repo
stringclasses 1
value | number
int64 1
25.3k
| state
stringclasses 2
values | title
stringlengths 1
487
| body
stringlengths 0
234k
⌀ | created_at
stringlengths 19
19
| closed_at
stringlengths 19
19
| comments
stringlengths 0
293k
|
---|---|---|---|---|---|---|---|
transformers | 1,890 | closed | Correction for the tuple problem | This fixes the problem described and corrected in https://github.com/huggingface/transformers/issues/831 | 11-20-2019 19:05:27 | 11-20-2019 19:05:27 | # [Codecov](https://codecov.io/gh/huggingface/transformers/pull/1890?src=pr&el=h1) Report
> Merging [#1890](https://codecov.io/gh/huggingface/transformers/pull/1890?src=pr&el=desc) into [xlnet](https://codecov.io/gh/huggingface/transformers/commit/1b35d05d4b3c121a9740544aa6f884f1039780b1?src=pr&el=desc) will **not change** coverage.
> The diff coverage is `n/a`.
[](https://codecov.io/gh/huggingface/transformers/pull/1890?src=pr&el=tree)
```diff
@@ Coverage Diff @@
## xlnet #1890 +/- ##
=====================================
Coverage 78.9% 78.9%
=====================================
Files 34 34
Lines 6181 6181
=====================================
Hits 4877 4877
Misses 1304 1304
```
------
[Continue to review full report at Codecov](https://codecov.io/gh/huggingface/transformers/pull/1890?src=pr&el=continue).
> **Legend** - [Click here to learn more](https://docs.codecov.io/docs/codecov-delta)
> `Δ = absolute <relative> (impact)`, `ø = not affected`, `? = missing data`
> Powered by [Codecov](https://codecov.io/gh/huggingface/transformers/pull/1890?src=pr&el=footer). Last update [1b35d05...5365fbd](https://codecov.io/gh/huggingface/transformers/pull/1890?src=pr&el=lastupdated). Read the [comment docs](https://docs.codecov.io/docs/pull-request-comments).
<|||||>Hi, we don't have finetune_on_pregenerated.py in the examples anymore but a simpler script, `run_lm_finetuning`. Closing for now. |
transformers | 1,889 | closed | explain how to successfully run examples in readme and doc | 11-20-2019 19:00:29 | 11-20-2019 19:00:29 | # [Codecov](https://codecov.io/gh/huggingface/transformers/pull/1889?src=pr&el=h1) Report
> Merging [#1889](https://codecov.io/gh/huggingface/transformers/pull/1889?src=pr&el=desc) into [master](https://codecov.io/gh/huggingface/transformers/commit/454455c695ff38df1ed3670a43677fdd1abcedf3?src=pr&el=desc) will **increase** coverage by `0.02%`.
> The diff coverage is `n/a`.
[](https://codecov.io/gh/huggingface/transformers/pull/1889?src=pr&el=tree)
```diff
@@ Coverage Diff @@
## master #1889 +/- ##
==========================================
+ Coverage 84.05% 84.08% +0.02%
==========================================
Files 97 97
Lines 14316 14316
==========================================
+ Hits 12034 12037 +3
+ Misses 2282 2279 -3
```
| [Impacted Files](https://codecov.io/gh/huggingface/transformers/pull/1889?src=pr&el=tree) | Coverage Δ | |
|---|---|---|
| [transformers/tests/modeling\_tf\_common\_test.py](https://codecov.io/gh/huggingface/transformers/pull/1889/diff?src=pr&el=tree#diff-dHJhbnNmb3JtZXJzL3Rlc3RzL21vZGVsaW5nX3RmX2NvbW1vbl90ZXN0LnB5) | `97.08% <0%> (+1.45%)` | :arrow_up: |
------
[Continue to review full report at Codecov](https://codecov.io/gh/huggingface/transformers/pull/1889?src=pr&el=continue).
> **Legend** - [Click here to learn more](https://docs.codecov.io/docs/codecov-delta)
> `Δ = absolute <relative> (impact)`, `ø = not affected`, `? = missing data`
> Powered by [Codecov](https://codecov.io/gh/huggingface/transformers/pull/1889?src=pr&el=footer). Last update [454455c...5cd8487](https://codecov.io/gh/huggingface/transformers/pull/1889?src=pr&el=lastupdated). Read the [comment docs](https://docs.codecov.io/docs/pull-request-comments).
<|||||>Cool, this is important. I feel like installing from source without cloning (e.g. using `pip install git+https://github.com/huggingface/transformers`) would put less strain on the users' set-up, is there a reason we prefer cloning (I know the aforementioned method also clones, but it puts it in a temporary folder)? This is fine as it is, I'm just curious.<|||||>That way you also cover the case of people who clone the repository to execute the examples (I would believe the majority of users), and encourage this behavior which is a much better practice than copy/pasting.
Doing so you are absolutely sure the version of the library is the exact same as the version of the examples. This may not be true for `pip install git+https://github.com/huggingface/transformers` if the library has since changed.<|||||>> That way you also cover the case of people who clone the repository to execute the examples (I would believe the majority of users), and encourage this behavior which is a much better practice than copy/pasting.
> Doing so you are absolutely sure the version of the library is the exact same as the version of the examples. This may not be true for `pip install git+https://github.com/huggingface/transformers` if the library has since changed.
in fact, passing a branch name, a commit hash, a tag name or a git ref is possible like so:
```
[-e] git://git.example.com/MyProject.git@master#egg=MyProject
[-e] git://git.example.com/[email protected]#egg=MyProject
[-e] git://git.example.com/MyProject.git@da39a3ee5e6b4b0d3255bfef95601890afd80709#egg=MyProject
[-e] git://git.example.com/MyProject.git@refs/pull/123/head#egg=MyProject
```
According to https://pip.pypa.io/en/stable/reference/pip_install/#git <|||||>@LysandreJik Maybe we can list both options i.e. also reference `pip install git+https://github.com/huggingface/transformers`
Also small nitpick, don't hesitate to squash commits when merging very related changes.<|||||>Fair nitpick |
|
transformers | 1,888 | closed | Is there a straightforward way to classify documents at the sentence level, while using surrounding sentences for context? | ## ❓ Questions & Help
I'm curious how one would go about classifying documents at the sentence level using the Sequence Classification classes where the classification of a document's sentence would use other sentences in the document for context.
For example, if a document says, "I truly hate this product. Thanks [company name]!" The two sentences should be classified as negative. However, if you classify each sentence separately, "I truly hate this product" would be classified as negative, while "Thanks [company name]!" would likely be classified as positive.
I understand I could classify the document as a whole, but I'm looking for a more granular level of text classification.
Any guidance on making adjustments for this need would be greatly appreciated.
Thanks!
| 11-20-2019 17:01:37 | 11-20-2019 17:01:37 | Maybe you should start with [XLNet](https://arxiv.org/abs/1906.08237).<|||||>@iedmrc Thanks, I'm sure that most of these models could do this. I'm looking for guidance on adjusting the architecture of these transformer models to look for context form surrounding sentences to classify the current sentence. XLNetForSequenceClassification takes in a document as a whole for classification.
I imagine many others have confronted this problem, so I'm reaching out for guidance on where to start.<|||||>@pydn You are probably looking for a model like this: https://github.com/allenai/sequential_sentence_classification<|||||>@armancohan Thank you! This is exactly what I was looking for. |
transformers | 1,887 | closed | Using GPU for gpt2-xl | I want to use gpt2-xl using my PC's GPU (NVIDIA GeForce GTX 1070). The usual way to do this is via conda, but it appears that the latest version of gpt-2 is not available for conda. For example, the following installation commands don't work:
```
conda install transformers
conda install git+https://github.com/huggingface/transformers
```
The latest version of transformers for conda is a[ month old,](https://anaconda.org/conda-forge/transformers) and therefore doesn't include gpt2-xl. How can I use my machine's GPU to run gpt2-xl without using conda? Will there be conda support soon?
| 11-20-2019 16:44:04 | 11-20-2019 16:44:04 | We have not released a new Pypi/conda version yet, but will do so in the next few days. You can install from source if you want to use GPT2-xl before that!<|||||>Via docker:
https://github.com/huggingface/transformers/blob/master/docker/Dockerfile
Via pip:
https://github.com/huggingface/transformers/blob/master/docker/Dockerfile
or
https://github.com/huggingface/transformers/issues/1837#issuecomment-554594306 |
transformers | 1,886 | closed | save_pretrained on CamembertTokenizer | ## 🐛 Bug
This is probably something you already know, but `save_pretrained` for `CamembertTokenizer` seems not working at the moment.
Here is the error message I get:
```
---------------------------------------------------------------------------
OSError Traceback (most recent call last)
<ipython-input-38-52393f32f8a0> in <module>
1 results = {}
2 if args.do_eval and args.local_rank in [-1, 0]:
----> 3 tokenizer = tokenizer_class.from_pretrained(args.output_dir, do_lower_case=args.do_lower_case)
4 checkpoints = [args.output_dir]
5 if args.eval_all_checkpoints:
/mnt/azmnt/code/Users/adm/transformers/transformers/tokenization_utils.py in from_pretrained(cls, *inputs, **kwargs)
281
282 """
--> 283 return cls._from_pretrained(*inputs, **kwargs)
284
285
/mnt/azmnt/code/Users/adm/transformers/transformers/tokenization_utils.py in _from_pretrained(cls, pretrained_model_name_or_path, *init_inputs, **kwargs)
410
411 # Instantiate tokenizer.
--> 412 tokenizer = cls(*init_inputs, **init_kwargs)
413
414 # Save inputs and kwargs for saving and re-loading with ``save_pretrained``
/mnt/azmnt/code/Users/adm/transformers/transformers/tokenization_camembert.py in __init__(self, vocab_file, bos_token, eos_token, sep_token, cls_token, unk_token, pad_token, mask_token, additional_special_tokens, **kwargs)
55 self.max_len_sentences_pair = self.max_len - 4 # take into account special tokens
56 self.sp_model = spm.SentencePieceProcessor()
---> 57 self.sp_model.Load(str(vocab_file))
58 # HACK: These tokens were added by fairseq but don't seem to be actually used when duplicated in the actual
59 # sentencepiece vocabulary (this is the case for <s> and </s>
/anaconda/envs/azureml_py36/lib/python3.6/site-packages/sentencepiece.py in Load(self, filename)
116
117 def Load(self, filename):
--> 118 return _sentencepiece.SentencePieceProcessor_Load(self, filename)
119
120 def LoadOrDie(self, filename):
OSError: Not found: "None": No such file or directory Error #2
```
Best | 11-20-2019 15:19:29 | 11-20-2019 15:19:29 | This should be fixed in #1860 which should be merged shortly |
transformers | 1,885 | closed | GPT2 Tokenizer Special Token ID Bug | ## 🐛 Bug
<!-- Important information -->
Model I am using (Bert, XLNet....): GPT2 Tokenizer
The problem arise when using:
my own modified script: The problem arises when I try to add special tokens to the GPT2 tokenizer, specifically a pad token and a sep token.
The tasks I am working on is:
* [ ] my own task or dataset: summarization on the xsum dataset, however, the current bug does not actually affect the model, but the pre-processing.
## To Reproduce
<!-- If you have a code sample, error messages, stack traces, please provide it here as well. -->
from transformers import AutoTokenizer
encoder = AutoTokenizer.from_pretrained('gpt2')
encoder.add_special_tokens({'pad_token': '[PAD]', 'sep_token': '[SEP]'})
print(encoder.sep_token, encoder.sep_token_id)
print(encoder.pad_token, encoder.pad_token_id)
print('All special tokens:', encoder.all_special_tokens)
print('All special ids:', encoder.all_special_ids)
## Expected behavior
The expected output of this script is below:
[SEP] 50258
[PAD] 50257
All special tokens: ['<|endoftext|>', '[PAD]', '[SEP]']
All special ids: [50256, 50257, 50258]
The actual output is:
[SEP] 50258
[PAD] 50257
All special tokens: ['<|endoftext|>', '[PAD]', '[SEP]']
All special ids: [50256, 50256, 50256]
As you can see, All special IDS do not match the actual ids of the [SEP] and [PAD] token. Not sure why this is the case. Am I misunderstanding something about how add_special_tokens works?
## Environment
* OS: Ubuntu 18.04
* Python version: 3.7
* PyTorch version: 1.0.0
* PyTorch Transformers version (or branch): 2.1.0
* Using GPU ? No
* Distributed of parallel setup ? No
* Any other relevant information: None
## Additional context
<!-- Add any other context about the problem here. -->
| 11-20-2019 14:52:08 | 11-20-2019 14:52:08 | Hi, there have been a few fixes done on the tokenizers recently that haven't been released on Pypi yet. When I run your script with the library installed from source, I obtain:
```py
[SEP] 50258
[PAD] 50257
All special tokens: ['[PAD]', '[SEP]', '<|endoftext|>']
All special ids: [50257, 50258, 50256]
```
Would you mind installing from source using `pip install git+https://github.com/huggingface/transformers` and let me know if it fixes your problem,?<|||||>That fixed it, thank you! |
transformers | 1,884 | closed | Wrong definition of the `logging_steps` parameter at the `run_lm_finetuning.py` | Hi,
`logging_steps` parameter defined as `"Log every X updates steps."` but it's been affected by `gradient_accumulation_steps` as follows:
https://github.com/huggingface/transformers/blob/f3386d938348628c91457fc7d8650c223317a053/examples/run_lm_finetuning.py#L243-L254
Therefore, if you, e.g., set logging_steps=1000 and gradient_accumulation_steps=5, it'll log in every 5000 steps. That affects `evaluate_during_training` in a not intended way. | 11-20-2019 14:23:19 | 11-20-2019 14:23:19 | This issue has been automatically marked as stale because it has not had recent activity. It will be closed if no further activity occurs. Thank you for your contributions.
|
transformers | 1,883 | closed | F score 0 in combining RoBERTa and BiLSTM | ## ❓ Questions & Help
<!-- A clear and concise description of the question. -->
I am trying to stack an LSTM on top of RoBERTa model for binary classification problem
I tried two moods , freezing BERT embedding and Fine-tuning
In case of freezing the embedding I get around 57% F-score compared to regular fine tune BERT which got me 81%
When I tried to unfreeze the embedding the f score is always 0 ,
Most probably I am doing something wrong, but I can't spot it I would appreciate some help
Model part
```
class RoBERTaLSTMClassifier(nn.Module):
def __init__(self, bert_config, num_classes, hidden_size=None, dropout=0.5):
"""
bert: pretrained bert model
num_classes: the number of num_classes
hidden_size: the number of hiddens which will be used by LSTM layer
dropout: dropout rate
"""
super(RoBERTaLSTMClassifier, self).__init__()
self.num_classes = num_classes
self.model = RobertaModel(bert_config)
if hidden_size is None: self.hidden_size = bert_config.hidden_size
else: self.hidden_size = hidden_size
self.lstm = nn.LSTM(bert_config.hidden_size, self.hidden_size, bidirectional=True,batch_first=True)
self.dropout = nn.Dropout(dropout)
self.classifier = nn.Linear(self.hidden_size * 2, 1)
self.softmax = nn.Softmax()
## add sigmoid non linearity for binary classification
self.sig = nn.Sigmoid()
def forward(self, input_ids, attention_mask, current_batch_size, hidden):
"""
all_layers: whether or not to return all encoded_layers
return: logits in the following format (batch_size, num_classes)
"""
#with torch.no_grad():
## freeze embedding from BERT
outputs = self.model(input_ids=input_ids, attention_mask=attention_mask)
# last hidden state is input to the LSTM
output, (hidden_h, hidden_c) = self.lstm(outputs[0], hidden)
output_hidden = torch.cat((hidden_h[0], hidden_h[1]), dim=1) #[B, H*2]
logits = self.classifier(self.dropout(output_hidden)) #[B, C]
sig_out = self.sig(logits).view(current_batch_size, -1)
## get the last batch output
sig_out = sig_out[:, -1] # get last batch of labels
hidden = (hidden_h, hidden_c)
return sig_out, hidden
def init_bilstm_hidden(self, batch_size):
h0 = torch.zeros(2, batch_size, self.hidden_size).to(device) # 2 for bidirection
c0 = torch.zeros(2, batch_size, self.hidden_size).to(device)
return (h0, c0)
```
The training loop part
```
from sklearn.metrics import f1_score
from tqdm import tqdm, trange
import numpy as np
lr=0.001
roberta_conf = RobertaConfig.from_pretrained('roberta-base')
num_classes = 2
hidden_size = 256
LSTMRoBERTaModel = RoBERTaLSTMClassifier(roberta_conf, num_classes=num_classes,hidden_size= hidden_size,dropout=0.5)
criterion = nn.BCELoss() ## binary cross entropy
optimizer = torch.optim.Adam(LSTMRoBERTaModel.parameters(), lr=lr)
epochs = 5
counter = 0
max_grad_norm = 1.0
nb_tr_examples, nb_tr_steps = 0, 0
for _ in trange(epochs, desc="Epoch"):
LSTMRoBERTaModel.cuda()
LSTMRoBERTaModel.train()
tr_loss = 0
y_preds = []
y_true = []
hidden_init = LSTMRoBERTaModel.init_bilstm_hidden(batch_size=bs)
h = hidden_init
for step, batch in enumerate(train_dataloader):
batch = tuple(t.to(device) for t in batch)
b_input_ids, b_input_mask, b_labels = batch
current_batch_size = b_input_ids.size()[0]
##
## need to ask why converting to tuple
h = tuple([each.data for each in h])
## forward pass
preds, h = LSTMRoBERTaModel.forward(b_input_ids, b_input_mask, current_batch_size,h)
loss = criterion(preds.squeeze(),b_labels.float())
# track train loss
tr_loss += loss.item()
nb_tr_examples += b_input_ids.size(0)
nb_tr_steps += 1
# gradient clipping
torch.nn.utils.clip_grad_norm_(parameters=LSTMRoBERTaModel.parameters(), max_norm=max_grad_norm)
loss.backward()
optimizer.step()
LSTMRoBERTaModel.zero_grad()
# print train loss per epoch
print("\nTrain loss: {}".format(tr_loss/nb_tr_steps))
LSTMRoBERTaModel.eval()
eval_loss, eval_accuracy = 0, 0
nb_eval_steps, nb_eval_examples = 0, 0
val_h = LSTMRoBERTaModel.init_bilstm_hidden(bs)
for batch in dev_dataloader:
batch = tuple(t.to(device) for t in batch)
b_input_ids, b_input_mask, b_labels = batch
current_batch_size = b_input_ids.size()[0]
with torch.no_grad():
preds, val_h = LSTMRoBERTaModel.forward(b_input_ids, b_input_mask, current_batch_size, val_h)
loss = criterion(preds.squeeze(),b_labels.float())
eval_loss += loss
y_preds.extend(np.round(preds.data.cpu()))
y_true.extend(b_labels.data.cpu())
#print(preds[2], b_labels[2] )
#eval_accuracy += f1_score(torch.tensor.numpy(b_labels.float), toch.tensor.numpy(preds))
nb_eval_examples += b_input_ids.size(0)
nb_eval_steps += 1
eval_loss = eval_loss/nb_eval_steps
print("Validation loss: {}".format(eval_loss))
print("F1 - Score: {}".format(f1_score(y_true,y_preds)))
#print("F1- Score: {}".format(eval_accuracy/nb_eval_steps))
```
| 11-20-2019 12:03:02 | 11-20-2019 12:03:02 | I got the same situation. However, when I changed the learning rate to 1e-5, the problem was solved.<|||||>This issue has been automatically marked as stale because it has not had recent activity. It will be closed if no further activity occurs. Thank you for your contributions.
|
transformers | 1,882 | closed | XLNetForSequenceClassification and CLS token | ## ❓ Questions & Help
<!-- A clear and concise description of the question. -->
Hi,
As we can see in the official XLNet code ([https://github.com/zihangdai/xlnet]()), the [SEP] and [CLS] tokens are added before the zero-padding. (in convert_single_sequence() in classifier_utils.py)
(example : "This is my sequence ! [SEP][CLS]")
What is strange is that in the XLNetModelForSequenceClassification, we use a SequenceSummary which will keep the last value from the hidden state, i.e. the last token from padding (which doesn't correspond to the real [CLS] token)? (As I can see, it's working like that in the official code too).
Maybe someone here can explain me if this is an error, or if I forgot something ? | 11-20-2019 10:23:25 | 11-20-2019 10:23:25 | Ok, I found why : the padding is done before the sentence, and not after. |
transformers | 1,881 | closed | convert list to set in tokenize().split_on_tokens() | As [issue 1830](https://github.com/huggingface/transformers/issues/1830), I meet the same question
when i add some special_tokens in Tokenizer.
But I think it is property **self.all_special_tokens** that slow the code.
property self.all_special_tokens will **be called so many time** when we added some special token.
An easy way to solve this problem is to create a temporary Set.
In my implementation, it faster about 10 times when 207 special tokens are added, I do not get a precise number because of multiprocessing : ) | 11-20-2019 07:08:40 | 11-20-2019 07:08:40 | # [Codecov](https://codecov.io/gh/huggingface/transformers/pull/1881?src=pr&el=h1) Report
> Merging [#1881](https://codecov.io/gh/huggingface/transformers/pull/1881?src=pr&el=desc) into [master](https://codecov.io/gh/huggingface/transformers/commit/f3386d938348628c91457fc7d8650c223317a053?src=pr&el=desc) will **increase** coverage by `1.35%`.
> The diff coverage is `100%`.
[](https://codecov.io/gh/huggingface/transformers/pull/1881?src=pr&el=tree)
```diff
@@ Coverage Diff @@
## master #1881 +/- ##
==========================================
+ Coverage 82.72% 84.08% +1.35%
==========================================
Files 97 97
Lines 14316 14316
==========================================
+ Hits 11843 12037 +194
+ Misses 2473 2279 -194
```
| [Impacted Files](https://codecov.io/gh/huggingface/transformers/pull/1881?src=pr&el=tree) | Coverage Δ | |
|---|---|---|
| [transformers/tokenization\_utils.py](https://codecov.io/gh/huggingface/transformers/pull/1881/diff?src=pr&el=tree#diff-dHJhbnNmb3JtZXJzL3Rva2VuaXphdGlvbl91dGlscy5weQ==) | `92.14% <100%> (ø)` | :arrow_up: |
| [transformers/modeling\_openai.py](https://codecov.io/gh/huggingface/transformers/pull/1881/diff?src=pr&el=tree#diff-dHJhbnNmb3JtZXJzL21vZGVsaW5nX29wZW5haS5weQ==) | `82% <0%> (+1.33%)` | :arrow_up: |
| [transformers/modeling\_ctrl.py](https://codecov.io/gh/huggingface/transformers/pull/1881/diff?src=pr&el=tree#diff-dHJhbnNmb3JtZXJzL21vZGVsaW5nX2N0cmwucHk=) | `96.46% <0%> (+2.21%)` | :arrow_up: |
| [transformers/modeling\_xlnet.py](https://codecov.io/gh/huggingface/transformers/pull/1881/diff?src=pr&el=tree#diff-dHJhbnNmb3JtZXJzL21vZGVsaW5nX3hsbmV0LnB5) | `73.61% <0%> (+2.43%)` | :arrow_up: |
| [transformers/modeling\_roberta.py](https://codecov.io/gh/huggingface/transformers/pull/1881/diff?src=pr&el=tree#diff-dHJhbnNmb3JtZXJzL21vZGVsaW5nX3JvYmVydGEucHk=) | `71.76% <0%> (+12.35%)` | :arrow_up: |
| [transformers/tests/modeling\_tf\_common\_test.py](https://codecov.io/gh/huggingface/transformers/pull/1881/diff?src=pr&el=tree#diff-dHJhbnNmb3JtZXJzL3Rlc3RzL21vZGVsaW5nX3RmX2NvbW1vbl90ZXN0LnB5) | `97.08% <0%> (+15.53%)` | :arrow_up: |
| [transformers/modeling\_tf\_pytorch\_utils.py](https://codecov.io/gh/huggingface/transformers/pull/1881/diff?src=pr&el=tree#diff-dHJhbnNmb3JtZXJzL21vZGVsaW5nX3RmX3B5dG9yY2hfdXRpbHMucHk=) | `92.95% <0%> (+83.09%)` | :arrow_up: |
------
[Continue to review full report at Codecov](https://codecov.io/gh/huggingface/transformers/pull/1881?src=pr&el=continue).
> **Legend** - [Click here to learn more](https://docs.codecov.io/docs/codecov-delta)
> `Δ = absolute <relative> (impact)`, `ø = not affected`, `? = missing data`
> Powered by [Codecov](https://codecov.io/gh/huggingface/transformers/pull/1881?src=pr&el=footer). Last update [f3386d9...821ba9e](https://codecov.io/gh/huggingface/transformers/pull/1881?src=pr&el=lastupdated). Read the [comment docs](https://docs.codecov.io/docs/pull-request-comments).
<|||||>Hi, thanks for looking into it! What's your use-case for adding 207 special tokens?<|||||>In the kaggle Tensorflow 2 Nature question competition. I try to add some additional Sequence Embedding Such as [Tableid=13] and split short sentence. <|||||>I may misunderstand, but why not use the `add_tokens` method rather than the `add_special_tokens` method, which is reserved for tokens like CLS or MASK?<|||||>Yes, `add_special_tokens` method is reserved for a limited number of tokens with special properties and usage like CLS or MASK. For other uses, go for `add_tokens`.
<|||||>Here is how we solved the performance issue when adding custom vocabulary: In the `add_tokens` method, we simply integrate `new_tokens` into the `self.vocab`.
```
from transformers import BertTokenizer, WordpieceTokenizer
from collections import OrderedDict
class CustomVocabBertTokenizer(BertTokenizer):
def add_tokens(self, new_tokens):
new_tokens = [token for token in tokens if not (token in self.vocab or token in self.all_special_tokens)]
self.vocab = OrderedDict([
*self.vocab.items(),
*[
(token, i + len(self.vocab))
for i, token in enumerate(new_tokens)
]
])
self.ids_to_tokens = OrderedDict([(ids, tok) for tok, ids in self.vocab.items()])
self.wordpiece_tokenizer = WordpieceTokenizer(vocab=self.vocab, unk_token=self.unk_token)
return len(new_tokens)
``` |
transformers | 1,880 | closed | question about 'add_prefix_space' of encode method | ## ❓ Questions & Help
<!-- A clear and concise description of the question. -->
Hi, I have a question about the 'add_prefix_space' parameter of encode method. I'll use small gpt2 for example.
> tokenizer = GPT2Tokenizer.from_pretrained('gpt2')
> print(tokenizer.encode("my",add_prefix_space=True)) #616
> print(tokenizer.encode("my",add_prefix_space=False)) #1820
I think 616 is the index for the word 'my'(' my') since there's a space before letter 'm'.
And 1820 is the index for '-me' which is part of a word. Am I right?
Thank you! | 11-20-2019 02:27:01 | 11-20-2019 02:27:01 | Actually, both 616 and 1820 are indices for `my`. The difference lies in the prefix space that was added: in the case that it was not added (1820), it is identified as being the beginning of the sentence or part of a word.
In the case that it was added (616), it is identified as being the beginning of a word in a sentence.
You can check the behavior by calling `tokenize` instead of `encode`, for example:
```py
tokenizer = GPT2Tokenizer.from_pretrained('gpt2')
print(tokenizer.tokenize("my son is jeremy",add_prefix_space=True))
# ['Ġmy', 'Ġson', 'Ġis', 'Ġj', 'ere', 'my']
print(tokenizer.tokenize("my son is my son",add_prefix_space=False))
# ['my', 'Ġson', 'Ġis', 'Ġmy', 'Ġson']
```<|||||>Thank you! <|||||>still don't understand what is the difference in
```
tokenizer = tokenizers.ByteLevelBPETokenizer(
vocab_file=PATH+'vocab-roberta-base.json',
merges_file=PATH+'merges-roberta-base.txt',
lowercase=True,
add_prefix_space=True
)
```<|||||>The argument you mention is for the initialization of a tokenizer from the `huggingface/tokenizers` library, you would have better luck opening an issue there. |
transformers | 1,879 | closed | run_squad hangs for small max_seq_length | ## 🐛 Bug
I am experimenting with minimal examples to understand how sequences are generated, setting `max_seq_length` very low to trigger document chunking, and I hit this bug:
When `max_seq_length` is smaller than the query, `max_tokens_for_doc` becomes negative causing an infinite loop.
https://github.com/huggingface/transformers/blob/f3386d938348628c91457fc7d8650c223317a053/examples/utils_squad.py#L241-L258
Obviously having `max_seq_length` smaller than the query isn't very useful, and I should be setting `max_query_length`, But it would still be nice to have an assertion catch this.
### Steps to reproduce
1. `rm $SQUAD_DIR/cached*`
2. train with `--max_seq_length 16`
```
python examples/run_squad.py \
--model_type bert \
--model_name_or_path bert-base-uncased \
--do_lower_case \
--do_train \
--train_file $SQUAD_DIR/train-v1.1.json \
--predict_file $SQUAD_DIR/dev-v1.1.json \
--per_gpu_train_batch_size 12 \
--learning_rate 3e-5 \
--num_train_epochs 2.0 \
--max_seq_length 16 \
--doc_stride 8 \
--output_dir /tmp/squad_seq_len_bug
```
| 11-20-2019 01:45:41 | 11-20-2019 01:45:41 | Great, thanks for letting us know! |
transformers | 1,878 | closed | Is this a bug? | Hello,
I tried to import this:
`from transformers import AdamW, get_linear_schedule_with_warmup`
but got error : model not found
but when i did this, it worked:
```
from transformers import AdamW
from transformers import WarmupLinearSchedule as get_linear_schedule_with_warmup
```
however when I set the scheduler like this :
```
scheduler = get_linear_schedule_with_warmup(optimizer, num_warmup_steps=args.warmup_steps, num_training_steps=t_total)
```
I got this error :
```
__init__() got an unexpected keyword argument 'num_warmup_steps'
``` | 11-20-2019 00:27:57 | 11-20-2019 00:27:57 | duplicated
https://github.com/huggingface/transformers/issues/1837#issue-523145270<|||||>I checked the other comment but did not work. Any idea ?
```
__init__() got an unexpected keyword argument 'num_warmup_steps'
```
<|||||>Hi, could you specify which versions of Python and Transformers is your environment running on?<|||||>Thanks
```
python: 3.6.8
Transformers: 2.1.1
```
<|||||>Did you install transformers from source or from pypi?<|||||>Could you try installing from source and telling me if it fixes your problem?<|||||>Thanks it worked<|||||>worked with building from the source and this
```
from transformers import AdamW,get_linear_schedule_with_warmup
```<|||||>Great to hear!<|||||>whats the meaning of installing from source ,i am a little bit confused. could you please tell me ,thank you.
i've tried 'pip install git+https://github.com/huggingface/transformers' or pip install transformers
or pip install pytorhc-transformers. But they all doesn't work.<|||||>> Hello,
> I tried to import this:
>
> `from transformers import AdamW, get_linear_schedule_with_warmup`
> but got error : model not found
> but when i did this, it worked:
>
> ```
> from transformers import AdamW
> from transformers import WarmupLinearSchedule as get_linear_schedule_with_warmup
> ```
>
> however when I set the scheduler like this :
>
> ```
> scheduler = get_linear_schedule_with_warmup(optimizer, num_warmup_steps=args.warmup_steps, num_training_steps=t_total)
> ```
>
> I got this error :
>
> ```
> __init__() got an unexpected keyword argument 'num_warmup_steps'
> ```
can you kindly tell me how to install from source.<|||||>@TLCFYBJJHYYSND See https://github.com/huggingface/transformers#from-source<|||||>In order to install Transformers library, you have to open your command line and enter:
```
pip install git+https://github.com/huggingface/transformers.git
```
> > Hello,
> > I tried to import this:
> > `from transformers import AdamW, get_linear_schedule_with_warmup`
> > but got error : model not found
> > but when i did this, it worked:
> > ```
> > from transformers import AdamW
> > from transformers import WarmupLinearSchedule as get_linear_schedule_with_warmup
> > ```
> >
> >
> > however when I set the scheduler like this :
> > ```
> > scheduler = get_linear_schedule_with_warmup(optimizer, num_warmup_steps=args.warmup_steps, num_training_steps=t_total)
> > ```
> >
> >
> > I got this error :
> > ```
> > __init__() got an unexpected keyword argument 'num_warmup_steps'
> > ```
>
> can you kindly tell me how to install from source.<|||||>Thanks for that, it really works!!! |
transformers | 1,877 | closed | Can the HuggingFace GPT2DoubleHeadsModel either for regular language modelling or solving multiple-choice questions? or is it only for solving multiple-choice questions? | Hello,
According to the HuggingFace Transformer's website (https://huggingface.co/transformers/model_doc/gpt2.html#gpt2doubleheadsmodel), GPT2DoubleHeadsModel is the GPT2 Model transformer with a language modelling and a multiple-choice classification head on top e.g. for RocStories/SWAG tasks.
Does this mean that the GPT2DoubleHeadsModel can be used for both the regular language modelling tasks (predicting the next token) and also for solving multiple-choice questions?
Or does this mean that GPT2DoubleHeadsModel can be used to test machines on the multiple-choice type questions only?
Thank you,
| 11-19-2019 23:36:58 | 11-19-2019 23:36:58 | |
transformers | 1,876 | closed | Mean does not exist in TF2 | 11-19-2019 23:15:25 | 11-19-2019 23:15:25 | # [Codecov](https://codecov.io/gh/huggingface/transformers/pull/1876?src=pr&el=h1) Report
> Merging [#1876](https://codecov.io/gh/huggingface/transformers/pull/1876?src=pr&el=desc) into [master](https://codecov.io/gh/huggingface/transformers/commit/f3386d938348628c91457fc7d8650c223317a053?src=pr&el=desc) will **increase** coverage by `1.35%`.
> The diff coverage is `0%`.
[](https://codecov.io/gh/huggingface/transformers/pull/1876?src=pr&el=tree)
```diff
@@ Coverage Diff @@
## master #1876 +/- ##
==========================================
+ Coverage 82.72% 84.08% +1.35%
==========================================
Files 97 97
Lines 14316 14316
==========================================
+ Hits 11843 12037 +194
+ Misses 2473 2279 -194
```
| [Impacted Files](https://codecov.io/gh/huggingface/transformers/pull/1876?src=pr&el=tree) | Coverage Δ | |
|---|---|---|
| [transformers/modeling\_tf\_utils.py](https://codecov.io/gh/huggingface/transformers/pull/1876/diff?src=pr&el=tree#diff-dHJhbnNmb3JtZXJzL21vZGVsaW5nX3RmX3V0aWxzLnB5) | `92.68% <0%> (ø)` | :arrow_up: |
| [transformers/modeling\_openai.py](https://codecov.io/gh/huggingface/transformers/pull/1876/diff?src=pr&el=tree#diff-dHJhbnNmb3JtZXJzL21vZGVsaW5nX29wZW5haS5weQ==) | `82% <0%> (+1.33%)` | :arrow_up: |
| [transformers/modeling\_ctrl.py](https://codecov.io/gh/huggingface/transformers/pull/1876/diff?src=pr&el=tree#diff-dHJhbnNmb3JtZXJzL21vZGVsaW5nX2N0cmwucHk=) | `96.46% <0%> (+2.21%)` | :arrow_up: |
| [transformers/modeling\_xlnet.py](https://codecov.io/gh/huggingface/transformers/pull/1876/diff?src=pr&el=tree#diff-dHJhbnNmb3JtZXJzL21vZGVsaW5nX3hsbmV0LnB5) | `73.61% <0%> (+2.43%)` | :arrow_up: |
| [transformers/modeling\_roberta.py](https://codecov.io/gh/huggingface/transformers/pull/1876/diff?src=pr&el=tree#diff-dHJhbnNmb3JtZXJzL21vZGVsaW5nX3JvYmVydGEucHk=) | `71.76% <0%> (+12.35%)` | :arrow_up: |
| [transformers/tests/modeling\_tf\_common\_test.py](https://codecov.io/gh/huggingface/transformers/pull/1876/diff?src=pr&el=tree#diff-dHJhbnNmb3JtZXJzL3Rlc3RzL21vZGVsaW5nX3RmX2NvbW1vbl90ZXN0LnB5) | `97.08% <0%> (+15.53%)` | :arrow_up: |
| [transformers/modeling\_tf\_pytorch\_utils.py](https://codecov.io/gh/huggingface/transformers/pull/1876/diff?src=pr&el=tree#diff-dHJhbnNmb3JtZXJzL21vZGVsaW5nX3RmX3B5dG9yY2hfdXRpbHMucHk=) | `92.95% <0%> (+83.09%)` | :arrow_up: |
------
[Continue to review full report at Codecov](https://codecov.io/gh/huggingface/transformers/pull/1876?src=pr&el=continue).
> **Legend** - [Click here to learn more](https://docs.codecov.io/docs/codecov-delta)
> `Δ = absolute <relative> (impact)`, `ø = not affected`, `? = missing data`
> Powered by [Codecov](https://codecov.io/gh/huggingface/transformers/pull/1876?src=pr&el=footer). Last update [f3386d9...3de31f8](https://codecov.io/gh/huggingface/transformers/pull/1876?src=pr&el=lastupdated). Read the [comment docs](https://docs.codecov.io/docs/pull-request-comments).
<|||||>Thanks a lot @LysandreJik! |
|
transformers | 1,875 | closed | Clarifications about the Quick-tour of the fine-tuning scripts? | ## ❓ Questions & Help
The [quick tour](https://github.com/huggingface/transformers#quick-tour-of-the-fine-tuningusage-scripts) mentions an example of fine-tuning on different GLUE tasks.
My task/trained model has nothing to do with GLUE tasks, i.e.: I already have a pre-trained pytorch model file and the formatted corpus files (train.tsv, dev.tsv and test.tsv).
Is the run_glue.py script meant to be edited by me for doing a new *NON GLUE* fine-tuning?
Or it is a matter of adjusting parameters on the CLI?
If none of them applies, where I can find an example of fine-tuning with support for "customized" task?
| 11-19-2019 22:56:11 | 11-19-2019 22:56:11 | Hi, what exactly is your task? Is it question answering, sequence classification, language modeling, other?<|||||>> Hi, what exactly is your task? Is it question answering, sequence classification, language modeling, other?
Hi LysandreJik
I have two tasks: First one is sequence classification. Second one is Relation Extraction.
<|||||>For sequence classification, you can indeed take inspiration from the script `run_glue.py`. Those scripts are meant as examples showcasing how to manage models for different tasks, so that users may train our models in any way they see fit. The models are standard PyTorch models so they can be trained like any other model.
For relation extraction, there are no models specifically targeting this task but feel free to adapt a standard model by adding a few layers on top. For training there are examples in our [HMTL repository](https://github.com/huggingface/hmtl) which may be of help. |
transformers | 1,874 | closed | Disparitry with Fairseq Roberta implementation for predicting the mask token | ## ❓ Questions & Help
When experimenting with the fill mask functionality on the fairseq repo I realized there is a disparity with the results I get from huggingface implementation. Wondering if there is a mismatch between the model released here and their latest release.
Thanks | 11-19-2019 20:49:34 | 11-19-2019 20:49:34 | We would need more information<|||||>This issue has been automatically marked as stale because it has not had recent activity. It will be closed if no further activity occurs. Thank you for your contributions.
<|||||>Also cc @joeddav <|||||>Ok, I've reproduced an issue here.
```python
sentence = ' My favorite type of cheese is <mask>!'
roberta_fairseq = torch.hub.load('pytorch/fairseq', 'roberta.base')
roberta_hf = pipeline('fill-mask', model='roberta-base')
roberta_fairseq.fill_mask(sentence)
"""[(' My favorite type of cheese is goat!', 0.10264863073825836, ' goat'),
(' My favorite type of cheese is cream!', 0.07233648002147675, ' cream'),
(' My favorite type of cheese is broccoli!', 0.057516228407621384,' broccoli'),
(' My favorite type of cheese is bacon!', 0.037444233894348145, ' bacon'),
(' My favorite type of cheese is ham!', 0.03281955048441887, ' ham')]"""
roberta_hf(sentence)
"""[{'sequence': '<s> My favorite type of cheese is goat!</s>',
'score': 0.09398240596055984,
'token': 24791},
{'sequence': '<s> My favorite type of cheese is cream!</s>',
'score': 0.07240654528141022,
'token': 6353},
{'sequence': '<s> My favorite type of cheese is broccoli!</s>',
'score': 0.06303773820400238,
'token': 34803},
{'sequence': '<s> My favorite type of cheese is bacon!</s>',
'score': 0.04124978929758072,
'token': 18599},
{'sequence': '<s> My favorite type of cheese is jack!</s>',
'score': 0.03125162795186043,
'token': 10267}]"""
```
They don't align when I use roberta large either. cc @sshleifer.<|||||>Probably want to compare the encoded input IDs. If they are the same, then there is a model difference (which is a big deal). Otherwise it can be attributed to a different tokenisation (e.g. difference in spaces or difference in adding special tokens).<|||||>The input IDs do match (again, when an empty space is prepended in fairseq's case): `[ 0, 1308, 2674, 1907, 9, 7134, 16, 50264, 328, 2]`.
I doesn't look like a pipelines issue:
```python
tokenizer = RobertaTokenizer.from_pretrained('roberta-base')
roberta_hf = RobertaForMaskedLM.from_pretrained('roberta-base')
roberta_fairseq = torch.hub.load('pytorch/fairseq', 'roberta.base')
roberta_fairseq.model.eval()
sequence = 'My favorite type of cheese is gouda!'
tokens = torch.tensor([tokenizer.encode(sequence)])
hf_out = roberta_hf.forward(tokens)[0]
fairseq_out = roberta_fairseq.model.forward(tokens)[0]
torch.mean(torch.abs(fairseq_out - hf_out)).item()
# 0.053489409387111664
```
Masked LM outputs differ by an average of `0.053`.<|||||>I don't have any time to look into this further, but from looking at the named parameters of both implementations, it seems that there is a difference in the LM head, that is, everything that comes after the encode (embedding + 12 layers). I can't pinpoint it exactly, but it seems note-worthy that the weight LM head in fairseq, comes from the token embedding weights
https://github.com/pytorch/fairseq/blob/4923f34790761f41170fd88cd06e4d00ab0c527c/fairseq/models/roberta/model.py#L286-L291
I am not sure in how far that is the same in transformers, but it seemed peculiar. Particularly, it seems that `transformers` does the 'None' case here, whereas it should actually take the token embedding weights.
https://github.com/pytorch/fairseq/blob/4923f34790761f41170fd88cd06e4d00ab0c527c/fairseq/models/roberta/model.py#L211-L213
I might be completely wrong, though. Other input welcome.
**Tl;dr**
Transformers does this
```python
self.decoder = nn.Linear(config.hidden_size, config.vocab_size, bias=False)
```
whereas fairseq does
```python
self.lm_head = RobertaLMHead(
embed_dim=args.encoder_embed_dim,
output_dim=len(dictionary),
activation_fn=args.activation_fn,
weight=self.sentence_encoder.embed_tokens.weight,
)
...
# `weight` is **not** None
if weight is None:
weight = nn.Linear(embed_dim, output_dim, bias=False).weight
self.weight = weight
```
I'd be very interested to know _why_ fairseq does this. Why would you want specifically those weights to be the same? <|||||>I implemented my findings in https://github.com/huggingface/transformers/pull/2928.
The following snippet (based on the problem stated above) runs as expected, with identical results for both `transformers` and `fairseq`.
```python
import torch
from transformers import RobertaTokenizer, RobertaForMaskedLM, pipeline
# init HuggingFace
tokenizer_hf = RobertaTokenizer.from_pretrained('roberta-base')
roberta_hf = RobertaForMaskedLM.from_pretrained('roberta-base')
# init fairseq
roberta_fairseq = torch.hub.load('pytorch/fairseq', 'roberta.base')
roberta_fairseq.model.eval()
# LM test
sequence = 'My favorite type of cheese is gouda!'
tokens = torch.tensor([tokenizer_hf.encode(sequence)])
hf_out = roberta_hf.forward(tokens)[0]
fairseq_out = roberta_fairseq.model.forward(tokens)[0]
print(torch.mean(torch.abs(fairseq_out - hf_out)).item())
# should be 0.0
# ---
# pipeline test fill-mask
sentence = ' My favorite type of cheese is <mask>!'
print(roberta_fairseq.fill_mask(sentence))
roberta_hf = pipeline('fill-mask', tokenizer=tokenizer_hf, model='roberta-base')
print(roberta_hf(sentence))
# should have identical predictions
```<|||||>Resolved by #2958 |
transformers | 1,873 | closed | German DistilBERT | Hi,
this PR adds the German DistilBERT to the library 🤗
Thanks to the Hugging Face team (incl. hardware support) the German DistilBERT was trained on 1/2 of the data that was used for training the [German DBMDZ BERT](https://github.com/dbmdz/german-bert) model for ~4 days.
Evaluation on NER tasks (German CoNLL and GermEval) shows a performance difference of 1.3% on average compared to the German BERT model.
---
Remaining tasks:
* [x] Model, configuration and vocab is already uploaded to S3, only file permissions need to be adjusted | 11-19-2019 19:04:27 | 11-19-2019 19:04:27 | # [Codecov](https://codecov.io/gh/huggingface/transformers/pull/1873?src=pr&el=h1) Report
> Merging [#1873](https://codecov.io/gh/huggingface/transformers/pull/1873?src=pr&el=desc) into [master](https://codecov.io/gh/huggingface/transformers/commit/f3386d938348628c91457fc7d8650c223317a053?src=pr&el=desc) will **increase** coverage by `1.35%`.
> The diff coverage is `n/a`.
[](https://codecov.io/gh/huggingface/transformers/pull/1873?src=pr&el=tree)
```diff
@@ Coverage Diff @@
## master #1873 +/- ##
==========================================
+ Coverage 82.72% 84.08% +1.35%
==========================================
Files 97 97
Lines 14316 14316
==========================================
+ Hits 11843 12037 +194
+ Misses 2473 2279 -194
```
| [Impacted Files](https://codecov.io/gh/huggingface/transformers/pull/1873?src=pr&el=tree) | Coverage Δ | |
|---|---|---|
| [transformers/tokenization\_distilbert.py](https://codecov.io/gh/huggingface/transformers/pull/1873/diff?src=pr&el=tree#diff-dHJhbnNmb3JtZXJzL3Rva2VuaXphdGlvbl9kaXN0aWxiZXJ0LnB5) | `100% <ø> (ø)` | :arrow_up: |
| [transformers/configuration\_distilbert.py](https://codecov.io/gh/huggingface/transformers/pull/1873/diff?src=pr&el=tree#diff-dHJhbnNmb3JtZXJzL2NvbmZpZ3VyYXRpb25fZGlzdGlsYmVydC5weQ==) | `89.74% <ø> (ø)` | :arrow_up: |
| [transformers/modeling\_distilbert.py](https://codecov.io/gh/huggingface/transformers/pull/1873/diff?src=pr&el=tree#diff-dHJhbnNmb3JtZXJzL21vZGVsaW5nX2Rpc3RpbGJlcnQucHk=) | `95.87% <ø> (ø)` | :arrow_up: |
| [transformers/modeling\_openai.py](https://codecov.io/gh/huggingface/transformers/pull/1873/diff?src=pr&el=tree#diff-dHJhbnNmb3JtZXJzL21vZGVsaW5nX29wZW5haS5weQ==) | `82% <0%> (+1.33%)` | :arrow_up: |
| [transformers/modeling\_ctrl.py](https://codecov.io/gh/huggingface/transformers/pull/1873/diff?src=pr&el=tree#diff-dHJhbnNmb3JtZXJzL21vZGVsaW5nX2N0cmwucHk=) | `96.46% <0%> (+2.21%)` | :arrow_up: |
| [transformers/modeling\_xlnet.py](https://codecov.io/gh/huggingface/transformers/pull/1873/diff?src=pr&el=tree#diff-dHJhbnNmb3JtZXJzL21vZGVsaW5nX3hsbmV0LnB5) | `73.61% <0%> (+2.43%)` | :arrow_up: |
| [transformers/modeling\_roberta.py](https://codecov.io/gh/huggingface/transformers/pull/1873/diff?src=pr&el=tree#diff-dHJhbnNmb3JtZXJzL21vZGVsaW5nX3JvYmVydGEucHk=) | `71.76% <0%> (+12.35%)` | :arrow_up: |
| [transformers/tests/modeling\_tf\_common\_test.py](https://codecov.io/gh/huggingface/transformers/pull/1873/diff?src=pr&el=tree#diff-dHJhbnNmb3JtZXJzL3Rlc3RzL21vZGVsaW5nX3RmX2NvbW1vbl90ZXN0LnB5) | `97.08% <0%> (+15.53%)` | :arrow_up: |
| [transformers/modeling\_tf\_pytorch\_utils.py](https://codecov.io/gh/huggingface/transformers/pull/1873/diff?src=pr&el=tree#diff-dHJhbnNmb3JtZXJzL21vZGVsaW5nX3RmX3B5dG9yY2hfdXRpbHMucHk=) | `92.95% <0%> (+83.09%)` | :arrow_up: |
------
[Continue to review full report at Codecov](https://codecov.io/gh/huggingface/transformers/pull/1873?src=pr&el=continue).
> **Legend** - [Click here to learn more](https://docs.codecov.io/docs/codecov-delta)
> `Δ = absolute <relative> (impact)`, `ø = not affected`, `? = missing data`
> Powered by [Codecov](https://codecov.io/gh/huggingface/transformers/pull/1873?src=pr&el=footer). Last update [f3386d9...da06afa](https://codecov.io/gh/huggingface/transformers/pull/1873?src=pr&el=lastupdated). Read the [comment docs](https://docs.codecov.io/docs/pull-request-comments).
<|||||>fyi I just adjusted the permissions on the s3 objects.<|||||>Perfect! |
transformers | 1,871 | closed | Understanding feature creation | ## ❓ Questions & Help
Hi,
I am trying to understand feature creation when using run_lm_finetuning.py. If I let the script download the tokenizer files, everything works fine. If I manually assign a folder for the tokenizer with vocab.json and merges.txt downloaded from Hugging Face, I get an empty dataframe during feature creation.
`11/19/2019 16:36:20 - INFO - __main__ - Creating features from dataset file at /data/test
11/19/2019 16:41:53 - INFO - __main__ - Saving features into cached file /data/test/roberta-base_cached_lm_999999999998_moleculenet_roberta_train.csv
Traceback (most recent call last):
File "run_lm_finetuning.py", line 558, in <module>
main()
File "run_lm_finetuning.py", line 510, in main
global_step, tr_loss = train(args, train_dataset, model, tokenizer)
File "run_lm_finetuning.py", line 175, in train
train_sampler = RandomSampler(train_dataset) if args.local_rank == -1 else DistributedSampler(train_dataset)
File "/usr/local/conda3/lib/python3.7/site-packages/torch/utils/data/sampler.py", line 94, in __init__
"value, but got num_samples={}".format(self.num_samples))
ValueError: num_samples should be a positive integer value, but got num_samples=0`
| 11-19-2019 16:48:46 | 11-19-2019 16:48:46 | Just to clarify, I am asking this because I originally tried to create a custom vocab/merges files by giving a new path for tokenizer.from_pretrained for my new files. Failure to do so led me to notice that giving a new path for the original files downloaded from S3 also fail in the new path. If I put my custom files to the path used for the S3 cache (with identical filenames used by the cache, everything works fine. In other words, if I replace the contents of the following files with my custom content, everything works:
`11/19/2019 18:31:15 - INFO - transformers.tokenization_utils - loading file https://s3.amazonaws.com/models.huggingface.co/bert/roberta-base-vocab.json from cache at /root/.cache/torch/transformers/d0c5776499adc1ded22493fae699da0971c1ee4c2587111707a4d177d20257a2.ef00af9e673c7160b4d41cfda1f48c5f4cba57d5142754525572a846a1ab1b9b
11/19/2019 18:31:15 - INFO - transformers.tokenization_utils - loading file https://s3.amazonaws.com/models.huggingface.co/bert/roberta-base-merges.txt from cache at /root/.cache/torch/transformers/b35e7cd126cd4229a746b5d5c29a749e8e84438b14bcdb575950584fe33207e8.70bec105b4158ed9a1747fea67a43f5dee97855c64d62b6ec3742f4cfdb5feda` <|||||>Have you learned anything about this? I'm currently running to the same issue trying to specify an existing model. I noticed that the cache_lm_* file that it creates is only 5 bytes, and gedit says the files is corrupt.<|||||>Yes, so bizarrely even if you have the original hugggingface files in a new location, an empty data frame (the cache_lm file) will be created. Try replacing the original hugging face files downloaded from S3 with your custom vocab/merges files. They should be in /root/.cache/torch/transformers
If you still run into the same problem, then either your data is too small or the vocab/merges files are not in the correct format.<|||||>@AVSuni I get the same issue when using a checkpoint generated by huggingface/transformers. Even when using cached versions of the merge/vocab files from `/root/.cache/torch/transformers/`. Even when I delete the `cache_lm_*` file, specifying the model rather than giving a model name to be used causes this issue.<|||||>Maybe I'm being superstitious, but setting a block size seems to alleviate the issue. I didn't bother tracing the code, but I did notice that when I set `block_size`, the cache file is set with the number of the block size. When I do not set the block size, the temp file has some absurdly long number in the cache file where the block size would be. I don't really see that documented anywhere, but here we are.<|||||>This issue has been automatically marked as stale because it has not had recent activity. It will be closed if no further activity occurs. Thank you for your contributions.
|
transformers | 1,870 | closed | XLNet for Token classification | Hi,
this PR adds a XLNet based token classifier for PyTorch `XLNetForTokenClassification` and TensorFlow `TFXLNetForTokenClassification` with unit test that allows performing sequence labeling tasks like NER or PoS tagging.
| 11-19-2019 11:54:05 | 11-19-2019 11:54:05 | # [Codecov](https://codecov.io/gh/huggingface/transformers/pull/1870?src=pr&el=h1) Report
> Merging [#1870](https://codecov.io/gh/huggingface/transformers/pull/1870?src=pr&el=desc) into [master](https://codecov.io/gh/huggingface/transformers/commit/f3386d938348628c91457fc7d8650c223317a053?src=pr&el=desc) will **increase** coverage by `1.37%`.
> The diff coverage is `88.4%`.
[](https://codecov.io/gh/huggingface/transformers/pull/1870?src=pr&el=tree)
```diff
@@ Coverage Diff @@
## master #1870 +/- ##
==========================================
+ Coverage 82.72% 84.09% +1.37%
==========================================
Files 97 97
Lines 14316 14383 +67
==========================================
+ Hits 11843 12096 +253
+ Misses 2473 2287 -186
```
| [Impacted Files](https://codecov.io/gh/huggingface/transformers/pull/1870?src=pr&el=tree) | Coverage Δ | |
|---|---|---|
| [transformers/tests/modeling\_tf\_xlnet\_test.py](https://codecov.io/gh/huggingface/transformers/pull/1870/diff?src=pr&el=tree#diff-dHJhbnNmb3JtZXJzL3Rlc3RzL21vZGVsaW5nX3RmX3hsbmV0X3Rlc3QucHk=) | `96.05% <100%> (+0.3%)` | :arrow_up: |
| [transformers/modeling\_tf\_xlnet.py](https://codecov.io/gh/huggingface/transformers/pull/1870/diff?src=pr&el=tree#diff-dHJhbnNmb3JtZXJzL21vZGVsaW5nX3RmX3hsbmV0LnB5) | `88.53% <100%> (+0.34%)` | :arrow_up: |
| [transformers/tests/modeling\_xlnet\_test.py](https://codecov.io/gh/huggingface/transformers/pull/1870/diff?src=pr&el=tree#diff-dHJhbnNmb3JtZXJzL3Rlc3RzL21vZGVsaW5nX3hsbmV0X3Rlc3QucHk=) | `94.28% <81.81%> (-1.85%)` | :arrow_down: |
| [transformers/modeling\_xlnet.py](https://codecov.io/gh/huggingface/transformers/pull/1870/diff?src=pr&el=tree#diff-dHJhbnNmb3JtZXJzL21vZGVsaW5nX3hsbmV0LnB5) | `73.95% <82.6%> (+2.77%)` | :arrow_up: |
| [transformers/modeling\_openai.py](https://codecov.io/gh/huggingface/transformers/pull/1870/diff?src=pr&el=tree#diff-dHJhbnNmb3JtZXJzL21vZGVsaW5nX29wZW5haS5weQ==) | `82% <0%> (+1.33%)` | :arrow_up: |
| [transformers/modeling\_ctrl.py](https://codecov.io/gh/huggingface/transformers/pull/1870/diff?src=pr&el=tree#diff-dHJhbnNmb3JtZXJzL21vZGVsaW5nX2N0cmwucHk=) | `96.46% <0%> (+2.21%)` | :arrow_up: |
| [transformers/modeling\_roberta.py](https://codecov.io/gh/huggingface/transformers/pull/1870/diff?src=pr&el=tree#diff-dHJhbnNmb3JtZXJzL21vZGVsaW5nX3JvYmVydGEucHk=) | `71.76% <0%> (+12.35%)` | :arrow_up: |
| [transformers/tests/modeling\_tf\_common\_test.py](https://codecov.io/gh/huggingface/transformers/pull/1870/diff?src=pr&el=tree#diff-dHJhbnNmb3JtZXJzL3Rlc3RzL21vZGVsaW5nX3RmX2NvbW1vbl90ZXN0LnB5) | `97.08% <0%> (+15.53%)` | :arrow_up: |
| [transformers/modeling\_tf\_pytorch\_utils.py](https://codecov.io/gh/huggingface/transformers/pull/1870/diff?src=pr&el=tree#diff-dHJhbnNmb3JtZXJzL21vZGVsaW5nX3RmX3B5dG9yY2hfdXRpbHMucHk=) | `92.95% <0%> (+83.09%)` | :arrow_up: |
------
[Continue to review full report at Codecov](https://codecov.io/gh/huggingface/transformers/pull/1870?src=pr&el=continue).
> **Legend** - [Click here to learn more](https://docs.codecov.io/docs/codecov-delta)
> `Δ = absolute <relative> (impact)`, `ø = not affected`, `? = missing data`
> Powered by [Codecov](https://codecov.io/gh/huggingface/transformers/pull/1870?src=pr&el=footer). Last update [f3386d9...4193aa9](https://codecov.io/gh/huggingface/transformers/pull/1870?src=pr&el=lastupdated). Read the [comment docs](https://docs.codecov.io/docs/pull-request-comments).
<|||||>This looks good to me, thank you @alexzubiaga !<|||||>This is great, thanks a lot @alexzubiaga (and nice work adding the tests!).
Merging |
transformers | 1,869 | closed | Pre-training a smaller version of BERT on own data | ## ❓ Questions & Help
Hello,
<!-- A clear and concise description of the question. -->
I want to load the first 3-4 layers of BERT, pre-train those on my own data and then fine-tune the model on the target task. Is this possible?
I took a look at the [run_lm_finetuning.py](https://github.com/huggingface/transformers/blob/master/examples/run_lm_finetuning.py) and changed the lines 478-479:
` config = config_class.from_pretrained(args.config_name if args.config_name else args.model_name_or_path,
cache_dir=args.cache_dir if args.cache_dir else None)`
to
` config = BertConfig(num_hidden_layers=3)
`
I'm not sure, if this change is sufficient and if the first or last three layers will be taken from the pre-trained BERT?
Is the _next sentence prediction_ pre-training task implemented somewhere ?
Thanks in Advance
| 11-19-2019 10:36:37 | 11-19-2019 10:36:37 | Hi, you might be interested in freezing some layers rather than initializing a model with only 3 layers. Freezing some layers mean that they won't be affected by the backpropagation.
If that's what you're looking for, @BramVanroy's answer may be of help: https://github.com/huggingface/transformers/issues/1431
We do not have an NSP example in our examples. |
transformers | 1,868 | closed | XLNet for Token classification | Hi,
this PR adds a `TFXLNetForTokenClassification` implementation and unit test that allows to perform sequence labeling tasks like NER or PoS tagging.
| 11-19-2019 10:02:07 | 11-19-2019 10:02:07 | |
transformers | 1,867 | closed | How to fine-tune BERT on a large training dataset? | ## ❓ Questions & Help
Hi,
I want to fine-tune BERT on a large training dataset. With around 1.5million training examples, this is currently consuming around 60GB of RAM. Is there any way to reduce the RAM usage or load the training examples in parts? Thanks!.
| 11-19-2019 09:28:30 | 11-19-2019 09:28:30 | I think you should check out pytorch dataloaders https://pytorch.org/docs/stable/data.html
Also, gradient accumulation is helpful.<|||||>This issue has been automatically marked as stale because it has not had recent activity. It will be closed if no further activity occurs. Thank you for your contributions.
|
transformers | 1,866 | closed | BertForTokenClassification for NER . what is the conclusion of this output ? | ## ❓ Questions & Help
<!-- A clear and concise description of the question. -->
Hi ,
Im trying to perform NER using BertForTokenClassification .I saw this sample code in transformers GIT page.
from transformers import BertForTokenClassification
tokenizer = BertTokenizer.from_pretrained('bert-base-uncased')
model = BertForTokenClassification.from_pretrained('bert-base-uncased')
input_ids = torch.tensor(tokenizer.encode("Hello, my dog is cute")).unsqueeze(0) # Batch size 1
labels = torch.tensor([1] * input_ids.size(1)).unsqueeze(0) # Batch size 1
print(labels)
outputs = model(input_ids, labels=labels)
loss, scores = outputs[:2]
output loss:
tensor(0.5975, grad_fn=<NllLossBackward>)
output scores:
tensor([[[-0.1622, 0.1824],
[-0.1552, -0.0534],
[-0.3032, -0.1166],
[-0.2453, -0.1182],
[-0.4388, -0.1898],
[-0.3159, -0.1067]]], grad_fn=<AddBackward0>)
1.When i printed the loss and score i got below values .Now how should i infer this output ? what dose these value represent for performing NER ? what should i do to get the NER tags for the sentence "Hello, my dog is cute" .
2.i referred few NER codes in GIT using BERT and they have humongous line of code written for performing the NER . Is there any simple way to perform NER using bert ? like how Flair library has very simple method for performing the NER task ?
| 11-19-2019 09:23:23 | 11-19-2019 09:23:23 | Why not just use flair? Flair has integrated BERT.<|||||>BERT was performing sentence embedding better than Flair(tired all different type of stacked embedding ) but less when compared to USC . Flair had a functionality that gave NER tagging directly . i was expecting the same will would be available in BERT but its not available directly .It would be good if BERT gives a direct plug and play functionality for NER task . <|||||>@ajbot2019
1. compute prediction list
https://github.com/huggingface/transformers/blob/master/examples/run_ner.py#L242
2. print it with
https://github.com/huggingface/transformers/blob/master/examples/run_ner.py#L503
shell scripts to train and evaluate for CoNLL 2003 (english) dataset.
https://github.com/dsindex/transformers_examples
this may help you.<|||||>This issue has been automatically marked as stale because it has not had recent activity. It will be closed if no further activity occurs. Thank you for your contributions.
|
transformers | 1,865 | closed | Run bert for multi-classification but loss never decrease | ## ❓ Questions & Help
<!-- A clear and concise description of the question. -->
I copy the code in example/run_glue.py for text classification task with bert model. All i change in this code is changed to a multi-classification task. I didn't change the main stream of this code. however, after 80 epoches running, i just found the eval result didn't change from the begging to the end. Next time i print the loss and i found the loss nearly unchanged from the begining to the end. i change loss from 1e-5 to 1e-3 and it is not the case, the loss is still nearly unchanged. i just sum the loss together in an single epoch, and after several epochs it only changed from 4703 to 4700 . So that's my problem and any ideas will be appreciated .
`
import torch
from transformers import BertForSequenceClassification, BertTokenizer, InputExample, AdamW, WarmupLinearSchedule
from torch.utils.data import DataLoader, Dataset, SequentialSampler, RandomSampler, TensorDataset
from torch.utils.data.distributed import DistributedSampler
import random
import numpy as np
import os, pickle
import argparse
from tqdm import tqdm, trange
import copy, json
import glob
from apex import amp
from transformers import glue_convert_examples_to_features as convert_examples_to_features
from Bert_eval import evaluate
def train(args, model, tokenizer):
with open("../../data/train_texts", "r") as fr:
texts = fr.readlines()
with open("../../data/train_labels", "r") as fr:
labels = fr.readlines()
examples = load_dataset(texts, labels)
label_list = ["0", "1", "2", "3", "4", "5", "6", "7", "8", "9"]
features = convert_examples_to_features(examples, tokenizer,
label_list=label_list,
output_mode="classification")
cached_path = "cached_file"
torch.save(features, cached_path)
all_input_ids = torch.tensor([f.input_ids for f in features], dtype=torch.long)
all_attention_mask = torch.tensor([f.attention_mask for f in features], dtype=torch.long)
all_token_type_ids = torch.tensor([f.token_type_ids for f in features], dtype=torch.long)
all_labels = torch.tensor([f.label for f in features], dtype=torch.long)
train_dataset = TensorDataset(all_input_ids, all_attention_mask, all_token_type_ids, all_labels)
train_dataloader = DataLoader(train_dataset, batch_size=args.train_batch_size)
no_decay = ['bias', 'LayerNorm.weight']
optimizer_grouped_parameters = [
{'params': [p for n, p in model.named_parameters() if not any(nd in n for nd in no_decay)], 'weight_decay': args.weight_decay},
{'params': [p for n, p in model.named_parameters() if any(nd in n for nd in no_decay)], 'weight_decay': 0.0}
]
optimizer = AdamW(optimizer_grouped_parameters, lr=args.learning_rate, eps=args.adam_epsilon)
scheduler = WarmupLinearSchedule(optimizer, warmup_steps=args.warmup_steps, t_total=args.t_total)
model, optimizer = amp.initialize(model, optimizer, opt_level=args.fp16_opt_level)
# multi-gpu training (should be after apex fp16 initialization)
if args.n_gpu > 1:
model = torch.nn.DataParallel(model)
# Distributed training (should be after apex fp16 initialization)
if args.local_rank != -1:
model = torch.nn.parallel.DistributedDataParallel(model, device_ids=[args.local_rank],
output_device=args.local_rank,
find_unused_parameters=True)
# Train!
print("***** Running training *****")
print(" Num examples = %d", len(train_dataset))
print(" Num Epochs = %d", args.num_train_epochs)
print(" Gradient Accumulation steps = %d", args.gradient_accumulation_steps)
print(" Total optimization steps = %d", args.t_total)
global_step = 0
train_iterator = trange(int(args.num_train_epochs), desc="Epoch")#, disable=args.local_rank not in [-1, 0])
set_seed(args)
for _ in train_iterator:
tr_loss = 0.0
print("global_step: ", global_step)
epoch_iterator = tqdm(train_dataloader, desc="Iteration", disable=args.local_rank not in [-1, 0])
print("optimizer: ", optimizer)
for step, batch in enumerate(epoch_iterator):
# print("batch: ", len(batch))
model.train()
batch = tuple(t.to(args.device) for t in batch)
inputs = {'input_ids': batch[0],
'attention_mask': batch[1],
'token_type_ids': batch[2],
'labels': batch[3]}
outputs = model(**inputs)
loss = outputs[0] # model outputs are always tuple in transformers (see doc)
print("loss:", loss)
loss.backward()
tr_loss += loss.item()
if (step + 1) % args.gradient_accumulation_steps == 0:
if args.fp16:
torch.nn.utils.clip_grad_norm_(amp.master_params(optimizer), args.max_grad_norm)
else:
torch.nn.utils.clip_grad_norm_(model.parameters(), args.max_grad_norm)
optimizer.step()
scheduler.step() # Update learning rate schedule
model.zero_grad()
global_step += 1
output_dir = os.path.join(args.output_dir, 'checkpoint-{}'.format(global_step))
if not os.path.exists(output_dir):
os.makedirs(output_dir)
result = evaluate(args, model, tokenizer)
print("result: ", result)
model_to_save = model.module if hasattr(model, 'module') else model # Take care of distributed/parallel training
model_to_save.save_pretrained(output_dir)
torch.save(args, os.path.join(output_dir, 'training_args.bin'))
def load_dataset(lines, labels):
"""
convert examples for the training sets for document classification
"""
examples = []
for (i, (line, label)) in enumerate(zip(lines, labels)):
line = line.strip()
label = label.strip()
# label = str(i % 2)
guid = i
examples.append(
InputExample(guid=guid, text_a=line, label=label)
)
return examples
if __name__ == "__main__":
parser = argparse.ArgumentParser()
parser.add_argument("--data_dir", type=str, default="./data/",
help="data path for train or test")
parser.add_argument("--train_batch_size", type=int, default=32, help="training batch size")
parser.add_argument("--t_total", type=int, default=100, help="training epoch")
parser.add_argument("--weight_decay", default=0.0, type=float,
help="Weight decay if we apply some.")
parser.add_argument("--output_mode", default="classification", type=str,
help="task name.")
parser.add_argument("--learning_rate", default=1e-3, type=float,
help="The initial learning rate for Adam.")
parser.add_argument("--max_grad_norm", default=1.0, type=float,
help="Max gradient norm.")
parser.add_argument("--num_train_epochs", default=100, type=float,
help="Total number of training epochs to perform.")
parser.add_argument("--max_steps", default=-1, type=int,
help="If > 0: set total number of training steps to perform. Override num_train_epochs.")
parser.add_argument("--warmup_steps", default=0, type=int,
help="Linear warmup over warmup_steps.")
parser.add_argument("--n_gpu", type=int, default=1, help="number of gpus to run")
parser.add_argument('--logging_steps', type=int, default=50,
help="Log every X updates steps.")
parser.add_argument('--save_steps', type=int, default=5000,
help="Save checkpoint every X updates steps.")
parser.add_argument("--per_gpu_eval_batch_size", default=256, type=int,
help="task name.")
parser.add_argument("--do_train", action="store_true", help="train model flag")
parser.add_argument("--do_eval", action="store_true", help="eval model flag")
parser.add_argument("--eval_all_checkpoints", action='store_true',
help="Evaluate all checkpoints starting with the same prefix as model_name ending and ending with step number")
parser.add_argument("--no_cuda", action='store_true',
help="Avoid using CUDA when available")
parser.add_argument('--overwrite_output_dir', action='store_true',
help="Overwrite the content of the output directory")
parser.add_argument('--overwrite_cache', action='store_true',
help="Overwrite the cached training and evaluation sets")
parser.add_argument('--seed', type=int, default=42,
help="random seed for initialization")
parser.add_argument("--adam_epsilon", default=1e-8, type=float,
help="Epsilon for Adam optimizer.")
parser.add_argument('--fp16', action='store_true',
help="Whether to use 16-bit (mixed) precision (through NVIDIA apex) instead of 32-bit")
parser.add_argument('--fp16_opt_level', type=str, default='O1',
help="For fp16: Apex AMP optimization level selected in ['O0', 'O1', 'O2', and 'O3']."
"See details at https://nvidia.github.io/apex/amp.html")
parser.add_argument("--local_rank", type=int, default=-1,
help="For distributed training: local_rank")
parser.add_argument('--gradient_accumulation_steps', type=int, default=1,
help="Number of updates steps to accumulate before performing a backward/update pass.")
parser.add_argument("--output_dir", default="./checkpoint", type=str,
help="The output directory where the model predictions and checkpoints will be written.")
parser.add_argument("--eval_checkpoint", type=str, default="1730", help="the checkpoint to reload")
# parser.add_argument("--gpu", type=int, default=0, help="choose gpu device")
args = parser.parse_args()
if args.local_rank == -1 or args.no_cuda:
device = torch.device("cuda" if torch.cuda.is_available() and not args.no_cuda else "cpu")
args.n_gpu = torch.cuda.device_count()
else: # Initializes the distributed backend which will take care of sychronizing nodes/GPUs
torch.cuda.set_device(args.local_rank)
device = torch.device("cuda", args.local_rank)
torch.distributed.init_process_group(backend='nccl')
args.n_gpu = 1
args.device = device
model_class = BertForSequenceClassification
tokenizer_class = BertTokenizer
pretrained_weights = "bert-base-chinese"
tokenizer = tokenizer_class.from_pretrained(pretrained_weights)
model = model_class.from_pretrained(pretrained_weights, num_labels=10)#, output_hidden_states=True, output_attentions=True)
if torch.cuda.is_available():
model.to(args.device)
train(args, model, tokenizer)
if True:
# Create output directory if needed
if not os.path.exists(args.output_dir) and args.local_rank in [-1, 0]:
os.makedirs(args.output_dir)
print("model saved")
model_to_save = model.module if hasattr(model, 'module') else model # Take care of distributed/parallel training
model_to_save.save_pretrained(args.output_dir)
tokenizer.save_pretrained(args.output_dir)
print("model saved into ", args.output_dir)
# Good practice: save your training arguments together with the trained model
torch.save(args, os.path.join(args.output_dir, 'training_args.bin'))
# Load a trained model and vocabulary that you have fine-tuned
model = model_class.from_pretrained(args.output_dir)
tokenizer = tokenizer_class.from_pretrained(args.output_dir)
model.to(args.device)
results = {}
if True:
tokenizer = tokenizer_class.from_pretrained(args.output_dir)#, do_lower_case=args.do_lower_case)
checkpoints = [args.output_dir]
if args.eval_all_checkpoints:
checkpoints = list(os.path.dirname(c) for c in sorted(glob.glob(args.output_dir + '/**/' + WEIGHTS_NAME, recursive=True)))
for checkpoint in checkpoints:
global_step = checkpoint.split('-')[-1] if len(checkpoints) > 1 else ""
prefix = checkpoint.split('/')[-1] if checkpoint.find('checkpoint') != -1 else ""
model = model_class.from_pretrained(checkpoint)
model.to(args.device)
result = evaluate(args, model, tokenizer, prefix=prefix)
result = dict((k + '_{}'.format(global_step), v) for k, v in result.items())
results.update(result)
print("result: ", results)
` | 11-19-2019 07:24:20 | 11-19-2019 07:24:20 | Your copy&pasta is broken. Perhaps Roberto can help you? [Text classification with RoBERTa](https://rsilveira79.github.io/fermenting_gradients/machine_learning/nlp/pytorch/text_classification_roberta/)<|||||>> Your copy&pasta is broken. Perhaps Roberto can help you? [Text classification with RoBERTa](https://rsilveira79.github.io/fermenting_gradients/machine_learning/nlp/pytorch/text_classification_roberta/)
hahaha, actually i solve this problem last night. It's because WarmupLinearSchedule, and I set a t_total args which is smaller than warmup steps , so the lr is nearly zero actually.<|||||>This issue has been automatically marked as stale because it has not had recent activity. It will be closed if no further activity occurs. Thank you for your contributions.
|
transformers | 1,864 | closed | tensorflow2.0 does not has mean, but reduce mean | ## 🐛 Bug
tensorflow2.0 does not has a mean function but only a reduce_mean().
```
output = tf.mean(hidden_states, axis=1)
```
should be:
```
output = tf.reduce_mean(hidden_states, axis=1)
```
<!-- Important information -->
Model I am using: TFXLMForSequenceClassification with summary_type == 'mean'
Language I am using the model on (English, Chinese....): any
The problem arise when using:
when loading the model, due to the lazy initialisation of tf2.0 you have to run the model with dummy input.
```
TFXLMForSequenceClassification.from_pretrained(os.path.join(args.model_path, "xlm-mlm-17-1280-tf_model.h5"), config=config)
```
The tasks I am working on is:
* [ x] my own task or dataset: I want to finetune the model on a custom english dataset and transfer it to multiple language
## To Reproduce
Steps to reproduce the behavior:
1. load config file and add summary info
```
config = XLMConfig.from_pretrained(os.path.join(args.data_path, "xlm-mlm-17-1280-config.json"))
config.summary_use_proj = True
config.summary_type = 'mean'
config.summary_proj_to_labels = True
config.num_labels = len(LABELS)
config.summary_activation = "tanh"
```
2. load the model
```
model = TFXLMForSequenceClassification.from_pretrained(os.path.join(args.model_path, "xlm-mlm-17-1280-tf_model.h5"), config=config)
```
<!-- If you have a code sample, error messages, stack traces, please provide it here as well. -->
## Expected behavior
You should see an error stating that tf2.0 does not has a mean function.
File "transformers/modeling_tf_utils.py", line 442:
``output = tf.mean(hidden_states, axis=1)`` should be ``output = tf.reduce_mean(hidden_states, axis=1)``
```AttributeError: module 'tensorflow' has no attribute 'mean'```
## Environment
* OS: Ubuntu16.04
* Python version: 3.7.5
* Tensorflow version: 2.0
* Transformers version (or branch): 2.1.1
* Using GPU: yes
* Distributed of parallel setup : not distributed, only single GPU
## Additional context
<!-- Add any other context about the problem here. -->
| 11-19-2019 04:46:32 | 11-19-2019 04:46:32 | This bug has been reviewed and corrected in this PR #1876!
Close this issue. |
transformers | 1,863 | closed | How do I train OpenAIGPTDoubleHeadsModel from scratch? | ## ❓ Questions & Help
It looks like I cannot train `OpenAIGPTDoubleHeadsModel` from scratch because it necessarily needs to be initialized using the `from_pretrained()` method. How should I initialize this model to be able to train it from scratch?
As a hack, perhaps I could initialize it using `from_pretrained()` and then reset the pre-trained initialization so any fine-tuning would essentially be the same as pre-training? | 11-19-2019 02:43:37 | 11-19-2019 02:43:37 | You can initialize a model by calling its constructor with a configuration:
```py
from transformers import OpenAIGPTDoubleHeadsModel, OpenAIGPTConfig
config = OpenAIGPTConfig()
model = OpenAIGPTDoubleHeadsModel(config)
```<|||||>Cool, so if I instantiate the model with the default config, it will use the same vocab as the pre-trained model but it won't initialize the pre-trained weights, is that correct?
Also, could you please point me to where the model weights are initialized when we don't use pre-trained weights?<|||||>If you instantiate a model with a default configuration without specifying any argument, it will instantiate your model according to the default configuration values, [visible here](https://github.com/huggingface/transformers/blob/master/transformers/configuration_openai.py#L59).
The model weights are initialized in the `OpenAIGPTPreTrainedModel`'s [`_init_weights` method](https://github.com/huggingface/transformers/blob/master/transformers/modeling_openai.py#L267). |
transformers | 1,862 | closed | save all 12 layers outputs for each token | Hi,
I am following [this](https://mccormickml.com/2019/05/14/BERT-word-embeddings-tutorial/) post to create sentence vectors from the last 4 layers or sum all 12 layers and so on.
Considering a given document has 10,000 tokens and I am planning to use "bert_base_uncased", I was wondering how to save all 12 layers for each token efficiently. Also, further, how to retrieve these layers in order to build sentence vectors.
Thanks! | 11-19-2019 00:05:57 | 11-19-2019 00:05:57 | Hi, to retrieve all layers you can set the `output_hidden_states` flag to `True` in your model configuration:
```py
from transformers import BertConfig, BertModel
config = BertConfig.from_pretrained("bert-base-uncased")
config.output_hidden_states = True
model = BertModel.from_pretrained("bert-base-uncased", config=config)
outputs = model(inputs)
hidden_states = outputs[-1]
```
The model will now output an additional value which will be a tuple of size 1 + n_layer:
`hidden_states[0]`: output of the embedding layer
`hidden_states[1]`: output of the first layer
...
`hidden_states[12]`: output of the last layer
|
transformers | 1,861 | closed | Better TensorFlow 2 examples | This PR aims to improve the TensorFlow examples of the library. It aims to use many tf-specific paradigms, including but not limited to Keras API, AMP, XLA, `tensorflow_datasets`, `tf.train.Features` and `tf.train.Examples`, model saving using Keras callbacks.
Two examples are specifically targeted:
- [x] `run_tf_glue.py`
- [ ] `run_tf_squad.py`
Aims to have a similar architecture to the PyTorch examples: using a parser with similar arguments so as to be used from a terminal.
Feedback is most welcome. | 11-18-2019 16:14:17 | 11-18-2019 16:14:17 | # [Codecov](https://codecov.io/gh/huggingface/transformers/pull/1861?src=pr&el=h1) Report
> Merging [#1861](https://codecov.io/gh/huggingface/transformers/pull/1861?src=pr&el=desc) into [master](https://codecov.io/gh/huggingface/transformers/commit/a5a06a851e1da79138e53978aa079a093f243dde?src=pr&el=desc) will **not change** coverage.
> The diff coverage is `n/a`.
[](https://codecov.io/gh/huggingface/transformers/pull/1861?src=pr&el=tree)
```diff
@@ Coverage Diff @@
## master #1861 +/- ##
=======================================
Coverage 81.43% 81.43%
=======================================
Files 122 122
Lines 18338 18338
=======================================
Hits 14933 14933
Misses 3405 3405
```
------
[Continue to review full report at Codecov](https://codecov.io/gh/huggingface/transformers/pull/1861?src=pr&el=continue).
> **Legend** - [Click here to learn more](https://docs.codecov.io/docs/codecov-delta)
> `Δ = absolute <relative> (impact)`, `ø = not affected`, `? = missing data`
> Powered by [Codecov](https://codecov.io/gh/huggingface/transformers/pull/1861?src=pr&el=footer). Last update [a5a06a8...0f730de](https://codecov.io/gh/huggingface/transformers/pull/1861?src=pr&el=lastupdated). Read the [comment docs](https://docs.codecov.io/docs/pull-request-comments).
<|||||>This issue has been automatically marked as stale because it has not had recent activity. It will be closed if no further activity occurs. Thank you for your contributions.
|
transformers | 1,860 | closed | [WIP] Add support for CamembertForTokenClassification | Hi,
this PR adds a `CamembertForTokenClassification` implementation, so that fine-tuning of NER models is possible.
Tasks:
* [x] Implement `CamembertForTokenClassification`
* [x] Add `CamembertForTokenClassification` to module import list
* [x] Add support for CamemBERT model in `run_ner.py` example | 11-18-2019 13:18:09 | 11-18-2019 13:18:09 | # [Codecov](https://codecov.io/gh/huggingface/transformers/pull/1860?src=pr&el=h1) Report
> Merging [#1860](https://codecov.io/gh/huggingface/transformers/pull/1860?src=pr&el=desc) into [master](https://codecov.io/gh/huggingface/transformers/commit/3916b334a86484af8442d1cfdb2f15695feae581?src=pr&el=desc) will **decrease** coverage by `0.03%`.
> The diff coverage is `55.55%`.
[](https://codecov.io/gh/huggingface/transformers/pull/1860?src=pr&el=tree)
```diff
@@ Coverage Diff @@
## master #1860 +/- ##
==========================================
- Coverage 84.08% 84.04% -0.04%
==========================================
Files 97 97
Lines 14316 14333 +17
==========================================
+ Hits 12037 12046 +9
- Misses 2279 2287 +8
```
| [Impacted Files](https://codecov.io/gh/huggingface/transformers/pull/1860?src=pr&el=tree) | Coverage Δ | |
|---|---|---|
| [transformers/modeling\_camembert.py](https://codecov.io/gh/huggingface/transformers/pull/1860/diff?src=pr&el=tree#diff-dHJhbnNmb3JtZXJzL21vZGVsaW5nX2NhbWVtYmVydC5weQ==) | `100% <100%> (ø)` | :arrow_up: |
| [transformers/tokenization\_camembert.py](https://codecov.io/gh/huggingface/transformers/pull/1860/diff?src=pr&el=tree#diff-dHJhbnNmb3JtZXJzL3Rva2VuaXphdGlvbl9jYW1lbWJlcnQucHk=) | `36.92% <38.46%> (+0.38%)` | :arrow_up: |
------
[Continue to review full report at Codecov](https://codecov.io/gh/huggingface/transformers/pull/1860?src=pr&el=continue).
> **Legend** - [Click here to learn more](https://docs.codecov.io/docs/codecov-delta)
> `Δ = absolute <relative> (impact)`, `ø = not affected`, `? = missing data`
> Powered by [Codecov](https://codecov.io/gh/huggingface/transformers/pull/1860?src=pr&el=footer). Last update [3916b33...56c8486](https://codecov.io/gh/huggingface/transformers/pull/1860?src=pr&el=lastupdated). Read the [comment docs](https://docs.codecov.io/docs/pull-request-comments).
<|||||>This looks good to me @stefan-it 👌
We'll also need to do the TF models but that can be in a different PR.
cc @LysandreJik @thomwolf <|||||>I tried to replicate the results for PoS tagging on ParTUT dataset (using the tagged 2.2 version from [here](https://github.com/UniversalDependencies/UD_French-ParTUT)). I could replicate the results mentioned in the CamemBERT paper for the multilingual (cased) BERT model: `run_ner` outputs F1-score, so I added the `accuracy_score` metric from the `seqeval` module for debugging ;)
However, the CamemBERT model is ~10% behind it. I'm trying to figure out why, loss is really high compared to mBERT...
<|||||>LGTM thanks! |
transformers | 1,859 | closed | Adds CamemBERT to Model architectures list | 11-18-2019 09:07:21 | 11-18-2019 09:07:21 | # [Codecov](https://codecov.io/gh/huggingface/transformers/pull/1859?src=pr&el=h1) Report
> Merging [#1859](https://codecov.io/gh/huggingface/transformers/pull/1859?src=pr&el=desc) into [master](https://codecov.io/gh/huggingface/transformers/commit/0477b307c7501ea76e01b03cb387a2312db752b3?src=pr&el=desc) will **not change** coverage.
> The diff coverage is `n/a`.
[](https://codecov.io/gh/huggingface/transformers/pull/1859?src=pr&el=tree)
```diff
@@ Coverage Diff @@
## master #1859 +/- ##
=======================================
Coverage 84.08% 84.08%
=======================================
Files 97 97
Lines 14316 14316
=======================================
Hits 12037 12037
Misses 2279 2279
```
------
[Continue to review full report at Codecov](https://codecov.io/gh/huggingface/transformers/pull/1859?src=pr&el=continue).
> **Legend** - [Click here to learn more](https://docs.codecov.io/docs/codecov-delta)
> `Δ = absolute <relative> (impact)`, `ø = not affected`, `? = missing data`
> Powered by [Codecov](https://codecov.io/gh/huggingface/transformers/pull/1859?src=pr&el=footer). Last update [0477b30...63af013](https://codecov.io/gh/huggingface/transformers/pull/1859?src=pr&el=lastupdated). Read the [comment docs](https://docs.codecov.io/docs/pull-request-comments).
<|||||>Thanks! Slightly reworded in next commit |
|
transformers | 1,858 | closed | Conversion of [Model]ForSequenceClassification logits to probabilities | ## ❓ Questions & Help
<!-- A clear and concise description of the question. -->
I noticed that whenever I would convert logits coming from the model to probabilities using the following equation:
probability = e^logit/(1 + e^logit)
The probabilities for my two classes do not add up to 1. Why is this?
| 11-17-2019 23:19:48 | 11-17-2019 23:19:48 | Logits are the classification scores before softmax.
I don't see why the results of your equation should add up to 1.
You may try to use the softmax function directly.<|||||>This issue has been automatically marked as stale because it has not had recent activity. It will be closed if no further activity occurs. Thank you for your contributions.
|
transformers | 1,857 | closed | XLM Masked Word Prediction | ## ❓ Questions & Help
Does anyone knows how to mask a word in a sentence and then get predictions with probabilities from XLM models | 11-17-2019 16:43:32 | 11-17-2019 16:43:32 | Duplicate of #1842 |
transformers | 1,856 | closed | multitask learning | ## ❓ Questions & Help
<!-- A clear and concise description of the question. -->
Hi, I would like to apply multitask learning, using for example two tasks, and the one (and maybe both) of the two tasks is sequential labeling like NER. To my understanding, in order to apply the library on such a task, the way to go is with BertForTokenClassification. Is that correct? But also what I think is that I do not have enough flexibility to use it/ adapt it to create a multitask model.
Could you share your thoughts on that? Any help would be much appreciated.
| 11-17-2019 14:35:28 | 11-17-2019 14:35:28 | from the documentation of the class,
https://github.com/huggingface/transformers/blob/933841d903a032d93b5100220dc72db9d1283eca/pytorch_transformers/modeling_bert.py#L1100
I understand that I could use the ```scores```, as input to an additional module that I could stack on top of BertForTokenClassification, for example for a second task. Is that correct?
```loss, scores = outputs[:2] ```
But what I am thinking right now, is that scores could have small dimensions, so probably I would need the weights of the last layer. How could I extract them?
Always your thoughts on that would be much appreciated!<|||||>for every other suffered person that needs an answer on that last question above, I think that the way to extract those weights, is if you open the black box of the implementation of this class, and here it is what you want:
outputs = self.bert(..)
so I think that reimplementation/enhancment is needed to support my needs as was given above.
Am I missing something if I reimplement this class adding more functionality? I think that no, and that it is safe. <|||||>Hi, there are several things you can do to obtain the last layer representation.
- First of all, you can use a standard `BertModel` on which you add your own classifier for token classification (that's what's done with `BertForTokenClassification`). This will allow you to easily switch the heads for your multi-task setup.
- You could also use the `BertForTokenClassification`, as you have said, and use the inner model (model.bert) to obtain the last layer.
- Finally, the cleanest way would be to output hidden states directly by specifying the option in the configuration:
```py
from transformers import BertConfig, BertForTokenClassification
import torch
config = BertConfig.from_pretrained("bert-base-cased")
config.output_hidden_states = True
model = BertForTokenClassification.from_pretrained("bert-base-cased", config=config)
inputs = torch.tensor([[1, 2, 3]])
outputs = model(inputs)
token_classification_outputs, hidden_states = outputs
last_layer_hidden_states = hidden_states[-1]
```
The variable `last_layer_hidden_states` is of shape `[batch_size, seq_len, hidden_size]` and is the output of the last transformer layer.
I hope this clears things up.<|||||>https://github.com/jiant-dev/jiant |
transformers | 1,855 | closed | Some questions about the abstractive summarization code. | ## ❓ Questions & Help
<!-- A clear and concise description of the question. -->
Hi, thanks for sharing the code, i was confused about some code and implements when i reading it.
a) In run_summarization_finetuning.py,

should cls_token_id be replaced by sep_token_id?
b) In utils_summarization.py
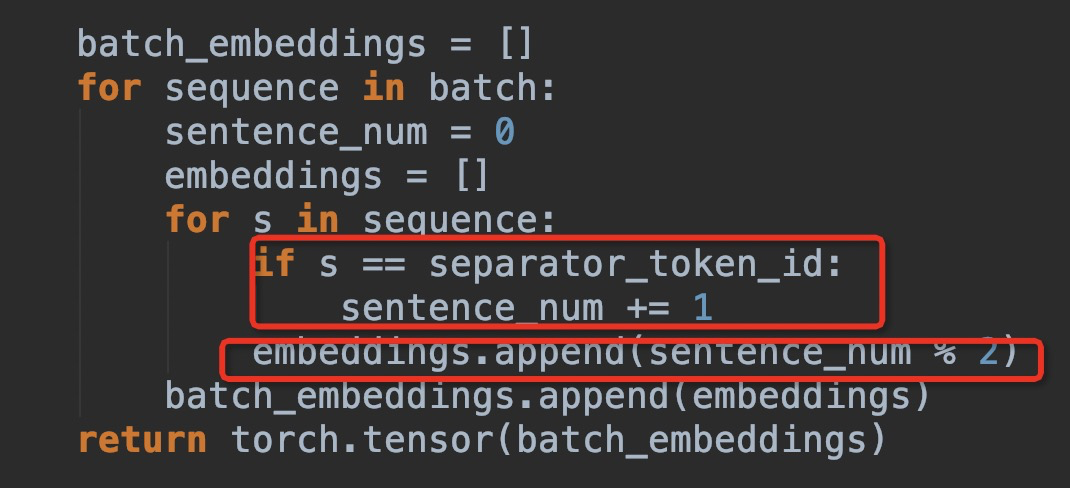
This will make sequence [cls] a b [sep] c d [sep] encoded as 0 0 0 1 1 1 0, should the order be changed?
c) In the decoder, the deocder_mask only mask the pad token, i think look_ahead_mask is more accurate for we can't see the future words in advance.
Looking forward to reply. Thanks!
| 11-17-2019 14:05:35 | 11-17-2019 14:05:35 | Hi!
First, please check the `run_summarization.py` script in the `example-summarization` branch as it is more up-to-date. To answer your questions:
a) We are following this implementation: https://arxiv.org/pdf/1908.08345.pdf where they add an extra [CLS] token for each new sentence.
b) I would need to triple-check the authors’ code, but I hope it does not matter.
c) Good point. If you look at the way the encoder-decoder is implemented you will see that masks passed to the decoder are automatically turned into causal (“look-ahead”), so the code does have the expected behavior :)<|||||>Thank you so much. |
transformers | 1,854 | closed | run glue problem | ## ❓ Questions & Help
How to fix this problem

| 11-17-2019 10:29:30 | 11-17-2019 10:29:30 | Could you please respect the issue template so that we can help you better? It's hard to help without seeing the versions or the full error. |
transformers | 1,853 | closed | typo "deay" -> "decay" | a typo has been fixed. | 11-17-2019 09:09:44 | 11-17-2019 09:09:44 | # [Codecov](https://codecov.io/gh/huggingface/transformers/pull/1853?src=pr&el=h1) Report
> Merging [#1853](https://codecov.io/gh/huggingface/transformers/pull/1853?src=pr&el=desc) into [master](https://codecov.io/gh/huggingface/transformers/commit/0477b307c7501ea76e01b03cb387a2312db752b3?src=pr&el=desc) will **decrease** coverage by `0.09%`.
> The diff coverage is `n/a`.
[](https://codecov.io/gh/huggingface/transformers/pull/1853?src=pr&el=tree)
```diff
@@ Coverage Diff @@
## master #1853 +/- ##
=========================================
- Coverage 84.08% 83.98% -0.1%
=========================================
Files 97 97
Lines 14316 14316
=========================================
- Hits 12037 12023 -14
- Misses 2279 2293 +14
```
| [Impacted Files](https://codecov.io/gh/huggingface/transformers/pull/1853?src=pr&el=tree) | Coverage Δ | |
|---|---|---|
| [transformers/tests/modeling\_tf\_auto\_test.py](https://codecov.io/gh/huggingface/transformers/pull/1853/diff?src=pr&el=tree#diff-dHJhbnNmb3JtZXJzL3Rlc3RzL21vZGVsaW5nX3RmX2F1dG9fdGVzdC5weQ==) | `96.36% <0%> (-1.82%)` | :arrow_down: |
| [transformers/tests/modeling\_tf\_transfo\_xl\_test.py](https://codecov.io/gh/huggingface/transformers/pull/1853/diff?src=pr&el=tree#diff-dHJhbnNmb3JtZXJzL3Rlc3RzL21vZGVsaW5nX3RmX3RyYW5zZm9feGxfdGVzdC5weQ==) | `93% <0%> (-1%)` | :arrow_down: |
| [transformers/tests/modeling\_tf\_ctrl\_test.py](https://codecov.io/gh/huggingface/transformers/pull/1853/diff?src=pr&el=tree#diff-dHJhbnNmb3JtZXJzL3Rlc3RzL21vZGVsaW5nX3RmX2N0cmxfdGVzdC5weQ==) | `93.06% <0%> (-1%)` | :arrow_down: |
| [transformers/tests/modeling\_tf\_distilbert\_test.py](https://codecov.io/gh/huggingface/transformers/pull/1853/diff?src=pr&el=tree#diff-dHJhbnNmb3JtZXJzL3Rlc3RzL21vZGVsaW5nX3RmX2Rpc3RpbGJlcnRfdGVzdC5weQ==) | `98.16% <0%> (-0.92%)` | :arrow_down: |
| [transformers/tests/modeling\_tf\_openai\_gpt\_test.py](https://codecov.io/gh/huggingface/transformers/pull/1853/diff?src=pr&el=tree#diff-dHJhbnNmb3JtZXJzL3Rlc3RzL21vZGVsaW5nX3RmX29wZW5haV9ncHRfdGVzdC5weQ==) | `93.85% <0%> (-0.88%)` | :arrow_down: |
| [transformers/tests/modeling\_tf\_gpt2\_test.py](https://codecov.io/gh/huggingface/transformers/pull/1853/diff?src=pr&el=tree#diff-dHJhbnNmb3JtZXJzL3Rlc3RzL21vZGVsaW5nX3RmX2dwdDJfdGVzdC5weQ==) | `93.85% <0%> (-0.88%)` | :arrow_down: |
| [transformers/tests/modeling\_tf\_xlm\_test.py](https://codecov.io/gh/huggingface/transformers/pull/1853/diff?src=pr&el=tree#diff-dHJhbnNmb3JtZXJzL3Rlc3RzL21vZGVsaW5nX3RmX3hsbV90ZXN0LnB5) | `94.35% <0%> (-0.81%)` | :arrow_down: |
| [transformers/tests/modeling\_tf\_roberta\_test.py](https://codecov.io/gh/huggingface/transformers/pull/1853/diff?src=pr&el=tree#diff-dHJhbnNmb3JtZXJzL3Rlc3RzL21vZGVsaW5nX3RmX3JvYmVydGFfdGVzdC5weQ==) | `74.4% <0%> (-0.8%)` | :arrow_down: |
| [transformers/tests/modeling\_tf\_xlnet\_test.py](https://codecov.io/gh/huggingface/transformers/pull/1853/diff?src=pr&el=tree#diff-dHJhbnNmb3JtZXJzL3Rlc3RzL21vZGVsaW5nX3RmX3hsbmV0X3Rlc3QucHk=) | `95.03% <0%> (-0.71%)` | :arrow_down: |
| [transformers/tests/modeling\_tf\_bert\_test.py](https://codecov.io/gh/huggingface/transformers/pull/1853/diff?src=pr&el=tree#diff-dHJhbnNmb3JtZXJzL3Rlc3RzL21vZGVsaW5nX3RmX2JlcnRfdGVzdC5weQ==) | `95.59% <0%> (-0.63%)` | :arrow_down: |
| ... and [2 more](https://codecov.io/gh/huggingface/transformers/pull/1853/diff?src=pr&el=tree-more) | |
------
[Continue to review full report at Codecov](https://codecov.io/gh/huggingface/transformers/pull/1853?src=pr&el=continue).
> **Legend** - [Click here to learn more](https://docs.codecov.io/docs/codecov-delta)
> `Δ = absolute <relative> (impact)`, `ø = not affected`, `? = missing data`
> Powered by [Codecov](https://codecov.io/gh/huggingface/transformers/pull/1853?src=pr&el=footer). Last update [0477b30...de3cb08](https://codecov.io/gh/huggingface/transformers/pull/1853?src=pr&el=lastupdated). Read the [comment docs](https://docs.codecov.io/docs/pull-request-comments).
|
transformers | 1,852 | closed | XLNet model params for Question answering | Model: XLNet for QA
model(parameters ...)
RuntimeError: CUDA error: device-side assert triggered | 11-16-2019 20:14:07 | 11-16-2019 20:14:07 | What parameters should I exactly pass for XLNet QA model?<|||||>More details would be nice but please check #1849 #1848 and #1805 . Also set `CUDA_LAUNCH_BLOCKING=1` environment variable to have more details about the error, from the CUDA.<|||||>Thanks. It worked after changing the input sequence limit to 128.<|||||>This issue has been automatically marked as stale because it has not had recent activity. It will be closed if no further activity occurs. Thank you for your contributions.
|
transformers | 1,851 | closed | The acc of RACE is always low by roberta model | ## ❓ Questions & Help
<!-- A clear and concise description of the question. -->
My scripts like follows:
python ./examples/run_multiple_choice.py \
--model_type roberta \
--task_name race \
--model_name_or_path roberta-base \
--do_train \
--do_eval \
--do_lower_case \
--do_test \
--data_dir $RACE_DIR \
--learning_rate 5e-5 \
--num_train_epochs 5 \
--max_seq_length 512 \
--output_dir ./race_base_1115 \
--per_gpu_eval_batch_size=2 \
--per_gpu_train_batch_size=2 \
--gradient_accumulation_steps 1 \
--overwrite_output_dir \
--logging_steps 1000\
--save_steps 1000\
--evaluate_during_training \
but my acc result is always under 30%, both test and evaluate :
eval_acc = 0.2833400891771382
eval_loss = 1.386294308898901
Maybe it be that the token length is too long? More than 3W input sentences' length is longer than 512. It would truncated context.
But this problem didn't happen in fairseq(original Roberta code). i wanna know why.
Ask for help. Thanks a lot. | 11-16-2019 16:20:58 | 11-16-2019 16:20:58 | Same.
model =roberta-base
total batch size=8
train num epochs=5
fp16 =False
max seq length =512
eval_acc = 0.29568242275424594
eval_loss = 1.3862943781258896
@spolu <|||||>This issue has been automatically marked as stale because it has not had recent activity. It will be closed if no further activity occurs. Thank you for your contributions.
<|||||>In my experience, it's because loss not getting down.
refer to fb repo issue https://github.com/pytorch/fairseq/issues/1114#issue-490144245
It seems that learning rate needs to be smaller and require larger batch size
Use the config below:
```
python ./examples/run_multiple_choice.py --model_type roberta --task_name race --model_name_or_path roberta-base-openai-detector --do_eval --data_dir $RACE_DIR --learning_rate 1e-5 --num_train_epochs 10 --max_seq_length 512 --output_dir ./roberta-base-openai-race --model_name_or_path ./roberta-base-openai-race/ --per_gpu_eval_batch_size=9 --per_gpu_train_batch_size=9 --gradient_accumulation_steps 2 --save_steps 4882 --eval_all_checkpoints --fp16 --seed 77 --do_lower_case
```
I can get
***** Eval results checkpoint-24410 is test:False *****
eval_acc = 0.6758747697974218
eval_loss = 0.9408586282721337
It‘s getting close, but still faraway from current result......<|||||>Thank you very much, I will try it.<|||||>This issue has been automatically marked as stale because it has not had recent activity. It will be closed if no further activity occurs. Thank you for your contributions.
|
transformers | 1,850 | closed | Can't run convert_roberta_original_pytorch_checkpoint_to_pytorch.py | ## ❓ Questions & Help
<!-- A clear and concise description of the question. -->
when i want to convert my model which prertrained in fairseq, the error like follows:
2019-11-16 23:53:48.119139: I tensorflow/core/platform/cpu_feature_guard.cc:142] Your CPU supports instructions that this TensorFlow binary was not compiled to use: AVX2 FMA
2019-11-16 23:53:48.148610: I tensorflow/core/platform/profile_utils/cpu_utils.cc:94] CPU Frequency: 2097740000 Hz
2019-11-16 23:53:48.151302: I tensorflow/compiler/xla/service/service.cc:168] XLA service 0x557dc08b8900 executing computations on platform Host. Devices:
2019-11-16 23:53:48.151342: I tensorflow/compiler/xla/service/service.cc:175] StreamExecutor device (0): Host, Default Version
loading archive file ./checkpoint_best.pt
extracting archive file ./checkpoint_best.pt to temp dir /tmp/tmpz46rlxeu
Traceback (most recent call last):
File "./transformers/convert_roberta_original_pytorch_checkpoint_to_pytorch.py", line 178, in <module>
args.classification_head
File "./transformers/convert_roberta_original_pytorch_checkpoint_to_pytorch.py", line 46, in convert_roberta_checkpoint_to_pytorch
roberta = FairseqRobertaModel.from_pretrained(roberta_checkpoint_path)
File "/home/liangchen/.conda/envs/py36lc/lib/python3.6/site-packages/fairseq/models/roberta/model.py", line 139, in from_pretrained
**kwargs,
File "/home/liangchen/.conda/envs/py36lc/lib/python3.6/site-packages/fairseq/hub_utils.py", line 33, in from_pretrained
model_path = file_utils.load_archive_file(model_name_or_path)
File "/home/liangchen/.conda/envs/py36lc/lib/python3.6/site-packages/fairseq/file_utils.py", line 80, in load_archive_file
with tarfile.open(resolved_archive_file, 'r:' + ext) as archive:
File "/home/liangchen/.conda/envs/py36lc/lib/python3.6/tarfile.py", line 1588, in open
raise CompressionError("unknown compression type %r" % comptype)
tarfile.CompressionError: unknown compression type 'pt'
Ask for help. Thanks a lot! | 11-16-2019 16:04:13 | 11-16-2019 16:04:13 | Hi! Do you succeed in loading your pre-trained model in Fairseq?<|||||>Yes, it work well in Fairseq~<|||||>My script:
python ./transformers/convert_roberta_original_pytorch_checkpoint_to_pytorch.py --roberta_checkpoint_path ./checkpoint_best.pt --pytorch_dump_folder_path ./race_base/roberta-convert-checkpoint/<|||||>you will need --classification-head to load the final layer i guess<|||||>Hi, I also come across this issue, have you solved it?<|||||>No, I give up :(<|||||>Hi, I found that --roberta_checkpoint_path should be ./ not ./checkpoint_best.pt<|||||>Is there a way to convert from a PyTorch bin (obtained with Huggin Face) to a Roberta model? When I run this script:
```
from fairseq.models.roberta import RobertaModel
roberta= RortaModel.from_pretrained('/path/to/checkpoint/folder/',checkpoint_file='pytorch_model.bin')
```
I got this error:
```
File "/usr/local/lib/python3.6/dist-packages/fairseq/checkpoint_utils.py", line 162, in load_checkpoint_to_cpu
args = state["args"]
KeyError: 'args'
```
I think I have the opposite problem, but I don't find a script for this.<|||||>@paulthemagno https://github.com/pytorch/fairseq/issues/1514#issuecomment-567934059<|||||>This issue has been automatically marked as stale because it has not had recent activity. It will be closed if no further activity occurs. Thank you for your contributions.
<|||||>`--roberta_checkpoint_path` should be path to folder with checkpoint named as model.pt.
I am getting:
```
Traceback (most recent call last):
File "convert_roberta_original_pytorch_checkpoint_to_pytorch.py", line 176, in <module>
convert_roberta_checkpoint_to_pytorch(
File "convert_roberta_original_pytorch_checkpoint_to_pytorch.py", line 54, in convert_roberta_checkpoint_to_pytorch
roberta = FairseqRobertaModel.from_pretrained(roberta_checkpoint_path)
File "venv/lib/python3.8/site-packages/fairseq/models/roberta/model.py", line 244, in from_pretrained
x = hub_utils.from_pretrained(
File "venv/lib/python3.8/site-packages/fairseq/hub_utils.py", line 70, in from_pretrained
models, args, task = checkpoint_utils.load_model_ensemble_and_task(
File "venv/lib/python3.8/site-packages/fairseq/checkpoint_utils.py", line 279, in load_model_ensemble_and_task
state = load_checkpoint_to_cpu(filename, arg_overrides)
File "venv/lib/python3.8/site-packages/fairseq/checkpoint_utils.py", line 231, in load_checkpoint_to_cpu
setattr(args, arg_name, arg_val)
AttributeError: 'NoneType' object has no attribute 'bpe'
```<|||||>@djstrong I'm getting the same issue. were you able to get around this problem? |
transformers | 1,849 | closed | run_finetuning resize token embeddings | Please see #1848 | 11-16-2019 14:49:39 | 11-16-2019 14:49:39 | # [Codecov](https://codecov.io/gh/huggingface/transformers/pull/1849?src=pr&el=h1) Report
> Merging [#1849](https://codecov.io/gh/huggingface/transformers/pull/1849?src=pr&el=desc) into [master](https://codecov.io/gh/huggingface/transformers/commit/0477b307c7501ea76e01b03cb387a2312db752b3?src=pr&el=desc) will **not change** coverage.
> The diff coverage is `n/a`.
[](https://codecov.io/gh/huggingface/transformers/pull/1849?src=pr&el=tree)
```diff
@@ Coverage Diff @@
## master #1849 +/- ##
=======================================
Coverage 84.08% 84.08%
=======================================
Files 97 97
Lines 14316 14316
=======================================
Hits 12037 12037
Misses 2279 2279
```
------
[Continue to review full report at Codecov](https://codecov.io/gh/huggingface/transformers/pull/1849?src=pr&el=continue).
> **Legend** - [Click here to learn more](https://docs.codecov.io/docs/codecov-delta)
> `Δ = absolute <relative> (impact)`, `ø = not affected`, `? = missing data`
> Powered by [Codecov](https://codecov.io/gh/huggingface/transformers/pull/1849?src=pr&el=footer). Last update [0477b30...a76db2e](https://codecov.io/gh/huggingface/transformers/pull/1849?src=pr&el=lastupdated). Read the [comment docs](https://docs.codecov.io/docs/pull-request-comments).
<|||||>Looks good to me, thanks for looking into it!<|||||>You are welcome and thanks @LysandreJik for interested. Do you mind approve and merge, if there is no problem? |
transformers | 1,848 | closed | CUDA runtime error (59) : device-side assert triggered | Hi,
When you run `run_lm_finetuning.py` with a custom tokenizer via `--tokenizer_name tokenizer` parameter, you need to resize model embedding according to the new tokenizer. Otherwise you are going to get `CUDA runtime error (59) : device-side assert triggered` or `Assertion 'srcIndex < srcSelectDimSize' failed`.
I fixed this issue and going to create a PR if it's okay?
Thanks! | 11-16-2019 14:37:49 | 11-16-2019 14:37:49 | Indeed, this seems to be a problem. Thanks for the PR!<|||||>fixed at #1849 |
transformers | 1,847 | closed | Update modeling_utils.py by adding "DUMMY_INPUTS" after "logger" variable. | This Pull Request fixes a probable bug found using Transformers in Anaconda, using TFBertForSequenceClassification, Tensorflow 2.0.0b0, according to:
https://github.com/huggingface/transformers/issues/1810#issuecomment-554572471
| 11-16-2019 14:34:21 | 11-16-2019 14:34:21 | # [Codecov](https://codecov.io/gh/huggingface/transformers/pull/1847?src=pr&el=h1) Report
> Merging [#1847](https://codecov.io/gh/huggingface/transformers/pull/1847?src=pr&el=desc) into [master](https://codecov.io/gh/huggingface/transformers/commit/0477b307c7501ea76e01b03cb387a2312db752b3?src=pr&el=desc) will **increase** coverage by `<.01%`.
> The diff coverage is `100%`.
[](https://codecov.io/gh/huggingface/transformers/pull/1847?src=pr&el=tree)
```diff
@@ Coverage Diff @@
## master #1847 +/- ##
==========================================
+ Coverage 84.08% 84.08% +<.01%
==========================================
Files 97 97
Lines 14316 14317 +1
==========================================
+ Hits 12037 12038 +1
Misses 2279 2279
```
| [Impacted Files](https://codecov.io/gh/huggingface/transformers/pull/1847?src=pr&el=tree) | Coverage Δ | |
|---|---|---|
| [transformers/modeling\_utils.py](https://codecov.io/gh/huggingface/transformers/pull/1847/diff?src=pr&el=tree#diff-dHJhbnNmb3JtZXJzL21vZGVsaW5nX3V0aWxzLnB5) | `89.67% <100%> (+0.02%)` | :arrow_up: |
------
[Continue to review full report at Codecov](https://codecov.io/gh/huggingface/transformers/pull/1847?src=pr&el=continue).
> **Legend** - [Click here to learn more](https://docs.codecov.io/docs/codecov-delta)
> `Δ = absolute <relative> (impact)`, `ø = not affected`, `? = missing data`
> Powered by [Codecov](https://codecov.io/gh/huggingface/transformers/pull/1847?src=pr&el=footer). Last update [0477b30...07bda77](https://codecov.io/gh/huggingface/transformers/pull/1847?src=pr&el=lastupdated). Read the [comment docs](https://docs.codecov.io/docs/pull-request-comments).
<|||||>I think this bug is already fixed on master.<|||||>It should be fixed the latest release (2.2.1).
Feel free to reopen if it's not the case. |
transformers | 1,846 | closed | fix summary_type value of SequenceSummary | from #1845
| 11-16-2019 09:48:56 | 11-16-2019 09:48:56 | # [Codecov](https://codecov.io/gh/huggingface/transformers/pull/1846?src=pr&el=h1) Report
> Merging [#1846](https://codecov.io/gh/huggingface/transformers/pull/1846?src=pr&el=desc) into [master](https://codecov.io/gh/huggingface/transformers/commit/0477b307c7501ea76e01b03cb387a2312db752b3?src=pr&el=desc) will **not change** coverage.
> The diff coverage is `100%`.
[](https://codecov.io/gh/huggingface/transformers/pull/1846?src=pr&el=tree)
```diff
@@ Coverage Diff @@
## master #1846 +/- ##
=======================================
Coverage 84.08% 84.08%
=======================================
Files 97 97
Lines 14316 14316
=======================================
Hits 12037 12037
Misses 2279 2279
```
| [Impacted Files](https://codecov.io/gh/huggingface/transformers/pull/1846?src=pr&el=tree) | Coverage Δ | |
|---|---|---|
| [transformers/modeling\_utils.py](https://codecov.io/gh/huggingface/transformers/pull/1846/diff?src=pr&el=tree#diff-dHJhbnNmb3JtZXJzL21vZGVsaW5nX3V0aWxzLnB5) | `89.64% <100%> (ø)` | :arrow_up: |
------
[Continue to review full report at Codecov](https://codecov.io/gh/huggingface/transformers/pull/1846?src=pr&el=continue).
> **Legend** - [Click here to learn more](https://docs.codecov.io/docs/codecov-delta)
> `Δ = absolute <relative> (impact)`, `ø = not affected`, `? = missing data`
> Powered by [Codecov](https://codecov.io/gh/huggingface/transformers/pull/1846?src=pr&el=footer). Last update [0477b30...d08a338](https://codecov.io/gh/huggingface/transformers/pull/1846?src=pr&el=lastupdated). Read the [comment docs](https://docs.codecov.io/docs/pull-request-comments).
<|||||>Yes, thanks! |
transformers | 1,845 | closed | summary_type value of SequenceSummary is incorrect | https://github.com/huggingface/transformers/blob/0477b307c7501ea76e01b03cb387a2312db752b3/transformers/modeling_utils.py#L731
I think `if hasattr(config, 'summary_use_proj')` is incorrect, `if hasattr(config, 'summary_type')` is correct. | 11-16-2019 09:46:44 | 11-16-2019 09:46:44 | Thanks! |
transformers | 1,844 | closed | Rebase and merge Louismartin/camembert | 11-16-2019 05:10:53 | 11-16-2019 05:10:53 | Please, continue the comments [here](https://github.com/huggingface/transformers/pull/1822#issuecomment-558095282)
> Great work!
>
> Could you show how to get the embedding vector of a sentence please?
>
> ```python
> from transformers import CamembertTokenizer
> import torch
>
> camembert_tokenizer = CamembertTokenizer.from_pretrained("camembert-base")
>
> camembert_tokenizer.encode("Salut, ça va ?") # How to get embedding of this sentence not just the ids of tokens ?
> ``` |
|
transformers | 1,843 | closed | "This tokenizer does not make use of special tokens." warning | ## ❓ Questions & Help
<!-- A clear and concise description of the question. -->
I just updated to the latest version transformers. Now when I use tokenizer to encode word, it always show the warning "This tokenizer does not make use of special tokens."
Is there any way to hide that warning? Thank you. | 11-15-2019 20:06:32 | 11-15-2019 20:06:32 | Same here. Is there any way to suppress this warning? I use `run_lm_finetuning.py` to finetune distilgpt2 and it outputs thousands "This tokenizer does not make use of special tokens.". It's so annoying :(<|||||>Here's how to suppress the warnings until this is fixed:
```py
import logging
logging.getLogger('transformers.tokenization_utils').setLevel(logging.ERROR)
```<|||||>> Here's how to suppress the warnings until this is fixed:
>
> ```python
> import logging
> logging.getLogger('transformers.tokenization_utils').disabled = True
> ```
Thank you!<|||||>Is this fixed? If not, I think it should be open until it's been fixed.<|||||>This has been fixed on the master and in the latest release (2.2.1)<|||||>Hi, I use the latest release but I still have this problem.<|||||>@iedmrc
I close it because the 'log' method works. I don't know whether it's a bug or not.<|||||>Hi @yeliu918, could you please show us what you obtain when running this script in your environment?
```py
from transformers import GPT2Tokenizer, __version__
print(__version__)
tokenizer = GPT2Tokenizer.from_pretrained("gpt2")
print(tokenizer.encode("What does this output?"))
```<|||||>I am getting warning despite trying everything mentioned above....
```
import torch
from transformers import GPT2LMHeadModel, GPT2Tokenizer, __version__
import logging
tokenizer = GPT2Tokenizer.from_pretrained("gpt2")
print(__version__)
logging.getLogger('transformers.tokenization_utils').disabled = True
tokens_tensor = torch.tensor([tokenizer.encode("some example sentence")])
greedy_output = model.generate(tokens_tensor, max_length=60, num_beams=16)
```
Version 2.8.0
Setting `pad_token_id` to 50256 (first `eos_token_id`) to generate sequence |
transformers | 1,842 | closed | xlm-mlm-17-1280 model masked word prediction | Hi
I would like some help with how to use pretrained xlm-mlm-17-1280 model to get predictions for masked word prediction. I have followed http://mayhewsw.github.io/2019/01/16/can-bert-generate-text/ for BERT mask prediction and it is working. Could you help me with how to use xlm-mlm-17-1280 model for word prediction. I need to get prediction for Turkish Language which is one of the languages in 17 languages | 11-15-2019 17:41:37 | 11-15-2019 17:41:37 | Would it be possible to use XML-R #1769 ? Its model has a simple description ( `Masked Language Models` in chapter 3) and is similar to BERT-Base besides tokenization, training configuration and language embeddings.<|||||>Hi
Thanks for the advice but idk if the model you mentioned has a pretrained one for Turkish because I need to use it for Turkish. Also it is kind of a need for me to use the model I asked for prediction. Any tips on how I could use that model for getting masked word prediction would be great. Thanks in advance<|||||>There are also multilingual, pretrained models for BERT, which we could try. Usually the quality decreases in large, multilingual models with very different languages.
But they have mostly the similar architecture like `bert-base`, so we could try to rerun the linked example with the line `modelpath = "bert-base-multilingual-cased"`.<|||||>I get the following warning and error when trying modelpath = "bert-base-multilingual-cased":
Sorry I am not familiar with the transformers so it may be an easy error to fix but Idk how
The pre-trained model you are loading is a cased model but you have not set `do_lower_case` to False. We are setting `do_lower_case=False` for you but you may want to check this behavior.
Traceback (most recent call last):
File "e.py", line 13, in <module>
masked_index = tokenized_text.index(target)
ValueError: 'hungry' is not in list
<|||||>'hungry' is in the list, but as two tokens since the multilingual model has a different vocabulary. Therefore, we have to tokenize the target word. Check this out:
```
#!/usr/bin/python3
#
# first Axiom: Aaron Swartz is everything
# second Axiom: The Schwartz Space is his discription of physical location
# first conclusion: His linear symmetry is the Fourier transform
# second conclusion: His location is the Montel space
# Third conclusion: His location is the Fréchet space
import torch
from transformers import BertModel, BertTokenizer, BertForMaskedLM
modelname = "bert-base-multilingual-cased"
tokenizer = BertTokenizer.from_pretrained(modelname)
model = BertModel.from_pretrained(modelname)
def predictMask(maskedText, masked_index):
# Convert token to vocabulary indices
indexed_tokens = tokenizer.convert_tokens_to_ids(maskedText)
# Define sentence A and B indices associated to 1st and 2nd sentences (see paper)
segments_ids = [1] * len(maskedText)
# Convert inputs to PyTorch tensors
tokens_tensor = torch.tensor([indexed_tokens])
segments_tensors = torch.tensor([segments_ids])
# Load pre-trained model (weights)
model = BertForMaskedLM.from_pretrained(modelname)
model.eval()
# Predict all tokens
predictions = model(tokens_tensor, segments_tensors)
predicted_index = torch.argmax(predictions[0][0][masked_index]).item()
predicted_token = tokenizer.convert_ids_to_tokens([predicted_index])
print("Original:", text)
print("Masked:", " ".join(maskedText))
print("Predicted token:", predicted_token)
maskedText[masked_index] = predicted_token[0]
# delete this section for faster inference
print("Other options:")
# just curious about what the next few options look like.
for i in range(10):
predictions[0][0][masked_index][predicted_index] = -11100000
predicted_index = torch.argmax(predictions[0][0][masked_index]).item()
predicted_token = tokenizer.convert_ids_to_tokens([predicted_index])
print(predicted_token)
print("Masked, tokenized text with the prediction:", maskedText)
return maskedText
text = "let´s go fly a kite!"
target = "kite"
tokenized_text = tokenizer.tokenize(text)
tokenized_target = tokenizer.tokenize(target)
print("tokenized text:", tokenized_text)
print("tokenized target:", tokenized_target)
# Mask a token that we will try to predict back with `BertForMaskedLM`
masked_index = tokenized_text.index(tokenized_target[0])
for i in range(len(tokenized_target)):
tokenized_text[masked_index+i] = '[MASK]'
for i in range(len(tokenized_target)):
tokenized_text = predictMask(tokenized_text, masked_index+i)
```<|||||>I tried the code but it's giving word pieces suggestions, not whole word. And the suggestions are poor. Thank you so much for your effort but this is not useful for me unless somehow I could get whole word suggestions. Also, I am still seeking for an implementation of xlm model to get prediction, of anyone could help, that would be great<|||||>Don't the pieces build complete words in the end?
Read my first answer for XML, the mentioned model supports the turkish language.<|||||>Hi, you can predict a masked word with XLM as you would do with any other MLM-based model. Here's an example using the checkpoint `xlm-mlm-17-1280` you mentioned:
```py
from transformers import XLMTokenizer, XLMWithLMHeadModel
import torch
# load tokenizer
tokenizer = XLMTokenizer.from_pretrained("xlm-mlm-17-1280")
# encode sentence with a masked token in the middle
sentence = torch.tensor([tokenizer.encode("This was the first time Nicolas ever saw a " + tokenizer.mask_token + ". It was huge.")])
# Identify the masked token position
masked_index = torch.where(sentence == tokenizer.mask_token_id)[1].tolist()[0]
# Load model
model = XLMWithLMHeadModel.from_pretrained("xlm-mlm-17-1280")
# Get the five top answers
result = model(sentence)
result = result[0][:, masked_index].topk(5).indices
result = result.tolist()[0]
print(tokenizer.decode(result))
# monster dragon snake wolf tiger
```<|||||>Thank you so much guys for the replies, they been very helpfull. |
transformers | 1,841 | closed | BPE error when fine-tuning a CTRL model | Hi,
cc @keskarnitish
I'm using a slightly modified version of the exapmles/run_lm_finetuning.py code to fine-tune a CTRL model and getting this BPE warning:
```
WARNING - transformers.tokenization_ctrl - Saving vocabulary to /home/ubuntu/data/ctrl/pytrained4/merges.txt: BPE merge indices are not consecutive. Please check that the tokenizer is not corrupted!
```
Is this something I should be concerned about?
| 11-15-2019 16:27:41 | 11-15-2019 16:27:41 | Can you post the code for the `run_lm_finetuning`? I am not as familiar with what this error message might entail. <|||||>Sure. Here it is.
[run_ctrl_finetuning.py.zip](https://github.com/huggingface/transformers/files/3852748/run_ctrl_finetuning.py.zip)
<|||||>This issue has been automatically marked as stale because it has not had recent activity. It will be closed if no further activity occurs. Thank you for your contributions.
|
transformers | 1,840 | closed | Sampling sequence generator for transformers | [@thomwolf version]: In this PR I introduce a flexible API to generate sequences for the models of the library provided with LM heads.
The API is designed to have a very flexible and quite exhaustive API:
- with/without a prompt
- with/without beam search
- with/without greedy decoding/sampling
- with any (and combination) of top-k/top-p/penalized repetitions
Only single-stack architectures are currently supported.
Here is an example generating 4 continuations of a prompt with using a beam search of 3 for each continuation and token sampling at each step with top-p filtering and repetition penalization until we reach a <unk> token or a max length of 50.
```python
import torch
from transformers import OpenAIGPTLMHeadModel, OpenAIGPTTokenizer
tokenizer = OpenAIGPTTokenizer.from_pretrained('openai-gpt')
model = OpenAIGPTLMHeadModel.from_pretrained('openai-gpt')
input_ids = tokenizer.encode("This is the", return_tensors='pt')
output = model.generate(input_ids,
max_length=50,
do_sample=True,
num_beams=3,
temperature=0.7,
top_k=0,
top_p=0.8,
repetition_penalty=1.4,
bos_token_id=None,
pad_token_id=None,
eos_token_ids=tok.unk_token_id,
batch_size=None,
length_penalty=None,
num_return_sequences=4)[0]
for j in range(len(output)):
print(tok.decode(output[j].tolist()))
```
To-add features:
- Add support for encoder-decoder architectures
- Add support for past and cached states | 11-15-2019 09:59:45 | 11-15-2019 09:59:45 | # [Codecov](https://codecov.io/gh/huggingface/transformers/pull/1840?src=pr&el=h1) Report
> Merging [#1840](https://codecov.io/gh/huggingface/transformers/pull/1840?src=pr&el=desc) into [master](https://codecov.io/gh/huggingface/transformers/commit/8618bf15d6edc8774cedc0aae021d259d89c91fc?src=pr&el=desc) will **increase** coverage by `0.32%`.
> The diff coverage is `13.93%`.
[](https://codecov.io/gh/huggingface/transformers/pull/1840?src=pr&el=tree)
```diff
@@ Coverage Diff @@
## master #1840 +/- ##
==========================================
+ Coverage 78.91% 79.23% +0.32%
==========================================
Files 131 131
Lines 19450 19680 +230
==========================================
+ Hits 15348 15593 +245
+ Misses 4102 4087 -15
```
| [Impacted Files](https://codecov.io/gh/huggingface/transformers/pull/1840?src=pr&el=tree) | Coverage Δ | |
|---|---|---|
| [transformers/tokenization\_utils.py](https://codecov.io/gh/huggingface/transformers/pull/1840/diff?src=pr&el=tree#diff-dHJhbnNmb3JtZXJzL3Rva2VuaXphdGlvbl91dGlscy5weQ==) | `92.1% <ø> (ø)` | :arrow_up: |
| [transformers/configuration\_utils.py](https://codecov.io/gh/huggingface/transformers/pull/1840/diff?src=pr&el=tree#diff-dHJhbnNmb3JtZXJzL2NvbmZpZ3VyYXRpb25fdXRpbHMucHk=) | `93.75% <100%> (+0.89%)` | :arrow_up: |
| [transformers/configuration\_xlm.py](https://codecov.io/gh/huggingface/transformers/pull/1840/diff?src=pr&el=tree#diff-dHJhbnNmb3JtZXJzL2NvbmZpZ3VyYXRpb25feGxtLnB5) | `96.42% <100%> (+0.13%)` | :arrow_up: |
| [transformers/modeling\_xlm.py](https://codecov.io/gh/huggingface/transformers/pull/1840/diff?src=pr&el=tree#diff-dHJhbnNmb3JtZXJzL21vZGVsaW5nX3hsbS5weQ==) | `86.49% <11.11%> (-1.81%)` | :arrow_down: |
| [transformers/modeling\_xlnet.py](https://codecov.io/gh/huggingface/transformers/pull/1840/diff?src=pr&el=tree#diff-dHJhbnNmb3JtZXJzL21vZGVsaW5nX3hsbmV0LnB5) | `73.72% <12.5%> (+1.51%)` | :arrow_up: |
| [transformers/modeling\_encoder\_decoder.py](https://codecov.io/gh/huggingface/transformers/pull/1840/diff?src=pr&el=tree#diff-dHJhbnNmb3JtZXJzL21vZGVsaW5nX2VuY29kZXJfZGVjb2Rlci5weQ==) | `27.9% <16.66%> (+1.98%)` | :arrow_up: |
| [transformers/modeling\_utils.py](https://codecov.io/gh/huggingface/transformers/pull/1840/diff?src=pr&el=tree#diff-dHJhbnNmb3JtZXJzL21vZGVsaW5nX3V0aWxzLnB5) | `64.29% <5.78%> (-28.74%)` | :arrow_down: |
| [transformers/modeling\_transfo\_xl.py](https://codecov.io/gh/huggingface/transformers/pull/1840/diff?src=pr&el=tree#diff-dHJhbnNmb3JtZXJzL21vZGVsaW5nX3RyYW5zZm9feGwucHk=) | `75.89% <80%> (-0.01%)` | :arrow_down: |
| [transformers/pipelines.py](https://codecov.io/gh/huggingface/transformers/pull/1840/diff?src=pr&el=tree#diff-dHJhbnNmb3JtZXJzL3BpcGVsaW5lcy5weQ==) | `67.94% <0%> (+0.58%)` | :arrow_up: |
| ... and [6 more](https://codecov.io/gh/huggingface/transformers/pull/1840/diff?src=pr&el=tree-more) | |
------
[Continue to review full report at Codecov](https://codecov.io/gh/huggingface/transformers/pull/1840?src=pr&el=continue).
> **Legend** - [Click here to learn more](https://docs.codecov.io/docs/codecov-delta)
> `Δ = absolute <relative> (impact)`, `ø = not affected`, `? = missing data`
> Powered by [Codecov](https://codecov.io/gh/huggingface/transformers/pull/1840?src=pr&el=footer). Last update [8618bf1...f86ed23](https://codecov.io/gh/huggingface/transformers/pull/1840?src=pr&el=lastupdated). Read the [comment docs](https://docs.codecov.io/docs/pull-request-comments).
<|||||>Notes:
1. XLM's CLM model gives good results for only one of the languages.
2. Although using XLM's MLM pretrained weights + a mask token at the end of sequence could have worked, it doesn't give sequences that make sense. I think we should instead display a warning when the user attempts to use a mlm.<|||||>Ok this looks good! It's quite a change to what we were doing previously, which was hosting all the generation logic inside the script. It may have become a bit too cluttered as models with different behaviors and attributes were added, so I believe this is a welcome change.
This also means that we're adding a level of abstraction for the user; it does not showcase the way the models should be used for generations with different inputs anymore. This is fine with me but I think we need to add some additional snippets showcasing how the models should be used when doing generation, perhaps in https://huggingface.co/transformers/quickstart.html?<|||||>Do you mean exposing the logic inside `SamplerXLM` and `SamplerXLNet`?<|||||>I think moving some decoding in the core library is a good idea.
Though I have doubts on a few aspects of this PR:
- the functional approach currently proposed for implementing this feature is very different from the way the rest of the library is designed, and
- having to maintain another location (`generation/sampler`) with model-specific peculiarities adds burden when implementing a new model and is not really the way we are trying to take right now (simplifying the addition of new models).
I'll try to think a little bit about how we could move this PR closer to the current and future directions of the library and come back with proposals.<|||||>> * the functional approach currently proposed for implementing this feature is very different from the way the rest of the library is designed, and
I agree it looks different from most of the code in the library, but I am no sure what you mean by functional. The code could not get a lot more OO than this. Plus I think it is very legible as is.
> * having to maintain another location (`generation/sampler`) with model-specific peculiarities adds burden when implementing a new model and is not really the way we are trying to take right now (simplifying the addition of new models).
Let's consider for a second what happens when we add model X to the library and X could be used to generate sequences.
1. X does not require specific pre-treatment or input, computed masks etc at each forward pass in which case we have nothing to do and it is supported out of the box (`SamplerSingleStack`).
2. X does require some specific preprocessing before each forward pass (hooks on the forward pass if you wish), you subclass `SamplerSingleStack` and reference it in the factory.
3. You're done
Taking a step back I don't think it is a great idea to add model-specific logic in `generate` either (this would belong in a more battery-included library). What we could do, however, is move `SamplerSingleStack` subclasses (`SamplerFroXLM` and `SamplerForXLNet`) in the example script. I am fine with keeping the `SamplerSingleStack` and the future `SamplerEncoderDecoder` there as they are very general.
And yes, please give me suggestions to future-proof the PR as I currently have little visibility on this.<|||||>After some thoughts, here is a proposal for re-writing the user experience.
Context:
- I'm ok with adding abstractions as long as they are used internally. For user-facing abstraction like here, we need a really strong reason to increase the learning curve for the user and I don't see one here.
- the second important element is to reduce the burden for us (and increasingly the community) of updating many things at many places when adding a new model (currently already too high).
Proposal:
- let's have the decoding logic be accessed by the user by calling a (new) `decode` method of `PretrainedModel` instead of a new class (so no new abstraction).
- a Decoder can be added to the model at instantiation (in the `PretrainedModel` class `__init__`), probably only if `get_output_embeddings` is not None (i.e. with have a model with a LM head of some kind), and selected with a configuration parameter (to add to `PretrainedConfig`).
- a specific `_prepare_input_for_decoding` method (can find a better name) can be in charge of preparing the inputs for decoding and overridden in the specific model classes with model-specific preprocessing if needed (ex in XLM and XLNet). This way all the specificities of the model architectures are in the model class. This method should also take care of PyTorch/TF 2.0 specificities (currently the decoding classes are only PyTorch specific). I think the samplers can even be in `model_utils.py` if they are PyTorch specific or in another file if they are framework agnostic.
Tell me if it's not clear, I can detail further.
Question:
- if we have a decoder with trained parameters in the future, we'll want to have the `decode` method behind the `forward` method to catch all PyTorch hooks (like in [XLM](https://github.com/facebookresearch/XLM/blob/master/src/model/transformer.py#L320-L330) for instance). Probably for now we can just not worry about that.<|||||>Clear for now, but the devil is in the details so I'll probably ask for clarification later.<|||||>I followed your idea to move the logic closer to the models, and simplified the implementation a little bit. As a result, the `sampler.py` only contains the `Sampler` and `SamplerSingleStack` class (`SamplerEncoderDecoder` coming, slightly different from the single stack case as we only want to pass through the encoder once). Here is a summary of changes:
1. Removed model-specific classes in the `generate` module. It is much nicer.
2. Added a `decode` and a `_prepare_input_for_decoding` method to `PretrainedModel`. By default, the first raises a `NotImplementedError`, the second one returns a dictionary `{"input_ids": input_ids}`
3. Overrided `decode` in `GPT2LMHeadModel,`OpenAIGPTLMHeadModel`,` XLNetLMHeadModel`, `TransfoXLLMHeadModel`, `CTRLLMHeadModel`, `XLMWithLMHeadModel`; If the user tries to initiate a sampler with another model, they will be greated with an explanatory error message.
As a result the design feels nicer and easier to maintain & to use.<|||||>I needed to add Encoder-Decoder support as a backup to the beam search in `example-summarization`, so I went on with it.
Adding encoder-decoder support with the previous changes was a breeze: I added a `decode` and an `encoder` method to the `PreTrainedEncoderDecoder` class and added a very simple `SamplerEncoderDecoder` class. I also moved the preparation of the encoder/decoder kwargs into a new method (which can thus be overriden).
Routing the factory function is based on the presence/absence of the `decode` and `encode` methods in the respective classes.
Improvements for another PR:
- Support batch size > 1
- Support num_samples > 1
@thomwolf I believe I implemented a simpler version of your recommendations. Let me know if there is something that I did not understand correctly.<|||||>Hi @thomwolf could you tell me if the changes (to the single-stack case) I just made are in the spirit of your proposal? I decided to remove the `SamplerSingleStack` class that doesn't really have a purpose now and `SamplerEncoderDecoder` will have the same fate. All the decoding logic is in the `decode` function in `PreTrainedModel`.
With this implementation I don't think we do need a `Sampler` class anymore. We can just have a collection of functions (its current methods) and pass the configuration as a dictionary. It is simpler, and makes `decode` truly indempotent. What do you think?
It am still afraid the `decode` function is going to become a big plate of spaghetti when we add new methods, but maybe there is a way to prevent that from happening?
(there may be a few errors here and there, I just quickly drafted the new implementation).<|||||>@thomwolf I followed your API recommendations. As a result:
- The `Sampler` class has been moved to `modeling_utils.py`. `SingleStackSampler` an `EncoderDecoderSampler` are not needed anymore so I removed them. I thus also removed the `generate` folder;
- `PreTrainedModel` and `PreTrainedEncoderDecoder` now have a `decode` method that raises an error if the model (decoder) does not have a `get_output_embeddings` method and raises an warning if the model is not on the same device as the prompt;
- I added a `get_output_embeddings` method to `TransfoXLLMHeadModel` **please check as I did not take the time to dive in the intricacies of adaptive softmax**
- I initialize `Sampler` each time `decode` is called; it is simpler than initializing it at the same time as the model and incurs little to no overhead;
- I add a `_prepare_inputs_for_decoding` method to `PreTrainedModel` that is overridden for every model that needs it;
- The examples in `run_generation.py` have been updated to reflect the API change. I ran the script for every model;
- I updated all tests;
- I documented the `decode` methods;
- `device` is only needed to check that the decoder and prompt are on the same device. It is not needed in the `Sampler` class at all, so I removed it there.
- I squashed my commits and rebased on master. |
transformers | 1,839 | closed | Add support for Japanese BERT models by cl-tohoku | This PR adds new BERT models for Japanese text.
Details of the models can be found in [this repository](https://github.com/cl-tohoku/bert-japanese).
Since the way of tokenization is Japanese-specific, a new file is added for the tokenizers.
And, the locations of the files (models, configs, etc.) should be updated when the files are uploaded to S3. (How could I do this?)
| 11-15-2019 08:42:48 | 11-15-2019 08:42:48 | This looks great @singletongue. For the file upload, I will send you a way to upload to our S3, early next week, if that works for you.<|||||>That will be great. Thank you!<|||||>I could try it on my side, it seems to work well!
I don't believe I've seen any benchmark on Japanese tasks in your repository, how did you evaluate the models? Did you use perplexity on portions of the Japanese wikipedia?<|||||># [Codecov](https://codecov.io/gh/huggingface/transformers/pull/1839?src=pr&el=h1) Report
> Merging [#1839](https://codecov.io/gh/huggingface/transformers/pull/1839?src=pr&el=desc) into [master](https://codecov.io/gh/huggingface/transformers/commit/6a73382706ce3c6905023872f63a680f0eb419a4?src=pr&el=desc) will **decrease** coverage by `0.19%`.
> The diff coverage is `61.73%`.
[](https://codecov.io/gh/huggingface/transformers/pull/1839?src=pr&el=tree)
```diff
@@ Coverage Diff @@
## master #1839 +/- ##
=========================================
- Coverage 80.07% 79.87% -0.2%
=========================================
Files 112 114 +2
Lines 16867 17058 +191
=========================================
+ Hits 13506 13625 +119
- Misses 3361 3433 +72
```
| [Impacted Files](https://codecov.io/gh/huggingface/transformers/pull/1839?src=pr&el=tree) | Coverage Δ | |
|---|---|---|
| [transformers/configuration\_bert.py](https://codecov.io/gh/huggingface/transformers/pull/1839/diff?src=pr&el=tree#diff-dHJhbnNmb3JtZXJzL2NvbmZpZ3VyYXRpb25fYmVydC5weQ==) | `87.09% <ø> (ø)` | :arrow_up: |
| [transformers/modeling\_bert.py](https://codecov.io/gh/huggingface/transformers/pull/1839/diff?src=pr&el=tree#diff-dHJhbnNmb3JtZXJzL21vZGVsaW5nX2JlcnQucHk=) | `87.75% <ø> (ø)` | :arrow_up: |
| [transformers/modeling\_tf\_bert.py](https://codecov.io/gh/huggingface/transformers/pull/1839/diff?src=pr&el=tree#diff-dHJhbnNmb3JtZXJzL21vZGVsaW5nX3RmX2JlcnQucHk=) | `96.31% <100%> (ø)` | :arrow_up: |
| [transformers/tokenization\_auto.py](https://codecov.io/gh/huggingface/transformers/pull/1839/diff?src=pr&el=tree#diff-dHJhbnNmb3JtZXJzL3Rva2VuaXphdGlvbl9hdXRvLnB5) | `44.18% <33.33%> (-0.82%)` | :arrow_down: |
| [...nsformers/tests/tokenization\_bert\_japanese\_test.py](https://codecov.io/gh/huggingface/transformers/pull/1839/diff?src=pr&el=tree#diff-dHJhbnNmb3JtZXJzL3Rlc3RzL3Rva2VuaXphdGlvbl9iZXJ0X2phcGFuZXNlX3Rlc3QucHk=) | `53.84% <53.84%> (ø)` | |
| [transformers/tokenization\_bert\_japanese.py](https://codecov.io/gh/huggingface/transformers/pull/1839/diff?src=pr&el=tree#diff-dHJhbnNmb3JtZXJzL3Rva2VuaXphdGlvbl9iZXJ0X2phcGFuZXNlLnB5) | `67.41% <67.41%> (ø)` | |
| [transformers/tests/utils.py](https://codecov.io/gh/huggingface/transformers/pull/1839/diff?src=pr&el=tree#diff-dHJhbnNmb3JtZXJzL3Rlc3RzL3V0aWxzLnB5) | `93.54% <83.33%> (+2.24%)` | :arrow_up: |
| [transformers/tokenization\_bert.py](https://codecov.io/gh/huggingface/transformers/pull/1839/diff?src=pr&el=tree#diff-dHJhbnNmb3JtZXJzL3Rva2VuaXphdGlvbl9iZXJ0LnB5) | `96.38% <0%> (+0.45%)` | :arrow_up: |
------
[Continue to review full report at Codecov](https://codecov.io/gh/huggingface/transformers/pull/1839?src=pr&el=continue).
> **Legend** - [Click here to learn more](https://docs.codecov.io/docs/codecov-delta)
> `Δ = absolute <relative> (impact)`, `ø = not affected`, `? = missing data`
> Powered by [Codecov](https://codecov.io/gh/huggingface/transformers/pull/1839?src=pr&el=footer). Last update [6a73382...0f53007](https://codecov.io/gh/huggingface/transformers/pull/1839?src=pr&el=lastupdated). Read the [comment docs](https://docs.codecov.io/docs/pull-request-comments).
<|||||>At the moment, there isn't an official publication regarding this work yet.
As a simple experiment, I applied the models to a Japanese document classification task using [livedoor news corpus](https://www.rondhuit.com/download.html#ldcc).
It is a 9-class classification, and I've confirmed that some of our models outperformed `bert-base-multilingual-cased` model.
| Model | Macro Precision | Macro Recall | Macro F1 |
| --- | --- | --- | --- |
| `bert-base-multilingual-cased` | 94.70 | 94.86 | 94.74 |
| `bert-base-japanese` | 95.64 | 95.58 | 95.60 |
| `bert-base-japanese-whole-word-masking` | 95.18 | 95.39 | 95.27 |
| `bert-base-japanese-char` | 94.32 | 93.96 | 94.09 |
| `bert-base-japanese-char-whole-word-masking` | 94.98 | 94.88 | 94.92 |
(Note that we expect the `char` models to be effective for SQuAD-like tasks, where span-level representation is needed. More comprehensive experiments are left for future work.)
Also, I've manually confirmed that our models predict reasonable tokens given a masked Japanese text.
Shall I add an example script for the classification task?<|||||>Alright, thanks for clarifying. I don't think adding an example script is necessary.
This looks good to me but I'll wait for @thomwolf to chime in and review the PR before merging as it adds a few core functionalities (like the tokenizer).<|||||>Hi @singletongue, the first step in uploading your weights to our S3 would be for you to create an account here: https://huggingface.co/join
The models will be namespaced under the chosen username or org name.
Can you let me know if you encounter any issue?<|||||>Thank you for your guidance, @julien-c.
I've just created an account. Then I was told to use a CLI tool to log in and upload the model. However, I couldn't find the specified CLI in the repository.<|||||>Yeah I should have been clearer, it's just that step 1 for now. What was clear or not clear in that page?
I will let you know here as soon as the CLI is operational.<|||||>This looks great to me, ok for merging.
Congratulations and thanks a lot @singletongue, this is an amazing work, both on preparing the data and training the model and on the super clean integration in the library. It's a masterpiece!<|||||>I think we add a test on the new tokenizer class though.
Do you think you could copy the file `tests/tokenization_bert_test` in a file `tests/tokenization_bert_japanese_test.py` and adapt a few English tests to Japanese specific characters @singletongue?<|||||>Thank you for your review, @thomwolf.
I added a test for the tokenizers.
The test on CircleCI failed since the tokenizers need [MeCab](https://taku910.github.io/mecab/) and `mecab-python3` to be installed.
I've checked that the test run successfully on my local environment where the dependencies are installed.<|||||>@singletongue The file upload CLI has been developed in https://github.com/huggingface/transformers/pull/2044 (soon to be merged to master)
Could you please upload your weights by doing:
```bash
git checkout cli_upload
pip install -e .
transformers-cli login
transformers-cli upload
```
Thanks!<|||||>(For the tests requirements, maybe @thomwolf or @LysandreJik can chime in – I think you can either add a `pip install mecab-python3 ` to the config.yml file, or maybe create a specific test target for JP)<|||||>Thank you, @julien-c. I've uploaded all the required files.
Also, I've updated `.circleci/config.yml` to successfully run tests on the tokenizers.
Thanks!<|||||>Ok LGTM |
transformers | 1,838 | closed | resize_token_embeddings not implemented for TFBert* | ## ❓ Questions & Help
<!-- A clear and concise description of the question. -->
Hey,
I noticed for the Tensorflow 2 implementation of BERT, the `resize_token_embeddings` function is not implemented, while the Pytorch BERT class does.
### Questions:
* Is the implementation of `resize_token_embeddings` for TF models expected?
* I'd be happy to help with this
* Any other solutions to resizing token embedding?
* Possibly manually updating the config, vocab file, and weights to support the added tokens
Example:
```
special_tokens = {
'additional_special_tokens': ['[SPC]']
}
tokenizer = BertTokenizer.from_pretrained('bert-base-cased')
tokens_added = tokenizer.add_special_tokens(special_tokens)
model = TFBertForSequenceClassification.from_pretrained('bert-base-cased')
model.resize_token_embeddings(len(tokenizer))
```
Output: ` File ".../transformers/modeling_tf_utils.py", line 115, in resize_token_embeddings
raise NotImplementedError`
### Source:
`TFPreTrainedModel`
https://github.com/huggingface/transformers/blob/74ce8de7d8e0375a9123f9542f3483f46cc8df9b/transformers/modeling_tf_utils.py#L117
Here I think the implementation would look similar to the PyTorch model. Updating the embeddings specifically for BERT would then occur in TFBertMainLayer.resize_token_embeddings.
```
def resize_token_embeddings(self, new_num_tokens=None):
""" Resize input token embeddings matrix of the model if new_num_tokens != config.vocab_size.
Take care of tying weights embeddings afterwards if the model class has a `tie_weights()` method.
Arguments:
new_num_tokens: (`optional`) int:
New number of tokens in the embedding matrix. Increasing the size will add newly initialized vectors at the end. Reducing the size will remove vectors from the end.
If not provided or None: does nothing and just returns a pointer to the input tokens ``tf.Variable`` Module of the model.
Return: ``tf.Variable``
Pointer to the input tokens Embeddings Module of the model
"""
base_model = getattr(self, self.base_model_prefix, self) # get the base model if needed
model_embeds = base_model._resize_token_embeddings(new_num_tokens)
if new_num_tokens is None:
return model_embeds
# Update base model and current model config
self.config.vocab_size = new_num_tokens
base_model.vocab_size = new_num_tokens
# Tie weights again if needed
if hasattr(self, 'tie_weights'):
self.tie_weights()
return model_embeds
```
`TFBertMainLayer`
https://github.com/huggingface/transformers/blob/74ce8de7d8e0375a9123f9542f3483f46cc8df9b/transformers/modeling_tf_bert.py#L472
Thanks,
Ali Momin | 11-15-2019 00:07:39 | 11-15-2019 00:07:39 | Did you get this to work for you? I'm currently trying to do the same thing but with TFGPT-2<|||||>Any update on when this would be completed?<|||||>I have the same issue, I wanted to use "resize_token_embeddings" with TFAlbert model but it's not implemented yet.<|||||>Hi, we have no plans of implementing this on our roadmap, but we are always open to PRs.<|||||>This issue has been automatically marked as stale because it has not had recent activity. It will be closed if no further activity occurs. Thank you for your contributions.
<|||||>Any updates on this? Trying to use tf gpt2 |
transformers | 1,837 | closed | Error started happening today: ImportError: cannot import name 'get_linear_schedule_with_warmup' | ## 🐛 Bug
<!-- Important information -->
Model I am using (Bert, XLNet....): Bert
Language I am using the model on (English, Chinese....): English
The problem arise when using:
* [ x] the official example scripts: (give details)
The squad script:
https://github.com/huggingface/transformers/blob/master/examples/run_squad.py
The tasks I am working on is:
* [X ] an official GLUE/SQUaD task: (give the name)
Squad 2.0 from https://rajpurkar.github.io/SQuAD-explorer/
* [ ] my own task or dataset: (give details)
## To Reproduce
```
!pip install transformers
import urllib.request
url = 'https://rajpurkar.github.io/SQuAD-explorer/dataset/train-v2.0.json'
urllib.request.urlretrieve(url, 'train-v2.0.json')
url = 'https://rajpurkar.github.io/SQuAD-explorer/dataset/dev-v2.0.json'
urllib.request.urlretrieve(url, 'dev-v2.0.json')
!wget 'https://raw.githubusercontent.com/huggingface/transformers/master/examples/run_squad.py'
!wget 'https://raw.githubusercontent.com/huggingface/pytorch-transformers/master/examples/utils_squad.py'
!wget 'https://raw.githubusercontent.com/huggingface/pytorch-transformers/master/examples/utils_squad_evaluate.py'
# $SQUAD_DIR/train-v1.1.json
SQUAD_Train = '/content/train-v2.0.json'
SQUAD_Dev = '/content/dev-v2.0.json'
!python run_squad.py \
--model_type bert \
--model_name_or_path bert-base-cased \
--do_train \
--do_eval \
--do_lower_case \
--train_file '$SQUAD_Train' \
--predict_file '$SQUAD_Dev' \
--per_gpu_train_batch_size 12 \
--learning_rate 3e-5 \
--num_train_epochs 2.0 \
--max_seq_length 384 \
--doc_stride 128 \
--version_2_with_negative \
--output_dir /tmp/debug_squad/
```
Results in
>ImportError: cannot import name 'get_linear_schedule_with_warmup'
I ran it yesterday and it worked fine, but today it's not working.
For convenience, here's a colab notebook with the code
https://colab.research.google.com/drive/1tNisXX5siuNnkuEQ-X_XdEdDTtdJ0qeL
| 11-14-2019 22:39:42 | 11-14-2019 22:39:42 | try edit:
from transformers import AdamW
from transformers import WarmupLinearSchedule as get_linear_schedule_with_warmup<|||||>I tried the WarmupLinearSchedule, but I have a problem no key num_warmup_steps and num_training_steps.
scheduler = WarmupLinearSchedule(optimizer, num_warmup_steps=args.warmup_steps,
num_training_steps=t_total)
I think get_linear_schedule_with_warmup and WarmupLinearSchedule are two different scheduler<|||||>The version of the lib you use is not in sync with the scripts you run (cc @rlouf, @LysandreJik)
If you run the scripts from `master`, then you also need to install the lib from `master`:
```bash
pip install git+https://github.com/huggingface/transformers
```
This is a frequent issue so maybe we should do something about it, @thomwolf <|||||>> I tried the WarmupLinearSchedule, but I have a problem no key num_warmup_steps and num_training_steps.
> scheduler = WarmupLinearSchedule(optimizer, num_warmup_steps=args.warmup_steps,
> num_training_steps=t_total)
> I think get_linear_schedule_with_warmup and WarmupLinearSchedule are two different scheduler
They are the same schedulers but we introduced breaking changes, and indeed renamed `warmup_steps` -> `num_warmup_steps` and `t_total` -> ˋnum_training_steps`.
And yes, to work on the same version of the lib as the examples, go in the root directory and:
```bash
makevirtualenv my-project && workon my-project # or anything else you use to create a virtual environnement
pip install . # or Julien-c’s command
```
@julien-c I asked for advice on this one.
<|||||>> The version of the lib you use is not in sync with the scripts you run (cc @rlouf, @LysandreJik)
>
> If you run the scripts from `master`, then you also need to install the lib from `master`:
>
> ```shell
> pip install git+https://github.com/huggingface/transformers
> ```
>
> This is a frequent issue so maybe we should do something about it, @thomwolf
Maybe we can indicate clearly on https://github.com/huggingface/transformers/blob/master/README.md#from-source or documentation.<|||||>same problem for me too :(<|||||>> same problem for me too :(
reinstall the package from local:
pip install .<|||||>We are documenting this in #1889. It is because you are trying to run bleeding-edge examples with a pip-installed version of the library, which corresponds to the last release. Do as @YuxiangLu says in a new virtual environment.<|||||>can be closed because solved in #1889 ?<|||||>when breaking changes are introduced the major number should increase to make the users aware.<|||||>Indeed @aminHatim ! This is why this was released in version 2.2.0 an hour ago. Breaking changes are bound to happen when installing from source or running bleeding edges examples (which are based on the source). |
transformers | 1,836 | closed | Model parallelism support? | Hi. I'm running into memory issues when trying to fine tune the CTRL language model on a 16 GB GPU. Is there any built-in support for splitting models across more than one GPU? I suppose that mapping the input embedding layer to a different GPU or even the CPU would do the trick.
| 11-14-2019 21:33:37 | 11-14-2019 21:33:37 | How do you training CTRL ? Did you check this: https://github.com/huggingface/transformers/blob/0477b307c7501ea76e01b03cb387a2312db752b3/examples/run_lm_finetuning.py#L198
You can also decrease batch size up to 1.
And also gradients can be accumulated:
https://github.com/huggingface/transformers/blob/0477b307c7501ea76e01b03cb387a2312db752b3/examples/run_lm_finetuning.py#L389<|||||>@iedmrc Thanks for the suggestions. I'm using a slightly modified version of run_lm_finetuning.py but for CTRL even with a batch size of 1 the fine tuning consumes more than 16 GB of memory. I tried also to fine tune with half precision (fp16), but for some reason that didn't help either (I have seen other people complain about similar issues here).<|||||>This issue has been automatically marked as stale because it has not had recent activity. It will be closed if no further activity occurs. Thank you for your contributions.
<|||||>See https://github.com/huggingface/transformers/pull/3578. |
transformers | 1,835 | closed | Parallell compute failing for finetuning GPT2 using GPU | ## ? Questions & Help
**SYSTEM**
OS: Ubuntu 18.04
Python version: 3.6.8
Torch version: 1.0.0
Transformers version: 2.1.1
I am trying to finetune GPT2 using a GPU and am getting an error. I run the `run_lm_finetuning.py` example script with the following parameters:
```python
python run_lm_finetuning.py \
--output_dir=/home/user/gpt2_finetuned_isr/model \
--model_type=gpt2 \
--model_name_or_path=gpt2 \
--do_train \
--train_data_file=/path/to/train/data/train_data.txt \
--do_eval \
--eval_data_file=/path/to/test/data/test_data.txt
```
Here is the error:
```python
/home/user/.local/lib/python3.6/site-packages/tensorflow/python/framework/dtypes.py:516: FutureWarning: Passing (type, 1) or '1type' as a synonym of type is deprecated; in a future version of numpy, it will be understood as (type, (1,)) / '(1,)type'.
_np_qint8 = np.dtype([("qint8", np.int8, 1)])
/home/user/.local/lib/python3.6/site-packages/tensorflow/python/framework/dtypes.py:517: FutureWarning: Passing (type, 1) or '1type' as a synonym of type is deprecated; in a future version of numpy, it will be understood as (type, (1,)) / '(1,)type'.
_np_quint8 = np.dtype([("quint8", np.uint8, 1)])
/home/user/.local/lib/python3.6/site-packages/tensorflow/python/framework/dtypes.py:518: FutureWarning: Passing (type, 1) or '1type' as a synonym of type is deprecated; in a future version of numpy, it will be understood as (type, (1,)) / '(1,)type'.
_np_qint16 = np.dtype([("qint16", np.int16, 1)])
/home/user/.local/lib/python3.6/site-packages/tensorflow/python/framework/dtypes.py:519: FutureWarning: Passing (type, 1) or '1type' as a synonym of type is deprecated; in a future version of numpy, it will be understood as (type, (1,)) / '(1,)type'.
_np_quint16 = np.dtype([("quint16", np.uint16, 1)])
/home/user/.local/lib/python3.6/site-packages/tensorflow/python/framework/dtypes.py:520: FutureWarning: Passing (type, 1) or '1type' as a synonym of type is deprecated; in a future version of numpy, it will be understood as (type, (1,)) / '(1,)type'.
_np_qint32 = np.dtype([("qint32", np.int32, 1)])
/home/user/.local/lib/python3.6/site-packages/tensorflow/python/framework/dtypes.py:525: FutureWarning: Passing (type, 1) or '1type' as a synonym of type is deprecated; in a future version of numpy, it will be understood as (type, (1,)) / '(1,)type'.
np_resource = np.dtype([("resource", np.ubyte, 1)])
/home/user/.local/lib/python3.6/site-packages/tensorboard/compat/tensorflow_stub/dtypes.py:541: FutureWarning: Passing (type, 1) or '1type' as a synonym of type is deprecated; in a future version of numpy, it will be understood as (type, (1,)) / '(1,)type'.
_np_qint8 = np.dtype([("qint8", np.int8, 1)])
/home/user/.local/lib/python3.6/site-packages/tensorboard/compat/tensorflow_stub/dtypes.py:542: FutureWarning: Passing (type, 1) or '1type' as a synonym of type is deprecated; in a future version of numpy, it will be understood as (type, (1,)) / '(1,)type'.
_np_quint8 = np.dtype([("quint8", np.uint8, 1)])
/home/user/.local/lib/python3.6/site-packages/tensorboard/compat/tensorflow_stub/dtypes.py:543: FutureWarning: Passing (type, 1) or '1type' as a synonym of type is deprecated; in a future version of numpy, it will be understood as (type, (1,)) / '(1,)type'.
_np_qint16 = np.dtype([("qint16", np.int16, 1)])
/home/user/.local/lib/python3.6/site-packages/tensorboard/compat/tensorflow_stub/dtypes.py:544: FutureWarning: Passing (type, 1) or '1type' as a synonym of type is deprecated; in a future version of numpy, it will be understood as (type, (1,)) / '(1,)type'.
_np_quint16 = np.dtype([("quint16", np.uint16, 1)])
/home/user/.local/lib/python3.6/site-packages/tensorboard/compat/tensorflow_stub/dtypes.py:545: FutureWarning: Passing (type, 1) or '1type' as a synonym of type is deprecated; in a future version of numpy, it will be understood as (type, (1,)) / '(1,)type'.
_np_qint32 = np.dtype([("qint32", np.int32, 1)])
/home/user/.local/lib/python3.6/site-packages/tensorboard/compat/tensorflow_stub/dtypes.py:550: FutureWarning: Passing (type, 1) or '1type' as a synonym of type is deprecated; in a future version of numpy, it will be understood as (type, (1,)) / '(1,)type'.
np_resource = np.dtype([("resource", np.ubyte, 1)])
To use data.metrics please install scikit-learn. See https://scikit-learn.org/stable/index.html
11/14/2019 11:01:48 - WARNING - __main__ - Process rank: -1, device: cuda, n_gpu: 1, distributed training: False, 16-bits training: False
11/14/2019 11:01:49 - INFO - transformers.configuration_utils - loading configuration file https://s3.amazonaws.com/models.huggingface.co/bert/gpt2-config.json from cache at /home/user/.cache/torch/transformers/4be02c5697d91738003fb1685c9872f284166aa32e061576bbe6aaeb95649fcf.085d5f6a8e7812ea05ff0e6ed0645ab2e75d80387ad55c1ad9806ee70d272f80
11/14/2019 11:01:49 - INFO - transformers.configuration_utils - Model config {
"attn_pdrop": 0.1,
"embd_pdrop": 0.1,
"finetuning_task": null,
"initializer_range": 0.02,
"layer_norm_epsilon": 1e-05,
"n_ctx": 1024,
"n_embd": 768,
"n_head": 12,
"n_layer": 12,
"n_positions": 1024,
"num_labels": 1,
"output_attentions": false,
"output_hidden_states": false,
"output_past": true,
"pruned_heads": {},
"resid_pdrop": 0.1,
"summary_activation": null,
"summary_first_dropout": 0.1,
"summary_proj_to_labels": true,
"summary_type": "cls_index",
"summary_use_proj": true,
"torchscript": false,
"use_bfloat16": false,
"vocab_size": 50257
}
11/14/2019 11:01:50 - INFO - transformers.tokenization_utils - loading file https://s3.amazonaws.com/models.huggingface.co/bert/gpt2-vocab.json from cache at /home/user/.cache/torch/transformers/f2808208f9bec2320371a9f5f891c184ae0b674ef866b79c58177067d15732dd.1512018be4ba4e8726e41b9145129dc30651ea4fec86aa61f4b9f40bf94eac71
11/14/2019 11:01:50 - INFO - transformers.tokenization_utils - loading file https://s3.amazonaws.com/models.huggingface.co/bert/gpt2-merges.txt from cache at /home/user/.cache/torch/transformers/d629f792e430b3c76a1291bb2766b0a047e36fae0588f9dbc1ae51decdff691b.70bec105b4158ed9a1747fea67a43f5dee97855c64d62b6ec3742f4cfdb5feda
11/14/2019 11:01:50 - INFO - transformers.modeling_utils - loading weights file https://s3.amazonaws.com/models.huggingface.co/bert/gpt2-pytorch_model.bin from cache at /home/user/.cache/torch/transformers/4295d67f022061768f4adc386234dbdb781c814c39662dd1662221c309962c55.778cf36f5c4e5d94c8cd9cefcf2a580c8643570eb327f0d4a1f007fab2acbdf1
/home/user/.local/lib/python3.6/site-packages/torch/cuda/__init__.py:117: UserWarning:
Found GPU0 Quadro 4000 which is of cuda capability 2.0.
PyTorch no longer supports this GPU because it is too old.
warnings.warn(old_gpu_warn % (d, name, major, capability[1]))
11/14/2019 11:02:10 - INFO - __main__ - Training/evaluation parameters Namespace(adam_epsilon=1e-08, block_size=1024, cache_dir='', config_name='', device=device(type='cuda'), do_eval=True, do_lower_case=False, do_train=True, eval_all_checkpoints=False, eval_data_file='/home/user/gpt2_finetuned_isr/finetune_gpt2_test.txt', evaluate_during_training=False, fp16=False, fp16_opt_level='O1', gradient_accumulation_steps=1, learning_rate=5e-05, local_rank=-1, logging_steps=50, max_grad_norm=1.0, max_steps=-1, mlm=False, mlm_probability=0.15, model_name_or_path='gpt2', model_type='gpt2', n_gpu=1, no_cuda=False, num_train_epochs=1.0, output_dir='/home/user/gpt2_finetuned_isr/model', overwrite_cache=False, overwrite_output_dir=False, per_gpu_eval_batch_size=4, per_gpu_train_batch_size=4, save_steps=50, save_total_limit=None, seed=42, server_ip='', server_port='', tokenizer_name='', train_data_file='/home/user/gpt2_finetuned_isr/finetune_gpt2_train.txt', warmup_steps=0, weight_decay=0.0)
11/14/2019 11:02:10 - INFO - __main__ - Creating features from dataset file at /home/user/gpt2_finetuned_isr
11/14/2019 11:02:16 - WARNING - transformers.tokenization_utils - Token indices sequence length is longer than the specified maximum sequence length for this model (1003332 > 1024). Running this sequence through the model will result in indexing errors
11/14/2019 11:02:16 - WARNING - transformers.tokenization_utils - This tokenizer does not make use of special tokens. Input is returned with no modification.
11/14/2019 11:02:16 - WARNING - transformers.tokenization_utils - This tokenizer does not make use of special tokens. Input is returned with no modification.
11/14/2019 11:02:16 - WARNING - transformers.tokenization_utils - This tokenizer does not make use of special tokens. Input is returned with no modification.
11/14/2019 11:02:16 - WARNING - transformers.tokenization_utils - This tokenizer does not make use of special tokens. Input is returned with no modification.
11/14/2019 11:02:16 - WARNING - transformers.tokenization_utils - This tokenizer does not make use of special tokens. Input is returned with no modification.
BTW, I get TONS of these tokenizer warnings... I won't paste them here as they say the same thing...
11/14/2019 11:02:16 - INFO - __main__ - Saving features into cached file /home/user/gpt2_finetuned_isr/cached_lm_1024_finetune_gpt2_train.txt
11/14/2019 11:02:16 - INFO - __main__ - ***** Running training *****
11/14/2019 11:02:16 - INFO - __main__ - Num examples = 979
11/14/2019 11:02:16 - INFO - __main__ - Num Epochs = 1
11/14/2019 11:02:16 - INFO - __main__ - Instantaneous batch size per GPU = 4
11/14/2019 11:02:16 - INFO - __main__ - Total train batch size (w. parallel, distributed & accumulation) = 4
11/14/2019 11:02:16 - INFO - __main__ - Gradient Accumulation steps = 1
11/14/2019 11:02:16 - INFO - __main__ - Total optimization steps = 245
Epoch: 0%| | 0/1 [00:00<?, ?it/sTraceback (most recent call last): | 0/245 [00:00<?, ?it/s]
File "run_lm_finetuning.py", line 545, in <module>
main()
File "run_lm_finetuning.py", line 497, in main
global_step, tr_loss = train(args, train_dataset, model, tokenizer)
File "run_lm_finetuning.py", line 228, in train
outputs = model(inputs, masked_lm_labels=labels) if args.mlm else model(inputs, labels=labels)
File "/home/user/.local/lib/python3.6/site-packages/torch/nn/modules/module.py", line 489, in __call__
result = self.forward(*input, **kwargs)
File "/home/user/.local/lib/python3.6/site-packages/transformers/modeling_gpt2.py", line 533, in forward
head_mask=head_mask)
File "/home/user/.local/lib/python3.6/site-packages/torch/nn/modules/module.py", line 489, in __call__
result = self.forward(*input, **kwargs)
File "/home/user/.local/lib/python3.6/site-packages/transformers/modeling_gpt2.py", line 385, in forward
position_ids = torch.arange(past_length, input_ids.size(-1) + past_length, dtype=torch.long, device=input_ids.device)
RuntimeError: parallel_for failed: no kernel image is available for execution on the device
Epoch: 0%| | 0/1 [00:00<?, ?it/s]
Iteration: 0%| | 0/245 [00:00<?, ?it/s]
```
I see the pytorch warning about the GPU, but it still seems available to use:
```python
Python 3.6.8 (default, Oct 7 2019, 12:59:55)
[GCC 8.3.0] on linux
Type "help", "copyright", "credits" or "license" for more information.
>>> import torch
>>> torch.cuda.current_device()
/home/user/.local/lib/python3.6/site-packages/torch/cuda/__init__.py:117: UserWarning:
Found GPU0 Quadro 4000 which is of cuda capability 2.0.
PyTorch no longer supports this GPU because it is too old.
warnings.warn(old_gpu_warn % (d, name, major, capability[1]))
0
>>> torch.cuda.device(0)
<torch.cuda.device object at 0x7fb833751d30>
>>> torch.cuda.device_count()
1
>>> torch.cuda.get_device_name(0)
'Quadro 4000'
>>> torch.cuda.is_available()
True
```
Any guidance is much appreciated!
| 11-14-2019 18:36:04 | 11-14-2019 18:36:04 | |
transformers | 1,834 | closed | Where is Model2Model PreTrainedEncoderDecoder in run_summerization_finetune | ## ❓ Questions & Help
<!-- A clear and concise description of the question. -->
| 11-14-2019 18:09:24 | 11-14-2019 18:09:24 | @yeliu918 was there any ```run_summerization_finetune``` script before? Since I cannot find it now.<|||||>This issue has been automatically marked as stale because it has not had recent activity. It will be closed if no further activity occurs. Thank you for your contributions.
|
transformers | 1,833 | closed | Token indices sequence length is longer than the specified maximum sequence length for this model | As of now the tokenizers output a specific warning when an encoded sequence is longer than the maximum specified sequence length, which is model-specific:
```
Token indices sequence length is longer than the specified maximum sequence length for this model (X > 1024). Running this sequence through the model will result in indexing errors
```
It is currently in the `convert_tokens_to_ids` and this leads to two issues:
- using `encode` or `encode_plus` methods with a `max_length` specified will still output that warning as the `convert_tokens_to_ids` method is used before the truncation is done. (cf #1791)
- since `prepare_for_model` was introduced, I personally feel that all modifications related to the model should happen in that method and not in `tokenize` or `convert_tokens_to_ids`.
This PR aims to slightly change the behavior so that both aforementioned issues may be solved by putting the warning in the `prepare_for_model` method if no `max_length` is specified. | 11-14-2019 15:39:05 | 11-14-2019 15:39:05 | |
transformers | 1,832 | closed | replace LambdaLR scheduler wrappers by function | Custom schedulers are currently initiated by wrapping Pytorch's LambdaLR
class and passing a method of the wrapping class to the __init__
function of LambdaLR. This approach is not appropriate for several
reasons:
1. one does not need to define a class when it only defines a
__init__() method;
2. instantiating the parent class by passing a method of the child class
creates a cyclical reference which leads to memory leaks. See issues #1742 and #1134.
In this commit we replace the wrapper classes with functions that
instantiate `LambdaLR` with a custom learning rate function. We use a
closure to specify the parameter of the latter. We also do a bit of
renaming within the function to explicit the behaviour and removed
docstrings that were subsequently not necessary. | 11-14-2019 14:41:10 | 11-14-2019 14:41:10 | Yes, great job tracking and fixing this.
Can you update all the examples as well?<|||||>Great job finding the issue!<|||||>I updated the docs and examples. I am confused because the tests fail on this function:
```
=================================== FAILURES ===================================
______________ TFDistilBertModelTest.test_pt_tf_model_equivalence ______________
self = <transformers.tests.modeling_tf_distilbert_test.TFDistilBertModelTest testMethod=test_pt_tf_model_equivalence>
def test_pt_tf_model_equivalence(self):
if not is_torch_available():
return
import torch
import transformers
config, inputs_dict = self.model_tester.prepare_config_and_inputs_for_common()
for model_class in self.all_model_classes:
pt_model_class_name = model_class.__name__[2:] # Skip the "TF" at the beggining
pt_model_class = getattr(transformers, pt_model_class_name)
config.output_hidden_states = True
tf_model = model_class(config)
pt_model = pt_model_class(config)
# Check we can load pt model in tf and vice-versa with model => model functions
tf_model = transformers.load_pytorch_model_in_tf2_model(tf_model, pt_model, tf_inputs=inputs_dict)
pt_model = transformers.load_tf2_model_in_pytorch_model(pt_model, tf_model)
# Check predictions on first output (logits/hidden-states) are close enought given low-level computational differences
pt_model.eval()
pt_inputs_dict = dict((name, torch.from_numpy(key.numpy()).to(torch.long))
for name, key in inputs_dict.items())
with torch.no_grad():
pto = pt_model(**pt_inputs_dict)
tfo = tf_model(inputs_dict)
max_diff = np.amax(np.abs(tfo[0].numpy() - pto[0].numpy()))
> self.assertLessEqual(max_diff, 2e-2)
E AssertionError: nan not less than or equal to 0.02
```
which has no apparent link with my changes.<|||||>This test has been failing on and off for a week or so now; I'll look into it soon. |
transformers | 1,831 | closed | sum() is replaced by itertools.chain.from_iterable() | sum() is the leanest method to flatten a string list, so it's been replaced by itertools.chain.from_iterable() . Please check #1830 | 11-14-2019 14:10:19 | 11-14-2019 14:10:19 | # [Codecov](https://codecov.io/gh/huggingface/transformers/pull/1831?src=pr&el=h1) Report
> Merging [#1831](https://codecov.io/gh/huggingface/transformers/pull/1831?src=pr&el=desc) into [master](https://codecov.io/gh/huggingface/transformers/commit/155c782a2ccd103cf63ad48a2becd7c76a7d2115?src=pr&el=desc) will **decrease** coverage by `1.36%`.
> The diff coverage is `100%`.
[](https://codecov.io/gh/huggingface/transformers/pull/1831?src=pr&el=tree)
```diff
@@ Coverage Diff @@
## master #1831 +/- ##
==========================================
- Coverage 84.16% 82.79% -1.37%
==========================================
Files 94 94
Lines 14185 14186 +1
==========================================
- Hits 11939 11746 -193
- Misses 2246 2440 +194
```
| [Impacted Files](https://codecov.io/gh/huggingface/transformers/pull/1831?src=pr&el=tree) | Coverage Δ | |
|---|---|---|
| [transformers/tokenization\_utils.py](https://codecov.io/gh/huggingface/transformers/pull/1831/diff?src=pr&el=tree#diff-dHJhbnNmb3JtZXJzL3Rva2VuaXphdGlvbl91dGlscy5weQ==) | `91.22% <100%> (+0.02%)` | :arrow_up: |
| [transformers/modeling\_tf\_pytorch\_utils.py](https://codecov.io/gh/huggingface/transformers/pull/1831/diff?src=pr&el=tree#diff-dHJhbnNmb3JtZXJzL21vZGVsaW5nX3RmX3B5dG9yY2hfdXRpbHMucHk=) | `9.85% <0%> (-83.1%)` | :arrow_down: |
| [transformers/tests/modeling\_tf\_common\_test.py](https://codecov.io/gh/huggingface/transformers/pull/1831/diff?src=pr&el=tree#diff-dHJhbnNmb3JtZXJzL3Rlc3RzL21vZGVsaW5nX3RmX2NvbW1vbl90ZXN0LnB5) | `81.55% <0%> (-15.54%)` | :arrow_down: |
| [transformers/modeling\_roberta.py](https://codecov.io/gh/huggingface/transformers/pull/1831/diff?src=pr&el=tree#diff-dHJhbnNmb3JtZXJzL21vZGVsaW5nX3JvYmVydGEucHk=) | `59.41% <0%> (-12.36%)` | :arrow_down: |
| [transformers/modeling\_xlnet.py](https://codecov.io/gh/huggingface/transformers/pull/1831/diff?src=pr&el=tree#diff-dHJhbnNmb3JtZXJzL21vZGVsaW5nX3hsbmV0LnB5) | `71.18% <0%> (-2.44%)` | :arrow_down: |
| [transformers/modeling\_ctrl.py](https://codecov.io/gh/huggingface/transformers/pull/1831/diff?src=pr&el=tree#diff-dHJhbnNmb3JtZXJzL21vZGVsaW5nX2N0cmwucHk=) | `94.24% <0%> (-2.22%)` | :arrow_down: |
| [transformers/modeling\_openai.py](https://codecov.io/gh/huggingface/transformers/pull/1831/diff?src=pr&el=tree#diff-dHJhbnNmb3JtZXJzL21vZGVsaW5nX29wZW5haS5weQ==) | `80.66% <0%> (-1.34%)` | :arrow_down: |
------
[Continue to review full report at Codecov](https://codecov.io/gh/huggingface/transformers/pull/1831?src=pr&el=continue).
> **Legend** - [Click here to learn more](https://docs.codecov.io/docs/codecov-delta)
> `Δ = absolute <relative> (impact)`, `ø = not affected`, `? = missing data`
> Powered by [Codecov](https://codecov.io/gh/huggingface/transformers/pull/1831?src=pr&el=footer). Last update [155c782...7627dde](https://codecov.io/gh/huggingface/transformers/pull/1831?src=pr&el=lastupdated). Read the [comment docs](https://docs.codecov.io/docs/pull-request-comments).
<|||||>Looks good to me, thank you @iedmrc!<|||||>Great, thanks a lot @iedmrc! |
transformers | 1,830 | closed | GPT2 tokenizer is so slow because of sum() | ## 🐛 Bug
Hi,
As the discussion started in that #1621 issue, GPT2 tokenization is so slow even with 50MB of the dataset.
I'm using `run_lm_finetuning.py` and here are the steps to reproduce the problem:
- Have a dataset not an even bigger one. 20MB of a dataset is enough.
- Call `run_lm_finetuning.py` to train (finetune) the dataset. Here are my parameters:
```
--train_data_file "/train/datafile" \
--eval_data_file "/eval/datafile" \
--output_dir "/train/model" \
--model_type gpt2 \
--model_name_or_path distilgpt2 \
--cache_dir "/train/cache" \
--do_train \
--evaluate_during_training \
--per_gpu_train_batch_size 1 \
--per_gpu_eval_batch_size 1 \
--gradient_accumulation_steps 5 \
--overwrite_output_dir \
--seed 99
```
- You'll see it'll spend 20+ mins (depends on your cpu) to tokenize just 50MB of a text file.
I dug into `huggingface/transformers` 's codebase and profiled the tokenization process. And it is obvious that this summation drains the time:
https://github.com/huggingface/transformers/blob/155c782a2ccd103cf63ad48a2becd7c76a7d2115/transformers/tokenization_utils.py#L644
I run profiler and here is the result:
```
73791524 function calls in 1566.379 seconds
Ordered by: standard name
ncalls tottime percall cumtime percall filename:lineno(function)
27157 0.083 0.000 0.109 0.000 <frozen importlib._bootstrap>:1009(_handle_fromlist)
27157 0.065 0.000 0.128 0.000 _bootlocale.py:33(getpreferredencoding)
81471 0.070 0.000 0.327 0.000 locale.py:589(setlocale)
27157 0.422 0.000 0.876 0.000 locale.py:647(getpreferredencoding)
27157 0.363 0.000 5.997 0.000 regex.py:328(findall)
27157 0.662 0.000 1.682 0.000 regex.py:434(_compile)
4815114 8.744 0.000 16.641 0.000 tokenization_gpt2.py:139(bpe)
2030532 1.116 0.000 1.887 0.000 tokenization_gpt2.py:149(<lambda>)
27157 22.702 0.001 110.038 0.004 tokenization_gpt2.py:180(_tokenize)
25459527 5.702 0.000 5.702 0.000 tokenization_gpt2.py:194(<genexpr>)
10242602 1.764 0.000 1.764 0.000 tokenization_gpt2.py:195(<genexpr>)
1377876 1.678 0.000 1.975 0.000 tokenization_gpt2.py:91(get_pairs)
95205 0.526 0.000 0.910 0.000 tokenization_utils.py:1043(special_tokens_map)
95205 0.932 0.000 1.987 0.000 tokenization_utils.py:1055(all_special_tokens)
1 0.119 0.119 1566.379 1566.379 tokenization_utils.py:615(tokenize)
40789 0.099 0.000 0.169 0.000 tokenization_utils.py:623(split_on_token)
1 0.287 0.287 1566.260 1566.260 tokenization_utils.py:641(split_on_tokens)
54417 0.698 0.000 112.123 0.002 tokenization_utils.py:659(<genexpr>)
27157 0.063 0.000 0.063 0.000 {built-in method _locale.nl_langinfo}
81471 0.252 0.000 0.252 0.000 {built-in method _locale.setlocale}
761640 0.384 0.000 0.384 0.000 {built-in method builtins.getattr}
54314 0.022 0.000 0.022 0.000 {built-in method builtins.hasattr}
516605 0.150 0.000 0.150 0.000 {built-in method builtins.isinstance}
1821447 0.159 0.000 0.159 0.000 {built-in method builtins.len}
472563 3.469 0.000 5.355 0.000 {built-in method builtins.min}
1 1453.081 1453.081 1565.204 1565.204 {built-in method builtins.sum}
2043214 0.297 0.000 0.297 0.000 {method 'add' of 'set' objects}
456488 0.055 0.000 0.055 0.000 {method 'append' of 'list' objects}
1 0.000 0.000 0.000 0.000 {method 'disable' of '_lsprof.Profiler' objects}
4815114 1.169 0.000 1.169 0.000 {method 'encode' of 'str' objects}
5550977 16.572 0.000 18.336 0.000 {method 'extend' of 'list' objects}
27157 3.952 0.000 3.952 0.000 {method 'findall' of '_regex.Pattern' objects}
2057689 0.784 0.000 0.784 0.000 {method 'get' of 'dict' objects}
735863 0.233 0.000 0.233 0.000 {method 'index' of 'tuple' objects}
4894984 38.307 0.000 44.010 0.000 {method 'join' of 'str' objects}
1 0.000 0.000 0.000 0.000 {method 'keys' of 'dict' objects}
4855903 1.365 0.000 1.365 0.000 {method 'split' of 'str' objects}
68048 0.009 0.000 0.009 0.000 {method 'strip' of 'str' objects}
95205 0.024 0.000 0.024 0.000 {method 'values' of 'dict' objects}
```
I turned it into this by removing `sum()`
```
(self._tokenize(token, **kwargs) if token not \
in self.added_tokens_encoder and token not in self.all_special_tokens \
else [token] for token in tokenized_text)
```
and here is the profiler result:
```
73275678 function calls in 121.030 seconds
Ordered by: standard name
ncalls tottime percall cumtime percall filename:lineno(function)
27157 0.058 0.000 0.076 0.000 <frozen importlib._bootstrap>:1009(_handle_fromlist)
27157 0.041 0.000 0.084 0.000 _bootlocale.py:33(getpreferredencoding)
81471 0.058 0.000 0.211 0.000 locale.py:589(setlocale)
27157 0.330 0.000 0.625 0.000 locale.py:647(getpreferredencoding)
27157 0.267 0.000 4.996 0.000 regex.py:328(findall)
27157 0.434 0.000 1.160 0.000 regex.py:434(_compile)
4815114 9.797 0.000 18.875 0.000 tokenization_gpt2.py:139(bpe)
2030532 1.270 0.000 2.100 0.000 tokenization_gpt2.py:149(<lambda>)
27157 24.693 0.001 119.272 0.004 tokenization_gpt2.py:180(_tokenize)
25459527 6.204 0.000 6.204 0.000 tokenization_gpt2.py:194(<genexpr>)
10242602 1.975 0.000 1.975 0.000 tokenization_gpt2.py:195(<genexpr>)
1377876 2.002 0.000 2.328 0.000 tokenization_gpt2.py:91(get_pairs)
68050 0.287 0.000 0.475 0.000 tokenization_utils.py:1043(special_tokens_map)
68050 0.507 0.000 1.061 0.000 tokenization_utils.py:1055(all_special_tokens)
1 0.031 0.031 121.030 121.030 tokenization_utils.py:615(tokenize)
27263 0.077 0.000 0.158 0.000 tokenization_utils.py:623(split_on_token)
1 0.178 0.178 120.999 120.999 tokenization_utils.py:641(split_on_tokens)
1 0.330 0.330 120.350 120.350 tokenization_utils.py:659(<listcomp>)
27157 0.043 0.000 0.043 0.000 {built-in method _locale.nl_langinfo}
81471 0.148 0.000 0.148 0.000 {built-in method _locale.setlocale}
544400 0.188 0.000 0.188 0.000 {built-in method builtins.getattr}
54314 0.014 0.000 0.014 0.000 {built-in method builtins.hasattr}
407985 0.092 0.000 0.092 0.000 {built-in method builtins.isinstance}
1807921 0.181 0.000 0.181 0.000 {built-in method builtins.len}
472563 3.992 0.000 6.092 0.000 {built-in method builtins.min}
2043214 0.326 0.000 0.326 0.000 {method 'add' of 'set' objects}
456488 0.064 0.000 0.064 0.000 {method 'append' of 'list' objects}
1 0.000 0.000 0.000 0.000 {method 'disable' of '_lsprof.Profiler' objects}
4815114 1.259 0.000 1.259 0.000 {method 'encode' of 'str' objects}
5550977 18.064 0.000 20.040 0.000 {method 'extend' of 'list' objects}
27157 3.569 0.000 3.569 0.000 {method 'findall' of '_regex.Pattern' objects}
2057689 0.839 0.000 0.839 0.000 {method 'get' of 'dict' objects}
735863 0.273 0.000 0.273 0.000 {method 'index' of 'tuple' objects}
4894984 41.821 0.000 48.026 0.000 {method 'join' of 'str' objects}
1 0.000 0.000 0.000 0.000 {method 'keys' of 'dict' objects}
4842377 1.597 0.000 1.597 0.000 {method 'split' of 'str' objects}
54522 0.007 0.000 0.007 0.000 {method 'strip' of 'str' objects}
68050 0.012 0.000 0.012 0.000 {method 'values' of 'dict' objects}
```
You can see 121 seconds vs 1566 seconds. It is 12x times faster without `sum()`. Okay lets discuss do we need `sum()`? Actually, not. Because the `sum()` just flattens the array with the leanest way and there are far more efficient ways. See that [answer](https://stackoverflow.com/a/953097) on StackOverflow.
Also as written in official python [doc](https://docs.python.org/3/library/functions.html#sum) , `sum()` is developed for numbers rather than strings.
So I replaced `sum()` with `list(itertools.chain.from_iterable(text))` as follows and run profiler.
```
return list(itertools.chain.from_iterable((self._tokenize(token, **kwargs) if token not \
in self.added_tokens_encoder and token not in self.all_special_tokens \
else [token] for token in tokenized_text)))
```
Here is the result:
```
73791524 function calls in 114.720 seconds
Ordered by: standard name
ncalls tottime percall cumtime percall filename:lineno(function)
27157 0.045 0.000 0.060 0.000 <frozen importlib._bootstrap>:1009(_handle_fromlist)
27157 0.035 0.000 0.067 0.000 _bootlocale.py:33(getpreferredencoding)
81471 0.045 0.000 0.159 0.000 locale.py:589(setlocale)
27157 0.277 0.000 0.502 0.000 locale.py:647(getpreferredencoding)
27157 0.237 0.000 4.258 0.000 regex.py:328(findall)
27157 0.346 0.000 0.929 0.000 regex.py:434(_compile)
4815114 8.703 0.000 16.973 0.000 tokenization_gpt2.py:139(bpe)
2030532 1.171 0.000 1.923 0.000 tokenization_gpt2.py:149(<lambda>)
27157 22.988 0.001 112.449 0.004 tokenization_gpt2.py:180(_tokenize)
25459527 5.708 0.000 5.708 0.000 tokenization_gpt2.py:194(<genexpr>)
10242602 1.755 0.000 1.755 0.000 tokenization_gpt2.py:195(<genexpr>)
1377876 1.595 0.000 1.900 0.000 tokenization_gpt2.py:91(get_pairs)
95205 0.345 0.000 0.565 0.000 tokenization_utils.py:1043(special_tokens_map)
95205 0.581 0.000 1.236 0.000 tokenization_utils.py:1055(all_special_tokens)
1 0.022 0.022 114.720 114.720 tokenization_utils.py:615(tokenize)
40789 0.103 0.000 0.182 0.000 tokenization_utils.py:623(split_on_token)
1 0.583 0.583 114.698 114.698 tokenization_utils.py:641(split_on_tokens)
54417 0.248 0.000 113.314 0.002 tokenization_utils.py:659(<genexpr>)
27157 0.032 0.000 0.032 0.000 {built-in method _locale.nl_langinfo}
81471 0.111 0.000 0.111 0.000 {built-in method _locale.setlocale}
761640 0.219 0.000 0.219 0.000 {built-in method builtins.getattr}
54314 0.012 0.000 0.012 0.000 {built-in method builtins.hasattr}
516605 0.097 0.000 0.097 0.000 {built-in method builtins.isinstance}
1821447 0.166 0.000 0.166 0.000 {built-in method builtins.len}
472563 3.855 0.000 5.777 0.000 {built-in method builtins.min}
1 0.000 0.000 0.000 0.000 {built-in method from_iterable}
2043214 0.305 0.000 0.305 0.000 {method 'add' of 'set' objects}
456488 0.058 0.000 0.058 0.000 {method 'append' of 'list' objects}
1 0.000 0.000 0.000 0.000 {method 'disable' of '_lsprof.Profiler' objects}
4815114 1.104 0.000 1.104 0.000 {method 'encode' of 'str' objects}
5550977 17.434 0.000 19.189 0.000 {method 'extend' of 'list' objects}
27157 3.092 0.000 3.092 0.000 {method 'findall' of '_regex.Pattern' objects}
2057689 0.759 0.000 0.759 0.000 {method 'get' of 'dict' objects}
735863 0.243 0.000 0.243 0.000 {method 'index' of 'tuple' objects}
4894984 41.030 0.000 46.738 0.000 {method 'join' of 'str' objects}
1 0.000 0.000 0.000 0.000 {method 'keys' of 'dict' objects}
4855903 1.396 0.000 1.396 0.000 {method 'split' of 'str' objects}
68048 0.009 0.000 0.009 0.000 {method 'strip' of 'str' objects}
95205 0.013 0.000 0.013 0.000 {method 'values' of 'dict' objects}
```
It significantly improves the speed as seen in the difference between 114 seconds and 1566 seconds.
I'm going to create a pull request if everything is clear?
Thank you for your effort. | 11-14-2019 12:47:18 | 11-14-2019 12:47:18 | Thank you for taking the time to look into this and opening a pull request!<|||||>You are welcome,
This thing has big effort and I always support as a community member :) .
If you merge PR I would be appreciated because I want to use it originally as provided in the master branch, for my ongoing project.
Thanks!<|||||>Your PR was merged, you can now use it from the master branch :)
Feel free to open other issues if you find other sub-optimal processes. |
transformers | 1,829 | closed | NotImplementedError when using TFDistilBertModel | ## 🐛 Bug
<!-- Important information -->
I have this error when I train a model with TFDistilBertModel. The same code works if I change to BERT for example. The transformers is loaded with `TFDistilBertModel.from_pretrained("distilbert-base-uncased")`
```
File "/home/<user>/miniconda3/envs/nlp/lib/python3.7/site-packages/tensorflow_core/python/autograph/impl/api.py", line 237, in wrapper
raise e.ag_error_metadata.to_exception(e)
NotImplementedError: in converted code:
relative to /home/<user>:
../models.py:68 call *
bert_hidden_states = self.model(
miniconda3/envs/nlp/lib/python3.7/site-packages/tensorflow_core/python/keras/engine/base_layer.py:842 __call__
outputs = call_fn(cast_inputs, *args, **kwargs)
miniconda3/envs/nlp/lib/python3.7/site-packages/transformers/modeling_tf_distilbert.py:545 call *
outputs = self.distilbert(inputs, **kwargs)
miniconda3/envs/nlp/lib/python3.7/site-packages/tensorflow_core/python/keras/engine/base_layer.py:842 __call__
outputs = call_fn(cast_inputs, *args, **kwargs)
miniconda3/envs/nlp/lib/python3.7/site-packages/transformers/modeling_tf_distilbert.py:431 call *
raise NotImplementedError
NotImplementedError:
```
## Environment
* OS: Manjaro
* Python version: 3.7.5
* TF: 2.0
* Using GPU ? Yes
<!-- Add any other context about the problem here. -->
| 11-14-2019 11:51:44 | 11-14-2019 11:51:44 | In my environemnt, it **works as expected**.
**Environment:**
- **O.S.** Linux Ubuntu
- **Python**: 3.6.9
- **HuggingFace's Transformers**: 2.1.1 (installed **today** from source with `pip install git+https://github.com/huggingface/transformers`)
- **PyTorch**: 1.3.1
- **TensorFlow**: 2.0
Below the execution:
```
Python 3.6.9 |Anaconda, Inc.| (default, Jul 30 2019, 19:07:31)
[GCC 7.3.0] on linux
Type "help", "copyright", "credits" or "license" for more information.
>>> import transformers
/home/<user>/anaconda3/lib/python3.6/site-packages/h5py/__init__.py:36: FutureWarning: Conversion of the second argument of issubdtype from `float` to `np.floating` is deprecated. In future, it will be treated as `np.float64 == np.dtype(float).type`.
from ._conv import register_converters as _register_converters
2019-11-14 13:56:38.199689: I tensorflow/core/platform/cpu_feature_guard.cc:142] Your CPU supports instructions that this TensorFlow binary was not compiled to use: AVX2 FMA
2019-11-14 13:56:38.337808: I tensorflow/core/platform/profile_utils/cpu_utils.cc:94] CPU Frequency: 3408000000 Hz
2019-11-14 13:56:38.338355: I tensorflow/compiler/xla/service/service.cc:168] XLA service 0x560bcbc86ae0 executing computations on platform Host. Devices:
2019-11-14 13:56:38.338375: I tensorflow/compiler/xla/service/service.cc:175] StreamExecutor device (0): Host, Default Version
>>> transformers.__version__
'2.1.1'
>>> import torch
>>> torch.__version__
'1.3.1'
>>> import tensorflow as tf
>>> tf.__version__
'2.0.0'
>>> import platform
>>> platform.platform()
'Linux-4.15.0-69-generic-x86_64-with-debian-buster-sid'
>>> platform.python_version()
'3.6.9'
>>> from transformers import DistilBertTokenizer, TFDistilBertModel
>>> tokenizer = DistilBertTokenizer.from_pretrained('distilbert-base-uncased')
>>> model = TFDistilBertModel.from_pretrained('distilbert-base-uncased')
100%|███████████████████████████████████████████████████████████████████████████████████████████████████████████████████████████████████████████████████████████████████████████████████████████████████████████████| 363423424/363423424 [00:39<00:00, 9179101.04B/s]
2019-11-14 13:58:58.633432: W tensorflow/python/util/util.cc:299] Sets are not currently considered sequences, but this may change in the future, so consider avoiding using them.
>>>
```
> ## Bug
> I have this error when I train a model with TFDistilBertModel. The same code works if I change to BERT for example. The transformers is loaded with `TFDistilBertModel.from_pretrained("distilbert-base-uncased")`
>
> ```
> File "/home/<user>/miniconda3/envs/nlp/lib/python3.7/site-packages/tensorflow_core/python/autograph/impl/api.py", line 237, in wrapper
> raise e.ag_error_metadata.to_exception(e)
> NotImplementedError: in converted code:
> relative to /home/<user>:
>
> ../models.py:68 call *
> bert_hidden_states = self.model(
> miniconda3/envs/nlp/lib/python3.7/site-packages/tensorflow_core/python/keras/engine/base_layer.py:842 __call__
> outputs = call_fn(cast_inputs, *args, **kwargs)
> miniconda3/envs/nlp/lib/python3.7/site-packages/transformers/modeling_tf_distilbert.py:545 call *
> outputs = self.distilbert(inputs, **kwargs)
> miniconda3/envs/nlp/lib/python3.7/site-packages/tensorflow_core/python/keras/engine/base_layer.py:842 __call__
> outputs = call_fn(cast_inputs, *args, **kwargs)
> miniconda3/envs/nlp/lib/python3.7/site-packages/transformers/modeling_tf_distilbert.py:431 call *
> raise NotImplementedError
>
> NotImplementedError:
> ```
>
> ## Environment
> * OS: Manjaro
> * Python version: 3.7.5
> * TF: 2.0
> * Using GPU ? Yes<|||||>@TheEdoardo93 thank you for showing me it's not a problem of the library. I uninstalled and reinstalled from source (like you did) and now it works. |
transformers | 1,828 | closed | GPT2 tokenizer is so slow because of regex.findall | ## 🐛 Bug
Hi,
As the discussion started in that #1621 issue, GPT2 tokenization is so slow even with 50MB of the dataset.
I'm using `run_lm_finetuning.py` and here are the steps to reproduce the problem:
- Have a dataset not an even bigger one. 50MB of a dataset is enough.
- Call `run_lm_finetuning.py` to train (finetune) the dataset. Here are my parameters:
```
--train_data_file "/train/datafile" \
--eval_data_file "/eval/datafile" \
--output_dir "/train/model" \
--model_type gpt2 \
--model_name_or_path distilgpt2 \
--cache_dir "/train/cache" \
--do_train \
--evaluate_during_training \
--per_gpu_train_batch_size 1 \
--per_gpu_eval_batch_size 1 \
--gradient_accumulation_steps 5 \
--overwrite_output_dir \
--seed 99
```
- You'll see it'll spend 20+ mins (depends on your cpu) to tokenize just 50MB of a text file.
I dug into `huggingface/transformers` 's codebase and profiled the tokenization process. And it seems like this regex drains the time:
https://github.com/huggingface/transformers/blob/155c782a2ccd103cf63ad48a2becd7c76a7d2115/transformers/tokenization_gpt2.py#L193
I run cProfiler and here is the result:
```
19537 function calls in 0.077 seconds
Ordered by: standard name
ncalls tottime percall cumtime percall filename:lineno(function)
1 0.000 0.000 0.000 0.000 <frozen importlib._bootstrap>:1009(_handle_fromlist)
1 0.000 0.000 0.077 0.077 <string>:1(<module>)
1 0.000 0.000 0.000 0.000 _bootlocale.py:33(getpreferredencoding)
3 0.000 0.000 0.000 0.000 locale.py:589(setlocale)
1 0.000 0.000 0.000 0.000 locale.py:647(getpreferredencoding)
1 0.000 0.000 0.035 0.035 regex.py:328(findall)
1 0.000 0.000 0.000 0.000 regex.py:434(_compile)
1457 0.004 0.000 0.006 0.000 tokenization_gpt2.py:139(bpe)
350 0.000 0.000 0.000 0.000 tokenization_gpt2.py:149(<lambda>)
1 0.009 0.009 0.077 0.077 tokenization_gpt2.py:191(plot)
6536 0.002 0.000 0.002 0.000 tokenization_gpt2.py:196(<genexpr>)
3240 0.003 0.000 0.003 0.000 tokenization_gpt2.py:197(<genexpr>)
607 0.001 0.000 0.001 0.000 tokenization_gpt2.py:91(get_pairs)
1 0.000 0.000 0.000 0.000 {built-in method _locale.nl_langinfo}
3 0.000 0.000 0.000 0.000 {built-in method _locale.setlocale}
1 0.000 0.000 0.077 0.077 {built-in method builtins.exec}
2 0.000 0.000 0.000 0.000 {built-in method builtins.hasattr}
5 0.000 0.000 0.000 0.000 {built-in method builtins.isinstance}
332 0.000 0.000 0.000 0.000 {built-in method builtins.len}
86 0.001 0.000 0.001 0.000 {built-in method builtins.min}
350 0.000 0.000 0.000 0.000 {method 'add' of 'set' objects}
85 0.000 0.000 0.000 0.000 {method 'append' of 'list' objects}
1 0.000 0.000 0.000 0.000 {method 'disable' of '_lsprof.Profiler' objects}
1457 0.000 0.000 0.000 0.000 {method 'encode' of 'str' objects}
1594 0.007 0.000 0.010 0.000 {method 'extend' of 'list' objects}
1 0.035 0.035 0.035 0.035 {method 'findall' of '_regex.Pattern' objects}
351 0.000 0.000 0.000 0.000 {method 'get' of 'dict' objects}
137 0.000 0.000 0.000 0.000 {method 'index' of 'tuple' objects}
1474 0.014 0.000 0.016 0.000 {method 'join' of 'str' objects}
1457 0.001 0.000 0.001 0.000 {method 'split' of 'str' objects}
```
P.s. Here `plot()` is the intermadiate function I named in order to profile and it covers that section:
```
for token in re.findall(self.pat, text):
if sys.version_info[0] == 2:
token = ''.join(self.byte_encoder[ord(b)] for b in token) # Maps all our bytes to unicode strings, avoiding controle tokens of the BPE (spaces in our case)
else:
token = ''.join(self.byte_encoder[b] for b in token.encode('utf-8')) # Maps all our bytes to unicode strings, avoiding controle tokens of the BPE (spaces in our case)
bpe_tokens.extend(bpe_token for bpe_token in self.bpe(token).split(' '))
```
Is there anyone having that issue? Haven't anybody tried to finetune GPT2 with a dataset larger than 300MB?
It's been 39 hours and my tokenization still in progress. Is it normal or could we optimize it?
Thanks! | 11-14-2019 09:55:57 | 11-14-2019 09:55:57 | |
transformers | 1,827 | closed | How to get all layers(12) hidden states of BERT? | ## ❓ Questions & Help
<!-- A clear and concise description of the question. -->
I tried to set the output_hidden_states=True, but only got 3 layers of the hidden states of model outputs for BERT, but theoricaly it should be 12, how can I get that? | 11-14-2019 08:21:18 | 11-14-2019 08:21:18 | You should have obtained the 12 layers as well as the embedding output. Are you sure you're not mistaking the output of the forward call (which is a tuple as well) with the hidden states?
Just to make sure, this is the correct way to obtain the hidden states:
```py
from transformers import BertModel, BertConfig
config = BertConfig.from_pretrained("xxx", output_hidden_states=True)
model = BertModel.from_pretrained("xxx", config=config)
outputs = model(inputs)
print(len(outputs)) # 3
hidden_states = outputs[2]
print(len(hidden_states)) # 13
embedding_output = hidden_states[0]
attention_hidden_states = hidden_states[1:]
```<|||||>> You should have obtained the 12 layers as well as the embedding output. Are you sure you're not mistaking the output of the forward call (which is a tuple as well) with the hidden states?
>
> Just to make sure, this is the correct way to obtain the hidden states:
>
> ```python
> from transformers import BertModel, BertConfig
>
> config = BertConfig.from_pretrained("xxx", output_hidden_states=True)
> model = BertModel.from_pretrained("xxx", config=config)
>
> outputs = model(inputs)
> print(len(outputs)) # 3
>
> hidden_states = outputs[2]
> print(len(hidden_states)) # 13
>
> embedding_output = hidden_states[0]
> attention_hidden_states = hidden_states[1:]
> ```
Thanks a lot, I think I just not find and realized the hidden states are stored at index 2 in the outputs.
By the way, where can I find the docs about the meaning of stored vectors at each index of the tuples? <|||||>In the doc for the `outputs` of `BertModel.
it's here: https://huggingface.co/transformers/model_doc/bert.html#transformers.BertModel<|||||>But for BERT model there is two input pooled_output and sequence_output.
pooled_output, sequence_output = bert_layer([input_word_id, input_mask, segment_id])
From here how can I get last 3 hidden layer outputs?<|||||>Hidden states will be returned if you will specify it in bert config, as noted above:
```
config = BertConfig.from_pretrained("xxx", output_hidden_states=True)
model = BertModel.from_pretrained("xxx", config=config)
```<|||||>@LysandreJik In ur code, what are `output[0]` and` output[1]`?<|||||>As it is mentioned in the [documentation](https://huggingface.co/transformers/model_doc/bert.html#bertmodel), the returns of the BERT model are `(last_hidden_state, pooler_output, hidden_states[optional], attentions[optional])`
`output[0]` is therefore the last hidden state and `output[1]` is the pooler output.<|||||>@LysandreJik What exactly pooler output is?<|||||>It's written in the documentation:
> Last layer hidden-state of the first token of the sequence (classification token) further processed by a Linear layer and a Tanh activation function. The Linear layer weights are trained from the next sentence prediction (classification) objective during pre-training.
>
> This output is usually not a good summary of the semantic content of the input, you’re often better with averaging or pooling the sequence of hidden-states for the whole input sequence.<|||||>@LysandreJik Sorry but I don't understand what this means. If I want to get a vector of a sentence - I use the hidden state (`output[0]`) right?
What could pooler output be used for?<|||||>`pooler: (batch, hidden_dim)`: Can be used when you want to have a representation for the whole sequence, like the last state of a RNN would give you. It is used for instance in Text Classification task where the predicted label doesn't depend on each token in the input.<|||||>@mfuntowicz So `output[0]` is for a separate representation of each word in the sequence, and the pooler is for a joint representation of the entire sequence?<|||||>Exactly 😊<|||||>@mfuntowicz Great thanks!
Two question please:
1. When taking hidden state, I can also access per-token representation of intermediate layers by adding config. Is it possible to do access pooler_output of an intermediate layer?
2. So if I want to analyse sentence similarity (so the sentence "this desk is green" will be more similar to "this chair is yellow" than to "We ate pizza") - Is it better to take pooler output or to average token representation in the hidden states?<|||||>@mfuntowicz Can you please help?<|||||>Hi @orko19,
1. No this is not possible to do so because the "pooler" is a layer in itself in BERT that depends [on the last representation](https://github.com/huggingface/transformers/blob/master/src/transformers/modeling_bert.py#L437).
2. The best would be to finetune the pooling representation for you task and use the pooler then. Using either the pooling layer or the averaged representation of the tokens **as it**, might be too biased towards the training objective it was initially trained for. These layers directly linked to the loss so very prone to high bias.<|||||>@mfuntowicz Great thanks!<|||||>如果是使用的trainer.train()这个是怎么获取它的中间层数据呢<|||||>Then, How to convert the output tensor to numpy?<|||||>> 如果是使用的trainer.train()这个是怎么获取它的中间层数据呢
老哥,我也遇到这个问题了,请问你最后解决了吗,怎么弄的呢。 |
transformers | 1,826 | closed | Regarding Fine-Tuning for Abstractive Summarization | Hi,
What result did you obtain with your Abstractive Summarization code for CNN-DM? Also, is it implemented based on this paper: https://arxiv.org/abs/1908.08345 ?
It will be great if you provide some details regarding it. | 11-14-2019 06:48:28 | 11-14-2019 06:48:28 | This issue has been automatically marked as stale because it has not had recent activity. It will be closed if no further activity occurs. Thank you for your contributions.
|
transformers | 1,825 | closed | 合并 | 11-14-2019 06:46:28 | 11-14-2019 06:46:28 | Is this related to this repository? |
|
transformers | 1,824 | closed | Issue testing run_squad.py example | ## ❓ Questions & Help
<!-- A clear and concise description of the question. -->
I am trying to run the squad example here
https://github.com/huggingface/transformers/blob/master/examples/run_squad.py
Using the Squad data from here
https://rajpurkar.github.io/SQuAD-explorer/
But I get this error
>ValueError: For training, each question should have exactly 1 answer.
Here is my code
```
!pip install transformers
import urllib.request
url = 'https://rajpurkar.github.io/SQuAD-explorer/dataset/train-v2.0.json'
urllib.request.urlretrieve(url, 'train-v2.0.json')
url = 'https://rajpurkar.github.io/SQuAD-explorer/dataset/dev-v2.0.json'
urllib.request.urlretrieve(url, 'dev-v2.0.json')
!wget 'https://raw.githubusercontent.com/huggingface/transformers/master/examples/run_squad.py'
!wget 'https://raw.githubusercontent.com/huggingface/pytorch-transformers/master/examples/utils_squad.py'
!wget 'https://raw.githubusercontent.com/huggingface/pytorch-transformers/master/examples/utils_squad_evaluate.py'
# $SQUAD_DIR/train-v1.1.json
SQUAD_Train = '/content/train-v2.0.json'
SQUAD_Dev = '/content/dev-v2.0.json'
!python run_squad.py \
--model_type bert \
--model_name_or_path bert-base-cased \
--do_train \
--do_eval \
--do_lower_case \
--train_file '$SQUAD_Train' \
--predict_file '$SQUAD_Dev' \
--per_gpu_train_batch_size 12 \
--learning_rate 3e-5 \
--num_train_epochs 2.0 \
--max_seq_length 384 \
--doc_stride 128 \
--output_dir /tmp/debug_squad/
```
Here is the error message
```
11/14/2019 05:01:20 - WARNING - __main__ - Process rank: -1, device: cuda, n_gpu: 1, distributed training: False, 16-bits training: False
11/14/2019 05:01:20 - INFO - transformers.configuration_utils - loading configuration file https://s3.amazonaws.com/models.huggingface.co/bert/bert-base-cased-config.json from cache at /root/.cache/torch/transformers/b945b69218e98b3e2c95acf911789741307dec43c698d35fad11c1ae28bda352.d7a3af18ce3a2ab7c0f48f04dc8daff45ed9a3ed333b9e9a79d012a0dedf87a6
11/14/2019 05:01:20 - INFO - transformers.configuration_utils - Model config {
"attention_probs_dropout_prob": 0.1,
"finetuning_task": null,
"hidden_act": "gelu",
"hidden_dropout_prob": 0.1,
"hidden_size": 768,
"initializer_range": 0.02,
"intermediate_size": 3072,
"layer_norm_eps": 1e-12,
"max_position_embeddings": 512,
"num_attention_heads": 12,
"num_hidden_layers": 12,
"num_labels": 2,
"output_attentions": false,
"output_hidden_states": false,
"output_past": true,
"pruned_heads": {},
"torchscript": false,
"type_vocab_size": 2,
"use_bfloat16": false,
"vocab_size": 28996
}
11/14/2019 05:01:21 - INFO - transformers.tokenization_utils - loading file https://s3.amazonaws.com/models.huggingface.co/bert/bert-base-cased-vocab.txt from cache at /root/.cache/torch/transformers/5e8a2b4893d13790ed4150ca1906be5f7a03d6c4ddf62296c383f6db42814db2.e13dbb970cb325137104fb2e5f36fe865f27746c6b526f6352861b1980eb80b1
11/14/2019 05:01:21 - INFO - transformers.modeling_utils - loading weights file https://s3.amazonaws.com/models.huggingface.co/bert/bert-base-cased-pytorch_model.bin from cache at /root/.cache/torch/transformers/35d8b9d36faaf46728a0192d82bf7d00137490cd6074e8500778afed552a67e5.3fadbea36527ae472139fe84cddaa65454d7429f12d543d80bfc3ad70de55ac2
11/14/2019 05:01:24 - INFO - transformers.modeling_utils - Weights of BertForQuestionAnswering not initialized from pretrained model: ['qa_outputs.weight', 'qa_outputs.bias']
11/14/2019 05:01:24 - INFO - transformers.modeling_utils - Weights from pretrained model not used in BertForQuestionAnswering: ['cls.predictions.bias', 'cls.predictions.transform.dense.weight', 'cls.predictions.transform.dense.bias', 'cls.predictions.decoder.weight', 'cls.seq_relationship.weight', 'cls.seq_relationship.bias', 'cls.predictions.transform.LayerNorm.weight', 'cls.predictions.transform.LayerNorm.bias']
11/14/2019 05:01:27 - INFO - __main__ - Training/evaluation parameters Namespace(adam_epsilon=1e-08, cache_dir='', config_name='', device=device(type='cuda'), do_eval=True, do_lower_case=True, do_train=True, doc_stride=128, eval_all_checkpoints=False, evaluate_during_training=False, fp16=False, fp16_opt_level='O1', gradient_accumulation_steps=1, learning_rate=3e-05, local_rank=-1, logging_steps=50, max_answer_length=30, max_grad_norm=1.0, max_query_length=64, max_seq_length=384, max_steps=-1, model_name_or_path='bert-base-cased', model_type='bert', n_best_size=20, n_gpu=1, no_cuda=False, null_score_diff_threshold=0.0, num_train_epochs=2.0, output_dir='/tmp/debug_squad/', overwrite_cache=False, overwrite_output_dir=False, per_gpu_eval_batch_size=8, per_gpu_train_batch_size=12, predict_file='/content/dev-v2.0.json', save_steps=50, seed=42, server_ip='', server_port='', tokenizer_name='', train_file='/content/train-v2.0.json', verbose_logging=False, version_2_with_negative=False, warmup_steps=0, weight_decay=0.0)
11/14/2019 05:01:27 - INFO - __main__ - Creating features from dataset file at /content/train-v2.0.json
Traceback (most recent call last):
File "run_squad.py", line 569, in <module>
main()
File "run_squad.py", line 514, in main
train_dataset = load_and_cache_examples(args, tokenizer, evaluate=False, output_examples=False)
File "run_squad.py", line 307, in load_and_cache_examples
version_2_with_negative=args.version_2_with_negative)
File "/content/utils_squad.py", line 152, in read_squad_examples
"For training, each question should have exactly 1 answer.")
ValueError: For training, each question should have exactly 1 answer.
```
For convenience, here's a colab notebook with the code that you can run
https://colab.research.google.com/drive/1tNisXX5siuNnkuEQ-X_XdEdDTtdJ0qeL | 11-14-2019 05:06:19 | 11-14-2019 05:06:19 | Try adding `--version_2_with_negative \` to your `run_squad.py `script.<|||||>That fixed it, thanks! |
transformers | 1,823 | closed | how to use convert_pytorch_checkpoint_to_tf2.py | ## ❓ Questions & Help
I am wondering how to use convert_pytorch_checkpoint_to_tf2.py to convert pytorch checkpoint to tensorflow checkpoint. I found Comparing-PT-and-TF-models.ipynb have an example to use pytorch_pretrained_bert. But It doesn't work for convert_pytorch_checkpoint_to_tf2. Could you please give me any help?
<!-- A clear and concise description of the question. -->
| 11-14-2019 02:48:06 | 11-14-2019 02:48:06 | Hi, you can show the help with the following command:
```
python transformers/convert_pytorch_checkpoint_to_tf2.py --help
```
What is your use-case?<|||||>> Hi, you can show the help with the following command:
>
> ```
> python transformers/convert_pytorch_checkpoint_to_tf2.py --help
> ```
>
> What is your use-case?
Thanks for your suggestion. I used convert_bert_pytorch_checkpoint_to_original_tf. It works for me. |
transformers | 1,822 | closed | CamemBERT | Code for the CamemBERT model, adapted from https://github.com/pytorch/fairseq/tree/master/examples/camembert | 11-14-2019 01:04:36 | 11-14-2019 01:04:36 | # [Codecov](https://codecov.io/gh/huggingface/transformers/pull/1822?src=pr&el=h1) Report
> Merging [#1822](https://codecov.io/gh/huggingface/transformers/pull/1822?src=pr&el=desc) into [master](https://codecov.io/gh/huggingface/transformers/commit/155c782a2ccd103cf63ad48a2becd7c76a7d2115?src=pr&el=desc) will **decrease** coverage by `0.14%`.
> The diff coverage is `62.5%`.
[](https://codecov.io/gh/huggingface/transformers/pull/1822?src=pr&el=tree)
```diff
@@ Coverage Diff @@
## master #1822 +/- ##
==========================================
- Coverage 84.16% 84.02% -0.15%
==========================================
Files 94 97 +3
Lines 14185 14281 +96
==========================================
+ Hits 11939 11999 +60
- Misses 2246 2282 +36
```
| [Impacted Files](https://codecov.io/gh/huggingface/transformers/pull/1822?src=pr&el=tree) | Coverage Δ | |
|---|---|---|
| [transformers/modeling\_camembert.py](https://codecov.io/gh/huggingface/transformers/pull/1822/diff?src=pr&el=tree#diff-dHJhbnNmb3JtZXJzL21vZGVsaW5nX2NhbWVtYmVydC5weQ==) | `100% <100%> (ø)` | |
| [transformers/configuration\_camembert.py](https://codecov.io/gh/huggingface/transformers/pull/1822/diff?src=pr&el=tree#diff-dHJhbnNmb3JtZXJzL2NvbmZpZ3VyYXRpb25fY2FtZW1iZXJ0LnB5) | `100% <100%> (ø)` | |
| [transformers/tokenization\_auto.py](https://codecov.io/gh/huggingface/transformers/pull/1822/diff?src=pr&el=tree#diff-dHJhbnNmb3JtZXJzL3Rva2VuaXphdGlvbl9hdXRvLnB5) | `45.94% <33.33%> (-1.12%)` | :arrow_down: |
| [transformers/tokenization\_camembert.py](https://codecov.io/gh/huggingface/transformers/pull/1822/diff?src=pr&el=tree#diff-dHJhbnNmb3JtZXJzL3Rva2VuaXphdGlvbl9jYW1lbWJlcnQucHk=) | `36.53% <36.53%> (ø)` | |
| [transformers/configuration\_auto.py](https://codecov.io/gh/huggingface/transformers/pull/1822/diff?src=pr&el=tree#diff-dHJhbnNmb3JtZXJzL2NvbmZpZ3VyYXRpb25fYXV0by5weQ==) | `59.45% <66.66%> (+0.63%)` | :arrow_up: |
| [transformers/modeling\_tf\_pytorch\_utils.py](https://codecov.io/gh/huggingface/transformers/pull/1822/diff?src=pr&el=tree#diff-dHJhbnNmb3JtZXJzL21vZGVsaW5nX3RmX3B5dG9yY2hfdXRpbHMucHk=) | `92.95% <0%> (ø)` | :arrow_up: |
| [transformers/tests/modeling\_tf\_common\_test.py](https://codecov.io/gh/huggingface/transformers/pull/1822/diff?src=pr&el=tree#diff-dHJhbnNmb3JtZXJzL3Rlc3RzL21vZGVsaW5nX3RmX2NvbW1vbl90ZXN0LnB5) | `97.08% <0%> (ø)` | :arrow_up: |
| [transformers/modeling\_roberta.py](https://codecov.io/gh/huggingface/transformers/pull/1822/diff?src=pr&el=tree#diff-dHJhbnNmb3JtZXJzL21vZGVsaW5nX3JvYmVydGEucHk=) | `71.76% <0%> (ø)` | :arrow_up: |
| [transformers/modeling\_xlnet.py](https://codecov.io/gh/huggingface/transformers/pull/1822/diff?src=pr&el=tree#diff-dHJhbnNmb3JtZXJzL21vZGVsaW5nX3hsbmV0LnB5) | `73.61% <0%> (ø)` | :arrow_up: |
| [transformers/modeling\_ctrl.py](https://codecov.io/gh/huggingface/transformers/pull/1822/diff?src=pr&el=tree#diff-dHJhbnNmb3JtZXJzL21vZGVsaW5nX2N0cmwucHk=) | `96.46% <0%> (ø)` | :arrow_up: |
| ... and [4 more](https://codecov.io/gh/huggingface/transformers/pull/1822/diff?src=pr&el=tree-more) | |
------
[Continue to review full report at Codecov](https://codecov.io/gh/huggingface/transformers/pull/1822?src=pr&el=continue).
> **Legend** - [Click here to learn more](https://docs.codecov.io/docs/codecov-delta)
> `Δ = absolute <relative> (impact)`, `ø = not affected`, `? = missing data`
> Powered by [Codecov](https://codecov.io/gh/huggingface/transformers/pull/1822?src=pr&el=footer). Last update [155c782...65549e3](https://codecov.io/gh/huggingface/transformers/pull/1822?src=pr&el=lastupdated). Read the [comment docs](https://docs.codecov.io/docs/pull-request-comments).
<|||||>Hi @louismartin many thanks for adding this :heart:
I tested the implementation a bit, and I got the following error message when I try to save the tokenizer object:
For RoBERTa this is working correctly:
```python
In [2]: roberta_tokenizer = RobertaTokenizer.from_pretrained("roberta-base")
In [3]: roberta_tokenizer.save_pretrained("/tmp")
Out[3]:
('/tmp/vocab.json',
'/tmp/merges.txt',
'/tmp/special_tokens_map.json',
'/tmp/added_tokens.json')
```
But for CamemBERT:
```python
In [6]: camembert_tokenizer = CamembertTokenizer.from_pretrained("camembert-base")
In [7]: camembert_tokenizer.save_pretrained("/tmp")
---------------------------------------------------------------------------
NotImplementedError Traceback (most recent call last)
<ipython-input-7-417ff9326aaf> in <module>
----> 1 camembert_tokenizer.save_pretrained("/tmp")
/mnt/transformers-camembert/transformers/tokenization_utils.py in save_pretrained(self, save_directory)
463 f.write(out_str)
464
--> 465 vocab_files = self.save_vocabulary(save_directory)
466
467 return vocab_files + (special_tokens_map_file, added_tokens_file)
/mnt/transformers-camembert/transformers/tokenization_utils.py in save_vocabulary(self, save_directory)
474 Please use :func:`~transformers.PreTrainedTokenizer.save_pretrained` `()` to save the full Tokenizer state if you want to reload it using the :func:`~transformers.PreTrainedTokenizer.from_pretrained` class method.
475 """
--> 476 raise NotImplementedError
477
478
```
`.save_vocabulary()` is used e.g. in the NER example script :)<|||||>> Hi @louismartin many thanks for adding this ❤️
>
> I tested the implementation a bit, and I got the following error message when I try to save the tokenizer object:
>
> For RoBERTa this is working correctly:
>
> ```python
> In [2]: roberta_tokenizer = RobertaTokenizer.from_pretrained("roberta-base")
>
> In [3]: roberta_tokenizer.save_pretrained("/tmp")
> Out[3]:
> ('/tmp/vocab.json',
> '/tmp/merges.txt',
> '/tmp/special_tokens_map.json',
> '/tmp/added_tokens.json')
> ```
>
> But for CamemBERT:
>
> ```python
> In [6]: camembert_tokenizer = CamembertTokenizer.from_pretrained("camembert-base")
>
> In [7]: camembert_tokenizer.save_pretrained("/tmp")
> ---------------------------------------------------------------------------
> NotImplementedError Traceback (most recent call last)
> <ipython-input-7-417ff9326aaf> in <module>
> ----> 1 camembert_tokenizer.save_pretrained("/tmp")
>
> /mnt/transformers-camembert/transformers/tokenization_utils.py in save_pretrained(self, save_directory)
> 463 f.write(out_str)
> 464
> --> 465 vocab_files = self.save_vocabulary(save_directory)
> 466
> 467 return vocab_files + (special_tokens_map_file, added_tokens_file)
>
> /mnt/transformers-camembert/transformers/tokenization_utils.py in save_vocabulary(self, save_directory)
> 474 Please use :func:`~transformers.PreTrainedTokenizer.save_pretrained` `()` to save the full Tokenizer state if you want to reload it using the :func:`~transformers.PreTrainedTokenizer.from_pretrained` class method.
> 475 """
> --> 476 raise NotImplementedError
> 477
> 478
> ```
>
> `.save_vocabulary()` is used e.g. in the NER example script :)
Hi Stefan,
Thanks for trying our model!
The reason is that I haven't implemented saving the tokenizer.
Two solutions: either don't use `tokenizer.save_pretrained`, or implement it by adapting the necessary methods from [tokenization_xlnet.py](https://github.com/huggingface/transformers/blob/master/transformers/tokenization_xlnet.py) to implement `save_pretrained()`. It is probably only necessary to copy and paste `__setstate__` and `__getstate__` methods :)
I don't have much time to do it in the next few weeks, but don't hesitate if you have more questions!
Louis
<|||||>Hi @louismartin!!
### **#1844**
I rebased on master, did the few discussed tweaks, and merged this: https://github.com/huggingface/transformers/pull/1844<|||||>Great work!
@stefan-it @julien-c @louismartin
Could you show how to get the embedding vector of a sentence please?
```python
from transformers import CamembertTokenizer
import torch
camembert_tokenizer = CamembertTokenizer.from_pretrained("camembert-base")
camembert_tokenizer.encode("Salut, ça va ?") # How to get embedding of this sentence not just the ids of tokens ?
```<|||||>@stefan-it @julien-c @louismartin @hzitoun
Question: the following weights I've extracted with the following code are the weights of the pre-trained CamemBERT model or the sentence embeddings?
```
from transformers import CamembertTokenizer
from transformers import CamembertModel
text="J'aime le camembert !"
tokenizer = CamembertTokenizer.from_pretrained('camembert-base')
model = CamembertModel.from_pretrained('camembert-base', output_hidden_states=True) # add output_hidden_states in order to retrieve ALL hidden states of the CamemBERT model (so the embeddings layer too!)
input_ids = torch.tensor(tokenizer.encode(text)).unsqueeze(0)
output = model(input_ids)
embeddings = output[2][0]
print('embeddings: \n{}'.format(embeddings))
>>> tensor([[[ 0.0399, -0.0788, 0.0552, ..., -0.0855, -0.0394, 0.0222],
[-0.1678, 0.1954, -0.2219, ..., 0.1590, -0.2967, -0.1723],
[ 0.0699, 0.2307, -0.0723, ..., 0.2294, 0.0463, -0.0167],
...,
[ 0.0766, 0.2548, 0.0690, ..., 0.0172, -0.3713, -0.0262],
[ 0.1681, 0.0253, -0.0386, ..., 0.1626, -0.1203, -0.2415],
[ 0.4876, -0.0714, -0.0020, ..., -0.0628, -0.2701, 0.2210]]],
grad_fn=<NativeLayerNormBackward>)
```
It has been tested by comparing with the official Camembert model found [here](https://camembert-model.fr/#contact).
```
import torch
camembert = torch.hub.load('pytorch/fairseq', 'camembert.v0')
camembert.eval() # disable dropout (or leave in train mode to finetune)
line = "J'aime le camembert!"
tokens = camembert.encode(line)
all_layers = camembert.extract_features(tokens, return_all_hiddens=True)
embeddings = all_layers[0]
print('embeddings: \n{}'.format(embeddings)
>>> tensor([[[ 0.0399, -0.0788, 0.0552, ..., -0.0855, -0.0394, 0.0222],
[-0.1678, 0.1954, -0.2219, ..., 0.1590, -0.2967, -0.1723],
[ 0.0699, 0.2307, -0.0723, ..., 0.2294, 0.0463, -0.0167],
...,
[ 0.0766, 0.2548, 0.0690, ..., 0.0172, -0.3713, -0.0262],
[ 0.1461, 0.0414, -0.0877, ..., -0.0577, -0.2219, -0.3685],
[ 0.4876, -0.0714, -0.0020, ..., -0.0628, -0.2701, 0.2210]]],
grad_fn=<TransposeBackward0>)
```
Most values correspond, but there are some values different (e.g. 0.1681 vs 0.1461 or 0.2415 vs -0.3685)..
> Great work!
>
> @stefan-it @julien-c @louismartin
>
> Could you show how to get the embedding vector of a sentence please?
>
> ```python
> from transformers import CamembertTokenizer
> import torch
>
> camembert_tokenizer = CamembertTokenizer.from_pretrained("camembert-base")
>
> camembert_tokenizer.encode("Salut, ça va ?") # How to get embedding of this sentence not just the ids of tokens ?
> ```<|||||>> @stefan-it @julien-c @louismartin @hzitoun
> Question: the following weights I've extracted with the following code are the weights of the pre-trained CamemBERT model or the sentence embeddings?
>
> ```
> from transformers import CamembertTokenizer
> from transformers import CamembertModel
>
> text="J'aime le camembert !"
> tokenizer = CamembertTokenizer.from_pretrained('camembert-base')
> model = CamembertModel.from_pretrained('camembert-base', output_hidden_states=True) # add output_hidden_states in order to retrieve ALL hidden states of the CamemBERT model (so the embeddings layer too!)
> input_ids = torch.tensor(tokenizer.encode(text)).unsqueeze(0)
> output = model(input_ids)
>
> embeddings = output[2][0]
> print('embeddings: \n{}'.format(embeddings))
> >>> tensor([[[ 0.0399, -0.0788, 0.0552, ..., -0.0855, -0.0394, 0.0222],
> [-0.1678, 0.1954, -0.2219, ..., 0.1590, -0.2967, -0.1723],
> [ 0.0699, 0.2307, -0.0723, ..., 0.2294, 0.0463, -0.0167],
> ...,
> [ 0.0766, 0.2548, 0.0690, ..., 0.0172, -0.3713, -0.0262],
> [ 0.1681, 0.0253, -0.0386, ..., 0.1626, -0.1203, -0.2415],
> [ 0.4876, -0.0714, -0.0020, ..., -0.0628, -0.2701, 0.2210]]],
> grad_fn=<NativeLayerNormBackward>)
> ```
>
> It has been tested by comparing with the official Camembert model found [here](https://camembert-model.fr/#contact).
>
> ```
> import torch
> camembert = torch.hub.load('pytorch/fairseq', 'camembert.v0')
> camembert.eval() # disable dropout (or leave in train mode to finetune)
>
> line = "J'aime le camembert!"
> tokens = camembert.encode(line)
>
> all_layers = camembert.extract_features(tokens, return_all_hiddens=True)
> embeddings = all_layers[0]
> print('embeddings: \n{}'.format(embeddings)
> >>> tensor([[[ 0.0399, -0.0788, 0.0552, ..., -0.0855, -0.0394, 0.0222],
> [-0.1678, 0.1954, -0.2219, ..., 0.1590, -0.2967, -0.1723],
> [ 0.0699, 0.2307, -0.0723, ..., 0.2294, 0.0463, -0.0167],
> ...,
> [ 0.0766, 0.2548, 0.0690, ..., 0.0172, -0.3713, -0.0262],
> [ 0.1461, 0.0414, -0.0877, ..., -0.0577, -0.2219, -0.3685],
> [ 0.4876, -0.0714, -0.0020, ..., -0.0628, -0.2701, 0.2210]]],
> grad_fn=<TransposeBackward0>)
> ```
>
> Most values correspond, but there are some values different (e.g. 0.1681 vs 0.1461 or 0.2415 vs -0.3685)..
>
> > Great work!
> > @stefan-it @julien-c @louismartin
> > Could you show how to get the embedding vector of a sentence please?
> > ```python
> > from transformers import CamembertTokenizer
> > import torch
> >
> > camembert_tokenizer = CamembertTokenizer.from_pretrained("camembert-base")
> >
> > camembert_tokenizer.encode("Salut, ça va ?") # How to get embedding of this sentence not just the ids of tokens ?
> > ```
@TheEdoardo93
Thanks for showing how to do the same thing with native `torch` and for pointing out the difference in embedding for some values!
Does any one have an idea how to get a fixed shape of embedding vector regardless the number of tokens in a sentence? (in order to do cosine distance for example between two sentences even if they have different tokens sizes)
Calculating the `mean on axis=1` for example ? I've asked the question on SOF too if you could answer https://stackoverflow.com/questions/59030907/nlp-transformers-how-to-get-a-fixed-embedding-vector-size :) |
transformers | 1,821 | closed | Generated text makes no sense. Trying to auto-generate sentences like https://transformer.huggingface.co/doc/distil-gpt2 | I would like to replicate the text-generation behavior of https://transformer.huggingface.co/doc/distil-gpt2 on my MacOS. I went to that URL and typed "A doula is a person" and kept tabbing for auto-text generation, and got this:
```
A doula is a person who cares for a child or infant. A doula is not a midwife. But the two are not entirely independent. Why did I chose a doula and not myself? There are two good reasons: First, since my daughter was still growing , I had the luxury of time to learn as much knowledge about breastfeeding as I could. Second, I have the opportunity
```
Quite impressive. But now, I want to replicate this in Python on my system so I can auto-generate text that makes sense. I did the following:
1. Installed tensor flow
2. installed pytorch
3. installed huggingface :D
4. Based on the recommendation in the [Examples section here](https://huggingface.co/transformers/examples.html#language-generation), I then ran:
```
python run_generation.py --model_type=gpt2 --model_name_or_path=gpt2
```
and gave it the same sample input:
```
Model prompt >>> A doula
100%|███████████████████████████████████████████████████████████████████████████████████████████████████████████████████████████████████████████████████████████████████████| 20/20 [00:01<00:00, 10.24it/s]
hatter with a broad laugh and a guffaw over her stomach are eminently adorable. In
Model prompt >>> A doula is a person
100%|███████████████████████████████████████████████████████████████████████████████████████████████████████████████████████████████████████████████████████████████████████| 20/20 [00:02<00:00, 8.94it/s]
who wishes to be free and independent while having her resources donated to society.
Gender Non-
```
As you can see, I get gibberish back, the sentences and context makes no sense. What am I missing? | 11-14-2019 00:17:50 | 11-14-2019 00:17:50 | Have you ever tried to finetune some hyper-paremeters, such as **temperature** or **seed**? You can also modify the **length** of the generated text by OpenAI GPT-2.
_Tip_: if you want to make OpenAI GPT-2 model more comfortable and sure about its results, please set the temperature value lower than 0,5 (e.g. 0,2 or 0,3).
> I would like to replicate the text-generation behavior of https://transformer.huggingface.co/doc/distil-gpt2 on my MacOS. I went to that URL and typed "A doula is a person" and kept tabbing for auto-text generation, and got this:
>
> ```
> A doula is a person who cares for a child or infant. A doula is not a midwife. But the two are not entirely independent. Why did I chose a doula and not myself? There are two good reasons: First, since my daughter was still growing , I had the luxury of time to learn as much knowledge about breastfeeding as I could. Second, I have the opportunity
> ```
>
> Quite impressive. But now, I want to replicate this in Python on my system so I can auto-generate text that makes sense. I did the following:
>
> 1. Installed tensor flow
> 2. installed pytorch
> 3. installed huggingface :D
> 4. Based on the recommendation in the [Examples section here](https://huggingface.co/transformers/examples.html#language-generation), I then ran:
>
> ```
> python run_generation.py --model_type=gpt2 --model_name_or_path=gpt2
> ```
>
> and gave it the same sample input:
>
> ```
> Model prompt >>> A doula
> 100%|███████████████████████████████████████████████████████████████████████████████████████████████████████████████████████████████████████████████████████████████████████| 20/20 [00:01<00:00, 10.24it/s]
> hatter with a broad laugh and a guffaw over her stomach are eminently adorable. In
> Model prompt >>> A doula is a person
> 100%|███████████████████████████████████████████████████████████████████████████████████████████████████████████████████████████████████████████████████████████████████████| 20/20 [00:02<00:00, 8.94it/s]
> who wishes to be free and independent while having her resources donated to society.
>
> Gender Non-
> ```
>
> As you can see, I get gibberish back, the sentences and context makes no sense. What am I missing?<|||||>This issue has been automatically marked as stale because it has not had recent activity. It will be closed if no further activity occurs. Thank you for your contributions.
|
transformers | 1,820 | closed | Is there a way of finetuning DistilGPT2? | ## ❓ Questions & Help
<!-- A clear and concise description of the question. -->
Hello! I am trying to finetune GPT2 for a project of mine but my aim is to deploy the model on a server and hence, I would like the final model file to be small. I was thinking of using the Distil* models especially the DistilGPT2 but couldnt find it in the run_lm_finetuning.py script.
Currently, is finetuning distilGPT2 supported? If yes, then how should I do it? | 11-13-2019 21:45:56 | 11-13-2019 21:45:56 | Yes you can, call `run_lm_finetuning` via these parameters:
```
--model_type gpt2 \
--model_name_or_path distilgpt2
```<|||||>This issue has been automatically marked as stale because it has not had recent activity. It will be closed if no further activity occurs. Thank you for your contributions.
<|||||>I can't find the file `run_lm_finetuning.py` under this repo. Could you please give the full file path please? Thanks a lot.<|||||>> I can't find the file `run_lm_finetuning.py` under this repo. Could you please give the full file path please? Thanks a lot.
Got it . it has been renamed to [run_language_modeling.py](https://github.com/huggingface/transformers/blob/master/examples/language-modeling/run_language_modeling.py) now. |
transformers | 1,819 | closed | CUDA out of memory on loss.backward when fine-tuning GPT2 (117M) | ## ❓ Questions & Help
File "run_lm_finetuning.py", line 551, in <module>
main()
File "run_lm_finetuning.py", line 503, in main
global_step, tr_loss = train(args, train_dataset, model, tokenizer)
File "run_lm_finetuning.py", line 240, in train
loss.backward()
File "/home/antonio/anaconda3/lib/python3.7/site-packages/torch/tensor.py", line 150, in backward
torch.autograd.backward(self, gradient, retain_graph, create_graph)
File "/home/antonio/anaconda3/lib/python3.7/site-packages/torch/autograd/__init__.py", line 99, in backward
allow_unreachable=True) # allow_unreachable flag
RuntimeError: CUDA out of memory. Tried to allocate 198.00 MiB (GPU 0; 5.93 GiB total capacity; 4.64 GiB already allocated; 54.94 MiB free; 233.05 MiB cached)
I encounter the above error with my **1060 GTX 6GB Nvidia, on the GPT-2 small model. The training configs are:
batch size = 1
gradient accumulation steps = 1024** (I've started without gradient accumulation, then tried accumulation based on an old issue from this repo, then from small values I went up to this value, but the error always occurs).
**If i run with no gradient accumulation, I get this instead:**
File "run_lm_finetuning.py", line 228, in train
outputs = model(inputs, masked_lm_labels=labels) if args.mlm else model(inputs, labels=labels)
File "/home/antonio/anaconda3/lib/python3.7/site-packages/torch/nn/modules/module.py", line 541, in __call__
result = self.forward(*input, **kwargs)
File "/home/antonio/anaconda3/lib/python3.7/site-packages/transformers/modeling_gpt2.py", line 549, in forward
inputs_embeds=inputs_embeds)
File "/home/antonio/anaconda3/lib/python3.7/site-packages/torch/nn/modules/module.py", line 541, in __call__
result = self.forward(*input, **kwargs)
File "/home/antonio/anaconda3/lib/python3.7/site-packages/transformers/modeling_gpt2.py", line 460, in forward
head_mask=head_mask[i])
File "/home/antonio/anaconda3/lib/python3.7/site-packages/torch/nn/modules/module.py", line 541, in __call__
result = self.forward(*input, **kwargs)
File "/home/antonio/anaconda3/lib/python3.7/site-packages/transformers/modeling_gpt2.py", line 232, in forward
head_mask=head_mask)
File "/home/antonio/anaconda3/lib/python3.7/site-packages/torch/nn/modules/module.py", line 541, in __call__
result = self.forward(*input, **kwargs)
File "/home/antonio/anaconda3/lib/python3.7/site-packages/transformers/modeling_gpt2.py", line 182, in forward
x = self.c_attn(x)
File "/home/antonio/anaconda3/lib/python3.7/site-packages/torch/nn/modules/module.py", line 541, in __call__
result = self.forward(*input, **kwargs)
File "/home/antonio/anaconda3/lib/python3.7/site-packages/transformers/modeling_utils.py", line 488, in forward
x = torch.addmm(self.bias, x.view(-1, x.size(-1)), self.weight)
RuntimeError: CUDA out of memory. Tried to allocate 20.00 MiB (GPU 0; 5.93 GiB total capacity; 4.77 GiB already allocated; 12.81 MiB free; 154.93 MiB cached)
Can you please give me a little hint on how to overcome this error, or a little hope to run gpt-2 small on 6 GB of GPU.
Thanks | 11-13-2019 20:48:47 | 11-13-2019 20:48:47 | If your gpu is out of memory, try decreasing the batch size (this will save memory). In order to retain the same effective batch size, use gradient accumulation.
Basically, do `loss.backward()` for each step, but only every, say, 10 steps, do `optimizer.step()` and `optimizer.backward()`.<|||||>Hi @aced125, batch size is already set to one and I have tried values from 1 to 1024 for gradient accumulation, it gives me always CUDA out of memory error.<|||||>Hijacking this issue to say that I have the exact same problem with `xlm-mlm-17-1280`. Even with `batch size = 1`, Apex enabled and two 1080Ti's it always give memory error in the first `loss.backward()` call.
<|||||>Which optimizer are you using? If you're using the default (AdamW) that may be part of your problem. Different optimizers have different memory requirements. Adam is one of the worst offenders. Give RMSProp a try since it has much less memory overhead. Every additional feature like using momentum will increase memory overhead.<|||||>Hi @dvaltchanov thanks but using RMSprop led me to the same errors<|||||>@antocapp Which block_size are you using? The default (512) or something else? Using a smaller block size (e.g. 256) will also use up less memory. On a smaller card like yours, you basically need to use batch size of 1 with small block size and memory efficient optimizer for it to fit into GPU memory. An alternative is to try running your test on Google Colab or another cloud service where you can get 12+ GB of GPU memory.<|||||>> Hijacking this issue to say that I have the exact same problem with `xlm-mlm-17-1280`. Even with `batch size = 1`, Apex enabled and two 1080Ti's it always give memory error in the first `loss.backward()` call.
hi,I have the exact same problem with `xlm-mlm-17-1280`.Have you solved this issue?<|||||>This issue has been automatically marked as stale because it has not had recent activity. It will be closed if no further activity occurs. Thank you for your contributions.
|
transformers | 1,818 | closed | Trouble running fine tuned language model script | ## 🐛 Bug
I'm having trouble running the finetuning script. When I run
```
TRAIN_FILE='/home/khev/research/transformers/data/wikitext-2-raw/wiki.train.raw'
TEST_FILE='/home/khev/research/transformers/data/wikitext-2-raw/wiki.test.raw'
%run run_lm_finetuning.py \
--output_dir=output \
--model_type=bert \
--model_name_or_path=bert-base-uncased\
--do_train \
--train_data_file=$TRAIN_FILE \
--do_eval \
--eval_data_file=$TEST_FILE \
--mlm
```
I get the following error:
```
---------------------------------------------------------------------------
RuntimeError Traceback (most recent call last)
~/research/transformers/examples/run_lm_finetuning.py in <module>
545
546 if __name__ == "__main__":
--> 547 main()
~/research/transformers/examples/run_lm_finetuning.py in main()
497 torch.distributed.barrier()
498
--> 499 global_step, tr_loss = train(args, train_dataset, model, tokenizer)
500 logger.info(" global_step = %s, average loss = %s", global_step, tr_loss)
501
~/research/transformers/examples/run_lm_finetuning.py in train(args, train_dataset, model, tokenizer)
222 epoch_iterator = tqdm(train_dataloader, desc="Iteration", disable=args.local_rank not in [-1, 0])
223 for step, batch in enumerate(epoch_iterator):
--> 224 inputs, labels = mask_tokens(batch, tokenizer, args) if args.mlm else (batch, batch)
225 inputs = inputs.to(args.device)
226 labels = labels.to(args.device)
~/research/transformers/examples/run_lm_finetuning.py in mask_tokens(inputs, tokenizer, args)
147 probability_matrix = torch.full(labels.shape, args.mlm_probability)
148 special_tokens_mask = [tokenizer.get_special_tokens_mask(val, already_has_special_tokens=True) for val in labels.tolist()]
--> 149 probability_matrix.masked_fill_(torch.tensor(special_tokens_mask, dtype=torch.bool), value=0.0)
150 masked_indices = torch.bernoulli(probability_matrix).bool()
151 labels[~masked_indices] = -1 # We only compute loss on masked tokens
RuntimeError: Expected object of scalar type Byte but got scalar type Bool for argument #2 'mask'
```
Info:
Python 3.7.3
Torch 1.1.0
Ubuntu 18.04.3 LTS
| 11-13-2019 17:08:14 | 11-13-2019 17:08:14 | Hi, could you specify the versions on which you are running? (especially PyTorch).
`bool` was introduced in PyTorch 1.2 so, unfortunately, this example will crash if using an anterior version.<|||||>Sorry, forgot to give that info. As you preempted, I'm running torch 1.1.0. Guess I should update?<|||||>The core library should run on PyTorch 1.0.1+, but examples usually require torch 1.2+. It would be better to upgrade if you want to try out the examples, indeed!<|||||>Excellent, will do, thanks! And Kudos on the fast response time BTW :-)<|||||>No problem, glad to help!<|||||>Ah, now I'm getting the following error. Any insight?
FYI: I'm now at pytorch 1.3.1 -- think I should downgrade to 1.2.0?
```
---------------------------------------------------------------------------
RuntimeError Traceback (most recent call last)
~/research/transformers/examples/run_lm_finetuning.py in <module>
546
547 if __name__ == "__main__":
--> 548 main()
~/research/transformers/examples/run_lm_finetuning.py in main()
498 torch.distributed.barrier()
499
--> 500 global_step, tr_loss = train(args, train_dataset, model, tokenizer)
501 logger.info(" global_step = %s, average loss = %s", global_step, tr_loss)
502
~/research/transformers/examples/run_lm_finetuning.py in train(args, train_dataset, model, tokenizer)
227 labels = labels.to(args.device)
228 model.train()
--> 229 outputs = model(inputs, masked_lm_labels=labels) if args.mlm else model(inputs, labels=labels)
230 loss = outputs[0] # model outputs are always tuple in transformers (see doc)
231
~/anaconda3/lib/python3.7/site-packages/torch/nn/modules/module.py in __call__(self, *input, **kwargs)
539 result = self._slow_forward(*input, **kwargs)
540 else:
--> 541 result = self.forward(*input, **kwargs)
542 for hook in self._forward_hooks.values():
543 hook_result = hook(self, input, result)
~/anaconda3/lib/python3.7/site-packages/transformers/modeling_bert.py in forward(self, input_ids, attention_mask, token_type_ids, position_ids, head_mask, masked_lm_labels)
768 token_type_ids=token_type_ids,
769 position_ids=position_ids,
--> 770 head_mask=head_mask)
771
772 sequence_output = outputs[0]
~/anaconda3/lib/python3.7/site-packages/torch/nn/modules/module.py in __call__(self, *input, **kwargs)
539 result = self._slow_forward(*input, **kwargs)
540 else:
--> 541 result = self.forward(*input, **kwargs)
542 for hook in self._forward_hooks.values():
543 hook_result = hook(self, input, result)
~/anaconda3/lib/python3.7/site-packages/transformers/modeling_bert.py in forward(self, input_ids, attention_mask, token_type_ids, position_ids, head_mask)
622 head_mask = [None] * self.config.num_hidden_layers
623
--> 624 embedding_output = self.embeddings(input_ids, position_ids=position_ids, token_type_ids=token_type_ids)
625 encoder_outputs = self.encoder(embedding_output,
626 extended_attention_mask,
~/anaconda3/lib/python3.7/site-packages/torch/nn/modules/module.py in __call__(self, *input, **kwargs)
539 result = self._slow_forward(*input, **kwargs)
540 else:
--> 541 result = self.forward(*input, **kwargs)
542 for hook in self._forward_hooks.values():
543 hook_result = hook(self, input, result)
~/anaconda3/lib/python3.7/site-packages/transformers/modeling_bert.py in forward(self, input_ids, token_type_ids, position_ids)
165 token_type_ids = torch.zeros_like(input_ids)
166
--> 167 words_embeddings = self.word_embeddings(input_ids)
168 position_embeddings = self.position_embeddings(position_ids)
169 token_type_embeddings = self.token_type_embeddings(token_type_ids)
~/anaconda3/lib/python3.7/site-packages/torch/nn/modules/module.py in __call__(self, *input, **kwargs)
539 result = self._slow_forward(*input, **kwargs)
540 else:
--> 541 result = self.forward(*input, **kwargs)
542 for hook in self._forward_hooks.values():
543 hook_result = hook(self, input, result)
~/anaconda3/lib/python3.7/site-packages/torch/nn/modules/sparse.py in forward(self, input)
112 return F.embedding(
113 input, self.weight, self.padding_idx, self.max_norm,
--> 114 self.norm_type, self.scale_grad_by_freq, self.sparse)
115
116 def extra_repr(self):
~/anaconda3/lib/python3.7/site-packages/torch/nn/functional.py in embedding(input, weight, padding_idx, max_norm, norm_type, scale_grad_by_freq, sparse)
1482 # remove once script supports set_grad_enabled
1483 _no_grad_embedding_renorm_(weight, input, max_norm, norm_type)
-> 1484 return torch.embedding(weight, input, padding_idx, scale_grad_by_freq, sparse)
1485
1486
RuntimeError: index out of range: Tried to access index 38889 out of table with 30521 rows. at /pytorch/aten/src/TH/generic/THTensorEvenMoreMath.cpp:418
```<|||||>Hmm that error should not be linked to a version problem. Did you use the same command:
```
run_lm_finetuning.py \
--output_dir=output \
--model_type=bert \
--model_name_or_path=bert-base-uncased\
--do_train \
--train_data_file=$TRAIN_FILE \
--do_eval \
--eval_data_file=$TEST_FILE \
--mlm
```
or did you use a different checkpoint?<|||||>I used the same command...<|||||>I also have same issue while finetuning distilgpt2:
```
File "train_docker/train", line 229, in train
outputs = model(inputs, masked_lm_labels=labels) if args.mlm else model(inputs, labels=labels)
File "/usr/local/lib/python3.7/site-packages/torch/nn/modules/module.py", line 547, in __call__
result = self.forward(*input, **kwargs)
File "/usr/local/lib/python3.7/site-packages/transformers/modeling_gpt2.py", line 533, in forward
head_mask=head_mask)
File "/usr/local/lib/python3.7/site-packages/torch/nn/modules/module.py", line 547, in __call__
result = self.forward(*input, **kwargs)
File "/usr/local/lib/python3.7/site-packages/transformers/modeling_gpt2.py", line 420, in forward
inputs_embeds = self.wte(input_ids)
File "/usr/local/lib/python3.7/site-packages/torch/nn/modules/module.py", line 547, in __call__
result = self.forward(*input, **kwargs)
File "/usr/local/lib/python3.7/site-packages/torch/nn/modules/sparse.py", line 114, in forward
self.norm_type, self.scale_grad_by_freq, self.sparse)
File "/usr/local/lib/python3.7/site-packages/torch/nn/functional.py", line 1467, in embedding
return torch.embedding(weight, input, padding_idx, scale_grad_by_freq, sparse)
RuntimeError: index out of range: Tried to access index 50257 out of table with 50256 rows. at ../aten/src/TH/generic/THTensorEvenMoreMath.cpp:237
```<|||||>Update: the following two commands, for GTP-2 and roberto, are working. So I will stick to these for now. Thanks for your help!
```
%run run_lm_finetuning.py \
--output_dir=output \
--model_type=gpt2 \
--model_name_or_path=gpt2 \
--do_train \
--train_data_file=$TRAIN_FILE \
--do_eval \
--eval_data_file=$TEST_FILE
```
```
TRAIN_FILE='/home/khev/research/transformers/data/wikitext-2-raw/wiki.train.raw'
TEST_FILE='/home/khev/research/transformers/data/wikitext-2-raw/wiki.test.raw'
%run run_lm_finetuning.py \
--output_dir=output \
--model_type=roberta \
--model_name_or_path=roberta-base \
--do_train \
--train_data_file=$TRAIN_FILE \
--do_eval \
--eval_data_file=$TEST_FILE \
--mlm
```<|||||>Thanks for reporting those bugs @Khev and @iedmrc, I'll check what's going on for BERT and DistilGPT-2.<|||||>I found that It occurs when we have a "cached feature file".<|||||>I have one last question. I want to use the fine tuning script on a custom dataset: the titles of scientific papers. So my dataset has form:
[title1, title2, ..., ]
where title_i = 'this is a paper title'
I'm wondering how this dataset should be structured. I assume it should mimic the wikitext dataset, but I'm not entirely sure of their structure. It looks like sections are delimited by " ==". For exampl, in the wiki.test.raw file, the first few lines are:
"""
= Robert Boulter =
TEXT
== Career ==
"""
Should I put my dataset in this form? So like:
== Paper1 ==
title 1
== Paper2
title 2?
<|||||>To me, you should use <|endoftext|> as a delimiter in the gpt2 case. Check page 4 of the original gpt paper openai published.
https://s3-us-west-2.amazonaws.com/openai-assets/research-covers/language-unsupervised/language_understanding_paper.pdf<|||||>As @iedmrc said, you should probably mimic the way the individual models were pre-trained instead.
For example, for GPT-2 you might want to add the suffix `<|endoftext|>` (available via the `tokenizer.eos_token` attribute) to indicate to the model you're joining different texts.
We're not doing that in the `run_lm_finetuning.py` script as we want to keep the example simple and the texts are long enough in the WikiText-2 dataset for it not to affect performance too much.<|||||>I understand. Thanks!<|||||>I just pushed a fix on `master` that should correct the second issue you faced. Feel free to re-open if it did not solve your issue so that I may investigate further. |
transformers | 1,817 | closed | Using multiple inputs for GPT-2 | ## ❓ Questions & Help
A current project I am working on, to utilize a tuned approach in text generation, is creating a cover letter based off of a job description and some personal qualifications. From researching, I found the convAI blog that you used and thought to apply the concept with OpenAIGPTDoubleHeadsModel. I added special tokens to the vocabulary for delimiters and segment indicators to include word, position and segment embeddings. I have the persona ('some personal qualifications'), turned history into job description and the reply was "I am qualified for this position because...". From my understanding, the attention will help relate the job description and persona to the text generation. Now I am stuck when it comes to fine tuning the model. I have a bunch of cover letters to use scraped from the web. I have fine tuned a base model and it outputs some coherent cover letters but I want to incorporate the skills needed in the job description for a more accurate an specific cover letter. Am I on the right track and if so how do I fine tune the model on the cover letters? Any suggestions or ideas are welcomed.
<!-- A clear and concise description of the question. -->
| 11-13-2019 14:57:05 | 11-13-2019 14:57:05 | This issue has been automatically marked as stale because it has not had recent activity. It will be closed if no further activity occurs. Thank you for your contributions.
|
transformers | 1,816 | closed | Best way to fine tune GPT-2 in order to create a custom text generator? | Hello to everyone, and thanks for this wonderful work.
I am new to this library and I would appreciate an help for a task that i want to accomplish, just to know if I am acting right, to create a custom english text generator, such that giving it an input (title/sentence) it would generate 200-300 words based on that input.
My questions are:
1) I have prepared my dataset (each input is composed basically by the title and the corpus), what file should I look for to fine-tune GPT-2: run_lm_finetuning.py ? How many epochs/iterations do you suggest to run to fine tune? How large the dataset should be?
2) Once I have fine-tuned GPT-2, how to generate my custom text giving as input a title/sentence and using the fine-tuned model?
Thanks a lot | 11-13-2019 09:36:25 | 11-13-2019 09:36:25 | Hi, you can use a combination of the scripts `run_lm_finetuning.py` and `run_generation.py` to accomplish what you want:
- Fine-tune GPT-2 to your dataset using `run_lm_finetuning.py`. The default parameters should work well enough, I usually use three epochs (rather than the default 1) when training on small datasets. I have had success with datasets as small as a few 10s of MBs, but I have never tried with less.
- Generate text by using `run_generation.py` and specifying your custom checkpoint. Specify a `--length` of 200 or 300.<|||||>Thanks @LysandreJik !
Can you point me out how to organize my dataset file(s) or where to look within the repository? Moreover, does fine-tuning handle OOV words?
Thanks again<|||||>Merge your files into one by splitting them via <|endoftext|> token. You could also split the dataset into two files in order to have a dataset for evaluation. I use %90 and %10 for training and evaluation, respectively. Don't remove any stopwords etc. GPT2 will do the rest. Don't forget that GPT2 is so powerful to learn from your dataset so it may slightly overfit if you have not enough data. For example, train GPT2 with just 10MB data and you'll see it won't generate anything other than learnt from the dataset.<|||||>This issue has been automatically marked as stale because it has not had recent activity. It will be closed if no further activity occurs. Thank you for your contributions.
<|||||>Did you use a special token to separate the title and the corpus?<|||||>I think this would depend on what specifically you are inputting. If it's a title that you want to be part of the body (part of the first sentence) then you wouldn't want to break that sentence up with a separate token. If it's a title that you want the document to derive the topic from but not include as part of the body then a separate token might be helpful to prevent the model from expanding the title to form the body. :)<|||||>> If it's a title that you want the document to derive the topic from but not include as part of the body then a separate token might be helpful to prevent the model from expanding the title to form the body. :)
So in essence, if you want to have a title should be used as context but not include as part of the body, you should structure data as:
```
Title: This is a great title
<|endoftext|>
Titles are one of the greatest inventions of humanity.
Well crafted titles continue to save countless man-years by not requiring readers to actually read the article.
<|endoftext|>
Title: ...
```
Is that what you mean? Because intuitively I would assume that this wouldn't work as intended: since the Title is separated in a different token, it should not influence the next token. Or am I missing something?<|||||>Same question. Is there different token for separating the title and the body?<|||||>You could add a special token to the tokenizer and train the dataset with that. |
transformers | 1,815 | closed | computing self-attention for tokens in a sentence | ## ❓ Questions & Help
Hi,
Please refer to this [issue](https://github.com/google-research/bert/issues/914).
Thanks!
| 11-13-2019 03:38:46 | 11-13-2019 03:38:46 | To get the attention score of each heads, refer to the [documentation](https://huggingface.co/transformers/model_doc/bert.html#transformers.BertModel) : see `outputs`.
---
> how do I know which heads give the best scores ?
You can't really know. Each heads will compute some kind of attention. It can be attention for `this token refer to that token`, or it can be attention for `this token is similar to that token` or even if can be any kind of relation, even something, we human, can't interpret.
If you want to extract some specific kind of attention, I see no other solution than manually inspect all the heads' attention and empirically choose one (or several).
Some papers did it : [Contrastive Attention Mechanism for Abstractive Sentence Summarization](https://arxiv.org/abs/1910.13114)<|||||>Thanks for your reply.
Please refer to [here](https://github.com/huggingface/transformers/issues/2054) for more details.
Thanks!<|||||>This issue has been automatically marked as stale because it has not had recent activity. It will be closed if no further activity occurs. Thank you for your contributions.
|
transformers | 1,814 | closed | Sample a constant number of tokens for masking in LM finetuning | @thomwolf @LysandreJik @julien-c Re-creating this PR as the original PR https://github.com/huggingface/transformers/pull/1555 that I created about a month ago has become quite stale. Please review.
For Masked LM fine-tuning, I think both the original BERT and RoBERTa implementations uniformly sample x number of tokens in *each* sequence for masking (where x = mlm_probability * 100 * sequence_length)
However, The current logic in run_lm_finetuning.py does an indepdendent sampling (from bernoulli distribution) for each token in the sequence. This leads to variance in the number of masked tokens (with the average number still close to x%).
The below example illustrates an extreme case, of the current logic, where no token in the input sequence is masked.
```
In [1]: import numpy as np
...: import torch
...: from transformers import BertTokenizer
...:
...: mlm_probability = 0.15
...: tokenizer = BertTokenizer.from_pretrained('bert-large-uncased')
...:
...: tokens = tokenizer.convert_ids_to_tokens(tokenizer.encode('please mask me, o lord!', add_special_tokens=True))
...:
...: input_ids = tokenizer.convert_tokens_to_ids(tokens)
...:
...: inputs = torch.Tensor([input_ids])
...:
...: labels = inputs.clone()
...:
...: probability_matrix = torch.full(labels.shape, mlm_probability)
...:
...: special_tokens_mask = [tokenizer.get_special_tokens_mask(val, already_has_special_tokens=True) for val in labels.tolist()]
...: probability_matrix.masked_fill_(torch.tensor(special_tokens_mask, dtype=torch.bool), value=0.0)
...: masked_indices = torch.bernoulli(probability_matrix).bool()
...:
...:
In [2]: masked_indices
Out[2]: tensor([[False, False, False, False, False, False, False, False, False]])
```
This PR modifies the logic so the percentage of masked tokens is constant (at x).
Separately, the existing and the new masking logic both rely on boolean tensors of pytorch.
So, this also updates README to include the minimum pytorch version needed. (1.2.0) | 11-13-2019 02:13:10 | 11-13-2019 02:13:10 | Hi @rakeshchada, we've thought about this. It is interesting and we might merge it, but will probably will be behind a flag :)
Thanks for contributing!<|||||>This issue has been automatically marked as stale because it has not had recent activity. It will be closed if no further activity occurs. Thank you for your contributions.
|
transformers | 1,813 | closed | LM Fine-tuning for XLNET? | ## ❓ Questions & Help
<!-- A clear and concise description of the question. -->
Hi there, is there any plan to add support for fine-tuning XLNET? This one currently isn't available in run_lm_finetuning.py.
Thanks! | 11-12-2019 22:09:11 | 11-12-2019 22:09:11 | I'm also looking forward to it! <|||||>I might be naive here, but will adding xlnet's config, LMHeadModel, and tokenizer to MODEL_CLASSES work?
https://github.com/huggingface/transformers/blob/74ce8de7d8e0375a9123f9542f3483f46cc8df9b/examples/run_lm_finetuning.py#L61<|||||>I don't think adding the Python classes for XLNet is enough. The code distinguishes between two modes of fine-tuning a language model: 1. masked language models (MLM), i.e. BERT and company 2. Sequential left-to-right LM, GPT and GPT2.
Check this, for example:
https://github.com/huggingface/transformers/blob/74ce8de7d8e0375a9123f9542f3483f46cc8df9b/examples/run_lm_finetuning.py#L224
XLNet is neither. Its LM task is to predict factorization permutations (check paper for more details) of a given sequence. In simple words, it splits in a sequence into two sequential sub-sequences in all possible ways and it uses the first one as input for predicting the second one.
The original implementation has sample code on how to produce the factorization permutation:
https://github.com/zihangdai/xlnet/blob/5cd50bc451436e188a8e7fea15358d5a8c916b72/data_utils.py#L579<|||||>This issue has been automatically marked as stale because it has not had recent activity. It will be closed if no further activity occurs. Thank you for your contributions.
<|||||>Pretty much looking forward to it |
transformers | 1,812 | closed | Update conversion script to convert XLM-R | It requires a few changes to the existing RoBERTa conversion script because `fairseq` has updated the RoBERTa model definition.
Specifically, the definition of multiheaded attention is changed at https://github.com/pytorch/fairseq/blob/2a9b4ec2374574cd0315f95e126788e4fe795f0d/fairseq/modules/multihead_attention.py#L42
With this new script, you should be able to convert the XLM-R model released at https://github.com/pytorch/fairseq/tree/master/examples/xlmr and requested by #1769.
I have successfully converted the two released model weights. | 11-12-2019 21:51:37 | 11-12-2019 21:51:37 | # [Codecov](https://codecov.io/gh/huggingface/transformers/pull/1812?src=pr&el=h1) Report
> Merging [#1812](https://codecov.io/gh/huggingface/transformers/pull/1812?src=pr&el=desc) into [master](https://codecov.io/gh/huggingface/transformers/commit/155c782a2ccd103cf63ad48a2becd7c76a7d2115?src=pr&el=desc) will **not change** coverage.
> The diff coverage is `n/a`.
[](https://codecov.io/gh/huggingface/transformers/pull/1812?src=pr&el=tree)
```diff
@@ Coverage Diff @@
## master #1812 +/- ##
=======================================
Coverage 84.16% 84.16%
=======================================
Files 94 94
Lines 14185 14185
=======================================
Hits 11939 11939
Misses 2246 2246
```
------
[Continue to review full report at Codecov](https://codecov.io/gh/huggingface/transformers/pull/1812?src=pr&el=continue).
> **Legend** - [Click here to learn more](https://docs.codecov.io/docs/codecov-delta)
> `Δ = absolute <relative> (impact)`, `ø = not affected`, `? = missing data`
> Powered by [Codecov](https://codecov.io/gh/huggingface/transformers/pull/1812?src=pr&el=footer). Last update [155c782...6d2a5bf](https://codecov.io/gh/huggingface/transformers/pull/1812?src=pr&el=lastupdated). Read the [comment docs](https://docs.codecov.io/docs/pull-request-comments).
<|||||>This is great indeed but we are losing backward compatibility to convert the original RoBERTa weights aren't we?
Maybe we can add a flag to control which version of fairseq RoBERTa the original weight come from? For instance a flag as a string selected between `roberta` and `XLM-R`.<|||||>I think the situation is a bit more complicated than I thought. We also need to change the vocabulary size, etc. I think it would be better to have a separate treatment for XLM-R. So I am closing this for now. |
transformers | 1,811 | closed | Fix special tokens addition in decoder #1807 | Fixes the issue detailed in #1807
Added special tokens should not be present when decoding with the `skip_special_tokens` flag set to `True`.
Using the `convert_tokens_to_ids` checks whether those added tokens are present and removes them if necessary. | 11-12-2019 20:32:11 | 11-12-2019 20:32:11 | # [Codecov](https://codecov.io/gh/huggingface/transformers/pull/1811?src=pr&el=h1) Report
> Merging [#1811](https://codecov.io/gh/huggingface/transformers/pull/1811?src=pr&el=desc) into [master](https://codecov.io/gh/huggingface/transformers/commit/155c782a2ccd103cf63ad48a2becd7c76a7d2115?src=pr&el=desc) will **decrease** coverage by `0.08%`.
> The diff coverage is `100%`.
[](https://codecov.io/gh/huggingface/transformers/pull/1811?src=pr&el=tree)
```diff
@@ Coverage Diff @@
## master #1811 +/- ##
==========================================
- Coverage 84.16% 84.08% -0.09%
==========================================
Files 94 94
Lines 14185 14047 -138
==========================================
- Hits 11939 11811 -128
+ Misses 2246 2236 -10
```
| [Impacted Files](https://codecov.io/gh/huggingface/transformers/pull/1811?src=pr&el=tree) | Coverage Δ | |
|---|---|---|
| [transformers/tests/tokenization\_tests\_commons.py](https://codecov.io/gh/huggingface/transformers/pull/1811/diff?src=pr&el=tree#diff-dHJhbnNmb3JtZXJzL3Rlc3RzL3Rva2VuaXphdGlvbl90ZXN0c19jb21tb25zLnB5) | `100% <100%> (ø)` | :arrow_up: |
| [transformers/tokenization\_utils.py](https://codecov.io/gh/huggingface/transformers/pull/1811/diff?src=pr&el=tree#diff-dHJhbnNmb3JtZXJzL3Rva2VuaXphdGlvbl91dGlscy5weQ==) | `92.12% <100%> (+0.92%)` | :arrow_up: |
| [transformers/modeling\_tf\_roberta.py](https://codecov.io/gh/huggingface/transformers/pull/1811/diff?src=pr&el=tree#diff-dHJhbnNmb3JtZXJzL21vZGVsaW5nX3RmX3JvYmVydGEucHk=) | `89.9% <0%> (-0.53%)` | :arrow_down: |
| [transformers/modeling\_tf\_xlnet.py](https://codecov.io/gh/huggingface/transformers/pull/1811/diff?src=pr&el=tree#diff-dHJhbnNmb3JtZXJzL21vZGVsaW5nX3RmX3hsbmV0LnB5) | `87.82% <0%> (-0.37%)` | :arrow_down: |
| [transformers/modeling\_tf\_transfo\_xl.py](https://codecov.io/gh/huggingface/transformers/pull/1811/diff?src=pr&el=tree#diff-dHJhbnNmb3JtZXJzL21vZGVsaW5nX3RmX3RyYW5zZm9feGwucHk=) | `92.21% <0%> (-0.33%)` | :arrow_down: |
| [transformers/tests/modeling\_tf\_common\_test.py](https://codecov.io/gh/huggingface/transformers/pull/1811/diff?src=pr&el=tree#diff-dHJhbnNmb3JtZXJzL3Rlc3RzL21vZGVsaW5nX3RmX2NvbW1vbl90ZXN0LnB5) | `96.8% <0%> (-0.28%)` | :arrow_down: |
| [transformers/modeling\_tf\_utils.py](https://codecov.io/gh/huggingface/transformers/pull/1811/diff?src=pr&el=tree#diff-dHJhbnNmb3JtZXJzL21vZGVsaW5nX3RmX3V0aWxzLnB5) | `92.4% <0%> (-0.28%)` | :arrow_down: |
| [transformers/modeling\_tf\_ctrl.py](https://codecov.io/gh/huggingface/transformers/pull/1811/diff?src=pr&el=tree#diff-dHJhbnNmb3JtZXJzL21vZGVsaW5nX3RmX2N0cmwucHk=) | `97.75% <0%> (-0.11%)` | :arrow_down: |
| [transformers/modeling\_tf\_xlm.py](https://codecov.io/gh/huggingface/transformers/pull/1811/diff?src=pr&el=tree#diff-dHJhbnNmb3JtZXJzL21vZGVsaW5nX3RmX3hsbS5weQ==) | `90.39% <0%> (-0.08%)` | :arrow_down: |
| [transformers/modeling\_tf\_distilbert.py](https://codecov.io/gh/huggingface/transformers/pull/1811/diff?src=pr&el=tree#diff-dHJhbnNmb3JtZXJzL21vZGVsaW5nX3RmX2Rpc3RpbGJlcnQucHk=) | `98.59% <0%> (-0.07%)` | :arrow_down: |
| ... and [6 more](https://codecov.io/gh/huggingface/transformers/pull/1811/diff?src=pr&el=tree-more) | |
------
[Continue to review full report at Codecov](https://codecov.io/gh/huggingface/transformers/pull/1811?src=pr&el=continue).
> **Legend** - [Click here to learn more](https://docs.codecov.io/docs/codecov-delta)
> `Δ = absolute <relative> (impact)`, `ø = not affected`, `? = missing data`
> Powered by [Codecov](https://codecov.io/gh/huggingface/transformers/pull/1811?src=pr&el=footer). Last update [155c782...74d0bcb](https://codecov.io/gh/huggingface/transformers/pull/1811?src=pr&el=lastupdated). Read the [comment docs](https://docs.codecov.io/docs/pull-request-comments).
<|||||>Nice fix, a lot cleaner as well |
transformers | 1,810 | closed | NameError: name 'DUMMY_INPUTS' is not defined - From TF to PyTorch | ## 🐛 Bug
I'm using TFBertForSequenceClassification, Tensorflow 2.0.0b0, PyTorch is up-to-date and the code from Hugging Face README.md:
```
import tensorflow as tf
import tensorflow_datasets
from transformers import *
tf.compat.v1.enable_eager_execution()
tokenizer = BertTokenizer.from_pretrained('bert-base-cased')
model = TFBertForSequenceClassification.from_pretrained('bert-base-cased')
data = tensorflow_datasets.load('glue/mrpc')
train_dataset = glue_convert_examples_to_features(data['train'], tokenizer, max_length=128, task='mrpc')
valid_dataset = glue_convert_examples_to_features(data['validation'], tokenizer, max_length=128, task='mrpc')
train_dataset = train_dataset.shuffle(100).batch(32).repeat(2)
valid_dataset = valid_dataset.batch(64)
optimizer = tf.keras.optimizers.Adam(learning_rate=3e-5, epsilon=1e-08, clipnorm=1.0)
loss = tf.keras.losses.SparseCategoricalCrossentropy(from_logits=True)
metric = tf.keras.metrics.SparseCategoricalAccuracy('accuracy')
model.compile(optimizer=optimizer, loss=loss, metrics=[metric])
history = model.fit(train_dataset, epochs=2, steps_per_epoch=115,
validation_data=valid_dataset, validation_steps=7)
model.save_pretrained('/home/rubens_gmail_com/tf')
```
The model trains properly, but after weights are saved as `.h5` and I try to load to `BertForSequenceClassification.from_pretrained`, the following error shows up:
```
pytorch_model = BertForSequenceClassification.from_pretrained('/home/rubens_gmail_com/tf',from_tf=True)
NameError Traceback (most recent call last)
<ipython-input-25-7a878059a298> in <module>
1 pytorch_model = BertForSequenceClassification.from_pretrained('/home/rubens_gmail_com/tf',
----> 2 from_tf=True)
~/.local/lib/python3.7/site-packages/transformers/modeling_utils.py in from_pretrained(cls, pretrained_model_name_or_path, *model_args, **kwargs)
357 try:
358 from transformers import load_tf2_checkpoint_in_pytorch_model
--> 359 model = load_tf2_checkpoint_in_pytorch_model(model, resolved_archive_file, allow_missing_keys=True)
360 except ImportError as e:
361 logger.error("Loading a TensorFlow model in PyTorch, requires both PyTorch and TensorFlow to be installed. Please see "
~/.local/lib/python3.7/site-packages/transformers/modeling_tf_pytorch_utils.py in load_tf2_checkpoint_in_pytorch_model(pt_model, tf_checkpoint_path, tf_inputs, allow_missing_keys)
199
200 if tf_inputs is None:
--> 201 tf_inputs = tf.constant(DUMMY_INPUTS)
202
203 if tf_inputs is not None:
NameError: name 'DUMMY_INPUTS' is not defined
```
If I run:
```
pytorch_model = BertForSequenceClassification.from_pretrained('/home/rubens_gmail_com/tf/tf_model.h5',from_tf=True)
```
I get:
```
UnicodeDecodeError Traceback (most recent call last)
<ipython-input-35-4146e5f25140> in <module>
----> 1 pytorch_model = BertForSequenceClassification.from_pretrained('/home/rubens_gmail_com/tf/tf_model.h5',from_tf=True)
~/.local/lib/python3.7/site-packages/transformers/modeling_utils.py in from_pretrained(cls, pretrained_model_name_or_path, *model_args, **kwargs)
285 cache_dir=cache_dir, return_unused_kwargs=True,
286 force_download=force_download,
--> 287 **kwargs
288 )
289 else:
~/.local/lib/python3.7/site-packages/transformers/configuration_utils.py in from_pretrained(cls, pretrained_model_name_or_path, **kwargs)
152
153 # Load config
--> 154 config = cls.from_json_file(resolved_config_file)
155
156 if hasattr(config, 'pruned_heads'):
~/.local/lib/python3.7/site-packages/transformers/configuration_utils.py in from_json_file(cls, json_file)
184 """Constructs a `BertConfig` from a json file of parameters."""
185 with open(json_file, "r", encoding='utf-8') as reader:
--> 186 text = reader.read()
187 return cls.from_dict(json.loads(text))
188
/opt/anaconda3/lib/python3.7/codecs.py in decode(self, input, final)
320 # decode input (taking the buffer into account)
321 data = self.buffer + input
--> 322 (result, consumed) = self._buffer_decode(data, self.errors, final)
323 # keep undecoded input until the next call
324 self.buffer = data[consumed:]
UnicodeDecodeError: 'utf-8' codec can't decode byte 0x89 in position 0: invalid start byte
```
I'm using Python 3.7.4, 8 x V100 GPU, Anaconda environment on a Debian on GCP. Any ideas on how to overcome this issue ? | 11-12-2019 20:23:55 | 11-12-2019 20:23:55 | Hi! I believe this is a bug that was fixed on master. Could you try and install from source and tell me if it fixes your issue?
You can do so with the following command in your python environment:
```
pip install git+https://github.com/huggingface/transformers
```<|||||>@LysandreJik Thanks for the hint. I used a workaround. I installed `transformers` using:
```
conda install -c conda-forge transformers
```
In Python 3.7.4, then I added `DUMMY_INPUTS = [[7, 6, 0, 0, 1], [1, 2, 3, 0, 0], [0, 0, 0, 4, 5]]` after variable `logger` in `/opt/anaconda3/lib/python3.7/site-packages/transformers/modeling_tf_utils.py`, because it was missing.
It was interesting, because in GCP, transformers were not showing in `python3` , only in `sudo python3`<|||||>@LysandreJik I updated the install from source but ran into the same issue. The "solution" was again as @RubensZimbres mentions: adding `DUMMY_INPUTS = [[7, 6, 0, 0, 1], [1, 2, 3, 0, 0], [0, 0, 0, 4, 5]]` after variable `logger`, however I needed it changed in `/opt/anaconda3/lib/python3.7/site-packages/transformers/modeling_tf_pytorch_utils.py`.
<|||||>I created a Pull Request at: https://github.com/huggingface/transformers/pull/1847<|||||>Can't reproduce on master and the latest release (2.2.1).
Feel free to reopen if the issue is still there.
|
transformers | 1,809 | closed | Why do language modeling heads not have activation functions? | ## ❓ Questions & Help
<!-- A clear and concise description of the question. -->
This is more of a question about the transformer architecture in general than anything else. I noticed that, in `modeling_openai.py`, for example, the `self.lm_head()` module is just a linear layer. Why is it sufficient to use a linear transformation here for the language modeling task? Would there by any advantages/disadvantages to throwing a `gelu` or something on the end and then calling the output of that the logits?
```python
class OpenAIGPTLMHeadModel(OpenAIGPTPreTrainedModel):
r"""
**labels**: (`optional`) ``torch.LongTensor`` of shape ``(batch_size, sequence_length)``:
Labels for language modeling.
Note that the labels **are shifted** inside the model, i.e. you can set ``labels = input_ids``
Indices are selected in ``[-1, 0, ..., config.vocab_size]``
All labels set to ``-1`` are ignored (masked), the loss is only
computed for labels in ``[0, ..., config.vocab_size]``
Outputs: `Tuple` comprising various elements depending on the configuration (config) and inputs:
**loss**: (`optional`, returned when ``labels`` is provided) ``torch.FloatTensor`` of shape ``(1,)``:
Language modeling loss.
**prediction_scores**: ``torch.FloatTensor`` of shape ``(batch_size, sequence_length, config.vocab_size)``
Prediction scores of the language modeling head (scores for each vocabulary token before SoftMax).
**hidden_states**: (`optional`, returned when ``config.output_hidden_states=True``)
list of ``torch.FloatTensor`` (one for the output of each layer + the output of the embeddings)
of shape ``(batch_size, sequence_length, hidden_size)``:
Hidden-states of the model at the output of each layer plus the initial embedding outputs.
**attentions**: (`optional`, returned when ``config.output_attentions=True``)
list of ``torch.FloatTensor`` (one for each layer) of shape ``(batch_size, num_heads, sequence_length, sequence_length)``:
Attentions weights after the attention softmax, used to compute the weighted average in the self-attention heads.
Examples::
tokenizer = OpenAIGPTTokenizer.from_pretrained('openai-gpt')
model = OpenAIGPTLMHeadModel.from_pretrained('openai-gpt')
input_ids = torch.tensor(tokenizer.encode("Hello, my dog is cute")).unsqueeze(0) # Batch size 1
outputs = model(input_ids, labels=input_ids)
loss, logits = outputs[:2]
"""
def __init__(self, config):
super(OpenAIGPTLMHeadModel, self).__init__(config)
self.transformer = OpenAIGPTModel(config)
self.lm_head = nn.Linear(config.n_embd, config.vocab_size, bias=False)
self.init_weights()
def get_output_embeddings(self):
return self.lm_head
def forward(self, input_ids=None, attention_mask=None, token_type_ids=None, position_ids=None, head_mask=None, inputs_embeds=None,
labels=None):
transformer_outputs = self.transformer(input_ids,
attention_mask=attention_mask,
token_type_ids=token_type_ids,
position_ids=position_ids,
head_mask=head_mask,
inputs_embeds=inputs_embeds)
hidden_states = transformer_outputs[0]
lm_logits = self.lm_head(hidden_states)
outputs = (lm_logits,) + transformer_outputs[1:]
if labels is not None:
# Shift so that tokens < n predict n
shift_logits = lm_logits[..., :-1, :].contiguous()
shift_labels = labels[..., 1:].contiguous()
# Flatten the tokens
loss_fct = CrossEntropyLoss(ignore_index=-1)
loss = loss_fct(shift_logits.view(-1, shift_logits.size(-1)),
shift_labels.view(-1))
outputs = (loss,) + outputs
return outputs # (loss), lm_logits, (all hidden states), (all attentions)
```
| 11-12-2019 19:19:03 | 11-12-2019 19:19:03 | Hi, some Transformers have activation in their heads, for instance, Bert.
See here: https://github.com/huggingface/transformers/blob/master/transformers/modeling_bert.py#L421
This is most likely a design choice with a minor effect for deep transformers as they learn to generate the current or next token along several of the output layers.
See the nice blog post and paper of Lena Voita for some intuition on this: https://lena-voita.github.io/posts/emnlp19_evolution.html<|||||>Thank you! That link was very helpful. |
transformers | 1,808 | closed | XLMForSequenceClassification - help with zero-shot cross-lingual classification | ## ❓ Questions & Help
Hi guys
According to XLM description (https://github.com/facebookresearch/XLM?fbclid=IwAR0-ZJpmWmIVfR20fA2KCHgrUU3k0cMUyx2n_V9-9C8g857-nhavrfBnVSI#pretrained-cross-lingual-language-models), we could potentially do XLNI by training in `en` dataset and do inference in other language:
```
XLMs can be used to build cross-lingual classifiers. After fine-tuning an XLM model
on an English training corpus for instance (e.g. of sentiment analysis, natural language
inference), the model is still able to make accurate predictions at test time in other
languages, for which there is very little or no training data. This approach is usually
referred to as "zero-shot cross-lingual classification".
```
I'm doing some tests w/ a dataset here at work, and I'm able to train the model with *XLMForSequenceClassification* and when I test it in `en`, performance looks great!
However, when I try to pass another language and do some inference, performance on other language (the so-called zero shot XLNI) does not look fine.
Here are the configurations I'm using:
```python
config = XLMConfig.from_pretrained('xlm-mlm-tlm-xnli15-1024')
config.num_labels = len(list(label_to_ix.values()))
config.n_layers = 6
tokenizer = XLMTokenizer.from_pretrained('xlm-mlm-tlm-xnli15-1024')
model = XLMForSequenceClassification(config)
```
I trained the forward pass passing the `lang` vector with `en` language:
```python
langs = torch.full(ids.shape, fill_value = language_id, dtype = torch.long).cuda()
output = model.forward(ids.cuda(), token_type_ids=tokens.cuda(), langs=langs, head_mask=None)[0]
```
For inference, I'm passing now other languages, by using following function:
```python
def get_reply(msg, language = 'en'):
features = prepare_features(msg, zero_pad = False)
language_id = tokenizer.lang2id[language]
ids = torch.tensor(features['input_ids']).unsqueeze(0).cuda()
langs = torch.full(ids.shape, fill_value = language_id, dtype = torch.long).cuda()
tokens = torch.tensor(features['token_type_ids']).unsqueeze(0).cuda()
output = model.forward(ids,token_type_ids=tokens, langs=langs)[0]
_, predicted = torch.max(output.data, 1)
return list(label_to_ix.keys())[predicted]
```
BTW, my `prepare_features` function is quite simple, just use the `encode_plus` method and then zero pad for a given sentence size:
```python
def prepare_features(seq_1, zero_pad = False, max_seq_length = 120):
enc_text = tokenizer.encode_plus(seq_1, add_special_tokens=True, max_length=300)
if zero_pad:
while len(enc_text['input_ids']) < max_seq_length:
enc_text['input_ids'].append(0)
enc_text['token_type_ids'].append(0)
return enc_text
```
I've noticed in file (https://github.com/huggingface/transformers/blob/master/transformers/modeling_xlm.py) that the forward loop for `XLMModel` does add `lang` vector as sort of an offset for the embeddings:
```python
tensor = tensor + self.lang_embeddings(langs)
```
I'm wondering if I'm passing the `lang` vector in right format. I choose the model with *tlm* language model on purpose because I was guessing that it benefits of the translation language model of the XLM. Have you guys experiment already with zero-shot text classification? Any clue my inference in other languages is not working?
Any insight will be greatly appreciated!
Cheers,
Roberto | 11-12-2019 18:47:47 | 11-12-2019 18:47:47 | Hi, we've added some details on the multi-modal models here: https://huggingface.co/transformers/multilingual.html
And an XNLI example here: https://github.com/huggingface/transformers/tree/master/examples#xnli |
transformers | 1,807 | closed | Whether it belongs to the bug of class trainedtokenizer decode? | ## ❓ Questions & Help
<!-- A clear and concise description of the question. -->
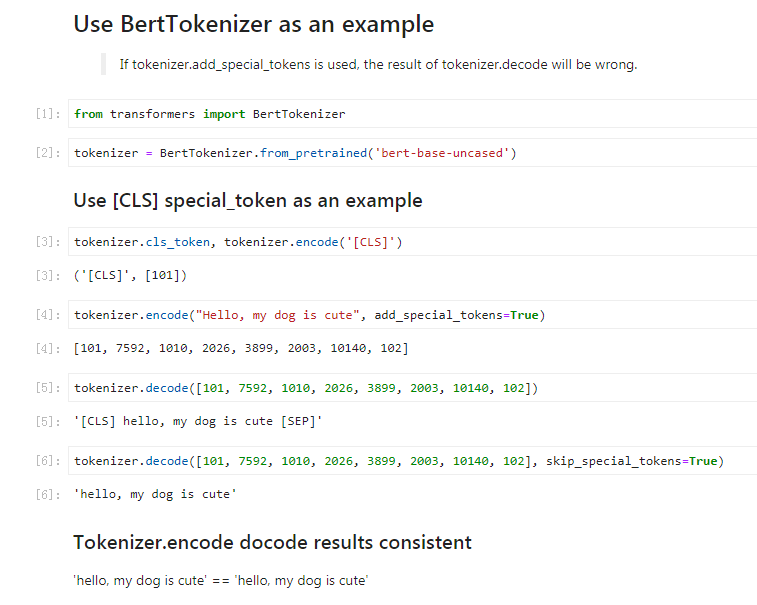
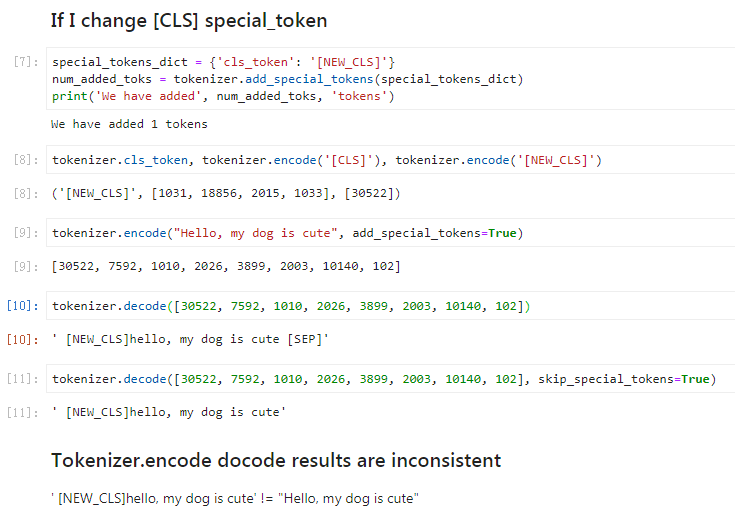
| 11-12-2019 15:25:40 | 11-12-2019 15:25:40 | You're right, this is an error. The PR #1811 aims to fix that issue!<|||||>It should be fixed now, thanks! Feel free to re-open if the error persists. |
transformers | 1,806 | closed | Extracting First Hidden States | ## ❓ Questions & Help
For example, right now in order to extract the first hidden states of DistilBert model,
tokenizer = DistilBertTokenizer.from_pretrained('distilbert-base-uncased')
model = DistilBertModel.from_pretrained('distilbert-base-uncased', **output_hidden_states=True**)
input_ids = torch.tensor(tokenizer.encode("Hello, my dog is cute")).unsqueeze(0) # Batch size 1
outputs = model(input_ids)
**first_hidden_states = outputs[1][0] # The first hidden-state**
However, I can only extract the first layer hidden states after running through the entire model; all 7 layers (which is unnecessary if I only want the first).
Is there a way I could use the model to only extract the hidden states of the first layer and stop there? I am looking to further optimize the inference time of DistilBert.
Thank you :)
| 11-12-2019 14:37:43 | 11-12-2019 14:37:43 | |
transformers | 1,805 | closed | RuntimeError: CUDA error: device-side assert triggered | ## ❓ Questions & Help
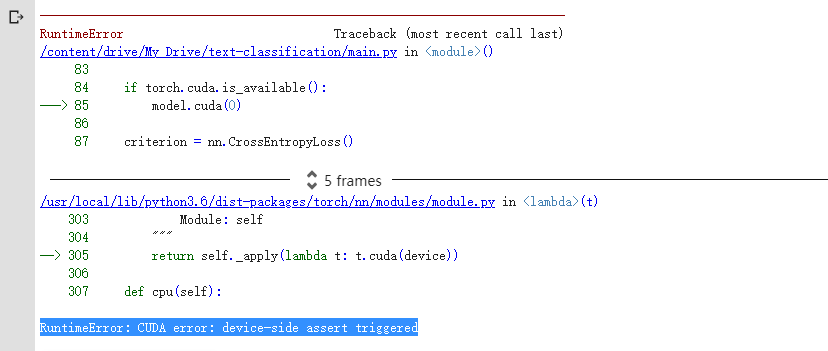. "RuntimeError: CUDA error: device-side assert triggered" occurs. My model is as follows:
```
class TextClassify(nn.Module):
def __init__(self, bert, kernel_size, word_dim, out_dim, num_class, dropout=0.5):
super(TextClassify, self).__init__()
self.bert = bert
self.cnn = nn.Sequential(nn.Conv1d(word_dim, out_dim, kernel_size=kernel_size, padding=1), nn.ReLU(inplace=True))
self.drop = nn.Dropout(dropout, inplace=True)
self.fc = nn.Linear(out_dim, num_class)
def forward(self, word_input):
batch_size = word_input.size(0)
represent = self.xlnet(word_input)[0]
represent = self.drop(represent)
represent = represent.transpose(1, 2)
contexts = self.cnn(represent)
feature = F.max_pool1d(contexts, contexts.size(2)).contiguous().view(batch_size, -1)
feature = self.drop(feature)
feature = self.fc(feature)
return feature
```
How can I solve it?
| 11-12-2019 14:10:39 | 11-12-2019 14:10:39 | Are you in a multi-GPU setup ?<|||||>I did not use multi-GPU setup, I used to `model.cuda()`, it ocuurs "RuntimeError: CUDA error: device-side assert triggered", so I changed to `model.cuda(0)`, but the error still occurs.<|||||>Do you mind showing how you initialize BERT and the code surrounding the error?<|||||>My project structure is as follows:
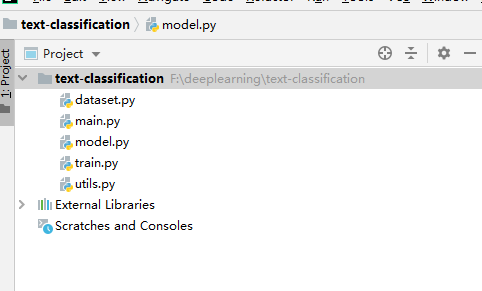
And `dataset.py` is as follows:
```
# -*- coding: utf-8 -*-
"""
@time: 2019/8/6 19:47
@author: wangjiawei
"""
from torch.utils.data import Dataset
import torch
import csv
class MyDataset(Dataset):
def __init__(self, data_path, tokenizer):
super(MyDataset, self).__init__()
self.tokenizer = tokenizer
texts, labels = [], []
with open(data_path, 'r', encoding='utf-8') as csv_file:
reader = csv.reader(csv_file, quotechar='"')
for idx, line in enumerate(reader):
text = ""
for tx in line[1:]:
text += tx
text += " "
text = self.tokenizer.tokenize(text)
if len(text) > 512:
text = text[:512]
text = self.tokenizer.encode(text, add_special_tokens=True)
text_id = torch.tensor(text)
texts.append(text_id)
label = int(line[0]) - 1
labels.append(label)
self.texts = texts
self.labels = labels
self.num_class = len(set(self.labels))
def __len__(self):
return len(self.labels)
def __getitem__(self, item):
label = self.labels[item]
text = self.texts[item]
return {'text': text, 'label': torch.tensor(label).long()}
```
`main.py` is as follows:
```
# -*- coding: utf-8 -*-
"""
@time: 2019/7/17 20:37
@author: wangjiawei
"""
import os
import torch
import torch.nn as nn
from torch.utils.data import DataLoader, random_split
from utils import get_default_folder, my_collate_fn
from dataset import MyDataset
from model import TextClassify
from torch.utils.tensorboard import SummaryWriter
import argparse
import shutil
import numpy as np
import random
import time
from train import train_model, evaluate
from transformers import BertModel, BertTokenizer
seed_num = 123
random.seed(seed_num)
torch.manual_seed(seed_num)
np.random.seed(seed_num)
if __name__ == "__main__":
parser = argparse.ArgumentParser("self attention for Text Categorization")
parser.add_argument("--batch_size", type=int, default=64)
parser.add_argument("--num_epoches", type=int, default=20)
parser.add_argument("--lr", type=float, default=0.001)
parser.add_argument("--kernel_size", type=int, default=3)
parser.add_argument("--word_dim", type=int, default=768)
parser.add_argument("--out_dim", type=int, default=300)
parser.add_argument("--dropout", default=0.5)
parser.add_argument("--es_patience", type=int, default=3)
parser.add_argument("--dataset", type=str,
choices=["agnews", "dbpedia", "yelp_review", "yelp_review_polarity", "amazon_review",
"amazon_polarity", "sogou_news", "yahoo_answers"], default="yelp_review")
parser.add_argument("--log_path", type=str, default="tensorboard/classify")
args = parser.parse_args()
input, output = get_default_folder(args.dataset)
train_path = input + os.sep + "train.csv"
if not os.path.exists(output):
os.makedirs(output)
# with open(input + os.sep + args.vocab_file, 'rb') as f1:
# vocab = pickle.load(f1)
# emb_begin = time.time()
# pretrain_word_embedding = build_pretrain_embedding(args.embedding_path, vocab, args.word_dim)
# emb_end = time.time()
# emb_min = (emb_end - emb_begin) % 3600 // 60
# print('build pretrain embed cost {}m'.format(emb_min))
model_class, tokenizer_class, pretrained_weights = BertModel, BertTokenizer, 'bert-base-uncased'
tokenizer = tokenizer_class.from_pretrained(pretrained_weights)
bert = model_class.from_pretrained(pretrained_weights)
train_dev_dataset = MyDataset(input + os.sep + "train.csv", tokenizer)
len_train_dev_dataset = len(train_dev_dataset)
dev_size = int(len_train_dev_dataset * 0.1)
train_size = len_train_dev_dataset - dev_size
train_dataset, dev_dataset = random_split(train_dev_dataset, [train_size, dev_size])
test_dataset = MyDataset(input + os.sep + "test.csv", tokenizer)
train_dataloader = DataLoader(train_dataset, batch_size=args.batch_size, shuffle=True, collate_fn=my_collate_fn)
dev_dataloader = DataLoader(dev_dataset, batch_size=args.batch_size, shuffle=False, collate_fn=my_collate_fn)
test_dataloader = DataLoader(test_dataset, batch_size=args.batch_size, shuffle=False, collate_fn=my_collate_fn)
model = TextClassify(bert, args.kernel_size, args.word_dim, args.out_dim, test_dataset.num_class, args.dropout)
log_path = "{}_{}".format(args.log_path, args.dataset)
if os.path.isdir(log_path):
shutil.rmtree(log_path)
os.makedirs(log_path)
writer = SummaryWriter(log_path)
if torch.cuda.is_available():
model.cuda(0)
criterion = nn.CrossEntropyLoss()
optimizer = torch.optim.Adam(model.parameters(), lr=args.lr)
best_acc = -1
early_stop = 0
model.train()
batch_num = -1
train_begin = time.time()
for epoch in range(args.num_epoches):
epoch_begin = time.time()
print('train {}/{} epoch'.format(epoch + 1, args.num_epoches))
batch_num = train_model(model, optimizer, criterion, batch_num, train_dataloader, writer)
dev_acc = evaluate(model, dev_dataloader)
writer.add_scalar('dev/Accuracy', dev_acc, epoch)
print('dev_acc:', dev_acc)
if dev_acc > best_acc:
early_stop = 0
best_acc = dev_acc
print('new best_acc on dev set:', best_acc)
torch.save(model.state_dict(), "{}{}".format(output, 'best.pt'))
else:
early_stop += 1
epoch_end = time.time()
cost_time = epoch_end - epoch_begin
print('train {}th epoch cost {}m {}s'.format(epoch + 1, int(cost_time / 60), int(cost_time % 60)))
print()
# Early stopping
if early_stop > args.es_patience:
print(
"Stop training at epoch {}. The best dev_acc achieved is {}".format(epoch - args.es_patience, best_acc))
break
train_end = time.time()
train_cost = train_end - train_begin
hour = int(train_cost / 3600)
min = int((train_cost % 3600) / 60)
second = int(train_cost % 3600 % 60)
print()
print()
print('train end', '-' * 50)
print('train total cost {}h {}m {}s'.format(hour, min, second))
print('-' * 50)
model_name = "{}{}".format(output, 'best.pt')
model.load_state_dict(torch.load(model_name))
test_acc = evaluate(model, test_dataloader)
print('test acc on test set:', test_acc)
```
`model.py` is as follows:
```
# -*- coding: utf-8 -*-
"""
@time: 2019/8/6 19:04
@author: wangjiawei
"""
import torch.nn as nn
import torch
import torch.nn.functional as F
class TextClassify(nn.Module):
def __init__(self, bert, kernel_size, word_dim, out_dim, num_class, dropout=0.5):
super(TextClassify, self).__init__()
self.bert = bert
self.cnn = nn.Sequential(nn.Conv1d(word_dim, out_dim, kernel_size=kernel_size, padding=1), nn.ReLU(inplace=True))
self.drop = nn.Dropout(dropout, inplace=True)
self.fc = nn.Linear(out_dim, num_class)
def forward(self, word_input):
batch_size = word_input.size(0)
represent = self.bert(word_input)[0]
represent = self.drop(represent)
represent = represent.transpose(1, 2)
contexts = self.cnn(represent)
feature = F.max_pool1d(contexts, contexts.size(2)).contiguous().view(batch_size, -1)
feature = self.drop(feature)
feature = self.fc(feature)
return feature
```
`train.py` is as follows:
```
# -*- coding: utf-8 -*-
"""
@time: 2019/8/6 20:14
@author: wangjiawei
"""
import torch
def train_model(model, optimizer, criterion, batch_num, dataloader, writer):
model.train()
for batch in dataloader:
model.zero_grad()
batch_num += 1
text = batch['text']
label = batch['label']
if torch.cuda.is_available():
text = text.cuda(0)
label = label.cuda(0)
feature = model(text)
loss = criterion(feature, label)
writer.add_scalar('Train/Loss', loss, batch_num)
loss.backward()
optimizer.step()
return batch_num
def evaluate(model, dataloader):
model.eval()
correct_num = 0
total_num = 0
for batch in dataloader:
text = batch['text']
label = batch['label']
if torch.cuda.is_available():
text = text.cuda(0)
label = label.cuda(0)
with torch.no_grad():
predictions = model(text)
_, preds = torch.max(predictions, 1)
correct_num += torch.sum((preds == label)).item()
total_num += len(label)
acc = (correct_num / total_num) * 100
return acc
```
`utils.py` is as follows:
```
# -*- coding: utf-8 -*-
"""
@time: 2019/8/6 19:54
@author: wangjiawei
"""
import csv
import nltk
import numpy as np
from torch.nn.utils.rnn import pad_sequence
import torch
def load_pretrain_emb(embedding_path, embedd_dim):
embedd_dict = dict()
with open(embedding_path, 'r', encoding="utf8") as file:
for line in file:
line = line.strip()
if len(line) == 0:
continue
tokens = line.split()
if not embedd_dim + 1 == len(tokens):
continue
embedd = np.empty([1, embedd_dim])
embedd[:] = tokens[1:]
first_col = tokens[0]
embedd_dict[first_col] = embedd
return embedd_dict
def build_pretrain_embedding(embedding_path, vocab, embedd_dim=300):
embedd_dict = dict()
if embedding_path is not None:
embedd_dict = load_pretrain_emb(embedding_path, embedd_dim)
alphabet_size = vocab.size()
scale = 0.1
pretrain_emb = np.empty([vocab.size(), embedd_dim])
perfect_match = 0
case_match = 0
not_match = 0
for word, index in vocab.items():
if word in embedd_dict:
pretrain_emb[index, :] = embedd_dict[word]
perfect_match += 1
elif word.lower() in embedd_dict:
pretrain_emb[index, :] = embedd_dict[word.lower()]
case_match += 1
else:
pretrain_emb[index, :] = np.random.uniform(-scale, scale, [1, embedd_dim])
not_match += 1
pretrain_emb[0, :] = np.zeros((1, embedd_dim))
pretrained_size = len(embedd_dict)
print('pretrained_size:', pretrained_size)
print("Embedding:\n pretrain word:%s, prefect match:%s, case_match:%s, oov:%s, oov%%:%s" % (
pretrained_size, perfect_match, case_match, not_match, (not_match + 0.) / alphabet_size))
return pretrain_emb
def get_default_folder(dataset):
if dataset == "agnews":
input = "data/ag_news_csv"
output = "output/ag_news/"
elif dataset == "dbpedia":
input = "data/dbpedia_csv"
output = "output/dbpedia/"
elif dataset == "yelp_review":
input = "data/yelp_review_full_csv"
output = "output/yelp_review_full/"
elif dataset == "yelp_review_polarity":
input = "data/yelp_review_polarity_csv"
output = "output/yelp_review_polarity/"
elif dataset == "amazon_review":
input = "data/amazon_review_full_csv"
output = "output/amazon_review_full/"
elif dataset == "amazon_polarity":
input = "data/amazon_review_polarity_csv"
output = "output/amazon_review_polarity/"
elif dataset == "sogou_news":
input = "data/sogou_news_csv"
output = "output/sogou_news/"
elif dataset == "yahoo_answers":
input = "data/yahoo_answers_csv"
output = "output/yahoo_answers/"
return input, output
def my_collate(batch_tensor, key):
if key == 'text':
batch_tensor = pad_sequence(batch_tensor, batch_first=True, padding_value=0)
else:
batch_tensor = torch.stack(batch_tensor)
return batch_tensor
def my_collate_fn(batch):
return {key: my_collate([d[key] for d in batch], key) for key in batch[0]}
class Vocabulary(object):
def __init__(self, filename):
self._id_to_word = []
self._word_to_id = {}
self._pad = -1
self._unk = -1
self.index = 0
self._id_to_word.append('<PAD>')
self._word_to_id['<PAD>'] = self.index
self._pad = self.index
self.index += 1
self._id_to_word.append('<UNK>')
self._word_to_id['<UNK>'] = self.index
self._unk = self.index
self.index += 1
word_num = dict()
with open(filename, 'r', encoding='utf-8') as f1:
reader = csv.reader(f1, quotechar='"')
for line in reader:
text = ""
for tx in line[1:]:
text += tx
text += " "
text = nltk.word_tokenize(text)
for word in text:
if word not in word_num:
word_num[word] = 0
word_num[word] += 1
for word, num in word_num.items():
if num >= 3:
self._id_to_word.append(word)
self._word_to_id[word] = self.index
self.index += 1
def unk(self):
return self._unk
def pad(self):
return self._pad
def size(self):
return len(self._id_to_word)
def word_to_id(self, word):
if word in self._word_to_id:
return self._word_to_id[word]
elif word.lower() in self._word_to_id:
return self._word_to_id[word.lower()]
return self.unk()
def id_to_word(self, cur_id):
return self._id_to_word[cur_id]
def items(self):
return self._word_to_id.items()
```
<|||||>when I use Google Cloud, it works. Before I used Google Colab. It is strange.<|||||>It's probably because your token embeddings size (vocab size) doesn't match with pre-trained model. Do `model.resize_token_embeddings(len(tokenizer))` before training. Please check #1848 and #1849 <|||||>This issue has been automatically marked as stale because it has not had recent activity. It will be closed if no further activity occurs. Thank you for your contributions.
<|||||>I also faced the same issue while encoding 20_newspaper dataset, After further investigation, I found there are some sentences which are not in the English language, for example :
`'subject romanbmp pa of from pwisemansalmonusdedu cliff replyto pwisemansalmonusdedu cliff distribution usa organization university of south dakota lines maxaxaxaxaxaxaxaxaxaxaxaxaxaxax maxaxaxax39f8z51 wwizbhjbhjbhjbhjgiz mgizgizbhjgizm 1tei0lv9f9f9fra 5z46q0475vo4 mu34u34u m34w 084oo aug 0y5180 mc p8v5555555555965hwgv 7uqgy gp452gvbdigiz maxaxaxaxaxaxaxaxaxaxaxaxaxaxax maxaxax34t2php2app12a34u34tm11 6um6 mum8 zbjf0kvlvc9vde5e9e5g9vg9v38vc o3o n5 mardi 24y2 g92li6e0q8axaxaxaxaxaxaxax maxaxaxaxaxaxaxas9ne1whjn 1tei4pmf9l3u3 mr hjpm75u4u34u34u nfyn 46uo m5ug 0y4518hr8y3m15556tdy65c8u 47y m7 hsxgjeuaxaxaxaxaxaxaxaxaxaxaxax maxaxaxaxaxaxaxax34t2pchp2a2a2a234u m34u3 zexf3w21fp4 wm3t f9d uzi0mf8axaxaxaxaxaxrxkrldajj mkjznbbwp0nvmkvmkvnrkmkvmhi axaxaxaxax maxaxaxaxaxaxaxks8vc9vfiue949h g9v38v6un5 mg83q3x w5 3t pi0wsr4c362l zkn2axaxaxaxaxaxaxaxaxaxax maxaxaxaxaxaxaxaxaxaxaxaxaxaxax maxax39f9fpli6e1t1'`
**This was causing the model to produce this error If you are facing this issue follow these steps:**
1. Catch Exception and store the sentences in the new list to see if the sentence is having weird characters
2. Reduce the batch size, If your batch size is too high (if you are using batch encoding)
3. Check if your sentence length is too long for the model to encode, trim the sentence length ( try 200 first then 128 )<|||||>I am continuously getting the runtime error: CUDA error: device-side assert triggered, I am new to transformer library.
I am creating the longformer classifier in the below format:
model = LongformerForSequenceClassification.from_pretrained('allenai/longformer-base-4096', gradient_checkpointing = True, num_labels = 5)
and tokenizer as :
tokenizer = LongformerTokenizer.from_pretrained('allenai/longformer-base-4096')
encoded_data_train = tokenizer.batch_encode_plus(
df[df.data_type=='train'].content.values,
add_special_tokens = True,
return_attention_mask=True,
padding = True,
max_length = 3800,
return_tensors='pt'
)
Can anyone please help? I am using latest version of transformer library and using google colab GPU for building my classifier.<|||||>> I also faced the same issue while encoding 20_newspaper dataset, After further investigation, I found there are some sentences which are not in the English language, for example :
>
> `'subject romanbmp pa of from pwisemansalmonusdedu cliff replyto pwisemansalmonusdedu cliff distribution usa organization university of south dakota lines maxaxaxaxaxaxaxaxaxaxaxaxaxaxax maxaxaxax39f8z51 wwizbhjbhjbhjbhjgiz mgizgizbhjgizm 1tei0lv9f9f9fra 5z46q0475vo4 mu34u34u m34w 084oo aug 0y5180 mc p8v5555555555965hwgv 7uqgy gp452gvbdigiz maxaxaxaxaxaxaxaxaxaxaxaxaxaxax maxaxax34t2php2app12a34u34tm11 6um6 mum8 zbjf0kvlvc9vde5e9e5g9vg9v38vc o3o n5 mardi 24y2 g92li6e0q8axaxaxaxaxaxaxax maxaxaxaxaxaxaxas9ne1whjn 1tei4pmf9l3u3 mr hjpm75u4u34u34u nfyn 46uo m5ug 0y4518hr8y3m15556tdy65c8u 47y m7 hsxgjeuaxaxaxaxaxaxaxaxaxaxaxax maxaxaxaxaxaxaxax34t2pchp2a2a2a234u m34u3 zexf3w21fp4 wm3t f9d uzi0mf8axaxaxaxaxaxrxkrldajj mkjznbbwp0nvmkvmkvnrkmkvmhi axaxaxaxax maxaxaxaxaxaxaxks8vc9vfiue949h g9v38v6un5 mg83q3x w5 3t pi0wsr4c362l zkn2axaxaxaxaxaxaxaxaxaxax maxaxaxaxaxaxaxaxaxaxaxaxaxaxax maxax39f9fpli6e1t1'`
>
> **This was causing the model to produce this error If you are facing this issue follow these steps:**
>
> 1. Catch Exception and store the sentences in the new list to see if the sentence is having weird characters
>
> 2. Reduce the batch size, If your batch size is too high (if you are using batch encoding)
>
> 3. Check if your sentence length is too long for the model to encode, trim the sentence length ( try 200 first then 128 )
What a bizzare dataset.
So, this error does occur if you use a an english tokenizer on some random garbage input!
```python3
from langdetect import detect
def detect_robust(x):
try:
out = detect(x) # 20 news managed to break this
except Exception:
out = 'not-en'
return out
lang_ = train_df['message'].map(detect_robust)
train_df = train_df[lang_=='en']
```
^ this is ridiculously slow, but after the cleaning the error is gone!
|
transformers | 1,804 | closed | fix multi-gpu eval in torch examples | Although batch_size for eval is updated to include multiple GPUs, DataParallel is missing from the model and hence doesn't use multi-GPUs. This PR allows DataParallel (multi-GPU) model in eval. | 11-12-2019 11:04:00 | 11-12-2019 11:04:00 | Indeed, good catch, thanks @ronakice |
transformers | 1,803 | closed | fix run_squad.py during fine-tuning xlnet on squad2.0 | The following is a piece of code in forward function of xlnet model, which obviously is the key point of training the model on unanswerable questions using cls token representations. But the default value of tensor `is_impossible`(using to indicate whether this example is answerable) is none, and we also hadn't passed this tensor into forward function. That's the problem.
```
if cls_index is not None and is_impossible is not None:
# Predict answerability from the representation of CLS and START
cls_logits = self.answer_class(hidden_states, start_positions=start_positions, cls_index=cls_index)
loss_fct_cls = nn.BCEWithLogitsLoss()
cls_loss = loss_fct_cls(cls_logits, is_impossible)
total_loss += cls_loss * 0.5
```
I added the `is_impossible` tensor to TensorDataset and model inputs, and got a reasonable result,
{
"exact": 80.4177545691906,
"f1": 84.07154997729623,
"total": 11873,
"HasAns_exact": 77.59784075573549,
"HasAns_f1": 84.83993323200234,
"HasAns_total": 5928,
"NoAns_exact": 84.0874684608915,
"NoAns_f1": 84.0874684608915,
"NoAns_total": 5945
}
My running command:
```
python run_squad.py \
--model_type xlnet \
--model_name_or_path xlnet-large-cased \
--do_train \
--do_eval \
--version_2_with_negative \
--train_file data/train-v2.0.json \
--predict_file data/dev-v2.0.json \
--learning_rate 3e-5 \
--num_train_epochs 4 \
--max_seq_length 384 \
--doc_stride 128 \
--output_dir ./wwm_cased_finetuned_squad/ \
--per_gpu_eval_batch_size=2 \
--per_gpu_train_batch_size=2 \
--save_steps 5000
```
I run my code with 4 nvidia GTX 1080ti gpus and pytorch==1.2.0.
| 11-12-2019 08:29:19 | 11-12-2019 08:29:19 | This looks good, do you want to add your command and the results you mention in the README of the examples in `examples/README.md`?<|||||># [Codecov](https://codecov.io/gh/huggingface/transformers/pull/1803?src=pr&el=h1) Report
> Merging [#1803](https://codecov.io/gh/huggingface/transformers/pull/1803?src=pr&el=desc) into [master](https://codecov.io/gh/huggingface/transformers/commit/8618bf15d6edc8774cedc0aae021d259d89c91fc?src=pr&el=desc) will **increase** coverage by `1.08%`.
> The diff coverage is `n/a`.
[](https://codecov.io/gh/huggingface/transformers/pull/1803?src=pr&el=tree)
```diff
@@ Coverage Diff @@
## master #1803 +/- ##
==========================================
+ Coverage 78.91% 79.99% +1.08%
==========================================
Files 131 131
Lines 19450 19450
==========================================
+ Hits 15348 15559 +211
+ Misses 4102 3891 -211
```
| [Impacted Files](https://codecov.io/gh/huggingface/transformers/pull/1803?src=pr&el=tree) | Coverage Δ | |
|---|---|---|
| [transformers/tests/utils.py](https://codecov.io/gh/huggingface/transformers/pull/1803/diff?src=pr&el=tree#diff-dHJhbnNmb3JtZXJzL3Rlc3RzL3V0aWxzLnB5) | `91.17% <0%> (-2.95%)` | :arrow_down: |
| [transformers/pipelines.py](https://codecov.io/gh/huggingface/transformers/pull/1803/diff?src=pr&el=tree#diff-dHJhbnNmb3JtZXJzL3BpcGVsaW5lcy5weQ==) | `67.94% <0%> (+0.58%)` | :arrow_up: |
| [transformers/modeling\_utils.py](https://codecov.io/gh/huggingface/transformers/pull/1803/diff?src=pr&el=tree#diff-dHJhbnNmb3JtZXJzL21vZGVsaW5nX3V0aWxzLnB5) | `94.1% <0%> (+1.07%)` | :arrow_up: |
| [transformers/modeling\_openai.py](https://codecov.io/gh/huggingface/transformers/pull/1803/diff?src=pr&el=tree#diff-dHJhbnNmb3JtZXJzL21vZGVsaW5nX29wZW5haS5weQ==) | `81.51% <0%> (+1.32%)` | :arrow_up: |
| [transformers/modeling\_ctrl.py](https://codecov.io/gh/huggingface/transformers/pull/1803/diff?src=pr&el=tree#diff-dHJhbnNmb3JtZXJzL21vZGVsaW5nX2N0cmwucHk=) | `96.47% <0%> (+2.2%)` | :arrow_up: |
| [transformers/modeling\_xlnet.py](https://codecov.io/gh/huggingface/transformers/pull/1803/diff?src=pr&el=tree#diff-dHJhbnNmb3JtZXJzL21vZGVsaW5nX3hsbmV0LnB5) | `74.54% <0%> (+2.32%)` | :arrow_up: |
| [transformers/modeling\_roberta.py](https://codecov.io/gh/huggingface/transformers/pull/1803/diff?src=pr&el=tree#diff-dHJhbnNmb3JtZXJzL21vZGVsaW5nX3JvYmVydGEucHk=) | `72.57% <0%> (+12%)` | :arrow_up: |
| [transformers/tests/modeling\_tf\_common\_test.py](https://codecov.io/gh/huggingface/transformers/pull/1803/diff?src=pr&el=tree#diff-dHJhbnNmb3JtZXJzL3Rlc3RzL21vZGVsaW5nX3RmX2NvbW1vbl90ZXN0LnB5) | `94.39% <0%> (+17.24%)` | :arrow_up: |
| [transformers/modeling\_tf\_pytorch\_utils.py](https://codecov.io/gh/huggingface/transformers/pull/1803/diff?src=pr&el=tree#diff-dHJhbnNmb3JtZXJzL21vZGVsaW5nX3RmX3B5dG9yY2hfdXRpbHMucHk=) | `90.06% <0%> (+80.79%)` | :arrow_up: |
------
[Continue to review full report at Codecov](https://codecov.io/gh/huggingface/transformers/pull/1803?src=pr&el=continue).
> **Legend** - [Click here to learn more](https://docs.codecov.io/docs/codecov-delta)
> `Δ = absolute <relative> (impact)`, `ø = not affected`, `? = missing data`
> Powered by [Codecov](https://codecov.io/gh/huggingface/transformers/pull/1803?src=pr&el=footer). Last update [8618bf1...8a2be93](https://codecov.io/gh/huggingface/transformers/pull/1803?src=pr&el=lastupdated). Read the [comment docs](https://docs.codecov.io/docs/pull-request-comments).
<|||||>ok, I have updated the readme.<|||||>@importpandas thanks for contributing this fix, works great! My results running this mod:
```
{
"exact": 82.07698138633876,
"f1": 85.898874470488,
"total": 11873,
"HasAns_exact": 79.60526315789474,
"HasAns_f1": 87.26000954590184,
"HasAns_total": 5928,
"NoAns_exact": 84.54163162321278,
"NoAns_f1": 84.54163162321278,
"NoAns_total": 5945,
"best_exact": 83.22243746315169,
"best_exact_thresh": -11.112004280090332,
"best_f1": 86.88541353813282,
"best_f1_thresh": -11.112004280090332
}
```
Distributed processing for batch size 48 using gradient accumulation consumes 10970 MiB on each of 2x NVIDIA 1080Ti.
```<|||||>@ahotrod As for your first results, I will suggest you watch my changes on file `run_squad.py` in this pr. It just added a another input to the model and only a few lines. Without this changing, I got the same results with you, which is nearly to 0 on unanswerable questions. I think Distributed processing isn't responsible for that. When it comes to the running script, it doesn't matter since I had hardly changed it.<|||||>@importpandas re-run just completed (36 hours) and results are posted above.
Works great, thanks again!<|||||>> @importpandas re-run just completed (36 hours) and results are posted above.
> Works great, thanks again!
okay, pleasure<|||||>LGTM, merging cc @LysandreJik (the `run_squad` master) |
transformers | 1,802 | closed | pip cannot install transformers with python version 3.8.0 | ## ❓ Questions & Help
The error message looks like this,
` ERROR: Command errored out with exit status 1:
command: 'c:\users\enderaoe\appdata\local\programs\python\python38-32\python.exe' -c 'import sys, setuptools, tokenize; sys.argv[0] = '"'"'C:\\Users\\Enderaoe\\AppData\\Local\\Temp\\pip-install-g7jzfokt\\sentencepiece\\setup.py'"'"'; __file__='"'"'C:\\Users\\Enderaoe\\AppData\\Local\\Temp\\pip-install-g7jzfokt\\sentencepiece\\setup.py'"'"';f=getattr(tokenize, '"'"'open'"'"', open)(__file__);code=f.read().replace('"'"'\r\n'"'"', '"'"'\n'"'"');f.close();exec(compile(code, __file__, '"'"'exec'"'"'))' egg_info --egg-base 'C:\Users\Enderaoe\AppData\Local\Temp\pip-install-g7jzfokt\sentencepiece\pip-egg-info'
cwd: C:\Users\Enderaoe\AppData\Local\Temp\pip-install-g7jzfokt\sentencepiece\
Complete output (7 lines):
Traceback (most recent call last):
File "<string>", line 1, in <module>
File "C:\Users\Enderaoe\AppData\Local\Temp\pip-install-g7jzfokt\sentencepiece\setup.py", line 29, in <module>
with codecs.open(os.path.join('..', 'VERSION'), 'r', 'utf-8') as f:
File "c:\users\enderaoe\appdata\local\programs\python\python38-32\lib\codecs.py", line 905, in open
file = builtins.open(filename, mode, buffering)
FileNotFoundError: [Errno 2] No such file or directory: '..\\VERSION'
----------------------------------------
ERROR: Command errored out with exit status 1: python setup.py egg_info Check the logs for full command output.`
Should I lower my python version, or there is any other solution? | 11-12-2019 08:23:08 | 11-12-2019 08:23:08 | This looks like an error related to Google SentencePiece and in particular this issue: https://github.com/google/sentencepiece/issues/411<|||||>https://github.com/google/sentencepiece/issues/411#issuecomment-557596691
```
pip install https://github.com/google/sentencepiece/releases/download/v0.1.84/sentencepiece-0.1.84-cp38-cp38-manylinux1_x86_64.whl
```<|||||>I've got problem installing matplotlib in python. While installing these error massage is shown. What to do now?

Command "C:\Users\tawfiq\PycharmProjects\untitled4\venv\Scripts\python.exe -u -c "import setuptools, tokenize;__file__='C:\\Users\\tawfiq\\AppData\\Local\\Temp\\pip-install-yfe_bqlr\\matplotlib\\setup.py';f=getatt
r(tokenize, 'open', open)(__file__);code=f.read().replace('\r\n', '\n');f.close();exec(compile(code, __file__, 'exec'))" install --record C:\Users\tawfiq\AppData\Local\Temp\pip-record-vmiaih4n\install-record.txt -
-single-version-externally-managed --compile --install-headers C:\Users\tawfiq\PycharmProjects\untitled4\venv\include\site\python3.8\matplotlib" failed with error code 1 in C:\Users\tawfiq\AppData\Local\Temp\pip-i
nstall-yfe_bqlr\matplotlib\
<|||||>This issue has been automatically marked as stale because it has not had recent activity. It will be closed if no further activity occurs. Thank you for your contributions.
<|||||>This is still an issue on MacOS: https://github.com/google/sentencepiece/issues/411#issuecomment-578509088<|||||>> google/sentencepiece#411 (comment)
This error comes up: ``` ERROR: sentencepiece-0.1.84-cp38-cp38-manylinux1_x86_64.whl is not a supported wheel on this platform.```
<|||||>This issue has been automatically marked as stale because it has not had recent activity. It will be closed if no further activity occurs. Thank you for your contributions.
|
transformers | 1,801 | closed | run_glue.py RuntimeError: module must have its parameters and buffers on device cuda:0 (device_ids[0]) but found one of them on device: cuda:3 | ## 🐛 Bug
<!-- Important information -->
Model I am using (Bert, XLNet....): Bert
Language I am using the model on (English, Chinese....): English
The problem arise when using:
* [ ] the official example scripts: (give details) : transformers/examples/run_glue.py
* [ ] my own modified scripts: (give details)
The tasks I am working on is:
* [ ] an official GLUE/SQUaD task: (give the name) : MRPC
* [ ] my own task or dataset: (give details)
## To Reproduce
Steps to reproduce the behavior:
1.
I've tested using
python -m pytest -sv ./transformers/tests/
python -m pytest -sv ./examples/
and it works fine without couple of tesks.
2.
after test, i downloaded glue datafile via
https://gist.github.com/W4ngatang/60c2bdb54d156a41194446737ce03e2e
and tried run_glue.py
pip install -r ./examples/requirements.txt
export GLUE_DIR=/path/to/glue
export TASK_NAME=MRPC
3.
python ./examples/run_glue.py \
--model_type bert \
--model_name_or_path bert-base-uncased \
--task_name $TASK_NAME \
--do_train \
--do_eval \
--do_lower_case \
--data_dir $GLUE_DIR/$TASK_NAME \
--max_seq_length 128 \
--per_gpu_eval_batch_size=8 \
--per_gpu_train_batch_size=8 \
--learning_rate 2e-5 \
--num_train_epochs 3.0 \
--output_dir /tmp/$TASK_NAME/
and i got this error.
`11/11/2019 21:10:50 - INFO - __main__ - Total optimization steps = 345
Epoch: 0%| | 0/3 [00:00<?, ?it/sTraceback (most recent call last): | 0/115 [00:00<?, ?it/s]
File "./examples/run_glue.py", line 552, in <module>
main()
File "./examples/run_glue.py", line 503, in main
global_step, tr_loss = train(args, train_dataset, model, tokenizer)
File "./examples/run_glue.py", line 146, in train
outputs = model(**inputs)
File "/home/insublee/anaconda3/envs/py_torch4/lib/python3.7/site-packages/torch/nn/modules/module.py", line 541, in __call__
result = self.forward(*input, **kwargs)
File "/home/insublee/anaconda3/envs/py_torch4/lib/python3.7/site-packages/torch/nn/parallel/data_parallel.py", line 146, in forward
"them on device: {}".format(self.src_device_obj, t.device))
RuntimeError: module must have its parameters and buffers on device cuda:0 (device_ids[0]) but found one of them on device: cuda:3`
<!-- A clear and concise description of what you expected to happen. -->
## Environment
* OS: ubuntu16.04LTS
* Python version: 3.7.5
* PyTorch version: 1.2.0
* PyTorch Transformers version (or branch): 2.1.1
* Using GPU ? 4-way 2080ti
* Distributed of parallel setup ? cuda10.0 cudnn 7.6.4
* Any other relevant information:
## Additional context
thank you. | 11-12-2019 05:22:52 | 11-12-2019 05:22:52 | This problem comes out from multiple GPUs usage. The error you have reported says that you have parameters or the buffers of the model in **two different locations**.
Said this, it's probably related to #1504 issue. Reading comments in the #1504 issue, i saw that @h-sugi suggests 4 days ago to modify the source code in `run_**.py` like this:
BEFORE: `device = torch.device("cuda" if torch.cuda.is_available() and not args.no_cuda else "cpu")`
AFTER: `device = torch.device("cuda:0" if torch.cuda.is_available() and not args.no_cuda else "cpu").`
Moreover, the person who opened the issue @ahotrod says this: _Have had many successful SQuAD fine-tuning runs on PyTorch 1.2.0 with Pytorch-Transformers 1.2.0, maybe even Transformers 2.0.0, and Apex 0.1. New environment built with the latest versions (Pytorch 1.3.0, Transformers 2.1.1) spawns data parallel related error above_
Please, keep us updated on this topic!
> ## Bug
> Model I am using (Bert, XLNet....): Bert
>
> Language I am using the model on (English, Chinese....): English
>
> The problem arise when using:
>
> * [ ] the official example scripts: (give details) : transformers/examples/run_glue.py
> * [ ] my own modified scripts: (give details)
>
> The tasks I am working on is:
>
> * [ ] an official GLUE/SQUaD task: (give the name) : MRPC
> * [ ] my own task or dataset: (give details)
>
> ## To Reproduce
> Steps to reproduce the behavior:
>
>
> I've tested using
> python -m pytest -sv ./transformers/tests/
> python -m pytest -sv ./examples/
> and it works fine without couple of tesks.
>
>
> after test, i downloaded glue datafile via
> https://gist.github.com/W4ngatang/60c2bdb54d156a41194446737ce03e2e
> and tried run_glue.py
>
> pip install -r ./examples/requirements.txt
> export GLUE_DIR=/path/to/glue
> export TASK_NAME=MRPC
>
>
> python ./examples/run_glue.py
> --model_type bert
> --model_name_or_path bert-base-uncased
> --task_name $TASK_NAME
> --do_train
> --do_eval
> --do_lower_case
> --data_dir $GLUE_DIR/$TASK_NAME
> --max_seq_length 128
> --per_gpu_eval_batch_size=8
> --per_gpu_train_batch_size=8
> --learning_rate 2e-5
> --num_train_epochs 3.0
> --output_dir /tmp/$TASK_NAME/
>
> and i got this error.
>
> `11/11/2019 21:10:50 - INFO - __main__ - Total optimization steps = 345 Epoch: 0%| | 0/3 [00:00<?, ?it/sTraceback (most recent call last): | 0/115 [00:00<?, ?it/s] File "./examples/run_glue.py", line 552, in <module> main() File "./examples/run_glue.py", line 503, in main global_step, tr_loss = train(args, train_dataset, model, tokenizer) File "./examples/run_glue.py", line 146, in train outputs = model(**inputs) File "/home/insublee/anaconda3/envs/py_torch4/lib/python3.7/site-packages/torch/nn/modules/module.py", line 541, in __call__ result = self.forward(*input, **kwargs) File "/home/insublee/anaconda3/envs/py_torch4/lib/python3.7/site-packages/torch/nn/parallel/data_parallel.py", line 146, in forward "them on device: {}".format(self.src_device_obj, t.device)) RuntimeError: module must have its parameters and buffers on device cuda:0 (device_ids[0]) but found one of them on device: cuda:3`
>
> ## Environment
> * OS: ubuntu16.04LTS
> * Python version: 3.7.5
> * PyTorch version: 1.2.0
> * PyTorch Transformers version (or branch): 2.1.1
> * Using GPU ? 4-way 2080ti
> * Distributed of parallel setup ? cuda10.0 cudnn 7.6.4
> * Any other relevant information:
>
> ## Additional context
> thank you.<|||||>thanks a lot. it works!!!! :)<|||||>@TheEdoardo93 After the change of `cuda` to `cuda:0`, will we still have multiple GPU usage for the jobs?<|||||>As stated in the official [docs](https://pytorch.org/tutorials/beginner/blitz/data_parallel_tutorial.html), if you use `torch.device('cuda:0')` you will use **only** a GPU. If you want to use **multiple** GPUs, you can easily run your operations on multiple GPUs by making your model run parallelly using DataParallel: `model = nn.DataParallel(model)`
You can read more information [here](https://discuss.pytorch.org/t/run-pytorch-on-multiple-gpus/20932/38) and [here](https://pytorch.org/tutorials/beginner/former_torchies/parallelism_tutorial.html).
> @TheEdoardo93 After the change of `cuda` to `cuda:0`, will we still have multiple GPU usage for the jobs?<|||||>@TheEdoardo93 I tested `run_glue.py` on a multi-gpu machine. Even, after changing `"cuda"` to `"cuda:0"` in this line https://github.com/huggingface/transformers/blob/b0ee7c7df3d49a819c4d6cef977214bd91f5c075/examples/run_glue.py#L425 , the training job will still use both GPUs relying on `torch.nn.DataParallel`. It means that `torch.nn.DataParallel` is smart enough even if you are defining `torch.device` to be `cuda:0`, if there are several gpus available, it will use all of them. <|||||>> @TheEdoardo93 I tested `run_glue.py` on a multi-gpu machine. Even, after changing `"cuda"` to `"cuda:0"` in this line
>
> https://github.com/huggingface/transformers/blob/b0ee7c7df3d49a819c4d6cef977214bd91f5c075/examples/run_glue.py#L425
>
> , the training job will still use both GPUs relying on `torch.nn.DataParallel`. It means that `torch.nn.DataParallel` is smart enough even if you are defining `torch.device` to be `cuda:0`, if there are several gpus available, it will use all of them.
manually set 'args.n_gpu = 1' works for me<|||||>Just a simple `os.environ['CUDA_VISIBLE_DEVICES'] = 'GPU_NUM'` at the beginning of the script should work.<|||||>> > @TheEdoardo93 I tested `run_glue.py` on a multi-gpu machine. Even, after changing `"cuda"` to `"cuda:0"` in this line
> > https://github.com/huggingface/transformers/blob/b0ee7c7df3d49a819c4d6cef977214bd91f5c075/examples/run_glue.py#L425
> >
> > , the training job will still use both GPUs relying on `torch.nn.DataParallel`. It means that `torch.nn.DataParallel` is smart enough even if you are defining `torch.device` to be `cuda:0`, if there are several gpus available, it will use all of them.
>
> manually set 'args.n_gpu = 1' works for me
but then you are not able to use more than 1 gpu, right?<|||||>> @TheEdoardo93 I tested `run_glue.py` on a multi-gpu machine. Even, after changing `"cuda"` to `"cuda:0"` in this line
>
> https://github.com/huggingface/transformers/blob/b0ee7c7df3d49a819c4d6cef977214bd91f5c075/examples/run_glue.py#L425
>
> , the training job will still use both GPUs relying on `torch.nn.DataParallel`. It means that `torch.nn.DataParallel` is smart enough even if you are defining `torch.device` to be `cuda:0`, if there are several gpus available, it will use all of them.
I have trid but it doesn't work for me.<|||||>This issue has been automatically marked as stale because it has not had recent activity. It will be closed if no further activity occurs. Thank you for your contributions.
|
transformers | 1,800 | closed | Exact and F1 score do not increase when fine-tunes XLM on the SQuAD dataset | ## ❓ Questions & Help
I am trying to fine-tune XLM on the SQuAD dataset.
The command is as following:
[CUDA_VISIBLE_DEVICES=0 python run_squad.py --model_type xlm --model_name_or_path xlm-mlm-tlm-xnli15-1024 --do_train --do_eval --train_file $SQUAD_DIR/train-v1.1.json --predict_file $SQUAD_DIR/dev-v1.1.json --per_gpu_train_batch_size 12 --learning_rate 3e-5 --num_train_epochs 100 --max_seq_length 384 --doc_stride 128 --eval_all_checkpoints --save_steps 50 --evaluate_during_training --output_dir /home/weihua/Sqad/transformers/xlm_out/ ]

All the parameters I set are in accordance with the example in the pytorch-transformers document. But Exact and F1 scores have barely increased. And the loss of each epoch also drops very slowly. Each epoch drops by about 0.01.
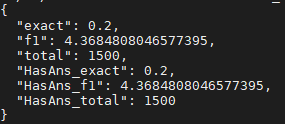
Is there a problem with my parameter settings? Or do I need to adjust some parts of the model when I use XLM?
| 11-12-2019 03:47:56 | 11-12-2019 03:47:56 | This issue has been automatically marked as stale because it has not had recent activity. It will be closed if no further activity occurs. Thank you for your contributions.
<|||||>duplicate #1799 |
transformers | 1,799 | closed | Exact and F1 score do not increase when fine-tunes XLM on the SQuAD dataset | ## 🐛 Bug
<!-- Important information -->
Model I am using XLM:
Language I am using the model on English:
The problem arise when using:
[CUDA_VISIBLE_DEVICES=0 python run_squad.py --model_type xlm --model_name_or_path xlm-mlm-tlm-xnli15-1024 --do_train --do_eval --train_file $SQUAD_DIR/train-v1.1.json --predict_file $SQUAD_DIR/dev-v1.1.json --per_gpu_train_batch_size 12 --learning_rate 3e-5 --num_train_epochs 100 --max_seq_length 384 --doc_stride 128 --eval_all_checkpoints --save_steps 50 --evaluate_during_training --output_dir /home/weihua/Sqad/transformers/xlm_out/ ]

The tasks I am working on is an official SQUaD task.
All the parameters I set are in accordance with the example in the pytorch-transformers document. But Exact and F1 scores have barely increased. And the loss of each epoch also drops very slowly. Each epoch drops by about 0.01.
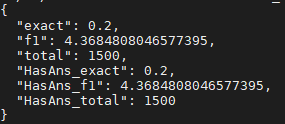
Is there a problem with my parameter settings? Or do I need to adjust some parts of the model when I use XLM?
| 11-12-2019 03:43:09 | 11-12-2019 03:43:09 | I haven't yet tried XLM for SQUad but I did try to finetune it on a similar (private) dataset. I managed to make it converge however the F1 is much worse than that of BERT (~0.75 vs ~0.80). XLM training seems to be very learning rate sensitive so you may want to tinker with that a bit.<|||||>Thank you for your answer.
Did you have any changes to the code released in pytorch-transformers when you test on your own dataset? Could you tell me if you did any changes? Or cloud you share your parameter settings when you test on your own data set?
Thank you.<|||||>> Thank you for your answer.
> Did you have any changes to the code released in pytorch-transformers when you test on your own dataset? Could you tell me if you did any changes? Or cloud you share your parameter settings when you test on your own data set?
> Thank you.
I think the only changes I made was adding language embeddings since I was finetuning `xlm-mlm-tlm-xnli15-1024` which requires that.<|||||>> > Thank you for your answer.
> > Did you have any changes to the code released in pytorch-transformers when you test on your own dataset? Could you tell me if you did any changes? Or cloud you share your parameter settings when you test on your own data set?
> > Thank you.
>
> I think the only changes I made was adding language embeddings since I was finetuning `xlm-mlm-tlm-xnli15-1024` which requires that.
Hi, I've tried all the XLM pre-training models and got random results. loss does not converge. can you help me with this problem? thanks @suicao @ZhengWeiH <|||||>> > Thank you for your answer.
> > Did you have any changes to the code released in pytorch-transformers when you test on your own dataset? Could you tell me if you did any changes? Or cloud you share your parameter settings when you test on your own data set?
> > Thank you.
>
> I think the only changes I made was adding language embeddings since I was finetuning `xlm-mlm-tlm-xnli15-1024` which requires that.
Thanks @suicao !
Where did you obtain language embeddings? Doesn't pre-trained `XLM` already come with a multilingual BPE embedding dictionary?<|||||>Ok, I think I may have found the issue in this particular model: it requires language IDs to be specified, but the default is `0`, which for `xlm-mlm-tlm-xnli15-1024` is Arabic, not English (which SQuAD is in). English is `4`, based on the `XLMConfig` class.
I think this will require passing an additional input tensor to all training and evaluation batches, that's all `4` `int64`s.<|||||>This issue has been automatically marked as stale because it has not had recent activity. It will be closed if no further activity occurs. Thank you for your contributions.
|
transformers | 1,798 | closed | Add an LSTM and CNN layer on top of BERT embeddings for sentiment analysis task | I am trying to add an LSTM and a convolutional layer on top of my BERT embeddings using the Transformers package in Tensorflow for a sentiment analysis task. Does someone know how I can go about that? | 11-12-2019 03:28:27 | 11-12-2019 03:28:27 | Hi @johnahug, I would recommend checking out how the TFBertFor* models work and trying a similar method to add the desired layers via Keras, for example: https://github.com/huggingface/transformers/blob/155c782a2ccd103cf63ad48a2becd7c76a7d2115/transformers/modeling_tf_bert.py#L834
I might be able to help out more at a later time, but I'm not sure exactly when just yet. So it might be worthwhile to go ahead and try it out :)<|||||>This issue has been automatically marked as stale because it has not had recent activity. It will be closed if no further activity occurs. Thank you for your contributions.
<|||||>Hi @watkinsm any update on this?<|||||>hey @johnahug have you got what you were looking for? I am also trying to add CNN to my bert embedding but have no idea how to |
transformers | 1,797 | closed | TF: model forwards can take an inputs_embeds param | see https://github.com/huggingface/transformers/pull/1695 (non-TF) | 11-12-2019 03:27:37 | 11-12-2019 03:27:37 | |
transformers | 1,796 | closed | Fix GPT2LMHeadModel.from_pretrained(from_tf=True) | GPT2LMHeadModel.from_pretrained(from_tf=True) doesn't work because pointer points to the GPT2LMHeadModel instance, not the GPT2Model instance.
This bug causes errors like:
'GPT2LMHeadModel' object has no attribute 'h' | 11-12-2019 01:36:51 | 11-12-2019 01:36:51 | # [Codecov](https://codecov.io/gh/huggingface/transformers/pull/1796?src=pr&el=h1) Report
> Merging [#1796](https://codecov.io/gh/huggingface/transformers/pull/1796?src=pr&el=desc) into [master](https://codecov.io/gh/huggingface/transformers/commit/b5d330d11820f4ac2cc8c909b1a6a77e0cd961e0?src=pr&el=desc) will **decrease** coverage by `0.01%`.
> The diff coverage is `0%`.
[](https://codecov.io/gh/huggingface/transformers/pull/1796?src=pr&el=tree)
```diff
@@ Coverage Diff @@
## master #1796 +/- ##
==========================================
- Coverage 84.03% 84.02% -0.02%
==========================================
Files 94 94
Lines 14032 14034 +2
==========================================
Hits 11792 11792
- Misses 2240 2242 +2
```
| [Impacted Files](https://codecov.io/gh/huggingface/transformers/pull/1796?src=pr&el=tree) | Coverage Δ | |
|---|---|---|
| [transformers/modeling\_gpt2.py](https://codecov.io/gh/huggingface/transformers/pull/1796/diff?src=pr&el=tree#diff-dHJhbnNmb3JtZXJzL21vZGVsaW5nX2dwdDIucHk=) | `83.91% <0%> (-0.54%)` | :arrow_down: |
------
[Continue to review full report at Codecov](https://codecov.io/gh/huggingface/transformers/pull/1796?src=pr&el=continue).
> **Legend** - [Click here to learn more](https://docs.codecov.io/docs/codecov-delta)
> `Δ = absolute <relative> (impact)`, `ø = not affected`, `? = missing data`
> Powered by [Codecov](https://codecov.io/gh/huggingface/transformers/pull/1796?src=pr&el=footer). Last update [b5d330d...60ebaa5](https://codecov.io/gh/huggingface/transformers/pull/1796?src=pr&el=lastupdated). Read the [comment docs](https://docs.codecov.io/docs/pull-request-comments).
<|||||>Looks good to me!<|||||>Are you loading from a TF 1.0 or a TF 2.0 model?<|||||>This issue has been automatically marked as stale because it has not had recent activity. It will be closed if no further activity occurs. Thank you for your contributions.
|
transformers | 1,795 | closed | RuntimeError: Connection timed out in Single node Multi GPU training | I am trying to pre-train DistilBERT with single node multigpu as given here https://github.com/huggingface/transformers/tree/master/examples/distillation.
I have set the IP address of my gcp instance and port number. I am getting this error. Any solutions?
> File "/opt/conda/lib/python3.6/site-packages/torch/distributed/distributed_c10d.py", line 400, in init_process_group
store, rank, world_size = next(rendezvous(url))
File "/opt/conda/lib/python3.6/site-packages/torch/distributed/rendezvous.py", line 143, in _env_rendezvous_handler
store = TCPStore(master_addr, master_port, world_size, start_daemon)
RuntimeError: Connection timed out | 11-12-2019 00:59:29 | 11-12-2019 00:59:29 | By any chance, did you open the port number you're using?<|||||>Yes, it is established. What is the master_addr and master_port when submitting a job with single node and multigpu config in GCP?<|||||>This issue has been automatically marked as stale because it has not had recent activity. It will be closed if no further activity occurs. Thank you for your contributions.
<|||||>Hi, @kamalravi were you able to solve this issue?? I am facing the same issue right now where my `init_process_group` freezes and doesn't move forward for training. Could you take a look at this and suggest any relevant solutions if you have found them??
Thanks!
https://github.com/pytorch/pytorch/issues/53395#issuecomment-954103393 |
transformers | 1,794 | closed | Confused by GPT2DoubleHeadsModel example | ## ❓ Questions & Help
<!-- A clear and concise description of the question. -->
Your library is really helpful. And I have 2 questions about the example of GPT2DoubleHeadsModel.
1.
In source code of model,
> choices = ["Hello, my dog is cute [CLS]", "Hello, my cat is cute [CLS]"]
Here the comment said that "number of choices: 2". So is it a single sample or two samples? What are the two label classes?
2.
What task is the GPT2DOUBLEHeadsModel trained on? I don't find the information from the document, issues or the original paper.
Please help me. Thank you in advance. | 11-11-2019 21:21:26 | 11-11-2019 21:21:26 | For 2: It's next word prediction: https://openai.com/blog/better-language-models/<|||||>> For 2: It's next word prediction: https://openai.com/blog/better-language-models/
Thank you for your reply. From my perspective, I believe the next word prediction is what the language model does, which means it is the "unsupervised" part in the first head.
The evidence is that gpt-2 is based on gpt. Based on figure 1 in gpt paper, the left head is for "text prediction"(next word prediction), and the right head is for "task prediction".<|||||>Hi, the GPT2DoubleHeadsModel, as defined in [the documentation](https://huggingface.co/transformers/model_doc/gpt2.html#gpt2doubleheadsmodel), is: "_The GPT2 Model transformer with a language modeling and a multiple-choice classification head on top e.g. for RocStories/SWAG tasks. The two heads are two linear layers. The language modeling head has its weights tied to the input embeddings, the classification head takes as input the input of a specified classification token index in the input sequence)._".
You use a double head model when you want to have two separate losses. In this case the two linear layers compute two different losses: one of the heads is a language modeling loss, with a linear layer of size `hidden_size` x `vocab_size` and the other is a classification loss with a linear layer of size `hidden size` x `number_of_choices`.
You can read [this blog post](https://medium.com/huggingface/how-to-build-a-state-of-the-art-conversational-ai-with-transfer-learning-2d818ac26313) by @thomwolf which uses GPT2DoubleHeadsModel with a previous version of this library (pytorch-pretrained-BERT).<|||||>@LysandreJik
Hi, thank you for your help! |
transformers | 1,793 | closed | MNLI: BERT No Training Progress | ## 🐛 Bug
I am using BERT and have successfully set up pipelines for 7/8 GLUE tasks, and I find comparably good accuracy on all of them. However, for MNLI task, training loss does not converge at all. I am correctly using 3 classes with `num_classes`. In fact, I have even tried reduced the scope of MNLI to a 2-class problem (entailment vs neutral/contradiction) for testing purposes to see if this is the issue, and the model fails to converge on this training task as well. However, the exact same code (with only the dataset switched) works for MRPC, for example.
Model I am using (Bert, XLNet....): **BERT**
Language I am using the model on (English, Chinese....): **English**
The problem arise when using:
* [ ] the official example scripts: (give details)
* [x] my own modified scripts: **see co-lab**
The tasks I am working on is:
* [x] an official GLUE/SQUaD task: **MNLI**
* [ ] my own task or dataset: (give details)
## To Reproduce
Steps to reproduce the behavior:
See linked co-lab for minimum viable example
https://colab.research.google.com/drive/1Thdrpvp0uX2TCaCpqsLoUVn7DuRLlILQ
## Expected behavior
I expect to see good training progress; instead, training loss does not converge.
## Environment
* OS: **Linux**
* Python version: **3.6**
* PyTorch version: **1.3.0**
* PyTorch Transformers version (or branch): **2.1.1**
* Using GPU ? **Yes**
* Distributed of parallel setup ? **No, single GPU**
* Any other relevant information: **None**
| 11-11-2019 21:17:32 | 11-11-2019 21:17:32 | I'm assuming you did multiple runs with ≠ seeds?<|||||>Yes -- I have tried with multiple different seeds.<|||||>This issue has been automatically marked as stale because it has not had recent activity. It will be closed if no further activity occurs. Thank you for your contributions.
<|||||>> Yes -- I have tried with multiple different seeds.
@evanweissburg Have you found a solution? I'm also having issues with MNLI data. <|||||>@anthonyfuller7 Nope. I think one resolution was to not use BERT large.<|||||>I too am facing the same issue with BERT and Albert as well. The model does not converge on fine-tuning and the loss does not decrease even over 5 epochs. Did someone manage to solve this issue? |
transformers | 1,792 | closed | DistilBERT for token classification | Hi,
this PR adds a `DistilBertForTokenClassification` implementation (mainly inspired by the BERT implementation) that allows to perform sequence labeling tasks like NER or PoS tagging.
Additionally, the `run_ner.py` example script was modified to fully support DistilBERT for NER tasks.
I did a small comparison between BERT (large, cased), RoBERTa (large, cased) and DistilBERT (base, uncased) with the same hyperparameters as specified in the [example documentation](https://huggingface.co/transformers/examples.html#named-entity-recognition) (one run):
| Model | F-Score Dev | F-Score Test
| --------------------------------- | ------- | --------
| `bert-large-cased` | 95.59 | 91.70
| `roberta-large` | 95.96 | 91.87
| `distilbert-base-uncased` | 94.34 | 90.32
Unit test for the `DistilBertForTokenClassification` implementation is also added. | 11-11-2019 16:35:53 | 11-11-2019 16:35:53 | This is great, thanks @stefan-it!<|||||>This is great, thanks a lot @stefan-it.
I've added your quick benchmark in the readme.<|||||># [Codecov](https://codecov.io/gh/huggingface/transformers/pull/1792?src=pr&el=h1) Report
> Merging [#1792](https://codecov.io/gh/huggingface/transformers/pull/1792?src=pr&el=desc) into [master](https://codecov.io/gh/huggingface/transformers/commit/b5d330d11820f4ac2cc8c909b1a6a77e0cd961e0?src=pr&el=desc) will **increase** coverage by `0.03%`.
> The diff coverage is `97.29%`.
[](https://codecov.io/gh/huggingface/transformers/pull/1792?src=pr&el=tree)
```diff
@@ Coverage Diff @@
## master #1792 +/- ##
==========================================
+ Coverage 84.03% 84.07% +0.03%
==========================================
Files 94 94
Lines 14032 14069 +37
==========================================
+ Hits 11792 11828 +36
- Misses 2240 2241 +1
```
| [Impacted Files](https://codecov.io/gh/huggingface/transformers/pull/1792?src=pr&el=tree) | Coverage Δ | |
|---|---|---|
| [transformers/tests/modeling\_distilbert\_test.py](https://codecov.io/gh/huggingface/transformers/pull/1792/diff?src=pr&el=tree#diff-dHJhbnNmb3JtZXJzL3Rlc3RzL21vZGVsaW5nX2Rpc3RpbGJlcnRfdGVzdC5weQ==) | `99.18% <100%> (+0.08%)` | :arrow_up: |
| [transformers/modeling\_distilbert.py](https://codecov.io/gh/huggingface/transformers/pull/1792/diff?src=pr&el=tree#diff-dHJhbnNmb3JtZXJzL21vZGVsaW5nX2Rpc3RpbGJlcnQucHk=) | `95.87% <96.15%> (+0.02%)` | :arrow_up: |
------
[Continue to review full report at Codecov](https://codecov.io/gh/huggingface/transformers/pull/1792?src=pr&el=continue).
> **Legend** - [Click here to learn more](https://docs.codecov.io/docs/codecov-delta)
> `Δ = absolute <relative> (impact)`, `ø = not affected`, `? = missing data`
> Powered by [Codecov](https://codecov.io/gh/huggingface/transformers/pull/1792?src=pr&el=footer). Last update [b5d330d...05db5bc](https://codecov.io/gh/huggingface/transformers/pull/1792?src=pr&el=lastupdated). Read the [comment docs](https://docs.codecov.io/docs/pull-request-comments).
<|||||>I just wanted to say thanks for this PR, it was just what I was looking for at the time. |
transformers | 1,791 | closed | token indices sequence length is longer than the specified maximum sequence length | ## ❓ Questions & Help
When I use Bert, the "token indices sequence length is longer than the specified maximum sequence length for this model (1017 > 512)" occurs. How can I solve this error? | 11-11-2019 14:05:26 | 11-11-2019 14:05:26 | This means you're encoding a sequence that is larger than the max sequence the model can handle (which is 512 tokens). This is not an error but a warning; if you pass that sequence to the model it will crash as it cannot handle such a long sequence.
You can truncate the sequence: `seq = seq[:512]` or use the `max_length` tokenizer parameter so that it handles it on its own.<|||||>Thank you. I truncate the sequence and it worked. But I use the parameter `max_length` of the method "encode" of the class of Tokenizer , it do not works.<|||||>Hi, could you show me how you're using the `max_length` parameter?
Edit:
The recommended way is to call the tokenizer directly instead of using the `encode` method, so the following is the recommended way of handling it:
```py
from transformers import GPT2Tokenizer
text = "This is a sequence"
tokenizer = GPT2Tokenizer.from_pretrained("gpt2")
x = tokenizer(text, truncation=True, max_length=2)
print(len(x)) # 2
```
---
Previous answer:
If you use it as such it should truncate your sequences:
```py
from transformers import GPT2Tokenizer
text = "This is a sequence"
tokenizer = GPT2Tokenizer.from_pretrained("gpt2")
x = tokenizer.encode(text, max_length=2)
print(len(x)) # 2
```<|||||>I use `max_length` is as follows:
```
model_class, tokenizer_class, pretrained_weights = BertModel, BertTokenizer, 'bert-base-uncased'
tokenizer = tokenizer_class.from_pretrained(pretrained_weights)
model = model_class.from_pretrained(pretrained_weights)
text = "After a morning of Thrift Store hunting, a friend and I were thinking of lunch, and he ... " #this sentence is very long, Its length is more than 512. In order to save space, not all of them are shown here
# text = tokenizer.tokenize(text)
# if len(text) > 512:
# text = text[:512]
#text = "After a morning of Thrift Store hunting, a friend and I were thinking of lunch"
text = tokenizer.encode(text, add_special_tokens=True, max_length=10)
print(text)
print(len(text))
```
It works. I previously set `max_length` to 512, just output the encoded list, so I didn't notice that the length has changed. But the warning still occurs:

<|||||>Glad it works! Indeed, we should do something about this warning, it shouldn't appear when a max length is specified.<|||||>Thank you very much!<|||||>What if I need the sequence length to be longer than 512 (e.g., to retrieve the answer in a QA model)?<|||||>Hi @LukasMut this question might be better suited to Stack Overflow.<|||||>I have the same doubt as @LukasMut . Did you open a Stack Overflow question?<|||||>did you got the solution @LukasMut @paulogaspar <|||||>Not really. All solutions point to using only the 512 tokens, and choosing what to place in those tokens (for example, picking which part of the text)<|||||>Having the same issue @paulogaspar any update on this? I'm having sequences with more than 512 tokens.<|||||>> Having the same issue @paulogaspar any update on this? I'm having sequences with more than 512 tokens.
Take a look at my last answer, that's the point I'm at.<|||||>Also dealing with this issue and thought I'd post what's going through my head, correct me if I'm wrong but I think the maximum sequence length is determined when the model is first trained? In which case training a model with a larger sequence length is the solution? And I'm wondering if fine-tuning can be used to increase the sequence length.<|||||>Same question. What to do if text is long?<|||||>That's a research questions guys<|||||>This might help people looking for further details https://github.com/pytorch/fairseq/issues/1685 & https://github.com/google-research/bert/issues/27<|||||>Hi,
The question i have is almost the same.
Bert has some configuration options. As far as i know about transformers, it's not constrain by sequence length at all.
Can I change the config to have more than 512 tokens ?<|||||>Most transformers are unfortunately completely constrained, which is the case for BERT (512 tokens max).
If you want to use transformers without being limited to a sequence length, you should take a look at Transformer-XL or XLNet.<|||||>@LysandreJik
I thought [XLNet](https://arxiv.org/pdf/1906.08237.pdf) has a max length of 512 as well.
[Transformer-XL](https://arxiv.org/pdf/1906.08237.pdf) is still is a mystery to me because it seems like the length is still 512 for downstream tasks, unlike language modeling (pre-training).
Please let me know if my understanding is incorrect.
Thanks!<|||||>XLNet was pre-trained/fine-tuned with a maximum length of 512, indeed. However, the model is not limited to such a length:
```py
from transformers import XLNetLMHeadModel, XLNetTokenizer
tokenizer = XLNetTokenizer.from_pretrained("xlnet-base-cased")
model = XLNetLMHeadModel.from_pretrained("xlnet-base-cased")
encoded = tokenizer.encode_plus("Alright, let's do this" * 500, return_tensors="pt")
print(encoded["input_ids"].shape) # torch.Size([1, 3503])
print(model(**encoded)[0].shape) # torch.Size([1, 3503, 32000])
```
The model is not limited to a specific length because it doesn't leverage absolute positional embeddings, instead leveraging the same relative positional embeddings that Transformer-XL used. Please note that since the model isn't trained on larger sequences thant 512, no results are guaranteed on larger sequences, even if the model can still handle them.<|||||>I was going to try this out, but after reading this out few times now, I still have no idea how I'm supposed to truncate the token stream for the pipeline.
I got some results by combining @cswangjiawei 's advice of running the tokenizer, but it returns a truncated sequence that is slightly longer than the limit I set.
Otherwise the results are good, although they come out slow and I may have to figure how to activate cuda on py torch.
Update: There is an article that shows how to run the summarizer on large texts, I got it to work with this one: https://www.thepythoncode.com/article/text-summarization-using-huggingface-transformers-python<|||||>> What if I need the sequence length to be longer than 512 (e.g., to retrieve the answer in a QA model)?
You can go for BigBird as it takes a input token size of 4096 tokens(but can take upto 16K size)<|||||>Let me help with what I have understood. Correct me if I am wrong. The reason you can't use sequence length more than max_length is because of the positional encoding. Let's have a look at the positional encoding in the original Transformer paper
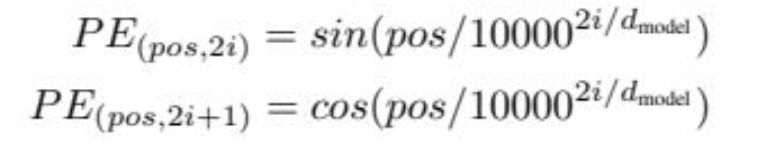
So the _pos_ in the formula is the index of the words, and they have set 10000 as the scale to cover the usual length of most of the sentences. Now, if you look at the visualization of these functions, you will notice until the _pos_ value is less than 10000 we will get a unique temporal representation of each word. But once it's length is more than 10000 representation won't be unique for each word (e.g. 1st and 10001 will have the same representation). So if max_length = scale (512 as discussed here) and sequence_length > max_length positional encoding will not work.
I didn't check what scale value (you can check it by yourself) BERT uses, but probably this may be the reason.
.<|||||>> > What if I need the sequence length to be longer than 512 (e.g., to retrieve the answer in a QA model)?
>
> You can go for BigBird as it takes a input token size of 4096 tokens(but can take upto 16K size)
The code and weights for BigBird haven't been published yet, am I right?<|||||>> > > What if I need the sequence length to be longer than 512 (e.g., to retrieve the answer in a QA model)?
> >
> >
> > You can go for BigBird as it takes a input token size of 4096 tokens(but can take upto 16K size)
>
> The code and weights for BigBird haven't been published yet, am I right?
Yes and in that case you have Longformers, Reformers which can handle the long sequences.<|||||>My model was pretrained with max_seq_len of 128 and max_posi_embeddings of 512 using the original BERT code release.
I am having the same problem here. I have tried a couple of fixes, but none of them is working for me.
```
export MAX_LENGTH=120
export MODEL="./bert-1.5M"
python3 preprocess.py ./data/train.txt $MODEL $MAX_LENGTH > train.txt
python3 preprocess.py ./data/dev.txt $MODEL $MAX_LENGTH > dev.txt
python3 preprocess.py ./data/test.txt $MODEL $MAX_LENGTH > test.txt
```
```export MAX_LENGTH=512 # I have tried 128, 256
I am running run_ner_old.py file.
Can anyone help.<|||||>> I use `max_length` is as follows:
>
> ```
> model_class, tokenizer_class, pretrained_weights = BertModel, BertTokenizer, 'bert-base-uncased'
> tokenizer = tokenizer_class.from_pretrained(pretrained_weights)
> model = model_class.from_pretrained(pretrained_weights)
>
> text = "After a morning of Thrift Store hunting, a friend and I were thinking of lunch, and he ... " #this sentence is very long, Its length is more than 512. In order to save space, not all of them are shown here
> # text = tokenizer.tokenize(text)
> # if len(text) > 512:
> # text = text[:512]
> #text = "After a morning of Thrift Store hunting, a friend and I were thinking of lunch"
>
> text = tokenizer.encode(text, add_special_tokens=True, max_length=10)
> print(text)
> print(len(text))
> ```
>
> It works. I previously set `max_length` to 512, just output the encoded list, so I didn't notice that the length has changed. But the warning still occurs:
> 
How to apply this method in csv file i have csv file "data.csv" in 2nd column it contains news that to be pass in bert of 512 length <|||||>I am trying to create an arbitrary length text summarizer using Huggingface; should I just partition the input text to the max model length, summarize each part to, say, half its original length, and repeat this procedure as long as necessary to reach the target length for the whole sequence?
It feels to me that this is quite a general problem. Shouldn't this be supported as part of the `pipeline` API itself? (I can do a PR if it's a good fit for the API.)<|||||>Not sure if this is the best approach, but I did something like this and it solves the problem ^
```python
summarizer = pipeline("summarization", model="facebook/bart-large-cnn")
def summarize_text(text: str, max_len: int) -> str:
try:
summary = summarizer(text, max_length=max_len, min_length=10, do_sample=False)
return summary[0]["summary_text"]
except IndexError as ex:
logging.warning("Sequence length too large for model, cutting text in half and calling again")
return summarize_text(text=text[:(len(text) // 2)], max_len=max_len//2) + summarize_text(text=text[(len(text) // 2):], max_len=max_len//2)
```<|||||>> ```python
> summarizer = pipeline("summarization", model="facebook/bart-large-cnn")
> def summarize_text(text: str, max_len: int) -> str:
> try:
> summary = summarizer(text, max_length=max_len, min_length=10, do_sample=False)
> return summary[0]["summary_text"]
> except IndexError as ex:
> logging.warning("Sequence length too large for model, cutting text in half and calling again")
> return summarize_text(text=text[:(len(text) // 2)], max_len=max_len//2) + summarize_text(text=text[(len(text) // 2):], max_len=max_len//2)
> ```
i have tested and works great awesome<|||||>Thanks to [sfbaker7](https://github.com/sfbaker7) recursive suggestion, i made a similar function for translating
```
model_name = "Helsinki-NLP/opus-mt-ja-en"
tokenizer = MarianTokenizer.from_pretrained(model_name)
model = MarianMTModel.from_pretrained(model_name)
def translate_text(text):
try:
translated = model.generate(**tokenizer(text, return_tensors="pt"))[0]
return tokenizer.decode(translated, skip_special_tokens=True)
except IndexError as err :
return translate_text(text[:(len(text) // 2)]) + translate_text(text[len(text) // 2:])
```
Hope this helps someone<|||||>Found an elegant solution.
If you study [this line](https://github.com/huggingface/transformers/blob/9417c924af539be5f941c8a709a96b60dfe29eb3/src/transformers/pipelines/base.py#L1061) of the implementation of the `pipeline` API, you can notice that **you can give the arguments for `tokenizer.encode` directly during the initialization of the pipeline**.
For a specific task, use:
```python
pipeline(
"summarization", # your preferred task
model="facebook/bart-large-cnn", # your preferred model
<other arguments>, # top_k, ....
"max_length" : 30, # or 512, or whatever your cut-off is
"padding" : 'max_length',
"truncation" : True,
)
```
For loading a pretrained model, use:
```python
model = <....>
tokenizer = <....>
pipeline(
model=model,
tokenizer=tokenizer,
<other arguments> # top_k, ....
"max_length" : 30, # or 512, or whatever your cut-off is
"padding" : 'max_length',
"truncation" : True,
)
```
This has worked for me with `TextClassificationPipeline` and `BertTokenizer`. I haven't tested other pipelines or tokenizers, but judging by the implementation, it should work.<|||||>I faced the same issue when using the **fill-mask pipeline**. The fill_mask.py does not consider any preprocess_params or keyword arguments, but can be fixed as follows:
https://github.com/huggingface/transformers/blob/main/src/transformers/pipelines/fill_mask.py#L96 -> `model_inputs = self.tokenizer(inputs, return_tensors=return_tensors, **preprocess_parameters)`
https://github.com/huggingface/transformers/blob/main/src/transformers/pipelines/fill_mask.py#L201 -> `def _sanitize_parameters(self, top_k=None, targets=None, **tokenizer_kwargs):`
` preprocess_params = tokenizer_kwargs`
https://github.com/huggingface/transformers/blob/main/src/transformers/pipelines/fill_mask.py#L215 -> `return preprocess_params, {}, postprocess_params`
After that you can create a pipeline as follows:
`from transformers import pipeline`
`fill_mask_pipeline = pipeline(`
` 'fill-mask', `
` model=model, `
` tokenizer=tokenizer, `
` device=0`
`)`
`tokenizer_kwargs = {'truncation':True, 'max_length':2048}`
`output = fill_mask_pipeline("Text to predict <mask>", **tokenizer_kwargs)`
I will add a pull request too!
|
transformers | 1,790 | closed | transformers vs pytorch_pretrained_bert giving different scores for BertForNextSentencePrediction | ## ❓ Questions & Help
<!-- A clear and concise description of the question. -->
import torch
from transformers import BertTokenizer, BertModel, BertForMaskedLM,BertForNextSentencePrediction
tokenizer=BertTokenizer.from_pretrained('bert-base-uncased')
BertNSP=BertForNextSentencePrediction.from_pretrained('bert-base-uncased')
text1 = "How old are you?"
text2 = "The Eiffel Tower is in Paris"
text1_toks = ["[CLS]"] + tokenizer.tokenize(text1) + ["[SEP]"]
text2_toks = tokenizer.tokenize(text2) + ["[SEP]"]
text=text1_toks+text2_toks
indexed_tokens = tokenizer.convert_tokens_to_ids(text)
segments_ids = [0]*len(text1_toks) + [1]*len(text2_toks)
tokens_tensor = torch.tensor([indexed_tokens])
segments_tensors = torch.tensor([segments_ids])
BertNSP.eval()
prediction = BertNSP(tokens_tensor, segments_tensors)
prediction=prediction[0] # tuple to tensor
print(predictions)
softmax = torch.nn.Softmax(dim=1)
prediction_sm = softmax(prediction)
print (prediction_sm)
output:
tensor([[ 2.1772, -0.8097]], grad_fn=)
tensor([[0.9923, 0.0077]], grad_fn=)
Now when trying with pytorch_pretrained_bert :
!pip install pytorch_pretrained_bert
import torch
import pytorch_pretrained_bert
from pytorch_pretrained_bert import BertTokenizer, BertAdam, BertForNextSentencePrediction
tokenizer = BertTokenizer.from_pretrained('bert-base-uncased')
model = BertForNextSentencePrediction.from_pretrained('bert-base-uncased')
text1 = "How old are you?"
text2 = "The Eiffel Tower is in Paris"
text1_toks = ["[CLS]"] + tokenizer.tokenize(text1) + ["[SEP]"]
text2_toks = tokenizer.tokenize(text2) + ["[SEP]"]
text=text1_toks+text2_toks
print(text)
indexed_tokens = tokenizer.convert_tokens_to_ids(text)
segments_ids = [0]*len(text1_toks) + [1]*len(text2_toks)
tokens_tensor = torch.tensor([indexed_tokens])
segments_tensors = torch.tensor([segments_ids])
print(indexed_tokens)
print(segments_ids)
model.eval()
prediction = model(tokens_tensor, segments_tensors)
print(prediction)
softmax = torch.nn.Softmax(dim=1)
prediction_sm = softmax(prediction)
print (prediction_sm)
output:
tensor([[-2.3808, 5.4018]], grad_fn=)
tensor([[4.1673e-04, 9.9958e-01]], grad_fn=)
1. which is the correct score here ? is the softmax score from transformers model correct or pytorch_pretrained_bert model correct ?
2.Also the output of model in pytorch_pretrained_bert is tensor but output of model in transformers is tuple .Why again is it like this ? | 11-11-2019 11:08:57 | 11-11-2019 11:08:57 | Hi, since `pytorch_pretrained_BERT`, many breaking changes have happened, two of which are causing confusion in your snippet:
- The order of arguments in the forward call has been slightly changed [(v2.0.0)](https://github.com/huggingface/transformers/releases/tag/v2.0.0)
- The models now always return tuples [(v1.0.0)](https://github.com/huggingface/transformers/releases/tag/v1.0.0).
The attention mask and token type ids order has been changed for the forward method to better respect the order of importance, which is important when compiling a model with torchscript.
Update the forward call of your model as such to obtain identical results on both:
```py
prediction = model(tokens_tensor, token_type_ids=segments_tensors)
```
To prevent further breaking changes from affecting your workflow, we recommend using named arguments when calling different methods, like it is done in the aforementioned snippet.<|||||>@LysandreJik thanks for the information .Dose this apply for all the bert models or only for the next sentence prediction alone ?<|||||>I applies to all models. |
Subsets and Splits
No community queries yet
The top public SQL queries from the community will appear here once available.