repo
stringclasses 1
value | number
int64 1
25.3k
| state
stringclasses 2
values | title
stringlengths 1
487
| body
stringlengths 0
234k
⌀ | created_at
stringlengths 19
19
| closed_at
stringlengths 19
19
| comments
stringlengths 0
293k
|
---|---|---|---|---|---|---|---|
transformers | 2,091 | closed | Error msg when running on the colab | ## ❓ Questions & Help
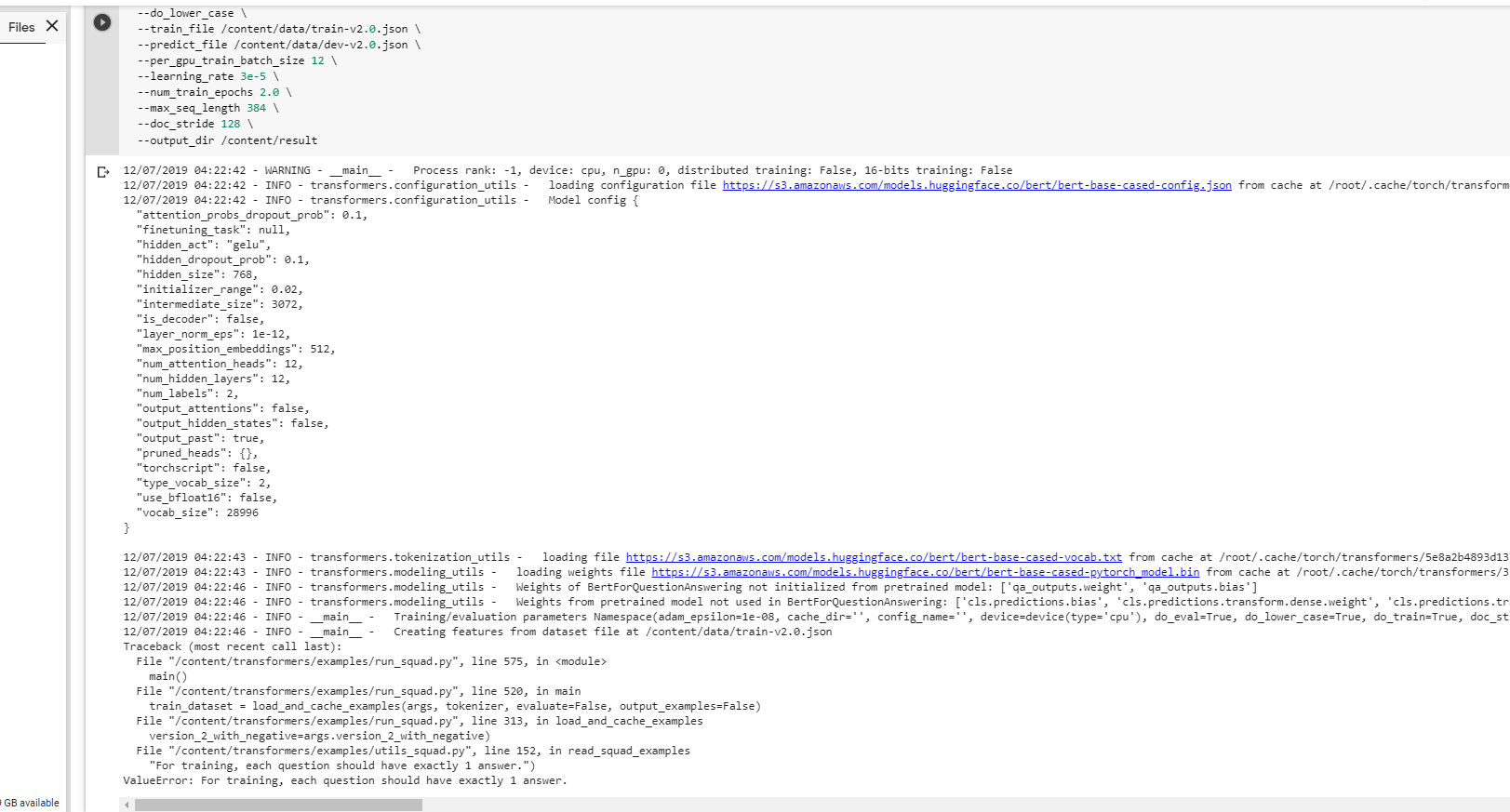
Can anyone tell me where am I wrong or it's not my problem?I cloned whole the files from huggingface. Is it can be fixed? I would appreciate for any suggestion. Thank you. | 12-07-2019 04:30:05 | 12-07-2019 04:30:05 | Hi! How did you obtain the train-v2.0 and dev-v2.0 files? Did you put the `--version_2_with_negative` flag to specify you're using SQuAD V2?<|||||>This issue has been automatically marked as stale because it has not had recent activity. It will be closed if no further activity occurs. Thank you for your contributions.
|
transformers | 2,090 | closed | AssertionError in official example | ## 🐛 Bug
<!-- Important information -->
Model I am using (Bert, XLNet....): Bert
Language I am using the model on (English, Chinese....): English
The problem arise when using:
* [x] the official example scripts: (give details)
* [ ] my own modified scripts: (give details)
The tasks I am working on is:
* [ ] an official GLUE/SQUaD task: (give the name)
* [ ] my own task or dataset: (give details)
## To Reproduce
Steps to reproduce the behavior:
in the [official example](https://huggingface.co/transformers/quickstart.html)
the tokenizer result raise an assertionError
```
text = "[CLS] Who was Jim Henson ? [SEP] Jim Henson was a puppeteer [SEP]"
tokenized_text = tokenizer.tokenize(text)
# Mask a token that we will try to predict back with `BertForMaskedLM`
masked_index = 8
tokenized_text[masked_index] = '[MASK]'
assert tokenized_text == ['[CLS]', 'who', 'was', 'jim', 'henson', '?', '[SEP]', 'jim', '[MASK]', 'was', 'a', 'puppet', '##eer', '[SEP]']
```
gives
```
AssertionError Traceback (most recent call last)
<ipython-input-1-6533b2fb8252> in <module>
16 masked_index = 8
17 tokenized_text[masked_index] = '[MASK]'
---> 18 assert tokenized_text == ['[CLS]', 'who', 'was', 'jim', 'henson', '?', '[SEP]', 'jim', '[MASK]', 'was', 'a', 'puppet', '##eer', '[SEP]']
19
20 # Convert token to vocabulary indices
AssertionError:
```
And when i print out the tokenized_text, i find the sepcial tokens had been tokenized in a wrong way.
This may caused by the lower operation to the speicial tokens
```
print(tokenized_text)
['[', 'cl', '##s', ']', 'who', 'was', 'jim', 'henson', '[MASK]', '[', 'sep', ']', 'jim', 'henson', 'was', 'a', 'puppet', '##eer', '[', 'sep', ']']
```
## Expected behavior
no exception.
## Environment
* OS: Ubuntu 19.04 / Centos ?
* Python version: 3.7.5 / 3.6.4
* PyTorch version: 1.2.0
* PyTorch Transformers version (or branch): 2.2.1
* Using GPU ? maybe
* Distributed of parallel setup ? No
* Any other relevant information:
The official example is ok in Transformers version 2.1.1, after i update my Transformers it goes wrong
## Additional context
| 12-07-2019 02:19:38 | 12-07-2019 02:19:38 | Dpulicated to #2052 and closed it .
|
transformers | 2,089 | closed | Use run_lm-finetuning on tpu | ## ❓ Questions & Help
<!-- A clear and concise description of the question. -->
Is it possible to use the script run_lm-finetuning on TPUs, if not, what do you recommend to fine-tune BERT language model on TPUs using the transformers library | 12-06-2019 18:42:17 | 12-06-2019 18:42:17 | Hello, the script would need to be adapted to run on TPU to take full advantage of the chips. We're actively working with the Cloud TPU team on scripts for fine-tuning on TPUs, which should be available in the coming weeks.<|||||>This issue has been automatically marked as stale because it has not had recent activity. It will be closed if no further activity occurs. Thank you for your contributions.
|
transformers | 2,088 | closed | Help with converting fine-tuned PT model to TF checkpoint | How do I convert PT model (.bin) to TF checkpoint successfully so that I can start serving using bert-as-a-service?
Below are the steps and errors:
Huggingface v2.2.1, Pytorch 1.2, TF 2.0
1. executed run_lm_finetuning.py to fine-tune an already finetuned model (clinicalBERT) on the target domain dataset. Successfully saved all the necessary files (.bin, config, vocab etc.)
2. To convert PT to TF, executed convert_pytorch_checkpoint_to_tf2.py with --tf_dump_path="/tf_test/" --model_type="bert" --pytorch_checkpoint_path="../pytorch_model.bin" --config_file='../config.json'
**below was the error**
```
Traceback (most recent call last):
File "/home/imagen/skc/bert/transformers-2.2.1/transformers/convert_pytorch_checkpoint_to_tf2.py", line 248, in
only_convert_finetuned_models=args.only_convert_finetuned_models)
File "/home/imagen/skc/bert/transformers-2.2.1/transformers/convert_pytorch_checkpoint_to_tf2.py", line 194, in convert_all_pt_checkpoints_to_tf
compare_with_pt_model=compare_with_pt_model)
File "/home/imagen/skc/bert/transformers-2.2.1/transformers/convert_pytorch_checkpoint_to_tf2.py", line 115, in convert_pt_checkpoint_to_tf
tf_model = load_pytorch_checkpoint_in_tf2_model(tf_model, pytorch_checkpoint_path)
File "/home/imagen/skc/environments/.virtualenvs/lstm_dev_tf2x/lib/python3.6/site-packages/transformers/modeling_tf_pytorch_utils.py", line 82, in load_pytorch_checkpoint_in_tf2_model
return load_pytorch_weights_in_tf2_model(tf_model, pt_state_dict, tf_inputs=tf_inputs, allow_missing_keys=allow_missing_keys)
File "/home/imagen/skc/environments/.virtualenvs/lstm_dev_tf2x/lib/python3.6/site-packages/transformers/modeling_tf_pytorch_utils.py", line 145, in load_pytorch_weights_in_tf2_model
assert name in pt_state_dict, "{} not found in PyTorch model".format(name)
**AssertionError: cls.seq_relationship.weight not found in PyTorch model**
```
3. I wanted to test PT to TF conversion, so I've pointed the script to original clinicalBERT model directory and it successfully converted. However, it was saved as .h5 model and not .ckpt
3.1 Ran below code to convert .h5 to save it as checkpoint - however, it seems not possible to save as checkpoint without creating the model architecture
ran below code for saving as .ckpt in tf2.0
```
import tensorflow as tf
from keras.models import load_model
saver = tf.train.Checkpoint()
model = load_model("../converted_model-tf_model.h5", compile=False)
sess = tf.compat.v1.keras.backend.get_session()
save_path = saver.save("../converted_model-tf_model.ckpt")
```
So, in order to successfully use a fine-tuned model in bert-as-a-service
1. Was there anything I am doing incorrectly when fine-tuning a model? because, somehow the PT to TF conversion goes smoothly for clinicalBERT, but not for fine-tuned version of it (AssertionError: cls.seq_relationship.weight not found in PyTorch model)
2. How to save as checkpoint (.ckpt) instead of .h5 model? this is for bert-as-a-service? if this is not possible, please suggest alternatives (is creating architecture a necessary step?)
#2069 - fwiw - I've used cleaned up version of the script
Thanks | 12-06-2019 16:45:55 | 12-06-2019 16:45:55 | Hi @thomwolf - any suggestion would be greatly appreciated.
I am looking forward to hosting one of the fine-tuned model (pytorch) using bert-as-a-service library. However, TF conversion seems to be the way to go, and I'm stuck as the script throws above errors that I am unable to understand.
<|||||>Hello! Indeed there seems to be a bug with the conversion script. In the meantime, here's how you can load your PyTorch checkpoint in a TF model:
```py
from transformers import BertForMaskedLM, TFBertForMaskedLM
# The script should have already done that
model = BertForMaskedLM.from_pretrained("bert-base-cased")
model.save_pretrained("here")
# Load the PyTorch model in TensorFlow
tf_model = TFBertForMaskedLM.from_pretrained("here", from_pt=True)
# Save the TensorFlow model
tf_model.save_pretrained("tf_test")
```
You can then convert the generated `.h5` model in a ckpt, like is described in [this issue](https://github.com/keras-team/keras/issues/9040) or this [stackoverflow issue](https://stackoverflow.com/questions/52650842/how-to-convert-hdf5-to-tensorflow-checkpoint)<|||||>Thanks for the suggestion @LysandreJik
I just tried this approach.
In my case, I fine tuned a model on MLM using run_lm_finetuning.py
```
from transformers import BertConfig, BertTokenizer, BertModel, BertForMaskedLM
import os
tokenizer = BertTokenizer.from_pretrained(ft_cbert)
model = BertModel.from_pretrained(ft_cbert)
model.save_pretrained(str(os.path.join(ft_cbert, "pt_bertmodel")))
model = BertForMaskedLM.from_pretrained(str(os.path.join(ft_cbert, "pt_bertmodel")))
model.save_pretrained(str(os.path.join(ft_cbert, "pt_maskedlm_bertmodel")))
model = TFBertModel.from_pretrained(os.path.join(ft_cbert, "pt_maskedlm_bertmodel"), from_pt=True)
model.save_pretrained(os.path.join(ft_cbert, "tf_maskedlm_bertmodel"))
```
Now, when loading the pytorch model, TF doesn't seem to find weights and initializing all of the layers to 0 (correct me if I am interpreting incorrectly); I see a list of weights not loaded from pytorch model at the end of the log.
> I1212 16:31:52.322784 139685136627520 modeling_utils.py:334] loading weights file /home/imagen/skc/bert/data/gold-regions/gold-finetune/cb-finetune-with-eval/pt_bertmodel/pytorch_model.bin
> I1212 16:31:55.378468 139685136627520 configuration_utils.py:71] Configuration saved in /home/imagen/skc/bert/data/gold-regions/gold-finetune/cb-finetune-with-eval/pt_maskedlm_bertmodel/config.json
> I1212 16:31:57.219412 139685136627520 modeling_utils.py:205] Model weights saved in /home/imagen/skc/bert/data/gold-regions/gold-finetune/cb-finetune-with-eval/pt_maskedlm_bertmodel/pytorch_model.bin
> I1212 16:31:57.220998 139685136627520 configuration_utils.py:148] loading configuration file /home/imagen/skc/bert/data/gold-regions/gold-finetune/cb-finetune-with-eval/pt_maskedlm_bertmodel/config.json
> I1212 16:31:57.222085 139685136627520 configuration_utils.py:168] Model config {
> "attention_probs_dropout_prob": 0.1,
> "finetuning_task": null,
> "hidden_act": "gelu",
> "hidden_dropout_prob": 0.1,
> "hidden_size": 768,
> "initializer_range": 0.02,
> "intermediate_size": 3072,
> "is_decoder": false,
> "layer_norm_eps": 1e-12,
> "max_position_embeddings": 512,
> "num_attention_heads": 12,
> "num_hidden_layers": 12,
> "num_labels": 2,
> "output_attentions": false,
> "output_hidden_states": false,
> "output_past": true,
> "pruned_heads": {},
> "torchscript": false,
> "type_vocab_size": 2,
> "use_bfloat16": false,
> "vocab_size": 28996
> }
>
> I1212 16:31:57.222966 139685136627520 modeling_tf_utils.py:255] loading weights file /home/imagen/skc/bert/data/gold-regions/gold-finetune/cb-finetune-with-eval/pt_maskedlm_bertmodel/pytorch_model.bin
> I1212 16:31:57.293533 139685136627520 modeling_tf_pytorch_utils.py:78] Loading PyTorch weights from /home/imagen/skc/bert/data/gold-regions/gold-finetune/cb-finetune-with-eval/pt_maskedlm_bertmodel/pytorch_model.bin
> I1212 16:31:58.017100 139685136627520 modeling_tf_pytorch_utils.py:159] Initialize TF weight tf_bert_model_3/bert/embeddings/word_embeddings/weight:0
> I1212 16:31:58.018263 139685136627520 modeling_tf_pytorch_utils.py:159] Initialize TF weight tf_bert_model_3/bert/embeddings/position_embeddings/embeddings:0
> I1212 16:31:58.019075 139685136627520 modeling_tf_pytorch_utils.py:159] Initialize TF weight tf_bert_model_3/bert/embeddings/token_type_embeddings/embeddings:0
> I1212 16:31:58.019884 139685136627520 modeling_tf_pytorch_utils.py:159] Initialize TF weight tf_bert_model_3/bert/embeddings/LayerNorm/gamma:0
> I1212 16:31:58.020372 139685136627520 modeling_tf_pytorch_utils.py:159] Initialize TF weight tf_bert_model_3/bert/embeddings/LayerNorm/beta:0
> I1212 16:31:58.020853 139685136627520 modeling_tf_pytorch_utils.py:159] Initialize TF weight tf_bert_model_3/bert/encoder/layer_._0/attention/self/query/kernel:0
> I1212 16:31:58.021338 139685136627520 modeling_tf_pytorch_utils.py:159] Initialize TF weight tf_bert_model_3/bert/encoder/layer_._0/attention/self/query/bias:0
> I1212 16:31:58.021814 139685136627520 modeling_tf_pytorch_utils.py:159] Initialize TF weight tf_bert_model_3/bert/encoder/layer_._0/attention/self/key/kernel:0
> I1212 16:31:58.022383 139685136627520 modeling_tf_pytorch_utils.py:159] Initialize TF weight tf_bert_model_3/bert/encoder/layer_._0/attention/self/key/bias:0
> I1212 16:31:58.022871 139685136627520 modeling_tf_pytorch_utils.py:159] Initialize TF weight tf_bert_model_3/bert/encoder/layer_._0/attention/self/value/kernel:0
> I1212 16:31:58.023389 139685136627520 modeling_tf_pytorch_utils.py:159] Initialize TF weight tf_bert_model_3/bert/encoder/layer_._0/attention/self/value/bias:0
> I1212 16:31:58.023855 139685136627520 modeling_tf_pytorch_utils.py:159] Initialize TF weight tf_bert_model_3/bert/encoder/layer_._0/attention/output/dense/kernel:0
> I1212 16:31:58.024335 139685136627520 modeling_tf_pytorch_utils.py:159] Initialize TF weight tf_bert_model_3/bert/encoder/layer_._0/attention/output/dense/bias:0
> I1212 16:31:58.024829 139685136627520 modeling_tf_pytorch_utils.py:159] Initialize TF weight tf_bert_model_3/bert/encoder/layer_._0/attention/output/LayerNorm/gamma:0
> I1212 16:31:58.025296 139685136627520 modeling_tf_pytorch_utils.py:159] Initialize TF weight tf_bert_model_3/bert/encoder/layer_._0/attention/output/LayerNorm/beta:0
> I1212 16:31:58.025762 139685136627520 modeling_tf_pytorch_utils.py:159] Initialize TF weight tf_bert_model_3/bert/encoder/layer_._0/intermediate/dense/kernel:0
> I1212 16:31:58.026222 139685136627520 modeling_tf_pytorch_utils.py:159] Initialize TF weight tf_bert_model_3/bert/encoder/layer_._0/intermediate/dense/bias:0
> I1212 16:31:58.026710 139685136627520 modeling_tf_pytorch_utils.py:159] Initialize TF weight tf_bert_model_3/bert/encoder/layer_._0/output/dense/kernel:0
> I1212 16:31:58.027182 139685136627520 modeling_tf_pytorch_utils.py:159] Initialize TF weight tf_bert_model_3/bert/encoder/layer_._0/output/dense/bias:0
> I1212 16:31:58.027667 139685136627520 modeling_tf_pytorch_utils.py:159] Initialize TF weight tf_bert_model_3/bert/encoder/layer_._0/output/LayerNorm/gamma:0
> I1212 16:31:58.028124 139685136627520 modeling_tf_pytorch_utils.py:159] Initialize TF weight tf_bert_model_3/bert/encoder/layer_._0/output/LayerNorm/beta:0
> I1212 16:31:58.028624 139685136627520 modeling_tf_pytorch_utils.py:159] Initialize TF weight tf_bert_model_3/bert/encoder/layer_._1/attention/self/query/kernel:0
> I1212 16:31:58.029091 139685136627520 modeling_tf_pytorch_utils.py:159] Initialize TF weight tf_bert_model_3/bert/encoder/layer_._1/attention/self/query/bias:0
> I1212 16:31:58.029582 139685136627520 modeling_tf_pytorch_utils.py:159] Initialize TF weight tf_bert_model_3/bert/encoder/layer_._1/attention/self/key/kernel:0
> I1212 16:31:58.030059 139685136627520 modeling_tf_pytorch_utils.py:159] Initialize TF weight tf_bert_model_3/bert/encoder/layer_._1/attention/self/key/bias:0
> I1212 16:31:58.030566 139685136627520 modeling_tf_pytorch_utils.py:159] Initialize TF weight tf_bert_model_3/bert/encoder/layer_._1/attention/self/value/kernel:0
> I1212 16:31:58.031049 139685136627520 modeling_tf_pytorch_utils.py:159] Initialize TF weight tf_bert_model_3/bert/encoder/layer_._1/attention/self/value/bias:0
> I1212 16:31:58.031528 139685136627520 modeling_tf_pytorch_utils.py:159] Initialize TF weight tf_bert_model_3/bert/encoder/layer_._1/attention/output/dense/kernel:0
> I1212 16:31:58.032037 139685136627520 modeling_tf_pytorch_utils.py:159] Initialize TF weight tf_bert_model_3/bert/encoder/layer_._1/attention/output/dense/bias:0
> I1212 16:31:58.032562 139685136627520 modeling_tf_pytorch_utils.py:159] Initialize TF weight tf_bert_model_3/bert/encoder/layer_._1/attention/output/LayerNorm/gamma:0
> I1212 16:31:58.033143 139685136627520 modeling_tf_pytorch_utils.py:159] Initialize TF weight tf_bert_model_3/bert/encoder/layer_._1/attention/output/LayerNorm/beta:0
> I1212 16:31:58.033643 139685136627520 modeling_tf_pytorch_utils.py:159] Initialize TF weight tf_bert_model_3/bert/encoder/layer_._1/intermediate/dense/kernel:0
> I1212 16:31:58.034140 139685136627520 modeling_tf_pytorch_utils.py:159] Initialize TF weight tf_bert_model_3/bert/encoder/layer_._1/intermediate/dense/bias:0
> I1212 16:31:58.034643 139685136627520 modeling_tf_pytorch_utils.py:159] Initialize TF weight tf_bert_model_3/bert/encoder/layer_._1/output/dense/kernel:0
> I1212 16:31:58.035099 139685136627520 modeling_tf_pytorch_utils.py:159] Initialize TF weight tf_bert_model_3/bert/encoder/layer_._1/output/dense/bias:0
> I1212 16:31:58.035623 139685136627520 modeling_tf_pytorch_utils.py:159] Initialize TF weight tf_bert_model_3/bert/encoder/layer_._1/output/LayerNorm/gamma:0
> I1212 16:31:58.036166 139685136627520 modeling_tf_pytorch_utils.py:159] Initialize TF weight tf_bert_model_3/bert/encoder/layer_._1/output/LayerNorm/beta:0
> I1212 16:31:58.036743 139685136627520 modeling_tf_pytorch_utils.py:159] Initialize TF weight tf_bert_model_3/bert/encoder/layer_._2/attention/self/query/kernel:0
> I1212 16:31:58.037309 139685136627520 modeling_tf_pytorch_utils.py:159] Initialize TF weight tf_bert_model_3/bert/encoder/layer_._2/attention/self/query/bias:0
> I1212 16:31:58.037782 139685136627520 modeling_tf_pytorch_utils.py:159] Initialize TF weight tf_bert_model_3/bert/encoder/layer_._2/attention/self/key/kernel:0
> I1212 16:31:58.038266 139685136627520 modeling_tf_pytorch_utils.py:159] Initialize TF weight tf_bert_model_3/bert/encoder/layer_._2/attention/self/key/bias:0
> I1212 16:31:58.038728 139685136627520 modeling_tf_pytorch_utils.py:159] Initialize TF weight tf_bert_model_3/bert/encoder/layer_._2/attention/self/value/kernel:0
> I1212 16:31:58.039192 139685136627520 modeling_tf_pytorch_utils.py:159] Initialize TF weight tf_bert_model_3/bert/encoder/layer_._2/attention/self/value/bias:0
> I1212 16:31:58.039664 139685136627520 modeling_tf_pytorch_utils.py:159] Initialize TF weight tf_bert_model_3/bert/encoder/layer_._2/attention/output/dense/kernel:0
> I1212 16:31:58.040130 139685136627520 modeling_tf_pytorch_utils.py:159] Initialize TF weight tf_bert_model_3/bert/encoder/layer_._2/attention/output/dense/bias:0
> I1212 16:31:58.040640 139685136627520 modeling_tf_pytorch_utils.py:159] Initialize TF weight tf_bert_model_3/bert/encoder/layer_._2/attention/output/LayerNorm/gamma:0
> I1212 16:31:58.041108 139685136627520 modeling_tf_pytorch_utils.py:159] Initialize TF weight tf_bert_model_3/bert/encoder/layer_._2/attention/output/LayerNorm/beta:0
> I1212 16:31:58.041579 139685136627520 modeling_tf_pytorch_utils.py:159] Initialize TF weight tf_bert_model_3/bert/encoder/layer_._2/intermediate/dense/kernel:0
> I1212 16:31:58.042079 139685136627520 modeling_tf_pytorch_utils.py:159] Initialize TF weight tf_bert_model_3/bert/encoder/layer_._2/intermediate/dense/bias:0
> I1212 16:31:58.042617 139685136627520 modeling_tf_pytorch_utils.py:159] Initialize TF weight tf_bert_model_3/bert/encoder/layer_._2/output/dense/kernel:0
> I1212 16:31:58.043088 139685136627520 modeling_tf_pytorch_utils.py:159] Initialize TF weight tf_bert_model_3/bert/encoder/layer_._2/output/dense/bias:0
> I1212 16:31:58.043587 139685136627520 modeling_tf_pytorch_utils.py:159] Initialize TF weight tf_bert_model_3/bert/encoder/layer_._2/output/LayerNorm/gamma:0
> I1212 16:31:58.044040 139685136627520 modeling_tf_pytorch_utils.py:159] Initialize TF weight tf_bert_model_3/bert/encoder/layer_._2/output/LayerNorm/beta:0
> I1212 16:31:58.044509 139685136627520 modeling_tf_pytorch_utils.py:159] Initialize TF weight tf_bert_model_3/bert/encoder/layer_._3/attention/self/query/kernel:0
> I1212 16:31:58.045005 139685136627520 modeling_tf_pytorch_utils.py:159] Initialize TF weight tf_bert_model_3/bert/encoder/layer_._3/attention/self/query/bias:0
> I1212 16:31:58.050858 139685136627520 modeling_tf_pytorch_utils.py:159] Initialize TF weight tf_bert_model_3/bert/encoder/layer_._3/attention/self/key/kernel:0
> I1212 16:31:58.051367 139685136627520 modeling_tf_pytorch_utils.py:159] Initialize TF weight tf_bert_model_3/bert/encoder/layer_._3/attention/self/key/bias:0
> I1212 16:31:58.051822 139685136627520 modeling_tf_pytorch_utils.py:159] Initialize TF weight tf_bert_model_3/bert/encoder/layer_._3/attention/self/value/kernel:0
> I1212 16:31:58.052374 139685136627520 modeling_tf_pytorch_utils.py:159] Initialize TF weight tf_bert_model_3/bert/encoder/layer_._3/attention/self/value/bias:0
> I1212 16:31:58.052869 139685136627520 modeling_tf_pytorch_utils.py:159] Initialize TF weight tf_bert_model_3/bert/encoder/layer_._3/attention/output/dense/kernel:0
> I1212 16:31:58.053370 139685136627520 modeling_tf_pytorch_utils.py:159] Initialize TF weight tf_bert_model_3/bert/encoder/layer_._3/attention/output/dense/bias:0
> I1212 16:31:58.053862 139685136627520 modeling_tf_pytorch_utils.py:159] Initialize TF weight tf_bert_model_3/bert/encoder/layer_._3/attention/output/LayerNorm/gamma:0
> I1212 16:31:58.054336 139685136627520 modeling_tf_pytorch_utils.py:159] Initialize TF weight tf_bert_model_3/bert/encoder/layer_._3/attention/output/LayerNorm/beta:0
> I1212 16:31:58.054825 139685136627520 modeling_tf_pytorch_utils.py:159] Initialize TF weight tf_bert_model_3/bert/encoder/layer_._3/intermediate/dense/kernel:0
> I1212 16:31:58.055315 139685136627520 modeling_tf_pytorch_utils.py:159] Initialize TF weight tf_bert_model_3/bert/encoder/layer_._3/intermediate/dense/bias:0
> I1212 16:31:58.055775 139685136627520 modeling_tf_pytorch_utils.py:159] Initialize TF weight tf_bert_model_3/bert/encoder/layer_._3/output/dense/kernel:0
> I1212 16:31:58.056253 139685136627520 modeling_tf_pytorch_utils.py:159] Initialize TF weight tf_bert_model_3/bert/encoder/layer_._3/output/dense/bias:0
> I1212 16:31:58.056724 139685136627520 modeling_tf_pytorch_utils.py:159] Initialize TF weight tf_bert_model_3/bert/encoder/layer_._3/output/LayerNorm/gamma:0
> I1212 16:31:58.057177 139685136627520 modeling_tf_pytorch_utils.py:159] Initialize TF weight tf_bert_model_3/bert/encoder/layer_._3/output/LayerNorm/beta:0
> I1212 16:31:58.057679 139685136627520 modeling_tf_pytorch_utils.py:159] Initialize TF weight tf_bert_model_3/bert/encoder/layer_._4/attention/self/query/kernel:0
> I1212 16:31:58.058135 139685136627520 modeling_tf_pytorch_utils.py:159] Initialize TF weight tf_bert_model_3/bert/encoder/layer_._4/attention/self/query/bias:0
> I1212 16:31:58.058606 139685136627520 modeling_tf_pytorch_utils.py:159] Initialize TF weight tf_bert_model_3/bert/encoder/layer_._4/attention/self/key/kernel:0
> I1212 16:31:58.059053 139685136627520 modeling_tf_pytorch_utils.py:159] Initialize TF weight tf_bert_model_3/bert/encoder/layer_._4/attention/self/key/bias:0
> I1212 16:31:58.059546 139685136627520 modeling_tf_pytorch_utils.py:159] Initialize TF weight tf_bert_model_3/bert/encoder/layer_._4/attention/self/value/kernel:0
> I1212 16:31:58.060031 139685136627520 modeling_tf_pytorch_utils.py:159] Initialize TF weight tf_bert_model_3/bert/encoder/layer_._4/attention/self/value/bias:0
> I1212 16:31:58.060508 139685136627520 modeling_tf_pytorch_utils.py:159] Initialize TF weight tf_bert_model_3/bert/encoder/layer_._4/attention/output/dense/kernel:0
> I1212 16:31:58.060971 139685136627520 modeling_tf_pytorch_utils.py:159] Initialize TF weight tf_bert_model_3/bert/encoder/layer_._4/attention/output/dense/bias:0
> I1212 16:31:58.061455 139685136627520 modeling_tf_pytorch_utils.py:159] Initialize TF weight tf_bert_model_3/bert/encoder/layer_._4/attention/output/LayerNorm/gamma:0
> I1212 16:31:58.061920 139685136627520 modeling_tf_pytorch_utils.py:159] Initialize TF weight tf_bert_model_3/bert/encoder/layer_._4/attention/output/LayerNorm/beta:0
> I1212 16:31:58.062463 139685136627520 modeling_tf_pytorch_utils.py:159] Initialize TF weight tf_bert_model_3/bert/encoder/layer_._4/intermediate/dense/kernel:0
> I1212 16:31:58.062933 139685136627520 modeling_tf_pytorch_utils.py:159] Initialize TF weight tf_bert_model_3/bert/encoder/layer_._4/intermediate/dense/bias:0
> I1212 16:31:58.063439 139685136627520 modeling_tf_pytorch_utils.py:159] Initialize TF weight tf_bert_model_3/bert/encoder/layer_._4/output/dense/kernel:0
> I1212 16:31:58.063920 139685136627520 modeling_tf_pytorch_utils.py:159] Initialize TF weight tf_bert_model_3/bert/encoder/layer_._4/output/dense/bias:0
> I1212 16:31:58.064412 139685136627520 modeling_tf_pytorch_utils.py:159] Initialize TF weight tf_bert_model_3/bert/encoder/layer_._4/output/LayerNorm/gamma:0
> I1212 16:31:58.064872 139685136627520 modeling_tf_pytorch_utils.py:159] Initialize TF weight tf_bert_model_3/bert/encoder/layer_._4/output/LayerNorm/beta:0
> I1212 16:31:58.066597 139685136627520 modeling_tf_pytorch_utils.py:159] Initialize TF weight tf_bert_model_3/bert/encoder/layer_._5/attention/self/query/kernel:0
> I1212 16:31:58.068921 139685136627520 modeling_tf_pytorch_utils.py:159] Initialize TF weight tf_bert_model_3/bert/encoder/layer_._5/attention/self/query/bias:0
> I1212 16:31:58.069412 139685136627520 modeling_tf_pytorch_utils.py:159] Initialize TF weight tf_bert_model_3/bert/encoder/layer_._5/attention/self/key/kernel:0
> I1212 16:31:58.069909 139685136627520 modeling_tf_pytorch_utils.py:159] Initialize TF weight tf_bert_model_3/bert/encoder/layer_._5/attention/self/key/bias:0
> I1212 16:31:58.070411 139685136627520 modeling_tf_pytorch_utils.py:159] Initialize TF weight tf_bert_model_3/bert/encoder/layer_._5/attention/self/value/kernel:0
> I1212 16:31:58.070859 139685136627520 modeling_tf_pytorch_utils.py:159] Initialize TF weight tf_bert_model_3/bert/encoder/layer_._5/attention/self/value/bias:0
> I1212 16:31:58.071335 139685136627520 modeling_tf_pytorch_utils.py:159] Initialize TF weight tf_bert_model_3/bert/encoder/layer_._5/attention/output/dense/kernel:0
> I1212 16:31:58.071808 139685136627520 modeling_tf_pytorch_utils.py:159] Initialize TF weight tf_bert_model_3/bert/encoder/layer_._5/attention/output/dense/bias:0
> I1212 16:31:58.072312 139685136627520 modeling_tf_pytorch_utils.py:159] Initialize TF weight tf_bert_model_3/bert/encoder/layer_._5/attention/output/LayerNorm/gamma:0
> I1212 16:31:58.072788 139685136627520 modeling_tf_pytorch_utils.py:159] Initialize TF weight tf_bert_model_3/bert/encoder/layer_._5/attention/output/LayerNorm/beta:0
> I1212 16:31:58.073315 139685136627520 modeling_tf_pytorch_utils.py:159] Initialize TF weight tf_bert_model_3/bert/encoder/layer_._5/intermediate/dense/kernel:0
> I1212 16:31:58.073767 139685136627520 modeling_tf_pytorch_utils.py:159] Initialize TF weight tf_bert_model_3/bert/encoder/layer_._5/intermediate/dense/bias:0
> I1212 16:31:58.074249 139685136627520 modeling_tf_pytorch_utils.py:159] Initialize TF weight tf_bert_model_3/bert/encoder/layer_._5/output/dense/kernel:0
> I1212 16:31:58.074745 139685136627520 modeling_tf_pytorch_utils.py:159] Initialize TF weight tf_bert_model_3/bert/encoder/layer_._5/output/dense/bias:0
> I1212 16:31:58.075211 139685136627520 modeling_tf_pytorch_utils.py:159] Initialize TF weight tf_bert_model_3/bert/encoder/layer_._5/output/LayerNorm/gamma:0
> I1212 16:31:58.075714 139685136627520 modeling_tf_pytorch_utils.py:159] Initialize TF weight tf_bert_model_3/bert/encoder/layer_._5/output/LayerNorm/beta:0
> I1212 16:31:58.076181 139685136627520 modeling_tf_pytorch_utils.py:159] Initialize TF weight tf_bert_model_3/bert/encoder/layer_._6/attention/self/query/kernel:0
> I1212 16:31:58.076673 139685136627520 modeling_tf_pytorch_utils.py:159] Initialize TF weight tf_bert_model_3/bert/encoder/layer_._6/attention/self/query/bias:0
> I1212 16:31:58.077143 139685136627520 modeling_tf_pytorch_utils.py:159] Initialize TF weight tf_bert_model_3/bert/encoder/layer_._6/attention/self/key/kernel:0
> I1212 16:31:58.077627 139685136627520 modeling_tf_pytorch_utils.py:159] Initialize TF weight tf_bert_model_3/bert/encoder/layer_._6/attention/self/key/bias:0
> I1212 16:31:58.078094 139685136627520 modeling_tf_pytorch_utils.py:159] Initialize TF weight tf_bert_model_3/bert/encoder/layer_._6/attention/self/value/kernel:0
> I1212 16:31:58.078586 139685136627520 modeling_tf_pytorch_utils.py:159] Initialize TF weight tf_bert_model_3/bert/encoder/layer_._6/attention/self/value/bias:0
> I1212 16:31:58.079055 139685136627520 modeling_tf_pytorch_utils.py:159] Initialize TF weight tf_bert_model_3/bert/encoder/layer_._6/attention/output/dense/kernel:0
> I1212 16:31:58.079540 139685136627520 modeling_tf_pytorch_utils.py:159] Initialize TF weight tf_bert_model_3/bert/encoder/layer_._6/attention/output/dense/bias:0
> I1212 16:31:58.080033 139685136627520 modeling_tf_pytorch_utils.py:159] Initialize TF weight tf_bert_model_3/bert/encoder/layer_._6/attention/output/LayerNorm/gamma:0
> I1212 16:31:58.080506 139685136627520 modeling_tf_pytorch_utils.py:159] Initialize TF weight tf_bert_model_3/bert/encoder/layer_._6/attention/output/LayerNorm/beta:0
> I1212 16:31:58.080977 139685136627520 modeling_tf_pytorch_utils.py:159] Initialize TF weight tf_bert_model_3/bert/encoder/layer_._6/intermediate/dense/kernel:0
> I1212 16:31:58.081467 139685136627520 modeling_tf_pytorch_utils.py:159] Initialize TF weight tf_bert_model_3/bert/encoder/layer_._6/intermediate/dense/bias:0
> I1212 16:31:58.081947 139685136627520 modeling_tf_pytorch_utils.py:159] Initialize TF weight tf_bert_model_3/bert/encoder/layer_._6/output/dense/kernel:0
> I1212 16:31:58.082474 139685136627520 modeling_tf_pytorch_utils.py:159] Initialize TF weight tf_bert_model_3/bert/encoder/layer_._6/output/dense/bias:0
> I1212 16:31:58.082974 139685136627520 modeling_tf_pytorch_utils.py:159] Initialize TF weight tf_bert_model_3/bert/encoder/layer_._6/output/LayerNorm/gamma:0
> I1212 16:31:58.083476 139685136627520 modeling_tf_pytorch_utils.py:159] Initialize TF weight tf_bert_model_3/bert/encoder/layer_._6/output/LayerNorm/beta:0
> I1212 16:31:58.083951 139685136627520 modeling_tf_pytorch_utils.py:159] Initialize TF weight tf_bert_model_3/bert/encoder/layer_._7/attention/self/query/kernel:0
> I1212 16:31:58.084461 139685136627520 modeling_tf_pytorch_utils.py:159] Initialize TF weight tf_bert_model_3/bert/encoder/layer_._7/attention/self/query/bias:0
> I1212 16:31:58.084934 139685136627520 modeling_tf_pytorch_utils.py:159] Initialize TF weight tf_bert_model_3/bert/encoder/layer_._7/attention/self/key/kernel:0
> I1212 16:31:58.085417 139685136627520 modeling_tf_pytorch_utils.py:159] Initialize TF weight tf_bert_model_3/bert/encoder/layer_._7/attention/self/key/bias:0
> I1212 16:31:58.085875 139685136627520 modeling_tf_pytorch_utils.py:159] Initialize TF weight tf_bert_model_3/bert/encoder/layer_._7/attention/self/value/kernel:0
> I1212 16:31:58.086349 139685136627520 modeling_tf_pytorch_utils.py:159] Initialize TF weight tf_bert_model_3/bert/encoder/layer_._7/attention/self/value/bias:0
> I1212 16:31:58.086802 139685136627520 modeling_tf_pytorch_utils.py:159] Initialize TF weight tf_bert_model_3/bert/encoder/layer_._7/attention/output/dense/kernel:0
> I1212 16:31:58.087476 139685136627520 modeling_tf_pytorch_utils.py:159] Initialize TF weight tf_bert_model_3/bert/encoder/layer_._7/attention/output/dense/bias:0
> I1212 16:31:58.087949 139685136627520 modeling_tf_pytorch_utils.py:159] Initialize TF weight tf_bert_model_3/bert/encoder/layer_._7/attention/output/LayerNorm/gamma:0
> I1212 16:31:58.088423 139685136627520 modeling_tf_pytorch_utils.py:159] Initialize TF weight tf_bert_model_3/bert/encoder/layer_._7/attention/output/LayerNorm/beta:0
> I1212 16:31:58.089007 139685136627520 modeling_tf_pytorch_utils.py:159] Initialize TF weight tf_bert_model_3/bert/encoder/layer_._7/intermediate/dense/kernel:0
> I1212 16:31:58.089831 139685136627520 modeling_tf_pytorch_utils.py:159] Initialize TF weight tf_bert_model_3/bert/encoder/layer_._7/intermediate/dense/bias:0
> I1212 16:31:58.090376 139685136627520 modeling_tf_pytorch_utils.py:159] Initialize TF weight tf_bert_model_3/bert/encoder/layer_._7/output/dense/kernel:0
> I1212 16:31:58.090837 139685136627520 modeling_tf_pytorch_utils.py:159] Initialize TF weight tf_bert_model_3/bert/encoder/layer_._7/output/dense/bias:0
> I1212 16:31:58.091311 139685136627520 modeling_tf_pytorch_utils.py:159] Initialize TF weight tf_bert_model_3/bert/encoder/layer_._7/output/LayerNorm/gamma:0
> I1212 16:31:58.091777 139685136627520 modeling_tf_pytorch_utils.py:159] Initialize TF weight tf_bert_model_3/bert/encoder/layer_._7/output/LayerNorm/beta:0
> I1212 16:31:58.092295 139685136627520 modeling_tf_pytorch_utils.py:159] Initialize TF weight tf_bert_model_3/bert/encoder/layer_._8/attention/self/query/kernel:0
> I1212 16:31:58.092808 139685136627520 modeling_tf_pytorch_utils.py:159] Initialize TF weight tf_bert_model_3/bert/encoder/layer_._8/attention/self/query/bias:0
> I1212 16:31:58.093313 139685136627520 modeling_tf_pytorch_utils.py:159] Initialize TF weight tf_bert_model_3/bert/encoder/layer_._8/attention/self/key/kernel:0
> I1212 16:31:58.093771 139685136627520 modeling_tf_pytorch_utils.py:159] Initialize TF weight tf_bert_model_3/bert/encoder/layer_._8/attention/self/key/bias:0
> I1212 16:31:58.094259 139685136627520 modeling_tf_pytorch_utils.py:159] Initialize TF weight tf_bert_model_3/bert/encoder/layer_._8/attention/self/value/kernel:0
> I1212 16:31:58.099888 139685136627520 modeling_tf_pytorch_utils.py:159] Initialize TF weight tf_bert_model_3/bert/encoder/layer_._8/attention/self/value/bias:0
> I1212 16:31:58.100401 139685136627520 modeling_tf_pytorch_utils.py:159] Initialize TF weight tf_bert_model_3/bert/encoder/layer_._8/attention/output/dense/kernel:0
> I1212 16:31:58.100865 139685136627520 modeling_tf_pytorch_utils.py:159] Initialize TF weight tf_bert_model_3/bert/encoder/layer_._8/attention/output/dense/bias:0
> I1212 16:31:58.101369 139685136627520 modeling_tf_pytorch_utils.py:159] Initialize TF weight tf_bert_model_3/bert/encoder/layer_._8/attention/output/LayerNorm/gamma:0
> I1212 16:31:58.101860 139685136627520 modeling_tf_pytorch_utils.py:159] Initialize TF weight tf_bert_model_3/bert/encoder/layer_._8/attention/output/LayerNorm/beta:0
> I1212 16:31:58.102412 139685136627520 modeling_tf_pytorch_utils.py:159] Initialize TF weight tf_bert_model_3/bert/encoder/layer_._8/intermediate/dense/kernel:0
> I1212 16:31:58.103574 139685136627520 modeling_tf_pytorch_utils.py:159] Initialize TF weight tf_bert_model_3/bert/encoder/layer_._8/intermediate/dense/bias:0
> I1212 16:31:58.104034 139685136627520 modeling_tf_pytorch_utils.py:159] Initialize TF weight tf_bert_model_3/bert/encoder/layer_._8/output/dense/kernel:0
> I1212 16:31:58.104549 139685136627520 modeling_tf_pytorch_utils.py:159] Initialize TF weight tf_bert_model_3/bert/encoder/layer_._8/output/dense/bias:0
> I1212 16:31:58.105008 139685136627520 modeling_tf_pytorch_utils.py:159] Initialize TF weight tf_bert_model_3/bert/encoder/layer_._8/output/LayerNorm/gamma:0
> I1212 16:31:58.105483 139685136627520 modeling_tf_pytorch_utils.py:159] Initialize TF weight tf_bert_model_3/bert/encoder/layer_._8/output/LayerNorm/beta:0
> I1212 16:31:58.105949 139685136627520 modeling_tf_pytorch_utils.py:159] Initialize TF weight tf_bert_model_3/bert/encoder/layer_._9/attention/self/query/kernel:0
> I1212 16:31:58.106442 139685136627520 modeling_tf_pytorch_utils.py:159] Initialize TF weight tf_bert_model_3/bert/encoder/layer_._9/attention/self/query/bias:0
> I1212 16:31:58.106897 139685136627520 modeling_tf_pytorch_utils.py:159] Initialize TF weight tf_bert_model_3/bert/encoder/layer_._9/attention/self/key/kernel:0
> I1212 16:31:58.107369 139685136627520 modeling_tf_pytorch_utils.py:159] Initialize TF weight tf_bert_model_3/bert/encoder/layer_._9/attention/self/key/bias:0
> I1212 16:31:58.107837 139685136627520 modeling_tf_pytorch_utils.py:159] Initialize TF weight tf_bert_model_3/bert/encoder/layer_._9/attention/self/value/kernel:0
> I1212 16:31:58.108303 139685136627520 modeling_tf_pytorch_utils.py:159] Initialize TF weight tf_bert_model_3/bert/encoder/layer_._9/attention/self/value/bias:0
> I1212 16:31:58.108789 139685136627520 modeling_tf_pytorch_utils.py:159] Initialize TF weight tf_bert_model_3/bert/encoder/layer_._9/attention/output/dense/kernel:0
> I1212 16:31:58.109263 139685136627520 modeling_tf_pytorch_utils.py:159] Initialize TF weight tf_bert_model_3/bert/encoder/layer_._9/attention/output/dense/bias:0
> I1212 16:31:58.109742 139685136627520 modeling_tf_pytorch_utils.py:159] Initialize TF weight tf_bert_model_3/bert/encoder/layer_._9/attention/output/LayerNorm/gamma:0
> I1212 16:31:58.110190 139685136627520 modeling_tf_pytorch_utils.py:159] Initialize TF weight tf_bert_model_3/bert/encoder/layer_._9/attention/output/LayerNorm/beta:0
> I1212 16:31:58.110669 139685136627520 modeling_tf_pytorch_utils.py:159] Initialize TF weight tf_bert_model_3/bert/encoder/layer_._9/intermediate/dense/kernel:0
> I1212 16:31:58.111116 139685136627520 modeling_tf_pytorch_utils.py:159] Initialize TF weight tf_bert_model_3/bert/encoder/layer_._9/intermediate/dense/bias:0
> I1212 16:31:58.111589 139685136627520 modeling_tf_pytorch_utils.py:159] Initialize TF weight tf_bert_model_3/bert/encoder/layer_._9/output/dense/kernel:0
> I1212 16:31:58.112125 139685136627520 modeling_tf_pytorch_utils.py:159] Initialize TF weight tf_bert_model_3/bert/encoder/layer_._9/output/dense/bias:0
> I1212 16:31:58.112630 139685136627520 modeling_tf_pytorch_utils.py:159] Initialize TF weight tf_bert_model_3/bert/encoder/layer_._9/output/LayerNorm/gamma:0
> I1212 16:31:58.113107 139685136627520 modeling_tf_pytorch_utils.py:159] Initialize TF weight tf_bert_model_3/bert/encoder/layer_._9/output/LayerNorm/beta:0
> I1212 16:31:58.113591 139685136627520 modeling_tf_pytorch_utils.py:159] Initialize TF weight tf_bert_model_3/bert/encoder/layer_._10/attention/self/query/kernel:0
> I1212 16:31:58.114055 139685136627520 modeling_tf_pytorch_utils.py:159] Initialize TF weight tf_bert_model_3/bert/encoder/layer_._10/attention/self/query/bias:0
> I1212 16:31:58.114537 139685136627520 modeling_tf_pytorch_utils.py:159] Initialize TF weight tf_bert_model_3/bert/encoder/layer_._10/attention/self/key/kernel:0
> I1212 16:31:58.115001 139685136627520 modeling_tf_pytorch_utils.py:159] Initialize TF weight tf_bert_model_3/bert/encoder/layer_._10/attention/self/key/bias:0
> I1212 16:31:58.115493 139685136627520 modeling_tf_pytorch_utils.py:159] Initialize TF weight tf_bert_model_3/bert/encoder/layer_._10/attention/self/value/kernel:0
> I1212 16:31:58.115964 139685136627520 modeling_tf_pytorch_utils.py:159] Initialize TF weight tf_bert_model_3/bert/encoder/layer_._10/attention/self/value/bias:0
> I1212 16:31:58.116458 139685136627520 modeling_tf_pytorch_utils.py:159] Initialize TF weight tf_bert_model_3/bert/encoder/layer_._10/attention/output/dense/kernel:0
> I1212 16:31:58.116904 139685136627520 modeling_tf_pytorch_utils.py:159] Initialize TF weight tf_bert_model_3/bert/encoder/layer_._10/attention/output/dense/bias:0
> I1212 16:31:58.117376 139685136627520 modeling_tf_pytorch_utils.py:159] Initialize TF weight tf_bert_model_3/bert/encoder/layer_._10/attention/output/LayerNorm/gamma:0
> I1212 16:31:58.117864 139685136627520 modeling_tf_pytorch_utils.py:159] Initialize TF weight tf_bert_model_3/bert/encoder/layer_._10/attention/output/LayerNorm/beta:0
> I1212 16:31:58.118321 139685136627520 modeling_tf_pytorch_utils.py:159] Initialize TF weight tf_bert_model_3/bert/encoder/layer_._10/intermediate/dense/kernel:0
> I1212 16:31:58.118805 139685136627520 modeling_tf_pytorch_utils.py:159] Initialize TF weight tf_bert_model_3/bert/encoder/layer_._10/intermediate/dense/bias:0
> I1212 16:31:58.119260 139685136627520 modeling_tf_pytorch_utils.py:159] Initialize TF weight tf_bert_model_3/bert/encoder/layer_._10/output/dense/kernel:0
> I1212 16:31:58.119747 139685136627520 modeling_tf_pytorch_utils.py:159] Initialize TF weight tf_bert_model_3/bert/encoder/layer_._10/output/dense/bias:0
> I1212 16:31:58.120195 139685136627520 modeling_tf_pytorch_utils.py:159] Initialize TF weight tf_bert_model_3/bert/encoder/layer_._10/output/LayerNorm/gamma:0
> I1212 16:31:58.120673 139685136627520 modeling_tf_pytorch_utils.py:159] Initialize TF weight tf_bert_model_3/bert/encoder/layer_._10/output/LayerNorm/beta:0
> I1212 16:31:58.121122 139685136627520 modeling_tf_pytorch_utils.py:159] Initialize TF weight tf_bert_model_3/bert/encoder/layer_._11/attention/self/query/kernel:0
> I1212 16:31:58.121608 139685136627520 modeling_tf_pytorch_utils.py:159] Initialize TF weight tf_bert_model_3/bert/encoder/layer_._11/attention/self/query/bias:0
> I1212 16:31:58.122125 139685136627520 modeling_tf_pytorch_utils.py:159] Initialize TF weight tf_bert_model_3/bert/encoder/layer_._11/attention/self/key/kernel:0
> I1212 16:31:58.122639 139685136627520 modeling_tf_pytorch_utils.py:159] Initialize TF weight tf_bert_model_3/bert/encoder/layer_._11/attention/self/key/bias:0
> I1212 16:31:58.123139 139685136627520 modeling_tf_pytorch_utils.py:159] Initialize TF weight tf_bert_model_3/bert/encoder/layer_._11/attention/self/value/kernel:0
> I1212 16:31:58.127967 139685136627520 modeling_tf_pytorch_utils.py:159] Initialize TF weight tf_bert_model_3/bert/encoder/layer_._11/attention/self/value/bias:0
> I1212 16:31:58.128448 139685136627520 modeling_tf_pytorch_utils.py:159] Initialize TF weight tf_bert_model_3/bert/encoder/layer_._11/attention/output/dense/kernel:0
> I1212 16:31:58.128974 139685136627520 modeling_tf_pytorch_utils.py:159] Initialize TF weight tf_bert_model_3/bert/encoder/layer_._11/attention/output/dense/bias:0
> I1212 16:31:58.129623 139685136627520 modeling_tf_pytorch_utils.py:159] Initialize TF weight tf_bert_model_3/bert/encoder/layer_._11/attention/output/LayerNorm/gamma:0
> I1212 16:31:58.130099 139685136627520 modeling_tf_pytorch_utils.py:159] Initialize TF weight tf_bert_model_3/bert/encoder/layer_._11/attention/output/LayerNorm/beta:0
> I1212 16:31:58.130589 139685136627520 modeling_tf_pytorch_utils.py:159] Initialize TF weight tf_bert_model_3/bert/encoder/layer_._11/intermediate/dense/kernel:0
> I1212 16:31:58.131052 139685136627520 modeling_tf_pytorch_utils.py:159] Initialize TF weight tf_bert_model_3/bert/encoder/layer_._11/intermediate/dense/bias:0
> I1212 16:31:58.131555 139685136627520 modeling_tf_pytorch_utils.py:159] Initialize TF weight tf_bert_model_3/bert/encoder/layer_._11/output/dense/kernel:0
> I1212 16:31:58.132040 139685136627520 modeling_tf_pytorch_utils.py:159] Initialize TF weight tf_bert_model_3/bert/encoder/layer_._11/output/dense/bias:0
> I1212 16:31:58.132566 139685136627520 modeling_tf_pytorch_utils.py:159] Initialize TF weight tf_bert_model_3/bert/encoder/layer_._11/output/LayerNorm/gamma:0
> I1212 16:31:58.133050 139685136627520 modeling_tf_pytorch_utils.py:159] Initialize TF weight tf_bert_model_3/bert/encoder/layer_._11/output/LayerNorm/beta:0
> I1212 16:31:58.133538 139685136627520 modeling_tf_pytorch_utils.py:159] Initialize TF weight tf_bert_model_3/bert/pooler/dense/kernel:0
> I1212 16:31:58.133999 139685136627520 modeling_tf_pytorch_utils.py:159] Initialize TF weight tf_bert_model_3/bert/pooler/dense/bias:0
> I1212 16:31:58.654147 139685136627520 modeling_tf_pytorch_utils.py:169] Weights or buffers not loaded from PyTorch model: {'cls.predictions.transform.dense.bias', 'cls.predictions.transform.LayerNorm.bias', 'cls.predictions.transform.dense.weight', 'cls.predictions.decoder.weight', 'cls.predictions.bias', 'cls.predictions.transform.LayerNorm.weight'}<|||||>Hmm it says it's initializing all the weights from the PyTorch model, so they're not initialized to zero.
It's indeed not loading some weights from the PyTorch models, which are not needed for the TF model you're initializing (you're loading a BertForMaskedLM in a TFBertModel, so some weights are not used).<|||||>Thanks for the clarification @LysandreJik
This way I am able to save to the model as .h5 version. However, since this step only saves model weights, converting .h5 to .ckpt is not straightforward as it requires the suitable architecture defined (when I am loading it in non-hface libs like tf.keras). It seems the model is not saved using model.save() instead with save_weights(). One needs to define the architecture to load weights and save as .ckpt. It would be great if there is an option to save the model including the necessary architecture to be loaded in TF. Let me know if I am missing something here.
Thank you.<|||||>> Thanks for the clarification @LysandreJik
>
> This way I am able to save to the model as .h5 version. However, since this step only saves model weights, converting .h5 to .ckpt is not straightforward as it requires the suitable architecture defined (when I am loading it in non-hface libs like tf.keras). It seems the model is not saved using model.save() instead with save_weights(). One needs to define the architecture to load weights and save as .ckpt. It would be great if there is an option to save the model including the necessary architecture to be loaded in TF. Let me know if I am missing something here.
>
> Thank you.
same question<|||||>
> Thanks for the clarification @LysandreJik
>
> This way I am able to save to the model as .h5 version. However, since this step only saves model weights, converting .h5 to .ckpt is not straightforward as it requires the suitable architecture defined (when I am loading it in non-hface libs like tf.keras). It seems the model is not saved using model.save() instead with save_weights(). One needs to define the architecture to load weights and save as .ckpt. It would be great if there is an option to save the model including the necessary architecture to be loaded in TF. Let me know if I am missing something here.
>
> Thank you.
Hi, I have the same question, been stuck with this, have you solved the issue?
Thanks you. |
transformers | 2,087 | closed | How can I get similarity matching ? | ## ❓ Questions & Help
Is there any way that can help me calculate the similarity between 2 questions ? Sometimes the questions is out of the scope of the data set questions. Using a simple similarity algorithm will always return the most similar even if it is not really correct.
It is the same thing as here :
https://github.com/deepmipt/dp_notebooks/blob/master/DP_BERT.ipynb
from deeppavlov import build_model, configs
model = build_model(configs.squad.squad_bert, download=True)
model(['DeepPavlov is a library for NLP and dialogue systems.'], ['What is DeepPavlov?'])
<!-- A clear and concise description of the question. -->
| 12-06-2019 15:40:21 | 12-06-2019 15:40:21 | Not sure to understand what you mean by `Using a simple similarity algorithm will always return the most similar even if it is not really correct`. What kind of simple similarity algo are you evoking here? What do you mean those simple algorithms aren't precise enough for your usecase?
Considering sentence similarity algorithms, I know:
- Statistical approach using bag-of-words TF-IDF-based methods like BM25 (better on longer docs than sentences).
- bag-of-word sentences and pooling (average-like) on word embeddings (word2vec-like) weighted by TF-IDF on a corpus for example.
- Full Sentence Embedding learnt directly on a similarity training set (maybe finetuned on your domain). It builds sentence embedding in a vector-space in which you can compute distance between sentences. Those models often uses siamese approach based on pre-trained language models such as BERT.
Sentence embedding technique requires more work and domain knowledge but is the one reaching the highest metrics in SOTA. Other techniques can be enough depending on your needs and domain.
<|||||>Excuse me for not being clear enough,
I wanted to say that if I have an FAQ data set and I want to get the most similar question for the user's question then, cosine similarity or TF-IDF will always give back a question even if it is not related.
This is about classifying either that question does have a similar one or not like does the snippet of code I posted earlier from deeppavlov. <|||||>No need to excuse ;)
Except an approach based on a dataset classifying "similar and non-similar" on your domain, any approach based on a score will require that you set a threshold to discriminate similar and non-similar. It can be fuzzy but sentence similarity is a very relative concept in any case.
<|||||>This issue has been automatically marked as stale because it has not had recent activity. It will be closed if no further activity occurs. Thank you for your contributions.
|
transformers | 2,086 | closed | "Only evaluate when single GPU otherwise metrics may not average well" | Hi,
The script examples/run_lm_finetuning.py skips evaluation on the validation dataset when run in distributed mode on multiple GPUs. The code includes this comment regarding this:
"Only evaluate when single GPU otherwise metrics may not average well"
I'd appreciate it if someone could explain this issue in a few words and a maybe suggest a way around it? Can I simply run the evaluation on a single GPU (e.g. only for local_rank==0)?
Thanks!
| 12-06-2019 14:16:34 | 12-06-2019 14:16:34 | I have wondered about this comment as well. I have implemented multi-GPU evaluation and it works perfectly fine. By evaluation I mean that the the work of evaluating is distributed and all results are then gathered to the main GPU (e.g. 0) or CPU which then calculates loss and secondary metrics (f1/pearson). I haven't experienced any issues with it but perhaps there is a reason that I don't know about. <|||||>Thanks, @BramVanroy. BTW, since you mentioned CPU, did you succeed in distributing LM fine-tuning on multiple CPUs? I tried that using torch.distributed and the 'gloo' backend and it seemed to be working fine, except that the total speed hardly improved.<|||||>Oh no, what I meant was doing some calculations such as correlations in the CPU. I've never done fine-tuning on CPU. I can imagine that it takes a long time. <|||||>This issue has been automatically marked as stale because it has not had recent activity. It will be closed if no further activity occurs. Thank you for your contributions.
<|||||>@BramVanroy I was thinking of doing the same. Can you point me to your training script which uses multi-gpu eval the way you have described? I am hoping that if I see that first, I can avoid common mistakes.
Thanks!<|||||>@dhruvdcoder Unfortunately, that code is in no state to be made public, in part because it is too complex and not incredibly well written. If I find the time, I plan to improve it and to add a PR here to update the example scripts to make use of multi-GPU evaluation. |
transformers | 2,085 | closed | Write With Transformer: PPLM document is stuck | The Uber PPLM on Write With Transformer does not generate anything, regardless of the parameters. It simply sits there, loading, forever. | 12-06-2019 13:51:14 | 12-06-2019 13:51:14 | nevermind, it suddenly started working. |
transformers | 2,084 | closed | CUDA out of memory for 8x V100 GPU | ```
python -m torch.distributed.launch --nproc_per_node=8 run_squad.py \
--model_type bert \
--model_name_or_path bert-base-cased \
--do_train \
--do_eval \
--do_lower_case \
--train_file $SQUAD_DIR/train-v1.1.json \
--predict_file $SQUAD_DIR/dev-v1.1.json \
--learning_rate 3e-5 \
--num_train_epochs 2 \
--max_seq_length 384 \
--doc_stride 128 \
--output_dir ../models/wwm_uncased_finetuned_squad/ \
--per_gpu_train_batch_size 24 \
--gradient_accumulation_steps 12
```
We are trying the same command (except bert-base-cased, we are using bert-large-uncased-whole-word-masking) on 8x V100 GPU but getting CUDA out of memory error (CUDA out of memory. Tried to allocate 216.00 MiB....)
As per the https://github.com/huggingface/transformers/tree/master/examples it should work but it's giving error and stopping in the middle. Any tips would be appreciated. | 12-06-2019 13:36:24 | 12-06-2019 13:36:24 | bert large is bigger than bert base. You're using a batch size of 24 (which is big, especially with 12 gradient accumulation steps).
Reduce your batch size in order for your model + your tensors to fit on the GPU and you won't experience the same error!<|||||>Right @LysandreJik , reducing the batch size did fix the error but it looks like the generated model we receive is not same as provided by huggingface.
In our demo of closed domain QnA, https://demos.pragnakalp.com/bert-chatbot-demo, the answers are pretty good where we are using the model provided by huggingface (bert-large-uncased-whole-word-masking-finetuned-squad). But when we finetune on our own and even though we get 93.XX f1 score the accuracy of the model is not same as demo.
What other parameters were set by huggingface to generate "bert-large-uncased-whole-word-masking-finetuned-squad" model? <|||||>If the only difference between the command you used and the command available [here](https://huggingface.co/transformers/examples.html#id1) is the batch size, you could try and adjust the gradient accumulation so that the resulting batch size is unchanged. For example if you put batch size equal to 6 (1/4 of the specified batch size, 24), you can multiply by 4 the gradient accumulation steps (-> 48) so that you keep the same batch size.
What `exact_match` result did you obtain alongside the 93.xx F1 score?<|||||>This issue has been automatically marked as stale because it has not had recent activity. It will be closed if no further activity occurs. Thank you for your contributions.
|
transformers | 2,083 | closed | ALBERT how to obtain the embedding matrix? | ## ❓ Questions & Help
Hi, I'm trying to use ALBERT for word embedding with this library.
ALBERT's doc mentioned an embedding size of 128 independently of the model version (base, large, ...) while the hidden_size changes.
I would like to obtain the 128 word (or subword) vectors but the model gives me only the output of the last hidden state (so for xxlarge a 4096 tensor for each token).
What am I doing wrong? | 12-06-2019 13:26:34 | 12-06-2019 13:26:34 | This issue has been automatically marked as stale because it has not had recent activity. It will be closed if no further activity occurs. Thank you for your contributions.
|
transformers | 2,082 | closed | ImportError: cannot import name 'WarmupLinearSchedule' | $ pip show transformers
Name: transformers
Version: 2.2.1
Summary: State-of-the-art Natural Language Processing for TensorFlow 2.0 and PyTorch
Home-page: https://github.com/huggingface/transformers
Author: Thomas Wolf, Lysandre Debut, Victor Sanh, Julien Chaumond, Google AI Language Team Authors, Open AI team Authors, Facebook AI Authors, Carnegie Mellon University Authors
Author-email: [email protected]
License: Apache
Location: /home/ubuntu/anaconda3/lib/python3.6/site-packages
Requires: numpy, requests, regex, sacremoses, tqdm, sentencepiece, boto3
Required-by:
| 12-06-2019 11:49:58 | 12-06-2019 11:49:58 | It is in the [optimization.py](https://github.com/huggingface/transformers/blob/df99f8c5a1c54d64fb013b43107011390c3be0d5/transformers/optimization.py), at line 45. It creates a schedule with a learning rate that decreases linearly after linearly increasing during a warmup period. In order to import it, you have to do the following:
```
> from transformers import get_linear_schedule_with_warmup
> ...
```
I've tested this statement with **Python 3.6.9**, **Transformers 2.2.1** (installed with `pip install transformers`), **PyTorch 1.3.1** and **TensorFlow 2.0**.
> $ pip show transformers
> Name: transformers
> Version: 2.2.1
> Summary: State-of-the-art Natural Language Processing for TensorFlow 2.0 and PyTorch
> Home-page: https://github.com/huggingface/transformers
> Author: Thomas Wolf, Lysandre Debut, Victor Sanh, Julien Chaumond, Google AI Language Team Authors, Open AI team Authors, Facebook AI Authors, Carnegie Mellon University Authors
> Author-email: [[email protected]](mailto:[email protected])
> License: Apache
> Location: /home/ubuntu/anaconda3/lib/python3.6/site-packages
> Requires: numpy, requests, regex, sacremoses, tqdm, sentencepiece, boto3
> Required-by:<|||||>I have the same error<|||||>Do you see my comment above? Did you try out?
> I have the same error<|||||>> Do you see my comment above? Did you try out?
>
> > I have the same error
I try to install from the git, fix the problem<|||||>I tried both pip and git, still having the issue<|||||>Still having this issue on 2.3.0 too<|||||>Use get_linear_schedule_with_warmup() instead of WarmupLinearSchedule. I think they have the same function.<|||||>> Use get_linear_schedule_with_warmup() instead of WarmupLinearSchedule. I think they have the same function.
The API is not quite the same, but it's similar enough that it should be easy enough to convert.
For example:
```python
scheduler = WarmupLinearSchedule(optimizer, warmup_steps=WARMUP_STEPS, t_total = -1)
```
becomes...
```python
scheduler = get_linear_schedule_with_warmup(optimizer, num_warmup_steps=WARMUP_STEPS, num_training_steps = -1)
```
For future visitors, see [docs](https://huggingface.co/transformers/main_classes/optimizer_schedules.html?highlight=get_linear_schedule_with_warmup#transformers.get_linear_schedule_with_warmup)<|||||>This issue has been automatically marked as stale because it has not had recent activity. It will be closed if no further activity occurs. Thank you for your contributions.
|
transformers | 2,081 | closed | handle string with only whitespaces as empty | #2027 | 12-06-2019 09:33:59 | 12-06-2019 09:33:59 | Does this fix the non-deterministic behavior mentioned in #2027 ?<|||||>Yes, this should return `[]` for every string that only contains whitespace characters. <|||||>Ok, great, merging then, thanks! |
transformers | 2,080 | closed | Encoding special tokens | ## 🐛 Bug
<!-- Important information -->
In version 2.2.1 encoding special tokens changed.
```python
from transformers import BertTokenizer
tokenizer = BertTokenizer.from_pretrained("bert-base-uncased")
tokenizer.decode(tokenizer.encode("[CLS] hello world [SEP]", add_special_tokens=False))
```
output: `'[ cls ] hello world [ sep ]'`
For version `transformers==2.2.0` the output is: `'[CLS] hello world [SEP]'`
| 12-06-2019 09:21:17 | 12-06-2019 09:21:17 | I got the same issue for version 2.2.1. <|||||>I also meet this issue and you may check out the possible root cause from #2052.
My workaround is backoff to 2.1.1 version.
<|||||>Should have been fixed with https://github.com/huggingface/transformers/pull/2051 |
transformers | 2,079 | closed | How to average sub-words embeddings to obtain word embeddings? | Hi~
How to average sub-words embeddings to obtain word embeddings?
I only want word-level embedding instead of sub-word-level, how can I get them?
Is there any tokenizer that provides a method that can output the index/mask of sub-words or something? | 12-06-2019 05:46:02 | 12-06-2019 05:46:02 | You may use the word as the input and make the sentence embedding as the word embedding.
for example, input is
"puppeteer"
tokens as
'[CLS]', 'puppet', '##eer', '[SEP]'
and then get embedding of this tokens list output.<|||||>I have similar usage as well, I did a simple experiment, and observe that the subword embedding [subword1, subword2, subword3...] when input a whole sentence,
the cosine similarity of [subword1,subword2],[subword1,subword3]... tends to above 90%.
So that sum and average subwords' embedding doesn't change much.
Btw, I tested this with Roberta models, and I observe quite different result for Bert models.<|||||>Take a look at how bert-sense does it :)
https://github.com/uhh-lt/bert-sense/blob/bfecb3c0e677d36ccfab4e2131ef9183995efaef/BERT_Model.py#L342<|||||>This issue has been automatically marked as stale because it has not had recent activity. It will be closed if no further activity occurs. Thank you for your contributions.
<|||||>Excuse me did someone solve it ?<|||||>@mathshangw I turn to use the `TransformerWordEmbeddings` of `flair` library to handle this. Here is an [example](https://github.com/flairNLP/flair/blob/master/examples/ner/run_ner.py#L119).<|||||>I found a way to obtain the subtoken mask.
There is an argument called `return_offsets_mapping`. When you pass the tokenized sequence to the tokenizer, the returned offsets mapping records the start position of each token instead of the entire sentence, for example.
```python
tokens: list[str] = 'this is a niceing work'.split()
# NOT THIS => tokens: str = 'this is a niceing work'
tokenizer.tokenize(tokens, add_special_tokens=True)
# ['▁this', '▁is', '▁a', '▁nice', 'ing', '▁work', '</s>', 'en_XX']
tokens = tokenizer(tokens, add_special_tokens=True, is_split_into_words=True,
return_offsets_mapping=True, return_tensors='pt')
# {
# 'input_ids': tensor([[903, 83, 10, 26267, 214, 4488, 2, 250004]]),
# 'attention_mask': tensor([[1, 1, 1, 1, 1, 1, 1, 1]]),
# 'offset_mapping': tensor([[[0, 4],
# [0, 2],
# [0, 1],
# [0, 4],
# [4, 7],
# [0, 4],
# [0, 0],
# [0, 0]]])}
subtoken_mask = tokens['offset_mapping'][..., 0] != 0
# tensor([[False, False, False, False, True, False, False, False]])
```
Forget about the weird word *niceing*, I just want to get some sub-tokens. Now, by simply checking if this token starts from the beginning of the given word, we can tell if it is a sub-token.
Hope this is helpful to you guys. |
transformers | 2,078 | closed | [cli] Uploads: add progress bar | see https://github.com/huggingface/transformers/pull/2044#discussion_r354057827 for context
There might be a more pythonic way (to do a "simple" method overriding) but I couldn't find it. | 12-06-2019 00:31:40 | 12-06-2019 00:31:40 | # [Codecov](https://codecov.io/gh/huggingface/transformers/pull/2078?src=pr&el=h1) Report
> Merging [#2078](https://codecov.io/gh/huggingface/transformers/pull/2078?src=pr&el=desc) into [master](https://codecov.io/gh/huggingface/transformers/commit/35ff345fc9df9e777b27903f11fa213e4052595b?src=pr&el=desc) will **increase** coverage by `0.01%`.
> The diff coverage is `100%`.
[](https://codecov.io/gh/huggingface/transformers/pull/2078?src=pr&el=tree)
```diff
@@ Coverage Diff @@
## master #2078 +/- ##
==========================================
+ Coverage 83.16% 83.18% +0.01%
==========================================
Files 109 109
Lines 15858 15874 +16
==========================================
+ Hits 13188 13204 +16
Misses 2670 2670
```
| [Impacted Files](https://codecov.io/gh/huggingface/transformers/pull/2078?src=pr&el=tree) | Coverage Δ | |
|---|---|---|
| [transformers/hf\_api.py](https://codecov.io/gh/huggingface/transformers/pull/2078/diff?src=pr&el=tree#diff-dHJhbnNmb3JtZXJzL2hmX2FwaS5weQ==) | `97.5% <100%> (+0.62%)` | :arrow_up: |
------
[Continue to review full report at Codecov](https://codecov.io/gh/huggingface/transformers/pull/2078?src=pr&el=continue).
> **Legend** - [Click here to learn more](https://docs.codecov.io/docs/codecov-delta)
> `Δ = absolute <relative> (impact)`, `ø = not affected`, `? = missing data`
> Powered by [Codecov](https://codecov.io/gh/huggingface/transformers/pull/2078?src=pr&el=footer). Last update [35ff345...5543617](https://codecov.io/gh/huggingface/transformers/pull/2078?src=pr&el=lastupdated). Read the [comment docs](https://docs.codecov.io/docs/pull-request-comments).
|
transformers | 2,077 | closed | corrected documentation for past tensor shape for ctrl and gpt2 model | fix issue #1904 | 12-06-2019 00:28:10 | 12-06-2019 00:28:10 | # [Codecov](https://codecov.io/gh/huggingface/transformers/pull/2077?src=pr&el=h1) Report
> Merging [#2077](https://codecov.io/gh/huggingface/transformers/pull/2077?src=pr&el=desc) into [master](https://codecov.io/gh/huggingface/transformers/commit/35ff345fc9df9e777b27903f11fa213e4052595b?src=pr&el=desc) will **not change** coverage.
> The diff coverage is `n/a`.
[](https://codecov.io/gh/huggingface/transformers/pull/2077?src=pr&el=tree)
```diff
@@ Coverage Diff @@
## master #2077 +/- ##
=======================================
Coverage 83.16% 83.16%
=======================================
Files 109 109
Lines 15858 15858
=======================================
Hits 13188 13188
Misses 2670 2670
```
| [Impacted Files](https://codecov.io/gh/huggingface/transformers/pull/2077?src=pr&el=tree) | Coverage Δ | |
|---|---|---|
| [transformers/modeling\_ctrl.py](https://codecov.io/gh/huggingface/transformers/pull/2077/diff?src=pr&el=tree#diff-dHJhbnNmb3JtZXJzL21vZGVsaW5nX2N0cmwucHk=) | `96.47% <ø> (ø)` | :arrow_up: |
| [transformers/modeling\_tf\_ctrl.py](https://codecov.io/gh/huggingface/transformers/pull/2077/diff?src=pr&el=tree#diff-dHJhbnNmb3JtZXJzL21vZGVsaW5nX3RmX2N0cmwucHk=) | `97.86% <ø> (ø)` | :arrow_up: |
| [transformers/modeling\_gpt2.py](https://codecov.io/gh/huggingface/transformers/pull/2077/diff?src=pr&el=tree#diff-dHJhbnNmb3JtZXJzL21vZGVsaW5nX2dwdDIucHk=) | `84.44% <ø> (ø)` | :arrow_up: |
| [transformers/modeling\_tf\_gpt2.py](https://codecov.io/gh/huggingface/transformers/pull/2077/diff?src=pr&el=tree#diff-dHJhbnNmb3JtZXJzL21vZGVsaW5nX3RmX2dwdDIucHk=) | `94.75% <ø> (ø)` | :arrow_up: |
------
[Continue to review full report at Codecov](https://codecov.io/gh/huggingface/transformers/pull/2077?src=pr&el=continue).
> **Legend** - [Click here to learn more](https://docs.codecov.io/docs/codecov-delta)
> `Δ = absolute <relative> (impact)`, `ø = not affected`, `? = missing data`
> Powered by [Codecov](https://codecov.io/gh/huggingface/transformers/pull/2077?src=pr&el=footer). Last update [35ff345...d0383e4](https://codecov.io/gh/huggingface/transformers/pull/2077?src=pr&el=lastupdated). Read the [comment docs](https://docs.codecov.io/docs/pull-request-comments).
<|||||>LGTM, merging! |
transformers | 2,076 | closed | Text Generation in Hebrew | ## ❓ Questions & Help
Hi all,
I have 30K tweets in Hebrew and I want to create a sort of chatbot that will answer in the style of those tweets, similar to [this](https://medium.com/huggingface/how-to-build-a-state-of-the-art-conversational-ai-with-transfer-learning-2d818ac26313). The only multilingual models that were trained on Hebrew are BERT and XLM, and they are both MLMs which are not too good at text generation. I thought I could fine-tune XLM and then run `run_generation.py`, but `run_lm_finetuning.py` doesn't support XLM.
Is there a way I can go about my task?
Thanks! | 12-05-2019 22:27:37 | 12-05-2019 22:27:37 | This issue has been automatically marked as stale because it has not had recent activity. It will be closed if no further activity occurs. Thank you for your contributions.
|
transformers | 2,075 | closed | Check link validity | We would like to make sure that every download link in the code base works. The best way to do this is to check automatically with the CI; this also prevents us from merging code with broken links.
This PR adds a small script that:
- Lists all source code files
- Extracts links with a regexp
- Performs HEAD requests to check the validity of each link
- Returns an error if at least one link is broken, along with the list of all broken links.
I also add a Circle CI workflow `repository-consistency` with a small machine that runs this script. It could be used to enforce things such as coding styles etc in the future.
For now the links are checked sequentially; if it turns out to take too long we can use `aiohttp` to run the queries concurrently.
_Edit:_ commits squashed | 12-05-2019 20:27:06 | 12-05-2019 20:27:06 | It works so well the CI failed because of a broken link :)<|||||>Ok great!
Maybe in the future, we would like to ensure model files can also be loaded without problems but this will suffice for now (and be fast)!
merging (when I've converted and added the missing model)<|||||>Yes it would be great too! The only limit is the RAM available and the bandwidth on Circle CI's side. Assuming they're big enough we can download and load all files at the same time, it is easy to do. Maybe next time a related issue pops up?<|||||># [Codecov](https://codecov.io/gh/huggingface/transformers/pull/2075?src=pr&el=h1) Report
> Merging [#2075](https://codecov.io/gh/huggingface/transformers/pull/2075?src=pr&el=desc) into [master](https://codecov.io/gh/huggingface/transformers/commit/9c58b236ef5fbbe5d0cbde4932eb342a73eaa0dc?src=pr&el=desc) will **increase** coverage by `0.01%`.
> The diff coverage is `n/a`.
[](https://codecov.io/gh/huggingface/transformers/pull/2075?src=pr&el=tree)
```diff
@@ Coverage Diff @@
## master #2075 +/- ##
==========================================
+ Coverage 80.35% 80.36% +0.01%
==========================================
Files 114 114
Lines 17091 17091
==========================================
+ Hits 13733 13736 +3
+ Misses 3358 3355 -3
```
| [Impacted Files](https://codecov.io/gh/huggingface/transformers/pull/2075?src=pr&el=tree) | Coverage Δ | |
|---|---|---|
| [transformers/tests/modeling\_common\_test.py](https://codecov.io/gh/huggingface/transformers/pull/2075/diff?src=pr&el=tree#diff-dHJhbnNmb3JtZXJzL3Rlc3RzL21vZGVsaW5nX2NvbW1vbl90ZXN0LnB5) | `74.53% <0%> (+0.55%)` | :arrow_up: |
------
[Continue to review full report at Codecov](https://codecov.io/gh/huggingface/transformers/pull/2075?src=pr&el=continue).
> **Legend** - [Click here to learn more](https://docs.codecov.io/docs/codecov-delta)
> `Δ = absolute <relative> (impact)`, `ø = not affected`, `? = missing data`
> Powered by [Codecov](https://codecov.io/gh/huggingface/transformers/pull/2075?src=pr&el=footer). Last update [9c58b23...d5712f7](https://codecov.io/gh/huggingface/transformers/pull/2075?src=pr&el=lastupdated). Read the [comment docs](https://docs.codecov.io/docs/pull-request-comments).
<|||||>Ok great, merging, thanks @rlouf |
transformers | 2,074 | closed | Check the validity of download links | We would like to make sure regularly that every download link in the codebase works. The best way to do this is to check automatically with the CI; this also prevents us from merging code with broken links.
This PR adds a small script that:
- Lists all source code files
- Extract links with a regexp
- Perform HEAD requests to check the validity of each links
- Returns an error if at least one link is broken, along with the list of all broken links.
I also add a Circle CI workflow `repository-consistency` with a small machine that runs this script. It could be used to enforce things such as coding styles etc in the future. | 12-05-2019 20:20:49 | 12-05-2019 20:20:49 | It works so well that the CI failed because of a broken link :) |
transformers | 2,073 | closed | How to structure text data to finetune distilGPT2 using tf.keras.model.fit()? | here is the relevant section of code where I get my text data via a txt file "file_path":
```
examples=[]
with open(file_path, encoding="utf-8") as f:
text = f.read()
tokenized_text = tokenizer.convert_tokens_to_ids(tokenizer.tokenize(text))
block_size = 256
for i in range(0, len(tokenized_text)-block_size+1, block_size): # Truncate in block of block_size
examples.append(tokenized_text[i:i+block_size])
```
This looks to be the way it is structured in the run_lm_finetuning.py script?
then:
```
dataset = tf.data.Dataset.from_tensor_slices(examples)
BATCH_SIZE = 32
BUFFER_SIZE = 10000
dataset = dataset.shuffle(BUFFER_SIZE).batch(BATCH_SIZE, drop_remainder=True)
optimizer = tf.keras.optimizers.Adam(learning_rate=3e-5, epsilon=1e-08, clipnorm=1.0)
loss = tf.keras.losses.SparseCategoricalCrossentropy(from_logits=True)
metric = tf.keras.metrics.SparseCategoricalAccuracy('accuracy')
model.compile(optimizer=optimizer, loss=[loss, None, None, None, None, None, None], metrics=[metric])
model.fit(dataset, epochs=1)
```
and I get this error:
```
---------------------------------------------------------------------------
IndexError Traceback (most recent call last)
<ipython-input-15-acd4de99eacb> in <module>
----> 1 model.fit(dataset1, epochs=10)
~/.local/lib/python3.7/site-packages/tensorflow_core/python/keras/engine/training.py in fit(self, x, y, batch_size, epochs, verbose, callbacks, validation_split, validation_data, shuffle, class_weight, sample_weight, initial_epoch, steps_per_epoch, validation_steps, validation_freq, max_queue_size, workers, use_multiprocessing, **kwargs)
726 max_queue_size=max_queue_size,
727 workers=workers,
--> 728 use_multiprocessing=use_multiprocessing)
729
730 def evaluate(self,
~/.local/lib/python3.7/site-packages/tensorflow_core/python/keras/engine/training_v2.py in fit(self, model, x, y, batch_size, epochs, verbose, callbacks, validation_split, validation_data, shuffle, class_weight, sample_weight, initial_epoch, steps_per_epoch, validation_steps, validation_freq, **kwargs)
322 mode=ModeKeys.TRAIN,
323 training_context=training_context,
--> 324 total_epochs=epochs)
325 cbks.make_logs(model, epoch_logs, training_result, ModeKeys.TRAIN)
326
~/.local/lib/python3.7/site-packages/tensorflow_core/python/keras/engine/training_v2.py in run_one_epoch(model, iterator, execution_function, dataset_size, batch_size, strategy, steps_per_epoch, num_samples, mode, training_context, total_epochs)
121 step=step, mode=mode, size=current_batch_size) as batch_logs:
122 try:
--> 123 batch_outs = execution_function(iterator)
124 except (StopIteration, errors.OutOfRangeError):
125 # TODO(kaftan): File bug about tf function and errors.OutOfRangeError?
~/.local/lib/python3.7/site-packages/tensorflow_core/python/keras/engine/training_v2_utils.py in execution_function(input_fn)
84 # `numpy` translates Tensors to values in Eager mode.
85 return nest.map_structure(_non_none_constant_value,
---> 86 distributed_function(input_fn))
87
88 return execution_function
~/.local/lib/python3.7/site-packages/tensorflow_core/python/eager/def_function.py in __call__(self, *args, **kwds)
455
456 tracing_count = self._get_tracing_count()
--> 457 result = self._call(*args, **kwds)
458 if tracing_count == self._get_tracing_count():
459 self._call_counter.called_without_tracing()
~/.local/lib/python3.7/site-packages/tensorflow_core/python/eager/def_function.py in _call(self, *args, **kwds)
501 # This is the first call of __call__, so we have to initialize.
502 initializer_map = object_identity.ObjectIdentityDictionary()
--> 503 self._initialize(args, kwds, add_initializers_to=initializer_map)
504 finally:
505 # At this point we know that the initialization is complete (or less
~/.local/lib/python3.7/site-packages/tensorflow_core/python/eager/def_function.py in _initialize(self, args, kwds, add_initializers_to)
406 self._concrete_stateful_fn = (
407 self._stateful_fn._get_concrete_function_internal_garbage_collected( # pylint: disable=protected-access
--> 408 *args, **kwds))
409
410 def invalid_creator_scope(*unused_args, **unused_kwds):
~/.local/lib/python3.7/site-packages/tensorflow_core/python/eager/function.py in _get_concrete_function_internal_garbage_collected(self, *args, **kwargs)
1846 if self.input_signature:
1847 args, kwargs = None, None
-> 1848 graph_function, _, _ = self._maybe_define_function(args, kwargs)
1849 return graph_function
1850
~/.local/lib/python3.7/site-packages/tensorflow_core/python/eager/function.py in _maybe_define_function(self, args, kwargs)
2148 graph_function = self._function_cache.primary.get(cache_key, None)
2149 if graph_function is None:
-> 2150 graph_function = self._create_graph_function(args, kwargs)
2151 self._function_cache.primary[cache_key] = graph_function
2152 return graph_function, args, kwargs
~/.local/lib/python3.7/site-packages/tensorflow_core/python/eager/function.py in _create_graph_function(self, args, kwargs, override_flat_arg_shapes)
2039 arg_names=arg_names,
2040 override_flat_arg_shapes=override_flat_arg_shapes,
-> 2041 capture_by_value=self._capture_by_value),
2042 self._function_attributes,
2043 # Tell the ConcreteFunction to clean up its graph once it goes out of
~/.local/lib/python3.7/site-packages/tensorflow_core/python/framework/func_graph.py in func_graph_from_py_func(name, python_func, args, kwargs, signature, func_graph, autograph, autograph_options, add_control_dependencies, arg_names, op_return_value, collections, capture_by_value, override_flat_arg_shapes)
913 converted_func)
914
--> 915 func_outputs = python_func(*func_args, **func_kwargs)
916
917 # invariant: `func_outputs` contains only Tensors, CompositeTensors,
~/.local/lib/python3.7/site-packages/tensorflow_core/python/eager/def_function.py in wrapped_fn(*args, **kwds)
356 # __wrapped__ allows AutoGraph to swap in a converted function. We give
357 # the function a weak reference to itself to avoid a reference cycle.
--> 358 return weak_wrapped_fn().__wrapped__(*args, **kwds)
359 weak_wrapped_fn = weakref.ref(wrapped_fn)
360
~/.local/lib/python3.7/site-packages/tensorflow_core/python/keras/engine/training_v2_utils.py in distributed_function(input_iterator)
71 strategy = distribution_strategy_context.get_strategy()
72 outputs = strategy.experimental_run_v2(
---> 73 per_replica_function, args=(model, x, y, sample_weights))
74 # Out of PerReplica outputs reduce or pick values to return.
75 all_outputs = dist_utils.unwrap_output_dict(
~/.local/lib/python3.7/site-packages/tensorflow_core/python/distribute/distribute_lib.py in experimental_run_v2(self, fn, args, kwargs)
758 fn = autograph.tf_convert(fn, ag_ctx.control_status_ctx(),
759 convert_by_default=False)
--> 760 return self._extended.call_for_each_replica(fn, args=args, kwargs=kwargs)
761
762 def reduce(self, reduce_op, value, axis):
~/.local/lib/python3.7/site-packages/tensorflow_core/python/distribute/distribute_lib.py in call_for_each_replica(self, fn, args, kwargs)
1785 kwargs = {}
1786 with self._container_strategy().scope():
-> 1787 return self._call_for_each_replica(fn, args, kwargs)
1788
1789 def _call_for_each_replica(self, fn, args, kwargs):
~/.local/lib/python3.7/site-packages/tensorflow_core/python/distribute/distribute_lib.py in _call_for_each_replica(self, fn, args, kwargs)
2130 self._container_strategy(),
2131 replica_id_in_sync_group=constant_op.constant(0, dtypes.int32)):
-> 2132 return fn(*args, **kwargs)
2133
2134 def _reduce_to(self, reduce_op, value, destinations):
~/.local/lib/python3.7/site-packages/tensorflow_core/python/autograph/impl/api.py in wrapper(*args, **kwargs)
290 def wrapper(*args, **kwargs):
291 with ag_ctx.ControlStatusCtx(status=ag_ctx.Status.DISABLED):
--> 292 return func(*args, **kwargs)
293
294 if inspect.isfunction(func) or inspect.ismethod(func):
~/.local/lib/python3.7/site-packages/tensorflow_core/python/keras/engine/training_v2_utils.py in train_on_batch(model, x, y, sample_weight, class_weight, reset_metrics)
262 y,
263 sample_weights=sample_weights,
--> 264 output_loss_metrics=model._output_loss_metrics)
265
266 if reset_metrics:
~/.local/lib/python3.7/site-packages/tensorflow_core/python/keras/engine/training_eager.py in train_on_batch(model, inputs, targets, sample_weights, output_loss_metrics)
309 sample_weights=sample_weights,
310 training=True,
--> 311 output_loss_metrics=output_loss_metrics))
312 if not isinstance(outs, list):
313 outs = [outs]
~/.local/lib/python3.7/site-packages/tensorflow_core/python/keras/engine/training_eager.py in _process_single_batch(model, inputs, targets, output_loss_metrics, sample_weights, training)
250 output_loss_metrics=output_loss_metrics,
251 sample_weights=sample_weights,
--> 252 training=training))
253 if total_loss is None:
254 raise ValueError('The model cannot be run '
~/.local/lib/python3.7/site-packages/tensorflow_core/python/keras/engine/training_eager.py in _model_loss(model, inputs, targets, output_loss_metrics, sample_weights, training)
164
165 if hasattr(loss_fn, 'reduction'):
--> 166 per_sample_losses = loss_fn.call(targets[i], outs[i])
167 weighted_losses = losses_utils.compute_weighted_loss(
168 per_sample_losses,
IndexError: list index out of range
```
Any ideas? it looks like maybe I'm supposed to provide labels? I could not find the relevant section of run_lm_finetuning.py that deals with that.
| 12-05-2019 18:55:21 | 12-05-2019 18:55:21 | |
transformers | 2,072 | closed | Accessing roberta embeddings | ## Finetune Setup
* Model: roberta-base
* Language: english
* OS: Ubuntu 18.04.3
* Python version: 3.7.3
* PyTorch version: 1.3.1+cpu
* PyTorch Transformers version (or branch): 2.2.0
* Using GPU ? No
* Distributed of parallel setup ? No
* Script inputs:
```
python run_lm_finetuning.py \
--output_dir=$OUTPUT_DIR \
--model_type=roberta \
--model_name_or_path=roberta_base \
--do_train \
--train_data_file=$TRAIN_FILE \
--do_eval \
--eval_data_file=$TEST_FILE \
--mlm \
--no_cuda
```
## ❓ Questions & Help
I would like to compare the embeddings of a sentence produced by `roberta-base` and my finetuned model (which is based on roberta-base using my domain specific data), but I am not sure how to access them. Any pointers on how to do this? Thanks in advance.
| 12-05-2019 18:43:47 | 12-05-2019 18:43:47 | Hi, there are several ways to check out the embeddings.
1 - The easy way is to get the `embeddings` and use it as a `torch.nn.Module` (which it inherits from):
For example, this is the output of the embedding layer of the sentence "Alright, let's do this", of dimension (batch_size, sequence_length, hidden_size):
```py
from transformers import RobertaTokenizer, RobertaModel
import torch
tok = RobertaTokenizer.from_pretrained("roberta-base")
model = RobertaModel.from_pretrained("roberta-base")
sentence = torch.tensor([tok.encode("Alright, let's do this")])
embedding_output = model.embeddings(sentence)
```
2 - A different way you can access them is by accessing the hidden states. You have to create a configuration object in order to specify that you would like the model to output its hidden states. You can then initialize the model from that configuration.
Using the example described above:
```py
from transformers import RobertaTokenizer, RobertaModel, RobertaConfig
import torch
config = RobertaConfig.from_pretrained("roberta-base")
config.output_hidden_states = True
tok = RobertaTokenizer.from_pretrained("roberta-base")
model = RobertaModel.from_pretrained("roberta-base", config=config)
sentence = torch.tensor([tok.encode("Alright, let's do this")])
output = model(sentence) # returns a tuple(sequence_output, pooled_output, hidden_states)
hidden_states = output[-1]
embedding_output = hidden_states[0]
```
Those are the embeddings using only the embeddings layer, which do not change much when fine-tuned. If you want to access the sentence representations of the two models, you can simply use the `sequence_outputs`:
```py
output = model(input)
sequence_output = output[0]
finetuned_output = finetuned_model(input)
finetuned_sequence_output = finetuned_output[0]
```
You can then compare those however you see fit!<|||||>which model from roberta i can use for RU lang?
or better using `xlm-mlm-17-1280` or `bert-base-multilingual-cased`?<|||||>@LysandreJik thank you very much for your response!
To check my understanding, I can access the output of the embedding layer of roberta using the procedures you described (1&2). I can also access the embeddings learned at the last layer of roberta (the final layer) doing the following:
```python
from transformers import RobertaTokenizer, RobertaModel, RobertaConfig
import torch
config = RobertaConfig.from_pretrained("roberta-base")
config.output_hidden_states = True
tok = RobertaTokenizer.from_pretrained("roberta-base")
model = RobertaModel.from_pretrained("roberta-base", config=config)
sentence = torch.tensor([tok.encode("Alright, let's do this")])
output = model(sentence)
final_embeddings = output[0]
```
Is my understanding correct or have I missed something?<|||||>@aclifton314 i think u should take `output[-1]` not a `output[0]`
btw, the 1st & 2nd example return similar vectors
also, the 2nd example has been working longer than 1st<|||||>@vtrokhymenko Do you know what the difference is between `output[-1]` and `output[0]`?<|||||>@aclifton314 the answer u can find here:
>You have to create a configuration object in order to specify that you would like the model to output its hidden states<|||||>@aclifton314 Referring to the output of the last layer as embeddings may be a bit ambiguous here, but yes, your `final_embeddings` variable holds the representation of your sequence at the uppermost layer (having gone through every model layer).
`output[-1]` returns the hidden states while `output[0]` returns the sequence output.<|||||>@LysandreJik @vtrokhymenko , thank you both for your replies! Closing this issue. |
transformers | 2,071 | closed | The generation script could fail when there's a double space in the prompt | ## 🚀 Feature
Hey, thanks for everything,
The generation script could fail when there's a double space in the prompt, e.g. " I go to"
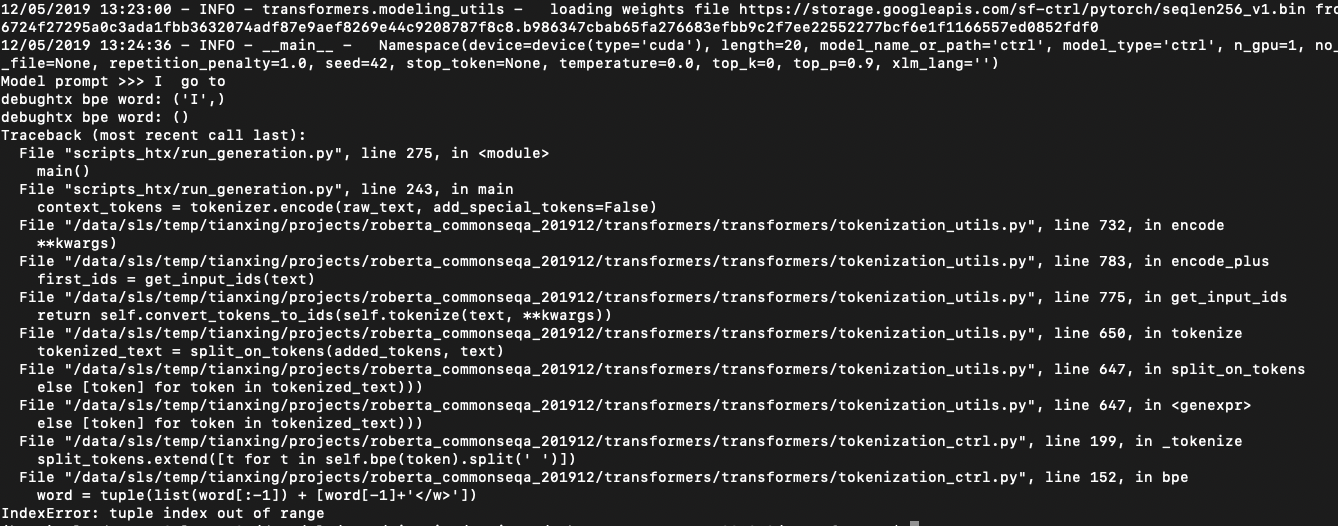
I know it's not important, but it would be good if the tokenize is more "robust" | 12-05-2019 18:27:35 | 12-05-2019 18:27:35 | Hi! Could you specify the command you used to launch `run_generation` as well as the versions in your environment? Pyton, pytorch, transformers? Thanks.<|||||>`python scripts_htx/run_generation.py --model_type ctrl --model_name ctrl --repetition 1.2`
python=3.7.3
torch=1.3.0
transformers=2.2.1
But I guess this issue is not related to the versions....<|||||>This is actually the same as #1920
Now fixed on master (will be in the next release). |
transformers | 2,070 | closed | XLMWithLMHeadModel forwarding questions | ## ❓ Questions & Help
<!-- A clear and concise description of the question. -->
1. Why is the labels argument named 'labels' instead of 'masked_lm_labels' like in BertForMaskedLM?
2. When I change labels for masked tokens to -1 as suggested in documentation, I got an error from NLLLoss for label being outside valid num_classes range. When I instead change labels for masked tokens to -100 (default ignore_index), it seems to work. Why is this happening? | 12-05-2019 15:20:04 | 12-05-2019 15:20:04 | This issue has been automatically marked as stale because it has not had recent activity. It will be closed if no further activity occurs. Thank you for your contributions.
|
transformers | 2,069 | closed | clean up PT <=> TF conversion | Cleaning up PT <=> TF conversion method.
cc @VictorSanh | 12-05-2019 14:20:24 | 12-05-2019 14:20:24 | Cool!<|||||># [Codecov](https://codecov.io/gh/huggingface/transformers/pull/2069?src=pr&el=h1) Report
> Merging [#2069](https://codecov.io/gh/huggingface/transformers/pull/2069?src=pr&el=desc) into [master](https://codecov.io/gh/huggingface/transformers/commit/ee53de7aac8312140e87d452718e15e3d42e27dd?src=pr&el=desc) will **not change** coverage.
> The diff coverage is `100%`.
[](https://codecov.io/gh/huggingface/transformers/pull/2069?src=pr&el=tree)
```diff
@@ Coverage Diff @@
## master #2069 +/- ##
=======================================
Coverage 83.16% 83.16%
=======================================
Files 109 109
Lines 15858 15858
=======================================
Hits 13188 13188
Misses 2670 2670
```
| [Impacted Files](https://codecov.io/gh/huggingface/transformers/pull/2069?src=pr&el=tree) | Coverage Δ | |
|---|---|---|
| [transformers/modeling\_utils.py](https://codecov.io/gh/huggingface/transformers/pull/2069/diff?src=pr&el=tree#diff-dHJhbnNmb3JtZXJzL21vZGVsaW5nX3V0aWxzLnB5) | `90.86% <100%> (ø)` | :arrow_up: |
------
[Continue to review full report at Codecov](https://codecov.io/gh/huggingface/transformers/pull/2069?src=pr&el=continue).
> **Legend** - [Click here to learn more](https://docs.codecov.io/docs/codecov-delta)
> `Δ = absolute <relative> (impact)`, `ø = not affected`, `? = missing data`
> Powered by [Codecov](https://codecov.io/gh/huggingface/transformers/pull/2069?src=pr&el=footer). Last update [ee53de7...1d87b37](https://codecov.io/gh/huggingface/transformers/pull/2069?src=pr&el=lastupdated). Read the [comment docs](https://docs.codecov.io/docs/pull-request-comments).
<|||||>@thomwolf and @VictorSanh - could you please look into #2088 (PT to TF)? tagging you guys in this thread as it seems relevant, please let me know otherwise.
Thanks<|||||>This thread is not relevant but I'll give a look at your issue soon.<|||||>thanks! |
transformers | 2,068 | closed | Nicer error message when Bert's input is missing batch size | Currently it fails in the computation of the attention_mask.
Let's fail with a shape error message instead. | 12-05-2019 13:39:26 | 12-05-2019 13:39:26 | # [Codecov](https://codecov.io/gh/huggingface/transformers/pull/2068?src=pr&el=h1) Report
> Merging [#2068](https://codecov.io/gh/huggingface/transformers/pull/2068?src=pr&el=desc) into [master](https://codecov.io/gh/huggingface/transformers/commit/2d5d86e03779b4b316698438caff0f675ee54abd?src=pr&el=desc) will **increase** coverage by `0.01%`.
> The diff coverage is `100%`.
[](https://codecov.io/gh/huggingface/transformers/pull/2068?src=pr&el=tree)
```diff
@@ Coverage Diff @@
## master #2068 +/- ##
==========================================
+ Coverage 83.15% 83.17% +0.01%
==========================================
Files 109 109
Lines 15869 15869
==========================================
+ Hits 13196 13199 +3
+ Misses 2673 2670 -3
```
| [Impacted Files](https://codecov.io/gh/huggingface/transformers/pull/2068?src=pr&el=tree) | Coverage Δ | |
|---|---|---|
| [transformers/modeling\_bert.py](https://codecov.io/gh/huggingface/transformers/pull/2068/diff?src=pr&el=tree#diff-dHJhbnNmb3JtZXJzL21vZGVsaW5nX2JlcnQucHk=) | `87.68% <100%> (ø)` | :arrow_up: |
| [transformers/tests/modeling\_common\_test.py](https://codecov.io/gh/huggingface/transformers/pull/2068/diff?src=pr&el=tree#diff-dHJhbnNmb3JtZXJzL3Rlc3RzL21vZGVsaW5nX2NvbW1vbl90ZXN0LnB5) | `74.23% <0%> (+0.57%)` | :arrow_up: |
------
[Continue to review full report at Codecov](https://codecov.io/gh/huggingface/transformers/pull/2068?src=pr&el=continue).
> **Legend** - [Click here to learn more](https://docs.codecov.io/docs/codecov-delta)
> `Δ = absolute <relative> (impact)`, `ø = not affected`, `? = missing data`
> Powered by [Codecov](https://codecov.io/gh/huggingface/transformers/pull/2068?src=pr&el=footer). Last update [2d5d86e...18fb935](https://codecov.io/gh/huggingface/transformers/pull/2068?src=pr&el=lastupdated). Read the [comment docs](https://docs.codecov.io/docs/pull-request-comments).
<|||||>lgtm<|||||>should this fix be added here as well? @thomwolf https://github.com/huggingface/transformers/blob/6cc06d17394f5715cdf2d13a1ef7680bedaee9e2/src/transformers/modeling_utils.py#L700 |
transformers | 2,067 | closed | Save model for tensorflow serving | Hello,
Thanks for the library. I tried your Multi label classification. I trained it with my data. It worked very accurate and fast. Now i want to use this model with tensorflow. I am new on pytorch and i looked some tutorials. As i understand i need to save model then convert to Onnx then to tensorflow. So I tried to save model first but it gave me this error;
```
AttributeError Traceback (most recent call last)
<ipython-input-10-4f1e1257e6e8> in <module>()
1
----> 2 torch.save(model.state_dict(), 'output/multilabel.pth')
AttributeError: 'MultiLabelClassificationModel' object has no attribute 'state_dict'
```
How can i save the model and export to onnx? | 12-05-2019 13:23:23 | 12-05-2019 13:23:23 | Did you get the solution to this? @elixium <|||||>Hi any update on this? I would like to deploy huggingface Transformers model with Tensorflow Serving too |
transformers | 2,066 | closed | CPU RAM out of memory when detach from GPU | ## ❓ Questions & Help
I am using the following code to get embedding layer from BERT:
```
class BertEmbedding():
def __init__(self, load_model=None, load_config=None, model='bert-base-uncased', max_len=512, batch_size=6):
self.pre_trained_model = model
self.max_len = max_len
self.batch_size = batch_size
self.model = BertModel.from_pretrained(self.pre_trained_model)
self.tokenizer = BertTokenizer.from_pretrained(self.pre_trained_model)
#self.optimizer = AdamW(params = self.model.parameters(), lr=1e-5)
def create_ids(self, sentences):
logging.getLogger("transformers.tokenization_utils").setLevel(logging.ERROR) #Disable tokenizer logs, it's really annoy
input_ids = []
for sen in tqdm_notebook(sentences, desc="Create Ids"):
tmp = self.tokenizer.encode(sen)
input_ids.append(tmp)
input_ids = pad_sequences(input_ids,
maxlen=self.max_len,
dtype='int64',
truncating='post',
padding='post')
return input_ids
def generate(self, inputs):
test_ids = self.create_ids(inputs)
test_dataloader = DataLoader(torch.tensor(test_ids), batch_size=self.batch_size)
embedding = []
self.model.to(device)
self.model.eval()
for input_ids in tqdm_notebook(test_dataloader, desc="Generating"):
with torch.no_grad():
last_state = self.model(input_ids.to(device))[0]
last_state = last_state.detach().cpu().numpy()
embedding.extend(last_state)
return embedding
bert_embedding = BertEmbedding(batch_size=100)
embedding = bert_embedding.generate(train.sentence.values)
```
The problem is when it generate embedding layer from model (train on GPU and detach to CPU), RAM is increasing significantly (1GB --> 30GB for a list of 25,000 arrays (512,768)). While I checked with `sys.getsizeof(embedding)` = `224208` and size of `bert_embedding` is `56` only.
If I delete both `embedding` and `bert_embedding`, RAM ~ 20GB. I think that the model is still existing. How can I optimize this for CPU RAM?
| 12-05-2019 12:38:05 | 12-05-2019 12:38:05 | This issue has been automatically marked as stale because it has not had recent activity. It will be closed if no further activity occurs. Thank you for your contributions.
|
transformers | 2,065 | closed | Fixing camembert tokenization | The original fairseq implmentation of Camembert has a bunch of duplicate tokens in the dictionary, in particular there are two `<unk>` tokens but only the index of the first `<unk>` should be used:
```
import torch
camembert = torch.hub.load('pytorch/fairseq', 'camembert.v0')
list(camembert.task.source_dictionary[i] for i in range(10))
>>> ['<s>', '<pad>', '</s>', '<unk>', '<unk>', '<s>', '</s>', ',', '▁de', '.']
```
This PR updates Camembert tokenizer to fix this behavior and as a consequence fixes #2019 and #2020 | 12-05-2019 12:30:27 | 12-05-2019 12:30:27 | Merging now to fix the xlnet test issue on master at the same time.<|||||>Also cc'ing @louismartin on this.<|||||>Thanks for fixing that.
This comes from a problem in fairseq where special tokens are added twice when using SentencePiece.
Cross-referencing the fairseq issue: [https://github.com/pytorch/fairseq/issues/1309](https://github.com/pytorch/fairseq/issues/1309) |
transformers | 2,064 | closed | [ Structure of LM vocab trained from scratch ] | ## ❓ Questions & Help
<!-- A clear and concise description of the question. -->
Hi,
I am trying to create a BERT LM trained from scratch and I have a question about the tokenizer.
I have a big text corpus and I trained a tokenizer with SentencePiece with 32K as dimension of the vocabulary. Then I applied a transformation from SentencePiece notation to WordPiece notation in order to be compatible with BERT.
The result is that my dictionary has this structure:
----------------------------------------
MY DICTIONARY
```
0 [PAD]
1 [UNK]
2 [CLS]
3 [SEP]
4 [MASK]
5 ,
6 .
7 '
...
.... [ ALL USED TOKENS ]
....
100 ##ndo
...
.... [ ALL USED TOKENS ]
....
31741 [ disgustat ]
31742 [ UNUSED TOKEN]
.......
....... [ ALL UNUSED TOKENS ]
.......
31999 [ UNUSED TOKEN]
```
And all the unused tokens are at the end of the vocab, in my case from 31742 to 31999.
---------------------------
And this is the STANDARD VOCAB for BERT:
BERT cased_L-12_H-768_A-12 VOCABULARY
```
0 [PAD]
1 [unused1]
2 ........
3 ........ [ ALL UNUSED TOKENS ]
4 .......
...........
100 [UNK]
101 [CLS]
102 [SEP]
103 [MASK]
[unused100]
[unused101]
!
"
..... [ ALL USED TOKENS ]
```
----------------------------
My question is: the fact that the SPECIAL TOKENS in MY DICTIONARY are in different positions than the Standard BERT VOCABULARY , can be a problem? Do you think I should keep the same positions of BERT vocab also for my dictionary? Also for unused token I have the same doubts.
( I saw that in the sentence piece training it's possibile to specify the exact positions of special tokens, but my question is if the position of special tokens will affect the LM training in some way )
Thank you
| 12-05-2019 11:58:59 | 12-05-2019 11:58:59 | I don't think it is a problem.
Your model will learn the embeddings of the words in your own dictionary.
Actually Nothing will be unchanged if you changed dictionary position as well as you keeped the embedding weight just the same order with your dictionary. <|||||>Thanks @karajan1001.
I am not sure if I understood well the last sentence.
You meant that I must not change the dictionary after I train the LM based on that?
After that I train the SentencePiece tokenizer and the relative vocabulary is given, than the vocab is an input of Language Model training and it cannot be changed anymore.<|||||>> After that I train the SentencePiece tokenizer and the relative vocabulary is given, than the vocab is an input of Language Model training and it cannot be changed anymore.
I think so. The vocabulary tells the model which array to get with the input tokens.
|
transformers | 2,063 | closed | special_tokens_mask value was unused and calculated twice | In the current master, in the `prepare_for_model` method of the `PreTrainedTokenizer` class, the sepcial_tokens_mask is calculated but not used: https://github.com/huggingface/transformers/blob/5bfcd0485ece086ebcbed2d008813037968a9e58/transformers/tokenization_utils.py#L904.
```python
# Handle special_tokens
if add_special_tokens:
sequence = self.build_inputs_with_special_tokens(ids, pair_ids)
token_type_ids = self.create_token_type_ids_from_sequences(ids, pair_ids)
special_tokens_mask = self.get_special_tokens_mask(ids, pair_ids)
else:
sequence = ids + pair_ids if pair else ids
token_type_ids = [0] * len(ids) + ([1] * len(pair_ids) if pair else [])
special_tokens_mask = [0] * (len(ids) + (len(pair_ids) if pair else 0))
if return_special_tokens_mask:
encoded_inputs["special_tokens_mask"] = self.get_special_tokens_mask(ids, pair_ids)
```
The proposed change is to use the `special_tokens_mask ` computed in the if/else statement in the output dictionary. | 12-05-2019 08:06:26 | 12-05-2019 08:06:26 | # [Codecov](https://codecov.io/gh/huggingface/transformers/pull/2063?src=pr&el=h1) Report
> Merging [#2063](https://codecov.io/gh/huggingface/transformers/pull/2063?src=pr&el=desc) into [master](https://codecov.io/gh/huggingface/transformers/commit/fb0d2f1da102d699c6457fd98be35f89852d08b9?src=pr&el=desc) will **not change** coverage.
> The diff coverage is `100%`.
[](https://codecov.io/gh/huggingface/transformers/pull/2063?src=pr&el=tree)
```diff
@@ Coverage Diff @@
## master #2063 +/- ##
=======================================
Coverage 83.58% 83.58%
=======================================
Files 105 105
Lines 15568 15568
=======================================
Hits 13012 13012
Misses 2556 2556
```
| [Impacted Files](https://codecov.io/gh/huggingface/transformers/pull/2063?src=pr&el=tree) | Coverage Δ | |
|---|---|---|
| [transformers/tokenization\_utils.py](https://codecov.io/gh/huggingface/transformers/pull/2063/diff?src=pr&el=tree#diff-dHJhbnNmb3JtZXJzL3Rva2VuaXphdGlvbl91dGlscy5weQ==) | `91.87% <100%> (ø)` | :arrow_up: |
------
[Continue to review full report at Codecov](https://codecov.io/gh/huggingface/transformers/pull/2063?src=pr&el=continue).
> **Legend** - [Click here to learn more](https://docs.codecov.io/docs/codecov-delta)
> `Δ = absolute <relative> (impact)`, `ø = not affected`, `? = missing data`
> Powered by [Codecov](https://codecov.io/gh/huggingface/transformers/pull/2063?src=pr&el=footer). Last update [fb0d2f1...7f998b1](https://codecov.io/gh/huggingface/transformers/pull/2063?src=pr&el=lastupdated). Read the [comment docs](https://docs.codecov.io/docs/pull-request-comments).
<|||||>Thanks for catching it!
@LysandreJik do we want to have `special_tokens_mask` returned as a tensor when `encode` is called with `return_tensors='pt' or 'tf'`. I would say no.<|||||>Thanks for that @guillaume-be, we can merge.
@thomwolf I don't really see a use-case where having it as a tensor would be useful. I believe its main use is be for pre-processing, maybe it would be useful to have it as a tensor then but I'm not convinced.<|||||>Ok, great! merging |
transformers | 2,062 | closed | TypeError: argument of type 'PosixPath' is not iterable (in modeling_utils.py) | ## 🐛 Bug
<!-- Important information -->
Model I am using (Bert, XLNet....):BERT
Language I am using the model on (English, Chinese....):English
The problem arise when using:
* [x] the official example scripts: (give details)
* [ ] my own modified scripts: (give details)
The tasks I am working on is:
* [ ] an official GLUE/SQUaD task: (give the name)
* [x] my own task or dataset: (give details)
## To Reproduce
TypeError: argument of type 'PosixPath' is not iterable
Steps to reproduce the behavior:
1.install transformers by pip
2.make test function with model=Bert..... line, put your own values as arguements
<!-- If you have a code sample, error messages, stack traces, please provide it here as well. -->
```
Traceback (most recent call last):
File "run_bert.py", line 226, in <module>
main()
File "run_bert.py", line 219, in main
run_train(args)
File "run_bert.py", line 70, in run_train
model = BertForMultiLable.from_pretrained(config['bert_model_dir'], num_labels=len(label_list))
File "/home/aditya/anaconda3/envs/RD/lib/python3.7/site-packages/transformers/modeling_utils.py", line 321, in from_pretrained
if "albert" in pretrained_model_name_or_path and "v2" in pretrained_model_name_or_path:
TypeError: argument of type 'PosixPath' is not iterable
```
run_train():
```
def run_train(args):
# --------- data
processor = BertProcessor(vocab_path=config['bert_vocab_path'], do_lower_case=args.do_lower_case)
label_list = processor.get_labels()
label2id = {label: i for i, label in enumerate(label_list)}
id2label = {i: label for i, label in enumerate(label_list)}
train_data = processor.get_train(config['data_dir'] / f"{args.data_name}.train.pkl")
train_examples = processor.create_examples(lines=train_data,
example_type='train',
cached_examples_file=config[
'data_dir'] / f"cached_train_examples_{args.arch}")
train_features = processor.create_features(examples=train_examples,
max_seq_len=args.train_max_seq_len,
cached_features_file=config[
'data_dir'] / "cached_train_features_{}_{}".format(
args.train_max_seq_len, args.arch
))
train_dataset = processor.create_dataset(train_features, is_sorted=args.sorted)
if args.sorted:
train_sampler = SequentialSampler(train_dataset)
else:
train_sampler = RandomSampler(train_dataset)
train_dataloader = DataLoader(train_dataset, sampler=train_sampler, batch_size=args.train_batch_size)
valid_data = processor.get_dev(config['data_dir'] / f"{args.data_name}.valid.pkl")
valid_examples = processor.create_examples(lines=valid_data,
example_type='valid',
cached_examples_file=config[
'data_dir'] / f"cached_valid_examples_{args.arch}")
valid_features = processor.create_features(examples=valid_examples,
max_seq_len=args.eval_max_seq_len,
cached_features_file=config[
'data_dir'] / "cached_valid_features_{}_{}".format(
args.eval_max_seq_len, args.arch
))
valid_dataset = processor.create_dataset(valid_features)
valid_sampler = SequentialSampler(valid_dataset)
valid_dataloader = DataLoader(valid_dataset, sampler=valid_sampler, batch_size=args.eval_batch_size)
# ------- model
logger.info("initializing model")
if args.resume_path:
args.resume_path = Path(args.resume_path)
model = BertForMultiLable.from_pretrained(args.resume_path, num_labels=len(label_list))
else:
model = BertForMultiLable.from_pretrained(config['bert_model_dir'], num_labels=len(label_list))
t_total = int(len(train_dataloader) / args.gradient_accumulation_steps * args.epochs)
param_optimizer = list(model.named_parameters())
no_decay = ['bias', 'LayerNorm.weight']
optimizer_grouped_parameters = [
{'params': [p for n, p in param_optimizer if not any(nd in n for nd in no_decay)],'weight_decay': args.weight_decay},
{'params': [p for n, p in param_optimizer if any(nd in n for nd in no_decay)], 'weight_decay': 0.0}
]
warmup_steps = int(t_total * args.warmup_proportion)
optimizer = AdamW(optimizer_grouped_parameters, lr=args.learning_rate, eps=args.adam_epsilon)
lr_scheduler = get_linear_schedule_with_warmup(optimizer, num_warmup_steps=warmup_steps, num_training_steps=t_total)
if args.fp16:
try:
from apex import amp
except ImportError:
raise ImportError("Please install apex from https://www.github.com/nvidia/apex to use fp16 training.")
model, optimizer = amp.initialize(model, optimizer, opt_level=args.fp16_opt_level)
# ---- callbacks
logger.info("initializing callbacks")
train_monitor = TrainingMonitor(file_dir=config['figure_dir'], arch=args.arch)
model_checkpoint = ModelCheckpoint(checkpoint_dir=config['checkpoint_dir'],mode=args.mode,
monitor=args.monitor,arch=args.arch,
save_best_only=args.save_best)
# **************************** training model ***********************
logger.info("***** Running training *****")
logger.info(" Num examples = %d", len(train_examples))
logger.info(" Num Epochs = %d", args.epochs)
logger.info(" Total train batch size (w. parallel, distributed & accumulation) = %d",
args.train_batch_size * args.gradient_accumulation_steps * (
torch.distributed.get_world_size() if args.local_rank != -1 else 1))
logger.info(" Gradient Accumulation steps = %d", args.gradient_accumulation_steps)
logger.info(" Total optimization steps = %d", t_total)
trainer = Trainer(n_gpu=args.n_gpu,
model=model,
epochs=args.epochs,
logger=logger,
criterion=BCEWithLogLoss(),
optimizer=optimizer,
lr_scheduler=lr_scheduler,
early_stopping=None,
training_monitor=train_monitor,
fp16=args.fp16,
resume_path=args.resume_path,
grad_clip=args.grad_clip,
model_checkpoint=model_checkpoint,
gradient_accumulation_steps=args.gradient_accumulation_steps,
batch_metrics=[AccuracyThresh(thresh=0.5)],
epoch_metrics=[AUC(average='micro', task_type='binary'),
MultiLabelReport(id2label=id2label)])
trainer.train(train_data=train_dataloader, valid_data=valid_dataloader, seed=args.seed)
```
## Expected behavior
<!-- A clear and concise description of what you expected to happen. -->
## Environment
* OS:Ubuntu 18.04
* Python version:3.6
* PyTorch version:
* PyTorch Transformers version (or branch):2.2.1
* Using GPU ?No
* Distributed of parallel setup ?Non Distrubuted
* Any other relevant information:
## Additional context
<!-- Add any other context about the problem here. -->
| 12-05-2019 07:16:11 | 12-05-2019 07:16:11 | Solved it by typecasting posixpath to string |
transformers | 2,061 | closed | BertForSequenceClassification' object has no attribute 'bias | ## 🐛 Bug
<!-- Important information -->
Model I am using (Bert, XLNet....):
Language I am using the model on (English, Chinese....):
The problem arise when using:
* [x] the official example scripts: (give details)
* [ ] my own modified scripts: (give details)
The tasks I am working on is:
* [ ] an official GLUE/SQUaD task: (give the name)
* [x] my own task or dataset: (give details)
<!-- Add any other context about the problem here. -->
I used this script to load the bert model i had finetuned for a classification task following google_research_bert.
I want to convert those TF checkpoints to pytorch.
```python
config = BertConfig.from_pretrained('bert-base-uncased')
config.num_labels=4
# You will need to load a BertForSequenceClassification model
model = BertForSequenceClassification(config)
tf_checkpoint_path = init_checkpoint
# Load weights from tf checkpoint
load_tf_weights_in_bert(model, config, tf_checkpoint_path)
pytorch_dump_path = "./pytorch_bert_output"
# Save pytorch-model
print("Save PyTorch model to {}".format(pytorch_dump_path))
torch.save(model.state_dict(), pytorch_dump_path)
```
When i execute this, i get the following error, BertForSequenceClassification' object has no attribute 'bias. Any leads would be helpful.
Thanks | 12-05-2019 05:53:01 | 12-05-2019 05:53:01 | Can you show us the full error message?<|||||>Can it be related to #2109 in some way?
> ## Bug
> Model I am using (Bert, XLNet....):
>
> Language I am using the model on (English, Chinese....):
>
> The problem arise when using:
>
> * [x] the official example scripts: (give details)
> * [ ] my own modified scripts: (give details)
>
> The tasks I am working on is:
>
> * [ ] an official GLUE/SQUaD task: (give the name)
> * [x] my own task or dataset: (give details)
>
> I used this script to load the bert model i had finetuned for a classification task following google_research_bert.
> I want to convert those TF checkpoints to pytorch.
>
> ```python
> config = BertConfig.from_pretrained('bert-base-uncased')
> config.num_labels=4
> # You will need to load a BertForSequenceClassification model
> model = BertForSequenceClassification(config)
>
> tf_checkpoint_path = init_checkpoint
> # Load weights from tf checkpoint
> load_tf_weights_in_bert(model, config, tf_checkpoint_path)
>
> pytorch_dump_path = "./pytorch_bert_output"
> # Save pytorch-model
> print("Save PyTorch model to {}".format(pytorch_dump_path))
> torch.save(model.state_dict(), pytorch_dump_path)
> ```
>
> When i execute this, i get the following error, BertForSequenceClassification' object has no attribute 'bias. Any leads would be helpful.
> Thanks<|||||>This issue has been automatically marked as stale because it has not had recent activity. It will be closed if no further activity occurs. Thank you for your contributions.
|
transformers | 2,060 | closed | Pr for pplm | Updated paper link and better commands to generate samples. | 12-05-2019 05:08:34 | 12-05-2019 05:08:34 | # [Codecov](https://codecov.io/gh/huggingface/transformers/pull/2060?src=pr&el=h1) Report
> Merging [#2060](https://codecov.io/gh/huggingface/transformers/pull/2060?src=pr&el=desc) into [master](https://codecov.io/gh/huggingface/transformers/commit/5bfcd0485ece086ebcbed2d008813037968a9e58?src=pr&el=desc) will **not change** coverage.
> The diff coverage is `n/a`.
[](https://codecov.io/gh/huggingface/transformers/pull/2060?src=pr&el=tree)
```diff
@@ Coverage Diff @@
## master #2060 +/- ##
=======================================
Coverage 83.58% 83.58%
=======================================
Files 105 105
Lines 15568 15568
=======================================
Hits 13012 13012
Misses 2556 2556
```
------
[Continue to review full report at Codecov](https://codecov.io/gh/huggingface/transformers/pull/2060?src=pr&el=continue).
> **Legend** - [Click here to learn more](https://docs.codecov.io/docs/codecov-delta)
> `Δ = absolute <relative> (impact)`, `ø = not affected`, `? = missing data`
> Powered by [Codecov](https://codecov.io/gh/huggingface/transformers/pull/2060?src=pr&el=footer). Last update [5bfcd04...12d18d4](https://codecov.io/gh/huggingface/transformers/pull/2060?src=pr&el=lastupdated). Read the [comment docs](https://docs.codecov.io/docs/pull-request-comments).
|
transformers | 2,059 | closed | How to run a batch of data through BERT model? | ## ❓ Questions & Help
<!-- A clear and concise description of the question. -->
I understand how to run **1 data** point of d words through a BERT model, but how can I run **n data** sequence of words through the BERT model?
Nvm solved this issue.
I can just pass sth like a 2xd data that looks like this:
tensor([[2182, 2003, 1996, 6251, 1045, 2215, 7861, 8270, 4667, 2015, 2005,
1012],
[2182, 2003, 1996, 6251, 1045, 2215, 7861, 8270, 4667, 2015, 2005,
1012]], device='cuda:0')
through the BERT forward function
| 12-05-2019 01:29:33 | 12-05-2019 01:29:33 | Did you solve it? I have the same problem as you. |
transformers | 2,058 | closed | Automatically allocates memory in GPU, always OOM when create TFALBERT model | ## 🐛 Bug
<!-- Important information -->
Model I am using :ALBERT
Language I am using the model on (English, Chinese....):English
> from transformers import TFAlbertModel
> model2=TFAlbertModel.from_pretrained('albert-base-v1')
Then:
> ---------------------------------------------------------------------------
OSError Traceback (most recent call last)
<ipython-input-2-a440a0748e94> in <module>
----> 1 model2=TFAlbertModel.from_pretrained('albert-base-v1')
~/anaconda3/lib/python3.7/site-packages/transformers/modeling_tf_utils.py in from_pretrained(cls, pretrained_model_name_or_path, *model_args, **kwargs)
287 # 'by_name' allow us to do transfer learning by skipping/adding layers
288 # see https://github.com/tensorflow/tensorflow/blob/00fad90125b18b80fe054de1055770cfb8fe4ba3/tensorflow/python/keras/engine/network.py#L1339-L1357
--> 289 model.load_weights(resolved_archive_file, by_name=True)
290
291 ret = model(model.dummy_inputs, training=False) # Make sure restore ops are run
~/anaconda3/lib/python3.7/site-packages/tensorflow_core/python/keras/engine/training.py in load_weights(self, filepath, by_name)
179 raise ValueError('Load weights is not yet supported with TPUStrategy '
180 'with steps_per_run greater than 1.')
--> 181 return super(Model, self).load_weights(filepath, by_name)
182
183 @trackable.no_automatic_dependency_tracking
~/anaconda3/lib/python3.7/site-packages/tensorflow_core/python/keras/engine/network.py in load_weights(self, filepath, by_name)
1169 'first, then load the weights.')
1170 self._assert_weights_created()
-> 1171 with h5py.File(filepath, 'r') as f:
1172 if 'layer_names' not in f.attrs and 'model_weights' in f:
1173 f = f['model_weights']
~/anaconda3/lib/python3.7/site-packages/h5py/_hl/files.py in __init__(self, name, mode, driver, libver, userblock_size, swmr, rdcc_nslots, rdcc_nbytes, rdcc_w0, track_order, **kwds)
392 fid = make_fid(name, mode, userblock_size,
393 fapl, fcpl=make_fcpl(track_order=track_order),
--> 394 swmr=swmr)
395
396 if swmr_support:
~/anaconda3/lib/python3.7/site-packages/h5py/_hl/files.py in make_fid(name, mode, userblock_size, fapl, fcpl, swmr)
168 if swmr and swmr_support:
169 flags |= h5f.ACC_SWMR_READ
--> 170 fid = h5f.open(name, flags, fapl=fapl)
171 elif mode == 'r+':
172 fid = h5f.open(name, h5f.ACC_RDWR, fapl=fapl)
h5py/_objects.pyx in h5py._objects.with_phil.wrapper()
h5py/_objects.pyx in h5py._objects.with_phil.wrapper()
h5py/h5f.pyx in h5py.h5f.open()
OSError: Unable to open file (file signature not found)
I tried to trace the GPU state, the memory usage is 15513MiB / 16130MiB, it is obvious that
when I create a model, it automatically allocates memory in GPU, but when I tried this in colab and use the same TF version, it works well, after creating model, there still are much free memory.
*OS: Linux version 4.9.0-11-amd64
* Python version:3.7
* TF version:TF2.0
* Transformers version (or branch):2.2
* Using GPU ?GPU
## Additional context
<!-- Add any other context about the problem here. -->
| 12-05-2019 00:53:21 | 12-05-2019 00:53:21 | What is the batch size you used?<|||||>The same bug occurs with Python 3.6.9, Transformers 2.2.1 (installed with `pip install transformers`), PyTorch 1.3.1 and TensorFlow 2.0.
Stack trace:
```
Python 3.6.9 |Anaconda, Inc.| (default, Jul 30 2019, 19:07:31)
[GCC 7.3.0] on linux
Type "help", "copyright", "credits" or "license" for more information.
>>> from transformers import TFAlbertModel
/home/<user>/anaconda3/envs/huggingface/lib/python3.6/site-packages/tensorboard/compat/tensorflow_stub/dtypes.py:541: FutureWarning: Passing (type, 1) or '1type' as a synonym of type is deprecated; in a future version of numpy, it will be understood as (type, (1,)) / '(1,)type'.
_np_qint8 = np.dtype([("qint8", np.int8, 1)])
/home/<user>/anaconda3/envs/huggingface/lib/python3.6/site-packages/tensorboard/compat/tensorflow_stub/dtypes.py:542: FutureWarning: Passing (type, 1) or '1type' as a synonym of type is deprecated; in a future version of numpy, it will be understood as (type, (1,)) / '(1,)type'.
_np_quint8 = np.dtype([("quint8", np.uint8, 1)])
/home/<user>/anaconda3/envs/huggingface/lib/python3.6/site-packages/tensorboard/compat/tensorflow_stub/dtypes.py:543: FutureWarning: Passing (type, 1) or '1type' as a synonym of type is deprecated; in a future version of numpy, it will be understood as (type, (1,)) / '(1,)type'.
_np_qint16 = np.dtype([("qint16", np.int16, 1)])
/home/<user>/anaconda3/envs/huggingface/lib/python3.6/site-packages/tensorboard/compat/tensorflow_stub/dtypes.py:544: FutureWarning: Passing (type, 1) or '1type' as a synonym of type is deprecated; in a future version of numpy, it will be understood as (type, (1,)) / '(1,)type'.
_np_quint16 = np.dtype([("quint16", np.uint16, 1)])
/home/<user>/anaconda3/envs/huggingface/lib/python3.6/site-packages/tensorboard/compat/tensorflow_stub/dtypes.py:545: FutureWarning: Passing (type, 1) or '1type' as a synonym of type is deprecated; in a future version of numpy, it will be understood as (type, (1,)) / '(1,)type'.
_np_qint32 = np.dtype([("qint32", np.int32, 1)])
/home/<user>/anaconda3/envs/huggingface/lib/python3.6/site-packages/tensorboard/compat/tensorflow_stub/dtypes.py:550: FutureWarning: Passing (type, 1) or '1type' as a synonym of type is deprecated; in a future version of numpy, it will be understood as (type, (1,)) / '(1,)type'.
np_resource = np.dtype([("resource", np.ubyte, 1)])
2019-12-05 09:55:19.006308: I tensorflow/core/platform/cpu_feature_guard.cc:142] Your CPU supports instructions that this TensorFlow binary was not compiled to use: AVX2 FMA
2019-12-05 09:55:19.027197: I tensorflow/core/platform/profile_utils/cpu_utils.cc:94] CPU Frequency: 3408000000 Hz
2019-12-05 09:55:19.027888: I tensorflow/compiler/xla/service/service.cc:168] XLA service 0x5582d4e6e4c0 executing computations on platform Host. Devices:
2019-12-05 09:55:19.027909: I tensorflow/compiler/xla/service/service.cc:175] StreamExecutor device (0): Host, Default Version
>>> model = TFAlbertModel.from_pretrained('albert-base-v1')
100%|████████████████████████████████████████████████████████████████████████████████████████████████████████████████████████████████████████████████████████████████████████████████████████████████████████████████████████████| 484/484 [00:00<00:00, 185172.23B/s]
299B [00:00, 131456.70B/s]
2019-12-05 09:55:28.628697: W tensorflow/python/util/util.cc:299] Sets are not currently considered sequences, but this may change in the future, so consider avoiding using them.
Traceback (most recent call last):
File "<stdin>", line 1, in <module>
File "/home/<user>/anaconda3/envs/huggingface/lib/python3.6/site-packages/transformers/modeling_tf_utils.py", line 289, in from_pretrained
model.load_weights(resolved_archive_file, by_name=True)
File "/home/<user>/anaconda3/envs/huggingface/lib/python3.6/site-packages/tensorflow_core/python/keras/engine/training.py", line 181, in load_weights
return super(Model, self).load_weights(filepath, by_name)
File "/home/<user>/anaconda3/envs/huggingface/lib/python3.6/site-packages/tensorflow_core/python/keras/engine/network.py", line 1171, in load_weights
with h5py.File(filepath, 'r') as f:
File "/home/<user>/anaconda3/envs/huggingface/lib/python3.6/site-packages/h5py/_hl/files.py", line 408, in __init__
swmr=swmr)
File "/home/<user>/anaconda3/envs/huggingface/lib/python3.6/site-packages/h5py/_hl/files.py", line 173, in make_fid
fid = h5f.open(name, flags, fapl=fapl)
File "h5py/_objects.pyx", line 54, in h5py._objects.with_phil.wrapper
File "h5py/_objects.pyx", line 55, in h5py._objects.with_phil.wrapper
File "h5py/h5f.pyx", line 88, in h5py.h5f.open
OSError: Unable to open file (file signature not found)
```
If I try to use the PyTorch version of Albert with _albert-base-v1_, it works as expected!
Stack trace:
```
>>> model = AlbertModel.from_pretrained('albert-base-v1')
100%|████████████████████████████████████████████████████████████████████████████████████████████████████████████████████████████████████████████████████████████████████████████████████████████████████████████████| 47376396/47376396 [00:04<00:00, 10033199.48B/s]
```
Said this, I suspect that the TensorFlow version of Albert is not developed correctly (it misses the config). Is it possible? Now I'm investigating on..
UPDATE 1: I've gone to the Transformers' source code in the [modeling_tf_albert.py](https://github.com/huggingface/transformers/blob/e85855f2c408f65a4aaf5d15baab6ca90fd26050/transformers/) and I've downloaded the .h5 model **correctly** (from [this link](https://s3.amazonaws.com/models.huggingface.co/bert/albert-base-v1-tf_model.h5)). So I suspect there is an internal error that is independent from the download of the .h5 file.
> ## Bug
> Model I am using :ALBERT
>
> Language I am using the model on (English, Chinese....):English
>
> > from transformers import TFAlbertModel
>
> > model2=TFAlbertModel.from_pretrained('albert-base-v1')
>
> Then:
>
> >
>
> OSError Traceback (most recent call last)
> in
> ----> 1 model2=TFAlbertModel.from_pretrained('albert-base-v1')
>
> ~/anaconda3/lib/python3.7/site-packages/transformers/modeling_tf_utils.py in from_pretrained(cls, pretrained_model_name_or_path, *model_args, **kwargs)
> 287 # 'by_name' allow us to do transfer learning by skipping/adding layers
> 288 # see https://github.com/tensorflow/tensorflow/blob/00fad90125b18b80fe054de1055770cfb8fe4ba3/tensorflow/python/keras/engine/network.py#L1339-L1357
> --> 289 model.load_weights(resolved_archive_file, by_name=True)
> 290
> 291 ret = model(model.dummy_inputs, training=False) # Make sure restore ops are run
>
> ~/anaconda3/lib/python3.7/site-packages/tensorflow_core/python/keras/engine/training.py in load_weights(self, filepath, by_name)
> 179 raise ValueError('Load weights is not yet supported with TPUStrategy '
> 180 'with steps_per_run greater than 1.')
> --> 181 return super(Model, self).load_weights(filepath, by_name)
> 182
> 183 @trackable.no_automatic_dependency_tracking
>
> ~/anaconda3/lib/python3.7/site-packages/tensorflow_core/python/keras/engine/network.py in load_weights(self, filepath, by_name)
> 1169 'first, then load the weights.')
> 1170 self._assert_weights_created()
> -> 1171 with h5py.File(filepath, 'r') as f:
> 1172 if 'layer_names' not in f.attrs and 'model_weights' in f:
> 1173 f = f['model_weights']
>
> ~/anaconda3/lib/python3.7/site-packages/h5py/_hl/files.py in **init**(self, name, mode, driver, libver, userblock_size, swmr, rdcc_nslots, rdcc_nbytes, rdcc_w0, track_order, **kwds)
> 392 fid = make_fid(name, mode, userblock_size,
> 393 fapl, fcpl=make_fcpl(track_order=track_order),
> --> 394 swmr=swmr)
> 395
> 396 if swmr_support:
>
> ~/anaconda3/lib/python3.7/site-packages/h5py/_hl/files.py in make_fid(name, mode, userblock_size, fapl, fcpl, swmr)
> 168 if swmr and swmr_support:
> 169 flags |= h5f.ACC_SWMR_READ
> --> 170 fid = h5f.open(name, flags, fapl=fapl)
> 171 elif mode == 'r+':
> 172 fid = h5f.open(name, h5f.ACC_RDWR, fapl=fapl)
>
> h5py/_objects.pyx in h5py._objects.with_phil.wrapper()
>
> h5py/_objects.pyx in h5py._objects.with_phil.wrapper()
>
> h5py/h5f.pyx in h5py.h5f.open()
>
> OSError: Unable to open file (file signature not found)
>
> I tried to trace the GPU state, the memory usage is 15513MiB / 16130MiB, it is obvious that
> when I create a model, it automatically allocates memory in GPU, but when I tried this in colab and use the same TF version, it works well, after creating model, there still are much free memory.
> *OS: Linux version 4.9.0-11-amd64
>
> * Python version:3.7
> * TF version:TF2.0
> * Transformers version (or branch):2.2
> * Using GPU ?GPU
>
> ## Additional context<|||||>> What is the batch size you used?
I haven't tried to train, I just run one line code to create the model, then problem happened.
> model2=TFAlbertModel.from_pretrained('albert-base-v1')
<|||||>This issue has been automatically marked as stale because it has not had recent activity. It will be closed if no further activity occurs. Thank you for your contributions.
|
transformers | 2,057 | closed | `distilroberta-base` link missing | ## ❓ Questions & Help
<!-- A clear and concise description of the question. -->
According to the current master code, link for `distilroberta-base` isn't provided.
https://github.com/huggingface/transformers/blob/1ab8dc44b3d84ed1894f5b6a6fab58fb39298fc7/transformers/configuration_distilbert.py#L28-L33 | 12-04-2019 23:35:36 | 12-04-2019 23:35:36 | It is located under `configuration_roberta.py`, see it [here](https://github.com/huggingface/transformers/blob/1c542df7e554a2014051dd09becf60f157fed524/transformers/configuration_roberta.py#L31) :)<|||||>Thanks @stefan-it ! Missed the readme part of calling `distilroberta-base` with `RobertaModel` instead of `DistilBertModel`. Closing. |
transformers | 2,056 | closed | cannot import name 'get_linear_schedule_with_warmup' from 'transformers.optimization' | ## ❓ Questions & Help
cannot import name 'get_linear_schedule_with_warmup' from 'transformers.optimization'
<!-- A clear and concise description of the question. -->
| 12-04-2019 23:17:27 | 12-04-2019 23:17:27 | This could be related to this issue here: https://github.com/huggingface/transformers/issues/1837 :)<|||||>I copied the get_linear_schedule_with_warmup function code add to my project in the transformers/optimization.py
and then it worked
Thank you for developing such an brilliant library.
------------------ 原始邮件 ------------------
发件人: "Stefan Schweter"<[email protected]>;
发送时间: 2019年12月5日(星期四) 上午7:19
收件人: "huggingface/transformers"<[email protected]>;
抄送: "FOXaaFOX"<[email protected]>;"Author"<[email protected]>;
主题: Re: [huggingface/transformers] cannot import name 'get_linear_schedule_with_warmup' from 'transformers.optimization' (#2056)
This could be related to this issue here: #1837 :)
—
You are receiving this because you authored the thread.
Reply to this email directly, view it on GitHub, or unsubscribe. |
transformers | 2,055 | closed | Remove dependency on pytest for running tests | 12-04-2019 20:45:18 | 12-04-2019 20:45:18 | # [Codecov](https://codecov.io/gh/huggingface/transformers/pull/2055?src=pr&el=h1) Report
> Merging [#2055](https://codecov.io/gh/huggingface/transformers/pull/2055?src=pr&el=desc) into [master](https://codecov.io/gh/huggingface/transformers/commit/35ff345fc9df9e777b27903f11fa213e4052595b?src=pr&el=desc) will **decrease** coverage by `0.45%`.
> The diff coverage is `95.45%`.
[](https://codecov.io/gh/huggingface/transformers/pull/2055?src=pr&el=tree)
```diff
@@ Coverage Diff @@
## master #2055 +/- ##
=========================================
- Coverage 83.16% 82.7% -0.46%
=========================================
Files 109 109
Lines 15858 15943 +85
=========================================
- Hits 13188 13186 -2
- Misses 2670 2757 +87
```
| [Impacted Files](https://codecov.io/gh/huggingface/transformers/pull/2055?src=pr&el=tree) | Coverage Δ | |
|---|---|---|
| [transformers/modeling\_openai.py](https://codecov.io/gh/huggingface/transformers/pull/2055/diff?src=pr&el=tree#diff-dHJhbnNmb3JtZXJzL21vZGVsaW5nX29wZW5haS5weQ==) | `81.45% <0%> (-0.55%)` | :arrow_down: |
| [transformers/tokenization\_xlnet.py](https://codecov.io/gh/huggingface/transformers/pull/2055/diff?src=pr&el=tree#diff-dHJhbnNmb3JtZXJzL3Rva2VuaXphdGlvbl94bG5ldC5weQ==) | `90.24% <100%> (ø)` | :arrow_up: |
| [transformers/tests/modeling\_tf\_auto\_test.py](https://codecov.io/gh/huggingface/transformers/pull/2055/diff?src=pr&el=tree#diff-dHJhbnNmb3JtZXJzL3Rlc3RzL21vZGVsaW5nX3RmX2F1dG9fdGVzdC5weQ==) | `36.36% <100%> (ø)` | :arrow_up: |
| [transformers/tokenization\_albert.py](https://codecov.io/gh/huggingface/transformers/pull/2055/diff?src=pr&el=tree#diff-dHJhbnNmb3JtZXJzL3Rva2VuaXphdGlvbl9hbGJlcnQucHk=) | `89.74% <100%> (ø)` | :arrow_up: |
| [transformers/tests/modeling\_tf\_openai\_gpt\_test.py](https://codecov.io/gh/huggingface/transformers/pull/2055/diff?src=pr&el=tree#diff-dHJhbnNmb3JtZXJzL3Rlc3RzL21vZGVsaW5nX3RmX29wZW5haV9ncHRfdGVzdC5weQ==) | `94.73% <100%> (ø)` | :arrow_up: |
| [transformers/tests/modeling\_tf\_bert\_test.py](https://codecov.io/gh/huggingface/transformers/pull/2055/diff?src=pr&el=tree#diff-dHJhbnNmb3JtZXJzL3Rlc3RzL21vZGVsaW5nX3RmX2JlcnRfdGVzdC5weQ==) | `96.22% <100%> (ø)` | :arrow_up: |
| [transformers/tests/tokenization\_auto\_test.py](https://codecov.io/gh/huggingface/transformers/pull/2055/diff?src=pr&el=tree#diff-dHJhbnNmb3JtZXJzL3Rlc3RzL3Rva2VuaXphdGlvbl9hdXRvX3Rlc3QucHk=) | `50% <100%> (ø)` | :arrow_up: |
| [transformers/tests/tokenization\_distilbert\_test.py](https://codecov.io/gh/huggingface/transformers/pull/2055/diff?src=pr&el=tree#diff-dHJhbnNmb3JtZXJzL3Rlc3RzL3Rva2VuaXphdGlvbl9kaXN0aWxiZXJ0X3Rlc3QucHk=) | `63.63% <100%> (ø)` | :arrow_up: |
| [transformers/tests/modeling\_tf\_roberta\_test.py](https://codecov.io/gh/huggingface/transformers/pull/2055/diff?src=pr&el=tree#diff-dHJhbnNmb3JtZXJzL3Rlc3RzL21vZGVsaW5nX3RmX3JvYmVydGFfdGVzdC5weQ==) | `75.2% <100%> (ø)` | :arrow_up: |
| [transformers/tests/tokenization\_transfo\_xl\_test.py](https://codecov.io/gh/huggingface/transformers/pull/2055/diff?src=pr&el=tree#diff-dHJhbnNmb3JtZXJzL3Rlc3RzL3Rva2VuaXphdGlvbl90cmFuc2ZvX3hsX3Rlc3QucHk=) | `97.43% <100%> (ø)` | :arrow_up: |
| ... and [39 more](https://codecov.io/gh/huggingface/transformers/pull/2055/diff?src=pr&el=tree-more) | |
------
[Continue to review full report at Codecov](https://codecov.io/gh/huggingface/transformers/pull/2055?src=pr&el=continue).
> **Legend** - [Click here to learn more](https://docs.codecov.io/docs/codecov-delta)
> `Δ = absolute <relative> (impact)`, `ø = not affected`, `? = missing data`
> Powered by [Codecov](https://codecov.io/gh/huggingface/transformers/pull/2055?src=pr&el=footer). Last update [35ff345...61978c1](https://codecov.io/gh/huggingface/transformers/pull/2055?src=pr&el=lastupdated). Read the [comment docs](https://docs.codecov.io/docs/pull-request-comments).
<|||||>Given that the PR touches the whole test suite and that tests pass, if there's no opposition,I'd like to merge it before master diverges.
We can figure out running tests on the GPU on CircleCI separately.<|||||>Agreed. Squashed and merged. |
|
transformers | 2,054 | closed | Find dot product of query and key vectors | Hi,
I am following [this popular article](http://jalammar.github.io/illustrated-transformer/) to understand the Transformers. Alongside this, I am using [huggingface transformers](https://huggingface.co/transformers/model_doc/bert.html#bertmodel) to get the attention scores.
On running the following code:
`from transformers import BertTokenizer, BertModel, BertConfig, BertForTokenClassification
import torch
config = BertConfig.from_pretrained('bert-base-uncased', output_hidden_states=True, output_attentions=True)
tokenizer = BertTokenizer.from_pretrained('bert-base-uncased')
model = BertModel.from_pretrained('bert-base-uncased', config=config)
input_ids = torch.tensor(tokenizer.encode("Hello my dog is cute", add_special_tokens=False)).unsqueeze(0) # Batch size 1
outputs = model(input_ids)
print(len(outputs))
last_hidden_states, pooler_outputs, hidden_states, attentions = outputs # The last hidden-state is the first element of the output tuple
print(attentions)`
I get the weighted sum attention matrix of size 5x5. I am actually trying to find the softmax values like 0.88 and 0.12. I was wondering if there is any way I can obtain the dot-product scores.
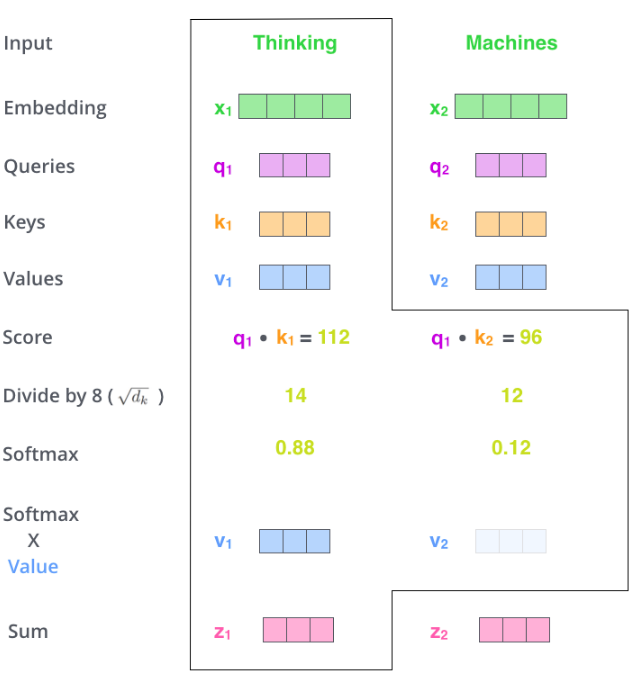
Thanks! | 12-04-2019 19:31:23 | 12-04-2019 19:31:23 | I found [this](https://huggingface.co/transformers/_modules/transformers/modeling_bert.html) code which has transpose_for_scores but I am not sure how this can be used with the above code.<|||||>Yes, the `attentions` outputs of the model are the softmax values.<|||||>This issue has been automatically marked as stale because it has not had recent activity. It will be closed if no further activity occurs. Thank you for your contributions.
|
transformers | 2,053 | closed | Crosslingual classification with XLM, loss does not converge | ## ❓ Questions & Help
<!-- A clear and concise description of the question. -->
I am trying to use the XLM pretrained model `xlm-mlm-tlm-xnli15-1024` for a cross lingual classification task, but I cannot get the loss to converge and the final accuracy is random.
To check this was not an implementation error of my own doing, I ran the `run_xnli.py` example and found using `xlm-mlm-tlm-xnli15-1024` results in an accuracy of 30% while using `bert-base-multilingual-cased` results in the expected accuracy of 70%.
system config:
```
Platform Linux-4.4.0-1098-aws-x86_64-with-debian-stretch-sid
Python 3.6.9 |Anaconda, Inc.| (default, Jul 30 2019, 19:07:31)
[GCC 7.3.0]
PyTorch 1.2.0+cu92
Tensorflow 2.0.0
``` | 12-04-2019 18:14:38 | 12-04-2019 18:14:38 | I had the same problem with different tasks . I've tried all the XLM pre-training models and got random results. Please let us know if you have solved this problem. I'm trying to figure it out. @DanKing1903 <|||||>I was able to reproduce the results of XLM on XNLI.
It was highly sensitive to hyper parameters.
I would suggest that you tune your learning_rate ~ 1.5e-6 and batch_size ~ 4.<|||||>> I was able to reproduce the results of XLM on XNLI.
> It was highly sensitive to hyper parameters.
> I would suggest that you tune your learning_rate ~ 1.5e-6 and batch_size ~ 4.
Thank you for your answer. I modified the parameters as you suggested. xlm-mlm-17-1280 and xlm-mlm-100-1280 batch_size only go up to 2, and others modified the parameters as before. I wonder if it has anything to do with the task. Looking forward to your reply<|||||>I got the same problem.
I suggest you to go with RMSprop (require less memory than Adam so you can have a bigger batch size) with learning rate 3e-5 (very important to use a small learning rate otherwise it diverge) and clipnorm of 1.0. Personally, I use a global batch size of 20 where each GPU has a batch size of 10. I haven't tested with accumulated gradient since tf2.0 does not have a wrapper for it at the moment, but I think it will help.
It might also help adding momentum to RMSprop or a scheduling learning rate, but haven't test it yet. If you have some hint or previous experience on it please let me know
<|||||>> > I was able to reproduce the results of XLM on XNLI.
> > It was highly sensitive to hyper parameters.
> > I would suggest that you tune your learning_rate ~ 1.5e-6 and batch_size ~ 4.
>
> Thank you for your answer. I modified the parameters as you suggested. xlm-mlm-17-1280 and xlm-mlm-100-1280 batch_size only go up to 2, and others modified the parameters as before. I wonder if it has anything to do with the task. Looking forward to your reply
In my limited experience, XLM is highly sensitive to HPs (it seems to be also the case with RoBERTa on GLUE, to a lesser extent). However, it is not something I observed with mBERT (and Distil-mBERT). So I don't think it has to do with XNLI since there is no consistent pattern across different models.<|||||>This issue has been automatically marked as stale because it has not had recent activity. It will be closed if no further activity occurs. Thank you for your contributions.
<|||||>Has anyone come up with a good set of hyper-parameters to train XLM models very well? Thanks for sharing the experience! |
transformers | 2,052 | closed | Missing "do_lower_case" action for special token (e.g. mask_token) | ## 🐛 Bug
Model I am using (Bert, XLNet....): 'bert-base-uncased'
Language I am using the model on (English, Chinese....): English
After upgrading to 2.2.1 version, the BERT tokenizer cannot tokenize special word while it works in 2.1.1 version.
According to [here](https://github.com/huggingface/transformers/blob/master/transformers/tokenization_bert.py#L71), 'bert-base-uncased' should perform lower case operation. Inputs follow this config to [perform lower case operation](https://github.com/huggingface/transformers/blob/master/transformers/tokenization_utils.py#L615), while no corresponding action for [special tokens](https://github.com/huggingface/transformers/blob/master/transformers/tokenization_utils.py#L1100). Eventually, it tokenizes '[MASK]' to 3 subwords (e.g. [, mask and ]) rather than skip the tokenization operation in [here](https://github.com/huggingface/transformers/blob/master/transformers/tokenization_utils.py#L658).
Error occurs after this [commit](https://github.com/huggingface/transformers/commit/7246d3c2f93c4461f3ec8ada7a26a002d8f196ea).
## To Reproduce
Steps to reproduce the behavior:
```
import torch
from transformers import BertTokenizer
tokenizer = BertTokenizer.from_pretrained('bert-base-uncased')
tokenizer.tokenize('The quick brown [MASK] jumps over the lazy dog.')
```
## Expected behavior
Expected output is
['the', 'quick', 'brown', **'[MASK]'**, 'jumps', 'over', 'the', 'lazy', 'dog', '.']
while actual output is
['the', 'quick', 'brown', **'[', 'mask', ']'**, 'jumps', 'over', 'the', 'lazy', 'dog', '.']
| 12-04-2019 17:54:53 | 12-04-2019 17:54:53 | With Transformers **2.2.0**, it works as expected!
```
Python 3.6.9 |Anaconda, Inc.| (default, Jul 30 2019, 19:07:31)
[GCC 7.3.0] on linux
Type "help", "copyright", "credits" or "license" for more information.
>>> import torch
>>> from transformers import BertTokenizer
/home/vidiemme/anaconda3/envs/huggingface/lib/python3.6/site-packages/tensorboard/compat/tensorflow_stub/dtypes.py:541: FutureWarning: Passing (type, 1) or '1type' as a synonym of type is deprecated; in a future version of numpy, it will be understood as (type, (1,)) / '(1,)type'.
_np_qint8 = np.dtype([("qint8", np.int8, 1)])
/home/vidiemme/anaconda3/envs/huggingface/lib/python3.6/site-packages/tensorboard/compat/tensorflow_stub/dtypes.py:542: FutureWarning: Passing (type, 1) or '1type' as a synonym of type is deprecated; in a future version of numpy, it will be understood as (type, (1,)) / '(1,)type'.
_np_quint8 = np.dtype([("quint8", np.uint8, 1)])
/home/vidiemme/anaconda3/envs/huggingface/lib/python3.6/site-packages/tensorboard/compat/tensorflow_stub/dtypes.py:543: FutureWarning: Passing (type, 1) or '1type' as a synonym of type is deprecated; in a future version of numpy, it will be understood as (type, (1,)) / '(1,)type'.
_np_qint16 = np.dtype([("qint16", np.int16, 1)])
/home/vidiemme/anaconda3/envs/huggingface/lib/python3.6/site-packages/tensorboard/compat/tensorflow_stub/dtypes.py:544: FutureWarning: Passing (type, 1) or '1type' as a synonym of type is deprecated; in a future version of numpy, it will be understood as (type, (1,)) / '(1,)type'.
_np_quint16 = np.dtype([("quint16", np.uint16, 1)])
/home/vidiemme/anaconda3/envs/huggingface/lib/python3.6/site-packages/tensorboard/compat/tensorflow_stub/dtypes.py:545: FutureWarning: Passing (type, 1) or '1type' as a synonym of type is deprecated; in a future version of numpy, it will be understood as (type, (1,)) / '(1,)type'.
_np_qint32 = np.dtype([("qint32", np.int32, 1)])
/home/vidiemme/anaconda3/envs/huggingface/lib/python3.6/site-packages/tensorboard/compat/tensorflow_stub/dtypes.py:550: FutureWarning: Passing (type, 1) or '1type' as a synonym of type is deprecated; in a future version of numpy, it will be understood as (type, (1,)) / '(1,)type'.
np_resource = np.dtype([("resource", np.ubyte, 1)])
2019-12-05 10:19:00.776555: I tensorflow/core/platform/cpu_feature_guard.cc:142] Your CPU supports instructions that this TensorFlow binary was not compiled to use: AVX2 FMA
2019-12-05 10:19:00.799189: I tensorflow/core/platform/profile_utils/cpu_utils.cc:94] CPU Frequency: 3408000000 Hz
2019-12-05 10:19:00.799911: I tensorflow/compiler/xla/service/service.cc:168] XLA service 0x55971fe06640 executing computations on platform Host. Devices:
2019-12-05 10:19:00.799929: I tensorflow/compiler/xla/service/service.cc:175] StreamExecutor device (0): Host, Default Version
>>> tokenizer = BertTokenizer.from_pretrained('bert-base-uncased')
>>> tokenizer.tokenize('The quick brown [MASK] jumps over the lazy dog.')
['the', 'quick', 'brown', '[MASK]', 'jumps', 'over', 'the', 'lazy', 'dog', '.']
```
With Transformers **2.2.1**, the bug you've highlighted occurs to me too!
> ## Bug
> Model I am using (Bert, XLNet....): 'bert-base-uncased'
>
> Language I am using the model on (English, Chinese....): English
>
> After upgrading to 2.2.1 version, the BERT tokenizer cannot tokenize special word while it works in 2.1.1 version.
>
> According to [here](https://github.com/huggingface/transformers/blob/master/transformers/tokenization_bert.py#L71), 'bert-base-uncased' should perform lower case operation. Inputs follow this config to [perform lower case operation](https://github.com/huggingface/transformers/blob/master/transformers/tokenization_utils.py#L615), while no corresponding action for [special tokens](https://github.com/huggingface/transformers/blob/master/transformers/tokenization_utils.py#L1100). Eventually, it tokenizes '[MASK]' to 3 subwords (e.g. [, mask and ]) rather than skip the tokenization operation in [here](https://github.com/huggingface/transformers/blob/master/transformers/tokenization_utils.py#L658).
>
> Error occurs after this [commit](https://github.com/huggingface/transformers/commit/7246d3c2f93c4461f3ec8ada7a26a002d8f196ea).
>
> ## To Reproduce
> Steps to reproduce the behavior:
>
> ```
> import torch
> from transformers import BertTokenizer
> tokenizer = BertTokenizer.from_pretrained('bert-base-uncased')
> tokenizer.tokenize('The quick brown [MASK] jumps over the lazy dog.')
> ```
>
> ## Expected behavior
> Expected output is
> ['the', 'quick', 'brown', **'[mask]'**, 'jumps', 'over', 'the', 'lazy', 'dog', '.']
> while actual output is
> ['the', 'quick', 'brown', **'[', 'mask', ']'**, 'jumps', 'over', 'the', 'lazy', 'dog', '.']<|||||>I've tracked it yesterday evening and I confirm all of that too. `PreTrainedTokenizer.add_tokens` forces added tokens to lower-case but tokens coming from BertTokenizer constructor aren't lower-cased.
Yet considering a viable patch, I tend to think there is an issue linked to current design of Tokenizers with respect to flags such as `do_lower_case`.
For example, current BertTokenizer is:
```python
class BertTokenizer(PreTrainedTokenizer):
...
def __init__(self, vocab_file, do_lower_case=True, do_basic_tokenize=True, never_split=None,
unk_token="[UNK]", sep_token="[SEP]", pad_token="[PAD]", cls_token="[CLS]",
mask_token="[MASK]", tokenize_chinese_chars=True, **kwargs):
super(BertTokenizer, self).__init__(unk_token=unk_token, sep_token=sep_token,
pad_token=pad_token, cls_token=cls_token,
mask_token=mask_token, **kwargs)
```
So `BertTokenizer` knows about `do_lower_case` but not the super class `PreTrainedTokenizer`. Moreover, by default `do_lower_case` is True but all tokens are defined in upper_case.
Then, in `PreTrainedTokenizer`, there are some `if self.init_kwargs.get('do_lower_case', False):` in different places of the code to force text or added_tokens to lower_case before tokenization. But this means you inject a knowledge of `lower_case` in a class that doesn't know it by construction. It works but as we see in the case of token case, it's error-prone and not so robust. Moreover, if there were several flags, it would become even harder to track.
A solution could be to provide a simple callback system in `PreTrainedTokenizer` with callbacks `prepare_tokens` and `prepare_text` provided by the implementing Tokenizer class which takes into account its own flags.
Yet it requires a bigger modification of code and a bit more reflection (I can propose a PR on this if we agree on something).
For now, an immediate solution to current issue would be to force BertTokenizer to lower_case its tokens by construction:
```python
class BertTokenizer(PreTrainedTokenizer):
...
def __init__(self, vocab_file, do_lower_case=True, do_basic_tokenize=True, never_split=None,
unk_token="[UNK]", sep_token="[SEP]", pad_token="[PAD]", cls_token="[CLS]",
mask_token="[MASK]", tokenize_chinese_chars=True, **kwargs):
if do_lower_case:
unk_token, sep_token, pad_token, cls_token, mask_token = unk_token.lower(), sep_token.lower(), pad_token.lower(), cls_token.lower(), mask_token.lower()
super(BertTokenizer, self).__init__(unk_token=unk_token, sep_token=sep_token,
pad_token=pad_token, cls_token=cls_token,
mask_token=mask_token, **kwargs)
```
WDYT?<|||||>Should have been fixed with #2051<|||||>I confirm it should solve the issue! It introduces a bit more external logic about `do_lower_case` in `PreTrainedTokenizer` as I explained. It's not critical but keep in mind for the future, there are solutions to improve that in the code ;)<|||||>This issue has been automatically marked as stale because it has not had recent activity. It will be closed if no further activity occurs. Thank you for your contributions.
|
transformers | 2,051 | closed | Fix bug which lowercases special tokens | A previous PR (#1592), which lowercases input and added tokens if `do_lower_case` is set to `True` for a given tokenizer, introduced a bug which lowercases text without considering whether parts of the input are special tokens. The result is that special tokens may not be tokenized properly, e.g. "[CLS]" becomes 4 separate tokens when using the BERT tokenizer: "[", "cl", "##s", "]".
This change fixes that by only applying lowercasing to non-special tokens. The do_lower_case test case has also been expanded to use some special token based on the subclass.
Closes #2047 | 12-04-2019 16:01:52 | 12-04-2019 16:01:52 | # [Codecov](https://codecov.io/gh/huggingface/transformers/pull/2051?src=pr&el=h1) Report
> Merging [#2051](https://codecov.io/gh/huggingface/transformers/pull/2051?src=pr&el=desc) into [master](https://codecov.io/gh/huggingface/transformers/commit/5bfcd0485ece086ebcbed2d008813037968a9e58?src=pr&el=desc) will **increase** coverage by `<.01%`.
> The diff coverage is `100%`.
[](https://codecov.io/gh/huggingface/transformers/pull/2051?src=pr&el=tree)
```diff
@@ Coverage Diff @@
## master #2051 +/- ##
==========================================
+ Coverage 83.58% 83.58% +<.01%
==========================================
Files 105 105
Lines 15568 15574 +6
==========================================
+ Hits 13012 13018 +6
Misses 2556 2556
```
| [Impacted Files](https://codecov.io/gh/huggingface/transformers/pull/2051?src=pr&el=tree) | Coverage Δ | |
|---|---|---|
| [transformers/tests/tokenization\_tests\_commons.py](https://codecov.io/gh/huggingface/transformers/pull/2051/diff?src=pr&el=tree#diff-dHJhbnNmb3JtZXJzL3Rlc3RzL3Rva2VuaXphdGlvbl90ZXN0c19jb21tb25zLnB5) | `100% <100%> (ø)` | :arrow_up: |
| [transformers/tokenization\_utils.py](https://codecov.io/gh/huggingface/transformers/pull/2051/diff?src=pr&el=tree#diff-dHJhbnNmb3JtZXJzL3Rva2VuaXphdGlvbl91dGlscy5weQ==) | `91.96% <100%> (+0.09%)` | :arrow_up: |
------
[Continue to review full report at Codecov](https://codecov.io/gh/huggingface/transformers/pull/2051?src=pr&el=continue).
> **Legend** - [Click here to learn more](https://docs.codecov.io/docs/codecov-delta)
> `Δ = absolute <relative> (impact)`, `ø = not affected`, `? = missing data`
> Powered by [Codecov](https://codecov.io/gh/huggingface/transformers/pull/2051?src=pr&el=footer). Last update [5bfcd04...0025a20](https://codecov.io/gh/huggingface/transformers/pull/2051?src=pr&el=lastupdated). Read the [comment docs](https://docs.codecov.io/docs/pull-request-comments).
<|||||>@LysandreJik @thomwolf my last PR seems to have introduced a nasty bug, which got into the most recent release. Could one of you (or someone else at 🤗) review this PR, which should fix it? Sorry for the regression and inconvenience :disappointed: <|||||>Indeed, this is an issue! No worries, bugs happen.
Using a regex may be a bit slow but we'll merge this as to fix the bug, and think of optimization afterward.<|||||>>Using a regex may be a bit slow but we'll merge this as to fix the bug, and think of optimization afterward.
@LysandreJik true! It just seemed like the fastest way to get to some fix for now. I can help improve it later if necessary :) |
transformers | 2,050 | closed | [CamemBert] About SentencePiece training | ## ❓ Questions & Help
According to the paper, SentencePiece uses a vocabulary of size of 32k subword tokens, learned on 107 sentences sampled from the pretraining dataset. How the sampling was performed? The chosen size of the vocabulary (32K subwords token) is related to the pretraining dataset in some way? Or it is an arbitrary choice?
Thank you. | 12-04-2019 13:41:08 | 12-04-2019 13:41:08 | ping author @louismartin :)<|||||>Hi @loretoparisi,
We sampled 10**7 lines randomly from the pretraining corpus.
The size of the vocabulary was chosen to somewhat match the original BERT paper which used a 30k wordpiece vocabulary, so yes it's mostly arbitrary. <|||||>@louismartin thanks a lot for the details. I was wondering about the 32k size if this could have been biased by the language...w<|||||>Yes maybe there is a more adapted vocabulary size, we did not investigate that :)
Can I close the issue now?<|||||>Adding a reference to https://github.com/google/sentencepiece/issues/415<|||||>@louismartin:
> We sampled 10**7 lines randomly from the pretraining corpus.
May I ask how did you come up with that number? I'm trying to figure out how many lines I should select to train a model. Assuming I have access to 1 billion rows of ngrams with mean length of 7 words; I'm not sure how many random lines/ngrams would be enough to train a tokenizer with fixed vocab of size 50k?<|||||>I think this is more of a resource allocation question. How much time or compute do you want to allocate to training your tokenizer? Alternate phrasing: why wouldn't you train your tokenizer on the full corpus?<|||||>@julien-c Well sure, that's a valid point. In theory I can train a tokenizer on full corpus by setting the fixed size for the vocabulary. It will just take more and more time (& possibly more compute resources) with increased size of dataset. I was wondering if there's any correlation between quality of fixed threshold vocabulary generated from increasing size of training dataset. I can see that this may be task dependent and requires iterative experiments. Is there any paper that I can look into regarding this? Thanks! <|||||>PS: did you check out [`tokenizers`](https://github.com/huggingface/tokenizers)? It is pretty fast 😄
I've trained a byte-level BPE on 10 GB of text in ~15 minutes.<|||||>@julien-c are you suggesting that, thanks to 🤗 amazing library `tokenizer` we could potentially train the sentence piece tokenizer without setting up a boundary?
This means that, potentially, current models could improve a lot: from 32K subwords tokens to let's say 1M, what will happen?<|||||>Those are two different things: size of vocab, and size of corpus that you train your tokenizer on.<|||||>@julien-c that's true, and it also seems to have no clear relation in terms of final overall accuracy. Let's say we take as metrics the PPL, and we consider a fixed corpus size and vary the vocab size in batches of 8K, like: 8K, 16K, 32K, 64K, 128K, until we are closer to the whole size of the non unique tokens vocabulary. What will be the related PPL for each training?
(PPL or BLEU, or other...) |
transformers | 2,049 | closed | ModuleNotFoundError: No module named 'git' | ## 🐛 Bug
`
Traceback (most recent call last):
File "train.py", line 32, in <module>
from distiller import Distiller
File "~/transformers/examples/distillation/distiller.py", line 40, in <module>
from utils import logger
File "~/transformers/examples/distillation/utils.py", line 18, in <module>
import git
ModuleNotFoundError: No module named 'git'
`
how to install git package?
| 12-04-2019 11:33:40 | 12-04-2019 11:33:40 | with referring to that file https://github.com/huggingface/transformers/blob/master/examples/distillation/requirements.txt
run:
`pip install -r requirements.txt`<|||||>> with referring to that file https://github.com/huggingface/transformers/blob/master/examples/distillation/requirements.txt
>
> run:
> `pip install -r requirements.txt`
thx, got it~ |
transformers | 2,048 | closed | Changing the number of hidden layers for BERT | ## ❓ Questions & Help
Hello,
when reducing the number of hidden layers for BERT, say from 12 to 3, which layers are loaded from the pretrained model, the first 3 layers or the last 3 ones? and is there a way to control this?
Thanks in advance
| 12-04-2019 10:29:53 | 12-04-2019 10:29:53 | Hi,
The first ones are loaded and there is currently no simple way to control this.<|||||>**Is there any evidence than the first layers is the best choice when reducing the number of layers ?**
For example in your article about Distil-Bert, you chose to initialize the student by taking the even layers. Why so ?<|||||>> For example in your article about Distil-Bert, you chose to initialize the student by taking the even layers. Why so ?
It empirically produces stronger performance.
There are some other empirical evidences in [this paper](https://arxiv.org/abs/1909.11556) from Angela Fan, Edouard Grave and Armand Joulin.<|||||>This issue has been automatically marked as stale because it has not had recent activity. It will be closed if no further activity occurs. Thank you for your contributions.
<|||||>I also want to ask this |
transformers | 2,047 | closed | Tokenization in quickstart guide fails | ## 🐛 Bug
<!-- Important information -->
The same issue as in #226 re-appears in transformers==2.2.1 (it works on 2.1!)
I just encountered the same issue as @dhirajmadan1 with `transformers==2.2.1`. Is this expected somehow?
I am following the quickstart guide: https://huggingface.co/transformers/quickstart.html
## To Reproduce
Steps to reproduce the behavior:
```
tokenizer = BertTokenizer.from_pretrained('bert-base-uncased')
# Run an example text through this:
text = "[CLS] Who was Jim Henson ? [SEP] Jim Henson was a puppeteer [SEP]"
tokenized_text = tokenizer.tokenize(text)
masked_index = 8
tokenized_text[masked_index] = '[MASK]'
predicted_tokenized_sentence = ['[CLS]', 'who', 'was', 'jim', 'henson', '?', '[SEP]', 'jim', '[MASK]', 'was', 'a', 'puppet', '##eer', '[SEP]']
```
## Expected behavior
This should not fail:
```assert tokenized_text == ['[CLS]', 'who', 'was', 'jim', 'henson', '?', '[SEP]', 'jim', '[MASK]', 'was', 'a', 'puppet', '##eer', '[SEP]']```
## Environment
* OS: Mac
* Python version: 3.6
* PyTorch version: 1.3.1
* PyTorch Transformers version (or branch): 2.1 (latest-minor)
* Using GPU no
* Distributed of parallel setup no
* Any other relevant information:
## Additional context
<!-- Add any other context about the problem here. -->
| 12-04-2019 09:12:17 | 12-04-2019 09:12:17 | Oops, that appears to be my fault. Should be a quick fix though, so I'll try to make a PR on it right away. Sorry about that! :grimacing: <|||||>Thanks man! :) yeah no worries, thought it may be a good idea to report haha |
transformers | 2,046 | closed | Add NER TF2 example. | Create a NER example similar to the Pytorch one. It takes the same options, and can be run the same way.
As you asked @julien-c I prefered I did a fresh new PR :) | 12-04-2019 08:44:38 | 12-04-2019 08:44:38 | # [Codecov](https://codecov.io/gh/huggingface/transformers/pull/2046?src=pr&el=h1) Report
> Merging [#2046](https://codecov.io/gh/huggingface/transformers/pull/2046?src=pr&el=desc) into [master](https://codecov.io/gh/huggingface/transformers/commit/7edb51f3a516ca533797fb2bb2f2b7ce86e0df70?src=pr&el=desc) will **increase** coverage by `0.05%`.
> The diff coverage is `78.17%`.
[](https://codecov.io/gh/huggingface/transformers/pull/2046?src=pr&el=tree)
```diff
@@ Coverage Diff @@
## master #2046 +/- ##
==========================================
+ Coverage 83.45% 83.51% +0.05%
==========================================
Files 105 107 +2
Lines 15568 15765 +197
==========================================
+ Hits 12993 13166 +173
- Misses 2575 2599 +24
```
| [Impacted Files](https://codecov.io/gh/huggingface/transformers/pull/2046?src=pr&el=tree) | Coverage Δ | |
|---|---|---|
| [transformers/modeling\_tf\_distilbert.py](https://codecov.io/gh/huggingface/transformers/pull/2046/diff?src=pr&el=tree#diff-dHJhbnNmb3JtZXJzL21vZGVsaW5nX3RmX2Rpc3RpbGJlcnQucHk=) | `95.22% <26.66%> (-3.44%)` | :arrow_down: |
| [transformers/optimization\_tf.py](https://codecov.io/gh/huggingface/transformers/pull/2046/diff?src=pr&el=tree#diff-dHJhbnNmb3JtZXJzL29wdGltaXphdGlvbl90Zi5weQ==) | `79.82% <79.82%> (ø)` | |
| [transformers/tests/optimization\_tf\_test.py](https://codecov.io/gh/huggingface/transformers/pull/2046/diff?src=pr&el=tree#diff-dHJhbnNmb3JtZXJzL3Rlc3RzL29wdGltaXphdGlvbl90Zl90ZXN0LnB5) | `86.76% <86.76%> (ø)` | |
| [transformers/tests/modeling\_common\_test.py](https://codecov.io/gh/huggingface/transformers/pull/2046/diff?src=pr&el=tree#diff-dHJhbnNmb3JtZXJzL3Rlc3RzL21vZGVsaW5nX2NvbW1vbl90ZXN0LnB5) | `74.23% <0%> (+0.19%)` | :arrow_up: |
| [transformers/tests/modeling\_bert\_test.py](https://codecov.io/gh/huggingface/transformers/pull/2046/diff?src=pr&el=tree#diff-dHJhbnNmb3JtZXJzL3Rlc3RzL21vZGVsaW5nX2JlcnRfdGVzdC5weQ==) | `96.5% <0%> (+0.5%)` | :arrow_up: |
| [transformers/tests/modeling\_xlnet\_test.py](https://codecov.io/gh/huggingface/transformers/pull/2046/diff?src=pr&el=tree#diff-dHJhbnNmb3JtZXJzL3Rlc3RzL21vZGVsaW5nX3hsbmV0X3Rlc3QucHk=) | `96.12% <0%> (+0.64%)` | :arrow_up: |
| [transformers/tests/modeling\_xlm\_test.py](https://codecov.io/gh/huggingface/transformers/pull/2046/diff?src=pr&el=tree#diff-dHJhbnNmb3JtZXJzL3Rlc3RzL21vZGVsaW5nX3hsbV90ZXN0LnB5) | `96% <0%> (+0.66%)` | :arrow_up: |
| [transformers/tests/modeling\_roberta\_test.py](https://codecov.io/gh/huggingface/transformers/pull/2046/diff?src=pr&el=tree#diff-dHJhbnNmb3JtZXJzL3Rlc3RzL21vZGVsaW5nX3JvYmVydGFfdGVzdC5weQ==) | `75.38% <0%> (+0.76%)` | :arrow_up: |
| ... and [11 more](https://codecov.io/gh/huggingface/transformers/pull/2046/diff?src=pr&el=tree-more) | |
------
[Continue to review full report at Codecov](https://codecov.io/gh/huggingface/transformers/pull/2046?src=pr&el=continue).
> **Legend** - [Click here to learn more](https://docs.codecov.io/docs/codecov-delta)
> `Δ = absolute <relative> (impact)`, `ø = not affected`, `? = missing data`
> Powered by [Codecov](https://codecov.io/gh/huggingface/transformers/pull/2046?src=pr&el=footer). Last update [7edb51f...9200a75](https://codecov.io/gh/huggingface/transformers/pull/2046?src=pr&el=lastupdated). Read the [comment docs](https://docs.codecov.io/docs/pull-request-comments).
<|||||>This looks great, amazing work @jplu!
Before merging we would need to add:
- a few tests on the optimizer (create a new file `./transformers/tests/optimization_tf_test.py` like in `./transformers/tests/optimization_test.py`)
- documentation for the optimizer (for instance in `./docs/source/main_classes/optimizer_schedules.rst.py`)
- an example of a command line to run the `run_tf_ner.py` script and the associated results you should obtain (in `./examples/README.md`)
Do you think you can do it?<|||||>Thanks a lots! :)
I can do these tasks, no problems!!<|||||>I have done what you asked @thomwolf, please let me know if I have to change something.<|||||>This is awesome, merging! <|||||>Amazing!! Thanks a lot ;) |
transformers | 2,045 | closed | Remove dead code in tests. | 12-04-2019 07:21:47 | 12-04-2019 07:21:47 | # [Codecov](https://codecov.io/gh/huggingface/transformers/pull/2045?src=pr&el=h1) Report
> Merging [#2045](https://codecov.io/gh/huggingface/transformers/pull/2045?src=pr&el=desc) into [master](https://codecov.io/gh/huggingface/transformers/commit/7edb51f3a516ca533797fb2bb2f2b7ce86e0df70?src=pr&el=desc) will **increase** coverage by `0.58%`.
> The diff coverage is `n/a`.
[](https://codecov.io/gh/huggingface/transformers/pull/2045?src=pr&el=tree)
```diff
@@ Coverage Diff @@
## master #2045 +/- ##
==========================================
+ Coverage 83.45% 84.04% +0.58%
==========================================
Files 105 105
Lines 15568 15544 -24
==========================================
+ Hits 12993 13064 +71
+ Misses 2575 2480 -95
```
| [Impacted Files](https://codecov.io/gh/huggingface/transformers/pull/2045?src=pr&el=tree) | Coverage Δ | |
|---|---|---|
| [transformers/tests/modeling\_tf\_common\_test.py](https://codecov.io/gh/huggingface/transformers/pull/2045/diff?src=pr&el=tree#diff-dHJhbnNmb3JtZXJzL3Rlc3RzL21vZGVsaW5nX3RmX2NvbW1vbl90ZXN0LnB5) | `96.92% <ø> (+1.77%)` | :arrow_up: |
| [transformers/tokenization\_utils.py](https://codecov.io/gh/huggingface/transformers/pull/2045/diff?src=pr&el=tree#diff-dHJhbnNmb3JtZXJzL3Rva2VuaXphdGlvbl91dGlscy5weQ==) | `91.91% <0%> (+0.03%)` | :arrow_up: |
| [transformers/tests/modeling\_common\_test.py](https://codecov.io/gh/huggingface/transformers/pull/2045/diff?src=pr&el=tree#diff-dHJhbnNmb3JtZXJzL3Rlc3RzL21vZGVsaW5nX2NvbW1vbl90ZXN0LnB5) | `74.23% <0%> (+0.19%)` | :arrow_up: |
| [transformers/tests/modeling\_bert\_test.py](https://codecov.io/gh/huggingface/transformers/pull/2045/diff?src=pr&el=tree#diff-dHJhbnNmb3JtZXJzL3Rlc3RzL21vZGVsaW5nX2JlcnRfdGVzdC5weQ==) | `96.5% <0%> (+0.5%)` | :arrow_up: |
| [transformers/file\_utils.py](https://codecov.io/gh/huggingface/transformers/pull/2045/diff?src=pr&el=tree#diff-dHJhbnNmb3JtZXJzL2ZpbGVfdXRpbHMucHk=) | `70.5% <0%> (+0.5%)` | :arrow_up: |
| [transformers/tests/modeling\_xlnet\_test.py](https://codecov.io/gh/huggingface/transformers/pull/2045/diff?src=pr&el=tree#diff-dHJhbnNmb3JtZXJzL3Rlc3RzL21vZGVsaW5nX3hsbmV0X3Rlc3QucHk=) | `96.12% <0%> (+0.64%)` | :arrow_up: |
| [transformers/tests/modeling\_xlm\_test.py](https://codecov.io/gh/huggingface/transformers/pull/2045/diff?src=pr&el=tree#diff-dHJhbnNmb3JtZXJzL3Rlc3RzL21vZGVsaW5nX3hsbV90ZXN0LnB5) | `96% <0%> (+0.66%)` | :arrow_up: |
| [transformers/tests/modeling\_roberta\_test.py](https://codecov.io/gh/huggingface/transformers/pull/2045/diff?src=pr&el=tree#diff-dHJhbnNmb3JtZXJzL3Rlc3RzL21vZGVsaW5nX3JvYmVydGFfdGVzdC5weQ==) | `75.38% <0%> (+0.76%)` | :arrow_up: |
| [transformers/tests/modeling\_distilbert\_test.py](https://codecov.io/gh/huggingface/transformers/pull/2045/diff?src=pr&el=tree#diff-dHJhbnNmb3JtZXJzL3Rlc3RzL21vZGVsaW5nX2Rpc3RpbGJlcnRfdGVzdC5weQ==) | `99.18% <0%> (+0.81%)` | :arrow_up: |
| [transformers/tests/modeling\_albert\_test.py](https://codecov.io/gh/huggingface/transformers/pull/2045/diff?src=pr&el=tree#diff-dHJhbnNmb3JtZXJzL3Rlc3RzL21vZGVsaW5nX2FsYmVydF90ZXN0LnB5) | `95.08% <0%> (+0.81%)` | :arrow_up: |
| ... and [15 more](https://codecov.io/gh/huggingface/transformers/pull/2045/diff?src=pr&el=tree-more) | |
------
[Continue to review full report at Codecov](https://codecov.io/gh/huggingface/transformers/pull/2045?src=pr&el=continue).
> **Legend** - [Click here to learn more](https://docs.codecov.io/docs/codecov-delta)
> `Δ = absolute <relative> (impact)`, `ø = not affected`, `? = missing data`
> Powered by [Codecov](https://codecov.io/gh/huggingface/transformers/pull/2045?src=pr&el=footer). Last update [7edb51f...40255ab](https://codecov.io/gh/huggingface/transformers/pull/2045?src=pr&el=lastupdated). Read the [comment docs](https://docs.codecov.io/docs/pull-request-comments).
<|||||>Yes, thanks @aaugustin! |
|
transformers | 2,044 | closed | CLI for authenticated file sharing | ping review @mfuntowicz & @thomwolf
(I'll fix the tests for Python 2 and Python 3.5 tomorrow)
To create an account in `staging` (used by the tests): https://moon-staging.huggingface.co/join
To create an account in `production` (used by the CLI): https://huggingface.co/join | 12-04-2019 05:56:16 | 12-04-2019 05:56:16 | Seen in person with @julien-c, really slick implementation!<|||||>Can't wait to test it 😊<|||||># [Codecov](https://codecov.io/gh/huggingface/transformers/pull/2044?src=pr&el=h1) Report
> Merging [#2044](https://codecov.io/gh/huggingface/transformers/pull/2044?src=pr&el=desc) into [master](https://codecov.io/gh/huggingface/transformers/commit/7edb51f3a516ca533797fb2bb2f2b7ce86e0df70?src=pr&el=desc) will **decrease** coverage by `0.33%`.
> The diff coverage is `50.46%`.
[](https://codecov.io/gh/huggingface/transformers/pull/2044?src=pr&el=tree)
```diff
@@ Coverage Diff @@
## master #2044 +/- ##
==========================================
- Coverage 83.45% 83.12% -0.34%
==========================================
Files 105 109 +4
Lines 15568 15784 +216
==========================================
+ Hits 12993 13121 +128
- Misses 2575 2663 +88
```
| [Impacted Files](https://codecov.io/gh/huggingface/transformers/pull/2044?src=pr&el=tree) | Coverage Δ | |
|---|---|---|
| [transformers/commands/\_\_init\_\_.py](https://codecov.io/gh/huggingface/transformers/pull/2044/diff?src=pr&el=tree#diff-dHJhbnNmb3JtZXJzL2NvbW1hbmRzL19faW5pdF9fLnB5) | `0% <0%> (ø)` | |
| [transformers/commands/user.py](https://codecov.io/gh/huggingface/transformers/pull/2044/diff?src=pr&el=tree#diff-dHJhbnNmb3JtZXJzL2NvbW1hbmRzL3VzZXIucHk=) | `0% <0%> (ø)` | |
| [transformers/hf\_api.py](https://codecov.io/gh/huggingface/transformers/pull/2044/diff?src=pr&el=tree#diff-dHJhbnNmb3JtZXJzL2hmX2FwaS5weQ==) | `96.87% <96.87%> (ø)` | |
| [transformers/tests/hf\_api\_test.py](https://codecov.io/gh/huggingface/transformers/pull/2044/diff?src=pr&el=tree#diff-dHJhbnNmb3JtZXJzL3Rlc3RzL2hmX2FwaV90ZXN0LnB5) | `97.91% <97.91%> (ø)` | |
| [transformers/tests/modeling\_common\_test.py](https://codecov.io/gh/huggingface/transformers/pull/2044/diff?src=pr&el=tree#diff-dHJhbnNmb3JtZXJzL3Rlc3RzL21vZGVsaW5nX2NvbW1vbl90ZXN0LnB5) | `74.23% <0%> (+0.19%)` | :arrow_up: |
| [transformers/tests/modeling\_bert\_test.py](https://codecov.io/gh/huggingface/transformers/pull/2044/diff?src=pr&el=tree#diff-dHJhbnNmb3JtZXJzL3Rlc3RzL21vZGVsaW5nX2JlcnRfdGVzdC5weQ==) | `96.5% <0%> (+0.5%)` | :arrow_up: |
| ... and [14 more](https://codecov.io/gh/huggingface/transformers/pull/2044/diff?src=pr&el=tree-more) | |
------
[Continue to review full report at Codecov](https://codecov.io/gh/huggingface/transformers/pull/2044?src=pr&el=continue).
> **Legend** - [Click here to learn more](https://docs.codecov.io/docs/codecov-delta)
> `Δ = absolute <relative> (impact)`, `ø = not affected`, `? = missing data`
> Powered by [Codecov](https://codecov.io/gh/huggingface/transformers/pull/2044?src=pr&el=footer). Last update [7edb51f...3ba417e](https://codecov.io/gh/huggingface/transformers/pull/2044?src=pr&el=lastupdated). Read the [comment docs](https://docs.codecov.io/docs/pull-request-comments).
<|||||>If anyone wants to try it out before it's merged, instructions are:
```bash
git checkout cli_upload
pip install -e .
transformers-cli login
transformers-cli upload
```<|||||>Perfect, I love it! Very slick |
transformers | 2,043 | closed | Missing xlm-mlm-100-1280 | ## 🐛 Bug
For some reason I can't download the xlm-mlm-100-1280 model for tensorflow 2.0
Model I am using (Bert, XLNet....): XLM
Language I am using the model on (English, Chinese....): 100 languages
The problem arise when using:
```TFXLMForSequenceClassification.from_pretrained("xlm-mlm-100-1280")```
## Expected behavior
Being able to download the model as for the other configuration
## Environment
* OS: Ubuntu 16.04
* Python version: 3.7.5
* Using GPU : yes
* Distributed of parallel setup : distributed
* Tensorflow 2.0
* transformers version 2.1.1
| 12-04-2019 01:34:47 | 12-04-2019 01:34:47 | It works with **PyTorch**, but not with **TensorFlow**. I'm using Python 3.6.9, Transformers 2.2.1 (installed with `pip install transformers`), PyTorch 1.3.1 and TensorFlow 2.0.0.
With TensorFlow, the stack trace is the following:
```
> from transformers import TFXLMForSequenceClassification
> model = TFXLMForSequenceClassification.from_pretrained("xlm-mlm-100-1280")
100%|████████████████████████████████████████████████████████████████████████████████████████████████████████████████████████████████████████████████████████████████████████████████████████████████████████████████████████| 41478/41478 [00:00<00:00, 365198.30B/s]
304B [00:00, 133069.13B/s]
2019-12-04 10:44:05.684050: W tensorflow/python/util/util.cc:299] Sets are not currently considered sequences, but this may change in the future, so consider avoiding using them.
Traceback (most recent call last):
File "<stdin>", line 1, in <module>
File "/home/<user>/anaconda3/envs/huggingface/lib/python3.6/site-packages/transformers/modeling_tf_utils.py", line 289, in from_pretrained
model.load_weights(resolved_archive_file, by_name=True)
File "/home/<user>/anaconda3/envs/huggingface/lib/python3.6/site-packages/tensorflow_core/python/keras/engine/training.py", line 181, in load_weights
return super(Model, self).load_weights(filepath, by_name)
File "/home/<user>/anaconda3/envs/huggingface/lib/python3.6/site-packages/tensorflow_core/python/keras/engine/network.py", line 1171, in load_weights
with h5py.File(filepath, 'r') as f:
File "/home/<user>/anaconda3/envs/huggingface/lib/python3.6/site-packages/h5py/_hl/files.py", line 408, in __init__
swmr=swmr)
File "/home/<user>/anaconda3/envs/huggingface/lib/python3.6/site-packages/h5py/_hl/files.py", line 173, in make_fid
fid = h5f.open(name, flags, fapl=fapl)
File "h5py/_objects.pyx", line 54, in h5py._objects.with_phil.wrapper
File "h5py/_objects.pyx", line 55, in h5py._objects.with_phil.wrapper
File "h5py/h5f.pyx", line 88, in h5py.h5f.open
OSError: Unable to open file (file signature not found)
```
If you want, with TensorFlow, it works the XLM model with config **xlm-mlm-17-1280**, which is a Masked Language Modeling with 17 languages.
```
> from transformers import TFXLMForSequenceClassification
> model = TFXLMForSequenceClassification.from_pretrained("xlm-mlm-17-1280")
100%|█████████████████████████████████████████████████████████████████████████████████████████████████████████████████████████████████████████████████████████████████████████████████████████████████████████████| 3311066864/3311066864 [05:40<00:00, 9737775.86B/s]
```
> ## Bug
> For some reason I can't download the xlm-mlm-100-1280 model for tensorflow 2.0
>
> Model I am using (Bert, XLNet....): XLM
>
> Language I am using the model on (English, Chinese....): 100 languages
>
> The problem arise when using:
> `TFXLMForSequenceClassification.from_pretrained("xlm-mlm-100-1280")`
>
> ## Expected behavior
> Being able to download the model as for the other configuration
>
> ## Environment
> * OS: Ubuntu 16.04
> * Python version: 3.7.5
> * Using GPU : yes
> * Distributed of parallel setup : distributed
> * Tensorflow 2.0
> * transformers version 2.1.1<|||||>Yes I'm refearing to TF2 and I'm currently using ``xlm-mlm-17-1280``, but I wanted to use the bigger model to see if I was able to achieve better performances.
At the moment I'm quite disappointed with xlm-mlm-17-1280, but it might be my fault.<|||||>If you suspect that you're in trouble, please copy and paste your code here and discuss together
> Yes I'm refearing to TF2 and I'm currently using `xlm-mlm-17-1280`, but I wanted to use the bigger model to see if I was able to achieve better performances.
>
> At the moment I'm quite disappointed with xlm-mlm-17-1280, but it might be my fault.
<|||||>Indeed, this one is missing from the S3. Adding it now!<|||||>This issue has been automatically marked as stale because it has not had recent activity. It will be closed if no further activity occurs. Thank you for your contributions.
|
transformers | 2,042 | closed | UnboundLocalError: local variable 'extended_attention_mask' referenced before assignment | ## Finetuning Setup
* Model: roberta-base
* Language: english
* OS: Ubuntu 18.04.3
* Python version: 3.7.3
* PyTorch version: 1.3.1+cpu
* PyTorch Transformers version (or branch): 2.2.0
* Using GPU ? No
* Distributed of parallel setup ? No
* Script inputs:
```
python run_lm_finetuning.py \
--output_dir=$OUTPUT_DIR \
--model_type=roberta \
--model_name_or_path=roberta_base \
--do_train \
--train_data_file=$TRAIN_FILE \
--do_eval \
--eval_data_file=$TEST_FILE \
--mlm \
--no_cuda
```
## ❓ Questions & Help
I finetuned roberta on some domain specific data I have and was trying to follow the example in the Quick Tour section for getting the output, however I get the following error:
```python
Traceback (most recent call last):
File "/path/to/code/roberta_compare.py", line 26, in <module>
last_hidden_states = model(input_ids)
File "/usr/local/anaconda3/lib/python3.7/site-packages/torch/nn/modules/module.py", line 489, in __call__
result = self.forward(*input, **kwargs)
File "/usr/local/anaconda3/lib/python3.7/site-packages/transformers/modeling_roberta.py", line 246, in forward
inputs_embeds=inputs_embeds)
File "/usr/local/anaconda3/lib/python3.7/site-packages/torch/nn/modules/module.py", line 489, in __call__
result = self.forward(*input, **kwargs)
File "/usr/local/anaconda3/lib/python3.7/site-packages/transformers/modeling_bert.py", line 688, in forward
extended_attention_mask = extended_attention_mask.to(dtype=next(self.parameters()).dtype) # fp16 compatibility
UnboundLocalError: local variable 'extended_attention_mask' referenced before assignment
```
Here is my code:
```python
import torch
from transformers import RobertaTokenizer, RobertaForMaskedLM
model_class = RobertaForMaskedLM
model = model_class.from_pretrained('/path/to/models/roberta_finetuned/model')
tokenizer_class = RobertaTokenizer
tokenizer = tokenizer_class.from_pretrained('/path/to/models/roberta_finetuned/model')
tmp = tokenizer.encode('A sentence to encode with roberta.', add_special_tokens=True)
input_ids = torch.tensor(tmp)
with torch.no_grad():
last_hidden_states = model(input_ids)[0]
```
Any thoughts on what I might be messing up? | 12-04-2019 01:29:14 | 12-04-2019 01:29:14 | You forgot to add the batch size.
You can either
- do `input_ids = tokenizer.encode('A sentence to encode with roberta.', add_special_tokens=True, return_tensors='pt')`
- or `input_ids = torch.tensor([tokenizer.encode('A sentence to encode with roberta.')])`
But for a specific reason, the current failure message is really not clear. Improving that in #2068<|||||>That fixes it! Thank you for the response! Closing the issue. |
transformers | 2,041 | closed | How do I load a pretrained file offline? | ## ❓ Questions & Help
Hi, when I use "RobertaModel.from_pretrained(roberta.large)" to load model. A progress bar appears to download the pre-training model. I've already downloaded files like "roberta-large-pytorch_model.bin ". How can I stop automatically downloading files to the ".cache" folder and instead specify these pre-training files I downloaded?
| 12-04-2019 01:14:32 | 12-04-2019 01:14:32 | You can do it, instead of loading `from_pretrained(roberta.large)` like this download the respective `config.json` and `<mode_name>.bin` and save it on your folder then just write
`.from_pretrained('Users/<location>/<your folder name>')` and thats about it.<|||||>OK, Thank you very much!<|||||>@shashankMadan-designEsthetics' solution may require git-lfs to download the files of some models. If you are not a sudoer, this can be a problem. The most reliable and easy solution I've found is this:
```
from transformers import AutoModel, AutoTokenizer
# Do this on a machine with internet access
model = AutoModel.from_pretrained("model-name")
tokenizer = AutoTokenizer.from_pretrained("model-name")
_ = model.save_pretrained("./model-dir")
_ = tokenizer.save_pretrained("./model-dir")
```
Then you can do whatever you want with your model -- send it to a computing cluster, put it on a flash drive etc. Then you just do:
```
model = AutoModel.from_pretrained("path/model-dir")
tokenizer = AutoTokenizer.from_pretrained("path/model-dir")
``` |
transformers | 2,040 | closed | XLM-R Support | ## ❓ Questions & Help
Hello!
Is there a way to use XLM-R (https://github.com/pytorch/fairseq/blob/master/examples/xlmr/README.md) with the library of transformers? maybe via RoBERTa? can you provide some guidance on this please?
Thank you in advance | 12-03-2019 19:34:09 | 12-03-2019 19:34:09 | The latest news about using XLM-R model with Transformers are discussed in #1769
Briefly, **at the moment it's not possible to use this model with Transformers directly**.
> ## Questions & Help
> Hello!
>
> Is there a way to use XLM-R (https://github.com/pytorch/fairseq/blob/master/examples/xlmr/README.md) with the library of transformers? maybe via RoBERTa? can you provide some guidance on this please?
>
> Thank you in advance<|||||>Thank you,
I'm closing this one and keep an eye in #1769 |
transformers | 2,039 | closed | Meaning of run_lm_finetuning.py output | ## ❓ Questions & Help
Is there documentation somewhere about what the various output files that get created when running `run_lm_finetuning.py` are and what the meaning of their contents is? Concretely, what are the files and directories:
```
added_tokens.json
checkpoint-50/
checkpoint-100/
checkpoint-150/
checkpoint-200/
checkpoint-250/
checkpoint-300/
checkpoint-350/
checkpoint-400/
config.json
eval_results.txt
merges.txt
pytorch_model.bin
runs/
special_tokens_map.json
tokenizer_config.json
training_args.bin
vocab.json
```
and what is the meaning of their contents? the `checkpoint` directories contain:
```
config.json
pytorch_model.bin
training_args.bin
```
and `runs/Dec03_09-15-51_MACHINENAME` contains:
```
events.out.tfevents.20414.0
```
## Finetuning Setup
* Model: roberta-base
* Language: english
* OS: Ubuntu 18.04.3
* Python version: 3.7.3
* PyTorch version: 1.3.1+cpu
* PyTorch Transformers version (or branch): 2.2.1, I believe
* Using GPU ? No
* Distributed of parallel setup ? No
* Script inputs:
```
python run_lm_finetuning.py \
--output_dir=$OUTPUT_DIR \
--model_type=roberta \
--model_name_or_path=roberta_base \
--do_train \
--train_data_file=$TRAIN_FILE \
--do_eval \
--eval_data_file=$TEST_FILE \
--mlm \
--no_cuda
```
Thanks in advance!
| 12-03-2019 19:18:29 | 12-03-2019 19:18:29 | I have a similar question. When using default settings, does anything change in the tokenizer? Is the tokenizer fine-tuned in anyway (or is any vocabulary added)? In other words, is the vocab.txt of use in any way, when using the default tokenizer? If not, I assume that you only need the `pytorch_model.bin` file and you're good to go?<|||||>This issue has been automatically marked as stale because it has not had recent activity. It will be closed if no further activity occurs. Thank you for your contributions.
|
transformers | 2,038 | closed | run_squad with xlm: Dataparallel has no attribute config. | ## 🐛 Bug
<!-- Important information -->
Model I am using XLM.
Language I am using the model on English:
The problem arise when using:
* [x] the official example scripts: run_squad.py
## To Reproduce
Steps to reproduce the behavior:
1. Azure VM with 2 GPUs
2. run_squad with XLM
3. Everything fine until evaluation step.
<!-- If you have a code sample, error messages, stack traces, please provide it here as well. -->
```
12/03/2019 15:54:26 - INFO - __main__ - ***** Running evaluation *****
12/03/2019 15:54:26 - INFO - __main__ - Num examples = 10918
12/03/2019 15:54:26 - INFO - __main__ - Batch size = 16
Evaluating: 100%|█████████████████████████████████████████████████████| 683/683 [05:16<00:00, 2.16it/s]
12/03/2019 15:59:42 - INFO - __main__ - Evaluation done in total 316.178766 secs (0.028959 sec per example)
Traceback (most recent call last):
File "transformers/examples/run_squad.py", line 575, in <module>
main()
File "transformers/examples/run_squad.py", line 564, in main
result = evaluate(args, model, tokenizer, prefix=global_step)
File "transformers/examples/run_squad.py", line 280, in evaluate
model.config.start_n_top, model.config.end_n_top,
File "/home/wallis/.local/lib/python3.7/site-packages/torch/nn/modules/module.py", line 585, in __getattr__
type(self).__name__, name))
AttributeError: 'DataParallel' object has no attribute 'config'
```
## Expected behavior
Calculate scores and prints them.
## Possible suggestion for parallel use:
Change that line 280 in run_squad.py to:
```
model.module.config.start_n_top, model.module.config.end_n_top,
```
as suggested [here](https://discuss.pytorch.org/t/dataparallel-throws-an-error-attributeerror-dataparallel-object-has-no-attribute-loss/34228).
With this change, it seems to progress but only reach another
[error](https://github.com/huggingface/transformers/issues/1771)
so not sure.
<!-- A clear and concise description of what you expected to happen. -->
## Environment
* OS: ubuntu 16.04 on azure
* Python version: 3.7
* PyTorch version: 1.3.1
* PyTorch Transformers version (or branch): 2.2.0
* Using GPU ? yes
* Distributed of parallel setup ? I think its trying to do parallel
* Any other relevant information:
## Additional context
<!-- Add any other context about the problem here. -->
| 12-03-2019 18:12:12 | 12-03-2019 18:12:12 | Having exactly same error when updating to transformers v2.2.1, have you fix the bug yet?
`12/05/2019 08:57:41 - INFO - __main__ - Saving features into cached file ./datasets/SQuAD/cached_dev_xlnet-base-cased_384
12/05/2019 08:57:53 - INFO - __main__ - ***** Running evaluation *****
12/05/2019 08:57:53 - INFO - __main__ - Num examples = 12551
12/05/2019 08:57:53 - INFO - __main__ - Batch size = 32
Evaluating: 100%|████████████████████████████████████████████████████████████████████████████████████████████████████████████████████████████████████████████████████████████████████████████████████████| 393/393 [04:08<00:00, 1.58it/s]
12/05/2019 09:02:01 - INFO - __main__ - Evaluation done in total 248.621486 secs (0.019809 sec per example)
Traceback (most recent call last):
File "./examples/run_squad.py", line 578, in <module>
main()
File "./examples/run_squad.py", line 567, in main
result = evaluate(args, model, tokenizer, prefix=global_step)
File "./examples/run_squad.py", line 283, in evaluate
model.config.start_n_top, model.config.end_n_top,
File "/root/workspace/renqian/kzs/anaconda3/lib/python3.7/site-packages/torch/nn/modules/module.py", line 585, in __getattr__
type(self).__name__, name))
AttributeError: 'DataParallel' object has no attribute 'config'`<|||||>The suggested fix gets pass that step.
However, the evaluation step errors (as linked) and the model output is junk.
By which I mean, I ran the output model on my own evaluation script and it gave junk answers.
I don't understand why the training script run_squad is organized like it is, why the comments refer only to xlnet, yet apply to xlm also (in a separate script it says the heads of xlnet and xlm are the same...), or why xlm has two classes: ".XLMForQuestionAnswering" and "XLMForQuestionAnsweringSimple", or etc...
I'm sure people had good reasons for all these things, but they aren't apparent to me.
I posted this as a solidarity search cos I couldn't find anyone else saying they ran into this problem.
If someone knows where to find the script that the XLM authors used to train for squad, please share.<|||||>Hi! A very big SQuAD refactor was done these past few weeks, and the issue you're talking about with `DataParallel` was fixed. You can try the new `run_squad` script (make sure you install the library from source beforehand as it leverages several important and recent abstractions).
As for your other questions, I'll try to answer as best as I can:
>With this change, it seems to progress but only reach another
error
This error was patched as well with the new `run_squad` script.
> I don't understand why the training script run_squad is organized like it is, why the comments refer only to xlnet, yet apply to xlm also
It was organized like it was because models were added separately. I agree that as more models were added, there was a discrepancy between the comments and the code. The comments should be more understandable as of now.
> why xlm has two classes: ".XLMForQuestionAnswering" and "XLMForQuestionAnsweringSimple"
This is the case for both XLNet and XLM. Models that are used with question answering heads (like BERT or RoBERTa) usually add a simple linear layer on top of the transformer model. This linear layer gets as input the transformer outputs, and outputs logits corresponding to the beginning and end of the predicted sequence.
This is not the case with either XLNet or XLM, which use much more complex question answering heads. For example, `XLNetForQuestionAnswering` has the [following architecture](https://github.com/huggingface/transformers/blob/master/transformers/modeling_xlnet.py#L1358).
This leads to a difference in outputs: traditional question answering heads output only two values: `(start_logits, end_logits)`, while XLNet and XLM output five values: `(start_top_log_probs, start_top_index, end_top_log_probs, end_top_index, cls_logits)`
This introduces a more complex post-processing, and explains why [two methods are necessary in the `run_squad.py` script](https://github.com/huggingface/transformers/blob/master/examples/run_squad.py#L305-L314): one needs to handle the two outputs and the other one the five outputs.
The models `XXXForQuestionAnsweringSimple` use a simple dense layer like the one used by BERT/RoBERTa. Those models are not currently supported by the `run_squad` script, but they eventually will.
------
We just released the new `run_squad` script this morning and do not have the time nor compute to test it extensively on all the models supported. We would gladly appreciate it if you could share your results when using this script so that we may be aware of improvements that need to be made, especially for newly supported models like XLM.
Let me know if you have any other questions.<|||||>Hey!!
Thanks LysandreJik for your detailed response.
I'm pretty sure that when I ran the code I pip installed transformers from the pypi repository,
but ran the run_squad from a clone of the git repo. Probably not the best idea.
Yeah I saw the refactor this morning and have been going through the code.
Its a huge improvement. Still, there are many bits in [this](https://github.com/huggingface/transformers/blob/master/examples/run_squad.py) like:
* Line 301 queries by model type (quite clear)
* Line 266 queries by number of output commenting on model type (huh, why?)
I was prepping a feature request to push more of the functionality into the ``XXXForQuestionAnswering`` classes.
And I still think it would be so much better if this was done.
Much of the answering cleaning in the squad scripts is useful in application,
and it would remove this persistent if... else conditioning.
I'll post the feature request and maybe we can discuss the merits/ demerits there.
I also saw you released distil-mbert. I tried finetuning that on monday, which worked more or less (I had to drop the --evaluating_during_training as it wasn't happy with it - i didnt record the error, sorry). (Oh, and the bootstrapping to other languages proved to be wishful thinking).
I have previously finetuned distilbert on squad using the example arguments in the docs. The model that is output, when you query it returns 2 tensors (start and end logits, maybe?)
My finetuned distil-Mbert gives those, plus an additional tuple of three other things...
(Which caused me issues integrating it into my own test framework.
And I didn't bother going to find out what they were by this point. )
But it seemed inconsistent for this to happen. <|||||>> do not have the time nor compute to test it extensively on all the models supported. We would gladly appreciate it if you could share your results when using this script so that we may be aware of improvements that need to be made, especially for newly supported models like XLM.
Very happy to. I'm current burning through my free trial accounts on various cloud compute services.
Rather than me saying "I set up a VM, installed these things, ran this code with these parameters, it took n hours and here are my copy and pasted results/ error messages".
How easy would it be to properly formulate/automate this?
Ie instead have a script that takes a ip, port, username, password, and automatically sets up and experiment and formats a report of the results?
This would probably give better quality reporting, and would make my life easier. <|||||>There's a new script, which doesn't get this far, so I'll close this and make a new one. |
transformers | 2,037 | closed | how to select best model in run_glue | ## ❓ Questions & Help
<!-- A clear and concise description of the question. -->
i'm a green hand and i know it is a rediculous problem.I just saw
` # Saving best-practices: if you use defaults names for the model, you can reload it using from_pretrained()
if args.do_train and (args.local_rank == -1 or torch.distributed.get_rank() == 0) and not args.tpu:
# Create output directory if needed
if not os.path.exists(args.output_dir) and args.local_rank in [-1, 0]:
os.makedirs(args.output_dir)`
in row 526,but it seems like that i can hardly find any word about selecting the best model.Would be very appriciate if you can tell me. | 12-03-2019 14:09:52 | 12-03-2019 14:09:52 | This issue has been automatically marked as stale because it has not had recent activity. It will be closed if no further activity occurs. Thank you for your contributions.
|
transformers | 2,036 | closed | error | ## ❓ Questions & Help
12/03/2019 09:12:25 - INFO - transformers.modeling_utils - loading weights file model_check_points112/pytorch_model.bin
12/03/2019 09:12:40 - INFO - __main__ - Creating features from dataset file at dev-v1.1.json
Traceback (most recent call last):
File "run_squad.py", line 558, in <module>
main()
File "run_squad.py", line 547, in main
result = evaluate(args, model, tokenizer, prefix=global_step)
File "run_squad.py", line 195, in evaluate
dataset, examples, features = load_and_cache_examples(args, tokenizer, evaluate=True, output_examples=True)
File "run_squad.py", line 296, in load_and_cache_examples
version_2_with_negative=args.version_2_with_negative)
File "/content/drive/My Drive/examples/utils_squad.py", line 97, in read_squad_examples
input_data = json.load(reader)["data"]
File "/usr/lib/python3.6/json/__init__.py", line 299, in load
parse_constant=parse_constant, object_pairs_hook=object_pairs_hook, **kw)
File "/usr/lib/python3.6/json/__init__.py", line 354, in loads
return _default_decoder.decode(s)
File "/usr/lib/python3.6/json/decoder.py", line 339, in decode
obj, end = self.raw_decode(s, idx=_w(s, 0).end())
File "/usr/lib/python3.6/json/decoder.py", line 355, in raw_decode
obj, end = self.scan_once(s, idx)
json.decoder.JSONDecodeError: Unterminated string starting at: line 1 column 4194293 (char 4194292)
| 12-03-2019 11:10:48 | 12-03-2019 11:10:48 | Please, post the command and all the parameters in order to understand deeply your problem. Moreover, please specify your environment (e.g. Python version, PyTorch version, TensorFlow version, Transformers version, OS).
> ## Questions & Help
> 12/03/2019 09:12:25 - INFO - transformers.modeling_utils - loading weights file model_check_points112/pytorch_model.bin
> 12/03/2019 09:12:40 - INFO - **main** - Creating features from dataset file at dev-v1.1.json
> Traceback (most recent call last):
> File "run_squad.py", line 558, in
> main()
> File "run_squad.py", line 547, in main
> result = evaluate(args, model, tokenizer, prefix=global_step)
> File "run_squad.py", line 195, in evaluate
> dataset, examples, features = load_and_cache_examples(args, tokenizer, evaluate=True, output_examples=True)
> File "run_squad.py", line 296, in load_and_cache_examples
> version_2_with_negative=args.version_2_with_negative)
> File "/content/drive/My Drive/examples/utils_squad.py", line 97, in read_squad_examples
> input_data = json.load(reader)["data"]
> File "/usr/lib/python3.6/json/**init**.py", line 299, in load
> parse_constant=parse_constant, object_pairs_hook=object_pairs_hook, **kw)
> File "/usr/lib/python3.6/json/**init**.py", line 354, in loads
> return _default_decoder.decode(s)
> File "/usr/lib/python3.6/json/decoder.py", line 339, in decode
> obj, end = self.raw_decode(s, idx=_w(s, 0).end())
> File "/usr/lib/python3.6/json/decoder.py", line 355, in raw_decode
> obj, end = self.scan_once(s, idx)
> json.decoder.JSONDecodeError: Unterminated string starting at: line 1 column 4194293 (char 4194292)<|||||>solved.its working now<|||||>> solved.its working now
How do you solve this issue? I have the same errors <|||||>I am also getting same error. How you solved it?
<|||||>just remove non-asciii character from your data-set
On Tue, 9 Jun 2020 at 20:29, Amar Wagh <[email protected]> wrote:
> I am also getting same error. How you solved it?
>
> —
> You are receiving this because you modified the open/close state.
> Reply to this email directly, view it on GitHub
> <https://github.com/huggingface/transformers/issues/2036#issuecomment-641353851>,
> or unsubscribe
> <https://github.com/notifications/unsubscribe-auth/AIPNMU4BGBNGCNIEFPHRITDRVZE6NANCNFSM4JUWHE5A>
> .
>
|
transformers | 2,035 | closed | Doubts on modeling_gpt2.py | ## ❓ Questions & Help
<!-- A clear and concise description of the question. -->
Hi, I've been going through the gpt2 source code and i was tracing out how the self attention and feed forward work basically we have `Block` which is a decoder consisting of other 2 segments `Attention` and `MLP`. I was also reading a blog where it mentions the `queries` has to be learned i finally saw the class responsible for that is `Conv1D`
```
class Conv1D(nn.Module):
def __init__(self, nf, nx):
""" Conv1D layer as defined by Radford et al. for OpenAI GPT (and also used in GPT-2)
Basically works like a Linear layer but the weights are transposed
"""
super(Conv1D, self).__init__()
self.nf = nf
w = torch.empty(nx, nf)
nn.init.normal_(w, std=0.02)
self.weight = nn.Parameter(w)
self.bias = nn.Parameter(torch.zeros(nf))
def forward(self, x):
size_out = x.size()[:-1] + (self.nf,)
x = torch.addmm(self.bias, x.view(-1, x.size(-1)), self.weight)
x = x.view(*size_out)
return x
```
Here we have a affine function `addmm` is how we do... but i was expecting `train optimer.step, loss` basically all the thing that goes into training in pytorch. Can anyone elaborate on it?
Then in `GPT2LMHeadModel` we use a linear layer to represent `vocab_embed` and we produce `logits` by multiplying it with the transformer output so if the linear layer is trained what is the use of `from_pretrained` anyway?
I am sure this may perhaps be silly questions but i'd like to get some help here.
Thanks a lot. | 12-03-2019 10:43:43 | 12-03-2019 10:43:43 | Can anyone answer it please...<|||||>This issue has been automatically marked as stale because it has not had recent activity. It will be closed if no further activity occurs. Thank you for your contributions.
|
transformers | 2,034 | closed | Updated examples/README and parser for run_summarization_finetuning | 1. Updated `examples/README.md` to change default `--model_type` and `--model_name_or_path` to `bert` and `bert_base_cased` because `bert2bert` just won't work
2. Updated `examples/run_summarization_finetuning.py` parser to take in `--do-train` instead of `--do-train=True` for consistency with other examples and `--model_type` + `--model_name_or_path`
3. Changed `add_special_tokens_single_sequence` to `build_inputs_with_special_tokens` in `examples/utils_summarization.py` | 12-03-2019 09:44:24 | 12-03-2019 09:44:24 | Let's wait that the summarization script is finalized before merging this. |
transformers | 2,033 | closed | run_lm_finetuning.py script CLM inputs and labels preparing | I'm trying to finetune the GPT-2 on my own dataset, while I'm reading the code in `run_lm_finetuning.py` script, I found a weird thing in line `227`. When the script preparing CLM batch inputs and labels, it gives the model the same `batch` variable as inputs and labels:
```
inputs, labels = mask_tokens(batch, tokenizer, args) if args.mlm else (batch, batch)
```
In this way, the model will learn to take the token as an input and predict it directly, right?
Can anyone explain what happens? | 12-03-2019 07:16:51 | 12-03-2019 07:16:51 | Ok, in [modeling_gpt2.py](https://github.com/huggingface/transformers/blob/master/transformers/modeling_gpt2.py) file I found this comment in line `495`:
```
Note that the labels **are shifted** inside the model, i.e. you can set ``lm_labels = input_ids``
```
So, the model takes care of the shifting process. |
transformers | 2,032 | closed | Any workaround to extend the embeddings on TFGPT2DoubleHeadsModel? | Getting access to Keras' `model.fit()` method makes life so much easier for transfer learning/fine-tuning, but TFGPT2DoubleHeadsModel doesn't currently support extending embeddings, so it really restricts practical applications. You almost always have to add something to the vocabulary / generate special tokens. Does anyone know of a workaround? | 12-03-2019 04:58:59 | 12-03-2019 04:58:59 | This issue has been automatically marked as stale because it has not had recent activity. It will be closed if no further activity occurs. Thank you for your contributions.
|
transformers | 2,031 | closed | Typo in modeling_albert.py for mask_token | ## 🐛 Bug
<!-- Important information -->
Model I am using (Bert, XLNet....): Albert
Language I am using the model on (English, Chinese....): English
## To Reproduce
```
tokenizer_class, pretrained_weights = AlbertTokenizer, "albert-base-v1"
tokenizer = tokenizer_class.from_pretrained(pretrained_weights)
print(tokenizer.mask_token) # [MASK]>
print(tokenizer.mask_token_id) # 1 (same as <unk>)
```
I think the typo lies here https://github.com/huggingface/transformers/blob/fbaf05bd92249b6dd961f5f8d60eb0892c541ac8/transformers/tokenization_albert.py#L69
<!-- If you have a code sample, error messages, stack traces, please provide it here as well. -->
## Expected behavior
mask_token should be "[MASK]" and mask_token_id should be 4
## Environment
* OS: Windows 10
* Python version: 3.6.9
* PyTorch version: 1.2.0
* PyTorch Transformers version (or branch): 2.2.0
| 12-03-2019 04:07:14 | 12-03-2019 04:07:14 | Indeed, good catch, thanks! Fixed on master. |
transformers | 2,030 | closed | cannot import name 'WEIGHTS_NAME' | ## 🐛 Bug
<!-- Important information -->
Model I am using (Bert, XLNet....): gpt2
Language I am using the model on (English, Chinese....): english
The problem arise when using:
* [X] the official example scripts: `run_lm_finetuning.py`
* [ ] my own modified scripts: (give details)
## To Reproduce
Steps to reproduce the behavior:
1. Obtain `transformers` from zip file on github.
2. try to run `run_lm_finetuning.py` using the example in the documentation.
<!-- If you have a code sample, error messages, stack traces, please provide it here as well. -->
``` python
Traceback (most recent call last):
File "run_lm_finetuning.py", line 45, in <module>
from transformers import (WEIGHTS_NAME, AdamW, get_linear_schedule_with_warmup,
ImportError: cannot import name 'WEIGHTS_NAME' from 'transformers' (unknown location)
```
## Environment
* OS: Ubuntu 18.04.3
* Python version: 3.7.3
* PyTorch version: 1.3.1+cpu
* PyTorch Transformers version (or branch): whichever version is included in the zip file off github
* Using GPU ? No
* Distributed of parallel setup ? No
* Any other relevant information: None.
## Additional context
<!-- Add any other context about the problem here. -->
I tried to obtain transformers from source using `git clone https://github.com/huggingface/transformers.git` but I got a timeout error (which is why I opted to try to zip file).
| 12-02-2019 23:43:40 | 12-02-2019 23:43:40 | This is **not** a bug! It works as expected.
```
> from transformers import WEIGHTS_NAME
>
```
I've tried with the latest version of Transformers, installed with `pip install transformers`
The variable _WEIGHTS_NAME_ is located in [file_utils.py](https://github.com/huggingface/transformers/blob/49108288ba6e6dcfe554d1af98699ae7a1e6f39c/transformers/file_utils.py)
> ## Bug
> Model I am using (Bert, XLNet....): gpt2
>
> Language I am using the model on (English, Chinese....): english
>
> The problem arise when using:
>
> * [x] the official example scripts: `run_lm_finetuning.py`
> * [ ] my own modified scripts: (give details)
>
> ## To Reproduce
> Steps to reproduce the behavior:
>
> 1. Obtain `transformers` from zip file on github.
> 2. try to run `run_lm_finetuning.py` using the example in the documentation.
>
> ```python
> Traceback (most recent call last):
> File "run_lm_finetuning.py", line 45, in <module>
> from transformers import (WEIGHTS_NAME, AdamW, get_linear_schedule_with_warmup,
> ImportError: cannot import name 'WEIGHTS_NAME' from 'transformers' (unknown location)
> ```
>
> ## Environment
> * OS: Ubuntu 18.04.3
> * Python version: 3.7.3
> * PyTorch version: 1.3.1+cpu
> * PyTorch Transformers version (or branch): whichever version is included in the zip file off github
> * Using GPU ? No
> * Distributed of parallel setup ? No
> * Any other relevant information: None.
>
> ## Additional context
> I tried to obtain transformers from source using `git clone https://github.com/huggingface/transformers.git` but I got a timeout error (which is why I opted to try to zip file).<|||||>Hmm, the error is removed when I use the `pip` version as well but remains with the zipped version. I'll close this out and rely on the version that comes from `pip`. |
transformers | 2,029 | closed | gpt-2 generation examples | ## ❓ Questions & Help
Hi! Thanks for everything, I want to try generation with the gpt-2 model, following:
```
python ./examples/run_generation.py \
--model_type=gpt2 \
--length=20 \
--model_name_or_path=gpt2 \
```
But it does not seem to work very well, for example (Prompt -> Generation):
i go to -> the Kailua Islands? Eh? Ahh. Although they did say the
i like reading -> -_-/- 40:25:13 7d 9h 25m We battle trainer. Before we
i like running -> from someone which can easily overwhelm your battery in those moments and through the rest of your day
I mean, the generation don't really look good to me, is that anything I should mind during trying this?
Thanks!
Additional info:
`12/02/2019 15:41:46 - INFO - __main__ - Namespace(device=device(type='cuda'), length=20, model_name_or_path='gpt2', model_type='gpt2', n_gpu=1, no_cuda=False, num_samples=1, padding_text='', prompt='', repetition_penalty=1.0, seed=42, stop_token=None, temperature=1.0, top_k=0, top_p=0.9, xlm_lang='')` | 12-02-2019 20:44:19 | 12-02-2019 20:44:19 | You can tune the value for **temperature** and **seed**. **Temperature** is a hyper-parameter used to control the randomness of predictions by scaling the logits before applying softmax.
- when temperature is a small value (e.g. 0,2), the GPT-2 model is more confident but also more conservative
- when temperature is a large value (e.g. 1), the GPT-2 model produces more diversity and also more mistakes
If I were you, I'll change the temperature value down to 0,2 or 0,3 and see what happens (i.e. the result is what you want).
N.B: if you want (and you can), it is more preferably to use CPUs over GPUs for inference.
> ## Questions & Help
> Hi! Thanks for everything, I want to try generation with the gpt-2 model, following:
>
> ```
> python ./examples/run_generation.py \
> --model_type=gpt2 \
> --length=20 \
> --model_name_or_path=gpt2 \
> ```
>
> But it does not seem to work very well, for example (Prompt -> Generation):
> i go to -> the Kailua Islands? Eh? Ahh. Although they did say the
> i like reading -> -_-/- 40:25:13 7d 9h 25m We battle trainer. Before we
> i like running -> from someone which can easily overwhelm your battery in those moments and through the rest of your day
>
> I mean, the generation don't really look good to me, is that anything I should mind during trying this?
> Thanks!
>
> Additional info:
> `12/02/2019 15:41:46 - INFO - __main__ - Namespace(device=device(type='cuda'), length=20, model_name_or_path='gpt2', model_type='gpt2', n_gpu=1, no_cuda=False, num_samples=1, padding_text='', prompt='', repetition_penalty=1.0, seed=42, stop_token=None, temperature=1.0, top_k=0, top_p=0.9, xlm_lang='')`<|||||>@TheEdoardo93
Thanks for the reply, I tried temperature 0.2 or topk 20, the generation does makes more sense to me.
But one thing that's still mysterious to me is that it loves to generate a lot of **line breaks**, do you have any intuition why that's happening?
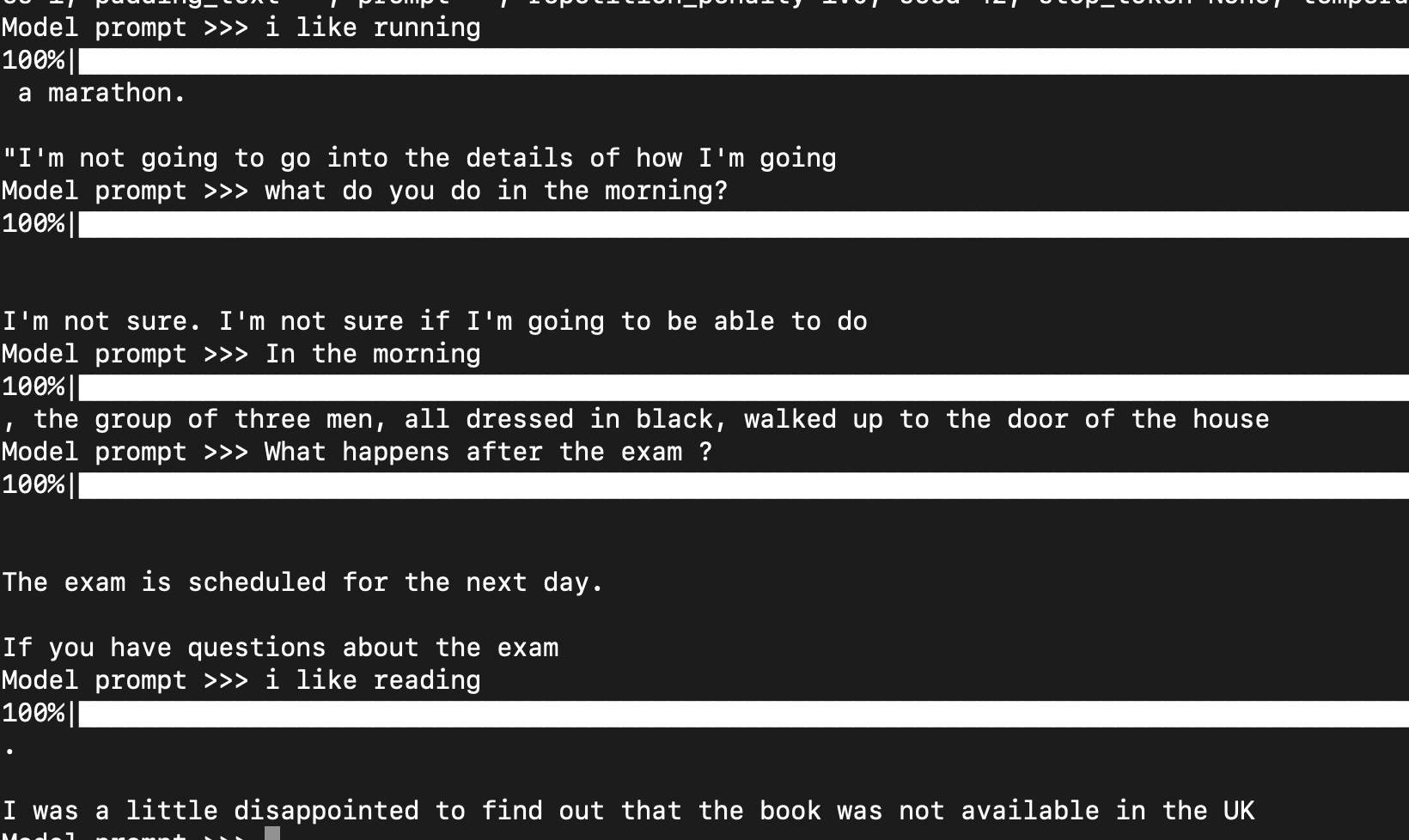
Also, could you also explain why it is more preferably to use CPUs over GPUs for inference?
Thanks!<|||||>Typically, if you have small-medium models (in terms of hyper-parameters), it's common to use CPUs for inference; GPUs are well suited for training large models. In general, it's up to you the choice to use CPU or GPU in inference mode. It depends on different factors: for example if you have a requirements of larger batches in the fastest way, you have to use GPU, but if you don't have such requirements of speed and batches, you can use CPU.
Source: my opinion on this topic :D
> @TheEdoardo93
> Thanks for the reply, I tried temperature 0.2 or topk 20, the generation does makes more sense to me.
> But one thing that's still mysterious to me is that it loves to generate a lot of **line breaks**, do you have any intuition why that's happening?
> 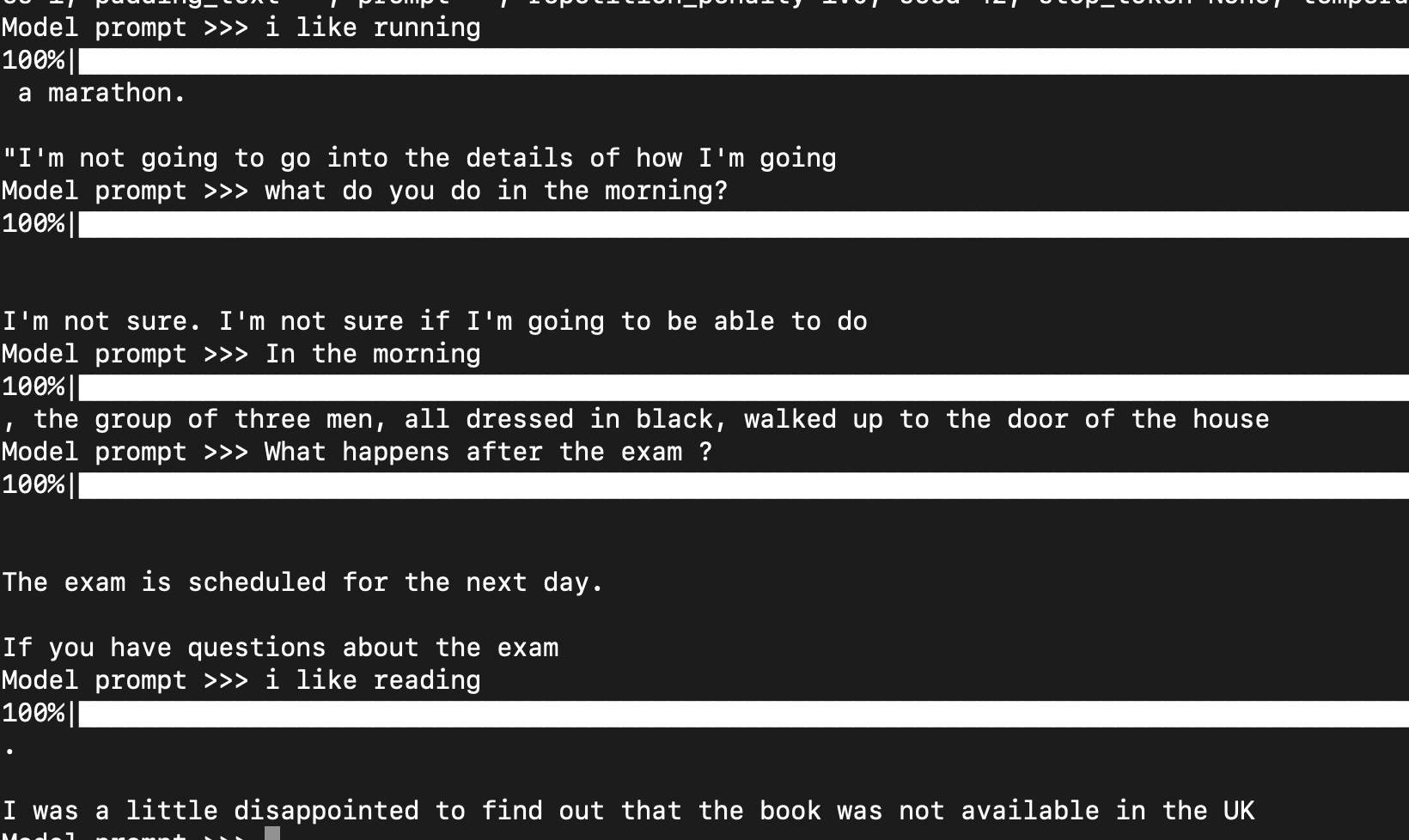
>
> Also, could you also explain why it is more preferably to use CPUs over GPUs for inference?
>
> Thanks!<|||||>I'm still wondering about the line breaks and whether there's any thing I can do about that. Thanks~<|||||>I believe the line breaks are due to your context. You're simulating dialog, which is often represented as a sentence followed by line breaks, followed by another entity's response.
If you give the model inputs that are similar to traditionally long texts (e.g. Wikipedia articles), you're bound to have generations not split by line returns.<|||||>>
>
> I'm still wondering about the line breaks and whether there's any thing I can do about that. Thanks~
You can actually use [bad_words_id](https://github.com/huggingface/transformers/blob/5ab21b072fa2a122da930386381d23f95de06e28/src/transformers/generation_tf_utils.py#L122) parameter with a line break, which will prevent [generate function](https://github.com/huggingface/transformers/blob/5ab21b072fa2a122da930386381d23f95de06e28/examples/text-generation/run_generation.py#L252) from giving you results, which contain "\n". (though you'd probably have to add every id from your vocab, which has line breaks in it, since I do think there tends to be more than one "breaking" sequence out there...) |
transformers | 2,028 | closed | [CamemBERT] Potential error in the docs | Thanks for the great work on this repo! As I was going through the details about the available pre-trained models (https://huggingface.co/transformers/v2.2.0/pretrained_models.html), I spotted what I think is an error in the description of camembert-base (12-layer, 768-hidden, 12-heads, 110M parameters; CamemBERT using the BERT-base architecture). Isn't it RoBERTa-based?
| 12-02-2019 17:00:42 | 12-02-2019 17:00:42 | RoBERTa and BERT (and CamemBERT) share mostly the same model architecture. Most of the differences lie in:
- the tokenizers
- the pre-training method
cc @LysandreJik <|||||>Cool, thanks for the reply! :) |
transformers | 2,027 | closed | Tokenization differs for different intepreter instances | ## 🐛 Bug
<!-- Important information -->
Tokenization of `" "` changes for each python interpreter instance.
## To Reproduce
```python
from transformers import BertTokenizer
tokenizer = BertTokenizer.from_pretrained('bert-base-uncased')
for i in range(5):
print(tokenizer.encode(" "))
```
## Environment
* Python version: 3.7.2
* PyTorch version: 1.3.1
* PyTorch Transformers version (or branch): 2.2.0 | 12-02-2019 14:20:38 | 12-02-2019 14:20:38 | By using **Python 3.6.9**, the results is the following:
```
> from transformers import BertTokenizer
> tokenizer = BertTokenizer.from_pretrained('bert-base-uncased')
> for i in range(5):
print(tokenizer.encode(" "))
>>> [101, 100, 102]
[101, 100, 102]
[101, 100, 102]
[101, 100, 102]
[101, 100, 102]
```
I don't understand your question very much. Are you saying that with Python v.X the output of the code you've posted is different from that with Python v.Y?
> ## Bug
> Tokenization of `" "` changes for each python interpreter instance.
>
> ## To Reproduce
> ```python
> from transformers import BertTokenizer
>
> tokenizer = BertTokenizer.from_pretrained('bert-base-uncased')
> for i in range(5):
> print(tokenizer.encode(" "))
> ```
>
> ## Environment
> * Python version: 3.7.2
> * PyTorch version: 1.3.1
> * PyTorch Transformers version (or branch): 2.2.0<|||||>No, if I execute this code in a script, the output differs each time.
For example:
```
# first run
[101, 0, 102]
[101, 0, 102]
[101, 0, 102]
[101, 0, 102]
[101, 0, 102]
# second run
[101, 100, 102]
[101, 100, 102]
[101, 100, 102]
[101, 100, 102]
[101, 100, 102]
```<|||||>I've noticed this "bug" right now.
Sometimes, the whitespace character " " is encoded with token with ID=100, other times with token with ID= 103. After looking "into" the `tokenizer.vocab` variable, I've seen that:
- token with ID = 0 is **'[PAD]'**
- token with ID = 100 is **'[UNK]'**
- token with ID = 103 is **'[MASK]'**
**1st run:**
```
> from transformers import BertTokenizer
> tokenizer = BertTokenizer.from_pretrained('bert-base-uncased')
> for i in range(5):
... print(tokenizer.encode(" "))
>>> [101, 100, 102]
[101, 100, 102]
[101, 100, 102]
[101, 100, 102]
[101, 100, 102]
```
**2nd run:**
```
> from transformers import BertTokenizer
> tokenizer = BertTokenizer.from_pretrained('bert-base-uncased')
> for i in range(5):
... print(tokenizer.encode(" "))
>>> [101, 103, 102]
[101, 103, 102]
[101, 103, 102]
[101, 103, 102]
[101, 103, 102]
```
> No, if I execute this code in a script, the output differs each time.
> For example:
>
> ```
> # first run
> [101, 0, 102]
> [101, 0, 102]
> [101, 0, 102]
> [101, 0, 102]
> [101, 0, 102]
>
> # second run
> [101, 100, 102]
> [101, 100, 102]
> [101, 100, 102]
> [101, 100, 102]
> [101, 100, 102]
> ```<|||||>Feel like this is a non-deterministic behavior arising from encoding an empty sentence.
Do you have a real-world use case for encoding empty sentence?<|||||>I guess we could catch this case before using the model, but a deterministic behaviour would still be neat.
If `" "` is the same as `""`, the tokenizer should just return an empty list right?
<|||||>fixed in #2081 |
transformers | 2,026 | closed | Does GPT2LMHeadModel need <|startoftext|> and <|endoftext|> tokens? | ## ❓ Does GPT2LMHeadModel need <|startoftext|> and <|endoftext|> tokens?
Hey!
I'm using GPT2LMHeadModel to get a good representation of a Language Model - I want to get probabilities for each word. The problem is - the **model predicts probabilities very well for all tokens except for the first one**. The first's token probability is often very small no matter what word I choose.
I've read that there is "<|startoftext|>" token, but have not found information on how to use it. It also doesn't exist in GPT2Tokenizer.vocabulary. Do we have to use it?
### Example code:
```
import torch
from pytorch_transformers import *
pretrained_weights='gpt2'
tokenizer = GPT2Tokenizer.from_pretrained(pretrained_weights)
model = GPT2LMHeadModel.from_pretrained(pretrained_weights)
model.eval()
def show_probabilities(INPUT_TEXT):
input_ids = torch.tensor([tokenizer.encode(INPUT_TEXT)])
with torch.no_grad():
index=0
outputs = model(input_ids=input_ids)
logits = outputs[0][0]
probs = torch.softmax(logits, 1)
for index in range(0, len(input_ids[0])):
token_id = input_ids[0][index]
probability = probs[index - 1][token_id].item()
print(f"Probability for the token \"{tokenizer.decode(token_id.item())}\" is {probability}")
print("\n")
show_probabilities('To be or not to be <|endoftext|>')
show_probabilities('<|startoftext|> To be or not to be <|endoftext|>')
show_probabilities('<|endoftext|> To be or not to be <|endoftext|>')
show_probabilities('Hello world is so wierd?')
```
### Output:
###### (so that you dont have to run it)
```
Probability for the token " To" is 6.045737421800368e-08
Probability for the token " be" is 0.01369183138012886
Probability for the token " or" is 0.0001948970602825284
Probability for the token " not" is 0.7490634322166443
Probability for the token " to" is 0.5098284482955933
Probability for the token " be" is 0.9639962911605835
Probability for the token "<|endoftext|>" is 0.00017062896222341806
Probability for the token " <" is 1.5030431086415774e-06
Probability for the token "|" is 0.0006586791132576764
Probability for the token "start" is 7.143173570511863e-05
Probability for the token "of" is 0.0012107481015846133
Probability for the token "text" is 0.0007207148591987789
Probability for the token "|" is 0.4524894058704376
Probability for the token ">" is 0.027218399569392204
Probability for the token " To" is 0.0003593114379327744
Probability for the token " be" is 0.015610950998961926
Probability for the token " or" is 0.0021431492641568184
Probability for the token " not" is 0.46310704946517944
Probability for the token " to" is 0.8615797162055969
Probability for the token " be" is 0.9770862460136414
Probability for the token "<|endoftext|>" is 0.0008418861543759704
Probability for the token "<|endoftext|>" is 3.0863736810715636e-06
Probability for the token " To" is 3.549279790604487e-05
Probability for the token " be" is 0.04548846557736397
Probability for the token " or" is 0.0003993543505202979
Probability for the token " not" is 0.8718274831771851
Probability for the token " to" is 0.9372356534004211
Probability for the token " be" is 0.9853253960609436
Probability for the token "<|endoftext|>" is 0.0009108908707275987
Probability for the token " Hello" is 0.00041539999074302614
Probability for the token " world" is 0.00014912338519934565
Probability for the token " is" is 0.029302824288606644
Probability for the token " so" is 0.01128558162599802
Probability for the token " w" is 0.00020273651171009988
Probability for the token "ier" is 0.008098911494016647
Probability for the token "d" is 0.8924543857574463
Probability for the token "?" is 0.0036612364929169416
```
| 12-02-2019 14:17:38 | 12-02-2019 14:17:38 | Huggingface GPT2's default beggining of sentence token is `<|endoftext|>`, not `<|startoftext|>` as mentioned [here](https://huggingface.co/transformers/model_doc/gpt2.html#gpt2tokenizer). So either just use `<|endoftext|>` or replace tokenizer's default `bos` attribute with `<|startoftext|>`. Or you may add `<|startoftext|>` as `additional_speacial_token` (read more [here](https://huggingface.co/transformers/main_classes/tokenizer.html#transformers.PreTrainedTokenizer.add_special_tokens)). As seen in the part you provide above, GPT2 tokenizer splits `<|startoftext|>` to byte-pairs. So you need to specify it as either one of special tokens or as additional special token.<|||||>Thank you, it really helps!
To give more insight for future dwellers:
I also found the [code](https://github.com/huggingface/transformers/blob/fbaf05bd92249b6dd961f5f8d60eb0892c541ac8/transformers/tokenization_gpt2.py#L119-L121) for tokenization_gpt2 that uses bos/eos/unk tokens and an [example](https://github.com/huggingface/transformers/blob/fbaf05bd92249b6dd961f5f8d60eb0892c541ac8/transformers/tokenization_utils.py#L577-L589) of using `<CLS>` token.
I've run tests with adding `<CLS>` token, `<|startoftext|>` token and `<|endoftext|>` token.
While adding `<CLS>` or `<|startoftext|>` at the beginning of the sentence raises the probability of the first token 10<sup>3</sup> times greater, results differ a little bit ("To" was 6.04e-8, now is 9.51e-5 or 7,67e-5). But it means we can just use `<|endofsentence|>` at the beginning and it will work too.
Adding `<|endoftext|>` token at the end in GPT2LMHeadModel doesn't change the resulting probabilities, but I haven't checked how it influences text prediction.
<|||||>> The problem is - the model predicts probabilities very well for all tokens except for the first one.
I think you should start the for loop from `1` instead of `0` otherwise you will access `probs[-1]` which is not correct. If you add the `bos` token, intuitively this means that you don't consider the probability of the `bos` token in your summation (which you can't have anyway).
I published a (hopefully) corrected and vectorized version of your code together with [`lm-scorer`](https://github.com/simonepri/lm-scorer).
> Adding <|endoftext|> token at the end in GPT2LMHeadModel doesn't change the resulting probabilities, but I haven't checked how it influences text prediction.
I actually observed the opposite.
In the following example, you can see that the sentence without the dot at the end of the sentence has a lower probability than the (correct) one with the correct punctuation. Without the `eos` the incorrect one would have higher probability instead.
```bash
$ lm-scorer -t - <<< """I like it.
I like it"""
I 0.018321
Ġlike 0.0066431
Ġit 0.042104
. 0.23876
<|endoftext|> 0.0067232
I 0.018321
Ġlike 0.0066431
Ġit 0.042104
<|endoftext|> 0.00023855
$lm-scorer - <<< """I like it.
I like it"""
I like it. 8.2257e-09
I like it 1.2224e-09
```
More tests [here](https://github.com/simonepri/lm-scorer/blob/master/tests/models/test_gpt2.py#L32-L239). |
transformers | 2,025 | closed | How to convert a tf2 pre-trained model to pytorch model? | ## ❓ Questions & Help
<!-- A clear and concise description of the question. -->
I have trained a pre-trained model from scratch using a tensorflow 2.0 official script (run_pretraining.py).
https://github.com/tensorflow/models/tree/master/official/nlp/bert
My question is how to convert the pre-trained model to pytorch model?
Thanks in advance. | 12-02-2019 13:45:14 | 12-02-2019 13:45:14 | Have you ever tried [convert_bert_original_tf_checkpoint_to_pytorch.py](https://github.com/huggingface/transformers/blob/1ab8dc44b3d84ed1894f5b6a6fab58fb39298fc7/transformers/convert_bert_original_tf_checkpoint_to_pytorch.py)?
> ## Questions & Help
> I have trained a pre-trained model from scratch using a tensorflow 2.0 official script (run_pretraining.py).
> https://github.com/tensorflow/models/tree/master/official/nlp/bert
>
> My question is how to convert the pre-trained model to pytorch model?
> Thanks in advance.<|||||>Thanks for your comment.
This script is for tensorflow 1.0.
https://github.com/google-research/bert
The weight names are different between tf 1.0 and 2.0, and this script does not work for a tf2 pre-trained model.<|||||>If you have enough time, you can implement it, open a PR and share your source code with us
> Thanks for your comment.
>
> This script is for tensorflow 1.0.
> https://github.com/google-research/bert
>
> The weight names are different between tf 1.0 and 2.0, and this script does not work for a tf2 pre-trained model.<|||||>OK, I will try it.
As a tentative workaround I will ask how to convert a tf2 pre-trained model to a tf1 model in the official tensorflow BERT project.<|||||>This issue has been automatically marked as stale because it has not had recent activity. It will be closed if no further activity occurs. Thank you for your contributions.
<|||||>@tomohideshibata did you ever succeed in converting your model to pytorch or tf1?
edit: seems it was added already to `transformers` in #5791<|||||>No.
Thanks for the information. |
transformers | 2,024 | closed | [ALBERT] : ValueError: Layer #1 (named "predictions") expects 11 weight(s), but the saved weights have 10 element(s). | ## 🐛 Bug
<!-- Important information -->
Model I am using (Bert, XLNet....): ALBERT
Language I am using the model on (English, Chinese....): English
The problem arise when using:
* [ ] the official example scripts: (give details)
* [ ] my own modified scripts: (give details)
import tensorflow as tf
from transformers import *
#Download AlbertMaskedLM model
model = TFAlbertForMaskedLM.from_pretrained('albert-large-v2')
The tasks I am working on is:
* [ ] an official GLUE/SQUaD task: (give the name)
* [ ] my own task or dataset: (give details) Initial validation
## To Reproduce
Steps to reproduce the behavior:
import tensorflow as tf
from transformers import *
#Download AlbertMaskedLM model
model = TFAlbertForMaskedLM.from_pretrained('albert-large-v2')
1.
2.
3.
<!-- If you have a code sample, error messages, stack traces, please provide it here as well. -->
The code throws an error as follows :
100%|██████████| 484/484 [00:00<00:00, 271069.99B/s]
100%|██████████| 87059544/87059544 [00:03<00:00, 28448930.07B/s]
---------------------------------------------------------------------------
ValueError Traceback (most recent call last)
<ipython-input-28-a2c768b76a32> in <module>()
----> 1 model = TFAlbertForMaskedLM.from_pretrained('albert-large-v2')
3 frames
/usr/local/lib/python3.6/dist-packages/transformers/modeling_tf_utils.py in from_pretrained(cls, pretrained_model_name_or_path, *model_args, **kwargs)
287 # 'by_name' allow us to do transfer learning by skipping/adding layers
288 # see https://github.com/tensorflow/tensorflow/blob/00fad90125b18b80fe054de1055770cfb8fe4ba3/tensorflow/python/keras/engine/network.py#L1339-L1357
--> 289 model.load_weights(resolved_archive_file, by_name=True)
290
291 ret = model(model.dummy_inputs, training=False) # Make sure restore ops are run
/usr/local/lib/python3.6/dist-packages/tensorflow_core/python/keras/engine/training.py in load_weights(self, filepath, by_name)
179 raise ValueError('Load weights is not yet supported with TPUStrategy '
180 'with steps_per_run greater than 1.')
--> 181 return super(Model, self).load_weights(filepath, by_name)
182
183 @trackable.no_automatic_dependency_tracking
/usr/local/lib/python3.6/dist-packages/tensorflow_core/python/keras/engine/network.py in load_weights(self, filepath, by_name)
1173 f = f['model_weights']
1174 if by_name:
-> 1175 saving.load_weights_from_hdf5_group_by_name(f, self.layers)
1176 else:
1177 saving.load_weights_from_hdf5_group(f, self.layers)
/usr/local/lib/python3.6/dist-packages/tensorflow_core/python/keras/saving/hdf5_format.py in load_weights_from_hdf5_group_by_name(f, layers)
749 '") expects ' + str(len(symbolic_weights)) +
750 ' weight(s), but the saved weights' + ' have ' +
--> 751 str(len(weight_values)) + ' element(s).')
752 # Set values.
753 for i in range(len(weight_values)):
ValueError: Layer #1 (named "predictions") expects 11 weight(s), but the saved weights have 10 element(s).
## Expected behavior
<!-- A clear and concise description of what you expected to happen. -->
TFAlbertMaskedLM model can not be loaded from pre-trained
## Environment
* OS: Linux (Colab)
* Python version: 3.6
* PyTorch version: Tensorflow 2.0
* PyTorch Transformers version (or branch):
* Using GPU ? Yes
* Distributed of parallel setup ?
* Any other relevant information:
## Additional context
<!-- Add any other context about the problem here. -->
| 12-02-2019 13:04:31 | 12-02-2019 13:04:31 | cc @LysandreJik <|||||>It should be fixed now, thanks for raising an issue.<|||||>Thanks @LysandreJik for your prompt response. The issue mentioned above is resolved but I am getting an error in converting predicted IDs back to token using AlbertTokenizer. Here is the error that I am seeing (pred_index value below is 29324). Please advise or let me know if I should open another issue as original issue has been resolved.
TypeError Traceback (most recent call last)
<ipython-input-26-0151f2884b58> in <module>()
----> 1 pred_token = tokenizer.convert_ids_to_tokens([pred_index])[0]
2 print('Predicted token:', pred_token)
2 frames
/usr/local/lib/python3.6/dist-packages/transformers/tokenization_utils.py in convert_ids_to_tokens(self, ids, skip_special_tokens)
1034 tokens.append(self.added_tokens_decoder[index])
1035 else:
-> 1036 tokens.append(self._convert_id_to_token(index))
1037 return tokens
1038
/usr/local/lib/python3.6/dist-packages/transformers/tokenization_albert.py in _convert_id_to_token(self, index, return_unicode)
172 def _convert_id_to_token(self, index, return_unicode=True):
173 """Converts an index (integer) in a token (string/unicode) using the vocab."""
--> 174 token = self.sp_model.IdToPiece(index)
175 if six.PY2 and return_unicode and isinstance(token, str):
176 token = token.decode('utf-8')
/usr/local/lib/python3.6/dist-packages/sentencepiece.py in IdToPiece(self, id)
185
186 def IdToPiece(self, id):
--> 187 return _sentencepiece.SentencePieceProcessor_IdToPiece(self, id)
188
189 def GetScore(self, id):
TypeError: in method 'SentencePieceProcessor_IdToPiece', argument 2 of type 'int'<|||||>Hmm, I have no issues running this code snippet:
```py
from transformers import AlbertTokenizer
tokenizer = AlbertTokenizer.from_pretrained("albert-large-v2")
print(tokenizer.convert_ids_to_tokens(29324))
# or
print(tokenizer.convert_ids_to_tokens([29324]))
```
Is there a way you could give us a short code sample that reproduces the problem, so that we may debug what's happening? Thank you.<|||||>@LysandreJik thanks for your response. I figured out the issue. Below is the code which reproduces the issue. In the below code, 'pred_index' comes out as numpy.int64 and when placed in 'convert_ids_to_tokens' method, it throws the error mentioned above. If I convert it to an int then it works fine.
Here is the example code to reproduce the issue
# Encode a text inputs
text = "What is the fastest car in the world."
tokenized_text = tokenizer.tokenize(text)
#Get tokenizer
tokenizer = AlbertTokenizer.from_pretrained('albert-base-v2')
#Lets mask 'world' and check if model can predict it
tokenized_text[7] = '[MASK]'
#Convert tokenized text to indexes
indexed_tokens = tokenizer.convert_tokens_to_ids(tokenized_text)
#Download AlbertMaskedLM model
model = TFAlbertForMaskedLM.from_pretrained('albert-base-v2')
#Prediction
inputs = tf.constant(indexed_tokens)[None,:]
outputs = model(inputs)
#Lets check the prediction at index 7 (in place of [MASK])
pred_index = tf.argmax(outputs[0][0,7]).numpy()
pred_token = tokenizer.convert_ids_to_tokens([pred_index])[0]
print('Predicted token:', pred_token)<|||||>Please note that above code works as is for BERT (but throws an error for Albert).<|||||>This is probably the exact same problem than https://github.com/huggingface/transformers/issues/945
If I understand correctly SentencePiece doesn't like numpy integers and crashes. Should we cast it to an int @thomwolf?<|||||>Yes I think so. We can probably just add a `int(idx)` in the base tokenizer class `PretrainedTokenizer` before the call to `_convert_id_to_tokens` so we can even input tensors in addition to np arrays.<|||||>This issue has been automatically marked as stale because it has not had recent activity. It will be closed if no further activity occurs. Thank you for your contributions.
|
transformers | 2,023 | closed | Is it possible to fine-tune models on TPUs using TensorFlow? | I have looked at the release notes and found out that:
"Training on TPU using free TPUs provided in the TensorFlow Research Cloud (TFRC) program is possible but requires to implement a custom training loop (not possible with keras.fit at the moment).
We will add an example of such a custom training loop soon." (Note from September 26).
Is this observation still true? Can we train the transformer models on TPUs in TF? | 12-02-2019 12:39:52 | 12-02-2019 12:39:52 | following, would love to know if this is possible<|||||>We have some code in the `tpu-experiment` branch, for instance here: https://github.com/huggingface/transformers/tree/tpu-experiments/examples/TPU/tensorflow
And planning to make it clean in the mid-term (not sure that will be before the end of the year though).
cc @LysandreJik <|||||>This issue has been automatically marked as stale because it has not had recent activity. It will be closed if no further activity occurs. Thank you for your contributions.
<|||||>Any updates on this one?<|||||>Same here<|||||>This issue has been automatically marked as stale because it has not had recent activity. It will be closed if no further activity occurs. Thank you for your contributions.
<|||||>Same here <|||||>bump! Would love this! If you guys have your hands full, let me know if I can help in anyway :)<|||||>Hi! We recently have updated all of our scripts with `Trainer` classes, for both TensorFlow and PyTorch. Both trainers now have TPU support!
The [examples README](https://github.com/huggingface/transformers/tree/master/examples#running-on-tpus) has been updated accordingly.<|||||>That's great! Will try it out and report! <|||||>This issue has been automatically marked as stale because it has not had recent activity. It will be closed if no further activity occurs. Thank you for your contributions.
|
transformers | 2,022 | closed | How to convert the ALBERT tfhub model to pytorch model? | ## ❓ Questions & Help
<!-- A clear and concise description of the question. -->
I want to apply ALBERT to other QA datasets. But the first question is how to convert the tf_hub model. I downloaded the tfhub model from the repo "google research". The script ```convert_albert_original_tf_checkpoint_to_pytorch.py``` need the parameter ```--tf_checkpoint_path```. I attempted the directory 'variables', 'assets' and the root directory but all failed. I haven't solve it by document. Is there some demos for ALBERT? | 12-02-2019 11:25:46 | 12-02-2019 11:25:46 | Hi, if you ran the script `run_pretraining.py` in the original ALBERT repo, you should have put as argument an `--output_dir=dir`. In that directory should be several files, among which `model.ckpt-xxx.index`, `model.ckpt-xxx.meta`, `checkpoint` and `model.ckpt-xxx.data-xxxxx-of-xxxxx`.
You can pass this as argument to `convert_albert_original_tf_checkpoint_to_pytorch`: `--tf_checkpoint_path=dir/model.ckpt-xxx`.
A few changes to the script were done today so you might want to install from source to be sure it loads fine.<|||||>But I don't have the resources to pretrain my own ALBERT model, I just want to fine-tune the pretrained ALBERT-base model for my task. Are there some other methods to use the google's tfhub model in pytorch? Or if there is other pretrained ckpt type models I can download.<|||||>Yeah you can just load them using our API:
```py
from transformers import AlbertModel
model = AlbertModel.from_pretrained("albert-base-v1")
``` |
transformers | 2,021 | closed | save as tensorflow saved model format and how to inference? | ## ❓ Questions & Help
<!-- A clear and concise description of the question. -->
Hi, l follow the script in readme, train a model and save as tensorflow saved_model format instead of h5 format.
When inferencing, I get some problem, I don't know how to feed the inputs to the model. Here is code.
```python
import tensorflow as tf
import tensorflow_datasets
from transformers import *
# Load dataset, tokenizer, model from pretrained model/vocabulary
tokenizer = BertTokenizer.from_pretrained('bert-base-cased')
model = TFBertForSequenceClassification.from_pretrained('bert-base-cased')
data = tensorflow_datasets.load('glue/mrpc')
# Prepare dataset for GLUE as a tf.data.Dataset instance
train_dataset = glue_convert_examples_to_features(data['train'], tokenizer, max_length=128, task='mrpc')
valid_dataset = glue_convert_examples_to_features(data['validation'], tokenizer, max_length=128, task='mrpc')
train_dataset = train_dataset.shuffle(100).batch(32).repeat(2)
valid_dataset = valid_dataset.batch(64)
# Prepare training: Compile tf.keras model with optimizer, loss and learning rate schedule
optimizer = tf.keras.optimizers.Adam(learning_rate=3e-5, epsilon=1e-08, clipnorm=1.0)
loss = tf.keras.losses.SparseCategoricalCrossentropy(from_logits=True)
metric = tf.keras.metrics.SparseCategoricalAccuracy('accuracy')
model.compile(optimizer=optimizer, loss=loss, metrics=[metric])
# Train and evaluate using tf.keras.Model.fit()
history = model.fit(train_dataset, epochs=2, steps_per_epoch=115,
validation_data=valid_dataset, validation_steps=7)
tf.saved_model.save(model,"/content/saved")
```
I change the last line code to get a tensorflow saved_model. I get a problem when inferencing.
```python
loaded = tf.saved_model.load("/content/saved")
inference_func = loaded.signatures["serving_default"]
for inputs,_ in valid_dataset:
print(inference_func(inputs))
```
Then I get:
```
---------------------------------------------------------------------------
TypeError Traceback (most recent call last)
<ipython-input-27-7c90c411776e> in <module>()
1 for inputs,_ in valid_dataset:
----> 2 print(inference_func(inputs))
1 frames
/usr/local/lib/python3.6/dist-packages/tensorflow_core/python/eager/function.py in _call_impl(self, args, kwargs, cancellation_manager)
1098 "of {}), got {}. When calling a concrete function, positional "
1099 "arguments may not be bound to Tensors within nested structures."
-> 1100 ).format(self._num_positional_args, self._arg_keywords, args))
1101 args = list(args)
1102 for keyword in self._arg_keywords[len(args):]:
TypeError: Expected at most 0 positional arguments (and the rest keywords, of ['attention_mask', 'input_ids', 'token_type_ids']), got ({'input_ids': <tf.Tensor: id=130383, shape=(64, 128), dtype=int32, numpy=
array([[ 101, 1284, 5376, ..., 0, 0, 0],
[ 101, 2061, 117, ..., 0, 0, 0],
[ 101, 1130, 1103, ..., 0, 0, 0],
...,
[ 101, 1109, 3302, ..., 0, 0, 0],
[ 101, 1556, 1292, ..., 0, 0, 0],
[ 101, 1109, 158, ..., 0, 0, 0]], dtype=int32)>, 'attention_mask': <tf.Tensor: id=130382, shape=(64, 128), dtype=int32, numpy=
array([[1, 1, 1, ..., 0, 0, 0],
[1, 1, 1, ..., 0, 0, 0],
[1, 1, 1, ..., 0, 0, 0],
...,
[1, 1, 1, ..., 0, 0, 0],
[1, 1, 1, ..., 0, 0, 0],
[1, 1, 1, ..., 0, 0, 0]], dtype=int32)>, 'token_type_ids': <tf.Tensor: id=130384, shape=(64, 128), dtype=int32, numpy=
array([[0, 0, 0, ..., 0, 0, 0],
[0, 0, 0, ..., 0, 0, 0],
[0, 0, 0, ..., 0, 0, 0],
...,
[0, 0, 0, ..., 0, 0, 0],
[0, 0, 0, ..., 0, 0, 0],
[0, 0, 0, ..., 0, 0, 0]], dtype=int32)>},). When calling a concrete function, positional arguments may not be bound to Tensors within nested structures.
```
Has anyone encountered this problem before? | 12-02-2019 11:25:06 | 12-02-2019 11:25:06 | It's a mix of 2 issues:
- you need to transform your input dict into function args
- you need to expand batch dimension in all tensors
Please try:
```
inference_func(**({k: tf.expand_dims(v, axis=0) for k, v in inputs.items()}))
```
<|||||>This issue has been automatically marked as stale because it has not had recent activity. It will be closed if no further activity occurs. Thank you for your contributions.
<|||||>@cbqin @mandubian Hi, do you solve this problem, Can you explain about this, I met similar problem.
By the way,
```
loaded = tf.saved_model.load("/content/saved")
inference_func = loaded.signatures["serving_default"]
# is this line necessary ??? why not just use loaded(inputs) when inferencing
for inputs,_ in valid_dataset:
print(inference_func(inputs))
```<|||||>@xiaoyangnihao I have some issue about incompatible shape too, have you solved the error ? |
transformers | 2,020 | closed | Camenbert length Tokenizer not equal config vocab_size | ## ❓ Questions & Help
<!-- A clear and concise description of the question. -->
Hi there,
when I load the pretrained Camenbert model and tokenizer via
`model = CamembertForMaskedLM.from_pretrained('camembert-base')
tokenizer = CamembertTokenizer.from_pretrained('camembert-base')`
the length of the tokenizer is 32004 but the vocab_size of the model is 32005.
`print(len(tokenizer))`
'print(model.config.vocab_size'
This throws me an error
> Index out of range
when I try to adapt the lm_finetuning example because of
`model.resize_token_embeddings(len(tokenizer))`
It runs when I comment out this line. So my question is, is this the intended behaviour resp. what's the reason for the unevenness between the tokenizer and the model vocab_size? | 12-02-2019 10:30:44 | 12-02-2019 10:30:44 | Indeed, upon deeper investigation, it appears that the original fairseq model has a bunch of duplicate tokens in the dictionary:
```
import torch
camembert = torch.hub.load('pytorch/fairseq', 'camembert.v0')
list(camembert.task.source_dictionary[i] for i in range(10))
>>> ['<s>', '<pad>', '</s>', '<unk>', '<unk>', '<s>', '</s>', ',', '▁de', '.']
```
I'm cleaning and updating for this in #2065<|||||>This issue has been automatically marked as stale because it has not had recent activity. It will be closed if no further activity occurs. Thank you for your contributions.
|
transformers | 2,019 | closed | [CamemBert] Tokenizer function add_tokens doesn't work | ## ❓ Questions & Help
Hi,
I am trying to add new tokens to the CamemBert tokenizer, but when I run the function tokenizer.add_tokens, it doesn't seem to add any token at all :
`from transformers import CamembertTokenizer`
`tokenizer = CamembertTokenizer.from_pretrained('camembert-base')`
`tokenizer.add_tokens(['notfrenchword'])`
`Out[12]: 0`
Whereas with Bert model it works perfectly. Is this a bug or am I doing something wrong ?
Thanks
| 12-02-2019 10:18:30 | 12-02-2019 10:18:30 | This method is **not** implemented into the CamemBERT tokenizer, at the moment.
> ## Questions & Help
> Hi,
>
> I am trying to add new tokens to the CamemBert tokenizer, but when I run the function tokenizer.add_tokens, it doesn't seem to add any token at all :
>
> `from transformers import CamembertTokenizer`
> `tokenizer = CamembertTokenizer.from_pretrained('camembert-base')`
> `tokenizer.add_tokens(['notfrenchword'])`
>
> `Out[12]: 0`
>
> Whereas with Bert model it works perfectly. Is this a bug or am I doing something wrong ?
>
> Thanks<|||||>Hi,
This method is actually implemented (it's a method in the base class of all tokenizer).
The reason is was failing in the present case is that the original fairseq model has a bunch of duplicate tokens in the dictionary:
```
import torch
camembert = torch.hub.load('pytorch/fairseq', 'camembert.v0')
list(camembert.task.source_dictionary[i] for i in range(10))
>>> ['<s>', '<pad>', '</s>', '<unk>', '<unk>', '<s>', '</s>', ',', '▁de', '.']
```
fixing this in #2065 |
transformers | 2,018 | closed | FileNotFoundError: [Errno 2] No such file or directory: 'data/dump.txt' |
@stefan-it
Hello, I am new learner in BERT and I want to have a try the excellent work - distilBert.
But the problem happened when I ran the training step, and Could you tell me where can I download the `dump.txt` file ?
Thank you very much!
| 12-02-2019 08:29:57 | 12-02-2019 08:29:57 | As stated [here](https://github.com/huggingface/transformers/blob/1ab8dc44b3d84ed1894f5b6a6fab58fb39298fc7/examples/distillation/README.md), the `dump.txt` file is **your training file**. This file will contain one sequence per line (a sequence being composed of one of several coherent sentences).
> @stefan-it
> Hello, I am new learner in BERT and I want to have a try the excellent work - distilBert.
> But the problem happened when I ran the training step, and Could you tell me where can I download the `dump.txt` file ?
> Thank you very much!<|||||>This issue has been automatically marked as stale because it has not had recent activity. It will be closed if no further activity occurs. Thank you for your contributions.
|
transformers | 2,017 | closed | How to use GPT-2 text generator in spanish | ## ❓ Questions & Help
<!-- A clear and concise description of the question. -->
I would like to know if there is a way to use the gpt-xl model for text generation in spanish.
The command I use to run the english text generation model is the following:
$ python ./examples/run_generation.py --model_type=gpt2 --length=50 --model_name_or_path=gpt2-xl
What other parameter must I use to allow spanish text generation?
| 12-01-2019 20:49:26 | 12-01-2019 20:49:26 | At the moment, there is **no pre-trained model in Spanish language**. If you want, you can use a **multi-lingual** pre-trained model, such as BERT or XLM. In particular, Transformers offer the following settings of multi-lingual models:
- **bert-base-multilingual-cased** (Masked language modeling + Next sentence prediction, 104 languages)
- **bert-base-multilingual-uncased** (Masked language modeling + Next sentence prediction, 102 languages)
- **xlm-mlm-17-1280** (Masked language modeling, 17 languages)
- **xlm-mlm-100-1280** (Masked language modeling, 100 languages)
You can find more information in the [official documentation](https://huggingface.co/transformers/multilingual.html). <|||||>Thank you. I will close the issue. |
transformers | 2,016 | closed | GPT-2 finetuning with run_lm_finetuning.py script | ## ❓ Questions & Help
I tried to finetune gpt-2 model using `run_lm_finetuning.py` script with the following parameters:
```
python run_lm_finetuning.py \
--train_data_file=text8.train \
--output_dir=/content/gpt2 \
--eval_data_file=text8.val \
--model_type=gpt2 \
--model_name_or_path=gpt2 \
--do_train \
--do_eval \
--per_gpu_train_batch_size=32 \
--per_gpu_eval_batch_size=32 \
--gradient_accumulation_steps=1 \
--num_train_epochs=3 \
--warmup_steps=200
```
and it throws memory error no matter what my machine type is. In extreme case I wanted to run this demo on Google Cloud with 32 cpus and 120GB RAM - not possible. It just eats the whole RAM and does not even make a single iteration. On the other hand, I was able to do finetuning from this project [gpt-2-simple](https://github.com/minimaxir/gpt-2-simple) on Google Colab with 124M model (CPU). What is going on? Am I doing something wrong?
| 12-01-2019 20:36:00 | 12-01-2019 20:36:00 | To me, you have set an extreme `batch_size`. Did you try it with e.g. `per_gpu_train_batch_size=1` and `per_gpu_eval_batch_size=1` ?<|||||>@iedmrc I finally managed to fine-tune it with `per_gpu_train_batch_size=1` and `gradient_accumulation_steps=32`. Indeed the batch size was the problem but I haven't realized it is such a big problem. Everything works fine now.
|
transformers | 2,015 | closed | [CamemBERT] Add CamembertForQuestionAnswering | Firstly, a huge thanks to Hugging Face team for their great work ! As we have now Camembert, it would be nice to use it for question answering using transformers ! You can find SQuAD in French on GitHub so it would be so easy to a lot of people to fine-tune Camembert for this task.
Please consider it in future releases 😉
| 12-01-2019 19:57:18 | 12-01-2019 19:57:18 | I wonder if loading as roberta really works for camembert<|||||>What do you mean with this statement?
> I wonder if loading as roberta really works for camembert<|||||>Happy to review a PR for this (should be pretty easy to add!)<|||||>>
>
> What do you mean with this statement?
>
> > I wonder if loading as roberta really works for camembert
you can load camembert config and checkpoints as roberta models but I'm not perfectly sure it's identical.
I did'nt checked if there was RobertaForQuestionAnswering so my comment is partly irrevelent. However both slould be added if possible. maybe some generic heads using PreTrainedModel could be possible even in not efficient<|||||>> > What do you mean with this statement?
> > > I wonder if loading as roberta really works for camembert
>
> you can load camembert config and checkpoints as roberta models but I'm not perfectly sure it's identical.
> I did'nt checked if there was RobertaForQuestionAnswering so my comment is partly irrevelent. However both slould be added if possible. maybe some generic heads using PreTrainedModel could be possible even in not efficient
At the moment, you **can't** use RoBERTa model for QuestionAnswering. You can use `RoBERTa` model for **token classification**, **multiple choice**, **sequence classification** and **MaskedLM** (with the usual `RobertaFor*` naming convention).<|||||>Hi, thanks to the Hugging Face team for the amazing work ! I think there is a PR here to add camembert for question answering : https://github.com/huggingface/transformers/pull/2746<|||||>This issue has been automatically marked as stale because it has not had recent activity. It will be closed if no further activity occurs. Thank you for your contributions.
|
transformers | 2,014 | closed | Mark tests in TFAutoModelTest as slow. | Each test forces downloading the same 536MB file, which is slow
even with a decent internet connection. | 12-01-2019 17:26:55 | 12-01-2019 17:26:55 | # [Codecov](https://codecov.io/gh/huggingface/transformers/pull/2014?src=pr&el=h1) Report
> Merging [#2014](https://codecov.io/gh/huggingface/transformers/pull/2014?src=pr&el=desc) into [master](https://codecov.io/gh/huggingface/transformers/commit/b0ee7c7df3d49a819c4d6cef977214bd91f5c075?src=pr&el=desc) will **decrease** coverage by `0.39%`.
> The diff coverage is `100%`.
[](https://codecov.io/gh/huggingface/transformers/pull/2014?src=pr&el=tree)
```diff
@@ Coverage Diff @@
## master #2014 +/- ##
=========================================
- Coverage 84.05% 83.66% -0.4%
=========================================
Files 105 105
Lines 15555 15555
=========================================
- Hits 13075 13014 -61
- Misses 2480 2541 +61
```
| [Impacted Files](https://codecov.io/gh/huggingface/transformers/pull/2014?src=pr&el=tree) | Coverage Δ | |
|---|---|---|
| [transformers/tests/modeling\_tf\_auto\_test.py](https://codecov.io/gh/huggingface/transformers/pull/2014/diff?src=pr&el=tree#diff-dHJhbnNmb3JtZXJzL3Rlc3RzL21vZGVsaW5nX3RmX2F1dG9fdGVzdC5weQ==) | `36.36% <100%> (-61.82%)` | :arrow_down: |
| [transformers/modeling\_tf\_auto.py](https://codecov.io/gh/huggingface/transformers/pull/2014/diff?src=pr&el=tree#diff-dHJhbnNmb3JtZXJzL21vZGVsaW5nX3RmX2F1dG8ucHk=) | `32.5% <0%> (-18.75%)` | :arrow_down: |
| [transformers/configuration\_auto.py](https://codecov.io/gh/huggingface/transformers/pull/2014/diff?src=pr&el=tree#diff-dHJhbnNmb3JtZXJzL2NvbmZpZ3VyYXRpb25fYXV0by5weQ==) | `45% <0%> (-15%)` | :arrow_down: |
| [transformers/configuration\_utils.py](https://codecov.io/gh/huggingface/transformers/pull/2014/diff?src=pr&el=tree#diff-dHJhbnNmb3JtZXJzL2NvbmZpZ3VyYXRpb25fdXRpbHMucHk=) | `88.31% <0%> (-3.9%)` | :arrow_down: |
| [transformers/modeling\_tf\_utils.py](https://codecov.io/gh/huggingface/transformers/pull/2014/diff?src=pr&el=tree#diff-dHJhbnNmb3JtZXJzL21vZGVsaW5nX3RmX3V0aWxzLnB5) | `91.51% <0%> (-1.22%)` | :arrow_down: |
| [transformers/file\_utils.py](https://codecov.io/gh/huggingface/transformers/pull/2014/diff?src=pr&el=tree#diff-dHJhbnNmb3JtZXJzL2ZpbGVfdXRpbHMucHk=) | `70% <0%> (-0.5%)` | :arrow_down: |
------
[Continue to review full report at Codecov](https://codecov.io/gh/huggingface/transformers/pull/2014?src=pr&el=continue).
> **Legend** - [Click here to learn more](https://docs.codecov.io/docs/codecov-delta)
> `Δ = absolute <relative> (impact)`, `ø = not affected`, `? = missing data`
> Powered by [Codecov](https://codecov.io/gh/huggingface/transformers/pull/2014?src=pr&el=footer). Last update [b0ee7c7...5ab9308](https://codecov.io/gh/huggingface/transformers/pull/2014?src=pr&el=lastupdated). Read the [comment docs](https://docs.codecov.io/docs/pull-request-comments).
<|||||>Predictably, this lowers code coverage, because CircleCI does coverage measurement without running the slow tests. Given that other tests with similar performance are skipped, I thought it would be consistent to skip these. If there's a specific reason for not doing so, I can document it in a comment instead.<|||||>I'm ok with that. |
transformers | 2,013 | closed | What is the real parameters to weight the triple loss (L_{ce}, L_{mlm}, L_{cos}) in DistilBert? | Hello! Thanks for your great work DistilBert. I want to ask what is the real parameters "alpha" you used in DistilBert to weight the triple loss (L_{ce}, L_{mlm}, L_{cos})?
You did not mention this detail in your NIPS workshop paper (http://arxiv.org/abs/1910.01108). In the [README](https://github.com/huggingface/transformers/blob/master/examples/distillation/README.md) file, you listed two different setups: `--alpha_ce 5.0 --alpha_mlm 2.0 --alpha_cos 1.0 --alpha_clm 0.0` for single GPU training and `--alpha_ce 0.33 --alpha_mlm 0.33 --alpha_cos 0.33 --alpha_clm 0.0` for distributed training. Can you tell me what is the best setting?
Actually, I have tried to reproduce your results of DistilBert. I trained the DistilBert with the corpus used by BERT, but the performance of GLUE seemed slightly fall behind your pre-trained `distilbert-base-uncased` by 2 points. I would be appreciated if you can tell me the parameters for reproducibility. Thanks!
| 12-01-2019 16:49:05 | 12-01-2019 16:49:05 | Hello @voidism,
Thank you for your interest!
The parameters we used for training DistilBERT are the first one you listed: `--alpha_ce 5.0 --alpha_mlm 2.0 --alpha_cos 1.0 --alpha_clm 0.0`.
Victor<|||||>@VictorSanh Thank you very much! |
transformers | 2,012 | closed | How to output the vectors of the last four layers of BERT_Model. | E.g
output = [the_last_one_layer_output, second_last_layer_output, ...] | 12-01-2019 08:58:54 | 12-01-2019 08:58:54 | TF or pytorch?
If what you want is TF, you can check #1936. |
transformers | 2,011 | closed | typo fix on the docs as per Pytorch v1.1+ | https://github.com/huggingface/transformers/issues/2010 | 12-01-2019 08:38:39 | 12-01-2019 08:38:39 | # [Codecov](https://codecov.io/gh/huggingface/transformers/pull/2011?src=pr&el=h1) Report
> Merging [#2011](https://codecov.io/gh/huggingface/transformers/pull/2011?src=pr&el=desc) into [master](https://codecov.io/gh/huggingface/transformers/commit/b0ee7c7df3d49a819c4d6cef977214bd91f5c075?src=pr&el=desc) will **not change** coverage.
> The diff coverage is `n/a`.
[](https://codecov.io/gh/huggingface/transformers/pull/2011?src=pr&el=tree)
```diff
@@ Coverage Diff @@
## master #2011 +/- ##
=======================================
Coverage 84.05% 84.05%
=======================================
Files 105 105
Lines 15555 15555
=======================================
Hits 13075 13075
Misses 2480 2480
```
------
[Continue to review full report at Codecov](https://codecov.io/gh/huggingface/transformers/pull/2011?src=pr&el=continue).
> **Legend** - [Click here to learn more](https://docs.codecov.io/docs/codecov-delta)
> `Δ = absolute <relative> (impact)`, `ø = not affected`, `? = missing data`
> Powered by [Codecov](https://codecov.io/gh/huggingface/transformers/pull/2011?src=pr&el=footer). Last update [b0ee7c7...c356290](https://codecov.io/gh/huggingface/transformers/pull/2011?src=pr&el=lastupdated). Read the [comment docs](https://docs.codecov.io/docs/pull-request-comments).
<|||||>Thanks!<|||||>Thanks a lot to the creators (and contributors) of this amazing lib for making our lives easier! |
transformers | 2,010 | closed | Changing the docs as per Pytorch v1.1+ | ## ❓ Questions & Help
[Docs Link](https://huggingface.co/transformers/migration.html#optimizers-bertadam-openaiadam-are-now-adamw-schedules-are-standard-pytorch-schedules)
```
# From the Docs
### In Transformers, optimizer and schedules are splitted and instantiated like this:
optimizer = AdamW(model.parameters(), lr=lr, correct_bias=False) # To reproduce BertAdam specific behavior set correct_bias=False
scheduler = get_linear_schedule_with_warmup(optimizer, num_warmup_steps=num_warmup_steps, num_training_steps=num_training_steps) # PyTorch scheduler
### and used like this:
for batch in train_data:
loss = model(batch)
loss.backward()
torch.nn.utils.clip_grad_norm_(model.parameters(), max_grad_norm) # Gradient clipping is not in AdamW anymore (so you can use amp without issue)
scheduler.step()
optimizer.step()
```
As per the Pytorch 1.1+,
>Prior to PyTorch 1.1.0, the learning rate scheduler was expected to be called before the optimizer’s update; 1.1.0 changed this behavior in a BC-breaking way. If you use the learning rate scheduler (calling scheduler.step()) before the optimizer’s update (calling optimizer.step()), this will skip the first value of the learning rate schedule. If you are unable to reproduce results after upgrading to PyTorch 1.1.0, please check if you are calling scheduler.step() at the wrong time.
[Pytorch Reference Link](https://pytorch.org/docs/stable/optim.html#how-to-adjust-learning-rate)
Thanks.
PS Not sure if the issue category selected is apt. | 12-01-2019 08:34:51 | 12-01-2019 08:34:51 | This issue has been automatically marked as stale because it has not had recent activity. It will be closed if no further activity occurs. Thank you for your contributions.
|
transformers | 2,009 | closed | Reason for using einsum in xlnet? | ## ❓ Questions & Help
Hello.
This might be a newbie question, so I apologize in advance.
While reading your implementation of xlnet, I ran into several usages of `torch.einsum` function.
example) `k_head_h = torch.einsum('ibh,hnd->ibnd', cat, self.k) `
After studying the definition of einsum, I came to a conclusion that the above statement is exactly like using a linear layer (without bias) (from dimension h to n*d), and then resizing the output to be ibnd.
So if I'm not wrong, is there any reason to prefer using `torch.einsum` over `nn.Linear`?
Is it related to performance issues?
I ran a simple test, and `nn.Linear` seems to be a bit faster than `torch.einsum`.
I would really appreciate your help.
Thank you. | 12-01-2019 05:30:39 | 12-01-2019 05:30:39 | This issue has been automatically marked as stale because it has not had recent activity. It will be closed if no further activity occurs. Thank you for your contributions.
|
transformers | 2,008 | closed | Expand run_lm_finetuning.py to all models | ## 🚀 Feature
[run_lm_finetuning.py](https://github.com/huggingface/transformers/blob/b0ee7c7df3d49a819c4d6cef977214bd91f5c075/examples/run_lm_finetuning.py) is a very useful tool for finetuning many models the library provided. But it doesn't cover all the models. Currently available models are:
- gpt2
- openai-gpt
- bert
- roberta
- distilbert
- camembert
And not available ones:
- ctrl
- xlm
- xlnet
- transfo-xl
- albert
## Motivation
Most important part of such a library is that it can be easily finetuned. `run_lm_finetuning.py` gives us that opportunity but why say no more :)
| 11-30-2019 22:19:57 | 11-30-2019 22:19:57 | Indeed, here are my 2 cents on that:
- ctrl: easy to add (should work out of the box)
- xlm: should also work out of the box (but need to check if the model is an mlm or a clm model to finetune)
- albert: should work out of the box
- transfo-xl: need to take care of history => a little more work
- xlnet: need to take care of history + permutations => quite more work.
Do you want to give it a try? We don't have that in our short term roadmap until the end of the year. <|||||>Okay, I'm gonna try to add `ctrl`, `xlm` and `albert`. Then I'll make pull request in order to discuss on it.
Isn't there any example of how to train `transfo-xl` and `xlnet`?<|||||>You have to look at both original repos<|||||>Out of curiosity, has any progress been made on a pull request for this?<|||||>+1 for this request, especially `transfo-xl` :)<|||||>Is this issue addressed with https://github.com/huggingface/transformers/commit/a8e3336a850e856188350a93e67d77c07c85b8af?<|||||>a8e3336a850e856188350a93e67d77c07c85b8af makes all those models accessible from `run_language_modeling.py`, but does not do anything special for models whose training has peculiarities, like `transfo-xl` or `xlnet`. I'm not familiar with those two so maybe someone else (@patrickvonplaten?) can chime in.<|||||>As far as I know:
Currently the `lun_language_modeling.py` script is not really made to train `transfo-xl` or `xlnet`
First as @thomwolf already said, the `mems` parameter (the "history") of the models is not taken care of during training. During training the model "caches" past sequences to effectively reuse them afterwards. It's described quite well in Figure 2 in the [Transfo-XL paper](https://arxiv.org/pdf/1901.02860.pdf). This should be rather easy to add though.
Second, `XLNet` samples from a permutation mask during training, which is one of the core ideas of the paper, see https://github.com/huggingface/transformers/issues/2822 or Equation 5 in the official [paper](https://arxiv.org/pdf/1906.08237.pdf) This is a very special case for `XLNet` and is not yet implemented in `run_language_modeling.py` (shouldn't be too hard though to implement it since there is only one additional sum per training sample).
Third, `Transfo-XL` uses adaptive word embeddings and adaptive softmax which also leads to some specialties when training. See also this issue #3310. This should be implemented in the model class itself though. <|||||>I'm assuming that `Albert` is fine out of the box. What about `T5`?<|||||>Is anybody still working on this currently?<|||||>We are currently working on it. Might still take ~2 weeks.<|||||>Any update?<|||||>I'd like to try this (#4739). I'd like to start with XLNet since that's relevant to my work right now.<|||||>I think you would just need to add a XLNet data collator to this file so that the trainer can be used with XLNet :-) So I would add a new XLNetLanguageModelingCollator here: https://github.com/huggingface/transformers/blob/1b5820a56540a2096daeb43a0cd8247c8c94a719/src/transformers/data/data_collator.py#L76<|||||>Thanks so much! I'll look into it :)<|||||>Any progress on XLNet? @shngt<|||||>Any updates regarding XLNet ?<|||||>@patrickvonplaten I added the data collator as you suggested - please review :) You also mentioned earlier "the `mems` parameter (the "history") of the models is not taken care of during training" - has that been taken care of, or does the logic need to be implemented separately?<|||||>I was looking into the other models requested:
- CTRL -> CLM, works out of the box, already added comments
- XLM -> can be trained with three different objectives - CLM, MLM and Translation LM, which is a supervised multilingual extension of MLM. The example script does not seem to require any changes (except for maybe a warning somewhere to use the right flag with the right checkpoint?). TLM does require a lot of data-specific preprocessing, but it seems relevant only in light of the multilingual setting. I feel it would be better to incorporate those in a separate `mulitlingual_language_modeling` example script if others would like an end-to-end example of how this would be properly done.
- Albert -> Instead of the random masking in BERT, the authors use a span-based masking system first seen in SpanBERT (section 3.1 of https://arxiv.org/pdf/1907.10529.pdf). It seems to be a mix of what I implemented in XLNet and the masking procedure in BERT, so should be kept in another function in the main `DataCollatorForLanguageModeling` class in my opinion
- TransformerXL -> seems to be CLM with reuse of previous states. I think this functionality has been added, so no additional work should be needed
In summary, I think all that needs to be done right now for XLM and TransformerXL is to add a line or two in the starting docstring mentioning which type of LM to use. For Albert, I think we need to incorporate the masking scheme as a separate procedure in `DataCollatorForLanguageModeling`, but am not sure if this is the cleanest way to do it. Let me know what you would like.
@patrickvonplaten <|||||>I agree very much with what you say. For `XLM` and `TransformerXL` the script should work pretty much out of the box, so we would just have to adapt some comments in `examples/language-modeling/run_language_modeling.py`.
For Albert, it would be nice to create a new `SpanMaskLanguageModeling` Data collator.<|||||>Great, I'll get started then. I'll try to finish it over the weekend :)<|||||>Awesome, no rush though ;-)<|||||>Maybe a stupid question, but where should I find `run_lm_finetuning.py`? [The docs](https://huggingface.co/transformers/v2.0.0/examples.html) point to a dead link, as the file doesn't exist in the master branch. <|||||>it's renamed and moved [there](https://github.com/huggingface/transformers/tree/master/examples/language-modeling).<|||||>Thanks for the notice @KristenMoore - The documentation was quite old. The new documentation should have fixed it :-) <|||||>This issue has been automatically marked as stale because it has not had recent activity. It will be closed if no further activity occurs. Thank you for your contributions.
|
transformers | 2,007 | closed | fixed XLNet attention output for both attention streams whenever target_mapping is provided | XLNet uses two separate attention streams, i.e. there are two separate tensors for representing the model's attention. Both of them need to have their dimensions permuted.
The problem has been described in #1994 . | 11-30-2019 15:11:14 | 11-30-2019 15:11:14 | # [Codecov](https://codecov.io/gh/huggingface/transformers/pull/2007?src=pr&el=h1) Report
> Merging [#2007](https://codecov.io/gh/huggingface/transformers/pull/2007?src=pr&el=desc) into [master](https://codecov.io/gh/huggingface/transformers/commit/b0ee7c7df3d49a819c4d6cef977214bd91f5c075?src=pr&el=desc) will **increase** coverage by `0.03%`.
> The diff coverage is `100%`.
[](https://codecov.io/gh/huggingface/transformers/pull/2007?src=pr&el=tree)
```diff
@@ Coverage Diff @@
## master #2007 +/- ##
==========================================
+ Coverage 84.05% 84.09% +0.03%
==========================================
Files 105 105
Lines 15555 15570 +15
==========================================
+ Hits 13075 13093 +18
+ Misses 2480 2477 -3
```
| [Impacted Files](https://codecov.io/gh/huggingface/transformers/pull/2007?src=pr&el=tree) | Coverage Δ | |
|---|---|---|
| [transformers/tests/modeling\_xlnet\_test.py](https://codecov.io/gh/huggingface/transformers/pull/2007/diff?src=pr&el=tree#diff-dHJhbnNmb3JtZXJzL3Rlc3RzL21vZGVsaW5nX3hsbmV0X3Rlc3QucHk=) | `96.42% <100%> (+0.29%)` | :arrow_up: |
| [transformers/modeling\_xlnet.py](https://codecov.io/gh/huggingface/transformers/pull/2007/diff?src=pr&el=tree#diff-dHJhbnNmb3JtZXJzL21vZGVsaW5nX3hsbmV0LnB5) | `74.22% <100%> (+0.61%)` | :arrow_up: |
------
[Continue to review full report at Codecov](https://codecov.io/gh/huggingface/transformers/pull/2007?src=pr&el=continue).
> **Legend** - [Click here to learn more](https://docs.codecov.io/docs/codecov-delta)
> `Δ = absolute <relative> (impact)`, `ø = not affected`, `? = missing data`
> Powered by [Codecov](https://codecov.io/gh/huggingface/transformers/pull/2007?src=pr&el=footer). Last update [b0ee7c7...76c0bc0](https://codecov.io/gh/huggingface/transformers/pull/2007?src=pr&el=lastupdated). Read the [comment docs](https://docs.codecov.io/docs/pull-request-comments).
<|||||>That's great, thanks for fixing the issue! Looks good to me.<|||||>Yes, this is great, thanks a lot @roskoN! |
transformers | 2,006 | closed | [ALBERT]: 'AlbertForMaskedLM' object has no attribute 'bias' | Hi,
I wanted to convert an own trained ALBERT model with the `convert_albert_original_tf_checkpoint_to_pytorch.py` script:
```bash
$ python3 convert_albert_original_tf_checkpoint_to_pytorch.py --tf_checkpoint_path /mnt/albert-base-secrect-language-cased/ --albert_config_file /mnt/albert-base-secrect-language-cased/config.json --pytorch_dump_path pytorch_model.bin
```
Unfortunately, the following error message is returned:
```bash
<--snip-->
bert/pooler/dense/bias
bert/pooler/dense/bias/adam_m
bert/pooler/dense/bias/adam_v
bert/pooler/dense/kernel
bert/pooler/dense/kernel/adam_m
bert/pooler/dense/kernel/adam_v
cls/predictions/output_bias
cls/predictions/output_bias/adam_m
cls/predictions/output_bias/adam_v
cls/predictions/transform/LayerNorm/beta
cls/predictions/transform/LayerNorm/beta/adam_m
cls/predictions/transform/LayerNorm/beta/adam_v
cls/predictions/transform/LayerNorm/gamma
cls/predictions/transform/LayerNorm/gamma/adam_m
cls/predictions/transform/LayerNorm/gamma/adam_v
cls/predictions/transform/dense/bias
cls/predictions/transform/dense/bias/adam_m
cls/predictions/transform/dense/bias/adam_v
cls/predictions/transform/dense/kernel
cls/predictions/transform/dense/kernel/adam_m
cls/predictions/transform/dense/kernel/adam_v
cls/seq_relationship/output_bias
cls/seq_relationship/output_bias/adam_m
cls/seq_relationship/output_bias/adam_v
cls/seq_relationship/output_weights
cls/seq_relationship/output_weights/adam_m
cls/seq_relationship/output_weights/adam_v
global_step
INFO:transformers.modeling_albert:Skipping bert/embeddings/attention/LayerNorm/beta
INFO:transformers.modeling_albert:Skipping bert/embeddings/attention/LayerNorm/beta
INFO:transformers.modeling_albert:Skipping bert/embeddings/attention/LayerNorm/beta
INFO:transformers.modeling_albert:Skipping bert/embeddings/attention/LayerNorm/beta
Traceback (most recent call last):
File "convert_albert_original_tf_checkpoint_to_pytorch.py", line 66, in <module>
args.pytorch_dump_path)
File "convert_albert_original_tf_checkpoint_to_pytorch.py", line 37, in convert_tf_checkpoint_to_pytorch
load_tf_weights_in_albert(model, config, tf_checkpoint_path)
File "/mnt/transformers/transformers/modeling_albert.py", line 92, in load_tf_weights_in_albert
pointer = getattr(pointer, 'bias')
File "/usr/local/lib/python3.6/dist-packages/torch/nn/modules/module.py", line 585, in __getattr__
type(self).__name__, name))
AttributeError: 'AlbertForMaskedLM' object has no attribute 'bias'
```
I'm using the latest commit in `google-research` for training the ALBERT model. Configuration is:
```json
{
"attention_probs_dropout_prob": 0.1,
"hidden_act": "gelu",
"hidden_dropout_prob": 0.1,
"embedding_size": 128,
"hidden_size": 768,
"initializer_range": 0.02,
"intermediate_size": 3072,
"max_position_embeddings": 512,
"num_attention_heads": 12,
"num_hidden_layers": 12,
"num_hidden_groups": 1,
"net_structure_type": 0,
"gap_size": 0,
"num_memory_blocks": 0,
"inner_group_num": 1,
"down_scale_factor": 1,
"type_vocab_size": 2,
"vocab_size": 32000
}
```
| 11-30-2019 14:15:25 | 11-30-2019 14:15:25 | Same issue here. I did slightly different steps, but same result.
```
model = AlbertModel(config=config)
model = load_tf_weights_in_albert(model,config,'sample_tf_checkpoint/model.ckpt-100000')
```
Then I get,
```
---------------------------------------------------------------------------
AttributeError Traceback (most recent call last)
<ipython-input-5-a47f5e7bff26> in <module>
----> 1 model = load_tf_weights_in_albert(model,config,'sample_tf_checkpoint/model.ckpt-100000')
~/anaconda3/envs/pytorch_py37/lib/python3.7/site-packages/transformers/modeling_albert.py in load_tf_weights_in_albert(model, config, tf_checkpoint_path)
90 pointer = getattr(pointer, 'weight')
91 elif l[0] == 'output_bias' or l[0] == 'beta':
---> 92 pointer = getattr(pointer, 'bias')
93 elif l[0] == 'output_weights':
94 pointer = getattr(pointer, 'weight')
~/anaconda3/envs/pytorch_py37/lib/python3.7/site-packages/torch/nn/modules/module.py in __getattr__(self, name)
589 return modules[name]
590 raise AttributeError("'{}' object has no attribute '{}'".format(
--> 591 type(self).__name__, name))
592
593 def __setattr__(self, name, value):
AttributeError: 'AlbertModel' object has no attribute 'bias'
```
Miserably waiting for the solution :(
The pretrained tensorflow checkpoints were generated using the codes in https://github.com/google-research/google-research/tree/master/albert
It seems the latest code update was 3 days ago (Nov. 27). My training was initiated after that.
Please help us.<|||||>Same issue here. <|||||>You can Try my repo convert Albert tf to torch .py
On Mon, Dec 2, 2019 at 11:28 SunYan <[email protected]> wrote:
> Same issue here.
>
> —
> You are receiving this because you are subscribed to this thread.
> Reply to this email directly, view it on GitHub
> <https://github.com/huggingface/transformers/issues/2006?email_source=notifications&email_token=AIEAE4BXOVAOQN7RGG35JHLQWR6GRA5CNFSM4JTGZEWKYY3PNVWWK3TUL52HS4DFVREXG43VMVBW63LNMVXHJKTDN5WW2ZLOORPWSZGOEFSCWFA#issuecomment-560212756>,
> or unsubscribe
> <https://github.com/notifications/unsubscribe-auth/AIEAE4DCFSKTP2GFFB3GYDTQWR6GRANCNFSM4JTGZEWA>
> .
>
<|||||>> You can Try my repo convert Albert tf to torch .py
> […](#)
> On Mon, Dec 2, 2019 at 11:28 SunYan ***@***.***> wrote: Same issue here. — You are receiving this because you are subscribed to this thread. Reply to this email directly, view it on GitHub <#2006?email_source=notifications&email_token=AIEAE4BXOVAOQN7RGG35JHLQWR6GRA5CNFSM4JTGZEWKYY3PNVWWK3TUL52HS4DFVREXG43VMVBW63LNMVXHJKTDN5WW2ZLOORPWSZGOEFSCWFA#issuecomment-560212756>, or unsubscribe <https://github.com/notifications/unsubscribe-auth/AIEAE4DCFSKTP2GFFB3GYDTQWR6GRANCNFSM4JTGZEWA> .
秀!<|||||>Hi, this should have been fixed with b3d834a, you can load the changes by installing from source.
Let me know if you still have an error.<|||||>@LysandreJik Thank you for your help. I am getting a different error saying that object Embedding doesn't have 'shape'
It seems the module is expecting numpy array, while the checkpoint contains object called Embedding, thus has no attribute "shape"
I am not sure how to correct it though.
Thank you again!
```
---------------------------------------------------------------------------
AttributeError Traceback (most recent call last)
<ipython-input-4-a47f5e7bff26> in <module>
----> 1 model = load_tf_weights_in_albert(model,config,'sample_tf_checkpoint/model.ckpt-100000')
~/anaconda3/envs/pytorch_py37/lib/python3.7/site-packages/transformers/modeling_albert.py in load_tf_weights_in_albert(model, config, tf_checkpoint_path)
130 array = np.transpose(array)
131 try:
--> 132 assert pointer.shape == array.shape
133 except AssertionError as e:
134 e.args += (pointer.shape, array.shape)
~/anaconda3/envs/pytorch_py37/lib/python3.7/site-packages/torch/nn/modules/module.py in __getattr__(self, name)
589 return modules[name]
590 raise AttributeError("'{}' object has no attribute '{}'".format(
--> 591 type(self).__name__, name))
592
593 def __setattr__(self, name, value):
AttributeError: 'Embedding' object has no attribute 'shape'
```
<|||||>Hi @hansaimlim, what is the size of the model you are loading? Could you paste here the 5-10 lines output by the conversion before the error was raised? <|||||>I could also reproduce that error:
```bash
global_step
Initialize PyTorch weight ['albert', 'embeddings', 'LayerNorm', 'beta'] from bert/embeddings/LayerNorm/beta
INFO:transformers.modeling_albert:Skipping albert/embeddings/LayerNorm/beta/adam_m
Initialize PyTorch weight ['albert', 'embeddings', 'LayerNorm', 'beta', 'adam_m'] from bert/embeddings/LayerNorm/beta/adam_m
INFO:transformers.modeling_albert:Skipping albert/embeddings/LayerNorm/beta/adam_v
Initialize PyTorch weight ['albert', 'embeddings', 'LayerNorm', 'beta', 'adam_v'] from bert/embeddings/LayerNorm/beta/adam_v
Initialize PyTorch weight ['albert', 'embeddings', 'LayerNorm', 'gamma'] from bert/embeddings/LayerNorm/gamma
INFO:transformers.modeling_albert:Skipping albert/embeddings/LayerNorm/gamma/adam_m
Initialize PyTorch weight ['albert', 'embeddings', 'LayerNorm', 'gamma', 'adam_m'] from bert/embeddings/LayerNorm/gamma/adam_m
INFO:transformers.modeling_albert:Skipping albert/embeddings/LayerNorm/gamma/adam_v
Initialize PyTorch weight ['albert', 'embeddings', 'LayerNorm', 'gamma', 'adam_v'] from bert/embeddings/LayerNorm/gamma/adam_v
Initialize PyTorch weight ['albert', 'embeddings', 'position_embeddings'] from bert/embeddings/position_embeddings
INFO:transformers.modeling_albert:Skipping albert/embeddings/position_embeddings/adam_m
Traceback (most recent call last):
File "convert_albert_original_tf_checkpoint_to_pytorch.py", line 66, in <module>
args.pytorch_dump_path)
File "convert_albert_original_tf_checkpoint_to_pytorch.py", line 37, in convert_tf_checkpoint_to_pytorch
load_tf_weights_in_albert(model, config, tf_checkpoint_path)
File "/mnt/transformers/transformers/modeling_albert.py", line 134, in load_tf_weights_in_albert
assert pointer.shape == array.shape
File "/usr/local/lib/python3.6/dist-packages/torch/nn/modules/module.py", line 585, in __getattr__
type(self).__name__, name))
AttributeError: 'Embedding' object has no attribute 'shape'
```<|||||>@LysandreJik Sure. Thanks for prompt feedback!
my_albert_config.json
```
attention_probs_dropout_prob:0
hidden_act:"gelu"
hidden_dropout_prob:0
embedding_size:128
hidden_size:312
initializer_range:0.02
intermediate_size:1248
max_position_embeddings:512
num_attention_heads:12
num_hidden_layers:4
num_hidden_groups:1
net_structure_type:0
gap_size:0
num_memory_blocks:0
inner_group_num:1
down_scale_factor:1
type_vocab_size:2
ln_type:"postln"
vocab_size:19686
```
```
bert/embeddings/LayerNorm/beta
bert/embeddings/LayerNorm/beta/adam_m
bert/embeddings/LayerNorm/beta/adam_v
bert/embeddings/LayerNorm/gamma
bert/embeddings/LayerNorm/gamma/adam_m
bert/embeddings/LayerNorm/gamma/adam_v
bert/embeddings/position_embeddings
bert/embeddings/position_embeddings/adam_m
bert/embeddings/position_embeddings/adam_v
bert/embeddings/token_type_embeddings
bert/embeddings/token_type_embeddings/adam_m
bert/embeddings/token_type_embeddings/adam_v
bert/embeddings/word_embeddings
bert/embeddings/word_embeddings/adam_m
bert/embeddings/word_embeddings/adam_v
bert/encoder/embedding_hidden_mapping_in/bias
bert/encoder/embedding_hidden_mapping_in/bias/adam_m
bert/encoder/embedding_hidden_mapping_in/bias/adam_v
bert/encoder/embedding_hidden_mapping_in/kernel
bert/encoder/embedding_hidden_mapping_in/kernel/adam_m
bert/encoder/embedding_hidden_mapping_in/kernel/adam_v
bert/encoder/transformer/group_0/inner_group_0/LayerNorm/beta
bert/encoder/transformer/group_0/inner_group_0/LayerNorm/beta/adam_m
bert/encoder/transformer/group_0/inner_group_0/LayerNorm/beta/adam_v
bert/encoder/transformer/group_0/inner_group_0/LayerNorm/gamma
bert/encoder/transformer/group_0/inner_group_0/LayerNorm/gamma/adam_m
bert/encoder/transformer/group_0/inner_group_0/LayerNorm/gamma/adam_v
bert/encoder/transformer/group_0/inner_group_0/LayerNorm_1/beta
bert/encoder/transformer/group_0/inner_group_0/LayerNorm_1/beta/adam_m
bert/encoder/transformer/group_0/inner_group_0/LayerNorm_1/beta/adam_v
bert/encoder/transformer/group_0/inner_group_0/LayerNorm_1/gamma
bert/encoder/transformer/group_0/inner_group_0/LayerNorm_1/gamma/adam_m
bert/encoder/transformer/group_0/inner_group_0/LayerNorm_1/gamma/adam_v
bert/encoder/transformer/group_0/inner_group_0/attention_1/output/dense/bias
bert/encoder/transformer/group_0/inner_group_0/attention_1/output/dense/bias/adam_m
bert/encoder/transformer/group_0/inner_group_0/attention_1/output/dense/bias/adam_v
bert/encoder/transformer/group_0/inner_group_0/attention_1/output/dense/kernel
bert/encoder/transformer/group_0/inner_group_0/attention_1/output/dense/kernel/adam_m
bert/encoder/transformer/group_0/inner_group_0/attention_1/output/dense/kernel/adam_v
bert/encoder/transformer/group_0/inner_group_0/attention_1/self/key/bias
bert/encoder/transformer/group_0/inner_group_0/attention_1/self/key/bias/adam_m
bert/encoder/transformer/group_0/inner_group_0/attention_1/self/key/bias/adam_v
bert/encoder/transformer/group_0/inner_group_0/attention_1/self/key/kernel
bert/encoder/transformer/group_0/inner_group_0/attention_1/self/key/kernel/adam_m
bert/encoder/transformer/group_0/inner_group_0/attention_1/self/key/kernel/adam_v
bert/encoder/transformer/group_0/inner_group_0/attention_1/self/query/bias
bert/encoder/transformer/group_0/inner_group_0/attention_1/self/query/bias/adam_m
bert/encoder/transformer/group_0/inner_group_0/attention_1/self/query/bias/adam_v
bert/encoder/transformer/group_0/inner_group_0/attention_1/self/query/kernel
bert/encoder/transformer/group_0/inner_group_0/attention_1/self/query/kernel/adam_m
bert/encoder/transformer/group_0/inner_group_0/attention_1/self/query/kernel/adam_v
bert/encoder/transformer/group_0/inner_group_0/attention_1/self/value/bias
bert/encoder/transformer/group_0/inner_group_0/attention_1/self/value/bias/adam_m
bert/encoder/transformer/group_0/inner_group_0/attention_1/self/value/bias/adam_v
bert/encoder/transformer/group_0/inner_group_0/attention_1/self/value/kernel
bert/encoder/transformer/group_0/inner_group_0/attention_1/self/value/kernel/adam_m
bert/encoder/transformer/group_0/inner_group_0/attention_1/self/value/kernel/adam_v
bert/encoder/transformer/group_0/inner_group_0/ffn_1/intermediate/dense/bias
bert/encoder/transformer/group_0/inner_group_0/ffn_1/intermediate/dense/bias/adam_m
bert/encoder/transformer/group_0/inner_group_0/ffn_1/intermediate/dense/bias/adam_v
bert/encoder/transformer/group_0/inner_group_0/ffn_1/intermediate/dense/kernel
bert/encoder/transformer/group_0/inner_group_0/ffn_1/intermediate/dense/kernel/adam_m
bert/encoder/transformer/group_0/inner_group_0/ffn_1/intermediate/dense/kernel/adam_v
bert/encoder/transformer/group_0/inner_group_0/ffn_1/intermediate/output/dense/bias
bert/encoder/transformer/group_0/inner_group_0/ffn_1/intermediate/output/dense/bias/adam_m
bert/encoder/transformer/group_0/inner_group_0/ffn_1/intermediate/output/dense/bias/adam_v
bert/encoder/transformer/group_0/inner_group_0/ffn_1/intermediate/output/dense/kernel
bert/encoder/transformer/group_0/inner_group_0/ffn_1/intermediate/output/dense/kernel/adam_m
bert/encoder/transformer/group_0/inner_group_0/ffn_1/intermediate/output/dense/kernel/adam_v
bert/pooler/dense/bias
bert/pooler/dense/bias/adam_m
bert/pooler/dense/bias/adam_v
bert/pooler/dense/kernel
bert/pooler/dense/kernel/adam_m
bert/pooler/dense/kernel/adam_v
cls/predictions/output_bias
cls/predictions/output_bias/adam_m
cls/predictions/output_bias/adam_v
cls/predictions/transform/LayerNorm/beta
cls/predictions/transform/LayerNorm/beta/adam_m
cls/predictions/transform/LayerNorm/beta/adam_v
cls/predictions/transform/LayerNorm/gamma
cls/predictions/transform/LayerNorm/gamma/adam_m
cls/predictions/transform/LayerNorm/gamma/adam_v
cls/predictions/transform/dense/bias
cls/predictions/transform/dense/bias/adam_m
cls/predictions/transform/dense/bias/adam_v
cls/predictions/transform/dense/kernel
cls/predictions/transform/dense/kernel/adam_m
cls/predictions/transform/dense/kernel/adam_v
cls/seq_relationship/output_bias
cls/seq_relationship/output_bias/adam_m
cls/seq_relationship/output_bias/adam_v
cls/seq_relationship/output_weights
cls/seq_relationship/output_weights/adam_m
cls/seq_relationship/output_weights/adam_v
global_step
Initialize PyTorch weight ['albert', 'embeddings', 'LayerNorm', 'beta'] from bert/embeddings/LayerNorm/beta
Initialize PyTorch weight ['albert', 'embeddings', 'LayerNorm', 'beta', 'adam_m'] from bert/embeddings/LayerNorm/beta/adam_m
Initialize PyTorch weight ['albert', 'embeddings', 'LayerNorm', 'beta', 'adam_v'] from bert/embeddings/LayerNorm/beta/adam_v
Initialize PyTorch weight ['albert', 'embeddings', 'LayerNorm', 'gamma'] from bert/embeddings/LayerNorm/gamma
Initialize PyTorch weight ['albert', 'embeddings', 'LayerNorm', 'gamma', 'adam_m'] from bert/embeddings/LayerNorm/gamma/adam_m
Initialize PyTorch weight ['albert', 'embeddings', 'LayerNorm', 'gamma', 'adam_v'] from bert/embeddings/LayerNorm/gamma/adam_v
Initialize PyTorch weight ['albert', 'embeddings', 'position_embeddings'] from bert/embeddings/position_embeddings
---------------------------------------------------------------------------
AttributeError Traceback (most recent call last)
<ipython-input-4-a47f5e7bff26> in <module>
----> 1 model = load_tf_weights_in_albert(model,config,'sample_tf_checkpoint/model.ckpt-100000')
~/anaconda3/envs/pytorch_py37/lib/python3.7/site-packages/transformers/modeling_albert.py in load_tf_weights_in_albert(model, config, tf_checkpoint_path)
130 array = np.transpose(array)
131 try:
--> 132 assert pointer.shape == array.shape
133 except AssertionError as e:
134 e.args += (pointer.shape, array.shape)
~/anaconda3/envs/pytorch_py37/lib/python3.7/site-packages/torch/nn/modules/module.py in __getattr__(self, name)
589 return modules[name]
590 raise AttributeError("'{}' object has no attribute '{}'".format(
--> 591 type(self).__name__, name))
592
593 def __setattr__(self, name, value):
AttributeError: 'Embedding' object has no attribute 'shape'
```<|||||>Alright, I see where the issue stems from, I'm patching it and will get back to you soon.<|||||>Alright, please let me know if e85855f fixed it. I tested it with models saved from `run_pretraning.py` (with `AlbertForMaskedLM` as the host model) and `run_classifier_sp.py` (with `AlbertForSequenceClassifiication`) and both seem to work fine now.
Please keep in mind that we have no albert model that can do next sentence prediction so the weights from `cls/seq_relationship` are dropped. <|||||>@LysandreJik
Works fine!! :)))) Thank you so much! 👍 <|||||>Glad I could help!<|||||>Thanks @LysandreJik ! I can also confirm that the conversion script is working now :+1: <|||||>Short update: I used the converted ALBERT model to perform NER. F-score was ~0.1%. I've seen this strange behaviour for v2 ALBERT models but still have no solution for that.
@hansaimlim have you done some evaluations with your trained model? Would be great to know if this problem also occurs for non-NER tasks!
<|||||>@stefan-it I'm working on drug activity prediction. In my case, I used v2 ALBERT as well, and its performance for masked LM was fine, and I haven't done downstream prediction tasks yet. Assuming you're working on human language, I believe our tasks are very different. How was it when you use BERT?<|||||>I used my trained model for predicting a masked token, and the model always returns `<unk>` (which is not the case for the English v1 and v2 models), so I guess I did something wrong in the pre-training steps... <|||||>Dear All,
I still ha ve an issue by converting an albert checkpoint to pytorch binary using this script. Here is the error:
```Traceback (most recent call last):
File "$WORK/Tools/miniconda3/envs/py309/lib/python3.9/site-packages/transformers/models/albert/convert_albert_original_tf_checkpoint_to_pytorch.py", line 63, in <module>
convert_tf_checkpoint_to_pytorch(args.tf_checkpoint_path, args.albert_config_file, args.pytorch_dump_path)
File "$WORK/Tools/miniconda3/envs/py309/lib/python3.9/site-packages/transformers/models/albert/convert_albert_original_tf_checkpoint_to_pytorch.py", line 36, in convert_tf_checkpoint_to_pytorch
load_tf_weights_in_albert(model, config, tf_checkpoint_path)
File "$WORK/Tools/miniconda3/envs/py309/lib/python3.9/site-packages/transformers/models/albert/modeling_albert.py", line 163, in load_tf_weights_in_albert
pointer = getattr(pointer, "bias")
File "$WORK/Tools/miniconda3/envs/py309/lib/python3.9/site-packages/torch/nn/modules/module.py", line 1269, in __getattr__
raise AttributeError("'{}' object has no attribute '{}'".format(
AttributeError: 'AlbertEmbeddings' object has no attribute 'bias'
```
Any idea?
Using python 3.9
transformers 4.26.1
under linux (ubuntu) |
transformers | 2,005 | closed | tf.keras.mixed_precision.experimental.Policy | ## ❓ Questions & Help
I want to use `mixed_precision`, and I found [tf.keras.mixed_precision.experimental.Policy](https://www.tensorflow.org/api_docs/python/tf/keras/mixed_precision/experimental/Policy).
So I put `tf.keras.mixed_precision.experimental.set_policy("mixed_float16")` before `TFBertModel.from_pretrained(pretrained_weights)`. When I run the code, I got the following error:
> InvalidArgumentError: cannot compute AddV2 as input #1(zero-based) was expected to be a half tensor but is a float tensor [Op:AddV2] name: tf_bert_model_1/bert/embeddings/add/
which happened at `ret = model(model.dummy_inputs, training=False) # build the network with dummy inputs`.
I am not sure if I used it correctly. I think `tf.keras.mixed_precision.experimental.set_policy` is supposed to be used before constructing / build the model, as the tf page says `Policies can be passed to the 'dtype' argument of layer constructors, or a global policy can be set with 'tf.keras.mixed_precision.experimental.set_policy'`.
I wonder if I can use AMP with tf based transformer models and how. Thanks.
[error.txt](https://github.com/huggingface/transformers/files/3907032/error.txt)
| 11-30-2019 11:01:39 | 11-30-2019 11:01:39 | Sorry, I created this duplicated issue as the previous one. Please delete this one, thank you. |
transformers | 2,004 | closed | Can we use tf.keras.mixed_precision.experimental.set_policy ? | ## ❓ Questions & Help
I want to use `mixed_precision`, and I found [tf.keras.mixed_precision.experimental.Policy](https://www.tensorflow.org/api_docs/python/tf/keras/mixed_precision/experimental/Policy).
So I put `tf.keras.mixed_precision.experimental.set_policy("mixed_float16")` before `TFBertModel.from_pretrained(pretrained_weights)`. When I run the code, I got the following error:
> InvalidArgumentError: cannot compute AddV2 as input #1(zero-based) was expected to be a half tensor but is a float tensor [Op:AddV2] name: tf_bert_model_1/bert/embeddings/add/
which happened at `ret = model(model.dummy_inputs, training=False) # build the network with dummy inputs`.
I am not sure if I used it correctly. I think `tf.keras.mixed_precision.experimental.set_policy` is supposed to be used before constructing / build the model, as the tf page says `Policies can be passed to the 'dtype' argument of layer constructors, or a global policy can be set with 'tf.keras.mixed_precision.experimental.set_policy'`.
I wonder if I can use AMP with tf based transformer models and how. Thanks.
[error.txt](https://github.com/huggingface/transformers/files/3907032/error.txt)
| 11-30-2019 10:55:30 | 11-30-2019 10:55:30 | For now we need to use:
```python
tf.config.optimizer.set_experimental_options({"auto_mixed_precision": True})
```
Please see [example here](https://github.com/huggingface/transformers/blob/master/examples/run_tf_glue.py).<|||||>Thanks. I tried it during waiting the answer, and it doesn't speed up the training. I probably can post my model later.<|||||>This issue has been automatically marked as stale because it has not had recent activity. It will be closed if no further activity occurs. Thank you for your contributions.
|
transformers | 2,003 | closed | Where I could find the vocab.json for XLNet | ## ❓ Questions & Help
<!-- A clear and concise description of the question. -->
I notice in tokenizer_xlnet.py there is not the vocab,json only spiece model. So I want to know where I could find the vocab.json? And what I should rename the file ? | 11-30-2019 09:01:24 | 11-30-2019 09:01:24 | If you want to have `xlnet_config.json`, which is a JSON file which specifies the hyper-parameters of the XLNet model, you can download the .zip file from [here](https://github.com/zihangdai/xlnet/blob/5cd50bc451436e188a8e7fea15358d5a8c916b72/README.md) which contains the pre-trained weights of XLNet model.
> ## Questions & Help
> I notice in tokenizer_xlnet.py there is not the vocab,json only spiece model. So I want to know where I could find the vocab.json? And what I should rename the file ?<|||||>I downloaded the pre-trained model, the config file and the sentence piece model, but when I run the code I found the vocab_size = -1. Did I miss something?<|||||>An example of the content of `xlnet_config.json` is the following:
```
{
"d_head": 64,
"d_inner": 3072,
"d_model": 768,
"ff_activation": "gelu",
"n_head": 12,
"n_layer": 12,
"n_token": 32000,
"untie_r": true
}
```
> When i run the code I found the vocab_size=-1
Which code you are talking about?
<|||||>You can either download from the S3 repo or the script is supposed to automatically download the vocab file. Make sure you have a working internet connection. |
transformers | 2,002 | closed | Always use SequentialSampler during evaluation | When evaluating, shouldn't we always use the SequentialSampler instead of DistributedSampler? Evaluation only runs on 1 GPU no matter what, so if you use the DistributedSampler with N GPUs, I think you'll only evaluate on 1/N of the evaluation set. That's at least what I'm finding when I run an older/modified version of this repo. | 11-30-2019 00:22:57 | 11-30-2019 00:22:57 | # [Codecov](https://codecov.io/gh/huggingface/transformers/pull/2002?src=pr&el=h1) Report
> Merging [#2002](https://codecov.io/gh/huggingface/transformers/pull/2002?src=pr&el=desc) into [master](https://codecov.io/gh/huggingface/transformers/commit/b0ee7c7df3d49a819c4d6cef977214bd91f5c075?src=pr&el=desc) will **not change** coverage.
> The diff coverage is `n/a`.
[](https://codecov.io/gh/huggingface/transformers/pull/2002?src=pr&el=tree)
```diff
@@ Coverage Diff @@
## master #2002 +/- ##
=======================================
Coverage 84.05% 84.05%
=======================================
Files 105 105
Lines 15555 15555
=======================================
Hits 13075 13075
Misses 2480 2480
```
------
[Continue to review full report at Codecov](https://codecov.io/gh/huggingface/transformers/pull/2002?src=pr&el=continue).
> **Legend** - [Click here to learn more](https://docs.codecov.io/docs/codecov-delta)
> `Δ = absolute <relative> (impact)`, `ø = not affected`, `? = missing data`
> Powered by [Codecov](https://codecov.io/gh/huggingface/transformers/pull/2002?src=pr&el=footer). Last update [b0ee7c7...508f939](https://codecov.io/gh/huggingface/transformers/pull/2002?src=pr&el=lastupdated). Read the [comment docs](https://docs.codecov.io/docs/pull-request-comments).
<|||||>Yes, this works! Thank you @ethanjperez. |
transformers | 2,001 | closed | GPT2: how to construct batch for Language Modeling | I am a little confused about how to prepare input bathces for GPT2LMHeadModel. I want to use GPT2 as an LM. For instance, I want to generate probability distributions over the vocabulary at each timestep, as well as computing the perplexities of sentences. It is important to note that I am working with sentences and not documents, so I will have to pad the inputs in the batch.
```python
from transformers import GPT2Tokenizer, GPT2LMHeadModel
# Prepare model
tokenizer = GPT2Tokenizer.from_pretrained('gpt2')
model = GPT2LMHeadModel.from_pretrained('gpt2')
model.eval()
model.to('cuda')
# input sentences
batch = ['this is a sentence.',
'this is another sentence.',
'this is another even longer sentence.']
```
## Question 1: Special tokens
a) Do I have to add a bos token id on my own or is it handled internally by GPT2Tokenizer? Same for the eos token.
```
# tokenize
tokens = [tokenizer.encode(x) for x in batch]
# add BOS and EOS
tokens = [[tokenizer.bos_token_id] + x + [tokenizer.eos_token_id] for x in tokens]
```
```
[[50256, 428, 318, 257, 6827, 13, 50256],
[50256, 428, 318, 1194, 6827, 13, 50256],
[50256, 428, 318, 1194, 772, 2392, 6827, 13, 50256]]
```
b) `tokenizer.encode(x)` gives me a warning "This tokenizer does not make use of special tokens. Input is returned with no modification." I replaced it with `tokenizer.convert_tokens_to_ids(tokenizer.tokenize(x, add_prefix_space=True))` and the warning went away, but I am not sure what is the difference. Which tokenization should I use?
c) By looking at the properties of an instance of GPT2Tokenizer, I see that `bos_token` and `eos_token` are the same. Is this correct?
## Question 2: Padding
I want to pad based on the longest sentence in the batch. This is how I usually do it.
```
batch = pad_sequence([torch.LongTensor(x) for x in tokens], batch_first=True).to('cuda')
```
```
tensor([[50256, 428, 318, 257, 6827, 13, 50256, 0, 0],
[50256, 428, 318, 1194, 6827, 13, 50256, 0, 0],
[50256, 428, 318, 1194, 772, 2392, 6827, 13, 50256]])
```
a) What id does the model expect for the padded tokens? Do I have to pass the token id as an argument to the model or the tokenizer or you have a predifined one?
## Question 3: Model input
How is GPT2 made aware of the padded steps? For instance for an RNN I would do something like this:
```
lengths = (batch != 0).sum(-1) # tensor([7, 7, 9])
packed = pack_padded_sequence(x, lengths, batch_first=True)
out_packed, hn = rnn(packed)
```
but for GPT2 I havent found an example. The only ones i found are with batch size 1. So something like this wont work as expected:
```
outputs = model(x, labels=x) # labels are shifted inside the model, right?
loss, logits = outputs[:2]
```
---
# Update
So there are still things unclear, but from reading other issues this is my current understanding:
- GPT2 has no padding token, as it was trained on documents and not sentences.
- In order to use GPT2 with variable length inputs, we can apply padding with an arbitrary token and ensure that those tokens are not used by the model with an `attention_mask`.
- As for the labels, we should replace **only** on the `labels` variable the padded token ids with `-1`.
So based on that, here is my current toy implementation:
```python
inputs = [
'this is a sentence.',
'this is another sentence.',
'this is another even longer sentence.', ]
# tokenize
# tokens = [tokenizer.encode(x) for x in batch]
tokens = [tokenizer.convert_tokens_to_ids(
tokenizer.tokenize(x, add_prefix_space=True))
for x in inputs]
# add BOS and EOS
tokens = [[tokenizer.bos_token_id] + x + [tokenizer.eos_token_id]
for x in tokens]
# padding_value can be whatever...
inputs = pad_sequence([torch.LongTensor(x) for x in tokens], batch_first=True, padding_value=0).to('cuda')
# 1 for real tokens and 0 for padded tokens
mask = (inputs != 0).float()
# replace the ids of the padded tokens (where token_id==padded_id) with `-1`
labels = inputs.masked_fill(inputs == 0, -1)
outputs = model(inputs, attention_mask=mask, labels=labels)
loss, logits = outputs[:2]
```
Is this correct??
### Bug: Padded tokens are not excluded from the loss
However, I computed the loss on my own and found your implementation does not take into account the padded tokens when averaging, unless I am missing something
```python
_logits = logits.view(-1, logits.size(-1)) # flatten logits
_labels = torch.cat([inputs[:, 1:], inputs[:, :1] * 0], dim=1).view(-1) # shift inputs one position to the left and flatten
loss_real_avg = F.cross_entropy(_logits, _labels, ignore_index=0, reduction='sum') / mask.sum() # ignore padded timesteps
loss_naive_avg = F.cross_entropy(_logits, _labels, ignore_index=0, reduction='mean')
print("GPT2 loss:", loss.item())
print("loss_naive_avg:", loss_naive_avg.item())
print("loss_real_avg:", loss_real_avg.item())
```
```
GPT2 loss: 4.664564609527588
loss_naive_avg: 4.664564609527588
loss_real_avg: 4.056143283843994
``` | 11-29-2019 20:28:13 | 11-29-2019 20:28:13 | > I am a little confused about how to prepare input bathces for GPT2LMHeadModel. I want to use GPT2 as an LM. For instance, I want to generate probability distributions over the vocabulary at each timestep, as well as computing the perplexities of sentences. It is important to note that I am working with sentences and not documents, so I will have to pad the inputs in the batch.
>
> ```python
> from transformers import GPT2Tokenizer, GPT2LMHeadModel
>
> # Prepare model
> tokenizer = GPT2Tokenizer.from_pretrained('gpt2')
> model = GPT2LMHeadModel.from_pretrained('gpt2')
> model.eval()
> model.to('cuda')
>
> # input sentences
> batch = ['this is a sentence.',
> 'this is another sentence.',
> 'this is another even longer sentence.']
> ```
>
> ## Question 1: Special tokens
> a) Do I have to add a bos token id on my own or is it handled internally by GPT2Tokenizer? Same for the eos token.
>
> ```
> # tokenize
> tokens = [tokenizer.encode(x) for x in batch]
>
> # add BOS and EOS
> tokens = [[tokenizer.bos_token_id] + x + [tokenizer.eos_token_id] for x in tokens]
> ```
>
> ```
> [[50256, 428, 318, 257, 6827, 13, 50256],
> [50256, 428, 318, 1194, 6827, 13, 50256],
> [50256, 428, 318, 1194, 772, 2392, 6827, 13, 50256]]
> ```
>
> b) `tokenizer.encode(x)` gives me a warning "This tokenizer does not make use of special tokens. Input is returned with no modification." I replaced it with `tokenizer.convert_tokens_to_ids(tokenizer.tokenize(x, add_prefix_space=True))` and the warning went away, but I am not sure what is the difference. Which tokenization should I use?
>
> c) By looking at the properties of an instance of GPT2Tokenizer, I see that `bos_token` and `eos_token` are the same. Is this correct?
>
> ## Question 2: Padding
> I want to pad based on the longest sentence in the batch. This is how I usually do it.
>
> ```
> batch = pad_sequence([torch.LongTensor(x) for x in tokens], batch_first=True).to('cuda')
> ```
>
> ```
> tensor([[50256, 428, 318, 257, 6827, 13, 50256, 0, 0],
> [50256, 428, 318, 1194, 6827, 13, 50256, 0, 0],
> [50256, 428, 318, 1194, 772, 2392, 6827, 13, 50256]])
> ```
>
> a) What id does the model expect for the padded tokens? Do I have to pass the token id as an argument to the model or the tokenizer or you have a predifined one?
>
> ## Question 3: Model input
> How is GPT2 made aware of the padded steps? For instance for an RNN I would do something like this:
>
> ```
> lengths = (batch != 0).sum(-1) # tensor([7, 7, 9])
> packed = pack_padded_sequence(x, lengths, batch_first=True)
> out_packed, hn = rnn(packed)
> ```
>
> but for GPT2 I havent found an example. The only ones i found are with batch size 1. So something like this wont work as expected:
>
> ```
> outputs = model(x, labels=x) # labels are shifted inside the model, right?
> loss, logits = outputs[:2]
> ```
>
> # Update
> So there are still things unclear, but from reading other issues this is my current understanding:
>
> * GPT2 has no padding token, as it was trained on documents and not sentences.
> * In order to use GPT2 with variable length inputs, we can apply padding with an arbitrary token and ensure that those tokens are not used by the model with an `attention_mask`.
> * As for the labels, we should replace **only** on the `labels` variable the padded token ids with `-1`.
> So based on that, here is my current toy implementation:
>
> ```python
> inputs = [
> 'this is a sentence.',
> 'this is another sentence.',
> 'this is another even longer sentence.', ]
>
> # tokenize
> # tokens = [tokenizer.encode(x) for x in batch]
> tokens = [tokenizer.convert_tokens_to_ids(
> tokenizer.tokenize(x, add_prefix_space=True))
> for x in inputs]
>
> # add BOS and EOS
> tokens = [[tokenizer.bos_token_id] + x + [tokenizer.eos_token_id]
> for x in tokens]
>
> # padding_value can be whatever...
> inputs = pad_sequence([torch.LongTensor(x) for x in tokens], batch_first=True, padding_value=0).to('cuda')
> # 1 for real tokens and 0 for padded tokens
> mask = (inputs != 0).float()
> # replace the ids of the padded tokens (where token_id==padded_id) with `-1`
> labels = inputs.masked_fill(inputs == 0, -1)
>
> outputs = model(inputs, attention_mask=mask, labels=labels)
> loss, logits = outputs[:2]
> ```
>
> Is this correct??
>
> ### Bug: Padded tokens are not excluded from the loss
> However, I computed the loss on my own and found your implementation does not take into account the padded tokens when averaging, unless I am missing something
>
> ```python
> _logits = logits.view(-1, logits.size(-1)) # flatten logits
> _labels = torch.cat([inputs[:, 1:], inputs[:, :1] * 0], dim=1).view(-1) # shift inputs one position to the left and flatten
> loss_real_avg = F.cross_entropy(_logits, _labels, ignore_index=0, reduction='sum') / mask.sum() # ignore padded timesteps
> loss_naive_avg = F.cross_entropy(_logits, _labels, ignore_index=0, reduction='mean')
>
> print("GPT2 loss:", loss.item())
> print("loss_naive_avg:", loss_naive_avg.item())
> print("loss_real_avg:", loss_real_avg.item())
> ```
>
> ```
> GPT2 loss: 4.664564609527588
> loss_naive_avg: 4.664564609527588
> loss_real_avg: 4.056143283843994
> ```
What you proposed seems a valid walk-around to me. Also, look at #1464, which talked about adding `pad_token` to `tokenizer` and `embedding`. Perhaps that will help as well.<|||||>This issue has been automatically marked as stale because it has not had recent activity. It will be closed if no further activity occurs. Thank you for your contributions.
<|||||>Check out [#3311](https://github.com/huggingface/transformers/issues/3311#issuecomment-601264426). GPT2 doesn't add BOS or EOS token, you must do it manually or use a tokenizer to do so. <|||||>> Bug: Padded tokens are not excluded from the loss
>
> However, I computed the loss on my own and found your implementation does not take into account the padded tokens when averaging, unless I am missing something
> ```py
> _logits = logits.view(-1, logits.size(-1)) # flatten logits
> _labels = torch.cat([inputs[:, 1:], inputs[:, :1] * 0], dim=1).view(-1) # shift inputs one position to the left and flatten
> loss_real_avg = F.cross_entropy(_logits, _labels, ignore_index=0, reduction='sum') / mask.sum() # ignore padded timesteps
> loss_naive_avg = F.cross_entropy(_logits, _labels, ignore_index=0, reduction='mean')
> print("GPT2 loss:", loss.item())
> print("loss_naive_avg:", loss_naive_avg.item())
> print("loss_real_avg:", loss_real_avg.item())
> GPT2 loss: 4.664564609527588
> loss_naive_avg: 4.664564609527588
> loss_real_avg: 4.056143283843994
For this bug, you may need to set `ignore_index` to -1 instead of 0 in `F.cross_entropy` according to this line:
> ```py
> # replace the ids of the padded tokens (where token_id==padded_id) with `-1`
> labels = inputs.masked_fill(inputs == 0, -1)
> ``` |
transformers | 2,000 | closed | Wrong tokenization in Transformer-XL documentation | ## 🐛 Bug
This is a documentation-related bug. In the [TransfoXL documentation](https://huggingface.co/transformers/model_doc/transformerxl.html), the tokenization example is wrong. The snippet goes:
```
tokenizer = TransfoXLTokenizer.from_pretrained('transfo-xl-wt103')
...
input_ids = torch.tensor(tokenizer.encode("Hello, my dog is cute")).unsqueeze(0) # Batch size 1
```
This code outputs the tokens `[24, 617, 3225, 23, 16072]`, of which `24` is `<unk>`.
The problem comes from the fact that Transformer-XL does **not** use a wordpiece vocabulary, but a regular (whole-word) one. Also, in WT-103, punctuation marks are split from the words. Consequently, the example should read instead (note that space in from of `,`):
```
tokenizer = TransfoXLTokenizer.from_pretrained('transfo-xl-wt103')
...
input_ids = torch.tensor(tokenizer.encode("Hello , my dog is cute")).unsqueeze(0) # Batch size 1
```
It would also be nice to warn the user about this fact in the documentation, perhaps in `TransfoXLTokenizer`'s docstring? | 11-29-2019 15:15:02 | 11-29-2019 15:15:02 | Indeed, do you want to fix this in a PR?<|||||>This issue has been automatically marked as stale because it has not had recent activity. It will be closed if no further activity occurs. Thank you for your contributions.
|
transformers | 1,999 | closed | Training masked language model with Tensorflow | ## ❓ Questions & Help
I'm trying to fine-tune a masked language model starting from bert-base-multilingual-cased with Tensorflow using the PyTorch-based example _examples/run_lm_finetuning_ as starting point. I'd like to take the multilingual model and adapt it to the Italian language.
Unfortunately I'm unable to find examples over the internet for the TFBertForMaskedLM model in training mode, so I hope this is the appropriate place for this question.
System and libraries
> Platform Linux-5.0.0-36-generic-x86_64-with-debian-buster-sid
> Python 3.7.5 (default, Oct 25 2019, 15:51:11)
> [GCC 7.3.0]
> PyTorch 1.3.1
> Tensorflow 2.0.0
> Transformers 2.2.0
I first convert my train sentences in 4 arrays:
1) train_ids_masked: tokens ids with special tokens and masking + padding up to max_seq_length = 10
2) train_attnmasks: masks for attention (padding masks)
3) train_segments: masks for sentence (constant array since sentences are independent)
4) train_labels: original masked tokens + UNK tokens everywhere else
Every array has shape (num sentences, max_seq_length) = (72,10)
Then I define the model and print the summary
```python
pre_trained_model = 'bert-base-multilingual-cased'
config = transformers.BertConfig.from_pretrained(pre_trained_model)
model = transformers.TFBertForMaskedLM.from_pretrained(pre_trained_model, config=config)
model.compile(optimizer=tf.optimizers.Adam(lr=params['learning_rate']), loss='binary_crossentropy')
print(model.summary())
```
which outputs
```
Model: "tf_bert_for_masked_lm_1"
_________________________________________________________________
Layer (type) Output Shape Param #
=================================================================
bert (TFBertMainLayer) multiple 177853440
_________________________________________________________________
mlm___cls (TFBertMLMHead) multiple 92920059
=================================================================
Total params: 178,565,115
Trainable params: 178,565,115
Non-trainable params: 0
```
Then I try to train the model
```python
model.fit([train_ids_masked, train_attnmasks, train_segments], train_labels, epochs=1, batch_size=20)
```
The model trains over the first batch but returns the following error
```
Train on 72 samples
20/72 [=======>......................] - ETA: 7sTraceback (most recent call last):
File "/home/andrea/anaconda3/envs/tf2/lib/python3.7/site-packages/tensorflow_core/python/framework/ops.py", line 1610, in _create_c_op
c_op = c_api.TF_FinishOperation(op_desc)
tensorflow.python.framework.errors_impl.InvalidArgumentError: Dimensions must be equal, but are 10 and 119547 for 'loss/output_1_loss/mul' (op: 'Mul') with input shapes: [?,10], [?,?,119547].
```
when calculating the loss, trying to compare the padding length max_seq_length (= 10) to the vocabulary size (= 119547).
I've also tried to define the model in the following way
```python
inp_ids = tf.keras.layers.Input(shape=(max_seq_length, ), dtype='int32', name="bert_input_ids")
inp_attnmasks = tf.keras.layers.Input(shape=(max_seq_length, ), dtype='int32', name="bert_input_attention_masks")
inp_segments = tf.keras.layers.Input(shape=(max_seq_length, ), dtype='int32', name="bert_input_segment_ids")
inputs = [inp_ids, inp_attnmasks, inp_segments]
outputs = transformers.TFBertForMaskedLM.from_pretrained(pre_trained_model)(inputs)
model = tf.keras.Model(inputs=inputs, outputs=outputs)
model.compile(optimizer=tf.optimizers.Adam(lr=params['learning_rate']), loss='binary_crossentropy')
```
but I get the same error.
My input and label arrays have the same shape as the ones in the _run_lm_finetuning_ example and my model is simply the Tensorflow equivalent to the model used there.
What am I doing wrong?
Is it possible that this is related to the loss calculation rather than the definition of the model?
I've noticed that in the _run_lm_finetuning_ example the model has an additional argument **masked_lm_labels**
```python
outputs = model(inputs, masked_lm_labels=labels) if args.mlm else model(inputs, labels=labels)
```
that allows to compute the loss only on masked tokens using PyTorch, but this option is not present in TFBertForMaskedLM, how can I achieve that? | 11-29-2019 11:49:41 | 11-29-2019 11:49:41 | > I've noticed that in the run_lm_finetuning example the model has an additional argument masked_lm_labels
Yes, I have the same issue here. Did you manage to port the example code to TF?
In the torch models the argument is interpreted as follows:
```
if masked_lm_labels is not None:
loss_fct = CrossEntropyLoss(ignore_index=-1) # -1 index = padding token
masked_lm_loss = loss_fct(prediction_scores.view(-1, self.config.vocab_size), masked_lm_labels.view(-1))
outputs = (masked_lm_loss,) + outputs
```
which means that one has to define a custom cross-entropy loss in Tensorflow.<|||||>Unfortunately no, I had a look around in order to implement the custom cross-entropy we are talking about. I switched to Pytorch since it wasn't clear to me whether switching to the custom loss would solve all the problems I had.<|||||>I see. I guess I will take the same road ;-) At least I can do the finetuning in torch and later convert the model to TF. Thanks for sharing the info!
BTW I found the implementation of the custom loss that we are talking about in google repo:
```python
# The `positions` tensor might be zero-padded (if the sequence is too
# short to have the maximum number of predictions). The `label_weights`
# tensor has a value of 1.0 for every real prediction and 0.0 for the
# padding predictions.
per_example_loss = -tf.reduce_sum(log_probs * one_hot_labels, axis=[-1])
numerator = tf.reduce_sum(label_weights * per_example_loss)
denominator = tf.reduce_sum(label_weights) + 1e-5
loss = numerator / denominator
```
Here is the link to the original code:
https://github.com/google-research/bert/blob/cc7051dc592802f501e8a6f71f8fb3cf9de95dc9/run_pretraining.py#L273-L280
<|||||>This issue has been automatically marked as stale because it has not had recent activity. It will be closed if no further activity occurs. Thank you for your contributions.
<|||||>While everyone correctly pointed out that you need a loss function which handles masks, the original error message posted here is actually unrelated to that.
> ```python
> model.compile(optimizer=tf.optimizers.Adam(lr=params['learning_rate']), loss='binary_crossentropy')
> ```
Your model is compiled with binary crossenropy, e.g. one hot encoded binary labels of shape (batch size x len x len(dict)), while you provide the labels as integers representing the token values (5673 etc) with shape (batchsize x len). This leads to a shape mismatch.
The error message comes from the comparison of the last values of the shapes len(dict) vs textlen.
> tensorflow.python.framework.errors_impl.InvalidArgumentError: Dimensions must be equal, but are 10 and 119547 for 'loss/output_1_loss/mul' (op: 'Mul') with input shapes: [?,10], [?,?,119547].
Using tf.keras.losses.SparseCategoricalCrossentropy solves the error message, but of course you will still need to implement a masked loss function to use it properly.
<|||||>Is there anyone who went on with tensorflow? I don't want to switch to pytorch. I will try to implement a masked loss function. If there is anyone already did this, I would be happy to know.<|||||>I made an attempt on kaggle: https://www.kaggle.com/riblidezso/finetune-xlm-roberta-on-jigsaw-test-data-with-mlm<|||||>> I made an attempt on kaggle: https://www.kaggle.com/riblidezso/finetune-xlm-roberta-on-jigsaw-test-data-with-mlm
It is a very interesting and usefull notebook. Thanks for sharing<|||||>> I made an attempt on kaggle: https://www.kaggle.com/riblidezso/finetune-xlm-roberta-on-jigsaw-test-data-with-mlm
Super useful, thank you! |
transformers | 1,998 | closed | Added Camembert to available models | Added Camembert to the available models in the `run_lm_finetuning.py` example. | 11-29-2019 11:34:08 | 11-29-2019 11:34:08 | # [Codecov](https://codecov.io/gh/huggingface/transformers/pull/1998?src=pr&el=h1) Report
> Merging [#1998](https://codecov.io/gh/huggingface/transformers/pull/1998?src=pr&el=desc) into [master](https://codecov.io/gh/huggingface/transformers/commit/1ab8dc44b3d84ed1894f5b6a6fab58fb39298fc7?src=pr&el=desc) will **increase** coverage by `1.28%`.
> The diff coverage is `n/a`.
[](https://codecov.io/gh/huggingface/transformers/pull/1998?src=pr&el=tree)
```diff
@@ Coverage Diff @@
## master #1998 +/- ##
==========================================
+ Coverage 82.83% 84.11% +1.28%
==========================================
Files 105 105
Lines 15545 15545
==========================================
+ Hits 12877 13076 +199
+ Misses 2668 2469 -199
```
| [Impacted Files](https://codecov.io/gh/huggingface/transformers/pull/1998?src=pr&el=tree) | Coverage Δ | |
|---|---|---|
| [transformers/modeling\_openai.py](https://codecov.io/gh/huggingface/transformers/pull/1998/diff?src=pr&el=tree#diff-dHJhbnNmb3JtZXJzL21vZGVsaW5nX29wZW5haS5weQ==) | `82% <0%> (+1.33%)` | :arrow_up: |
| [transformers/modeling\_ctrl.py](https://codecov.io/gh/huggingface/transformers/pull/1998/diff?src=pr&el=tree#diff-dHJhbnNmb3JtZXJzL21vZGVsaW5nX2N0cmwucHk=) | `96.47% <0%> (+2.2%)` | :arrow_up: |
| [transformers/modeling\_xlnet.py](https://codecov.io/gh/huggingface/transformers/pull/1998/diff?src=pr&el=tree#diff-dHJhbnNmb3JtZXJzL21vZGVsaW5nX3hsbmV0LnB5) | `73.61% <0%> (+2.43%)` | :arrow_up: |
| [transformers/modeling\_roberta.py](https://codecov.io/gh/huggingface/transformers/pull/1998/diff?src=pr&el=tree#diff-dHJhbnNmb3JtZXJzL21vZGVsaW5nX3JvYmVydGEucHk=) | `71.76% <0%> (+12.35%)` | :arrow_up: |
| [transformers/tests/modeling\_tf\_common\_test.py](https://codecov.io/gh/huggingface/transformers/pull/1998/diff?src=pr&el=tree#diff-dHJhbnNmb3JtZXJzL3Rlc3RzL21vZGVsaW5nX3RmX2NvbW1vbl90ZXN0LnB5) | `97.08% <0%> (+15.53%)` | :arrow_up: |
| [transformers/modeling\_tf\_pytorch\_utils.py](https://codecov.io/gh/huggingface/transformers/pull/1998/diff?src=pr&el=tree#diff-dHJhbnNmb3JtZXJzL21vZGVsaW5nX3RmX3B5dG9yY2hfdXRpbHMucHk=) | `95.13% <0%> (+85.41%)` | :arrow_up: |
------
[Continue to review full report at Codecov](https://codecov.io/gh/huggingface/transformers/pull/1998?src=pr&el=continue).
> **Legend** - [Click here to learn more](https://docs.codecov.io/docs/codecov-delta)
> `Δ = absolute <relative> (impact)`, `ø = not affected`, `? = missing data`
> Powered by [Codecov](https://codecov.io/gh/huggingface/transformers/pull/1998?src=pr&el=footer). Last update [1ab8dc4...a80f3cd](https://codecov.io/gh/huggingface/transformers/pull/1998?src=pr&el=lastupdated). Read the [comment docs](https://docs.codecov.io/docs/pull-request-comments).
<|||||>Thanks! |
transformers | 1,997 | closed | How to get a spiece.model from customize chinese vocab.txt in Albert xlnet ? | ## ❓ Questions & Help
How to get a spiece.model from customize chinese vocab.txt in Albert xlnet ? | 11-29-2019 11:14:24 | 11-29-2019 11:14:24 | Have you taken a look at [sentencepiece](https://github.com/google/sentencepiece)?<|||||>请问这个问题您解决了吗<|||||>I have the same problem. Have you solved it?<|||||>> Have you taken a look at [sentencepiece](https://github.com/google/sentencepiece)?
I have taken a look at sentencepiece documents, but found nothing to build a spiece.model from customized chinese vocab.txt in ALBERT. Do you have any solution to solve this problem?<|||||>I think that the chinese version of albert uses wordpiece model instead of sentencepiece model.
[https://github.com/google-research/ALBERT/issues/58](url)
> For Chinese models, we use word piece model provided by Jacob as sentence piece get worse performance on reading comprehension tasks for Chinese.
https://github.com/google-research/ALBERT/blob/master/tokenization.py
```python
class FullTokenizer(object):
"""Runs end-to-end tokenziation."""
def __init__(self, vocab_file, do_lower_case=True, spm_model_file=None):
self.vocab = None
self.sp_model = None
if spm_model_file:
self.sp_model = spm.SentencePieceProcessor()
tf.logging.info("loading sentence piece model")
self.sp_model.Load(spm_model_file)
# Note(mingdachen): For the purpose of consisent API, we are
# generating a vocabulary for the sentence piece tokenizer.
self.vocab = {self.sp_model.IdToPiece(i): i for i
in range(self.sp_model.GetPieceSize())}
else:
self.vocab = load_vocab(vocab_file)
self.basic_tokenizer = BasicTokenizer(do_lower_case=do_lower_case)
self.wordpiece_tokenizer = WordpieceTokenizer(vocab=self.vocab)
self.inv_vocab = {v: k for k, v in self.vocab.items()}
```
When the sentencepiece model is None, the full tokenizer is initialized with a basic tokenizer and a workpiece tokenizer.<|||||>This issue has been automatically marked as stale because it has not had recent activity. It will be closed if no further activity occurs. Thank you for your contributions.
<|||||>This issue has been automatically marked as stale because it has not had recent activity. It will be closed if no further activity occurs. Thank you for your contributions.
|
transformers | 1,996 | closed | ALBERT is missing from AutoClasses | Pull request to fix this: https://github.com/huggingface/transformers/pull/1995
| 11-29-2019 10:57:56 | 11-29-2019 10:57:56 | |
transformers | 1,995 | closed | Add ALBERT to AutoClasses | Adds ALBERT to AutoClasses and also fixes some documentation mistakes along the way | 11-29-2019 10:53:52 | 11-29-2019 10:53:52 | # [Codecov](https://codecov.io/gh/huggingface/transformers/pull/1995?src=pr&el=h1) Report
> Merging [#1995](https://codecov.io/gh/huggingface/transformers/pull/1995?src=pr&el=desc) into [master](https://codecov.io/gh/huggingface/transformers/commit/1ab8dc44b3d84ed1894f5b6a6fab58fb39298fc7?src=pr&el=desc) will **increase** coverage by `1.22%`.
> The diff coverage is `31.25%`.
[](https://codecov.io/gh/huggingface/transformers/pull/1995?src=pr&el=tree)
```diff
@@ Coverage Diff @@
## master #1995 +/- ##
==========================================
+ Coverage 82.83% 84.06% +1.22%
==========================================
Files 105 105
Lines 15545 15561 +16
==========================================
+ Hits 12877 13081 +204
+ Misses 2668 2480 -188
```
| [Impacted Files](https://codecov.io/gh/huggingface/transformers/pull/1995?src=pr&el=tree) | Coverage Δ | |
|---|---|---|
| [transformers/modeling\_auto.py](https://codecov.io/gh/huggingface/transformers/pull/1995/diff?src=pr&el=tree#diff-dHJhbnNmb3JtZXJzL21vZGVsaW5nX2F1dG8ucHk=) | `30.61% <20%> (-1.21%)` | :arrow_down: |
| [transformers/tokenization\_auto.py](https://codecov.io/gh/huggingface/transformers/pull/1995/diff?src=pr&el=tree#diff-dHJhbnNmb3JtZXJzL3Rva2VuaXphdGlvbl9hdXRvLnB5) | `45% <33.33%> (-0.95%)` | :arrow_down: |
| [transformers/configuration\_auto.py](https://codecov.io/gh/huggingface/transformers/pull/1995/diff?src=pr&el=tree#diff-dHJhbnNmb3JtZXJzL2NvbmZpZ3VyYXRpb25fYXV0by5weQ==) | `60% <66.66%> (+0.54%)` | :arrow_up: |
| [transformers/modeling\_openai.py](https://codecov.io/gh/huggingface/transformers/pull/1995/diff?src=pr&el=tree#diff-dHJhbnNmb3JtZXJzL21vZGVsaW5nX29wZW5haS5weQ==) | `82% <0%> (+1.33%)` | :arrow_up: |
| [transformers/modeling\_ctrl.py](https://codecov.io/gh/huggingface/transformers/pull/1995/diff?src=pr&el=tree#diff-dHJhbnNmb3JtZXJzL21vZGVsaW5nX2N0cmwucHk=) | `96.47% <0%> (+2.2%)` | :arrow_up: |
| [transformers/modeling\_xlnet.py](https://codecov.io/gh/huggingface/transformers/pull/1995/diff?src=pr&el=tree#diff-dHJhbnNmb3JtZXJzL21vZGVsaW5nX3hsbmV0LnB5) | `73.61% <0%> (+2.43%)` | :arrow_up: |
| [transformers/modeling\_roberta.py](https://codecov.io/gh/huggingface/transformers/pull/1995/diff?src=pr&el=tree#diff-dHJhbnNmb3JtZXJzL21vZGVsaW5nX3JvYmVydGEucHk=) | `71.76% <0%> (+12.35%)` | :arrow_up: |
| [transformers/tests/modeling\_tf\_common\_test.py](https://codecov.io/gh/huggingface/transformers/pull/1995/diff?src=pr&el=tree#diff-dHJhbnNmb3JtZXJzL3Rlc3RzL21vZGVsaW5nX3RmX2NvbW1vbl90ZXN0LnB5) | `97.08% <0%> (+15.53%)` | :arrow_up: |
| [transformers/modeling\_tf\_pytorch\_utils.py](https://codecov.io/gh/huggingface/transformers/pull/1995/diff?src=pr&el=tree#diff-dHJhbnNmb3JtZXJzL21vZGVsaW5nX3RmX3B5dG9yY2hfdXRpbHMucHk=) | `95.13% <0%> (+85.41%)` | :arrow_up: |
------
[Continue to review full report at Codecov](https://codecov.io/gh/huggingface/transformers/pull/1995?src=pr&el=continue).
> **Legend** - [Click here to learn more](https://docs.codecov.io/docs/codecov-delta)
> `Δ = absolute <relative> (impact)`, `ø = not affected`, `? = missing data`
> Powered by [Codecov](https://codecov.io/gh/huggingface/transformers/pull/1995?src=pr&el=footer). Last update [1ab8dc4...a415156](https://codecov.io/gh/huggingface/transformers/pull/1995?src=pr&el=lastupdated). Read the [comment docs](https://docs.codecov.io/docs/pull-request-comments).
<|||||>Thank you, that's great! |
transformers | 1,994 | closed | XLnet output_attentions=True raises an exception | ## 🐛 Bug
I am working on conditional sentences probabilities based on [this code](https://github.com/huggingface/transformers/issues/917#issuecomment-525297746) and whenever `output_attentions=True` and `target_mapping` is provided, there is an exception thrown.
Model I am using (Bert, XLNet....): XLNet ('xlnet-base-cased')
Language I am using the model on (English, Chinese....): English
The problem arise when using:
* [x] my own modified scripts: Setting `output_attentions=True`, throws an exception: `AttributeError: 'tuple' object has no attribute 'permute'`.
The tasks I am working on is:
* [x] my own task or dataset: Using just some sample text
## To Reproduce
Here is a [Google Colab notebook](https://colab.research.google.com/drive/1fkNB0Aqlhtvo3CcHWQ6IqxmCm2Qt9Etn) where the issue can be reproduced as well. Just run all cells.
**Code:**
```python
# https://github.com/huggingface/transformers/issues/917#issuecomment-525297746
import torch
from transformers import XLNetTokenizer, XLNetLMHeadModel
import numpy as np
from scipy.special import softmax
PADDING_TEXT = """In 1991, the remains of Russian Tsar Nicholas II and his family
(except for Alexei and Maria) are discovered.
The voice of Nicholas's young son, Tsarevich Alexei Nikolaevich, narrates the
remainder of the story. 1883 Western Siberia,
a young Grigori Rasputin is asked by his father and a group of men to perform magic.
Rasputin has a vision and denounces one of the men as a horse thief. Although his
father initially slaps him for making such an accusation, Rasputin watches as the
man is chased outside and beaten. Twenty years later, Rasputin sees a vision of
the Virgin Mary, prompting him to become a priest. Rasputin quickly becomes famous,
with people, even a bishop, begging for his blessing. <eod> """
text = "The dog is very cute."
tokenizer = XLNetTokenizer.from_pretrained('xlnet-base-cased')
model = XLNetLMHeadModel.from_pretrained('xlnet-base-cased', output_attentions=True)
tokenize_input = tokenizer.tokenize(PADDING_TEXT + text)
tokenize_text = tokenizer.tokenize(text)
sum_lp = 0.0
for max_word_id in range((len(tokenize_input)-len(tokenize_text)), (len(tokenize_input))):
sent = tokenize_input[:]
input_ids = torch.tensor([tokenizer.convert_tokens_to_ids(sent)])
perm_mask = torch.zeros((1, input_ids.shape[1], input_ids.shape[1]), dtype=torch.float)
perm_mask[:, :, max_word_id:] = 1.0
target_mapping = torch.zeros((1, 1, input_ids.shape[1]), dtype=torch.float)
target_mapping[0, 0, max_word_id] = 1.0
with torch.no_grad():
next_token_logits, attentions = model(input_ids, perm_mask=perm_mask, target_mapping=target_mapping)
word_id = tokenizer.convert_tokens_to_ids([tokenize_input[max_word_id]])[0]
predicted_prob = softmax(np.array(next_token_logits[0][-1]))
lp = np.log(predicted_prob[word_id])
sum_lp += lp
print("sentence logprob =", sum_lp)
```
**Stacktrace:**
```shell
AttributeError Traceback (most recent call last)
<ipython-input-5-6490f5f4333c> in <module>()
38
39 with torch.no_grad():
---> 40 next_token_logits, attentions = model(input_ids, perm_mask=perm_mask, target_mapping=target_mapping)
41
42 word_id = tokenizer.convert_tokens_to_ids([tokenize_input[max_word_id]])[0]
4 frames
/usr/local/lib/python3.6/dist-packages/torch/nn/modules/module.py in __call__(self, *input, **kwargs)
539 result = self._slow_forward(*input, **kwargs)
540 else:
--> 541 result = self.forward(*input, **kwargs)
542 for hook in self._forward_hooks.values():
543 hook_result = hook(self, input, result)
/usr/local/lib/python3.6/dist-packages/transformers/modeling_xlnet.py in forward(self, input_ids, attention_mask, mems, perm_mask, target_mapping, token_type_ids, input_mask, head_mask, inputs_embeds, labels)
952 input_mask=input_mask,
953 head_mask=head_mask,
--> 954 inputs_embeds=inputs_embeds)
955
956 logits = self.lm_loss(transformer_outputs[0])
/usr/local/lib/python3.6/dist-packages/torch/nn/modules/module.py in __call__(self, *input, **kwargs)
539 result = self._slow_forward(*input, **kwargs)
540 else:
--> 541 result = self.forward(*input, **kwargs)
542 for hook in self._forward_hooks.values():
543 hook_result = hook(self, input, result)
/usr/local/lib/python3.6/dist-packages/transformers/modeling_xlnet.py in forward(self, input_ids, attention_mask, mems, perm_mask, target_mapping, token_type_ids, input_mask, head_mask, inputs_embeds)
879 outputs = outputs + (hidden_states,)
880 if self.output_attentions:
--> 881 attentions = tuple(t.permute(2, 3, 0, 1).contiguous() for t in attentions)
882 outputs = outputs + (attentions,)
883
/usr/local/lib/python3.6/dist-packages/transformers/modeling_xlnet.py in <genexpr>(.0)
879 outputs = outputs + (hidden_states,)
880 if self.output_attentions:
--> 881 attentions = tuple(t.permute(2, 3, 0, 1).contiguous() for t in attentions)
882 outputs = outputs + (attentions,)
883
AttributeError: 'tuple' object has no attribute 'permute'
```
## Expected behavior
The model should output the logits for each token and the attention values across layers, heads, and tokens.
## Environment
* OS: 18.04.3 LTS (Bionic Beaver)
* Python version: 3.6.8
* PyTorch version: 1.3.1
* PyTorch Transformers version (or branch): 2.2.0
* Using GPU ? No
* Distributed of parallel setup ? N/A
* Any other relevant information:
## Additional context
<!-- Add any other context about the problem here. -->
| 11-29-2019 10:39:43 | 11-29-2019 10:39:43 | The issue is fixed in #2007 . |
transformers | 1,993 | closed | Why is the weight of linear layer tied to the input embeddings in OpenAIGPTLMHeadModel? | ## ❓ Questions & Help
Yes the original GPT paper also uses same `W_e` as both token embedding matrix and linear weight, and seems that many succeeding models like GPT-2, XLNet also use the same matrix. In my perspective, the token embedding matrix and the weight in linear layer have nothing related (though they have both the same shape). Could you please explain a bit of that?
| 11-29-2019 07:54:12 | 11-29-2019 07:54:12 | The token embedding matrix and the linear layer of the **language modeling head** are indeed tied. The embedding matrix is used to map the vocabulary to vectors of last dimension `hidden_size`.
The linear layer is used to do the exact same thing, just the other way around -> mapping the model output of last dimension `hidden_size` to the vocabulary, so that the output may be converted into vocabulary tokens.<|||||>First of all, thanks for the replay!
I know that the last linear layer is to mapping `hidden_state` with the size `hidden_size` to the size of the vocabulary, but the linear layer does not need to output concrete tokens, right? It just needs to output a group of probabilities (with the size of vocabulary) with softmax, and these probabilities seem to have nothing to do with the token embedding matrix?
I have read some other papers, like the CBOW model in word2vec, which uses a linear layer with separate parameters before softmax to train the language model. As a result, the way that GPT does makes me feel confused.<|||||>This issue has been automatically marked as stale because it has not had recent activity. It will be closed if no further activity occurs. Thank you for your contributions.
|
transformers | 1,992 | closed | Worse F1 on squad2 with finetune+distil distilroberta-base than just finetune | Hi there,
I am trying to finetune distilroberta on squad2. First, I simply used the _distilroberta-base_ model and finetuned it on the squad2 dataset using `run_squad.py`, which gave me **74/71 F1/EM**. It's a lot worse than the roberta-base accuracy.
Currently, I am trying to finetune+distil (from roberta-base squad2 finetuned model) using `run_squad_w_distillation.py`. My roberta-base squad2 finetuned model has around 83/80 F1/EM. However, when I try to finetune+distil _distilroberta-base_ with the finetuned roberta-base as teacher, I only get around **63/60 F1/EM**. Maybe my hyperparams are way off or I need to train longer? Here's my current config:
- learning_rate=3e-5
- total_batch_size=16
- num_train_epochs=2
- max_seq_length=384
I left all other hyperparams as default.
I also checked out some predictions and it seems the model most of the time predicts _no answer_ as the best answer. In cases where it actually predicts an answer, the accuracy is not that bad.
Would be awesome to get some feedback on this, as I am trying to do inference on CPU and a distilled model would greatly benefit me in this case.
Cheers | 11-29-2019 07:32:14 | 11-29-2019 07:32:14 | cc @VictorSanh <|||||>This issue has been automatically marked as stale because it has not had recent activity. It will be closed if no further activity occurs. Thank you for your contributions.
|
Subsets and Splits
No community queries yet
The top public SQL queries from the community will appear here once available.