir=models/roberta_wikitext --model_type=roberta --model_name_or_path=roberta-base --do_train --train_data_file=/Users/oadams/corpora/wikitext-2/wiki.train.tokens --mlm
```
This is basically what's recommended [in the examples](https://huggingface.co/transformers/examples.html)
## Expected behavior
Warning-free training, or a warning that's easy to interpret for the user.
## Environment
* OS: MacOS and Ubuntu.
* Python version: 3.7.4
* PyTorch version: 1.2.0
* PyTorch Transformers version (or branch): 2.0
* Using GPU ? No.
* Distributed of parallel setup ? No
* Any other relevant information: | 10-01-2019 15:36:21 | 10-01-2019 15:36:21 | The same issue here, using 4 RTX 2080Ti with Ubuntu 18.04.<|||||>This issue exists as the `mask_tokens` function will sometimes replace `<s>` with a random word. Not sure whether `<s>` should be masked. A workaround would be adding a line
```
masked_indices[:, 0] = 0 # tokenizer.bos_token_id
```
right below
```
masked_indices = torch.bernoulli(torch.full(labels.shape, args.mlm_probability)).bool()
```
https://github.com/huggingface/transformers/blob/master/examples/run_lm_finetuning.py#L111.
<|||||>This issue has been automatically marked as stale because it has not had recent activity. It will be closed if no further activity occurs. Thank you for your contributions.
<|||||>So the [CLS] and [SEP] are being masked during the training or not? |
transformers | 1,394 | closed | Change gpt2 language model loss function | Hi all,
I want to include a new loss term for the gpt2 training loss. I am using the script run_lm_finetuning from the examples. This is my command:
python examples/run_lm_finetuning.py --output_dir=output --model_type=gpt2 --model_name_or_path=gpt2 --do_train --train_data_file=$TRAIN_FILE --eval_data_file=$TEST_FILE --overwrite_output_dir --max_steps 50
but I really can't figure out which loss function is being used. If i print inside the GPT2LMHeadModel forward function, nothing happens. Could you please tell me which loss function should I change?
Thank you a lot. | 10-01-2019 15:26:59 | 10-01-2019 15:26:59 | Afaik the "default" loss function that gets computed if you pass your labels to `GPT2LMHeadModel` is `torch.nn.CrossEntropyLoss`. If you want to use a different loss function, can't you just grab the logits from the model and apply your own?
Source:
https://github.com/huggingface/transformers/blob/391db836ab7ed2ca61c51a7cf1b135b6ab92be58/transformers/modeling_gpt2.py#L539<|||||>Unfortunately if I print a string inside the forward function, and then I run the training script, I don't get anything printed, so it seems like the training script is not using that function at all.<|||||>Hello! The `GPT2LMHeadModel` does have a way to compute its own cross-entropy loss, but only when the `labels` are specified -> you're providing the values like so:
```
model(inputs, labels=inputs)
```
and the model takes care of shifting the inputs to calculate a causal language modeling loss on them with cross-entropy.
If you wish to use your own loss function, don't specify the labels and the model will return a tuple containing the language modeling logits as the first value.<|||||>Hi,
thanks for your answer.
Can you tell me why a print statement inside the forward fuction of the GPT2LMHeadModel doesn't print anything when I run the run_lm_finetuning script ?
Which is the forward function I need to change?
Thanks.<|||||>Where have you put your print statement? Do you have `transformers` installed in your environment or is it relying on the cloned repository? You could try to add a breakpoint and debug it to see which function calls are made and how the loss is calculated.
Once again, if you wish to use your own loss function, don't specify the labels and the model will return a tuple containing the language modeling logits as the first value.<|||||>This issue has been automatically marked as stale because it has not had recent activity. It will be closed if no further activity occurs. Thank you for your contributions.
|
transformers | 1,393 | closed | With GPT-2 is it possible to get previous word prediction? |
Feature/Question: With GPT-2 is it possible to get previous word prediction?
Hi,
I say this after seeing this https://towardsdatascience.com/deconstructing-bert-distilling-6-patterns-from-100-million-parameters-b49113672f77
And wondering how I could maybe write a method that would allow me to predict the previous word? (ideally for GPT2)
Many thanks,
Vince. | 10-01-2019 12:39:24 | 10-01-2019 12:39:24 | Hi! There is one big difference between BERT and GPT-2, in that BERT is trained using masked language modeling, whereas GPT-2 is trained using causal language modeling.
During pre-training, BERT learns to predict masked words given a bi-directional context. GPT-2, on the other hand, learns to predict a word given only its left context. This is why GPT-2 is very good at text generation (it only needs the left-hand side context), while BERT isn't.
Given this, GPT-2 won't be able to do previous word prediction, as it does not handle the right-hand side context.<|||||>If you want to train your own GPT-2 model to predict previous words, you could feed in your entire training set in reverse word order. Then GPT-2 would learn to predict text backwards, and that model would then be able to tell you what word should come before a piece of text. |
transformers | 1,392 | closed | Bert's keyword argument 'output_all_encoded_layers' does not exist anymore? | ## 📚 Migration
Model I am using (Bert, XLNet....): Bert
Language I am using the model on (English, Chinese....): English
The problem arise when using:
* [ ] the official example scripts: (give details)
* [X] my own modified scripts: (give details)
The tasks I am working on is:
* [ ] an official GLUE/SQUaD task: (give the name)
* [X] my own task or dataset: (give details)
Details of the issue:
I was using the keyword argument `output_all_encoded_layers` before. Now the code throws an error, since it seems that this argument was removed. How can I still set `output_all_encoded_layers` to either True or False, e.g.:
```
context, _ = self.bert(context, output_all_encoded_layers=False)
```
## Checklist
- [X] I have read the migration guide in the readme.
- [X] I checked if a related official extension example runs on my machine.
| 10-01-2019 09:56:29 | 10-01-2019 09:56:29 | Hi! @thomwolf can correct me if I'm wrong, but I believe this keyword was changed to `output_hidden_states` in version 1.0.0.<|||||>I can confirm what @LysandreJik suggests. The output of the embeddings is now also included as the first element. <|||||>Alright, thank you very much! |
transformers | 1,391 | closed | Built-in pretrained models location | My laptop was run out of disk space while loading built-in pre-trained model.
Now BertForTokenClassification.from_pretrained("bert-base-cased") gives me RuntimeError: unexpected EOF, expected 5896093 more bytes. The file might be corrupted.
Where can I find that incomplete model and delete it so I can download the model from the start again? | 10-01-2019 08:45:30 | 10-01-2019 08:45:30 | I've found it. It's a binary file. It's in ~/.cache/torch/transformers |
transformers | 1,390 | closed | ❓ How to use cached hidden states in run_generation ? | ## ❓ Questions & Help
https://github.com/huggingface/transformers/blob/5c3b32d44d0164aaa9b91405f48e53cf53a82b35/examples/run_generation.py#L124
This line states that we could use `cached hidden states`. Correct me if I'm wrong :
* **Without using `cached hidden states`** : every step, the next token is predicted, but also all previous tokens are re-computed (which is useless because we already predicted it !)
* **Using `cached hidden states`** : every step, the next token is predicted, but previous tokens are not re-computed, because we are using their cached states.
So using cached hidden states would greatly increase the inference speed, specially for long generations.
---
My question is : **How to do that ?**
From the documentation I understand how to get the `cached hidden states` from the forward pass of the model, but I don't understand how to use it at the following step ? | 10-01-2019 08:11:34 | 10-01-2019 08:11:34 | Hi! Yes, you understood the gist of it. The self-attention related to already computed tokens is not computed again.
In order to use the past, you would get the past from the model pass (I'm using GPT-2 in this example, XLNet would have `mems` instead of `past`):
```py
logits, past = model(**inputs)
```
and you would then use the past on the following pass as follow:
```py
logits, past = model(**inputs, past=past)
```<|||||>Thank you for your fast response @LysandreJik !
Now it's very clear, but I have one more question :
For XLNet and TransfoXL, we need to use memory in order to not recompute previously generated token. This is ok when not using the memory for something else.
**But what if the memory is already used for something else ?**
Like we have a memory of 256 for XLNet, representing previous segments (or whatever), if we update the memory everytime a new token is generated, it means we are loosing part of the memory (and after generating 256 tokens, we will not be able to see anymore the memory of previous segment !).
**Is there a way around this problem in the current API ?**<|||||>This issue has been automatically marked as stale because it has not had recent activity. It will be closed if no further activity occurs. Thank you for your contributions.
|
Subsets and Splits
No community queries yet
The top public SQL queries from the community will appear here once available.