repo
stringclasses 1
value | number
int64 1
25.3k
| state
stringclasses 2
values | title
stringlengths 1
487
| body
stringlengths 0
234k
⌀ | created_at
stringlengths 19
19
| closed_at
stringlengths 19
19
| comments
stringlengths 0
293k
|
---|---|---|---|---|---|---|---|
transformers | 1,689 | closed | Can't export TransfoXLModel model | ## 🐛 Bug
<!-- Important information -->
I am trying to export TransfoXLModel and use it for inference from C++ API.
I tried torch.jit.trace(), torch.jit.script() and torch.onnx.export(). But none of these work.
Model I am using - TransfoXLModel:
Language I am using the model on - English
The problem arise when using:
```
model = TransfoXLModel.from_pretrained("transfo-xl-wt103", torchscript=True)
model.eval()
tokenizer = TransfoXLTokenizer.from_pretrained("transfo-xl-wt103", torchscript=True)
input_ids = torch.tensor(tokenizer.encode("Hello, my dog is cute")).unsqueeze(0) # Batch size 1
torch.jit.script(model, (input_ids))
```
The tasks I am working on is:
Running inference using C++ API
## To Reproduce
Executing above python code throws error.
<!-- If you have a code sample, error messages, stack traces, please provide it here as well. -->
```
/home/user/anaconda3/lib/python3.7/site-packages/torch/jit/__init__.py:1200: UserWarning: `optimize` is deprecated and has no effect. Use `with torch.jit.optimized_execution() instead
warnings.warn("`optimize` is deprecated and has no effect. Use `with torch.jit.optimized_execution() instead")
Traceback (most recent call last):
File "test_bert_jit.py", line 28, in <module>
torch.jit.script(model, (input_ids))
File "/home/user/anaconda3/lib/python3.7/site-packages/torch/jit/__init__.py", line 1203, in script
return torch.jit.torch.jit._recursive.recursive_script(obj)
File "/home/user/anaconda3/lib/python3.7/site-packages/torch/jit/_recursive.py", line 172, in recursive_script
stubs = list(map(make_stub, filtered_methods))
File "/home/user/anaconda3/lib/python3.7/site-packages/torch/jit/_recursive.py", line 169, in make_stub
return torch.jit.script_method(func, _jit_internal.createResolutionCallbackFromClosure(func))
File "/home/user/anaconda3/lib/python3.7/site-packages/torch/jit/__init__.py", line 1280, in script_method
ast = get_jit_def(fn, self_name="ScriptModule")
File "/home/user/anaconda3/lib/python3.7/site-packages/torch/jit/frontend.py", line 169, in get_jit_def
return build_def(ctx, py_ast.body[0], type_line, self_name)
File "/home/user/anaconda3/lib/python3.7/site-packages/torch/jit/frontend.py", line 209, in build_def
build_stmts(ctx, body))
File "/home/user/anaconda3/lib/python3.7/site-packages/torch/jit/frontend.py", line 127, in build_stmts
stmts = [build_stmt(ctx, s) for s in stmts]
File "/home/user/anaconda3/lib/python3.7/site-packages/torch/jit/frontend.py", line 127, in <listcomp>
stmts = [build_stmt(ctx, s) for s in stmts]
File "/home/user/anaconda3/lib/python3.7/site-packages/torch/jit/frontend.py", line 185, in __call__
return method(ctx, node)
File "/home/user/anaconda3/lib/python3.7/site-packages/torch/jit/frontend.py", line 352, in build_If
build_stmts(ctx, stmt.body),
File "/home/user/anaconda3/lib/python3.7/site-packages/torch/jit/frontend.py", line 127, in build_stmts
stmts = [build_stmt(ctx, s) for s in stmts]
File "/home/user/anaconda3/lib/python3.7/site-packages/torch/jit/frontend.py", line 127, in <listcomp>
stmts = [build_stmt(ctx, s) for s in stmts]
File "/home/user/anaconda3/lib/python3.7/site-packages/torch/jit/frontend.py", line 185, in __call__
return method(ctx, node)
File "/home/user/anaconda3/lib/python3.7/site-packages/torch/jit/frontend.py", line 283, in build_Assign
rhs = build_expr(ctx, stmt.value)
File "/home/user/anaconda3/lib/python3.7/site-packages/torch/jit/frontend.py", line 185, in __call__
return method(ctx, node)
File "/home/user/anaconda3/lib/python3.7/site-packages/torch/jit/frontend.py", line 442, in build_Call
args = [build_expr(ctx, py_arg) for py_arg in expr.args]
File "/home/user/anaconda3/lib/python3.7/site-packages/torch/jit/frontend.py", line 442, in <listcomp>
args = [build_expr(ctx, py_arg) for py_arg in expr.args]
File "/home/user/anaconda3/lib/python3.7/site-packages/torch/jit/frontend.py", line 184, in __call__
raise UnsupportedNodeError(ctx, node)
torch.jit.frontend.UnsupportedNodeError: GeneratorExp aren't supported:
at /home/user/transformers/transformers/modeling_transfo_xl.py:767:24
core_out = self.drop(core_out)
new_mems = self._update_mems(hids, mems, mlen, qlen)
# We transpose back here to shape [bsz, len, hidden_dim]
outputs = [core_out.transpose(0, 1).contiguous(), new_mems]
if self.output_hidden_states:
# Add last layer and transpose to library standard shape [bsz, len, hidden_dim]
hids.append(core_out)
hids = list(t.transpose(0, 1).contiguous() for t in hids)
~ <--- HERE
outputs.append(hids)
if self.output_attentions:
# Transpose to library standard shape [bsz, n_heads, query_seq_len, key_seq_len]
attentions = list(t.permute(2, 3, 0, 1).contiguous() for t in attentions)
outputs.append(attentions)
return outputs # last hidden state, new_mems, (all hidden states), (all attentions)
```
## Expected behavior
torch.jit.script() succeeds without any error
## Environment
* OS: Ubunut 18.04
* Python version: 3.7.4
* PyTorch version: 1.3.0
* PyTorch Transformers version (or branch): master @ ae1d03fc51bb22ed59517ee6f92c560417fdb049
* Using GPU ? Yes
* Distributed of parallel setup ? No.
* Any other relevant information:
Using torch.onnx.export() throws below error:
```
/home/user/transformers/transformers/modeling_transfo_xl.py:452: TracerWarning: There are 2 live references to the data region being modified when tracing in-place operator mul_. This might cause the trace to be incorrect, because all other views that also reference this data will not reflect this change in the trace! On the other hand, if all other views use the same memory chunk, but are disjoint (e.g. are outputs of torch.split), this might still be safe.
embed.mul_(self.emb_scale)
/home/user/transformers/transformers/modeling_transfo_xl.py:725: TracerWarning: Converting a tensor to a Python boolean might cause the trace to be incorrect. We can't record the data flow of Python values, so this value will be treated as a constant in the future. This means that the trace might not generalize to other inputs!
if mask_len > 0:
/home/user/transformers/transformers/modeling_transfo_xl.py:729: TracerWarning: Converting a tensor to a Python integer might cause the trace to be incorrect. We can't record the data flow of Python values, so this value will be treated as a constant in the future. This means that the trace might not generalize to other inputs!
dec_attn_mask = (torch.triu(all_ones, 1+mlen)
/home/user/transformers/transformers/modeling_transfo_xl.py:730: TracerWarning: Converting a tensor to a Python integer might cause the trace to be incorrect. We can't record the data flow of Python values, so this value will be treated as a constant in the future. This means that the trace might not generalize to other inputs!
+ torch.tril(all_ones, -mask_shift_len))[:, :, None] # -1
/home/user/transformers/transformers/modeling_transfo_xl.py:290: TracerWarning: Converting a tensor to a Python index might cause the trace to be incorrect. We can't record the data flow of Python values, so this value will be treated as a constant in the future. This means that the trace might not generalize to other inputs!
w_head_q = w_head_q[-qlen:]
/home/user/transformers/transformers/modeling_transfo_xl.py:321: TracerWarning: Converting a tensor to a Python number might cause the trace to be incorrect. We can't record the data flow of Python values, so this value will be treated as a constant in the future. This means that the trace might not generalize to other inputs!
if attn_mask is not None and torch.sum(attn_mask).item():
/home/user/transformers/transformers/modeling_transfo_xl.py:684: TracerWarning: Converting a tensor to a Python boolean might cause the trace to be incorrect. We can't record the data flow of Python values, so this value will be treated as a constant in the future. This means that the trace might not generalize to other inputs!
end_idx = mlen + max(0, qlen - 0 - self.ext_len)
/home/user/transformers/transformers/modeling_transfo_xl.py:685: TracerWarning: Converting a tensor to a Python boolean might cause the trace to be incorrect. We can't record the data flow of Python values, so this value will be treated as a constant in the future. This means that the trace might not generalize to other inputs!
beg_idx = max(0, end_idx - self.mem_len)
/home/user/transformers/transformers/modeling_transfo_xl.py:689: TracerWarning: Converting a tensor to a Python index might cause the trace to be incorrect. We can't record the data flow of Python values, so this value will be treated as a constant in the future. This means that the trace might not generalize to other inputs!
new_mems.append(cat[beg_idx:end_idx].detach())
/home/user/anaconda3/lib/python3.7/site-packages/torch/onnx/utils.py:617: UserWarning: ONNX export failed on ATen operator triu because torch.onnx.symbolic_opset10.triu does not exist
.format(op_name, opset_version, op_name))
Traceback (most recent call last):
File "test_bert_jit.py", line 37, in <module>
output_names = ['output']) # the model's output names
File "/home/user/anaconda3/lib/python3.7/site-packages/torch/onnx/__init__.py", line 143, in export
strip_doc_string, dynamic_axes, keep_initializers_as_inputs)
File "/home/user/anaconda3/lib/python3.7/site-packages/torch/onnx/utils.py", line 66, in export
dynamic_axes=dynamic_axes, keep_initializers_as_inputs=keep_initializers_as_inputs)
File "/home/user/anaconda3/lib/python3.7/site-packages/torch/onnx/utils.py", line 382, in _export
fixed_batch_size=fixed_batch_size)
File "/home/user/anaconda3/lib/python3.7/site-packages/torch/onnx/utils.py", line 262, in _model_to_graph
fixed_batch_size=fixed_batch_size)
File "/home/user/anaconda3/lib/python3.7/site-packages/torch/onnx/utils.py", line 132, in _optimize_graph
graph = torch._C._jit_pass_onnx(graph, operator_export_type)
File "/home/user/anaconda3/lib/python3.7/site-packages/torch/onnx/__init__.py", line 174, in _run_symbolic_function
return utils._run_symbolic_function(*args, **kwargs)
File "/home/user/anaconda3/lib/python3.7/site-packages/torch/onnx/utils.py", line 618, in _run_symbolic_function
op_fn = sym_registry.get_registered_op(op_name, '', opset_version)
File "/home/user/anaconda3/lib/python3.7/site-packages/torch/onnx/symbolic_registry.py", line 91, in get_registered_op
return _registry[(domain, version)][opname]
KeyError: 'triu'
```
## Additional context
<!-- Add any other context about the problem here. -->
| 11-01-2019 12:03:24 | 11-01-2019 12:03:24 | Yes, this is a known issue, `TransformerXL` is not traceable.
Fixing this is not on our short-term roadmap (cc @LysandreJik) but feel free to investigate and propose a solution in a PR if you want.<|||||>This issue has been automatically marked as stale because it has not had recent activity. It will be closed if no further activity occurs. Thank you for your contributions.
<|||||>Any update? Does it work successfully?<|||||>Same problem here.<|||||>`hids = [t.transpose(0, 1).contiguous() for t in hids]` maybe work. |
transformers | 1,688 | closed | fine tuning bert and roberta_base model | could you please let me know how to fine tune the BERT/ ROBERTA_Base models? | 11-01-2019 11:17:38 | 11-01-2019 11:17:38 | There is an example in [the documentation](https://huggingface.co/transformers/examples.html#roberta-bert-and-masked-language-modeling). |
transformers | 1,687 | closed | request for a Bert_base uncase model.bin file | ## 🚀 Feature
currently we r using BERT_Large uncased which slows down our application we like to use BERT_Base uncased model but BERT_base uncased model does not contain bin file.could you please let me know where I get model.bin file. | 11-01-2019 09:51:23 | 11-01-2019 09:51:23 | As with any model hosted on our S3, you can do as follows to load one of the checkpoints:
```py
from transformers import BertModel
model = BertModel.from_pretrained("bert-base-uncased")
```
You can find the list of pre-trained models in [the documentation](https://huggingface.co/transformers/pretrained_models.html).<|||||>can we use bert-based-uncased model for QA(question-answer) if yes then how
because model.predict(doc,q) giving error( **BertModel has no attribute predict**)
<|||||>The usage u need to reference on examples/run_squad.py and u will know
everything on that code
On Mon, Nov 4, 2019 at 02:53 rhl2k <[email protected]> wrote:
> can we use bert-based-uncased model for QA(question-answer) if yes then how
>
> because model.predict(doc,q) giving error( *BertModel has no attribute
> predict*)
>
> —
> You are receiving this because you are subscribed to this thread.
> Reply to this email directly, view it on GitHub
> <https://github.com/huggingface/transformers/issues/1687?email_source=notifications&email_token=AIEAE4FNAI7STEJQG4J6GYDQR4M2XA5CNFSM4JHYQUQ2YY3PNVWWK3TUL52HS4DFVREXG43VMVBW63LNMVXHJKTDN5WW2ZLOORPWSZGOEC52AAA#issuecomment-549167104>,
> or unsubscribe
> <https://github.com/notifications/unsubscribe-auth/AIEAE4GZOEPRXAX6NLWSAX3QR4M2XANCNFSM4JHYQUQQ>
> .
>
<|||||>You can find the documentation [here](https://huggingface.co/transformers/). The [quickstart](https://huggingface.co/transformers/quickstart.html) may be especially useful for you. As @pohanchi said, looking at the examples can also help in understanding the usage. |
transformers | 1,686 | closed | OpenAIGPTDoubleHeadsModel Not working (even with the official example...) | ## 🐛 Bug
<!-- Important information -->
Model I am using : OpenAI GPT (DoubleHeadsModel)
Language I am using the model on : English
The problem arise when using:
* [ ] the official example scripts: [link](https://huggingface.co/transformers/model_doc/gpt.html)
```
tokenizer = OpenAIGPTTokenizer.from_pretrained('openai-gpt')
model = OpenAIGPTDoubleHeadsModel.from_pretrained('openai-gpt')
tokenizer.add_special_tokens({'cls_token': '[CLS]'}) # Add a [CLS] to the vocabulary (we should train it also!)
choices = ["Hello, my dog is cute [CLS]", "Hello, my cat is cute [CLS]"]
input_ids = torch.tensor([tokenizer.encode(s) for s in choices]).unsqueeze(0) # Batch size 1, 2 choices
mc_token_ids = torch.tensor([input_ids.size(-1), input_ids.size(-1)]).unsqueeze(0) # Batch size 1
outputs = model(input_ids, mc_token_ids=mc_token_ids)
lm_prediction_scores, mc_prediction_scores = outputs[:2]
```
This codes doesn't work.
Maybe need to add `model.resize_token_embeddings(len(tokenizer))`
However, it still doesn't work with the following error
```
---------------------------------------------------------------------------
RuntimeError Traceback (most recent call last)
<ipython-input-20-53e79c250ad3> in <module>
7 input_ids = torch.tensor([tokenizer.encode(s) for s in choices]).unsqueeze(0) # Batch size 1, 2 choices
8 mc_token_ids = torch.tensor([input_ids.size(-1), input_ids.size(-1)]).unsqueeze(0) # Batch size 1
----> 9 outputs = model(input_ids, mc_token_ids=mc_token_ids)
10 lm_prediction_scores, mc_prediction_scores = outputs[:2]
/usr/local/lib/python3.6/dist-packages/torch/nn/modules/module.py in __call__(self, *input, **kwargs)
539 result = self._slow_forward(*input, **kwargs)
540 else:
--> 541 result = self.forward(*input, **kwargs)
542 for hook in self._forward_hooks.values():
543 hook_result = hook(self, input, result)
/usr/local/lib/python3.6/dist-packages/transformers/modeling_openai.py in forward(self, input_ids, attention_mask, token_type_ids, position_ids, head_mask, mc_token_ids, lm_labels, mc_labels)
603
604 lm_logits = self.lm_head(hidden_states)
--> 605 mc_logits = self.multiple_choice_head(hidden_states, mc_token_ids).squeeze(-1)
606
607 outputs = (lm_logits, mc_logits) + transformer_outputs[1:]
/usr/local/lib/python3.6/dist-packages/torch/nn/modules/module.py in __call__(self, *input, **kwargs)
539 result = self._slow_forward(*input, **kwargs)
540 else:
--> 541 result = self.forward(*input, **kwargs)
542 for hook in self._forward_hooks.values():
543 hook_result = hook(self, input, result)
/usr/local/lib/python3.6/dist-packages/transformers/modeling_utils.py in forward(self, hidden_states, cls_index)
728 cls_index = cls_index.expand((-1,) * (cls_index.dim()-1) + (hidden_states.size(-1),))
729 # shape of cls_index: (bsz, XX, 1, hidden_size) where XX are optional leading dim of hidden_states
--> 730 output = hidden_states.gather(-2, cls_index).squeeze(-2) # shape (bsz, XX, hidden_size)
731 elif self.summary_type == 'attn':
732 raise NotImplementedError
RuntimeError: Invalid index in gather at /pytorch/aten/src/TH/generic/THTensorEvenMoreMath.cpp:657
```
## Environment
* OS: Linux
* Python version: 3.6
* PyTorch version: 1.3
* Using GPU ? yes
| 11-01-2019 04:09:12 | 11-01-2019 04:09:12 | Indeed thanks, fixed |
transformers | 1,685 | closed | Unpickling errors when running examples | ## ❓ Questions & Help
Hi there, when I run the examples
```
%run run_generation.py \
--model_type=gpt2 \
--model_name_or_path=gpt2
```
I keep getting the following errors:
```
---------------------------------------------------------------------------
UnpicklingError Traceback (most recent call last)
~/research/transformers/examples/run_generation.py in <module>
258
259 if __name__ == '__main__':
--> 260 main()
~/research/transformers/examples/run_generation.py in main()
186 model_class, tokenizer_class = MODEL_CLASSES[args.model_type]
187 tokenizer = tokenizer_class.from_pretrained(args.model_name_or_path)
--> 188 model = model_class.from_pretrained(args.model_name_or_path)
189 model.to(args.device)
190 model.eval()
~/anaconda3/lib/python3.7/site-packages/transformers/modeling_utils.py in from_pretrained(cls, pretrained_model_name_or_path, *model_args, **kwargs)
343
344 if state_dict is None and not from_tf:
--> 345 state_dict = torch.load(resolved_archive_file, map_location='cpu')
346
347 missing_keys = []
~/anaconda3/lib/python3.7/site-packages/torch/serialization.py in load(f, map_location, pickle_module, **pickle_load_args)
385 f = f.open('rb')
386 try:
--> 387 return _load(f, map_location, pickle_module, **pickle_load_args)
388 finally:
389 if new_fd:
~/anaconda3/lib/python3.7/site-packages/torch/serialization.py in _load(f, map_location, pickle_module, **pickle_load_args)
562 f.seek(0)
563
--> 564 magic_number = pickle_module.load(f, **pickle_load_args)
565 if magic_number != MAGIC_NUMBER:
566 raise RuntimeError("Invalid magic number; corrupt file?")
UnpicklingError: invalid load key, '<'.
```
| 10-31-2019 21:01:30 | 10-31-2019 21:01:30 | Closing this in favor of #1684 |
transformers | 1,684 | closed | Access denied to pretrained GPT2 model | ## 🐛 Bug
<!-- Important information -->
Model I am using (Bert, XLNet....): GPT2
Language I am using the model on (English, Chinese....): English
The problem arise when using:
* [ ] the official example scripts: (give details)
* [x] my own modified scripts: I cannot load the GPT2 small pretrained model.
The tasks I am working on is:
* [ ] an official GLUE/SQUaD task: (give the name)
* [x] my own task or dataset: I am trying to instantiate a GPT2 pretrained model.
## To Reproduce
Steps to reproduce the behavior:
```
from pytorch_transformers import AutoModel
model = AutoModel.from_pretrained('gpt2')
```
This only happens with the 'gpt2' shortcut, not others ('gpt2-medium', etc.)
<!-- If you have a code sample, error messages, stack traces, please provide it here as well. -->
Error message:
```
---------------------------------------------------------------------------
UnpicklingError Traceback (most recent call last)
<ipython-input-14-e057f5f0ba3e> in <module>
1 from pytorch_transformers import AutoModel
----> 2 model = AutoModel.from_pretrained('gpt2')
~/pipeline/.venv/lib/python3.7/site-packages/pytorch_transformers/modeling_auto.py in from_pretrained(cls, pretrained_model_name_or_path, *model_args, **kwargs)
246 return OpenAIGPTModel.from_pretrained(pretrained_model_name_or_path, *model_args, **kwargs)
247 elif 'gpt2' in pretrained_model_name_or_path:
--> 248 return GPT2Model.from_pretrained(pretrained_model_name_or_path, *model_args, **kwargs)
249 elif 'transfo-xl' in pretrained_model_name_or_path:
250 return TransfoXLModel.from_pretrained(pretrained_model_name_or_path, *model_args, **kwargs)
~/pipeline/.venv/lib/python3.7/site-packages/pytorch_transformers/modeling_utils.py in from_pretrained(cls, pretrained_model_name_or_path, *model_args, **kwargs)
537
538 if state_dict is None and not from_tf:
--> 539 state_dict = torch.load(resolved_archive_file, map_location='cpu')
540 if from_tf:
541 # Directly load from a TensorFlow checkpoint
~/pipeline/.venv/lib/python3.7/site-packages/torch/serialization.py in load(f, map_location, pickle_module, **pickle_load_args)
385 f = f.open('rb')
386 try:
--> 387 return _load(f, map_location, pickle_module, **pickle_load_args)
388 finally:
389 if new_fd:
~/pipeline/.venv/lib/python3.7/site-packages/torch/serialization.py in _load(f, map_location, pickle_module, **pickle_load_args)
562 f.seek(0)
563
--> 564 magic_number = pickle_module.load(f, **pickle_load_args)
565 if magic_number != MAGIC_NUMBER:
566 raise RuntimeError("Invalid magic number; corrupt file?")
UnpicklingError: invalid load key, '<'.
```
Contents of the downloaded file:
```
<?xml version="1.0" encoding="UTF-8"?>
<Error><Code>AccessDenied</Code><Message>Access Denied</Message><RequestId>D027DE3363BB3D26</RequestId><HostId>GPDcAN+fZerpFZ5ZR9ZnATk3XIJ4GgLjCDMLnzvs48MRKG8soooyb8HM+zjBA0Gnn7HJc4CRqpA=</HostId></Error>%
```
## Expected behavior
Successfully load the pretrained model.
## Environment
* OS: macOS Catalina
* Python version: 3.7.4
* PyTorch version: 1.1.0
* PyTorch Transformers version (or branch): 1.1.0
* Using GPU ? No
* Distributed of parallel setup ? No
* Any other relevant information:
## Additional context
<!-- Add any other context about the problem here. -->
| 10-31-2019 21:00:47 | 10-31-2019 21:00:47 | I'm having the same error<|||||>There is a known (temporary) issue with our `gpt2` model – can you guys use `gpt2-medium` or `distilgpt2` instead for now?
cc @LysandreJik @thomwolf @n1t0 @clmnt <|||||>Sure thing! Thanks for letting us know :)<|||||>(should be fixed now)<|||||>Thank you!<|||||>This issue has been automatically marked as stale because it has not had recent activity. It will be closed if no further activity occurs. Thank you for your contributions.
|
transformers | 1,683 | closed | Add ALBERT to the library | This PR adds ALBERT to the library. It offers two new model architectures:
- AlbertModel
- AlbertForMaskedLM
AlbertModel acts in a similar way to BertModel as it returns a sequence output as well as a pooled output. AlbertForMaskedLM exposes an additional language modeling head.
A total of four pre-trained checkpoints are available, which are the checkpoints discussed in the official ALBERT paper, available on the TensorFlow hub page:
- albert-base
- albert-large
- albert-xlarge
- albert-xxlarge
These are currently available on the S3 bucket: an ALBERT model may be loaded like other models with the following code.
```py
from transformers import AlbertTokenizer, AlbertModel
tokenizer = AlbertTokenizer.from_pretrained("albert-base")
model = AlbertModel.from_pretrained("albert-base")
```
What is left to implement:
- ~PyTorch model & tests~
- ~Tokenizer & tests~
- ~Export PyTorch checkpoints~
- ~TensorFlow 2 model & tests~
- ~Export TensorFlow 2 checkpoints~
- Replicate the results obtained in the paper; **currently obtained 81 acc on MNLI with albert-base**
# Workflow for including a model from [README.md](https://github.com/huggingface/transformers/blob/master/templates/adding_a_new_model/README.md)
Here an overview of the general workflow:
- [ ] add model/configuration/tokenization classes
- [ ] add conversion scripts
- [ ] add tests
- [ ] finalize
Let's details what should be done at each step
## Adding model/configuration/tokenization classes
Here is the workflow for adding model/configuration/tokenization classes:
- [x] copy the python files from the present folder to the main folder and rename them, replacing `xxx` with your model name,
- [x] edit the files to replace `XXX` (with various casing) with your model name
- [x] copy-past or create a simple configuration class for your model in the `configuration_...` file
- [x] copy-past or create the code for your model in the `modeling_...` files (PyTorch and TF 2.0)
- [x] copy-past or create a tokenizer class for your model in the `tokenization_...` file
# Adding conversion scripts
Here is the workflow for the conversion scripts:
- [x] copy the conversion script (`convert_...`) from the present folder to the main folder.
- [x] edit this script to convert your original checkpoint weights to the current pytorch ones.
# Adding tests:
Here is the workflow for the adding tests:
- [x] copy the python files from the `tests` sub-folder of the present folder to the `tests` subfolder of the main folder and rename them, replacing `xxx` with your model name,
- [x] edit the tests files to replace `XXX` (with various casing) with your model name
- [x] edit the tests code as needed
# Final steps
You can then finish the addition step by adding imports for your classes in the common files:
- [x] add import for all the relevant classes in `__init__.py`
- [x] add your configuration in `configuration_auto.py`
- [x] add your PyTorch and TF 2.0 model respectively in `modeling_auto.py` and `modeling_tf_auto.py`
- [x] add your tokenizer in `tokenization_auto.py`
- [x] [high-level-API] add your models and tokenizer to `pipeline.py`
- [x] [high-level-API] add a link to your conversion script in the main conversion utility (currently in `__main__` but will be moved to the `commands` subfolder in the near future)
- [x] edit the PyTorch to TF 2.0 conversion script to add your model in the `convert_pytorch_checkpoint_to_tf2.py` file
- [x] add a mention of your model in the doc: `README.md` and the documentation it-self at `docs/source/pretrained_models.rst`.
- [x] upload the pretrained weigths, configurations and vocabulary files.
| 10-31-2019 18:17:08 | 10-31-2019 18:17:08 | # [Codecov](https://codecov.io/gh/huggingface/transformers/pull/1683?src=pr&el=h1) Report
> Merging [#1683](https://codecov.io/gh/huggingface/transformers/pull/1683?src=pr&el=desc) into [master](https://codecov.io/gh/huggingface/transformers/commit/fa735208c96c18283b8d2f3fcbfc3157bbd12b1e?src=pr&el=desc) will **increase** coverage by `0.9%`.
> The diff coverage is `87.16%`.
[](https://codecov.io/gh/huggingface/transformers/pull/1683?src=pr&el=tree)
```diff
@@ Coverage Diff @@
## master #1683 +/- ##
=========================================
+ Coverage 85.08% 85.99% +0.9%
=========================================
Files 94 98 +4
Lines 13920 14713 +793
=========================================
+ Hits 11844 12652 +808
+ Misses 2076 2061 -15
```
| [Impacted Files](https://codecov.io/gh/huggingface/transformers/pull/1683?src=pr&el=tree) | Coverage Δ | |
|---|---|---|
| [transformers/tokenization\_xlnet.py](https://codecov.io/gh/huggingface/transformers/pull/1683/diff?src=pr&el=tree#diff-dHJhbnNmb3JtZXJzL3Rva2VuaXphdGlvbl94bG5ldC5weQ==) | `90.24% <ø> (ø)` | :arrow_up: |
| [transformers/configuration\_albert.py](https://codecov.io/gh/huggingface/transformers/pull/1683/diff?src=pr&el=tree#diff-dHJhbnNmb3JtZXJzL2NvbmZpZ3VyYXRpb25fYWxiZXJ0LnB5) | `100% <100%> (ø)` | |
| [transformers/modeling\_albert.py](https://codecov.io/gh/huggingface/transformers/pull/1683/diff?src=pr&el=tree#diff-dHJhbnNmb3JtZXJzL21vZGVsaW5nX2FsYmVydC5weQ==) | `81.73% <81.73%> (ø)` | |
| [transformers/modeling\_tf\_albert.py](https://codecov.io/gh/huggingface/transformers/pull/1683/diff?src=pr&el=tree#diff-dHJhbnNmb3JtZXJzL21vZGVsaW5nX3RmX2FsYmVydC5weQ==) | `84.46% <84.46%> (ø)` | |
| [transformers/tokenization\_albert.py](https://codecov.io/gh/huggingface/transformers/pull/1683/diff?src=pr&el=tree#diff-dHJhbnNmb3JtZXJzL3Rva2VuaXphdGlvbl9hbGJlcnQucHk=) | `89.74% <89.74%> (ø)` | |
| [transformers/tests/modeling\_tf\_albert\_test.py](https://codecov.io/gh/huggingface/transformers/pull/1683/diff?src=pr&el=tree#diff-dHJhbnNmb3JtZXJzL3Rlc3RzL21vZGVsaW5nX3RmX2FsYmVydF90ZXN0LnB5) | `94.39% <94.39%> (ø)` | |
| [transformers/tests/modeling\_albert\_test.py](https://codecov.io/gh/huggingface/transformers/pull/1683/diff?src=pr&el=tree#diff-dHJhbnNmb3JtZXJzL3Rlc3RzL21vZGVsaW5nX2FsYmVydF90ZXN0LnB5) | `95.04% <95.04%> (ø)` | |
| [transformers/tests/tokenization\_albert\_test.py](https://codecov.io/gh/huggingface/transformers/pull/1683/diff?src=pr&el=tree#diff-dHJhbnNmb3JtZXJzL3Rlc3RzL3Rva2VuaXphdGlvbl9hbGJlcnRfdGVzdC5weQ==) | `97.43% <97.43%> (ø)` | |
| [transformers/tests/modeling\_bert\_test.py](https://codecov.io/gh/huggingface/transformers/pull/1683/diff?src=pr&el=tree#diff-dHJhbnNmb3JtZXJzL3Rlc3RzL21vZGVsaW5nX2JlcnRfdGVzdC5weQ==) | `96.38% <0%> (-0.54%)` | :arrow_down: |
| [transformers/configuration\_utils.py](https://codecov.io/gh/huggingface/transformers/pull/1683/diff?src=pr&el=tree#diff-dHJhbnNmb3JtZXJzL2NvbmZpZ3VyYXRpb25fdXRpbHMucHk=) | `98.66% <0%> (-0.02%)` | :arrow_down: |
| ... and [20 more](https://codecov.io/gh/huggingface/transformers/pull/1683/diff?src=pr&el=tree-more) | |
------
[Continue to review full report at Codecov](https://codecov.io/gh/huggingface/transformers/pull/1683?src=pr&el=continue).
> **Legend** - [Click here to learn more](https://docs.codecov.io/docs/codecov-delta)
> `Δ = absolute <relative> (impact)`, `ø = not affected`, `? = missing data`
> Powered by [Codecov](https://codecov.io/gh/huggingface/transformers/pull/1683?src=pr&el=footer). Last update [fa73520...afef0ac](https://codecov.io/gh/huggingface/transformers/pull/1683?src=pr&el=lastupdated). Read the [comment docs](https://docs.codecov.io/docs/pull-request-comments).
<|||||>Will there be models for classification?<|||||>Yes, at the moment there is AlbertForSequenceClassification and there may be more soon<|||||>@LysandreJik Thanks for adding this :+1:
I've one question: the ALBERT team did release version 2 of their models yesterday, see:
https://github.com/google-research/google-research/commit/2ba150bef51fcedcfda31f16321264300f201a8d
Are these updated models available on S3 yet 🤔<|||||>V2 just use 0 dropout and lr to 1e-5, the architecture didn’t change, so
maybe it just need time to transfer model to here.
On Sat, Nov 2, 2019 at 21:06 Stefan Schweter <[email protected]>
wrote:
> @LysandreJik <https://github.com/LysandreJik> Thanks for adding this 👍
>
> I've one question: the ALBERT team did release version 2 of their models
> yesterday, see:
>
> google-research/google-research@2ba150b
> <https://github.com/google-research/google-research/commit/2ba150bef51fcedcfda31f16321264300f201a8d>
>
> Are these updated models available on S3 yet 🤔
>
> —
> You are receiving this because you are subscribed to this thread.
> Reply to this email directly, view it on GitHub
> <https://github.com/huggingface/transformers/pull/1683?email_source=notifications&email_token=AIEAE4FY2TZTBZ5YV6P5ARLQRV3ONA5CNFSM4JHPMB22YY3PNVWWK3TUL52HS4DFVREXG43VMVBW63LNMVXHJKTDN5WW2ZLOORPWSZGOEC43PFI#issuecomment-549042069>,
> or unsubscribe
> <https://github.com/notifications/unsubscribe-auth/AIEAE4BGB37TAZRZI6CQ3HLQRV3ONANCNFSM4JHPMB2Q>
> .
>
<|||||>I'm not sure how heavily you want to take advantage of Apex when available, but Apex does provide a fused implementation of Lamb. https://nvidia.github.io/apex/optimizers.html#apex.optimizers.FusedLAMB<|||||>@stefan-it the ALBERT v2 models are now available on the S3. You can access them using `albert-{base,large,xlarge,xxlarge}-v2` identifiers!
@BramVanroy Indeed, thanks! For now we're focusing more on the model implementation rather than the optimizers; the optimizers can be obtained from other libraries (such as apex) and used with the models from `transformers` so it is not a priority right now.<|||||>Hi @LysandreJik thanks for the model versioning :)
Just a few notes from my (early) experiments with this ALBERT implementation.
I used a feature-based approach in Flair for NER on English CoNLL dataset. More precisely I used embeddings from all layers (incl. word embedding layer) + scalar mix over all layers to get an embedding for the first subtoken of each token. Results for the base model are "ok": 93.13 (dev) and 89.17 (test) compared to BERT base: 94.74 (dev) and 91.38 (test).
After work I implemented an `AlbertForTokenClassification` class and added it to the `run_ner.py` example script. With default parameters 88.06 (dev) and 82.94 (test) could be achieved (so there's large room for improvement in my implementation 😅).
But: I also tested the `large` and `xlarge` models. Using Flair (and all 24 + 1 layers with scalar mix) the F-score dropped to 45% on test set?! The fine-tuning experiment (with `run_ner.py`) yields 0% for F-score 😂
I'm not sure what's going on with the > `large` models 🤔 (I did experiments for NER only)<|||||>HI @stefan-it,
I won't suggest ALBERT for NER task. As of now, all the released weights are trained using lowering the sentence. NER model is usually built using Cased models. BERT NER is based on bert-base/large-cased. <|||||>For BERT the difference was ~ 0.2 to 0.3% on CoNLL (base and large model, feature-base approach) - but I'll further investigate the large ALBERT models 😅<|||||>Hi @stefan-it, thanks for your study! I could indeed replicate the issue with ALBERT-large which has very bad results on SQuAD after being fine-tuned on it. I'm looking into it today and I'll update you on the progress.<|||||>I curious about how worse for squad
On Wed, Nov 6, 2019 at 21:57 Lysandre Debut <[email protected]>
wrote:
> Hi @stefan-it <https://github.com/stefan-it>, thanks for your study! I
> could indeed replicate the issue with ALBERT-large which has very bad
> results on SQuAD after being fine-tuned on it. I'm looking into it today
> and I'll update you on the progress.
>
> —
> You are receiving this because you commented.
>
>
> Reply to this email directly, view it on GitHub
> <https://github.com/huggingface/transformers/pull/1683?email_source=notifications&email_token=AIEAE4G7RYHSXRPZ5Y2MWJ3QSLENDA5CNFSM4JHPMB22YY3PNVWWK3TUL52HS4DFVREXG43VMVBW63LNMVXHJKTDN5WW2ZLOORPWSZGOEDGTPTQ#issuecomment-550320078>,
> or unsubscribe
> <https://github.com/notifications/unsubscribe-auth/AIEAE4AOIEITNSQM6EO55FDQSLENDANCNFSM4JHPMB2Q>
> .
>
<|||||>I've looked into it and there seems to be an error with the models `large`, `xlarge` and `xxlarge` version 2. The `base` models of both versions as well as the larger models of version 1 seem to work correctly (there was an issue that was fixed this morning).
@pohanchi based on a single epoch just to check the models were learning, `albert-base-v2` obtains 82.5 exact and 89.9 F1 and `albert-large-v1` obtains 82.8 exact and 90 F1
I'm looking into the V2 models now.<|||||>Side question: for how long are you planning to support Python 2? Considering it's as good as EOL and all that.<|||||>@BramVanroy, as long as Google outputs models in Python 2 we'll continue to maintain it, and probably for a few months after that!<|||||>(That's only for the core code though. Examples and scripts are already Python 3 only AFAIK)<|||||>@LysandreJik Great job! Could you elaborate on why you added and removed the Lamb optimizer? Is there any issue with this implementation?<|||||>Great work all. Tried it and noticed a few things, that may or may not be issues, but I'll post the details here just in case.:
- doesn't work in pytorch 1.1.0, does in 1.2.0. This is probobly OK as 1.2.0 is the version listed in requirements.dev.txt
- The error is for line [`w = self.dense.weight.T` "Parameter self.dense.weight has not attribute T"](https://github.com/huggingface/transformers/blob/06fc337815/transformers/modeling_albert.py#L206)
- You may be aware of this but it doesn't work with fp16 O1 yet
- `RuntimeError: Expected object of scalar type Half but got scalar type Float for argument #2 'mat2'`
- reffering to line [`projected_context_layer = torch.einsum("bfnd,ndh->bfh", context_layer, w) + b`](https://github.com/huggingface/transformers/blob/06fc337815/transformers/modeling_albert.py#L209). Specifically context_layer is half, w while b are float.
- these changes fix fp16 O1:
- `w = self.dense.weight.T.view(self.num_attention_heads, self.attention_head_size, self.hidden_size).to(context_layer.dtype)`
- `b = self.dense.bias.to(context_layer.dtype)`
- it does run without fp16 :)<|||||>Thank you very much for your great work!@LysandreJik
I have tried running with the run_glue.py file to obtain the test accuracy for MNLI task.
**Without training, just evaluation**. Using the **albert-base-v1** model from the S3, I have obtained **31.8% accuracy** for MNLI, which differs greatly from the ALBERT paper. However, after training with the default hyperparameters specified in the run_glue.py file, I obtained an accuracy which is similar to the paper.
I am a new guy to NLP, previously working in CV. I am wondering does the S3 model contains the pretrained weight for ALBERT? Since without training, the result differs greatly from the papers.
<|||||>@panaali LAMB was first added but I couldn't manage to make it work immediately, so as the authors said that there was not a huge difference between ADAM and LAMB, I removed it and fine-tuned with ADAM instead. As I told Bram a few messages ago: "For now we're focusing more on the model implementation rather than the optimizers; the optimizers can be obtained from other libraries (such as apex) and used with the models from transformers so it is not a priority right now.". I believe you can use existing LAMB implementations with our models and it will work out of the box, such as [cybertronai's implementation.](https://github.com/cybertronai/pytorch-lamb), or from apex.
@wassname Thank you for your comments, I'm looking into that.
@astrongstorm the model as it is saved on our S3 only contains the base model, without the classification head (similarly to most of the models hosted on our S3). Before using them, it is essential to fine-tune them so that the classification head may be trained on the actual dataset.<|||||>@LysandreJik Thanks for your reply! There is still one confusing point about the S3 model. I am wondering in S3 model, does it contain both hyperparameter and the parameters for the model, or it only contains one of them. <|||||>The S3 holds several files:
- The configuration files which holds what you might call the hyper-parameters: number of inner group, hidden size, vocabulary size, etc.
- The model files which contain parameters for pytorch (albert-xxx-pytorch_model.bin) and for tensorflow (albert-xxx-tf_model.h5)
- the tokenizer vocabulary files<|||||>Thanks @LysandreJik for the great work! I am looking forward to use it. When will this branch be merged to the master or is there a timeline?<|||||>Hi @jimmycode, it should be merged at the end of the week.<|||||>I think the v1 models are looking good (v2 are currently very bad) - I did some comparisons for NER (CoNLL-2003):
| Model | Run 1 | Run 2 | Run 3 | Run 4 | Run 5 | Avg.
| ------------------------ | ----- | ----- | ----- | ----- | ----- | ---------
| BERT large, cased (Dev) | 95.69 | 95.47 | 95.77 | 95.86 | 95.91 | 95.74
| BERT large, cased (Test) | 91.73 | 91.17 | 91.77 | 91.22 | 91.46 | **91.47**
| ALBERT xxlarge, uncased, v1 (Dev) | 95.35 | 95.42 | 95.17 | 95.16 | 95.39 | 95.30
| ALBERT xxlarge, uncased, v1 (Test) | 91.49 | 91.60 | 91.69 | 90.88 | 91.27 | 91.39
(although cased vs. uncased is not really a fair comparison)
I'll prepare a PR when the ALBERT code was merged to support a "for-token-classification" interface that can be used in the `run_ner.py` example.<|||||>Hi Thanks for the quick addition.
Does ALBERT require the usage of AlbertTokenizer? or can we simply use BERTTokenizer?
Because otherwise, there might be a need to re-process all data using AlbertTokenizer.<|||||>Hi @jshin49. yes the `AlbertTokenizer` should be used: BERT uses word pieces, ALBERT uses a sentence piece model. The output of the tokenizer implementations is totally different:
```python
from transformers import AlbertTokenizer, BertTokenizer
bert_tokenizer = BertTokenizer.from_pretrained("bert-base-uncased")
albert_tokenizer = AlbertTokenizer.from_pretrained("albert-base-v1")
sentence = "neuschwanstein near munich"
```
Outputs:
```python
In [9]: bert_tokenizer.tokenize(sentence)
Out[9]: ['ne', '##us', '##ch', '##wan', '##stein', 'near', 'munich']
In [10]: albert_tokenizer.tokenize(sentence)
Out[10]: ['▁neu', 'sch', 'wan', 'stein', '▁near', '▁munich']
```<|||||>Thank you @LysandreJik for the great work! , Do you have any plans to add multilingual ALBERT?<|||||>> After work I implemented an `AlbertForTokenClassification` class and added it to the `run_ner.py` example script.
@stefan-it could you add this as PR?
<|||||>Oh, I totally forgot that 😅
I can look into it the next days :) |
transformers | 1,682 | closed | xnli benchmark | adapted from `run_glue.py` | 10-31-2019 16:31:24 | 10-31-2019 16:31:24 | # [Codecov](https://codecov.io/gh/huggingface/transformers/pull/1682?src=pr&el=h1) Report
> Merging [#1682](https://codecov.io/gh/huggingface/transformers/pull/1682?src=pr&el=desc) into [master](https://codecov.io/gh/huggingface/transformers/commit/7daacf00df433621e3d3872a9f3bb574d1b00f5a?src=pr&el=desc) will **increase** coverage by `1.67%`.
> The diff coverage is `35.84%`.
[](https://codecov.io/gh/huggingface/transformers/pull/1682?src=pr&el=tree)
```diff
@@ Coverage Diff @@
## master #1682 +/- ##
=========================================
+ Coverage 84.03% 85.7% +1.67%
=========================================
Files 94 92 -2
Lines 14021 13704 -317
=========================================
- Hits 11782 11745 -37
+ Misses 2239 1959 -280
```
| [Impacted Files](https://codecov.io/gh/huggingface/transformers/pull/1682?src=pr&el=tree) | Coverage Δ | |
|---|---|---|
| [transformers/configuration\_distilbert.py](https://codecov.io/gh/huggingface/transformers/pull/1682/diff?src=pr&el=tree#diff-dHJhbnNmb3JtZXJzL2NvbmZpZ3VyYXRpb25fZGlzdGlsYmVydC5weQ==) | `89.74% <ø> (ø)` | :arrow_up: |
| [transformers/tokenization\_distilbert.py](https://codecov.io/gh/huggingface/transformers/pull/1682/diff?src=pr&el=tree#diff-dHJhbnNmb3JtZXJzL3Rva2VuaXphdGlvbl9kaXN0aWxiZXJ0LnB5) | `100% <ø> (ø)` | :arrow_up: |
| [transformers/modeling\_distilbert.py](https://codecov.io/gh/huggingface/transformers/pull/1682/diff?src=pr&el=tree#diff-dHJhbnNmb3JtZXJzL21vZGVsaW5nX2Rpc3RpbGJlcnQucHk=) | `95.8% <ø> (-0.03%)` | :arrow_down: |
| [transformers/data/\_\_init\_\_.py](https://codecov.io/gh/huggingface/transformers/pull/1682/diff?src=pr&el=tree#diff-dHJhbnNmb3JtZXJzL2RhdGEvX19pbml0X18ucHk=) | `100% <100%> (ø)` | :arrow_up: |
| [transformers/data/processors/\_\_init\_\_.py](https://codecov.io/gh/huggingface/transformers/pull/1682/diff?src=pr&el=tree#diff-dHJhbnNmb3JtZXJzL2RhdGEvcHJvY2Vzc29ycy9fX2luaXRfXy5weQ==) | `100% <100%> (ø)` | :arrow_up: |
| [transformers/tokenization\_xlm.py](https://codecov.io/gh/huggingface/transformers/pull/1682/diff?src=pr&el=tree#diff-dHJhbnNmb3JtZXJzL3Rva2VuaXphdGlvbl94bG0ucHk=) | `83.6% <100%> (+0.39%)` | :arrow_up: |
| [transformers/data/metrics/\_\_init\_\_.py](https://codecov.io/gh/huggingface/transformers/pull/1682/diff?src=pr&el=tree#diff-dHJhbnNmb3JtZXJzL2RhdGEvbWV0cmljcy9fX2luaXRfXy5weQ==) | `34.04% <25%> (-0.85%)` | :arrow_down: |
| [transformers/data/processors/xnli.py](https://codecov.io/gh/huggingface/transformers/pull/1682/diff?src=pr&el=tree#diff-dHJhbnNmb3JtZXJzL2RhdGEvcHJvY2Vzc29ycy94bmxpLnB5) | `31.11% <31.11%> (ø)` | |
| [transformers/modeling\_ctrl.py](https://codecov.io/gh/huggingface/transformers/pull/1682/diff?src=pr&el=tree#diff-dHJhbnNmb3JtZXJzL21vZGVsaW5nX2N0cmwucHk=) | `95.45% <0%> (-1.01%)` | :arrow_down: |
| [transformers/modeling\_transfo\_xl.py](https://codecov.io/gh/huggingface/transformers/pull/1682/diff?src=pr&el=tree#diff-dHJhbnNmb3JtZXJzL21vZGVsaW5nX3RyYW5zZm9feGwucHk=) | `75.16% <0%> (-0.75%)` | :arrow_down: |
| ... and [39 more](https://codecov.io/gh/huggingface/transformers/pull/1682/diff?src=pr&el=tree-more) | |
------
[Continue to review full report at Codecov](https://codecov.io/gh/huggingface/transformers/pull/1682?src=pr&el=continue).
> **Legend** - [Click here to learn more](https://docs.codecov.io/docs/codecov-delta)
> `Δ = absolute <relative> (impact)`, `ø = not affected`, `? = missing data`
> Powered by [Codecov](https://codecov.io/gh/huggingface/transformers/pull/1682?src=pr&el=footer). Last update [7daacf0...828058a](https://codecov.io/gh/huggingface/transformers/pull/1682?src=pr&el=lastupdated). Read the [comment docs](https://docs.codecov.io/docs/pull-request-comments).
<|||||>Great addition! As you may have seen, we've been slowly moving the utils from our examples folder to the actual transformer library.
We've done so with GLUE and we have put the processors directly in `transformers/data/processors/glue.py`. This way the processors may be used as a component of the library rather than as a utility class/function.
Do you think you could do the same for XNLI? It would require you to create a file `transformers/data/processors/xnli.py` and put the `XnliProcessor` there.<|||||>Concerning the documentation, if you choose to add `XnliProcessor` to the processors it would be great to add it to the processors documentation in `docs/source/main_classes/processors.rst`<|||||>This one looks ready to be merged. @thomwolf ? |
transformers | 1,681 | closed | Wrong Roberta special tokens in releases on GitHub | ## 🐛 Bug
Model I am using (Bert, XLNet....): Roberta
Language I am using the model on (English, Chinese....): Potentially wrong on any language
The problem arise when using the official example scripts: see https://github.com/huggingface/transformers/releases/tag/1.1.0
In the section `Tokenizer sequence pair handling` the special tokens for Roberta are wrong if I'm not mistaken. The example reads:
```
[CLS] SEQUENCE_0 [SEP] [SEP] SEQUENCE_1 [SEP]
```
whereas Roberta's actual representation for a sequence pair including special tokens should be (also following transformer's official documentation, cf. https://huggingface.co/transformers/model_doc/roberta.html):
```
<s> SEQUENCE_0 </s> <s> SEQUENCE_1 </s>
```
Note the <s> or </s> instead of [SEP]. I am not sure about the [CLS], though, but I think for Roberta it should not be there.
| 10-31-2019 16:21:38 | 10-31-2019 16:21:38 | You are right the release is wrong, it should be `<s> SEQUENCE_0 </s></s> SEQUENCE_1 </s>`. I just updated it; thank you! |
transformers | 1,680 | closed | Error when creating RobertTokenizer for distilroberta-base | ## 🐛 Bug
Model I am using (Bert, XLNet....): DistilRoberta
Language I am using the model on (English, Chinese....): EN
The problem arise when using the official example scripts: https://github.com/huggingface/transformers/tree/master/examples/distillation
## To Reproduce
```
RobertaTokenizer.from_pretrained('distilroberta-base')
```
this will yield an error:
```
OSError: Model name 'distilroberta-base' was not found in tokenizers model name list (roberta-base, roberta-large, roberta-large-mnli). We assumed 'distilroberta-base' was a path or url to a directory containing vocabulary files named ['vocab.json', 'merges.txt'] but couldn't find such vocabulary files at this path or url.
```
## Expected behavior
should produce a RobertaTokenizer instance, which can also be used for `distilroberta-base`.
## Environment
* OS: MacOS
* Python version: 3.7
* PyTorch version: 1.3.0
* PyTorch Transformers version (or branch): 2.1.1
* Using GPU ? no
| 10-31-2019 10:26:20 | 10-31-2019 10:26:20 | It's not in a pip released version yet so you need to pull from master if you want to use it for now.
We'll do a release soon.<|||||>Thanks for the info. Do you have an estimation when that pip release would be, @julien-c ?<|||||>Reviving this thread. I just cloned 2.2.2 from the master and updated `transformers`. `distilroberta-base` is still not available. Am I missing something? Thanks, you all!..
> OSError: Model name 'distilroberta-base' was not found in model name list (distilbert-base-uncased, distilbert-base-uncased-distilled-squad, distilbert-base-german-cased, distilbert-base-multilingual-cased)
<|||||>What are the exact commands you typed @oersoy1? <|||||>@julien-c OK, I found out what is happening and will document here just in case someone else falls into the same trap. I wrote my custom script similar to run_glue.py. I was passing `distilbert` to the `args.model_type` argument and the `model_name` got `distilroberta-base` assigned. I assumed that it was a subclass of `distilbert` models so the type intuitively looked as if it should have been distilbert. [This list](https://huggingface.co/transformers/pretrained_models.html) certainly gives me that impression.
However, looking at the distillation [examples](https://github.com/huggingface/transformers/tree/master/examples/distillation), I realized the model type needs to be `roberta` not `distilbert`.
It is a little bit confusing but regardless, I got `distilroberta-base` working and it gave me great results.
Thanks a lot!<|||||>Ah, yeah, you are correct. Moved the model shortcut in the table in ac1b449
<|||||>No worries. I see that you have corrected the pretrained models list and moved `distilroberta-base` under `roberta` which was the main problem for me. Updating all documentation when you make the changes could be difficult, especially when the gatekeepers for a specific document is different than the ones making the change. |
transformers | 1,679 | closed | Fix https://github.com/huggingface/transformers/issues/1673 | 10-31-2019 09:09:24 | 10-31-2019 09:09:24 | # [Codecov](https://codecov.io/gh/huggingface/transformers/pull/1679?src=pr&el=h1) Report
> Merging [#1679](https://codecov.io/gh/huggingface/transformers/pull/1679?src=pr&el=desc) into [master](https://codecov.io/gh/huggingface/transformers/commit/fa735208c96c18283b8d2f3fcbfc3157bbd12b1e?src=pr&el=desc) will **increase** coverage by `0.05%`.
> The diff coverage is `n/a`.
[](https://codecov.io/gh/huggingface/transformers/pull/1679?src=pr&el=tree)
```diff
@@ Coverage Diff @@
## master #1679 +/- ##
==========================================
+ Coverage 85.08% 85.14% +0.05%
==========================================
Files 94 94
Lines 13920 13920
==========================================
+ Hits 11844 11852 +8
+ Misses 2076 2068 -8
```
| [Impacted Files](https://codecov.io/gh/huggingface/transformers/pull/1679?src=pr&el=tree) | Coverage Δ | |
|---|---|---|
| [transformers/modeling\_utils.py](https://codecov.io/gh/huggingface/transformers/pull/1679/diff?src=pr&el=tree#diff-dHJhbnNmb3JtZXJzL21vZGVsaW5nX3V0aWxzLnB5) | `92.44% <ø> (+1.45%)` | :arrow_up: |
| [transformers/tests/modeling\_common\_test.py](https://codecov.io/gh/huggingface/transformers/pull/1679/diff?src=pr&el=tree#diff-dHJhbnNmb3JtZXJzL3Rlc3RzL21vZGVsaW5nX2NvbW1vbl90ZXN0LnB5) | `76.49% <0%> (+0.59%)` | :arrow_up: |
------
[Continue to review full report at Codecov](https://codecov.io/gh/huggingface/transformers/pull/1679?src=pr&el=continue).
> **Legend** - [Click here to learn more](https://docs.codecov.io/docs/codecov-delta)
> `Δ = absolute <relative> (impact)`, `ø = not affected`, `? = missing data`
> Powered by [Codecov](https://codecov.io/gh/huggingface/transformers/pull/1679?src=pr&el=footer). Last update [fa73520...ac29353](https://codecov.io/gh/huggingface/transformers/pull/1679?src=pr&el=lastupdated). Read the [comment docs](https://docs.codecov.io/docs/pull-request-comments).
<|||||>This looks good to me!<|||||>Yes indeed, thanks! |
|
transformers | 1,678 | closed | Download assets directly to the specified cache_dir | ## 🚀 Feature
```
import torch
from transformers import *
TRANSFORMERS_CACHE='/path/to/my/transformers-cache'
tokenizer = BertTokenizer.from_pretrained('bert-base-uncased', cache_dir=TRANSFORMERS_CACHE)
```
Actual behavior: It downloads the asset into a temp folder and then copies it to the specified cache_dir.
Proposed behavior: Download the asset directly to the specified cache_dir.
Impacted code part: https://github.com/huggingface/transformers/blob/master/transformers/file_utils.py#L295-L322
## Motivation
We have an environment setup where the tmp folders have limited space, because it is not a mounted docker volume. If the `"asset size" > 10GB - "docker image size"` then it won't be able to download the asset. (The 10GB limitation is a docker limitation)
| 10-31-2019 08:39:19 | 10-31-2019 08:39:19 | I have read the comment part of the reference code:
```
# Download to temporary file, then copy to cache dir once finished.
# Otherwise you get corrupt cache entries if the download gets interrupted.
```
So I would change my proposal:
* Either let it be configurable to skip the tmp folder and download directly to the cache folder -> the user will know what he is doing and will know that the asset could get corrupted
* Or check the file in the cache before usage - e.g. using checksums
* Or write "download has started" and "download has finished" information to the meta data file that can be checked before asset usage.
<|||||>I would propose to download to the cache_dir with a specific temporary name (like a `.part` suffix) and copy + rename at the end.
Probably best to activate that with an option `use_cache_dir_as_tmp`. To not clutter the cache dir with temporary files in the default settings.
Do you want to submit a PR for that? Would be happy to review it<|||||>Yes that is also a good approach. For now, we seem to be okay with this limitation, but I'll do a pr if we face this as an issue or have some free time.<|||||>Same problem here. On cluster, /tmp folder is small. Keep getting no space on device.<|||||>I fixed this in b67fa1a8d2302d808ecb9d95355181eaf21ee3b6.<|||||>Until there's a release with this fix, you can set $TMPDIR to an appropriate location if /tmp is too small.<|||||>Cool, thank you @aaugustin ! |
transformers | 1,677 | closed | i want to use bert pre-trained modle in a text classification problem which the text with Multi-label. But,there are some problems . | ## ❓ Questions & Help
<!-- A clear and concise description of the question. -->
i want to use bert pre-trained modle in a text classification problem which the text with Multi-labels. Which program and task should i select between run_glue.py、run_multiple_choice.py、run_squad.py and so on?
for example of my text:“I'd like 2 tickets to see Zoolander 2 tomorrow at Regal Meridian 16 theater in Seattle at 9:25 PM”
in this text include this labels: request_ticket;inform_moviename;inform_date;inform_theater;inform_city;inform_starttime;inform_numberofpeople
which program should i select? Thanks very much! | 10-31-2019 04:42:14 | 10-31-2019 04:42:14 | run_multiple_choice.py will be a good choice.
in case ‘bert’, it uses
https://github.com/huggingface/transformers/blob/master/transformers/modeling_bert.py#L1021
but i think your problem formulation is odd.
what about classifying ‘request_*’ intent as normal classification problem and slot tagging ‘inform_*’ as sequence classification?
<|||||>hi. i just start to learn bert for a very short time,so i could not have learned it clearly.
‘request_’ intent classifying can be very different with the normal classification problem?
i have not understand this.<|||||>This issue has been automatically marked as stale because it has not had recent activity. It will be closed if no further activity occurs. Thank you for your contributions.
|
transformers | 1,676 | closed | 🌟 BART | # 🌟New model addition
## Model description
method for pre-training seq2seq models by de-noising text. BART outperforms previous work on a bunch of generation tasks (summarization/dialogue/QA), while getting similar performance to RoBERTa on SQuAD/GLUE
[BART: Denoising Sequence-to-Sequence Pre-training for Natural Language Generation, Translation, and Comprehension](https://arxiv.org/pdf/1910.13461.pdf)
Amazing results on text summarization.
## Open Source status
* [x] the model implementation is available: not yet
* [x] the model weights are available: not yet
* [ ] who are the authors: @yinhanliu @ernamangoyal | 10-31-2019 02:41:24 | 10-31-2019 02:41:24 | @thomwolf another encoder-decoder<|||||>Was released today: https://github.com/pytorch/fairseq/tree/master/examples/bart 🎉<|||||>Let me know if you guys plan to add xsum/eli5/cnn-dm ft with our released bart into hugging face. <|||||>Is there any news on this?<|||||>any progress on this one? also thanks :)<|||||>I'm getting started on this Feb 4! |
transformers | 1,675 | closed | Any example of how to do multi-class classification with TFBertSequenceClassification | ## ❓ Questions & Help
I am trying to create a multi-class text classification model using BertSequenceClassifier for Tensorflow 2.0. Any help with the implementation strategy would be appreciated. Also, are there any recommendations as to how to convert a simple CSV file containing text and labels into TF dataset format such as Glue? | 10-31-2019 00:14:58 | 10-31-2019 00:14:58 | im asking for the same thing<|||||>I also need this. experts, please help/guide.<|||||>This issue has been automatically marked as stale because it has not had recent activity. It will be closed if no further activity occurs. Thank you for your contributions.
|
transformers | 1,674 | closed | possible issues with run_summarization_finetuning.py | Hi,
thanks for pushing summarization codes, here are my comments on this file:
- line 473: checkpoints = [] is empty and will not be evaluated. Also, the evaluation script is not called.
- line 482: results = "placeholder" is set to the placeholder, I was wondering if the function could return the generated text,so user could see the performance of the method visually.
- line 463: the model is only saved after the training, would be great to have the saving option like run_glue, "eval_during_training" also during the training activated.
- line 272 /transformers/modeling_encoder_decoder.py, the tie of weights is not done, this is a part of the model, would be great to have it implemented
- line 139 of transformers/modeling_auto.py, here you check the "path" if this starts with the name of bert, ..., you load the relevant one, but in run_summarization_finetuning, the user does not need to save the model in args.output_dir which starts with the name of model, so the codes wont work if the model is not saved with the path starting with the name of the model
- line 152 of /run_summarization_finetuning.py for param_group in optimizer.param_groups: I think this should be optimizer[stack] not optimizer alone
- utils_summarization.py: line 180, the comparison should not work, since s is a sequence, and then it is compared with special token,
- utils_summarization.py: line 182: embeddings.append(sentence_num % 2), to me you need to add sentence_sum%2 for the length of the sentence, but not 1 for each sentence.
thanks. | 10-30-2019 20:20:52 | 10-30-2019 20:20:52 | Hi!
Thanks for pointing these out. The summarization is still work in progress and should be included in the next release. Latest changes are in the `example-summarization` branch.<|||||>This issue has been automatically marked as stale because it has not had recent activity. It will be closed if no further activity occurs. Thank you for your contributions.
|
transformers | 1,673 | closed | BertModel.from_pretrained is failing with "HTTP 407 Proxy Authentication Required" during model weight download when running behing a proxy | ## 🐛 Bug
<!-- Important information -->
Hello,
I'am using transformers behind a proxy. `BertConfig.from_pretrained(..., proxies=proxies)` is working as expected, where `BertModel.from_pretrained(..., proxies=proxies)` gets a
`OSError: Tunnel connection failed: 407 Proxy Authentication Required` . This could be the symptom of `proxies` parameter not being passed through the `request` package commands.
Model I am using (Bert, XLNet....): Bert, base, cased.
Language I am using the model on (English, Chinese....): English
The problem arise when using:
* [X] the official example scripts: (give details)
* [ ] my own modified scripts: (give details)
The tasks I am working on is:
* [X] an official GLUE/SQUaD task: (give the name)
* [ ] my own task or dataset: (give details)
## To Reproduce
Steps to reproduce the behavior:
1. put your endpoint behind a proxy
2. configure the proxies variable accordingly `proxies={"https": 'foo.bar:3128'}
3. run any script calling BertConfig.from_pretrained( ...,proxies=proxies)
<!-- If you have a code sample, error messages, stack traces, please provide it here as well. -->
Stack Trace :
```
bash-3.2$ cd /Users/xxxx/_Data.science/NLP ; env PYTHONIOENCODING=UTF-8 PYTHONUNBUFFERED=1 /Users/xxxx/anaconda3/envs/farm-nlp/bin/python /Users/xxxx/FARM/examples/embeddings_extraction.py
10/29/2019 13:10:21 - INFO - transformers.file_utils - PyTorch version 1.2.0 available.
10/29/2019 13:10:22 - INFO - transformers.modeling_xlnet - Better speed can be achieved with apex installed from https://www.github.com/nvidia/apex .
10/29/2019 13:10:22 - WARNING - farm.utils - TensorboardX not installed. Required if you use tensorboard logger.
10/29/2019 13:10:22 - INFO - farm.utils - device: cpu n_gpu: 0, distributed training: False, 16-bits training: False
10/29/2019 13:10:22 - INFO - farm.modeling.tokenization - Loading tokenizer of type 'BertTokenizer'
10/29/2019 13:10:23 - INFO - transformers.file_utils - https://s3.amazonaws.com/models.huggingface.co/bert/bert-base-cased-vocab.txt not found in cache or force_download set to True, downloading to /var/folders/kg/qbqf751d6r13qchghq3vs15w0000gn/T/tmpwaag8tam
100%|█████████████████████████████████████████████████████████████████████████████████████████████████████████████████████████████████████████████████████████████████████████████████████████████████████████████████████████████████████████████████| 231508/231508 [00:01<00:00, 154673.07B/s]
10/29/2019 13:10:25 - INFO - transformers.file_utils - copying /var/folders/kg/qbqf751d6r13qchghq3vs15w0000gn/T/tmpwaag8tam to cache at /Users/xxxx/.cache/torch/transformers/26bc1ad6c0ac742e9b52263248f6d0f00068293b33709fae12320c0e35ccfbbb.542ce4285a40d23a559526243235df47c5f75c197f04f37d1a0c124c32c9a084
10/29/2019 13:10:25 - INFO - transformers.file_utils - creating metadata file for /Users/xxxx/.cache/torch/transformers/26bc1ad6c0ac742e9b52263248f6d0f00068293b33709fae12320c0e35ccfbbb.542ce4285a40d23a559526243235df47c5f75c197f04f37d1a0c124c32c9a084
10/29/2019 13:10:25 - INFO - transformers.file_utils - removing temp file /var/folders/kg/qbqf751d6r13qchghq3vs15w0000gn/T/tmpwaag8tam
10/29/2019 13:10:25 - INFO - transformers.tokenization_utils - loading file https://s3.amazonaws.com/models.huggingface.co/bert/bert-base-cased-vocab.txt from cache at /Users/xxxx/.cache/torch/transformers/26bc1ad6c0ac742e9b52263248f6d0f00068293b33709fae12320c0e35ccfbbb.542ce4285a40d23a559526243235df47c5f75c197f04f37d1a0c124c32c9a084
10/29/2019 13:10:26 - INFO - transformers.file_utils - https://s3.amazonaws.com/models.huggingface.co/bert/bert-base-cased-config.json not found in cache or force_download set to True, downloading to /var/folders/kg/qbqf751d6r13qchghq3vs15w0000gn/T/tmprex2n__s
Traceback (most recent call last):
File "/Users/xxxx/anaconda3/envs/farm-nlp/lib/python3.6/site-packages/urllib3/connectionpool.py", line 662, in urlopen
self._prepare_proxy(conn)
File "/Users/xxxx/anaconda3/envs/farm-nlp/lib/python3.6/site-packages/urllib3/connectionpool.py", line 948, in _prepare_proxy
conn.connect()
File "/Users/xxxx/anaconda3/envs/farm-nlp/lib/python3.6/site-packages/urllib3/connection.py", line 342, in connect
self._tunnel()
File "/Users/xxxx/anaconda3/envs/farm-nlp/lib/python3.6/http/client.py", line 919, in _tunnel
message.strip()))
OSError: Tunnel connection failed: 407 Proxy Authentication Required
During handling of the above exception, another exception occurred:
Traceback (most recent call last):
File "/Users/xxxx/anaconda3/envs/farm-nlp/lib/python3.6/site-packages/requests/adapters.py", line 449, in send
timeout=timeout
File "/Users/xxxx/anaconda3/envs/farm-nlp/lib/python3.6/site-packages/urllib3/connectionpool.py", line 720, in urlopen
method, url, error=e, _pool=self, _stacktrace=sys.exc_info()[2]
File "/Users/xxxx/anaconda3/envs/farm-nlp/lib/python3.6/site-packages/urllib3/util/retry.py", line 436, in increment
raise MaxRetryError(_pool, url, error or ResponseError(cause))
urllib3.exceptions.MaxRetryError: HTTPSConnectionPool(host='s3.amazonaws.com', port=443): Max retries exceeded with url: /models.huggingface.co/bert/bert-base-cased-config.json (Caused by ProxyError('Cannot connect to proxy.', OSError('Tunnel connection failed: 407 Proxy Authentication Required',)))
During handling of the above exception, another exception occurred:
Traceback (most recent call last):
File "/Users/xxxx/anaconda3/envs/farm-nlp/lib/python3.6/site-packages/transformers/configuration_utils.py", line 133, in from_pretrained
resolved_config_file = cached_path(config_file, cache_dir=cache_dir, force_download=force_download, proxies=proxies)
File "/Users/xxxx/anaconda3/envs/farm-nlp/lib/python3.6/site-packages/transformers/file_utils.py", line 176, in cached_path
return get_from_cache(url_or_filename, cache_dir=cache_dir, force_download=force_download, proxies=proxies)
File "/Users/xxxx/anaconda3/envs/farm-nlp/lib/python3.6/site-packages/transformers/file_utils.py", line 302, in get_from_cache
http_get(url, temp_file, proxies=proxies)
File "/Users/xxxx/anaconda3/envs/farm-nlp/lib/python3.6/site-packages/transformers/file_utils.py", line 238, in http_get
req = requests.get(url, stream=True, proxies=proxies)
File "/Users/xxxx/anaconda3/envs/farm-nlp/lib/python3.6/site-packages/requests/api.py", line 75, in get
return request('get', url, params=params, **kwargs)
File "/Users/xxxx/anaconda3/envs/farm-nlp/lib/python3.6/site-packages/requests/api.py", line 60, in request
return session.request(method=method, url=url, **kwargs)
File "/Users/xxxx/anaconda3/envs/farm-nlp/lib/python3.6/site-packages/requests/sessions.py", line 533, in request
resp = self.send(prep, **send_kwargs)
File "/Users/xxxx/anaconda3/envs/farm-nlp/lib/python3.6/site-packages/requests/sessions.py", line 646, in send
r = adapter.send(request, **kwargs)
File "/Users/xxxx/anaconda3/envs/farm-nlp/lib/python3.6/site-packages/requests/adapters.py", line 510, in send
raise ProxyError(e, request=request)
requests.exceptions.ProxyError: HTTPSConnectionPool(host='s3.amazonaws.com', port=443): Max retries exceeded with url: /models.huggingface.co/bert/bert-base-cased-config.json (Caused by ProxyError('Cannot connect to proxy.', OSError('Tunnel connection failed: 407 Proxy Authentication Required',)))
During handling of the above exception, another exception occurred:
Traceback (most recent call last):
File "/Users/xxxx/.vscode/extensions/ms-python.python-2019.9.34911/pythonFiles/ptvsd_launcher.py", line 43, in <module>
main(ptvsdArgs)
File "/Users/xxxx/.vscode/extensions/ms-python.python-2019.9.34911/pythonFiles/lib/python/ptvsd/__main__.py", line 432, in main
run()
File "/Users/xxxx/.vscode/extensions/ms-python.python-2019.9.34911/pythonFiles/lib/python/ptvsd/__main__.py", line 316, in run_file
runpy.run_path(target, run_name='__main__')
File "/Users/xxxx/anaconda3/envs/farm-nlp/lib/python3.6/runpy.py", line 263, in run_path
pkg_name=pkg_name, script_name=fname)
File "/Users/xxxx/anaconda3/envs/farm-nlp/lib/python3.6/runpy.py", line 96, in _run_module_code
mod_name, mod_spec, pkg_name, script_name)
File "/Users/xxxx/anaconda3/envs/farm-nlp/lib/python3.6/runpy.py", line 85, in _run_code
exec(code, run_globals)
File "/Users/xxxx/_Data.science/NLP/FARM/examples/embeddings_extraction.py", line 38, in <module>
language_model = Bert.load(lang_model_conf)
File "/Users/xxxx/_Data.science/NLP/FARM/farm/modeling/language_model.py", line 253, in load
bert.model = BertModel.from_pretrained(pretrained_model_name_or_path)
File "/Users/xxxx/anaconda3/envs/farm-nlp/lib/python3.6/site-packages/transformers/modeling_utils.py", line 287, in from_pretrained
**kwargs
File "/Users/xxxx/anaconda3/envs/farm-nlp/lib/python3.6/site-packages/transformers/configuration_utils.py", line 145, in from_pretrained
raise EnvironmentError(msg)
OSError: Couldn't reach server at 'https://s3.amazonaws.com/models.huggingface.co/bert/bert-base-cased-config.json' to download pretrained model configuration file.
Terminated: 15
```
## Expected behavior
<!-- A clear and concise description of what you expected to happen. -->
Model-weights.bin file download, after silent, behind-the-scene correct proxy authentication :
```
cd /Users/xxxxx/_Data.science/NLP ; env PYTHONIOENCODING=UTF-8 PYTHONUNBUFFERED=1 /Users/xxxxx/anaconda3/envs/farm-nlp/bin/python /Users/xxxxx/FARM/examples/embeddings_extraction.py
10/29/2019 15:28:48 - INFO - transformers.file_utils - PyTorch version 1.2.0 available.
10/29/2019 15:28:48 - INFO - transformers.modeling_xlnet - Better speed can be achieved with apex installed from https://www.github.com/nvidia/apex .
10/29/2019 15:29:00 - WARNING - farm.utils - TensorboardX not installed. Required if you use tensorboard logger.
10/29/2019 15:29:00 - INFO - farm.utils - device: cpu n_gpu: 0, distributed training: False, 16-bits training: False
10/29/2019 15:29:00 - INFO - farm.modeling.tokenization - Loading tokenizer of type 'BertTokenizer'
10/29/2019 15:29:00 - INFO - transformers.tokenization_utils - loading file https://s3.amazonaws.com/models.huggingface.co/bert/bert-base-cased-vocab.txt from cache at /Users/xxxxx/.cache/torch/transformers/5e8a2b4893d13790ed4150ca1906be5f7a03d6c4ddf62296c383f6db42814db2.e13dbb970cb325137104fb2e5f36fe865f27746c6b526f6352861b1980eb80b1
10/29/2019 15:29:03 - INFO - transformers.file_utils - https://s3.amazonaws.com/models.huggingface.co/bert/bert-base-cased-config.json not found in cache or force_download set to True, downloading to /var/folders/kg/qbqf751d6r13qchghq3vs15w0000gn/T/tmpxtz55r5f
100%|██████████████████████████████████████████████████████████████████████████████████████████████████████████████████████████████████████████████████████████████████████████████████████████████████████████████████████████████████████████████████████████████████████████████████████████████████████████████████████████████████████████████████████| 313/313 [00:00<00:00, 88643.97B/s]
10/29/2019 15:29:04 - INFO - transformers.file_utils - copying /var/folders/kg/qbqf751d6r13qchghq3vs15w0000gn/T/tmpxtz55r5f to cache at /Users/xxxxx/.cache/torch/transformers/b945b69218e98b3e2c95acf911789741307dec43c698d35fad11c1ae28bda352.d7a3af18ce3a2ab7c0f48f04dc8daff45ed9a3ed333b9e9a79d012a0dedf87a6
10/29/2019 15:29:04 - INFO - transformers.file_utils - creating metadata file for /Users/xxxxx/.cache/torch/transformers/b945b69218e98b3e2c95acf911789741307dec43c698d35fad11c1ae28bda352.d7a3af18ce3a2ab7c0f48f04dc8daff45ed9a3ed333b9e9a79d012a0dedf87a6
10/29/2019 15:29:04 - INFO - transformers.file_utils - removing temp file /var/folders/kg/qbqf751d6r13qchghq3vs15w0000gn/T/tmpxtz55r5f
10/29/2019 15:29:04 - INFO - transformers.configuration_utils - loading configuration file https://s3.amazonaws.com/models.huggingface.co/bert/bert-base-cased-config.json from cache at /Users/xxxxx/.cache/torch/transformers/b945b69218e98b3e2c95acf911789741307dec43c698d35fad11c1ae28bda352.d7a3af18ce3a2ab7c0f48f04dc8daff45ed9a3ed333b9e9a79d012a0dedf87a6
10/29/2019 15:29:04 - INFO - transformers.configuration_utils - Model config {
"attention_probs_dropout_prob": 0.1,
"finetuning_task": null,
"hidden_act": "gelu",
"hidden_dropout_prob": 0.1,
"hidden_size": 768,
"initializer_range": 0.02,
"intermediate_size": 3072,
"layer_norm_eps": 1e-12,
"max_position_embeddings": 512,
"num_attention_heads": 12,
"num_hidden_layers": 12,
"num_labels": 2,
"output_attentions": false,
"output_hidden_states": false,
"output_past": true,
"pruned_heads": {},
"torchscript": false,
"type_vocab_size": 2,
"use_bfloat16": false,
"vocab_size": 28996
}
10/29/2019 15:29:05 - INFO - transformers.file_utils - https://s3.amazonaws.com/models.huggingface.co/bert/bert-base-cased-pytorch_model.bin not found in cache or force_download set to True, downloading to /var/folders/kg/qbqf751d6r13qchghq3vs15w0000gn/T/tmpaz0jbgo4
100%|████████████████████████████████████████████████████████████████████████████████████████████████████████████████████████████████████████████████████████████████████████████████████████████████████████████████████████████████████████████████████████████████████████████████████████████████████████████████████████████████████████| 435779157/435779157 [04:19<00:00, 1677901.46B/s]
10/29/2019 15:33:25 - INFO - transformers.file_utils - copying /var/folders/kg/qbqf751d6r13qchghq3vs15w0000gn/T/tmpaz0jbgo4 to cache at /Users/xxxxx/.cache/torch/transformers/35d8b9d36faaf46728a0192d82bf7d00137490cd6074e8500778afed552a67e5.3fadbea36527ae472139fe84cddaa65454d7429f12d543d80bfc3ad70de55ac2
10/29/2019 15:33:26 - INFO - transformers.file_utils - creating metadata file for /Users/xxxxx/.cache/torch/transformers/35d8b9d36faaf46728a0192d82bf7d00137490cd6074e8500778afed552a67e5.3fadbea36527ae472139fe84cddaa65454d7429f12d543d80bfc3ad70de55ac2
10/29/2019 15:33:26 - INFO - transformers.file_utils - removing temp file /var/folders/kg/qbqf751d6r13qchghq3vs15w0000gn/T/tmpaz0jbgo4
10/29/2019 15:33:26 - INFO - transformers.modeling_utils - loading weights file https://s3.amazonaws.com/models.huggingface.co/bert/bert-base-cased-pytorch_model.bin from cache at /Users/xxxxx/.cache/torch/transformers/35d8b9d36faaf46728a0192d82bf7d00137490cd6074e8500778afed552a67e5.3fadbea36527ae472139fe84cddaa65454d7429f12d543d80bfc3ad70de55ac2
```
## Environment
* OS: MacOS
* Python version: 3.6
* PyTorch version: 1.2.0
* PyTorch Transformers version (or branch): 2.1.1
* Using GPU ? Yes
* Distributed of parallel setup ? No
* Any other relevant information: Proxy
## Additional context
<!-- Add any other context about the problem here. -->
| 10-30-2019 12:53:40 | 10-30-2019 12:53:40 | |
transformers | 1,672 | closed | Is HuggingFace TransfoXLLMHeadModels trainable from scratch? | Hello,
Is HuggingFace TransfoXLLMHeadModels trainable from scratch? The documentation makes it look like it is possible to train the TransfoXLLMHeadModel from scratch, since (according to the documentation) the loss can be returned via TransfoXLLMHeadModel( ) as long as labels are provided (https://huggingface.co/transformers/model_doc/transformerxl.html#transfoxllmheadmodel) .
However, the code for TransfoXLLMHeadModels shown in the Github repository
(https://github.com/huggingface/transformers/blob/master/transformers/modeling_transfo_xl.py#L780) seem to suggest that the loss is, in fact, not returned even when the labels are provided.
Is HuggingFace TransfoXLLMHeadModels trainable from scratch?
Thank you,
| 10-30-2019 12:42:17 | 10-30-2019 12:42:17 | Hi @h56cho,
The loss is actually returned if labels are present.
Check https://github.com/huggingface/transformers/blob/master/transformers/modeling_transfo_xl.py#L793<|||||>This issue has been automatically marked as stale because it has not had recent activity. It will be closed if no further activity occurs. Thank you for your contributions.
|
transformers | 1,671 | closed | Quick Tour TF2.0 Training Script has Control Flow Error when Replacing TFBERT with TFRoberta | ## 📚 Migration
<!-- Important information -->
Model I am using (Bert, XLNet....): TFRoberta.
Language I am using the model on (English, Chinese....): English
The problem arise when using:
* [x] the official example scripts: Quick Tour TF2.0 Training Script.
* [ ] my own modified scripts:
Details of the issue:
When replacing TF BERT with TF Roberta (and the relevant tokenizer) in the quick tour script, I get the following error:
```
TypeError: You are attempting to use Python control flow in a layer that was not declared to be dynamic. Pass `dynamic=True` to the class constructor.
Encountered error:
"""
using a `tf.Tensor` as a Python `bool` is not allowed: AutoGraph is disabled in this function. Try decorating it directly with @tf.function.
"""
```
I suspect this extends to all models, though I haven't verified this. Any thoughts?
## Environment
* OS: Catalina
* Python version: 3.7.14
* PyTorch version: NA
* PyTorch Transformers version (or branch): Transformers
* Using GPU ? No
* Distributed of parallel setup ? No
* Any other relevant information:
## Checklist
- [x] I have read the migration guide in the readme.
- [x] I checked if a related official extension example runs on my machine.
## Additional context
<!-- Add any other context about the problem here. -->
| 10-30-2019 11:06:16 | 10-30-2019 11:06:16 | Hi, I think this was fixed by #1601, could you try now by cloning and installing from master?<|||||>This issue has been automatically marked as stale because it has not had recent activity. It will be closed if no further activity occurs. Thank you for your contributions.
|
transformers | 1,670 | closed | Templates and explanation for adding a new model and example script | This PR adds:
- templates and explantations for all the steps needed to add a new model
- a simple template for adding a new example script (basically the current `run_squad` example).
- links to them in the `README` and `CONTRIBUTING` docs.
@LysandreJik and @rlouf, feel free to update if you want to add stuff or fix the wording. | 10-30-2019 10:40:38 | 10-30-2019 10:40:38 | Thanks @stefan-it, feel free to give your opinion on the explanation/templates as well, always happy to have your feedback |
transformers | 1,669 | closed | How to load trained model of distilbert | ## ❓ Questions & Help
Hi,
I have trained distilbert using the steps mentioned in example/distillation.
saved the checkpoints into one directory.
But i cant use run_glue.py using the checkpoint path i saved for distilbert.
It throws error for tokenizer missing.
Would you please help me, how to achieve that. If i am doing any mistake in my step.
TIA!!!
<!-- A clear and concise description of the question. -->
| 10-30-2019 09:55:08 | 10-30-2019 09:55:08 | Hello @ANSHUMAN87,
Could you share the command you're using (and the error you get)?
You should have at least these arguments: `--model_type distilbert --model_name_or_path <your_model_path>`.
Victor<|||||>I have mentioned below the steps i followed.
Step 1: python3 train.py --student_type distilbert --student_config training_configs/distilbert-base-uncased.json --teacher_type bert --teacher_name bert-base-uncased --alpha_ce 5.0 --alpha_mlm 2.0 --alpha_cos 1.0 --mlm --freeze_pos_embs --dump_path serialization_dir/my_first_training --data_file binarized_text.bert-base-uncased.pickle --token_counts token_counts.bert-base-uncased.pickle --force
Result: Successful
Step 2: python3 run_glue.py --model_type distilbert --model_name_or_path distillation/serialization_dir/my_first_training/ --task_name CoLA --do_eval --do_lower_case --data_dir /home/anshuman/3/GLUE-Dataset/glue_data/CoLA/ --max_seq_length 128 --output_dir distillation/serialization_dir/my_first_training/
Error:
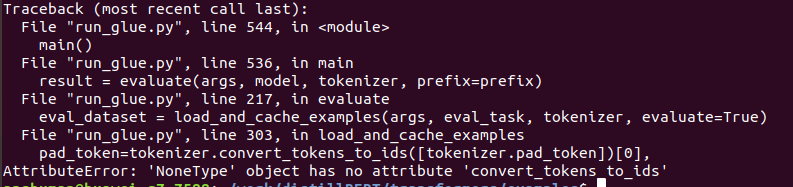
<|||||>Thank you, I understand what's happening now.
It happens because you're not launching any training (`--do_train`) before evaluating. What happens in `run_glue.py` is that when you do the evaluation, the tokenizer is loaded from the one saved in `output_dir` (see [here](https://github.com/huggingface/transformers/blob/master/examples/run_glue.py#L524)). The latter has been saved a few lines before ([here](https://github.com/huggingface/transformers/blob/master/examples/run_glue.py#L510)) in `do_train`... So basically, you're trying to load something that doesn't exist yet...
One way to quickly bypass this is: a/ adding `--do_train --num_train_epochs 0.0`, b/ set the return to `global_step, tr_loss / 1` (see [here](https://github.com/huggingface/transformers/blob/master/examples/run_glue.py#L207)) to avoid division by 0.
Of course, by doing that, you're evaluating on a GLUE task a model that hasn't been finetuned for the GLUE task in question (i.e. you're doing zero-shot).
Also, I recommend to use a different `output_dir` in the `run_glue.py` command: run_glue will overwrite your pre-training (step 1) when saving the model under the name `pytorch_model.bin`.<|||||>This issue has been automatically marked as stale because it has not had recent activity. It will be closed if no further activity occurs. Thank you for your contributions.
<|||||>> Thank you, I understand what's happening now.
>
> It happens because you're not launching any training (`--do_train`) before evaluating. What happens in `run_glue.py` is that when you do the evaluation, the tokenizer is loaded from the one saved in `output_dir` (see [here](https://github.com/huggingface/transformers/blob/master/examples/run_glue.py#L524)). The latter has been saved a few lines before ([here](https://github.com/huggingface/transformers/blob/master/examples/run_glue.py#L510)) in `do_train`... So basically, you're trying to load something that doesn't exist yet...
>
> One way to quickly bypass this is: a/ adding `--do_train --num_train_epochs 0.0`, b/ set the return to `global_step, tr_loss / 1` (see [here](https://github.com/huggingface/transformers/blob/master/examples/run_glue.py#L207)) to avoid division by 0.
>
> Of course, by doing that, you're evaluating on a GLUE task a model that hasn't been finetuned for the GLUE task in question (i.e. you're doing zero-shot).
>
> Also, I recommend to use a different `output_dir` in the `run_glue.py` command: run_glue will overwrite your pre-training (step 1) when saving the model under the name `pytorch_model.bin`.
Hello,@VictorSanh,
I have completed model train in pytorch. But how can I use the trained model to do some new test on a new test.tvs? my run.sh is:
export TASK_NAME=mytask
python src/run_glue.py \
--model_name_or_path ch/ \
--task_name $TASK_NAME \
--do_predict \
--data_dir data/ \
--max_seq_length 128 \
--output_dir saved_test_moels/ \
--overwrite_cache
it doesn't work. What should I change? Thank you. |
transformers | 1,668 | closed | Fixed training for TF XLM | This PR fixes `model.fit()` training for TF XLM model, and tested in a script similar to `run_tf_glue.py`. It also is tested and works with AMP and tf.distribute for mixed precision and multi-GPU training.
This changes some Python `assert` statements to `tf.debugging.assert_equal` both in `TFXLMMainLayer.call()` and `gen_mask()`
Otherwise, errors encountered:
* `TypeError: You are attempting to use Python control flow in a layer that was not declared to be dynamic. Pass 'dynamic=True' to the class constructor.`
* `OperatorNotAllowedInGraphError: using a 'tf.Tensor' as a Python 'bool' is not allowed in Graph execution. Use Eager execution or decorate this function with @tf.function.` | 10-30-2019 01:35:20 | 10-30-2019 01:35:20 | # [Codecov](https://codecov.io/gh/huggingface/transformers/pull/1668?src=pr&el=h1) Report
> Merging [#1668](https://codecov.io/gh/huggingface/transformers/pull/1668?src=pr&el=desc) into [master](https://codecov.io/gh/huggingface/transformers/commit/079bfb32fba4f2b39d344ca7af88d79a3ff27c7c?src=pr&el=desc) will **not change** coverage.
> The diff coverage is `75%`.
[](https://codecov.io/gh/huggingface/transformers/pull/1668?src=pr&el=tree)
```diff
@@ Coverage Diff @@
## master #1668 +/- ##
======================================
Coverage 85.9% 85.9%
======================================
Files 91 91
Lines 13653 13653
======================================
Hits 11728 11728
Misses 1925 1925
```
| [Impacted Files](https://codecov.io/gh/huggingface/transformers/pull/1668?src=pr&el=tree) | Coverage Δ | |
|---|---|---|
| [transformers/modeling\_tf\_xlm.py](https://codecov.io/gh/huggingface/transformers/pull/1668/diff?src=pr&el=tree#diff-dHJhbnNmb3JtZXJzL21vZGVsaW5nX3RmX3hsbS5weQ==) | `90.39% <75%> (ø)` | :arrow_up: |
------
[Continue to review full report at Codecov](https://codecov.io/gh/huggingface/transformers/pull/1668?src=pr&el=continue).
> **Legend** - [Click here to learn more](https://docs.codecov.io/docs/codecov-delta)
> `Δ = absolute <relative> (impact)`, `ø = not affected`, `? = missing data`
> Powered by [Codecov](https://codecov.io/gh/huggingface/transformers/pull/1668?src=pr&el=footer). Last update [079bfb3...842f3bf](https://codecov.io/gh/huggingface/transformers/pull/1668?src=pr&el=lastupdated). Read the [comment docs](https://docs.codecov.io/docs/pull-request-comments).
<|||||>Awesome, thanks a lot @tlkh |
transformers | 1,667 | closed | Added FP16 support to benchmarks.py | This PR adds in FP16 support for the inference benchmarks for TensorFlow and PyTorch, and presents the collected results. This is a "re-do" of a previous PR (#1567) taking into account changes to `benchmark.py` and also adding in the PyTorch component with additional results collected.
**TensorFlow**
Added a automatic mixed precision (AMP) option to the benchmark script. As you can see, we can get between 1.2x to up to 4.5x inference speed depending on model, batch size and sequence length. (1.0x refers to no change in speed)
| Batch Size | Speedup (XLA only) | Speedup (XLA + AMP) | Min. Seq Len* |
| -------------- | --------------------------- | ------------------------------- | ------------------ |
| 1 | 1.1 ~ 1.9 | 1.4 ~ 2.9 | 512 |
| 2 | 1.1 ~ 1.9 | 1.4 ~ 3.4 | 256 |
| 4 | 1.1 ~ 2.1 | 1.2 ~ 3.8 | 128 |
| 8 | 1.1 ~ 3.1 | 1.2 ~ 4.5 | 64 |
*Min. Seq Len refers to minimum sequence length required to not see **any** performance regression at all. For example, at batch size 1:
* Seq Len of 512 tokens see speed up of 1.4~2.1x depending on model
* Seq Len of 256 tokens see speed up of 0.8~1.2x depending on model
**PyTorch**
Added a FP16 (half precision) option to the benchmark script. As you can see, we can get between up to 4.2x inference speed depending on model, batch size and sequence length. (1.0x refers to no change in speed)
| Batch Size | Speedup (TorchScript only) | Speedup (FP16 Only) |
| -------------- | ------------------------------------- | ----------------------------- |
| 1 | 1.0 ~ 1.7 | 1.0 ~ 3.0 |
| 2 | 1.0 ~ 1.8 | 1.0 ~ 3.5 |
| 4 | 1.0 ~ 1.7 | 1.0 ~ 4.0 |
| 8 | 1.0 ~ 1.7 | 1.4 ~ 4.2 |
*FP16 and CTRL result in performance regression below 1x256, 2x128, 4x64.
**Summary of Collected Results**
Google Sheets with the TF/PyTorch results [here](https://docs.google.com/spreadsheets/d/1IW7Xbv-yfE8j-T0taqdyoSehca4mNcsyx6u0IXTzSJ4/edit#gid=1307979840). GPU used is a single V100 (16GB). | 10-30-2019 01:16:05 | 10-30-2019 01:16:05 | # [Codecov](https://codecov.io/gh/huggingface/transformers/pull/1667?src=pr&el=h1) Report
> Merging [#1667](https://codecov.io/gh/huggingface/transformers/pull/1667?src=pr&el=desc) into [master](https://codecov.io/gh/huggingface/transformers/commit/079bfb32fba4f2b39d344ca7af88d79a3ff27c7c?src=pr&el=desc) will **increase** coverage by `0.02%`.
> The diff coverage is `n/a`.
[](https://codecov.io/gh/huggingface/transformers/pull/1667?src=pr&el=tree)
```diff
@@ Coverage Diff @@
## master #1667 +/- ##
==========================================
+ Coverage 85.9% 85.92% +0.02%
==========================================
Files 91 91
Lines 13653 13653
==========================================
+ Hits 11728 11732 +4
+ Misses 1925 1921 -4
```
| [Impacted Files](https://codecov.io/gh/huggingface/transformers/pull/1667?src=pr&el=tree) | Coverage Δ | |
|---|---|---|
| [transformers/file\_utils.py](https://codecov.io/gh/huggingface/transformers/pull/1667/diff?src=pr&el=tree#diff-dHJhbnNmb3JtZXJzL2ZpbGVfdXRpbHMucHk=) | `76.37% <0%> (+2.19%)` | :arrow_up: |
------
[Continue to review full report at Codecov](https://codecov.io/gh/huggingface/transformers/pull/1667?src=pr&el=continue).
> **Legend** - [Click here to learn more](https://docs.codecov.io/docs/codecov-delta)
> `Δ = absolute <relative> (impact)`, `ø = not affected`, `? = missing data`
> Powered by [Codecov](https://codecov.io/gh/huggingface/transformers/pull/1667?src=pr&el=footer). Last update [079bfb3...2669079](https://codecov.io/gh/huggingface/transformers/pull/1667?src=pr&el=lastupdated). Read the [comment docs](https://docs.codecov.io/docs/pull-request-comments).
<|||||>Great, thank you @tlkh ! Feel free to add a link to your spreadsheet in the documentation. |
transformers | 1,666 | closed | Question: Token sequence length longer maximum sequence length | ## ❓ Questions & Help
I'm using `run_glue.py` with a task name of `SST-2` to fine-tune a binary classifier on my data, which I put into the required format. However, some of my data's sentences are longer than the `max_seq_length` of `512` for `BERT` and `RoBERTa`; so, I get
`WARNING - transformers.tokenization_utils - Token indices sequence length is longer than the specified maximum sequence length for this model (length_of_my_string > 512). Running this sequence through the model will result in indexing errors`.
What exactly is happening here? Are the training examples with more than `510` tokens still being used? If so, is the string being truncated down to `[CLS]` + the `first 510 tokens` + `[SEP]`? Is there any way to increase the `max_seq_length` or implement something like `head+tail`, which selects the `first 128` and the `last 382` tokens like suggested in this [paper](https://arxiv.org/pdf/1905.05583.pdf). That paper also uses `discriminative learning rate` as suggested [here](https://arxiv.org/pdf/1801.06146.pdf). Is there any plan to implement this?
| 10-29-2019 23:41:39 | 10-29-2019 23:41:39 | Going through the source code, the sequence is actually truncated.
https://github.com/huggingface/transformers/blob/079bfb32fba4f2b39d344ca7af88d79a3ff27c7c/transformers/tokenization_utils.py#L846-L853
The warning occurs because `encode_plus` calls `convert_tokens_to_ids` _first_ and only then the IDs are truncated. The warning originates from `convert_tokens_to_ids` before truncation has happened. This is quite confusing indeed, since in the end result the IDs _are_ truncated.
Perhaps one of the maintainers can chip in.<|||||>> Going through the source code, the sequence is actually truncated.
>
> https://github.com/huggingface/transformers/blob/079bfb32fba4f2b39d344ca7af88d79a3ff27c7c/transformers/tokenization_utils.py#L846-L853
>
> The warning occurs because `encode_plus` calls `convert_tokens_to_ids` _first_ and only then the IDs are truncated. The warning originates from `convert_tokens_to_ids` before truncation has happened. This is quite confusing indeed, since in the end result the IDs _are_ truncated.
>
> Perhaps one of the maintainers can chip in.
So, it safe to use or not?<|||||>This should have been patched in release 2.2.0.<|||||>This issue has been automatically marked as stale because it has not had recent activity. It will be closed if no further activity occurs. Thank you for your contributions.
|
transformers | 1,665 | closed | Allowing PR#1455 to be merged in the master | Hi Thomas
Remi was saying in PR:#1455 it has the bert seq2seq ready, could you move in a gradual way please and allow this PR to be merged at this stage that is working for BERT? Then people can use the BERT one, this is already great, then after a while when this is ready for also other encoders, you can add them later, I really appreciate adding the BERT ones thanks | 10-29-2019 21:19:22 | 10-29-2019 21:19:22 | Please don't post issues like this. I'm sure the maintainers work as hard as they can. Asking them to _work faster_ doesn't help. In fact, adding these kind of non-issues only distract the maintainers from actually working on the actual issues at hand. Please close this question. |
transformers | 1,664 | closed | Moving model from GPU -> CPU doesn't work | ## 🐛 Bug
Hi,
I tried creating a model (doesn't matter which one from my experiments), moving it first to multiple GPUs and then back to CPU. But I think it doesn't work as intended.
The following is the code to reproduce the error:
```python
import torch
import torch.nn as nn
from transformers import BertTokenizer, BertModel
>>> model = BertModel.from_pretrained('bert-base-uncased')
>>> model.to('cuda:0')
>>> model = nn.DataParallel(model, device_ids=range(torch.cuda.device_count()))
>>> print(model.device_ids)
[0, 1]
>>> model.to('cpu')
>>> print(model.device_ids) # Still on GPUs
[0, 1]
``` | 10-29-2019 21:15:40 | 10-29-2019 21:15:40 | `.to()` is not an in-place operation. You should use `model = model.to('cpu')`. If that doesn't work, it might be that you need to access the module as part of the DataParallel object, like this:
```python
model = model.module.to('cpu')
```<|||||>Ahh gotcha. Thanks for the quick reply! |
transformers | 1,663 | closed | Problem with restoring GPT-2 weights | Hello, I've been debugging an issue for a while and it seem it's a model-specific issue.
I'm training GPT-2 on a TPU and I can't save and restore it. It looks like there is a code that silently changes parameter values right in `load_state_dict()`.
```
print(state_dict['transformer.wte.weight'])
print(state_dict['transformer.wte.weight'].shape)
cpu_model = model_class(config=config)
cpu_model.load_state_dict(state_dict)
print(cpu_model.state_dict()['transformer.wte.weight'])
print(cpu_model.state_dict()['transformer.wte.weight'].shape)
```
```
tensor([[-0.1101, -0.0393, 0.0331, ..., -0.1364, 0.0151, 0.0453],
[ 0.0417, -0.0488, 0.0485, ..., 0.0827, 0.0097, 0.0454],
[-0.1275, 0.0479, 0.1841, ..., 0.0899, -0.1297, -0.0879],
...,
[-0.0439, -0.0579, 0.0103, ..., 0.1113, 0.0919, -0.0724],
[ 0.1846, 0.0156, 0.0444, ..., -0.0974, 0.0785, -0.0211],
[ 0.0471, -0.0284, 0.0492, ..., 0.0048, 0.1511, 0.1202]])
torch.Size([50257, 768])
tensor([[-0.1317, -0.0305, 0.0339, ..., -0.1310, 0.0113, 0.0262],
[ 0.0413, -0.0491, 0.0451, ..., 0.0930, -0.0019, 0.0457],
[-0.1465, 0.0565, 0.1839, ..., 0.0962, -0.1339, -0.1074],
...,
[-0.0432, -0.0628, 0.0088, ..., 0.1002, 0.1045, -0.0654],
[ 0.1725, 0.0160, 0.0444, ..., -0.0944, 0.0760, -0.0289],
[ 0.0330, -0.0182, 0.0455, ..., 0.0136, 0.1487, 0.0975]])
torch.Size([50257, 768])
```
For the context
https://github.com/pytorch/xla/issues/1245
https://discuss.pytorch.org/t/problem-with-model-accuracy-after-restore-on-tpu/59304/3
Full code is here
https://github.com/mgrankin/ru_transformers/blob/9d52a4caef16df5b921c386f4841c879877d03a4/debug_lm_finetuning.py
| 10-29-2019 18:21:21 | 10-29-2019 18:21:21 | I found a bug, it's TPU related. For some reason, after I move the mode to TPU, using `model = model.to(device)`, the weights become decoupled. Then I save decoupled weights and during restore it ties them again. It loads correctly, it just doesn't expect tied weights to be different.
The workaround is to tie weights again after moving model to the TPU.
```
model = model.to(args.device)
model.tie_weights()
```
<|||||>I'm sorry to reopen this issue, Davide Libenzi suggesting this is the model issue, not the PyTorch XLA issue. I'm a bit of a tired from debugging and I'm happy with the workaround.
You can find details here https://github.com/pytorch/xla/issues/1245
<|||||>Ok, do you think we should fix this upstream in our library?
I'm not super excited about overwriting PyTorch built-in `nn.Module.apply()` method.<|||||>It feels to me, the Pytorch/XLA is more appropriate place for fix, since Pytorch/Cuda have that behavior and the fix will make two libraries consistent.
But I don't feel competent neither in Pytorch/XLA nor in Transformers to insist.
It would be great to have somebody from Transformers to talk to Pytorch/XLA over this issue. <|||||>Ok, we'll try to push this up-stream<|||||>The PyTorch community decided it's more appropriate to tie weights after moving the model to the device (TPU/GPU/CPU). I believe it's worth to fix the model accordingly.
https://github.com/pytorch/xla/issues/1245#issuecomment-552559970
https://github.com/pytorch/xla/pull/1335<|||||>This issue has been automatically marked as stale because it has not had recent activity. It will be closed if no further activity occurs. Thank you for your contributions.
<|||||>Hello, stale bot, it would be great to keep it open.<|||||>This issue has been automatically marked as stale because it has not had recent activity. It will be closed if no further activity occurs. Thank you for your contributions.
|
transformers | 1,662 | closed | Tokenizer.tokenize return none on some utf8 string in current pypi version | Tokenizer.tokenize return none on some utf8 string in current pypi version
## 🐛 Bug
<!-- Important information -->
Model I am using (Bert):
Language I am using the model on (English, Chinese....):
The problem arise when using:
* [ ] the official example scripts:
The tasks I am working on is:
* [ ] my own task or dataset: SQUaD format, Chinese, DRCD
## To Reproduce
Current seems not updated
Cause returning null result in Tokenizer.tokenize when input some special utf8 string
Steps to reproduce the behavior:
1.
```
tokenizer = BertTokenizer.from_pretrained('bert-base-multilingual-uncased')
```
2.
```
text = "ุ"
tokenized_text = tokenizer.tokenize(text)
print(len(text.split()),len(text.strip().split()),text,tokenized_text,"\n")
```
3.
```
1 1 ุ []
```
## Expected behavior
I have try the implementation from GitHub, It seems to be fine :
```
def whitespace_tokenize(text):
"""Runs basic whitespace cleaning and splitting on a piece of text."""
text = text.strip()
if not text:
return []
tokens = text.split()
return tokens
def tokenize(text):
output_tokens = []
for token in whitespace_tokenize(text):
chars = list(token)
if len(chars) > 100:
output_tokens.append("[UNK]")
continue
is_bad = False
start = 0
sub_tokens = []
while start < len(chars):
end = len(chars)
cur_substr = None
while start < end:
substr = "".join(chars[start:end])
if start > 0:
substr = "##" + substr
if substr in tokenizer.vocab:
cur_substr = substr
break
end -= 1
if cur_substr is None:
is_bad = True
break
sub_tokens.append(cur_substr)
start = end
if is_bad:
output_tokens.append("[UNK]")
else:
output_tokens.extend(sub_tokens)
return output_tokens
print(len(text.split()),len(text.strip().split()),text,tokenize(text),"\n")
```
Return
```
1 1 ุ ['[UNK]']
```
## Colab demo : https://colab.research.google.com/drive/1WGu4dYLWtaPRPBq_YZEPvrmMALEFlCBn
| 10-29-2019 17:07:58 | 10-29-2019 17:07:58 | We've seen this issues also with other tokenizers, like XLNet.
It would be awesome to have a unified tokenization strategy (across all `Tokenizer` classes) that return `unk_token` in these cases. And of course we should discuss other possibilities here :)
<|||||>@voidful this behavior arises because `bert-base-multilingual-uncased` is lower-casing the input (as the name indicates) and as such remove accents.
Your character is classified as an accent in the Unicode category database (see "Mn" [here](https://www.fileformat.info/info/unicode/category/index.htm)).
To fix this behavior, use the recommended multilingual model for Bert: `bert-base-multilingual-cased` instead of the one you are using (see the list of models and the recommended ones [here](https://huggingface.co/transformers/pretrained_models.html))
@stefan-it I think the other issues you are referring to are likely different from this one.
Feel free to open another issue if you want us to investigate them in detail.<|||||>Thank you for your help! It really solve the problem ! |
transformers | 1,661 | closed | BERT multi heads attentions | Hello,
I would like to analysis the effect of specific heads' attention.
Is it possible to turn off some heads attentions in a particular layer?
if yes, can you please tell me how to do that or share any helpful document?
Thank you in advance | 10-29-2019 15:21:03 | 10-29-2019 15:21:03 | I think the [BERTology](https://huggingface.co/transformers/bertology.html) section could help, especially the [run_bertology.py](https://github.com/huggingface/transformers/blob/master/examples/run_bertology.py) script can perform pruning and includes other useful functions :)<|||||>I am beginner to BERT, can you please tell me to turn off the second head in the ninth layer for example.
Here is my model config
config = BertConfig.from_pretrained("bert-base-uncased",output_attentions=True,output_hidden_states=True, num_labels=2)
model = BertForSequenceClassification.from_pretrained("bert-base-uncased", config= config)
model.cuda()<|||||>This issue has been automatically marked as stale because it has not had recent activity. It will be closed if no further activity occurs. Thank you for your contributions.
|
transformers | 1,660 | closed | How to fine-tune CTRL? | How to fine-tune CTRL on a custom dataset with custom control codes using the transformers package?
I'm aware of the [guide](https://github.com/salesforce/ctrl/tree/master/training_utils) for tensorflow users. However, as a PyTorch user, the guide is not friendly to me.
I'm also aware of the language modelling fine-tuning script [here](https://github.com/huggingface/transformers/blob/master/examples/run_lm_finetuning.py). However, it does not support CTRL right now.
Thanks,
Peixiang | 10-29-2019 12:43:44 | 10-29-2019 12:43:44 | This issue has been automatically marked as stale because it has not had recent activity. It will be closed if no further activity occurs. Thank you for your contributions.
<|||||>@zhongpeixiang do you have any info about finetuning ctrl?<|||||>@saippuakauppias No, in the end I chose the original CTRL repo from Salesforce to finetune.<|||||>Hey @zhongpeixiang, could you share some more information on how you fine-tuned the CTRL? I am also struggling to fine tune it using transformers. <|||||>@ludoro I followed this repo to fine-tune the CTRL: https://github.com/salesforce/ctrl<|||||>also struggling through the fine-tuning of CTRL, if someone can show a notebook or just the code to do that, it will help a lot!<|||||>> also struggling through the fine-tuning of CTRL, if someone can show a notebook or just the code to do that, it will help a lot!
https://github.com/salesforce/ctrl/tree/master/training_utils |
transformers | 1,659 | closed | How is the interactive GPT-2 implemented? | ## ❓ Questions & Help
I came across this online demo from HuggingFace for GPT-2 writing: https://transformer.huggingface.co/doc/gpt2-large. The demo is really amazing, both accurate and fast. My major observation is that the service actually uses user's earlier writing examples in later prediction, almost instantly. I'm very curious how it is implemented? It seems to me that it is not fine-tuned in real-time, then is there some other mechanism behind it? Any ideas are appreciated.
Context examples I typed in:
> Set the a c to low level = the room is very cold
> turn down the volume = the music is too loud
Then when i try:
> turn on the lights =
It gives me
> the room is too bright
Also, I tried fine-tuning the entire model with much more than 2 examples (around 30), however the result for "turn on the lights = " after fine-tuning is a lot worse than the demo:
> about 0.016 (lit a second and a 100 pixels)
Is it that the demo only fine-tune, e.g. the very last layer of the model?
| 10-29-2019 04:55:57 | 10-29-2019 04:55:57 | Hi, the models are not fine-tuned on the fly. Language models like GPT-2 are very context-aware and are strong at generating words related to the inputs they were given.
We are not training the models in that demo, we are only using them for inference.<|||||>This issue has been automatically marked as stale because it has not had recent activity. It will be closed if no further activity occurs. Thank you for your contributions.
|
transformers | 1,658 | closed | How to fine tune xlm-mlm-100-128 model. | ## ❓ Questions & Help
How to fine tune xlm-mlm-17-128 model for own dataset. Since, run_lm_finetuning.py has no option to fine tune XLM models.
| 10-29-2019 00:56:22 | 10-29-2019 00:56:22 | If you're looking to fine-tune it on an MLM task you could simply re-use some parts of the `run_lm_finetuning.py` script to do it. <|||||>This issue has been automatically marked as stale because it has not had recent activity. It will be closed if no further activity occurs. Thank you for your contributions.
|
transformers | 1,657 | closed | [WIP] Raise error if larger sequences | Hi,
I suggest to improve a bit user experience when using pretrained models by raising more errors if some of parameters are incoherent.
For example, in this PR, there is a suggestion to raise error and thus inform user about potential error as "RuntimeError: cublas runtime error ..." which can be harder to find if running on GPU.
What do you think ? | 10-29-2019 00:02:12 | 10-29-2019 00:02:12 | # [Codecov](https://codecov.io/gh/huggingface/transformers/pull/1657?src=pr&el=h1) Report
> Merging [#1657](https://codecov.io/gh/huggingface/transformers/pull/1657?src=pr&el=desc) into [master](https://codecov.io/gh/huggingface/transformers/commit/079bfb32fba4f2b39d344ca7af88d79a3ff27c7c?src=pr&el=desc) will **decrease** coverage by `1.39%`.
> The diff coverage is `100%`.
[](https://codecov.io/gh/huggingface/transformers/pull/1657?src=pr&el=tree)
```diff
@@ Coverage Diff @@
## master #1657 +/- ##
=========================================
- Coverage 85.9% 84.51% -1.4%
=========================================
Files 91 91
Lines 13653 13654 +1
=========================================
- Hits 11728 11539 -189
- Misses 1925 2115 +190
```
| [Impacted Files](https://codecov.io/gh/huggingface/transformers/pull/1657?src=pr&el=tree) | Coverage Δ | |
|---|---|---|
| [transformers/modeling\_bert.py](https://codecov.io/gh/huggingface/transformers/pull/1657/diff?src=pr&el=tree#diff-dHJhbnNmb3JtZXJzL21vZGVsaW5nX2JlcnQucHk=) | `88.2% <100%> (+0.02%)` | :arrow_up: |
| [transformers/modeling\_tf\_pytorch\_utils.py](https://codecov.io/gh/huggingface/transformers/pull/1657/diff?src=pr&el=tree#diff-dHJhbnNmb3JtZXJzL21vZGVsaW5nX3RmX3B5dG9yY2hfdXRpbHMucHk=) | `9.85% <0%> (-83.1%)` | :arrow_down: |
| [transformers/tests/modeling\_tf\_common\_test.py](https://codecov.io/gh/huggingface/transformers/pull/1657/diff?src=pr&el=tree#diff-dHJhbnNmb3JtZXJzL3Rlc3RzL21vZGVsaW5nX3RmX2NvbW1vbl90ZXN0LnB5) | `79.78% <0%> (-17.03%)` | :arrow_down: |
| [transformers/modeling\_roberta.py](https://codecov.io/gh/huggingface/transformers/pull/1657/diff?src=pr&el=tree#diff-dHJhbnNmb3JtZXJzL21vZGVsaW5nX3JvYmVydGEucHk=) | `58.68% <0%> (-12.58%)` | :arrow_down: |
| [transformers/modeling\_xlnet.py](https://codecov.io/gh/huggingface/transformers/pull/1657/diff?src=pr&el=tree#diff-dHJhbnNmb3JtZXJzL21vZGVsaW5nX3hsbmV0LnB5) | `70.82% <0%> (-2.47%)` | :arrow_down: |
| [transformers/modeling\_ctrl.py](https://codecov.io/gh/huggingface/transformers/pull/1657/diff?src=pr&el=tree#diff-dHJhbnNmb3JtZXJzL21vZGVsaW5nX2N0cmwucHk=) | `93.18% <0%> (-2.28%)` | :arrow_down: |
| [transformers/modeling\_openai.py](https://codecov.io/gh/huggingface/transformers/pull/1657/diff?src=pr&el=tree#diff-dHJhbnNmb3JtZXJzL21vZGVsaW5nX29wZW5haS5weQ==) | `80.4% <0%> (-1.36%)` | :arrow_down: |
| [transformers/file\_utils.py](https://codecov.io/gh/huggingface/transformers/pull/1657/diff?src=pr&el=tree#diff-dHJhbnNmb3JtZXJzL2ZpbGVfdXRpbHMucHk=) | `76.37% <0%> (+2.19%)` | :arrow_up: |
------
[Continue to review full report at Codecov](https://codecov.io/gh/huggingface/transformers/pull/1657?src=pr&el=continue).
> **Legend** - [Click here to learn more](https://docs.codecov.io/docs/codecov-delta)
> `Δ = absolute <relative> (impact)`, `ø = not affected`, `? = missing data`
> Powered by [Codecov](https://codecov.io/gh/huggingface/transformers/pull/1657?src=pr&el=footer). Last update [079bfb3...cbd0696](https://codecov.io/gh/huggingface/transformers/pull/1657?src=pr&el=lastupdated). Read the [comment docs](https://docs.codecov.io/docs/pull-request-comments).
<|||||>This issue has been automatically marked as stale because it has not had recent activity. It will be closed if no further activity occurs. Thank you for your contributions.
|
transformers | 1,656 | closed | Parallel data preprocessing for distillation | ## 🚀 Feature
Use the `multiprocessing.Pool` function to parallelize the text tokenization and uint16 conversion in `transformers/examples/distillation/scripts/binarized_data.py`.
## Motivation
I tried to preprocess a 2.6 GB txt file using the python script, but the expected time is 2.4 hours. I tried to parallelize it myself and the total time decreased to 10 minutes on my server.
## Additional context
My code is something like this:
```
def process_data(text):
return tokenizer.encode(f'{bos} {text.strip()} {sep}')
pool = Pool()
rslt = pool.map(process_data, data)
rslt_ = pool.map(np.uint16, rslt)
``` | 10-28-2019 23:20:20 | 10-28-2019 23:20:20 | What is your suggestion, then? Adding a mp_encode function? Perhaps this is something that should stay at the user's side. <|||||>Hello @jianwolf,
Yes indeed, I've never taken the time to do it (mainly because most of the I do pre-processing are one-shot: I launch it before leaving the office 😴).
If you feel like opening a pull request with your suggestion, I would happy to add it.
@BramVanroy do you see any drawbacks of having parallelized pre-processing by default?
I tried to integrate your few lines and had this error:
```
File "/usr/lib/python3.6/multiprocessing/reduction.py", line 51, in dumps
cls(buf, protocol).dump(obj)
AttributeError: Can't pickle local object 'main.<locals>.process_data'
```
It seems like `process_data` should be outside of the `main`, that shouldn't be too complicated.
(Also, how many parallel processes/cpus do you have on your server for this order of magnitude in reduction?)
Victor
<|||||>@VictorSanh At first reading I thought the suggestion was to implement a default multiprocessing encoding for tokenizers. That would seem like a large change that needs a lot of testing across multiple platforms (note the different between fork and spawn) as well as a possible reproducibility issue when retrieving results from different threads, and thus different batch orders. Of course these problems could be mitigated but it seemed like a lot of work to suddenly overhaul all tokenizers in this way.
Now that it's clear that it's only for the distillation script, I'm sure there's no big issue here even though I would like to see this implemented in a deterministic way, i.e. order of return values should always be identical. <|||||>Hi! Yeah I will create a pull request for this code! On my machine there are 80 CPU threads available!<|||||>This issue has been automatically marked as stale because it has not had recent activity. It will be closed if no further activity occurs. Thank you for your contributions.
|
transformers | 1,655 | closed | Missing a line in examples/distillation/README.md | In How to train Distil* -> B, in both of the training commands, you should add `--alpha_clm 0.0 \`, otherwise an assertion error will be triggered (https://github.com/huggingface/transformers/blob/079bfb32fba4f2b39d344ca7af88d79a3ff27c7c/examples/distillation/train.py#L49). | 10-28-2019 23:09:44 | 10-28-2019 23:09:44 | Oh yes indeed. Let me correct it. Thank you for pointing that out @jianwolf! |
transformers | 1,654 | closed | Can I load a CTRL model that was fine-tuned using the Salesforce code? | ## ❓ Questions & Help
I have a custom CTRL model that I trained using the Salesforce TF code and I was hoping that I could convert it into the transformers format and load it there. Any advice?
| 10-28-2019 21:18:17 | 10-28-2019 21:18:17 | cc @keskarnitish :)<|||||>Sure, I'll get on this soon. I'll push it to https://github.com/salesforce/ctrl and link here once I'm done. <|||||>Thanks @keskarnitish! That would be great!<|||||>Added in https://github.com/salesforce/ctrl/commit/a0d0b4d2f38ae55a1396dfad4d6bff7cc9435c2d , see updated `README.md` for usage. <|||||>That is awesome @keskarnitish! We'll add it to our doc here as well. |
transformers | 1,653 | closed | No way to control ID of special chars e.g. mask IDs | ## Summary
Hi, many thanks for the library - this is a fantastic tool for the NLP community!
I notice there are a number of constants defined in code that the user cannot inject whilst initialising.
Some examples are:
- `padding_idx = 1` in `RobertaEmbeddings`
- `CrossEntropyLoss(ignore_index=-1)` in `RobertaForMaskedLM`.
- `padding_idx=0` in `BertEmbeddings`
`RobertaModel` also raises a warning if there are no tokens with index `0`, but it is not clear which control character this corresponds to.
1. Would it be a good idea to allow these parameters to be injectable so a user can control the ID of the special tokens?
2. Is it possible to provide a list of what the expected indices for special characters are?
I think for example:
```
-1 => Ignore target during loss
0 => `[CLS]`
1 => `[PAD]`
```
but 0 could also be `[SEP]` as I believe both are always used in roBERTa.
Is there an index I must respect other than these? E.g. does `[SEP]` need a specific index?
3. Why is the ignore index -1? Is this just to stay true to the original papers? Wouldn't the index of the `[PAD]` token make sense? I notice this index is different in the different embedding classes.
Many thanks for your thoughts,
Dom
| 10-28-2019 20:28:44 | 10-28-2019 20:28:44 | To answer question 2:
You can see the assumed indices for special characters by loading a tokenizer and inspecting the `vocab` attribute.
For example:
```
from transformers import BertTokenizer
tokenizer = BertTokenizer.from_pretrained('bert-base-uncased')
print(tokenizer.vocab)
```
This shows that for the `bert-base-uncased` model it is assumed that:
| Special Token | Index |
| --- | --- |
| [PAD] | 0 |
| [UNK] | 100 |
| [CLS] | 101 |
| [SEP] | 102 |
| [MASK] | 103 |<|||||>This issue has been automatically marked as stale because it has not had recent activity. It will be closed if no further activity occurs. Thank you for your contributions.
|
transformers | 1,652 | closed | Missing required argument 'mode' in run_ner. | ## 🐛 Bug
<!-- Important information -->
Model I am using: BERT
Language I am using the model on: Polish (irrelevant for the error)
The problem arise when using:
* [x] the official example scripts: run_ner.py
The tasks I am working on is:
* [x] my own task or dataset: token classification (aka NER)
## To Reproduce
Steps to reproduce the behavior:
1. Start run_ner.py with --evaluate_during_training
2. During evaluation the error will happen
## Expected behavior
Evaluation should run fine
## Additional context
There is a missing argument in line 156 `mode`, which (I belive) should be `"dev"`.
I can provide a PR if the above solution is confirmed. | 10-28-2019 19:43:11 | 10-28-2019 19:43:11 | yes sure, happy to welcome a PR on this<|||||>This issue has been automatically marked as stale because it has not had recent activity. It will be closed if no further activity occurs. Thank you for your contributions.
|
transformers | 1,651 | closed | How to set local_rank argument in run_squad.py | Hi!
I would like to try out the run_squad.py script (with AWS SageMaker in a PyTorch container).
I will use 8 x 100V 16 GB GPUs for the training.
How should I set the the local_rank parameter in this case?
( I tried to understand it from the code, but I couldn't really.)
Thank you for the help! | 10-28-2019 15:51:47 | 10-28-2019 15:51:47 | The easiest way is to use the torch launch script. It will automatically set the local rank correctly. It would look something like this (can't test, am on phone) :
```bash
python -m torch.distributed.launch --nproc_per_node 8 run_squad.py <your arguments>
```<|||||>Hi,
Thanks for the fast answer!
Yes I saw this solution in the examples, but I am interested in the case when I am using PyTorch container and I have to set up an entry point for the training (= run_squad.py) and its parameters . And so in that case how should I set it? Or just let it to be -1?
(Or you recommend in that case to create a bash file as entry where I start this torch lunch.)
Thanks again! <|||||>If you want to run it manually, you'll have to run the script once for each GPU, and set the local rank to the GPU ID for each process. It might help to look at the contents of the launch script that I mentioned before. It shows you how to set the local rank automatically for multiple processes, which I think is what you want. <|||||>Ok, thanks for the response! I will try that!<|||||>If your problem is fixed, please do close this issue. <|||||>@tothniki Did you have to modify the script very much to run with SM? Attempting to do so now, as well. <|||||>@petulla No, at the end i didn't modify anything regarding to the multiple GPU problem. ( of course I had to modify the read-in and the save to a S3 Bucket).I tried with SageMaker as it was, and it seemed to me that the distribution between GPUs worked.<|||||>> The easiest way is to use the torch launch script. It will automatically set the local rank correctly. It would look something like this (can't test, am on phone) :
>
> ```shell
> python -m torch.distributed.launch --nproc_per_node 8 run_squad.py <your arguments>
> ```
Hi @ugent
what about ( run_language_modeling.py ) ?
Does passing local_rank = 0 to it means it will automatically do the task on 4 GPUs (for ex.) which we have available ? and our speed will be 4 times faster ? (by distributed training)
or we have to run script by ( python -m torch.distributed.launch .....)
<|||||>@mahdirezaey
Please use the correct tag when tagging...
No, it will not do this automatically, you have to use the launch utility. |
transformers | 1,650 | closed | Custom language text generation | ## ❓ Questions & Help
How to generate text in non english languages. Is xlm-mlm-100-1280 best for this, I tried but results are too worst.
Also tried things mentioned here:
https://github.com/huggingface/transformers/issues/1414
https://github.com/huggingface/transformers/issues/1068
https://github.com/huggingface/transformers/issues/1407
https://github.com/Morizeyao/GPT2-Chinese
Any better suggestion Please.
| 10-28-2019 05:05:36 | 10-28-2019 05:05:36 | This issue has been automatically marked as stale because it has not had recent activity. It will be closed if no further activity occurs. Thank you for your contributions.
|
transformers | 1,649 | closed | ALBERT | # 🌟New model addition
## Model description
ALBERT is "A Lite" version of BERT, a popular unsupervised language representation learning algorithm. ALBERT uses parameter-reduction techniques that allow for large-scale configurations, overcome previous memory limitations, and achieve better behavior with respect to model degradation.
For a technical description of the algorithm, see our paper:
ALBERT: A Lite BERT for Self-supervised Learning of Language Representations
Zhenzhong Lan, Mingda Chen, Sebastian Goodman, Kevin Gimpel, Piyush Sharma, Radu Soricut
## Open Source status
* [ ] the model implementation is available: https://github.com/google-research/google-research/tree/master/albert.
I just want to ask whether you guys have plan to add ALBERT in near future.
| 10-28-2019 03:56:45 | 10-28-2019 03:56:45 | Merging with #1370 |
transformers | 1,648 | closed | Changing LM loss function | Hi all,
I am modifying gpt2 loss function. My new code looks like that:
lm_logits = self.lm_head(hidden_states)
outputs = (lm_logits,) + transformer_outputs[1:]
if labels is not None:
# Shift so that tokens < n predict n
shift_logits = lm_logits[..., :-1, :].contiguous()
shift_labels = labels[..., 1:].contiguous()
all_logits = shift_logits[0].cpu().data.numpy()
tokenizer = GPT2Tokenizer.from_pretrained("gpt2")
sampled_words = []
for elem in all_logits:
logits = elem.reshape(-1)
exps = np.exp(numbers - np.max(logits))
output_logits_normalized = exps / np.sum(exps)
sampled_word = np.array(np.argmax(output_logits_normalized)).reshape([1,1])
sampled_words.append(sampled_word)
text = tokenizer.decode(np.array(sampled_words).reshape(-1))
# Flatten the tokens
loss_fct = CrossEntropyLoss(ignore_index=-1)
loss = loss_fct(shift_logits.view(-1, shift_logits.size(-1)),
shift_labels.view(-1))
print("CE Loss:", loss.cpu().data.numpy())
l = Lyrics(text=text, language='en-us', lookback=15)
rl = l.get_avg_rhyme_length()
beta = 1
rl_loss = rl * beta
print("RL loss: ", rl_loss)
total_loss = loss * 1/rl_loss
print("Total loss: ", total_loss.cpu().data.numpy())
outputs = (total_loss,) + outputs
return outputs # (loss), lm_logits, presents, (all hidden_states), (attentions)
But after evaluation, every model checkpoint returns the same loss on the test set, so it seems that parameters are never updated. Could you please tell me why and how I could solve this?
Thank you a lot. | 10-28-2019 01:15:11 | 10-28-2019 01:15:11 | This is quite a general question. Perhaps it's more useful to put this on Stack Overflow. <|||||>We plan to have a forum associated to the repo to discuss these types of general questions.
In the meantime, we are still happy to welcome them in the PR but the visibility is limited indeed.<|||||>This issue has been automatically marked as stale because it has not had recent activity. It will be closed if no further activity occurs. Thank you for your contributions.
|
transformers | 1,647 | closed | distilroberta-base unavailable in pip install transformers | ## ❓ Questions & Help
<!-- A clear and concise description of the question. -->
Hi,
Would you please update the `pip install transformers` with the addition of `distilroberta-base`?
As of 28 Nov 2019, I tried `pip install transformers` or `pip install --upgrade transformers` but the `distilroberta-base` model is not available. But I can see it from the master branch and if I install it from source, it will work btw.
Thanks!
| 10-28-2019 00:00:42 | 10-28-2019 00:00:42 | Yes, we should push a new pip release this coming week. In the meantime please use master. |
transformers | 1,646 | closed | Undefined behavior | ## 🐛 Bug
There is an undefined behavior in `get_from_cache()` method in `transformers/transformers/file_utils.py`:
```python3
if not os.path.exists(cache_path) and etag is None:
matching_files = fnmatch.filter(os.listdir(cache_dir), filename + '.*')
matching_files = list(filter(lambda s: not s.endswith('.json'), matching_files))
if matching_files:
cache_path = os.path.join(cache_dir, matching_files[-1])
```
According to [docs](https://docs.python.org/3/library/os.html) `os.listdir()`
> Return a list containing the names of the entries in the directory given by path. The list is in **arbitrary order**, ...
so taking last element from list returned by `os.listdir()` in the last row of snippet doesn't make sense because of arbitrary order. A possible solution is to add `sorted()`:
```python3
cache_path = os.path.join(cache_dir, sorted(matching_files)[-1])
```
I can make a PR if you agree. | 10-27-2019 20:10:51 | 10-27-2019 20:10:51 | Yes thanks would be happy to welcome a PR.
Thanks for ca(t😂)ching that<|||||>This issue has been automatically marked as stale because it has not had recent activity. It will be closed if no further activity occurs. Thank you for your contributions.
|
transformers | 1,645 | closed | Error while importing RoBERTa model | I tried to import RoBERTa model.
But running the following snippet:
# Load the model in fairseq
`from fairseq.models.roberta import RobertaModel`
`roberta = RobertaModel.from_pretrained('./roberta.large', checkpoint_file='model.pt')`
`roberta.eval() # disable dropout (or leave in train mode to finetune)`
I got the following error:
`RuntimeError: Error(s) in loading state_dict for RobertaModel: Missing key(s) in state_dict: "decoder.sentence_encoder.layers.0.self_attn.k_proj.weight", "decoder.sentence_encoder.layers.0.self_attn.k_proj.bias", "decoder.sentence_encoder.layers.0.self_attn.v_proj.weight", "decoder.sentence_encoder.layers.0.self_attn.v_proj.bias", "decoder.sentence_encoder.layers.0.self_attn.q_proj.weight", "decoder.sentence_encoder.layers.0.self_attn.q_proj.bias", "decoder.sentence_encoder.layers.1.self_attn.k_proj.weight", "decoder.sentence_encoder.layers.1.self_attn.k_proj.bias", "decoder.sentence_encoder.layers.1.self_attn.v_proj.weight", "decoder.sentence_encoder.layers.1.self_attn.v_proj.bias", "decoder.sentence_encoder.layers.1.self_attn.q_proj.weight", "decoder.sentence_encoder.layers.1.self_attn.q_proj.bias", "decoder.sentence_encoder.layers.2.self_attn.k_proj.weight", "decoder.sentence_encoder.layers.2.self_attn.k_proj.bias", "decoder.sentence_encoder.layers.2.self_attn.v_proj.weight", "decoder.sentence_encoder.layers.2.self_attn.v_proj.bias", "decoder.sentence_encoder.layers.2.self_attn.q_proj.weight", "decoder.sentence_encoder.layers.2.self_attn.q_proj.bias", "decoder.sentence_encoder.layers.3.self_attn.k_proj.weight", "decoder.sentence_encoder.layers.3.self_attn.k_proj.bias", "decoder.sentence_encoder.layers.3.self_attn.v_proj.weight", "decoder.sentence_encoder.layers.3.self_attn.v_proj.bias", "decoder.sentence_encoder.layers.3.self_attn.q_proj.weight", "decoder.sentence_encoder.layers.3.self_attn.q_proj.bias", "decoder.sentence_encoder.... Unexpected key(s) in state_dict: "decoder.sentence_encoder.layers.0.self_attn.in_proj_weight", "decoder.sentence_encoder.layers.0.self_attn.in_proj_bias", "decoder.sentence_encoder.layers.1.self_attn.in_proj_weight", "decoder.sentence_encoder.layers.1.self_attn.in_proj_bias", "decoder.sentence_encoder.layers.2.self_attn.in_proj_weight", "decoder.sentence_encoder.layers.2.self_attn.in_proj_bias", "decoder.sentence_encoder.layers.3.self_attn.in_proj_weight", "decoder.sentence_encoder.layers.3.self_attn.in_proj_bias", "decoder.sentence_encoder.layers.4.self_attn.in_proj_weight", "decoder.sentence_encoder.layers.4.self_attn.in_proj_bias", "decoder.sentence_encoder.layers.5.self_attn.in_proj_weight", "decoder.sentence_encoder.layers.5.self_attn.in_proj_bias", "decoder.sentence_encoder.layers.6.self_attn.in_proj_weight", "decoder.sentence_encoder.layers.6.self_attn.in_proj_bias", "decoder.sentence_encoder.layers.7.self_attn.in_proj_weight", "decoder.sentence_encoder.layers.7.self_attn.in_proj_bias", "decoder.sentence_encoder.layers.8.self_attn.in_proj_weight", "decoder.sentence_encoder.layers.8.self_attn.in_proj_bias", "decoder.sentence_encoder.layers.9.self_attn.in_proj_weight", "decoder.sentence_encoder.layers.9.self_attn.in_proj_bias", "decoder.sentence_encoder.layers.10.self_attn.in_proj_weight", "decoder.sentence_encoder.layers.10.self_attn.in_proj_bias", "decoder.sentence_encoder.layers.11.self_attn.in_proj_weight", "decoder.sentence_encoder.layers.11.self_attn.in_proj_bi...`
Is it related to the above error? How can we fix it? Using the hub I get the same error. | 10-27-2019 19:37:31 | 10-27-2019 19:37:31 | You have opened an issue for the transformers repository but executed code from fairseq. Don't you think you should create an issue there [1]?
[1] https://github.com/pytorch/fairseq/blob/master/examples/roberta/README.md<|||||>Right. Sorry! I made a mistake.<|||||>I have error when i run this code : please how to fix it?????
# Load the model in fairseq
from fairseq.models.roberta import RobertaModel
roberta = RobertaModel.from_pretrained('/path/to/roberta.large', checkpoint_file='model.pt')
roberta.eval() # disable dropout (or leave in train mode to finetune)
---------------------------------------------------------------------------
TypeError Traceback (most recent call last)
[<ipython-input-23-cd858fcec71b>](https://localhost:8080/#) in <module>
1 # Load the model in fairseq
2 from fairseq.models.roberta import RobertaModel
----> 3 roberta = RobertaModel.from_pretrained('/path/to/roberta.large', checkpoint_file='model.pt')
4 roberta.eval() # disable dropout (or leave in train mode to finetune)
2 frames
[/usr/lib/python3.8/posixpath.py](https://localhost:8080/#) in join(a, *p)
74 will be discarded. An empty last part will result in a path that
75 ends with a separator."""
---> 76 a = os.fspath(a)
77 sep = _get_sep(a)
78 path = a
TypeError: expected str, bytes or os.PathLike object, not NoneType<|||||>Same comment as above, please open your issue in the correct repository.<|||||>i did not understand this.. can you write the code for this, please?
note i run the previous code as following:
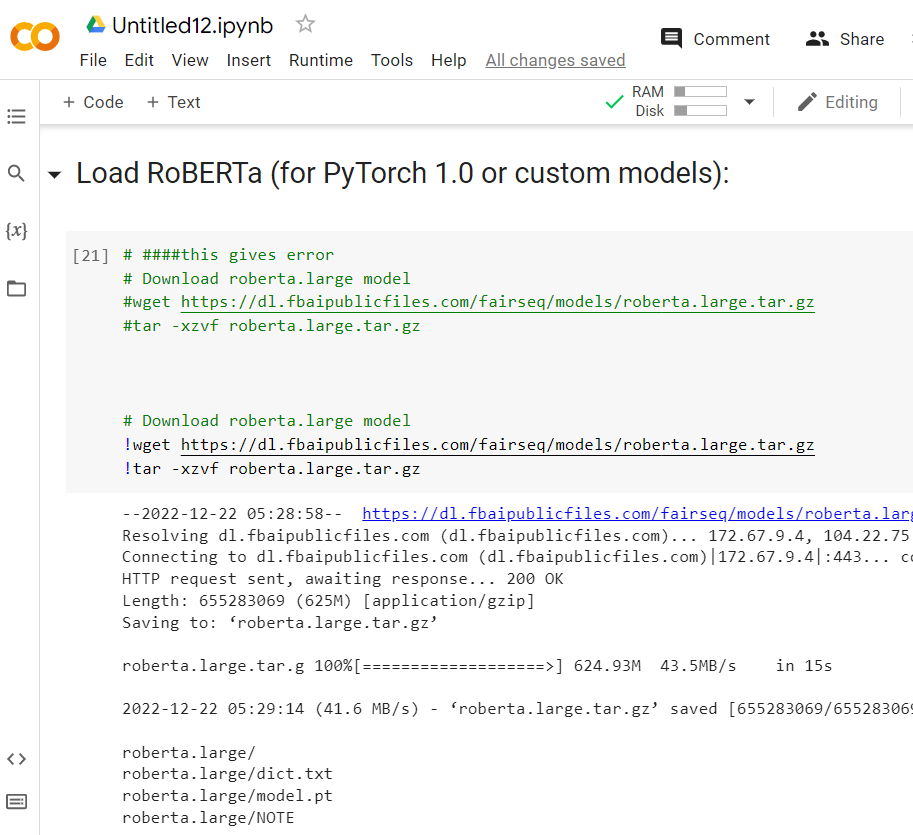
<|||||>can you write the correct code please for that?<|||||>I fixed it ... thanks
|
transformers | 1,644 | closed | Maximum length of out put generated in run_generation.py is of length (1021 ) despite changing position id length and length parameter | I would like to generate a text of about 3000 words.
However the run_generation.py file limits it to 1024, and produces only 1021 words. I have tried changing the internal parameters for the same but in vain. | 10-27-2019 19:01:36 | 10-27-2019 19:01:36 | What model are you using?<|||||>This issue has been automatically marked as stale because it has not had recent activity. It will be closed if no further activity occurs. Thank you for your contributions.
|
transformers | 1,643 | closed | how to use BertForMaskedLM | Hi
I want to use BertForMaskedLM as a decoder, apparently I need to give ids and then this function generates the ids and computes the loss. could you tell me how the generation with this function work? I see for instance in run_generation.py codes you use neucleus sampling or beam search, I see none of them used here, could you explain how this works. Also I want to see the generated sequence as text, could you tell me how from the output of this function I can get this information? thanks | 10-27-2019 17:25:59 | 10-27-2019 17:25:59 | This issue has been automatically marked as stale because it has not had recent activity. It will be closed if no further activity occurs. Thank you for your contributions.
|
transformers | 1,642 | closed | How to compute loss with HuggingFace transformers? | Hello,
Is it possible to train HuggingFace TransfoXLLMHeadModel on a dataset different than WikiText103, say, on the combined WikiText2 and WikiText103 dataset?
Below are my code:
```js
# Import packages
import torch
import torch.nn as nn
import torch.nn.functional as F
from transformers import TransfoXLConfig, TransfoXLTokenizer, TransfoXLLMHeadModel
from transformers import AdamW, WarmupLinearSchedule
import spacy
import torchtext
from torchtext.data.utils import get_tokenizer
from torchtext.data import Field, BPTTIterator, TabularDataset
import tensorflow as tf
import math
import random
import numpy as np
import pandas as pd
import time
# set hyperparameters for this experiment
bptt = 30
batch_size = 64
lr = 0.01 # learning rate
criterion = nn.CrossEntropyLoss() # loss criterion
# define tokenizer
en = spacy.load('en')
def Sp_Tokenizer(text):
return [tok.text for tok in en.tokenizer(text)]
# define the English text field
TEXT = Field(tokenize = Sp_Tokenizer,
init_token='< sos >',
eos_token='< eos >',
unk_token='< unk >',
tokenizer_language='en',
lower=True)
# load the datasets
train_Wiki2, val_Wiki2, test_Wiki2 = torchtext.datasets.WikiText2.splits(TEXT)
train_Wiki103, val_Wiki103, test_Wiki103 = torchtext.datasets.WikiText103.splits(TEXT)
# Define device
device = torch.device("cuda" if torch.cuda.is_available() else "cpu")
# build vocabulary based on the defined field and
# the combined WikiText2 and WikiText103 datasets.
TEXT.build_vocab(train_Wiki2, val_Wiki2, test_Wiki2,
train_Wiki103, val_Wiki103, test_Wiki103)
# set hyperparameter ntokens
ntokens = len(TEXT.vocab.stoi)
## specify the transformer-XL model that we are going to use.
#
# define transformer-XL configuration.
transfoXLconfig = TransfoXLConfig(vocab_size_or_config_json_file = ntokens,
cutoffs = [20000, 40000, 200000],
d_model = 64,
d_embed = 64,
n_head = 16,
d_head = 64,
n_layer = 5,
attn_type = 0,
dropout = 0.1,
output_hidden_states = True,
output_attentions = True)
# define the transformer-XL model based on the specified configuration.
model = TransfoXLLMHeadModel(transfoXLconfig)
# add new tokens to the embeddings of our model
model.resize_token_embeddings(ntokens)
# define BPTTiterators
#
train_iter, val_iter, test_iter = BPTTIterator.splits(
(train_Wiki2, val_Wiki2, test_Wiki2),
batch_size = batch_size,
bptt_len= bptt,
sort_key=lambda x: len(x.text),
sort_within_batch = True,
shuffle = False,
device= device,
repeat=False)
train = next(iter(train_iter))
val = next(iter(train_iter))
test = next(iter(test_iter))
```
and now I am trying to write the train function but I am not sure how exactly I should proceed.
Below is what I tried:
```js
# define the hyperparameters for running the train function.
train = train
optimizer = AdamW(model.parameters())
scheduler = WarmupLinearSchedule(optimizer = optimizer,
warmup_steps = 200,
t_total = 1000,
last_epoch = -1)
model.train()
# define the train function
def train(model, train, bptt, criterion, optimizer, scheduler, ntokens, log_interval):
# initialize total_loss to 0
total_loss = 0
# measure the computation time
start_time = time.time()
# number of tokens in the vocabulary
ntokens = ntokens
for i in range(train.text.size()[1]):
batch = i
input_ids, targets = train.text[:,i], train.target[:,i]
input_ids = torch.tensor(input_ids.tolist()).unsqueeze(0)
targets = torch.tensor(targets.tolist()).unsqueeze(0)
optimizer.zero_grad()
# I intend this 'output' to be the final output of the Transformer-XL....
output = model(input_ids)
#... to execute this line
loss = criterion(output.view(-1, ntokens), targets)
loss.backward()
torch.nn.utils.clip_grad_norm_(model.parameters(), 0.5)
optimizer.step()
```
But I don't think the line `loss = criterion(output.view(-1, ntokens), targets)` shouldn't work since the line `output = model(input_ids)` does not actually give out the final output from the model, but it rather gives out (according to the HuggingFace documentation) prediction_scores, mems, attention, etc. How can I train TransfoXLLMHeadModel on a dataset different than just WikiText103?
Thank you, | 10-27-2019 12:35:19 | 10-27-2019 12:35:19 | This issue has been automatically marked as stale because it has not had recent activity. It will be closed if no further activity occurs. Thank you for your contributions.
|
transformers | 1,641 | closed | How to use custom built Torchtext vocabulary with HuggingFace TransfoXLLMHeadModel? | Hello,
I am trying to use my custom built vocabulary which I defined using Torchtext functions with the HuggingFace TransfoXLLMHeadModel, and I am having some troubles with it.
I defined my text field as below:
```js
# Import packages
import torch
import torch.nn as nn
import torch.nn.functional as F
from transformers import TransfoXLConfig, TransfoXLTokenizer, TransfoXLLMHeadModel
from transformers import AdamW, WarmupLinearSchedule
import spacy
import torchtext
from torchtext.data.utils import get_tokenizer
from torchtext.data import Field, BPTTIterator, TabularDataset
import tensorflow as tf
#import lineflow as lf
#import lineflow.datasets as lfds
import math
import random
import numpy as np
import pandas as pd
import time
# define tokenizer
en = spacy.load('en')
def Sp_Tokenizer(text):
return [tok.text for tok in en.tokenizer(text)]
# define the English text field
TEXT = Field(tokenize = Sp_Tokenizer,
init_token='< sos >',
eos_token='< eos >',
unk_token='< unk >',
tokenizer_language='en',
lower=True)
# load WikiText-2 dataset and split it into train and test set
train_Wiki2, val_Wiki2, test_Wiki2 = torchtext.datasets.WikiText2.splits(TEXT)
train_Wiki103, val_Wiki103, test_Wiki103 = torchtext.datasets.WikiText103.splits(TEXT)
train_Penn, val_Penn, test_Penn = torchtext.datasets.PennTreebank.splits(TEXT)
# build custom vocabulary based on the field that we just defined.
TEXT.build_vocab(train_Wiki2, val_Wiki2, test_Wiki2,
train_Wiki103, val_Wiki103, test_Wiki103,
train_Penn, val_Penn, test_Penn)
```
and then I defined the HuggingFace transformer's configuration as below:
```js
# set hyperparameter ntokens
ntokens = len(TEXT.vocab.stoi)
# define transformer-XL configuration.
transfoXLconfig = TransfoXLConfig(vocab_size_or_config_json_file = ntokens,
cutoffs = [20000, 40000, 200000],
d_model = 64,
d_embed = 64,
n_head = 16,
d_head = 64,
n_layer = 5,
attn_type = 0,
dropout = 0.1,
output_hidden_states = True,
output_attentions = True)
# define the transformer-XL model based on the specified configuration.
model = TransfoXLLMHeadModel(transfoXLconfig)
# add new tokens to the embeddings of our model
model.resize_token_embeddings(ntokens)
```
and then I want to somehow specify that I want to use my `TEXT.vocab` that I defined earlier via Torchtext for my vocabulary along with the TransfoXLLMHeadModel, but I am not sure how to do this. Can someone help me on this? Thank you! | 10-27-2019 08:59:51 | 10-27-2019 08:59:51 | This issue has been automatically marked as stale because it has not had recent activity. It will be closed if no further activity occurs. Thank you for your contributions.
|
transformers | 1,640 | closed | Why DistilBertTokenizer and BertTokenizer are creating different number of features?? | Hi,
I tried working with DistilBertTokenizer and BertTokenizer from transformers. And according to documentation DistilBertTokenizer was identical to the BertTokenizer . But while creating features for a particular Dataset it creates different number of examples. Why? I also tried using distilbert model with BertTokenizer but still it doesnot work.
could you please explain me this ?? or How can i get same number of features ??
| 10-27-2019 07:43:27 | 10-27-2019 07:43:27 | |
transformers | 1,639 | closed | Add Transformer-XL fine-tuning support. | ## 🚀 Feature
Add Transformer-XL fine-tuning support.
## Motivation
This model archieves good language modeling result while having a "saner" number of parameters compared with GPT-2 or other language modeling.
| 10-26-2019 10:25:48 | 10-26-2019 10:25:48 | We don't have the bandwidth for that at the moment. But if somebody in the community is interested in working on that, happy to welcome a PR.<|||||>This issue has been automatically marked as stale because it has not had recent activity. It will be closed if no further activity occurs. Thank you for your contributions.
|
transformers | 1,638 | closed | how can I pre-training my own model from the existed model or from scratch | ## ❓ Questions & Help
I want to load the pre-training model like bert offered by google,and train language model on more corpus,how can I do it ?thanks | 10-26-2019 05:09:24 | 10-26-2019 05:09:24 | Hi, you can see how to use the library in the [documentation](https://huggingface.co/transformers/). You might be interested in the library philosophy and the way to load pre-trained models, which is [described here](https://huggingface.co/transformers/quickstart.html). You might also be interested in the [examples](https://huggingface.co/transformers/examples.html), which showcase [how to fine-tune a language model](https://huggingface.co/transformers/examples.html#language-model-fine-tuning).<|||||>@hischen did you find solution for pre-training BERT on your corpus?
@LysandreJik fine tuning is different from pre-training. I could not find documentation about pre-training the model on a corpus. Can you please help me with that.
Regards,
D. Ravi Theja.<|||||>This issue has been automatically marked as stale because it has not had recent activity. It will be closed if no further activity occurs. Thank you for your contributions.
|
transformers | 1,637 | closed | Installation error :Command "python setup.py egg_info" failed with error code 1 | [puttyerrortransformers2.log](https://github.com/huggingface/transformers/files/3774258/puttyerrortransformers2.log)
## 🐛 Bug
Hello Everyone,
I am trying to install transformers using the command:
pip3 install -v --no-binary :all: --prefix=/short/oe7/uk1594 transformers
* Python version: Python 3.6.7
* PyTorch version:1.12.0
* CentOS release 6.10 (Final)
I get the below error:
Using cached https://files.pythonhosted.org/packages/1b/87/c3c2fa8cbec61fffe031ca9f0da512747520bec9be7f886f748457daac31/sentencepiece-0.1.83.tar.gz
Downloading from URL https://files.pythonhosted.org/packages/1b/87/c3c2fa8cbec61fffe031ca9f0da512747520bec9be7f886f748457daac31/sentencepiece-0.1.83.tar.gz#sha256=d194cf7431dd87798963ff998380f1c02ff0f9e380cc922a07926b69e21c4e2b (from https://pypi.org/simple/sentencepiece/)
Running setup.py (path:/short/oe7/uk1594/tmp/pip-install-5c0k51ol/sentencepiece/setup.py) egg_info for package sentencepiece
Running command python setup.py egg_info
Traceback (most recent call last):
File "<string>", line 1, in <module>
File "/short/oe7/uk1594/tmp/pip-install-5c0k51ol/sentencepiece/setup.py", line 29, in <module>
with codecs.open(os.path.join('..', 'VERSION'), 'r', 'utf-8') as f:
File "/apps/python3/3.6.7/lib/python3.6/codecs.py", line 897, in open
file = builtins.open(filename, mode, buffering)
FileNotFoundError: [Errno 2] No such file or directory: '../VERSION'
Cleaning up...
Removing source in /short/oe7/uk1594/tmp/pip-install-5c0k51ol/transformers
Removing source in /short/oe7/uk1594/tmp/pip-install-5c0k51ol/boto3
Removing source in /short/oe7/uk1594/tmp/pip-install-5c0k51ol/requests
Removing source in /short/oe7/uk1594/tmp/pip-install-5c0k51ol/tqdm
Removing source in /short/oe7/uk1594/tmp/pip-install-5c0k51ol/regex
Removing source in /short/oe7/uk1594/tmp/pip-install-5c0k51ol/sentencepiece
Command "python setup.py egg_info" failed with error code 1 in /short/oe7/uk1594/tmp/pip-install-5c0k51ol/sentencepiece/
Exception information:
Traceback (most recent call last):
File "/apps/python3/3.6.7/lib/python3.6/site-packages/pip/_internal/basecommand.py", line 228, in main
status = self.run(options, args)
File "/apps/python3/3.6.7/lib/python3.6/site-packages/pip/_internal/commands/install.py", line 291, in run
resolver.resolve(requirement_set)
File "/apps/python3/3.6.7/lib/python3.6/site-packages/pip/_internal/resolve.py", line 103, in resolve
self._resolve_one(requirement_set, req)
File "/apps/python3/3.6.7/lib/python3.6/site-packages/pip/_internal/resolve.py", line 257, in _resolve_one
abstract_dist = self._get_abstract_dist_for(req_to_install)
File "/apps/python3/3.6.7/lib/python3.6/site-packages/pip/_internal/resolve.py", line 210, in _get_abstract_dist_for
self.require_hashes
File "/apps/python3/3.6.7/lib/python3.6/site-packages/pip/_internal/operations/prepare.py", line 324, in prepare_linked_requirement
abstract_dist.prep_for_dist(finder, self.build_isolation)
File "/apps/python3/3.6.7/lib/python3.6/site-packages/pip/_internal/operations/prepare.py", line 154, in prep_for_dist
self.req.run_egg_info()
File "/apps/python3/3.6.7/lib/python3.6/site-packages/pip/_internal/req/req_install.py", line 486, in run_egg_info
command_desc='python setup.py egg_info')
File "/apps/python3/3.6.7/lib/python3.6/site-packages/pip/_internal/utils/misc.py", line 698, in call_subprocess
% (command_desc, proc.returncode, cwd))
pip._internal.exceptions.InstallationError: Command "python setup.py egg_info" failed with error code 1 in /short/oe7/uk1594/tmp/pip-install-5c0k51ol/sentencepiece/
Please find the logs attached.
Appreciate your help.
Thanks.
[puttyerrortransformers2.log](https://github.com/huggingface/transformers/files/3774257/puttyerrortransformers2.log)
| 10-26-2019 00:43:53 | 10-26-2019 00:43:53 | @thomwolf Please if you could provide your insights on the issue.
Thanks<|||||>https://github.com/google/sentencepiece/issues/386 |
transformers | 1,636 | closed | AttributeError: 'CTRLTokenizer' object has no attribute 'control_codes' | ## 🐛 Bug
I can't seem to get ctrl generation working.
This is with a pull of the repo from master, and pip3 install as recommended during installation:
The problem arise when using:
* [ X ] the official example scripts:
```bash
$ uname -a
Linux ctrl 4.9.0-11-amd64 #1 SMP Debian 4.9.189-3+deb9u1 (2019-09-20) x86_64 GNU/Linux
```
<!-- If you have a code sample, error messages, stack traces, please provide it here as well. -->
```bash
python3 ./examples/run_generation.py --model_type=ctrl --length=20 --model_name_or_path=ctrl --temperature=0 --repetition_penalty=1.2
/home/vessenes/.local/lib/python3.5/site-packages/tensorflow/python/framework/dtypes.py:516: FutureWarning: Passing (type, 1) or '1type' as a synonym of type is deprecated; in a future version of numpy, it will be understood as (type, (1,)) / '(1,)type'.
_np_qint8 = np.dtype([("qint8", np.int8, 1)])
/home/vessenes/.local/lib/python3.5/site-packages/tensorflow/python/framework/dtypes.py:517: FutureWarning: Passing (type, 1) or '1type' as a synonym of type is deprecated; in a future version of numpy, it will be understood as (type, (1,)) / '(1,)type'.
_np_quint8 = np.dtype([("quint8", np.uint8, 1)])
/home/vessenes/.local/lib/python3.5/site-packages/tensorflow/python/framework/dtypes.py:518: FutureWarning: Passing (type, 1) or '1type' as a synonym of type is deprecated; in a future version of numpy, it will be understood as (type, (1,)) / '(1,)type'.
_np_qint16 = np.dtype([("qint16", np.int16, 1)])
/home/vessenes/.local/lib/python3.5/site-packages/tensorflow/python/framework/dtypes.py:519: FutureWarning: Passing (type, 1) or '1type' as a synonym of type is deprecated; in a future version of numpy, it will be understood as (type, (1,)) / '(1,)type'.
_np_quint16 = np.dtype([("quint16", np.uint16, 1)])
/home/vessenes/.local/lib/python3.5/site-packages/tensorflow/python/framework/dtypes.py:520: FutureWarning: Passing (type, 1) or '1type' as a synonym of type is deprecated; in a future version of numpy, it will be understood as (type, (1,)) / '(1,)type'.
_np_qint32 = np.dtype([("qint32", np.int32, 1)])
/home/vessenes/.local/lib/python3.5/site-packages/tensorflow/python/framework/dtypes.py:525: FutureWarning: Passing (type, 1) or '1type' as a synonym of type is deprecated; in a future version of numpy, it will be understood as (type, (1,)) / '(1,)type'.
np_resource = np.dtype([("resource", np.ubyte, 1)])
/home/vessenes/.local/lib/python3.5/site-packages/tensorboard/compat/tensorflow_stub/dtypes.py:541: FutureWarning: Passing (type, 1) or '1type' as a synonym of type is deprecated; in a future version of numpy, it will be understood as (type, (1,)) / '(1,)type'.
_np_qint8 = np.dtype([("qint8", np.int8, 1)])
/home/vessenes/.local/lib/python3.5/site-packages/tensorboard/compat/tensorflow_stub/dtypes.py:542: FutureWarning: Passing (type, 1) or '1type' as a synonym of type is deprecated; in a future version of numpy, it will be understood as (type, (1,)) / '(1,)type'.
_np_quint8 = np.dtype([("quint8", np.uint8, 1)])
/home/vessenes/.local/lib/python3.5/site-packages/tensorboard/compat/tensorflow_stub/dtypes.py:543: FutureWarning: Passing (type, 1) or '1type' as a synonym of type is deprecated; in a future version of numpy, it will be understood as (type, (1,)) / '(1,)type'.
_np_qint16 = np.dtype([("qint16", np.int16, 1)])
/home/vessenes/.local/lib/python3.5/site-packages/tensorboard/compat/tensorflow_stub/dtypes.py:544: FutureWarning: Passing (type, 1) or '1type' as a synonym of type is deprecated; in a future version of numpy, it will be understood as (type, (1,)) / '(1,)type'.
_np_quint16 = np.dtype([("quint16", np.uint16, 1)])
/home/vessenes/.local/lib/python3.5/site-packages/tensorboard/compat/tensorflow_stub/dtypes.py:545: FutureWarning: Passing (type, 1) or '1type' as a synonym of type is deprecated; in a future version of numpy, it will be understood as (type, (1,)) / '(1,)type'.
_np_qint32 = np.dtype([("qint32", np.int32, 1)])
/home/vessenes/.local/lib/python3.5/site-packages/tensorboard/compat/tensorflow_stub/dtypes.py:550: FutureWarning: Passing (type, 1) or '1type' as a synonym of type is deprecated; in a future version of numpy, it will be understood as (type, (1,)) / '(1,)type'.
np_resource = np.dtype([("resource", np.ubyte, 1)])
10/25/2019 22:11:28 - INFO - transformers.tokenization_utils - loading file https://raw.githubusercontent.com/salesforce/ctrl/master/ctrl-vocab.json from cache at /home/vessenes/.cache/torch/transformers/a858ad854d3847b02da3aac63555142de6a05f2a26d928bb49e881970514e186.285c96a541cf6719677cfb634929022b56b76a0c9a540186ba3d8bbdf02bca42
10/25/2019 22:11:28 - INFO - transformers.tokenization_utils - loading file https://raw.githubusercontent.com/salesforce/ctrl/master/ctrl-merges.txt from cache at /home/vessenes/.cache/torch/transformers/aa2c569e6648690484ade28535a8157aa415f15202e84a62e82cc36ea0c20fa9.26153bf569b71aaf15ae54be4c1b9254dbeff58ca6fc3e29468c4eed078ac142
10/25/2019 22:11:29 - INFO - transformers.configuration_utils - loading configuration file https://storage.googleapis.com/sf-ctrl/pytorch/ctrl-config.json from cache at /home/vessenes/.cache/torch/transformers/d6492ca334c2a4e079f43df30956acf935134081b2b3844dc97457be69b623d0.1ebc47eb44e70492e0c20494a084f108332d20fea7fe5ad408ef5e7a8f2baef4
10/25/2019 22:11:29 - INFO - transformers.configuration_utils - Model config {
"attn_pdrop": 0.1,
"dff": 8192,
"embd_pdrop": 0.1,
"finetuning_task": null,
"from_tf": false,
"initializer_range": 0.02,
"layer_norm_epsilon": 1e-06,
"n_ctx": 512,
"n_embd": 1280,
"n_head": 16,
"n_layer": 48,
"n_positions": 50000,
"num_labels": 1,
"output_attentions": false,
"output_hidden_states": false,
"output_past": true,
"pruned_heads": {},
"resid_pdrop": 0.1,
"summary_activation": null,
"summary_first_dropout": 0.1,
"summary_proj_to_labels": true,
"summary_type": "cls_index",
"summary_use_proj": true,
"torchscript": false,
"use_bfloat16": false,
"vocab_size": 246534
}
10/25/2019 22:00:19 - INFO - transformers.modeling_utils - loading weights file https://storage.googleapis.com/sf
-ctrl/pytorch/seqlen256_v1.bin from cache at /home/vessenes/.cache/torch/transformers/c146cc96724f27295a0c3ada1fbb3
632074adf87e9aef8269e44c9208787f8c8.b986347cbab65fa276683efbb9c2f7ee22552277bcf6e1f1166557ed0852fdf0
10/25/2019 22:01:17 - INFO - __main__ - Namespace(device=device(type='cpu'), length=20, model_name_or_path='ctrl'
, model_type='ctrl', n_gpu=0, no_cuda=False, padding_text='', prompt='', repetition_penalty=1.2, seed=42, stop_toke
n=None, temperature=0.0, top_k=0, top_p=0.9, xlm_lang='')
Model prompt >>> Link Thid is a test article
Traceback (most recent call last):
File "./examples/run_generation.py", line 256, in <module>
main()
File "./examples/run_generation.py", line 228, in main
if not any(context_tokens[0] == x for x in tokenizer.control_codes.values()):
AttributeError: 'CTRLTokenizer' object has no attribute 'control_codes'
```
## Expected behavior
I expect to be able to type in a prompt and see text generated.
## Environment
* OS: Debian 4.9
* Python version: 3.5.3
* PyTorch version: 1.3.0
* PyTorch Transformers version (or branch): not sure how to find this info
* Using GPU ? Yes - V100 on GCP
* Distributed of parallel setup ? No
* Any other relevant information:
## Additional context
<!-- Add any other context about the problem here. -->
| 10-25-2019 22:08:36 | 10-25-2019 22:08:36 | I had the same issue. As a temporary workaround you can simply comment out the following lines as long as you remember to use a control token at the beginning of every prompt that you supply to ctrl:
if args.model_type == "ctrl":
if not any(context_tokens[0] == x for x in tokenizer.control_codes.values()):
logger.info("WARNING! You are not starting your generation from a control code so you won't get good results")<|||||>Hmm, not sure what happens there. Have you tried doing:
```python
from transformers.tokenization_ctrl import CTRLTokenizer
tokenizer = CTRLTokenizer.from_pretrained('ctrl')
print(tokenizer.control_codes)
```
?
Your version of Python is 3.5.x?
Is there a `control_codes = CONTROL_CODES` attributed defined inside your `CTRLTokenizer` class?<|||||>can you also paste your `pip list`?
<|||||> @julien-c
I've encountered the same bug too! I don't know how to resolve this problem!
Keep reading my description below because it could be very interesting what I wrote!
### WHEN THE BUG HAS BEEN FOUND
First of all, I've created a virtual environment dedicated to trying out Transformers library. After that, I've installed _tensorflow-gpu 2.0_ and _PyTorch 1.3.0_. Finally, I've installed transformers today with the following command:
`pip install transformers`
I'm trying to use the CTRL by SalesForce model for text generation purposes. I've gone to the **examples** directory and after that I've executed the script called _run_generation.py_ with the following statement:
`python run_generation.py --model_type ctrl --model_name_or_path ctrl --temperature 0.5 --repetition_penalty 1.2 --no_cuda`.
### EXPECTED BEHAVIOUR
I expect to be able to type in a prompt and insert a control code I like and see the text generated by CTRL model.
### A BIT OF REVERSE ENGINEERING
After I've found this error, I've opened a command line launching **python** (**version 3.6.9**) and I've written the following code lines:
```
from transformers.tokenization_ctrl import CTRLTokenizer
tokenizer = CTRLTokenizer.from_pretrained('ctrl')
tokenizer.control_codes
Traceback (most recent call last):
File "<stdin>", line 1, in <module>
AttributeError: 'CTRLTokenizer' object has no attribute 'control_codes'
```
After found the same error, I've used the **inspect** module of Python in order to view internally what **CTRLTokenizer** class contains. The result opens a doubts:
`'class CTRLTokenizer(PreTrainedTokenizer):\n """\n CTRL BPE tokenizer. Peculiarities:\n - Byte-level Byte-Pair-Encoding\n - Requires a space to start the input string => the encoding methods should be called with the\n ``add_prefix_space`` flag set to ``True``.\n Otherwise, this tokenizer ``encode`` and ``decode`` method will not conserve\n the absence of a space at the beginning of a string: `tokenizer.decode(tokenizer.encode("Hello")) = " Hello"`\n """\n vocab_files_names = VOCAB_FILES_NAMES\n pretrained_vocab_files_map = PRETRAINED_VOCAB_FILES_MAP\n max_model_input_sizes = PRETRAINED_POSITIONAL_EMBEDDINGS_SIZES\n\n def __init__(self, vocab_file, merges_file, unk_token="<unk>", **kwargs):\n super(CTRLTokenizer, self).__init__(unk_token=unk_token, **kwargs)\n self.max_len_single_sentence = self.max_len # no default special tokens - you can update this value if you add special tokens\n self.max_len_sentences_pair = self.max_len # no default special tokens - you can update this value if you add special tokens\n\n self.encoder = json.load(open(vocab_file, encoding="utf-8"))\n self.decoder = {v:k for k,v in self.encoder.items()}\n merges = open(merges_file, encoding=\'utf-8\').read().split(\'\\n\')[1:-1]\n merges = [tuple(merge.split()) for merge in merges]\n self.bpe_ranks = dict(zip(merges, range(len(merges))))\n self.cache = {}\n\n @property\n def vocab_size(self):\n return len(self.encoder)\n\n def bpe(self, token):\n if token in self.cache:\n return self.cache[token]\n word = tuple(token)\n word = tuple(list(word[:-1]) + [word[-1]+\'</w>\'])\n pairs = get_pairs(word)\n\n if not pairs:\n return token\n\n while True:\n bigram = min(pairs, key = lambda pair: self.bpe_ranks.get(pair, float(\'inf\')))\n if bigram not in self.bpe_ranks:\n break\n first, second = bigram\n new_word = []\n i = 0\n while i < len(word):\n try:\n j = word.index(first, i)\n new_word.extend(word[i:j])\n i = j\n except:\n new_word.extend(word[i:])\n break\n\n if word[i] == first and i < len(word)-1 and word[i+1] == second:\n new_word.append(first+second)\n i += 2\n else:\n new_word.append(word[i])\n i += 1\n new_word = tuple(new_word)\n word = new_word\n if len(word) == 1:\n break\n else:\n pairs = get_pairs(word)\n word = \'@@ \'.join(word)\n word = word[:-4]\n self.cache[token] = word\n return word\n\n def _tokenize(self, text):\n """ Tokenize a string.\n """\n split_tokens = []\n\n text = text.split(\' \')\n\n for token in text:\n split_tokens.extend([t for t in self.bpe(token).split(\' \')])\n return split_tokens\n\n def _convert_token_to_id(self, token):\n """ Converts a token (str/unicode) in an id using the vocab. """\n return self.encoder.get(token, self.encoder.get(self.unk_token))\n\n def _convert_id_to_token(self, index):\n """Converts an index (integer) in a token (string/unicode) using the vocab."""\n return self.decoder.get(index, self.unk_token)\n\n def convert_tokens_to_string(self, tokens):\n """ Converts a sequence of tokens (string) in a single string. """\n out_string = \' \'.join(tokens).replace(\'@@ \', \'\').strip()\n return out_string\n\n def save_vocabulary(self, save_directory):\n """Save the tokenizer vocabulary and merge files to a directory."""\n if not os.path.isdir(save_directory):\n logger.error("Vocabulary path ({}) should be a directory".format(save_directory))\n return\n vocab_file = os.path.join(save_directory, VOCAB_FILES_NAMES[\'vocab_file\'])\n merge_file = os.path.join(save_directory, VOCAB_FILES_NAMES[\'merges_file\'])\n\n with open(vocab_file, \'w\', encoding=\'utf-8\') as f:\n f.write(json.dumps(self.encoder, ensure_ascii=False))\n\n index = 0\n with open(merge_file, "w", encoding="utf-8") as writer:\n writer.write(u\'#version: 0.2\\n\')\n for bpe_tokens, token_index in sorted(self.bpe_ranks.items(), key=lambda kv: kv[1]):\n if index != token_index:\n logger.warning("Saving vocabulary to {}: BPE merge indices are not consecutive."\n " Please check that the tokenizer is not corrupted!".format(merge_file))\n index = token_index\n writer.write(\' \'.join(bpe_tokens) + u\'\\n\')\n index += 1\n\n return vocab_file, merge_file\n'
`
It is strange because it is **different from the source code reported in GitHub of the CTRLTokenizer class** [https://github.com/huggingface/transformers/blob/master/transformers/tokenization_ctrl.py](url). Maybe the code is an old version of this Python script?
Moreover, by using the **inspect** module another time, I've found that the _tokenization_ctrl.py_ Python script contains the following source code (no "CONTROL_CODES" is into this script). It seems to be a bug problem of not using the correct Python class (i.e. not the same script in GitHub):
`'# coding=utf-8\n# Copyright 2018 Salesforce and The HuggingFace Inc. team.\n#\n# Licensed under the Apache License, Version 2.0 (the "License");\n# you may not use this file except in compliance with the License.\n# You may obtain a copy of the License at\n#\n# http://www.apache.org/licenses/LICENSE-2.0\n#\n# Unless required by applicable law or agreed to in writing, software\n# distributed under the License is distributed on an "AS IS" BASIS,\n# WITHOUT WARRANTIES OR CONDITIONS OF ANY KIND, either express or implied.\n# See the License for the specific language governing permissions and\n# limitations under the License.\n"""Tokenization classes for Salesforce CTRL."""\nfrom __future__ import (absolute_import, division, print_function,\n unicode_literals)\n\nimport json\nimport logging\nimport os\nimport regex as re\nfrom io import open\n\nfrom .tokenization_utils import PreTrainedTokenizer\n\nlogger = logging.getLogger(__name__)\n\nVOCAB_FILES_NAMES = {\n \'vocab_file\': \'vocab.json\',\n \'merges_file\': \'merges.txt\',\n}\n\nPRETRAINED_VOCAB_FILES_MAP = {\n \'vocab_file\':\n {\n \'ctrl\': "https://raw.githubusercontent.com/salesforce/ctrl/master/ctrl-vocab.json",\n },\n \'merges_file\':\n {\n \'ctrl\': "https://raw.githubusercontent.com/salesforce/ctrl/master/ctrl-merges.txt",\n },\n}\n\nPRETRAINED_POSITIONAL_EMBEDDINGS_SIZES = {\n \'ctrl\': 256,\n}\n\ndef get_pairs(word):\n """Return set of symbol pairs in a word.\n\n Word is represented as tuple of symbols (symbols being variable-length strings).\n """\n pairs = set()\n prev_char = word[0]\n for char in word[1:]:\n pairs.add((prev_char, char))\n prev_char = char\n\n pairs = set(pairs)\n return pairs\n\nclass CTRLTokenizer(PreTrainedTokenizer):\n """\n CTRL BPE tokenizer. Peculiarities:\n - Byte-level Byte-Pair-Encoding\n - Requires a space to start the input string => the encoding methods should be called with the\n ``add_prefix_space`` flag set to ``True``.\n Otherwise, this tokenizer ``encode`` and ``decode`` method will not conserve\n the absence of a space at the beginning of a string: `tokenizer.decode(tokenizer.encode("Hello")) = " Hello"`\n """\n vocab_files_names = VOCAB_FILES_NAMES\n pretrained_vocab_files_map = PRETRAINED_VOCAB_FILES_MAP\n max_model_input_sizes = PRETRAINED_POSITIONAL_EMBEDDINGS_SIZES\n\n def __init__(self, vocab_file, merges_file, unk_token="<unk>", **kwargs):\n super(CTRLTokenizer, self).__init__(unk_token=unk_token, **kwargs)\n self.max_len_single_sentence = self.max_len # no default special tokens - you can update this value if you add special tokens\n self.max_len_sentences_pair = self.max_len # no default special tokens - you can update this value if you add special tokens\n\n self.encoder = json.load(open(vocab_file, encoding="utf-8"))\n self.decoder = {v:k for k,v in self.encoder.items()}\n merges = open(merges_file, encoding=\'utf-8\').read().split(\'\\n\')[1:-1]\n merges = [tuple(merge.split()) for merge in merges]\n self.bpe_ranks = dict(zip(merges, range(len(merges))))\n self.cache = {}\n\n @property\n def vocab_size(self):\n return len(self.encoder)\n\n def bpe(self, token):\n if token in self.cache:\n return self.cache[token]\n word = tuple(token)\n word = tuple(list(word[:-1]) + [word[-1]+\'</w>\'])\n pairs = get_pairs(word)\n\n if not pairs:\n return token\n\n while True:\n bigram = min(pairs, key = lambda pair: self.bpe_ranks.get(pair, float(\'inf\')))\n if bigram not in self.bpe_ranks:\n break\n first, second = bigram\n new_word = []\n i = 0\n while i < len(word):\n try:\n j = word.index(first, i)\n new_word.extend(word[i:j])\n i = j\n except:\n new_word.extend(word[i:])\n break\n\n if word[i] == first and i < len(word)-1 and word[i+1] == second:\n new_word.append(first+second)\n i += 2\n else:\n new_word.append(word[i])\n i += 1\n new_word = tuple(new_word)\n word = new_word\n if len(word) == 1:\n break\n else:\n pairs = get_pairs(word)\n word = \'@@ \'.join(word)\n word = word[:-4]\n self.cache[token] = word\n return word\n\n def _tokenize(self, text):\n """ Tokenize a string.\n """\n split_tokens = []\n\n text = text.split(\' \')\n\n for token in text:\n split_tokens.extend([t for t in self.bpe(token).split(\' \')])\n return split_tokens\n\n def _convert_token_to_id(self, token):\n """ Converts a token (str/unicode) in an id using the vocab. """\n return self.encoder.get(token, self.encoder.get(self.unk_token))\n\n def _convert_id_to_token(self, index):\n """Converts an index (integer) in a token (string/unicode) using the vocab."""\n return self.decoder.get(index, self.unk_token)\n\n def convert_tokens_to_string(self, tokens):\n """ Converts a sequence of tokens (string) in a single string. """\n out_string = \' \'.join(tokens).replace(\'@@ \', \'\').strip()\n return out_string\n\n def save_vocabulary(self, save_directory):\n """Save the tokenizer vocabulary and merge files to a directory."""\n if not os.path.isdir(save_directory):\n logger.error("Vocabulary path ({}) should be a directory".format(save_directory))\n return\n vocab_file = os.path.join(save_directory, VOCAB_FILES_NAMES[\'vocab_file\'])\n merge_file = os.path.join(save_directory, VOCAB_FILES_NAMES[\'merges_file\'])\n\n with open(vocab_file, \'w\', encoding=\'utf-8\') as f:\n f.write(json.dumps(self.encoder, ensure_ascii=False))\n\n index = 0\n with open(merge_file, "w", encoding="utf-8") as writer:\n writer.write(u\'#version: 0.2\\n\')\n for bpe_tokens, token_index in sorted(self.bpe_ranks.items(), key=lambda kv: kv[1]):\n if index != token_index:\n logger.warning("Saving vocabulary to {}: BPE merge indices are not consecutive."\n " Please check that the tokenizer is not corrupted!".format(merge_file))\n index = token_index\n writer.write(\' \'.join(bpe_tokens) + u\'\\n\')\n index += 1\n\n return vocab_file, merge_file\n\n # def decode(self, token_ids, skip_special_tokens=False, clean_up_tokenization_spaces=True):\n # filtered_tokens = \' \'.join(self.convert_ids_to_tokens(token_ids, skip_special_tokens=skip_special_tokens))\n # tokens_generated_so_far = re.sub(\'(@@ )\', \'\', string=filtered_tokens)\n # tokens_generated_so_far = re.sub(\'(@@ ?$)\', \'\', string=tokens_generated_so_far)\n # return \'\'.join(tokens_generated_so_far)\n'
`
### STACK TRACE
```
2019-10-31 15:02:03.443162: I tensorflow/stream_executor/platform/default/dso_loader.cc:44] Successfully opened dynamic library libcuda.so.1
2019-10-31 15:02:03.455996: I tensorflow/stream_executor/cuda/cuda_gpu_executor.cc:1006] successful NUMA node read from SysFS had negative value (-1), but there must be at least one NUMA node, so returning NUMA node zero
2019-10-31 15:02:03.456755: I tensorflow/core/common_runtime/gpu/gpu_device.cc:1618] Found device 0 with properties:
name: GeForce GTX 980 Ti major: 5 minor: 2 memoryClockRate(GHz): 1.076
pciBusID: 0000:01:00.0
2019-10-31 15:02:03.456943: I tensorflow/stream_executor/platform/default/dso_loader.cc:44] Successfully opened dynamic library libcudart.so.10.0
2019-10-31 15:02:03.457919: I tensorflow/stream_executor/platform/default/dso_loader.cc:44] Successfully opened dynamic library libcublas.so.10.0
2019-10-31 15:02:03.458684: I tensorflow/stream_executor/platform/default/dso_loader.cc:44] Successfully opened dynamic library libcufft.so.10.0
2019-10-31 15:02:03.458868: I tensorflow/stream_executor/platform/default/dso_loader.cc:44] Successfully opened dynamic library libcurand.so.10.0
2019-10-31 15:02:03.460032: I tensorflow/stream_executor/platform/default/dso_loader.cc:44] Successfully opened dynamic library libcusolver.so.10.0
2019-10-31 15:02:03.460829: I tensorflow/stream_executor/platform/default/dso_loader.cc:44] Successfully opened dynamic library libcusparse.so.10.0
2019-10-31 15:02:03.460921: W tensorflow/stream_executor/platform/default/dso_loader.cc:55] Could not load dynamic library 'libcudnn.so.7'; dlerror: libcudnn.so.7: cannot open shared object file: No such file or directory; LD_LIBRARY_PATH: :/usr/local/cuda/lib64:/usr/local/cuda/extras/CUPTI/lib64
2019-10-31 15:02:03.460930: W tensorflow/core/common_runtime/gpu/gpu_device.cc:1641] Cannot dlopen some GPU libraries. Please make sure the missing libraries mentioned above are installed properly if you would like to use GPU. Follow the guide at https://www.tensorflow.org/install/gpu for how to download and setup the required libraries for your platform.
Skipping registering GPU devices...
2019-10-31 15:02:03.461171: I tensorflow/core/platform/cpu_feature_guard.cc:142] Your CPU supports instructions that this TensorFlow binary was not compiled to use: AVX2 FMA
2019-10-31 15:02:03.485286: I tensorflow/core/platform/profile_utils/cpu_utils.cc:94] CPU Frequency: 3408000000 Hz
2019-10-31 15:02:03.485895: I tensorflow/compiler/xla/service/service.cc:168] XLA service 0x559a4bb637a0 executing computations on platform Host. Devices:
2019-10-31 15:02:03.485911: I tensorflow/compiler/xla/service/service.cc:175] StreamExecutor device (0): Host, Default Version
2019-10-31 15:02:03.525426: I tensorflow/stream_executor/cuda/cuda_gpu_executor.cc:1006] successful NUMA node read from SysFS had negative value (-1), but there must be at least one NUMA node, so returning NUMA node zero
2019-10-31 15:02:03.525984: I tensorflow/compiler/xla/service/service.cc:168] XLA service 0x559a4bb3de90 executing computations on platform CUDA. Devices:
2019-10-31 15:02:03.525999: I tensorflow/compiler/xla/service/service.cc:175] StreamExecutor device (0): GeForce GTX 980 Ti, Compute Capability 5.2
2019-10-31 15:02:03.526083: I tensorflow/core/common_runtime/gpu/gpu_device.cc:1159] Device interconnect StreamExecutor with strength 1 edge matrix:
2019-10-31 15:02:03.526090: I tensorflow/core/common_runtime/gpu/gpu_device.cc:1165]
10/31/2019 15:02:05 - INFO - transformers.tokenization_utils - loading file https://raw.githubusercontent.com/salesforce/ctrl/master/ctrl-vocab.json from cache at /home/vidiemme/.cache/torch/transformers/a858ad854d3847b02da3aac63555142de6a05f2a26d928bb49e881970514e186.285c96a541cf6719677cfb634929022b56b76a0c9a540186ba3d8bbdf02bca42
10/31/2019 15:02:05 - INFO - transformers.tokenization_utils - loading file https://raw.githubusercontent.com/salesforce/ctrl/master/ctrl-merges.txt from cache at /home/vidiemme/.cache/torch/transformers/aa2c569e6648690484ade28535a8157aa415f15202e84a62e82cc36ea0c20fa9.26153bf569b71aaf15ae54be4c1b9254dbeff58ca6fc3e29468c4eed078ac142
10/31/2019 15:02:05 - INFO - transformers.configuration_utils - loading configuration file https://storage.googleapis.com/sf-ctrl/pytorch/ctrl-config.json from cache at /home/vidiemme/.cache/torch/transformers/d6492ca334c2a4e079f43df30956acf935134081b2b3844dc97457be69b623d0.1ebc47eb44e70492e0c20494a084f108332d20fea7fe5ad408ef5e7a8f2baef4
10/31/2019 15:02:05 - INFO - transformers.configuration_utils - Model config {
"attn_pdrop": 0.1,
"dff": 8192,
"embd_pdrop": 0.1,
"finetuning_task": null,
"from_tf": false,
"initializer_range": 0.02,
"layer_norm_epsilon": 1e-06,
"n_ctx": 512,
"n_embd": 1280,
"n_head": 16,
"n_layer": 48,
"n_positions": 50000,
"num_labels": 1,
"output_attentions": false,
"output_hidden_states": false,
"output_past": true,
"pruned_heads": {},
"resid_pdrop": 0.1,
"summary_activation": null,
"summary_first_dropout": 0.1,
"summary_proj_to_labels": true,
"summary_type": "cls_index",
"summary_use_proj": true,
"torchscript": false,
"use_bfloat16": false,
"vocab_size": 246534
}
10/31/2019 15:02:05 - INFO - transformers.modeling_utils - loading weights file https://storage.googleapis.com/sf-ctrl/pytorch/seqlen256_v1.bin from cache at /home/vidiemme/.cache/torch/transformers/c146cc96724f27295a0c3ada1fbb3632074adf87e9aef8269e44c9208787f8c8.b986347cbab65fa276683efbb9c2f7ee22552277bcf6e1f1166557ed0852fdf0
10/31/2019 15:02:37 - INFO - __main__ - Namespace(device=device(type='cpu'), length=20, model_name_or_path='ctrl', model_type='ctrl', n_gpu=1, no_cuda=True, padding_text='', prompt='', repetition_penalty=1.2, seed=42, stop_token=None, temperature=0.5, top_k=0, top_p=0.9, xlm_lang='')
Model prompt >>> Hi, my name is Edward and i'm 26 years old
Traceback (most recent call last):
File "run_generation.py", line 256, in <module>
main()
File "run_generation.py", line 228, in main
if not any(context_tokens[0] == x for x in tokenizer.control_codes.values()):
AttributeError: 'CTRLTokenizer' object has no attribute 'control_codes'
```
### REQUIREMENTS.TXT OF MY VIRTUAL ENVIRONMENT
```
Package Version
-------------------- ---------
absl-py 0.8.1
astor 0.8.0
boto3 1.10.6
botocore 1.13.6
cachetools 3.1.1
certifi 2019.9.11
chardet 3.0.4
Click 7.0
docutils 0.15.2
gast 0.2.2
google-auth 1.6.3
google-auth-oauthlib 0.4.1
google-pasta 0.1.7
grpcio 1.24.3
h5py 2.10.0
idna 2.8
jmespath 0.9.4
joblib 0.14.0
Keras-Applications 1.0.8
Keras-Preprocessing 1.1.0
Markdown 3.1.1
numpy 1.17.3
oauthlib 3.1.0
opt-einsum 3.1.0
pandas 0.25.2
Pillow 6.2.1
pip 19.3.1
protobuf 3.10.0
pyasn1 0.4.7
pyasn1-modules 0.2.7
python-dateutil 2.8.0
pytz 2019.3
PyYAML 5.1.2
regex 2019.8.19
requests 2.22.0
requests-oauthlib 1.2.0
rsa 4.0
s3transfer 0.2.1
sacremoses 0.0.35
scikit-learn 0.21.3
scipy 1.3.1
sentencepiece 0.1.83
setuptools 41.4.0
six 1.12.0
tensorboard 2.0.1
tensorflow-estimator 2.0.1
tensorflow-gpu 2.0.0
termcolor 1.1.0
torch 1.3.0
torchtext 0.4.0
torchvision 0.4.1
tqdm 4.36.1
transformers 2.1.1
urllib3 1.25.6
Werkzeug 0.16.0
wheel 0.33.6
wrapt 1.11.2
```
### ENVIRONMENT
```
>>> import platform; print("Platform", platform.platform())
Platform Linux-4.15.0-66-generic-x86_64-with-debian-buster-sid
>>> import sys; print("Python", sys.version)
Python 3.6.9 |Anaconda, Inc.| (default, Jul 30 2019, 19:07:31)
[GCC 7.3.0]
>>> import torch; print("PyTorch", torch.__version__)
PyTorch 1.3.0
>>> import tensorflow; print("Tensorflow", tensorflow.__version__)
Tensorflow 2.0.0
```<|||||>Oh ok I think I know what happens to you guys. This repo contains both a **lib** (pushed to Pypi) and a set of **example scripts**. To reliably run the versions of the scripts that are on master, you also need to install the lib from master (i.e. not the last pypi release).
Can you run `pip install -e .` from master? This will ensure the lib's code and the scripts are in sync.
cc @thomwolf @LysandreJik
Closing this as I don't think it's a bug per se.<|||||>As suggested correctly by @julien-c, in order to solve the problem pointed out in #1636, you have to:
1. download the entire GitHub repository with `git clone https://github.com/huggingface/transformers.git` command
2. enter to the directory you have just downloaded with `cd transformers` command
3. install the repo by running `pip install -e .` command
4. go to "examples" directory
5. now you can run `run_generation.py` script
Hoping it is helpful for developers that want to trying out CTRL model by HuggingFace.<|||||>> As suggested correctly by @julien-c, in order to solve the problem pointed out in #1636, you have to:
>
> 1. download the entire GitHub repository with `git clone https://github.com/huggingface/transformers.git` command
> 2. enter to the directory you have just downloaded with `cd transformers` command
> 3. install the repo by running `pip install -e .` command
> 4. go to "examples" directory
> 5. now you can run `run_generation.py` script
>
> Hoping it is helpful for developers that want to trying out CTRL model by HuggingFace.
I using anaconda. When `pip install -e`, it ran but only installed certain package.<|||||>> > As suggested correctly by @julien-c, in order to solve the problem pointed out in #1636, you have to:
> >
> > 1. download the entire GitHub repository with `git clone https://github.com/huggingface/transformers.git` command
> > 2. enter to the directory you have just downloaded with `cd transformers` command
> > 3. install the repo by running `pip install -e .` command
> > 4. go to "examples" directory
> > 5. now you can run `run_generation.py` script
> >
> > Hoping it is helpful for developers that want to trying out CTRL model by HuggingFace.
>
> I using anaconda. When `pip install -e`, it ran but only installed certain package.
@mzjuin please be more detailed about your problem |
transformers | 1,635 | closed | Training DistilBert - RuntimeError: index out of range at /pytorch/aten/src/TH/generic/THTensorEvenMoreMath.cpp:237 | ## 🐛 Bug
Hello,
<!-- Important information -->
Model I am using (Bert, XLNet....): DistilBert
Language I am using the model on (English, Chinese....): French
The problem arise when using:
* [ ] the official example scripts: examples/distillation/train.py
The tasks I am working on is:
* [ ] the official training DistilBert from scratch task
## To Reproduce
I followed the required steps to train distil* from scratch :
```bash
python ./scripts/binarized_data.py \
--file_path ./data/dataset.txt \
--tokenizer_type bert \
--tokenizer_name bert-base-multilingual-cased \
--dump_file ./data_output/binarized_text &
```
The only modification I made was to increase the vocab_size, otherwise I had a bug:
```bash
python ./scripts/token_counts.py \
--data_file ./data/binarized_text.bert-base-multilingual-cased.pickle \
--token_counts_dump ./data/token_counts.bert-base-multilingual-cased.pickle \
--vocab_size 65536
```
Then, I launched the training with the following :
```bash
python train.py \
--student_type distilbert \
--student_config ./training_configs/distilbert-base-uncased.json \
--teacher_type bert \
--teacher_name bert-base-multilingual-cased \
--alpha_ce 5.0 --alpha_mlm 2.0 --alpha_cos 1.0 --mlm \
--dump_path ./serialization_dir/my_first_training \
--data_file ./data/binarized_text.bert-base-multilingual-cased.pickle \
--token_counts ./data/token_counts.bert-base-multilingual-cased.pickle \
--force
```
<!-- If you have a code sample, error messages, stack traces, please provide it here as well. -->
Error message :
```bash
-Iter: 0% 0/586181 [00:00<?, ?it/s]Traceback (most recent call last):
File "train.py", line 289, in <module>
main()
File "train.py", line 284, in main
distiller.train()
File "/dds/work/distil/transformers/examples/distillation/distiller.py", line 339, in train
self.step(input_ids=token_ids, attention_mask=attn_mask, lm_labels=lm_labels)
File "/dds/work/distil/transformers/examples/distillation/distiller.py", line 369, in step
s_logits, s_hidden_states = self.student(input_ids=input_ids, attention_mask=attention_mask) # (bs, seq_length, voc_size)
File "/dds/miniconda/envs/dds/lib/python3.5/site-packages/torch/nn/modules/module.py", line 547, in __call__
result = self.forward(*input, **kwargs)
File "/dds/miniconda/envs/dds/lib/python3.5/site-packages/transformers/modeling_distilbert.py", line 528, in forward
head_mask=head_mask)
File "/dds/miniconda/envs/dds/lib/python3.5/site-packages/torch/nn/modules/module.py", line 547, in __call__
result = self.forward(*input, **kwargs)
File "/dds/miniconda/envs/dds/lib/python3.5/site-packages/transformers/modeling_distilbert.py", line 461, in forward
embedding_output = self.embeddings(input_ids) # (bs, seq_length, dim)
File "/dds/miniconda/envs/dds/lib/python3.5/site-packages/torch/nn/modules/module.py", line 547, in __call__
result = self.forward(*input, **kwargs)
File "/dds/miniconda/envs/dds/lib/python3.5/site-packages/transformers/modeling_distilbert.py", line 92, in forward
word_embeddings = self.word_embeddings(input_ids) # (bs, max_seq_length, dim)
File "/dds/miniconda/envs/dds/lib/python3.5/site-packages/torch/nn/modules/module.py", line 547, in __call__
result = self.forward(*input, **kwargs)
File "/dds/miniconda/envs/dds/lib/python3.5/site-packages/torch/nn/modules/sparse.py", line 114, in forward
self.norm_type, self.scale_grad_by_freq, self.sparse)
File "/dds/miniconda/envs/dds/lib/python3.5/site-packages/torch/nn/functional.py", line 1467, in embedding
return torch.embedding(weight, input, padding_idx, scale_grad_by_freq, sparse)
RuntimeError: index out of range: Tried to access index 61578 out of table with 30521 rows. at /pytorch/aten/src/TH/generic/THTensorEvenMoreMath.cpp:237
-Iter: 0% 0/586181 [00:00<?, ?it/s]
```
## Environment
* OS: Debian
* Python version: 3.5
* PyTorch version: 1.2.0
* PyTorch Transformers version (or branch): 1.2.0
* Using GPU ? No
* Distributed of parallel setup ?
* Any other relevant information:
Thanks in advance !
| 10-25-2019 20:02:20 | 10-25-2019 20:02:20 | I re-downloaded PyTorch 1.2.0 and the problem was fixed for some reason... |
transformers | 1,634 | closed | How to initialize AdamW optimizer in HuggingFace transformers? | Hello,
I am new to Python and NLP and so I have some questions that may sound a bit funny to the experts.
I had been trying to set my optimizer by setting
`optimizer = AdamW()`
but of course it failed, because I did not specify the required parameter `'param'` (for lr, betas, eps, weight_decay, and correct_bias, I am just going to use the default values).
As a beginner, I am not so clear on what `'param'` stands for in this case. What kind of input should I provide for `'param'`?
Thank you,
| 10-25-2019 13:58:20 | 10-25-2019 13:58:20 | I had the same issue, apparently it should be model.params()<|||||>Thank you! This is helpful<|||||>You have to tell the optimizer which parameters it should optimize. Theoretically you could use multiple optimizers for different parameters. This is useful if you want to use different learning rates or different weight decays.
If your question is answered, please close the question. <|||||>> You have to tell the optimizer which parameters it should optimize. Theoretically you could use multiple optimizers for different parameters. This is useful if you want to use different learning rates or different weight decays.
>
> If your question is answered, please close the question.
I'm guessing this may have something to do with how the params are set in this code from the squad example
```
# Prepare optimizer and schedule (linear warmup and decay)
no_decay = ["bias", "LayerNorm.weight"]
optimizer_grouped_parameters = [
{
"params": [p for n, p in model.named_parameters() if not any(nd in n for nd in no_decay)],
"weight_decay": args.weight_decay,
},
{"params": [p for n, p in model.named_parameters() if any(nd in n for nd in no_decay)], "weight_decay": 0.0},
]
optimizer = AdamW(optimizer_grouped_parameters, lr=args.learning_rate, eps=args.adam_epsilon)
scheduler = get_linear_schedule_with_warmup(
optimizer, num_warmup_steps=args.warmup_steps, num_training_steps=t_total
)
```
https://github.com/huggingface/transformers/blob/master/examples/run_squad.py
|
transformers | 1,633 | closed | Fix for mlm evaluation in run_lm_finetuning.py | No masking is done in the original evaluation code, so the resulting perplexity is always something like 1.0. In this PR a simple fix is proposed, using just the same masked scheme as in a training code. | 10-25-2019 10:31:29 | 10-25-2019 10:31:29 | # [Codecov](https://codecov.io/gh/huggingface/transformers/pull/1633?src=pr&el=h1) Report
> Merging [#1633](https://codecov.io/gh/huggingface/transformers/pull/1633?src=pr&el=desc) into [master](https://codecov.io/gh/huggingface/transformers/commit/ae1d03fc51bb22ed59517ee6f92c560417fdb049?src=pr&el=desc) will **not change** coverage.
> The diff coverage is `n/a`.
[](https://codecov.io/gh/huggingface/transformers/pull/1633?src=pr&el=tree)
```diff
@@ Coverage Diff @@
## master #1633 +/- ##
======================================
Coverage 85.9% 85.9%
======================================
Files 91 91
Lines 13653 13653
======================================
Hits 11728 11728
Misses 1925 1925
```
------
[Continue to review full report at Codecov](https://codecov.io/gh/huggingface/transformers/pull/1633?src=pr&el=continue).
> **Legend** - [Click here to learn more](https://docs.codecov.io/docs/codecov-delta)
> `Δ = absolute <relative> (impact)`, `ø = not affected`, `? = missing data`
> Powered by [Codecov](https://codecov.io/gh/huggingface/transformers/pull/1633?src=pr&el=footer). Last update [ae1d03f...a9b7ec4](https://codecov.io/gh/huggingface/transformers/pull/1633?src=pr&el=lastupdated). Read the [comment docs](https://docs.codecov.io/docs/pull-request-comments).
<|||||>This is great, thanks! |
transformers | 1,632 | closed | Loading from ckpt is not possible for bert, neither tf to pytorch conversion works in 2.1.1 | ## 🐛 Bug
<!-- Important information -->
- I am trying to load a BERT model (for simplicity assume the original uncased-base from google's repo) using instructions in: https://github.com/huggingface/transformers/blob/ae1d03fc51bb22ed59517ee6f92c560417fdb049/transformers/modeling_tf_utils.py#L195
and more specifically:
`self.tokenizer = BertTokenizer.from_pretrained('bert-base-uncased')`
`config = BertConfig.from_json_file('data/uncased_L-12_H-768_A-12/config.json')`
`self.model = TFBertForSequenceClassification.from_pretrained( pretrained_model_name_or_path='data/uncased_L-12_H-768_A-12/model.ckpt.index', config=config, from_pt=True)`
- This fails as expected since you need to change line https://github.com/huggingface/transformers/blob/ae1d03fc51bb22ed59517ee6f92c560417fdb049/transformers/modeling_tf_utils.py#L225
with this `elif os.path.isfile(os.path.join(pretrained_model_name_or_path, TF_WEIGHTS_NAME)):`
and set from_pt=False.
- Even then it fails in https://github.com/huggingface/transformers/blob/ae1d03fc51bb22ed59517ee6f92c560417fdb049/transformers/modeling_tf_utils.py#L274 with some tf notImplementedError.
Then I decided to use the converter and turn the tf ckpt into pytorch:
https://github.com/huggingface/transformers/blob/master/transformers/convert_bert_original_tf_checkpoint_to_pytorch.py
This seems to do the conversion correctly but then fails when loading it (I follow precisely the same steps from https://github.com/huggingface/transformers/issues/457#issuecomment-518403170 )
it fails with `AssertionError: classifier.weight not found in PyTorch model`.
So if I am not missing sth, at this point it does not seem possible to load somehow a tf ckpt?
Would it make sense to convert ckpt to h5 and use that?
Thanks!
Model I am using (Bert, XLNet....):
Bert
Language I am using the model on (English, Chinese....):
English
The problem arise when using:
* [ x] the official example scripts: (give details)
* [ ] my own modified scripts: (give details)
The tasks I am working on is:
* [ ] an official GLUE/SQUaD task: (give the name)
* [x ] my own task or dataset: (give details)
## To Reproduce
Steps to reproduce the behavior:
1. convert_tf_checkpoint_to_pytorch('data/uncased_L-12_H-768_A-12/model.ckpt',
'data/uncased_L-12_H-768_A-12/config.json',
'data/uncased_L-12_H-768_A-12/pytorch_model.bin')
2. model = TFBertForSequenceClassification.from_pretrained('data/uncased_L-12_H-768_A-12/', from_pt=True)
<!-- If you have a code sample, error messages, stack traces, please provide it here as well. -->
## Expected behavior
<!-- A clear and concise description of what you expected to happen. -->
## Environment
* OS: Debian Linux
* Python version: 3.6.8
* PyTorch version: 1.3.0
* PyTorch Transformers version (or branch): 2.1.1
* Using GPU ? yes
* Distributed of parallel setup ?
* Any other relevant information:
tf 2.0
## Additional context
<!-- Add any other context about the problem here. -->
| 10-25-2019 10:09:29 | 10-25-2019 10:09:29 | This issue has been automatically marked as stale because it has not had recent activity. It will be closed if no further activity occurs. Thank you for your contributions.
|
transformers | 1,631 | closed | cannot import name 'RobertaForTokenClassification' | ## 🐛 Bug
<!-- Important information -->
Model I am using (Bert, XLNet....): BERT
Language I am using the model on (English, Chinese....): English
The problem arise when using:
* [x] the official example scripts: (give details) examples/run_ner.py
* [ ] my own modified scripts: (give details)
The tasks I am working on is:
* [x] an official GLUE/SQUaD task: (give the name) NER on CoNLL2003 ENG
* [ ] my own task or dataset: (give details)
## To Reproduce
Steps to reproduce the behavior:
1. $ pip install transformers
2. $ python
3. > from transformers import RobertaConfig, RobertaForTokenClassification, RobertaTokenizer
Traceback (most recent call last):
File "<stdin>", line 1, in <module>
ImportError: cannot import name 'RobertaForTokenClassification'
<!-- If you have a code sample, error messages, stack traces, please provide it here as well. -->
## Expected behavior
<!-- A clear and concise description of what you expected to happen. -->
## Environment
* OS: Ubuntu 18.04.3
* Python version: 3.6
* PyTorch version: 1.2.0
* PyTorch Transformers version (or branch): pip install transformers
* Using GPU ? Yes, CUDA 10
* Distributed of parallel setup ? No
* Any other relevant information:
## Additional context
<!-- Add any other context about the problem here. -->
| 10-25-2019 09:06:51 | 10-25-2019 09:06:51 | + other bug
- with '--evaluate_during_training'
```
File "/path-to/run_ner.py", line 167, in train
results, _ = evaluate(args, model, tokenizer, labels, pad_token_label_id)
TypeError: evaluate() missing 1 required positional argument: 'mode'
```<|||||>- update
- using `pip3 install git+https://github.com/huggingface/transformers.git --upgrade` command, the first bug got away. <|||||>This issue has been automatically marked as stale because it has not had recent activity. It will be closed if no further activity occurs. Thank you for your contributions.
<|||||>I am also facing the same issue, but even installing from git (as stated by dsindex) did not help.<|||||>It could be because torch is not installed. transformers doesn't install torch automatically but needs the same to load the models. try `pip install torch` and import again!<|||||>I am having the same issue, neither installing from git, nor `pip install torch` have fixed the issue<|||||>Could you provide your software versions, by running `transformers-cli env` in your environment? |
transformers | 1,630 | closed | rename _has_sklearn to _sklearn_available | Rename `_has_sklearn` to `_sklearn_available`, because variables that act like `_has_sklearn` in `transformers/file_utils.py` are named like `_{module}_available` | 10-25-2019 07:18:02 | 10-25-2019 07:18:02 | This issue has been automatically marked as stale because it has not had recent activity. It will be closed if no further activity occurs. Thank you for your contributions.
|
transformers | 1,629 | closed | Perm Mask in XLNet | ## ❓ Questions & Help
<!-- A clear and concise description of the question. -->
"Mask to indicate the attention pattern for each input token with values selected in [0, 1]: **If perm_mask[k, i, j] = 0, i attend to j in batch k; if perm_mask[k, i, j] = 1**, i does not attend to j in batch k. If None, each token attends to all the others (full bidirectional attention). Only used during pretraining (to define factorization order) or for sequential decoding (generation)."
Can you confirm this is not the reverse i attend to j in batch k **if perm_mask[k, i, j] = 1** ?
Thanks a lot for your great work! | 10-25-2019 07:06:54 | 10-25-2019 07:06:54 | This issue has been automatically marked as stale because it has not had recent activity. It will be closed if no further activity occurs. Thank you for your contributions.
|
transformers | 1,628 | closed | run_tf_glue works with all tasks | Slightly changed the logic of the DataProcessor so that it can handle GLUE data coming from the `tensorflow_datasets` package.
Updated the script so that all tasks are now available, with regression tasks (STS-B) as well as all classification tasks.
Should update the import into PyTorch that currently tests if two sentences are paraphrases of each other; as the glue script handles all GLUE tasks it should be a different test. | 10-24-2019 21:44:57 | 10-24-2019 21:44:57 | |
transformers | 1,627 | closed | Loading pretrained RobertaForSequenceClassification fails, size missmatch error | ## 🐛 Bug
<!-- Important information -->
Model I am using `RobertaForSequenceClassification` and when I tried to load `'roberta-base'` model using this code on Google Colab:
```from transformers import RobertaForSequenceClassification, RobertaConfig
config = RobertaConfig()
model = RobertaForSequenceClassification.from_pretrained(
"roberta-base", config = config)
model
```
I get the following error:
```
RuntimeError: Error(s) in loading state_dict for RobertaForSequenceClassification:
size mismatch for roberta.embeddings.word_embeddings.weight: copying a param with shape torch.Size([50265, 768]) from checkpoint, the shape in current model is torch.Size([30522, 768]).
size mismatch for roberta.embeddings.position_embeddings.weight: copying a param with shape torch.Size([514, 768]) from checkpoint, the shape in current model is torch.Size([512, 768]).
size mismatch for roberta.embeddings.token_type_embeddings.weight: copying a param with shape torch.Size([1, 768]) from checkpoint, the shape in current model is torch.Size([2, 768]).
```
Maybe related to #1340
## Environment
* Google Colab Platform Linux-4.14.137+-x86_64-with-Ubuntu-18.04-bionic
Python 3.6.8 (default, Oct 7 2019, 12:59:55)
[GCC 8.3.0]
PyTorch 1.3.0+cu100
Transformers 2.1.1
| 10-24-2019 21:21:45 | 10-24-2019 21:21:45 | Hi! You're initializing RoBERTa with a blank configuration, which results in a very BERT-like configuration. BERT has different attributes than RoBERTa (different vocabulary size, positional embeddings size etc) so this indeed results in an error.
To instantiate RoBERTa you can simply do:
```py
model = RobertaForSequenceClassification.from_pretrained("roberta-base")
```
If you wish to have a configuration file so that you can change attributes like outputting the hidden states, you could do it like this:
```py
config = RobertaConfig.from_pretrained("roberta-base", output_hidden_states=True)
model = RobertaForSequenceClassification.from_pretrained("roberta-base", config=config)
```<|||||>Hi @LysandreJik ,
Thanks a lot for the clarification, this is indeed much clearer. I tried the code again and it is working. |
transformers | 1,626 | closed | What is currently the best way to add a custom dictionary to a neural machine translator that uses the transformer architecture? | ## ❓ Questions & Help
It's common to add a custom dictionary to a machine translator to ensure that terminology from a specific domain is correctly translated. For example, the term server should be translated differently when the document is about data centers, vs when the document is about restaurants.
With a transformer model, this is not very obvious to do, since words are not aligned 1:1. I've seen a couple of papers on this topic, but I'm not sure which would be the best one to use. What are the best practices for this problem?
One paper I found that seem to describe what I'm looking for is [here](aclweb.org/anthology/W18-6318.pdf ) - I have a bunch of questions regarding the paper, which I'm happy to discuss here as well. I'm also wondering if there are other approaches.
| 10-24-2019 17:48:10 | 10-24-2019 17:48:10 | This question is too general for this repo. It's not specific to anything this repository offers. Perhaps it's better to ask this on one of the Stack Exchange sites. <|||||>This issue has been automatically marked as stale because it has not had recent activity. It will be closed if no further activity occurs. Thank you for your contributions.
|
transformers | 1,625 | closed | Update run_ner.py example with RoBERTa | PR for #1534
The `run_ner.py` script in the examples directory only used BERT based models. The main objective was to utilize the new DistilRoBERTa model for NER as it is cased by default, potentially leading to better results (at least for the English language). This PR is based on #1613, I will rebase after it is merged.
The command used for the results below:
```
# Bert (cased)
python run_ner.py --data_dir ./data --model_type bert --model_name_or_path bert-base-cased --output_dir ./bert-cased --do_train --do_eval --do_predict
# Bert (uncased)
python run_ner.py --data_dir ./data --model_type bert --model_name_or_path bert-base-uncased --output_dir ./bert --do_train --do_eval --do_predict
# RoBERTa
python run_ner.py --data_dir ./data --model_type roberta --model_name_or_path roberta-base --output_dir ./roberta-base --do_train --do_eval --do_predict
# DistilRoBERTa
python run_ner.py --data_dir ./data --model_type roberta --model_name_or_path distilroberta-base --output_dir ./roberta --do_train --do_eval --do_predict
```
```
BERT cased (for comparison)
dev
***** Eval results *****
f1 = 0.9531893436423229
loss = 0.03520505422085489
precision = 0.9510313600536643
recall = 0.9553571428571429
test
***** Eval results *****
f1 = 0.911254075967216
loss = 0.12860409794469702
precision = 0.9065404173242153
recall = 0.9160170092133239
BERT uncased (for comparison)
dev
***** Eval results *****
f1 = 0.7946049454666556
loss = 0.13505880897513595
precision = 0.7862909869830285
recall = 0.8030966004712218
test
***** Eval results *****
f1 = 0.7315113943944818
loss = 0.2360093453855909
precision = 0.7216192937123169
recall = 0.7416784702549575
RoBERTa base
dev
***** Eval results *****
f1 = 0.9486079569349818
loss = 0.04320113215679077
precision = 0.9466174248782945
recall = 0.9506068779501011
test
***** Eval results *****
f1 = 0.8999385047878415
loss = 0.15529698813410237
precision = 0.8917130919220055
recall = 0.90831707749601
recall = 0.9160170092133239
DistilRoBERTa
dev
***** Eval results *****
f1 = 0.9384563645535564
loss = 0.04439822954706492
precision = 0.9360952700436095
recall = 0.9408293998651382
test
***** Eval results *****
f1 = 0.8873288873288874
loss = 0.15643812390490658
precision = 0.8782351919402467
recall = 0.8966128746231601
``` | 10-24-2019 17:38:31 | 10-24-2019 17:38:31 | Only issue I saw when running the prediction for RoBERTa, I noticed some `Maximum sequence length exceeded` warnings.<|||||>That's awesome! We can merge as soon as tests pass, unless you plan on pushing something else before.
For reference, do you think you could add Eval results for `bert-base-cased` too?<|||||>Ya I can run it right now. Might take an hour or two as it's all via colab.<|||||>Updated main comment with `bert-base-cased` results. Thanks again!<|||||>Updated main comment to clarify that it's `DistilRoBERTa`, not `RoBERTa`. I'll try to add those results to our [examples/README.md](https://github.com/huggingface/transformers/blob/master/examples/README.md).
Thanks again!<|||||>I think because it's extending the main Roberta config that all models are available to it correct? If not I'm ok with just distilRoberta.<|||||>Oh yeah with what you pushed run_ner should work out of the box with all RoBERTa models, I'm just pointing out that the eval results you list are for `distilroberta-base` (so a way smaller model than roberta-base)<|||||>Oh I see now. Ha. That's what I get for looking at it with my phone. Totally get it now :). Thanks for the edit. I can add the default Roberta as well today if I get the chance. |
transformers | 1,624 | closed | Add support for resumable downloads for HTTP protocol. | Hi. This PR adds support for resumable downloads for HTTP protocol (`resume_download` flag, disabled by default). It solved my problems with unreliable network connection and may also prevent issues like
* https://github.com/huggingface/transformers/issues/985
* https://github.com/huggingface/transformers/issues/1303
* https://github.com/huggingface/transformers/issues/1423 | 10-24-2019 15:25:04 | 10-24-2019 15:25:04 | # [Codecov](https://codecov.io/gh/huggingface/transformers/pull/1624?src=pr&el=h1) Report
> Merging [#1624](https://codecov.io/gh/huggingface/transformers/pull/1624?src=pr&el=desc) into [master](https://codecov.io/gh/huggingface/transformers/commit/10bd1ddb39235b2f58594e48867595e7d38cd619?src=pr&el=desc) will **increase** coverage by `27.37%`.
> The diff coverage is `67.56%`.
[](https://codecov.io/gh/huggingface/transformers/pull/1624?src=pr&el=tree)
```diff
@@ Coverage Diff @@
## master #1624 +/- ##
===========================================
+ Coverage 56.21% 83.58% +27.37%
===========================================
Files 105 105
Lines 15507 15528 +21
===========================================
+ Hits 8717 12979 +4262
+ Misses 6790 2549 -4241
```
| [Impacted Files](https://codecov.io/gh/huggingface/transformers/pull/1624?src=pr&el=tree) | Coverage Δ | |
|---|---|---|
| [transformers/configuration\_auto.py](https://codecov.io/gh/huggingface/transformers/pull/1624/diff?src=pr&el=tree#diff-dHJhbnNmb3JtZXJzL2NvbmZpZ3VyYXRpb25fYXV0by5weQ==) | `59.45% <ø> (+13.51%)` | :arrow_up: |
| [transformers/modeling\_auto.py](https://codecov.io/gh/huggingface/transformers/pull/1624/diff?src=pr&el=tree#diff-dHJhbnNmb3JtZXJzL21vZGVsaW5nX2F1dG8ucHk=) | `31.81% <ø> (ø)` | :arrow_up: |
| [transformers/tokenization\_auto.py](https://codecov.io/gh/huggingface/transformers/pull/1624/diff?src=pr&el=tree#diff-dHJhbnNmb3JtZXJzL3Rva2VuaXphdGlvbl9hdXRvLnB5) | `45.94% <ø> (ø)` | :arrow_up: |
| [transformers/modeling\_tf\_auto.py](https://codecov.io/gh/huggingface/transformers/pull/1624/diff?src=pr&el=tree#diff-dHJhbnNmb3JtZXJzL21vZGVsaW5nX3RmX2F1dG8ucHk=) | `51.25% <ø> (+51.25%)` | :arrow_up: |
| [transformers/tokenization\_utils.py](https://codecov.io/gh/huggingface/transformers/pull/1624/diff?src=pr&el=tree#diff-dHJhbnNmb3JtZXJzL3Rva2VuaXphdGlvbl91dGlscy5weQ==) | `92.23% <100%> (+0.24%)` | :arrow_up: |
| [transformers/configuration\_utils.py](https://codecov.io/gh/huggingface/transformers/pull/1624/diff?src=pr&el=tree#diff-dHJhbnNmb3JtZXJzL2NvbmZpZ3VyYXRpb25fdXRpbHMucHk=) | `92.2% <100%> (+4.04%)` | :arrow_up: |
| [transformers/modeling\_tf\_utils.py](https://codecov.io/gh/huggingface/transformers/pull/1624/diff?src=pr&el=tree#diff-dHJhbnNmb3JtZXJzL21vZGVsaW5nX3RmX3V0aWxzLnB5) | `92.72% <100%> (+92.72%)` | :arrow_up: |
| [transformers/modeling\_utils.py](https://codecov.io/gh/huggingface/transformers/pull/1624/diff?src=pr&el=tree#diff-dHJhbnNmb3JtZXJzL21vZGVsaW5nX3V0aWxzLnB5) | `89.45% <100%> (+0.02%)` | :arrow_up: |
| [transformers/file\_utils.py](https://codecov.io/gh/huggingface/transformers/pull/1624/diff?src=pr&el=tree#diff-dHJhbnNmb3JtZXJzL2ZpbGVfdXRpbHMucHk=) | `66.5% <58.62%> (-5.48%)` | :arrow_down: |
| [transformers/tokenization\_albert.py](https://codecov.io/gh/huggingface/transformers/pull/1624/diff?src=pr&el=tree#diff-dHJhbnNmb3JtZXJzL3Rva2VuaXphdGlvbl9hbGJlcnQucHk=) | `82.9% <0%> (-6.84%)` | :arrow_down: |
| ... and [41 more](https://codecov.io/gh/huggingface/transformers/pull/1624/diff?src=pr&el=tree-more) | |
------
[Continue to review full report at Codecov](https://codecov.io/gh/huggingface/transformers/pull/1624?src=pr&el=continue).
> **Legend** - [Click here to learn more](https://docs.codecov.io/docs/codecov-delta)
> `Δ = absolute <relative> (impact)`, `ø = not affected`, `? = missing data`
> Powered by [Codecov](https://codecov.io/gh/huggingface/transformers/pull/1624?src=pr&el=footer). Last update [10bd1dd...5340d1f](https://codecov.io/gh/huggingface/transformers/pull/1624?src=pr&el=lastupdated). Read the [comment docs](https://docs.codecov.io/docs/pull-request-comments).
<|||||>Hi @grwlf, that's a nice addition. Do you think you could add the new arguments in the `from_pretrained` methods calling `cached_path` (and their docstrings)?<|||||>> Hi @grwlf, that's a nice addition. Do you think you could add the new arguments in the `from_pretrained` methods calling `cached_path` (and their docstrings)?
Sure. I've redesigned the solution. Now if users pass `resume_download=True`, the downloader explicitly stores the data in a file with '.incomplete' suffix, and reads it if it already exists.
This version currently doesn't protect us from strange and rare network situations where the connection is broken, but `request.get` thinks that download is completed normally. For this case I think that request handling code should be patched somehow.
But I hope that most network problems really end with an exception and that is the case which should be handled now. <|||||>Ok great, merging! |
transformers | 1,623 | closed | --cache_dir argument in run_lm_finetuning.py not used at all | ## 🐛 Bug
<!-- Important information -->
Model I am using (Bert, XLNet....): GPT-2
Language I am using the model on (English, Chinese....): English
The problem arise when using:
* [ ] the official example scripts: run_lm_finetuning.py
The tasks I am working on is:
* [ ] my own task or dataset: Language model finetuning on custom dataset from human resources domain
## To Reproduce
Steps to reproduce the behavior:
1. Clone the repo
2. Navigate to transformers/examples directory
3. Prepare custom train and test datasets (.txt files)
4. Create ./cache directory
3. Run the following command in terminal (with replaced custom_ arguments):
```
python run_lm_finetuning.py \
--output_dir=<custom_output_dir_path> \
--model_type=gpt2 \
--model_name_or_path=gpt2 \
--do_train \
--train_data_file=<custom_train_data_file> \
--do_eval \
--eval_data_file=<custom_eval_data_file> \
--per_gpu_eval_batch_size=1 \
--per_gpu_train_batch_size=1 \
--save_total_limit=2 \
--num_train_epochs=1 \
--cache_dir=./cache
```
<!-- If you have a code sample, error messages, stack traces, please provide it here as well. -->
## Expected behavior
When the model is downloaded from S3, it is stored to default cache directory in `<user_home>/.cache/transformers/` directory, instead to `./cache`, as specified in `--cache_dir` argument. Seems like `--cache_dir` argument isn't used in `.from_pretrained()` methods in lines 472, 473 and 477 in the run_lm_finetuning.py script.
## Environment
* OS: Ubuntu 18.04
* Python version: 3.6.6
* PyTorch version: 1.3
* PyTorch Transformers version (or branch): 2.1.1
* Using GPU ? Yes
* Distributed of parallel setup ? No
* Any other relevant information:
## Additional context
<!-- Add any other context about the problem here. -->
| 10-24-2019 14:21:52 | 10-24-2019 14:21:52 | |
transformers | 1,622 | closed | Fine-tuning BERT using Next sentence prediction loss | In `pytorch_pretrained_bert`, there is an example for fine-tuning BERT using next sentence prediction loss. In the new version, how shall we fine-tune BERT on the next sentence prediction task?
Thank you. | 10-24-2019 13:37:11 | 10-24-2019 13:37:11 | We do not have any scripts that display how to do next sentence prediction as it was shown with RoBERTa to be of little importance during training.
We had some scripts until version 1.1.0 that allowed this, you can find them [here](https://github.com/huggingface/transformers/tree/1.1.0/examples/lm_finetuning). They are deprecated but can give you an idea of the process.<|||||>Ah, gotcha. Thanks! |
transformers | 1,621 | closed | tokenization slow | ## ❓ Questions & Help
<!-- A clear and concise description of the question. -->
Hi,
I want to fine-tune the gpt2 model with a very large corpus (~9GB text data)
However, the tokenization of run_lm_finetuning.py takes forever (what is not surprising with a 9GB text file)
My question is: is there any way to speed up the tokenization like multiprocessing, or do I have to break up my training file and train with a sample?
Best regards | 10-24-2019 12:34:02 | 10-24-2019 12:34:02 | Hi, with the current implementation of the `run_lm_finetuning.py` file there is no way to speed up the tokenization. It is an example to showcase how to use the library and is therefore not completely optimized especially concerning the data pre-processing.
You could modify the script a bit to setup multiprocessing and tokenize the whole dataset at once. You could then re-use these features and fine-tune your model using these.<|||||>Perhaps something can be done with Dataloader's num_workzrs and collate_fn. <|||||>@EndruK I'm actually working on applying ```multiprocessing``` to parallelize the tokenization process of ```transformers``` workflows as well. I can share my fork with you as soon I get this started.<|||||>Nice,
I'm also working on a multiprocessing approach.
Looking forward to share it when its done.<|||||>@BramVanroy How are you thinking about using ```collate_fn```? The bottleneck from my understanding is at the tokenization and numericalization step which is before the data is converted to a tensor, and so speedup will have to be implemented pre-Dataloader.<|||||>Well, since `collate_fn` is basically a callback between loading the data and returning the data. I admit I haven't looked into this in detail, but from my brief reading into it, it should be possible to do some processing in there. Something like this (pseudo-code, un-tested)
```python
def collate_fn(batch):
tokens = [tokenizer.tokenize(text) for text in batch]
ids = [[tokenizer.convert_tokens_to_ids(tok) for tok in seq] for seq in tokens]
return ids
```
See [this section](https://pytorch.org/docs/stable/data.html#dataloader-collate-fn) for more information. A typical use-case for collate_fn, according to the documentation, is padding a sequence up to some max_len. Therefore I'd think that it's also useful for tokenisation and other things.<|||||>Got it yes this makes sense<|||||>Would love to see the multiprocessing fork as well<|||||>Hi @enzoampil @BramVanroy , I need to speed up the tokenization process, too. I'm not a pytorch guy and not sure the things you mentioned. Could you please provide a little more ? Thanks!<|||||>I haven't done anything like this since I didn't have a performance issue, but theoretically you can add a custom collate function to your Dataloader. A batch will then be passed to that collate_fn and the result will be returned. The following is an example, but it's untested.
```python
def tokenize(batch):
sentences, labels = batch
input_ids = torch.Tensor([tokenizer.encode(s) for s in sentences])
# generate masks ...
# add padding ...
return input_ids, mask_ids, labels
DataLoader(dataset, batch_size=64, collate_fn=tokenize, num_workers=4)
```
Of course it depends on your dataset what will be fed to the collate_fn.<|||||>Rapids AI CuDF GPU data science library?
https://github.com/rapidsai/cudf<|||||>> Rapids AI CuDF GPU data science library?
>
> https://github.com/rapidsai/cudf
Perhaps elaborate on how this is useful in this context?<|||||>> Rapids AI CuDF GPU data science library?
> https://github.com/rapidsai/cudf
>
> Perhaps elaborate on how this is useful in this context?
GPU-accelerated word tokenization. Expand on this basic example:
https://medium.com/rapids-ai/show-me-the-word-count-3146e1173801
High-speed data loading & processing of textual dataframes on GPU with CUDA. Moving panda dfs to GPU is several lines of code or perhaps data loading straight to GPU. Stand-alone string library cuStrings & python-wrapper nvStrings are available: https://github.com/rapidsai/custrings<|||||>I should mention that I'm trying to finetune distilgpt2 on my 880MB dataset and in this sense I use `run_lm_finetuning.py`. It takes so many times to tokenize and I could say that it stucks [here](https://github.com/huggingface/transformers/blob/master/examples/run_lm_finetuning.py#L82). It's been 20 hours and I'm still waiting. I know there is something wrong and It shouldn't have taken this much time because I tokenized 470MB dataset before via [gpt2-simple](https://github.com/minimaxir/gpt-2-simple) and it took less than 5 mins.
I run `run_lm_finetuning.py` with a truncated 1 MB version of my dataset and It took ~1 mins. But when I tried a 50MB version, it's already exceeded 30 mins. That means, there is something causing `tokenizer.convert_tokens_to_ids(tokenizer.tokenize(text))` to run in exponentially much more time.<|||||>> I should mention that I'm trying to finetune distilgpt2 on my 880MB dataset and in this sense I use `run_lm_finetuning.py`. It takes so many times to tokenize and I could say that it stucks [here](https://github.com/huggingface/transformers/blob/master/examples/run_lm_finetuning.py#L82). It's been 20 hours and I'm still waiting. I know there is something wrong and It shouldn't have taken this much time because I tokenized 470MB dataset before via [gpt2-simple](https://github.com/minimaxir/gpt-2-simple) and it took less than 5 mins.
>
> I run `run_lm_finetuning.py` with a truncated 1 MB version of my dataset and It took ~1 mins. But when I tried a 50MB version, it's already exceeded 30 mins. That means, there is something causing `tokenizer.convert_tokens_to_ids(tokenizer.tokenize(text))` to run in exponentially much more time.
Do you perhaps have any strange data? Sentences that are particularly long or contain strange characters, stuff like that?<|||||>What should be the most strange characters? I scanned for non-ascii chars and found nothing. It's full of ascii chars and I think that makes it usual :) . (Btw, the dataset just consists of emails.)
Any other suggestions? Because that is too annoying. <|||||>Hm, no. No idea. You can try profiling and see where it goes wrong.<|||||>I dug into `transformers` codebase and found the problem:
https://github.com/huggingface/transformers/blob/master/transformers/tokenization_utils.py#L644
That for loop lasts almost forever. Seems like It just splits the text into tokens. How could we optimize it?<|||||>Okay, here is more details. This function takes so many time:
https://github.com/huggingface/transformers/blob/155c782a2ccd103cf63ad48a2becd7c76a7d2115/transformers/tokenization_gpt2.py#L183
That means, BPE takes a long time. Here is a quick benchmark in my 4th gen i7 CPU:
```
0 0.002872943878173828
100 0.2857849597930908
200 0.46935296058654785
300 0.7295417785644531
400 0.8204867839813232
500 0.965552806854248
600 1.0516178607940674
700 1.1927227973937988
800 1.3081107139587402
900 1.354628086090088
1000 1.4476778507232666
```
the first column is the iteration number and the second one is elapsed time. 1000 iteration takes 1.44 seconds. If we think that I have 2068444 tokens, it'll last ~50 hours. Isn't there anyone tried to train such a big (?) dataset?<|||||>This issue has been automatically marked as stale because it has not had recent activity. It will be closed if no further activity occurs. Thank you for your contributions.
<|||||>Please check out our [`tokenizers`](https://github.com/huggingface/tokenizers) repo. We rebuilt the tokenizers from scratch in Rust for performance and extensibility.
Feedback (and contributions) welcome 🤗<|||||>I used multiprocessing to tokenize my dataset, and after adding tokens in vocab it took nearly 6hrs to tokenize ~2 million sentences, while without adding vocab It took only 2 min.<|||||>@DarshanPatel11 Can you share the code how you did it?
<|||||>> @DarshanPatel11 Can you share the code how you did it?
What exactly you need the code for?
For multiprocessing here is the code:
https://www.ppaste.org/XbVqp6VzJ
Btw, Now you should use FastTokenizers only, they are insanely fast.<|||||>@DarshanPatel11 what do you mean by "adding tokens in vocab"?<|||||>> @DarshanPatel11 what do you mean by "adding tokens in vocab"?
By "adding tokens in vocab", I meant Adding my custom domain-specific words into the existing vocabulary.<|||||>@DarshanPatel11 Running into the same problem. It is odd that using the default tokenizer seems to be much faster than using the same tokenizer, but with an expanded vocabulary.<|||||>> I should mention that I'm trying to finetune distilgpt2 on my 880MB dataset and in this sense I use `run_lm_finetuning.py`. It takes so many times to tokenize and I could say that it stucks [here](https://github.com/huggingface/transformers/blob/master/examples/run_lm_finetuning.py#L82). It's been 20 hours and I'm still waiting. I know there is something wrong and It shouldn't have taken this much time because I tokenized 470MB dataset before via [gpt2-simple](https://github.com/minimaxir/gpt-2-simple) and it took less than 5 mins.
>
> I run `run_lm_finetuning.py` with a truncated 1 MB version of my dataset and It took ~1 mins. But when I tried a 50MB version, it's already exceeded 30 mins. That means, there is something causing `tokenizer.convert_tokens_to_ids(tokenizer.tokenize(text))` to run in exponentially much more time.
my training file has a size of around 880 MB but when I'm training a tokenizer (BPE), it getting halt, and **Killed** is coming on the terminal. Any suggestion? <|||||>I had a similar experience with XLM-R Tokenizer:
I wanted to make the XLM-R Longformer according to https://github.com/allenai/longformer/blob/master/scripts/convert_model_to_long.ipynb, I was working with a train text file around 1GB. The issue was that tokenization got stuck at some point and even after several days there was no sign of progress. According to my tracking it got stuck in the _split_on_token function_ in the _split_ here [tokenization_utils.py#L287](https://github.com/huggingface/transformers/blob/023f0f3708f73e4fdffb92505296cd7d3d928aef/src/transformers/tokenization_utils.py#L287) even though there should not be any of the special tokens in my text. At the end I have processed the text line by line (like in the minimal example below) which did the trick for me.
Note: The conversion guide above requires version 3.0.2 of transformers, but same thing seems to happen also using the new version, see the minimal example for illustration: https://colab.research.google.com/drive/1gIfcQ4XcWCRrPfGCGF8rHR6UViZAgoIS?usp=sharing
At first, it seemed to me that it is just incredibly slow. But I am still suspicious that something is off. Any explanation/comment on that would be appreciated! :)
|
transformers | 1,620 | closed | 'utf-8' codec can't decode byte 0x80 in position 0: invalid start byte | When I load the pretrained model from the local bin file, there is a decoding problem. | 10-24-2019 12:12:28 | 10-24-2019 12:12:28 | Hi, could you provide more information: **e.g. respect the template**? Please tell us which model, which bin file, with which command?<|||||>> Hi, could you provide more information: **e.g. respect the template**? Please tell us which model, which bin file, with which command?
tokenizer = BertTokenizer.from_pretrained("/home/liping/liping/bert/bert-base-cased-pytorch_model.bin")
XLNetModel.from_pretrained("/data2/liping/xlnet/xlnet-base-cased-pytorch_model.bin")
Those two command will make the problem occur.<|||||> @lipingbj With the latest versions of `transformers` you need to pass the path to the PyTorch-compatible model, so in your example use:
```
tokenizer = BertTokenizer.from_pretrained("/home/liping/liping/bert/")
```
The following files must be located in that folder:
* `vocab.txt` - vocabulary file
* `pytorch_model.bin` - the PyTorch-compatible (and converted) model
* `config.json` - json-based model configuration
Please make sure that these files exist and e.g. rename `bert-base-cased-pytorch_model.bin` to `pytorch_model.bin`.
That should work :)<|||||>> @lipingbj With the latest versions of `transformers` you need to pass the path to the PyTorch-compatible model, so in your example use:
>
> ```
> tokenizer = BertTokenizer.from_pretrained("/home/liping/liping/bert/")
> ```
>
> The following files must be located in that folder:
>
> * `vocab.txt` - vocabulary file
> * `pytorch_model.bin` - the PyTorch-compatible (and converted) model
> * `config.json` - json-based model configuration
>
> Please make sure that these files exist and e.g. rename `bert-base-cased-pytorch_model.bin` to `pytorch_model.bin`.
>
> That should work :)
encoder_model = BertModel.from_pretrained("/home/liping/liping/bert/pytorch-bert-model")
tokenizer = BertTokenizer.from_pretrained("/home/liping/liping/bert/pytorch-bert-model")
vocab.txt, pytorch_model.bin, config.json have included in directory bert/pytorch-bert-model
OSError: Model name '/home/liping/liping/bert/pytorch-bert-model' was not found in model name list (bert-base-uncased, bert-large-uncased, bert-base-cased, bert-large-cased, bert-base-multilingual-uncased, bert-base-multilingual-cased, bert-base-chinese, bert-base-german-cased, bert-large-uncased-whole-word-masking, bert-large-cased-whole-word-masking, bert-large-uncased-whole-word-masking-finetuned-squad, bert-large-cased-whole-word-masking-finetuned-squad, bert-base-cased-finetuned-mrpc, bert-base-german-dbmdz-cased, bert-base-german-dbmdz-uncased). We assumed '/home/liping/liping/bert/pytorch-bert-model/config.json' was a path or url to a configuration file named config.json or a directory containing such a file but couldn't find any such file at this path or url.<|||||>As the error says, "We assumed '/home/liping/liping/bert/pytorch-bert-model/config.json' was a path or url to a configuration file named config.json or a directory containing such a file but couldn't find any such file at this path or url."
Your data does not seem to be in "/home/liping/liping/bert/pytorch-bert-model"<|||||>Hello,
I'm trying to load biobert into pytorch, seeing a different error:
UnicodeDecodeError: 'utf-8' codec can't decode byte 0x8b in position 1: invalid start byte
any hints? @LysandreJik <|||||>> Hello,
>
> I'm trying to load biobert into pytorch, seeing a different error:
> UnicodeDecodeError: 'utf-8' codec can't decode byte 0x8b in position 1: invalid start byte
>
> any hints? @LysandreJik
Can you show the code that you are running to load from pre-trained weights?
For example
```
model = BertForSequenceClassification.from_pretrained('/path/to/directory/containing/model_artifacts/')
```
As stefan-it mentioned above, the directory must contain the 3 required files.
|
transformers | 1,619 | closed | AttributeError: 'BertForPreTraining' object has no attribute 'classifier' | I was trying to convert my fine tuned model to pytorch using the following command.
`
tf_checkpoint_path='models/model.ckpt-21'
bert_config_file='PRETRAINED_MODELS/uncased_L-12_H-768_A-12/bert_config.json'
pytorch_dump_path='pytorch_models/pytorch_model.bin'
python convert_bert_original_tf_checkpoint_to_pytorch.py --tf_checkpoint_path=$tf_checkpoint_path --bert_config_file=$bert_config_file --pytorch_dump_path=$pytorch_dump_path `
The issue that I face is given below. Any help would be appreciated
Traceback (most recent call last):
File "convert_bert_original_tf_checkpoint_to_pytorch.py", line 65, in <module>
args.pytorch_dump_path)
File "convert_bert_original_tf_checkpoint_to_pytorch.py", line 36, in convert_tf_checkpoint_to_pytorch
load_tf_weights_in_bert(model, config, tf_checkpoint_path)
File "/home/cibin/virtual_envs/pytorch/lib/python3.7/site-packages/transformers/modeling_bert.py", line 98, in load_tf_weights_in_bert
pointer = getattr(pointer, 'classifier')
File "/home/cibin/virtual_envs/pytorch/lib/python3.7/site-packages/torch/nn/modules/module.py", line 585, in __getattr__
type(self).__name__, name))
AttributeError: 'BertPreTrainingHeads' object has no attribute 'classifier'
| 10-24-2019 09:34:18 | 10-24-2019 09:34:18 | Hi! Are your fine-tuned models in the format of the original BERT, or were they fine-tuned using our library?<|||||>@LysandreJik It is fine tuned in the format of the original BERT.<|||||>This issue has been automatically marked as stale because it has not had recent activity. It will be closed if no further activity occurs. Thank you for your contributions.
<|||||>Hello, I'm having the same issue too. Also trying to load a model finetuned in original BERT format. I 'm getting the same error message.<|||||>I was able to fix this issue while converting a BERT Model trained on SQuAD by patching the convert_bert_original_tf_checkpoint_to_pytorch.py file
```
from transformers import BertConfig, BertForQuestionAnswering, load_tf_weights_in_bert
model = BertForQuestionAnswering(config)
```
and then in the modeling_bert.py file
_Note - my config file had '__num_labels' as the config for that, whereas yours might be num_labels_
```
class BertForQuestionAnswering(BertPreTrainedModel):
def __init__(self, config):
super(BertForQuestionAnswering, self).__init__(config)
self.num_labels = config._num_labels
self.bert = BertModel(config)
self.classifier = nn.Linear(config.hidden_size, config._num_labels)
#self.qa_outputs = nn.Linear(config.hidden_size, config.num_labels)
self.init_weights()
@add_start_docstrings_to_callable(BERT_INPUTS_DOCSTRING)
def forward(
self,
input_ids=None,
attention_mask=None,
token_type_ids=None,
position_ids=None,
head_mask=None,
inputs_embeds=None,
start_positions=None,
end_positions=None,
):
r"""
start_positions (:obj:`torch.LongTensor` of shape :obj:`(batch_size,)`, `optional`, defaults to :obj:`None`):
Labels for position (index) of the start of the labelled span for computing the token classification loss.
Positions are clamped to the length of the sequence (`sequence_length`).
Position outside of the sequence are not taken into account for computing the loss.
end_positions (:obj:`torch.LongTensor` of shape :obj:`(batch_size,)`, `optional`, defaults to :obj:`None`):
Labels for position (index) of the end of the labelled span for computing the token classification loss.
Positions are clamped to the length of the sequence (`sequence_length`).
Position outside of the sequence are not taken into account for computing the loss.
Returns:
:obj:`tuple(torch.FloatTensor)` comprising various elements depending on the configuration (:class:`~transformers.BertConfig`) and inputs:
loss (:obj:`torch.FloatTensor` of shape :obj:`(1,)`, `optional`, returned when :obj:`labels` is provided):
Total span extraction loss is the sum of a Cross-Entropy for the start and end positions.
start_scores (:obj:`torch.FloatTensor` of shape :obj:`(batch_size, sequence_length,)`):
Span-start scores (before SoftMax).
end_scores (:obj:`torch.FloatTensor` of shape :obj:`(batch_size, sequence_length,)`):
Span-end scores (before SoftMax).
hidden_states (:obj:`tuple(torch.FloatTensor)`, `optional`, returned when ``config.output_hidden_states=True``):
Tuple of :obj:`torch.FloatTensor` (one for the output of the embeddings + one for the output of each layer)
of shape :obj:`(batch_size, sequence_length, hidden_size)`.
Hidden-states of the model at the output of each layer plus the initial embedding outputs.
attentions (:obj:`tuple(torch.FloatTensor)`, `optional`, returned when ``config.output_attentions=True``):
Tuple of :obj:`torch.FloatTensor` (one for each layer) of shape
:obj:`(batch_size, num_heads, sequence_length, sequence_length)`.
Attentions weights after the attention softmax, used to compute the weighted average in the self-attention
heads.
Examples::
from transformers import BertTokenizer, BertForQuestionAnswering
import torch
tokenizer = BertTokenizer.from_pretrained('bert-base-uncased')
model = BertForQuestionAnswering.from_pretrained('bert-large-uncased-whole-word-masking-finetuned-squad')
question, text = "Who was Jim Henson?", "Jim Henson was a nice puppet"
input_ids = tokenizer.encode(question, text)
token_type_ids = [0 if i <= input_ids.index(102) else 1 for i in range(len(input_ids))]
start_scores, end_scores = model(torch.tensor([input_ids]), token_type_ids=torch.tensor([token_type_ids]))
all_tokens = tokenizer.convert_ids_to_tokens(input_ids)
answer = ' '.join(all_tokens[torch.argmax(start_scores) : torch.argmax(end_scores)+1])
assert answer == "a nice puppet"
"""
outputs = self.bert(
input_ids,
attention_mask=attention_mask,
token_type_ids=token_type_ids,
position_ids=position_ids,
head_mask=head_mask,
inputs_embeds=inputs_embeds,
)
sequence_output = outputs[0]
logits = self.classifier(sequence_output)
start_logits, end_logits = logits.split(1, dim=-1)
start_logits = start_logits.squeeze(-1)
end_logits = end_logits.squeeze(-1)
outputs = (start_logits, end_logits,) + outputs[2:]
if start_positions is not None and end_positions is not None:
# If we are on multi-GPU, split add a dimension
if len(start_positions.size()) > 1:
start_positions = start_positions.squeeze(-1)
if len(end_positions.size()) > 1:
end_positions = end_positions.squeeze(-1)
# sometimes the start/end positions are outside our model inputs, we ignore these terms
ignored_index = start_logits.size(1)
start_positions.clamp_(0, ignored_index)
end_positions.clamp_(0, ignored_index)
loss_fct = CrossEntropyLoss(ignore_index=ignored_index)
start_loss = loss_fct(start_logits, start_positions)
end_loss = loss_fct(end_logits, end_positions)
total_loss = (start_loss + end_loss) / 2
outputs = (total_loss,) + outputs
return outputs # (loss), start_logits, end_logits, (hidden_states), (attentions)
```
After which, you'll need to reinstall transformers and install it from the source where you edited it
```
pip uninstall -y transformers
%cd ~/transformers
pip install .
export BERT_BASE_DIR=/your/model
cd ~/transformers/src/transformers
python convert_bert_original_tf_checkpoint_to_pytorch.py \
--tf_checkpoint_path $BERT_BASE_DIR/model.ckpt \
--bert_config_file $BERT_BASE_DIR/bert_config.json \
--pytorch_dump_path $BERT_BASE_DIR/pytorch_model.bin
```
This would likely work for other models that run into the same issue - just need to fix the layers names and import model. |
transformers | 1,618 | closed | Format problem when training DistilBert | ## Format problem when training DistilBert
Hello,
I'm trying to train DistilBert from scratch on French language with the official "trainin with distillation task" script.
## To Reproduce
Steps to reproduce the behavior:
The problem arise when I invoke the script :
https://github.com/huggingface/transformers/blob/master/examples/distillation/distiller.py
With the command line :
```bash
python train.py --student_type distilbert --student_config training_configs/distilbert-base-uncased.json \
--teacher_type bert --teacher_name bert-base-uncased --mlm --dump_path train_model/my_first_training --data_file data/binarized_text.bert-base-multilingual-cased.pickle \
--token_counts data/token_counts.bert-base-uncased.pickle --force --n_gpu 1
```
I did not modify the script in any way, and I get the error :
```bash
Traceback (most recent call last):
File "train.py", line 286, in <module>
main()
File "train.py", line 281, in main
distiller.train()
File "/dds/work/distil/transformers/examples/distillation/distiller.py", line 335, in train
token_ids, attn_mask, lm_labels = self.prepare_batch_mlm(batch=batch)
File "/dds/work/distil/transformers/examples/distillation/distiller.py", line 227, in prepare_batch_mlm
token_ids = token_ids.masked_scatter(pred_mask, _token_ids)
RuntimeError: Expected object of scalar type Byte but got scalar type Bool for argument #2 'mask'
```
## Environment
* OS: Windows
* Python version: 3.6
* PyTorch version: 1.1.0
* PyTorch Transformers version (or branch): 2.1.1
* Using 1 GPU
Could you help me resolve this ?
| 10-24-2019 09:25:43 | 10-24-2019 09:25:43 | Hi, I believe that `torch.bool` was introduced in PyTorch 1.2.0. Do you think you could try to upgrade it to 1.2.0 to try out the distillation scripts?<|||||>Problem fixed, the problem was the PyTorch version as you said, thank you so much! :) |
transformers | 1,617 | closed | Add T5 model | # 🌟New model addition
## Model description
Google released paper + code + dataset + pre-trained model about their new **T5**, beating state-of-the-art in 17/24 tasks.
[Paper link](https://arxiv.org/pdf/1910.10683.pdf)
## Open Source status
* [x] the model implementation and weights are available: [Official codebase](https://github.com/google-research/text-to-text-transfer-transformer)
| 10-24-2019 09:25:37 | 10-24-2019 09:25:37 | +1, it is a very impressive work<|||||>https://github.com/google-research/text-to-text-transfer-transformer
However i would prefer seeing Albert implemented before T5.<|||||>This issue has been automatically marked as stale because it has not had recent activity. It will be closed if no further activity occurs. Thank you for your contributions.
<|||||>Please<|||||>It's not super-well documented, but it's clearly present:
https://github.com/huggingface/transformers/blob/dc17f2a1110aed8d1729e77b0619601e3d96b84e/src/transformers/modeling_tf_t5.py |
transformers | 1,616 | closed | run_generation.py example for a batch | Hi
I want to use example/run_generation.py to enter a batch of sentences and get a batch of generated outputs. could you please assist me and provide me with the commands how I can do it, is this possible with this code, if not I really appreciate adding this feature. thanks | 10-24-2019 09:16:15 | 10-24-2019 09:16:15 | This issue has been automatically marked as stale because it has not had recent activity. It will be closed if no further activity occurs. Thank you for your contributions.
|
transformers | 1,615 | closed | CUDA error: device-side assert triggered(pretrained_model.cuda()) | ## 🐛 Bug
<!-- Important information -->
Model I am using (XLNet....):
Language I am using the model on (English):
The problem arise when using:
config = XLNetConfig.from_json_file('/data2/liping/xlnet/xlnet_cased_L-12_H-768_A-12/xlnet_config.json')
encoder_model = XLNetModel.from_pretrained("/data2/liping/xlnet/xlnet_cased_L-12_H-768_A-12/xlnet_model.ckpt.index", config=config, from_tf=True)
encoder_model.cuda("cuda:0")
The problem:
~/anaconda3/lib/python3.7/site-packages/torch/nn/modules/module.py in _apply(self, fn)
228 # `with torch.no_grad():`
229 with torch.no_grad():
--> 230 param_applied = fn(param)
231 should_use_set_data = compute_should_use_set_data(param, param_applied)
232 if should_use_set_data:
~/anaconda3/lib/python3.7/site-packages/torch/nn/modules/module.py in <lambda>(t)
309 Module: self
310 """
--> 311 return self._apply(lambda t: t.cuda(device))
312
313 def cpu(self):
RuntimeError: CUDA error: device-side assert triggered | 10-24-2019 09:12:43 | 10-24-2019 09:12:43 | Hello! Is your checkpoint the original one from the XLNet repository or one of our TensorFlow checkpoints hosted on S3?<|||||>> Hello! Is your checkpoint the original one from the XLNet repository or one of our TensorFlow checkpoints hosted on S3?
The checkpoint is from the XLNet repository.<|||||>Could you then convert it to one a checkpoint readable by our models by using the script [convert_xlnet_original_tf_checkpoint_to_pytorch.py](https://github.com/huggingface/transformers/blob/master/transformers/convert_xlnet_original_tf_checkpoint_to_pytorch.py)?<|||||>> Could you then convert it to one a checkpoint readable by our models by using the script [convert_xlnet_original_tf_checkpoint_to_pytorch.py](https://github.com/huggingface/transformers/blob/master/transformers/convert_xlnet_original_tf_checkpoint_to_pytorch.py)?
I have tried with the script, but the problem is still exiting.
encoder_model = XLNetModel.from_pretrained("/data2/liping/xlnet/produce/")
encoder_model.cuda()
-> 230 param_applied = fn(param)
231 should_use_set_data = compute_should_use_set_data(param, param_applied)
232 if should_use_set_data:
~/anaconda3/lib/python3.7/site-packages/torch/nn/modules/module.py in <lambda>(t)
309 Module: self
310 """
--> 311 return self._apply(lambda t: t.cuda(device))
312
313 def cpu(self):
RuntimeError: CUDA error: device-side assert triggered<|||||>What happens once you have converted the original checkpoint to PyTorch? What is inside the folder "/data2/liping/xlnet/produce/" ?<|||||>> What happens once you have converted the original checkpoint to PyTorch? What is inside the folder "/data2/liping/xlnet/produce/" ?
Thank you for your help and i have convert the original checkpoint to PyTorch and load the xlnet pre-training model successful.<|||||>@lipingbj Good to hear that you've fixed the problem. i just met the same problem when i use run_lm_finetuning.py and when i try convert with convert_bertabs_original_pytorch_checkpoint.py it just returned no module named'model_bertabs'. By the way i put the pytorch_model.bin that i have trained before in convert file ,is that right and how you fix the problem.Will be really appreciate for your reply! |
transformers | 1,614 | closed | Slight different output between transformers and pytorch-transformers | I am now working on a Chinese NER tagging task. I applied BertForTokenClassification. The original library I used is pytorch-transformers 1.2.0. Then I migrated to Tranformers 2.1.1. But I found the output is slightly different between two versions. See the pics below
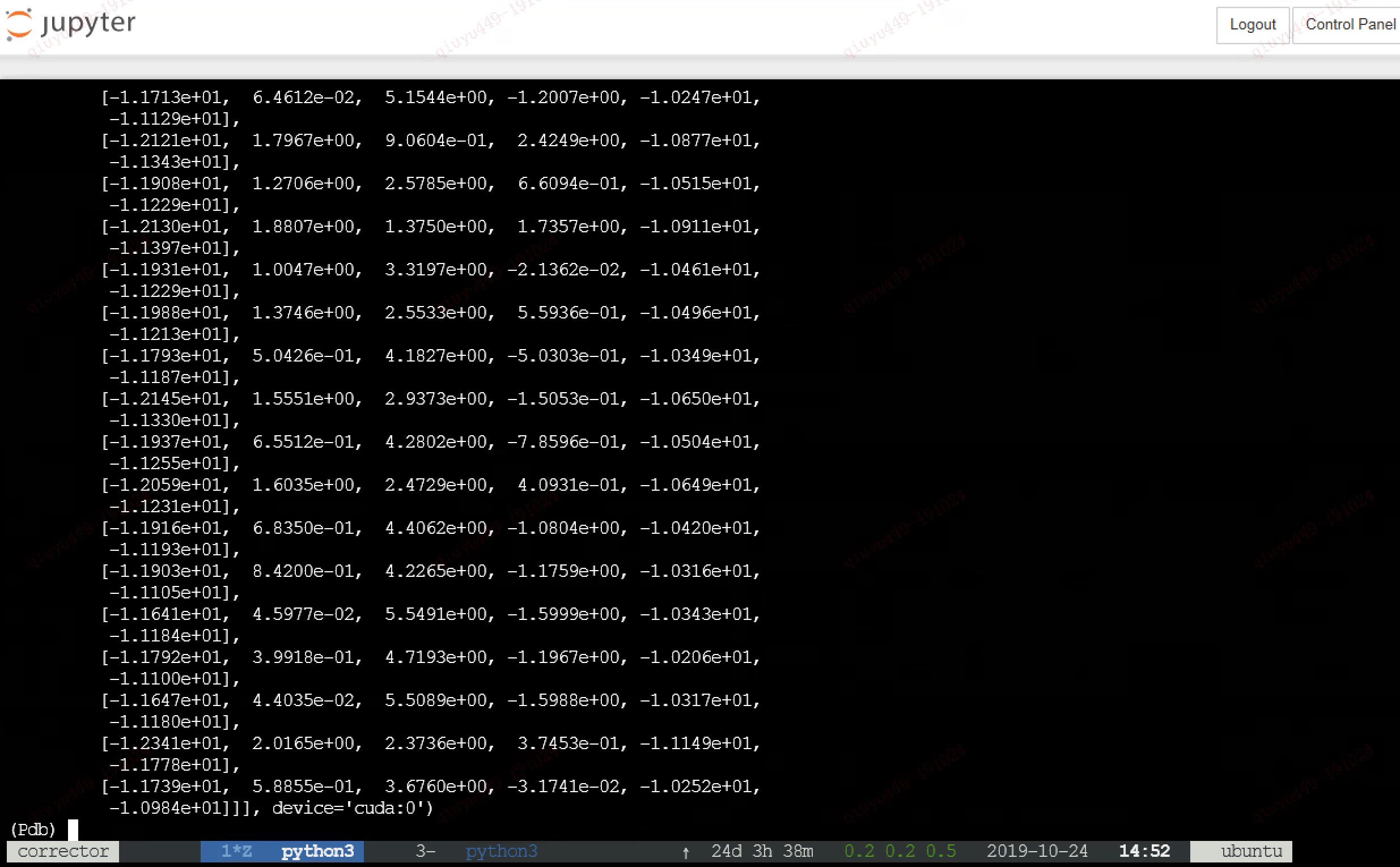
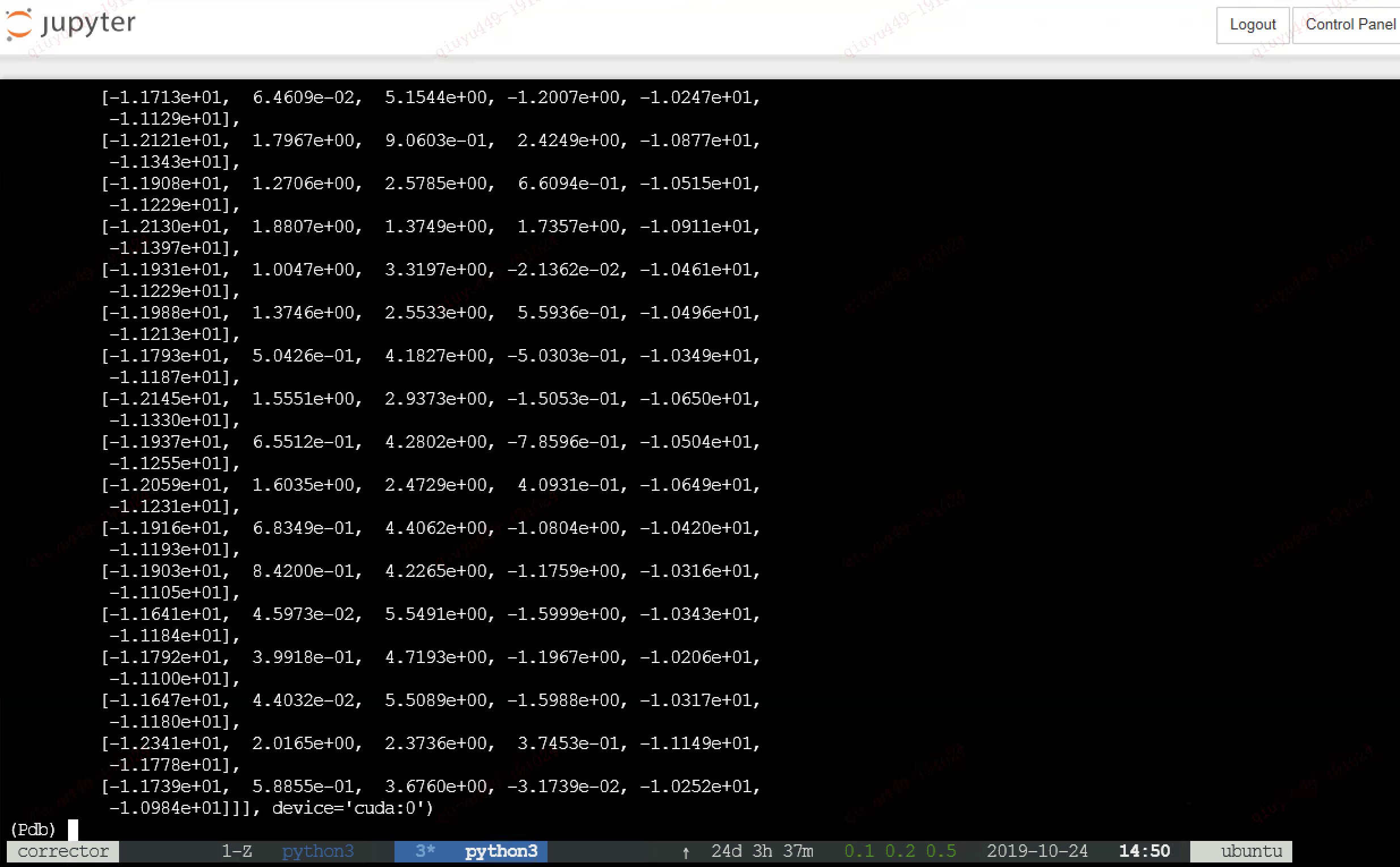
I wondered what potentially caused this difference?
## 🐛 Bug
<!-- Important information -->
Model I am using (Bert, XLNet....):
BertForTokenClassification
Language I am using the model on (English, Chinese....):
Chinese
The problem arise when using:
* [ ] the official example scripts: (give details)
* [x] my own modified scripts: (give details)
The tasks I am working on is:
* [ ] an official GLUE/SQUaD task: (give the name)
* [x] my own task or dataset: (give details)
## To Reproduce
Steps to reproduce the behavior:
1.
2.
3.
<!-- If you have a code sample, error messages, stack traces, please provide it here as well. -->
## Expected behavior
<!-- A clear and concise description of what you expected to happen. -->
## Environment
* OS:
* Python version:
* PyTorch version:
* PyTorch Transformers version (or branch):
* Using GPU ?
* Distributed of parallel setup ?
* Any other relevant information:
## Additional context
<!-- Add any other context about the problem here. -->
| 10-24-2019 06:56:02 | 10-24-2019 06:56:02 | Maybe you didn't put the model in evaluation mode in one of the tests and the DropOut modules were not deactivated as such.<|||||>This issue has been automatically marked as stale because it has not had recent activity. It will be closed if no further activity occurs. Thank you for your contributions.
|
transformers | 1,613 | closed | Roberta token classification | Roberta is missing the token classification that is already available in the BERT models. Per discussion in #1166 they mention it should be more or less a copy paste of the current `BertForTokenClassification` and `TFBertForTokenClassification `. I noticed this is also missing as I hope to update the `run_ner.py` file to include DistilRoberta, which needs these new classes (#1534).
Changes
* Simple copy paste of the related Bert models for Roberta. I added tests that also reflect the same changes. Minor tweaks were made that are different in the Roberta models (inheriting from Bert and changing the configs to Roberta).
Tests seem to pass but as this is my first PR, I would like some more feedback if this in fact works correctly. | 10-24-2019 05:12:36 | 10-24-2019 05:12:36 | # [Codecov](https://codecov.io/gh/huggingface/transformers/pull/1613?src=pr&el=h1) Report
> Merging [#1613](https://codecov.io/gh/huggingface/transformers/pull/1613?src=pr&el=desc) into [master](https://codecov.io/gh/huggingface/transformers/commit/5b6cafb11b39e78724dc13b57b81bd73c9a66b49?src=pr&el=desc) will **decrease** coverage by `0.27%`.
> The diff coverage is `23.72%`.
[](https://codecov.io/gh/huggingface/transformers/pull/1613?src=pr&el=tree)
```diff
@@ Coverage Diff @@
## master #1613 +/- ##
=========================================
- Coverage 86.17% 85.9% -0.28%
=========================================
Files 91 91
Lines 13595 13653 +58
=========================================
+ Hits 11715 11728 +13
- Misses 1880 1925 +45
```
| [Impacted Files](https://codecov.io/gh/huggingface/transformers/pull/1613?src=pr&el=tree) | Coverage Δ | |
|---|---|---|
| [transformers/tests/modeling\_tf\_roberta\_test.py](https://codecov.io/gh/huggingface/transformers/pull/1613/diff?src=pr&el=tree#diff-dHJhbnNmb3JtZXJzL3Rlc3RzL21vZGVsaW5nX3RmX3JvYmVydGFfdGVzdC5weQ==) | `75.2% <14.28%> (-3.62%)` | :arrow_down: |
| [transformers/tests/modeling\_roberta\_test.py](https://codecov.io/gh/huggingface/transformers/pull/1613/diff?src=pr&el=tree#diff-dHJhbnNmb3JtZXJzL3Rlc3RzL21vZGVsaW5nX3JvYmVydGFfdGVzdC5weQ==) | `75.38% <22.22%> (-4.13%)` | :arrow_down: |
| [transformers/modeling\_roberta.py](https://codecov.io/gh/huggingface/transformers/pull/1613/diff?src=pr&el=tree#diff-dHJhbnNmb3JtZXJzL21vZGVsaW5nX3JvYmVydGEucHk=) | `71.25% <25%> (-9.32%)` | :arrow_down: |
| [transformers/modeling\_tf\_roberta.py](https://codecov.io/gh/huggingface/transformers/pull/1613/diff?src=pr&el=tree#diff-dHJhbnNmb3JtZXJzL21vZGVsaW5nX3RmX3JvYmVydGEucHk=) | `90.67% <26.66%> (-9.33%)` | :arrow_down: |
------
[Continue to review full report at Codecov](https://codecov.io/gh/huggingface/transformers/pull/1613?src=pr&el=continue).
> **Legend** - [Click here to learn more](https://docs.codecov.io/docs/codecov-delta)
> `Δ = absolute <relative> (impact)`, `ø = not affected`, `? = missing data`
> Powered by [Codecov](https://codecov.io/gh/huggingface/transformers/pull/1613?src=pr&el=footer). Last update [5b6cafb...d555603](https://codecov.io/gh/huggingface/transformers/pull/1613?src=pr&el=lastupdated). Read the [comment docs](https://docs.codecov.io/docs/pull-request-comments).
<|||||>Closing this as superseded by #1625 |
transformers | 1,612 | closed | add model & config address in appendix, and add link to appendix.md i… | Support certain model & config download address in appendix. | 10-24-2019 03:05:16 | 10-24-2019 03:05:16 | I don't think we want to commit to maintaining an exhaustive, centralized list of models in the future.
Will close this unless further comments |
transformers | 1,611 | closed | How can I get the probability of a word which fits the masked place? | ## ❓ Questions & Help
I want to get the probability of a word which fits the masked place.
```
tokenizer = BertTokenizer.from_pretrained('bert-base-uncased')
model = BertForMaskedLM.from_pretrained('bert-base-uncased')
model.eval()
text = '[CLS] I want to [MASK] the car because it is cheap . [SEP]'
tokenized_text = tokenizer.tokenize(text)
indexed_tokens = tokenizer.convert_tokens_to_ids(tokenized_text)
segments_ids = [0] * len(tokenized_text)
tokens_tensor = torch.tensor([indexed_tokens])
segments_tensors = torch.tensor([segments_ids])
with torch.no_grad():
predictions = model(tokens_tensor, segments_tensors)
masked_index = tokenized_text.index('[MASK]')
predicted_score, predicted_indexes = torch.topk(predictions[0][0, masked_index], k=5)
predicted_tokens = tokenizer.convert_ids_to_tokens(predicted_indexes.tolist())
```
`predicted_tokens`
> `['buy', 'sell', 'rent', 'take', 'drive']`
'predicted_score'
> 'tensor([10.9675, 10.4480, 9.5352, 9.5170, 9.3046])'
`predicted_score` is not a probability. I want a pair of the word and its probability (the total score for all words is 1) which goes in the [MASK]. | 10-24-2019 02:16:47 | 10-24-2019 02:16:47 | |
transformers | 1,610 | closed | Update setup.py | changed update setup file | 10-23-2019 12:41:51 | 10-23-2019 12:41:51 | # [Codecov](https://codecov.io/gh/huggingface/transformers/pull/1610?src=pr&el=h1) Report
> Merging [#1610](https://codecov.io/gh/huggingface/transformers/pull/1610?src=pr&el=desc) into [master](https://codecov.io/gh/huggingface/transformers/commit/ef1b8b2ae5ad1057154a126879f7eb8de685f862?src=pr&el=desc) will **not change** coverage.
> The diff coverage is `n/a`.
[](https://codecov.io/gh/huggingface/transformers/pull/1610?src=pr&el=tree)
```diff
@@ Coverage Diff @@
## master #1610 +/- ##
=======================================
Coverage 86.17% 86.17%
=======================================
Files 91 91
Lines 13595 13595
=======================================
Hits 11715 11715
Misses 1880 1880
```
------
[Continue to review full report at Codecov](https://codecov.io/gh/huggingface/transformers/pull/1610?src=pr&el=continue).
> **Legend** - [Click here to learn more](https://docs.codecov.io/docs/codecov-delta)
> `Δ = absolute <relative> (impact)`, `ø = not affected`, `? = missing data`
> Powered by [Codecov](https://codecov.io/gh/huggingface/transformers/pull/1610?src=pr&el=footer). Last update [ef1b8b2...2248c6b](https://codecov.io/gh/huggingface/transformers/pull/1610?src=pr&el=lastupdated). Read the [comment docs](https://docs.codecov.io/docs/pull-request-comments).
<|||||>Why should we change this? |
transformers | 1,609 | closed | Can the prefix for GPT-2 conditional sampling be very long (longer than context window size)? | Can the prefix for GPT-2 conditional sampling be very long (longer than context window size)? | 10-23-2019 12:38:33 | 10-23-2019 12:38:33 | This issue has been automatically marked as stale because it has not had recent activity. It will be closed if no further activity occurs. Thank you for your contributions.
|
transformers | 1,608 | closed | Error raised by "tmp_eval_loss += tmp_eval_loss.item()" when using multi-gpu | fixed the bug raised by "tmp_eval_loss += tmp_eval_loss.item()" when parallelly using multi-gpu. | 10-23-2019 12:30:24 | 10-23-2019 12:30:24 | # [Codecov](https://codecov.io/gh/huggingface/transformers/pull/1608?src=pr&el=h1) Report
> Merging [#1608](https://codecov.io/gh/huggingface/transformers/pull/1608?src=pr&el=desc) into [master](https://codecov.io/gh/huggingface/transformers/commit/ef1b8b2ae5ad1057154a126879f7eb8de685f862?src=pr&el=desc) will **increase** coverage by `0.02%`.
> The diff coverage is `n/a`.
[](https://codecov.io/gh/huggingface/transformers/pull/1608?src=pr&el=tree)
```diff
@@ Coverage Diff @@
## master #1608 +/- ##
=========================================
+ Coverage 86.17% 86.2% +0.02%
=========================================
Files 91 91
Lines 13595 13595
=========================================
+ Hits 11715 11719 +4
+ Misses 1880 1876 -4
```
| [Impacted Files](https://codecov.io/gh/huggingface/transformers/pull/1608?src=pr&el=tree) | Coverage Δ | |
|---|---|---|
| [transformers/file\_utils.py](https://codecov.io/gh/huggingface/transformers/pull/1608/diff?src=pr&el=tree#diff-dHJhbnNmb3JtZXJzL2ZpbGVfdXRpbHMucHk=) | `76.37% <0%> (+2.19%)` | :arrow_up: |
------
[Continue to review full report at Codecov](https://codecov.io/gh/huggingface/transformers/pull/1608?src=pr&el=continue).
> **Legend** - [Click here to learn more](https://docs.codecov.io/docs/codecov-delta)
> `Δ = absolute <relative> (impact)`, `ø = not affected`, `? = missing data`
> Powered by [Codecov](https://codecov.io/gh/huggingface/transformers/pull/1608?src=pr&el=footer). Last update [ef1b8b2...bd847ce](https://codecov.io/gh/huggingface/transformers/pull/1608?src=pr&el=lastupdated). Read the [comment docs](https://docs.codecov.io/docs/pull-request-comments).
<|||||>Thanks @focox! |
transformers | 1,607 | closed | failed to download pretrained weights | ## 🐛 Bug
<!-- Important information -->
Model I am using (Bert, XLNet....):BERT
Language I am using the model on (English, Chinese....):English
During downloading pretrained weights with code of modeling_bert.BertForMaskedLM.from_pretrained('bert-base-uncased'),another exception occurred:
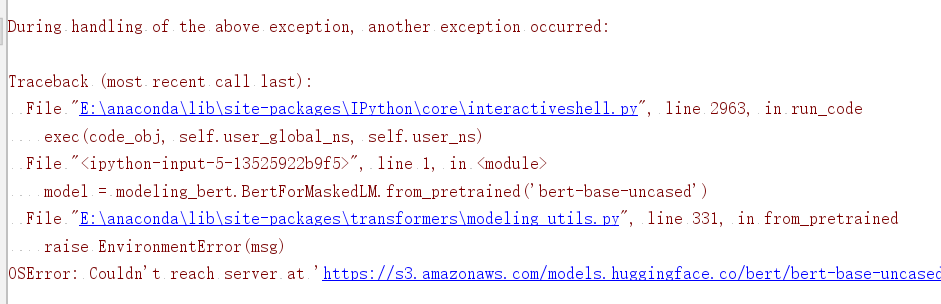
| 10-23-2019 09:49:37 | 10-23-2019 09:49:37 | Hi, this seems to be a network error. Are you sure you have access to the internet on this machine, or is it behind a firewall?<|||||>I had exactly the same problem Yesterday and s3.amazonaws.com just was not reachable. We also had the same problem with another service as well. After trying for some time it just started working again. |
transformers | 1,606 | closed | Show pretrained model and config file download address directly in README.md & doc | ## 🚀 Feature
<!-- A clear and concise description of the feature proposal. Please provide a link to the paper and code in case they exist. -->
Show download address of pretrained model address directly in markdown file.
## Motivation
Since my server cannot directly go to aws server, and it's not configed to proxy too. Intuitively i need to download pretrained model in my computer and then upload it to my server. The question is I need to checkout source code for download address. It's a really bad experience.
<!-- Please outline the motivation for the proposal. Is your feature request related to a problem? e.g., I'm always frustrated when [...]. If this is related to another GitHub issue, please link here too. -->
## Additional context
<!-- Add any other context or screenshots about the feature request here. -->
| 10-23-2019 07:29:51 | 10-23-2019 07:29:51 | I feel that this would clutter the README, leading to a bad experience for 99.99% of users. But you can always submit a PR and see what the maintainers think.<|||||>Just make a PR. |
transformers | 1,605 | closed | Support for gpt2-medium, gpt2-large and distilgpt2 in pytorch-pretrained-bert 0.6.2 | ## 🚀 Feature
Request: Inclusion of the below 3 lines in pytorch-pretrained-bert 0.6.2
https://github.com/huggingface/transformers/blob/ef1b8b2ae5ad1057154a126879f7eb8de685f862/transformers/modeling_gpt2.py#L40-L42
## Motivation
Currently, the above 3 lines exist in the latest version of transformers in PyPI, but not in pytorch-pretrained-bert 0.6.2 (also available in PyPI). Consequently, folks wanting to experiment with the above 3 pre-trained models need to necessarily upgrade to the latest version of transformers immediately. As a relief for such folks who plan to migrate eventually but not immediately, it would be great if the above 3 lines are added in pytorch-pretrained-bert 0.6.2.
| 10-23-2019 05:36:54 | 10-23-2019 05:36:54 | As far as I know pytorch-pretrained-bert development has been discontinued. That makes sense. If you want the new features, you have to upgrade.<|||||>Well technically what I'm asking for isn't a new feature, it's just backwards-compatibility for the above three model artifacts.
I can manually add them to the `modeling_gpt2.py` in my conda environment containing pytorch-pretrained-bert 0.6.2 and verify if these model artifacts work with the old package by invoking the `from_pretrained()` method with each of these three artifact names. I am guessing they would work, but I haven't tried yet.
I feel like this dictionary containing pre-trained artifact names should itself reside in S3, and in `modeling_gpt2.py`, the dictionary should be pulled from S3. Then you could continually add new artifact sizes to that dictionary in S3 and it will work with all versions of this repo, not just some versions. Does that make sense?<|||||>This issue has been automatically marked as stale because it has not had recent activity. It will be closed if no further activity occurs. Thank you for your contributions.
|
transformers | 1,604 | closed | Versioning in documentation | Several versions of the documentation can now be accessed:
`huggingface.co/transformers` for the master release
`huggingface.co/transformers/v2.1.1` for the 2.1.1 official release and so on. | 10-22-2019 22:04:21 | 10-22-2019 22:04:21 | # [Codecov](https://codecov.io/gh/huggingface/transformers/pull/1604?src=pr&el=h1) Report
> Merging [#1604](https://codecov.io/gh/huggingface/transformers/pull/1604?src=pr&el=desc) into [master](https://codecov.io/gh/huggingface/transformers/commit/ef1b8b2ae5ad1057154a126879f7eb8de685f862?src=pr&el=desc) will **decrease** coverage by `<.01%`.
> The diff coverage is `n/a`.
[](https://codecov.io/gh/huggingface/transformers/pull/1604?src=pr&el=tree)
```diff
@@ Coverage Diff @@
## master #1604 +/- ##
==========================================
- Coverage 86.17% 86.16% -0.01%
==========================================
Files 91 91
Lines 13595 13593 -2
==========================================
- Hits 11715 11713 -2
Misses 1880 1880
```
| [Impacted Files](https://codecov.io/gh/huggingface/transformers/pull/1604?src=pr&el=tree) | Coverage Δ | |
|---|---|---|
| [transformers/tokenization\_ctrl.py](https://codecov.io/gh/huggingface/transformers/pull/1604/diff?src=pr&el=tree#diff-dHJhbnNmb3JtZXJzL3Rva2VuaXphdGlvbl9jdHJsLnB5) | `96.03% <0%> (-0.08%)` | :arrow_down: |
------
[Continue to review full report at Codecov](https://codecov.io/gh/huggingface/transformers/pull/1604?src=pr&el=continue).
> **Legend** - [Click here to learn more](https://docs.codecov.io/docs/codecov-delta)
> `Δ = absolute <relative> (impact)`, `ø = not affected`, `? = missing data`
> Powered by [Codecov](https://codecov.io/gh/huggingface/transformers/pull/1604?src=pr&el=footer). Last update [ef1b8b2...6e85bcc](https://codecov.io/gh/huggingface/transformers/pull/1604?src=pr&el=lastupdated). Read the [comment docs](https://docs.codecov.io/docs/pull-request-comments).
<|||||>Ready to merge<|||||>Awesome! |
transformers | 1,603 | closed | [scripts] Proposal: add a specific device flag | wdyt?
Will do in other scripts if this gets merged.
My use case is I have an instance with multiple GPUs and want to run one generation on `cuda:0`, another one on `cuda:1`, etc. | 10-22-2019 19:34:25 | 10-22-2019 19:34:25 | # [Codecov](https://codecov.io/gh/huggingface/transformers/pull/1603?src=pr&el=h1) Report
> Merging [#1603](https://codecov.io/gh/huggingface/transformers/pull/1603?src=pr&el=desc) into [master](https://codecov.io/gh/huggingface/transformers/commit/e16d46843a19ab289b82138e4eccec5610a76de7?src=pr&el=desc) will **not change** coverage.
> The diff coverage is `n/a`.
[](https://codecov.io/gh/huggingface/transformers/pull/1603?src=pr&el=tree)
```diff
@@ Coverage Diff @@
## master #1603 +/- ##
=======================================
Coverage 86.16% 86.16%
=======================================
Files 91 91
Lines 13593 13593
=======================================
Hits 11713 11713
Misses 1880 1880
```
| [Impacted Files](https://codecov.io/gh/huggingface/transformers/pull/1603?src=pr&el=tree) | Coverage Δ | |
|---|---|---|
| [transformers/tokenization\_ctrl.py](https://codecov.io/gh/huggingface/transformers/pull/1603/diff?src=pr&el=tree#diff-dHJhbnNmb3JtZXJzL3Rva2VuaXphdGlvbl9jdHJsLnB5) | `96.03% <0%> (ø)` | :arrow_up: |
------
[Continue to review full report at Codecov](https://codecov.io/gh/huggingface/transformers/pull/1603?src=pr&el=continue).
> **Legend** - [Click here to learn more](https://docs.codecov.io/docs/codecov-delta)
> `Δ = absolute <relative> (impact)`, `ø = not affected`, `? = missing data`
> Powered by [Codecov](https://codecov.io/gh/huggingface/transformers/pull/1603?src=pr&el=footer). Last update [e16d468...b0af23c](https://codecov.io/gh/huggingface/transformers/pull/1603?src=pr&el=lastupdated). Read the [comment docs](https://docs.codecov.io/docs/pull-request-comments).
<|||||>In my script, I take another approach for this. I assume that distributed training can only be instantiated by using torch's launch script 'or that at least the WORLD_SIZE env variable is set). `local_rank` will be used for the GPU id, even when not in distributed mode.
```python
# torch.distributed.launch adds a world_size environment variable
distributed = int(os.environ['WORLD_SIZE']) > 1 if 'WORLD_SIZE' in os.environ else False
```
Based on that, you can decide what you want to do with `local_rank`. If we're in distributed mode, start the process group, if we're not: use the `local_rank` cuda device.
```python
if local_rank == -1 or not torch.cuda.is_available():
device = torch.device('cpu')
else:
device = torch.device(f"cuda:{local_rank}")
if distributed:
dist.init_process_group(backend='nccl', init_method='env://')
```
As a bonus, to ensure that all processes such as validating only happen on the main device, even if that's not cuda:0 (even though personally I do that on all devices, too):
```python
is_first_process = not distributed or local_rank in [0, -1]
# ...
if args.do_eval and is_first_process:
# do eval
```
I merely post this for possible inspiration, of course!<|||||>Sounds good to me let's add this to all the examples (and the template in `templates/adding_a_new_example_script`)<|||||>> Sounds good to me let's add this to all the examples
The other scripts maybe make less sense as you would want to train on all available devices? Not 100% sure yet.<|||||>Ok I see, then maybe let's have the device flag on `run_generation` instead of `run_squad` (as currently proposed in the PR)?<|||||>This issue has been automatically marked as stale because it has not had recent activity. It will be closed if no further activity occurs. Thank you for your contributions.
|
transformers | 1,602 | closed | Fix architectures count | 10-22-2019 19:11:22 | 10-22-2019 19:11:22 | Great, thanks!<|||||># [Codecov](https://codecov.io/gh/huggingface/transformers/pull/1602?src=pr&el=h1) Report
> Merging [#1602](https://codecov.io/gh/huggingface/transformers/pull/1602?src=pr&el=desc) into [master](https://codecov.io/gh/huggingface/transformers/commit/1cfd9748683db43af2c98da1a19d39f0efc8cc3b?src=pr&el=desc) will **not change** coverage.
> The diff coverage is `n/a`.
[](https://codecov.io/gh/huggingface/transformers/pull/1602?src=pr&el=tree)
```diff
@@ Coverage Diff @@
## master #1602 +/- ##
=======================================
Coverage 86.16% 86.16%
=======================================
Files 91 91
Lines 13593 13593
=======================================
Hits 11713 11713
Misses 1880 1880
```
------
[Continue to review full report at Codecov](https://codecov.io/gh/huggingface/transformers/pull/1602?src=pr&el=continue).
> **Legend** - [Click here to learn more](https://docs.codecov.io/docs/codecov-delta)
> `Δ = absolute <relative> (impact)`, `ø = not affected`, `? = missing data`
> Powered by [Codecov](https://codecov.io/gh/huggingface/transformers/pull/1602?src=pr&el=footer). Last update [1cfd974...25d32f4](https://codecov.io/gh/huggingface/transformers/pull/1602?src=pr&el=lastupdated). Read the [comment docs](https://docs.codecov.io/docs/pull-request-comments).
|
|
transformers | 1,601 | closed | Clean roberta model & all tokenizers now add special tokens by default (breaking change) | The RoBERTa model checks that special tokens are in the input sequence as it cannot function as expected if they are not here. This is not the best practice:
- The print method is not handled on TPU, and the check is problematic when tracing the models
- RoBERTa is the only model to print this warning while other models that require special tokens (BERT, XLNet) don't.
The warning was removed and the encode/encode_plus/prepare_for_model methods now have `add_special_tokens` set to `True` by default. This is a **breaking change**, but it is a better practice. | 10-22-2019 18:20:01 | 10-22-2019 18:20:01 | # [Codecov](https://codecov.io/gh/huggingface/transformers/pull/1601?src=pr&el=h1) Report
> Merging [#1601](https://codecov.io/gh/huggingface/transformers/pull/1601?src=pr&el=desc) into [master](https://codecov.io/gh/huggingface/transformers/commit/079bfb32fba4f2b39d344ca7af88d79a3ff27c7c?src=pr&el=desc) will **decrease** coverage by `0.01%`.
> The diff coverage is `100%`.
[](https://codecov.io/gh/huggingface/transformers/pull/1601?src=pr&el=tree)
```diff
@@ Coverage Diff @@
## master #1601 +/- ##
==========================================
- Coverage 85.9% 85.88% -0.02%
==========================================
Files 91 91
Lines 13653 13640 -13
==========================================
- Hits 11728 11715 -13
Misses 1925 1925
```
| [Impacted Files](https://codecov.io/gh/huggingface/transformers/pull/1601?src=pr&el=tree) | Coverage Δ | |
|---|---|---|
| [transformers/modeling\_roberta.py](https://codecov.io/gh/huggingface/transformers/pull/1601/diff?src=pr&el=tree#diff-dHJhbnNmb3JtZXJzL21vZGVsaW5nX3JvYmVydGEucHk=) | `70.55% <ø> (-0.71%)` | :arrow_down: |
| [transformers/modeling\_tf\_roberta.py](https://codecov.io/gh/huggingface/transformers/pull/1601/diff?src=pr&el=tree#diff-dHJhbnNmb3JtZXJzL21vZGVsaW5nX3RmX3JvYmVydGEucHk=) | `89.9% <ø> (-0.77%)` | :arrow_down: |
| [transformers/tests/tokenization\_bert\_test.py](https://codecov.io/gh/huggingface/transformers/pull/1601/diff?src=pr&el=tree#diff-dHJhbnNmb3JtZXJzL3Rlc3RzL3Rva2VuaXphdGlvbl9iZXJ0X3Rlc3QucHk=) | `98.66% <100%> (ø)` | :arrow_up: |
| [transformers/tokenization\_utils.py](https://codecov.io/gh/huggingface/transformers/pull/1601/diff?src=pr&el=tree#diff-dHJhbnNmb3JtZXJzL3Rva2VuaXphdGlvbl91dGlscy5weQ==) | `91.43% <100%> (ø)` | :arrow_up: |
| [transformers/tests/tokenization\_roberta\_test.py](https://codecov.io/gh/huggingface/transformers/pull/1601/diff?src=pr&el=tree#diff-dHJhbnNmb3JtZXJzL3Rlc3RzL3Rva2VuaXphdGlvbl9yb2JlcnRhX3Rlc3QucHk=) | `92.45% <100%> (ø)` | :arrow_up: |
| [transformers/tests/tokenization\_xlnet\_test.py](https://codecov.io/gh/huggingface/transformers/pull/1601/diff?src=pr&el=tree#diff-dHJhbnNmb3JtZXJzL3Rlc3RzL3Rva2VuaXphdGlvbl94bG5ldF90ZXN0LnB5) | `97.91% <100%> (ø)` | :arrow_up: |
| [transformers/tests/tokenization\_tests\_commons.py](https://codecov.io/gh/huggingface/transformers/pull/1601/diff?src=pr&el=tree#diff-dHJhbnNmb3JtZXJzL3Rlc3RzL3Rva2VuaXphdGlvbl90ZXN0c19jb21tb25zLnB5) | `100% <100%> (ø)` | :arrow_up: |
| [transformers/tests/tokenization\_xlm\_test.py](https://codecov.io/gh/huggingface/transformers/pull/1601/diff?src=pr&el=tree#diff-dHJhbnNmb3JtZXJzL3Rlc3RzL3Rva2VuaXphdGlvbl94bG1fdGVzdC5weQ==) | `97.72% <100%> (ø)` | :arrow_up: |
| [transformers/tests/tokenization\_distilbert\_test.py](https://codecov.io/gh/huggingface/transformers/pull/1601/diff?src=pr&el=tree#diff-dHJhbnNmb3JtZXJzL3Rlc3RzL3Rva2VuaXphdGlvbl9kaXN0aWxiZXJ0X3Rlc3QucHk=) | `95.23% <100%> (ø)` | :arrow_up: |
------
[Continue to review full report at Codecov](https://codecov.io/gh/huggingface/transformers/pull/1601?src=pr&el=continue).
> **Legend** - [Click here to learn more](https://docs.codecov.io/docs/codecov-delta)
> `Δ = absolute <relative> (impact)`, `ø = not affected`, `? = missing data`
> Powered by [Codecov](https://codecov.io/gh/huggingface/transformers/pull/1601?src=pr&el=footer). Last update [079bfb3...3617469](https://codecov.io/gh/huggingface/transformers/pull/1601?src=pr&el=lastupdated). Read the [comment docs](https://docs.codecov.io/docs/pull-request-comments).
<|||||>Great, LGTM |
transformers | 1,600 | closed | None in openAi-gpt tokenization | Hi
I want to concat two sentences, and give it to openAI-gpt, I use cl sentence1 sep sentence2 sep
I got none with openai-gpt in the first position, could you tell me what is the expected format? thanks | 10-22-2019 16:43:20 | 10-22-2019 16:43:20 | Are you using the GPT tokenizer? If not try
```
tokenizer = transformers.OpenAIGTPTTokenizer()
input_ids = tokenizer.encode(your_text)
```
<|||||>This issue has been automatically marked as stale because it has not had recent activity. It will be closed if no further activity occurs. Thank you for your contributions.
|
transformers | 1,599 | closed | Issue in Cost Function | ## 🐛 Bug
<!-- Important information -->
Model I am using (Bert, XLNet....): GPT2
Language I am using the model on (English, Chinese....): English
The problem arise when using:
* [X] the official example scripts: (give details)
* [ ] my own modified scripts: (give details)
The tasks I am working on is:
* [ ] an official GLUE/SQUaD task: (give the name)
* [X] my own task or dataset: (give details)
## To Reproduce
And in cost function, in logits we are ignoring the last element, why is that? though we're not using any padding
```
shift_logits = lm_logits[..., :-1, :].contiguous()
shift_labels = labels[..., 1:].contiguous()
```
and for labels we're dropping the first token why is that ?
<!-- If you have a code sample, error messages, stack traces, please provide it here as well. -->
## Expected behavior
Loss function shouldn't drop the last and first element in logits and labels unless it is padded, correct me if I'm wrong.
| 10-22-2019 15:31:49 | 10-22-2019 15:31:49 | Hi @anandhperumal. Remember that you train GPT-2 by doing next-token prediction, therefore you need to compare the i-th input label--the truth--with what the model predicted: the (i-1)th output. Hence the indices shift.<|||||>@rlouf oh yeah. Thanks for the input.
if you don't mind can you answer this question as well [transformers](https://github.com/huggingface/transfer-learning-conv-ai/issues/43) it's not directly related to transformers.
Thanks again.<|||||>You're welcome. I haven't worked on the other codebase, but I'll try to help if I can. |
transformers | 1,598 | closed | changing "out_features" of final linear layer | calling `resize_token_embeddings` changes the dimensions of the final linear layer. so changed `out_features` | 10-22-2019 13:00:48 | 10-22-2019 13:00:48 | # [Codecov](https://codecov.io/gh/huggingface/transformers/pull/1598?src=pr&el=h1) Report
> Merging [#1598](https://codecov.io/gh/huggingface/transformers/pull/1598?src=pr&el=desc) into [master](https://codecov.io/gh/huggingface/transformers/commit/b8c9ea0010a09cca8173e5bdf4af855123aebfc7?src=pr&el=desc) will **decrease** coverage by `4.94%`.
> The diff coverage is `100%`.
[](https://codecov.io/gh/huggingface/transformers/pull/1598?src=pr&el=tree)
```diff
@@ Coverage Diff @@
## master #1598 +/- ##
==========================================
- Coverage 86.16% 81.22% -4.95%
==========================================
Files 91 57 -34
Lines 13593 8028 -5565
==========================================
- Hits 11713 6521 -5192
+ Misses 1880 1507 -373
```
| [Impacted Files](https://codecov.io/gh/huggingface/transformers/pull/1598?src=pr&el=tree) | Coverage Δ | |
|---|---|---|
| [pytorch\_transformers/modeling\_utils.py](https://codecov.io/gh/huggingface/transformers/pull/1598/diff?src=pr&el=tree#diff-cHl0b3JjaF90cmFuc2Zvcm1lcnMvbW9kZWxpbmdfdXRpbHMucHk=) | `90.3% <100%> (ø)` | |
| [transformers/modeling\_tf\_xlm.py](https://codecov.io/gh/huggingface/transformers/pull/1598/diff?src=pr&el=tree#diff-dHJhbnNmb3JtZXJzL21vZGVsaW5nX3RmX3hsbS5weQ==) | | |
| [transformers/configuration\_distilbert.py](https://codecov.io/gh/huggingface/transformers/pull/1598/diff?src=pr&el=tree#diff-dHJhbnNmb3JtZXJzL2NvbmZpZ3VyYXRpb25fZGlzdGlsYmVydC5weQ==) | | |
| [transformers/configuration\_bert.py](https://codecov.io/gh/huggingface/transformers/pull/1598/diff?src=pr&el=tree#diff-dHJhbnNmb3JtZXJzL2NvbmZpZ3VyYXRpb25fYmVydC5weQ==) | | |
| [transformers/tests/tokenization\_transfo\_xl\_test.py](https://codecov.io/gh/huggingface/transformers/pull/1598/diff?src=pr&el=tree#diff-dHJhbnNmb3JtZXJzL3Rlc3RzL3Rva2VuaXphdGlvbl90cmFuc2ZvX3hsX3Rlc3QucHk=) | | |
| [transformers/tests/modeling\_bert\_test.py](https://codecov.io/gh/huggingface/transformers/pull/1598/diff?src=pr&el=tree#diff-dHJhbnNmb3JtZXJzL3Rlc3RzL21vZGVsaW5nX2JlcnRfdGVzdC5weQ==) | | |
| [transformers/tests/tokenization\_utils\_test.py](https://codecov.io/gh/huggingface/transformers/pull/1598/diff?src=pr&el=tree#diff-dHJhbnNmb3JtZXJzL3Rlc3RzL3Rva2VuaXphdGlvbl91dGlsc190ZXN0LnB5) | | |
| [transformers/tests/modeling\_tf\_ctrl\_test.py](https://codecov.io/gh/huggingface/transformers/pull/1598/diff?src=pr&el=tree#diff-dHJhbnNmb3JtZXJzL3Rlc3RzL21vZGVsaW5nX3RmX2N0cmxfdGVzdC5weQ==) | | |
| [transformers/modeling\_xlnet.py](https://codecov.io/gh/huggingface/transformers/pull/1598/diff?src=pr&el=tree#diff-dHJhbnNmb3JtZXJzL21vZGVsaW5nX3hsbmV0LnB5) | | |
| [transformers/tokenization\_utils.py](https://codecov.io/gh/huggingface/transformers/pull/1598/diff?src=pr&el=tree#diff-dHJhbnNmb3JtZXJzL3Rva2VuaXphdGlvbl91dGlscy5weQ==) | | |
| ... and [139 more](https://codecov.io/gh/huggingface/transformers/pull/1598/diff?src=pr&el=tree-more) | |
------
[Continue to review full report at Codecov](https://codecov.io/gh/huggingface/transformers/pull/1598?src=pr&el=continue).
> **Legend** - [Click here to learn more](https://docs.codecov.io/docs/codecov-delta)
> `Δ = absolute <relative> (impact)`, `ø = not affected`, `? = missing data`
> Powered by [Codecov](https://codecov.io/gh/huggingface/transformers/pull/1598?src=pr&el=footer). Last update [b8c9ea0...9388320](https://codecov.io/gh/huggingface/transformers/pull/1598?src=pr&el=lastupdated). Read the [comment docs](https://docs.codecov.io/docs/pull-request-comments).
<|||||>Thanks you for this, I actually had this fix included in #1721 |
transformers | 1,597 | closed | _tokenize() got an unexpected keyword argument 'add_prefix_space' in CTRL | ## 🐛 Bug
If you look at [the search results in this repo](https://github.com/huggingface/transformers/search?q=add_prefix_space) for `add_prefix_space`, you'll find gpt2, roberta, and ctrl all document that
> `add_prefix_space`: Requires a space to start the input string => the encoding methods should be called with the --``add_prefix_space`` flag set to ``True``.
However, this attribute is only implemented in the GPT2Tokenizer. Since RobertaTokenizer subclasses GPT2Tokenizer, that is fine. However, CTRLTokenizer just subclasses the PretrainedTokenizer. As such, it does not have a `_tokenize()` method that accepts the `add_prefix_space` keyword.
I would fix this in a PR, but I am not sure what the actual correct fix is: does CTRL need the added space, or not? And can it subclass GPT2's tokenizer, or should it implement its own `_tokenize(*, add_prefix_space)` method?
| 10-22-2019 12:24:31 | 10-22-2019 12:24:31 | Hi @BramVanroy, thanks for reporting this. There was an issue in the docstring. It does not use prefix spaces and it does not use a byte-level BPE like GPT-2 does. The docstring should be fixed now. |
transformers | 1,596 | closed | How to use BERT for ENTITY extraction from a Sequence without classification in the NER task ? | ## ❓ Questions & Help
<!-- A clear and concise description of the question. -->
My requirement here is given a sentence(sequence), I would like to just extract the entities present in the sequence without classifying them to a type in the NER task. I see that BERT has BertForTokenClassification for NER which does the classification.
So, can somebody give me an idea of how to do **entity extraction/identification using BERT**? | 10-22-2019 09:51:24 | 10-22-2019 09:51:24 | I'm a bit confused: you're basically defining the broad case of named entity recognition. Is it not enough to have a binary NER (token-level classification) task for entity vs non-entity?<|||||>Assuming you have 3-class (PER, ORG, LOC) data with labels:
B-PER, I-PER, B-ORG, I-ORG, B-LOC, I-LOC, as well as O
Replace PER, ORG, LOC with ENT.
This leaves you with these labels:
B-ENT, I-ENT, O
You can do this before training, and then train a model specifically for 1-class named entity detection only.
Or you can do this as a post-processing step on the output of the normal 3-class model.<|||||>@bheinzerling Thank you!!
I will try this. |
transformers | 1,595 | closed | Using HuggingFace TransfoXLLMHeadModel() with custom Torchtext vocabulary | Hello,
I am trying to use the HuggingFace TransfoXLLMHeadModel on WikiText2 dataset under a customized TransfoXLConfig with different vocabulary, and it causing an error. I am not sure how to fix it. Below are my code:
```js
# Import packages
import torch
import torch.nn as nn
import torch.nn.functional as F
from transformers import TransfoXLConfig, TransfoXLTokenizer, TransfoXLModel, TransfoXLLMHeadModel, TFTransfoXLModel, TFTransfoXLLMHeadModel
import spacy
import torchtext
from torchtext.data.utils import get_tokenizer
from torchtext.data import Field, BPTTIterator, TabularDataset
import math
import random
import numpy as np
import pandas as pd
import time
# define the English text field
TEXT = Field(tokenize = 'spacy',
init_token='<sos>',
eos_token='<eos>',
tokenizer_language='en',
lower=True)
# load WikiText-2 dataset and split it into train and test set
train_Wiki2, val_Wiki2, test_Wiki2 = torchtext.datasets.WikiText2.splits(TEXT)
# build vocabulary based on the field that we just defined.
TEXT.build_vocab(train_Wiki2, val_Wiki2, test_Wiki2)
# get number of tokens
ntokens = len(TEXT.vocab.stoi) # ntokens = 28871
# define transformer-XL configuration.
transfoXLconfig = TransfoXLConfig(vocab_size_or_config_json_file = ntokens,
cutoffs = [20000, 40000, 200000],
d_model = 64,
d_embed = 64,
n_head = 16,
d_head = 64,
n_layer = 5,
attn_type = 0,
dropout = 0.1,
output_hidden_states = True,
output_attentions = True)
# define the transformer-XL model based on the specified configuration.
model = TransfoXLLMHeadModel(transfoXLconfig) # this line is causing an error.
"""
Error message:
Traceback (most recent call last):
File "<ipython-input-14-fa91df67f439>", line 1, in <module>
model = TransfoXLLMHeadModel(transfoXLconfig)
File "/Users/jin-dominique/anaconda3/lib/python3.7/site-packages/transformers/modeling_transfo_xl.py", line 818, in __init__
self.transformer = TransfoXLModel(config)
File "/Users/jin-dominique/anaconda3/lib/python3.7/site-packages/transformers/modeling_transfo_xl.py", line 599, in __init__
div_val=config.div_val)
File "/Users/jin-dominique/anaconda3/lib/python3.7/site-packages/transformers/modeling_transfo_xl.py", line 421, in __init__
self.emb_layers.append(nn.Embedding(r_idx-l_idx, d_emb_i))
File "/Users/jin-dominique/anaconda3/lib/python3.7/site-packages/torch/nn/modules/sparse.py", line 97, in __init__
self.weight = Parameter(torch.Tensor(num_embeddings, embedding_dim))
RuntimeError: Trying to create tensor with negative dimension -171129: [-171129, 1]
model = TransfoXLLMHeadModel(transfoXLconfig)
"""
```
How can I use HuggingFace TransfoXLLMHeadModel( ) with a custom vocabulary of different size?
Thank you,
| 10-22-2019 08:26:27 | 10-22-2019 08:26:27 | This issue has been automatically marked as stale because it has not had recent activity. It will be closed if no further activity occurs. Thank you for your contributions.
<|||||>I faced the same problem:I solved it by passing the size of your vocabulary (from your custom tokenizer) as a parameter.
I proceeded as follows:
`vocabulary_size = tokenizer.vocab_size`
```
configuration = tf.TransfoXLConfig(vocab_size_or_config_json_file=vocabulary_size, cutoffs=cutoffs,
d_model=512, d_embed=512, n_head=8, d_head=64, n_layer=12, d_inner=2048)
```
I hope that helped :)<|||||>P.s. What do you pass as inputs and labels?
For now, I create a batch as follows:
"The quick brown fox jumps over the lazy dog"
If I have batch_size=2, and sequence length=4:
["The quick brown fox",
"jumps over the lazy"]
What do you feed to the Transformer-XL as input? |
transformers | 1,594 | closed | Make benchmark more flexible (TF or PT) | I've been trying to run the benchmark, but I gave up after running into a trillion compatibility issues with tensorflow and bazel. To be fair, I just want to contribute and test all there is to test on PyTorch with 4x Tesla V100. It would be great if only the required modules are needed, and not all of them. So only try to import PyTorch or Tensorflow when the tester actually wants to test those frameworks. | 10-22-2019 07:43:02 | 10-22-2019 07:43:02 | I believe a quick workaround is to just install the pre-built, CPU version of TensorFlow 2.0. If you won't be running the TF benchmarks, it wouldn't affect anything.<|||||>True, but still not quite flexible. Since the goal of the benchmark script is to, I believe, encourage the community to add there runtimes, it's a good to make this as easy-to-use as possible.<|||||>You're right that we shouldn't require to have both libraries installed in order to benchmark only one of them. I've updated the Benchmark code so that you can run it with only a single library installed.<|||||>That's great, Lysandre. Thanks for pushing out changes so quickly! |
transformers | 1,593 | closed | Fix AdamW import error for <1.2 | closes #1585 | 10-22-2019 07:35:08 | 10-22-2019 07:35:08 | # [Codecov](https://codecov.io/gh/huggingface/transformers/pull/1593?src=pr&el=h1) Report
> Merging [#1593](https://codecov.io/gh/huggingface/transformers/pull/1593?src=pr&el=desc) into [master](https://codecov.io/gh/huggingface/transformers/commit/702f589848baba97ea4897aa3f0bb937e1ec3bcf?src=pr&el=desc) will **decrease** coverage by `0.77%`.
> The diff coverage is `82.23%`.
[](https://codecov.io/gh/huggingface/transformers/pull/1593?src=pr&el=tree)
```diff
@@ Coverage Diff @@
## master #1593 +/- ##
==========================================
- Coverage 84.73% 83.95% -0.78%
==========================================
Files 84 94 +10
Lines 12573 13951 +1378
==========================================
+ Hits 10654 11713 +1059
- Misses 1919 2238 +319
```
| [Impacted Files](https://codecov.io/gh/huggingface/transformers/pull/1593?src=pr&el=tree) | Coverage Δ | |
|---|---|---|
| [transformers/modeling\_tf\_transfo\_xl.py](https://codecov.io/gh/huggingface/transformers/pull/1593/diff?src=pr&el=tree#diff-dHJhbnNmb3JtZXJzL21vZGVsaW5nX3RmX3RyYW5zZm9feGwucHk=) | `92.21% <ø> (+0.97%)` | :arrow_up: |
| [transformers/modeling\_tf\_bert.py](https://codecov.io/gh/huggingface/transformers/pull/1593/diff?src=pr&el=tree#diff-dHJhbnNmb3JtZXJzL21vZGVsaW5nX3RmX2JlcnQucHk=) | `96.6% <ø> (+0.89%)` | :arrow_up: |
| [transformers/modeling\_tf\_gpt2.py](https://codecov.io/gh/huggingface/transformers/pull/1593/diff?src=pr&el=tree#diff-dHJhbnNmb3JtZXJzL21vZGVsaW5nX3RmX2dwdDIucHk=) | `94.79% <ø> (+1.31%)` | :arrow_up: |
| [transformers/tokenization\_gpt2.py](https://codecov.io/gh/huggingface/transformers/pull/1593/diff?src=pr&el=tree#diff-dHJhbnNmb3JtZXJzL3Rva2VuaXphdGlvbl9ncHQyLnB5) | `96.72% <ø> (ø)` | :arrow_up: |
| [transformers/configuration\_gpt2.py](https://codecov.io/gh/huggingface/transformers/pull/1593/diff?src=pr&el=tree#diff-dHJhbnNmb3JtZXJzL2NvbmZpZ3VyYXRpb25fZ3B0Mi5weQ==) | `88.63% <ø> (ø)` | :arrow_up: |
| [transformers/configuration\_roberta.py](https://codecov.io/gh/huggingface/transformers/pull/1593/diff?src=pr&el=tree#diff-dHJhbnNmb3JtZXJzL2NvbmZpZ3VyYXRpb25fcm9iZXJ0YS5weQ==) | `100% <ø> (ø)` | :arrow_up: |
| [transformers/configuration\_bert.py](https://codecov.io/gh/huggingface/transformers/pull/1593/diff?src=pr&el=tree#diff-dHJhbnNmb3JtZXJzL2NvbmZpZ3VyYXRpb25fYmVydC5weQ==) | `87.09% <ø> (ø)` | :arrow_up: |
| [transformers/modeling\_tf\_openai.py](https://codecov.io/gh/huggingface/transformers/pull/1593/diff?src=pr&el=tree#diff-dHJhbnNmb3JtZXJzL21vZGVsaW5nX3RmX29wZW5haS5weQ==) | `96.04% <ø> (+1.43%)` | :arrow_up: |
| [transformers/modeling\_tf\_distilbert.py](https://codecov.io/gh/huggingface/transformers/pull/1593/diff?src=pr&el=tree#diff-dHJhbnNmb3JtZXJzL21vZGVsaW5nX3RmX2Rpc3RpbGJlcnQucHk=) | `98.59% <ø> (+1.98%)` | :arrow_up: |
| [transformers/tests/modeling\_tf\_gpt2\_test.py](https://codecov.io/gh/huggingface/transformers/pull/1593/diff?src=pr&el=tree#diff-dHJhbnNmb3JtZXJzL3Rlc3RzL21vZGVsaW5nX3RmX2dwdDJfdGVzdC5weQ==) | `94.73% <0%> (ø)` | :arrow_up: |
| ... and [79 more](https://codecov.io/gh/huggingface/transformers/pull/1593/diff?src=pr&el=tree-more) | |
------
[Continue to review full report at Codecov](https://codecov.io/gh/huggingface/transformers/pull/1593?src=pr&el=continue).
> **Legend** - [Click here to learn more](https://docs.codecov.io/docs/codecov-delta)
> `Δ = absolute <relative> (impact)`, `ø = not affected`, `? = missing data`
> Powered by [Codecov](https://codecov.io/gh/huggingface/transformers/pull/1593?src=pr&el=footer). Last update [702f589...3408e84](https://codecov.io/gh/huggingface/transformers/pull/1593?src=pr&el=lastupdated). Read the [comment docs](https://docs.codecov.io/docs/pull-request-comments).
<|||||>I just realized that it's better to try to import AdamW in optimization, and if not available define the custom AdamW class. |
transformers | 1,592 | closed | Consider do_lower_case in PreTrainedTokenizer | As pointed out in #1545, when using an uncased model, and adding a new uncased token, the tokenizer does not correctly identify this in the case that the input text contains the token in a cased format.
For instance, if we load bert-base-uncased into BertTokenizer, and then use .add_tokens() to add "cool-token", we get the expected result for .tokenize('this is a cool-token'). However, we get a possibly unexpected result for .tokenize('this is a cOOl-Token'), which in fact mirrors the result for the former from before the new token was added.
This PR adds
- functionality to PreTrainedTokenizer to handle this situation in case a tokenizer (currently Bert, DistilBert, and XLNet) has the do_lower_case=True kwarg by:
1) lowercasing tokens added with .add_tokens()
2) lowercasing text at the beginning of .tokenize()
- new common test case for tokenizers
XLMTokenizer's `do_lowercase_and_remove_accent` is a bit more complicated and is not included in this PR. | 10-22-2019 07:06:00 | 10-22-2019 07:06:00 | this lgtm but let's wait for @thomwolf and @LysandreJik to chime in<|||||>I'd also like to improve the test cases. I'll try to find some time for that this weekend<|||||>Nice improvement, it would be even better with tests for DistilBERT and XLNet as both those models make use of the `do_lower_case` argument. TransfoXL also uses the `lower_case` argument and XLM the `do_lowercase_and_remove_accent` argument so it might be a good idea to test that those models have the correct behavior.
Putting tests in the `tokenization_tests_common` would probably be cleaner than in each model's test file, if we test all models rather than a single one.<|||||>@LysandreJik good points.
~Now that I'm thinking about it, it seems like it would make more sense to do the lowercasing/accent removal directly in the subclasses (`BertTokenizer`, `XLMtokenizer`, etc.) by overriding the `tokenize()` method from `PreTrainedTokenizer`, performing the normalization there, then calling the super `tokenize()` with the now-normalized text.~
Never mind, this would result in some silly code duplication.<|||||>Alright that looks good to me!<|||||># [Codecov](https://codecov.io/gh/huggingface/transformers/pull/1592?src=pr&el=h1) Report
> Merging [#1592](https://codecov.io/gh/huggingface/transformers/pull/1592?src=pr&el=desc) into [master](https://codecov.io/gh/huggingface/transformers/commit/de2696f68e20019fef3a5e1b54de10351abb4145?src=pr&el=desc) will **decrease** coverage by `1.22%`.
> The diff coverage is `100%`.
[](https://codecov.io/gh/huggingface/transformers/pull/1592?src=pr&el=tree)
```diff
@@ Coverage Diff @@
## master #1592 +/- ##
==========================================
- Coverage 84.26% 83.03% -1.23%
==========================================
Files 104 104
Lines 15431 15456 +25
==========================================
- Hits 13003 12834 -169
- Misses 2428 2622 +194
```
| [Impacted Files](https://codecov.io/gh/huggingface/transformers/pull/1592?src=pr&el=tree) | Coverage Δ | |
|---|---|---|
| [transformers/tests/tokenization\_tests\_commons.py](https://codecov.io/gh/huggingface/transformers/pull/1592/diff?src=pr&el=tree#diff-dHJhbnNmb3JtZXJzL3Rlc3RzL3Rva2VuaXphdGlvbl90ZXN0c19jb21tb25zLnB5) | `100% <100%> (ø)` | :arrow_up: |
| [transformers/tokenization\_utils.py](https://codecov.io/gh/huggingface/transformers/pull/1592/diff?src=pr&el=tree#diff-dHJhbnNmb3JtZXJzL3Rva2VuaXphdGlvbl91dGlscy5weQ==) | `92.21% <100%> (+0.07%)` | :arrow_up: |
| [transformers/modeling\_tf\_pytorch\_utils.py](https://codecov.io/gh/huggingface/transformers/pull/1592/diff?src=pr&el=tree#diff-dHJhbnNmb3JtZXJzL21vZGVsaW5nX3RmX3B5dG9yY2hfdXRpbHMucHk=) | `9.85% <0%> (-83.1%)` | :arrow_down: |
| [transformers/tests/modeling\_tf\_common\_test.py](https://codecov.io/gh/huggingface/transformers/pull/1592/diff?src=pr&el=tree#diff-dHJhbnNmb3JtZXJzL3Rlc3RzL21vZGVsaW5nX3RmX2NvbW1vbl90ZXN0LnB5) | `81.55% <0%> (-15.54%)` | :arrow_down: |
| [transformers/modeling\_roberta.py](https://codecov.io/gh/huggingface/transformers/pull/1592/diff?src=pr&el=tree#diff-dHJhbnNmb3JtZXJzL21vZGVsaW5nX3JvYmVydGEucHk=) | `59.41% <0%> (-12.36%)` | :arrow_down: |
| [transformers/modeling\_xlnet.py](https://codecov.io/gh/huggingface/transformers/pull/1592/diff?src=pr&el=tree#diff-dHJhbnNmb3JtZXJzL21vZGVsaW5nX3hsbmV0LnB5) | `71.18% <0%> (-2.44%)` | :arrow_down: |
| [transformers/modeling\_ctrl.py](https://codecov.io/gh/huggingface/transformers/pull/1592/diff?src=pr&el=tree#diff-dHJhbnNmb3JtZXJzL21vZGVsaW5nX2N0cmwucHk=) | `94.24% <0%> (-2.22%)` | :arrow_down: |
| [transformers/modeling\_openai.py](https://codecov.io/gh/huggingface/transformers/pull/1592/diff?src=pr&el=tree#diff-dHJhbnNmb3JtZXJzL21vZGVsaW5nX29wZW5haS5weQ==) | `80.66% <0%> (-1.34%)` | :arrow_down: |
------
[Continue to review full report at Codecov](https://codecov.io/gh/huggingface/transformers/pull/1592?src=pr&el=continue).
> **Legend** - [Click here to learn more](https://docs.codecov.io/docs/codecov-delta)
> `Δ = absolute <relative> (impact)`, `ø = not affected`, `? = missing data`
> Powered by [Codecov](https://codecov.io/gh/huggingface/transformers/pull/1592?src=pr&el=footer). Last update [de2696f...21637d4](https://codecov.io/gh/huggingface/transformers/pull/1592?src=pr&el=lastupdated). Read the [comment docs](https://docs.codecov.io/docs/pull-request-comments).
<|||||>Ok merging! |
transformers | 1,591 | closed | Error when trying to reuse hidden states in CTRL | ## 🐛 Bug
<!-- Important information -->
Model I am using (Bert, XLNet....): CTRL
Language I am using the model on (English, Chinese....): English
The problem arise when using:
My own script, the colab link is available [here](https://colab.research.google.com/drive/143T4sBda4r2nDYzmuNwi-ZFTbhJWfeOW)
The stack trace is:
```
---------------------------------------------------------------------------
RuntimeError Traceback (most recent call last)
<ipython-input-9-ac2d93f8c410> in <module>()
3 for i in range(3):
4 print(i)
----> 5 logits, past = model(**inputs, past=past)
6 logits = logits[0, -1]
7
8 frames
/usr/local/lib/python3.6/dist-packages/transformers/modeling_ctrl.py in scaled_dot_product_attention(q, k, v, mask, attention_mask, head_mask)
64
65 if mask is not None:
---> 66 scaled_attention_logits += (mask * -1e4)
67
68 if attention_mask is not None:
RuntimeError: The size of tensor a (13) must match the size of tensor b (7) at non-singleton dimension 3
```
The tasks I am working on is:
Generating text with CTRL
## To Reproduce
Just run the colab from the link I posted above
The main part of the code is:
```
input_ids = torch.tensor(tokenizer.encode("Links Hello, my dog is cute")).unsqueeze(0).to(device)
inputs = {'input_ids': input_ids}
with torch.no_grad():
past = None
for i in range(3):
print(i)
logits, past = model(**inputs, past=past)
logits = logits[0, -1]
next_token = logits.argmax()
input_ids = torch.cat([input_ids, next_token.view(1, 1)], dim=1)
inputs = {'input_ids': input_ids}
```
## Expected behavior
passing in `past` should not throw an error and should speed up generation
## Environment
* OS: Linux
* Python version: 3.6.8
* PyTorch version: 1.3.0+cu100
* PyTorch Transformers version (or branch): 2.1.1
* Using GPU: yes
* Distributed of parallel setup: No
* Any other relevant information: I'm running on an extended memory colab instance with a K80
| 10-22-2019 03:01:07 | 10-22-2019 03:01:07 | The same error occurs with the library installed with `git clone` (_master_ version) + torch v1.3.0 + python v3.6.8.
[Here](https://colab.research.google.com/drive/1nawWX6Lrfh9ZVKyRfTLgFSIG355xkPRy#scrollTo=n93UZjq5EIE_) is a more verbose version of the Colab Notebook posted by @bkkaggle with Google Colab.<|||||>I've just pushed a fix on the branch `fix-ctrl-past`. It should be in the next release.<|||||>Thanks, closing |
transformers | 1,590 | closed | [WIP] Fixes for TF Roberta (and other models WIP) | When converting the `run_tf_glue.py` example to the same format at `benchmarks.py` to create a standardized benchmark for training, I ran into errors with **training** the non-BERT models with the normal `model.fit()` method. I am attempting to resolve all the errors I encountered in this PR. In particular, I have fixed the errors I have encountered with `TFRobertaForSequenceClassification`, `TFXLMForSequenceClassification`, and `TFXLNetForSequenceClassification`.
### Changes
**Roberta**
* Roberta requires `@tf.function()` on `TFRobertaMainLayer.call()`
* Otherwise, errors encountered:
* `TypeError: You are attempting to use Python control flow in a layer that was not declared to be dynamic. Pass 'dynamic=True' to the class constructor.`
* `OperatorNotAllowedInGraphError: using a 'tf.Tensor' as a Python 'bool' is not allowed in Graph execution. Use Eager execution or decorate this function with @tf.function.`
* Issues:
* Fails test `TFRobertaModelTest.test_pt_tf_model_equivalence`: `AssertionError: layer.0.attention.self.query.weight not found in PyTorch model`.
**XLM**
* XLX requires changing some Python `assert` statements to `tf.debugging.assert_equal` both in `TFXLMMainLayer.call()` and `gen_mask()`
* Otherwise, errors encountered:
* `TypeError: You are attempting to use Python control flow in a layer that was not declared to be dynamic. Pass 'dynamic=True' to the class constructor.`
* `OperatorNotAllowedInGraphError: using a 'tf.Tensor' as a Python 'bool' is not allowed in Graph execution. Use Eager execution or decorate this function with @tf.function.`
**XLNet**
* XLNet had a dtype error (float vs int) in line `input_mask = 1.0 - attention_mask`. Since `input_mask` and `attention_mask` are both supposed (afaik) to be int32, I've replace `1.0` with `1`.
* Still has shape error (see below) that I have not managed to track down. **This is particularly confusion because the training works in eager mode!**
* Solution is to simply provide a workaround `model.run_eagerly = True`.
* Of course, this will make the model train much slower (~140s for first epoch). Decorating `TFXLNetForSequenceClassification`'s `call()` method with `tf.function` works, and results in ~80s per first epoch. We cannot decorate the individual `call()` methods (aka create "overlapping" `tf.function`) as that will cause model saving to not work.
* Irregardless of my changes, there is a warning `gradients do not exist for variables ['transformer/mask_emb:0'] when minimizing the loss.` But from my observation the model trains fine. Is this embedding supposed to be trainable in the first place?
* Issues:
* Fails test `TFXLNetModelTest.test_pt_tf_model_equivalence`: `AssertionError: mask_emb not found in PyTorch model`.
Shape error:
```
tensorflow.python.framework.errors_impl.InvalidArgumentError: Incompatible shapes: [128,128,16,12] vs. [128,255,16,12]
[[node tfxl_net_for_sequence_classification/transformer/layer_._0/rel_attn/add_3 (defined at /opt/conda/lib/python3.6/site-packages/tensorflow_core/python/framework/ops.py:1751) ]] [Op:__inference_distributed_function_72170]
```
Do let me know if there are any feedback on the changes I made. | 10-22-2019 02:53:33 | 10-22-2019 02:53:33 | # [Codecov](https://codecov.io/gh/huggingface/transformers/pull/1590?src=pr&el=h1) Report
> Merging [#1590](https://codecov.io/gh/huggingface/transformers/pull/1590?src=pr&el=desc) into [master](https://codecov.io/gh/huggingface/transformers/commit/4d456542e9d381090f9a00b2bcc5a4cb07f6f3f7?src=pr&el=desc) will **not change** coverage.
> The diff coverage is `n/a`.
[](https://codecov.io/gh/huggingface/transformers/pull/1590?src=pr&el=tree)
```diff
@@ Coverage Diff @@
## master #1590 +/- ##
=======================================
Coverage 86.16% 86.16%
=======================================
Files 91 91
Lines 13593 13593
=======================================
Hits 11713 11713
Misses 1880 1880
```
------
[Continue to review full report at Codecov](https://codecov.io/gh/huggingface/transformers/pull/1590?src=pr&el=continue).
> **Legend** - [Click here to learn more](https://docs.codecov.io/docs/codecov-delta)
> `Δ = absolute <relative> (impact)`, `ø = not affected`, `? = missing data`
> Powered by [Codecov](https://codecov.io/gh/huggingface/transformers/pull/1590?src=pr&el=footer). Last update [4d45654...0322842](https://codecov.io/gh/huggingface/transformers/pull/1590?src=pr&el=lastupdated). Read the [comment docs](https://docs.codecov.io/docs/pull-request-comments).
<|||||>Fixing TF Roberta and TF XLNet seem to be much trickier than XLM. **I will open a separate PR for XLM alone since that works fine.**
For TF Roberta and TF XLNet, the solution might be to simply run them eagerly at a rather severe performance penalty. `tf.function` speeds it up a lot but seems to introduce some inconsistency in the weight saving, which might be a TensorFlow issue and I don't yet have the time to investigate.<|||||>@tlkh did you look into the shape errors any further? I'm getting similar errors in eager mode on tf-nightly, didn't try 2.0 (need some other fixes in 2.1)
```
tensorflow.python.framework.errors_impl.InvalidArgumentError: Incompatible shapes: [91,91,64,12] vs. [91,181,64,12]
[[node model/tfxl_net_lm_head_model/transformer/layer_._0/rel_attn/add_2 (defined at .../transformers/modeling_tf_xlnet.py:148) ]] [Op:__inference_distributed_function_17027]
```<|||||>@NathanHowell sorry, I don't have any ideas about that! Seems to be the same error, but oddly running it in eager mode fixed it for me.<|||||>Thanks a lot @tlkh
So I think RoBERTa is now fixed on master (removed the faulty check in the forward pass) and XLM as well (with your other PR).
Do you want to make a new PR with fixes for XLNet and we close the present one maybe?<|||||>@thomwolf thanks, I'll close the current PR and open a new one for XLNet after I validate it again. |
Subsets and Splits
No saved queries yet
Save your SQL queries to embed, download, and access them later. Queries will appear here once saved.