repo
stringclasses 1
value | number
int64 1
25.3k
| state
stringclasses 2
values | title
stringlengths 1
487
| body
stringlengths 0
234k
⌀ | created_at
stringlengths 19
19
| closed_at
stringlengths 19
19
| comments
stringlengths 0
293k
|
---|---|---|---|---|---|---|---|
transformers | 1,389 | closed | Fix compatibility issue with PyTorch 1.2 | Using PyTorch 1.2.0 give an error when running XLNet.
We should use the new way to reverse mask : instead of using `1 - mask`, we should use `~mask` | 10-01-2019 07:44:23 | 10-01-2019 07:44:23 | Hi,
We can accept this since it breaks lower versions of PyTorch.
You can just feed your mask as a FloatTensor (as indicated in the docstrings I think). |
transformers | 1,388 | closed | Add Roberta SQuAD model | There is the realisation of a RoBERTa SQuAD finetuning.
On 2x1080Ti on RoBERTa Base it gives:
python3 run_squad.py \
--model_type roberta \
--model_name_or_path roberta-base \
--do_train \
--do_eval \
--train_file $SQUAD_DIR/train-v1.1.json \
--predict_file $SQUAD_DIR/dev-v1.1.json \
--per_gpu_train_batch_size 8 \
--per_gpu_eval_batch_size 8 \
--learning_rate 3e-5 \
--num_train_epochs 2.0 \
--max_seq_length 384 \
--doc_stride 128 \
--save_steps 2000 \
--overwrite_output_dir \
--verbose_logging \
--output_dir /tmp/debug_squad/
Results: {'exact': 85.80889309366131, 'f1': 92.09291402361669, 'total': 10570, 'HasAns_exact': 85.80889309366131, 'HasAns_f1': 92.09291402361669, 'HasAns_total': 10570}
On RoBERTa Large:
python3 run_squad.py \
--model_type roberta \
--model_name_or_path roberta-large \
--do_train \
--do_eval \
--train_file $SQUAD_DIR/train-v1.1.json \
--predict_file $SQUAD_DIR/dev-v1.1.json \
--per_gpu_train_batch_size 2 \
--per_gpu_eval_batch_size 2 \
--learning_rate 3e-5 \
--num_train_epochs 2.0 \
--max_seq_length 384 \
--doc_stride 128 \
--save_steps 2000 \
--overwrite_output_dir \
--verbose_logging \
--output_dir /tmp/debug_squad/
Results: {'exact': 87.04824976348155, 'f1': 93.14253401654709, 'total': 10570, 'HasAns_exact': 87.04824976348155, 'HasAns_f1': 93.14253401654709, 'HasAns_total': 10570} | 10-01-2019 04:50:09 | 10-01-2019 04:50:09 | # [Codecov](https://codecov.io/gh/huggingface/transformers/pull/1388?src=pr&el=h1) Report
> Merging [#1388](https://codecov.io/gh/huggingface/transformers/pull/1388?src=pr&el=desc) into [master](https://codecov.io/gh/huggingface/transformers/commit/5c3b32d44d0164aaa9b91405f48e53cf53a82b35?src=pr&el=desc) will **decrease** coverage by `0.16%`.
> The diff coverage is `23.52%`.
[](https://codecov.io/gh/huggingface/transformers/pull/1388?src=pr&el=tree)
```diff
@@ Coverage Diff @@
## master #1388 +/- ##
==========================================
- Coverage 84.69% 84.52% -0.17%
==========================================
Files 84 84
Lines 12596 12627 +31
==========================================
+ Hits 10668 10673 +5
- Misses 1928 1954 +26
```
| [Impacted Files](https://codecov.io/gh/huggingface/transformers/pull/1388?src=pr&el=tree) | Coverage Δ | |
|---|---|---|
| [transformers/modeling\_roberta.py](https://codecov.io/gh/huggingface/transformers/pull/1388/diff?src=pr&el=tree#diff-dHJhbnNmb3JtZXJzL21vZGVsaW5nX3JvYmVydGEucHk=) | `61.17% <23.52%> (-10.05%)` | :arrow_down: |
------
[Continue to review full report at Codecov](https://codecov.io/gh/huggingface/transformers/pull/1388?src=pr&el=continue).
> **Legend** - [Click here to learn more](https://docs.codecov.io/docs/codecov-delta)
> `Δ = absolute <relative> (impact)`, `ø = not affected`, `? = missing data`
> Powered by [Codecov](https://codecov.io/gh/huggingface/transformers/pull/1388?src=pr&el=footer). Last update [5c3b32d...1ba42ca](https://codecov.io/gh/huggingface/transformers/pull/1388?src=pr&el=lastupdated). Read the [comment docs](https://docs.codecov.io/docs/pull-request-comments).
<|||||>I am also working on reproducing the results reported in the Roberta paper and found two issues in this PR. One issue is explained in the comment above. The other issue is that it is required to insert two sep_tokens between question tokens and answer tokens for Roberta as implemented [here](https://github.com/huggingface/transformers/blob/master/transformers/tokenization_roberta.py#L101). Therefore, `max_tokens_for_doc` should be `max_seq_length - len(query_tokens) - 4`.<|||||>In the Fairseq realisation of RoBERTa on Commonsense QA:
https://github.com/pytorch/fairseq/tree/master/examples/roberta/commonsense_qa
https://github.com/pytorch/fairseq/blob/master/examples/roberta/commonsense_qa/commonsense_qa_task.py
There is the only one sep_token between question and answer:
`<s> Q: Where would I not want a fox? </s> A: hen house </s>`<|||||>> In the Fairseq realisation of RoBERTa on Commonsense QA:
> There is the only one sep_token between question and answer:
> `<s> Q: Where would I not want a fox? </s> A: hen house </s>`
Thank you very much for your prompt reply. I did not know this. It seems to be appropriate to use single `sep_token` here because Commonsense QA is somewhat more similar to SQuAD than other tasks (e.g., GLUE).<|||||>Thanks for this @vlarine! (and @ikuyamada)
Would you agree to share the weights on our S3 as well?
Also, did you try with the same separators encoding scheme as the other RoBERTa models?
`<s> Q: Where would I not want a fox? </s> </s> A: hen house </s>` – did the results differ significantly?<|||||>No, I have not tried. But why there are two `</s>` tokens? I think more natural way is:
`<s> Q: Where would I not want a fox? </s> <s> A: hen house </s>`<|||||>@vlarine See this docstring in `fairseq`: https://github.com/pytorch/fairseq/pull/969/files
Do you think you could try with this sep encoding scheme? Otherwise I'll do it in the next couple of days.
I would like to merge your PR soon. Any way you can give me write access to your fork, cf. https://help.github.com/en/articles/committing-changes-to-a-pull-request-branch-created-from-a-fork – so that i can add commits on top of your PR?
<|||||>@vlarine @julien-c thanks for the amazing work! I can try it on SQuAD 2.0 and let you know if anything pops up there<|||||>Nice work. I also tried to add roberta into run_squad.py several days ago. Hope that my implementation would be useful. [run_squad.py with roberta](https://github.com/erenup/pytorch-transformers/pull/4) <|||||>Folding this PR into #1386, which is close to being ready to being merged.
@vlarine @ikuyamada @pminervini @erenup Can you guys please check it out?<|||||>Closing in favor of #1386. |
transformers | 1,387 | closed | TFTransfoXLLMHeadModel doesn't accept lm_labels parameter | ## 🐛 Bug
<!-- Important information -->
Model I am using (Bert, XLNet....): TFTransfoXLLMHeadModel
Language I am using the model on (English, Chinese....): Other
The problem arise when using:
* [ ] the official example scripts: (give details)
* [ X ] my own modified scripts: I have a script that trains a new TransformerXL
The tasks I am working on is:
* [ ] an official GLUE/SQUaD task: (give the name)
* [ X ] my own task or dataset: The dataset is a language modeling dataset of novel symbolic data.
## To Reproduce
Steps to reproduce the behavior:
Call the TFTransfoXLLMHeadModel as such:
mems = transformer(data,
lm_labels = lm_labels,
mems = mems,
training=True)
File "/home/tom/.local/lib/python3.7/site-packages/tensorflow_core/python/keras/engine/base_layer.py", line 891, in __call__
outputs = self.call(cast_inputs, *args, **kwargs)
TypeError: call() got an unexpected keyword argument 'lm_labels'
If I instead include lm_labels in a dict, it is simply ignored.
## Expected behavior
The model documentation says that including lm_labels is recommended for training because it allows the adaptive softmax to be calculated more efficiently
## Environment
* OS: Ubuntu 19
* PyTorch Transformers version (or branch): 2.0.0
* Using GPU Yes
| 09-30-2019 23:19:16 | 09-30-2019 23:19:16 | I see now that I missed something. The documentation uses the parameter 'lm_labels' but the correct parameter is just 'labels'. The documentation says that when this parameter is present, prediction logits will not be output, but this is incorrect. They are output regardless of the presence of 'labels'.<|||||>This issue has been automatically marked as stale because it has not had recent activity. It will be closed if no further activity occurs. Thank you for your contributions.
|
transformers | 1,386 | closed | Add RoBERTa question answering & Update SQuAD runner to support RoBERTa | 09-30-2019 22:26:33 | 09-30-2019 22:26:33 | @thomwolf / @LysandreJik / @VictorSanh / @julien-c Could you help review this PR? Thanks!<|||||># [Codecov](https://codecov.io/gh/huggingface/transformers/pull/1386?src=pr&el=h1) Report
> Merging [#1386](https://codecov.io/gh/huggingface/transformers/pull/1386?src=pr&el=desc) into [master](https://codecov.io/gh/huggingface/transformers/commit/be916cb3fb4579e278ceeaec11a6524662797d7f?src=pr&el=desc) will **decrease** coverage by `0.15%`.
> The diff coverage is `21.21%`.
[](https://codecov.io/gh/huggingface/transformers/pull/1386?src=pr&el=tree)
```diff
@@ Coverage Diff @@
## master #1386 +/- ##
==========================================
- Coverage 86.16% 86.01% -0.16%
==========================================
Files 91 91
Lines 13593 13626 +33
==========================================
+ Hits 11713 11720 +7
- Misses 1880 1906 +26
```
| [Impacted Files](https://codecov.io/gh/huggingface/transformers/pull/1386?src=pr&el=tree) | Coverage Δ | |
|---|---|---|
| [transformers/modeling\_roberta.py](https://codecov.io/gh/huggingface/transformers/pull/1386/diff?src=pr&el=tree#diff-dHJhbnNmb3JtZXJzL21vZGVsaW5nX3JvYmVydGEucHk=) | `69.18% <21.21%> (-11.39%)` | :arrow_down: |
------
[Continue to review full report at Codecov](https://codecov.io/gh/huggingface/transformers/pull/1386?src=pr&el=continue).
> **Legend** - [Click here to learn more](https://docs.codecov.io/docs/codecov-delta)
> `Δ = absolute <relative> (impact)`, `ø = not affected`, `? = missing data`
> Powered by [Codecov](https://codecov.io/gh/huggingface/transformers/pull/1386?src=pr&el=footer). Last update [be916cb...ee83f98](https://codecov.io/gh/huggingface/transformers/pull/1386?src=pr&el=lastupdated). Read the [comment docs](https://docs.codecov.io/docs/pull-request-comments).
<|||||>Hi @thomwolf / @LysandreJik / @VictorSanh / @julien-c
I have also run experiments using RoBERT large setting in original paper and reproduced their results,
- **SQuAD v1.1**
{
"exact": 88.25922421948913,
"f1": 94.43790487416292,
"total": 10570,
"HasAns_exact": 88.25922421948913,
"HasAns_f1": 94.43790487416292,
"HasAns_total": 10570
}
- **SQuAD v2.0**
{
"exact": 86.05238777057188,
"f1": 88.99602665148535,
"total": 11873,
"HasAns_exact": 83.38394062078272,
"HasAns_f1": 89.27965999208608,
"HasAns_total": 5928,
"NoAns_exact": 88.71320437342304,
"NoAns_f1": 88.71320437342304,
"NoAns_total": 5945,
"best_exact": 86.5914259243662,
"best_exact_thresh": -2.146007537841797,
"best_f1": 89.43104312625539,
"best_f1_thresh": -2.146007537841797
}<|||||>Awesome @stevezheng23. Can I push on top of your PR to change a few things before we merge?
(We refactored the tokenizer to handle the encoding of sequence pairs, including special tokens. So we don't need to do it inside each example script anymore)<|||||>@julien-c sure, please add changes in this PR if needed 👍 <|||||>@julien-c I've also upload the roberta large model finetuned on squad v2.0 data together with its prediction & evaluation results to public cloud storage https://storage.googleapis.com/mrc_data/squad/roberta.large.squad.v2.zip<|||||>Can you check my latest commit @stevezheng23? Main change is that I removed the `add_prefix_space` for RoBERTa (which the RoBERTa authors don't use, as far as I know) which doesn't seem to make a significant difference.
@thomwolf @LysandreJik this is ready for review.<|||||>Everything looks good.
As for the `add_prefix_space` flag,
- For `add_prefix_space=True`, I have run the experiment, the F1 score is around 89.4
- For `add_prefix_space=False`, I have also run the experiment, the F1 score is around 88.2<|||||>Great! Good job on reimplementing the cross-entropy loss when start/end positions are given.<|||||>Look good to me.
We'll probably be able to simplify `utils_squad` a lot soon but that will be fine for now.
Do you want to add your experimental results with RoBERTa in `examples/readme`, with a recommendation to use `add_prefix_space=True` (fyi it's the opposite for NER)?<|||||>@julien-c do you want to add the roberta model finetuned on squad by @stevezheng23 in our library?<|||||>Yep @thomwolf <|||||>@thomwolf I have updated README file as you suggested, you can merge this PR when you think it's good to go. BTW, it seems CI build is broken<|||||>Ok thanks, I'll let @julien-c finish to handle this PR when he's back.<|||||>> @julien-c I've also upload the roberta large model finetuned on squad v2.0 data together with its prediction & evaluation results to public cloud storage https://storage.googleapis.com/mrc_data/squad/roberta.large.squad.v2.zip
Hey @stevezheng23 !
I just tried to reproduce your model with slightly different hyperparameters (`batch_size=2` and `gradient_accumulation=6` instead of `batch_size=12`), and I am currently getting worse results.
Results with your model:
```
{
"exact": 86.05238777057188,
"f1": 88.99602665148535,
"total": 11873,
"HasAns_exact": 83.38394062078272,
"HasAns_f1": 89.27965999208608,
"HasAns_total": 5928,
"NoAns_exact": 88.71320437342304,
"NoAns_f1": 88.71320437342304,
"NoAns_total": 5945
}
```
Results with the model I trained, on the best checkpoint I was able to obtain after training for 8 epochs:
```
{
"exact": 82.85184873241809,
"f1": 85.85477834702593,
"total": 11873,
"HasAns_exact": 77.80026990553306,
"HasAns_f1": 83.8147407750069,
"HasAns_total": 5928,
"NoAns_exact": 87.88898233809924,
"NoAns_f1": 87.88898233809924,
"NoAns_total": 5945
}
```
Your hyperparameters:
```
Namespace(adam_epsilon=1e-08, cache_dir='', config_name='', device=device(type='cuda', index=0), do_eval=True, do_lower_case=False, do_train=True, doc_stride=128, eval_all_checkpoints=False, evaluate_during_training=False, fp16=False, fp16_opt_level='O1', gradient_accumulation_steps=1, learning_rate=1.5e-05, local_rank=0, logging_steps=50, max_answer_length=30, max_grad_norm=1.0, max_query_length=64, max_seq_length=512, max_steps=-1, model_name_or_path='roberta-large', model_type='roberta', n_best_size=20, n_gpu=1, no_cuda=False, null_score_diff_threshold=0.0, num_train_epochs=2.0, output_dir='output/squad/v2.0/roberta.large', overwrite_cache=False, overwrite_output_dir=False, per_gpu_eval_batch_size=12, per_gpu_train_batch_size=12, predict_file='data/squad/v2.0/dev-v2.0.json', save_steps=500, seed=42, server_ip='', server_port='', tokenizer_name='', train_batch_size=12, train_file='data/squad/v2.0/train-v2.0.json', verbose_logging=False, version_2_with_negative=True, warmup_steps=500, weight_decay=0.01)
```
My hyperparameters:
```
Namespace(adam_epsilon=1e-08, cache_dir='', config_name='', device=device(type='cuda'), do_eval=True, do_lower_case=False, do_train=True, doc_stride=128, eval_all_checkpoints=False, evaluate_during_training=False, fp16=False, fp16_opt_level='O1', gradient_accumulation_steps=6, learning_rate=1.5e-05, local_rank=-1, logging_steps=50, max_answer_length=30, max_grad_norm=1.0, max_query_length=64, max_seq_length=512, max_steps=-1, model_name_or_path='roberta-large', model_type='roberta', n_best_size=20, n_gpu=1, no_cuda=False, null_score_diff_threshold=0.0, num_train_epochs=8.0, output_dir='../roberta.large.squad2.v1p', overwrite_cache=False, overwrite_output_dir=False, per_gpu_eval_batch_size=2, per_gpu_train_batch_size=2, predict_file='/home/testing/drive/invariance//workspace/data/squad/dev-v2.0.json', save_steps=500, seed=42, server_ip='', server_port='', tokenizer_name='', train_batch_size=2, train_file='/home/testing/drive/invariance//workspace/data/squad/train-v2.0.json', verbose_logging=False, version_2_with_negative=True, warmup_steps=500, weight_decay=0.01)
```
Do you have any ideas why this is happening ?
One thing that may be happening is that, when using `max_grad_norm` and `gradient_accumulation=n`, the clipping of the gradient norm seems to be done `n` times rather than just 1, but I need to look deeper into this.
I'd like to see what happens without the need of gradient accumulation - anyone with a spare TPU to share? 😬<|||||>> Ok thanks, I'll let @julien-c finish to handle this PR when he's back.
thanks, @thomwolf <|||||>@pminervini I haven't tried out using `max_grad_norm` and `gradient_accumulation=n` combination before. One thing you could pay attention to is that the checkpoint is trained with `add_prefix_space=True` for RoBERTa tokenizer.<|||||>@stevezheng23 if you look at it, the `max_grad_norm` is performed on all the gradients in the accumulation - I think it should be done just before the `optimizer.step()` call.
https://github.com/huggingface/transformers/blob/master/examples/run_squad.py#L163
@thomwolf what do you think ? should I go and do a PR ?<|||||>@LysandreJik just significantly rewrote our SQuAD integration in https://github.com/huggingface/transformers/pull/1984 so we were holding out on merging this.
Does anyone here want to revisit this PR with the changes from #1984? Otherwise, we'll do it, time permitting.<|||||>cool, I'm willing to revisit it. I will take a look at your changes and tansformers' recent updates today (have been away from the Master branch for some time😊).<|||||>> > @julien-c I've also upload the roberta large model finetuned on squad v2.0 data together with its prediction & evaluation results to public cloud storage https://storage.googleapis.com/mrc_data/squad/roberta.large.squad.v2.zip
>
> Hey @stevezheng23 !
>
> I just tried to reproduce your model with slightly different hyperparameters (`batch_size=2` and `gradient_accumulation=6` instead of `batch_size=12`), and I am currently getting worse results.
> Your hyperparameters:
>
> ```
> Namespace(adam_epsilon=1e-08, cache_dir='', config_name='', device=device(type='cuda', index=0), do_eval=True, do_lower_case=False, do_train=True, doc_stride=128, eval_all_checkpoints=False, evaluate_during_training=False, fp16=False, fp16_opt_level='O1', gradient_accumulation_steps=1, learning_rate=1.5e-05, local_rank=0, logging_steps=50, max_answer_length=30, max_grad_norm=1.0, max_query_length=64, max_seq_length=512, max_steps=-1, model_name_or_path='roberta-large', model_type='roberta', n_best_size=20, n_gpu=1, no_cuda=False, null_score_diff_threshold=0.0, num_train_epochs=2.0, output_dir='output/squad/v2.0/roberta.large', overwrite_cache=False, overwrite_output_dir=False, per_gpu_eval_batch_size=12, per_gpu_train_batch_size=12, predict_file='data/squad/v2.0/dev-v2.0.json', save_steps=500, seed=42, server_ip='', server_port='', tokenizer_name='', train_batch_size=12, train_file='data/squad/v2.0/train-v2.0.json', verbose_logging=False, version_2_with_negative=True, warmup_steps=500, weight_decay=0.01)
> ```
>
> My hyperparameters:
>
> ```
> Namespace(adam_epsilon=1e-08, cache_dir='', config_name='', device=device(type='cuda'), do_eval=True, do_lower_case=False, do_train=True, doc_stride=128, eval_all_checkpoints=False, evaluate_during_training=False, fp16=False, fp16_opt_level='O1', gradient_accumulation_steps=6, learning_rate=1.5e-05, local_rank=-1, logging_steps=50, max_answer_length=30, max_grad_norm=1.0, max_query_length=64, max_seq_length=512, max_steps=-1, model_name_or_path='roberta-large', model_type='roberta', n_best_size=20, n_gpu=1, no_cuda=False, null_score_diff_threshold=0.0, num_train_epochs=8.0, output_dir='../roberta.large.squad2.v1p', overwrite_cache=False, overwrite_output_dir=False, per_gpu_eval_batch_size=2, per_gpu_train_batch_size=2, predict_file='/home/testing/drive/invariance//workspace/data/squad/dev-v2.0.json', save_steps=500, seed=42, server_ip='', server_port='', tokenizer_name='', train_batch_size=2, train_file='/home/testing/drive/invariance//workspace/data/squad/train-v2.0.json', verbose_logging=False, version_2_with_negative=True, warmup_steps=500, weight_decay=0.01)
> ```
>
> Do you have any ideas why this is happening ?
You're using num_train_epochs=8 instead of 2, which makes the learning rate decay more slowly. Maybe that is causing the difference?<|||||>Regarding `max_grad_norm` - RoBERTa doesn't use gradient clipping, so the `max_grad_norm` changes aren't strictly necessary here
RoBERTa also uses `adam_epsilon=1e-06` as I understand, but I'm not sure if it would change the results here<|||||>Hi @stevezheng23 @julien-c @thomwolf @ethanjperez , I updated the run squad with roberta in #2173
based on #1984 and #1386. Could you please help to review it? Thank you very much.<|||||>Closed in favor of #2173 which should be merged soon.
|
|
transformers | 1,385 | closed | [multiple-choice] Simplify and use tokenizer.encode_plus | Our base tokenizer `PreTrainedTokenizer` now has the ability to encode a sentence pair up to a `max_length`, adding special tokens for each model and returning a mask of `token_type_ids`.
In this PR we upgrade `run_multiple_choice` by adopting this factorized tokenizer API.
To ensure the results are strictly the same as before, we implement a new `TruncatingStrategy` (ideally this could be an enum).
@erenup as you spent a lot of time on this script, would you be able to review this PR?
Result of eval with parameters from [examples/readme](https://github.com/huggingface/transformers/blob/julien_multiple-choice/examples/README.md#multiple-choice):
```
eval_acc = 0.8352494251724483
eval_loss = 0.42866929549320487
``` | 09-30-2019 20:10:23 | 09-30-2019 20:10:23 | Great addition. I feel like using enums would be especially helpful for the truncating strategy, indeed.<|||||># [Codecov](https://codecov.io/gh/huggingface/transformers/pull/1385?src=pr&el=h1) Report
> Merging [#1385](https://codecov.io/gh/huggingface/transformers/pull/1385?src=pr&el=desc) into [master](https://codecov.io/gh/huggingface/transformers/commit/5c3b32d44d0164aaa9b91405f48e53cf53a82b35?src=pr&el=desc) will **decrease** coverage by `0.07%`.
> The diff coverage is `41.66%`.
[](https://codecov.io/gh/huggingface/transformers/pull/1385?src=pr&el=tree)
```diff
@@ Coverage Diff @@
## master #1385 +/- ##
==========================================
- Coverage 84.69% 84.61% -0.08%
==========================================
Files 84 84
Lines 12596 12610 +14
==========================================
+ Hits 10668 10670 +2
- Misses 1928 1940 +12
```
| [Impacted Files](https://codecov.io/gh/huggingface/transformers/pull/1385?src=pr&el=tree) | Coverage Δ | |
|---|---|---|
| [transformers/tokenization\_utils.py](https://codecov.io/gh/huggingface/transformers/pull/1385/diff?src=pr&el=tree#diff-dHJhbnNmb3JtZXJzL3Rva2VuaXphdGlvbl91dGlscy5weQ==) | `87.73% <41.66%> (-2.46%)` | :arrow_down: |
------
[Continue to review full report at Codecov](https://codecov.io/gh/huggingface/transformers/pull/1385?src=pr&el=continue).
> **Legend** - [Click here to learn more](https://docs.codecov.io/docs/codecov-delta)
> `Δ = absolute <relative> (impact)`, `ø = not affected`, `? = missing data`
> Powered by [Codecov](https://codecov.io/gh/huggingface/transformers/pull/1385?src=pr&el=footer). Last update [5c3b32d...9e136ff](https://codecov.io/gh/huggingface/transformers/pull/1385?src=pr&el=lastupdated). Read the [comment docs](https://docs.codecov.io/docs/pull-request-comments).
<|||||>I have reviewed this PR and It looks good to me. Thank you! @julien-c . I added two lines of comments above. Hope they are useful. Thank you. <|||||>Merged in, and superseded by, #1384 |
transformers | 1,384 | closed | Quality of life enhancements in encoding + patch MLM masking | This PR aims to add quality of life features to the encoding mechanism and patches an issue with the masked language modeling masking function.
1 - ~It introduces an `always_truncate` argument to the `encode` method.~ The `always_truncate` argument is now used as default, with no option to set it to `False` when a `max_length` is specified. Currently, if a `max_length` is specified to the `encode` method with a sequence pair, with both sequences being longer than the max length, then the sequence pair won't be truncated. This may then result in a sequence longer than the specified max length, which may crash the preprocessing mechanism (see current `run_glue.py` with the QNLI task). This argument may be further improved by truncating according to the pair of sequences length ratio.
2 - It adds a new return to the `encode_plus` return dictionary: `sequence_ids`. This is a list of numbers corresponding to the position of special/sequence ids. As an example:
```py
sequence = "This is a sequence"
input_ids_no_special = tok.encode(sequence) # [1188, 1110, 170, 4954]
input_ids = tok.encode(sequence, add_special_tokens=True) # [101, 1188, 1110, 170, 4954, 102]
# Special tokens ─────────────────────────────────────────────┴───────────────────────────┘
```
The new method offers several choices: single sequence (with or without special tokens), sequence pairs, and already existing special tokens:
```py
tok.get_sequence_ids(input_ids_no_special) # [0, 1, 1, 1, 1, 0]
tok.get_sequence_ids(input_ids, special_tokens_present=True) # [0, 1, 1, 1, 1, 0]
```
This offers several quality of life changes:
1 - The users are now aware of the location of the encoded sequences in their input ids: they can have custom truncating methods while leveraging model agnostic encoding
2 - Being aware of the location of special tokens is essential in the case of masked language modeling: we do not want to mask special tokens. An example of this is shown in the modified `run_lm_finetuning.py` script.
Considering sequence ids, the naming may not be optimal, therefore I'm especially open to propositions @thomwolf. Furthermore, I'm not sure it is necessary to consider the cases where no special tokens are currently in the sequence. | 09-30-2019 18:43:32 | 09-30-2019 18:43:32 | I think we should drop the `always_truncate` param, and just set it to `True` iff `max_length is not None`<|||||>Other than that I like it.<|||||>As seen with @julien-c , `always_truncate` really should be enabled by default when a `max_length` is specified. |
transformers | 1,383 | closed | Adding CTRL | EDIT 10/04
Almost complete (tests pass / generation makes sense).
Please comment with issues if you find them.
**Incomplete - Adding to facilitate collaboration**
This PR would add functionality to perform inference on CTRL (https://github.com/salesforce/ctrl) in the `🤗/transformers` repo.
Commits will be squashed later before merging. | 09-30-2019 18:25:55 | 09-30-2019 18:25:55 | # [Codecov](https://codecov.io/gh/huggingface/transformers/pull/1383?src=pr&el=h1) Report
> Merging [#1383](https://codecov.io/gh/huggingface/transformers/pull/1383?src=pr&el=desc) into [master](https://codecov.io/gh/huggingface/transformers/commit/1c5079952f5f10eeac4cb6801b4fd1f36b0eff73?src=pr&el=desc) will **increase** coverage by `1.63%`.
> The diff coverage is `92.38%`.
[](https://codecov.io/gh/huggingface/transformers/pull/1383?src=pr&el=tree)
```diff
@@ Coverage Diff @@
## master #1383 +/- ##
==========================================
+ Coverage 83.79% 85.42% +1.63%
==========================================
Files 84 91 +7
Lines 12587 13464 +877
==========================================
+ Hits 10547 11502 +955
+ Misses 2040 1962 -78
```
| [Impacted Files](https://codecov.io/gh/huggingface/transformers/pull/1383?src=pr&el=tree) | Coverage Δ | |
|---|---|---|
| [transformers/modeling\_roberta.py](https://codecov.io/gh/huggingface/transformers/pull/1383/diff?src=pr&el=tree#diff-dHJhbnNmb3JtZXJzL21vZGVsaW5nX3JvYmVydGEucHk=) | `80.57% <ø> (+15.1%)` | :arrow_up: |
| [transformers/tests/modeling\_tf\_gpt2\_test.py](https://codecov.io/gh/huggingface/transformers/pull/1383/diff?src=pr&el=tree#diff-dHJhbnNmb3JtZXJzL3Rlc3RzL21vZGVsaW5nX3RmX2dwdDJfdGVzdC5weQ==) | `94.73% <0%> (ø)` | :arrow_up: |
| [transformers/tests/modeling\_tf\_common\_test.py](https://codecov.io/gh/huggingface/transformers/pull/1383/diff?src=pr&el=tree#diff-dHJhbnNmb3JtZXJzL3Rlc3RzL21vZGVsaW5nX3RmX2NvbW1vbl90ZXN0LnB5) | `95.38% <100%> (+7.88%)` | :arrow_up: |
| [transformers/modeling\_openai.py](https://codecov.io/gh/huggingface/transformers/pull/1383/diff?src=pr&el=tree#diff-dHJhbnNmb3JtZXJzL21vZGVsaW5nX29wZW5haS5weQ==) | `81.75% <100%> (+1.35%)` | :arrow_up: |
| [transformers/file\_utils.py](https://codecov.io/gh/huggingface/transformers/pull/1383/diff?src=pr&el=tree#diff-dHJhbnNmb3JtZXJzL2ZpbGVfdXRpbHMucHk=) | `74.17% <100%> (ø)` | :arrow_up: |
| [transformers/modeling\_auto.py](https://codecov.io/gh/huggingface/transformers/pull/1383/diff?src=pr&el=tree#diff-dHJhbnNmb3JtZXJzL21vZGVsaW5nX2F1dG8ucHk=) | `51.85% <20%> (-2.1%)` | :arrow_down: |
| [transformers/configuration\_auto.py](https://codecov.io/gh/huggingface/transformers/pull/1383/diff?src=pr&el=tree#diff-dHJhbnNmb3JtZXJzL2NvbmZpZ3VyYXRpb25fYXV0by5weQ==) | `58.82% <33.33%> (-2.47%)` | :arrow_down: |
| [transformers/tokenization\_auto.py](https://codecov.io/gh/huggingface/transformers/pull/1383/diff?src=pr&el=tree#diff-dHJhbnNmb3JtZXJzL3Rva2VuaXphdGlvbl9hdXRvLnB5) | `67.64% <33.33%> (-3.33%)` | :arrow_down: |
| [transformers/tokenization\_ctrl.py](https://codecov.io/gh/huggingface/transformers/pull/1383/diff?src=pr&el=tree#diff-dHJhbnNmb3JtZXJzL3Rva2VuaXphdGlvbl9jdHJsLnB5) | `83.6% <83.6%> (ø)` | |
| [transformers/configuration\_ctrl.py](https://codecov.io/gh/huggingface/transformers/pull/1383/diff?src=pr&el=tree#diff-dHJhbnNmb3JtZXJzL2NvbmZpZ3VyYXRpb25fY3RybC5weQ==) | `88.88% <88.88%> (ø)` | |
| ... and [18 more](https://codecov.io/gh/huggingface/transformers/pull/1383/diff?src=pr&el=tree-more) | |
------
[Continue to review full report at Codecov](https://codecov.io/gh/huggingface/transformers/pull/1383?src=pr&el=continue).
> **Legend** - [Click here to learn more](https://docs.codecov.io/docs/codecov-delta)
> `Δ = absolute <relative> (impact)`, `ø = not affected`, `? = missing data`
> Powered by [Codecov](https://codecov.io/gh/huggingface/transformers/pull/1383?src=pr&el=footer). Last update [1c50799...d9e60f4](https://codecov.io/gh/huggingface/transformers/pull/1383?src=pr&el=lastupdated). Read the [comment docs](https://docs.codecov.io/docs/pull-request-comments).
<|||||>Ok for merge<|||||>Thanks for adding this! I'm currently doing some experiments with the CTRL model, and I've a question about the tokenization:
```bash
tokenizer.tokenize("Munich and Berlin are nice cities.")
Out[6]: ['m@@', 'unic@@', 'h', 'and', 'ber@@', 'lin', 'are', 'nice', 'cities', '.']
```
Do you have any idea, why the output returns lowercased tokens only - `Berlin` and `Munich` do both appear in the vocab file (cased, and the splitting of `Munich` looks really weird 😅).<|||||>Yes, we are aware of the issue.
We are fixing this problem in #1480. |
transformers | 1,382 | closed | Issue with `decode` in the presence of special tokens | ## 🐛 Bug
<!-- Important information -->
Model I am using (Bert, XLNet....): GPT-2
Language I am using the model on (English, Chinese....): English
The problem arise when using:
* [ ] the official example scripts: (give details)
* [x] my own modified scripts: (give details)
The tasks I am working on is:
* [ ] an official GLUE/SQUaD task: (give the name)
* [x] my own task or dataset: (give details)
## To Reproduce
Steps to reproduce the behavior:
Run the following:
```bash
from transformers.tokenization_gpt2 import GPT2Tokenizer
tokenizer = GPT2Tokenizer.from_pretrained('gpt2')
tokenizer.add_special_tokens({"sep_token": "[SEP]"})
# this works, outputting "[SEP]"
tokenizer.convert_tokens_to_string(tokenizer.convert_ids_to_tokens(tokenizer.encode("[SEP]")))
# this fails
tokenizer.decode(tokenizer.encode("[SEP]"))
```
The last command gives this error:
```
miniconda3/envs/deepnlg/lib/python3.7/site-packages/transformers/tokenization_utils.py", line 937, in decode
text = text.replace(self._cls_token, self._sep_token)
TypeError: replace() argument 1 must be str, not None
```
<!-- If you have a code sample, error messages, stack traces, please provide it here as well. -->
## Expected behavior
The expectation is that [SEP] is output from the `decode` function.
## Environment
* OS: OSX
* Python version: 3.7
* PyTorch version: 1.1.0
* PyTorch Transformers version (or branch): Master (2dc8cb87341223e86220516951bb4ad84f880b4a)
* Using GPU ? No
* Distributed of parallel setup ? No
* Any other relevant information:
## Additional context
<!-- Add any other context about the problem here. --> | 09-30-2019 14:18:37 | 09-30-2019 14:18:37 | Can't reproduce this on master now. Seems to be fixed.<|||||>Thanks a lot. It seems to be fixed. Now I get `'[SEP]'` and `' [SEP]'` consecutively with the first and the second command above. So we can close this issue. |
transformers | 1,381 | closed | how to train RoBERTa from scratch | ## ❓ Questions & Help
<!-- A clear and concise description of the question. -->
I want to train RoBERTa model from scratch on different language. Is there any implementation available here to do this? | 09-30-2019 14:09:17 | 09-30-2019 14:09:17 | https://github.com/pytorch/fairseq/blob/master/examples/roberta/README.pretraining.md<|||||>This issue has been automatically marked as stale because it has not had recent activity. It will be closed if no further activity occurs. Thank you for your contributions.
<|||||>You can now leave `--model_name_or_path` to None in `run_language_modeling.py` to train a model from scratch.
See also https://huggingface.co/blog/how-to-train<|||||>When I put new --config_name and --tokenizer_name. It shows me that
json.decoder.JSONDecodeError: Expecting value: line 1 column 1 (char 0)
Anyone can help me? |
transformers | 1,380 | closed | Confusing tokenizer result on single word | Not sure if this is expected, but it seems confusing to me:
```python
import transformers
t=transformers.AutoTokenizer.from_pretrained('roberta-base')
t.tokenize("mystery")
```
yields two tokens, `['my', 'stery']`.
Yet
```
t.tokenize("a mystery")
```
*also* yields two tokens, `['a', 'Ġmystery']`. I would have thought this should yield one more token than tokenizing "mystery" alone.
| 09-30-2019 04:38:54 | 09-30-2019 04:38:54 | Hey @malmaud I think this #1196 can help you. The Roberta/GPT2 tokenizer expect a space to start. Without that, it sounds like you'll get strange behaviors.
To get the same output, in your first example, change it to
```
t.tokenize("mystery", add_prefix_space=True)
['Ġmystery']
```<|||||>That does work, thanks. I'm still confused why this doesn't work, though:
```
t.tokenize("<s> mystery </s>")
```
gives `['<s>', 'my', 'stery', '</s>']`<|||||>Hey @malmaud, spent some time going through the source code. So like above this gives the correct result:
```
t.tokenize("mystery", add_prefix_space=True)
['Ġmystery']
```
However
```
t.tokenizer(" mystery")
['my', 'stery']
```
I thought these should be doing the same thing. In the tokenization_gpt2.py file, it says:
```
if add_prefix_space:
text = ' ' + text
```
This should give the same results in both files then however when I add a print(text) statement before and after that I noticed I got these results. (using your example now)
```
t.tokenize("<s> mystery")
mystery
mystery
['<s>', 'my', 'stery']
t.tokenize("<s> mystery", add_prefix_space=True)
mystery
mystery
['<s>', 'Ġmystery']
```
This means that even though we are putting a single word in with a leading space, something in the preprocessing is getting rid of the initial space(s). So we need to use the add_prefix_space=True in order to get the space back or else the function won't be using the string we are expecting it will be using.<|||||>This issue has been automatically marked as stale because it has not had recent activity. It will be closed if no further activity occurs. Thank you for your contributions.
|
transformers | 1,379 | closed | TransfoXLCorpus requires pytorch to tokenize files | ## 🐛 Bug
The current TransfoXLCorpus code requires pytorch, and fails if it is not installed.
Model I am using (Bert, XLNet....): Transformer-XL
Language I am using the model on (English, Chinese....): Other
The problem arise when using:
* [ X ] my own modified scripts: I'm using a very simple script to read in text files, see code below
The tasks I am working on is:
* [ X ] my own task or dataset: I am attempting to build a corpus from my own dataset of long text sentences.
## To Reproduce
Steps to reproduce the behavior:
corpus = TransfoXLCorpus(lower_case=True, delimiter=" ")
corpus.build_corpus(EXAMPLE_DIR, "text8")
Traceback (most recent call last):
File "build_xl_corpus.py", line 26, in <module>
corpus.build_corpus(EXAMPLE_DIR, "text8")
File "/home/tom/.local/lib/python3.7/site-packages/transformers/tokenization_transfo_xl.py", line 521, in build_corpus
os.path.join(path, 'train.txt'), ordered=True, add_eos=False)
File "/home/tom/.local/lib/python3.7/site-packages/transformers/tokenization_transfo_xl.py", line 187, in encode_file
encoded.append(self.convert_to_tensor(symbols))
File "/home/tom/.local/lib/python3.7/site-packages/transformers/tokenization_transfo_xl.py", line 246, in convert_to_tensor
return torch.LongTensor(self.convert_tokens_to_ids(symbols))
NameError: name 'torch' is not defined
## Expected behavior
I did not expect this behavior to require pytorch
## Environment
* OS: Ubuntu
* Python version:
* PyTorch version: None
* PyTorch Transformers version (or branch): 2.0.0
* Using GPU ? Yes
* Distributed of parallel setup ? No
* Any other relevant information:
| 09-30-2019 00:23:32 | 09-30-2019 00:23:32 | This issue has been automatically marked as stale because it has not had recent activity. It will be closed if no further activity occurs. Thank you for your contributions.
|
transformers | 1,378 | closed | TFDistilBertForSequenceClassification - TypeError: len is not well defined for symbolic Tensors during model.fit() | ## 🐛 Bug
<!-- Important information -->
Model I am using (TFDistilBertForSequenceClassification):
Language I am using the model on (English):
The problem arise when using: model.fit()
* [ ] the official example scripts:
* [x] my own modified scripts:
The tasks I am working on is:
* [ ] an official GLUE/SQUaD task:
* [x] my own task or dataset:
## To Reproduce
Steps to reproduce the behavior:
1. create a random classification train,test set
2. get the pretrained TFDistilBertForSequenceClassification model
3. call fit() on the model for finetuning
```python
x_train = np.random.randint(2000, size=(100, 12))
x_train[:,0]=101
x_train[:,11]=102
y_train = np.random.randint(2, size=100)
model = TFDistilBertForSequenceClassification.from_pretrained('distilbert-base-uncased',num_labels = 2)
model.compile()
model.fit(x_train,y_train,epochs = 1,batch_size = 32,verbose=1)
```
```
TypeError: in converted code:
relative to /usr/local/lib/python3.6/dist-packages:
transformers/modeling_tf_distilbert.py:680 call *
distilbert_output = self.distilbert(inputs, **kwargs)
tensorflow_core/python/keras/engine/base_layer.py:842 __call__
outputs = call_fn(cast_inputs, *args, **kwargs)
transformers/modeling_tf_distilbert.py:447 call *
tfmr_output = self.transformer([embedding_output, attention_mask, head_mask], training=training)
tensorflow_core/python/keras/engine/base_layer.py:891 __call__
outputs = self.call(cast_inputs, *args, **kwargs)
transformers/modeling_tf_distilbert.py:382 call
layer_outputs = layer_module([hidden_state, attn_mask, head_mask[i]], training=training)
tensorflow_core/python/keras/engine/base_layer.py:891 __call__
outputs = self.call(cast_inputs, *args, **kwargs)
transformers/modeling_tf_distilbert.py:324 call
sa_output = self.attention([x, x, x, attn_mask, head_mask], training=training)
tensorflow_core/python/keras/engine/base_layer.py:891 __call__
outputs = self.call(cast_inputs, *args, **kwargs)
transformers/modeling_tf_distilbert.py:229 call
assert 2 <= len(tf.shape(mask)) <= 3
tensorflow_core/python/framework/ops.py:741 __len__
"shape information.".format(self.name))
TypeError: len is not well defined for symbolic Tensors. (tf_distil_bert_for_sequence_classification/distilbert/transformer/layer_._0/attention/Shape_2:0) Please call `x.shape` rather than `len(x)` for shape information.
```
## Expected behavior
<!-- A clear and concise description of what you expected to happen. -->
## Environment
* OS: Colab Notebook
* Python version:3.6.8
* PyTorch version:N/A
* Tensorflow version:tf-nightly-gpu-2.0-preview
* PyTorch Transformers version (or branch): 2.0/0
* Using GPU ? yes
* Distributed of parallel setup ? No
## Additional context
Calling the model directly with the input as mentioned in the example model doc works fine | 09-29-2019 18:44:18 | 09-29-2019 18:44:18 | so, how to solve this problem?<|||||>Should be solved on master and the latest release. |
transformers | 1,377 | closed | Error when calculate tokens_id and Mask LM | ## 🐛 Bug
<!-- Important information -->
Model I am using (DistilBert):
Language I am using the model on (English):
The problem arise when using: Distiller.prepare_batch( )
Error when token_ids is masked by mask LM matrix
* the official example scripts:
_token_ids_real = token_ids[pred_mask]
* my own modified scripts:
_token_ids_real=torch.mul(token_ids, pred_mask)
The tasks I am working on is:
* [GLUE ] an official GLUE/SQUaD task: (give the name)
* [ ] my own task or dataset: (give details)
## To Reproduce
Steps to reproduce the behavior:
1. pred_mask is matrix with 0,1.
Operation token_ids[pred_mask] seems to make some same matrix, instead of masking token_ids
<!-- If you have a code sample, error messages, stack traces, please provide it here as well. -->
## Expected behavior
<!-- A clear and concise description of what you expected to happen. -->
## Environment
* OS: Win10
* Python version: 3.6
* PyTorch version: 1.1
* PyTorch Transformers version (or branch): 2.0/0
* Using GPU ? Yes
* Distributed of parallel setup ? No
* Any other relevant information:
## Additional context
<!-- Add any other context about the problem here. --> | 09-29-2019 15:28:18 | 09-29-2019 15:28:18 | This issue has been automatically marked as stale because it has not had recent activity. It will be closed if no further activity occurs. Thank you for your contributions.
|
transformers | 1,376 | closed | Is it save the best model when used example like run_glue? | ## ❓ Questions & Help
<!-- A clear and concise description of the question. -->
I read the code of `run_glue.py`, I think it just save model checkpoint and the last step.
Is it wrong for me, or do I have to do some other operations? | 09-29-2019 13:21:15 | 09-29-2019 13:21:15 | |
transformers | 1,375 | closed | cannot import name 'TFBertForSequenceClassification' | I am unable to import TFBertForSequenceClassification.
from transformers import TFBertForSequenceClassification shows an error of cannot import name 'TFBertForSequenceClassification' | 09-29-2019 12:43:32 | 09-29-2019 12:43:32 | Hi! The TensorFlow components are only available when you have TF2 installed on your system. Could you please check that you have it in the environment in which you're running your code?<|||||>It worked. Thanks |
transformers | 1,374 | closed | Fix run_glue.py on QNLI part | In QNLI task, the ids should be truncated is the pair cuz that is the huge one. Or we can't load QNLI dataset successfully. | 09-29-2019 12:39:19 | 09-29-2019 12:39:19 | This issue has been automatically marked as stale because it has not had recent activity. It will be closed if no further activity occurs. Thank you for your contributions.
|
transformers | 1,373 | closed | Fixed critical css font-family issues | Fixed critical css font-family issues to ensure compatibility with multiple web browsers | 09-29-2019 11:51:32 | 09-29-2019 11:51:32 | Amazing! |
transformers | 1,372 | closed | Simplify code by using six.string_types | https://six.readthedocs.io/#six.string_types | 09-29-2019 08:40:51 | 09-29-2019 08:40:51 | # [Codecov](https://codecov.io/gh/huggingface/transformers/pull/1372?src=pr&el=h1) Report
> Merging [#1372](https://codecov.io/gh/huggingface/transformers/pull/1372?src=pr&el=desc) into [master](https://codecov.io/gh/huggingface/transformers/commit/fd97761c5a977fd22df789d2851cf57c7c9c0930?src=pr&el=desc) will **increase** coverage by `1.42%`.
> The diff coverage is `83.33%`.
[](https://codecov.io/gh/huggingface/transformers/pull/1372?src=pr&el=tree)
```diff
@@ Coverage Diff @@
## master #1372 +/- ##
==========================================
+ Coverage 84.74% 86.16% +1.42%
==========================================
Files 91 91
Lines 13593 13593
==========================================
+ Hits 11519 11713 +194
+ Misses 2074 1880 -194
```
| [Impacted Files](https://codecov.io/gh/huggingface/transformers/pull/1372?src=pr&el=tree) | Coverage Δ | |
|---|---|---|
| [transformers/tokenization\_utils.py](https://codecov.io/gh/huggingface/transformers/pull/1372/diff?src=pr&el=tree#diff-dHJhbnNmb3JtZXJzL3Rva2VuaXphdGlvbl91dGlscy5weQ==) | `91.43% <83.33%> (ø)` | :arrow_up: |
| [transformers/modeling\_openai.py](https://codecov.io/gh/huggingface/transformers/pull/1372/diff?src=pr&el=tree#diff-dHJhbnNmb3JtZXJzL21vZGVsaW5nX29wZW5haS5weQ==) | `81.75% <0%> (+1.35%)` | :arrow_up: |
| [transformers/modeling\_ctrl.py](https://codecov.io/gh/huggingface/transformers/pull/1372/diff?src=pr&el=tree#diff-dHJhbnNmb3JtZXJzL21vZGVsaW5nX2N0cmwucHk=) | `95.45% <0%> (+2.27%)` | :arrow_up: |
| [transformers/modeling\_xlnet.py](https://codecov.io/gh/huggingface/transformers/pull/1372/diff?src=pr&el=tree#diff-dHJhbnNmb3JtZXJzL21vZGVsaW5nX3hsbmV0LnB5) | `73.28% <0%> (+2.46%)` | :arrow_up: |
| [transformers/modeling\_roberta.py](https://codecov.io/gh/huggingface/transformers/pull/1372/diff?src=pr&el=tree#diff-dHJhbnNmb3JtZXJzL21vZGVsaW5nX3JvYmVydGEucHk=) | `80.57% <0%> (+15.1%)` | :arrow_up: |
| [transformers/tests/modeling\_tf\_common\_test.py](https://codecov.io/gh/huggingface/transformers/pull/1372/diff?src=pr&el=tree#diff-dHJhbnNmb3JtZXJzL3Rlc3RzL21vZGVsaW5nX3RmX2NvbW1vbl90ZXN0LnB5) | `96.8% <0%> (+17.02%)` | :arrow_up: |
| [transformers/modeling\_tf\_pytorch\_utils.py](https://codecov.io/gh/huggingface/transformers/pull/1372/diff?src=pr&el=tree#diff-dHJhbnNmb3JtZXJzL21vZGVsaW5nX3RmX3B5dG9yY2hfdXRpbHMucHk=) | `92.95% <0%> (+83.09%)` | :arrow_up: |
------
[Continue to review full report at Codecov](https://codecov.io/gh/huggingface/transformers/pull/1372?src=pr&el=continue).
> **Legend** - [Click here to learn more](https://docs.codecov.io/docs/codecov-delta)
> `Δ = absolute <relative> (impact)`, `ø = not affected`, `? = missing data`
> Powered by [Codecov](https://codecov.io/gh/huggingface/transformers/pull/1372?src=pr&el=footer). Last update [fd97761...ba6f2d6](https://codecov.io/gh/huggingface/transformers/pull/1372?src=pr&el=lastupdated). Read the [comment docs](https://docs.codecov.io/docs/pull-request-comments).
<|||||>This issue has been automatically marked as stale because it has not had recent activity. It will be closed if no further activity occurs. Thank you for your contributions.
<|||||>We'll handle this by dropping python2 support in the next release (and using flake8) cc @aaugustin |
transformers | 1,371 | closed | Make activation functions available from modeling_utils (PyTorch) | * This commit replaces references to PyTorch activation functions/modules by a dict of functions that lives in `modeling_utils`. This ensures that all activation functions are available to all modules, praticularly custom functions such as swish and new_gelu.
* In addition, when available (PT1.2) the native PyTorch gelu function will be used - it supports a CPP/CUDA implementation.
**NOTE** that this replaces all `nn.Module`'s by bare functions except for one which was required for testing to be of the type `nn.Module`. If requested, this can be reverted so that only function calls are replaced by ACT2FN functions, and that existing `nn.Module`s are untouched.
**NOTE** that one would thus also expect that _all_ usages of activation functions are taken from `ACT2FN` for consistency's sake.
**NOTE** since the Module counter-part of PyTorch's GeLU [isn't available (yet)](https://github.com/pytorch/pytorch/pull/20665#issuecomment-536359684), it might be worth waiting to implement this pull, and then use Modules and functions in the right places where one would expect, i.e. `Module` when part of architecture, function when processing other kinds of data. | 09-29-2019 07:40:10 | 09-29-2019 07:40:10 | # [Codecov](https://codecov.io/gh/huggingface/transformers/pull/1371?src=pr&el=h1) Report
> Merging [#1371](https://codecov.io/gh/huggingface/transformers/pull/1371?src=pr&el=desc) into [master](https://codecov.io/gh/huggingface/transformers/commit/ae50ad91ea2fedb64ecd2e7c8e2d0d4778dc03aa?src=pr&el=desc) will **increase** coverage by `0.97%`.
> The diff coverage is `85.71%`.
[](https://codecov.io/gh/huggingface/transformers/pull/1371?src=pr&el=tree)
```diff
@@ Coverage Diff @@
## master #1371 +/- ##
==========================================
+ Coverage 83.76% 84.74% +0.97%
==========================================
Files 84 84
Lines 12596 12559 -37
==========================================
+ Hits 10551 10643 +92
+ Misses 2045 1916 -129
```
| [Impacted Files](https://codecov.io/gh/huggingface/transformers/pull/1371?src=pr&el=tree) | Coverage Δ | |
|---|---|---|
| [transformers/modeling\_openai.py](https://codecov.io/gh/huggingface/transformers/pull/1371/diff?src=pr&el=tree#diff-dHJhbnNmb3JtZXJzL21vZGVsaW5nX29wZW5haS5weQ==) | `80.41% <100%> (ø)` | :arrow_up: |
| [transformers/modeling\_xlnet.py](https://codecov.io/gh/huggingface/transformers/pull/1371/diff?src=pr&el=tree#diff-dHJhbnNmb3JtZXJzL21vZGVsaW5nX3hsbmV0LnB5) | `72.02% <100%> (+0.77%)` | :arrow_up: |
| [transformers/modeling\_gpt2.py](https://codecov.io/gh/huggingface/transformers/pull/1371/diff?src=pr&el=tree#diff-dHJhbnNmb3JtZXJzL21vZGVsaW5nX2dwdDIucHk=) | `83.88% <100%> (-0.11%)` | :arrow_down: |
| [transformers/modeling\_roberta.py](https://codecov.io/gh/huggingface/transformers/pull/1371/diff?src=pr&el=tree#diff-dHJhbnNmb3JtZXJzL21vZGVsaW5nX3JvYmVydGEucHk=) | `71.01% <100%> (+5.54%)` | :arrow_up: |
| [transformers/modeling\_distilbert.py](https://codecov.io/gh/huggingface/transformers/pull/1371/diff?src=pr&el=tree#diff-dHJhbnNmb3JtZXJzL21vZGVsaW5nX2Rpc3RpbGJlcnQucHk=) | `95.8% <100%> (-0.03%)` | :arrow_down: |
| [transformers/modeling\_transfo\_xl.py](https://codecov.io/gh/huggingface/transformers/pull/1371/diff?src=pr&el=tree#diff-dHJhbnNmb3JtZXJzL21vZGVsaW5nX3RyYW5zZm9feGwucHk=) | `75.16% <100%> (ø)` | :arrow_up: |
| [transformers/modeling\_bert.py](https://codecov.io/gh/huggingface/transformers/pull/1371/diff?src=pr&el=tree#diff-dHJhbnNmb3JtZXJzL21vZGVsaW5nX2JlcnQucHk=) | `88.41% <100%> (+0.23%)` | :arrow_up: |
| [transformers/modeling\_xlm.py](https://codecov.io/gh/huggingface/transformers/pull/1371/diff?src=pr&el=tree#diff-dHJhbnNmb3JtZXJzL21vZGVsaW5nX3hsbS5weQ==) | `88.36% <100%> (-0.07%)` | :arrow_down: |
| [transformers/modeling\_transfo\_xl\_utilities.py](https://codecov.io/gh/huggingface/transformers/pull/1371/diff?src=pr&el=tree#diff-dHJhbnNmb3JtZXJzL21vZGVsaW5nX3RyYW5zZm9feGxfdXRpbGl0aWVzLnB5) | `54.16% <37.5%> (+0.27%)` | :arrow_up: |
| [transformers/modeling\_utils.py](https://codecov.io/gh/huggingface/transformers/pull/1371/diff?src=pr&el=tree#diff-dHJhbnNmb3JtZXJzL21vZGVsaW5nX3V0aWxzLnB5) | `92.57% <90%> (-0.12%)` | :arrow_down: |
| ... and [7 more](https://codecov.io/gh/huggingface/transformers/pull/1371/diff?src=pr&el=tree-more) | |
------
[Continue to review full report at Codecov](https://codecov.io/gh/huggingface/transformers/pull/1371?src=pr&el=continue).
> **Legend** - [Click here to learn more](https://docs.codecov.io/docs/codecov-delta)
> `Δ = absolute <relative> (impact)`, `ø = not affected`, `? = missing data`
> Powered by [Codecov](https://codecov.io/gh/huggingface/transformers/pull/1371?src=pr&el=footer). Last update [ae50ad9...716d783](https://codecov.io/gh/huggingface/transformers/pull/1371?src=pr&el=lastupdated). Read the [comment docs](https://docs.codecov.io/docs/pull-request-comments).
<|||||>This issue has been automatically marked as stale because it has not had recent activity. It will be closed if no further activity occurs. Thank you for your contributions.
<|||||>Unstale. <|||||>Would feel syntactically cleaner if we could do `ACT2FN.gelu()` instead of a dict (also gives some IDE goodness like autocomplete) (I guess through a class or namespace or something), what do you guys think?<|||||>> Would feel syntactically cleaner if we could do `ACT2FN.gelu()` instead of a dict (also gives some IDE goodness like autocomplete) (I guess through a class or namespace or something), what do you guys think?
Sounds good but note that this is not something I introduced. The ACT2FN dict already existed, but wasn't used consistently it seemed.<|||||>Ah yeah, I see. Would you want to do this change, if you have the time/bandwidth? (+ rebasing on current master so we can merge easily?)<|||||>This issue has been automatically marked as stale because it has not had recent activity. It will be closed if no further activity occurs. Thank you for your contributions.
<|||||>AFAICT, this has been done by @sshleifer on master. Re-open if necessary! |
transformers | 1,370 | closed | considerd to add albert? | ## 🚀 Feature
<!-- A clear and concise description of the feature proposal. Please provide a link to the paper and code in case they exist. -->
## Motivation
<!-- Please outline the motivation for the proposal. Is your feature request related to a problem? e.g., I'm always frustrated when [...]. If this is related to another GitHub issue, please link here too. -->
## Additional context
<!-- Add any other context or screenshots about the feature request here. --> | 09-29-2019 02:21:47 | 09-29-2019 02:21:47 | Would definitely love to see an implementation of ALBERT added to this repository. Just for completeness:
* paper: https://arxiv.org/abs/1909.11942
* reddit: https://www.reddit.com/r/MachineLearning/comments/d9tdfo/albert_a_lite_bert_for_selfsupervised_learning_of/
* medium: https://medium.com/syncedreview/googles-albert-is-a-leaner-bert-achieves-sota-on-3-nlp-benchmarks-f64466dd583
That said, it could be even more interesting to implement the core improvements (factorized embedding parameterization, cross-layer parameter sharing) from ALBERT in (some?/all?) other transformers as optional features?
<|||||>Knowing how fast the team works, I would expect ALBERT to be implemented quite soon. That being said, I haven't had time to read the ALBERT paper yet, so it might be more difficult than previous BERT iterations such as distilbert and RoBERTa.<|||||>I think ALBERT is very cool! Expect...<|||||>And in pytorch (using code from this repo and weights from brightmart) https://github.com/lonePatient/albert_pytorch<|||||>Any Update on the progress?<|||||>The ALBERT paper will be presented at ICLR in April 2020. From what I last heard, the huggingface team has been talking with the people over at Google AI to share the details of the model, but I can imagine that the researchers rather wait until the paper has been presented. One of those reasons being that they want to get citations from their ICLR talk rather than an arXiv citation which, in the field, is "worth less" than a big conference proceeding.
For now, just be patient. I am sure that the huggingface team will have a big announcement (follow their Twitter/LinkedIn channels) with a new version bump. No need to keep bumping this topic.<|||||>https://github.com/interviewBubble/Google-ALBERT<|||||>The official code and models got released :slightly_smiling_face:
https://github.com/google-research/google-research/tree/master/albert <|||||>[WIP]
ALBERT in tensorflow 2.0
https://github.com/kamalkraj/ALBERT-TF2.0
<|||||>https://github.com/lonePatient/albert_pytorch
Dataset: MNLI
Model: ALBERT_BASE_V2
Dev accuracy : 0.8418
Dataset: SST-2
Model: ALBERT_BASE_V2
Dev accuracy :0.926<|||||>PR was created, see here:
https://github.com/huggingface/transformers/pull/1683<|||||>> [WIP]
> ALBERT in tensorflow 2.0
> https://github.com/kamalkraj/ALBERT-TF2.0
Verison 2 weights added.
Support for SQuAD 1.1 and 2.0 added.
Reproduces the same results from paper. From my experiments, ALBERT model is very sensitive to hyperparameter like Batch Size. FineTuning using AdamW as Default in Original Repo. AdamW performs better than LAMB on Model finetuning. <|||||>This issue has been automatically marked as stale because it has not had recent activity. It will be closed if no further activity occurs. Thank you for your contributions.
|
transformers | 1,369 | closed | Update README.md | Lines 183 - 200, fixed indentation. Line 198, replaced `tokenizer_class` with `BertTokenizer`, since `tokenizer_class` is not defined in the loop it belongs to. | 09-28-2019 23:37:05 | 09-28-2019 23:37:05 | # [Codecov](https://codecov.io/gh/huggingface/transformers/pull/1369?src=pr&el=h1) Report
> Merging [#1369](https://codecov.io/gh/huggingface/transformers/pull/1369?src=pr&el=desc) into [master](https://codecov.io/gh/huggingface/transformers/commit/ae50ad91ea2fedb64ecd2e7c8e2d0d4778dc03aa?src=pr&el=desc) will **increase** coverage by `0.92%`.
> The diff coverage is `n/a`.
[](https://codecov.io/gh/huggingface/transformers/pull/1369?src=pr&el=tree)
```diff
@@ Coverage Diff @@
## master #1369 +/- ##
==========================================
+ Coverage 83.76% 84.69% +0.92%
==========================================
Files 84 84
Lines 12596 12596
==========================================
+ Hits 10551 10668 +117
+ Misses 2045 1928 -117
```
| [Impacted Files](https://codecov.io/gh/huggingface/transformers/pull/1369?src=pr&el=tree) | Coverage Δ | |
|---|---|---|
| [transformers/modeling\_xlnet.py](https://codecov.io/gh/huggingface/transformers/pull/1369/diff?src=pr&el=tree#diff-dHJhbnNmb3JtZXJzL21vZGVsaW5nX3hsbmV0LnB5) | `72.14% <0%> (+0.89%)` | :arrow_up: |
| [transformers/modeling\_roberta.py](https://codecov.io/gh/huggingface/transformers/pull/1369/diff?src=pr&el=tree#diff-dHJhbnNmb3JtZXJzL21vZGVsaW5nX3JvYmVydGEucHk=) | `71.22% <0%> (+5.75%)` | :arrow_up: |
| [transformers/tests/modeling\_tf\_common\_test.py](https://codecov.io/gh/huggingface/transformers/pull/1369/diff?src=pr&el=tree#diff-dHJhbnNmb3JtZXJzL3Rlc3RzL21vZGVsaW5nX3RmX2NvbW1vbl90ZXN0LnB5) | `95% <0%> (+7.5%)` | :arrow_up: |
| [transformers/modeling\_tf\_pytorch\_utils.py](https://codecov.io/gh/huggingface/transformers/pull/1369/diff?src=pr&el=tree#diff-dHJhbnNmb3JtZXJzL21vZGVsaW5nX3RmX3B5dG9yY2hfdXRpbHMucHk=) | `76.92% <0%> (+66.43%)` | :arrow_up: |
------
[Continue to review full report at Codecov](https://codecov.io/gh/huggingface/transformers/pull/1369?src=pr&el=continue).
> **Legend** - [Click here to learn more](https://docs.codecov.io/docs/codecov-delta)
> `Δ = absolute <relative> (impact)`, `ø = not affected`, `? = missing data`
> Powered by [Codecov](https://codecov.io/gh/huggingface/transformers/pull/1369?src=pr&el=footer). Last update [ae50ad9...d1176d5](https://codecov.io/gh/huggingface/transformers/pull/1369?src=pr&el=lastupdated). Read the [comment docs](https://docs.codecov.io/docs/pull-request-comments).
<|||||>Great, thanks for updating the README! |
transformers | 1,368 | closed | Tried to import TFBertForPreTraining in google colab | Tried to import TFBertForPreTraining and received an error
from transformers import BertTokenizer, TFBertForPreTraining
---------------------------------------------------------------------------
ImportError Traceback (most recent call last)
<ipython-input-24-91f8709e090f> in <module>()
----> 1 from transformers import BertTokenizer, TFBertForPreTraining
ImportError: cannot import name 'TFBertForPreTraining'
---------------------------------------------------------------------------
NOTE: If your import is failing due to a missing package, you can
manually install dependencies using either !pip or !apt.
To view examples of installing some common dependencies, click the
"Open Examples" button below.
--------------------------------------------------------------------------- | 09-28-2019 23:34:22 | 09-28-2019 23:34:22 | Hey @mandavachetana its not just a google colab thing. Take a look here #1375 You need to make sure you are using tensorflow 2.0 and it should work.<|||||>This issue has been automatically marked as stale because it has not had recent activity. It will be closed if no further activity occurs. Thank you for your contributions.
|
transformers | 1,367 | closed | Model does not train when using new BertModel, but does with old BertModel | ## 📚 Migration
I am currently working on using Transformers with Snorkel's classification library [https://github.com/snorkel-team/snorkel](https://github.com/snorkel-team/snorkel) (for MTL learning in the future). I currently am trying to troubleshoot why the model is not learning, and so have my experiment set up such that the Snorkel library learns one task, essentially training a BERT model and linear layer.
The code for this experiment can be found at [https://github.com/Peter-Devine/test_cls_snorkel_mtl]( https://github.com/Peter-Devine/test_cls_snorkel_mtl ). To run it, you will need torch, snorkel, numpy, pytorch_pretrained_bert and transformers.
My problem is as follows.
When I run the code in `test_cls_snorkel_mtl/tutorials/ISEAR_pretrain_tutorial.py`, my code runs fine and the model's validation accuracy scores are good. This is because I am using the old pytorch_pretrained_bert BertModel in `test_cls_snorkel_mtl/modules/bert_module.py`. If you uncomment line 6 of `test_cls_snorkel_mtl/modules/bert_module.py` and use the new transformers BertModel, then running `test_cls_snorkel_mtl/tutorials/ISEAR_pretrain_tutorial.py` will result in a model that never converges and bad validation accuracy.
From reading the code on Snorkel, I cannot seem to find the reason as to why this would be. What are the major changes in training a model between versions of pytorch_pretrained_bert and transformers. Do back-passes etc. work the same way in both models?
Thanks | 09-28-2019 20:04:12 | 09-28-2019 20:04:12 | You can check the two migration guides, they explain all the differences:
- https://github.com/huggingface/transformers#Migrating-from-pytorch-transformers-to-transformers
- https://github.com/huggingface/transformers#migrating-from-pytorch-pretrained-bert-to-transformers <|||||>This issue has been automatically marked as stale because it has not had recent activity. It will be closed if no further activity occurs. Thank you for your contributions.
|
transformers | 1,366 | closed | fix redundant initializations of Embeddings in RobertaEmbeddings | Based on the discussion with @julien-c in #1258, this PR fixes the issue of redundant multiple initializations of the embeddings in the constructor of `RobertaEmbeddings` by removing the constructor call of its parent class (i.e., `BertEmbeddings`) and creating `token_type_embeddings`, `LayerNorm`, and `dropout` in the constructor. | 09-28-2019 16:29:23 | 09-28-2019 16:29:23 | Sorry, I will fix this |
transformers | 1,365 | closed | Why add the arguments 'head_mask' and when to use this arguments | ## ❓ Questions & Help
<!-- A clear and concise description of the question. -->
**head_mask**: (`optional`) ``torch.FloatTensor`` of shape ``(num_heads,)`` or ``(num_layers, num_heads)``:
Mask to nullify selected heads of the self-attention modules.
Mask values selected in ``[0, 1]``:
``1`` indicates the head is **not masked**, ``0`` indicates the head is **masked**. | 09-28-2019 13:40:38 | 09-28-2019 13:40:38 | This issue has been automatically marked as stale because it has not had recent activity. It will be closed if no further activity occurs. Thank you for your contributions.
|
transformers | 1,364 | closed | Is there any plan for Roberta in SQuAD? | ## 🚀 Feature
<!-- A clear and concise description of the feature proposal. Please provide a link to the paper and code in case they exist. -->
Hello, thx for the RoBERTa implementation. But I want to know is there any plan for the RoBERTa in SQuAD, because it is complex. And I simple changed the run_squad code as the run_gule code, I got some bugs. And the fairseq doesn't give a official code, too. I really want to know how to use the RoBERTa in SQuAD use the transformers.
## Motivation
<!-- Please outline the motivation for the proposal. Is your feature request related to a problem? e.g., I'm always frustrated when [...]. If this is related to another GitHub issue, please link here too. -->
## Additional context
<!-- Add any other context or screenshots about the feature request here. --> | 09-28-2019 12:56:27 | 09-28-2019 12:56:27 | |
transformers | 1,363 | closed | Why the RoBERTa's max_position_embeddings size is 512+2=514? | ## ❓ Questions & Help
<!-- A clear and concise description of the question. -->
When I see the code of Roberta, I have a question about the padding_idx = 1, I don't know very well. And the comment is still confused for me. | 09-28-2019 11:52:45 | 09-28-2019 11:52:45 | What's your precise question?<|||||>> What's your precise question?
the self.padding_idx's meaning in modeling_roberta.py<|||||>It's the position of the padding vector. It's not unique to RoBERTa but far more general, especially for embeddings. Take a look at [the PyTorch documentation](https://pytorch.org/docs/stable/nn.html#embedding).<|||||>> It's the position of the padding vector. It's not unique to RoBERTa but far more general, especially for embeddings. Take a look at [the PyTorch documentation](https://pytorch.org/docs/stable/nn.html#embedding).
I know that, but I confuse about why there is 1 and the \<s\> is 0, is it ignore and why the max_position_embeddings size is 512+2=514?<|||||>Because that's their index [in the vocab](https://s3.amazonaws.com/models.huggingface.co/bert/roberta-base-vocab.json). The max_position_embeddings size is indeed 514, I'm not sure why. The tokenizer seems to handle text correctly with a max of 512. Perhaps someone of the developers can help with that. I would advise you to change the title of your topic.
https://github.com/huggingface/transformers/blob/ae50ad91ea2fedb64ecd2e7c8e2d0d4778dc03aa/transformers/tokenization_roberta.py#L84-L85<|||||>@LysandreJik can chime in if I’m wrong, but afaik `max_position_embeddings` is just the name of the variable that we use to encode the size of the embedding matrix. Max_len is correctly set to 512.<|||||>This issue has been automatically marked as stale because it has not had recent activity. It will be closed if no further activity occurs. Thank you for your contributions.
<|||||>Answer here in case anyone from the future is curious: https://github.com/pytorch/fairseq/issues/1187<|||||>> Answer here in case anyone from the future is curious: [pytorch/fairseq#1187](https://github.com/pytorch/fairseq/issues/1187)
@morganmcg1 Tks for this, was getting all kinds of CUDA errors because i setted `max_position_embeddings=512`, now that i setted 514 it's running ok... |
transformers | 1,362 | closed | fix link | 09-28-2019 08:22:16 | 09-28-2019 08:22:16 | # [Codecov](https://codecov.io/gh/huggingface/transformers/pull/1362?src=pr&el=h1) Report
> Merging [#1362](https://codecov.io/gh/huggingface/transformers/pull/1362?src=pr&el=desc) into [master](https://codecov.io/gh/huggingface/transformers/commit/a6a6d9e6382961dc92a1a08d1bab05a52dc815f9?src=pr&el=desc) will **not change** coverage.
> The diff coverage is `n/a`.
[](https://codecov.io/gh/huggingface/transformers/pull/1362?src=pr&el=tree)
```diff
@@ Coverage Diff @@
## master #1362 +/- ##
=======================================
Coverage 84.69% 84.69%
=======================================
Files 84 84
Lines 12596 12596
=======================================
Hits 10668 10668
Misses 1928 1928
```
------
[Continue to review full report at Codecov](https://codecov.io/gh/huggingface/transformers/pull/1362?src=pr&el=continue).
> **Legend** - [Click here to learn more](https://docs.codecov.io/docs/codecov-delta)
> `Δ = absolute <relative> (impact)`, `ø = not affected`, `? = missing data`
> Powered by [Codecov](https://codecov.io/gh/huggingface/transformers/pull/1362?src=pr&el=footer). Last update [a6a6d9e...60f7916](https://codecov.io/gh/huggingface/transformers/pull/1362?src=pr&el=lastupdated). Read the [comment docs](https://docs.codecov.io/docs/pull-request-comments).
<|||||>👍 |
|
transformers | 1,361 | closed | distil-finetuning in run_squad | - Add the option for double loss: fine-tuning + distillation from a larger squad-finetune model.
- Fix `inputs` for `DistilBERT` (also see fix in `run_glue.py` 702f589848baba97ea4897aa3f0bb937e1ec3bcf) | 09-27-2019 21:47:59 | 09-27-2019 21:47:59 | cf https://github.com/huggingface/transformers/issues/1193#issuecomment-534740929<|||||>Ok, as discussed let's copy this script to the `examples/distillation` folder and keep `run_squad` barebone for now as it's going to evolve in the short term.<|||||># [Codecov](https://codecov.io/gh/huggingface/transformers/pull/1361?src=pr&el=h1) Report
> Merging [#1361](https://codecov.io/gh/huggingface/transformers/pull/1361?src=pr&el=desc) into [master](https://codecov.io/gh/huggingface/transformers/commit/2dc8cb87341223e86220516951bb4ad84f880b4a?src=pr&el=desc) will **not change** coverage.
> The diff coverage is `n/a`.
[](https://codecov.io/gh/huggingface/transformers/pull/1361?src=pr&el=tree)
```diff
@@ Coverage Diff @@
## master #1361 +/- ##
=======================================
Coverage 84.69% 84.69%
=======================================
Files 84 84
Lines 12596 12596
=======================================
Hits 10668 10668
Misses 1928 1928
```
------
[Continue to review full report at Codecov](https://codecov.io/gh/huggingface/transformers/pull/1361?src=pr&el=continue).
> **Legend** - [Click here to learn more](https://docs.codecov.io/docs/codecov-delta)
> `Δ = absolute <relative> (impact)`, `ø = not affected`, `? = missing data`
> Powered by [Codecov](https://codecov.io/gh/huggingface/transformers/pull/1361?src=pr&el=footer). Last update [2dc8cb8...b4df865](https://codecov.io/gh/huggingface/transformers/pull/1361?src=pr&el=lastupdated). Read the [comment docs](https://docs.codecov.io/docs/pull-request-comments).
<|||||>no squash @VictorSanh? 😬 |
transformers | 1,360 | closed | Chunking Long Documents for Classification Tasks | ## 🚀 Feature
A way to process long documents for downstream classification tasks. One approach is to chunk long sequences with a specific stride similar to what is done in the run_squad example.
## Motivation
For classification tasks using datasets that are on average longer than 512 tokens, I believe it would improve performance.
## Additional context
https://github.com/google-research/bert/issues/27#issuecomment-435265194 | 09-27-2019 20:03:14 | 09-27-2019 20:03:14 | I'm not sure that I understand. As you say, you can see it implemented in the run_squad example. What else would you like? <|||||>Hello Bram,
I mean I want to apply it with a sequence classification task like BertForSequenceClassification, for example, versus what is being done in squad.
I don't think it should be too hard but I'm not exactly sure how a long document that is being chunked gets trained. Do we ignore the fact that these are chunks of the same document and just treat them as independent docs? Or do we do some sort of trick to join the tokens/embeddings with the first chunk?
How would this be implemented for sequence classification?<|||||>I quickly glared over the `convert_examples_to_features` function, and it seems that given some stride different parts are used as input. So, yes, as far as I can see they are treated as independent docs.
https://github.com/huggingface/transformers/blob/ae50ad91ea2fedb64ecd2e7c8e2d0d4778dc03aa/examples/utils_squad.py#L189-L397
<|||||>isn't there a way to deal with long documents without ignoring the fact that the chunks represent the same doc?
Maybe something along the lines of https://finetune.indico.io/chunk.html?highlight=long or https://explosion.ai/blog/spacy-pytorch-transformers#batching<|||||>After a first look, I don't see how `spacy-pytorch-transformers` does anything special rather than processing a document sentence-per-sentence. `finetune`'s approach might be what you after (taking the mean over all the slided windows), but as always: "a mean is just a mean", so the question remains how representative it is of the whole document. I am not saying that slicing is _better_ by any means, but averaging can distort "real" values greatly.<|||||>Yeah I see your point. I'm starting to think that maybe trying out chunking with a couple of different strides and maybe at inference time taking a voting approach would be a better option.
In any case, thank you for your feedback!<|||||>I agree that that might be the more efficient approach. No worries, thanks for the interesting question. If you think it's okay the question, please close it so it's easy to keep track of all open issues.<|||||>Hi, just to let you know that there is an option to manage strides in the `encode_plus` method. It handles special tokens and returns the overflowing elements in the `overflowing_tokens` field of the returned dictionary. |
transformers | 1,359 | closed | Update run_lm_finetuning.py | The previous method, just as phrased, did not exist in the class. | 09-27-2019 18:19:34 | 09-27-2019 18:19:34 | # [Codecov](https://codecov.io/gh/huggingface/transformers/pull/1359?src=pr&el=h1) Report
> Merging [#1359](https://codecov.io/gh/huggingface/transformers/pull/1359?src=pr&el=desc) into [master](https://codecov.io/gh/huggingface/transformers/commit/ca559826c4188be8713e46f191ddf5f379c196e7?src=pr&el=desc) will **not change** coverage.
> The diff coverage is `n/a`.
[](https://codecov.io/gh/huggingface/transformers/pull/1359?src=pr&el=tree)
```diff
@@ Coverage Diff @@
## master #1359 +/- ##
=======================================
Coverage 84.73% 84.73%
=======================================
Files 84 84
Lines 12573 12573
=======================================
Hits 10654 10654
Misses 1919 1919
```
------
[Continue to review full report at Codecov](https://codecov.io/gh/huggingface/transformers/pull/1359?src=pr&el=continue).
> **Legend** - [Click here to learn more](https://docs.codecov.io/docs/codecov-delta)
> `Δ = absolute <relative> (impact)`, `ø = not affected`, `? = missing data`
> Powered by [Codecov](https://codecov.io/gh/huggingface/transformers/pull/1359?src=pr&el=footer). Last update [ca55982...9478590](https://codecov.io/gh/huggingface/transformers/pull/1359?src=pr&el=lastupdated). Read the [comment docs](https://docs.codecov.io/docs/pull-request-comments).
<|||||>Thanks @dennymarcels! |
transformers | 1,358 | closed | How to contribute to “Write with transformer”? | ## 🚀 I would like to contribute to a French version of this App
I’m French, I write short stories, and I’m also a software engineer
## Motivation
I’ll retire in 6 months and I wanted to build such an app before I stumbled on your demo.
## Additional context
https://www.linkedin.com/in/mauceri/
| 09-27-2019 17:59:29 | 09-27-2019 17:59:29 | What is it that you can contribute? The only (yet impressive) thing that is going on is language modeling. Can you contribute a pre-trained French model for one of the frameworks? That's (as far as I know) the only way to contribute. <|||||>Thanks Bram, I’m going to investigate what the cost could be for XLNet on clevergrid https://www.clevergrid.io/?pk_campaign=ga-gpu-1&pk_source=adwords&pk_medium=sem&pk_content=gpuasaservicefr&gclid=CjwKCAjwibzsBRAMEiwA1pHZrvm8ozRMrbcDR7YoYiKqsq6gEnPo9AecJwjKzBxa8L-4_hB6ny4uARoCwfMQAvD_BwE
Envoyé de mon iPad
> Le 28 sept. 2019 à 09:44, Bram Vanroy <[email protected]> a écrit :
>
> What is it that you can contribute? The only (yet impressive) thing that is going on is language modeling. Can you contribute a pre-trained French model for one of the frameworks? That's (as far as I know) the only way to contribute.
>
> —
> You are receiving this because you authored the thread.
> Reply to this email directly, view it on GitHub, or mute the thread.
<|||||>Hi all,
We (ovh) are open to calculate it for free.<|||||>Not sure if a new French language model is still necessary after Camembert has been introduced.<|||||>That's awesome news, @jqueguiner! Let us know if we can help.
@BramVanroy To work well with Write With Transformer, we would want more like a FR-pretrained GPT-2-like model. CamemBERT wouldn't do on generation out of the box.
See also the more specific issue: https://github.com/huggingface/transformers/issues/1356<|||||>For generation CamemBERT is of no use I think...
Envoyé de mon iPad
> Le 22 nov. 2019 à 14:02, Bram Vanroy <[email protected]> a écrit :
>
>
> Not sure if a new French language model is still necessary after Camembert has been introduced.
>
> —
> You are receiving this because you authored the thread.
> Reply to this email directly, view it on GitHub, or unsubscribe.
<|||||>Yes, CamemBERT is awesome, but for WWT we need a FR-Pretrained GPT-2 model!
Envoyé de mon iPad
> Le 22 nov. 2019 à 14:55, Julien Chaumond <[email protected]> a écrit :
>
>
> That's awesome news, @jqueguiner! Let us know if we can help.
>
> @BramVanroy To work well with Write With Transformer, we would want more like a FR-pretrained GPT-2-like model. CamemBERT wouldn't do on generation out of the box.
>
> See also the more specific issue: #1356
>
> —
> You are receiving this because you authored the thread.
> Reply to this email directly, view it on GitHub, or unsubscribe.
<|||||>Camembert doesnt offer generation, only syntax analysis and masking due to the nature of the network. Multiple mask generation (<mask><mask><mask>) gives really uggly results as you cna test here : https://market-place.ai.ovh.net/#!/apis/43323c37-59e7-4092-b23c-3759e7c09288/pages/94d31892-4e64-446f-9318-924e64346f9e
IMO we should start training using OSCAR dataset
https://traces1.inria.fr/oscar/
@julien-c yes we can start with a collab GPT2 french training ipynb together then I'll prepare the env for a DGX1 or something similar. I didn't train a GPT2 before. IS it scaling over multiple GPU's ? do we need horovod adaptation ?<|||||>Oops, sorry everyone. I thought this was a general French model question. My bad. <|||||>This issue has been automatically marked as stale because it has not had recent activity. It will be closed if no further activity occurs. Thank you for your contributions.
|
transformers | 1,357 | closed | Support for SuperGLUE fine-tune/eval? | ## 🚀 Feature
https://super.gluebenchmark.com/
Current canonical implem is https://github.com/nyu-mll/jiant/
## Motivation
https://twitter.com/_florianmai/status/1177489945918722050
| 09-27-2019 17:33:54 | 09-27-2019 17:33:54 | This issue has been automatically marked as stale because it has not had recent activity. It will be closed if no further activity occurs. Thank you for your contributions.
<|||||>So is HuggingFace going to write the finetuning implementation for SuperGlue?<|||||>Hi @jiachangliu, did you have any news about support for superglue?<|||||>> Hi @jiachangliu, did you have any news about support for superglue?
No I have not heard any HugginFace support on SuperGlue. It was not urgent for me to run those experiments. However, if you want to run SuperGlue, I guess you need to install JIANT, which uses the model structures built by HuggingFace.<|||||>> > Hi @jiachangliu, did you have any news about support for superglue?
>
> No I have not heard any HugginFace support on SuperGlue. It was not urgent for me to run those experiments. However, if you want to run SuperGlue, I guess you need to install JIANT, which uses the model structures built by HuggingFace.
Thank you !! |
transformers | 1,356 | closed | GPT and BERT pretrained models in French | ## 🚀 Need for GPT and BERT pretrained models in French
All models are in English only and the multilingual models are quite poor
## Motivation
Applications like tools for writers and linguists need fully dedicated language support
## Additional context
The computation cost to pretrain models in French is still high and it’s difficult for individuals to afford it, I would be glad to take a part of the burden | 09-27-2019 16:01:31 | 09-27-2019 16:01:31 | Pre-training is indeed a tough pill to swallow. First of all you need a good dataset (does such dataset exist for French?), second you need a lot of processing power. A lot. If a dataset is available (preprocessed, ready to train) then I'd be willing to look into training the model on hardware that I have available. <|||||>Have you an example of a good dataset prepared for the english language (my experience on such things is limited to training Glove on a cleaned dump of the french wikipedia) ?<|||||>English BERT was trained on Wikipedia and BookCorpus for 1M steps.
After reading throug hthe BERT readme, I have to retract my previous statement, though. I do not have the resources to pretrain such a model. I thought it would be max one week on a V100, but they speak of four days on *4 to 16 cloud TPUs*. I do not possess such power!<|||||>Hi Bram,
I planned to use the French Wikipedia and some Gutenberg famous French works like La comédie humaine for a start, I let you know when I finish to preprocess them. Concerning the hardware I would like to use gpu ec2 spot instances but I do not know how long I’ll have to run them and if it exceeds my meagre financial resources.
Envoyé de mon iPad
> Le 28 sept. 2019 à 10:53, Nestor Demeure <[email protected]> a écrit :
>
> Have you an example of a good dataset prepared for the english language (my experience on such things is limited to training Glove on a cleaned dump of the french wikipedia) ?
>
> —
> You are receiving this because you authored the thread.
> Reply to this email directly, view it on GitHub, or mute the thread.
<|||||>Reading [this](https://cloud.google.com/blog/products/ai-machine-learning/now-you-can-train-ml-models-faster-and-lower-cost-cloud-tpu-pods) comparison post, 16 TPUv2's are about twice as fast as 8x V100's that are in the ec2 instances. I would then guess that you'd have to run training for a week.<|||||>Order of magnitude for the compute cost (on cloud platforms) of pre-training a large model is anywhere between $10k and $100k. That's for one pre-training, and you usually at least start multiple ones to search the hyperparameter space.
RoBERTa was pre-trained for 24 hours on 1,024 (full size, 32GB) V100s.<|||||>> Order of magnitude for the compute cost (on cloud platforms) of pre-training a large model is anywhere between $10k and $100k. That's for one pre-training, and you usually at least start multiple ones to search the hyperparameter space.
>
> RoBERTa was pre-trained for 24 hours on 1,024 (full size, 32GB) V100s.
Pretty sure that [this](https://media1.tenor.com/images/dbf3ee8c8e92b4c1bd3492636a774dc7/tenor.gif) is applicable for everyone here.<|||||>i made a dataset by converting books from [bibebook](http://www.bibebook.com/) package to text files.
it's a package of 1 700 Créative Commons BY-SA and public domain book in french
[livre en francais kaggle dataset](https://www.kaggle.com/cedriclacrambe/livres-en-francais)<|||||>Wonderful! Thank you very much!
> Le 30 sept. 2019 à 12:33, cedspam <[email protected]> a écrit :
>
> i made a dataset by converting books from bibebook <http://www.bibebook.com/> to text files.
> it's a package of 1 700 Créative Commons BY-SA and public domain book in french
>
> livre francais kaggle dataset <https://www.kaggle.com/cedriclacrambe/livres-en-francais>
> —
> You are receiving this because you authored the thread.
> Reply to this email directly, view it on GitHub <https://github.com/huggingface/transformers/issues/1356?email_source=notifications&email_token=AAHXAP2JVSBU2KSTRLJI6HDQMHIZJA5CNFSM4I3IELGKYY3PNVWWK3TUL52HS4DFVREXG43VMVBW63LNMVXHJKTDN5WW2ZLOORPWSZGOD75GLQY#issuecomment-536503747>, or mute the thread <https://github.com/notifications/unsubscribe-auth/AAHXAP7ER7H4ERVY7J7JS7LQMHIZJANCNFSM4I3IELGA>.
>
<|||||>Hi all,
I'm currently preparing the `.tfrecords` (both cased and uncased) for a French BERT model (corpus is mainly taken from Wikipedia + OPUS corpora, resulting in ~20GB of text).
I'll share the results (TF checkpoints + Transformers weights) whenever the training on TPU has finished.
Evaluation tasks for that model are a bit limited, so I would evaluate the model for PoS tagging and NER (Universal Dependencies and WikiANN) and compare the model with mBERT.<|||||>Great news!
Envoyé de mon iPad
> Le 5 oct. 2019 à 20:20, Stefan Schweter <[email protected]> a écrit :
>
>
> Hi all,
>
> I'm currently preparing the .tfrecords (both cased and uncased) for a French BERT model (corpus is mainly taken from Wikipedia + OPUS corpora, resulting in ~20GB of text).
>
> I'll share the results (TF checkpoints + Transformers weights) whenever the training on TPU has finished.
>
> Evaluation tasks for that model are a bit limited, so I would evaluate the model for PoS tagging and NER (Universal Dependencies and WikiANN) and compare the model with mBERT.
>
> —
> You are receiving this because you authored the thread.
> Reply to this email directly, view it on GitHub, or mute the thread.
<|||||>That's awesome @stefan-it. Let us know if we can help.<|||||>I'm training the GPT-2 on corpus of Russian classical literature. I've modified training script to make it more robust and useful. You can find it [here](https://github.com/mgrankin/ru_transformers). <|||||>Thanks for sharing Mikhail :)
Envoyé de mon iPad
> Le 7 oct. 2019 à 17:53, Mikhail Grankin <[email protected]> a écrit :
>
>
> I'm training the GPT-2 on corpus of Russian classical literature. I've modified training script to make it more robust and useful. You can find it here.
>
> —
> You are receiving this because you authored the thread.
> Reply to this email directly, view it on GitHub, or mute the thread.
<|||||>> Hi all,
>
> I'm currently preparing the `.tfrecords` (both cased and uncased) for a French BERT model (corpus is mainly taken from Wikipedia + OPUS corpora, resulting in ~20GB of text).
>
> I'll share the results (TF checkpoints + Transformers weights) whenever the training on TPU has finished.
>
> Evaluation tasks for that model are a bit limited, so I would evaluate the model for PoS tagging and NER (Universal Dependencies and WikiANN) and compare the model with mBERT.
@stefan-it Could you explain to me how you trained your model from scratch without using Bert multilingual?
I would like to train BERT from scratch for a textual base in PT-BR (8GB data). Is it possible to use the run_lm_finetuning.py code to perform this process without using the multi-language bert model?
I already have a vocab.txt for the PT-BR base and I don't want to load initial weights.
Is there any script or tutorial to perform this process step by step?<|||||>I don’t know if this link https://github.com/facebookresearch/XLM can answer your question.
Envoyé de mon iPad
> Le 17 oct. 2019 à 20:03, calusbr <[email protected]> a écrit :
>
>
> Hi all,
>
> I'm currently preparing the .tfrecords (both cased and uncased) for a French BERT model (corpus is mainly taken from Wikipedia + OPUS corpora, resulting in ~20GB of text).
>
> I'll share the results (TF checkpoints + Transformers weights) whenever the training on TPU has finished.
>
> Evaluation tasks for that model are a bit limited, so I would evaluate the model for PoS tagging and NER (Universal Dependencies and WikiANN) and compare the model with mBERT.
>
> @stefan-it Could you explain to me how you trained your model from scratch without using Bert multilingual?
>
> I would like to train BERT from scratch for a textual base in PT-BR (8GB data). Is it possible to use the run_lm_finetuning.py code to perform this process without using the multi-language bert model?
>
> I already have a vocab.txt for the PT-BR base and I don't want to load initial weights.
>
> Is there any script or tutorial to perform this process step by step?
>
> —
> You are receiving this because you authored the thread.
> Reply to this email directly, view it on GitHub, or unsubscribe.
<|||||>Hi @calusbr,
I'm using the official Google BERT implementation from [this repository](https://github.com/google-research/bert) on a TPU. Then the trained model TensorFlow model can easily be converted into a Transformers-compatible one (so I can be used with this library).
Regarding to your question: if you don't want to use and fine-tune the multi-lingual BERT model, you could try to train a model with the official BERT implementation for a few steps (Google Colab has TPU support). Then you can fine-tune this model with `transformers` (or you can try to use the Colab instance) :)<|||||>> Hi all,
>
> I'm currently preparing the `.tfrecords` (both cased and uncased) for a French BERT model (corpus is mainly taken from Wikipedia + OPUS corpora, resulting in ~20GB of text).
>
> I'll share the results (TF checkpoints + Transformers weights) whenever the training on TPU has finished.
>
> Evaluation tasks for that model are a bit limited, so I would evaluate the model for PoS tagging and NER (Universal Dependencies and WikiANN) and compare the model with mBERT.
Hi @stefan-it !
Very happy to know that you will possibly able to share this model with us!
Do you have any update on it?
Many thanks!! :)<|||||>Sure, no problem :)
I did some experiments with a training corpus size from 16 to 40 GB. I used the same fine-tuning parameters as used in the SciBERT paper/repository. That means training with a sequence length of 128, then fine-tuning with a sequence length of 512.
Unfortunately, the model trained from scratch is ~ 0.5% worse than the multilingual model on a WikiNER split (80/10/10). In another experiment I used the TensorFlow checkpoint from the multilingual cased model and did training with a sequence length of 128. This results in a +0.2% "boost" on WikiNER.
However, for PoS tagging the model (trained from scratch) is always better (~0.3%) than the BERT multilingual cased model (I used 4 PoS tagging datasets).
I'm currently doing more experiments (mainly focussing on training corpus cleaning...) and will report back here :)<|||||>Thanks Stefan !
> Le 4 nov. 2019 à 11:33, Stefan Schweter <[email protected]> a écrit :
>
> Sure, no problem :)
>
> I did some experiments with a training corpus size from 16 to 40 GB. I used the same fine-tuning parameters as used in the SciBERT paper/repository. That means training with a sequence length of 128, then fine-tuning with a sequence length of 512.
>
> Unfortunately, the model trained from scratch is ~ 0.5% worse than the multilingual model on a WikiNER split (80/10/10). In another experiment I used the TensorFlow checkpoint from the multilingual cased model and did training with a sequence length of 128. This results in a +0.2% "boost" on WikiNER.
>
> However, for PoS tagging the model (trained from scratch) is always better (~0.3%) than the BERT multilingual cased model (I used 4 PoS tagging datasets).
>
> I'm currently doing more experiments (mainly focussing on training corpus cleaning...) and will report back here :)
>
> —
> You are receiving this because you authored the thread.
> Reply to this email directly, view it on GitHub <https://github.com/huggingface/transformers/issues/1356?email_source=notifications&email_token=AAHXAP7ZVWXK4GP236MLDIDQR726NA5CNFSM4I3IELGKYY3PNVWWK3TUL52HS4DFVREXG43VMVBW63LNMVXHJKTDN5WW2ZLOORPWSZGOEC6ZPXY#issuecomment-549296095>, or unsubscribe <https://github.com/notifications/unsubscribe-auth/AAHXAP3PKUDBDELDVEGMUT3QR726NANCNFSM4I3IELGA>.
>
<|||||>Thanks for your work @stefan-it. It's nice, but perhaps disappointing, to see that the multilingual models aren't that bad after all. From what I read, the multilingual models were said to perform poorly but from your tests it seems that is not (laways?) the case.<|||||>I think we should wait for CamemBERT then 😅
https://camembert-model.fr/<|||||>Coming soon! cc @louismartin @LysandreJik <|||||>Two days ago they released on arXiv the [https://128.84.21.199/pdf/1911.03894.pdf](url)
> I think we should wait for CamemBERT then
>
> https://camembert-model.fr/<|||||>CamemBERT was merged into master: https://github.com/huggingface/transformers/pull/1822
I'll keep this issue open for GPT.<|||||>Hello, this thread is what I was looking for but I'm not sure I found the answer to my questions:
- how long does it take to go through GPT-2 and BERT in French?
- what configuration of GPUs?
- what size of corpus?
Thanks a lot in advance.<|||||>We trained CamemBERT on 138GB of raw text on 256 GPUs (32 GB Tesla V100) for 1 day.<|||||>Thank you very much for this valuable information !
Christian Mauceri, PhD
Le 4 déc. 2019 à 16:17 +0100, Louis Martin <[email protected]>, a écrit :
> We trained CamemBERT on 138GB of raw text on 258 GPUs (32 GB Tesla V100) for 1 day.
> —
> You are receiving this because you authored the thread.
> Reply to this email directly, view it on GitHub, or unsubscribe.
<|||||>> We trained CamemBERT on 138GB of raw text on 258 GPUs (32 GB Tesla V100) for 1 day.
Thanks @louismartin. I find great what your did and published with CamemBERT (I'm French :-) ) and the fact you share as well this kind of information.
About your answer: 258 GPUs Tesla V100... waoooooo!!!!!
Where did you find this power of computation? In [Facebook AI](https://ai.facebook.com)?
I read in the [Download section of CamemBERT site](https://camembert-model.fr/#download
) that the model has only 110 millions of parameters. Was it worth to train it on 132 GB of data? <|||||>> Hi all,
>
> I'm currently preparing the `.tfrecords` (both cased and uncased) for a French BERT model (corpus is mainly taken from Wikipedia + OPUS corpora, resulting in ~20GB of text).
>
> I'll share the results (TF checkpoints + Transformers weights) whenever the training on TPU has finished.
>
> Evaluation tasks for that model are a bit limited, so I would evaluate the model for PoS tagging and NER (Universal Dependencies and WikiANN) and compare the model with mBERT.
Hi @stefan-it , do you mind to upload your French Bert check point ? I am interested in your model for generation task. Thanks<|||||>This issue has been automatically marked as stale because it has not had recent activity. It will be closed if no further activity occurs. Thank you for your contributions.
<|||||>Hi, any news about a French GPT?<|||||>> Hi, any news about a French GPT?
You can use the model hub to search for this. One such model is [belgpt2](https://huggingface.co/antoiloui/belgpt2). |
transformers | 1,355 | closed | Fix tensorflow_dataset glue support | This PR fixes issue #1354 .
`glue_convert_examples_to_features` assumed that tensorflow_dataset examples contains the features `'sentence1'` and `'sentence2'`. This commit encapsulates the choice of features in the glue processor and uses that to parse examples.
Built with @philipp-eisen . | 09-27-2019 15:21:40 | 09-27-2019 15:21:40 | # [Codecov](https://codecov.io/gh/huggingface/transformers/pull/1355?src=pr&el=h1) Report
> Merging [#1355](https://codecov.io/gh/huggingface/transformers/pull/1355?src=pr&el=desc) into [master](https://codecov.io/gh/huggingface/transformers/commit/ca559826c4188be8713e46f191ddf5f379c196e7?src=pr&el=desc) will **decrease** coverage by `0.04%`.
> The diff coverage is `50%`.
[](https://codecov.io/gh/huggingface/transformers/pull/1355?src=pr&el=tree)
```diff
@@ Coverage Diff @@
## master #1355 +/- ##
==========================================
- Coverage 84.73% 84.68% -0.05%
==========================================
Files 84 84
Lines 12573 12592 +19
==========================================
+ Hits 10654 10664 +10
- Misses 1919 1928 +9
```
| [Impacted Files](https://codecov.io/gh/huggingface/transformers/pull/1355?src=pr&el=tree) | Coverage Δ | |
|---|---|---|
| [transformers/data/processors/utils.py](https://codecov.io/gh/huggingface/transformers/pull/1355/diff?src=pr&el=tree#diff-dHJhbnNmb3JtZXJzL2RhdGEvcHJvY2Vzc29ycy91dGlscy5weQ==) | `46.66% <100%> (+1.21%)` | :arrow_up: |
| [transformers/data/processors/glue.py](https://codecov.io/gh/huggingface/transformers/pull/1355/diff?src=pr&el=tree#diff-dHJhbnNmb3JtZXJzL2RhdGEvcHJvY2Vzc29ycy9nbHVlLnB5) | `27.98% <47.36%> (+1.76%)` | :arrow_up: |
------
[Continue to review full report at Codecov](https://codecov.io/gh/huggingface/transformers/pull/1355?src=pr&el=continue).
> **Legend** - [Click here to learn more](https://docs.codecov.io/docs/codecov-delta)
> `Δ = absolute <relative> (impact)`, `ø = not affected`, `? = missing data`
> Powered by [Codecov](https://codecov.io/gh/huggingface/transformers/pull/1355?src=pr&el=footer). Last update [ca55982...795b3e7](https://codecov.io/gh/huggingface/transformers/pull/1355?src=pr&el=lastupdated). Read the [comment docs](https://docs.codecov.io/docs/pull-request-comments).
<|||||>Nice and clean, thanks a lot @agrinh and @philipp-eisen!<|||||>@thomwolf Happy to help, we're finding this package super useful! |
transformers | 1,354 | closed | run_tf_glue.py breaks when changing to a glue dataset different from mrpc | ## 🐛 Bug - run_tf_glue.py breaks when changing to a glue dataset different from mrpc
<!-- Important information -->
[run_tf_glue.py](https://github.com/huggingface/transformers/blob/master/examples/run_tf_glue.py) breaks when changing to a glue dataset different from `mrpc`, where the features are not called `'sentence1'` and `'sentence2'`. That happens because of the hard coded accesses in the tensor_dict https://github.com/huggingface/transformers/blob/ca559826c4188be8713e46f191ddf5f379c196e7/transformers/data/processors/glue.py#L83
The tasks I am working on is:
* [x] an official GLUE/SQUaD task: SST-2
* [ ] my own task or dataset: (give details)
## To Reproduce
Steps to reproduce the behavior:
1. Go to https://github.com/huggingface/transformers/blob/master/examples/run_tf_glue.py#L11
2. Change `mrpc` to `sst-2`
3. 💥BOOM! broken
## Expected behavior
* [ ] Handle all glue datasets from `tensorflow_datasets` correctly
P.S.: A colleague and I are currently working on a fix and will submit a PR for this issue in the next couple of minutes.
| 09-27-2019 15:03:28 | 09-27-2019 15:03:28 | Fixed with #1355 |
transformers | 1,353 | closed | Fix some typos | 09-27-2019 14:56:01 | 09-27-2019 14:56:01 | 👍 |
|
transformers | 1,352 | closed | wwm-bert lm_finetune | ## 🚀 Feature
in run_lm_finetuning.py
present how to finetune language model with dataset
## Motivation
But there isn't option to finetune whole word masking bert models
I suggest to add it | 09-27-2019 14:02:51 | 09-27-2019 14:02:51 | This issue has been automatically marked as stale because it has not had recent activity. It will be closed if no further activity occurs. Thank you for your contributions.
|
transformers | 1,351 | closed | SQUAD: V2 referenced at top of Readme; V1 referenced in usage instructions | ## ❓ Questions & Help
There seems to be an inconsistency in the README, namely that run_squad.py is cited to be trained on SQUAD v2 towards the top, but scrolling down to view the command shows that v1 is used. Running the command cited over a copy of the v2 dataset on my machine yields the following error:
```
Traceback (most recent call last):
File "./examples/run_squad.py", line 533, in <module>
main()
File "./examples/run_squad.py", line 478, in main
train_dataset = load_and_cache_examples(args, tokenizer, evaluate=False, output_examples=False)
File "./examples/run_squad.py", line 291, in load_and_cache_examples
version_2_with_negative=args.version_2_with_negative)
File "./examples/utils_squad.py", line 151, in read_squad_examples
"For training, each question should have exactly 1 answer.")
ValueError: For training, each question should have exactly 1 answer.
``` | 09-27-2019 13:38:08 | 09-27-2019 13:38:08 | Need to use the flag
--version_2_with_negative |
transformers | 1,350 | closed | Custom models: MixUp Transformers with TF.Keras code | Ideally I would like to use `TFRobertaModel` or any other model (BERT, XLNet) as parts (modules) of a bigger model. For example, it could be nice to start with Roberta as a document encoder and then build a multi-label classifier on top of that. Possibly there are ways to hack `TFRobertaForSequenceClassification` in order to do multi-label classification using custom configurations, but the point is:
**How we could leverage Roberta and any other pre-trained model and stack other layers on top (e.g., I may want to add a custom attention layer or do a hierarchical version of Roberta with a shared Roberta encoder)?**
```
import tensorflow as tf
import numpy as np
from transformers import TFRobertaModel, RobertaTokenizer
from tensorflow.keras.layers import Input, Dense
from tensorflow.keras.models import Model
# Define input layer
inputs = Input(shape=(None, ))
# Define Roberta a document encoder
roberta_model = TFRobertaModel.from_pretrained('roberta-base')
# Collect hidden state representations
roberta_encodings = roberta_model(inputs)[0]
# Collect CLS representations
document_encodings = tf.squeeze(roberta_encodings[:, 0:1, :], axis=1)
# Add classification layer (Linear + Sigmoid)
outputs = Dense(10, activation='sigmoid')(document_encodings)
# Build meta-model
model = Model(inputs=[inputs], outputs=[outputs])
# Compile model
model.compile(optimizer='adam', loss='binary_crossentropy')
# Train model
tokenizer = RobertaTokenizer.from_pretrained('roberta-base')
x = np.asarray(tokenizer.encode("Hello, my dog is cute", add_special_tokens=True))[None, :]
y = tf.convert_to_tensor(np.zeros((1,10)), dtype=tf.float32)
model.fit(x, y)
```
The main issue here is that we can't use an `Input` layer to feed Roberta... Any ideas for a workaround to make this piece of code working...? | 09-27-2019 12:22:13 | 09-27-2019 12:22:13 | The main issue is at line 85 on the forward pass of `TFRobertaMainLayer`:
https://github.com/huggingface/transformers/blob/ca559826c4188be8713e46f191ddf5f379c196e7/transformers/modeling_tf_roberta.py#L85
It seems that passing Input placeholders mess up this comparison:
> OperatorNotAllowedInGraphError: using a `tf.Tensor` as a Python `bool` is not allowed in Graph execution. Use Eager execution or decorate this function with @tf.function.
When I comment-out this block of code, the training process works... I can't find any way to by-pass this error without commenting-out though....<|||||>@iliaschalkidis I've also run into this issue when trying to make a plug-and-play wrapper around the numerous TF-compatible models. Like you, I was able to get the RoBERTa model working by hacking around it a bit. Not ideal, but it works.
For anyone else that's interested, the line above that raises the error occurs in `TFRobertaMainLayer.call`. You can get around it by wrapping the `call` as a TensorFlow 2.0 `function` whenever you want to use a model that depends on `TFRobertaMainLayer` (which is all of them?). Here I'm using `TFRobertaForSequenceClassification`:
```python
from transformers import TFRobertaForSequenceClassification
import tensorflow as tf
# Establish a RoBERTa-based classifier.
clf = TFRobertaForSequenceClassification.from_pretrained("roberta-base", num_labels=5)
# "Decorate" the `call` method as a TensorFlow2.0 function.
clf.roberta.call = tf.function(clf.transformer.roberta.call)
```
Using that, I was successfully able to fine-tune the classifier on a multi-GPU setup without much trouble. I still get a ton of warnings from ZMQ and TensorFlow, but I'm not yet sure they're official `transformer` issues.
**Note:** I suspect you'll have to wrap the `call` instance method any time you initialize this model (e.g., if you save your pre-trained model and re-load for prediction/inference, you may not be able to just use `TFRobertaForSequenceClassification`). In that case, it may be simpler to define a minimal subclass that does it for you. This is untested code, but I suspect it'd work alright:
```python
import tensorflow as tf
import transformers
class _TFRobertaForSequenceClassification(transformers.TFRobertaForSequenceClassification):
def __init__(self, config, *inputs, **kwargs):
super(_TFRobertaForSequenceClassification, self).__init__(config, *inputs, **kwargs)
self.roberta.call = tf.function(self.roberta.call)
```
Hope this helps!
---
Unrelated tip: I also had a bit of trouble using TFv2 metrics (e.g., `tf.keras.metrics.[Precision/Recall/AUC]` because the `TFRobertaClassificationHead` outputs logits (no softmax activation). If anybody else is wondering, you can set the classifier head's output layer to use softmax quite easily:
```python
# Continuing from the previous setup.
clf.classifier.out_proj.activation = tf.keras.activations.softmax
```
This way, you can monitor Precision/Recall/AUC in the call to `clf.compile`:
```python
# Compile our model.
clf.compile(
optimizer=...,
loss=...,
metrics=[
tf.keras.metrics.CategoricalCrossentropy(from_logits=False),
tf.keras.metrics.Precision(thresholds=.50, name="precision"),
tf.keras.metrics.Recall(thresholds=.50, name="recall"),
tf.keras.metrics.AUC(curve="PR", name="auc-pr")
]
)
```
Furthermore, if you want to just fine-tune the classifier layer, you can easily freeze the core RoBERTa layers:
```python
# Note you have ~125M trainable parameters. This'll take a while!
clf.summary()
# Freeze core RoBERTa model (embeddings, encoder, pooler).
clf.roberta.trainable = False
# Note you have ~600K trainable parameters. Much better!
clf.summary()
```<|||||>@dataframing thanx a lot, this was really helpful! I opted to go with a very similar solution...
Define a meta-model on top of `TFRobertaModel`:
```python
import tensorflow as tf
import transformers
class ROBERTA(transformers.TFRobertaModel):
def __init__(self, config, *inputs, **kwargs):
super(ROBERTA, self).__init__(config, *inputs, **kwargs)
self.roberta.call = tf.function(self.roberta.call)
```
Build a wrapper `tf.keras.Model`:
```python
# Define inputs (token_ids, mask_ids, seg_ids)
token_inputs = Input(shape=(None,), name='word_inputs', dtype='int32')
mask_inputs = Input(shape=(None,), name='mask_inputs', dtype='int32')
seg_inputs = Input(shape=(None,), name='seg_inputs', dtype='int32')
# Load model and collect encodings
roberta = ROBERTA.from_pretrained('roberta-base')
roberta_encodings = roberta([token_inputs, mask_inputs, seg_inputs])[0]
# Keep [CLS] token encoding
doc_encoding = tf.squeeze(roberta_encodings[:, 0:1, :], axis=1)
# Apply dropout
doc_encoding = Dropout(0.1)(doc_encoding)
# Final output (projection) layer
outputs = Dense(self.n_classes, activation='sigmoid', name='outputs')(doc_encoding)
# Wrap-up model
model = Model(inputs=[word_inputs, mask_inputs, seg_inputs], outputs=[outputs])
model.compile(optimizer=Adam(lr=3e-4), loss='binary_crossentropy')
```
Everything works like a charm, except the annoying warnings. Although working on a single RTX 2080Ti or any other 12GB GPU has a limitation of batch size up to 4-5 samples of 512 subword units (the same applies for BERT), while I was able to go up to 8 when I was calling `bert-base` via Tensorflow Hub and wrap it as Keras layer, which is really weird... Any idea why, moving to transformers library and TF2 will make such a different?<|||||>Thanks for the report.
We can probably get rid of this test in the TF version of RoBERTa if it's a blocking element for integrating with other Keras modules.
I've never been a huge fan of this hacky solution anyway. In the future, we should probably move forward with a breaking change in the tokenizers and have control tokens included by default in the tokenizer encoding output instead of having them as an option.
cc @LysandreJik @julien-c <|||||>@thomwolf Having the tokenizers include special tokens in the call to `tokenizer.encode[_plus]` seems like a pretty safe default, but I think it also makes sense to have this inline inspection to make sure that the end user has properly encoded their tokens. Wrapping the method in a `tf.function` like above call seems to make it work fine as-is, so maybe there's a way to have the best of both worlds?<|||||>@dataframing BERT and ROBERTa work like a charm with the tweaks you proposed, although with XLNet I still have issues:
```python
# Define token ids as inputs
word_inputs = Input(batch_shape=(2, 2000), name='word_inputs', dtype='int32')
# Call XLNet model
xlnet = TFXLNetModel.from_pretrained('xlnet-base-cased')
xlnet_encodings = xlnet(word_inputs)
# Collect last hidden step (CLS)
doc_encoding = tf.squeeze(xlnet_encodings[:, -1:, :], axis=1)
# Apply dropout
doc_encoding = Dropout(dropout_rate)(doc_encoding)
# Final output (projection) layer
outputs = Dense(n_classes, activation='softmax', name='outputs')(doc_encoding)
# Compile model
model = Model(inputs=[word_inputs], outputs=[outputs])
model.compile(optimizer=Adam(lr=lr, loss='categorical_crossentropy'))
```
> xlnet_encodings = xlnet(word_inputs)
> .../tensorflow_core/python/keras/engine/base_layer.py", line 842, in __call__
> outputs = call_fn(cast_inputs, *args, **kwargs)
> .../tensorflow_core/python/autograph/impl/api.py", line 237, in wrapper
> raise e.ag_error_metadata.to_exception(e) AttributeError: in converted code:
> relative to .../transformers/modeling_tf_xlnet.py:810 call *
> outputs = self.transformer(inputs, **kwargs)
> tensorflow_core/python/keras/engine/base_layer.py:874 __call__
> inputs, outputs, args, kwargs)
> tensorflow_core/python/keras/engine/base_layer.py:2038 _set_connectivity_metadata_
> input_tensors=inputs, output_tensors=outputs, arguments=arguments)
> tensorflow_core/python/keras/engine/base_layer.py:2068 _add_inbound_node
> arguments=arguments)
> tensorflow_core/python/keras/engine/node.py:110 __init__
> self.output_shapes = nest.map_structure(backend.int_shape, output_tensors)
> tensorflow_core/python/util/nest.py:535 map_structure
> structure[0], [func(*x) for x in entries],
> tensorflow_core/python/util/nest.py:535 <listcomp>
> structure[0], [func(*x) for x in entries],
> tensorflow_core/python/keras/backend.py:1185 int_shape
> shape = x.shape
> AttributeError: 'NoneType' object has no attribute 'shape'
Pretty much the same story happens using the `TFXLNetForSequenceClassification` class:
```python
# Call TFXLNetForSequenceClassification model
model = TFXLNetForSequenceClassification.from_pretrained('xlnet-base-cased', num_labels=n_classes)
# Amend activation functions
model.logits_proj.activation = tf.keras.activations.softmax
# Compile model
model.compile(optimizer=Adam(lr=lr, loss='categorical_crossentropy'))
```
> File .../tensorflow_core/python/keras/engine/training.py", line 2709, in _set_inputs
> outputs = self(inputs, **kwargs)
> File .../tensorflow_core/python/keras/engine/base_layer.py", line 842, in __call__
> outputs = call_fn(cast_inputs, *args, **kwargs)
> File .../tensorflow_core/python/autograph/impl/api.py", line 237, in wrapper
> raise e.ag_error_metadata.to_exception(e)
> TypeError: in converted code:
> transformers/modeling_tf_xlnet.py:916 call *
> output = self.sequence_summary(output)
> tensorflow_core/python/keras/engine/base_layer.py:842 __call__
> outputs = call_fn(cast_inputs, *args, **kwargs)
> transformers/modeling_tf_utils.py:459 call *
> output = self.first_dropout(output)
> tensorflow_core/python/autograph/impl/api.py:396 converted_call
> return py_builtins.overload_of(f)(*args)
> TypeError: 'NoneType' object is not callable<|||||>In your case, it might be because you are not extracting the hidden states from the model tuple output.
This line: `xlnet_encodings = xlnet(word_inputs)`
Should be like this:
```
outputs = xlnet(word_inputs)
xlnet_encodings = outputs[0]
```
I'm working on adding some tests on this integration with other Keras modules here: #1482<|||||>Hi @thomwolf,
Even with this update it keeps producing the exact same error. The actual error happens internally on TF2, when the abstract `keras.Layer` calls the Autograph API to do some adjustments. This actually parse the whole network layer by layer and convert the `call()` functions for some reason. It fails in the very end, when it tries to convert the final (outer) call of the `TFXLNetMainLayer`:
```python
outputs = self.transformer(inputs, **kwargs)
```
The main reason, as I see it through debugging, is the fact that you return by default as part of the outputs a list called `new_mems`. This returns a list of `None`, if the user do not provide such an input, that later the internal Keras engine cannot handle, because the elements of this list lack of shape and lead to the aforementioned error `AttributeError: 'NoneType' object has no attribute 'shape'`.
The only way to surpass this at this stage, is again with some hacking in line 653 of `modeling_tf_xlnet.py`:
```python
outputs = (tf.transpose(output, perm=(1, 0, 2)), new_mems)
```
to
```python
outputs = tf.transpose(output, perm=(1, 0, 2))
```
Probably, if I pass memories as an input in `TFXLNetModel`, this won't happen any more and I'll avoid hacking. Could you please remind me the notion of memories and how should I pass this information when I'm calling the model? Is this a single integer denoting how many steps back can the Transformer-XL use?<|||||>In two words memories are cached hidden-states to be reused to speed up or allow for longer sentence inputs. The best to understand the notion of memory is to read the Transformer-XL paper which is here: http://arxiv.org/abs/1901.02860
We have a couple of models outputting memories and it seems to be a problem for Keras indeed (GPT-2) has the same.
So the best (non-breaking) solution is probably to add a flag in the configuration that you can set to False to avoid outputting memories or cache. <|||||>Great, I read Transformer-XL a few months ago. Maybe if I pass memories as input, I'll avoid this error, and probably I have to do so, if i want the model to act as a real Transformer-XL and not forget all previous timesteps at each segment... What's the specification for `mems`: a tensor of shape (batch_size, ) including integers (e.g., 200 steps back) for the memory length?<|||||>This issue has been automatically marked as stale because it has not had recent activity. It will be closed if no further activity occurs. Thank you for your contributions.
<|||||>Hi,
Why not just take the language model layer from the transformer using `roberta_model = roberta_model.layers[0]` and then build on top of it?
|
transformers | 1,349 | closed | Just some typos | 09-27-2019 10:09:50 | 09-27-2019 10:09:50 | 👍 <|||||># [Codecov](https://codecov.io/gh/huggingface/transformers/pull/1349?src=pr&el=h1) Report
> Merging [#1349](https://codecov.io/gh/huggingface/transformers/pull/1349?src=pr&el=desc) into [master](https://codecov.io/gh/huggingface/transformers/commit/d83d295763b738aa0c071f8b63ad6e155b6cf515?src=pr&el=desc) will **not change** coverage.
> The diff coverage is `n/a`.
[](https://codecov.io/gh/huggingface/transformers/pull/1349?src=pr&el=tree)
```diff
@@ Coverage Diff @@
## master #1349 +/- ##
=======================================
Coverage 84.73% 84.73%
=======================================
Files 84 84
Lines 12573 12573
=======================================
Hits 10654 10654
Misses 1919 1919
```
------
[Continue to review full report at Codecov](https://codecov.io/gh/huggingface/transformers/pull/1349?src=pr&el=continue).
> **Legend** - [Click here to learn more](https://docs.codecov.io/docs/codecov-delta)
> `Δ = absolute <relative> (impact)`, `ø = not affected`, `? = missing data`
> Powered by [Codecov](https://codecov.io/gh/huggingface/transformers/pull/1349?src=pr&el=footer). Last update [d83d295...d2de5b9](https://codecov.io/gh/huggingface/transformers/pull/1349?src=pr&el=lastupdated). Read the [comment docs](https://docs.codecov.io/docs/pull-request-comments).
|
|
transformers | 1,348 | closed | Urgent: RoBERTa-Large-MNLI does not work for 2-way classification anymore | ## 🐛 Bug
<!-- Important information -->
Model I am using (RoBERTa):
Language I am using the model on (English):
The problem arise when using:
* [ ] the official example scripts: (give details)
* [ ] my own modified scripts: (give details)
The tasks I am working on is:
* [ ] an official GLUE/SQUaD task: (RTE)
## To Reproduce
Steps to reproduce the behavior:
pretrain_model_dir = 'roberta-large-mnli' #'roberta-large' , 'roberta-large-mnli'
model = RobertaForSequenceClassification.from_pretrained(pretrain_model_dir, num_labels=2)
It will have error message as follows:
> model = RobertaForSequenceClassification.from_pretrained(pretrain_model_dir, num_labels=num_labels)
File "/opt/conda/lib/python3.6/site-packages/transformers/modeling_utils.py", line 411, in from_pretrained
model.__class__.__name__, "\n\t".join(error_msgs)))
RuntimeError: Error(s) in loading state_dict for RobertaForSequenceClassification:
size mismatch for classifier.out_proj.weight: copying a param with shape torch.Size([3, 1024]) from checkpoint, the shape in current model is torch.Size([2, 1024]).
size mismatch for classifier.out_proj.bias: copying a param with shape torch.Size([3]) from checkpoint, the shape in current model is torch.Size([2]).
This only happened yesterday when I used the pretrained 3-way roberta-large-mnli model for a 2-way classification task; seems like the a bug in initializating or neglecting the classifier's parameters
<!-- Add any other context about the problem here. --> | 09-27-2019 09:20:06 | 09-27-2019 09:20:06 | Please choose a better title for your post and specify (or remove) the first part of your post.
As far as I can tell this is an issue specific to the mnli model. As you say it's pre-trained with three final out features. When loading the state dict into the model, all weights from the pretrained model are "transferred" to the initialized model, this is a one-to-one mapping. Since RobertaForSequenceClassification has a classification head, which you can configure a.o. with `num_labels` it _can_ clash with the classification head of the pretrained model.
The intuitive solution would be to just load all weight excluding the classifier - so that torch doesn't try to load those mis-matching states, if and only if the num_labels specified in `from_pretrained` are not the same as the ones inside the models `self.config`. **However**, I'm not sure if that is the right approach, since one method (from_pretrained) then does different things with the same given pretrained model. In one case you use it completely, in the other you only use part.<|||||>Thanks for the hint. But I do not think this is the mnli model problem, because it worked before even the label size is not 3. This is my old log:
09/11/2019 22:37:46 - INFO - pytorch_transformers.modeling_utils - loading configuration file https://s3.amazonaws.com/models.huggingface.co/bert/roberta-large-mnli-config.json from cache at /root/.cache/torch/pytorch_transformers/54eef9bf74f919edd81b765fee413c8229620f3e271a51bdcdc67797422ef3f3.233bd69ec613d2ebcb1d55823dfc5b1e109157918e13bdbde6db7f694e1a0039
09/11/2019 22:37:46 - INFO - pytorch_transformers.modeling_utils - Model config {
"attention_probs_dropout_prob": 0.1,
"finetuning_task": null,
"hidden_act": "gelu",
"hidden_dropout_prob": 0.1,
"hidden_size": 1024,
"initializer_range": 0.02,
"intermediate_size": 4096,
"layer_norm_eps": 1e-05,
"max_position_embeddings": 514,
"num_attention_heads": 16,
"num_hidden_layers": 24,
"num_labels": 2,
"output_attentions": false,
"output_hidden_states": false,
"pruned_heads": {},
"torchscript": false,
"type_vocab_size": 1,
"vocab_size": 50265
}
09/11/2019 22:37:46 - INFO - pytorch_transformers.modeling_utils - loading weights file https://s3.amazonaws.com/models.huggingface.co/bert/roberta-large-mnli-pytorch_model.bin from cache at /root/.cache/torch/pytorch_transformers/1c2e185bc053ae7261ce2289653438a4c05b871ff7f30eaee1cdb787154410e0.c1823b934e18e923174ff260ba955eef25b2205f48fe2655c432a5fb805f8c8a
09/11/2019 22:38:02 - INFO - pytorch_transformers.modeling_utils - Weights of RobertaForSequenceClassification not initialized from pretrained model: ['classifier.dense.weight', 'classifier.dense.bias', 'classifier.out_proj.weight', 'classifier.out_proj.bias']
09/11/2019 22:38:02 - INFO - pytorch_transformers.modeling_utils - Weights from pretrained model not used in RobertaForSequenceClassification: ['lm_head.weight', 'lm_head.bias', 'lm_head.dense.weight', 'lm_head.dense.bias', 'lm_head.layer_norm.weight', 'lm_head.layer_norm.bias']
You can see that the system can automatically detect which part of the mnli parameters not used to initialize, then it will neglect it; but now the transformers only output error message, I found it from yesterday.
My code is the same, but behave differently now<|||||>It's odd since `modeling_utils` hasn't seen any updates apart from the naming update. I'm not sure where else to look for this issue.<|||||>My "pytorch_transformers" was installed maybe 3 weeks ago, but the latest "transformers" was installed yesterday. But both will make the same error, in different lines:
"transformers" in line 411:
File "/opt/conda/lib/python3.6/site-packages/transformers/modeling_utils.py", line 411, in from_pretrained
model.__class__.__name__, "\n\t".join(error_msgs)))
RuntimeError: Error(s) in loading state_dict for RobertaForSequenceClassification:
size mismatch for classifier.out_proj.weight: copying a param with shape torch.Size([3, 1024]) from checkpoint, the shape in current model is torch.Size([2, 1024]).
size mismatch for classifier.out_proj.bias: copying a param with shape torch.Size([3]) from checkpoint, the shape in current model is torch.Size([2]).
"pytorch_transformers" in line 594:
File "/opt/conda/lib/python3.6/site-packages/pytorch_transformers/modeling_utils.py", line 594, in from_pretrained
model.__class__.__name__, "\n\t".join(error_msgs)))
RuntimeError: Error(s) in loading state_dict for RobertaForSequenceClassification:
size mismatch for classifier.out_proj.weight: copying a param with shape torch.Size([3, 1024]) from checkpoint, the shape in current model is torch.Size([2, 1024]).
size mismatch for classifier.out_proj.bias: copying a param with shape torch.Size([3]) from checkpoint, the shape in current model is torch.Size([2]).
But both come from the same reason. Since the "pytorch_transformers" worked for me a couple days ago (even though i found "transformer" did not work for me yesterday, but very likely something changed before yesterday, since I haven't run the piece of code for some days).
Now, I kind of agree with you that the "roberta-large-mnli" model itself had something changed recently, which makes it unable to neglect the mismatch of hyperparameters.
<|||||>Also having this issue using the `roberta-large-mnli` model on a single-document (not paired) multiclass classification task.<|||||>I guess the simplest solution would be to load the model with previous num_labels and than directly change its num_labels and initialize a new classifier layer in the `run_glue.py` script. This way you won't need to modify any of the `transformers` code.
This is what I do:
```
# for num_labels(mnli)
num_labels_old = config_class.from_pretrained(args.model_name_or_path).num_labels
config = config_class.from_pretrained(args.config_name if args.config_name else args.model_name_or_path,
num_labels=num_labels_old,
finetuning_task=args.task_name,
cache_dir=args.cache_dir if args.cache_dir else None)
tokenizer = tokenizer_class.from_pretrained(args.tokenizer_name if args.tokenizer_name else args.model_name_or_path,
do_lower_case=args.do_lower_case,
cache_dir=args.cache_dir if args.cache_dir else None)
if num_labels != num_labels_old:
config.num_labels = num_labels_old
model = model_class.from_pretrained(args.model_name_or_path,
from_tf=bool('.ckpt' in args.model_name_or_path),
config=config,
cache_dir=args.cache_dir if args.cache_dir else None)
config.num_labels = num_labels
logger.info('Reintializing model classifier layer...')
model.num_labels = num_labels
model.classifier = RobertaClassificationHead(config)
else:
model = model_class.from_pretrained(args.model_name_or_path,
from_tf=bool('.ckpt' in args.model_name_or_path),
config=config,
cache_dir=args.cache_dir if args.cache_dir else None)
```
Of course, it would be better to modify the `transformers` code directly.
<|||||>Hi felicity,
Sorry for the late reply. I actually have forgotten how i solve that, or
gave up. I will check my code when I finish a deadline in the next couple
of days. Thanks for sharing your experience.
Best.
On Thu, Nov 28, 2019 at 8:01 AM felicitywang <[email protected]>
wrote:
> I'm getting the same error. Did you solve this problem? @wyin-Salesforce
> <https://github.com/wyin-Salesforce> @pmbaumgartner
> <https://github.com/pmbaumgartner> Would really appreciate it if you
> could share your solutions. Thank you.
>
> —
> You are receiving this because you authored the thread.
> Reply to this email directly, view it on GitHub
> <https://github.com/huggingface/transformers/issues/1348?email_source=notifications&email_token=AM2XN4JEMO7KYWJ4OFXFSYDQV7TPFA5CNFSM4I3D6BP2YY3PNVWWK3TUL52HS4DFVREXG43VMVBW63LNMVXHJKTDN5WW2ZLOORPWSZGOEFM7XIA#issuecomment-559545248>,
> or unsubscribe
> <https://github.com/notifications/unsubscribe-auth/AM2XN4IUZKSK4D22E2WI3ZDQV7TPFANCNFSM4I3D6BPQ>
> .
>
--
Wenpeng Yin
Research Scientist @ Salesforce Research, Palo Alto
https://sites.google.com/site/yinwenpeng1987/
<|||||>Thanks @wyin-Salesforce . If people are still having trouble with this, the solution above worked. <|||||>This issue has been automatically marked as stale because it has not had recent activity. It will be closed if no further activity occurs. Thank you for your contributions.
<|||||>I want to evaluate the pre-trained **roberta-large-mnli** model on a **2-way classification task**. I tried to imitate what @felicitywang posted by adding these four lines after calling config/tokenizer/model in run_glue.py (after line 134):
```
num_labels = 2 # ADDED
config.num_labels = num_labels # ADDED
model.num_labels = num_labels # ADDED
model.classifier = RobertaClassificationHead(config) # ADDED
```
However, I'm still getting the following error (from line 131) when I run my modified run_glue.py:
```
RuntimeError: Error(s) in loading state_dict for RobertaForSequenceClassification:
size mismatch for classifier.out_proj.weight: copying a param with shape torch.Size([3, 1024]) from checkpoint, the shape in current model is torch.Size([2, 1024]).
size mismatch for classifier.out_proj.bias: copying a param with shape torch.Size([3]) from checkpoint, the shape in current model is torch.Size([2]).
```
where the line 131 is the last line ('cache_dir=...') of this code block:
```
model = AutoModelForSequenceClassification.from_pretrained(
model_args.model_name_or_path,
from_tf=bool(".ckpt" in model_args.model_name_or_path),
config=config,
cache_dir=model_args.cache_dir,
)
```
Does anyone know how to make it work?<|||||>> I want to evaluate the pre-trained **roberta-large-mnli** model on a **2-way classification task**. I tried to imitate what @felicitywang posted by adding these four lines after calling config/tokenizer/model in run_glue.py (after line 134):
>
> ```
> num_labels = 2 # ADDED
> config.num_labels = num_labels # ADDED
> model.num_labels = num_labels # ADDED
> model.classifier = RobertaClassificationHead(config) # ADDED
> ```
>
> However, I'm still getting the following error (from line 131) when I run my modified run_glue.py:
>
> ```
> RuntimeError: Error(s) in loading state_dict for RobertaForSequenceClassification:
> size mismatch for classifier.out_proj.weight: copying a param with shape torch.Size([3, 1024]) from checkpoint, the shape in current model is torch.Size([2, 1024]).
> size mismatch for classifier.out_proj.bias: copying a param with shape torch.Size([3]) from checkpoint, the shape in current model is torch.Size([2]).
> ```
>
> where the line 131 is the last line ('cache_dir=...') of this code block:
>
> ```
> model = AutoModelForSequenceClassification.from_pretrained(
> model_args.model_name_or_path,
> from_tf=bool(".ckpt" in model_args.model_name_or_path),
> config=config,
> cache_dir=model_args.cache_dir,
> )
> ```
>
> Does anyone know how to make it work?
@scarletcho
If you just want to evaluate the pretrained roberta-large-mnli on a new dataset without any fine-tuning; let's say your new dataset has two classes "entail" and "non_entail", then you just manually combine the outputs "neutral" and "contradict" as a single output "non_entail".
If you want to load this pretrained model and fine-tune on your 2-way dataset, today I just found the following approach works for using N-way fine-tuning:
` model_config = BartConfig.from_pretrained(pretrain_model_dir)
model_config.num_labels=new_num_labels
model = BartForSequenceClassification.from_pretrained(pretrain_model_dir, config=model_config)`
I tried Bart, but it should work for roberta too (here "pretrain_model_dir" is string "facebook/bart-large", you can use "roberta-large-mnli" instead)<|||||>> I guess the simplest solution would be to load the model with previous num_labels and than directly change its num_labels and initialize a new classifier layer in the `run_glue.py` script. This way you won't need to modify any of the `transformers` code.
>
> This is what I do:
>
> ```
> # for num_labels(mnli)
> num_labels_old = config_class.from_pretrained(args.model_name_or_path).num_labels
> config = config_class.from_pretrained(args.config_name if args.config_name else args.model_name_or_path,
> num_labels=num_labels_old,
> finetuning_task=args.task_name,
> cache_dir=args.cache_dir if args.cache_dir else None)
> tokenizer = tokenizer_class.from_pretrained(args.tokenizer_name if args.tokenizer_name else args.model_name_or_path,
> do_lower_case=args.do_lower_case,
> cache_dir=args.cache_dir if args.cache_dir else None)
> if num_labels != num_labels_old:
> config.num_labels = num_labels_old
> model = model_class.from_pretrained(args.model_name_or_path,
> from_tf=bool('.ckpt' in args.model_name_or_path),
> config=config,
> cache_dir=args.cache_dir if args.cache_dir else None)
> config.num_labels = num_labels
> logger.info('Reintializing model classifier layer...')
> model.num_labels = num_labels
> model.classifier = RobertaClassificationHead(config)
>
> else:
> model = model_class.from_pretrained(args.model_name_or_path,
> from_tf=bool('.ckpt' in args.model_name_or_path),
> config=config,
> cache_dir=args.cache_dir if args.cache_dir else None)
> ```
>
> Of course, it would be better to modify the `transformers` code directly.
Hi,
I am using this code to solve this issue. What is `RobertaClassificationHead(config)` ? I cannot find this from huggingface.<|||||>> > I want to evaluate the pre-trained **roberta-large-mnli** model on a **2-way classification task**. I tried to imitate what @felicitywang posted by adding these four lines after calling config/tokenizer/model in run_glue.py (after line 134):
> > ```
> > num_labels = 2 # ADDED
> > config.num_labels = num_labels # ADDED
> > model.num_labels = num_labels # ADDED
> > model.classifier = RobertaClassificationHead(config) # ADDED
> > ```
> >
> >
> >
> >
> >
> >
> >
> >
> >
> >
> >
> > However, I'm still getting the following error (from line 131) when I run my modified run_glue.py:
> > ```
> > RuntimeError: Error(s) in loading state_dict for RobertaForSequenceClassification:
> > size mismatch for classifier.out_proj.weight: copying a param with shape torch.Size([3, 1024]) from checkpoint, the shape in current model is torch.Size([2, 1024]).
> > size mismatch for classifier.out_proj.bias: copying a param with shape torch.Size([3]) from checkpoint, the shape in current model is torch.Size([2]).
> > ```
> >
> >
> >
> >
> >
> >
> >
> >
> >
> >
> >
> > where the line 131 is the last line ('cache_dir=...') of this code block:
> > ```
> > model = AutoModelForSequenceClassification.from_pretrained(
> > model_args.model_name_or_path,
> > from_tf=bool(".ckpt" in model_args.model_name_or_path),
> > config=config,
> > cache_dir=model_args.cache_dir,
> > )
> > ```
> >
> >
> >
> >
> >
> >
> >
> >
> >
> >
> >
> > Does anyone know how to make it work?
>
> @scarletcho
> If you just want to evaluate the pretrained roberta-large-mnli on a new dataset without any fine-tuning; let's say your new dataset has two classes "entail" and "non_entail", then you just manually combine the outputs "neutral" and "contradict" as a single output "non_entail".
>
> If you want to load this pretrained model and fine-tune on your 2-way dataset, today I just found the following approach works for using N-way fine-tuning:
>
> ` model_config = BartConfig.from_pretrained(pretrain_model_dir) model_config.num_labels=new_num_labels model = BartForSequenceClassification.from_pretrained(pretrain_model_dir, config=model_config)`
>
> I tried Bart, but it should work for roberta too (here "pretrain_model_dir" is string "facebook/bart-large", you can use "roberta-large-mnli" instead)
It is ok to use roberta-large, but it stills has the error in roberta-large-mnli.<|||||>I fix the issue when I use `transformers=2.3.0` and I put num_labels in the config and then put the config into the model.<|||||>I'm using `transformers=4.20.1` and [this example code](https://github.com/huggingface/transformers/blob/24a85cca61fda92b9376fe45da1dcb10c8853066/examples/pytorch/text-classification/run_glue.py) (the most recent commit that passed all the automated testing) and I'm still running into this error.
In the code, it looks like they do [add the num_labels](https://github.com/huggingface/transformers/issues/1348#issuecomment-888779209) to the config, and then put the config into the model, but I'm still getting the error.
The exact command I'm running is `python run_glue.py --train_file sg_train_dataset.csv --validation_file sg_test_dataset.csv --do_train --do_eval --model_name roberta-large-mnli --output_dir output --overwrite_output_dir` where `sg_test_dataset.csv` is a CSV file with three columns, "sentence1", "sentence2" and "label" and "label" is either 0 or 1.
Any suggestions on how to fix it? |
transformers | 1,347 | closed | Use PyTorch's GELU activation | ## 🚀 Feature
PyTorch 1.2 provides a built-in, GPU-accelerated GELU function at `torch.nn.functional.gelu`. Reading through the merged pull request (https://github.com/pytorch/pytorch/pull/20665) it seems that this is optimised for CUDA, too. Therefore I would propose trying to import the built-in gelu function first, and use the back-off gelu definition if it's not found for torch < 1.2.
## Additional context
I started _very_ basic changes over at https://github.com/BramVanroy/transformers/tree/pytorch_gelu by changing the gelu definition in e.g. BERT to something like
```python
def gelu(x):
""" Original Implementation of the gelu activation function in Google Bert repo when initialy created.
For information: OpenAI GPT's gelu is slightly different (and gives slightly different results):
0.5 * x * (1 + torch.tanh(math.sqrt(2 / math.pi) * (x + 0.044715 * torch.pow(x, 3))))
Also see https://arxiv.org/abs/1606.08415
"""
return x * 0.5 * (1.0 + torch.erf(x / math.sqrt(2.0)))
ACT2FN = {"relu": torch.nn.functional.relu, "swish": swish, "gelu_new": gelu_new}
try:
ACT2FN["gelu"] = torch.nn.functional.gelu
except AttributeError:
ACT2FN["gelu"] = gelu
```
However, I wonder whether it wouldn't be cleaner to have all activation functions in an importable constant `ACT2FN` somewhere. Maybe under `modeling_utils`? This should make it easier to keep a good overview of all activation functions that can be used. If requested, I can put some time in refactoring this. | 09-27-2019 08:53:51 | 09-27-2019 08:53:51 | This issue has been automatically marked as stale because it has not had recent activity. It will be closed if no further activity occurs. Thank you for your contributions.
|
transformers | 1,346 | closed | Add small note about the output of hidden states (closes #1332) | Closes huggingface/transformers#1332 | 09-27-2019 08:03:43 | 09-27-2019 08:03:43 | # [Codecov](https://codecov.io/gh/huggingface/transformers/pull/1346?src=pr&el=h1) Report
> Merging [#1346](https://codecov.io/gh/huggingface/transformers/pull/1346?src=pr&el=desc) into [master](https://codecov.io/gh/huggingface/transformers/commit/da2e47ad15e552b84815da20daf3282b517103f7?src=pr&el=desc) will **not change** coverage.
> The diff coverage is `n/a`.
[](https://codecov.io/gh/huggingface/transformers/pull/1346?src=pr&el=tree)
```diff
@@ Coverage Diff @@
## master #1346 +/- ##
=======================================
Coverage 84.73% 84.73%
=======================================
Files 84 84
Lines 12573 12573
=======================================
Hits 10654 10654
Misses 1919 1919
```
------
[Continue to review full report at Codecov](https://codecov.io/gh/huggingface/transformers/pull/1346?src=pr&el=continue).
> **Legend** - [Click here to learn more](https://docs.codecov.io/docs/codecov-delta)
> `Δ = absolute <relative> (impact)`, `ø = not affected`, `? = missing data`
> Powered by [Codecov](https://codecov.io/gh/huggingface/transformers/pull/1346?src=pr&el=footer). Last update [da2e47a...15749bf](https://codecov.io/gh/huggingface/transformers/pull/1346?src=pr&el=lastupdated). Read the [comment docs](https://docs.codecov.io/docs/pull-request-comments).
<|||||>Awesome, thanks @BramVanroy! |
transformers | 1,345 | closed | Ram utilisation of DistilBERT | ## ❓ Questions & Help
<!-- A clear and concise description of the question. -->
I was checking the memory consumption of RoBERTa and DistilBERT. I found there is no significant change in memory usage. Although Inference time is around 1sec for DistilBERT and for RoBERTa is 2sec.
Memory usage on CPU:
Port 9000: DistilBERT
Port 9002: RoBERTa

Have you guys seen any significant change in memory usage or am I missing something here? | 09-27-2019 07:46:33 | 09-27-2019 07:46:33 | This issue has been automatically marked as stale because it has not had recent activity. It will be closed if no further activity occurs. Thank you for your contributions.
|
transformers | 1,344 | closed | Errors when using fp16 with traced models | ## 🐛 Bug
When I run
```
roberta_model = RobertaForMaskedLM.from_pretrained("roberta-base", torchscript=True)
roberta_model.cuda()
roberta_model.half()
traced_model = torch.jit.trace(roberta_model, (r_input_ids))
```
I get the following error
`
Expected object of scalar type Float but got scalar type Half for argument #2 'mat2'
`
When I attempt to load a normally traced model
```
loaded_model = torch.jit.load("traced_roberta_cuda.pt")
loaded_model.cuda()
loaded_model.half()
loaded_model(r_input_ids )
```
I get
`RuntimeError: expected device cuda:0 and dtype Float but got device cuda:0 and dtype Half
`
Is there a way to use fp16 with traced models? It happened with BertForSequenceClassification, RobertaForSequenceClassification and RobertaForMaskedLM.
## Environment
* Models tested on: Bert and Roberta:
* Language: English
* OS: Ubuntu 18.04
* Python version: 3.6.9
* PyTorch version: 1.2.0
* PyTorch Transformers version (or branch): 2.0.0
| 09-27-2019 00:25:19 | 09-27-2019 00:25:19 | This issue has been automatically marked as stale because it has not had recent activity. It will be closed if no further activity occurs. Thank you for your contributions.
<|||||>Has this ever been solved? I have the same issue<|||||>I think maybe the reason is `.half()` only change(cast) the data, but it is not traceable. And there is no fp32 -> fp16 in python code, the function will expect fp32 input instead of fp16.<|||||>Just confirming I am still using this in production and never found a solution. If there is an easy solution here I'd happily pay a small bounty for the information.<|||||>Just stumbled across this trying to look for anything talking about using fp16 precision with torchscript. Converting the model and inputs to half seems to work. I get a lot higher warnings about loss of precision with torchscript when I have `use_fp16=True`. Not sure if I'm being paranoid with the `torch.no_grad()` statement, I don't know if it'll do that internally within `torch.jit.trace` but I couldn't see anything about it.
```python
model = model.cuda()
model.eval()
with torch.no_grad():
inputs = torch.randn(input_shape, device='cuda')
if use_fp16:
model = model.half()
inputs = inputs.half()
traced_model = torch.jit.trace(model, inputs)
```<|||||>It's kind of strange. When I don't check the trace during tracing and call inference without ``torch.no_grad()`` it does actually work (but consumes way too much memory of course because of gradient computations).
```
model.half()
model = torch.jit.trace(model, (dummy_input, dummy_input, dummy_input), check_trace=False)
outputs = model(inputs)
```
Actually I also have another issue with TorchScript, because I cannot feed the inputs as dict during tracing. In the case of BERT it then somehow uses ``input_ids, attention_mask, inputs`` as input names instead of ``input_ids, attention_mask, token_type_ids``.<|||||>For someone who encounters the same problem, this issue is fixed in torch 1.5 and present back in torch 1.6. |
transformers | 1,343 | closed | RobertaTokenizer documentation is off with the new transformers library | ## 🐛 Bug
<!-- Important information -->
Model I am using (Bert, XLNet....): Roberta
Language I am using the model on (English, Chinese....): NA
The problem arise when using:
* [ ] the official example scripts:
The tasks I am working on is:
NA
## To Reproduce
Steps to reproduce the behavior:
In the documentation for the tokenization_roberta.py, it says in the RobertaTokenizer class
```
RoBERTa BPE tokenizer, derived from the GPT-2 tokenizer. Peculiarities:
- Byte-level Byte-Pair-Encoding
- Requires a space to start the input string => will add a space is there isn't.
As a consequence, this tokenizer `encode` and `decode` method will not conserve
the absence of a space at the beginning of a string: `tokenizer.decode(tokenizer.encode("Hello")) = " Hello"
```
However, with using the new transformers library, when I run this example I get
```
from transformers import RobertaTokenizer
tokenizer = RobertaTokenizer.from_pretrained("roberta-base")
tokenizer.decode(tokenizer.encode("Hello"))
"Hello"
```
The leading space seems to no longer present as it was in pytorch_transformers, however if (per the source code) if I add the arg add_prefix_space = True, then it outputs with the leading space. Just a tiny fix to hopefully help out anyone else who gets confused by it.
Thanks and love the new updates to the library!
| 09-26-2019 20:11:49 | 09-26-2019 20:11:49 | You're right! Thanks for letting us know. |
transformers | 1,342 | closed | AttributeError: 'RobertaTokenizer' object has no attribute 'add_special_tokens_sentences_pair' | With the latest update to `Transformers`, has the function been removed? I still see it in the code, but I run into the error:
`AttributeError: 'RobertaTokenizer' object has no attribute 'add_special_tokens_sentences_pair'` | 09-26-2019 18:19:40 | 09-26-2019 18:19:40 | Hey @frankfka
Perhaps you are looking for tokenizer.add_special_tokens_sequence_pair instead of tokenizer.add_special_tokens_sentences_pair?
```
from transformers import RobertaTokenizer
tokenizer = RobertaTokenizer.from_pretrained("roberta-base")
tokenizer.add_special_tokens_sequence_pair([31414], [31414])
```
This returns <br>
```
[0, 31414, 2, 2, 31414, 2]
```<|||||>> Hey @frankfka
>
> Perhaps you are looking for tokenizer.add_special_tokens_sequence_pair instead of tokenizer.add_special_tokens_sentences_pair?
>
> ```
> from transformers import RobertaTokenizer
> tokenizer = RobertaTokenizer.from_pretrained("roberta-base")
>
> tokenizer.add_special_tokens_sequence_pair([31414], [31414])
> ```
>
> This returns
>
> ```
> [0, 31414, 2, 2, 31414, 2]
> ```
Good catch, thanks! I suppose this was renamed in this release? |
transformers | 1,341 | closed | Examples in Colab | Hi all , does anyone have a Colab sample to share ? | 09-26-2019 17:49:19 | 09-26-2019 17:49:19 | Why not simply run the example scripts in colab yourself?<|||||>I'm not exactly sure how to set it up , this is a pretty popular library so I was thinking their might be a blog post out there <|||||>https://huggingface.co/transformers/notebooks.html<|||||>This issue has been automatically marked as stale because it has not had recent activity. It will be closed if no further activity occurs. Thank you for your contributions.
|
transformers | 1,340 | closed | Size mismatch when loading pretrained model | I'm seeing this:
```
In [1]: import pytorch_transformers
In [2]: m=pytorch_transformers.AutoModel.from_pretrained('roberta-base')
---------------------------------------------------------------------------
RuntimeError Traceback (most recent call last)
<ipython-input-2-7a33f5ecb345> in <module>
----> 1 m=pytorch_transformers.AutoModel.from_pretrained('roberta-base')
/opt/anaconda3/lib/python3.7/site-packages/pytorch_transformers/modeling_auto.py in from_pretrained(cls, pretrained
_model_name_or_path, *model_args, **kwargs)
240 return DistilBertModel.from_pretrained(pretrained_model_name_or_path, *model_args, **kwargs)
241 elif 'roberta' in pretrained_model_name_or_path:
--> 242 return RobertaModel.from_pretrained(pretrained_model_name_or_path, *model_args, **kwargs)
243 elif 'bert' in pretrained_model_name_or_path:
244 return BertModel.from_pretrained(pretrained_model_name_or_path, *model_args, **kwargs)
/opt/anaconda3/lib/python3.7/site-packages/pytorch_transformers/modeling_utils.py in from_pretrained(cls, pretraine
d_model_name_or_path, *model_args, **kwargs)
592 if len(error_msgs) > 0:
593 raise RuntimeError('Error(s) in loading state_dict for {}:\n\t{}'.format(
--> 594 model.__class__.__name__, "\n\t".join(error_msgs)))
595
596 if hasattr(model, 'tie_weights'):
RuntimeError: Error(s) in loading state_dict for RobertaModel:
size mismatch for roberta.embeddings.position_embeddings.weight: copying a param with shape torch.Size([514
, 768]) from checkpoint, the shape in current model is torch.Size([512, 768]).
``` | 09-26-2019 16:52:53 | 09-26-2019 16:52:53 | I'm having the same problem with RoBERTa, it didn't happen until a few hours.<|||||>Hi, thanks for pointing it out, I made a mistake with a config object hosted on our S3. It should be fixed now.<|||||>Running the following snippet:
`# Load the model in fairseq`
`from fairseq.models.roberta import RobertaModel`
`roberta = RobertaModel.from_pretrained('./roberta.large', checkpoint_file='model.pt')`
`roberta.eval() # disable dropout (or leave in train mode to finetune)`
I got the following error:
`RuntimeError: Error(s) in loading state_dict for RobertaModel:
Missing key(s) in state_dict: "decoder.sentence_encoder.layers.0.self_attn.k_proj.weight", "decoder.sentence_encoder.layers.0.self_attn.k_proj.bias", "decoder.sentence_encoder.layers.0.self_attn.v_proj.weight", "decoder.sentence_encoder.layers.0.self_attn.v_proj.bias", "decoder.sentence_encoder.layers.0.self_attn.q_proj.weight", "decoder.sentence_encoder.layers.0.self_attn.q_proj.bias", "decoder.sentence_encoder.layers.1.self_attn.k_proj.weight", "decoder.sentence_encoder.layers.1.self_attn.k_proj.bias", "decoder.sentence_encoder.layers.1.self_attn.v_proj.weight", "decoder.sentence_encoder.layers.1.self_attn.v_proj.bias", "decoder.sentence_encoder.layers.1.self_attn.q_proj.weight", "decoder.sentence_encoder.layers.1.self_attn.q_proj.bias", "decoder.sentence_encoder.layers.2.self_attn.k_proj.weight", "decoder.sentence_encoder.layers.2.self_attn.k_proj.bias", "decoder.sentence_encoder.layers.2.self_attn.v_proj.weight", "decoder.sentence_encoder.layers.2.self_attn.v_proj.bias", "decoder.sentence_encoder.layers.2.self_attn.q_proj.weight", "decoder.sentence_encoder.layers.2.self_attn.q_proj.bias", "decoder.sentence_encoder.layers.3.self_attn.k_proj.weight", "decoder.sentence_encoder.layers.3.self_attn.k_proj.bias", "decoder.sentence_encoder.layers.3.self_attn.v_proj.weight", "decoder.sentence_encoder.layers.3.self_attn.v_proj.bias", "decoder.sentence_encoder.layers.3.self_attn.q_proj.weight", "decoder.sentence_encoder.layers.3.self_attn.q_proj.bias", "decoder.sentence_encoder....
Unexpected key(s) in state_dict: "decoder.sentence_encoder.layers.0.self_attn.in_proj_weight", "decoder.sentence_encoder.layers.0.self_attn.in_proj_bias", "decoder.sentence_encoder.layers.1.self_attn.in_proj_weight", "decoder.sentence_encoder.layers.1.self_attn.in_proj_bias", "decoder.sentence_encoder.layers.2.self_attn.in_proj_weight", "decoder.sentence_encoder.layers.2.self_attn.in_proj_bias", "decoder.sentence_encoder.layers.3.self_attn.in_proj_weight", "decoder.sentence_encoder.layers.3.self_attn.in_proj_bias", "decoder.sentence_encoder.layers.4.self_attn.in_proj_weight", "decoder.sentence_encoder.layers.4.self_attn.in_proj_bias", "decoder.sentence_encoder.layers.5.self_attn.in_proj_weight", "decoder.sentence_encoder.layers.5.self_attn.in_proj_bias", "decoder.sentence_encoder.layers.6.self_attn.in_proj_weight", "decoder.sentence_encoder.layers.6.self_attn.in_proj_bias", "decoder.sentence_encoder.layers.7.self_attn.in_proj_weight", "decoder.sentence_encoder.layers.7.self_attn.in_proj_bias", "decoder.sentence_encoder.layers.8.self_attn.in_proj_weight", "decoder.sentence_encoder.layers.8.self_attn.in_proj_bias", "decoder.sentence_encoder.layers.9.self_attn.in_proj_weight", "decoder.sentence_encoder.layers.9.self_attn.in_proj_bias", "decoder.sentence_encoder.layers.10.self_attn.in_proj_weight", "decoder.sentence_encoder.layers.10.self_attn.in_proj_bias", "decoder.sentence_encoder.layers.11.self_attn.in_proj_weight", "decoder.sentence_encoder.layers.11.self_attn.in_proj_bi...`
Is it related to the above error? How can we fix it?<|||||>I am seeing the same error as @pbabvey is seeing. I suspect the S3 object is out-of-sync with the code?<|||||>I am running into the same issue as @pbabvey while loading the model. Is there any fix available for this?<|||||>Hi, if you're running the following code:
```py
# Load the model in fairseq
from fairseq.models.roberta import RobertaModel
roberta = RobertaModel.from_pretrained('./roberta.large', checkpoint_file='model.pt')
roberta.eval() # disable dropout (or leave in train mode to finetune)
```
Then you are not using our library, but [fairseq](https://github.com/pytorch/fairseq).
To use our library you would do it as follows:
```py
from transformers import RobertaModel
model = RobertaModel.from_pretrained("roberta-large")
```<|||||>There is one argument called `ignore_mismatched_sizes` in `from_pretrained` method. ISSUE: [#13187](https://github.com/huggingface/transformers/issues/13187) |
transformers | 1,339 | closed | Why is the vocabulary of token_type_ids and input_ids shared? | ## ❓ Questions & Help
<!-- A clear and concise description of the question. -->
https://github.com/huggingface/transformers/blob/17ea43cf985829634bd86b36b44e5410c6f83e36/transformers/modeling_gpt2.py#L421
In GPT2Model, forward method, it seems the vocabulary of token_type_ids and input_ids is shared. I checked the vocabulary table, 0 and 1 corresponds to the exclamation sign and the quote sign. What is the reason of sharing the vocabulary? Is it on purpose?
| 09-26-2019 15:25:33 | 09-26-2019 15:25:33 | This issue has been automatically marked as stale because it has not had recent activity. It will be closed if no further activity occurs. Thank you for your contributions.
|
transformers | 1,338 | closed | Extending `examples/` to TensorFlow | ## 🚀 Feature
Hi, thanks for putting in the tremendous effort for TensorFlow-PyTorch interoperability! Would those scripts in the `examples/` be soon extended to Tensorflow as well?
## Motivation
I (and presumably many others) rely on the examples to quickly experiment with models and ideas. Extending the examples to Tensorflow would be hugely helpful, and should help the codebase reach a broader set of audiences.
## Additional context
<!-- Add any other context or screenshots about the feature request here. --> | 09-26-2019 14:29:20 | 09-26-2019 14:29:20 | Indeed, there is currently one example for tensorflow, `run_tf_glue` and it doesn't have command-line arguments. We'll update this one to make it as flexible as the PyTorch one and add other examples when we have the bandwidth.
Do you want to help in this project? Happy to welcome a PR on this topic (for instance to add command line argument similar to `run_glue` in `run_tf_glue`).<|||||>Thanks for the response. I'm not an expert in this field but I'm happy to help and review codes.<|||||>This issue has been automatically marked as stale because it has not had recent activity. It will be closed if no further activity occurs. Thank you for your contributions.
|
transformers | 1,337 | closed | faster dataset building | Now it takes around 1 minute to process 20mb and it takes forever for 200mb dataset (it's non-linear). This is a fix to make it linear. | 09-26-2019 13:56:25 | 09-26-2019 13:56:25 | # [Codecov](https://codecov.io/gh/huggingface/transformers/pull/1337?src=pr&el=h1) Report
> Merging [#1337](https://codecov.io/gh/huggingface/transformers/pull/1337?src=pr&el=desc) into [master](https://codecov.io/gh/huggingface/transformers/commit/a3e0dbba9512866064c20e9bc99c62725f6c36fb?src=pr&el=desc) will **not change** coverage.
> The diff coverage is `n/a`.
[](https://codecov.io/gh/huggingface/transformers/pull/1337?src=pr&el=tree)
```diff
@@ Coverage Diff @@
## master #1337 +/- ##
=======================================
Coverage 84.73% 84.73%
=======================================
Files 84 84
Lines 12573 12573
=======================================
Hits 10654 10654
Misses 1919 1919
```
------
[Continue to review full report at Codecov](https://codecov.io/gh/huggingface/transformers/pull/1337?src=pr&el=continue).
> **Legend** - [Click here to learn more](https://docs.codecov.io/docs/codecov-delta)
> `Δ = absolute <relative> (impact)`, `ø = not affected`, `? = missing data`
> Powered by [Codecov](https://codecov.io/gh/huggingface/transformers/pull/1337?src=pr&el=footer). Last update [a3e0dbb...f71a457](https://codecov.io/gh/huggingface/transformers/pull/1337?src=pr&el=lastupdated). Read the [comment docs](https://docs.codecov.io/docs/pull-request-comments).
<|||||>I was about to make this PR myself and then saw this!<|||||>Thanks a lot @mgrankin (was meaning to fix this as well haha)! |
transformers | 1,336 | closed | Completed the documentation with TF2 | 09-26-2019 11:44:50 | 09-26-2019 11:44:50 | ||
transformers | 1,335 | closed | Optimize XLNet model to generate embedding of long documents | We experiment generating embeddings with TransformerXL and XLnet. Our documents have 5000 to 80000 characters each.
We got an average of 0.8 second per document with TransformerXL and 1.3 second per document with XLNet.
To optimize XLNet we found that using only 200 tokens per call is optimal.
A ratio around 350 tokens/second with xlnet-base-cased and 100 tokens/second with large.
Any idea if XLNet can be optimize?
tokenizer = XLNetTokenizer.from_pretrained("xlnet-base-cased")
model = XLNetModel.from_pretrained("xlnet-base-cased")
outputs = model(text_tokens, mems=mems) | 09-25-2019 20:44:48 | 09-25-2019 20:44:48 | |
transformers | 1,334 | closed | Typo in modeling_bert file | I was looking at the code of BertModel adapted for different tasks here
https://github.com/huggingface/pytorch-transformers/blob/master/pytorch_transformers/modeling_bert.py
I noticed a small typo in line 882
`self.classifier = nn.Linear(config.hidden_size, self.config.num_labels)`
I think it should be either `self.num_labels` or `config.num_labels` in the second argument
The complete function
```
def __init__(self, config):
super(BertForSequenceClassification, self).__init__(config)
self.num_labels = config.num_labels
self.bert = BertModel(config)
self.dropout = nn.Dropout(config.hidden_dropout_prob)
self.classifier = nn.Linear(config.hidden_size, self.config.num_labels)
self.init_weights()
``` | 09-25-2019 13:21:30 | 09-25-2019 13:21:30 | Hi! Indeed it is inconsistent, but it doesn't really change anything as the superclass `PreTrainedModel` assigns the config as one of its attributes: `self.config = config`. Referencing `config` or `self.config` therefore references the same object! |
transformers | 1,333 | closed | [FIX] fix run_generation.py to work with batch_size > 1 | I expended the `top_k_top_p_filtering` function, and by that the`run_generation.py` script to work with num_samples > 1.
This can be expended by scattering the sorted tensors.
First pull request in this repository, so let me know if I need to do anything else :)
Cheers, Matan. | 09-25-2019 12:57:05 | 09-25-2019 12:57:05 | @thomwolf
I created this PR to deal with the `top p` generations.
Should I have opened an issue first to check if it is needed?
Should I deal with the conflicts?
Cheers.<|||||>Hi @mataney, thanks.
This was rebased, fixed by https://github.com/huggingface/transformers/commit/f96ce1c24151349251880c95e9a9fb144b62367c, and merged to master by 2a5663c28043dc6d2746e69f0fb89e0d5872c63d.
Check that everything looks good on your side if you can. |
transformers | 1,332 | closed | pytorch-transformers returns output of 13 layers? | ## 📚 Migration
<!-- Important information -->
Model I am using (Bert, XLNet....): BertModel
Language I am using the model on (English, Chinese....): English
The problem arise when using:
* [x] my own modified scripts: (give details)
The tasks I am working on is:
* [x] my own task or dataset: (give details)
Details of the issue:
I am using pytorch-transformers for the rather unconventional task of regression (one output). In my research I use BERT and I'm planning to try out the other transformers as well. When I started, I got good results with `pytorch-pretrained-bert`. However, running the same code with `pytorch-transformers` gives me results that are a lot worse.
In the original code, I use the output of the model, and concatenate the last four layers - as was proposed in the BERT paper. The architecture that I used looks like this:
```python
from pytorch_pretrained_bert.modeling import BertModel
import torch
from torch import nn
class Net(nn.Module):
def __init__(self):
super(Net, self).__init__()
self.bert_model = BertModel.from_pretrained('bert-base-uncased')
self.pre_classifier = nn.Linear(3072, 512)
self.dropout = nn.Dropout(0.2)
self.classifier = nn.Linear(512, 1)
def forward(self, bert_ids, bert_mask):
all_bert_layers, _ = self.bert_model(bert_ids, attention_mask=bert_mask)
print('hidden_states', len(all_bert_layers))
# concat last four layers
out = torch.cat(tuple([all_bert_layers[i] for i in [-1, -2, -3, -4]]), dim=-1)
print('output', out.size())
# Pooling by also setting masked items to zero
bert_mask = bert_mask.unsqueeze(2)
# Multiply output with mask to only retain non-paddding tokens
out = torch.mul(out, bert_mask)
print('output', out.size())
# First item ['CLS'] is sentence representation
out = out[:, 0, :]
print('pooled_output', out.size())
out = self.pre_classifier(out)
print('pre_classifier', out.size())
out = self.dropout(out)
print('dropout', out.size())
out = self.classifier(out)
print('classifier', out.size())
return out
```
When porting this to `pytorch-transformers`, the main thing was that now we get a tuple back from the model *and* we have to explicitly ask to get all hidden states back. As such, the converted code looks like this:
```python
from pytorch_transformers import BertModel
import torch
from torch import nn
class Net(nn.Module):
def __init__(self):
super(Net, self).__init__()
self.bert_model = BertModel.from_pretrained('bert-base-uncased', output_hidden_states=True)
self.pre_classifier = nn.Linear(3072, 512)
self.dropout = nn.Dropout(0.2)
self.classifier = nn.Linear(512, 1)
def forward(self, bert_ids, bert_mask):
out, _ = self.bert_model(input_ids=bert_ids, attention_mask=bert_mask)
hidden_states = out[2]
print('hidden_states', len(hidden_states))
out = torch.cat(tuple([hidden_states[i] for i in [-1, -2, -3, -4]]), dim=-1)
print('output', out.size())
# Pooling by also setting masked items to zero
bert_mask = bert_mask.unsqueeze(2)
# Multiply output with mask to only retain non-paddding tokens
out = torch.mul(out, bert_mask)
print('output', out.size())
# First item ['CLS'] is sentence representation
out = out[:, 0, :]
print('pooled_output', out.size())
out = self.pre_classifier(out)
print('pre_classifier', out.size())
out = self.dropout(out)
print('dropout', out.size())
out = self.classifier(out)
print('classifier', out.size())
return out
```
As I said before, this leads to *very* different results. Seeding cannot be the issue, since I set all seeds manually in both cases, like this:
```python
def set_seed():
torch.manual_seed(3)
torch.cuda.manual_seed_all(3)
torch.backends.cudnn.deterministic = True
torch.backends.cudnn.benchmark = False
np.random.seed(3)
random.seed(3)
os.environ['PYTHONHASHSEED'] = str(3)
```
I have added the print statements as a sort of debugging and I quickly found that there is a fundamental difference between the two architectures. The *hidden_states* print statement will yield `12` for pytorch-pretrained-bert and `13` for `pytorch-transformers`! I am not sure how that relates, but I would assume that this could be the starting point to start looking.
I have tried comparing the created models, but in both cases the encoder consists of 12 layers, so I am not sure why `pytorch-transformers` returns 13? What's the extra one?
Going through the source code, it seems that the first hidden_state (= last hidden_state from the embeddings) is included. Is that true?
https://github.com/huggingface/pytorch-transformers/blob/7c0f2d0a6a8937063bb310fceb56ac57ce53811b/pytorch_transformers/modeling_bert.py#L340-L352
Even so, since the embeddings would be the first item in all_hidden_states, the last four layers should be the same still. Therefore, I am not sure why there is such a big difference in the results of the above two. If you spot any faults, please advise.
## Environment
* OS: Win 10
* Python version: 3.7
* PyTorch version: 1.2
* PyTorch Transformers version (or branch):
* Using GPU ? Yes, CUDA 10
* Distributed of parallel setup ? No
## Checklist
- [x] I have read the migration guide in the readme. | 09-25-2019 09:51:36 | 09-25-2019 09:51:36 | I am looking at this too and I believe (might be wrong) that the embedding layer sits in the last position. So I guess you should do [-2:-5] <|||||>> I am looking at this too and I believe (might be wrong) that the embedding layer sits in the last position. So I guess you should do [-2:-5]
Hm, I don't think so. The embedding state is passed to the forward function, and that state is used to initialize the `all_hidden_states` variable. Then you iterate over all layers and append to the tuple sequentially.
https://github.com/huggingface/pytorch-transformers/blob/7c0f2d0a6a8937063bb310fceb56ac57ce53811b/pytorch_transformers/modeling_bert.py#L337-L359<|||||>Hi Bram,
Please read the details of `BertModel`'s outputs in the docstring or the doc here: https://huggingface.co/pytorch-transformers/model_doc/bert.html#pytorch_transformers.BertModel
The first element of the output tuple of Bert is always the last hidden-state and the full list of hidden-states is the last element of the output tuple in your case.
These lines:
```
out, _ = self.bert_model(input_ids=bert_ids, attention_mask=bert_mask)
hidden_states = out[2]
```
should be changed in:
```
model_outputs = self.bert_model(input_ids=bert_ids, attention_mask=bert_mask)
hidden_states = model_outputs[-1]
```<|||||>> Hi Bram,
>
> Please read the details of `BertModel`'s outputs in the docstring or the doc here: https://huggingface.co/pytorch-transformers/model_doc/bert.html#pytorch_transformers.BertModel
>
> The first element of the output tuple of Bert is always the last hidden-state and the full list of hidden-states is the last element of the output tuple in your case.
>
> These lines:
>
> ```
> out, _ = self.bert_model(input_ids=bert_ids, attention_mask=bert_mask)
> hidden_states = out[2]
> ```
>
> should be changed in:
>
> ```
> model_outputs = self.bert_model(input_ids=bert_ids, attention_mask=bert_mask)
> hidden_states = model_outputs[-1]
> ```
Hi Thomas, thank you for your time
Apparently a mistake crept into my comment on GitHub. In my code, I do have the correct version, i.e.
```python
out = self.bert_model(input_ids=bert_ids, attention_mask=bert_mask)
hidden_states = out[2]
```
The question that I have is, when you then print the length of those hidden states, you get different numbers.
```python
print(len(hidden_states))
# 13 for pytorch_transformers, 12 for pytorch_pretrained_bert
```
Going through the source code, it seems that the input hidden state (final hidden state of the embeddings) is included when using `pytorch_transformers`, but not for `pytorch_pretrained_bert`.
https://github.com/huggingface/pytorch-transformers/blob/7c0f2d0a6a8937063bb310fceb56ac57ce53811b/pytorch_transformers/modeling_bert.py#L337-L352
I couldn't find this documented anywhere, but I am curious to see the reasoning behind this - since the embedding state is _not_ an encoder state, so it might not be what one expects to get back from the model. On the other hand, it does make it easy for users to get the embeddings.<|||||>Hi Bram,
It's written in the link to the doc that I've sent you above and also in the docstring of the model:

I'll see if I can find a way to make it more visible.
There are a few reasons we did that, one is this great paper by Tenney et al (http://arxiv.org/abs/1905.05950) which use the output of the embeddings as well at the hidden states to study Bert's performances. Another is to have easy access to the embeddings as you mention.<|||||>> # Add last layer
> if self.output_hidden_states:
> all_hidden_states = all_hidden_states + (hidden_states,)
https://github.com/huggingface/transformers/blob/7c0f2d0a6a8937063bb310fceb56ac57ce53811b/pytorch_transformers/modeling_bert.py#L350-L352
But on line 350-352, it adds the "hidden states" (last layer of embedding) to the "all_hidden_states", so the last item is the embedding output. <|||||>> > # Add last layer
> > ```
> > if self.output_hidden_states:
> > all_hidden_states = all_hidden_states + (hidden_states,)
> > ```
>
> https://github.com/huggingface/transformers/blob/7c0f2d0a6a8937063bb310fceb56ac57ce53811b/pytorch_transformers/modeling_bert.py#L350-L352
>
> But on line 350-352, it adds the "hidden states" (last layer of embedding) to the "all_hidden_states", so the last item is the embedding output.
No, by that time the initial `hidden_states` variable has already been reassigned in the for loop. So at each step hidden_states is:
enter function: it is the embeddings
on each iteration in the loop: `hidden_states = layer_outputs[0]`
Perhaps the not-so-intuitive part is that the `hidden_states` are appended to `all_hidden_states` as the first thing in the loop. That means that in the at the end of the first iteration; `all_hidden_states` consists *only* of the embeddings, and at the end of the last iteration, it does not contain the last hidden state yet (because appending happens *before* getting the layer_outputs). Therefore, the hidden states of the last layer (iteration) have to be added manually still, on the lines that you mentioned.<|||||>> > > # Add last layer
> > > ```
> > > if self.output_hidden_states:
> > > all_hidden_states = all_hidden_states + (hidden_states,)
> > > ```
> >
> >
> > https://github.com/huggingface/transformers/blob/7c0f2d0a6a8937063bb310fceb56ac57ce53811b/pytorch_transformers/modeling_bert.py#L350-L352
> >
> > But on line 350-352, it adds the "hidden states" (last layer of embedding) to the "all_hidden_states", so the last item is the embedding output.
>
> No, by that time the initial `hidden_states` variable has already been reassigned in the for loop. So at each step hidden_states is:
>
> ```
> enter function: it is the embeddings
> on each iteration in the loop: `hidden_states = layer_outputs[0]`
> ```
>
> Perhaps the not-so-intuitive part is that the `hidden_states` are appended to `all_hidden_states` as the first thing in the loop. That means that in the at the end of the first iteration; `all_hidden_states` consists _only_ of the embeddings, and at the end of the last iteration, it does not contain the last hidden state yet (because appending happens _before_ getting the layer_outputs). Therefore, the hidden states of the last layer (iteration) have to be added manually still, on the lines that you mentioned.
You are right, thanks for the clarification!<|||||>@thomwolf Thanks for the clarification. I was looking in all the wrong places, it appears. Particularly, I had expected this in the README's [migration part](https://github.com/huggingface/transformers#migrating-from-pytorch-pretrained-bert-to-transformers). If you want I can do a small doc pull request for that.
Re-opened. Will close after doc change if requested. |
transformers | 1,331 | closed | Is the UI code for https://transformer.huggingface.co open source? | ## ❓ Questions & Help
Is the UI code for https://transformer.huggingface.co open source? | 09-25-2019 04:18:43 | 09-25-2019 04:18:43 | No we haven't open sourced the UI code.<|||||>Are there plans to open source the UI or there's no plan for it?<|||||>No short term plans to do it! |
transformers | 1,330 | closed | Loading errors for BERT base on GPU with PyTorch 0.4.1 | 09-25-2019 03:15:34 | 09-25-2019 03:15:34 | ||
transformers | 1,329 | closed | GLUE Script for Tensorflow | 09-25-2019 02:05:50 | 09-25-2019 02:05:50 | # [Codecov](https://codecov.io/gh/huggingface/pytorch-transformers/pull/1329?src=pr&el=h1) Report
> Merging [#1329](https://codecov.io/gh/huggingface/pytorch-transformers/pull/1329?src=pr&el=desc) into [tf2](https://codecov.io/gh/huggingface/pytorch-transformers/commit/e8e956dbb2a6df696d79e2f4dc154849a8e06611?src=pr&el=desc) will **decrease** coverage by `0.05%`.
> The diff coverage is `n/a`.
[](https://codecov.io/gh/huggingface/pytorch-transformers/pull/1329?src=pr&el=tree)
```diff
@@ Coverage Diff @@
## tf2 #1329 +/- ##
==========================================
- Coverage 86.01% 85.95% -0.06%
==========================================
Files 79 79
Lines 12041 12028 -13
==========================================
- Hits 10357 10339 -18
- Misses 1684 1689 +5
```
| [Impacted Files](https://codecov.io/gh/huggingface/pytorch-transformers/pull/1329?src=pr&el=tree) | Coverage Δ | |
|---|---|---|
| [pytorch\_transformers/modeling\_utils.py](https://codecov.io/gh/huggingface/pytorch-transformers/pull/1329/diff?src=pr&el=tree#diff-cHl0b3JjaF90cmFuc2Zvcm1lcnMvbW9kZWxpbmdfdXRpbHMucHk=) | `91.44% <0%> (-1.48%)` | :arrow_down: |
| [pytorch\_transformers/tests/modeling\_common\_test.py](https://codecov.io/gh/huggingface/pytorch-transformers/pull/1329/diff?src=pr&el=tree#diff-cHl0b3JjaF90cmFuc2Zvcm1lcnMvdGVzdHMvbW9kZWxpbmdfY29tbW9uX3Rlc3QucHk=) | `73.61% <0%> (-0.41%)` | :arrow_down: |
| [...orch\_transformers/tests/modeling\_tf\_common\_test.py](https://codecov.io/gh/huggingface/pytorch-transformers/pull/1329/diff?src=pr&el=tree#diff-cHl0b3JjaF90cmFuc2Zvcm1lcnMvdGVzdHMvbW9kZWxpbmdfdGZfY29tbW9uX3Rlc3QucHk=) | `94.73% <0%> (-0.27%)` | :arrow_down: |
------
[Continue to review full report at Codecov](https://codecov.io/gh/huggingface/pytorch-transformers/pull/1329?src=pr&el=continue).
> **Legend** - [Click here to learn more](https://docs.codecov.io/docs/codecov-delta)
> `Δ = absolute <relative> (impact)`, `ø = not affected`, `? = missing data`
> Powered by [Codecov](https://codecov.io/gh/huggingface/pytorch-transformers/pull/1329?src=pr&el=footer). Last update [e8e956d...cc73950](https://codecov.io/gh/huggingface/pytorch-transformers/pull/1329?src=pr&el=lastupdated). Read the [comment docs](https://docs.codecov.io/docs/pull-request-comments).
|
|
transformers | 1,328 | closed | Sequence Classification pooled output vs last hidden state | ## ❓ Questions & Help
Why in BertForSequenceClassification do we pass the pooled output to the classifier as below from the source code
```python
outputs = self.bert(input_ids,
attention_mask=attention_mask,
token_type_ids=token_type_ids,
position_ids=position_ids,
head_mask=head_mask)
pooled_output = outputs[1]
pooled_output = self.dropout(pooled_output)
logits = self.classifier(pooled_output)
```
but in RobertaForSequenceClassification we do not seem to pass the pooler output?
```python
outputs = self.roberta(input_ids,
attention_mask=attention_mask,
token_type_ids=token_type_ids,
position_ids=position_ids,
head_mask=head_mask)
sequence_output = outputs[0]
logits = self.classifier(sequence_output)
```
I thought we would pass the pooled_output in both cases to the classifier? | 09-24-2019 20:30:19 | 09-24-2019 20:30:19 | Both would probably work, but I agree that streamlining is a good idea. In their paper, BERT gets the best results by concatenating the last four layers, so what I always use is something like this (from the top of my head):
```python
outputs = self.bert(input_ids,
attention_mask=attention_mask,
token_type_ids=token_type_ids,
position_ids=position_ids,
head_mask=head_mask)
hidden_states = outputs[1]
pooled_output = torch.cat(tuple([hidden_states[i] for i in [-4, -3, -2, -1]]), dim=-1)
pooled_output = pooled_output[:, 0, :]
pooled_output = self.dropout(pooled_output)
# classifier of course has to be 4 * hidden_dim, because we concat 4 layers
logits = self.classifier(pooled_output)
```
I might put a pre_classifier and an activation function before the drop out depending on the case.<|||||>This is very helpful. Thanks @BramVanroy for the ideas<|||||>@BramVanroy Thanks for the solution, but I think you meant writing `hidden_states = outputs[2]` instead of `pooled_output = outputs[1]`, right?<|||||>@mkaze I think you are talking about `TFBertModel` which has `hidden_states` at index `2`, but OP is talking about `TFBertForSequenceClassification` which has `hidden_states` at index `1`, so we need to use index `1`. @BramVanroy is this correct?<|||||>@BramVanroy also, is it useful to use `outputs[1]` as in your code example with the `RobertaForSequenceClassification` and `TFDistilBertForSequenceClassification` models?<|||||>@mkaze @don-prog My variables were badly named, indeed. In BertForSequenceClassification, the hidden_states are at index 1 (if you provided the option to return all hidden_states) and if you are not using labels. At index 2 if you did pass the labels.
I do not know the position of hidden states for the other models by heart. Just read through the documentation and look at the `forward` method. There you can see under "returns" what is returned at which index.<|||||>@BramVanroy @don-prog The weird thing is that the documentation claims that the `pooler_output` of BERT model is not a good semantic representation of the input, one time in "Returns" section of `forward` method of `BertModel` ([here](https://huggingface.co/transformers/model_doc/bert.html#transformers.BertModel)):

and another one at the third tip in "Tips" section of "Overview" ([here](https://huggingface.co/transformers/model_doc/bert.html)):
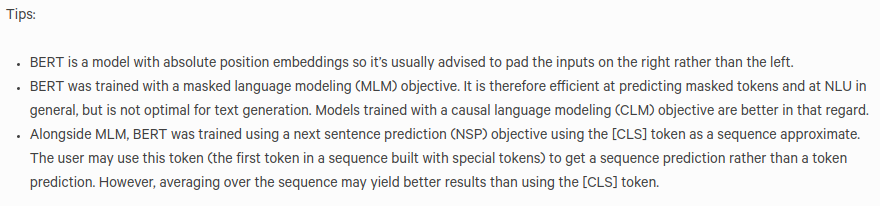
However, despite these two tips, the pooler output is used in implementation of `BertForSequenceClassification` ([here](https://github.com/huggingface/transformers/blob/a39dfe4fb122c11be98a563fb8ca43b322e01036/src/transformers/modeling_bert.py#L1284-L1287)).
Interestingly, when I used their suggestion, i.e. using the average of hidden-states for sequence classification instead of pooler output, I got a worse result. I asked about this a few months ago in issue #4048, but unfortunately no one provided an explanation.<|||||>@BramVanroy Many thanks for the quick reply! So, this is my usage of the last `TFDistilBertModel` 4 hidden states in the TensorFlow:
```
def create_model():
input_ids = tf.keras.Input(shape=(100,), dtype='int32')
transformer = TFDistilBertModel.from_pretrained('distilbert-base-uncased', output_hidden_states=True)(input_ids)
print(len(transformer)) #2
print(len(transformer[1])) #7
hidden_states = transformer[1]
merged = tf.keras.layers.concatenate(tuple([hidden_states[i] for i in [-4, -3, -2, -1]]))
output = tf.keras.layers.Dense(32,activation='relu')(merged)
output = tf.keras.layers.Dropout(0.1)(output)
output = tf.keras.layers.Dense(1, activation='sigmoid')(output)
model = tf.keras.models.Model(inputs = input_ids, outputs = output)
model.compile(tf.keras.optimizers.Adam(lr=6e-6), loss='binary_crossentropy', metrics=['accuracy'])
return model
```
Is this this correct representation of your PyTorch code in the TensorFlow(except for the difference in additional layers)?<|||||>@mkaze Yes, this is always something that comes up for discussion. I think the only correct answer here is (as so often): try it out and see what works best in your scemario. Results will differ between different projects, depending on the task, training steps, dataset, and so on. There is no one right answer. You may even decide to use maxpooling rather than average pooling. There are loads of things to try if you really want to. But generally speaking, you should get good results with either CLS or averaging over tokens.
@don-prog Unfortunately I am not very familiar with TF so I fear I cannot help you with that. Try it out, and keep track of the sizes of the tensors that are passed through (or just have a look at the graph of your model). If those are correct, then I think it's fine. You can ask your question on the [forums,](https://discuss.huggingface.co/) maybe someone can help you out there.<|||||>I think the classification for robertaforsequenceclassification is the RobertaClassificationHead, which takes the CLS embedding for classification
https://github.com/huggingface/transformers/blob/master/src/transformers/modeling_roberta.py#L957
https://github.com/huggingface/transformers/blob/13c185771847370d695b8eee3cbf12f4edc2111c/src/transformers/modeling_roberta.py#L1205-L1221
I also found that AlBERT takes pooler result as bert, but distillbert has something different
https://github.com/huggingface/transformers/blob/master/src/transformers/modeling_distilbert.py#L607-L610
just wondering if huggingface plans to consolidate this part for the sequence classification?<|||||>@DanqingZ Probably not. Most often these implementation are specific to how the original paper implemented them for downstream tasks. In that sense, it is normal that they differ. If you want to create your own one, as I did before, you can simply create a custom SequenceClassificationHead that works with any `PretrainedModel`'s output. It is quite simple, so I don't think the library should provide this.<|||||>@BramVanroy yeah, I can do that.
But imagine a scenario. If I want to inherit the AutoModelForSequenceClassification, and add my own components to different types of model(bert, roberta, distillbert). If huggingface could make classifier have the same meaning and usage, it will be easier for other people to make downstream changes for multiple models at the same time, like adding label attention layer etc. The classifier is a bit misleading now, like roberta has pooler within the classifier while bert has pooled output.
Yeah I agree that if one has enough time to dig into details then it should be easy for them to make changes, but it is just less intuitive for people who just start using huggingface transformers.<|||||>@DanqingZ I understand what you mean, but these implementations are not necessarily chosen by HuggingFace. Those are the original implementations in the paper by the authors. It is therefore not possible that they are all the same and they will not be changed.
If you want to add the functionality that you want, I would recommend writing your own extension to transformers. The process will teach you a lot about how PyTorch models work in general and how this library functions specifically. Yes, it will take a while, but it is the only solution.<|||||>> @BramVanroy Many thanks for the quick reply! So, this is my usage of the last `TFDistilBertModel` 4 hidden states in the TensorFlow:
>
> ```
> def create_model():
> input_ids = tf.keras.Input(shape=(100,), dtype='int32')
>
> transformer = TFDistilBertModel.from_pretrained('distilbert-base-uncased', output_hidden_states=True)(input_ids)
>
> print(len(transformer)) #2
> print(len(transformer[1])) #7
>
> hidden_states = transformer[1]
>
> merged = tf.keras.layers.concatenate(tuple([hidden_states[i] for i in [-4, -3, -2, -1]]))
>
> output = tf.keras.layers.Dense(32,activation='relu')(merged)
> output = tf.keras.layers.Dropout(0.1)(output)
>
> output = tf.keras.layers.Dense(1, activation='sigmoid')(output)
> model = tf.keras.models.Model(inputs = input_ids, outputs = output)
> model.compile(tf.keras.optimizers.Adam(lr=6e-6), loss='binary_crossentropy', metrics=['accuracy'])
> return model
> ```
>
> Is this this correct representation of your PyTorch code in the TensorFlow(except for the difference in additional layers)?
it throwing some errors
<|||||>Hi, @mkaze, regarding your question:
> @BramVanroy @don-prog The weird thing is that the documentation claims that the `pooler_output` of BERT model is not a good semantic representation of the input, one time in "Returns" section of `forward` method of `BertModel` ([here](https://huggingface.co/transformers/model_doc/bert.html#transformers.BertModel)):
> However, despite these two tips, the pooler output is used in implementation of `BertForSequenceClassification` ([here](https://github.com/huggingface/transformers/blob/a39dfe4fb122c11be98a563fb8ca43b322e01036/src/transformers/modeling_bert.py#L1284-L1287)).
> Interestingly, when I used their suggestion, i.e. using the average of hidden-states for sequence classification instead of pooler output, I got a worse result. I asked about this a few months ago in issue #4048, but unfortunately no one provided an explanation.
The BERT paper explicitly says the following:
_The vector C is not a meaningful sentence representation **without fine-tuning**, since it was trained with NSP._
That means, it only says the CLS output token (pooler output) is not useful on its own from the pre-trained model (used without funetuning), but if you fine tune the model, it is useful for classification purposes.<|||||>> > @BramVanroy Many thanks for the quick reply! So, this is my usage of the last `TFDistilBertModel` 4 hidden states in the TensorFlow:
> > ```
> > def create_model():
> > input_ids = tf.keras.Input(shape=(100,), dtype='int32')
> >
> > transformer = TFDistilBertModel.from_pretrained('distilbert-base-uncased', output_hidden_states=True)(input_ids)
> >
> > print(len(transformer)) #2
> > print(len(transformer[1])) #7
> >
> > hidden_states = transformer[1]
> >
> > merged = tf.keras.layers.concatenate(tuple([hidden_states[i] for i in [-4, -3, -2, -1]]))
> >
> > output = tf.keras.layers.Dense(32,activation='relu')(merged)
> > output = tf.keras.layers.Dropout(0.1)(output)
> >
> > output = tf.keras.layers.Dense(1, activation='sigmoid')(output)
> > model = tf.keras.models.Model(inputs = input_ids, outputs = output)
> > model.compile(tf.keras.optimizers.Adam(lr=6e-6), loss='binary_crossentropy', metrics=['accuracy'])
> > return model
> > ```
> >
> >
> >
> >
> >
> >
> >
> >
> >
> >
> >
> > Is this this correct representation of your PyTorch code in the TensorFlow(except for the difference in additional layers)?
>
> it throwing some errors
"merged" one would have a shape like [None(batch_size), max_seq_len, hidden_size].
in order to follow concatenating the last four layers strategy, you may need to add the code something like "merged = merged[:, 0, :]" before the output dense layer.<|||||>Hi,
In my small project, I got significantly better results by _flattening the last hidden states of all tokens_. I wonder if people have tried it, and what you think of this approach.
I'm using an auto-regressive model (a.k.a "decoder only", or GPT-like), where each token can only pay attention to the past tokens.
The way the classification head is currently implemented in the huggingface (causal) models I looked at, is to take the hidden state of the last token, for example:
https://github.com/huggingface/transformers/blob/849367ccf741d8c58aa88ccfe1d52d8636eaf2b7/src/transformers/models/llama/modeling_llama.py#L770-L771
or
https://github.com/huggingface/transformers/blob/849367ccf741d8c58aa88ccfe1d52d8636eaf2b7/src/transformers/models/gpt2/modeling_gpt2.py#L1364-L1365
What worked best for me, is to flatten the last hidden state of all tokens.
So:
* The pretrained model returns the last `hidden_states` for all tokens, with shape `(batch_size, seq_length, hidden_size)`.
* I flatten it along the last 2 dimensions (`hidden_states.view(batch_size, seq_lenght*hidden_size)`), which results in one long vector for each batch - with the last hidden states of all the tokens in the sequence concatenated.
* The classification head projects it back to the num_labels: `nn.Linear(seq_lenght*hidden_size, num_labels)`
The downside I can see is that the classifier is fixed to a specific sequence length, but this is not a problem in my case.
Would love any comments about this approach.
Edit:
I should mention that I'm working with semi-structured data and tokens are not text, but instead coded items in patient's medical history.
My theory of why this approach works better in my case: the classification task is very different from the pre-training objective, so the pre-training (next token prediction) has no good reason to propagate the relevant context to the last token. |
transformers | 1,327 | closed | Pytorch/TF2 determinism | Check to see if the models have the same results when in eval mode (pt) or when training=False (tf) | 09-24-2019 19:04:02 | 09-24-2019 19:04:02 | # [Codecov](https://codecov.io/gh/huggingface/pytorch-transformers/pull/1327?src=pr&el=h1) Report
> Merging [#1327](https://codecov.io/gh/huggingface/pytorch-transformers/pull/1327?src=pr&el=desc) into [tf2](https://codecov.io/gh/huggingface/pytorch-transformers/commit/128bdd4c3549e2a1401af87493ff6be467c79c14?src=pr&el=desc) will **increase** coverage by `0.01%`.
> The diff coverage is `100%`.
[](https://codecov.io/gh/huggingface/pytorch-transformers/pull/1327?src=pr&el=tree)
```diff
@@ Coverage Diff @@
## tf2 #1327 +/- ##
==========================================
+ Coverage 85.99% 86.01% +0.01%
==========================================
Files 79 79
Lines 12028 12041 +13
==========================================
+ Hits 10344 10357 +13
Misses 1684 1684
```
| [Impacted Files](https://codecov.io/gh/huggingface/pytorch-transformers/pull/1327?src=pr&el=tree) | Coverage Δ | |
|---|---|---|
| [pytorch\_transformers/tests/modeling\_common\_test.py](https://codecov.io/gh/huggingface/pytorch-transformers/pull/1327/diff?src=pr&el=tree#diff-cHl0b3JjaF90cmFuc2Zvcm1lcnMvdGVzdHMvbW9kZWxpbmdfY29tbW9uX3Rlc3QucHk=) | `74.01% <100%> (+0.4%)` | :arrow_up: |
| [...orch\_transformers/tests/modeling\_tf\_common\_test.py](https://codecov.io/gh/huggingface/pytorch-transformers/pull/1327/diff?src=pr&el=tree#diff-cHl0b3JjaF90cmFuc2Zvcm1lcnMvdGVzdHMvbW9kZWxpbmdfdGZfY29tbW9uX3Rlc3QucHk=) | `95% <100%> (+0.26%)` | :arrow_up: |
------
[Continue to review full report at Codecov](https://codecov.io/gh/huggingface/pytorch-transformers/pull/1327?src=pr&el=continue).
> **Legend** - [Click here to learn more](https://docs.codecov.io/docs/codecov-delta)
> `Δ = absolute <relative> (impact)`, `ø = not affected`, `? = missing data`
> Powered by [Codecov](https://codecov.io/gh/huggingface/pytorch-transformers/pull/1327?src=pr&el=footer). Last update [128bdd4...1761d20](https://codecov.io/gh/huggingface/pytorch-transformers/pull/1327?src=pr&el=lastupdated). Read the [comment docs](https://docs.codecov.io/docs/pull-request-comments).
<|||||>Yes, good! |
transformers | 1,326 | closed | RuntimeError: expected scalar type Half but found Float | ## 🐛 Bug
<!-- Important information -->
Model I am using (Bert, XLNet....):
bert-large-uncased
Language I am using the model on (English, Chinese....):
English
The problem arise when using:
* [ ] the official example scripts: (give details)
* [x] my own modified scripts: (give details)
I am using a modified version of the run_lm_finetuning.py and amp at optimization level o1. Level O2 runs without issue.
The tasks I am working on is:
* [ ] an official GLUE/SQUaD task: (give the name)
* [x] my own task or dataset: (give details)
I am finetuning on a dataset of sentences, with a min length of 10 tokens, using padding and the mask_tokens function given by the repo. Input and labels are padded per specs and of type=LongTensor (torch.Size([4, 200]) torch.Size([4, 200])) with batch size of 4.
## To Reproduce
Steps to reproduce the behavior:
1. When I run without amp, training works as intended. If I train at amp level O2, training runs as intended.
2. Running with amp level O1 leads to an error: RuntimeError: expected scalar type Half but found Float
3. I have verified that there is no model.eval() and that scaled_loss and clip_grad_norm_ calls are the same as prescribed in the example.
3. Examples of model initialization, train loop, and error are below.
<!-- If you have a code sample, error messages, stack traces, please provide it here as well. -->
## Expected behavior
Training to complete without error.
<!-- A clear and concise description of what you expected to happen. -->
## Environment
* OS: Google Colab
* Python version: 3.6
* PyTorch version: 1.2.0
* PyTorch Transformers version (or branch): 1.2.0
* Using GPU ? Y
* Distributed of parallel setup ? No
* Any other relevant information:
## Additional context
<!-- Add any other context about the problem here. -->
**Model/Optimizer code:**
```
model = model_class.from_pretrained(args.model_name_or_path, from_tf=bool('.ckpt' in args.model_name_or_path), config=config)
model.to(args.device)
# Prepare optimizer and schedule (linear warmup and decay)
#no_decay = ['bias', 'LayerNorm.weight']
no_decay = ['bias', 'LayerNorm.bias', 'LayerNorm.weight']
optimizer_grouped_parameters = [
{'params': [p for n, p in model.named_parameters() if not any(nd in n for nd in no_decay)], 'weight_decay': args.weight_decay},
{'params': [p for n, p in model.named_parameters() if any(nd in n for nd in no_decay)], 'weight_decay': 0.0}
]
optimizer = AdamW(optimizer_grouped_parameters, lr=args.learning_rate, weight_decay=args.weight_decay)
scheduler = WarmupLinearSchedule(optimizer, warmup_steps=args.warmup_steps, t_total=t_total)
if args.fp16:
model, optimizer = amp.initialize(model, optimizer, opt_level=args.fp16_opt_level)
```
**Import Part of Train Loop:**
```
for step, batch in enumerate(epoch_iterator):
inputs, labels = mask_tokens(batch, tokenizer, args) if args.mlm else mask_labels(batch, tokenizer, args)
inputs = inputs.to(args.device)
labels = labels.to(args.device)
model.train()
loss, _ = model(inputs, masked_lm_labels=labels) if args.mlm else model(inputs, labels=labels)
if args.n_gpu > 1:
loss = loss.mean() # mean() to average on multi-gpu parallel training
if args.gradient_accumulation_steps > 1:
loss = loss / args.gradient_accumulation_steps
if args.fp16:
with amp.scale_loss(loss, optimizer) as scaled_loss:
scaled_loss.backward()
else:
loss.backward()
tr_loss += loss.item()
if (step + 1) % args.gradient_accumulation_steps == 0:
print('Clipping Grad Norm. fp16:', args.fp16)
if args.fp16:
torch.nn.utils.clip_grad_norm_(amp.master_params(optimizer), args.max_grad_norm)
else:
torch.nn.utils.clip_grad_norm_(model.parameters(), args.max_grad_norm)
scheduler.step() # Update learning rate schedule
optimizer.step()
optimizer.zero_grad()
global_step += 1
```
**Error:**
```
RuntimeError Traceback (most recent call last)
<ipython-input-7-627529edcd83> in <module>()
10 ft_data.fp16_opt_level = 'O1'
11
---> 12 run_finetune(ft_data)
13
14 print('Finetuned BERT model loaded.')
12 frames
<ipython-input-6-39df7e7a4180> in run_finetune(args)
337 torch.distributed.barrier()
338
--> 339 global_step, tr_loss = train(args, train_dataset, model, tokenizer)
340 logger.info(" global_step = %s, average loss = %s", global_step, tr_loss)
341
<ipython-input-6-39df7e7a4180> in train(args, train_dataset, model, tokenizer)
193 model.train()
194
--> 195 loss, _ = model(inputs, masked_lm_labels=labels) if args.mlm else model(inputs, labels=labels)
196
197 if args.n_gpu > 1:
/usr/local/lib/python3.6/dist-packages/torch/nn/modules/module.py in __call__(self, *input, **kwargs)
545 result = self._slow_forward(*input, **kwargs)
546 else:
--> 547 result = self.forward(*input, **kwargs)
548 for hook in self._forward_hooks.values():
549 hook_result = hook(self, input, result)
/usr/local/lib/python3.6/dist-packages/pytorch_transformers/modeling_bert.py in forward(self, input_ids, attention_mask, token_type_ids, position_ids, head_mask, masked_lm_labels)
767
768 sequence_output = outputs[0]
--> 769 prediction_scores = self.cls(sequence_output)
770
771 outputs = (prediction_scores,) + outputs[2:] # Add hidden states and attention if they are here
/usr/local/lib/python3.6/dist-packages/torch/nn/modules/module.py in __call__(self, *input, **kwargs)
545 result = self._slow_forward(*input, **kwargs)
546 else:
--> 547 result = self.forward(*input, **kwargs)
548 for hook in self._forward_hooks.values():
549 hook_result = hook(self, input, result)
/usr/local/lib/python3.6/dist-packages/pytorch_transformers/modeling_bert.py in forward(self, sequence_output)
417
418 def forward(self, sequence_output):
--> 419 prediction_scores = self.predictions(sequence_output)
420 return prediction_scores
421
/usr/local/lib/python3.6/dist-packages/torch/nn/modules/module.py in __call__(self, *input, **kwargs)
545 result = self._slow_forward(*input, **kwargs)
546 else:
--> 547 result = self.forward(*input, **kwargs)
548 for hook in self._forward_hooks.values():
549 hook_result = hook(self, input, result)
/usr/local/lib/python3.6/dist-packages/pytorch_transformers/modeling_bert.py in forward(self, hidden_states)
406
407 def forward(self, hidden_states):
--> 408 hidden_states = self.transform(hidden_states)
409 hidden_states = self.decoder(hidden_states) + self.bias
410 return hidden_states
/usr/local/lib/python3.6/dist-packages/torch/nn/modules/module.py in __call__(self, *input, **kwargs)
545 result = self._slow_forward(*input, **kwargs)
546 else:
--> 547 result = self.forward(*input, **kwargs)
548 for hook in self._forward_hooks.values():
549 hook_result = hook(self, input, result)
/usr/local/lib/python3.6/dist-packages/pytorch_transformers/modeling_bert.py in forward(self, hidden_states)
388 hidden_states = self.dense(hidden_states)
389 hidden_states = self.transform_act_fn(hidden_states)
--> 390 hidden_states = self.LayerNorm(hidden_states)
391 return hidden_states
392
/usr/local/lib/python3.6/dist-packages/torch/nn/modules/module.py in __call__(self, *input, **kwargs)
545 result = self._slow_forward(*input, **kwargs)
546 else:
--> 547 result = self.forward(*input, **kwargs)
548 for hook in self._forward_hooks.values():
549 hook_result = hook(self, input, result)
/usr/local/lib/python3.6/dist-packages/apex/normalization/fused_layer_norm.py in forward(self, input)
157 if self.elementwise_affine:
158 return FusedLayerNormAffineFunction.apply(
--> 159 input, self.weight, self.bias, self.normalized_shape,self.eps)
160 else:
161 return FusedLayerNormFunction.apply(input, self.normalized_shape, self.eps)
/usr/local/lib/python3.6/dist-packages/apex/normalization/fused_layer_norm.py in forward(ctx, input, weight, bias, normalized_shape, eps)
23 bias_ = bias.contiguous()
24 output, mean, invvar = fused_layer_norm_cuda.forward_affine(
---> 25 input_, ctx.normalized_shape, weight_, bias_, ctx.eps)
26 ctx.save_for_backward(input_, weight_, bias_, mean, invvar)
27 return output
RuntimeError: expected scalar type Half but found Float (data<c10::Half> at /usr/local/lib/python3.6/dist-packages/torch/include/ATen/core/TensorMethods.h:1821)
frame #0: c10::Error::Error(c10::SourceLocation, std::string const&) + 0x33 (0x7f0840be9273 in /usr/local/lib/python3.6/dist-packages/torch/lib/libc10.so)
frame #1: c10::Half* at::Tensor::data<c10::Half>() const + 0x3ee (0x7f08298ccf8e in /usr/local/lib/python3.6/dist-packages/fused_layer_norm_cuda.cpython-36m-x86_64-linux-gnu.so)
frame #2: cuda_layer_norm(at::Tensor*, at::Tensor*, at::Tensor*, at::Tensor*, int, int, c10::ArrayRef<long>, at::Tensor*, at::Tensor*, double) + 0x4c5 (0x7f08298ca745 in /usr/local/lib/python3.6/dist-packages/fused_layer_norm_cuda.cpython-36m-x86_64-linux-gnu.so)
```
There are a total of 63 frames which output in the error that have been truncated here.
| 09-24-2019 14:46:25 | 09-24-2019 14:46:25 | I've encountered this problem as well<|||||>Seems like an apex error (apex should be converting the tensors to half).
Maybe try to update or reinstall apex following carefully the required step for installation? <|||||>This issue has been automatically marked as stale because it has not had recent activity. It will be closed if no further activity occurs. Thank you for your contributions.
|
transformers | 1,325 | closed | [Proposal] GLUE processors included in library | 09-24-2019 13:48:05 | 09-24-2019 13:48:05 | # [Codecov](https://codecov.io/gh/huggingface/pytorch-transformers/pull/1325?src=pr&el=h1) Report
> Merging [#1325](https://codecov.io/gh/huggingface/pytorch-transformers/pull/1325?src=pr&el=desc) into [glue-example](https://codecov.io/gh/huggingface/pytorch-transformers/commit/a6981076eca5494b9d230f13217c14b93443888a?src=pr&el=desc) will **decrease** coverage by `1.63%`.
> The diff coverage is `34.48%`.
[](https://codecov.io/gh/huggingface/pytorch-transformers/pull/1325?src=pr&el=tree)
```diff
@@ Coverage Diff @@
## glue-example #1325 +/- ##
================================================
- Coverage 81.07% 79.44% -1.64%
================================================
Files 57 62 +5
Lines 8207 8489 +282
================================================
+ Hits 6654 6744 +90
- Misses 1553 1745 +192
```
| [Impacted Files](https://codecov.io/gh/huggingface/pytorch-transformers/pull/1325?src=pr&el=tree) | Coverage Δ | |
|---|---|---|
| [pytorch\_transformers/data/processors/\_\_init\_\_.py](https://codecov.io/gh/huggingface/pytorch-transformers/pull/1325/diff?src=pr&el=tree#diff-cHl0b3JjaF90cmFuc2Zvcm1lcnMvZGF0YS9wcm9jZXNzb3JzL19faW5pdF9fLnB5) | `100% <100%> (ø)` | |
| [pytorch\_transformers/data/\_\_init\_\_.py](https://codecov.io/gh/huggingface/pytorch-transformers/pull/1325/diff?src=pr&el=tree#diff-cHl0b3JjaF90cmFuc2Zvcm1lcnMvZGF0YS9fX2luaXRfXy5weQ==) | `100% <100%> (ø)` | |
| [pytorch\_transformers/data/processors/glue.py](https://codecov.io/gh/huggingface/pytorch-transformers/pull/1325/diff?src=pr&el=tree#diff-cHl0b3JjaF90cmFuc2Zvcm1lcnMvZGF0YS9wcm9jZXNzb3JzL2dsdWUucHk=) | `27.45% <15.78%> (ø)` | |
| [pytorch\_transformers/data/metrics/\_\_init\_\_.py](https://codecov.io/gh/huggingface/pytorch-transformers/pull/1325/diff?src=pr&el=tree#diff-cHl0b3JjaF90cmFuc2Zvcm1lcnMvZGF0YS9tZXRyaWNzL19faW5pdF9fLnB5) | `34.88% <34.88%> (ø)` | |
| [pytorch\_transformers/data/processors/utils.py](https://codecov.io/gh/huggingface/pytorch-transformers/pull/1325/diff?src=pr&el=tree#diff-cHl0b3JjaF90cmFuc2Zvcm1lcnMvZGF0YS9wcm9jZXNzb3JzL3V0aWxzLnB5) | `42.85% <42.85%> (ø)` | |
------
[Continue to review full report at Codecov](https://codecov.io/gh/huggingface/pytorch-transformers/pull/1325?src=pr&el=continue).
> **Legend** - [Click here to learn more](https://docs.codecov.io/docs/codecov-delta)
> `Δ = absolute <relative> (impact)`, `ø = not affected`, `? = missing data`
> Powered by [Codecov](https://codecov.io/gh/huggingface/pytorch-transformers/pull/1325?src=pr&el=footer). Last update [a698107...789ea72](https://codecov.io/gh/huggingface/pytorch-transformers/pull/1325?src=pr&el=lastupdated). Read the [comment docs](https://docs.codecov.io/docs/pull-request-comments).
|
|
transformers | 1,324 | closed | A Micro BERT | ## ❓ Questions & Help
Hello,
Has anyone solved a problem like this, or knows of a solution:
I want to pre-train BERT on a custom dataset, but this data is much smaller than the one used by Google.
So is it possible to train it on a "micro" bert with much lesser layers, etc.
Thanks in advance | 09-24-2019 12:04:24 | 09-24-2019 12:04:24 | I am using a much smaller dataset with my project, but it doesn't mean I need a bert with lesser layers. Otherwise, I have no way to utilize the pre-trained model.
What is the problem you have with the smaller dataset?<|||||>My dataset is very esoteric, in the sense that BERTs pretrained weights will almost be like noise.<|||||>YOU NEED ALBERT<|||||>Einstein?<|||||>They are referring to this new [ALBERT paper](https://old.reddit.com/r/MachineLearning/comments/d9tdfo/albert_a_lite_bert_for_selfsupervised_learning_of/). No weights are available however so give it a few months.
Definitely try fine-tuning a pre-trained BERT first, you can also just edit the BertConfig class to get a smaller network, but you probably can't train it from scratch on a small amount of data.<|||||>Interesting. Can't I train a very small BERT as you said(maybe 2 layers) on like 4million tokens<|||||>I'm not sure what the minimum tokens and layers are, I'm not sure anyone has published that. Best to try it out.<|||||>This issue has been automatically marked as stale because it has not had recent activity. It will be closed if no further activity occurs. Thank you for your contributions.
|
transformers | 1,323 | closed | How to build a Text-to-Feature Extractor based on Fine-Tuned BERT Model | I have now tried for several days to solve an issue I have...
I need to make a feature extractor for a project I am doing, so I am able to translate a given sentence e.g. "My hat is blue" into a vector of a given length e.g. 768. That vector will then later on be combined with several other values for the final prediction in e.g. a random forest algorithm.
My dataset contains a text column + a label column (with 0 and 1 values) + several other columns that are not of interest for this problem.
I know how to do make that feature extractor using word2vec, Glove, FastText and pre-trained BERT/Elmo Models. That works okay.
Now I want to improve the text-to-feature extractor by using a FINE-TUNED BERT model, instead of a PRE-TRAINED BERT MODEL. I want to fine-tune the BERT model on my dataset and then use that new BERT model to do the feature extraction. I am NOT INTERESTED in using the bert model for the predictions themselves! Only for the feature extraction. How can i do that? I think i need the run_lm_finetuning.py somehow, but simply cant figure out how to do it.
I could really need some help...
P.S. I have already created a binary classifier using the text information to predict the label (0/1), by adding an additional layer. Could I in principle use the output of the previous layers, in evaluation mode, as word embeddings? If I can, then I am not sure how to get the output of those in evaluation mode.
| 09-24-2019 09:35:17 | 09-24-2019 09:35:17 | The explanation for fine-tuning is in the README https://github.com/huggingface/pytorch-transformers#quick-tour-of-the-fine-tuningusage-scripts.<|||||>Thanks, but as far as i understands its about "Fine-tuning on GLUE tasks for **sequence classification**". I want to do "Fine-tuning on My Data for **word-to-features extraction**". I am not interested in building a classifier, just a fine-tuned word-to-features extraction. I am not sure how to get there, from the GLUE example?? I need to somehow do the fine-tuning and then find a way to extract the output from e.g. the last four layers in evalution mode for each sentence i want to extract features from. But how to do that?
<|||||>You can only fine-tune a model if you have a task, of course, otherwise the model doesn't know whether it is improving over some baseline or not. Since 'feature extraction', as you put it, doesn't come with a predefined correct result, that doesn't make since. In your case it might be better to fine-tune the masked LM on your dataset. https://github.com/huggingface/pytorch-transformers/blob/master/pytorch_transformers/modeling_bert.py#L713 <|||||>But wouldnt it be possible to proceed like thus:
1) fine-tune the BERT model on my labelled data by adding a layer with two nodes (for 0 and 1) [ALREADY DONE]
2) Run all my data/sentences through the fine-tuned model in evalution, and use the output of the last layers (before the classification layer) as the word-embeddings instead of the predictons? Then I can use that feature vector in my further analysis of my problem and I have created a feature extractor fine-tuned on my data.
What do you think of that approach?
<|||||>But what do you wish to use these word representations for? It's a bit odd using word representations from deep learning as features in other kinds of systems.
But, yes, what you say is theoretically possible. But take into account that those are *not* word embeddings what you are extracting. They are the final *task specific* representation of words. In other words, if you finetune the model on another task, you'll get other word representations.<|||||>The idea is that I have several columns in my dataset. Most of them have numerical values and then I have ONE text column. The idea is to extract features from the text, so I can represent the text fields as numerical values.
Now that all my columns have numerical values (after feature extraction) I can use e.g. a neural network or random forest algorithm to do the predictions based on both the text column and the other columns with numerical values
By the way, do you know - after I fine-tune the model - how do I get the output from the last four layers in evalution mode? My model is BertForSequenceClassification.from_pretrained("bert-base-uncased", num_labels=2) but i can only figure out how to get the final predictions (model.eval() -> predictions = model(b_input_ids, token_type_ids=None, attention_mask=b_input_mask), not the output from all the layers...
<|||||>If I were you, I would just extend BERT and add the features there, so that everything is optimised in one go. That will give you the cleanest pipeline and most reproducible. But of course you can do what you want. I also once tried Sent2Vec as features in SVR and that worked pretty well. So what I'm saying is, it might _work_ but the pipeline might get messy. So make sure that your code is well structured and easy to follow along. The more broken up your pipeline, the easier it is for errors the sneak in.
I advise you to read through the whole BERT process. Especially its config counterpart. Down the line you'll find that there's this option that can be used:
https://github.com/huggingface/pytorch-transformers/blob/7c0f2d0a6a8937063bb310fceb56ac57ce53811b/pytorch_transformers/configuration_utils.py#L55
When you enable `output_hidden_states` all layers' final states will be returned.
```python
bert = BertModel.from_pretrained('bert-base-uncased', output_hidden_states=True)
out = bert.(input_ids=input_ids, attention_mask=attention_mask
# out is a tuple, the hidden states are the third element (cf. source code)
hidden_states = out[2]
```<|||||>Thanks alot! Now my only problem is that, when I do:
model = BertForSequenceClassification.from_pretrained("bert-base-uncased", num_labels=2, output_hidden_states=True)
I get:
TypeError: __init__() got an unexpected keyword argument 'output_hidden_states'<|||||>@pvester what version of pytorch-transformers are you using? I'm on 1.2.0 and it seems to be working with output_hidden_states = True.<|||||>@cformosa I am using 1.2.0
This is the full output
TypeError Traceback (most recent call last)
<ipython-input-39-06d5140bbc0a> in <module>()
1
----> 2 model = BertForSequenceClassification.from_pretrained("bert-base-uncased", num_labels=2, output_hidden_states=True)
3 model.cuda()
4
/usr/local/lib/python3.6/dist-packages/pytorch_pretrained_bert/modeling.py in from_pretrained(cls, pretrained_model_name_or_path, *inputs, **kwargs)
598 logger.info("Model config {}".format(config))
599 # Instantiate model.
--> 600 model = cls(config, *inputs, **kwargs)
601 if state_dict is None and not from_tf:
602 weights_path = os.path.join(serialization_dir, WEIGHTS_NAME)
TypeError: __init__() got an unexpected keyword argument 'output_hidden_states'<|||||>@pvester perhaps this will help?
[#1073 ](https://github.com/huggingface/pytorch-transformers/issues/1073)<|||||>thanks @cformosa
I think I got more confused than before. I hope you guys are able to help me making this work. My latest try is:
config = BertConfig.from_pretrained("bert-base-uncased", output_hidden_states=True)
model = BertForSequenceClassification.from_pretrained("bert-base-uncased", num_labels=2, config=config)
ERROR:
AttributeError: type object 'BertConfig' has no attribute 'from_pretrained'<|||||>No, don't do it like that. Your first approach was correct. (You don't need to use config manually when using a pre-trained model.) So
```python
model = BertForSequenceClassification.from_pretrained('bert-base-uncased', num_labels=2, output_hidden_states=True)
```
is correct. I tested it and it works. I would assume that you are on an older version of pytorch-transformers. Try updating the package to the latest pip release.
EDIT: I just read the reference by cformosa. Apparently there are different ways. But if they don't work, it might indicate a version issue.<|||||>Are you sure you have a recent version of pytorch_transformers ?
```
import pytorch_transformers
pytorch_transformers.__version__
```
On Wed, 25 Sep 2019 at 15:47, pvester <[email protected]> wrote:
> I think I got more confused than before. I hope you guys are able to help
> me making this work. My latest try is:
>
> config = BertConfig.from_pretrained("bert-base-uncased",
> output_hidden_states=True)
> model = BertForSequenceClassification.from_pretrained("bert-base-uncased",
> num_labels=2, config=config)
>
> ERROR:
> AttributeError: type object 'BertConfig' has no attribute 'from_pretrained'
>
> —
> You are receiving this because you are subscribed to this thread.
> Reply to this email directly, view it on GitHub
> <https://github.com/huggingface/pytorch-transformers/issues/1323?email_source=notifications&email_token=ABYDIHOSVHXKBF5PTRPEYHDQLNTWBA5CNFSM4IZ5GVFKYY3PNVWWK3TUL52HS4DFVREXG43VMVBW63LNMVXHJKTDN5WW2ZLOORPWSZGOD7R64AY#issuecomment-535031299>,
> or mute the thread
> <https://github.com/notifications/unsubscribe-auth/ABYDIHPW7ZATNPB2MYISKVTQLNTWBANCNFSM4IZ5GVFA>
> .
>
<|||||>@BramVanroy, @thomwolf
pytorch_transformers.__version__ gives me "1.2.0"
Everything works when i do a it **without** output_hidden_states=True
I do a pip install of pytorch-transformers right before, with the output
Requirement already satisfied: pytorch-transformers in /usr/local/lib/python3.6/dist-packages (1.2.0)
Requirement already satisfied: requests in /usr/local/lib/python3.6/dist-packages (from pytorch-transformers) (2.21.0)
Requirement already satisfied: tqdm in /usr/local/lib/python3.6/dist-packages (from pytorch-transformers) (4.28.1)
Requirement already satisfied: regex in /usr/local/lib/python3.6/dist-packages (from pytorch-transformers) (2019.8.19)
Requirement already satisfied: torch>=1.0.0 in /usr/local/lib/python3.6/dist-packages (from pytorch-transformers) (1.1.0)
Requirement already satisfied: sacremoses in /usr/local/lib/python3.6/dist-packages (from pytorch-transformers) (0.0.34)
Requirement already satisfied: sentencepiece in /usr/local/lib/python3.6/dist-packages (from pytorch-transformers) (0.1.83)
Requirement already satisfied: boto3 in /usr/local/lib/python3.6/dist-packages (from pytorch-transformers) (1.9.224)
Requirement already satisfied: numpy in /usr/local/lib/python3.6/dist-packages (from pytorch-transformers) (1.16.5)
Requirement already satisfied: certifi>=2017.4.17 in /usr/local/lib/python3.6/dist-packages (from requests->pytorch-transformers) (2019.6.16)
Requirement already satisfied: chardet<3.1.0,>=3.0.2 in /usr/local/lib/python3.6/dist-packages (from requests->pytorch-transformers) (3.0.4)
Requirement already satisfied: idna<2.9,>=2.5 in /usr/local/lib/python3.6/dist-packages (from requests->pytorch-transformers) (2.8)
Requirement already satisfied: urllib3<1.25,>=1.21.1 in /usr/local/lib/python3.6/dist-packages (from requests->pytorch-transformers) (1.24.3)
Requirement already satisfied: six in /usr/local/lib/python3.6/dist-packages (from sacremoses->pytorch-transformers) (1.12.0)
Requirement already satisfied: click in /usr/local/lib/python3.6/dist-packages (from sacremoses->pytorch-transformers) (7.0)
Requirement already satisfied: joblib in /usr/local/lib/python3.6/dist-packages (from sacremoses->pytorch-transformers) (0.13.2)
Requirement already satisfied: botocore<1.13.0,>=1.12.224 in /usr/local/lib/python3.6/dist-packages (from boto3->pytorch-transformers) (1.12.224)
Requirement already satisfied: s3transfer<0.3.0,>=0.2.0 in /usr/local/lib/python3.6/dist-packages (from boto3->pytorch-transformers) (0.2.1)
Requirement already satisfied: jmespath<1.0.0,>=0.7.1 in /usr/local/lib/python3.6/dist-packages (from boto3->pytorch-transformers) (0.9.4)
Requirement already satisfied: python-dateutil<3.0.0,>=2.1; python_version >= "2.7" in /usr/local/lib/python3.6/dist-packages (from botocore<1.13.0,>=1.12.224->boto3->pytorch-transformers) (2.5.3)
Requirement already satisfied: docutils<0.16,>=0.10 in /usr/local/lib/python3.6/dist-packages (from botocore<1.13.0,>=1.12.224->boto3->pytorch-transformers) (0.15.2)<|||||>I tried with two different python setups now and always the same error:
TypeError: __init__() got an unexpected keyword argument 'output_hidden_states'
I can upload a Google Colab notesbook, if it helps to find the error??<|||||>You're sure that you are passing in the keyword argument *after* the 'bert-base-uncased' argument, right? Yes, you can try a Colab.<|||||>@BramVanroy
Okat thanks, the Colab link is here:
https://colab.research.google.com/drive/1tIFeHITri6Au8jb4c64XyVH7DhyEOeMU
scroll down to the end for the error message<|||||>You're loading it from the old pytorch_pretrained_bert, not from the new pytorch_transformers. Why are you importing `pytorch_pretrained_bert` in the first place? Using both at the same time will definitely lead to mistakes or at least confusion. Stick to one.
This line
```python
from pytorch_pretrained_bert import BertAdam, BertForSequenceClassification
```
should be
```python
from pytorch_transformers import BertAdam, BertForSequenceClassification
```<|||||>@BramVanroy
Now i get
ImportError: cannot import name 'BertAdam'<|||||>I'm sorry but this is getting annoying. If you'd just _read_, you'd understand what's wrong. In the README it is stated that there have been changes to the optimizers. Now you can use AdamW and it's in optimizer.py. It's not hard to find out why an import goes wrong. Just look through the source code here.<|||||>@BramVanroy @thomwolf @cformosa
Thanks for your help. I now managed to do my task as intended with a quite good performance and I am very happy with the results.
Thank to all of you for your valuable help and patience
I am sorry I did not understand everything in the documentation right away - it has been a learning experience for as well for me :) I now feel more at ease with these packages and manipulating an existing neural network.<|||||>No worries. Just remember that reading the documentation and particularly the source code will help you a lot. Not only for your current problem, but also for better understanding the bigger picture.
Glad that your results are as good as you expected.<|||||>I'm trying to extract the features from FlaubertForSequenceClassification. My concern is the huge size of embeddings being extracted. Is there any work you can point me to which involves compressing the embeddings/features extracted from the model.
Thanks in advance! <|||||>> I'm trying to extract the features from FlaubertForSequenceClassification. My concern is the huge size of embeddings being extracted. Is there any work you can point me to which involves compressing the embeddings/features extracted from the model.
> Thanks in advance!
You can use pooling for this. Typically average or maxpooling. You'll find a lot of info if you google it.<|||||>> If I were you, I would just extend BERT and add the features there, so that everything is optimised in one go. That will give you the cleanest pipeline and most reproducible. But of course you can do what you want. I also once tried Sent2Vec as features in SVR and that worked pretty well. So what I'm saying is, it might _work_ but the pipeline might get messy. So make sure that your code is well structured and easy to follow along. The more broken up your pipeline, the easier it is for errors the sneak in.
>
> I advise you to read through the whole BERT process. Especially its config counterpart. Down the line you'll find that there's this option that can be used:
>
> https://github.com/huggingface/pytorch-transformers/blob/7c0f2d0a6a8937063bb310fceb56ac57ce53811b/pytorch_transformers/configuration_utils.py#L55
>
> When you enable `output_hidden_states` all layers' final states will be returned.
>
> ```python
> bert = BertModel.from_pretrained('bert-base-uncased', output_hidden_states=True)
> out = bert.(input_ids=input_ids, attention_mask=attention_mask
> # out is a tuple, the hidden states are the third element (cf. source code)
> hidden_states = out[2]
> ```
Hi @BramVanroy , I'm relatively new to neural network and I'm using 🤗transformer to fine-tune a BERT for my research thesis.
The major challenge I'm having now happens to be mentioned in your comment here, that's _"extend BERT and add features"_. Is it possible to integrate the fine-tuned BERT model into a bigger network? Something like appending some more features in the output layer of BERT then continue forward to the next layer in the bigger network.
I know it's more of a ML question than a specific question toward this package, but it would be MUCH MUCH appreciated if you can refer some material/blog that explain similar practice. Thanks!<|||||>@BenjiTheC I don't have any blog post to link to, but I wrote a small snippet that could help get you started. You just have to make sure the dimensions are correct for the features that you want to include. For more help you may want to get in touch via [the forum](https://discuss.huggingface.co/). You can tag me there as well.
```python
import torch
import torch.nn as nn
from torch.nn import GELU
from transformers import BertModel
class ExtendedBert(nn.Module):
def __init__(self):
super().__init__()
self.bert = BertModel.from_pretrained("bert-base-cased")
self.linear = nn.Linear(1024, 1024)
self.act = GELU()
# regression problem: one label
self.classifier = nn.Linear(1024, 1)
def forward(self, encoded, other_feats):
# get the hidden state of the last layer
last_hidden = self.bert(**encoded)[0]
# concatenate with the other given features
cat = torch.cat([last_hidden, other_feats], dim=-1)
# pass through linear layer
output = self.linear(cat)
# pass through non-linear activation and final classifier layer
return self.classifier(self.act(output))
```<|||||>> @BenjiTheC I don't have any blog post to link to, but I wrote a small smippet that could help get you started. You just have to make sure the dimensions are correct for the features that you want to include. For more help you may want to get in touch via [the forum](https://discuss.huggingface.co/). You can tag me there as well.
>
> ```python
> import torch
> import torch.nn as nn
> from torch.nn import GELU
> from transformers import BertModel
>
>
> class ExtendedBert(nn.Module):
> def __init__(self):
> super().__init__()
>
> self.bert = BertModel.from_pretrained("bert-base-cased")
> self.linear = nn.Linear(1024, 1024)
> self.act = GELU()
> # regression problem: one label
> self.classifier = nn.Linear(1024, 1)
>
> def forward(self, encoded, other_feats):
> # get the hidden state of the last layer
> last_hidden = self.bert(**encoded)[0]
> # concatenate with the other given features
> cat = torch.cat([last_hidden, other_feats], dim=-1)
> # pass through linear layer
> output = self.linear(cat)
> # pass through non-linear activation and final classifier layer
> return self.classifier(self.act(output))
> ```
Thank you so much for such a timely response!
I'm a TF2 user but your snippet definitely point me to the right direction - to concat the last layer's state and new features to forward. One more follow up question though: I saw in the previous discussion, to get the hidden state of the model, you need to set `output_hidden_state` to `True`, do I need this flag to be True to get what I want?<|||||>@BenjiTheC That flag is needed if you want the hidden states of _all_ layers. If you just want the last layer's hidden state (as in my example), then you do not need that flag.<|||||>> @BenjiTheC That flag is needed if you want the hidden states of _all_ layers. If you just want the last layer's hidden state (as in my example), then you do not need that flag.
Thanks so much! Will stay tuned in the forum and continue the discussion there if needed.<|||||>hi @BramVanroy, I am relatively new to 🤗transformers. I would like to know is it possible to use a fine-tuned model to be retrained/reused on a different set of labels? The new set of labels may be a subset of the old labels or the old labels + some additional labels. I already ask this on the [forum](https://discuss.huggingface.co/t/retrain-reuse-fine-tuned-models-on-different-set-of-labels/346) but no reply yet. AFAIK now it is not possible to use the fine-tuned model to be retrained on a new set of labels. A workaround for this is to fine-tune a pre-trained model use whole (old + new) data with a superset of the old + new labels. Is true?
I know it's more of an ML question than a specific question toward this package, but I will really appreciate it if you can refer me to some reference that explains this. Thank you in advance.<|||||>Is it possible to use RoBERTa as the feature extractor and not train it while fine-tuning a model on my dataset? |
transformers | 1,322 | closed | parameter never_split not added in BasicTokenizer's tokenize | ## 🐛 Bug
<!-- Important information -->
Model I am using (Bert, XLNet....):
Bert
Language I am using the model on (English, Chinese....):
English
The problem arise when using:
* [ ] my own modified scripts: I need to add some special tokens that will not been split during tokenizing. And my special tokens contain punc, like [E1]. This will be split in _run_split_on_punc() if omit parameter never_split in this function.
## To Reproduce
Steps to reproduce the behavior:
1.
omit parameter never_split when invoke self._run_split_on_punc(token)
<!-- If you have a code sample, error messages, stack traces, please provide it here as well. -->
```python
# tokenization_bert.py
def tokenize(self, text, never_split=None):
....
orig_tokens = whitespace_tokenize(text)
split_tokens = []
for token in orig_tokens:
if self.do_lower_case and token not in never_split:
token = token.lower()
token = self._run_strip_accents(token)
split_tokens.extend(self._run_split_on_punc(token))
output_tokens = whitespace_tokenize(" ".join(split_tokens))
return output_tokens
```
Modify
```python
split_tokens.extend(self._run_split_on_punc(token))
```
to
```python
split_tokens.extend(self._run_split_on_punc(token, never_split))
```
wolud solve this problem.
| 09-24-2019 08:48:21 | 09-24-2019 08:48:21 | This issue has been automatically marked as stale because it has not had recent activity. It will be closed if no further activity occurs. Thank you for your contributions.
|
transformers | 1,321 | closed | Using pytorch-transformer to reimplement the "Attention is all you need" paper | ## ❓ Questions & Help
I use this repo for a long time but I realized even though the name is PyTorch transformers I can't find an easy way to re-implement the original paper of "Attention is all you need" with pretrained model. Can someone help me? | 09-23-2019 20:51:36 | 09-23-2019 20:51:36 | Hi, this repository's objective is mainly to host **pretrained** models, not really to build a model from scratch.
You could use some of this library's components though, like multi-headed attention, to help you in your endeavor. |
transformers | 1,320 | closed | Why does padding affect the embedding results for XLNet? Pre-padding returns different embeddings than post-padding. Which one should be used? | ## ❓ Questions & Help
Hello, I am confused with different results of XLNet depending on padding. For Bert, padding doesn't affect the outputs, but for XLNet with **pre** padding (which I saw in https://github.com/huggingface/pytorch-transformers/blob/master/examples/run_glue.py#L281), returns very different results for the same sentence, with and without padding. The difference between no padding and post padding for XLNet returns similar results. Why did "pre" padding is used for run_glue.py? Does XLNet expect post padding or pre padding? Is there any document I am missing to clarify those important distinctions?
Here is a demonstration of the differences for pre, post padding for BERT and XLNet: https://colab.research.google.com/drive/1PCiU3icdfUB-nrLbrKAhgpePoIcFRlqX
Thanks,
Osman | 09-23-2019 18:56:10 | 09-23-2019 18:56:10 | I might be wrong, but intuitively I would say that that makes things easier. XLNet expects single sequences that look like this `tok1 tok2 ... SEP CLS`. So in contrast with BERT, the classification token is at the end of a sentence rather than beginning. This is before padding. So if you use post-padding, the position of the CLS element can differ for each element in your batch, but if you use pre-padding, then you can access the CLS element by its `-1` index.
That's not to say that it's not possible to retrieve the CLS element in XLNet when you've used post-padding. Something like this should work. Find the position (indices) where the input IDs are the classification token, then use those indices to slice the output.
```python
output = output[torch.where(input_ids == tokenizer.cls_token_id)]
```
If you've used pre-padding, this can be simplified to
```python
output = output[:, -1]
```<|||||>Hey @BramVanroy, thanks for the answer. You may be right that pre padding for XLNet makes things easier (i.e., getting `[cls]` token from the last index) but the question I want to be answered is not why we would like "pre" padding but why pre padding and post padding gives different answers. Maybe I should change the title further. If you see the notebook I shared, depending on padding you're getting different results.<|||||>Sorry, I fear I can't help with that. I am also wondering when padding is necessary and when it isn't. <|||||>This issue has been automatically marked as stale because it has not had recent activity. It will be closed if no further activity occurs. Thank you for your contributions.
<|||||>Maybe too late for this thread, but any answers to this issue?<|||||>Any answer on this issue?<|||||>Any answer on this issue? |
transformers | 1,319 | closed | BertForQuestionAnswering output to predict text |
<!-- A clear and concise description of the question. -->
In predict mode, BertForQuestionAnswering model output a tuple like below, how to get the text answer interactively
```
tensor([[ 0.4691, 0.3912, -0.3447, 0.9756, 0.7171, 0.3746, 0.5273, 0.3756,
0.2083, 0.4130, 0.2145, 0.1327, 0.7265, 0.4678, 0.6294, 0.3284]])
```
| 09-23-2019 14:31:51 | 09-23-2019 14:31:51 | |
transformers | 1,318 | closed | A sequence with no special tokens has been passed to the RoBERTa model. This model requires special tokens in order to work. Please specify add_special_tokens=True in your encoding. | This is my code for Roberta:
```
# coding: utf-8
# In[4]:
import pandas as pd
import numpy as np
import json, re
from tqdm import tqdm_notebook
from uuid import uuid4
## Torch Modules
import torch
import torch.optim as optim
import torch.nn as nn
import torch.nn.functional as F
from torch.autograd import Variable
from torch.utils.data import Dataset, DataLoader
## PyTorch Transformer
from pytorch_transformers import RobertaModel, RobertaTokenizer
from pytorch_transformers import RobertaForSequenceClassification, RobertaConfig
# In[21]:
import pandas as pd
train_data = pd.read_csv("walmart_input/test/train.csv")
test_data = pd.read_csv("walmart_input/test/test.csv")
dataset = pd.concat([train_data, test_data])
test_data.head()
# In[46]:
total_length = len(dataset)
# In[47]:
label_to_ix = {}
for label in dataset.PT:
if label not in label_to_ix:
label_to_ix[label]=len(label_to_ix)
total_pt_count = len(list(set(list(train_data["PT"]))))
# In[48]:
config = RobertaConfig.from_pretrained('roberta-base')
config.num_labels = len(list(set(list(train_data["PT"]))))
print(f"Total length of dataset: {total_length} \n Total PT count: {total_pt_count} \n {config}")
# In[36]:
tokenizer = RobertaTokenizer.from_pretrained('roberta-base')
model = RobertaForSequenceClassification(config)
def prepare_features(seq_1, max_seq_length = 300,
zero_pad = False, include_CLS_token = True, include_SEP_token = True):
## Tokenzine Input
tokens_a = tokenizer.tokenize(seq_1)
## Truncate
if len(tokens_a) > max_seq_length - 2:
tokens_a = tokens_a[0:(max_seq_length - 2)]
## Initialize Tokens
tokens = []
if include_CLS_token:
tokens.append(tokenizer.cls_token)
## Add Tokens and separators
for token in tokens_a:
tokens.append(token)
if include_SEP_token:
tokens.append(tokenizer.sep_token)
input_ids = tokenizer.convert_tokens_to_ids(tokens)
## Input Mask
input_mask = [1] * len(input_ids)
## Zero-pad sequence lenght
if zero_pad:
while len(input_ids) < max_seq_length:
input_ids.append(0)
input_mask.append(0)
return torch.tensor(input_ids).unsqueeze(0), input_mask
# In[38]:
class Intents(Dataset):
def __init__(self, dataframe):
self.len = len(dataframe)
self.data = dataframe
def __getitem__(self, index):
title = self.data.title[index]
label = self.data.PT[index]
X, _ = prepare_features(title)
y = label_to_ix[self.data.PT[index]]
return X, y
def __len__(self):
return self.len
print("FULL Dataset: {}".format(dataset.shape))
print("TRAIN Dataset: {}".format(train_data.shape))
print("TEST Dataset: {}".format(test_data.shape))
training_set = Intents(train_data)
testing_set = Intents(test_data)
training_set.__getitem__(0)[0].shape
model(training_set.__getitem__(0)[0])
# In[65]:
## Training Params
if torch.cuda.device_count() > 1:
print("Let's use", torch.cuda.device_count(), "GPUs!")
# dim = 0 [30, xxx] -> [10, ...], [10, ...], [10, ...] on 3 GPUs
#model = nn.DataParallel(model)
## Training Params
device = torch.device("cuda:0,1" if torch.cuda.is_available() else "cpu")
model = model.cuda()
model = nn.DataParallel(model,device_ids=[0,1],dim=1)
model.to(device)
# Parameters
params = {'batch_size': 1,
'shuffle': True,
'num_workers': 2}
training_loader = DataLoader(training_set, **params)
testing_loader = DataLoader(testing_set, **params)
# In[66]:
loss_function = nn.CrossEntropyLoss()
learning_rate = 1e-02
optimizer = optim.Adam(params = model.parameters(), lr=learning_rate)
## Test Forward Pass
inp = training_set.__getitem__(0)[0].cuda()
#print(inp)
output = model(inp)[0]
torch.max(output.data, 1)
# In[ ]:
import time
start_time = time.time()
max_epochs = 2
model = model.train()
for epoch in tqdm_notebook(range(max_epochs)):
print("EPOCH -- {}".format(epoch))
for i, (sent, label) in enumerate(training_loader):
optimizer.zero_grad()
sent = sent.squeeze(0)
if torch.cuda.is_available():
sent = sent.cuda()
label = label.cuda()
print("CUDA detail:")
print(sent)
print(label)
output = model.forward(sent)[0]
_, predicted = torch.max(output, 1)
print(f" - {i}.) {predicted}")
loss = loss_function(output, label)
loss.backward()
optimizer.step()
if i%100 == 0:
correct = 0
total = 0
for sent, label in testing_loader:
sent = sent.squeeze(0)
if torch.cuda.is_available():
sent = sent.cuda()
label = label.cuda()
output = model.forward(sent)[0]
_, predicted = torch.max(output.data, 1)
total += label.size(0)
correct += (predicted.cpu() == label.cpu()).sum()
accuracy = 100.00 * correct.numpy() / total
print('Iteration: {}. Loss: {}. Accuracy: {}%'.format(i, loss.item(), accuracy))
timetaken = format(float((time.time() - start_time)),'.3f')
print(timetaken)
torch.save(model.state_dict(), 'roberta_state_dict_on_new_data_MAY2019.pth')
```
Here I am trying to run the code on Multiple GPUs(2-P100). But I keep getting this warning:
```
A sequence with no special tokens has been passed to the RoBERTa model. This model
requires special tokens in order to work. Please specify add_special_tokens=True in
your encoding.
```
I am not sure, what causing this issue. But when I run it using single (i.e: after removing DataParallel wrapper), it doesn't give this warning.
Any help would be appreciated.
-thanks.
| 09-23-2019 11:02:30 | 09-23-2019 11:02:30 | @yaroslavvb @cynthia @myleott <|||||>Hi, this error springs when you're passing an input to the model which doesn't have the special tokens it needs (cls token and sep token).
The `encode` method accepts the argument `add_special_tokens`, which will take care of adding the special tokens to your sequence.<|||||>I have exactly the same problem, when running on a single GPU it works well, but on the 2-GPUS it got this warning and an index error then caused cuDNN error: CUDNN_STATUS_NOT_INITIALIZED<|||||>I found the problem, when running on multi-gpus, all inputs in forward well divided into n-gpus, for an input tensor with shape (batch, x, y), it will divided into (n-gpus, batch/n-gpus, x, y), if tensor doesn't have the batch dim, then caused this error.<|||||>This issue has been automatically marked as stale because it has not had recent activity. It will be closed if no further activity occurs. Thank you for your contributions.
<|||||>I have the same issue on just loading the model
```
tokenizer = RobertaTokenizer.from_pretrained('roberta-base',add_special_tokens=True)
model = TFRobertaForSequenceClassification.from_pretrained('roberta-base')
```
Returns
```
A sequence with no special tokens has been passed to the RoBERTa model. This model requires special tokens in order to work. Please specify add_special_tokens=True in your encoding.
A sequence with no special tokens has been passed to the RoBERTa model. This model requires special tokens in order to work. Please specify add_special_tokens=True in your encoding.
```<|||||>Can you try specifying it in `encode()` not in `from_pretrained()`?<|||||>Yes but it surprises me that it throws the warning and i did not pass any data to the model. So far in the code there is nothing to encode.<|||||>TensorFlow models need to be "built" by first passing inputs through their layers. This warning occurs then.
This warning was removed in the recent versions of transformers. |
transformers | 1,317 | closed | BertTokenizer provides wrong encode function for Japanese BERT | ## 🐛 Bug
<!-- Important information -->
Model I am using (Bert, XLNet....): BertTokenizer
Language I am using the model on (English, Chinese....): Japanese
I tried to load the tokenizer for Bert from pretrained [Bert for Japanese](http://nlp.ist.i.kyoto-u.ac.jp/index.php?BERT%E6%97%A5%E6%9C%AC%E8%AA%9EPretrained%E3%83%A2%E3%83%87%E3%83%AB). The tokenizer recognize "が" the same as "か" although both appears in vocab file. Here is example code:
```
from pytorch_transformers import BertTokenizer
tokenizer = BertTokenizer.from_pretrained('vocab.txt')
token_ids = tokenizer.encode('が')
print('token_ids: ', token_ids)
print(tokenizer.decode(token_ids))
token_ids = tokenizer.encode('か')
print('token_ids: ', token_ids)
print(tokenizer.decode(token_ids))
```
the result:
```
token_ids: [90]
か
token_ids: [90]
か
```
The vocab.txt file can be downloaded from [here](https://drive.google.com/open?id=1f3k9GcyqEIjjFo8EgqqaOQmiSXxT1hqF):
I also found that BertTokenizer also mis-recognized: 'て' and 'で', 'ば' and 'は' | 09-23-2019 08:07:27 | 09-23-2019 08:07:27 | I had discovered that this phenomenon is due to function: _run_strip_accents(token) in class: BasicTokenizer. Perhaps, the authors should give an option to choose whether to remove accents or not because in some language such as Japanese, removing accents makes a new word<|||||>Hi, I have trained this Japanese BERT model, and made it public.
Please set `do_lower_case` option to false so that the function `_run_strip_accents` is disabled. |
transformers | 1,316 | closed | How to predict missing word [MASK] using Robert | I am reading the docs and I still cannot figure out how to I predict missing word in a sentence using Robert. With bert this is described at https://huggingface.co/pytorch-transformers/quickstart.html
# Mask a token that we will try to predict back with `BertForMaskedLM`
masked_index = 8
tokenized_text[masked_index] = '[MASK]'
assert tokenized_text == ['[CLS]', 'who', 'was', 'jim', 'henson', '?', '[SEP]', 'jim', '[MASK]', 'was', 'a', 'puppet', '##eer', '[SEP]']
Robert example is something I do not understand. What is the output of that?
import torch
from pytorch_transformers import RobertaTokenizer, RobertaForMaskedLM
tokenizer = RobertaTokenizer.from_pretrained('roberta-
base',cache_dir="/var/software/Models/robert/")
model = RobertaForMaskedLM.from_pretrained('roberta-
base',cache_dir="/var/software/Models/robert/")
input_ids = torch.tensor(tokenizer.encode("Hello, my dog is cute")).unsqueeze(0) # Batch size 1
outputs = model(input_ids, masked_lm_labels=input_ids)
loss, prediction_scores = outputs[:2]
print("prediction_scores",prediction_scores,len(prediction_scores))
output:
A sequence with no special tokens has been passed to the RoBERTa model. This model requires
special tokens in order to work. Please specify add_special_tokens=True in your encoding.
prediction_scores tensor([[[33.6519, -3.9080, 24.2591, ..., 2.8165, 4.9966, 12.8938],
[ 5.8086, -4.2237, 16.1383, ..., -1.0431, -0.8348, 3.5343],
[ 0.3336, -4.1881, 10.7825, ..., 0.7295, 0.9056, 3.7928],
[ 0.2897, -4.4614, 8.1219, ..., -3.9978, 0.1261, -1.4313],
[ 3.3684, -4.0727, 10.7862, ..., 1.7704, -2.2975, 3.9174],
[ 2.0526, -4.9519, 18.1501, ..., -4.2190, -5.0759, 1.4990]]],
grad_fn=<AddBackward0>) 1
| 09-23-2019 02:41:12 | 09-23-2019 02:41:12 | Basically, the problem is that the model is called masked language model, but it does not mask anything.
If i want to get token distribution for word "dog", but the model sees word dog, because its not masked, so it use the word in prediction. Input should not be "Hello, my dog is cute", but something like that "Hello, my [MASK] is cute".
How do I do this?
Maybe there is another way to specify that the word is masked by index or something?
When I put:
input_ids = torch.tensor(tokenizer.encode("Hello, my dog is cute")).unsqueeze(0)
top_predicted_words: dog puppy pet pup Dog dogs guy husband kid housedog brother girl friend job cat post
input_ids = torch.tensor(tokenizer.encode("Hello, my wife is cute")).unsqueeze(0)
top_predicted_words: wife spouse marriage Wife bride husband head house family wives culture throat
<|||||>Ok, i got it, I should use <mask> instead of [MASK] and <pad> instead of [PAD].
I find robera-base to be around 7% faster than bert-base, but a bit less precise.<|||||>> Ok, i got it, I should use instead of [MASK] and instead of [PAD].
>
> I find robera-base to be around 7% faster than bert-base, but a bit less precise.
@Oxi84 Hi, do you mean should use [PAD] instead of [MASK]? I am having the same problem here. Could you plz share the whole code snippet? Thanks very much. |
transformers | 1,315 | closed | Remove unnecessary use of FusedLayerNorm | Fix #1172 | 09-23-2019 00:33:16 | 09-23-2019 00:33:16 | # [Codecov](https://codecov.io/gh/huggingface/pytorch-transformers/pull/1315?src=pr&el=h1) Report
> Merging [#1315](https://codecov.io/gh/huggingface/pytorch-transformers/pull/1315?src=pr&el=desc) into [master](https://codecov.io/gh/huggingface/pytorch-transformers/commit/a2d4950f5c909f7bb4ea7c06afa6cdecde7e8750?src=pr&el=desc) will **decrease** coverage by `<.01%`.
> The diff coverage is `100%`.
[](https://codecov.io/gh/huggingface/pytorch-transformers/pull/1315?src=pr&el=tree)
```diff
@@ Coverage Diff @@
## master #1315 +/- ##
==========================================
- Coverage 80.77% 80.76% -0.01%
==========================================
Files 57 57
Lines 8092 8091 -1
==========================================
- Hits 6536 6535 -1
Misses 1556 1556
```
| [Impacted Files](https://codecov.io/gh/huggingface/pytorch-transformers/pull/1315?src=pr&el=tree) | Coverage Δ | |
|---|---|---|
| [pytorch\_transformers/modeling\_bert.py](https://codecov.io/gh/huggingface/pytorch-transformers/pull/1315/diff?src=pr&el=tree#diff-cHl0b3JjaF90cmFuc2Zvcm1lcnMvbW9kZWxpbmdfYmVydC5weQ==) | `88.3% <100%> (-0.03%)` | :arrow_down: |
------
[Continue to review full report at Codecov](https://codecov.io/gh/huggingface/pytorch-transformers/pull/1315?src=pr&el=continue).
> **Legend** - [Click here to learn more](https://docs.codecov.io/docs/codecov-delta)
> `Δ = absolute <relative> (impact)`, `ø = not affected`, `? = missing data`
> Powered by [Codecov](https://codecov.io/gh/huggingface/pytorch-transformers/pull/1315?src=pr&el=footer). Last update [a2d4950...98dd19b](https://codecov.io/gh/huggingface/pytorch-transformers/pull/1315?src=pr&el=lastupdated). Read the [comment docs](https://docs.codecov.io/docs/pull-request-comments).
<|||||>Note this change makes the codebase to be compatible with apex amp O1.<|||||>Ok great, thanks @bryant1410! |
transformers | 1,314 | closed | How to preprocess my own data to use RoBERTa of Multiple GPUs | Hey,
I am bit naive using deep learning of text-classification, my data **(.csv)** consist of basically two columns:
- Text
- Labels
As per basic objective, model should take unseen text and predict label _(variable y)_ from the trained labels.
**I followed this tutorial to train RoBERTa algorithm:**
- [https://colab.research.google.com/drive/1xg4UMQmXjDik3v9w-dAsk4kq7dXX_0Fm](https://colab.research.google.com/drive/1xg4UMQmXjDik3v9w-dAsk4kq7dXX_0Fm)
Here the input format is universal (Train.tsv and Test.tsv) with 2 columns (which I mentioned above). The only problem is that this code doesn't utilize the multiple GPUs **(I even tried the DataParallel wrapper)**.
Somehow, I found the repository of the pytorch-transformer where they have given an example of how to utilize multiple GPUs and train the RoBERTa model i.e:
- [https://github.com/huggingface/pytorch-transformers/tree/master/examples](https://github.com/huggingface/pytorch-transformers/tree/master/examples)
This repository example takes the input-data in the wiki.text data format and they have provided the link [ Pretraining RoBERTa using your own data ](https://github.com/pytorch/fairseq/blob/master/examples/roberta/README.pretraining.md#pretraining-roberta-using-your-own-data) and **yet I don't find it of any use as it just talks about the format what they think is right not about the transforming of the standard format (i.e: .CSV)**
Here is the sample:
```
= Valkyria Chronicles III =
Senjō no Valkyria 3 : <unk> Chronicles ( Japanese : 戦場のヴァルキュリア3 , lit . Valkyria of the Battlefield 3 ) , commonly referred to as Valkyria Chronicles III outside Japan , is a tactical role @-@ playing video game developed by Sega and Media.Vision for the PlayStation Portable . Released in January 2011 in Japan , it is the third game in the Valkyria series . <unk> the same fusion of tactical and real @-@ time gameplay as its predecessors , the story runs parallel to the first game and follows the " Nameless " , a penal military unit serving the nation of Gallia during the Second Europan War who perform secret black operations and are pitted against the Imperial unit " <unk> Raven " .
```
I mean, why? do they have to use this format, why can't they go with standard format of classification algorithm? and if they want to then why they don't provide the detail regarding the data and adjusting the personal data corresponding to the algorithm intake?
Just wanted to know, how to preprocess the data according to multiple GPUs RoBERTa example, any help would be appreciated.
thanks.
| 09-22-2019 18:46:43 | 09-22-2019 18:46:43 | @spolu @cynthia @myleott <|||||>Hi, you can follow the `run_glue` example which is better for text classification.
But you will have to modify it for your needs, it's not plug and play.<|||||>This issue has been automatically marked as stale because it has not had recent activity. It will be closed if no further activity occurs. Thank you for your contributions.
|
transformers | 1,313 | closed | Add option to use a 'stop token' | This will be used to truncate the output text to everything till right before the 'stop token'. If the 'stop token' is not found, then the whole text will be returned based on the specified 'length'. | 09-22-2019 13:42:37 | 09-22-2019 13:42:37 | # [Codecov](https://codecov.io/gh/huggingface/transformers/pull/1313?src=pr&el=h1) Report
> :exclamation: No coverage uploaded for pull request base (`master@ecc4f1b`). [Click here to learn what that means](https://docs.codecov.io/docs/error-reference#section-missing-base-commit).
> The diff coverage is `n/a`.
[](https://codecov.io/gh/huggingface/transformers/pull/1313?src=pr&el=tree)
```diff
@@ Coverage Diff @@
## master #1313 +/- ##
=========================================
Coverage ? 84.72%
=========================================
Files ? 84
Lines ? 12591
Branches ? 0
=========================================
Hits ? 10668
Misses ? 1923
Partials ? 0
```
------
[Continue to review full report at Codecov](https://codecov.io/gh/huggingface/transformers/pull/1313?src=pr&el=continue).
> **Legend** - [Click here to learn more](https://docs.codecov.io/docs/codecov-delta)
> `Δ = absolute <relative> (impact)`, `ø = not affected`, `? = missing data`
> Powered by [Codecov](https://codecov.io/gh/huggingface/transformers/pull/1313?src=pr&el=footer). Last update [ecc4f1b...d3f24df](https://codecov.io/gh/huggingface/transformers/pull/1313?src=pr&el=lastupdated). Read the [comment docs](https://docs.codecov.io/docs/pull-request-comments).
<|||||>Great, thanks! |
transformers | 1,312 | closed | In BertForSequenceClassification, why is loss initialised in every forward? | Looking at [the source](https://github.com/huggingface/pytorch-transformers/blob/master/pytorch_transformers/modeling_bert.py#L902-L910) I can see that the correct loss function is initialized in each call to forward.
https://github.com/huggingface/pytorch-transformers/blob/master/pytorch_transformers/modeling_bert.py#L902-L910
Can you explain why? Why isn't the loss function set up as part of `init()`? Is there any advantage of always re-initialising it on each forward?
Edit: I see that you do this in other parts as well, e.g. the ReLU layer in distilbert: https://github.com/huggingface/pytorch-transformers/blob/master/pytorch_transformers/modeling_distilbert.py#L598 | 09-22-2019 08:45:51 | 09-22-2019 08:45:51 | Also it will be nice if the user gets to use the loss_func itself, Like currently i am using that class with slight modifications to match the pipeline with different losses rather than only CrossEntropy loss. (plus add class_weights etc as well to it)
Though this is what i did actually to use a different loss function, just grab the logits from the model and apply your own..<|||||>You can always subclass the class, to make it your own.
Some extra information for this issue: in an issue over at pytorch, it came to light that loss functions are actually meant to be imported as functions (from nn.functional) rather than modules (from nn). <|||||>This issue has been automatically marked as stale because it has not had recent activity. It will be closed if no further activity occurs. Thank you for your contributions.
<|||||>Unstale. <|||||>This issue has been automatically marked as stale because it has not had recent activity. It will be closed if no further activity occurs. Thank you for your contributions.
<|||||>This issue has been automatically marked as stale because it has not had recent activity. It will be closed if no further activity occurs. Thank you for your contributions.
|
transformers | 1,311 | closed | RoBERTa : add_special_tokens=True | I set add_special_tokens=True
but I still get:
A sequence with no special tokens has been passed to the RoBERTa model. This model requires special tokens in order to work. Please specify add_special_tokens=True in your encoding. | 09-22-2019 07:29:04 | 09-22-2019 07:29:04 | I'm getting the same warning, also here: https://github.com/huggingface/pytorch-transformers/issues/1318<|||||>I think you should add < s > without spaces before as well as after sentences.<|||||>Please share a self contained script exhibiting the behavior and allthe information on the python/pytorch/pytorch-transformers versions you are using.<|||||>This issue has been automatically marked as stale because it has not had recent activity. It will be closed if no further activity occurs. Thank you for your contributions.
|
transformers | 1,310 | closed | Redundant sep_token_extra option for RoBERTa Fine-tuning | ## 🐛 Bug
<!-- Important information -->
Model I am using (Bert, XLNet....): RoBERTa
Language I am using the model on (English, Chinese....): English
## Context
I was reading the code on RoBERTa fine-tuning and noticed the [`sep_token_extra` option](https://github.com/huggingface/pytorch-transformers/search?q=sep_token_extra&unscoped_q=sep_token_extra), which looks like a misinterpretation of a sentence from the original paper.
The current implementation [added an extra `[SEP]` to the RoBERTa input compared to BERT](https://github.com/huggingface/pytorch-transformers/blob/d8923270e6c497862f990a3c72e40cc1ddd01d4e/examples/utils_glue.py#L453), which seems wrong. Check out:
1. The [Facebook language model format](https://github.com/pytorch/fairseq/blob/e75cff5f2c1d62f12dc911e0bf420025eb1a4e33/fairseq/data/legacy/masked_lm_dataset.py#L193)
2. A related [Twitter discussion](https://twitter.com/VictoriaLinML/status/1175596109009321986)
The tasks I am working on is:
Fine-tuning RoBERTa on downstream tasks
## Code Sample
https://github.com/huggingface/pytorch-transformers/search?q=sep_token_extra&unscoped_q=sep_token_extra | 09-22-2019 03:49:35 | 09-22-2019 03:49:35 | Myle Ott from Facebook commented on the Twitter thread (https://twitter.com/myleott/status/1175750596630056961) confirming that there is an extra separator being used, so there should be details I did not understand well.
I will revisit this issue when I understand it better.<|||||>The `sep_token_extra` param is deprecated, as we have simpler ways to do this now (thanks to @LysandreJik). Closing this for now, feel free to re-open if needed. |
transformers | 1,309 | closed | Best loss | I am building a classifier by adopting codes from `run_glue.py`. There is plenty of optimization in training and tuning hyperparameters. Could anyone explain the difference between loss, tr_loss and logging_loss in these parts?
https://github.com/huggingface/pytorch-transformers/blob/a2d4950f5c909f7bb4ea7c06afa6cdecde7e8750/examples/run_glue.py#L120
https://github.com/huggingface/pytorch-transformers/blob/a2d4950f5c909f7bb4ea7c06afa6cdecde7e8750/examples/run_glue.py#L134
Thank you. | 09-21-2019 20:19:37 | 09-21-2019 20:19:37 | |
transformers | 1,308 | closed | Planned support for new Grover 1.5B models? | Thanks for the great repo.
Just wondering if there's any planned support for the new Grover 1.5B models?
https://github.com/rowanz/grover (original 1.5B now available via download_model.py)
https://github.com/vanyacohen/opengpt2-1.5B-gpu-inference (slightly different variation)
Cheers
| 09-21-2019 14:15:12 | 09-21-2019 14:15:12 | No short-term plan to implement this ourselves, but we'd welcome a PR (especially one involving the original authors for validation).<|||||>This issue has been automatically marked as stale because it has not had recent activity. It will be closed if no further activity occurs. Thank you for your contributions.
|
transformers | 1,307 | closed | mask_tokens sometimes masks special tokens | ## 🐛 Bug
<!-- Important information -->
Model I am using (Bert, XLNet....): RoBERTa
Language I am using the model on (English, Chinese....):
The problem arise when using:
* [ ] the official example scripts: (give details) run_lm_finetuning
* [ ] my own modified scripts: (give details)
The tasks I am working on is:
* [ ] an official GLUE/SQUaD task: (give the name)
* [ ] my own task or dataset: (give details)
## To Reproduce
Steps to reproduce the behavior:
1.
2.
3.
the mask_token function in run_lm_finetuning script sometimes masks the special tokens. This leads roberta model to throw a warning message:
A sequence with no special tokens has been passed to the RoBERTa model. "
"This model requires special tokens in order to work. "
"Please specify add_special_tokens=True in your encoding
I would prevent the first and last tokens being masked by adding the below lines immediately after masked_indices are calculated.
masked_indices[:, 0] = False
masked_indices[:, -1] = False
<!-- If you have a code sample, error messages, stack traces, please provide it here as well. -->
## Expected behavior
<!-- A clear and concise description of what you expected to happen. -->
## Environment
* OS:
* Python version:
* PyTorch version:
* PyTorch Transformers version (or branch):
* Using GPU ?
* Distributed of parallel setup ?
* Any other relevant information:
## Additional context
<!-- Add any other context about the problem here. --> | 09-20-2019 22:01:49 | 09-20-2019 22:01:49 | Hi, thank you for the bug report. Indeed, this does seem problematic. I'm looking into it.<|||||>This issue has been automatically marked as stale because it has not had recent activity. It will be closed if no further activity occurs. Thank you for your contributions.
|
transformers | 1,306 | closed | Which model is best to used for language model rescoring for ASR | ## ❓ Questions & Help
<!-- A clear and concise description of the question. -->
Hello all, I am wanting to use this library to rescore an output from an automatic speech recognition model. Still learning a lot about language model so out of curiosity for anyone who's tried, which model has given you the best performance? Looking to a language model that can predict score the probability of a sentence most effectively. | 09-20-2019 16:23:09 | 09-20-2019 16:23:09 | Same as https://github.com/google-research/bert/issues/35<|||||>And https://github.com/huggingface/transformers/issues/37<|||||>This issue has been automatically marked as stale because it has not had recent activity. It will be closed if no further activity occurs. Thank you for your contributions.
<|||||>GPT2 can be used for rescoring https://arxiv.org/pdf/1910.11450.pdf<|||||>[I tested](https://github.com/simonepri/lm-scorer/blob/master/tests/models/test_gpt2.py#L52-L239) GPT2 on different english error types (I used the one defined in the [ERRANT framework](https://www.aclweb.org/anthology/P17-1074/)) and it seems that is able to give a lower probability to the wrong version of a sentence (At least for simple examples). |
transformers | 1,305 | closed | Dataset format and Best Practices For Language Model Fine-tuning | ## ❓ Questions & Help
Hi, thanks for making this code base available!
I have two questions, one on the input format of for fine-tuning the language model on custom dataset, and one on (unreasonably-)long data preprocessing time. Thanks in advance for any help!
- I'm trying to fine-tune the BERT Model on an extra dataset, and am using the `run_lm_finetuning.py` script in the `examples/` directory. However, I'm having trouble locating instructions on the proper format of the input data. There used to be some instructions in the `examples/lm_finetuning/` directory, but they seem deprecated now.
- As a start, I followed the `run_lm_finetuning.py` example and changed nothing but `--train_data_file` argument with a bigger text file (arbitrary format). The training, however, hangs on the data preprocessing part for about 10 hours, and the last standard output is shown below.
```
pytorch_transformers.tokenization_utils - Token indices sequence length is longer than the specified maximum sequence length for this model (164229992 > 512). Running this sequence through the model will result in indexing errors
``` | 09-20-2019 15:12:26 | 09-20-2019 15:12:26 | I am facing the same issue as there is no proper format available for defining the train and test dataset.
As usual, I use .csv file in a format of columns with (UID, Text, and Labels). But according to the Wiki.txt its more of arbitrary format.
Any help would be appreciated.<|||||>I'm having the same issue. I think it's counting the total length of the tokenized corpus not only the tokenized document length. I tried to run the wiki raw files as mentioned in the read me and still get this warning of total tokenized corpus length.
I tried to the following formats with no success:
1. sentence per line with a blank line in between docs
2. document per line with a blank line in between docs
Update:
After looking at the code again it looks like even though this warning is showing the sequence length being longer than 512 it is still chunking the corpus into 512 tokens and training it that way. This raises the question of whether it is problematic to just separate the corpus based on token length alone especially that BERT for example is training on predicting next sentence. What happens to the probably recurring case of the data being chunked mid-way the sentence?<|||||>This issue has been automatically marked as stale because it has not had recent activity. It will be closed if no further activity occurs. Thank you for your contributions.
|
transformers | 1,304 | closed | max_len_single_sentence should be max_len - 2 for RoBERTa | ## 🐛 Bug
<!-- Important information -->
Model I am using (Bert, XLNet....): RoBERTa
Language I am using the model on (English, Chinese....): English
The problem arise when using:
* [ ] the official example scripts: (give details) run_lm_finetuning
* [ ] my own modified scripts: (give details)
The tasks I am working on is:
* [ ] an official GLUE/SQUaD task: (give the name)
* [ ] my own task or dataset: (give details) . Language model finetuning
## To Reproduce
Steps to reproduce the behavior:
1.
2.
3.
<!-- If you have a code sample, error messages, stack traces, please provide it here as well. -->
## Expected behavior
<!-- A clear and concise description of what you expected to happen. -->
While using language model finetuning for roberta-base, I got an error cublas runtime error : resource allocation failed at /pytorch/aten/src/THC/THCGeneral.cpp:216.
When I checked the tokenized dataset, I observed that it had 514 tokens, i.e. 512 coming from max_len_single_sentence plus 2 special tokens. RoBERTa tokenizer should have max_len_single_sentence set to 510 just like the one in BERT.
max_len_single_sentence = max_len - 2
## Environment
* OS: Ubuntu
* Python version: 3.7
* PyTorch version: 1.2.0
* PyTorch Transformers version (or branch): Master
* Using GPU ? Yes
* Distributed of parallel setup ? No
* Any other relevant information:
## Additional context
<!-- Add any other context about the problem here. -->
R | 09-20-2019 13:20:46 | 09-20-2019 13:20:46 | I think you may be right and we've been meaning to fix this. cf recent discussion @LysandreJik @VictorSanh <|||||>thanks. Adding LM fine-tuning to fast-bert. Have added a workaround for now :)<|||||>Also see https://github.com/pytorch/fairseq/issues/1187 |
transformers | 1,303 | closed | Getting an unexpected EOF when trying to download 'bert-large-uncased-whole-word-masking-finetuned-squad' model. | ## 🐛 Bug
<!-- Important information -->
Model I am using (Bert, XLNet....): Bert
Language I am using the model on (English, Chinese....): English
The problem arise when using:
* [ ] the official example scripts: (give details): BertForQuestionAnswering
* [ ] my own modified scripts: (give details)
The tasks I am working on is:
* [ ] an official GLUE/SQUaD task: (give the name): SQuaD
* [ ] my own task or dataset: (give details)
## To Reproduce
Steps to reproduce the behavior:
1. from pytorch_transformers import BertForQuestionAnswering
2. model = BertForQuestionAnswering.from_pretrained('bert-large-uncased-whole-word-masking-finetuned-squad')
<!-- If you have a code sample, error messages, stack traces, please provide it here as well. -->
## Expected behavior
<!-- A clear and concise description of what you expected to happen. -->
## Environment
* OS:
* Python version:
* PyTorch version:
* PyTorch Transformers version (or branch):
* Using GPU ?
* Distributed of parallel setup ?
* Any other relevant information:
## Additional context
<!-- Add any other context about the problem here. --> | 09-20-2019 13:09:18 | 09-20-2019 13:09:18 | This issue has been automatically marked as stale because it has not had recent activity. It will be closed if no further activity occurs. Thank you for your contributions.
<|||||>In my environment, **it works as expected**!
_Environment_:
- **Python**: 3.6.9
- **O.S.** : Linux-4.15.0-70-generic-x86_64-with-debian-buster-sid
- **Transformers**: 2.1.1 (installed from source with `pip install git+https://github.com/huggingface/transformers.git`)
- **Torch**: 1.3.1
_Example code_:
```
>>> import transformers
>>> from transformers import BertForQuestionAnswering
>>> model = BertForQuestionAnswering.from_pretrained('bert-large-uncased-whole-word-masking-finetuned-squad')
100%|████████████████████████████████████████████████████████████████████████████████████████████████████████████████████████████████████████████████████████████████████████████████████████████████████████████████████████████| 341/341 [00:00<00:00, 152821.63B/s]
100%|████████████████████████████████████████████████████████████████████████████████████████████████████████████████████████████████████████████████████████████████████████████████████████████████████████████| 1340675298/1340675298 [02:04<00:00, 10793596.06B/s]
>>> ...
```
The same correct behavior occurs with TensorFlow 2.0:
```
>>> import transformers
>>> from transformers import TFBertForQuestionAnswering
>>> model = TFBertForQuestionAnswering.from_pretrained('bert-large-uncased-whole-word-masking-finetuned-squad')
████████████████████████████████████████████████████████████████████████████████████████████████████████████████████████████████████████████████████████████████████████████████████████████████████████████| 1340675298/1340675298 [02:30<00:00, 8890808.45B/s]
>>> ...
```
Now, you can close this issue!
> ## Bug
> Model I am using (Bert, XLNet....): Bert
>
> Language I am using the model on (English, Chinese....): English
>
> The problem arise when using:
>
> * [ ] the official example scripts: (give details): BertForQuestionAnswering
> * [ ] my own modified scripts: (give details)
>
> The tasks I am working on is:
>
> * [ ] an official GLUE/SQUaD task: (give the name): SQuaD
> * [ ] my own task or dataset: (give details)
>
> ## To Reproduce
> Steps to reproduce the behavior:
>
> 1. from pytorch_transformers import BertForQuestionAnswering
> 2. model = BertForQuestionAnswering.from_pretrained('bert-large-uncased-whole-word-masking-finetuned-squad')
>
> ## Expected behavior
> ## Environment
> * OS:
> * Python version:
> * PyTorch version:
> * PyTorch Transformers version (or branch):
> * Using GPU ?
> * Distributed of parallel setup ?
> * Any other relevant information:
>
> ## Additional context<|||||>This is usually because of
- a network error or
- not enough space on the disk
while downloading the file. To make sure it isn't the first, you can try running the `from_pretrained` method with the `force_download` option set to `True`:
```py
model = BertForQuestionAnswering.from_pretrained('bert-large-uncased-whole-word-masking-finetuned-squad', force_download=True)
```<|||||>This issue has been automatically marked as stale because it has not had recent activity. It will be closed if no further activity occurs. Thank you for your contributions.
|
transformers | 1,302 | closed | Rectified Adam + LARS | ## 🚀 Feature
There has been a lot of buzz around the new Radam and Ralamb (Radam + LARS) optimizers, and I was wondering if it could also be implemented in pytorch-transformers.
## Motivation
It seems to have consistent performance improvements. It also seems to handle different learning rates a lot better. And with LARS it also allows for really large batch sizes without regressing.
## Additional context
https://gist.github.com/redknightlois/c4023d393eb8f92bb44b2ab582d7ec20
https://github.com/mgrankin/over9000
https://twitter.com/jeremyphoward/status/1162118545095852032
https://medium.com/@lessw/new-state-of-the-art-ai-optimizer-rectified-adam-radam-5d854730807b | 09-20-2019 13:00:54 | 09-20-2019 13:00:54 | From what I can tell, Radam makes automatic warmup and LARS is good but requires more calculations per batch. Before implementing it here it's worth do testing to tell if it's a good idea.<|||||>This issue has been automatically marked as stale because it has not had recent activity. It will be closed if no further activity occurs. Thank you for your contributions.
<|||||>any update?<|||||>For anyone interested in testing, I've created a fork that uses Radam+LARS+LookAhead, https://github.com/i404788/transformers<|||||>This issue has been automatically marked as stale because it has not had recent activity. It will be closed if no further activity occurs. Thank you for your contributions.
|
transformers | 1,301 | closed | RBERT implementation | As per #1250, this PR describes an additional classification head for BERT for relationship classification tasks. This work is originally documented in [this paper](https://arxiv.org/pdf/1905.08284.pdf). In addition, the new head can be used with RoBERTa, producing a new SOTA as far as I know....
I have included a new example script and associated utils file that demonstrate how it can be used:
```run_semeval.py```, and updated the README.md in ```examples``` accordingly.
Note, contrary to what I said in the original issue, there is no need for new tokenisation classes - rather, strings simply need to be preprocessed with entity delimiting characters prior to tokenisation, and the input ID's of these characters passed to the classification head (see included example for details) | 09-20-2019 11:39:13 | 09-20-2019 11:39:13 | # [Codecov](https://codecov.io/gh/huggingface/transformers/pull/1301?src=pr&el=h1) Report
> Merging [#1301](https://codecov.io/gh/huggingface/transformers/pull/1301?src=pr&el=desc) into [master](https://codecov.io/gh/huggingface/transformers/commit/2dc8cb87341223e86220516951bb4ad84f880b4a?src=pr&el=desc) will **increase** coverage by `0.22%`.
> The diff coverage is `96.35%`.
[](https://codecov.io/gh/huggingface/transformers/pull/1301?src=pr&el=tree)
```diff
@@ Coverage Diff @@
## master #1301 +/- ##
==========================================
+ Coverage 84.69% 84.91% +0.22%
==========================================
Files 84 85 +1
Lines 12596 12840 +244
==========================================
+ Hits 10668 10903 +235
- Misses 1928 1937 +9
```
| [Impacted Files](https://codecov.io/gh/huggingface/transformers/pull/1301?src=pr&el=tree) | Coverage Δ | |
|---|---|---|
| [transformers/tests/modeling\_bert\_test.py](https://codecov.io/gh/huggingface/transformers/pull/1301/diff?src=pr&el=tree#diff-dHJhbnNmb3JtZXJzL3Rlc3RzL21vZGVsaW5nX2JlcnRfdGVzdC5weQ==) | `97.47% <100%> (+1.09%)` | :arrow_up: |
| [transformers/configuration\_rbert.py](https://codecov.io/gh/huggingface/transformers/pull/1301/diff?src=pr&el=tree#diff-dHJhbnNmb3JtZXJzL2NvbmZpZ3VyYXRpb25fcmJlcnQucHk=) | `100% <100%> (ø)` | |
| [transformers/modeling\_roberta.py](https://codecov.io/gh/huggingface/transformers/pull/1301/diff?src=pr&el=tree#diff-dHJhbnNmb3JtZXJzL21vZGVsaW5nX3JvYmVydGEucHk=) | `74.54% <92.59%> (+3.32%)` | :arrow_up: |
| [transformers/tests/modeling\_roberta\_test.py](https://codecov.io/gh/huggingface/transformers/pull/1301/diff?src=pr&el=tree#diff-dHJhbnNmb3JtZXJzL3Rlc3RzL21vZGVsaW5nX3JvYmVydGFfdGVzdC5weQ==) | `85% <93.67%> (+5.49%)` | :arrow_up: |
| [transformers/modeling\_bert.py](https://codecov.io/gh/huggingface/transformers/pull/1301/diff?src=pr&el=tree#diff-dHJhbnNmb3JtZXJzL21vZGVsaW5nX2JlcnQucHk=) | `88.92% <96.15%> (+0.75%)` | :arrow_up: |
------
[Continue to review full report at Codecov](https://codecov.io/gh/huggingface/transformers/pull/1301?src=pr&el=continue).
> **Legend** - [Click here to learn more](https://docs.codecov.io/docs/codecov-delta)
> `Δ = absolute <relative> (impact)`, `ø = not affected`, `? = missing data`
> Powered by [Codecov](https://codecov.io/gh/huggingface/transformers/pull/1301?src=pr&el=footer). Last update [2dc8cb8...4bcfa63](https://codecov.io/gh/huggingface/transformers/pull/1301?src=pr&el=lastupdated). Read the [comment docs](https://docs.codecov.io/docs/pull-request-comments).
<|||||>Ok, I went through the PR.
This is a very nice work @RichJackson!
One thing we should simplify though is to not have a separate configuration for RBERT and roberta. I will update a bit the configuration classes so we can safely add new parameters in them and have them initialized to defaults values when loading from pretrained config.
Let me do that now in this PR.<|||||>Actually I can't push on your PR so I'll create a new one to update that. |
transformers | 1,300 | closed | ❓ Why the criterion of XLNet LMHeadModel use ignore_index = -1 ? | In the XLNetLMHeadModel, the criterion used to compute the loss uses `ignore_index=-1` :
https://github.com/huggingface/pytorch-transformers/blob/9f995b99d4c4067662c3bd4f1274315c0839deeb/pytorch_transformers/modeling_xlnet.py#L927-L931
**Why ?**
Isn't it supposed to ignore the padding index ID, i.e. 5 ? | 09-20-2019 07:53:20 | 09-20-2019 07:53:20 | you should set padding and all the labels to be ignored to -1. In Bert/XLNet training, we usually only use 15% of the tokens as labels. |
transformers | 1,299 | closed | What is the best CPU inference acceleration solution for BERT now? | Thank you very much.
Thank you very much.
Thank you very much. | 09-20-2019 02:50:55 | 09-20-2019 02:50:55 | Give us a little more details about your `(latency, compute)` constraints.<|||||>This issue has been automatically marked as stale because it has not had recent activity. It will be closed if no further activity occurs. Thank you for your contributions.
|
transformers | 1,298 | closed | fix annotation | 09-20-2019 02:09:26 | 09-20-2019 02:09:26 | # [Codecov](https://codecov.io/gh/huggingface/pytorch-transformers/pull/1298?src=pr&el=h1) Report
> Merging [#1298](https://codecov.io/gh/huggingface/pytorch-transformers/pull/1298?src=pr&el=desc) into [master](https://codecov.io/gh/huggingface/pytorch-transformers/commit/9f995b99d4c4067662c3bd4f1274315c0839deeb?src=pr&el=desc) will **not change** coverage.
> The diff coverage is `n/a`.
[](https://codecov.io/gh/huggingface/pytorch-transformers/pull/1298?src=pr&el=tree)
```diff
@@ Coverage Diff @@
## master #1298 +/- ##
=======================================
Coverage 80.77% 80.77%
=======================================
Files 57 57
Lines 8092 8092
=======================================
Hits 6536 6536
Misses 1556 1556
```
| [Impacted Files](https://codecov.io/gh/huggingface/pytorch-transformers/pull/1298?src=pr&el=tree) | Coverage Δ | |
|---|---|---|
| [pytorch\_transformers/tokenization\_xlnet.py](https://codecov.io/gh/huggingface/pytorch-transformers/pull/1298/diff?src=pr&el=tree#diff-cHl0b3JjaF90cmFuc2Zvcm1lcnMvdG9rZW5pemF0aW9uX3hsbmV0LnB5) | `89.18% <ø> (ø)` | :arrow_up: |
------
[Continue to review full report at Codecov](https://codecov.io/gh/huggingface/pytorch-transformers/pull/1298?src=pr&el=continue).
> **Legend** - [Click here to learn more](https://docs.codecov.io/docs/codecov-delta)
> `Δ = absolute <relative> (impact)`, `ø = not affected`, `? = missing data`
> Powered by [Codecov](https://codecov.io/gh/huggingface/pytorch-transformers/pull/1298?src=pr&el=footer). Last update [9f995b9...51decd5](https://codecov.io/gh/huggingface/pytorch-transformers/pull/1298?src=pr&el=lastupdated). Read the [comment docs](https://docs.codecov.io/docs/pull-request-comments).
<|||||>Thanks! |
|
transformers | 1,297 | closed | add support for file I/O | sometimes we need to process multiple prompts from a file and generate multiple sequences. also, writing results to a file would be less verbose and fast. | 09-19-2019 15:20:38 | 09-19-2019 15:20:38 | # [Codecov](https://codecov.io/gh/huggingface/pytorch-transformers/pull/1297?src=pr&el=h1) Report
> Merging [#1297](https://codecov.io/gh/huggingface/pytorch-transformers/pull/1297?src=pr&el=desc) into [master](https://codecov.io/gh/huggingface/pytorch-transformers/commit/0d1dad6d5323cf627cb8d7ddd428856ab8475f6b?src=pr&el=desc) will **not change** coverage.
> The diff coverage is `n/a`.
[](https://codecov.io/gh/huggingface/pytorch-transformers/pull/1297?src=pr&el=tree)
```diff
@@ Coverage Diff @@
## master #1297 +/- ##
=======================================
Coverage 80.77% 80.77%
=======================================
Files 57 57
Lines 8092 8092
=======================================
Hits 6536 6536
Misses 1556 1556
```
------
[Continue to review full report at Codecov](https://codecov.io/gh/huggingface/pytorch-transformers/pull/1297?src=pr&el=continue).
> **Legend** - [Click here to learn more](https://docs.codecov.io/docs/codecov-delta)
> `Δ = absolute <relative> (impact)`, `ø = not affected`, `? = missing data`
> Powered by [Codecov](https://codecov.io/gh/huggingface/pytorch-transformers/pull/1297?src=pr&el=footer). Last update [0d1dad6...2a11412](https://codecov.io/gh/huggingface/pytorch-transformers/pull/1297?src=pr&el=lastupdated). Read the [comment docs](https://docs.codecov.io/docs/pull-request-comments).
<|||||>Hi @rajarsheem ! Thank you for your PR. With the example scripts, we are really reaching for **simple scripts that showcase how the library works** and how it interacts with different elements of the Pytorch codebase (ex: distributed learning, gradient clipping, ...).
Using a text file as input may be useful in some cases, however, I don't feel like it really gives a deeper understanding of the library, as it is just a different way to obtain a context string. I don't think it would be particularly worth it in terms of the added complexity/deeper understanding of the lib ratio.
Please don't let that discourage you from opening other PRs. |
transformers | 1,296 | closed | Added ValueError for duplicates in list of added tokens | Very small addition to raise an error if the list of tokens passed to `add_tokens` contains duplicates. This otherwise raises cryptic errors down the line. Happy to update it to `Warning` if someone believes there's any reason for duplicates to be allowed here. | 09-19-2019 14:46:32 | 09-19-2019 14:46:32 | # [Codecov](https://codecov.io/gh/huggingface/transformers/pull/1296?src=pr&el=h1) Report
> Merging [#1296](https://codecov.io/gh/huggingface/transformers/pull/1296?src=pr&el=desc) into [master](https://codecov.io/gh/huggingface/transformers/commit/391db836ab7ed2ca61c51a7cf1b135b6ab92be58?src=pr&el=desc) will **not change** coverage.
> The diff coverage is `n/a`.
[](https://codecov.io/gh/huggingface/transformers/pull/1296?src=pr&el=tree)
```diff
@@ Coverage Diff @@
## master #1296 +/- ##
=======================================
Coverage 84.72% 84.72%
=======================================
Files 84 84
Lines 12591 12591
=======================================
Hits 10668 10668
Misses 1923 1923
```
| [Impacted Files](https://codecov.io/gh/huggingface/transformers/pull/1296?src=pr&el=tree) | Coverage Δ | |
|---|---|---|
| [transformers/tokenization\_utils.py](https://codecov.io/gh/huggingface/transformers/pull/1296/diff?src=pr&el=tree#diff-dHJhbnNmb3JtZXJzL3Rva2VuaXphdGlvbl91dGlscy5weQ==) | `91.48% <ø> (ø)` | :arrow_up: |
------
[Continue to review full report at Codecov](https://codecov.io/gh/huggingface/transformers/pull/1296?src=pr&el=continue).
> **Legend** - [Click here to learn more](https://docs.codecov.io/docs/codecov-delta)
> `Δ = absolute <relative> (impact)`, `ø = not affected`, `? = missing data`
> Powered by [Codecov](https://codecov.io/gh/huggingface/transformers/pull/1296?src=pr&el=footer). Last update [391db83...a951585](https://codecov.io/gh/huggingface/transformers/pull/1296?src=pr&el=lastupdated). Read the [comment docs](https://docs.codecov.io/docs/pull-request-comments).
<|||||>Ok, great, thanks @danai-antoniou! |
transformers | 1,295 | closed | Where are BERT's pretrained Embeddings loaded? | I am trying to better understand the difference between the different types of embeddings that BERT uses (from the BERT paper: token, segment, position). For this purpose, I was hoping to put some print statement in the `pytorch_transformers` source code to see how the IDs change into vector representations for each type of embedding.
First of all I am confused about the embeddings that `pytorch_transformers` uses. Going through the source code for [`BertEmbeddings`](https://github.com/huggingface/pytorch-transformers/blob/master/pytorch_transformers/modeling_bert.py#L142-L171) I can see
- word embeddings
- position embeddings
- token type embeddings
What are these _token type_ embeddings? Are they the same as segment embeddings?
Secondly, during my quest for better understanding what's going on, I couldn't figure out where the pretrained embedding models are loaded, or even where they are downloaded. I am curious to see the vocab list of all types of embeddings, but I couldn't find them anywhere.
Any pointers? | 09-19-2019 12:48:50 | 09-19-2019 12:48:50 | - "token type embeddings" are the BERT paper's segment embeddings
- embeddings are inside the pretrained weights<|||||>Ah that makes sense. So there are no "separate" word2vec-style pretrained embedding models for the different types of embeddings which one could load with `nn.Embedding().from_pretrained`. Rather, they are loaded in a bunch as a set of pretrained weights. Theoretically, though, one could extract the weights for each embedding, and extract the vocab from the tokenizer, and create a simple lookup (`token\tvector`)?
Thanks for the reply and your work.<|||||>Sure you could, but I suspect it wouldn’t work too well.
You could say that a large language model’s hidden states are the new way to do word/sentence embeddings (see Sebastian Ruder’s imageNet moment).<|||||>Apologies if this is taking too much of your time, but I have a follow-up question. Why wouldn't it work too well? I understand that they are not typical word2vec word representations, since they have been trained together with the whole language model, but why would extracting the embeddings and using them in another task not work well? In other words, what makes the token embeddings of BERT fundamentally different from a typical word2vec model?<|||||>I think you'll find this repo (and associated EMNLP 2019 paper) by @nriemers interesting:
https://github.com/UKPLab/sentence-transformers (built on top of `transformers`)<|||||>> * "token type embeddings" are the BERT paper's segment embeddings
> * embeddings are inside the pretrained weights
hi, could you tell where the code about BertEmbedding loaded with the pre-trained weights is? |
transformers | 1,294 | closed | Delete n_special reference in docstring | I don't think the `n_special` param is used, even in `**kwargs`. | 09-19-2019 08:37:27 | 09-19-2019 08:37:27 | # [Codecov](https://codecov.io/gh/huggingface/pytorch-transformers/pull/1294?src=pr&el=h1) Report
> Merging [#1294](https://codecov.io/gh/huggingface/pytorch-transformers/pull/1294?src=pr&el=desc) into [master](https://codecov.io/gh/huggingface/pytorch-transformers/commit/0d1dad6d5323cf627cb8d7ddd428856ab8475f6b?src=pr&el=desc) will **not change** coverage.
> The diff coverage is `n/a`.
[](https://codecov.io/gh/huggingface/pytorch-transformers/pull/1294?src=pr&el=tree)
```diff
@@ Coverage Diff @@
## master #1294 +/- ##
=======================================
Coverage 80.77% 80.77%
=======================================
Files 57 57
Lines 8092 8092
=======================================
Hits 6536 6536
Misses 1556 1556
```
| [Impacted Files](https://codecov.io/gh/huggingface/pytorch-transformers/pull/1294?src=pr&el=tree) | Coverage Δ | |
|---|---|---|
| [pytorch\_transformers/configuration\_openai.py](https://codecov.io/gh/huggingface/pytorch-transformers/pull/1294/diff?src=pr&el=tree#diff-cHl0b3JjaF90cmFuc2Zvcm1lcnMvY29uZmlndXJhdGlvbl9vcGVuYWkucHk=) | `89.13% <ø> (ø)` | :arrow_up: |
------
[Continue to review full report at Codecov](https://codecov.io/gh/huggingface/pytorch-transformers/pull/1294?src=pr&el=continue).
> **Legend** - [Click here to learn more](https://docs.codecov.io/docs/codecov-delta)
> `Δ = absolute <relative> (impact)`, `ø = not affected`, `? = missing data`
> Powered by [Codecov](https://codecov.io/gh/huggingface/pytorch-transformers/pull/1294?src=pr&el=footer). Last update [0d1dad6...119610b](https://codecov.io/gh/huggingface/pytorch-transformers/pull/1294?src=pr&el=lastupdated). Read the [comment docs](https://docs.codecov.io/docs/pull-request-comments).
<|||||>Indeed, thanks Sam |
transformers | 1,293 | closed | cannot import name 'XLNetForMultipleChoice' but python can import | ## 🐛 Bug
<!-- Important information -->
Model I am using (Bert):
Language I am using the model on (English):
when I use the following command to run run_multiple_choice.py, like:
'''
python examples/run_multiple_choice.py --model_type bert --task_name race --model_name_or_path bert_large --do_train --do_eval --do_lower_case --data_dir $RACE_DIR --learning_rate 5e-5 --num_train_epochs 3 --max_seq_length 80 --output_dir models_bert/race_base --per_gpu_eval_batch_size=16 --per_gpu_train_batch_size=16 --gradient_accumulation_steps 2 --overwrite_output
'''
it gives the error information:

but when I in my python environment to import the package , it has no problem!
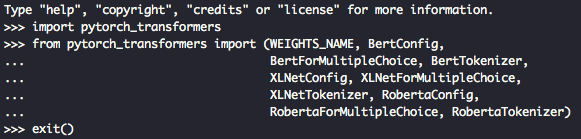
what's wrong with the run_multiple_choice.py?
## Environment
* OS:
* Python version: 3.6.2
* PyTorch version: 1.1.0
* PyTorch Transformers version (or branch): 1.2.0
* Using GPU ? yes
* Distributed of parallel setup ?
* Any other relevant information:
## Additional context
<!-- Add any other context about the problem here. --> | 09-19-2019 06:48:40 | 09-19-2019 06:48:40 | I found maybe the current code is not consistent with the pip package pytorch_transformers, so when use the pip package it does't work, but when just run the code without the pip package, it can work, but you need change some path to make the code work correctly!<|||||>Hi, I believe this was fixed with @VictorSanh's commit ae50ad9 |
transformers | 1,292 | closed | Fine Tuning GPT2 on wikitext-103-raw | ## ❓ Questions & Help
<!-- A clear and concise description of the question. -->
Running pytorch-transformers\examples\run_lm_finetuning.py. This is stuck at load_and_cache_examples step. I just see message like
WARNING - pytorch_transformers.tokenization_utils - This tokenizer does not make use of special tokens. The sequence has been returned with no modification.
The train file has 1.8M rows with this rate it would take few days to just tokenize and cache training data.
Is this expected? Did anyone face this before?
Thanks in advance for your help. | 09-19-2019 06:00:03 | 09-19-2019 06:00:03 | @snaik2016 I ran into the same issue and had to parallelize my code to make it faster. Also getting rid of the while loop and list splicing in the TextDataset class with a for loop made it much quicker.<|||||>Please check #1830 . I made some tuning on a training part. But I guess it'll still take many days for 1.8M rows dataset (in fact, talking about the token count rather than rows count is more meaningful) .<|||||>This issue has been automatically marked as stale because it has not had recent activity. It will be closed if no further activity occurs. Thank you for your contributions.
|
transformers | 1,291 | closed | traced_model | when I ran: traced_model = torch.jit.trace(model, (input_ids,))
I got:
/home/jhy/py3.6/lib/python3.6/site-packages/torch/tensor.py:389: RuntimeWarning: Iterating over a tensor might cause the trace to be incorrect. Passing a tensor of different shape won't change the number of iterations executed (and might lead to errors or silently give incorrect results).
'incorrect results).', category=RuntimeWarning)
Traceback (most recent call last):
File "/home/jhy/project/xlnet/src/xlnet_test.py", line 13, in <module>
traced_model = torch.jit.trace(model, (input_ids,))
File "/home/jhy/py3.6/lib/python3.6/site-packages/torch/jit/__init__.py", line 772, in trace
check_tolerance, _force_outplace, _module_class)
File "/home/jhy/py3.6/lib/python3.6/site-packages/torch/jit/__init__.py", line 904, in trace_module
module._c._create_method_from_trace(method_name, func, example_inputs, var_lookup_fn, _force_outplace)
RuntimeError: Tracer cannot infer type of (tensor([[[-0.9993, 0.2632, -0.6305, ..., -0.3520, -1.2041, -1.5944],
[ 4.5358, 2.6032, -1.4790, ..., 2.1211, 1.6621, -0.9913],
[ 2.0586, 2.1398, 0.6811, ..., 1.9191, 0.0836, -1.2848],
...,
[-1.4818, 0.5329, 0.5212, ..., 0.6176, 1.7843, -1.8773],
[-2.8784, 1.9871, 0.5379, ..., 1.3778, 1.0554, -1.3039],
[-4.1723, 1.3071, 0.6565, ..., 1.2515, 1.6618, -0.8640]]],
grad_fn=<PermuteBackward>), (None, None, None, None, None, None, None, None, None, None, None, None, None, None, None, None, None, None, None, None, None, None, None, None))
:Cannot infer type of a None value (toTraceableIValue at /pytorch/torch/csrc/jit/pybind_utils.h:268)
frame #0: c10::Error::Error(c10::SourceLocation, std::string const&) + 0x33 (0x7f8ea599c273 in /home/jhy/py3.6/lib/python3.6/site-packages/torch/lib/libc10.so)
frame #1: <unknown function> + 0x44e288 (0x7f8ea69db288 in /home/jhy/py3.6/lib/python3.6/site-packages/torch/lib/libtorch_python.so)
frame #2: <unknown function> + 0x4bdda2 (0x7f8ea6a4ada2 in /home/jhy/py3.6/lib/python3.6/site-packages/torch/lib/libtorch_python.so)
frame #3: <unknown function> + 0x4d1d81 (0x7f8ea6a5ed81 in /home/jhy/py3.6/lib/python3.6/site-packages/torch/lib/libtorch_python.so)
frame #4: <unknown function> + 0x1d3ef4 (0x7f8ea6760ef4 in /home/jhy/py3.6/lib/python3.6/site-packages/torch/lib/libtorch_python.so)
frame #5: _PyCFunction_FastCallDict + 0x288 (0x566ad8 in /home/jhy/py3.6/bin/python)
frame #6: /home/jhy/py3.6/bin/python() [0x5067b0]
frame #7: _PyEval_EvalFrameDefault + 0x4de (0x50729e in /home/jhy/py3.6/bin/python)
frame #8: /home/jhy/py3.6/bin/python() [0x504232]
frame #9: /home/jhy/py3.6/bin/python() [0x505e83]
frame #10: /home/jhy/py3.6/bin/python() [0x5066f0]
frame #11: _PyEval_EvalFrameDefault + 0x4de (0x50729e in /home/jhy/py3.6/bin/python)
frame #12: /home/jhy/py3.6/bin/python() [0x504232]
frame #13: /home/jhy/py3.6/bin/python() [0x505e83]
frame #14: /home/jhy/py3.6/bin/python() [0x5066f0]
frame #15: _PyEval_EvalFrameDefault + 0x4de (0x50729e in /home/jhy/py3.6/bin/python)
frame #16: /home/jhy/py3.6/bin/python() [0x504232]
frame #17: PyEval_EvalCode + 0x23 (0x6022e3 in /home/jhy/py3.6/bin/python)
frame #18: /home/jhy/py3.6/bin/python() [0x647fa2]
frame #19: PyRun_FileExFlags + 0x9a (0x64806a in /home/jhy/py3.6/bin/python)
frame #20: PyRun_SimpleFileExFlags + 0x197 (0x649d97 in /home/jhy/py3.6/bin/python)
frame #21: Py_Main + 0x5c2 (0x63c352 in /home/jhy/py3.6/bin/python)
frame #22: main + 0xe9 (0x4dbcb9 in /home/jhy/py3.6/bin/python)
frame #23: __libc_start_main + 0xf0 (0x7f8eabcff830 in /lib/x86_64-linux-gnu/libc.so.6)
frame #24: _start + 0x29 (0x5cb639 in /home/jhy/py3.6/bin/python)
| 09-19-2019 01:12:59 | 09-19-2019 01:12:59 | Which model did you use?<|||||>> Which model did you use?
xlnet<|||||>Hi! Could you show the inputs you use to trace your model?<|||||>This issue has been automatically marked as stale because it has not had recent activity. It will be closed if no further activity occurs. Thank you for your contributions.
|
transformers | 1,290 | closed | MemoryError on run_lm_finetuning.py | Previous versions of finetune_on_pregenerated.py had a `--reduce_memory` parameter to keep memory requirements from going overboard, it seems like it is no longer available in the new run_lm_finetuning.py file? | 09-18-2019 22:05:45 | 09-18-2019 22:05:45 | This issue has been automatically marked as stale because it has not had recent activity. It will be closed if no further activity occurs. Thank you for your contributions.
<|||||>I also have the same problem.... |
Subsets and Splits
No community queries yet
The top public SQL queries from the community will appear here once available.