repo
stringclasses 1
value | number
int64 1
25.3k
| state
stringclasses 2
values | title
stringlengths 1
487
| body
stringlengths 0
234k
⌀ | created_at
stringlengths 19
19
| closed_at
stringlengths 19
19
| comments
stringlengths 0
293k
|
---|---|---|---|---|---|---|---|
transformers | 1,589 | closed | Fix architectures count | 10-22-2019 00:30:00 | 10-22-2019 00:30:00 | Hi! Actually, if we count DistilGPT-2 as a standalone architecture, it should be 10. Do you think you could update it to 10 before we merge? Thanks. |
|
transformers | 1,588 | closed | Using HuggingFace pre-trained transformer to tokenize and generate iterator for a different text than the one it was trained on | Hello,
I am trying to do NLP by using HuggingFace transformers, and I have a question. Is it possible to use the pre-trained HuggingFace Transformer-XL and its pre-trained vocabulary to tokenize and generate BPTTIterator for the WikiText2 dataset instead of the WikiText103 that the transformer was originally trained on? If yes, could someone provide me example codes to illustrate how to 1.tokenize and 2. generate BPTTIterator to analyze the WikiText2, based on the pre-trained HuggingFace transformer XL model and its vocabulary?
NOTE: the WikiText2 can be obtained via
```js
import torchtext
# load WikiText-2 dataset and split it into train and test set
train_Wiki2, val_Wiki2, test_Wiki2 = torchtext.datasets.WikiText2.splits(TEXT)
```
or
```js
import lineflow as lf
import lineflow.datasets as lfds
# load WikiText-103 dataset
train_Wiki103 = lfds.WikiText2('train')
test_Wiki103 = lfds.WikiText2('test')
```
Thank you, | 10-21-2019 18:59:42 | 10-21-2019 18:59:42 | This issue has been automatically marked as stale because it has not had recent activity. It will be closed if no further activity occurs. Thank you for your contributions.
|
transformers | 1,587 | closed | Sequence to sequence with GPT model | Hi, I really appreciate if you could tell me if I can build a seq2seq model with gpt2 like this:
I am getting GPT2 run_generation codes, and I want to finetune it in a way, that I give a
sequence as a context, and then generate another sequence with gpt2, and then I minimize
the cross-entropy loss between the generated sequence and the expected out, and I want to
modify the run_finetune_lm in a way to do it, I was wondering if this way I can make a seq2seq
model with GPT, thank you. | 10-21-2019 08:31:25 | 10-21-2019 08:31:25 | We are currently working on implementing seq2seq for most models in the library (see https://github.com/huggingface/transformers/pull/1455). I won't be ready before a week or two.<|||||>I'm closing this issue, but feel free to reply in #1506 that we leave open for comments on this implementation. |
transformers | 1,586 | closed | Add special tokens to documentation for bert examples to resolve issue: #1561 | **Currently the BERT examples only show the strings encoded without the inclusion of special tokens (e.g. [CLS] and [SEP]) as illustrated below:**
```
tokenizer = BertTokenizer.from_pretrained('bert-base-uncased')
sentence = "Hello there, General Kenobi!"
print(tokenizer.encode(sentence))
print(tokenizer.cls_token_id, tokenizer.sep_token_id)
# [7592, 2045, 1010, 2236, 6358, 16429, 2072, 999]
# 101 102
```
**In this pull request i set add_special_tokens=True in order to include special tokens in the documented examples as illustrated below:**
```
tokenizer = BertTokenizer.from_pretrained('bert-base-uncased')
sentence = "Hello there, General Kenobi!"
print(tokenizer.encode(sentence, add_special_tokens=True))
print(tokenizer.cls_token_id, tokenizer.sep_token_id)
# [101, 7592, 2045, 1010, 2236, 6358, 16429, 2072, 999, 102]
# 101 102
``` | 10-21-2019 04:59:55 | 10-21-2019 04:59:55 | # [Codecov](https://codecov.io/gh/huggingface/transformers/pull/1586?src=pr&el=h1) Report
> Merging [#1586](https://codecov.io/gh/huggingface/transformers/pull/1586?src=pr&el=desc) into [master](https://codecov.io/gh/huggingface/transformers/commit/82f6abd98aaa691ca0adfe21e85a17dc6f386497?src=pr&el=desc) will **not change** coverage.
> The diff coverage is `n/a`.
[](https://codecov.io/gh/huggingface/transformers/pull/1586?src=pr&el=tree)
```diff
@@ Coverage Diff @@
## master #1586 +/- ##
=======================================
Coverage 86.16% 86.16%
=======================================
Files 91 91
Lines 13593 13593
=======================================
Hits 11713 11713
Misses 1880 1880
```
| [Impacted Files](https://codecov.io/gh/huggingface/transformers/pull/1586?src=pr&el=tree) | Coverage Δ | |
|---|---|---|
| [transformers/modeling\_gpt2.py](https://codecov.io/gh/huggingface/transformers/pull/1586/diff?src=pr&el=tree#diff-dHJhbnNmb3JtZXJzL21vZGVsaW5nX2dwdDIucHk=) | `84.19% <ø> (ø)` | :arrow_up: |
| [transformers/modeling\_tf\_openai.py](https://codecov.io/gh/huggingface/transformers/pull/1586/diff?src=pr&el=tree#diff-dHJhbnNmb3JtZXJzL21vZGVsaW5nX3RmX29wZW5haS5weQ==) | `96.04% <ø> (ø)` | :arrow_up: |
| [transformers/modeling\_tf\_transfo\_xl.py](https://codecov.io/gh/huggingface/transformers/pull/1586/diff?src=pr&el=tree#diff-dHJhbnNmb3JtZXJzL21vZGVsaW5nX3RmX3RyYW5zZm9feGwucHk=) | `92.21% <ø> (ø)` | :arrow_up: |
| [transformers/modeling\_roberta.py](https://codecov.io/gh/huggingface/transformers/pull/1586/diff?src=pr&el=tree#diff-dHJhbnNmb3JtZXJzL21vZGVsaW5nX3JvYmVydGEucHk=) | `80.57% <ø> (ø)` | :arrow_up: |
| [transformers/modeling\_transfo\_xl.py](https://codecov.io/gh/huggingface/transformers/pull/1586/diff?src=pr&el=tree#diff-dHJhbnNmb3JtZXJzL21vZGVsaW5nX3RyYW5zZm9feGwucHk=) | `75.16% <ø> (ø)` | :arrow_up: |
| [transformers/modeling\_tf\_ctrl.py](https://codecov.io/gh/huggingface/transformers/pull/1586/diff?src=pr&el=tree#diff-dHJhbnNmb3JtZXJzL21vZGVsaW5nX3RmX2N0cmwucHk=) | `97.75% <ø> (ø)` | :arrow_up: |
| [transformers/modeling\_xlm.py](https://codecov.io/gh/huggingface/transformers/pull/1586/diff?src=pr&el=tree#diff-dHJhbnNmb3JtZXJzL21vZGVsaW5nX3hsbS5weQ==) | `88.42% <ø> (ø)` | :arrow_up: |
| [transformers/modeling\_bert.py](https://codecov.io/gh/huggingface/transformers/pull/1586/diff?src=pr&el=tree#diff-dHJhbnNmb3JtZXJzL21vZGVsaW5nX2JlcnQucHk=) | `88.17% <ø> (ø)` | :arrow_up: |
| [transformers/modeling\_ctrl.py](https://codecov.io/gh/huggingface/transformers/pull/1586/diff?src=pr&el=tree#diff-dHJhbnNmb3JtZXJzL21vZGVsaW5nX2N0cmwucHk=) | `95.45% <ø> (ø)` | :arrow_up: |
| [transformers/modeling\_openai.py](https://codecov.io/gh/huggingface/transformers/pull/1586/diff?src=pr&el=tree#diff-dHJhbnNmb3JtZXJzL21vZGVsaW5nX29wZW5haS5weQ==) | `81.75% <ø> (ø)` | :arrow_up: |
| ... and [7 more](https://codecov.io/gh/huggingface/transformers/pull/1586/diff?src=pr&el=tree-more) | |
------
[Continue to review full report at Codecov](https://codecov.io/gh/huggingface/transformers/pull/1586?src=pr&el=continue).
> **Legend** - [Click here to learn more](https://docs.codecov.io/docs/codecov-delta)
> `Δ = absolute <relative> (impact)`, `ø = not affected`, `? = missing data`
> Powered by [Codecov](https://codecov.io/gh/huggingface/transformers/pull/1586?src=pr&el=footer). Last update [82f6abd...d36680d](https://codecov.io/gh/huggingface/transformers/pull/1586?src=pr&el=lastupdated). Read the [comment docs](https://docs.codecov.io/docs/pull-request-comments).
<|||||>This is great thanks. Actually we should be adding this to all the examples for all the models...<|||||>@thomwolf Would be happy to make the changes for the rest of the models.<|||||>@thomwolf added changes for the rest of the pytorch model examples and all the tensorflow model examples
I used the two bash scripts below to identify files to edit:
```
# Pytorch model examples
grep -iR "input_ids = torch.tensor(tokenizer.encode(" .
# Tensorflow model examples
grep -iR "input_ids = tf.constant(tokenizer.encode(" .
```
**UPDATE:** Example documentation changes were implemented for all tensorflow models except for ```modeling_tf_distilbert.py``` since ```TFDistilBertModelTest.test_pt_tf_model_equivalence``` would fail under **build_py3_torch_and_tf** (details in error [logs](https://circleci.com/gh/huggingface/transformers/5245?utm_campaign=vcs-integration-link&utm_medium=referral&utm_source=github-build-link) for commit: ec276d6abad7eae800f1a1a039ddc78fde406009)<|||||>Thanks for that.
@LysandreJik even though we will have special tokens added by default in the coming release, maybe we still want to update the doc for the current release with this? (not sure this is possible)<|||||>I agree that the necessity to add special tokens should be explicit. However, the documentation is based on previous commits so changing the previous documentation would require to change the commit history of the repo (which we should not do).
We might need to think of a way to work around that to update the misleading documentation of previous versions like in this case. |
transformers | 1,585 | closed | AdamW requires torch>=1.2.0 | ## 🐛 Bug
<!-- Important information -->
AdamW requires torch>=1.2.0, torch < 1.2.0 will cause an importError: cannot import name 'AdamW'
Model I am using (Bert, XLNet....):
Language I am using the model on (English, Chinese....):
The problem arise when using:
* [ ] the official example scripts: (give details)
* [ ] my own modified scripts: (give details)
The tasks I am working on is:
* [x] an official GLUE/SQUaD task: (give the name)
* [ ] my own task or dataset: (give details)
## To Reproduce
Steps to reproduce the behavior:
1.
2.
3.
<!-- If you have a code sample, error messages, stack traces, please provide it here as well. -->
## Expected behavior
<!-- A clear and concise description of what you expected to happen. -->
## Environment
* OS:
* Python version:
* PyTorch version:
* PyTorch Transformers version (or branch):
* Using GPU ?
* Distributed of parallel setup ?
* Any other relevant information:
## Additional context
<!-- Add any other context about the problem here. -->
| 10-21-2019 03:52:13 | 10-21-2019 03:52:13 | I don't think that's right. AdamW is implemented in transformers.optimization
https://github.com/huggingface/transformers/blob/82f6abd98aaa691ca0adfe21e85a17dc6f386497/transformers/optimization.py#L107
As far as I can see that does not require anything specific to torch 1.2. _However_, if you are trying to import [AdamW from torch ](https://pytorch.org/docs/stable/optim.html#torch.optim.AdamW), you may indeed be required to use torch 1.2.
I haven't compared the implementation in torch vs. transformers, but I'd go with torch's native implementation if you can and otherwise fallback to transformers' implementation.<|||||>sorry, I didn't show the details:
the error is from 29 line in transformers/examples/distillation/distiller.py
from torch.optim import AdamW
this AdamW is imported from torch.optim<|||||>does this really need torch >=1.2? I met this problem<|||||>my torch version is 1.1<|||||>No. Importing AdamW from transformers should work with earlier versions. If you're trying to import it directly from torch, then you'll need 1.2+.<|||||>This issue has been automatically marked as stale because it has not had recent activity. It will be closed if no further activity occurs. Thank you for your contributions.
<|||||>Unstale. <|||||>This issue has been automatically marked as stale because it has not had recent activity. It will be closed if no further activity occurs. Thank you for your contributions.
|
transformers | 1,584 | closed | Add special tokens to documentation for bert examples to resolve issue: #1561 | **Currently the BERT examples only show the strings encoded without the inclusion of special tokens (e.g. [CLS] and [SEP]) as illustrated below:**
```
tokenizer = BertTokenizer.from_pretrained('bert-base-uncased')
sentence = "Hello there, General Kenobi!"
print(tokenizer.encode(sentence))
print(tokenizer.cls_token_id, tokenizer.sep_token_id)
# [7592, 2045, 1010, 2236, 6358, 16429, 2072, 999]
# 101 102
```
**In this pull request i set ```add_special_tokens=True``` in order to include special tokens in the documented examples as illustrated below:**
```
tokenizer = BertTokenizer.from_pretrained('bert-base-uncased')
sentence = "Hello there, General Kenobi!"
print(tokenizer.encode(sentence, add_special_tokens=True))
print(tokenizer.cls_token_id, tokenizer.sep_token_id)
# [101, 7592, 2045, 1010, 2236, 6358, 16429, 2072, 999, 102]
# 101 102
``` | 10-21-2019 03:51:09 | 10-21-2019 03:51:09 | # [Codecov](https://codecov.io/gh/huggingface/transformers/pull/1584?src=pr&el=h1) Report
> Merging [#1584](https://codecov.io/gh/huggingface/transformers/pull/1584?src=pr&el=desc) into [master](https://codecov.io/gh/huggingface/transformers/commit/82f6abd98aaa691ca0adfe21e85a17dc6f386497?src=pr&el=desc) will **not change** coverage.
> The diff coverage is `n/a`.
[](https://codecov.io/gh/huggingface/transformers/pull/1584?src=pr&el=tree)
```diff
@@ Coverage Diff @@
## master #1584 +/- ##
=======================================
Coverage 86.16% 86.16%
=======================================
Files 91 91
Lines 13593 13593
=======================================
Hits 11713 11713
Misses 1880 1880
```
| [Impacted Files](https://codecov.io/gh/huggingface/transformers/pull/1584?src=pr&el=tree) | Coverage Δ | |
|---|---|---|
| [transformers/modeling\_bert.py](https://codecov.io/gh/huggingface/transformers/pull/1584/diff?src=pr&el=tree#diff-dHJhbnNmb3JtZXJzL21vZGVsaW5nX2JlcnQucHk=) | `88.17% <ø> (ø)` | :arrow_up: |
------
[Continue to review full report at Codecov](https://codecov.io/gh/huggingface/transformers/pull/1584?src=pr&el=continue).
> **Legend** - [Click here to learn more](https://docs.codecov.io/docs/codecov-delta)
> `Δ = absolute <relative> (impact)`, `ø = not affected`, `? = missing data`
> Powered by [Codecov](https://codecov.io/gh/huggingface/transformers/pull/1584?src=pr&el=footer). Last update [82f6abd...1972e0e](https://codecov.io/gh/huggingface/transformers/pull/1584?src=pr&el=lastupdated). Read the [comment docs](https://docs.codecov.io/docs/pull-request-comments).
|
transformers | 1,583 | closed | Question answering for SQuAD with XLNet | ## ❓ Questions & Help
Dear huggingface,
Thank you very much for your great implementation of NLP architectures! I'm currently trying to train an XLNet model for question answering in French.
I studied your code to understand how question answering is done with XLNet, but I am struggling to follow how it works. Especially, I would like to understand the reasoning behind `PoolerStartLogits`, `PoolerStartLogits` and `PoolerAnswerClass`.
I also don't quite understand how prediction of the answer indices works during inference time.
I know this is a lot of questions, I appreciate any help you can give me!
Thank you very much!
| 10-21-2019 02:25:08 | 10-21-2019 02:25:08 | This issue has been automatically marked as stale because it has not had recent activity. It will be closed if no further activity occurs. Thank you for your contributions.
|
transformers | 1,582 | closed | How does arg --vocab_transform help in extract_distilbert.py? | ## ❓ Questions & Help
<!-- A clear and concise description of the question. -->
Hi everyone, I'm new to experiment with bert model distillation. When running extract_distilbert.py on my fine tuned bert model, I came across the argusment vocat_transform.
```
if args.vocab_transform:
for w in ['weight', 'bias']:
compressed_sd[f'vocab_transform.{w}'] = state_dict[f'cls.predictions.transform.dense.{w}']
compressed_sd[f'vocab_layer_norm.{w}'] = state_dict[f'cls.predictions.transform.LayerNorm.{w}']
```
When should we use this argument in running extract_distilbert? Any scenario we could benefit doing so?
Thanks! | 10-21-2019 01:01:33 | 10-21-2019 01:01:33 | Hello @evehsu,
BERT uses an additional non-linearity before the vocabulary projection (see [here](https://github.com/huggingface/transformers/blob/master/transformers/modeling_bert.py#L381)).
It's a design choice, as far as I know, XLM doesn't a non-linearity right before the vocab projection (the language modeling head).
I left this option because I experimented with it, but if you want to keep the BERT architecture as unchanged as possible, you should use the `--vocab_transform` to ensure you also extract the pre-trained weights for this non-linearity.
Victor<|||||>This issue has been automatically marked as stale because it has not had recent activity. It will be closed if no further activity occurs. Thank you for your contributions.
|
transformers | 1,581 | closed | Is there a computation/speed advantage to batching inputs into `TransformerModel` to reduce its number calls | ## ❓ Questions & Help
<!-- A clear and concise description of the question. -->
For my particular application, I need to have several `output = TransformerModel(inputIDs)` calls per step, from different datasets.
so
```
output1 = TransformerModel(inputIDs_dataset1)
output2 = TransformerModel(inputIDs_dataset2)
output3 = TransformerModel(inputIDs_dataset3)
```
Initially I preferred to have these calls separate, as each dataset has a different average and distribution of sequence length, so keeping these separated would decrease the number of paddings I need to do within each batch.
On the other hand, I imagine that the TransformerModel objects have some optimizations which would make it overall more computationally efficient just to concatenate all the datasets, and make only one call to the `TransformerModel`.
My intuition is towards the latter approach, but would hear takes from those who designed it. | 10-20-2019 23:07:27 | 10-20-2019 23:07:27 | This issue has been automatically marked as stale because it has not had recent activity. It will be closed if no further activity occurs. Thank you for your contributions.
|
transformers | 1,580 | closed | Gradient norm clipping should be done right before calling the optimiser | Right now it's done after each step in the gradient accumulation. What do you think? | 10-20-2019 21:36:20 | 10-20-2019 21:36:20 | # [Codecov](https://codecov.io/gh/huggingface/transformers/pull/1580?src=pr&el=h1) Report
> Merging [#1580](https://codecov.io/gh/huggingface/transformers/pull/1580?src=pr&el=desc) into [master](https://codecov.io/gh/huggingface/transformers/commit/82f6abd98aaa691ca0adfe21e85a17dc6f386497?src=pr&el=desc) will **not change** coverage.
> The diff coverage is `n/a`.
[](https://codecov.io/gh/huggingface/transformers/pull/1580?src=pr&el=tree)
```diff
@@ Coverage Diff @@
## master #1580 +/- ##
=======================================
Coverage 86.16% 86.16%
=======================================
Files 91 91
Lines 13593 13593
=======================================
Hits 11713 11713
Misses 1880 1880
```
------
[Continue to review full report at Codecov](https://codecov.io/gh/huggingface/transformers/pull/1580?src=pr&el=continue).
> **Legend** - [Click here to learn more](https://docs.codecov.io/docs/codecov-delta)
> `Δ = absolute <relative> (impact)`, `ø = not affected`, `? = missing data`
> Powered by [Codecov](https://codecov.io/gh/huggingface/transformers/pull/1580?src=pr&el=footer). Last update [82f6abd...abd7110](https://codecov.io/gh/huggingface/transformers/pull/1580?src=pr&el=lastupdated). Read the [comment docs](https://docs.codecov.io/docs/pull-request-comments).
<|||||>Oh yes, great, thanks, Pasquale. Would you mind fixing the `run_glue` and `run_ner` examples as well?<|||||>@thomwolf done! what's the best way to check this code before merging?<|||||>Thanks a lot!
It should be fine, we have continuous integration tests on `run_glue` and `run_squad` so if it passed at least the code run. |
transformers | 1,579 | closed | seq2seq with gpt2 | Hi,
I want to have a seq2seq model from gpt2, if I change the script of "run_lm_finetuning.py" in a way that it gets a sequence, then make it a context ids, and let it generate another sequence, like "run_generation.py" code, then minimize the cross-entropy loss, does it this way, create a seq2seq model? I rgreatly appreciate your help. thanks a lot. | 10-20-2019 17:40:48 | 10-20-2019 17:40:48 | Merging with #1506 |
transformers | 1,578 | closed | distilled gpt2 to be added to run_generation and run_lm_fintuning | Hi
I greatly appreciated also adding distilled GPT2 to the codes above, thanks | 10-20-2019 15:37:12 | 10-20-2019 15:37:12 | Hi, DistilGPT-2 is considered to be a checkpoint of GPT-2 in our library (differently to DistilBERT). You can already use DistilGPT-2 for both of these scripts with the following:
```bash
python run_generation --model_type=gpt2 --model_name_or_path=distilgpt2
``` |
transformers | 1,577 | closed | Add feature #1572 which gives support for multiple candidate sequences | **Multiple candidate sequences can be generated by setting ```num_samples > 1``` (still 1 by default).**
EXAMPLE with ```num_samples == 2``` for a GPT2 model:
```
INPUT:
Why did the chicken
OUTPUT:
cross the road <eoq> To go to the other side. <eoa>
eat food <eoq> Because it was hungry <eoa>
```
(above is illustrative w some words changed from the actual output)
**UPDATE:** Multiple candidate sequences can now be generated with _repetition penalty_ and ```top_k_top_p_filtering``` applied separately to each candidate. This allows for independent probability distributions across candidate sequences.
~Samples are generated with replacement to allow for sequences that have similar tokens at the same index (e.g. [CLS], stopwords, punctuations).~
~When ```temperature == 0```, the tokens returned are the top ```num_samples``` logits (first sample gets top 1, second gets top 2, and so on). I realize this might not be the best implementation because it doesn't allow for similar tokens at the same index across samples. I will consider later changing this to just returning ```num_samples``` copies of the top 1 logits (argmax).~ | 10-20-2019 15:27:47 | 10-20-2019 15:27:47 | Please note that main() now returns a list with ```num_samples``` elements inside.
Because of this, the test for run_generation.py should be updated to test for ```length``` for each element within the list. This explains why **build_py3_torch** test failed.
I will update ```ExamplesTests.test_generation``` to reflect the new output format.<|||||># [Codecov](https://codecov.io/gh/huggingface/transformers/pull/1577?src=pr&el=h1) Report
> Merging [#1577](https://codecov.io/gh/huggingface/transformers/pull/1577?src=pr&el=desc) into [master](https://codecov.io/gh/huggingface/transformers/commit/ef1b8b2ae5ad1057154a126879f7eb8de685f862?src=pr&el=desc) will **not change** coverage.
> The diff coverage is `n/a`.
[](https://codecov.io/gh/huggingface/transformers/pull/1577?src=pr&el=tree)
```diff
@@ Coverage Diff @@
## master #1577 +/- ##
=======================================
Coverage 86.17% 86.17%
=======================================
Files 91 91
Lines 13595 13595
=======================================
Hits 11715 11715
Misses 1880 1880
```
------
[Continue to review full report at Codecov](https://codecov.io/gh/huggingface/transformers/pull/1577?src=pr&el=continue).
> **Legend** - [Click here to learn more](https://docs.codecov.io/docs/codecov-delta)
> `Δ = absolute <relative> (impact)`, `ø = not affected`, `? = missing data`
> Powered by [Codecov](https://codecov.io/gh/huggingface/transformers/pull/1577?src=pr&el=footer). Last update [ef1b8b2...17dd64e](https://codecov.io/gh/huggingface/transformers/pull/1577?src=pr&el=lastupdated). Read the [comment docs](https://docs.codecov.io/docs/pull-request-comments).
<|||||>Latest commit 17dd64e now applies repetition penalty and ```top_k_top_p_filtering``` to each candidate sequence separately.<|||||>Thanks @enzoampil
Superseded by https://github.com/huggingface/transformers/pull/1333 which was just merged to master.
Let me know if this works for your use case. |
transformers | 1,576 | closed | evaluating on race dataset with checkpoints fine tuned on roberta with fairseq | ## ❓ Questions & Help
<!-- A clear and concise description of the question. -->
I fine tuned a model on race dataset with reberta, following the fairseq instruction, got the result:
| epoch 004 | valid on 'valid' subset: | loss 0.913 | nll_loss 0.003 | ppl 1.00 | num_updates 21849 | best_accuracy 0.846563 | accuracy 0.836129
| epoch 004 | valid on 'valid' subset: | loss 0.913 | nll_loss 0.003 | ppl 1.00 | num_updates 21849 | best_accuracy 0.846563 | accuracy 0.836129
| epoch 004 | valid on 'valid' subset: | loss 0.913 | nll_loss 0.003 | ppl 1.00 | num_updates 21849 | best_accuracy 0.846563 | accuracy 0.836129
| epoch 004 | valid on 'valid' subset: | loss 0.913 | nll_loss 0.003 | ppl 1.00 | num_updates 21849 | best_accuracy 0.846563 | accuracy 0.836129
| saved checkpoint checkpoints/checkpoint4.pt (epoch 4 @ 21849 updates) (writing took 145.8246190547943 seconds)
| done training in 76377.9 seconds
But I load the weight to the transformers with convert_roberta_original_pytorch_checkpoint_to_pytorch script:
python convert_roberta_original_pytorch_checkpoint_to_pytorch.py --roberta_checkpoint_path ../pytorch-transformers-master/data/roberta-best-checkpoint/ --pytorch_dump_folder_path ../pytorch-transformers-master/data/roberta-best-checkpoint/
then evaluate on RACE dataset, I got terrible results on dev set:
model =data/models_roberta_race/
total batch size=8
train num epochs=5
fp16 =False
max seq length =512
eval_acc = 0.4808676079394311
eval_loss = 1.352066347319484
and on test set:
model =data/models_roberta_race/
total batch size=8
train num epochs=5
fp16 =False
max seq length =512
eval_acc = 0.6015403323875153
eval_loss = 1.3087183478393092
I don't know why. Could anyone can help? Thank you! | 10-20-2019 13:37:25 | 10-20-2019 13:37:25 | Do you get any improvement?
The ACC of eval and test has a huge gap.
<|||||> --classification-head when converting the models <|||||>This issue has been automatically marked as stale because it has not had recent activity. It will be closed if no further activity occurs. Thank you for your contributions.
<|||||>I am unable to reproduce the results on the RACE dataset. If anyone has been able to reproduce it, could you kindly share the weights of the fine-tuned model ? |
transformers | 1,575 | closed | use gpt2 as a seq2seq model | Hi
could you assist me please and show me with example on how I can use GPT-2 language model decoding method so train seq2seq model? thanks a lot | 10-20-2019 12:04:19 | 10-20-2019 12:04:19 | Merging with #1506 |
transformers | 1,574 | closed | Why the output is same within a batch use BertForSequenceClassification? | ## ❓ Questions & Help
I use BertForSequenceClassification for classification task, but the output witin a batch became same after just 2 or 3 batch, the value between different batch is different, really strange.
batch 1 output:
[-0.5966, 0.6081],
[-0.4659, 0.3766],
[-0.3595, 0.1334],
[-0.4178, 0.6873],
[-0.3884, 0.2640],
[-0.5017, 0.3465],
[-0.5978, 0.4961],
[-0.3146, 0.6879],
[-0.6525, 0.2702],
[-0.2500, 0.1232],
[-0.3137, 0.4212],
[-0.2663, 0.5169],
[-0.5225, 0.7992],
[-0.4844, 0.1942],
[-0.1459, 0.4033],
[-0.9007, 0.5122],
[-0.5833, 0.8187],
[-0.5552, 0.1253],
[-0.5420, -0.1123]], device='cuda:0', grad_fn=<AddmmBackward>))
2:
(tensor(1.2256, device='cuda:0', grad_fn=<NllLossBackward>), tensor([[ 0.7105, -0.8978],
[ 0.7925, -0.9382],
[ 0.6098, -0.9100],
[ 0.7522, -0.9534],
[ 0.7706, -0.9142],
[ 0.7778, -0.9246],
[ 0.7703, -0.8327],
[ 0.5850, -0.8817],
[ 0.6266, -0.9271],
[ 0.8061, -0.8157],
[ 0.8036, -0.9927],
[ 0.7619, -0.9277],
[ 0.7773, -0.7931],
[ 0.8458, -0.8186],
[ 0.6291, -0.8925],
[ 0.5919, -0.8709],
[ 0.6222, -0.9173],
[ 0.8290, -0.9817],
[ 0.7155, -0.9171],
[ 0.8107, -0.9364]], device='cuda:0', grad_fn=<AddmmBackward>))
3
(tensor(0.7688, device='cuda:0', grad_fn=<NllLossBackward>), tensor([[-0.7892, 0.5464],
[-0.7873, 0.5431],
[-0.7914, 0.5424],
[-0.7938, 0.5448],
[-0.7934, 0.5449],
[-0.7876, 0.5430],
[-0.7973, 0.5446],
[-0.7905, 0.5430],
[-0.7924, 0.5451],
[-0.7900, 0.5438],
[-0.7879, 0.5449],
[-0.7869, 0.5408],
[-0.7924, 0.5458],
[-0.7928, 0.5436],
[-0.7954, 0.5469],
[-0.7900, 0.5429],
[-0.7945, 0.5453],
[-0.8027, 0.5492],
[-0.7937, 0.5437],
[-0.7934, 0.5506]], device='cuda:0', grad_fn=<AddmmBackward>))
(tensor(1.1733, device='cuda:0', grad_fn=<NllLossBackward>), tensor([[ 1.3647, -0.3074],
[ 1.3588, -0.2927],
[ 1.3581, -0.2915],
[ 1.3628, -0.3009],
[ 1.3625, -0.3001],
[ 1.3630, -0.3016],
[ 1.3666, -0.3157],
[ 1.3604, -0.2953],
[ 1.3655, -0.3108],
[ 1.3604, -0.2942],
[ 1.3623, -0.3041],
[ 1.3555, -0.2866],
[ 1.3600, -0.2943],
[ 1.3654, -0.3091],
[ 1.3628, -0.3004],
[ 1.3658, -0.3080],
[ 1.3643, -0.3041],
[ 1.3599, -0.2967],
[ 1.3629, -0.3024],
[ 1.3688, -0.3206]], device='cuda:0', grad_fn=<AddmmBackward>))
<!-- A clear and concise description of the question. -->
| 10-20-2019 07:54:24 | 10-20-2019 07:54:24 | Hello, could you provide a script so that we may better understand the problem here?<|||||>This issue has been automatically marked as stale because it has not had recent activity. It will be closed if no further activity occurs. Thank you for your contributions.
|
transformers | 1,573 | closed | GPT2 attention mask and output masking | ## ❓ Questions & Help
<!-- A clear and concise description of the question. -->
I have couple of questions:
1. In the original gpt2 they didn't pad the sequence, so they didn't need a attention mask, but in other cases where we our input sequence is small and we pad the input, don't we need a attention mask?
2. I have padded the labels in the left with -1, in the cost function how do I skip the padded elements in labels? and same for logits how do I skip the padded elements ? | 10-20-2019 05:31:35 | 10-20-2019 05:31:35 | Closing this because I found my answer<|||||>Hi, would you mind sharing what the answer you found is? Thank you so much!<|||||>Sorry for the delay. Gpt2 was trained as a CLM model with a fixed block size of data. So there was no need for attention mask. (That is what I understood). |
transformers | 1,572 | closed | Can we generate multiple possible sentences using GPT? | Hi,
Is there any way to generate multiple candidate text sequences using the pretrained generators? | 10-20-2019 04:46:35 | 10-20-2019 04:46:35 | @zhaoxy92 I happen to have a use case for this as well. I'll add in this feature to the ```run_generation.py```<|||||>@zhaoxy92 Added this functionality in ```run_generation.py```. You can set the number of candidates generated by setting the argument ```num_samples``` which is set to 1 by default.<|||||>I think you need to change `top_k_top_p_filtering()` as well.<|||||>@s-js not sure why we'd have to change```top_k_top_p_filtering()``` since the sampling only happens at ```sample_sequence()```. ```top_k_top_p_filtering()``` only filters the logits, so we can still generate multiple candidate sequences (independent of the filtered distribution).<|||||>@enzoampil Sorry, I meant repetition penalty (https://github.com/enzoampil/transformers/blob/7facbbe9871fe458b530ae8ce1b4bfefabd47c74/examples/run_generation.py#L142). Each sample has a different set of seen tokens.
At first I thought you were doing it inside `top_k_top_p_filtering()`.<|||||>Hi, thanks very much for adding this functionality – I'm trying to implement this into my own notebook and hitting a tensor mismatch error I can't figure out. I hope this is the right forum to post this question, since I'm using the new functionality you created.
At line 150: `generated = torch.cat((generated, next_token.unsqueeze(0)), dim=1)`
I'm getting this error:
```RuntimeError: invalid argument 0: Sizes of tensors must match except in dimension 1. Got 1 and 3 in dimension 0 at /opt/conda/conda-bld/pytorch_1556653114079/work/aten/src/THC/generic/THCTensorMath.cu:71```
The debugger shows me the sizes:
generated = (3,37)
next_token.unsqueeze(0) = (1,3)
So I figure that next_token tensor shape ought to be (3,1) instead, so I tried changing the line to `next_token.unsqueeze(1)` instead. When I do that I get a `CUDA error: device-side assert triggered`. Did that change fix my problem or just cause a new one?
Any ideas are greatly appreciated, thank you!<|||||>hi @buttchurch , did you run ```run_generation.py``` (with the multiple sentence functionality) as a CLI? It should work if you run it from my [fork](https://github.com/enzoampil/transformers/blob/7facbbe9871fe458b530ae8ce1b4bfefabd47c74/examples/run_generation.py#L142). Can you please post here the exact script you ran and the complete error message.
Also, my pull [request](https://github.com/enzoampil/transformers/blob/7facbbe9871fe458b530ae8ce1b4bfefabd47c74/examples/run_generation.py#L142) shows ```generated = torch.cat((generated, next_token.unsqueeze(1)), dim=1)``` in the last line of ```sample_sequence``` so I'm not sure where you got that line 150 code snippet.<|||||>@s-js noted on repetition penalty support. I'll try to find time for this within the next week.<|||||>hi @enzoampil, thanks for such a quick response! I still don't understand navigating git forks and branches and the different versions of git projects very well, so I have been just going off the main code I find in the transformers github.
It's probably not the 'right' way to do it, but I've pulled my own jupyter notebook together from a couple of the transformer example.py files, rather than using the run_generation.py. I think it might be way too long to post here, but I will now try implementing the changes in your fork to my notebook. I'll report back – thanks again for your help, and for creating this new functionality :)
Edit: It works! Seems like the important bit I was missing was `replacement=True` on the previous line.<|||||>@buttchurch glad it works for you :) Very welcome!<|||||>@s-js Latest [commit](https://github.com/huggingface/transformers/commit/17dd64ed939e09c1c9b1fa666390dd69a4731387) now implements _repetition penalty_ and ```top_k_top_p_filtering``` separately per candidate sequence generated.<|||||>We just merged https://github.com/huggingface/transformers/pull/1333 to master (+ subsequent fixes), can you check that it does what you guys want?
I'll close the issue for now, re-open if needed. |
transformers | 1,571 | closed | Pytorch Transformers no longer loads SciBert weights, getting `UnicodeDecodeError`. Worked in pytorch_pretrained_bert | ## 🐛 Bug
<!-- Important information -->
When using the old pytorch_pretrained_bert library, I could point the model with `from_pretrained` to the SciBert weights.tar.gz file, and it would load this just. However, if I try this with the Pytorch Transformers, I get this error.
```
UnicodeDecodeError: 'utf-8' codec can't decode byte 0x8b in position 1: invalid start byte
```
Model I am using: Bert
Language I am using the model on (English, Chinese....): English
The problem arise when using:
* [ X] my own modified scripts: (give details)
I have a colab notebook that loads the SciBert weights using the old pytorch_pretrained_bert library, and the new Transformers library.
## To Reproduce
Steps to reproduce the behavior:
Here is the code
```
import requests
import os
import tarfile
import zipfile
import multiprocess
import json
if not os.path.exists('TempDir'):
os.makedirs('TempDir')
#Download SciBert weights and vocab file
import urllib.request
# Download the file from `url` and save it locally under `file_name`:
urllib.request.urlretrieve('https://s3-us-west-2.amazonaws.com/ai2-s2-research/scibert/pytorch_models/scibert_scivocab_uncased.tar', 'scibert.tar')
#Untar weights
import tarfile
tar = tarfile.open('scibert.tar', "r:")
tar.extractall()
tar.close()
#Extract weights
tar = tarfile.open('scibert_scivocab_uncased/weights.tar.gz', "r:gz")
tar.extractall('scibert_scivocab_uncased')
tar.close()
os.listdir('scibert_scivocab_uncased')
!pip install pytorch-pretrained-bert
from pytorch_pretrained_bert import BertModel as OldBertModel
#Works
oldBert = OldBertModel.from_pretrained('/content/scibert_scivocab_uncased/weights.tar.gz', cache_dir= 'TempDir')
!pip install transformers
from transformers import BertModel as NewBertModel
#Doesn't work
newBert = NewBertModel.from_pretrained('/content/scibert_scivocab_uncased/weights.tar.gz', cache_dir= 'TempDir')
```
<!-- If you have a code sample, error messages, stack traces, please provide it here as well. -->
Here is the error
```
---------------------------------------------------------------------------
UnicodeDecodeError Traceback (most recent call last)
<ipython-input-14-7e88a8c51c18> in <module>()
----> 1 newBert = NewBertModel.from_pretrained('/content/scibert_scivocab_uncased/weights.tar.gz', cache_dir= 'TempDir')
3 frames
/usr/local/lib/python3.6/dist-packages/transformers/modeling_utils.py in from_pretrained(cls, pretrained_model_name_or_path, *model_args, **kwargs)
285 cache_dir=cache_dir, return_unused_kwargs=True,
286 force_download=force_download,
--> 287 **kwargs
288 )
289 else:
/usr/local/lib/python3.6/dist-packages/transformers/configuration_utils.py in from_pretrained(cls, pretrained_model_name_or_path, **kwargs)
152
153 # Load config
--> 154 config = cls.from_json_file(resolved_config_file)
155
156 if hasattr(config, 'pruned_heads'):
/usr/local/lib/python3.6/dist-packages/transformers/configuration_utils.py in from_json_file(cls, json_file)
184 """Constructs a `BertConfig` from a json file of parameters."""
185 with open(json_file, "r", encoding='utf-8') as reader:
--> 186 text = reader.read()
187 return cls.from_dict(json.loads(text))
188
/usr/lib/python3.6/codecs.py in decode(self, input, final)
319 # decode input (taking the buffer into account)
320 data = self.buffer + input
--> 321 (result, consumed) = self._buffer_decode(data, self.errors, final)
322 # keep undecoded input until the next call
323 self.buffer = data[consumed:]
UnicodeDecodeError: 'utf-8' codec can't decode byte 0x8b in position 1: invalid start byte
```
For convenience, here is a colab notebook with the code that you can run
https://colab.research.google.com/drive/1xzYYM1_Vo4wRMicBfnfzfAg_47SitwQi
## Expected behavior
pretrained weights should load just fine.
## Environment
* OS: Google Colab
* Python version:
* PyTorch version:
* PyTorch Transformers version (or branch): Current
* Using GPU ?
* Distributed of parallel setup ?
* Any other relevant information:
| 10-19-2019 22:11:59 | 10-19-2019 22:11:59 | `from_pretrained` expects the following files: `vocab.txt`, `config.json` and `pytorch_model.bin`.
Thus, you only need to extract the `weights.tar.gz` archive.
Then rename `bert_config.json` to `config.json` and pass the path name to the `from_pretrained` method: this should be `/content/scibert_scivocab_uncased` in your example :) |
transformers | 1,570 | closed | Fix Roberta on TPU | Fixes #1569
- Revert tf.print() to logger , since tf.print() is an unsupported TPU ops. | 10-19-2019 21:38:10 | 10-19-2019 21:38:10 | # [Codecov](https://codecov.io/gh/huggingface/transformers/pull/1570?src=pr&el=h1) Report
> Merging [#1570](https://codecov.io/gh/huggingface/transformers/pull/1570?src=pr&el=desc) into [master](https://codecov.io/gh/huggingface/transformers/commit/82f6abd98aaa691ca0adfe21e85a17dc6f386497?src=pr&el=desc) will **not change** coverage.
> The diff coverage is `100%`.
[](https://codecov.io/gh/huggingface/transformers/pull/1570?src=pr&el=tree)
```diff
@@ Coverage Diff @@
## master #1570 +/- ##
=======================================
Coverage 86.16% 86.16%
=======================================
Files 91 91
Lines 13593 13593
=======================================
Hits 11713 11713
Misses 1880 1880
```
| [Impacted Files](https://codecov.io/gh/huggingface/transformers/pull/1570?src=pr&el=tree) | Coverage Δ | |
|---|---|---|
| [transformers/modeling\_tf\_roberta.py](https://codecov.io/gh/huggingface/transformers/pull/1570/diff?src=pr&el=tree#diff-dHJhbnNmb3JtZXJzL21vZGVsaW5nX3RmX3JvYmVydGEucHk=) | `100% <100%> (ø)` | :arrow_up: |
------
[Continue to review full report at Codecov](https://codecov.io/gh/huggingface/transformers/pull/1570?src=pr&el=continue).
> **Legend** - [Click here to learn more](https://docs.codecov.io/docs/codecov-delta)
> `Δ = absolute <relative> (impact)`, `ø = not affected`, `? = missing data`
> Powered by [Codecov](https://codecov.io/gh/huggingface/transformers/pull/1570?src=pr&el=footer). Last update [82f6abd...55c3ae1](https://codecov.io/gh/huggingface/transformers/pull/1570?src=pr&el=lastupdated). Read the [comment docs](https://docs.codecov.io/docs/pull-request-comments).
<|||||>Hum this situation is a bit annoying because we switched from `logger.error` to `tf.print` to solve #1350<|||||>Is there a specific reason why we have such a warning message for Roberta but not for other models? All models based on BERT are require the special tokens.
I was having the same issues as #1350 on my end using the logger (lots of zmq , operationnotallowed errors) . The solution for me was to remove the entire warning message altogether.
Is that viable in this scenario? <|||||>This issue has been automatically marked as stale because it has not had recent activity. It will be closed if no further activity occurs. Thank you for your contributions.
|
transformers | 1,569 | closed | TFRobertaForSequenceClassification fails on TPU on Transformers >2.0.0 | ## 🐛 Bug
<!-- Important information -->
Model I am using (TFRobertaForSequenceClassification):
Language I am using the model on (English):
The problem arise when using:
* [ ] the official example scripts: (give details)
* [x] my own modified scripts: (give details)
The tasks I am working on is:
* [ ] an official GLUE/SQUaD task: (give the name)
* [x] my own task or dataset: (give details)
## To Reproduce
Steps to reproduce the behavior:
1. Use a TPU runtime on colab
2. ```python
resolver = tf.distribute.cluster_resolver.TPUClusterResolver()
tf.config.experimental_connect_to_cluster(resolver)
tf.tpu.experimental.initialize_tpu_system(resolver)
strategy = tf.distribute.experimental.TPUStrategy(resolver)
with tf.device('/job:worker'):
with strategy.scope():
# model = TFRobertaForSequenceClassification.from_pretrained('bert-large-uncased-whole-word-masking',num_labels = (len(le.classes_)))
model = TFRobertaForSequenceClassification.from_pretrained('roberta-large',num_labels = 2)
print('model loaded')
inp = np.random.randint(10,100, size=(12800, 64))
inp[:,0]=0
inp[:,63]=2
labels = np.random.randint(2,size = (12800,1))
print('data generated')
optimizer = tf.keras.optimizers.Adam(learning_rate=3e-5, epsilon=1e-08, clipnorm=1.0)
loss = tf.keras.losses.SparseCategoricalCrossentropy(from_logits=True)
metric = tf.keras.metrics.SparseCategoricalAccuracy('accuracy')
model.compile(optimizer=optimizer, loss=loss, metrics=[metric])
print('starting fitting')
# model.fit([train_input_ids,train_input_masks],y_train,epochs = 3,batch_size = 64,validation_data=([test_input_ids,test_input_masks], y_test),verbose=1)
model.fit(inp,labels,epochs = 2,batch_size = 64,verbose=1)
## Environment
* OS: Google Colab TPU runtime
* Python version:3.6
* PyTorch version:NA
* PyTorch Transformers version (or branch):2.1.1
* Using GPU ? No
* Distributed of parallel setup ? Yes
## Additional context
The following error gets thrown when calling model.fit()
```
InvalidArgumentError Traceback (most recent call last)
<ipython-input-4-b77065bb89ae> in <module>()
15 print('starting fitting')
16 # model.fit([train_input_ids,train_input_masks],y_train,epochs = 3,batch_size = 64,validation_data=([test_input_ids,test_input_masks], y_test),verbose=1)
---> 17 model.fit(inp,labels,epochs = 2,batch_size = 64,verbose=1)
11 frames
/usr/local/lib/python3.6/dist-packages/six.py in raise_from(value, from_value)
InvalidArgumentError: Compilation failure: Detected unsupported operations when trying to compile graph tf_roberta_for_sequence_classification_roberta_cond_true_122339[] on XLA_TPU_JIT: PrintV2 (No registered 'PrintV2' OpKernel for XLA_TPU_JIT devices compatible with node {{node PrintV2}}
. Registered: device='CPU'
){{node PrintV2}}
[[tf_roberta_for_sequence_classification/roberta/cond]]
TPU compilation failed
[[tpu_compile_succeeded_assert/_5504150486074133972/_3]]
Additional GRPC error information:
{"created":"@1571518085.015232162","description":"Error received from peer","file":"external/grpc/src/core/lib/surface/call.cc","file_line":1039,"grpc_message":" Compilation failure: Detected unsupported operations when trying to compile graph tf_roberta_for_sequence_classification_roberta_cond_true_122339[] on XLA_TPU_JIT: PrintV2 (No registered 'PrintV2' OpKernel for XLA_TPU_JIT devices compatible with node {{node PrintV2}}\n\t. Registered: device='CPU'\n){{node PrintV2}}\n\t [[tf_roberta_for_sequence_classification/roberta/cond]]\n\tTPU compilation failed\n\t [[tpu_compile_succeeded_assert/_5504150486074133972/_3]]","grpc_status":3} [Op:__inference_distributed_function_154989]
Function call stack:
distributed_function -> distributed_function
```
The reason behind this error seems to be the tf.print() in the following code , which is not supported on TPU.
https://github.com/huggingface/transformers/blob/82f6abd98aaa691ca0adfe21e85a17dc6f386497/transformers/modeling_tf_roberta.py#L78-L80
| 10-19-2019 21:30:37 | 10-19-2019 21:30:37 | This issue has been automatically marked as stale because it has not had recent activity. It will be closed if no further activity occurs. Thank you for your contributions.
|
transformers | 1,568 | closed | Fix hanging when loading pretrained models | - Fix hanging when loading pretrained models from the cache without having internet access. This is a widespread issue on supercomputers whose internal compute nodes are firewalled. | 10-19-2019 20:20:02 | 10-19-2019 20:20:02 | # [Codecov](https://codecov.io/gh/huggingface/transformers/pull/1568?src=pr&el=h1) Report
> Merging [#1568](https://codecov.io/gh/huggingface/transformers/pull/1568?src=pr&el=desc) into [master](https://codecov.io/gh/huggingface/transformers/commit/82f6abd98aaa691ca0adfe21e85a17dc6f386497?src=pr&el=desc) will **decrease** coverage by `0.02%`.
> The diff coverage is `100%`.
[](https://codecov.io/gh/huggingface/transformers/pull/1568?src=pr&el=tree)
```diff
@@ Coverage Diff @@
## master #1568 +/- ##
==========================================
- Coverage 86.16% 86.14% -0.03%
==========================================
Files 91 91
Lines 13593 13593
==========================================
- Hits 11713 11710 -3
- Misses 1880 1883 +3
```
| [Impacted Files](https://codecov.io/gh/huggingface/transformers/pull/1568?src=pr&el=tree) | Coverage Δ | |
|---|---|---|
| [transformers/file\_utils.py](https://codecov.io/gh/huggingface/transformers/pull/1568/diff?src=pr&el=tree#diff-dHJhbnNmb3JtZXJzL2ZpbGVfdXRpbHMucHk=) | `74.17% <100%> (ø)` | :arrow_up: |
| [transformers/tests/modeling\_tf\_common\_test.py](https://codecov.io/gh/huggingface/transformers/pull/1568/diff?src=pr&el=tree#diff-dHJhbnNmb3JtZXJzL3Rlc3RzL21vZGVsaW5nX3RmX2NvbW1vbl90ZXN0LnB5) | `95.21% <0%> (-1.6%)` | :arrow_down: |
------
[Continue to review full report at Codecov](https://codecov.io/gh/huggingface/transformers/pull/1568?src=pr&el=continue).
> **Legend** - [Click here to learn more](https://docs.codecov.io/docs/codecov-delta)
> `Δ = absolute <relative> (impact)`, `ø = not affected`, `? = missing data`
> Powered by [Codecov](https://codecov.io/gh/huggingface/transformers/pull/1568?src=pr&el=footer). Last update [82f6abd...a2c8c8e](https://codecov.io/gh/huggingface/transformers/pull/1568?src=pr&el=lastupdated). Read the [comment docs](https://docs.codecov.io/docs/pull-request-comments).
<|||||>Ok, LGTM thanks |
transformers | 1,567 | closed | Added mixed precision (AMP) to inference benchmark | I added a mixed precision option to the benchmark script and ran it on a DGX Station to get the results. As you can see, we can get between 1.2x to up to 4.5x inference speed depending on model, batch size and sequence length.
**Summary**
| Batch Size | Speedup (XLA only) | Speedup (XLA + AMP) | Min. Seq Len* |
| -------------- | --------------------------- | ------------------------------- | ------------------ |
| 1 | 1.1 ~ 1.9 | 1.4 ~ 2.9 | 512 |
| 2 | 1.1 ~ 1.9 | 1.4 ~ 3.4 | 256 |
| 4 | 1.1 ~ 2.1 | 1.2 ~ 3.8 | 128 |
| 8 | 1.1 ~ 3.1 | 1.2 ~ 4.5 | 64 |
*Min. Seq Len refers to minimum sequence length required to not see **any** performance regression at all. For example, at batch size 1:
* Seq Len of 512 tokens see speed up of 1.4~2.1x depending on model
* Seq Len of 256 tokens see speed up of 0.8~1.2x depending on model
Google Sheets with the results [here](https://docs.google.com/spreadsheets/d/1IW7Xbv-yfE8j-T0taqdyoSehca4mNcsyx6u0IXTzSJ4/edit#gid=0). GPU used is a single V100 (16GB). | 10-19-2019 09:02:26 | 10-19-2019 09:02:26 | # [Codecov](https://codecov.io/gh/huggingface/transformers/pull/1567?src=pr&el=h1) Report
> Merging [#1567](https://codecov.io/gh/huggingface/transformers/pull/1567?src=pr&el=desc) into [master](https://codecov.io/gh/huggingface/transformers/commit/079bfb32fba4f2b39d344ca7af88d79a3ff27c7c?src=pr&el=desc) will **not change** coverage.
> The diff coverage is `n/a`.
[](https://codecov.io/gh/huggingface/transformers/pull/1567?src=pr&el=tree)
```diff
@@ Coverage Diff @@
## master #1567 +/- ##
======================================
Coverage 85.9% 85.9%
======================================
Files 91 91
Lines 13653 13653
======================================
Hits 11728 11728
Misses 1925 1925
```
------
[Continue to review full report at Codecov](https://codecov.io/gh/huggingface/transformers/pull/1567?src=pr&el=continue).
> **Legend** - [Click here to learn more](https://docs.codecov.io/docs/codecov-delta)
> `Δ = absolute <relative> (impact)`, `ø = not affected`, `? = missing data`
> Powered by [Codecov](https://codecov.io/gh/huggingface/transformers/pull/1567?src=pr&el=footer). Last update [079bfb3...079bfb3](https://codecov.io/gh/huggingface/transformers/pull/1567?src=pr&el=lastupdated). Read the [comment docs](https://docs.codecov.io/docs/pull-request-comments).
<|||||>Any reason why you kept the batch sizes so small? With a V100, you should be able to easily pull of a batch size of 64 for seq len 64. (Perhaps that's what the benchmark script uses as default, I don't know EDIT: yes, those are the values from the benchmark script. Not sure why, though.) I'm a bit surprised by the relatively small speed up. I've experienced **much** greater speed ups when using AMP, but that was on PyTorch with apex.<|||||>@BramVanroy this is for **inference** hence the emphasis on low batch size.<|||||>Oh, my bad. I was under the impression that the benchmark script included training profiling with PyProf. |
transformers | 1,566 | closed | error load bert model :not found model file | Error content:
OSError: Error no file named ['pytorch_model.bin', 'tf_model.h5', 'model.ckpt.index'] found in directory ./uncased_L-12_H-768_A-12_transformers or `from_tf` set to False
but file exists
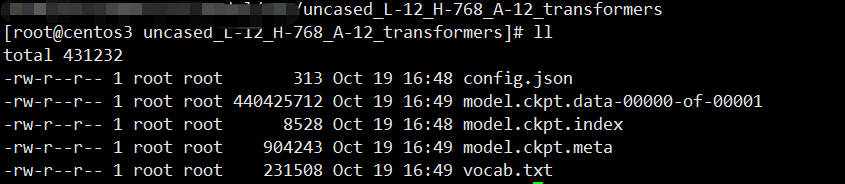
| 10-19-2019 08:56:17 | 10-19-2019 08:56:17 | I don't understand when you get this error?<|||||>In order to understand when you've encountered this bug, as suggested by @iedmrc , you've to write down the source code that generates the bug! And please show your environment (Python, Transformers, PyTorch, TensorFlow versions) too!
> Error content:
> OSError: Error no file named ['pytorch_model.bin', 'tf_model.h5', 'model.ckpt.index'] found in directory ./uncased_L-12_H-768_A-12_transformers or `from_tf` set to False
> but file exists
> 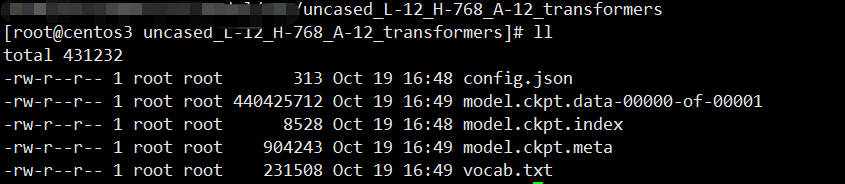<|||||>This issue has been automatically marked as stale because it has not had recent activity. It will be closed if no further activity occurs. Thank you for your contributions.
<|||||>I got the same error when loading a TF BERT model:
```
dir = "/Users/danielk/ideaProjects/farsi-language-models/src/models/perbert_L-12_H-768_A-12/"
tokenizer = BertTokenizer.from_pretrained(dir)
config = BertConfig.from_json_file(dir + '/bert_config.json')
model = TFBertForMaskedLM.from_pretrained(dir, config=config)
```
The error happens in the last line.
```
Traceback (most recent call last):
File "6.2.try_tf_bert_transformers.py", line 8, in <module>
model = TFBertForMaskedLM.from_pretrained(dir, config=config)
File "/Users/danielk/opt/anaconda3/lib/python3.7/site-packages/transformers/modeling_tf_utils.py", line 353, in from_pretrained
[WEIGHTS_NAME, TF2_WEIGHTS_NAME], pretrained_model_name_or_path
OSError: Error no file named ['pytorch_model.bin', 'tf_model.h5'] found in directory /Users/danielk/ideaProjects/farsi-language-models/src/models/perbert_L-12_H-768_A-12/ or `from_pt` set to False
``` |
transformers | 1,565 | closed | How to add the output word vector of bert to my model | ## ❓ Questions & Help
Hello, I am a student who is learning nlp.
Now I want to use the word vector output by bert to apply to my model, but **I can't connect the word vector to the network**. Could you give me an example program or tutorial about this which use textCNN or LSTM. You can sent e-mail to **[email protected]** or reply me, please.
Thank you for your kind cooperation!
<!-- A clear and concise description of the question. -->
| 10-19-2019 03:14:58 | 10-19-2019 03:14:58 | This issue has been automatically marked as stale because it has not had recent activity. It will be closed if no further activity occurs. Thank you for your contributions.
<|||||>I think you can see [here](https://jalammar.github.io/a-visual-guide-to-using-bert-for-the-first-time/). In more details, this tutorial uses **BERT** as **feature extractor** and on-top they have used a **Logistic Regression** model from [Scikit-learn](https://scikit-learn.org/stable/) for the **sentiment analysis** task.
Question: which is the problem in details? Are you not able to connect the feature vector extracted by BERT to a custom classifier on-top? Is the shape of the feature vector fixed?
> ## Questions & Help
> Hello, I am a student who is learning nlp.
> Now I want to use the word vector output by bert to apply to my model, but **I can't connect the word vector to the network**. Could you give me an example program or tutorial about this which use textCNN or LSTM. You can sent e-mail to **[[email protected]](mailto:[email protected])** or reply me, please.
> Thank you for your kind cooperation!<|||||>This issue has been automatically marked as stale because it has not had recent activity. It will be closed if no further activity occurs. Thank you for your contributions.
|
transformers | 1,564 | closed | ALBERT: will it be supported? | will you release an ALBERT model?
it sets the new state of art;
# 🌟New model addition
ALBERT: A LITE BERT FOR SELF-SUPERVISED
LEARNING OF LANGUAGE REPRESENTATIONS
https://arxiv.org/pdf/1909.11942.pdf
## Model description
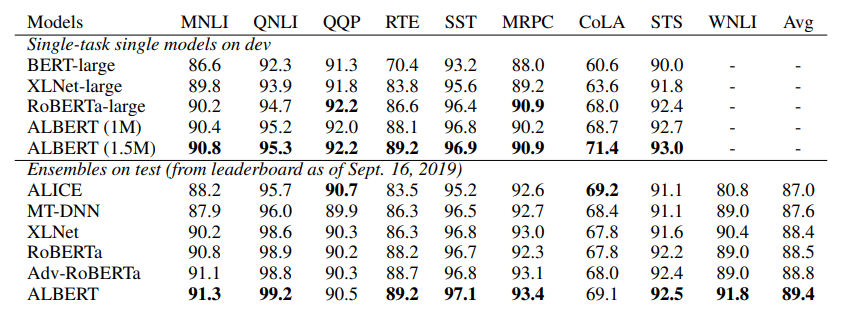
<!-- Important information -->
## Open Source status
* [ ] the model implementation is available: (give details)
* [ ] the model weights are available: (give details)
## Additional context
<!-- Add any other context about the problem here. -->
| 10-19-2019 02:42:16 | 10-19-2019 02:42:16 | https://github.com/brightmart/albert_zh<|||||>Please direct all your questions to the main albert topic. https://github.com/huggingface/transformers/issues/1370
Please close this current topic. It does not add anything.<|||||>... We should extend the issue template and redirect all ALBERT questions to #1370 😂 |
transformers | 1,563 | closed | The implementation of grad clipping is not correct when gradient accumulation is enabled | torch.nn.utils.clip_grad_norm_ should be applied before optimizer.step() not after each backward
## 🐛 Bug
<!-- Important information -->
Model I am using (Bert, XLNet....):
Language I am using the model on (English, Chinese....):
The problem arise when using:
* [ ] the official example scripts: (give details)
* [ ] my own modified scripts: (give details)
The tasks I am working on is:
* [ ] an official GLUE/SQUaD task: (give the name)
* [ ] my own task or dataset: (give details)
## To Reproduce
Steps to reproduce the behavior:
1.
2.
3.
<!-- If you have a code sample, error messages, stack traces, please provide it here as well. -->
## Expected behavior
<!-- A clear and concise description of what you expected to happen. -->
## Environment
* OS:
* Python version:
* PyTorch version:
* PyTorch Transformers version (or branch):
* Using GPU ?
* Distributed of parallel setup ?
* Any other relevant information:
## Additional context
<!-- Add any other context about the problem here. -->
| 10-19-2019 00:12:56 | 10-19-2019 00:12:56 | This should be fixed in https://github.com/huggingface/transformers/pull/1580<|||||>yeah @yangyiben please let me know if that merge fixes it<|||||>#1580 is now merged |
transformers | 1,562 | closed | training BERT on coreference resolution | Hi
I really appreciate if you could add codes to train BERT on coref resolution dataset of CONLL-2012, thanks | 10-18-2019 14:32:57 | 10-18-2019 14:32:57 | This issue has been automatically marked as stale because it has not had recent activity. It will be closed if no further activity occurs. Thank you for your contributions.
<|||||>There's a newer [approach](https://github.com/mandarjoshi90/coref) using BERT but it's using tensorflow 1.14. I wish if we could get this into hugginface.
|
transformers | 1,561 | closed | [CLS] & [SEP] tokens missing in documentation | https://github.com/huggingface/transformers/blob/fd97761c5a977fd22df789d2851cf57c7c9c0930/transformers/modeling_bert.py#L1017-L1023
In this example of bert for token classification input sentence is encoded, but [CLS] & [SEP] tokens are not added. Is this intentional or just a typo?
Do I need to add [CLS] & [SEP] tokens when I fine tune base bert for sequence classification or token classification? | 10-18-2019 14:12:41 | 10-18-2019 14:12:41 | @hawkeoni [CLS] and [SEP] tokens are added automatically as long as you use the tokenizer, ```BertTokenizer```<|||||>@enzoampil It doesn't seem to work.
The following code
```python
from transformers import BertTokenizer
tokenizer = BertTokenizer.from_pretrained('bert-base-uncased')
sentence = "Hello there, General Kenobi!"
print(tokenizer.encode(sentence))
print(tokenizer.cls_token_id, tokenizer.sep_token_id)
```
produces the next output:
[7592, 2045, 1010, 2236, 6358, 16429, 2072, 999]
101 102
As you can see, cls and sep tokens are not in the list.<|||||>@hawkeoni please try ```print(tokenizer.encode(sentence, add_special_tokens=True))```<|||||>@enzoampil I'm sorry, but you're missing the point that the documentation is plainly wrong and misleading. That's why I asked whether the tokens should be added at all.<|||||>@hawkeoni Apologies, yes I did miss your point.
Is this intentional or just a typo? **Looks like a typo since special tokens weren't added. Setting ```add_special_tokens=True``` should make this correct (will add this in).**
Do I need to add [CLS] & [SEP] tokens when I fine tune base bert for sequence classification or token classification? **Yes, I believe this is currently handled by ```load_and_cache_examples``` in the sample training scripts (e.g. ```run_ner.py```)**<|||||>@enzoampil Thanks for your answer! If you plan on fixing this typo, please, fix it everywhere, so this issue never occurs again.
You can find it with
```bash
grep -iR "input_ids = torch.tensor(tokenizer.encode(" .
```
<|||||>@hawkeoni thanks for the bash script reco. Ended up using it :)<|||||>Thank you both for that.
Please note that the special tokens will be added by default from now on (already on master and in the coming release). |
transformers | 1,560 | closed | Finetuning OpenAI GPT-2 for another language. | ## ❓ Questions & Help
Hi,
Is there any option to finetune and use OpenAI GPT-2 for another language except English? | 10-18-2019 11:54:33 | 10-18-2019 11:54:33 | Hello, if you want to try and fine-tune GPT-2 to another language, you can just give the `run_lm_finetuning` script your text in the other language on which you want to fine-tune your model.
However, please be aware that according to the language and its distance to the English language (language on which GPT-2 was pre-trained), you may find it hard to obtain good results. <|||||>@0x01h
GPT-2 can produce great results given a proper vocabulary. If you just run `run_lm_finetuning` on your lang dataset it will give you poor results, regardless of language distance from English because the vocab.
I'd suggest that you train your tokenizer model first and then fine-tune GPT-2 with it. I'm doing that here
https://github.com/mgrankin/ru_transformers
<|||||>This issue has been automatically marked as stale because it has not had recent activity. It will be closed if no further activity occurs. Thank you for your contributions.
|
transformers | 1,559 | closed | Compatibility between DistilBert and Bert models | ## ❓ Questions & Help
I have a regular classification task for sentences in russian language.
I used to train `BertForSequenceClassification` with pretrained Bert from [DeepPavlov](http://docs.deeppavlov.ai/en/master/features/models/bert.html) [RuBERT](http://files.deeppavlov.ai/deeppavlov_data/bert/rubert_cased_L-12_H-768_A-12_v2.tar.gz) (using PyTorch). Then I switched to `DistilBertForSequenceClassification`, but still using pretrained RuBert (because there is no pretrained DistilBert with russian language). And it worked.
Then after [this changing](https://github.com/huggingface/transformers/pull/1203/commits/465870c33fe4ade66863ca0edfe13616f9d24da5#diff-9dc1f6db4a89dbf13c19d02a9f27093dL178) there is impossible to load `DistilBertConfig` from `BertConfig` config.json. `DistilBertConfig` uses property decorator for compatibility between `DistilBertConfig` and `BertConfig`, that's why using setattr() causes error `AttributeError: can't set attribute`.
So my question is following: Is it a bug or feature? Is it OK to load DistilBert from pretrained Bert or not? Or maybe the best way for me is to distill RuBERT by myself using your script for distillation? | 10-18-2019 11:05:16 | 10-18-2019 11:05:16 | This issue has been automatically marked as stale because it has not had recent activity. It will be closed if no further activity occurs. Thank you for your contributions.
|
transformers | 1,558 | closed | unable to parse E:/litao/bert/bert-base-cased\config.json as a URL or as a local path | ## ❓ Questions & Help
<!-- A clear and concise description of the question. -->
train_file = 'E:/litao/bert/SQuAD 1.1/train-v1.1.json'
predict_file = 'E:/litao/bert/SQuAD 1.1/dev-v1.1.json'
model_type = 'bert'
model_name_or_path = 'E:/litao/bert/bert-base-cased'
output_dir = 'E:/litao/bert/transformers-master/examples/output'
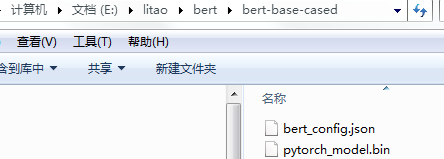
| 10-18-2019 10:06:42 | 10-18-2019 10:06:42 | This issue has been automatically marked as stale because it has not had recent activity. It will be closed if no further activity occurs. Thank you for your contributions.
<|||||>Did you find a solution?<|||||>> Did you find a solution?
Rename "bert_config.json" to "config.json". |
transformers | 1,557 | closed | Tuning BERT on our own data set for multi-class classification problem | I want to tune pre-trained BERT for multi-class classification with **6 million class, 30 million rows & highly imbalance data set.**
Can we tune BERT in batch of classes?
For example, I will take 15 classes (last layer will have only 15 neuron) and train my BERT model & in next batch use that trained model to train batch of next 15 classes, I just want to understand cons of this process. | 10-18-2019 06:53:23 | 10-18-2019 06:53:23 | This issue has been automatically marked as stale because it has not had recent activity. It will be closed if no further activity occurs. Thank you for your contributions.
|
transformers | 1,556 | closed | Does the function of 'evaluate()' change the result? | ## ❓ Questions & Help
<!-- A clear and concise description of the question. -->
when i run RTE task , and logging steps=50: the result is:
gloabl_step 50: 0.8953
global_step 100: 0.8953
gloabl_step 150: 0.8916
global_step 200: 0.8736
but when logging steps =100:
global_step 100: 0.8953
global_step 200: 0.8880
when global_step is 200,what cause the difference? i didn't modify any code
| 10-18-2019 05:10:50 | 10-18-2019 05:10:50 | Could you specify what script you're running, with which parameters? Did you set a random seed?<|||||>> Could you specify what script you're running, with which parameters? Did you set a random seed?
for SEEDS in 99
do
CUDA_VISIBLE_DEVICES=2 python run_glue.py \
--data_dir '/data/transformers/data/RTE/' \
--model_type 'roberta' \
--model_name_or_path '/data/transformers/examples/pretrained_model/roberta-mnli/' \
--task_name 'rte' \
--output_dir ./$SEEDS \
--overwrite_output_dir \
--max_seq_length 128 \
--do_train \
--do_eval \
--evaluate_during_training \
--per_gpu_train_batch_size 8 \
--per_gpu_eval_batch_size 8 \
--gradient_accumulation_steps 2 \
--learning_rate 1e-5 \
--num_train_epochs 10 \
--logging_steps 50 \
--save_steps -1 \
--seed $SEEDS \
done
<|||||>> Could you specify what script you're running, with which parameters? Did you set a random seed?
i change 'roberta' to 'bert' and set the same seed, the result is also different, Is there any wrong with my shell script? |
transformers | 1,555 | closed | Sample a constant number of tokens for masking in LM finetuning | For Masked LM fine-tuning, I think both the original BERT and RoBERTa implementations uniformly sample x number of tokens in *each* sequence for masking (where x = mlm_probability * 100 * sequence_length)
However, The current logic in run_lm_finetuning.py does an indepdendent sampling (from bernoulli distribution) for each token in the sequence. This leads to variance in the number of masked tokens (with the average number still close to x%).
The below example illustrates an extreme case, of the current logic, where no token in the input sequence is masked.
```
In [1]: import numpy as np
...: import torch
...: from transformers import BertTokenizer
...:
...: mlm_probability = 0.15
...: tokenizer = BertTokenizer.from_pretrained('bert-large-uncased')
...:
...: tokens = tokenizer.convert_ids_to_tokens(tokenizer.encode('please mask me, o lord!', add_special_tokens=True))
...:
...: input_ids = tokenizer.convert_tokens_to_ids(tokens)
...:
...: inputs = torch.Tensor([input_ids])
...:
...: labels = inputs.clone()
...:
...: probability_matrix = torch.full(labels.shape, mlm_probability)
...:
...: special_tokens_mask = [tokenizer.get_special_tokens_mask(val, already_has_special_tokens=True) for val in labels.tolist()]
...: probability_matrix.masked_fill_(torch.tensor(special_tokens_mask, dtype=torch.bool), value=0.0)
...: masked_indices = torch.bernoulli(probability_matrix).bool()
...:
...:
In [2]: masked_indices
Out[2]: tensor([[False, False, False, False, False, False, False, False, False]])
```
This PR modifies the logic so the percentage of masked tokens is constant (at x).
Separately, the existing and the new masking logic both rely on boolean tensors of pytorch.
So, this also updates README to include the minimum pytorch version needed. (1.2.0) | 10-18-2019 01:54:02 | 10-18-2019 01:54:02 | # [Codecov](https://codecov.io/gh/huggingface/transformers/pull/1555?src=pr&el=h1) Report
> Merging [#1555](https://codecov.io/gh/huggingface/transformers/pull/1555?src=pr&el=desc) into [master](https://codecov.io/gh/huggingface/transformers/commit/fd97761c5a977fd22df789d2851cf57c7c9c0930?src=pr&el=desc) will **increase** coverage by `1.42%`.
> The diff coverage is `n/a`.
[](https://codecov.io/gh/huggingface/transformers/pull/1555?src=pr&el=tree)
```diff
@@ Coverage Diff @@
## master #1555 +/- ##
==========================================
+ Coverage 84.74% 86.16% +1.42%
==========================================
Files 91 91
Lines 13593 13593
==========================================
+ Hits 11519 11713 +194
+ Misses 2074 1880 -194
```
| [Impacted Files](https://codecov.io/gh/huggingface/transformers/pull/1555?src=pr&el=tree) | Coverage Δ | |
|---|---|---|
| [transformers/modeling\_openai.py](https://codecov.io/gh/huggingface/transformers/pull/1555/diff?src=pr&el=tree#diff-dHJhbnNmb3JtZXJzL21vZGVsaW5nX29wZW5haS5weQ==) | `81.75% <0%> (+1.35%)` | :arrow_up: |
| [transformers/modeling\_ctrl.py](https://codecov.io/gh/huggingface/transformers/pull/1555/diff?src=pr&el=tree#diff-dHJhbnNmb3JtZXJzL21vZGVsaW5nX2N0cmwucHk=) | `95.45% <0%> (+2.27%)` | :arrow_up: |
| [transformers/modeling\_xlnet.py](https://codecov.io/gh/huggingface/transformers/pull/1555/diff?src=pr&el=tree#diff-dHJhbnNmb3JtZXJzL21vZGVsaW5nX3hsbmV0LnB5) | `73.28% <0%> (+2.46%)` | :arrow_up: |
| [transformers/modeling\_roberta.py](https://codecov.io/gh/huggingface/transformers/pull/1555/diff?src=pr&el=tree#diff-dHJhbnNmb3JtZXJzL21vZGVsaW5nX3JvYmVydGEucHk=) | `80.57% <0%> (+15.1%)` | :arrow_up: |
| [transformers/tests/modeling\_tf\_common\_test.py](https://codecov.io/gh/huggingface/transformers/pull/1555/diff?src=pr&el=tree#diff-dHJhbnNmb3JtZXJzL3Rlc3RzL21vZGVsaW5nX3RmX2NvbW1vbl90ZXN0LnB5) | `96.8% <0%> (+17.02%)` | :arrow_up: |
| [transformers/modeling\_tf\_pytorch\_utils.py](https://codecov.io/gh/huggingface/transformers/pull/1555/diff?src=pr&el=tree#diff-dHJhbnNmb3JtZXJzL21vZGVsaW5nX3RmX3B5dG9yY2hfdXRpbHMucHk=) | `92.95% <0%> (+83.09%)` | :arrow_up: |
------
[Continue to review full report at Codecov](https://codecov.io/gh/huggingface/transformers/pull/1555?src=pr&el=continue).
> **Legend** - [Click here to learn more](https://docs.codecov.io/docs/codecov-delta)
> `Δ = absolute <relative> (impact)`, `ø = not affected`, `? = missing data`
> Powered by [Codecov](https://codecov.io/gh/huggingface/transformers/pull/1555?src=pr&el=footer). Last update [fd97761...090cbd6](https://codecov.io/gh/huggingface/transformers/pull/1555?src=pr&el=lastupdated). Read the [comment docs](https://docs.codecov.io/docs/pull-request-comments).
<|||||>Thanks @rakeshchada. Closing as superseded by #1814 |
transformers | 1,554 | closed | GPT2 not in modeltype | ## 🐛 Bug
<!-- Important information -->
Model I am using (Bert, XLNet....): GPT2
Language I am using the model on (English, Chinese....): ENGLISH
The problem arise when using:
* [ ] the official example scripts: (give details) run_glue.py
* [ ] my own modified scripts: (give details)
The tasks I am working on is:
* [ ] an official GLUE/SQUaD task: (give the name) MRPC GLUE
* [ ] my own task or dataset: (give details)
## To Reproduce
Steps to reproduce the behavior:
1.
2.
3.
python ./examples/run_glue.py --model_type gpt2 --model_name_or_path gp
t2 --task_name MRPC --do_train --do_eval --do_lower_case --data_dir ./fake --max_seq_length 512 --per_gpu_eval_batc
h_size=8 --per_gpu_train_batch_size=8 --learning_rate 2e-5 --num_train_epochs 3.0 --output_dir /tmp/bot/
10/18/2019 00:22:28 - WARNING - __main__ - Process rank: -1, device: cuda, n_gpu: 1, distributed training: False,
16-bits training: False
Traceback (most recent call last):
File "./examples/run_glue.py", line 541, in <module>
main()
File "./examples/run_glue.py", line 476, in main
config_class, model_class, tokenizer_class = MODEL_CLASSES[args.model_type]
KeyError: 'gpt2'
## Environment
* OS: UBUNTU LINUX
* Python version: 3.7
* PyTorch version: LATEST
* PyTorch Transformers version (or branch): LATEST
* Using GPU ? YES
* Distributed of parallel setup ? NO
* Any other relevant information:
## Additional context
<!-- Add any other context about the problem here. -->
| 10-18-2019 00:24:40 | 10-18-2019 00:24:40 | Hey @tuhinjubcse gpt2 is a text generation model. If you look in the run_glue.py file you will see your options for model selection for using the run_glue.py script.
```
MODEL_CLASSES = {
'bert': (BertConfig, BertForSequenceClassification, BertTokenizer),
'xlnet': (XLNetConfig, XLNetForSequenceClassification, XLNetTokenizer),
'xlm': (XLMConfig, XLMForSequenceClassification, XLMTokenizer),
'roberta': (RobertaConfig, RobertaForSequenceClassification, RobertaTokenizer),
'distilbert': (DistilBertConfig, DistilBertForSequenceClassification, DistilBertTokenizer)
}
```<|||||>gpt2 is a transformer model. Why would it be limited to only generation ??<|||||>It's not that you can't use it for classification, etc. It's that you would need to make a few changes to the code and model. #1248 . Right now the changes are not made for gpt2. Generally speaking, people use gpt2 for text generation.<|||||>Autoregressive models are not as good as mlms, at classification tasks. You should check masked language models (mlm) or similars. But it's not impossible, Open AI has shown some example use cases on original [GPT paper](https://s3-us-west-2.amazonaws.com/openai-assets/research-covers/language-unsupervised/language_understanding_paper.pdf). Check the figure 1 and page 6 for more details.
Also, this is not a bug but just not implemented. <|||||>This issue has been automatically marked as stale because it has not had recent activity. It will be closed if no further activity occurs. Thank you for your contributions.
|
transformers | 1,553 | closed | Add speed log to examples/run_squad.py | Add a speed estimate log (time per example)
for evaluation to examples/run_squad.py | 10-17-2019 21:44:08 | 10-17-2019 21:44:08 | # [Codecov](https://codecov.io/gh/huggingface/transformers/pull/1553?src=pr&el=h1) Report
> Merging [#1553](https://codecov.io/gh/huggingface/transformers/pull/1553?src=pr&el=desc) into [master](https://codecov.io/gh/huggingface/transformers/commit/fd97761c5a977fd22df789d2851cf57c7c9c0930?src=pr&el=desc) will **increase** coverage by `1.42%`.
> The diff coverage is `n/a`.
[](https://codecov.io/gh/huggingface/transformers/pull/1553?src=pr&el=tree)
```diff
@@ Coverage Diff @@
## master #1553 +/- ##
==========================================
+ Coverage 84.74% 86.16% +1.42%
==========================================
Files 91 91
Lines 13593 13593
==========================================
+ Hits 11519 11713 +194
+ Misses 2074 1880 -194
```
| [Impacted Files](https://codecov.io/gh/huggingface/transformers/pull/1553?src=pr&el=tree) | Coverage Δ | |
|---|---|---|
| [transformers/modeling\_openai.py](https://codecov.io/gh/huggingface/transformers/pull/1553/diff?src=pr&el=tree#diff-dHJhbnNmb3JtZXJzL21vZGVsaW5nX29wZW5haS5weQ==) | `81.75% <0%> (+1.35%)` | :arrow_up: |
| [transformers/modeling\_ctrl.py](https://codecov.io/gh/huggingface/transformers/pull/1553/diff?src=pr&el=tree#diff-dHJhbnNmb3JtZXJzL21vZGVsaW5nX2N0cmwucHk=) | `95.45% <0%> (+2.27%)` | :arrow_up: |
| [transformers/modeling\_xlnet.py](https://codecov.io/gh/huggingface/transformers/pull/1553/diff?src=pr&el=tree#diff-dHJhbnNmb3JtZXJzL21vZGVsaW5nX3hsbmV0LnB5) | `73.28% <0%> (+2.46%)` | :arrow_up: |
| [transformers/modeling\_roberta.py](https://codecov.io/gh/huggingface/transformers/pull/1553/diff?src=pr&el=tree#diff-dHJhbnNmb3JtZXJzL21vZGVsaW5nX3JvYmVydGEucHk=) | `80.57% <0%> (+15.1%)` | :arrow_up: |
| [transformers/tests/modeling\_tf\_common\_test.py](https://codecov.io/gh/huggingface/transformers/pull/1553/diff?src=pr&el=tree#diff-dHJhbnNmb3JtZXJzL3Rlc3RzL21vZGVsaW5nX3RmX2NvbW1vbl90ZXN0LnB5) | `96.8% <0%> (+17.02%)` | :arrow_up: |
| [transformers/modeling\_tf\_pytorch\_utils.py](https://codecov.io/gh/huggingface/transformers/pull/1553/diff?src=pr&el=tree#diff-dHJhbnNmb3JtZXJzL21vZGVsaW5nX3RmX3B5dG9yY2hfdXRpbHMucHk=) | `92.95% <0%> (+83.09%)` | :arrow_up: |
------
[Continue to review full report at Codecov](https://codecov.io/gh/huggingface/transformers/pull/1553?src=pr&el=continue).
> **Legend** - [Click here to learn more](https://docs.codecov.io/docs/codecov-delta)
> `Δ = absolute <relative> (impact)`, `ø = not affected`, `? = missing data`
> Powered by [Codecov](https://codecov.io/gh/huggingface/transformers/pull/1553?src=pr&el=footer). Last update [fd97761...0919389](https://codecov.io/gh/huggingface/transformers/pull/1553?src=pr&el=lastupdated). Read the [comment docs](https://docs.codecov.io/docs/pull-request-comments).
<|||||>up ?<|||||>Why not, indeed. Ok to merge it. |
transformers | 1,552 | closed | There is not space after generating an 'special token' and the next word using gpt2. | ## ❓ Questions & Help
Hi,
I have used ```run_lm_finetuning.py``` to finetune gpt2 and then tried to do some generation. I have added a couple of special token to dictionary and the finetuned gpt2 without any problem.
Then when I am doing the generation using ```run_generation.py```, I realized whenever the model generates a special_token , it clubs it with the next generated token. For example, consider [SEP] is a special token and this is an output:
[SEP]and it has been a very , ....
And this happens with all of my special_tokens. That is if the next token of an special token isn't an special token, then there is no space between them.
Does anyone know the reason?
Best | 10-17-2019 20:09:33 | 10-17-2019 20:09:33 | Can you show exactly how you ran ```run_generation.py```?<|||||>@enzoampil thanks, this is my command:
```python run_generation.py --model_type=gpt2 --model_name_or_path=gpt2_finetuned/ --top_k 10 --temperature 0.8 --top_p 0.0 --stop_token "<|endoftext|>" ```<|||||>Can you try running ```python run_generation.py --model_type=gpt2 --model_name_or_path=gpt2_finetuned/ --top_k 10 --temperature 0.8 --top_p 0.0``` and post the output here.
If the output looks fine at this point, just exclude the ```stop_token``` argument when running ```run_generation.py```<|||||>I see the problem. At the decoding step using ```tokenizer.decode```, we set ```skip_special_tokens=True```.
In other words, special tokens are removed at the decoding step, so you the ```stop_token``` argument should not be a special token.<|||||>@enzoampil
1) I tried without --stop_token and the output is the same.
2) I don't think ``` skip_special_tokens=True ``` is the problem, since I had already set that to **False**.
Did you mean --stop_token is a problem? Am I doing something wrong?<|||||>Can you try to set ```clean_up_tokenization_spaces=False```<|||||>Yeah I actually tried that one too and still the output doesn't change. @enzoampil <|||||>Would you mind sending over your model files? Can't seem to replicate the issue.<|||||>This issue has been automatically marked as stale because it has not had recent activity. It will be closed if no further activity occurs. Thank you for your contributions.
<|||||>Is it solved?<|||||>This issue has been automatically marked as stale because it has not had recent activity. It will be closed if no further activity occurs. Thank you for your contributions.
<|||||>I know this is old, but I'm having the same issue (and I've never had it before). It was introduced after I added a Tokenizers `BPEDecoder` to my tokenizer. I'm using special tokens as a way of logically parsing my (non-language) input, and I need the special tokens in the output so that another stage of processing can use them for understanding the structure of the prediction. But there are no spaces between my special tokens and the next word. It's not a huge deal, I suppose, because I could fix it in post-processing, but I'd like to know what's up.
[EDIT] Just to note; in my case it has nothing to do with prediction. I'm just testing the encode/decode of my tokenizer and noticing these spaces missing in the decoded output. |
transformers | 1,551 | closed | [FIX] fix repetition penalty in `examples/run_generation.py` | The repetition penalty in `examples/run_generation.py` is incorrectly implemented due to the following snippet.
```python
for _ in set(generated):
next_token_logits[_] /= repetition_penalty
```
`generated` is a tensor, and python built-in `set` does not compare tensors correctly, e.g.:
```python
>>> import torch
>>> set(torch.cat([torch.arange(2),torch.arange(3)]))
{tensor(0), tensor(1), tensor(1), tensor(0), tensor(2)}
```
This PR fixes this subtle error. | 10-17-2019 18:14:20 | 10-17-2019 18:14:20 | # [Codecov](https://codecov.io/gh/huggingface/transformers/pull/1551?src=pr&el=h1) Report
> Merging [#1551](https://codecov.io/gh/huggingface/transformers/pull/1551?src=pr&el=desc) into [master](https://codecov.io/gh/huggingface/transformers/commit/c5441946112e68441b46866d114bf8d3c29b0c1d?src=pr&el=desc) will **not change** coverage.
> The diff coverage is `n/a`.
[](https://codecov.io/gh/huggingface/transformers/pull/1551?src=pr&el=tree)
```diff
@@ Coverage Diff @@
## master #1551 +/- ##
=======================================
Coverage 86.16% 86.16%
=======================================
Files 91 91
Lines 13593 13593
=======================================
Hits 11713 11713
Misses 1880 1880
```
------
[Continue to review full report at Codecov](https://codecov.io/gh/huggingface/transformers/pull/1551?src=pr&el=continue).
> **Legend** - [Click here to learn more](https://docs.codecov.io/docs/codecov-delta)
> `Δ = absolute <relative> (impact)`, `ø = not affected`, `? = missing data`
> Powered by [Codecov](https://codecov.io/gh/huggingface/transformers/pull/1551?src=pr&el=footer). Last update [c544194...4f05239](https://codecov.io/gh/huggingface/transformers/pull/1551?src=pr&el=lastupdated). Read the [comment docs](https://docs.codecov.io/docs/pull-request-comments).
<|||||>Hello! That's great, thank you! |
transformers | 1,550 | closed | training BERT from scratch for native language PT-BR? Without init weight | I would like to train BERT from scratch for a textual base in PT-BR (8GB data). Is it possible to use the run_lm_finetuning.py code to perform this process without using the multi-language bert model?
I already have a vocab.txt for the PT-BR base and I don't want to load initial weights.
Is there any script or tutorial to perform this process step by step? | 10-17-2019 17:30:58 | 10-17-2019 17:30:58 | This issue has been automatically marked as stale because it has not had recent activity. It will be closed if no further activity occurs. Thank you for your contributions.
|
transformers | 1,549 | closed | Fix token order in xlnet preprocessing for SQuAD | #947
My current result on SQuAD 1.1
{
"exact": 85.45884578997162,
"f1": 92.5974600601065,
"total": 10570,
"HasAns_exact": 85.45884578997162,
"HasAns_f1": 92.59746006010651,
"HasAns_total": 10570
}
My code validation command
```
python /data/home/hlu/transformers/examples/run_squad.py \
--model_type xlnet \
--model_name_or_path xlnet-large-cased \
--do_train \
--do_eval \
--do_lower_case \
--train_file /data/home/hlu/notebooks/NLP/examples/question_answering/train-v1.1.json \
--predict_file /data/home/hlu/notebooks/NLP/examples/question_answering/dev-v1.1.json \
--learning_rate 3e-5 \
--num_train_epochs 2 \
--max_seq_length 384 \
--doc_stride 128 \
--output_dir ./wwm_cased_finetuned_squad/ \
--per_gpu_eval_batch_size=4 \
--per_gpu_train_batch_size=4 \
--save_steps 5000
``` | 10-17-2019 15:14:28 | 10-17-2019 15:14:28 | # [Codecov](https://codecov.io/gh/huggingface/transformers/pull/1549?src=pr&el=h1) Report
> Merging [#1549](https://codecov.io/gh/huggingface/transformers/pull/1549?src=pr&el=desc) into [master](https://codecov.io/gh/huggingface/transformers/commit/8a62835577a2a93642546858b21372e43c1a1ff8?src=pr&el=desc) will **not change** coverage.
> The diff coverage is `n/a`.
[](https://codecov.io/gh/huggingface/transformers/pull/1549?src=pr&el=tree)
```diff
@@ Coverage Diff @@
## master #1549 +/- ##
=======================================
Coverage 85.14% 85.14%
=======================================
Files 94 94
Lines 13920 13920
=======================================
Hits 11852 11852
Misses 2068 2068
```
------
[Continue to review full report at Codecov](https://codecov.io/gh/huggingface/transformers/pull/1549?src=pr&el=continue).
> **Legend** - [Click here to learn more](https://docs.codecov.io/docs/codecov-delta)
> `Δ = absolute <relative> (impact)`, `ø = not affected`, `? = missing data`
> Powered by [Codecov](https://codecov.io/gh/huggingface/transformers/pull/1549?src=pr&el=footer). Last update [8a62835...9a3b173](https://codecov.io/gh/huggingface/transformers/pull/1549?src=pr&el=lastupdated). Read the [comment docs](https://docs.codecov.io/docs/pull-request-comments).
<|||||>This is great, thanks @hlums.
I'm adding information on the runs/results in the example's readme and merging.<|||||>@ShengeBelmendo I think we set them to the right values here as well (cf lines 309-310 in `run_squad`).<|||||>Ok LGTM, merging this PR, thanks @hlums <|||||>@thomwolf oh, I'm sorry for missing that.
And another small question. @hlums Have you tested whether set the `do_lower_case` option or not ? It seems that we shouldn't set this option cuz the model is the cased version and it also didn't be set in official code.
I'm trying to reproduce the results of xlnet but obviously there are some problems especially for squad2.0, it's still a long way to go.<|||||>> @thomwolf oh, I'm sorry for missing that.
>
> And another small question. @hlums Have you tested whether set the `do_lower_case` option or not ? It seems that we shouldn't set this option cuz the model is the cased version and it also didn't be set in official code.
>
> I'm trying to reproduce the results of xlnet but obviously there are some problems especially for squad2.0, it's still a long way to go.
You are right. I shouldn't have set the do_lower_case flag. I can give it a try some time this week.
Have you tried the latest code on squad 2.0? I thought put the cls token at the right place would help a lot because it's used for unanswerable question classification. <|||||>> > @thomwolf oh, I'm sorry for missing that.
> > And another small question. @hlums Have you tested whether set the `do_lower_case` option or not ? It seems that we shouldn't set this option cuz the model is the cased version and it also didn't be set in official code.
> > I'm trying to reproduce the results of xlnet but obviously there are some problems especially for squad2.0, it's still a long way to go.
>
> You are right. I shouldn't have set the do_lower_case flag. I can give it a try some time this week.
> Have you tried the latest code on squad 2.0? I thought put the cls token at the right place would help a lot because it's used for unanswerable question classification.
@hlums Sorry for late reply. The latest code doesn't work, you will get a f1 score closed to 0 on unanswerable questions. But I have found the reason.
The following is a piece of code in forward function of xlnet model, which obviously is the key point of training the model on unanswerable questions using cls token representations. But the default value of tensor `is_impossible`(using to indicate whether this example is answerable) is none, and we also hadn't passed this tensor into forward function. That's the problem.
```
if cls_index is not None and is_impossible is not None:
# Predict answerability from the representation of CLS and START
cls_logits = self.answer_class(hidden_states, start_positions=start_positions, cls_index=cls_index)
loss_fct_cls = nn.BCEWithLogitsLoss()
cls_loss = loss_fct_cls(cls_logits, is_impossible)
total_loss += cls_loss * 0.5
```
I added the `is_impossible` tensor to TensorDataset and model inputs, and got a reasonable result, f1: 84, EM: 80. Maybe I can creat a PR for this, maybe after I find more discrepancies and get better results. I'm working hard to reproduce the results of xlnet on squad2.0, so I hope you can tell me if you have some new ideas or finds.Thanks! <|||||>@hlums before your fix:
`xlnet-large-cased`, SQuAD 1.1, 2 epochs, MSL: 512, BS: 48
{
"exact": 75.01419110690634,
"f1": 82.13017516396678,
"total": 10570,
"HasAns_exact": 75.01419110690634,
"HasAns_f1": 82.13017516396678,
"HasAns_total": 10570
}
Post fix, awesome:
{
"exact": 85.1371807000946,
"f1": 92.24219729313499,
"total": 10570,
"HasAns_exact": 85.1371807000946,
"HasAns_f1": 92.24219729313499,
"HasAns_total": 10570
}
Thanks again for your fix!
`xlnet-large-cased`, SQuAD 2.0, max_steps: 8000, MSL: 512, BS: 48
{
"exact": 40.95005474606249,
"f1": 45.305949189220875,
"total": 11873,
"HasAns_exact": 81.96693657219973,
"HasAns_f1": 90.69121705864026,
"HasAns_total": 5928,
"NoAns_exact": 0.050462573591253154,
"NoAns_f1": 0.050462573591253154,
"NoAns_total": 5945
}
Hopefully `xlnet-large-cased` on _SQuAD 2.0_ for the holidays: @ShengeBelmendo https://github.com/huggingface/transformers/pull/1803
Limited what I can contribute to the code/logic, but I can run tests 24 x 7.<|||||>@ShengeBelmendo I tried turning do_lower_case off, but the model performance didn't change much.
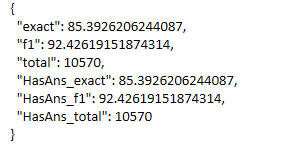
Sorry I'm not actively working QA anymore so probably won't be able to contribute to the improvement on squad 2.0
Another thing mentioned in the XLNet paper is layer-wise learning rate decay. I actually tried implementing it, but it didn't help with the performance on 1.1 for me. See #1198
The pre-processing code in the XLNet repo also looks much more complicated than here. I'm not sure if it has anything to do with the performance discrepancy though.
<|||||>@hlums ok, tks again. I will check more carefully about the pre-processing part and maybe read all the official code for comparison. |
transformers | 1,548 | closed | [2.2] - Command-line interface - Pipeline class | Adding a `Pipeline` class that encapsulates a `Tokenizer` and a `Model`.
`Pipelines` take python objects as inputs (lists/dict of string/int/float) and output python objects as well (lists/dict of string/int/float).
`Pipelines` can be used to query and train models and should be framework agnostic (default to TF 2.0 if installed, fallback to PyTorch).
ex:
```python
# load/initialize a text classification model from Bert-base-uncased
pipeline = TextClassificationPipeline.from_pretrained('bert-base-uncased')
# Train the text classification model with lists of strings and associated labels
pipeline.fit(list_of_texts, list_of_labels)
# Predict with the trained classification model
# (input: list of strings, output: list of int)
batched_predictions = pipeline(list_of_texts)
```
Also adding a simple CLI based on these pipeline models. | 10-17-2019 15:04:10 | 10-17-2019 15:04:10 | # [Codecov](https://codecov.io/gh/huggingface/transformers/pull/1548?src=pr&el=h1) Report
> Merging [#1548](https://codecov.io/gh/huggingface/transformers/pull/1548?src=pr&el=desc) into [master](https://codecov.io/gh/huggingface/transformers/commit/33adab2b91697b3e78af618a21ab9f1176281165?src=pr&el=desc) will **decrease** coverage by `1.44%`.
> The diff coverage is `44.59%`.
[](https://codecov.io/gh/huggingface/transformers/pull/1548?src=pr&el=tree)
```diff
@@ Coverage Diff @@
## master #1548 +/- ##
==========================================
- Coverage 81.47% 80.03% -1.45%
==========================================
Files 122 128 +6
Lines 18342 19325 +983
==========================================
+ Hits 14945 15467 +522
- Misses 3397 3858 +461
```
| [Impacted Files](https://codecov.io/gh/huggingface/transformers/pull/1548?src=pr&el=tree) | Coverage Δ | |
|---|---|---|
| [transformers/commands/download.py](https://codecov.io/gh/huggingface/transformers/pull/1548/diff?src=pr&el=tree#diff-dHJhbnNmb3JtZXJzL2NvbW1hbmRzL2Rvd25sb2FkLnB5) | `0% <0%> (ø)` | |
| [transformers/commands/run.py](https://codecov.io/gh/huggingface/transformers/pull/1548/diff?src=pr&el=tree#diff-dHJhbnNmb3JtZXJzL2NvbW1hbmRzL3J1bi5weQ==) | `0% <0%> (ø)` | |
| [transformers/commands/train.py](https://codecov.io/gh/huggingface/transformers/pull/1548/diff?src=pr&el=tree#diff-dHJhbnNmb3JtZXJzL2NvbW1hbmRzL3RyYWluLnB5) | `0% <0%> (ø)` | |
| [transformers/commands/convert.py](https://codecov.io/gh/huggingface/transformers/pull/1548/diff?src=pr&el=tree#diff-dHJhbnNmb3JtZXJzL2NvbW1hbmRzL2NvbnZlcnQucHk=) | `0% <0%> (ø)` | |
| [transformers/tests/model\_card\_test.py](https://codecov.io/gh/huggingface/transformers/pull/1548/diff?src=pr&el=tree#diff-dHJhbnNmb3JtZXJzL3Rlc3RzL21vZGVsX2NhcmRfdGVzdC5weQ==) | `97.5% <100%> (ø)` | :arrow_up: |
| [transformers/data/\_\_init\_\_.py](https://codecov.io/gh/huggingface/transformers/pull/1548/diff?src=pr&el=tree#diff-dHJhbnNmb3JtZXJzL2RhdGEvX19pbml0X18ucHk=) | `100% <100%> (ø)` | :arrow_up: |
| [transformers/data/processors/\_\_init\_\_.py](https://codecov.io/gh/huggingface/transformers/pull/1548/diff?src=pr&el=tree#diff-dHJhbnNmb3JtZXJzL2RhdGEvcHJvY2Vzc29ycy9fX2luaXRfXy5weQ==) | `100% <100%> (ø)` | :arrow_up: |
| [transformers/modeling\_utils.py](https://codecov.io/gh/huggingface/transformers/pull/1548/diff?src=pr&el=tree#diff-dHJhbnNmb3JtZXJzL21vZGVsaW5nX3V0aWxzLnB5) | `93.06% <100%> (+1.37%)` | :arrow_up: |
| [transformers/data/processors/utils.py](https://codecov.io/gh/huggingface/transformers/pull/1548/diff?src=pr&el=tree#diff-dHJhbnNmb3JtZXJzL2RhdGEvcHJvY2Vzc29ycy91dGlscy5weQ==) | `19.37% <12.5%> (-25.53%)` | :arrow_down: |
| [transformers/modeling\_tf\_auto.py](https://codecov.io/gh/huggingface/transformers/pull/1548/diff?src=pr&el=tree#diff-dHJhbnNmb3JtZXJzL21vZGVsaW5nX3RmX2F1dG8ucHk=) | `32.55% <19.04%> (-5.08%)` | :arrow_down: |
| ... and [34 more](https://codecov.io/gh/huggingface/transformers/pull/1548/diff?src=pr&el=tree-more) | |
------
[Continue to review full report at Codecov](https://codecov.io/gh/huggingface/transformers/pull/1548?src=pr&el=continue).
> **Legend** - [Click here to learn more](https://docs.codecov.io/docs/codecov-delta)
> `Δ = absolute <relative> (impact)`, `ø = not affected`, `? = missing data`
> Powered by [Codecov](https://codecov.io/gh/huggingface/transformers/pull/1548?src=pr&el=footer). Last update [33adab2...db0795b](https://codecov.io/gh/huggingface/transformers/pull/1548?src=pr&el=lastupdated). Read the [comment docs](https://docs.codecov.io/docs/pull-request-comments).
|
transformers | 1,547 | closed | Is it possible/is there a plan to enable continued pretraining? | ## 🚀 Feature
Standardised interface to pretrain various Transformers with standardised expectations with regards to formatting training data.
## Motivation
To achieve state of the art within a given domain it is not sufficient to take models pretrained on nonspecific literature (wikipedia/books/etc). The ideal situation would be able to leverage all the compute put into this training and then further train on domain literature before fine tuning on a specific task. The great strength of this library is having a standard interface to use new SOTA models and it would be very helpful if this was extended to include further pretraining to help rapidly push domain SOTAs. | 10-17-2019 14:46:56 | 10-17-2019 14:46:56 | Hi @oligiles0, you can actually use ```run_lm_finetuning.py``` for this. You can find more details in the **RoBERTa/BERT and masked language modeling** section in the README<|||||>> Hi @oligiles0, you can actually use `run_lm_finetuning.py` for this. You can find more details in the **RoBERTa/BERT and masked language modeling** section in the README
Thanks very much @enzoampil . Is there a reason this uses a single text file as opposed to taking a folder of text files? I wouldn't want to combine multiple documents because some chunks will then cross documents and interfere with training, but I also wouldn't want to rerun the script for individual documents. <|||||>> Thanks very much @enzoampil . Is there a reason this uses a single text file as opposed to taking a folder of text files? I wouldn't want to combine multiple documents because some chunks will then cross documents and interfere with training, but I also wouldn't want to rerun the script for individual documents.
Please check https://github.com/huggingface/transformers/issues/1896#issuecomment-557222822
<|||||>This issue has been automatically marked as stale because it has not had recent activity. It will be closed if no further activity occurs. Thank you for your contributions.
|
transformers | 1,546 | closed | Q / Note: BERT Masked-LM fails to predict last token in sequence if it is not punctuation | ## ❓ Questions & Help
I am playing with BERT to see what the distributions of the prediction for a MASK token are. I wrote a quick script that successively masks all words in an input sequence.
This is based on the implementation in the examples (e.g. the lm finetuning script and the examples in the documentation).
In doing so, I found out that BERT generally fails to predict the last word in the sentence if it is not punctuation. With overwhelmingly high likelihood, BERT expects a normal SVO sentence to end with a full stop. While it can predict the correct word (the correct word usually appears in the top 10 most likely tokens), the likelihood as given by softmax is very low.
So by itself this is perhaps not surprising, because the large majority of examples in pre-training will have punctuation, especially if pre-training is not just the MLM but also the sentence prediction.
But I wonder if it should be best-practice to ensure every sentence is punctuated? If the MLM part of BERT consistently predicts punctuation, then a sentence without it will not be efficiently classified compared to one with punctuation, even on downstream tasks, right?
One thing to confirm, of course, would be that this is not an issue of the pyTorch implementation and how attention masks the <SEP> token or something?
What do you think?
Attached is the script, you should just be able to run it.
[lm_test.py.txt](https://github.com/huggingface/transformers/files/3739434/lm_test.py.txt)
| 10-17-2019 13:19:11 | 10-17-2019 13:19:11 | If you play with the script a bit, you can see that the loss for BERT with the MLM head is actually quite high, as someone suggested elsewhere, this may be due to pre-training on different tasks than just MLM<|||||>This issue has been automatically marked as stale because it has not had recent activity. It will be closed if no further activity occurs. Thank you for your contributions.
|
transformers | 1,545 | closed | Adding new tokens to uncased tokenizers - case insensitivity is lost | ## ❓ Questions & Help
Hello!
I'm trying to add new tokens to bert-base-uncased. Let's say my token is '**cool-token**' and it was not present in the original vocab
```
from transformers import BertTokenizer
tokenizer = BertTokenizer.from_pretrained('bert-base-uncased')
print(tokenizer.tokenize('Sentence with cool-token'))
```
Prints as expected:
`['sentence', 'with', 'cool', '-', 'token']`
Now I add this token
`tokenizer.add_tokens(['cool-token'])`
Once again, prints as expected:
`['sentence', 'with', 'cool-token']`
However, when I try to utilize case-insensitivity my new token seems to be not recognized:
`print(tokenizer.tokenize('SenTenCE wIth cOOl-token'))`
prints
`['sentence', 'with', 'cool', '-', 'token']`
I would expect:
`['sentence', 'with', 'cool-token']`
It seems that custom tokens are not lowercased. Is it expected behavior and I have to `.lower()` my text manually or am I doing something wrong?
Anyway, I <3 your library
| 10-17-2019 12:57:13 | 10-17-2019 12:57:13 | Hmm looks like BertTokenizer's super class handles `.add_tokens()` and the first steps of `.tokenize()`, and doesn't really seem to consider whether the tokens should be made lowercase. I'm not sure whether it's intentional, but I'll make a PR and find out :smile:
In the meantime, it might be a good idea to manually lowercase your text before tokenization.<|||||>This issue has been automatically marked as stale because it has not had recent activity. It will be closed if no further activity occurs. Thank you for your contributions.
|
transformers | 1,544 | closed | the num_labels in run_squad | ## ❓ Questions & Help
In the run_squad, I have not find any code to define the num_labels. In the modeling_utils, the num_labels have been default as 2, but in the Question & Answer task it may predict the start_position and end_position in the inputs. Is there the code have missing consider the reset of num_labels, or 2 is the right setting of num_labels for the task?
<!-- A clear and concise description of the question. -->
| 10-17-2019 11:34:51 | 10-17-2019 11:34:51 | Hey @zhujun5164, 2 is the right setting of num_labels for the task. If you look at the model they use (say Bert is BertForQuestionAnswering), you'll see that they get two outputs for each position which is from the num_labels = 2. The two outputs correspond to the start_logits position and the end_logits position.
```
outputs = self.bert(input_ids,
attention_mask=attention_mask,
token_type_ids=token_type_ids,
position_ids=position_ids,
head_mask=head_mask)
sequence_output = outputs[0]
logits = self.qa_outputs(sequence_output)
start_logits, end_logits = logits.split(1, dim=-1)
start_logits = start_logits.squeeze(-1)
end_logits = end_logits.squeeze(-1)
```
Does that make sense?<|||||>thanks @cformosa, and i find that in the untils_squad.py(341-357) the start_position and end_position have defined as a position number in the sentence (may be in token by word piece), it means that the run_squad predict the position number of start_position and end_position. The split(1, dim = -1) in your copy code is split half of the data in the last dim, so it easier to make me misunderstand it have predict one-hot of the start_position and end_position.<|||||>This issue has been automatically marked as stale because it has not had recent activity. It will be closed if no further activity occurs. Thank you for your contributions.
|
transformers | 1,543 | closed | Where is pytorch-pretrained-BERT? | ## ❓ Questions & Help
<!-- A clear and concise description of the question. -->
As the title shows, where is pytorch-pretrained-BERT? Please tell me the path, THX. | 10-17-2019 07:46:13 | 10-17-2019 07:46:13 | `pytorch-pretrained-BERT` is this library, but four or five months ago. It evolved into `pytorch-transformers` as more models were added to the library, before becoming `transformers` as we now have a front-end for both pytorch and tensorflow.<|||||>is this still an issue?<|||||>I don't think so. In my opinion, @ShallTearchen would know where it goes pytorch-pretrained-BERT, but I think that @LysandreJik explains very well the transformation of this library to Transformers.
In my opinion, I'll close this "issue"!
> is this still an issue? |
transformers | 1,542 | closed | Running CTRL Model On Google Colab Environment | ## ❓ Questions & Help
As you know Google Colab environment has ****12 GB** ram** limit . When i want to run [run_generation.py](https://github.com/huggingface/transformers/blob/master/examples/run_generation.py) file , Colab automatically stops the process. How much ram does the CTRL Model need? Where can I learn that? Or is there another way to run it?
| 10-17-2019 07:12:27 | 10-17-2019 07:12:27 | [In the official repo,](https://github.com/salesforce/ctrl) you can find a 'lower_memory' branch. You can take a look there. As always, you can try to make the batch size and max sequence length smaller, too.<|||||>Thank you for your help<|||||>Please close this topic if you have no further questions. |
transformers | 1,541 | closed | Type of model for each GLUE task | ## ❓ Questions & Help
There are nine GLUE tasks, and I wanted to verify which BERT model type is best suited for each task. Can anyone confirm these matchings? I am not sure what to do for STS-B especially, and am unsure if BertForMultipleChoice is perhaps the correct option for MNLI.
| 10-17-2019 04:41:25 | 10-17-2019 04:41:25 | All models on GLUE should use BertForSequenceClassification (MNLI is 3 class, STS-B is 1 class).<|||||>As specified in the documentation, for `XxxForSequenceClassification` models:
```
If ``config.num_labels == 1`` a regression loss is computed (Mean-Square loss),
If ``config.num_labels > 1`` a classification loss is computed (Cross-Entropy).
```
So you can use `BertForSequenceClassification` for a regression task such as STS-B. |
transformers | 1,540 | closed | Should the option to run on TPU in run_glue.py use some sort of xla data parallelizer ? | ## ❓ Questions & Help
<!-- A clear and concise description of the question. -->
The xla API ( https://github.com/pytorch/xla/blob/master/API_GUIDE.md ) and the TPU colab examples ( https://github.com/pytorch/xla/tree/master/contrib/colab ) each parallelize their data, either using a `torch_xla.distributed.parallel_loader.c` object or a `torch_xla.distributed.data_parallel.DataParallel` object (which uses `ParallelLoader`).
The `run_glue.py` example (https://github.com/huggingface/transformers/blob/master/examples/run_glue.py ) doesn't do this. I am wondering if there was a reason not to use xla's data parallelizers. | 10-17-2019 00:38:51 | 10-17-2019 00:38:51 | Indeed, it would be great to improve the current TPU script to include better optimization, such as using the TPU DataParallel from Pytorch. We haven't gotten to it yet and we'll probably do so soon.
We'd be very happy to welcome a PR too! :)<|||||>Sounds good, I'm trying to figure it out. The part I'm stuck on is that the colab TPU examples use a multi-threading approach, but the official API recommends using multi-processing over multi-threading, so I am wonder if the TPUs require a multi-threading approach.
Once I figure this out I think I can update the code. I asked a question on the xla repo about this
https://github.com/pytorch/xla/issues/1217
<|||||>This issue has been automatically marked as stale because it has not had recent activity. It will be closed if no further activity occurs. Thank you for your contributions.
|
transformers | 1,539 | closed | A couple of noob-to-transformers questions | ## ❓ Questions & Help
#### If you don't want to or don't know the answer to all of these, just answer some that you know!
1. How is it that you can provide context to these models? Say, if you want to summarize or pull data from a text. Do you have to train it on that text or just put it somehow in the prompt?
2. Can you train each model? Like, if you wanted to, completely unfreeze and retrain each model?
4. How can I train? I ran into a bunch of issues including [this one](https://github.com/huggingface/transformers/issues/1517) when just running the official sample scripts in Colab.
5. Are certain models better at certain tasks? Which ones are good for what? | 10-17-2019 00:29:34 | 10-17-2019 00:29:34 | This issue has been automatically marked as stale because it has not had recent activity. It will be closed if no further activity occurs. Thank you for your contributions.
<|||||>@GrahamboJangles
1) If you want to provide _context_ into any model offered by Transformers, you can **fine-tune** the model you've chosen with your custom data in such a way that the model can learn the context.
I suggest you to look the _CTRL_ model developed by SalesForce. By using this model, you can pass a parameter called _control_code_ which specify the domain of the generated text by the model itself, for example Fitness, Funny, Diet, etc. [Here](https://github.com/huggingface/transformers/blob/master/transformers/modeling_ctrl.py) you can find the source code of the model implemented in Transformers, and [here](https://arxiv.org/pdf/1909.05858.pdf) you can find the scientific paper about it.
2) **Yes**, you can train the model you've chosen from scratch. You can import it with the pre-trained weights, unfreeze all layers and set random weights and starting to train the model.
3) There are many Python scripts written in TensorFlow 2.0 or PyTorch for fine-tuning the model. The from-scratch training scripts are missing, at the moment (in my best knowledge). You can find maybe some advide on the _Issues_ page.
4) **Yes**, and I think that in the Python scripts in this library they suggest to you to use a particular set of models for a certain task. In more details, they have developed the base model architecture, and they have added some layers on-top for addressing a particular task, e.g. you can use _BertForTokenClassification_, _RobertaForTokenClassification_, _DistilBertForTokenClassification_, _CamembertForTokenClassification_ for token classification. More details [here](https://github.com/huggingface/transformers/blob/master/examples/run_ner.py).
> ## Questions & Help
> #### If you don't want to or don't know the answer to all of these, just answer some that you know!
> 1. How is it that you can provide context to these models? Say, if you want to summarize or pull data from a text. Do you have to train it on that text or just put it somehow in the prompt?
> 2. Can you train each model? Like, if you wanted to, completely unfreeze and retrain each model?
> 3. How can I train? I ran into a bunch of issues including [this one](https://github.com/huggingface/transformers/issues/1517) when just running the official sample scripts in Colab.
> 4. Are certain models better at certain tasks? Which ones are good for what?<|||||>This issue has been automatically marked as stale because it has not had recent activity. It will be closed if no further activity occurs. Thank you for your contributions.
|
transformers | 1,538 | closed | Fine-tune RoBERTa on WikiText-2 | ## ❓ Questions & Help
I am trying to train Roberta using the run_lm_finetuning.py script and TRAIN_FILE=wiki.train.raw, TEST_FILE=wiki.test.raw, basically, I use the demo data (wikiText-2) as described at https://huggingface.co/transformers/examples.html
CUDA_LAUNCH_BLOCKING=1 python run_lm_finetuning.py \
--output_dir=output \
--model_type=roberta \
--model_name_or_path=roberta-base \
--do_train \
--train_data_file=$TRAIN_FILE \
--do_eval \
--eval_data_file=$TEST_FILE \
--mlm
/pytorch/aten/src/THC/THCTensorIndex.cu:361: void indexSelectLargeIndex(TensorInfo<T, IndexType>, TensorInfo<T, IndexType>, TensorInfo<long, IndexType>, int, int, IndexType, IndexType, long) [with T = float, IndexType = unsigned int, DstDim = 2, SrcDim = 2, IdxDim = -2, IndexIsMajor = true]: block: [386,0,0], thread: [63,0,0] Assertion `srcIndex < srcSelectDimSize` failed.
Debugging, I saw it fails to get an embedding that exceeds the max size, but I am not sure in which module to correct. Also, I assume this should have run correctly given that it is the dataset used in the example at https://huggingface.co/transformers/examples.html.
Any help is greatly appreciated.
Thanks.
| 10-16-2019 22:52:42 | 10-16-2019 22:52:42 | Hi, could you give us a bit more information? For example, you seem to be running this on a GPU, are you running on a distributed setting? Could you list your software versions (python, torch, transformers)?<|||||>Thank you for your response. I am running on a single machine with one gpu,
Python 3.6.8, pytorch_transformers 1.2.0 (from setup.py), torch>=1.0.0
(from requirements.txt). Linux 4.15.0-1044-gcp, NVIDIA-SMI 418.40.04,
Driver Version: 418.40.04, CUDA Version: 10.1. Thank you for your help.
On Thu, Oct 17, 2019 at 11:26 AM Lysandre Debut <[email protected]>
wrote:
> Hi, could you give us a bit more information? For example, you seem to be
> running this on a GPU, are you running on a distributed setting? Could you
> list your software versions (python, torch, transformers)?
>
> —
> You are receiving this because you authored the thread.
> Reply to this email directly, view it on GitHub
> <https://github.com/huggingface/transformers/issues/1538?email_source=notifications&email_token=ANQITTRRWMQRRYU7CBCTFXLQPCU6ZA5CNFSM4JBR7JA2YY3PNVWWK3TUL52HS4DFVREXG43VMVBW63LNMVXHJKTDN5WW2ZLOORPWSZGOEBRCGXQ#issuecomment-543302494>,
> or unsubscribe
> <https://github.com/notifications/unsubscribe-auth/ANQITTTS3UTD4XDKO5MGVTTQPCU6ZANCNFSM4JBR7JAQ>
> .
>
<|||||>Does the error still happen if you remove `CUDA_LAUNCH_BLOCKING=1` ?<|||||>yes, the error happens at
File "run_lm_finetuning.py", line 472, in main
global_step, tr_loss = train(args, train_dataset, model, tokenizer)
File "run_lm_finetuning.py", line 209, in train
outputs = model(inputs, masked_lm_labels=labels) if args.mlm else
model(inputs, labels=labels)
.......................(other info) .......
result = self.forward(*input, **kwargs)
output = input.matmul(weight.t())
RuntimeError: cublas runtime error : resource allocation failed at
/pytorch/aten/src/THC/THCGeneral.cpp:216
Epoch: 0%|
| 0/1 [00:00<?, ?it/s]
Iteration: 0%|
Thank you.
On Thu, Oct 17, 2019 at 1:46 PM Lysandre Debut <[email protected]>
wrote:
> Does the error still happen if you remove CUDA_LAUNCH_BLOCKING=1 ?
>
> —
> You are receiving this because you authored the thread.
> Reply to this email directly, view it on GitHub
> <https://github.com/huggingface/transformers/issues/1538?email_source=notifications&email_token=ANQITTS7JZTEFDMUUEHJ3NDQPDFJTA5CNFSM4JBR7JA2YY3PNVWWK3TUL52HS4DFVREXG43VMVBW63LNMVXHJKTDN5WW2ZLOORPWSZGOEBRO53Q#issuecomment-543354606>,
> or unsubscribe
> <https://github.com/notifications/unsubscribe-auth/ANQITTWFFITTOT7DILCJQMDQPDFJTANCNFSM4JBR7JAQ>
> .
>
<|||||>I have also noticed this issue when trying to fine-tune a RoBERTa language model.
Part of the issue appears to be in the the calculation of the maximum sequence length in `run_lm_finetuning.py`
```
if args.block_size <= 0:
args.block_size = tokenizer.max_len_single_sentence # Our input block size will be the max possible for the model
```
This produces a cached file like this: `cached_lm_999999999998_wiki.train.raw`
Manually checking shows that it is indeed setting the `args.block_size` parameter to 999999999998
Adding the `--block-size = 512` argument prevents this, but then leads to a similar index error to the one @estoica111 is experiencing.
Strangely, if I reduce to `--block-size = 500`, the model trains successfully, but the reported perplexity on the test set seems far too low:
```
10/18/2019 15:35:44 - INFO - __main__ - Saving features into cached file ~/wikitext-2-raw/cached_lm_500_wiki.test.raw
10/18/2019 15:35:44 - INFO - __main__ - ***** Running evaluation *****
10/18/2019 15:35:44 - INFO - __main__ - Num examples = 572
10/18/2019 15:35:44 - INFO - __main__ - Batch size = 32
Evaluating: 100%|█████████████████████████████████████████████████████████████████████████████████████████████████████████████████████████████████████████████████████████████████████████| 18/18 [00:08<00:00, 2.14it/s]
10/18/2019 15:35:53 - INFO - __main__ - ***** Eval results *****
10/18/2019 15:35:53 - INFO - __main__ - perplexity = tensor(1.0631)
```
**Update:** I get the exact same perplexity (1.0631) even with the standard pre-trained RoBERTa model on wikitext-2-raw test set. Very confused.<|||||>I'm having a hard time replicating this error in transformers 2.1.1. Would it be possible for you to try this on the latest version and let me know your results?
I get a 1.03 perplexity fine-tuning on `wiki.train.raw` and evaluating on `wiki.test.raw`, vs 1.45 without fine-tuning.<|||||>@LysandreJik, I was on 2.1.1, but just in case I did a full-reinstall of the environment from master and that seems to have fixed the perplexity issue (now getting 1.03 - 1.06 after finetuning in `wiki.train.raw`.
However, the default behavior for block_size still does not work with the provided example. I have to set `block_size 500`, or I get the errors I described above. `block_size 512` also still produces a similar error to @estoica111 .
```
/pytorch/aten/src/THC/THCTensorIndex.cu:361: void indexSelectLargeIndex(TensorInfo<T, IndexType>, TensorInfo<T, IndexType>, TensorInfo<long, IndexType>, int, int, IndexType, IndexType, long) [with T = float, IndexType = unsigned int, DstDim = 2, SrcDim = 2, IdxDim = -2, IndexIsMajor = true]: block: [171,0,0], thread: [127,0,0] Assertion `srcIndex < srcSelectDimSize` failed.
Evaluating: 0%| | 0/18 [00:00<?, ?it/s]
Traceback (most recent call last):
File "run_lm_finetuning.py", line 543, in <module>
main()
File "run_lm_finetuning.py", line 535, in main
result = evaluate(args, model, tokenizer, prefix=prefix)
File "run_lm_finetuning.py", line 315, in evaluate
outputs = model(batch, masked_lm_labels=batch) if args.mlm else model(batch, labels=batch)
File "/home/dscripka/software/venv_transformers/lib/python3.6/site-packages/torch/nn/modules/module.py", line 547, in __call__
result = self.forward(*input, **kwargs)
File "/home/dscripka/software/transformers/transformers/modeling_roberta.py", line 242, in forward
head_mask=head_mask)
File "/home/dscripka/software/venv_transformers/lib/python3.6/site-packages/torch/nn/modules/module.py", line 547, in __call__
result = self.forward(*input, **kwargs)
File "/home/dscripka/software/transformers/transformers/modeling_roberta.py", line 182, in forward
head_mask=head_mask)
File "/home/dscripka/software/transformers/transformers/modeling_bert.py", line 627, in forward
head_mask=head_mask)
File "/home/dscripka/software/venv_transformers/lib/python3.6/site-packages/torch/nn/modules/module.py", line 547, in __call__
result = self.forward(*input, **kwargs)
File "/home/dscripka/software/transformers/transformers/modeling_bert.py", line 348, in forward
layer_outputs = layer_module(hidden_states, attention_mask, head_mask[i])
File "/home/dscripka/software/venv_transformers/lib/python3.6/site-packages/torch/nn/modules/module.py", line 547, in __call__
result = self.forward(*input, **kwargs)
File "/home/dscripka/software/transformers/transformers/modeling_bert.py", line 326, in forward
attention_outputs = self.attention(hidden_states, attention_mask, head_mask)
File "/home/dscripka/software/venv_transformers/lib/python3.6/site-packages/torch/nn/modules/module.py", line 547, in __call__
result = self.forward(*input, **kwargs)
File "/home/dscripka/software/transformers/transformers/modeling_bert.py", line 283, in forward
self_outputs = self.self(input_tensor, attention_mask, head_mask)
File "/home/dscripka/software/venv_transformers/lib/python3.6/site-packages/torch/nn/modules/module.py", line 547, in __call__
result = self.forward(*input, **kwargs)
File "/home/dscripka/software/transformers/transformers/modeling_bert.py", line 202, in forward
mixed_query_layer = self.query(hidden_states)
File "/home/dscripka/software/venv_transformers/lib/python3.6/site-packages/torch/nn/modules/module.py", line 547, in __call__
result = self.forward(*input, **kwargs)
File "/home/dscripka/software/venv_transformers/lib/python3.6/site-packages/torch/nn/modules/linear.py", line 87, in forward
return F.linear(input, self.weight, self.bias)
File "/home/dscripka/software/venv_transformers/lib/python3.6/site-packages/torch/nn/functional.py", line 1371, in linear
output = input.matmul(weight.t())
RuntimeError: cublas runtime error : resource allocation failed at /pytorch/aten/src/THC/THCGeneral.cpp:216
```
Software versions:
Python: 3.6.5
Transformers: 2.1.1 (master)
Cuda: 10.0
Torch: 1.2.0<|||||>Maybe this can be caused by `RuntimeError: index out of range: Tried to access index 512 out of table with 511 rows`. I got the same error on cuda. But trying to compute a single iteration on CPU, I get more clear error description: `RuntimeError: index out of range: Tried to access index 512 out of table with 511 rows`.<|||||>@LysandreJik I am trying to fine-tune roberta following in the examples for using run_lm_finetuning.py. The only change I am making is using gradient accumulation as 2 and a gpu batch size of 2 as I was running into cuda memory issues. I am using the raw wiki data from the link provided.
I did a fresh install and have these on aws:
Python: 3.6.5
Transformers: 2.1.1 (master)
Cuda: 10.0
Torch: 1.2.0
1 V100 GPU
After fine-tuning on roberta-large I am getting a perplexity of 2.88 and when I do it on roberta-base I am getting a perplexity of 3.4.
Do you have any ideas on what I might be doing wrong or my setup or possible solutions?<|||||>> /pytorch/aten/src/THC/THCTensorIndex.cu:361: void indexSelectLargeIndex(TensorInfo<T, IndexType>, TensorInfo<T, IndexType>, TensorInfo<long, IndexType>, int, int, IndexType, IndexType, long) [with T = float, IndexType = unsigned int, DstDim = 2, SrcDim = 2, IdxDim = -2, IndexIsMajor = true]: block: [386,0,0], thread: [63,0,0] Assertion `srcIndex < srcSelectDimSize` failed.
>
> Debugging, I saw it fails to get an embedding that exceeds the max size, but I am not sure in which module to correct. Also, I assume this should have run correctly given that it is the dataset used in the example at https://huggingface.co/transformers/examples.html.
> Any help is greatly appreciated.
> Thanks.
I encountered essentially the same error when using RoBERTa for SQuAD.
What I found was that the Tokenizer.encode_plus() generates a token_type_ids vector that contains 1s and 0s when two sequences are fed in (question and passage tokens in the case of SQuAD).
The RobertaModel tries to look up these indices in RobertaModel.embeddings.token_type_embeddings. However, the size of the token_type_embeddings is [1,768] and so the error that started this issue arises when it tries to look up the index 1.
I think one solution would be to set token_type_ids to None in the forward method of RobertaModel<|||||>Also having this issue training RoBERTa on MNLI. Similar to @brandenchan's observations, if I set the `token_type_ids` to all 0, then I don't have a a problem, but if I use `encode_plus` to generate the segment ids, then it triggers that error.
Additionally, it seems like `RobertaConfig` sets `type_vocab_size=2`, which seems like it should handle multiple segment ids? But the segment embeddings (currently) only have space for 1.<|||||>> Maybe this can be caused by `RuntimeError: index out of range: Tried to access index 512 out of table with 511 rows`. I got the same error on cuda. But trying to compute a single iteration on CPU, I get more clear error description: `RuntimeError: index out of range: Tried to access index 512 out of table with 511 rows`.
This is pretty weird as I was getting the same error when running the bert_lm_finetuning, I guess it's because the sentence's length is greater than 512, but as in the script TextDataset truncation is done via the parameter block_size, so this isn't supposed to appear... I set block_size<511(510,500...) and the error's gone.<|||||>From what read in [this thread](https://github.com/huggingface/transformers/issues/1234), it seems the cause for the issue @shreydesai points to is the absence of pre-trained token_type_id beyond a single [1, 768] parameter (explains why passing 0 doesn't trigger index out of range). The thread above offers a hack to get around this (i.e. modifying this parameter ad hoc) if multi-segment inputs are a must (which _is_ the case in my task).
To make this more useful, the hack snippet is (credit: [Colanim](https://github.com/Colanim))
```
model = RobertaModel.from_pretrained('roberta-base')
model.config.type_vocab_size = 2
single_emb = model.embeddings.token_type_embeddings
model.embeddings.token_type_embeddings = torch.nn.Embedding(2, single_emb.embedding_dim)
model.embeddings.token_type_embeddings.weight = torch.nn.Parameter(single_emb.weight.repeat([2, 1]))
```
If a headed model wrapper is used (e.g. RobertaForSequenceClassification), add .roberta after model to modify the RobertaModel object in the wrapper.
Having experimented in my classifier, I can contribute one evidence point that it doesn't break anything and works as intended.<|||||>In my case (using ver 2.3), [this hard coded padding_idx](https://github.com/huggingface/transformers/blob/a436574bfde4f75f518a107f45f987579d813ce5/transformers/modeling_roberta.py#L48) caused the problem.
If `position_ids=None ^ seq_length = 512`, the max value of position_ids exceeds 511 [here](https://github.com/huggingface/transformers/blob/a436574bfde4f75f518a107f45f987579d813ce5/transformers/modeling_roberta.py#L62-L66), which is the largest index the embedding matrix can use.
The code in the latest version is different from the one above, but **setting position_ids manually** fixed the problem for me.<|||||>This issue has been automatically marked as stale because it has not had recent activity. It will be closed if no further activity occurs. Thank you for your contributions.
|
transformers | 1,537 | closed | Behavior of Masked-LM BERT, dependence on masked token | I am experimenting with the masked-LM for BERT to understand how the masking affects predictions of the other tokens.
Of course using no [MASK] is not the intended usage, nor is it to predict each token in the sentence. But my understanding is that the LM head is a separate softmax classifier, taking the final embeddings of BERT for the whole sequence as an input. Therefore the model outputs predictions for all tokens, including the masked token.
I would have expected, that when no token is masked, the prediction should be pretty much perfect. The embedding of any word is then a function of both context and its own position-adjusted initial encoding.
If a token is masked, however, BERT essentially needs to predict it from context and position.
Interestingly, I have run across a sentence
(Donald Trump is the president of the United States), where the first word is not predicted whenever no [MASK] token is set, but is predicted correctly if a later token is masked.
Consider the sequence without any masking
`['[CLS]', 'donald', 'trump', 'is', 'the', 'president', 'of', 'the', 'united', 'states', '[SEP]']`
The output of the masked LM model is
`['.', '.', 'trump', 'is', 'the', 'president', 'of', 'the', 'united', 'states', '.']`
where the first token is missing
If we input the sequence
`['[CLS]', 'donald', 'trump', 'is', 'the', '[MASK]', 'of', 'the', 'united', 'states', '[SEP]']`
Then the output is correctly
`['.', 'donald', 'trump', 'is', 'the', 'president', 'of', 'the', 'united', 'states', '.']`
But this behavior also occurs with masking: further experimentation shows that the position of the [MASK] token determines whether the sentence is correctly predicted. If the [MASK] is early in the sequence (position 2-4 in this case), the first word is mispredicted. If it is later, after position 4, then the first token is predicted correctly.
There are other sentences, where this "problem" does not occur (for example if the sentence starts with "the").
I am trying to understand this behavior. Why does BERT fail prediction of the first non-masked token in some cases, in particular, when no token is masked and the model should have "full information"?
Am I misunderstanding the model or the implementation?
Attached is a small example based on the github readme that replicates this behavior
[lm_test.py.txt](https://github.com/huggingface/transformers/files/3735740/lm_test.py.txt)
Edit: In case you are wondering why the heck I would want to do this. I am working with a model that uses (part of the) logits from the LM head repeatedly for different positions. The corpus is fixed. So the correct way would be to run the LM each time, but if I could run BERT instead once for every sequence in the corpus and save the relevant predictions, it would save a lot of time.
| 10-16-2019 17:56:06 | 10-16-2019 17:56:06 | Yes, this is nice illustration of the discrepancy between Bert's training (in which masked tokens are provided) and Bert's testing (in which no masked token is provided).<|||||>I've noticed that this also frequently occurs when the last token in the sentence is masked.
For example,
`['[CLS]', 'donald', 'trump', 'is', 'the', 'president', 'of', 'the', 'united', '[MASK]', '[SEP]']`
is predicted as
`['.', 'donald', 'trump', 'is', 'the', 'president', 'of', 'the', 'united', '.', '.']`
But if we mask a middle token, anything besides the last, then it works well:
`['[CLS]', 'donald', 'trump', 'is', 'the', 'president', 'of', 'the', '[MASK]', 'states', '[SEP]']`
is predicted as
`['.', 'donald', 'trump', 'is', 'the', 'president', 'of', 'the', 'united', 'states', '.']`
@thomwolf Any insight on why this is the case?
<|||||>We are also interested to work with the prediction of each token (not just the masked ones) and are wondering what is happening there.
As you closed this issue, @IngoMarquart , have you found an explanation, or some link, which sheds more light on this?<|||||>Hey guys,
I would like to investigate how good the top k predictions are. But I don't know how you can generate more than one prediction.
Can anyone help with this?
|
transformers | 1,536 | closed | Penalize high confident false negative classifications? | ## ❓ Questions & Help
I added the line `logits = torch.nn.functional.softmax(logits)` to convert binary classifications to a confidence score between 0.0 - 1.0. However, the predictions are very harsh being really close to either 0.0 or 1.0 and not somewhere in between. Is there a way to penalize the model from being so categorical? I especially want to minimize high confidence scores on false negatives. | 10-16-2019 12:37:36 | 10-16-2019 12:37:36 | The softmax function specifically uses exponentiation to exacerbate the differences in scores (to get the soft 'max'). You can normalize scores by other means than a softmax.
Related to your title: using a log loss will penalize wrong predictions with high confidence more (e.g. BCE).<|||||>Related read is the section entitled "Don’t Mistake Class Probabilities for Confidence" here: https://www.inovex.de/blog/uncertainty-quantification-deep-learning/<|||||>This issue has been automatically marked as stale because it has not had recent activity. It will be closed if no further activity occurs. Thank you for your contributions.
|
transformers | 1,535 | closed | Why the output of DistilBertModel is inconsistent with BertModel?! | [The output of DistilBertModel](https://github.com/huggingface/transformers/blob/be916cb3fb4579e278ceeaec11a6524662797d7f/transformers/modeling_distilbert.py#L468) does not contain [pooled_output as available in BERT model](https://github.com/huggingface/transformers/blob/be916cb3fb4579e278ceeaec11a6524662797d7f/transformers/modeling_bert.py#L632).
I'm going to replace BERT with DistilBert in a classification task, if pooled_output is not a proper way in DistillBertModel? -Currently, I'm using pooled_output of BERT in my experiments. | 10-16-2019 12:30:57 | 10-16-2019 12:30:57 | Hello @amirj,
The "pooled_output" is the hidden state of the `[CLS]`. It is this hidden state that is used for classification tasks for instance (see DistilBertForSequenceClassification). So you could retrieve it by filtering out `hidden_states `.
The reason why there is no linear transformation in the pooler of DistilBERT is because I'm removing the next sentence prediction (see RoBERTa which also do the same). However, this linear transformation is still in `DistilBertForSequenceClassification` so that the classification heads for DistilBERT and BERT have the same number of parameters.
I hope it answers your question.
Victor<|||||>Hello @VictorSanh,
DistilBertForSequenceClassification is the rescue.
Thanks.
Amir<|||||>def forward(self, input_ids, attention_mask, labels=None):
output = self.bert(input_ids, attention_mask = attention_mask)
output = self.classifier(output.hidden_states)
output = torch.sigmoid(output)
loss = 0
if labels is not None:
loss = self.criterion(output, labels)
return loss, output
for BERT model self.classifier will take output.pooler_output as its input
But for DistilBERT this doesnt happen.
I used "DistilBertForSequenceClassification " and replaced pooler_output with hidden_states, still it doesnt work
Error I get is: linear(): argument 'input' (position 1) must be Tensor, not NoneType |
transformers | 1,534 | closed | run_ner.py file with Distill Bert | ## ❓ Questions & Help
<!-- A clear and concise description of the question. -->
I wish to use the Distill Bert model for NER. I am not sure if it will work with it directly. Any suggestions on that end would be great.
Also, what values should the parameters **--model_type** and **--model_name_or_path** take for Distill Bert?
Other parameters per my understanding would be the same. | 10-16-2019 11:45:53 | 10-16-2019 11:45:53 | I have the same issue. Did you end up using Distilbert?<|||||>Not sure how well it will perform. Casing is an important feature used in many NER tasks. So I would say it _could_ work, but ymmv. For reference: https://stackoverflow.com/questions/56384231/case-sensitive-entity-recognition<|||||>RoBERTa is cased so you guys can try using DistilRoBERTa, released today by @VictorSanh:
```
--model_name_or_path distilroberta-base
```
You'll probably need to adapt run_ner.py (PR welcome)<|||||>Actually working on that today so I'll let you know how it goes. <|||||>This is actually really cool. I was looking today at the models and had no idea DistilRoBERTa was released today. Awesome @VictorSanh!<|||||>@amankedia I think this issue is resolved? If so we can resolve it :)<|||||>Solved indeed. Thanks everyone for contributing! |
transformers | 1,533 | closed | Add vocabulary gives sequence length warning | ## ❓ Questions & Help
I'm trying to add extra vocabulary to RoBERTa using the `tokenizer.add_tokens()` function. However, when training I get the following warning message:
`WARNING - transformers.tokenization_utils - Token indices sequence length is longer than the specified maximum sequence length for this model (751 > 512). Running this sequence through the model will result in indexing errors`
What's going on here? Should I be concerned about this or should I ignore it? The function that calls this error is `tokenizer.convert_tokens_to_ids()`. | 10-16-2019 11:34:30 | 10-16-2019 11:34:30 | Hi, this warning means that the sequence you have encoded is longer than the maximum sequence length the model can handle. It isn't related to the tokens you have added.
RoBERTa can only handle sequences of a maximum of 512 tokens, so you should make sure you only pass sequences of a max length of 512 or else it will crash. You can truncate your sequence so that it fits, or you can use another model that can accept longer sequences.<|||||>> Hi, this warning means that the sequence you have encoded is longer than the maximum sequence length the model can handle. It isn't related to the tokens you have added.
>
> RoBERTa can only handle sequences of a maximum of 512 tokens, so you should make sure you only pass sequences of a max length of 512 or else it will crash. You can truncate your sequence so that it fits, or you can use another model that can accept longer sequences.
Thanks! My bad, completely misunderstood the warning, all fixed. However, my problem now seems to be that the function `tokenizer.encode_plus()` used in `glue_convert_examples_to_features()` gets exponentially slower the more words I add to the tokenizer's vocabulary.
For example, starting with a tokenizer vocabulary size of 50265, the `tokenizer.encode_plus()` takes ~0.00048 sec per call. If I add 1200 more words, giving me a tokenizer vocabulary size of 51465, the `tokenizer.encode_plus()` now takes ~0.05729 sec per call, which is ~120x slower. It gets even worse the more words I add, causing me waiting times up to 1h just to pre-process the dataset. What causes this exponential (or extreme linear) growth to happen? Is it possible to optimize it?<|||||>> > Hi, this warning means that the sequence you have encoded is longer than the maximum sequence length the model can handle. It isn't related to the tokens you have added.
> > RoBERTa can only handle sequences of a maximum of 512 tokens, so you should make sure you only pass sequences of a max length of 512 or else it will crash. You can truncate your sequence so that it fits, or you can use another model that can accept longer sequences.
>
> Thanks! My bad, completely misunderstood the warning, all fixed. However, my problem now seems to be that the function `tokenizer.encode_plus()` used in `glue_convert_examples_to_features()` gets exponentially slower the more words I add to the tokenizer's vocabulary.
>
> For example, starting with a tokenizer vocabulary size of 50265, the `tokenizer.encode_plus()` takes ~0.00048 sec per call. If I add 1200 more words, giving me a tokenizer vocabulary size of 51465, the `tokenizer.encode_plus()` now takes ~0.05729 sec per call, which is ~120x slower. It gets even worse the more words I add, causing me waiting times up to 1h just to pre-process the dataset. What causes this exponential (or extreme linear) growth to happen? Is it possible to optimize it?
Please see https://github.com/huggingface/transformers/issues/1830 , https://github.com/huggingface/transformers/issues/1621 and https://github.com/huggingface/transformers/pull/1881<|||||>This issue has been automatically marked as stale because it has not had recent activity. It will be closed if no further activity occurs. Thank you for your contributions.
|
transformers | 1,532 | closed | 'BertForSequenceClassification' is not defined 'DUMMY_INPUTS' is not defined | transformers-2.1.1
https://github.com/huggingface/transformers#quick-tour-tf-20-training-and-pytorch-interoperability
i just copied and paste and run the code.
it showed
```
NameError: name 'BertForSequenceClassification' is not defined
```
i can't even
```
from transformers import BertForSequenceClassification
```
```
---------------------------------------------------------------------------
ImportError Traceback (most recent call last)
<ipython-input-27-7a027f32a339> in <module>
----> 1 from transformers import BertForSequenceClassification
ImportError: cannot import name 'BertForSequenceClassification' from 'transformers'
``` | 10-16-2019 08:53:40 | 10-16-2019 08:53:40 | next time i
```
import torch
```
it showed
```
---------------------------------------------------------------------------
NameError Traceback (most recent call last)
<ipython-input-14-71a5c3f94250> in <module>
----> 1 pytorch_model = BertForSequenceClassification.from_pretrained('model', from_tf=True)
~/miniconda3/envs/tfenv2/lib/python3.7/site-packages/transformers/modeling_utils.py in from_pretrained(cls, pretrained_model_name_or_path, *model_args, **kwargs)
357 try:
358 from transformers import load_tf2_checkpoint_in_pytorch_model
--> 359 model = load_tf2_checkpoint_in_pytorch_model(model, resolved_archive_file, allow_missing_keys=True)
360 except ImportError as e:
361 logger.error("Loading a TensorFlow model in PyTorch, requires both PyTorch and TensorFlow to be installed. Please see "
~/miniconda3/envs/tfenv2/lib/python3.7/site-packages/transformers/modeling_tf_pytorch_utils.py in load_tf2_checkpoint_in_pytorch_model(pt_model, tf_checkpoint_path, tf_inputs, allow_missing_keys)
199
200 if tf_inputs is None:
--> 201 tf_inputs = tf.constant(DUMMY_INPUTS)
202
203 if tf_inputs is not None:
NameError: name 'DUMMY_INPUTS' is not defined
```<|||||>That's right, you can't import the PyTorch models if you don't have PyTorch installed in your environment. The `DUMMY_INPUTS` is a bug that was fixed with #1509. Could you please install it from source and let me know if you still have the error?<|||||>> Indeed, you can't import the PyTorch models if you don't have PyTorch installed in your environment. The `DUMMY_INPUTS` indeed is a bug that was fixed with #1509. Could you please install it from source and let me know if you still have the error?
does```pip install https://github.com/huggingface/transformers``` mean install from source?<|||||>I believe the correct way would be to specify it is a git url:
`pip install git+https://github.com/huggingface/transformers.git`<|||||>> I believe the correct way would be to specify it is a git url:
>
> `pip install git+https://github.com/huggingface/transformers.git`
it worked, but another issues showed up
```
---------------------------------------------------------------------------
TypeError Traceback (most recent call last)
<ipython-input-16-5f3cd63765b9> in <module>
6 inputs_2 = tokenizer.encode_plus(sentence_0, sentence_2, add_special_tokens=True, return_tensors='pt')
7
----> 8 pred_1 = pytorch_model(**inputs_1)[0].argmax().item()
9 pred_2 = pytorch_model(**inputs_2)[0].argmax().item()
10 print("sentence_1 is", "a paraphrase" if pred_1 else "not a paraphrase", "of sentence_0")
~/miniconda3/envs/tfenv2/lib/python3.7/site-packages/torch/nn/modules/module.py in __call__(self, *input, **kwargs)
539 result = self._slow_forward(*input, **kwargs)
540 else:
--> 541 result = self.forward(*input, **kwargs)
542 for hook in self._forward_hooks.values():
543 hook_result = hook(self, input, result)
TypeError: forward() got an unexpected keyword argument 'special_tokens_mask'
```<|||||>Indeed, this is a bug, it seems the readme is not up-to-date since we added the `special_tokens_mask` in 2.1. Thank you for reporting it!
If you add the two lines mentioned below, it should work:
```py
inputs_1 = tokenizer.encode_plus(sentence_0, sentence_1, add_special_tokens=True, return_tensors='pt')
inputs_2 = tokenizer.encode_plus(sentence_0, sentence_2, add_special_tokens=True, return_tensors='pt')
del inputs_1["special_tokens_mask"] # <---- add this
del inputs_2["special_tokens_mask"] # <---- add this
pred_1 = pytorch_model(**inputs_1)[0].argmax().item()
pred_2 = pytorch_model(**inputs_2)[0].argmax().item()
print("sentence_1 is", "a paraphrase" if pred_1 else "not a paraphrase", "of sentence_0")
print("sentence_2 is", "a paraphrase" if pred_2 else "not a paraphrase", "of sentence_0")
```<|||||>> Indeed, this is a bug, it seems the readme is not up-to-date since we added the `special_tokens_mask` in 2.1. Thank you for reporting it!
>
> If you add the two lines mentioned below, it should work:
>
> ```python
> inputs_1 = tokenizer.encode_plus(sentence_0, sentence_1, add_special_tokens=True, return_tensors='pt')
> inputs_2 = tokenizer.encode_plus(sentence_0, sentence_2, add_special_tokens=True, return_tensors='pt')
>
> del inputs_1["special_tokens_mask"] # <---- add this
> del inputs_2["special_tokens_mask"] # <---- add this
>
> pred_1 = pytorch_model(**inputs_1)[0].argmax().item()
> pred_2 = pytorch_model(**inputs_2)[0].argmax().item()
> print("sentence_1 is", "a paraphrase" if pred_1 else "not a paraphrase", "of sentence_0")
> print("sentence_2 is", "a paraphrase" if pred_2 else "not a paraphrase", "of sentence_0")
> ```
thanks it worked!!<|||||>We updated the README accordingly, feel free to open other issues if you encounter other bugs.<|||||>@LysandreJik
i got a similar error when i run `run_tf_glue.py`.
```
...
Dataset glue downloaded and prepared to /root/tensorflow_datasets/glue/mrpc/0.0.2. Subsequent calls will reuse this data.
INFO:absl:Constructing tf.data.Dataset for split None, from /root/tensorflow_datasets/glue/mrpc/0.0.2
Train for 114 steps, validate for 6 steps
Epoch 1/2
114/114 [==============================] - 69s 601ms/step - loss: 0.5447 - accuracy: 0.7314 - val_loss: 0.4515 - val_accuracy: 0.7943
Epoch 2/2
114/114 [==============================] - 35s 306ms/step - loss: 0.2919 - accuracy: 0.8872 - val_loss: 0.4064 - val_accuracy: 0.8542
Traceback (most recent call last):
File "run_tf_glue.py", line 51, in <module>
pytorch_model = BertForSequenceClassification.from_pretrained('./save/', from_tf=True)
File "/usr/local/lib/python3.6/dist-packages/transformers/modeling_utils.py", line 359, in from_pretrained
model = load_tf2_checkpoint_in_pytorch_model(model, resolved_archive_file, allow_missing_keys=True)
File "/usr/local/lib/python3.6/dist-packages/transformers/modeling_tf_pytorch_utils.py", line 201, in load_tf2_checkpoint_in_pytorch_model
tf_inputs = tf.constant(DUMMY_INPUTS)
NameError: name 'DUMMY_INPUTS' is not defined
```
tf version : 2.0 ( via pip )
torch version : 1.2.0 (via pip )<|||||>Have you tried installing from source, as was mentioned in the comment before yourts? `pip install git+https://github.com/huggingface/transformers.git`<|||||>@LysandreJik
i got another error ;;
```
$ pip3 install git+https://github.com/huggingface/transformers.git --upgrade
$ python run_tf_glue.py
...
Train for 114 steps, validate for 6 steps
Epoch 1/2
114/114 [==============================] - 60s 525ms/step - loss: 0.5817 - accuracy: 0.6911 - val_loss: 0.3961 - val_accuracy: 0.8229
Epoch 2/2
114/114 [==============================] - 34s 300ms/step - loss: 0.3505 - accuracy: 0.8460 - val_loss: 0.3403 - val_accuracy: 0.8516
Traceback (most recent call last):
File "run_tf_glue.py", line 60, in <module>
pred_1 = pytorch_model(**inputs_1)[0].argmax().item()
File "/usr/local/lib/python3.6/dist-packages/torch/nn/modules/module.py", line 547, in __call__
result = self.forward(*input, **kwargs)
TypeError: forward() got an unexpected keyword argument 'special_tokens_mask'
```<|||||>try importing
from transformers.modeling_tf_bert import TFBertForSequenceClassification
<|||||>> try importing
>
> from transformers.modeling_tf_bert import TFBertForSequenceClassification
It worked! thanks.<|||||>Has transformers.modeling_tf_bert been changed? I tried it and got:
> ModuleNotFoundError: No module named 'transformers.modeling_tf_bert'
even though I've successfully imported transformers
what is the proper call to import BertForSequenceClassification?<|||||>To import `BertForSequenceClassification` (you need to have PyTorch installed),
```py
from transformers import BertForSequenceClassification
```
To import `TFBertForSequenceClassification` (you need to have TensorFlow installed):
```py
from transformers import TFBertForSequenceClassification
``` |
transformers | 1,531 | closed | why xlnet requires a long prompt for short inputs while Bert does not ? | hey guys,
Q1)
can someone give some more insight what @thomwolf explaining about?
'''
#846
The main reason you get bad performance is that XLNet is not good on short inputs (comes from the way it is pretrained, always having a long memory and only guessing a few words in the sequence).
The run_generation example here will show you how to get better performances by adding a random text as initiator.
Aman Rusia also wrote a blog post about that here. We are using his solution in the run_generation example.
'''
I can't understand the difference the way both Bert and XLnetLM works for LMhead task.
Aren't both model having disadvantages if they have short sentence?
It seems he said XLnet has huge disadvantage on short input sentence
while Bert does not(or has less disadvantage). Any detail explanation could be useful !
Q2)
Also, I can't get the point of adding extra padding or adding random padding things to improve XLnetLMHead model. Any snippet or explanation could be appreciated too...(saw the link but could not fully understood). I experimented by just adding extra strings of line:'I believe my sister is because she is a blonde ' + ' ' and it gives much better result than not having at the end....
Q3)
#846 (comment)
Lastly, why do we have better result when we don't use perm_mask ? above link response shows that
not having perm_mask option does give at least better result...But isn't perm_mask supposed to help to get better prediction and what author of paper used for SOTA ?
isn't perm_mask allow model to not seeing the next tokens in the given input while can see the previous tokens? According to the paper and the original code, I could see that if permute order is 3->4->1->2, mask=1,3, then model cannot see masked<1> when it tried to predict masked<3> but the reverse is possible.
Many thanks in advance ! | 10-16-2019 06:05:51 | 10-16-2019 06:05:51 | This issue has been automatically marked as stale because it has not had recent activity. It will be closed if no further activity occurs. Thank you for your contributions.
|
transformers | 1,530 | closed | Plan to support UniLM ? | # 🌟New model addition
## Model description
**UniLM** : Pre-trained transformer for sequence to sequence generation.
Paper : https://arxiv.org/pdf/1905.03197.pdf
## Open Source status
* [x] the model implementation is available: **[official Pytorch](https://github.com/microsoft/unilm)**
* [x] the model weights are available: For now only english : [UniLMv1-large-cased](https://github.com/microsoft/unilm#pre-trained-models)
## Additional context
The official implementation is based on a modified version of this repository (version 0.4.0).
That would be nice to have a unified API :)
*Note : They didn't release the code for pretraining yet.* | 10-16-2019 02:57:26 | 10-16-2019 02:57:26 | This is on our mid-term roadmap.
We have a project adding Seq2seq models and UniLM will be part of this project. |
transformers | 1,529 | closed | Hight CPU and low GPU on XLNet | ## 🐛 Bug
I am running Bert, GPT, GPT2, XLNET. I got very high CPU usage (e.g. 16 cores) with XLNet while the others (Bert, GPT, GPT2) dont.
For BERT, GPT, GPT2: CPU 1 cores, 100%GPU
For XLNet: CPU 16 cores, 50 to 60% GPU
Is there any hidden implementation which requires CPU?
<!-- Important information -->
Model I am using (XLNet....): XLNET
Language I am using the model on: English
The problem arise when using:
* [ ] the official example scripts: (give details)
* [ ] my own modified scripts: (give details)
The tasks I am working on is:
* [ ] an official GLUE/SQUaD task: (give the name)
* [ ] my own task or dataset: (give details)
## To Reproduce
Steps to reproduce the behavior:
1.
2.
3.
<!-- If you have a code sample, error messages, stack traces, please provide it here as well. -->
## Expected behavior
<!-- A clear and concise description of what you expected to happen. -->
## Environment
* OS: Ubuntu 18.04
* Python version: 3.6
* PyTorch version: 1.0
* PyTorch Transformers version (or branch): pytorch_transformers 1.2.0
* Using GPU ? RTX 2080 TI
* Distributed of parallel setup ? No
* Any other relevant information:
## Additional context
<!-- Add any other context about the problem here. -->
| 10-16-2019 01:56:28 | 10-16-2019 01:56:28 | I also meet the above problem with XLNet. The GPU usage is very low and unstable, but the CPU usage is very high. The running speed is very low.
Are there any ops running on CPU rather than GPU in your XLNet implementation? How to improve the GPU usage and speed up the running speed ? Thanks!
Environment:
Pytorch: 1.1.0
GPU: V100, 16G
pytorch_transformers: 1.2.0
OS: centos 7.6
Python: 3.6<|||||>Does it occur while training or predicting? Are you sure your gpu is available (to PyTorch or TensorFlow)? What do logs say? <|||||>It is likely that this is caused by the tokenisation rather than the model training. Tokenisation typically happens on the CPU, the token_ids are then transferred to the GPU and from then on out the training happens on the GPU. <|||||>This issue has been automatically marked as stale because it has not had recent activity. It will be closed if no further activity occurs. Thank you for your contributions.
<|||||>The same problem. How to solve it? |
transformers | 1,528 | closed | Question about hidden states in GPT2 | ## ❓ Questions & Help
<!-- A clear and concise description of the question. -->
>tokenizer = GPT2Tokenizer.from_pretrained('gpt2')
>model = GPT2LMHeadModel.from_pretrained('gpt2',output_hidden_states=True)
>model.eval()
>input_ids = torch.tensor(tokenizer.encode("Hello, my dog is cute")).unsqueeze(0) # Batch size 1
>outputs = model(input_ids, labels=input_ids)
>hidden_states = outputs[3]
Here the shape of hidden_states is (13,6,768). I have 2 questions.
1. Which one is the vector for the top layer, hidden_states[0] or hidden_states[12]?
2. Suppose hidden_states[12] is for the top layer, then I extract hidden_states[12][0][0], whose size is 768. Is it the vector for prediction based on the word "hello"? But since I know the next word is ",", why do I need hidden_states[12][0][0]? In my opinion, the shape of hidden_states should be (13,1,768), which is only used for predicting next word after "cute". I'm quite confused of the "6" here.
Please help me with the questions. Thank you in advance! | 10-15-2019 21:57:18 | 10-15-2019 21:57:18 | Hi! The vector of the `hidden_states` is indeed of shape `(13, seq_len, 768)`. The first value (`hidden_states[0]`), of shape `(seq_len, 768)` corresponds to the sum of the word + positional embeddings. The subsequent values are added every time the model goes through an attention layer.
Without taking into account the dropout, you would therefore have:
```
hidden_states[0] | 0 -> word_embeddings(inputs) + positional_embeds(outputs)
hidden_states[1] | 1 -> first_attention_layer(0)
hidden_states[2] | 2 -> second_attention_layer(1)
...
```
If by top layer you mean first attention layer of the model, then it would be `hidden_states[1]`. If by top you mean last, it would be `hidden_states[12]`, which would be the same as `outputs[0] `.
The size of those is of `(13, seq_len, 768)` and not `(13, 1, 768)` because the model computes every token and not only the last token.
<|||||>> The size of those is of `(13, seq_len, 768)` and not `(13, 1, 768)` because the model computes every token and not only the last token.
Hi! Thank you for your reply. I wonder if the states for the previous token will be used for calculating the attention when predicting the later token? Is that the reason that you store the states for the previous tokens?
<|||||>The models keep the key-value pairs so that they're not recomputed on the next model pass. These are stored in the `past`, and can reduce the amount of computing for each following model pass if you pass them to the next forward pass (like we do in run_generation).
The hidden states won't be used for this though, but you can use them to extract intermediate features from the transformer.<|||||>> The models keep the key-value pairs so that they're not recomputed on the next model pass. These are stored in the `past`, and can reduce the amount of computing for each following model pass if you pass them to the next forward pass (like we do in run_generation).
>
> The hidden states won't be used for this though, but you can use them to extract intermediate features from the transformer.
Hi! Thank you for your reply. That really helps.
So now I want to make sure that in the code block in question:
Since hidden_states[12] is for the top layer, then I extract hidden_states[12][0][5], whose size is 768. Is it the vector for prediction based on the word "cute" (and all previous 5 words)?
<|||||>Yes, you're right. You could also retrieve this vector by using a `GPT2Model` instead of a `GPT2LMHeadModel`, which is the base transformer:
```py
from transformers import GPT2Tokenizer, GPT2LMHeadModel, GPT2Model
import torch
tokenizer = GPT2Tokenizer.from_pretrained('gpt2')
lm_model = GPT2LMHeadModel.from_pretrained("gpt2", output_hidden_states=True)
lm_model.eval()
model = GPT2Model.from_pretrained('gpt2')
model.eval()
input_ids = torch.tensor(tokenizer.encode("Hello, my dog is cute")).unsqueeze(0) # Batch size 1
outputs = model(input_ids)
lm_outputs = lm_model(input_ids, labels=input_ids)
transformer_output = outputs[0]
transformer_hidden_states = lm_outputs[3]
print(transformer_hidden_states[12][:, -1, :] - transformer_output[:, -1, :])
```
This should output a tensor of 0s as the two tensors are equal.<|||||>@LysandreJik Thank you so much for your help! That works. |
transformers | 1,527 | closed | Training GPT or GPT-2 from scratch | ## ❓ Questions & Help
<!-- A clear and concise description of the question. -->
I am trying to retrain GPT or GPT-2 from scratch, is there any implementation for this? | 10-15-2019 18:24:10 | 10-15-2019 18:24:10 | I think, just create an instance of the model (without loading from pretrained one), switch it to train mode and run. That's all.<|||||>This issue has been automatically marked as stale because it has not had recent activity. It will be closed if no further activity occurs. Thank you for your contributions.
<|||||>I am currently trying to implement this as well. Once I figured it out i'll let you know ! |
transformers | 1,526 | closed | Alignment of tokens - 'extract_features_aligned_to_words' from fairseq roberta? | ## ❓ Questions & Help
I'm using RoBERTa pretrained model to get embeddings for a dataset. But I want to get the embeddings as per the tokenization which is already present in my dataset. So basically I would want to average the embeddings of a token's BPE if that token in my dataset is getting split into different BPEs. fairseq roberta has a method for this as follows:
```
import torch
from fairseq.models.roberta import alignment_utils
roberta = torch.hub.load('pytorch/fairseq', 'roberta.large')
roberta.eval()
example_string_tokens = ['Dr', 'Greenwalt', 'fixed', 'my', 'neck', 'from', 'a', 'snowboard', 'injury', 'and', 'was', 'way', 'more', 'effective', 'that', 'a', 'regular', 'doctor', '.']
doc = roberta.extract_features_aligned_to_words(" ".join(example_string_tokens))
for tok in doc:
print('{:10}{} (...)'.format(str(tok), tok.vector[:5]))
```
The output is:
```
<s> tensor([-0.0656, 0.0189, -0.0003, -0.0907, 0.0550], grad_fn=<SliceBackward>) (...)
Dr tensor([ 0.2180, -0.0530, -0.3689, -0.0619, -0.6243], grad_fn=<SliceBackward>) (...)
Greenwalt tensor([ 0.3744, 0.0741, -0.7149, 0.0654, -0.1234], grad_fn=<SliceBackward>) (...)
fixed tensor([ 0.2132, 0.0841, -0.2535, -0.1404, -0.0060], grad_fn=<SliceBackward>) (...)
my tensor([ 0.1313, -0.0466, -0.1373, 0.1730, 0.1771], grad_fn=<SliceBackward>) (...)
neck tensor([ 0.0674, -0.3413, -0.0192, 0.0290, -0.3497], grad_fn=<SliceBackward>) (...)
from tensor([-0.0301, -0.3562, -0.3798, 0.0687, 0.0290], grad_fn=<SliceBackward>) (...)
a tensor([-0.2496, -0.1036, 0.0270, -0.0819, -0.2146], grad_fn=<SliceBackward>) (...)
snowboard tensor([ 0.4018, 0.1432, -0.0499, 0.2095, -0.0520], grad_fn=<SliceBackward>) (...)
injury tensor([ 0.0010, -0.6273, -0.0312, -0.1957, -0.4832], grad_fn=<SliceBackward>) (...)
and tensor([ 0.0747, -0.3335, -0.0593, -0.3805, 0.0930], grad_fn=<SliceBackward>) (...)
was tensor([ 0.1501, -0.1334, -0.4789, -0.1974, -0.3096], grad_fn=<SliceBackward>) (...)
way tensor([-0.2803, 0.3204, -0.1663, -0.4420, -0.2641], grad_fn=<SliceBackward>) (...)
more tensor([-0.1037, 0.1878, -0.5839, -0.4437, -0.1683], grad_fn=<SliceBackward>) (...)
effective tensor([-0.1794, 0.2419, -0.3182, -0.2252, -0.1534], grad_fn=<SliceBackward>) (...)
that tensor([-0.1146, -0.1935, -0.3615, -0.4998, -0.1000], grad_fn=<SliceBackward>) (...)
a tensor([-0.2107, -0.2103, -0.1996, 0.0046, -0.1112], grad_fn=<SliceBackward>) (...)
regular tensor([ 0.2236, -0.0613, -0.5496, -0.3562, 0.1022], grad_fn=<SliceBackward>) (...)
doctor tensor([ 0.1275, -0.0589, -0.0283, -0.1557, -0.9282], grad_fn=<SliceBackward>) (...)
. tensor([ 0.1765, 0.0812, -0.1684, -0.2818, 0.0134], grad_fn=<SliceBackward>) (...)
</s> tensor([-0.0409, -0.0024, 0.0107, -0.0183, -0.0479], grad_fn=<SliceBackward>) (...)
```
I want to be able to do something similar with the hugging transformers library, but I can't find any alignment methods.
Any suggestions? | 10-15-2019 16:02:44 | 10-15-2019 16:02:44 | This issue has been automatically marked as stale because it has not had recent activity. It will be closed if no further activity occurs. Thank you for your contributions.
<|||||>It seems this is still unsupported by Huggingface? |
transformers | 1,525 | closed | Understanding run_glue in distributed mode | ## ❓ Questions & Help
In my own project I am building on top of `transformers` and I'd like to take advantage of DDP. For inspiration I've been looking at how different libraries implement that, as well as how `transformers` handles it. In particular, I've been looking at [`run_glue`](https://github.com/huggingface/transformers/blob/master/examples/run_glue.py).
I guess the main issue that I have, is that I don't quite understand how the forward/backward is synced across processes. **Does it mean that before each forward and backward pass the processes are synced, and that the backward pass averages over all gradients for all processes?**
In addition, I am a bit confused about how `run_glue` presents its results. It seems that the logger is only active for the first process
https://github.com/huggingface/transformers/blob/be916cb3fb4579e278ceeaec11a6524662797d7f/examples/run_glue.py#L453-L455
**Does this mean that `tr_loss` in the following fragment is a generalization**, i.e. it's the training loss of only the first process and NOT an average over all processes. It's to give users _some_ idea of the results, but it is not a factual representation of the full training loss, since it only represents the loss of one process?
https://github.com/huggingface/transformers/blob/be916cb3fb4579e278ceeaec11a6524662797d7f/examples/run_glue.py#L493
I noticed that you do the testing with only one device:
https://github.com/huggingface/transformers/blob/be916cb3fb4579e278ceeaec11a6524662797d7f/examples/run_glue.py#L520
**What is the reasoning behind this?** Exactly what I suggested before, namely that you do not get all results back easily when running in distributed mode? In a setting with training, validating, and testing, would you recommend that only training is done distributed, and testing and validating on one device?
Thanks in advance for your time. | 10-15-2019 14:26:41 | 10-15-2019 14:26:41 | To understand how DDP synchronize across processes, you can read:
- the official doc for DDP: https://pytorch.org/docs/stable/nn.html?highlight=distributed%20data%20parallel#torch.nn.parallel.DistributedDataParallel
- this detailed blog post I did a few months ago: https://medium.com/huggingface/training-larger-batches-practical-tips-on-1-gpu-multi-gpu-distributed-setups-ec88c3e51255
Yes, `tr_loss` is only for the first device, this is just an information to follow the training. To get the average loss over all devices, you can add a step of synchronization of losses. It's pretty simple to do, here is an example I wrote this summer for our NAACL tutorial: https://github.com/huggingface/naacl_transfer_learning_tutorial/blob/master/utils.py#L53-L59
Doing evaluation on only one device is better since the total metrics are not always averages of metrics on each node. For instance F1 uses a non linear fonction of the samples and as thus can't be computed in a distributed setting just by averaging F1 on all devices.
As a general note: these points can be improved (and we are aware of that and how to do it). Not including more complexities in examples like `run_glue` is a conscious decision to keep the examples simple to understand (I already think distributed training makes them a bit complex but honestly we can't really do without it on our big models). <|||||>I did indeed read through the official documentation as well as your blog post and other (official and non-official) tutorials. However, many were outdated or didn't go into details about setting up the actual training loop and all the intricacies that are involved. It often seems to be explained as "wow, you can do so much cool stuff with this because it is _D I S T R I B U T E D_", but then details are absolutely lacking and often you're left to figure out how things work by going through source code. I did set up initialisation and training, but then I wasn't sure how to deal with the gathered loss and validating/testing.
That being said, thank you very much for your response, this is very helpful! I had also never heard about ignite. Great. Now I'll have to refactor all my code! (Kidding, even though it might be worth my time to look into it.)
Closing this. Thanks again for the information.<|||||>@thomwolf As a small update: I have decided that I will still use distributed testing and validating. However, the final metric (per epoch for validating) will be calculated on the collected results of all processes. In other words, after a validating iteration where the loss for all steps is saved as a tensor, gather ALL losses, and then average those. That seems like a good compromise to me. Something like this.
```python
def gather_cat(x):
gather = [torch.empty_like(x) for _ in range(dist.get_world_size())]
dist.all_gather(gather, x)
return torch.cat(gather)
# ...
# distributed:
loss = model(...)
loss = gather_cat(loss)
avg_loss = torch.mean(loss)
# ... track avg_loss only in the first process for instance
```
II think this would be especially useful when you have a lot of data and you want your validation and test to run smoothly as well. If anything's wrong with approach, I'd be happy to hear about it. |
transformers | 1,524 | closed | Question on AllenNLP vocabulary and huggingface BERT out of sync | Perhaps this should also be posted in the allennlp repo.
I'm currently trying to use a pretrained model (clinicalBERT) with a different set of vocabulary with huggingface's BertModel. Even though the code runs, I'm not 100% convinced that the vocabulary index to weight mappings are synced between the allennlp vocabulalry object and the BertModel. The tokenizer used for clinicalBERT is scispacy, not wordpiece. First of all, are there any examples in this repo or other places online that does this (has a stack of allennlp vocab + huggingface BERT, and loading a pretrained BERT model with vocab.txt?).
This is what I do:
` model = pytorch_transformers.BertModel.from_pretrained("my/path/to/clinicalBERT", output_hidden_states=True)`
Then, for the vocabulary, I do:
` vocab = Vocabulary(counter=None, max_vocab_size=max_v_sizes) `
` vocab.set_from_file(filename="path/to/clinicalBERT/vocab.txt", is_padded=1, namespace="scispacy")`
I then use resize_tokens on the model, and use a TokenIndexer from allennlp and index my instances with that vocabulary. Even at this point though, ` vocab.get_token_from_index(0, "scispacy")`
returns ` @@PADDING@@` , and ` @@UNKNOWN@@` is at index 101 for the vocab (even though allennlp's vocabulary to my understanding sets ` @@UNKNOWN@@` to 1).
What should I do to realign the Vocabulary back to the vocab index -> weight embedding mapping in the model?
| 10-15-2019 14:08:10 | 10-15-2019 14:08:10 | This issue has been automatically marked as stale because it has not had recent activity. It will be closed if no further activity occurs. Thank you for your contributions.
|
transformers | 1,523 | closed | Why the codes of training BERT from scratch are deprecated | ## ❓ Questions & Help
I'm wondering why the team removed the codes of training BERT from scratch, including pregenerate_training_data.py and finetune_on_pregenerated.py. They're very helpful and I still continue developing them to train the BERT as well as Roberta from scratch. | 10-15-2019 11:30:11 | 10-15-2019 11:30:11 | They were community provided and the core team didn't have the bandwidth to maintain them.
Also we want to limit the number of single-model examples now and favor examples that work for a range of models.
If you want to update them to the current version of the repo and add the various models (for instance all the models currently in `run_lm_finetuning`), happy to review a PR.<|||||>This issue has been automatically marked as stale because it has not had recent activity. It will be closed if no further activity occurs. Thank you for your contributions.
|
transformers | 1,522 | closed | When to support Albert? | ## ❓ Questions & Help
<!-- A clear and concise description of the question. -->
Do you have any plan to support Google's new model-ALBERT? | 10-15-2019 09:21:25 | 10-15-2019 09:21:25 | Please use the search function. There's an open issue about albert here: https://github.com/huggingface/transformers/issues/1370 |
transformers | 1,521 | closed | Downloading model in distributed mode | ## 🐛 Bug
When running in distributed mode with `n` processes, a new model will be download `n` times. I don't think that's what you want. I found [this related issue](https://github.com/huggingface/transformers/issues/44) but that only fixed the race condition. Downloads still happen in parallel. Is there a way to only download the model once? Perhaps by passing a `local_rank` parameter and only downloading when `local_rank==0`?
Especially for large models this is not ideal as i. they take up a lot of space (multiplied by the number of processes) ii. downloading is extra slow because it happens multiple times in parallel, limiting bandwidth.
```bash
15-Oct 03:08:45 - [INFO]: https://storage.googleapis.com/sf-ctrl/pytorch/seqlen256_v1.bin not found in cache or force_download set to True, downloading to /tmp/tmp0amm9x2s
15-Oct 03:08:45 - [INFO]: https://storage.googleapis.com/sf-ctrl/pytorch/seqlen256_v1.bin not found in cache or force_download set to True, downloading to /tmp/tmp7wpg48uj
15-Oct 03:08:45 - [INFO]: https://storage.googleapis.com/sf-ctrl/pytorch/seqlen256_v1.bin not found in cache or force_download set to True, downloading to /tmp/tmp89svv055
15-Oct 03:08:45 - [INFO]: https://storage.googleapis.com/sf-ctrl/pytorch/seqlen256_v1.bin not found in cache or force_download set to True, downloading to /tmp/tmp7yk94f8s
100%|█████████████████████████████████████████████████████████████████████████████████████████████████████████████████████████████████| 6552025106/6552025106 [03:57<00:00, 27631147.05B/s]
15-Oct 03:12:42 - [INFO]: copying /tmp/tmp89svv055 to cache at /home/bram/.cache/torch/transformers/c146cc96724f27295a0c3ada1fbb3632074adf87e9aef8269e44c9208787f8c8.b986347cbab65fa276683efbb9c2f7ee22552277bcf6e1f1166557ed0852fdf0
100%|█████████████████████████████████████████████████████████████████████████████████████████████████████████████████████████████████| 6552025106/6552025106 [03:57<00:00, 27614197.65B/s]
15-Oct 03:12:43 - [INFO]: copying /tmp/tmp7wpg48uj to cache at /home/bram/.cache/torch/transformers/c146cc96724f27295a0c3ada1fbb3632074adf87e9aef8269e44c9208787f8c8.b986347cbab65fa276683efbb9c2f7ee22552277bcf6e1f1166557ed0852fdf0
100%|█████████████████████████████████████████████████████████████████████████████████████████████████████████████████████████████████| 6552025106/6552025106 [03:57<00:00, 27605553.23B/s]
15-Oct 03:12:43 - [INFO]: copying /tmp/tmp0amm9x2s to cache at /home/bram/.cache/torch/transformers/c146cc96724f27295a0c3ada1fbb3632074adf87e9aef8269e44c9208787f8c8.b986347cbab65fa276683efbb9c2f7ee22552277bcf6e1f1166557ed0852fdf0
100%|█████████████████████████████████████████████████████████████████████████████████████████████████████████████████████████████████| 6552025106/6552025106 [03:57<00:00, 27599668.53B/s]
15-Oct 03:12:43 - [INFO]: copying /tmp/tmp7yk94f8s to cache at /home/bram/.cache/torch/transformers/c146cc96724f27295a0c3ada1fbb3632074adf87e9aef8269e44c9208787f8c8.b986347cbab65fa276683efbb9c2f7ee22552277bcf6e1f1166557ed0852fdf0
```
An alternative would be to already 'touch' the file in the .cache _before_ downloading, and when it exists, not initiate a new download. (Taking into account sudden abortions.)
| 10-15-2019 08:14:12 | 10-15-2019 08:14:12 | This should be fixed in most of the examples through the use of `torch.distributed.barrier`.
E.g. here: https://github.com/huggingface/transformers/blob/master/examples/run_glue.py#L473
Don't hesitate to submit a PR if some examples don't make use of this technique yet.<|||||>Thanks for the quick reply! So to ensure that I understand this correctly: barrier blocks until all processes are synchronized (i.e. have reached that point). So before we enter the loading of the model, we block and only the first process continues (and downloads the model and vocab). After successfully downloading the required files, the first process also reaches barrier() and thus satisfying the need for all processes to have called the function and lifting the block. Then the other processes also continue (but find that the model has already been downloaded, so get it from cache). <|||||>Yes |
transformers | 1,520 | closed | Changelog | ## 🚀 Add changelog between versions
New versions are pushed to PyPi at a steady pace, but it's not evident to find the changes that new versions bring. Is there a changelog anywhere? Something similar to a HISTORY file would be nice. I think it would definitely contribute to better documentation!
| 10-15-2019 07:48:21 | 10-15-2019 07:48:21 | Hi @BramVanroy, we detail the changes in the ["Releases" section](https://github.com/huggingface/transformers/releases). Are you thinking of something different?
Having a documentation per-version is on our roadmap, it should help tremendously regarding version changes.<|||||>Ah, I was looking inside different major version commits for some sort of changelog file - which still might be useful in itself, as you indicate. But having the Github releases is exactly what I was after! Apologies, should've thought this through. |
transformers | 1,519 | closed | Accuracy drop in finetuning roBERTa | ## ❓ How to achieve GLUE leaderboard acc for QQP task trained with roBERTa?
I am trying to finetune roberta-base model for Quora Question Pair task. In the [GLUE Leaderboard](url) the accuracy claimed is F1 / Accuracy is 74.3/90.2
I am training with the following command to fine tune the roberta-base model,
> CUDA_VISIBLE_DEVICES=0 python run_glue.py --data_dir /home/arjun/datasets/quora_roberta --model_type roberta --model_name_or_path /home/arjun/transformers/models/roberta --task_name qqp --output_dir /home/arjun/transformers/output_models/run-2/ --do_train --do_eval --do_lower_case --logging_steps 250 --save_steps 5000
All other params are default params.
I get the accuracy after 3 epochs as (taken from the eval_results.txt file),
acc = 0.6329982984448578
acc_and_f1 = 0.3164991492224289
f1 = 0.0
Also I ran evaluation for all the checkpoints stored, for every checkpoint I got the same accuracy which is quite confusing.
I'm I doing something wrong here? How to reproduce the accuracy mentioned in GLUE leaderboard?
Thanks in advance. | 10-15-2019 06:16:20 | 10-15-2019 06:16:20 | This issue has been automatically marked as stale because it has not had recent activity. It will be closed if no further activity occurs. Thank you for your contributions.
|
transformers | 1,518 | closed | Predefined token classification | Hello,
I am just wondering if "BertForTokenClassification" can be modified to classify predefined tokens (just targeted tokens). E.g. in NER it identifies the entity and then classify it, but in my case I want to classify the targeted tokens only in a sentence with a predefined labels. I thought of adding the targeted token in the sentence to the end of the sentence to let the model knows this is the targeted token but I am sure it is not a good idea because I need to again add a label for the added token. (silly Q I know :)
Any idea on how to approach it? I feel that NER task "BertForTokenClassification"
model can be modified to achieve it but do not know how.
Thanks | 10-15-2019 01:02:57 | 10-15-2019 01:02:57 | This issue has been automatically marked as stale because it has not had recent activity. It will be closed if no further activity occurs. Thank you for your contributions.
|
transformers | 1,517 | closed | Unable to import TF models | ## 🐛 Bug
<!-- Important information -->
Model I am using (Bert, XLNet....): Bert
Language I am using the model on (English, Chinese....): English
The problem arise when using:
* [x] the official example scripts: Quick tour TF 2.0 training and PyTorch interoperability from github homepage
## To Reproduce
Steps to reproduce the behavior:
1. Install libraries (update tensorflow to 2.0.0)
```
!pip install tensorflow-gpu
!pip install torch
!pip install transformers
```
2. Run example
```import tensorflow as tf
import tensorflow_datasets
from transformers import *
# Load dataset, tokenizer, model from pretrained model/vocabulary
tokenizer = BertTokenizer.from_pretrained('bert-base-cased')
model = TFBertForSequenceClassification.from_pretrained('bert-base-cased')
data = tensorflow_datasets.load('glue/mrpc')
# Prepare dataset for GLUE as a tf.data.Dataset instance
train_dataset = glue_convert_examples_to_features(data['train'], tokenizer, max_length=128, task='mrpc')
valid_dataset = glue_convert_examples_to_features(data['validation'], tokenizer, max_length=128, task='mrpc')
train_dataset = train_dataset.shuffle(100).batch(32).repeat(2)
valid_dataset = valid_dataset.batch(64)
# Prepare training: Compile tf.keras model with optimizer, loss and learning rate schedule
optimizer = tf.keras.optimizers.Adam(learning_rate=3e-5, epsilon=1e-08, clipnorm=1.0)
loss = tf.keras.losses.SparseCategoricalCrossentropy(from_logits=True)
metric = tf.keras.metrics.SparseCategoricalAccuracy('accuracy')
model.compile(optimizer=optimizer, loss=loss, metrics=[metric])
# Train and evaluate using tf.keras.Model.fit()
history = model.fit(train_dataset, epochs=2, steps_per_epoch=115,
validation_data=valid_dataset, validation_steps=7)
# Load the TensorFlow model in PyTorch for inspection
model.save_pretrained('./save/')
pytorch_model = BertForSequenceClassification.from_pretrained('./save/', from_tf=True)
# Quickly test a few predictions - MRPC is a paraphrasing task, let's see if our model learned the task
sentence_0 = "This research was consistent with his findings."
sentence_1 = "His findings were compatible with this research."
sentence_2 = "His findings were not compatible with this research."
inputs_1 = tokenizer.encode_plus(sentence_0, sentence_1, add_special_tokens=True, return_tensors='pt')
inputs_2 = tokenizer.encode_plus(sentence_0, sentence_2, add_special_tokens=True, return_tensors='pt')
pred_1 = pytorch_model(**inputs_1)[0].argmax().item()
pred_2 = pytorch_model(**inputs_2)[0].argmax().item()
print("sentence_1 is", "a paraphrase" if pred_1 else "not a paraphrase", "of sentence_0")
print("sentence_2 is", "a paraphrase" if pred_2 else "not a paraphrase", "of sentence_0")
```
3. Get error
```
5 # Load dataset, tokenizer, model from pretrained model/vocabulary
6 tokenizer = BertTokenizer.from_pretrained('bert-base-cased')
----> 7 model = TFBertForSequenceClassification.from_pretrained('bert-base-cased')
8 data = tensorflow_datasets.load('glue/mrpc')
9
NameError: name 'TFBertForSequenceClassification' is not defined
```
## Environment
Google collab
I get the same error when trying to use any TF version of the transformers. | 10-14-2019 22:58:46 | 10-14-2019 22:58:46 | Can you run the following and report back? It might be that you have some namespace conflict.
```python
! pip list | grep "tensorflow" # Check tensorflow==2.0.0, tensorflow-gpu==2.0.0
! pip list | grep "transformers" # Check transformers>=2.0.0
```
<|||||>Cleaning the environment fixed the issue. You are right, there was a namespace conflict.<|||||>@tylerjthomas9 - I'm having the same problem. Can you elaborate on what you did to fix the namespace conflict?<|||||>@GrahamboJangles If you have issues with the import of tensorflow models on a blank colab notebook, please make sure you have the correct tensorflow version installed in your colab environment (2.0+). You can do so by overriding the already-installed TensorFlow with the following command:
```
!pip install tensorflow==2.0.0
```<|||||>@LysandreJik - I made sure I had Tensorflow 2.0.0 and I still get the same error.
```
100%|██████████| 231508/231508 [00:00<00:00, 2665916.96B/s]
100%|██████████| 313/313 [00:00<00:00, 195011.46B/s]
100%|██████████| 440473133/440473133 [00:05<00:00, 73953508.44B/s]
100%|██████████| 815973/815973 [00:00<00:00, 5548125.39B/s]
100%|██████████| 458495/458495 [00:00<00:00, 3162846.19B/s]
ftfy or spacy is not installed using BERT BasicTokenizer instead of SpaCy & ftfy.
100%|██████████| 273/273 [00:00<00:00, 154235.59B/s]
100%|██████████| 478750579/478750579 [00:08<00:00, 56444018.22B/s]
This tokenizer does not make use of special tokens. Input is returned with no modification.
This tokenizer does not make use of special tokens. Input is returned with no modification.
This tokenizer does not make use of special tokens.
100%|██████████| 1042301/1042301 [00:00<00:00, 7120216.12B/s]
100%|██████████| 456318/456318 [00:00<00:00, 3926917.54B/s]
100%|██████████| 176/176 [00:00<00:00, 110459.00B/s]
100%|██████████| 548118077/548118077 [00:09<00:00, 59420986.50B/s]
This tokenizer does not make use of special tokens. Input is returned with no modification.
This tokenizer does not make use of special tokens. Input is returned with no modification.
This tokenizer does not make use of special tokens.
4608350B [00:00, 42689870.73B/s]
2257285B [00:00, 28527684.80B/s]
100%|██████████| 611/611 [00:00<00:00, 408988.15B/s]
100%|██████████| 6552025106/6552025106 [03:27<00:00, 31645156.91B/s]
This tokenizer does not make use of special tokens. Input is returned with no modification.
This tokenizer does not make use of special tokens. Input is returned with no modification.
This tokenizer does not make use of special tokens.
100%|██████████| 9143613/9143613 [00:00<00:00, 29615841.04B/s]
100%|██████████| 606/606 [00:00<00:00, 397210.22B/s]
100%|██████████| 1140884800/1140884800 [00:21<00:00, 53037879.64B/s]
This tokenizer does not make use of special tokens. Input is returned with no modification.
This tokenizer does not make use of special tokens. Input is returned with no modification.
This tokenizer does not make use of special tokens.
100%|██████████| 798011/798011 [00:00<00:00, 5526095.41B/s]
100%|██████████| 641/641 [00:00<00:00, 405390.36B/s]
100%|██████████| 467042463/467042463 [00:08<00:00, 52695048.04B/s]
100%|██████████| 1452741/1452741 [00:00<00:00, 8067948.45B/s]
100%|██████████| 1008321/1008321 [00:00<00:00, 5690556.88B/s]
100%|██████████| 396/396 [00:00<00:00, 225243.34B/s]
100%|██████████| 830122454/830122454 [00:24<00:00, 33868891.23B/s]
100%|██████████| 492/492 [00:00<00:00, 307311.63B/s]
100%|██████████| 267967963/267967963 [00:14<00:00, 18543027.08B/s]
100%|██████████| 898823/898823 [00:00<00:00, 6115044.08B/s]
100%|██████████| 456318/456318 [00:00<00:00, 3196420.05B/s]
100%|██████████| 473/473 [00:00<00:00, 295048.45B/s]
100%|██████████| 501200538/501200538 [00:06<00:00, 77291522.27B/s]
---------------------------------------------------------------------------
OSError Traceback (most recent call last)
/usr/local/lib/python3.6/dist-packages/transformers/configuration_utils.py in from_pretrained(cls, pretrained_model_name_or_path, **kwargs)
132 try:
--> 133 resolved_config_file = cached_path(config_file, cache_dir=cache_dir, force_download=force_download, proxies=proxies)
134 except EnvironmentError:
3 frames
OSError: file roberta-base not found
During handling of the above exception, another exception occurred:
OSError Traceback (most recent call last)
/usr/local/lib/python3.6/dist-packages/transformers/configuration_utils.py in from_pretrained(cls, pretrained_model_name_or_path, **kwargs)
143 ', '.join(cls.pretrained_config_archive_map.keys()),
144 config_file, CONFIG_NAME)
--> 145 raise EnvironmentError(msg)
146
147 if resolved_config_file == config_file:
OSError: Model name 'roberta-base' was not found in model name list (bert-base-uncased, bert-large-uncased, bert-base-cased, bert-large-cased, bert-base-multilingual-uncased, bert-base-multilingual-cased, bert-base-chinese, bert-base-german-cased, bert-large-uncased-whole-word-masking, bert-large-cased-whole-word-masking, bert-large-uncased-whole-word-masking-finetuned-squad, bert-large-cased-whole-word-masking-finetuned-squad, bert-base-cased-finetuned-mrpc, bert-base-german-dbmdz-cased, bert-base-german-dbmdz-uncased). We assumed 'roberta-base' was a path or url to a configuration file named config.json or a directory containing such a file but couldn't find any such file at this path or url.
```<|||||>@GrahamboJangles this does not seem to be the same error. It seems to me that you're trying to load a RoBERTa checkpoint in a BERT model/tokenizer.<|||||>@LysandreJik - Maybe that is the problem, but `tokenizer = BertTokenizer.from_pretrained('bert-base-uncased')` so I don't see why it would be trying to use a RoBERTa checkpoint unless there's something I'm missing. Also, when I try with the RobertaModel I get the same error.<|||||>Could you provide a script so that we can try and reproduce the error on our side?<|||||>@LysandreJik - [Here's my Colab notebook.](https://colab.research.google.com/drive/1TeCwrGAzEH4IMcgLewR8OJRVwlnmJxdd) |
transformers | 1,516 | closed | Fused optimizer and gradient clipper using apex | Significant (40ms / iter for XLNet squad finetuning) performance increase.
Also adds fused grad clipping, gives further ~30ms / iter saving in the same XLNet squad case.
Redefines the `AdamW` implementation such that the existing code will be used if apex's multi_tensor_apply code isn't available, and should drop-in speedup all existing scripts using `AdamW`. Also abstracts the gradient clipping (in order to keep run scripts concise and move apex-specific logic into `optimizations.py`) | 10-14-2019 20:31:54 | 10-14-2019 20:31:54 | # [Codecov](https://codecov.io/gh/huggingface/transformers/pull/1516?src=pr&el=h1) Report
> Merging [#1516](https://codecov.io/gh/huggingface/transformers/pull/1516?src=pr&el=desc) into [master](https://codecov.io/gh/huggingface/transformers/commit/1d4d07025635c998acf8c7abab426b013e87206c?src=pr&el=desc) will **decrease** coverage by `0.18%`.
> The diff coverage is `25%`.
[](https://codecov.io/gh/huggingface/transformers/pull/1516?src=pr&el=tree)
```diff
@@ Coverage Diff @@
## master #1516 +/- ##
==========================================
- Coverage 85.17% 84.98% -0.19%
==========================================
Files 94 94
Lines 13920 13953 +33
==========================================
+ Hits 11856 11858 +2
- Misses 2064 2095 +31
```
| [Impacted Files](https://codecov.io/gh/huggingface/transformers/pull/1516?src=pr&el=tree) | Coverage Δ | |
|---|---|---|
| [transformers/tests/optimization\_test.py](https://codecov.io/gh/huggingface/transformers/pull/1516/diff?src=pr&el=tree#diff-dHJhbnNmb3JtZXJzL3Rlc3RzL29wdGltaXphdGlvbl90ZXN0LnB5) | `99.02% <100%> (ø)` | :arrow_up: |
| [transformers/optimization.py](https://codecov.io/gh/huggingface/transformers/pull/1516/diff?src=pr&el=tree#diff-dHJhbnNmb3JtZXJzL29wdGltaXphdGlvbi5weQ==) | `75.2% <18.18%> (-21.43%)` | :arrow_down: |
| [transformers/file\_utils.py](https://codecov.io/gh/huggingface/transformers/pull/1516/diff?src=pr&el=tree#diff-dHJhbnNmb3JtZXJzL2ZpbGVfdXRpbHMucHk=) | `74.17% <0%> (-2.2%)` | :arrow_down: |
------
[Continue to review full report at Codecov](https://codecov.io/gh/huggingface/transformers/pull/1516?src=pr&el=continue).
> **Legend** - [Click here to learn more](https://docs.codecov.io/docs/codecov-delta)
> `Δ = absolute <relative> (impact)`, `ø = not affected`, `? = missing data`
> Powered by [Codecov](https://codecov.io/gh/huggingface/transformers/pull/1516?src=pr&el=footer). Last update [1d4d070...a359214](https://codecov.io/gh/huggingface/transformers/pull/1516?src=pr&el=lastupdated). Read the [comment docs](https://docs.codecov.io/docs/pull-request-comments).
<|||||>Does this apply that apex's FusedAdam is a fused implementation of AdamW rather than Adam (vanilla)?
It might be nice to first try to import [torch's native AdamW](https://pytorch.org/docs/stable/_modules/torch/optim/adamw.html#AdamW) (from 1.2), and if not available fallback to the transformers implementation. Cf. https://github.com/huggingface/transformers/pull/1593<|||||>https://github.com/huggingface/transformers/pull/1516/files#diff-59de7b854fbd60c6ba87f68027a2db36R208 enables AdamW support in the `FusedAdam` optimizer.
I see you've done the work to check for native PyT implementation & defining if necessary, it should be an easy rebase & resolve if/when these individual PRs get merged.<|||||>Oh, my bad. I hadn't noticed this `adam_w_mode=True` in Apex's Adam before. Good to know!<|||||>Updated to clip gradient outside of gradient accumulation inner loop as we are trying to do now.
We should update the other training scripts as well (`run_glue` for instance). I think it may be soon time to refactor and gather the common portions of the examples (which are numerous) so we spend less time synchronizing them.
What do you think @LysandreJik?<|||||>I agree that some scripts definitely need some refactoring and that having shared pieces of code like that gradient clipping seems like the way to go.<|||||>This issue has been automatically marked as stale because it has not had recent activity. It will be closed if no further activity occurs. Thank you for your contributions.
|
transformers | 1,515 | closed | Main and train for CTRL model | ## 🚀 Feature
I have seen the CTRL model has been added to the repo but I don't see any script to run or train it. Is this going to be added soon?
| 10-14-2019 16:24:33 | 10-14-2019 16:24:33 | The CTRL model has been added to the [run_generation](https://github.com/huggingface/transformers/blob/master/examples/run_generation.py) script as of now. We will implement it in other scripts as time goes on, but as it has the same API as the other models hosted on our repo it the training script would be very similar to the current training scripts.<|||||>This issue has been automatically marked as stale because it has not had recent activity. It will be closed if no further activity occurs. Thank you for your contributions.
|
transformers | 1,514 | closed | /pytorch/aten/src/THC/THCTensorScatterGather.cu:100: void THCudaTensor_gatherKernel(TensorInfo<Real, IndexType>, TensorInfo<Real, IndexType>, TensorInfo<long, IndexType>, int, IndexType) [with IndexType = unsigned int, Real = float, Dims = 3]: block: [4,0,0], thread: [319,0,0] Assertion `indexValue >= 0 && indexValue < src.sizes[dim]` failed. | ## ❓ Questions & Help
<!-- A clear and concise description of the question. -->
I have no idea about this error。 | 10-14-2019 15:48:49 | 10-14-2019 15:48:49 | Could you please provide more information? Where does this error occur? Are you using one of our example scripts? I believe there are templates you can use so we may help you more efficiently.<|||||>> Could you please provide more information? Where does this error occur? Are you using one of our example scripts? I believe there are templates you can use so we may help you more efficiently.
I run run_squad.py in a chinese reading comprehension dataset. I change sereval places in utils_squad.py ,I throw all examples which have no answer because my passage is so longth. if i don't throw all examples without answer, my trained model will predict no answer in test dataset. This error occur in the begining of training. I have no idea why this error happen ?<|||||>This issue has been automatically marked as stale because it has not had recent activity. It will be closed if no further activity occurs. Thank you for your contributions.
<|||||>This issue has been automatically marked as stale because it has not had recent activity. It will be closed if no further activity occurs. Thank you for your contributions.
|
transformers | 1,513 | closed | Force einsum to run in fp16 | As noted in the comments, this will force `torch.einsum` to run in fp16 for the squad finetuning task (it should be valid for other tasks, but I haven't verified that) when run with `--fp16_opt_level="O1"` which is the default.
Otherwise, `torch.einsum` is treated as a "promote" operation by `apex.amp`, and if any argument is fp32, all arguments will be cast to fp32, and the answer will return in fp32. This will happen at any point when a parameter is used (XLNet in particular suffers here). Given all uses I've seen for einsum are to express gemm, batched-gemm and transpose, operations we'd normally consider to be safe in fp16, this should be a safe change. From a performance standpoint it allows TensorCore usage which can significantly boost achieved performance.
This change doesn't affect accuracy in my testing, and gives ~20-25% higher throughput on XLNet-based finetuning. | 10-14-2019 15:14:03 | 10-14-2019 15:14:03 | # [Codecov](https://codecov.io/gh/huggingface/transformers/pull/1513?src=pr&el=h1) Report
> Merging [#1513](https://codecov.io/gh/huggingface/transformers/pull/1513?src=pr&el=desc) into [master](https://codecov.io/gh/huggingface/transformers/commit/f62f992cf7aa7f1e4eb0d1ef912bd06d26c4dd8c?src=pr&el=desc) will **not change** coverage.
> The diff coverage is `n/a`.
[](https://codecov.io/gh/huggingface/transformers/pull/1513?src=pr&el=tree)
```diff
@@ Coverage Diff @@
## master #1513 +/- ##
=======================================
Coverage 85.98% 85.98%
=======================================
Files 91 91
Lines 13579 13579
=======================================
Hits 11676 11676
Misses 1903 1903
```
------
[Continue to review full report at Codecov](https://codecov.io/gh/huggingface/transformers/pull/1513?src=pr&el=continue).
> **Legend** - [Click here to learn more](https://docs.codecov.io/docs/codecov-delta)
> `Δ = absolute <relative> (impact)`, `ø = not affected`, `? = missing data`
> Powered by [Codecov](https://codecov.io/gh/huggingface/transformers/pull/1513?src=pr&el=footer). Last update [f62f992...4e6a557](https://codecov.io/gh/huggingface/transformers/pull/1513?src=pr&el=lastupdated). Read the [comment docs](https://docs.codecov.io/docs/pull-request-comments).
<|||||>Great, thanks a lot for your work on that @slayton58!<|||||>Why not adopt this change to other finetuning tasks? Currently, I only see the code snippet in the squad task.<|||||>Einsum is tricky because it can express both tasks that are very likely to be good in fp16 (gemm, batch-gemm) and some that are not (large summations).
It could be adopted for other tasks but it needs to be done task-by-task (with testing) to ensure that no problems are caused.
> On Jul 12, 2021, at 2:01 AM, Gordon Lee ***@***.***> wrote:
>
>
> Why not adopt this change to other finetuning tasks? Currently, I only see the code snippet in the squad task.
>
> —
> You are receiving this because you were mentioned.
> Reply to this email directly, view it on GitHub, or unsubscribe.
|
transformers | 1,512 | closed | Fix import error in script to convert faisreq roberta checkpoints | Fix ImportError in `convert_roberta_original_pytorch_checkpoint_to_pytorch.py`, see #1459.
| 10-14-2019 08:41:21 | 10-14-2019 08:41:21 | # [Codecov](https://codecov.io/gh/huggingface/transformers/pull/1512?src=pr&el=h1) Report
> Merging [#1512](https://codecov.io/gh/huggingface/transformers/pull/1512?src=pr&el=desc) into [master](https://codecov.io/gh/huggingface/transformers/commit/a701c9b32126f1e6974d9fcb3a5c3700527d8559?src=pr&el=desc) will **not change** coverage.
> The diff coverage is `n/a`.
[](https://codecov.io/gh/huggingface/transformers/pull/1512?src=pr&el=tree)
```diff
@@ Coverage Diff @@
## master #1512 +/- ##
=======================================
Coverage 85.98% 85.98%
=======================================
Files 91 91
Lines 13579 13579
=======================================
Hits 11676 11676
Misses 1903 1903
```
------
[Continue to review full report at Codecov](https://codecov.io/gh/huggingface/transformers/pull/1512?src=pr&el=continue).
> **Legend** - [Click here to learn more](https://docs.codecov.io/docs/codecov-delta)
> `Δ = absolute <relative> (impact)`, `ø = not affected`, `? = missing data`
> Powered by [Codecov](https://codecov.io/gh/huggingface/transformers/pull/1512?src=pr&el=footer). Last update [a701c9b...49cba6e](https://codecov.io/gh/huggingface/transformers/pull/1512?src=pr&el=lastupdated). Read the [comment docs](https://docs.codecov.io/docs/pull-request-comments).
<|||||>Looks good to me, thanks! |
transformers | 1,511 | closed | Run squad with all model lq | 10-14-2019 06:32:17 | 10-14-2019 06:32:17 | This does not seem to be related to our repo. Closing. Please don't reopen unless you want to submit a real PR. |
|
transformers | 1,510 | closed | CalledProcessError | I'm running
```
python /content/transformers/examples/run_lm_finetuning.py \
--output_dir=output \
--model_type=gpt2 \
--model_name_or_path=gpt2 \
--do_train \
--train_data_file=TRAIN_FILE \
--do_eval \
--eval_data_file=TEST_FILE
```
in my [Colab notebook](https://colab.research.google.com/drive/1T3fUHHWPAgWKEEITOKZJFGvNp9332RW3) and it returns this:
```
10/14/2019 03:30:53 - WARNING - __main__ - Process rank: -1, device: cpu, n_gpu: 0, distributed training: False, 16-bits training: False
10/14/2019 03:30:53 - INFO - transformers.configuration_utils - loading configuration file https://s3.amazonaws.com/models.huggingface.co/bert/gpt2-config.json from cache at /root/.cache/torch/transformers/4be02c5697d91738003fb1685c9872f284166aa32e061576bbe6aaeb95649fcf.085d5f6a8e7812ea05ff0e6ed0645ab2e75d80387ad55c1ad9806ee70d272f80
10/14/2019 03:30:53 - INFO - transformers.configuration_utils - Model config {
"attn_pdrop": 0.1,
"embd_pdrop": 0.1,
"finetuning_task": null,
"initializer_range": 0.02,
"layer_norm_epsilon": 1e-05,
"n_ctx": 1024,
"n_embd": 768,
"n_head": 12,
"n_layer": 12,
"n_positions": 1024,
"num_labels": 1,
"output_attentions": false,
"output_hidden_states": false,
"output_past": true,
"pruned_heads": {},
"resid_pdrop": 0.1,
"summary_activation": null,
"summary_first_dropout": 0.1,
"summary_proj_to_labels": true,
"summary_type": "cls_index",
"summary_use_proj": true,
"torchscript": false,
"use_bfloat16": false,
"vocab_size": 50257
}
10/14/2019 03:30:53 - INFO - transformers.tokenization_utils - loading file https://s3.amazonaws.com/models.huggingface.co/bert/gpt2-vocab.json from cache at /root/.cache/torch/transformers/f2808208f9bec2320371a9f5f891c184ae0b674ef866b79c58177067d15732dd.1512018be4ba4e8726e41b9145129dc30651ea4fec86aa61f4b9f40bf94eac71
10/14/2019 03:30:53 - INFO - transformers.tokenization_utils - loading file https://s3.amazonaws.com/models.huggingface.co/bert/gpt2-merges.txt from cache at /root/.cache/torch/transformers/d629f792e430b3c76a1291bb2766b0a047e36fae0588f9dbc1ae51decdff691b.70bec105b4158ed9a1747fea67a43f5dee97855c64d62b6ec3742f4cfdb5feda
10/14/2019 03:30:54 - INFO - transformers.modeling_utils - loading weights file https://s3.amazonaws.com/models.huggingface.co/bert/gpt2-pytorch_model.bin from cache at /root/.cache/torch/transformers/4295d67f022061768f4adc386234dbdb781c814c39662dd1662221c309962c55.778cf36f5c4e5d94c8cd9cefcf2a580c8643570eb327f0d4a1f007fab2acbdf1
10/14/2019 03:30:58 - INFO - __main__ - Training/evaluation parameters Namespace(adam_epsilon=1e-08, block_size=1024, cache_dir='', config_name='', device=device(type='cpu'), do_eval=True, do_lower_case=False, do_train=True, eval_all_checkpoints=False, eval_data_file='TEST_FILE', evaluate_during_training=False, fp16=False, fp16_opt_level='O1', gradient_accumulation_steps=1, learning_rate=5e-05, local_rank=-1, logging_steps=50, max_grad_norm=1.0, max_steps=-1, mlm=False, mlm_probability=0.15, model_name_or_path='gpt2', model_type='gpt2', n_gpu=0, no_cuda=False, num_train_epochs=1.0, output_dir='output', overwrite_cache=False, overwrite_output_dir=False, per_gpu_eval_batch_size=4, per_gpu_train_batch_size=4, save_steps=50, save_total_limit=None, seed=42, server_ip='', server_port='', tokenizer_name='', train_data_file='TRAIN_FILE', warmup_steps=0, weight_decay=0.0)
Traceback (most recent call last):
File "/content/transformers/examples/run_lm_finetuning.py", line 543, in <module>
main()
File "/content/transformers/examples/run_lm_finetuning.py", line 490, in main
train_dataset = load_and_cache_examples(args, tokenizer, evaluate=False)
File "/content/transformers/examples/run_lm_finetuning.py", line 102, in load_and_cache_examples
dataset = TextDataset(tokenizer, file_path=args.eval_data_file if evaluate else args.train_data_file, block_size=args.block_size)
File "/content/transformers/examples/run_lm_finetuning.py", line 67, in __init__
assert os.path.isfile(file_path)
AssertionError
---------------------------------------------------------------------------
CalledProcessError Traceback (most recent call last)
<ipython-input-21-2156f3b9e4fc> in <module>()
----> 1 get_ipython().run_cell_magic('shell', '', 'cd /content/transformers\nexport TRAIN_FILE=/content/wikitext-103-raw/wiki.train.raw\nexport TEST_FILE=/content/wikitext-103-raw/wiki.test.raw\n \npython /content/transformers/examples/run_lm_finetuning.py \\\n --output_dir=output \\\n --model_type=gpt2 \\\n --model_name_or_path=gpt2 \\\n --do_train \\\n --train_data_file=TRAIN_FILE \\\n --do_eval \\\n --eval_data_file=TEST_FILE')
2 frames
/usr/local/lib/python3.6/dist-packages/google/colab/_system_commands.py in check_returncode(self)
136 if self.returncode:
137 raise subprocess.CalledProcessError(
--> 138 returncode=self.returncode, cmd=self.args, output=self.output)
139
140 def _repr_pretty_(self, p, cycle): # pylint:disable=unused-argument
CalledProcessError: Command 'cd /content/transformers
export TRAIN_FILE=/content/wikitext-103-raw/wiki.train.raw
export TEST_FILE=/content/wikitext-103-raw/wiki.test.raw
python /content/transformers/examples/run_lm_finetuning.py \
--output_dir=output \
--model_type=gpt2 \
--model_name_or_path=gpt2 \
--do_train \
--train_data_file=TRAIN_FILE \
--do_eval \
--eval_data_file=TEST_FILE' returned non-zero exit status 1.
``` | 10-14-2019 03:34:01 | 10-14-2019 03:34:01 | Hello, does this still crash if you replace `TRAIN_FILE` with `$TRAIN_FILE` and `TEST_FILE` with `$TEST_FILE` in your command ?<|||||>@LysandreJik - Yes, it does.
```
10/14/2019 16:55:10 - WARNING - __main__ - Process rank: -1, device: cpu, n_gpu: 0, distributed training: False, 16-bits training: False
10/14/2019 16:55:10 - INFO - transformers.file_utils - https://s3.amazonaws.com/models.huggingface.co/bert/gpt2-config.json not found in cache or force_download set to True, downloading to /tmp/tmpi7g0lm6a
100% 176/176 [00:00<00:00, 131305.14B/s]
10/14/2019 16:55:10 - INFO - transformers.file_utils - copying /tmp/tmpi7g0lm6a to cache at /root/.cache/torch/transformers/4be02c5697d91738003fb1685c9872f284166aa32e061576bbe6aaeb95649fcf.085d5f6a8e7812ea05ff0e6ed0645ab2e75d80387ad55c1ad9806ee70d272f80
10/14/2019 16:55:10 - INFO - transformers.file_utils - creating metadata file for /root/.cache/torch/transformers/4be02c5697d91738003fb1685c9872f284166aa32e061576bbe6aaeb95649fcf.085d5f6a8e7812ea05ff0e6ed0645ab2e75d80387ad55c1ad9806ee70d272f80
10/14/2019 16:55:10 - INFO - transformers.file_utils - removing temp file /tmp/tmpi7g0lm6a
10/14/2019 16:55:10 - INFO - transformers.configuration_utils - loading configuration file https://s3.amazonaws.com/models.huggingface.co/bert/gpt2-config.json from cache at /root/.cache/torch/transformers/4be02c5697d91738003fb1685c9872f284166aa32e061576bbe6aaeb95649fcf.085d5f6a8e7812ea05ff0e6ed0645ab2e75d80387ad55c1ad9806ee70d272f80
10/14/2019 16:55:10 - INFO - transformers.configuration_utils - Model config {
"attn_pdrop": 0.1,
"embd_pdrop": 0.1,
"finetuning_task": null,
"initializer_range": 0.02,
"layer_norm_epsilon": 1e-05,
"n_ctx": 1024,
"n_embd": 768,
"n_head": 12,
"n_layer": 12,
"n_positions": 1024,
"num_labels": 1,
"output_attentions": false,
"output_hidden_states": false,
"output_past": true,
"pruned_heads": {},
"resid_pdrop": 0.1,
"summary_activation": null,
"summary_first_dropout": 0.1,
"summary_proj_to_labels": true,
"summary_type": "cls_index",
"summary_use_proj": true,
"torchscript": false,
"use_bfloat16": false,
"vocab_size": 50257
}
10/14/2019 16:55:11 - INFO - transformers.file_utils - https://s3.amazonaws.com/models.huggingface.co/bert/gpt2-vocab.json not found in cache or force_download set to True, downloading to /tmp/tmp103hb26e
100% 1042301/1042301 [00:00<00:00, 3111277.27B/s]
10/14/2019 16:55:11 - INFO - transformers.file_utils - copying /tmp/tmp103hb26e to cache at /root/.cache/torch/transformers/f2808208f9bec2320371a9f5f891c184ae0b674ef866b79c58177067d15732dd.1512018be4ba4e8726e41b9145129dc30651ea4fec86aa61f4b9f40bf94eac71
10/14/2019 16:55:11 - INFO - transformers.file_utils - creating metadata file for /root/.cache/torch/transformers/f2808208f9bec2320371a9f5f891c184ae0b674ef866b79c58177067d15732dd.1512018be4ba4e8726e41b9145129dc30651ea4fec86aa61f4b9f40bf94eac71
10/14/2019 16:55:11 - INFO - transformers.file_utils - removing temp file /tmp/tmp103hb26e
10/14/2019 16:55:12 - INFO - transformers.file_utils - https://s3.amazonaws.com/models.huggingface.co/bert/gpt2-merges.txt not found in cache or force_download set to True, downloading to /tmp/tmpp3c_047z
100% 456318/456318 [00:00<00:00, 1830222.06B/s]
10/14/2019 16:55:12 - INFO - transformers.file_utils - copying /tmp/tmpp3c_047z to cache at /root/.cache/torch/transformers/d629f792e430b3c76a1291bb2766b0a047e36fae0588f9dbc1ae51decdff691b.70bec105b4158ed9a1747fea67a43f5dee97855c64d62b6ec3742f4cfdb5feda
10/14/2019 16:55:12 - INFO - transformers.file_utils - creating metadata file for /root/.cache/torch/transformers/d629f792e430b3c76a1291bb2766b0a047e36fae0588f9dbc1ae51decdff691b.70bec105b4158ed9a1747fea67a43f5dee97855c64d62b6ec3742f4cfdb5feda
10/14/2019 16:55:12 - INFO - transformers.file_utils - removing temp file /tmp/tmpp3c_047z
10/14/2019 16:55:12 - INFO - transformers.tokenization_utils - loading file https://s3.amazonaws.com/models.huggingface.co/bert/gpt2-vocab.json from cache at /root/.cache/torch/transformers/f2808208f9bec2320371a9f5f891c184ae0b674ef866b79c58177067d15732dd.1512018be4ba4e8726e41b9145129dc30651ea4fec86aa61f4b9f40bf94eac71
10/14/2019 16:55:12 - INFO - transformers.tokenization_utils - loading file https://s3.amazonaws.com/models.huggingface.co/bert/gpt2-merges.txt from cache at /root/.cache/torch/transformers/d629f792e430b3c76a1291bb2766b0a047e36fae0588f9dbc1ae51decdff691b.70bec105b4158ed9a1747fea67a43f5dee97855c64d62b6ec3742f4cfdb5feda
10/14/2019 16:55:13 - INFO - transformers.file_utils - https://s3.amazonaws.com/models.huggingface.co/bert/gpt2-pytorch_model.bin not found in cache or force_download set to True, downloading to /tmp/tmpfgx0mkjn
100% 548118077/548118077 [00:15<00:00, 34424870.34B/s]
10/14/2019 16:55:29 - INFO - transformers.file_utils - copying /tmp/tmpfgx0mkjn to cache at /root/.cache/torch/transformers/4295d67f022061768f4adc386234dbdb781c814c39662dd1662221c309962c55.778cf36f5c4e5d94c8cd9cefcf2a580c8643570eb327f0d4a1f007fab2acbdf1
10/14/2019 16:55:31 - INFO - transformers.file_utils - creating metadata file for /root/.cache/torch/transformers/4295d67f022061768f4adc386234dbdb781c814c39662dd1662221c309962c55.778cf36f5c4e5d94c8cd9cefcf2a580c8643570eb327f0d4a1f007fab2acbdf1
10/14/2019 16:55:31 - INFO - transformers.file_utils - removing temp file /tmp/tmpfgx0mkjn
10/14/2019 16:55:31 - INFO - transformers.modeling_utils - loading weights file https://s3.amazonaws.com/models.huggingface.co/bert/gpt2-pytorch_model.bin from cache at /root/.cache/torch/transformers/4295d67f022061768f4adc386234dbdb781c814c39662dd1662221c309962c55.778cf36f5c4e5d94c8cd9cefcf2a580c8643570eb327f0d4a1f007fab2acbdf1
10/14/2019 16:55:35 - INFO - __main__ - Training/evaluation parameters Namespace(adam_epsilon=1e-08, block_size=1024, cache_dir='', config_name='', device=device(type='cpu'), do_eval=True, do_lower_case=False, do_train=True, eval_all_checkpoints=False, eval_data_file='/content/wikitext-103-raw/wiki.test.raw', evaluate_during_training=False, fp16=False, fp16_opt_level='O1', gradient_accumulation_steps=1, learning_rate=5e-05, local_rank=-1, logging_steps=50, max_grad_norm=1.0, max_steps=-1, mlm=False, mlm_probability=0.15, model_name_or_path='gpt2', model_type='gpt2', n_gpu=0, no_cuda=False, num_train_epochs=1.0, output_dir='output', overwrite_cache=False, overwrite_output_dir=False, per_gpu_eval_batch_size=4, per_gpu_train_batch_size=4, save_steps=50, save_total_limit=None, seed=42, server_ip='', server_port='', tokenizer_name='', train_data_file='/content/wikitext-103-raw/wiki.train.raw', warmup_steps=0, weight_decay=0.0)
10/14/2019 16:55:35 - INFO - __main__ - Creating features from dataset file at /content/wikitext-103-raw
tcmalloc: large alloc 1081139200 bytes == 0x8b1a4000 @ 0x7f2fddd0b1e7 0x50ca4f 0x50440b 0x504bff 0x52d7c2 0x59aa60 0x4f858d 0x4f98c7 0x4f6128 0x4f42e7 0x5a1481 0x57c57c 0x57e6ae 0x583d97 0x627fff 0x4f858d 0x4f98c7 0x4f6128 0x4f426e 0x5a1481 0x512a60 0x53ee21 0x57ec0c 0x4f88ba 0x4fa6c0 0x4f6128 0x4f7d60 0x4f876d 0x4fa6c0 0x4f6128 0x4f7d60
tcmalloc: large alloc 2162278400 bytes == 0xcb8b2000 @ 0x7f2fddd0b1e7 0x50ca4f 0x50440b 0x504bff 0x52d7c2 0x59aa60 0x4f858d 0x4f98c7 0x4f6128 0x4f42e7 0x5a1481 0x57c57c 0x57e6ae 0x583d97 0x627fff 0x4f858d 0x4f98c7 0x4f6128 0x4f426e 0x5a1481 0x512a60 0x53ee21 0x57ec0c 0x4f88ba 0x4fa6c0 0x4f6128 0x4f7d60 0x4f876d 0x4fa6c0 0x4f6128 0x4f7d60
tcmalloc: large alloc 2158272512 bytes == 0x14c6ce000 @ 0x7f2fddd0b1e7 0x5bd1cb 0x583f51 0x627fff 0x4f858d 0x4f98c7 0x4f6128 0x4f426e 0x5a1481 0x512a60 0x53ee21 0x57ec0c 0x4f88ba 0x4fa6c0 0x4f6128 0x4f7d60 0x4f876d 0x4fa6c0 0x4f6128 0x4f7d60 0x4f876d 0x4f98c7 0x4f6128 0x4f9023 0x6415b2 0x64166a 0x643730 0x62b26e 0x4b4cb0 0x7f2fdd908b97 0x5bdf6a
tcmalloc: large alloc 2158272512 bytes == 0x6ae1c000 @ 0x7f2fddd0b1e7 0x50ca4f 0x50de4a 0x58405c 0x627fff 0x4f858d 0x4f98c7 0x4f6128 0x4f426e 0x5a1481 0x512a60 0x53ee21 0x57ec0c 0x4f88ba 0x4fa6c0 0x4f6128 0x4f7d60 0x4f876d 0x4fa6c0 0x4f6128 0x4f7d60 0x4f876d 0x4f98c7 0x4f6128 0x4f9023 0x6415b2 0x64166a 0x643730 0x62b26e 0x4b4cb0 0x7f2fdd908b97
tcmalloc: large alloc 2158272512 bytes == 0xeb866000 @ 0x7f2fddd0b1e7 0x50ca4f 0x50de4a 0x5aebf9 0x4f858d 0x4f98c7 0x4f6128 0x4f7d60 0x4f876d 0x4f98c7 0x4f6128 0x4f7d60 0x4f876d 0x4f98c7 0x4f6128 0x4f7d60 0x4f876d 0x4f98c7 0x4f6128 0x4f426e 0x5a1481 0x512a60 0x53ee21 0x57ec0c 0x4f88ba 0x4fa6c0 0x4f6128 0x4f7d60 0x4f876d 0x4fa6c0 0x4f6128
---------------------------------------------------------------------------
CalledProcessError Traceback (most recent call last)
<ipython-input-5-cbb21af32de2> in <module>()
----> 1 get_ipython().run_cell_magic('shell', '', 'cd /content/transformers\nexport TRAIN_FILE=/content/wikitext-103-raw/wiki.train.raw\nexport TEST_FILE=/content/wikitext-103-raw/wiki.test.raw\n \npython /content/transformers/examples/run_lm_finetuning.py \\\n --output_dir=output \\\n --model_type=gpt2 \\\n --model_name_or_path=gpt2 \\\n --do_train \\\n --train_data_file=$TRAIN_FILE \\\n --do_eval \\\n --eval_data_file=$TEST_FILE')
2 frames
/usr/local/lib/python3.6/dist-packages/google/colab/_system_commands.py in check_returncode(self)
136 if self.returncode:
137 raise subprocess.CalledProcessError(
--> 138 returncode=self.returncode, cmd=self.args, output=self.output)
139
140 def _repr_pretty_(self, p, cycle): # pylint:disable=unused-argument
CalledProcessError: Command 'cd /content/transformers
export TRAIN_FILE=/content/wikitext-103-raw/wiki.train.raw
export TEST_FILE=/content/wikitext-103-raw/wiki.test.raw
python /content/transformers/examples/run_lm_finetuning.py \
--output_dir=output \
--model_type=gpt2 \
--model_name_or_path=gpt2 \
--do_train \
--train_data_file=$TRAIN_FILE \
--do_eval \
--eval_data_file=$TEST_FILE' died with <Signals.SIGKILL: 9>.
```
I've tried: with GPU, without GPU, TPU. All have the same error.<|||||>I currently face the same issue<|||||>I have the same problem:
Im running on GoogleColab:
!python run_language_modeling.py \
--output_dir=output \
--model_type=gpt2 \
--model_name_or_path=gpt2 \
--do_train \
--train_data_file="../../drive/My Drive/HuggingFace/train.txt" \
--per_gpu_train_batch_size=1
And I get this:
`03/03/2020 06:46:34 - INFO - __main__ - Creating features from dataset file at ../../drive/My Drive/HuggingFace
tcmalloc: large alloc 1684217856 bytes == 0x14e26c000 @ 0x7f80cc4a21e7 0x5450df 0x52e319 0x52f3cf 0x53e701 0x4f2b30 0x50a8af 0x50c5b9 0x508245 0x5096b7 0x595311 0x5a522c 0x5a670a 0x4bb19c 0x5bd993 0x50a8af 0x50c5b9 0x508245 0x509642 0x595311 0x54a6ff 0x551b81 0x5aa6ec 0x50abb3 0x50d390 0x508245 0x50a080 0x50aa7d 0x50d390 0x508245 0x50a080
tcmalloc: large alloc 3368435712 bytes == 0x7f7f0339c000 @ 0x7f80cc4a21e7 0x5450df 0x52e319 0x52f3cf 0x53e701 0x4f2b30 0x50a8af 0x50c5b9 0x508245 0x5096b7 0x595311 0x5a522c 0x5a670a 0x4bb19c 0x5bd993 0x50a8af 0x50c5b9 0x508245 0x509642 0x595311 0x54a6ff 0x551b81 0x5aa6ec 0x50abb3 0x50d390 0x508245 0x50a080 0x50aa7d 0x50d390 0x508245 0x50a080
tcmalloc: large alloc 3344367616 bytes == 0x7f7e3be2c000 @ 0x7f80cc4a21e7 0x5ad4cb 0x4bb356 0x5bd993 0x50a8af 0x50c5b9 0x508245 0x509642 0x595311 0x54a6ff 0x551b81 0x5aa6ec 0x50abb3 0x50d390 0x508245 0x50a080 0x50aa7d 0x50d390 0x508245 0x50a080 0x50aa7d 0x50c5b9 0x508245 0x50b403 0x635222 0x6352d7 0x638a8f 0x639631 0x4b0f40 0x7f80cc09fb97 0x5b2fda
tcmalloc: large alloc 3343319040 bytes == 0x7f7f0339c000 @ 0x7f80cc4a21e7 0x5450df 0x5464ca 0x4bb455 0x5bd993 0x50a8af 0x50c5b9 0x508245 0x509642 0x595311 0x54a6ff 0x551b81 0x5aa6ec 0x50abb3 0x50d390 0x508245 0x50a080 0x50aa7d 0x50d390 0x508245 0x50a080 0x50aa7d 0x50c5b9 0x508245 0x50b403 0x635222 0x6352d7 0x638a8f 0x639631 0x4b0f40 0x7f80cc09fb97
tcmalloc: large alloc 3343319040 bytes == 0x7f7e3be2c000 @ 0x7f80cc4a21e7 0x5450df 0x5464ca 0x536c89 0x50a8af 0x50c5b9 0x508245 0x50a080 0x50aa7d 0x50c5b9 0x508245 0x50a080 0x50aa7d 0x50c5b9 0x508245 0x509642 0x595311 0x54a6ff 0x551b81 0x5aa6ec 0x50abb3 0x50d390 0x508245 0x50a080 0x50aa7d 0x50d390 0x508245 0x50a080 0x50aa7d 0x50c5b9 0x508245
tcmalloc: large alloc 3343319040 bytes == 0x7f7e3be2c000 @ 0x7f80cc4a21e7 0x5450df 0x5464ca 0x536808 0x50a8af 0x50c5b9 0x508245 0x50a080 0x50aa7d 0x50c5b9 0x508245 0x50a080 0x50aa7d 0x50c5b9 0x508245 0x50a080 0x50aa7d 0x50c5b9 0x508245 0x509642 0x595311 0x54a6ff 0x551b81 0x5aa6ec 0x50abb3 0x50d390 0x508245 0x50a080 0x50aa7d 0x50d390 0x508245
^C`<|||||>This issue has been automatically marked as stale because it has not had recent activity. It will be closed if no further activity occurs. Thank you for your contributions.
<|||||>I still get this problem. Are there any updates?<|||||>I also got this issue! Are there any updates?
|
transformers | 1,509 | closed | remove leftover usage of DUMMY_INPUTS | Hey @thomwolf
This change https://github.com/huggingface/transformers/commit/da26bae61b8c1e741fdc6735d46c61b43f649561#diff-8ddce309e88e8eb5b4d02228fd8881daL28 removed the constant `DUMMY_INPUTS`, but one usage of that constant remains in the code.
So any call to `load_tf2_checkpoint_in_pytorch_model` is currently throwing: `NameError: name 'DUMMY_INPUTS' is not defined`
```
/usr/local/lib/python3.6/dist-packages/transformers/modeling_tf_pytorch_utils.py in load_tf2_checkpoint_in_pytorch_model(pt_model, tf_checkpoint_path, tf_inputs, allow_missing_keys)
199
200 if tf_inputs is None:
--> 201 tf_inputs = tf.constant(DUMMY_INPUTS)
202
203 if tf_inputs is not None:
NameError: name 'DUMMY_INPUTS' is not defined
``` | 10-13-2019 23:10:47 | 10-13-2019 23:10:47 | # [Codecov](https://codecov.io/gh/huggingface/transformers/pull/1509?src=pr&el=h1) Report
> Merging [#1509](https://codecov.io/gh/huggingface/transformers/pull/1509?src=pr&el=desc) into [master](https://codecov.io/gh/huggingface/transformers/commit/a701c9b32126f1e6974d9fcb3a5c3700527d8559?src=pr&el=desc) will **decrease** coverage by `1.24%`.
> The diff coverage is `6.25%`.
[](https://codecov.io/gh/huggingface/transformers/pull/1509?src=pr&el=tree)
```diff
@@ Coverage Diff @@
## master #1509 +/- ##
==========================================
- Coverage 85.98% 84.74% -1.25%
==========================================
Files 91 91
Lines 13579 13594 +15
==========================================
- Hits 11676 11520 -156
- Misses 1903 2074 +171
```
| [Impacted Files](https://codecov.io/gh/huggingface/transformers/pull/1509?src=pr&el=tree) | Coverage Δ | |
|---|---|---|
| [transformers/modeling\_tf\_pytorch\_utils.py](https://codecov.io/gh/huggingface/transformers/pull/1509/diff?src=pr&el=tree#diff-dHJhbnNmb3JtZXJzL21vZGVsaW5nX3RmX3B5dG9yY2hfdXRpbHMucHk=) | `9.85% <0%> (-66.91%)` | :arrow_down: |
| [transformers/tests/modeling\_tf\_common\_test.py](https://codecov.io/gh/huggingface/transformers/pull/1509/diff?src=pr&el=tree#diff-dHJhbnNmb3JtZXJzL3Rlc3RzL21vZGVsaW5nX3RmX2NvbW1vbl90ZXN0LnB5) | `79.78% <6.66%> (-16.75%)` | :arrow_down: |
| [transformers/modeling\_roberta.py](https://codecov.io/gh/huggingface/transformers/pull/1509/diff?src=pr&el=tree#diff-dHJhbnNmb3JtZXJzL21vZGVsaW5nX3JvYmVydGEucHk=) | `65.46% <0%> (-15.11%)` | :arrow_down: |
| [transformers/modeling\_xlnet.py](https://codecov.io/gh/huggingface/transformers/pull/1509/diff?src=pr&el=tree#diff-dHJhbnNmb3JtZXJzL21vZGVsaW5nX3hsbmV0LnB5) | `70.87% <0%> (-2.46%)` | :arrow_down: |
| [transformers/modeling\_ctrl.py](https://codecov.io/gh/huggingface/transformers/pull/1509/diff?src=pr&el=tree#diff-dHJhbnNmb3JtZXJzL21vZGVsaW5nX2N0cmwucHk=) | `93.18% <0%> (-2.28%)` | :arrow_down: |
| [transformers/modeling\_openai.py](https://codecov.io/gh/huggingface/transformers/pull/1509/diff?src=pr&el=tree#diff-dHJhbnNmb3JtZXJzL21vZGVsaW5nX29wZW5haS5weQ==) | `80.4% <0%> (-1.36%)` | :arrow_down: |
------
[Continue to review full report at Codecov](https://codecov.io/gh/huggingface/transformers/pull/1509?src=pr&el=continue).
> **Legend** - [Click here to learn more](https://docs.codecov.io/docs/codecov-delta)
> `Δ = absolute <relative> (impact)`, `ø = not affected`, `? = missing data`
> Powered by [Codecov](https://codecov.io/gh/huggingface/transformers/pull/1509?src=pr&el=footer). Last update [a701c9b...898ce06](https://codecov.io/gh/huggingface/transformers/pull/1509?src=pr&el=lastupdated). Read the [comment docs](https://docs.codecov.io/docs/pull-request-comments).
<|||||>Oh nice catch. Let's add a test on `load_tf2_checkpoint_in_pytorch_model` to catch such errors going forward.<|||||>Ok, merging, thanks<|||||>I was wondering where I should add that test and saw you just did it yourself :) thanks
|
transformers | 1,508 | closed | Added performance enhancements (XLA, AMP) to examples | Summary of changes
- Minor enhancements to `run_tf_glue.py` (e.g. calculate train/val steps from number of train/val examples, standardize quotes etc.)
- Added option for mixed precision (Automatic Mixed Precision / AMP) to run models on Tensor Cores (NVIDIA Volta/Turing GPUs) and future hardware
- Added option for XLA, which uses the XLA compiler to reduce model runtime
- Options are toggled using `USE_XLA` or `USE_AMP`
Quick benchmarks from the script (no other modifications):
| GPU | Mode | Time (2nd epoch) | Val Acc (3 runs) |
| --------- | -------- | ----------------------- | ----------------------|
| Titan V | FP32 | 41s | 0.8438/0.8281/0.8333 |
| Titan V | AMP | 26s | 0.8281/0.8568/0.8411 |
| V100 | FP32 | 35s | 0.8646/0.8359/0.8464 |
| V100 | AMP | 22s | 0.8646/0.8385/0.8411 |
| 1080 Ti | FP32 | 55s | - |
Mixed precision (AMP) reduces the training time considerably for the same hardware and hyper-parameters (same batch size was used).
>**Important Note**
>
>Unrelated to this PR, but restoring the PyTorch model for the TF2 saved model does not work. This does not work in the original, unmodified example script. [Here](https://github.com/huggingface/transformers/blob/master/transformers/modeling_tf_pytorch_utils.py#L201) is the offending line in the Transformers library that references a uninitialized variable. This is fixed by PR #1509
Feedback and comments welcome!
Related: Issue #1441 | 10-13-2019 13:17:50 | 10-13-2019 13:17:50 | # [Codecov](https://codecov.io/gh/huggingface/transformers/pull/1508?src=pr&el=h1) Report
> Merging [#1508](https://codecov.io/gh/huggingface/transformers/pull/1508?src=pr&el=desc) into [master](https://codecov.io/gh/huggingface/transformers/commit/a701c9b32126f1e6974d9fcb3a5c3700527d8559?src=pr&el=desc) will **not change** coverage.
> The diff coverage is `n/a`.
[](https://codecov.io/gh/huggingface/transformers/pull/1508?src=pr&el=tree)
```diff
@@ Coverage Diff @@
## master #1508 +/- ##
=======================================
Coverage 85.98% 85.98%
=======================================
Files 91 91
Lines 13579 13579
=======================================
Hits 11676 11676
Misses 1903 1903
```
------
[Continue to review full report at Codecov](https://codecov.io/gh/huggingface/transformers/pull/1508?src=pr&el=continue).
> **Legend** - [Click here to learn more](https://docs.codecov.io/docs/codecov-delta)
> `Δ = absolute <relative> (impact)`, `ø = not affected`, `? = missing data`
> Powered by [Codecov](https://codecov.io/gh/huggingface/transformers/pull/1508?src=pr&el=footer). Last update [a701c9b...2c1d556](https://codecov.io/gh/huggingface/transformers/pull/1508?src=pr&el=lastupdated). Read the [comment docs](https://docs.codecov.io/docs/pull-request-comments).
<|||||>Great, looks good to me cc @LysandreJik.
Adding the info of the PR in the example README.<|||||>Ok, merging, thanks @tlkh! |
transformers | 1,507 | closed | GPU Usage? | **Question**
> Note the query/issue might not have anything to do with the library as such, just looking for info as to why it will happen. Thanks for understanding.
- Why would the GPU show a usage[verified using `nvidia-smi`] of 420MB/32GB when i import `transformers`?
Note this only happens when i have `tensorflow-gpu 2.0` version in the same enviornment, otherwise it just works normally.
Pytorch v1.3.0
Transformers v2.1.1
tf-gpu v2.0.0
apex 0.1
GPU Tesla V100-SXM2-32GB
NVIDIA-SMI 410.79
Driver Version: 410.79
CUDA Version: 10.0
Python 3.7.3
Thanks.
(Sorry for a vague title and a quey)
Extra Outputs when i import transformers
```
Python 3.7.3 (default, Mar 27 2019, 22:11:17)
[GCC 7.3.0] :: Anaconda, Inc. on linux
Type "help", "copyright", "credits" or "license" for more information.
>>> import transformers
2019-10-12 18:33:00.840558: I tensorflow/stream_executor/platform/default/dso_loader.cc:44] Successfully opened dynamic library libcuda.so.1
2019-10-12 18:33:00.881658: I tensorflow/core/common_runtime/gpu/gpu_device.cc:1618] Found device 0 with properties:
name: Tesla V100-SXM2-32GB major: 7 minor: 0 memoryClockRate(GHz): 1.53
pciBusID: 0000:b5:00.0
2019-10-12 18:33:00.881762: W tensorflow/stream_executor/platform/default/dso_loader.cc:55] Could not load dynamic library 'libcudart.so.10.0'; dlerror: libcudart.so.10.0: cannot open shared object file: No such file or directory
2019-10-12 18:33:00.881816: W tensorflow/stream_executor/platform/default/dso_loader.cc:55] Could not load dynamic library 'libcublas.so.10.0'; dlerror: libcublas.so.10.0: cannot open shared object file: No such file or directory
2019-10-12 18:33:00.881854: W tensorflow/stream_executor/platform/default/dso_loader.cc:55] Could not load dynamic library 'libcufft.so.10.0'; dlerror: libcufft.so.10.0: cannot open shared object file: No such file or directory
2019-10-12 18:33:00.881892: W tensorflow/stream_executor/platform/default/dso_loader.cc:55] Could not load dynamic library 'libcurand.so.10.0'; dlerror: libcurand.so.10.0: cannot open shared object file: No such file or directory
2019-10-12 18:33:00.881931: W tensorflow/stream_executor/platform/default/dso_loader.cc:55] Could not load dynamic library 'libcusolver.so.10.0'; dlerror: libcusolver.so.10.0: cannot open shared object file: No such file or directory
2019-10-12 18:33:00.881967: W tensorflow/stream_executor/platform/default/dso_loader.cc:55] Could not load dynamic library 'libcusparse.so.10.0'; dlerror: libcusparse.so.10.0: cannot open shared object file: No such file or directory
2019-10-12 18:33:00.882003: W tensorflow/stream_executor/platform/default/dso_loader.cc:55] Could not load dynamic library 'libcudnn.so.7'; dlerror: libcudnn.so.7: cannot open shared object file: No such file or directory
2019-10-12 18:33:00.882015: W tensorflow/core/common_runtime/gpu/gpu_device.cc:1641] Cannot dlopen some GPU libraries. Please make sure the missing libraries mentioned above are installed properly if you would like to use GPU. Follow the guide at https://www.tensorflow.org/install/gpu for how to download and setup the required libraries for your platform.
Skipping registering GPU devices...
2019-10-12 18:33:00.882328: I tensorflow/core/platform/cpu_feature_guard.cc:142] Your CPU supports instructions that this TensorFlow binary was not compiled to use: AVX2 AVX512F FMA
2019-10-12 18:33:00.909789: I tensorflow/core/platform/profile_utils/cpu_utils.cc:94] CPU Frequency: 2400000000 Hz
2019-10-12 18:33:00.916559: I tensorflow/compiler/xla/service/service.cc:168] XLA service 0x55b9ec5e0b90 executing computations on platform Host. Devices:
2019-10-12 18:33:00.916618: I tensorflow/compiler/xla/service/service.cc:175] StreamExecutor device (0): Host, Default Version
2019-10-12 18:33:01.606656: I tensorflow/compiler/xla/service/service.cc:168] XLA service 0x55b9ec644220 executing computations on platform CUDA. Devices:
2019-10-12 18:33:01.606754: I tensorflow/compiler/xla/service/service.cc:175] StreamExecutor device (0): Tesla V100-SXM2-32GB, Compute Capability 7.0
2019-10-12 18:33:01.607078: I tensorflow/core/common_runtime/gpu/gpu_device.cc:1159] Device interconnect StreamExecutor with strength 1 edge matrix:
2019-10-12 18:33:01.607115: I tensorflow/core/common_runtime/gpu/gpu_device.cc:1165]
>>>
```
| 10-12-2019 18:33:13 | 10-12-2019 18:33:13 | the same here.
installing last apex code from repository: git clone https://github.com/NVIDIA/apex
it says it's apex-0.1 version, but i think it should say apex-1.0<|||||>I run into this problem while trying to create virtual GPU devices:
```python
import tensorflow as tf
import transformers
devices = tf.config.experimental.list_physical_devices('GPU')
tf.config.experimental.set_virtual_device_configuration(devices[0], [tf.config.experimental.VirtualDeviceConfiguration(memory_limit=1024)])
```
that ends up with error:
```
RuntimeError Traceback (most recent call last)
<ipython-input-4-d51e3d242817> in <module>
----> 1 tf.config.experimental.set_virtual_device_configuration(devices[0], [tf.config.experimental.VirtualDeviceConfiguration(memory_limit=1024)])
~/miniconda3/envs/transformers/lib/python3.7/site-packages/tensorflow_core/python/framework/config.py in set_virtual_device_configuration(device, virtual_devices)
554 virtual_devices: (optional) Need to update
555 """
--> 556 context.context().set_virtual_device_configuration(device, virtual_devices)
~/miniconda3/envs/transformers/lib/python3.7/site-packages/tensorflow_core/python/eager/context.py in set_virtual_device_configuration(self, dev, virtual_devices)
1269 if self._context_handle is not None:
1270 raise RuntimeError(
-> 1271 "Virtual devices cannot be modified after being initialized")
1272
1273 self._virtual_device_map[dev] = virtual_devices
RuntimeError: Virtual devices cannot be modified after being initialized
```
versions:
* Platform Linux-5.0.9-050009-generic-x86_64-with-debian-buster-sid
* Python 3.7.5 (default, Oct 25 2019, 15:51:11)
* [GCC 7.3.0]
* PyTorch 1.2.0
* Tensorflow 2.0.0
The reason is the use of class variable `dummy_inputs` in `transformers/modeling_tf_utils.py:54` where tensorflow is initialized (and starts using GPU) at import time. I created a [PR](https://github.com/huggingface/transformers/pull/1735) that should fix this.<|||||>Thanks for the PR and figuring it where the issue lied.<|||||>This issue has been automatically marked as stale because it has not had recent activity. It will be closed if no further activity occurs. Thank you for your contributions.
|
transformers | 1,506 | closed | Seq2Seq model with HugginFace | Hi
I am looking for a Seq2Seq model which is based on HuggingFace BERT model, I know fairseq has some implementation, but they are generally to me not very clean or easy to use, and I am looking for some good implementation based on HuggingFace work, thanks a lot for your help | 10-12-2019 12:43:14 | 10-12-2019 12:43:14 | Hey @juliahane, glad you’re asking: I am currently working on this (See PR #1455) Stay tuned! Closing this as it is not an issue per se.<|||||>Hi Remi
thanks a lot for the great work, since I need it for a deadline approaching
very soon, I would really appreciate
if you may know approximately when could be possible to use?
thanks a lot again for your efforts.
Best regards
Julia
On Sun, Oct 13, 2019 at 7:29 PM Rémi Louf <[email protected]> wrote:
> Closed #1506 <https://github.com/huggingface/transformers/issues/1506>.
>
> —
> You are receiving this because you were mentioned.
> Reply to this email directly, view it on GitHub
> <https://github.com/huggingface/transformers/issues/1506?email_source=notifications&email_token=AM3GZM3GKOHDP6CEUFCVFY3QONLGJA5CNFSM4JACXTZKYY3PNVWWK3TUL52HS4DFWZEXG43VMVCXMZLOORHG65DJMZUWGYLUNFXW5KTDN5WW2ZLOORPWSZGOUF2MLRY#event-2708784583>,
> or unsubscribe
> <https://github.com/notifications/unsubscribe-auth/AM3GZM5SYZMX4IAXP4YJNUDQONLGJANCNFSM4JACXTZA>
> .
>
<|||||>Hi, probably not in time for your deadline. We are expecting a first working version in a few weeks.<|||||>Hi Thomas,
I really need to make this code working for a deadline, I really appreciate to point me to the current existing implementations you may be aware of which I could use for now, thank you so much for your help<|||||>@thomwolf , I see you have run_lm_finetuning.py script, can I use this script for seq2seq generation task? Does it work for this purpose? thanks<|||||>Hi @juliahane, no you cannot use `run_lm_finetuning` for seq2seq generation.
If you cannot wait, I think this repo is a good place to start. It's based on our library and specifically target seq2seq for summarization: https://github.com/nlpyang/PreSumm<|||||>Let's keep this issue open to gather all threads asking about seq2seq in the repo.<|||||>> Hi Thomas,
> I really need to make this code working for a deadline, I really appreciate to point me to the current existing implementations you may be aware of which I could use for now, thank you so much for your help
You can have a look at PR #1455 . What you're looking for is in the `modeling_seq2seq.py` and `run_seq2seq_finetuning.py` scripts. This only works for Bert at the moment.<|||||>Hi
thanks a lot for the response, I cannot see the files, I really
appreciate sharing the files with me, thanks
On Tue, Oct 22, 2019 at 9:21 PM Rémi Louf <[email protected]> wrote:
> Hi Thomas,
> I really need to make this code working for a deadline, I really
> appreciate to point me to the current existing implementations you may be
> aware of which I could use for now, thank you so much for your help
>
> You can have a look at PR #1455
> <https://github.com/huggingface/transformers/pull/1455> . What you're
> looking for is in the modeling_seq2seq.py and run_seq2seq_finetuning.py
> scripts. This only works for Bert at the moment.
>
> —
> You are receiving this because you were mentioned.
> Reply to this email directly, view it on GitHub
> <https://github.com/huggingface/transformers/issues/1506?email_source=notifications&email_token=AM3GZM6WIWPHCKAUXPHXTM3QP5HCDA5CNFSM4JACXTZKYY3PNVWWK3TUL52HS4DFVREXG43VMVBW63LNMVXHJKTDN5WW2ZLOORPWSZGOEB64OFI#issuecomment-545113877>,
> or unsubscribe
> <https://github.com/notifications/unsubscribe-auth/AM3GZMZ4LJJNLRIT6LOL5GDQP5HCDANCNFSM4JACXTZA>
> .
>
<|||||>BERT is sufficient for me, I really appreciate sharing the files and
telling me the commands to run them, thanks
On Wed, Oct 23, 2019 at 11:25 PM julia hane <[email protected]> wrote:
> Hi
> thanks a lot for the response, I cannot see the files, I really
> appreciate sharing the files with me, thanks
>
> On Tue, Oct 22, 2019 at 9:21 PM Rémi Louf <[email protected]>
> wrote:
>
>> Hi Thomas,
>> I really need to make this code working for a deadline, I really
>> appreciate to point me to the current existing implementations you may be
>> aware of which I could use for now, thank you so much for your help
>>
>> You can have a look at PR #1455
>> <https://github.com/huggingface/transformers/pull/1455> . What you're
>> looking for is in the modeling_seq2seq.py and run_seq2seq_finetuning.py
>> scripts. This only works for Bert at the moment.
>>
>> —
>> You are receiving this because you were mentioned.
>> Reply to this email directly, view it on GitHub
>> <https://github.com/huggingface/transformers/issues/1506?email_source=notifications&email_token=AM3GZM6WIWPHCKAUXPHXTM3QP5HCDA5CNFSM4JACXTZKYY3PNVWWK3TUL52HS4DFVREXG43VMVBW63LNMVXHJKTDN5WW2ZLOORPWSZGOEB64OFI#issuecomment-545113877>,
>> or unsubscribe
>> <https://github.com/notifications/unsubscribe-auth/AM3GZMZ4LJJNLRIT6LOL5GDQP5HCDANCNFSM4JACXTZA>
>> .
>>
>
<|||||>Hi Remi
I really appreciate providing me with the command that I could get this
pull request in my installed huggingface library, thanks
Best
Julia
On Tue, Oct 22, 2019 at 9:21 PM Rémi Louf <[email protected]> wrote:
> Hi Thomas,
> I really need to make this code working for a deadline, I really
> appreciate to point me to the current existing implementations you may be
> aware of which I could use for now, thank you so much for your help
>
> You can have a look at PR #1455
> <https://github.com/huggingface/transformers/pull/1455> . What you're
> looking for is in the modeling_seq2seq.py and run_seq2seq_finetuning.py
> scripts. This only works for Bert at the moment.
>
> —
> You are receiving this because you were mentioned.
> Reply to this email directly, view it on GitHub
> <https://github.com/huggingface/transformers/issues/1506?email_source=notifications&email_token=AM3GZM6WIWPHCKAUXPHXTM3QP5HCDA5CNFSM4JACXTZKYY3PNVWWK3TUL52HS4DFVREXG43VMVBW63LNMVXHJKTDN5WW2ZLOORPWSZGOEB64OFI#issuecomment-545113877>,
> or unsubscribe
> <https://github.com/notifications/unsubscribe-auth/AM3GZMZ4LJJNLRIT6LOL5GDQP5HCDANCNFSM4JACXTZA>
> .
>
<|||||>```
git checkout --track origin/conditional-generation
```
Should work if you cloned the original repository.
However I am afraid we cannot provide support for work that has not made its way into the library yet as the interface is very likely to change.<|||||>Hi Remi
I was trying to run the bert seq2seq based codes, It gots a lot of errors,
I really appreciate if you could run it, and
making sure BERT one works, thanks a lot
On Tue, Oct 22, 2019 at 9:21 PM Rémi Louf <[email protected]> wrote:
> Hi Thomas,
> I really need to make this code working for a deadline, I really
> appreciate to point me to the current existing implementations you may be
> aware of which I could use for now, thank you so much for your help
>
> You can have a look at PR #1455
> <https://github.com/huggingface/transformers/pull/1455> . What you're
> looking for is in the modeling_seq2seq.py and run_seq2seq_finetuning.py
> scripts. This only works for Bert at the moment.
>
> —
> You are receiving this because you were mentioned.
> Reply to this email directly, view it on GitHub
> <https://github.com/huggingface/transformers/issues/1506?email_source=notifications&email_token=AM3GZM6WIWPHCKAUXPHXTM3QP5HCDA5CNFSM4JACXTZKYY3PNVWWK3TUL52HS4DFVREXG43VMVBW63LNMVXHJKTDN5WW2ZLOORPWSZGOEB64OFI#issuecomment-545113877>,
> or unsubscribe
> <https://github.com/notifications/unsubscribe-auth/AM3GZMZ4LJJNLRIT6LOL5GDQP5HCDANCNFSM4JACXTZA>
> .
>
<|||||>Hi Remi
Sure, I understand you cannot provide support for ongoing work, I anyway
have a deadline and will need to use it,
could you tell me please just how much this code is tested? Does it work
for BERT? what I saw the code had
several bugs in the optimizer part and does not run, I really appreciate if
you could just tell me how much this
code is tested
thanks
On Fri, Oct 25, 2019 at 12:15 PM Rémi Louf <[email protected]> wrote:
> https://stackoverflow.com/questions/9537392/git-fetch-remote-branch
>
> The name of the branch is conditional-generation. However I am afraid we
> cannot provide support for ongoing work that has not made its way into the
> library yet.
>
> —
> You are receiving this because you were mentioned.
> Reply to this email directly, view it on GitHub
> <https://github.com/huggingface/transformers/issues/1506?email_source=notifications&email_token=AM3GZM77Y7LXZSDFIIOE26TQQLBL7A5CNFSM4JACXTZKYY3PNVWWK3TUL52HS4DFVREXG43VMVBW63LNMVXHJKTDN5WW2ZLOORPWSZGOECH4WBQ#issuecomment-546294534>,
> or unsubscribe
> <https://github.com/notifications/unsubscribe-auth/AM3GZM5CMVSXO56YSYEYPILQQLBL7ANCNFSM4JACXTZA>
> .
>
<|||||>Hi Remi
I made this work, could you please tell me how can I get the generated
sequence from decoder please?
thanks
<|||||>Hi Thomas
Remi was saying in PR:#1455 it has the bert seq2seq ready, could you move in a gradual way please? So merging the codes for BERT already so people can use the BERT one, this is already great, then after a while when this is ready for also other encoders, add them later, I really appreciate adding the BERT ones thanks <|||||>#1455 was merged and it is now possible to define and train encoder-decoder models. Only Bert is supported at the moment.<|||||>Hi Remi and thomas
Thank you so much for the great help, this is awesome, and I would like to
really appreciate your hard work,
Best regards
Julia
On Wed, Oct 30, 2019 at 5:47 PM Rémi Louf <[email protected]> wrote:
> #1455 <https://github.com/huggingface/transformers/pull/1455> was merged
> and it is now possible to define and train encoder-decoder models. Only
> Bert is supported at the moment.
>
> —
> You are receiving this because you were mentioned.
> Reply to this email directly, view it on GitHub
> <https://github.com/huggingface/transformers/issues/1506?email_source=notifications&email_token=AM3GZM6IFGCLINEDGL6ELWLQRG3CLA5CNFSM4JACXTZKYY3PNVWWK3TUL52HS4DFVREXG43VMVBW63LNMVXHJKTDN5WW2ZLOORPWSZGOECU5W6A#issuecomment-548002680>,
> or unsubscribe
> <https://github.com/notifications/unsubscribe-auth/AM3GZMY5O7QGB55RCHUSCP3QRG3CLANCNFSM4JACXTZA>
> .
>
<|||||>Hi
I was wondering if you could give some explanations how the decoder part
work, I see this is a masked language model head BERT,
used as decoder, I think masked language model head bert mask some part and
predict specific masked tokens,
I am not sure how this work as a generation module, thanks for clarifying.
On Wed, Oct 30, 2019 at 8:47 PM julia hane <[email protected]> wrote:
> Hi Remi and thomas
> Thank you so much for the great help, this is awesome, and I would like to
> really appreciate your hard work,
> Best regards
> Julia
>
> On Wed, Oct 30, 2019 at 5:47 PM Rémi Louf <[email protected]>
> wrote:
>
>> #1455 <https://github.com/huggingface/transformers/pull/1455> was merged
>> and it is now possible to define and train encoder-decoder models. Only
>> Bert is supported at the moment.
>>
>> —
>> You are receiving this because you were mentioned.
>> Reply to this email directly, view it on GitHub
>> <https://github.com/huggingface/transformers/issues/1506?email_source=notifications&email_token=AM3GZM6IFGCLINEDGL6ELWLQRG3CLA5CNFSM4JACXTZKYY3PNVWWK3TUL52HS4DFVREXG43VMVBW63LNMVXHJKTDN5WW2ZLOORPWSZGOECU5W6A#issuecomment-548002680>,
>> or unsubscribe
>> <https://github.com/notifications/unsubscribe-auth/AM3GZMY5O7QGB55RCHUSCP3QRG3CLANCNFSM4JACXTZA>
>> .
>>
>
<|||||>> #1455 was merged and it is now possible to define and train encoder-decoder models. Only Bert is supported at the moment.
Hi Remi, I posted some bugs/suggestions about this code at #1674, thanks <|||||>Hi
when I run this code I got this erorr, thanks for help.
File "/user/julia/dev/temp/transformers/examples/utils_summarization.py",
line 143, in encode_for_summarization
for line in story_lines
File "/user/julia/dev/temp/transformers/examples/utils_summarization.py",
line 143, in <listcomp>
for line in story_lines
AttributeError: 'BertTokenizer' object has no attribute
'add_special_tokens_single_sequence'
On Fri, Nov 1, 2019 at 12:08 PM Rabeeh Karimi Mahabadi <
[email protected]> wrote:
> #1455 <https://github.com/huggingface/transformers/pull/1455> was merged
> and it is now possible to define and train encoder-decoder models. Only
> Bert is supported at the moment.
>
> Hi Remi, I posted some bugs/suggestions about this code at #1674
> <https://github.com/huggingface/transformers/issues/1674>, thanks
>
> —
> You are receiving this because you were mentioned.
> Reply to this email directly, view it on GitHub
> <https://github.com/huggingface/transformers/issues/1506?email_source=notifications&email_token=AM3GZM6BVCAVV3DJCFEBAJ3QRQE3PA5CNFSM4JACXTZKYY3PNVWWK3TUL52HS4DFVREXG43VMVBW63LNMVXHJKTDN5WW2ZLOORPWSZGOEC2UPNQ#issuecomment-548751286>,
> or unsubscribe
> <https://github.com/notifications/unsubscribe-auth/AM3GZMYWFHZTBG7CP2BGOR3QRQE3PANCNFSM4JACXTZA>
> .
>
<|||||>Hi
can you please also add a way to see the generated sequences? thanks
On Fri, Nov 1, 2019 at 3:19 PM julia hane <[email protected]> wrote:
> Hi
> when I run this code I got this erorr, thanks for help.
>
> File "/user/julia/dev/temp/transformers/examples/utils_summarization.py",
> line 143, in encode_for_summarization
> for line in story_lines
> File
> "/user/julia/dev/temp/transformers/examples/utils_summarization.py", line
> 143, in <listcomp>
> for line in story_lines
> AttributeError: 'BertTokenizer' object has no attribute
> 'add_special_tokens_single_sequence'
>
> On Fri, Nov 1, 2019 at 12:08 PM Rabeeh Karimi Mahabadi <
> [email protected]> wrote:
>
>> #1455 <https://github.com/huggingface/transformers/pull/1455> was merged
>> and it is now possible to define and train encoder-decoder models. Only
>> Bert is supported at the moment.
>>
>> Hi Remi, I posted some bugs/suggestions about this code at #1674
>> <https://github.com/huggingface/transformers/issues/1674>, thanks
>>
>> —
>> You are receiving this because you were mentioned.
>> Reply to this email directly, view it on GitHub
>> <https://github.com/huggingface/transformers/issues/1506?email_source=notifications&email_token=AM3GZM6BVCAVV3DJCFEBAJ3QRQE3PA5CNFSM4JACXTZKYY3PNVWWK3TUL52HS4DFVREXG43VMVBW63LNMVXHJKTDN5WW2ZLOORPWSZGOEC2UPNQ#issuecomment-548751286>,
>> or unsubscribe
>> <https://github.com/notifications/unsubscribe-auth/AM3GZMYWFHZTBG7CP2BGOR3QRQE3PANCNFSM4JACXTZA>
>> .
>>
>
<|||||>If both your source are target belong to same language (summarization etc.):
Well, with a next word prediction language model like GPT2, you can just create a dataset like "source [SEP] target" and the run the LM (```run_lm_finetuning.py```) on it. During test time, you can provide "source [SEP]" as your prompt and you will get "target" as your prediction.
One small thing that you can do is mask your source tokens in the loss computation because you don't want to predict the source tokens as well! This will give you better performance and results.
This is not much different that Seq2Seq I believe. You are sharing the same parameters for source and target.<|||||>> #1455 was merged and it is now possible to define and train encoder-decoder models. Only Bert is supported at the moment.
could you tell me how to get the two file modeling_seq2seq.py and run_seq2seq_finetuning.py,
so l could fine tune seq2seq model with pretrained encode model like bert?<|||||>any news about seq2seq training script using transformers? |
transformers | 1,505 | closed | Fixed the sample code in the title 'Quick tour'. | The variable pretrained_weights was fixed to 'bert-base-uncased' to be used in each model to experiment. Otherwise, the last value of this variable in the previous loop was unintentionally effective in this loop which was causing throwing error. | 10-12-2019 11:20:22 | 10-12-2019 11:20:22 | # [Codecov](https://codecov.io/gh/huggingface/transformers/pull/1505?src=pr&el=h1) Report
> Merging [#1505](https://codecov.io/gh/huggingface/transformers/pull/1505?src=pr&el=desc) into [master](https://codecov.io/gh/huggingface/transformers/commit/a701c9b32126f1e6974d9fcb3a5c3700527d8559?src=pr&el=desc) will **not change** coverage.
> The diff coverage is `n/a`.
[](https://codecov.io/gh/huggingface/transformers/pull/1505?src=pr&el=tree)
```diff
@@ Coverage Diff @@
## master #1505 +/- ##
=======================================
Coverage 85.98% 85.98%
=======================================
Files 91 91
Lines 13579 13579
=======================================
Hits 11676 11676
Misses 1903 1903
```
------
[Continue to review full report at Codecov](https://codecov.io/gh/huggingface/transformers/pull/1505?src=pr&el=continue).
> **Legend** - [Click here to learn more](https://docs.codecov.io/docs/codecov-delta)
> `Δ = absolute <relative> (impact)`, `ø = not affected`, `? = missing data`
> Powered by [Codecov](https://codecov.io/gh/huggingface/transformers/pull/1505?src=pr&el=footer). Last update [a701c9b...5a8c6e7](https://codecov.io/gh/huggingface/transformers/pull/1505?src=pr&el=lastupdated). Read the [comment docs](https://docs.codecov.io/docs/pull-request-comments).
<|||||>Thanks! |
transformers | 1,504 | closed | Fine-tuning with run_squad.py, Transformers 2.1.1 & PyTorch 1.3.0 Data Parallel Error | ## 🐛 Bug
Error message when fine-tuning BERT or XLNet on SQuAD1.1 or 2.0 with dual 1080Ti GPUs:
_"RuntimeError: module must have its parameters and buffers on device cuda:0 (device_ids[0]) but found one of them on device: cuda:1"_
Model I am using: BERT & XLNet
Language I am using the model on: English
The problem arise when using:
* [X] my own modified scripts: example script file below which ran successfully under previous PyTorch, PyTorch-Transformers, & Transformers versions.
The tasks I am working on is:
* [X] an official GLUE/SQUaD task: (give the name) SQuAD 1.1 & 2.0
## To Reproduce
One shell script (there are others) that had worked before:
SQUAD_DIR=/media/dn/dssd/nlp/squad1.1
python ./run_squad.py \
--model_type bert \
--model_name_or_path bert-base-uncased \
--do_train \
--do_eval \
--do_lower_case \
--train_file=${SQUAD_DIR}/train-v1.1.json \
--predict_file=${SQUAD_DIR}/dev-v1.1.json \
--per_gpu_eval_batch_size=8 \
--per_gpu_train_batch_size=8 \
--gradient_accumulation_steps=1 \
--learning_rate=3e-5 \
--num_train_epochs=2 \
--max_seq_length=384 \
--doc_stride=128 \
--save_steps=2000 \
--output_dir=./runs/bert_base_squad1_dp_ft_3 \
## Environment
* OS: Ubuntu 18.04, Linux kernel 4.15.0-65-generic
* Python version: 3.7.4
* PyTorch version: 1.3.0
* Transformers version: 2.1.1 built from latest source
* Using GPU? NVIDIA 1080Ti x 2
* Distributed or parallel setup? Data Parallel
* Any other relevant information: Have had many successful SQuAD fine-tuning runs on PyTorch 1.2.0 with Pytorch-Transformers 1.2.0, maybe even Transformers 2.0.0, and Apex 0.1. New environment built with the latest versions (Pytorch 1.3.0, Transformers 2.1.1) spawns data parallel related error above
| 10-12-2019 08:12:54 | 10-12-2019 08:12:54 | Runs are in a dedicated environment with only the following packages:
python 3.7.4
pytorch 1.3.0, install includes cudatoolkit 10.1
tensorflow_gpu 2.0 and dependencies
apex 0.1
transformers 2.1.1
Complete terminal output:
[output_term_ERROR.TXT](https://github.com/huggingface/transformers/files/3720906/output_term_ERROR.TXT)
<|||||>Change the line in run_**.py
device = torch.device("cuda" if torch.cuda.is_available() and not args.no_cuda else "cpu")
to
device = torch.device("cuda:0" if torch.cuda.is_available() and not args.no_cuda else "cpu").
In my environment, it works.<|||||>> Change the line in run_**.py
> device = torch.device("cuda" if torch.cuda.is_available() and not args.no_cuda else "cpu")
> to
> device = torch.device("cuda:0" if torch.cuda.is_available() and not args.no_cuda else "cpu").
>
> In my environment, it works.
It seems that all GPUs will still be used even if we specify "cuda:0" here. But I am not sure how much the other GPUs contribute to the computation. In my case, I have 8-way 1080ti but the other 7 are hardly fully loaded.
Does anyone compare the training speed with/without this error?<|||||>In my case, the solution is changing
```python
if args.n_gpu > 1:
model = torch.nn.DataParallel(model)
```
to
```python
if args.n_gpu > 1 and not isinstance(model, torch.nn.DataParallel):
model = torch.nn.DataParallel(model)
```
<|||||>> In my case, the solution is changing
>
> ```python
> if args.n_gpu > 1:
> model = torch.nn.DataParallel(model)
> ```
>
> to
>
> ```python
> if args.n_gpu > 1 and not isinstance(model, torch.nn.DataParallel):
> model = torch.nn.DataParallel(model)
> ```
changing this in evaluate function fixes the error, when i run with ```--evaluate_during_training```<|||||>This issue has been automatically marked as stale because it has not had recent activity. It will be closed if no further activity occurs. Thank you for your contributions.
<|||||>> In my case, the solution is changing
>
> ```python
> if args.n_gpu > 1:
> model = torch.nn.DataParallel(model)
> ```
>
> to
>
> ```python
> if args.n_gpu > 1 and not isinstance(model, torch.nn.DataParallel):
> model = torch.nn.DataParallel(model)
> ```
Agree, also notice that
`args.eval_batch_size = args.per_gpu_eval_batch_size * max(1, args.n_gpu)` is now multiplied by n_gpu again which is undesired <|||||>> In my case, the solution is changing
>
> ```python
> if args.n_gpu > 1:
> model = torch.nn.DataParallel(model)
> ```
>
> to
>
> ```python
> if args.n_gpu > 1 and not isinstance(model, torch.nn.DataParallel):
> model = torch.nn.DataParallel(model)
> ```
Thanks! I have met the same error in evaluation function. It works for me.<|||||>> > In my case, the solution is changing
> > ```python
> > if args.n_gpu > 1:
> > model = torch.nn.DataParallel(model)
> > ```
> >
> >
> > to
> > ```python
> > if args.n_gpu > 1 and not isinstance(model, torch.nn.DataParallel):
> > model = torch.nn.DataParallel(model)
> > ```
>
> changing this in evaluate function fixes the error, when i run with `--evaluate_during_training`
This solution fixed the issue for me. I am observing this while training a new LM using transformers 2.5.1. The issue happened during evaluation.<|||||>One more comment about this fixing. If you use a validation set with odd number of instances, it will raise an error on line`outputs = model(inputs, masked_lm_labels=labels) if args.mlm else model(inputs, labels=labels)`, if using run_language_modeling.py. This happens because the parall gpu needs two instances to be fed into.
I dont know how to fix properly. All I do is add a copy of instance of the last one to meet the number requirement.
> In my case, the solution is changing
>
> ```python
> if args.n_gpu > 1:
> model = torch.nn.DataParallel(model)
> ```
>
> to
>
> ```python
> if args.n_gpu > 1 and not isinstance(model, torch.nn.DataParallel):
> model = torch.nn.DataParallel(model)
> ```
<|||||>This issue has been automatically marked as stale because it has not had recent activity. It will be closed if no further activity occurs. Thank you for your contributions.
|
transformers | 1,503 | closed | What is the best way to handle sequences > max_len for tasks like abstract summarization? | What is the best way to handle situations where a sequence in your dataset exceeds the max length defined for a model?
For example, if I'm working on an abstract summarization task with a Bert model having a `max_position_embeddings=512` and tokenizer with `max_len=512`, how should I handle documents where the tokens to evaluate exceed 512?
Is there a recommended practice for this situation?
Thanks | 10-12-2019 00:40:50 | 10-12-2019 00:40:50 | Most people truncate the document at 512 tokens.
Most of the time it is enough. For example on CNNDM dataset, the lead-3 baseline give a pretty strong score, for simply using the first 3 sentences of the article as summary.
It indicates that most salient information are located at the beginning of the document (in this particular case).
---
But I'm also curious of the possible solutions to **really** handle longer sequences (truncating is not really handling it...)<|||||>Good information ... thanks.
Are any of the Transformer models available capable of summarization
tasks?
From what I can tell they all seem geared for classification, Language
modeling, question/answering type tasks.
On Sun, Oct 13, 2019 at 7:42 PM Cola <[email protected]> wrote:
> Most people truncate the document at 512 tokens.
>
> Most of the time it is enough. For example on CNNDM dataset, the lead-3
> baseline give a pretty strong score, for simply using the first 3 sentences
> of the article as summary.
>
> It indicates that most salient information are located at the beginning of
> the document (in this particular case).
>
> —
> You are receiving this because you authored the thread.
> Reply to this email directly, view it on GitHub
> <https://github.com/huggingface/transformers/issues/1503?email_source=notifications&email_token=AAADNMH4PSVJXDZXNZVS5STQOPL75A5CNFSM4JAAO2K2YY3PNVWWK3TUL52HS4DFVREXG43VMVBW63LNMVXHJKTDN5WW2ZLOORPWSZGOEBDHAEQ#issuecomment-541487122>,
> or unsubscribe
> <https://github.com/notifications/unsubscribe-auth/AAADNMHXAPKQLZKPX2DOALTQOPL75ANCNFSM4JAAO2KQ>
> .
>
<|||||>You can take a look at this repo :
https://github.com/nlpyang/PreSumm<|||||>Nice paper/code ... thanks much for your time and the link!
-wg
On Mon, Oct 14, 2019 at 4:29 PM Cola <[email protected]> wrote:
> You can take a look at this repo :
> https://github.com/nlpyang/PreSumm
>
> —
> You are receiving this because you authored the thread.
> Reply to this email directly, view it on GitHub
> <https://github.com/huggingface/transformers/issues/1503?email_source=notifications&email_token=AAADNMAWWMBWPF4K5EKTMMDQOT6HLA5CNFSM4JAAO2K2YY3PNVWWK3TUL52HS4DFVREXG43VMVBW63LNMVXHJKTDN5WW2ZLOORPWSZGOEBG5S6A#issuecomment-541972856>,
> or unsubscribe
> <https://github.com/notifications/unsubscribe-auth/AAADNMAKZ4LKHQJOUZKCOU3QOT6HLANCNFSM4JAAO2KQ>
> .
>
<|||||>@Colanim Indeed, for newspaper articles most of the information is contained in the first sentences. This is how journalists are taught to write! The dataset does not really push the models to their limits. If only longer pieces like New Yorker articles were available in a big dataset...
@ohmeow I am currently working on the implementation of several seq2seq models that use transformers, and our first example will be abstractive summarization (PR #1455 )
I am also curious about solutions to the finite number of tokens limit :)<|||||>> Good information ... thanks. Are any of the Transformer models available capable of summarization tasks? From what I can tell they all seem geared for classification, Language modeling, question/answering type tasks.
> […](#)
> On Sun, Oct 13, 2019 at 7:42 PM Cola ***@***.***> wrote: Most people truncate the document at 512 tokens. Most of the time it is enough. For example on CNNDM dataset, the lead-3 baseline give a pretty strong score, for simply using the first 3 sentences of the article as summary. It indicates that most salient information are located at the beginning of the document (in this particular case). — You are receiving this because you authored the thread. Reply to this email directly, view it on GitHub <#1503?email_source=notifications&email_token=AAADNMH4PSVJXDZXNZVS5STQOPL75A5CNFSM4JAAO2K2YY3PNVWWK3TUL52HS4DFVREXG43VMVBW63LNMVXHJKTDN5WW2ZLOORPWSZGOEBDHAEQ#issuecomment-541487122>, or unsubscribe <https://github.com/notifications/unsubscribe-auth/AAADNMHXAPKQLZKPX2DOALTQOPL75ANCNFSM4JAAO2KQ> .
maybe this repo also helps
https://github.com/caitian521/RCTransformer<|||||>Thanks Remi!
Yah I'm playing with your summarization code in huggingface as we speak.
Looking great! Would be nice to have fine-tuning scripts included for
reference as well.
Are you all working on implementing the extractive summarization and the
double-fine-tuning example for abstractive in the paper?
Thanks - wg
On Tue, Oct 15, 2019 at 12:32 PM Rémi Louf <[email protected]> wrote:
> @Colanim <https://github.com/Colanim> Indeed, for newspaper articles most
> of the information is contained in the first sentences. This is how
> journalists are taught to write! The dataset does not really push the
> models to their limits. If only longer pieces like New Yorker articles were
> available in a big dataset...
>
> @ohmeow <https://github.com/ohmeow> I am currently working on the
> implementation of several seq2seq models that use transformers, and our
> first example will be abstractive summarization (PR #1455
> <https://github.com/huggingface/transformers/pull/1455> )
>
> I am also curious about solutions to the finite number of tokens limit :)
>
> —
> You are receiving this because you were mentioned.
> Reply to this email directly, view it on GitHub
> <https://github.com/huggingface/transformers/issues/1503?email_source=notifications&email_token=AAADNMEZGOZPKRB4RN43H73QOYLEDA5CNFSM4JAAO2K2YY3PNVWWK3TUL52HS4DFVREXG43VMVBW63LNMVXHJKTDN5WW2ZLOORPWSZGOEBJ6M6A#issuecomment-542369400>,
> or unsubscribe
> <https://github.com/notifications/unsubscribe-auth/AAADNMAIQQQMAPB3F7BK5FLQOYLEDANCNFSM4JAAO2KQ>
> .
>
<|||||>Glad it works! This is not on the roadmap at the moment, but we may come back to it later.<|||||>This issue has been automatically marked as stale because it has not had recent activity. It will be closed if no further activity occurs. Thank you for your contributions.
|
transformers | 1,502 | closed | the working example code to use BertForQuestionAnswering | so we can use the pre-trained and fine-tuned on SQUAD Bert model to get an answer from a text and a question, similar to the way the CoreML model BERTSQUADFP16.mlmodel is used in the iOS example [Finding Answers to Questions in a Text Document](https://developer.apple.com/documentation/coreml/finding_answers_to_questions_in_a_text_document) | 10-12-2019 00:10:47 | 10-12-2019 00:10:47 | # [Codecov](https://codecov.io/gh/huggingface/transformers/pull/1502?src=pr&el=h1) Report
> Merging [#1502](https://codecov.io/gh/huggingface/transformers/pull/1502?src=pr&el=desc) into [master](https://codecov.io/gh/huggingface/transformers/commit/a701c9b32126f1e6974d9fcb3a5c3700527d8559?src=pr&el=desc) will **increase** coverage by `<.01%`.
> The diff coverage is `n/a`.
[](https://codecov.io/gh/huggingface/transformers/pull/1502?src=pr&el=tree)
```diff
@@ Coverage Diff @@
## master #1502 +/- ##
==========================================
+ Coverage 85.98% 85.98% +<.01%
==========================================
Files 91 91
Lines 13579 13574 -5
==========================================
- Hits 11676 11672 -4
+ Misses 1903 1902 -1
```
| [Impacted Files](https://codecov.io/gh/huggingface/transformers/pull/1502?src=pr&el=tree) | Coverage Δ | |
|---|---|---|
| [transformers/modeling\_bert.py](https://codecov.io/gh/huggingface/transformers/pull/1502/diff?src=pr&el=tree#diff-dHJhbnNmb3JtZXJzL21vZGVsaW5nX2JlcnQucHk=) | `88.17% <ø> (ø)` | :arrow_up: |
| [transformers/tests/modeling\_tf\_common\_test.py](https://codecov.io/gh/huggingface/transformers/pull/1502/diff?src=pr&el=tree#diff-dHJhbnNmb3JtZXJzL3Rlc3RzL21vZGVsaW5nX3RmX2NvbW1vbl90ZXN0LnB5) | `94.79% <0%> (-1.74%)` | :arrow_down: |
| [transformers/modeling\_tf\_auto.py](https://codecov.io/gh/huggingface/transformers/pull/1502/diff?src=pr&el=tree#diff-dHJhbnNmb3JtZXJzL21vZGVsaW5nX3RmX2F1dG8ucHk=) | `53.33% <0%> (+2.08%)` | :arrow_up: |
------
[Continue to review full report at Codecov](https://codecov.io/gh/huggingface/transformers/pull/1502?src=pr&el=continue).
> **Legend** - [Click here to learn more](https://docs.codecov.io/docs/codecov-delta)
> `Δ = absolute <relative> (impact)`, `ø = not affected`, `? = missing data`
> Powered by [Codecov](https://codecov.io/gh/huggingface/transformers/pull/1502?src=pr&el=footer). Last update [a701c9b...e76d715](https://codecov.io/gh/huggingface/transformers/pull/1502?src=pr&el=lastupdated). Read the [comment docs](https://docs.codecov.io/docs/pull-request-comments).
<|||||>Nice, thanks! |
transformers | 1,501 | closed | Issue with XLNet pretrained model | ## 🐛 Bug
<!-- Important information -->
Model I am using (Bert, XLNet....): XLNet
Language I am using the model on (English, Chinese....): English
The problem arise when using:
* [ ] the official example scripts: (give details)
* [X] my own modified scripts: (give details)
The tasks I am working on is:
* [ ] an official GLUE/SQUaD task: (give the name)
* [X] my own task or dataset: (give details)
## To Reproduce
I'm trying to train the XLNet dropping the last layer, I get error stating that
below is my code :
```
class xlmodel(nn.Module):
def __init__(self, xlnetModell):
super(xlmodel, self).__init__()
self.xlnetfeatures = nn.Sequential(*list(xlnetModel.children())[:-1])
self.concat = nn.Linear(786, 200)
self.predict = nn.Linear(200,2)
def forward(self, xlinput_ids, xlattention_mask, labels, xltoken_type_ids) :
inputs = { 'input_ids' : xlinput_ids , 'attention_mask' : xlattention_mask, 'token_type_ids' : xltoken_type_ids }
xlnet_output = self.xlnetfeatures(**inputs)
xl = nn.functional.relu((xlnet_output))
output = self.predict(xl)
return output
pretrained_weights = 'xlnet-base-cased'
xlnetmodel = XLNetForSequenceClassification.from_pretrained(pretrained_weights, num_labels=2)
model(xlnetmodel)
for _ in trange(num_train_epochs, desc="Epochs"):
ep_tr_loss, nb_tr_steps, eval_accuracy = 0, 0, 0
for step, batch in enumerate(train_data):
model.train()
batch = tuple(t.to(device) for t in batch)
# model.
inputs = {'xlinput_ids': batch[0], 'xlattention_mask': batch[1], 'labels': batch[3],
'xltoken_type_ids': batch[2] }
optimizer.zero_grad()
output = model(**inputs) -----> this is where error occurs
```
error stack :
```
xlnet_output = self.xlnetfeatures(**inputs)
File "E:\PycharmProjects\CommonSense\venv\lib\site-packages\torch\nn\modules\module.py", line 547, in __call__
result = self.forward(*input, **kwargs)
TypeError: forward() got an unexpected keyword argument 'input_ids'
Epochs: 0%| | 0/10 [00:10<?, ?it/s]
```
I even tried not passing the inputs as dictionary but still i get this error.
I verified the input variable name in pytorch xlnet transformer it has input_ids
Any lead will be appreciated thanks.
But if I try to run xlnet as it is works fine.
## Environment
* OS: Windows 10
* Python version: 3.7
* PyTorch version: 1.2.0
* PyTorch Transformers version (or branch): master
* Using GPU ? YEs
* Distributed of parallel setup ? No
* Any other relevant information:
| 10-11-2019 21:23:35 | 10-11-2019 21:23:35 | I think you have the wrong Base-Class imported? [Ref](https://github.com/huggingface/transformers/blob/a701c9b32126f1e6974d9fcb3a5c3700527d8559/transformers/modeling_xlnet.py#L959)
```
from transformers.modeling_xlnet import XLNetPreTrainedModel
class XLNetForSequenceClassification(XLNetPreTrainedModel):
def __init__(self, config):
super(XLNetForSequenceClassification, self).__init__(config)
....
....
```<|||||>@AdityaSoni19031997 Sorry for the delay.
I fixed the issue but I don't remember what was the issue though, anyways Thanks.
|
transformers | 1,500 | closed | How to load a different domain BERT-based pre-trained model? | I am trying to load the pre-trained model at pred/FinBERT-Pre2K_128MSL-500K [FinBERT](https://github.com/psnonis/FinBERT) and trying to run the basic task of SST-2 (sentiment classification) using run_glue.py (https://huggingface.co/transformers/examples.html#glue).
But I run into the following error:
OSError: Model name '/data/ftm/xgb_regr/FinBERT/pred/FinBERT-Pre2K_128MSL-250K' was not found in model name list (bert-base-uncased, bert-large-uncased, bert-base-cased, bert-large-cased, bert-base-multilingual-uncased, bert-base-multilingual-cased, bert-base-chinese, bert-base-german-cased, bert-large-uncased-whole-word-masking, bert-large-cased-whole-word-masking, bert-large-uncased-whole-word-masking-finetuned-squad, bert-large-cased-whole-word-masking-finetuned-squad, bert-base-cased-finetuned-mrpc, bert-base-german-dbmdz-cased, bert-base-german-dbmdz-uncased). We assumed '/data/ftm/xgb_regr/FinBERT/pred/FinBERT-Pre2K_128MSL-250K' was a path or url to a configuration file named config.json or a directory containing such a file but couldn't find any such file at this path or url.
Also, since this seems to be trained using TF, I was wondering if I can use PyTorch to load it.
Thanks. | 10-11-2019 20:11:49 | 10-11-2019 20:11:49 | You can use torch to load it, convert the weights using the helper files;
Not sure about your task, but for mine, i was using a BertModel with different pre-trained weights,
```
model = BertForSequenceClassification(MODEL_PATH, num_labels=len(np.unique(y_train_torch)))
```
(iirc from_tf is also a param to the function)
where `MODEL_PATH` is a directory that has
- config.json.
- your model [checkpoint/bin file].
- a vocab file as well.<|||||>Thank you for your reply.
The issue is a little different. All the 3 files: config.json, checkpoint, and vocab.txt are linked by a symbolic link in their repo. I am not sure how to get the actual files. Any suggestions for such a case?<|||||>Well if you are running the experiments yourself, you will be downloading them either ways, just make changes where ever needed?
(i haven't tried passing a symbolic link to this func so not sure myself but it should work imo as well)<|||||>This issue has been automatically marked as stale because it has not had recent activity. It will be closed if no further activity occurs. Thank you for your contributions.
|
transformers | 1,499 | closed | model.to(args.device) in run_glue.py taking around 10 minutes. Is this normal? | ## ❓ Questions & Help
Currently line 484 of run_glue.py `model.to(args.device)` is taking close to 10 minutes to complete when loading the bert-base pretrained model. This seems like a long time compared to what I was seeing in pytorch-transformers.
My configuration:
Tesla V100 - Driver 418.87.00
Cuda toolkit 10.1
PyTorch 1.3.0
The code I am running is:
`python example/run_glue.py \
--model_type bert \
--model_name_or_path bert-base-uncased \
--task_name $(MY TASK) \
--do_train \
--do_eval \
--do_lower_case \
--data_dir $(MY_DIR) \
--max_seq_length 128 \
--per_gpu_eval_batch_size=64 \
--per_gpu_train_batch_size=64 \
--learning_rate 2e-5 \
--num_train_epochs 3.0 \
--output_dir $(MY_OUTDIR) \
--overwrite_output_dir \
--fp16`
Is this behavior expected or am I doing something wrong? Thanks!
| 10-11-2019 17:22:36 | 10-11-2019 17:22:36 | This seems weird, I'm looking into this.<|||||>By running the run_glue.py script as it is right now with your exact parameters, I timed to model.to and it took 6.4 seconds<|||||>Ok, thanks for looking into that! I'm using my own dataset so I made adjustments to the processor, but I don't think that should be causing the issue when transferring the model to the GPU. I'll run a few more tests and see if I can pinpoint what is going on. It's super helpful to know that you are seeing it take only 6.4 seconds. Thank you!<|||||>I just tested again using the SST-2 data keeping the run_glue.py code as is and I'm still having the same issue. My guess is that there is something with my VM set up that's causing the hanging issue. I'm having a hard time identifying what might be the exact cause of the issue.<|||||>Hmm do you think you can reproduce it on another VM? Are you running into the same issue if you simply put the model on the device in a standalone script?<|||||>Ok, it's definitely an issue with my setup. I have the same issue when running the following:
`from torchvision import models
model = models.densenet121(pretrained=True)
model.to('cuda')`
I'll close the issue and keep troublehsooting on my end. Thanks!<|||||>Reopening because I found the issue and hopefully it can help someone else. I was comparing model loading times to what I was seeing on the hosted runtimes in Google Colab notebooks.
Even through they have cuda toolkit 10.1 installed as you can see when running the command !nvidia-smi, when you run torch.version.cuda they have 10.0.130 installed instead of the 10.1 version. They are also running pytorch 1.2.0.
I downgraded my environment to match and the model from models.densenet121(pretrained=True) loaded in 4.9 seconds.
Thanks for the help! |
transformers | 1,498 | closed | Merge pull request #1 from huggingface/master | from 1.0->1.1 | 10-11-2019 12:27:23 | 10-11-2019 12:27:23 | Can you check your workflow to stop opening/closing these PRs?<|||||>@thomwolf Yeah. I have checked it. It's really embarrased to opening/closing these PRs. |
transformers | 1,497 | closed | Merge pull request #1 from huggingface/master | from 1.0->1.1 | 10-11-2019 12:01:13 | 10-11-2019 12:01:13 | |
transformers | 1,496 | closed | Merge pull request #1 from huggingface/master | from 1.0->1.1 | 10-11-2019 12:00:13 | 10-11-2019 12:00:13 | |
transformers | 1,495 | closed | Merge pull request #1 from huggingface/master | from 1.0->1.1 | 10-11-2019 11:33:19 | 10-11-2019 11:33:19 | |
transformers | 1,494 | closed | Merge pull request #1 from huggingface/master | from 1.0->1.1 | 10-11-2019 11:26:00 | 10-11-2019 11:26:00 | # [Codecov](https://codecov.io/gh/huggingface/transformers/pull/1494?src=pr&el=h1) Report
> Merging [#1494](https://codecov.io/gh/huggingface/transformers/pull/1494?src=pr&el=desc) into [master](https://codecov.io/gh/huggingface/transformers/commit/700331b5ece63381ad1b775fc8661cf3ae4493fd?src=pr&el=desc) will **decrease** coverage by `5.94%`.
> The diff coverage is `n/a`.
[](https://codecov.io/gh/huggingface/transformers/pull/1494?src=pr&el=tree)
```diff
@@ Coverage Diff @@
## master #1494 +/- ##
==========================================
- Coverage 85.56% 79.61% -5.95%
==========================================
Files 91 42 -49
Lines 13534 6898 -6636
==========================================
- Hits 11580 5492 -6088
+ Misses 1954 1406 -548
```
| [Impacted Files](https://codecov.io/gh/huggingface/transformers/pull/1494?src=pr&el=tree) | Coverage Δ | |
|---|---|---|
| [transformers/modeling\_tf\_xlm.py](https://codecov.io/gh/huggingface/transformers/pull/1494/diff?src=pr&el=tree#diff-dHJhbnNmb3JtZXJzL21vZGVsaW5nX3RmX3hsbS5weQ==) | | |
| [transformers/configuration\_distilbert.py](https://codecov.io/gh/huggingface/transformers/pull/1494/diff?src=pr&el=tree#diff-dHJhbnNmb3JtZXJzL2NvbmZpZ3VyYXRpb25fZGlzdGlsYmVydC5weQ==) | | |
| [transformers/configuration\_bert.py](https://codecov.io/gh/huggingface/transformers/pull/1494/diff?src=pr&el=tree#diff-dHJhbnNmb3JtZXJzL2NvbmZpZ3VyYXRpb25fYmVydC5weQ==) | | |
| [transformers/tests/tokenization\_transfo\_xl\_test.py](https://codecov.io/gh/huggingface/transformers/pull/1494/diff?src=pr&el=tree#diff-dHJhbnNmb3JtZXJzL3Rlc3RzL3Rva2VuaXphdGlvbl90cmFuc2ZvX3hsX3Rlc3QucHk=) | | |
| [transformers/tests/modeling\_bert\_test.py](https://codecov.io/gh/huggingface/transformers/pull/1494/diff?src=pr&el=tree#diff-dHJhbnNmb3JtZXJzL3Rlc3RzL21vZGVsaW5nX2JlcnRfdGVzdC5weQ==) | | |
| [transformers/tests/tokenization\_utils\_test.py](https://codecov.io/gh/huggingface/transformers/pull/1494/diff?src=pr&el=tree#diff-dHJhbnNmb3JtZXJzL3Rlc3RzL3Rva2VuaXphdGlvbl91dGlsc190ZXN0LnB5) | | |
| [transformers/tests/modeling\_tf\_ctrl\_test.py](https://codecov.io/gh/huggingface/transformers/pull/1494/diff?src=pr&el=tree#diff-dHJhbnNmb3JtZXJzL3Rlc3RzL21vZGVsaW5nX3RmX2N0cmxfdGVzdC5weQ==) | | |
| [transformers/modeling\_xlnet.py](https://codecov.io/gh/huggingface/transformers/pull/1494/diff?src=pr&el=tree#diff-dHJhbnNmb3JtZXJzL21vZGVsaW5nX3hsbmV0LnB5) | | |
| [transformers/tokenization\_utils.py](https://codecov.io/gh/huggingface/transformers/pull/1494/diff?src=pr&el=tree#diff-dHJhbnNmb3JtZXJzL3Rva2VuaXphdGlvbl91dGlscy5weQ==) | | |
| [transformers/tests/modeling\_tf\_transfo\_xl\_test.py](https://codecov.io/gh/huggingface/transformers/pull/1494/diff?src=pr&el=tree#diff-dHJhbnNmb3JtZXJzL3Rlc3RzL21vZGVsaW5nX3RmX3RyYW5zZm9feGxfdGVzdC5weQ==) | | |
| ... and [123 more](https://codecov.io/gh/huggingface/transformers/pull/1494/diff?src=pr&el=tree-more) | |
------
[Continue to review full report at Codecov](https://codecov.io/gh/huggingface/transformers/pull/1494?src=pr&el=continue).
> **Legend** - [Click here to learn more](https://docs.codecov.io/docs/codecov-delta)
> `Δ = absolute <relative> (impact)`, `ø = not affected`, `? = missing data`
> Powered by [Codecov](https://codecov.io/gh/huggingface/transformers/pull/1494?src=pr&el=footer). Last update [700331b...a2cfe98](https://codecov.io/gh/huggingface/transformers/pull/1494?src=pr&el=lastupdated). Read the [comment docs](https://docs.codecov.io/docs/pull-request-comments).
|
transformers | 1,493 | closed | FR: Tokenizer function that can handle arbitrary number of sequences | ## 🚀 Feature
Currently Tokenizers only support 1 or 2 sequences being added together, and them being concatenated with the appropriate SEP and CLS tokens for each model. My use case requires more sequences being added together, all separated by SEP tokens and having one CLS token at the start (or end for XLNet) of the entire sequence.
E.g., for BERT:
```
[CLS] This is my first sentence [SEP] This is my second sentence [SEP] And finally my third sentence [SEP]
```
Would it be possible to have a function supported for current and future models that can take a list of strings in and process them as outlined above.
I would be especially happy if there was an accompanying feature for token type ids, which would alternate between successive sequences.
Using the above example, this would mean:
```
0 0 0 0 0 0 0 1 1 1 1 1 1 0 0 0 0 0 0
```
Thanks!
| 10-11-2019 11:19:02 | 10-11-2019 11:19:02 | Looks like a pretty simple and natural extension, what do you think @LysandreJik?<|||||>It would be easy to implement indeed, do you use this for dialog (because of the alternating token type ids)?<|||||>Not particularly, but I can certainly imagine that being a useful use-case.
I am more interested in adding extra features directly to the language model when classifying a piece of text. For example:
`[Location] + [Occupation] + [Social media post]`<|||||>This issue has been automatically marked as stale because it has not had recent activity. It will be closed if no further activity occurs. Thank you for your contributions.
|
transformers | 1,492 | closed | Add new BERT models for German (cased and uncased) | Hi,
this PR adds new BERT models for German (both cased and uncased) from @dbmdz.
Details can be found in [this repository](https://github.com/dbmdz/german-bert).
Tasks:
* [x] Models are stored on S3, only permissions need to be adjusted by @julien-c | 10-11-2019 08:25:29 | 10-11-2019 08:25:29 | Great, ok all the models should be public. Merging this now.
Awesome work @stefan-it! |
transformers | 1,491 | closed | RuntimeError: unexpected EOF, expected 7491165 more bytes. The file might be corrupted. | ## ❓ Questions & Help
<!-- A clear and concise description of the question. -->
I tried a small chunk of code from the Readme.md
```
import torch
from transformers import *
MODELS = [(BertModel, BertTokenizer, 'bert-base-uncased')]
for model_class, tokenizer_class, pretrained_weights in MODELS:
# Load pretrained model/tokenizer
tokenizer = tokenizer_class.from_pretrained(pretrained_weights)
model = model_class.from_pretrained(pretrained_weights)
input_ids = torch.tensor([tokenizer.encode("Here is some text to encode", add_special_tokens=True)]) # Add special tokens takes care of adding [CLS], [SEP], <s>... tokens in the right way for each model.
with torch.no_grad():
last_hidden_states = model(input_ids)[0]
```
It is giving me the following error
```
RuntimeError Traceback (most recent call last)
<ipython-input-3-6528fe9b0472> in <module>
3 tokenizer = tokenizer_class.from_pretrained(pretrained_weights)
----> 4 model = model_class.from_pretrained(pretrained_weights)
~/.conda/envs/transformers/lib/python3.7/site-packages/transformers/modeling_utils.py in from_pretrained(cls, pretrained_model_name_or_path, *model_args, **kwargs)
343
344 if state_dict is None and not from_tf:
--> 345 state_dict = torch.load(resolved_archive_file, map_location='cpu')
346
347 missing_keys = []
~/.conda/envs/transformers/lib/python3.7/site-packages/torch/serialization.py in load(f, map_location, pickle_module, **pickle_load_args)
424 if sys.version_info >= (3, 0) and 'encoding' not in pickle_load_args.keys():
425 pickle_load_args['encoding'] = 'utf-8'
--> 426 return _load(f, map_location, pickle_module, **pickle_load_args)
427 finally:
428 if new_fd:
~/.conda/envs/transformers/lib/python3.7/site-packages/torch/serialization.py in _load(f, map_location, pickle_module, **pickle_load_args)
618 for key in deserialized_storage_keys:
619 assert key in deserialized_objects
--> 620 deserialized_objects[key]._set_from_file(f, offset, f_should_read_directly)
621 if offset is not None:
622 offset = f.tell()
RuntimeError: unexpected EOF, expected 7491165 more bytes. The file might be corrupted.
```
Haven't modified anything in the library. | 10-11-2019 05:46:58 | 10-11-2019 05:46:58 | Hi! It seems to me that the file that was downloaded was corrupted, probably because of lacking space or a network error. Could you try using the `from_pretrained` with the `force_download` option ?<|||||>That worked. Thanks!<|||||>If you are using Window 10 machine, deleting `vgg16-something` in folder `C:\Users\UserName\.cache\torch\checkpoints` would solve probelm.<|||||>Using `force_download` option also works for me.<|||||>> Hi! It seems to me that the file that was downloaded was corrupted, probably because of lacking space or a network error. Could you try using the `from_pretrained` with the `force_download` option ?
where to use this in the code?
> Using `force_download` option also works for me.
> Using `force_download` option also works for me.
> Hi! It seems to me that the file that was downloaded was corrupted, probably because of lacking space or a network error. Could you try using the `from_pretrained` with the `force_download` option ?
how or where to use this in my code
<|||||>Well, what's your code? `from_pretrained` should be the method you use to load models/configurations/tokenizers.
```py
model = model_class.from_pretrained(pretrained_weights, force_download=True)
```<|||||>I want to run mmdetection demo image_demo.py but has this problems
I use google colab pytorch 1.3.1 .
Traceback (most recent call last):
File "demo/image_demo.py", line 26, in <module>
main()
File "demo/image_demo.py", line 18, in main
model = init_detector(args.config, args.checkpoint, device=args.device)
File "/content/mmdetection/mmdet/apis/inference.py", line 35, in init_detector
checkpoint = load_checkpoint(model, checkpoint)
File "/root/mmcv/mmcv/runner/checkpoint.py", line 224, in load_checkpoint
checkpoint = _load_checkpoint(filename, map_location)
File "/root/mmcv/mmcv/runner/checkpoint.py", line 200, in _load_checkpoint
checkpoint = torch.load(filename, map_location=map_location)
File "/content/anaconda3/lib/python3.7/site-packages/torch/serialization.py", line 426, in load
return _load(f, map_location, pickle_module, **pickle_load_args)
File "/content/anaconda3/lib/python3.7/site-packages/torch/serialization.py", line 620, in _load
deserialized_objects[key]._set_from_file(f, offset, f_should_read_directly)
RuntimeError: storage has wrong size: expected -4934180888905747925 got 64<|||||>if you are loading any weights in code, there might be problem with that just redownload the weights.. worked for me.<|||||>> Using `force_download` option also works for me.
Where to add this argument ?<|||||>See this comment https://github.com/huggingface/transformers/issues/1491#issuecomment-618626059<|||||>
here is my code:
` model = torchvision.models.detection.fasterrcnn_resnet50_fpn(pretrained=True)`
and I encountered the same problem, i delete the relevant files in "C:\Users\UserName\.cache\torch\checkpoints" then solve the problem.<|||||>I am experiencing the same issue, I am using Ubuntu 18 WSL. When adding the `force_download=True` I am getting the following error:
`/tape/models/modeling_utils.py", line 506, in from_pretrained
model = cls(config, *model_args, **model_kwargs)
TypeError: __init__() got an unexpected keyword argument 'force_download'`
Any solutions will be highly appreciated. <|||||>> If you are using Window 10 machine, deleting `vgg16-something` in folder `C:\Users\UserName\.cache\torch\checkpoints` would solve probelm.
This worked for me<|||||>so how to solve this problem? @Geraldene |
transformers | 1,490 | closed | Is encode_plus supposed to pad to max_length? | ## ❓ Questions & Help
I am using AutoTokenizer and AutoModelForSequenceClassification and `encode_plus` to encode text. I am calling it like this:
`
tokenizer = AutoTokenizer.from_pretrained(self.model_name)
encoded_inputs = tokenizer.encode_plus(text,add_special_tokens=True,max_length=max_seq_length)
input_ids = encoded_inputs["input_ids"]
special_tokens_mask = encoded_inputs["special_tokens_mask"]
token_type_ids = encoded_inputs["token_type_ids"]
loggerinfo(logger, "len of encoded vals {} {} {}".format(len(input_ids),len(special_tokens_mask),len(token_type_ids)))
`
The output indicates that the encoded values are of different length. I expected them to all be = max_length which is 100 in this case.
Output:
> max seq len = 100
> len of encoded vals 39 39 39
> len of encoded vals 24 24 24
> len of encoded vals 11 11 11
Is that an incorrect expectation? | 10-11-2019 05:42:45 | 10-11-2019 05:42:45 | From what I remember (can't check now), padding up to max model seq length is not done and not necessary. The tokenizer will limit longer sequences to the max seq length, but otherwise you can just make sure the batch sizes are equal (so pad up to max _batch_ length, so you can actually create m-dimensional tensors (all rows in a matrix have to have the same length).<|||||>@BramVanroy Not sure I understand. The above code does the encoding for one row of a text column. The encodings are appended to a list to capture all the encoding for the text column. If I convert that list to a tensor, without any padding it errors out due to different vector lengths.
<|||||>Exactly. So the tokenizer limits the length (to a max seq length) but doesn't pad it. You'll have to do that manually. You can pad up to the largest sequence _in the batch_ (rather than the max seq length) so that all items in the batch are the same size, which you can then convert to a tensor. A general usage could look like this. The padding happens at the end. Here I pad up to the MAX_SEQ_LEN if available, and otherwise up to the largest sequence in the batch.
```python
def tokenize(text):
all_input_ids = []
all_input_mask = []
for sentence in text:
tokens = tokenizer.tokenize(sentence)
# limit size to make room for special tokens
if MAX_SEQ_LEN:
tokens = tokens[0:(MAX_SEQ_LEN - 2)]
# add special tokens
tokens = [tokenizer.cls_token, *tokens, tokenizer.sep_token]
# convert tokens to IDs
input_ids = tokenizer.convert_tokens_to_ids(tokens)
# create mask same size of input
input_mask = [1] * len(input_ids)
all_input_ids.append(input_ids)
all_input_mask.append(input_mask)
# pad up to max length
# up to max_seq_len if provided, otherwise the max of current batch
max_length = MAX_SEQ_LEN if MAX_SEQ_LEN else max([len(ids) for ids in all_input_ids])
all_input_ids = torch.LongTensor([i + [tokenizer.pad_token_id] * (max_length - len(i))
for i in all_input_ids])
all_input_mask = torch.FloatTensor([m + [0] * (max_length - len(m)) for m in all_input_mask])
return all_input_ids, all_input_mask
```<|||||>Thanks, that clarifies things. I will close this issue. <|||||>> From what I remember (can't check now), padding up to max model seq length is not done and not necessary. The tokenizer will limit longer sequences to the max seq length, but otherwise you can just make sure the batch sizes are equal (so pad up to max _batch_ length, so you can actually create m-dimensional tensors (all rows in a matrix have to have the same length).
I am wondering if there are any disadvantages to just padding all inputs to 512. It would certainly cut down on batch processing time. But standard practice seems to be dynamically padding to the largest sequence length. <|||||>I have wondered about this myself but I have no answer. Perhaps someone else can help. <|||||>I think it comes down to loading CPU computation vs LA GPU computation. It might be worth doing some statistical analysis on the inputIDs length and checking if 1 standard limit is possible. For example if at least sample of 512 occurs in 90% of your batches, it would be worth just setting to padding to 512 for all batches. |
Subsets and Splits
No community queries yet
The top public SQL queries from the community will appear here once available.