hexsha
stringlengths 40
40
| size
int64 6
14.9M
| ext
stringclasses 1
value | lang
stringclasses 1
value | max_stars_repo_path
stringlengths 6
260
| max_stars_repo_name
stringlengths 6
119
| max_stars_repo_head_hexsha
stringlengths 40
41
| max_stars_repo_licenses
sequence | max_stars_count
int64 1
191k
⌀ | max_stars_repo_stars_event_min_datetime
stringlengths 24
24
⌀ | max_stars_repo_stars_event_max_datetime
stringlengths 24
24
⌀ | max_issues_repo_path
stringlengths 6
260
| max_issues_repo_name
stringlengths 6
119
| max_issues_repo_head_hexsha
stringlengths 40
41
| max_issues_repo_licenses
sequence | max_issues_count
int64 1
67k
⌀ | max_issues_repo_issues_event_min_datetime
stringlengths 24
24
⌀ | max_issues_repo_issues_event_max_datetime
stringlengths 24
24
⌀ | max_forks_repo_path
stringlengths 6
260
| max_forks_repo_name
stringlengths 6
119
| max_forks_repo_head_hexsha
stringlengths 40
41
| max_forks_repo_licenses
sequence | max_forks_count
int64 1
105k
⌀ | max_forks_repo_forks_event_min_datetime
stringlengths 24
24
⌀ | max_forks_repo_forks_event_max_datetime
stringlengths 24
24
⌀ | avg_line_length
float64 2
1.04M
| max_line_length
int64 2
11.2M
| alphanum_fraction
float64 0
1
| cells
sequence | cell_types
sequence | cell_type_groups
sequence |
---|---|---|---|---|---|---|---|---|---|---|---|---|---|---|---|---|---|---|---|---|---|---|---|---|---|---|---|---|---|---|
e7648d6c26bc6ab7bd6f49b7e1122f787b502f6d | 22,532 | ipynb | Jupyter Notebook | Python/DeepLearning/python/01_sample1_tf/sample/chap05/05_08/keras/SaveModelAndWeights_Keras.ipynb | DongriPocket/study | f9d50f34102c5cf2b5391a9bb8c099bac4248e35 | [
"MIT"
] | null | null | null | Python/DeepLearning/python/01_sample1_tf/sample/chap05/05_08/keras/SaveModelAndWeights_Keras.ipynb | DongriPocket/study | f9d50f34102c5cf2b5391a9bb8c099bac4248e35 | [
"MIT"
] | null | null | null | Python/DeepLearning/python/01_sample1_tf/sample/chap05/05_08/keras/SaveModelAndWeights_Keras.ipynb | DongriPocket/study | f9d50f34102c5cf2b5391a9bb8c099bac4248e35 | [
"MIT"
] | null | null | null | 56.049751 | 148 | 0.495961 | [
[
[
"'''\n1. データセットの読み込みと正規化\n'''\n# tensorflow.keras のインポート\nfrom tensorflow import keras\n\n# Fashion-MNISTデータセットの読み込み\n(x_train, t_train), (x_test, t_test) = keras.datasets.fashion_mnist.load_data()\n\n# 訓練データを正規化\nx_train = x_train / 255\n# テストデータを正規化\nx_test = x_test / 255",
"_____no_output_____"
],
[
"'''\n2.モデルの生成\n'''\n# モデルオブジェクトを生成\nmodel = keras.Sequential([\n # 入力するテンソルの形状を(28, 28)から(784)にフラット化する\n keras.layers.Flatten(input_shape=(28, 28)),\n # 隠れ層のユニット数:256\n # 活性化関数:ReLU\n keras.layers.Dense(256, activation='relu'),\n # 出力層のニューロン数:10\n # 活性化関数:ソフトマックス \n keras.layers.Dense(10, activation='softmax')\n])\n\n# 学習率\nlearning_rate = 0.1 \n# モデルのコンパイル\nmodel.compile(\n # 損失関数はスパースラベル対応クロスエントロピー誤差\n loss='sparse_categorical_crossentropy',\n # オプティマイザーはAdam\n optimizer=keras.optimizers.Adam(learning_rate=0.001),\n # 学習評価として正解率を指定\n metrics=['accuracy']\n )\n\nmodel.summary() # モデルのサマリー(概要)を出力",
"Model: \"sequential\"\n_________________________________________________________________\nLayer (type) Output Shape Param # \n=================================================================\nflatten (Flatten) (None, 784) 0 \n_________________________________________________________________\ndense (Dense) (None, 256) 200960 \n_________________________________________________________________\ndense_1 (Dense) (None, 10) 2570 \n=================================================================\nTotal params: 203,530\nTrainable params: 203,530\nNon-trainable params: 0\n_________________________________________________________________\n"
],
[
"%%time\n'''\n3. モデルの学習\n'''\nepoch = 100\n# ミニバッチのサイズ\nbatch_size = 64\n\nhistory = model.fit(\n x_train, # 訓練データ\n t_train, # 正解ラベル\n epochs=epoch, # エポック数を設定\n batch_size=batch_size, # ミニバッチのサイズを設定\n verbose=1, # 進捗状況を出力\n validation_split=0.2 # 20パーセントのデータを検証に使用\n )",
"Train on 48000 samples, validate on 12000 samples\nEpoch 1/100\n48000/48000 [==============================] - 3s 69us/sample - loss: 0.5225 - accuracy: 0.8196 - val_loss: 0.4493 - val_accuracy: 0.8384\nEpoch 2/100\n48000/48000 [==============================] - 3s 57us/sample - loss: 0.3866 - accuracy: 0.8615 - val_loss: 0.3729 - val_accuracy: 0.8646\nEpoch 3/100\n48000/48000 [==============================] - 3s 57us/sample - loss: 0.3489 - accuracy: 0.8723 - val_loss: 0.3472 - val_accuracy: 0.8768\nEpoch 4/100\n48000/48000 [==============================] - 3s 60us/sample - loss: 0.3213 - accuracy: 0.8838 - val_loss: 0.3457 - val_accuracy: 0.8753\nEpoch 5/100\n48000/48000 [==============================] - 3s 60us/sample - loss: 0.2985 - accuracy: 0.8908 - val_loss: 0.3340 - val_accuracy: 0.8803\nEpoch 6/100\n48000/48000 [==============================] - 3s 57us/sample - loss: 0.2828 - accuracy: 0.8955 - val_loss: 0.3280 - val_accuracy: 0.8842\nEpoch 7/100\n48000/48000 [==============================] - 3s 58us/sample - loss: 0.2710 - accuracy: 0.8993 - val_loss: 0.3212 - val_accuracy: 0.8832\nEpoch 8/100\n48000/48000 [==============================] - 3s 61us/sample - loss: 0.2574 - accuracy: 0.9040 - val_loss: 0.3068 - val_accuracy: 0.8904\nEpoch 9/100\n48000/48000 [==============================] - 3s 61us/sample - loss: 0.2448 - accuracy: 0.9097 - val_loss: 0.3187 - val_accuracy: 0.8874\nEpoch 10/100\n48000/48000 [==============================] - 3s 58us/sample - loss: 0.2373 - accuracy: 0.9121 - val_loss: 0.3225 - val_accuracy: 0.8838\nEpoch 11/100\n48000/48000 [==============================] - 3s 64us/sample - loss: 0.2267 - accuracy: 0.9157 - val_loss: 0.3161 - val_accuracy: 0.8907\nEpoch 12/100\n48000/48000 [==============================] - 3s 60us/sample - loss: 0.2183 - accuracy: 0.9185 - val_loss: 0.3175 - val_accuracy: 0.8882\nEpoch 13/100\n48000/48000 [==============================] - 3s 63us/sample - loss: 0.2128 - accuracy: 0.9200 - val_loss: 0.3283 - val_accuracy: 0.8857\nEpoch 14/100\n48000/48000 [==============================] - 3s 58us/sample - loss: 0.2026 - accuracy: 0.9240 - val_loss: 0.3047 - val_accuracy: 0.8933\nEpoch 15/100\n48000/48000 [==============================] - 3s 58us/sample - loss: 0.1956 - accuracy: 0.9268 - val_loss: 0.3186 - val_accuracy: 0.8906\nEpoch 16/100\n48000/48000 [==============================] - 3s 58us/sample - loss: 0.1878 - accuracy: 0.9310 - val_loss: 0.3176 - val_accuracy: 0.8932\nEpoch 17/100\n48000/48000 [==============================] - 3s 60us/sample - loss: 0.1846 - accuracy: 0.9304 - val_loss: 0.3595 - val_accuracy: 0.8840\nEpoch 18/100\n48000/48000 [==============================] - 3s 58us/sample - loss: 0.1773 - accuracy: 0.9336 - val_loss: 0.3187 - val_accuracy: 0.8928\nEpoch 19/100\n48000/48000 [==============================] - 3s 57us/sample - loss: 0.1704 - accuracy: 0.9361 - val_loss: 0.3304 - val_accuracy: 0.8930\nEpoch 20/100\n48000/48000 [==============================] - 3s 65us/sample - loss: 0.1654 - accuracy: 0.9388 - val_loss: 0.3245 - val_accuracy: 0.8933\nEpoch 21/100\n48000/48000 [==============================] - 3s 62us/sample - loss: 0.1586 - accuracy: 0.9413 - val_loss: 0.3344 - val_accuracy: 0.8982\nEpoch 22/100\n48000/48000 [==============================] - 3s 66us/sample - loss: 0.1555 - accuracy: 0.9432 - val_loss: 0.3436 - val_accuracy: 0.8902\nEpoch 23/100\n48000/48000 [==============================] - 3s 64us/sample - loss: 0.1497 - accuracy: 0.9439 - val_loss: 0.3504 - val_accuracy: 0.8912\nEpoch 24/100\n48000/48000 [==============================] - 3s 61us/sample - loss: 0.1440 - accuracy: 0.9462 - val_loss: 0.3541 - val_accuracy: 0.8917\nEpoch 25/100\n48000/48000 [==============================] - 3s 59us/sample - loss: 0.1414 - accuracy: 0.9471 - val_loss: 0.3552 - val_accuracy: 0.8880\nEpoch 26/100\n48000/48000 [==============================] - 3s 57us/sample - loss: 0.1376 - accuracy: 0.9485 - val_loss: 0.3611 - val_accuracy: 0.8892\nEpoch 27/100\n48000/48000 [==============================] - 3s 59us/sample - loss: 0.1341 - accuracy: 0.9496 - val_loss: 0.3492 - val_accuracy: 0.8972\nEpoch 28/100\n48000/48000 [==============================] - 4s 74us/sample - loss: 0.1271 - accuracy: 0.9532 - val_loss: 0.3735 - val_accuracy: 0.8928\nEpoch 29/100\n48000/48000 [==============================] - 3s 64us/sample - loss: 0.1221 - accuracy: 0.9547 - val_loss: 0.3925 - val_accuracy: 0.8909\nEpoch 30/100\n48000/48000 [==============================] - 3s 57us/sample - loss: 0.1235 - accuracy: 0.9540 - val_loss: 0.3851 - val_accuracy: 0.8892\nEpoch 31/100\n48000/48000 [==============================] - 3s 60us/sample - loss: 0.1168 - accuracy: 0.9569 - val_loss: 0.3671 - val_accuracy: 0.8953\nEpoch 32/100\n48000/48000 [==============================] - 3s 60us/sample - loss: 0.1147 - accuracy: 0.9574 - val_loss: 0.3761 - val_accuracy: 0.8969\nEpoch 33/100\n48000/48000 [==============================] - 3s 66us/sample - loss: 0.1107 - accuracy: 0.9588 - val_loss: 0.4050 - val_accuracy: 0.8912\nEpoch 34/100\n48000/48000 [==============================] - 3s 61us/sample - loss: 0.1091 - accuracy: 0.9605 - val_loss: 0.3798 - val_accuracy: 0.8937\nEpoch 35/100\n48000/48000 [==============================] - 3s 68us/sample - loss: 0.1052 - accuracy: 0.9613 - val_loss: 0.4008 - val_accuracy: 0.8893\nEpoch 36/100\n48000/48000 [==============================] - 3s 60us/sample - loss: 0.1025 - accuracy: 0.9621 - val_loss: 0.3919 - val_accuracy: 0.8942\nEpoch 37/100\n48000/48000 [==============================] - 3s 64us/sample - loss: 0.1006 - accuracy: 0.9619 - val_loss: 0.4097 - val_accuracy: 0.8922\nEpoch 38/100\n48000/48000 [==============================] - 3s 65us/sample - loss: 0.0966 - accuracy: 0.9642 - val_loss: 0.4126 - val_accuracy: 0.8933\nEpoch 39/100\n48000/48000 [==============================] - 3s 58us/sample - loss: 0.0960 - accuracy: 0.9646 - val_loss: 0.4178 - val_accuracy: 0.8923\nEpoch 40/100\n48000/48000 [==============================] - 3s 58us/sample - loss: 0.0910 - accuracy: 0.9654 - val_loss: 0.4257 - val_accuracy: 0.8950\nEpoch 41/100\n48000/48000 [==============================] - 3s 58us/sample - loss: 0.0911 - accuracy: 0.9667 - val_loss: 0.4437 - val_accuracy: 0.8898\nEpoch 42/100\n48000/48000 [==============================] - 3s 59us/sample - loss: 0.0929 - accuracy: 0.9656 - val_loss: 0.4364 - val_accuracy: 0.8907\nEpoch 43/100\n48000/48000 [==============================] - 3s 60us/sample - loss: 0.0840 - accuracy: 0.9698 - val_loss: 0.4295 - val_accuracy: 0.8949\nEpoch 44/100\n48000/48000 [==============================] - 3s 61us/sample - loss: 0.0859 - accuracy: 0.9679 - val_loss: 0.4542 - val_accuracy: 0.8927\nEpoch 45/100\n48000/48000 [==============================] - 3s 60us/sample - loss: 0.0813 - accuracy: 0.9700 - val_loss: 0.4451 - val_accuracy: 0.8943\nEpoch 46/100\n48000/48000 [==============================] - 3s 60us/sample - loss: 0.0840 - accuracy: 0.9680 - val_loss: 0.4926 - val_accuracy: 0.8901\nEpoch 47/100\n48000/48000 [==============================] - 3s 59us/sample - loss: 0.0791 - accuracy: 0.9704 - val_loss: 0.4431 - val_accuracy: 0.8960\nEpoch 48/100\n48000/48000 [==============================] - 3s 60us/sample - loss: 0.0803 - accuracy: 0.9702 - val_loss: 0.4448 - val_accuracy: 0.8935\nEpoch 49/100\n48000/48000 [==============================] - 3s 66us/sample - loss: 0.0744 - accuracy: 0.9728 - val_loss: 0.4530 - val_accuracy: 0.8972\nEpoch 50/100\n48000/48000 [==============================] - 3s 66us/sample - loss: 0.0716 - accuracy: 0.9736 - val_loss: 0.4719 - val_accuracy: 0.8922\nEpoch 51/100\n48000/48000 [==============================] - 3s 62us/sample - loss: 0.0671 - accuracy: 0.9754 - val_loss: 0.4800 - val_accuracy: 0.8946\nEpoch 52/100\n48000/48000 [==============================] - 4s 79us/sample - loss: 0.0722 - accuracy: 0.9731 - val_loss: 0.4659 - val_accuracy: 0.8923\nEpoch 53/100\n48000/48000 [==============================] - 3s 61us/sample - loss: 0.0711 - accuracy: 0.9746 - val_loss: 0.4678 - val_accuracy: 0.8949\nEpoch 54/100\n48000/48000 [==============================] - 3s 59us/sample - loss: 0.0681 - accuracy: 0.9756 - val_loss: 0.4999 - val_accuracy: 0.8930\n"
],
[
"# モデルとパラメーターの値を保存\n# モデルをmodel.jasonとして保存\nwith open('model.json', 'w') as json_file:\n json_file.write(model.to_json())\n# パラメーターをweight.h5として保存\nmodel.save_weights('weight.h5')",
"_____no_output_____"
],
[
"from tensorflow.keras.models import model_from_json\n\n# モデルの読み込み\nmodel_r = model_from_json(open('model.json', 'r').read())\n\n# 復元したモデルのコンパイル\nmodel_r.compile(loss='sparse_categorical_crossentropy',\n optimizer=keras.optimizers.Adam(learning_rate=0.001),\n metrics=['accuracy']\n )\n# 重みの読み込み\nmodel_r.load_weights('weight.h5')",
"_____no_output_____"
],
[
"# テストデータで保存済みのモデルを評価\ntest_loss, test_acc = model_r.evaluate(x_test, t_test, verbose=1)",
"10000/10000 [==============================] - 0s 47us/sample - loss: 0.8120 - accuracy: 0.8853\n"
]
]
] | [
"code"
] | [
[
"code",
"code",
"code",
"code",
"code",
"code"
]
] |
e7649d876d0de2de721cc81805a9e855371edea7 | 8,835 | ipynb | Jupyter Notebook | Ch5/01_KPE.ipynb | Samyak2/practical-nlp | 15b9b278e958f1b98f21702b6889861747d41eeb | [
"MIT"
] | null | null | null | Ch5/01_KPE.ipynb | Samyak2/practical-nlp | 15b9b278e958f1b98f21702b6889861747d41eeb | [
"MIT"
] | null | null | null | Ch5/01_KPE.ipynb | Samyak2/practical-nlp | 15b9b278e958f1b98f21702b6889861747d41eeb | [
"MIT"
] | null | null | null | 52.589286 | 3,667 | 0.693718 | [
[
[
"#We need texacy, which inturn loads spacy library\n#!pip install textacy==0.9.1\n\nimport spacy\nimport textacy.ke\nfrom textacy import *\n",
"_____no_output_____"
],
[
"#Load a spacy model, which will be used for all further processing.\nen = textacy.load_spacy_lang(\"en_core_web_sm\")\n\n#Let us use a sample text file, nlphistory.txt, which is the text from the history section of Wikipedia's\n#page on Natural Language Processing \n#https://en.wikipedia.org/wiki/Natural_language_processing\nmytext = open('nlphistory.txt').read()\n\n#convert the text into a spacy document.\ndoc = textacy.make_spacy_doc(mytext, lang=en)",
"_____no_output_____"
],
[
"textacy.ke.textrank(doc, topn=5)",
"_____no_output_____"
],
[
"#Print the keywords using TextRank algorithm, as implemented in Textacy.\nprint(\"Textrank output: \", [kps for kps, weights in textacy.ke.textrank(doc, normalize=\"lemma\", topn=5)])\\\n#Print the key words and phrases, using SGRank algorithm, as implemented in Textacy\nprint(\"SGRank output: \", [kps for kps, weights in textacy.ke.sgrank(doc, topn=5)])\\\n",
"Textrank output: ['successful natural language processing system', 'statistical machine translation system', 'natural language system', 'statistical natural language processing', 'natural language task']\nSGRank output: ['natural language processing system', 'statistical machine translation', 'research', 'late 1980', 'early']\n"
],
[
"#TODO: More examples on using SGRank parameter options to show variants.\n",
"_____no_output_____"
],
[
"#To address the issue of overlapping key phrases, textacy has a function: aggregage_term_variants.\n#Choosing one of the grouped terms per item will give us a list of non-overlapping key phrases!\nterms = set([term for term,weight in textacy.ke.sgrank(doc)])\nprint(textacy.ke.utils.aggregate_term_variants(terms))",
"[{'natural language processing system'}, {'statistical machine translation'}, {'statistical model'}, {'late 1980'}, {'research'}, {'example'}, {'early'}, {'ELIZA'}, {'world'}, {'real'}]\n"
],
[
"#A way to look at key phrases is just consider all noun chunks as potential ones. \n#However, keep in mind this will result in a lot of phrases, and no way to rank them!\n\nprint([chunk for chunk in textacy.extract.noun_chunks(doc)])",
"[history, natural language processing, 1950s, work, earlier periods, Alan Turing, article, what, criterion, intelligence, Georgetown experiment, fully automatic translation, more than sixty Russian sentences, English, authors, three or five years, machine translation, solved problem.[2, real progress, ALPAC report, ten-year-long research, expectations, machine translation, Little further research, machine translation, late 1980s, first statistical machine translation systems, notably successful natural language processing systems, 1960s, SHRDLU, natural language system, restricted \"blocks worlds, restricted vocabularies, ELIZA, simulation, Rogerian psychotherapist, Joseph Weizenbaum, almost no information, human thought, emotion, ELIZA, startlingly human-like interaction, \"patient, very small knowledge base, ELIZA, generic response, example, \"My head, you, head, 1970s, many programmers, \"conceptual ontologies, real-world information, computer-understandable data, Examples, MARGIE, (Schank, SAM, (Cullingford, PAM, (Wilensky, TaleSpin, (Meehan, QUALM, (Lehnert, Politics, (Carbonell, Plot Units, Lehnert, time, many chatterbots, PARRY, Racter, Jabberwacky, 1980s, most natural language processing systems, complex sets, hand-written rules, late 1980s, revolution, natural language processing, introduction, algorithms, language processing, the steady increase, computational power, Moore's law, gradual lessening, dominance, Chomskyan theories, linguistics, e.g. transformational grammar, theoretical underpinnings, sort, corpus linguistics, machine-learning approach, language, earliest-used machine learning algorithms, decision trees, produced systems, existing hand-written rules, speech, use, hidden Markov models, natural language processing, research, statistical models, soft, probabilistic decisions, real-valued weights, features, input data, cache language models, many speech recognition systems, examples, such statistical models, Such models, unfamiliar input, errors, real-world data, more reliable results, larger system, multiple subtasks, notable early successes, field, machine translation, IBM Research, more complicated statistical models, systems, advantage, existing multilingual textual corpora, Parliament, Canada, European Union, result, laws, translation, governmental proceedings, official languages, corresponding systems, government, However, most other systems, corpora, tasks, systems, major limitation, success, systems, result, great deal, research, methods, limited amounts, data, Recent research, unsupervised and semi-supervised learning algorithms, Such algorithms, data, desired answers, combination, annotated and non-annotated data, task, supervised learning, less accurate results, given amount, input data, enormous amount, non-annotated data, other things, entire content, World Wide Web, inferior results, algorithm, low enough time complexity, representation learning, deep neural network-style machine learning methods, natural language processing, part, flurry, results, such techniques[4][5, -art, many natural language tasks, example, language, Popular techniques, use, word embeddings, semantic properties, words, increase, end, higher-level task, (e.g., question, pipeline, separate intermediate tasks, speech, dependency parsing, areas, shift, substantial changes, NLP systems, deep neural network-based approaches, new paradigm, statistical natural language processing, instance, term neural machine translation, NMT, fact, deep learning-based approaches, machine translation, sequence, need, intermediate steps, word alignment, language modeling, statistical machine translation, SMT]\n"
]
],
[
[
"Textacy also has a bunch of other information extraction functions, many of them based on regular expression patterns and heuristics to address extracting specific expressions such as acronyms and quotations. Apart from these, we can also extract matching custom regular expressions including POS tag patterns, or look for statements involving an entity, subject-verb-object tuples etc. We will discuss some of these as they come, in this chapter. \n\nDocumentation: https://chartbeat-labs.github.io/textacy/api_reference.html",
"_____no_output_____"
]
]
] | [
"code",
"markdown"
] | [
[
"code",
"code",
"code",
"code",
"code",
"code",
"code"
],
[
"markdown"
]
] |
e764a2e204f003bbdc97bba5d1e9e7ebf6ba5697 | 73,082 | ipynb | Jupyter Notebook | Python-for-Signal-Processing/Projection.ipynb | gopala-kr/ds-notebooks | bc35430ecdd851f2ceab8f2437eec4d77cb59423 | [
"MIT"
] | 10 | 2016-11-19T14:10:23.000Z | 2020-08-28T18:10:42.000Z | Projection.ipynb | wshaow/Python-for-Signal-Processing | a2565b75600359c244b694274bb03e4a1df934d6 | [
"CC-BY-3.0"
] | null | null | null | Projection.ipynb | wshaow/Python-for-Signal-Processing | a2565b75600359c244b694274bb03e4a1df934d6 | [
"CC-BY-3.0"
] | 5 | 2018-02-26T06:14:46.000Z | 2019-09-04T07:23:13.000Z | 261.942652 | 26,268 | 0.884814 | [
[
[
"empty"
]
]
] | [
"empty"
] | [
[
"empty"
]
] |
e764a7a9d066ee0cd54c361f93979e9caba3af7a | 52,496 | ipynb | Jupyter Notebook | Jupyter/SIT742P04A-ExploratoryDA.ipynb | jllemusc/sit742 | a88bc5d0a73caee62c000526ec276f596999234b | [
"MIT"
] | null | null | null | Jupyter/SIT742P04A-ExploratoryDA.ipynb | jllemusc/sit742 | a88bc5d0a73caee62c000526ec276f596999234b | [
"MIT"
] | null | null | null | Jupyter/SIT742P04A-ExploratoryDA.ipynb | jllemusc/sit742 | a88bc5d0a73caee62c000526ec276f596999234b | [
"MIT"
] | null | null | null | 26.811032 | 376 | 0.535279 | [
[
[
"# SIT742: Modern Data Science \n**(Module 04: Exploratory Data Analysis)**\n\n\n---\n- Materials in this module include resources collected from various open-source online repositories.\n- You are free to use, change and distribute this package.\n- If you found any issue/bug for this document, please submit an issue at [tulip-lab/sit742](https://github.com/tulip-lab/sit742/issues)\n\nPrepared by **SIT742 Teaching Team**\n\n---\n\n\n## Session 4A - Exploratory Data Analysis\n\n\nThis practical session will show you how to use packages for data exploration.\n\n\n## Content\n\n### Part 1 Matplotlib Module\n\n\n### Part 2 Plotting a Histogram\n\n2.1 [Dataset](#ds)\n\n2.2 [Histogram](#hist)\n\n2.3 [Boxplot](#boxplot)\n\n\n### Part 3: Data Understanding\n\n1.1 [Pie Chart](#pie)\n\n1.2 [Bar Chart](#bar)\n\n1.3 [Word Cloud](#wordcloud)\n\n1.4 [Step Plot](#stepplot)\n\n1.5 [Histogram](#histogram)\n\n1.6 [Box Plot](#box)\n\n1.7 [Scatter Plot](#scatter)\n\n### Part 4: Exercise\n\n",
"_____no_output_____"
],
[
"## <span style=\"color:#0b486b\">1. Matplotlib</span>\n",
"_____no_output_____"
],
[
"matplotlib is a python plotting library which produces publication quality figures in a variety of hardcopy formats and interactive environments across platforms. You can generate plots, histograms, power spectra, bar charts, errorcharts, scatterplots, etc, with just a few lines of code. \n\nFor simple plotting the pyplot interface provides a MATLAB-like interface, particularly when combined with IPython. You have full control of line styles, font properties, axes properties, etc, via an object oriented interface or via a set of functions familiar to MATLAB users.",
"_____no_output_____"
],
[
"### <span style=\"color:#0b486b\">1.1 Get started</span>\n",
"_____no_output_____"
],
[
"To get started with `'matplotlib'` you can either execute:",
"_____no_output_____"
]
],
[
[
"from pylab import *",
"_____no_output_____"
]
],
[
[
"or",
"_____no_output_____"
]
],
[
[
"import matplotlib.pyplot",
"_____no_output_____"
]
],
[
[
"In fact it is a convention to import it under the name of `'plt'`:",
"_____no_output_____"
]
],
[
[
"import matplotlib.pyplot as plt",
"_____no_output_____"
]
],
[
[
"**note: The method, 'import matplotlib.pyplot as plt', is preferred.**",
"_____no_output_____"
]
],
[
[
"import matplotlib.pyplot as plt\nimport numpy as np",
"_____no_output_____"
]
],
[
[
"Regardless of the method you use, it is better to configure matplotlib to embed figures in the notebook instead of opening them in a new window for each figure. To do this use the magic function:",
"_____no_output_____"
]
],
[
[
"%matplotlib inline",
"_____no_output_____"
]
],
[
[
"### <span style=\"color:#0b486b\">1.2 `plot`</span>\n",
"_____no_output_____"
],
[
"By using `'subplots()'` you have access to both figure and axes objects. ",
"_____no_output_____"
]
],
[
[
"#To define the x-Axis and y-Axis\nx = np.linspace(0, 10)\ny = np.sin(x)",
"_____no_output_____"
],
[
"#fig, ax = plt.subplots() is more consice the below code\n#If you use it,you unpack this tuple into the variables fig and ax\n#Actually, it equals the below 2 lines code. \n#fig = plt.figure()\n#ax = fig.add_subplot(111) #the 111 menas 1x1 grid, first subplot\n\nfig, ax = plt.subplots()\nax.plot(x, y)",
"_____no_output_____"
]
],
[
[
"### <span style=\"color:#0b486b\">1.3 title and labels</span>\n",
"_____no_output_____"
]
],
[
[
"#To set the title, xlabel and ylabel \nax.set_title('title here!')\nax.set_xlabel('x')\nax.set_ylabel('sin(x)')\nfig",
"_____no_output_____"
]
],
[
[
"You can also use [$\\LaTeX$](https://www.latex-project.org/about/) in title or labels, or change the font size or font family.",
"_____no_output_____"
]
],
[
[
"#To set the domain of X-Axis from -10 to 10\nx = np.linspace(-10, 10)\n\n#Parameter figsize : (float, float), optional, default: None\n#width, height in inches. If not provided, defaults to rcParams[\"figure.figsize\"] = [6.4, 4.8].\n\n#Parameter dpi : integer, optional, default: None\n#resolution of the figure. If not provided, defaults to rcParams[\"figure.dpi\"] = 100.\nfig, ax = plt.subplots(figsize=(6, 4), dpi=100)\n\n#x, x**3-x**2 are used to define the horizontal / vertical coordinates of the data points. x values are optional. If not given, they default to [0, ..., N-1].\n#'bo-' means blue circle with the solid line style. Its name pattern follow the '[color][marker][line]' structure.\n#linewidth defines the line width\n#markersize defines the circle size\nax.plot(x, x**3-x**2, 'bo-', linewidth=1, markersize=5)\n\n\n#To set title, xlabel, ylabel and their fontsize\nax.set_title('$x^3-x^2$', fontsize=18)\nax.set_xlabel('$x$', fontsize=18)\nax.set_ylabel('$y$', fontsize=18)\nax",
"_____no_output_____"
]
],
[
[
"### <span style=\"color:#0b486b\">1.4 Subplots</span>\n\nYou can pass the number of subplots to `'subplots()'`. In this case, `'axes'` will be an array that each of its elements associates with one of the subgraphs. You can set properties of each `'ax'` object separately like the cell below. \n\nObviously you caould use a loop to iterate over `'axes'`.",
"_____no_output_____"
]
],
[
[
"#nrows, ncols : int, optional, default: 1\n#They are used to define the number of rows/columns of the subplot grid.\n#You can try to comment the below code out and run the 'fig, axes = plt.subplots(nrows=1, ncols=2)', then you will find the difference.\nfig, axes = plt.subplots(nrows=2, ncols=1)\n\n#To define the domain of the x-Axis from 0 to 10\nx = np.linspace(0, 10)\n\n#x, np.sin(x) are used to define the horizontal / vertical coordinates of the data points. x values are optional. If not given, they default to [0, ..., N-1]. \naxes[0].plot(x, np.sin(x))\naxes[0].set_xlabel('x for sin(x)')\naxes[0].set_ylabel('sin(x)')\n\n#x, np.cos(x) are used to define the horizontal / vertical coordinates of the data points. x values are optional. If not given, they default to [0, ..., N-1]. \naxes[1].plot(x, np.cos(x))\naxes[1].set_xlabel('x for cos(x)')\naxes[1].set_ylabel('cos(x)')\n\naxes",
"_____no_output_____"
]
],
[
[
"`'cos(x)'` label is overlapping with the `'sin'` graph. You can adjust the size of the graph or space between the subplots to fix it.",
"_____no_output_____"
]
],
[
[
"#nrows, ncols : int, optional, default: 1\n#They are used to define the number of rows/columns of the subplot grid.\n#You can try to comment the below code out and run the 'fig, axes = plt.subplots(nrows=1, ncols=2)', then you will find the difference.\n#You also can use the fig, axes = plt.subplots(nrows=1, ncols=2, figsize=(10,5)) to resize the graph\nfig, axes = plt.subplots(nrows=2, ncols=1)\n\n#To adjust the space between the subpots\nfig.subplots_adjust(hspace=1)\n\n#To define the domain of the x-Axis from 0 to 10\nx = np.linspace(0, 10)\n\n#x, np.sin(x) are used to define the horizontal / vertical coordinates of the data points. x values are optional. If not given, they default to [0, ..., N-1]. \naxes[0].plot(x, np.sin(x))\naxes[0].set_xlabel('x for sin(x)')\naxes[0].set_ylabel('sin(x)')\n\n#x, np.cos(x) are used to define the horizontal / vertical coordinates of the data points. x values are optional. If not given, they default to [0, ..., N-1]. \naxes[1].plot(x, np.cos(x))\naxes[1].set_xlabel('x for cos(x)')\naxes[1].set_ylabel('cos(x)')\n\naxes",
"_____no_output_____"
]
],
[
[
"### <span style=\"color:#0b486b\">1.5 Legend</span>\n",
"_____no_output_____"
]
],
[
[
"##To define the domain of the x-Axis from 0 to 10\nx = np.linspace(0, 10)\n\n#To define the figsize\nfig, ax = plt.subplots(figsize=(7, 5))\n\n#To define the x-Axis and y-Axis and label\nax.plot(x, np.sin(x), label='$sin(x)$')\nax.plot(x, np.cos(x), label='$cos(x)$')\n\n#To place the legend for the two plot and define their fontsize and the location of the legend. \n#Possible codes for the 'loc' are from 0 to 10:\nax.legend(fontsize=16, loc=3)\n",
"_____no_output_____"
]
],
[
[
"### <span style=\"color:#0b486b\">1.6 Customizing ticks</span>\n",
"_____no_output_____"
],
[
"In many cases you want to customize the ticks and their labels on x or y axis. First draw a simple graph and look at the ticks on x-axis. ",
"_____no_output_____"
]
],
[
[
"x = np.linspace(0, 10, num=100)\n\nfig, ax = plt.subplots(figsize=(10, 5))\nax.plot(x, np.sin(x), x, np.cos(x), linewidth=2)",
"_____no_output_____"
]
],
[
[
"You can change the ticks easily with passing a list (or array) to `'set_xticks()'` or `'set_yticks()'`:",
"_____no_output_____"
]
],
[
[
"xticks = [0, 1, 2, 5, 8, 8.5, 10]\nax.set_xticks(xticks)\nfig",
"_____no_output_____"
]
],
[
[
"Or even you can change the labels:",
"_____no_output_____"
]
],
[
[
"xticklabels = ['$\\gamma$', '$\\delta$', 'apple', 'b', '', 'c'] \nax.set_xticklabels(xticklabels, fontsize=18)\nfig",
"_____no_output_____"
]
],
[
[
"### <span style=\"color:#0b486b\">1.7 Saving figures</span>\n",
"_____no_output_____"
]
],
[
[
"x = np.linspace(0, 10)\nfig, ax = plt.subplots(figsize=(7, 5))\nax.plot(x, np.sin(x), label='$sin(x)$')\nax.plot(x, np.cos(x), label='$cos(x)$')\nax.legend(fontsize=16, loc=3)\nfig.savefig('P03Saved.pdf', format='PDF', dpi=300)",
"_____no_output_____"
]
],
[
[
"### <span style=\"color:#0b486b\">1.8 Other plot styles</span>\n\nThere are many other plot types in addition to simple `'plot'` supported by `'matplotlib'`. You will find a complete list of them on [matplotlib gallery](http://matplotlib.org/gallery.html).",
"_____no_output_____"
],
[
"#### <span style=\"color:#0b486b\">1.8.1 Scatter plot</span>\n\n",
"_____no_output_____"
]
],
[
[
"fig, ax = plt.subplots(figsize=(10,5))\n\nx = np.linspace(-0.75, 1., 100)\n\n\n#s is the marker size in points**2. Default is rcParams['lines.markersize'] ** 2.\n#alpha is the alpha blending value, between 0 (transparent) and 1 (opaque).\n\n#edgecolor it the edge color of the marker. Possible values:\n# 'face': The edge color will always be the same as the face color.\n# 'none': No patch boundary will be drawn.\n# A matplotib color.\nax.scatter(x, np.random.randn(x.shape[0]), \n s = 250*np.abs(np.random.randn(x.shape[0])), \n alpha=0.5,\n facecolor='green',\n edgecolor='face')\nax.set_title('scatter')\nax",
"_____no_output_____"
]
],
[
[
"#### <span style=\"color:#0b486b\">1.8.2 Bar plot</span>\n",
"_____no_output_____"
]
],
[
[
"fig, ax = plt.subplots()\n\nx = np.arange(1, 6)\nax.bar(x, x**2, align=\"center\")\nax.set_title('bar')",
"_____no_output_____"
]
],
[
[
"---\n## <span style=\"color:#0b486b\"> 2. Plotting a histogram</span>\n",
"_____no_output_____"
],
[
"<a id = \"ds\"></a>\n\n\n### <span style=\"color:#0b486b\">2.1 Dataset</span>\n",
"_____no_output_____"
],
[
"You are provided with a dataset of percentage of body fat and 10 simple body measurements recoreded for 252 men (courtesy of Journal of Statistics Education - JSE). You can read about this and other [JSE datasets here](http://www.amstat.org/publications/jse/jse_data_archive.htm).\n\nFirst load the data set into an array:",
"_____no_output_____"
]
],
[
[
"!pip install wget\n",
"_____no_output_____"
],
[
"import numpy as np\nimport wget\n\nlink_to_data = 'https://github.com/tulip-lab/sit742/raw/master/Jupyter/data/fat.dat.txt'\nDataSet = wget.download(link_to_data)",
"_____no_output_____"
],
[
"data = np.genfromtxt(\"fat.dat.txt\")\ndata.shape",
"_____no_output_____"
]
],
[
[
"Based on the [dataset description](http://www.amstat.org/publications/jse/datasets/fat.txt), 5th column represents the weight in lbs. Index the weight column and call it `'weights'`:",
"_____no_output_____"
]
],
[
[
"weights = data[:, 5]\nweights",
"_____no_output_____"
]
],
[
[
"Use array operators to convert the weigts into kg. 1 lb equals to 0.453592 kg.",
"_____no_output_____"
]
],
[
[
"# weights *= 0.453592 is equivalent to weights = weights * 0.453592\n#It multiplies right operand with the left operand and assign the result to left operand\nweights *= 0.453592\n\n#Round the converted weights to only two decimals:\nweights = weights.round(2)\n\nweights",
"_____no_output_____"
]
],
[
[
"<a id = \"hist\"></a>\n\n### <span style=\"color:#0b486b\">2.2 Histogram</span>\n",
"_____no_output_____"
],
[
"A histogtram is a bar plot that shows you the statistical distribution of the data over a variable. The bars represent the frequency of occurenve by classess of data. We use the package `'matplotlib'` and the function `'hist()'` for plotting the histogram. To learn more about `'matplotlib'` make sure you have read tutorial.\n\nThe first line of the cell below if for showing the figure in the notebook and not opening it in a separate window.",
"_____no_output_____"
]
],
[
[
"%matplotlib inline\nimport matplotlib.pyplot as plt",
"_____no_output_____"
],
[
"fig, ax = plt.subplots()\nax.hist(weights)\nax",
"_____no_output_____"
]
],
[
[
"The `'hist()'` functions automatically group the data over 10 bins. Usually you need to tweek the number of bins to obtain a more expressive histogram.",
"_____no_output_____"
]
],
[
[
"fig, ax = plt.subplots(figsize=(7, 5))\nax.hist(weights, bins=20)\n\n# title\nax.set_title('Weight Distribution Diagram ')\n\n# label\nax.set_xlabel('Weight (kg) ')\nax.set_ylabel('The number of people')\n\nax",
"_____no_output_____"
]
],
[
[
"<a id = \"boxplot\"></a>\n\n### <span style=\"color:#0b486b\">2.3 Boxplot</span>\n\nA `Boxplot` is a convenient way to graphically display numerical data. ",
"_____no_output_____"
]
],
[
[
"import matplotlib\n\nfig, ax = matplotlib.pyplot.subplots(figsize=(7, 5))\n\nmatplotlib.rcParams.update({'font.size': 14})\n\nax.boxplot(weights, 0, labels=['group1'])\n\nax.set_ylabel('weight (kg)', fontsize=16)\nax.set_title('Weights BoxPlot', fontsize=16)",
"_____no_output_____"
]
],
[
[
"You have already been thought about different sorts of plots, how they help to get a better understanding of the data, and when to use which. In this practical session we will work with `matplotlib` package to learn more about plotting in Python.",
"_____no_output_____"
],
[
"---\n## <span style=\"color:#0b486b\">3. Data Understanding </span>\n\n\nYou have already been thought about different sorts of plots, how they help to get a better understanding of the data, and when to use which. In this practical session we will work with `matplotlib` package to learn more about plotting in Python.",
"_____no_output_____"
]
],
[
[
"import numpy as np\nimport csv\n\nimport matplotlib.pyplot as plt\n%matplotlib inline",
"_____no_output_____"
]
],
[
[
"<a id = \"pie\"></a>\n\n### <span style=\"color:#0b486b\">3.1 Pie Chart</span>\n\nSuppose you have the frequency count of a variable (e.g. hair_colour). Draw a pie chart to explain it.",
"_____no_output_____"
]
],
[
[
"labels = 'Black', 'Red', 'Brown'\n\n# frequency count\nhair_colour_freq = [5, 3, 2] # Black, Red, Brown\n\n# colors\ncolors = ['yellowgreen', 'gold', 'lightskyblue']\n\n# explode the third one\nexplode = (0, 0, 0.1)\n\nfig, ax = plt.subplots(figsize=(5, 5))\nax.pie(hair_colour_freq, labels=labels, explode=explode, colors=colors, \n autopct='%1.1f', shadow=True, startangle=90);",
"_____no_output_____"
]
],
[
[
"What if we have too many tags and sectors?",
"_____no_output_____"
]
],
[
[
"# Excellence in Reasearch Australia\nlabels = ['HEALTH', 'ENGINEERING', 'COMPUTER SCIENCES', 'HUMAN SOCIETY', \n 'TOURISM SERVICES', 'EDUCATION', 'CHEMISTRY', 'BIOLOGY', 'PSYCHOLOGY', \n 'CREATIVE ARTS', 'LINGUISTICS', 'BUILT ENVIRONMENT', 'HISTORY', \n 'ECONOMICS', 'PHILOSOPHY', 'AGRICULTURE', 'ENVIRONMENT', 'TECHNOLOGY', \n 'LAW', 'MATHS', 'EARTH SCIENCES', 'PHYSICS']\n\n\n# frequency count\nxx = [2625.179999, 1306.259999, 1187.039999, 1166.04, 980.8599997, 810.5999998,\n 725.6399996, 678.7899998, 436.5999997, 404.3299999, 348.01, 304.33, 294.19, \n 293.02, 282.31, 228.21, 197.3399999, 164.0599998, 157, 50.49999998, 49.60999999, 48.08000005]\n\nfig, ax = plt.subplots(figsize=(10, 10))\nax.pie(xx, labels=labels, autopct=\"%1.1f\");",
"_____no_output_____"
]
],
[
[
"<a id = \"bar\"></a>\n\n### <span style=\"color:#0b486b\">3.2 Bar Chart</span>\n\nUse the hair colour data to draw a bar chart.",
"_____no_output_____"
]
],
[
[
"labels = ['Black', 'Red', 'Brown']\nhair_colour_freq = [5, 3, 2]\n\nfig, ax = plt.subplots(figsize=(7, 5), dpi=100)\n\nx_pos = np.arange(len(hair_colour_freq))\ncolors = ['black', 'red', 'brown']\n\nax.bar(x_pos, hair_colour_freq, align='center', color=colors)\n\nax.set_xlabel(\"Hair Colour\")\nax.set_ylabel(\"Number of participants\")\nax.set_title(\"Hair Colour Distribution\")\n\nax.set_xticks(x_pos)\nax.set_xticklabels(labels)\nax",
"_____no_output_____"
]
],
[
[
"Now suppose we have the hair colour distribution across genders, so we can plot grouped bar charts. Plot a grouped bar chart to show the distribution of colours acros genders.",
"_____no_output_____"
]
],
[
[
"\"\"\"\n black red brown\nMale 4 1 3\nFemale 1 2 2\n\n\"\"\"\n\ndata = np.array([[4, 1, 3], \n [1, 2, 3]])\n\nx_pos = np.arange(2)\nwidth = 0.2\n\nfig, ax = plt.subplots(figsize=(7, 5), dpi=100)\nax.bar(x_pos, data[:, 0], width=width, color='black', label='Black', align='center')\nax.bar(x_pos+width, data[:, 1], width=width, color='red', label='Red', align='center')\nax.bar(x_pos+2*width, data[:, 2], width=width, color='brown', label='Brown', align='center')\n\nax.legend()\n\nax.set_xlabel(\"Gender\")\nax.set_ylabel(\"Frequency\")\nax.set_title(\"Distribution of hair colour amongst genders\")\n\nax.set_xticks(x_pos+width)\nax.set_xticklabels(['Male', 'Female'])\nax",
"_____no_output_____"
]
],
[
[
"Can we plot it more intelligently? We are doing the same thing multiple times! Is it a good idea to use a loop?",
"_____no_output_____"
]
],
[
[
"\"\"\"\n black red brown\nMale 4 1 3\nFemale 1 2 2\n\n\"\"\"\n\ndata = np.array([[4, 1, 3], \n [1, 2, 3]])\n\nn_groups, n_colours = data.shape\n\nx_pos = np.arange(n_groups)\nwidth = 0.2\nfig, ax = plt.subplots(figsize=(7, 5), dpi=100)\n\ncolours = ['black', 'red', 'brown']\nlabels = ['Black', 'Red', 'Brown']\nfor i in range(n_colours):\n ax.bar(x_pos + i*width, data[:, i], width=width, color=colours[i], label=labels[i], align='center')\n \nax.legend()\n\nax.set_xlabel(\"Gender\")\nax.set_ylabel(\"Frequency\")\nax.set_title(\"Distribution of hair colour amongst genders\")\n\nax.set_xticks(x_pos+width)\nax.set_xticklabels(['Male', 'Female'])\nax",
"_____no_output_____"
]
],
[
[
"What if we want to group the bar charts based on the hair colour?",
"_____no_output_____"
]
],
[
[
"\"\"\"\n black red brown\nMale 4 1 3\nFemale 1 2 2\n\n\"\"\"\n\nlabels = ['Black', 'Red', 'Brown']\ncolours = ['r', 'y']\ndata = np.array([[4, 1, 3], \n [1, 2, 3]])\n\nn_groups, n_colours = data.shape\nwidth = 0.2\nx_pos = np.arange(n_colours)\n\nfig, ax = plt.subplots(figsize=(7, 5), dpi=100)\nfor i in range(n_groups):\n ax.bar(x_pos + i*width, data[i, :], width, align='center', label=labels[i], color=colours[i])\nax.set_xlabel(\"Hair Colour\")\nax.set_ylabel(\"Frequency\")\nax.set_title(\"Distribution of gender amongst hair colours\")\n\nax.set_xticks(x_pos+width/2)\nax.set_xticklabels(labels)\n\nax.legend()\nax",
"_____no_output_____"
]
],
[
[
"#### Stacked bar chart\n\nThe other type of bar chart is stacked bar chart. draw a stacked bar plot of the hair colour data grouped on hair colours.",
"_____no_output_____"
]
],
[
[
"\"\"\"\n black red brown\nMale 4 1 3\nFemale 1 2 2\n\n\"\"\"\n\nlabels = ['Black', 'Red', 'Brown']\ndata = np.array([[4, 1, 3], \n [1, 2, 3]])\n\nmale_freq = data[0,:]\n\nwidth = 0.4\nx_pos = np.arange(n_colours)\n\nfig, ax = plt.subplots(figsize=(7, 5), dpi=100)\nax.bar(x_pos, data[0, :], width, align='center', label='Male', color='r')\nax.bar(x_pos, data[1, :], width, bottom=male_freq, align='center', label='Female', color='y')\n\nax.set_xlabel(\"Hair Colour\")\nax.set_ylabel(\"Frequency\")\nax.set_title(\"Distribution of gender amongst hair colours\")\n\nax.set_xticks(x_pos)\nax.set_xticklabels(labels)\n\nax.legend(loc=0)",
"_____no_output_____"
]
],
[
[
"draw a stacked bar plot grouped on the gender.",
"_____no_output_____"
]
],
[
[
"\"\"\"\n black red brown\nMale 4 1 3\nFemale 1 2 2\n\n\"\"\"\n\nlabels = ['Black', 'Red', 'Brown']\ndata = np.array([[4, 1, 3], \n [1, 2, 3]])\n\nblack = data[:,0]\nred = data[:,1]\nbrown = data[:,2]\n\n\nx_pos = np.arange(2)\nwidth = 0.4\nfig, ax = plt.subplots(figsize=(7, 5), dpi=100)\nax.bar(x_pos, data[:, 0], width=width, color='black', label='Black', align='center')\nax.bar(x_pos, data[:, 1], width=width, bottom=black, color='red', label='Red', align='center')\nax.bar(x_pos, data[:, 2], width=width, color='brown', bottom=black+red, label='Brown', align='center')\n\nax.legend(loc=0)\n\nax.set_xlabel(\"Gender\")\nax.set_ylabel(\"Frequency\")\nax.set_title(\"Distribution of hair colour amongst genders\")\n\nax.set_xticks(x_pos)\nax.set_xticklabels(['Male', 'Female'])",
"_____no_output_____"
],
[
"labels = ['Black', 'Red', 'Brown']\nmale_freq = [4, 1, 3]\nfemale_freq = [1, 2, 2]\n\nx_pos = np.arange(3)\n\nfig, ax = plt.subplots(nrows=1, ncols=2, figsize=(14, 5), dpi=100)\n\nw1 = 0.2\nw2 = 0.4\n\nax[0].bar(x_pos, male_freq, width=w1, align='center', label='Male', color='r')\nax[0].bar(x_pos+width, female_freq, width=w1, align='center', label='Female', color='y')\nax[1].bar(x_pos, male_freq, width=w2, align='center', label='Male', color='r')\nax[1].bar(x_pos, female_freq, width=w2, bottom=male_freq, align='center', label='Female', color='y')\n\n\nax[0].set_xlabel(\"Hair Colour\")\nax[0].set_ylabel(\"Frequency\")\nax[0].set_title(\"Distribution of gender amongst hair colours\")\nax[1].set_xlabel(\"Hair Colour\")\nax[1].set_ylabel(\"Frequency\")\nax[1].set_title(\"Distribution of gender amongst hair colours\")\n\nax[0].set_xticks(x_pos+width/2)\nax[0].set_xticklabels(labels)\nax[1].set_xticks(x_pos)\nax[1].set_xticklabels(labels)\n\nax[0].legend()\nax[1].legend(loc=0)",
"_____no_output_____"
]
],
[
[
"What if we have too many groups? Draw a bar chart for the Excellence in Research Australia data. ",
"_____no_output_____"
]
],
[
[
"import matplotlib.pyplot as plt\nimport numpy as np\n\n# Excellence in Research Australia\nlabels = ['HEALTH', 'ENGINEERING', 'COMPUTER SCIENCES', 'HUMAN SOCIETY', \n 'TOURISM SERVICES', 'EDUCATION', 'CHEMISTRY', 'BIOLOGY', 'PSYCHOLOGY', \n 'CREATIVE ARTS', 'LINGUISTICS', 'BUILT ENVIRONMENT', 'HISTORY', \n 'ECONOMICS', 'PHILOSOPHY', 'AGRICULTURE', 'ENVIRONMENT', 'TECHNOLOGY', \n 'LAW', 'MATHS', 'EARTH SCIENCES', 'PHYSICS']\n\n\n# frequency count\nxx = [2625.179999, 1306.259999, 1187.039999, 1166.04, 980.8599997, 810.5999998,\n 725.6399996, 678.7899998, 436.5999997, 404.3299999, 348.01, 304.33, 294.19, \n 293.02, 282.31, 228.21, 197.3399999, 164.0599998, 157, 50.49999998, 49.60999999, 48.08000005]\n\nxx_pos = np.arange(len(xx))\n\nfig, ax = plt.subplots(figsize=(15, 5))\nax.bar(xx_pos, xx, align='center')\nax.set_xlabel(\"research subject\")\nax.set_ylabel(\"score\")\nax.set_xticks(xx_pos)\nax.set_xticklabels(labels, rotation=90)\nax.set_xlim(-1, len(xx))",
"_____no_output_____"
]
],
[
[
"You can also refer to one online [post](http://queirozf.com/entries/add-labels-and-text-to-matplotlib-plots-annotation-examples) for different customization of those plots.",
"_____no_output_____"
],
[
"<a id = \"wordcloud\"></a>\n\n### <span style=\"color:#0b486b\">3.3 Wordcloud</span>\n\nAs you saw, pie-chart is not very helpful when we have too many sectors. It is hard to read and visually ugly. Instead we can use wordcloud representation. A useful tool is [wordle.net](http://wordle.net). Go to [wordle.net](http://wordle.net) and use it to create a wordcloud for the previous data.",
"_____no_output_____"
]
],
[
[
"for i in range(len(labels)):\n print(\"{}:{}\".format(labels[i], xx[i]))",
"_____no_output_____"
],
[
"!pip install wordcloud",
"_____no_output_____"
],
[
"import wget\n\nlink_to_data = 'https://github.com/tulip-lab/sit742/raw/master/Jupyter/data/constitution.txt'\nDataSet = wget.download(link_to_data)",
"_____no_output_____"
],
[
"from os import path\nfrom wordcloud import WordCloud\n\n# Read the whole text.\ntext = open('constitution.txt').read()\n\n# Generate a word cloud image\nwordcloud = WordCloud().generate(text)\n\n# Display the generated image:\n# the matplotlib way:\nimport matplotlib.pyplot as plt\nplt.imshow(wordcloud, interpolation='bilinear')\nplt.axis(\"off\")\nplt.show()\n\n# lower max_font_size\nwordcloud = WordCloud(max_font_size=30).generate(text)\nplt.figure()\nplt.imshow(wordcloud, interpolation=\"bilinear\")\nplt.axis(\"off\")\nplt.show()\n\n# The PIL way (if you don't have matplotlib)\n#image = wordcloud.to_image()\n#image.show()",
"_____no_output_____"
]
],
[
[
"<a id = \"stepplot\"></a>\n\n### <span style=\"color:#0b486b\">3.4 Step plot</span>\n\nDraw a step plot for the seatbelt data.",
"_____no_output_____"
]
],
[
[
"freq = np.array([0, 2, 1, 5, 7])\nlabels = ['Never', 'Rarely', 'Sometimes', 'Most-times', 'Always']\nfreq_cumsum = np.cumsum(freq)\nx_pos = np.arange(len(freq))\n\nfig, ax = plt.subplots()\nax.step(x_pos, freq_cumsum, where='mid')\nax.set_xlabel(\"Fastening seatbelt behaviour\")\nax.set_ylabel(\"Cumulative frequency\")\nax.set_xticks(x_pos)\nax.set_xticklabels(labels)\nax",
"_____no_output_____"
]
],
[
[
"<a id = \"histogram\"></a>\n\n### <span style=\"color:#0b486b\">3.5 Histogram</span>\n\nGoogle for this paper:\n\n``Johnson, Roger W. \"Fitting percentage of body fat to simple body measurements.\" Journal of Statistics Education 4.1 (1996): 265-266.``\n\nDownload the dataset and read the dataset description. Draw a histogram of male weights and female weights.",
"_____no_output_____"
]
],
[
[
"import wget\n\nlink_to_data = 'https://github.com/tulip-lab/sit742/raw/master/Jupyter/data/body.dat.txt'\nDataSet = wget.download(link_to_data)",
"_____no_output_____"
],
[
"data = np.genfromtxt('body.dat.txt')\nm_w = data[data[:, -1] == 1][:, -3]\nf_w = data[data[:, -1] == 0][:, -3]\n\nfig, ax = plt.subplots(figsize=(7, 5), dpi=100)\nax.hist(m_w, bins=15, alpha=0.6, label='male')\nax.hist(f_w, bins=15, alpha=0.6, label='female')\nax.set_xlabel(\"weight (kg)\")\nax.set_title(\"Weight Distribution amongst gXenders\")\nax.legend()",
"_____no_output_____"
]
],
[
[
"<a id = \"box\"></a>\n\n### <span style=\"color:#0b486b\">3.6 Boxplot</span>\nDraw a box plot for male and female weights of the previous dataset.",
"_____no_output_____"
]
],
[
[
"fig, ax = plt.subplots(figsize=(7, 5), dpi=100)\n\n#To define the style of the fliers.\n#red_square = dict(markerfacecolor='r', marker='s') is another example, you can try define you own style.\ngreen_diamond = dict(markerfacecolor='g', marker='D')\n\n#Set the value of showfliers with True to show the outliers beyond the caps.\nax.boxplot([m_w, f_w], labels=['male', 'female'],showfliers=True, flierprops=green_diamond)\n\nax.set_title(\"weight distribution amongst genders\")\nax",
"_____no_output_____"
]
],
[
[
"<a id = \"scatter\"></a>\n\n### <span style=\"color:#0b486b\">3.7 Scatter plot</span>\n\nDraw a scatter plot of the car weights and their fuel consumption as displayed in the lecture.",
"_____no_output_____"
]
],
[
[
"import wget\n\nlink_to_data = 'https://github.com/tulip-lab/sit742/raw/master/Jupyter/data/Auto.csv'\nDataSet = wget.download(link_to_data)",
"_____no_output_____"
],
[
"datafile = 'Auto.csv'\ndata = np.genfromtxt(datafile, delimiter=',')\ndata = []\nwith open(datafile, 'r') as fp:\n reader = csv.reader(fp, delimiter=',')\n for row in reader:\n data.append(row)\nmiles = [dd[1] for dd in data[1:]]\nweights = [dd[5] for dd in data[1:]]",
"_____no_output_____"
],
[
"fig, ax = plt.subplots(figsize=(15, 5), dpi=100)\nax.scatter(weights,miles, alpha=0.6, edgecolor='none', s=100)\nax.set_xlabel('Car Weight (tons)')\nax.set_ylabel('Miles Per Gallon')\n",
"_____no_output_____"
]
],
[
[
"Can I also show the number of cylinders on this graph? In other words use the scatter plot to show three variable?",
"_____no_output_____"
]
],
[
[
"cylinder = 75 * np.array([int(dd[2]) for dd in data[1:]])",
"_____no_output_____"
],
[
"fig, ax = plt.subplots(figsize=(15, 5), dpi=100)\nax.scatter(weights,miles, alpha=0.6, edgecolor='none', s=cylinder)\nax.set_xlabel('Car Weight (tons)')\nax.set_ylabel('Miles Per Gallon')\nax",
"_____no_output_____"
]
],
[
[
"---\n## 4. Mini Exercise\n\n\nIn 1970, US Congress instituted a random selection process for the military draft. All 366 possible birth dates were placed in plastic capsules in a rotating drum and were selected one by one. The first date drawn from the drum received draft number one and eligible men born on that date were drafted first. The data is provided in a text file with a structure like:\n\n```\nDay Month MO.NUMBER DAY_OF_YEAR DRAFT_NO.\n1 JAN 1 1 305\n2 JAN 1 2 159\n.\n31 JAN 1 31 221\n1 FEB 2 32 86\n.\n31 Dec 12 366 100\n```\n",
"_____no_output_____"
],
[
"Using what you have learnt by now, can you tell if it was a fair lottary or not?",
"_____no_output_____"
],
[
"Read the data file and save the values in a 2D array.",
"_____no_output_____"
]
],
[
[
"import wget\n\nlink_to_data = 'https://github.com/tulip-lab/sit742/raw/master/Jupyter/data/DraftLottery.txt'\nDataSet = wget.download(link_to_data)",
"_____no_output_____"
],
[
"data = []\nwith open('DraftLottery.txt', 'r') as fp:\n reader = csv.reader(fp, delimiter='\\t')\n for row in reader:\n data.append(row)\n \nbirthdays = np.array([int(row[3]) for row in data[1:]])\ndraft_no = np.array([int(row[4]) for row in data[1:]])\nmonths = np.array([int(row[2]) for row in data[1:]])",
"_____no_output_____"
]
],
[
[
"Plot a `'scatter plot'` of the draft priority vs birthdays.",
"_____no_output_____"
]
],
[
[
"fig, ax = plt.subplots(figsize=(10, 7), dpi=100)\nax.scatter(birthdays, draft_no, alpha=0.7, s = 100, edgecolor='none')\nax.set_xlabel(\"Birthday (day of the year)\", fontsize=12)\nax.set_ylabel(\"Draft priority value\", fontsize=12)\nax.set_title(\"USA Draft Lottery Data\", fontsize=14)\nax",
"_____no_output_____"
]
],
[
[
"In a truly random lottery there should be no relationship between the date and the draft number. To investigate this further we draw boxplots by months and compare them together.",
"_____no_output_____"
]
],
[
[
"fig, ax = plt.subplots(figsize=(10, 7), dpi=100)\nmonths_range = range(1, 13)\n\n# boxplot data\nboxplot_data = [draft_no[months == mm] for mm in months_range]\nax.boxplot(boxplot_data)\n\n# medians\nmedians = [np.median(dd) for dd in boxplot_data]\nax.plot(months_range, medians, \"g--\", lw=2)\n\n# means\nmeans = [dd.mean() for dd in boxplot_data]\nax.plot(months_range, means, \"k--\", lw=2)\n\nmonth_labels = [\"Jan\", \"Feb\", \"Mar\", \"Apr\", \"May\", \"Jun\",\n \"Jul\", \"Aug\", \"Sep\", \"Oct\", \"Nov\", \"Dec\"]\nax.set_xlabel(\"Month\", fontsize=12)\nax.set_xticklabels(month_labels)\nax.set_ylabel(\"Draft priority value\", fontsize=12)\nax.set_title(\"USA Draft Lottery Data\", fontsize=14)\nax",
"_____no_output_____"
]
],
[
[
"While it is impossible to view this trend in a scatterplot of draft number vs. birth date, a series of side-by-side boxplots by month illustrate it clearly. A further investigation of the lottery revealed that the birthdates were placed in the drum by month and were not thoroughly mixed.",
"_____no_output_____"
]
]
] | [
"markdown",
"code",
"markdown",
"code",
"markdown",
"code",
"markdown",
"code",
"markdown",
"code",
"markdown",
"code",
"markdown",
"code",
"markdown",
"code",
"markdown",
"code",
"markdown",
"code",
"markdown",
"code",
"markdown",
"code",
"markdown",
"code",
"markdown",
"code",
"markdown",
"code",
"markdown",
"code",
"markdown",
"code",
"markdown",
"code",
"markdown",
"code",
"markdown",
"code",
"markdown",
"code",
"markdown",
"code",
"markdown",
"code",
"markdown",
"code",
"markdown",
"code",
"markdown",
"code",
"markdown",
"code",
"markdown",
"code",
"markdown",
"code",
"markdown",
"code",
"markdown",
"code",
"markdown",
"code",
"markdown",
"code",
"markdown",
"code",
"markdown",
"code",
"markdown",
"code",
"markdown",
"code",
"markdown",
"code",
"markdown",
"code",
"markdown",
"code",
"markdown",
"code",
"markdown",
"code",
"markdown"
] | [
[
"markdown",
"markdown",
"markdown",
"markdown",
"markdown"
],
[
"code"
],
[
"markdown"
],
[
"code"
],
[
"markdown"
],
[
"code"
],
[
"markdown"
],
[
"code"
],
[
"markdown"
],
[
"code"
],
[
"markdown",
"markdown"
],
[
"code",
"code"
],
[
"markdown"
],
[
"code"
],
[
"markdown"
],
[
"code"
],
[
"markdown"
],
[
"code"
],
[
"markdown"
],
[
"code"
],
[
"markdown"
],
[
"code"
],
[
"markdown",
"markdown"
],
[
"code"
],
[
"markdown"
],
[
"code"
],
[
"markdown"
],
[
"code"
],
[
"markdown"
],
[
"code"
],
[
"markdown",
"markdown"
],
[
"code"
],
[
"markdown"
],
[
"code"
],
[
"markdown",
"markdown",
"markdown"
],
[
"code",
"code",
"code"
],
[
"markdown"
],
[
"code"
],
[
"markdown"
],
[
"code"
],
[
"markdown",
"markdown"
],
[
"code",
"code"
],
[
"markdown"
],
[
"code"
],
[
"markdown"
],
[
"code"
],
[
"markdown",
"markdown"
],
[
"code"
],
[
"markdown"
],
[
"code"
],
[
"markdown"
],
[
"code"
],
[
"markdown"
],
[
"code"
],
[
"markdown"
],
[
"code"
],
[
"markdown"
],
[
"code"
],
[
"markdown"
],
[
"code"
],
[
"markdown"
],
[
"code"
],
[
"markdown"
],
[
"code",
"code"
],
[
"markdown"
],
[
"code"
],
[
"markdown",
"markdown"
],
[
"code",
"code",
"code",
"code"
],
[
"markdown"
],
[
"code"
],
[
"markdown"
],
[
"code",
"code"
],
[
"markdown"
],
[
"code"
],
[
"markdown"
],
[
"code",
"code",
"code"
],
[
"markdown"
],
[
"code",
"code"
],
[
"markdown",
"markdown",
"markdown"
],
[
"code",
"code"
],
[
"markdown"
],
[
"code"
],
[
"markdown"
],
[
"code"
],
[
"markdown"
]
] |
e764b384520aedc349d13f8a929b7f68cd7a0e23 | 873,374 | ipynb | Jupyter Notebook | pong-PPO(1).ipynb | vigneshyaadav27/Pong-DRL | f0db20c50dd9d46b8432db3db4faa1a1b0e1a489 | [
"MIT"
] | null | null | null | pong-PPO(1).ipynb | vigneshyaadav27/Pong-DRL | f0db20c50dd9d46b8432db3db4faa1a1b0e1a489 | [
"MIT"
] | null | null | null | pong-PPO(1).ipynb | vigneshyaadav27/Pong-DRL | f0db20c50dd9d46b8432db3db4faa1a1b0e1a489 | [
"MIT"
] | null | null | null | 533.847188 | 10,284 | 0.953493 | [
[
[
"# Welcome!\nBelow, we will learn to implement and train a policy to play atari-pong, using only the pixels as input. We will use convolutional neural nets, multiprocessing, and pytorch to implement and train our policy. Let's get started!",
"_____no_output_____"
]
],
[
[
"# install package for displaying animation\n!pip install JSAnimation\n\n# custom utilies for displaying animation, collecting rollouts and more\nimport pong_utils\n\n%matplotlib inline\n\n# check which device is being used. \n# I recommend disabling gpu until you've made sure that the code runs\ndevice = pong_utils.device\nprint(\"using device: \",device)",
"Collecting JSAnimation\n Downloading https://files.pythonhosted.org/packages/3c/e6/a93a578400c38a43af8b4271334ed2444b42d65580f1d6721c9fe32e9fd8/JSAnimation-0.1.tar.gz\nBuilding wheels for collected packages: JSAnimation\n Running setup.py bdist_wheel for JSAnimation ... \u001b[?25ldone\n\u001b[?25h Stored in directory: /root/.cache/pip/wheels/3c/c2/b2/b444dffc3eed9c78139288d301c4009a42c0dd061d3b62cead\nSuccessfully built JSAnimation\nInstalling collected packages: JSAnimation\nSuccessfully installed JSAnimation-0.1\nusing device: cuda:0\n"
],
[
"# render ai gym environment\nimport gym\nimport time\n\n# PongDeterministic does not contain random frameskip\n# so is faster to train than the vanilla Pong-v4 environment\nenv = gym.make('PongDeterministic-v4')\n\nprint(\"List of available actions: \", env.unwrapped.get_action_meanings())\n\n# we will only use the actions 'RIGHTFIRE' = 4 and 'LEFTFIRE\" = 5\n# the 'FIRE' part ensures that the game starts again after losing a life\n# the actions are hard-coded in pong_utils.py",
"List of available actions: ['NOOP', 'FIRE', 'RIGHT', 'LEFT', 'RIGHTFIRE', 'LEFTFIRE']\n"
]
],
[
[
"# Preprocessing\nTo speed up training, we can simplify the input by cropping the images and use every other pixel\n\n",
"_____no_output_____"
]
],
[
[
"import matplotlib\nimport matplotlib.pyplot as plt\n\n# show what a preprocessed image looks like\nenv.reset()\n_, _, _, _ = env.step(0)\n# get a frame after 20 steps\nfor _ in range(20):\n frame, _, _, _ = env.step(1)\n\nplt.subplot(1,2,1)\nplt.imshow(frame)\nplt.title('original image')\n\nplt.subplot(1,2,2)\nplt.title('preprocessed image')\n\n# 80 x 80 black and white image\nplt.imshow(pong_utils.preprocess_single(frame), cmap='Greys')\nplt.show()\n\n",
"_____no_output_____"
]
],
[
[
"# Policy\n\n## Exercise 1: Implement your policy\n \nHere, we define our policy. The input is the stack of two different frames (which captures the movement), and the output is a number $P_{\\rm right}$, the probability of moving left. Note that $P_{\\rm left}= 1-P_{\\rm right}$",
"_____no_output_____"
]
],
[
[
"import torch\nimport torch.nn as nn\nimport torch.nn.functional as F\n\n\n\n# set up a convolutional neural net\n# the output is the probability of moving right\n# P(left) = 1-P(right)\nclass Policy(nn.Module):\n\n def __init__(self):\n super(Policy, self).__init__()\n \n \n ########\n ## \n ## Modify your neural network\n ##\n ########\n \n # 80x80 to outputsize x outputsize\n # outputsize = (inputsize - kernel_size + stride)/stride \n # (round up if not an integer)\n\n # output = 20x20 here\n self.conv_1 = nn.Conv2d(2, 4, kernel_size=2, stride=2)\n self.conv_2 = nn.Conv2d(4, 8, kernal_size=2, stride=2)\n self.conv_3 = nn.Conv2d(8, 16, kernal_size=2, stride=2)\n self.conv_4 = nn.Conv2d(16, 32, kernel_size=2, stride=2)\n self.conv_5 = nn.Conv2d(32, 64, kernel_size=2, stride=2)\n self.conv_6 = nn.Conv2d(64, 128, kernel_size=2, stride=2)\n self.size1 = 35 * 5 *5\n \n # 1 fully connected layer\n self.fc_1 = nn.Linear(self.size1, 128)\n self.fc_2 = nn.Linear(128, 64)\n self.fc_3 = nn.Linear(64, 8)\n self.fc_4 = nn.Linear(8, 1)\n self.sig = nn.Sigmoid()\n \n def forward(self, x):\n \n ########\n ## \n ## Modify your neural network\n ##\n ########\n \n x = F.relu(self.conv_1(x))\n x = F.relu(self.conv_2(x))\n x = F.relu(self.conv_3(x))\n x = F.relu(self.conv_4(x))\n # flatten the tensor\n x = x.view(-1,self.size)\n x = F.relu(self.fc_1(x))\n x = F.relu(self.fc_2(x))\n x = F.relu(self.fc_3(x))\n return self.sig(self.fc(x))\n\n\n# run your own policy!\n# policy=Policy().to(device)\npolicy=pong_utils.Policy().to(device)\n\n# we use the adam optimizer with learning rate 2e-4\n# optim.SGD is also possible\nimport torch.optim as optim\noptimizer = optim.Adam(policy.parameters(), lr=1e-4)",
"_____no_output_____"
]
],
[
[
"# Game visualization\npong_utils contain a play function given the environment and a policy. An optional preprocess function can be supplied. Here we define a function that plays a game and shows learning progress",
"_____no_output_____"
]
],
[
[
"pong_utils.play(env, policy, time=200) \n# try to add the option \"preprocess=pong_utils.preprocess_single\"\n# to see what the agent sees",
"_____no_output_____"
]
],
[
[
"# Function Definitions\nHere you will define key functions for training. \n\n## Exercise 2: write your own function for training\n(what I call scalar function is the same as policy_loss up to a negative sign)\n\n### PPO\nLater on, you'll implement the PPO algorithm as well, and the scalar function is given by\n$\\frac{1}{T}\\sum^T_t \\min\\left\\{R_{t}^{\\rm future}\\frac{\\pi_{\\theta'}(a_t|s_t)}{\\pi_{\\theta}(a_t|s_t)},R_{t}^{\\rm future}{\\rm clip}_{\\epsilon}\\!\\left(\\frac{\\pi_{\\theta'}(a_t|s_t)}{\\pi_{\\theta}(a_t|s_t)}\\right)\\right\\}$\n\nthe ${\\rm clip}_\\epsilon$ function is implemented in pytorch as ```torch.clamp(ratio, 1-epsilon, 1+epsilon)```",
"_____no_output_____"
]
],
[
[
"def discounted_future_rewards(rewards, ratio=0.99):\n n = rewards.shape[1]\n step = torch.arange(n)[:,None] - torch.arange(n)[:,None]\n ones = torch.ones_like(step)\n zeros = torch.zeros_like(step)\n target = torch.where(step >= 0, ones, zeros)\n step = torch.where(step >= 0, step, zeros)\n discount = target * (ratio ** step)\n discount = discount.to(device)\n \n rewards_discounted = torch.mm(rewards, discount)\n return rewards_discounted\n\n\n\ndef clipped_surrogate(policy, old_probs, states, actions, rewards,\n discount = 0.995, epsilon=0.1, beta=0.01):\n\n ########\n ## \n ## WRITE YOUR OWN CODE HERE\n ##\n ########\n \n actions = torch.tensor(actions, dtype=torch.int8, device=device)\n rewards = torch.tensor(rewards, dtype=torch.float, device=device)\n old_probs = torch.tensor(old_probs, dtype=torch.float, device=device)\n\n\n # convert states to policy (or probability)\n new_probs = pong_utils.states_to_prob(policy, states)\n new_probs = torch.where(actions == pong_utils.RIGHT, new_probs, 1.0-new_probs)\n \n # discounted cumalitive rewards\n R_future = discounted_future_rewards(rewards, discount)\n \n R_mean = torch.mean(R_future)\n R_future -= R_mean\n \n ratio = new_probs / (old_probs + 1e-6)\n ratio_clamped = torch.clamp(ratio, 1-epsilon, 1+epsilon)\n ratio_PPO = torch.where(ratio < ratio_clamped, ratio, ratio_clamped)\n \n # policy gradient maxmize target\n surrogates = (R_future * ratio_PPO).mean()\n \n\n # include a regularization term\n # this steers new_policy towards 0.5\n # prevents policy to become exactly 0 or 1 helps exploration\n # add in 1.e-10 to avoid log(0) which gives nan\n entropy = -(new_probs*torch.log(old_probs+1.e-10)+ \\\n (1.0-new_probs)*torch.log(1.0-old_probs+1.e-10))\n\n return torch.mean(beta*entropy+clipped_surrogate)\n",
"_____no_output_____"
]
],
[
[
"# Training\nWe are now ready to train our policy!\nWARNING: make sure to turn on GPU, which also enables multicore processing. It may take up to 45 minutes even with GPU enabled, otherwise it will take much longer!",
"_____no_output_____"
]
],
[
[
"from parallelEnv import parallelEnv\nimport numpy as np\n# keep track of how long training takes\n# WARNING: running through all 800 episodes will take 30-45 minutes\n\n# training loop max iterations\nepisode = 500\n\n# widget bar to display progress\n!pip install progressbar\nimport progressbar as pb\nwidget = ['training loop: ', pb.Percentage(), ' ', \n pb.Bar(), ' ', pb.ETA() ]\ntimer = pb.ProgressBar(widgets=widget, maxval=episode).start()\n\n\nenvs = parallelEnv('PongDeterministic-v4', n=8, seed=1234)\n\ndiscount_rate = .99\nepsilon = 0.1\nbeta = .01\ntmax = 320\nSGD_epoch = 4\n\n# keep track of progress\nmean_rewards = []\n\nfor e in range(episode):\n\n # collect trajectories\n old_probs, states, actions, rewards = \\\n pong_utils.collect_trajectories(envs, policy, tmax=tmax)\n \n total_rewards = np.sum(rewards, axis=0)\n\n\n # gradient ascent step\n for _ in range(SGD_epoch):\n \n # uncomment to utilize your own clipped function!\n # L = -clipped_surrogate(policy, old_probs, states, actions, rewards, epsilon=epsilon, beta=beta)\n\n L = -pong_utils.clipped_surrogate(policy, old_probs, states, actions, rewards,\n epsilon=epsilon, beta=beta)\n optimizer.zero_grad()\n L.backward()\n optimizer.step()\n del L\n \n # the clipping parameter reduces as time goes on\n epsilon*=.999\n \n # the regulation term also reduces\n # this reduces exploration in later runs\n beta*=.995\n \n # get the average reward of the parallel environments\n mean_rewards.append(np.mean(total_rewards))\n \n # display some progress every 20 iterations\n if (e+1)%20 ==0 :\n print(\"Episode: {0:d}, score: {1:f}\".format(e+1,np.mean(total_rewards)))\n print(total_rewards)\n \n # update progress widget bar\n timer.update(e+1)\n \ntimer.finish()",
"Collecting progressbar\n Downloading https://files.pythonhosted.org/packages/a3/a6/b8e451f6cff1c99b4747a2f7235aa904d2d49e8e1464e0b798272aa84358/progressbar-2.5.tar.gz\nBuilding wheels for collected packages: progressbar\n Running setup.py bdist_wheel for progressbar ... \u001b[?25ldone\n\u001b[?25h Stored in directory: /root/.cache/pip/wheels/c0/e9/6b/ea01090205e285175842339aa3b491adeb4015206cda272ff0\nSuccessfully built progressbar\nInstalling collected packages: progressbar\nSuccessfully installed progressbar-2.5\n"
],
[
"pong_utils.play(env, policy, time=200) ",
"_____no_output_____"
],
[
"\n# save your policy!\ntorch.save(policy, 'PPO.policy')\n\n# load policy if needed\n# policy = torch.load('PPO.policy')\n\n# try and test out the solution \n# make sure GPU is enabled, otherwise loading will fail\n# (the PPO verion can win more often than not)!\n#\n# policy_solution = torch.load('PPO_solution.policy')\n# pong_utils.play(env, policy_solution, time=2000) ",
"_____no_output_____"
]
]
] | [
"markdown",
"code",
"markdown",
"code",
"markdown",
"code",
"markdown",
"code",
"markdown",
"code",
"markdown",
"code"
] | [
[
"markdown"
],
[
"code",
"code"
],
[
"markdown"
],
[
"code"
],
[
"markdown"
],
[
"code"
],
[
"markdown"
],
[
"code"
],
[
"markdown"
],
[
"code"
],
[
"markdown"
],
[
"code",
"code",
"code"
]
] |
e764b38c523fdce1a1a6a0d61fcda5f57ab84204 | 5,664 | ipynb | Jupyter Notebook | 2.workspace/Heeran/YOLO_Training.ipynb | Heeran-cloud/FaceDetection_Blurring_YOLO | 480541b384bf6d92e613e6d672415b2afe06cddf | [
"MIT"
] | null | null | null | 2.workspace/Heeran/YOLO_Training.ipynb | Heeran-cloud/FaceDetection_Blurring_YOLO | 480541b384bf6d92e613e6d672415b2afe06cddf | [
"MIT"
] | null | null | null | 2.workspace/Heeran/YOLO_Training.ipynb | Heeran-cloud/FaceDetection_Blurring_YOLO | 480541b384bf6d92e613e6d672415b2afe06cddf | [
"MIT"
] | 2 | 2021-03-07T05:55:31.000Z | 2021-03-07T05:56:42.000Z | 5,664 | 5,664 | 0.700565 | [
[
[
"from google.colab import drive\ndrive.mount('/content/gdrive')",
"_____no_output_____"
],
[
"!tar xzvf /content/gdrive/MyDrive/darknet/cudnn/cudnn-10.1-linux-x64-v8.0.4.30.tgz -C /usr/local/",
"_____no_output_____"
],
[
"!cat /usr/local/cuda/include/cudnn_version.h | grep CUDNN_MAJOR -A 2",
"_____no_output_____"
],
[
"%cd /content/gdrive/MyDrive/darknet/bin/darknet",
"_____no_output_____"
],
[
"!chmod +x ./darknet",
"_____no_output_____"
],
[
"%ls",
"_____no_output_____"
],
[
"# Train\r\n!LD_LIBRARY_PATH=/usr/local/cuda/lib64 ./darknet detector train train_2.1_600_frames_tuned_v4/r1mini.data train_2.1_600_frames_tuned_v4/yolov4.cfg -dont_show",
"_____no_output_____"
],
[
"# mAP 확인\r\n!LD_LIBRARY_PATH=/usr/local/cuda/lib64 ./darknet detector map train_2.1_600_frames_tuned_v4/r1mini.data train_2.1_600_frames_tuned_v4/yolov4.cfg train_2.1_600_frames_tuned_v4/backup/yolov4_6000.weights",
"_____no_output_____"
],
[
"# img Test\r\n!LD_LIBRARY_PATH=/usr/local/cuda/lib64 ./darknet detector test train_2.1/r1mini.data train_2.1/yolov3-tiny.cfg train_2.1/joon.weights train_2.1/scary_4.JPG",
"_____no_output_____"
],
[
"# video test\r\n!LD_LIBRARY_PATH=/usr/local/cuda/lib64 ./darknet detector demo train_2.1_600_frames_tuned_v4/r1mini.data train_2.1_600_frames_tuned_v4/yolov4.cfg train_2.1_600_frames_tuned_v4/backup/yolov4_4000.weights ./fastandfurious_short.mov -i 0 -out_filename 600fr_v4.mov -dont_show",
"_____no_output_____"
],
[
"# ROI\r\n!LD_LIBRARY_PATH=/usr/local/cuda/lib64 ./darknet detector test train_2/r1mini.data train_2/yolov3.cfg train_2/backup/yolov3_last.weights -ext_output train_2/r1mini/test.jpg",
"_____no_output_____"
],
[
"# 폴더에 있는 모든 img를 predict하기\r\n\r\n# 변수 선언\r\n\r\n#1. predict할 파일 들어있는 폴더 (txt파일에 입력될 path기 때문에 절대경로 추천, 마지막에 꼭 '/' 넣어줘야함.)\r\nimage_directory = '/content/gdrive/MyDrive/darknet/bin/darknet/train_2.1_600_frames_tuned_v4/final_test/'\r\n#2. 확장자\r\nextension = \"*.jpg\"\r\n#3. txt파일 저장경로 및 파일 이름\r\nsave_at = './re.txt'\r\n#4. predicted_pics 저장 경로+ 파일 이름 ex) predicted_img0.jpg,predicted_img1, ~ predicted_img10 로 저장할거면 아래처럼\r\npredicted_pics_path ='/content/gdrive/MyDrive/darknet/bin/darknet/predicted_img/600_frames_v4_wt2000/'\r\n\r\n# ***************************************************************************************************\r\n# 아래는 걍 이것저것 편하게 넣어보려고 함수화한 것, 불필요하면 해당 자리에 그냥 사용하시는 커맨드 + $img_path 입력하면 됨 (.py로 선언 불가)\r\n# ex) !./darknet detector test train/r1mini.data train/yolov3.cfg train/backup/yolov3_final.weights $img_path\r\n#5. .data 파일 경로\r\ndata_path = '/content/gdrive/MyDrive/darknet/bin/darknet/train_2.1_600_frames_tuned_v4/r1mini.data'\r\n#6. config 파일 경로\r\ncfg_path = '/content/gdrive/MyDrive/darknet/bin/darknet/train_2.1_600_frames_tuned_v4/yolov4.cfg'\r\n#7. weights 파일 경로\r\nweights_path = '/content/gdrive/MyDrive/darknet/bin/darknet/train_2.1_600_frames_tuned_v4/backup/yolov4_2000.weights'\r\n\r\n#command 함수\r\ndef darknet_predict_img(data_path,cfg_path,weights_path,img_path):\r\n !LD_LIBRARY_PATH=/usr/local/cuda/lib64 ./darknet detector test $data_path $cfg_path $weights_path $img_path\r\n\r\n\r\nimport glob\r\nimport PIL\r\nimport PIL.Image as Image\r\nimport make_path_txt\r\nimport os\r\n\r\n\r\n\r\nd=0\r\nwith open(make_path_txt.make_data_path(image_directory,extension, save_at),'r') as fobj:\r\n image_List = [[num for num in line.split()] for line in fobj]\r\n for images in image_List:\r\n # print(images[0])\r\n img_path=images[0]\r\n darknet_predict_img(data_path,cfg_path,weights_path,img_path)\r\n #다크넷 경로 + prdictions.jpg\r\n predicted_image = Image.open(str(os.getcwd())+'/'+'predictions'+extension[1:])\r\n #predict 결과 저장할 경로+ 파일이름\r\n output = predicted_pics_path + '{}{}'.format(d,extension[1:])\r\n predicted_image.save(output) \r\n d+=1",
"_____no_output_____"
],
[
"# Train 중단뒤 다시 연결하기\r\n!LD_LIBRARY_PATH=/usr/local/cuda/lib64 ./darknet detector train train_3/r1mini.data train_3/yolov3.cfg train_3/backup/yolov3_2000.weights -dont_show",
"_____no_output_____"
]
]
] | [
"code"
] | [
[
"code",
"code",
"code",
"code",
"code",
"code",
"code",
"code",
"code",
"code",
"code",
"code",
"code"
]
] |
e764b6d5ace583f0dfff4b7f5e16f0c94b1201c7 | 23,470 | ipynb | Jupyter Notebook | site/en/guide/migrate/migrating_feature_columns.ipynb | jiankaiwang/docs | ab165fda010d80d82548b3797c1eaaf5803a00e8 | [
"Apache-2.0"
] | 13 | 2021-08-09T20:23:49.000Z | 2022-02-15T12:28:13.000Z | site/en/guide/migrate/migrating_feature_columns.ipynb | jiankaiwang/docs | ab165fda010d80d82548b3797c1eaaf5803a00e8 | [
"Apache-2.0"
] | null | null | null | site/en/guide/migrate/migrating_feature_columns.ipynb | jiankaiwang/docs | ab165fda010d80d82548b3797c1eaaf5803a00e8 | [
"Apache-2.0"
] | null | null | null | 35.668693 | 614 | 0.545761 | [
[
[
"##### Copyright 2021 The TensorFlow Authors.",
"_____no_output_____"
]
],
[
[
"#@title Licensed under the Apache License, Version 2.0 (the \"License\");\n# you may not use this file except in compliance with the License.\n# You may obtain a copy of the License at\n#\n# https://www.apache.org/licenses/LICENSE-2.0\n#\n# Unless required by applicable law or agreed to in writing, software\n# distributed under the License is distributed on an \"AS IS\" BASIS,\n# WITHOUT WARRANTIES OR CONDITIONS OF ANY KIND, either express or implied.\n# See the License for the specific language governing permissions and\n# limitations under the License.",
"_____no_output_____"
]
],
[
[
"# Migrating feature_columns to TF2\n\n<table class=\"tfo-notebook-buttons\" align=\"left\">\n <td>\n <a target=\"_blank\" href=\"https://www.tensorflow.org/guide/migrate/migrating_feature_columns\">\n <img src=\"https://www.tensorflow.org/images/tf_logo_32px.png\" />\n View on TensorFlow.org</a>\n </td>\n <td>\n <a target=\"_blank\" href=\"https://colab.research.google.com/github/tensorflow/docs/blob/master/site/en/guide/migrate/migrating_feature_columns.ipynb\">\n <img src=\"https://www.tensorflow.org/images/colab_logo_32px.png\" />\n Run in Google Colab</a>\n </td>\n <td>\n <a target=\"_blank\" href=\"https://github.com/tensorflow/docs/blob/master/site/en/guide/migrate/migrating_feature_columns.ipynb\">\n <img src=\"https://www.tensorflow.org/images/GitHub-Mark-32px.png\" />\n View source on GitHub</a>\n </td>\n <td>\n <a href=\"https://storage.googleapis.com/tensorflow_docs/docs/site/en/guide/migrate/migrating_feature_columns.ipynb\"><img src=\"https://www.tensorflow.org/images/download_logo_32px.png\" />Download notebook</a>\n </td>\n</table>",
"_____no_output_____"
]
],
[
[
"# Temporarily install tf-nightly as the notebook depends on symbols in 2.6.\n!pip uninstall -q -y tensorflow keras\n!pip install -q tf-nightly",
"_____no_output_____"
]
],
[
[
"Training a model will usually come with some amount of feature preprocessing, particularly when dealing with structured data. When training an `tf.estimator.Estimator` in TF1, this feature preprocessing is done with the `tf.feature_column` API. In TF2, this preprocessing can be done directly with Keras layers, called _preprocessing layers_.\n\nIn this migration guide, you will perform some common feature transformations using both feature columns and preprocessing layers, followed by training a complete model with both APIs.\n\nFirst, start with a couple of necessary imports,",
"_____no_output_____"
]
],
[
[
"import tensorflow as tf\nimport tensorflow.compat.v1 as tf1\nimport math",
"_____no_output_____"
]
],
[
[
"and add a utility for calling a feature column for demonstration:",
"_____no_output_____"
]
],
[
[
"def call_feature_columns(feature_columns, inputs):\n # This is a convenient way to call a `feature_column` outside of an estimator\n # to display its output.\n feature_layer = tf1.keras.layers.DenseFeatures(feature_columns)\n return feature_layer(inputs)",
"_____no_output_____"
]
],
[
[
"## One-hot encoding integer IDs\n\nA common feature transformation is one-hot encoding integer inputs of a known range. Here is an example using feature columns:",
"_____no_output_____"
]
],
[
[
"categorical_col = tf1.feature_column.categorical_column_with_identity(\n 'type', num_buckets=3)\nindicator_col = tf1.feature_column.indicator_column(categorical_col)\ncall_feature_columns(indicator_col, {'type': [0, 1, 2]})",
"_____no_output_____"
]
],
[
[
"Using Keras preprocessing layers, these columns can be replaced by a single `tf.keras.layers.CategoryEncoding` layer with `output_mode` set to `'one_hot'`:",
"_____no_output_____"
]
],
[
[
"one_hot_layer = tf.keras.layers.CategoryEncoding(\n num_tokens=3, output_mode='one_hot')\none_hot_layer([0, 1, 2])",
"_____no_output_____"
]
],
[
[
"## One-hot encoding string data with a vocabulary\n\nHandling string features often requires a vocabulary lookup to translate strings into indices. Here is an example using feature columns to lookup strings and then one-hot encode the indices:",
"_____no_output_____"
]
],
[
[
"vocab_col = tf1.feature_column.categorical_column_with_vocabulary_list(\n 'sizes',\n vocabulary_list=['small', 'medium', 'large'],\n num_oov_buckets=0)\nindicator_col = tf1.feature_column.indicator_column(vocab_col)\ncall_feature_columns(indicator_col, {'sizes': ['small', 'medium', 'large']})",
"_____no_output_____"
]
],
[
[
"Using Keras preprocessing layers, use the `tf.keras.layers.StringLookup` layer with `output_mode` set to `'one_hot'`:",
"_____no_output_____"
]
],
[
[
"string_lookup_layer = tf.keras.layers.StringLookup(\n vocabulary=['small', 'medium', 'large'],\n num_oov_indices=0,\n output_mode='one_hot')\nstring_lookup_layer(['small', 'medium', 'large'])",
"_____no_output_____"
]
],
[
[
"## Embedding string data with a vocabulary\n\nFor larger vocabularies, an embedding is often needed for good performance. Here is an example embedding a string feature using feature columns:",
"_____no_output_____"
]
],
[
[
"vocab_col = tf1.feature_column.categorical_column_with_vocabulary_list(\n 'col',\n vocabulary_list=['small', 'medium', 'large'],\n num_oov_buckets=0)\nembedding_col = tf1.feature_column.embedding_column(vocab_col, 4)\ncall_feature_columns(embedding_col, {'col': ['small', 'medium', 'large']})",
"_____no_output_____"
]
],
[
[
"Using Keras preprocessing layers, this can be achieved by combining a `tf.keras.layers.StringLookup` layer and an `tf.keras.layers.Embedding` layer. The default output for the `StringLookup` will be integer indices which can be fed directly into an embedding.\n\nNote that the `Embedding` layer contains trainable parameters. While the `StringLookup` layer can be applied to data inside or outside of a model, the `Embedding` must always be part of a trainable Keras model to function correctly.",
"_____no_output_____"
]
],
[
[
"string_lookup_layer = tf.keras.layers.StringLookup(\n vocabulary=['small', 'medium', 'large'], num_oov_indices=0)\nembedding = tf.keras.layers.Embedding(3, 4)\nembedding(string_lookup_layer(['small', 'medium', 'large']))",
"_____no_output_____"
]
],
[
[
"## Complete training example\n\nTo show a complete training workflow, first prepare some data with three features of different types:",
"_____no_output_____"
]
],
[
[
"features = {\n 'type': [0, 1, 1],\n 'size': ['small', 'small', 'medium'],\n 'weight': [2.7, 1.8, 1.6],\n}\nlabels = [1, 1, 0]\npredict_features = {'type': [0], 'size': ['foo'], 'weight': [-0.7]}",
"_____no_output_____"
]
],
[
[
"Define some common constants for both TF1 and TF2 workflows:",
"_____no_output_____"
]
],
[
[
"vocab = ['small', 'medium', 'large']\none_hot_dim = 3\nembedding_dim = 4\nweight_mean = 2.0\nweight_variance = 1.0",
"_____no_output_____"
]
],
[
[
"### With feature columns\n\nFeature columns must be passed as a list to the estimator on creation, and will be called implicitly during training.",
"_____no_output_____"
]
],
[
[
"categorical_col = tf1.feature_column.categorical_column_with_identity(\n 'type', num_buckets=one_hot_dim)\n# Convert index to one-hot; e.g. [2] -> [0,0,1].\nindicator_col = tf1.feature_column.indicator_column(categorical_col)\n\n# Convert strings to indices; e.g. ['small'] -> [1].\nvocab_col = tf1.feature_column.categorical_column_with_vocabulary_list(\n 'size', vocabulary_list=vocab, num_oov_buckets=1)\n# Embed the indices.\nembedding_col = tf1.feature_column.embedding_column(vocab_col, embedding_dim)\n\nnormalizer_fn = lambda x: (x - weight_mean) / math.sqrt(weight_variance)\n# Normalize the numeric inputs; e.g. [2.0] -> [0.0].\nnumeric_col = tf1.feature_column.numeric_column(\n 'weight', normalizer_fn=normalizer_fn)\n\nestimator = tf1.estimator.DNNClassifier(\n feature_columns=[indicator_col, embedding_col, numeric_col],\n hidden_units=[1])\n\ndef _input_fn():\n return tf1.data.Dataset.from_tensor_slices((features, labels)).batch(1)\n\nestimator.train(_input_fn)",
"_____no_output_____"
]
],
[
[
"The feature columns will also be used to transform input data when running inference on the model.",
"_____no_output_____"
]
],
[
[
"def _predict_fn():\n return tf1.data.Dataset.from_tensor_slices(predict_features).batch(1)\n\nnext(estimator.predict(_predict_fn))",
"_____no_output_____"
]
],
[
[
"### With Keras preprocessing layers\n\nKeras preprocessing layers are more flexible in where they can be called. A layer can be applied directly to tensors, used inside a `tf.data` input pipeline, or built directly into a trainable Keras model.\n\nIn this example, we will apply preprocessing layers inside a `tf.data` input pipeline. To do this, you can define a separate `tf.keras.Model` to preprocess your input features. This model is not trainable, but is a convenient way to group preprocessing layers.",
"_____no_output_____"
]
],
[
[
"inputs = {\n 'type': tf.keras.Input(shape=(), dtype='int64'),\n 'size': tf.keras.Input(shape=(), dtype='string'),\n 'weight': tf.keras.Input(shape=(), dtype='float32'),\n}\noutputs = {\n # Convert index to one-hot; e.g. [2] -> [0,0,1].\n 'type': tf.keras.layers.CategoryEncoding(\n one_hot_dim, output_mode='one_hot')(inputs['type']),\n # Convert size strings to indices; e.g. ['small'] -> [1].\n 'size': tf.keras.layers.StringLookup(vocabulary=vocab)(inputs['size']),\n # Normalize the numeric inputs; e.g. [2.0] -> [0.0].\n 'weight': tf.keras.layers.Normalization(\n axis=None, mean=weight_mean, variance=weight_variance)(inputs['weight']),\n}\npreprocessing_model = tf.keras.Model(inputs, outputs)",
"_____no_output_____"
]
],
[
[
"You can now apply this model inside a call to `tf.data.Dataset.map`. Please note that the function passed to `map` will automatically be converted into\na `tf.function`, and usual caveats for writing `tf.function` code apply (no side effects).",
"_____no_output_____"
]
],
[
[
"# Apply the preprocessing in tf.data.Dataset.map.\ndataset = tf.data.Dataset.from_tensor_slices((features, labels)).batch(1)\ndataset = dataset.map(lambda x, y: (preprocessing_model(x), y),\n num_parallel_calls=tf.data.AUTOTUNE)\n# Display a preprocessed input sample.\nnext(dataset.take(1).as_numpy_iterator())",
"_____no_output_____"
]
],
[
[
"Next, you can define a separate `Model` containing the trainable layers. Note how the inputs to this model now reflect the preprocessed feature types and shapes.",
"_____no_output_____"
]
],
[
[
"inputs = {\n 'type': tf.keras.Input(shape=(one_hot_dim,), dtype='float32'),\n 'size': tf.keras.Input(shape=(), dtype='int64'),\n 'weight': tf.keras.Input(shape=(), dtype='float32'),\n}\n# Since the embedding is trainable, it needs to be part of the training model.\nembedding = tf.keras.layers.Embedding(len(vocab), embedding_dim)\noutputs = tf.keras.layers.Concatenate()([\n inputs['type'],\n embedding(inputs['size']),\n tf.expand_dims(inputs['weight'], -1),\n])\noutputs = tf.keras.layers.Dense(1)(outputs)\ntraining_model = tf.keras.Model(inputs, outputs)",
"_____no_output_____"
]
],
[
[
"You can now train the `training_model` with `tf.keras.Model.fit`.",
"_____no_output_____"
]
],
[
[
"# Train on the preprocessed data.\ntraining_model.compile(\n loss=tf.keras.losses.BinaryCrossentropy(from_logits=True))\ntraining_model.fit(dataset)",
"_____no_output_____"
]
],
[
[
"Finally, at inference time, it can be useful to combine these separate stages into a single model that handles raw feature inputs.",
"_____no_output_____"
]
],
[
[
"inputs = preprocessing_model.input\noutpus = training_model(preprocessing_model(inputs))\ninference_model = tf.keras.Model(inputs, outpus)\n\npredict_dataset = tf.data.Dataset.from_tensor_slices(predict_features).batch(1)\ninference_model.predict(predict_dataset)",
"_____no_output_____"
]
],
[
[
"Note: Preprocessing layers are not trainable, which allows you to apply them *asynchronously* using with `tf.data`. This has performence benefits, as you can both [prefetch](https://www.tensorflow.org/guide/data_performance#prefetching) batches with preprocessing applied, and free up any accelerators to focus on the differentiable parts of a model. However, when training performance is not important, it is sometimes simpler to add preprocessing layers directly into a complete model. This is often the case during inference, and may also be true for smaller models or when training entirely on a CPU.",
"_____no_output_____"
],
[
"## Feature column equivalence table\n\nFor reference, here is an approximate correspondence between feature columns and\npreprocessing layers:<table>\n <tr>\n <th>Feature Column</th>\n <th>Keras Layer</th>\n </tr>\n <tr>\n <td>`feature_column.bucketized_column`</td>\n <td>`layers.Discretization`</td>\n </tr>\n <tr>\n <td>`feature_column.categorical_column_with_hash_bucket`</td>\n <td>`layers.Hashing`</td>\n </tr>\n <tr>\n <td>`feature_column.categorical_column_with_identity`</td>\n <td>`layers.CategoryEncoding`</td>\n </tr>\n <tr>\n <td>`feature_column.categorical_column_with_vocabulary_file`</td>\n <td>`layers.StringLookup` or `layers.IntegerLookup`</td>\n </tr>\n <tr>\n <td>`feature_column.categorical_column_with_vocabulary_list`</td>\n <td>`layers.StringLookup` or `layers.IntegerLookup`</td>\n </tr>\n <tr>\n <td>`feature_column.crossed_column`</td>\n <td>Not implemented.</td>\n </tr>\n <tr>\n <td>`feature_column.embedding_column`</td>\n <td>`layers.Embedding`</td>\n </tr>\n <tr>\n <td>`feature_column.indicator_column`</td>\n <td>`output_mode='one_hot'` or `output_mode='multi_hot'`*</td>\n </tr>\n <tr>\n <td>`feature_column.numeric_column`</td>\n <td>`layers.Normalization`</td>\n </tr>\n <tr>\n <td>`feature_column.sequence_categorical_column_with_hash_bucket`</td>\n <td>`layers.Hashing`</td>\n </tr>\n <tr>\n <td>`feature_column.sequence_categorical_column_with_identity`</td>\n <td>`layers.CategoryEncoding`</td>\n </tr>\n <tr>\n <td>`feature_column.sequence_categorical_column_with_vocabulary_file`</td>\n <td>`layers.StringLookup` or `layers.IntegerLookup`</td>\n </tr>\n <tr>\n <td>`feature_column.sequence_categorical_column_with_vocabulary_list`</td>\n <td>`layers.StringLookup` or `layers.IntegerLookup`</td>\n </tr>\n <tr>\n <td>`feature_column.sequence_numeric_column`</td>\n <td>`layers.Normalization`</td>\n </tr>\n <tr>\n <td>`feature_column.weighted_categorical_column`</td>\n <td>`layers.CategoryEncoding`</td>\n </tr>\n</table>\n\n\\* `output_mode` can be passed to `layers.CategoryEncoding`, `layers.StringLookup`, and `layers.IntegerLookup`.\n",
"_____no_output_____"
],
[
"## Next Steps\n\n - For more information on keras preprocessing layers, see [the guide to preprocessing layers](https://www.tensorflow.org/guide/keras/preprocessing_layers).\n - For a more in-depth example of applying preprocessing layers to structured data, see [the structured data tutorial](https://www.tensorflow.org/tutorials/structured_data/preprocessing_layers).",
"_____no_output_____"
]
]
] | [
"markdown",
"code",
"markdown",
"code",
"markdown",
"code",
"markdown",
"code",
"markdown",
"code",
"markdown",
"code",
"markdown",
"code",
"markdown",
"code",
"markdown",
"code",
"markdown",
"code",
"markdown",
"code",
"markdown",
"code",
"markdown",
"code",
"markdown",
"code",
"markdown",
"code",
"markdown",
"code",
"markdown",
"code",
"markdown",
"code",
"markdown",
"code",
"markdown"
] | [
[
"markdown"
],
[
"code"
],
[
"markdown"
],
[
"code"
],
[
"markdown"
],
[
"code"
],
[
"markdown"
],
[
"code"
],
[
"markdown"
],
[
"code"
],
[
"markdown"
],
[
"code"
],
[
"markdown"
],
[
"code"
],
[
"markdown"
],
[
"code"
],
[
"markdown"
],
[
"code"
],
[
"markdown"
],
[
"code"
],
[
"markdown"
],
[
"code"
],
[
"markdown"
],
[
"code"
],
[
"markdown"
],
[
"code"
],
[
"markdown"
],
[
"code"
],
[
"markdown"
],
[
"code"
],
[
"markdown"
],
[
"code"
],
[
"markdown"
],
[
"code"
],
[
"markdown"
],
[
"code"
],
[
"markdown"
],
[
"code"
],
[
"markdown",
"markdown",
"markdown"
]
] |
e764ba59679b5d3a558fc479ca4c53a9ee0f18db | 585,098 | ipynb | Jupyter Notebook | assignments/2019/assignment1/two_layer_net.ipynb | comratvlad/cs231n.github.io | 63c72c3e8e88a6edfea7db7df604d715416ba15b | [
"MIT"
] | null | null | null | assignments/2019/assignment1/two_layer_net.ipynb | comratvlad/cs231n.github.io | 63c72c3e8e88a6edfea7db7df604d715416ba15b | [
"MIT"
] | null | null | null | assignments/2019/assignment1/two_layer_net.ipynb | comratvlad/cs231n.github.io | 63c72c3e8e88a6edfea7db7df604d715416ba15b | [
"MIT"
] | null | null | null | 844.297258 | 256,140 | 0.952317 | [
[
[
"# Implementing a Neural Network\nIn this exercise we will develop a neural network with fully-connected layers to perform classification, and test it out on the CIFAR-10 dataset.",
"_____no_output_____"
]
],
[
[
"# A bit of setup\n\nimport numpy as np\nimport matplotlib.pyplot as plt\n\nfrom cs231n.classifiers.neural_net import TwoLayerNet\n\n%matplotlib inline\nplt.rcParams['figure.figsize'] = (10.0, 8.0) # set default size of plots\nplt.rcParams['image.interpolation'] = 'nearest'\nplt.rcParams['image.cmap'] = 'gray'\n\n# for auto-reloading external modules\n# see http://stackoverflow.com/questions/1907993/autoreload-of-modules-in-ipython\n%load_ext autoreload\n%autoreload 2\n\ndef rel_error(x, y):\n \"\"\" returns relative error \"\"\"\n return np.max(np.abs(x - y) / (np.maximum(1e-8, np.abs(x) + np.abs(y))))",
"_____no_output_____"
]
],
[
[
"We will use the class `TwoLayerNet` in the file `cs231n/classifiers/neural_net.py` to represent instances of our network. The network parameters are stored in the instance variable `self.params` where keys are string parameter names and values are numpy arrays. Below, we initialize toy data and a toy model that we will use to develop your implementation.",
"_____no_output_____"
]
],
[
[
"# Create a small net and some toy data to check your implementations.\n# Note that we set the random seed for repeatable experiments.\n\ninput_size = 4\nhidden_size = 10\nnum_classes = 3\nnum_inputs = 5\n\ndef init_toy_model():\n np.random.seed(0)\n return TwoLayerNet(input_size, hidden_size, num_classes, std=1e-1)\n\ndef init_toy_data():\n np.random.seed(1)\n X = 10 * np.random.randn(num_inputs, input_size)\n y = np.array([0, 1, 2, 2, 1])\n return X, y\n\nnet = init_toy_model()\nX, y = init_toy_data()",
"_____no_output_____"
]
],
[
[
"# Forward pass: compute scores\nOpen the file `cs231n/classifiers/neural_net.py` and look at the method `TwoLayerNet.loss`. This function is very similar to the loss functions you have written for the SVM and Softmax exercises: It takes the data and weights and computes the class scores, the loss, and the gradients on the parameters. \n\nImplement the first part of the forward pass which uses the weights and biases to compute the scores for all inputs.",
"_____no_output_____"
]
],
[
[
"scores = net.loss(X)\nprint('Your scores:')\nprint(scores)\nprint()\nprint('correct scores:')\ncorrect_scores = np.asarray([\n [-0.81233741, -1.27654624, -0.70335995],\n [-0.17129677, -1.18803311, -0.47310444],\n [-0.51590475, -1.01354314, -0.8504215 ],\n [-0.15419291, -0.48629638, -0.52901952],\n [-0.00618733, -0.12435261, -0.15226949]])\nprint(correct_scores)\nprint()\n\n# The difference should be very small. We get < 1e-7\nprint('Difference between your scores and correct scores:')\nprint(np.sum(np.abs(scores - correct_scores)))",
"Your scores:\n[[-0.81233741 -1.27654624 -0.70335995]\n [-0.17129677 -1.18803311 -0.47310444]\n [-0.51590475 -1.01354314 -0.8504215 ]\n [-0.15419291 -0.48629638 -0.52901952]\n [-0.00618733 -0.12435261 -0.15226949]]\n\ncorrect scores:\n[[-0.81233741 -1.27654624 -0.70335995]\n [-0.17129677 -1.18803311 -0.47310444]\n [-0.51590475 -1.01354314 -0.8504215 ]\n [-0.15419291 -0.48629638 -0.52901952]\n [-0.00618733 -0.12435261 -0.15226949]]\n\nDifference between your scores and correct scores:\n3.6802720745909845e-08\n"
]
],
[
[
"# Forward pass: compute loss\nIn the same function, implement the second part that computes the data and regularization loss.",
"_____no_output_____"
]
],
[
[
"loss, _ = net.loss(X, y, reg=0.05)\ncorrect_loss = 1.30378789133\n\n# should be very small, we get < 1e-12\nprint('Difference between your loss and correct loss:')\nprint(np.sum(np.abs(loss - correct_loss)))",
"Difference between your loss and correct loss:\n1.7985612998927536e-13\n"
]
],
[
[
"# Backward pass\nImplement the rest of the function. This will compute the gradient of the loss with respect to the variables `W1`, `b1`, `W2`, and `b2`. Now that you (hopefully!) have a correctly implemented forward pass, you can debug your backward pass using a numeric gradient check:",
"_____no_output_____"
]
],
[
[
"from cs231n.gradient_check import eval_numerical_gradient\n\n# Use numeric gradient checking to check your implementation of the backward pass.\n# If your implementation is correct, the difference between the numeric and\n# analytic gradients should be less than 1e-8 for each of W1, W2, b1, and b2.\n\nloss, grads = net.loss(X, y, reg=0.05)\n\n# these should all be less than 1e-8 or so\nfor param_name in grads:\n f = lambda W: net.loss(X, y, reg=0.05)[0]\n param_grad_num = eval_numerical_gradient(f, net.params[param_name], verbose=False)\n print('%s max relative error: %e' % (param_name, rel_error(param_grad_num, grads[param_name])))",
"W2 max relative error: 3.440708e-09\nb2 max relative error: 4.447677e-11\nW1 max relative error: 3.561318e-09\nb1 max relative error: 2.738421e-09\n"
]
],
[
[
"# Train the network\nTo train the network we will use stochastic gradient descent (SGD), similar to the SVM and Softmax classifiers. Look at the function `TwoLayerNet.train` and fill in the missing sections to implement the training procedure. This should be very similar to the training procedure you used for the SVM and Softmax classifiers. You will also have to implement `TwoLayerNet.predict`, as the training process periodically performs prediction to keep track of accuracy over time while the network trains.\n\nOnce you have implemented the method, run the code below to train a two-layer network on toy data. You should achieve a training loss less than 0.02.",
"_____no_output_____"
]
],
[
[
"net = init_toy_model()\nstats = net.train(X, y, X, y,\n learning_rate=1e-1, reg=5e-6,\n num_iters=100, verbose=False)\n\nprint('Final training loss: ', stats['loss_history'][-1])\n\n# plot the loss history\nplt.plot(stats['loss_history'])\nplt.xlabel('iteration')\nplt.ylabel('training loss')\nplt.title('Training Loss history')\nplt.show()",
"Final training loss: 0.01714960793873204\n"
]
],
[
[
"# Load the data\nNow that you have implemented a two-layer network that passes gradient checks and works on toy data, it's time to load up our favorite CIFAR-10 data so we can use it to train a classifier on a real dataset.",
"_____no_output_____"
]
],
[
[
"from cs231n.data_utils import load_CIFAR10\n\ndef get_CIFAR10_data(num_training=49000, num_validation=1000, num_test=1000):\n \"\"\"\n Load the CIFAR-10 dataset from disk and perform preprocessing to prepare\n it for the two-layer neural net classifier. These are the same steps as\n we used for the SVM, but condensed to a single function. \n \"\"\"\n # Load the raw CIFAR-10 data\n cifar10_dir = 'cs231n/datasets/cifar-10-batches-py'\n \n # Cleaning up variables to prevent loading data multiple times (which may cause memory issue)\n try:\n del X_train, y_train\n del X_test, y_test\n print('Clear previously loaded data.')\n except:\n pass\n\n X_train, y_train, X_test, y_test = load_CIFAR10(cifar10_dir)\n \n # Subsample the data\n mask = list(range(num_training, num_training + num_validation))\n X_val = X_train[mask]\n y_val = y_train[mask]\n mask = list(range(num_training))\n X_train = X_train[mask]\n y_train = y_train[mask]\n mask = list(range(num_test))\n X_test = X_test[mask]\n y_test = y_test[mask]\n\n # Normalize the data: subtract the mean image\n mean_image = np.mean(X_train, axis=0)\n X_train -= mean_image\n X_val -= mean_image\n X_test -= mean_image\n\n # Reshape data to rows\n X_train = X_train.reshape(num_training, -1)\n X_val = X_val.reshape(num_validation, -1)\n X_test = X_test.reshape(num_test, -1)\n\n return X_train, y_train, X_val, y_val, X_test, y_test\n\n\n# Invoke the above function to get our data.\nX_train, y_train, X_val, y_val, X_test, y_test = get_CIFAR10_data()\nprint('Train data shape: ', X_train.shape)\nprint('Train labels shape: ', y_train.shape)\nprint('Validation data shape: ', X_val.shape)\nprint('Validation labels shape: ', y_val.shape)\nprint('Test data shape: ', X_test.shape)\nprint('Test labels shape: ', y_test.shape)",
"Train data shape: (49000, 3072)\nTrain labels shape: (49000,)\nValidation data shape: (1000, 3072)\nValidation labels shape: (1000,)\nTest data shape: (1000, 3072)\nTest labels shape: (1000,)\n"
]
],
[
[
"# Train a network\nTo train our network we will use SGD. In addition, we will adjust the learning rate with an exponential learning rate schedule as optimization proceeds; after each epoch, we will reduce the learning rate by multiplying it by a decay rate.",
"_____no_output_____"
]
],
[
[
"input_size = 32 * 32 * 3\nhidden_size = 50\nnum_classes = 10\nnet = TwoLayerNet(input_size, hidden_size, num_classes)\n\n# Train the network\nstats = net.train(X_train, y_train, X_val, y_val,\n num_iters=1000, batch_size=200,\n learning_rate=1e-4, learning_rate_decay=0.95,\n reg=0.25, verbose=True)\n\n# Predict on the validation set\nval_acc = (net.predict(X_val) == y_val).mean()\nprint('Validation accuracy: ', val_acc)\n",
"iteration 0 / 1000: loss 2.302954\niteration 100 / 1000: loss 2.302550\niteration 200 / 1000: loss 2.297648\niteration 300 / 1000: loss 2.259602\niteration 400 / 1000: loss 2.204170\niteration 500 / 1000: loss 2.118565\niteration 600 / 1000: loss 2.051535\niteration 700 / 1000: loss 1.988466\niteration 800 / 1000: loss 2.006591\niteration 900 / 1000: loss 1.951473\nValidation accuracy: 0.287\n"
]
],
[
[
"# Debug the training\nWith the default parameters we provided above, you should get a validation accuracy of about 0.29 on the validation set. This isn't very good.\n\nOne strategy for getting insight into what's wrong is to plot the loss function and the accuracies on the training and validation sets during optimization.\n\nAnother strategy is to visualize the weights that were learned in the first layer of the network. In most neural networks trained on visual data, the first layer weights typically show some visible structure when visualized.",
"_____no_output_____"
]
],
[
[
"# Plot the loss function and train / validation accuracies\nplt.subplot(2, 1, 1)\nplt.plot(stats['loss_history'])\nplt.title('Loss history')\nplt.xlabel('Iteration')\nplt.ylabel('Loss')\n\nplt.subplot(2, 1, 2)\nplt.plot(stats['train_acc_history'], label='train')\nplt.plot(stats['val_acc_history'], label='val')\nplt.title('Classification accuracy history')\nplt.xlabel('Epoch')\nplt.ylabel('Classification accuracy')\nplt.legend()\nplt.show()",
"_____no_output_____"
],
[
"from cs231n.vis_utils import visualize_grid\n\n# Visualize the weights of the network\n\ndef show_net_weights(net):\n W1 = net.params['W1']\n W1 = W1.reshape(32, 32, 3, -1).transpose(3, 0, 1, 2)\n plt.imshow(visualize_grid(W1, padding=3).astype('uint8'))\n plt.gca().axis('off')\n plt.show()\n\nshow_net_weights(net)",
"_____no_output_____"
]
],
[
[
"# Tune your hyperparameters\n\n**What's wrong?**. Looking at the visualizations above, we see that the loss is decreasing more or less linearly, which seems to suggest that the learning rate may be too low. Moreover, there is no gap between the training and validation accuracy, suggesting that the model we used has low capacity, and that we should increase its size. On the other hand, with a very large model we would expect to see more overfitting, which would manifest itself as a very large gap between the training and validation accuracy.\n\n**Tuning**. Tuning the hyperparameters and developing intuition for how they affect the final performance is a large part of using Neural Networks, so we want you to get a lot of practice. Below, you should experiment with different values of the various hyperparameters, including hidden layer size, learning rate, numer of training epochs, and regularization strength. You might also consider tuning the learning rate decay, but you should be able to get good performance using the default value.\n\n**Approximate results**. You should be aim to achieve a classification accuracy of greater than 48% on the validation set. Our best network gets over 52% on the validation set.\n\n**Experiment**: You goal in this exercise is to get as good of a result on CIFAR-10 as you can (52% could serve as a reference), with a fully-connected Neural Network. Feel free implement your own techniques (e.g. PCA to reduce dimensionality, or adding dropout, or adding features to the solver, etc.).",
"_____no_output_____"
],
[
"**Explain your hyperparameter tuning process below.**\n\n$\\color{blue}{\\textit Your Answer:}$ First of all we should pick up approximate range of hyperparameters and then just check all of them in some grid by val score.",
"_____no_output_____"
]
],
[
[
"best_net = None # store the best model into this \n\n\nlearning_rates = [5e-5, 1e-4, 1e-3]\nregularization_strengths = [0.0001, 0.001, 0.1]\n\n \nresults = {}\nbest_val = -1\nbest_net = None\n\n#################################################################################\n# TODO: Tune hyperparameters using the validation set. Store your best trained #\n# model in best_net. #\n# #\n# To help debug your network, it may help to use visualizations similar to the #\n# ones we used above; these visualizations will have significant qualitative #\n# differences from the ones we saw above for the poorly tuned network. #\n# #\n# Tweaking hyperparameters by hand can be fun, but you might find it useful to #\n# write code to sweep through possible combinations of hyperparameters #\n# automatically like we did on the previous exercises. #\n#################################################################################\n# *****START OF YOUR CODE (DO NOT DELETE/MODIFY THIS LINE)*****\n\nfor lr in learning_rates:\n for reg in regularization_strengths:\n print('lr: {}, reg: {}'.format(lr, reg))\n net = TwoLayerNet(input_size, hidden_size, num_classes)\n stats = net.train(X_train, y_train, X_val, y_val,\n num_iters=1600, batch_size=256,\n learning_rate=lr, learning_rate_decay=0.95,\n reg=reg, verbose=False)\n y_train_pred = net.predict(X_train)\n train_acc = np.mean(y_train == y_train_pred)\n\n val_acc = (net.predict(X_val) == y_val).mean()\n print('Validation accuracy: ', val_acc)\n results[(lr, reg)] = val_acc\n if val_acc > best_val:\n best_val = val_acc\n best_net = net \n\n# *****END OF YOUR CODE (DO NOT DELETE/MODIFY THIS LINE)*****",
"lr: 5e-05, reg: 0.0001\nValidation accuracy: 0.264\nlr: 5e-05, reg: 0.001\nValidation accuracy: 0.272\nlr: 5e-05, reg: 0.1\nValidation accuracy: 0.277\nlr: 0.0001, reg: 0.0001\nValidation accuracy: 0.35\nlr: 0.0001, reg: 0.001\nValidation accuracy: 0.35\nlr: 0.0001, reg: 0.1\nValidation accuracy: 0.362\nlr: 0.001, reg: 0.0001\nValidation accuracy: 0.48\nlr: 0.001, reg: 0.001\nValidation accuracy: 0.508\nlr: 0.001, reg: 0.1\nValidation accuracy: 0.488\n"
],
[
"# visualize the weights of the best network\nshow_net_weights(best_net)",
"_____no_output_____"
]
],
[
[
"# Run on the test set\nWhen you are done experimenting, you should evaluate your final trained network on the test set; you should get above 48%.",
"_____no_output_____"
]
],
[
[
"test_acc = (best_net.predict(X_test) == y_test).mean()\nprint('Test accuracy: ', test_acc)",
"Test accuracy: 0.491\n"
]
],
[
[
"**Inline Question**\n\nNow that you have trained a Neural Network classifier, you may find that your testing accuracy is much lower than the training accuracy. In what ways can we decrease this gap? Select all that apply.\n\n1. Train on a larger dataset.\n2. Add more hidden units.\n3. Increase the regularization strength.\n4. None of the above.\n\n$\\color{blue}{\\textit Your Answer:}$ 1, 3\n\n$\\color{blue}{\\textit Your Explanation:}$ we can decrease the gap between train and test accuracy via use more data because it will more difficult to overfit. Bigger capacity (more hidden units) could increase train and test accuracy but not likely to decrease gap between them. More regularization also can help with overfitting, but in our case when the train accuracy is low it's more likely that model with good capacity or more suitable architecture will help better.",
"_____no_output_____"
]
]
] | [
"markdown",
"code",
"markdown",
"code",
"markdown",
"code",
"markdown",
"code",
"markdown",
"code",
"markdown",
"code",
"markdown",
"code",
"markdown",
"code",
"markdown",
"code",
"markdown",
"code",
"markdown",
"code",
"markdown"
] | [
[
"markdown"
],
[
"code"
],
[
"markdown"
],
[
"code"
],
[
"markdown"
],
[
"code"
],
[
"markdown"
],
[
"code"
],
[
"markdown"
],
[
"code"
],
[
"markdown"
],
[
"code"
],
[
"markdown"
],
[
"code"
],
[
"markdown"
],
[
"code"
],
[
"markdown"
],
[
"code",
"code"
],
[
"markdown",
"markdown"
],
[
"code",
"code"
],
[
"markdown"
],
[
"code"
],
[
"markdown"
]
] |
e764bc3dd45c6f374a1eaca84ec0c381042328ed | 25,205 | ipynb | Jupyter Notebook | extract_ddos_feature.ipynb | nyu-tandon-hsn-ai/tcp-trace-feature-selection | 90126ffd37d7a000ca5f9266c5d0dea225d757a0 | [
"Apache-2.0"
] | 1 | 2019-11-29T14:07:46.000Z | 2019-11-29T14:07:46.000Z | extract_ddos_feature.ipynb | nyu-tandon-hsn-ai/trace-feature-selection | 90126ffd37d7a000ca5f9266c5d0dea225d757a0 | [
"Apache-2.0"
] | 19 | 2018-04-06T00:34:46.000Z | 2018-07-19T17:40:29.000Z | extract_ddos_feature.ipynb | nyu-tandon-hsn-ai/tcp-trace-feature-selection | 90126ffd37d7a000ca5f9266c5d0dea225d757a0 | [
"Apache-2.0"
] | 1 | 2018-07-08T20:21:05.000Z | 2018-07-08T20:21:05.000Z | 38.016591 | 420 | 0.458719 | [
[
[
"import os\nimport os.path\nimport sys\nimport pandas as pd\nimport numpy as np",
"_____no_output_____"
],
[
"LOCAL_PATH = 'data'\nRAW_TRACE = 'ashakan_raw.pcapng'\nTRACE_FEATURE_FILE = 'tcp_flow_features.csv'\nBUCKET_NAME = 'edu.nyu.hsn.ddos-data' # replace with your bucket name\nKEY = 'CAP_NIC1_00931_20130727230801.dms' # replace with your object key",
"_____no_output_____"
],
[
"if not os.path.exists(os.path.join(LOCAL_PATH, RAW_TRACE)):\n if not os.path.exists(LOCAL_PATH):\n os.mkdir(LOCAL_PATH)\n \n import boto3\n import botocore\n\n s3 = boto3.resource('s3')\n\n try:\n s3.Bucket(BUCKET_NAME).download_file(KEY, os.path.join(LOCAL_PATH, RAW_TRACE))\n except botocore.exceptions.ClientError as e:\n if e.response['Error']['Code'] == \"404\":\n print(\"The object does not exist.\")\n else:\n raise",
"_____no_output_____"
],
[
"import subprocess\nif not os.path.exists(os.path.join(LOCAL_PATH, TRACE_FEATURE_FILE)):\n tshark_command = subprocess.Popen('tshark -r {} -Y tcp -T fields -e ip.src -e ip.dst -e tcp.srcport -e tcp.dstport -e tcp.len -e frame.time_relative -e tcp.seq -e tcp.ack -e tcp.flags.ack -e tcp.flags.syn -e tcp.flags.fin -e tcp.stream -Eheader=y -Eseparator=, > {}'.format(LOCAL_PATH + \"/\" + RAW_TRACE, LOCAL_PATH + \"/\" + TRACE_FEATURE_FILE), shell=True, stdout=subprocess.PIPE, stderr=subprocess.PIPE)\n out_data, err_data = tshark_command.communicate()\n out_data, err_data = out_data.decode('utf-8'), err_data.decode('utf-8')\n if err_data != '':\n pass\n# print(err_data)",
"_____no_output_____"
],
[
"trace_df = pd.read_csv(os.path.join(LOCAL_PATH, TRACE_FEATURE_FILE))\ntrace_df['src_addr'] = trace_df['ip.src'] + \":\" + trace_df['tcp.srcport'].apply(str)\ntrace_df['dst_addr'] = trace_df['ip.dst'] + \":\" + trace_df['tcp.dstport'].apply(str)",
"_____no_output_____"
],
[
"trace_df.shape",
"_____no_output_____"
],
[
"trace_df.dtypes",
"_____no_output_____"
],
[
"trace_df.describe()",
"_____no_output_____"
],
[
"trace_df.head()",
"_____no_output_____"
],
[
"# run 'jupyter nbextension enable --py --sys-prefix widgetsnbextension' first\nfrom tqdm import tqdm\ndef to_feature_df(raw_trace_df,sampling_rate=1.0,upsampled=False):\n def calculate_two_way_tcp(df):\n def get_statistical_features(df, criter, feature_name,name_pred):\n # upsampling\n feature_avg = df[criter][feature_name].mean()\n feature_avg = -1 if pd.isnull(feature_avg) else feature_avg\n feature_min = df[criter][feature_name].min()\n feature_min = -1 if pd.isnull(feature_min) else feature_min\n feature_max = df[criter][feature_name].max()\n feature_max = -1 if pd.isnull(feature_max) else feature_max\n feature_std = df[criter][feature_name].std()\n feature_std = -1 if pd.isnull(feature_std) else feature_std\n feature_sum = df[criter][feature_name].sum()\n feature_sum = -1 if pd.isnull(feature_sum) else feature_sum / sampling_rate if upsampled else feature_sum\n feature_count = df[criter][feature_name].count()\n feature_count = feature_count / sampling_rate if upsampled else feature_count\n return {'avg('+name_pred+')':feature_avg,'std('+name_pred+')':feature_std,'min('+name_pred+')':feature_min,'max('+name_pred+')':feature_max,'count('+name_pred[0:8]+')':feature_count, 'sum('+name_pred+')':feature_sum}\n \n addrs = list(set(np.append(df['src_addr'].unique(), df['dst_addr'].unique())))\n if len(addrs) != 2:\n raise\n stat = get_statistical_features(df, df['src_addr'] == addrs[0],'tcp.len','forw_pkt_len')\n stat.update(get_statistical_features(df, df['src_addr'] == addrs[1],'tcp.len','back_pkt_len'))\n return pd.Series(stat)\n\n trace_df = raw_trace_df\n tcp_flow_df = pd.DataFrame()\n # upsampling\n tcp_flow_df['avg(tcp_pkt_len)'] = trace_df.groupby('tcp.stream')['tcp.len'].mean()\n tcp_flow_df['stddev(tcp_pkt_len)'] = trace_df.groupby('tcp.stream')['tcp.len'].std().fillna(-1)\n tcp_flow_df['min(tcp_pkt_len)'] = trace_df.groupby('tcp.stream')['tcp.len'].min()\n tcp_flow_df['max(tcp_pkt_len)'] = trace_df.groupby('tcp.stream')['tcp.len'].max()\n tcp_flow_df['tot_pkt'] = trace_df.groupby('tcp.stream')['tcp.len'].count()\n tcp_flow_df['tot_byte'] = trace_df.groupby('tcp.stream')['tcp.len'].sum()\n tcp_flow_df['rel_start'] = trace_df.groupby('tcp.stream')['frame.time_relative'].min()\n tcp_flow_df['duration'] = trace_df.groupby('tcp.stream')['frame.time_relative'].max() - tcp_flow_df['rel_start']\n if not upsampled:\n tqdm.pandas(desc='{} samp rate no upsampling'.format(sampling_rate))\n else:\n tcp_flow_df['tot_pkt'] /= sampling_rate\n tcp_flow_df['tot_byte'] /= sampling_rate\n tqdm.pandas(desc='{} samp rate with upsampling'.format(sampling_rate))\n two_way_flow_df = trace_df.groupby('tcp.stream')[['tcp.len','src_addr','dst_addr']].progress_apply(calculate_two_way_tcp)\n tcp_flow_df = pd.concat([tcp_flow_df,two_way_flow_df],axis=1)\n return tcp_flow_df\n\ndef sample_trace(raw_trace_df,sampling_rate):\n import time\n return raw_trace_df.sample(frac=sampling_rate, random_state=int(time.time()))",
"_____no_output_____"
],
[
"SAMPLING_RATES = [5,10,15,20,40,60,80,100]",
"_____no_output_____"
],
[
"# run 'jupyter nbextension enable --py --sys-prefix widgetsnbextension' first\nfrom tqdm import tqdm_notebook\n# packet-based random sampling\nfor sampling_percent in tqdm_notebook(SAMPLING_RATES,desc='Sampling'):\n sampling_rate = sampling_percent / 100.0\n sampled_df = sample_trace(trace_df, sampling_rate)\n if sampling_percent < 100:\n to_feature_df(sampled_df, sampling_rate, upsampled=False).to_csv(os.path.join(LOCAL_PATH,'packet_rand_{PERCENT}%_no_upsampling.csv'.format(PERCENT=sampling_percent)))\n to_feature_df(sampled_df, sampling_rate, upsampled=True).to_csv(os.path.join(LOCAL_PATH,'packet_rand_{PERCENT}%_with_upsampling.csv'.format(PERCENT=sampling_percent)))\n elif sampling_percent == 100:\n to_feature_df(sampled_df, sampling_rate, upsampled=False).to_csv('{PATH}/packet_full.csv'.format(PATH=LOCAL_PATH))\n else:\n raise",
"_____no_output_____"
]
]
] | [
"code"
] | [
[
"code",
"code",
"code",
"code",
"code",
"code",
"code",
"code",
"code",
"code",
"code",
"code"
]
] |
e764c14e97f4d4c5ab778ea6d845401954f397aa | 26,918 | ipynb | Jupyter Notebook | dimer_pipe.ipynb | asy1113/NLP_PE | b0a31bb2c80754da20ee68ec4073013a18767577 | [
"Apache-2.0"
] | null | null | null | dimer_pipe.ipynb | asy1113/NLP_PE | b0a31bb2c80754da20ee68ec4073013a18767577 | [
"Apache-2.0"
] | null | null | null | dimer_pipe.ipynb | asy1113/NLP_PE | b0a31bb2c80754da20ee68ec4073013a18767577 | [
"Apache-2.0"
] | null | null | null | 31.446262 | 179 | 0.437663 | [
[
[
"# dimer pipeline",
"_____no_output_____"
],
[
"### Read filenames",
"_____no_output_____"
]
],
[
[
"import os\nunid = 'u0496358'\npath = \"/home/\"+str(unid)+\"/BRAT/\"+str(unid)+\"/Project_pe_test\" \nfiles = os.listdir(path)\n\nlen(files)",
"_____no_output_____"
]
],
[
[
"## define regular expression",
"_____no_output_____"
]
],
[
[
"import re\n \nrule=r'(?P<name>(d-dimer|ddimer))(?P<n1>.{1,25}?)(?P<value>[0-9]{1,4}(\\.[0-9]{0,3})?\\s*)(?P<n2>[^\\n\\w\\d]*)(?P<unit>(ug\\/l|ng\\/ml|mg\\/l|nmol\\/l)?)'\nrule1=r'(elevated|pos|positive|increased|high|\\+)(.{1,20})?(\\n)?\\s?(d-dimer|d\\s?dimer)' \nrule2=r'(d-dimer|d\\s?dimer)([^0-9-:]{1,15})?(positive|pos)'\nneg_regex = '(\\\\bno\\\\b|denies)'\n",
"_____no_output_____"
]
],
[
[
"## d-dimer pipeline apply rules",
"_____no_output_____"
]
],
[
[
"def ddimer_val(rule='rule', rule1='rule1', rule2='rule2', file_txt='note'):\n\n # import libraries\n import re\n from pipeUtils import Annotation\n from pipeUtils import Document\n\n # initite Document obj\n file1a = ''\n doc = Document()\n doc.load_document_from_file(file_txt) \n\n # change to lower case\n doc.text = doc.text.lower()\n \n####################################################################################### \n# match name value unit in note e.g. d-dimer 123.456 ng/mL\n \n # for rule in rules: # different process, cannot repeat.\n # compile and match in note text \n pattern=re.compile(rule)\n matches=pattern.finditer(doc.text) \n\n ann_index=0\n for match in matches:\n ann_id = 'NLP_'+ str(ann_index)\n ann_index=ann_index+1\n\n # check value and unit, then nomalize value\n if match.group('value') != None:\n value = float(match.group('value')) # mg/L*1000, ug/L, ng/mL, nmol/L*186\n if match.group('unit')=='mg/l':\n value = value * 1000\n if match.group('unit')=='nmol/l':\n value = value * 186\n # compare the value\n if value < 500:\n label = 'low_ddimer' \n else:\n label = 'high_ddimer' \n\n # Add new annotation\n new_annotation = Annotation(start_index=int(match.start()), \n end_index=int(match.end()), \n type=label,\n ann_id = ann_id\n )\n new_annotation.spanned_text = doc.text[new_annotation.start_index:new_annotation.end_index]\n\n # Check negation right before the found target up to 35 charachers before, \n # making sure that the pre-text does not cross the text boundary and is valid\n if new_annotation.start_index - 35 > 0:\n pre_text_start = new_annotation.start_index - 35\n else:\n pre_text_start = 0\n\n # ending index of the pre_text is the beginning of the found target \n pre_text_end = new_annotation.start_index \n\n # substring the document text to identify the pre_text string\n pre_text = doc.text[pre_text_start: pre_text_end]\n\n if value < 500:\n new_annotation.attributes[\"Negation\"] ='Negated'\n doc.annotations.append(new_annotation)\n \n\n#######################################################################################\n# annotate Target 2: Modifier + Name\n\n # compile and match in note text \n pattern1=re.compile(rule1)\n matches1=pattern1.finditer(doc.text) # match positive/+ d-dimer in note\n\n for match1 in matches1:\n ann_id = 'NLP_'+ str(ann_index)\n ann_index=ann_index+1\n new_annotation = Annotation(start_index=int(match1.start()), \n end_index=int(match1.end()), \n type='high_ddimer',\n ann_id = ann_id\n )\n new_annotation.spanned_text = doc.text[new_annotation.start_index:new_annotation.end_index]\n\n # Check negation right before the found target up to 30 charachers before, \n # making sure that the pre-text does not cross the text boundary and is valid\n\n if new_annotation.start_index - 30 > 0:\n pre_text_start = new_annotation.start_index - 30\n else:\n pre_text_start = 0\n\n # ending index of the pre_text is the beginning of the found target \n pre_text_end = new_annotation.start_index \n\n # substring the document text to identify the pre_text string\n pre_text = doc.text[pre_text_start: pre_text_end]\n\n # We do not need to know the exact location of the negation keyword, so re.search is acceptable\n if re.search(neg_regex, pre_text , re.IGNORECASE):\n new_annotation.attributes[\"Negation\"] ='Negated'\n doc.annotations.append(new_annotation)\n \n#######################################################################################\n# match d-dimer + positive in note\n\n pattern2=re.compile(rule2)\n matches2=pattern2.finditer(doc.text) \n\n for match2 in matches2:\n ann_id = 'NLP_'+ str(ann_index)\n ann_index=ann_index+1\n new_annotation = Annotation(start_index=int(match2.start()), \n end_index=int(match2.end()), \n type='high_ddimer',\n ann_id = ann_id\n )\n new_annotation.spanned_text = doc.text[new_annotation.start_index:new_annotation.end_index]\n\n # Check negation right before the found target up to 30 charachers before, \n # making sure that the pre-text does not cross the text boundary and is valid\n\n if new_annotation.start_index - 30 > 0:\n pre_text_start = new_annotation.start_index - 30\n else:\n pre_text_start = 0\n\n # ending index of the pre_text is the beginning of the found target \n pre_text_end = new_annotation.start_index \n\n # substring the document text to identify the pre_text string\n pre_text = doc.text[pre_text_start: pre_text_end]\n\n # We do not need to know the exact location of the negation keyword, so re.search is acceptable\n if re.search(neg_regex, pre_text , re.IGNORECASE):\n new_annotation.attributes[\"Negation\"] ='Negated'\n doc.annotations.append(new_annotation)\n \n return doc.annotations ",
"_____no_output_____"
]
],
[
[
"## Apply the pipeline",
"_____no_output_____"
]
],
[
[
"import chardet\n\ndoc_annotations=dict()\n\nnote_count = 0 # count the number of text notes want to process ***\nfor i in files[:]:\n if \".txt\" in i:\n doc_file = os.path.join(path,i)\n #note_count = note_count + 1 #\n #if note_count > 2: # count the number of text notes want to process ***\n # break #\n \n note_annotations = ddimer_val(rule=rule, rule1=rule1, rule2=rule2, file_txt=doc_file)\n\n doc_annotations[i] = note_annotations\n",
"_____no_output_____"
]
],
[
[
"## Append annotation dataframes to annotation files",
"_____no_output_____"
]
],
[
[
"\nfor k in doc_annotations: # dict of annotations\n \n k0=k.split('.')[0]\n k1=k0+'.nlp' \n\n nlp_ann=''\n for doc_ann in doc_annotations[k]: # doc_ann is line of mention ann in doc annotation\n \n nlp_ann = nlp_ann + doc_ann.ann_id +'\\t' \n nlp_ann = nlp_ann + doc_ann.type +' '\n nlp_ann = nlp_ann + str(doc_ann.start_index) +' '\n nlp_ann = nlp_ann + str(doc_ann.end_index) +'\\t'\n nlp_ann = nlp_ann + doc_ann.spanned_text +'\\n' \n\n nlpann='nlpann/'+k1\n with open(nlpann, 'a') as myfile:\n myfile.write(nlp_ann)\n",
"_____no_output_____"
]
],
[
[
"## doc classification",
"_____no_output_____"
]
],
[
[
"\ndoc_cls_results={}\nfor k in doc_annotations: # dict of annotations\n doc_cls_results[k]='low_ddimer'\n for doc_ann in doc_annotations[k]:\n if doc_ann.type =='high_ddimer':\n doc_cls_results[k]='high_ddimer'\nfor k in doc_cls_results:\n print(k, '-----', doc_cls_results[k])",
"90688_292.txt ----- high_ddimer\n65675_64.txt ----- high_ddimer\n48640_63.txt ----- low_ddimer\n86087_123.txt ----- high_ddimer\n83838_106.txt ----- high_ddimer\n72554_306.txt ----- high_ddimer\n15899_182.txt ----- high_ddimer\n13867_266.txt ----- high_ddimer\n61180_73.txt ----- high_ddimer\n32113_141.txt ----- high_ddimer\n59381_293.txt ----- high_ddimer\n58515_159.txt ----- high_ddimer\n6878_279.txt ----- high_ddimer\n820_14.txt ----- high_ddimer\n32113_109.txt ----- high_ddimer\n49079_68.txt ----- high_ddimer\n10568_20.txt ----- high_ddimer\n25764_268.txt ----- high_ddimer\n1498_225.txt ----- high_ddimer\n82326_55.txt ----- high_ddimer\n"
]
],
[
[
"## Select one doc annotation for mention level evaluation",
"_____no_output_____"
]
],
[
[
"k = '90688_292.txt'\nann1 = doc_annotations[k]\nprint(ann1)",
"[<pipeUtils.Annotation object at 0x7fa074ff0ef0>, <pipeUtils.Annotation object at 0x7fa074ebe7b8>]\n"
]
],
[
[
"## read annotation and convert to dataframe",
"_____no_output_____"
]
],
[
[
"import numpy as np\nimport pandas as pd\n\nnlp_list=[]\nfor a in ann1: \n list1=[a.ann_id, a.type, a.start_index, a.end_index, a.spanned_text]\n nlp_list.append(list1)\nnlp_list \n\nnlp_df = pd.DataFrame(nlp_list, columns=['markup_id','type','start','end','txt']) \nnlp_df",
"_____no_output_____"
]
],
[
[
"## convert df to annotation object, compare two annotations",
"_____no_output_____"
]
],
[
[
"def df2ann(df=[], pdoc_type='', ndoc_type=''):\n from pipeUtils import Annotation\n from pipeUtils import Document\n\n #ann_obj=Annotation()\n Annotations1=[]\n for index, row in df.iterrows() :\n\n if (pdoc_type == row['type'] or ndoc_type == row['type']):\n continue\n ann_obj=Annotation(start_index=row['start'], end_index=row['end'], type=row['type'], spanned_text=row['txt'], ann_id=row['markup_id'])\n Annotations1.append(ann_obj)\n\n return Annotations1\n\n###############################################################################################\n\ndef compare2ann_types_by_span(ref_ann=[], sys_ann=[], ref_type ='Annotation', sys_type='Annotation', exact=True):\n tp, fp, fn = 0,0,0\n tp_list = []\n fp_list = []\n fn_list = []\n ref_anns = []\n sys_anns = []\n\n # Split annotations of different types into two lists\n for a in ref_ann:\n if(a.type == ref_type):\n ref_anns.append(a)\n for a in sys_ann:\n if(a.type == sys_type):\n sys_anns.append(a)\n\n # Count tp and fp\n for sys_ann in sys_anns:\n tp_flag = False\n matching_ref = None\n for ref_ann in ref_anns:\n if exact:\n if(sys_ann.exactMatch(ref_ann)):\n tp_flag=True\n matching_ref = ref_ann\n else:\n if sys_ann.overlaps(ref_ann):\n tp_flag = True\n matching_ref = ref_ann\n if tp_flag:\n tp = tp + 1\n tp_list.append([sys_ann, matching_ref])\n else:\n fp = fp + 1\n fp_list.append(sys_ann)\n\n # Count fn\n for ref_ann in ref_anns:\n tp_flag = False\n for sys_ann in sys_anns:\n if exact:\n if(ref_ann.exactMatch(sys_ann)):\n tp_flag=True\n else:\n if ref_ann.overlaps(sys_ann):\n tp_flag = True\n if not tp_flag:\n fn = fn + 1\n fn_list.append(ref_ann)\n\n return tp, fp, fn, tp_list, fp_list, fn_list",
"_____no_output_____"
]
],
[
[
"## Convert d-dimer nlp to annotation obj",
"_____no_output_____"
]
],
[
[
"Annotations2=df2ann(df=nlp_df, pdoc_type='positive_DOC', ndoc_type='negative_DOC')\nfor a in Annotations2:\n print (a.ann_id, a.type, a.start_index, a.end_index, a.spanned_text)",
"NLP_0 high_ddimer 398 414 elevated d-dimer\nNLP_1 high_ddimer 2023 2039 elevated d-dimer\n"
]
],
[
[
"## read manual ref_ann and convert to df",
"_____no_output_____"
]
],
[
[
"import os\nunid = 'u0496358'\npath = \"/home/\"+str(unid)+\"/BRAT/\"+str(unid)+\"/Project_pe_test\" \n\nann_file='90688_292.ann'",
"_____no_output_____"
],
[
"# read ann and convert to df\n\nimport numpy as np\nimport pandas as pd\n\nannoList = []\n\nwith open(os.path.join(path,ann_file)) as f:\n ann_file = f.read()\nann_file=ann_file.split('\\n')\n\nfor line in ann_file:\n\n if(line.startswith('T')):\n line=line.replace('\\n', '')\n line=line.split('\\t')\n\n line0=line[0]\n line2=line[2]\n line1=line[1].split(' ')\n \n if (';' in line1[2]):\n line1.remove(line1[2]) # remove middle span of annotated phrase seprated in 2 line, keep the annotation.\n \n annList = []\n annList.append(line[0])\n annList.extend(line1)\n annList.append(line[2])\n annoList.append(annList)\n#print(annoList) \n \nann_df = pd.DataFrame(annoList, columns=['markup_id','type','start','end','txt']) \nann_df\n#ann_file",
"_____no_output_____"
]
],
[
[
"## convert manual ref_ann df to annotation obj",
"_____no_output_____"
]
],
[
[
"Annotations3=df2ann(ann_df, pdoc_type='positive_DOC', ndoc_type='negative_DOC')\nfor a in Annotations2:\n print (a.ann_id, a.type, a.start_index, a.end_index, a.spanned_text)",
"NLP_0 high_ddimer 398 414 elevated d-dimer\nNLP_1 high_ddimer 2023 2039 elevated d-dimer\n"
]
],
[
[
"## Mention Level Evaluation",
"_____no_output_____"
]
],
[
[
"tp, fp, fn, tp_list, fp_list, fn_list = compare2ann_types_by_span(ref_ann=Annotations2, sys_ann=Annotations3, ref_type ='high_ddimer', sys_type='high_ddimer', exact=True)\nprint(\"tp, fp, fn\")\nprint(tp, fp, fn)\nprint(\"-----fn_list-----\")\nfor i in fn_list:\n print(i.ann_id, i.start_index, i.end_index)",
"tp, fp, fn\n0 0 2\n-----fn_list-----\nNLP_0 398 414\nNLP_1 2023 2039\n"
]
]
] | [
"markdown",
"code",
"markdown",
"code",
"markdown",
"code",
"markdown",
"code",
"markdown",
"code",
"markdown",
"code",
"markdown",
"code",
"markdown",
"code",
"markdown",
"code",
"markdown",
"code",
"markdown",
"code",
"markdown",
"code",
"markdown",
"code"
] | [
[
"markdown",
"markdown"
],
[
"code"
],
[
"markdown"
],
[
"code"
],
[
"markdown"
],
[
"code"
],
[
"markdown"
],
[
"code"
],
[
"markdown"
],
[
"code"
],
[
"markdown"
],
[
"code"
],
[
"markdown"
],
[
"code"
],
[
"markdown"
],
[
"code"
],
[
"markdown"
],
[
"code"
],
[
"markdown"
],
[
"code"
],
[
"markdown"
],
[
"code",
"code"
],
[
"markdown"
],
[
"code"
],
[
"markdown"
],
[
"code"
]
] |
e764ca55fd4af97346094dfcbd8a39e8816ae74e | 16,549 | ipynb | Jupyter Notebook | notebook/_PCA/KernelPCA_Censo.ipynb | victorblois/py_datascience | bf5d05a0a4d9e26f55f9259d973f7f4f54432e24 | [
"MIT"
] | 1 | 2020-05-11T22:22:55.000Z | 2020-05-11T22:22:55.000Z | notebook/_PCA/KernelPCA_Censo.ipynb | victorblois/py_datascience | bf5d05a0a4d9e26f55f9259d973f7f4f54432e24 | [
"MIT"
] | null | null | null | notebook/_PCA/KernelPCA_Censo.ipynb | victorblois/py_datascience | bf5d05a0a4d9e26f55f9259d973f7f4f54432e24 | [
"MIT"
] | null | null | null | 62.214286 | 1,464 | 0.659677 | [
[
[
"import pandas as pd\npd.options.display.float_format = '{:.5f}'.format\nimport numpy as np\nnp.set_printoptions(precision=4)\nnp.set_printoptions(suppress=True)\nimport warnings\nwarnings.filterwarnings(\"ignore\")",
"_____no_output_____"
],
[
"import os.path\ndef path_base(base_name):\n current_dir = os.path.abspath(os.path.join(os.getcwd(), os.pardir))\n print(current_dir)\n data_dir = current_dir.replace('notebook','data')\n print(data_dir)\n data_base = data_dir + '\\\\' + base_name\n print(data_base)\n return data_base",
"_____no_output_____"
],
[
"base = pd.read_csv(path_base('db_censo.csv'))",
"C:\\MyPhyton\\DataScience\\notebook\nC:\\MyPhyton\\DataScience\\data\nC:\\MyPhyton\\DataScience\\data\\db_censo.csv\n"
],
[
"previsores = base.iloc[:, 0:14].values\nclasse = base.iloc[:, 14].values",
"_____no_output_____"
],
[
"from sklearn.preprocessing import LabelEncoder\nlabelencoder_previsores = LabelEncoder()\nprevisores[:, 1] = labelencoder_previsores.fit_transform(previsores[:, 1])\nprevisores[:, 3] = labelencoder_previsores.fit_transform(previsores[:, 3])\nprevisores[:, 5] = labelencoder_previsores.fit_transform(previsores[:, 5])\nprevisores[:, 6] = labelencoder_previsores.fit_transform(previsores[:, 6])\nprevisores[:, 7] = labelencoder_previsores.fit_transform(previsores[:, 7])\nprevisores[:, 8] = labelencoder_previsores.fit_transform(previsores[:, 8])\nprevisores[:, 9] = labelencoder_previsores.fit_transform(previsores[:, 9])\nprevisores[:, 13] = labelencoder_previsores.fit_transform(previsores[:, 13])",
"_____no_output_____"
],
[
"from sklearn.preprocessing import StandardScaler\nscaler = StandardScaler()\nprevisores = scaler.fit_transform(previsores)",
"_____no_output_____"
],
[
"from sklearn.model_selection import train_test_split\nprevisores_treinamento, previsores_teste, classe_treinamento, classe_teste = train_test_split(previsores, classe, test_size=0.15, random_state=0)",
"_____no_output_____"
],
[
"from sklearn.decomposition import KernelPCA\nkpca = KernelPCA(n_components = 6,kernel='rbf')\nprevisores_treinamento = kpca.fit_transform(previsores_treinamento)\nprevisores_teste = kpca.transform(previsores_teste)\n",
"_____no_output_____"
],
[
"len(previsores_treinamento)\n",
"_____no_output_____"
],
[
"previsores_treinamento[0:3,:]",
"_____no_output_____"
],
[
"from sklearn.ensemble import RandomForestClassifier\nclassificador = RandomForestClassifier(n_estimators = 40, criterion = 'entropy', random_state = 0)\nclassificador.fit(previsores_treinamento, classe_treinamento)\nprevisoes = classificador.predict(previsores_teste)\nprevisoes",
"_____no_output_____"
],
[
"from sklearn.metrics import confusion_matrix, accuracy_score\nprecisao = accuracy_score(classe_teste, previsoes)",
"_____no_output_____"
],
[
"precisao",
"_____no_output_____"
]
]
] | [
"code"
] | [
[
"code",
"code",
"code",
"code",
"code",
"code",
"code",
"code",
"code",
"code",
"code",
"code",
"code"
]
] |
e764cc9065a650957a86c76dbdc0ddd39498e345 | 171,351 | ipynb | Jupyter Notebook | notebooks/.ipynb_checkpoints/deep_learning_object_recognition-checkpoint.ipynb | daniel-acuna/ist718 | 0a83f373aa00dc9cd1ff2e8da74d0255f04c9728 | [
"BSD-4-Clause-UC"
] | 13 | 2018-09-17T14:02:59.000Z | 2021-08-31T19:08:07.000Z | notebooks/.ipynb_checkpoints/deep_learning_object_recognition-checkpoint.ipynb | wozhouwozhou/ist718 | 565e9767f6f35f77f9c14f2a94b2d75a0a6e2c02 | [
"BSD-4-Clause-UC"
] | 5 | 2020-03-24T15:51:10.000Z | 2021-12-13T19:48:14.000Z | notebooks/.ipynb_checkpoints/deep_learning_object_recognition-checkpoint.ipynb | wozhouwozhou/ist718 | 565e9767f6f35f77f9c14f2a94b2d75a0a6e2c02 | [
"BSD-4-Clause-UC"
] | 9 | 2018-09-25T13:35:39.000Z | 2021-09-04T15:29:42.000Z | 139.878367 | 77,896 | 0.875139 | [
[
[
"# Object recognition with deep learning",
"_____no_output_____"
],
[
"Load preliminary packages and launch a Spark connection",
"_____no_output_____"
]
],
[
[
"from pyspark.sql import SparkSession\nfrom pyspark.ml import feature\nfrom pyspark.ml import classification\nfrom pyspark.sql import functions as fn\nfrom pyspark.ml import Pipeline\nfrom pyspark.ml.evaluation import BinaryClassificationEvaluator, \\\n MulticlassClassificationEvaluator, \\\n RegressionEvaluator\nfrom pyspark.ml.tuning import CrossValidator, ParamGridBuilder\nimport matplotlib.pyplot as plt\nimport seaborn as sns\nimport pandas as pd\nimport numpy as np\n\nfrom pyspark.sql import SparkSession\n\n\nspark = SparkSession.builder.getOrCreate()\nsc = spark.sparkContext\n%matplotlib inline",
"_____no_output_____"
],
[
"# utility function to display the first element of a Spark dataframe as an image\ndef display_first_as_img(df):\n plt.imshow(df.first().raw_pixels.toArray().reshape([60,40]), 'gray', aspect=0.5);\n display()",
"_____no_output_____"
]
],
[
[
"# Read the data",
"_____no_output_____"
]
],
[
[
"caltech101_df = spark.read.parquet('datasets/caltech101_60_40_ubyte.parquet')",
"_____no_output_____"
]
],
[
[
"The dataframe contains images from the [Caltech 101 dataset](https://www.vision.caltech.edu/Image_Datasets/Caltech101/). These images have been downsized and transformed to gray scale by the professor. Let's look at the content of the dataset:",
"_____no_output_____"
]
],
[
[
"caltech101_df.printSchema()",
"root\n |-- category: string (nullable = true)\n |-- filename: string (nullable = true)\n |-- raw_pixels: vector (nullable = true)\n\n"
]
],
[
[
"The column `raw_pixels` contains a flattened version of the 60 by 40 images. The dataset has 101 categories plus 1 distracting category:",
"_____no_output_____"
]
],
[
[
"caltech101_df.select(fn.countDistinct('category')).show()",
"+------------------------+\n|count(DISTINCT category)|\n+------------------------+\n| 102|\n+------------------------+\n\n"
]
],
[
[
"The number of examples for each category is not balanced. For example the _airplanes_ and _motorbikes_ categories have nearly 10 times more examples than kangaroo and starfish:",
"_____no_output_____"
]
],
[
[
"caltech101_df.groupby('category').agg(fn.count('*').alias('n_images')).orderBy(fn.desc('n_images')).show()",
"+-----------------+--------+\n| category|n_images|\n+-----------------+--------+\n| airplanes| 800|\n| Motorbikes| 798|\n|BACKGROUND_Google| 468|\n| Faces_easy| 435|\n| Faces| 435|\n| watch| 239|\n| Leopards| 200|\n| bonsai| 128|\n| car_side| 123|\n| ketch| 114|\n| chandelier| 107|\n| hawksbill| 100|\n| grand_piano| 99|\n| brain| 98|\n| butterfly| 91|\n| helicopter| 88|\n| menorah| 87|\n| trilobite| 86|\n| kangaroo| 86|\n| starfish| 86|\n+-----------------+--------+\nonly showing top 20 rows\n\n"
]
],
[
[
"We will use the helper function `display_first_as_img` to display some images on the notebook. This function takes the first row of the dataframe and displays the `raw_pixels` as an image.",
"_____no_output_____"
]
],
[
[
"display_first_as_img(caltech101_df.where(fn.col('category') == \"Motorbikes\").sample(True, 0.1))",
"_____no_output_____"
],
[
"display_first_as_img(caltech101_df.where(fn.col('category') == \"Faces_easy\").sample(True, 0.5))",
"_____no_output_____"
],
[
"display_first_as_img(caltech101_df.where(fn.col('category') == \"airplanes\").sample(True, 1.))",
"_____no_output_____"
]
],
[
[
"## Multilayer perceptron in SparkML",
"_____no_output_____"
],
[
"In this homework, we will use the multilayer perceptron as a learning model. Our idea is to take the raw pixels of an image and predict the category of such image. This is therefore a classification problem. A multilayer perceptron for classification is [available in Spark ML](http://spark.apache.org/docs/latest/ml-classification-regression.html#multilayer-perceptron-classifier).",
"_____no_output_____"
],
[
"In this homework, we will focus on only three categories: airplanes, faces (easy), and motorbikes. We will split the dataset into training, validation, and testing as usual:",
"_____no_output_____"
]
],
[
[
"training_df, validation_df, testing_df = caltech101_df.\\\n where(fn.col('category').isin(['airplanes', 'Faces_easy', 'Motorbikes'])).\\\n randomSplit([0.6, 0.2, 0.2], seed=0)",
"_____no_output_____"
],
[
"[training_df.count(), validation_df.count(), testing_df.count()]",
"_____no_output_____"
]
],
[
[
"It is important to check the distribution of cateogires in the validation and testing dataframes to see if they have a similar distribution as the training dataset:",
"_____no_output_____"
]
],
[
[
"validation_df.groupBy('category').agg(fn.count('*')).show()\ntesting_df.groupBy('category').agg(fn.count('*')).show()",
"+----------+--------+\n| category|count(1)|\n+----------+--------+\n|Motorbikes| 152|\n|Faces_easy| 87|\n| airplanes| 166|\n+----------+--------+\n\n+----------+--------+\n| category|count(1)|\n+----------+--------+\n|Motorbikes| 151|\n|Faces_easy| 83|\n| airplanes| 163|\n+----------+--------+\n\n"
]
],
[
[
"They are similar.",
"_____no_output_____"
],
[
"## Transforming string labels into numerical labels",
"_____no_output_____"
],
[
"To use a multilayer perceptron, we first need to transform the input data to be fitted by Spark ML. One of the things that Spark ML needs is a numerical representation of the category (`label` as they call it). In our case, we only have a string representation of such category. Luckily, the [`StringIndexer`](http://spark.apache.org/docs/latest/ml-features.html#stringindexer) estimator allows us to do that.\n\nWe first need to fit the estimator to the data so that it learns the distribution of labels:",
"_____no_output_____"
]
],
[
[
"from pyspark.ml import feature",
"_____no_output_____"
],
[
"category_to_number_model = feature.StringIndexer(inputCol='category', outputCol='label').\\\n fit(training_df)",
"_____no_output_____"
]
],
[
[
"Now, we can see how it transforms the category into a `label`:",
"_____no_output_____"
]
],
[
[
"category_to_number_model.transform(training_df).show()",
"+----------+--------------+--------------------+-----+\n| category| filename| raw_pixels|label|\n+----------+--------------+--------------------+-----+\n|Faces_easy|image_0013.jpg|[254.0,221.0,110....| 2.0|\n|Faces_easy|image_0034.jpg|[82.0,79.0,80.0,7...| 2.0|\n|Faces_easy|image_0091.jpg|[125.0,125.0,125....| 2.0|\n|Faces_easy|image_0112.jpg|[155.0,141.0,142....| 2.0|\n|Faces_easy|image_0121.jpg|[33.0,20.0,31.0,3...| 2.0|\n|Faces_easy|image_0132.jpg|[53.0,11.0,32.0,8...| 2.0|\n|Faces_easy|image_0138.jpg|[200.0,201.0,203....| 2.0|\n|Faces_easy|image_0158.jpg|[113.0,113.0,114....| 2.0|\n|Faces_easy|image_0198.jpg|[74.0,31.0,44.0,8...| 2.0|\n|Faces_easy|image_0199.jpg|[154.0,182.0,194....| 2.0|\n|Faces_easy|image_0211.jpg|[117.0,117.0,121....| 2.0|\n|Faces_easy|image_0213.jpg|[77.0,121.0,117.0...| 2.0|\n|Faces_easy|image_0226.jpg|[150.0,161.0,156....| 2.0|\n|Faces_easy|image_0250.jpg|[167.0,167.0,168....| 2.0|\n|Faces_easy|image_0279.jpg|[111.0,111.0,115....| 2.0|\n|Faces_easy|image_0306.jpg|[167.0,147.0,170....| 2.0|\n|Faces_easy|image_0407.jpg|[198.0,195.0,123....| 2.0|\n|Motorbikes|image_0009.jpg|[255.0,255.0,255....| 0.0|\n|Motorbikes|image_0012.jpg|[255.0,255.0,255....| 0.0|\n|Motorbikes|image_0014.jpg|[255.0,255.0,255....| 0.0|\n+----------+--------------+--------------------+-----+\nonly showing top 20 rows\n\n"
]
],
[
[
"There are the categories found the estimator:",
"_____no_output_____"
]
],
[
[
"list(enumerate(category_to_number_model.labels))",
"_____no_output_____"
]
],
[
[
"## Multi-layer perceptron",
"_____no_output_____"
],
[
"The multi-layer perceptron will take the inputs as a flattened list of pixels and it will have three output neurons, each representing a label:",
"_____no_output_____"
]
],
[
[
"from pyspark.ml import classification",
"_____no_output_____"
],
[
"mlp = classification.MultilayerPerceptronClassifier(seed=0).\\\n setStepSize(0.2).\\\n setMaxIter(200).\\\n setFeaturesCol('raw_pixels')",
"_____no_output_____"
]
],
[
[
"The parameter `stepSize` is the learning rate for stochastic gradient descent and we set the maximum number of stochastic gradient descent to 200.",
"_____no_output_____"
],
[
"Now, to define the layers, the multilayer perceptron needs to receive the number of neurons of each intermediate layer (hidden layers).\n\nAs we saw in class, however, if we don't have hidden layers, then the model is simply logistic regression. In this case, it will be logistic regression for multiple outputs.",
"_____no_output_____"
],
[
"For this case, the number of input neurons will be equal to the number of pixels (60*40) and the output will be equal to the categories in the dataset:",
"_____no_output_____"
]
],
[
[
"mlp = mlp.setLayers([60*40, 3])",
"_____no_output_____"
]
],
[
[
"Now, we are ready to fit this simple multi-class logistic regression to the data. We need to create a pipeline that will take the training data, transform the category column, and apply the perceptron.",
"_____no_output_____"
]
],
[
[
"from pyspark.ml import Pipeline",
"_____no_output_____"
],
[
"mlp_simple_model = Pipeline(stages=[category_to_number_model, mlp]).fit(training_df)",
"_____no_output_____"
]
],
[
[
"Now we can apply the model to the validation data to do some simple tests:",
"_____no_output_____"
]
],
[
[
"mlp_simple_model.transform(validation_df).show(10)",
"+----------+--------------+--------------------+-----+--------------------+--------------------+----------+\n| category| filename| raw_pixels|label| rawPrediction| probability|prediction|\n+----------+--------------+--------------------+-----+--------------------+--------------------+----------+\n|Faces_easy|image_0068.jpg|[158.0,139.0,90.0...| 2.0|[-267.14463869778...|[1.22171606014870...| 2.0|\n|Faces_easy|image_0099.jpg|[169.0,172.0,172....| 2.0|[79.4862586535628...|[1.0,1.2098074256...| 0.0|\n|Faces_easy|image_0246.jpg|[83.0,85.0,89.0,8...| 2.0|[-148.31663213448...|[3.34716951535113...| 2.0|\n|Faces_easy|image_0426.jpg|[155.0,141.0,151....| 2.0|[-229.73840332275...|[8.67088070085318...| 2.0|\n|Motorbikes|image_0018.jpg|[255.0,255.0,255....| 0.0|[142.100086340399...|[0.99999999999999...| 0.0|\n|Motorbikes|image_0036.jpg|[255.0,255.0,255....| 0.0|[186.130385324797...|[1.0,1.4695150567...| 0.0|\n|Motorbikes|image_0041.jpg|[255.0,255.0,255....| 0.0|[163.246963734832...|[1.0,1.4472064883...| 0.0|\n|Motorbikes|image_0042.jpg|[255.0,253.0,228....| 0.0|[174.399348568706...|[1.0,5.9486642145...| 0.0|\n|Motorbikes|image_0044.jpg|[255.0,246.0,243....| 0.0|[96.8114868427504...|[5.05752744984478...| 1.0|\n|Motorbikes|image_0049.jpg|[255.0,255.0,255....| 0.0|[151.204268771771...|[1.0,5.6309107960...| 0.0|\n+----------+--------------+--------------------+-----+--------------------+--------------------+----------+\nonly showing top 10 rows\n\n"
]
],
[
[
"As we can see, the label and prediction mostly coincides.",
"_____no_output_____"
],
[
"We can be a more systematic by computing the accuracy of the prediction:",
"_____no_output_____"
]
],
[
[
"mlp_simple_model.transform(validation_df).select(fn.expr('avg(float(label=prediction))').alias('accuracy')).show()",
"+------------------+\n| accuracy|\n+------------------+\n|0.7728395061728395|\n+------------------+\n\n"
]
],
[
[
"Alternatively, we can take advantage of the [evaluators shipped with Spark ML](https://spark.apache.org/docs/latest/mllib-evaluation-metrics.html) as follows:",
"_____no_output_____"
]
],
[
[
"from pyspark.ml import evaluation\nevaluator = evaluation.MulticlassClassificationEvaluator(metricName=\"accuracy\")",
"_____no_output_____"
],
[
"evaluator.evaluate(mlp_simple_model.transform(validation_df))",
"_____no_output_____"
]
],
[
[
"Which gives us the same number as before.",
"_____no_output_____"
],
[
"## More layers (warning: this will take a long time)",
"_____no_output_____"
],
[
"Now, let's see if we add one hidden layer, which adds non-linearity and interactions to the input. The definition will be similar, but we need to define how many hidden layers and how many neurons per hiden layer. This is a field of its own and we can use cross validation to see which direction is better. However, for now, we will only add one hidden layer, and play with the number of neurons.",
"_____no_output_____"
],
[
"Let's define a Multilayer Perceptron (MLP) with 1 hidden layer with 100 neurons:",
"_____no_output_____"
]
],
[
[
"mlp2 = classification.MultilayerPerceptronClassifier(seed=0).\\\n setStepSize(0.2).\\\n setMaxIter(200).\\\n setFeaturesCol('raw_pixels').\\\n setLayers([60*40, 100, 3])",
"_____no_output_____"
]
],
[
[
"Now, __fitting this model will take significantly more time because we are adding 2 orders of magnitude more parameters than the previous model__:",
"_____no_output_____"
]
],
[
[
"mlp2_model = Pipeline(stages=[category_to_number_model, mlp2]).fit(training_df)",
"_____no_output_____"
]
],
[
[
"But the model is significantly more powerful and fits the data better:",
"_____no_output_____"
]
],
[
[
"evaluator.evaluate(mlp2_model.transform(validation_df))",
"_____no_output_____"
]
],
[
[
"# Complexity of the model with more data (warning: this will take a long time)",
"_____no_output_____"
],
[
"We will evaluate how the multilayer percetron learns",
"_____no_output_____"
],
[
"Let's evaluate how the performance changes with 1) the amount of training data and 2) the number of neurons in the hidden layer",
"_____no_output_____"
]
],
[
[
"evaluation_info = []\n\nfor training_size in [0.1, 0.5, 1.]:\n for n_neurons in [1, 3, 10, 20]:\n print(\"Training size: \", training_size, \"; # Neurons: \", n_neurons)\n training_sample_df = training_df.sample(False, training_size, seed=0)\n mlp_template = classification.MultilayerPerceptronClassifier(seed=0).\\\n setStepSize(0.2).\\\n setMaxIter(200).\\\n setFeaturesCol('raw_pixels').\\\n setLayers([60*40, n_neurons, 3])\n mlp_template_model = Pipeline(stages=[category_to_number_model, mlp_template]).fit(training_sample_df)\n # append training performance\n evaluation_info.append({'dataset': 'training', \n 'training_size': training_size,\n 'n_neurons': n_neurons,\n 'accuracy': evaluator.evaluate(mlp_template_model.transform(training_sample_df))})\n evaluation_info.append({'dataset': 'validation', \n 'training_size': training_size,\n 'n_neurons': n_neurons,\n 'accuracy': evaluator.evaluate(mlp_template_model.transform(validation_df))})",
"_____no_output_____"
]
],
[
[
"You will try to understand some trends based on these numbers and plots:",
"_____no_output_____"
]
],
[
[
"import pandas as pd",
"_____no_output_____"
],
[
"evaluation_df = pd.DataFrame(evaluation_info)\nevaluation_df",
"_____no_output_____"
],
[
"for training_size in sorted(evaluation_df.training_size.unique()):\n fig, ax = plt.subplots(1, 1);\n evaluation_df.query('training_size == ' + str(training_size)).groupby(['dataset']).\\\n plot(x='n_neurons', y='accuracy', ax=ax);\n plt.legend(['training', 'validation'], loc='upper left');\n plt.title('Training size: ' + str(int(training_size*100)) + '%');\n plt.ylabel('accuracy');\n plt.ylim([0, 1]);\n display()",
"_____no_output_____"
]
],
[
[
"Another way to look at it is by varying the training size",
"_____no_output_____"
]
],
[
[
"for n_neurons in sorted(evaluation_df.n_neurons.unique()):\n fig, ax = plt.subplots(1, 1);\n evaluation_df.query('n_neurons == ' + str(n_neurons)).groupby(['dataset']).\\\n plot(x='training_size', y='accuracy', ax=ax);\n plt.legend(['training', 'validation'], loc='upper left');\n plt.title('# Neurons: ' + str(n_neurons));\n plt.ylabel('accuracy');\n plt.ylim([0, 1]);\n display()",
"_____no_output_____"
]
],
[
[
"# Predicting",
"_____no_output_____"
],
[
"We will load an image from the Internet:",
"_____no_output_____"
]
],
[
[
"from PIL import Image\nimport requests\nfrom io import BytesIO\n# response = requests.get(\"http://images.all-free-download.com/images/graphicthumb/airplane_311727.jpg\")\n# response = requests.get(\"https://www.tugraz.at/uploads/pics/Alexander_by_Kanizaj_02.jpg\")\n\n# face\nresponse = requests.get(\"https://www.sciencenewsforstudents.org/sites/default/files/scald-image/350_.inline2_beauty_w.png\")\n# motorbujke\nresponse = requests.get(\"https://www.cubomoto.co.uk/img-src/_themev2-cubomoto-1613/theme/panel-1.png\")\nimg = Image.open(BytesIO(response.content))",
"_____no_output_____"
]
],
[
[
"We need to transform this image to grayscale and shrink it to the size that the neural network expects. We will use these steps using several packages:",
"_____no_output_____"
],
[
"Transform to grayscale:",
"_____no_output_____"
]
],
[
[
"# convert to grayscale\ngray_img = np.array(img.convert('P'))\nplt.imshow(255-gray_img, 'gray');",
"_____no_output_____"
]
],
[
[
"Shrink it to 60 by 40:",
"_____no_output_____"
]
],
[
[
"shrinked_img = np.array((img.resize([40, 60]).convert('P')))\nplt.imshow(shrinked_img, 'gray');",
"_____no_output_____"
]
],
[
[
"Flatten it and put it in a Spark dataframe:",
"_____no_output_____"
]
],
[
[
"# from pyspark.ml.linalg import Vectors\n# new_image = shrinked_img.flatten()\n# true_label = int(np.where(np.array(category_to_number_model.labels) == 'motorcyles')[0][0])\n# new_img_df = spark.createDataFrame([[Vectors.dense(new_image), true_label]], ['raw_pixels', 'label'])",
"_____no_output_____"
],
[
"from pyspark.ml.linalg import Vectors\nnew_image = shrinked_img.flatten()\nnew_img_df = spark.createDataFrame([[Vectors.dense(new_image)]], ['raw_pixels'])",
"_____no_output_____"
],
[
"display_first_as_img(new_img_df)",
"_____no_output_____"
]
],
[
[
"Now `new_img_df` has one image",
"_____no_output_____"
]
],
[
[
"display(new_img_df)",
"_____no_output_____"
]
],
[
[
"Use the `mlp2_model` and `new_img_df` to predict the category that the new image belongs to. Show the code you use for such prediction.",
"_____no_output_____"
]
],
[
[
"mlp_simple_model.transform(new_img_df).show()",
"+--------------------+--------------------+--------------------+----------+\n| raw_pixels| rawPrediction| probability|prediction|\n+--------------------+--------------------+--------------------+----------+\n|[0.0,0.0,0.0,0.0,...|[-308.65365268629...|[6.86004075999194...| 2.0|\n+--------------------+--------------------+--------------------+----------+\n\n"
],
[
"from pyspark.ml.feature import OneHotEncoder, StringIndexer, VectorAssembler\n\ndf = spark.createDataFrame([\n (0, \"a\"),\n (1, \"b\"),\n (2, \"c\"),\n (3, \"a\"),\n (4, \"a\"),\n (5, \"c\")\n], [\"id\", \"category\"])\n\nstringIndexer = StringIndexer(inputCol=\"category\", outputCol=\"categoryIndex\")\nmodel = stringIndexer.fit(df)\nindexed = model.transform(df)\n\nencoder = OneHotEncoder(inputCol=\"categoryIndex\", outputCol=\"categoryVec\")\nencoded = encoder.transform(indexed)\nencoded.show()",
"+---+--------+-------------+-------------+\n| id|category|categoryIndex| categoryVec|\n+---+--------+-------------+-------------+\n| 0| a| 0.0|(2,[0],[1.0])|\n| 1| b| 2.0| (2,[],[])|\n| 2| c| 1.0|(2,[1],[1.0])|\n| 3| a| 0.0|(2,[0],[1.0])|\n| 4| a| 0.0|(2,[0],[1.0])|\n| 5| c| 1.0|(2,[1],[1.0])|\n+---+--------+-------------+-------------+\n\n"
],
[
"VectorAssembler(inputCols=['categoryIndex', 'categoryVec']).transform(encoded).show()",
"+---+--------+-------------+-------------+--------------------------------------------+\n| id|category|categoryIndex| categoryVec|VectorAssembler_44d095f846fbbd1b9186__output|\n+---+--------+-------------+-------------+--------------------------------------------+\n| 0| a| 0.0|(2,[0],[1.0])| [0.0,1.0,0.0]|\n| 1| b| 2.0| (2,[],[])| [2.0,0.0,0.0]|\n| 2| c| 1.0|(2,[1],[1.0])| [1.0,0.0,1.0]|\n| 3| a| 0.0|(2,[0],[1.0])| [0.0,1.0,0.0]|\n| 4| a| 0.0|(2,[0],[1.0])| [0.0,1.0,0.0]|\n| 5| c| 1.0|(2,[1],[1.0])| [1.0,0.0,1.0]|\n+---+--------+-------------+-------------+--------------------------------------------+\n\n"
]
]
] | [
"markdown",
"code",
"markdown",
"code",
"markdown",
"code",
"markdown",
"code",
"markdown",
"code",
"markdown",
"code",
"markdown",
"code",
"markdown",
"code",
"markdown",
"code",
"markdown",
"code",
"markdown",
"code",
"markdown",
"code",
"markdown",
"code",
"markdown",
"code",
"markdown",
"code",
"markdown",
"code",
"markdown",
"code",
"markdown",
"code",
"markdown",
"code",
"markdown",
"code",
"markdown",
"code",
"markdown",
"code",
"markdown",
"code",
"markdown",
"code",
"markdown",
"code",
"markdown",
"code",
"markdown",
"code",
"markdown",
"code",
"markdown",
"code"
] | [
[
"markdown",
"markdown"
],
[
"code",
"code"
],
[
"markdown"
],
[
"code"
],
[
"markdown"
],
[
"code"
],
[
"markdown"
],
[
"code"
],
[
"markdown"
],
[
"code"
],
[
"markdown"
],
[
"code",
"code",
"code"
],
[
"markdown",
"markdown",
"markdown"
],
[
"code",
"code"
],
[
"markdown"
],
[
"code"
],
[
"markdown",
"markdown",
"markdown"
],
[
"code",
"code"
],
[
"markdown"
],
[
"code"
],
[
"markdown"
],
[
"code"
],
[
"markdown",
"markdown"
],
[
"code",
"code"
],
[
"markdown",
"markdown",
"markdown"
],
[
"code"
],
[
"markdown"
],
[
"code",
"code"
],
[
"markdown"
],
[
"code"
],
[
"markdown",
"markdown"
],
[
"code"
],
[
"markdown"
],
[
"code",
"code"
],
[
"markdown",
"markdown",
"markdown",
"markdown"
],
[
"code"
],
[
"markdown"
],
[
"code"
],
[
"markdown"
],
[
"code"
],
[
"markdown",
"markdown",
"markdown"
],
[
"code"
],
[
"markdown"
],
[
"code",
"code",
"code"
],
[
"markdown"
],
[
"code"
],
[
"markdown",
"markdown"
],
[
"code"
],
[
"markdown",
"markdown"
],
[
"code"
],
[
"markdown"
],
[
"code"
],
[
"markdown"
],
[
"code",
"code",
"code"
],
[
"markdown"
],
[
"code"
],
[
"markdown"
],
[
"code",
"code",
"code"
]
] |
e764cd468fe0dba722929476e730c97dd522efdb | 26,051 | ipynb | Jupyter Notebook | app/notebooks/labeled_identities/shooters/robert_lewis_dear.ipynb | scanner-research/esper-tv | 179ef57d536ebd52f93697aab09bf5abec19ce93 | [
"Apache-2.0"
] | 5 | 2019-04-17T01:01:46.000Z | 2021-07-11T01:32:50.000Z | app/notebooks/labeled_identities/shooters/robert_lewis_dear.ipynb | DanFu09/esper | ccc5547de3637728b8aaab059b6781baebc269ec | [
"Apache-2.0"
] | 4 | 2019-11-12T08:35:03.000Z | 2021-06-10T20:37:04.000Z | app/notebooks/labeled_identities/shooters/robert_lewis_dear.ipynb | DanFu09/esper | ccc5547de3637728b8aaab059b6781baebc269ec | [
"Apache-2.0"
] | 1 | 2020-09-01T01:15:44.000Z | 2020-09-01T01:15:44.000Z | 33.570876 | 2,941 | 0.621396 | [
[
[
"<h1>Table of Contents<span class=\"tocSkip\"></span></h1>\n<div class=\"toc\" style=\"margin-top: 1em;\"><ul class=\"toc-item\"><li><span><a href=\"#Name\" data-toc-modified-id=\"Name-1\"><span class=\"toc-item-num\">1 </span>Name</a></span></li><li><span><a href=\"#Search\" data-toc-modified-id=\"Search-2\"><span class=\"toc-item-num\">2 </span>Search</a></span><ul class=\"toc-item\"><li><span><a href=\"#Load-Cached-Results\" data-toc-modified-id=\"Load-Cached-Results-2.1\"><span class=\"toc-item-num\">2.1 </span>Load Cached Results</a></span></li><li><span><a href=\"#Build-Model-From-Google-Images\" data-toc-modified-id=\"Build-Model-From-Google-Images-2.2\"><span class=\"toc-item-num\">2.2 </span>Build Model From Google Images</a></span></li></ul></li><li><span><a href=\"#Analysis\" data-toc-modified-id=\"Analysis-3\"><span class=\"toc-item-num\">3 </span>Analysis</a></span><ul class=\"toc-item\"><li><span><a href=\"#Gender-cross-validation\" data-toc-modified-id=\"Gender-cross-validation-3.1\"><span class=\"toc-item-num\">3.1 </span>Gender cross validation</a></span></li><li><span><a href=\"#Face-Sizes\" data-toc-modified-id=\"Face-Sizes-3.2\"><span class=\"toc-item-num\">3.2 </span>Face Sizes</a></span></li><li><span><a href=\"#Screen-Time-Across-All-Shows\" data-toc-modified-id=\"Screen-Time-Across-All-Shows-3.3\"><span class=\"toc-item-num\">3.3 </span>Screen Time Across All Shows</a></span></li><li><span><a href=\"#Appearances-on-a-Single-Show\" data-toc-modified-id=\"Appearances-on-a-Single-Show-3.4\"><span class=\"toc-item-num\">3.4 </span>Appearances on a Single Show</a></span></li><li><span><a href=\"#Other-People-Who-Are-On-Screen\" data-toc-modified-id=\"Other-People-Who-Are-On-Screen-3.5\"><span class=\"toc-item-num\">3.5 </span>Other People Who Are On Screen</a></span></li></ul></li><li><span><a href=\"#Persist-to-Cloud\" data-toc-modified-id=\"Persist-to-Cloud-4\"><span class=\"toc-item-num\">4 </span>Persist to Cloud</a></span><ul class=\"toc-item\"><li><span><a href=\"#Save-Model-to-Google-Cloud-Storage\" data-toc-modified-id=\"Save-Model-to-Google-Cloud-Storage-4.1\"><span class=\"toc-item-num\">4.1 </span>Save Model to Google Cloud Storage</a></span></li><li><span><a href=\"#Save-Labels-to-DB\" data-toc-modified-id=\"Save-Labels-to-DB-4.2\"><span class=\"toc-item-num\">4.2 </span>Save Labels to DB</a></span><ul class=\"toc-item\"><li><span><a href=\"#Commit-the-person-and-labeler\" data-toc-modified-id=\"Commit-the-person-and-labeler-4.2.1\"><span class=\"toc-item-num\">4.2.1 </span>Commit the person and labeler</a></span></li><li><span><a href=\"#Commit-the-FaceIdentity-labels\" data-toc-modified-id=\"Commit-the-FaceIdentity-labels-4.2.2\"><span class=\"toc-item-num\">4.2.2 </span>Commit the FaceIdentity labels</a></span></li></ul></li></ul></li></ul></div>",
"_____no_output_____"
]
],
[
[
"from esper.prelude import *\nfrom esper.identity import *\nfrom esper.plot_util import *\nfrom esper.topics import *\nfrom esper import embed_google_images",
"_____no_output_____"
]
],
[
[
"# Name",
"_____no_output_____"
],
[
"Please add the person's name and their expected gender below (Male/Female).",
"_____no_output_____"
]
],
[
[
"name = 'Robert Lewis Dear Jr'\ngender = 'Male'",
"_____no_output_____"
]
],
[
[
"# Search",
"_____no_output_____"
],
[
"## Load Cached Results",
"_____no_output_____"
],
[
"Reads cached identity model from local disk. Run this if the person has been labelled before and you only wish to regenerate the graphs. Otherwise, if you have never created a model for this person, please see the next section.",
"_____no_output_____"
]
],
[
[
"assert name != ''\nresults = FaceIdentityModel.load(name=name) # or load_from_gcs(name=name)\nimshow(tile_images([cv2.resize(x[1][0], (200, 200)) for x in results.model_params['images']], cols=10))\nplt.show()\nplot_precision_and_cdf(results)",
"_____no_output_____"
]
],
[
[
"## Build Model From Google Images",
"_____no_output_____"
],
[
"Run this section if you do not have a cached model and precision curve estimates. This section will grab images using Google Image Search and score each of the faces in the dataset. We will interactively build the precision vs score curve.\n\nIt is important that the images that you select are accurate. If you make a mistake, rerun the cell below.",
"_____no_output_____"
]
],
[
[
"assert name != ''\n# Grab face images from Google\nimg_dir = embed_google_images.fetch_images(name)\n\n# If the images returned are not satisfactory, rerun the above with extra params:\n# query_extras='' # additional keywords to add to search\n# force=True # ignore cached images\n\nface_imgs = load_and_select_faces_from_images(img_dir)\nface_embs = embed_google_images.embed_images(face_imgs)\nassert(len(face_embs) == len(face_imgs))\n\nreference_imgs = tile_images([cv2.resize(x[0], (200, 200)) for x in face_imgs if x], cols=10)\ndef show_reference_imgs():\n print('User selected reference images for {}.'.format(name))\n imshow(reference_imgs)\n plt.show()\nshow_reference_imgs()",
"_____no_output_____"
],
[
"# Score all of the faces in the dataset (this can take a minute)\nface_ids_by_bucket, face_ids_to_score = face_search_by_embeddings(face_embs)",
"_____no_output_____"
],
[
"precision_model = PrecisionModel(face_ids_by_bucket)",
"_____no_output_____"
]
],
[
[
"Now we will validate which of the images in the dataset are of the target identity.\n\n__Hover over with mouse and press S to select a face. Press F to expand the frame.__",
"_____no_output_____"
]
],
[
[
"show_reference_imgs()\nprint(('Mark all images that ARE NOT {}. Thumbnails are ordered by DESCENDING distance '\n 'to your selected images. (The first page is more likely to have non \"{}\" images.) '\n 'There are a total of {} frames. (CLICK THE DISABLE JUPYTER KEYBOARD BUTTON '\n 'BEFORE PROCEEDING.)').format(\n name, name, precision_model.get_lower_count()))\nlower_widget = precision_model.get_lower_widget()\nlower_widget",
"_____no_output_____"
],
[
"show_reference_imgs()\nprint(('Mark all images that ARE {}. Thumbnails are ordered by ASCENDING distance '\n 'to your selected images. (The first page is more likely to have \"{}\" images.) '\n 'There are a total of {} frames. (CLICK THE DISABLE JUPYTER KEYBOARD BUTTON '\n 'BEFORE PROCEEDING.)').format(\n name, name, precision_model.get_lower_count()))\nupper_widget = precision_model.get_upper_widget()\nupper_widget",
"_____no_output_____"
]
],
[
[
"Run the following cell after labelling to compute the precision curve. Do not forget to re-enable jupyter shortcuts.",
"_____no_output_____"
]
],
[
[
"# Compute the precision from the selections\nlower_precision = precision_model.compute_precision_for_lower_buckets(lower_widget.selected)\nupper_precision = precision_model.compute_precision_for_upper_buckets(upper_widget.selected)\nprecision_by_bucket = {**lower_precision, **upper_precision}\n\nresults = FaceIdentityModel(\n name=name, \n face_ids_by_bucket=face_ids_by_bucket, \n face_ids_to_score=face_ids_to_score,\n precision_by_bucket=precision_by_bucket, \n model_params={\n 'images': list(zip(face_embs, face_imgs))\n }\n)\nplot_precision_and_cdf(results)",
"_____no_output_____"
]
],
[
[
"The next cell persists the model locally.",
"_____no_output_____"
]
],
[
[
"results.save()",
"_____no_output_____"
]
],
[
[
"# Analysis",
"_____no_output_____"
],
[
"## Gender cross validation\n\nSituations where the identity model disagrees with the gender classifier may be cause for alarm. We would like to check that instances of the person have the expected gender as a sanity check. This section shows the breakdown of the identity instances and their labels from the gender classifier.",
"_____no_output_____"
]
],
[
[
"gender_breakdown = compute_gender_breakdown(results)\n\nprint('Expected counts by gender:')\nfor k, v in gender_breakdown.items():\n print(' {} : {}'.format(k, int(v)))\nprint()\n\nprint('Percentage by gender:')\ndenominator = sum(v for v in gender_breakdown.values())\nfor k, v in gender_breakdown.items():\n print(' {} : {:0.1f}%'.format(k, 100 * v / denominator))\nprint()",
"_____no_output_____"
]
],
[
[
"Situations where the identity detector returns high confidence, but where the gender is not the expected gender indicate either an error on the part of the identity detector or the gender detector. The following visualization shows randomly sampled images, where the identity detector returns high confidence, grouped by the gender label. ",
"_____no_output_____"
]
],
[
[
"high_probability_threshold = 0.8\nshow_gender_examples(results, high_probability_threshold)",
"_____no_output_____"
]
],
[
[
"## Face Sizes\n\nFaces shown on-screen vary in size. For a person such as a host, they may be shown in a full body shot or as a face in a box. Faces in the background or those part of side graphics might be smaller than the rest. When calculuating screentime for a person, we would like to know whether the results represent the time the person was featured as opposed to merely in the background or as a tiny thumbnail in some graphic.\n\nThe next cell, plots the distribution of face sizes. Some possible anomalies include there only being very small faces or large faces. ",
"_____no_output_____"
]
],
[
[
"plot_histogram_of_face_sizes(results)",
"_____no_output_____"
]
],
[
[
"The histogram above shows the distribution of face sizes, but not how those sizes occur in the dataset. For instance, one might ask why some faces are so large or whhether the small faces are actually errors. The following cell groups example faces, which are of the target identity with probability, by their sizes in terms of screen area.",
"_____no_output_____"
]
],
[
[
"high_probability_threshold = 0.8\nshow_faces_by_size(results, high_probability_threshold, n=10)",
"_____no_output_____"
]
],
[
[
"## Screen Time Across All Shows\n\nOne question that we might ask about a person is whether they received a significantly different amount of screentime on different shows. The following section visualizes the amount of screentime by show in total minutes and also in proportion of the show's total time. For a celebrity or political figure such as Donald Trump, we would expect significant screentime on many shows. For a show host such as Wolf Blitzer, we expect that the screentime be high for shows hosted by Wolf Blitzer.",
"_____no_output_____"
]
],
[
[
"screen_time_by_show = get_screen_time_by_show(results)",
"_____no_output_____"
],
[
"plot_screen_time_by_show(name, screen_time_by_show)",
"_____no_output_____"
]
],
[
[
"We might also wish to validate these findings by comparing to the whether the person's name is mentioned in the subtitles. This might be helpful in determining whether extra or lack of screentime for a person may be due to a show's aesthetic choices. The following plots show compare the screen time with the number of caption mentions.",
"_____no_output_____"
]
],
[
[
"caption_mentions_by_show = get_caption_mentions_by_show([name.upper()])\nplot_screen_time_and_other_by_show(name, screen_time_by_show, caption_mentions_by_show, \n 'Number of caption mentions', 'Count')",
"_____no_output_____"
]
],
[
[
"## Appearances on a Single Show\n\nFor people such as hosts, we would like to examine in greater detail the screen time allotted for a single show. First, fill in a show below.",
"_____no_output_____"
]
],
[
[
"show_name = 'INSERT-SHOW-HERE'",
"_____no_output_____"
],
[
"# Compute the screen time for each video of the show\nscreen_time_by_video_id = get_screen_time_by_video(results, show_name)",
"_____no_output_____"
]
],
[
[
"One question we might ask about a host is \"how long they are show on screen\" for an episode. Likewise, we might also ask for how many episodes is the host not present due to being on vacation or on assignment elsewhere. The following cell plots a histogram of the distribution of the length of the person's appearances in videos of the chosen show.",
"_____no_output_____"
]
],
[
[
"plot_histogram_of_screen_times_by_video(name, show_name, screen_time_by_video_id)",
"_____no_output_____"
]
],
[
[
"For a host, we expect screentime over time to be consistent as long as the person remains a host. For figures such as Hilary Clinton, we expect the screentime to track events in the real world such as the lead-up to 2016 election and then to drop afterwards. The following cell plots a time series of the person's screentime over time. Each dot is a video of the chosen show. Red Xs are videos for which the face detector did not run.",
"_____no_output_____"
]
],
[
[
"plot_screentime_over_time(name, show_name, screen_time_by_video_id)",
"_____no_output_____"
]
],
[
[
"We hypothesized that a host is more likely to appear at the beginning of a video and then also appear throughout the video. The following plot visualizes the distibution of shot beginning times for videos of the show.",
"_____no_output_____"
]
],
[
[
"plot_distribution_of_appearance_times_by_video(results, show_name)",
"_____no_output_____"
]
],
[
[
"In the section 3.3, we see that some shows may have much larger variance in the screen time estimates than others. This may be because a host or frequent guest appears similar to the target identity. Alternatively, the images of the identity may be consistently low quality, leading to lower scores. The next cell plots a histogram of the probabilites for for faces in a show.",
"_____no_output_____"
]
],
[
[
"plot_distribution_of_identity_probabilities(results, show_name)",
"_____no_output_____"
]
],
[
[
"## Other People Who Are On Screen\n\nFor some people, we are interested in who they are often portrayed on screen with. For instance, the White House press secretary might routinely be shown with the same group of political pundits. A host of a show, might be expected to be on screen with their co-host most of the time. The next cell takes an identity model with high probability faces and displays clusters of faces that are on screen with the target person.",
"_____no_output_____"
]
],
[
[
"get_other_people_who_are_on_screen(results, k=25, precision_thresh=0.8)",
"_____no_output_____"
]
],
[
[
"# Persist to Cloud\n\nThe remaining code in this notebook uploads the built identity model to Google Cloud Storage and adds the FaceIdentity labels to the database.",
"_____no_output_____"
],
[
"## Save Model to Google Cloud Storage",
"_____no_output_____"
]
],
[
[
"gcs_model_path = results.save_to_gcs()",
"_____no_output_____"
]
],
[
[
"To ensure that the model stored to Google Cloud is valid, we load it and print the precision and cdf curve below. ",
"_____no_output_____"
]
],
[
[
"gcs_results = FaceIdentityModel.load_from_gcs(name=name)\nimshow(tile_images([cv2.resize(x[1][0], (200, 200)) for x in gcs_results.model_params['images']], cols=10))\nplt.show()\nplot_precision_and_cdf(gcs_results)",
"_____no_output_____"
]
],
[
[
"## Save Labels to DB\n\nIf you are satisfied with the model, we can commit the labels to the database.",
"_____no_output_____"
]
],
[
[
"from django.core.exceptions import ObjectDoesNotExist\n\ndef standardize_name(name):\n return name.lower()\n\nperson_type = ThingType.objects.get(name='person')\n\ntry:\n person = Thing.objects.get(name=standardize_name(name), type=person_type)\n print('Found person:', person.name)\nexcept ObjectDoesNotExist:\n person = Thing(name=standardize_name(name), type=person_type)\n print('Creating person:', person.name)\n\nlabeler = Labeler(name='face-identity:{}'.format(person.name), data_path=gcs_model_path)",
"_____no_output_____"
]
],
[
[
"### Commit the person and labeler\n\nThe labeler and person have been created but not set saved to the database. If a person was created, please make sure that the name is correct before saving.",
"_____no_output_____"
]
],
[
[
"person.save()\nlabeler.save()",
"_____no_output_____"
]
],
[
[
"### Commit the FaceIdentity labels\n\nNow, we are ready to add the labels to the database. We will create a FaceIdentity for each face whose probability exceeds the minimum threshold.",
"_____no_output_____"
]
],
[
[
"commit_face_identities_to_db(results, person, labeler, min_threshold=0.001)",
"_____no_output_____"
],
[
"print('Committed {} labels to the db'.format(FaceIdentity.objects.filter(labeler=labeler).count()))",
"_____no_output_____"
]
]
] | [
"markdown",
"code",
"markdown",
"code",
"markdown",
"code",
"markdown",
"code",
"markdown",
"code",
"markdown",
"code",
"markdown",
"code",
"markdown",
"code",
"markdown",
"code",
"markdown",
"code",
"markdown",
"code",
"markdown",
"code",
"markdown",
"code",
"markdown",
"code",
"markdown",
"code",
"markdown",
"code",
"markdown",
"code",
"markdown",
"code",
"markdown",
"code",
"markdown",
"code",
"markdown",
"code",
"markdown",
"code",
"markdown",
"code",
"markdown",
"code"
] | [
[
"markdown"
],
[
"code"
],
[
"markdown",
"markdown"
],
[
"code"
],
[
"markdown",
"markdown",
"markdown"
],
[
"code"
],
[
"markdown",
"markdown"
],
[
"code",
"code",
"code"
],
[
"markdown"
],
[
"code",
"code"
],
[
"markdown"
],
[
"code"
],
[
"markdown"
],
[
"code"
],
[
"markdown",
"markdown"
],
[
"code"
],
[
"markdown"
],
[
"code"
],
[
"markdown"
],
[
"code"
],
[
"markdown"
],
[
"code"
],
[
"markdown"
],
[
"code",
"code"
],
[
"markdown"
],
[
"code"
],
[
"markdown"
],
[
"code",
"code"
],
[
"markdown"
],
[
"code"
],
[
"markdown"
],
[
"code"
],
[
"markdown"
],
[
"code"
],
[
"markdown"
],
[
"code"
],
[
"markdown"
],
[
"code"
],
[
"markdown",
"markdown"
],
[
"code"
],
[
"markdown"
],
[
"code"
],
[
"markdown"
],
[
"code"
],
[
"markdown"
],
[
"code"
],
[
"markdown"
],
[
"code",
"code"
]
] |
e764cd5c52e5d9753f34b5fb19cd630f5c29743d | 632,657 | ipynb | Jupyter Notebook | Code/IPython/bootcamp_graphics.ipynb | ljsun88/data_bootcamp_nyu | abc2486060672e19f5e4a71342cb8ca05155db83 | [
"MIT"
] | 74 | 2015-01-14T22:51:39.000Z | 2021-01-31T17:23:58.000Z | Code/IPython/bootcamp_graphics.ipynb | ljsun88/data_bootcamp_nyu | abc2486060672e19f5e4a71342cb8ca05155db83 | [
"MIT"
] | 13 | 2015-03-18T20:24:40.000Z | 2016-05-06T13:44:33.000Z | Code/IPython/bootcamp_graphics.ipynb | ljsun88/data_bootcamp_nyu | abc2486060672e19f5e4a71342cb8ca05155db83 | [
"MIT"
] | 60 | 2015-03-24T00:05:50.000Z | 2021-05-12T15:15:32.000Z | 288.752624 | 53,046 | 0.914325 | [
[
[
"# Python graphics: Matplotlib fundamentals\n\nWe illustrate three approaches to graphing data with Python's Matplotlib package: \n\n* Approach #1: Apply a `plot()` method to a dataframe\n* Approach #2: Use the `plot(x,y)` function \n* Approach #3: Create a figure object and apply methods to it\n\nThe last one is the least intuitive but also the most useful. We work up to it gradually. This [book chapter](https://davebackus.gitbooks.io/test/content/graphs1.html) covers the same material with more words and fewer pictures. \n\nThis IPython notebook was created by Dave Backus for the NYU Stern course [Data Bootcamp](http://databootcamp.nyuecon.com/). ",
"_____no_output_____"
],
[
"## Reminders\n\n* **Packages**: collections of tools that we access with `import` statements\n* **Pandas**: Python's data package \n* **Objects** and **methods**: we apply the method `justdoit` to the object `x` with `x.justdoit`\n* **Dataframe**: a spreadsheet-like data structure \n* **Series**: a single variable \n* **Jupyter**: an environment for combining code with text and graphics \n",
"_____no_output_____"
],
[
"## Preliminaries \n\n### Jupyter \n\nLook around, what do you see? Check out the **menubar** at the top: File, Edit, etc. Also the **toolbar** below it. Click on Help -> User Interface Tour for a tour of the landscape. \n\nThe **cells** below come in two forms. Those labeled Code (see the menu in the toolbar) are Python code. Those labeled Markdown are text. ",
"_____no_output_____"
],
[
"### Markdown\n\nMarkdown is a user-friendly language for text formatting. You can see how it works by clicking on any of the Markdown cells and looking at the raw text that underlies it. In addition to just plain text, we'll use three things a lot:\n\n* Bold and italics. The raw text `**bold**` displays as **bold**. The raw text `*italics*` displays as *italics*. \n* Bullet lists. If we want a list of items marked by bullets, we start with a blank line and mark each item with an asterisk on a new line. Double click on this cell for an example. \n* Headings. We create section headings by putting a hash in front of the text. `# Heading` gives us a large heading. Two hashes a smaller heading, three hashes smaller still, up to four hashes. In this cell there's a two-hash heading at the top. \n\n**Exercise.** Click on this cell, then click the `+` in the toolbar to create a new empty cell below. \n\n**Exercise.** Click on the new cell below. Choose Markdown in the menubar at the top. Add your name and a description of what we're doing. Execute the cell by either (i) clicking on the \"run cell\" button in the toolbar or (ii) clicking on \"Cell\" in the menubar and choosing Run. ",
"_____no_output_____"
],
[
"### Import packages",
"_____no_output_____"
]
],
[
[
"import sys # system module \nimport pandas as pd # data package\nimport matplotlib as mpl # graphics package\nimport matplotlib.pyplot as plt # graphics module \nimport datetime as dt # date and time module\n\n# check versions (overkill, but why not?)\nprint('Python version:', sys.version)\nprint('Pandas version: ', pd.__version__)\nprint('Matplotlib version: ', mpl.__version__)\nprint('Today: ', dt.date.today())",
"Python version: 3.5.1 |Anaconda 2.5.0 (x86_64)| (default, Dec 7 2015, 11:24:55) \n[GCC 4.2.1 (Apple Inc. build 5577)]\nPandas version: 0.17.1\nMatplotlib version: 1.5.1\nToday: 2016-03-10\n"
]
],
[
[
"**Comment.** When you run the code cell above, its output appears below it. \n\n**Exercise.** Enter `pd.read_csv?` in the empty cell below. Run the cell (Cell at the top, or shift-enter). Do you see the documentation? This is the Jupyter version of help in Spyder's IPython console. ",
"_____no_output_____"
]
],
[
[
"# This is an IPython command. It puts plots here in the notebook, rather than a separate window.\n%matplotlib inline",
"_____no_output_____"
]
],
[
[
"### Create dataframes to play with \n\n* US GDP and consumption \n* World Bank GDP per capita for several countries \n* Fama-French equity returns ",
"_____no_output_____"
]
],
[
[
"# US GDP and consumption \ngdp = [13271.1, 13773.5, 14234.2, 14613.8, 14873.7, 14830.4, 14418.7,\n 14783.8, 15020.6, 15369.2, 15710.3]\npce = [8867.6, 9208.2, 9531.8, 9821.7, 10041.6, 10007.2, 9847.0, 10036.3,\n 10263.5, 10449.7, 10699.7]\nyear = list(range(2003,2014)) # use range for years 2003-2013 \n\n# create dataframe from dictionary \nus = pd.DataFrame({'gdp': gdp, 'pce': pce}, index=year) \nprint(us.head(3))",
" gdp pce\n2003 13271.1 8867.6\n2004 13773.5 9208.2\n2005 14234.2 9531.8\n"
],
[
"# GDP per capita (World Bank data, 2013, thousands of USD) \ncode = ['USA', 'FRA', 'JPN', 'CHN', 'IND', 'BRA', 'MEX']\ncountry = ['United States', 'France', 'Japan', 'China', 'India',\n 'Brazil', 'Mexico']\ngdppc = [53.1, 36.9, 36.3, 11.9, 5.4, 15.0, 16.5]\n\nwbdf = pd.DataFrame({'gdppc': gdppc, 'country': country}, index=code)\nwbdf",
"_____no_output_____"
]
],
[
[
"**Comment.** In the previous cell, we used the `print()` function to produce output. Here we just put the name of the dataframe. The latter displays the dataframe -- and formats it nicely -- **if it's the last statement in the cell**. ",
"_____no_output_____"
]
],
[
[
"# Fama-French \nimport pandas.io.data as web\n\n# read annual data from website and rename variables \nff = web.DataReader('F-F_Research_Data_factors', 'famafrench')[1]\nff.columns = ['xsm', 'smb', 'hml', 'rf']\nff['rm'] = ff['xsm'] + ff['rf']\nff = ff[['rm', 'rf']] # extract rm and rf (return on market, riskfree rate, percent)\nff.head(5)",
"/Users/sglyon/anaconda3/lib/python3.5/site-packages/pandas/io/data.py:33: FutureWarning: \nThe pandas.io.data module is moved to a separate package (pandas-datareader) and will be removed from pandas in a future version.\nAfter installing the pandas-datareader package (https://github.com/pydata/pandas-datareader), you can change the import ``from pandas.io import data, wb`` to ``from pandas_datareader import data, wb``.\n FutureWarning)\n"
]
],
[
[
"**Comment.** The warning in pink tells us that the Pandas DataReader will be spun off into a separate package in the near future. ",
"_____no_output_____"
],
[
"**Exercise.** What kind of object is `wbdf`? What are its column and row labels? \n\n**Exercise.** What is `ff.index`? What does that tell us? ",
"_____no_output_____"
]
],
[
[
"# This is an IPython command: it puts plots here in the notebook, rather than a separate window.\n%matplotlib inline",
"_____no_output_____"
]
],
[
[
"## Digression: Graphing in Excel\n\nRemind yourself that we need to choose: \n \n* Data. Typically a block of cells in a spreadsheet. \n* Chart type. Lines, bars, scatter, or something else. \n* x and y variables. What is the x axis? What is y? \n\nWe'll see the same in Matplotlib. ",
"_____no_output_____"
],
[
"## Approach #1: Apply `plot()` method to dataframe\n\nGood simple approach, we use it a lot. It comes with some useful defaults:\n\n* Data. The whole dataframe. \n* Chart type. We have options for lines, bars, or other things. \n* `x` and `y` variables. By default, the `x` variable is the dataframe's index and the `y` variables are all the columns of the dataframe. \n\nAll of these things can be changed, but this is the starting point. \n\nLet's do some examples, see how they work. ",
"_____no_output_____"
],
[
"### US GDP and consumption",
"_____no_output_____"
]
],
[
[
"# try this with US GDP\nus.plot()",
"_____no_output_____"
],
[
"# do GDP alone\nus['gdp'].plot()",
"_____no_output_____"
],
[
"# bar chart \nus.plot(kind='bar')",
"_____no_output_____"
]
],
[
[
"**Exercise.** Show that we get the output from `us.plot.bar()`. ",
"_____no_output_____"
]
],
[
[
"us.plot",
"_____no_output_____"
],
[
"# scatter plot \n# we need to be explicit about the x and y variables: x = 'gdp', y = 'pce'\nus.plot.scatter('gdp', 'pce')",
"_____no_output_____"
]
],
[
[
"**Exercise.** Enter `us.plot(kind='bar')` and `us.plot.bar()` in separate cells. Show that they produce the same bar chart. \n\n**Exercise.** Add each of these arguments, one at a time, to `us.plot()`: \n\n* `kind='area'`\n* `subplots=True`\n* `sharey=True`\n* `figsize=(3,6)`\n* `ylim=(0,16000)`\n\nWhat do they do?\n\n**Exercise.** Type `us.plot?` in a new cell. Run the cell (shift-enter or click on the run cell icon). What options do you see for the `kind=` argument? Which ones have we tried? What are the other ones? ",
"_____no_output_____"
],
[
"### Fama-French asset returns ",
"_____no_output_____"
]
],
[
[
"# now try a few things with the Fama-French data\nff.plot()",
"_____no_output_____"
],
[
"ff.plot()",
"_____no_output_____"
]
],
[
[
"**Exercise.** What do each of the arguments do in the code below? ",
"_____no_output_____"
]
],
[
[
"ff.plot(kind='hist', bins=20, subplots=True)",
"_____no_output_____"
],
[
"# \"smoothed\" histogram \nff.plot(kind='kde', subplots=True, sharex=True) # smoothed histogram (\"kernel density estimate\")",
"_____no_output_____"
]
],
[
[
"**Exercise.** Let's see if we can dress up the histogram a little. Try adding, one at a time, the arguments `title='Fama-French returns'`, `grid=True`, and `legend=False`. What does the documentation say about them? What do they do? \n\n**Exercise.** What do the histograms tell us about the two returns? How do they differ? \n\n\n**Exercise.** Use the World Bank dataframe `wbdf` to create a bar chart of GDP per capita, the variable `'gdppc'`. *Bonus points:* Create a horizontal bar chart. Which do you prefer? ",
"_____no_output_____"
],
[
"## Approach #2: the `plot(x,y)` function \n\nHere we plot variable `y` against variable `x`. This comes closest to what we would do in Excel: identify a dataset, a plot type, and the `x` and `y` variables, then press play. ",
"_____no_output_____"
]
],
[
[
"# import pyplot module of Matplotlib \nimport matplotlib.pyplot as plt ",
"_____no_output_____"
],
[
"plt.plot(us.index, us['gdp'])",
"_____no_output_____"
]
],
[
[
"**Exercise.** What is the `x` variable here? The `y` variable? ",
"_____no_output_____"
]
],
[
[
"# we can do two lines together\nplt.plot(us.index, us['gdp'])\nplt.plot(us.index, us['pce'])",
"_____no_output_____"
],
[
"# or a bar chart \nplt.bar(us.index, us['gdp'], align='center')",
"_____no_output_____"
]
],
[
[
"**Exercise.** Experiment with \n```python\nplt.bar(us.index, us['gdp'], \n align='center', \n alpha=0.65, \n color='red', \n edgecolor='green')\n```\nPlay with the arguments one by one to see what they do. Or use `plt.bar?` to look them up. Add comments to remind yourself. *Bonus points:* Can you make this graph even uglier? ",
"_____no_output_____"
]
],
[
[
"# we can also add things to plots \nplt.plot(us.index, us['gdp']) \nplt.plot(us.index, us['pce']) \n\nplt.title('US GDP', fontsize=14, loc='left') # add title\nplt.ylabel('Billions of 2009 USD') # y axis label \nplt.xlim(2002.5, 2013.5) # shrink x axis limits\nplt.tick_params(labelcolor='red') # change tick labels to red\nplt.legend(['GDP', 'Consumption']) # more descriptive variable names",
"_____no_output_____"
]
],
[
[
"**Comment.** All of these statements must be in the same cell for this to work. ",
"_____no_output_____"
],
[
"**Comment.** This is overkill -- it looks horrible -- but it makes the point that we control everything in the plot. We recommend you do very little of this until you're more comfortable with the basics. ",
"_____no_output_____"
],
[
"**Exercise.** Add a `plt.ylim()` statement to make the `y` axis start at zero, as it did in the bar charts. *Bonus points:* Change the color to magenta and the linewidth to 2. *Hint:* Use `plt.ylim?` and `plt.plot?` to get the documentation. ",
"_____no_output_____"
],
[
"**Exercise.** Create a line plot for the Fama-French dataframe `ff` that includes both returns. *Bonus points:* Add a title and label the y axis. ",
"_____no_output_____"
],
[
"## Approach #3: Create figure objects and apply methods\n\nThis approach is the most foreign to beginners, but now that we’re used to it we like it a lot. The idea is to generate an object – two objects, in fact – and apply methods to them to produce the various elements of a graph: the data, their axes, their labels, and so on.",
"_____no_output_____"
]
],
[
[
"# create fig and ax objects\nfig, ax = plt.subplots()",
"_____no_output_____"
]
],
[
[
"**Exercise.** What do we have here? What `type` are `fig` and `ax`? ",
"_____no_output_____"
],
[
"We say `fig` is a **figure object** and `ax` is an **axis object**. This means:\n \n* `fig` is a blank canvas for creating a figure.\n* `ax` is everything in it: axes, labels, lines or bars, and so on. ",
"_____no_output_____"
],
[
"**Exercise.** Use tab completion to see what methods are available for `fig` and `ax`. What do you see? Do you feel like screaming?",
"_____no_output_____"
]
],
[
[
"# let's try that again, this time with content \n# create objects \nfig, axe = plt.subplots()\n\n# add things by applying methods to ax \nus.plot(ax=axe) ",
"_____no_output_____"
]
],
[
[
"**Comment.** Both of these statements must be in the same cell. ",
"_____no_output_____"
]
],
[
[
"# Fama-French example \nfig, ax = plt.subplots()\nff.plot(ax=ax, \n kind='line', # line plot \n color=['blue', 'magenta'], # line color \n title='Fama-French market and riskfree returns')",
"_____no_output_____"
]
],
[
[
"**Exercise.** Let's see if we can teach ourselves the rest: \n\n* Add the argument `kind='bar'` to convert this into a bar chart. \n* Add the argument `alpha=0.65` to the bar chart. What does it do? \n* What would you change in the bar chart to make it look better? Use the help facility to find options that might help. Which ones appeal to you? \n\n**Exercise (somewhat challenging).** Use the same approach to reproduce our earlier histograms of the Fama-French series. ",
"_____no_output_____"
],
[
"## Quick review of the bidding\n\nTake a deep breath. We've covered a lot of ground, let's take stock. \n\nWe looked at three ways to use Matplotlib:\n\n* Approach #1: apply plot method to dataframe\n* Approach #2: use `plot(x,y)` function \n* Approach #3: create `fig, ax` objects, apply plot methods to them\n\nSame result, different syntax. This is what each of them looks like applied to US GDP: \n\n```python\nus['gdp'].plot() # Approach #1\n\nplt.plot(us.index, us['gdp']) # Approach #2\n\nfig, ax = plt.subplots() # Approach #3 \nax.plot(us.index, us['gdp']) \n```",
"_____no_output_____"
],
[
"## Bells and whistles ",
"_____no_output_____"
],
[
"### Adding things to graphs\n\nWe have lots of choices. Here's an example.",
"_____no_output_____"
]
],
[
[
"fig, ax = plt.subplots()\n\nus.plot(ax=ax) \nax.set_title('US GDP and Consumption', fontsize=14, loc='left')\nax.set_ylabel('Billions of 2013 USD')\nax.legend(['Real GDP', 'Consumption'], loc=0) # more descriptive variable names \nax.set_xlim(2002.5, 2013.5) # expand x axis limits\nax.tick_params(labelcolor='red') # change tick labels to red\nax.set_ylim(0)",
"_____no_output_____"
]
],
[
[
"(Your results may differ, but we really enjoyed that.) ",
"_____no_output_____"
],
[
"**Exercise.** Use the `set_xlabel()` method to add an x-axis label. What would you choose? Or would you prefer to leave it empty? \n\n**Exercise.** Enter `ax.set_legend?` to access the documentation for the `set_legend` method. What options appeal to you? \n\n**Exercise.** Change the line width to 2 and the line colors to blue and magenta. *Hint:* Use `us.plot?` to get the documentation. \n\n**Exercise (challenging).** Use the `set_ylim()` method to start the `y` axis at zero. *Hint:* Use `ax.set_ylim?` to get the documentation. \n\n**Exercise.** Create a line plot for the Fama-French dataframe `ff` that includes both returns. *Bonus points:* Add a title with the `set_title` method. ",
"_____no_output_____"
],
[
"### Multiple subplots \n\nSame idea, but we create a multidimensional `ax` and apply methods to each component. Here we redo the plots of US GDP and consumption. ",
"_____no_output_____"
]
],
[
[
"# this creates a 2-dimensional ax \nfig, ax = plt.subplots(nrows=2, ncols=1, sharex=True) \nprint('Object ax has dimension', len(ax))",
"Object ax has dimension 2\n"
],
[
"# now add some content \nfig, ax = plt.subplots(nrows=2, ncols=1, sharex=True, sharey=True)\n\nus['gdp'].plot(ax=ax[0], color='green') # first plot\nus['pce'].plot(ax=ax[1], color='red') # second plot",
"_____no_output_____"
]
],
[
[
"## Examples\n\nWe conclude with examples that take the data from the previous chapter and make better graphs with it. ",
"_____no_output_____"
],
[
"### Student test scores (PISA) \n\nThe international test scores often used to compare quality of education across countries. ",
"_____no_output_____"
]
],
[
[
"# data input \nimport pandas as pd\nurl = 'http://dx.doi.org/10.1787/888932937035'\npisa = pd.read_excel(url, \n skiprows=18, # skip the first 18 rows \n skipfooter=7, # skip the last 7 \n parse_cols=[0,1,9,13], # select columns \n index_col=0, # set index = first column\n header=[0,1] # set variable names \n )\npisa = pisa.dropna() # drop blank lines \npisa.columns = ['Math', 'Reading', 'Science'] # simplify variable names ",
"_____no_output_____"
],
[
"# bar chart of math scores \nfig, ax = plt.subplots()\npisa['Math'].plot(kind='barh', ax=ax) ",
"_____no_output_____"
]
],
[
[
"**Comment.** Yikes! That's horrible! What can we do about it? \n\nLet's make the figure taller. The `figsize` argument has the form `(width, height)`. The default is `(6, 4)`. We want a tall figure, so we need to increase the height setting. ",
"_____no_output_____"
]
],
[
[
"fig.",
"_____no_output_____"
],
[
"# make the plot taller \nfig, ax = plt.subplots(figsize=(4, 13)) # note figsize \npisa['Math'].plot(kind='barh', ax=ax) \nax.set_title('PISA Math Score', loc='left')",
"_____no_output_____"
]
],
[
[
"**Comment.** What if we wanted to make the US bar red? This is ridiculously complicated, but we used our Google fu and found [a solution](http://stackoverflow.com/questions/18973404/setting-different-bar-color-in-matplotlib-python). Remember: The solution to many problems is Google fu + patience. ",
"_____no_output_____"
]
],
[
[
"fig, ax = plt.subplots()\npisa['Math'].plot(kind='barh', ax=ax, figsize=(4,13))\nax.set_title('PISA Math Score', loc='left')\nax.get_children()[36].set_color('r')",
"_____no_output_____"
]
],
[
[
"**Exercise.** Create the same graph for the Reading score. ",
"_____no_output_____"
],
[
"### World Bank data\n\nWe'll use World Bank data for GDP, GDP per capita, and life expectancy to produce a few graphs and illsutrate some methods we haven't seen yet. \n\n* Bar charts of GDP and GDP per capita \n* Scatter plot (bubble plot) of life expectancy v GDP per capita ",
"_____no_output_____"
]
],
[
[
"# load packages (redundancy is ok)\nimport pandas as pd # data management tools\nfrom pandas.io import wb # World Bank api\nimport matplotlib.pyplot as plt # plotting tools\n\n# variable list (GDP, GDP per capita, life expectancy)\nvar = ['NY.GDP.PCAP.PP.KD', 'NY.GDP.MKTP.PP.KD', 'SP.DYN.LE00.IN'] \n# country list (ISO codes)\niso = ['USA', 'FRA', 'JPN', 'CHN', 'IND', 'BRA', 'MEX']\nyear = 2013\n\n# get data from World Bank \ndf = wb.download(indicator=var, country=iso, start=year, end=year)\n\n# massage data\ndf = df.reset_index(level='year', drop=True)\ndf.columns = ['gdppc', 'gdp', 'life'] # rename variables\ndf['pop'] = df['gdp']/df['gdppc'] # population \ndf['gdp'] = df['gdp']/10**12 # convert to trillions\ndf['gdppc'] = df['gdppc']/10**3 # convert to thousands\ndf['order'] = [5, 3, 1, 4, 2, 6, 0] # reorder countries\ndf = df.sort_values(by='order', ascending=False)\ndf",
"/Users/sglyon/anaconda3/lib/python3.5/site-packages/pandas/io/wb.py:19: FutureWarning: \nThe pandas.io.wb module is moved to a separate package (pandas-datareader) and will be removed from pandas in a future version.\nAfter installing the pandas-datareader package (https://github.com/pydata/pandas-datareader), you can change the import ``from pandas.io import data, wb`` to ``from pandas_datareader import data, wb``.\n FutureWarning)\n"
],
[
"# GDP bar chart\nfig, ax = plt.subplots()\ndf['gdp'].plot(ax=ax, kind='barh', alpha=0.5)\nax.set_title('GDP', loc='left', fontsize=14)\nax.set_xlabel('Trillions of US Dollars')\nax.set_ylabel('')",
"_____no_output_____"
],
[
"# ditto for GDP per capita (per person)\nfig, ax = plt.subplots()\ndf['gdppc'].plot(ax=ax, kind='barh', color='m', alpha=0.50) # 'm' == 'magenta'\nax.set_title('GDP Per Capita', loc='left', fontsize=14)\nax.set_xlabel('Thousands of US Dollars')\nax.set_ylabel('')",
"_____no_output_____"
]
],
[
[
"And just because it's fun, here's an example of Tufte-like axes from [Matplotlib examples](http://matplotlib.org/examples/ticks_and_spines/spines_demo_dropped.html). If you want to do this yourself, copy the last six line and prepare yourself to sink some time into it. ",
"_____no_output_____"
]
],
[
[
"# ditto for GDP per capita (per person)\nfig, ax = plt.subplots()\ndf['gdppc'].plot(ax=ax, kind='barh', color='b', alpha=0.5)\nax.set_title('GDP Per Capita', loc='left', fontsize=14)\nax.set_xlabel('Thousands of US Dollars')\nax.set_ylabel('')\n\n# Tufte-like axes\nax.spines['left'].set_position(('outward', 7))\nax.spines['bottom'].set_position(('outward', 7))\nax.spines['right'].set_visible(False)\nax.spines['top'].set_visible(False)\nax.yaxis.set_ticks_position('left')\nax.xaxis.set_ticks_position('bottom')",
"_____no_output_____"
]
],
[
[
"**Exercise (challenging).** Make the ticks point out. ",
"_____no_output_____"
]
],
[
[
"# scatterplot of life expectancy vs gdp per capita\nfig, ax = plt.subplots()\nax.scatter(df['gdppc'], df['life'], # x,y variables\n s=df['pop']/10**6, # size of bubbles\n alpha=0.5) \nax.set_title('Life expectancy vs. GDP per capita', loc='left', fontsize=14)\nax.set_xlabel('GDP Per Capita')\nax.set_ylabel('Life Expectancy')\nax.text(58, 66, 'Bubble size represents population', horizontalalignment='right')",
"_____no_output_____"
]
],
[
[
"**Exercise.** Make the bubble a little larger. ",
"_____no_output_____"
],
[
"## Styles (optional)\n\nGraph settings you might like. ",
"_____no_output_____"
]
],
[
[
"# We'll look at this chart under a variety of styles.\n# Let's make a function so we don't have to repeat the\n# code to create \ndef gdp_bar():\n fig, ax = plt.subplots()\n df['gdp'].plot(ax=ax, kind='barh', alpha=0.5)\n ax.set_title('Real GDP', loc='left', fontsize=14)\n ax.set_xlabel('Trillions of US Dollars')\n ax.set_ylabel('')\n \ngdp_bar()",
"_____no_output_____"
]
],
[
[
"**Exercise.** Create the same graph with this statement at the top:\n```python\nplt.style.use('fivethirtyeight')\n```\n(Once we execute this statement, it stays executed.) ",
"_____no_output_____"
],
[
"**Comment.** We can get a list of files from `plt.style.available`. ",
"_____no_output_____"
]
],
[
[
"plt.style.available",
"_____no_output_____"
]
],
[
[
"**Comment.** Ignore the seaborn styles, that's a package we don't have yet. \n\n**Exercise.** Try another one by editing the code below. ",
"_____no_output_____"
]
],
[
[
"plt.style.use('fivethirtyeight')\ngdp_bar()",
"_____no_output_____"
]
],
[
[
"**Comment.** For aficionados, the always tasteful [xkcd style](http://xkcd.com/1235/). ",
"_____no_output_____"
]
],
[
[
"plt.style.use('ggplot')\ngdp_bar()",
"_____no_output_____"
],
[
"plt.xkcd()\ngdp_bar()",
"_____no_output_____"
]
],
[
[
"**Comment.** We reset the style with these two lines: ",
"_____no_output_____"
]
],
[
[
"mpl.rcParams.update(mpl.rcParamsDefault)\n%matplotlib inline",
"_____no_output_____"
]
],
[
[
"## Review\n\nConsider the data from Randal Olson's [blog post](http://www.randalolson.com/2014/06/28/how-to-make-beautiful-data-visualizations-in-python-with-matplotlib/): \n\n```python\nimport pandas as pd \ndata = {'Food': ['French Fries', 'Potato Chips', 'Bacon', 'Pizza', 'Chili Dog'], \n 'Calories per 100g': [607, 542, 533, 296, 260]}\ncals = pd.DataFrame(data)\n```\n\nThe dataframe `cals` contains the calories in 100 grams of several different foods. \n\n\n**Exercise.** We'll create and modify visualizations of this data: \n\n* Set `'Food'` as the index of `cals`. \n* Create a bar chart with `cals` using figure and axis objects. \n* Add a title. \n* Change the color of the bars. What color do you prefer? \n* Add the argument `alpha=0.5`. What does it do? \n* Change your chart to a horizontal bar chart. Which do you prefer? \n* *Challenging.* Eliminate the legend. \n* *Challenging.* Skim the top of Olson's [blog post](http://www.randalolson.com/2014/06/28/how-to-make-beautiful-data-visualizations-in-python-with-matplotlib/). What do you see that you'd like to imitate? ",
"_____no_output_____"
],
[
"## Where does that leave us?\n\n* We now have several ways to produce graphs. \n* Next up: think about what we want to graph and why. The tools serve that higher purpose. ",
"_____no_output_____"
]
]
] | [
"markdown",
"code",
"markdown",
"code",
"markdown",
"code",
"markdown",
"code",
"markdown",
"code",
"markdown",
"code",
"markdown",
"code",
"markdown",
"code",
"markdown",
"code",
"markdown",
"code",
"markdown",
"code",
"markdown",
"code",
"markdown",
"code",
"markdown",
"code",
"markdown",
"code",
"markdown",
"code",
"markdown",
"code",
"markdown",
"code",
"markdown",
"code",
"markdown",
"code",
"markdown",
"code",
"markdown",
"code",
"markdown",
"code",
"markdown",
"code",
"markdown",
"code",
"markdown",
"code",
"markdown",
"code",
"markdown",
"code",
"markdown"
] | [
[
"markdown",
"markdown",
"markdown",
"markdown",
"markdown"
],
[
"code"
],
[
"markdown"
],
[
"code"
],
[
"markdown"
],
[
"code",
"code"
],
[
"markdown"
],
[
"code"
],
[
"markdown",
"markdown"
],
[
"code"
],
[
"markdown",
"markdown",
"markdown"
],
[
"code",
"code",
"code"
],
[
"markdown"
],
[
"code",
"code"
],
[
"markdown",
"markdown"
],
[
"code",
"code"
],
[
"markdown"
],
[
"code",
"code"
],
[
"markdown",
"markdown"
],
[
"code",
"code"
],
[
"markdown"
],
[
"code",
"code"
],
[
"markdown"
],
[
"code"
],
[
"markdown",
"markdown",
"markdown",
"markdown",
"markdown"
],
[
"code"
],
[
"markdown",
"markdown",
"markdown"
],
[
"code"
],
[
"markdown"
],
[
"code"
],
[
"markdown",
"markdown",
"markdown",
"markdown"
],
[
"code"
],
[
"markdown",
"markdown",
"markdown"
],
[
"code",
"code"
],
[
"markdown",
"markdown"
],
[
"code",
"code"
],
[
"markdown"
],
[
"code",
"code"
],
[
"markdown"
],
[
"code"
],
[
"markdown",
"markdown"
],
[
"code",
"code",
"code"
],
[
"markdown"
],
[
"code"
],
[
"markdown"
],
[
"code"
],
[
"markdown",
"markdown"
],
[
"code"
],
[
"markdown",
"markdown"
],
[
"code"
],
[
"markdown"
],
[
"code"
],
[
"markdown"
],
[
"code",
"code"
],
[
"markdown"
],
[
"code"
],
[
"markdown",
"markdown"
]
] |
e764fabbbe9c77748d944e39b4556eaec737ae3b | 230,230 | ipynb | Jupyter Notebook | notebooks/graph_algos/node2vec/corpus 2018 audience_overlap lvl data 1.ipynb | Panayot9/News-Media-Peers | 3a45aabd2c04c15dc16a43633898efbc7cbc5baa | [
"MIT"
] | null | null | null | notebooks/graph_algos/node2vec/corpus 2018 audience_overlap lvl data 1.ipynb | Panayot9/News-Media-Peers | 3a45aabd2c04c15dc16a43633898efbc7cbc5baa | [
"MIT"
] | null | null | null | notebooks/graph_algos/node2vec/corpus 2018 audience_overlap lvl data 1.ipynb | Panayot9/News-Media-Peers | 3a45aabd2c04c15dc16a43633898efbc7cbc5baa | [
"MIT"
] | 1 | 2021-12-26T17:12:53.000Z | 2021-12-26T17:12:53.000Z | 112.802548 | 469 | 0.634049 | [
[
[
"import pandas as pd\nimport sys\nimport os\nsys.path.insert(0, '../../../')\n\nfrom notebooks.utils import (\n _ALEXA_DATA_PATH, load_node_features, load_level_data,\n create_audience_overlap_nodes, export_model_as_feature, create_node2vec_model\n)\nfrom train import run_experiment",
"2022-01-14 10:29:34.576483: I tensorflow/core/platform/cpu_feature_guard.cc:151] This TensorFlow binary is optimized with oneAPI Deep Neural Network Library (oneDNN) to use the following CPU instructions in performance-critical operations: AVX2 FMA\nTo enable them in other operations, rebuild TensorFlow with the appropriate compiler flags.\n"
]
],
[
[
"# Load audience overlap edges for level 1",
"_____no_output_____"
]
],
[
[
"level = 1\naudience_overlap_sites = load_level_data(os.path.join(_ALEXA_DATA_PATH, 'corpus_2018_audience_overlap_sites_scrapping_result.json'), level=level)\naudience_overlap_sites_NODES = create_audience_overlap_nodes(audience_overlap_sites)\n\nprint(audience_overlap_sites_NODES[:5])",
"01-14 10:29:36 notebooks.utils INFO Loaded 4238 nodes with records level <= 1 and child size:20335\n"
],
[
"edge_df = pd.DataFrame(audience_overlap_sites_NODES, columns=['source', 'target'])\n\nedge_df.head()",
"_____no_output_____"
]
],
[
[
"# Create Graph",
"_____no_output_____"
]
],
[
[
"import stellargraph as sg\n\nG = sg.StellarGraph(edges=edge_df)\n\nprint(G.info())",
"StellarGraph: Undirected multigraph\n Nodes: 11865, Edges: 20399\n\n Node types:\n default: [11865]\n Features: none\n Edge types: default-default->default\n\n Edge types:\n default-default->default: [20399]\n Weights: all 1 (default)\n Features: none\n"
]
],
[
[
"# Create Node2Vec models",
"_____no_output_____"
]
],
[
[
"models = create_node2vec_model(G, dimensions=[64, 128, 256, 512, 1024], is_weighted=False,\n prefix='corpus_2018_audience_overlap_lvl_one')",
"Start creating random walks\nNumber of random walks: 118650\n"
]
],
[
[
"# Export embeddings as feature",
"_____no_output_____"
]
],
[
[
"for model_name, model in models.items():\n print(f'Processing model: {model_name}')\n embeddings_wv = {site: model.wv.get_vector(site).tolist() for site in G.nodes()}\n export_model_as_feature(embeddings_wv, model_name, data_year='2018')\n run_experiment(features=model_name, dataset='emnlp2018', task='fact')\n print('\\n', '-'*50, '\\n')\n run_experiment(features=model_name, dataset='emnlp2018', task='bias')\n print('\\n', '='*50, '\\n')",
"Processing model: corpus_2018_audience_overlap_lvl_one_unweighted_64D.model\n+------+---------------------+---------------+--------------------+-----------------------------------------------------------+\n| task | classification_mode | type_training | normalize_features | features |\n+------+---------------------+---------------+--------------------+-----------------------------------------------------------+\n| fact | single classifier | combine | False | corpus_2018_audience_overlap_lvl_one_unweighted_64D.model |\n+------+---------------------+---------------+--------------------+-----------------------------------------------------------+\n"
]
]
] | [
"code",
"markdown",
"code",
"markdown",
"code",
"markdown",
"code",
"markdown",
"code"
] | [
[
"code"
],
[
"markdown"
],
[
"code",
"code"
],
[
"markdown"
],
[
"code"
],
[
"markdown"
],
[
"code"
],
[
"markdown"
],
[
"code"
]
] |
e764fda8f21beab4180e3c5ed565c25bcd15f51e | 290,153 | ipynb | Jupyter Notebook | demo.ipynb | skad00sh/gsudmlab-mvtsdata_toolkit | 2c5495deb6d31eef556e7f410ac1c1632bffa961 | [
"MIT"
] | 7 | 2020-07-07T10:27:02.000Z | 2021-04-02T13:20:24.000Z | demo.ipynb | skad00sh/gsudmlab-mvtsdata_toolkit | 2c5495deb6d31eef556e7f410ac1c1632bffa961 | [
"MIT"
] | 3 | 2020-03-31T09:35:53.000Z | 2021-08-23T20:46:33.000Z | demo.ipynb | skad00sh/gsudmlab-mvtsdata_toolkit | 2c5495deb6d31eef556e7f410ac1c1632bffa961 | [
"MIT"
] | 3 | 2020-08-31T10:21:21.000Z | 2021-12-01T11:38:17.000Z | 74.820268 | 36,164 | 0.687499 | [
[
[
"# <center>MVTS Data Toolkit</center>\n## <center>A Toolkit for Pre-processing Multivariate Time Series Data</center>\n\n<img src=\"https://bitbucket.org/gsudmlab/mvtsdata_toolkit/downloads/MVTS_Data_Toolkit_icon2.png\">\n\n* **Title:** MVTS Data Toolkit: A Toolkit for Pre-processing Multivariate Time Series Data\n* **Journal:** SoftwareX Journal [$\\triangleright$](https://www.journals.elsevier.com/softwarex)(Elsevier)\n* **Authors:** Azim Ahmadzadeh [$\\oplus$](https://www.azim-a.com/), Kankana Sinha [$\\ominus$](https://www.linkedin.com/in/kankana-sinha-4b4b13131/), Berkay Aydin [$\\otimes$](https://grid.cs.gsu.edu/~baydin2/), Rafal A. Angryk [$\\oslash$](https://grid.cs.gsu.edu/~rangryk/)\n* **Demo Author:** Azim Ahmadzadeh\n* **Last Modified:** May 02, 2020\n\n## Intro\n\nThis demo gives a quick tour over the toolkit's funcionalities. Here I will:\n 1. download a multi-class dataset of 2000 multivariate time series (mvts) instances,\n 2. show how the configuration file can be prepared,\n 3. get some basic statistics about the data,\n 4. extract multiple statistical features from the mvts instances, and visualize the results,\n 5. analyze the extracted-feature data,\n 6. normalize the extracted features,\n 7. sample the extracted data to obtain a different ratios of the five classes.",
"_____no_output_____"
]
],
[
[
"import os\nimport yaml\nimport urllib.request\nfrom mvtsdatatoolkit.data.data_retriever import DataRetriever # for downloading data\nimport CONSTANTS as CONST\n%matplotlib inline",
"_____no_output_____"
]
],
[
[
"## 1. Download the Dataset\nIn this demo I use an example dataset. In the following cells, it will be automatically downloaded. But in case something goes wrong, here is the direct link:\nhttps://bitbucket.org/gsudmlab/mvtsdata_toolkit/downloads/petdataset_01.zip\n. Please note that here I am using the class `DataRetriever` that I implemented. This is only implemented for the purpose of this demo and is not one of the generic tools to be used for any other datasets.\n\nBefore we download it, let's take a quick look:",
"_____no_output_____"
]
],
[
[
"dr = DataRetriever(1)\ndr.print_info()",
"URL:\t\thttps://bitbucket.org/gsudmlab/mvtsdata_toolkit/downloads/petdataset_01.zip\nNAME:\t\tpetdataset_01.zip\nTYPE:\t\tapplication/zip\nSIZE:\t\t32M\n"
]
],
[
[
"Ready to download? This may take a few seconds, depending on your internet bandwidth. Wait for the progress bar.",
"_____no_output_____"
]
],
[
[
"where_to = './temp/' # Don't change this path.\ndr.retrieve(target_path = where_to)",
"Extracting: 100%|██████████| 2001/2001 [00:01<00:00, 1869.24it/s]\n"
]
],
[
[
"OK. Let's see how many files are available to us now.",
"_____no_output_____"
]
],
[
[
"dr.get_total_number_of_files()",
"_____no_output_____"
]
],
[
[
"## 2. Setup Configurations\nFor tasks such as feature extraction (module: `features.feature_extractor`) and data analysis (modules: `data_analysis.mvts_data_analysis` and `data_analysis.extracted_features_analysis`) a configuration file must be provided by the user.\n\nThis configuration file is a `yml` file, specificly defined for the dataset of interest and can be stored anywhere the user wish.\n\nHere, we download and use our own configuration file as an example.",
"_____no_output_____"
]
],
[
[
"conf_url = 'https://bitbucket.org/gsudmlab/mvtsdata_toolkit/downloads/demo_configs.yml'\npath_to_config = './demo_configs.yml'\nurllib.request.urlretrieve(conf_url, path_to_config)",
"_____no_output_____"
]
],
[
[
"Let's take a look at the content of the config file which is located at `./demo_configs.yml`:",
"_____no_output_____"
]
],
[
[
"with open(path_to_config, 'r') as f:\n print(f.read())",
"PATH_TO_MVTS: './temp/petdataset_01/'\nPATH_TO_EXTRACTED_FEATURES: './temp/extracted_features/'\nMETA_DATA_TAGS: ['id', 'lab', 'st', 'et']\nMVTS_PARAMETERS:\n - 'TOTUSJH'\n - 'TOTBSQ'\n - 'TOTPOT'\n - 'TOTUSJZ'\n - 'ABSNJZH'\n - 'SAVNCPP'\n - 'USFLUX'\n - 'TOTFZ'\n - 'MEANPOT'\n - 'EPSZ'\n - 'MEANSHR'\n - 'SHRGT45'\n - 'MEANGAM'\n - 'MEANGBT'\n - 'MEANGBZ'\n - 'MEANGBH'\n - 'MEANJZH'\n - 'TOTFY'\n - 'MEANJZD'\n - 'MEANALP'\n - 'TOTFX'\n - 'EPSY'\n - 'EPSX'\n - 'R_VALUE'\nSTATISTICAL_FEATURES:\n - 'get_min'\n - 'get_max'\n - 'get_median'\n - 'get_mean'\n - 'get_stddev'\n - 'get_var'\n - 'get_skewness'\n - 'get_kurtosis'\n - 'get_no_local_maxima'\n - 'get_no_local_minima'\n - 'get_no_local_extrema'\n - 'get_no_zero_crossings'\n - 'get_mean_local_maxima_value'\n - 'get_mean_local_minima_value'\n - 'get_no_mean_local_maxima_upsurges'\n - 'get_no_mean_local_minima_downslides'\n - 'get_difference_of_mins'\n - 'get_difference_of_maxs'\n - 'get_difference_of_means'\n - 'get_difference_of_stds'\n - 'get_difference_of_vars'\n - 'get_difference_of_medians'\n - 'get_dderivative_mean'\n - 'get_gderivative_mean'\n - 'get_dderivative_stddev'\n - 'get_gderivative_stddev'\n - 'get_dderivative_skewness'\n - 'get_gderivative_skewness'\n - 'get_dderivative_kurtosis'\n - 'get_gderivative_kurtosis'\n - 'get_linear_weighted_average'\n - 'get_quadratic_weighted_average'\n - 'get_average_absolute_change'\n - 'get_average_absolute_derivative_change'\n - 'get_positive_fraction'\n - 'get_negative_fraction'\n - 'get_last_value'\n - 'get_sum_of_last_K'\n - 'get_mean_last_K'\n - 'get_slope_of_longest_mono_increase'\n - 'get_slope_of_longest_mono_decrease'\n - 'get_avg_mono_increase_slope'\n - 'get_avg_mono_decrease_slope'\n\n\n"
]
],
[
[
"Here is the break-down of the pieces:\n - `PATH_to_MVTS`: A relative or absolute path to where the multivariate time series dataset is stored at.\n - `PATH_TO_EXTRACTED`: A relative or absolute path to where the extracted features should be stored at, using the Feature Extraction component of the package.\n - `META_DATA_TAGS`: A list of tags based on which some pieces of information can be extracted from the file-names of the multivariate time series. For example, if timestamps are encoded in the file-names, e.g., `_st[YYYY-MM-DD HH:MM:SS]`, then the string `st` (without brackets) is a tag that can be included in this list. In the feature extraction process, this will add the corresponding metadata (i.e., what is wrapped in the square brackets) in each filename, as an extra column to the data-frame of the extracted features. Generally, using this functionality, any extra metadata (e.g., class labels, start-time, end-time, id's, etc) can be encoded in the file-names and consequently passed into the extracted features.\n - `MVTS_PARAMETERS`: A list of parameter-names that are used in the multivariate time series dataset, whose statistical features are of interest. These are, in other words, the column-names in the multivariate time series files.\n - `STATISTICAL_FEATURES`: A list of statistical features of interest to be extracted from the multivariate time series. They must be chosen from the provided methods in the module `features.feature_collection.`. For example, `get_min` is a valid feature-name as this method is implemented in the package.\n \n In the following steps, you will see how this can be used.",
"_____no_output_____"
],
[
"## 3. Analysis of Raw Data (MVTS Data Analysis)\n\n- #### How many files? How large of a dataset?\n\nUsing `mvts_data_analysis` module users can get an idea of the dataset they are going to work on. I start with creating an instance of the `MVTSDataAnalysis` class. This right away gives me some high-level information about the dataset.",
"_____no_output_____"
]
],
[
[
"from mvtsdatatoolkit.data_analysis.mvts_data_analysis import MVTSDataAnalysis\n\nmvda = MVTSDataAnalysis(path_to_config)\nmvda.print_stat_of_directory()",
"----------------------------------------\nDirectory:\t\t\t./temp/petdataset_01/\nTotal no. of files:\t2000\nTotal size:\t\t\t76M\nTotal average:\t\t38K\n----------------------------------------\n"
]
],
[
[
"- #### Get a summary stats of the data.\n\nLet's now get some statistics from the content of the files. To speed up the demo, I analyze only 3 time series parameters (namely `TOTUSJH`, `TOTBSQ`, and `TOTPOT`), and only the first 50 mvts files.",
"_____no_output_____"
]
],
[
[
"params = ['TOTUSJH', 'TOTBSQ', 'TOTPOT']\nn = 50\nmvda.compute_summary(params_name=params, first_k=n)\nmvda.summary",
"_____no_output_____"
]
],
[
[
"... which says the length of the time series, across the 50 mvts files is 3000, including 0 `NA/NAN` values. In addition, `mean`, `min`, `max`, and three quantiles are calculated for each time series.",
"_____no_output_____"
],
[
" - #### You have a LARGE dataset?\n A parallel version of this function is also provided to help process much larger datasets efficiently. Below, we will have 4 processes to compute the summary statistics.",
"_____no_output_____"
]
],
[
[
"mvda.compute_summary_in_parallel(n_jobs=4, first_k=50, verbose=False,\n params_name=['TOTUSJH', 'TOTBSQ', 'TOTPOT'])\nmvda.summary",
"_____no_output_____"
]
],
[
[
"**Note**: The results of the parallel and sequential versions of `mvts_data_analysis` are not exactly identical. This discrepency is due to the fact that in the parallel version, the program has to approximate the percentiles. More specifically, it is designed to avoid loading the entire dataset into memory so that it is not confined to any particular data size. Therefore, it relies on some statistical estimators to approximate the percentiles with some acceptable error. The errors decrease significantly as the number of mvts files increases. In conclusion, for small datasets I recommend using the sequential version.",
"_____no_output_____"
],
[
"## 4. Feature Extraction\n\n- #### What statistical features are available?\n\nNow that we have an idea about our raw data, let's extract some features from the data. A list of 48 statistical features are implemented in `feature_collection`. Let's take a look at them.",
"_____no_output_____"
]
],
[
[
"import mvtsdatatoolkit.features.feature_collection as fc\nhelp(fc)",
"Help on module mvtsdatatoolkit.features.feature_collection in mvtsdatatoolkit.features:\n\nNAME\n mvtsdatatoolkit.features.feature_collection\n\nFUNCTIONS\n get_average_absolute_change(uni_ts:Union[pandas.core.series.Series, numpy.ndarray]) -> numpy.float64\n :return: The average absolute first difference of a univariate time series.\n \n get_average_absolute_derivative_change(uni_ts:Union[pandas.core.series.Series, numpy.ndarray]) -> numpy.float64\n :return: The average absolute first difference of a derivative of univariate time series.\n \n get_avg_mono_decrease_slope(uni_ts:Union[pandas.core.series.Series, numpy.ndarray]) -> numpy.float64\n :return: The average slope of monotonically decreasing segments.\n \n get_avg_mono_increase_slope(uni_ts:Union[pandas.core.series.Series, numpy.ndarray]) -> numpy.float64\n :return: The average slope of monotonically increasing segments.\n \n get_dderivative_kurtosis(uni_ts:Union[pandas.core.series.Series, numpy.ndarray], step_size:int=1) -> numpy.float64\n :return: The kurtosis of the difference derivative of univariate time series within the\n function we use step_size to find derivative (default value of step_size is 1).\n \n get_dderivative_mean(uni_ts:Union[pandas.core.series.Series, numpy.ndarray], step_size:int=1) -> numpy.float64\n :return: The mean of the difference-derivative of univariate time series within the function\n we use step_size to find derivative (default value of step_size is 1).\n \n get_dderivative_skewness(uni_ts:Union[pandas.core.series.Series, numpy.ndarray], step_size:int=1) -> numpy.float64\n :return: The skewness of the difference derivative of univariate time series within the\n function we use step_size to find derivative (default value of step_size is 1).\n \n get_dderivative_stddev(uni_ts:Union[pandas.core.series.Series, numpy.ndarray], step_size:int=1) -> numpy.float64\n :return: The std.dev of the difference derivative of univariate time series within the\n function we use step_size to find derivative (default value of step_size is 1).\n \n get_difference_of_maxs(uni_ts:Union[pandas.core.series.Series, numpy.ndarray]) -> numpy.float64\n :return: The absolute difference between the maximums of the first and the second halves of a\n given univariate time series.\n \n get_difference_of_means(uni_ts:Union[pandas.core.series.Series, numpy.ndarray]) -> numpy.float64\n :return: The absolute difference between the means of the first and the second halves of a\n given univariate time series.\n \n get_difference_of_medians(uni_ts:Union[pandas.core.series.Series, numpy.ndarray]) -> numpy.float64\n :return: The absolute difference between the medians of the first and the second halves of a\n given univariate time series.\n \n get_difference_of_mins(uni_ts:Union[pandas.core.series.Series, numpy.ndarray]) -> numpy.float64\n :return: The absolute difference between the minimums of the first and the second halves of a\n given univariate time series.\n \n get_difference_of_stds(uni_ts:Union[pandas.core.series.Series, numpy.ndarray]) -> numpy.float64\n :return: The absolute difference between the standard dev. of the first and the second halves\n of a given univariate time series.\n \n get_difference_of_vars(uni_ts:Union[pandas.core.series.Series, numpy.ndarray]) -> numpy.float64\n :return: The absolute difference between the variances of the first and the second halves of\n a given univariate time series.\n \n get_gderivative_kurtosis(uni_ts:Union[pandas.core.series.Series, numpy.ndarray]) -> numpy.float64\n :return: The kurtosis of the gradient derivative of the univariate time series.\n \n get_gderivative_mean(uni_ts:Union[pandas.core.series.Series, numpy.ndarray]) -> numpy.float64\n :return: The mean of the gradient-derivative of univariate time series.\n \n get_gderivative_skewness(uni_ts:Union[pandas.core.series.Series, numpy.ndarray]) -> numpy.float64\n :return: The skewness of the gradient derivative of the univariate time series.\n \n get_gderivative_stddev(uni_ts:Union[pandas.core.series.Series, numpy.ndarray]) -> numpy.float64\n :return: The std.dev of the gradient derivative of univariate time series.\n \n get_kurtosis(uni_ts:Union[pandas.core.series.Series, numpy.ndarray])\n :return: The kurtosis of a given univariate time series.\n \n get_last_K(uni_ts:Union[pandas.core.series.Series, numpy.ndarray], k:int) -> pandas.core.series.Series\n :return: The last k values in a univariate time series.\n \n get_last_value(uni_ts) -> numpy.float64\n :return: The last value in a univariate time series. This seems redundant since `get_last_K`\n already does this job, but it is necessary because the return type is different (\n `numpy.int64`) than what `get_last_K` returns (`numpy.ndarray`). This is especially\n important if the methods in this module are going to be called from a list.\n \n get_linear_weighted_average(uni_ts:Union[pandas.core.series.Series, numpy.ndarray]) -> numpy.float64\n Computes the linear weighted average of a univariate time series. It simply, for each `x_i` in\n `uni_ts` computes the following::\n \n 2/(n*(n+1)) * sum(i* x_i)\n \n where `n` is the length of the time series.\n \n :return: The linear weighted average of `uni_ts`.\n \n get_longest_monotonic_decrease(uni_ts:Union[pandas.core.series.Series, numpy.ndarray]) -> numpy.int64\n :return: The length of the time series segment with the longest monotonic increase.\n \n get_longest_monotonic_increase(uni_ts:Union[pandas.core.series.Series, numpy.ndarray]) -> numpy.int64\n :return: The length of the time series segment with the longest monotonic increase.\n \n get_longest_negative_run(uni_ts:Union[pandas.core.series.Series, numpy.ndarray]) -> numpy.int64\n :return: The longest negative run in a univariate time series.\n \n get_longest_positive_run(uni_ts:Union[pandas.core.series.Series, numpy.ndarray]) -> numpy.int64\n :return: The longest positive run in a univariate time series.\n \n get_max(uni_ts:Union[pandas.core.series.Series, numpy.ndarray]) -> numpy.float64\n :return: The maximum value of a given univariate time series.\n \n get_mean(uni_ts:Union[pandas.core.series.Series, numpy.ndarray]) -> numpy.float64\n :return: The arithmetic mean value of a given univariate time series.\n \n get_mean_last_K(uni_ts:Union[pandas.core.series.Series, numpy.ndarray], k:int=10) -> numpy.float64\n :return: The mean of last k-values in a univariate time series.\n \n get_mean_local_maxima_value(uni_ts:Union[pandas.core.series.Series, numpy.ndarray], only_positive:bool=False) -> numpy.float64\n Returns the mean of local maxima values.\n \n :param uni_ts: Univariate time series.\n :param only_positive: Only positive flag for local maxima. When True only positive local\n maxima are considered. Default is False.\n \n :return: Mean of local maxima values.\n \n get_mean_local_minima_value(uni_ts:Union[pandas.core.series.Series, numpy.ndarray], only_negative:bool=False) -> numpy.float64\n Returns the mean of local minima values.\n \n :param uni_ts: Univariate time series.\n :param only_negative: Only negative flag for local minima. When True only negative local\n minima are considered. Default is False.\n \n :return: Mean of local minima values.\n \n get_median(uni_ts:Union[pandas.core.series.Series, numpy.ndarray]) -> numpy.float64\n :return: The median value of a given univariate time series.\n \n get_min(uni_ts:Union[pandas.core.series.Series, numpy.ndarray]) -> numpy.float64\n :return: The minimum value of a given univariate time series.\n \n get_negative_fraction(uni_ts:Union[pandas.core.series.Series, numpy.ndarray]) -> numpy.float64\n :return: The fraction of negative numbers in uni_ts.\n \n get_no_local_extrema(uni_ts:Union[pandas.core.series.Series, numpy.ndarray])\n :return: The number of local extrema in a given univariate time series.\n \n get_no_local_maxima(uni_ts:Union[pandas.core.series.Series, numpy.ndarray])\n :return: The number of local maxima in a given univariate time series.\n \n get_no_local_minima(uni_ts:Union[pandas.core.series.Series, numpy.ndarray])\n :return: The number of local minima in a given univariate time series.\n \n get_no_mean_local_maxima_upsurges(uni_ts:Union[pandas.core.series.Series, numpy.ndarray], only_positive:bool=False) -> numpy.int64\n Returns the number of values in a given time series whose value is greater than the mean of\n local maxima values (# of upsurges).\n \n :param uni_ts: Univariate time series.\n :param only_positive: Only positive flag for mean local maxima. When True only positive local\n maxima are considered. Default is False.\n \n :return: Number of points whose value is greater than mean local maxima.\n \n get_no_mean_local_minima_downslides(uni_ts:Union[pandas.core.series.Series, numpy.ndarray], only_negative:bool=False) -> numpy.int64\n Returns the number of values in a given time series whose value is less than the mean of\n local minima values (# of downslides).\n \n :param uni_ts: Univariate time series.\n :param only_negative: Only negative flag for mean local minima. When True only negative local\n minima are considered. Default is False.\n \n :return: Number of points whose value is less than mean local minima.\n \n get_no_zero_crossings(uni_ts:Union[pandas.core.series.Series, numpy.ndarray])\n :return: The number of zero-crossings in a given univariate time series.\n \n get_positive_fraction(uni_ts:Union[pandas.core.series.Series, numpy.ndarray]) -> numpy.float64\n :return: The fraction of positive numbers in uni_ts.\n \n get_quadratic_weighted_average(uni_ts:Union[pandas.core.series.Series, numpy.ndarray]) -> numpy.float64\n Computes the quadratic weighted average of a univariate time series. It simply, for each `x_i` in\n `uni_ts`, computes the following::\n \n 6/(n*(n+1)(2*n+1)) * sum(i^2 * x_i)\n \n where `n` is the length of the time seires.\n \n :return: The quadratic weighted average of `uni_ts`.\n \n get_skewness(uni_ts:Union[pandas.core.series.Series, numpy.ndarray])\n :return: The skewness of a given univariate time series.\n \n get_slope_of_longest_mono_decrease(uni_ts:Union[pandas.core.series.Series, numpy.ndarray]) -> numpy.float64\n Identifies the longest monotonic decrease and gets the slope.\n \n :return: The slope of the longest monotonic decrease in `uni_ts`.\n \n get_slope_of_longest_mono_increase(uni_ts:Union[pandas.core.series.Series, numpy.ndarray]) -> numpy.float64\n Identifies the longest monotonic increase and gets the slope.\n \n :return: The slope of the longest monotonic increase in `uni_ts`.\n \n get_stddev(uni_ts:Union[pandas.core.series.Series, numpy.ndarray]) -> numpy.float64\n :return: The standard deviation of a given univariate time series.\n \n get_sum_of_last_K(uni_ts:Union[pandas.core.series.Series, numpy.ndarray], k:int=10) -> numpy.float64\n :return: The sum of last k-values in a univariate time series.\n \n get_var(uni_ts:Union[pandas.core.series.Series, numpy.ndarray]) -> numpy.float64\n :return: The variance of a given univariate time series.\n \n tee(...)\n tee(iterable, n=2) --> tuple of n independent iterators.\n\nDATA\n Union = typing.Union\n\nFILE\n /home/azim/CODES/PyWorkspace/mvtsdata_toolkit/mvtsdatatoolkit/features/feature_collection.py\n\n\n"
]
],
[
[
"- #### How to extract these features from the data?\n\nLet's extract 3 simple statistical features, namely `min`, `max`, and `median`, from 3 parameters, such as `TOTUSJH`, `TOTBSQ`, and `TOTPOT`. Again, to speed up the process in this demo, we only process the first 50 mvts files.",
"_____no_output_____"
]
],
[
[
"from mvtsdatatoolkit.features.feature_extractor import FeatureExtractor\n\nfe = FeatureExtractor(path_to_config)\nfe.do_extraction(features_name=['get_min', 'get_max', 'get_median'],\n params_name=['TOTUSJH', 'TOTBSQ', 'TOTPOT'], first_k=50)\nfe.df_all_features",
"_____no_output_____"
]
],
[
[
"... where each row corresponds to one mvts file, and the first 4 columns represent the extracted information from the file-names using the tags specified in the configuration file (i.e., `id`, `lab`, `st`, and `et`). The remaining columns contains the extracted features. They are named by appending each statistical-feature name to the end of a parameter-name, e.g., `TOTUSJH_min`.",
"_____no_output_____"
],
[
" - #### Need to visulaize it?\n There are multiple visualizations incorporated in this package that are called in the `FeatureExtractor` class. Here is how you can used them:",
"_____no_output_____"
]
],
[
[
"fe.plot_boxplot(feature_names=['TOTUSJH_median', 'TOTBSQ_median', 'TOTPOT_median'])",
"_____no_output_____"
],
[
"fe.plot_violinplot(feature_names=['TOTUSJH_median', 'TOTBSQ_median', 'TOTPOT_median'])",
"_____no_output_____"
],
[
"fe.plot_splom(feature_names=['TOTUSJH_median', 'TOTBSQ_median', 'TOTPOT_median'])",
"_____no_output_____"
],
[
"fe.plot_correlation_heatmap(feature_names=['TOTUSJH_median', 'TOTBSQ_median', 'TOTPOT_median'])",
"_____no_output_____"
],
[
"fe.plot_covariance_heatmap(feature_names=['TOTUSJH_median', 'TOTBSQ_median', 'TOTPOT_median'])",
"_____no_output_____"
]
],
[
[
"For all of these plots, it is a common practice to have the data normalized before generating such plots. This is automatically done in the above steps. Iin rare cases that normaliztion should not take place, using the `StatVisualizer` class in `stat_visualizer` module, this can be avoided. Simply, set the argument `normalize` in the class constructor to `False`.\n\nMoreover, in any of the above visulization methods, by setting a path to the argument `output_path`, the generated plots can be stored as *png* files, instead of being shown through the GUI.\n\n----",
"_____no_output_____"
],
[
" - #### You have a LARGE dataset?\n No worries. Using the parallel implementation of the feature extraction process, this can be significantly sped up. Let's have 4 processes to extract the features.\n \n **Note** This time we work on all 2000 mvts files, not just the first 50! This is 40 times larger but it takes only 10 times longer (40 times / 4 jobs) than the sequential version.",
"_____no_output_____"
]
],
[
[
"fe.do_extraction_in_parallel(n_jobs=4,\n features_name=['get_min', 'get_max', 'get_median'],\n params_name=['TOTUSJH', 'TOTBSQ', 'TOTPOT'])\nfe.df_all_features[:5]",
"_____no_output_____"
]
],
[
[
"**Note**: Here I am showing only the first 5 rows of the extracted features. Also, keep in mind that the column `id` should be used if we want to compare the results of this table with the one that was sequentially calculated.",
"_____no_output_____"
],
[
"## 5. Extracted Features Analysis\n\n- #### A quick look over the results?\n\nThe extracted features can be easily summarized using descriptive statistics such as `meam`, `std`, `min`, `max`, and first, second and third quartiles. In addition, any missing value can also be spotted.",
"_____no_output_____"
]
],
[
[
"from mvtsdatatoolkit.data_analysis.extracted_features_analysis import ExtractedFeaturesAnalysis\n\nefa = ExtractedFeaturesAnalysis(fe.df_all_features, exclude=['id'])\nefa.compute_summary()\nefa.summary",
"_____no_output_____"
]
],
[
[
"... which gives a summary statistics over every extracted feature. For instance, in row `0` that corresponds to the extracted feature `TOTUSJH_min`, the changes of the minimum values of the parameter `TUOTUSJH`, across 2000 mvts files, is described in terms of `mean`, `std`, `min`, `max`, and percentiles `25th` , `50th` (i.e., median), and `75th`. It also indicates that no `NA/NAN` or missing value was generated in the process.",
"_____no_output_____"
],
[
"## 6. Data Normalization\n\nThe extracted features can also be normalized using four different methods. Below, I use the zero-one normalization to transform values of each time-series (independently) to the `[0,1]` interval.",
"_____no_output_____"
]
],
[
[
"from mvtsdatatoolkit.normalizing import normalizer\n\ndf_norm = normalizer.zero_one_normalize(df=fe.df_all_features, excluded_colnames=['id'])\ndf_norm",
"_____no_output_____"
]
],
[
[
"**Note**: The argument `excluded_colnames` is used to keep the column `id` unchanged in the normalization process. Although this column is numeric, normalization of its values would be meaningless. Moreover, any other column with non-numeric values were automatically preserved in the output.",
"_____no_output_____"
],
[
"## 7. Data Sampling\n\nVery often our dataset suffers from the class-imbalance issue, especially when we are dealing with forecast/classification of natural phenomena. There are several generic methods in `sampling.sampler` module that allow a variety of different undersampling and oversampling techniques. Below, I will show some of them.\n\n**Note**: Our dataset has 5 class labels, namely `X`, `M`, `C`, `B`, and `NF`.\n\nFirst, I create a `Sampler` and check out the population of each class.",
"_____no_output_____"
]
],
[
[
"from mvtsdatatoolkit.sampling.sampler import Sampler\n\nsampler = Sampler(extracted_features_df=fe.df_all_features, label_col_name='lab')\nsampler.original_class_populations",
"_____no_output_____"
]
],
[
[
"- #### Sampling by size?\n\nSuppose I want only 100 instances of `NF` class, nothing from `M`, all of the `X` and `C` instances, 20 of the `B` class.",
"_____no_output_____"
]
],
[
[
"desired_populations = {'X': -1, 'M': 0, 'C': -1, 'B': 20, 'NF': 100}\nsampler.sample(desired_populations=desired_populations)\nsampler.sampled_class_populations",
"_____no_output_____"
]
],
[
[
"Which gives me exactly what I asked for. Note that I used *-1* to indicate that I want *all* instances of the `X` and `C` classes. Also, see how I received 20 instances of `B` class while there was originally only 10 insances of that class in the dataset. This allows a seamless *undersampling* and *oversampling*.\n\nLet's make sure that the sampled dataframe has changed as I wanted.",
"_____no_output_____"
]
],
[
[
"print('Original shape: {}'.format(sampler.original_mvts.shape))\nprint('Sampled shape: {}'.format(sampler.sampled_mvts.shape))",
"Original shape: (2000, 13)\nSampled shape: (471, 13)\n"
]
],
[
[
"471 rows (= 100 + 335 + 0 + 20 + 16) is indeed what I wanted.",
"_____no_output_____"
],
[
"- #### Sampling by ratio?\n\nThe `Sampler` class allows sampling using the desired *ratio*s as well. This is particularly handy when a specific balance ratio is desired.\n\nSuppose I need 50% of the entire population to come from `NF` class, nothing from `M` or `B` class, all of `X` class, and 20% from the `C` class.",
"_____no_output_____"
]
],
[
[
"desired_ratios = {'X': -1, 'M': 0.0, 'C': 0.20, 'B': 0.0,'NF': 0.50}\nsampler.sample(desired_ratios=desired_ratios)\nsampler.sampled_class_populations",
"_____no_output_____"
]
],
[
[
"Which is the exact ratios I asked for.\n\n**Note**: I received 400 `C` instances while the dataset contains only 335 instances of `C` class.\n\n**Note**: The desired ratios do not have to add up to 1. This allows users to do both *undersampling* and *oversampling* using ratios.",
"_____no_output_____"
],
[
"For more sampling methodologies implemented in `Sampler` class, see the documentation of the class.\n\n----",
"_____no_output_____"
],
[
"## Final Note\n\nIn case\n* you noticed any issue/bug in this demo or in the *MVTSData Toolkit* package, or\n* there are other functionalities that you found it useful for your domain, but they are nor implemented here, or\n* you have some ideas and you are willing to help us improve this package through a *pull request*,\n\nplease don't hesitate to contanct me (*aahmadzadeh1[at]cs[dot]gsu[dot].com*).\n\n----\n\n### Citation\n\nPleace cite this work if it comes handy in your research.\n\nCurrently, this package is under review in [SoftwareX journal](https://www.journals.elsevier.com/softwarex). If you are interested in using this, I can share the manuscrip with you. Till it is published, it can be cited as follows:\n\n```\n@article{ahmadzadeh2020mvts,\n title={MVTS-Data Toolkit: A Python Package for Preprocessing Multivariate Time Series Data}},\n author={Azim Ahmadzadeh, Kankana Sinha, Berkay Aydin, Rafal A. Angryk},\n journal={SoftwareX},\n volume={},\n pages={},\n year={under-review},\n publisher={Elsevier}\n}\n```",
"_____no_output_____"
]
]
] | [
"markdown",
"code",
"markdown",
"code",
"markdown",
"code",
"markdown",
"code",
"markdown",
"code",
"markdown",
"code",
"markdown",
"code",
"markdown",
"code",
"markdown",
"code",
"markdown",
"code",
"markdown",
"code",
"markdown",
"code",
"markdown",
"code",
"markdown",
"code",
"markdown",
"code",
"markdown",
"code",
"markdown",
"code",
"markdown",
"code",
"markdown",
"code",
"markdown"
] | [
[
"markdown"
],
[
"code"
],
[
"markdown"
],
[
"code"
],
[
"markdown"
],
[
"code"
],
[
"markdown"
],
[
"code"
],
[
"markdown"
],
[
"code"
],
[
"markdown"
],
[
"code"
],
[
"markdown",
"markdown"
],
[
"code"
],
[
"markdown"
],
[
"code"
],
[
"markdown",
"markdown"
],
[
"code"
],
[
"markdown",
"markdown"
],
[
"code"
],
[
"markdown"
],
[
"code"
],
[
"markdown",
"markdown"
],
[
"code",
"code",
"code",
"code",
"code"
],
[
"markdown",
"markdown"
],
[
"code"
],
[
"markdown",
"markdown"
],
[
"code"
],
[
"markdown",
"markdown"
],
[
"code"
],
[
"markdown",
"markdown"
],
[
"code"
],
[
"markdown"
],
[
"code"
],
[
"markdown"
],
[
"code"
],
[
"markdown",
"markdown"
],
[
"code"
],
[
"markdown",
"markdown",
"markdown"
]
] |
e76503ffc1ec91a133a850c4599b0a3a1298f262 | 2,679 | ipynb | Jupyter Notebook | GettingStarted.ipynb | SofianeB/jh-prod-image | 267dd2173f58f20da2a6dfad27adf5ab8be766dd | [
"BSD-3-Clause"
] | null | null | null | GettingStarted.ipynb | SofianeB/jh-prod-image | 267dd2173f58f20da2a6dfad27adf5ab8be766dd | [
"BSD-3-Clause"
] | null | null | null | GettingStarted.ipynb | SofianeB/jh-prod-image | 267dd2173f58f20da2a6dfad27adf5ab8be766dd | [
"BSD-3-Clause"
] | 1 | 2018-10-24T12:08:56.000Z | 2018-10-24T12:08:56.000Z | 21.432 | 235 | 0.549832 | [
[
[
"# *Examples*\n## Import PyOphidia \n\nImport *client* module from *PyOphidia* package",
"_____no_output_____"
]
],
[
[
"from PyOphidia import client",
"_____no_output_____"
]
],
[
[
"## Instantiate a client\n\nCreate a new *Client()* using the login parameters *username*, *password*, *host* and *port*. It will also try to resume the last session the user was connected to, as well as the last working directory and the last produced cube",
"_____no_output_____"
]
],
[
[
"ophclient = client.Client(username=\"*oph-user*\", password=\"*password*\", server=\"ecas-server.dkrz.de\", port=\"11732\")",
"_____no_output_____"
]
],
[
[
"### Submit a request \nExecute the request oph_list level=2:",
"_____no_output_____"
]
],
[
[
"ophclient.submit(\"oph_list level=2\", display=True)",
"_____no_output_____"
]
],
[
[
"## Set a Client for the Cube class\nInstantiate a new Client common to all Cube instances:",
"_____no_output_____"
]
],
[
[
"from PyOphidia import cube\ncube.Cube.setclient(username=\"oph-user\",password=\"oph-passwd\",server=\"ecas-server.dkrz.de\",port=\"11732\")",
"_____no_output_____"
]
],
[
[
"### Create a Cube object with an existing cube identifier\nInstantiate a new Cube using the PID of an existing cube:",
"_____no_output_____"
]
],
[
[
"mycube = cube.Cube(pid='http://127.0.0.1/1/2')",
"_____no_output_____"
]
]
] | [
"markdown",
"code",
"markdown",
"code",
"markdown",
"code",
"markdown",
"code",
"markdown",
"code"
] | [
[
"markdown"
],
[
"code"
],
[
"markdown"
],
[
"code"
],
[
"markdown"
],
[
"code"
],
[
"markdown"
],
[
"code"
],
[
"markdown"
],
[
"code"
]
] |
e7650d37f045002fbfa7109fc35f6ee7a125e1d0 | 52,288 | ipynb | Jupyter Notebook | HW3.ipynb | ihasanreza/HW3-ADM | 3fcfc50294a9da7ee0f2da3aa719ce0ee904d361 | [
"MIT"
] | null | null | null | HW3.ipynb | ihasanreza/HW3-ADM | 3fcfc50294a9da7ee0f2da3aa719ce0ee904d361 | [
"MIT"
] | null | null | null | HW3.ipynb | ihasanreza/HW3-ADM | 3fcfc50294a9da7ee0f2da3aa719ce0ee904d361 | [
"MIT"
] | null | null | null | 35.715847 | 331 | 0.47967 | [
[
[
"# HW3",
"_____no_output_____"
],
[
"### Importing Libraries ",
"_____no_output_____"
]
],
[
[
"import requests\nfrom bs4 import BeautifulSoup as bs\nimport os\nimport pickle\nimport numpy as np\nimport time\nimport datetime as dt\nimport csv\nimport pandas as pd\nimport nltk\nimport re\nfrom nltk.corpus import stopwords\nimport nltk\nimport string\nimport heapq",
"_____no_output_____"
],
[
"# nltk.download('stopwords')\n# nltk.download('punkt')",
"[nltk_data] Downloading package punkt to /Users/hassan/nltk_data...\n[nltk_data] Unzipping tokenizers/punkt.zip.\n"
]
],
[
[
"## 1. Data collection",
"_____no_output_____"
],
[
"### 1.1.",
"_____no_output_____"
]
],
[
[
"URL = \"https://myanimelist.net/topanime.php\"\nurls = [] # list for storing urls of all the anime\n\ndef get_urls():\n \n \"\"\"get_urls() returns the list of the urls for each anime\"\"\"\n \n for lim in range(0, 20000, 50):\n r = requests.get(URL, params={\"limit\": lim})\n\n if r.status_code == 404: # in case page is inaccessable\n print(\"Unfortunately, page {} is inaccessable. We're interrupting the operation and returning the pages found.\".format(lim))\n\n soup = bs(r.content, 'html5lib')\n\n for res in soup.find_all('a', class_='hoverinfo_trigger fl-l ml12 mr8'):\n url = res['href']\n if url not in urls:\n urls.append(url)\n\n return urls",
"_____no_output_____"
],
[
"filename = 'urls.txt'\n\nif filename not in os.listdir(): # create file if not already created\n with open(filename, 'w') as f:\n f.write('\\n'.join(list(map(str, urls))))\n\nelse: # load file\n with open(filename, 'r', encoding=\"utf8\") as f:\n urls = f.read().split(\"\\n\")\n print(\"urls.txt loaded.\")",
"urls.txt loaded.\n"
],
[
"get_urls()",
"_____no_output_____"
],
[
"print(len(urls)) # number of urls loaded",
"19218\n"
]
],
[
[
"### 1.2",
"_____no_output_____"
]
],
[
[
"def crawl_animes(urls_):\n \n \"\"\"crawl_animes function fetches html of every anime found by the get_url() method. It then\n saves them in an 'htmls' directory. Inside 'htmls' directory, it saves htmls wrt to the page folder\n it belongs to with the fashion 'htmls/page_rank_i/article_j.html'. In order to avoid repeatedly\n downloading the htmls file, a binary file named as 'counter' is created to start from where\n we left off in case of any interruption.\"\"\"\n \n if 'counter' not in os.listdir(): # initialize counter in case not already created\n start = 0\n else:\n with open('counter', 'rb') as c: # load counter\n start = pickle.load(c) + 1\n print(\"Starting from anime no. {}\".format(start))\n\n for i in range(start, len(urls_)):\n page_rank = str(int(np.floor(i/50)))\n \n if i%50 == 0 or f\"page_rank_{page_rank}\" not in os.listdir('./htmls'):\n os.mkdir('htmls/page_rank_{}'.format(page_rank))\n\n html = requests.get(urls_[i])\n sleep = 20\n\n while html.status_code != 200:\n print(\"Waiting {} seconds as we reach request limit while retrieving page no. {}.\\n\".format(sleep, i))\n html.close()\n time.sleep(sleep)\n html = requests.get(urls_[i])\n sleep += 5\n\n with open(\"htmls/page_rank_{}/article_{}.html\".format(page_rank, i), \"w\", encoding=\"utf-8\") as f:\n f.write(html.text)\n\n with open(\"counter\", \"wb\") as c:\n pickle.dump(i, c)",
"_____no_output_____"
],
[
"if 'htmls' not in os.listdir():\n os.mkdir('htmls')",
"_____no_output_____"
],
[
"crawl_animes(urls)",
"Starting from anime no. 19218\n"
]
],
[
[
"### 1.3",
"_____no_output_____"
]
],
[
[
"def parse_pages(i_, folder_name=\"anime_tsvs\"):\n \n \"\"\"This routine parses the htmls we downloaded and fetches the information we are required in the homework\n and saves them in an article_i.tsv file inside anime_tsvs directory.\"\"\"\n \n print(\"Working on page {}\".format(i_))\n page_rank = str(int(np.floor(i_/50)))\n article_path = \"htmls/page_rank_{}/article_{}.html\".format(page_rank, i_)\n\n with open(article_path, 'r', encoding='utf-8') as f:\n article = bs(f.read(), 'html.parser')\n\n animeTitle = article.find(\"h1\", {\"class\":\"title-name h1_bold_none\"}).string\n # print(animeTitle)\n\n animeType = article.find(\"span\", {\"class\":\"information type\"}).string\n # print(animeType)\n\n contents = article.find_all('div', {'class': \"spaceit_pad\"}) \n for c in contents:\n span_ = c.find('span', {'class': \"dark_text\"})\n if span_ is not None:\n if span_.string == \"Episodes:\":\n if c.contents[2] != '\\n Unknown\\n ':\n animeNumEpisode = int(c.contents[2])\n else:\n animeNumEpisode = '' \n # print(animeNumEpisode)\n\n if span_.string == \"Aired:\":\n dates_ = c.contents[2].string.replace('\\n', '').strip().split(' to ')\n # print(dates_)\n if dates_[0] == 'Not available':\n releaseDate = ''\n endDate = ''\n else:\n if len(dates_) == 2 and '?' not in dates_: \n releaseDate = dates_[0]\n endDate = dates_[1]\n\n if len(releaseDate.split(' ')) == 3:\n releaseDate = dt.datetime.strptime(releaseDate, \"%b %d, %Y\") # Datetime conversion\n\n elif len(releaseDate.split(' ')) == 2:\n releaseDate = dt.datetime.strptime(releaseDate, \"%b %Y\")\n\n else:\n releaseDate = print(dt.datetime.strptime(releaseDate, \"%Y\"))\n\n if len(endDate.split(' ')) == 3:\n endDate = dt.datetime.strptime(endDate, \"%b %d, %Y\")\n\n elif len(endDate.split(' ')) == 2:\n endDate = dt.datetime.strptime(endDate, \"%b %Y\")\n\n else:\n endDate = dt.datetime.strptime(endDate, \"%Y\")\n else:\n endDate = ''\n releaseDate = dates_[0]\n\n if len(releaseDate.split(' ')) == 3:\n releaseDate = dt.datetime.strptime(releaseDate, \"%b %d, %Y\")\n\n elif len(releaseDate.split(' ')) == 2:\n releaseDate = dt.datetime.strptime(releaseDate, \"%b %Y\")\n\n else:\n releaseDate = dt.datetime.strptime(releaseDate, \"%Y\")\n\n animeNumMembers = int(article.find(\"span\", {\"class\": \"numbers members\"}).contents[1].string.replace(',', ''))\n # print(animeNumMembers)\n\n if article.find(\"div\", {\"class\": \"score-label score-9\"}) is not None:\n animeScore = float(article.find(\"div\", {\"class\": \"score-label score-9\"}).contents[0])\n else:\n animeScore = ''\n # print(animeScore)\n\n if article.find(\"span\", {\"itemprop\": {\"ratingCount\"}}) is not None:\n animeUsers = int(article.find(\"span\", {\"itemprop\": {\"ratingCount\"}}).contents[0])\n else:\n animeUsers = ''\n # print(animeUsers)\n\n if (article.find(\"span\", {\"class\": \"numbers ranked\"}) is not None):\n try:\n animeRank = int(article.find(\"span\", {\"class\": \"numbers ranked\"}).contents[1].string[1:])\n except:\n animeRank = ''\n else:\n animeRank = ''\n # print(animeRank)\n\n if article.find(\"span\", {\"class\": \"numbers popularity\"}) is not None:\n animePopularity = int(article.find(\"span\", {\"class\": \"numbers popularity\"}).contents[1].string[1:])\n else:\n animePopularity = ''\n # print(animePopularity)\n\n if article.find(\"p\", {\"itemprop\": {\"description\"}}) is not None:\n animeDescription = article.find(\"p\", {\"itemprop\": {\"description\"}}).contents[0]\n else:\n animeDescription = ''\n # print(animeDescription)\n\n animeRelated = []\n\n tbl_anime = article.find(\"table\", {\"class\": \"anime_detail_related_anime\"})\n if tbl_anime is not None:\n anime_links = tbl_anime.find_all(\"a\")\n for e in anime_links:\n animeRelated.append(str(e.text))\n\n animeRelated = list(set(animeRelated))\n if '' in animeRelated:\n animeRelated.remove('')\n if ' ' in animeRelated:\n animeRelated.remove(' ')\n else:\n animeRelated = ''\n # print(animeRelated)\n\n animeCharacters = []\n\n tbl_characters = article.find_all(\"h3\", {\"class\": \"h3_characters_voice_actors\"})\n if tbl_characters is not None:\n for e in tbl_characters:\n a_ = e.find(\"a\")\n animeCharacters.append((a_.text))\n else:\n animeCharacters = ''\n # print(animeCharacters)\n\n animeVoices = []\n\n tbl_voices = article.find_all(\"td\", {\"class\": \"va-t ar pl4 pr4\"})\n if tbl_voices is not None:\n for e in tbl_voices:\n a_ = e.find(\"a\")\n animeVoices.append((a_.text))\n else:\n animeVoices = ''\n\n # print(animeVoices)\n\n animeStaff = []\n \n if len(article.find_all('div', {'class': \"detail-characters-list clearfix\"})) > 1:\n staff = article.find_all('div', {'class': \"detail-characters-list clearfix\"})[1]\n td = staff.find_all('td', {'class': \"borderClass\"})\n \n for td_ in td:\n if td_.get('width') == None:\n animeStaff.append([td_.find('a').string, td_.find('small').string])\n else:\n animeStaff = ''\n \n# print(animeStaff)\n\n with open('{}/anime_{}.tsv'.format(folder_name, i_), 'wt', e # save parsed info. into a tsv file\n ncoding=\"utf8\") as f_:\n tsv_wt = csv.writer(f_, delimiter='\\t')\n tsv_wt.writerow([animeTitle, animeType, animeNumEpisode, releaseDate, endDate, animeNumMembers,animeScore, \\\n animeUsers, animeRank, animePopularity, animeDescription, animeRelated, animeCharacters, \\\n animeVoices, animeStaff])",
"_____no_output_____"
],
[
"if \"anime_tsvs\" not in os.listdir():\n os.mkdir(\"anime_tsvs\")\n for i in range(len(urls)):\n parse_pages(i)\n \nfor i in range(len(urls)):\n parse_pages(i)",
"_____no_output_____"
]
],
[
[
"## 2. Search Engine",
"_____no_output_____"
],
[
"### Pre processing steps",
"_____no_output_____"
],
[
"The steps that follow involves the merging of all the tsv, resulting in a dataframe. We then process this dataframe by working on its description (synopsis) field. We do tokenization, removing of stopwords & punctuation, and stemming. The resulting dataframe is saved in the csv format and in binary format for its use later.",
"_____no_output_____"
]
],
[
[
"def sort_files(t):\n\n \"\"\"This method sorts all the tsv files in the following fashion\n anime_0.tsv, anime_1.tsv, anime_2.tsv, anime_3.tsv, .....\"\"\"\n\n return [a(x) for x in re.split(r'(\\d+)', t)]\n\ndef a(t):\n return int(t) if t.isdigit() else t",
"_____no_output_____"
],
[
"def merge_tsvs(path, column_names):\n \n \"\"\"Here we merge the tsv files into a single dataframe.\"\"\"\n\n list_of_files = sorted(os.listdir(path), key=sort_files)\n df = pd.read_csv(path+list_of_files[0],\n names=column_names,\n sep=\"\\t\", engine='c')\n \n for f in list_of_files[1:]:\n df_ = pd.read_csv(path+f,\n names=column_names,\n sep=\"\\t\", engine='c')\n df = pd.concat([df, df_], ignore_index=True)\n \n return df",
"_____no_output_____"
],
[
"path = \"./anime_tsvs/\"\ncolumns = [\"animeTitle\", \"animeType\", \"animeNumEpisode\", \"releaseDate\", \"endDate\", \"animeNumMembers\",\n \"animeScore\", \"animeUsers\", \"animeRank\", \"animePopularity\", \"animeDescription\", \"animeRelated\",\n \"animeCharacters\", \"animeVoices\", \"animeStaff\"]\n\nif \"df.csv\" not in os.listdir(): # then create and pre-process dataset\n df = merge_tsvs(path, columns)\n df = df.drop([0], axis=0)\n df = df.reset_index(drop=True)\n df[\"animeNumMembers\"].fillna(0)\n df[\"animePopularity\"].fillna(0)\n df[\"animeNumMembers\"] = df[\"animeNumMembers\"].astype(int)\n df[\"animePopularity\"] = df[\"animePopularity\"].astype(int)\n\n df.to_csv(\"./df.csv\")\n\nelse:\n df = pd.read_csv(\"df.csv\")",
"_____no_output_____"
],
[
"def text_process(text_, type_stemmer=\"porter\"): # we use porter stemmer by default\n\n \"\"\"Here we process the synopsis as mentioned above. We return a list containing words which are\n stemmed, tokenized, removed fom punctuation and stopwords.\"\"\"\n\n stopwords_english = stopwords.words(\"english\")\n\n if type_stemmer == \"porter\":\n stemmer = nltk.stem.PorterStemmer()\n elif type_stemmer == \"lancaster\":\n stemmer = nltk.stem.LancasterStemmer()\n \n try:\n text_tokenized = nltk.word_tokenize(text_) # tokenization\n stemmed = [stemmer.stem(word) for word in text_tokenized if ((word.lower() not in stopwords_english) and (word not in string.punctuation))] # stemming\n except TypeError as e:\n print(text_)\n raise e\n \n return stemmed",
"_____no_output_____"
],
[
"# Load or create (if not already) the dataframe with an additional column of preprocessed description\n\nif \"tokenized_df.p\" not in os.listdir():\n df_tokenized = df.assign(description_tokenized=df[\"animeDescription\"].fillna('').apply(lambda m: text_process(m)))\n with open(\"tokenized_df.p\", \"wb\") as f:\n pickle.dump(df_tokenized, f)\nelse:\n with open(\"tokenized_df.p\", \"rb\") as f:\n df_tokenized = pickle.load(f)",
"_____no_output_____"
]
],
[
[
"## 2.1",
"_____no_output_____"
],
[
"### 2.1.1",
"_____no_output_____"
]
],
[
[
"def get_vocabulary(synopsis, vocabulary_file = \"vocabulary.pkl\"):\n \n \"\"\"Here we generate a vocab of all words from the description. We tag each word with an integer term_id\n and then save it in a binary file.\"\"\"\n\n vocab = set()\n\n for desc in synopsis:\n vocab = vocab.union(set(desc))\n\n vocab_dict = dict(zip(sorted(vocab), range(len(vocab))))\n with open(vocabulary_file, \"wb\") as f:\n pickle.dump(vocab_dict, f)\n \n return vocab_dict",
"_____no_output_____"
],
[
"def inverted_idx(synopsis, vocab, inverted_idx_file):\n \n \"\"\"Here we create a dictionary (inverted index) in which against each term id we have a list of documents no.\n which contain that specific word.\"\"\"\n\n inverted_idx = dict()\n for term, term_id in vocab.items():\n inverted_idx[term_id] = set() # create and initialize the dictionary with a set against each key to avoid duplicates\n\n descriptions = zip(synopsis, range(len(synopsis))) # tokenized description against doc no. \n for desc, doc_n in descriptions:\n checked_words = []\n for word in desc:\n if word not in checked_words: # check if we have already worked on this word\n checked_words.append(word)\n term_id = vocab[word]\n inverted_idx[term_id] = inverted_idx[term_id].union(set([doc_n]))\n\n for term_id, docs_set in inverted_idx.items():\n inverted_idx[term_id] = sorted(list(inverted_idx[term_id]))\n\n # create and save the inv_idx in a binary file\n with open(inverted_idx_file, \"wb\") as f:\n pickle.dump(inverted_idx, f)\n\n return inverted_idx",
"_____no_output_____"
],
[
"def get_synopsis(synopsis_file = \"tokenized_df.p\"):\n\n \"\"\"Here we load the descriptions.\"\"\"\n\n print('Loading synopsis... ', end ='')\n with open(synopsis_file, 'rb') as f:\n df = pickle.load(f)\n\n synopsis = list(df['description_tokenized'])\n print('\\nSuccessfully loaded.\\n')\n return synopsis",
"_____no_output_____"
],
[
"def get_vocab(synopsis, vocabulary_file = \"vocabulary.pkl\"):\n \n \"\"\"Load vocabulary (in case it's present) otherwise create it.\"\"\"\n\n print('Loading vocabulary... ', end ='')\n if vocabulary_file not in os.listdir():\n vocab = get_vocabulary(synopsis, vocabulary_file)\n else:\n with open(vocabulary_file, \"rb\") as f:\n vocab = pickle.load(f)\n print('\\nSuccessfully loaded.\\n')\n \n return vocab",
"_____no_output_____"
],
[
"def get_inverted_idx(synopsis, vocab, inverted_idx_file = \"inverted_index.pkl\"):\n \n \"\"\"Load inverted index (in case it's present) otherwise create it.\"\"\"\n\n print('Loading inverted index... ', end ='')\n if inverted_idx_file not in os.listdir():\n inverted_idx = inverted_idx(synopsis, vocab, inverted_idx_file)\n else:\n with open(inverted_idx_file, \"rb\") as f:\n inverted_idx = pickle.load(f)\n print('\\nSuccessfully loaded.\\n')\n \n return inverted_idx",
"_____no_output_____"
],
[
"vocabulary_file = \"vocabulary.pkl\"\nsynopsis_file = \"tokenized_df.p\"\ninverted_idx_file = \"inverted_index.pkl\"\n\n# Load synopsis, vocabulary, and inverted index\nsynopsis = get_synopsis(synopsis_file)\nvocab = get_vocab(synopsis, vocabulary_file)\ninverted_idx = get_inverted_idx(synopsis, vocab, inverted_idx_file)",
"Loading synopsis... \nSuccessfully loaded.\n\nLoading vocabulary... \nSuccessfully loaded.\n\nLoading inverted index... \nSuccessfully loaded.\n\n"
],
[
"def search_engine(vocab, inverted_idx, urls):\n \n \"\"\"Search engine receives an input query and gives back the result of all anime documents that contain\n every word of the query inputted.\"\"\"\n\n query = input('Please enter your query...\\nquery: ') # Input query here\n\n q = query.lower()\n query = text_process(q) # pre-processing step\n\n # if first word not in our vocab, then no need to search for later words (since it's an AND query)\n if query[0] in vocab:\n term_id_1 = vocab[query[0]]\n docs_set = set(inverted_idx[term_id_1])\n\n for word in query[1:]:\n if word in vocab:\n term_id = vocab[word]\n docs = inverted_idx[term_id]\n\n # Intersection is necassary to ensure all words of the query are in the synopsis\n docs_set = docs_set.intersection(set(docs))\n\n # In case no intersection found\n if len(docs_set) == 0:\n print(\"No result found.\")\n return\n\n else:\n print(\"No result found.\")\n return\n\n df = pd.read_csv(\"./df.csv\") # df containing the processed snypsis\n \n res = df.iloc[sorted(list(docs_set))][[\"animeTitle\", \"animeDescription\"]]\n \n for i in sorted(list(docs_set)):\n res['URL'] = urls[i]\n\n return res\n\n else:\n print('No result found.')\n return\n",
"_____no_output_____"
],
[
"search_engine(vocab, inverted_idx, urls)",
"Please enter your query...\nquery: saiyan race\n"
]
],
[
[
"## 2.2",
"_____no_output_____"
],
[
"### 2.2.1",
"_____no_output_____"
]
],
[
[
"def find_tfidf(word, desc, synopsis, idf=None):\n \n \"\"\"Here we calculate tfidf score corresponding the inputted word.\"\"\"\n\n counter = 0\n if idf == None: # calculate idf if not provided\n for desc in synopsis:\n if word in desc:\n counter += 1\n \n idf = np.log(len(synopsis)/counter)\n \n tfidf = desc.count(word)/len(desc) * idf\n \n return idf, tfidf",
"_____no_output_____"
],
[
"def inverted_idx_2(synopsis, vocab, inverted_idx_tfidf_file=\"inverted_index_2.p\", idfs_file=\"idfs.p\"):\n \n \"\"\"Here we generate a dictionary for our inverted index \"\"\"\n \n second_inverted_idx = dict()\n for term_id in vocab.values():\n second_inverted_idx[term_id] = list()\n\n calculated_idfs = {}\n \n descriptions = zip(synopsis, range(len(synopsis)))\n for desc, doc_n in descriptions:\n checked_words = []\n for word in desc:\n # avoid redundancy of checking already checked words\n if word not in checked_words:\n checked_words.append(word)\n term_id = vocab[word]\n \n if word not in calculated_idfs.keys():\n idf, tfidf = find_tfidf(word, desc, synopsis) # calculate idf and tfidf for this new word\n calculated_idfs[word] = idf\n \n else:\n _, tfidf = find_tfidf(word, desc, synopsis, idf)\n\n second_inverted_idx[term_id].append([doc_n, tfidf]) # append document id and corresponding tfidf score\n\n for term_id, lists in second_inverted_idx.items():\n second_inverted_idx[term_id] = sorted(second_inverted_idx[term_id], key=lambda m: m[1]) # sort by tfidf score\n\n with open(inverted_idx_tfidf_file, \"wb\") as f:\n pickle.dump(second_inverted_idx, f)\n\n with open(idfs_file, \"wb\") as f:\n pickle.dump(calculated_idfs, f)\n\n return second_inverted_idx, calculated_idfs\n",
"_____no_output_____"
],
[
"def get_inverted_idx_tfidf(synopsis, vocab, inverted_idx_tfidf_file, idfs_file):\n\n \"\"\"Load inverted index with tfidfs (in case it's present) otherwise create it.\"\"\"\n\n print('Loading inverted index tfidf... \\n', end ='')\n if (idfs_file not in os.listdir()) or (inverted_idx_tfidf_file not in os.listdir()):\n inv_idx_2, idfs = inverted_idx_2(synopsis, vocab, inverted_idx_tfidf_file, idfs_file)\n \n else:\n with open(inverted_idx_tfidf_file, \"rb\") as f:\n inv_idx_2 = pickle.load(f)\n \n with open(idfs_file, \"rb\") as f:\n idfs = pickle.load(f)\n print('Successfully loaded.')\n return inv_idx_2, idfs",
"_____no_output_____"
],
[
"inverted_idx_tfidf_file = \"inverted_index_2.p\"\nidfs_file = \"idfs.p\"\n\ninv_idx_2, idfs = get_inverted_idx_tfidf(synopsis, vocab, inverted_idx_tfidf_file, idfs_file)",
"Loading inverted index tfidf... \nSuccessfully loaded.\n"
],
[
"def find_cos_similarity(vector_1, vector_2):\n\n \"\"\"Computes cosine similarity between two vectors\"\"\"\n \n return (np.dot(vector_1, vector_2))/(np.linalg.norm(vector_1) * np.linalg.norm(vector_2))",
"_____no_output_____"
],
[
"def find_top_k_docs(query, synopsis, vocab, inv_idx_2, idfs, urls, k=10):\n\n \"\"\"Here we create max-heap of the documents containing words of the input query,\n we then arrange them wrt cosine similarity of these documents with the query and\n return top k documents only.\"\"\"\n\n df = pd.read_csv(\"./df.csv\")\n\n query = text_process(query.lower()) # query pre-processing\n\n res_dict = {} # result dictionary\n\n for word in query:\n if word in vocab.keys():\n term_id = vocab[word]\n for list_ in inv_idx_2[term_id]:\n if list_[0] not in res_dict.keys():\n res_dict[list_[0]] = []\n res_dict[list_[0]].append(list_[1])\n# else:\n# print(\"No result found.\")\n\n vector_query = [(query.count(q)/len(query)) * idfs[q] for q in query if q in idfs.keys()]\n \n dists = []\n \n for key in res_dict.keys():\n vec = res_dict[key]\n if len(vec) == len(vector_query):\n dists.append((-find_cos_similarity(vector_query, vec), key))\n\n heapq.heapify(dists) # using heap data structure\n dists_len = len(dists)\n res = []\n for i in range(min(k, dists_len)):\n e = heapq.heappop(dists)\n res.append([e[1], -e[0]])\n\n indices = [i[0] for i in res]\n dists = [i[1] for i in res]\n\n df_1 = df.iloc[indices][[\"animeTitle\", \"animeDescription\"]]\n \n df_res = df_1.assign(URL=[urls[i] for i in indices],\n Similarity=dists)\n return df_res",
"_____no_output_____"
],
[
"query = \"first anime\"\noutput = find_top_k_docs(query, synopsis, vocab, inv_idx_2, idfs, urls)\noutput",
"_____no_output_____"
],
[
"query = \"famous story\"\noutput = find_top_k_docs(query, synopsis, vocab, inv_idx_2, idfs, urls)\noutput",
"_____no_output_____"
]
],
[
[
"## 5. Algorithmic question",
"_____no_output_____"
],
[
"Steps to follow:\n1. Given an input list of appointments, find all combinations of the possible solutions\n2. Check each combination if it is valid or not (no consecutive appointments)\n3. For all valid combinations, find their durations\n4. Find the combination with the maximum duration\n5. Return list of the last step and its duration\n\nInput: appointments_list of length n and distinct values\\\n\nroutine max_len_appointments(appointments_list):\\\n validCombinations = [all combinations in which ther are no consecutive appointments]\\\n appointmentDurations = [durations of every instance of validCombinations]\\\n maxDuration = max(appointmentDurations)\\\n maxLenappointments = [instances of appointmentDurations where duration is maxDuration]\n \n return maxLenappointments, maxDuration\n ",
"_____no_output_____"
]
]
] | [
"markdown",
"code",
"markdown",
"code",
"markdown",
"code",
"markdown",
"code",
"markdown",
"code",
"markdown",
"code",
"markdown",
"code",
"markdown"
] | [
[
"markdown",
"markdown"
],
[
"code",
"code"
],
[
"markdown",
"markdown"
],
[
"code",
"code",
"code",
"code"
],
[
"markdown"
],
[
"code",
"code",
"code"
],
[
"markdown"
],
[
"code",
"code"
],
[
"markdown",
"markdown",
"markdown"
],
[
"code",
"code",
"code",
"code",
"code"
],
[
"markdown",
"markdown"
],
[
"code",
"code",
"code",
"code",
"code",
"code",
"code",
"code"
],
[
"markdown",
"markdown"
],
[
"code",
"code",
"code",
"code",
"code",
"code",
"code",
"code"
],
[
"markdown",
"markdown"
]
] |
e765256c7a2d73d807eb19781c02dca48ce164d7 | 341,110 | ipynb | Jupyter Notebook | 02-Project-Luther/Model_Creation_and_Analysis.ipynb | igabr/Metis_Projects_Chicago_2017 | ffb8ae0246fce77d023ea4fdae5c58693c2be789 | [
"MIT"
] | 26 | 2017-11-07T21:28:20.000Z | 2022-01-01T06:42:01.000Z | 02-Project-Luther/Model_Creation_and_Analysis.ipynb | AmineMassaabi/Metis_Projects_Chicago_2017 | ffb8ae0246fce77d023ea4fdae5c58693c2be789 | [
"MIT"
] | 1 | 2018-01-28T04:00:35.000Z | 2018-03-10T18:34:57.000Z | 02-Project-Luther/Model_Creation_and_Analysis.ipynb | AmineMassaabi/Metis_Projects_Chicago_2017 | ffb8ae0246fce77d023ea4fdae5c58693c2be789 | [
"MIT"
] | 19 | 2017-08-30T21:41:59.000Z | 2021-11-01T18:27:32.000Z | 544.035088 | 134,490 | 0.936613 | [
[
[
"%run imports.py\n%run helper_functions.py\n%matplotlib inline\n%run grid.py\n%autosave 120\nplt.rcParams[\"xtick.labelsize\"] = 20\nplt.rcParams[\"ytick.labelsize\"] = 20\nplt.rcParams[\"axes.labelsize\"] = 20",
"_____no_output_____"
]
],
[
[
"The objective of this notebook, and more broadly, this project is to see whether we can discern a linear relationship between metrics found on Rotton Tomatoes and Box Office performance.\n\nBox office performance is measured in millions as is budget.\n\nBecause we have used scaling, interpretation of the raw coefficients will be difficult. Luckily, sklearns standard scaler has an inverse_transform method, thus, if we had to, we could reverse transform the coefficients (```sc_X_train``` for the holdout group and ```sc_x``` for the non-holdout group) to get some interpretation. The same logic follows for interpreting target variables should we use the model for prediction.\n",
"_____no_output_____"
],
[
"The year, country, language and month will all be made into dummy variables. I will do this with the built in `pd.get_dummies()` function! This will turn all columns into type `object` into dummies! I will also use the optional parameter `drop_first` to avoid the dummy variable trap!\n\nI will use sklearn's standard scaler on all of my variables, except for the dummies! This is important since we will be using regularized regression i.e. Lasso, Ridge, Elastic Net\n\nI will shuffle my dataframe before the train, test split. I will utilise the X_train, y_train, X_test and y_test variables in GridsearchCV. This is an example of employing cross-validation with a holdout set. This will help guard against overfitting.\n\nTo be truly honest, I do not have enough data to justify using a hold out set, however, I want to implement it as an academic exercise! It also give me more code to write!\n\nI will then re-implement the models with using the hold out set to compare results!\n\nLet's get to it!",
"_____no_output_____"
],
[
"# CROSS-VALIDATION WITH HOLDOUT SECTION",
"_____no_output_____"
]
],
[
[
"df = unpickle_object(\"final_dataframe_for_analysis.pkl\") #dataframe we got from webscraping and cleaning!\n#see other notebooks for more info.",
"_____no_output_____"
],
[
"df.dtypes # there are all our features. Our target variable is Box_office",
"_____no_output_____"
],
[
"df.shape",
"_____no_output_____"
]
],
[
[
"Upon further thought, it doesnt make sense to have rank_in_genre as a predictor variable for box office budget. When the movie is release, it is not ranked immeadiately. The ranks assigned often occur many years after the movie is released and so it not related to the amount of money accrued at the box office. We will drop this variable.\n\nRight now, our index is the name of the movie! We dont need these to be indicies, it would be cleaner to have a numeric index\n\nThe month and year columns are currently in numerical form, however, for our analysis, we require that these be of type object!",
"_____no_output_____"
]
],
[
[
"df['Month'] = df['Month'].astype(object)\ndf['Year'] = df['Year'].astype(object)\ndel df['Rank_in_genre']\ndf.reset_index(inplace=True)\ndel df['index']",
"_____no_output_____"
],
[
"percentage_missing(df)",
"No data missing in rotton_rating_(/10) column\nNo data missing in No._of_reviews_rotton column\nNo data missing in Tomato_Freshness_(%) column\nNo data missing in audience_rating_(/5) column\nNo data missing in Runtime column\nNo data missing in Country column\nNo data missing in Language column\nNo data missing in Month column\nNo data missing in Box_office column\nNo data missing in Budget_final column\nNo data missing in Year column\n"
],
[
"df.hist(layout=(4,2), figsize=(50,50))",
"_____no_output_____"
]
],
[
[
"From the above plots, we see that we have heavy skewness in all of our features and our target variable.\n\nThe features will be scaled using standard scaler.\n\nWhen splitting the data into training and test. I will fit my scaler according to the training data!\n\nThere is no sign of multi-collinearity $(>= 0.9)$ - good to go!",
"_____no_output_____"
]
],
[
[
"plot_corr_matrix(df)",
"_____no_output_____"
],
[
"X = unpickle_object(\"X_features_selection.pkl\") #all features from the suffled dataframe. Numpy array\ny = unpickle_object(\"y_variable_selection.pkl\") #target variable from shuffled dataframe. Numpy array\nfinal_df = unpickle_object(\"analysis_dataframe.pkl\") #this is the shuffled dataframe!",
"_____no_output_____"
]
],
[
[
"## Baseline Model and Cross-Validation with Holdout Sets",
"_____no_output_____"
],
[
"### Creation of Holdout Set",
"_____no_output_____"
]
],
[
[
"X_train, X_test, y_train, y_test = train_test_split(X, y, test_size=0.25, random_state = 0) #train on 75% of data",
"_____no_output_____"
],
[
"sc_X_train = StandardScaler()\nsc_y_train = StandardScaler()\n\nsc_X_train.fit(X_train[:,:6])#only need to learn fit of first 6 - rest are dummies\nsc_y_train.fit(y_train)\n\nX_train[:,:6] = sc_X_train.transform(X_train[:,:6]) #only need to transform first 6 columns - rest are dummies\nX_test[:,:6] = sc_X_train.transform(X_test[:,:6]) #same as above\n\ny_train = sc_y_train.transform(y_train)\ny_test = sc_y_train.transform(y_test)",
"_____no_output_____"
]
],
[
[
"# Baseline Model",
"_____no_output_____"
],
[
"As we can see - the baseline model of regular linear regression is dreadful! Let's move on to more sophisticated methods!",
"_____no_output_____"
]
],
[
[
"baseline_model(X_train, X_test, y_train, y_test)",
"The R2 score of a basline regression model is -1.9775011421280398e+23\n\nMean squared error: 139458111276315616215040.00\n\n\nThe top 3 features for predictive power according to the baseline model is ['Country_argentina', 'Country_finland', 'Country_mexico']\n"
]
],
[
[
"# Ridge, Lasso and Elastic Net regression - Holdouts",
"_____no_output_____"
]
],
[
[
"holdout_results = holdout_grid([\"Ridge\", \"Lasso\", \"Elastic Net\"], X_train, X_test, y_train, y_test)",
"_____no_output_____"
],
[
"pickle_object(holdout_results, \"holdout_model_results\")",
"_____no_output_____"
]
],
[
[
"# Cross-Validation - No Holdout Sets",
"_____no_output_____"
]
],
[
[
"sc_X = StandardScaler()\nsc_y = StandardScaler()",
"_____no_output_____"
],
[
"sc_X.fit(X[:,:6])#only need to learn fit of first 6 - rest are dummies\nsc_y.fit(y)\n\nX[:,:6] = sc_X.transform(X[:,:6]) #only need to transform first 6 columns - rest are dummies\ny = sc_y.transform(y)",
"_____no_output_____"
],
[
"no_holdout_results = regular_grid([\"Ridge\", \"Lasso\", \"Elastic Net\"], X, y)",
"_____no_output_____"
],
[
"pickle_object(no_holdout_results, \"no_holdout_model_results\")",
"_____no_output_____"
]
],
[
[
"# Analysis of Results!",
"_____no_output_____"
],
[
"# Ridge Analysis",
"_____no_output_____"
]
],
[
[
"extract_model_comparisons(holdout_results, no_holdout_results, \"Ridge\")",
"\nThe Model with no holdout set has a higher R2 of 0.4581898049882941. This is higher by 0.020384382171813153\n\nThe optimal parameters for this model are {'alpha': 107.5}\n\nThe mean cross validation score for all of the data is: 0.426813529439751\n\nThe most important features accordning to this model is ['Budget_final', 'No._of_reviews_rotton', 'Month_12']\n\nGraphical Comparison below: \n\n"
]
],
[
[
"# Lasso Analysis",
"_____no_output_____"
]
],
[
[
"extract_model_comparisons(holdout_results, no_holdout_results, \"Lasso\")",
"\nThe Model with the holdout set has a higher R2 of 0.4290751892395685. This is higher by 0.013850206355912664\n\nThe optimal parameters for this model are {'alpha': 0.10000000000000001}\n\nThe mean cross validation score on the test set is: 0.41774774276015764\n\nThe most important features accordning to this model is ['Budget_final', 'No._of_reviews_rotton', 'rotton_rating_(/10)']\n\nGraphical Comparison below: \n\n"
]
],
[
[
"# Elastic Net Analysis",
"_____no_output_____"
]
],
[
[
"extract_model_comparisons(holdout_results, no_holdout_results, \"Elastic Net\")",
"\nThe Model with no holdout set has a higher R2 of 0.4459659739120837. This is higher by 0.0027491346588406906\n\nThe optimal parameters for this model are {'alpha': 0.1, 'l1_ratio': 0.1}\n\nThe mean cross validation score for all of the data is: 0.42861943354426085\n\nThe most important features accordning to this model is ['Budget_final', 'No._of_reviews_rotton', 'rotton_rating_(/10)']\n\nGraphical Comparison below: \n\n"
]
],
[
[
"From the above, we can see that 2/3 models had a higher $R^{2}$ without the use of a holdout set. This fit in line with the theory that when you use less data (i.e. use a hold-out set), you model will perform worse. As such, hold -out sets should only be implemented when a plethora of training data is available!\n\nWe also see that the budget feature and No. of Reviews on Rotton tomatoes were the strongest feature when predicting Box Office Revenue!\n\nPlease note: The data collection process was a nightmare with lots of missing data, as such, various methods of imputation were employed. This is probably most apparent from the fact that our highest $R^{2}$ across all models was $~0.45$.\n\nWhile I did not obtain the strongest results, this project was fantastic in exposing me to methods of regularization, standardization and introductory machine learning techniques.\n\nI am especially ecstatic that I got to use the GridSearchCV class! This class will make it very easy for me to take on more advanced machine learning topics in future projects.\n\nOther than the focus on Machine Learning - this project was an excellent exercise in data collection and cleaning!",
"_____no_output_____"
]
]
] | [
"code",
"markdown",
"code",
"markdown",
"code",
"markdown",
"code",
"markdown",
"code",
"markdown",
"code",
"markdown",
"code",
"markdown",
"code",
"markdown",
"code",
"markdown",
"code",
"markdown",
"code",
"markdown"
] | [
[
"code"
],
[
"markdown",
"markdown",
"markdown"
],
[
"code",
"code",
"code"
],
[
"markdown"
],
[
"code",
"code",
"code"
],
[
"markdown"
],
[
"code",
"code"
],
[
"markdown",
"markdown"
],
[
"code",
"code"
],
[
"markdown",
"markdown"
],
[
"code"
],
[
"markdown"
],
[
"code",
"code"
],
[
"markdown"
],
[
"code",
"code",
"code",
"code"
],
[
"markdown",
"markdown"
],
[
"code"
],
[
"markdown"
],
[
"code"
],
[
"markdown"
],
[
"code"
],
[
"markdown"
]
] |
e76530274b809386084b265541dffe6f3e2f8cd9 | 156,550 | ipynb | Jupyter Notebook | params/MS/MS_Distribution.ipynb | NREL/human-metrics-explore | 205d0f18e3630c72bc6b826efbb4984e1738e1e0 | [
"BSD-3-Clause"
] | null | null | null | params/MS/MS_Distribution.ipynb | NREL/human-metrics-explore | 205d0f18e3630c72bc6b826efbb4984e1738e1e0 | [
"BSD-3-Clause"
] | 1 | 2022-01-12T21:19:12.000Z | 2022-01-12T23:50:53.000Z | params/MS/MS_Distribution.ipynb | NREL/human-metrics-explore | 205d0f18e3630c72bc6b826efbb4984e1738e1e0 | [
"BSD-3-Clause"
] | null | null | null | 307.563851 | 25,664 | 0.926662 | [
[
[
"import pandas as pd\nimport numpy as np\nimport matplotlib.pyplot as plt\n%matplotlib inline\nfrom datetime import datetime, timedelta\nimport scipy.optimize as opt\nimport scipy.stats\nimport generate_bus_ms\nimport generate_pvt_ms\nimport generate_wb_ms",
"_____no_output_____"
],
[
"###add prob values if needed later and update variable names\n#plot_df_pvt = pd.read_csv(\"./PvtOccupancyProb.csv\")\n#plot_df_bus = pd.read_csv(\"./BusOccProb.csv\")\n#plot_df_wb = pd.read_csv(\"./BusOccProb.csv\")\n\n# plot_df_wb_MS_freq = pd.read_csv(\"./WB_MS_freq.csv\") \n# plot_df_pvt_MS_freq = pd.read_csv(\"./PVT_MS_freq.csv\") \n# plot_df_bus_MS_freq = pd.read_csv(\"./BUS_MS_freq.csv\")\n\nms_pvt_gen = generate_pvt_ms.Generator(\"PVT_MS_freq.csv\")\nms_wb_gen = generate_wb_ms.Generator(\"WB_MS_freq.csv\")\nms_bus_gen = generate_bus_ms.Generator(\"BUS_MS_freq.csv\")",
"_____no_output_____"
],
[
"###update this block with variable names if there is a need to plot the histogram/bar/line plot\n\n# #View initial distribution\n# fig = plt.figure()\n# ax = plt.axes()\n# x_ax = plot_df_pvt['pvt_occ_means_vec']\n# y_ax = plot_df_pvt['Freq']\n\n\n# #x = np.linspace(0, 10, 1000)\n# #ax.bar(plot_df['pvt_occ_means_vec'],plot_df['Freq']) ##i dont know why this refuses to plot a decent bar chart!!!!!!!\n# plt.plot(x_ax,y_ax)\n# plt.xlabel('Occupancy of private vehicles',size=18)\n# plt.ylabel('Probability',size=18)\n# plt.xticks(size=14)\n# plt.yticks(size=14)",
"_____no_output_____"
],
[
"###update this block with variable names if there is a need to plot the histogram/bar/line plot\n\n# fig = plt.figure()\n# ax = plt.axes()\n# x_ax = plot_df_bus['bus_main_data_mod']\n# y_ax = plot_df_bus['Freq']\n\n\n# #x = np.linspace(0, 10, 1000)\n# #ax.bar(plot_df['pvt_occ_means_vec'],plot_df['Freq']) ##i dont know why this refuses to plot a decent bar chart!!!!!!!\n# plt.plot(x_ax,y_ax)\n# plt.xlabel('Bus Occupancy',size=18)\n# plt.ylabel('Probability',size=18)\n# plt.xticks(size=14)\n# plt.yticks(size=14)\n",
"_____no_output_____"
],
[
"# #Create series with values for walk+bike \n# vals_wb_MS= []\n# for i,row in plot_df_wb_MS_freq.iterrows():\n# freq = int(row['Freq'])\n# #print(freq)\n# for num in range(0,freq):\n# vals_wb_MS.append(row['non_motorized_vec'])\n# vals_wb_MS = pd.Series(vals_wb_MS)\n# print(vals_wb_MS)\n\n# #Create series with values for pvt vehicle PMT \n# vals_pvt_MS= []\n# for i,row in plot_df_pvt_MS_freq.iterrows():\n# freq = int(row['Freq'])\n# #print(freq)\n# for num in range(0,freq):\n# vals_pvt_MS.append(row['private_vec'])\n# vals_pvt_MS = pd.Series(vals_pvt_MS)\n\n# #Create series with values for bus PMT \n# vals_bus_MS= []\n# for i,row in plot_df_bus_MS_freq.iterrows():\n# freq = int(row['Freq'])\n# #print(freq)\n# for num in range(0,freq):\n# vals_bus_MS.append(row['bus_vec'])\n# vals_bus_MS = pd.Series(vals_bus_MS)",
"0 0.070\n1 0.095\n2 0.095\n3 0.095\n4 0.095\n ... \n19995 0.280\n19996 0.285\n19997 0.285\n19998 0.295\n19999 0.295\nLength: 20000, dtype: float64\n"
],
[
"#KDE plot documentation: https://pandas.pydata.org/pandas-docs/stable/reference/api/pandas.DataFrame.plot.kde.html\n\nms_pvt_gen.plot_df_pvt_ms_series.plot.kde()",
"_____no_output_____"
],
[
"#KDE plot documentation: https://pandas.pydata.org/pandas-docs/stable/reference/api/pandas.DataFrame.plot.kde.html\n\nms_bus_gen.plot_df_bus_ms_series.plot.kde()",
"_____no_output_____"
],
[
"#KDE plot documentation: https://pandas.pydata.org/pandas-docs/stable/reference/api/pandas.DataFrame.plot.kde.html\n\nms_wb_gen.plot_df_wb_ms_series.plot.kde()",
"_____no_output_____"
],
[
"#Code from here: https://stackoverflow.com/questions/35434363/python-generate-random-values-from-empirical-distribution\n\nsample_pdf_wb_MS = ms_wb_gen.plot_df_wb_ms_pdf\n\n# Sample new datapoints from the KDE\nnew_sample_data_wb_MS = sample_pdf_wb_MS.resample(10000).T[:,0]\n\n# Histogram of initial empirical sample\ncnts, bins, p = plt.hist(ms_wb_gen.plot_df_wb_ms_series, label='original sample', bins=20,\n histtype='step', linewidth=1.5, density=True)\n\n# Histogram of datapoints sampled from KDE\nplt.hist(new_sample_data_wb_MS, label='sample from KDE', bins=bins,\n histtype='step', linewidth=1.5, density=True)\n\n# Visualize the kde itself\ny_kde = sample_pdf_wb_MS(bins)\nplt.plot(bins, y_kde, label='KDE')\nplt.legend()\nplt.show(block=False)\n\n",
"_____no_output_____"
],
[
"#Code from here: https://stackoverflow.com/questions/35434363/python-generate-random-values-from-empirical-distribution\n\nsample_pdf_pvt_MS = ms_pvt_gen.plot_df_pvt_ms_pdf\n\n# Sample new datapoints from the KDE\nnew_sample_data_pvt_MS = sample_pdf_pvt_MS.resample(10000).T[:,0]\n\n# Histogram of initial empirical sample\ncnts, bins, p = plt.hist(ms_pvt_gen.plot_df_pvt_ms_series, label='original sample', bins=20,\n histtype='step', linewidth=1.5, density=True)\n\n# Histogram of datapoints sampled from KDE\nplt.hist(new_sample_data_pvt_MS, label='sample from KDE', bins=bins,\n histtype='step', linewidth=1.5, density=True)\n\n# Visualize the kde itself\ny_kde = sample_pdf_pvt_MS(bins)\nplt.plot(bins, y_kde, label='KDE')\nplt.legend()\nplt.show(block=False)",
"_____no_output_____"
],
[
"#Code from here: https://stackoverflow.com/questions/35434363/python-generate-random-values-from-empirical-distribution\n\nsample_pdf_bus_MS = ms_bus_gen.plot_df_bus_ms_pdf\n\n# Sample new datapoints from the KDE\nnew_sample_data_bus_MS = sample_pdf_bus_MS.resample(10000).T[:,0]\n\n# Histogram of initial empirical sample\ncnts, bins, p = plt.hist(ms_bus_gen.plot_df_bus_ms_series, label='original sample', bins=20,\n histtype='step', linewidth=1.5, density=True)\n\n# Histogram of datapoints sampled from KDE\nplt.hist(new_sample_data_bus_MS, label='sample from KDE', bins=bins,\n histtype='step', linewidth=1.5, density=True)\n\n# Visualize the kde itself\ny_kde = sample_pdf_bus_MS(bins)\nplt.plot(bins, y_kde, label='KDE')\nplt.legend()\nplt.show(block=False)",
"_____no_output_____"
],
[
"#To generate 100 samples from MPG distribution:sample_pdf_wb_MS.resample(100)\nsample_pdf_wb_MS.resample(100)",
"_____no_output_____"
],
[
"#To generate 100 samples from MPG distribution:\nsample_pdf_pvt_MS.resample(100)",
"_____no_output_____"
],
[
"#To generate 100 samples from MPG distribution:\nsample_pdf_bus_MS.resample(100)",
"_____no_output_____"
]
]
] | [
"code"
] | [
[
"code",
"code",
"code",
"code",
"code",
"code",
"code",
"code",
"code",
"code",
"code",
"code",
"code",
"code"
]
] |
e7653143fc80f612119d1baea800dd45aca9f2b0 | 20,132 | ipynb | Jupyter Notebook | implicit/explore.ipynb | ranieri-unimi/notes-ferrara-2022 | 505736d6492d30f63e39b76f688bc028fecda056 | [
"MIT"
] | 10 | 2021-02-16T09:25:44.000Z | 2022-03-11T10:08:50.000Z | implicit/explore.ipynb | ranieri-unimi/notes-ferrara-2022 | 505736d6492d30f63e39b76f688bc028fecda056 | [
"MIT"
] | null | null | null | implicit/explore.ipynb | ranieri-unimi/notes-ferrara-2022 | 505736d6492d30f63e39b76f688bc028fecda056 | [
"MIT"
] | 7 | 2020-02-22T14:10:02.000Z | 2022-02-06T16:55:54.000Z | 38.56705 | 246 | 0.601331 | [
[
[
"# Explore a dataset of argumentative and dialog texts\n> Christian Stab and Iryna Gurevych (2014) Annotating Argument Components and Relations in Persuasive Essays. In: Proceedings of the the 25th International Conference on Computational Linguistics (COLING 2014), p.1501-1510, Ireland, Dublin.",
"_____no_output_____"
]
],
[
[
"import numpy as np\nimport pandas as pd\nfrom tqdm.notebook import tqdm",
"_____no_output_____"
],
[
"import spacy\nimport os",
"_____no_output_____"
],
[
"nlp = spacy.load(\"en_core_web_sm\")",
"_____no_output_____"
],
[
"dataset_folder = 'data/brat-project/'\ndocs = []\nfor f in tqdm(os.listdir(dataset_folder)):\n if f.endswith('.txt'):\n with open(os.path.join(dataset_folder, f), 'r') as data:\n text = data.read()\n docs += [s for s in nlp(text).sents]",
"_____no_output_____"
]
],
[
[
"## Surveys",
"_____no_output_____"
]
],
[
[
"c = 'but'\nfor z, doc in enumerate(docs):\n i = [j for j, w in enumerate(doc) if w.lemma_ == c]\n if len(i) > 0:\n pos = i[0]\n if len(doc[:pos]) > 0:\n print(doc[:pos])\n print(c)\n print(doc[pos+1:], '\\n')\n else:\n print(docs[z-1])\n print(c)\n print(doc[pos+1:], '\\n')",
"However, on the other side of the coin are voices in the opposition, saying that universities provide not only skills for careers,\nbut\nalso academic knowledge for the human race, such as bio science, politics, and medicine. \n\nNowadays, the popularity of mobile phones has brought about a lot of convenience\nbut\nat the meanwhile a variety of problems as well, such as social, medical and technical problems. \n\nThis possibility exists all the time.\nbut\na more efficient and reliable system can also be invented to guarantee the private information of users.\n \n\nNot only used for communication,\nbut\nit also functions as an Internet browser, a music player , a personal organiser and so forth.\n \n\nIt's done nothing\nbut\nwaste tax payer dollars, fill prisons with non violent criminals, and create a black market. \n\nand that's why it's illegal.\nbut\nthen you'd have to explain why alcohol and tobacco are legal because those substances are way more harmful than cannabis. \n\nMost of the time, it is not about the function or quality of the commodities themselves\nbut\nreally the promotion of the good feeling of possessing the product. \n\nTherefore, scientists should not place less emphasis on technological solutions,\nbut\ntry to pay more attention to develop new technologies instead. \n\nSome people think that computer is good for children and it should be used daily by children\nbut\nsome others think differently. \n\nPeople should learn how to use it properly to make it an effective tool because computer should be used not only for entertaining\nbut\nalso for working and studying purpose.\n \n\nIt not only raises the notice the meaning of harmony and love,\nbut\nalso be a good chance to learn humane doctrine of globalization.\n \n\nAt the increasing living pace, the majority of people tend to choose microwave as their unique cooker that help them prepare a dish in five minutes.\nbut\nrare people have been aware that this has contributed to a modification of cooking habits, which may cause the loss of our custom and culture about cooking.\n \n\nIn the second place, the revolution in females' right has brought about many benefits not only to themselves\nbut\nsociety as well. \n\nTo sum up, students can learn outside the textbook and classroom with a real working experiences that can be a great opportunity for future job consideration whereas organizations earn much more as they can not only save time and money\nbut\nalso be enlightened by what young people have to say.\n \n\nIn conclusion, after analyzing the pros and cons of advertising, both of the views have strong support,\nbut\nit is felt that more good comes from advertising than bad. \n\nLast\nbut\nnot least, while generating energy from any source be it hydro power or oil \n\nTo sum it up, from above mentioned facts it can easily be deduced that nuclear power may apear silver bullet for energy crisis\nbut\nits disadvantages far outweigh the adavantages. \n\nIn conclusion, I admit that modern technology has provided a more convenient and comfortable manner for people to watch exhibitions,\nbut\nmuseums and art galleries are necessary to be preserved for its importance of education and culture.\n \n\nAs a result, students who get part time jobs have not only academic knowledge\nbut\nalso hands-on experience. \n\nAs a result, they have no choice\nbut\nchoosing English as a second language.\n \n\nI suggest all youths should learn English owing to its key role in globalization.\nbut\nat the same time, actions, like recording, can be taken to protect native languages and cultures.\n \n\nFor instance, junior high school teachers have great responsibility not only for students' private lives as they are going through adolescence\nbut\nalso their school work due to the fact that it is an important phase for them to study hard and get into the high school they want. \n\nIn conclusion, both of the arguments have strong supports,\nbut\nin my own view, more good come from zoos than bad. \n\nIn conclusion, after analysing the effects of world games both for countries at war and for organising countries, it is clear that they not only constitute a tremendous appeal towards a peaceful society,\nbut\nalso guide the public demonstrations of national proudness. \n\nnot only because they have the right to enjoy freedom\nbut\nthey also escape from isolation and depression.\n \n\nIt is, therefore, not necessary for a certain course or type of training to be offered to the students by a university,\nbut\ninstead, these must be made available when needed. \n\nPersonally, English as an elegant language should be advocated in many fields to expand the global trade and communications.\nbut\n, we still need preserve other languages for sake of keeping the linguistic and cultural diversity.\n \n\nIn our hectic life, however, demand is not for musically orientated students,\nbut\nfor academically developed employees. \n\nFor instance, individuals had to pay much more for fuel if they possess more than three vehicles; secondly, the government should develop the public transport to meet the residents' demand; last\nbut\nnot least, the people should be encouraged to use electric car to reduce the car emissions.\n \n\nNevertheless, universities are not only continuation of schooling\nbut\na platform where people start knowing more about society. \n\nThis means preserving local arts may promote the preservation of the local cultural heritage, which not only helps the local people have a sense of belonging\nbut\nalso contributes to the booming local tourism industry. \n\nThanks to the economical online-teaching, universities and colleges are able to offer grants for students who have outstanding academic achievements\nbut\nare unable to attend schools because of financial constrains.\n \n\nStudents can also indulge in smoking or drinking during this time.\nbut\n, the break should be an optional thing. \n\nSome people, therefore, harbour a view saying that governments should preserve those by spending some budgets on them.\nbut\nthe others do not believe it is possible. \n\nArt is not the key determination of quality of life,\nbut\neducation is. \n\nTo conclude, art could play an active role in improving the quality of people's lives,\nbut\nI think that governments should attach heavier weight to other social issues such as education and housing needs because those are the most essential ways enable to make people a decent life.\n \n\nFor example, in earlier days , if one had to send a message to someone in other country, it used to take months.\nbut\ntoday, it can be sent in few minutes by typing a email and few clicks. \n\nTo begin with, mobile phones and other tools of modern communication facilitate not only contact with friends and relatives in faraway places\nbut\nalso global business. \n\nAnyone who aims to use these innovations have to not only pay for the appliances such as a mobile phone or a computer\nbut\nalso cover up costs for communication services. \n\nConsequently, it is quite easy to have a nutritious meal with guaranteed quality,\nbut\ndo not waste a lot of time.\n \n\nThey argue that people, especially youngsters, are crazier about fresh and advanced things, such as digital products, thus becoming indifferent to traditional techniques.\nbut\nI believe this is not the issue because people tend to follow the trend back to the tradition in the recent years.\n \n\nIn fact, they are not in the purpose of making profits\nbut\ntaking a role of disseminating valued culture, good moral traits and calling for helps for the disabled and the poor.\n \n\nMeanwhile, cultivating a sense of environmental protection would also work out, though it might not be into effect immediately,\nbut\nin the long run, it would be a wise choice.\n \n\nSociety does need an advertising\nbut\nit is our responsibility to control the content and what kind of goods and services we would like to offer to our customers.\n \n\nThese days, not only many businesses,\nbut\nalso governments have to rely on advertising. \n\nAds will keep as well informed about new products and services,\nbut\nwe should also bear in mind that advertising cigarettes and alcohol will definitely affect our children in negative way.\n \n\nBecause not only do teachers teach us knowlegde\nbut\nalso the skills to tell right from wrong. \n\nHowever, when we discuss the issue of competition or cooperation, what we are concerned about is not the whole society,\nbut\nthe development of an individual's whole life. \n\nWhat we acquired from team work is not only how to achieve the same goal with others\nbut\nmore importantly, how to get along with others. \n\nThe winner is the athlete\nbut\nthe success belongs to the whole team. \n\nLast\nbut\nnot least, nowadays, there are many supporting conditions that help women balance housework and work. \n\nCamping doesn't discriminate, doesn't have deadlines and has nothing\nbut\nfun around each corner. \n\nOne who is living overseas will of course struggle with loneliness, living away from family and friends\nbut\nthose difficulties will turn into valuable experiences in the following steps of life. \n\nOxygen tanks, for instance, are proved useful not only in outer space\nbut\nalso underwater. \n\nExercise not only reduces the risk of health problems and various diseases,\nbut\nit also has an effect on overall appearance. \n\nExercising not only helps prevent cardiovascular diseases,\nbut\nit also helps prevent strokes, type 2 diabetes, and even certain types of cancer. \n \n\nMost people think of exercising as only being a physical activity,\nbut\nit's also a mental activity. \n\nRandom locker checks are not done to torment and/or invade the privacy of the students,\nbut\nfor many other important reasons which include school security. \n\nYou even have the choice of printing your own ticket or just pick it up at the airport at the day of your departure.\nbut\nof course, there would still be several customers that prefer to talk with an agent in completing their purchase of a ticket. \n\nThey may have several reasons to it\nbut\nthen maybe they are just comfortable dealing with a human being than a computer.\n \n\nIt's true that technology and computers do make their jobs easier\nbut\nit cannot definitely replace them. \n\nLast,\nbut\nnot least, when taking environment into consideration, people must conceive that the more newspapers are published, the more trees are cut down. \n\n"
]
],
[
[
"## Dialogues",
"_____no_output_____"
]
],
[
[
"dataset = '/Users/alfio/Dati/cornell movie-dialogs corpus/data/movie_lines.txt'\nwith open(dataset, 'r', encoding='latin-1') as gf:\n lines = gf.readlines()",
"_____no_output_____"
],
[
"raw = [nlp(l.split(' +++$+++ ')[-1].rstrip()) for l in lines[:200]]",
"_____no_output_____"
],
[
"for z, doc in enumerate(raw):\n i = [j for j, w in enumerate(doc) if w.lemma_ == c]\n if len(i) > 0:\n pos = i[0]\n print(raw[z-1])\n print(doc, '\\n')",
"You always been this selfish?\nBut \n\nI was?\nI looked for you back at the party, but you always seemed to be \"occupied\". \n\nShe's not a...\nI'm workin' on it. But she doesn't seem to be goin' for him. \n\nI'm workin' on it. But she doesn't seem to be goin' for him.\nI really, really, really wanna go, but I can't. Not unless my sister goes. \n\nIs he oily or dry?\nHe practically proposed when he found out we had the same dermatologist. I mean. Dr. Bonchowski is great an all, but he's not exactly relevant party conversation. \n\nNeat...\nIt's a gay cruise line, but I'll be, like, wearing a uniform and stuff. \n\nAfter that, I swore I'd never do anything just because \"everyone else\" was doing it. And I haven't since. Except for Bogey's party, and my stunning gastro-intestinal display --\nBut \n\nNow I do. Back then, was a different story.\nBut you hate Joey \n\nI wish I had that luxury. I'm the only sophomore that got asked to the prom and I can't go, because you won ' t.\nI do care. But I'm a firm believer in doing something for your own reasons, not someone else ' s . \n\nCan't you forget for just one night that you're completely wretched?\nBogey Lowenstein's party is normal, but you're too busy listening to Bitches Who Need Prozac to know that. \n\nWhat do you think?\nOh, I thought you might have a date I don't know why I'm bothering to ask, but are you going to Bogey Lowenstein's party Saturday night? \n\nIt's that hot rod Joey, right? That ' s who you want me to bend my rules for?\nNo, but \n\nDaddy, people expect me to be there!\nIt's just a party. Daddy, but I knew you'd forbid me to go since \"Gloria Steinem\" over there isn't going -- \n\nExactly my point\nBut she doesn't want to date. \n\nBut she doesn't want to date.\nBut it's not fair -- she's a mutant, Daddy! \n\nNo! You're not dating until your sister starts dating. End of discussion.\nNow don't get upset. Daddy, but there's this boy... and I think he might ask... \n\n"
]
]
] | [
"markdown",
"code",
"markdown",
"code",
"markdown",
"code"
] | [
[
"markdown"
],
[
"code",
"code",
"code",
"code"
],
[
"markdown"
],
[
"code"
],
[
"markdown"
],
[
"code",
"code",
"code"
]
] |
e7653827db099a61669ade75e1c227d2cfd8f429 | 295,270 | ipynb | Jupyter Notebook | analyses/suppl_simulations/brain_data_vs_brainsize.ipynb | lukassnoek/MVCA | dd194140a5babb4605b9248d34508b9d9e4f799c | [
"MIT"
] | 11 | 2018-03-29T09:39:28.000Z | 2021-09-09T15:49:53.000Z | analyses/suppl_simulations/brain_data_vs_brainsize.ipynb | lukassnoek/MVCA | dd194140a5babb4605b9248d34508b9d9e4f799c | [
"MIT"
] | 2 | 2021-02-04T11:10:34.000Z | 2022-03-07T14:41:54.000Z | analyses/suppl_simulations/brain_data_vs_brainsize.ipynb | lukassnoek/MVCA | dd194140a5babb4605b9248d34508b9d9e4f799c | [
"MIT"
] | 3 | 2018-04-12T09:11:31.000Z | 2018-11-30T10:17:54.000Z | 305.979275 | 171,436 | 0.908755 | [
[
[
"from __future__ import print_function\nimport nibabel as nib\nimport sklearn\nimport numpy as np\nimport pandas as pd\nimport matplotlib.pyplot as plt\nimport os\nimport seaborn as sns\nfrom sklearn.externals import joblib\nfrom sklearn.preprocessing import PolynomialFeatures, StandardScaler\nfrom sklearn.linear_model import LinearRegression\nfrom sklearn.model_selection import KFold\nfrom sklearn.pipeline import make_pipeline\nfrom sklearn.model_selection import cross_val_score\nimport statsmodels\nfrom statsmodels.regression.linear_model import OLS # includes AIC, BIC\nimport tqdm\n\n%matplotlib inline\nsns.set()\nsns.set_style(\"ticks\")\n",
"_____no_output_____"
]
],
[
[
"### Load brain data",
"_____no_output_____"
]
],
[
[
"mvp_VBM = joblib.load('mvp/mvp_vbm.jl')\nmvp_TBSS = joblib.load('mvp/mvp_tbss.jl')",
"_____no_output_____"
]
],
[
[
"... and brain size",
"_____no_output_____"
]
],
[
[
"brain_size = pd.read_csv('./mvp/PIOP1_behav_2017_MVCA_with_brainsize.tsv', sep='\\t', index_col=0)\n\n# Remove subjects without known brain size (or otherwise excluded from dataset)\ninclude_subs = np.in1d(brain_size.index.values, mvp_VBM.subject_list)\nbrain_size = brain_size.loc[include_subs]\n\nbrain_size_VBM = brain_size.brain_size_GM.values\nbrain_size_TBSS = brain_size.brain_size_WM.values",
"_____no_output_____"
]
],
[
[
"#### Calculate correlations between voxels & brain size\nCheck-out linear, cubic, and quadratic correlation",
"_____no_output_____"
]
],
[
[
"if os.path.isfile('./cache/r2s_VBM.tsv'):\n r2s_VBM = pd.read_csv('./cache/r2s_VBM.tsv', sep='\\t', index_col=0).values\nelse:\n # Calculate R2 by-voxel with polynomial models of degrees 1, 2, 3 (linear, quadratic, cubic)\n\n r2s_VBM = np.empty((mvp_VBM.X.shape[1], 3)) # col 1 = Linear, col 2 = Poly2, col 3 = Poly3\n\n # make feature vecs\n X_linear = PolynomialFeatures(degree=1).fit_transform(brain_size_VBM.reshape(-1,1))\n X_poly2 = PolynomialFeatures(degree=2).fit_transform(brain_size_VBM.reshape(-1,1))\n X_poly3 = PolynomialFeatures(degree=3).fit_transform(brain_size_VBM.reshape(-1,1))\n\n for i in tqdm.tqdm_notebook(range(r2s_VBM.shape[0])):\n r2s_VBM[i,0] = OLS(mvp_VBM.X[:,i], X_linear).fit().rsquared\n r2s_VBM[i,1] = OLS(mvp_VBM.X[:,i], X_poly2).fit().rsquared\n r2s_VBM[i,2] = OLS(mvp_VBM.X[:,i], X_poly3).fit().rsquared\n\n # save to disk\n pd.DataFrame(r2s_VBM).to_csv('./cache/r2s_VBM.tsv', sep='\\t')",
"_____no_output_____"
],
[
"# Repeat for TBSS\nif os.path.isfile('./cache/r2s_TBSS.tsv'):\n r2s_TBSS = pd.read_csv('./cache/r2s_TBSS.tsv', sep='\\t', index_col=0).values\nelse:\n r2s_TBSS = np.empty((mvp_TBSS.X.shape[1], 3))\n\n # make feature vecs\n X_linear = PolynomialFeatures(degree=1).fit_transform(brain_size_TBSS.reshape(-1,1))\n X_poly2 = PolynomialFeatures(degree=2).fit_transform(brain_size_TBSS.reshape(-1,1))\n X_poly3 = PolynomialFeatures(degree=3).fit_transform(brain_size_TBSS.reshape(-1,1))\n\n for i in tqdm.tqdm_notebook(range(r2s_TBSS.shape[0])):\n r2s_TBSS[i,0] = OLS(mvp_TBSS.X[:,i], X_linear).fit().rsquared\n r2s_TBSS[i,1] = OLS(mvp_TBSS.X[:,i], X_poly2).fit().rsquared\n r2s_TBSS[i,2] = OLS(mvp_TBSS.X[:,i], X_poly3).fit().rsquared\n \n # save to disk\n pd.DataFrame(r2s_TBSS).to_csv('./cache/r2s_TBSS.tsv', sep='\\t')",
"_____no_output_____"
]
],
[
[
"#### Function for plotting",
"_____no_output_____"
]
],
[
[
"def plot_voxel(voxel_idx, data, brain_size, ax=None, add_title=False, scale_bs=False, **kwargs):\n \n try:\n len(voxel_idx)\n except:\n voxel_idx = [voxel_idx]\n \n # Useful for plotting regression lines later\n # scale brain size first\n if scale_bs:\n brain_size = StandardScaler().fit_transform(brain_size.reshape(-1,1))\n\n bs_range = np.linspace(np.min(brain_size), np.max(brain_size), num=500)\n bs_range_poly2 = PolynomialFeatures(degree=2).fit_transform(bs_range.reshape(-1,1))\n bs_range_poly3 = PolynomialFeatures(degree=3).fit_transform(bs_range.reshape(-1,1))\n bs_range_intercept = PolynomialFeatures(degree=1).fit_transform(bs_range.reshape(-1,1))\n \n model_names = ['Linear', 'Poly2', 'Poly3']\n X_linear = PolynomialFeatures(degree=1).fit_transform(brain_size.reshape(-1,1))\n X_poly2 = PolynomialFeatures(degree=2).fit_transform(brain_size.reshape(-1,1))\n X_poly3 = PolynomialFeatures(degree=3).fit_transform(brain_size.reshape(-1,1))\n \n n_voxels = len(voxel_idx)\n nrow = int(np.ceil(n_voxels/2.))\n ncol = 2 if n_voxels > 1 else 1\n\n if ax is None:\n # create fig and axis if not passed to function\n f, axis = plt.subplots(nrow, ncol)\n else:\n axis = ax\n\n for i, idx in enumerate(voxel_idx):\n # Get data\n y = data[:,idx]\n\n # Fit overall model (no CV)\n lr_linear = OLS(y, X_linear).fit()\n lr_poly2 = OLS(y, X_poly2).fit()\n lr_poly3 = OLS(y, X_poly3).fit()\n\n # Get axis\n this_ax = axis if n_voxels == 1 else plt.subplot(nrow, ncol, i+1)\n\n sns.regplot(x=X_linear[:,1], y=y, ax=this_ax,\n dropna=True, fit_reg=False, lowess=False, scatter=True, **kwargs)\n\n this_ax.plot(bs_range, lr_linear.predict(bs_range_intercept), 'r-', label='Linear')\n this_ax.plot(bs_range, lr_poly2.predict(bs_range_poly2), 'b-', label='Quadratic')\n this_ax.plot(bs_range, lr_poly3.predict(bs_range_poly3), 'y-', label='Cubic')\n\n# this_ax.legend()\n \n if add_title:\n this_ax.set_title('Voxel %d' %i)\n if scale_bs:\n this_ax.set_xlabel('Brain size (scaled)')\n else:\n this_ax.set_xlabel('Brain size')\n this_ax.set_ylabel('Intensity')\n\n if ax is None:\n return f, axis",
"_____no_output_____"
]
],
[
[
"#### Inner function for cross-validation. This does the work.",
"_____no_output_____"
]
],
[
[
"def do_crossval(voxel_idx, data, brain_size, n_iter=100, n_fold=10):\n \n # make sure voxel_idx has a len\n try:\n len(voxel_idx)\n except:\n voxel_idx = [voxel_idx]\n \n # Create feature vectors out of brain size\n X_linear = PolynomialFeatures(degree=1).fit_transform(brain_size.reshape(-1,1))\n X_poly2 = PolynomialFeatures(degree=2).fit_transform(brain_size.reshape(-1,1))\n X_poly3 = PolynomialFeatures(degree=3).fit_transform(brain_size.reshape(-1,1))\n \n # Output dataframes for crossvalidation and BIC\n results_CV = pd.DataFrame(columns=['voxel_idx', 'iter', 'Linear', 'Poly2', 'Poly3'])\n results_CV['voxel_idx'] = np.repeat(voxel_idx, n_iter)\n results_CV['iter'] = np.tile(np.arange(n_iter), len(voxel_idx))\n\n results_BIC = pd.DataFrame(columns=['voxel_idx', 'Linear', 'Poly2', 'Poly3'])\n results_BIC['voxel_idx'] = voxel_idx\n \n for i, idx in enumerate(voxel_idx):\n \n # Get target data (vbm intensity)\n y = data[:,idx]\n\n # Make pipeline\n pipe = make_pipeline(StandardScaler(), LinearRegression())\n\n Xdict = {'Linear': X_linear, 'Poly2': X_poly2, 'Poly3': X_poly3}\n\n for iteration in range(n_iter):\n if n_iter > 10:\n if iteration % int(n_iter/10) == 0:\n print('.', end='')\n\n # KFold inside loop for shuffling\n cv = KFold(n_splits=n_fold, random_state=iteration, shuffle=True)\n\n # get row idx in results DataFrame\n row_idx = (results_CV['voxel_idx']==idx)&(results_CV['iter']==iteration)\n\n for model_type, X in Xdict.items():\n r2 = cross_val_score(pipe, X=X, y=y, cv=cv).mean()\n results_CV.loc[row_idx, model_type] = cross_val_score(pipe, X=X, y=y, cv=cv).mean()\n\n # add BIC info\n # Fit overall model (no CV)\n lr_linear = OLS(y, X_linear).fit()\n lr_poly2 = OLS(y, X_poly2).fit()\n lr_poly3 = OLS(y, X_poly3).fit()\n\n # get BICs of fitted models, add to output dataframe\n bics = [lr_linear.bic, lr_poly2.bic, lr_poly3.bic]\n results_BIC.loc[results_BIC['voxel_idx']==idx, 'Linear'] = lr_linear.bic\n results_BIC.loc[results_BIC['voxel_idx']==idx, 'Poly2'] = lr_poly2.bic\n results_BIC.loc[results_BIC['voxel_idx']==idx, 'Poly3'] = lr_poly3.bic\n \n return [results_CV, results_BIC]",
"_____no_output_____"
]
],
[
[
"#### Wrapper function for multiprocessing",
"_____no_output_____"
]
],
[
[
"import multiprocessing\nfrom functools import partial\nimport tqdm\n\ndef run_CV_MP(data, voxel_idx, brain_size, n_iter, n_processes=10, n_fold=10, pool=None):\n if pool is None:\n private_pool = True\n pool = multiprocessing.Pool(processes=n_processes)\n else:\n private_pool = False\n \n results_all_vox = []\n with tqdm.tqdm(total=len(voxel_idx)) as pbar:\n for i, res in tqdm.tqdm(enumerate(pool.imap_unordered(partial(do_crossval,\n data=data,\n brain_size=brain_size,\n n_fold=10,\n n_iter=n_iter), voxel_idx))):\n results_all_vox.append(res)\n \n if private_pool:\n pool.terminate()\n return results_all_vox",
"_____no_output_____"
]
],
[
[
"## Cross-validation",
"_____no_output_____"
]
],
[
[
"# How many iterations do we want? Iterations are repeats of KFold CV with random partitioning of the data, \n# to ensure that the results are not dependent on the (random) partitioning\nn_iter = 50\n\n# How many voxels should we take?\nn_voxels = 500\n\n# # What modality are we in?\n# modality = 'VBM'\n\n# # Which voxels are selected?\n# voxel_type = 1 # 0 = linear, 1 = poly2, 2 = poly3",
"_____no_output_____"
]
],
[
[
"#### Cross-validate for all combinations of modality & relation type\n2 modalitites (TBSS, VBM) x 4 relation types (linear, quadratic, cubic, random) = 8 options in total. Each takes about an hour here",
"_____no_output_____"
]
],
[
[
"# set-up pool here so we can terminate if necessary\npool = multiprocessing.Pool(processes=10)\n\nfor modality in ['VBM', 'TBSS']:\n # select right mvp & brain size\n mvp = mvp_VBM if modality == 'VBM' else mvp_TBSS\n brain_size = brain_size_VBM if modality == 'VBM' else brain_size_TBSS\n\n for relation_type in [0, 1, 2, 3]:\n print('Processing %s, type %d' %(modality, relation_type))\n if relation_type < 3:\n # linear, quadratic, cubic relations?\n corrs = r2s_VBM[:,relation_type] if modality == 'VBM' else r2s_TBSS[:,relation_type]\n vox_idx = corrs.argsort()[-n_voxels:] # these are the voxel idx with highest r2\n else:\n # random voxels\n vox_idx = np.random.choice(np.arange(mvp.X.shape[1]), replace=False, size=n_voxels)\n \n # Run multiprocessed\n output = run_CV_MP(data=mvp.X, voxel_idx=vox_idx, brain_size=brain_size, n_iter=n_iter, pool=pool)\n\n # Save results\n tmp = pd.concat([x[0] for x in output], ignore_index=True)\n tmp.to_csv('./cache/results_%s_type-%d_nvox-%d.tsv' %(modality, relation_type, n_voxels), sep='\\t')",
" 0%| | 0/500 [00:00<?, ?it/s]\n"
]
],
[
[
"Load all results, make Supplementary Figures S7 (VBM) and S9 (TBSS)",
"_____no_output_____"
]
],
[
[
"modality = 'VBM'\nmvp = mvp_VBM if modality == 'VBM' else mvp_TBSS\nbrain_size = brain_size_VBM if modality == 'VBM' else brain_size_TBSS\nresults_linear_vox = pd.read_csv('./cache/results_%s_type-%d_nvox-%d.tsv' %(modality, 0, n_voxels), sep='\\t')\nresults_poly2_vox = pd.read_csv('./cache/results_%s_type-%d_nvox-%d.tsv' %(modality, 1, n_voxels), sep='\\t')\nresults_poly3_vox = pd.read_csv('./cache/results_%s_type-%d_nvox-%d.tsv' %(modality, 2, n_voxels), sep='\\t')\nresults_random_vox = pd.read_csv('./cache/results_%s_type-%d_nvox-%d.tsv' %(modality, 3, n_voxels), sep='\\t')",
"_____no_output_____"
],
[
"sns.set_style('ticks')\nimport warnings\nwarnings.filterwarnings(\"ignore\", category=FutureWarning)\nwarnings.filterwarnings(\"ignore\", category=UserWarning)\n\n# set seed\nseed = 2 if modality == 'TBSS' else 4\nnp.random.seed(seed)\n\nf, ax = plt.subplots(2, 4)\n\nto_plot = [{'Random voxels': results_random_vox}, \n {'Cubic correlating voxels': results_poly2_vox},\n {'Quadratic correlating voxels': results_poly3_vox},\n {'Linearly correlating voxels': results_linear_vox}]\nlabels = ['Linear-Quadratic', 'Linear-Cubic']\n\nfor col, d in enumerate(to_plot):\n title = list(d.keys())[0]\n results = d[title]\n \n # For every voxel & iter, how much better does the linear model do compared to the polynomial models?\n results['Linear-Poly2'] = results['Linear']-results['Poly2']\n results['Linear-Poly3'] = results['Linear']-results['Poly3']\n\n # Mean over iterations to get results by voxel\n results_by_voxel = results.groupby('voxel_idx')[['Linear-Poly2', 'Linear-Poly3']].mean()\n\n # plot histogram\n this_ax = ax[0, col]\n this_ax.axvline(x=0, color='k', ls='--', label='$\\Delta R^2=0$') # add line at 0\n for i, model in enumerate(['Linear-Poly2', 'Linear-Poly3']):\n sns.distplot(results_by_voxel[model], ax=this_ax, kde=True, hist=True, label=labels[i], bins=n_voxels/10)\n\n # Set some stuff (labels, title, legend)\n this_ax.set_xlabel('$\\Delta R^2$')\n this_ax.set_ylabel('Density')\n this_ax.set_title(title)\n \n if col == 0 and modality == 'VBM':\n this_ax.set_xlim(-.1, .1)\n\n # Select random voxel\n plot_vox_idx = np.random.choice(results_by_voxel.index.values, size=1)\n\n # plot this voxel's correlation with brain size, plus the three models\n plot_voxel(plot_vox_idx, mvp.X, brain_size, ax=ax[1, col], color=sns.color_palette()[0], scale_bs=True)\n # scale bs here for axis ticks readability\n \n # add text\n # increase ylm by 10%\n ax[1,col].set_ylim(ax[1,col].get_ylim()[0], ax[1,col].get_ylim()[0]+(ax[1,col].get_ylim()[1]-ax[1,col].get_ylim()[0])*1.1)\n r2_lq = results_by_voxel.loc[plot_vox_idx, 'Linear-Poly2']\n r2_lc = results_by_voxel.loc[plot_vox_idx, 'Linear-Poly3']\n ax[1,col].text(0.025, .975,'$\\Delta R^2_{Linear-Quadratic}=%.3f$\\n$\\Delta R^2_{Linear-Cubic}=%.3f$' %(r2_lq, r2_lc),\n horizontalalignment='left',\n verticalalignment='top', \n transform=ax[1,col].transAxes)\n \n # add grid\n this_ax.grid(ls='--', lw=.5)\n ax[1,col].grid(ls='--', lw=.5)\n \n if col == len(to_plot)-1:\n this_ax.legend(bbox_to_anchor=(1.05, 1), loc=2, borderaxespad=0.)\n ax[1, col].legend(bbox_to_anchor=(1.05, 1), loc=2, borderaxespad=0.)\n\nsns.despine()\nf.set_size_inches(14,7)\nf.tight_layout()\nf.savefig('./figs/brain_size_vs_%s_intensity.png' %modality, bbox_type='tight', dpi=200)",
"_____no_output_____"
]
],
[
[
"And descriptives?",
"_____no_output_____"
]
],
[
[
"to_plot = [{'Random voxels': results_random_vox}, \n {'Cubic correlating voxels': results_poly2_vox},\n {'Quadratic correlating voxels': results_poly3_vox},\n {'Linearly correlating voxels': results_linear_vox}]\nlabels = ['Linear-Quadratic', 'Linear-Cubic']\n\nfor col, d in enumerate(to_plot):\n title = list(d.keys())[0]\n results = d[title]\n \n # For every voxel & iter, how much better does the linear model do compared to the polynomial models?\n results['Linear-Poly2'] = results['Linear']-results['Poly2']\n results['Linear-Poly3'] = results['Linear']-results['Poly3']\n\n # Mean over iterations to get results by voxel\n results_by_voxel = results.groupby('voxel_idx')[['Linear-Poly2', 'Linear-Poly3']].mean()\n \n \n print('For the %s:\\n-linear models have a mean +%.3f R^2 (SD %.3f, min: %.3f) than quadratic models, '\n '\\n-and %.3f (SD %.3f, max: %.3f) over cubic models. \\n-A proportion of %.3f of voxels prefers a quadratic model, '\n 'and a proportion of %.3f prefers a cubic model\\n\\n'%(title, \n results_by_voxel['Linear-Poly2'].mean(), \n results_by_voxel['Linear-Poly2'].std(),\n results_by_voxel['Linear-Poly2'].min(),\n results_by_voxel['Linear-Poly3'].mean(),\n results_by_voxel['Linear-Poly3'].std(),\n results_by_voxel['Linear-Poly3'].min(),\n np.mean(results_by_voxel['Linear-Poly2']<0),\n np.mean(results_by_voxel['Linear-Poly3']<0)))",
"For the Random voxels:\n-linear models have a mean +0.009 R^2 (SD 0.014, min: -0.024) than quadratic models, \n-and 0.019 (SD 0.027, max: -0.036) over cubic models. \n-A proportion of 0.134 of voxels prefers a quadratic model, and a proportion of 0.092 prefers a cubic model\n\n\nFor the Cubic correlating voxels:\n-linear models have a mean +0.006 R^2 (SD 0.007, min: -0.040) than quadratic models, \n-and 0.003 (SD 0.015, max: -0.039) over cubic models. \n-A proportion of 0.106 of voxels prefers a quadratic model, and a proportion of 0.422 prefers a cubic model\n\n\nFor the Quadratic correlating voxels:\n-linear models have a mean +0.006 R^2 (SD 0.006, min: -0.036) than quadratic models, \n-and 0.003 (SD 0.014, max: -0.039) over cubic models. \n-A proportion of 0.096 of voxels prefers a quadratic model, and a proportion of 0.424 prefers a cubic model\n\n\nFor the Linearly correlating voxels:\n-linear models have a mean +0.007 R^2 (SD 0.004, min: -0.006) than quadratic models, \n-and 0.004 (SD 0.014, max: -0.039) over cubic models. \n-A proportion of 0.058 of voxels prefers a quadratic model, and a proportion of 0.408 prefers a cubic model\n\n\n"
]
],
[
[
"Supplementary Figure S8",
"_____no_output_____"
]
],
[
[
"import warnings\nwarnings.filterwarnings(\"ignore\", category=FutureWarning)\nwarnings.filterwarnings(\"ignore\", category=UserWarning)\n\nf, ax = plt.subplots(1, 3)\n\nto_plot = [{'Random voxels': results_random_vox}, \n {'Cubic correlating voxels': results_poly2_vox}]\nlabels = ['Linear-Quadratic', 'Linear-Cubic']\n\nfor col, d in enumerate(to_plot):\n title = list(d.keys())[0]\n results = d[title]\n \n # For every voxel & iter, how much better does the linear model do compared to the polynomial models?\n results['Linear-Poly2'] = results['Linear']-results['Poly2']\n results['Linear-Poly3'] = results['Linear']-results['Poly3']\n\n # Mean over iterations to get results by voxel\n results_by_voxel = results.groupby('voxel_idx')[['Linear-Poly2', 'Linear-Poly3']].mean()\n\n # plot histogram\n this_ax = ax[col]\n\n # Select voxels where poly3 wins by largest margin, for plotting\n plot_vox = np.argmin(results_by_voxel['Linear-Poly3'])\n print(results_by_voxel.loc[plot_vox, 'Linear-Poly3'])\n\n # plot this voxel's correlation with brain size, plus the three models\n plot_voxel(plot_vox, mvp.X, brain_size, ax=ax[col], color=sns.color_palette()[0])\n\n ax[col].legend()\n ax[col].grid(ls='--', lw=.5)\n\n\n# Add a voxel where a linear model fits nicely\nresults = results_linear_vox\nresults_by_voxel = results.groupby('voxel_idx')[['Linear-Poly2', 'Linear-Poly3']].mean()\nplot_vox = np.argmax(results_by_voxel['Linear-Poly3'])\n\n# plot this voxel's correlation with brain size, plus the three models\nplot_voxel(plot_vox, mvp.X, brain_size, ax=ax[2], color=sns.color_palette()[0])\nax[2].legend()\nax[2].grid(ls='--', lw=.5)\nprint(results_by_voxel.loc[plot_vox, 'Linear-Poly3'])\n\nsns.despine()\nf.set_size_inches(14,3.5)\nf.tight_layout()\nf.savefig('./figs/bs_vs_vox.png', bbox_type='tight', dpi=200)",
"-0.0357580830296\n-0.0387817539704\n0.032486994328\n"
]
],
[
[
"### Conclusions\n\nTogether, the results show that a linear model is a good approximation of the relation between VBM/TBSS voxel intensity and brain size",
"_____no_output_____"
]
]
] | [
"code",
"markdown",
"code",
"markdown",
"code",
"markdown",
"code",
"markdown",
"code",
"markdown",
"code",
"markdown",
"code",
"markdown",
"code",
"markdown",
"code",
"markdown",
"code",
"markdown",
"code",
"markdown",
"code",
"markdown"
] | [
[
"code"
],
[
"markdown"
],
[
"code"
],
[
"markdown"
],
[
"code"
],
[
"markdown"
],
[
"code",
"code"
],
[
"markdown"
],
[
"code"
],
[
"markdown"
],
[
"code"
],
[
"markdown"
],
[
"code"
],
[
"markdown"
],
[
"code"
],
[
"markdown"
],
[
"code"
],
[
"markdown"
],
[
"code",
"code"
],
[
"markdown"
],
[
"code"
],
[
"markdown"
],
[
"code"
],
[
"markdown"
]
] |
e765396d4da5b2765825a88fda35993993e6c969 | 42,271 | ipynb | Jupyter Notebook | simulations/notebooks_sim_bin/0.4_sim_ind_compLasso_binary_update.ipynb | shihuang047/stability-analyses | 692609befb2bd8fe2cc12e7730cd94530b7c6b43 | [
"BSD-3-Clause"
] | null | null | null | simulations/notebooks_sim_bin/0.4_sim_ind_compLasso_binary_update.ipynb | shihuang047/stability-analyses | 692609befb2bd8fe2cc12e7730cd94530b7c6b43 | [
"BSD-3-Clause"
] | 2 | 2021-04-16T17:24:10.000Z | 2021-04-16T18:27:56.000Z | simulations/notebooks_sim_bin/0.4_sim_ind_compLasso_binary_update.ipynb | shihuang047/stability-analyses | 692609befb2bd8fe2cc12e7730cd94530b7c6b43 | [
"BSD-3-Clause"
] | 2 | 2020-10-19T17:23:00.000Z | 2020-10-28T16:38:42.000Z | 62.346608 | 422 | 0.439971 | [
[
[
"### summarize compositional lasso results on Independent Simulation Scenarios for binary outcome",
"_____no_output_____"
]
],
[
[
"dir = '/panfs/panfs1.ucsd.edu/panscratch/lij014/Stability_2020/sim_data'",
"_____no_output_____"
],
[
"dim.list = list()\nsize = c(50, 100, 500, 1000)\nidx = 0\nfor (P in size){\n for (N in size){\n idx = idx + 1\n dim.list[[idx]] = c(P=P, N=N)\n }\n}\n\nfiles = NULL\nfor (dim in dim.list){\n p = dim[1]\n n = dim[2]\n files = cbind(files, paste0(dir, '/sim_independent_', paste('P', p, 'N', n, sep='_'), '.RData'))\n}",
"_____no_output_____"
],
[
"length(files)",
"_____no_output_____"
],
[
"avg_FDR = NULL\ntable_toe = NULL\ntmp_num_select = rep(0, length(files))\nfor (i in 1:length(files)){\n print(paste0('indx: ', i))\n load(paste0(dir, '/binary_update/ind_GenCompLasso_binary_', i, '.RData')) \n \n table_toe = rbind(table_toe, results_ind_GenCompLasso[c('n', 'p', 'rou', 'FP', 'FN', 'ROC', 'Stab')])\n tmp_num_select[i] = mean(rowSums(results_ind_GenCompLasso$Stab.table))\n \n # calculate FDR\n load(file_name, dat <- new.env())\n sub = dat$sim_array[[i]]\n p = sub$p # take true values from 1st replicate of each simulated data\n coef = sub$beta\n coef.true = which(coef != 0)\n \n tt = results_ind_GenCompLasso$Stab.table\n FDR = NULL # false positive rate\n for (r in 1:nrow(tt)){\n FDR = c(FDR, length(setdiff(which(tt[r, ] !=0), coef.true))/sum(tt[r, ]))\n\n }\n \n avg_FDR = c(avg_FDR, mean(FDR, na.rm=T))\n}\ntable_toe = as.data.frame(table_toe)\ntable_toe$num_select = tmp_num_select\ntable_toe$FDR = round(avg_FDR,2)",
"[1] \"indx: 1\"\n[1] \"indx: 2\"\n[1] \"indx: 3\"\n[1] \"indx: 4\"\n[1] \"indx: 5\"\n[1] \"indx: 6\"\n[1] \"indx: 7\"\n[1] \"indx: 8\"\n[1] \"indx: 9\"\n[1] \"indx: 10\"\n[1] \"indx: 11\"\n[1] \"indx: 12\"\n[1] \"indx: 13\"\n[1] \"indx: 14\"\n[1] \"indx: 15\"\n[1] \"indx: 16\"\n"
],
[
"head(table_toe)",
"_____no_output_____"
],
[
"tail(table_toe)",
"_____no_output_____"
],
[
"# export result\nresult.table_toe <- apply(table_toe,2,as.character)\nrownames(result.table_toe) = rownames(table_toe)\nresult.table_toe = as.data.frame(result.table_toe)\n\n# extract numbers only for 'n' & 'p'\nresult.table_toe$n = tidyr::extract_numeric(result.table_toe$n)\nresult.table_toe$p = tidyr::extract_numeric(result.table_toe$p)\nresult.table_toe$ratio = result.table_toe$p / result.table_toe$n\n\nresult.table_toe = result.table_toe[c('n', 'p', 'rou', 'ratio', 'Stab', 'ROC', 'FP', 'FN', 'num_select', 'FDR')]\ncolnames(result.table_toe)[1:4] = c('N', 'P', 'Corr', 'Ratio')",
"extract_numeric() is deprecated: please use readr::parse_number() instead\n\nextract_numeric() is deprecated: please use readr::parse_number() instead\n\n"
],
[
"# convert interested measurements to be numeric\nresult.table_toe$Stab = as.numeric(as.character(result.table_toe$Stab))\n# result.table_toe$ROC_mean = as.numeric(substr(result.table_toe$ROC, start=1, stop=4))\n# result.table_toe$FP_mean = as.numeric(substr(result.table_toe$FP, start=1, stop=4))\n# result.table_toe$FN_mean = as.numeric(substr(result.table_toe$FN, start=1, stop=4))\n# result.table_toe$FN_mean[is.na(result.table_toe$FN_mean)] = 0\nresult.table_toe$num_select = as.numeric(as.character(result.table_toe$num_select))\n\nresult.table_toe$ROC_mean = as.numeric(sub(\"\\\\(.*\", \"\", result.table_toe$ROC))\nresult.table_toe$FP_mean = as.numeric(sub(\"\\\\(.*\", \"\", result.table_toe$FP))\nresult.table_toe$FN_mean = as.numeric(sub(\"\\\\(.*\", \"\", result.table_toe$FN))",
"_____no_output_____"
],
[
"# check whether missing values exists\nresult.table_toe[rowSums(is.na(result.table_toe)) > 0,]",
"_____no_output_____"
],
[
"head(result.table_toe)",
"_____no_output_____"
],
[
"tail(result.table_toe)",
"_____no_output_____"
],
[
"result.table_toe\n\n## export\nwrite.table(result.table_toe, '../results_summary_updated/sim_ind_GencompLasso_binary.txt', sep='\\t', row.names=F)",
"_____no_output_____"
]
]
] | [
"markdown",
"code"
] | [
[
"markdown"
],
[
"code",
"code",
"code",
"code",
"code",
"code",
"code",
"code",
"code",
"code",
"code",
"code"
]
] |
e7653eec3d34df781ff2c4fc961c3780758ef0ee | 4,039 | ipynb | Jupyter Notebook | file_not_found.ipynb | tanubuddi988/Errors | 1deca138f1e06891cabb05b1a1dbe8c5a3d8fed2 | [
"MIT"
] | null | null | null | file_not_found.ipynb | tanubuddi988/Errors | 1deca138f1e06891cabb05b1a1dbe8c5a3d8fed2 | [
"MIT"
] | null | null | null | file_not_found.ipynb | tanubuddi988/Errors | 1deca138f1e06891cabb05b1a1dbe8c5a3d8fed2 | [
"MIT"
] | null | null | null | 43.902174 | 755 | 0.581827 | [
[
[
"import os\n\nos.remove('C:\\workspace\\python\\data.txt')\nprint('The file is removed.')",
"_____no_output_____"
]
]
] | [
"code"
] | [
[
"code"
]
] |
e7654bd067af797b6d175a57cf1f4086a484eb7a | 12,594 | ipynb | Jupyter Notebook | docs/example.ipynb | jamesktkim/pycounts_jkim | 3c627da06b694aab8099ad69d0d4b2798aaab7fd | [
"MIT"
] | null | null | null | docs/example.ipynb | jamesktkim/pycounts_jkim | 3c627da06b694aab8099ad69d0d4b2798aaab7fd | [
"MIT"
] | null | null | null | docs/example.ipynb | jamesktkim/pycounts_jkim | 3c627da06b694aab8099ad69d0d4b2798aaab7fd | [
"MIT"
] | null | null | null | 91.927007 | 10,008 | 0.87232 | [
[
[
"# Example usage\n",
"_____no_output_____"
],
[
"Here we will demonstrate how to use `pycounts_jkim` to count the words in a text file and plot the top 5 results.",
"_____no_output_____"
],
[
"## Imports",
"_____no_output_____"
]
],
[
[
"from pycounts_jkim.pycounts_jkim import count_words\nfrom pycounts_jkim.plotting import plot_words",
"_____no_output_____"
]
],
[
[
"## Create a text file",
"_____no_output_____"
]
],
[
[
"quote = \"\"\"Insanity is doing the same thing \nover and over and expecting different results.\"\"\"\nwith open(\"einstein.txt\", \"w\") as file:\n file.write(quote)",
"_____no_output_____"
]
],
[
[
"## Count words",
"_____no_output_____"
]
],
[
[
"counts = count_words(\"einstein.txt\")\nprint(counts)",
"Counter({'over': 2, 'and': 2, 'insanity': 1, 'is': 1, 'doing': 1, 'the': 1, 'same': 1, 'thing': 1, 'expecting': 1, 'different': 1, 'results': 1})\n"
]
],
[
[
"## Plot words",
"_____no_output_____"
]
],
[
[
"fig = plot_words(counts, n=5)",
"_____no_output_____"
]
]
] | [
"markdown",
"code",
"markdown",
"code",
"markdown",
"code",
"markdown",
"code"
] | [
[
"markdown",
"markdown",
"markdown"
],
[
"code"
],
[
"markdown"
],
[
"code"
],
[
"markdown"
],
[
"code"
],
[
"markdown"
],
[
"code"
]
] |
e7654ebb05d78103fde67091bfea79a3bf79ee53 | 128,919 | ipynb | Jupyter Notebook | task2/textClassification_RNN.ipynb | luxuantao/FduNLP-beginner-task | 884ae8277e303cfda881fd06e374cd20b2428947 | [
"MIT"
] | 4 | 2020-06-11T02:47:57.000Z | 2022-01-24T09:12:22.000Z | task2/textClassification_RNN.ipynb | luxuantao/FduNLP-beginner-task | 884ae8277e303cfda881fd06e374cd20b2428947 | [
"MIT"
] | null | null | null | task2/textClassification_RNN.ipynb | luxuantao/FduNLP-beginner-task | 884ae8277e303cfda881fd06e374cd20b2428947 | [
"MIT"
] | null | null | null | 86.291165 | 165 | 0.687719 | [
[
[
"import os\nimport time\nimport torch\nimport torch.nn as nn\nfrom torch.nn import init\nimport torch.nn.functional as F\nimport numpy as np\nimport pandas as pd\nimport sklearn\n\n\ndir_all_data='data/train.tsv'\n\n#超参数设置\nBATCH_SIZE = 32\nDEVICE = torch.device(\"cuda\" if torch.cuda.is_available() else \"cpu\")",
"_____no_output_____"
],
[
"#从文件中读取数据\ndata_all = pd.read_csv(dir_all_data, sep='\\t')\nprint(data_all.shape) #(156060, 4)\nprint(data_all.keys()) #['PhraseId', 'SentenceId', 'Phrase', 'Sentiment']\nprint(data_all.head())",
"(156060, 4)\nIndex(['PhraseId', 'SentenceId', 'Phrase', 'Sentiment'], dtype='object')\n PhraseId SentenceId Phrase \\\n0 1 1 A series of escapades demonstrating the adage ... \n1 2 1 A series of escapades demonstrating the adage ... \n2 3 1 A series \n3 4 1 A \n4 5 1 series \n\n Sentiment \n0 1 \n1 2 \n2 2 \n3 2 \n4 2 \n"
],
[
"#shuffle、划分验证集、测试集,并保存\nidx = np.arange(data_all.shape[0])\nseed = 0\nnp.random.seed(seed)\nnp.random.shuffle(idx) \n\ntrain_size = int(len(idx) * 0.6)\ntest_size = int(len(idx) * 0.8)\n\ndata_all.iloc[idx[:train_size], :].to_csv('data/task2_train.csv', index=False)\ndata_all.iloc[idx[train_size:test_size], :].to_csv(\"data/task2_test.csv\", index=False)\ndata_all.iloc[idx[test_size:], :].to_csv(\"data/task2_dev.csv\", index=False)",
"_____no_output_____"
],
[
"#使用Torchtext采用声明式方法加载数据\n#参考https://blog.csdn.net/JWoswin/article/details/92821752\nfrom torchtext import data\nPAD_TOKEN = '<pad>'\nTEXT = data.Field(sequential=True, batch_first=True, lower=True, pad_token=PAD_TOKEN)\nLABEL = data.Field(sequential=False, batch_first=True, unk_token=None)",
"_____no_output_____"
],
[
"#读取数据\ndatafields = [(\"PhraseId\", None), # 不需要的filed设置为None\n (\"SentenceId\", None),\n ('Phrase', TEXT),\n ('Sentiment', LABEL)]\ntrain_data = data.TabularDataset(path='data/task2_train.csv', format='csv', fields=datafields)\ndev_data = data.TabularDataset(path='data/task2_dev.csv', format='csv', fields=datafields)\ntest_data = data.TabularDataset(path='data/task2_test.csv', format='csv', fields=datafields)",
"_____no_output_____"
],
[
"#构建词典,字符映射到embedding\n#TEXT.vocab.vectors 就是词向量\nTEXT.build_vocab(train_data, vectors='glove.6B.50d', \n unk_init= lambda x:torch.nn.init.uniform_(x, a=-0.25, b=0.25))\nLABEL.build_vocab(train_data)\n#得到索引,PAD_TOKEN='<pad>'\nPAD_INDEX = TEXT.vocab.stoi[PAD_TOKEN]\nTEXT.vocab.vectors[PAD_INDEX] = 0.0",
"_____no_output_____"
],
[
"print(TEXT.vocab.itos[1510])\nprint(TEXT.vocab.stoi['bore'])\n# 词向量矩阵: TEXT.vocab.vectors\nprint(TEXT.vocab.vectors.shape)\nword_vec = TEXT.vocab.vectors[TEXT.vocab.stoi['bore']]\nprint(word_vec.shape)\nprint(word_vec)",
"succeeds\n1486\ntorch.Size([16473, 50])\ntorch.Size([50])\ntensor([ 0.7493, 0.7730, 0.5915, -0.3801, 0.4761, 1.3279, 0.3476, 0.0737,\n -0.0291, -0.2731, -0.3928, -0.1822, -0.0110, -0.3036, -0.5352, -0.4523,\n -0.8613, -0.0940, -0.3921, -0.3335, -0.6319, -0.2460, 0.3667, -0.9392,\n 0.3502, -0.9397, -1.1096, 0.8062, 0.5669, -0.3130, 1.5001, -0.1960,\n 0.3081, 0.1727, 0.5624, 0.2619, 0.4756, -0.5688, -0.5013, 0.1903,\n 0.0685, -0.0869, -0.1641, -0.2432, 0.3557, -0.1629, -0.1993, -0.1561,\n 0.3508, -0.9423])\n"
],
[
"word_vec = TEXT.vocab.vectors[TEXT.vocab.stoi['<pad>']]\nprint(word_vec.shape)\nprint(word_vec)",
"torch.Size([50])\ntensor([0., 0., 0., 0., 0., 0., 0., 0., 0., 0., 0., 0., 0., 0., 0., 0., 0., 0., 0., 0., 0., 0., 0., 0.,\n 0., 0., 0., 0., 0., 0., 0., 0., 0., 0., 0., 0., 0., 0., 0., 0., 0., 0., 0., 0., 0., 0., 0., 0.,\n 0., 0.])\n"
],
[
"#构建迭代器\ntrain_iterator = data.BucketIterator(train_data, batch_size=BATCH_SIZE, train=True, shuffle=True, device=DEVICE)\ndev_iterator = data.Iterator(dev_data, batch_size=BATCH_SIZE, train=False, sort=False, device=DEVICE) #batch_size应该为len(dev_data) \ntest_iterator = data.Iterator(test_data, batch_size=BATCH_SIZE, train=False, sort=False, device=DEVICE)# 在 test_iter , sort一定要设置成 False, 要不然会被 torchtext 搞乱样本顺序",
"_____no_output_____"
],
[
"embedding_choice = 'glove' # 'static' 'non-static'\nnum_embeddings = len(TEXT.vocab)\nembedding_dim = 50\ndropout_p = 0.5\nhidden_size = 50 #隐藏单元数\nnum_layers = 2 #层数\nvocab_size = len(TEXT.vocab)\nlabel_num = len(LABEL.vocab)\nprint(vocab_size, label_num)",
"16473 6\n"
],
[
"class LSTM(nn.Module):\n def __init__(self):\n super(LSTM, self).__init__()\n self.embedding_choice = embedding_choice \n self.hidden_size = hidden_size\n self.num_layers = num_layers\n if self.embedding_choice == 'rand':\n self.embedding = nn.Embedding(num_embeddings, embedding_dim)\n if self.embedding_choice == 'glove':\n self.embedding = nn.Embedding(num_embeddings, embedding_dim, padding_idx = PAD_INDEX) \\\n .from_pretrained(TEXT.vocab.vectors, freeze=True)\n self.lstm = nn.LSTM(embedding_dim, hidden_size, num_layers,\n batch_first=True, dropout=dropout_p, bidirectional=True)\n self.dropout = nn.Dropout(dropout_p) \n self.fc = nn.Linear(hidden_size * 2, label_num) # 2 for bidirection\n \n def forward(self, x): # (Batch_size, Length) \n # h_n (num_layers * num_directions, batch, hidden_size) 注意第一维不是Batch_size\n h0 = torch.zeros(self.num_layers * 2, x.size(0), self.hidden_size).to(DEVICE) \n # c_n (num_layers * num_directions, batch, hidden_size): \n c0 = torch.zeros(self.num_layers * 2, x.size(0), self.hidden_size).to(DEVICE)\n x = self.embedding(x) #(Batch_size, Length, Dimention) \n out, _ = self.lstm(x, (h0, c0)) # (batch_size, Length, hidden_size * 2) \n out = self.dropout(out)\n out = torch.cat((out[:,0,self.hidden_size:], out[:,-1,:self.hidden_size]), dim=1)\n out = self.fc(out) # (batch_size, label_num) \n return out ",
"_____no_output_____"
],
[
"#构建模型\nmodel = LSTM()\noptimizer = torch.optim.Adam(model.parameters(), lr=0.001)#创建优化器SGD\ncriterion = nn.CrossEntropyLoss() #损失函数\nmodel.to(DEVICE)",
"_____no_output_____"
],
[
"#开始训练\nepoch = 1\nbest_accuracy = 0.0\nstart_time = time.time()\n\nfor i in range(epoch):\n model.train()\n total_loss = 0.0\n accuracy = 0.0\n total_correct = 0.0\n total_data_num = len(train_iterator.dataset)\n steps = 0.0\n for batch in train_iterator:\n steps += 1\n optimizer.zero_grad() # 梯度缓存清零\n batch_text = batch.Phrase\n batch_label = batch.Sentiment\n out = model(batch_text) #[batch_size, label_num]\n loss = criterion(out, batch_label)\n total_loss += loss.item() \n loss.backward()\n optimizer.step() \n correct = (torch.max(out, dim=1)[1] == batch_label).sum()\n total_correct += correct.item()\n if steps % 100 == 0:\n print(\"Epoch %d_%.3f%%: Training average Loss: %f\" \n % (i, steps * train_iterator.batch_size * 100 / len(train_iterator.dataset), total_loss / steps)) \n #每个epoch都验证一下\n model.eval()\n total_loss = 0.0\n accuracy = 0.0\n total_correct = 0.0\n total_data_num = len(dev_iterator.dataset)\n steps = 0.0 \n for batch in dev_iterator:\n steps += 1\n batch_text = batch.Phrase\n batch_label = batch.Sentiment\n out = model(batch_text)\n loss = criterion(out, batch_label)\n total_loss += loss.item()\n correct = (torch.max(out, dim=1)[1] == batch_label).sum()\n total_correct += correct.item()\n print(\"Epoch %d : Verification average Loss: %f, Verification accuracy: %f%%, Total Time:%f\"\n %(i, total_loss / steps, total_correct * 100 / total_data_num, time.time() - start_time)) \n if best_accuracy < total_correct / total_data_num:\n best_accuracy = total_correct / total_data_num \n torch.save(model, 'model_saved/epoch_%d_accuracy_%f' % (i, total_correct / total_data_num))\n print('Model is saved in model_saved/epoch_%d_accuracy_%f' % (i, total_correct / total_data_num))\n #推荐使用 torch.save(net.state_dict(),path) net.load_state_dict(torch.load(path)):",
"Epoch 0_3.417%: Training average Loss: 1.202789\nEpoch 0_6.835%: Training average Loss: 1.175800\nEpoch 0_10.252%: Training average Loss: 1.154274\nEpoch 0_13.670%: Training average Loss: 1.141255\nEpoch 0_17.087%: Training average Loss: 1.125114\nEpoch 0_20.505%: Training average Loss: 1.114919\nEpoch 0_23.922%: Training average Loss: 1.105873\nEpoch 0_27.340%: Training average Loss: 1.100409\nEpoch 0_30.757%: Training average Loss: 1.096192\nEpoch 0_34.175%: Training average Loss: 1.092510\nEpoch 0_37.592%: Training average Loss: 1.084081\nEpoch 0_41.009%: Training average Loss: 1.081887\nEpoch 0_44.427%: Training average Loss: 1.080616\nEpoch 0_47.844%: Training average Loss: 1.077013\nEpoch 0_51.262%: Training average Loss: 1.074782\nEpoch 0_54.679%: Training average Loss: 1.071123\nEpoch 0_58.097%: Training average Loss: 1.069022\nEpoch 0_61.514%: Training average Loss: 1.067044\nEpoch 0_64.932%: Training average Loss: 1.064643\nEpoch 0_68.349%: Training average Loss: 1.061310\nEpoch 0_71.767%: Training average Loss: 1.057823\nEpoch 0_75.184%: Training average Loss: 1.055667\nEpoch 0_78.601%: Training average Loss: 1.053250\nEpoch 0_82.019%: Training average Loss: 1.051809\nEpoch 0_85.436%: Training average Loss: 1.050216\nEpoch 0_88.854%: Training average Loss: 1.047876\nEpoch 0_92.271%: Training average Loss: 1.046180\nEpoch 0_95.689%: Training average Loss: 1.045012\nEpoch 0_99.106%: Training average Loss: 1.044251\nEpoch 0 : Verification average Loss: 1.259765, Verification accuracy: 0.057668%, Total Time:61.184696\nEpoch 0 : Verification average Loss: 1.203250, Verification accuracy: 0.108929%, Total Time:61.191674\nEpoch 0 : Verification average Loss: 1.034372, Verification accuracy: 0.179412%, Total Time:61.197670\nEpoch 0 : Verification average Loss: 1.063587, Verification accuracy: 0.233877%, Total Time:61.205675\nEpoch 0 : Verification average Loss: 0.987396, Verification accuracy: 0.307564%, Total Time:61.212661\nEpoch 0 : Verification average Loss: 0.987572, Verification accuracy: 0.358825%, Total Time:61.220657\nEpoch 0 : Verification average Loss: 1.008748, Verification accuracy: 0.416493%, Total Time:61.227653\nEpoch 0 : Verification average Loss: 1.002652, Verification accuracy: 0.477365%, Total Time:61.234649\nEpoch 0 : Verification average Loss: 1.005636, Verification accuracy: 0.544645%, Total Time:61.241645\nEpoch 0 : Verification average Loss: 0.999864, Verification accuracy: 0.605517%, Total Time:61.246644\nEpoch 0 : Verification average Loss: 1.029147, Verification accuracy: 0.663185%, Total Time:61.254643\nEpoch 0 : Verification average Loss: 1.021467, Verification accuracy: 0.717650%, Total Time:61.260643\nEpoch 0 : Verification average Loss: 0.999549, Verification accuracy: 0.784929%, Total Time:61.268639\nEpoch 0 : Verification average Loss: 0.983919, Verification accuracy: 0.865024%, Total Time:61.274627\nEpoch 0 : Verification average Loss: 0.976434, Verification accuracy: 0.922692%, Total Time:61.281622\nEpoch 0 : Verification average Loss: 0.963450, Verification accuracy: 0.999584%, Total Time:61.287620\nEpoch 0 : Verification average Loss: 0.951515, Verification accuracy: 1.070067%, Total Time:61.293616\nEpoch 0 : Verification average Loss: 0.938011, Verification accuracy: 1.143754%, Total Time:61.299612\nEpoch 0 : Verification average Loss: 0.939967, Verification accuracy: 1.204626%, Total Time:61.307607\nEpoch 0 : Verification average Loss: 0.933363, Verification accuracy: 1.275110%, Total Time:61.312605\nEpoch 0 : Verification average Loss: 0.946646, Verification accuracy: 1.316759%, Total Time:61.318601\nEpoch 0 : Verification average Loss: 0.951412, Verification accuracy: 1.374427%, Total Time:61.326596\nEpoch 0 : Verification average Loss: 0.953787, Verification accuracy: 1.438503%, Total Time:61.332603\nEpoch 0 : Verification average Loss: 0.957847, Verification accuracy: 1.505783%, Total Time:61.340590\nEpoch 0 : Verification average Loss: 0.964233, Verification accuracy: 1.550636%, Total Time:61.346586\nEpoch 0 : Verification average Loss: 0.976862, Verification accuracy: 1.605100%, Total Time:61.353582\nEpoch 0 : Verification average Loss: 0.980285, Verification accuracy: 1.665973%, Total Time:61.359578\nEpoch 0 : Verification average Loss: 0.982172, Verification accuracy: 1.726845%, Total Time:61.366573\nEpoch 0 : Verification average Loss: 0.977595, Verification accuracy: 1.800532%, Total Time:61.374572\nEpoch 0 : Verification average Loss: 0.975434, Verification accuracy: 1.867811%, Total Time:61.380566\nEpoch 0 : Verification average Loss: 0.972615, Verification accuracy: 1.931887%, Total Time:61.388561\nEpoch 0 : Verification average Loss: 0.970159, Verification accuracy: 2.002371%, Total Time:61.395557\nEpoch 0 : Verification average Loss: 0.963919, Verification accuracy: 2.069650%, Total Time:61.402558\nEpoch 0 : Verification average Loss: 0.964012, Verification accuracy: 2.133726%, Total Time:61.409563\nEpoch 0 : Verification average Loss: 0.967546, Verification accuracy: 2.191395%, Total Time:61.417545\nEpoch 0 : Verification average Loss: 0.966286, Verification accuracy: 2.252267%, Total Time:61.424541\nEpoch 0 : Verification average Loss: 0.973002, Verification accuracy: 2.293916%, Total Time:61.430563\nEpoch 0 : Verification average Loss: 0.964357, Verification accuracy: 2.374011%, Total Time:61.440545\nEpoch 0 : Verification average Loss: 0.967421, Verification accuracy: 2.428475%, Total Time:61.447527\nEpoch 0 : Verification average Loss: 0.967987, Verification accuracy: 2.479736%, Total Time:61.454537\nEpoch 0 : Verification average Loss: 0.971454, Verification accuracy: 2.524589%, Total Time:61.461521\nEpoch 0 : Verification average Loss: 0.975653, Verification accuracy: 2.572646%, Total Time:61.468516\nEpoch 0 : Verification average Loss: 0.971609, Verification accuracy: 2.646333%, Total Time:61.475511\nEpoch 0 : Verification average Loss: 0.966719, Verification accuracy: 2.710409%, Total Time:61.482509\nEpoch 0 : Verification average Loss: 0.963781, Verification accuracy: 2.771281%, Total Time:61.490503\nEpoch 0 : Verification average Loss: 0.962756, Verification accuracy: 2.825746%, Total Time:61.496506\nEpoch 0 : Verification average Loss: 0.960424, Verification accuracy: 2.899433%, Total Time:61.503498\nEpoch 0 : Verification average Loss: 0.958078, Verification accuracy: 2.966713%, Total Time:61.510492\nEpoch 0 : Verification average Loss: 0.957016, Verification accuracy: 3.024381%, Total Time:61.516502\nEpoch 0 : Verification average Loss: 0.956247, Verification accuracy: 3.082049%, Total Time:61.524484\nEpoch 0 : Verification average Loss: 0.951483, Verification accuracy: 3.158940%, Total Time:61.529481\nEpoch 0 : Verification average Loss: 0.950114, Verification accuracy: 3.226220%, Total Time:61.535478\nEpoch 0 : Verification average Loss: 0.950163, Verification accuracy: 3.290296%, Total Time:61.543473\nEpoch 0 : Verification average Loss: 0.948849, Verification accuracy: 3.354372%, Total Time:61.549469\nEpoch 0 : Verification average Loss: 0.947805, Verification accuracy: 3.415244%, Total Time:61.557466\nEpoch 0 : Verification average Loss: 0.948190, Verification accuracy: 3.482523%, Total Time:61.563461\nEpoch 0 : Verification average Loss: 0.947134, Verification accuracy: 3.556211%, Total Time:61.569458\nEpoch 0 : Verification average Loss: 0.946987, Verification accuracy: 3.626694%, Total Time:61.576463\nEpoch 0 : Verification average Loss: 0.948649, Verification accuracy: 3.681158%, Total Time:61.582460\nEpoch 0 : Verification average Loss: 0.949866, Verification accuracy: 3.738827%, Total Time:61.590446\nEpoch 0 : Verification average Loss: 0.948630, Verification accuracy: 3.809310%, Total Time:61.595443\nEpoch 0 : Verification average Loss: 0.948893, Verification accuracy: 3.879794%, Total Time:61.601439\nEpoch 0 : Verification average Loss: 0.947912, Verification accuracy: 3.931054%, Total Time:61.608436\nEpoch 0 : Verification average Loss: 0.953263, Verification accuracy: 3.975907%, Total Time:61.615442\nEpoch 0 : Verification average Loss: 0.952674, Verification accuracy: 4.036780%, Total Time:61.623427\n"
],
[
"#测试-重新读取文件\nPATH = 'model_saved/epoch_0_accuracy_0.596707'\nmodel = torch.load(PATH)\nmodel.to(DEVICE)\ntotal_loss = 0.0\naccuracy = 0.0\ntotal_correct = 0.0\ntotal_data_num = len(test_iterator.dataset)\nsteps = 0.0 \nstart_time = time.time()\nfor batch in test_iterator:\n steps += 1\n batch_text = batch.Phrase\n batch_label = batch.Sentiment\n out = model(batch_text)\n loss = criterion(out, batch_label)\n total_loss += loss.item()\n correct = (torch.max(out, dim=1)[1] == batch_label).sum()\n total_correct += correct.item()\nprint(\"Test average Loss: %f, Test accuracy: %f,Total time: %f\"\n %(total_loss/steps, total_correct/total_data_num, time.time()-start_time) ) ",
"Test average Loss: 0.964893, Test accuracy: 0.597796,Total time: 4.077614\n"
]
]
] | [
"code"
] | [
[
"code",
"code",
"code",
"code",
"code",
"code",
"code",
"code",
"code",
"code",
"code",
"code",
"code",
"code"
]
] |
e7656037378d90623f1607abdded31a28ca2f4e5 | 6,726 | ipynb | Jupyter Notebook | DataScience/Services.ipynb | marcoparenzan/DotNetInteractive | 91f515782e5490075dddd591d983950f72c939f4 | [
"MIT"
] | 1 | 2022-01-12T13:23:21.000Z | 2022-01-12T13:23:21.000Z | DataScience/Services.ipynb | marcoparenzan/DotNetInteractive | 91f515782e5490075dddd591d983950f72c939f4 | [
"MIT"
] | null | null | null | DataScience/Services.ipynb | marcoparenzan/DotNetInteractive | 91f515782e5490075dddd591d983950f72c939f4 | [
"MIT"
] | null | null | null | 35.21466 | 128 | 0.424621 | [
[
[
"\ndocker run -d -p 8080:8080 -p 8081:8081 -p 5567:5567 -p 4040:4040 3rdman/dotnet-spark:latest",
"_____no_output_____"
],
[
"ls WordCount/input\n",
"\n Directory: D:\\repos\\DotNetInteractive\\DataScience\\WordCount\\input\n\nMode LastWriteTime Length Name\n---- ------------- ------ ----\n-a--- 13/02/2021 04:23 8395 input.txt\n\n"
]
]
] | [
"code"
] | [
[
"code",
"code"
]
] |
e76565cd11b3c7c7f795e7073c75267b36ee219b | 2,045 | ipynb | Jupyter Notebook | Coursera/Cisco Networking Basics Specializations/Course_3-Data_Communications_and_Network_Services/Week-1/Quiz/Week-1-Quiz.ipynb | manipiradi/Online-Courses-Learning | 2a4ce7590d1f6d1dfa5cfde632660b562fcff596 | [
"MIT"
] | 331 | 2019-10-22T09:06:28.000Z | 2022-03-27T13:36:03.000Z | Coursera/Cisco Networking Basics Specializations/Course_3-Data_Communications_and_Network_Services/Week-1/Quiz/Week-1-Quiz.ipynb | manipiradi/Online-Courses-Learning | 2a4ce7590d1f6d1dfa5cfde632660b562fcff596 | [
"MIT"
] | 8 | 2020-04-10T07:59:06.000Z | 2022-02-06T11:36:47.000Z | Coursera/Cisco Networking Basics Specializations/Course_3-Data_Communications_and_Network_Services/Week-1/Quiz/Week-1-Quiz.ipynb | manipiradi/Online-Courses-Learning | 2a4ce7590d1f6d1dfa5cfde632660b562fcff596 | [
"MIT"
] | 572 | 2019-07-28T23:43:35.000Z | 2022-03-27T22:40:08.000Z | 20.656566 | 156 | 0.539853 | [
[
[
"#### 1. What is the destination MAC address that is used in a DHCP Discover frame?",
"_____no_output_____"
],
[
"##### Ans: FF-FF-FF-FF-FF-FF",
"_____no_output_____"
],
[
"#### 2. Which destination IPv4 address does a DHCPv4 client use to send the initial DHCP Discover packet when the client is looking for a DHCP server?",
"_____no_output_____"
],
[
"##### Ans: 255.255.255.255",
"_____no_output_____"
],
[
"#### 3. Which type of packet is sent by a DHCP server after receiving a DHCP Discover message?",
"_____no_output_____"
],
[
"##### Ans: DHCP Offer",
"_____no_output_____"
],
[
"#### 4. Which three addresses are not allowed to be in the DCHP pool for clients? (Choose 3.)",
"_____no_output_____"
],
[
"##### Ans: \n- network broadcast address\n- router interface address\n- network address",
"_____no_output_____"
],
[
"#### 5. In which order do the DHCP messages occur when a client and server are negotiating address configuration?",
"_____no_output_____"
],
[
"##### Ans: DHCPDISCOVER, DHCPOFFER, DHCPREQUEST, DHCPACK",
"_____no_output_____"
]
]
] | [
"markdown"
] | [
[
"markdown",
"markdown",
"markdown",
"markdown",
"markdown",
"markdown",
"markdown",
"markdown",
"markdown",
"markdown"
]
] |
e76566d528405f394a22c00d59b4574cbc38957c | 86,735 | ipynb | Jupyter Notebook | doc/etc/hough.ipynb | pshobowale/SudokuSolver | 24e26b3d7ecdffa01e1b2a47914cbe3fe3cebb1a | [
"MIT"
] | 1 | 2021-08-03T07:44:09.000Z | 2021-08-03T07:44:09.000Z | doc/etc/hough.ipynb | pshobowale/SudokuSolver | 24e26b3d7ecdffa01e1b2a47914cbe3fe3cebb1a | [
"MIT"
] | null | null | null | doc/etc/hough.ipynb | pshobowale/SudokuSolver | 24e26b3d7ecdffa01e1b2a47914cbe3fe3cebb1a | [
"MIT"
] | null | null | null | 264.435976 | 62,968 | 0.908388 | [
[
[
"import cv2 as cv\nimport numpy as np\nimport matplotlib\nimport matplotlib.pyplot as plt",
"_____no_output_____"
],
[
"plt.rc('image', cmap='gray')",
"_____no_output_____"
],
[
"Org=cv.imread(\"Samples/1.jpg\")\nOrg=cv.resize(Org,(400,round(Org.shape[0]/Org.shape[1]*400)))\nI=cv.cvtColor(Org,cv.COLOR_RGB2GRAY)\nI= cv.GaussianBlur(I,(7,7),3)\n#I= cv.Canny(I,50,150,apertureSize = 3)\n\n#Filter horizontal Lines\nI_Hor= cv.morphologyEx(I, cv.MORPH_OPEN, cv.getStructuringElement(cv.MORPH_RECT,(3,1)))\nI_Hor= cv.morphologyEx(I_Hor, cv.MORPH_CLOSE, cv.getStructuringElement(cv.MORPH_RECT,(5,1)))\n\nI_Vert= cv.morphologyEx(I, cv.MORPH_OPEN, cv.getStructuringElement(cv.MORPH_RECT,(1,3)))\nI_Vert= cv.morphologyEx(I_Vert, cv.MORPH_CLOSE, cv.getStructuringElement(cv.MORPH_RECT,(1,5)))\n\nI=(I_Hor+I_Vert)/2\nI=np.array(abs(I-np.min(I)),dtype=np.uint8)\n#I=cv.colorChange()\n#I= cv.GaussianBlur(I,(7,7),1)\nprint(np.min(I))",
"0\n"
],
[
"plt.imshow(I_Hor)\nplt.colorbar()\n\nsum=0\nfor r in I:\n for p in r:\n if p:\n sum+=1\nprint(sum)",
"11999\n"
],
[
"linesP = cv.HoughLinesP(I, 1, np.pi / 180, 50, None, 50, 10)\n\nif linesP is not None:\n for i in range(0, len(linesP)):\n l = linesP[i][0]\n cv.line(Org, (l[0], l[1]), (l[2], l[3]), (0,0,255), 2, cv.LINE_AA)\nplt.imshow(Org)",
"_____no_output_____"
],
[
"def drawline(I,p1,p2,val=255):\n\n dy = p2[0] - p1[0];\n dx = p2[1] - p1[1];\n\n\n step=dy\n if np.abs(dx)>np.abs(dy):\n step=dx\n\n\n #print(step)\n incX = dx/step\n incY = dy/step\n #print(incX,incY)\n \n y =yi= p1[0]\n x =xi= p1[1]\n\n if(step>0):\n for i in range(step+1):\n #print(xi,yi)\n I[xi,yi]=val\n x += incX;\n y += incY;\n \n xi,yi=np.round(x),np.round(y)\n xi,yi=np.int(xi),np.int(yi)\n \n \n else:\n y =yi= p2[0]\n x =xi= p2[1]\n for i in range(step,1):\n #print(xi,yi)\n I[xi,yi]=val\n x += incX;\n y += incY;\n \n xi,yi=np.round(x),np.round(y)\n xi,yi=np.int(xi),np.int(yi)\n\n",
"_____no_output_____"
],
[
"def line_energy(I,p1,p2):\n energy=0\n dy = p2[0] - p1[0];\n dx = p2[1] - p1[1];\n\n\n step=dy\n if np.abs(dx)>np.abs(dy):\n step=dx\n\n\n #print(step)\n incX = dx/step\n incY = dy/step\n #print(incX,incY)\n \n y =yi= p1[0]\n x =xi= p1[1]\n\n if(step>0):\n for i in range(step+1):\n #print(xi,yi)\n energy+=I[xi,yi]\n x += incX;\n y += incY;\n \n xi,yi=np.round(x),np.round(y)\n xi,yi=np.int(xi),np.int(yi)\n \n \n else:\n y =yi= p2[0]\n x =xi= p2[1]\n for i in range(step,1):\n #print(xi,yi)\n energy+=I[xi,yi]\n x += incX;\n y += incY;\n \n xi,yi=np.round(x),np.round(y)\n xi,yi=np.int(xi),np.int(yi)\n \n return energy/(np.max(I)*sq_distance((0,0),I.shape))\n\n \ndef distance(p1,p2):\n if (p1[0]-p2[0])*(p1[0]-p2[0])+(p1[1]-p2[1])*(p1[1]-p2[1]):\n return np.sqrt((p1[0]-p2[0])*(p1[0]-p2[0])+(p1[1]-p2[1])*(p1[1]-p2[1]))\n else:\n return 0.5\n\ndef e_func(p1,p2,I):\n return line_energy(I,p1,p2)+1/distance(p1,p2)",
"_____no_output_____"
],
[
"s1,s2=(0,9),(100,100)\nI=np.ones((110,110))*255\n\ndrawline(I,(0,0),(100,100),0)\nprint(1000*e_func(s1,s2,I))\n\nexample=np.zeros((10,10))\n\nfor i in range(10,0,-1):\n print()\n for j in range(10,0,-1):\n print(\"%.2f\" % e_func(I,(j,i),s2),i,j,end=\"|\")\n example[i-1,j-1]=e_func(I,(j,i),s2)\n \ndrawline(I,s1,s2,200)\n#plt.imshow(I)\nplt.matshow(example,cmap=\"Pastel1\")\n",
"_____no_output_____"
],
[
"I=np.ones((110,110))*255\ndrawline(I,(0,0),(100,100),0)\n\nfrom scipy.optimize import minimize\nfrom scipy.optimize import Bounds\n\nbounds = Bounds([0, 0], [I.shape[1], I.shape[0]])",
"_____no_output_____"
]
]
] | [
"code"
] | [
[
"code",
"code",
"code",
"code",
"code",
"code",
"code",
"code",
"code"
]
] |
e7656f12448d6c08306eba2c8344796591995a58 | 10,052 | ipynb | Jupyter Notebook | random.ipynb | kangyeolyoun2/python-coding-practice-folder | f7808b47d0ead3e273008bd5641b5b90fc4173fb | [
"MIT"
] | null | null | null | random.ipynb | kangyeolyoun2/python-coding-practice-folder | f7808b47d0ead3e273008bd5641b5b90fc4173fb | [
"MIT"
] | null | null | null | random.ipynb | kangyeolyoun2/python-coding-practice-folder | f7808b47d0ead3e273008bd5641b5b90fc4173fb | [
"MIT"
] | null | null | null | 19.405405 | 110 | 0.44618 | [
[
[
"import random\n\nnum1 = random.random()\nnum2 = random.randrange(1,4)\nprint(num1)\nprint(num2)",
"0.635466803841304\n3\n"
],
[
"from random import randrange",
"_____no_output_____"
],
[
"n = randrange(1, 100)",
"_____no_output_____"
],
[
"from random import *\n\nn = randrange(1, 100)",
"_____no_output_____"
],
[
"n",
"_____no_output_____"
],
[
"#158\nn = randrange(1, 100)\nwhile True:\n ans1 = input(\"Guess number (Q to exit): \")\n \n ans2 = int(ans1)\n if (n == ans2):\n print(\"Correct!\")\n break\n elif (n > ans2):\n print(\"Choose higher number\")\n else:\n print(\"Choose lower number\")",
"Guess number (Q to exit): 47\nChoose higher number\nGuess number (Q to exit): 60\nChoose higher number\nGuess number (Q to exit): 80\nChoose lower number\nGuess number (Q to exit): 70\nChoose lower number\nGuess number (Q to exit): 65\nChoose lower number\nGuess number (Q to exit): 63\nChoose lower number\nGuess number (Q to exit): 62\nCorrect!\n"
],
[
"#159\nfrom random import randrange\n\na = randrange(10, 100)\na",
"_____no_output_____"
],
[
"random()*10,1\nimport math",
"_____no_output_____"
],
[
"b = round(random()*10,1)\nb",
"_____no_output_____"
],
[
"num = input(\"insert two integers, two integers must be separated by space\").split(\" \")\nnum = list(map(int, num))\n",
"insert two numbers, two numbers must be separated by space15 35\n"
],
[
"num = input(\"insert two integers, two integers must be separated by space\").split(\" \")\nnum = list(map(int, num))\n\nif abs(num[0] - num[1]) <= 1:\n print(\"No integer between two numbers\")\nelse:\n ran_gen = randrange(min(num),max(num))\n\nran_gen",
"insert two integers, two integers must be separated by space-5 -5\nNo integer between two numbers\n"
],
[
"ran_gen",
"_____no_output_____"
],
[
"#161\nnum = input(\"insert two integers, two integers must be separated by space. You put: \").split(\" \")\nnum = list(map(int, num))\n\nif abs(num[0] - num[1]) <= 1:\n print(\"No integer between two numbers\")\nelse:\n ran_gen = randrange(min(num),max(num))\n",
"insert two integers, two integers must be separated by space. You put: 90 90\nNo integer between two numbers\n"
],
[
"num",
"_____no_output_____"
],
[
"#162\n\nnum = []\nfor i in range(1, 5):\n a = randrange(10, 20)\n num.append(a)\n \nif sum(num)/len(num) >= 15:\n print(\"Big\")\n print(sum(num)/len(num))\nelse:\n print(\"small\")\n print(sum(num)/len(num))",
"Big\n17.25\n"
],
[
"sum(num)/len(num)",
"_____no_output_____"
],
[
"from random import choice\n\n\nnumbers = range(10,21)\n\nrand_nums = []\n\nfor i in range(0,4):\n a = choice(numbers)\n rand_nums.append(a)\n \nrand_nums\n\n",
"_____no_output_____"
],
[
"#162\n\nfrom random import choice\n\nnumbers = range(10,21)\nrand_nums = []\n\nfor i in range(0,4):\n a = choice(numbers)\n rand_nums.append(a)\n \nprint(\"4가지수 : \", rand_nums)\n\nmean = sum(rand_nums) / len(rand_nums)\nprint(\"평균 : \", mean)\n\nif mean >= 15:\n print(\"Big\")\nelse:\n print(\"small\")",
"4가지수 : [20, 16, 13, 19]\n평균 : 17.0\nBig\n"
],
[
"### 163\n\nmin_n = 1\nmax_n = 2\nlevel = 0\n\nwhile level<3:\n level+=1\n ans = randrange(min_n, max_n+1)\n num = int(input(\"level{} (choose between {} and {}): \".format(level, min_n, max_n)))\n if ans == num:\n print(\"Correct!\")\n max_n = max_n *2\n else:\n print(\"Failure!\")\n print(\"Answer is :\", ans)\n break\n if max_n > 8:\n print(\"Lucky\")",
"level1 (choose between 1 and 2): 1\nCorrect!\nlevel2 (choose between 1 and 4): 1\nCorrect!\nlevel3 (choose between 1 and 8): 3\nCorrect!\nLucky\n"
],
[
"#164\nfrom random import shuffle\ncars = [\"Hyundai\", \"Kia\", \"BMW\", \"Benz\"]\n\nshuffle(cars)\nprint(cars[0] == \"Hyundai\", cars)",
"False ['Benz', 'Hyundai', 'BMW', 'Kia']\n"
]
]
] | [
"code"
] | [
[
"code",
"code",
"code",
"code",
"code",
"code",
"code",
"code",
"code",
"code",
"code",
"code",
"code",
"code",
"code",
"code",
"code",
"code",
"code",
"code"
]
] |
e76572f263eac5e0afd69e41f3a2b37e1bbcf5b4 | 6,033 | ipynb | Jupyter Notebook | tutorials/large_scale_LEM/large_scale_LEMs.ipynb | BCampforts/hylands_modeling | 2bf99ee7938b6b132829b5269792231eb7798443 | [
"CC-BY-4.0"
] | 5 | 2021-11-30T17:50:42.000Z | 2022-02-02T13:59:05.000Z | tutorials/large_scale_LEM/large_scale_LEMs.ipynb | elizama1/hylands_modeling | 2bf99ee7938b6b132829b5269792231eb7798443 | [
"CC-BY-4.0"
] | null | null | null | tutorials/large_scale_LEM/large_scale_LEMs.ipynb | elizama1/hylands_modeling | 2bf99ee7938b6b132829b5269792231eb7798443 | [
"CC-BY-4.0"
] | 3 | 2021-11-30T17:51:06.000Z | 2022-02-08T22:19:16.000Z | 27.422727 | 264 | 0.559423 | [
[
[
"<a href=\"http://landlab.github.io\"><img style=\"float: left\" src=\"../../media/landlab_header.png\"></a>",
"_____no_output_____"
],
[
"# Large scale landscape evolution model with Priority flood flow router and Space_v2\n<hr>\n\nThe priority flood flow director is designed to calculate flow properties over large scale grids. In the following notebook we illustrate how the priority flood flow accumulator can be used to simulate landscape evolution using the SPAVE_V2 Landlab component",
"_____no_output_____"
]
],
[
[
"import numpy as np\nfrom matplotlib import pyplot as plt\nfrom tqdm import tqdm\nimport time\n\nfrom landlab import imshow_grid, RasterModelGrid\nfrom landlab.components import (\n FlowAccumulator,\n DepressionFinderAndRouter,\n Space,\n SpaceLargeScaleEroder,\n PriorityFloodFlowRouter,\n)",
"_____no_output_____"
]
],
[
[
"Create raster grid",
"_____no_output_____"
]
],
[
[
"# nr = 20\n# nc = 20\nnr = 75\nnc = 75\nxy_spacing = 10.0\nmg = RasterModelGrid((nr, nc), xy_spacing=xy_spacing)\nz = mg.add_zeros(\"topographic__elevation\", at=\"node\")\nmg.at_node[\"topographic__elevation\"][mg.core_nodes] += np.random.rand(\n mg.number_of_core_nodes\n)\n\ns = mg.add_zeros(\"soil__depth\", at=\"node\", dtype=float)\nmg.at_node[\"soil__depth\"][mg.core_nodes] += 0.5\nmg.at_node[\"topographic__elevation\"] += mg.at_node[\"soil__depth\"]\n\nfr = FlowAccumulator(mg, flow_director='D8')\ndf = DepressionFinderAndRouter(mg)\n\nha = Space(mg, K_sed=0.00005, K_br=0.00005, phi=0.3, H_star=1)\n\nbr = mg.at_node[\"bedrock__elevation\"]\nz = mg.at_node[\"topographic__elevation\"]\n\nspace_dt = 500",
"_____no_output_____"
],
[
"z_ori = np.array(z)\nt1 = time.time()\nfor i in tqdm(range(50)):\n # Uplift\n br[mg.core_nodes] += 0.001 * space_dt\n z[mg.core_nodes] = br[mg.core_nodes] + s[mg.core_nodes]\n fr.run_one_step()\n df.map_depressions()\n ha.run_one_step(dt=space_dt)\n\nt_span1 = time.time() - t1\nprint('Total run time is %.f s' %t_span1)",
"_____no_output_____"
],
[
"plt.figure(figsize=(10,10))\nimshow_grid(mg, \"topographic__elevation\", cmap=\"terrain\")\nplt.title(\"Final topographic__elevation\")",
"_____no_output_____"
],
[
"mg2 = RasterModelGrid((nr, nc), xy_spacing=xy_spacing)\nz2 = mg2.add_zeros(\"topographic__elevation\", at=\"node\")\nmg2.at_node[\"topographic__elevation\"][mg2.core_nodes] += np.random.rand(\n mg2.number_of_core_nodes\n)\n\ns2 = mg2.add_zeros(\"soil__depth\", at=\"node\", dtype=float)\nmg2.at_node[\"soil__depth\"][mg2.core_nodes] += 0.5\nmg2.at_node[\"topographic__elevation\"] += mg2.at_node[\"soil__depth\"]\n\nfr2 = PriorityFloodFlowRouter(mg2, flow_metric=\"D8\", update_flow_depressions=True)\n\nha2 = SpaceLargeScaleEroder(mg2, K_sed=0.00005, K_br=0.00005, phi=0.3, H_star=1)\n\nbr2 = mg2.at_node[\"bedrock__elevation\"]\nz2 = mg2.at_node[\"topographic__elevation\"]",
"_____no_output_____"
],
[
"z_ori = np.array(z2)\nt2 = time.time()\nfor i in tqdm(range(50)):\n # Uplift\n br2[mg2.core_nodes] += 0.001 * space_dt\n z2[mg2.core_nodes] = br2[mg2.core_nodes] + s2[mg2.core_nodes]\n fr2.run_one_step()\n ha2.run_one_step(dt=space_dt)\n\nt_span2 = time.time() - t2\n\nprint('Total run time is %.f s' %t_span2)",
"_____no_output_____"
],
[
"plt.figure(figsize=(10,10))\nimshow_grid(mg2, \"topographic__elevation\", cmap=\"terrain\")\nplt.title(\"Final topographic__elevation\")",
"_____no_output_____"
],
[
"plt.figure()\nplt.bar(['Default flow accumulator','Priority Flood flow accumulator'],[t_span1,t_span2])\nplt.ylabel('Seconds')",
"_____no_output_____"
]
],
[
[
"## Back to HyLands tutorial page\n[Click here to go back to the tutorial overview page](../index.ipynb)",
"_____no_output_____"
]
]
] | [
"markdown",
"code",
"markdown",
"code",
"markdown"
] | [
[
"markdown",
"markdown"
],
[
"code"
],
[
"markdown"
],
[
"code",
"code",
"code",
"code",
"code",
"code",
"code"
],
[
"markdown"
]
] |
e7658f7a25d1d5424cfe26a47f43ae412ea814b0 | 28,156 | ipynb | Jupyter Notebook | notebooks/archive/__compliance.ipynb | hgzech/trr265 | 11807677d782ce5ef9e0e59e10be55f1da4e3371 | [
"Apache-2.0"
] | null | null | null | notebooks/archive/__compliance.ipynb | hgzech/trr265 | 11807677d782ce5ef9e0e59e10be55f1da4e3371 | [
"Apache-2.0"
] | 1 | 2021-11-18T16:42:24.000Z | 2021-11-18T17:11:09.000Z | notebooks/archive/__compliance.ipynb | hgzech/trr265 | 11807677d782ce5ef9e0e59e10be55f1da4e3371 | [
"Apache-2.0"
] | null | null | null | 31.494407 | 167 | 0.356016 | [
[
[
"#export\n%load_ext autoreload\n%autoreload 2\nfrom trr265.gbe.ist.data_provider import ISTDataProvider\nfrom trr265.gbe.wm.data_provider import WMDataProvider\nfrom trr265.gbe.sst.data_provider import SSTDataProvider\nfrom trr265.gbe.rtt.data_provider import RTTDataProvider\n\nimport trr265.gbe.ist.scoring as ist_scoring \nimport trr265.gbe.wm.scoring as wm_scoring \nimport trr265.gbe.sst.scoring as sst_scoring \nimport trr265.gbe.rtt.scoring as rtt_scoring \n\nimport pandas as pd",
"_____no_output_____"
],
[
"# Getting raw data\ndp = ISTDataProvider('/Users/hilmarzech/Projects/trr265/trr265/data/')\ndf = dp.get_ist_data()\n# Adding data from redcap\ndf = df.merge(dp.get_gbe_data(columns = ['participant','session_number','is_initial','is_baseline']), left_on = 'gbe_index', right_index = True, how = 'left')\n# Filtering out replication and ema data\ndf = df.query(\"is_initial\")\nist = ist_scoring.get_oversampling_predicted_joint(df)[0]\nist.columns = ['ist_oversampling']\nist",
"_____no_output_____"
],
[
"# Getting raw data\ndp = WMDataProvider('/Users/hilmarzech/Projects/trr265/trr265/data/')\ndf = dp.get_wm_data()\n# Adding data from redcap\ndf = df.merge(dp.get_gbe_data(columns = ['participant','session_number','is_initial','is_baseline']), left_on = 'gbe_index', right_index = True, how = 'left')\n# Filtering out replication and ema data\ndf = df.query(\"is_initial\")\n# Filtering participants with old app\ndf = dp.filter_old_app_sessions(df)\ndf = dp.filter_level_two_failures(df)\nwm = wm_scoring.get_perc_correct_predicted_sep_trial(df)[0]\nwm = wm.rename(columns={'perc_predicted_sep_trial_no_distractor_1': 'wm_no_1',\n 'perc_predicted_sep_trial_no_distractor_2': 'wm_no_2',\n 'perc_predicted_sep_trial_encoding_distractor': 'wm_encoding',\n 'perc_predicted_sep_trial_delayed_distractor':'wm_delayed'})",
"9 participants used an old version of the task in some of their sessions. 30 sessions (1.09%) were removed from the dataset.\n31 sessions (1.14%) were removed because participants failed a level two trial.\n"
],
[
"# Getting raw data\ndp = RTTDataProvider('/Users/hilmarzech/Projects/trr265/trr265/data/')\ndf = dp.get_rtt_data()\n# Adding data from redcap\ndf = df.merge(dp.get_gbe_data(columns = ['participant','session_number','is_initial','is_baseline']), left_on = 'gbe_index', right_index = True, how = 'left')\n# Filtering out replication and ema data\ndf = df.query(\"is_initial\")\nrtt = rtt_scoring.get_perc_gamble_predicted_joint(df)[0]\nrtt = rtt.rename(columns={'perc_gamble_joint_win': 'rtt_win',\n 'perc_gamble_joint_loss': 'rtt_loss',\n 'perc_gamble_joint_mixed': 'rtt_mixed'})",
"_____no_output_____"
],
[
"# Getting raw data\ndp = SSTDataProvider('/Users/hilmarzech/Projects/trr265/trr265/data/')\ndf = dp.get_sst_data()\n# Adding data from redcap\ndf = df.merge(dp.get_gbe_data(columns = ['participant','session_number','is_initial','is_baseline']), left_on = 'gbe_index', right_index = True, how = 'left')\n# Filtering out replication and ema data\ndf = df.query(\"is_initial\")\nsst = sst_scoring.get_ssrt_predicted_joint(df)[0]\nsst.columns = ['ssrt']",
"_____no_output_____"
],
[
"tasks = pd.concat([wm[['wm_no_1']], sst, rtt[['rtt_win']],ist],axis = 1).reset_index()",
"_____no_output_____"
],
[
"tasks['session'] = tasks.gbe_index.str.split('_').apply(lambda x: x[1]).astype(int)\ntasks['participant'] = tasks.gbe_index.str.split('_').apply(lambda x: x[0])\n\ntasks",
"_____no_output_____"
],
[
"len(tasks)",
"_____no_output_____"
],
[
"tasks.groupby('session').agg(lambda x: x.isnull().sum()).sum()",
"_____no_output_____"
],
[
"tasks.groupby('session').agg(lambda x: x.isnull().sum())",
"_____no_output_____"
],
[
"tasks",
"_____no_output_____"
],
[
"sessions = 8\n(tasks.query('session<%d'%(sessions+1)).groupby('participant').agg(lambda x: len(x.dropna()))==sessions).sum()",
"_____no_output_____"
]
]
] | [
"code"
] | [
[
"code",
"code",
"code",
"code",
"code",
"code",
"code",
"code",
"code",
"code",
"code",
"code"
]
] |
e7658fa3c3676c55ba4a6036173b458da9e223bb | 23,285 | ipynb | Jupyter Notebook | 03 Misc/02 keras_basics.ipynb | alphaolomi/colab | 19e4eb1bed56346dd18ba65638cda2d17a960d0c | [
"Apache-2.0"
] | null | null | null | 03 Misc/02 keras_basics.ipynb | alphaolomi/colab | 19e4eb1bed56346dd18ba65638cda2d17a960d0c | [
"Apache-2.0"
] | null | null | null | 03 Misc/02 keras_basics.ipynb | alphaolomi/colab | 19e4eb1bed56346dd18ba65638cda2d17a960d0c | [
"Apache-2.0"
] | null | null | null | 49.967811 | 402 | 0.656474 | [
[
[
"# Keras Tutorial: Develop Your First Neural Network in Python Step-By-Step\nby Alpha Olomi\nOn August 15, 2019 in Deep Learning",
"_____no_output_____"
],
[
"Keras is a powerful and easy-to-use free open source Python library for developing and evaluating deep learning models.\n\nIt wraps the efficient numerical computation libraries Theano and TensorFlow and allows you to define and train neural network models in just a few lines of code.\n\nIn this tutorial, you will discover how to create your first deep learning neural network model in Python using Keras.\n\nLet’s get started.\n\nThere is not a lot of code required, but we are going to step over it slowly so that you will know how to create your own models in the future.\n\nThe steps you are going to cover in this tutorial are as follows:\n\n- Load Data.\n- Define Keras Model.\n- Compile Keras Model.\n- Fit Keras Model.\n- Evaluate Keras Model.\n- Tie It All Together.\n- Make Predictions\n- This Keras tutorial has a few requirements:",
"_____no_output_____"
],
[
"## 1. Load Data\nThe first step is to define the functions and classes we intend to use in this tutorial.\n\nWe will use the NumPy library to load our dataset and we will use two classes from the Keras library to define our model.\n\nThe imports required are listed below.\n\n# first neural network with keras tutorial\nfrom numpy import loadtxt\nfrom keras.models import Sequential\nfrom keras.layers import Dense\n#...\nWe can now load our dataset.\n\nIn this Keras tutorial, we are going to use the Pima Indians onset of diabetes dataset. This is a standard machine learning dataset from the UCI Machine Learning repository. It describes patient medical record data for Pima Indians and whether they had an onset of diabetes within five years.\n\nAs such, it is a binary classification problem (onset of diabetes as 1 or not as 0). All of the input variables that describe each patient are numerical. This makes it easy to use directly with neural networks that expect numerical input and output values, and ideal for our first neural network in Keras.\n\nThe dataset us available from here:\n\nDataset CSV File (pima-indians-diabetes.csv)\nDataset Details\nDownload the dataset and place it in your local working directory, the same location as your python file.\n\nSave it with the filename:\n\npima-indians-diabetes.csv\n\n\nTake a look inside the file, you should see rows of data like the following:\n\nWe can now load the file as a matrix of numbers using the NumPy function loadtxt().\n\nThere are eight input variables and one output variable (the last column). We will be learning a model to map rows of input variables (X) to an output variable (y), which we often summarize as y = f(X).\n\nThe variables can be summarized as follows:\n\nInput Variables (X):\n\nNumber of times pregnant\nPlasma glucose concentration a 2 hours in an oral glucose tolerance test\nDiastolic blood pressure (mm Hg)\nTriceps skin fold thickness (mm)\n2-Hour serum insulin (mu U/ml)\nBody mass index (weight in kg/(height in m)^2)\nDiabetes pedigree function\nAge (years)\nOutput Variables (y):\n\nClass variable (0 or 1)\nOnce the CSV file is loaded into memory, we can split the columns of data into input and output variables.\n\nThe data will be stored in a 2D array where the first dimension is rows and the second dimension is columns, e.g. [rows, columns].\n\nWe can split the array into two arrays by selecting subsets of columns using the standard NumPy slice operator or “:” We can select the first 8 columns from index 0 to index 7 via the slice 0:8. We can then select the output column (the 9th variable) via index 8.\n\n...\n# load the dataset\ndataset = loadtxt('pima-indians-diabetes.csv', delimiter=',')\n# split into input (X) and output (y) variables\nX = dataset[:,0:8]\ny = dataset[:,8]\n...\n\nWe are now ready to define our neural network model.\n\nNote, the dataset has 9 columns and the range 0:8 will select columns from 0 to 7, stopping before index 8. If this is new to you, then you can learn more about array slicing and ranges in this post:\n\nHow to Index, Slice and Reshape NumPy Arrays for Machine Learning in Python\n",
"_____no_output_____"
],
[
"## 2. Define Keras Model\nModels in Keras are defined as a sequence of layers.\n\nWe create a Sequential model and add layers one at a time until we are happy with our network architecture.\n\nThe first thing to get right is to ensure the input layer has the right number of input features. This can be specified when creating the first layer with the input_dim argument and setting it to 8 for the 8 input variables.\n\nHow do we know the number of layers and their types?\n\nThis is a very hard question. There are heuristics that we can use and often the best network structure is found through a process of trial and error experimentation (I explain more about this here). Generally, you need a network large enough to capture the structure of the problem.\n\nIn this example, we will use a fully-connected network structure with three layers.\n\nFully connected layers are defined using the Dense class. We can specify the number of neurons or nodes in the layer as the first argument, and specify the activation function using the activation argument.\n\nWe will use the rectified linear unit activation function referred to as ReLU on the first two layers and the Sigmoid function in the output layer.\n\nIt used to be the case that Sigmoid and Tanh activation functions were preferred for all layers. These days, better performance is achieved using the ReLU activation function. We use a sigmoid on the output layer to ensure our network output is between 0 and 1 and easy to map to either a probability of class 1 or snap to a hard classification of either class with a default threshold of 0.5.\n\nWe can piece it all together by adding each layer:\n\nThe model expects rows of data with 8 variables (the input_dim=8 argument)\nThe first hidden layer has 12 nodes and uses the relu activation function.\nThe second hidden layer has 8 nodes and uses the relu activation function.\nThe output layer has one node and uses the sigmoid activation function.\n...\n# define the keras model\nmodel = Sequential()\nmodel.add(Dense(12, input_dim=8, activation='relu'))\nmodel.add(Dense(8, activation='relu'))\nmodel.add(Dense(1, activation='sigmoid'))\n\n\nNote, the most confusing thing here is that the shape of the input to the model lis defined as an argument on the first hidden layer. This means that the line of code that adds the first Dense layer is doing 2 things, defining the input or visible layer and the first hidden layer.",
"_____no_output_____"
],
[
"## 3. Compile Keras Model\nNow that the model is defined, we can compile it.\n\nCompiling the model uses the efficient numerical libraries under the covers (the so-called backend) such as Theano or TensorFlow. The backend automatically chooses the best way to represent the network for training and making predictions to run on your hardware, such as CPU or GPU or even distributed.\n\nWhen compiling, we must specify some additional properties required when training the network. Remember training a network means finding the best set of weights to map inputs to outputs in our dataset.\n\nWe must specify the loss function to use to evaluate a set of weights, the optimizer is used to search through different weights for the network and any optional metrics we would like to collect and report during training.\n\nIn this case, we will use cross entropy as the loss argument. This loss is for a binary classification problems and is defined in Keras as “binary_crossentropy“. You can learn more about choosing loss functions based on your problem here:\n\nHow to Choose Loss Functions When Training Deep Learning Neural Networks\nWe will define the optimizer as the efficient stochastic gradient descent algorithm “adam“. This is a popular version of gradient descent because it automatically tunes itself and gives good results in a wide range of problems. To learn more about the Adam version of stochastic gradient descent see the post:\n\nGentle Introduction to the Adam Optimization Algorithm for Deep Learning\nFinally, because it is a classification problem, we will collect and report the classification accuracy, defined via the metrics argument.\n\n...\n# compile the keras model\nmodel.compile(loss='binary_crossentropy', optimizer='adam', metrics=['accuracy'])\n",
"_____no_output_____"
],
[
"## 4. Fit Keras Model\nWe have defined our model and compiled it ready for efficient computation.\n\nNow it is time to execute the model on some data.\n\nWe can train or fit our model on our loaded data by calling the fit() function on the model.\n\nTraining occurs over epochs and each epoch is split into batches.\n\nEpoch: One pass through all of the rows in the training dataset.\nBatch: One or more samples considered by the model within an epoch before weights are updated.\nOne epoch is comprised of one or more batches, based on the chosen batch size and the model is fit for many epochs. For more on the difference between epochs and batches, see the post:\n\nWhat is the Difference Between a Batch and an Epoch in a Neural Network?\nThe training process will run for a fixed number of iterations through the dataset called epochs, that we must specify using the epochs argument. We must also set the number of dataset rows that are considered before the model weights are updated within each epoch, called the batch size and set using the batch_size argument.\n\nFor this problem, we will run for a small number of epochs (150) and use a relatively small batch size of 10. This means that each epoch will involve (150/10) 15 updates to the model weights.\n\nThese configurations can be chosen experimentally by trial and error. We want to train the model enough so that it learns a good (or good enough) mapping of rows of input data to the output classification. The model will always have some error, but the amount of error will level out after some point for a given model configuration. This is called model convergence.\n\n...\n# fit the keras model on the dataset\nmodel.fit(X, y, epochs=150, batch_size=10)\n...\n\n\nThis is where the work happens on your CPU or GPU.\n\nNo GPU is required for this example, but if you’re interested in how to run large models on GPU hardware cheaply in the cloud, see this post:\n\nHow to Setup Amazon AWS EC2 GPUs to Train Keras Deep Learning Models\n",
"_____no_output_____"
],
[
"## 5. Evaluate Keras Model\nWe have trained our neural network on the entire dataset and we can evaluate the performance of the network on the same dataset.\n\nThis will only give us an idea of how well we have modeled the dataset (e.g. train accuracy), but no idea of how well the algorithm might perform on new data. We have done this for simplicity, but ideally, you could separate your data into train and test datasets for training and evaluation of your model.\n\nYou can evaluate your model on your training dataset using the evaluate() function on your model and pass it the same input and output used to train the model.\n\nThis will generate a prediction for each input and output pair and collect scores, including the average loss and any metrics you have configured, such as accuracy.\n\nThe evaluate() function will return a list with two values. The first will be the loss of the model on the dataset and the second will be the accuracy of the model on the dataset. We are only interested in reporting the accuracy, so we will ignore the loss value.\n\n...\n# evaluate the keras model\n_, accuracy = model.evaluate(X, y)\n\n## 6. Tie It All Together\nYou have just seen how you can easily create your first neural network model in Keras.\n\nLet’s tie it all together into a complete code example.\n\n# first neural network with keras tutorial\nfrom numpy import loadtxt\nfrom keras.models import Sequential\nfrom keras.layers import Dense\n# load the dataset\ndataset = loadtxt('pima-indians-diabetes.csv', delimiter=',')\n# split into input (X) and output (y) variables\nX = dataset[:,0:8]\ny = dataset[:,8]\n# define the keras model\nmodel = Sequential()\nmodel.add(Dense(12, input_dim=8, activation='relu'))\nmodel.add(Dense(8, activation='relu'))\nmodel.add(Dense(1, activation='sigmoid'))\n# compile the keras model\nmodel.compile(loss='binary_crossentropy', optimizer='adam', metrics=['accuracy'])\n# fit the keras model on the dataset\nmodel.fit(X, y, epochs=150, batch_size=10)\n# evaluate the keras model\n_, accuracy = model.evaluate(X, y)\nprint('Accuracy: %.2f' % (accuracy*100)) \n\n\nYou can copy all of the code into your Python file and save it as “keras_first_network.py” in the same directory as your data file “pima-indians-diabetes.csv“. You can then run the Python file as a script from your command line (command prompt) as follows:\n\npython keras_first_network.py\n\n\n\nRunning this example, you should see a message for each of the 150 epochs printing the loss and accuracy, followed by the final evaluation of the trained model on the training dataset.\n\nIt takes about 10 seconds to execute on my workstation running on the CPU.\n\nIdeally, we would like the loss to go to zero and accuracy to go to 1.0 (e.g. 100%). This is not possible for any but the most trivial machine learning problems. Instead, we will always have some error in our model. The goal is to choose a model configuration and training configuration that achieve the lowest loss and highest accuracy possible for a given dataset.\n\n...\n768/768 [==============================] - 0s 63us/step - loss: 0.4817 - acc: 0.7708\nEpoch 147/150\n768/768 [==============================] - 0s 63us/step - loss: 0.4764 - acc: 0.7747\nEpoch 148/150\n768/768 [==============================] - 0s 63us/step - loss: 0.4737 - acc: 0.7682\nEpoch 149/150\n768/768 [==============================] - 0s 64us/step - loss: 0.4730 - acc: 0.7747\nEpoch 150/150\n768/768 [==============================] - 0s 63us/step - loss: 0.4754 - acc: 0.7799\n768/768 [==============================] - 0s 38us/step\nAccuracy: 76.56\n\n\nNote, if you try running this example in an IPython or Jupyter notebook you may get an error.\n\nThe reason is the output progress bars during training. You can easily turn these off by setting verbose=0 in the call to the fit() and evaluate() functions, for example:\n\n...\n# fit the keras model on the dataset without progress bars\nmodel.fit(X, y, epochs=150, batch_size=10, verbose=0)\n# evaluate the keras model\n_, accuracy = model.evaluate(X, y, verbose=0)\n\n\nNote, the accuracy of your model will vary.\n\nNeural networks are a stochastic algorithm, meaning that the same algorithm on the same data can train a different model with different skill each time the code is run. This is a feature, not a bug. You can learn more about this in the post:\n\nEmbrace Randomness in Machine Learning\nThe variance in the performance of the model means that to get a reasonable approximation of how well your model is performing, you may need to fit it many times and calculate the average of the accuracy scores. For more on this approach to evaluating neural networks, see the post:\n\nHow to Evaluate the Skill of Deep Learning Models\nFor example, below are the accuracy scores from re-running the example 5 times:\n\nAccuracy: 75.00\nAccuracy: 77.73\nAccuracy: 77.60\nAccuracy: 78.12\nAccuracy: 76.17\n\n\nWe can see that all accuracy scores are around 77% and the average is 76.924%.",
"_____no_output_____"
],
[
"## 7. Make Predictions\nThe number one question I get asked is:\n\nAfter I train my model, how can I use it to make predictions on new data?\n\nGreat question.\n\nWe can adapt the above example and use it to generate predictions on the training dataset, pretending it is a new dataset we have not seen before.\n\nMaking predictions is as easy as calling the predict() function on the model. We are using a sigmoid activation function on the output layer, so the predictions will be a probability in the range between 0 and 1. We can easily convert them into a crisp binary prediction for this classification task by rounding them.\n\nFor example:\n\n...\n# make probability predictions with the model\npredictions = model.predict(X)\n# round predictions \nrounded = [round(x[0]) for x in predictions]\n\n\nAlternately, we can call the predict_classes() function on the model to predict crisp classes directly, for example:\n\n...\n# make class predictions with the model\npredictions = model.predict_classes(X)\n\n\nThe complete example below makes predictions for each example in the dataset, then prints the input data, predicted class and expected class for the first 5 examples in the dataset.\n\n# first neural network with keras make predictions\nfrom numpy import loadtxt\nfrom keras.models import Sequential\nfrom keras.layers import Dense\n# load the dataset\ndataset = loadtxt('pima-indians-diabetes.csv', delimiter=',')\n# split into input (X) and output (y) variables\nX = dataset[:,0:8]\ny = dataset[:,8]\n# define the keras model\nmodel = Sequential()\nmodel.add(Dense(12, input_dim=8, activation='relu'))\nmodel.add(Dense(8, activation='relu'))\nmodel.add(Dense(1, activation='sigmoid'))\n# compile the keras model\nmodel.compile(loss='binary_crossentropy', optimizer='adam', metrics=['accuracy'])\n# fit the keras model on the dataset\nmodel.fit(X, y, epochs=150, batch_size=10, verbose=0)\n# make class predictions with the model\npredictions = model.predict_classes(X)\n# summarize the first 5 cases\nfor i in range(5):\n\tprint('%s => %d (expected %d)' % (X[i].tolist(), predictions[i], y[i]))",
"_____no_output_____"
],
[
"Running the example does not show the progress bar as before as we have set the verbose argument to 0.\n\nAfter the model is fit, predictions are made for all examples in the dataset, and the input rows and predicted class value for the first 5 examples is printed and compared to the expected class value.\n\nWe can see that most rows are correctly predicted. In fact, we would expect about 76.9% of the rows to be correctly predicted based on our estimated performance of the model in the previous section.\n\n[6.0, 148.0, 72.0, 35.0, 0.0, 33.6, 0.627, 50.0] => 0 (expected 1)\n[1.0, 85.0, 66.0, 29.0, 0.0, 26.6, 0.351, 31.0] => 0 (expected 0)\n[8.0, 183.0, 64.0, 0.0, 0.0, 23.3, 0.672, 32.0] => 1 (expected 1)\n[1.0, 89.0, 66.0, 23.0, 94.0, 28.1, 0.167, 21.0] => 0 (expected 0)\n[0.0, 137.0, 40.0, 35.0, 168.0, 43.1, 2.288, 33.0] => 1 (expected 1)\n",
"_____no_output_____"
],
[
"## Keras Tutorial Summary\nIn this post, you discovered how to create your first neural network model using the powerful Keras Python library for deep learning.\n\nSpecifically, you learned the six key steps in using Keras to create a neural network or deep learning model, step-by-step including:\n\n- How to load data.\n- How to define a neural network in Keras.\n- How to compile a Keras model using the efficient numerical backend.\n- How to train a model on data.\n- How to evaluate a model on data.\n- How to make predictions with the model.",
"_____no_output_____"
]
]
] | [
"markdown"
] | [
[
"markdown",
"markdown",
"markdown",
"markdown",
"markdown",
"markdown",
"markdown",
"markdown",
"markdown",
"markdown"
]
] |
e765a2a4fc65b38796cdec66f9696d63477640ba | 145,293 | ipynb | Jupyter Notebook | Classification/Linear Models/Perceptron_StandardScaler_QuantileTransformer.ipynb | shreepad-nade/ds-seed | 93ddd3b73541f436b6832b94ca09f50872dfaf10 | [
"Apache-2.0"
] | 53 | 2021-08-28T07:41:49.000Z | 2022-03-09T02:20:17.000Z | Classification/Linear Models/Perceptron_StandardScaler_QuantileTransformer.ipynb | shreepad-nade/ds-seed | 93ddd3b73541f436b6832b94ca09f50872dfaf10 | [
"Apache-2.0"
] | 142 | 2021-07-27T07:23:10.000Z | 2021-08-25T14:57:24.000Z | Classification/Linear Models/Perceptron_StandardScaler_QuantileTransformer.ipynb | shreepad-nade/ds-seed | 93ddd3b73541f436b6832b94ca09f50872dfaf10 | [
"Apache-2.0"
] | 38 | 2021-07-27T04:54:08.000Z | 2021-08-23T02:27:20.000Z | 204.350211 | 40,065 | 0.714281 | [
[
[
"# Perceptron with StandardScaler & Quantile Transformer",
"_____no_output_____"
],
[
"This Code template is for the Classification task using simple Perceptron. Which is a simple classification algorithm suitable for large scale learning where data rescaling is done using StandardScaler and feature transformation is done is using QuantileTransformer in a pipeline.",
"_____no_output_____"
],
[
"### Required Packages",
"_____no_output_____"
]
],
[
[
"!pip install imblearn",
"_____no_output_____"
],
[
"import warnings \r\nimport numpy as np\r\nimport pandas as pd \r\nimport matplotlib.pyplot as plt \r\nimport seaborn as se \r\nfrom imblearn.over_sampling import RandomOverSampler\r\nfrom sklearn.preprocessing import LabelEncoder \r\nfrom sklearn.model_selection import train_test_split \r\nfrom sklearn.linear_model import Perceptron\r\nfrom sklearn.pipeline import make_pipeline\r\nfrom sklearn.preprocessing import QuantileTransformer\r\nfrom sklearn.preprocessing import StandardScaler\r\nfrom sklearn.metrics import classification_report,plot_confusion_matrix\r\nwarnings.filterwarnings('ignore')",
"_____no_output_____"
]
],
[
[
"### Initialization\n\nFilepath of CSV file",
"_____no_output_____"
]
],
[
[
"#filepath\r\nfile_path= \"\"",
"_____no_output_____"
]
],
[
[
"List of features which are required for model training .",
"_____no_output_____"
]
],
[
[
"#x_values\r\nfeatures=[] ",
"_____no_output_____"
]
],
[
[
"Target feature for prediction.",
"_____no_output_____"
]
],
[
[
"#y_value\r\ntarget= ''",
"_____no_output_____"
]
],
[
[
"### Data Fetching\n\nPandas is an open-source, BSD-licensed library providing high-performance, easy-to-use data manipulation and data analysis tools.\n\nWe will use panda's library to read the CSV file using its storage path.And we use the head function to display the initial row or entry.",
"_____no_output_____"
]
],
[
[
"df=pd.read_csv(file_path)\r\ndf.head()",
"_____no_output_____"
]
],
[
[
"### Feature Selections\n\nIt is the process of reducing the number of input variables when developing a predictive model. Used to reduce the number of input variables to both reduce the computational cost of modelling and, in some cases, to improve the performance of the model.\n\nWe will assign all the required input features to X and target/outcome to Y.",
"_____no_output_____"
]
],
[
[
"X = df[features]\r\nY = df[target]",
"_____no_output_____"
]
],
[
[
"### Data Preprocessing\n\nSince the majority of the machine learning models in the Sklearn library doesn't handle string category data and Null value, we have to explicitly remove or replace null values. The below snippet have functions, which removes the null value if any exists. And convert the string classes data in the datasets by encoding them to integer classes.\n",
"_____no_output_____"
]
],
[
[
"def NullClearner(df):\r\n if(isinstance(df, pd.Series) and (df.dtype in [\"float64\",\"int64\"])):\r\n df.fillna(df.mean(),inplace=True)\r\n return df\r\n elif(isinstance(df, pd.Series)):\r\n df.fillna(df.mode()[0],inplace=True)\r\n return df\r\n else:return df\r\ndef EncodeX(df):\r\n return pd.get_dummies(df)\r\ndef EncodeY(df):\r\n if len(df.unique())<=2:\r\n return df\r\n else:\r\n un_EncodedT=np.sort(pd.unique(df), axis=-1, kind='mergesort')\r\n df=LabelEncoder().fit_transform(df)\r\n EncodedT=[xi for xi in range(len(un_EncodedT))]\r\n print(\"Encoded Target: {} to {}\".format(un_EncodedT,EncodedT))\r\n return df",
"_____no_output_____"
],
[
"x=X.columns.to_list()\r\nfor i in x:\r\n X[i]=NullClearner(X[i]) \r\nX=EncodeX(X)\r\nY=EncodeY(NullClearner(Y))\r\nX.head()",
"_____no_output_____"
]
],
[
[
"#### Correlation Map\n\nIn order to check the correlation between the features, we will plot a correlation matrix. It is effective in summarizing a large amount of data where the goal is to see patterns.",
"_____no_output_____"
]
],
[
[
"f,ax = plt.subplots(figsize=(18, 18))\r\nmatrix = np.triu(X.corr())\r\nse.heatmap(X.corr(), annot=True, linewidths=.5, fmt= '.1f',ax=ax, mask=matrix)\r\nplt.show()",
"_____no_output_____"
]
],
[
[
"#### Distribution Of Target Variable",
"_____no_output_____"
]
],
[
[
"plt.figure(figsize = (10,6))\r\nse.countplot(Y)",
"_____no_output_____"
]
],
[
[
"### Data Splitting\n\nThe train-test split is a procedure for evaluating the performance of an algorithm. The procedure involves taking a dataset and dividing it into two subsets. The first subset is utilized to fit/train the model. The second subset is used for prediction. The main motive is to estimate the performance of the model on new data.",
"_____no_output_____"
]
],
[
[
"x_train,x_test,y_train,y_test=train_test_split(X,Y,test_size=0.2,random_state=123)",
"_____no_output_____"
]
],
[
[
"#### Handling Target Imbalance\n\nThe challenge of working with imbalanced datasets is that most machine learning techniques will ignore, and in turn have poor performance on, the minority class, although typically it is performance on the minority class that is most important.\n\nOne approach to addressing imbalanced datasets is to oversample the minority class. The simplest approach involves duplicating examples in the minority class.We will perform overspampling using imblearn library. ",
"_____no_output_____"
]
],
[
[
"x_train,y_train = RandomOverSampler(random_state=123).fit_resample(x_train, y_train)",
"_____no_output_____"
]
],
[
[
"### Model\n the perceptron is an algorithm for supervised learning of binary classifiers.\n The algorithm learns the weights for the input signals in order to draw a linear decision boundary.This enables you to distinguish between the two linearly separable classes +1 and -1.\n#### Model Tuning Parameters\n\n> **penalty** ->The penalty (aka regularization term) to be used. {‘l2’,’l1’,’elasticnet’}\n\n> **alpha** -> Constant that multiplies the regularization term if regularization is used.\n\n> **l1_ratio** -> The Elastic Net mixing parameter, with 0 <= l1_ratio <= 1. l1_ratio=0 corresponds to L2 penalty, l1_ratio=1 to L1. Only used if penalty='elasticnet'.\n\n> **tol** -> The stopping criterion. If it is not None, the iterations will stop when (loss > previous_loss - tol).\n\n> **early_stopping**-> Whether to use early stopping to terminate training when validation. score is not improving. If set to True, it will automatically set aside a stratified fraction of training data as validation and terminate training when validation score is not improving by at least tol for n_iter_no_change consecutive epochs.\n\n> **validation_fraction** -> The proportion of training data to set aside as validation set for early stopping. Must be between 0 and 1. Only used if early_stopping is True.\n\n> **n_iter_no_change** -> Number of iterations with no improvement to wait before early stopping.\n\nScaling\nStandardize features by removing the mean and scaling to unit variance\nThe standard score of a sample x is calculated as:\nz = (x - u) / s\nRefer [API](https://scikit-learn.org/stable/modules/generated/sklearn.preprocessing.StandardScaler.html) for parameters.\n\nFeature Transformation\nPower transforms are a family of parametric, monotonic transformations that are applied to make data more Gaussian-like. This is useful for modeling issues related to heteroscedasticity (non-constant variance), or other situations where normality is desired.\n\nCurrently, PowerTransformer supports the Box-Cox transform and the Yeo-Johnson transform. The optimal parameter for stabilizing variance and minimizing skewness is estimated through maximum likelihood.\n\n\nRefer [API](https://scikit-learn.org/stable/modules/generated/sklearn.preprocessing.PowerTransformer.html) for the parameters",
"_____no_output_____"
],
[
"### Data Rescaling\r\n\r\nStandardize features by removing the mean and scaling to unit variance\r\n\r\nThe standard score of a sample x is calculated as:\r\n\r\n z = (x - u) / s\r\n\r\nwhere u is the mean of the training samples or zero if with_mean=False, and s is the standard deviation of the training samples or one if with_std=False.",
"_____no_output_____"
],
[
"### Feature Transformation\r\n\r\nTransform features using quantiles information.\r\n\r\nThis method transforms the features to follow a uniform or a normal distribution. Therefore, for a given feature, this transformation tends to spread out the most frequent values. It also reduces the impact of (marginal) outliers: this is therefore a robust preprocessing scheme.The transformation is applied on each feature independently.",
"_____no_output_____"
]
],
[
[
"# Build Model here\r\nmodel=make_pipeline(StandardScaler(),QuantileTransformer(),Perceptron(random_state=123))\r\nmodel.fit(x_train, y_train)",
"_____no_output_____"
]
],
[
[
"#### Model Accuracy\n\nscore() method return the mean accuracy on the given test data and labels.\n\nIn multi-label classification, this is the subset accuracy which is a harsh metric since you require for each sample that each label set be correctly predicted.",
"_____no_output_____"
]
],
[
[
"print(\"Accuracy score {:.2f} %\\n\".format(model.score(x_test,y_test)*100))",
"Accuracy score 68.75 %\n\n"
]
],
[
[
"#### Confusion Matrix\n\nA confusion matrix is utilized to understand the performance of the classification model or algorithm in machine learning for a given test set where results are known.",
"_____no_output_____"
]
],
[
[
"plot_confusion_matrix(model,x_test,y_test,cmap=plt.cm.Blues)",
"_____no_output_____"
]
],
[
[
"#### Classification Report\nA Classification report is used to measure the quality of predictions from a classification algorithm. How many predictions are True, how many are False.\n\n* **where**:\n - Precision:- Accuracy of positive predictions.\n - Recall:- Fraction of positives that were correctly identified.\n - f1-score:- percent of positive predictions were correct\n - support:- Support is the number of actual occurrences of the class in the specified dataset.",
"_____no_output_____"
]
],
[
[
"print(classification_report(y_test,model.predict(x_test)))",
" precision recall f1-score support\n\n 0 0.93 0.54 0.68 50\n 1 0.55 0.93 0.69 30\n\n accuracy 0.69 80\n macro avg 0.74 0.74 0.69 80\nweighted avg 0.79 0.69 0.69 80\n\n"
]
],
[
[
"#### Creator: Vamsi Mukkamala , Github: [Profile](https://github.com/vmc99)\r\n",
"_____no_output_____"
]
]
] | [
"markdown",
"code",
"markdown",
"code",
"markdown",
"code",
"markdown",
"code",
"markdown",
"code",
"markdown",
"code",
"markdown",
"code",
"markdown",
"code",
"markdown",
"code",
"markdown",
"code",
"markdown",
"code",
"markdown",
"code",
"markdown",
"code",
"markdown",
"code",
"markdown",
"code",
"markdown"
] | [
[
"markdown",
"markdown",
"markdown"
],
[
"code",
"code"
],
[
"markdown"
],
[
"code"
],
[
"markdown"
],
[
"code"
],
[
"markdown"
],
[
"code"
],
[
"markdown"
],
[
"code"
],
[
"markdown"
],
[
"code"
],
[
"markdown"
],
[
"code",
"code"
],
[
"markdown"
],
[
"code"
],
[
"markdown"
],
[
"code"
],
[
"markdown"
],
[
"code"
],
[
"markdown"
],
[
"code"
],
[
"markdown",
"markdown",
"markdown"
],
[
"code"
],
[
"markdown"
],
[
"code"
],
[
"markdown"
],
[
"code"
],
[
"markdown"
],
[
"code"
],
[
"markdown"
]
] |
e765af430c2b73191fa7ea9f760c55e720ef5d5e | 942,709 | ipynb | Jupyter Notebook | experiments/notebooks/VectorRepresentation_tests_V2.ipynb | leomrocha/minibrain | e243f7742495c50104ee13ddc6929b1f3cacfc97 | [
"MIT"
] | 9 | 2018-10-18T18:42:20.000Z | 2021-04-17T14:23:22.000Z | experiments/notebooks/VectorRepresentation_tests_V2.ipynb | leomrocha/minibrain | e243f7742495c50104ee13ddc6929b1f3cacfc97 | [
"MIT"
] | null | null | null | experiments/notebooks/VectorRepresentation_tests_V2.ipynb | leomrocha/minibrain | e243f7742495c50104ee13ddc6929b1f3cacfc97 | [
"MIT"
] | 1 | 2020-04-22T15:13:26.000Z | 2020-04-22T15:13:26.000Z | 643.487372 | 124,896 | 0.935427 | [
[
[
"import tensorflow as tf\nimport random\nimport matplotlib.pyplot as plt\n\nfrom sklearn import linear_model\nfrom sklearn.model_selection import train_test_split\n\nimport numpy as np\nimport pandas as pd\n\n%matplotlib inline",
"_____no_output_____"
],
[
"#importing funcitons from ScalarRepresentation-tests notebook\n#import nbimporter\n#import ScalarRepresentation_tests as ScalarRep",
"_____no_output_____"
],
[
"#from ScalarRepresentation_tests import Ensemble, Neuron, neuron_creation, neuron_evaluator, param_creation",
"_____no_output_____"
]
],
[
[
"## Goal\n\nAs with the previous scalar representation the goal here is to be able to randomly create neurons and synapses and be able to find a linear decoder that is able to correctly decode the non-linear encoded value.\n\nTo do this, in this section, instead of considering that the neuron has only one input and one output, I'll start playing with synapse weights. \n\nThe goal is to understand how to generate different synapse weights (with some random values of a certain distribution) without the need to train the system, and only finding (training) the linear decoder.",
"_____no_output_____"
],
[
"## First Vector Representation Experience\n\nThe main point of this notebook is to understand how to represent nicely vectors with the same neuron populations as the Scalars.\n\nFor this exercise I'll start considering that the input current is limited to the range [-1;1] and this implies that bigger currents (module) than this can not be represented.\nThis means that if the input is saturated by one of the synapses, the other synapses contributions to the neural input current will be maybe ignored.\n\nI propose the following ideas to study the behaviour:\n\n* All synapse values are equal and the sum is 1\n* Uniform random distribution of the variables, where all synapse values add to a maximum of 1 (one), this might be done doing the synapse weights first [0,1], then [0,1-s1], [0,1-s1-s2], .... ,[0,1-s1-s2-....-sn] .. this might be good to take into account negative numbers too\n* Gaussian distribution (attention) for each neuron on the input values of the input vector\n* Try other distributions (as attention) to input values of the vector\n\n**Questions**:\n\n - Should I use NEGATIVE synapse values? -> tried uniform random and NO, it does not work better in simple scenarios\n - If so: How to take care of NEGATIVE synapse values?",
"_____no_output_____"
],
[
"### Case Study - All synapses sum 1\n\n\n",
"_____no_output_____"
]
],
[
[
"class VectorNeuron(object):\n def __init__(self, a, b, sat, weights):\n self.a = a\n self.b = b\n self.saturation = sat\n self.weights = weights",
"_____no_output_____"
],
[
"# redefining the vector creation function to allow for the neurons different totally random synapse weights\n\ndef rand_vector_neuron_creation(n_synapses, min_y=0.5, max_y=1.5,\n min_weight=0.0, max_weight=0.8, # to experiment with the weight limits\n min_x=-1.0, max_x=1.0, saturation=None):\n \"\"\"\n This model creates two points, one in x range, one in y range, then decides if a >0 or a<0.\n Depending on the sign (which will be the sign of a), will resolve the linear equation to find \n a and b\n \"\"\"\n max_w = 0.9 # I don understand the error in random when passing the param by arg\n if(saturation is None):\n saturation = random.uniform(0.8, 1.0)\n s = random.choice([-1, 1])\n a = b = 0\n # the first point is for y=0\n x1 = random.uniform(-1, 1)\n y1 = 0\n # the second point is for x=+-1\n x2 = s\n y2 = random.uniform(min_y, max_y)\n\n a = (y1 - y2) / (x1 - x2)\n b = y1 - a * x1\n # weights = [random.uniform(0, 0.8) for s in range(n_synapses)] #do the test with synapse weight = 1\n weights = [random.uniform(min_weight, max_w) for s in range(\n n_synapses)] # do the test with synapse weight = 1\n return VectorNeuron(a, b, saturation, np.array(weights))\n\n\n# now redefine the weights function here, so I can redo the study\nvector_neuron_creation = rand_vector_neuron_creation",
"_____no_output_____"
]
],
[
[
"## Important NOTE:\n\nI tried with min_weight values of -1.0, -0.9 and 0.0\n\nThe value 0 clearly outperforms the negative values, giving min_weight to the synapse weight should be then the value 0.0 (zero)",
"_____no_output_____"
]
],
[
[
"min_weight = -0.8\nmax_weight = 0.8\n\nrandom.uniform(min_weight, max_weight)",
"_____no_output_____"
],
[
"# Definition of function evaluation\n\ndef limited_vector_neuron_evaluator(x, neuron ):\n a = neuron.a\n b = neuron.b\n sat=neuron.saturation\n current = np.minimum(x,np.ones(x.shape)).transpose().dot(neuron.weights)\n return max(0, min(a*current + b, sat))\n \n\ndef vector_neuron_evaluator(x, neuron ):\n a = neuron.a\n b = neuron.b\n sat = neuron.saturation\n current = np.minimum(x,np.ones(x.shape)).transpose().dot(neuron.weights)\n return max(0, a*current + b)",
"_____no_output_____"
],
[
"class VectorEnsemble(object):\n def __init__(self, vect_dimension, n_neurons, min_y=0.5, max_y=1.5, max_x=1,min_x=-1,saturation=None):\n self.neurons = [vector_neuron_creation(vect_dimension, min_y, max_y, max_x, saturation) for i in range(n_neurons)]\n\n def encode_saturation(self, inputs):\n \"\"\"\n For every point in the input will calculate all the outputs for all the neurons\n \"\"\"\n output = []\n for x in inputs:\n outpoint = []\n for n in self.neurons:\n v = limited_vector_neuron_evaluator(x, n)\n outpoint.append(v)\n output.append(outpoint)\n return np.array(output)\n \n def encode(self, inputs):\n \"\"\"\n For every point in the input will calculate all the outputs for all the neurons\n \"\"\"\n output = []\n for x in inputs:\n outpoint = []\n for n in self.neurons:\n v = vector_neuron_evaluator(x, n)\n outpoint.append(v)\n output.append(outpoint)\n return np.array(output)",
"_____no_output_____"
],
[
"#make ensembles for different dimensions, the ensambles will have 1000 neurons each\n#dimensions is the input dimension of the function\nensembles = []\n\n#dimensions = [2,3,5,10,20,50,100,1000, 10000]\ndimensions = [2,3,4,10]\n\nnneurons=500\n\nfor d in dimensions:\n ensembles.append(VectorEnsemble(d, nneurons))",
"_____no_output_____"
],
[
"npoints = 10000\nxt = np.linspace(0, 8*np.pi, npoints)",
"_____no_output_____"
],
[
"%%time\n\nfsin = np.sin(xt)\nfcos = np.cos(xt)\nxlasc = np.linspace(0,1,npoints)\nxldes = np.linspace(1,0,npoints)\n\nind4 = np.column_stack((fsin, fcos, xlasc, xldes))\n\nplt.plot(range(npoints), ind4);",
"CPU times: user 24 ms, sys: 0 ns, total: 24 ms\nWall time: 21.9 ms\n"
],
[
"%%time\n\nins = ind4 #inputs[0]\nens = ensembles[2]\n\nencs = ens.encode_saturation(ind4)\nencns = ens.encode(ind4)",
"_____no_output_____"
],
[
"n_vectors = npoints \n\ninputs = [np.random.rand(n_vectors,d) for d in dimensions]",
"_____no_output_____"
],
[
"plt.plot(range(n_vectors), encs);",
"_____no_output_____"
],
[
"plt.plot(range(n_vectors), encns);",
"_____no_output_____"
]
],
[
[
"Seems that a few particular values are taking control of all the elements.\n\nI think I should ALSO limit the Post Synaptic Current (PSC) for each connexion",
"_____no_output_____"
]
],
[
[
"decoders = []\n\nntrain = 50\n\nlm = linear_model.LinearRegression()\nmodel = lm.fit(encs[:ntrain],ins[:ntrain])\n \n\n#now evaluate the results with those numbers\nprint (model.score(encs[ntrain:], ins[ntrain:]))\n \nplt.plot(range(n_vectors), model.predict(encs));",
"0.370233091488\n"
],
[
"decoders = []\n\nntrain = 200\n\nlm = linear_model.LinearRegression()\nmodel = lm.fit(encs[:ntrain],ins[:ntrain])\n \n\n#now evaluate the results with those numbers\nprint (model.score(encs[ntrain:], ins[ntrain:]))\n \nplt.plot(range(n_vectors), model.predict(encs));",
"0.97525499879\n"
],
[
"decoders = []\n\nntrain = 300\n\nlm = linear_model.LinearRegression()\nmodel = lm.fit(encs[:ntrain],ins[:ntrain])\n \n\n#now evaluate the results with those numbers\nprint (model.score(encs[ntrain:], ins[ntrain:]))\n \nplt.plot(range(n_vectors), model.predict(encs));",
"0.802730774837\n"
],
[
"decoders = []\n\nntrain = 500\n\nlm = linear_model.LinearRegression()\nmodel = lm.fit(encs[:ntrain],ins[:ntrain])\n \n\n#now evaluate the results with those numbers\nprint (model.score(encs[ntrain:], ins[ntrain:]))\n \nplt.plot(range(n_vectors), model.predict(encs));",
"-34.2821286008\n"
],
[
"decoders = []\n\nntrain = 1000\n\nlm = linear_model.LinearRegression()\nmodel = lm.fit(encs[:ntrain],ins[:ntrain])\n \n\n#now evaluate the results with those numbers\nprint (model.score(encs[ntrain:], ins[ntrain:]))\n \nplt.plot(range(n_vectors), model.predict(encs));",
"-0.789882305034\n"
],
[
"decoders = []\n\nntrain = 1500\n\nlm = linear_model.LinearRegression()\nmodel = lm.fit(encs[:ntrain],ins[:ntrain])\n \n\n#now evaluate the results with those numbers\nprint (model.score(encs[ntrain:], ins[ntrain:]))\n \nplt.plot(range(n_vectors), model.predict(encs));",
"0.999970693261\n"
],
[
"decoders = []\n\nntrain = 1750\n\nlm = linear_model.LinearRegression()\nmodel = lm.fit(encs[:ntrain],ins[:ntrain])\n \n\n#now evaluate the results with those numbers\nprint (model.score(encs[ntrain:], ins[ntrain:]))\n \nplt.plot(range(n_vectors), model.predict(encs));",
"0.999972795578\n"
],
[
"decoders = []\n\nntrain = 2000\n\nlm = linear_model.LinearRegression()\nmodel = lm.fit(encs[:ntrain],ins[:ntrain])\n \n\n#now evaluate the results with those numbers\nprint (model.score(encs[ntrain:], ins[ntrain:]))\n \nplt.plot(range(n_vectors), model.predict(encs));",
"0.999947133064\n"
],
[
"decoders = []\n\nntrain = 3000\n\nlm = linear_model.LinearRegression()\nmodel = lm.fit(encs[:ntrain],ins[:ntrain])\n \n\n#now evaluate the results with those numbers\nprint (model.score(encs[ntrain:], ins[ntrain:]))\n \nplt.plot(range(n_vectors), model.predict(encs));",
"1.0\n"
],
[
"decoders = []\n\nntrain = 4000\n\nlm = linear_model.LinearRegression()\nmodel = lm.fit(encs[:ntrain],ins[:ntrain])\n \n\n#now evaluate the results with those numbers\nprint (model.score(encs[ntrain:], ins[ntrain:]))\n \nplt.plot(range(n_vectors), model.predict(encs));",
"1.0\n"
],
[
"decoders = []\n\nntrain = 5000\n\nlm = linear_model.LinearRegression()\nmodel = lm.fit(encs[:ntrain],ins[:ntrain])\n \n\n#now evaluate the results with those numbers\nprint (model.score(encs[ntrain:], ins[ntrain:]))\n \nplt.plot(range(n_vectors), model.predict(encs));",
"0.999999995646\n"
],
[
"decoders = []\n\nntrain = 6000\n\nlm = linear_model.LinearRegression()\nmodel = lm.fit(encs[:ntrain],ins[:ntrain])\n \n\n#now evaluate the results with those numbers\nprint (model.score(encs[ntrain:], ins[ntrain:]))\n \nplt.plot(range(n_vectors), model.predict(encs));",
"0.999999998984\n"
],
[
"#and now I try with unlimited neuron output\n\ndecoders = []\n\nntrain = 500\n\nlm = linear_model.LinearRegression()\nmodel = lm.fit(encns[:ntrain],ins[:ntrain])\n \n\n#now evaluate the results with those numbers\nprint (model.score(encns[ntrain:], ins[ntrain:]))\n \nplt.plot(range(n_vectors), model.predict(encns));",
"0.162450117581\n"
],
[
"#and now I try with unlimited neuron output\n\ndecoders = []\n\nntrain = 2000\n\nlm = linear_model.LinearRegression()\nmodel = lm.fit(encns[:ntrain],ins[:ntrain])\n \n\n#now evaluate the results with those numbers\nprint (model.score(encns[ntrain:], ins[ntrain:]))\n \nplt.plot(range(n_vectors), model.predict(encns));",
"0.999980705409\n"
]
],
[
[
"Limited current neuron outperforms the non limited one, but the difference does not seems to be too much.\n\nThe main point of limiting the neuron output I think is to avoid divergence, I'd rather have more noise than divergence",
"_____no_output_____"
],
[
"## Important NOTE\n\nThe number of neurons in the ensemble, plus the number of training elements plays a huge rol in the results of the decoder",
"_____no_output_____"
],
[
"I'll try now with random points in higer dimension spaces",
"_____no_output_____"
]
],
[
[
"%%time\n\n#make ensembles for different dimensions, the ensambles will have 1000 neurons each\n#dimensions is the input dimension of the function\nensembles = {}\nrand_values = {}\n#dimensions = [10,20,50,100,1000, 5000, 10000]\ndimensions = [2, 3, 4, 10, 20, 100]\nnsamples=10000\nnneurons = [10,20,50,100,1000]\n\nfor d in dimensions:\n for ns in nneurons:\n if d not in ensembles.keys():\n ensembles[d] = {}\n if d not in rand_values.keys():\n rand_values[d] = {}\n ensembles[d][ns] = VectorEnsemble(d, ns)\n rand_values[d][ns] = np.random.rand(nsamples,d)\n\n",
"CPU times: user 120 ms, sys: 0 ns, total: 120 ms\nWall time: 116 ms\n"
],
[
"%%time\n\nencoded = {}\n\nfor d in dimensions:\n for ns in nneurons:\n if d not in encoded.keys():\n rnd = rand_values[d][ns]\n encoded[d] = {}\n ens = ensembles[d][ns]\n encoded[d][ns] = ens.encode_saturation(rnd)\n ",
"CPU times: user 3min 44s, sys: 1.33 s, total: 3min 45s\nWall time: 3min 43s\n"
],
[
"%%time\n#I'm curious about the \n#and now I do a grid search to check on the performances on random numbers for each of the \n\nmodels = []\ndecoded = []\nscores = []\n\n\nresults = []\n\nntrain = [50, 100, 200, 300, 400, 500, 750, 1000, 1500, 2000, 2500, 3000, 4000, 5000, 7000]\n\n\nfor n in ntrain:\n for d in dimensions:\n for ns in nneurons:\n encs = encoded[d][ns].copy()\n ins = rand_values[d][ns].copy()\n lm = linear_model.LinearRegression()\n model = lm.fit(encs[:n].copy(),ins[:n])\n s = model.score(encs[n:], ins[n:])\n dec = model.predict(encs[n:])\n models.append([n, d, ns, model])\n scores.append([n, d, ns, s])\n decoded.append([n, d, ns, dec])\n",
"CPU times: user 2min 12s, sys: 1min 56s, total: 4min 8s\nWall time: 32.5 s\n"
],
[
"scoresdf = pd.DataFrame(scores)",
"_____no_output_____"
],
[
"scoresdf.describe()",
"_____no_output_____"
]
],
[
[
"I am not sure how to interpret this just yet, I'll have to double check on the results and try to make some sens of it.\n\nIt seems that for certain values, the regression of RANDOM values is really great, but for the others, is really bad. This seems to be related to the number of dimensions .... 2 dimensions is great but more seems bad?\n\nI don get the difference of results with the previous graphs ...\n\n- Is it related with the number of neurons?\n- Is it related to the number of training samples?\n- Is it some other thing that I don't understand?\n\n\n",
"_____no_output_____"
]
],
[
[
"scoresdf[abs(scoresdf[3]) <= 1][abs(scoresdf[3]) >= 0.5]\n",
"/home/leo/DeepLearning/venv3/lib/python3.5/site-packages/ipykernel_launcher.py:1: UserWarning: Boolean Series key will be reindexed to match DataFrame index.\n \"\"\"Entry point for launching an IPython kernel.\n"
]
],
[
[
"It seems that the random values do not give too much meaning .... there is something to see here then.\n\nIt might be able to decode functions, but random points dont seem to make sense ... ?\n\nI have to dig that more in depth ...\n\nAlso, it seems that the higher the dimension the more difficult is to find a nice decoder",
"_____no_output_____"
]
]
] | [
"code",
"markdown",
"code",
"markdown",
"code",
"markdown",
"code",
"markdown",
"code",
"markdown",
"code",
"markdown"
] | [
[
"code",
"code",
"code"
],
[
"markdown",
"markdown",
"markdown"
],
[
"code",
"code"
],
[
"markdown"
],
[
"code",
"code",
"code",
"code",
"code",
"code",
"code",
"code",
"code",
"code"
],
[
"markdown"
],
[
"code",
"code",
"code",
"code",
"code",
"code",
"code",
"code",
"code",
"code",
"code",
"code",
"code",
"code"
],
[
"markdown",
"markdown",
"markdown"
],
[
"code",
"code",
"code",
"code",
"code"
],
[
"markdown"
],
[
"code"
],
[
"markdown"
]
] |
e765dbd451e520eaedd70fc17dc1cc36925a0979 | 464,345 | ipynb | Jupyter Notebook | Lecture34- seaborn.ipynb | dajebbar/AI-Programming-with-python | 11ec5638513f1cb4f7a3f45b61494e863033d614 | [
"MIT"
] | null | null | null | Lecture34- seaborn.ipynb | dajebbar/AI-Programming-with-python | 11ec5638513f1cb4f7a3f45b61494e863033d614 | [
"MIT"
] | null | null | null | Lecture34- seaborn.ipynb | dajebbar/AI-Programming-with-python | 11ec5638513f1cb4f7a3f45b61494e863033d614 | [
"MIT"
] | null | null | null | 413.118327 | 109,796 | 0.9321 | [
[
[
"from IPython.display import YouTubeVideo, Image\nimport warnings\nwarnings.filterwarnings('ignore')",
"_____no_output_____"
],
[
"YouTubeVideo(id='TLdXM0A7SR8', width=900, height=400)",
"_____no_output_____"
]
],
[
[
"---",
"_____no_output_____"
],
[
"# `seaborn`",
"_____no_output_____"
]
],
[
[
"# The normal imports\nimport numpy as np\nfrom numpy.random import randn\nimport pandas as pd\n\n# Import the stats library from numpy\nfrom scipy import stats\n\n# These are the plotting modules adn libraries we'll use:\nimport matplotlib as mpl\nimport matplotlib.pyplot as plt\nimport seaborn as sns\n\n# Declare a pre-difinerd style\n# you can define your own style\nplt.style.use('fivethirtyeight')\n\n# Useful for plots to display in cells\n%matplotlib inline",
"_____no_output_____"
]
],
[
[
"## What is seaborn?",
"_____no_output_____"
],
[
"[Seaborn](https://seaborn.pydata.org/) is a library specially built for data visualization in python. It is like the plotting functions of pandas built on top of matplotlib. It has a lot of nice features for easy visualization and styling.",
"_____no_output_____"
],
[
"## What is the difference between categorical, ordinal and numerical variables?",
"_____no_output_____"
],
[
"### Categorical",
"_____no_output_____"
],
[
"A categorical variable (sometimes called a nominal variable) is one that has two or more categories, but there is no intrinsic ordering to the categories. For example, gender is a categorical variable having two categories (male and female) and there is no intrinsic ordering to the categories. Hair color is also a categorical variable having a number of categories (blonde, brown, brunette, red, etc.) and again, there is no agreed way to order these from highest to lowest. A purely categorical variable is one that simply allows you to assign categories but you cannot clearly order the categories. If the variable has a clear ordering, then that variable would be an ordinal variable, as described below.",
"_____no_output_____"
],
[
"### Ordinal",
"_____no_output_____"
],
[
"An ordinal variable is similar to a categorical variable. The difference between the two is that there is a clear ordering of the categories. For example, suppose you have a variable, economic status, with three categories (low, medium and high). In addition to being able to classify people into these three categories, you can order the categories as low, medium and high. Now consider a variable like educational experience (with values such as elementary school graduate, high school graduate, some college and college graduate). These also can be ordered as elementary school, high school, some college, and college graduate. Even though we can order these from lowest to highest, the spacing between the values may not be the same across the levels of the variables. Say we assign scores 1, 2, 3 and 4 to these four levels of educational experience and we compare the difference in education between categories one and two with the difference in educational experience between categories two and three, or the difference between categories three and four. The difference between categories one and two (elementary and high school) is probably much bigger than the difference between categories two and three (high school and some college). In this example, we can order the people in level of educational experience but the size of the difference between categories is inconsistent (because the spacing between categories one and two is bigger than categories two and three). If these categories were equally spaced, then the variable would be an numerical variable.",
"_____no_output_____"
],
[
"### Numerical",
"_____no_output_____"
],
[
"An numerical variable is similar to an ordinal variable, except that the intervals between the values of the numerical variable are equally spaced. For example, suppose you have a variable such as annual income that is measured in dollars, and we have three people who make (10,000), (15,000) and (20,000). The second person makes (5,000) more than the first person and (5,000) less than the third person, and the size of these intervals is the same. If there were two other people who make (90,000) and (95,000), the size of that interval between these two people is also the same (5,000).",
"_____no_output_____"
],
[
"---",
"_____no_output_____"
],
[
"# Applying the seaborn visualization to a movies rating dataset",
"_____no_output_____"
],
[
"### Reading dataset",
"_____no_output_____"
]
],
[
[
"movie = pd.read_csv('movie_raitings.csv')\nmovie.head()",
"_____no_output_____"
],
[
"# let's check the columns name\nmovie.columns",
"_____no_output_____"
],
[
"# We see that there are column names with spaces, \n# which can be tedious when calling these names.\n# let's change them\nmovie.columns = ['film', 'genre', 'critic_rating',\\\n 'audience_rating', 'budget', 'year']\nmovie.head()",
"_____no_output_____"
],
[
"# let's explore movie dataframe and \n# see the types of each column\nmovie.info()",
"<class 'pandas.core.frame.DataFrame'>\nRangeIndex: 559 entries, 0 to 558\nData columns (total 6 columns):\n # Column Non-Null Count Dtype \n--- ------ -------------- ----- \n 0 film 559 non-null object\n 1 genre 559 non-null object\n 2 critic_rating 559 non-null int64 \n 3 audience_rating 559 non-null int64 \n 4 budget 559 non-null int64 \n 5 year 559 non-null int64 \ndtypes: int64(4), object(2)\nmemory usage: 26.3+ KB\n"
],
[
"# we can see that film and genre \n#are of type object whereas they are of type category\nmovie.loc[:,'genre'].unique()",
"_____no_output_____"
],
[
"movie.loc[:,'genre'].nunique()",
"_____no_output_____"
],
[
"# for example genre contains 7 different categories\n# also we see that the column year is of type int64 \n# whereas it should be of type category\nmovie.describe()",
"_____no_output_____"
],
[
"# there is no point in the year variable\n# having an average and quantiles\n# let's make a change:\n\nmovie.loc[:, 'film'] = movie.loc[:,'film'].astype('category')\nmovie.loc[:, 'genre'] = movie.loc[:, 'genre'].astype('category')\nmovie.loc[:, 'year'] = movie.loc[:,'year'].astype('category')\n\n# we can proceed as follows but it is not recommended\n# movie.film = movie.film.astype('category')",
"_____no_output_____"
],
[
"movie.info()",
"<class 'pandas.core.frame.DataFrame'>\nRangeIndex: 559 entries, 0 to 558\nData columns (total 6 columns):\n # Column Non-Null Count Dtype \n--- ------ -------------- ----- \n 0 film 559 non-null category\n 1 genre 559 non-null category\n 2 critic_rating 559 non-null int64 \n 3 audience_rating 559 non-null int64 \n 4 budget 559 non-null int64 \n 5 year 559 non-null category\ndtypes: category(3), int64(3)\nmemory usage: 40.3 KB\n"
],
[
"movie.describe()",
"_____no_output_____"
]
],
[
[
"---",
"_____no_output_____"
],
[
"### `countplot`",
"_____no_output_____"
],
[
"A count plot can be thought of as a histogram across a categorical, instead of quantitative, variable. The basic API and options are identical to those for `barplot()`, so you can compare counts across nested variables.",
"_____no_output_____"
]
],
[
[
"ax = sns.countplot(movie.loc[:, 'genre'])",
"_____no_output_____"
]
],
[
[
"### `jointplot`",
"_____no_output_____"
],
[
"Draw a plot of two variables with bivariate and univariate graphs.",
"_____no_output_____"
]
],
[
[
"j = sns.jointplot(data=movie, x='critic_rating', y='audience_rating')",
"_____no_output_____"
],
[
"# we can change the style of the joint\nj = sns.jointplot(data=movie, x='critic_rating', y='audience_rating', kind='hex')",
"_____no_output_____"
]
],
[
[
"### `Histograms`",
"_____no_output_____"
]
],
[
[
"sns.distplot(movie.loc[:,'audience_rating'], bins=15, label='audience_rating')\nsns.distplot(movie.loc[:,'critic_rating'], bins=15, label='critic_rating')\nplt.legend()\nplt.show()",
"_____no_output_____"
]
],
[
[
"#### Stacked histograms",
"_____no_output_____"
]
],
[
[
"# Let's compare the income of the movies by genre\nlst = []\nlabels = []\nfor genre in movie.loc[:, 'genre'].unique():\n lst.append(movie.loc[movie.loc[:,'genre']==genre, 'budget'])\n labels.append(genre)\nplt.figure(figsize=(10,8))\nplt.hist(lst, bins=50, stacked=True, rwidth=1, label=labels)\nplt.legend()\nplt.show()",
"_____no_output_____"
]
],
[
[
"### `kdeplot`",
"_____no_output_____"
],
[
"A kernel density estimate (KDE) plot is a method for visualizing the distribution of observations in a dataset, analagous to a histogram. KDE represents the data using a continuous probability density curve in one or more dimensions.",
"_____no_output_____"
]
],
[
[
"sns.kdeplot(movie.loc[:,'critic_rating'], movie.loc[:, 'audience_rating'])",
"_____no_output_____"
]
],
[
[
"### `violinplot`",
"_____no_output_____"
]
],
[
[
"comedy = movie.loc[movie.loc[:,'genre']=='Comedy',:]\nsns.violinplot(data=comedy, x='year', y='critic_rating')",
"_____no_output_____"
]
],
[
[
"### Creating a `FacetGrid`",
"_____no_output_____"
]
],
[
[
"sns.FacetGrid(movie, row='genre', col='year', hue='genre')\\\n.map(plt.scatter, 'critic_rating','audience_rating')",
"_____no_output_____"
],
[
"# can populate with any type of chart. Example: histograms\nsns.FacetGrid(movie, row='genre', col='year', hue='genre')\\\n.map(plt.hist, 'budget')",
"_____no_output_____"
]
]
] | [
"code",
"markdown",
"code",
"markdown",
"code",
"markdown",
"code",
"markdown",
"code",
"markdown",
"code",
"markdown",
"code",
"markdown",
"code",
"markdown",
"code",
"markdown",
"code"
] | [
[
"code",
"code"
],
[
"markdown",
"markdown"
],
[
"code"
],
[
"markdown",
"markdown",
"markdown",
"markdown",
"markdown",
"markdown",
"markdown",
"markdown",
"markdown",
"markdown",
"markdown",
"markdown"
],
[
"code",
"code",
"code",
"code",
"code",
"code",
"code",
"code",
"code",
"code"
],
[
"markdown",
"markdown",
"markdown"
],
[
"code"
],
[
"markdown",
"markdown"
],
[
"code",
"code"
],
[
"markdown"
],
[
"code"
],
[
"markdown"
],
[
"code"
],
[
"markdown",
"markdown"
],
[
"code"
],
[
"markdown"
],
[
"code"
],
[
"markdown"
],
[
"code",
"code"
]
] |
e765e2fd8a926361f3fb11c9ee3b7028861fb6df | 22,405 | ipynb | Jupyter Notebook | notebooks/json_to_docx_v1.ipynb | JainyJoy/document-formatting | 5510e3553c17b3f6207d1f018829830f2140c474 | [
"MIT"
] | null | null | null | notebooks/json_to_docx_v1.ipynb | JainyJoy/document-formatting | 5510e3553c17b3f6207d1f018829830f2140c474 | [
"MIT"
] | null | null | null | notebooks/json_to_docx_v1.ipynb | JainyJoy/document-formatting | 5510e3553c17b3f6207d1f018829830f2140c474 | [
"MIT"
] | null | null | null | 33.793363 | 120 | 0.39634 | [
[
[
"import os\nimport sys\nimport time\nimport base64\nimport uuid\nimport json\nimport codecs\nimport pandas as pd\n",
"_____no_output_____"
],
[
"base_dir = os.path.dirname(os.getcwd())\ninput_dir = os.path.join(base_dir, 'data', 'input')\noutput_dir = os.path.join(base_dir, 'data', 'output')\nfilename = 'judgement.json'\n\ninput_filepath = os.path.join(input_dir, filename)\noutput_filepath = os.path.join(output_dir, os.path.splitext(os.path.basename(filename))[0]+'.docx')\n",
"_____no_output_____"
],
[
"def get_pages(filepath):\n data = json.load(codecs.open(filepath, 'r', 'utf-8-sig'))\n pages = data['data']\n return pages",
"_____no_output_____"
],
[
"pages = get_pages(input_filepath) \nprint('document has %d pages' % (len(pages)))",
"document has 54 pages\n"
],
[
"dfs = []\npage_width = None\npage_height = None\n\nfor page in pages:\n text_tops = []\n text_lefts = []\n text_widths = []\n text_heights = []\n font_sizes = []\n font_families = []\n font_colors = []\n text_values = []\n b64_images = []\n\n images = page['images']\n texts = page['text_blocks']\n page_num = page['page_no']\n page_width = page['page_width']\n page_height = page['page_height']\n \n for text in texts:\n text_tops.append(text['text_top'])\n text_lefts.append(text['text_left'])\n text_widths.append(text['text_width'])\n text_heights.append(text['text_height'])\n font_sizes.append(text['font_size'])\n font_families.append(text['font_family'])\n font_colors.append(text['font_color'])\n b64_images.append(None)\n \n text_value = []\n for processed_text in text['tokenized_sentences']:\n text_value.append(processed_text['src_text']) \n text_values.append(' '.join(text_value))\n \n for image in images:\n text_tops.append(image['text_top'])\n text_lefts.append(image['text_left'])\n text_widths.append(image['text_width'])\n text_heights.append(image['text_height'])\n b64_images.append(image['base64'])\n text_values.append(None)\n font_sizes.append(None)\n font_families.append(None)\n font_colors.append(None)\n \n df = pd.DataFrame(list(zip(text_tops, text_lefts, text_widths, text_heights,\n text_values, font_sizes, font_families, font_colors, b64_images)), \n columns =['text_top', 'text_left', 'text_width', 'text_height',\n 'text', 'font_size', 'font_family', 'font_color', 'base64'])\n df.sort_values('text_top', axis = 0, ascending = True, inplace=True) \n dfs.append(df)\n",
"_____no_output_____"
],
[
"from docx import Document\nfrom docx.shared import Pt\nfrom docx.shared import Twips, Cm\nfrom docx.enum.text import WD_ALIGN_PARAGRAPH, WD_BREAK\nfrom docx.enum.section import WD_SECTION, WD_ORIENT\nfrom docx.shared import Length\n\n\ndef get_pixel_twips(pixels):\n PIXEL_TO_TWIPS = 14.999903622654\n return int(PIXEL_TO_TWIPS * pixels)\n\ndef get_font_point(pixels):\n return pixels * 0.75\n\ndef get_cms(pixels):\n PPI = 108\n INCH_TO_CM = 2.54\n PIXEL_PER_CM = PPI / 2.54\n \n return pixels / PIXEL_PER_CM\n\ndef get_path_from_base64(work_dir, b64_data):\n filepath = os.path.join(work_dir, str(uuid.uuid4().hex) + '.jpg')\n with open(filepath, 'wb') as file:\n file.write(base64.b64decode(b64_data))\n return filepath\n\ndef pixel_to_twips(px, dpi=108):\n INCH_TO_TWIPS = 1440\n px_to_inches = 1.0 / float(dpi)\n return int(px * px_to_inches * INCH_TO_TWIPS)",
"_____no_output_____"
],
[
"df_index = 0\ndf = dfs[df_index]\ndf",
"_____no_output_____"
],
[
"document = Document()\nsection = document.sections[-1]\nsection.left_margin = Cm(1.27)\nsection.right_margin = Cm(1.27)\nsection.top_margin = Cm(1.27)\nsection.bottom_margin = Cm(1.27)\n\nrow = df.iloc[1]\n# empty p at start of page\np1 = document.add_paragraph()\np1_format = p1.paragraph_format\np1_format.line_spacing = Pt(18)\n\n# text p\np2 = document.add_paragraph()\np2_format = p2.paragraph_format\np2_format.left_indent = Twips(pixel_to_twips(row['text_left']))\n\nrun = p2.add_run()\nfont = run.font\nfont.name = 'Arial'\nfont.size = Twips(pixel_to_twips(row['font_size']))\nrun.add_text(row['text'])\n\n# next text on the same page\nrow = df.iloc[2]\n\np3 = document.add_paragraph()\np3_format = p3.paragraph_format\np3_format.left_indent = Cm(get_cms(row['text_left']))\n\nrun = p3.add_run()\nfont = run.font\nfont.name = 'Arial'\nfont.size = Twips(pixel_to_twips(row['font_size']))\nrun.add_text(row['text'])\n\n# next text on the same page\nrow = df.iloc[3]\n\np3 = document.add_paragraph()\np3_format = p3.paragraph_format\np3_format.left_indent = Twips(pixel_to_twips(row['text_left']))\np4_format.space_before = Twips(pixel_to_twips(row['text_top'] - df.iloc[2]['text_top']) )\n\n\nrun = p3.add_run()\nfont = run.font\nfont.name = 'Arial'\nfont.size = Twips(pixel_to_twips(row['font_size']))\nrun.add_text(row['text'])\n\n# next text on the same page\nrow = df.iloc[4]\np4 = document.add_paragraph()\np4_format = p4.paragraph_format\np4_format.left_indent = Twips(pixel_to_twips(row['text_left']))\np4_format.space_before = Twips(pixel_to_twips(row['text_top'] - df.iloc[3]['text_top']) )\n\nrun = p4.add_run()\nfont = run.font\nfont.name = 'Arial'\nfont.size = Twips(pixel_to_twips(row['font_size']))\nrun.add_text(row['text'])\n\n\ndocument.save(output_filepath)",
"_____no_output_____"
],
[
"page_width, page_height, get_pixel_twips(page_width)",
"_____no_output_____"
],
[
"width_dpi = 892\nheight_dpi = 1263\n\n",
"_____no_output_____"
],
[
"dpi = 108\npixel_to_twips(width_dpi, dpi), pixel_to_twips(height_dpi, dpi) \n",
"_____no_output_____"
],
[
"document = Document()\n\nfor index, df in enumerate(dfs[:1]):\n section = document.sections[-1]\n section.orientation = WD_ORIENT.PORTRAIT\n section.page_width = Cm(get_cms(page_width))\n section.page_height = Cm(get_cms(page_height))\n\n section.left_margin = Cm(1.27)\n section.right_margin = Cm(1.27)\n section.top_margin = Cm(1.27)\n section.bottom_margin = Cm(1.27)\n\n for index, row in df.iterrows():\n if row['text'] == None and row['base64'] != None:\n pass\n# image_path = get_path_from_base64(output_dir, row['base64'])\n# paragraph = document.add_paragraph()\n# run = paragraph.add_run()\n# run.add_drawing(image_path, width=Cm(get_cms(row['text_width'])), \n# height=Cm(get_cms(row['text_height'])))\n# os.remove(image_path)\n else:\n paragraph = document.add_paragraph()\n\n paragraph_format = paragraph.paragraph_format\n paragraph_format.left_indent = Cm(get_cms(row['text_left']))\n\n run = paragraph.add_run()\n font = run.font\n font.name = 'Arial'\n font.size = Cm(get_cms(row['font_size']))\n run.add_text(row['text'])\n \n paragraph = document.add_paragraph()\n run = paragraph.add_run()\n run.add_break(WD_BREAK.PAGE)\n \n \ndocument.save(output_filepath)",
"_____no_output_____"
]
]
] | [
"code"
] | [
[
"code",
"code",
"code",
"code",
"code",
"code",
"code",
"code",
"code",
"code",
"code",
"code"
]
] |
e76608a99ba8cb0c08a940a364ab0a2d0c7c0a04 | 473,688 | ipynb | Jupyter Notebook | DataAnalysis_Desafio_mod02.ipynb | vinicius-mattoso/Wine_classifier | cf210490d0cfe7ae09da6dbe3108992724d7b5ba | [
"MIT"
] | null | null | null | DataAnalysis_Desafio_mod02.ipynb | vinicius-mattoso/Wine_classifier | cf210490d0cfe7ae09da6dbe3108992724d7b5ba | [
"MIT"
] | null | null | null | DataAnalysis_Desafio_mod02.ipynb | vinicius-mattoso/Wine_classifier | cf210490d0cfe7ae09da6dbe3108992724d7b5ba | [
"MIT"
] | null | null | null | 245.180124 | 110,720 | 0.896453 | [
[
[
"# Análise exploratória da base de dados \"Winequality-red.csv\" do Módulo 02 do IGIT BOOTCAMP",
"_____no_output_____"
]
],
[
[
"#importando as bibliotecas\nimport pandas as pd #biblioteca para utilizar os dataframes\nimport numpy as np #biblioteca para trabalhar de forma otimizada com matrizes e vetores\nimport matplotlib.pylab as plt #biblioteca para a construção de gráficos\nimport seaborn as sn #biblioteca para gráficos mais \"bonitos\"",
"_____no_output_____"
],
[
"#criando o nosso dataframe a partir dos dados\ndf_vinhos=pd.read_csv(\"winequality-red.csv\", sep=';')",
"_____no_output_____"
],
[
"#mostrando o dataset dos vinhos\ndf_vinhos.head()",
"_____no_output_____"
]
],
[
[
"**Quantas instâncias e atributos possuem o dataset?**",
"_____no_output_____"
]
],
[
[
"instancias,atributos = df_vinhos.shape",
"_____no_output_____"
],
[
"print(\"O dataset possue {} instâncias e {} atributos\".format(instancias,atributos))",
"O dataset possue 1599 instâncias e 12 atributos\n"
]
],
[
[
"**Existem valores nulos?**",
"_____no_output_____"
]
],
[
[
"df_vinhos.info()",
"<class 'pandas.core.frame.DataFrame'>\nRangeIndex: 1599 entries, 0 to 1598\nData columns (total 12 columns):\n # Column Non-Null Count Dtype \n--- ------ -------------- ----- \n 0 fixed acidity 1599 non-null float64\n 1 volatile acidity 1599 non-null float64\n 2 citric acid 1599 non-null float64\n 3 residual sugar 1599 non-null float64\n 4 chlorides 1599 non-null float64\n 5 free sulfur dioxide 1599 non-null float64\n 6 total sulfur dioxide 1599 non-null float64\n 7 density 1599 non-null float64\n 8 pH 1599 non-null float64\n 9 sulphates 1599 non-null float64\n 10 alcohol 1599 non-null float64\n 11 quality 1599 non-null int64 \ndtypes: float64(11), int64(1)\nmemory usage: 150.0 KB\n"
],
[
"#contando os valores\ndf_vinhos.isnull().sum() ",
"_____no_output_____"
],
[
"print(\"Existem {} atributos do tipo {} e {} atributos do tipo {}.\".format(df_vinhos.dtypes.value_counts()[0],df_vinhos.dtypes.value_counts().index[0],df_vinhos.dtypes.value_counts()[1], df_vinhos.dtypes.value_counts().index[1]))",
"Existem 11 atributos do tipo float64 e 1 atributos do tipo int64.\n"
],
[
"#aplicando as \"estatísticas\" para o dataset\ndf_vinhos.describe()",
"_____no_output_____"
],
[
"#encontrando a mediana\ndf_vinhos.median()",
"_____no_output_____"
]
],
[
[
"**Aplicando a matriz de correlação**",
"_____no_output_____"
]
],
[
[
"#matriz de correlação não gráfica\ndf_vinhos.corr()",
"_____no_output_____"
]
],
[
[
"### Observando o heatmap de correlação abaixo que a qualidade do vinho tem grande correlação com a quantidade de alcool, os sufactantes, a volatilidade da acidez, a acidez crítica",
"_____no_output_____"
]
],
[
[
"#matriz de correlação plotada\nplt.figure(figsize=(12,9))\nmatriz_correlacao=df_vinhos.corr()\nsn.heatmap(matriz_correlacao, annot=True)\nplt.show()",
"_____no_output_____"
]
],
[
[
"## Agora vamos fazer uma série de plot para melhor visualizar a correlação entre as variaveis",
"_____no_output_____"
]
],
[
[
"%matplotlib inline\nfrom matplotlib import style\nstyle.use(\"seaborn-colorblind\")\n#df_vinhos.plot(x='pH', y='alcohol', c='quality', kind='scatter' , colormap='Reds')\nplt.plot(x=df_vinhos['pH'], y=df_vinhos['alcohol'], c=df_vinhos['quality'], kind='scatter' , colormap='Reds')",
"_____no_output_____"
]
],
[
[
"### Podemos observar que existe um correlação inversa entre a densidade do vinho e a quantidade de alcool nele",
"_____no_output_____"
]
],
[
[
"fig, ax = plt.subplots()\nplt.scatter(df_vinhos['alcohol'], df_vinhos['density'],marker='.')\n\nplt.grid(True, linestyle='-.')\nplt.tick_params(labelcolor='r', labelsize='medium', width=3)\nplt.xlabel(\"alcohol\")\nplt.ylabel(\"density\")\nplt.show()",
"_____no_output_____"
]
],
[
[
"### Podemos observar que existe um correlação entre os sufactantes e \"chlorides\", vemos que temos uma grande concentração de \"Chlorides\" em 0.1",
"_____no_output_____"
]
],
[
[
"fig, ax = plt.subplots()\nplt.scatter(df_vinhos['sulphates'], df_vinhos['chlorides'],marker='.')\n\nplt.grid(True, linestyle='-.')\nplt.tick_params(labelcolor='r', labelsize='medium', width=3)\nplt.xlabel(\"sulphates\")\nplt.ylabel(\"chlorides\")\nplt.show()",
"_____no_output_____"
]
],
[
[
"### Podemos observar que existe uma correlação negativa entre a acidez crítica e a \"Volatilie acidity\"",
"_____no_output_____"
]
],
[
[
"fig, ax = plt.subplots()\nplt.scatter(df_vinhos['volatile acidity'], df_vinhos['citric acid'],marker='.')\n\nplt.grid(True, linestyle='-.')\nplt.tick_params(labelcolor='r', labelsize='medium', width=3)\nplt.xlabel(\"volatile acidity\")\nplt.ylabel(\"citric acid\")\nplt.show()",
"_____no_output_____"
]
],
[
[
"## Separação em Features (Entradas) e Target (Saída)",
"_____no_output_____"
]
],
[
[
"#dividindo o dataset entre entrada e saída\nentradas=df_vinhos.iloc[:,:-1] #seleciona todas as colunas menor a última\nsaida=df_vinhos.iloc[:,-1] #seleciona apenas a coluna de qualidade do vinho ",
"_____no_output_____"
],
[
"entradas.head(5)",
"_____no_output_____"
],
[
"saida.head(5)",
"_____no_output_____"
]
],
[
[
"**Quantas instâncias existem para a qualidade do vinho igual a 5?**",
"_____no_output_____"
]
],
[
[
"#identificando as instâncias existentes para os dados\ndf_vinhos['quality'].value_counts()",
"_____no_output_____"
],
[
"df_vinhos['quality'].unique()",
"_____no_output_____"
]
],
[
[
"## Criando uma nova base de dados, na qual a classificação é binária onde vinhos com qualidade superior a 5 é considerado bom (1) ",
"_____no_output_____"
]
],
[
[
"#modificando o dataset\nnew_df=df_vinhos.copy()\nnew_df['nova_qualidade']=new_df['quality'].apply(lambda x: 0 if x<=5 else 1)",
"_____no_output_____"
],
[
"new_df.tail()",
"_____no_output_____"
],
[
"# Como criamos um novo target, podemos retirar o quality anterior\nnew_df.drop('quality', axis=1, inplace=True)",
"_____no_output_____"
]
],
[
[
"### Após a criação do novo target, podemos observar que o alcool, \"volatile acidity\", \"sulphates\" e \"total sufur dioxide\"\n\n#### Podemos observar que nessa nova base houve uma mudança de importancia entre \"citric acid\" para o \"total sufur dioxide\"",
"_____no_output_____"
]
],
[
[
"#matriz de correlação plotada da nova base de dados\nplt.figure(figsize=(12,9))\nmatriz_correlacao=new_df.corr()\nsn.heatmap(matriz_correlacao, annot=True)\nplt.show()",
"_____no_output_____"
]
],
[
[
"### Podemos observar que existe uma correlação positiva entre a quantidade total de ácido sufúrico e a quantidade de ácido livre, ou seja, quanto maior a quantidade de ácido maior a quantidade de ácido livre",
"_____no_output_____"
]
],
[
[
"fig, ax = plt.subplots()\nplt.scatter(df_vinhos['total sulfur dioxide'], df_vinhos['free sulfur dioxide'],marker='.')\n\nplt.grid(True, linestyle='-.')\nplt.tick_params(labelcolor='r', labelsize='medium', width=3)\nplt.xlabel(\"total sulfur dioxide\")\nplt.ylabel(\"free sulfur dioxide\")\nplt.show()",
"_____no_output_____"
]
]
] | [
"markdown",
"code",
"markdown",
"code",
"markdown",
"code",
"markdown",
"code",
"markdown",
"code",
"markdown",
"code",
"markdown",
"code",
"markdown",
"code",
"markdown",
"code",
"markdown",
"code",
"markdown",
"code",
"markdown",
"code",
"markdown",
"code",
"markdown",
"code"
] | [
[
"markdown"
],
[
"code",
"code",
"code"
],
[
"markdown"
],
[
"code",
"code"
],
[
"markdown"
],
[
"code",
"code",
"code",
"code",
"code"
],
[
"markdown"
],
[
"code"
],
[
"markdown"
],
[
"code"
],
[
"markdown"
],
[
"code"
],
[
"markdown"
],
[
"code"
],
[
"markdown"
],
[
"code"
],
[
"markdown"
],
[
"code"
],
[
"markdown"
],
[
"code",
"code",
"code"
],
[
"markdown"
],
[
"code",
"code"
],
[
"markdown"
],
[
"code",
"code",
"code"
],
[
"markdown"
],
[
"code"
],
[
"markdown"
],
[
"code"
]
] |
e7660cb72a7d83456e96ae407a4b730c0c567350 | 150,726 | ipynb | Jupyter Notebook | demo_plot/plotnine-examples/tutorials/miscellaneous-order-plot-series.ipynb | hermanzhaozzzz/DataScienceScripts | 39cca0b76e669d348dbbe3860c7177e62829aa99 | [
"MIT"
] | 86 | 2017-04-25T21:55:55.000Z | 2022-03-27T22:03:54.000Z | demo_plot/plotnine-examples/tutorials/miscellaneous-order-plot-series.ipynb | hermanzhaozzzz/DataScienceScripts | 39cca0b76e669d348dbbe3860c7177e62829aa99 | [
"MIT"
] | 15 | 2017-05-21T03:50:19.000Z | 2021-03-10T14:32:43.000Z | demo_plot/plotnine-examples/tutorials/miscellaneous-order-plot-series.ipynb | hermanzhaozzzz/DataScienceScripts | 39cca0b76e669d348dbbe3860c7177e62829aa99 | [
"MIT"
] | 34 | 2017-05-27T22:20:41.000Z | 2022-02-11T07:42:47.000Z | 459.530488 | 35,664 | 0.945437 | [
[
[
"# Custom sorting of plot series",
"_____no_output_____"
]
],
[
[
"import pandas as pd\nimport numpy as np\nfrom pandas.api.types import CategoricalDtype\nfrom plotnine import *\nfrom plotnine.data import mpg\n\n%matplotlib inline",
"_____no_output_____"
]
],
[
[
"## Bar plot of manufacturer - Default Output",
"_____no_output_____"
]
],
[
[
"(ggplot(mpg)\n + aes(x='manufacturer') \n + geom_bar(size=20)\n + coord_flip()\n + labs(y='Count', x='Manufacturer', title='Number of Cars by Make')\n)",
"_____no_output_____"
]
],
[
[
"## Bar plot of manufacturer - Ordered by count (Categorical)\n\nBy default the discrete values along axis are ordered alphabetically. If we want a\nspecific ordering\nwe use a pandas.Categorical variable with categories ordered to our \npreference.",
"_____no_output_____"
]
],
[
[
"# Determine order and create a categorical type\n# Note that value_counts() is already sorted\nmanufacturer_list = mpg['manufacturer'].value_counts().index.tolist()\nmanufacturer_cat = pd.Categorical(mpg['manufacturer'], categories=manufacturer_list)\n\n# assign to a new column in the DataFrame\nmpg = mpg.assign(manufacturer_cat = manufacturer_cat)\n\n(ggplot(mpg)\n + aes(x='manufacturer_cat')\n + geom_bar(size=20)\n + coord_flip()\n + labs(y='Count', x='Manufacturer', title='Number of Cars by Make')\n)",
"_____no_output_____"
]
],
[
[
"We could also modify the **existing manufacturer category** to set it as ordered\ninstead of having to create a new CategoricalDtype and apply that to the data.",
"_____no_output_____"
]
],
[
[
"mpg = mpg.assign(manufacturer_cat = \n mpg['manufacturer'].cat.reorder_categories(manufacturer_list))",
"_____no_output_____"
]
],
[
[
"## Bar plot of manufacturer - Ordered by count (limits)\n\nAnother method to quickly reorder a discrete axis without changing the data \nis to change it's limits",
"_____no_output_____"
]
],
[
[
"# Determine order and create a categorical type\n# Note that value_counts() is already sorted\nmanufacturer_list = mpg['manufacturer'].value_counts().index.tolist()\n\n(ggplot(mpg)\n + aes(x='manufacturer_cat')\n + geom_bar(size=20)\n + scale_x_discrete(limits=manufacturer_list)\n + coord_flip()\n + labs(y='Count', x='Manufacturer', title='Number of Cars by Make')\n)",
"_____no_output_____"
]
],
[
[
"You can 'flip' an axis (independent of limits) by reversing the order of the limits.",
"_____no_output_____"
]
],
[
[
"# Determine order and create a categorical type\n# Note that value_counts() is already sorted\nmanufacturer_list = mpg['manufacturer'].value_counts().index.tolist()[::-1]\n\n(ggplot(mpg)\n + aes(x='manufacturer_cat')\n + geom_bar(size=20)\n + scale_x_discrete(limits=manufacturer_list)\n + coord_flip()\n + labs(y='Count', x='Manufacturer', title='Number of Cars by Make')\n)",
"_____no_output_____"
]
],
[
[
"### Further Reading\n\n+ [Pandas documentation of how to use categorical data in practice](https://pandas.pydata.org/pandas-docs/stable/categorical.html)\n+ [Pandas API Reference for categorical](http://pandas.pydata.org/pandas-docs/stable/api.html#api-categorical)\n+ [Pandas documentation of pd.Categorical](http://pandas.pydata.org/pandas-docs/stable/generated/pandas.Categorical.html)",
"_____no_output_____"
]
]
] | [
"markdown",
"code",
"markdown",
"code",
"markdown",
"code",
"markdown",
"code",
"markdown",
"code",
"markdown",
"code",
"markdown"
] | [
[
"markdown"
],
[
"code"
],
[
"markdown"
],
[
"code"
],
[
"markdown"
],
[
"code"
],
[
"markdown"
],
[
"code"
],
[
"markdown"
],
[
"code"
],
[
"markdown"
],
[
"code"
],
[
"markdown"
]
] |
e76617f816688a803314913b38e7061a642ca7d9 | 308,490 | ipynb | Jupyter Notebook | Breast Cancer .ipynb | suvhradipghosh07/Breast-Cancer-prediction-using-Machine-Learning-Various-Algorithms | 895040b914273ef2b7bc8034f9166d5e4fb2abae | [
"MIT"
] | 7 | 2019-04-11T15:05:13.000Z | 2020-03-23T17:59:20.000Z | Breast Cancer .ipynb | helloai19/Breast-Cancer-prediction-using-Machine-Learning-Various-Algorithms | 895040b914273ef2b7bc8034f9166d5e4fb2abae | [
"MIT"
] | null | null | null | Breast Cancer .ipynb | helloai19/Breast-Cancer-prediction-using-Machine-Learning-Various-Algorithms | 895040b914273ef2b7bc8034f9166d5e4fb2abae | [
"MIT"
] | 3 | 2019-04-11T15:05:39.000Z | 2019-04-11T15:10:08.000Z | 207.597577 | 140,292 | 0.888259 | [
[
[
"# Breast Cancer Wisconsin (Diagnostic) Prediction\n*Predict whether the cancer is benign or malignant*\n\nFeatures are computed from a digitized image of a fine needle aspirate (FNA) of a breast mass. They describe characteristics of the cell nuclei present in the image. n the 3-dimensional space is that described in: [K. P. Bennett and O. L. Mangasarian: \"Robust Linear Programming Discrimination of Two Linearly Inseparable Sets\", Optimization Methods and Software 1, 1992, 23-34].\n\nThis database is also available through the UW CS ftp server: ftp ftp.cs.wisc.edu cd math-prog/cpo-dataset/machine-learn/WDBC/\n\nAlso can be found on UCI Machine Learning Repository: https://archive.ics.uci.edu/ml/datasets/Breast+Cancer+Wisconsin+%28Diagnostic%29\n\n**Attribute Information:**\n\n*1) ID number 2) Diagnosis (M = malignant, B = benign) 3-32)*\n\n**Ten real-valued features are computed for each cell nucleus:**\n\n*a) radius (mean of distances from center to points on the perimeter) b) texture (standard deviation of gray-scale values) c) perimeter d) area e) smoothness (local variation in radius lengths) f) compactness (perimeter^2 / area - 1.0) g) concavity (severity of concave portions of the contour) h) concave points (number of concave portions of the contour) i) symmetry j) fractal dimension (\"coastline approximation\" - 1)*\n\n*he mean, standard error and \"worst\" or largest (mean of the three largest values) of these features were computed for each image, resulting in 30 features. For instance, field 3 is Mean Radius, field 13 is Radius SE, field 23 is Worst Radius.*\n\nAll feature values are recoded with four significant digits.\n\nMissing attribute values: none\n\nClass distribution: 357 benign, 212 malignant",
"_____no_output_____"
]
],
[
[
"import numpy as np\nimport matplotlib.pyplot as plt\nimport pandas as pd\nimport seaborn as sns\nfrom sklearn.model_selection import train_test_split\nimport seaborn as sns # used for plot interactive graph. I like it most for plot\nfrom sklearn.linear_model import LogisticRegression # to apply the Logistic regression\nfrom sklearn.model_selection import train_test_split # to split the data into two parts\nfrom sklearn.cross_validation import KFold # use for cross validation\nfrom sklearn.model_selection import GridSearchCV# for tuning parameter\nfrom sklearn.ensemble import RandomForestClassifier # for random forest classifier\nfrom sklearn.naive_bayes import GaussianNB\nfrom sklearn.neighbors import KNeighborsClassifier\nfrom sklearn.tree import DecisionTreeClassifier\nfrom sklearn import svm # for Support Vector Machine\nfrom sklearn import metrics # for the check the error and accuracy of the model\n%matplotlib inline\nimport warnings\nwarnings.filterwarnings(\"ignore\")",
"_____no_output_____"
]
],
[
[
"# Data Cleaning",
"_____no_output_____"
]
],
[
[
"#df is dataframe and its self a variable & here i import the dataset into this variable\ndf=pd.read_csv(\"cancer.csv\")\n#it will show top 5 data rows\ndf.head()",
"_____no_output_____"
],
[
"#deleting the useless columns\ndf.drop(['id'], 1, inplace=True)\ndf.drop(['Unnamed: 32'], 1, inplace=True)",
"_____no_output_____"
],
[
"df.info()",
"<class 'pandas.core.frame.DataFrame'>\nRangeIndex: 569 entries, 0 to 568\nData columns (total 31 columns):\ndiagnosis 569 non-null object\nradius_mean 569 non-null float64\ntexture_mean 569 non-null float64\nperimeter_mean 569 non-null float64\narea_mean 569 non-null float64\nsmoothness_mean 569 non-null float64\ncompactness_mean 569 non-null float64\nconcavity_mean 569 non-null float64\nconcave points_mean 569 non-null float64\nsymmetry_mean 569 non-null float64\nfractal_dimension_mean 569 non-null float64\nradius_se 569 non-null float64\ntexture_se 569 non-null float64\nperimeter_se 569 non-null float64\narea_se 569 non-null float64\nsmoothness_se 569 non-null float64\ncompactness_se 569 non-null float64\nconcavity_se 569 non-null float64\nconcave points_se 569 non-null float64\nsymmetry_se 569 non-null float64\nfractal_dimension_se 569 non-null float64\nradius_worst 569 non-null float64\ntexture_worst 569 non-null float64\nperimeter_worst 569 non-null float64\narea_worst 569 non-null float64\nsmoothness_worst 569 non-null float64\ncompactness_worst 569 non-null float64\nconcavity_worst 569 non-null float64\nconcave points_worst 569 non-null float64\nsymmetry_worst 569 non-null float64\nfractal_dimension_worst 569 non-null float64\ndtypes: float64(30), object(1)\nmemory usage: 137.9+ KB\n"
],
[
"# lets now start with features_mean \n# now as ou know our diagnosis column is a object type so we can map it to integer value\ndf['diagnosis']=df['diagnosis'].map({'M':1,'B':0})",
"_____no_output_____"
],
[
"y=df['diagnosis']\ny.head()",
"_____no_output_____"
],
[
"# it will describe the all statistical function of our data\ndf.describe()",
"_____no_output_____"
]
],
[
[
"# Data Analysis",
"_____no_output_____"
]
],
[
[
"# plotting the diagonisis result \nsns.countplot(df['diagnosis'],label=\"Count\")",
"_____no_output_____"
],
[
"feat_mean= list(df.columns[1:11])\nfeat_se= list(df.columns[11:20])\nfeat_worst=list(df.columns[21:31])\n\ncorr = df[feat_mean].corr() # .corr is used for find corelation\nplt.figure(figsize=(14,14))\nsns.heatmap(corr, cbar = True, square = True, annot=True, fmt= '.2f',annot_kws={'size': 15},\n xticklabels= feat_mean, yticklabels= feat_mean,\n cmap= 'coolwarm') # for more on heatmap you can visit Link(http://seaborn.pydata.org/generated/seaborn.heatmap.html)",
"_____no_output_____"
],
[
"#Box Plot for the feature texture_mean\nsns.boxplot(x = 'diagnosis', y ='texture_mean', data = df)\nplt.show()\n\n#Box Plot for the feature perimeter_mean\nsns.boxplot(x = 'diagnosis',y = 'perimeter_mean', data = df)\nplt.show()\n\n#Box Plot for the feature smoothness_mean\nsns.boxplot(x = 'diagnosis', y = 'smoothness_mean', data = df)\nplt.show()\n\n#Box Plot for the feature compactness_mean\nsns.boxplot(x = 'diagnosis', y = 'compactness_mean', data = df)\nplt.show()\n\n#Box Plot for the feature symmetry_mean\nsns.boxplot(x = 'diagnosis', y = 'symmetry_mean', data = df)\nplt.show()",
"_____no_output_____"
],
[
"#Violin Plots for texture_mean\nsns.violinplot(x = 'diagnosis', y ='texture_mean', data = df, size = 8)\nplt.show()\n\n#Violin Plots for compactness_mean\nsns.violinplot(x = 'diagnosis', y = 'compactness_mean', data = df, size = 8)\nplt.show()",
"_____no_output_____"
],
[
"#Histogram of Axillary_Nodes\nsns.FacetGrid(df, hue = \"diagnosis\", size=5).map(sns.distplot, \"symmetry_worst\").add_legend();\nplt.show();",
"_____no_output_____"
],
[
"#taking the main parameters in a single variable \nmain_pred_var = ['texture_mean','perimeter_mean','smoothness_mean','compactness_mean','symmetry_mean']",
"_____no_output_____"
]
],
[
[
"# Spliting the Dataset into two part",
"_____no_output_____"
]
],
[
[
"# spliting the dataset into two part\ntrain_set,test_set=train_test_split(df, test_size=0.2)\n#printing the data shape\nprint(train_set.shape)\nprint(test_set.shape)",
"(455, 31)\n(114, 31)\n"
]
],
[
[
"**Training Set :**",
"_____no_output_____"
]
],
[
[
"x_train=train_set[main_pred_var]\ny_train=train_set.diagnosis",
"_____no_output_____"
],
[
"print(y_train.shape)\nprint(x_train.shape)",
"(455,)\n(455, 5)\n"
]
],
[
[
"**Test Set :**",
"_____no_output_____"
]
],
[
[
"x_test=test_set[main_pred_var]\ny_test=test_set.diagnosis\nprint(y_test.shape)\nprint(x_test.shape)",
"(114,)\n(114, 5)\n"
]
],
[
[
"<h1 align=\"center\">Various Algorithm</h1>\n<br>Now i will train this Breast cancer dataset using various Algorithm from scratch see how each of them behaves with respect to one another.\n\n<ul>\n <li>Random Forest</li>\n <li>SVM</li>\n</ul>",
"_____no_output_____"
],
[
"# RandomForst Algorithm ",
"_____no_output_____"
]
],
[
[
"#define the algorithm class into the algo_one variable\nalgo_one=RandomForestClassifier()\nalgo_one.fit(x_train,y_train)",
"_____no_output_____"
],
[
"#predicting the algorithm into the non trained dataset that is test set \nprediction = algo_one.predict(x_test)\nmetrics.accuracy_score(prediction,y_test)",
"_____no_output_____"
]
],
[
[
"# SupportVector Machine Algorithm (SVM)",
"_____no_output_____"
]
],
[
[
"algo_two=svm.SVC()\nalgo_two.fit(x_train,y_train)",
"_____no_output_____"
],
[
"#predicting the algorithm into the non trained dataset that is test set \nprediction = algo_two.predict(x_test)\nmetrics.accuracy_score(prediction,y_test)",
"_____no_output_____"
]
],
[
[
"# Decision Tree Classifier Algorithm",
"_____no_output_____"
]
],
[
[
"algo_three=DecisionTreeClassifier()\nalgo_three.fit(x_train,y_train)",
"_____no_output_____"
],
[
"#predicting the algorithm into the non trained dataset that is test set \nprediction = algo_three.predict(x_test)\nmetrics.accuracy_score(prediction,y_test)",
"_____no_output_____"
]
],
[
[
"# K-Nearest NeighborsClassifier Algorithm",
"_____no_output_____"
]
],
[
[
"algo_four=KNeighborsClassifier()\nalgo_four.fit(x_train,y_train)",
"_____no_output_____"
],
[
"#predicting the algorithm into the non trained dataset that is test set \nprediction = algo_four.predict(x_test)\nmetrics.accuracy_score(prediction,y_test)",
"_____no_output_____"
]
],
[
[
"# GaussianNB Algorithm",
"_____no_output_____"
]
],
[
[
"algo_five=GaussianNB()\nalgo_five.fit(x_train,y_train)",
"_____no_output_____"
],
[
"prediction = algo_five.predict(x_test)\nmetrics.accuracy_score(prediction,y_test)",
"_____no_output_____"
]
],
[
[
"<h1 align=\"center\">Tuning Parameters using grid search CV</h1>\n\nLets Start with Random Forest Classifier Tuning the parameters means using the best parameter for predict there are many parameters need to model a Machine learning Algorithm for RandomForestClassifier.",
"_____no_output_____"
]
],
[
[
"pred_var = ['radius_mean','perimeter_mean','area_mean','compactness_mean','concave points_mean']\n#creating new variable\nx_grid= df[pred_var]\ny_grid= df[\"diagnosis\"]",
"_____no_output_____"
],
[
"# lets Make a function for Grid Search CV\ndef Classification_model_gridsearchCV(model,param_grid,x_grid,y_grid):\n clf = GridSearchCV(model,param_grid,cv=10,scoring=\"accuracy\")\n # this is how we use grid serch CV we are giving our model\n # the we gave parameters those we want to tune\n # Cv is for cross validation\n # scoring means to score the classifier\n \n clf.fit(x_train,y_train)\n print(\"The best parameter found on development set is :\")\n # this will gie us our best parameter to use\n print(clf.best_params_)\n print(\"the bset estimator is \")\n print(clf.best_estimator_)\n print(\"The best score is \")\n # this is the best score that we can achieve using these parameters#\n print(clf.best_score_)",
"_____no_output_____"
],
[
"# you will understand these terms once you follow the link above\nparam_grid = {'max_features': ['auto', 'sqrt', 'log2'],\n 'min_samples_split': [2,3,4,5,6,7,8,9,10], \n 'min_samples_leaf':[2,3,4,5,6,7,8,9,10] }\n# here our gridasearchCV will take all combinations of these parameter and apply it to model \n# and then it will find the best parameter for model\nmodel= RandomForestClassifier()\nClassification_model_gridsearchCV(model,param_grid,x_grid,y_grid)",
"The best parameter found on development set is :\n{'max_features': 'log2', 'min_samples_leaf': 2, 'min_samples_split': 6}\nthe bset estimator is \nRandomForestClassifier(bootstrap=True, class_weight=None, criterion='gini',\n max_depth=None, max_features='log2', max_leaf_nodes=None,\n min_impurity_decrease=0.0, min_impurity_split=None,\n min_samples_leaf=2, min_samples_split=6,\n min_weight_fraction_leaf=0.0, n_estimators=10, n_jobs=1,\n oob_score=False, random_state=None, verbose=0,\n warm_start=False)\nThe best score is \n0.9384615384615385\n"
]
],
[
[
"# Observation\n<html>\n<body>\n <br>\n <b>Here are the results of our Five Algorithm observation</b> \n<table border=1>\n <tr>\n <th>Model</th>\n <th>Algorithm</th>\n <th>Test Accuracy</th>\n </tr>\n <tr>\n <td>Model 1</td>\n <td>Random Forest Algorithm</td>\n <td>95%</td>\n </tr>\n <tr>\n <td>Model 2</td>\n <td>SupportVector Machine Algorithm (SVM)</td>\n <td>90%</td>\n </tr>\n <tr>\n <td>Model 3</td>\n <td>Decision Tree Classifier Algorithm</td>\n <td>92%</td>\n </tr>\n <tr>\n <td>Model 4</td>\n <td>K-Nearest NeighborsClassifier Algorithm</td>\n <td>94.7%</td>\n </tr>\n <tr>\n <td>Model 5</td>\n <td>GaussianNB Algorithm</td>\n <td>93.8%</td>\n </tr>\n</table>\n</body>\n</html>",
"_____no_output_____"
]
]
] | [
"markdown",
"code",
"markdown",
"code",
"markdown",
"code",
"markdown",
"code",
"markdown",
"code",
"markdown",
"code",
"markdown",
"code",
"markdown",
"code",
"markdown",
"code",
"markdown",
"code",
"markdown",
"code",
"markdown",
"code",
"markdown"
] | [
[
"markdown"
],
[
"code"
],
[
"markdown"
],
[
"code",
"code",
"code",
"code",
"code",
"code"
],
[
"markdown"
],
[
"code",
"code",
"code",
"code",
"code",
"code"
],
[
"markdown"
],
[
"code"
],
[
"markdown"
],
[
"code",
"code"
],
[
"markdown"
],
[
"code"
],
[
"markdown",
"markdown"
],
[
"code",
"code"
],
[
"markdown"
],
[
"code",
"code"
],
[
"markdown"
],
[
"code",
"code"
],
[
"markdown"
],
[
"code",
"code"
],
[
"markdown"
],
[
"code",
"code"
],
[
"markdown"
],
[
"code",
"code",
"code"
],
[
"markdown"
]
] |
e7662eaad054ffe03d9979a7c75647524808b10d | 2,731 | ipynb | Jupyter Notebook | Linear Regression Analysis/Calculate Gradients.ipynb | TaehoLi/Pytorch-secondstep | acb5378951385c2d52b4a89945a98016b993d0d9 | [
"MIT"
] | null | null | null | Linear Regression Analysis/Calculate Gradients.ipynb | TaehoLi/Pytorch-secondstep | acb5378951385c2d52b4a89945a98016b993d0d9 | [
"MIT"
] | null | null | null | Linear Regression Analysis/Calculate Gradients.ipynb | TaehoLi/Pytorch-secondstep | acb5378951385c2d52b4a89945a98016b993d0d9 | [
"MIT"
] | null | null | null | 20.533835 | 99 | 0.499085 | [
[
[
"# 단순한 기울기 계산 \n\n- z = 2x^2+3\n\n",
"_____no_output_____"
]
],
[
[
"# 먼저 파이토치를 불러옵니다.\nimport torch",
"_____no_output_____"
],
[
"# x를 [2.0,3.0]의 값을 가진 텐서로 초기화 해주고 기울기 계산을 True로 켜 놓습니다. \n# z = 2x^2+3\n\nx = torch.tensor(data=[2.0,3.0],requires_grad=True)\ny = x**2\nz = 2*y +3",
"_____no_output_____"
],
[
"# https://pytorch.org/docs/stable/autograd.html?highlight=backward#torch.autograd.backward\n\n# 목표값을 지정합니다. \ntarget = torch.tensor([3.0,4.0])\n\n# z와 목표값의 절대값 차이를 계산합니다. \n# backward는 스칼라 값에 대해서 동작하기 때문에 길이 2짜리 텐서인 loss를 torch.sum을 통해 하나의 숫자로 바꿔줍니다.\nloss = torch.sum(torch.abs(z-target))\n\n# 그리고 스칼라 값이 된 loss에 대해 backward를 적용합니다.\nloss.backward()\n\n# 여기서 y와 z는 기울기가 None으로 나오는데 이는 x,y,z중에 x만이 leaf node이기 때문입니다.\nprint(x.grad, y.grad, z.grad)",
"tensor([ 8., 12.]) None None\n"
]
]
] | [
"markdown",
"code"
] | [
[
"markdown"
],
[
"code",
"code",
"code"
]
] |
e76634f70edfc0fdd99e103a14f52f9db875c5ec | 10,709 | ipynb | Jupyter Notebook | integration.ipynb | henrymorenoespitia/numerical_methods_and_analysis | 494b5503cef01dc0d6b745edb454bb77e03481e5 | [
"MIT"
] | null | null | null | integration.ipynb | henrymorenoespitia/numerical_methods_and_analysis | 494b5503cef01dc0d6b745edb454bb77e03481e5 | [
"MIT"
] | null | null | null | integration.ipynb | henrymorenoespitia/numerical_methods_and_analysis | 494b5503cef01dc0d6b745edb454bb77e03481e5 | [
"MIT"
] | null | null | null | 31.497059 | 183 | 0.462975 | [
[
[
"# Step1.\n### Define the function wich integrate with.",
"_____no_output_____"
]
],
[
[
"\nimport numpy as np\n\ndef f(x):\n return np.log(3+x)\n",
"_____no_output_____"
]
],
[
[
"#Step 2\n\ndefine functions for diferent methods",
"_____no_output_____"
]
],
[
[
"\n\n# Simple Trapezoid \n\ndef simpleTrapezoid(a, b):\n return (b-a) * (f(a) + f(b))\n\ndef simpleTrapezoidError(realIntegrate, a, b):\n return (abs(realIntegrate - simpleTrapezoid(a, b)) / realIntegrate ) * 100\n\n\n# compound trapezoid\n\ndef compoundTrapezoid(a, b, x):\n sum = 0\n for i in range(1, n):\n sum += 2 * f(x[i])\n return (b-a) / (2*n) * (f(a) + sum + f(b))\n\ndef compoundTrapezoidError(realIntegrate, a, b, x):\n return (abs(realIntegrate - compoundTrapezoid(a, b, x)) / realIntegrate ) * 100\n\n\n# simple Simpson 1/3\n\ndef simpleSimpson1_3(x):\n return (x[2] - x[0]) / 6 * (f(x[0]) + 4 * f(x[1]) + f(x[2]))\n\ndef simpleSimpson1_3Error(realIntegrate, x):\n return (abs(realIntegrate - simpleSimpson1_3(x)) / realIntegrate)\n\n\n# compound Sompson 1/3\n\ndef compoundSimpson1_3(a, b, x):\n sum = 0\n for i in range(1, n, 2):\n sum += 4 * f(x[i])\n sum_2 = 0\n for i in range(2, n-1, 2):\n sum_2 += 2 * f(x[i])\n return (b-a)/(3*n) * (f(a) + sum + sum_2 + f(b))\n\n\ndef compoundSimpson1_3Error(realIntegrate, a, b, x):\n return (abs(realIntegrate - compoundSimpson1_3(a, b, x)) / realIntegrate)\n\n\n# simple Simpson 3/8\n\ndef simpleSimpson1_8(x):\n return (x[3] - x[0]) / 8* (f(x[0]) + 3 *f(x[1]) +3 * f(x[2]) + f(x[3]))\n\ndef simpleSimpson1_8Error(realIntegrate, x):\n return (abs(realIntegrate - simpleSimpson1_8(x)) / realIntegrate)\n\n\n# compound Simpson 3/8\n\ndef compoundSimpsin3_8(a,b,x):\n sum = 0\n m = int(n/3)\n for i in range(0, m):\n sum += 3 * f(x[3*i+1]) + 3*f(x[3*i+2])\n for i in range(0, m-1):\n sum += 2 * f(x[3*i+3])\n return (3/8)*((b-a)/n) * (f(a) + sum + f(b))\n\ndef compoundSimpsin1_8Error(realIntegrate, a, b, x):\n return (abs(realIntegrate - compoundSimpsin3_8(a, b, x)) / realIntegrate) * 100\n\n\n\n\n",
"_____no_output_____"
]
],
[
[
"#Step 3\n### Define entry values",
"_____no_output_____"
]
],
[
[
"# code proof\n\na = 0.1\nb = 3.1\nrealIntegrate = -5.82773\n\n",
"_____no_output_____"
]
],
[
[
"a. proof of simple rules",
"_____no_output_____"
]
],
[
[
"\n# simple trapezoid\nprint(f\"La integral aproximada por la regla de trapecio simple es: {simpleTrapezoid(a, b)} ; error (%): {simpleTrapezoidError(realIntegrate, a, b)} \")\n\n#simple Simpson 1/3\nn= 2 \nx = np.linspace(a, b, n+1)\nprint(f\"La integral aproximada por la regla de Simpson 1/3 simle es: {simpleSimpson1_3(x)} : error (%): {simpleSimpson1_3Error(realIntegrate, x)} \")\n\n# simple Simpson 3/8\nn = 3\nx = np.linspace(a, b, n+1)\nprint(f\"La integral aproximada por la regla de Simpson 3/8 simple es: {simpleSimpson1_8(x)} ; error (%): {simpleSimpson1_8Error(realIntegrate, x)}\")\n\n\n",
"La integral aproximada por la regla de trapecio simple es: 8.819072648011097 ; error (%): -251.32946529799932 \nLa integral aproximada por la regla de Simpson 1/3 simle es: 4.521958048325281 : error (%): -1.7759381523037754 \nLa integral aproximada por la regla de Simpson 3/8 simple es: 4.522640033621997 ; error (%): -1.7760551764790058\n"
]
],
[
[
"a. proof of compound rules",
"_____no_output_____"
]
],
[
[
"\n# compound trapezoid\nn= 18 # number of segments\nx = np.linspace(a, b, n+1)\nprint(f\"La integral aproximada por la regla de trapecio compuesto es: {compoundTrapezoid(a, b, x)} ; error (%): {compoundTrapezoidError(realIntegrate, a, b, x)} \")\n\n#compound Simpson 1/3\nn= 18 # even number\nx = np.linspace(a, b, n+1)\nprint(f\"La integral aproximada por la regla de Simpson 1/3 compuesta es: {compoundSimpson1_3(a, b, x)} : error (%): {compoundSimpson1_3Error(realIntegrate, a, b, x)} \")\n\n# compound Simpson 3/8\nn = 18 # integer:: n%3 = 0\nx = np.linspace(a, b, n+1)\nprint(f\"La integral aproximada por la regla de Simpson 3/8 compuesta es: {compoundSimpsin3_8(a,b,x)} ; error (%): {compoundSimpsin1_8Error(realIntegrate, a, b, x)}\")\n",
"La integral aproximada por la regla de trapecio compuesto es: 4.522847784399211 ; error (%): -177.6090825141043 \nLa integral aproximada por la regla de Simpson 1/3 compuesta es: 4.523214709695509 : error (%): -1.776153787099867 \nLa integral aproximada por la regla de Simpson 3/8 compuesta es: 4.523214401106994 ; error (%): -177.61537341481147\n"
]
],
[
[
"#Step 5: Gauss quadrature\n to 2 and 3 points\n ",
"_____no_output_____"
]
],
[
[
"\n# 2 points\nxd_0 = -0.577350269\nxd_1 = 0.577350269\nC0 = 1\nC1 = 1\n\ndx = ( b- a ) / 2\nx0 = ((b+a) + (b-a) * xd_0 )/ 2 \nx1 = ((b+a) + (b-a) * xd_1)/ 2 \n\nF0 = f(x0) * dx\nF1 = f(x1) * dx\nintegralGaussQuadrature = C0 *F0 + C1 * F1\nintegralGaussQuadratureError = (abs(realIntegrate - integralGaussQuadrature) / realIntegrate) * 100\n\n\nprint(f\"la integral aproximada por el metodo de cuadratura de Gauss con 2 puntos es: {integralGaussQuadrature} ; y el error (%): {integralGaussQuadratureError}\")\n\n\n# 3 points\nxd_0 = -0.774596669\nxd_1 = 0\nxd_2 = 0.774596669\nC0 = 0.55555555\nC1 = 0.88888888\nC2 = 0.55555555\n\ndx = ( b- a ) / 2\nx0 = ((b+a) + (b-a) * xd_0 )/ 2 \nx1 = ((b+a) + (b-a) * xd_1)/ 2 \nx2 = ((b+a) + (b-a) * xd_2)/ 2 \n\nF0 = f(x0) * dx\nF1 = f(x1) * dx\nF2 = f(x2) * dx\nintegralGaussQuadrature_3 = C0 *F0 + C1 * F1 + C2 * F2\nintegralGaussQuadratureError_3 = (abs(realIntegrate - integralGaussQuadrature_3) / realIntegrate) * 100\n\n\n\n\nprint(f\"la integral aproximada por el metodo de cuadratura de Gauss con 3 puntos es: {integralGaussQuadrature_3} ; y el error (%): {integralGaussQuadratureError_3}\")\n\n",
"la integral aproximada por el metodo de cuadratura de Gauss con 2 puntos es: 4.5240374652688375 ; y el error (%): -177.62949665253603\nla integral aproximada por el metodo de cuadratura de Gauss con 3 puntos es: 4.52323074338855 ; y el error (%): -177.6156538375757\n"
]
]
] | [
"markdown",
"code",
"markdown",
"code",
"markdown",
"code",
"markdown",
"code",
"markdown",
"code",
"markdown",
"code"
] | [
[
"markdown"
],
[
"code"
],
[
"markdown"
],
[
"code"
],
[
"markdown"
],
[
"code"
],
[
"markdown"
],
[
"code"
],
[
"markdown"
],
[
"code"
],
[
"markdown"
],
[
"code"
]
] |
e7663631deed5a09c82d02ddb859a397d66dc767 | 6,066 | ipynb | Jupyter Notebook | examples/WPE_Numpy_online.ipynb | mdeegen/nara_wpe | 6ab06b6f34bcf7b5e4030dea468e6bcd3f623133 | [
"MIT"
] | 344 | 2018-05-03T00:27:46.000Z | 2022-03-28T02:13:54.000Z | examples/WPE_Numpy_online.ipynb | mdeegen/nara_wpe | 6ab06b6f34bcf7b5e4030dea468e6bcd3f623133 | [
"MIT"
] | 47 | 2018-06-27T07:22:53.000Z | 2022-02-12T01:18:39.000Z | examples/WPE_Numpy_online.ipynb | mdeegen/nara_wpe | 6ab06b6f34bcf7b5e4030dea468e6bcd3f623133 | [
"MIT"
] | 135 | 2018-05-24T09:14:58.000Z | 2022-03-25T02:55:17.000Z | 26.034335 | 311 | 0.549786 | [
[
[
"%reload_ext autoreload\n%autoreload 2\n%matplotlib inline\n\nimport IPython\nimport matplotlib.pyplot as plt\nimport numpy as np\nimport soundfile as sf\nimport time\nfrom tqdm import tqdm\n\nfrom nara_wpe.wpe import online_wpe_step, get_power_online, OnlineWPE\nfrom nara_wpe.utils import stft, istft, get_stft_center_frequencies\nfrom nara_wpe import project_root",
"_____no_output_____"
],
[
"stft_options = dict(size=512, shift=128)",
"_____no_output_____"
]
],
[
[
"# Example with real audio recordings\nThe iterations are dropped in contrast to the offline version. To use past observations the correlation matrix and the correlation vector are calculated recursively with a decaying window. $\\alpha$ is the decay factor.",
"_____no_output_____"
],
[
"### Setup",
"_____no_output_____"
]
],
[
[
"channels = 8\nsampling_rate = 16000\ndelay = 3\nalpha=0.9999\ntaps = 10\nfrequency_bins = stft_options['size'] // 2 + 1",
"_____no_output_____"
]
],
[
[
"### Audio data",
"_____no_output_____"
]
],
[
[
"file_template = 'AMI_WSJ20-Array1-{}_T10c0201.wav'\nsignal_list = [\n sf.read(str(project_root / 'data' / file_template.format(d + 1)))[0]\n for d in range(channels)\n]\ny = np.stack(signal_list, axis=0)\nIPython.display.Audio(y[0], rate=sampling_rate)",
"_____no_output_____"
]
],
[
[
"### Online buffer\nFor simplicity the STFT is performed before providing the frames.\n\nShape: (frames, frequency bins, channels)\n\nframes: K+delay+1",
"_____no_output_____"
]
],
[
[
"Y = stft(y, **stft_options).transpose(1, 2, 0)\nT, _, _ = Y.shape\n\ndef aquire_framebuffer():\n buffer = list(Y[:taps+delay+1, :, :])\n for t in range(taps+delay+1, T):\n yield np.array(buffer)\n buffer.append(Y[t, :, :])\n buffer.pop(0)",
"_____no_output_____"
]
],
[
[
"### Non-iterative frame online approach\nA frame online example requires, that certain state variables are kept from frame to frame. That is the inverse correlation matrix $\\text{R}_{t, f}^{-1}$ which is stored in Q and initialized with an identity matrix, as well as filter coefficient matrix that is stored in G and initialized with zeros. \n\nAgain for simplicity the ISTFT is applied afterwards.",
"_____no_output_____"
]
],
[
[
"Z_list = []\nQ = np.stack([np.identity(channels * taps) for a in range(frequency_bins)])\nG = np.zeros((frequency_bins, channels * taps, channels))\n\nfor Y_step in tqdm(aquire_framebuffer()):\n Z, Q, G = online_wpe_step(\n Y_step,\n get_power_online(Y_step.transpose(1, 2, 0)),\n Q,\n G,\n alpha=alpha,\n taps=taps,\n delay=delay\n )\n Z_list.append(Z)\n\nZ_stacked = np.stack(Z_list)\nz = istft(np.asarray(Z_stacked).transpose(2, 0, 1), size=stft_options['size'], shift=stft_options['shift'])\n\nIPython.display.Audio(z[0], rate=sampling_rate)",
"_____no_output_____"
]
],
[
[
"## Frame online WPE in class fashion:",
"_____no_output_____"
],
[
"Online WPE class holds the correlation Matrix and the coefficient matrix. ",
"_____no_output_____"
]
],
[
[
"Z_list = []\nonline_wpe = OnlineWPE(\n taps=taps,\n delay=delay,\n alpha=alpha\n)\nfor Y_step in tqdm(aquire_framebuffer()):\n Z_list.append(online_wpe.step_frame(Y_step))\n\nZ = np.stack(Z_list)\nz = istft(np.asarray(Z).transpose(2, 0, 1), size=stft_options['size'], shift=stft_options['shift'])\n\nIPython.display.Audio(z[0], rate=sampling_rate)",
"_____no_output_____"
]
],
[
[
"# Power spectrum\nBefore and after applying WPE.",
"_____no_output_____"
]
],
[
[
"fig, [ax1, ax2] = plt.subplots(1, 2, figsize=(20, 8))\nim1 = ax1.imshow(20 * np.log10(np.abs(Y[200:400, :, 0])).T, origin='lower')\nax1.set_xlabel('')\n_ = ax1.set_title('reverberated')\nim2 = ax2.imshow(20 * np.log10(np.abs(Z_stacked[200:400, :, 0])).T, origin='lower')\n_ = ax2.set_title('dereverberated')\ncb = fig.colorbar(im1)",
"_____no_output_____"
]
]
] | [
"code",
"markdown",
"code",
"markdown",
"code",
"markdown",
"code",
"markdown",
"code",
"markdown",
"code",
"markdown",
"code"
] | [
[
"code",
"code"
],
[
"markdown",
"markdown"
],
[
"code"
],
[
"markdown"
],
[
"code"
],
[
"markdown"
],
[
"code"
],
[
"markdown"
],
[
"code"
],
[
"markdown",
"markdown"
],
[
"code"
],
[
"markdown"
],
[
"code"
]
] |
e76641df960979c6c53bac64432f1e79d635702b | 1,607 | ipynb | Jupyter Notebook | algorithms/88-Merge-Sorted-Array.ipynb | DjangoPeng/leetcode-solutions | 9aa2b911b0278e743448f04241828d33182c9d76 | [
"Apache-2.0"
] | 10 | 2019-03-23T15:15:55.000Z | 2020-07-12T02:37:31.000Z | algorithms/88-Merge-Sorted-Array.ipynb | DjangoPeng/leetcode-solutions | 9aa2b911b0278e743448f04241828d33182c9d76 | [
"Apache-2.0"
] | null | null | null | algorithms/88-Merge-Sorted-Array.ipynb | DjangoPeng/leetcode-solutions | 9aa2b911b0278e743448f04241828d33182c9d76 | [
"Apache-2.0"
] | 3 | 2019-06-21T12:13:23.000Z | 2020-12-08T07:49:33.000Z | 21.426667 | 81 | 0.415059 | [
[
[
"class Solution:\n def merge(self, nums1: [int], m: int, nums2: [int], n: int) -> None:\n \"\"\"\n Do not return anything, modify nums1 in-place instead.\n \"\"\"\n while m > 0 and n > 0:\n if nums1[m-1] > nums2[n-1]:\n nums1[m+n-1] = nums1[m-1]\n m = m - 1\n else:\n nums1[m+n-1] = nums2[n-1]\n n = n - 1\n while n > 0:\n nums1[n-1] = nums2[n-1]\n n = n - 1\n return None",
"_____no_output_____"
],
[
"s = Solution()\ns.merge([1,2,3], 0, [4,5,6], 3)",
"[4, 5, 6]\n"
]
]
] | [
"code"
] | [
[
"code",
"code"
]
] |
e7664231a8b359370c50c0a9bf3221abef7110f3 | 134,451 | ipynb | Jupyter Notebook | module1-scrape-and-process-data/LS_DS_121_Scrape_and_process_data.ipynb | wjarvis2/DS-Unit-1-Sprint-2-Data-Wrangling | 605c1447abd6625a77e5f443c4a99cce154fbf82 | [
"MIT"
] | null | null | null | module1-scrape-and-process-data/LS_DS_121_Scrape_and_process_data.ipynb | wjarvis2/DS-Unit-1-Sprint-2-Data-Wrangling | 605c1447abd6625a77e5f443c4a99cce154fbf82 | [
"MIT"
] | null | null | null | module1-scrape-and-process-data/LS_DS_121_Scrape_and_process_data.ipynb | wjarvis2/DS-Unit-1-Sprint-2-Data-Wrangling | 605c1447abd6625a77e5f443c4a99cce154fbf82 | [
"MIT"
] | null | null | null | 101.243223 | 83,486 | 0.682412 | [
[
[
"_Lambda School Data Science_\n\n# Scrape and process data\n\nObjectives\n- scrape and parse web pages\n- use list comprehensions\n- select rows and columns with pandas\n\nLinks\n- [Automate the Boring Stuff with Python, Chapter 11](https://automatetheboringstuff.com/chapter11/)\n - Requests\n - Beautiful Soup\n- [Python List Comprehensions: Explained Visually](https://treyhunner.com/2015/12/python-list-comprehensions-now-in-color/)\n- [Pandas Cheat Sheet](https://github.com/pandas-dev/pandas/blob/master/doc/cheatsheet/Pandas_Cheat_Sheet.pdf)\n - Subset Observations (Rows)\n - Subset Variables (Columns)\n- Python Data Science Handbook\n - [Chapter 3.1](https://jakevdp.github.io/PythonDataScienceHandbook/03.01-introducing-pandas-objects.html), Introducing Pandas Objects\n - [Chapter 3.2](https://jakevdp.github.io/PythonDataScienceHandbook/03.02-data-indexing-and-selection.html), Data Indexing and Selection\n",
"_____no_output_____"
],
[
"## Scrape the titles of PyCon 2018 talks",
"_____no_output_____"
]
],
[
[
"url = 'https://us.pycon.org/2018/schedule/talks/list/'",
"_____no_output_____"
],
[
"",
"_____no_output_____"
]
],
[
[
"",
"_____no_output_____"
],
[
"## 5 ways to look at long titles\n\nLet's define a long title as greater than 80 characters",
"_____no_output_____"
],
[
"### 1. For Loop",
"_____no_output_____"
]
],
[
[
"",
"_____no_output_____"
]
],
[
[
"### 2. List Comprehension",
"_____no_output_____"
]
],
[
[
"",
"_____no_output_____"
]
],
[
[
"### 3. Filter with named function",
"_____no_output_____"
]
],
[
[
"",
"_____no_output_____"
]
],
[
[
"### 4. Filter with anonymous function",
"_____no_output_____"
]
],
[
[
"",
"_____no_output_____"
]
],
[
[
"### 5. Pandas\n\npandas documentation: [Working with Text Data](https://pandas.pydata.org/pandas-docs/stable/text.html)",
"_____no_output_____"
]
],
[
[
"",
"_____no_output_____"
]
],
[
[
"## Make new dataframe columns\n\npandas documentation: [apply](https://pandas.pydata.org/pandas-docs/stable/generated/pandas.Series.apply.html)",
"_____no_output_____"
]
],
[
[
"",
"_____no_output_____"
]
],
[
[
"### title length",
"_____no_output_____"
]
],
[
[
"",
"_____no_output_____"
]
],
[
[
"### long title",
"_____no_output_____"
]
],
[
[
"",
"_____no_output_____"
]
],
[
[
"### first letter",
"_____no_output_____"
]
],
[
[
"",
"_____no_output_____"
]
],
[
[
"### word count\n\nUsing [`textstat`](https://github.com/shivam5992/textstat)",
"_____no_output_____"
]
],
[
[
"#!pip install textstat",
"Collecting textstat\n Downloading https://files.pythonhosted.org/packages/9b/78/a050fa0f13c04db10c891167204e2cd0c0ae1be40842a10eaf5348360f94/textstat-0.5.4.tar.gz\nCollecting pyphen (from textstat)\n\u001b[?25l Downloading https://files.pythonhosted.org/packages/15/82/08a3629dce8d1f3d91db843bb36d4d7db6b6269d5067259613a0d5c8a9db/Pyphen-0.9.5-py2.py3-none-any.whl (3.0MB)\n\u001b[K 100% |████████████████████████████████| 3.0MB 8.1MB/s \n\u001b[?25hCollecting repoze.lru (from textstat)\n Downloading https://files.pythonhosted.org/packages/b0/30/6cc0c95f0b59ad4b3b9163bff7cdcf793cc96fac64cf398ff26271f5cf5e/repoze.lru-0.7-py3-none-any.whl\nBuilding wheels for collected packages: textstat\n Running setup.py bdist_wheel for textstat ... \u001b[?25l-\b \bdone\n\u001b[?25h Stored in directory: /root/.cache/pip/wheels/04/ac/d7/a05c0ad7825899f11eacd5f9a5a78534808c8159281e65863c\nSuccessfully built textstat\nInstalling collected packages: pyphen, repoze.lru, textstat\nSuccessfully installed pyphen-0.9.5 repoze.lru-0.7 textstat-0.5.4\n"
],
[
"",
"_____no_output_____"
]
],
[
[
"## Rename column\n\n`title length` --> `title character count`\n\npandas documentation: [rename](https://pandas.pydata.org/pandas-docs/stable/generated/pandas.DataFrame.rename.html)",
"_____no_output_____"
]
],
[
[
"",
"_____no_output_____"
]
],
[
[
"## Analyze the dataframe",
"_____no_output_____"
],
[
"### Describe\n\npandas documentation: [describe](https://pandas.pydata.org/pandas-docs/stable/generated/pandas.DataFrame.describe.html)",
"_____no_output_____"
]
],
[
[
"",
"_____no_output_____"
]
],
[
[
"### Sort values\n\npandas documentation: [sort_values](https://pandas.pydata.org/pandas-docs/stable/generated/pandas.DataFrame.sort_values.html)",
"_____no_output_____"
],
[
"Five shortest titles, by character count",
"_____no_output_____"
]
],
[
[
"",
"_____no_output_____"
]
],
[
[
"Titles sorted reverse alphabetically",
"_____no_output_____"
]
],
[
[
"",
"_____no_output_____"
]
],
[
[
"### Get value counts\n\npandas documentation: [value_counts](https://pandas.pydata.org/pandas-docs/stable/generated/pandas.Series.value_counts.html)\n",
"_____no_output_____"
],
[
"Frequency counts of first letters",
"_____no_output_____"
]
],
[
[
"",
"_____no_output_____"
]
],
[
[
"Percentage of talks with long titles",
"_____no_output_____"
]
],
[
[
"",
"_____no_output_____"
]
],
[
[
"### Plot\n\npandas documentation: [Visualization](https://pandas.pydata.org/pandas-docs/stable/visualization.html)\n\n\n\n",
"_____no_output_____"
],
[
"Top 5 most frequent first letters",
"_____no_output_____"
]
],
[
[
"",
"_____no_output_____"
]
],
[
[
"Histogram of title lengths, in characters",
"_____no_output_____"
]
],
[
[
"",
"_____no_output_____"
]
],
[
[
"# Assignment\n\n**Scrape** the talk descriptions. Hint: `soup.select('.presentation-description')`\n\n**Make** new columns in the dataframe:\n- description\n- description character count\n- description word count\n- description grade level (use [this `textstat` function](https://github.com/shivam5992/textstat#the-flesch-kincaid-grade-level) to get the Flesh-Kincaid grade level)\n\n**Describe** all the dataframe's columns. What's the average description word count? The minimum? The maximum?\n\n**Answer** these questions:\n- Which descriptions could fit in a tweet?\n- What's the distribution of grade levels? Plot a histogram.\n\n",
"_____no_output_____"
]
],
[
[
"import bs4\nimport requests\nimport numpy as np\nimport pandas as pd\nimport matplotlib.pyplot as plt",
"_____no_output_____"
],
[
"result = requests.get(url)\nsoup = bs4.BeautifulSoup(result.text)\ndescriptions = [tag.text.strip()\n for tag in soup.select('.presentation-description')]\nprint (len(descriptions))\nprint (descriptions)",
"95\n[\"At some point every Python programmer sees Python bytecode files -- they're those '.pyc' files Python likes to leave behind after it runs. But have you ever wondered what's really going on in those files? Well, wonder no more! In this talk you'll learn what Python bytecode is and how it's used to execute your code, as well as how to decipher and read it, and how to reason about bytecode to understand the performance of your Python code.\", \"Until very recently, Apache Spark has been a de facto standard choice of a framework for batch data processing. For Python developers, diving into Spark is challenging, because it requires learning the Java infrastructure, memory management, configuration management. The multiple layers of indirection also make it harder to debug things, especially when throwing the Pyspark wrapper into the equation.\\r\\n\\r\\nWith Dask emerging as a pure Python framework for parallel computing, Python developers might be looking at it with new hope, wondering if it might work for them in place of Spark. In this talk, Iâ\\x80\\x99m using a data aggregation example to highlight the important differences between the two frameworks, and make it clear how involved the switch may be.\\r\\n\\r\\nNote: Just in case it's unclear, there's no Java of any kind in this talk. All the code / examples use Python (PySpark).\", 'In this talk, youâ\\x80\\x99ll learn about a category of security issue known as side channel attacks. Youâ\\x80\\x99ll be amused to see how features like automatic data compression, short-circuit execution, and deterministic hashing can be abused to bypass security systems. No security background knowledge is required. The talk assumes at least intermediate Python experience.\\r\\n\\r\\nWeâ\\x80\\x99ll take a tour of real side channel vulnerabilities in open source Python codebases, including the patches that fixed them. It also offers practical advice for avoiding these issues. My goal is to demystify this topic, even if you arenâ\\x80\\x99t writing security-critical software.', 'â\\x80\\x9cSo tell me,â\\x80\\x9d my manager said, â\\x80\\x9cwhat is an average?â\\x80\\x9d\\r\\n\\r\\nThereâ\\x80\\x99s probably nothing worse than that sinking feeling when you finish an analysis, email it to your manager or client to review, and they point out a mistake so basic you canâ\\x80\\x99t even fathom how you missed it. \\r\\n\\r\\nThis talk is about mine: how to take an average.\\r\\n\\r\\nAverages are something we use everywhere - itâ\\x80\\x99s a simple np.mean() in pandas or AVG() in SQL. But recently Iâ\\x80\\x99ve come to appreciate just how easy it is to calculate this statistic incorrectly. We learn once - in middle school no less - how to take an average, and never revisit it. Then, when we are faced with multidimensional datasets (ie. pretty much every dataset out there), we never reconsider whether we should be taking an average the same way.\\r\\n\\r\\nIn this talk, we follow my arduous and humbling journey of learning how to properly take an average with multidimensional data. We will cover how improperly calculating it can produce grossly incorrect figures, which can slip into publications, research analyses and management reports.', 'Recommender systems have become increasingly popular in recent years, and are used by some of the largest websites in the world to predict the likelihood of a user taking an action on an item. In the world of Netflix, this means recommending similar movies to the ones you have seen. In the world of dating, this means suggesting matches similar to people you already showed interest in!\\r\\n\\r\\nMy path to recommenders has been an unusual one: from a Software Engineer to working on matching algorithms at a dating company, with a little background on machine learning. With my knowledge of Python and the use of basic SVD (Singular Value Decomposition) frameworks, I was able to understand SVDs from a practical standpoint of what you can do with them, instead of focusing on the science.\\r\\n\\r\\nIn my talk, you will learn 2 practical ways of generating recommendations using SVDs: matrix factorization and item similarity. We will be learning the high-level components of SVD the \"doer way\": we will be implementing a simple movie recommendation engine with the help of Jupiter notebooks, the MovieLens database, and the Surprise recommendation package.', \"Do we even need humans? Humans and data science are flawed on their own. Humans lack the ability to process large volumes of information. Machines lack intuition, empathy, and nuance. You'll learn how to guide users of expert-use systems by applying data science to their user experience. This allows us to take advantage of the human-touch while leveraging our large datasets. What is the relationship between human decisions and algorithms? Are we thinking about data science all wrong? In this talk, you'll learn the ways we balance human decisions and data science throughout our applications, the challenges we have faced along the way and the future of the relationship between humans and data.\", 'Writing quality Python code can be both tough and tedious. On top of the general design, there are many code quality aspects that you need to watch out for when writing and reviewing code such as adherence to PEP8, docstring quality, test quality, etc. Furthermore, everyone is human. If you are catching these code quality issues by hand, there is a good chance that at some point you will miss an easy opportunity to improve code quality. If the quality check can be done by a machine, then why would you even try to catch the code quality issue by hand? In the end, the machine will be able to perform the quality check with much more speed, accuracy, and consistency than a person.\\r\\n\\r\\nThis talk will dive into how existing open source projects offload and automate many of these code quality checks resulting in:\\r\\n\\r\\n- A higher quality and a more consistent codebase\\r\\n- Maintainers being able to focus more on the higher level design and interfaces\\r\\n of a project.\\r\\n- An improved contribution process and higher quality pull requests from\\r\\n external contributors\\r\\n\\r\\nBy diving into how these open source projects automate code quality checks, you will learn about:\\r\\n\\r\\n- The available tooling related to checking code quality such as `flake8`,\\r\\n `pylint`, `coverage`, etc.\\r\\n- How to automate code quality checks for both a development and team \\r\\n setting.\\r\\n- First-hand accounts of the benefits and lessons learned from automating\\r\\n code quality checks in real-life open source projects.', 'Nowadays, there are many ways of building data science models using Python, including statistical and machine learning methods. I will introduce probabilistic models, which use Bayesian statistical methods to quantify all aspects of uncertainty relevant to your problem, and provide inferences in simple, interpretable terms using probabilities. A particularly flexible form of probabilistic models uses Bayesian *non-parametric* methods, which allow models to vary in complexity depending on how much data are available. In doing so, they avoid the over-fitting that is common in machine learning and statistical modeling. I will demonstrate the basics of Bayesian non-parametric modeling in Python, using the PyMC3 package. Specifically, I will introduce two common types, Gaussian processes and Dirichlet processes, and show how they can be applied easily to real-world problems using two examples.', 'Behavior-Driven Development (BDD) is gaining popularity as an improved way to collaborate over product features and tests. In Python, **behave** is one of the leading BDD test frameworks. Using **behave**, teams write Gherkin behavior scenarios (e.g., tests) in plain language, and then programmers write Python code to automate the steps. BDD testing is great because tests are self-documenting and steps abide by the DRY principle. An example test could be:\\r\\n\\r\\n> Given the DuckDuckGo home page is displayed\\r\\n> When the user searches the phrase \"Python\"\\r\\n> Then search results for \"Python\" are shown\\r\\n\\r\\nThis talk will teach how to use **behave** to develop well-designed test scenarios and a robust automation framework. It will focus on the layers of the behave framework: feature files, step definitions, support classes, and config files. A full example project will be hosted on GitHub for audience members to reference after the talk.', 'Scraping one web site for information is easy, scraping 10000 different sites is hard. Beyond page-specific scraping, how do you build a program than can extract the publication date of (almost) any news article online, no matter the web site?\\r\\n\\r\\nWeâ\\x80\\x99ll cover when to use machine learning vs. humans or heuristics for data extraction, the different steps of how to phrase the problem in terms of machine learning, including feature selection on HTML documents, and issues that arise when turning research into production code.', \"You've used pytest and you've used mypy, but bugs are still slipping through your code. What's next? In this talk, we cover two simple but powerful tools for keeping your code problem-free. Property-based testing, provided by the [Hypothesis](https://hypothesis.readthedocs.io/en/latest/) library, lets you run hundreds of tests from a single template. Contracts, via [dpcontracts](https://github.com/deadpixi/contracts), make your program test itself. You'll learn how and why to use these tools and how to combine them with the rest of your testing suite.\", \"Big-O is a computer science technique for analyzing how code performs as data gets larger. It's a very handy tool for the working programmer, but it's often shrouded in off-putting mathematics.\\r\\n\\r\\nIn this talk, I'll teach you what you need to know about Big-O, and how to use it to keep your programs running well. Big-O helps you choose the data structures and algorithms that will let your code work efficiently even on large data sets.\\r\\n\\r\\nYou can understand Big-O even if you aren't a theoretical computer science math nerd. Big-O isn't as mystical as it appears. It's wrapped in mathematical trappings, but doesn't have to be more than a common-sense assessment of how your code will behave.\", \"In the past few years, the power of computer vision has exploded. In this talk, we'll apply a deep learning model to a bird feeder. We'll use that model to detect, identify, and record birds that come to a smart bird feeder.\\r\\n\\r\\nAlong the way, we'll learn about different platforms to deploy deep learning cameras on, from the lowly Raspberry PI all the way up to the powerful NVIDIA Jetson embedded computer with a built in GPU.\", 'Facebook, Google, Uber, LinkedIn, and friends are the rarefied heights of software engineering. They encounter and solve problems at scales shared by few others, and as a result, their priorities in production engineering and architecture are just a bit different from the rest of us down here in the other 99% of services. Through deconstructing a few blog posts from these giants, weâ\\x80\\x99ll evaluate just what is it that theyâ\\x80\\x99re thinking about when they build systems and whether any of their choices are relevant to those of us operating at high scale yet still something less than millions of requests per second.\\r\\n\\r\\nThis talk will go into depth on how to make technological decisions to meet your customersâ\\x80\\x99 requirements without requiring a small army of engineers to answer 2 AM pages, and how to set realistic goals for your team around operations, uptime, communications, and disaster recovery.\\r\\n\\r\\nWith these guidelines in mind, you should be better equipped to say no (or yes!) the next time your teamâ\\x80\\x99s software hipster proposes moving everything to the Next Big Thing.', \"Have you ever wanted to write a GUI application you can run on your laptop? What about an app that you can run on your phone? Historically, these have been difficult to achieve with Python, and impossible to achieve without learning a different API for each platform. But no more.\\r\\n\\r\\nBeeWare is a collection of tools and libraries that allows you to build cross-platform native GUI applications in pure Python, targeting desktop, mobile and web platforms. In this talk, you'll be introduced to the BeeWare suite of tools and libraries, and see how you can use them to develop, from scratch, a GUI ChatBot application that can be deployed as a standalone desktop application, a mobile phone application, and a single page webapp - without making any changes to the application's codebase.\", 'Itâ\\x80\\x99s one thing to build a robust data pipeline process in python but a whole other challenge to find tooling and build out the framework that allows for testing a data process. In order to truly iterate and develop a codebase, one has to be able to confidently test during the development process and monitor the production system. \\r\\n\\r\\nIn this talk, I hope to address the key components for building out end to end testing for data pipelines by borrowing concepts from how we test python web services. Just like how we want to check for healthy status codes from our API responses, we want to be able to check that a pipeline is working as expected given the correct inputs. Weâ\\x80\\x99ll talk about key features that allows a data pipeline to be easily testable and how to identify timeseries metrics that can be used to monitor the health of a data pipeline.', 'We build product and software as teams. And as anyone who as worked on a team knows, thereâ\\x80\\x99s often a lot more that goes into working together to build that product than actually just building the product itself. A highly functional team is not as elusive it may seem. Software engineering is a skill weâ\\x80\\x99ve developed, but even more importantly software engineering on teams is another skill weâ\\x80\\x99ve been practicing and improving on as an industry. Software engineering principles and best practices may seem to have very little to do with teamwork, but being able to thoughtfully apply some of what weâ\\x80\\x99ve learned as engineers towards teamwork, we can help move towards creating such success with our teams.', 'Want to know about the latest trends in the Python community and see the the big picture of how things have changed over the last few years? Interested in the results of the latest official Python Developers Survey 2017 which was supported by the Python Software Foundation and gathered responses from more than 10.000 Python developers? Come learn about the most popular types of Python development, trending frameworks, libraries and tools, additional languages being used by Python developers, Python versions usage statistics and many other insights from the world of Python. All derived from the actual data and professional research such as the Python Developers Survey 2017 which collected responses from over 10.000 Python developers, organized in partnership between the Python Software Foundation and JetBrains, the Python Developers Survey 2016, 3rd party surveys and supplementary analytical research.', \"Python now offers static types! Companies like Dropbox and Facebook, and open-source projects like Zulip, use static types (with [PEP 484](https://www.python.org/dev/peps/pep-0484/) and [mypy](https://github.com/python/mypy)) to make Python more productive and fun to work with â\\x80\\x94 in existing codebases from 40k lines to 4 million, in Python 2 and 3, and while preserving the conciseness and flexibility that make Python a great language in the first place. Iâ\\x80\\x99ll describe how.\\r\\n\\r\\nReading and understanding code is a huge part of what we do as software developers. If we make it easier to understand our codebases, we make everyone more productive, help each other write fewer bugs, and lower barriers for new contributors. That's why Python now features optional static types, and why Dropbox, [Facebook](https://engineering.instagram.com/let-your-code-type-hint-itself-introducing-open-source-monkeytype-a855c7284881), and [Zulip](https://blog.zulip.org/2016/10/13/static-types-in-python-oh-mypy/) use them on part or all of their Python code.\\r\\n\\r\\nIn this talk, Iâ\\x80\\x99ll share lessons from Zulipâ\\x80\\x99s and Dropboxâ\\x80\\x99s experience â\\x80\\x94 having led the mypy team at Dropbox and working now on the Zulip core team â\\x80\\x94 for how you can start using static types in your own codebases, large or small. Weâ\\x80\\x99ll discuss how to make it a seamless part of your projectâ\\x80\\x99s tooling; what order to approach things in; and powerful new tools that make it even easier today to add static types to your Python codebase than ever before.\", 'As engineers, we care a lot about the reliability of our applications. When a website falls over, pagers go off, and engineers burst into action to bring a site back to life. Postmortems are written, and teams develop strategies to prevent similar failures in the future.\\r\\n\\r\\nBut what about the reliability of our data? Would _you_ trust financial reports built on your data? \\r\\n\\r\\nIf not, what can you do to improve data health? If you _would_ trust these reports, how can you prove to customers, investors, and auditors alike that they should too?\\r\\n\\r\\nIn this talk, youâ\\x80\\x99ll learn to apply strategies from the world of dev-ops to data. Youâ\\x80\\x99ll learn about questions auditors ask that can help you pinpoint data problems. Youâ\\x80\\x99ll also learn some accounting-specific tools for accurate and timely record keeping that Iâ\\x80\\x99ve found fascinating and helpful!', \"Code reviews don't have to be a time consuming, morale zapping, arduous tasks. Not only can they catch bugs and errors but they can contribute in positive ways to the individual developer, the team, management and company as a whole. \\r\\n\\r\\nArt critiques have existed in academia for hundreds of years. The methodology of the critique has evolved to be time sensitive and productive, while keeping the enthusiasm of the student artist intact. \\r\\n\\r\\nThe purpose of the art critique is to get peers and mentors to look at the work and raise any problems they may see. It's also time where people with more experience could contribute their knowledge in a helpful way. This process is about producing the best work, quickly and in a productive and constructive way. \\r\\n \\r\\nThese methods can be applied to code review.\", 'In 2017, I was released from prison after serving 17 years. One of the most transformational experiences I had while incarcerated was learning to code, through a pioneering new program called Code.7370 â\\x80\\x94 the first coding curriculum in a United States prison.\\r\\n\\r\\nIn this talk, Iâ\\x80\\x99d like to share my experiences learning to code in prison and getting a software engineering job after my release, with the goals of:\\r\\n\\r\\nInspiring new programmers to stick with it and be confident in their abilities\\r\\n\\r\\nInspiring educators to think about how to support new coders in a broad range of learning environments (thereâ\\x80\\x99s no internet in prison!)\\r\\n\\r\\nInspiring everyone to think about the potential for rehabilitation in prison in a new way', \"Colossal Cave, also known as Adventure or ADVENT, is the original text adventure. It was written in FORTRAN IV and there is practically no way to run the original program without translating it. We'll explore software archeology to write a Python interpreter to run the FORTRAN code as-is, without translating it. Come learn about pre-ASCII and 36-bit integers and writing interpreters in Python!\\r\\n\\r\\nAnd, we'll show how to use BeeWare's Batavia Python interpreter (in JavaScript) to execute the program. FORTRAN IV in Python in JavaScript in your browser!\", 'Testing mobile applications is hard. Testing manually is nearly impossible.\\r\\nThatâ\\x80\\x99s where automated testing shines. Just sit back and watch the machine go!\\r\\nPython is a very powerful language for writing automated tests, but since Python is not installed on mobile platforms, we need to find a way to remotely control and monitor the device.\\r\\nBut how do we automate a device remotely? The answer is Appium.\\r\\n\\r\\nIn this talk I will go over the process of deploying and testing iOS (or Android) applications, and how to work with Appium to easily generate Python 3 code for testing your application.', \"Setting up application monitoring is often an afterthought, and in the speaker's opinion can be a bit overwhelming to get started with. What is a `metric`? What is a `gauge`? What is a `counter`? What's that `upper 90` metric you have up on your `dashboard`? And what *all* metrics should I monitor?\\r\\n\\r\\nThis talk aims to get you started on the monitoring journey in Python. In addition to clearing up some of the jargon, we will look at `statsd` and `prometheus` monitoring systems and how to integrate our applications with these.\\r\\n\\r\\nWithout the numbers, we are really flying blind!\", \"![Logo][1]\\r\\n\\r\\n[**Website**](https://cupy.chainer.org/) | [**Docs**](https://docs-cupy.chainer.org/en/stable/) | [**Install Guide**](https://docs-cupy.chainer.org/en/stable/install.html) | [**Tutorial**](https://docs-cupy.chainer.org/en/stable/tutorial/) | **Examples** ([Official](https://github.com/cupy/cupy/blob/master/examples)) | [**Forum**](https://groups.google.com/forum/#!forum/cupy)\\r\\n\\r\\nCuPy is an open-source library with NumPy syntax that increases speed by doing matrix operations on NVIDIA GPUs. It is accelerated with the CUDA platform from NVIDIA and also uses CUDA-related libraries, including cuBLAS, cuDNN, cuRAND, cuSOLVER, cuSPARSE, and NCCL, to make full use of the GPU architecture. CuPy's interface is highly compatible with NumPy; in most cases it can be used as a drop-in replacement. CuPy supports various methods, data types, indexing, broadcasting, and more.\\r\\n\\r\\n [1]: https://raw.githubusercontent.com/cupy/cupy/master/docs/image/cupy_logo_1000px.png\", \"The PEP 557 dataclasses module is available in starting in Python 3.7. It will become an essential part of every Python programmer's toolkit. This talk shows what problem the module solves, explains its key design decisions, and provides practical examples of how to put it to work.\\r\\n\\r\\nDataclasses are shown to be the next step in a progression of data aggregation tools: tuple, dict, simple class, bunch recipe, named tuples, records, attrs, and then dataclasses. Each builds upon the one that came before, adding expressiveness at the expense of complexity.\\r\\n\\r\\nDataclasses are unique in that they let you selectively turn-on or turn-off its various capabilities and it lets the user choose the underlying data store (either instance dictionary, instance slots, or an inherited base class).\\r\\n\\r\\nDataclasses and typing.NamedTuple both use variable annotations which were new in Python 3.6.\", 'Data Visualization charts are supposed to be our map to information. However, when making charts, customarily we are just re-sizing lines and circles based on metrics instead of creating data-driven version of reality. The contemporary charting techniques have a few shortcomings (especially when dealing with high-dimensional dataset): \\r\\n\\r\\n* **Context Reduction**: in order to fit a high-dimensional dataset into a chart one needs to filter/ aggregate/ flatten data which results in reduction of full context of information. Without context most of the charts show only a part of the story, that can potentially lead to data misinterpretation/misunderstanding. \\r\\n* **Numeric Thinking**: naturally humans have hard time perceiving big numbers. While data visualization is suppose to help us to conceptualize large volumes, unless the dataset is carefully prepared, 2D charts rarely give us the intuitive grasp of magnitude. \\r\\n* **Perceptual de-humanization**: when examining charts it is easy to forget that we are dealing with activity in real world instead of lines/bars. \\r\\n\\r\\nAugmented/Mixed Reality can potentially solve all of the issues listed above by presenting an intuitive and interactive environment for data exploration. Three dimensional space provides conditions to create complex data stories with more â\\x80\\x9crealistic assetsâ\\x80\\x9d (beyond lines and bars). The talk would present the architecture required to create MR data visualization story with Python (70% of architecture), starting with drawing 3D assets in a data-driven way and finishing with deployment on MR devices.', 'Apache Spark is one of the most popular big data projects, offering greatly improved performance over traditional MapReduce models. Much of Apache Sparkâ\\x80\\x99s power comes from lazy evaluation along with intelligent pipelining, which can make debugging more challenging. This talk will examine how to debug Apache Spark applications, the different options for logging in PySpark, as well as some common errors and how to detect them.\\r\\n\\r\\nSparkâ\\x80\\x99s own internal logging can often be quite verbose, and this talk will examine how to effectively search logs from Apache Spark to spot common problems. In addition to the internal logging, this talk will look at options for logging from within our program itself.\\r\\n\\r\\nSparkâ\\x80\\x99s accumulators have gotten a bad rap because of how they interact in the event of cache misses or partial recomputes, but this talk will look at how to effectively use Sparkâ\\x80\\x99s current accumulators for debugging as well as a look to future for data property type accumulators which may be coming to Spark in future version.\\r\\n\\r\\nIn addition to reading logs, and instrumenting our program with accumulators, Sparkâ\\x80\\x99s UI can be of great help for quickly detecting certain types of problems.\\r\\n\\r\\nDebuggers are a wonderful tool, however when you have 100 computers the â\\x80\\x9cwonderâ\\x80\\x9d can be a bit more like â\\x80\\x9cpainâ\\x80\\x9d. This talk will look at how to connect remote debuggers, but also remind you that itâ\\x80\\x99s probably not the easiest path forward.', 'In 2011 I gave a talk about \"Killing Patents with Python\" - finding the right piece of prior art by using statistical natural language processing techniques on the US Patent Database. A number of unexpected benefits came out of that exploration, including the ability to describe large patent portfolios and businesses in a way that had not been done before.\\r\\n\\r\\nSince then, the state of the art has advanced - and so has the ability to do strange and wonderful things by applying the latest neural network-based analysis to the nine million patents and patent applications that people have submitted to the USPTO. Not only can we learn new things about what people have invented, we might just be able to get the computer to do a little \"inventing\" itself.', 'We use JupyterHub, XArray, Dask, and Kubernetes to build a cloud-based system to enable scientists to analyze and manage large datasets. We use this in practice to serve a broad community of atmospheric and climate scientists.\\r\\n\\r\\nAtmospheric and climate scientists analyze large volumes of observational and simulated data to better understand our planet. They have historically used tools like NumPy and SciPy along with Jupyter notebooks to combine efficient computation with accessibility. However, as datasets increase in size and collaboration extends to new populations of scientists these tools begin to feel their age. In this talk we use more recent libraries to build a modern deployment for academic scientists. In particular we use the following tools:\\r\\n\\r\\n- **Dask:** to parallelize and scale NumPy computations\\r\\n- **XArray**: as a self-discribing data model and tool kit for labeled and index arrays\\r\\n- **JupyterLab:** to enable more APIs for users beyond the classic notebook\\r\\n- **JupyterHub:** to manage users and maintain environments for a new population of cloud-friendly users\\r\\n- **Kubernetes:** to manage everything and deploy easily on cloud hardware\\r\\n\\r\\nThis talk will focus less on how these libraries work and will instead be a case study of using them together in an operational setting. During the talk we will build up and deploy a running system that the audience can then use to access distributed computing resources.', 'One of the most challenging and important thing fors for Python developers learn is the unittest mock library. The patch function is in particular confusing- there are many different ways to use it. Should I use a context manager? Decorator? When would I use it manually? Improperly used patch functions can make unit tests useless, all the while making them look as if they are correctly testing code.Letâ\\x80\\x99s learn how to wield patch with confidence!', 'In the 1850s, Edward Orange Wildman Whitehouse was appointed the lead engineer of the first attempt to build a trans-Atlantic telegraph cable. With the entire population of two continents waiting for his go-live, their handlebar moustaches aquiver, he demonstrated in fine form just how spectacularly a big project can be a bigger disaster.\\r\\n\\r\\nThis is a tale of long-winded rants, spectacular sideburns, and gentlemen scientists behaving badly. It is also a lesson about the importance of honest reflection in technical teamwork. Lilly outlines some of the mistakes made during one of the biggest tech delivery projects in history, and how a constructive view of failure helped to turn it all around. Through the public meltdowns of Wildman Whitehouse you will learn the importance of feedback, how to handle complex tasks gracefully, and the best way to recover from having your pipeline eaten by a whale.', 'Want to have fun with Python? Do something visual? Get started today? Learn how to draw, animate, and use sprites for games with the [Python Arcade](http://arcade.academy/) library.\\r\\n\\r\\n\"Arcade\" is an easy-to-use Python library for creating 2D arcade games. We\\'ll show you how to get started creating your own game, and find plenty of example code to get an idea of what you can do with this library. If you are familiar with PyGame, Arcade is easier, more powerful, and uses recent Python features like type hinting and decorators.\\r\\n\\r\\nThis talk is great for beginners, educators, and people who want to create their own arcade games.', \"Multithreading makes shared memory easy, but true parallelism next to impossible. Multiprocessing gives us true parallelism, but it makes sharing memory very difficult, and high overhead. In this talk, we'll explore techniques to share memory between processes efficiently, with a focus on sharing read-only massive data structures.\", 'Logs are our best friend, especially on those late nights when we try to troubleshoot a problem in production that was written by a co-worker who is on vacation. Logs are the main way to know what is happening with an application at runtime, but we donâ\\x80\\x99t realize how important they are until we actually need them. Unfortunately, they are usually an under-estimated part of the development process.\\r\\n\\r\\nThis talk aims to transmit the need for the logging module, briefly explains how to use it and how it is built, and dives into all the complexity that is hidden to us. This will help attendees not just understand all the magic that allows us to inspect our applications at runtime, but also to avoid mistakes and adapt the module to our needs for more esoteric scenarios.\\r\\n\\r\\nThe talk is structured to simplify the understanding of the logging module. Many people have read the documentation, but still struggle to fully understand what is happening under the hood. This talk aims to eliminate that barrier by presenting it in an easier-to-digest manner.', \"Are you an intermediate python developer looking to level up? Luckily, python provides us with a unique set of tools to make our code more elegant and readable by providing language features that make your code more intuitive and cut down on repetition. In this talk, Iâ\\x80\\x99ll share practical pythonic solutions for supercharging your code. \\r\\n\\r\\nSpecifically, I'll cover:\\r\\n\\r\\n- What magic methods are, and show you how to use them in your own code.\\r\\n- When and how to use partial methods.\\r\\n- An explanation of ContextManagers and Decorators, as well as multiple techniques for implementing them.\\r\\n- How to effectively use `NamedTuples`, and even subclass and extend them!\\r\\n\\r\\nLastly, I'll go over some example code that ties many of these techniques together in a cohesive way. You'll leave this talk feeling confident about using these tools and techniques in your next python project!\", \"Anyone who is interested in deep learning has gotten their hands dirty playing around with Tensorflow, Google's open source deep learning framework. Tensorflow has its benefits like wide scale adoption, deployment on mobile, and support for distributed computing, but it also has a somewhat challenging learning curve, is difficult to debug, and hard to deploy in production. PyTorch is a new deep learning framework that solves a lot of those problems.\\r\\n\\r\\nPyTorch is only in beta, but users are rapidly adopting this modular deep learning framework. PyTorch supports tensor computation and dynamic computation graphs that allow you to change how the network behaves on the fly unlike static graphs that are used in frameworks such as Tensorflow. PyTorch offers modularity which enhances the ability to debug or see within the network and for many, is more intuitive to learn than Tensorflow.\\r\\n\\r\\nThis talk will objectively look at PyTorch and why it might be the best fit for your deep learning use case and we'll look at use cases that will showcase why you might want consider using Tensorflow instead.\", 'At the end of 2017, there were seven states with ongoing redistricting litigation. We will discuss a statistical model that the United States Supreme Court declared to be appropriate in cases of racial gerrymandering, and show how it can be implemented and used with the library `PyMC3`. We will also discuss what the model tells us about racial gerrymandering in North Carolina.', 'Today, services built on Python 3.6.3 are widely used at Facebook. But as recently as May of 2014 it was actually impossible at all to use Python 3 at Facebook. Come learn how we cut the Gordian Knot of dependencies and social aversion to the point where new services are now being written in Python 3 while older Python 2 projects are actively migrated to Python 3. All accomplished by a small group of individual contributors in their spare time. Learn to fight the good fight and upgrade your organization to Python 3 like we did at Facebook.', 'You maintain an Open Source project with great code? Yet your project isnâ\\x80\\x99t succeeding in the ways you want? Maybe youâ\\x80\\x99re struggling with funding or documentation? Or you just canâ\\x80\\x99t find new contributors and youâ\\x80\\x99re drowning in issues and pull requests?\\r\\nOpen Source is made up of many components and we are often better-trained in methods for writing good code, than in methods for succeeding in the other dimensions we want our project to grow. \\r\\nIn this talk weâ\\x80\\x99ll explore the different components of an Open Source project and how they work together. After this talk youâ\\x80\\x99ll be well-equipped with a ideas and strategies for growing, cultivating, and nourishing your Open Source project. \\r\\n\\r\\nFor your project to succeed, all of its non-code components must be well-maintained. What are these different components and what methods can we learn to maintain them?\\r\\n\\r\\n* Build real relationships with your sponsors and determine ways how both sides can benefit from this relationship, donâ\\x80\\x99t just ask people for money. \\r\\n* Establish a good communication system with your contributors: Keep them informed, listen to their feedback and input, make them feel heard. \\r\\n* Thank the people who worked on ticket triage or marketing, not just those who wrote code, in your release notes. \\r\\n* Make it easy for new contributors to get started: Write and maintain good documentation, answer questions in a friendly and timely manner. \\r\\n* Market and evangelize in the right places and at the right time: Give conference talks, organize sprints, keep your projectâ\\x80\\x99s Twitter account active, always curate new and interesting content on your blog or website.\\r\\n* Implement a Code of Conduct and enforce it if needed: Make your project a safe space to contribute for everyone. \\r\\n\\r\\nWith these methods and a half-dozen others, youâ\\x80\\x99ll handle beautifully all the components your project needs to succeed.', \"Resources are files that live within Python packages. Think test data files, certificates, templates, translation catalogs, and other static files you want to access from Python code. Sometimes you put these static files in a package directory within your source tree, and then locate them by importing the package and using its `__file__` attribute. But this doesn't work for zip files!\\r\\n\\r\\nYou could use `pkg_resources`, an API that comes with `setuptools` and hides the differences between files on the file system and files in a zip file. This is great because you don't have to use `__file__`, but it's not so great because `pkg_resources` is a big library and can have potentially severe performance problems, even at import time.\\r\\n\\r\\nWelcome to `importlib.resources`, a new module and API in Python 3.7 that is also available as a standalone library for older versions of Python. `importlib.resources` is build on top of Python's existing import system, so it is very efficient. It also defines an abstract base class which loaders can implement to provide their own resource access. Python's built-in zipimporter uses this to provide efficient access to resources within a zip file. Third party import hooks can do the same, so resources can come from anything that is importable by Python.\\r\\n\\r\\nThis talk will step through the motivations behind `importlib.resources`, the library's usage, its interfaces, and the hooks made available to third party packages. It will also talk about the minor differences between the standalone version and the version in Python 3.7's standard library. Hopefully audience members will come away with compelling reasons to port their code to this much more efficient library.\", 'Have you ever considered how many relationships you have in your virtual life? Every friend or page liked on Facebook, each connection in LinkedIn or Twitter account followed is a new relationship not only between two people, but also between their data. In Brazil only, we have 160 millions Facebook users. How can we represent and manipulate all these relationships? Graph Databases are storage systems that use graph structure (nodes and edges) to represent and store data in a semantic way.\\r\\n\\r\\nThis talk will begin approaching the challenge in representing relationships in Relational Databases and introducing a more friendly solution using graph. The definition of Graph Database, its pros and cons and some available tools (Neo4J, OrientDB and TitanDB) will be shown during the presentation, as well as how these tools can be integrated with Python.', \"During peak hours, Netflix video streams make up more than one third of internet traffic. Netflix must stream uninterrupted in the face of widespread network issues, bad code deploys, AWS service outages, and much more. Failovers make this possible.\\r\\n\\r\\nFailover is the process of transferring all of our traffic from one region in AWS to another. While most of Netflix runs on Java, failovers are powered entirely by Python. Python's versatility and rich ecosystem means we can use it for everything from predicting our traffic patterns to orchestrating traffic movement, while dealing with the eventual consistency of AWS.\\r\\n\\r\\nToday, we can shift all of our 100 million+ users in under seven minutes. A lot of engineering work went into making this possible. The issues we faced and solutions we created have broad application to availability strategies in the cloud or the datacenter.\", 'A function is a small chunk of code that does useful work. Your job when writing a function is to do it in a way that it easy to read. Based on over 15 years of code reviews here are some tips and guidelines I give again and again.', 'The DevOps movement gave us many ways to put Python applications into production. But should your *application* care? Should it need to know whether itâ\\x80\\x99s running on your notebook, on a server, in a Docker container, or in some cloud platform as a service?\\r\\n\\r\\nIt should not, because environment-agnostic applications are easier to **test**, easier to **deploy**, easier to **handle**, and easier to **scale**.\\r\\n\\r\\nBut how can you *practically* structure and configure your applications to make them indifferent to the environment they run in? How do secrets fit into the picture? And where do you put that log file?\\r\\n\\r\\nBy the end of this talk youâ\\x80\\x99ll know the tools and techniques that enable you to write such Python applications and youâ\\x80\\x99ll be ready for the next big change.', 'New conferences rarely have resources to run the sort of outreach and inclusion programs that big conferences have. Itâ\\x80\\x99s hard to guess how much money youâ\\x80\\x99ll have to spend, how many attendees youâ\\x80\\x99ll have, and what your new community will look like. With so many things to worry about, itâ\\x80\\x99s no surprise that most events donâ\\x80\\x99t prioritise outreach until theyâ\\x80\\x99ve got a few years under their belt, if at all.\\r\\n\\r\\nIt doesnâ\\x80\\x99t have to be this way, and it can even be easier to build a new event around outreach and inclusion than it is to build it in later on!\\r\\n\\r\\nThis talk shares the story of North Bay Pythonâ\\x80\\x99s inaugural conference, which we planned in under 6 months, ran on a $40,000 budget, and built a welcoming community to make it real. We made inclusivity a founding principle and did so without compromising our speaker lineup while still attracting great sponsorship and hosted an event that almost every attendee wants to return to.\\r\\n\\r\\nIn this talk, weâ\\x80\\x99re going to share with you how we built a conference, from the ground up, to be as inclusive as we could make it. Weâ\\x80\\x99ll touch on early organisation, marketing, and on-the ground logistics. Throughout the talk, youâ\\x80\\x99ll learn:\\r\\n\\r\\n* How we designed a budget that let us prioritise outreach and inclusion activities\\r\\n* How we built the community that we wanted before the conference even started\\r\\n* How we ran an event that proved that we meant everything we said\\r\\n\\r\\nYou too can host a new conference with a great lineup on a shoestring budget and short timeline, and you can do it while being inclusive, welcoming, and putting attendee safety first. Find out how you can have your cake, eat it, and still have lots to share with your new community.', \"Most software has a user. Depending on the software, the user may need to provide various details about themselves for proper operation -- their name, their date of birth, where they live. However, it is quite common for software systems such as these to ask the wrong questions, collect too much data, and when it comes down to it, serialise the parts of the user's identity wrongly. This talk will discuss common ways that real-world systems store identity wrong, what questions you shouldn't ask, and how you can fix it in your own projects.\", \"Timezones are one of those things every programmer loves to hate. Most of us, at\\r\\nleast in the US, just try to ignore them and hope nobody notices. Then twice a\\r\\nyear, we fear with impending doom those 3 small words: Daylight Saving Time.\\r\\n\\r\\nIt doesn't have to be this way. Armed with some best practices and a little help\\r\\nfrom supporting libraries, timezone-related bugs can be a thing of the past.\\r\\n\\r\\nThis talk explores standard library and 3rd party library timezone support, as\\r\\nwell as persistence and serialization techniques for timezone-aware datetimes.\\r\\nBy the end of the talk, the listener should feel confident in their ability to\\r\\ncorrectly store, send, receive, and manipulate datetime objects in any timezone.\", \"Questions and confusion about the Python packaging ecosystem abound. What is this `setup.py` file? What's the difference between wheels and eggs? Do I use setuptools or distutils? Why should I use twine? Do I put my projects dependencies in a `requirements.txt` or in `setup.py`? How do I just get my module up on PyPI? Wait, what is Warehouse?\\r\\n\\r\\nThis talk will identify the key tools one might encounter when trying to distribute Python software, what they are used for, why they exist, and their history (including where their weird names come from). In addition, we'll see how they all work together, what it takes to make them work, and what the future has in store for Python packaging.\", \"Unless you work on pacemakers or at NASA, you've probably accepted the fact that you will make mistakes in your code, and those mistakes will creep into production. This talk will introduce you to post-mortems, and how to use them as a vehicle for improving your code and your process.\", 'Imagine you have an appointment in a large building you do not know. Your host sent instructions describing how to reach their office. Though the instructions were fairly clear, in a few places, such as at the end, you had to infer what to do. How does a _robot (agent)_ interpret an instruction in the environment to infer the correct course of action? Enabling harmonious _Human - Robot Interaction_ is of primary importance if they are to work seamlessly alongside people.\\r\\n\\r\\nDealing with natural language instructions in hard because of two main reasons, first being, Humans - through their prior experience know how to interpret natural language but agents canâ\\x80\\x99t, and second is overcoming the ambiguity that is inherently associated with natural language instructions. This talk is about how deep learning models were used to solve such complex and ambiguous problem of converting natural language instruction into its corresponding action sequence.\\r\\n\\r\\nFollowing verbal route instructions requires knowledge of language, space, action and perception. In this talk I shall be presenting, a neural sequence-to-sequence model for direction following, a task that is essential to realize effective autonomous agents.\\r\\n\\r\\nAt a high level, a sequence-to- sequence model is an end-to-end model made up of two recurrent neural networks: \\r\\n\\r\\n - **Encoder** - which takes the modelâ\\x80\\x99s input sequence as input and encodes it into a fixed-size context vector.\\r\\n - **Decoder** - which uses the context vector from above as a seed from which to generate an output sequence. \\r\\n\\r\\nFor this reason, sequence-to-sequence models are often referred to as _encoder-decoder_ models. The alignment based encoder-decoder model would translate the natural language instructions into corresponding action sequences. This model does not assume any prior linguistic knowledge: syntactic, semantic or lexical. The model learns the meaning of every word, including object names, verbs, spatial relations as well as syntax and the compositional semantics of the language on its own.\\r\\n\\r\\nIn this talk, steps involved in pre-processing of data, training the model, testing the model and final simulation of the model in the virtual environment will be discussed. This talk will also cover some of the challenges and trade-offs made while designing the model.', \"Wrestling bugs can be one of the most frustrating parts of programming - but with the right framing, bugs can also be our best allies. I'll tell the tales of two of my favorite bugs, including the time I triggered a DDOS of a logging cluster, and explain why I love them. I'll also give you concrete strategies for approaching tricky bugs and making them easier and more fun.\", 'Those of us who have worked in software development for longer than a few years probably feel we have an intuitive sense of what a great developer is. Some traits come more easily to mind than others when it comes to identifying a great developer. In this talk we will take a slightly different approach to evaluating software development best practices, and identify one underrated skill common to great software developers: empathy. I hope to demonstrate that cognitive and emotional empathy skills are critical to good software development. We will explore ways to cultivate this trait in order to become better developers, both for our own sakes and for the sake of the teams in which we work.', \"What do AWS, GitHub, Travis CI, DockerHub, Google, Stripe, New Relic, and the rest of the myriad of services that make our developer life easier have in common?\\r\\n They all give you secret keys to authenticate with. Did you ever commit one of these to source control by mistake? That happened to me more times than I'm willing to admit!\\r\\n\\r\\nIn this talk I'm going to go over the best practices to follow when writing Python applications that prevent this type of accident.\", 'Python provides a powerful platform for working with data, but often the most straightforward data analysis can be painfully slow. When used effectively, though, Python can be as fast as even compiled languages like C. This talk presents an overview of how to effectively approach optimization of numerical code in Python, touching on tools like numpy, pandas, scipy, cython, numba, and more.', 'This talk is about the history of Python packaging, the tools that have been historically available for application deployment, the problems/constraints presented by them, and presents a holistic solution to many of these problems: Pipenv.\\r\\n\\r\\nA live demo of the tool will be presented, as well as a Q&A session.', \"Each member of your project team uses something different to document\\r\\ntheir work -- RestructuredText, Markdown, and Jupyter Notebooks. How do\\r\\nyou combine all of these into useful documentation for your project's users.\\r\\nSphinx and friends to the rescue!\\r\\n\\r\\nLearn how to integrate documentation into your everyday development\\r\\nworkflow, apply best practices, and use modern development tools and\\r\\nservices, like Travis CI and ReadTheDocs, to create engaging and up-to-date\\r\\ndocumentation which users and contributors will love.\", 'The genome of a typical microbe contains roughly 5 million base pairs of DNA including > 4000 genes, which provide the instructions for cellular replication, energy metabolism, and other biological processes. At Zymergen, we edit DNA to design microbes with improved ability to produce valuable materials and molecules. Microbes with these edits are built and tested in high throughput by our fleet of robots. Genomes are far too large for exhaustive search, so identifying which edits to make requires machine learning on non-standard features. Our task to extract information from trees, networks, and graphs of independently representable knowledge bases (metabolism, genomics, regulation), in ways that respect the strongly causal relationships between systems. In this talk, I will describe how we use Pythonâ\\x80\\x99s biological packages (e.g. BioPython, CobraPy, Escher, goatools) and other packages (NetworkX, TensorFlow, PyStan, AirFlow) to extract machine learning features and predict which genetic edits will produce high-performance microbes.', 'If youâ\\x80\\x99ve spent much time writing (or debugging) Python performance problems, youâ\\x80\\x99ve probably had a hard time managing memory with its limited language support. \\r\\n\\r\\nIn this talk, we venture deep into the belly of the Rust Language to uncover the secret incantations for building high performance and memory safe Python extensions using Rust. \\r\\n\\r\\nRust has a lot to offer in terms of safety and performance for high-level programming languages such Python, Ruby, Js and more with its easy Foreign Function Interface capabilities which enables developers to easily develop bindings for foreign code.', \"The end of life for Python 2 is 2020. Python 3 is the future and you'll need to consider both your upgrade plan and what steps you'll take after upgrading to start leveraging Python 3 features.\\r\\n\\r\\nDuring this talk we'll briefly discuss how to start **the process of upgrading your code to Python 3**. We'll then dive into some of **the most useful Python 3 features** that you'll be able to start embracing once you drop Python 2 support.\\r\\n\\r\\nA number of the most powerful Python 3 features are syntactic features that are **Python 3 only**. You won't get any experience using these features until you fully upgrade. These features are an incentive to drop Python 2 support in existing 2 and 3 compatible code. You can consider this talk as a teaser of Python 3 features that you may have never used.\\r\\n\\r\\nAfter this talk I hope you'll be inspired to fully upgrade your code to Python 3.\", \"Looking back at Python evolutions over the last 10 years.\\r\\n\\r\\nPython 3.0 was released ten years ago (December 2008). It's time to look back: analyze the migration from Python 2 to Python 3, see the progress we made on the language, list bugs by cannot be fixed in Python 2 because of the backward compatibility, and discuss if it's time or not to bury Python 2.\\r\\n\\r\\nPython became the defacto language in the scientific world and the favorite programming language as the first language to learn programming.\", 'For 2 years, a family of three has traveled on a converted school bus from conference to conference, building tooling for the road in Python and visiting Python families in every corner of the country.', 'What do geiger counters, black holes, heart monitors, and volcanoes have in common? They all can use sound to convey information! This talk will explore using python for sonification: the process of translating data into sound that could otherwise be represented visually. Have you ever wondered how to use python to represent data other than making charts and graphs? Are you a musician looking for inspiration in the world around you? This talk will go over how to use python to translate time series data to MIDI that can be played back in real time. Weâ\\x80\\x99ll sonically interpret light-curve data from the Kepler space telescope using pygame, MIDIUtil, and astropy, turning points on a graph into a musical masterpiece! Come learn about how data sonification is used to help people, to expand the reach of scientific research, and to create music from data.', 'Quantum computers are slowly turning in to reality more than 30 years after they were first theorized. The need for quantum computers have become clear as we reach the limits of Mooreâ\\x80\\x99s law and yet we need more computational power. We are at a very early stage of quantum computing. Yet Python is slowly becoming a defacto language for programming quantum computers. \\r\\n\\r\\nIn this talk, we will discuss the difference a traditional computer and a quantum computer. We will learn about the two architectures namely Quantum annealing and Quantum gate. Finally, we will learn to program quantum computers using Python.', 'Python 3 removes a lot of the confusion around Unicode handling in Python, but that by no means fixes everything. Different locales and writing systems have unique behaviours that can trip you up. Hereâ\\x80\\x99s some of the worst ones and how to handle them correctly.', 'Occasionally weâ\\x80\\x99ll find that some bit of Python weâ\\x80\\x99ve written doesnâ\\x80\\x99t run as fast as weâ\\x80\\x99d like, what can we do? Performance bottlenecks arenâ\\x80\\x99t always intuitive or easy to spot by reading code so we need to collect data with [profiling](https://docs.python.org/3.6/library/profile.html). Once weâ\\x80\\x99ve identified the bottleneck weâ\\x80\\x99ll need to change our approach, but what options are faster than others?\\r\\n\\r\\nThis talk illustrates a Python performance investigation and improvements using an [Advent of Code](http://www.adventofcode.com/) programming challenge. Iâ\\x80\\x99ll walk through starting from a slow (but correct) solution, look at profiling data to investigate _why_ itâ\\x80\\x99s slow, and explore multiple paths for improving performance, including more efficient algorithms and using third-party tools like [Cython](http://cython.org/). Youâ\\x80\\x99ll leave this talk with a recipe for analyzing Python performance and information about some options for improved performance.', 'There are many computational needs for randomness--from creating a game to building a simulation involving naturally occurring randomness similar to the physical world. For most purposes using the python math module to create random numbers within a specific range can be done with no further questions, but sometimes we require a more nuanced implementation. \\r\\n\\r\\nWe will look at both pseudo-random number generators, which use statistically repeatable processes to generate seemingly random series and true random number generators, which inject physical processes like atmospheric noise to generate sequences of numbers. We will discuss the benefits and drawbacks of both approaches and common methods of implementing these two types of generators in python. \\r\\n\\r\\nFinally, we will look at several real applications for randomness and discuss the best method for generating â\\x80\\x9crandomnessâ\\x80\\x9d in each scenario.', \"Web applications contains lots of database operations, network calls, nested callbacks and other computationally expensive tasks that might take a long time to complete or even block other threads until it's done, here is where ReactiveX enters, it doesn't only gives us the facility to convert almost anything to a stream; variables, properties, user inputs, caches, etc to manage it asynchronously. But it also gives us an easy way to handle errors which is a hard task within asynchronous programming. ReactiveX makes our code more flexible, readable, maintainable and easy to write.\\r\\n\\r\\nWe will be exploring how ReactiveX help us to make things easier with its operators toolbox that can be used to filter, create, transform or unify any of those streams. We will learn that in just a few lines of maintainable code, we can have multiple web sockets which recieves multiple requests all handled by an asynchronous process that serves a filtered output.\\r\\n\\r\\nTo do that I decided to explain an example of the use with an example by implementing observables, observers/subscribers and subjects. We will start by requesting our data stream from the Github API with a Tornado web socket and then filtering and processing it asynchrounosly.\", \"Writing lexers and parsers is a complex problem that often involves the use of special tools and domain specific languages (e.g., the lex/yacc tools on Unix). In 2001, I wrote Python versions of these tools which can be found in the PLY project. PLY predates a huge number of modern Python features including the iteration protocol, generators, decorators, metaclasses, and more. As such, it relied on a variety of clever hacks to layer a domain specific parser specification language on top of Python itself. \\r\\n\\r\\nIn this talk, I discuss a modernization of the PLY project that abandons its past and freely abuses modern Python features including advanced metaclasses, guaranteed dictionary ordering, class decorators, type hints, and more. The result of this work can be found in the SLY project. However, this talk isn't so much about SLY as it is focused on how far you can push Python metaprogramming features to create domain-specific languages. Prepare to be horrified--and to write code that will break your IDE.\", \"The WSGI (Web Server Gateway Interface) specification for hosting Python web applications was created in 2003. Measured in Internet time, it is ancient. The oldest main stream implementation of the WSGI specification is mod_wsgi, for the Apache HTTPD server and it is over 10 years old.\\r\\n\\r\\nWSGI is starting to be regarded as not up to the job, with technologies such as HTTP/2, web sockets and async dispatching being the way forward. Reality is that WSGI will be around for quite some time yet and for the majority of use cases is more than adequate.\\r\\n\\r\\nThe real problem is not that we need to move to these new technologies, but that we aren't using the current WSGI servers to their best advantage. Moving to a new set of technologies will not necessarily make things better and will only create a new set of problems you have to solve.\\r\\n\\r\\nAs one of the oldest WSGI server implementations, Apache and mod\\\\_wsgi may be regarded as boring and not cool, but it is still the most stable option for hosting WSGI applications available. It also hasn't been sitting still, with a considerable amount of development work being done on mod\\\\_wsgi in the last few years to make it even more robust and easier to use in a development environment as well as production, including in containerised environments.\\r\\n\\r\\nIn this talk you will learn about many features of mod\\\\_wsgi which you probably didn't even know existed, features which can help towards ensuring your Python web application deployment performs to its best, is secure, and has a low maintenance burden.\\r\\n\\r\\nTopics which will be covered include:\\r\\n\\r\\n* Easy deployment of Python web applications using mod\\\\_wsgi-express.\\r\\n* Integration of mod_wsgi-express with a Django web application.\\r\\n* Using mod\\\\_wsgi-express in a development environment.\\r\\n* How to make use of mod\\\\_wsgi-express in a production environment.\\r\\n* Using mod_wsgi-express in a containerised runtime environment.\\r\\n* Ensuring consistency between development and production environments using warpdrive.\\r\\n* Using mod\\\\_wsgi-express to bootstrap a system Apache installation for hosting WSGI applications.\\r\\n* Why you should be using daemon mode of mod\\\\_wsgi and not embedded mode.\\r\\n* How to properly associate mod\\\\_wsgi with a Python virtual environment.\\r\\n* Building a robust deployment that can recover from misbehaving application code, backend services, or request overloading.\\r\\n* Using hooks provided by mod\\\\_wsgi to monitor the performance of your Python web application.\\r\\n\\r\\nIf you are a beginner, come learn why mod\\\\_wsgi is still a good option for deploying your Python web applications. If you are an old time user of mod\\\\_wsgi, find out about all the features you probably didn't know existed, revisit your current Python web application deployment and make it even better.\", 'When you think of an API, youâ\\x80\\x99re probably thinking about a web service. But itâ\\x80\\x99s important to think about your developer interface when designing a software library as well! Iâ\\x80\\x99ll talk about the scikit-learn package, and how its API makes it easy to construct complex models from simple building blocks, using three basic pieces: transformers, estimators, and meta-estimators. Then Iâ\\x80\\x99ll show how this interface enabled us to construct our own meta-estimator for model stacking. This will demonstrate how to implement new modeling techniques in a scikit-learn style, and more generally, the value of writing libraries with the developer interface in mind.', \"Stop writing crappy shell scriptsâ\\x80\\x94write crappy Python scripts instead!\\r\\n\\r\\nOther talks will show you how to write clean, performant, robust Python. But that's not always necessary. When writing personal automation or solving one-shot problems, it can be safe (and fun!) to quickly hack something together.\\r\\n\\r\\nThis talk will show examples of problems suitable for this approach, scenarios where it's reasonable to cut corners, novel techniques that can help break a problem down, and shortcuts that can speed development.\", \"At some point, we all find ourselves at a SQL prompt making edits to the production database. We know it's a bad practice and we always intend to put in place safer infrastructure before we need to do it again â\\x80\\x94 what does a better system actually look like?\\r\\n\\r\\nThis talk progresses through 5 strategies for teams using a Python stack to do SQL writes against a database, to achieve increasing safety and auditability:\\r\\n\\r\\n(1) Develop a process for raw SQL edits \\r\\n(2) Run scripts locally\\r\\n(3) Deploy and run scripts on an existing server\\r\\n(4) Use a task runner\\r\\n(5) Build a Script Runner service\\r\\n\\r\\nWeâ\\x80\\x99ll talk about the pros and cons of each strategy and help you determine which one is right for your specific needs.\\r\\n\\r\\nBy the end of this talk youâ\\x80\\x99ll be ready to start upgrading your infrastructure for making changes to your production database safely!\", 'Taking on leadership roles always includes new demands on your attention and time. Inevitably, your finite work week will conflict with the sheer amount of tasks you have to do. How can we as leaders keep stepping up to new responsibilities while balancing our pre-existing ones?\\r\\n\\r\\nThis talk will focus on strategies for managing a too-large workload without abandoning important tasks or doing a shoddy job. Weâ\\x80\\x99ll look at techniques to prioritize what work matters most, identify tasks we should be doing ourselves, and finally delegate the rest to build our teamâ\\x80\\x99s skills while reducing our own workload.', \"Done! Your shiny new application is functionally complete and ready to be deployed to production! But how exactly do you deploy properly on Linux? Wonder no more! In 30 minutes, this talk explains how you can harness the power of the init system and systemd to solve common deployment problems, including some that you didn't even know you had. Examples of things we will cover:\\r\\n\\r\\n* How to secure your system by having: private /tmp for your process, read-only paths so that your process can not write to them, inaccessible paths, protect users home, network access, bin directories, etc.\\r\\n* How to limit the resources you app can consume.\\r\\n* How to interact directly with systemd, so it can start transient units, start/stop services, mount disks, resolve addresses.\\r\\n* How to isolate your service without containers.\\r\\n* How to isolate your service using containers (using systemd to spawn a namespace).\\r\\n\\r\\nAll this will be covered from a Python developer's perspective.\", \"The Django Channels project has taken a major turn with version 2.0, embracing Python's async functionality and building applications around an async event loop rather than worker processes.\\r\\n\\r\\nDoing this, however, wasn't easy. We'll look through some of the techniques used to make Django coexist in this async world, including handing off between async and sync code, writing fully asynchronous HTTP and WebSocket handling, and what this means for the future of Django, and maybe Python web frameworks in general.\", \"Get under the hood and learn about Python's beloved Abstract Syntax Tree. Ever wonder how Python code is run? Overheard people arguing about whether Python is interpreted or compiled? In this talk, we will delve into the lifecycle of a piece of Python code in order to understand the role that Python's Abstract Syntax Tree plays in shaping the runtime of your code. Utilizing your newfound knowledge of Python's AST, you'll get a taste of how you probably already rely on ASTs and how they can be used to build awesome tools.\", \"As web apps grow increasingly complex, distributing asynchronous work across multiple background workers is often a basic requirement of a performant app. While there are a variety of tools that exist to solve this issue, one common feature among them is the need for a robust messaging platform.\\r\\n\\r\\n[RabbitMQ][1] is a stable, full-featured, and mature solution that is usually found in the Python ecosystem backing [Celery][2] implementations. While Celery's utilization of RabbitMQ works just fine out of the gate, users with complex workflows, unique constraints, or tight budgets can take advantage of the flexibility of RabbitMQ to streamline their data pipelines and get the most out of their infrastructure.\\r\\n\\r\\nThis talk will provide an overview of RabbitMQ, review its varied message-routing capabilities, and demonstrate some of the ways in which these features can be utilized in Python applications to solve common yet difficult use-cases.\\r\\n\\r\\n [1]: https://www.rabbitmq.com/\\r\\n [2]: http://www.celeryproject.org/\", 'Projects fail in droves. Systems hiccup and hours of downtime follows. Screws fall out all the time; the world is an imperfect place.\\r\\n\\r\\nWe talk a lot about building resilient systems, but all systems are (at least for now) built by humans. Humans who have been making the same types of mistakes for thousands of years. \\r\\n\\r\\nJust because failure happens doesnâ\\x80\\x99t mean we canâ\\x80\\x99t do our best to prevent it orâ\\x80\\x94at the very leastâ\\x80\\x94to minimize the damage when it does. As a matter of fact, embracing failure can be one of the best things you do for your system. Failure is a vital part of evolution. By learning to love failure we learn how to take the next step forward. Ignoring or punishing failure leads to stagnation and wasted potential.\\r\\n\\r\\nThis talk distills 3000 pages of failure research into 40 minutes of knowledge about the human factors of failure, how it can be recognised, and how you can work around it to create more resilient systems.\\r\\n\\r\\nBy the end of this talk the audience will have an awareness of the most common psychological reasons for mistakes and failures and how to develop systems and processes to protect against them.', 'All the data in the world is useless if you cannot understand it. EDA and data visualization are the most crucial yet overlooked stage in analytics process. This is because they give insights on the most relevant features in a particular data set required to build an accurate model. It is often said that the more the data, the better the model but sometimes, this can be counter-productive as more data can be a disadvantage. EDA helps avoid that.\\r\\n\\r\\nEDA is useful for professionals while data visualization is useful for end-users. \\r\\n\\r\\nFor end-users: \\r\\nA good sketch is better than a long speech. The value of a machine learning model is not known unless it is used to make data driven decisions. It is therefore necessary for data scientists to master the act of telling a story for their work to stay relevant. This is where data visualization is extremely useful. \\r\\nWe must remember that the end-users of the results are not professionals like us but people who know little or nothing about data analysis. For effective communication of our analysis, there is need for a detailed yet simple data visualization because the work of a data scientist is not done if data-driven insights and decisions are not made.\\r\\n\\r\\nFor professionals:\\r\\nHow do you ensure you are ready to use machine learning algorithms in a project? How do you choose the most suitable algorithms for your data set? How do you define the feature variables that can potentially be used for machine learning? Most data scientists ask these questions. EDA answers these questions explicitly.\\r\\nAlso, EDA helps in understanding the data. Understanding the data brings familiarity with the data, giving insights on the best models that fit the data set, the features in the dataset that will be useful for building an accurate machine learning model, making feature engineering an easy process.\\r\\n\\r\\nIn this talk, I will give a detailed explanation on what EDA and data visualization are and why they are very helpful in building accurate machine learning models for analytics as well as enhancing productivity and better understanding for clients. I will also discuss the risks of not mastering EDA and data visualization as a data scientist.', 'Congratulations on finishing your first tutorials or classes in python! In the parlance of the heroâ\\x80\\x99s journey myth, youâ\\x80\\x99ve had your â\\x80\\x98threshold momentâ\\x80\\x9d: youâ\\x80\\x99ve started down a path that could lead to a long and fulfilling career. But the road to this glorious future is frustratingly obscured by a lack of guidance in the present. You know enough to realize that you donâ\\x80\\x99t have all the skills you need yet, but itâ\\x80\\x99s hard to know how to learn those skills, or even articulate what they are. There are no easy solutions to this problem. There are, however, a few fundamental things to know and advice to keep in mind. Drawing from my own experience and with input from others, Iâ\\x80\\x99ve compiled some helpful hints about the skills, tools, and guiding questions that will get you to mastery.', \"Python's cyclic garbage collector wonderfully hides the complexity of memory management from the programmer. But we pay the price in performance. Ever wondered how that works? In this talk, you'll learn how garbage collection is designed in Python, what the tradeoffs are and how Instagram battled copy-on-write memory issues by disabling the garbage collector entirely.\\r\\n\\r\\nYou'll also learn why that isn't such a great idea after all and how we ended up extending the garbage collector API which allowed us to (mostly) re-enable garbage collection. We'll discuss our upstream contributions to the garbage collector that landed in Python 3.6 and 3.7.\\r\\n\\r\\nThis is an in-depth talk about memory management but no prior experience with CPython internals is necessary to follow it.\", \"Have you ever written a small, elegant application that couldn't keep up with the growth of your data or user demand? Did your beautiful design end up buried in threads and locks? Did Python's very special Global Interpreter Lock make all of this an exercise in futility?\\r\\n\\r\\nThis talk is for you! With the combined powers of AsyncIO and multiprocessing, we'll redesign an old multithreaded application limited by the GIL into a modern solution that scales with the demand using only the standard library. No prior AsyncIO or multiprocessing experience required.\", \"Concurrent programs are super useful: think of web apps juggling lots of simultaneous downloads and websocket connections, chat bots tracking multiple concurrent conversations, or web spiders fetching pages in parallel. But *writing* concurrent programs is complicated, intimidating to newcomers, and often challenging even for experts.\\r\\n\\r\\nDoes it have to be? Python is famous for being simple and straightforward; can Python make concurrent programming simple and straightforward too? I think so. By carefully analyzing usability pitfalls in other libraries, and taking advantage of new Python 3 features, I've come up with a new set of primitives that make it dramatically easier to write correct concurrent programs, and implemented them in a new library called [Trio](https://trio.readthedocs.io). In this talk, I'll describe these primitives, and demonstrate how to use them to implement a basic algorithm for speeding up TCP connections. Compared to the best previous Python implementation, our version turns out to be easier to understand, more correct, and dramatically shorter.\\r\\n\\r\\nThis talk assumes basic familiarity with Python, but does *not* require any prior experience with concurrency, async/await, or networking.\", \"You've heard about Python type annotations, but wondered if they're useful in the real world? Worried you've got too much code and can't afford to annotate it? Type-checked Python is here, it's for real, and it can help you catch bugs and make your code easier to understand. Come learn from our experience gradually typing a million-LOC production Python application!\\r\\n\\r\\nType checking solves real world problems in production Python systems. We'll cover the benefits, how type checking in Python works, how to introduce it gradually and sustainably in a production Python application, and how to measure success and avoid common pitfalls. We'll even demonstrate how modern Python typechecking goes hand-in-hand with duck-typing! Join us for a deep dive into type-checked Python in the real world.\", \"Many projects already take advantage of static analysis tools like flake8, PyLint, and MyPy. Can we do better? In this talk, I'll discuss how to take a type checker, bolt on an interprocedural static analyzer, and delight your security team with high quality results.\\r\\n\\r\\nAbstract \\r\\n\\r\\nIt is incredibly challenging to build a halfway decent static analysis tool for a dynamic language like Python. Fortunately, it gets quite a bit easier with Python type annotations. To explain why, I'll present a tool that finds security vulnerabilities by tracking dangerous flows of information interprocedurally across an entire codebase. **Then,** I'll demonstrate how that tool is really just a slightly slower, more sophisticated, type checker.\", \"When we talk about Web API Design, we're usually driven to think in architecture, verbs, and nouns. But we often forget our user: the developer.\\r\\n\\r\\nUX designers rely on many techniques to create great experiences. User research, User Testing, Personas, Usage Data Analysis and others. However when creating `invisible products` weâ\\x80\\x99re not used to think in usability. So why donâ\\x80\\x99t we take advantage of this background to improve our APIs experiences?\", \"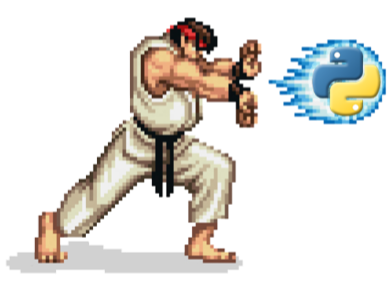\\r\\n\\r\\nHear the story of how we used Python to build an AI that plays Super StreetFighter II on the Super NES. We'll cover how Python provided the key glue between the SNES emulator and AI, and how the AI was built with `gym`, `keras-rl` and `tensorflow`. We'll show examples of game play and training, and talk about which bot beat which bot in the bot-v-bot tournament we ran. \\r\\n\\r\\nAfter this talk you'll know how easy it is to use Python and Python's machine learning libraries to teach a computer to play games. You'll see a practical example of the same type of machine learning used by AlphaGo, and also get to find out which character in StreetFighter II is best to pick when playing your friends.\\r\\n\\r\\n [1]: https://lh3.googleusercontent.com/Mh9uzCm4JeevMN5w-SWJgzWabrqOClAVMsa4jJtMRm-il1dP6oVTsRstJSQlbgKf4qh3A08yMZ36pwezsITA=w3230-h1786\\r\\n [2]: http://www.thesimplelogic.com/wordpress/wp-content/uploads/2017/12/ryu-python.png\", \"Recently, a new LED strip specification, APA102, has been released which allows these strips to be driven by a general purpose CPU instead of a dedicated microcontroller. This allows us the luxury of controlling them with Python!\\r\\n\\r\\nI'll teach you about how to get the the hardware, how to think about programming for lights and how to build anything from a psychedelic art installation to home lighting to an educational tool. \\r\\n\\r\\nProgramming with lights is awesome because you can SEE bugs with your eyes. I think the use of these LED's have great potential as a teaching tool because of the immediacy of the feedback.\\r\\n\\r\\nLIVE hardware demos! See Quicksort in brilliant colors!\", 'Know you should be doing testing but havenâ\\x80\\x99t gotten over the hurdle to learn it? pytest is Pythonâ\\x80\\x99s modern, friendly, and powerful testing framework. When paired with an IDE, testing gets a visual interface, making it much easier to get started.\\r\\n\\r\\nIn this talk we cover â\\x80\\x9cvisual testingâ\\x80\\x9d: starting, learning, using, and mastering test-driven development (TDD) with the help of a nice UI. Weâ\\x80\\x99ll show PyCharm Community Edition, a free and open-source Python IDE, as a productive TDD environment for pytest. Specifically, weâ\\x80\\x99ll show a workflow using pytest and PyCharm that helps make tests speed up development, or at the very least help to make testing seem less \"in the way\" of other development activities', \"How do you become a Python core developer? How can I become one? What is it like to be a Python core developer?\\r\\n\\r\\nThese are the questions I often receive ever since I became a Python core developer a year ago. Contributing to Python is a long journey that does not end when one earns the commit privilege. There are responsibilities to bear and expectations to live up to.\\r\\n\\r\\nIn the past year, I've been learning more about what it really means to be a Python core developer. Let me share all of that with you.\", 'Many of us practice test driven development, and pride ourselves in our code coverage. This is relatively easy to do when you begin a new project, but what happens when you take over an existing code base with little to no tests? Where and how do you start writing tests? This task can be very intimidating and frustrating, but can be accomplished!\\r\\n\\r\\nThis talk will run through some common approaches and methodologies for adding test coverage to pre-existing code (that you might not even be familiar with at all). The next time you take over an untested monolith, you will be able to do the right thing and start writing tests instead of hoping for the best!', 'RESTful has been the go-to choice of API world. Why another API approach? To support more data-driven applications, to provide more flexibility and ease unnecessary code and calls, to address a wide variety of large-scale development problems, **GraphQL** comes with HTTP, JSON, Versioning, Nullability, Pagination, and Server-side Batching & Caching in mind to make API \"Simple yet Powerful\".\\r\\n\\r\\nBy applying [Graphene-Python](http://graphene-python.org/), a library for building GraphQL APIs in Python easily, this talk will go through the background and challenges of applying GraphQL as the new API service in a restaurant POS (point of sale) system within complex cloud infrastructure in Python. Introduction, testing, and live demo is included for sure.', \"Knowing how to code and being able to teach it are two separate skills. When we have expertise in a subject, it's common to take for granted that we'll be able to effectively communicate our expertise to someone else. Come learn (or re-learn!) how to teach and discover practical examples you can put to work right away.\\r\\n\\r\\nBy sharpening your teaching skills, you'll be a more effective mentor, trainer, and team member.\"]\n"
],
[
"df = pd.DataFrame({'description': descriptions})\ndf['char count'] = df.description.apply(len)\ndf.head()",
"_____no_output_____"
],
[
"import textstat\ndf['descr. word count'] = df['description'].apply(textstat.lexicon_count)\ndf.head()",
"_____no_output_____"
],
[
"df['grade level'] = df['description'].apply(textstat.flesch_kincaid_grade)\ndf.head()\n",
"_____no_output_____"
],
[
"df.describe()",
"_____no_output_____"
],
[
"df.describe(exclude=np.number)",
"_____no_output_____"
],
[
"df['tweetable'] = df['char count']<=280\ndf[df['tweetable'] == True]",
"_____no_output_____"
],
[
"plt.hist(df['grade level'])\nplt.title('Histogram of Description Grade Levels')\nplt.show();",
"_____no_output_____"
],
[
"",
"_____no_output_____"
]
]
] | [
"markdown",
"code",
"markdown",
"code",
"markdown",
"code",
"markdown",
"code",
"markdown",
"code",
"markdown",
"code",
"markdown",
"code",
"markdown",
"code",
"markdown",
"code",
"markdown",
"code",
"markdown",
"code",
"markdown",
"code",
"markdown",
"code",
"markdown",
"code",
"markdown",
"code",
"markdown",
"code",
"markdown",
"code",
"markdown",
"code",
"markdown",
"code",
"markdown",
"code"
] | [
[
"markdown",
"markdown"
],
[
"code",
"code"
],
[
"markdown",
"markdown",
"markdown"
],
[
"code"
],
[
"markdown"
],
[
"code"
],
[
"markdown"
],
[
"code"
],
[
"markdown"
],
[
"code"
],
[
"markdown"
],
[
"code"
],
[
"markdown"
],
[
"code"
],
[
"markdown"
],
[
"code"
],
[
"markdown"
],
[
"code"
],
[
"markdown"
],
[
"code"
],
[
"markdown"
],
[
"code",
"code"
],
[
"markdown"
],
[
"code"
],
[
"markdown",
"markdown"
],
[
"code"
],
[
"markdown",
"markdown"
],
[
"code"
],
[
"markdown"
],
[
"code"
],
[
"markdown",
"markdown"
],
[
"code"
],
[
"markdown"
],
[
"code"
],
[
"markdown",
"markdown"
],
[
"code"
],
[
"markdown"
],
[
"code"
],
[
"markdown"
],
[
"code",
"code",
"code",
"code",
"code",
"code",
"code",
"code",
"code",
"code"
]
] |
e766453cef329b181a3e186658f1b6efedd6671c | 32,759 | ipynb | Jupyter Notebook | gan_toy_example.ipynb | acse-wx319/gans-on-multimodal-data | 81466f5fae228b7a6cdf4c0915911604d24e5c70 | [
"MIT"
] | null | null | null | gan_toy_example.ipynb | acse-wx319/gans-on-multimodal-data | 81466f5fae228b7a6cdf4c0915911604d24e5c70 | [
"MIT"
] | null | null | null | gan_toy_example.ipynb | acse-wx319/gans-on-multimodal-data | 81466f5fae228b7a6cdf4c0915911604d24e5c70 | [
"MIT"
] | null | null | null | 87.590909 | 19,860 | 0.778015 | [
[
[
"from lib.dependencies import *\nfrom lib.time_series_dependencies import *\nfrom lib.models import *\nimport os\nimport timeit",
"_____no_output_____"
]
],
[
[
"## Specifications\n\nThese parameters need to be specified prior to running the predictive GAN.",
"_____no_output_____"
]
],
[
[
"# modes\ngp = False\nsn = True\n\n# steps\ntrain = False\nfixed_input = True\neph = '9999' # the model to read if not training\n\n# paramters\nDATASET = 'sine' # sine, moon, 2spirals, circle, helix\nsuffix = '_sn' # suffix of output folder \nLATENT_DIM = 2 # latent space dimension\nDIM = 512 # 512 Model dimensionality\nINPUT_DIM = 2 # input dimension\nLAMBDA = 0.1 # smaller lambda seems to help for toy tasks specifically\nDROPOUT_RATE = 0.1 # rate of dropout \nlr = 1e-4 # learning rate for the optimizer\nCRITIC_ITERS = 5 # how many critic iterations per generator iteration\nBATCH_SIZE = 256 # batch size\nITERS = 30000 # 100000, how many generator iterations to train for\nlog_interval = 1000 # how frequent to write to log and save models \nuse_cuda = False\nplot_3d = (DATASET == 'helix')\nTMP_PATH = 'tmp/' + DATASET + suffix + '/'\n\nif not os.path.isdir(TMP_PATH):\n os.makedirs(TMP_PATH)",
"_____no_output_____"
]
],
[
[
"## Make generator and discriminator\n\nInitializing generator and discriminator objects. The architectures have been declared in lib.models. ",
"_____no_output_____"
]
],
[
[
"netG = Generator(LATENT_DIM, DIM, DROPOUT_RATE, INPUT_DIM)\nif sn:\n netD = DiscriminatorSN(DIM, INPUT_DIM)\nelse:\n netD = Discriminator(DIM, INPUT_DIM)\n\nnetG.apply(weights_init)\nnetD.apply(weights_init)",
"_____no_output_____"
]
],
[
[
"## Train or load model\n\nIf in training mode, a WGAN with either SN or GP will be trained on the specified type of synthetic data (sine, circle, half-moon, helix or double-spirals). A log file will be created with all specifications to keep track of the runs. Loss will be plotted against the number of epochs. Randomly generated samples will also be plotted. The frequency at which to save the plots is specified by the parameter 'log_interval'.\n\nIf not training, the pre-trained models saved in the tmp path will be loaded. Use 'eph' to specify from which epoch to load the models. ",
"_____no_output_____"
]
],
[
[
"if train:\n # start writing log\n f = open(TMP_PATH + \"log.txt\", \"w\")\n # print specifications\n f.write('gradient penalty: ' + str(gp))\n f.write('\\n spectral normalization: ' + str(sn))\n f.write('\\n datasest: ' + DATASET)\n f.write('\\n hidden layer dimension: ' + str(DIM))\n f.write('\\n latent space dimension: ' + str(LATENT_DIM))\n f.write('\\n gradient penalty lambda: ' + str(LAMBDA))\n f.write('\\n dropout rate: ' + str(DROPOUT_RATE))\n f.write('\\n critic iterations per generator iteration: ' + str(CRITIC_ITERS))\n f.write('\\n batch size: ' + str(BATCH_SIZE))\n f.write('\\n total iterations: ' + str(ITERS))\n f.write('\\n')\n # print model structures\n f.write(str(netG))\n f.write(str(netD))\n f.write('\\n')\n \n # option of using GPU\n if use_cuda:\n netD = netD.cuda()\n netG = netG.cuda()\n \n # declare optimizers for generator and discriminator\n optimizerD = optim.Adam(netD.parameters(), lr=lr, betas=(0.5, 0.9))\n optimizerG = optim.Adam(netG.parameters(), lr=lr, betas=(0.5, 0.9))\n \n # helper tensors for backpropogation\n one = torch.FloatTensor([1])\n mone = one * -1\n if use_cuda:\n one = one.cuda()\n mone = mone.cuda()\n \n # make synthetic data \n data = make_data_iterator(DATASET, BATCH_SIZE)\n \n # record loss and wasserstein-1 estimate\n losses = []\n wass_dist = []\n \n # start timing\n start = timeit.default_timer()\n \n # start training \n for iteration in range(ITERS):\n ############################\n # (1) Update D network\n ###########################\n for iter_d in range(CRITIC_ITERS):\n _data = next(data).float()\n if use_cuda:\n _data = _data.cuda()\n \n netD.zero_grad()\n \n # train with real\n D_real = netD(_data)\n D_real = D_real.mean().unsqueeze(0)\n D_real.backward(mone)\n \n # train with fake\n noise = torch.randn(BATCH_SIZE, LATENT_DIM)\n if use_cuda:\n noise = noise.cuda()\n fake = netG(noise)\n D_fake = netD(fake.detach())\n D_fake = D_fake.mean().unsqueeze(0)\n D_fake.backward(one)\n \n # train with gradient penalty\n if gp:\n gradient_penalty = calc_gradient_penalty(netD, _data, fake, BATCH_SIZE, LAMBDA, use_cuda)\n gradient_penalty.backward()\n \n if gp:\n D_cost = abs(D_fake - D_real) + gradient_penalty\n else:\n D_cost = abs(D_fake - D_real)\n \n Wasserstein_D = abs(D_real - D_fake)\n optimizerD.step()\n \n ############################\n # (2) Update G network\n ############################\n netG.zero_grad()\n \n _data = next(data).float()\n if use_cuda:\n _data = _data.cuda()\n \n noise = torch.randn(BATCH_SIZE, LATENT_DIM)\n if use_cuda:\n noise = noise.cuda()\n fake = netG(noise)\n G = netD(fake)\n G = G.mean().unsqueeze(0)\n G.backward(mone)\n G_cost = -G\n optimizerG.step()\n \n losses.append([G_cost.cpu().item(), D_cost.cpu().item()])\n wass_dist.append(Wasserstein_D.cpu().item())\n \n if iteration % log_interval == log_interval - 1:\n # save discriminator model\n torch.save(netD.state_dict(), TMP_PATH + 'disc_model' + str(iteration) + '.pth')\n # save generator model\n torch.save(netG.state_dict(), TMP_PATH + 'gen_model' + str(iteration) + '.pth')\n # report iteration number\n f.write('Iteration ' + str(iteration) + '\\n')\n # report time\n stop = timeit.default_timer()\n f.write(' Time spent: ' + str(stop - start) + '\\n')\n # report loss\n f.write(' Generator loss: ' + str(G_cost.cpu().item()) + '\\n')\n f.write(' Discriminator loss: ' + str(D_cost.cpu().item()) + '\\n')\n f.write(' Wasserstein distance: ' + str(Wasserstein_D.cpu().item()) + '\\n')\n # save frame plot\n noise = torch.randn(BATCH_SIZE, LATENT_DIM)\n if use_cuda:\n noise = noise.cuda()\n\n plot_data(_data.cpu().numpy(), netG(noise).cpu().data.numpy(), str(iteration), TMP_PATH, plot_3d=plot_3d)\n # save loss plot\n fig, ax = plt.subplots(1, 1, figsize=[10, 5])\n ax.plot(losses)\n ax.legend(['Generator', 'Discriminator'])\n plt.title('Generator Loss v.s Discriminator Loss')\n ax.grid()\n plt.savefig(TMP_PATH + 'loss_trend' + str(iteration) + '.png')\n # save wassertein loss plot\n fig, ax = plt.subplots(1, 1, figsize=[10, 5])\n ax.plot(wass_dist)\n plt.title('Wassertein Distance')\n ax.grid()\n plt.savefig(TMP_PATH + 'wass_dist' + str(iteration) + '.png')\n \n # close log file\n f.close()\nelse:\n # if not training, load pre-trained models from local files\n netG.load_state_dict(torch.load(TMP_PATH + 'gen_model' + eph + '.pth'))\n netD.load_state_dict(torch.load(TMP_PATH + 'disc_model' + eph + '.pth')) ",
"_____no_output_____"
]
],
[
[
"## Prediction\n\nFor a list of x, use the trained GAN to make multiple predictions for y. For each x, many predictions will be made. A subset is taken depending on the similarity between the generated x and the specified x. ",
"_____no_output_____"
]
],
[
[
"if fixed_input:\n data = make_data_iterator(DATASET, BATCH_SIZE)\n # sine data \n preds = None\n for x in np.linspace(-4., 4., 17):\n print(x)\n out = predict_fixed(netG, x, 80, 8, INPUT_DIM, LATENT_DIM, use_cuda)\n if preds == None:\n preds = out\n else:\n preds = torch.cat((preds, out))\n# plt.scatter(preds[:, 0], preds[:, 1])\n# plt.show()\n true_dist = next(data)\n fig, ax = plt.subplots(1, 1, figsize=(6, 4))\n plt.scatter(true_dist[:, 0], true_dist[:, 1], c='orange', label='Real data')\n plt.scatter(preds[:, 0], preds[:, 1], c='blue', label='Predictions')\n plt.savefig(TMP_PATH + 'fixed_input' + eph + '.jpg')\n",
"-4.0\n-3.5\n-3.0\n-2.5\n-2.0\n-1.5\n-1.0\n-0.5\n0.0\n0.5\n1.0\n1.5\n2.0\n2.5\n3.0\n3.5\n4.0\n"
]
]
] | [
"code",
"markdown",
"code",
"markdown",
"code",
"markdown",
"code",
"markdown",
"code"
] | [
[
"code"
],
[
"markdown"
],
[
"code"
],
[
"markdown"
],
[
"code"
],
[
"markdown"
],
[
"code"
],
[
"markdown"
],
[
"code"
]
] |
e7664571caeee2b7749929eb298313c21e4da6e7 | 8,865 | ipynb | Jupyter Notebook | examples/Dev.ipynb | AnthonyHorton/asteroid-marker | 5e53c043c97f6e31d63737d41df8ad5a2248918b | [
"MIT"
] | null | null | null | examples/Dev.ipynb | AnthonyHorton/asteroid-marker | 5e53c043c97f6e31d63737d41df8ad5a2248918b | [
"MIT"
] | null | null | null | examples/Dev.ipynb | AnthonyHorton/asteroid-marker | 5e53c043c97f6e31d63737d41df8ad5a2248918b | [
"MIT"
] | null | null | null | 33.707224 | 119 | 0.467343 | [
[
[
"# Core\nfrom pathlib import Path\nimport gzip\nfrom warnings import warn\nimport os\nimport time\n\n# 3rd party\nfrom wget import download\nimport numpy as np\nimport pandas as pd\nimport astropy.units as u\nimport ephem",
"_____no_output_____"
],
[
"def min_magnitude_estimate(minor_planet):\n return minor_planet.H + 2.5 * np.log(minor_planet.Perihelion_dist**2 * (1 - minor_planet.Perihelion_dist)**2)",
"_____no_output_____"
],
[
"class MinorPlanetDB():\n def __init__(self,\n path='../data/mpcorb_extended.json.gz',\n download_url='http://minorplanetcenter.net/Extended_Files/mpcorb_extended.json.gz',\n max_age=1 * u.day):\n \n self.path = Path(path)\n self.url = download_url\n self.max_age = max_age\n \n self.refresh_db()\n \n def refresh_db(self):\n \n # Check if MPCORB database already downloaded. If not attempt to download it\n if not self.path.exists():\n warn(\"MPC Orbit Database file not found. Downloading...\")\n download(self.url, out=self.path.as_posix())\n warn(\"Done.\")\n # Check age of MPCORB database. If exceeds max_age download again\n elif (time.time() - os.path.getmtime(self.path.as_posix())) * u.second > self.max_age:\n warm(\"MPC Orbit Database file stale. Downloading a fresh copy...\")\n download(self.url, out=self.path.as_posix())\n warn(\"Done.\")\n \n # Load MPCORB database as a pandas DataFrame\n with gzip.open(self.path.as_posix(), 'rt') as db:\n self.db = pd.read_json(db)\n \n # Create a unique ID column from Number & Name (if present) or Principal_desig if not\n self.db['ID'] = (self.db.Number + ' ' + self.db.Name).combine_first(self.db.Principal_desig)\n \n # Set unique ID as the index\n self.db.set_index('ID', inplace=True)\n \n def make_bodies(self, magnitude_limit=12):\n \n # Filter minor planets that definitely would be fainter than magnitude limit\n pruned_db = self.db[np.logical_or(lambda x: x.Perihelion_dist < 1.0, \n lambda x: max_magnitude_estimate(x) < magnitude_limit)]\n \n self.bodies = {}\n for ID, minor_planet in pruned_db.iterrows():\n body = ephem.EllipticalBody()\n body.name = ID\n body._inc = minor_planet.i\n body._Om = minor_planet.Node\n body._om = minor_planet.Peri\n body._a = minor_planet.a\n body._M = minor_planet.M\n body._epoch_M = minor_planet.Epoch\n body._e = minor_planet.e\n body._epoch = minor_planet.Epoch\n body._H = minor_planet.H\n body._G = minor_planet.G\n \n self.bodies[ID] = body",
"_____no_output_____"
],
[
"minor_planets = MinorPlanetDB()",
"_____no_output_____"
],
[
"minor_planets.db.info()",
"<class 'pandas.core.frame.DataFrame'>\nIndex: 747239 entries, (1) Ceres to 5154 T-3\nData columns (total 38 columns):\nAphelion_dist 747239 non-null float64\nArc_length 139560 non-null float64\nArc_years 607679 non-null object\nComputer 747239 non-null object\nCritical_list_numbered_object_flag 578 non-null float64\nEpoch 747239 non-null float64\nG 743886 non-null float64\nH 743886 non-null float64\nHex_flags 747239 non-null object\nLast_obs 747239 non-null object\nM 747239 non-null float64\nNEO_flag 16949 non-null float64\nName 21111 non-null object\nNode 747239 non-null float64\nNum_obs 747224 non-null float64\nNum_opps 747239 non-null int64\nNumber 503850 non-null object\nOne_km_NEO_flag 1361 non-null float64\nOne_opposition_object_flag 135573 non-null float64\nOrbit_type 747239 non-null object\nOrbital_period 747239 non-null float64\nOther_desigs 63782 non-null object\nPHA_flag 1861 non-null float64\nPeri 747239 non-null float64\nPerihelion_dist 747239 non-null float64\nPerturbers 631402 non-null object\nPerturbers_2 631402 non-null object\nPrincipal_desig 747136 non-null object\nRef 747239 non-null object\nSemilatus_rectum 747239 non-null float64\nSynodic_period 747239 non-null float64\nTp 747239 non-null float64\nU 639174 non-null object\na 747239 non-null float64\ne 747239 non-null float64\ni 747239 non-null float64\nn 747239 non-null float64\nrms 747056 non-null float64\ndtypes: float64(24), int64(1), object(13)\nmemory usage: 222.3+ MB\n"
],
[
"minor_planets.make_bodies()",
"_____no_output_____"
],
[
"minor_planets.bodies['(1566) Icarus']",
"_____no_output_____"
],
[
"minor_planets.bodies['(1566) Icarus'].compute()",
"_____no_output_____"
],
[
"minor_planets.bodies['(1566) Icarus'].a_ra",
"_____no_output_____"
]
]
] | [
"code"
] | [
[
"code",
"code",
"code",
"code",
"code",
"code",
"code",
"code",
"code"
]
] |
e76646307f09e7e88ec5a8c3984efb07f1a0e204 | 2,409 | ipynb | Jupyter Notebook | tests/nb_builds/nb_preheader/03.03..Part-Interception_and_Rendezvous.ipynb | rmsrosa/nbjoint | 7019ff336e4a7bb1f6ed20da5fd12b9f702c424a | [
"MIT"
] | null | null | null | tests/nb_builds/nb_preheader/03.03..Part-Interception_and_Rendezvous.ipynb | rmsrosa/nbjoint | 7019ff336e4a7bb1f6ed20da5fd12b9f702c424a | [
"MIT"
] | null | null | null | tests/nb_builds/nb_preheader/03.03..Part-Interception_and_Rendezvous.ipynb | rmsrosa/nbjoint | 7019ff336e4a7bb1f6ed20da5fd12b9f702c424a | [
"MIT"
] | null | null | null | 23.851485 | 320 | 0.574927 | [
[
[
"empty"
]
]
] | [
"empty"
] | [
[
"empty"
]
] |
e76655191a276f218b0ca82ec23941650c6e0fd6 | 79,698 | ipynb | Jupyter Notebook | Python_For_DSandAI_5_1_Intro_API.ipynb | ornob39/Python_For_DataScience_AI-IBM- | a6b3462d004425c7e80cc3dbdb2aa6c0f0354f23 | [
"MIT"
] | 1 | 2020-08-12T07:17:45.000Z | 2020-08-12T07:17:45.000Z | Python_For_DSandAI_5_1_Intro_API.ipynb | ornob39/Python_For_DataScience_AI-IBM- | a6b3462d004425c7e80cc3dbdb2aa6c0f0354f23 | [
"MIT"
] | null | null | null | Python_For_DSandAI_5_1_Intro_API.ipynb | ornob39/Python_For_DataScience_AI-IBM- | a6b3462d004425c7e80cc3dbdb2aa6c0f0354f23 | [
"MIT"
] | null | null | null | 55.5 | 33,274 | 0.64171 | [
[
[
"<a href=\"https://colab.research.google.com/github/ornob39/Python_For_DataScience_AI-IBM/blob/master/Python_For_DSandAI_5_1_Intro_API.ipynb\" target=\"_parent\"><img src=\"https://colab.research.google.com/assets/colab-badge.svg\" alt=\"Open In Colab\"/></a>",
"_____no_output_____"
],
[
"<a href=\"https://cognitiveclass.ai/\">\n <img src=\"https://s3-api.us-geo.objectstorage.softlayer.net/cf-courses-data/CognitiveClass/PY0101EN/Ad/CCLog.png\" width=\"200\" align=\"center\">\n</a>\n\n\n\n<h1 align=center><font size = 5><b>A </b>pplication <b>P</b>rogramming <b>I</b>nterface</font> (API)</h1>",
"_____no_output_____"
],
[
"An API lets two pieces of software talk to each other. Just like a function, you don’t have to know how the API works only its inputs and outputs. An essential type of API is a REST API that allows you to access resources via the internet. In this lab, we will review the Pandas Library in the context of an API, we will also review a basic REST API ",
"_____no_output_____"
],
[
"<div class=\"alert alert-block alert-info\" style=\"margin-top: 20px\">\n <a href=\"https://cocl.us/topNotebooksPython101Coursera\">\n <img src=\"https://s3-api.us-geo.objectstorage.softlayer.net/cf-courses-data/CognitiveClass/PY0101EN/Ad/TopAd.png\" width=\"750\" align=\"center\">\n </a>\n</div>",
"_____no_output_____"
],
[
"## Table of Contents\n<div class=\"alert alert-block alert-info\" style=\"margin-top: 20px\">\n<li><a href=\"#ref0\">Pandas is an API</a></li>\n<li><a href=\"#ref1\">REST APIs Basics </a></li>\n<li><a href=\"#ref2\">Quiz on Tuples</a></li>\n\n<p></p>\nEstimated Time Needed: <strong>15 min</strong>\n</div>\n\n<hr>",
"_____no_output_____"
]
],
[
[
"!pip install nba_api",
"Collecting nba_api\n\u001b[?25l Downloading https://files.pythonhosted.org/packages/fd/94/ee060255b91d945297ebc2fe9a8672aee07ce83b553eef1c5ac5b974995a/nba_api-1.1.8-py3-none-any.whl (217kB)\n\u001b[K |████████████████████████████████| 225kB 2.7MB/s \n\u001b[?25hRequirement already satisfied: requests in /usr/local/lib/python3.6/dist-packages (from nba_api) (2.23.0)\nRequirement already satisfied: certifi>=2017.4.17 in /usr/local/lib/python3.6/dist-packages (from requests->nba_api) (2020.6.20)\nRequirement already satisfied: urllib3!=1.25.0,!=1.25.1,<1.26,>=1.21.1 in /usr/local/lib/python3.6/dist-packages (from requests->nba_api) (1.24.3)\nRequirement already satisfied: idna<3,>=2.5 in /usr/local/lib/python3.6/dist-packages (from requests->nba_api) (2.10)\nRequirement already satisfied: chardet<4,>=3.0.2 in /usr/local/lib/python3.6/dist-packages (from requests->nba_api) (3.0.4)\nInstalling collected packages: nba-api\nSuccessfully installed nba-api-1.1.8\n"
]
],
[
[
"<h2 id=\"PandasAPI\">Pandas is an API </h2>",
"_____no_output_____"
],
[
"You will use this function in the lab:",
"_____no_output_____"
]
],
[
[
"def one_dict(list_dict):\n keys=list_dict[0].keys()\n out_dict={key:[] for key in keys}\n for dict_ in list_dict:\n for key, value in dict_.items():\n out_dict[key].append(value)\n return out_dict ",
"_____no_output_____"
]
],
[
[
"<h2 id=\"PandasAPI\">Pandas is an API </h2>",
"_____no_output_____"
],
[
"Pandas is actually set of software components , much of witch is not even written in Python.\n",
"_____no_output_____"
]
],
[
[
"import pandas as pd\nimport matplotlib.pyplot as plt",
"_____no_output_____"
]
],
[
[
"You create a dictionary, this is just data.",
"_____no_output_____"
]
],
[
[
"dict_={'a':[11,21,31],'b':[12,22,32]}",
"_____no_output_____"
]
],
[
[
"When you create a Pandas object with the Dataframe constructor in API lingo, this is an \"instance\". The data in the dictionary is passed along to the pandas API. You then use the dataframe to communicate with the API.",
"_____no_output_____"
]
],
[
[
"df=pd.DataFrame(dict_)\ntype(df)",
"_____no_output_____"
]
],
[
[
"<img src = \"https://s3-api.us-geo.objectstorage.softlayer.net/cf-courses-data/CognitiveClass/PY0101EN/Chapter%206/images/pandas_api.png\" width = 800, align = \"center\" alt=\"logistic regression block diagram\" />",
"_____no_output_____"
],
[
"When you call the method head the dataframe communicates with the API displaying the first few rows of the dataframe.\n\n\n",
"_____no_output_____"
]
],
[
[
"df.head()",
"_____no_output_____"
]
],
[
[
"When you call the method mean,the API will calculate the mean and return the value.",
"_____no_output_____"
]
],
[
[
"df.mean()",
"_____no_output_____"
]
],
[
[
"<h2 id=\"ref1\">REST APIs</h2>",
"_____no_output_____"
],
[
"<p>Rest API’s function by sending a <b>request</b>, the request is communicated via HTTP message. The HTTP message usually contains a JSON file. This contains instructions for what operation we would like the service or <b>resource</b> to perform. In a similar manner, API returns a <b>response</b>, via an HTTP message, this response is usually contained within a JSON.</p>\n<p>In this lab, we will use the <a href=https://pypi.org/project/nba-api/>NBA API</a> to determine how well the Golden State Warriors performed against the Toronto Raptors. We will use the API do the determined number of points the Golden State Warriors won or lost by for each game. So if the value is three, the Golden State Warriors won by three points. Similarly it the Golden State Warriors lost by two points the result will be negative two. The API is reltivly will handle a lot of the details such a Endpoints and Authentication </p>",
"_____no_output_____"
],
[
"In the nba api to make a request for a specific team, it's quite simple, we don't require a JSON all we require is an id. This information is stored locally in the API we import the module teams ",
"_____no_output_____"
]
],
[
[
"from nba_api.stats.static import teams\nimport matplotlib.pyplot as plt",
"_____no_output_____"
],
[
"#https://pypi.org/project/nba-api/",
"_____no_output_____"
]
],
[
[
"The method <code>get_teams()</code> returns a list of dictionaries the dictionary key id has a unique identifier for each team as a value ",
"_____no_output_____"
]
],
[
[
"nba_teams = teams.get_teams()",
"_____no_output_____"
]
],
[
[
"The dictionary key id has a unique identifier for each team as a value, let's look at the first three elements of the list:",
"_____no_output_____"
]
],
[
[
"nba_teams[0:3]",
"_____no_output_____"
]
],
[
[
"To make things easier, we can convert the dictionary to a table. First, we use the function <code>one dict</code>, to create a dictionary. We use the common keys for each team as the keys, the value is a list; each element of the list corresponds to the values for each team.\nWe then convert the dictionary to a dataframe, each row contains the information for a different team.",
"_____no_output_____"
]
],
[
[
"dict_nba_team=one_dict(nba_teams)\ndf_teams=pd.DataFrame(dict_nba_team)\ndf_teams.head()",
"_____no_output_____"
]
],
[
[
"Will use the team's nickname to find the unique id, we can see the row that contains the warriors by using the column nickname as follows:",
"_____no_output_____"
]
],
[
[
"df_warriors=df_teams[df_teams['nickname']=='Warriors']\ndf_warriors",
"_____no_output_____"
]
],
[
[
"we can use the following line of code to access the first column of the dataframe:",
"_____no_output_____"
]
],
[
[
"id_warriors=df_warriors[['id']].values[0][0]\n#we now have an integer that can be used to request the Warriors information \nid_warriors",
"_____no_output_____"
]
],
[
[
"The function \"League Game Finder \" will make an API call, its in the module <code>stats.endpoints</code> ",
"_____no_output_____"
]
],
[
[
"from nba_api.stats.endpoints import leaguegamefinder",
"_____no_output_____"
]
],
[
[
"The parameter <code>team_id_nullable</code> is the unique ID for the warriors. Under the hood, the NBA API is making a HTTP request. \nThe information requested is provided and is transmitted via an HTTP response this is assigned to the object <code>gamefinder</code>.",
"_____no_output_____"
]
],
[
[
"# Since https://stats.nba.com does lot allow api calls from Cloud IPs and Skills Network Labs uses a Cloud IP.\n# The following code is comment out, you can run it on jupyter labs on your own computer.\n# gamefinder = leaguegamefinder.LeagueGameFinder(team_id_nullable=id_warriors)",
"_____no_output_____"
]
],
[
[
"we can see the json file by running the following line of code. ",
"_____no_output_____"
]
],
[
[
"# Since https://stats.nba.com does lot allow api calls from Cloud IPs and Skills Network Labs uses a Cloud IP.\n# The following code is comment out, you can run it on jupyter labs on your own computer.\n# gamefinder.get_json()",
"_____no_output_____"
]
],
[
[
"The game finder object has a method <code>get_data_frames()</code>, that returns a dataframe. If we view the dataframe, we can see it contains information about all the games the Warriors played. The <code>PLUS_MINUS</code> column contains information on the score, if the value is negative the Warriors lost by that many points, if the value is positive, the warriors one by that amount of points. The column <code>MATCHUP </code>had the team the Warriors were playing, GSW stands for golden state and TOR means Toronto Raptors; <code>vs</code> signifies it was a home game and the <code>@ </code>symbol means an away game.",
"_____no_output_____"
]
],
[
[
"# Since https://stats.nba.com does lot allow api calls from Cloud IPs and Skills Network Labs uses a Cloud IP.\n# The following code is comment out, you can run it on jupyter labs on your own computer.\n# games = gamefinder.get_data_frames()[0]\n# games.head()",
"_____no_output_____"
]
],
[
[
"you can download the dataframe from the API call for Golden State and run the rest like a video.",
"_____no_output_____"
]
],
[
[
"! wget https://s3-api.us-geo.objectstorage.softlayer.net/cf-courses-data/CognitiveClass/PY0101EN/Chapter%205/Labs/Golden_State.pkl",
"--2020-08-12 07:10:18-- https://s3-api.us-geo.objectstorage.softlayer.net/cf-courses-data/CognitiveClass/PY0101EN/Chapter%205/Labs/Golden_State.pkl\nResolving s3-api.us-geo.objectstorage.softlayer.net (s3-api.us-geo.objectstorage.softlayer.net)... 67.228.254.196\nConnecting to s3-api.us-geo.objectstorage.softlayer.net (s3-api.us-geo.objectstorage.softlayer.net)|67.228.254.196|:443... connected.\nHTTP request sent, awaiting response... 200 OK\nLength: 811065 (792K) [application/octet-stream]\nSaving to: ‘Golden_State.pkl’\n\n\rGolden_State.pkl 0%[ ] 0 --.-KB/s \rGolden_State.pkl 100%[===================>] 792.06K --.-KB/s in 0.1s \n\n2020-08-12 07:10:19 (5.50 MB/s) - ‘Golden_State.pkl’ saved [811065/811065]\n\n"
],
[
"file_name = \"Golden_State.pkl\"\ngames = pd.read_pickle(file_name)\ngames.head()",
"_____no_output_____"
]
],
[
[
"We can create two dataframes, one for the games that the Warriors faced the raptors at home and the second for away games.",
"_____no_output_____"
]
],
[
[
"games_home=games [games ['MATCHUP']=='GSW vs. TOR']\ngames_away=games [games ['MATCHUP']=='GSW @ TOR']",
"_____no_output_____"
]
],
[
[
"We can calculate the mean for the column <code>PLUS_MINUS</code> for the dataframes <code>games_home</code> and <code> games_away</code>:",
"_____no_output_____"
]
],
[
[
"games_home.mean()['PLUS_MINUS']",
"_____no_output_____"
],
[
"games_away.mean()['PLUS_MINUS']",
"_____no_output_____"
]
],
[
[
"We can plot out the <code>PLUS MINUS</code> column for for the dataframes <code>games_home</code> and <code> games_away</code>.\nWe see the warriors played better at home.",
"_____no_output_____"
]
],
[
[
"fig, ax = plt.subplots()\n\ngames_away.plot(x='GAME_DATE',y='PLUS_MINUS', ax=ax)\ngames_home.plot(x='GAME_DATE',y='PLUS_MINUS', ax=ax)\nax.legend([\"away\", \"home\"])\nplt.show()",
"_____no_output_____"
]
],
[
[
" <a href=\"http://cocl.us/NotebooksPython101bottom\"><img src = \"https://ibm.box.com/shared/static/irypdxea2q4th88zu1o1tsd06dya10go.png\" width = 750, align = \"center\"></a>\n",
"_____no_output_____"
],
[
"#### About the Authors: \n\n [Joseph Santarcangelo]( https://www.linkedin.com/in/joseph-s-50398b136/) has a PhD in Electrical Engineering, his research focused on using machine learning, signal processing, and computer vision to determine how videos impact human cognition. Joseph has been working for IBM since he completed his PhD.\n",
"_____no_output_____"
],
[
"Copyright © 2017 [cognitiveclass.ai](https:cognitiveclass.ai). This notebook and its source code are released under the terms of the [MIT License](cognitiveclass.ai).",
"_____no_output_____"
]
],
[
[
"",
"_____no_output_____"
]
]
] | [
"markdown",
"code",
"markdown",
"code",
"markdown",
"code",
"markdown",
"code",
"markdown",
"code",
"markdown",
"code",
"markdown",
"code",
"markdown",
"code",
"markdown",
"code",
"markdown",
"code",
"markdown",
"code",
"markdown",
"code",
"markdown",
"code",
"markdown",
"code",
"markdown",
"code",
"markdown",
"code",
"markdown",
"code",
"markdown",
"code",
"markdown",
"code",
"markdown",
"code",
"markdown",
"code",
"markdown",
"code"
] | [
[
"markdown",
"markdown",
"markdown",
"markdown",
"markdown"
],
[
"code"
],
[
"markdown",
"markdown"
],
[
"code"
],
[
"markdown",
"markdown"
],
[
"code"
],
[
"markdown"
],
[
"code"
],
[
"markdown"
],
[
"code"
],
[
"markdown",
"markdown"
],
[
"code"
],
[
"markdown"
],
[
"code"
],
[
"markdown",
"markdown",
"markdown"
],
[
"code",
"code"
],
[
"markdown"
],
[
"code"
],
[
"markdown"
],
[
"code"
],
[
"markdown"
],
[
"code"
],
[
"markdown"
],
[
"code"
],
[
"markdown"
],
[
"code"
],
[
"markdown"
],
[
"code"
],
[
"markdown"
],
[
"code"
],
[
"markdown"
],
[
"code"
],
[
"markdown"
],
[
"code"
],
[
"markdown"
],
[
"code",
"code"
],
[
"markdown"
],
[
"code"
],
[
"markdown"
],
[
"code",
"code"
],
[
"markdown"
],
[
"code"
],
[
"markdown",
"markdown",
"markdown"
],
[
"code"
]
] |
e7669dd6ec7d2da36e9ff9fe6d8ac66dd55dc4a9 | 781,555 | ipynb | Jupyter Notebook | customer_segments/customer_segments.ipynb | nk101/Machine-Learning-ND | 3273a5ff35b51a5b41db03150a7e688ebbdbfa6a | [
"MIT"
] | null | null | null | customer_segments/customer_segments.ipynb | nk101/Machine-Learning-ND | 3273a5ff35b51a5b41db03150a7e688ebbdbfa6a | [
"MIT"
] | null | null | null | customer_segments/customer_segments.ipynb | nk101/Machine-Learning-ND | 3273a5ff35b51a5b41db03150a7e688ebbdbfa6a | [
"MIT"
] | null | null | null | 242.342636 | 263,408 | 0.877222 | [
[
[
"# Machine Learning Engineer Nanodegree\n## Unsupervised Learning\n## Project: Creating Customer Segments",
"_____no_output_____"
],
[
"Welcome to the third project of the Machine Learning Engineer Nanodegree! In this notebook, some template code has already been provided for you, and it will be your job to implement the additional functionality necessary to successfully complete this project. Sections that begin with **'Implementation'** in the header indicate that the following block of code will require additional functionality which you must provide. Instructions will be provided for each section and the specifics of the implementation are marked in the code block with a `'TODO'` statement. Please be sure to read the instructions carefully!\n\nIn addition to implementing code, there will be questions that you must answer which relate to the project and your implementation. Each section where you will answer a question is preceded by a **'Question X'** header. Carefully read each question and provide thorough answers in the following text boxes that begin with **'Answer:'**. Your project submission will be evaluated based on your answers to each of the questions and the implementation you provide. \n\n>**Note:** Code and Markdown cells can be executed using the **Shift + Enter** keyboard shortcut. In addition, Markdown cells can be edited by typically double-clicking the cell to enter edit mode.",
"_____no_output_____"
],
[
"## Getting Started\n\nIn this project, you will analyze a dataset containing data on various customers' annual spending amounts (reported in *monetary units*) of diverse product categories for internal structure. One goal of this project is to best describe the variation in the different types of customers that a wholesale distributor interacts with. Doing so would equip the distributor with insight into how to best structure their delivery service to meet the needs of each customer.\n\nThe dataset for this project can be found on the [UCI Machine Learning Repository](https://archive.ics.uci.edu/ml/datasets/Wholesale+customers). For the purposes of this project, the features `'Channel'` and `'Region'` will be excluded in the analysis — with focus instead on the six product categories recorded for customers.\n\nRun the code block below to load the wholesale customers dataset, along with a few of the necessary Python libraries required for this project. You will know the dataset loaded successfully if the size of the dataset is reported.",
"_____no_output_____"
]
],
[
[
"# Import libraries necessary for this project\nimport numpy as np\nimport pandas as pd\nfrom IPython.display import display # Allows the use of display() for DataFrames\nfrom scipy.stats import skew\n# Import supplementary visualizations code visuals.py\nimport visuals as vs\n\n# Pretty display for notebooks\n%matplotlib inline\n\n# Load the wholesale customers dataset\ntry:\n data = pd.read_csv(\"customers.csv\")\n data.drop(['Region', 'Channel'], axis = 1, inplace = True)\n print \"Wholesale customers dataset has {} samples with {} features each.\".format(*data.shape)\nexcept:\n print \"Dataset could not be loaded. Is the dataset missing?\"\ndisplay(data.head(4))\nprint data.skew()\n",
"Wholesale customers dataset has 440 samples with 6 features each.\n"
]
],
[
[
"## Data Exploration\nIn this section, you will begin exploring the data through visualizations and code to understand how each feature is related to the others. You will observe a statistical description of the dataset, consider the relevance of each feature, and select a few sample data points from the dataset which you will track through the course of this project.\n\nRun the code block below to observe a statistical description of the dataset. Note that the dataset is composed of six important product categories: **'Fresh'**, **'Milk'**, **'Grocery'**, **'Frozen'**, **'Detergents_Paper'**, and **'Delicatessen'**. Consider what each category represents in terms of products you could purchase.",
"_____no_output_____"
]
],
[
[
"# Display a description of the dataset\ndisplay(data.describe())\n#by looking at statistics, mean and 50% i.e. median are too far away for every colum. So, skew is found. apply log to every column\n#or use box cox test",
"_____no_output_____"
]
],
[
[
"### Implementation: Selecting Samples\nTo get a better understanding of the customers and how their data will transform through the analysis, it would be best to select a few sample data points and explore them in more detail. In the code block below, add **three** indices of your choice to the `indices` list which will represent the customers to track. It is suggested to try different sets of samples until you obtain customers that vary significantly from one another.",
"_____no_output_____"
]
],
[
[
"import seaborn as sns\n\n# TODO: Select three indices of your choice you wish to sample from the dataset\nindices = [23, 57,234]\n\n# Create a DataFrame of the chosen samples\nsamples = pd.DataFrame(data.loc[indices], columns = data.keys()).reset_index(drop = True)\nprint \"Chosen samples of wholesale customers dataset:\"\ndisplay(samples)\nsns.heatmap((samples-data.mean())/data.std(ddof=0), annot=True, cbar=False, square=True)",
"Chosen samples of wholesale customers dataset:\n"
]
],
[
[
"### Question 1\nConsider the total purchase cost of each product category and the statistical description of the dataset above for your sample customers. \n*What kind of establishment (customer) could each of the three samples you've chosen represent?* \n**Hint:** Examples of establishments include places like markets, cafes, and retailers, among many others. Avoid using names for establishments, such as saying *\"McDonalds\"* when describing a sample customer as a restaurant.",
"_____no_output_____"
],
[
"**Answer:**I have considered 3 categories to divide the sample.<br> \n1)Supermarket: It has extremely higher values of fresh, milk, Grocery and frozen which surely implies the big store.The spendings on Fresh, Grocery and Delicatessen is quite high than mean spending of each item. Hence, a super market<br>\n2)Grocerstore: The first sample shows high consumption of grocery which can be a outlet nearby human settlements.Convenience store would be a better name. Statistics show that spending on Grocery, Delicatessen and milk is quite higher than mean value of each. Hence, this can simply be assumed as Grocerstore since relevant items to grocery have high spending.<br>\n3)Hotel: The consumption of all the given things is quite lesser than Supermarket. It represents a cafe type hotel since detergents_paper , Fresh and Milk have greater spedings than mean of each from statistics.<br>\n",
"_____no_output_____"
],
[
"### Implementation: Feature Relevance\nOne interesting thought to consider is if one (or more) of the six product categories is actually relevant for understanding customer purchasing. That is to say, is it possible to determine whether customers purchasing some amount of one category of products will necessarily purchase some proportional amount of another category of products? We can make this determination quite easily by training a supervised regression learner on a subset of the data with one feature removed, and then score how well that model can predict the removed feature.\n\nIn the code block below, you will need to implement the following:\n - Assign `new_data` a copy of the data by removing a feature of your choice using the `DataFrame.drop` function.\n - Use `sklearn.cross_validation.train_test_split` to split the dataset into training and testing sets.\n - Use the removed feature as your target label. Set a `test_size` of `0.25` and set a `random_state`.\n - Import a decision tree regressor, set a `random_state`, and fit the learner to the training data.\n - Report the prediction score of the testing set using the regressor's `score` function.",
"_____no_output_____"
]
],
[
[
"'''# TODO: Make a copy of the DataFrame, using the 'drop' function to drop the given feature\nnew_data = None\n\n# TODO: Split the data into training and testing sets using the given feature as the target\nX_train, X_test, y_train, y_test = (None, None, None, None)\n\n# TODO: Create a decision tree regressor and fit it to the training set\nregressor = None\n\n# TODO: Report the score of the prediction using the testing set\nscore = None\n'''\nfrom scipy.stats import skew\nfrom sklearn.tree import DecisionTreeRegressor \nfrom sklearn.cross_validation import train_test_split\nfrom sklearn.metrics import accuracy_score\nscores = []\nnames = data.columns.values\nfor i, name in enumerate(data.columns.values):\n x = data.drop(name,axis=1).values\n y = data[name].values\n #print (x,y)\n X_train, X_test, y_train, y_test = train_test_split(x,y,test_size=0.25, random_state =10)\n #print (len(X_train), len(X_test),len(y_train),len(y_test))\n clf = DecisionTreeRegressor(random_state =10)\n clf.fit(X_train, y_train)\n scores.append(clf.score(X_test,y_test))\n#print scores\ndf = pd.DataFrame(np.array([scores,names]).T, columns = ['score','feature'])#take transpose of scores and names for vertical\ndisplay(df)\n#print data.skew()\n ",
"_____no_output_____"
]
],
[
[
"### Question 2\n*Which feature did you attempt to predict? What was the reported prediction score? Is this feature necessary for identifying customers' spending habits?* \n**Hint:** The coefficient of determination, `R^2`, is scored between 0 and 1, with 1 being a perfect fit. A negative `R^2` implies the model fails to fit the data.",
"_____no_output_____"
],
[
"**Answer:** Higher R^2 score implies that label or dependent variable are easily predicted by independent variables or training feature which is unnecessary for predictin customers spending habits<br>\nHowever, the one with lower R^2 score like Delicatessen, Fresh , Milk indicates that it is necessary for learning algorithm.<br>Also, the high R^2 values of Grocery and Frozen are not needed.\n",
"_____no_output_____"
],
[
"### Visualize Feature Distributions\nTo get a better understanding of the dataset, we can construct a scatter matrix of each of the six product features present in the data. If you found that the feature you attempted to predict above is relevant for identifying a specific customer, then the scatter matrix below may not show any correlation between that feature and the others. Conversely, if you believe that feature is not relevant for identifying a specific customer, the scatter matrix might show a correlation between that feature and another feature in the data. Run the code block below to produce a scatter matrix.",
"_____no_output_____"
]
],
[
[
"# Produce a scatter matrix for each pair of features in the data\npd.scatter_matrix(data, alpha = 0.3, figsize = (14,8), diagonal = 'kde');",
"_____no_output_____"
],
[
"import seaborn as sns\nsns.heatmap(data.corr(), annot=True)",
"_____no_output_____"
]
],
[
[
"### Question 3\n*Are there any pairs of features which exhibit some degree of correlation? Does this confirm or deny your suspicions about the relevance of the feature you attempted to predict? How is the data for those features distributed?* \n**Hint:** Is the data normally distributed? Where do most of the data points lie? ",
"_____no_output_____"
],
[
"**Answer:**How to identify correlation in scattermatrix plot? The plot should show a linear regression line surrounded closely by datapoints.<br>\nDetergents_paper and Grocery, Milk and Grocery, Detergent_paper and milk exhibit high correlation.\nIt confirms about my suspicion about relevance of feature.<br>\nThe data is distributed lognormal\nReveiewer Note: I am quite doubtful about the way I looked into graph and found correlation. Mention some other ways to find correlation.",
"_____no_output_____"
],
[
"## Data Preprocessing\nIn this section, you will preprocess the data to create a better representation of customers by performing a scaling on the data and detecting (and optionally removing) outliers. Preprocessing data is often times a critical step in assuring that results you obtain from your analysis are significant and meaningful.",
"_____no_output_____"
],
[
"### Implementation: Feature Scaling\nIf data is not normally distributed, especially if the mean and median vary significantly (indicating a large skew), it is most [often appropriate](http://econbrowser.com/archives/2014/02/use-of-logarithms-in-economics) to apply a non-linear scaling — particularly for financial data. One way to achieve this scaling is by using a [Box-Cox test](http://scipy.github.io/devdocs/generated/scipy.stats.boxcox.html), which calculates the best power transformation of the data that reduces skewness. A simpler approach which can work in most cases would be applying the natural logarithm.\n\nIn the code block below, you will need to implement the following:\n - Assign a copy of the data to `log_data` after applying logarithmic scaling. Use the `np.log` function for this.\n - Assign a copy of the sample data to `log_samples` after applying logarithmic scaling. Again, use `np.log`.",
"_____no_output_____"
]
],
[
[
"# TODO: Scale the data using the natural logarithm\nlog_data = np.log(data)\n\n# TODO: Scale the sample data using the natural logarithm\nlog_samples = np.log(samples)\n\n# Produce a scatter matrix for each pair of newly-transformed features\npd.scatter_matrix(log_data, alpha = 0.3, figsize = (14,8), diagonal = 'kde');\nlog_data.skew()\n# skew values are between -1<=skew value<=1 while fresh and delicatessen are almost satisfying condition.\n#Reviewer can explain how to use boxcox test. Please mention the code.",
"_____no_output_____"
]
],
[
[
"### Observation\nAfter applying a natural logarithm scaling to the data, the distribution of each feature should appear much more normal. For any pairs of features you may have identified earlier as being correlated, observe here whether that correlation is still present (and whether it is now stronger or weaker than before).\n\nRun the code below to see how the sample data has changed after having the natural logarithm applied to it.",
"_____no_output_____"
]
],
[
[
"# Display the log-transformed sample data\ndisplay(log_samples)",
"_____no_output_____"
]
],
[
[
"### Implementation: Outlier Detection\nDetecting outliers in the data is extremely important in the data preprocessing step of any analysis. The presence of outliers can often skew results which take into consideration these data points. There are many \"rules of thumb\" for what constitutes an outlier in a dataset. Here, we will use [Tukey's Method for identfying outliers](http://datapigtechnologies.com/blog/index.php/highlighting-outliers-in-your-data-with-the-tukey-method/): An *outlier step* is calculated as 1.5 times the interquartile range (IQR). A data point with a feature that is beyond an outlier step outside of the IQR for that feature is considered abnormal.\n\nIn the code block below, you will need to implement the following:\n - Assign the value of the 25th percentile for the given feature to `Q1`. Use `np.percentile` for this.\n - Assign the value of the 75th percentile for the given feature to `Q3`. Again, use `np.percentile`.\n - Assign the calculation of an outlier step for the given feature to `step`.\n - Optionally remove data points from the dataset by adding indices to the `outliers` list.\n\n**NOTE:** If you choose to remove any outliers, ensure that the sample data does not contain any of these points! \nOnce you have performed this implementation, the dataset will be stored in the variable `good_data`.",
"_____no_output_____"
]
],
[
[
"# For each feature find the data points with extreme high or low values\noutindex = {}\nfor feature in log_data.keys():\n \n # TODO: Calculate Q1 (25th percentile of the data) for the given feature\n Q1 = np.percentile(log_data[feature],25)\n \n # TODO: Calculate Q3 (75th percentile of the data) for the given feature\n Q3 = np.percentile(log_data[feature], 75)\n \n # TODO: Use the interquartile range to calculate an outlier step (1.5 times the interquartile range)\n step = 1.5*float((Q3-Q1))\n \n # Display the outliers\n print \"Data points considered outliers for the feature '{}':\".format(feature)\n display(log_data[~((log_data[feature] >= Q1 - step) & (log_data[feature] <= Q3 + step))])\n for i,r in log_data[feature].iteritems():\n if not((r >= Q1 -step) & (r <= Q3 + step)):\n if i not in outindex:\n outindex[i]=1\n else:\n outindex[i]=outindex[i]+1 \noutliers = [] \n# OPTIONAL: Select the indices for data points you wish to remove\nfor i in outindex:\n if outindex[i]>=2:\n outliers.append(i)\nprint outliers\n# Remove the outliers, if any were specified\ngood_data = log_data.drop(log_data.index[outliers]).reset_index(drop = True)",
"Data points considered outliers for the feature 'Fresh':\n"
]
],
[
[
"### Question 4\n*Are there any data points considered outliers for more than one feature based on the definition above? Should these data points be removed from the dataset? If any data points were added to the `outliers` list to be removed, explain why.* ",
"_____no_output_____"
],
[
"**Answer:**[128, 154, 65, 66, 75] are the outliers and should be removed from dataset. These are the points which are outlier for more than one feature. Outliers only in single feature can be neglected since removing them may cause loss in information.However, those which are caught as outlier in multiple features set are more likely to be real outliers. Hence, the should be removed.",
"_____no_output_____"
],
[
"## Feature Transformation\nIn this section you will use principal component analysis (PCA) to draw conclusions about the underlying structure of the wholesale customer data. Since using PCA on a dataset calculates the dimensions which best maximize variance, we will find which compound combinations of features best describe customers.",
"_____no_output_____"
],
[
"### Implementation: PCA\n\nNow that the data has been scaled to a more normal distribution and has had any necessary outliers removed, we can now apply PCA to the `good_data` to discover which dimensions about the data best maximize the variance of features involved. In addition to finding these dimensions, PCA will also report the *explained variance ratio* of each dimension — how much variance within the data is explained by that dimension alone. Note that a component (dimension) from PCA can be considered a new \"feature\" of the space, however it is a composition of the original features present in the data.\n\nIn the code block below, you will need to implement the following:\n - Import `sklearn.decomposition.PCA` and assign the results of fitting PCA in six dimensions with `good_data` to `pca`.\n - Apply a PCA transformation of `log_samples` using `pca.transform`, and assign the results to `pca_samples`.",
"_____no_output_____"
]
],
[
[
"from sklearn.decomposition import PCA\n# TODO: Apply PCA by fitting the good data with the same number of dimensions as features\npca = PCA(n_components = 6)\npca.fit(good_data)\n# TODO: Transform log_samples using the PCA fit above\npca_samples = pca.transform(log_samples)\n\n# Generate PCA results plot\npca_results = vs.pca_results(good_data, pca)",
"_____no_output_____"
]
],
[
[
"### Question 5\n*How much variance in the data is explained* ***in total*** *by the first and second principal component? What about the first four principal components? Using the visualization provided above, discuss what the first four dimensions best represent in terms of customer spending.* \n**Hint:** A positive increase in a specific dimension corresponds with an *increase* of the *positive-weighted* features and a *decrease* of the *negative-weighted* features. The rate of increase or decrease is based on the individual feature weights.",
"_____no_output_____"
],
[
"**Answer:**1) First and second principal component explains about 0.7068 variance in data.\n<br>2)The first four explains about 0.9311 variance in data.\n3)Dimension 1 has large increases for features Milk, Grocery and Detergents_Paper, a small increase for Delicatessen, and small decreases for features Fresh and Frozen.<br>\nDimension 2 has large increases for Fresh, Frozen and Delicatessen, and small increase for Milk, Grocery and Detergents_Paper.<br>\nDimension 3 has large increases for Frozen and Delicatessen, and large decreases for Fresh and Detergents_Paper.<br>\nDimension 4 has large increases for Frozen and Detergents_Paper, and large a decrease for Fish and Delicatessen.<br>",
"_____no_output_____"
],
[
"### Observation\nRun the code below to see how the log-transformed sample data has changed after having a PCA transformation applied to it in six dimensions. Observe the numerical value for the first four dimensions of the sample points. Consider if this is consistent with your initial interpretation of the sample points.",
"_____no_output_____"
]
],
[
[
"# Display sample log-data after having a PCA transformation applied\ndisplay(pd.DataFrame(np.round(pca_samples, 4), columns = pca_results.index.values))",
"_____no_output_____"
]
],
[
[
"### Implementation: Dimensionality Reduction\nWhen using principal component analysis, one of the main goals is to reduce the dimensionality of the data — in effect, reducing the complexity of the problem. Dimensionality reduction comes at a cost: Fewer dimensions used implies less of the total variance in the data is being explained. Because of this, the *cumulative explained variance ratio* is extremely important for knowing how many dimensions are necessary for the problem. Additionally, if a signifiant amount of variance is explained by only two or three dimensions, the reduced data can be visualized afterwards.\n\nIn the code block below, you will need to implement the following:\n - Assign the results of fitting PCA in two dimensions with `good_data` to `pca`.\n - Apply a PCA transformation of `good_data` using `pca.transform`, and assign the results to `reduced_data`.\n - Apply a PCA transformation of `log_samples` using `pca.transform`, and assign the results to `pca_samples`.",
"_____no_output_____"
]
],
[
[
"# TODO: Apply PCA by fitting the good data with only two dimensions\npca = PCA(n_components =2)\npca.fit(good_data)\n# TODO: Transform the good data using the PCA fit above\nreduced_data = pca.transform(good_data)\n\n# TODO: Transform log_samples using the PCA fit above\npca_samples = pca.transform(log_samples)\n\n# Create a DataFrame for the reduced data\nreduced_data = pd.DataFrame(reduced_data, columns = ['Dimension 1', 'Dimension 2'])\ndisplay(reduced_data)",
"_____no_output_____"
]
],
[
[
"### Observation\nRun the code below to see how the log-transformed sample data has changed after having a PCA transformation applied to it using only two dimensions. Observe how the values for the first two dimensions remains unchanged when compared to a PCA transformation in six dimensions.",
"_____no_output_____"
]
],
[
[
"# Display sample log-data after applying PCA transformation in two dimensions\ndisplay(pd.DataFrame(np.round(pca_samples, 4), columns = ['Dimension 1', 'Dimension 2']))",
"_____no_output_____"
]
],
[
[
"## Visualizing a Biplot\nA biplot is a scatterplot where each data point is represented by its scores along the principal components. The axes are the principal components (in this case `Dimension 1` and `Dimension 2`). In addition, the biplot shows the projection of the original features along the components. A biplot can help us interpret the reduced dimensions of the data, and discover relationships between the principal components and original features.\n\nRun the code cell below to produce a biplot of the reduced-dimension data.",
"_____no_output_____"
]
],
[
[
"# Create a biplot\nvs.biplot(good_data, reduced_data, pca)",
"_____no_output_____"
]
],
[
[
"### Observation\n\nOnce we have the original feature projections (in red), it is easier to interpret the relative position of each data point in the scatterplot. For instance, a point the lower right corner of the figure will likely correspond to a customer that spends a lot on `'Milk'`, `'Grocery'` and `'Detergents_Paper'`, but not so much on the other product categories. \n\nFrom the biplot, which of the original features are most strongly correlated with the first component? What about those that are associated with the second component? Do these observations agree with the pca_results plot you obtained earlier?",
"_____no_output_____"
],
[
"## Clustering\n\nIn this section, you will choose to use either a K-Means clustering algorithm or a Gaussian Mixture Model clustering algorithm to identify the various customer segments hidden in the data. You will then recover specific data points from the clusters to understand their significance by transforming them back into their original dimension and scale. ",
"_____no_output_____"
],
[
"### Question 6\n*What are the advantages to using a K-Means clustering algorithm? What are the advantages to using a Gaussian Mixture Model clustering algorithm? Given your observations about the wholesale customer data so far, which of the two algorithms will you use and why?*",
"_____no_output_____"
],
[
"**Answer:**1)kmeans:It is hard clustering type. It is simpler and minimizes the (x-u)^2 i.e. euclidean distance and it always converges.Its easy to implement.u=mean\n2)GMM:It is soft clustering type. It is used when there is possiblity of 2 overlapping clusters. It takes variance into account.\n3)Intuition is that there might be possibility of overlapping clusters with this dataset. Thus, soft clustering is good to use.Hence, GMM.",
"_____no_output_____"
],
[
"### Implementation: Creating Clusters\nDepending on the problem, the number of clusters that you expect to be in the data may already be known. When the number of clusters is not known *a priori*, there is no guarantee that a given number of clusters best segments the data, since it is unclear what structure exists in the data — if any. However, we can quantify the \"goodness\" of a clustering by calculating each data point's *silhouette coefficient*. The [silhouette coefficient](http://scikit-learn.org/stable/modules/generated/sklearn.metrics.silhouette_score.html) for a data point measures how similar it is to its assigned cluster from -1 (dissimilar) to 1 (similar). Calculating the *mean* silhouette coefficient provides for a simple scoring method of a given clustering.\n\nIn the code block below, you will need to implement the following:\n - Fit a clustering algorithm to the `reduced_data` and assign it to `clusterer`.\n - Predict the cluster for each data point in `reduced_data` using `clusterer.predict` and assign them to `preds`.\n - Find the cluster centers using the algorithm's respective attribute and assign them to `centers`.\n - Predict the cluster for each sample data point in `pca_samples` and assign them `sample_preds`.\n - Import `sklearn.metrics.silhouette_score` and calculate the silhouette score of `reduced_data` against `preds`.\n - Assign the silhouette score to `score` and print the result.",
"_____no_output_____"
]
],
[
[
"from sklearn.metrics import silhouette_score\nfrom sklearn.mixture import GMM\nscorer = {}#for n sample points 2 to n-1 clusters can be created.\nfor i in range(2,10):\n clusterer = GMM(n_components = i)\n clusterer.fit(reduced_data)\n pred = clusterer.predict(reduced_data)\n score = silhouette_score(reduced_data, pred)\n scorer[i]=score\nprint (scorer) \noptimal_components = 2\n# TODO: Apply your clustering algorithm of choice to the reduced data \nclusterer = GMM(n_components=optimal_components).fit(reduced_data)\n\n# TODO: Predict the cluster for each data point\npreds = clusterer.predict(reduced_data)\n\n# TODO: Find the cluster centers\ncenters = clusterer.means_\n\n# TODO: Predict the cluster for each transformed sample data point\nsample_preds = clusterer.predict(pca_samples)\n\n# TODO: Calculate the mean silhouette coefficient for the number of clusters chosen\nscore = silhouette_score(reduced_data, preds)\nprint 'Best number of clusters : %s and score : %s'%(str(optimal_components), str(score))\nprint '--------------------------------------------------------------------------------------------------------------------------' ",
"C:\\Users\\admin\\Anaconda2\\lib\\site-packages\\sklearn\\utils\\deprecation.py:52: DeprecationWarning: Class GMM is deprecated; The class GMM is deprecated in 0.18 and will be removed in 0.20. Use class GaussianMixture instead.\n warnings.warn(msg, category=DeprecationWarning)\nC:\\Users\\admin\\Anaconda2\\lib\\site-packages\\sklearn\\utils\\deprecation.py:70: DeprecationWarning: Function distribute_covar_matrix_to_match_covariance_type is deprecated; The functon distribute_covar_matrix_to_match_covariance_typeis deprecated in 0.18 and will be removed in 0.20.\n warnings.warn(msg, category=DeprecationWarning)\nC:\\Users\\admin\\Anaconda2\\lib\\site-packages\\sklearn\\utils\\deprecation.py:70: DeprecationWarning: Function log_multivariate_normal_density is deprecated; The function log_multivariate_normal_density is deprecated in 0.18 and will be removed in 0.20.\n warnings.warn(msg, category=DeprecationWarning)\nC:\\Users\\admin\\Anaconda2\\lib\\site-packages\\sklearn\\utils\\deprecation.py:70: DeprecationWarning: Function log_multivariate_normal_density is deprecated; The function log_multivariate_normal_density is deprecated in 0.18 and will be removed in 0.20.\n warnings.warn(msg, category=DeprecationWarning)\nC:\\Users\\admin\\Anaconda2\\lib\\site-packages\\sklearn\\utils\\deprecation.py:70: DeprecationWarning: Function log_multivariate_normal_density is deprecated; The function log_multivariate_normal_density is deprecated in 0.18 and will be removed in 0.20.\n warnings.warn(msg, category=DeprecationWarning)\nC:\\Users\\admin\\Anaconda2\\lib\\site-packages\\sklearn\\utils\\deprecation.py:70: DeprecationWarning: Function log_multivariate_normal_density is deprecated; The function log_multivariate_normal_density is deprecated in 0.18 and will be removed in 0.20.\n warnings.warn(msg, category=DeprecationWarning)\nC:\\Users\\admin\\Anaconda2\\lib\\site-packages\\sklearn\\utils\\deprecation.py:70: DeprecationWarning: Function log_multivariate_normal_density is deprecated; The function log_multivariate_normal_density is deprecated in 0.18 and will be removed in 0.20.\n warnings.warn(msg, category=DeprecationWarning)\nC:\\Users\\admin\\Anaconda2\\lib\\site-packages\\sklearn\\utils\\deprecation.py:70: DeprecationWarning: Function log_multivariate_normal_density is deprecated; The function log_multivariate_normal_density is deprecated in 0.18 and will be removed in 0.20.\n warnings.warn(msg, category=DeprecationWarning)\nC:\\Users\\admin\\Anaconda2\\lib\\site-packages\\sklearn\\utils\\deprecation.py:70: DeprecationWarning: Function log_multivariate_normal_density is deprecated; The function log_multivariate_normal_density is deprecated in 0.18 and will be removed in 0.20.\n warnings.warn(msg, category=DeprecationWarning)\nC:\\Users\\admin\\Anaconda2\\lib\\site-packages\\sklearn\\utils\\deprecation.py:70: DeprecationWarning: Function log_multivariate_normal_density is deprecated; The function log_multivariate_normal_density is deprecated in 0.18 and will be removed in 0.20.\n warnings.warn(msg, category=DeprecationWarning)\nC:\\Users\\admin\\Anaconda2\\lib\\site-packages\\sklearn\\utils\\deprecation.py:52: DeprecationWarning: Class GMM is deprecated; The class GMM is deprecated in 0.18 and will be removed in 0.20. Use class GaussianMixture instead.\n warnings.warn(msg, category=DeprecationWarning)\nC:\\Users\\admin\\Anaconda2\\lib\\site-packages\\sklearn\\utils\\deprecation.py:70: DeprecationWarning: Function distribute_covar_matrix_to_match_covariance_type is deprecated; The functon distribute_covar_matrix_to_match_covariance_typeis deprecated in 0.18 and will be removed in 0.20.\n warnings.warn(msg, category=DeprecationWarning)\nC:\\Users\\admin\\Anaconda2\\lib\\site-packages\\sklearn\\utils\\deprecation.py:70: DeprecationWarning: Function log_multivariate_normal_density is deprecated; The function log_multivariate_normal_density is deprecated in 0.18 and will be removed in 0.20.\n warnings.warn(msg, category=DeprecationWarning)\nC:\\Users\\admin\\Anaconda2\\lib\\site-packages\\sklearn\\utils\\deprecation.py:70: DeprecationWarning: Function log_multivariate_normal_density is deprecated; The function log_multivariate_normal_density is deprecated in 0.18 and will be removed in 0.20.\n warnings.warn(msg, category=DeprecationWarning)\nC:\\Users\\admin\\Anaconda2\\lib\\site-packages\\sklearn\\utils\\deprecation.py:70: DeprecationWarning: Function log_multivariate_normal_density is deprecated; The function log_multivariate_normal_density is deprecated in 0.18 and will be removed in 0.20.\n warnings.warn(msg, category=DeprecationWarning)\nC:\\Users\\admin\\Anaconda2\\lib\\site-packages\\sklearn\\utils\\deprecation.py:70: DeprecationWarning: Function log_multivariate_normal_density is deprecated; The function log_multivariate_normal_density is deprecated in 0.18 and will be removed in 0.20.\n warnings.warn(msg, category=DeprecationWarning)\nC:\\Users\\admin\\Anaconda2\\lib\\site-packages\\sklearn\\utils\\deprecation.py:70: DeprecationWarning: Function log_multivariate_normal_density is deprecated; The function log_multivariate_normal_density is deprecated in 0.18 and will be removed in 0.20.\n warnings.warn(msg, category=DeprecationWarning)\nC:\\Users\\admin\\Anaconda2\\lib\\site-packages\\sklearn\\utils\\deprecation.py:70: DeprecationWarning: Function log_multivariate_normal_density is deprecated; The function log_multivariate_normal_density is deprecated in 0.18 and will be removed in 0.20.\n warnings.warn(msg, category=DeprecationWarning)\nC:\\Users\\admin\\Anaconda2\\lib\\site-packages\\sklearn\\utils\\deprecation.py:70: DeprecationWarning: Function log_multivariate_normal_density is deprecated; The function log_multivariate_normal_density is deprecated in 0.18 and will be removed in 0.20.\n warnings.warn(msg, category=DeprecationWarning)\nC:\\Users\\admin\\Anaconda2\\lib\\site-packages\\sklearn\\utils\\deprecation.py:70: DeprecationWarning: Function log_multivariate_normal_density is deprecated; The function log_multivariate_normal_density is deprecated in 0.18 and will be removed in 0.20.\n warnings.warn(msg, category=DeprecationWarning)\nC:\\Users\\admin\\Anaconda2\\lib\\site-packages\\sklearn\\utils\\deprecation.py:70: DeprecationWarning: Function log_multivariate_normal_density is deprecated; The function log_multivariate_normal_density is deprecated in 0.18 and will be removed in 0.20.\n warnings.warn(msg, category=DeprecationWarning)\nC:\\Users\\admin\\Anaconda2\\lib\\site-packages\\sklearn\\utils\\deprecation.py:70: DeprecationWarning: Function log_multivariate_normal_density is deprecated; The function log_multivariate_normal_density is deprecated in 0.18 and will be removed in 0.20.\n warnings.warn(msg, category=DeprecationWarning)\nC:\\Users\\admin\\Anaconda2\\lib\\site-packages\\sklearn\\utils\\deprecation.py:70: DeprecationWarning: Function log_multivariate_normal_density is deprecated; The function log_multivariate_normal_density is deprecated in 0.18 and will be removed in 0.20.\n warnings.warn(msg, category=DeprecationWarning)\nC:\\Users\\admin\\Anaconda2\\lib\\site-packages\\sklearn\\utils\\deprecation.py:70: DeprecationWarning: Function log_multivariate_normal_density is deprecated; The function log_multivariate_normal_density is deprecated in 0.18 and will be removed in 0.20.\n warnings.warn(msg, category=DeprecationWarning)\nC:\\Users\\admin\\Anaconda2\\lib\\site-packages\\sklearn\\utils\\deprecation.py:70: DeprecationWarning: Function log_multivariate_normal_density is deprecated; The function log_multivariate_normal_density is deprecated in 0.18 and will be removed in 0.20.\n warnings.warn(msg, category=DeprecationWarning)\nC:\\Users\\admin\\Anaconda2\\lib\\site-packages\\sklearn\\utils\\deprecation.py:70: DeprecationWarning: Function log_multivariate_normal_density is deprecated; The function log_multivariate_normal_density is deprecated in 0.18 and will be removed in 0.20.\n warnings.warn(msg, category=DeprecationWarning)\nC:\\Users\\admin\\Anaconda2\\lib\\site-packages\\sklearn\\utils\\deprecation.py:70: DeprecationWarning: Function log_multivariate_normal_density is deprecated; The function log_multivariate_normal_density is deprecated in 0.18 and will be removed in 0.20.\n warnings.warn(msg, category=DeprecationWarning)\nC:\\Users\\admin\\Anaconda2\\lib\\site-packages\\sklearn\\utils\\deprecation.py:70: DeprecationWarning: Function log_multivariate_normal_density is deprecated; The function log_multivariate_normal_density is deprecated in 0.18 and will be removed in 0.20.\n warnings.warn(msg, category=DeprecationWarning)\nC:\\Users\\admin\\Anaconda2\\lib\\site-packages\\sklearn\\utils\\deprecation.py:70: DeprecationWarning: Function log_multivariate_normal_density is deprecated; The function log_multivariate_normal_density is deprecated in 0.18 and will be removed in 0.20.\n warnings.warn(msg, category=DeprecationWarning)\nC:\\Users\\admin\\Anaconda2\\lib\\site-packages\\sklearn\\utils\\deprecation.py:70: DeprecationWarning: Function log_multivariate_normal_density is deprecated; The function log_multivariate_normal_density is deprecated in 0.18 and will be removed in 0.20.\n warnings.warn(msg, category=DeprecationWarning)\nC:\\Users\\admin\\Anaconda2\\lib\\site-packages\\sklearn\\utils\\deprecation.py:70: DeprecationWarning: Function log_multivariate_normal_density is deprecated; The function log_multivariate_normal_density is deprecated in 0.18 and will be removed in 0.20.\n warnings.warn(msg, category=DeprecationWarning)\nC:\\Users\\admin\\Anaconda2\\lib\\site-packages\\sklearn\\utils\\deprecation.py:70: DeprecationWarning: Function log_multivariate_normal_density is deprecated; The function log_multivariate_normal_density is deprecated in 0.18 and will be removed in 0.20.\n warnings.warn(msg, category=DeprecationWarning)\nC:\\Users\\admin\\Anaconda2\\lib\\site-packages\\sklearn\\utils\\deprecation.py:70: DeprecationWarning: Function log_multivariate_normal_density is deprecated; The function log_multivariate_normal_density is deprecated in 0.18 and will be removed in 0.20.\n warnings.warn(msg, category=DeprecationWarning)\nC:\\Users\\admin\\Anaconda2\\lib\\site-packages\\sklearn\\utils\\deprecation.py:70: DeprecationWarning: Function log_multivariate_normal_density is deprecated; The function log_multivariate_normal_density is deprecated in 0.18 and will be removed in 0.20.\n warnings.warn(msg, category=DeprecationWarning)\nC:\\Users\\admin\\Anaconda2\\lib\\site-packages\\sklearn\\utils\\deprecation.py:52: DeprecationWarning: Class GMM is deprecated; The class GMM is deprecated in 0.18 and will be removed in 0.20. Use class GaussianMixture instead.\n warnings.warn(msg, category=DeprecationWarning)\nC:\\Users\\admin\\Anaconda2\\lib\\site-packages\\sklearn\\utils\\deprecation.py:70: DeprecationWarning: Function distribute_covar_matrix_to_match_covariance_type is deprecated; The functon distribute_covar_matrix_to_match_covariance_typeis deprecated in 0.18 and will be removed in 0.20.\n warnings.warn(msg, category=DeprecationWarning)\nC:\\Users\\admin\\Anaconda2\\lib\\site-packages\\sklearn\\utils\\deprecation.py:70: DeprecationWarning: Function log_multivariate_normal_density is deprecated; The function log_multivariate_normal_density is deprecated in 0.18 and will be removed in 0.20.\n warnings.warn(msg, category=DeprecationWarning)\nC:\\Users\\admin\\Anaconda2\\lib\\site-packages\\sklearn\\utils\\deprecation.py:70: DeprecationWarning: Function log_multivariate_normal_density is deprecated; The function log_multivariate_normal_density is deprecated in 0.18 and will be removed in 0.20.\n warnings.warn(msg, category=DeprecationWarning)\nC:\\Users\\admin\\Anaconda2\\lib\\site-packages\\sklearn\\utils\\deprecation.py:70: DeprecationWarning: Function log_multivariate_normal_density is deprecated; The function log_multivariate_normal_density is deprecated in 0.18 and will be removed in 0.20.\n warnings.warn(msg, category=DeprecationWarning)\nC:\\Users\\admin\\Anaconda2\\lib\\site-packages\\sklearn\\utils\\deprecation.py:70: DeprecationWarning: Function log_multivariate_normal_density is deprecated; The function log_multivariate_normal_density is deprecated in 0.18 and will be removed in 0.20.\n warnings.warn(msg, category=DeprecationWarning)\nC:\\Users\\admin\\Anaconda2\\lib\\site-packages\\sklearn\\utils\\deprecation.py:70: DeprecationWarning: Function log_multivariate_normal_density is deprecated; The function log_multivariate_normal_density is deprecated in 0.18 and will be removed in 0.20.\n warnings.warn(msg, category=DeprecationWarning)\nC:\\Users\\admin\\Anaconda2\\lib\\site-packages\\sklearn\\utils\\deprecation.py:70: DeprecationWarning: Function log_multivariate_normal_density is deprecated; The function log_multivariate_normal_density is deprecated in 0.18 and will be removed in 0.20.\n warnings.warn(msg, category=DeprecationWarning)\nC:\\Users\\admin\\Anaconda2\\lib\\site-packages\\sklearn\\utils\\deprecation.py:70: DeprecationWarning: Function log_multivariate_normal_density is deprecated; The function log_multivariate_normal_density is deprecated in 0.18 and will be removed in 0.20.\n warnings.warn(msg, category=DeprecationWarning)\nC:\\Users\\admin\\Anaconda2\\lib\\site-packages\\sklearn\\utils\\deprecation.py:70: DeprecationWarning: Function log_multivariate_normal_density is deprecated; The function log_multivariate_normal_density is deprecated in 0.18 and will be removed in 0.20.\n warnings.warn(msg, category=DeprecationWarning)\nC:\\Users\\admin\\Anaconda2\\lib\\site-packages\\sklearn\\utils\\deprecation.py:70: DeprecationWarning: Function log_multivariate_normal_density is deprecated; The function log_multivariate_normal_density is deprecated in 0.18 and will be removed in 0.20.\n warnings.warn(msg, category=DeprecationWarning)\nC:\\Users\\admin\\Anaconda2\\lib\\site-packages\\sklearn\\utils\\deprecation.py:70: DeprecationWarning: Function log_multivariate_normal_density is deprecated; The function log_multivariate_normal_density is deprecated in 0.18 and will be removed in 0.20.\n warnings.warn(msg, category=DeprecationWarning)\nC:\\Users\\admin\\Anaconda2\\lib\\site-packages\\sklearn\\utils\\deprecation.py:70: DeprecationWarning: Function log_multivariate_normal_density is deprecated; The function log_multivariate_normal_density is deprecated in 0.18 and will be removed in 0.20.\n warnings.warn(msg, category=DeprecationWarning)\nC:\\Users\\admin\\Anaconda2\\lib\\site-packages\\sklearn\\utils\\deprecation.py:70: DeprecationWarning: Function log_multivariate_normal_density is deprecated; The function log_multivariate_normal_density is deprecated in 0.18 and will be removed in 0.20.\n warnings.warn(msg, category=DeprecationWarning)\nC:\\Users\\admin\\Anaconda2\\lib\\site-packages\\sklearn\\utils\\deprecation.py:70: DeprecationWarning: Function log_multivariate_normal_density is deprecated; The function log_multivariate_normal_density is deprecated in 0.18 and will be removed in 0.20.\n warnings.warn(msg, category=DeprecationWarning)\nC:\\Users\\admin\\Anaconda2\\lib\\site-packages\\sklearn\\utils\\deprecation.py:70: DeprecationWarning: Function log_multivariate_normal_density is deprecated; The function log_multivariate_normal_density is deprecated in 0.18 and will be removed in 0.20.\n warnings.warn(msg, category=DeprecationWarning)\nC:\\Users\\admin\\Anaconda2\\lib\\site-packages\\sklearn\\utils\\deprecation.py:70: DeprecationWarning: Function log_multivariate_normal_density is deprecated; The function log_multivariate_normal_density is deprecated in 0.18 and will be removed in 0.20.\n warnings.warn(msg, category=DeprecationWarning)\nC:\\Users\\admin\\Anaconda2\\lib\\site-packages\\sklearn\\utils\\deprecation.py:70: DeprecationWarning: Function log_multivariate_normal_density is deprecated; The function log_multivariate_normal_density is deprecated in 0.18 and will be removed in 0.20.\n warnings.warn(msg, category=DeprecationWarning)\nC:\\Users\\admin\\Anaconda2\\lib\\site-packages\\sklearn\\utils\\deprecation.py:70: DeprecationWarning: Function log_multivariate_normal_density is deprecated; The function log_multivariate_normal_density is deprecated in 0.18 and will be removed in 0.20.\n warnings.warn(msg, category=DeprecationWarning)\nC:\\Users\\admin\\Anaconda2\\lib\\site-packages\\sklearn\\utils\\deprecation.py:70: DeprecationWarning: Function log_multivariate_normal_density is deprecated; The function log_multivariate_normal_density is deprecated in 0.18 and will be removed in 0.20.\n warnings.warn(msg, category=DeprecationWarning)\nC:\\Users\\admin\\Anaconda2\\lib\\site-packages\\sklearn\\utils\\deprecation.py:70: DeprecationWarning: Function log_multivariate_normal_density is deprecated; The function log_multivariate_normal_density is deprecated in 0.18 and will be removed in 0.20.\n warnings.warn(msg, category=DeprecationWarning)\nC:\\Users\\admin\\Anaconda2\\lib\\site-packages\\sklearn\\utils\\deprecation.py:52: DeprecationWarning: Class GMM is deprecated; The class GMM is deprecated in 0.18 and will be removed in 0.20. Use class GaussianMixture instead.\n warnings.warn(msg, category=DeprecationWarning)\nC:\\Users\\admin\\Anaconda2\\lib\\site-packages\\sklearn\\utils\\deprecation.py:70: DeprecationWarning: Function distribute_covar_matrix_to_match_covariance_type is deprecated; The functon distribute_covar_matrix_to_match_covariance_typeis deprecated in 0.18 and will be removed in 0.20.\n warnings.warn(msg, category=DeprecationWarning)\nC:\\Users\\admin\\Anaconda2\\lib\\site-packages\\sklearn\\utils\\deprecation.py:70: DeprecationWarning: Function log_multivariate_normal_density is deprecated; The function log_multivariate_normal_density is deprecated in 0.18 and will be removed in 0.20.\n warnings.warn(msg, category=DeprecationWarning)\nC:\\Users\\admin\\Anaconda2\\lib\\site-packages\\sklearn\\utils\\deprecation.py:70: DeprecationWarning: Function log_multivariate_normal_density is deprecated; The function log_multivariate_normal_density is deprecated in 0.18 and will be removed in 0.20.\n warnings.warn(msg, category=DeprecationWarning)\nC:\\Users\\admin\\Anaconda2\\lib\\site-packages\\sklearn\\utils\\deprecation.py:70: DeprecationWarning: Function log_multivariate_normal_density is deprecated; The function log_multivariate_normal_density is deprecated in 0.18 and will be removed in 0.20.\n warnings.warn(msg, category=DeprecationWarning)\nC:\\Users\\admin\\Anaconda2\\lib\\site-packages\\sklearn\\utils\\deprecation.py:70: DeprecationWarning: Function log_multivariate_normal_density is deprecated; The function log_multivariate_normal_density is deprecated in 0.18 and will be removed in 0.20.\n warnings.warn(msg, category=DeprecationWarning)\nC:\\Users\\admin\\Anaconda2\\lib\\site-packages\\sklearn\\utils\\deprecation.py:70: DeprecationWarning: Function log_multivariate_normal_density is deprecated; The function log_multivariate_normal_density is deprecated in 0.18 and will be removed in 0.20.\n warnings.warn(msg, category=DeprecationWarning)\nC:\\Users\\admin\\Anaconda2\\lib\\site-packages\\sklearn\\utils\\deprecation.py:70: DeprecationWarning: Function log_multivariate_normal_density is deprecated; The function log_multivariate_normal_density is deprecated in 0.18 and will be removed in 0.20.\n warnings.warn(msg, category=DeprecationWarning)\nC:\\Users\\admin\\Anaconda2\\lib\\site-packages\\sklearn\\utils\\deprecation.py:70: DeprecationWarning: Function log_multivariate_normal_density is deprecated; The function log_multivariate_normal_density is deprecated in 0.18 and will be removed in 0.20.\n warnings.warn(msg, category=DeprecationWarning)\nC:\\Users\\admin\\Anaconda2\\lib\\site-packages\\sklearn\\utils\\deprecation.py:70: DeprecationWarning: Function log_multivariate_normal_density is deprecated; The function log_multivariate_normal_density is deprecated in 0.18 and will be removed in 0.20.\n warnings.warn(msg, category=DeprecationWarning)\nC:\\Users\\admin\\Anaconda2\\lib\\site-packages\\sklearn\\utils\\deprecation.py:70: DeprecationWarning: Function log_multivariate_normal_density is deprecated; The function log_multivariate_normal_density is deprecated in 0.18 and will be removed in 0.20.\n warnings.warn(msg, category=DeprecationWarning)\nC:\\Users\\admin\\Anaconda2\\lib\\site-packages\\sklearn\\utils\\deprecation.py:70: DeprecationWarning: Function log_multivariate_normal_density is deprecated; The function log_multivariate_normal_density is deprecated in 0.18 and will be removed in 0.20.\n warnings.warn(msg, category=DeprecationWarning)\nC:\\Users\\admin\\Anaconda2\\lib\\site-packages\\sklearn\\utils\\deprecation.py:70: DeprecationWarning: Function log_multivariate_normal_density is deprecated; The function log_multivariate_normal_density is deprecated in 0.18 and will be removed in 0.20.\n warnings.warn(msg, category=DeprecationWarning)\nC:\\Users\\admin\\Anaconda2\\lib\\site-packages\\sklearn\\utils\\deprecation.py:70: DeprecationWarning: Function log_multivariate_normal_density is deprecated; The function log_multivariate_normal_density is deprecated in 0.18 and will be removed in 0.20.\n warnings.warn(msg, category=DeprecationWarning)\nC:\\Users\\admin\\Anaconda2\\lib\\site-packages\\sklearn\\utils\\deprecation.py:70: DeprecationWarning: Function log_multivariate_normal_density is deprecated; The function log_multivariate_normal_density is deprecated in 0.18 and will be removed in 0.20.\n warnings.warn(msg, category=DeprecationWarning)\nC:\\Users\\admin\\Anaconda2\\lib\\site-packages\\sklearn\\utils\\deprecation.py:70: DeprecationWarning: Function log_multivariate_normal_density is deprecated; The function log_multivariate_normal_density is deprecated in 0.18 and will be removed in 0.20.\n warnings.warn(msg, category=DeprecationWarning)\nC:\\Users\\admin\\Anaconda2\\lib\\site-packages\\sklearn\\utils\\deprecation.py:70: DeprecationWarning: Function log_multivariate_normal_density is deprecated; The function log_multivariate_normal_density is deprecated in 0.18 and will be removed in 0.20.\n warnings.warn(msg, category=DeprecationWarning)\nC:\\Users\\admin\\Anaconda2\\lib\\site-packages\\sklearn\\utils\\deprecation.py:70: DeprecationWarning: Function log_multivariate_normal_density is deprecated; The function log_multivariate_normal_density is deprecated in 0.18 and will be removed in 0.20.\n warnings.warn(msg, category=DeprecationWarning)\nC:\\Users\\admin\\Anaconda2\\lib\\site-packages\\sklearn\\utils\\deprecation.py:52: DeprecationWarning: Class GMM is deprecated; The class GMM is deprecated in 0.18 and will be removed in 0.20. Use class GaussianMixture instead.\n warnings.warn(msg, category=DeprecationWarning)\nC:\\Users\\admin\\Anaconda2\\lib\\site-packages\\sklearn\\utils\\deprecation.py:70: DeprecationWarning: Function distribute_covar_matrix_to_match_covariance_type is deprecated; The functon distribute_covar_matrix_to_match_covariance_typeis deprecated in 0.18 and will be removed in 0.20.\n warnings.warn(msg, category=DeprecationWarning)\nC:\\Users\\admin\\Anaconda2\\lib\\site-packages\\sklearn\\utils\\deprecation.py:70: DeprecationWarning: Function log_multivariate_normal_density is deprecated; The function log_multivariate_normal_density is deprecated in 0.18 and will be removed in 0.20.\n warnings.warn(msg, category=DeprecationWarning)\nC:\\Users\\admin\\Anaconda2\\lib\\site-packages\\sklearn\\utils\\deprecation.py:70: DeprecationWarning: Function log_multivariate_normal_density is deprecated; The function log_multivariate_normal_density is deprecated in 0.18 and will be removed in 0.20.\n warnings.warn(msg, category=DeprecationWarning)\nC:\\Users\\admin\\Anaconda2\\lib\\site-packages\\sklearn\\utils\\deprecation.py:70: DeprecationWarning: Function log_multivariate_normal_density is deprecated; The function log_multivariate_normal_density is deprecated in 0.18 and will be removed in 0.20.\n warnings.warn(msg, category=DeprecationWarning)\nC:\\Users\\admin\\Anaconda2\\lib\\site-packages\\sklearn\\utils\\deprecation.py:70: DeprecationWarning: Function log_multivariate_normal_density is deprecated; The function log_multivariate_normal_density is deprecated in 0.18 and will be removed in 0.20.\n warnings.warn(msg, category=DeprecationWarning)\nC:\\Users\\admin\\Anaconda2\\lib\\site-packages\\sklearn\\utils\\deprecation.py:70: DeprecationWarning: Function log_multivariate_normal_density is deprecated; The function log_multivariate_normal_density is deprecated in 0.18 and will be removed in 0.20.\n warnings.warn(msg, category=DeprecationWarning)\nC:\\Users\\admin\\Anaconda2\\lib\\site-packages\\sklearn\\utils\\deprecation.py:70: DeprecationWarning: Function log_multivariate_normal_density is deprecated; The function log_multivariate_normal_density is deprecated in 0.18 and will be removed in 0.20.\n warnings.warn(msg, category=DeprecationWarning)\nC:\\Users\\admin\\Anaconda2\\lib\\site-packages\\sklearn\\utils\\deprecation.py:70: DeprecationWarning: Function log_multivariate_normal_density is deprecated; The function log_multivariate_normal_density is deprecated in 0.18 and will be removed in 0.20.\n warnings.warn(msg, category=DeprecationWarning)\nC:\\Users\\admin\\Anaconda2\\lib\\site-packages\\sklearn\\utils\\deprecation.py:70: DeprecationWarning: Function log_multivariate_normal_density is deprecated; The function log_multivariate_normal_density is deprecated in 0.18 and will be removed in 0.20.\n warnings.warn(msg, category=DeprecationWarning)\nC:\\Users\\admin\\Anaconda2\\lib\\site-packages\\sklearn\\utils\\deprecation.py:70: DeprecationWarning: Function log_multivariate_normal_density is deprecated; The function log_multivariate_normal_density is deprecated in 0.18 and will be removed in 0.20.\n warnings.warn(msg, category=DeprecationWarning)\nC:\\Users\\admin\\Anaconda2\\lib\\site-packages\\sklearn\\utils\\deprecation.py:70: DeprecationWarning: Function log_multivariate_normal_density is deprecated; The function log_multivariate_normal_density is deprecated in 0.18 and will be removed in 0.20.\n warnings.warn(msg, category=DeprecationWarning)\nC:\\Users\\admin\\Anaconda2\\lib\\site-packages\\sklearn\\utils\\deprecation.py:70: DeprecationWarning: Function log_multivariate_normal_density is deprecated; The function log_multivariate_normal_density is deprecated in 0.18 and will be removed in 0.20.\n warnings.warn(msg, category=DeprecationWarning)\nC:\\Users\\admin\\Anaconda2\\lib\\site-packages\\sklearn\\utils\\deprecation.py:70: DeprecationWarning: Function log_multivariate_normal_density is deprecated; The function log_multivariate_normal_density is deprecated in 0.18 and will be removed in 0.20.\n warnings.warn(msg, category=DeprecationWarning)\nC:\\Users\\admin\\Anaconda2\\lib\\site-packages\\sklearn\\utils\\deprecation.py:70: DeprecationWarning: Function log_multivariate_normal_density is deprecated; The function log_multivariate_normal_density is deprecated in 0.18 and will be removed in 0.20.\n warnings.warn(msg, category=DeprecationWarning)\nC:\\Users\\admin\\Anaconda2\\lib\\site-packages\\sklearn\\utils\\deprecation.py:70: DeprecationWarning: Function log_multivariate_normal_density is deprecated; The function log_multivariate_normal_density is deprecated in 0.18 and will be removed in 0.20.\n warnings.warn(msg, category=DeprecationWarning)\nC:\\Users\\admin\\Anaconda2\\lib\\site-packages\\sklearn\\utils\\deprecation.py:70: DeprecationWarning: Function log_multivariate_normal_density is deprecated; The function log_multivariate_normal_density is deprecated in 0.18 and will be removed in 0.20.\n warnings.warn(msg, category=DeprecationWarning)\nC:\\Users\\admin\\Anaconda2\\lib\\site-packages\\sklearn\\utils\\deprecation.py:70: DeprecationWarning: Function log_multivariate_normal_density is deprecated; The function log_multivariate_normal_density is deprecated in 0.18 and will be removed in 0.20.\n warnings.warn(msg, category=DeprecationWarning)\nC:\\Users\\admin\\Anaconda2\\lib\\site-packages\\sklearn\\utils\\deprecation.py:70: DeprecationWarning: Function log_multivariate_normal_density is deprecated; The function log_multivariate_normal_density is deprecated in 0.18 and will be removed in 0.20.\n warnings.warn(msg, category=DeprecationWarning)\nC:\\Users\\admin\\Anaconda2\\lib\\site-packages\\sklearn\\utils\\deprecation.py:52: DeprecationWarning: Class GMM is deprecated; The class GMM is deprecated in 0.18 and will be removed in 0.20. Use class GaussianMixture instead.\n warnings.warn(msg, category=DeprecationWarning)\nC:\\Users\\admin\\Anaconda2\\lib\\site-packages\\sklearn\\utils\\deprecation.py:70: DeprecationWarning: Function distribute_covar_matrix_to_match_covariance_type is deprecated; The functon distribute_covar_matrix_to_match_covariance_typeis deprecated in 0.18 and will be removed in 0.20.\n warnings.warn(msg, category=DeprecationWarning)\nC:\\Users\\admin\\Anaconda2\\lib\\site-packages\\sklearn\\utils\\deprecation.py:70: DeprecationWarning: Function log_multivariate_normal_density is deprecated; The function log_multivariate_normal_density is deprecated in 0.18 and will be removed in 0.20.\n warnings.warn(msg, category=DeprecationWarning)\nC:\\Users\\admin\\Anaconda2\\lib\\site-packages\\sklearn\\utils\\deprecation.py:70: DeprecationWarning: Function log_multivariate_normal_density is deprecated; The function log_multivariate_normal_density is deprecated in 0.18 and will be removed in 0.20.\n warnings.warn(msg, category=DeprecationWarning)\nC:\\Users\\admin\\Anaconda2\\lib\\site-packages\\sklearn\\utils\\deprecation.py:70: DeprecationWarning: Function log_multivariate_normal_density is deprecated; The function log_multivariate_normal_density is deprecated in 0.18 and will be removed in 0.20.\n warnings.warn(msg, category=DeprecationWarning)\nC:\\Users\\admin\\Anaconda2\\lib\\site-packages\\sklearn\\utils\\deprecation.py:70: DeprecationWarning: Function log_multivariate_normal_density is deprecated; The function log_multivariate_normal_density is deprecated in 0.18 and will be removed in 0.20.\n warnings.warn(msg, category=DeprecationWarning)\nC:\\Users\\admin\\Anaconda2\\lib\\site-packages\\sklearn\\utils\\deprecation.py:70: DeprecationWarning: Function log_multivariate_normal_density is deprecated; The function log_multivariate_normal_density is deprecated in 0.18 and will be removed in 0.20.\n warnings.warn(msg, category=DeprecationWarning)\nC:\\Users\\admin\\Anaconda2\\lib\\site-packages\\sklearn\\utils\\deprecation.py:70: DeprecationWarning: Function log_multivariate_normal_density is deprecated; The function log_multivariate_normal_density is deprecated in 0.18 and will be removed in 0.20.\n warnings.warn(msg, category=DeprecationWarning)\nC:\\Users\\admin\\Anaconda2\\lib\\site-packages\\sklearn\\utils\\deprecation.py:70: DeprecationWarning: Function log_multivariate_normal_density is deprecated; The function log_multivariate_normal_density is deprecated in 0.18 and will be removed in 0.20.\n warnings.warn(msg, category=DeprecationWarning)\nC:\\Users\\admin\\Anaconda2\\lib\\site-packages\\sklearn\\utils\\deprecation.py:70: DeprecationWarning: Function log_multivariate_normal_density is deprecated; The function log_multivariate_normal_density is deprecated in 0.18 and will be removed in 0.20.\n warnings.warn(msg, category=DeprecationWarning)\nC:\\Users\\admin\\Anaconda2\\lib\\site-packages\\sklearn\\utils\\deprecation.py:70: DeprecationWarning: Function log_multivariate_normal_density is deprecated; The function log_multivariate_normal_density is deprecated in 0.18 and will be removed in 0.20.\n warnings.warn(msg, category=DeprecationWarning)\nC:\\Users\\admin\\Anaconda2\\lib\\site-packages\\sklearn\\utils\\deprecation.py:70: DeprecationWarning: Function log_multivariate_normal_density is deprecated; The function log_multivariate_normal_density is deprecated in 0.18 and will be removed in 0.20.\n warnings.warn(msg, category=DeprecationWarning)\nC:\\Users\\admin\\Anaconda2\\lib\\site-packages\\sklearn\\utils\\deprecation.py:70: DeprecationWarning: Function log_multivariate_normal_density is deprecated; The function log_multivariate_normal_density is deprecated in 0.18 and will be removed in 0.20.\n warnings.warn(msg, category=DeprecationWarning)\nC:\\Users\\admin\\Anaconda2\\lib\\site-packages\\sklearn\\utils\\deprecation.py:70: DeprecationWarning: Function log_multivariate_normal_density is deprecated; The function log_multivariate_normal_density is deprecated in 0.18 and will be removed in 0.20.\n warnings.warn(msg, category=DeprecationWarning)\nC:\\Users\\admin\\Anaconda2\\lib\\site-packages\\sklearn\\utils\\deprecation.py:70: DeprecationWarning: Function log_multivariate_normal_density is deprecated; The function log_multivariate_normal_density is deprecated in 0.18 and will be removed in 0.20.\n warnings.warn(msg, category=DeprecationWarning)\nC:\\Users\\admin\\Anaconda2\\lib\\site-packages\\sklearn\\utils\\deprecation.py:70: DeprecationWarning: Function log_multivariate_normal_density is deprecated; The function log_multivariate_normal_density is deprecated in 0.18 and will be removed in 0.20.\n warnings.warn(msg, category=DeprecationWarning)\nC:\\Users\\admin\\Anaconda2\\lib\\site-packages\\sklearn\\utils\\deprecation.py:70: DeprecationWarning: Function log_multivariate_normal_density is deprecated; The function log_multivariate_normal_density is deprecated in 0.18 and will be removed in 0.20.\n warnings.warn(msg, category=DeprecationWarning)\nC:\\Users\\admin\\Anaconda2\\lib\\site-packages\\sklearn\\utils\\deprecation.py:70: DeprecationWarning: Function log_multivariate_normal_density is deprecated; The function log_multivariate_normal_density is deprecated in 0.18 and will be removed in 0.20.\n warnings.warn(msg, category=DeprecationWarning)\nC:\\Users\\admin\\Anaconda2\\lib\\site-packages\\sklearn\\utils\\deprecation.py:70: DeprecationWarning: Function log_multivariate_normal_density is deprecated; The function log_multivariate_normal_density is deprecated in 0.18 and will be removed in 0.20.\n warnings.warn(msg, category=DeprecationWarning)\nC:\\Users\\admin\\Anaconda2\\lib\\site-packages\\sklearn\\utils\\deprecation.py:52: DeprecationWarning: Class GMM is deprecated; The class GMM is deprecated in 0.18 and will be removed in 0.20. Use class GaussianMixture instead.\n warnings.warn(msg, category=DeprecationWarning)\nC:\\Users\\admin\\Anaconda2\\lib\\site-packages\\sklearn\\utils\\deprecation.py:70: DeprecationWarning: Function distribute_covar_matrix_to_match_covariance_type is deprecated; The functon distribute_covar_matrix_to_match_covariance_typeis deprecated in 0.18 and will be removed in 0.20.\n warnings.warn(msg, category=DeprecationWarning)\nC:\\Users\\admin\\Anaconda2\\lib\\site-packages\\sklearn\\utils\\deprecation.py:70: DeprecationWarning: Function log_multivariate_normal_density is deprecated; The function log_multivariate_normal_density is deprecated in 0.18 and will be removed in 0.20.\n warnings.warn(msg, category=DeprecationWarning)\nC:\\Users\\admin\\Anaconda2\\lib\\site-packages\\sklearn\\utils\\deprecation.py:70: DeprecationWarning: Function log_multivariate_normal_density is deprecated; The function log_multivariate_normal_density is deprecated in 0.18 and will be removed in 0.20.\n warnings.warn(msg, category=DeprecationWarning)\nC:\\Users\\admin\\Anaconda2\\lib\\site-packages\\sklearn\\utils\\deprecation.py:70: DeprecationWarning: Function log_multivariate_normal_density is deprecated; The function log_multivariate_normal_density is deprecated in 0.18 and will be removed in 0.20.\n warnings.warn(msg, category=DeprecationWarning)\nC:\\Users\\admin\\Anaconda2\\lib\\site-packages\\sklearn\\utils\\deprecation.py:70: DeprecationWarning: Function log_multivariate_normal_density is deprecated; The function log_multivariate_normal_density is deprecated in 0.18 and will be removed in 0.20.\n warnings.warn(msg, category=DeprecationWarning)\nC:\\Users\\admin\\Anaconda2\\lib\\site-packages\\sklearn\\utils\\deprecation.py:70: DeprecationWarning: Function log_multivariate_normal_density is deprecated; The function log_multivariate_normal_density is deprecated in 0.18 and will be removed in 0.20.\n warnings.warn(msg, category=DeprecationWarning)\nC:\\Users\\admin\\Anaconda2\\lib\\site-packages\\sklearn\\utils\\deprecation.py:70: DeprecationWarning: Function log_multivariate_normal_density is deprecated; The function log_multivariate_normal_density is deprecated in 0.18 and will be removed in 0.20.\n warnings.warn(msg, category=DeprecationWarning)\nC:\\Users\\admin\\Anaconda2\\lib\\site-packages\\sklearn\\utils\\deprecation.py:70: DeprecationWarning: Function log_multivariate_normal_density is deprecated; The function log_multivariate_normal_density is deprecated in 0.18 and will be removed in 0.20.\n warnings.warn(msg, category=DeprecationWarning)\nC:\\Users\\admin\\Anaconda2\\lib\\site-packages\\sklearn\\utils\\deprecation.py:70: DeprecationWarning: Function log_multivariate_normal_density is deprecated; The function log_multivariate_normal_density is deprecated in 0.18 and will be removed in 0.20.\n warnings.warn(msg, category=DeprecationWarning)\nC:\\Users\\admin\\Anaconda2\\lib\\site-packages\\sklearn\\utils\\deprecation.py:70: DeprecationWarning: Function log_multivariate_normal_density is deprecated; The function log_multivariate_normal_density is deprecated in 0.18 and will be removed in 0.20.\n warnings.warn(msg, category=DeprecationWarning)\nC:\\Users\\admin\\Anaconda2\\lib\\site-packages\\sklearn\\utils\\deprecation.py:70: DeprecationWarning: Function log_multivariate_normal_density is deprecated; The function log_multivariate_normal_density is deprecated in 0.18 and will be removed in 0.20.\n warnings.warn(msg, category=DeprecationWarning)\nC:\\Users\\admin\\Anaconda2\\lib\\site-packages\\sklearn\\utils\\deprecation.py:70: DeprecationWarning: Function log_multivariate_normal_density is deprecated; The function log_multivariate_normal_density is deprecated in 0.18 and will be removed in 0.20.\n warnings.warn(msg, category=DeprecationWarning)\nC:\\Users\\admin\\Anaconda2\\lib\\site-packages\\sklearn\\utils\\deprecation.py:70: DeprecationWarning: Function log_multivariate_normal_density is deprecated; The function log_multivariate_normal_density is deprecated in 0.18 and will be removed in 0.20.\n warnings.warn(msg, category=DeprecationWarning)\nC:\\Users\\admin\\Anaconda2\\lib\\site-packages\\sklearn\\utils\\deprecation.py:70: DeprecationWarning: Function log_multivariate_normal_density is deprecated; The function log_multivariate_normal_density is deprecated in 0.18 and will be removed in 0.20.\n warnings.warn(msg, category=DeprecationWarning)\nC:\\Users\\admin\\Anaconda2\\lib\\site-packages\\sklearn\\utils\\deprecation.py:70: DeprecationWarning: Function log_multivariate_normal_density is deprecated; The function log_multivariate_normal_density is deprecated in 0.18 and will be removed in 0.20.\n warnings.warn(msg, category=DeprecationWarning)\nC:\\Users\\admin\\Anaconda2\\lib\\site-packages\\sklearn\\utils\\deprecation.py:70: DeprecationWarning: Function log_multivariate_normal_density is deprecated; The function log_multivariate_normal_density is deprecated in 0.18 and will be removed in 0.20.\n warnings.warn(msg, category=DeprecationWarning)\nC:\\Users\\admin\\Anaconda2\\lib\\site-packages\\sklearn\\utils\\deprecation.py:70: DeprecationWarning: Function log_multivariate_normal_density is deprecated; The function log_multivariate_normal_density is deprecated in 0.18 and will be removed in 0.20.\n warnings.warn(msg, category=DeprecationWarning)\nC:\\Users\\admin\\Anaconda2\\lib\\site-packages\\sklearn\\utils\\deprecation.py:70: DeprecationWarning: Function log_multivariate_normal_density is deprecated; The function log_multivariate_normal_density is deprecated in 0.18 and will be removed in 0.20.\n warnings.warn(msg, category=DeprecationWarning)\nC:\\Users\\admin\\Anaconda2\\lib\\site-packages\\sklearn\\utils\\deprecation.py:52: DeprecationWarning: Class GMM is deprecated; The class GMM is deprecated in 0.18 and will be removed in 0.20. Use class GaussianMixture instead.\n warnings.warn(msg, category=DeprecationWarning)\nC:\\Users\\admin\\Anaconda2\\lib\\site-packages\\sklearn\\utils\\deprecation.py:70: DeprecationWarning: Function distribute_covar_matrix_to_match_covariance_type is deprecated; The functon distribute_covar_matrix_to_match_covariance_typeis deprecated in 0.18 and will be removed in 0.20.\n warnings.warn(msg, category=DeprecationWarning)\nC:\\Users\\admin\\Anaconda2\\lib\\site-packages\\sklearn\\utils\\deprecation.py:70: DeprecationWarning: Function log_multivariate_normal_density is deprecated; The function log_multivariate_normal_density is deprecated in 0.18 and will be removed in 0.20.\n warnings.warn(msg, category=DeprecationWarning)\nC:\\Users\\admin\\Anaconda2\\lib\\site-packages\\sklearn\\utils\\deprecation.py:70: DeprecationWarning: Function log_multivariate_normal_density is deprecated; The function log_multivariate_normal_density is deprecated in 0.18 and will be removed in 0.20.\n warnings.warn(msg, category=DeprecationWarning)\nC:\\Users\\admin\\Anaconda2\\lib\\site-packages\\sklearn\\utils\\deprecation.py:70: DeprecationWarning: Function log_multivariate_normal_density is deprecated; The function log_multivariate_normal_density is deprecated in 0.18 and will be removed in 0.20.\n warnings.warn(msg, category=DeprecationWarning)\nC:\\Users\\admin\\Anaconda2\\lib\\site-packages\\sklearn\\utils\\deprecation.py:70: DeprecationWarning: Function log_multivariate_normal_density is deprecated; The function log_multivariate_normal_density is deprecated in 0.18 and will be removed in 0.20.\n warnings.warn(msg, category=DeprecationWarning)\nC:\\Users\\admin\\Anaconda2\\lib\\site-packages\\sklearn\\utils\\deprecation.py:70: DeprecationWarning: Function log_multivariate_normal_density is deprecated; The function log_multivariate_normal_density is deprecated in 0.18 and will be removed in 0.20.\n warnings.warn(msg, category=DeprecationWarning)\nC:\\Users\\admin\\Anaconda2\\lib\\site-packages\\sklearn\\utils\\deprecation.py:70: DeprecationWarning: Function log_multivariate_normal_density is deprecated; The function log_multivariate_normal_density is deprecated in 0.18 and will be removed in 0.20.\n warnings.warn(msg, category=DeprecationWarning)\nC:\\Users\\admin\\Anaconda2\\lib\\site-packages\\sklearn\\utils\\deprecation.py:70: DeprecationWarning: Function log_multivariate_normal_density is deprecated; The function log_multivariate_normal_density is deprecated in 0.18 and will be removed in 0.20.\n warnings.warn(msg, category=DeprecationWarning)\nC:\\Users\\admin\\Anaconda2\\lib\\site-packages\\sklearn\\utils\\deprecation.py:70: DeprecationWarning: Function log_multivariate_normal_density is deprecated; The function log_multivariate_normal_density is deprecated in 0.18 and will be removed in 0.20.\n warnings.warn(msg, category=DeprecationWarning)\nC:\\Users\\admin\\Anaconda2\\lib\\site-packages\\sklearn\\utils\\deprecation.py:70: DeprecationWarning: Function log_multivariate_normal_density is deprecated; The function log_multivariate_normal_density is deprecated in 0.18 and will be removed in 0.20.\n warnings.warn(msg, category=DeprecationWarning)\n"
]
],
[
[
"### Question 7\n*Report the silhouette score for several cluster numbers you tried. Of these, which number of clusters has the best silhouette score?* ",
"_____no_output_____"
],
[
"**Answer:**{2: 0.41181886438624482, 3: 0.37616616509083634, 4: 0.34168407828470648, 5: 0.28001985722335737, 6: 0.26923051036000389, 7: 0.32398601556485884, 8: 0.30410685766208839, 9: 0.27229645992822205} is dictionary with <br>\nnumber of clusters and their silhouette score as a tuple. <br>\nBest score is given by number of clusters = 2 and its score is 0.41181886438624482",
"_____no_output_____"
],
[
"### Cluster Visualization\nOnce you've chosen the optimal number of clusters for your clustering algorithm using the scoring metric above, you can now visualize the results by executing the code block below. Note that, for experimentation purposes, you are welcome to adjust the number of clusters for your clustering algorithm to see various visualizations. The final visualization provided should, however, correspond with the optimal number of clusters. ",
"_____no_output_____"
]
],
[
[
"# Display the results of the clustering from implementation\nvs.cluster_results(reduced_data, preds, centers, pca_samples)",
"_____no_output_____"
]
],
[
[
"### Implementation: Data Recovery\nEach cluster present in the visualization above has a central point. These centers (or means) are not specifically data points from the data, but rather the *averages* of all the data points predicted in the respective clusters. For the problem of creating customer segments, a cluster's center point corresponds to *the average customer of that segment*. Since the data is currently reduced in dimension and scaled by a logarithm, we can recover the representative customer spending from these data points by applying the inverse transformations.\n\nIn the code block below, you will need to implement the following:\n - Apply the inverse transform to `centers` using `pca.inverse_transform` and assign the new centers to `log_centers`.\n - Apply the inverse function of `np.log` to `log_centers` using `np.exp` and assign the true centers to `true_centers`.\n",
"_____no_output_____"
]
],
[
[
"# TODO: Inverse transform the centers\nlog_centers = pca.inverse_transform(centers)\n\n# TODO: Exponentiate the centers\ntrue_centers = np.exp(log_centers)\n\n# Display the true centers\nsegments = ['Segment {}'.format(i) for i in range(0,len(centers))]\ntrue_centers = pd.DataFrame(np.round(true_centers), columns = data.keys())\ntrue_centers.index = segments\ndisplay(true_centers)\ndata.describe()",
"_____no_output_____"
]
],
[
[
"### Question 8\nConsider the total purchase cost of each product category for the representative data points above, and reference the statistical description of the dataset at the beginning of this project. *What set of establishments could each of the customer segments represent?* \n**Hint:** A customer who is assigned to `'Cluster X'` should best identify with the establishments represented by the feature set of `'Segment X'`.",
"_____no_output_____"
],
[
"**Answer:**Seeing the statistics and taking segment 0 into account, Fresh is above median while all others are below median.\nThus segment 0 can be considered as Fresh Produce or Corner store.<br>\nWhereas for segment 1, all products except Fresh are close or above the median. Thus it possibly comes into convenience store.",
"_____no_output_____"
],
[
"### Question 9\n*For each sample point, which customer segment from* ***Question 8*** *best represents it? Are the predictions for each sample point consistent with this?*\n\nRun the code block below to find which cluster each sample point is predicted to be.",
"_____no_output_____"
]
],
[
[
"# Display the predictions\nfor i, pred in enumerate(sample_preds):\n print \"Sample point\", i, \"predicted to be in Cluster\", pred\ndisplay(samples)",
"Sample point 0 predicted to be in Cluster 0\nSample point 1 predicted to be in Cluster 0\nSample point 2 predicted to be in Cluster 1\n"
]
],
[
[
"**Answer:** The third datapoint is correctly classified by both me and gmm. The first two aren't exactly misclassfied since I have put them into two categories Supermarket and Convenience Store which can be also called as Retailer",
"_____no_output_____"
],
[
"## Conclusion",
"_____no_output_____"
],
[
"In this final section, you will investigate ways that you can make use of the clustered data. First, you will consider how the different groups of customers, the ***customer segments***, may be affected differently by a specific delivery scheme. Next, you will consider how giving a label to each customer (which *segment* that customer belongs to) can provide for additional features about the customer data. Finally, you will compare the ***customer segments*** to a hidden variable present in the data, to see whether the clustering identified certain relationships.",
"_____no_output_____"
],
[
"### Question 10\nCompanies will often run [A/B tests](https://en.wikipedia.org/wiki/A/B_testing) when making small changes to their products or services to determine whether making that change will affect its customers positively or negatively. The wholesale distributor is considering changing its delivery service from currently 5 days a week to 3 days a week. However, the distributor will only make this change in delivery service for customers that react positively. *How can the wholesale distributor use the customer segments to determine which customers, if any, would react positively to the change in delivery service?* \n**Hint:** Can we assume the change affects all customers equally? How can we determine which group of customers it affects the most?",
"_____no_output_____"
],
[
"**Answer:**Select random customers from each clusters.This should be a small subset. The owner can then change the services to these cutomers and find the customer satisfaction in range of 0 and 1. Care should be taken that the chosen subset should be statistically stable. Thus, if the customers behave positively. the delivery services could be extended by increasing the number of random customers. The prepared model can then be cross validated. The process is repeated until market goals are achieved.",
"_____no_output_____"
],
[
"### Question 11\nAdditional structure is derived from originally unlabeled data when using clustering techniques. Since each customer has a ***customer segment*** it best identifies with (depending on the clustering algorithm applied), we can consider *'customer segment'* as an **engineered feature** for the data. Assume the wholesale distributor recently acquired ten new customers and each provided estimates for anticipated annual spending of each product category. Knowing these estimates, the wholesale distributor wants to classify each new customer to a ***customer segment*** to determine the most appropriate delivery service. \n*How can the wholesale distributor label the new customers using only their estimated product spending and the* ***customer segment*** *data?* \n**Hint:** A supervised learner could be used to train on the original customers. What would be the target variable?",
"_____no_output_____"
],
[
"**Answer:**Running a supervised learning model which classifies the customer segments as (0:Grocer, Supermarket:2 and so on)can be done. The target would be segments(0:Grocer, 1:supermarket) generated by clusters and their features would be fresh, frozen, GRocery, Detergents_paper,Delicatessen, Milk. \n",
"_____no_output_____"
],
[
"### Visualizing Underlying Distributions\n\nAt the beginning of this project, it was discussed that the `'Channel'` and `'Region'` features would be excluded from the dataset so that the customer product categories were emphasized in the analysis. By reintroducing the `'Channel'` feature to the dataset, an interesting structure emerges when considering the same PCA dimensionality reduction applied earlier to the original dataset.\n\nRun the code block below to see how each data point is labeled either `'HoReCa'` (Hotel/Restaurant/Cafe) or `'Retail'` the reduced space. In addition, you will find the sample points are circled in the plot, which will identify their labeling.",
"_____no_output_____"
]
],
[
[
"# Display the clustering results based on 'Channel' data\nvs.channel_results(reduced_data, outliers, pca_samples)",
"_____no_output_____"
]
],
[
[
"### Question 12\n*How well does the clustering algorithm and number of clusters you've chosen compare to this underlying distribution of Hotel/Restaurant/Cafe customers to Retailer customers? Are there customer segments that would be classified as purely 'Retailers' or 'Hotels/Restaurants/Cafes' by this distribution? Would you consider these classifications as consistent with your previous definition of the customer segments?*",
"_____no_output_____"
],
[
"**Answer:** 1) The clustering algorithm GMM works well with 2 centers and is consistent.\n<br>2)No, there is an overlapping of clusters. Thus, they cannot be perfectly classified as Hotels, Restaurants and Cafes.\n3)Yes, the classifications are consistent with previous definition of customer segments.",
"_____no_output_____"
],
[
"> **Note**: Once you have completed all of the code implementations and successfully answered each question above, you may finalize your work by exporting the iPython Notebook as an HTML document. You can do this by using the menu above and navigating to \n**File -> Download as -> HTML (.html)**. Include the finished document along with this notebook as your submission.",
"_____no_output_____"
]
]
] | [
"markdown",
"code",
"markdown",
"code",
"markdown",
"code",
"markdown",
"code",
"markdown",
"code",
"markdown",
"code",
"markdown",
"code",
"markdown",
"code",
"markdown",
"code",
"markdown",
"code",
"markdown",
"code",
"markdown",
"code",
"markdown",
"code",
"markdown",
"code",
"markdown",
"code",
"markdown",
"code",
"markdown",
"code",
"markdown",
"code",
"markdown"
] | [
[
"markdown",
"markdown",
"markdown"
],
[
"code"
],
[
"markdown"
],
[
"code"
],
[
"markdown"
],
[
"code"
],
[
"markdown",
"markdown",
"markdown"
],
[
"code"
],
[
"markdown",
"markdown",
"markdown"
],
[
"code",
"code"
],
[
"markdown",
"markdown",
"markdown",
"markdown"
],
[
"code"
],
[
"markdown"
],
[
"code"
],
[
"markdown"
],
[
"code"
],
[
"markdown",
"markdown",
"markdown",
"markdown"
],
[
"code"
],
[
"markdown",
"markdown",
"markdown"
],
[
"code"
],
[
"markdown"
],
[
"code"
],
[
"markdown"
],
[
"code"
],
[
"markdown"
],
[
"code"
],
[
"markdown",
"markdown",
"markdown",
"markdown",
"markdown"
],
[
"code"
],
[
"markdown",
"markdown",
"markdown"
],
[
"code"
],
[
"markdown"
],
[
"code"
],
[
"markdown",
"markdown",
"markdown"
],
[
"code"
],
[
"markdown",
"markdown",
"markdown",
"markdown",
"markdown",
"markdown",
"markdown",
"markdown"
],
[
"code"
],
[
"markdown",
"markdown",
"markdown"
]
] |
e766b24d92c6f5ca9fd8cd40d4dd08493ce4715e | 341,221 | ipynb | Jupyter Notebook | ssd300_evaluation.ipynb | rogeryan/ssd_keras | 45c364a122d2aa894ce8ba687876b02223a83cd0 | [
"Apache-2.0"
] | null | null | null | ssd300_evaluation.ipynb | rogeryan/ssd_keras | 45c364a122d2aa894ce8ba687876b02223a83cd0 | [
"Apache-2.0"
] | null | null | null | ssd300_evaluation.ipynb | rogeryan/ssd_keras | 45c364a122d2aa894ce8ba687876b02223a83cd0 | [
"Apache-2.0"
] | null | null | null | 577.362098 | 312,664 | 0.924911 | [
[
[
"# SSD Evaluation Tutorial\n\nThis is a brief tutorial that explains how compute the average precisions for any trained SSD model using the `Evaluator` class. The `Evaluator` computes the average precisions according to the Pascal VOC pre-2010 or post-2010 detection evaluation algorithms. You can find details about these computation methods [here](http://host.robots.ox.ac.uk/pascal/VOC/voc2012/htmldoc/devkit_doc.html#sec:ap).\n\nAs an example we'll evaluate an SSD300 on the Pascal VOC 2007 `test` dataset, but note that the `Evaluator` works for any SSD model and any dataset that is compatible with the `DataGenerator`. If you would like to run the evaluation on a different model and/or dataset, the procedure is analogous to what is shown below, you just have to build the appropriate model and load the relevant dataset.\n\nNote: I that in case you would like to evaluate a model on MS COCO, I would recommend to follow the [MS COCO evaluation notebook](https://github.com/pierluigiferrari/ssd_keras/blob/master/ssd300_evaluation_COCO.ipynb) instead, because it can produce the results format required by the MS COCO evaluation server and uses the official MS COCO evaluation code, which computes the mAP slightly differently from the Pascal VOC method.\n\nNote: In case you want to evaluate any of the provided trained models, make sure that you build the respective model with the correct set of scaling factors to reproduce the official results. The models that were trained on MS COCO and fine-tuned on Pascal VOC require the MS COCO scaling factors, not the Pascal VOC scaling factors.",
"_____no_output_____"
]
],
[
[
"from keras import backend as K\nfrom tensorflow.keras.models import load_model\nfrom tensorflow.keras.optimizers import Adam\nfrom matplotlib.pyplot import imread\nimport numpy as np\nfrom matplotlib import pyplot as plt\n\nfrom models.keras_ssd300 import ssd_300\nfrom keras_loss_function.keras_ssd_loss import SSDLoss\nfrom keras_layers.keras_layer_AnchorBoxes import AnchorBoxes\nfrom keras_layers.keras_layer_DecodeDetections import DecodeDetections\nfrom keras_layers.keras_layer_DecodeDetectionsFast import DecodeDetectionsFast\nfrom keras_layers.keras_layer_L2Normalization import L2Normalization\nfrom data_generator.object_detection_2d_data_generator import DataGenerator\nfrom eval_utils.average_precision_evaluator import Evaluator\n\n%matplotlib inline",
"_____no_output_____"
],
[
"# Set a few configuration parameters.\nimg_height = 300\nimg_width = 300\nn_classes = 20\nmodel_mode = 'inference'",
"_____no_output_____"
]
],
[
[
"## 1. Load a trained SSD\n\nEither load a trained model or build a model and load trained weights into it. Since the HDF5 files I'm providing contain only the weights for the various SSD versions, not the complete models, you'll have to go with the latter option when using this implementation for the first time. You can then of course save the model and next time load the full model directly, without having to build it.\n\nYou can find the download links to all the trained model weights in the README.",
"_____no_output_____"
],
[
"### 1.1. Build the model and load trained weights into it",
"_____no_output_____"
]
],
[
[
"# 1: Build the Keras model\n\nK.clear_session() # Clear previous models from memory.\n\nmodel = ssd_300(image_size=(img_height, img_width, 3),\n n_classes=n_classes,\n mode=model_mode,\n l2_regularization=0.0005,\n scales=[0.1, 0.2, 0.37, 0.54, 0.71, 0.88, 1.05], # The scales for MS COCO [0.07, 0.15, 0.33, 0.51, 0.69, 0.87, 1.05]\n aspect_ratios_per_layer=[[1.0, 2.0, 0.5],\n [1.0, 2.0, 0.5, 3.0, 1.0/3.0],\n [1.0, 2.0, 0.5, 3.0, 1.0/3.0],\n [1.0, 2.0, 0.5, 3.0, 1.0/3.0],\n [1.0, 2.0, 0.5],\n [1.0, 2.0, 0.5]],\n two_boxes_for_ar1=True,\n steps=[8, 16, 32, 64, 100, 300],\n offsets=[0.5, 0.5, 0.5, 0.5, 0.5, 0.5],\n clip_boxes=False,\n variances=[0.1, 0.1, 0.2, 0.2],\n normalize_coords=True,\n subtract_mean=[123, 117, 104],\n swap_channels=[2, 1, 0],\n confidence_thresh=0.01,\n iou_threshold=0.45,\n top_k=200,\n nms_max_output_size=400)\n\n# 2: Load the trained weights into the model.\n\n# TODO: Set the path of the trained weights.\nweights_path = 'path/to/trained/weights/VGG_VOC0712_SSD_300x300_ft_iter_120000.h5'\n\nmodel.load_weights(weights_path, by_name=True)\n\n# 3: Compile the model so that Keras won't complain the next time you load it.\n\nadam = Adam(lr=0.001, beta_1=0.9, beta_2=0.999, epsilon=1e-08, decay=0.0)\n\nssd_loss = SSDLoss(neg_pos_ratio=3, alpha=1.0)\n\nmodel.compile(optimizer=adam, loss=ssd_loss.compute_loss)",
"_____no_output_____"
]
],
[
[
"Or",
"_____no_output_____"
],
[
"### 1.2. Load a trained model\n\nWe set `model_mode` to 'inference' above, so the evaluator expects that you load a model that was built in 'inference' mode. If you're loading a model that was built in 'training' mode, change the `model_mode` parameter accordingly.",
"_____no_output_____"
]
],
[
[
"# TODO: Set the path to the `.h5` file of the model to be loaded.\nmodel_path = 'path/to/trained/model.h5'\n\n# We need to create an SSDLoss object in order to pass that to the model loader.\nssd_loss = SSDLoss(neg_pos_ratio=3, alpha=1.0)\n\nK.clear_session() # Clear previous models from memory.\n\nmodel = load_model(model_path, custom_objects={'AnchorBoxes': AnchorBoxes,\n 'L2Normalization': L2Normalization,\n 'DecodeDetections': DecodeDetections,\n 'compute_loss': ssd_loss.compute_loss})",
"_____no_output_____"
]
],
[
[
"## 2. Create a data generator for the evaluation dataset\n\nInstantiate a `DataGenerator` that will serve the evaluation dataset during the prediction phase.",
"_____no_output_____"
]
],
[
[
"dataset = DataGenerator()\n\n# TODO: Set the paths to the dataset here.\nPascal_VOC_dataset_images_dir = '../../datasets/VOCdevkit/VOC2007/JPEGImages/'\nPascal_VOC_dataset_annotations_dir = '../../datasets/VOCdevkit/VOC2007/Annotations/'\nPascal_VOC_dataset_image_set_filename = '../../datasets/VOCdevkit/VOC2007/ImageSets/Main/test.txt'\n\n# The XML parser needs to now what object class names to look for and in which order to map them to integers.\nclasses = ['background',\n 'aeroplane', 'bicycle', 'bird', 'boat',\n 'bottle', 'bus', 'car', 'cat',\n 'chair', 'cow', 'diningtable', 'dog',\n 'horse', 'motorbike', 'person', 'pottedplant',\n 'sheep', 'sofa', 'train', 'tvmonitor']\n\ndataset.parse_xml(images_dirs=[Pascal_VOC_dataset_images_dir],\n image_set_filenames=[Pascal_VOC_dataset_image_set_filename],\n annotations_dirs=[Pascal_VOC_dataset_annotations_dir],\n classes=classes,\n include_classes='all',\n exclude_truncated=False,\n exclude_difficult=False,\n ret=False)",
"test.txt: 100%|██████████| 4952/4952 [00:13<00:00, 373.84it/s]\n"
]
],
[
[
"## 3. Run the evaluation\n\nNow that we have instantiated a model and a data generator to serve the dataset, we can set up the evaluator and run the evaluation.\n\nThe evaluator is quite flexible: It can compute the average precisions according to the Pascal VOC pre-2010 algorithm, which samples 11 equidistant points of the precision-recall curves, or according to the Pascal VOC post-2010 algorithm, which integrates numerically over the entire precision-recall curves instead of sampling a few individual points. You could also change the number of sampled recall points or the required IoU overlap for a prediction to be considered a true positive, among other things. Check out the `Evaluator`'s documentation for details on all the arguments.\n\nIn its default settings, the evaluator's algorithm is identical to the official Pascal VOC pre-2010 Matlab detection evaluation algorithm, so you don't really need to tweak anything unless you want to.\n\nThe evaluator roughly performs the following steps: It runs predictions over the entire given dataset, then it matches these predictions to the ground truth boxes, then it computes the precision-recall curves for each class, then it samples 11 equidistant points from these precision-recall curves to compute the average precision for each class, and finally it computes the mean average precision over all classes.",
"_____no_output_____"
]
],
[
[
"evaluator = Evaluator(model=model,\n n_classes=n_classes,\n data_generator=dataset,\n model_mode=model_mode)\n\nresults = evaluator(img_height=img_height,\n img_width=img_width,\n batch_size=8,\n data_generator_mode='resize',\n round_confidences=False,\n matching_iou_threshold=0.5,\n border_pixels='include',\n sorting_algorithm='quicksort',\n average_precision_mode='sample',\n num_recall_points=11,\n ignore_neutral_boxes=True,\n return_precisions=True,\n return_recalls=True,\n return_average_precisions=True,\n verbose=True)\n\nmean_average_precision, average_precisions, precisions, recalls = results",
"Number of images in the evaluation dataset: 4952\n\nProducing predictions batch-wise: 100%|██████████| 619/619 [02:17<00:00, 4.50it/s]\nMatching predictions to ground truth, class 1/20.: 100%|██████████| 7902/7902 [00:00<00:00, 19253.00it/s]\nMatching predictions to ground truth, class 2/20.: 100%|██████████| 4276/4276 [00:00<00:00, 23249.07it/s]\nMatching predictions to ground truth, class 3/20.: 100%|██████████| 19126/19126 [00:00<00:00, 28311.89it/s]\nMatching predictions to ground truth, class 4/20.: 100%|██████████| 25291/25291 [00:01<00:00, 21126.87it/s]\nMatching predictions to ground truth, class 5/20.: 100%|██████████| 33520/33520 [00:00<00:00, 34410.41it/s]\nMatching predictions to ground truth, class 6/20.: 100%|██████████| 4395/4395 [00:00<00:00, 20824.68it/s]\nMatching predictions to ground truth, class 7/20.: 100%|██████████| 41833/41833 [00:01<00:00, 20956.01it/s]\nMatching predictions to ground truth, class 8/20.: 100%|██████████| 2740/2740 [00:00<00:00, 24270.08it/s]\nMatching predictions to ground truth, class 9/20.: 100%|██████████| 91992/91992 [00:03<00:00, 25723.87it/s]\nMatching predictions to ground truth, class 10/20.: 100%|██████████| 4085/4085 [00:00<00:00, 23969.80it/s]\nMatching predictions to ground truth, class 11/20.: 100%|██████████| 6912/6912 [00:00<00:00, 26573.85it/s]\nMatching predictions to ground truth, class 12/20.: 100%|██████████| 4294/4294 [00:00<00:00, 24942.89it/s]\nMatching predictions to ground truth, class 13/20.: 100%|██████████| 2779/2779 [00:00<00:00, 20814.98it/s]\nMatching predictions to ground truth, class 14/20.: 100%|██████████| 3003/3003 [00:00<00:00, 17807.53it/s]\nMatching predictions to ground truth, class 15/20.: 100%|██████████| 183522/183522 [00:09<00:00, 19243.38it/s]\nMatching predictions to ground truth, class 16/20.: 100%|██████████| 35198/35198 [00:01<00:00, 21565.75it/s]\nMatching predictions to ground truth, class 17/20.: 100%|██████████| 10535/10535 [00:00<00:00, 19680.06it/s]\nMatching predictions to ground truth, class 18/20.: 100%|██████████| 4371/4371 [00:00<00:00, 11523.11it/s]\nMatching predictions to ground truth, class 19/20.: 100%|██████████| 5768/5768 [00:00<00:00, 9747.21it/s]\nMatching predictions to ground truth, class 20/20.: 100%|██████████| 10860/10860 [00:00<00:00, 13970.50it/s]\nComputing precisions and recalls, class 1/20\nComputing precisions and recalls, class 2/20\nComputing precisions and recalls, class 3/20\nComputing precisions and recalls, class 4/20\nComputing precisions and recalls, class 5/20\nComputing precisions and recalls, class 6/20\nComputing precisions and recalls, class 7/20\nComputing precisions and recalls, class 8/20\nComputing precisions and recalls, class 9/20\nComputing precisions and recalls, class 10/20\nComputing precisions and recalls, class 11/20\nComputing precisions and recalls, class 12/20\nComputing precisions and recalls, class 13/20\nComputing precisions and recalls, class 14/20\nComputing precisions and recalls, class 15/20\nComputing precisions and recalls, class 16/20\nComputing precisions and recalls, class 17/20\nComputing precisions and recalls, class 18/20\nComputing precisions and recalls, class 19/20\nComputing precisions and recalls, class 20/20\nComputing average precision, class 1/20\nComputing average precision, class 2/20\nComputing average precision, class 3/20\nComputing average precision, class 4/20\nComputing average precision, class 5/20\nComputing average precision, class 6/20\nComputing average precision, class 7/20\nComputing average precision, class 8/20\nComputing average precision, class 9/20\nComputing average precision, class 10/20\nComputing average precision, class 11/20\nComputing average precision, class 12/20\nComputing average precision, class 13/20\nComputing average precision, class 14/20\nComputing average precision, class 15/20\nComputing average precision, class 16/20\nComputing average precision, class 17/20\nComputing average precision, class 18/20\nComputing average precision, class 19/20\nComputing average precision, class 20/20\n"
]
],
[
[
"## 4. Visualize the results\n\nLet's take a look:",
"_____no_output_____"
]
],
[
[
"for i in range(1, len(average_precisions)):\n print(\"{:<14}{:<6}{}\".format(classes[i], 'AP', round(average_precisions[i], 3)))\nprint()\nprint(\"{:<14}{:<6}{}\".format('','mAP', round(mean_average_precision, 3)))",
"aeroplane AP 0.788\nbicycle AP 0.84\nbird AP 0.758\nboat AP 0.693\nbottle AP 0.509\nbus AP 0.868\ncar AP 0.858\ncat AP 0.886\nchair AP 0.601\ncow AP 0.822\ndiningtable AP 0.764\ndog AP 0.862\nhorse AP 0.875\nmotorbike AP 0.842\nperson AP 0.796\npottedplant AP 0.526\nsheep AP 0.779\nsofa AP 0.795\ntrain AP 0.875\ntvmonitor AP 0.773\n\n mAP 0.776\n"
],
[
"m = max((n_classes + 1) // 2, 2)\nn = 2\n\nfig, cells = plt.subplots(m, n, figsize=(n*8,m*8))\nfor i in range(m):\n for j in range(n):\n if n*i+j+1 > n_classes: break\n cells[i, j].plot(recalls[n*i+j+1], precisions[n*i+j+1], color='blue', linewidth=1.0)\n cells[i, j].set_xlabel('recall', fontsize=14)\n cells[i, j].set_ylabel('precision', fontsize=14)\n cells[i, j].grid(True)\n cells[i, j].set_xticks(np.linspace(0,1,11))\n cells[i, j].set_yticks(np.linspace(0,1,11))\n cells[i, j].set_title(\"{}, AP: {:.3f}\".format(classes[n*i+j+1], average_precisions[n*i+j+1]), fontsize=16)",
"_____no_output_____"
]
],
[
[
"## 5. Advanced use\n\n`Evaluator` objects maintain copies of all relevant intermediate results like predictions, precisions and recalls, etc., so in case you want to experiment with different parameters, e.g. different IoU overlaps, there is no need to compute the predictions all over again every time you make a change to a parameter. Instead, you can only update the computation from the point that is affected onwards.\n\nThe evaluator's `__call__()` method is just a convenience wrapper that executes its other methods in the correct order. You could just call any of these other methods individually as shown below (but you have to make sure to call them in the correct order).\n\nNote that the example below uses the same evaluator object as above. Say you wanted to compute the Pascal VOC post-2010 'integrate' version of the average precisions instead of the pre-2010 version computed above. The evaluator object still has an internal copy of all the predictions, and since computing the predictions makes up the vast majority of the overall computation time and since the predictions aren't affected by changing the average precision computation mode, we skip computing the predictions again and instead only compute the steps that come after the prediction phase of the evaluation. We could even skip the matching part, since it isn't affected by changing the average precision mode either. In fact, we would only have to call `compute_average_precisions()` `compute_mean_average_precision()` again, but for the sake of illustration we'll re-do the other computations, too.",
"_____no_output_____"
]
],
[
[
"evaluator.get_num_gt_per_class(ignore_neutral_boxes=True,\n verbose=False,\n ret=False)\n\nevaluator.match_predictions(ignore_neutral_boxes=True,\n matching_iou_threshold=0.5,\n border_pixels='include',\n sorting_algorithm='quicksort',\n verbose=True,\n ret=False)\n\nprecisions, recalls = evaluator.compute_precision_recall(verbose=True, ret=True)\n\naverage_precisions = evaluator.compute_average_precisions(mode='integrate',\n num_recall_points=11,\n verbose=True,\n ret=True)\n\nmean_average_precision = evaluator.compute_mean_average_precision(ret=True)",
"Matching predictions to ground truth, class 1/20.: 100%|██████████| 7902/7902 [00:00<00:00, 19849.68it/s]\nMatching predictions to ground truth, class 2/20.: 100%|██████████| 4276/4276 [00:00<00:00, 21798.36it/s]\nMatching predictions to ground truth, class 3/20.: 100%|██████████| 19126/19126 [00:00<00:00, 28263.72it/s]\nMatching predictions to ground truth, class 4/20.: 100%|██████████| 25291/25291 [00:01<00:00, 20847.78it/s]\nMatching predictions to ground truth, class 5/20.: 100%|██████████| 33520/33520 [00:00<00:00, 34610.95it/s]\nMatching predictions to ground truth, class 6/20.: 100%|██████████| 4395/4395 [00:00<00:00, 23612.98it/s]\nMatching predictions to ground truth, class 7/20.: 100%|██████████| 41833/41833 [00:02<00:00, 20821.01it/s]\nMatching predictions to ground truth, class 8/20.: 100%|██████████| 2740/2740 [00:00<00:00, 25909.74it/s]\nMatching predictions to ground truth, class 9/20.: 100%|██████████| 91992/91992 [00:03<00:00, 25150.58it/s]\nMatching predictions to ground truth, class 10/20.: 100%|██████████| 4085/4085 [00:00<00:00, 22590.90it/s]\nMatching predictions to ground truth, class 11/20.: 100%|██████████| 6912/6912 [00:00<00:00, 28966.61it/s]\nMatching predictions to ground truth, class 12/20.: 100%|██████████| 4294/4294 [00:00<00:00, 23105.94it/s]\nMatching predictions to ground truth, class 13/20.: 100%|██████████| 2779/2779 [00:00<00:00, 20409.40it/s]\nMatching predictions to ground truth, class 14/20.: 100%|██████████| 3003/3003 [00:00<00:00, 17314.28it/s]\nMatching predictions to ground truth, class 15/20.: 100%|██████████| 183522/183522 [00:09<00:00, 18903.68it/s]\nMatching predictions to ground truth, class 16/20.: 100%|██████████| 35198/35198 [00:01<00:00, 26489.65it/s]\nMatching predictions to ground truth, class 17/20.: 100%|██████████| 10535/10535 [00:00<00:00, 28867.54it/s]\nMatching predictions to ground truth, class 18/20.: 100%|██████████| 4371/4371 [00:00<00:00, 22087.65it/s]\nMatching predictions to ground truth, class 19/20.: 100%|██████████| 5768/5768 [00:00<00:00, 17063.02it/s]\nMatching predictions to ground truth, class 20/20.: 100%|██████████| 10860/10860 [00:00<00:00, 25999.09it/s]\nComputing precisions and recalls, class 1/20\nComputing precisions and recalls, class 2/20\nComputing precisions and recalls, class 3/20\nComputing precisions and recalls, class 4/20\nComputing precisions and recalls, class 5/20\nComputing precisions and recalls, class 6/20\nComputing precisions and recalls, class 7/20\nComputing precisions and recalls, class 8/20\nComputing precisions and recalls, class 9/20\nComputing precisions and recalls, class 10/20\nComputing precisions and recalls, class 11/20\nComputing precisions and recalls, class 12/20\nComputing precisions and recalls, class 13/20\nComputing precisions and recalls, class 14/20\nComputing precisions and recalls, class 15/20\nComputing precisions and recalls, class 16/20\nComputing precisions and recalls, class 17/20\nComputing precisions and recalls, class 18/20\nComputing precisions and recalls, class 19/20\nComputing precisions and recalls, class 20/20\nComputing average precision, class 1/20\nComputing average precision, class 2/20\nComputing average precision, class 3/20\nComputing average precision, class 4/20\nComputing average precision, class 5/20\nComputing average precision, class 6/20\nComputing average precision, class 7/20\nComputing average precision, class 8/20\nComputing average precision, class 9/20\nComputing average precision, class 10/20\nComputing average precision, class 11/20\nComputing average precision, class 12/20\nComputing average precision, class 13/20\nComputing average precision, class 14/20\nComputing average precision, class 15/20\nComputing average precision, class 16/20\nComputing average precision, class 17/20\nComputing average precision, class 18/20\nComputing average precision, class 19/20\nComputing average precision, class 20/20\n"
],
[
"for i in range(1, len(average_precisions)):\n print(\"{:<14}{:<6}{}\".format(classes[i], 'AP', round(average_precisions[i], 3)))\nprint()\nprint(\"{:<14}{:<6}{}\".format('','mAP', round(mean_average_precision, 3)))",
"aeroplane AP 0.822\nbicycle AP 0.874\nbird AP 0.787\nboat AP 0.713\nbottle AP 0.505\nbus AP 0.899\ncar AP 0.89\ncat AP 0.923\nchair AP 0.61\ncow AP 0.845\ndiningtable AP 0.79\ndog AP 0.899\nhorse AP 0.903\nmotorbike AP 0.875\nperson AP 0.825\npottedplant AP 0.526\nsheep AP 0.811\nsofa AP 0.83\ntrain AP 0.906\ntvmonitor AP 0.797\n\n mAP 0.802\n"
]
]
] | [
"markdown",
"code",
"markdown",
"code",
"markdown",
"code",
"markdown",
"code",
"markdown",
"code",
"markdown",
"code",
"markdown",
"code"
] | [
[
"markdown"
],
[
"code",
"code"
],
[
"markdown",
"markdown"
],
[
"code"
],
[
"markdown",
"markdown"
],
[
"code"
],
[
"markdown"
],
[
"code"
],
[
"markdown"
],
[
"code"
],
[
"markdown"
],
[
"code",
"code"
],
[
"markdown"
],
[
"code",
"code"
]
] |
e766c1b3410df7a8a3e5db01a3e47466139a62aa | 20,262 | ipynb | Jupyter Notebook | reinforcement_learning/rl_roboschool_ray/rl_roboschool_ray.ipynb | Amirosimani/amazon-sagemaker-examples | bc35e7a9da9e2258e77f98098254c2a8e308041a | [
"Apache-2.0"
] | 2,610 | 2020-10-01T14:14:53.000Z | 2022-03-31T18:02:31.000Z | reinforcement_learning/rl_roboschool_ray/rl_roboschool_ray.ipynb | Amirosimani/amazon-sagemaker-examples | bc35e7a9da9e2258e77f98098254c2a8e308041a | [
"Apache-2.0"
] | 1,959 | 2020-09-30T20:22:42.000Z | 2022-03-31T23:58:37.000Z | reinforcement_learning/rl_roboschool_ray/rl_roboschool_ray.ipynb | Amirosimani/amazon-sagemaker-examples | bc35e7a9da9e2258e77f98098254c2a8e308041a | [
"Apache-2.0"
] | 2,052 | 2020-09-30T22:11:46.000Z | 2022-03-31T23:02:51.000Z | 33.490909 | 556 | 0.606406 | [
[
[
"# Roboschool simulations of physical robotics with Amazon SageMaker\n\n---\n## Introduction\n\nRoboschool is an [open source](https://github.com/openai/roboschool/tree/master/roboschool) physics simulator that is commonly used to train RL policies for simulated robotic systems. Roboschool provides 3D visualization of physical systems with multiple joints in contact with each other and their environment.\n\nThis notebook will show how to install Roboschool into the SageMaker RL container, and train pre-built robotics applications that are included with Roboschool.",
"_____no_output_____"
],
[
"## Pick which Roboschool problem to solve\n\nRoboschool defines a [variety](https://github.com/openai/roboschool/blob/master/roboschool/__init__.py) of Gym environments that correspond to different robotics problems. Here we're highlighting a few of them at varying levels of difficulty:\n\n- **Reacher (easy)** - a very simple robot with just 2 joints reaches for a target\n- **Hopper (medium)** - a simple robot with one leg and a foot learns to hop down a track \n- **Humanoid (difficult)** - a complex 3D robot with two arms, two legs, etc. learns to balance without falling over and then to run on a track\n\nThe simpler problems train faster with less computational resources. The more complex problems are more fun.",
"_____no_output_____"
]
],
[
[
"# Uncomment the problem to work on\nroboschool_problem = \"reacher\"\n# roboschool_problem = 'hopper'\n# roboschool_problem = 'humanoid'",
"_____no_output_____"
]
],
[
[
"## Pre-requisites \n\n### Imports\n\nTo get started, we'll import the Python libraries we need, set up the environment with a few prerequisites for permissions and configurations.",
"_____no_output_____"
]
],
[
[
"import sagemaker\nimport boto3\nimport sys\nimport os\nimport glob\nimport re\nimport subprocess\nimport numpy as np\nfrom IPython.display import HTML\nimport time\nfrom time import gmtime, strftime\n\nsys.path.append(\"common\")\nfrom misc import get_execution_role, wait_for_s3_object\nfrom docker_utils import build_and_push_docker_image\nfrom sagemaker.rl import RLEstimator, RLToolkit, RLFramework",
"_____no_output_____"
]
],
[
[
"### Setup S3 bucket\n\nSet up the linkage and authentication to the S3 bucket that you want to use for checkpoint and the metadata. ",
"_____no_output_____"
]
],
[
[
"sage_session = sagemaker.session.Session()\ns3_bucket = sage_session.default_bucket()\ns3_output_path = \"s3://{}/\".format(s3_bucket)\nprint(\"S3 bucket path: {}\".format(s3_output_path))",
"_____no_output_____"
]
],
[
[
"### Define Variables \n\nWe define variables such as the job prefix for the training jobs *and the image path for the container (only when this is BYOC).*",
"_____no_output_____"
]
],
[
[
"# create a descriptive job name\njob_name_prefix = \"rl-roboschool-\" + roboschool_problem",
"_____no_output_____"
]
],
[
[
"### Configure where training happens\n\nYou can train your RL training jobs using the SageMaker notebook instance or local notebook instance. In both of these scenarios, you can run the following in either local or SageMaker modes. The local mode uses the SageMaker Python SDK to run your code in a local container before deploying to SageMaker. This can speed up iterative testing and debugging while using the same familiar Python SDK interface. You just need to set `local_mode = True`.",
"_____no_output_____"
]
],
[
[
"# run in local_mode on this machine, or as a SageMaker TrainingJob?\nlocal_mode = False\n\nif local_mode:\n instance_type = \"local\"\nelse:\n # If on SageMaker, pick the instance type\n instance_type = \"ml.c5.2xlarge\"",
"_____no_output_____"
]
],
[
[
"### Create an IAM role\n\nEither get the execution role when running from a SageMaker notebook instance `role = sagemaker.get_execution_role()` or, when running from local notebook instance, use utils method `role = get_execution_role()` to create an execution role.",
"_____no_output_____"
]
],
[
[
"try:\n role = sagemaker.get_execution_role()\nexcept:\n role = get_execution_role()\n\nprint(\"Using IAM role arn: {}\".format(role))",
"_____no_output_____"
]
],
[
[
"### Install docker for `local` mode\n\nIn order to work in `local` mode, you need to have docker installed. When running from you local machine, please make sure that you have docker and docker-compose (for local CPU machines) and nvidia-docker (for local GPU machines) installed. Alternatively, when running from a SageMaker notebook instance, you can simply run the following script to install dependenceis.\n\nNote, you can only run a single local notebook at one time.",
"_____no_output_____"
]
],
[
[
"# only run from SageMaker notebook instance\nif local_mode:\n !/bin/bash ./common/setup.sh",
"_____no_output_____"
]
],
[
[
"## Build docker container\n\nWe must build a custom docker container with Roboschool installed. This takes care of everything:\n\n1. Fetching base container image\n2. Installing Roboschool and its dependencies\n3. Uploading the new container image to ECR\n\nThis step can take a long time if you are running on a machine with a slow internet connection. If your notebook instance is in SageMaker or EC2 it should take 3-10 minutes depending on the instance type.\n",
"_____no_output_____"
]
],
[
[
"%%time\n\ncpu_or_gpu = \"gpu\" if instance_type.startswith(\"ml.p\") else \"cpu\"\nrepository_short_name = \"sagemaker-roboschool-ray-%s\" % cpu_or_gpu\ndocker_build_args = {\n \"CPU_OR_GPU\": cpu_or_gpu,\n \"AWS_REGION\": boto3.Session().region_name,\n}\ncustom_image_name = build_and_push_docker_image(repository_short_name, build_args=docker_build_args)\nprint(\"Using ECR image %s\" % custom_image_name)",
"_____no_output_____"
]
],
[
[
"## Write the Training Code\n\nThe training code is written in the file “train-coach.py” which is uploaded in the /src directory. \nFirst import the environment files and the preset files, and then define the main() function. ",
"_____no_output_____"
]
],
[
[
"!pygmentize src/train-{roboschool_problem}.py",
"_____no_output_____"
]
],
[
[
"## Train the RL model using the Python SDK Script mode\n\nIf you are using local mode, the training will run on the notebook instance. When using SageMaker for training, you can select a GPU or CPU instance. The RLEstimator is used for training RL jobs. \n\n1. Specify the source directory where the environment, presets and training code is uploaded.\n2. Specify the entry point as the training code \n3. Specify the choice of RL toolkit and framework. This automatically resolves to the ECR path for the RL Container. \n4. Define the training parameters such as the instance count, job name, S3 path for output and job name. \n5. Specify the hyperparameters for the RL agent algorithm. The RLCOACH_PRESET or the RLRAY_PRESET can be used to specify the RL agent algorithm you want to use. \n6. Define the metrics definitions that you are interested in capturing in your logs. These can also be visualized in CloudWatch and SageMaker Notebooks. ",
"_____no_output_____"
]
],
[
[
"%%time\n\nmetric_definitions = RLEstimator.default_metric_definitions(RLToolkit.RAY)\n\nestimator = RLEstimator(\n entry_point=\"train-%s.py\" % roboschool_problem,\n source_dir=\"src\",\n dependencies=[\"common/sagemaker_rl\"],\n image_uri=custom_image_name,\n role=role,\n instance_type=instance_type,\n instance_count=1,\n output_path=s3_output_path,\n base_job_name=job_name_prefix,\n metric_definitions=metric_definitions,\n hyperparameters={\n # Attention scientists! You can override any Ray algorithm parameter here:\n # \"rl.training.config.horizon\": 5000,\n # \"rl.training.config.num_sgd_iter\": 10,\n },\n)\n\nestimator.fit(wait=local_mode)\njob_name = estimator.latest_training_job.job_name\nprint(\"Training job: %s\" % job_name)",
"_____no_output_____"
]
],
[
[
"## Visualization\n\nRL training can take a long time. So while it's running there are a variety of ways we can track progress of the running training job. Some intermediate output gets saved to S3 during training, so we'll set up to capture that.",
"_____no_output_____"
]
],
[
[
"print(\"Job name: {}\".format(job_name))\n\ns3_url = \"s3://{}/{}\".format(s3_bucket, job_name)\n\nintermediate_folder_key = \"{}/output/intermediate/\".format(job_name)\nintermediate_url = \"s3://{}/{}\".format(s3_bucket, intermediate_folder_key)\n\nprint(\"S3 job path: {}\".format(s3_url))\nprint(\"Intermediate folder path: {}\".format(intermediate_url))\n\ntmp_dir = \"/tmp/{}\".format(job_name)\nos.system(\"mkdir {}\".format(tmp_dir))\nprint(\"Create local folder {}\".format(tmp_dir))",
"_____no_output_____"
]
],
[
[
"### Fetch videos of training rollouts\nVideos of certain rollouts get written to S3 during training. Here we fetch the last 10 videos from S3, and render the last one.",
"_____no_output_____"
]
],
[
[
"recent_videos = wait_for_s3_object(\n s3_bucket,\n intermediate_folder_key,\n tmp_dir,\n fetch_only=(lambda obj: obj.key.endswith(\".mp4\") and obj.size > 0),\n limit=10,\n training_job_name=job_name,\n)",
"_____no_output_____"
],
[
"last_video = sorted(recent_videos)[-1] # Pick which video to watch\nos.system(\"mkdir -p ./src/tmp_render/ && cp {} ./src/tmp_render/last_video.mp4\".format(last_video))\nHTML('<video src=\"./src/tmp_render/last_video.mp4\" controls autoplay></video>')",
"_____no_output_____"
]
],
[
[
"### Plot metrics for training job\nWe can see the reward metric of the training as it's running, using algorithm metrics that are recorded in CloudWatch metrics. We can plot this to see the performance of the model over time.",
"_____no_output_____"
]
],
[
[
"%matplotlib inline\nfrom sagemaker.analytics import TrainingJobAnalytics\n\nif not local_mode:\n df = TrainingJobAnalytics(job_name, [\"episode_reward_mean\"]).dataframe()\n num_metrics = len(df)\n if num_metrics == 0:\n print(\"No algorithm metrics found in CloudWatch\")\n else:\n plt = df.plot(x=\"timestamp\", y=\"value\", figsize=(12, 5), legend=True, style=\"b-\")\n plt.set_ylabel(\"Mean reward per episode\")\n plt.set_xlabel(\"Training time (s)\")\nelse:\n print(\"Can't plot metrics in local mode.\")",
"_____no_output_____"
]
],
[
[
"### Monitor training progress\nYou can repeatedly run the visualization cells to get the latest videos or see the latest metrics as the training job proceeds.",
"_____no_output_____"
],
[
"## Evaluation of RL models\n\nWe use the last checkpointed model to run evaluation for the RL Agent. \n\n### Load checkpointed model\n\nCheckpointed data from the previously trained models will be passed on for evaluation / inference in the checkpoint channel. In local mode, we can simply use the local directory, whereas in the SageMaker mode, it needs to be moved to S3 first.",
"_____no_output_____"
]
],
[
[
"if local_mode:\n model_tar_key = \"{}/model.tar.gz\".format(job_name)\nelse:\n model_tar_key = \"{}/output/model.tar.gz\".format(job_name)\n\nlocal_checkpoint_dir = \"{}/model\".format(tmp_dir)\n\nwait_for_s3_object(s3_bucket, model_tar_key, tmp_dir, training_job_name=job_name)\n\nif not os.path.isfile(\"{}/model.tar.gz\".format(tmp_dir)):\n raise FileNotFoundError(\"File model.tar.gz not found\")\n\nos.system(\"mkdir -p {}\".format(local_checkpoint_dir))\nos.system(\"tar -xvzf {}/model.tar.gz -C {}\".format(tmp_dir, local_checkpoint_dir))\n\nprint(\"Checkpoint directory {}\".format(local_checkpoint_dir))",
"_____no_output_____"
],
[
"if local_mode:\n checkpoint_path = \"file://{}\".format(local_checkpoint_dir)\n print(\"Local checkpoint file path: {}\".format(local_checkpoint_dir))\nelse:\n checkpoint_path = \"s3://{}/{}/checkpoint/\".format(s3_bucket, job_name)\n if not os.listdir(local_checkpoint_dir):\n raise FileNotFoundError(\"Checkpoint files not found under the path\")\n os.system(\"aws s3 cp --recursive {} {}\".format(local_checkpoint_dir, checkpoint_path))\n print(\"S3 checkpoint file path: {}\".format(checkpoint_path))",
"_____no_output_____"
],
[
"%%time\n\nestimator_eval = RLEstimator(\n entry_point=\"evaluate-ray.py\",\n source_dir=\"src\",\n dependencies=[\"common/sagemaker_rl\"],\n image_uri=custom_image_name,\n role=role,\n instance_type=instance_type,\n instance_count=1,\n base_job_name=job_name_prefix + \"-evaluation\",\n hyperparameters={\n \"evaluate_episodes\": 5,\n \"algorithm\": \"PPO\",\n \"env\": \"Roboschool%s-v1\" % roboschool_problem.capitalize(),\n },\n)\n\nestimator_eval.fit({\"model\": checkpoint_path})\njob_name = estimator_eval.latest_training_job.job_name\nprint(\"Evaluation job: %s\" % job_name)",
"_____no_output_____"
]
],
[
[
"### Visualize the output \n\nOptionally, you can run the steps defined earlier to visualize the output.",
"_____no_output_____"
],
[
"# Model deployment\n\nNow let us deploy the RL policy so that we can get the optimal action, given an environment observation.",
"_____no_output_____"
]
],
[
[
"from sagemaker.tensorflow.model import TensorFlowModel\n\nmodel = TensorFlowModel(model_data=estimator.model_data, framework_version=\"2.1.0\", role=role)\n\npredictor = model.deploy(initial_instance_count=1, instance_type=instance_type)",
"_____no_output_____"
],
[
"# Mapping of environments to observation space\nobservation_space_mapping = {\"reacher\": 9, \"hopper\": 15, \"humanoid\": 44}",
"_____no_output_____"
]
],
[
[
"Now let us predict the actions using a dummy observation",
"_____no_output_____"
]
],
[
[
"# ray 0.8.2 requires all the following inputs\n# 'prev_action', 'is_training', 'prev_reward' and 'seq_lens' are placeholders for this example\n# they won't affect prediction results\n\ninput = {\n \"inputs\": {\n \"observations\": np.ones(shape=(1, observation_space_mapping[roboschool_problem])).tolist(),\n \"prev_action\": [0, 0],\n \"is_training\": False,\n \"prev_reward\": -1,\n \"seq_lens\": -1,\n }\n}",
"_____no_output_____"
],
[
"result = predictor.predict(input)\n\nresult[\"outputs\"][\"actions\"]",
"_____no_output_____"
]
],
[
[
"### Clean up endpoint",
"_____no_output_____"
]
],
[
[
"predictor.delete_endpoint()",
"_____no_output_____"
]
]
] | [
"markdown",
"code",
"markdown",
"code",
"markdown",
"code",
"markdown",
"code",
"markdown",
"code",
"markdown",
"code",
"markdown",
"code",
"markdown",
"code",
"markdown",
"code",
"markdown",
"code",
"markdown",
"code",
"markdown",
"code",
"markdown",
"code",
"markdown",
"code",
"markdown",
"code",
"markdown",
"code",
"markdown",
"code"
] | [
[
"markdown",
"markdown"
],
[
"code"
],
[
"markdown"
],
[
"code"
],
[
"markdown"
],
[
"code"
],
[
"markdown"
],
[
"code"
],
[
"markdown"
],
[
"code"
],
[
"markdown"
],
[
"code"
],
[
"markdown"
],
[
"code"
],
[
"markdown"
],
[
"code"
],
[
"markdown"
],
[
"code"
],
[
"markdown"
],
[
"code"
],
[
"markdown"
],
[
"code"
],
[
"markdown"
],
[
"code",
"code"
],
[
"markdown"
],
[
"code"
],
[
"markdown",
"markdown"
],
[
"code",
"code",
"code"
],
[
"markdown",
"markdown"
],
[
"code",
"code"
],
[
"markdown"
],
[
"code",
"code"
],
[
"markdown"
],
[
"code"
]
] |
e766c8bef44b43a7fbad85fc50767238afa4a6d9 | 3,897 | ipynb | Jupyter Notebook | HW_Plotting.ipynb | UWashington-Astro300/Astro300-A21 | 3bfa058a09c40444a8cc89ca0f5a4162c44d5a30 | [
"MIT"
] | null | null | null | HW_Plotting.ipynb | UWashington-Astro300/Astro300-A21 | 3bfa058a09c40444a8cc89ca0f5a4162c44d5a30 | [
"MIT"
] | null | null | null | HW_Plotting.ipynb | UWashington-Astro300/Astro300-A21 | 3bfa058a09c40444a8cc89ca0f5a4162c44d5a30 | [
"MIT"
] | null | null | null | 23.618182 | 162 | 0.536053 | [
[
[
"## Plotting Asteroids",
"_____no_output_____"
]
],
[
[
"import matplotlib.pyplot as plt\n\nimport numpy as np\nimport pandas as pd",
"_____no_output_____"
]
],
[
[
"#### The file `./Data/SDSS_MainBelt.csv` contains data on objects in the asteroid belt collected by the [Sloan Digital Sky Survey](http://www.sdss.org/).\n\nThe columns are:\n- **`Name`** - Object name\n- **`semi_major`** - semi-major axis\n- **`a_color`** - SDSS a$^*$ color\n- **`i_color`** - SDSS i color (near infrared)\n- **`z_color`** - SDSS z color (infrared)",
"_____no_output_____"
],
[
"## Read in the file `./Data/SDSS_MainBelt.csv` as a pandas `DataFrame`",
"_____no_output_____"
],
[
"## The Color of the Asteroids\n- Make three (3) plots in one row\n- In each panel, plot a histogram of the semi-major axis (`semi_major`) for **ALL** of the asteroids.\n- Bins = 100.\n- Use `.set_xlim` to only show 2.0 AU < `semi_major` < 3.6 AU\n- In the first panel, overplot a histogram of `semi_major` for **C-Type** asteroids only.\n- In the second panel, overplot a histogram of `semi_major` for **S-Type** asteroids only.\n- In the third panel, overplot a histogram of `semi_major` for **V-Type** asteroids only.\n- In each panel, draw a vertical line at `semi_major` = mean(`semi_major`) for **that type** of asteroid.\n- Adjust the `color` and `histtype` to make the overplot easy to see.\n- The asteroid types can be determined from their SDSS-colors (see image below).\n- Output size width : 15 inches, height : 5 inches\n- Make the plot look nice (including clear labels)",
"_____no_output_____"
],
[
"---\n\n### Asteroid classes - SDSS Colors\n\n---\n\n<center><img src=\"images/Colors.jpg\" width=600px></center>",
"_____no_output_____"
],
[
"### Due Wed Nov 10 - 1 pm\n- `File -> Download as -> HTML (.html)`\n- `upload your .html file to the class Canvas page`",
"_____no_output_____"
]
]
] | [
"markdown",
"code",
"markdown"
] | [
[
"markdown"
],
[
"code"
],
[
"markdown",
"markdown",
"markdown",
"markdown",
"markdown"
]
] |
e766d184ebd7b0d2e73ed13a0fbafd53781696f2 | 969,424 | ipynb | Jupyter Notebook | Week 1/3_Natural_Disaster_Tweets_EDA_Visuals.ipynb | theaslo/NLP_Workshop_WAIA_Sept_2021 | 9c193c1cd7795e8f054d9b9970553a368359e140 | [
"MIT"
] | 2 | 2021-09-08T17:36:26.000Z | 2022-01-12T02:37:55.000Z | Week 1/3_Natural_Disaster_Tweets_EDA_Visuals.ipynb | theaslo/NLP_Workshop_WAIA_Sept_2021 | 9c193c1cd7795e8f054d9b9970553a368359e140 | [
"MIT"
] | null | null | null | Week 1/3_Natural_Disaster_Tweets_EDA_Visuals.ipynb | theaslo/NLP_Workshop_WAIA_Sept_2021 | 9c193c1cd7795e8f054d9b9970553a368359e140 | [
"MIT"
] | 4 | 2021-09-08T17:37:27.000Z | 2021-09-15T17:40:10.000Z | 732.746788 | 347,184 | 0.909825 | [
[
[
"## Imports",
"_____no_output_____"
]
],
[
[
"import pandas as pd\nimport numpy as np\n\npd.set_option('display.max_colwidth', None)\n\nimport re\n\nfrom wordcloud import WordCloud\nimport contractions\n\nimport matplotlib.pyplot as plt\nimport seaborn as sns\nplt.style.use('ggplot')\nplt.rcParams['font.size'] = 15\n\nimport nltk\nfrom nltk.stem.porter import PorterStemmer\nfrom nltk import sent_tokenize, word_tokenize\n\nfrom nltk.corpus import stopwords\nSTOPWORDS = set(stopwords.words('english'))",
"_____no_output_____"
]
],
[
[
"## Data Load",
"_____no_output_____"
]
],
[
[
"df_train = pd.read_csv('../Datasets/disaster_tweet/train.csv')\ndf_train.head(20)",
"_____no_output_____"
],
[
"df_train.tail(20)",
"_____no_output_____"
]
],
[
[
"### Observation\n\n1. Mixed case\n2. Contractions\n3. Hashtags and mentions\n4. Incorrect spellings\n5. Punctuations\n6. websites and urls",
"_____no_output_____"
],
[
"## Functions",
"_____no_output_____"
]
],
[
[
"all_text = ' '.join(list(df_train['text']))\n\ndef check_texts(check_item, all_text):\n return check_item in all_text",
"_____no_output_____"
],
[
"print(check_texts('<a', all_text))\nprint(check_texts('<div', all_text))\nprint(check_texts('<p', all_text))",
"False\nFalse\nFalse\n"
],
[
"print(check_texts('#x', all_text))",
"False\n"
],
[
"print(check_texts(':)', all_text))\nprint(check_texts('<3', all_text))\nprint(check_texts('heard', all_text))",
"True\nFalse\nTrue\n"
],
[
"def remove_urls(text):\n ''' This method takes in text to remove urls and website links, if any'''\n url_pattern = r'(www.|http[s]?://)(?:[a-zA-Z]|[0-9]|[$-_@.&+]|[!*\\(\\),]|(?:%[0-9a-fA-F][0-9a-fA-F]))+'\n text = re.sub(url_pattern, '', text)\n return text\n\ndef remove_html_entities(text):\n ''' This method removes html tags'''\n html_entities = r'<.*?>|&([a-z0-9]+|#[0-9]{1,6}|#x[0-9a-f]{1,6});'\n text = re.sub(html_entities, '', text)\n return text\n\ndef convert_lower_case(text):\n return text.lower()\n\ndef detect_news(text):\n if 'news' in text:\n text = text + ' news'\n return text\n\ndef remove_social_media_tags(text):\n ''' This method removes @ and # tags'''\n tag_pattern = r'@([a-z0-9]+)|#'\n text = re.sub(tag_pattern, '', text)\n return text\n\n# Count it before I remove them altogether\ndef count_punctuations(text):\n getpunctuation = re.findall('[.?\"\\'`\\,\\-\\!:;\\(\\)\\[\\]\\\\/“”]+?',text)\n return len(getpunctuation)\n\n\ndef preprocess_text(x):\n cleaned_text = re.sub(r'[^a-zA-Z\\d\\s]+', '', x)\n word_list = []\n for each_word in cleaned_text.split(' '):\n word_list.append(contractions.fix(each_word).lower())\n word_list = [porter_stemmer.stem(each_word.replace('\\n', '').strip()) for each_word in word_list]\n word_list = set(word_list) - set(STOPWORDS)\n return \" \".join(word_list)",
"_____no_output_____"
],
[
"porter_stemmer = PorterStemmer()\n\ndf_train['text'] = df_train['text'].apply(remove_urls)\ndf_train['text'] = df_train['text'].apply(remove_html_entities)\ndf_train['text'] = df_train['text'].apply(convert_lower_case)\ndf_train['text'] = df_train['text'].apply(detect_news)\ndf_train['text'] = df_train['text'].apply(remove_social_media_tags)\ndf_train['punctuation_count'] = df_train['text'].apply(count_punctuations)\ndf_train['text'] = df_train['text'].apply(preprocess_text)\n\ndf_train['text_tokenized'] = df_train['text'].apply(word_tokenize)\ndf_train['words_per_tweet'] = df_train['text_tokenized'].apply(len)",
"_____no_output_____"
],
[
"df_train",
"_____no_output_____"
]
],
[
[
"## Tweet Length Analysis",
"_____no_output_____"
]
],
[
[
"sns.histplot(x='words_per_tweet', hue='target', data=df_train, kde=True)\nplt.show()",
"_____no_output_____"
]
],
[
[
"## Punctuation Analysis",
"_____no_output_____"
]
],
[
[
"sns.countplot(x='target', hue='punctuation_count', data=df_train)\nplt.legend([])\nplt.show()",
"_____no_output_____"
]
],
[
[
"## Tweet Text Analysis using WordCloud",
"_____no_output_____"
]
],
[
[
"real_disaster_tweets = ' '.join(list(df_train[df_train['target'] == 1]['text']))",
"_____no_output_____"
],
[
"real_disaster_tweets",
"_____no_output_____"
],
[
"non_real_disaster_tweets = ' '. join(list(df_train[df_train['target'] == 0]['text']))",
"_____no_output_____"
],
[
"wc = WordCloud(background_color=\"black\", \n max_words=100, \n width=1000, \n height=600, \n random_state=1).generate(real_disaster_tweets)\n\nplt.figure(figsize=(15,15))\nplt.imshow(wc)\nplt.axis(\"off\")\nplt.title(\"Wordcloud of Tweets about Real Disasters\")\nplt.show()",
"_____no_output_____"
],
[
"wc = WordCloud(background_color=\"black\", \n max_words=100, \n width=1000, \n height=600,\n font_step=1,\n random_state=1).generate(non_real_disaster_tweets)\n\nplt.figure(figsize=(15,15))\nplt.imshow(wc)\nplt.axis(\"off\")\nplt.title(\"Wordcloud of Tweets NOT about Real Disasters\")\nplt.show()",
"_____no_output_____"
]
]
] | [
"markdown",
"code",
"markdown",
"code",
"markdown",
"code",
"markdown",
"code",
"markdown",
"code",
"markdown",
"code"
] | [
[
"markdown"
],
[
"code"
],
[
"markdown"
],
[
"code",
"code"
],
[
"markdown",
"markdown"
],
[
"code",
"code",
"code",
"code",
"code",
"code",
"code"
],
[
"markdown"
],
[
"code"
],
[
"markdown"
],
[
"code"
],
[
"markdown"
],
[
"code",
"code",
"code",
"code",
"code"
]
] |
e766f90718ba86a1bf0351fd39a1ac6283aaf5e6 | 703,822 | ipynb | Jupyter Notebook | Exploratory Data Analysis.ipynb | abhishek291994/Twitter-Sentiment-Analysis-for-Indian-Elections | d1ee4aee2b4ef06657f3381c360c0300c699ed16 | [
"MIT"
] | 1 | 2019-05-30T05:10:03.000Z | 2019-05-30T05:10:03.000Z | Exploratory Data Analysis.ipynb | abhishek291994/Twitter-Sentiment-Analysis-for-Indian-Elections | d1ee4aee2b4ef06657f3381c360c0300c699ed16 | [
"MIT"
] | null | null | null | Exploratory Data Analysis.ipynb | abhishek291994/Twitter-Sentiment-Analysis-for-Indian-Elections | d1ee4aee2b4ef06657f3381c360c0300c699ed16 | [
"MIT"
] | 1 | 2021-12-05T16:54:46.000Z | 2021-12-05T16:54:46.000Z | 3,319.915094 | 373,432 | 0.962159 | [
[
[
"import pandas as pd\nfrom wordcloud import WordCloud\nimport matplotlib.pyplot as plt \nimport seaborn as sns",
"_____no_output_____"
],
[
"bjp_df = pd.read_csv('bjp_final.csv')\ncongress_df = pd.read_csv('congress_final.csv')",
"_____no_output_____"
],
[
"sns.countplot(x = 'polarity', data = congress_df).set_title('Congress')",
"_____no_output_____"
],
[
"sns.countplot(x = 'polarity', data = bjp_df).set_title('BJP')",
"_____no_output_____"
]
],
[
[
"For both the parties the proportion of negative tweets is slightly greater than the positive tweets.",
"_____no_output_____"
],
[
"Let's create a Word Cloud to identify which words occur frequetly in the tweets and try to derive what is their significance.",
"_____no_output_____"
]
],
[
[
"bjp_tweets = bjp_df['clean_text']\nbjp_string =[]\nfor t in bjp_tweets:\n bjp_string.append(t)\nbjp_string = pd.Series(bjp_string).str.cat(sep=' ')\nfrom wordcloud import WordCloud\n\nwordcloud = WordCloud(width=1600, height=800,max_font_size=200).generate(bjp_string)\nplt.figure(figsize=(12,10))\nplt.title('BJP Word Cloud')\n#matplotlib.pyplot.title(label, fontdict=None, loc='center', pad=None, **kwargs)[source]\nplt.imshow(wordcloud, interpolation=\"bilinear\")\nplt.axis(\"off\")\nplt.show()",
"_____no_output_____"
]
],
[
[
"The words like 'JhaSanjay', 'ArvindKejriwal', 'Delhi', 'Govindraj' occur frequently in our corpus and are highlighted by our Word Cloud.<br>\n<br>In the context of the BJP. Arivind Kejriwal are staunch opponents of the BJP government. Delhi is the Capital of India and also a state that the BJP has suffered heavy losses in the previous elections. Hence, winning the polls in Delhi seems to be a major discussion in the BJP realated tweets.<br><br>\nThe South Indian States are all opposed to the BJP government. The clashed between the political idealogies have been causing violence in some cases which resulted in the death of a BJP supporter from the state of Tamil Nadu. This again was one of the major discussions on the Twitter<br><br> The Word Cloud Also shows 'https' which indicated that that tweets are not yet cleaned properly. I will further clean the tweets before building the Models.",
"_____no_output_____"
]
],
[
[
"cong_tweets = congress_df['clean_text']\ncong_string =[]\nfor t in cong_tweets:\n cong_string.append(t)\ncong_string = pd.Series(cong_string).str.cat(sep=' ')\nfrom wordcloud import WordCloud\n\nwordcloud = WordCloud(width=1600, height=800,max_font_size=200).generate(cong_string)\nplt.figure(figsize=(12,10))\nplt.title('Congress Word Cloud')\n#matplotlib.pyplot.title(label, fontdict=None, loc='center', pad=None, **kwargs)[source]\nplt.imshow(wordcloud, interpolation=\"bilinear\")\nplt.axis(\"off\")\nplt.show()",
"_____no_output_____"
]
],
[
[
"Unlike the BJP Word Cloud, the Congress Word Cloud doesn't convey much information or maybe it could be that due to my limited political knowlege I couldnt't make sense of the information provided. The main keywords that can be traced are 'Amethi' and 'utkarsh_aanand','chitraSD and 'RatanSharda'.<br><br> Amethi is a Consitituency in Uttar Pradesh, North India. It has traditional been a Congress Strong hold since 1966. It is also where Congress president and candidate to the Prime Minister position Rahul Gandhi will be contesting from. <br><br> RataSharda is a Right wing writer and an opponent of the Congress. Utkarsh Aanand and ChitraSD are journalists and their alliance is unclear from their writings.",
"_____no_output_____"
]
]
] | [
"code",
"markdown",
"code",
"markdown",
"code",
"markdown"
] | [
[
"code",
"code",
"code",
"code"
],
[
"markdown",
"markdown"
],
[
"code"
],
[
"markdown"
],
[
"code"
],
[
"markdown"
]
] |
e76720e13c323abdece38ecf7fb9d52555af8396 | 2,015 | ipynb | Jupyter Notebook | src/2.numpy/PRACTICE_2_numpy_04.ipynb | LTurret/NFU-Biotech-MachineLearning | 3b73467846ec04e46a94a38e0c1e76bba3d1b369 | [
"MIT"
] | null | null | null | src/2.numpy/PRACTICE_2_numpy_04.ipynb | LTurret/NFU-Biotech-MachineLearning | 3b73467846ec04e46a94a38e0c1e76bba3d1b369 | [
"MIT"
] | null | null | null | src/2.numpy/PRACTICE_2_numpy_04.ipynb | LTurret/NFU-Biotech-MachineLearning | 3b73467846ec04e46a94a38e0c1e76bba3d1b369 | [
"MIT"
] | null | null | null | 23.988095 | 79 | 0.440199 | [
[
[
"import pandas as pd\nimport numpy as np\n\n# Create element using arrange function\ndic = {\n \"A\": np.arange(1, 11),\n \"B\": np.arange(11, 21),\n \"C\": np.arange(21, 31),\n \"D\": np.arange(31, 41),\n \"E\": np.arange(41, 51)\n}\n\ndf = pd.DataFrame(dic)\n\nprint(f\"{df}\\n\")\nprint(f\"{df.head(3)}\\n\") # Print the first three columns of content\nprint(f\"{df.tail(3)}\\n\") # Print the end three columns of content",
" A B C D E\n0 1 11 21 31 41\n1 2 12 22 32 42\n2 3 13 23 33 43\n3 4 14 24 34 44\n4 5 15 25 35 45\n5 6 16 26 36 46\n6 7 17 27 37 47\n7 8 18 28 38 48\n8 9 19 29 39 49\n9 10 20 30 40 50\n\n A B C D E\n0 1 11 21 31 41\n1 2 12 22 32 42\n2 3 13 23 33 43\n\n A B C D E\n7 8 18 28 38 48\n8 9 19 29 39 49\n9 10 20 30 40 50\n\n"
]
]
] | [
"code"
] | [
[
"code"
]
] |
e7673643c7df6fc1c13211faf74c67cfc9d82186 | 8,164 | ipynb | Jupyter Notebook | nbs/010_rocket_functions.ipynb | williamsdoug/timeseriesAI | 7fc2b801621aa11ccea0ffbde593100a9360961e | [
"Apache-2.0"
] | 1 | 2020-07-29T01:27:45.000Z | 2020-07-29T01:27:45.000Z | nbs/010_rocket_functions.ipynb | williamsdoug/timeseriesAI | 7fc2b801621aa11ccea0ffbde593100a9360961e | [
"Apache-2.0"
] | null | null | null | nbs/010_rocket_functions.ipynb | williamsdoug/timeseriesAI | 7fc2b801621aa11ccea0ffbde593100a9360961e | [
"Apache-2.0"
] | null | null | null | 37.972093 | 120 | 0.539441 | [
[
[
"# default_exp rocket_functions",
"_____no_output_____"
]
],
[
[
"# rocket functions\n\n> ROCKET (RandOm Convolutional KErnel Transform) functions for univariate and multivariate time series using GPU.",
"_____no_output_____"
]
],
[
[
"#export\nfrom tsai.imports import *\nfrom tsai.data.external import *",
"_____no_output_____"
],
[
"#export\nfrom sklearn.linear_model import RidgeClassifierCV\nfrom numba import njit, prange",
"_____no_output_____"
],
[
"#export\n# Angus Dempster, Francois Petitjean, Geoff Webb\n\n# Dempster A, Petitjean F, Webb GI (2019) ROCKET: Exceptionally fast and\n# accurate time series classification using random convolutional kernels.\n# arXiv:1910.13051\n\n# changes: \n# - added kss parameter to generate_kernels\n# - convert X to np.float64\n\ndef generate_kernels(input_length, num_kernels, kss=[7, 9, 11], pad=True, dilate=True):\n candidate_lengths = np.array((kss))\n # initialise kernel parameters\n weights = np.zeros((num_kernels, candidate_lengths.max())) # see note\n lengths = np.zeros(num_kernels, dtype = np.int32) # see note\n biases = np.zeros(num_kernels)\n dilations = np.zeros(num_kernels, dtype = np.int32)\n paddings = np.zeros(num_kernels, dtype = np.int32)\n # note: only the first *lengths[i]* values of *weights[i]* are used\n for i in range(num_kernels):\n length = np.random.choice(candidate_lengths)\n _weights = np.random.normal(0, 1, length)\n bias = np.random.uniform(-1, 1)\n if dilate: dilation = 2 ** np.random.uniform(0, np.log2((input_length - 1) // (length - 1)))\n else: dilation = 1\n if pad: padding = ((length - 1) * dilation) // 2 if np.random.randint(2) == 1 else 0\n else: padding = 0\n weights[i, :length] = _weights - _weights.mean()\n lengths[i], biases[i], dilations[i], paddings[i] = length, bias, dilation, padding\n return weights, lengths, biases, dilations, paddings\n\n@njit(fastmath = True)\ndef apply_kernel(X, weights, length, bias, dilation, padding):\n # zero padding\n if padding > 0:\n _input_length = len(X)\n _X = np.zeros(_input_length + (2 * padding))\n _X[padding:(padding + _input_length)] = X\n X = _X\n input_length = len(X)\n output_length = input_length - ((length - 1) * dilation)\n _ppv = 0 # \"proportion of positive values\"\n _max = np.NINF\n for i in range(output_length):\n _sum = bias\n for j in range(length):\n _sum += weights[j] * X[i + (j * dilation)]\n if _sum > 0:\n _ppv += 1\n if _sum > _max:\n _max = _sum\n return _ppv / output_length, _max\n\n@njit(parallel = True, fastmath = True)\ndef apply_kernels(X, kernels):\n X = X.astype(np.float64)\n weights, lengths, biases, dilations, paddings = kernels\n num_examples = len(X)\n num_kernels = len(weights)\n # initialise output\n _X = np.zeros((num_examples, num_kernels * 2)) # 2 features per kernel\n for i in prange(num_examples):\n for j in range(num_kernels):\n _X[i, (j * 2):((j * 2) + 2)] = \\\n apply_kernel(X[i], weights[j][:lengths[j]], lengths[j], biases[j], dilations[j], paddings[j])\n return _X",
"_____no_output_____"
],
[
"#hide\nX_train, y_train, X_valid, y_valid = get_UCR_data('OliveOil')\nseq_len = X_train.shape[-1]\nX_train = X_train[:, 0].astype(np.float64)\nX_valid = X_valid[:, 0].astype(np.float64)\nlabels = np.unique(y_train)\ntransform = {}\nfor i, l in enumerate(labels): transform[l] = i\ny_train = np.vectorize(transform.get)(y_train).astype(np.int32)\ny_valid = np.vectorize(transform.get)(y_valid).astype(np.int32)\nX_train = (X_train - X_train.mean(axis = 1, keepdims = True)) / (X_train.std(axis = 1, keepdims = True) + 1e-8)\nX_valid = (X_valid - X_valid.mean(axis = 1, keepdims = True)) / (X_valid.std(axis = 1, keepdims = True) + 1e-8)\n\n# only univariate time series of shape (samples, len)\nkernels = generate_kernels(seq_len, 10000)\nX_train_tfm = apply_kernels(X_train, kernels)\nX_valid_tfm = apply_kernels(X_valid, kernels)\nclassifier = RidgeClassifierCV(alphas=np.logspace(-3, 3, 7), normalize=True)\nclassifier.fit(X_train_tfm, y_train)\nscore = classifier.score(X_valid_tfm, y_valid)\ntest_eq(ge(score,.9), True)",
"_____no_output_____"
],
[
"#export\nclass ROCKET(nn.Module):\n def __init__(self, c_in, seq_len, n_kernels=10000, kss=[7, 9, 11]):\n \n '''\n ROCKET is a GPU Pytorch implementation of the ROCKET methods generate_kernels \n and apply_kernels that can be used with univariate and multivariate time series.\n Input: is a 3d torch tensor of type torch.float32. When used with univariate TS, \n make sure you transform the 2d to 3d by adding unsqueeze(1).\n c_in: number of channels or features. For univariate c_in is 1.\n seq_len: sequence length\n '''\n super().__init__()\n kss = [ks for ks in kss if ks < seq_len]\n convs = nn.ModuleList()\n for i in range(n_kernels):\n ks = np.random.choice(kss)\n dilation = 2**np.random.uniform(0, np.log2((seq_len - 1) // (ks - 1)))\n padding = int((ks - 1) * dilation // 2) if np.random.randint(2) == 1 else 0\n weight = torch.randn(1, c_in, ks)\n weight -= weight.mean()\n bias = 2 * (torch.rand(1) - .5)\n layer = nn.Conv1d(c_in, 1, ks, padding=2 * padding, dilation=int(dilation), bias=True)\n layer.weight = torch.nn.Parameter(weight, requires_grad=False)\n layer.bias = torch.nn.Parameter(bias, requires_grad=False)\n convs.append(layer)\n self.convs = convs\n self.n_kernels = n_kernels\n self.kss = kss\n\n def forward(self, x):\n for i in range(self.n_kernels):\n out = self.convs[i](x)\n _max = out.max(dim=-1).values\n _ppv = torch.gt(out, 0).sum(dim=-1).float() / out.shape[-1]\n cat = torch.cat((_max, _ppv), dim=-1)\n output = cat if i == 0 else torch.cat((output, cat), dim=-1)\n return output",
"_____no_output_____"
],
[
"#hide\nout = create_scripts()\nbeep(out)",
"_____no_output_____"
]
]
] | [
"code",
"markdown",
"code"
] | [
[
"code"
],
[
"markdown"
],
[
"code",
"code",
"code",
"code",
"code",
"code"
]
] |
e767410b8b92a64988c4dabbc7a459967f5d862a | 2,862 | ipynb | Jupyter Notebook | local/experiment_4_learning_rate_high.ipynb | b09/Deep-Learning-101 | 050f173b05b75553ba27cf3555ad529d7fde6680 | [
"MIT"
] | null | null | null | local/experiment_4_learning_rate_high.ipynb | b09/Deep-Learning-101 | 050f173b05b75553ba27cf3555ad529d7fde6680 | [
"MIT"
] | null | null | null | local/experiment_4_learning_rate_high.ipynb | b09/Deep-Learning-101 | 050f173b05b75553ba27cf3555ad529d7fde6680 | [
"MIT"
] | 1 | 2021-06-30T22:50:37.000Z | 2021-06-30T22:50:37.000Z | 34.481928 | 87 | 0.61775 | [
[
[
"from __future__ import division, print_function, absolute_import\n\nimport tflearn\nfrom tflearn.data_utils import shuffle, to_categorical\nfrom tflearn.layers.core import input_data, dropout, fully_connected\nfrom tflearn.layers.conv import conv_2d, max_pool_2d\nfrom tflearn.layers.estimator import regression\nfrom tflearn.data_preprocessing import ImagePreprocessing\nfrom tflearn.data_augmentation import ImageAugmentation\n\n# Data loading and preprocessing\nfrom tflearn.datasets import cifar10\n(X, Y), (X_test, Y_test) = cifar10.load_data()\nX, Y = shuffle(X, Y)\nY = to_categorical(Y, 10)\nY_test = to_categorical(Y_test, 10)\n\n# Real-time data preprocessing\nimg_prep = ImagePreprocessing()\nimg_prep.add_featurewise_zero_center()\nimg_prep.add_featurewise_stdnorm()\n\n# Real-time data augmentation\nimg_aug = ImageAugmentation()\nimg_aug.add_random_flip_leftright()\nimg_aug.add_random_rotation(max_angle=25.)\n\n# Convolutional network building\nnetwork = input_data(shape=[None, 32, 32, 3],\n data_preprocessing=img_prep,\n data_augmentation=img_aug)\nnetwork = conv_2d(network, 32, 3, activation='relu')\nnetwork = max_pool_2d(network, 2)\nnetwork = conv_2d(network, 64, 3, activation='relu')\nnetwork = conv_2d(network, 64, 3, activation='relu')\nnetwork = max_pool_2d(network, 2)\nnetwork = fully_connected(network, 512, activation='relu')\nnetwork = dropout(network, 0.5)\nnetwork = fully_connected(network, 10, activation='softmax')\nnetwork = regression(network, optimizer='adam',\n loss='categorical_crossentropy',\n learning_rate=0.01)\n\n# Train using classifier\nmodel = tflearn.DNN(network, tensorboard_verbose=0, tensorboard_dir='/output')\nmodel.fit(X, Y, n_epoch=50, shuffle=True, validation_set=(X_test, Y_test),\n show_metric=True, batch_size=96, run_id='cifar_learning_rate_high')",
"_____no_output_____"
]
]
] | [
"code"
] | [
[
"code"
]
] |
e767414d2d86de4c4bbf4860805d7bb8d8c938d0 | 9,419 | ipynb | Jupyter Notebook | Languages/Python/00 - Python Object and Data Structure/05-Dictionaries.ipynb | Tikam02/ReBuild | a5766dabb232e0bc6729cdbb5b0b0411db8b73be | [
"MIT"
] | null | null | null | Languages/Python/00 - Python Object and Data Structure/05-Dictionaries.ipynb | Tikam02/ReBuild | a5766dabb232e0bc6729cdbb5b0b0411db8b73be | [
"MIT"
] | null | null | null | Languages/Python/00 - Python Object and Data Structure/05-Dictionaries.ipynb | Tikam02/ReBuild | a5766dabb232e0bc6729cdbb5b0b0411db8b73be | [
"MIT"
] | 1 | 2020-08-09T14:15:28.000Z | 2020-08-09T14:15:28.000Z | 21.358277 | 276 | 0.519588 | [
[
[
"# Dictionaries\n\nWe've been learning about *sequences* in Python but now we're going to switch gears and learn about *mappings* in Python. If you're familiar with other languages you can think of these Dictionaries as hash tables. \n\nThis section will serve as a brief introduction to dictionaries and consist of:\n\n 1.) Constructing a Dictionary\n 2.) Accessing objects from a dictionary\n 3.) Nesting Dictionaries\n 4.) Basic Dictionary Methods\n\nSo what are mappings? Mappings are a collection of objects that are stored by a *key*, unlike a sequence that stored objects by their relative position. This is an important distinction, since mappings won't retain order since they have objects defined by a key.\n\nA Python dictionary consists of a key and then an associated value. That value can be almost any Python object.\n\n\n## Constructing a Dictionary\nLet's see how we can construct dictionaries to get a better understanding of how they work!",
"_____no_output_____"
]
],
[
[
"# Make a dictionary with {} and : to signify a key and a value\nmy_dict = {'key1':'value1','key2':'value2'}",
"_____no_output_____"
],
[
"# Call values by their key\nmy_dict['key2']",
"_____no_output_____"
]
],
[
[
"Its important to note that dictionaries are very flexible in the data types they can hold. For example:",
"_____no_output_____"
]
],
[
[
"my_dict = {'key1':123,'key2':[12,23,33],'key3':['item0','item1','item2']}",
"_____no_output_____"
],
[
"# Let's call items from the dictionary\nmy_dict['key3']",
"_____no_output_____"
],
[
"# Can call an index on that value\nmy_dict['key3'][0]",
"_____no_output_____"
],
[
"# Can then even call methods on that value\nmy_dict['key3'][0].upper()",
"_____no_output_____"
]
],
[
[
"We can affect the values of a key as well. For instance:",
"_____no_output_____"
]
],
[
[
"my_dict['key1']",
"_____no_output_____"
],
[
"# Subtract 123 from the value\nmy_dict['key1'] = my_dict['key1'] - 123",
"_____no_output_____"
],
[
"#Check\nmy_dict['key1']",
"_____no_output_____"
]
],
[
[
"A quick note, Python has a built-in method of doing a self subtraction or addition (or multiplication or division). We could have also used += or -= for the above statement. For example:",
"_____no_output_____"
]
],
[
[
"# Set the object equal to itself minus 123 \nmy_dict['key1'] -= 123\nmy_dict['key1']",
"_____no_output_____"
]
],
[
[
"We can also create keys by assignment. For instance if we started off with an empty dictionary, we could continually add to it:",
"_____no_output_____"
]
],
[
[
"# Create a new dictionary\nd = {}",
"_____no_output_____"
],
[
"# Create a new key through assignment\nd['animal'] = 'Dog'",
"_____no_output_____"
],
[
"# Can do this with any object\nd['answer'] = 42",
"_____no_output_____"
],
[
"#Show\nd",
"_____no_output_____"
]
],
[
[
"## Nesting with Dictionaries\n\nHopefully you're starting to see how powerful Python is with its flexibility of nesting objects and calling methods on them. Let's see a dictionary nested inside a dictionary:",
"_____no_output_____"
]
],
[
[
"# Dictionary nested inside a dictionary nested inside a dictionary\nd = {'key1':{'nestkey':{'subnestkey':'value'}}}",
"_____no_output_____"
]
],
[
[
"Wow! That's a quite the inception of dictionaries! Let's see how we can grab that value:",
"_____no_output_____"
]
],
[
[
"# Keep calling the keys\nd['key1']['nestkey']['subnestkey']",
"_____no_output_____"
]
],
[
[
"## A few Dictionary Methods\n\nThere are a few methods we can call on a dictionary. Let's get a quick introduction to a few of them:",
"_____no_output_____"
]
],
[
[
"# Create a typical dictionary\nd = {'key1':1,'key2':2,'key3':3}",
"_____no_output_____"
],
[
"# Method to return a list of all keys \nd.keys()",
"_____no_output_____"
],
[
"# Method to grab all values\nd.values()",
"_____no_output_____"
],
[
"# Method to return tuples of all items (we'll learn about tuples soon)\nd.items()",
"_____no_output_____"
]
],
[
[
"Hopefully you now have a good basic understanding how to construct dictionaries. There's a lot more to go into here, but we will revisit dictionaries at later time. After this section all you need to know is how to create a dictionary and how to retrieve values from it.",
"_____no_output_____"
]
]
] | [
"markdown",
"code",
"markdown",
"code",
"markdown",
"code",
"markdown",
"code",
"markdown",
"code",
"markdown",
"code",
"markdown",
"code",
"markdown",
"code",
"markdown"
] | [
[
"markdown"
],
[
"code",
"code"
],
[
"markdown"
],
[
"code",
"code",
"code",
"code"
],
[
"markdown"
],
[
"code",
"code",
"code"
],
[
"markdown"
],
[
"code"
],
[
"markdown"
],
[
"code",
"code",
"code",
"code"
],
[
"markdown"
],
[
"code"
],
[
"markdown"
],
[
"code"
],
[
"markdown"
],
[
"code",
"code",
"code",
"code"
],
[
"markdown"
]
] |
e7674eafd93cace6959e22cab36e1555049064c3 | 10,345 | ipynb | Jupyter Notebook | notebooks/Mobility measures.ipynb | mikiec84/giddy | b2ff44a2d0348daf0dec1b239b8df1861a8caa56 | [
"BSD-3-Clause"
] | null | null | null | notebooks/Mobility measures.ipynb | mikiec84/giddy | b2ff44a2d0348daf0dec1b239b8df1861a8caa56 | [
"BSD-3-Clause"
] | null | null | null | notebooks/Mobility measures.ipynb | mikiec84/giddy | b2ff44a2d0348daf0dec1b239b8df1861a8caa56 | [
"BSD-3-Clause"
] | 1 | 2020-02-24T11:45:37.000Z | 2020-02-24T11:45:37.000Z | 31.539634 | 864 | 0.573224 | [
[
[
"# Measures of Income Mobility \n\n**Author: Wei Kang <[email protected]>, Serge Rey <[email protected]>**\n\nIncome mobility could be viewed as a reranking pheonomenon where regions switch income positions while it could also be considered to be happening as long as regions move away from the previous income levels. The former is named absolute mobility and the latter relative mobility.\n\nThis notebook introduces how to estimate income mobility measures from longitudinal income data using methods in **giddy**. Currently, five summary mobility estimators are implemented in **giddy.mobility**. All of them are Markov-based, meaning that they are closely related to the discrete Markov Chains methods introduced in [Markov Based Methods notebook](Markov Based Methods.ipynb). More specifically, each of them is derived from a transition probability matrix $P$. Whether the final estimate is absolute or reletive mobility depends on how the original continuous income data are discretized.",
"_____no_output_____"
],
[
"The five Markov-based summary measures of mobility (Formby et al., 2004) are listed below:\n\n| Num| Measures | Symbol | \n|-------------| :-------------: |:-------------:|\n|1| $M_P(P) = \\frac{m-\\sum_{i=1}^m p_{ii}}{m-1} $ | P |\n|2| $M_D(P) = 1-|det(P)|$ |D | \n|3| $M_{L2}(P)=1-|\\lambda_2|$| L2| \n|4| $M_{B1}(P) = \\frac{m-m \\sum_{i=1}^m \\pi_i P_{ii}}{m-1} $ | B1 | \n|5| $M_{B2}(P)=\\frac{1}{m-1} \\sum_{i=1}^m \\sum_{j=1}^m \\pi_i P_{ij} |i-j|$| B2| \n\n$\\pi$ is the inital income distribution. For any transition probability matrix with a quasi-maximal diagonal, all of these mobility measures take values on $[0,1]$. $0$ means immobility and $1$ perfect mobility. If the transition probability matrix takes the form of the identity matrix, every region is stuck in its current state implying complete immobility. On the contrary, when each row of $P$ is identical, current state is irrelevant to the probability of moving away to any class. Thus, the transition matrix with identical rows is considered perfect mobile. The larger the mobility estimate, the more mobile the regional income system is. However, it should be noted that these measures try to reveal mobility pattern from different aspects and are thus not comparable to each other. Actually the mean and variance of these measures are different. ",
"_____no_output_____"
],
[
"We implemented all the above five summary mobility measures in a single method $markov\\_mobility$. A parameter $measure$ could be specified to select which measure to calculate. By default, the mobility measure 'P' will be estimated.\n\n```python\ndef markov_mobility(p, measure = \"P\",ini=None)\n```",
"_____no_output_____"
]
],
[
[
"from giddy import markov,mobility\nmobility.markov_mobility?",
"_____no_output_____"
]
],
[
[
"### US income mobility example\nSimilar to [Markov Based Methods notebook](Markov Based Methods.ipynb), we will demonstrate the usage of the mobility methods by an application to data on per capita incomes observed annually from 1929 to 2009 for the lower 48 US states.",
"_____no_output_____"
]
],
[
[
"import libpysal\nimport numpy as np\nimport mapclassify as mc",
"_____no_output_____"
],
[
"income_path = libpysal.examples.get_path(\"usjoin.csv\")\nf = libpysal.io.open(income_path)\npci = np.array([f.by_col[str(y)] for y in range(1929, 2010)]) #each column represents an state's income time series 1929-2010\nq5 = np.array([mc.Quantiles(y).yb for y in pci]).transpose() #each row represents an state's income time series 1929-2010\nm = markov.Markov(q5)\nm.p",
"/Users/weikang/anaconda3/lib/python3.6/site-packages/scipy/stats/stats.py:1713: FutureWarning: Using a non-tuple sequence for multidimensional indexing is deprecated; use `arr[tuple(seq)]` instead of `arr[seq]`. In the future this will be interpreted as an array index, `arr[np.array(seq)]`, which will result either in an error or a different result.\n return np.add.reduce(sorted[indexer] * weights, axis=axis) / sumval\n"
]
],
[
[
"After acquiring the estimate of transition probability matrix, we could call the method $markov\\_mobility$ to estimate any of the five Markov-based summary mobility indice. ",
"_____no_output_____"
],
[
"### 1. Shorrock1's mobility measure\n\n$$M_{P} = \\frac{m-\\sum_{i=1}^m P_{ii}}{m-1}$$\n\n```python\nmeasure = \"P\"```",
"_____no_output_____"
]
],
[
[
"mobility.markov_mobility(m.p, measure=\"P\")",
"_____no_output_____"
]
],
[
[
"### 2. Shorroks2's mobility measure\n\n$$M_{D} = 1 - |\\det(P)|$$\n```python\nmeasure = \"D\"```",
"_____no_output_____"
]
],
[
[
"mobility.markov_mobility(m.p, measure=\"D\")",
"_____no_output_____"
]
],
[
[
"### 3. Sommers and Conlisk's mobility measure\n$$M_{L2} = 1 - |\\lambda_2|$$\n\n```python\nmeasure = \"L2\"```",
"_____no_output_____"
]
],
[
[
"mobility.markov_mobility(m.p, measure = \"L2\")",
"_____no_output_____"
]
],
[
[
"### 4. Bartholomew1's mobility measure\n\n$$M_{B1} = \\frac{m-m \\sum_{i=1}^m \\pi_i P_{ii}}{m-1}$$\n\n$\\pi$: the inital income distribution\n\n```python\nmeasure = \"B1\"```",
"_____no_output_____"
]
],
[
[
"pi = np.array([0.1,0.2,0.2,0.4,0.1])\nmobility.markov_mobility(m.p, measure = \"B1\", ini=pi)",
"_____no_output_____"
]
],
[
[
"### 5. Bartholomew2's mobility measure\n\n$$M_{B2} = \\frac{1}{m-1} \\sum_{i=1}^m \\sum_{j=1}^m \\pi_i P_{ij} |i-j|$$\n\n$\\pi$: the inital income distribution\n\n```python\nmeasure = \"B1\"```",
"_____no_output_____"
]
],
[
[
"pi = np.array([0.1,0.2,0.2,0.4,0.1])\nmobility.markov_mobility(m.p, measure = \"B2\", ini=pi)",
"_____no_output_____"
]
],
[
[
"## Next steps\n\n* Markov-based partial mobility measures\n* Other mobility measures:\n * Inequality reduction mobility measures (Trede, 1999)\n* Statistical inference for mobility measures",
"_____no_output_____"
],
[
"## References\n\n* Formby, J. P., W. J. Smith, and B. Zheng. 2004. “[Mobility Measurement, Transition Matrices and Statistical Inference](http://www.sciencedirect.com/science/article/pii/S0304407603002112).” Journal of Econometrics 120 (1). Elsevier: 181–205.\n* Trede, Mark. 1999. “[Statistical Inference for Measures of Income Mobility / Statistische Inferenz Zur Messung Der Einkommensmobilität](https://www.jstor.org/stable/23812388).” Jahrbücher Für Nationalökonomie Und Statistik / Journal of Economics and Statistics 218 (3/4). Lucius & Lucius Verlagsgesellschaft mbH: 473–90.",
"_____no_output_____"
]
]
] | [
"markdown",
"code",
"markdown",
"code",
"markdown",
"code",
"markdown",
"code",
"markdown",
"code",
"markdown",
"code",
"markdown",
"code",
"markdown"
] | [
[
"markdown",
"markdown",
"markdown"
],
[
"code"
],
[
"markdown"
],
[
"code",
"code"
],
[
"markdown",
"markdown"
],
[
"code"
],
[
"markdown"
],
[
"code"
],
[
"markdown"
],
[
"code"
],
[
"markdown"
],
[
"code"
],
[
"markdown"
],
[
"code"
],
[
"markdown",
"markdown"
]
] |
e767531bec272b6466675257de6f8cb09a246210 | 3,985 | ipynb | Jupyter Notebook | Parte1/books/FinalLInkClf.ipynb | mbs8/RI_smartphone | 30ed5ca76842bf904b48d3bbb46f89b7632af7a9 | [
"MIT"
] | null | null | null | Parte1/books/FinalLInkClf.ipynb | mbs8/RI_smartphone | 30ed5ca76842bf904b48d3bbb46f89b7632af7a9 | [
"MIT"
] | null | null | null | Parte1/books/FinalLInkClf.ipynb | mbs8/RI_smartphone | 30ed5ca76842bf904b48d3bbb46f89b7632af7a9 | [
"MIT"
] | null | null | null | 24.751553 | 248 | 0.529486 | [
[
[
"import pickle\nimport numpy as np\nimport pandas as pd\nfrom sklearn.pipeline import Pipeline\nfrom sklearn.preprocessing import MinMaxScaler\nfrom sklearn.ensemble import RandomForestClassifier\nfrom sklearn.feature_extraction.text import CountVectorizer\nfrom sklearn.utils import resample\n\nclass DenseTransformer(MinMaxScaler):\n\n def fit(self, X, y=None, **fit_params):\n return self\n\n def transform(self, X, y=None, **fit_params):\n return X.todense()",
"/Users/Matheus/anaconda3/lib/python3.7/site-packages/sklearn/ensemble/weight_boosting.py:29: DeprecationWarning: numpy.core.umath_tests is an internal NumPy module and should not be imported. It will be removed in a future NumPy release.\n from numpy.core.umath_tests import inner1d\n"
],
[
"df = pd.read_csv('text_store_label.csv')\ndf = df.fillna(\" \")\nu_class = df['store'].values\nl = df['link'].values\nX = df['text'].values\ny = df['label'].values",
"_____no_output_____"
],
[
"df.label.value_counts()",
"_____no_output_____"
],
[
"df_majority = df[df.label==0]\ndf_minority = df[df.label==1]\n \ndf_minority_upsampled = resample(df_minority, \n replace=True, # sample with replacement\n n_samples=len(df_majority), # to match majority class\n random_state=123) # reproducible results\n \ndf_upsampled = pd.concat([df_majority, df_minority_upsampled])\ndf_upsampled.label.value_counts()",
"_____no_output_____"
],
[
"u_class = df_upsampled['store'].values\nl = df_upsampled['link'].values\nX = df_upsampled['text'].values\ny = df_upsampled['label'].values",
"_____no_output_____"
],
[
"text_clf = Pipeline([\n ('tfidf', CountVectorizer()),\n ('tranf', DenseTransformer()),\n ('clf', RandomForestClassifier(n_estimators=200, n_jobs=3)),\n])\ntext_clf.fit(l, y)\nfilename = 'link_clf3.sav'\npickle.dump(text_clf, open(filename, 'wb'))",
"_____no_output_____"
]
]
] | [
"code"
] | [
[
"code",
"code",
"code",
"code",
"code",
"code"
]
] |
e767574f99b631e9cb1d5efa324d6684c6c5eb63 | 6,882 | ipynb | Jupyter Notebook | dev/Lid-Driv-Ipython/Lid Driven Cavity em Python.ipynb | Ricardoleite/TCC-RLM-LBM | 28fd8c47543c729fa685942e21e1ec42c19976fd | [
"CC-BY-4.0"
] | null | null | null | dev/Lid-Driv-Ipython/Lid Driven Cavity em Python.ipynb | Ricardoleite/TCC-RLM-LBM | 28fd8c47543c729fa685942e21e1ec42c19976fd | [
"CC-BY-4.0"
] | null | null | null | dev/Lid-Driv-Ipython/Lid Driven Cavity em Python.ipynb | Ricardoleite/TCC-RLM-LBM | 28fd8c47543c729fa685942e21e1ec42c19976fd | [
"CC-BY-4.0"
] | null | null | null | 29.663793 | 97 | 0.362976 | [
[
[
"empty"
]
]
] | [
"empty"
] | [
[
"empty"
]
] |
e7675b7d9ff2f71fa7c3f70e01b9f58ac890fc5b | 643 | ipynb | Jupyter Notebook | 3.algoritmo_ciclo_mientras/Ejercicio_12.ipynb | diemgomez/algoritmos-python | 797a824f100afca01c35c70b3172870caef96811 | [
"MIT"
] | null | null | null | 3.algoritmo_ciclo_mientras/Ejercicio_12.ipynb | diemgomez/algoritmos-python | 797a824f100afca01c35c70b3172870caef96811 | [
"MIT"
] | null | null | null | 3.algoritmo_ciclo_mientras/Ejercicio_12.ipynb | diemgomez/algoritmos-python | 797a824f100afca01c35c70b3172870caef96811 | [
"MIT"
] | null | null | null | 18.371429 | 79 | 0.528771 | [
[
[
"#Calcular la suma siguiente: 100 + 98 + 96 + 94 + . . . + 0 en este orden",
"_____no_output_____"
]
]
] | [
"code"
] | [
[
"code"
]
] |
e7675d50812f954308bd574558149b13a775e57f | 613,510 | ipynb | Jupyter Notebook | notebooks/demos/demo_embeddings.ipynb | psturmfels/adversarial_faces | e193a8a5b16a1085ddfe52150aa7f7a57bfa7a31 | [
"MIT"
] | null | null | null | notebooks/demos/demo_embeddings.ipynb | psturmfels/adversarial_faces | e193a8a5b16a1085ddfe52150aa7f7a57bfa7a31 | [
"MIT"
] | null | null | null | notebooks/demos/demo_embeddings.ipynb | psturmfels/adversarial_faces | e193a8a5b16a1085ddfe52150aa7f7a57bfa7a31 | [
"MIT"
] | null | null | null | 2,415.393701 | 499,876 | 0.962198 | [
[
[
"# FaceNet Keras Demo\nThis notebook demos the usage of the FaceNet model, and shows\nhow to preprocess images before feeding them into the model.",
"_____no_output_____"
]
],
[
[
"%load_ext autoreload\n%autoreload 2",
"_____no_output_____"
],
[
"import sys\nsys.path.append('../')",
"_____no_output_____"
],
[
"import os\nimport tensorflow as tf\nimport numpy as np\nimport matplotlib.pyplot as plt\nimport matplotlib as mpl\n\nfrom PIL import Image\nfrom skimage.transform import resize\nfrom skimage.util import img_as_ubyte, img_as_float\nfrom sklearn.metrics import pairwise_distances\nfrom utils import set_up_environment, prewhiten, maximum_center_crop, l2_normalize\nfrom plot.heatmap import heatmap, annotate_heatmap",
"_____no_output_____"
],
[
"set_up_environment(visible_devices='1')",
"_____no_output_____"
]
],
[
[
"## Loading the Model\nFirst you need to download the keras weights from https://github.com/nyoki-mtl/keras-facenet and put the downloaded weights file in the parent directory.",
"_____no_output_____"
]
],
[
[
"model_path = '../facenet_keras.h5'\nmodel = tf.keras.models.load_model(model_path)",
"WARNING:tensorflow:No training configuration found in save file: the model was *not* compiled. Compile it manually.\n"
]
],
[
[
"## Preprocessing the Input\nThis next cell preprocesses the input using Pillow and skimage, both of which can be installed using pip. We center crop the image to avoid scaling issues, then resize the image to 160 x 160, and then we standardize the images using the utils module in this repository.",
"_____no_output_____"
]
],
[
[
"images = []\nimages_whitened = []\nimage_path = '../images/'\nimage_files = os.listdir(image_path)\nimage_files = [image_file for image_file in image_files if image_file.endswith('.png')]\nfor image_file in image_files:\n image = np.array(Image.open(os.path.join(image_path, image_file)))\n image = image[:, :, :3]\n image = maximum_center_crop(image)\n image = np.array(Image.fromarray(image).resize(size=(160, 160)))\n image = img_as_ubyte(image)\n image_whitened = prewhiten(image.astype(np.float32))\n\n images.append(image)\n images_whitened.append(image_whitened)",
"_____no_output_____"
],
[
"mpl.rcParams['figure.dpi'] = 50\nfig, axs = plt.subplots(1, len(images), figsize=(5 * len(images), 5))\nfor i in range(len(images)):\n axs[i].imshow(images[i])\n axs[i].set_title(image_files[i], fontsize=24)\n axs[i].axis('off')",
"_____no_output_____"
]
],
[
[
"## Computing Embeddings\nFinally, we compute the embeddings and pairwise distances of the images. We can see that the model is able to distinguish the same identity from different identities!",
"_____no_output_____"
]
],
[
[
"image_batch = tf.convert_to_tensor(np.array(images_whitened))",
"_____no_output_____"
],
[
"embedding_batch = model.predict(image_batch)\nnormalized_embedding_batch = l2_normalize(embedding_batch)",
"_____no_output_____"
],
[
"np.sqrt(np.sum(np.square(normalized_embedding_batch[0] - normalized_embedding_batch[1])))",
"_____no_output_____"
],
[
"pairwise_distance_matrix = pairwise_distances(normalized_embedding_batch)",
"_____no_output_____"
],
[
"mpl.rcParams['figure.dpi'] = 150\nax, cbar = heatmap(pairwise_distance_matrix,\n image_files,\n image_files,\n cmap=\"seismic\",\n cbarlabel=\"Normalized L2 Distance\")\ntexts = annotate_heatmap(ax, valfmt=\"{x:.2f}\")\n\nfig.tight_layout()",
"_____no_output_____"
]
]
] | [
"markdown",
"code",
"markdown",
"code",
"markdown",
"code",
"markdown",
"code"
] | [
[
"markdown"
],
[
"code",
"code",
"code",
"code"
],
[
"markdown"
],
[
"code"
],
[
"markdown"
],
[
"code",
"code"
],
[
"markdown"
],
[
"code",
"code",
"code",
"code",
"code"
]
] |
e7676edc7d527328b280901e051b0e5ec2b36edc | 210,545 | ipynb | Jupyter Notebook | temporal-difference/Temporal_Difference.ipynb | kdliaokueida/Deep_Reinforcement_Learning | 8a990ddf140591c45a58c38e7ea657f9125b4ee3 | [
"MIT"
] | null | null | null | temporal-difference/Temporal_Difference.ipynb | kdliaokueida/Deep_Reinforcement_Learning | 8a990ddf140591c45a58c38e7ea657f9125b4ee3 | [
"MIT"
] | 3 | 2020-11-13T18:57:55.000Z | 2022-02-10T02:07:15.000Z | temporal-difference/Temporal_Difference.ipynb | kdliaokueida/Deep_Reinforcement_Learning | 8a990ddf140591c45a58c38e7ea657f9125b4ee3 | [
"MIT"
] | null | null | null | 355.650338 | 51,920 | 0.921841 | [
[
[
"# Temporal-Difference Methods\n\nIn this notebook, you will write your own implementations of many Temporal-Difference (TD) methods.\n\nWhile we have provided some starter code, you are welcome to erase these hints and write your code from scratch.\n\n---\n\n### Part 0: Explore CliffWalkingEnv\n\nWe begin by importing the necessary packages.",
"_____no_output_____"
]
],
[
[
"import sys\nimport gym\nimport numpy as np\nimport random as rn\nfrom collections import defaultdict, deque\nimport matplotlib.pyplot as plt\n%matplotlib inline\n\nimport check_test\nfrom plot_utils import plot_values",
"_____no_output_____"
]
],
[
[
"Use the code cell below to create an instance of the [CliffWalking](https://github.com/openai/gym/blob/master/gym/envs/toy_text/cliffwalking.py) environment.",
"_____no_output_____"
]
],
[
[
"env = gym.make('CliffWalking-v0')",
"_____no_output_____"
]
],
[
[
"The agent moves through a $4\\times 12$ gridworld, with states numbered as follows:\n```\n[[ 0, 1, 2, 3, 4, 5, 6, 7, 8, 9, 10, 11],\n [12, 13, 14, 15, 16, 17, 18, 19, 20, 21, 22, 23],\n [24, 25, 26, 27, 28, 29, 30, 31, 32, 33, 34, 35],\n [36, 37, 38, 39, 40, 41, 42, 43, 44, 45, 46, 47]]\n```\nAt the start of any episode, state `36` is the initial state. State `47` is the only terminal state, and the cliff corresponds to states `37` through `46`.\n\nThe agent has 4 potential actions:\n```\nUP = 0\nRIGHT = 1\nDOWN = 2\nLEFT = 3\n```\n\nThus, $\\mathcal{S}^+=\\{0, 1, \\ldots, 47\\}$, and $\\mathcal{A} =\\{0, 1, 2, 3\\}$. Verify this by running the code cell below.",
"_____no_output_____"
]
],
[
[
"print(env.action_space)\nprint(env.observation_space)",
"Discrete(4)\nDiscrete(48)\n"
]
],
[
[
"In this mini-project, we will build towards finding the optimal policy for the CliffWalking environment. The optimal state-value function is visualized below. Please take the time now to make sure that you understand _why_ this is the optimal state-value function.\n\n_**Note**: You can safely ignore the values of the cliff \"states\" as these are not true states from which the agent can make decisions. For the cliff \"states\", the state-value function is not well-defined._",
"_____no_output_____"
]
],
[
[
"# define the optimal state-value function\nV_opt = np.zeros((4,12))\nV_opt[0:13][0] = -np.arange(3, 15)[::-1]\nV_opt[0:13][1] = -np.arange(3, 15)[::-1] + 1\nV_opt[0:13][2] = -np.arange(3, 15)[::-1] + 2\nV_opt[3][0] = -13\n\nplot_values(V_opt)",
"_____no_output_____"
]
],
[
[
"### Part 1: TD Control: Sarsa\n\nIn this section, you will write your own implementation of the Sarsa control algorithm.\n\nYour algorithm has four arguments:\n- `env`: This is an instance of an OpenAI Gym environment.\n- `num_episodes`: This is the number of episodes that are generated through agent-environment interaction.\n- `alpha`: This is the step-size parameter for the update step.\n- `gamma`: This is the discount rate. It must be a value between 0 and 1, inclusive (default value: `1`).\n\nThe algorithm returns as output:\n- `Q`: This is a dictionary (of one-dimensional arrays) where `Q[s][a]` is the estimated action value corresponding to state `s` and action `a`.\n\nPlease complete the function in the code cell below.\n\n(_Feel free to define additional functions to help you to organize your code._)",
"_____no_output_____"
]
],
[
[
"def greedy_eps_action(Q, state, nA, eps):\n if rn.random()> eps:\n return np.argmax(Q[state])\n else:\n return rn.choice(np.arange(nA))",
"_____no_output_____"
],
[
"def sarsa(env, num_episodes, alpha, gamma=1.0, eps_start = 1, eps_decay = .95, eps_min = 1e-2):\n # initialize action-value function (empty dictionary of arrays)\n nA = env.action_space.n\n Q = defaultdict(lambda: np.zeros(nA))\n # initialize performance monitor\n # loop over episodes\n eps = eps_start\n for i_episode in range(1, num_episodes+1):\n # monitor progress\n if i_episode % 100 == 0:\n print(\"\\rEpisode {}/{}\".format(i_episode, num_episodes), end=\"\")\n sys.stdout.flush() \n \n ## TODO: complete the function\n eps = max(eps_min,eps*eps_decay)\n state = env.reset()\n score = 0\n action = greedy_eps_action(Q, state, nA, eps)\n \n while True:\n next_state, reward, done, info = env.step(action)\n score += reward\n if not done:\n next_action = greedy_eps_action(Q, next_state, nA, eps)\n this_V = Q[state][action]\n next_V = Q[next_state][next_action]\n Q[state][action] = this_V + alpha*(reward + gamma*next_V - this_V)\n \n state = next_state\n action = next_action\n if done:\n Q[state][action] = Q[state][action] + alpha*(reward - Q[state][action])\n tmp_scores.append(score)\n break\n \n return Q",
"_____no_output_____"
]
],
[
[
"Use the next code cell to visualize the **_estimated_** optimal policy and the corresponding state-value function. \n\nIf the code cell returns **PASSED**, then you have implemented the function correctly! Feel free to change the `num_episodes` and `alpha` parameters that are supplied to the function. However, if you'd like to ensure the accuracy of the unit test, please do not change the value of `gamma` from the default.",
"_____no_output_____"
]
],
[
[
"# obtain the estimated optimal policy and corresponding action-value function\nQ_sarsa = sarsa(env, 5000, .01)\n\n# print the estimated optimal policy\npolicy_sarsa = np.array([np.argmax(Q_sarsa[key]) if key in Q_sarsa else -1 for key in np.arange(48)]).reshape(4,12)\ncheck_test.run_check('td_control_check', policy_sarsa)\nprint(\"\\nEstimated Optimal Policy (UP = 0, RIGHT = 1, DOWN = 2, LEFT = 3, N/A = -1):\")\nprint(policy_sarsa)\n\n# plot the estimated optimal state-value function\nV_sarsa = ([np.max(Q_sarsa[key]) if key in Q_sarsa else 0 for key in np.arange(48)])\nplot_values(V_sarsa)",
"Episode 5000/5000"
]
],
[
[
"### Part 2: TD Control: Q-learning\n\nIn this section, you will write your own implementation of the Q-learning control algorithm.\n\nYour algorithm has four arguments:\n- `env`: This is an instance of an OpenAI Gym environment.\n- `num_episodes`: This is the number of episodes that are generated through agent-environment interaction.\n- `alpha`: This is the step-size parameter for the update step.\n- `gamma`: This is the discount rate. It must be a value between 0 and 1, inclusive (default value: `1`).\n\nThe algorithm returns as output:\n- `Q`: This is a dictionary (of one-dimensional arrays) where `Q[s][a]` is the estimated action value corresponding to state `s` and action `a`.\n\nPlease complete the function in the code cell below.\n\n(_Feel free to define additional functions to help you to organize your code._)",
"_____no_output_____"
]
],
[
[
"def q_learning(env, num_episodes, alpha, gamma=1.0, eps_start = 1, eps_decay = .95, eps_min = 1e-2):\n # initialize empty dictionary of arrays\n nA = env.action_space.n\n Q = defaultdict(lambda: np.zeros(env.nA))\n eps = eps_start\n # loop over episodes\n for i_episode in range(1, num_episodes+1):\n # monitor progress\n if i_episode % 100 == 0:\n print(\"\\rEpisode {}/{}\".format(i_episode, num_episodes), end=\"\")\n sys.stdout.flush()\n \n ## TODO: complete the function\n eps = max(eps_min,eps*eps_decay)\n state = env.reset()\n score = 0\n action = greedy_eps_action(Q, state, nA, eps)\n \n while True:\n next_state, reward, done, info = env.step(action)\n score += reward\n if not done:\n next_action = greedy_eps_action(Q, next_state, nA, eps)\n this_V = Q[state][action]\n next_V = Q[next_state][next_action]\n Q[state][action] = this_V + alpha*(reward + gamma*max(Q[next_state]) - this_V)\n \n state = next_state\n action = next_action\n if done:\n Q[state][action] = Q[state][action] + alpha*(reward - Q[state][action])\n break\n \n return Q",
"_____no_output_____"
]
],
[
[
"Use the next code cell to visualize the **_estimated_** optimal policy and the corresponding state-value function. \n\nIf the code cell returns **PASSED**, then you have implemented the function correctly! Feel free to change the `num_episodes` and `alpha` parameters that are supplied to the function. However, if you'd like to ensure the accuracy of the unit test, please do not change the value of `gamma` from the default.",
"_____no_output_____"
]
],
[
[
"# obtain the estimated optimal policy and corresponding action-value function\nQ_sarsamax = q_learning(env, 5000, .01)\n\n# print the estimated optimal policy\npolicy_sarsamax = np.array([np.argmax(Q_sarsamax[key]) if key in Q_sarsamax else -1 for key in np.arange(48)]).reshape((4,12))\ncheck_test.run_check('td_control_check', policy_sarsamax)\nprint(\"\\nEstimated Optimal Policy (UP = 0, RIGHT = 1, DOWN = 2, LEFT = 3, N/A = -1):\")\nprint(policy_sarsamax)\n\n# plot the estimated optimal state-value function\nplot_values([np.max(Q_sarsamax[key]) if key in Q_sarsamax else 0 for key in np.arange(48)])",
"Episode 5000/5000"
]
],
[
[
"### Part 3: TD Control: Expected Sarsa\n\nIn this section, you will write your own implementation of the Expected Sarsa control algorithm.\n\nYour algorithm has four arguments:\n- `env`: This is an instance of an OpenAI Gym environment.\n- `num_episodes`: This is the number of episodes that are generated through agent-environment interaction.\n- `alpha`: This is the step-size parameter for the update step.\n- `gamma`: This is the discount rate. It must be a value between 0 and 1, inclusive (default value: `1`).\n\nThe algorithm returns as output:\n- `Q`: This is a dictionary (of one-dimensional arrays) where `Q[s][a]` is the estimated action value corresponding to state `s` and action `a`.\n\nPlease complete the function in the code cell below.\n\n(_Feel free to define additional functions to help you to organize your code._)",
"_____no_output_____"
]
],
[
[
"def expected_sarsa(env, num_episodes, alpha, gamma=1.0, eps_start = 1, eps_decay = .9, eps_min = 1e-2):\n # initialize empty dictionary of arrays\n nA = env.action_space.n\n Q = defaultdict(lambda: np.zeros(env.nA))\n eps = eps_start\n # loop over episodes\n for i_episode in range(1, num_episodes+1):\n # monitor progress\n if i_episode % 100 == 0:\n print(\"\\rEpisode {}/{}\".format(i_episode, num_episodes), end=\"\")\n sys.stdout.flush()\n \n ## TODO: complete the function\n eps = .001\n state = env.reset()\n score = 0\n action = greedy_eps_action(Q, state, nA, eps)\n \n while True:\n next_state, reward, done, info = env.step(action)\n score += reward\n if not done:\n next_action = greedy_eps_action(Q, next_state, nA, eps)\n this_V = Q[state][action]\n prob_s = np.ones(nA)*eps/nA\n prob_s[np.argmax(Q[next_state])] = 1 - eps + eps/nA\n Q[state][action] = this_V + alpha*(reward + gamma*np.dot(Q[next_state], prob_s) - this_V)\n \n state = next_state\n action = next_action\n if done:\n Q[state][action] = Q[state][action] + alpha*(reward - Q[state][action])\n break\n \n \n return Q",
"_____no_output_____"
]
],
[
[
"Use the next code cell to visualize the **_estimated_** optimal policy and the corresponding state-value function. \n\nIf the code cell returns **PASSED**, then you have implemented the function correctly! Feel free to change the `num_episodes` and `alpha` parameters that are supplied to the function. However, if you'd like to ensure the accuracy of the unit test, please do not change the value of `gamma` from the default.",
"_____no_output_____"
]
],
[
[
"# obtain the estimated optimal policy and corresponding action-value function\nQ_expsarsa = expected_sarsa(env, 10000, 1)\n\n# print the estimated optimal policy\npolicy_expsarsa = np.array([np.argmax(Q_expsarsa[key]) if key in Q_expsarsa else -1 for key in np.arange(48)]).reshape(4,12)\ncheck_test.run_check('td_control_check', policy_expsarsa)\nprint(\"\\nEstimated Optimal Policy (UP = 0, RIGHT = 1, DOWN = 2, LEFT = 3, N/A = -1):\")\nprint(policy_expsarsa)\n\n# plot the estimated optimal state-value function\nplot_values([np.max(Q_expsarsa[key]) if key in Q_expsarsa else 0 for key in np.arange(48)])",
"Episode 10000/10000"
]
]
] | [
"markdown",
"code",
"markdown",
"code",
"markdown",
"code",
"markdown",
"code",
"markdown",
"code",
"markdown",
"code",
"markdown",
"code",
"markdown",
"code",
"markdown",
"code",
"markdown",
"code"
] | [
[
"markdown"
],
[
"code"
],
[
"markdown"
],
[
"code"
],
[
"markdown"
],
[
"code"
],
[
"markdown"
],
[
"code"
],
[
"markdown"
],
[
"code",
"code"
],
[
"markdown"
],
[
"code"
],
[
"markdown"
],
[
"code"
],
[
"markdown"
],
[
"code"
],
[
"markdown"
],
[
"code"
],
[
"markdown"
],
[
"code"
]
] |
e767707a73995fc8affeacb23999799473063490 | 11,149 | ipynb | Jupyter Notebook | Calculator.ipynb | VintageGold/Data690_MathML | ca9d37f2f4126d361b14d2219e3b2c9539977f5c | [
"MIT"
] | 3 | 2021-10-04T23:14:55.000Z | 2021-10-04T23:18:46.000Z | Calculator.ipynb | VintageGold/Data690_MathML | ca9d37f2f4126d361b14d2219e3b2c9539977f5c | [
"MIT"
] | 8 | 2021-09-30T22:56:40.000Z | 2021-11-08T01:41:23.000Z | Calculator.ipynb | VintageGold/Data690_MathML | ca9d37f2f4126d361b14d2219e3b2c9539977f5c | [
"MIT"
] | 1 | 2021-10-08T00:03:02.000Z | 2021-10-08T00:03:02.000Z | 26.232941 | 112 | 0.413221 | [
[
[
"# Author of calculator object: Tom Kelly\n\nimport numpy as np\nfrom dataclasses import dataclass\n\n# Create object/class\n\nclass calculator(object):\n \n # initialize object variables\n def __init__(self):\n # Create an empty list\n self.results = []\n\n# Decorator method for summation, subtraction, division, multiplication and power\n def decoratorfunc(func):\n def wrapper(self, a, b):\n try:\n func(self, a, b)\n print('Results Saved')\n except:\n print('Error: Results Not Saved')\n return wrapper\n\n# Decorator method for square root\n def decoratorsqrt(func):\n def wrapper(self, a):\n try:\n func(self, a)\n print('Results Saved')\n except:\n print('Error: Results Not Saved')\n return wrapper\n \n# Create methods for summation, subtraction, division, multiplication, power, and sqrt\n\n# Each method checks the length of the list. If it is less than 2, return the entire list \n# Otherwise, set the value of the list to the last two elements of the list and return the new list\n @decoratorfunc\n def summation(self, a, b):\n self.results.append(a + b)\n if len(self.results) > 2:\n self.results = self.results[-2:]\n else:\n self.results = self.results[:]\n return a + b\n\n @decoratorfunc\n def subtraction(self, a, b):\n self.results.append(a - b)\n if len(self.results) > 2:\n self.results = self.results[-2:]\n else:\n self.results = self.results[:]\n return a - b\n\n @decoratorfunc\n def division(self, a, b):\n self.results.append(a/b)\n if len(self.results) > 2:\n self.results = self.results[-2:]\n else:\n self.results = self.results[:]\n return a/b\n\n @decoratorfunc\n def multiplication(self, a, b):\n \n self.results.append(a*b)\n if len(self.results) > 2:\n self.results = self.results[-2:]\n else:\n self.results = self.results[:]\n return a*b\n\n @decoratorfunc\n def power(self, a, b):\n self.results.append(a**b)\n if len(self.results) > 2:\n self.results = self.results[-2:]\n else:\n self.results = self.results[:]\n return a**b\n\n @decoratorsqrt\n def sqrt(self, a):\n self.results.append(np.sqrt(a))\n if len(self.results) > 2:\n self.results = self.results[-2:]\n else:\n self.results = self.results[:]\n return np.sqrt(a)\n \n \n# Method to return latest two results \n def get_latest_results(self): \n return self.results\n\n \n# Method to clear list\n def clear_results(self):\n self.results = []\n return self.results\n\n# Square root using Newtons Method\n def sqrtnm(self, guess, a):\n xn = guess\n for i in range (0, 10):\n fxn = xn*xn-a\n Dfxn = 2*xn\n xn = xn - fxn/Dfxn\n return xn\n\n ",
"_____no_output_____"
],
[
"# Instantiate calculator object\ncomputation = calculator()",
"_____no_output_____"
],
[
"# compute divident of two numbers\n\ncomputation.division(10,5)",
"Results Saved\n"
],
[
"# compute quotient of two numbers\n\ncomputation.multiplication(4,6)",
"Results Saved\n"
],
[
"# compute a**b\n\ncomputation.power(2,3)",
"Results Saved\n"
],
[
"# Compute the square root of a number\ncomputation.sqrt(16)",
"Results Saved\n"
],
[
"# Compute sum of two numbers\ncomputation.summation(3,4)",
"Results Saved\n"
],
[
"# Compute difference of two numbers\ncomputation.subtraction(10,5)",
"Results Saved\n"
],
[
"# Get latest two results\ncomputation.get_latest_results()",
"_____no_output_____"
],
[
"# Clear results from list\ncomputation.clear_results()",
"_____no_output_____"
],
[
"computation.sqrtnm(4,25)",
"_____no_output_____"
],
[
"computation.sqrt(25)",
"_____no_output_____"
]
]
] | [
"code"
] | [
[
"code",
"code",
"code",
"code",
"code",
"code",
"code",
"code",
"code",
"code",
"code",
"code"
]
] |
e7677a29bb7850ebc363b0966b3e4ae3b847928c | 7,950 | ipynb | Jupyter Notebook | SuperdenseCoding/SuperdenseCoding.ipynb | ivylee/QuantumKatas | 3b9134908ba263478382eeeb353a37e3944a547c | [
"MIT"
] | null | null | null | SuperdenseCoding/SuperdenseCoding.ipynb | ivylee/QuantumKatas | 3b9134908ba263478382eeeb353a37e3944a547c | [
"MIT"
] | null | null | null | SuperdenseCoding/SuperdenseCoding.ipynb | ivylee/QuantumKatas | 3b9134908ba263478382eeeb353a37e3944a547c | [
"MIT"
] | null | null | null | 37.5 | 296 | 0.606038 | [
[
[
"empty"
]
]
] | [
"empty"
] | [
[
"empty"
]
] |
e7677c0de277b9c221f62a4d53b3aa6da3d49d24 | 5,701 | ipynb | Jupyter Notebook | w1d1_Primer_Notebook_de_Jupyter_en_GoogleColab_120521.ipynb | talitacardenas/The_Bridge_School_DataScience_PT | 7c059d06a0eb53c0370d1db8868e0e7cb88c857b | [
"Apache-2.0"
] | null | null | null | w1d1_Primer_Notebook_de_Jupyter_en_GoogleColab_120521.ipynb | talitacardenas/The_Bridge_School_DataScience_PT | 7c059d06a0eb53c0370d1db8868e0e7cb88c857b | [
"Apache-2.0"
] | null | null | null | w1d1_Primer_Notebook_de_Jupyter_en_GoogleColab_120521.ipynb | talitacardenas/The_Bridge_School_DataScience_PT | 7c059d06a0eb53c0370d1db8868e0e7cb88c857b | [
"Apache-2.0"
] | null | null | null | 23.953782 | 380 | 0.446764 | [
[
[
"<a href=\"https://colab.research.google.com/github/talitacardenas/The_Bridge_School_DataScience_PT/blob/Develop/w1d1_Primer_Notebook_de_Jupyter_en_GoogleColab_120521.ipynb\" target=\"_parent\"><img src=\"https://colab.research.google.com/assets/colab-badge.svg\" alt=\"Open In Colab\"/></a>",
"_____no_output_____"
],
[
"",
"_____no_output_____"
]
],
[
[
"",
"_____no_output_____"
]
],
[
[
"# Encabezado\n## Encabezado tipo 2\n\nSe llama ***Markdown***\n\n1. Elemento de lista\n2. Elemento de lista\n\n\n",
"_____no_output_____"
],
[
"En Jupyter oara ejecutar una celda tecleamos>\n\n- Ctrl + Enter\n\nO si queremos anadir una nueva línea de codigo y a la vez ejecutar\n- Alt + Enter\n\n\n\n",
"_____no_output_____"
],
[
"",
"_____no_output_____"
]
],
[
[
"# Si quiero escribir un cmentario en una celda utilizamos el fragmento #\n# Si sin más líneas también\n",
"_____no_output_____"
]
],
[
[
"30\n\n\n",
"_____no_output_____"
]
],
[
[
"1 + 1\n\n",
"_____no_output_____"
],
[
"",
"_____no_output_____"
],
[
"print(\"Es resultado de una operacion es:\" + suma)\n",
"_____no_output_____"
],
[
"",
"_____no_output_____"
]
],
[
[
"Veamos que nos devolverá un error porque estamos intentando imorimir una cadena de texto y un valor numerico\nLa solucion seria trnsformar nuestro valor numerico en String\n",
"_____no_output_____"
]
],
[
[
"print(\"El resultado de esta operacion es> + str (suma)\")\n",
"_____no_output_____"
],
[
"",
"_____no_output_____"
]
],
[
[
"",
"_____no_output_____"
]
]
] | [
"markdown",
"code",
"markdown",
"code",
"markdown",
"code",
"markdown",
"code",
"markdown"
] | [
[
"markdown",
"markdown"
],
[
"code"
],
[
"markdown",
"markdown",
"markdown"
],
[
"code"
],
[
"markdown"
],
[
"code",
"code",
"code",
"code"
],
[
"markdown"
],
[
"code",
"code"
],
[
"markdown"
]
] |
e7678cfdaf90ba367d14c247febd73177d9cfbed | 15,392 | ipynb | Jupyter Notebook | course6/course6.ipynb | razorcd/ml-training | 1ef5bbe4abd74ccefb896733b5bcfd98d68835ee | [
"MIT"
] | 3 | 2021-11-04T17:41:34.000Z | 2021-12-29T15:01:19.000Z | course6/course6.ipynb | razorcd/ml-training | 1ef5bbe4abd74ccefb896733b5bcfd98d68835ee | [
"MIT"
] | null | null | null | course6/course6.ipynb | razorcd/ml-training | 1ef5bbe4abd74ccefb896733b5bcfd98d68835ee | [
"MIT"
] | 1 | 2021-12-06T03:57:51.000Z | 2021-12-06T03:57:51.000Z | 15,392 | 15,392 | 0.706926 | [
[
[
"# This Python 3 environment comes with many helpful analytics libraries installed\n# It is defined by the kaggle/python Docker image: https://github.com/kaggle/docker-python\n# For example, here's several helpful packages to load\n\nimport numpy as np # linear algebra\nimport pandas as pd # data processing, CSV file I/O (e.g. pd.read_csv)\n\n# Input data files are available in the read-only \"../input/\" directory\n# For example, running this (by clicking run or pressing Shift+Enter) will list all files under the input directory\nimport os\nfor dirname, _, filenames in os.walk('/kaggle/input'):\n for filename in filenames:\n print(os.path.join(dirname, filename))\n\n# You can write up to 20GB to the current directory (/kaggle/working/) that gets preserved as output when you create a version using \"Save & Run All\" \n# You can also write temporary files to /kaggle/temp/, but they won't be saved outside of the current session",
"_____no_output_____"
],
[
"# !head /kaggle/input/creditscoring-course6/CreditScoring.csv\ndf = pd.read_csv(\"/kaggle/input/creditscoring-course6/CreditScoring.csv\")\ndf.columns = df.columns.str.lower()\n\n# convert numbers to string categories:\ndf.status = df.status.map({1: \"ok\", 2:\"default\", 0: \"unk\"})\n\nhome_values = {1: 'rent',2: 'owner',3: 'private',4: 'ignore',5: 'parents',6: 'other',0: 'unk'}\ndf.home = df.home.map(home_values)\n\nmarital_values = {1: 'single',2: 'married',3: 'widow',4: 'separated',5: 'divorced',0: 'unk'}\ndf.marital = df.marital.map(marital_values)\n\nrecords_values = {1: 'no',2: 'yes',0: 'unk'}\ndf.records = df.records.map(records_values)\n\njob_values = {1: 'fixed',2: 'partime',3: 'freelance',4: 'others',0: 'unk'}\ndf.job = df.job.map(job_values)\n\n\n# replace max value with NA:\nfor c in ['income', 'assets', 'debt']:\n df[c] = df[c].replace(to_replace=99999999, value=np.nan)\n \n# drop lines with unkown status:\ndf = df[df.status != 'unk'].reset_index(drop=True) \n \ndf.head()\ndf.describe().round()\n",
"_____no_output_____"
],
[
"from sklearn.model_selection import train_test_split\n\ndf_full_train, df_test = train_test_split(df, test_size=0.2, random_state=11)\ndf_train, df_val = train_test_split(df_full_train, test_size=0.25, random_state=11)\n\ndf_train = df_train.reset_index(drop=True)\ndf_val = df_val.reset_index(drop=True)\ndf_test = df_test.reset_index(drop=True)\n\ny_train = (df_train.status == 'default').astype('int').values\ny_val = (df_val.status == 'default').astype('int').values\ny_test = (df_test.status == 'default').astype('int').values\n\ndel df_train[\"status\"]\ndel df_val[\"status\"]\ndel df_test[\"status\"]\n\ndf_train",
"_____no_output_____"
],
[
"# Decision Trees\nfrom sklearn.feature_extraction import DictVectorizer\nfrom sklearn.tree import DecisionTreeClassifier\nfrom sklearn.metrics import roc_auc_score\n\ntrain_dict = df_train.fillna(0).to_dict(orient='records')\ntrain_dict[:5]\ndv = DictVectorizer(sparse=False)\nX_train = dv.fit_transform(train_dict)\n\ndv.get_feature_names()\n\ndt = DecisionTreeClassifier(max_depth=3)\ndt.fit(X_train, y_train)\n\n\n\nval_dict = df_val.fillna(0).to_dict(orient='records')\nX_val = dv.transform(val_dict)\ny_pred = dt.predict_proba(X_val)[:,1]\n\nroc_auc_score(y_val, y_pred)\n",
"_____no_output_____"
],
[
"#print decision tree\nfrom sklearn.tree import export_text\n\nprint(export_text(dt, feature_names=dv.get_feature_names()))\n",
"_____no_output_____"
],
[
"#AUC for different tree depth\n\n#find best depth:\nfor d in [1,2,3,4,5,6,10,20,None]:\n dt = DecisionTreeClassifier(max_depth=d)\n dt.fit(X_train, y_train)\n \n y_pred = dt.predict_proba(X_val)[:,1]\n auc = roc_auc_score(y_val, y_pred)\n \n print(\"auc: %.3f, depth: %4s\" % (auc, d))\nprint()\n\n#find min_samples_leaf:\nfor d in [4,5,6]:\n for s in [1,2,5,10,15,20,100,200,500]:\n dt = DecisionTreeClassifier(max_depth=d, min_samples_leaf=s)\n dt.fit(X_train, y_train)\n\n y_pred = dt.predict_proba(X_val)[:,1]\n auc = roc_auc_score(y_val, y_pred)\n\n print(\"auc: %.3f, depth: %4s, min_samples_leaf: %4s\" % (auc, d, s))\n\n ",
"_____no_output_____"
],
[
"# Random Forest of decision trees\nfrom sklearn.feature_extraction import DictVectorizer\nfrom sklearn.ensemble import RandomForestClassifier\nfrom sklearn.metrics import roc_auc_score\n\ntrain_dict = df_train.fillna(0).to_dict(orient='records')\ndv = DictVectorizer(sparse=False)\nX_train = dv.fit_transform(train_dict)\n\nrf = RandomForestClassifier(n_estimators=10)\nrf.fit(X_train, y_train)\n\nval_dict = df_val.fillna(0).to_dict(orient='records')\nX_val = dv.transform(val_dict)\ny_pred = rf.predict_proba(X_val)[:,1]\n\nroc_auc_score(y_val, y_pred)\n",
"_____no_output_____"
],
[
"from IPython.display import display\nimport matplotlib.pyplot as plt\n\n# Simulate multiple Random Forests to find best 'n_estimators' and 'max_depth'\n\nscores = []\nfor d in [5, 10, 15]:\n for n in range(10, 201, 20):\n rf = RandomForestClassifier(n_estimators=n, max_depth=d, random_state=1)\n rf.fit(X_train, y_train)\n\n y_pred = rf.predict_proba(X_val)[:,1]\n auc = roc_auc_score(y_val, y_pred)\n scores.append((d,n,auc))\n\ndf_scores = pd.DataFrame(scores, columns=['max_depth','n_estimators','auc']) \ndisplay(df_scores)\n\n# print all auc for each max_depth:\nfor d in [5, 10, 15]:\n df_scores_subset = df_scores[df_scores.max_depth==d]\n plt.plot(df_scores_subset.n_estimators, df_scores_subset.auc, label='max_depth=%d'%d)\n\nplt.legend() ",
"_____no_output_____"
],
[
"best_max_depth = 10 #from previous graph\n\n\nfrom IPython.display import display\nimport matplotlib.pyplot as plt\n\n# Simulate multiple Random Forests to find best 'n_estimators' and 'max_depth'\n\nscores = []\nfor s in [1, 3, 5, 10, 50]:\n for n in range(10, 201, 20):\n rf = RandomForestClassifier(n_estimators=n, \n max_depth=best_max_depth, \n min_samples_leaf=s,\n random_state=1)\n rf.fit(X_train, y_train)\n\n y_pred = rf.predict_proba(X_val)[:,1]\n auc = roc_auc_score(y_val, y_pred)\n scores.append((s,n,auc))\n\ndf_scores = pd.DataFrame(scores, columns=['min_samples_leaf','n_estimators','auc']) \ndisplay(df_scores)\n\n# print all auc for each max_depth:\nfor s in [1, 3, 5, 10, 50]:\n df_scores_subset = df_scores[df_scores.min_samples_leaf==s]\n plt.plot(df_scores_subset.n_estimators, df_scores_subset.auc, label='min_samples_leaf=%d'%s)\n\nplt.legend() ",
"_____no_output_____"
],
[
"best_max_depth = 10 #from previous graph\nbest_min_samples_leaf = 3 #from previous graph\nbest_n_estimators = 200 # from all graphs above\n\nrf_final = RandomForestClassifier(n_estimators=best_n_estimators, \n max_depth=best_max_depth, \n min_samples_leaf=best_min_samples_leaf,\n random_state=1)\nrf_final.fit(X_train, y_train)\n\n",
"_____no_output_____"
],
[
"# XGboost\n# !pip install xgboost\nimport xgboost as xgb\n\n# prepare XGboost data structure:\nfeatures = dv.get_feature_names()\ndtrain = xgb.DMatrix(X_train, label=y_train, feature_names=features)\ndval = xgb.DMatrix(X_val, label=y_val, feature_names=features)\n\n# default xgboost params:\nxgb_params = {\n 'eta': 0.3,\n 'max_debth': 6,\n 'min_child_weight': 1,\n 'objective': 'binary:logistic',\n 'nthread': 8,\n 'seed':1,\n 'verbosity':1\n}\nxgb_model = xgb.train(xgb_params, dtrain, num_boost_round=10)\n\ny_pred = xgb_model.predict(dval)\n\nroc_auc_score(y_val, y_pred)\n\n\n",
"_____no_output_____"
],
[
"%%capture output\n# captures stdout ^\n\n# xbgoost auc:\n\nwatchlist = [(dtrain, 'train'), (dval, 'val')]\n\nxgb_params = {\n 'eta': 0.3,\n 'max_debth': 6,\n 'min_child_weight': 1,\n 'objective': 'binary:logistic',\n 'eval_metric': 'auc',\n 'nthread': 8,\n 'seed':1,\n 'verbosity':0\n}\nxgb_model = xgb.train(xgb_params, \n dtrain, \n evals=watchlist,\n verbose_eval=5,\n num_boost_round=200)\n",
"_____no_output_____"
],
[
"# parse xgboost AUC values from stdout\ndef parse_xgb_output(output):\n result = []\n num_iter_arr = []\n train_auc_arr = []\n val_auc_arr = []\n\n for line in output.stdout.strip().split('\\n'):\n num_iter, train_auc, val_auc = line.split('\\t')\n num_iter = int(num_iter.strip(\"[]\"))\n train_auc = float(train_auc.split(\":\")[1])\n val_auc = float(val_auc.split(\":\")[1])\n result.append((num_iter, train_auc, val_auc))\n\n columns = [\"num_iter\", \"train_auc\", \"val_auc\"] \n return pd.DataFrame(result, columns=columns)\n\ndf_model_score = parse_xgb_output(output)\n\nplt.plot(df_model_score.num_iter, df_model_score.train_auc, label=\"train data\")\nplt.plot(df_model_score.num_iter, df_model_score.val_auc, label=\"val data\")\nplt.legend()\n\n",
"_____no_output_____"
],
[
"# best model\n\n# decision tree\ndt = DecisionTreeClassifier(max_depth=6, min_samples_leaf=15)\ndt.fit(X_train, y_train)\ny_pred = dt.predict_proba(X_val)[:,1]\nauc = roc_auc_score(y_val, y_pred)\ndisplay(\"DecisionTreeClassifier: %s\" %(auc))\n\n# random forest\nrf = RandomForestClassifier(n_estimators=200, \n max_depth=best_max_depth, \n min_samples_leaf=best_min_samples_leaf,\n random_state=1)\nrf.fit(X_train, y_train)\ny_pred = rf.predict_proba(X_val)[:,1]\nauc = roc_auc_score(y_val, y_pred)\ndisplay(\"RandomForestClassifier: %s\" %(auc))\n\n# gradient boosting\nxgb_params = {\n 'eta': 0.3,\n 'max_debth': 6,\n 'min_child_weight': 1,\n 'objective': 'binary:logistic',\n 'nthread': 8,\n 'seed':1,\n 'verbosity':0\n}\nxgb_model = xgb.train(xgb_params, dtrain, num_boost_round=10)\ny_pred = xgb_model.predict(dval)\nauc = roc_auc_score(y_val, y_pred)\ndisplay(\"Xgboost: %s\" %(auc))",
"_____no_output_____"
]
]
] | [
"code"
] | [
[
"code",
"code",
"code",
"code",
"code",
"code",
"code",
"code",
"code",
"code",
"code",
"code",
"code",
"code"
]
] |
e7679bb0ff7147d0595d131ec806664cce53d2fa | 4,789 | ipynb | Jupyter Notebook | src/results_method.ipynb | rahulsrma26/phonetic-word-embedding | d81edef39c1534ca007cd6514a58ae914d72d0cf | [
"MIT"
] | null | null | null | src/results_method.ipynb | rahulsrma26/phonetic-word-embedding | d81edef39c1534ca007cd6514a58ae914d72d0cf | [
"MIT"
] | null | null | null | src/results_method.ipynb | rahulsrma26/phonetic-word-embedding | d81edef39c1534ca007cd6514a58ae914d72d0cf | [
"MIT"
] | null | null | null | 35.474074 | 116 | 0.570892 | [
[
[
"! python -V\nimport os\nimport numpy as np\nfrom scipy import stats\nimport pandas as pd\nimport matplotlib.colors as mcolors\nimport matplotlib.pyplot as plt\nimport seaborn as sns\nfrom experiment import Experiment",
"_____no_output_____"
],
[
"experiment_words = ['sit', 'plant', 'wonder', 'relation']\nexp = Experiment(\n mapping='mapping_english.txt',\n dictionary='cmudict-0.7b-with-vitz-nonce',\n encoding='latin1',\n words=experiment_words)\ndataset = exp.get_dataset()",
"_____no_output_____"
],
[
"def similarity_scores(df, methods, words, rank=False):\n columns = {}\n for method in methods:\n scores = []\n for word in words:\n obtained = df[df['actual'] == word]['obtained'].to_numpy()\n vw_predicted = df[df['actual'] == word][method].to_numpy()\n pearson = np.corrcoef(obtained, vw_predicted)[0, 1]\n spearman = stats.spearmanr(obtained, vw_predicted)[0]\n scores.append(spearman if rank else pearson)\n columns[method] = scores\n return pd.DataFrame(columns, index=words)\n\ndef draw_plot(dataset, methods, rank=False):\n scores = similarity_scores(dataset, methods, experiment_words, rank=rank)\n # colors = list(mcolors.TABLEAU_COLORS)[::-1]\n colors = ['orange', 'lightskyblue', 'darkseagreen', 'palevioletred', 'silver', 'gold']\n fig, ax = plt.subplots(facecolor='w')\n scores.plot.bar(ax=ax, width=0.8, legend=False, figsize=(12,5), color=colors, fontsize=18)\n ax.patch.set_facecolor('w')\n title = 'Spearman' if rank else 'Pearson'\n ax.set_ylabel(f'{title}\\ncorrelation\\ncoefficient\\n', fontsize=18)\n ax.set_xlim(-0.5, len(scores)-.5)\n ax.set_ylim(np.around(scores.min(numeric_only=True).to_numpy().min()-0.05, decimals=1), 1)\n ax.axes.get_xaxis().set_visible(False)\n # ax.legend(loc='center right', bbox_to_anchor=(1.35, 0.5), shadow=True, ncol=1)\n table = pd.plotting.table(ax, np.round(scores.T, 5), loc='bottom', cellLoc='center', rowColours=colors)\n # table.update({'text.color' : \"blue\", 'axes.labelcolor' : \"blue\"})\n # print(dir(table.rcParams))\n table.set_fontsize(18)\n table.scale(1, 2)\n\ndraw_plot(dataset, ['unigram', 'bigram', 'bigram p=2.5', 'bigram p=2.5 VW'])\n# draw_plot(dataset, ['unigram', 'bigram', 'bigram p=2.5'], True)\ndraw_plot(dataset, ['PSSVec', 'bigram p=2.5 VW'])\n# draw_plot(dataset, ['vw_predicted', 'PSSVec', 'bigram p=2.5', 'bigram p=2.5 VW'], True)\ndraw_plot(dataset, ['PSSVec', 'Ours'])\n# draw_plot(dataset, ['PSSVec', 'Ours'], True)",
"_____no_output_____"
],
[
"penalties = exp.penalty_analysis(experiment_words, 1, 5, 33, bigram=True, vowel=False)\nbest_penalty = penalties['avg'].idxmax()\nprint(best_penalty, penalties['avg'].max())\npenalties = penalties.drop(columns=['avg'])\nfig, ax = plt.subplots(facecolor='w')\npenalties.plot.line(ax=ax, figsize=(10,4), fontsize=16)\nax.set_xlabel('Penalty', fontsize=16)\nax.set_ylabel('Pearson\\ncorrelation\\ncoefficient\\n', fontsize=16)\nax.legend(loc='center right', bbox_to_anchor=(1.25, 0.5), shadow=True, ncol=1, fontsize=16)\nax.axvline(best_penalty, color='k', linestyle='--')\nplt.text(best_penalty, 0.5, ' max of average', rotation=0, fontsize=12)",
"_____no_output_____"
]
]
] | [
"code"
] | [
[
"code",
"code",
"code",
"code"
]
] |
e767a50c9bb3d56c491ae4781e5031a68a9a436e | 117,643 | ipynb | Jupyter Notebook | python/coursera_gluonCV_class/notebook/image-classification-step-by-step.ipynb | rtp-aws/devpost_aws_disaster_recovery | 2ccfff2d8b85614f3043f09d98c9981dedf43c05 | [
"MIT"
] | 1 | 2022-01-13T23:36:05.000Z | 2022-01-13T23:36:05.000Z | python/coursera_gluonCV_class/notebook/image-classification-step-by-step.ipynb | rtp-aws/devpost_aws_disaster_recovery | 2ccfff2d8b85614f3043f09d98c9981dedf43c05 | [
"MIT"
] | 9 | 2022-01-13T19:34:34.000Z | 2022-01-14T19:41:18.000Z | python/coursera_gluonCV_class/notebook/image-classification-step-by-step.ipynb | rtp-aws/devpost_aws_disaster_recovery | 2ccfff2d8b85614f3043f09d98c9981dedf43c05 | [
"MIT"
] | null | null | null | 619.173684 | 107,128 | 0.940948 | [
[
[
"%%bash\npip install --upgrade mxnet gluoncv\n# optional - for displaying the image in notebook\npip install ipyplot\n# After you run this cell, you need to restart\n# the notebook",
"Requirement already up-to-date: mxnet in /home/ec2-user/anaconda3/envs/mxnet_p36/lib/python3.6/site-packages (1.6.0)\nRequirement already up-to-date: gluoncv in /home/ec2-user/anaconda3/envs/mxnet_p36/lib/python3.6/site-packages (0.7.0)\nRequirement not upgraded as not directly required: graphviz<0.9.0,>=0.8.1 in /home/ec2-user/anaconda3/envs/mxnet_p36/lib/python3.6/site-packages (from mxnet) (0.8.4)\nRequirement not upgraded as not directly required: requests<3,>=2.20.0 in /home/ec2-user/anaconda3/envs/mxnet_p36/lib/python3.6/site-packages (from mxnet) (2.20.0)\nRequirement not upgraded as not directly required: numpy<2.0.0,>1.16.0 in /home/ec2-user/anaconda3/envs/mxnet_p36/lib/python3.6/site-packages (from mxnet) (1.16.4)\nRequirement not upgraded as not directly required: portalocker in /home/ec2-user/anaconda3/envs/mxnet_p36/lib/python3.6/site-packages (from gluoncv) (1.7.0)\nRequirement not upgraded as not directly required: scipy in /home/ec2-user/anaconda3/envs/mxnet_p36/lib/python3.6/site-packages (from gluoncv) (1.2.1)\nRequirement not upgraded as not directly required: tqdm in /home/ec2-user/anaconda3/envs/mxnet_p36/lib/python3.6/site-packages (from gluoncv) (4.46.1)\nRequirement not upgraded as not directly required: Pillow in /home/ec2-user/anaconda3/envs/mxnet_p36/lib/python3.6/site-packages (from gluoncv) (5.1.0)\nRequirement not upgraded as not directly required: matplotlib in /home/ec2-user/anaconda3/envs/mxnet_p36/lib/python3.6/site-packages (from gluoncv) (3.0.3)\nRequirement not upgraded as not directly required: idna<2.8,>=2.5 in /home/ec2-user/anaconda3/envs/mxnet_p36/lib/python3.6/site-packages (from requests<3,>=2.20.0->mxnet) (2.6)\nRequirement not upgraded as not directly required: certifi>=2017.4.17 in /home/ec2-user/anaconda3/envs/mxnet_p36/lib/python3.6/site-packages (from requests<3,>=2.20.0->mxnet) (2019.11.28)\nRequirement not upgraded as not directly required: urllib3<1.25,>=1.21.1 in /home/ec2-user/anaconda3/envs/mxnet_p36/lib/python3.6/site-packages (from requests<3,>=2.20.0->mxnet) (1.23)\nRequirement not upgraded as not directly required: chardet<3.1.0,>=3.0.2 in /home/ec2-user/anaconda3/envs/mxnet_p36/lib/python3.6/site-packages (from requests<3,>=2.20.0->mxnet) (3.0.4)\nRequirement not upgraded as not directly required: pyparsing!=2.0.4,!=2.1.2,!=2.1.6,>=2.0.1 in /home/ec2-user/anaconda3/envs/mxnet_p36/lib/python3.6/site-packages (from matplotlib->gluoncv) (2.2.0)\nRequirement not upgraded as not directly required: cycler>=0.10 in /home/ec2-user/anaconda3/envs/mxnet_p36/lib/python3.6/site-packages (from matplotlib->gluoncv) (0.10.0)\nRequirement not upgraded as not directly required: kiwisolver>=1.0.1 in /home/ec2-user/anaconda3/envs/mxnet_p36/lib/python3.6/site-packages (from matplotlib->gluoncv) (1.0.1)\nRequirement not upgraded as not directly required: python-dateutil>=2.1 in /home/ec2-user/anaconda3/envs/mxnet_p36/lib/python3.6/site-packages (from matplotlib->gluoncv) (2.7.3)\nRequirement not upgraded as not directly required: six in /home/ec2-user/anaconda3/envs/mxnet_p36/lib/python3.6/site-packages (from cycler>=0.10->matplotlib->gluoncv) (1.11.0)\nRequirement not upgraded as not directly required: setuptools in /home/ec2-user/anaconda3/envs/mxnet_p36/lib/python3.6/site-packages (from kiwisolver>=1.0.1->matplotlib->gluoncv) (39.1.0)\nRequirement already satisfied: ipyplot in /home/ec2-user/anaconda3/envs/mxnet_p36/lib/python3.6/site-packages (1.0.5)\nRequirement already satisfied: numpy in /home/ec2-user/anaconda3/envs/mxnet_p36/lib/python3.6/site-packages (from ipyplot) (1.16.4)\nRequirement already satisfied: pillow in /home/ec2-user/anaconda3/envs/mxnet_p36/lib/python3.6/site-packages (from ipyplot) (5.1.0)\nRequirement already satisfied: IPython in /home/ec2-user/anaconda3/envs/mxnet_p36/lib/python3.6/site-packages (from ipyplot) (6.4.0)\nRequirement already satisfied: pygments in /home/ec2-user/anaconda3/envs/mxnet_p36/lib/python3.6/site-packages (from IPython->ipyplot) (2.2.0)\nRequirement already satisfied: pexpect; sys_platform != \"win32\" in /home/ec2-user/anaconda3/envs/mxnet_p36/lib/python3.6/site-packages (from IPython->ipyplot) (4.5.0)\nRequirement already satisfied: prompt-toolkit<2.0.0,>=1.0.15 in /home/ec2-user/anaconda3/envs/mxnet_p36/lib/python3.6/site-packages (from IPython->ipyplot) (1.0.15)\nRequirement already satisfied: jedi>=0.10 in /home/ec2-user/anaconda3/envs/mxnet_p36/lib/python3.6/site-packages (from IPython->ipyplot) (0.12.0)\nRequirement already satisfied: setuptools>=18.5 in /home/ec2-user/anaconda3/envs/mxnet_p36/lib/python3.6/site-packages (from IPython->ipyplot) (39.1.0)\nRequirement already satisfied: traitlets>=4.2 in /home/ec2-user/anaconda3/envs/mxnet_p36/lib/python3.6/site-packages (from IPython->ipyplot) (4.3.2)\nRequirement already satisfied: decorator in /home/ec2-user/anaconda3/envs/mxnet_p36/lib/python3.6/site-packages (from IPython->ipyplot) (4.3.0)\nRequirement already satisfied: pickleshare in /home/ec2-user/anaconda3/envs/mxnet_p36/lib/python3.6/site-packages (from IPython->ipyplot) (0.7.4)\nRequirement already satisfied: backcall in /home/ec2-user/anaconda3/envs/mxnet_p36/lib/python3.6/site-packages (from IPython->ipyplot) (0.1.0)\nRequirement already satisfied: simplegeneric>0.8 in /home/ec2-user/anaconda3/envs/mxnet_p36/lib/python3.6/site-packages (from IPython->ipyplot) (0.8.1)\nRequirement already satisfied: ptyprocess>=0.5 in /home/ec2-user/anaconda3/envs/mxnet_p36/lib/python3.6/site-packages (from pexpect; sys_platform != \"win32\"->IPython->ipyplot) (0.5.2)\nRequirement already satisfied: six>=1.9.0 in /home/ec2-user/anaconda3/envs/mxnet_p36/lib/python3.6/site-packages (from prompt-toolkit<2.0.0,>=1.0.15->IPython->ipyplot) (1.11.0)\nRequirement already satisfied: wcwidth in /home/ec2-user/anaconda3/envs/mxnet_p36/lib/python3.6/site-packages (from prompt-toolkit<2.0.0,>=1.0.15->IPython->ipyplot) (0.1.7)\nRequirement already satisfied: parso>=0.2.0 in /home/ec2-user/anaconda3/envs/mxnet_p36/lib/python3.6/site-packages (from jedi>=0.10->IPython->ipyplot) (0.2.0)\nRequirement already satisfied: ipython_genutils in /home/ec2-user/anaconda3/envs/mxnet_p36/lib/python3.6/site-packages (from traitlets>=4.2->IPython->ipyplot) (0.2.0)\n"
],
[
"#import gluoncv as gcv\nimport mxnet as mx\n# optional for displaying the image\nimport ipyplot\nimport numpy as np\n# class method for displaying images\nimport matplotlib.pyplot as plt\n\n\n\n\nfrom mxnet import nd, image\n\nimport gluoncv as gcv\ngcv.utils.check_version('0.6.0')\nfrom gluoncv.data import ImageNet1kAttr\nfrom gluoncv.data.transforms.presets.imagenet import transform_eval\nfrom gluoncv.model_zoo import get_model\n\n",
"_____no_output_____"
],
[
"# will mxnet work with a nparray. Using the original simple script as a guide\n\n\n# Load Model\nnetwork = gcv.model_zoo.get_model('ResNet50_v1d', pretrained=True)\n# or\n#network = gcv.model_zoo.resnet50_v1d(pretrained=True)\n# Load Image\nimage = mx.image.image.imread('mt_baker.jpg')\n# plot Image\nplt.imshow(image.asnumpy())\n# Tranform Image into mxnext array and NCHW format\nimage = gcv.data.transforms.presets.imagenet.transform_eval(image)\n# Generate predictions\nprediction = network(image)\n# Print the top 5\ntopK = 5\nind = nd.topk(prediction, k=topK)[0].astype('int')\nclasses = network.classes\nprint('The input picture is classified to be')\nfor i in range(topK):\n print('\\t[%s], with probability %.3f.'% (classes[ind[i].asscalar()], nd.softmax(prediction)[0][ind[i]].asscalar()))\n",
"The input picture is classified to be\n\t[volcano], with probability 0.832.\n\t[alp], with probability 0.051.\n\t[valley], with probability 0.006.\n\t[mountain tent], with probability 0.005.\n\t[lakeside], with probability 0.005.\n"
]
]
] | [
"code"
] | [
[
"code",
"code",
"code"
]
] |
e767a86566665286a38da6f0ef510db6eb43c2c0 | 79,011 | ipynb | Jupyter Notebook | Solution/Day_38_Solution.ipynb | YenLinWu/DataScienceMarathon | 06ac92af91c02c5a2f9530fbfe68339df4fe177f | [
"MIT"
] | 8 | 2021-02-25T08:26:52.000Z | 2022-01-01T07:51:52.000Z | Solution/Day_38_Solution.ipynb | YenLinWu/DataScienceMarathon | 06ac92af91c02c5a2f9530fbfe68339df4fe177f | [
"MIT"
] | null | null | null | Solution/Day_38_Solution.ipynb | YenLinWu/DataScienceMarathon | 06ac92af91c02c5a2f9530fbfe68339df4fe177f | [
"MIT"
] | 6 | 2021-01-28T14:26:21.000Z | 2022-03-21T12:58:46.000Z | 75.753595 | 23,266 | 0.754224 | [
[
[
"## 作業\n在鐵達尼資料集中,今天我們專注觀察變數之間的相關性,以Titanic_train.csv 中,首先將有遺失值的數值刪除,並回答下列問題。\n* Q1: 透過數值法計算 Age 和 Survived 是否有相關性?\n* Q2:透過數值法計算 Sex 和 Survived 是否有相關性?\n* Q3: 透過數值法計算 Age 和 Fare 是否有相關性? \n* 提示: \n1.產稱一個新的變數 Survived_cate ,資料型態傳換成類別型態 \n2.把題目中的 Survived 用 Survived_cate 來做分析 \n3.首先觀察一下這些變數的資料型態後,再來想要以哪一種判斷倆倆的相關性。 \n",
"_____no_output_____"
]
],
[
[
"# import library\nimport matplotlib.pyplot as plt\nimport numpy as np\nimport pandas as pd\nfrom scipy import stats\nimport math\nimport statistics\nimport seaborn as sns\nfrom IPython.display import display\n\nimport pingouin as pg\nimport researchpy \n%matplotlib inline",
"_____no_output_____"
]
],
[
[
"## 讀入資料",
"_____no_output_____"
]
],
[
[
"df_train = pd.read_csv(\"Titanic_train.csv\")\nprint(df_train.info())",
"<class 'pandas.core.frame.DataFrame'>\nRangeIndex: 891 entries, 0 to 890\nData columns (total 12 columns):\n # Column Non-Null Count Dtype \n--- ------ -------------- ----- \n 0 PassengerId 891 non-null int64 \n 1 Survived 891 non-null int64 \n 2 Pclass 891 non-null int64 \n 3 Name 891 non-null object \n 4 Sex 891 non-null object \n 5 Age 714 non-null float64\n 6 SibSp 891 non-null int64 \n 7 Parch 891 non-null int64 \n 8 Ticket 891 non-null object \n 9 Fare 891 non-null float64\n 10 Cabin 204 non-null object \n 11 Embarked 889 non-null object \ndtypes: float64(2), int64(5), object(5)\nmemory usage: 83.7+ KB\nNone\n"
],
[
"## 這邊我們做一個調整,把 Survived 變成離散型變數 Survived_cate",
"_____no_output_____"
],
[
"df_train['Survived_cate']=df_train['Survived']\ndf_train['Survived_cate']=df_train['Survived_cate'].astype('object')\nprint(df_train.info())",
"<class 'pandas.core.frame.DataFrame'>\nRangeIndex: 891 entries, 0 to 890\nData columns (total 13 columns):\n # Column Non-Null Count Dtype \n--- ------ -------------- ----- \n 0 PassengerId 891 non-null int64 \n 1 Survived 891 non-null int64 \n 2 Pclass 891 non-null int64 \n 3 Name 891 non-null object \n 4 Sex 891 non-null object \n 5 Age 714 non-null float64\n 6 SibSp 891 non-null int64 \n 7 Parch 891 non-null int64 \n 8 Ticket 891 non-null object \n 9 Fare 891 non-null float64\n 10 Cabin 204 non-null object \n 11 Embarked 889 non-null object \n 12 Survived_cate 891 non-null object \ndtypes: float64(2), int64(5), object(6)\nmemory usage: 90.6+ KB\nNone\n"
],
[
"display(df_train.head(5))",
"_____no_output_____"
]
],
[
[
"### Q1: 透過數值法計算 Age 和 Survived 是否有相關性?\n",
"_____no_output_____"
]
],
[
[
"## Age:連續型 Survived_cate 為離散型,所以採用 Eta Squared",
"_____no_output_____"
]
],
[
[
"### 計算相關係數,不能允許有遺失值,所以必須先補值,或者把遺失值刪除",
"_____no_output_____"
]
],
[
[
"## 取出資料後,把遺失值刪除\ncomplete_data=df_train[['Age','Survived_cate']].dropna()\ndisplay(complete_data)",
"_____no_output_____"
],
[
"aov = pg.anova(dv='Age', between='Survived_cate', data=complete_data, detailed=True)\naov",
"_____no_output_____"
],
[
"etaSq = aov.SS[0] / (aov.SS[0] + aov.SS[1])\netaSq",
"_____no_output_____"
],
[
"def judgment_etaSq(etaSq):\n if etaSq < .01:\n qual = 'Negligible'\n elif etaSq < .06:\n qual = 'Small'\n elif etaSq < .14:\n qual = 'Medium'\n else:\n qual = 'Large'\n return(qual)\njudgment_etaSq(etaSq)",
"_____no_output_____"
],
[
"g = sns.catplot(x=\"Survived_cate\", y=\"Age\", hue=\"Survived_cate\",\n data=complete_data, kind=\"violin\")",
"_____no_output_____"
]
],
[
[
"### 結論: 年紀和存活沒有相關性(complete_data),思考是否需要放入模型,或者要深入觀察特性,是否需要做特徵轉換",
"_____no_output_____"
],
[
"### Q2:透過數值法計算 Sex 和 Survived 是否有相關性?\n",
"_____no_output_____"
]
],
[
[
"## Sex:離散型 Survived_cate 為離散型,所以採用 Cramér's V",
"_____no_output_____"
],
[
"contTable = pd.crosstab(df_train['Sex'], df_train['Survived_cate'])\ncontTable",
"_____no_output_____"
],
[
"df = min(contTable.shape[0], contTable.shape[1]) - 1\ndf",
"_____no_output_____"
],
[
"crosstab, res = researchpy.crosstab(df_train['Survived_cate'], df_train['Sex'], test='chi-square')\n#print(res)\nprint(\"Cramer's value is\",res.loc[2,'results'])\n\n#這邊用卡方檢定獨立性,所以採用的 test 參數為卡方 \"test =\" argument.\n# 採用的變數在這個模組中,會自己根據資料集來判斷,Cramer's Phi if it a 2x2 table, or Cramer's V is larger than 2x2.",
"Cramer's value is 0.5434\n"
],
[
"## 寫一個副程式判斷相關性的強度\ndef judgment_CramerV(df,V):\n if df == 1:\n if V < 0.10:\n qual = 'negligible'\n elif V < 0.30:\n qual = 'small'\n elif V < 0.50:\n qual = 'medium'\n else:\n qual = 'large'\n elif df == 2:\n if V < 0.07:\n qual = 'negligible'\n elif V < 0.21:\n qual = 'small'\n elif V < 0.35:\n qual = 'medium'\n else:\n qual = 'large'\n elif df == 3:\n if V < 0.06:\n qual = 'negligible'\n elif V < 0.17:\n qual = 'small'\n elif V < 0.29:\n qual = 'medium'\n else:\n qual = 'large'\n elif df == 4:\n if V < 0.05:\n qual = 'negligible'\n elif V < 0.15:\n qual = 'small'\n elif V < 0.25:\n qual = 'medium'\n else:\n qual = 'large'\n else:\n if V < 0.05:\n qual = 'negligible'\n elif V < 0.13:\n qual = 'small'\n elif V < 0.22:\n qual = 'medium'\n else:\n qual = 'large'\n return(qual)\n\njudgment_CramerV(df,res.loc[2,'results'])",
"_____no_output_____"
],
[
"g= sns.countplot(x=\"Sex\", hue=\"Survived_cate\", data=df_train)",
"_____no_output_____"
]
],
[
[
"### 數值型態和圖形, 存活和性別存在高度的相關性,要預測存活,一定要把性別加上去。",
"_____no_output_____"
],
[
"### Q3: 透過數值法計算 Age 和 Fare 是否有相關性? ",
"_____no_output_____"
]
],
[
[
"## Age 連續 , Fare 連續,用 Pearson 相關係數",
"_____no_output_____"
],
[
"## 取出資料後,把遺失值刪除\ncomplete_data=df_train[['Age','Fare']].dropna()\ndisplay(complete_data)",
"_____no_output_____"
],
[
"# 由於 pearsonr 有兩個回傳結果,我們只需取第一個回傳值為相關係數\ncorr, _=stats.pearsonr(complete_data['Age'],complete_data['Fare'])\nprint(corr)\n#代表身高和體重有高度線性相關",
"0.0960666917690389\n"
],
[
"g = sns.regplot(x=\"Age\", y=\"Fare\", color=\"g\",data=complete_data)\n#年齡和身高有關連",
"_____no_output_____"
]
],
[
[
"### 年紀和票價沒有線性相關姓,圖形上也觀察到沒有相關性",
"_____no_output_____"
]
]
] | [
"markdown",
"code",
"markdown",
"code",
"markdown",
"code",
"markdown",
"code",
"markdown",
"code",
"markdown",
"code",
"markdown"
] | [
[
"markdown"
],
[
"code"
],
[
"markdown"
],
[
"code",
"code",
"code",
"code"
],
[
"markdown"
],
[
"code"
],
[
"markdown"
],
[
"code",
"code",
"code",
"code",
"code"
],
[
"markdown",
"markdown"
],
[
"code",
"code",
"code",
"code",
"code",
"code"
],
[
"markdown",
"markdown"
],
[
"code",
"code",
"code",
"code"
],
[
"markdown"
]
] |
e767ae3b6724990210b23c6f1c4d548cc0b65213 | 266,349 | ipynb | Jupyter Notebook | MultinomialNB.ipynb | djendara/heart-disease-classification | cac78d49ab56a99fce48683c79278b32db28d83c | [
"MIT"
] | null | null | null | MultinomialNB.ipynb | djendara/heart-disease-classification | cac78d49ab56a99fce48683c79278b32db28d83c | [
"MIT"
] | null | null | null | MultinomialNB.ipynb | djendara/heart-disease-classification | cac78d49ab56a99fce48683c79278b32db28d83c | [
"MIT"
] | null | null | null | 134.451792 | 118,964 | 0.861392 | [
[
[
"## Imports",
"_____no_output_____"
]
],
[
[
"import pandas as pd\nimport numpy as np\nimport matplotlib.pyplot as plt\nimport seaborn as sns\n\nfrom sklearn.model_selection import train_test_split, GridSearchCV, cross_val_score, validation_curve\n\nfrom sklearn.naive_bayes import MultinomialNB\n\nfrom sklearn.metrics import accuracy_score, confusion_matrix, classification_report, plot_roc_curve, plot_confusion_matrix, f1_score",
"_____no_output_____"
]
],
[
[
"## Loading the data",
"_____no_output_____"
]
],
[
[
"df = pd.read_csv('../input/heart-disease-uci/heart.csv')\ndf.head()",
"_____no_output_____"
],
[
"df.shape",
"_____no_output_____"
]
],
[
[
"#### as we can see this data this data is about 303 rows and 14 column",
"_____no_output_____"
],
[
"## Exploring our dataset ",
"_____no_output_____"
]
],
[
[
"df.sex.value_counts(normalize=True)",
"_____no_output_____"
]
],
[
[
"#### this mean we have more female then male.",
"_____no_output_____"
],
[
"#### let's plot only people who got disease by sex",
"_____no_output_____"
]
],
[
[
"df.sex[df.target==1].value_counts().plot(kind=\"bar\")\n# commenting the plot \nplt.title(\"people who got disease by sex\")\nplt.xlabel(\"sex\")\nplt.ylabel(\"effected\"); \nplt.xticks(rotation = 0);",
"_____no_output_____"
],
[
"df.target.value_counts(normalize=True)",
"_____no_output_____"
]
],
[
[
"#### the two classes are almost equal",
"_____no_output_____"
],
[
"### Ploting Heart Disease by Age / Max Heart Rate",
"_____no_output_____"
]
],
[
[
"sns.scatterplot(x=df.age, y=df.thalach, hue = df.target);\n# commenting the plot \nplt.title(\"Heart Disease by Age / Max Heart Rate\")\nplt.xlabel(\"Age\")\nplt.legend([\"Disease\", \"No Disease\"])\nplt.ylabel(\"Max Heart Rate\");",
"_____no_output_____"
]
],
[
[
"### Correlation matrix ",
"_____no_output_____"
]
],
[
[
"corr = df.corr()\nf, ax = plt.subplots(figsize=(12, 10))\nsns.heatmap(corr, annot=True, fmt='.2f', ax=ax);",
"_____no_output_____"
],
[
"df.head()",
"_____no_output_____"
]
],
[
[
"## Modeling",
"_____no_output_____"
]
],
[
[
"df.head()",
"_____no_output_____"
]
],
[
[
"#### Features / Lable",
"_____no_output_____"
]
],
[
[
"X = df.drop('target', axis=1)\nX.head()",
"_____no_output_____"
],
[
"y = df.target\ny.head()",
"_____no_output_____"
]
],
[
[
"#### Spliting our dataset with 20% for test",
"_____no_output_____"
]
],
[
[
"np.random.seed(42)\nX_train, X_test, y_train, y_test = train_test_split(X, y, test_size=0.2)",
"_____no_output_____"
],
[
"y_train.head()",
"_____no_output_____"
]
],
[
[
"## Evaluation metrics",
"_____no_output_____"
],
[
"#### Function for geting score (f1 and acc) and ploting the confusion metrix",
"_____no_output_____"
]
],
[
[
"def getScore(model, X_test, y_test):\n y_pred = model.predict(X_test)\n print('f1_score')\n print(f1_score(y_test,y_pred,average='binary'))\n print('accuracy')\n acc = accuracy_score(y_test,y_pred, normalize=True)\n print(acc)\n print('Confusion Matrix :')\n plot_confusion_matrix(model, X_test, y_test)\n plt.show()\n return acc",
"_____no_output_____"
],
[
"np.random.seed(42)\nclf = MultinomialNB()\nclf.fit(X_train, y_train);\nclf_accuracy = getScore(clf, X_test, y_test)",
"f1_score\n0.8524590163934426\naccuracy\n0.8524590163934426\nConfusion Matrix :\n"
]
],
[
[
"#### Classification report",
"_____no_output_____"
]
],
[
[
"print(classification_report(y_test, clf.predict(X_test)))",
" precision recall f1-score support\n\n 0 0.81 0.90 0.85 29\n 1 0.90 0.81 0.85 32\n\n accuracy 0.85 61\n macro avg 0.85 0.85 0.85 61\nweighted avg 0.86 0.85 0.85 61\n\n"
]
],
[
[
"### ROC curve",
"_____no_output_____"
]
],
[
[
"plot_roc_curve(clf, X_test, y_test);",
"_____no_output_____"
]
],
[
[
"## Feature importance",
"_____no_output_____"
]
],
[
[
"clf.coef_",
"_____no_output_____"
],
[
"f_dict = dict(zip(X.columns , clf.coef_[0]))\nf_data = pd.DataFrame(f_dict, index=[0])\nf_data.T.plot.bar(title=\"Feature Importance\", legend=False, figsize=(10,4));\nplt.xticks(rotation = 0);",
"_____no_output_____"
]
],
[
[
"#### from this plot we can see features who have importance or not",
"_____no_output_____"
],
[
"## Cross-validation",
"_____no_output_____"
]
],
[
[
"cv_precision = np.mean(cross_val_score(MultinomialNB(),\n X,\n y,\n cv=5)) \ncv_precision",
"_____no_output_____"
]
],
[
[
"## GreadSearcheCV",
"_____no_output_____"
]
],
[
[
"np.random.seed(42)\nparam_grid = {\n 'alpha': [0.01, 0.1, 0.5, 1.0, 10.0]\n \n} \ngrid_search = GridSearchCV(estimator = MultinomialNB(), param_grid = param_grid, \n cv = 10, n_jobs = -1, verbose = 2)\ngrid_search.fit(X_train, y_train)\nbest_grid = grid_search.best_params_\nprint('best grid = ', best_grid)\ngrid_accuracy = grid_search.score(X_test, y_test)\nprint('Grid Score = ', grid_accuracy)",
"Fitting 10 folds for each of 5 candidates, totalling 50 fits\n"
],
[
"best_grid\n",
"_____no_output_____"
],
[
"grid_accuracy",
"_____no_output_____"
]
],
[
[
"## Comparing results",
"_____no_output_____"
]
],
[
[
"model_scores = {'MNB': clf_accuracy, 'grid_searche': grid_accuracy}\nmodel_compare = pd.DataFrame(model_scores, index=['accuracy'])\nmodel_compare.T.plot.bar();\nplt.xticks(rotation = 0);",
"_____no_output_____"
]
]
] | [
"markdown",
"code",
"markdown",
"code",
"markdown",
"code",
"markdown",
"code",
"markdown",
"code",
"markdown",
"code",
"markdown",
"code",
"markdown",
"code",
"markdown",
"code",
"markdown",
"code",
"markdown",
"code",
"markdown",
"code",
"markdown",
"code",
"markdown",
"code",
"markdown",
"code",
"markdown",
"code"
] | [
[
"markdown"
],
[
"code"
],
[
"markdown"
],
[
"code",
"code"
],
[
"markdown",
"markdown"
],
[
"code"
],
[
"markdown",
"markdown"
],
[
"code",
"code"
],
[
"markdown",
"markdown"
],
[
"code"
],
[
"markdown"
],
[
"code",
"code"
],
[
"markdown"
],
[
"code"
],
[
"markdown"
],
[
"code",
"code"
],
[
"markdown"
],
[
"code",
"code"
],
[
"markdown",
"markdown"
],
[
"code",
"code"
],
[
"markdown"
],
[
"code"
],
[
"markdown"
],
[
"code"
],
[
"markdown"
],
[
"code",
"code"
],
[
"markdown",
"markdown"
],
[
"code"
],
[
"markdown"
],
[
"code",
"code",
"code"
],
[
"markdown"
],
[
"code"
]
] |
e767b3821277ec44721e2851b028e5e26abd4a11 | 361,014 | ipynb | Jupyter Notebook | Fig2b_HitDetection.ipynb | menchelab/BioProfilingNotebooks | a6b646ed91d1b3ae031298bade4246b20f696e9b | [
"MIT"
] | null | null | null | Fig2b_HitDetection.ipynb | menchelab/BioProfilingNotebooks | a6b646ed91d1b3ae031298bade4246b20f696e9b | [
"MIT"
] | null | null | null | Fig2b_HitDetection.ipynb | menchelab/BioProfilingNotebooks | a6b646ed91d1b3ae031298bade4246b20f696e9b | [
"MIT"
] | 1 | 2021-08-16T09:48:05.000Z | 2021-08-16T09:48:05.000Z | 205.354949 | 84,541 | 0.863903 | [
[
[
"empty"
]
]
] | [
"empty"
] | [
[
"empty"
]
] |
e767c392b1d64e1ebae168dade18b1e9beca8985 | 47,206 | ipynb | Jupyter Notebook | notebooks/180418 - FCM SpatioTemporal.ipynb | cseveriano/spatio-temporal-forecasting | 8391f3de72b840edb2b35148537502ec5d2ac888 | [
"MIT"
] | 5 | 2018-05-25T17:43:52.000Z | 2021-12-22T13:13:43.000Z | notebooks/180418 - FCM SpatioTemporal.ipynb | cseveriano/spatio-temporal-forecasting | 8391f3de72b840edb2b35148537502ec5d2ac888 | [
"MIT"
] | null | null | null | notebooks/180418 - FCM SpatioTemporal.ipynb | cseveriano/spatio-temporal-forecasting | 8391f3de72b840edb2b35148537502ec5d2ac888 | [
"MIT"
] | 2 | 2021-07-20T12:32:00.000Z | 2021-12-13T05:32:21.000Z | 210.741071 | 40,780 | 0.909037 | [
[
[
"%matplotlib inline\nimport pandas as pd\nimport numpy as np\nimport matplotlib.pyplot as plt\nimport datetime\nimport math\n\nimport os\nimport sys\nfrom numpy.fft import fft, ifft\nimport glob",
"_____no_output_____"
],
[
"def remove_periodic(X, df_index, detrending=True, model='additive', frequency_threshold=0.1e12):\n rad = np.array(X)\n \n if detrending:\n det_rad = rad - np.average(rad)\n else:\n det_rad = rad\n \n det_rad_fft = fft(det_rad)\n\n # Get the power spectrum\n rad_ps = [np.abs(rd)**2 for rd in det_rad_fft]\n \n clean_rad_fft = [det_rad_fft[i] if rad_ps[i] > frequency_threshold else 0 \n for i in range(len(det_rad_fft))]\n \n rad_series_clean = ifft(clean_rad_fft)\n rad_series_clean = [value.real for value in rad_series_clean]\n \n if detrending:\n rad_trends = rad_series_clean + np.average(rad)\n else:\n rad_trends = rad_series_clean\n \n rad_clean_ts = pd.Series(rad_trends, index=df_index)\n \n #rad_clean_ts[(rad_clean_ts.index.hour < 6) | (rad_clean_ts.index.hour > 20)] = 0\n residual = rad - rad_clean_ts.values\n clean = rad_clean_ts.values\n return residual, clean",
"_____no_output_____"
],
[
"def normalized_rmse(targets, forecasts):\n if isinstance(targets, list):\n targets = np.array(targets)\n if isinstance(forecasts, list):\n forecasts = np.array(forecasts)\n return ((np.sqrt(np.nanmean((targets - forecasts) ** 2))) / np.nanmean(targets) ) * 100",
"_____no_output_____"
],
[
"def load_data(path, resampling=None):\n ## some resampling options: 'H' - hourly, '15min' - 15 minutes, 'M' - montlhy\n ## more options at:\n ## http://benalexkeen.com/resampling-time-series-data-with-pandas/\n allFiles = glob.iglob(path + \"/**/*.txt\", recursive=True)\n frame = pd.DataFrame()\n list_ = []\n for file_ in allFiles:\n #print(\"Reading: \",file_)\n df = pd.read_csv(file_,index_col=\"datetime\",parse_dates=['datetime'], header=0, sep=\",\")\n if frame.columns is None :\n frame.columns = df.columns\n list_.append(df)\n frame = pd.concat(list_)\n if resampling is not None:\n frame = frame.resample(resampling).mean()\n frame = frame.fillna(method='ffill')\n return frame",
"_____no_output_____"
],
[
"path = '/Users/cseveriano/spatio-temporal-forecasting/data/processed/NREL/Oahu'\n\ndf = load_data(path)\n\n# Corrigir ordem das colunas\ndf.columns = ['DHHL_3','DHHL_4', 'DHHL_5', 'DHHL_10', 'DHHL_11', 'DHHL_9', 'DHHL_2', 'DHHL_1', 'DHHL_1_Tilt', 'AP_6', 'AP_6_Tilt', 'AP_1', 'AP_3', 'AP_5', 'AP_4', 'AP_7', 'DHHL_6', 'DHHL_7', 'DHHL_8']",
"_____no_output_____"
],
[
"#inicio dos dados possui falhas na medicao\ndf = df.loc[df.index > '2010-03-20']",
"_____no_output_____"
]
],
[
[
"## Preparação dos dados",
"_____no_output_____"
]
],
[
[
"clean_df = pd.DataFrame(columns=df.columns, index=df.index)\nresidual_df = pd.DataFrame(columns=df.columns, index=df.index)\n\nfor col in df.columns:\n residual, clean = remove_periodic(df[col].tolist(), df.index, frequency_threshold=0.01e12)\n clean_df[col] = clean.tolist()\n residual_df[col] = residual.tolist()",
"_____no_output_____"
],
[
"train_df = df[(df.index >= '2010-09-01') & (df.index <= '2011-09-01')]\ntrain_clean_df = clean_df[(clean_df.index >= '2010-09-01') & (clean_df.index <= '2011-09-01')]\ntrain_residual_df = residual_df[(residual_df.index >= '2010-09-01') & (residual_df.index <= '2011-09-01')]\n\n\ntest_df = df[(df.index >= '2010-08-05')& (df.index < '2010-08-06')]\ntest_clean_df = clean_df[(clean_df.index >= '2010-08-05')& (clean_df.index < '2010-08-06')]\ntest_residual_df = residual_df[(residual_df.index >= '2010-08-05')& (residual_df.index < '2010-08-06')]",
"_____no_output_____"
],
[
"fig = plt.figure(figsize=(12, 8))\nplt.plot(test_clean_df.DHHL_3.values, color='blue')\nplt.plot(test_df.DHHL_3.values, color='red')\nplt.show()",
"_____no_output_____"
]
],
[
[
"## Fuzzy C-Means",
"_____no_output_____"
]
],
[
[
"from pyFTS.partitioners import FCM",
"_____no_output_____"
]
]
] | [
"code",
"markdown",
"code",
"markdown",
"code"
] | [
[
"code",
"code",
"code",
"code",
"code",
"code"
],
[
"markdown"
],
[
"code",
"code",
"code"
],
[
"markdown"
],
[
"code"
]
] |
e767d9d2df8d38e6509fa95ae8b87f015f0a380f | 31,462 | ipynb | Jupyter Notebook | chapter06_optimization/adagrad-scratch.ipynb | sgeos/mxnet_the_straight_dope | 5af16ade3dad964eb48d4c4fd6c09176cfe68f66 | [
"Apache-2.0"
] | null | null | null | chapter06_optimization/adagrad-scratch.ipynb | sgeos/mxnet_the_straight_dope | 5af16ade3dad964eb48d4c4fd6c09176cfe68f66 | [
"Apache-2.0"
] | 1 | 2020-03-30T20:36:17.000Z | 2020-03-30T20:36:17.000Z | chapter06_optimization/adagrad-scratch.ipynb | sgeos/mxnet_the_straight_dope | 5af16ade3dad964eb48d4c4fd6c09176cfe68f66 | [
"Apache-2.0"
] | null | null | null | 158.89899 | 25,796 | 0.877312 | [
[
[
"# Adagrad from scratch\n",
"_____no_output_____"
]
],
[
[
"from mxnet import ndarray as nd\n\n# Adagrad.\ndef adagrad(params, sqrs, lr, batch_size):\n eps_stable = 1e-7\n for param, sqr in zip(params, sqrs):\n g = param.grad / batch_size\n sqr[:] += nd.square(g)\n div = lr * g / nd.sqrt(sqr + eps_stable)\n param[:] -= div\n\nimport mxnet as mx\nfrom mxnet import autograd\nfrom mxnet import gluon\nimport random\n\nmx.random.seed(1)\nrandom.seed(1)\n\n# Generate data.\nnum_inputs = 2\nnum_examples = 1000\ntrue_w = [2, -3.4]\ntrue_b = 4.2\nX = nd.random_normal(scale=1, shape=(num_examples, num_inputs))\ny = true_w[0] * X[:, 0] + true_w[1] * X[:, 1] + true_b\ny += .01 * nd.random_normal(scale=1, shape=y.shape)\ndataset = gluon.data.ArrayDataset(X, y)\n\n\n# Construct data iterator.\ndef data_iter(batch_size):\n idx = list(range(num_examples))\n random.shuffle(idx)\n for batch_i, i in enumerate(range(0, num_examples, batch_size)):\n j = nd.array(idx[i: min(i + batch_size, num_examples)])\n yield batch_i, X.take(j), y.take(j)\n\n# Initialize model parameters.\ndef init_params():\n w = nd.random_normal(scale=1, shape=(num_inputs, 1))\n b = nd.zeros(shape=(1,))\n params = [w, b]\n sqrs = []\n for param in params:\n param.attach_grad()\n # \n sqrs.append(param.zeros_like())\n return params, sqrs\n\n# Linear regression.\ndef net(X, w, b):\n return nd.dot(X, w) + b\n\n# Loss function.\ndef square_loss(yhat, y):\n return (yhat - y.reshape(yhat.shape)) ** 2 / 2",
"_____no_output_____"
],
[
"%matplotlib inline\nimport matplotlib as mpl\nmpl.rcParams['figure.dpi']= 120\nimport matplotlib.pyplot as plt\nimport numpy as np\n\ndef train(batch_size, lr, epochs, period):\n assert period >= batch_size and period % batch_size == 0\n [w, b], sqrs = init_params()\n total_loss = [np.mean(square_loss(net(X, w, b), y).asnumpy())]\n\n # Epoch starts from 1.\n for epoch in range(1, epochs + 1):\n for batch_i, data, label in data_iter(batch_size):\n with autograd.record():\n output = net(data, w, b)\n loss = square_loss(output, label)\n loss.backward()\n adagrad([w, b], sqrs, lr, batch_size)\n if batch_i * batch_size % period == 0:\n total_loss.append(np.mean(square_loss(net(X, w, b), y).asnumpy()))\n print(\"Batch size %d, Learning rate %f, Epoch %d, loss %.4e\" % \n (batch_size, lr, epoch, total_loss[-1]))\n print('w:', np.reshape(w.asnumpy(), (1, -1)), \n 'b:', b.asnumpy()[0], '\\n')\n x_axis = np.linspace(0, epochs, len(total_loss), endpoint=True)\n plt.semilogy(x_axis, total_loss)\n plt.xlabel('epoch')\n plt.ylabel('loss')\n plt.show()",
"_____no_output_____"
],
[
"train(batch_size=10, lr=0.9, epochs=3, period=10)",
"Batch size 10, Learning rate 0.900000, Epoch 1, loss 5.3231e-05\nBatch size 10, Learning rate 0.900000, Epoch 2, loss 4.9388e-05\nBatch size 10, Learning rate 0.900000, Epoch 3, loss 4.9256e-05\nw: [[ 1.99946415 -3.39996123]] b: 4.19967 \n\n"
]
],
[
[
"## Next\n[Adagrad with Gluon](../chapter06_optimization/adagrad-gluon.ipynb)",
"_____no_output_____"
],
[
"For whinges or inquiries, [open an issue on GitHub.](https://github.com/zackchase/mxnet-the-straight-dope)",
"_____no_output_____"
]
]
] | [
"markdown",
"code",
"markdown"
] | [
[
"markdown"
],
[
"code",
"code",
"code"
],
[
"markdown",
"markdown"
]
] |
e767e2faebc49badbcabc3f0bb2da9cba3de33f2 | 2,909 | ipynb | Jupyter Notebook | Chapter11/_json_to_table.ipynb | Drtaylor1701/Learn-Python-by-Building-Data-Science-Applications | c97bc1b7d5b1e7070706832de364fa9337e32d58 | [
"MIT"
] | 65 | 2019-09-01T16:19:22.000Z | 2022-03-12T09:07:57.000Z | Chapter11/_json_to_table.ipynb | synchrony10/Learn-Python-by-Building-Data-Science-Applications | 317c5c1e82052e2a7da19a61e6b88c4c98440528 | [
"MIT"
] | 2 | 2019-09-23T14:27:00.000Z | 2020-08-26T07:31:10.000Z | Chapter11/_json_to_table.ipynb | synchrony10/Learn-Python-by-Building-Data-Science-Applications | 317c5c1e82052e2a7da19a61e6b88c4c98440528 | [
"MIT"
] | 55 | 2019-09-11T13:40:41.000Z | 2022-03-26T19:51:35.000Z | 20.631206 | 85 | 0.496391 | [
[
[
"%matplotlib inline\nimport pylab as plt\nplt.style.use('fivethirtyeight')\n\nimport pandas as pd\nimport json\nfrom copy import copy",
"_____no_output_____"
],
[
"path = '../Chapter07/all_battles_parsed.json'\nwith open(path, 'r') as f:\n battles = json.load(f)",
"_____no_output_____"
],
[
"from pandas.io.json import json_normalize",
"_____no_output_____"
]
],
[
[
"## Flatten all battles, campains, etc.",
"_____no_output_____"
]
],
[
[
"def _flatten_battles(battles, root=None):\n buttles_to_run = copy(battles)\n records = []\n \n for name, data in battles.items():\n if 'children' in data:\n children = data.pop('children')\n records.extend(_flatten_battles(children, root=name))\n else:\n data['level'] = 100\n \n data['name'] = name\n data['parent'] = root\n records.append(data)\n\n return records ",
"_____no_output_____"
],
[
"records = {k: _flatten_battles(v, root=k) for k, v in battles.items()} # fronts",
"_____no_output_____"
],
[
"records = {k: pd.DataFrame(json_normalize(v)) for k, v in records.items()}",
"_____no_output_____"
]
],
[
[
"# Store as CSV",
"_____no_output_____"
]
],
[
[
"for front, data in records.items():\n data.to_csv(f'./data/{front}.csv', index=None)",
"_____no_output_____"
]
]
] | [
"code",
"markdown",
"code",
"markdown",
"code"
] | [
[
"code",
"code",
"code"
],
[
"markdown"
],
[
"code",
"code",
"code"
],
[
"markdown"
],
[
"code"
]
] |
e767ec0b6e0382032f8ba64f66923f1419575dac | 66,825 | ipynb | Jupyter Notebook | stanza/stanza_depparse.ipynb | steysie/parse-xplore | 2f79f9eb76e1b2f59e0bdacb9cb4bb618fad7df4 | [
"CC0-1.0"
] | null | null | null | stanza/stanza_depparse.ipynb | steysie/parse-xplore | 2f79f9eb76e1b2f59e0bdacb9cb4bb618fad7df4 | [
"CC0-1.0"
] | null | null | null | stanza/stanza_depparse.ipynb | steysie/parse-xplore | 2f79f9eb76e1b2f59e0bdacb9cb4bb618fad7df4 | [
"CC0-1.0"
] | 1 | 2020-08-06T06:16:10.000Z | 2020-08-06T06:16:10.000Z | 51.963453 | 256 | 0.234523 | [
[
[
"# Stanza Dependency Parsing",
"_____no_output_____"
],
[
"## Navigation:\n* [General Info](#info)\n* [Setting up Stanza for training](#setup)\n* [Preparing Dataset for DEPPARSE](#prepare)\n* [Training a Dependency Parser with Stanza](#depparse)\n* [Using Trained Model for Prediction](#predict)\n* [Prediction and Saving to CONLL-U](#save)",
"_____no_output_____"
],
[
"## General Info <a class=\"anchor\" id=\"info\"></a>\n\n[`Link to Manual`](https://stanfordnlp.github.io/stanza/index.html) [`Training Page`](https://stanfordnlp.github.io/stanza/training.html)\n\n[`Link to GitHub Repository`](https://github.com/stanfordnlp/stanza) (git clone this repo)\n\n`Libraries needed:` `corpuscula` (conllu parsing); `stanza` (training); `tqdm` (displaying progress); `junky` (loading datasets); `mordl` (conllu evaluation script).\n\n`Pre-Trained Embeddings used in this example:` Recommended vectors are downloaded from [here](https://lindat.mff.cuni.cz/repository/xmlui/bitstream/handle/11234/1-1989/word-embeddings-conll17.tar?sequence=9&isAllowed=y)(~30GB, 60+ languages)\n\n`Pipeline Input:` CONLL-U file.\n\n`Pipeline Output:` CONLL-U file with predicitons.\n\n`Sample pipeline output:`\n```\n>>> nlp = stanza.Pipeline('ru',\n processors='tokenize,pos,lemma,ner,depparse',\n depparse_model_path='stanza/saved_models/depparse/ru_syntagrus_parser.pt',\n tokenize_pretokenized=True)\n\n>>> doc = nlp(' '.join(test[0]))\n\n>>> print(*[f'id: {word.id}\\tword: {word.text}\\thead id: {word.head}\\t\\\n head: {sent.words[word.head-1].text if word.head > 0 else \"root\"}\\tdeprel: {word.deprel}'\n for sent in doc.sentences for word in sent.words], sep='\\n')\n \nid: 1\tword: В\thead id: 3\t head: период\tdeprel: case\nid: 2\tword: советский\thead id: 3\t head: период\tdeprel: amod\nid: 3\tword: период\thead id: 11\t head: составляло\tdeprel: obl\nid: 4\tword: времени\thead id: 3\t head: период\tdeprel: nmod\nid: 5\tword: число\thead id: 11\t head: составляло\tdeprel: nsubj\nid: 6\tword: ИТ\thead id: 5\t head: число\tdeprel: nmod\nid: 7\tword: -\thead id: 8\t head: специалистов\tdeprel: punct\nid: 8\tword: специалистов\thead id: 6\t head: ИТ\tdeprel: appos\nid: 9\tword: в\thead id: 10\t head: Армении\tdeprel: case\nid: 10\tword: Армении\thead id: 5\t head: число\tdeprel: nmod\nid: 11\tword: составляло\thead id: 0\t head: root\tdeprel: root\nid: 12\tword: около\thead id: 14\t head: тысяч\tdeprel: case\nid: 13\tword: десяти\thead id: 14\t head: тысяч\tdeprel: nummod\nid: 14\tword: тысяч\thead id: 11\t head: составляло\tdeprel: obl\nid: 15\tword: .\thead id: 11\t head: составляло\tdeprel: punct\n```",
"_____no_output_____"
],
[
"## Setting up Stanza for training<a class=\"anchor\" id=\"setup\"></a>",
"_____no_output_____"
]
],
[
[
"#!pip install stanza",
"_____no_output_____"
],
[
"# !pip install -U stanza",
"_____no_output_____"
]
],
[
[
"Run in terminal.\n\n1. Clone Stanza GitHub repository\n```\n$git clone https://github.com/stanfordnlp/stanza\n```\n\n2. Move to cloned git repository & download embeddings ({lang}.vectors.xz format)\n(run in a screen, takes up several hours, depending on the Internet speed). Make sure the vectors are in `/extern_data/word2vec` folder. You will probably need to create this folder and move the downloaded folders with word vectors there manually.\n```\n$ cd stanza\n$ ./scripts/download_vectors.sh ./extern_data/\n```\n\n3. Make sure your `./stanza/scripts/config.sh` is set up like below. Modify if necessary (pay attention to UDBASE and NERBASE).\n\n```\nexport UDBASE=./udbase\n\nexport NERBASE=./nerbase\n\n# Set directories to store processed training/evaluation files\nexport DATA_ROOT=./data\nexport TOKENIZE_DATA_DIR=$DATA_ROOT/tokenize\nexport MWT_DATA_DIR=$DATA_ROOT/mwt\nexport POS_DATA_DIR=$DATA_ROOT/pos\nexport LEMMA_DATA_DIR=$DATA_ROOT/lemma\nexport DEPPARSE_DATA_DIR=$DATA_ROOT/depparse\nexport ETE_DATA_DIR=$DATA_ROOT/ete\nexport NER_DATA_DIR=$DATA_ROOT/ner\nexport CHARLM_DATA_DIR=$DATA_ROOT/charlm\n\n# Set directories to store external word vector data\nexport WORDVEC_DIR=./extern_data/\n```\n**NB!** Make sure `WORDVEC_DIR=./extern_data/` if your vectors are in `/extern_data/word2vec` folder.\nIf you leave `WORDVEC_DIR=./extern_data/`, your vectors should be stored in `/extern_data/word2vec/word2vec` folder.\n\n4. Download language resources:",
"_____no_output_____"
]
],
[
[
"import stanza\nstanza.download('ru')",
"Downloading https://raw.githubusercontent.com/stanfordnlp/stanza-resources/master/resources_1.0.0.json: 115kB [00:00, 2.47MB/s] \n2020-07-24 11:27:31 INFO: Downloading default packages for language: ru (Russian)...\n2020-07-24 11:27:32 INFO: File exists: /home/steysie/stanza_resources/ru/default.zip.\n2020-07-24 11:27:38 INFO: Finished downloading models and saved to /home/steysie/stanza_resources.\n"
]
],
[
[
"## Preparing Dataset for DEPPARSE<a class=\"anchor\" id=\"prepare\"></a>",
"_____no_output_____"
]
],
[
[
"from corpuscula.corpus_utils import syntagrus, download_ud, Conllu\nfrom corpuscula import corpus_utils\nimport junky\n\nimport corpuscula.corpus_utils as cu\nimport stanza\n# cu.set_root_dir('.')",
"_____no_output_____"
],
[
"# !pip install -U junky",
"_____no_output_____"
],
[
"corpus_utils.download_syntagrus(root_dir=corpus_utils.get_root_dir(), overwrite=True)",
"Downloading SynTagRus 1 of 3\n>##################] 100% \ndone: 81043533 bytes\nDownloading SynTagRus 2 of 3\n[###########] 100% \ndone: 10903424 bytes\nDownloading SynTagRus 3 of 3\n[###########] 100% \ndone: 10798207 bytes\n"
],
[
"junky.clear_tqdm()\n# train, train_heads, train_deprels = junky.get_conllu_fields(syntagrus.train, fields=['HEAD', 'DEPREL'])\n# dev, train_heads, dev_deprels = junky.get_conllu_fields(syntagrus.dev, fields=['HEAD', 'DEPREL'])\ntest, test_heads, test_deprels = junky.get_conllu_fields(syntagrus.test, fields=['HEAD', 'DEPREL'])",
"Load corpus\n"
]
],
[
[
"## Training a Dependency Parser with Stanza<a class=\"anchor\" id=\"depparse\"></a>",
"_____no_output_____"
],
[
"**`STEP 1`**\n\n`Input files for DEPPARSE model training should be placed here:` \n\n**`{UDBASE}/{corpus}/{corpus_short}-ud-{train,dev,test}.conllu`**, where \n* **`{UDBASE}`** is `./stanza/udbase/` (specified in `config.sh`), \n* **`{corpus}`** is full corpus name (e.g. `UD_Russian-SynTagRus` or `UD_English-EWT`, case-sensitive), and \n* **`{corpus_short}`** is the treebank code, can be [found here](https://stanfordnlp.github.io/stanza/model_history.html) (e.g. `ru_syntagrus`).\n\n**`STEP 2`**\n\n**Important:** Create `./data/depparse/` folder, otherwise the code below will fail to run.\n\n\n**`STEP 3`** To prepare data, run:\n```\n$ cd stanza\n$ ./scripts/prep_depparse_data.sh UD_Russian-SynTagRus gold\n```\nThe script above prepares the train-dev-test.conllu data which is located in `./udbase/UD_Russian-SynTagRus/`.\n\n**`STEP 4`**\nTo start training, run:\n```\n$ ./scripts/run_depparse.sh UD_Russian-SynTagRus gold\n```\nThe model will be saved to `saved_models/depparse/ru_syntagrus_parser.pt`.\n\n**`HOW TO USE`** \n#### Loading Trained Models to Pipeline\n\n\nTo load the model for prediction, when setting up Tagger Pipeline, specify path to the model:\n```\nnlp = stanza.Pipeline('ru', \n processors='tokenize,pos,lemma,ner,depparse',\n pos_model_path=<path to model>,\n lemma_model_path=<path to model>,\n ner_model_path=<path to model>,\n depparse_model_path=<path to model>)\n```",
"_____no_output_____"
],
[
"## Using Trained Model for Prediction <a class=\"anchor\" id=\"predict\"></a>",
"_____no_output_____"
],
[
"If you want to disable Stanza built-in tokenizer, specify `tokenize_pretokenized=True` parameter in Pipeline.\n\nInput should still be a list of strings, but tokens will be separated by spaces, no multi-word tokens will appear.",
"_____no_output_____"
]
],
[
[
"nlp = stanza.Pipeline('ru',\n processors='tokenize,pos,lemma,ner,depparse',\n depparse_model_path='stanza/saved_models/depparse/ru_syntagrus_parser.pt',\n tokenize_pretokenized=True)",
"2020-07-28 13:14:34 INFO: Loading these models for language: ru (Russian):\n=======================================\n| Processor | Package |\n---------------------------------------\n| tokenize | syntagrus |\n| pos | syntagrus |\n| lemma | syntagrus |\n| depparse | stanza/sav..._parser.pt |\n| ner | wikiner |\n=======================================\n\n2020-07-28 13:14:36 INFO: Use device: cpu\n2020-07-28 13:14:36 INFO: Loading: tokenize\n2020-07-28 13:14:36 INFO: Loading: pos\n2020-07-28 13:14:37 INFO: Loading: lemma\n2020-07-28 13:14:37 INFO: Loading: depparse\n2020-07-28 13:14:38 INFO: Loading: ner\n2020-07-28 13:14:38 INFO: Done loading processors!\n"
],
[
"doc = nlp(' '.join(test[0]))",
"_____no_output_____"
],
[
"print(*[f'id: {word.id}\\tword: {word.text}\\thead id: {word.head}\\t\\\n head: {sent.words[word.head-1].text if word.head > 0 else \"root\"}\\tdeprel: {word.deprel}'\n for sent in doc.sentences for word in sent.words], sep='\\n')",
"id: 1\tword: В\thead id: 3\t head: период\tdeprel: case\nid: 2\tword: советский\thead id: 3\t head: период\tdeprel: amod\nid: 3\tword: период\thead id: 11\t head: составляло\tdeprel: obl\nid: 4\tword: времени\thead id: 3\t head: период\tdeprel: nmod\nid: 5\tword: число\thead id: 11\t head: составляло\tdeprel: nsubj\nid: 6\tword: ИТ\thead id: 5\t head: число\tdeprel: nmod\nid: 7\tword: -\thead id: 8\t head: специалистов\tdeprel: punct\nid: 8\tword: специалистов\thead id: 6\t head: ИТ\tdeprel: appos\nid: 9\tword: в\thead id: 10\t head: Армении\tdeprel: case\nid: 10\tword: Армении\thead id: 5\t head: число\tdeprel: nmod\nid: 11\tword: составляло\thead id: 0\t head: root\tdeprel: root\nid: 12\tword: около\thead id: 14\t head: тысяч\tdeprel: case\nid: 13\tword: десяти\thead id: 14\t head: тысяч\tdeprel: nummod\nid: 14\tword: тысяч\thead id: 11\t head: составляло\tdeprel: obl\nid: 15\tword: .\thead id: 11\t head: составляло\tdeprel: punct\n"
],
[
"doc",
"_____no_output_____"
],
[
"from collections import OrderedDict\nimport stanza\nfrom tqdm import tqdm\n\ndef stanza_parse(sents,\n depparse_model='stanza/saved_models/depparse/ru_syntagrus_parser.pt'\n ):\n \n sents = [' '.join(sent) for sent in sents]\n nlp = stanza.Pipeline('ru',\n processors='tokenize,pos,lemma,ner,depparse',\n# pos_model_path=pos_model,\n# lemma_model_path=lemma_model,\n# ner_model_path=ner_model,\n depparse_model_path=depparse_model,\n tokenize_pretokenized=True)\n \n for idx, sent in enumerate(tqdm(sents)):\n doc = nlp(sent) \n res = []\n\n assert len(doc.sentences) == 1, \\\n 'ERROR: incorrect lengths of sentences ({}) for sent {}' \\\n .format(len(doc.sentences), idx)\n sent = doc.sentences[0]\n tokens, words = sent.tokens, sent.words\n assert len(tokens) == len(words), \\\n 'ERROR: inconsistent lengths of tokens and words for sent {}' \\\n .format(idx)\n for token, word in zip(tokens, words):\n res.append({\n 'ID': token.id,\n 'FORM': token.text,\n 'LEMMA': word.lemma,\n 'UPOS': word.upos,\n 'XPOS': word.xpos,\n 'FEATS': OrderedDict(\n [(k, v) for k, v in [\n t.split('=', 1) for t in word.feats.split('|')\n ]] if word.feats else []\n ),\n 'HEAD': str(word.head),\n 'DEPREL': word.deprel,\n 'DEPS': str(word.head)+':'+ word.deprel,\n 'MISC': OrderedDict(\n [('NE', token.ner[2:])] if token.ner != 'O' else []\n )\n })\n\n yield res",
"_____no_output_____"
]
],
[
[
"## Prediction and Saving Results to CONLL-U<a class=\"anchor\" id=\"save\"></a>",
"_____no_output_____"
]
],
[
[
"junky.clear_tqdm()",
"_____no_output_____"
],
[
"Conllu.save(stanza_parse(test), 'stanza_syntagrus.conllu',\n fix=True, log_file=None)",
"2020-07-28 13:24:45 INFO: Loading these models for language: ru (Russian):\n=======================================\n| Processor | Package |\n---------------------------------------\n| tokenize | syntagrus |\n| pos | syntagrus |\n| lemma | syntagrus |\n| depparse | stanza/sav..._parser.pt |\n| ner | wikiner |\n=======================================\n\n2020-07-28 13:24:45 INFO: Use device: cpu\n2020-07-28 13:24:45 INFO: Loading: tokenize\n2020-07-28 13:24:45 INFO: Loading: pos\n2020-07-28 13:24:46 INFO: Loading: lemma\n2020-07-28 13:24:46 INFO: Loading: depparse\n2020-07-28 13:24:47 INFO: Loading: ner\n2020-07-28 13:24:48 INFO: Done loading processors!\n100%|██████████| 6491/6491 [25:39<00:00, 4.22it/s]\n"
]
],
[
[
"## Inference on Test Corpus",
"_____no_output_____"
]
],
[
[
"# !pip install mordl",
"_____no_output_____"
],
[
"from mordl import conll18_ud_eval",
"_____no_output_____"
],
[
"gold_file = 'corpus/_UD/UD_Russian-SynTagRus/ru_syntagrus-ud-test.conllu'\nsystem_file = 'stanza_syntagrus.conllu'",
"_____no_output_____"
],
[
"conll18_ud_eval(gold_file, system_file, verbose=True, counts=False)",
" 0%| | 0/6491 [34:50<?, ?it/s]\n"
]
]
] | [
"markdown",
"code",
"markdown",
"code",
"markdown",
"code",
"markdown",
"code",
"markdown",
"code",
"markdown",
"code"
] | [
[
"markdown",
"markdown",
"markdown",
"markdown"
],
[
"code",
"code"
],
[
"markdown"
],
[
"code"
],
[
"markdown"
],
[
"code",
"code",
"code",
"code"
],
[
"markdown",
"markdown",
"markdown",
"markdown"
],
[
"code",
"code",
"code",
"code",
"code"
],
[
"markdown"
],
[
"code",
"code"
],
[
"markdown"
],
[
"code",
"code",
"code",
"code"
]
] |
e768017a36214cb600d2845327a5a16dde1afd11 | 867,303 | ipynb | Jupyter Notebook | Examples/ShortestPath.ipynb | gchqdev56268/annchor | 2705bc5073a1364d0f892077d37cfde435c4871b | [
"BSD-3-Clause"
] | 22 | 2021-08-02T17:29:52.000Z | 2022-03-11T10:21:28.000Z | Examples/ShortestPath.ipynb | gchqdev56268/annchor | 2705bc5073a1364d0f892077d37cfde435c4871b | [
"BSD-3-Clause"
] | 3 | 2021-08-04T16:16:11.000Z | 2021-08-31T13:19:44.000Z | Examples/ShortestPath.ipynb | gchqdev56268/annchor | 2705bc5073a1364d0f892077d37cfde435c4871b | [
"BSD-3-Clause"
] | 6 | 2021-08-03T04:23:09.000Z | 2021-09-27T06:50:08.000Z | 5,071.947368 | 863,184 | 0.965733 | [
[
[
"# requires networkx (pip install networkx)\n# requires matplotlib (pip install matplotlib)\n\nimport numpy as np\nimport time\nimport networkx as nkx\nimport matplotlib.pyplot as plt\nfrom annchor.datasets import load_graph_sp\nfrom annchor import compare_neighbor_graphs\n\n\nk=15\n\n\ngraph_sp_data = load_graph_sp()\nX = graph_sp_data['X']\ny = graph_sp_data['y']\nneighbor_graph = graph_sp_data['neighbor_graph']\nG = graph_sp_data['G']\nnx = X.shape[0]\n\nedges,weights = zip(*nkx.get_edge_attributes(G,'w').items())\n\npos = nkx.spring_layout(G)\n\nfig,ax = plt.subplots(figsize=(12,12))\nnkx.draw(G, \n pos,\n node_color='k',\n node_size=5,\n edgelist=edges,\n edge_color=weights,\n width=1.0,\n edge_cmap=plt.cm.viridis,\n ax=ax)\nplt.show()",
"_____no_output_____"
],
[
"def sp_dist(i,j):\n return nkx.dijkstra_path_length(G,i,j,weight='w')\n\n\nrandX = lambda : X[np.random.randint(nx)]\n%timeit sp_dist(randX(),randX())",
"2.94 ms ± 246 µs per loop (mean ± std. dev. of 7 runs, 100 loops each)\n"
],
[
"from annchor import BruteForce\n\nstart_time = time.time()\n\nbruteforce = BruteForce(X,sp_dist)\nbruteforce.fit()\n\nprint('Brute Force Time: %5.3f seconds' % (time.time()-start_time))\n\nerror = compare_neighbor_graphs(neighbor_graph,\n bruteforce.neighbor_graph,\n k)\n\nprint('Brute Force Accuracy: %d incorrect NN pairs (%5.3f%%)' % (error,error/(k*nx)))",
"_____no_output_____"
],
[
"k=15\n\nstart_time = time.time()\n\n# Call ANNchor\nann = Annchor(X,\n sp_dist,\n n_anchors=20,\n n_neighbors=k,\n random_seed=5,\n n_samples=5000,\n p_work=0.15)\n\nann.fit()\nprint('ANNchor Time: %5.3f seconds' % (time.time()-start_time))\n\n\n# Test accuracy\nerror = compare_neighbor_graphs(neighbor_graph,\n ann.neighbor_graph,\n k)\nprint('ANNchor Accuracy: %d incorrect NN pairs (%5.3f%%)' % (error,100*error/(k*nx)))",
"ANNchor Time: 32.454 seconds\nANNchor Accuracy: 1 incorrect NN pairs (0.008%)\n"
]
]
] | [
"code"
] | [
[
"code",
"code",
"code",
"code"
]
] |
e76805de0173f32c579caab239ea27cdbf9d1e3e | 912,119 | ipynb | Jupyter Notebook | analysis/isfog-2020-linear-model-demo.ipynb | alexandershires/offshore-geo | 259cf8768d813c4d10b7e7978892dc4b51b4463c | [
"Apache-2.0"
] | null | null | null | analysis/isfog-2020-linear-model-demo.ipynb | alexandershires/offshore-geo | 259cf8768d813c4d10b7e7978892dc4b51b4463c | [
"Apache-2.0"
] | null | null | null | analysis/isfog-2020-linear-model-demo.ipynb | alexandershires/offshore-geo | 259cf8768d813c4d10b7e7978892dc4b51b4463c | [
"Apache-2.0"
] | null | null | null | 452.43998 | 184,632 | 0.932708 | [
[
[
"# ISFOG 2020 - Pile driving prediction event\n\nData science techniques are rapidly transforming businesses in a broad range of sectors. While marketing and social applications have received most attention to date, geotechnical engineering can also benefit from data science tools that are now readily available. \n\nIn the context of the ISFOG2020 conference in Austin, TX, a prediction event is launched which invites geotechnical engineers to share knowledge and gain hands-on experience with machine learning models.\n\nThis Jupyter notebook shows you how to get started with machine learning (ML) tools and creates a simple ML model for pile driveability. Participants are encouraged to work through this initial notebook to get familiar with the dataset and the basics of ML.",
"_____no_output_____"
],
[
"## 1. Importing packages\n\nThe Python programming language works with a number of packages. We will work with the ```Pandas``` package for data processing, ```Matplotlib``` for data visualisation and ```scikit-learn``` for the ML. We will also make use of the numerical package ```Numpy```. These package come pre-installed with the Anaconda distribution (see installation guide). Each package is extensively documented with online documentation, tutorials and examples. We can import the necessary packages with the following code.\n\n<b>Note</b>: Code cells can be executed with <i>Shift+Enter</i> or by using the run button in the toolbar at the top. Note that code cells need to be executed from top to bottom. The order of execution is important.",
"_____no_output_____"
]
],
[
[
"import pandas as pd\nimport numpy as np\nimport matplotlib.pyplot as plt\nimport sklearn",
"_____no_output_____"
],
[
"%matplotlib inline",
"_____no_output_____"
]
],
[
[
"## 2. Pile driving data\n\nThe dataset is kindly provided by [Cathie Group](http://www.cathiegroup.com).\n\n### 2.1. Importing data\n\nThe first step in any data science exercise is to get familiar with the data. The data is provided in a csv file (```training_data.csv```). We can import the data with Pandas and display the first five rows using the ```head()``` function.",
"_____no_output_____"
]
],
[
[
"data = pd.read_csv(\"/kaggle/input/training_data.csv\") # Store the contents of the csv file in the variable 'data'\ndata.head()",
"_____no_output_____"
]
],
[
[
"The data has 12 columns, containing PCPT data ($ q_c $, $ f_s $ and $ u_2 $), recorded hammer data (blowcount, normalised hammer energy, normalised ENTHRU and total number of blows), pile data (diameter, bottom wall thickness and pile final penetration). A unique ID identifies the location and $ z $ defines the depth below the mudline.\n\nThe data has already been resampled to a regular grid with 0.5m grid intervals to facilitate the further data handling.\n\nThe hammer energy has been normalised using the same reference energy for all piles in this prediction exercise.\n\nWe can see that there is no driving data in the first five rows (NaN values), this is because driving only started after a given self-weight penetration of the pile.",
"_____no_output_____"
],
[
"### 2.2. Summary statistics\n\nWe can easily create summary statistics of each column using the ```describe()``` function on the data. This gives us the number of elements, mean, standard deviation, minimum, maximum and percentiles of each column of the data.\n\nWe can see that there are more PCPT data points than hammer data points. This makes sense as there is soil data available above the pile self-weight penetration and below the final pile penetration. The pile data is defined in the self-weight penetration part of the profile, so there are slightly more pile data points than hammer record data points.",
"_____no_output_____"
]
],
[
[
"data.describe()",
"_____no_output_____"
]
],
[
[
"### 2.3. Plotting\n\nWe can plot the cone tip resistance, blowcount and normalised ENTHRU energy for all locations to show how the data varies with depth. We can generate this plot using the ```Matplotlib``` package.",
"_____no_output_____"
]
],
[
[
"fig, ((ax1, ax2, ax3)) = plt.subplots(1, 3, sharey=True, figsize=(16,9))\nax1.scatter(data[\"qc [MPa]\"], data[\"z [m]\"], s=5) # Create the cone tip resistance vs depth plot\nax2.scatter(data[\"Blowcount [Blows/m]\"], data[\"z [m]\"], s=5) # Create the Blowcount vs depth plot \nax3.scatter(data[\"Normalised ENTRHU [-]\"], data[\"z [m]\"], s=5) # Create the ENTHRU vs depth plot\n# Format the axes (position, labels and ranges)\nfor ax in (ax1, ax2, ax3):\n ax.xaxis.tick_top()\n ax.xaxis.set_label_position('top')\n ax.grid()\n ax.set_ylim(50, 0)\nax1.set_xlabel(r\"Cone tip resistance, $ q_c $ (MPa)\")\nax1.set_xlim(0, 120)\nax2.set_xlabel(r\"Blowcount (Blows/m)\")\nax2.set_xlim(0, 200)\nax3.set_xlabel(r\"Normalised ENTRHU (-)\")\nax3.set_xlim(0, 1)\nax1.set_ylabel(r\"Depth below mudline, $z$ (m)\")\n# Show the plot\nplt.show()",
"_____no_output_____"
]
],
[
[
"The cone resistance data shows that the site mainly consists of sand of varying relative density. In certain profiles, clay is present below 10m. There are also locations with very high cone resistance (>70MPa).\n\nThe blowcount profile shows that blowcount is relatively well clustered around a generally increasing trend with depth. The normalised ENTHRU energy is also increasing with depth.",
"_____no_output_____"
],
[
"We can isolate the data for a single location by selecting this data from the dataframe with all data. As an example, we can do this for location <i>EK</i>.",
"_____no_output_____"
]
],
[
[
"# Select the data where the column 'Location ID' is equal to the location name\nlocation_data = data[data[\"Location ID\"] == \"EK\"]",
"_____no_output_____"
]
],
[
[
"We can plot the data for this location on top of the general data cloud.",
"_____no_output_____"
]
],
[
[
"fig, ((ax1, ax2, ax3)) = plt.subplots(1, 3, sharey=True, figsize=(16,9))\n# All data\nax1.scatter(data[\"qc [MPa]\"], data[\"z [m]\"], s=5)\nax2.scatter(data[\"Blowcount [Blows/m]\"], data[\"z [m]\"], s=5)\nax3.scatter(data[\"Normalised ENTRHU [-]\"], data[\"z [m]\"], s=5)\n# Location-specific data\nax1.plot(location_data[\"qc [MPa]\"], location_data[\"z [m]\"], color='red')\nax2.plot(location_data[\"Blowcount [Blows/m]\"], location_data[\"z [m]\"], color='red')\nax3.plot(location_data[\"Normalised ENTRHU [-]\"], location_data[\"z [m]\"], color='red')\nfor ax in (ax1, ax2, ax3):\n ax.xaxis.tick_top()\n ax.xaxis.set_label_position('top')\n ax.grid()\n ax.set_ylim(50, 0)\nax1.set_xlabel(r\"Cone tip resistance (MPa)\")\nax1.set_xlim(0, 120)\nax2.set_xlabel(r\"Blowcount (Blows/m)\")\nax2.set_xlim(0, 200)\nax3.set_xlabel(r\"Normalised ENTRHU (-)\")\nax3.set_xlim(0, 1)\nax1.set_ylabel(r\"Depth below mudline (m)\")\nplt.show()",
"_____no_output_____"
]
],
[
[
"We can see that pile driving started from 5m depth and continued until a depth of 30m, when the pile tip reached a sand layer with $ q_c $ > 60MPa.\n\nFeel free to investigate the soil profile and driving data for the other locations by changing the location ID.",
"_____no_output_____"
],
[
"For the purpose of the prediction event, we are interested in the variation of blowcount with $ q_c $, hammer energy, ... We can also generate plots to see the correlations. The data shows significant scatter and non-linear behaviour. We will take this into account for our machine learning model.",
"_____no_output_____"
]
],
[
[
"fig, ((ax1, ax2, ax3)) = plt.subplots(1, 3, figsize=(15,6))\n# All data\nax1.scatter(data[\"qc [MPa]\"], data[\"Blowcount [Blows/m]\"], s=5)\nax2.scatter(data[\"Normalised ENTRHU [-]\"], data[\"Blowcount [Blows/m]\"], s=5)\nax3.scatter(data[\"z [m]\"], data[\"Blowcount [Blows/m]\"], s=5)\n# Location-specific data\nax1.scatter(location_data[\"qc [MPa]\"], location_data[\"Blowcount [Blows/m]\"], color='red')\nax2.scatter(location_data[\"Normalised ENTRHU [-]\"], location_data[\"Blowcount [Blows/m]\"], color='red')\nax3.scatter(location_data[\"z [m]\"], location_data[\"Blowcount [Blows/m]\"], color='red')\nfor ax in (ax1, ax2, ax3):\n ax.grid()\n ax.set_ylim(0, 200)\n ax.set_ylabel(r\"Blowcount (Blows/m)\")\nax1.set_xlabel(r\"Cone tip resistance (MPa)\")\nax1.set_xlim(0, 120)\nax2.set_xlabel(r\"Normalised ENTRHU (-)\")\nax2.set_xlim(0, 1)\nax3.set_xlabel(r\"Depth below mudline (m)\")\nax3.set_xlim(0, 50)\nplt.show()",
"_____no_output_____"
]
],
[
[
"## 3. Basics of machine learning\n\nThe goal of the prediction exercise is to define a model relating the input (soil data, hammer energy, pile data) with the output (blowcount).\n\nIn ML terminology, we call the inputs (the columns of the dataset except for the blowcount) <i>features</i>. The blowcount is the <i>target variable</i>. Each row in the dataframe represents a <i>sample</i>, a combination of feature values for which the output is known. Data for which a value of the target variable is not yet available is called <i>unseen data</i>.\n\nBefore we dive into the code for generating ML models, let's discuss some of the concepts in more detail.",
"_____no_output_____"
],
[
"### 3.1. Machine learning techniques\n\nML combines several data science techniques under one general denominator. We can discern the following families:\n\n - Classification: Predict the value of a discrete target variable of a data point based on its features\n - Regression: Predict the value of a continuous target variable based on its features\n - Clustering: Identify groups of similar data points based on their features\n - Dimensionality reduction: Identify the features with the greatest influence on the data\n \nThe first techniques are examples of <i>supervised learning</i>. We will use data where the output has been observed and use that to <i>train</i> the ML model. Training a model is essentially the optimisation of the coefficients of a mathematical model to minimise the difference between model predictions and observed values. Such a trained algorithm is then capable of making predictions for unseen data.\n\nThis concept is not fundamentally different from any other type of data-driven modelling. The main advantage of the ML approach is the speed at which the models can be trained and the many types of models available to the engineer.\n\nIn our example of pile driving, we have a <b>regression</b> problem where we are training a model to relate features (soil data, hammer energy and pile data) with a continuous target variable (blowcount).",
"_____no_output_____"
],
[
"### 3.2. Model fitting\n\nMachine learning has disadvantages which can lead to problematic situations if the techniques are misused. One of these disadvantages is that the ML algorithm will always find a fit, even if it is a poor one.\n\nThe figure below shows an example with data showing a non-linear trend between input and output with some scatter around a trend. We can identify the following situations:\n\n - Underfitting: If we use a linear model for this data, we are not capturing the trend. The model predictions will be poor;\n - Good fit: If we formulate a model (quadratic in this case) which captures the general trend but allows variations around the trend, we obtain a good fit. In geotechnical problems, we will never get a perfect a fit but if we identify the influence of the input parameters in a consistent manner, we can build good-quality models;\n - Overfitting: If we have a model which perfectly fits all known data points, the prediction for an unseen data point will be poor. The influence of each measurement on the model is too important. The model overfits the data and does not capture the general trends. It just represents the data on which it was trained.",
"_____no_output_____"
],
[
"### 3.3. Model metrics\n\nTo prevent misuse of ML models, we will look at certain model metrics to check the quality. There are several model metrics. Two of the more common ones are the <b>Mean Squared Error (MSE)</b> and the <b>coefficient of determination ($ R^2 $)</b>.\n\nThe MSE-value is the normalised sum of quadratic differences. The closer it is to 0, the better the fit.\n\n$$ \\text{MSE}(y, \\hat{y}) = \\frac{1}{n_\\text{samples}} \\sum_{i=0}^{n_\\text{samples} - 1} (y_i - \\hat{y}_i)^2. $$\n\n$ \\hat{y}_i $ is the predicted value of the i-th sample and $ y_i $ is the true (measured) value.\n\nThe coefficient of determination ($ R^2 $) is a measure for a measure of how well future samples are likely to be predicted by the model. A good model has an $ R^2 $-value which is close to 1.\n\n$$ R^2(y, \\hat{y}) = 1 - \\frac{\\sum_{i=0}^{n_{\\text{samples}} - 1} (y_i - \\hat{y}_i)^2}{\\sum_{i=0}^{n_\\text{samples} - 1} (y_i - \\bar{y})^2} \\quad \\text{where} \\ \\bar{y} = \\frac{1}{n_{\\text{samples}}} \\sum_{i=0}^{n_{\\text{samples}} - 1} y_i$$\n\nIn the example, we will see how we can easily calculate these metrics from the data using the functions available in the ML Python package ```scikit-learn```.",
"_____no_output_____"
],
[
"### 3.4. Model validation\n\nWhen building a ML model, we will only use a subset of the data for training the model. The other subset is deliberately excluded from the learning process and used to <i>validate</i> the model. The trained model is applied on the unseen data of the validation dataset and the accuracy of the predictions is checked, resulting in a validation score representing the accuracy of the model for the validation dataset.\n\nIf our trained model is of good quality, the predictions for the validation dataset will be close to the measured values.\n\nWe will partition our data in a training dataset and a validation dataset. For the validation data set, we use seven piles. The other piles will be used as the training dataset.",
"_____no_output_____"
]
],
[
[
"validation_ids = ['EL', 'CB', 'AV', 'BV', 'EF', 'DL', 'BM']\n# Training data - ID not in validation_ids\ntraining_data = data[~data['Location ID'].isin(validation_ids)]\n# Validation data - ID in validation_ids\nvalidation_data = data[data['Location ID'].isin(validation_ids)]",
"_____no_output_____"
]
],
[
[
"With these concepts in mind, we can start building up a simple ML model.",
"_____no_output_____"
],
[
"## 4. Basic machine learning example: Linear modelling\n\nThe most basic type of ML model is a linear model. We are already using linear models in a variety of applications and often fit them without making use of ML techniques. The general equation for a linear model is given below for a model with $ N $ features:\n\n$$ y = a_0 + a_1 \\cdot x_1 + a_2 \\cdot x_2 + ... + a_N \\cdot x_N + \\epsilon $$\n\nwhere $ \\epsilon $ is the estimation error.\n\nBased on the training dataset, the value of the coefficients ($ a_0, a_1, ..., a_N $) is determined using optimisation techniques to minimise the difference between measured and predicted values. As the equation shows, a good fit will be obtained when the relation between output and inputs is truly linear. If there are non-linearities in the data, the fit will be less good.\n\nWe will illustrate how a linear regression machine learning model is generated from the available driving data.",
"_____no_output_____"
],
[
"### 4.1. Linear model based on normalised ENTHRU only\n\nThe simplest linear model depends on only one feature. We can select the normalised energy transmitted to the pile (ENTRHU) as the only feature for illustration purposes.\n\nThe mathematical form of the model can be written as:\n\n$$ BLCT = a_o + a_1 \\cdot \\text{ENTRHU}_{norm} + \\epsilon $$\n\nWe will create a dataframe $ X $ with only the normalised ENTHRU feature data and we will put the observed values of the target variable (blowcount) in the vector $ y $.\n\nNote that machine learning algorithms will raise errors when NaN values are provided. We need to ensure that we remove such values. We can creata a dataframe ```cleaned_training_data``` which only contains rows with no NaN values.",
"_____no_output_____"
]
],
[
[
"features = ['Normalised ENTRHU [-]']\ncleaned_training_data = training_data.dropna() # Remove NaN values\nX = cleaned_training_data[features]\ny = cleaned_training_data[\"Blowcount [Blows/m]\"]",
"_____no_output_____"
]
],
[
[
"We can now create a linear model. We need to import this type of model from the scikit-learn package. We can fit the linear model to the data using the ```fit()``` method.",
"_____no_output_____"
]
],
[
[
"from sklearn.linear_model import LinearRegression\nmodel_1 = LinearRegression().fit(X,y)",
"_____no_output_____"
]
],
[
[
"At this point, our model has been trained with the data and the coefficients are known. $ a_0 $ is called the intercept and $ a_1 $ to $ a_n $ are stored in ```coef_```. Because we only have one feature, ```coef_``` only returns a single value.",
"_____no_output_____"
]
],
[
[
"model_1.coef_, model_1.intercept_",
"_____no_output_____"
]
],
[
[
"We can plot the data with our trained fit. We can see that the fit follows a general trend but the quality is not great.",
"_____no_output_____"
]
],
[
[
"plt.scatter(X, y)\nx = np.linspace(0.0, 1, 50)\nplt.plot(x, model_1.intercept_ + model_1.coef_ * x, color='red')\nplt.xlabel(\"Normalised ENTHRU (-)\")\nplt.ylabel(\"Blowcount (Blows/m)\")\nplt.show()",
"_____no_output_____"
]
],
[
[
"We can also calculate the $ R^2 $ score for our training data. The score is below 0.5 and it goes without saying that this model needs improvement.",
"_____no_output_____"
]
],
[
[
"model_1.score(X,y)",
"_____no_output_____"
]
],
[
[
"In the following sections, we will explore ways to improve our model.",
"_____no_output_____"
],
[
"### 4.2. Linearizing features\n\nWhen using ENTRHU as our model feature, we can see that a linear model is not the most appropriate choice as the relation between blowcount and ENTRHU is clearly non-linear. However, we can <i>linearize</i> features.\n\nFor example, we can propose a relation using using a tangent hyperbolic law, which seems to fit better with the data.",
"_____no_output_____"
]
],
[
[
"plt.scatter(training_data[\"Normalised ENTRHU [-]\"], training_data[\"Blowcount [Blows/m]\"])\nx = np.linspace(0, 1, 100)\nplt.plot(x, 80 * np.tanh(5 * x - 0.5), color='red')\nplt.xlabel(\"Normalised ENTHRU (-)\")\nplt.ylabel(\"Blowcount (Blows/m)\")\nplt.ylim([0.0, 175.0])\nplt.show()",
"_____no_output_____"
]
],
[
[
"We can create a linearized feature:\n\n$$ (\\text{ENTHRU})_{lin} = \\tanh(5 \\cdot \\text{ENTHRU}_{norm} - 0.5) $$",
"_____no_output_____"
]
],
[
[
"Xlin = np.tanh(5 * cleaned_training_data[[\"Normalised ENTRHU [-]\"]] - 0.5)",
"_____no_output_____"
]
],
[
[
"When plotting the linearized data against the blowcount, we can see that a linear relation is much more appropriate.",
"_____no_output_____"
]
],
[
[
"plt.scatter(Xlin, y)\nplt.xlabel(r\"$ \\tanh(5 \\cdot ENTRHU_{norm} - 0.5) $\")\nplt.ylabel(\"Blowcount (Blows/m)\")\nplt.ylim([0.0, 175.0])\nplt.show()",
"_____no_output_____"
]
],
[
[
"We can fit another linear model using this linearized feature.",
"_____no_output_____"
]
],
[
[
"model_2 = LinearRegression().fit(Xlin, y)",
"_____no_output_____"
]
],
[
[
"We can check the intercept and the model coefficient:",
"_____no_output_____"
]
],
[
[
"model_2.coef_, model_2.intercept_",
"_____no_output_____"
]
],
[
[
"The model with the linearized feature can then be written as:\n\n$$ BLCT = a_0 + a_1 \\cdot (\\text{ENTHRU})_{lin} $$ \n\nWe can visualize the fit.",
"_____no_output_____"
]
],
[
[
"plt.scatter(X, y)\nx = np.linspace(0.0, 1, 50)\nplt.plot(x, model_2.intercept_ + model_2.coef_ * (np.tanh(5*x - 0.5)), color='red')\nplt.xlabel(\"Normalised ENTHRU (-)\")\nplt.ylabel(\"Blowcount (Blows/m)\")\nplt.ylim([0.0, 175])\nplt.show()",
"_____no_output_____"
]
],
[
[
"We can check the $ R^2 $ model score. By linearizing the normalised ENTHRU energy, we have improved our $ R^2 $ score and are thus fitting a model which better describes our data.",
"_____no_output_____"
]
],
[
[
"model_2.score(Xlin, y)",
"_____no_output_____"
]
],
[
[
"### 4.3. Using engineering knowledge\n\nWe know from engineering considerations on the pile driving problem that the soil resistance to driving (SRD) can be expressed as the sum of shaft friction and end bearing resistance. The shaft friction can be expressed as the integral of the unit shaft friction over the pile circumference and length.\n\nIf we make the simplifying assumption that there is a proportionality between the cone resistance and the unit shaft friction ($ f_s = \\alpha \\cdot q_c $), we can write the shaft resistance as follows:\n\n$$ R_s = \\int_{0}^{L} \\alpha \\cdot q_c \\cdot \\pi \\cdot D \\cdot dz \\approx \\alpha \\cdot \\pi \\cdot D \\cdot \\sum q_{c,i} \\cdot \\Delta z $$\n\nWe can create an additional feature for this. Creating features based on our engineering knowledge will often help us to introduce experience in a machine learning algorithm.\n\nTo achieve this, we will create a new dataframe using our training data. We will iteration over all locations in the training data and calculate the $ R_s $ feature using a cumulative sum function. We will then put this data together for all locations.",
"_____no_output_____"
]
],
[
[
"enhanced_data = pd.DataFrame() # Create a dataframe for the data enhanced with the shaft friction feature\nfor location in training_data['Location ID'].unique(): # Loop over all unique locations\n locationdata = training_data[training_data['Location ID']==location].copy() # Select the location-specific data\n # Calculate the shaft resistance feature\n locationdata[\"Rs [kN]\"] = \\\n (np.pi * locationdata[\"Diameter [m]\"] * locationdata[\"z [m]\"].diff() * locationdata[\"qc [MPa]\"]).cumsum()\n enhanced_data = pd.concat([enhanced_data, locationdata]) # Combine data for the different locations in 1 dataframe",
"_____no_output_____"
]
],
[
[
"We can plot the data to see that the clustering of our SRD shaft resistance feature vs blowcount is much better than the clustering of $ q_c $ vs blowcount. We can also linearize the relation between shaft resistance and blowcount.\n\nWe can propose the following relation:\n\n$$ BLCT = 85 \\cdot \\tanh \\left( \\frac{R_s}{1000} - 1 \\right) $$",
"_____no_output_____"
]
],
[
[
"fig, ((ax1, ax2)) = plt.subplots(1, 2, sharey=True, figsize=(12,6))\nax1.scatter(enhanced_data[\"qc [MPa]\"], enhanced_data[\"Blowcount [Blows/m]\"])\nax2.scatter(enhanced_data[\"Rs [kN]\"], enhanced_data[\"Blowcount [Blows/m]\"])\nx = np.linspace(0.0, 12000, 50)\nax2.plot(x, 85 * (np.tanh(0.001*x-1)), color='red')\nax1.set_xlabel(\"Cone tip resistance (MPa)\")\nax2.set_xlabel(\"Shaft resistance (kN)\")\nax1.set_ylabel(\"Blowcount (Blows/m)\")\nax2.set_ylabel(\"Blowcount (Blows/m)\")\nax1.set_ylim([0.0, 175])\nplt.show()",
"_____no_output_____"
]
],
[
[
"We then proceed to filter the NaN values from the data and fit a linear model.",
"_____no_output_____"
]
],
[
[
"features = [\"Rs [kN]\"]\nX = enhanced_data.dropna()[features]\ny = enhanced_data.dropna()[\"Blowcount [Blows/m]\"]\nXlin = np.tanh((0.001 * X) - 1)",
"_____no_output_____"
],
[
"model_3 = LinearRegression().fit(Xlin, y)",
"_____no_output_____"
]
],
[
[
"We can print the coefficients of the linear model and visualise the fit.",
"_____no_output_____"
]
],
[
[
"model_3.intercept_, model_3.coef_",
"_____no_output_____"
],
[
"plt.scatter(X, y)\nx = np.linspace(0.0, 12000, 50)\nplt.plot(x, model_3.intercept_ + model_3.coef_ * (np.tanh(0.001*x - 1)), color='red')\nplt.xlabel(\"Shaft resistance (kN)\")\nplt.ylabel(\"Blowcount (Blows/m)\")\nplt.ylim([0.0, 175])\nplt.show()",
"_____no_output_____"
]
],
[
[
"The fit looks reasonable and this is also reflected in the $ R^2 $ score which is just greater than 0.6. We have shown that using engineering knowledge can greatly improve model quality.",
"_____no_output_____"
]
],
[
[
"model_3.score(Xlin, y)",
"_____no_output_____"
]
],
[
[
"### 4.4. Using multiple features\n\nThe power of machine learning algorithms is that you can experiment with adding multiple features. Adding a feature can improve you model if it has a meaningful relation with the output.\n\nWe can use our linearized relation with normalised ENTHRU, shaft resistance and we can also linearize the variation of blowcount with depth:\n\n$$ BLCT = 100 \\cdot \\tanh \\left( \\frac{z}{10} - 0.5 \\right) $$",
"_____no_output_____"
]
],
[
[
"plt.scatter(data[\"z [m]\"], data[\"Blowcount [Blows/m]\"])\nz = np.linspace(0,35,100)\nplt.plot(z, 100 * np.tanh(0.1 * z - 0.5), color='red')\nplt.ylim([0, 175])\nplt.xlabel(\"Depth (m)\")\nplt.ylabel(\"Blowcount (Blows/m)\")\nplt.show()",
"_____no_output_____"
]
],
[
[
"Our model with the combined features will take the following mathematical form:\n\n$$ BLCT = a_0 + a_1 \\cdot \\tanh \\left( 5 \\cdot \\text{ENTHRU}_{norm} - 0.5 \\right) + a_2 \\cdot \\tanh \\left( \\frac{R_s}{1000} - 1 \\right) + a_3 \\cdot \\tanh \\left( \\frac{z}{10} - 0.5 \\right) $$\n\nWe can create the necessary features in our dataframe:",
"_____no_output_____"
]
],
[
[
"enhanced_data[\"linearized ENTHRU\"] = np.tanh(5 * enhanced_data[\"Normalised ENTRHU [-]\"] - 0.5)\nenhanced_data[\"linearized Rs\"] = np.tanh(0.001 * enhanced_data[\"Rs [kN]\"] - 1)\nenhanced_data[\"linearized z\"] = np.tanh(0.1 * enhanced_data[\"z [m]\"] - 0.5)\nlinearized_features = [\"linearized ENTHRU\", \"linearized Rs\", \"linearized z\"]",
"_____no_output_____"
]
],
[
[
"We can now fit a linear model with three features. The matrix $ X $ is now an $ n \\times 3 $ matrix ($ n $ samples and 3 features).",
"_____no_output_____"
]
],
[
[
"X = enhanced_data.dropna()[linearized_features]\ny = enhanced_data.dropna()[\"Blowcount [Blows/m]\"]\nmodel_4 = LinearRegression().fit(X,y)",
"_____no_output_____"
]
],
[
[
"We can calculate the $ R^2 $ score. The score is slightly better compared to our previous model. Given the scatter in the data, this score is already a reasonable value.",
"_____no_output_____"
]
],
[
[
"model_4.score(X, y)",
"_____no_output_____"
]
],
[
[
"### 4.4. Model predictions\n\nThe linear regression model always allows us to write down the mathematical form of the model. We can do so here by filling in the intercept ($ a_0 $) a coefficients $ a_1 $, $ a_2 $ and $ a_3 $ in the equation above.",
"_____no_output_____"
]
],
[
[
"model_4.intercept_, model_4.coef_",
"_____no_output_____"
]
],
[
[
"However, we don't need to explicitly write down the mathematical shape of the model to use it in the code. We can make predictions using the fitted model straightaway.",
"_____no_output_____"
]
],
[
[
"predictions = model_4.predict(X)\npredictions",
"_____no_output_____"
]
],
[
[
"We can plot these predictions together with the data. We can see that the model follows the general trend of the data fairly well. There is still significant scatter around the trend.",
"_____no_output_____"
]
],
[
[
"fig, ((ax1, ax2, ax3)) = plt.subplots(1, 3, figsize=(15,6))\n# Measurements\nax1.scatter(enhanced_data[\"Rs [kN]\"], enhanced_data[\"Blowcount [Blows/m]\"], s=5)\nax2.scatter(enhanced_data[\"Normalised ENTRHU [-]\"], enhanced_data[\"Blowcount [Blows/m]\"], s=5)\nax3.scatter(enhanced_data[\"z [m]\"], enhanced_data[\"Blowcount [Blows/m]\"], s=5)\n# Predictions\nax1.scatter(enhanced_data.dropna()[\"Rs [kN]\"], predictions, color='red')\nax2.scatter(enhanced_data.dropna()[\"Normalised ENTRHU [-]\"], predictions, color='red')\nax3.scatter(enhanced_data.dropna()[\"z [m]\"], predictions, color='red')\nfor ax in (ax1, ax2, ax3):\n ax.grid()\n ax.set_ylim(0, 175)\n ax.set_ylabel(r\"Blowcount (Blows/m)\")\nax1.set_xlabel(r\"Shaft resistance (kN)\")\nax1.set_xlim(0, 12000)\nax2.set_xlabel(r\"Normalised ENTRHU (-)\")\nax2.set_xlim(0, 1)\nax3.set_xlabel(r\"Depth below mudline (m)\")\nax3.set_xlim(0, 50)\nplt.show()",
"_____no_output_____"
]
],
[
[
"During the prediction event, the goal is to fit a machine learning model which further refines the model developed above.",
"_____no_output_____"
],
[
"### 4.5 Model validation\n\nAt the start of the exercise, we excluded a couple of locations from the fitting to check how well the model would perform for these unseen locations.\n\nWe can now perform this validation exercise by calculating the shaft resistance and linearizing the model features. We can then make predictions with our model developed above.\n\nWe will illustrate this for location CB.",
"_____no_output_____"
]
],
[
[
"# Create a copy of the dataframe with location-specific data\nvalidation_data_CB = validation_data[validation_data[\"Location ID\"] == \"CB\"].copy()",
"_____no_output_____"
],
[
"# Calculate the shaft resistance feature and put it in the column 'Rs [kN]'\nvalidation_data_CB[\"Rs [kN]\"] = \\\n (np.pi * validation_data_CB[\"Diameter [m]\"] * \\\n validation_data_CB[\"z [m]\"].diff() * validation_data_CB[\"qc [MPa]\"]).cumsum()",
"_____no_output_____"
],
[
"# Calculate linearized ENTHRU, Rs and z\nvalidation_data_CB[\"linearized ENTHRU\"] = np.tanh(5 * validation_data_CB[\"Normalised ENTRHU [-]\"] - 0.5)\nvalidation_data_CB[\"linearized Rs\"] = np.tanh(0.001 * validation_data_CB[\"Rs [kN]\"] - 1)\nvalidation_data_CB[\"linearized z\"] = np.tanh(0.1 * validation_data_CB[\"z [m]\"] - 0.5)",
"_____no_output_____"
],
[
"# Create the matrix with n samples and 3 features\nX_validation = validation_data_CB.dropna()[linearized_features]\n# Create the vector with n observations of blowcount\ny_validation = validation_data_CB.dropna()[\"Blowcount [Blows/m]\"]",
"_____no_output_____"
]
],
[
[
"Given our fitted model, we can now calculate the $ R^2 $ score for our validation data. The score is relatively high and we can conclude that the model generalises well. If this validation score would be low, we would have to re-evaluate our feature selection.",
"_____no_output_____"
]
],
[
[
"# Calculate the R2 score for the validation data\nmodel_4.score(X_validation, y_validation)",
"_____no_output_____"
]
],
[
[
"We can calculate the predicted blowcounts for our validation data.",
"_____no_output_____"
]
],
[
[
"validation_predictions = model_4.predict(X_validation)",
"_____no_output_____"
]
],
[
[
"The predictions (red dots) can be plotted against the actual observed blowcounts. The cone resistance and normalised ENTHRU are also plotted for information.\n\nThe predictions are reasonable and follow the general trend fairly well. In the layer with lower cone resistance below (10-15m depth), there is an overprediction of blowcount. This is due to the relatively limited amount of datapoints with low cone resistance in the training data. Further model refinement could address this issue. ",
"_____no_output_____"
]
],
[
[
"fig, ((ax1, ax2, ax3)) = plt.subplots(1, 3, figsize=(15,6))\n# All data\nax1.plot(validation_data_CB[\"qc [MPa]\"], validation_data_CB[\"z [m]\"])\nax2.plot(validation_data_CB[\"Normalised ENTRHU [-]\"], validation_data_CB[\"z [m]\"])\nax3.plot(validation_data_CB[\"Blowcount [Blows/m]\"], validation_data_CB[\"z [m]\"])\n# Location-specific data\nax3.scatter(validation_predictions, validation_data_CB.dropna()[\"z [m]\"], color='red')\nfor ax in (ax1, ax2, ax3):\n ax.grid()\n ax.xaxis.tick_top()\n ax.xaxis.set_label_position('top')\n ax.set_ylim(30, 0)\n ax.set_ylabel(r\"Depth below mudline (m)\")\nax1.set_xlabel(r\"Cone tip resistance (MPa)\")\nax1.set_xlim(0, 120)\nax2.set_xlabel(r\"Normalised ENTRHU (-)\")\nax2.set_xlim(0, 1)\nax3.set_xlabel(r\"Blowcount (Blows/m)\")\nax3.set_xlim(0, 175)\nplt.show()",
"_____no_output_____"
]
],
[
[
"The process of validation can be automated. The [scikit-learn documentation](https://scikit-learn.org/stable/modules/cross_validation.html) has further details on this.",
"_____no_output_____"
],
[
"## 5. Prediction event submission\n\nWhile a number of locations are held out during the training process to check if the model generalises well, the model will have to be applied to unseen data and predictions will need to be submitted.\n\nThe validation data which will be used for the ranking of submissions is provided in the file ```validation_data.csv```.",
"_____no_output_____"
]
],
[
[
"final_data = pd.read_csv(\"/kaggle/input/validation_data.csv\")\nfinal_data.head()",
"_____no_output_____"
]
],
[
[
"We can see that the target variable (```Blowcount [Blows/m]```) is not provided and we need to predict it.\n\nSimilary to the previous process, we will calculate the shaft resistance to enhance our data.",
"_____no_output_____"
]
],
[
[
"enhanced_final_data = pd.DataFrame() # Create a dataframe for the final data enhanced with the shaft friction feature\nfor location in final_data['Location ID'].unique(): # Loop over all unique locations\n locationdata = final_data[final_data['Location ID']==location].copy() # Select the location-specific data\n # Calculate the shaft resistance feature\n locationdata[\"Rs [kN]\"] = \\\n (np.pi * locationdata[\"Diameter [m]\"] * locationdata[\"z [m]\"].diff() * locationdata[\"qc [MPa]\"]).cumsum()\n enhanced_final_data = pd.concat(\n [enhanced_final_data, locationdata]) # Combine data for the different locations in 1 dataframe",
"_____no_output_____"
]
],
[
[
"A NaN value is generated at the pile top, we can remove any NaN values using the ```dropna``` method on the DataFrame.",
"_____no_output_____"
]
],
[
[
"enhanced_final_data.dropna(inplace=True) # Drop the rows containing NaN values and overwrite the dataframe",
"_____no_output_____"
]
],
[
[
"We can then linearize the features as before:",
"_____no_output_____"
]
],
[
[
"enhanced_final_data[\"linearized ENTHRU\"] = np.tanh(5 * enhanced_final_data[\"Normalised ENTRHU [-]\"] - 0.5)\nenhanced_final_data[\"linearized Rs\"] = np.tanh(0.001 * enhanced_final_data[\"Rs [kN]\"] - 1)\nenhanced_final_data[\"linearized z\"] = np.tanh(0.1 * enhanced_final_data[\"z [m]\"] - 0.5)",
"_____no_output_____"
]
],
[
[
"We can extract the linearized features which are required for the predictions:",
"_____no_output_____"
]
],
[
[
"# Create the matrix with n samples and 3 features\nX = enhanced_final_data[linearized_features]",
"_____no_output_____"
]
],
[
[
"We can make the predictions using our final model:",
"_____no_output_____"
]
],
[
[
"final_predictions = model_4.predict(X)",
"_____no_output_____"
]
],
[
[
"We can assign these predictions to the column ```Blowcount [Blows/m]``` in our resulting dataframe.",
"_____no_output_____"
]
],
[
[
"enhanced_final_data[\"Blowcount [Blows/m]\"] = final_predictions",
"_____no_output_____"
]
],
[
[
"We can write this file to a csv file. For the submission, we only need the ```ID``` and ```Blowcount [Blows/m]``` column. ",
"_____no_output_____"
]
],
[
[
"enhanced_final_data[[\"ID\", \"Blowcount [Blows/m]\"]].to_csv(\"sample_submission_linearmodel.csv\", index=False)",
"_____no_output_____"
]
],
[
[
"## 6. Conclusions\n\nThis tutorial shows how a basic machine learning model can be built up. The workflow shows the importance of integrating engineering knowledge and to ensure that the models make physical sense.\n\nA machine learning workflow is not fundamentally different from a conventional workflow for fitting a semi-empirical model. The methods available in scikit-learn make the process scaleable to large datasets with only a few lines of code.\n\nThe workflow will always consist of the following steps:\n\n - Select features for the machine learning. Use engineering knowledge to construct features which have a better correlation with the target variable under consideration;\n - Split the dataset in a training dataset and a validation dataset;\n - Select the type of machine learning model you want to use (e.g. Linear regression, Support Vector Machines, Neural Nets, ...);\n - Train the model using the training data;\n - Validate the model on the validation data;\n\nIf the validation is successful, the model can be used for predictions on unseen data. These predictions can then be submitted as an entry in the competition.",
"_____no_output_____"
],
[
"## 7. Can you do better?\n\nThis tutorial shows the creation of a simple linear model. This is only one possible model from the [many possible regression models available in scikit-learn](https://scikit-learn.org/stable/supervised_learning.html).\n\nAfter getting familiar with the basic concepts, participants are invited to suggest improved blowcount prediction models.",
"_____no_output_____"
],
[
"## 8. Further reading\n\nThe internet has a large amount of information available on machine learning. Here are a couple of suggestions to guide your reading:\n\n - [scikit-learn documentation](https://scikit-learn.org/stable/tutorial/basic/tutorial.html): The scikit-learn package has extensive documentation and provides good general-purpose tutorials;\n - [10 minutes to Pandas](http://pandas.pydata.org/pandas-docs/stable/getting_started/10min.html): The Pandas package is used extensively in this tutorial to facilitate the data processing. Getting to grips with Pandas is a good idea for every aspiring data enthousiast. The Pandas documentation is extensive and this guide provides you with a good overview of the capabilities of the package;\n - [Towards data science](https://towardsdatascience.com/): A blog with frequent posts on data science topics with contributions for the novice and advanced data scientist. ",
"_____no_output_____"
]
]
] | [
"markdown",
"code",
"markdown",
"code",
"markdown",
"code",
"markdown",
"code",
"markdown",
"code",
"markdown",
"code",
"markdown",
"code",
"markdown",
"code",
"markdown",
"code",
"markdown",
"code",
"markdown",
"code",
"markdown",
"code",
"markdown",
"code",
"markdown",
"code",
"markdown",
"code",
"markdown",
"code",
"markdown",
"code",
"markdown",
"code",
"markdown",
"code",
"markdown",
"code",
"markdown",
"code",
"markdown",
"code",
"markdown",
"code",
"markdown",
"code",
"markdown",
"code",
"markdown",
"code",
"markdown",
"code",
"markdown",
"code",
"markdown",
"code",
"markdown",
"code",
"markdown",
"code",
"markdown",
"code",
"markdown",
"code",
"markdown",
"code",
"markdown",
"code",
"markdown",
"code",
"markdown",
"code",
"markdown",
"code",
"markdown",
"code",
"markdown",
"code",
"markdown",
"code",
"markdown",
"code",
"markdown",
"code",
"markdown",
"code",
"markdown"
] | [
[
"markdown",
"markdown"
],
[
"code",
"code"
],
[
"markdown"
],
[
"code"
],
[
"markdown",
"markdown"
],
[
"code"
],
[
"markdown"
],
[
"code"
],
[
"markdown",
"markdown"
],
[
"code"
],
[
"markdown"
],
[
"code"
],
[
"markdown",
"markdown"
],
[
"code"
],
[
"markdown",
"markdown",
"markdown",
"markdown",
"markdown"
],
[
"code"
],
[
"markdown",
"markdown",
"markdown"
],
[
"code"
],
[
"markdown"
],
[
"code"
],
[
"markdown"
],
[
"code"
],
[
"markdown"
],
[
"code"
],
[
"markdown"
],
[
"code"
],
[
"markdown",
"markdown"
],
[
"code"
],
[
"markdown"
],
[
"code"
],
[
"markdown"
],
[
"code"
],
[
"markdown"
],
[
"code"
],
[
"markdown"
],
[
"code"
],
[
"markdown"
],
[
"code"
],
[
"markdown"
],
[
"code"
],
[
"markdown"
],
[
"code"
],
[
"markdown"
],
[
"code"
],
[
"markdown"
],
[
"code",
"code"
],
[
"markdown"
],
[
"code",
"code"
],
[
"markdown"
],
[
"code"
],
[
"markdown"
],
[
"code"
],
[
"markdown"
],
[
"code"
],
[
"markdown"
],
[
"code"
],
[
"markdown"
],
[
"code"
],
[
"markdown"
],
[
"code"
],
[
"markdown"
],
[
"code"
],
[
"markdown"
],
[
"code"
],
[
"markdown",
"markdown"
],
[
"code",
"code",
"code",
"code"
],
[
"markdown"
],
[
"code"
],
[
"markdown"
],
[
"code"
],
[
"markdown"
],
[
"code"
],
[
"markdown",
"markdown"
],
[
"code"
],
[
"markdown"
],
[
"code"
],
[
"markdown"
],
[
"code"
],
[
"markdown"
],
[
"code"
],
[
"markdown"
],
[
"code"
],
[
"markdown"
],
[
"code"
],
[
"markdown"
],
[
"code"
],
[
"markdown"
],
[
"code"
],
[
"markdown",
"markdown",
"markdown"
]
] |
e76817677d9bab65e339adabcd5c20b267e79bd8 | 26,530 | ipynb | Jupyter Notebook | app/notebooks/labeled_identities/shooters/syed_rizwan_farook.ipynb | scanner-research/esper-tv | 179ef57d536ebd52f93697aab09bf5abec19ce93 | [
"Apache-2.0"
] | 5 | 2019-04-17T01:01:46.000Z | 2021-07-11T01:32:50.000Z | app/notebooks/labeled_identities/shooters/syed_rizwan_farook.ipynb | DanFu09/esper | ccc5547de3637728b8aaab059b6781baebc269ec | [
"Apache-2.0"
] | 4 | 2019-11-12T08:35:03.000Z | 2021-06-10T20:37:04.000Z | app/notebooks/labeled_identities/shooters/syed_rizwan_farook.ipynb | DanFu09/esper | ccc5547de3637728b8aaab059b6781baebc269ec | [
"Apache-2.0"
] | 1 | 2020-09-01T01:15:44.000Z | 2020-09-01T01:15:44.000Z | 33.329146 | 2,941 | 0.620241 | [
[
[
"<h1>Table of Contents<span class=\"tocSkip\"></span></h1>\n<div class=\"toc\" style=\"margin-top: 1em;\"><ul class=\"toc-item\"><li><span><a href=\"#Name\" data-toc-modified-id=\"Name-1\"><span class=\"toc-item-num\">1 </span>Name</a></span></li><li><span><a href=\"#Search\" data-toc-modified-id=\"Search-2\"><span class=\"toc-item-num\">2 </span>Search</a></span><ul class=\"toc-item\"><li><span><a href=\"#Load-Cached-Results\" data-toc-modified-id=\"Load-Cached-Results-2.1\"><span class=\"toc-item-num\">2.1 </span>Load Cached Results</a></span></li><li><span><a href=\"#Build-Model-From-Google-Images\" data-toc-modified-id=\"Build-Model-From-Google-Images-2.2\"><span class=\"toc-item-num\">2.2 </span>Build Model From Google Images</a></span></li></ul></li><li><span><a href=\"#Analysis\" data-toc-modified-id=\"Analysis-3\"><span class=\"toc-item-num\">3 </span>Analysis</a></span><ul class=\"toc-item\"><li><span><a href=\"#Gender-cross-validation\" data-toc-modified-id=\"Gender-cross-validation-3.1\"><span class=\"toc-item-num\">3.1 </span>Gender cross validation</a></span></li><li><span><a href=\"#Face-Sizes\" data-toc-modified-id=\"Face-Sizes-3.2\"><span class=\"toc-item-num\">3.2 </span>Face Sizes</a></span></li><li><span><a href=\"#Screen-Time-Across-All-Shows\" data-toc-modified-id=\"Screen-Time-Across-All-Shows-3.3\"><span class=\"toc-item-num\">3.3 </span>Screen Time Across All Shows</a></span></li><li><span><a href=\"#Appearances-on-a-Single-Show\" data-toc-modified-id=\"Appearances-on-a-Single-Show-3.4\"><span class=\"toc-item-num\">3.4 </span>Appearances on a Single Show</a></span></li><li><span><a href=\"#Other-People-Who-Are-On-Screen\" data-toc-modified-id=\"Other-People-Who-Are-On-Screen-3.5\"><span class=\"toc-item-num\">3.5 </span>Other People Who Are On Screen</a></span></li></ul></li><li><span><a href=\"#Persist-to-Cloud\" data-toc-modified-id=\"Persist-to-Cloud-4\"><span class=\"toc-item-num\">4 </span>Persist to Cloud</a></span><ul class=\"toc-item\"><li><span><a href=\"#Save-Model-to-Google-Cloud-Storage\" data-toc-modified-id=\"Save-Model-to-Google-Cloud-Storage-4.1\"><span class=\"toc-item-num\">4.1 </span>Save Model to Google Cloud Storage</a></span></li><li><span><a href=\"#Save-Labels-to-DB\" data-toc-modified-id=\"Save-Labels-to-DB-4.2\"><span class=\"toc-item-num\">4.2 </span>Save Labels to DB</a></span><ul class=\"toc-item\"><li><span><a href=\"#Commit-the-person-and-labeler\" data-toc-modified-id=\"Commit-the-person-and-labeler-4.2.1\"><span class=\"toc-item-num\">4.2.1 </span>Commit the person and labeler</a></span></li><li><span><a href=\"#Commit-the-FaceIdentity-labels\" data-toc-modified-id=\"Commit-the-FaceIdentity-labels-4.2.2\"><span class=\"toc-item-num\">4.2.2 </span>Commit the FaceIdentity labels</a></span></li></ul></li></ul></li></ul></div>",
"_____no_output_____"
]
],
[
[
"from esper.prelude import *\nfrom esper.identity import *\nfrom esper.topics import *\nfrom esper.plot_util import *\nfrom esper import embed_google_images",
"_____no_output_____"
]
],
[
[
"# Name",
"_____no_output_____"
],
[
"Please add the person's name and their expected gender below (Male/Female).",
"_____no_output_____"
]
],
[
[
"name = 'Syed Rizwan Farook'\ngender = 'Male'",
"_____no_output_____"
]
],
[
[
"# Search",
"_____no_output_____"
],
[
"## Load Cached Results",
"_____no_output_____"
],
[
"Reads cached identity model from local disk. Run this if the person has been labelled before and you only wish to regenerate the graphs. Otherwise, if you have never created a model for this person, please see the next section.",
"_____no_output_____"
]
],
[
[
"assert name != ''\nresults = FaceIdentityModel.load(name=name)\nimshow(tile_images([cv2.resize(x[1][0], (200, 200)) for x in results.model_params['images']], cols=10))\nplt.show()\nplot_precision_and_cdf(results)",
"_____no_output_____"
]
],
[
[
"## Build Model From Google Images",
"_____no_output_____"
],
[
"Run this section if you do not have a cached model and precision curve estimates. This section will grab images using Google Image Search and score each of the faces in the dataset. We will interactively build the precision vs score curve.\n\nIt is important that the images that you select are accurate. If you make a mistake, rerun the cell below.",
"_____no_output_____"
]
],
[
[
"assert name != ''\n# Grab face images from Google\nimg_dir = embed_google_images.fetch_images(name)\n\n# If the images returned are not satisfactory, rerun the above with extra params:\n# query_extras='' # additional keywords to add to search\n# force=True # ignore cached images\n\nface_imgs = load_and_select_faces_from_images(img_dir)\nface_embs = embed_google_images.embed_images(face_imgs)\nassert(len(face_embs) == len(face_imgs))\n\nreference_imgs = tile_imgs([cv2.resize(x[0], (200, 200)) for x in face_imgs if x], cols=10)\ndef show_reference_imgs():\n print('User selected reference images for {}.'.format(name))\n imshow(reference_imgs)\n plt.show()\nshow_reference_imgs()",
"_____no_output_____"
],
[
"# Score all of the faces in the dataset (this can take a minute)\nface_ids_by_bucket, face_ids_to_score = face_search_by_embeddings(face_embs)",
"_____no_output_____"
],
[
"precision_model = PrecisionModel(face_ids_by_bucket)",
"_____no_output_____"
]
],
[
[
"Now we will validate which of the images in the dataset are of the target identity.\n\n__Hover over with mouse and press S to select a face. Press F to expand the frame.__",
"_____no_output_____"
]
],
[
[
"show_reference_imgs()\nprint(('Mark all images that ARE NOT {}. Thumbnails are ordered by DESCENDING distance '\n 'to your selected images. (The first page is more likely to have non \"{}\" images.) '\n 'There are a total of {} frames. (CLICK THE DISABLE JUPYTER KEYBOARD BUTTON '\n 'BEFORE PROCEEDING.)').format(\n name, name, precision_model.get_lower_count()))\nlower_widget = precision_model.get_lower_widget()\nlower_widget",
"_____no_output_____"
],
[
"show_reference_imgs()\nprint(('Mark all images that ARE {}. Thumbnails are ordered by ASCENDING distance '\n 'to your selected images. (The first page is more likely to have \"{}\" images.) '\n 'There are a total of {} frames. (CLICK THE DISABLE JUPYTER KEYBOARD BUTTON '\n 'BEFORE PROCEEDING.)').format(\n name, name, precision_model.get_lower_count()))\nupper_widget = precision_model.get_upper_widget()\nupper_widget",
"_____no_output_____"
]
],
[
[
"Run the following cell after labelling to compute the precision curve. Do not forget to re-enable jupyter shortcuts.",
"_____no_output_____"
]
],
[
[
"# Compute the precision from the selections\nlower_precision = precision_model.compute_precision_for_lower_buckets(lower_widget.selected)\nupper_precision = precision_model.compute_precision_for_upper_buckets(upper_widget.selected)\nprecision_by_bucket = {**lower_precision, **upper_precision}\n\nresults = FaceIdentityModel(\n name=name, \n face_ids_by_bucket=face_ids_by_bucket, \n face_ids_to_score=face_ids_to_score,\n precision_by_bucket=precision_by_bucket, \n model_params={\n 'images': list(zip(face_embs, face_imgs))\n }\n)\nplot_precision_and_cdf(results)",
"_____no_output_____"
]
],
[
[
"The next cell persists the model locally.",
"_____no_output_____"
]
],
[
[
"results.save()",
"_____no_output_____"
]
],
[
[
"# Analysis",
"_____no_output_____"
],
[
"## Gender cross validation\n\nSituations where the identity model disagrees with the gender classifier may be cause for alarm. We would like to check that instances of the person have the expected gender as a sanity check. This section shows the breakdown of the identity instances and their labels from the gender classifier.",
"_____no_output_____"
]
],
[
[
"gender_breakdown = compute_gender_breakdown(results)\n\nprint('Expected counts by gender:')\nfor k, v in gender_breakdown.items():\n print(' {} : {}'.format(k, int(v)))\nprint()\n\nprint('Percentage by gender:')\ndenominator = sum(v for v in gender_breakdown.values())\nfor k, v in gender_breakdown.items():\n print(' {} : {:0.1f}%'.format(k, 100 * v / denominator))\nprint()",
"_____no_output_____"
]
],
[
[
"Situations where the identity detector returns high confidence, but where the gender is not the expected gender indicate either an error on the part of the identity detector or the gender detector. The following visualization shows randomly sampled images, where the identity detector returns high confidence, grouped by the gender label. ",
"_____no_output_____"
]
],
[
[
"high_probability_threshold = 0.8\nshow_gender_examples(results, high_probability_threshold)",
"_____no_output_____"
]
],
[
[
"## Face Sizes\n\nFaces shown on-screen vary in size. For a person such as a host, they may be shown in a full body shot or as a face in a box. Faces in the background or those part of side graphics might be smaller than the rest. When calculuating screentime for a person, we would like to know whether the results represent the time the person was featured as opposed to merely in the background or as a tiny thumbnail in some graphic.\n\nThe next cell, plots the distribution of face sizes. Some possible anomalies include there only being very small faces or large faces. ",
"_____no_output_____"
]
],
[
[
"plot_histogram_of_face_sizes(results)",
"_____no_output_____"
]
],
[
[
"The histogram above shows the distribution of face sizes, but not how those sizes occur in the dataset. For instance, one might ask why some faces are so large or whhether the small faces are actually errors. The following cell groups example faces, which are of the target identity with probability, by their sizes in terms of screen area.",
"_____no_output_____"
]
],
[
[
"high_probability_threshold = 0.8\nshow_faces_by_size(results, high_probability_threshold, n=10)",
"_____no_output_____"
]
],
[
[
"## Screen Time Across All Shows\n\nOne question that we might ask about a person is whether they received a significantly different amount of screentime on different shows. The following section visualizes the amount of screentime by show in total minutes and also in proportion of the show's total time. For a celebrity or political figure such as Donald Trump, we would expect significant screentime on many shows. For a show host such as Wolf Blitzer, we expect that the screentime be high for shows hosted by Wolf Blitzer.",
"_____no_output_____"
]
],
[
[
"screen_time_by_show = get_screen_time_by_show(results)",
"_____no_output_____"
],
[
"plot_screen_time_by_show(name, screen_time_by_show)",
"_____no_output_____"
]
],
[
[
"We might also wish to validate these findings by comparing to the whether the person's name is mentioned in the subtitles. This might be helpful in determining whether extra or lack of screentime for a person may be due to a show's aesthetic choices. The following plots show compare the screen time with the number of caption mentions.",
"_____no_output_____"
]
],
[
[
"caption_mentions_by_show = get_caption_mentions_by_show([name.upper()])\nplot_screen_time_and_other_by_show(name, screen_time_by_show, caption_mentions_by_show, \n 'Number of caption mentions', 'Count')",
"_____no_output_____"
]
],
[
[
"## Appearances on a Single Show\n\nFor people such as hosts, we would like to examine in greater detail the screen time allotted for a single show. First, fill in a show below.",
"_____no_output_____"
]
],
[
[
"show_name = 'FOX and Friends'",
"_____no_output_____"
],
[
"# Compute the screen time for each video of the show\nscreen_time_by_video_id = compute_screen_time_by_video(results, show_name)",
"_____no_output_____"
]
],
[
[
"One question we might ask about a host is \"how long they are show on screen\" for an episode. Likewise, we might also ask for how many episodes is the host not present due to being on vacation or on assignment elsewhere. The following cell plots a histogram of the distribution of the length of the person's appearances in videos of the chosen show.",
"_____no_output_____"
]
],
[
[
"plot_histogram_of_screen_times_by_video(name, show_name, screen_time_by_video_id)",
"_____no_output_____"
]
],
[
[
"For a host, we expect screentime over time to be consistent as long as the person remains a host. For figures such as Hilary Clinton, we expect the screentime to track events in the real world such as the lead-up to 2016 election and then to drop afterwards. The following cell plots a time series of the person's screentime over time. Each dot is a video of the chosen show. Red Xs are videos for which the face detector did not run.",
"_____no_output_____"
]
],
[
[
"plot_screentime_over_time(name, show_name, screen_time_by_video_id)",
"_____no_output_____"
]
],
[
[
"We hypothesized that a host is more likely to appear at the beginning of a video and then also appear throughout the video. The following plot visualizes the distibution of shot beginning times for videos of the show.",
"_____no_output_____"
]
],
[
[
"plot_distribution_of_appearance_times_by_video(results, show_name)",
"_____no_output_____"
]
],
[
[
"In the section 3.3, we see that some shows may have much larger variance in the screen time estimates than others. This may be because a host or frequent guest appears similar to the target identity. Alternatively, the images of the identity may be consistently low quality, leading to lower scores. The next cell plots a histogram of the probabilites for for faces in a show.",
"_____no_output_____"
]
],
[
[
"plot_distribution_of_identity_probabilities(results, show_name)",
"_____no_output_____"
]
],
[
[
"## Other People Who Are On Screen\n\nFor some people, we are interested in who they are often portrayed on screen with. For instance, the White House press secretary might routinely be shown with the same group of political pundits. A host of a show, might be expected to be on screen with their co-host most of the time. The next cell takes an identity model with high probability faces and displays clusters of faces that are on screen with the target person.",
"_____no_output_____"
]
],
[
[
"get_other_people_who_are_on_screen(results, k=25, precision_thresh=0.8)",
"_____no_output_____"
]
],
[
[
"# Persist to Cloud\n\nThe remaining code in this notebook uploads the built identity model to Google Cloud Storage and adds the FaceIdentity labels to the database.",
"_____no_output_____"
],
[
"## Save Model to Google Cloud Storage",
"_____no_output_____"
]
],
[
[
"gcs_model_path = results.save_to_gcs()",
"_____no_output_____"
]
],
[
[
"To ensure that the model stored to Google Cloud is valid, we load it and print the precision and cdf curve below. ",
"_____no_output_____"
]
],
[
[
"gcs_results = FaceIdentityModel.load_from_gcs(name=name)\nimshow(tile_imgs([cv2.resize(x[1][0], (200, 200)) for x in gcs_results.model_params['images']], cols=10))\nplt.show()\nplot_precision_and_cdf(gcs_results)",
"_____no_output_____"
]
],
[
[
"## Save Labels to DB\n\nIf you are satisfied with the model, we can commit the labels to the database.",
"_____no_output_____"
]
],
[
[
"from django.core.exceptions import ObjectDoesNotExist\n\ndef standardize_name(name):\n return name.lower()\n\nperson_type = ThingType.objects.get(name='person')\n\ntry:\n person = Thing.objects.get(name=standardize_name(name), type=person_type)\n print('Found person:', person.name)\nexcept ObjectDoesNotExist:\n person = Thing(name=standardize_name(name), type=person_type)\n print('Creating person:', person.name)\n\nlabeler = Labeler(name='face-identity:{}'.format(person.name), data_path=gcs_model_path)",
"_____no_output_____"
]
],
[
[
"### Commit the person and labeler\n\nThe labeler and person have been created but not set saved to the database. If a person was created, please make sure that the name is correct before saving.",
"_____no_output_____"
]
],
[
[
"person.save()\nlabeler.save()",
"_____no_output_____"
]
],
[
[
"### Commit the FaceIdentity labels\n\nNow, we are ready to add the labels to the database. We will create a FaceIdentity for each face whose probability exceeds the minimum threshold.",
"_____no_output_____"
]
],
[
[
"commit_face_identities_to_db(results, person, labeler, min_threshold=0.001)",
"_____no_output_____"
],
[
"print('Committed {} labels to the db'.format(FaceIdentity.objects.filter(labeler=labeler).count()))",
"_____no_output_____"
]
]
] | [
"markdown",
"code",
"markdown",
"code",
"markdown",
"code",
"markdown",
"code",
"markdown",
"code",
"markdown",
"code",
"markdown",
"code",
"markdown",
"code",
"markdown",
"code",
"markdown",
"code",
"markdown",
"code",
"markdown",
"code",
"markdown",
"code",
"markdown",
"code",
"markdown",
"code",
"markdown",
"code",
"markdown",
"code",
"markdown",
"code",
"markdown",
"code",
"markdown",
"code",
"markdown",
"code",
"markdown",
"code",
"markdown",
"code",
"markdown",
"code"
] | [
[
"markdown"
],
[
"code"
],
[
"markdown",
"markdown"
],
[
"code"
],
[
"markdown",
"markdown",
"markdown"
],
[
"code"
],
[
"markdown",
"markdown"
],
[
"code",
"code",
"code"
],
[
"markdown"
],
[
"code",
"code"
],
[
"markdown"
],
[
"code"
],
[
"markdown"
],
[
"code"
],
[
"markdown",
"markdown"
],
[
"code"
],
[
"markdown"
],
[
"code"
],
[
"markdown"
],
[
"code"
],
[
"markdown"
],
[
"code"
],
[
"markdown"
],
[
"code",
"code"
],
[
"markdown"
],
[
"code"
],
[
"markdown"
],
[
"code",
"code"
],
[
"markdown"
],
[
"code"
],
[
"markdown"
],
[
"code"
],
[
"markdown"
],
[
"code"
],
[
"markdown"
],
[
"code"
],
[
"markdown"
],
[
"code"
],
[
"markdown",
"markdown"
],
[
"code"
],
[
"markdown"
],
[
"code"
],
[
"markdown"
],
[
"code"
],
[
"markdown"
],
[
"code"
],
[
"markdown"
],
[
"code",
"code"
]
] |
e76818307f1038c7796533012378df651cb333e4 | 98,240 | ipynb | Jupyter Notebook | lesson_2/gradient-descent/GradientDescent.ipynb | danielbruno301/pytorch-scholarship-challenge | f411dc93663ca22d80e0a541e04030160eca1ad4 | [
"MIT"
] | null | null | null | lesson_2/gradient-descent/GradientDescent.ipynb | danielbruno301/pytorch-scholarship-challenge | f411dc93663ca22d80e0a541e04030160eca1ad4 | [
"MIT"
] | null | null | null | lesson_2/gradient-descent/GradientDescent.ipynb | danielbruno301/pytorch-scholarship-challenge | f411dc93663ca22d80e0a541e04030160eca1ad4 | [
"MIT"
] | null | null | null | 308.930818 | 64,208 | 0.922404 | [
[
[
"# Implementing the Gradient Descent Algorithm\n\nIn this lab, we'll implement the basic functions of the Gradient Descent algorithm to find the boundary in a small dataset. First, we'll start with some functions that will help us plot and visualize the data.",
"_____no_output_____"
]
],
[
[
"import matplotlib.pyplot as plt\nimport numpy as np\nimport pandas as pd\n\n#Some helper functions for plotting and drawing lines\n\ndef plot_points(X, y):\n admitted = X[np.argwhere(y==1)]\n rejected = X[np.argwhere(y==0)]\n plt.scatter([s[0][0] for s in rejected], [s[0][1] for s in rejected], s = 25, color = 'blue', edgecolor = 'k')\n plt.scatter([s[0][0] for s in admitted], [s[0][1] for s in admitted], s = 25, color = 'red', edgecolor = 'k')\n\ndef display(m, b, color='g--'):\n plt.xlim(-0.05,1.05)\n plt.ylim(-0.05,1.05)\n x = np.arange(-10, 10, 0.1)\n plt.plot(x, m*x+b, color)",
"_____no_output_____"
]
],
[
[
"## Reading and plotting the data",
"_____no_output_____"
]
],
[
[
"data = pd.read_csv('data.csv', header=None)\nX = np.array(data[[0,1]])\ny = np.array(data[2])\nplot_points(X,y)\nplt.show()",
"_____no_output_____"
]
],
[
[
"## TODO: Implementing the basic functions\nHere is your turn to shine. Implement the following formulas, as explained in the text.\n- Sigmoid activation function\n\n$$\\sigma(x) = \\frac{1}{1+e^{-x}}$$\n\n- Output (prediction) formula\n\n$$\\hat{y} = \\sigma(w_1 x_1 + w_2 x_2 + b)$$\n\n- Error function\n\n$$Error(y, \\hat{y}) = - y \\log(\\hat{y}) - (1-y) \\log(1-\\hat{y})$$\n\n- The function that updates the weights\n\n$$ w_i \\longrightarrow w_i + \\alpha (y - \\hat{y}) x_i$$\n\n$$ b \\longrightarrow b + \\alpha (y - \\hat{y})$$",
"_____no_output_____"
]
],
[
[
"# Implement the following functions\n\n# Activation (sigmoid) function\ndef sigmoid(x):\n return 1/(1 + np.exp(-x))\n\n# Output (prediction) formula\ndef output_formula(features, weights, bias):\n y = np.dot(features,weights ) + bias\n y_hat = sigmoid(y) \n return y_hat\n\n# Error (log-loss) formula\ndef error_formula(y, output):\n error = -y*np.log(output) - (1-y)*np.log(1-output) \n return error\n\n# Gradient descent step\ndef update_weights(x, y, weights, bias, learnrate):\n y_hat = output_formula(x, weights, bias)\n learned_error = learnrate * (y-y_hat)\n weights = weights + learned_error * x\n bias = bias + learned_error \n return weights, bias",
"_____no_output_____"
]
],
[
[
"## Training function\nThis function will help us iterate the gradient descent algorithm through all the data, for a number of epochs. It will also plot the data, and some of the boundary lines obtained as we run the algorithm.",
"_____no_output_____"
]
],
[
[
"np.random.seed(44)\n\nepochs = 1000\nlearnrate = 0.01\n\ndef train(features, targets, epochs, learnrate, graph_lines=False):\n \n errors = []\n n_records, n_features = features.shape\n last_loss = None\n weights = np.random.normal(scale=1 / n_features**.5, size=n_features)\n bias = 0\n for e in range(epochs):\n del_w = np.zeros(weights.shape)\n for x, y in zip(features, targets):\n output = output_formula(x, weights, bias)\n error = error_formula(y, output)\n weights, bias = update_weights(x, y, weights, bias, learnrate)\n \n # Printing out the log-loss error on the training set\n out = output_formula(features, weights, bias)\n loss = np.mean(error_formula(targets, out))\n errors.append(loss)\n if e % (epochs / 10) == 0:\n print(\"\\n========== Epoch\", e,\"==========\")\n if last_loss and last_loss < loss:\n print(\"Train loss: \", loss, \" WARNING - Loss Increasing\")\n else:\n print(\"Train loss: \", loss)\n last_loss = loss\n predictions = out > 0.5\n accuracy = np.mean(predictions == targets)\n print(\"Accuracy: \", accuracy)\n if graph_lines and e % (epochs / 100) == 0:\n display(-weights[0]/weights[1], -bias/weights[1])\n \n\n # Plotting the solution boundary\n plt.title(\"Solution boundary\")\n display(-weights[0]/weights[1], -bias/weights[1], 'black')\n\n # Plotting the data\n plot_points(features, targets)\n plt.show()\n\n # Plotting the error\n plt.title(\"Error Plot\")\n plt.xlabel('Number of epochs')\n plt.ylabel('Error')\n plt.plot(errors)\n plt.show()",
"_____no_output_____"
]
],
[
[
"## Time to train the algorithm!\nWhen we run the function, we'll obtain the following:\n- 10 updates with the current training loss and accuracy\n- A plot of the data and some of the boundary lines obtained. The final one is in black. Notice how the lines get closer and closer to the best fit, as we go through more epochs.\n- A plot of the error function. Notice how it decreases as we go through more epochs.",
"_____no_output_____"
]
],
[
[
"train(X, y, epochs, learnrate, True)",
"\n========== Epoch 0 ==========\nTrain loss: 0.7135845195381634\nAccuracy: 0.4\n\n========== Epoch 100 ==========\nTrain loss: 0.3235511002047678\nAccuracy: 0.94\n\n========== Epoch 200 ==========\nTrain loss: 0.2445014537977157\nAccuracy: 0.94\n\n========== Epoch 300 ==========\nTrain loss: 0.21128008952075578\nAccuracy: 0.93\n\n========== Epoch 400 ==========\nTrain loss: 0.19288993789458375\nAccuracy: 0.93\n\n========== Epoch 500 ==========\nTrain loss: 0.18118268826379075\nAccuracy: 0.91\n\n========== Epoch 600 ==========\nTrain loss: 0.17307306304520367\nAccuracy: 0.92\n\n========== Epoch 700 ==========\nTrain loss: 0.16712852408679463\nAccuracy: 0.92\n\n========== Epoch 800 ==========\nTrain loss: 0.16259061436092043\nAccuracy: 0.92\n\n========== Epoch 900 ==========\nTrain loss: 0.15901909628351343\nAccuracy: 0.92\n"
]
]
] | [
"markdown",
"code",
"markdown",
"code",
"markdown",
"code",
"markdown",
"code",
"markdown",
"code"
] | [
[
"markdown"
],
[
"code"
],
[
"markdown"
],
[
"code"
],
[
"markdown"
],
[
"code"
],
[
"markdown"
],
[
"code"
],
[
"markdown"
],
[
"code"
]
] |
e76820430bbf436ff3b93858f62d82c0a8e15111 | 193,490 | ipynb | Jupyter Notebook | howard/playlist_recommendation/playlist_recommandation.ipynb | hmkim312/recommendation_project | 0d7f165c73b5ab037de347ee1149c3c97c2e0c39 | [
"MIT"
] | null | null | null | howard/playlist_recommendation/playlist_recommandation.ipynb | hmkim312/recommendation_project | 0d7f165c73b5ab037de347ee1149c3c97c2e0c39 | [
"MIT"
] | null | null | null | howard/playlist_recommendation/playlist_recommandation.ipynb | hmkim312/recommendation_project | 0d7f165c73b5ab037de347ee1149c3c97c2e0c39 | [
"MIT"
] | null | null | null | 48.083996 | 57,316 | 0.566096 | [
[
[
"## import",
"_____no_output_____"
]
],
[
[
"from tqdm.notebook import tqdm\n",
"_____no_output_____"
],
[
"raw_genre_gn_all = pd.read_json('./raw_data/genre_gn_all.json', typ = 'seriese')\nraw_song_meta = pd.read_json('./raw_data/song_meta.json')\nraw_test = pd.read_json('./raw_data/test.json')\nraw_train = pd.read_json('./raw_data/train.json')\nraw_val = pd.read_json('./raw_data/val.json')",
"_____no_output_____"
]
],
[
[
"## 장르",
"_____no_output_____"
]
],
[
[
"genre_gn_all = pd.DataFrame(raw_genre_gn_all, columns = ['genre_name']).reset_index().rename(columns={\"index\" : \"genre_code\"})",
"_____no_output_____"
],
[
"genre_gn_all.head()",
"_____no_output_____"
],
[
"genre_gn_all['genre_name'].unique()",
"_____no_output_____"
]
],
[
[
"### genre_code : 대분류",
"_____no_output_____"
]
],
[
[
"genre_code = genre_gn_all[genre_gn_all['genre_code'].str[-2:] == \"00\"]\ngenre_code.head()",
"_____no_output_____"
],
[
"import requests\nfrom bs4 import BeautifulSoup",
"_____no_output_____"
]
],
[
[
"### dtl_genre_code : 소분류",
"_____no_output_____"
]
],
[
[
"dtl_genre_code = genre_gn_all[genre_gn_all['genre_code'].str[-2:] != \"00\"]\ndtl_genre_code.columns = ['dtl_genre_code','dtl_genre_name']\ndtl_genre_code.head()",
"_____no_output_____"
]
],
[
[
"### genre : 장르 전체 df",
"_____no_output_____"
]
],
[
[
"genre_code['join_code'] = genre_code['genre_code'].str[:4]\ndtl_genre_code['join_code'] = dtl_genre_code['dtl_genre_code'].str[:4]\n\ngenre = pd.merge(genre_code, dtl_genre_code, how = 'left', on = 'join_code')\ngenre = genre[['genre_code','genre_name','dtl_genre_code','dtl_genre_name']]\ngenre",
"_____no_output_____"
]
],
[
[
"## 곡\n- list안에 들어있는 값들은 유니크한 값이 아님",
"_____no_output_____"
]
],
[
[
"raw_song_meta.head()",
"_____no_output_____"
],
[
"# 장르 분류가 이상한듯\nraw_song_meta[raw_song_meta['song_name']==\"그남자 그여자\"]",
"_____no_output_____"
],
[
"raw_song_meta.info()",
"<class 'pandas.core.frame.DataFrame'>\nRangeIndex: 707989 entries, 0 to 707988\nData columns (total 9 columns):\nsong_gn_dtl_gnr_basket 707989 non-null object\nissue_date 707989 non-null int64\nalbum_name 707985 non-null object\nalbum_id 707989 non-null int64\nartist_id_basket 707989 non-null object\nsong_name 707989 non-null object\nsong_gn_gnr_basket 707989 non-null object\nartist_name_basket 707989 non-null object\nid 707989 non-null int64\ndtypes: int64(3), object(6)\nmemory usage: 48.6+ MB\n"
],
[
"# 곡 아이디(id)와 대분류 장르코드 리스트(song_gn_gnr_basket) 추출\nsong_gnr_map = raw_song_meta.loc[:, ['id', 'song_gn_gnr_basket']]\n\n# 빈 list에 None값을 넣어줌\nsong_gnr_map['song_gn_gnr_basket'] = song_gnr_map.song_gn_gnr_basket.apply(lambda x: x if len(x) >= 1 else [None])\n\n\n# unnest song_gn_gnr_basket\nsong_gnr_map_unnest = np.dstack(\n (\n np.repeat(song_gnr_map.id.values, list(map(len, song_gnr_map.song_gn_gnr_basket))), \n np.concatenate(song_gnr_map.song_gn_gnr_basket.values)\n )\n)\n\n# unnested 데이터프레임 생성 : song_gnr_map\nsong_gnr_map = pd.DataFrame(data = song_gnr_map_unnest[0], columns = song_gnr_map.columns)\nsong_gnr_map['id'] = song_gnr_map['id'].astype(str)\nsong_gnr_map.rename(columns = {'id' : 'song_id', 'song_gn_gnr_basket' : 'gnr_code'}, inplace = True)\n\n# unnest 객체 제거\ndel song_gnr_map_unnest\nsong_gnr_map",
"_____no_output_____"
],
[
"# 1. 곡 별 장르 개수 count 테이블 생성 : song_gnr_count\nsong_gnr_count = song_gnr_map.groupby('song_id').gnr_code.nunique().reset_index(name = 'mapping_gnr_cnt')\n\n# 2. 1번에서 생성한 테이블을 가지고 매핑된 장르 개수 별 곡 수 count 테이블 생성 : gnr_song_count\ngnr_song_count = song_gnr_count.groupby('mapping_gnr_cnt').song_id.nunique().reset_index(name = '매핑된 곡 수')\n\n# 3. 2번 테이블에 비율 값 추가\ngnr_song_count.loc[:,'비율(%)'] = round(gnr_song_count['매핑된 곡 수']/sum(gnr_song_count['매핑된 곡 수'])*100, 2)\ngnr_song_count = gnr_song_count.reset_index().rename(columns = {'mapping_gnr_cnt' : '장르 수'})\ngnr_song_count[['장르 수', '매핑된 곡 수', '비율(%)']]",
"_____no_output_____"
],
[
"raw_song_meta[(raw_song_meta['song_gn_gnr_basket'].apply(len) == 0)]",
"_____no_output_____"
]
],
[
[
"# train & test data",
"_____no_output_____"
]
],
[
[
"raw_song_meta['song_gn_dtl_gnr']= raw_song_meta['song_gn_dtl_gnr_basket'].apply(','.join)",
"_____no_output_____"
],
[
"raw_song_meta['artist_name']= raw_song_meta['artist_name_basket'].apply(','.join)",
"_____no_output_____"
],
[
"id_gnr_df = raw_song_meta[['id','song_gn_dtl_gnr']]\nid_gnr_df.head()",
"_____no_output_____"
],
[
"raw_train",
"_____no_output_____"
],
[
"ls2 = []\nfor i in range(len(raw_train)):\n ls2.append(len(raw_train['songs'][i]))",
"_____no_output_____"
],
[
"ls = []\nfor i in range(len(raw_train)):\n ls.append(len(raw_train['tags'][i]))",
"_____no_output_____"
],
[
"a = pd.DataFrame(ls)",
"_____no_output_____"
],
[
"a[0].describe()",
"_____no_output_____"
],
[
"b = pd.DataFrame(ls2)\nb[0].describe()",
"_____no_output_____"
],
[
"raw_train.sort_values(by=\"like_cnt\",ascending=False)[:10]",
"_____no_output_____"
],
[
"raw_test.sort_values(by='like_cnt',ascending=False)",
"_____no_output_____"
]
],
[
[
"# validation",
"_____no_output_____"
]
],
[
[
"raw_val.tail()",
"_____no_output_____"
],
[
"msno.matrix(val)",
"_____no_output_____"
],
[
"ls = []\nfor i in range(len(raw_val['plylst_title'])):\n ls.append(len(raw_val['plylst_title'][i]))",
"_____no_output_____"
],
[
"pd.DataFrame(ls).describe(percentiles=[0.805])",
"_____no_output_____"
]
],
[
[
"## songs to grn",
"_____no_output_____"
]
],
[
[
"raw_song_meta['song_gn_dtl_gnr']= raw_song_meta['song_gn_dtl_gnr_basket'].apply(','.join)",
"_____no_output_____"
],
[
"raw_song_meta['artist_name']= raw_song_meta['artist_name_basket'].apply(','.join)",
"_____no_output_____"
],
[
"id_gnr_df = raw_song_meta[['id','song_gn_dtl_gnr_basket']]\nid_gnr_df.head()",
"_____no_output_____"
],
[
"song_tag = pd.read_csv('./raw_data/song_tags.csv')",
"_____no_output_____"
],
[
"song_tag",
"_____no_output_____"
],
[
"raw_train['tags']",
"_____no_output_____"
],
[
"raw_train['songs'].apply(lambda x : [ for i in x])",
"_____no_output_____"
],
[
"song_tag",
"_____no_output_____"
],
[
"song_tag.iloc[615137]",
"_____no_output_____"
],
[
"song_tag[song_tag['tags'] == \"['월드뮤직']\"]",
"_____no_output_____"
],
[
"raw_train",
"_____no_output_____"
],
[
"# 플레이리스트 아이디(id)와 매핑된 태그(tags) 추출\nplylst_tag_map = raw_train[['id', 'tags']]\n\n# unnest tags\nplylst_tag_map_unnest = np.dstack(\n (\n np.repeat(plylst_tag_map.id.values, list(map(len, plylst_tag_map.tags))), \n np.concatenate(plylst_tag_map.tags.values)\n )\n)\n\n# unnested 데이터프레임 생성 : plylst_tag_map\nplylst_tag_map = pd.DataFrame(data = plylst_tag_map_unnest[0], columns = plylst_tag_map.columns)\nplylst_tag_map['id'] = plylst_tag_map['id'].astype(str)\n\n# unnest 객체 제거\ndel plylst_tag_map_unnest\nplylst_tag_map",
"_____no_output_____"
],
[
"plylst_tag_map.drop_duplicates('tags')",
"_____no_output_____"
],
[
"# train_uniq_song_cnt = plylst_song_map.songs.nunique() # 유니크 곡 수\ntrain_uniq_tag_cnt = plylst_tag_map.tags.nunique() # 유니크 태그 수\n\n# print('곡 수 : %s' %train_uniq_song_cnt)\nprint('태그 수 : %s' %train_uniq_tag_cnt)",
"태그 수 : 29160\n"
],
[
"ls = []\nfor i in raw_train['songs'].iloc[:5]:\n for j in i:\n ls.append(id_gnr_df[id_gnr_df['id'] == j]['song_gn_dtl_gnr_basket'])",
"_____no_output_____"
],
[
"pd.DataFrame(ls",
"_____no_output_____"
]
]
] | [
"markdown",
"code",
"markdown",
"code",
"markdown",
"code",
"markdown",
"code",
"markdown",
"code",
"markdown",
"code",
"markdown",
"code",
"markdown",
"code",
"markdown",
"code"
] | [
[
"markdown"
],
[
"code",
"code"
],
[
"markdown"
],
[
"code",
"code",
"code"
],
[
"markdown"
],
[
"code",
"code"
],
[
"markdown"
],
[
"code"
],
[
"markdown"
],
[
"code"
],
[
"markdown"
],
[
"code",
"code",
"code",
"code",
"code",
"code"
],
[
"markdown"
],
[
"code",
"code",
"code",
"code",
"code",
"code",
"code",
"code",
"code",
"code",
"code"
],
[
"markdown"
],
[
"code",
"code",
"code",
"code"
],
[
"markdown"
],
[
"code",
"code",
"code",
"code",
"code",
"code",
"code",
"code",
"code",
"code",
"code",
"code",
"code",
"code",
"code",
"code"
]
] |
e76828cc6f5c49dcbd735b2b97fc48cf27641f89 | 164,982 | ipynb | Jupyter Notebook | research_code/sentiment_analysis/Untitled.ipynb | nicolaslesimple/Investing_For_Social_Good | 8db43eecb68b4df95ff3bcab052dace7c9deee30 | [
"MIT"
] | null | null | null | research_code/sentiment_analysis/Untitled.ipynb | nicolaslesimple/Investing_For_Social_Good | 8db43eecb68b4df95ff3bcab052dace7c9deee30 | [
"MIT"
] | 24 | 2018-11-09T16:19:21.000Z | 2018-11-28T13:30:14.000Z | research_code/sentiment_analysis/Untitled.ipynb | nicolaslesimple/Investing_For_Social_Good | 8db43eecb68b4df95ff3bcab052dace7c9deee30 | [
"MIT"
] | 2 | 2018-11-04T17:34:25.000Z | 2018-12-16T23:15:57.000Z | 56.13542 | 2,590 | 0.454468 | [
[
[
"import pandas as pd\nimport numpy as np\nlist_df = []\nfor i in range (10):\n list_df.append(pd.read_json(f'../cluster_data/0{i}.json', lines=True))\nfor i in (np.arange(10, 60, 1)):\n list_df.append(pd.read_json(f'../cluster_data/{i}.json', lines=True))\n\ndf = pd.concat(list_df)\nsentences = df.text.values.astype(str)\nsentences.shape",
"/anaconda3/lib/python3.6/site-packages/ipykernel_launcher.py:9: FutureWarning: Sorting because non-concatenation axis is not aligned. A future version\nof pandas will change to not sort by default.\n\nTo accept the future behavior, pass 'sort=True'.\n\nTo retain the current behavior and silence the warning, pass sort=False\n\n if __name__ == '__main__':\n"
],
[
"df.columns",
"_____no_output_____"
],
[
"df.text[0].reset_index().text[24]",
"_____no_output_____"
],
[
"list_hashtags = []\ndict_tot = dict()\nfor i in range(df.entities.index.max()):\n if (type(df.entities[i])==dict):\n if (len(df.entities[i]['hashtags'])!=0):\n #list_hashtags.append(df.entities[i]['hashtags'][0]['text'])\n dict_tot[i,0]['hashtags'] = df.entities[i]['hashtags'][0]['text']\n dict_tot[i,0]['text'] = df.text[i]\n elif type(df.entities[i])==pd.core.series.Series: \n for j in range(len(df.entities[i])): \n print(i,j)\n if (type(df.entities[i].reset_index().entities[j])==dict):\n if len(df.entities[i].reset_index().entities[j]['hashtags'])!=0:\n #list_hashtags.append(df.entities[i].reset_index().entities[j]['hashtags'][0]['text'])\n dict_tot[i,j]['hashtags'] = df.entities[i].reset_index().entities[j]['hashtags'][0]['text']\n dict_tot[i,j]['text'] = df.text[i].reset_index()['text'][j]\nlist_hashtags",
"0 0\n0 1\n0 2\n0 3\n0 4\n0 5\n0 6\n0 7\n0 8\n0 9\n0 10\n0 11\n0 12\n0 13\n0 14\n0 15\n0 16\n0 17\n0 18\n0 19\n0 20\n0 21\n0 22\n0 23\n0 24\n"
],
[
"df.text[0]",
"_____no_output_____"
],
[
"list_hashtags = []\ndict_tot = dict()\nfor i in range(df.text.index.max()):\n dict_tmp = dict()\n if (type(df.text[i])==str):\n if (len(df.entities[i]['hashtags'])!=0):\n dict_tmp['hashtags'] = df.entities[i]['hashtags'][0]['text']\n dict_tmp['text'] = df.text[i]\n else :\n dict_tmp['hashtags'] = df.entities[i]['hashtags']\n dict_tmp['text'] = df.text[i]\n dict_tot[(i,-1)]=dict_tmp\n elif type(df.text[i])==pd.core.series.Series: \n for j in range(len(df.text[i])): \n dict_tmp = dict()\n if (type(df.text[i].reset_index().text[j])==str):\n if len(df.entities[i].reset_index().entities[j]['hashtags'])!=0:\n dict_tmp['hashtags'] = df.entities[i].reset_index().entities[j]['hashtags'][0]['text']\n dict_tmp['text'] = df.text[i].reset_index()['text'][j]\n else : \n dict_tmp['hashtags'] = df.entities[i].reset_index().entities[j]['hashtags']\n dict_tmp['text'] = df.text[i].reset_index()['text'][j]\n dict_tot[(i,j)]=dict_tmp",
"_____no_output_____"
],
[
"pickle.dump(dict_tot, open('dictionary_with_all_tweets_and_hashtags.p', 'wb'))",
"_____no_output_____"
],
[
"import pickle\ncompagny = pickle.load(open('compagny.p', 'rb'))\ninvestor = pickle.load(open('investor.p', 'rb'))",
"_____no_output_____"
],
[
"compagny = compagny[0:5]\ninvestor = investor[0:5]",
"_____no_output_____"
],
[
"investor",
"_____no_output_____"
],
[
"dict_tot[(0,10)]['text'] = 'ALTABA INC will kill the world'",
"_____no_output_____"
],
[
"dict_tot[(0,8)]['hashtags'] = 'CATERPILLAR INC DEL' # allow to test if the program is working",
"_____no_output_____"
],
[
"dict_per_compagny = dict()\n\nfor name_compagny in compagny :\n list_tweet = []\n for key in list(dict_tot.keys()) :\n if (str(dict_tot[key]['hashtags']).lower().find(name_compagny.lower()) != -1) | (dict_tot[key]['text'].lower().find(name_compagny.lower()) != -1):\n list_tweet.append(dict_tot[key]['text'])\n dict_per_compagny[name_compagny] = list_tweet\npickle.dump(dict_tot, open('dictionary_per_compagny_tweet.p', 'wb'))\n\n",
"_____no_output_____"
],
[
"dict_per_compagny",
"_____no_output_____"
],
[
"dict_per_investor = dict()\n\nfor name_investor in investor :\n list_tweet = []\n for key in list(dict_tot.keys()) :\n if (str(dict_tot[key]['hashtags']).lower().find(name_investor.lower()) != -1) | (dict_tot[key]['text'].lower().find(name_investor.lower()) != -1):\n list_tweet.append(dict_tot[key]['text'])\n dict_per_investor[name_investor] = list_tweet\npickle.dump(dict_tot, open('dictionary_per_investor_tweet.p', 'wb'))\n\n",
"_____no_output_____"
],
[
"dict_per_investor",
"_____no_output_____"
],
[
"analyzer = SentimentIntensityAnalyzer()\ndict_score = dict()\nfor key in list(dict_per_compagny.keys()):\n neg, pos, neu, compound, tmp_dict = [], [], [], [], dict()\n for sentence in dict_per_compagny[key]:\n vs = analyzer.polarity_scores(sentence)\n neg.append(vs['neg'])\n neu.append(vs['neu'])\n pos.append(vs['pos'])\n compound.append(vs['compound'])\n tmp_dict['neg'] = np.mean(neg)\n tmp_dict['pos'] = np.mean(pos)\n tmp_dict['neu'] = np.mean(neu)\n tmp_dict['compound'] = np.mean(compound)\n dict_score[key] = tmp_dict ",
"/anaconda3/lib/python3.6/site-packages/numpy/core/fromnumeric.py:2957: RuntimeWarning: Mean of empty slice.\n out=out, **kwargs)\n/anaconda3/lib/python3.6/site-packages/numpy/core/_methods.py:80: RuntimeWarning: invalid value encountered in double_scalars\n ret = ret.dtype.type(ret / rcount)\n"
],
[
"dict_score",
"_____no_output_____"
],
[
"analyzer.polarity_scores('this is so nice')['pos']",
"_____no_output_____"
],
[
"analyzer = SentimentIntensityAnalyzer()\nresults = dict()\ni=0\nfor sentence in sentences:\n vs = analyzer.polarity_scores(sentence)\n results[i] = vs\n i=i+1\n #print(\"{:-<65} {}\".format(sentence, str(vs)))\n #print(str(vs))",
"_____no_output_____"
],
[
"results",
"_____no_output_____"
],
[
"from vaderSentiment.vaderSentiment import SentimentIntensityAnalyzer\n #note: depending on how you installed (e.g., using source code download versus pip install), you may need to import like this:\n #from vaderSentiment import SentimentIntensityAnalyzer\n\n# --- examples -------\nsentences = [\"NESTLE is smart, handsome, and funny.\", # positive sentence example\n \"NESTLE is smart, handsome, and funny!\", # punctuation emphasis handled correctly (sentiment intensity adjusted)\n \"NESTLE is very smart, handsome, and funny.\", # booster words handled correctly (sentiment intensity adjusted)\n \"NESTLE is VERY SMART, handsome, and FUNNY.\", # emphasis for ALLCAPS handled\n \"NESTLE is VERY SMART, handsome, and FUNNY!!!\", # combination of signals - VADER appropriately adjusts intensity\n \"NESTLE is VERY SMART, uber handsome, and FRIGGIN FUNNY!!!\", # booster words & punctuation make this close to ceiling for score\n \"NESTLE is not smart, handsome, nor funny.\", # negation sentence example\n \"The compagny was good.\", # positive sentence\n \"At least it isn't a horrible compagny.\", # negated negative sentence with contraction\n \"The compagny was only kind of good.\", # qualified positive sentence is handled correctly (intensity adjusted)\n \"The compagny was good, but the investors are bad.\", # mixed negation sentence\n \"NESTLE is horrible compagny!\", # negative slang with capitalization emphasis\n \"Today only kinda sux! But I'll get by, lol\", # mixed sentiment example with slang and constrastive conjunction \"but\"\n \"NESTLE is good :) or :D not so bad!\", # emoticons handled\n \"Catch utf-8 emoji such as such as 💘 and 💋 and 😁\", # emojis handled\n \"Not bad at all\", # Capitalized negation\n \"My cat was swimming.\" \n ]\n\nanalyzer = SentimentIntensityAnalyzer()\nfor sentence in sentences:\n vs = analyzer.polarity_scores(sentence)\n print(\"{:-<65} {}\".format(sentence, str(vs)))",
"NESTLE is smart, handsome, and funny.---------------------------- {'neg': 0.0, 'neu': 0.254, 'pos': 0.746, 'compound': 0.8316}\nNESTLE is smart, handsome, and funny!---------------------------- {'neg': 0.0, 'neu': 0.248, 'pos': 0.752, 'compound': 0.8439}\nNESTLE is very smart, handsome, and funny.----------------------- {'neg': 0.0, 'neu': 0.299, 'pos': 0.701, 'compound': 0.8545}\nNESTLE is VERY SMART, handsome, and FUNNY.----------------------- {'neg': 0.0, 'neu': 0.246, 'pos': 0.754, 'compound': 0.9227}\nNESTLE is VERY SMART, handsome, and FUNNY!!!--------------------- {'neg': 0.0, 'neu': 0.233, 'pos': 0.767, 'compound': 0.9342}\nNESTLE is VERY SMART, uber handsome, and FRIGGIN FUNNY!!!-------- {'neg': 0.0, 'neu': 0.294, 'pos': 0.706, 'compound': 0.9469}\nNESTLE is not smart, handsome, nor funny.------------------------ {'neg': 0.646, 'neu': 0.354, 'pos': 0.0, 'compound': -0.7424}\nThe compagny was good.------------------------------------------- {'neg': 0.0, 'neu': 0.508, 'pos': 0.492, 'compound': 0.4404}\nAt least it isn't a horrible compagny.--------------------------- {'neg': 0.0, 'neu': 0.637, 'pos': 0.363, 'compound': 0.431}\nThe compagny was only kind of good.------------------------------ {'neg': 0.0, 'neu': 0.697, 'pos': 0.303, 'compound': 0.3832}\nThe compagny was good, but the investors are bad.---------------- {'neg': 0.347, 'neu': 0.511, 'pos': 0.142, 'compound': -0.5859}\nNESTLE is horrible compagny!------------------------------------- {'neg': 0.558, 'neu': 0.442, 'pos': 0.0, 'compound': -0.5848}\nToday only kinda sux! But I'll get by, lol----------------------- {'neg': 0.127, 'neu': 0.556, 'pos': 0.317, 'compound': 0.5249}\nNESTLE is good :) or :D not so bad!------------------------------ {'neg': 0.0, 'neu': 0.273, 'pos': 0.727, 'compound': 0.923}\nCatch utf-8 emoji such as such as 💘 and 💋 and 😁------------------ {'neg': 0.0, 'neu': 0.746, 'pos': 0.254, 'compound': 0.7003}\nNot bad at all--------------------------------------------------- {'neg': 0.0, 'neu': 0.513, 'pos': 0.487, 'compound': 0.431}\nMy cat was swimming.--------------------------------------------- {'neg': 0.0, 'neu': 1.0, 'pos': 0.0, 'compound': 0.0}\n"
],
[
"import fastText",
"_____no_output_____"
],
[
"import os\n\n\nMODEL_DIR_PATH = \"../../Downloads/\"\n\nmodel = fastText.load_model(os.path.join(MODEL_DIR_PATH, \"amazon_review_full.bin\"))",
"_____no_output_____"
],
[
"\nmodel.predict(\"This compagny is amazing.\")",
"_____no_output_____"
],
[
"import re\nimport string\nmaketrans = str.maketrans\n\n\ndef clean_text(text):\n \"\"\"\n Applies some pre-processing to clean text data.\n \n In particular:\n - lowers the string\n - removes the character [']\n - replaces punctuation characters with spaces\n\n \"\"\"\n \n text = text.lower()\n\n text = re.sub(r\"\\'\", \"\", text) # remove the character [']\n\n # removing the punctuation\n filters='!\"#$%&()*+,-./:;<=>?@[\\\\]^_`{|}~\\t\\n'\n split = \" \"\n\n if isinstance(text, str):\n translate_map = dict((ord(c), str(split)) for c in filters)\n text = text.translate(translate_map)\n elif len(split) == 1:\n translate_map = maketrans(filters, split * len(filters))\n text = text.translate(translate_map)\n else:\n for c in filters:\n text = text.replace(c, split)\n return text",
"_____no_output_____"
],
[
"predict_sentiment = lambda s: model.predict(clean_text(s))",
"_____no_output_____"
],
[
"dict_score = dict()\nfor key in list(dict_per_compagny.keys()):\n label, confidence, tmp_dict = [], [], dict()\n for sentence in dict_per_compagny[key]:\n res = predict_sentiment(sentence)\n print(res[0][0][9])\n label.append(int(res[0][0][9]))\n confidence.append(res[1][0])\n tmp_dict['label'] = np.mean(label)\n tmp_dict['confidence'] = np.mean(confidence)\n dict_score[key] = tmp_dict \npickle.dump(dict_tot, open('dictionary_per_investor_tweet.p', 'wb'))",
"3\n1\n3\n3\n3\n"
],
[
"dict_score",
"_____no_output_____"
],
[
"predict_sentiment(\"This compagny is amazing.\")",
"_____no_output_____"
],
[
"predict_sentiment(\"This compagny is okay.\")",
"_____no_output_____"
],
[
"predict_sentiment(\"This compagny is horrible.\")",
"_____no_output_____"
],
[
"predict_sentiment(\"My cat was swimming.\")",
"_____no_output_____"
],
[
"predict_sentiment(\"The compagny killed people \")",
"_____no_output_____"
],
[
"results = dict()\ni=0\nfor sentence in sentences:\n results[i] = predict_sentiment(sentence)\n i=i+1",
"_____no_output_____"
],
[
"results",
"_____no_output_____"
]
]
] | [
"code"
] | [
[
"code",
"code",
"code",
"code",
"code",
"code",
"code",
"code",
"code",
"code",
"code",
"code",
"code",
"code",
"code",
"code",
"code",
"code",
"code",
"code",
"code",
"code",
"code",
"code",
"code",
"code",
"code",
"code",
"code",
"code",
"code",
"code",
"code",
"code",
"code",
"code"
]
] |
e76836fad1e3244190e6f9c35c1fd08424fbd0ad | 120,037 | ipynb | Jupyter Notebook | ComplexModel.ipynb | sawyerap/PythonDataViz | 6dad7ee89e24665d0de8e649c77e6fbd6bc8ffdc | [
"MIT"
] | null | null | null | ComplexModel.ipynb | sawyerap/PythonDataViz | 6dad7ee89e24665d0de8e649c77e6fbd6bc8ffdc | [
"MIT"
] | null | null | null | ComplexModel.ipynb | sawyerap/PythonDataViz | 6dad7ee89e24665d0de8e649c77e6fbd6bc8ffdc | [
"MIT"
] | null | null | null | 383.504792 | 39,324 | 0.943601 | [
[
[
"# Developing a Complex Model for Regression Testing\n\nThe purpose of this notebook is to establish a complex model that we can generate training and test data to practice our regression skills. We want some number of inputs, which can range from 0 to 10. Some are more important than others. Some will have dependence. Let's start with importing the necessary modules.",
"_____no_output_____"
]
],
[
[
"%matplotlib inline\nimport numpy as np\nimport scipy.stats as st\nimport matplotlib.pyplot as plt",
"_____no_output_____"
]
],
[
[
"Our first parameter will be alpha. It varies 0->10. It will be the first order parameter for the model. A weibull is added for some 'spice'",
"_____no_output_____"
]
],
[
[
"xa = np.linspace(0,10,100)\nw1 = st.weibull_min(1.79, loc=6.0, scale=2.0)\ndef alpha(x):\n return(0.1 * x - 0.5 * w1.cdf(x))",
"_____no_output_____"
],
[
"f = plt.plot(xa,alpha(xa))",
"_____no_output_____"
]
],
[
[
"Now we'll introduce beta, another parameter.",
"_____no_output_____"
]
],
[
[
"xb = np.linspace(0,10,100)\nn1 = st.norm(loc=5.0, scale=2.0)\ndef beta(y):\n return(1.5 * n1.pdf(y))\nf = plt.plot(xb,beta(xb))",
"_____no_output_____"
],
[
"xx, yy = np.meshgrid(xa,xb)",
"_____no_output_____"
],
[
"z = alpha(xx) + beta(yy)",
"_____no_output_____"
],
[
"fig, ax = plt.subplots()\nCS = ax.contour(xa, xb, z)\nl = ax.clabel(CS, inline=1, fontsize=10)",
"_____no_output_____"
],
[
"plt.plot(xa,alpha(xa)+beta(9))\nplt.plot(xa,alpha(xa)+beta(5))",
"_____no_output_____"
]
],
[
[
"Now to add a third variable, gamma.",
"_____no_output_____"
]
],
[
[
"xg = np.linspace(0,10,100)\ndef gamma(z):\n return((np.exp(0.036*z) - 1.0) * np.cos(2*z/np.pi))",
"_____no_output_____"
],
[
"plt.plot(xg, gamma(xg))",
"_____no_output_____"
]
],
[
[
"# The Response\n\nNow we have our function.",
"_____no_output_____"
]
],
[
[
"def response(a,b,g):\n out = alpha(a) + beta(b) + gamma(g)\n return(out)",
"_____no_output_____"
],
[
"plt.plot(xa,response(xa,8,0))\nplt.plot(xa,response(xa,8,5))",
"_____no_output_____"
]
]
] | [
"markdown",
"code",
"markdown",
"code",
"markdown",
"code",
"markdown",
"code",
"markdown",
"code"
] | [
[
"markdown"
],
[
"code"
],
[
"markdown"
],
[
"code",
"code"
],
[
"markdown"
],
[
"code",
"code",
"code",
"code",
"code"
],
[
"markdown"
],
[
"code",
"code"
],
[
"markdown"
],
[
"code",
"code"
]
] |
e76846af0023ce27878503f60114fa3193bde917 | 2,470 | ipynb | Jupyter Notebook | test-blogs.ipynb | SlothWorks-Hackathon/stylometrics | 3ff03826edbfdeb0f9f34eaefceb5119ebc3ee44 | [
"MIT"
] | 1 | 2018-01-25T09:37:30.000Z | 2018-01-25T09:37:30.000Z | test-blogs.ipynb | SlothWorks-Hackathon/stylometrics | 3ff03826edbfdeb0f9f34eaefceb5119ebc3ee44 | [
"MIT"
] | null | null | null | test-blogs.ipynb | SlothWorks-Hackathon/stylometrics | 3ff03826edbfdeb0f9f34eaefceb5119ebc3ee44 | [
"MIT"
] | null | null | null | 29.058824 | 82 | 0.527126 | [
[
[
"from vectorization import Vectorizer\nfrom glob import glob\nfrom sklearn.preprocessing import StandardScaler\nfrom scipy.spatial.distance import pdist, squareform\nfrom scipy.cluster.hierarchy import linkage, dendrogram\n\n%matplotlib inline\n\ndiv = 9\nimport random\nrandom.seed()\nrnd = random.randint(0, div - 1)",
"_____no_output_____"
],
[
"def preprocess(text, max_len=30000):\n return ''.join([c for c in text.lower()\n if c.isalpha() or c.isspace()])[:max_len]\n\nwith open('data/stopwords.txt', 'r', encoding='utf-8') as stopwords_file:\n stopwords_list = preprocess(stopwords_file.read()).split()\n\nfilenames, texts = [], []\nfor i, filename in enumerate(glob('data/blogs/**/*.txt')):\n with open(filename, 'r', encoding='utf-8') as f:\n text = preprocess(f.read())\n \n if i % div == rnd:\n filenames.append(filename)\n texts.append(text)\n \nwrappedVectorizer = Vectorizer(mfi=30,\n vector_space='tf',\n ngram_type='words',\n ngram_size=2,\n min_df=0.1)\nscaler = StandardScaler(with_mean=False)\nX = scaler.fit_transform(wrappedVectorizer.vectorize(texts)).toarray()\nprint(wrappedVectorizer.feature_names)\n\npd = pdist(X, 'euclidean')\n# dm = squareform(pd)\nlinkage_object = linkage(pd, method='ward')\nd = dendrogram(Z=linkage_object, labels=filenames, orientation='left')",
"_____no_output_____"
]
]
] | [
"code"
] | [
[
"code",
"code"
]
] |
e76847483f32c705663f27b56b17b7cac1c89781 | 248,549 | ipynb | Jupyter Notebook | lessons/python/ep1b-plotting-intro.ipynb | emichan14/2019-12-03-intro-to-python-workshop | a109897ef89bbc69a99dd3d365a080ec449bc7e1 | [
"MIT"
] | null | null | null | lessons/python/ep1b-plotting-intro.ipynb | emichan14/2019-12-03-intro-to-python-workshop | a109897ef89bbc69a99dd3d365a080ec449bc7e1 | [
"MIT"
] | null | null | null | lessons/python/ep1b-plotting-intro.ipynb | emichan14/2019-12-03-intro-to-python-workshop | a109897ef89bbc69a99dd3d365a080ec449bc7e1 | [
"MIT"
] | null | null | null | 170.589568 | 46,008 | 0.902486 | [
[
[
"# Programming with Python\n\n## Episode 1b - Introduction to Plotting\n\nTeaching: 60 min, \nExercises: 30 min \n",
"_____no_output_____"
],
[
"Objectives\n\n- Perform operations on arrays of data.\n\n- Plot simple graphs from data.",
"_____no_output_____"
],
[
"### Array operations\nOften, we want to do more than add, subtract, multiply, and divide array elements. NumPy knows how to do more complex operations, too. If we want to find the average inflammation for all patients on all days, for example, we can ask NumPy to compute data's mean value:\n\n```\nprint(numpy.mean(data))\n```",
"_____no_output_____"
]
],
[
[
"import numpy\ndata = numpy.loadtxt(fname='data/inflammation-01.csv', delimiter=',')\nprint(numpy.mean(data))\nprint(data)",
"6.14875\n[[0. 0. 1. ... 3. 0. 0.]\n [0. 1. 2. ... 1. 0. 1.]\n [0. 1. 1. ... 2. 1. 1.]\n ...\n [0. 1. 1. ... 1. 1. 1.]\n [0. 0. 0. ... 0. 2. 0.]\n [0. 0. 1. ... 1. 1. 0.]]\n"
]
],
[
[
"`mean()` is a function that takes an array as an argument.\n\nHowever, not all functions have input.\n\nGenerally, a function uses inputs to produce outputs. However, some functions produce outputs without needing any input. For example, checking the current time doesn't require any input.\n\n```\nimport time\nprint(time.ctime())\n```",
"_____no_output_____"
]
],
[
[
"import time\nprint(time.ctime())",
"Tue Dec 3 02:26:33 2019\n"
]
],
[
[
"For functions that don't take in any arguments, we still need parentheses `()` to tell Python to go and do something for us.\n\nNumPy has lots of useful functions that take an array as input. Let's use three of those functions to get some descriptive values about the dataset. We'll also use *multiple assignment*, a convenient Python feature that will enable us to do this all in one line.\n\n```\nmaxval, minval, stdval = numpy.max(data), numpy.min(data), numpy.std(data)\n```",
"_____no_output_____"
]
],
[
[
"maxval, minval, stdval = numpy.max(data), numpy.min(data), numpy.std(data)",
"_____no_output_____"
]
],
[
[
"Here we've assigned the return value from `numpy.max(data)` to the variable `maxval`, the return value from `numpy.min(data)` to `minval`, and so on. \n\nLet's have a look at the results:\n\n```\nprint('maximum inflammation:', maxval)\nprint('minimum inflammation:', minval)\nprint('standard deviation:', stdval)\n```",
"_____no_output_____"
]
],
[
[
"print('maximum inflammation:', maxval)\nprint('minimum inflammation:', minval)\nprint('standard deviation:', stdval)",
"maximum inflammation: 20.0\nminimum inflammation: 0.0\nstandard deviation: 4.613833197118566\n"
]
],
[
[
"#### Mystery Functions in IPython\n\nHow did we know what functions NumPy has and how to use them? \n\nIf you are working in IPython or in a Jupyter Notebook (which we are), there is an easy way to find out. If you type the name of something followed by a dot `.`, then you can use `Tab` completion (e.g. type `numpy.` and then press `tab`) to see a list of all functions and attributes that you can use. \n# tabを押して数秒待つ",
"_____no_output_____"
]
],
[
[
"numpy.",
"_____no_output_____"
]
],
[
[
"After selecting one, you can also add a question mark `?` (e.g. `numpy.cumprod?`), and IPython will return an explanation of the method! \n\nThis is the same as running `help(numpy.cumprod)`.",
"_____no_output_____"
]
],
[
[
"#help(numpy.cumprod)",
"_____no_output_____"
]
],
[
[
"When analysing data, though, we often want to look at variations in statistical values, such as the maximum inflammation per patient or the average inflammation per day. One way to do this is to create a new temporary array of the data we want, then ask it to do the calculation:\n\n```\npatient_0 = data[0, :] # Comment: 0 on the first axis (rows), everything on the second (columns)\nprint('maximum inflammation for patient 0:', numpy.max(patient_0))\n```",
"_____no_output_____"
]
],
[
[
"patient_0 = data[0, :]\nprint('maximum inflammation for patient 0:', numpy.max(patient_0))",
"maximum inflammation for patient 0: 18.0\n"
]
],
[
[
"Everything in a line of code following the `#` symbol is a comment that is ignored by Python. Comments allow programmers to leave explanatory notes for other programmers or their future selves.",
"_____no_output_____"
],
[
"We don't actually need to store the row in a variable of its own. Instead, we can combine the selection and the function call:\n\n```\nprint('maximum inflammation for patient 2:', numpy.max(data[2, :]))\n```",
"_____no_output_____"
]
],
[
[
"print('maximum inflammation for patient 2:', numpy.max(data[2, :]))",
"maximum inflammation for patient 2: 19.0\n"
]
],
[
[
"Operations Across Axes\n\nWhat if we need the maximum inflammation for each patient over all days or the average for each day ? In other words want to perform the operation across a different axis.\n\nTo support this functionality, most array functions allow us to specify the axis we want to work on. If we ask for the average across axis 0 (rows in our 2D example), we get:\n\n```\nprint(numpy.mean(data, axis=0))\n```",
"_____no_output_____"
]
],
[
[
"print(numpy.mean(data, axis=0))",
"[ 0. 0.45 1.11666667 1.75 2.43333333 3.15\n 3.8 3.88333333 5.23333333 5.51666667 5.95 5.9\n 8.35 7.73333333 8.36666667 9.5 9.58333333 10.63333333\n 11.56666667 12.35 13.25 11.96666667 11.03333333 10.16666667\n 10. 8.66666667 9.15 7.25 7.33333333 6.58333333\n 6.06666667 5.95 5.11666667 3.6 3.3 3.56666667\n 2.48333333 1.5 1.13333333 0.56666667]\n"
],
[
"print(numpy.mean(data, axis=0).shape)",
"(40,)\n"
]
],
[
[
"As a quick check, we can ask this array what its shape is:\n\n```\nprint(numpy.mean(data, axis=0).shape)\n```",
"_____no_output_____"
],
[
"The results (40,) tells us we have an N×1 vector, so this is the average inflammation per day for all 40 patients. If we average across axis 1 (columns in our example), we use:\n\n```\nprint(numpy.mean(data, axis=1))\n```",
"_____no_output_____"
]
],
[
[
"print(numpy.mean(data, axis=1).shape)\n# each patient - mean",
"(60,)\n"
]
],
[
[
"which is the average inflammation per patient across all days.\n\nAnd if you are now confused, here's a simpler example:\n\n```\ntiny = [[1, 2, 3, 4],\n [10, 20, 30, 40],\n [100, 200, 300, 400]]\n \nprint(tiny)\nprint('Sum the entire matrix: ', numpy.sum(tiny))\n```",
"_____no_output_____"
]
],
[
[
"tiny = [[1, 2, 3, 4],\n [10, 20, 30, 40],\n [100, 200, 300, 400]]\n\nprint(tiny)\nprint('Sum the entire matrix: ', numpy.sum(tiny))",
"[[1, 2, 3, 4], [10, 20, 30, 40], [100, 200, 300, 400]]\nSum the entire matrix: 1110\n"
]
],
[
[
"Now let's add the rows (first axis, i.e. zeroth)\n\n```\nprint('Sum the columns (i.e. add the rows): ', numpy.sum(tiny, axis=0))\n```",
"_____no_output_____"
]
],
[
[
"print('Sum the columns (i.e. add the rows): ', numpy.sum(tiny, axis=0))\n# axis=0 means 'sum of the columns'\n# 1+10+100, 2+20+200, 3+30+300, 4+40+400",
"Sum the columns (i.e. add the rows): [111 222 333 444]\n"
]
],
[
[
"and now on the other dimension (axis=1, i.e. the second dimension)\n\n```\nprint('Sum the rows (i.e. add the columns): ', numpy.sum(tiny, axis=1))\n```",
"_____no_output_____"
]
],
[
[
"print('Sum the rows (i.e. add the columns): ', numpy.sum(tiny, axis=1))\n# 1+2+3+4, 10+20+30+40, 100+200+300+400",
"Sum the rows (i.e. add the columns): [ 10 100 1000]\n"
]
],
[
[
"Here's a diagram to demonstrate how array axes work in NumPy:\n\n\n\n- `numpy.sum(data)` --> Sum all elements in data\n- `numpy.sum(data, axis=0)` --> Sum vertically (down, axis=0)\n- `numpy.sum(data, axis=1)` --> Sum horizontally (across, axis=1)\n",
"_____no_output_____"
],
[
"### Visualising data\n\nThe mathematician Richard Hamming once said, “The purpose of computing is insight, not numbers,” and the best way to develop insight is often to visualise data.\n\nVisualisation deserves an entire workshop of its own, but we can explore a few features of Python's `matplotlib` library here. While there is no official plotting library, `matplotlib` is the de facto standard. First, we will import the `pyplot` module from `matplotlib` and use two of its functions to create and display a heat map of our data:\n\n```\nimport matplotlib.pyplot\nplot = matplotlib.pyplot.imshow(data)\n```",
"_____no_output_____"
]
],
[
[
"import matplotlib.pyplot",
"_____no_output_____"
],
[
"plot = matplotlib.pyplot.imshow(data)\n# heat map",
"_____no_output_____"
]
],
[
[
"#### Heatmap of the Data\n\nBlue pixels in this heat map represent low values, while yellow pixels represent high values. As we can see, inflammation rises and falls over a 40-day period.\n\n#### Some IPython Magic\n\nIf you're using a Jupyter notebook, you'll need to execute the following command in order for your matplotlib images to appear in the notebook when show() is called:\n\n```\n%matplotlib inline\n```",
"_____no_output_____"
]
],
[
[
"%matplotlib inline\n# magic function only in the notebook",
"_____no_output_____"
]
],
[
[
"The `%` indicates an IPython magic function - a function that is only valid within the notebook environment. Note that you only have to execute this function once per notebook.",
"_____no_output_____"
],
[
"Let's take a look at the average inflammation over time:\n\n```\nave_inflammation = numpy.mean(data, axis=0)\nave_plot = matplotlib.pyplot.plot(ave_inflammation)\n```",
"_____no_output_____"
]
],
[
[
"ave_inflammation = numpy.mean(data, axis=0)\nave_plot = matplotlib.pyplot.plot(ave_inflammation)",
"_____no_output_____"
]
],
[
[
"Here, we have put the average per day across all patients in the variable `ave_inflammation`, then asked `matplotlib.pyplot` to create and display a line graph of those values. The result is a roughly linear rise and fall, which is suspicious: we might instead expect a sharper rise and slower fall. \n\nLet's have a look at two other statistics, the maximum inflammation of all the patients each day:\n```\nmax_plot = matplotlib.pyplot.plot(numpy.max(data, axis=0))\n```",
"_____no_output_____"
]
],
[
[
"max_plot = matplotlib.pyplot.plot(numpy.max(data, axis=0))",
"_____no_output_____"
]
],
[
[
"... and the minimum inflammation across all patient each day ...\n```\nmin_plot = matplotlib.pyplot.plot(numpy.min(data, axis=0))\nmatplotlib.pyplot.show()\n```",
"_____no_output_____"
]
],
[
[
"min_plot = matplotlib.pyplot.plot(numpy.min(data, axis=0))\nmatplotlib.pyplot.show()",
"_____no_output_____"
]
],
[
[
"The maximum value rises and falls smoothly, while the minimum seems to be a step function. Neither trend seems particularly likely, so either there's a mistake in our calculations or something is wrong with our data. This insight would have been difficult to reach by examining the numbers themselves without visualisation tools.",
"_____no_output_____"
]
],
[
[
"min_plot = matplotlib.pyplot.plot(numpy.min(data, axis=0))",
"_____no_output_____"
]
],
[
[
"### Grouping plots\n\nYou can group similar plots in a single figure using subplots. This script below uses a number of new commands. The function `matplotlib.pyplot.figure()` creates a space into which we will place all of our plots. The parameter `figsize` tells Python how big to make this space. \n\nEach subplot is placed into the figure using its `add_subplot` method. The `add_subplot` method takes 3 parameters. The first denotes how many total rows of subplots there are, the second parameter refers to the total number of subplot columns, and the final parameter denotes which subplot your variable is referencing (left-to-right, top-to-bottom). Each subplot is stored in a different variable (`axes1`, `axes2`, `axes3`). \n\nOnce a subplot is created, the axes can be labelled using the `set_xlabel()` command (or `set_ylabel()`). Here are our three plots side by side:\n",
"_____no_output_____"
]
],
[
[
"import numpy\nimport matplotlib.pyplot\n\ndata = numpy.loadtxt(fname='data/inflammation-01.csv', delimiter=',')\n\nfig = matplotlib.pyplot.figure(figsize=(15.0, 5.0))\n\naxes1 = fig.add_subplot(1, 3, 1) #(1,3,1 1つめのグラフ)\naxes2 = fig.add_subplot(1, 3, 2)\naxes3 = fig.add_subplot(1, 3, 3)\n\n#label の設定\naxes1.set_ylabel('average')\nplot = axes1.plot(numpy.mean(data, axis=0))\n\naxes2.set_ylabel('max')\nplot = axes2.plot(numpy.max(data, axis=0))\n\naxes3.set_ylabel('min')\naxes3.plot(numpy.min(data, axis=0))\n\n#fig.tight_layout() #makes it not too spread, but tighter\n",
"_____no_output_____"
]
],
[
[
"##### The Previous Plots as Subplots\n\nThe call to `loadtxt` reads our data, and the rest of the program tells the plotting library how large we want the figure to be, that we're creating three subplots, what to draw for each one, and that we want a tight layout. (If we leave out that call to `fig.tight_layout()`, the graphs will actually be squeezed together more closely.)",
"_____no_output_____"
],
[
"Exercise: See if you can add the label `Days` to the X-Axis of each subplot",
"_____no_output_____"
]
],
[
[
"import numpy\nimport matplotlib.pyplot\n\ndata = numpy.loadtxt(fname='data/inflammation-01.csv', delimiter=',')\n\nfig = matplotlib.pyplot.figure(figsize=(5.0, 5.0))\n\naxes1 = fig.add_subplot(1, 1, 1) #(1,3,1 1つめのグラフ)\n\n\n#label の設定 + #Days の設定\naxes1.set_ylabel('average')\naxes1.set_xlabel('days')\n\n\nplot = axes1.plot(numpy.mean(data, axis=0), label='mean')\nplot = axes1.plot(numpy.max(data, axis=0), label='max')\nplot = axes1.plot(numpy.min(data, axis=0), label='min')\naxes1.legend()\n\n#fig.tight_layout() #makes it not too spread, but tighter",
"_____no_output_____"
]
],
[
[
"##### Scientists Dislike Typing. \nWe will always use the syntax `import numpy` to import NumPy. However, in order to save typing, it is often suggested to make a shortcut like so: `import numpy as np`. If you ever see Python code online using a NumPy function with np (for example, `np.loadtxt(...))`, it's because they've used this shortcut. When working with other people, it is important to agree on a convention of how common libraries are imported.\n\nIn other words:\n\n```\nimport numpy\nnumpy.random.rand()\n```\n\nis the same as:\n\n```\nimport numpy as np\nnp.random.rand()\n```\n",
"_____no_output_____"
]
],
[
[
"import numpy as np\nnp.random.rand()\n#",
"_____no_output_____"
]
],
[
[
"## Exercises",
"_____no_output_____"
],
[
"### Plot Scaling\nWhy do all of our plots stop just short of the upper end of our graph?",
"_____no_output_____"
],
[
"Solution:",
"_____no_output_____"
],
[
"If we want to change this, we can use the `set_ylim(min, max)` method of each ‘axes’, for example:\n```\naxes3.set_ylim(0,6)\n```\nUpdate your plotting code to automatically set a more appropriate scale. (Hint: you can make use of the max and min methods to help.)",
"_____no_output_____"
]
],
[
[
"#see above",
"_____no_output_____"
]
],
[
[
"### Drawing Straight Lines\nIn the centre and right subplots above, we expect all lines to look like step functions because non-integer value are not realistic for the minimum and maximum values. However, you can see that the lines are not always vertical or horizontal, and in particular the step function in the subplot on the right looks slanted. Why is this?\n\nTry adding a `drawstyle` parameter to your plotting:\n```\naxes2.set_ylabel('average')\naxes2.plot(numpy.mean(data, axis=0), drawstyle='steps-mid')\n```",
"_____no_output_____"
]
],
[
[
"import numpy\nimport matplotlib.pyplot\n\ndata = numpy.loadtxt(fname='data/inflammation-01.csv', delimiter=',')\n\nfig = matplotlib.pyplot.figure(figsize=(15.0, 5.0))\n\naxes1 = fig.add_subplot(1, 3, 1) #(1,3,1 1つめのグラフ)\naxes2 = fig.add_subplot(1, 3, 2)\naxes3 = fig.add_subplot(1, 3, 3)\n\n#label の設定\naxes1.set_ylabel('average')\naxes1.set_xlabel('days')\nplot = axes1.plot(numpy.mean(data, axis=0))\n\naxes2.set_ylabel('max')\naxes2.set_xlabel('days')\nplot = axes2.plot(numpy.max(data, axis=0))\n\naxes3.set_ylabel('min')\naxes3.set_xlabel('days')\naxes3.plot(numpy.min(data, axis=0))\n\n#fig.tight_layout() #makes it not too spread, but tighter\n",
"_____no_output_____"
]
],
[
[
"Solution:",
"_____no_output_____"
],
[
"### Make Your Own Plot\nCreate a plot showing the standard deviation (using `numpy.std`) of the inflammation data for each day across all patients.",
"_____no_output_____"
]
],
[
[
"#standard deviation\n#help(numpy.std)\n# Example\n#a = np.array([[1, 2], [3, 4]])\n#>>> np.std(a)\n # 1.1180339887498949 # may vary\n # >>> np.std(a, axis=0)\n # array([1., 1.])\n #>>> np.std(a, axis=1)\n #array([0.5, 0.5])",
"_____no_output_____"
],
[
"numpy.std(data)",
"_____no_output_____"
],
[
"numpy.std(data, axis=0)",
"_____no_output_____"
],
[
"numpy.std(data, axis=1)",
"_____no_output_____"
]
],
[
[
"### Moving Plots Around\nModify the program to display the three plots vertically rather than side by side.",
"_____no_output_____"
]
],
[
[
"import numpy\nimport matplotlib.pyplot\n\ndata = numpy.loadtxt(fname='data/inflammation-01.csv', delimiter=',')\n\nfig = matplotlib.pyplot.figure(figsize=(15.0, 15.0))\n\naxes1 = fig.add_subplot(3, 3, 1) #(total no. of row,total no. of column, order starting from top left 1つめのグラフ)\naxes2 = fig.add_subplot(3, 3, 5)\naxes3 = fig.add_subplot(3, 3, 9)\n\n#label の設定\naxes1.set_ylabel('average')\naxes1.set_xlabel('days')\nplot = axes1.plot(numpy.mean(data, axis=0))\n\naxes2.set_ylabel('max')\naxes2.set_xlabel('days')\nplot = axes2.plot(numpy.max(data, axis=0))\n\naxes3.set_ylabel('min')\naxes3.set_xlabel('days')\naxes3.plot(numpy.min(data, axis=0))\n\n#fig.tight_layout() #makes it not too spread, but tighter",
"_____no_output_____"
]
],
[
[
"### Stacking Arrays\nArrays can be concatenated and stacked on top of one another, using NumPy’s `vstack` and `hstack` functions for vertical and horizontal stacking, respectively.\n\nRun the following code to view `A`, `B` and `C`\n",
"_____no_output_____"
]
],
[
[
"import numpy\n\nA = numpy.array([[1,2,3], [4,5,6], [7, 8, 9]])\nprint('A = ')\nprint(A)\n\nB = numpy.hstack([A, A])\nprint('B = ')\nprint(B)\n\nC = numpy.vstack([A, A])\nprint('C = ')\nprint(C)",
"A = \n[[1 2 3]\n [4 5 6]\n [7 8 9]]\nB = \n[[1 2 3 1 2 3]\n [4 5 6 4 5 6]\n [7 8 9 7 8 9]]\nC = \n[[1 2 3]\n [4 5 6]\n [7 8 9]\n [1 2 3]\n [4 5 6]\n [7 8 9]]\n"
]
],
[
[
"Write some additional code that slices the first and last columns of `A`,\nand stacks them into a 3x2 array. Make sure to print the results to verify your solution.",
"_____no_output_____"
]
],
[
[
"print(A[:,0]) # all rows from first column\n\n#print(result)",
"[1 4 7]\n"
]
],
[
[
"### Change In Inflammation\nThis patient data is longitudinal in the sense that each row represents a series of observations relating to one individual. This means that the change in inflammation over time is a meaningful concept.\n\nThe `numpy.diff()` function takes a NumPy array and returns the differences between two successive values along a specified axis. For example, with the following `numpy.array`:\n\n```\nnpdiff = numpy.array([ 0, 2, 5, 9, 14])\n```\n\nCalling `numpy.diff(npdiff)` would do the following calculations \n\n`2 - 0`, `5 - 2`, `9 - 5`, `14 - 9`\n\nand produce the following array.\n\n`[2, 3, 4, 5]`",
"_____no_output_____"
]
],
[
[
"npdiff = numpy.array([ 0, 2, 5, 9, 14])\nnumpy.diff(npdiff)",
"_____no_output_____"
]
],
[
[
"In our `data` Which axis would it make sense to use this function along?",
"_____no_output_____"
]
],
[
[
"npdiff = numpy.array(data[0][0:4])\nprint(npdiff)",
"[0. 0. 1. 3.]\n"
]
],
[
[
"Solution",
"_____no_output_____"
],
[
"If the shape of an individual data file is (60, 40) (60 rows and 40 columns), what would the shape of the array be after you run the diff() function and why?",
"_____no_output_____"
]
],
[
[
"npdiff = numpy.array(data[0:60][0:39])\nprint(npdiff)",
"[[0. 0. 1. ... 3. 0. 0.]\n [0. 1. 2. ... 1. 0. 1.]\n [0. 1. 1. ... 2. 1. 1.]\n ...\n [0. 1. 2. ... 3. 2. 1.]\n [0. 1. 1. ... 0. 1. 0.]\n [0. 1. 0. ... 3. 0. 1.]]\n"
]
],
[
[
"Solution",
"_____no_output_____"
],
[
"How would you find the largest change in inflammation for each patient? Does it matter if the change in inflammation is an increase or a decrease? Hint: NumPy has a function called `numpy.absolute()`,",
"_____no_output_____"
]
],
[
[
"numpy.absolute(data)",
"_____no_output_____"
]
],
[
[
"Solution:",
"_____no_output_____"
],
[
"## Key Points\nUse `numpy.mean(array)`, `numpy.max(array)`, and `numpy.min(array)` to calculate simple statistics.\n\nUse `numpy.mean(array, axis=0)` or `numpy.mean(array, axis=1)` to calculate statistics across the specified axis.\n\nUse the `pyplot` library from `matplotlib` for creating simple visualizations.",
"_____no_output_____"
],
[
"# Save, and version control your changes\n\n- save your work: `File -> Save`\n- add all your changes to your local repository: `Terminal -> git add .`\n- commit your updates a new Git version: `Terminal -> git commit -m \"End of Episode 1b\"`\n- push your latest commits to GitHub: `Terminal -> git push`",
"_____no_output_____"
]
]
] | [
"markdown",
"code",
"markdown",
"code",
"markdown",
"code",
"markdown",
"code",
"markdown",
"code",
"markdown",
"code",
"markdown",
"code",
"markdown",
"code",
"markdown",
"code",
"markdown",
"code",
"markdown",
"code",
"markdown",
"code",
"markdown",
"code",
"markdown",
"code",
"markdown",
"code",
"markdown",
"code",
"markdown",
"code",
"markdown",
"code",
"markdown",
"code",
"markdown",
"code",
"markdown",
"code",
"markdown",
"code",
"markdown",
"code",
"markdown",
"code",
"markdown",
"code",
"markdown",
"code",
"markdown",
"code",
"markdown",
"code",
"markdown",
"code",
"markdown",
"code",
"markdown",
"code",
"markdown",
"code",
"markdown"
] | [
[
"markdown",
"markdown",
"markdown"
],
[
"code"
],
[
"markdown"
],
[
"code"
],
[
"markdown"
],
[
"code"
],
[
"markdown"
],
[
"code"
],
[
"markdown"
],
[
"code"
],
[
"markdown"
],
[
"code"
],
[
"markdown"
],
[
"code"
],
[
"markdown",
"markdown"
],
[
"code"
],
[
"markdown"
],
[
"code",
"code"
],
[
"markdown",
"markdown"
],
[
"code"
],
[
"markdown"
],
[
"code"
],
[
"markdown"
],
[
"code"
],
[
"markdown"
],
[
"code"
],
[
"markdown",
"markdown"
],
[
"code",
"code"
],
[
"markdown"
],
[
"code"
],
[
"markdown",
"markdown"
],
[
"code"
],
[
"markdown"
],
[
"code"
],
[
"markdown"
],
[
"code"
],
[
"markdown"
],
[
"code"
],
[
"markdown"
],
[
"code"
],
[
"markdown",
"markdown"
],
[
"code"
],
[
"markdown"
],
[
"code"
],
[
"markdown",
"markdown",
"markdown",
"markdown"
],
[
"code"
],
[
"markdown"
],
[
"code"
],
[
"markdown",
"markdown"
],
[
"code",
"code",
"code",
"code"
],
[
"markdown"
],
[
"code"
],
[
"markdown"
],
[
"code"
],
[
"markdown"
],
[
"code"
],
[
"markdown"
],
[
"code"
],
[
"markdown"
],
[
"code"
],
[
"markdown",
"markdown"
],
[
"code"
],
[
"markdown",
"markdown"
],
[
"code"
],
[
"markdown",
"markdown",
"markdown"
]
] |
e7684755a37d8f2cac317fc3274376a11d52a49a | 86,425 | ipynb | Jupyter Notebook | hw1/hw1.ipynb | ultrareality/mat201a | 9f08fa4475096bfdc277e5bb1520198962477359 | [
"MIT"
] | null | null | null | hw1/hw1.ipynb | ultrareality/mat201a | 9f08fa4475096bfdc277e5bb1520198962477359 | [
"MIT"
] | null | null | null | hw1/hw1.ipynb | ultrareality/mat201a | 9f08fa4475096bfdc277e5bb1520198962477359 | [
"MIT"
] | null | null | null | 831.009615 | 83,469 | 0.937726 | [
[
[
"empty"
]
]
] | [
"empty"
] | [
[
"empty"
]
] |
e76850ca19ca47551e393358c9ea28c00cb1a334 | 33,152 | ipynb | Jupyter Notebook | exemple.ipynb | nathandecaux/napari-labelprop | 626a305c300725f3bc044b6c0ba4206382d90b7d | [
"BSD-3-Clause"
] | null | null | null | exemple.ipynb | nathandecaux/napari-labelprop | 626a305c300725f3bc044b6c0ba4206382d90b7d | [
"BSD-3-Clause"
] | null | null | null | exemple.ipynb | nathandecaux/napari-labelprop | 626a305c300725f3bc044b6c0ba4206382d90b7d | [
"BSD-3-Clause"
] | null | null | null | 436.210526 | 30,910 | 0.944347 | [
[
[
"#%%\nfrom skimage import data\nimport nibabel as ni\nimport napari\nimport numpy as np\nfrom kornia.geometry.transform import warp_perspective\nimport torch\nimport matplotlib.pyplot as plt\n# viewer = napari.view_path('/home/nathan/PLEX/norm/sub-002/img.nii.gz')\nimg=ni.load('img.nii.gz').get_fdata()\naffine=torch.eye(3)\ntest=img[:,:,40].astype('float32')\ntest=warp_perspective(torch.from_numpy(test[None,None]),affine[None],test.shape).numpy()[0,0]\nplt.imshow(test)\nplt.show()\n",
"/home/nathan/miniconda3/envs/test/lib/python3.9/site-packages/tqdm/auto.py:22: TqdmWarning: IProgress not found. Please update jupyter and ipywidgets. See https://ipywidgets.readthedocs.io/en/stable/user_install.html\n from .autonotebook import tqdm as notebook_tqdm\n/home/nathan/miniconda3/envs/test/lib/python3.9/site-packages/torch/functional.py:445: UserWarning: torch.meshgrid: in an upcoming release, it will be required to pass the indexing argument. (Triggered internally at ../aten/src/ATen/native/TensorShape.cpp:2157.)\n return _VF.meshgrid(tensors, **kwargs) # type: ignore[attr-defined]\n"
]
]
] | [
"code"
] | [
[
"code"
]
] |
e768584aa4f705d3eb0c524266c7db235bfbcdca | 38,027 | ipynb | Jupyter Notebook | NNs-and-deep-learning/jupyter/Week 2/Python Basics with Numpy/Python Basics With Numpy v3.ipynb | HaleTom/deeplearning.ai_notes | 63e4e33434ccbe71d9c0231390f35e9d1117b8b3 | [
"MIT"
] | 1 | 2022-03-13T12:31:54.000Z | 2022-03-13T12:31:54.000Z | NNs-and-deep-learning/jupyter/Week 2/Python Basics with Numpy/Python Basics With Numpy v3.ipynb | HaleTom/deeplearning.ai_notes | 63e4e33434ccbe71d9c0231390f35e9d1117b8b3 | [
"MIT"
] | null | null | null | NNs-and-deep-learning/jupyter/Week 2/Python Basics with Numpy/Python Basics With Numpy v3.ipynb | HaleTom/deeplearning.ai_notes | 63e4e33434ccbe71d9c0231390f35e9d1117b8b3 | [
"MIT"
] | null | null | null | 35.706103 | 921 | 0.533358 | [
[
[
"# Python Basics with Numpy (optional assignment)\n\nWelcome to your first assignment. This exercise gives you a brief introduction to Python. Even if you've used Python before, this will help familiarize you with functions we'll need. \n\n**Instructions:**\n- You will be using Python 3.\n- Avoid using for-loops and while-loops, unless you are explicitly told to do so.\n- Do not modify the (# GRADED FUNCTION [function name]) comment in some cells. Your work would not be graded if you change this. Each cell containing that comment should only contain one function.\n- After coding your function, run the cell right below it to check if your result is correct.\n\n**After this assignment you will:**\n- Be able to use iPython Notebooks\n- Be able to use numpy functions and numpy matrix/vector operations\n- Understand the concept of \"broadcasting\"\n- Be able to vectorize code\n\nLet's get started!",
"_____no_output_____"
],
[
"## About iPython Notebooks ##\n\niPython Notebooks are interactive coding environments embedded in a webpage. You will be using iPython notebooks in this class. You only need to write code between the ### START CODE HERE ### and ### END CODE HERE ### comments. After writing your code, you can run the cell by either pressing \"SHIFT\"+\"ENTER\" or by clicking on \"Run Cell\" (denoted by a play symbol) in the upper bar of the notebook. \n\nWe will often specify \"(≈ X lines of code)\" in the comments to tell you about how much code you need to write. It is just a rough estimate, so don't feel bad if your code is longer or shorter.\n\n**Exercise**: Set test to `\"Hello World\"` in the cell below to print \"Hello World\" and run the two cells below.",
"_____no_output_____"
]
],
[
[
"import numpy as np\n### START CODE HERE ### (≈ 1 line of code)\ntest = \"Hello World\"\n### END CODE HERE ###",
"_____no_output_____"
],
[
"print (\"test: \" + test)",
"test: Hello World\n"
]
],
[
[
"**Expected output**:\ntest: Hello World",
"_____no_output_____"
],
[
"<font color='blue'>\n**What you need to remember**:\n- Run your cells using SHIFT+ENTER (or \"Run cell\")\n- Write code in the designated areas using Python 3 only\n- Do not modify the code outside of the designated areas",
"_____no_output_____"
],
[
"## 1 - Building basic functions with numpy ##\n\nNumpy is the main package for scientific computing in Python. It is maintained by a large community (www.numpy.org). In this exercise you will learn several key numpy functions such as np.exp, np.log, and np.reshape. You will need to know how to use these functions for future assignments.\n\n### 1.1 - sigmoid function, np.exp() ###\n\nBefore using np.exp(), you will use math.exp() to implement the sigmoid function. You will then see why np.exp() is preferable to math.exp().\n\n**Exercise**: Build a function that returns the sigmoid of a real number x. Use math.exp(x) for the exponential function.\n\n**Reminder**:\n$sigmoid(x) = \\frac{1}{1+e^{-x}}$ is sometimes also known as the logistic function. It is a non-linear function used not only in Machine Learning (Logistic Regression), but also in Deep Learning.\n\n<img src=\"images/Sigmoid.png\" style=\"width:500px;height:228px;\">\n\nTo refer to a function belonging to a specific package you could call it using package_name.function(). Run the code below to see an example with math.exp().",
"_____no_output_____"
]
],
[
[
"# GRADED FUNCTION: basic_sigmoid\n\nimport math\n\ndef basic_sigmoid(x):\n \"\"\"\n Compute sigmoid of x.\n\n Arguments:\n x -- A scalar\n\n Return:\n s -- sigmoid(x)\n \"\"\"\n \n ### START CODE HERE ### (≈ 1 line of code)\n s = 1 / (1 + math.exp(-x))\n ### END CODE HERE ###\n \n return s",
"_____no_output_____"
],
[
"basic_sigmoid(3)",
"_____no_output_____"
]
],
[
[
"**Expected Output**: \n<table style = \"width:40%\">\n <tr>\n <td>** basic_sigmoid(3) **</td> \n <td>0.9525741268224334 </td> \n </tr>\n\n</table>",
"_____no_output_____"
],
[
"Actually, we rarely use the \"math\" library in deep learning because the inputs of the functions are real numbers. In deep learning we mostly use matrices and vectors. This is why numpy is more useful. ",
"_____no_output_____"
]
],
[
[
"### One reason why we use \"numpy\" instead of \"math\" in Deep Learning ###\nx = [1, 2, 3]\nbasic_sigmoid(x) # you will see this give an error when you run it, because x is a vector.",
"_____no_output_____"
]
],
[
[
"In fact, if $ x = (x_1, x_2, ..., x_n)$ is a row vector then $np.exp(x)$ will apply the exponential function to every element of x. The output will thus be: $np.exp(x) = (e^{x_1}, e^{x_2}, ..., e^{x_n})$",
"_____no_output_____"
]
],
[
[
"import numpy as np\n\n# example of np.exp\nx = np.array([1, 2, 3])\nprint(np.exp(x)) # result is (exp(1), exp(2), exp(3))",
"_____no_output_____"
]
],
[
[
"Furthermore, if x is a vector, then a Python operation such as $s = x + 3$ or $s = \\frac{1}{x}$ will output s as a vector of the same size as x.",
"_____no_output_____"
]
],
[
[
"# example of vector operation\nx = np.array([1, 2, 3])\nprint (x + 3)\n",
"_____no_output_____"
]
],
[
[
"Any time you need more info on a numpy function, we encourage you to look at [the official documentation](https://docs.scipy.org/doc/numpy-1.10.1/reference/generated/numpy.exp.html). \n\nYou can also create a new cell in the notebook and write `np.exp?` (for example) to get quick access to the documentation.\n\n**Exercise**: Implement the sigmoid function using numpy. \n\n**Instructions**: x could now be either a real number, a vector, or a matrix. The data structures we use in numpy to represent these shapes (vectors, matrices...) are called numpy arrays. You don't need to know more for now.\n$$ \\text{For } x \\in \\mathbb{R}^n \\text{, } sigmoid(x) = sigmoid\\begin{pmatrix}\n x_1 \\\\\n x_2 \\\\\n ... \\\\\n x_n \\\\\n\\end{pmatrix} = \\begin{pmatrix}\n \\frac{1}{1+e^{-x_1}} \\\\\n \\frac{1}{1+e^{-x_2}} \\\\\n ... \\\\\n \\frac{1}{1+e^{-x_n}} \\\\\n\\end{pmatrix}\\tag{1} $$",
"_____no_output_____"
]
],
[
[
"# GRADED FUNCTION: sigmoid\n\nimport numpy as np # this means you can access numpy functions by writing np.function() instead of numpy.function()\n\ndef sigmoid(x):\n \"\"\"\n Compute the sigmoid of x\n\n Arguments:\n x -- A scalar or numpy array of any size\n\n Return:\n s -- sigmoid(x)\n \"\"\"\n \n ### START CODE HERE ### (≈ 1 line of code)\n s = 1 / (1 + np.exp(-x))\n ### END CODE HERE ###\n \n return s",
"_____no_output_____"
],
[
"x = np.array([1, 2, 3])\nsigmoid(x)",
"_____no_output_____"
]
],
[
[
"**Expected Output**: \n<table>\n <tr> \n <td> **sigmoid([1,2,3])**</td> \n <td> array([ 0.73105858, 0.88079708, 0.95257413]) </td> \n </tr>\n</table> \n",
"_____no_output_____"
],
[
"### 1.2 - Sigmoid gradient\n\nAs you've seen in lecture, you will need to compute gradients to optimize loss functions using backpropagation. Let's code your first gradient function.\n\n**Exercise**: Implement the function sigmoid_grad() to compute the gradient of the sigmoid function with respect to its input x. The formula is: $$sigmoid\\_derivative(x) = \\sigma'(x) = \\sigma(x) (1 - \\sigma(x))\\tag{2}$$\nYou often code this function in two steps:\n1. Set s to be the sigmoid of x. You might find your sigmoid(x) function useful.\n2. Compute $\\sigma'(x) = s(1-s)$",
"_____no_output_____"
]
],
[
[
"# GRADED FUNCTION: sigmoid_derivative\n\ndef sigmoid_derivative(x):\n \"\"\"\n Compute the gradient (also called the slope or derivative) of the sigmoid function with respect to its input x.\n You can store the output of the sigmoid function into variables and then use it to calculate the gradient.\n \n Arguments:\n x -- A scalar or numpy array\n\n Return:\n ds -- Your computed gradient.\n \"\"\"\n \n ### START CODE HERE ### (≈ 2 lines of code)\n s = 1 / (1 + np.exp(-x))\n ds = s * (1 - s)\n ### END CODE HERE ###\n \n return ds",
"_____no_output_____"
],
[
"x = np.array([1, 2, 3])\nprint (\"sigmoid_derivative(x) = \" + str(sigmoid_derivative(x)))",
"_____no_output_____"
]
],
[
[
"**Expected Output**: \n\n\n<table>\n <tr> \n <td> **sigmoid_derivative([1,2,3])**</td> \n <td> [ 0.19661193 0.10499359 0.04517666] </td> \n </tr>\n</table> \n\n",
"_____no_output_____"
],
[
"### 1.3 - Reshaping arrays ###\n\nTwo common numpy functions used in deep learning are [np.shape](https://docs.scipy.org/doc/numpy/reference/generated/numpy.ndarray.shape.html) and [np.reshape()](https://docs.scipy.org/doc/numpy/reference/generated/numpy.reshape.html). \n- X.shape is used to get the shape (dimension) of a matrix/vector X. \n- X.reshape(...) is used to reshape X into some other dimension. \n\nFor example, in computer science, an image is represented by a 3D array of shape $(length, height, depth = 3)$. However, when you read an image as the input of an algorithm you convert it to a vector of shape $(length*height*3, 1)$. In other words, you \"unroll\", or reshape, the 3D array into a 1D vector.\n\n<img src=\"images/image2vector_kiank.png\" style=\"width:500px;height:300;\">\n\n**Exercise**: Implement `image2vector()` that takes an input of shape (length, height, 3) and returns a vector of shape (length\\*height\\*3, 1). For example, if you would like to reshape an array v of shape (a, b, c) into a vector of shape (a*b,c) you would do:\n``` python\nv = v.reshape((v.shape[0]*v.shape[1], v.shape[2])) # v.shape[0] = a ; v.shape[1] = b ; v.shape[2] = c\n```\n- Please don't hardcode the dimensions of image as a constant. Instead look up the quantities you need with `image.shape[0]`, etc. ",
"_____no_output_____"
]
],
[
[
"# GRADED FUNCTION: image2vector\ndef image2vector(image):\n \"\"\"\n Argument:\n image -- a numpy array of shape (length, height, depth)\n \n Returns:\n v -- a vector of shape (length*height*depth, 1)\n \"\"\"\n \n ### START CODE HERE ### (≈ 1 line of code)\n v = image\n v = v.reshape(v.shape[0] * v.shape[1] * v.shape[2], 1)\n ### END CODE HERE ###\n \n return v",
"_____no_output_____"
],
[
"# This is a 3 by 3 by 2 array, typically images will be (num_px_x, num_px_y,3) where 3 represents the RGB values\nimport numpy as np\nimage = np.array([[[ 0.67826139, 0.29380381],\n [ 0.90714982, 0.52835647],\n [ 0.4215251 , 0.45017551]],\n\n [[ 0.92814219, 0.96677647],\n [ 0.85304703, 0.52351845],\n [ 0.19981397, 0.27417313]],\n\n [[ 0.60659855, 0.00533165],\n [ 0.10820313, 0.49978937],\n [ 0.34144279, 0.94630077]]])\n\nprint (\"image2vector(image) = \" + str(image2vector(image)))",
"_____no_output_____"
]
],
[
[
"**Expected Output**: \n\n\n<table style=\"width:100%\">\n <tr> \n <td> **image2vector(image)** </td> \n <td> [[ 0.67826139]\n [ 0.29380381]\n [ 0.90714982]\n [ 0.52835647]\n [ 0.4215251 ]\n [ 0.45017551]\n [ 0.92814219]\n [ 0.96677647]\n [ 0.85304703]\n [ 0.52351845]\n [ 0.19981397]\n [ 0.27417313]\n [ 0.60659855]\n [ 0.00533165]\n [ 0.10820313]\n [ 0.49978937]\n [ 0.34144279]\n [ 0.94630077]]</td> \n </tr>\n \n \n</table>",
"_____no_output_____"
],
[
"### 1.4 - Normalizing rows\n\nAnother common technique we use in Machine Learning and Deep Learning is to normalize our data. It often leads to a better performance because gradient descent converges faster after normalization. Here, by normalization we mean changing x to $ \\frac{x}{\\| x\\|} $ (dividing each row vector of x by its norm).\n\nFor example, if $$x = \n\\begin{bmatrix}\n 0 & 3 & 4 \\\\\n 2 & 6 & 4 \\\\\n\\end{bmatrix}\\tag{3}$$ then $$\\| x\\| = np.linalg.norm(x, axis = 1, keepdims = True) = \\begin{bmatrix}\n 5 \\\\\n \\sqrt{56} \\\\\n\\end{bmatrix}\\tag{4} $$and $$ x\\_normalized = \\frac{x}{\\| x\\|} = \\begin{bmatrix}\n 0 & \\frac{3}{5} & \\frac{4}{5} \\\\\n \\frac{2}{\\sqrt{56}} & \\frac{6}{\\sqrt{56}} & \\frac{4}{\\sqrt{56}} \\\\\n\\end{bmatrix}\\tag{5}$$ Note that you can divide matrices of different sizes and it works fine: this is called broadcasting and you're going to learn about it in part 5.\n\n\n**Exercise**: Implement normalizeRows() to normalize the rows of a matrix. After applying this function to an input matrix x, each row of x should be a vector of unit length (meaning length 1).",
"_____no_output_____"
]
],
[
[
"# GRADED FUNCTION: normalizeRows\n\ndef normalizeRows(x):\n \"\"\"\n Implement a function that normalizes each row of the matrix x (to have unit length).\n \n Argument:\n x -- A numpy matrix of shape (n, m)\n \n Returns:\n x -- The normalized (by row) numpy matrix. You are allowed to modify x.\n \"\"\"\n \n ### START CODE HERE ### (≈ 2 lines of code)\n # Compute x_norm as the norm 2 of x. Use np.linalg.norm(..., ord = 2, axis = ..., keepdims = True)\n x_norm = np.linalg.norm(x, ord = 2, axis = 1, keepdims = True) \n \n # Divide x by its norm.\n x = x / x_norm\n ### END CODE HERE ###\n\n return x",
"_____no_output_____"
],
[
"x = np.array([\n [0, 3, 4],\n [1, 6, 4]])\nprint(\"normalizeRows(x) = \" + str(normalizeRows(x)))",
"_____no_output_____"
]
],
[
[
"**Expected Output**: \n\n<table style=\"width:60%\">\n\n <tr> \n <td> **normalizeRows(x)** </td> \n <td> [[ 0. 0.6 0.8 ]\n [ 0.13736056 0.82416338 0.54944226]]</td> \n </tr>\n \n \n</table>",
"_____no_output_____"
],
[
"**Note**:\nIn normalizeRows(), you can try to print the shapes of x_norm and x, and then rerun the assessment. You'll find out that they have different shapes. This is normal given that x_norm takes the norm of each row of x. So x_norm has the same number of rows but only 1 column. So how did it work when you divided x by x_norm? This is called broadcasting and we'll talk about it now! ",
"_____no_output_____"
],
[
"### 1.5 - Broadcasting and the softmax function ####\nA very important concept to understand in numpy is \"broadcasting\". It is very useful for performing mathematical operations between arrays of different shapes. For the full details on broadcasting, you can read the official [broadcasting documentation](http://docs.scipy.org/doc/numpy/user/basics.broadcasting.html).",
"_____no_output_____"
],
[
"**Exercise**: Implement a softmax function using numpy. You can think of softmax as a normalizing function used when your algorithm needs to classify two or more classes. You will learn more about softmax in the second course of this specialization.\n\n**Instructions**:\n- $ \\text{for } x \\in \\mathbb{R}^{1\\times n} \\text{, } softmax(x) = softmax(\\begin{bmatrix}\n x_1 &&\n x_2 &&\n ... &&\n x_n \n\\end{bmatrix}) = \\begin{bmatrix}\n \\frac{e^{x_1}}{\\sum_{j}e^{x_j}} &&\n \\frac{e^{x_2}}{\\sum_{j}e^{x_j}} &&\n ... &&\n \\frac{e^{x_n}}{\\sum_{j}e^{x_j}} \n\\end{bmatrix} $ \n\n- $\\text{for a matrix } x \\in \\mathbb{R}^{m \\times n} \\text{, $x_{ij}$ maps to the element in the $i^{th}$ row and $j^{th}$ column of $x$, thus we have: }$ $$softmax(x) = softmax\\begin{bmatrix}\n x_{11} & x_{12} & x_{13} & \\dots & x_{1n} \\\\\n x_{21} & x_{22} & x_{23} & \\dots & x_{2n} \\\\\n \\vdots & \\vdots & \\vdots & \\ddots & \\vdots \\\\\n x_{m1} & x_{m2} & x_{m3} & \\dots & x_{mn}\n\\end{bmatrix} = \\begin{bmatrix}\n \\frac{e^{x_{11}}}{\\sum_{j}e^{x_{1j}}} & \\frac{e^{x_{12}}}{\\sum_{j}e^{x_{1j}}} & \\frac{e^{x_{13}}}{\\sum_{j}e^{x_{1j}}} & \\dots & \\frac{e^{x_{1n}}}{\\sum_{j}e^{x_{1j}}} \\\\\n \\frac{e^{x_{21}}}{\\sum_{j}e^{x_{2j}}} & \\frac{e^{x_{22}}}{\\sum_{j}e^{x_{2j}}} & \\frac{e^{x_{23}}}{\\sum_{j}e^{x_{2j}}} & \\dots & \\frac{e^{x_{2n}}}{\\sum_{j}e^{x_{2j}}} \\\\\n \\vdots & \\vdots & \\vdots & \\ddots & \\vdots \\\\\n \\frac{e^{x_{m1}}}{\\sum_{j}e^{x_{mj}}} & \\frac{e^{x_{m2}}}{\\sum_{j}e^{x_{mj}}} & \\frac{e^{x_{m3}}}{\\sum_{j}e^{x_{mj}}} & \\dots & \\frac{e^{x_{mn}}}{\\sum_{j}e^{x_{mj}}}\n\\end{bmatrix} = \\begin{pmatrix}\n softmax\\text{(first row of x)} \\\\\n softmax\\text{(second row of x)} \\\\\n ... \\\\\n softmax\\text{(last row of x)} \\\\\n\\end{pmatrix} $$",
"_____no_output_____"
]
],
[
[
"# GRADED FUNCTION: softmax\n\ndef softmax(x):\n \"\"\"Calculates the softmax for each row of the input x.\n\n Your code should work for a row vector and also for matrices of shape (n, m).\n\n Argument:\n x -- A numpy matrix of shape (n,m)\n\n Returns:\n s -- A numpy matrix equal to the softmax of x, of shape (n,m)\n \"\"\"\n \n ### START CODE HERE ### (≈ 3 lines of code)\n # Apply exp() element-wise to x. Use np.exp(...).\n x_exp = np.exp(x)\n\n # Create a vector x_sum that sums each row of x_exp. Use np.sum(..., axis = 1, keepdims = True).\n x_sum = np.sum(x_exp, axis=1, keepdims=True)\n \n \n # Compute softmax(x) by dividing x_exp by x_sum. It should automatically use numpy broadcasting.\n s = x_exp / x_sum\n\n s_sum = np.sum(s, axis=1, keepdims=True)\n print(\"s_sum=\" + str(s_sum) + \"\\n\")\n \n ### END CODE HERE ###\n \n return s",
"_____no_output_____"
],
[
"x = np.array([\n [9, 2, 5, 0, 0],\n [7, 5, 0, 0 ,0]])\nprint(\"softmax(x) = \" + str(softmax(x)))",
"_____no_output_____"
]
],
[
[
"**Expected Output**:\n\n<table style=\"width:60%\">\n\n <tr> \n <td> **softmax(x)** </td> \n <td> [[ 9.80897665e-01 8.94462891e-04 1.79657674e-02 1.21052389e-04\n 1.21052389e-04]\n [ 8.78679856e-01 1.18916387e-01 8.01252314e-04 8.01252314e-04\n 8.01252314e-04]]</td> \n </tr>\n</table>\n",
"_____no_output_____"
],
[
"**Note**:\n- If you print the shapes of x_exp, x_sum and s above and rerun the assessment cell, you will see that x_sum is of shape (2,1) while x_exp and s are of shape (2,5). **x_exp/x_sum** works due to python broadcasting.\n\nCongratulations! You now have a pretty good understanding of python numpy and have implemented a few useful functions that you will be using in deep learning.",
"_____no_output_____"
],
[
"<font color='blue'>\n**What you need to remember:**\n- np.exp(x) works for any np.array x and applies the exponential function to every coordinate\n- the sigmoid function and its gradient\n- image2vector is commonly used in deep learning\n- np.reshape is widely used. In the future, you'll see that keeping your matrix/vector dimensions straight will go toward eliminating a lot of bugs. \n- numpy has efficient built-in functions\n- broadcasting is extremely useful",
"_____no_output_____"
],
[
"## 2) Vectorization",
"_____no_output_____"
],
[
"\nIn deep learning, you deal with very large datasets. Hence, a non-computationally-optimal function can become a huge bottleneck in your algorithm and can result in a model that takes ages to run. To make sure that your code is computationally efficient, you will use vectorization. For example, try to tell the difference between the following implementations of the dot/outer/elementwise product.",
"_____no_output_____"
]
],
[
[
"import time\n\nx1 = [9, 2, 5, 0, 0, 7, 5, 0, 0, 0, 9, 2, 5, 0, 0]\nx2 = [9, 2, 2, 9, 0, 9, 2, 5, 0, 0, 9, 2, 5, 0, 0]\n\n### CLASSIC DOT PRODUCT OF VECTORS IMPLEMENTATION ###\ntic = time.process_time()\ndot = 0\nfor i in range(len(x1)):\n dot+= x1[i]*x2[i]\ntoc = time.process_time()\nprint (\"dot = \" + str(dot) + \"\\n ----- Computation time = \" + str(1000*(toc - tic)) + \"ms\")\n\n### CLASSIC OUTER PRODUCT IMPLEMENTATION ###\ntic = time.process_time()\nouter = np.zeros((len(x1),len(x2))) # we create a len(x1)*len(x2) matrix with only zeros\nfor i in range(len(x1)):\n for j in range(len(x2)):\n outer[i,j] = x1[i]*x2[j]\ntoc = time.process_time()\nprint (\"outer = \" + str(outer) + \"\\n ----- Computation time = \" + str(1000*(toc - tic)) + \"ms\")\n\n### CLASSIC ELEMENTWISE IMPLEMENTATION ###\ntic = time.process_time()\nmul = np.zeros(len(x1))\nfor i in range(len(x1)):\n mul[i] = x1[i]*x2[i]\ntoc = time.process_time()\nprint (\"elementwise multiplication = \" + str(mul) + \"\\n ----- Computation time = \" + str(1000*(toc - tic)) + \"ms\")\n\n### CLASSIC GENERAL DOT PRODUCT IMPLEMENTATION ###\nW = np.random.rand(3,len(x1)) # Random 3*len(x1) numpy array\ntic = time.process_time()\ngdot = np.zeros(W.shape[0])\nfor i in range(W.shape[0]):\n for j in range(len(x1)):\n gdot[i] += W[i,j]*x1[j]\ntoc = time.process_time()\nprint (\"gdot = \" + str(gdot) + \"\\n ----- Computation time = \" + str(1000*(toc - tic)) + \"ms\")",
"_____no_output_____"
],
[
"x1 = [9, 2, 5, 0, 0, 7, 5, 0, 0, 0, 9, 2, 5, 0, 0]\nx2 = [9, 2, 2, 9, 0, 9, 2, 5, 0, 0, 9, 2, 5, 0, 0]\n\n### VECTORIZED DOT PRODUCT OF VECTORS ###\ntic = time.process_time()\ndot = np.dot(x1,x2)\ntoc = time.process_time()\nprint (\"dot = \" + str(dot) + \"\\n ----- Computation time = \" + str(1000*(toc - tic)) + \"ms\")\n\n### VECTORIZED OUTER PRODUCT ###\ntic = time.process_time()\nouter = np.outer(x1,x2)\ntoc = time.process_time()\nprint (\"outer = \" + str(outer) + \"\\n ----- Computation time = \" + str(1000*(toc - tic)) + \"ms\")\n\n### VECTORIZED ELEMENTWISE MULTIPLICATION ###\ntic = time.process_time()\nmul = np.multiply(x1,x2)\ntoc = time.process_time()\nprint (\"elementwise multiplication = \" + str(mul) + \"\\n ----- Computation time = \" + str(1000*(toc - tic)) + \"ms\")\n\n### VECTORIZED GENERAL DOT PRODUCT ###\ntic = time.process_time()\ndot = np.dot(W,x1)\ntoc = time.process_time()\nprint (\"gdot = \" + str(dot) + \"\\n ----- Computation time = \" + str(1000*(toc - tic)) + \"ms\")",
"_____no_output_____"
]
],
[
[
"As you may have noticed, the vectorized implementation is much cleaner and more efficient. For bigger vectors/matrices, the differences in running time become even bigger. \n\n**Note** that `np.dot()` performs a matrix-matrix or matrix-vector multiplication. This is different from `np.multiply()` and the `*` operator (which is equivalent to `.*` in Matlab/Octave), which performs an element-wise multiplication.",
"_____no_output_____"
],
[
"### 2.1 Implement the L1 and L2 loss functions\n\n**Exercise**: Implement the numpy vectorized version of the L1 loss. You may find the function abs(x) (absolute value of x) useful.\n\n**Reminder**:\n- The loss is used to evaluate the performance of your model. The bigger your loss is, the more different your predictions ($ \\hat{y} $) are from the true values ($y$). In deep learning, you use optimization algorithms like Gradient Descent to train your model and to minimize the cost.\n- L1 loss is defined as:\n$$\\begin{align*} & L_1(\\hat{y}, y) = \\sum_{i=0}^m|y^{(i)} - \\hat{y}^{(i)}| \\end{align*}\\tag{6}$$",
"_____no_output_____"
]
],
[
[
"# GRADED FUNCTION: L1\n\ndef L1(yhat, y):\n \"\"\"\n Arguments:\n yhat -- vector of size m (predicted labels)\n y -- vector of size m (true labels)\n \n Returns:\n loss -- the value of the L1 loss function defined above\n \"\"\"\n \n ### START CODE HERE ### (≈ 1 line of code)\n loss = np.sum(np.abs(yhat - y))\n ### END CODE HERE ###\n \n return loss",
"_____no_output_____"
],
[
"yhat = np.array([.9, 0.2, 0.1, .4, .9])\ny = np.array([1, 0, 0, 1, 1])\nprint(\"L1 = \" + str(L1(yhat,y)))",
"L1 = 1.1\n"
]
],
[
[
"**Expected Output**:\n\n<table style=\"width:20%\">\n\n <tr> \n <td> **L1** </td> \n <td> 1.1 </td> \n </tr>\n</table>\n",
"_____no_output_____"
],
[
"**Exercise**: Implement the numpy vectorized version of the L2 loss. There are several way of implementing the L2 loss but you may find the function np.dot() useful. As a reminder, if $x = [x_1, x_2, ..., x_n]$, then `np.dot(x,x)` = $\\sum_{j=0}^n x_j^{2}$. \n\n- L2 loss is defined as $$\\begin{align*} & L_2(\\hat{y},y) = \\sum_{i=0}^m(y^{(i)} - \\hat{y}^{(i)})^2 \\end{align*}\\tag{7}$$",
"_____no_output_____"
]
],
[
[
"# GRADED FUNCTION: L2\n\ndef L2(yhat, y):\n \"\"\"\n Arguments:\n yhat -- vector of size m (predicted labels)\n y -- vector of size m (true labels)\n \n Returns:\n loss -- the value of the L2 loss function defined above\n \"\"\"\n \n ### START CODE HERE ### (≈ 1 line of code)\n loss = np.sum((y - yhat) ** 2)\n ### END CODE HERE ###\n \n return loss",
"_____no_output_____"
],
[
"yhat = np.array([.9, 0.2, 0.1, .4, .9])\ny = np.array([1, 0, 0, 1, 1])\nprint(\"L2 = \" + str(L2(yhat,y)))",
"L2 = 0.43\n"
]
],
[
[
"**Expected Output**: \n<table style=\"width:20%\">\n <tr> \n <td> **L2** </td> \n <td> 0.43 </td> \n </tr>\n</table>",
"_____no_output_____"
],
[
"Congratulations on completing this assignment. We hope that this little warm-up exercise helps you in the future assignments, which will be more exciting and interesting!",
"_____no_output_____"
],
[
"<font color='blue'>\n**What to remember:**\n- Vectorization is very important in deep learning. It provides computational efficiency and clarity.\n- You have reviewed the L1 and L2 loss.\n- You are familiar with many numpy functions such as np.sum, np.dot, np.multiply, np.maximum, etc...",
"_____no_output_____"
]
]
] | [
"markdown",
"code",
"markdown",
"code",
"markdown",
"code",
"markdown",
"code",
"markdown",
"code",
"markdown",
"code",
"markdown",
"code",
"markdown",
"code",
"markdown",
"code",
"markdown",
"code",
"markdown",
"code",
"markdown",
"code",
"markdown",
"code",
"markdown"
] | [
[
"markdown",
"markdown"
],
[
"code",
"code"
],
[
"markdown",
"markdown",
"markdown"
],
[
"code",
"code"
],
[
"markdown",
"markdown"
],
[
"code"
],
[
"markdown"
],
[
"code"
],
[
"markdown"
],
[
"code"
],
[
"markdown"
],
[
"code",
"code"
],
[
"markdown",
"markdown"
],
[
"code",
"code"
],
[
"markdown",
"markdown"
],
[
"code",
"code"
],
[
"markdown",
"markdown"
],
[
"code",
"code"
],
[
"markdown",
"markdown",
"markdown",
"markdown"
],
[
"code",
"code"
],
[
"markdown",
"markdown",
"markdown",
"markdown",
"markdown"
],
[
"code",
"code"
],
[
"markdown",
"markdown"
],
[
"code",
"code"
],
[
"markdown",
"markdown"
],
[
"code",
"code"
],
[
"markdown",
"markdown",
"markdown"
]
] |
e768605529f93c2492d450550ecae94627cc26c8 | 105,627 | ipynb | Jupyter Notebook | python/bokeh/notebooks/heatmap-unemployment.ipynb | gnkm/cheetsheet | 037fdf53dbe2d3663964c59de8d328989c1d3536 | [
"Apache-2.0"
] | null | null | null | python/bokeh/notebooks/heatmap-unemployment.ipynb | gnkm/cheetsheet | 037fdf53dbe2d3663964c59de8d328989c1d3536 | [
"Apache-2.0"
] | null | null | null | python/bokeh/notebooks/heatmap-unemployment.ipynb | gnkm/cheetsheet | 037fdf53dbe2d3663964c59de8d328989c1d3536 | [
"Apache-2.0"
] | null | null | null | 52.316493 | 36,271 | 0.452129 | [
[
[
"from math import pi\nimport pandas as pd\n\nfrom bokeh.io import output_notebook, show\nfrom bokeh.models import LinearColorMapper, BasicTicker, PrintfTickFormatter, ColorBar\nfrom bokeh.plotting import figure\nfrom bokeh.sampledata.unemployment1948 import data",
"_____no_output_____"
],
[
"output_notebook()",
"_____no_output_____"
],
[
"type(data)",
"_____no_output_____"
],
[
"data.tail(10)",
"_____no_output_____"
],
[
"data['Year'] = data['Year'].astype(str)\ndata.tail(10)",
"_____no_output_____"
],
[
"data = data.set_index('Year')\ndata.tail(10)",
"_____no_output_____"
],
[
"data.drop('Annual', axis=1, inplace=True)\ndata.tail(10)",
"_____no_output_____"
],
[
"data.columns.name = 'Month'\ndata.tail(10)",
"_____no_output_____"
],
[
"years = list(data.index)\nyears",
"_____no_output_____"
],
[
"months = list(data.columns)\nmonths",
"_____no_output_____"
],
[
"data.stack()",
"_____no_output_____"
],
[
"# reshape to 1D array or rates with a month and year for each row.\ndf = pd.DataFrame(data.stack(), columns=['rate']).reset_index()\ndf.tail(10)",
"_____no_output_____"
],
[
"# this is the colormap from the original NYTimes plot\ncolors = [\"#75968f\", \"#a5bab7\", \"#c9d9d3\", \"#e2e2e2\", \"#dfccce\", \"#ddb7b1\", \"#cc7878\", \"#933b41\", \"#550b1d\"]\nmapper = LinearColorMapper(palette=colors, low=df.rate.min(), high=df.rate.max())",
"_____no_output_____"
],
[
"TOOLS = \"hover,save,pan,box_zoom,reset,wheel_zoom\"",
"_____no_output_____"
],
[
"p = figure(title=\"US Unemployment ({0} - {1})\".format(years[0], years[-1]),\n x_range=years, y_range=list(reversed(months)),\n x_axis_location=\"above\", plot_width=900, plot_height=400,\n tools=TOOLS, toolbar_location='below',\n tooltips=[('date', '@Month @Year'), ('rate', '@rate%')])\n\np.grid.grid_line_color = None\np.axis.axis_line_color = None\np.axis.major_tick_line_color = None\np.axis.major_label_text_font_size = \"5pt\"\np.axis.major_label_standoff = 0\np.xaxis.major_label_orientation = pi / 3\n\np.rect(x=\"Year\", y=\"Month\", width=1, height=1,\n source=df,\n fill_color={'field': 'rate', 'transform': mapper},\n line_color=None)\n\ncolor_bar = ColorBar(\n color_mapper=mapper,\n major_label_text_font_size=\"5pt\",\n ticker=BasicTicker(desired_num_ticks=len(colors)),\n formatter=PrintfTickFormatter(format=\"%d%%\"),\n label_standoff=6,\n border_line_color=None,\n location=(0, 0)\n)\np.add_layout(color_bar, 'right')\n\nshow(p) # show the plot",
"_____no_output_____"
]
]
] | [
"code"
] | [
[
"code",
"code",
"code",
"code",
"code",
"code",
"code",
"code",
"code",
"code",
"code",
"code",
"code",
"code",
"code"
]
] |
e768769ed566153aa02ac9c0edbb024108041460 | 27,864 | ipynb | Jupyter Notebook | 02_PYTHON/week02/week02_numpy_neural_network.ipynb | milaan9/Deep_Learning_Algorithms_from_Scratch | a50fb800402755a044d1d9407c4c330ee4e335a8 | [
"MIT"
] | 102 | 2021-12-20T14:59:37.000Z | 2022-01-03T18:57:01.000Z | 02_PYTHON/week02/week02_numpy_neural_network.ipynb | chen181016/Deep_Learning_Algorithms_from_Scratch | 01c64769da4de9f789d8e6f87c303a6f1a968a08 | [
"MIT"
] | null | null | null | 02_PYTHON/week02/week02_numpy_neural_network.ipynb | chen181016/Deep_Learning_Algorithms_from_Scratch | 01c64769da4de9f789d8e6f87c303a6f1a968a08 | [
"MIT"
] | 122 | 2021-12-20T14:59:29.000Z | 2022-02-28T00:22:24.000Z | 33.692866 | 438 | 0.571311 | [
[
[
"### Your very own neural network\n\nIn this programming assignment we're going to build a neural network using naught but pure numpy and steel nerves. It's going to be fun, we promise!\n\n__Disclaimer:__ This assignment is ungraded.",
"_____no_output_____"
]
],
[
[
"%%bash\n\nshred -u setup_colab.py\n\nwget https://raw.githubusercontent.com/hse-aml/intro-to-dl-pytorch/main/utils/setup_colab.py -O setup_colab.py",
"_____no_output_____"
],
[
"import setup_colab\n\nsetup_colab.setup_week02_honor()",
"_____no_output_____"
],
[
"import tqdm_utils",
"_____no_output_____"
],
[
"from __future__ import print_function\nimport numpy as np\nnp.random.seed(42)",
"_____no_output_____"
]
],
[
[
"Here goes our main class: a layer that can do .forward() and .backward() passes.",
"_____no_output_____"
]
],
[
[
"class Layer:\n \"\"\"\n A building block. Each layer is capable of performing two things:\n \n - Process input to get output: output = layer.forward(input)\n \n - Propagate gradients through itself: grad_input = layer.backward(input, grad_output)\n \n Some layers also have learnable parameters which they update during layer.backward.\n \"\"\"\n def __init__(self):\n \"\"\"Here you can initialize layer parameters (if any) and auxiliary stuff.\"\"\"\n # A dummy layer does nothing\n pass\n \n def forward(self, input):\n \"\"\"\n Takes input data of shape [batch, input_units], returns output data [batch, output_units]\n \"\"\"\n # A dummy layer just returns whatever it gets as input.\n return input\n\n def backward(self, input, grad_output):\n \"\"\"\n Performs a backpropagation step through the layer, with respect to the given input.\n \n To compute loss gradients w.r.t input, you need to apply chain rule (backprop):\n \n d loss / d x = (d loss / d layer) * (d layer / d x)\n \n Luckily, you already receive d loss / d layer as input, so you only need to multiply it by d layer / d x.\n \n If your layer has parameters (e.g. dense layer), you also need to update them here using d loss / d layer\n \"\"\"\n # The gradient of a dummy layer is precisely grad_output, but we'll write it more explicitly\n num_units = input.shape[1]\n \n d_layer_d_input = np.eye(num_units)\n \n return np.dot(grad_output, d_layer_d_input) # chain rule",
"_____no_output_____"
]
],
[
[
"### The road ahead\n\nWe're going to build a neural network that classifies MNIST digits. To do so, we'll need a few building blocks:\n- Dense layer - a fully-connected layer, $f(X)=W \\cdot X + \\vec{b}$\n- ReLU layer (or any other nonlinearity you want)\n- Loss function - crossentropy\n- Backprop algorithm - a stochastic gradient descent with backpropageted gradients\n\nLet's approach them one at a time.\n",
"_____no_output_____"
],
[
"### Nonlinearity layer\n\nThis is the simplest layer you can get: it simply applies a nonlinearity to each element of your network.",
"_____no_output_____"
]
],
[
[
"class ReLU(Layer):\n def __init__(self):\n \"\"\"ReLU layer simply applies elementwise rectified linear unit to all inputs\"\"\"\n pass\n \n def forward(self, input):\n \"\"\"Apply elementwise ReLU to [batch, input_units] matrix\"\"\"\n # <your code. Try np.maximum>\n \n def backward(self, input, grad_output):\n \"\"\"Compute gradient of loss w.r.t. ReLU input\"\"\"\n relu_grad = input > 0\n return grad_output*relu_grad ",
"_____no_output_____"
],
[
"# some tests\nfrom util import eval_numerical_gradient\nx = np.linspace(-1,1,10*32).reshape([10,32])\nl = ReLU()\ngrads = l.backward(x,np.ones([10,32])/(32*10))\nnumeric_grads = eval_numerical_gradient(lambda x: l.forward(x).mean(), x=x)\nassert np.allclose(grads, numeric_grads, rtol=1e-3, atol=0),\\\n \"gradient returned by your layer does not match the numerically computed gradient\"",
"_____no_output_____"
]
],
[
[
"#### Instant primer: lambda functions\n\nIn python, you can define functions in one line using the `lambda` syntax: `lambda param1, param2: expression`\n\nFor example: `f = lambda x, y: x+y` is equivalent to a normal function:\n\n```\ndef f(x,y):\n return x+y\n```\nFor more information, click [here](http://www.secnetix.de/olli/Python/lambda_functions.hawk). ",
"_____no_output_____"
],
[
"### Dense layer\n\nNow let's build something more complicated. Unlike nonlinearity, a dense layer actually has something to learn.\n\nA dense layer applies affine transformation. In a vectorized form, it can be described as:\n$$f(X)= W \\cdot X + \\vec b $$\n\nWhere \n* X is an object-feature matrix of shape [batch_size, num_features],\n* W is a weight matrix [num_features, num_outputs] \n* and b is a vector of num_outputs biases.\n\nBoth W and b are initialized during layer creation and updated each time backward is called.",
"_____no_output_____"
]
],
[
[
"class Dense(Layer):\n def __init__(self, input_units, output_units, learning_rate=0.1):\n \"\"\"\n A dense layer is a layer which performs a learned affine transformation:\n f(x) = <W*x> + b\n \"\"\"\n self.learning_rate = learning_rate\n \n # initialize weights with small random numbers. We use normal initialization, \n # but surely there is something better. Try this once you got it working: http://bit.ly/2vTlmaJ\n self.weights = np.random.randn(input_units, output_units)*0.01\n self.biases = np.zeros(output_units)\n \n def forward(self,input):\n \"\"\"\n Perform an affine transformation:\n f(x) = <W*x> + b\n \n input shape: [batch, input_units]\n output shape: [batch, output units]\n \"\"\"\n return #<your code here>\n \n def backward(self,input,grad_output):\n \n # compute d f / d x = d f / d dense * d dense / d x\n # where d dense/ d x = weights transposed\n grad_input = #<your code here>\n \n # compute gradient w.r.t. weights and biases\n grad_weights = #<your code here>\n grad_biases = #<your code here>\n \n assert grad_weights.shape == self.weights.shape and grad_biases.shape == self.biases.shape\n # Here we perform a stochastic gradient descent step. \n # Later on, you can try replacing that with something better.\n self.weights = self.weights - self.learning_rate * grad_weights\n self.biases = self.biases - self.learning_rate * grad_biases\n \n return grad_input",
"_____no_output_____"
]
],
[
[
"### Testing the dense layer\n\nHere we have a few tests to make sure your dense layer works properly. You can just run them, get 3 \"well done\"s and forget they ever existed.\n\n... or not get 3 \"well done\"s and go fix stuff. If that is the case, here are some tips for you:\n* Make sure you compute gradients for W and b as __sum of gradients over batch__, not mean over gradients. Grad_output is already divided by batch size.\n* If you're debugging, try saving gradients in class fields, like \"self.grad_w = grad_w\" or print first 3-5 weights. This helps debugging.\n* If nothing else helps, try ignoring tests and proceed to network training. If it trains alright, you may be off by something that does not affect network training.",
"_____no_output_____"
]
],
[
[
"l = Dense(128, 150)\n\nassert -0.05 < l.weights.mean() < 0.05 and 1e-3 < l.weights.std() < 1e-1,\\\n \"The initial weights must have zero mean and small variance. \"\\\n \"If you know what you're doing, remove this assertion.\"\nassert -0.05 < l.biases.mean() < 0.05, \"Biases must be zero mean. Ignore if you have a reason to do otherwise.\"\n\n# To test the outputs, we explicitly set weights with fixed values. DO NOT DO THAT IN ACTUAL NETWORK!\nl = Dense(3,4)\n\nx = np.linspace(-1,1,2*3).reshape([2,3])\nl.weights = np.linspace(-1,1,3*4).reshape([3,4])\nl.biases = np.linspace(-1,1,4)\n\nassert np.allclose(l.forward(x),np.array([[ 0.07272727, 0.41212121, 0.75151515, 1.09090909],\n [-0.90909091, 0.08484848, 1.07878788, 2.07272727]]))\nprint(\"Well done!\")",
"_____no_output_____"
],
[
"# To test the grads, we use gradients obtained via finite differences\n\nfrom util import eval_numerical_gradient\n\nx = np.linspace(-1,1,10*32).reshape([10,32])\nl = Dense(32,64,learning_rate=0)\n\nnumeric_grads = eval_numerical_gradient(lambda x: l.forward(x).sum(),x)\ngrads = l.backward(x,np.ones([10,64]))\n\nassert np.allclose(grads,numeric_grads,rtol=1e-3,atol=0), \"input gradient does not match numeric grad\"\nprint(\"Well done!\")",
"_____no_output_____"
],
[
"#test gradients w.r.t. params\ndef compute_out_given_wb(w,b):\n l = Dense(32,64,learning_rate=1)\n l.weights = np.array(w)\n l.biases = np.array(b)\n x = np.linspace(-1,1,10*32).reshape([10,32])\n return l.forward(x)\n \ndef compute_grad_by_params(w,b):\n l = Dense(32,64,learning_rate=1)\n l.weights = np.array(w)\n l.biases = np.array(b)\n x = np.linspace(-1,1,10*32).reshape([10,32])\n l.backward(x,np.ones([10,64]) / 10.)\n return w - l.weights, b - l.biases\n \nw,b = np.random.randn(32,64), np.linspace(-1,1,64)\n\nnumeric_dw = eval_numerical_gradient(lambda w: compute_out_given_wb(w,b).mean(0).sum(),w )\nnumeric_db = eval_numerical_gradient(lambda b: compute_out_given_wb(w,b).mean(0).sum(),b )\ngrad_w,grad_b = compute_grad_by_params(w,b)\n\nassert np.allclose(numeric_dw,grad_w,rtol=1e-3,atol=0), \"weight gradient does not match numeric weight gradient\"\nassert np.allclose(numeric_db,grad_b,rtol=1e-3,atol=0), \"weight gradient does not match numeric weight gradient\"\nprint(\"Well done!\")",
"_____no_output_____"
]
],
[
[
"### The loss function\n\nSince we want to predict probabilities, it would be logical for us to define softmax nonlinearity on top of our network and compute loss given predicted probabilities. However, there is a better way to do so.\n\nIf you write down the expression for crossentropy as a function of softmax logits (a), you'll see:\n\n$$ loss = - log \\space {e^{a_{correct}} \\over {\\underset i \\sum e^{a_i} } } $$\n\nIf you take a closer look, ya'll see that it can be rewritten as:\n\n$$ loss = - a_{correct} + log {\\underset i \\sum e^{a_i} } $$\n\nIt's called Log-softmax and it's better than naive log(softmax(a)) in all aspects:\n* Better numerical stability\n* Easier to get derivative right\n* Marginally faster to compute\n\nSo why not just use log-softmax throughout our computation and never actually bother to estimate probabilities.\n\nHere you are! We've defined the both loss functions for you so that you could focus on neural network part.",
"_____no_output_____"
]
],
[
[
"def softmax_crossentropy_with_logits(logits,reference_answers):\n \"\"\"Compute crossentropy from logits[batch,n_classes] and ids of correct answers\"\"\"\n logits_for_answers = logits[np.arange(len(logits)),reference_answers]\n \n xentropy = - logits_for_answers + np.log(np.sum(np.exp(logits),axis=-1))\n \n return xentropy\n\ndef grad_softmax_crossentropy_with_logits(logits,reference_answers):\n \"\"\"Compute crossentropy gradient from logits[batch,n_classes] and ids of correct answers\"\"\"\n ones_for_answers = np.zeros_like(logits)\n ones_for_answers[np.arange(len(logits)),reference_answers] = 1\n \n softmax = np.exp(logits) / np.exp(logits).sum(axis=-1,keepdims=True)\n \n return (- ones_for_answers + softmax) / logits.shape[0]",
"_____no_output_____"
],
[
"logits = np.linspace(-1,1,500).reshape([50,10])\nanswers = np.arange(50)%10\n\nsoftmax_crossentropy_with_logits(logits,answers)\ngrads = grad_softmax_crossentropy_with_logits(logits,answers)\nnumeric_grads = eval_numerical_gradient(lambda l: softmax_crossentropy_with_logits(l,answers).mean(),logits)\n\nassert np.allclose(numeric_grads,grads,rtol=1e-3,atol=0), \"The reference implementation has just failed. Someone has just changed the rules of math.\"",
"_____no_output_____"
]
],
[
[
"### Full network\n\nNow let's combine what we've just built into a working neural network. As we announced, we're gonna use this monster to classify handwritten digits, so let's get them loaded.",
"_____no_output_____"
],
[
"We will download the data using pythorch. ",
"_____no_output_____"
]
],
[
[
"!pip install torchvision",
"_____no_output_____"
],
[
"# import numpy and matplotlib\n%pylab inline\n\nimport torchvision\n\ntransform = torchvision.transforms.Compose([\n torchvision.transforms.ToTensor(),\n torchvision.transforms.Lambda(lambda x: x.flatten())\n])\n\ntrain_dataset = torchvision.datasets.MNIST(root='.', train=True,\n download=True, transform=transform)\ntest_dataset = torchvision.datasets.MNIST(root='.', train=True,\n download=True, transform=transform)",
"_____no_output_____"
],
[
"X_train, y_train = [], []\nfor i in range(len(train_dataset)):\n x, y = train_dataset[i]\n X_train.append(x.numpy())\n y_train.append(y)\n\nX_train = np.array(X_train)\ny_train = np.array(y_train)\n\n# we reserve the last 10000 training examples for validation\nX_train, X_val = X_train[:-10000], X_train[-10000:]\ny_train, y_val = y_train[:-10000], y_train[-10000:]\n\nX_test, y_test = [], []\nfor i in range(len(test_dataset)):\n x, y = test_dataset[i]\n X_test.append(x.numpy())\n y_test.append(y)\n\nX_test = np.array(X_test)\ny_test = np.array(y_test)",
"_____no_output_____"
],
[
"plt.figure(figsize=[6, 6])\n\nfor i in range(4):\n plt.subplot(2, 2, i + 1)\n plt.title(f\"Label: {y_train[i]}\")\n plt.imshow(X_train[i].reshape([28, 28]), cmap='gray')",
"_____no_output_____"
]
],
[
[
"We'll define network as a list of layers, each applied on top of previous one. In this setting, computing predictions and training becomes trivial.",
"_____no_output_____"
]
],
[
[
"network = []\nnetwork.append(Dense(X_train.shape[1],100))\nnetwork.append(ReLU())\nnetwork.append(Dense(100,200))\nnetwork.append(ReLU())\nnetwork.append(Dense(200,10))",
"_____no_output_____"
],
[
"def forward(network, X):\n \"\"\"\n Compute activations of all network layers by applying them sequentially.\n Return a list of activations for each layer. \n Make sure last activation corresponds to network logits.\n \"\"\"\n activations = []\n input = X\n\n # <your code here>\n \n assert len(activations) == len(network)\n return activations\n\ndef predict(network,X):\n \"\"\"\n Compute network predictions.\n \"\"\"\n logits = forward(network,X)[-1]\n return logits.argmax(axis=-1)\n\ndef train(network,X,y):\n \"\"\"\n Train your network on a given batch of X and y.\n You first need to run forward to get all layer activations.\n Then you can run layer.backward going from last to first layer.\n \n After you called backward for all layers, all Dense layers have already made one gradient step.\n \"\"\"\n \n # Get the layer activations\n layer_activations = forward(network,X)\n layer_inputs = [X]+layer_activations #layer_input[i] is an input for network[i]\n logits = layer_activations[-1]\n \n # Compute the loss and the initial gradient\n loss = softmax_crossentropy_with_logits(logits,y)\n loss_grad = grad_softmax_crossentropy_with_logits(logits,y)\n \n # <your code: propagate gradients through the network>\n \n return np.mean(loss)",
"_____no_output_____"
]
],
[
[
"Instead of tests, we provide you with a training loop that prints training and validation accuracies on every epoch.\n\nIf your implementation of forward and backward are correct, your accuracy should grow from 90~93% to >97% with the default network.",
"_____no_output_____"
],
[
"### Training loop\n\nAs usual, we split data into minibatches, feed each such minibatch into the network and update weights.",
"_____no_output_____"
]
],
[
[
"def iterate_minibatches(inputs, targets, batchsize, shuffle=False):\n assert len(inputs) == len(targets)\n if shuffle:\n indices = np.random.permutation(len(inputs))\n for start_idx in tqdm_utils.tqdm_notebook_failsafe(range(0, len(inputs) - batchsize + 1, batchsize)):\n if shuffle:\n excerpt = indices[start_idx:start_idx + batchsize]\n else:\n excerpt = slice(start_idx, start_idx + batchsize)\n yield inputs[excerpt], targets[excerpt]",
"_____no_output_____"
],
[
"from IPython.display import clear_output\ntrain_log = []\nval_log = []",
"_____no_output_____"
],
[
"for epoch in range(25):\n\n for x_batch,y_batch in iterate_minibatches(X_train,y_train,batchsize=32,shuffle=True):\n train(network,x_batch,y_batch)\n \n train_log.append(np.mean(predict(network,X_train)==y_train))\n val_log.append(np.mean(predict(network,X_val)==y_val))\n \n clear_output()\n print(\"Epoch\",epoch)\n print(\"Train accuracy:\",train_log[-1])\n print(\"Val accuracy:\",val_log[-1])\n plt.plot(train_log,label='train accuracy')\n plt.plot(val_log,label='val accuracy')\n plt.legend(loc='best')\n plt.grid()\n plt.show()\n ",
"_____no_output_____"
]
],
[
[
"### Try it out!\n\nCongradulations, you managed to get this far! Now you can chose one or more options what to do next. \n\n\n#### Option I: initialization\n* Implement Dense layer with Xavier initialization as explained [here](http://bit.ly/2vTlmaJ). Compare xavier initialization to default initialization on deep networks (5+ layers).\n\n#### Option II: regularization\n* Implement a version of Dense layer with L2 regularization penalty: when updating Dense Layer weights, adjust gradients to minimize\n\n$$ Loss = Crossentropy + \\alpha \\cdot \\underset i \\sum {w_i}^2 $$\n\nCheck that regularization mitigates overfitting in case of abundantly large number of neurons. Consider tuning $\\alpha$ for better results.\n\n#### Option III: optimization\n* Implement a version of Dense layer that uses momentum/rmsprop or whatever method worked best for you last time.\n\nMost of those methods require persistent parameters like momentum direction or moving average grad norm, but you can easily store those params inside your layers.\n\nCompare your chosen method performance with vanilla SGD's one.\n\n### Some advanced stuff\nIf you are still with us and want more, consider implementing Batch Normalization ([guide](https://gab41.lab41.org/batch-normalization-what-the-hey-d480039a9e3b)) or Dropout ([guide](https://medium.com/@amarbudhiraja/https-medium-com-amarbudhiraja-learning-less-to-learn-better-dropout-in-deep-machine-learning-74334da4bfc5)). Note, however, that those \"layers\" behave differently when training and when predicting on test set.\n\n* Dropout:\n * During training: drop units randomly with probability __p__ and multiply everything by __1/(1-p)__\n * During final predicton: do nothing; pretend there's no dropout\n \n* Batch normalization\n * During training, it substracts mean-over-batch and divides by std-over-batch and updates mean and variance.\n * During final prediction, it uses accumulated mean and variance.\n",
"_____no_output_____"
]
]
] | [
"markdown",
"code",
"markdown",
"code",
"markdown",
"code",
"markdown",
"code",
"markdown",
"code",
"markdown",
"code",
"markdown",
"code",
"markdown",
"code",
"markdown",
"code",
"markdown"
] | [
[
"markdown"
],
[
"code",
"code",
"code",
"code"
],
[
"markdown"
],
[
"code"
],
[
"markdown",
"markdown"
],
[
"code",
"code"
],
[
"markdown",
"markdown"
],
[
"code"
],
[
"markdown"
],
[
"code",
"code",
"code"
],
[
"markdown"
],
[
"code",
"code"
],
[
"markdown",
"markdown"
],
[
"code",
"code",
"code",
"code"
],
[
"markdown"
],
[
"code",
"code"
],
[
"markdown",
"markdown"
],
[
"code",
"code",
"code"
],
[
"markdown"
]
] |
e7687b1471598abcfc6e9957778222ec0aa0bed8 | 15,343 | ipynb | Jupyter Notebook | notebooks/community/ml_ops/stage4/get_started_with_google_artifact_registry.ipynb | prodonjs/vertex-ai-samples | 4970aabb2f940a7c7157cfdfc20ee427a9173d52 | [
"Apache-2.0"
] | null | null | null | notebooks/community/ml_ops/stage4/get_started_with_google_artifact_registry.ipynb | prodonjs/vertex-ai-samples | 4970aabb2f940a7c7157cfdfc20ee427a9173d52 | [
"Apache-2.0"
] | null | null | null | notebooks/community/ml_ops/stage4/get_started_with_google_artifact_registry.ipynb | prodonjs/vertex-ai-samples | 4970aabb2f940a7c7157cfdfc20ee427a9173d52 | [
"Apache-2.0"
] | null | null | null | 31.635052 | 293 | 0.534511 | [
[
[
"# Copyright 2022 Google LLC\n#\n# Licensed under the Apache License, Version 2.0 (the \"License\");\n# you may not use this file except in compliance with the License.\n# You may obtain a copy of the License at\n#\n# https://www.apache.org/licenses/LICENSE-2.0\n#\n# Unless required by applicable law or agreed to in writing, software\n# distributed under the License is distributed on an \"AS IS\" BASIS,\n# WITHOUT WARRANTIES OR CONDITIONS OF ANY KIND, either express or implied.\n# See the License for the specific language governing permissions and\n# limitations under the License.",
"_____no_output_____"
]
],
[
[
"# E2E ML on GCP: MLOps stage 4 : formalization: get started with Google Artifact Registry\n\n<table align=\"left\">\n <td>\n <a href=\"https://github.com/GoogleCloudPlatform/vertex-ai-samples/blob/main/notebooks/community/ml_ops/stage4/get_started_with_google_artifact_registry.ipynb\">\n <img src=\"https://cloud.google.com/ml-engine/images/github-logo-32px.png\" alt=\"GitHub logo\">\n View on GitHub\n </a>\n </td>\n <td>\n <a href=\"https://console.cloud.google.com/vertex-ai/workbench/deploy-notebook?download_url=https://github.com/GoogleCloudPlatform/vertex-ai-samples/blob/main/notebooks/community/ml_ops/stage4/get_started_with_google_artifact_registry.ipynb\">\n Open in Vertex AI Workbench\n </a>\n </td>\n</table>\n<br/><br/><br/>",
"_____no_output_____"
],
[
"## Overview\n\n\nThis tutorial demonstrates how to use Vertex AI for E2E MLOps on Google Cloud in production. This tutorial covers stage 4 : formalization: get started with Google Artifact Registry.",
"_____no_output_____"
],
[
"### Objective\n\nIn this tutorial, you learn how to use `Google Artifact Registry`.\n\nThis tutorial uses the following Google Cloud ML services:\n\n- `Google Artifact Registry`\n\nThe steps performed include:\n\n- Creating a private Docker repository.\n- Tagging a container image, specific to the private Docker repository.\n- Pushing a container image to the private Docker repository.\n- Pulling a container image from the private Docker repository.\n- Deleting a private Docker repository.",
"_____no_output_____"
],
[
"## Installations\n\nInstall *one time* the packages for executing the MLOps notebooks.",
"_____no_output_____"
]
],
[
[
"ONCE_ONLY = False\nif ONCE_ONLY:\n ! pip3 install -U tensorflow==2.5 $USER_FLAG\n ! pip3 install -U tensorflow-data-validation==1.2 $USER_FLAG\n ! pip3 install -U tensorflow-transform==1.2 $USER_FLAG\n ! pip3 install -U tensorflow-io==0.18 $USER_FLAG\n ! pip3 install --upgrade google-cloud-aiplatform[tensorboard] $USER_FLAG\n ! pip3 install --upgrade google-cloud-pipeline-components $USER_FLAG\n ! pip3 install --upgrade google-cloud-bigquery $USER_FLAG\n ! pip3 install --upgrade google-cloud-logging $USER_FLAG\n ! pip3 install --upgrade apache-beam[gcp] $USER_FLAG\n ! pip3 install --upgrade pyarrow $USER_FLAG\n ! pip3 install --upgrade cloudml-hypertune $USER_FLAG\n ! pip3 install --upgrade kfp $USER_FLAG\n ! pip3 install --upgrade torchvision $USER_FLAG\n ! pip3 install --upgrade rpy2 $USER_FLAG",
"_____no_output_____"
]
],
[
[
"### Restart the kernel\n\nOnce you've installed the additional packages, you need to restart the notebook kernel so it can find the packages.",
"_____no_output_____"
]
],
[
[
"import os\n\nif not os.getenv(\"IS_TESTING\"):\n # Automatically restart kernel after installs\n import IPython\n\n app = IPython.Application.instance()\n app.kernel.do_shutdown(True)",
"_____no_output_____"
]
],
[
[
"#### Set your project ID\n\n**If you don't know your project ID**, you may be able to get your project ID using `gcloud`.",
"_____no_output_____"
]
],
[
[
"PROJECT_ID = \"[your-project-id]\" # @param {type:\"string\"}",
"_____no_output_____"
],
[
"if PROJECT_ID == \"\" or PROJECT_ID is None or PROJECT_ID == \"[your-project-id]\":\n # Get your GCP project id from gcloud\n shell_output = ! gcloud config list --format 'value(core.project)' 2>/dev/null\n PROJECT_ID = shell_output[0]\n print(\"Project ID:\", PROJECT_ID)",
"_____no_output_____"
],
[
"! gcloud config set project $PROJECT_ID",
"_____no_output_____"
]
],
[
[
"#### Region\n\nYou can also change the `REGION` variable, which is used for operations\nthroughout the rest of this notebook. Below are regions supported for Vertex AI. We recommend that you choose the region closest to you.\n\n- Americas: `us-central1`\n- Europe: `europe-west4`\n- Asia Pacific: `asia-east1`\n\nYou may not use a multi-regional bucket for training with Vertex AI. Not all regions provide support for all Vertex AI services.\n\nLearn more about [Vertex AI regions](https://cloud.google.com/vertex-ai/docs/general/locations).",
"_____no_output_____"
]
],
[
[
"REGION = \"us-central1\" # @param {type: \"string\"}",
"_____no_output_____"
]
],
[
[
"#### Timestamp\n\nIf you are in a live tutorial session, you might be using a shared test account or project. To avoid name collisions between users on resources created, you create a timestamp for each instance session, and append the timestamp onto the name of resources you create in this tutorial.",
"_____no_output_____"
]
],
[
[
"from datetime import datetime\n\nTIMESTAMP = datetime.now().strftime(\"%Y%m%d%H%M%S\")",
"_____no_output_____"
]
],
[
[
"### Set up variables\n\nNext, set up some variables used throughout the tutorial.\n### Import libraries and define constants",
"_____no_output_____"
],
[
"## Introduction to Google Artifact Registry\n\nThe `Google Artifact Registry` is a service for storing and managing artifacts in private repositories, including container images, Helm charts, and language packages. It is the recommended container image registry for Google Cloud.\n\nLearn more about [Quick start for Docker](https://cloud.google.com/artifact-registry/docs/docker/quickstart)",
"_____no_output_____"
],
[
"### Enable Artifact Registry API\n\nFirst, you must enable the Artifact Registry API service for your project.\n\nLearn more about [Enabling service](https://cloud.google.com/artifact-registry/docs/enable-service).",
"_____no_output_____"
]
],
[
[
"! gcloud services enable artifactregistry.googleapis.com",
"_____no_output_____"
]
],
[
[
"## Create a private Docker repository\n\nYour first step is to create your own Docker repository in Google Artifact Registry.\n\n1. Run the `gcloud artifacts repositories create` command to create a new Docker repository with your region with the description \"docker repository\".\n\n2. Run the `gcloud artifacts repositories list` command to verify that your repository was created.",
"_____no_output_____"
]
],
[
[
"PRIVATE_REPO = \"my-docker-repo\"\n\n! gcloud artifacts repositories create {PRIVATE_REPO} --repository-format=docker --location={REGION} --description=\"Docker repository\"\n\n! gcloud artifacts repositories list",
"_____no_output_____"
]
],
[
[
"### Configure authentication to your private repo\n\nBefore you push or pull container images, configure Docker to use the `gcloud` command-line tool to authenticate requests to `Artifact Registry` for your region.",
"_____no_output_____"
]
],
[
[
"! gcloud auth configure-docker {REGION}-docker.pkg.dev --quiet",
"_____no_output_____"
]
],
[
[
"### Obtain an example container image\n\nFor demonstration purposes, you obtain (pull) a local copy of our demonstration container image: `hello-app:1.0`",
"_____no_output_____"
]
],
[
[
"! docker pull us-docker.pkg.dev/google-samples/containers/gke/hello-app:1.0",
"_____no_output_____"
]
],
[
[
"## Tagging your container image\n\nNow that you have your own container image, the first step is to tag your image.\n\n- Tagging the Docker image with a repository name configures the docker push command to push the image to a specific location, e.g., us-central1-docker.pkg.dev.\n\n- `:my-tag` is a tag you're adding to the Docker image. If a tag is not specified, it defaults to `:latest`.",
"_____no_output_____"
]
],
[
[
"CONTAINER_NAME = \"my-image:my-tag\"\n\n! docker tag us-docker.pkg.dev/google-samples/containers/gke/hello-app:1.0 us-central1-docker.pkg.dev/{PROJECT_ID}/{PRIVATE_REPO}/{CONTAINER_NAME}",
"_____no_output_____"
]
],
[
[
"## Push your image to your private Docker repository\n\nNext, push your container to your private Docker repository.",
"_____no_output_____"
]
],
[
[
"! docker push {REGION}-docker.pkg.dev/{PROJECT_ID}/{PRIVATE_REPO}/{CONTAINER_NAME}",
"_____no_output_____"
]
],
[
[
"## Pull your image from your private Docker repostory\n\nNow pull your container from your private Docker repository.",
"_____no_output_____"
]
],
[
[
"! docker pull {REGION}-docker.pkg.dev/{PROJECT_ID}/{PRIVATE_REPO}/{CONTAINER_NAME}",
"_____no_output_____"
]
],
[
[
"### Deleting your private Docker repostory\n\nFinally, once your private repository becomes obsolete, use the command `gcloud artifacts repositories delete` to delete it `Google Artifact Registry`.",
"_____no_output_____"
]
],
[
[
"! gcloud artifacts repositories delete {PRIVATE_REPO} --location={REGION} --quiet",
"_____no_output_____"
]
]
] | [
"code",
"markdown",
"code",
"markdown",
"code",
"markdown",
"code",
"markdown",
"code",
"markdown",
"code",
"markdown",
"code",
"markdown",
"code",
"markdown",
"code",
"markdown",
"code",
"markdown",
"code",
"markdown",
"code",
"markdown",
"code",
"markdown",
"code"
] | [
[
"code"
],
[
"markdown",
"markdown",
"markdown",
"markdown"
],
[
"code"
],
[
"markdown"
],
[
"code"
],
[
"markdown"
],
[
"code",
"code",
"code"
],
[
"markdown"
],
[
"code"
],
[
"markdown"
],
[
"code"
],
[
"markdown",
"markdown",
"markdown"
],
[
"code"
],
[
"markdown"
],
[
"code"
],
[
"markdown"
],
[
"code"
],
[
"markdown"
],
[
"code"
],
[
"markdown"
],
[
"code"
],
[
"markdown"
],
[
"code"
],
[
"markdown"
],
[
"code"
],
[
"markdown"
],
[
"code"
]
] |
e7687c1881153c4bd6a632a7a83f3a2b49643679 | 29,167 | ipynb | Jupyter Notebook | L02-datatypes.ipynb | dariomalchiodi/python-DS4EBF | e8134f890f9053d2dacabf4acbde3e5a8116fa0a | [
"Apache-2.0"
] | null | null | null | L02-datatypes.ipynb | dariomalchiodi/python-DS4EBF | e8134f890f9053d2dacabf4acbde3e5a8116fa0a | [
"Apache-2.0"
] | null | null | null | L02-datatypes.ipynb | dariomalchiodi/python-DS4EBF | e8134f890f9053d2dacabf4acbde3e5a8116fa0a | [
"Apache-2.0"
] | null | null | null | 29.343058 | 699 | 0.525525 | [
[
[
"# Introduzione pratica ai tipi di dati in Python\nPer ulteriori esempi e riferimenti, si veda [An Informal Introduction to Python](https://docs.python.org/2/tutorial/introduction.html#)",
"_____no_output_____"
]
],
[
[
"metadata = \"Name;Identity;Birth place;Publisher;Height;Weight;Gender;First appearance;Eye color;Hair color;Strength;Intelligence\"\nrowdata = 'Silver Surfer;Norrin Radd;Zenn-La;Marvel Comics;193.00999999999999;101.34;M;;White;No Hair;100;average'",
"_____no_output_____"
]
],
[
[
"## Stringhe\nLe stringhe in Python sono collezioni di caratteri incluse fra i simboli \"...\" o '...'. Le due notazioni non hanno significative differenze se usiamo \"...\" non dobbiamo quotare il simbolo ' e viceversa.",
"_____no_output_____"
]
],
[
[
"print 'Ero \"felice\"'\nprint 'Ero \\'felice\\''\nprint \"Ero 'felice'\"\nprint \"Ero \\\"felice\\\"\\nDavvero!\"",
"Ero \"felice\"\nEro 'felice'\nEro 'felice'\nEro \"felice\"\nDavvero!\n"
]
],
[
[
"Per evitare di interpretare il simbolo \\ come un marcatore possiamo ricorrere alle 'raw strings'. In generale, le stringhe Python possono essere qialificate come stringhe speciali, anteponendo alla stringa un descrittore. Per conoscere il tipo di un dato esiste la funzione `type`.",
"_____no_output_____"
]
],
[
[
"print 'Bianco\\nero'\nprint r'Bianco\\nero'\nprint type('Bianco\\nero'), type(r'Bianco\\nero'), type(u'Bianco\\nero')",
"Bianco\nero\nBianco\\nero\n<type 'str'> <type 'str'> <type 'unicode'>\n"
]
],
[
[
"Stringhe che occupano più linee e conservano la formattazione sono denotate dai simboli \"\"\"...\"\"\" o '''...'''.",
"_____no_output_____"
]
],
[
[
"silverbio = \"\"\"\nSilver Surfer, alter ego di Norrin Radd, è un personaggio immaginario dei fumetti \ncreato da Stan Lee e Jack Kirby nel 1966 \\\ne pubblicato dalla casa editrice statunitense Marvel Comics.\n\nEsordisce nella serie The Fantastic Four (Vol. 1[1]) n. 48 del 1966, nella Trilogia di Galactus,\n\\\nil cui riscontro positivo da parte del pubblico porta a dedicargli una sua serie personale nel 1968.\n\nDate importanti:\n - 1966\n - 1968\n\"\"\"",
"_____no_output_____"
],
[
"print silverbio",
"\nSilver Surfer, alter ego di Norrin Radd, è un personaggio immaginario dei fumetti \ncreato da Stan Lee e Jack Kirby nel 1966 e pubblicato dalla casa editrice statunitense Marvel Comics.\n\nEsordisce nella serie The Fantastic Four (Vol. 1[1]) n. 48 del 1966, nella Trilogia di Galactus,\nil cui riscontro positivo da parte del pubblico porta a dedicargli una sua serie personale nel 1968.\n\nDate importanti:\n - 1966\n - 1968\n\n"
]
],
[
[
"### Concatenazione di stringhe\nLe stringhe si concatenano con l'operatore `+`, si ripetono con `*`. I 'string literals' (le stringhe con '...' si concatenano anche solo giustapponendole",
"_____no_output_____"
]
],
[
[
"print 3 * \"super \" + \"silver\"\nprint 'con' 'catenato'\nsentence = ('Se uso le parentesi e '\n 'i literals '\n 'è super comodo!')\nprint sentence",
"super super super silver\nconcatenato\nSe uso le parentesi e i literals è super comodo!\n"
]
],
[
[
"Ma non si possono concatenare variabili e literals!",
"_____no_output_____"
]
],
[
[
"one = 'a '",
"_____no_output_____"
],
[
"print one 'string'",
"_____no_output_____"
],
[
"print one + 'string'\nprint 1 + 'string'",
"a string\n"
]
],
[
[
"# Indicizzazione delle stringhe\nI caratteri di una stringa sono indicizzati e accessibili come in una lista, secondo lo schema:\n\n|S|i|l|v|e|r|\n|:---:|:---:|:---:|:---:|:---:|:---:|\n|0|1|2|3|4|5|\n|-6|-5|-4|-3|-2|-1|\n",
"_____no_output_____"
]
],
[
[
"silver = 'Silver'\nprint len(silver)\nprint silver[0]\nprint silver[5]\nprint silver[-1]\nprint silver[-6]\nprint silver[6]",
"6\nS\nr\nr\nS\n"
]
],
[
[
"Possiamo perciò usare con le stringhe una tecnica molto usata anche per le liste e fondamentale in Python: lo 'slicing'",
"_____no_output_____"
]
],
[
[
"print silver[2:]\nprint silver[:3]\nprint silver[2:4]\nprint silver[3:22]\nprint silver[:-2]",
"lver\nSil\nlv\nver\nSilv\n"
]
],
[
[
"Tuttavia, a differenza delle liste, le stringhe python sono **immutabili**",
"_____no_output_____"
]
],
[
[
"silver[1] = 'o'",
"_____no_output_____"
],
[
"solver = silver[:1] + 'o' + silver[2:]",
"_____no_output_____"
],
[
"print solver",
"Solver\n"
],
[
"print \" - ; | \".join(['A', 'B', 'C'])",
"A - ; | B - ; | C\n"
]
],
[
[
"### Metodi di utilità per le stringhe\nIn Python, le stringhe sono oggetti dotati di un'ampia gamma di metodi per diverse funzioni (vedi [string methods](https://docs.python.org/2/library/stdtypes.html#string-methods)):",
"_____no_output_____"
]
],
[
[
"print 'find()', '->', silver.find('ver')\nprint 'endswith()/startswith()', '->', silver.endswith('er'), silver.startswith('er')\nprint 'lower()', '->', silver.lower()\nprint 'lstrip()/rstrip()', '->', silver.lstrip('S'), silver.rstrip('S')\nprint 'replace()', '->', silver.replace('er', 'an')\nprint 'split()', '->', silver.split('v')\nprint 'upper()', '->', silver.upper()\nprint 'join()', '->', silver.join(['A ', ' hero'])",
"find() -> 3\nendswith()/startswith() -> True False\nlower() -> silver\nlstrip()/rstrip() -> ilver Silver\nreplace() -> Silvan\nsplit() -> ['Sil', 'er']\nupper() -> SILVER\njoin() -> A Silver hero\n"
]
],
[
[
"### Formattazione di stringhe\nLe stringhe offrono anche l'operatore `%` (modulo). Si tratta di un operstore che consente di formattare e interpolare una stringa con diversi tipi di dato. ",
"_____no_output_____"
]
],
[
[
"print u\"%(superhero)s è stato creato da %(creator)s nel %(year)i\" % {\n 'superhero': 'Silver Surfer', 'creator': ' e '.join(['Stan Lee', 'Jack Kirby']), 'year': 1966\n}",
"_____no_output_____"
],
[
"print u\"{} è stato creato da {} nel {}\".format('Silver Surfer', ' e '.join(['Stan Lee', 'Jack Kirby']), 1966)",
"_____no_output_____"
]
],
[
[
"### Unicode\nIn Python 2.* le stringhe sono intese come succesione di caratteri ASCII dove non esplicitamente codificate per mezzo dei metodi `encode()` e `decode()`. Le stringhe `unicode` vanno dichiarate come tali col carattere `u`.",
"_____no_output_____"
]
],
[
[
"u = u'Silver Surfer è nato a Zenn-La'",
"_____no_output_____"
],
[
"u",
"_____no_output_____"
],
[
"str(u)",
"_____no_output_____"
],
[
"u.encode('utf-8')",
"_____no_output_____"
],
[
"str(u.encode('utf-8'))",
"_____no_output_____"
]
],
[
[
"# Numeri\nIn Python ci sono 4 tipi numerici principali: `plain integers`, `long integers`, `floating point numbers`, e `complex numbers`. I booleani sono un sottotipo di interi. \n\n- I `plain integers` hanno sempre almeno 32 bit di precisione (l'intero massimo è `sys.maxint` e il minimo `-sys.maxint - 1`). \n- I `Long integers` sono illimitati. \n- I `Floating point numbers` sono implementati come i tipi `double` in `C` (vedi `sys.float_info`) \n- I `Complex numbers` hanno una parte reale e una immaginaria entrambe rappresentate come `float`.",
"_____no_output_____"
]
],
[
[
"import sys\nprint sys.maxint\nprint sys.float_info",
"_____no_output_____"
]
],
[
[
"### Operatori aritmetici",
"_____no_output_____"
]
],
[
[
"print 2 + 2\nprint 2 * 3\nprint 17 / 3\nprint 17 / 3.0\nprint 17 // 3.0\nprint 17 % 3\nprint 17 ** 3",
"4\n6\n5\n5.66666666667\n5.0\n2\n4913\n"
]
],
[
[
"### Conversioni",
"_____no_output_____"
]
],
[
[
"a, b, c = 2, 3.0, 3.5\nprint float(a), int(b), int(c), str(c), bool(c), bool(c - 3.5)",
"2.0 3 3 3.5 True False\n"
]
],
[
[
"# Gestione delle date\nLe principali funzionalità per la gestione delle date sono incluse nei moduli `datetime`, `time` e `calendar` che si basano sullo standard Coordinated Universal Time (UTC). Le date sono **immutabili**.\n\n- `datetime.date` : date secondo il calendario gregoriano\n- `datetime.time` : tempo, considerando per ogni giorno 24*60*60 secondi\n- `datetime.datetime` : date + tempo\n- `datetime.timedelta` : durate con risoluzione al millisecondo\n- `datetime.tzinfo` : informazione sul time zone",
"_____no_output_____"
]
],
[
[
"import datetime as dtt\nimport time",
"_____no_output_____"
],
[
"when = dtt.date(1966, 4, 28)\nnow = dtt.time(16, 36)\ntemp = dtt.datetime(when.year, when.month, when.day, now.hour, now.minute, now.second, now.microsecond)\ntoday = dtt.datetime.today()",
"_____no_output_____"
],
[
"print when.day\nprint when.month\nprint when.year\nprint now.hour, now.minute, now.second, now.microsecond, now.tzinfo\nprint temp",
"28\n4\n1966\n16 36 0 0 None\n1966-04-28 16:36:00\n"
],
[
"delta = temp - today",
"_____no_output_____"
],
[
"print delta, type(delta), delta.total_seconds()\nprint today.isocalendar()\nprint today.isoformat(' ')",
"-18971 days, 4:40:04.205052 <type 'datetime.timedelta'> -1639077595.79\n(2018, 14, 5)\n2018-04-06 11:55:55.794948\n"
]
],
[
[
"### Conversione tra stringhe e date\n`strftime()` e `strptime()` convertono date in stringhe e viceversa secondo la seguente convenzione di formato.\n\n<table>\n<colgroup>\n<col width=\"15%\">\n<col width=\"43%\">\n<col width=\"32%\">\n<col width=\"9%\">\n</colgroup>\n<thead valign=\"bottom\">\n<tr class=\"row-odd\"><th class=\"head\">Directive</th>\n<th class=\"head\">Meaning</th>\n<th class=\"head\">Example</th>\n</tr>\n</thead>\n<tbody valign=\"top\">\n<tr class=\"row-even\"><td><code class=\"docutils literal\"><span class=\"pre\">%a</span></code></td>\n<td>Weekday as locale’s\nabbreviated name.</td>\n<td><div class=\"first last line-block\">\n<div class=\"line\">Sun, Mon, …, Sat\n(en_US);</div>\n<div class=\"line\">So, Mo, …, Sa\n(de_DE)</div>\n</div>\n</td>\n</tr>\n<tr class=\"row-odd\"><td><code class=\"docutils literal\"><span class=\"pre\">%A</span></code></td>\n<td>Weekday as locale’s full name.</td>\n<td><div class=\"first last line-block\">\n<div class=\"line\">Sunday, Monday, …,\nSaturday (en_US);</div>\n<div class=\"line\">Sonntag, Montag, …,\nSamstag (de_DE)</div>\n</div>\n</td>\n</tr>\n<tr class=\"row-even\"><td><code class=\"docutils literal\"><span class=\"pre\">%w</span></code></td>\n<td>Weekday as a decimal number,\nwhere 0 is Sunday and 6 is\nSaturday.</td>\n<td>0, 1, …, 6</td>\n</tr>\n<tr class=\"row-odd\"><td><code class=\"docutils literal\"><span class=\"pre\">%d</span></code></td>\n<td>Day of the month as a\nzero-padded decimal number.</td>\n<td>01, 02, …, 31</td>\n</tr>\n<tr class=\"row-even\"><td><code class=\"docutils literal\"><span class=\"pre\">%b</span></code></td>\n<td>Month as locale’s abbreviated\nname.</td>\n<td><div class=\"first last line-block\">\n<div class=\"line\">Jan, Feb, …, Dec\n(en_US);</div>\n<div class=\"line\">Jan, Feb, …, Dez\n(de_DE)</div>\n</div>\n</td>\n</tr>\n<tr class=\"row-odd\"><td><code class=\"docutils literal\"><span class=\"pre\">%B</span></code></td>\n<td>Month as locale’s full name.</td>\n<td><div class=\"first last line-block\">\n<div class=\"line\">January, February,\n…, December (en_US);</div>\n<div class=\"line\">Januar, Februar, …,\nDezember (de_DE)</div>\n</div>\n</td>\n</tr>\n<tr class=\"row-even\"><td><code class=\"docutils literal\"><span class=\"pre\">%m</span></code></td>\n<td>Month as a zero-padded\ndecimal number.</td>\n<td>01, 02, …, 12</td>\n</tr>\n<tr class=\"row-odd\"><td><code class=\"docutils literal\"><span class=\"pre\">%y</span></code></td>\n<td>Year without century as a\nzero-padded decimal number.</td>\n<td>00, 01, …, 99</td>\n</tr>\n<tr class=\"row-even\"><td><code class=\"docutils literal\"><span class=\"pre\">%Y</span></code></td>\n<td>Year with century as a decimal\nnumber.</td>\n<td>1970, 1988, 2001, 2013</td>\n</tr>\n<tr class=\"row-odd\"><td><code class=\"docutils literal\"><span class=\"pre\">%H</span></code></td>\n<td>Hour (24-hour clock) as a\nzero-padded decimal number.</td>\n<td>00, 01, …, 23</td>\n</tr>\n<tr class=\"row-even\"><td><code class=\"docutils literal\"><span class=\"pre\">%I</span></code></td>\n<td>Hour (12-hour clock) as a\nzero-padded decimal number.</td>\n<td>01, 02, …, 12</td>\n</tr>\n<tr class=\"row-odd\"><td><code class=\"docutils literal\"><span class=\"pre\">%p</span></code></td>\n<td>Locale’s equivalent of either\nAM or PM.</td>\n<td><div class=\"first last line-block\">\n<div class=\"line\">AM, PM (en_US);</div>\n<div class=\"line\">am, pm (de_DE)</div>\n</div>\n</td>\n</tr>\n<tr class=\"row-even\"><td><code class=\"docutils literal\"><span class=\"pre\">%M</span></code></td>\n<td>Minute as a zero-padded\ndecimal number.</td>\n<td>00, 01, …, 59</td>\n</tr>\n<tr class=\"row-odd\"><td><code class=\"docutils literal\"><span class=\"pre\">%S</span></code></td>\n<td>Second as a zero-padded\ndecimal number.</td>\n<td>00, 01, …, 59</td>\n</tr>\n<tr class=\"row-even\"><td><code class=\"docutils literal\"><span class=\"pre\">%f</span></code></td>\n<td>Microsecond as a decimal\nnumber, zero-padded on the\nleft.</td>\n<td>000000, 000001, …,\n999999</td>\n</tr>\n<tr class=\"row-odd\"><td><code class=\"docutils literal\"><span class=\"pre\">%z</span></code></td>\n<td>UTC offset in the form +HHMM\nor -HHMM (empty string if the\nthe object is naive).</td>\n<td>(empty), +0000, -0400,\n+1030</td>\n</tr>\n<tr class=\"row-even\"><td><code class=\"docutils literal\"><span class=\"pre\">%Z</span></code></td>\n<td>Time zone name (empty string\nif the object is naive).</td>\n<td>(empty), UTC, EST, CST</td>\n</tr>\n<tr class=\"row-odd\"><td><code class=\"docutils literal\"><span class=\"pre\">%j</span></code></td>\n<td>Day of the year as a\nzero-padded decimal number.</td>\n<td>001, 002, …, 366</td>\n</tr>\n<tr class=\"row-even\"><td><code class=\"docutils literal\"><span class=\"pre\">%U</span></code></td>\n<td>Week number of the year\n(Sunday as the first day of\nthe week) as a zero padded\ndecimal number. All days in a\nnew year preceding the first\nSunday are considered to be in\nweek 0.</td>\n<td>00, 01, …, 53</td>\n</tr>\n<tr class=\"row-odd\"><td><code class=\"docutils literal\"><span class=\"pre\">%W</span></code></td>\n<td>Week number of the year\n(Monday as the first day of\nthe week) as a decimal number.\nAll days in a new year\npreceding the first Monday\nare considered to be in\nweek 0.</td>\n<td>00, 01, …, 53</td>\n</tr>\n<tr class=\"row-even\"><td><code class=\"docutils literal\"><span class=\"pre\">%c</span></code></td>\n<td>Locale’s appropriate date and\ntime representation.</td>\n<td><div class=\"first last line-block\">\n<div class=\"line\">Tue Aug 16 21:30:00\n1988 (en_US);</div>\n<div class=\"line\">Di 16 Aug 21:30:00\n1988 (de_DE)</div>\n</div>\n</td>\n</tr>\n<tr class=\"row-odd\"><td><code class=\"docutils literal\"><span class=\"pre\">%x</span></code></td>\n<td>Locale’s appropriate date\nrepresentation.</td>\n<td><div class=\"first last line-block\">\n<div class=\"line\">08/16/88 (None);</div>\n<div class=\"line\">08/16/1988 (en_US);</div>\n<div class=\"line\">16.08.1988 (de_DE)</div>\n</div>\n</td>\n</tr>\n<tr class=\"row-even\"><td><code class=\"docutils literal\"><span class=\"pre\">%X</span></code></td>\n<td>Locale’s appropriate time\nrepresentation.</td>\n<td><div class=\"first last line-block\">\n<div class=\"line\">21:30:00 (en_US);</div>\n<div class=\"line\">21:30:00 (de_DE)</div>\n</div>\n</td>\n</tr>\n<tr class=\"row-odd\"><td><code class=\"docutils literal\"><span class=\"pre\">%%</span></code></td>\n<td>A literal <code class=\"docutils literal\"><span class=\"pre\">'%'</span></code> character.</td>\n<td>%</td>\n</tr>\n</tbody>\n</table>",
"_____no_output_____"
]
],
[
[
"print dtt.datetime.strftime(today, \"%d/%m/%Y %I:%M:%S %p %z\")",
"06/04/2018 11:55:55 AM \n"
],
[
"from_string = dtt.datetime.strptime('6/12/1978 12:36:46',\n '%d/%m/%Y %H:%M:%S')",
"_____no_output_____"
],
[
"print from_string",
"1978-12-06 12:36:46\n"
]
],
[
[
"# Put things together",
"_____no_output_____"
]
],
[
[
"silver_data = rowdata.split(';')",
"_____no_output_____"
],
[
"print metadata.split(';')\nprint silver_data",
"_____no_output_____"
]
]
] | [
"markdown",
"code",
"markdown",
"code",
"markdown",
"code",
"markdown",
"code",
"markdown",
"code",
"markdown",
"code",
"markdown",
"code",
"markdown",
"code",
"markdown",
"code",
"markdown",
"code",
"markdown",
"code",
"markdown",
"code",
"markdown",
"code",
"markdown",
"code",
"markdown",
"code",
"markdown",
"code",
"markdown",
"code",
"markdown",
"code"
] | [
[
"markdown"
],
[
"code"
],
[
"markdown"
],
[
"code"
],
[
"markdown"
],
[
"code"
],
[
"markdown"
],
[
"code",
"code"
],
[
"markdown"
],
[
"code"
],
[
"markdown"
],
[
"code",
"code",
"code"
],
[
"markdown"
],
[
"code"
],
[
"markdown"
],
[
"code"
],
[
"markdown"
],
[
"code",
"code",
"code",
"code"
],
[
"markdown"
],
[
"code"
],
[
"markdown"
],
[
"code",
"code"
],
[
"markdown"
],
[
"code",
"code",
"code",
"code",
"code"
],
[
"markdown"
],
[
"code"
],
[
"markdown"
],
[
"code"
],
[
"markdown"
],
[
"code"
],
[
"markdown"
],
[
"code",
"code",
"code",
"code",
"code"
],
[
"markdown"
],
[
"code",
"code",
"code"
],
[
"markdown"
],
[
"code",
"code"
]
] |
e7687e5e3d138816b40dafdfea4298afb0dc95e0 | 32,043 | ipynb | Jupyter Notebook | qa.ipynb | thingumajig/qa-prototype | a16216b3f37557a8efc7ad5d912d2693e9bd7b9b | [
"Unlicense"
] | 1 | 2020-04-19T13:26:31.000Z | 2020-04-19T13:26:31.000Z | qa.ipynb | thingumajig/qa-prototype | a16216b3f37557a8efc7ad5d912d2693e9bd7b9b | [
"Unlicense"
] | null | null | null | qa.ipynb | thingumajig/qa-prototype | a16216b3f37557a8efc7ad5d912d2693e9bd7b9b | [
"Unlicense"
] | null | null | null | 43.77459 | 1,360 | 0.520114 | [
[
[
"<a href=\"https://colab.research.google.com/github/thingumajig/qa-prototype/blob/master/qa.ipynb\" target=\"_parent\"><img src=\"https://colab.research.google.com/assets/colab-badge.svg\" alt=\"Open In Colab\"/></a>",
"_____no_output_____"
],
[
"# Init",
"_____no_output_____"
],
[
"## Install packages",
"_____no_output_____"
]
],
[
[
"!pip install spacy\n\n!pip3 uninstall --quiet --yes tensorflow\n!pip3 install --quiet tensorflow-gpu==1.14.0\n!pip3 install --quiet tensorflow-hub\n!pip3 install --quiet sentencepiece==0.1.83\n!pip3 install --quiet tf-sentencepiece==0.1.83\n!pip3 install --quiet simpleneighbors\n",
"Requirement already satisfied: spacy in /usr/local/lib/python3.6/dist-packages (2.1.8)\nRequirement already satisfied: murmurhash<1.1.0,>=0.28.0 in /usr/local/lib/python3.6/dist-packages (from spacy) (1.0.2)\nRequirement already satisfied: preshed<2.1.0,>=2.0.1 in /usr/local/lib/python3.6/dist-packages (from spacy) (2.0.1)\nRequirement already satisfied: srsly<1.1.0,>=0.0.6 in /usr/local/lib/python3.6/dist-packages (from spacy) (0.1.0)\nRequirement already satisfied: cymem<2.1.0,>=2.0.2 in /usr/local/lib/python3.6/dist-packages (from spacy) (2.0.2)\nRequirement already satisfied: blis<0.3.0,>=0.2.2 in /usr/local/lib/python3.6/dist-packages (from spacy) (0.2.4)\nRequirement already satisfied: plac<1.0.0,>=0.9.6 in /usr/local/lib/python3.6/dist-packages (from spacy) (0.9.6)\nRequirement already satisfied: thinc<7.1.0,>=7.0.8 in /usr/local/lib/python3.6/dist-packages (from spacy) (7.0.8)\nRequirement already satisfied: requests<3.0.0,>=2.13.0 in /usr/local/lib/python3.6/dist-packages (from spacy) (2.21.0)\nRequirement already satisfied: wasabi<1.1.0,>=0.2.0 in /usr/local/lib/python3.6/dist-packages (from spacy) (0.3.0)\nRequirement already satisfied: numpy>=1.15.0 in /usr/local/lib/python3.6/dist-packages (from spacy) (1.17.3)\nRequirement already satisfied: tqdm<5.0.0,>=4.10.0 in /usr/local/lib/python3.6/dist-packages (from thinc<7.1.0,>=7.0.8->spacy) (4.28.1)\nRequirement already satisfied: urllib3<1.25,>=1.21.1 in /usr/local/lib/python3.6/dist-packages (from requests<3.0.0,>=2.13.0->spacy) (1.24.3)\nRequirement already satisfied: idna<2.9,>=2.5 in /usr/local/lib/python3.6/dist-packages (from requests<3.0.0,>=2.13.0->spacy) (2.8)\nRequirement already satisfied: chardet<3.1.0,>=3.0.2 in /usr/local/lib/python3.6/dist-packages (from requests<3.0.0,>=2.13.0->spacy) (3.0.4)\nRequirement already satisfied: certifi>=2017.4.17 in /usr/local/lib/python3.6/dist-packages (from requests<3.0.0,>=2.13.0->spacy) (2019.9.11)\n\u001b[K |████████████████████████████████| 377.0MB 46kB/s \n\u001b[K |████████████████████████████████| 3.2MB 32.5MB/s \n\u001b[K |████████████████████████████████| 491kB 39.7MB/s \n\u001b[K |████████████████████████████████| 1.0MB 2.8MB/s \n\u001b[K |████████████████████████████████| 2.7MB 2.6MB/s \n\u001b[K |████████████████████████████████| 645kB 3.9MB/s \n\u001b[?25h Building wheel for annoy (setup.py) ... \u001b[?25l\u001b[?25hdone\n"
]
],
[
[
"## Initialize grammar parser",
"_____no_output_____"
]
],
[
[
"import spacy\nnlp = spacy.load(\"en_core_web_sm\")\n# doc = nlp(\"With respect to all losses caused by the peril of Flood, the Company shall not be liable, in the aggregate for any one Policy year, for more than its proportionate share of US$25,000,000.\")\n# doc = nlp(\"The Program limit of liability is US$400,000,000.\")\n# print(doc)\n# for token in doc:\n# print(\"{2}-{1}({3}-{6}, {0}-{5})\".format(token.text, token.tag_, token.dep_, token.head.text, token.head.tag_, token.i+1, token.head.i+1))\n# for np in doc.noun_chunks:\n# print(np.text)\n\n\nfrom spacy.symbols import *\n\nnp_labels = set([nsubj, nsubjpass, dobj, iobj, pobj]) # Probably others too\nnp_labels_full = set([nsubj, nsubjpass, dobj, iobj, pobj, csubj, csubjpass, attr]) # Probably others too\n# print(dir(spacy.symbols))\n# subj - subject\n# nsubj - nominal subject\n# nsubjpass - passive nominal subject\n# csubj - clausal subject\n# csubjpass - passive clausal subject\n\ndef iter_nps(doc):\n for word in doc:\n if word.dep in np_labels_full:\n yield word\n\ndef iter_nps_str(doc):\n s = ''\n for np in iter_nps(doc):\n for t in np.subtree:\n s += str(t)+' '\n yield s.strip()\n s = ''\n\n\n# print('='*20)\n# for np in iter_nps(doc):\n# print(np)\n# for t in np.subtree:\n# print(f'\\t{t}')\n\n# print('='*20)\n# for np in iter_nps_str(doc):\n# print(np)\n\n\n# print('='*20)\n# for token in doc:\n# print(f'{token.text} {token.tag_} {token.dep_} {str(token.dep)} \\t\\t\\thead: {token.head.tag_} {token.head.dep_} {token.head.text}') \n# for t in token.subtree:\n# print(f'\\t{t}')\n",
"_____no_output_____"
]
],
[
[
"## Set up Tensorflow graph",
"_____no_output_____"
]
],
[
[
"%%time\nimport tensorflow as tf\nimport tensorflow_hub as hub\nimport numpy as np\nimport tf_sentencepiece\n\n# Set up graph.\ng = tf.Graph()\nwith g.as_default():\n questions_input = tf.placeholder(dtype=tf.string, shape=[None])\n responses_input = tf.placeholder(dtype=tf.string, shape=[None])\n contexts_input = tf.placeholder(dtype=tf.string, shape=[None])\n\n\n module = hub.Module(\"https://tfhub.dev/google/universal-sentence-encoder-multilingual-qa/1\")\n question_embeddings = module(\n dict(input=questions_input),\n signature=\"question_encoder\", as_dict=True)\n\n response_embeddings = module(\n dict(input=responses_input,\n context=contexts_input),\n signature=\"response_encoder\", as_dict=True)\n\n init_op = tf.group([tf.global_variables_initializer(), tf.tables_initializer()])\ng.finalize()\n",
"/usr/local/lib/python3.6/dist-packages/tensorflow/python/framework/dtypes.py:516: FutureWarning: Passing (type, 1) or '1type' as a synonym of type is deprecated; in a future version of numpy, it will be understood as (type, (1,)) / '(1,)type'.\n _np_qint8 = np.dtype([(\"qint8\", np.int8, 1)])\n/usr/local/lib/python3.6/dist-packages/tensorflow/python/framework/dtypes.py:517: FutureWarning: Passing (type, 1) or '1type' as a synonym of type is deprecated; in a future version of numpy, it will be understood as (type, (1,)) / '(1,)type'.\n _np_quint8 = np.dtype([(\"quint8\", np.uint8, 1)])\n/usr/local/lib/python3.6/dist-packages/tensorflow/python/framework/dtypes.py:518: FutureWarning: Passing (type, 1) or '1type' as a synonym of type is deprecated; in a future version of numpy, it will be understood as (type, (1,)) / '(1,)type'.\n _np_qint16 = np.dtype([(\"qint16\", np.int16, 1)])\n/usr/local/lib/python3.6/dist-packages/tensorflow/python/framework/dtypes.py:519: FutureWarning: Passing (type, 1) or '1type' as a synonym of type is deprecated; in a future version of numpy, it will be understood as (type, (1,)) / '(1,)type'.\n _np_quint16 = np.dtype([(\"quint16\", np.uint16, 1)])\n/usr/local/lib/python3.6/dist-packages/tensorflow/python/framework/dtypes.py:520: FutureWarning: Passing (type, 1) or '1type' as a synonym of type is deprecated; in a future version of numpy, it will be understood as (type, (1,)) / '(1,)type'.\n _np_qint32 = np.dtype([(\"qint32\", np.int32, 1)])\n/usr/local/lib/python3.6/dist-packages/tensorflow/python/framework/dtypes.py:525: FutureWarning: Passing (type, 1) or '1type' as a synonym of type is deprecated; in a future version of numpy, it will be understood as (type, (1,)) / '(1,)type'.\n np_resource = np.dtype([(\"resource\", np.ubyte, 1)])\n/usr/local/lib/python3.6/dist-packages/tensorboard/compat/tensorflow_stub/dtypes.py:541: FutureWarning: Passing (type, 1) or '1type' as a synonym of type is deprecated; in a future version of numpy, it will be understood as (type, (1,)) / '(1,)type'.\n _np_qint8 = np.dtype([(\"qint8\", np.int8, 1)])\n/usr/local/lib/python3.6/dist-packages/tensorboard/compat/tensorflow_stub/dtypes.py:542: FutureWarning: Passing (type, 1) or '1type' as a synonym of type is deprecated; in a future version of numpy, it will be understood as (type, (1,)) / '(1,)type'.\n _np_quint8 = np.dtype([(\"quint8\", np.uint8, 1)])\n/usr/local/lib/python3.6/dist-packages/tensorboard/compat/tensorflow_stub/dtypes.py:543: FutureWarning: Passing (type, 1) or '1type' as a synonym of type is deprecated; in a future version of numpy, it will be understood as (type, (1,)) / '(1,)type'.\n _np_qint16 = np.dtype([(\"qint16\", np.int16, 1)])\n/usr/local/lib/python3.6/dist-packages/tensorboard/compat/tensorflow_stub/dtypes.py:544: FutureWarning: Passing (type, 1) or '1type' as a synonym of type is deprecated; in a future version of numpy, it will be understood as (type, (1,)) / '(1,)type'.\n _np_quint16 = np.dtype([(\"quint16\", np.uint16, 1)])\n/usr/local/lib/python3.6/dist-packages/tensorboard/compat/tensorflow_stub/dtypes.py:545: FutureWarning: Passing (type, 1) or '1type' as a synonym of type is deprecated; in a future version of numpy, it will be understood as (type, (1,)) / '(1,)type'.\n _np_qint32 = np.dtype([(\"qint32\", np.int32, 1)])\n/usr/local/lib/python3.6/dist-packages/tensorboard/compat/tensorflow_stub/dtypes.py:550: FutureWarning: Passing (type, 1) or '1type' as a synonym of type is deprecated; in a future version of numpy, it will be understood as (type, (1,)) / '(1,)type'.\n np_resource = np.dtype([(\"resource\", np.ubyte, 1)])\n"
]
],
[
[
"# Run",
"_____no_output_____"
],
[
"##Initialize tensorflow session.",
"_____no_output_____"
]
],
[
[
"%%time\n# Initialize session.\nsession = tf.Session(graph=g)\nsession.run(init_op)",
"CPU times: user 10.5 s, sys: 2 s, total: 12.5 s\nWall time: 14.3 s\n"
]
],
[
[
"## Tests",
"_____no_output_____"
]
],
[
[
"# %%time\nsentences = '''\nF.\tPROGRAM LIMITS OF LIABILITY\n\n(1)\tThe Program limit of liability is US$400,000,000. \n\n(2)\tSublimits below are applicable to all direct physical loss, damage or destruction insured against, except an Accident. \n\n(a)\tWith respect to all losses caused by the peril of Flood, the Company shall not be liable, in the aggregate for any one Policy year, for more than its proportionate share of US$25,000,000. \n\nBut not to exceed US$5,000,000 in the aggregate for any one Policy year\nfor the peril of Flood occurring in Special Flood Hazard Areas. However,\nthis Special Flood Hazard Area sublimit shall not apply to loss involving \npersonal property, buildings, or structures wholly located outside an area\ndesignated as a Special Flood Hazard Area.\n\nThe aggregate limit stated in the paragraph above shall be part of the overall aggregate limit stated in the introduction to this paragraph.\n\nEven if the peril of Flood is the predominant cause of direct physical loss, damage or destruction, any ensuing physical loss, damage or destruction arising from a peril not otherwise excluded herein shall not be subject to the sublimit or aggregate specified in this paragraph (a).\n'''\nsentence1 = 'With respect to all losses caused by the peril of Earthquake, the Company shall not be liable, in the aggregate for any one Policy year, for more than its proportionate share of US$50,000,000.'\n\nsentence2 = '''\nСуд освободил из-под стражи следователя Алексея Шиманского и полицейского Кирилла Лисового из Санкт-Петербурга, которых подозревают в посредничестве при передаче взятки в 19 миллионов рублей. Об этом сообщает «Фонтанка».\n'''\n\nimport simpleneighbors\n\ndef qa(sentences, queries, use_parser = True):\n index = simpleneighbors.SimpleNeighbors(\n 512, metric='angular')\n\n def candidate_generation(t, from_n=1, to_n=6):\n l = t.split()\n grams = []\n for n in range(from_n, to_n):\n grams += [' '.join(l[i:i+n]) for i in range(len(l)-n+1)]\n \n return grams\n\n\n def display_nearest_neighbors(query_text):\n print(f'Query: {query_text}')\n query_embedding = session.run(question_embeddings, feed_dict={questions_input: [query_text]})['outputs'][0]\n # print(\"query_embedding:\")\n # print(query_embedding)\n search_results = index.nearest(query_embedding, n=10)\n print('Top-10 Responses:')\n for s in search_results:\n print(f'\\t{s}')\n\n\n def calculate_sentences_emb(sentences, use_parser=True):\n responses = []\n contexts = []\n if use_parser:\n doc = nlp(sentences)\n for sent in doc.sents:\n # print('='*40)\n # print(sent)\n # print('-- Noun groups & attributes: --')\n for np in iter_nps_str(sent):\n # print(f'\\t{np}')\n responses.append(str(np).strip())\n contexts.append(str(sent).strip())\n else:\n grams = candidate_generation(sentences, 1, 5)\n for g in grams:\n responses.append(g.strip())\n contexts.append(sentences.strip())\n\n\n candidate_embeddings = session.run(\n response_embeddings,\n feed_dict={\n responses_input: responses,\n contexts_input: contexts\n })\n print(f'Shape: {candidate_embeddings[\"outputs\"].shape}')\n # print(candidate_embeddings[\"outputs\"][0])\n print('Candidates:')\n for i in range(len(responses)):\n print(f'\\t{responses[i]}')\n index.add_one(responses[i], candidate_embeddings['outputs'][i])\n\n index.build()\n \n calculate_sentences_emb(sentences, use_parser)\n # display_nearest_neighbors('Какие имена у освобождённых')\n if isinstance(queries, list):\n for q in queries:\n display_nearest_neighbors(q)\n else:\n display_nearest_neighbors(queries)\n\n\n\n# qa(sentences, 'имена отпущенных')\nqa(sentence1, ['peril','sum'])\nqa(sentence2, ['имена отпущенных', 'сумма взятки'])\n ",
"Shape: (10, 512)\nCandidates:\n\trespect to all losses caused by the peril of Earthquake\n\tall losses caused by the peril of Earthquake\n\tthe peril of Earthquake\n\tEarthquake\n\tthe Company\n\tthe aggregate for any one Policy year\n\tany one Policy year\n\tmore than its proportionate share of US$ 50,000,000\n\tits proportionate share of US$ 50,000,000\n\tUS$ 50,000,000\nQuery: peril\nTop-10 Responses:\n\tthe peril of Earthquake\n\trespect to all losses caused by the peril of Earthquake\n\tall losses caused by the peril of Earthquake\n\tthe Company\n\tEarthquake\n\tany one Policy year\n\tUS$ 50,000,000\n\tmore than its proportionate share of US$ 50,000,000\n\tthe aggregate for any one Policy year\n\tits proportionate share of US$ 50,000,000\nQuery: sum\nTop-10 Responses:\n\tmore than its proportionate share of US$ 50,000,000\n\tits proportionate share of US$ 50,000,000\n\tthe aggregate for any one Policy year\n\tUS$ 50,000,000\n\tEarthquake\n\tthe Company\n\trespect to all losses caused by the peril of Earthquake\n\tthe peril of Earthquake\n\tall losses caused by the peril of Earthquake\n\tany one Policy year\nShape: (8, 512)\nCandidates:\n\tСуд\n\tиз - под стражи\n\tАлексея Шиманского\n\tКирилла Лисового из Санкт - Петербурга ,\n\tкоторых\n\tпосредничестве\n\tпри передаче\n\tв 19 миллионов рублей\nQuery: имена отпущенных\nTop-10 Responses:\n\tАлексея Шиманского\n\tКирилла Лисового из Санкт - Петербурга ,\n\tкоторых\n\tСуд\n\tпри передаче\n\tпосредничестве\n\tиз - под стражи\n\tв 19 миллионов рублей\nQuery: сумма взятки\nTop-10 Responses:\n\tв 19 миллионов рублей\n\tСуд\n\tкоторых\n\tпосредничестве\n\tпри передаче\n\tиз - под стражи\n\tАлексея Шиманского\n\tКирилла Лисового из Санкт - Петербурга ,\n"
]
],
[
[
"# Form",
"_____no_output_____"
]
],
[
[
"%%time\n\n#@title Question and answer\ntext = \"\\u0410\\u0441\\u0442\\u0440\\u043E\\u043D\\u043E\\u043C\\u044B \\u0418\\u043D\\u0441\\u0442\\u0438\\u0442\\u0443\\u0442\\u0430 \\u0432\\u043D\\u0435\\u0437\\u0435\\u043C\\u043D\\u043E\\u0439 \\u0444\\u0438\\u0437\\u0438\\u043A\\u0438 \\u041E\\u0431\\u0449\\u0435\\u0441\\u0442\\u0432\\u0430 \\u041C\\u0430\\u043A\\u0441\\u0430 \\u041F\\u043B\\u0430\\u043D\\u043A\\u0430 \\u0432 \\u0413\\u0435\\u0440\\u043C\\u0430\\u043D\\u0438\\u0438 \\u0441\\u043E\\u043E\\u0431\\u0449\\u0438\\u043B\\u0438 \\u043E \\u0432\\u0441\\u043F\\u043B\\u0435\\u0441\\u043A\\u0435 \\u0430\\u043A\\u0442\\u0438\\u0432\\u043D\\u043E\\u0441\\u0442\\u0438 \\u043D\\u0435\\u0438\\u0437\\u0432\\u0435\\u0441\\u0442\\u043D\\u043E\\u0433\\u043E \\u0438\\u0441\\u0442\\u043E\\u0447\\u043D\\u0438\\u043A\\u0430 \\u0440\\u0435\\u043D\\u0442\\u0433\\u0435\\u043D\\u043E\\u0432\\u0441\\u043A\\u0438\\u0445 \\u043B\\u0443\\u0447\\u0435\\u0439 \\u0432 \\u0433\\u0430\\u043B\\u0430\\u043A\\u0442\\u0438\\u043A\\u0435 NGC 300, \\u0440\\u0430\\u0441\\u043F\\u043E\\u043B\\u043E\\u0436\\u0435\\u043D\\u043D\\u043E\\u0439 \\u0432 \\u0441\\u0435\\u043C\\u0438 \\u043C\\u0438\\u043B\\u043B\\u0438\\u043E\\u043D\\u0430\\u0445 \\u0441\\u0432\\u0435\\u0442\\u043E\\u0432\\u044B\\u0445 \\u043B\\u0435\\u0442 \\u043E\\u0442 \\u0417\\u0435\\u043C\\u043B\\u0438.\" #@param {type:\"string\"}\nquery = \"\\u043D\\u0430 \\u043A\\u0430\\u043A\\u043E\\u043C \\u0440\\u0430\\u0441\\u0441\\u0442\\u043E\\u044F\\u043D\\u0438\\u0438?\" #@param {type:\"string\"}\nuse_token_ngram = True #@param {type:\"boolean\"}\n\nqa(text, [query], not use_token_ngram)\n",
"Shape: (110, 512)\nCandidates:\n\tАстрономы\n\tИнститута\n\tвнеземной\n\tфизики\n\tОбщества\n\tМакса\n\tПланка\n\tв\n\tГермании\n\tсообщили\n\tо\n\tвсплеске\n\tактивности\n\tнеизвестного\n\tисточника\n\tрентгеновских\n\tлучей\n\tв\n\tгалактике\n\tNGC\n\t300,\n\tрасположенной\n\tв\n\tсеми\n\tмиллионах\n\tсветовых\n\tлет\n\tот\n\tЗемли.\n\tАстрономы Института\n\tИнститута внеземной\n\tвнеземной физики\n\tфизики Общества\n\tОбщества Макса\n\tМакса Планка\n\tПланка в\n\tв Германии\n\tГермании сообщили\n\tсообщили о\n\tо всплеске\n\tвсплеске активности\n\tактивности неизвестного\n\tнеизвестного источника\n\tисточника рентгеновских\n\tрентгеновских лучей\n\tлучей в\n\tв галактике\n\tгалактике NGC\n\tNGC 300,\n\t300, расположенной\n\tрасположенной в\n\tв семи\n\tсеми миллионах\n\tмиллионах световых\n\tсветовых лет\n\tлет от\n\tот Земли.\n\tАстрономы Института внеземной\n\tИнститута внеземной физики\n\tвнеземной физики Общества\n\tфизики Общества Макса\n\tОбщества Макса Планка\n\tМакса Планка в\n\tПланка в Германии\n\tв Германии сообщили\n\tГермании сообщили о\n\tсообщили о всплеске\n\tо всплеске активности\n\tвсплеске активности неизвестного\n\tактивности неизвестного источника\n\tнеизвестного источника рентгеновских\n\tисточника рентгеновских лучей\n\tрентгеновских лучей в\n\tлучей в галактике\n\tв галактике NGC\n\tгалактике NGC 300,\n\tNGC 300, расположенной\n\t300, расположенной в\n\tрасположенной в семи\n\tв семи миллионах\n\tсеми миллионах световых\n\tмиллионах световых лет\n\tсветовых лет от\n\tлет от Земли.\n\tАстрономы Института внеземной физики\n\tИнститута внеземной физики Общества\n\tвнеземной физики Общества Макса\n\tфизики Общества Макса Планка\n\tОбщества Макса Планка в\n\tМакса Планка в Германии\n\tПланка в Германии сообщили\n\tв Германии сообщили о\n\tГермании сообщили о всплеске\n\tсообщили о всплеске активности\n\tо всплеске активности неизвестного\n\tвсплеске активности неизвестного источника\n\tактивности неизвестного источника рентгеновских\n\tнеизвестного источника рентгеновских лучей\n\tисточника рентгеновских лучей в\n\tрентгеновских лучей в галактике\n\tлучей в галактике NGC\n\tв галактике NGC 300,\n\tгалактике NGC 300, расположенной\n\tNGC 300, расположенной в\n\t300, расположенной в семи\n\tрасположенной в семи миллионах\n\tв семи миллионах световых\n\tсеми миллионах световых лет\n\tмиллионах световых лет от\n\tсветовых лет от Земли.\nQuery: на каком расстоянии?\nTop-10 Responses:\n\tвнеземной\n\tот Земли.\n\tлучей в\n\tрасположенной\n\tрасположенной в семи миллионах\n\tлет от Земли.\n\tрасположенной в\n\tв галактике\n\tрасположенной в семи\n\tрентгеновских лучей в\nCPU times: user 161 ms, sys: 20 ms, total: 181 ms\nWall time: 178 ms\n"
]
]
] | [
"markdown",
"code",
"markdown",
"code",
"markdown",
"code",
"markdown",
"code",
"markdown",
"code",
"markdown",
"code"
] | [
[
"markdown",
"markdown",
"markdown"
],
[
"code"
],
[
"markdown"
],
[
"code"
],
[
"markdown"
],
[
"code"
],
[
"markdown",
"markdown"
],
[
"code"
],
[
"markdown"
],
[
"code"
],
[
"markdown"
],
[
"code"
]
] |
e7688c816ca10e4da2bc4913e1e76958115538b6 | 46,694 | ipynb | Jupyter Notebook | notebooks/Metro styling.ipynb | WimYedema/dagviz | 8fe70a3a7e5fe89b157767a61a6bd9a6b868a341 | [
"Apache-2.0"
] | 4 | 2021-07-01T12:29:17.000Z | 2022-03-25T05:09:24.000Z | notebooks/Metro styling.ipynb | WimYedema/dagviz | 8fe70a3a7e5fe89b157767a61a6bd9a6b868a341 | [
"Apache-2.0"
] | null | null | null | notebooks/Metro styling.ipynb | WimYedema/dagviz | 8fe70a3a7e5fe89b157767a61a6bd9a6b868a341 | [
"Apache-2.0"
] | null | null | null | 94.141129 | 3,405 | 0.541783 | [
[
[
"# DAGVIZ Metro styling options\nThis notebook demonstrates the various Metro styling options.\n\nIn order to apply styling we need to call `render_svg` by hand with the appropriate renderer and style configuration.",
"_____no_output_____"
]
],
[
[
"from dagviz import render_svg\nfrom dagviz.style.metro import svg_renderer, StyleConfig\nfrom IPython.display import HTML\nimport networkx as nx",
"_____no_output_____"
]
],
[
[
"First we construct a simple graph that demonstrates all the visual aspects a rendering may have.",
"_____no_output_____"
]
],
[
[
"g = nx.DiGraph()\ng.add_node(\"a\", label=\"switch(value)\")\ng.add_node(\"b\", label=\"case 1\")\ng.add_node(\"c\", label=\"case 2\")\ng.add_node(\"d\", label=\"case 3\")\ng.add_node(\"e\", label=\"end\")\n\ng.add_edge(\"a\", \"b\")\ng.add_edge(\"a\", \"c\")\ng.add_edge(\"a\", \"d\")\n\ng.add_edge(\"b\", \"e\")\ng.add_edge(\"c\", \"e\")\ng.add_edge(\"d\", \"e\")",
"_____no_output_____"
]
],
[
[
"## Default rendering\nWithout any configuration, we get (of course) the default rendering:",
"_____no_output_____"
]
],
[
[
"HTML(render_svg(g))",
"_____no_output_____"
]
],
[
[
"## Scale\nThe *scale* setting determines the amount of space each node has:",
"_____no_output_____"
]
],
[
[
"HTML(render_svg(g, style=svg_renderer(StyleConfig(scale=20))))",
"_____no_output_____"
]
],
[
[
"## Node radius\nThe *node radius* determines the size of the bubbles:",
"_____no_output_____"
]
],
[
[
"HTML(render_svg(g, style=svg_renderer(StyleConfig(node_radius=10))))",
"_____no_output_____"
]
],
[
[
"## Node fill\nBy default the node fill color is automatically selected. This can be overriden by specifying a fixed color:",
"_____no_output_____"
]
],
[
[
"HTML(render_svg(g, style=svg_renderer(StyleConfig(node_fill=\"black\"))))",
"_____no_output_____"
]
],
[
[
"## Node stroke\nThe *node stroke* specifies the color of the border of the bubble:",
"_____no_output_____"
]
],
[
[
"HTML(render_svg(g, style=svg_renderer(StyleConfig(node_stroke=\"black\"))))",
"_____no_output_____"
]
],
[
[
"## Node stroke width\nThe *node stroke width* determines the width of the border of the bubbles:",
"_____no_output_____"
]
],
[
[
"HTML(render_svg(g, style=svg_renderer(StyleConfig(node_stroke=\"black\", node_stroke_width=4))))",
"_____no_output_____"
]
],
[
[
"## Edge stroke width\nThe *edge stroke width* determines the width of the edges:",
"_____no_output_____"
]
],
[
[
"HTML(render_svg(g, style=svg_renderer(StyleConfig(node_fill=\"black\", edge_stroke_width=10))))",
"_____no_output_____"
]
],
[
[
"## Label font family\nThe default font family for labels is \"sans-serif\". This can be changes too:",
"_____no_output_____"
]
],
[
[
"HTML(render_svg(g, style=svg_renderer(StyleConfig(label_font_family=\"serif\"))))",
"_____no_output_____"
]
],
[
[
"## Label arrow stroke\nThe *label arrow stroke* determines the color of the line from node to label:",
"_____no_output_____"
]
],
[
[
"HTML(render_svg(g, style=svg_renderer(StyleConfig(label_arrow_stroke=\"black\"))))",
"_____no_output_____"
]
],
[
[
"## Label arrow dash array\nThe *label arrow dash array* determines how the label arrow is dashed:",
"_____no_output_____"
]
],
[
[
"HTML(render_svg(g, style=svg_renderer(StyleConfig(label_arrow_dash_array=\"0\"))))",
"_____no_output_____"
]
],
[
[
"## Arc radius\nThe *arc radius* determines the radius of the arc from vertical line to a node:",
"_____no_output_____"
]
],
[
[
"HTML(render_svg(g, style=svg_renderer(StyleConfig(arc_radius=5))))",
"_____no_output_____"
],
[
"[\"..\"]*4",
"_____no_output_____"
],
[
"\"../\"*4",
"_____no_output_____"
]
]
] | [
"markdown",
"code",
"markdown",
"code",
"markdown",
"code",
"markdown",
"code",
"markdown",
"code",
"markdown",
"code",
"markdown",
"code",
"markdown",
"code",
"markdown",
"code",
"markdown",
"code",
"markdown",
"code",
"markdown",
"code",
"markdown",
"code"
] | [
[
"markdown"
],
[
"code"
],
[
"markdown"
],
[
"code"
],
[
"markdown"
],
[
"code"
],
[
"markdown"
],
[
"code"
],
[
"markdown"
],
[
"code"
],
[
"markdown"
],
[
"code"
],
[
"markdown"
],
[
"code"
],
[
"markdown"
],
[
"code"
],
[
"markdown"
],
[
"code"
],
[
"markdown"
],
[
"code"
],
[
"markdown"
],
[
"code"
],
[
"markdown"
],
[
"code"
],
[
"markdown"
],
[
"code",
"code",
"code"
]
] |
e768972eb4dae10a9da6c1353ec044fc18f56c85 | 8,166 | ipynb | Jupyter Notebook | site/en-snapshot/addons/tutorials/time_stopping.ipynb | ilyaspiridonov/docs-l10n | a061a44e40d25028d0a4458094e48ab717d3565c | [
"Apache-2.0"
] | 2 | 2021-02-22T12:15:33.000Z | 2021-05-02T15:22:13.000Z | site/en-snapshot/addons/tutorials/time_stopping.ipynb | ilyaspiridonov/docs-l10n | a061a44e40d25028d0a4458094e48ab717d3565c | [
"Apache-2.0"
] | null | null | null | site/en-snapshot/addons/tutorials/time_stopping.ipynb | ilyaspiridonov/docs-l10n | a061a44e40d25028d0a4458094e48ab717d3565c | [
"Apache-2.0"
] | 1 | 2020-06-23T13:30:15.000Z | 2020-06-23T13:30:15.000Z | 30.58427 | 245 | 0.492775 | [
[
[
"##### Copyright 2020 The TensorFlow Authors.",
"_____no_output_____"
]
],
[
[
"#@title Licensed under the Apache License, Version 2.0 (the \"License\");\n# you may not use this file except in compliance with the License.\n# You may obtain a copy of the License at\n#\n# https://www.apache.org/licenses/LICENSE-2.0\n#\n# Unless required by applicable law or agreed to in writing, software\n# distributed under the License is distributed on an \"AS IS\" BASIS,\n# WITHOUT WARRANTIES OR CONDITIONS OF ANY KIND, either express or implied.\n# See the License for the specific language governing permissions and\n# limitations under the License.",
"_____no_output_____"
]
],
[
[
"# TensorFlow Addons Callbacks: TimeStopping",
"_____no_output_____"
],
[
"<table class=\"tfo-notebook-buttons\" align=\"left\">\n <td>\n <a target=\"_blank\" href=\"https://www.tensorflow.org/addons/tutorials/time_stopping\"><img src=\"https://www.tensorflow.org/images/tf_logo_32px.png\" />View on TensorFlow.org</a>\n </td>\n <td>\n <a target=\"_blank\" href=\"https://colab.research.google.com/github/tensorflow/addons/blob/master/docs/tutorials/time_stopping.ipynb\"><img src=\"https://www.tensorflow.org/images/colab_logo_32px.png\" />Run in Google Colab</a>\n </td>\n <td>\n <a target=\"_blank\" href=\"https://github.com/tensorflow/addons/blob/master/docs/tutorials/time_stopping.ipynb\"><img src=\"https://www.tensorflow.org/images/GitHub-Mark-32px.png\" />View source on GitHub</a>\n </td>\n <td>\n <a href=\"https://storage.googleapis.com/tensorflow_docs/addons/docs/tutorials/time_stopping.ipynb\"><img src=\"https://www.tensorflow.org/images/download_logo_32px.png\" />Download notebook</a>\n </td>\n</table>",
"_____no_output_____"
],
[
"## Overview\nThis notebook will demonstrate how to use TimeStopping Callback in TensorFlow Addons.",
"_____no_output_____"
],
[
"## Setup",
"_____no_output_____"
]
],
[
[
"import tensorflow_addons as tfa\n\nfrom tensorflow.keras.datasets import mnist\nfrom tensorflow.keras.models import Sequential\nfrom tensorflow.keras.layers import Dense, Dropout, Flatten",
"_____no_output_____"
]
],
[
[
"## Import and Normalize Data",
"_____no_output_____"
]
],
[
[
"# the data, split between train and test sets\n(x_train, y_train), (x_test, y_test) = mnist.load_data()\n# normalize data\nx_train, x_test = x_train / 255.0, x_test / 255.0",
"Downloading data from https://storage.googleapis.com/tensorflow/tf-keras-datasets/mnist.npz\n11493376/11490434 [==============================] - 0s 0us/step\n"
]
],
[
[
"## Build Simple MNIST CNN Model",
"_____no_output_____"
]
],
[
[
"# build the model using the Sequential API\nmodel = Sequential()\nmodel.add(Flatten(input_shape=(28, 28)))\nmodel.add(Dense(128, activation='relu'))\nmodel.add(Dropout(0.2))\nmodel.add(Dense(10, activation='softmax'))\n\nmodel.compile(optimizer='adam',\n loss = 'sparse_categorical_crossentropy',\n metrics=['accuracy'])",
"_____no_output_____"
]
],
[
[
"## Simple TimeStopping Usage",
"_____no_output_____"
]
],
[
[
"# initialize TimeStopping callback \ntime_stopping_callback = tfa.callbacks.TimeStopping(seconds=5, verbose=1)\n\n# train the model with tqdm_callback\n# make sure to set verbose = 0 to disable\n# the default progress bar.\nmodel.fit(x_train, y_train,\n batch_size=64,\n epochs=100,\n callbacks=[time_stopping_callback],\n validation_data=(x_test, y_test))",
"Train on 60000 samples, validate on 10000 samples\nEpoch 1/100\n60000/60000 [==============================] - 5s 81us/sample - loss: 0.3432 - accuracy: 0.9003 - val_loss: 0.1601 - val_accuracy: 0.9529\nEpoch 2/100\n60000/60000 [==============================] - 4s 67us/sample - loss: 0.1651 - accuracy: 0.9515 - val_loss: 0.1171 - val_accuracy: 0.9642\nTimed stopping at epoch 2 after training for 0:00:05\n"
]
]
] | [
"markdown",
"code",
"markdown",
"code",
"markdown",
"code",
"markdown",
"code",
"markdown",
"code"
] | [
[
"markdown"
],
[
"code"
],
[
"markdown",
"markdown",
"markdown",
"markdown"
],
[
"code"
],
[
"markdown"
],
[
"code"
],
[
"markdown"
],
[
"code"
],
[
"markdown"
],
[
"code"
]
] |
e768a2d9c36fbfef14fc210f0c1d87b2104cf3fe | 30,899 | ipynb | Jupyter Notebook | notebooks/521_subset_human_retina_genes.ipynb | czbiohub/kh-analysis | bf598b54b18a10344a08a65889b54fa11d52701c | [
"MIT"
] | 1 | 2019-08-19T23:05:43.000Z | 2019-08-19T23:05:43.000Z | notebooks/521_subset_human_retina_genes.ipynb | czbiohub/kh-analysis | bf598b54b18a10344a08a65889b54fa11d52701c | [
"MIT"
] | 5 | 2019-10-08T23:05:57.000Z | 2020-03-03T17:39:26.000Z | notebooks/521_subset_human_retina_genes.ipynb | czbiohub/kh-analysis | bf598b54b18a10344a08a65889b54fa11d52701c | [
"MIT"
] | null | null | null | 27.465778 | 832 | 0.455419 | [
[
[
"import glob\n\nimport pandas as pd\nimport screed",
"_____no_output_____"
]
],
[
[
"# Change to Quest for Orthologs 2019 data directory",
"_____no_output_____"
]
],
[
[
"cd ~/data_sm/kmer-hashing/quest-for-orthologs/data/2019/",
"_____no_output_____"
],
[
"ls -lha",
"total 2.6G\ndrwxr-xr-x 5 olga root 4.0K Jan 10 08:02 \u001b[0m\u001b[01;34m.\u001b[0m/\ndrwxr-xr-x 3 olga root 4.0K Dec 25 17:48 \u001b[01;34m..\u001b[0m/\ndrwxr-xr-x 5 olga czb 4.0K Dec 26 19:44 \u001b[01;34mArchaea\u001b[0m/\ndrwxr-xr-x 5 olga czb 16K Dec 26 19:44 \u001b[01;34mBacteria\u001b[0m/\ndrwxr-xr-x 8 olga czb 32K Jan 8 08:13 \u001b[01;34mEukaryota\u001b[0m/\n-rw-r--r-- 1 olga czb 754K Jan 10 07:50 human_transcription_factors_with_uniprot_ids.csv\n-rw-r--r-- 1 olga czb 68K Jan 10 07:50 \u001b[01;31mhuman_transcription_factors_with_uniprot_ids.csv.gz\u001b[0m\n-rw-r--r-- 1 olga czb 133K Jan 10 07:50 human_transcription_factors_with_uniprot_ids.parquet\n-rw-r--r-- 1 olga czb 64M Jan 10 07:43 opisthokont_not_human_transcription_factors_ensembl_compara.csv\n-rw-r--r-- 1 olga czb 1.8M Jan 10 08:02 \u001b[01;31mopisthokont_not_human_transcription_factors_ensembl_compara_merged_uniprot.csv.gz\u001b[0m\u001b[K\n-rw-r--r-- 1 olga czb 2.2M Jan 10 08:02 opisthokont_not_human_transcription_factors_ensembl_compara_merged_uniprot.parquet\n-rw-r--r-- 1 olga czb 12M Jan 10 07:43 opisthokont_not_human_transcription_factors_ensembl_compara.parquet\n-rw-r--r-- 1 olga czb 661 Jan 8 17:33 qfo_human_vs_opisthokont_tfs.sh\n-rw-r--r-- 1 olga czb 2.6G Dec 25 18:46 \u001b[01;31mQfO_release_2019_04.tar.gz\u001b[0m\n-rw-r--r-- 1 olga czb 18K May 10 2019 README\n-rw-r--r-- 1 olga czb 12K Jan 8 07:47 species_metadata.csv\n"
]
],
[
[
"# Get Retinal gene names from ",
"_____no_output_____"
]
],
[
[
"s = 'RHO\tGNAT1\tGNB1\tGNGT1\tOPN1SW\tOPN1MW\tGNAT2\tGNB3\tGNGT2 PDE6A\tPDE6B\tPDE6G PDE6C\tPDE6H SAG\tARR3\tRGS9 CNGA1\tCNGA3\tCNGB1\tCNGB3 GRK1\tGRK7\tRCVRN\tGUCA1A\t\tGUCA1B\tGUCY2D\tGUCY2F'\ngenes = s.split()\ngenes",
"_____no_output_____"
],
[
"len(genes)",
"_____no_output_____"
],
[
"ensembl_rest.lookup_post(genes[0])",
"_____no_output_____"
],
[
"s = 'P08100\tP11488\tP62873\tP63211\tP03999\tP04001\tP19087\tP16520\tO14610 PDE6A\tPDE6B\tPDE6G PDE6C\tPDE6H P10523\tP36575\tO75916\t\t\tP29973\tQ16281\tQ14028\tB9EK43 Q15835\tQ8WTQ7\tP35243\tP43080\t\tQ9UMX6\tQ02846\tP51841'\nuniprot_ids = [x for x in s.split() if x]\nuniprot_ids",
"_____no_output_____"
],
[
"genes_to_ids = pd.DataFrame({'symbol': genes, 'uniprot_id': uniprot_ids})\nprint(genes_to_ids.shape)\ngenes_to_ids.head()",
"(28, 2)\n"
],
[
"genes_to_ids.tail()",
"_____no_output_____"
],
[
"len(uniprot_ids)",
"_____no_output_____"
]
],
[
[
"# Read Human ID mapping",
"_____no_output_____"
]
],
[
[
"human_id_mapping = pd.read_csv('Eukaryota/UP000005640_9606.idmapping', sep='\\t', header=None, names=['uniprot_id', 'id_type', 'db_id'])\nhuman_id_mapping.columns = 'source__' + human_id_mapping.columns\nprint(human_id_mapping.shape)\nhuman_id_mapping.head()",
"(2668934, 3)\n"
]
],
[
[
"## Extract with gene symbsls",
"_____no_output_____"
]
],
[
[
"human_id_mapping_symbols = human_id_mapping.query('source__db_id in @genes_to_ids.symbol')\nprint(human_id_mapping_symbols.shape)\nhuman_id_mapping_symbols.head()",
"(194, 3)\n"
],
[
"human_id_mapping_symbols.source__db_id.nunique()",
"_____no_output_____"
]
],
[
[
"## Extract with uniprot ids",
"_____no_output_____"
]
],
[
[
"human_id_mapping_uniprot = human_id_mapping.query('source__db_id in @genes_to_ids.uniprot_id')\nprint(human_id_mapping_uniprot.shape)\nhuman_id_mapping_uniprot.head()",
"(47, 3)\n"
],
[
"\"RHO\" in human_id_mapping.source__db_id.values",
"_____no_output_____"
]
],
[
[
"## Get ENSMBL ids",
"_____no_output_____"
]
],
[
[
"visual_uniprot_ids = set(human_id_mapping_uniprot.source__uniprot_id) | set(human_id_mapping_symbols.source__uniprot_id)\nlen(visual_uniprot_ids)",
"_____no_output_____"
],
[
"human_id_mapping_visual_system = human_id_mapping.query('source__uniprot_id in @visual_uniprot_ids')\nhuman_id_mapping_visual_system.head()",
"_____no_output_____"
]
],
[
[
"# Concatenate all human mappings",
"_____no_output_____"
]
],
[
[
"human_retina_ids = pd.concat([human_id_mapping_symbols, human_id_mapping_uniprot], ignore_index=True)\nhuman_retina_ids = human_retina_ids.drop_duplicates()\nprint(human_retina_ids.shape)\nhuman_retina_ids.head()",
"(202, 3)\n"
]
],
[
[
"## Write human retinal genes with uniprot IDs to disk",
"_____no_output_____"
]
],
[
[
"pwd",
"_____no_output_____"
],
[
"human_id_mapping_visual_system.to_csv(\"human_visual_transduction_with_uniprot_ids.csv\", index=False)\nhuman_id_mapping_visual_system.to_csv(\"human_visual_transduction_with_uniprot_ids.csv.gz\", index=False)\nhuman_id_mapping_visual_system.to_parquet(\"human_visual_transduction_with_uniprot_ids.parquet\", index=False)",
"_____no_output_____"
]
],
[
[
"# Read human proteins and subset to human tfs",
"_____no_output_____"
]
],
[
[
"retinal_uniprot = set(human_retina_ids.source__uniprot_id)\nlen(retinal_uniprot)",
"_____no_output_____"
],
[
"tf_records = []\n\n\nfor filename in glob.iglob('Eukaryota/human-protein-fastas/*.fasta'):\n with screed.open(filename) as records:\n for record in records:\n name = record['name']\n record_id = name.split()[0]\n uniprot_id = record_id.split('|')[1]\n if uniprot_id in retinal_uniprot:\n tf_records.append(record)\nprint(len(tf_records))\n",
"67\n"
],
[
"tf_records[:3]",
"_____no_output_____"
]
],
[
[
"## Write output",
"_____no_output_____"
]
],
[
[
"human_outdir = 'Eukaryota/human-visual-transduction-fastas/'\n! mkdir $human_outdir\n\n\nwith open(f'{human_outdir}/human_visual_transduction_proteins.fasta', 'w') as f:\n for record in tf_records:\n f.write(\">{name}\\n{sequence}\\n\".format(**record))",
"mkdir: cannot create directory ‘Eukaryota/human-visual-transduction-fastas/’: File exists\n"
]
]
] | [
"code",
"markdown",
"code",
"markdown",
"code",
"markdown",
"code",
"markdown",
"code",
"markdown",
"code",
"markdown",
"code",
"markdown",
"code",
"markdown",
"code",
"markdown",
"code",
"markdown",
"code"
] | [
[
"code"
],
[
"markdown"
],
[
"code",
"code"
],
[
"markdown"
],
[
"code",
"code",
"code",
"code",
"code",
"code",
"code"
],
[
"markdown"
],
[
"code"
],
[
"markdown"
],
[
"code",
"code"
],
[
"markdown"
],
[
"code",
"code"
],
[
"markdown"
],
[
"code",
"code"
],
[
"markdown"
],
[
"code"
],
[
"markdown"
],
[
"code",
"code"
],
[
"markdown"
],
[
"code",
"code",
"code"
],
[
"markdown"
],
[
"code"
]
] |
e768a75971c8fe65fd0d84d3554ba861a99ae0ce | 86,135 | ipynb | Jupyter Notebook | Course5 - Sequence Models/week1 Recurrent Neural Networks/Building a recurrent neural network/Building+a+Recurrent+Neural+Network+-+Step+by+Step+-+v3.ipynb | bheil123/DeepLearning | ff24ee21176d8085bcdc21121bdddcf39f867c19 | [
"MIT"
] | null | null | null | Course5 - Sequence Models/week1 Recurrent Neural Networks/Building a recurrent neural network/Building+a+Recurrent+Neural+Network+-+Step+by+Step+-+v3.ipynb | bheil123/DeepLearning | ff24ee21176d8085bcdc21121bdddcf39f867c19 | [
"MIT"
] | null | null | null | Course5 - Sequence Models/week1 Recurrent Neural Networks/Building a recurrent neural network/Building+a+Recurrent+Neural+Network+-+Step+by+Step+-+v3.ipynb | bheil123/DeepLearning | ff24ee21176d8085bcdc21121bdddcf39f867c19 | [
"MIT"
] | null | null | null | 40.2312 | 683 | 0.464213 | [
[
[
"# Building your Recurrent Neural Network - Step by Step\n\nWelcome to Course 5's first assignment! In this assignment, you will implement your first Recurrent Neural Network in numpy.\n\nRecurrent Neural Networks (RNN) are very effective for Natural Language Processing and other sequence tasks because they have \"memory\". They can read inputs $x^{\\langle t \\rangle}$ (such as words) one at a time, and remember some information/context through the hidden layer activations that get passed from one time-step to the next. This allows a uni-directional RNN to take information from the past to process later inputs. A bidirection RNN can take context from both the past and the future. \n\n**Notation**:\n- Superscript $[l]$ denotes an object associated with the $l^{th}$ layer. \n - Example: $a^{[4]}$ is the $4^{th}$ layer activation. $W^{[5]}$ and $b^{[5]}$ are the $5^{th}$ layer parameters.\n\n- Superscript $(i)$ denotes an object associated with the $i^{th}$ example. \n - Example: $x^{(i)}$ is the $i^{th}$ training example input.\n\n- Superscript $\\langle t \\rangle$ denotes an object at the $t^{th}$ time-step. \n - Example: $x^{\\langle t \\rangle}$ is the input x at the $t^{th}$ time-step. $x^{(i)\\langle t \\rangle}$ is the input at the $t^{th}$ timestep of example $i$.\n \n- Lowerscript $i$ denotes the $i^{th}$ entry of a vector.\n - Example: $a^{[l]}_i$ denotes the $i^{th}$ entry of the activations in layer $l$.\n\nWe assume that you are already familiar with `numpy` and/or have completed the previous courses of the specialization. Let's get started!",
"_____no_output_____"
],
[
"Let's first import all the packages that you will need during this assignment.",
"_____no_output_____"
]
],
[
[
"import numpy as np\nfrom rnn_utils import *",
"_____no_output_____"
]
],
[
[
"## 1 - Forward propagation for the basic Recurrent Neural Network\n\nLater this week, you will generate music using an RNN. The basic RNN that you will implement has the structure below. In this example, $T_x = T_y$. ",
"_____no_output_____"
],
[
"<img src=\"images/RNN.png\" style=\"width:500;height:300px;\">\n<caption><center> **Figure 1**: Basic RNN model </center></caption>",
"_____no_output_____"
],
[
"Here's how you can implement an RNN: \n\n**Steps**:\n1. Implement the calculations needed for one time-step of the RNN.\n2. Implement a loop over $T_x$ time-steps in order to process all the inputs, one at a time. \n\nLet's go!\n\n## 1.1 - RNN cell\n\nA Recurrent neural network can be seen as the repetition of a single cell. You are first going to implement the computations for a single time-step. The following figure describes the operations for a single time-step of an RNN cell. \n\n<img src=\"images/rnn_step_forward.png\" style=\"width:700px;height:300px;\">\n<caption><center> **Figure 2**: Basic RNN cell. Takes as input $x^{\\langle t \\rangle}$ (current input) and $a^{\\langle t - 1\\rangle}$ (previous hidden state containing information from the past), and outputs $a^{\\langle t \\rangle}$ which is given to the next RNN cell and also used to predict $y^{\\langle t \\rangle}$ </center></caption>\n\n**Exercise**: Implement the RNN-cell described in Figure (2).\n\n**Instructions**:\n1. Compute the hidden state with tanh activation: $a^{\\langle t \\rangle} = \\tanh(W_{aa} a^{\\langle t-1 \\rangle} + W_{ax} x^{\\langle t \\rangle} + b_a)$.\n2. Using your new hidden state $a^{\\langle t \\rangle}$, compute the prediction $\\hat{y}^{\\langle t \\rangle} = softmax(W_{ya} a^{\\langle t \\rangle} + b_y)$. We provided you a function: `softmax`.\n3. Store $(a^{\\langle t \\rangle}, a^{\\langle t-1 \\rangle}, x^{\\langle t \\rangle}, parameters)$ in cache\n4. Return $a^{\\langle t \\rangle}$ , $y^{\\langle t \\rangle}$ and cache\n\nWe will vectorize over $m$ examples. Thus, $x^{\\langle t \\rangle}$ will have dimension $(n_x,m)$, and $a^{\\langle t \\rangle}$ will have dimension $(n_a,m)$. ",
"_____no_output_____"
]
],
[
[
"# GRADED FUNCTION: rnn_cell_forward\n\ndef rnn_cell_forward(xt, a_prev, parameters):\n \"\"\"\n Implements a single forward step of the RNN-cell as described in Figure (2)\n\n Arguments:\n xt -- your input data at timestep \"t\", numpy array of shape (n_x, m).\n a_prev -- Hidden state at timestep \"t-1\", numpy array of shape (n_a, m)\n parameters -- python dictionary containing:\n Wax -- Weight matrix multiplying the input, numpy array of shape (n_a, n_x)\n Waa -- Weight matrix multiplying the hidden state, numpy array of shape (n_a, n_a)\n Wya -- Weight matrix relating the hidden-state to the output, numpy array of shape (n_y, n_a)\n ba -- Bias, numpy array of shape (n_a, 1)\n by -- Bias relating the hidden-state to the output, numpy array of shape (n_y, 1)\n Returns:\n a_next -- next hidden state, of shape (n_a, m)\n yt_pred -- prediction at timestep \"t\", numpy array of shape (n_y, m)\n cache -- tuple of values needed for the backward pass, contains (a_next, a_prev, xt, parameters)\n \"\"\"\n \n # Retrieve parameters from \"parameters\"\n Wax = parameters[\"Wax\"]\n Waa = parameters[\"Waa\"]\n Wya = parameters[\"Wya\"]\n ba = parameters[\"ba\"]\n by = parameters[\"by\"]\n \n ### START CODE HERE ### (≈2 lines)\n # compute next activation state using the formula given above\n a_next = np.tanh(np.dot(Wax,xt) + np.dot(Waa, a_prev) + ba)\n # compute output of the current cell using the formula given above\n yt_pred = softmax(np.dot(Wya, a_next) + by) \n ### END CODE HERE ###\n \n # store values you need for backward propagation in cache\n cache = (a_next, a_prev, xt, parameters)\n \n return a_next, yt_pred, cache",
"_____no_output_____"
],
[
"np.random.seed(1)\nxt = np.random.randn(3,10)\na_prev = np.random.randn(5,10)\nWaa = np.random.randn(5,5)\nWax = np.random.randn(5,3)\nWya = np.random.randn(2,5)\nba = np.random.randn(5,1)\nby = np.random.randn(2,1)\nparameters = {\"Waa\": Waa, \"Wax\": Wax, \"Wya\": Wya, \"ba\": ba, \"by\": by}\n\na_next, yt_pred, cache = rnn_cell_forward(xt, a_prev, parameters)\nprint(\"a_next[4] = \", a_next[4])\nprint(\"a_next.shape = \", a_next.shape)\nprint(\"yt_pred[1] =\", yt_pred[1])\nprint(\"yt_pred.shape = \", yt_pred.shape)",
"a_next[4] = [ 0.59584544 0.18141802 0.61311866 0.99808218 0.85016201 0.99980978\n -0.18887155 0.99815551 0.6531151 0.82872037]\na_next.shape = (5, 10)\nyt_pred[1] = [ 0.9888161 0.01682021 0.21140899 0.36817467 0.98988387 0.88945212\n 0.36920224 0.9966312 0.9982559 0.17746526]\nyt_pred.shape = (2, 10)\n"
]
],
[
[
"**Expected Output**: \n\n<table>\n <tr>\n <td>\n **a_next[4]**:\n </td>\n <td>\n [ 0.59584544 0.18141802 0.61311866 0.99808218 0.85016201 0.99980978\n -0.18887155 0.99815551 0.6531151 0.82872037]\n </td>\n </tr>\n <tr>\n <td>\n **a_next.shape**:\n </td>\n <td>\n (5, 10)\n </td>\n </tr>\n <tr>\n <td>\n **yt[1]**:\n </td>\n <td>\n [ 0.9888161 0.01682021 0.21140899 0.36817467 0.98988387 0.88945212\n 0.36920224 0.9966312 0.9982559 0.17746526]\n </td>\n </tr>\n <tr>\n <td>\n **yt.shape**:\n </td>\n <td>\n (2, 10)\n </td>\n </tr>\n\n</table>",
"_____no_output_____"
],
[
"## 1.2 - RNN forward pass \n\nYou can see an RNN as the repetition of the cell you've just built. If your input sequence of data is carried over 10 time steps, then you will copy the RNN cell 10 times. Each cell takes as input the hidden state from the previous cell ($a^{\\langle t-1 \\rangle}$) and the current time-step's input data ($x^{\\langle t \\rangle}$). It outputs a hidden state ($a^{\\langle t \\rangle}$) and a prediction ($y^{\\langle t \\rangle}$) for this time-step.\n\n\n<img src=\"images/rnn.png\" style=\"width:800px;height:300px;\">\n<caption><center> **Figure 3**: Basic RNN. The input sequence $x = (x^{\\langle 1 \\rangle}, x^{\\langle 2 \\rangle}, ..., x^{\\langle T_x \\rangle})$ is carried over $T_x$ time steps. The network outputs $y = (y^{\\langle 1 \\rangle}, y^{\\langle 2 \\rangle}, ..., y^{\\langle T_x \\rangle})$. </center></caption>\n\n\n\n**Exercise**: Code the forward propagation of the RNN described in Figure (3).\n\n**Instructions**:\n1. Create a vector of zeros ($a$) that will store all the hidden states computed by the RNN.\n2. Initialize the \"next\" hidden state as $a_0$ (initial hidden state).\n3. Start looping over each time step, your incremental index is $t$ :\n - Update the \"next\" hidden state and the cache by running `rnn_cell_forward`\n - Store the \"next\" hidden state in $a$ ($t^{th}$ position) \n - Store the prediction in y\n - Add the cache to the list of caches\n4. Return $a$, $y$ and caches",
"_____no_output_____"
]
],
[
[
"# GRADED FUNCTION: rnn_forward\n\ndef rnn_forward(x, a0, parameters):\n \"\"\"\n Implement the forward propagation of the recurrent neural network described in Figure (3).\n\n Arguments:\n x -- Input data for every time-step, of shape (n_x, m, T_x).\n a0 -- Initial hidden state, of shape (n_a, m)\n parameters -- python dictionary containing:\n Waa -- Weight matrix multiplying the hidden state, numpy array of shape (n_a, n_a)\n Wax -- Weight matrix multiplying the input, numpy array of shape (n_a, n_x)\n Wya -- Weight matrix relating the hidden-state to the output, numpy array of shape (n_y, n_a)\n ba -- Bias numpy array of shape (n_a, 1)\n by -- Bias relating the hidden-state to the output, numpy array of shape (n_y, 1)\n\n Returns:\n a -- Hidden states for every time-step, numpy array of shape (n_a, m, T_x)\n y_pred -- Predictions for every time-step, numpy array of shape (n_y, m, T_x)\n caches -- tuple of values needed for the backward pass, contains (list of caches, x)\n \"\"\"\n \n # Initialize \"caches\" which will contain the list of all caches\n caches = []\n \n # Retrieve dimensions from shapes of x and parameters[\"Wya\"]\n n_x, m, T_x = x.shape\n n_y, n_a = parameters[\"Wya\"].shape\n \n ### START CODE HERE ###\n \n # initialize \"a\" and \"y\" with zeros (≈2 lines)\n a = np.zeros((n_a, m, T_x))\n y_pred = np.zeros((n_y, m, T_x))\n \n # Initialize a_next (≈1 line)\n a_next = a0\n \n # loop over all time-steps\n for t in range(T_x):\n # Update next hidden state, compute the prediction, get the cache (≈1 line)\n a_next, yt_pred, cache = rnn_cell_forward(x[:,:,t], a_next, parameters)\n # Save the value of the new \"next\" hidden state in a (≈1 line)\n a[:,:,t] = a_next\n # Save the value of the prediction in y (≈1 line)\n y_pred[:,:,t] = yt_pred\n # Append \"cache\" to \"caches\" (≈1 line)\n caches.append(cache)\n \n ### END CODE HERE ###\n \n # store values needed for backward propagation in cache\n caches = (caches, x)\n \n return a, y_pred, caches",
"_____no_output_____"
],
[
"np.random.seed(1)\nx = np.random.randn(3,10,4)\na0 = np.random.randn(5,10)\nWaa = np.random.randn(5,5)\nWax = np.random.randn(5,3)\nWya = np.random.randn(2,5)\nba = np.random.randn(5,1)\nby = np.random.randn(2,1)\nparameters = {\"Waa\": Waa, \"Wax\": Wax, \"Wya\": Wya, \"ba\": ba, \"by\": by}\n\na, y_pred, caches = rnn_forward(x, a0, parameters)\nprint(\"a[4][1] = \", a[4][1])\nprint(\"a.shape = \", a.shape)\nprint(\"y_pred[1][3] =\", y_pred[1][3])\nprint(\"y_pred.shape = \", y_pred.shape)\nprint(\"caches[1][1][3] =\", caches[1][1][3])\nprint(\"len(caches) = \", len(caches))",
"a[4][1] = [-0.99999375 0.77911235 -0.99861469 -0.99833267]\na.shape = (5, 10, 4)\ny_pred[1][3] = [ 0.79560373 0.86224861 0.11118257 0.81515947]\ny_pred.shape = (2, 10, 4)\ncaches[1][1][3] = [-1.1425182 -0.34934272 -0.20889423 0.58662319]\nlen(caches) = 2\n"
]
],
[
[
"**Expected Output**:\n\n<table>\n <tr>\n <td>\n **a[4][1]**:\n </td>\n <td>\n [-0.99999375 0.77911235 -0.99861469 -0.99833267]\n </td>\n </tr>\n <tr>\n <td>\n **a.shape**:\n </td>\n <td>\n (5, 10, 4)\n </td>\n </tr>\n <tr>\n <td>\n **y[1][3]**:\n </td>\n <td>\n [ 0.79560373 0.86224861 0.11118257 0.81515947]\n </td>\n </tr>\n <tr>\n <td>\n **y.shape**:\n </td>\n <td>\n (2, 10, 4)\n </td>\n </tr>\n <tr>\n <td>\n **cache[1][1][3]**:\n </td>\n <td>\n [-1.1425182 -0.34934272 -0.20889423 0.58662319]\n </td>\n </tr>\n <tr>\n <td>\n **len(cache)**:\n </td>\n <td>\n 2\n </td>\n </tr>\n\n</table>",
"_____no_output_____"
],
[
"Congratulations! You've successfully built the forward propagation of a recurrent neural network from scratch. This will work well enough for some applications, but it suffers from vanishing gradient problems. So it works best when each output $y^{\\langle t \\rangle}$ can be estimated using mainly \"local\" context (meaning information from inputs $x^{\\langle t' \\rangle}$ where $t'$ is not too far from $t$). \n\nIn the next part, you will build a more complex LSTM model, which is better at addressing vanishing gradients. The LSTM will be better able to remember a piece of information and keep it saved for many timesteps. ",
"_____no_output_____"
],
[
"## 2 - Long Short-Term Memory (LSTM) network\n\nThis following figure shows the operations of an LSTM-cell.\n\n<img src=\"images/LSTM.png\" style=\"width:500;height:400px;\">\n<caption><center> **Figure 4**: LSTM-cell. This tracks and updates a \"cell state\" or memory variable $c^{\\langle t \\rangle}$ at every time-step, which can be different from $a^{\\langle t \\rangle}$. </center></caption>\n\nSimilar to the RNN example above, you will start by implementing the LSTM cell for a single time-step. Then you can iteratively call it from inside a for-loop to have it process an input with $T_x$ time-steps. \n\n### About the gates\n\n#### - Forget gate\n\nFor the sake of this illustration, lets assume we are reading words in a piece of text, and want use an LSTM to keep track of grammatical structures, such as whether the subject is singular or plural. If the subject changes from a singular word to a plural word, we need to find a way to get rid of our previously stored memory value of the singular/plural state. In an LSTM, the forget gate lets us do this: \n\n$$\\Gamma_f^{\\langle t \\rangle} = \\sigma(W_f[a^{\\langle t-1 \\rangle}, x^{\\langle t \\rangle}] + b_f)\\tag{1} $$\n\nHere, $W_f$ are weights that govern the forget gate's behavior. We concatenate $[a^{\\langle t-1 \\rangle}, x^{\\langle t \\rangle}]$ and multiply by $W_f$. The equation above results in a vector $\\Gamma_f^{\\langle t \\rangle}$ with values between 0 and 1. This forget gate vector will be multiplied element-wise by the previous cell state $c^{\\langle t-1 \\rangle}$. So if one of the values of $\\Gamma_f^{\\langle t \\rangle}$ is 0 (or close to 0) then it means that the LSTM should remove that piece of information (e.g. the singular subject) in the corresponding component of $c^{\\langle t-1 \\rangle}$. If one of the values is 1, then it will keep the information. \n\n#### - Update gate\n\nOnce we forget that the subject being discussed is singular, we need to find a way to update it to reflect that the new subject is now plural. Here is the formulat for the update gate: \n\n$$\\Gamma_u^{\\langle t \\rangle} = \\sigma(W_u[a^{\\langle t-1 \\rangle}, x^{\\{t\\}}] + b_u)\\tag{2} $$ \n\nSimilar to the forget gate, here $\\Gamma_u^{\\langle t \\rangle}$ is again a vector of values between 0 and 1. This will be multiplied element-wise with $\\tilde{c}^{\\langle t \\rangle}$, in order to compute $c^{\\langle t \\rangle}$.\n\n#### - Updating the cell \n\nTo update the new subject we need to create a new vector of numbers that we can add to our previous cell state. The equation we use is: \n\n$$ \\tilde{c}^{\\langle t \\rangle} = \\tanh(W_c[a^{\\langle t-1 \\rangle}, x^{\\langle t \\rangle}] + b_c)\\tag{3} $$\n\nFinally, the new cell state is: \n\n$$ c^{\\langle t \\rangle} = \\Gamma_f^{\\langle t \\rangle}* c^{\\langle t-1 \\rangle} + \\Gamma_u^{\\langle t \\rangle} *\\tilde{c}^{\\langle t \\rangle} \\tag{4} $$\n\n\n#### - Output gate\n\nTo decide which outputs we will use, we will use the following two formulas: \n\n$$ \\Gamma_o^{\\langle t \\rangle}= \\sigma(W_o[a^{\\langle t-1 \\rangle}, x^{\\langle t \\rangle}] + b_o)\\tag{5}$$ \n$$ a^{\\langle t \\rangle} = \\Gamma_o^{\\langle t \\rangle}* \\tanh(c^{\\langle t \\rangle})\\tag{6} $$\n\nWhere in equation 5 you decide what to output using a sigmoid function and in equation 6 you multiply that by the $\\tanh$ of the previous state. ",
"_____no_output_____"
],
[
"### 2.1 - LSTM cell\n\n**Exercise**: Implement the LSTM cell described in the Figure (3).\n\n**Instructions**:\n1. Concatenate $a^{\\langle t-1 \\rangle}$ and $x^{\\langle t \\rangle}$ in a single matrix: $concat = \\begin{bmatrix} a^{\\langle t-1 \\rangle} \\\\ x^{\\langle t \\rangle} \\end{bmatrix}$\n2. Compute all the formulas 1-6. You can use `sigmoid()` (provided) and `np.tanh()`.\n3. Compute the prediction $y^{\\langle t \\rangle}$. You can use `softmax()` (provided).",
"_____no_output_____"
]
],
[
[
"# GRADED FUNCTION: lstm_cell_forward\n\ndef lstm_cell_forward(xt, a_prev, c_prev, parameters):\n \"\"\"\n Implement a single forward step of the LSTM-cell as described in Figure (4)\n\n Arguments:\n xt -- your input data at timestep \"t\", numpy array of shape (n_x, m).\n a_prev -- Hidden state at timestep \"t-1\", numpy array of shape (n_a, m)\n c_prev -- Memory state at timestep \"t-1\", numpy array of shape (n_a, m)\n parameters -- python dictionary containing:\n Wf -- Weight matrix of the forget gate, numpy array of shape (n_a, n_a + n_x)\n bf -- Bias of the forget gate, numpy array of shape (n_a, 1)\n Wi -- Weight matrix of the update gate, numpy array of shape (n_a, n_a + n_x)\n bi -- Bias of the update gate, numpy array of shape (n_a, 1)\n Wc -- Weight matrix of the first \"tanh\", numpy array of shape (n_a, n_a + n_x)\n bc -- Bias of the first \"tanh\", numpy array of shape (n_a, 1)\n Wo -- Weight matrix of the output gate, numpy array of shape (n_a, n_a + n_x)\n bo -- Bias of the output gate, numpy array of shape (n_a, 1)\n Wy -- Weight matrix relating the hidden-state to the output, numpy array of shape (n_y, n_a)\n by -- Bias relating the hidden-state to the output, numpy array of shape (n_y, 1)\n \n Returns:\n a_next -- next hidden state, of shape (n_a, m)\n c_next -- next memory state, of shape (n_a, m)\n yt_pred -- prediction at timestep \"t\", numpy array of shape (n_y, m)\n cache -- tuple of values needed for the backward pass, contains (a_next, c_next, a_prev, c_prev, xt, parameters)\n \n Note: ft/it/ot stand for the forget/update/output gates, cct stands for the candidate value (c tilde),\n c stands for the memory value\n \"\"\"\n\n # Retrieve parameters from \"parameters\"\n Wf = parameters[\"Wf\"]\n bf = parameters[\"bf\"]\n Wi = parameters[\"Wi\"]\n bi = parameters[\"bi\"]\n Wc = parameters[\"Wc\"]\n bc = parameters[\"bc\"]\n Wo = parameters[\"Wo\"]\n bo = parameters[\"bo\"]\n Wy = parameters[\"Wy\"]\n by = parameters[\"by\"]\n \n # Retrieve dimensions from shapes of xt and Wy\n n_x, m = xt.shape\n n_y, n_a = Wy.shape\n\n ### START CODE HERE ###\n # Concatenate a_prev and xt (≈3 lines)\n concat = np.zeros((n_a+n_x, m))\n concat[:n_a, :] = a_prev\n concat[n_a:, :] = xt\n\n # Compute values for ft, it, cct, c_next, ot, a_next using the formulas given figure (4) (≈6 lines)\n ft = sigmoid(np.dot(Wf, concat) + bf)\n it = sigmoid(np.dot(Wi, concat) + bi)\n ctilda = np.tanh(np.dot(Wc, concat) + bc)\n c_next = ft*c_prev + it* ctilda\n ot = sigmoid(np.dot(Wo, concat) + bo)\n a_next = ot*np.tanh(c_next)\n \n # Compute prediction of the LSTM cell (≈1 line)\n yt_pred = softmax(np.dot(Wy, a_next) + by)\n ### END CODE HERE ###\n\n # store values needed for backward propagation in cache\n #cache = (a_next, c_next, a_prev, c_prev, ft, it, cct, ot, xt, parameters)\n cache = (a_next, c_next, a_prev, c_prev, ft, it, ctilda, ot, xt, parameters)\n\n return a_next, c_next, yt_pred, cache",
"_____no_output_____"
],
[
"np.random.seed(1)\nxt = np.random.randn(3,10)\na_prev = np.random.randn(5,10)\nc_prev = np.random.randn(5,10)\nWf = np.random.randn(5, 5+3)\nbf = np.random.randn(5,1)\nWi = np.random.randn(5, 5+3)\nbi = np.random.randn(5,1)\nWo = np.random.randn(5, 5+3)\nbo = np.random.randn(5,1)\nWc = np.random.randn(5, 5+3)\nbc = np.random.randn(5,1)\nWy = np.random.randn(2,5)\nby = np.random.randn(2,1)\n\nparameters = {\"Wf\": Wf, \"Wi\": Wi, \"Wo\": Wo, \"Wc\": Wc, \"Wy\": Wy, \"bf\": bf, \"bi\": bi, \"bo\": bo, \"bc\": bc, \"by\": by}\n\na_next, c_next, yt, cache = lstm_cell_forward(xt, a_prev, c_prev, parameters)\nprint(\"a_next[4] = \", a_next[4])\nprint(\"a_next.shape = \", c_next.shape)\nprint(\"c_next[2] = \", c_next[2])\nprint(\"c_next.shape = \", c_next.shape)\nprint(\"yt[1] =\", yt[1])\nprint(\"yt.shape = \", yt.shape)\nprint(\"cache[1][3] =\", cache[1][3])\nprint(\"len(cache) = \", len(cache))",
"a_next[4] = [-0.66408471 0.0036921 0.02088357 0.22834167 -0.85575339 0.00138482\n 0.76566531 0.34631421 -0.00215674 0.43827275]\na_next.shape = (5, 10)\nc_next[2] = [ 0.63267805 1.00570849 0.35504474 0.20690913 -1.64566718 0.11832942\n 0.76449811 -0.0981561 -0.74348425 -0.26810932]\nc_next.shape = (5, 10)\nyt[1] = [ 0.79913913 0.15986619 0.22412122 0.15606108 0.97057211 0.31146381\n 0.00943007 0.12666353 0.39380172 0.07828381]\nyt.shape = (2, 10)\ncache[1][3] = [-0.16263996 1.03729328 0.72938082 -0.54101719 0.02752074 -0.30821874\n 0.07651101 -1.03752894 1.41219977 -0.37647422]\nlen(cache) = 10\n"
]
],
[
[
"**Expected Output**:\n\n<table>\n <tr>\n <td>\n **a_next[4]**:\n </td>\n <td>\n [-0.66408471 0.0036921 0.02088357 0.22834167 -0.85575339 0.00138482\n 0.76566531 0.34631421 -0.00215674 0.43827275]\n </td>\n </tr>\n <tr>\n <td>\n **a_next.shape**:\n </td>\n <td>\n (5, 10)\n </td>\n </tr>\n <tr>\n <td>\n **c_next[2]**:\n </td>\n <td>\n [ 0.63267805 1.00570849 0.35504474 0.20690913 -1.64566718 0.11832942\n 0.76449811 -0.0981561 -0.74348425 -0.26810932]\n </td>\n </tr>\n <tr>\n <td>\n **c_next.shape**:\n </td>\n <td>\n (5, 10)\n </td>\n </tr>\n <tr>\n <td>\n **yt[1]**:\n </td>\n <td>\n [ 0.79913913 0.15986619 0.22412122 0.15606108 0.97057211 0.31146381\n 0.00943007 0.12666353 0.39380172 0.07828381]\n </td>\n </tr>\n <tr>\n <td>\n **yt.shape**:\n </td>\n <td>\n (2, 10)\n </td>\n </tr>\n <tr>\n <td>\n **cache[1][3]**:\n </td>\n <td>\n [-0.16263996 1.03729328 0.72938082 -0.54101719 0.02752074 -0.30821874\n 0.07651101 -1.03752894 1.41219977 -0.37647422]\n </td>\n </tr>\n <tr>\n <td>\n **len(cache)**:\n </td>\n <td>\n 10\n </td>\n </tr>\n\n</table>",
"_____no_output_____"
],
[
"### 2.2 - Forward pass for LSTM\n\nNow that you have implemented one step of an LSTM, you can now iterate this over this using a for-loop to process a sequence of $T_x$ inputs. \n\n<img src=\"images/LSTM_rnn.png\" style=\"width:500;height:300px;\">\n<caption><center> **Figure 4**: LSTM over multiple time-steps. </center></caption>\n\n**Exercise:** Implement `lstm_forward()` to run an LSTM over $T_x$ time-steps. \n\n**Note**: $c^{\\langle 0 \\rangle}$ is initialized with zeros.",
"_____no_output_____"
]
],
[
[
"# GRADED FUNCTION: lstm_forward\n\ndef lstm_forward(x, a0, parameters):\n \"\"\"\n Implement the forward propagation of the recurrent neural network using an LSTM-cell described in Figure (3).\n\n Arguments:\n x -- Input data for every time-step, of shape (n_x, m, T_x).\n a0 -- Initial hidden state, of shape (n_a, m)\n parameters -- python dictionary containing:\n Wf -- Weight matrix of the forget gate, numpy array of shape (n_a, n_a + n_x)\n bf -- Bias of the forget gate, numpy array of shape (n_a, 1)\n Wi -- Weight matrix of the update gate, numpy array of shape (n_a, n_a + n_x)\n bi -- Bias of the update gate, numpy array of shape (n_a, 1)\n Wc -- Weight matrix of the first \"tanh\", numpy array of shape (n_a, n_a + n_x)\n bc -- Bias of the first \"tanh\", numpy array of shape (n_a, 1)\n Wo -- Weight matrix of the output gate, numpy array of shape (n_a, n_a + n_x)\n bo -- Bias of the output gate, numpy array of shape (n_a, 1)\n Wy -- Weight matrix relating the hidden-state to the output, numpy array of shape (n_y, n_a)\n by -- Bias relating the hidden-state to the output, numpy array of shape (n_y, 1)\n \n Returns:\n a -- Hidden states for every time-step, numpy array of shape (n_a, m, T_x)\n y -- Predictions for every time-step, numpy array of shape (n_y, m, T_x)\n caches -- tuple of values needed for the backward pass, contains (list of all the caches, x)\n \"\"\"\n\n # Initialize \"caches\", which will track the list of all the caches\n caches = []\n \n ### START CODE HERE ###\n # Retrieve dimensions from shapes of x and parameters['Wy'] (≈2 lines)\n n_x, m, T_x = x.shape\n n_y, n_a = parameters['Wy'].shape\n \n # initialize \"a\", \"c\" and \"y\" with zeros (≈3 lines)\n a = np.zeros((n_a, m, T_x))\n c = np.zeros((n_a, m, T_x))\n y = np.zeros((n_y, m, T_x))\n \n # Initialize a_next and c_next (≈2 lines)\n a_next = a0\n c_next = np.zeros((n_a, m))\n \n # loop over all time-steps\n for t in range(T_x):\n # Update next hidden state, next memory state, compute the prediction, get the cache (≈1 line)\n a_next, c_next, yt, cache = lstm_cell_forward(x[:,:,t], a_next, c_next, parameters)\n # Save the value of the new \"next\" hidden state in a (≈1 line)\n a[:,:,t] = a_next\n # Save the value of the prediction in y (≈1 line)\n y[:,:,t] = yt\n # Save the value of the next cell state (≈1 line)\n c[:,:,t] = c_next\n # Append the cache into caches (≈1 line)\n caches.append(cache)\n \n ### END CODE HERE ###\n \n # store values needed for backward propagation in cache\n caches = (caches, x)\n\n return a, y, c, caches",
"_____no_output_____"
],
[
"np.random.seed(1)\nx = np.random.randn(3,10,7)\na0 = np.random.randn(5,10)\nWf = np.random.randn(5, 5+3)\nbf = np.random.randn(5,1)\nWi = np.random.randn(5, 5+3)\nbi = np.random.randn(5,1)\nWo = np.random.randn(5, 5+3)\nbo = np.random.randn(5,1)\nWc = np.random.randn(5, 5+3)\nbc = np.random.randn(5,1)\nWy = np.random.randn(2,5)\nby = np.random.randn(2,1)\n\nparameters = {\"Wf\": Wf, \"Wi\": Wi, \"Wo\": Wo, \"Wc\": Wc, \"Wy\": Wy, \"bf\": bf, \"bi\": bi, \"bo\": bo, \"bc\": bc, \"by\": by}\n\na, y, c, caches = lstm_forward(x, a0, parameters)\nprint(\"a[4][3][6] = \", a[4][3][6])\nprint(\"a.shape = \", a.shape)\nprint(\"y[1][4][3] =\", y[1][4][3])\nprint(\"y.shape = \", y.shape)\nprint(\"caches[1][1[1]] =\", caches[1][1][1])\nprint(\"c[1][2][1]\", c[1][2][1])\nprint(\"len(caches) = \", len(caches))",
"a[4][3][6] = 0.172117767533\na.shape = (5, 10, 7)\ny[1][4][3] = 0.95087346185\ny.shape = (2, 10, 7)\ncaches[1][1[1]] = [ 0.82797464 0.23009474 0.76201118 -0.22232814 -0.20075807 0.18656139\n 0.41005165]\nc[1][2][1] -0.855544916718\nlen(caches) = 2\n"
]
],
[
[
"**Expected Output**:\n\n<table>\n <tr>\n <td>\n **a[4][3][6]** =\n </td>\n <td>\n 0.172117767533\n </td>\n </tr>\n <tr>\n <td>\n **a.shape** =\n </td>\n <td>\n (5, 10, 7)\n </td>\n </tr>\n <tr>\n <td>\n **y[1][4][3]** =\n </td>\n <td>\n 0.95087346185\n </td>\n </tr>\n <tr>\n <td>\n **y.shape** =\n </td>\n <td>\n (2, 10, 7)\n </td>\n </tr>\n <tr>\n <td>\n **caches[1][1][1]** =\n </td>\n <td>\n [ 0.82797464 0.23009474 0.76201118 -0.22232814 -0.20075807 0.18656139\n 0.41005165]\n </td>\n \n </tr>\n <tr>\n <td>\n **c[1][2][1]** =\n </td>\n <td>\n -0.855544916718\n </td>\n </tr> \n \n </tr>\n <tr>\n <td>\n **len(caches)** =\n </td>\n <td>\n 2\n </td>\n </tr>\n\n</table>",
"_____no_output_____"
],
[
"Congratulations! You have now implemented the forward passes for the basic RNN and the LSTM. When using a deep learning framework, implementing the forward pass is sufficient to build systems that achieve great performance. \n\nThe rest of this notebook is optional, and will not be graded.",
"_____no_output_____"
],
[
"## 3 - Backpropagation in recurrent neural networks (OPTIONAL / UNGRADED)\n\nIn modern deep learning frameworks, you only have to implement the forward pass, and the framework takes care of the backward pass, so most deep learning engineers do not need to bother with the details of the backward pass. If however you are an expert in calculus and want to see the details of backprop in RNNs, you can work through this optional portion of the notebook. \n\nWhen in an earlier course you implemented a simple (fully connected) neural network, you used backpropagation to compute the derivatives with respect to the cost to update the parameters. Similarly, in recurrent neural networks you can to calculate the derivatives with respect to the cost in order to update the parameters. The backprop equations are quite complicated and we did not derive them in lecture. However, we will briefly present them below. ",
"_____no_output_____"
],
[
"### 3.1 - Basic RNN backward pass\n\nWe will start by computing the backward pass for the basic RNN-cell.\n\n<img src=\"images/rnn_cell_backprop.png\" style=\"width:500;height:300px;\"> <br>\n<caption><center> **Figure 5**: RNN-cell's backward pass. Just like in a fully-connected neural network, the derivative of the cost function $J$ backpropagates through the RNN by following the chain-rule from calculas. The chain-rule is also used to calculate $(\\frac{\\partial J}{\\partial W_{ax}},\\frac{\\partial J}{\\partial W_{aa}},\\frac{\\partial J}{\\partial b})$ to update the parameters $(W_{ax}, W_{aa}, b_a)$. </center></caption>",
"_____no_output_____"
],
[
"#### Deriving the one step backward functions: \n\nTo compute the `rnn_cell_backward` you need to compute the following equations. It is a good exercise to derive them by hand. \n\nThe derivative of $\\tanh$ is $1-\\tanh(x)^2$. You can find the complete proof [here](https://www.wyzant.com/resources/lessons/math/calculus/derivative_proofs/tanx). Note that: $ \\text{sech}(x)^2 = 1 - \\tanh(x)^2$\n\nSimilarly for $\\frac{ \\partial a^{\\langle t \\rangle} } {\\partial W_{ax}}, \\frac{ \\partial a^{\\langle t \\rangle} } {\\partial W_{aa}}, \\frac{ \\partial a^{\\langle t \\rangle} } {\\partial b}$, the derivative of $\\tanh(u)$ is $(1-\\tanh(u)^2)du$. \n\nThe final two equations also follow same rule and are derived using the $\\tanh$ derivative. Note that the arrangement is done in a way to get the same dimensions to match.",
"_____no_output_____"
]
],
[
[
"def rnn_cell_backward(da_next, cache):\n \"\"\"\n Implements the backward pass for the RNN-cell (single time-step).\n\n Arguments:\n da_next -- Gradient of loss with respect to next hidden state\n cache -- python dictionary containing useful values (output of rnn_cell_forward())\n\n Returns:\n gradients -- python dictionary containing:\n dx -- Gradients of input data, of shape (n_x, m)\n da_prev -- Gradients of previous hidden state, of shape (n_a, m)\n dWax -- Gradients of input-to-hidden weights, of shape (n_a, n_x)\n dWaa -- Gradients of hidden-to-hidden weights, of shape (n_a, n_a)\n dba -- Gradients of bias vector, of shape (n_a, 1)\n \"\"\"\n \n # Retrieve values from cache\n (a_next, a_prev, xt, parameters) = cache\n \n # Retrieve values from parameters\n Wax = parameters[\"Wax\"]\n Waa = parameters[\"Waa\"]\n Wya = parameters[\"Wya\"]\n ba = parameters[\"ba\"]\n by = parameters[\"by\"]\n\n ### START CODE HERE ###\n # compute the gradient of tanh with respect to a_next (≈1 line)\n dtanh = (1 - a_next**2) * da_next\n\n # compute the gradient of the loss with respect to Wax (≈2 lines)\n dxt = np.dot(Wax.T, dtanh)\n dWax = np.dot(dtanh, xt.T)\n\n # compute the gradient with respect to Waa (≈2 lines)\n da_prev = np.dot(Waa.T, dtanh)\n dWaa = np.dot(dtanh, a_prev.T)\n\n # compute the gradient with respect to b (≈1 line)\n dba = np.sum(dtanh, 1, keepdims=True)\n\n ### END CODE HERE ###\n \n # Store the gradients in a python dictionary\n gradients = {\"dxt\": dxt, \"da_prev\": da_prev, \"dWax\": dWax, \"dWaa\": dWaa, \"dba\": dba}\n \n return gradients",
"_____no_output_____"
],
[
"np.random.seed(1)\nxt = np.random.randn(3,10)\na_prev = np.random.randn(5,10)\nWax = np.random.randn(5,3)\nWaa = np.random.randn(5,5)\nWya = np.random.randn(2,5)\nb = np.random.randn(5,1)\nby = np.random.randn(2,1)\nparameters = {\"Wax\": Wax, \"Waa\": Waa, \"Wya\": Wya, \"ba\": ba, \"by\": by}\n\na_next, yt, cache = rnn_cell_forward(xt, a_prev, parameters)\n\nda_next = np.random.randn(5,10)\ngradients = rnn_cell_backward(da_next, cache)\nprint(\"gradients[\\\"dxt\\\"][1][2] =\", gradients[\"dxt\"][1][2])\nprint(\"gradients[\\\"dxt\\\"].shape =\", gradients[\"dxt\"].shape)\nprint(\"gradients[\\\"da_prev\\\"][2][3] =\", gradients[\"da_prev\"][2][3])\nprint(\"gradients[\\\"da_prev\\\"].shape =\", gradients[\"da_prev\"].shape)\nprint(\"gradients[\\\"dWax\\\"][3][1] =\", gradients[\"dWax\"][3][1])\nprint(\"gradients[\\\"dWax\\\"].shape =\", gradients[\"dWax\"].shape)\nprint(\"gradients[\\\"dWaa\\\"][1][2] =\", gradients[\"dWaa\"][1][2])\nprint(\"gradients[\\\"dWaa\\\"].shape =\", gradients[\"dWaa\"].shape)\nprint(\"gradients[\\\"dba\\\"][4] =\", gradients[\"dba\"][4])\nprint(\"gradients[\\\"dba\\\"].shape =\", gradients[\"dba\"].shape)",
"gradients[\"dxt\"][1][2] = -0.460564103059\ngradients[\"dxt\"].shape = (3, 10)\ngradients[\"da_prev\"][2][3] = 0.0842968653807\ngradients[\"da_prev\"].shape = (5, 10)\ngradients[\"dWax\"][3][1] = 0.393081873922\ngradients[\"dWax\"].shape = (5, 3)\ngradients[\"dWaa\"][1][2] = -0.28483955787\ngradients[\"dWaa\"].shape = (5, 5)\ngradients[\"dba\"][4] = [ 0.80517166]\ngradients[\"dba\"].shape = (5, 1)\n"
]
],
[
[
"**Expected Output**:\n\n<table>\n <tr>\n <td>\n **gradients[\"dxt\"][1][2]** =\n </td>\n <td>\n -0.460564103059\n </td>\n </tr>\n <tr>\n <td>\n **gradients[\"dxt\"].shape** =\n </td>\n <td>\n (3, 10)\n </td>\n </tr>\n <tr>\n <td>\n **gradients[\"da_prev\"][2][3]** =\n </td>\n <td>\n 0.0842968653807\n </td>\n </tr>\n <tr>\n <td>\n **gradients[\"da_prev\"].shape** =\n </td>\n <td>\n (5, 10)\n </td>\n </tr>\n <tr>\n <td>\n **gradients[\"dWax\"][3][1]** =\n </td>\n <td>\n 0.393081873922\n </td>\n </tr>\n <tr>\n <td>\n **gradients[\"dWax\"].shape** =\n </td>\n <td>\n (5, 3)\n </td>\n </tr>\n <tr>\n <td>\n **gradients[\"dWaa\"][1][2]** = \n </td>\n <td>\n -0.28483955787\n </td>\n </tr>\n <tr>\n <td>\n **gradients[\"dWaa\"].shape** =\n </td>\n <td>\n (5, 5)\n </td>\n </tr>\n <tr>\n <td>\n **gradients[\"dba\"][4]** = \n </td>\n <td>\n [ 0.80517166]\n </td>\n </tr>\n <tr>\n <td>\n **gradients[\"dba\"].shape** = \n </td>\n <td>\n (5, 1)\n </td>\n </tr>\n</table>",
"_____no_output_____"
],
[
"#### Backward pass through the RNN\n\nComputing the gradients of the cost with respect to $a^{\\langle t \\rangle}$ at every time-step $t$ is useful because it is what helps the gradient backpropagate to the previous RNN-cell. To do so, you need to iterate through all the time steps starting at the end, and at each step, you increment the overall $db_a$, $dW_{aa}$, $dW_{ax}$ and you store $dx$.\n\n**Instructions**:\n\nImplement the `rnn_backward` function. Initialize the return variables with zeros first and then loop through all the time steps while calling the `rnn_cell_backward` at each time timestep, update the other variables accordingly.",
"_____no_output_____"
]
],
[
[
"def rnn_backward(da, caches):\n \"\"\"\n Implement the backward pass for a RNN over an entire sequence of input data.\n\n Arguments:\n da -- Upstream gradients of all hidden states, of shape (n_a, m, T_x)\n caches -- tuple containing information from the forward pass (rnn_forward)\n \n Returns:\n gradients -- python dictionary containing:\n dx -- Gradient w.r.t. the input data, numpy-array of shape (n_x, m, T_x)\n da0 -- Gradient w.r.t the initial hidden state, numpy-array of shape (n_a, m)\n dWax -- Gradient w.r.t the input's weight matrix, numpy-array of shape (n_a, n_x)\n dWaa -- Gradient w.r.t the hidden state's weight matrix, numpy-arrayof shape (n_a, n_a)\n dba -- Gradient w.r.t the bias, of shape (n_a, 1)\n \"\"\"\n \n ### START CODE HERE ###\n \n # Retrieve values from the first cache (t=1) of caches (≈2 lines)\n (caches, x) = caches\n (a1, a0, x1, parameters) = caches[0]\n \n # Retrieve dimensions from da's and x1's shapes (≈2 lines)\n n_a, m, T_x = da.shape\n n_x, m = x1.shape\n \n # initialize the gradients with the right sizes (≈6 lines)\n dx = np.zeros((n_x, m, T_x))\n dWax = np.zeros((n_a, n_x))\n dWaa = np.zeros((n_a, n_a))\n dba = np.zeros((n_a, 1))\n da0 = np.zeros((n_a, m))\n da_prevt = np.zeros((n_a, m))\n \n # Loop through all the time steps\n for t in reversed(range(T_x)):\n # Compute gradients at time step t. Choose wisely the \"da_next\" and the \"cache\" to use in the backward propagation step. (≈1 line)\n gradients = rnn_cell_backward(da[:,:, t] + da_prevt, caches[t])\n # Retrieve derivatives from gradients (≈ 1 line)\n dxt, da_prevt, dWaxt, dWaat, dbat = gradients[\"dxt\"], gradients[\"da_prev\"], gradients[\"dWax\"], gradients[\"dWaa\"], gradients[\"dba\"]\n # Increment global derivatives w.r.t parameters by adding their derivative at time-step t (≈4 lines)\n dx[:, :, t] = dxt\n dWax += dWaxt\n dWaa += dWaat\n dba += dbat\n \n # Set da0 to the gradient of a which has been backpropagated through all time-steps (≈1 line) \n da0 = da_prevt\n ### END CODE HERE ###\n\n # Store the gradients in a python dictionary\n gradients = {\"dx\": dx, \"da0\": da0, \"dWax\": dWax, \"dWaa\": dWaa,\"dba\": dba}\n \n return gradients",
"_____no_output_____"
],
[
"np.random.seed(1)\nx = np.random.randn(3,10,4)\na0 = np.random.randn(5,10)\nWax = np.random.randn(5,3)\nWaa = np.random.randn(5,5)\nWya = np.random.randn(2,5)\nba = np.random.randn(5,1)\nby = np.random.randn(2,1)\nparameters = {\"Wax\": Wax, \"Waa\": Waa, \"Wya\": Wya, \"ba\": ba, \"by\": by}\na, y, caches = rnn_forward(x, a0, parameters)\nda = np.random.randn(5, 10, 4)\ngradients = rnn_backward(da, caches)\n\nprint(\"gradients[\\\"dx\\\"][1][2] =\", gradients[\"dx\"][1][2])\nprint(\"gradients[\\\"dx\\\"].shape =\", gradients[\"dx\"].shape)\nprint(\"gradients[\\\"da0\\\"][2][3] =\", gradients[\"da0\"][2][3])\nprint(\"gradients[\\\"da0\\\"].shape =\", gradients[\"da0\"].shape)\nprint(\"gradients[\\\"dWax\\\"][3][1] =\", gradients[\"dWax\"][3][1])\nprint(\"gradients[\\\"dWax\\\"].shape =\", gradients[\"dWax\"].shape)\nprint(\"gradients[\\\"dWaa\\\"][1][2] =\", gradients[\"dWaa\"][1][2])\nprint(\"gradients[\\\"dWaa\\\"].shape =\", gradients[\"dWaa\"].shape)\nprint(\"gradients[\\\"dba\\\"][4] =\", gradients[\"dba\"][4])\nprint(\"gradients[\\\"dba\\\"].shape =\", gradients[\"dba\"].shape)",
"gradients[\"dx\"][1][2] = [-2.07101689 -0.59255627 0.02466855 0.01483317]\ngradients[\"dx\"].shape = (3, 10, 4)\ngradients[\"da0\"][2][3] = -0.314942375127\ngradients[\"da0\"].shape = (5, 10)\ngradients[\"dWax\"][3][1] = 11.2641044965\ngradients[\"dWax\"].shape = (5, 3)\ngradients[\"dWaa\"][1][2] = 2.30333312658\ngradients[\"dWaa\"].shape = (5, 5)\ngradients[\"dba\"][4] = [-0.74747722]\ngradients[\"dba\"].shape = (5, 1)\n"
]
],
[
[
"**Expected Output**:\n\n<table>\n <tr>\n <td>\n **gradients[\"dx\"][1][2]** =\n </td>\n <td>\n [-2.07101689 -0.59255627 0.02466855 0.01483317]\n </td>\n </tr>\n <tr>\n <td>\n **gradients[\"dx\"].shape** =\n </td>\n <td>\n (3, 10, 4)\n </td>\n </tr>\n <tr>\n <td>\n **gradients[\"da0\"][2][3]** =\n </td>\n <td>\n -0.314942375127\n </td>\n </tr>\n <tr>\n <td>\n **gradients[\"da0\"].shape** =\n </td>\n <td>\n (5, 10)\n </td>\n </tr>\n <tr>\n <td>\n **gradients[\"dWax\"][3][1]** =\n </td>\n <td>\n 11.2641044965\n </td>\n </tr>\n <tr>\n <td>\n **gradients[\"dWax\"].shape** =\n </td>\n <td>\n (5, 3)\n </td>\n </tr>\n <tr>\n <td>\n **gradients[\"dWaa\"][1][2]** = \n </td>\n <td>\n 2.30333312658\n </td>\n </tr>\n <tr>\n <td>\n **gradients[\"dWaa\"].shape** =\n </td>\n <td>\n (5, 5)\n </td>\n </tr>\n <tr>\n <td>\n **gradients[\"dba\"][4]** = \n </td>\n <td>\n [-0.74747722]\n </td>\n </tr>\n <tr>\n <td>\n **gradients[\"dba\"].shape** = \n </td>\n <td>\n (5, 1)\n </td>\n </tr>\n</table>",
"_____no_output_____"
],
[
"## 3.2 - LSTM backward pass",
"_____no_output_____"
],
[
"### 3.2.1 One Step backward\n\nThe LSTM backward pass is slighltly more complicated than the forward one. We have provided you with all the equations for the LSTM backward pass below. (If you enjoy calculus exercises feel free to try deriving these from scratch yourself.) \n\n### 3.2.2 gate derivatives\n\n$$d \\Gamma_o^{\\langle t \\rangle} = da_{next}*\\tanh(c_{next}) * \\Gamma_o^{\\langle t \\rangle}*(1-\\Gamma_o^{\\langle t \\rangle})\\tag{7}$$\n\n$$d\\tilde c^{\\langle t \\rangle} = dc_{next}*\\Gamma_u^{\\langle t \\rangle}+ \\Gamma_o^{\\langle t \\rangle} (1-\\tanh(c_{next})^2) * i_t * da_{next} * \\tilde c^{\\langle t \\rangle} * (1-\\tanh(\\tilde c)^2) \\tag{8}$$\n\n$$d\\Gamma_u^{\\langle t \\rangle} = dc_{next}*\\tilde c^{\\langle t \\rangle} + \\Gamma_o^{\\langle t \\rangle} (1-\\tanh(c_{next})^2) * \\tilde c^{\\langle t \\rangle} * da_{next}*\\Gamma_u^{\\langle t \\rangle}*(1-\\Gamma_u^{\\langle t \\rangle})\\tag{9}$$\n\n$$d\\Gamma_f^{\\langle t \\rangle} = dc_{next}*\\tilde c_{prev} + \\Gamma_o^{\\langle t \\rangle} (1-\\tanh(c_{next})^2) * c_{prev} * da_{next}*\\Gamma_f^{\\langle t \\rangle}*(1-\\Gamma_f^{\\langle t \\rangle})\\tag{10}$$\n\n### 3.2.3 parameter derivatives \n\n$$ dW_f = d\\Gamma_f^{\\langle t \\rangle} * \\begin{pmatrix} a_{prev} \\\\ x_t\\end{pmatrix}^T \\tag{11} $$\n$$ dW_u = d\\Gamma_u^{\\langle t \\rangle} * \\begin{pmatrix} a_{prev} \\\\ x_t\\end{pmatrix}^T \\tag{12} $$\n$$ dW_c = d\\tilde c^{\\langle t \\rangle} * \\begin{pmatrix} a_{prev} \\\\ x_t\\end{pmatrix}^T \\tag{13} $$\n$$ dW_o = d\\Gamma_o^{\\langle t \\rangle} * \\begin{pmatrix} a_{prev} \\\\ x_t\\end{pmatrix}^T \\tag{14}$$\n\nTo calculate $db_f, db_u, db_c, db_o$ you just need to sum across the horizontal (axis= 1) axis on $d\\Gamma_f^{\\langle t \\rangle}, d\\Gamma_u^{\\langle t \\rangle}, d\\tilde c^{\\langle t \\rangle}, d\\Gamma_o^{\\langle t \\rangle}$ respectively. Note that you should have the `keep_dims = True` option.\n\nFinally, you will compute the derivative with respect to the previous hidden state, previous memory state, and input.\n\n$$ da_{prev} = W_f^T*d\\Gamma_f^{\\langle t \\rangle} + W_u^T * d\\Gamma_u^{\\langle t \\rangle}+ W_c^T * d\\tilde c^{\\langle t \\rangle} + W_o^T * d\\Gamma_o^{\\langle t \\rangle} \\tag{15}$$\nHere, the weights for equations 13 are the first n_a, (i.e. $W_f = W_f[:n_a,:]$ etc...)\n\n$$ dc_{prev} = dc_{next}\\Gamma_f^{\\langle t \\rangle} + \\Gamma_o^{\\langle t \\rangle} * (1- \\tanh(c_{next})^2)*\\Gamma_f^{\\langle t \\rangle}*da_{next} \\tag{16}$$\n$$ dx^{\\langle t \\rangle} = W_f^T*d\\Gamma_f^{\\langle t \\rangle} + W_u^T * d\\Gamma_u^{\\langle t \\rangle}+ W_c^T * d\\tilde c_t + W_o^T * d\\Gamma_o^{\\langle t \\rangle}\\tag{17} $$\nwhere the weights for equation 15 are from n_a to the end, (i.e. $W_f = W_f[n_a:,:]$ etc...)\n\n**Exercise:** Implement `lstm_cell_backward` by implementing equations $7-17$ below. Good luck! :)",
"_____no_output_____"
]
],
[
[
"def lstm_cell_backward(da_next, dc_next, cache):\n \"\"\"\n Implement the backward pass for the LSTM-cell (single time-step).\n\n Arguments:\n da_next -- Gradients of next hidden state, of shape (n_a, m)\n dc_next -- Gradients of next cell state, of shape (n_a, m)\n cache -- cache storing information from the forward pass\n\n Returns:\n gradients -- python dictionary containing:\n dxt -- Gradient of input data at time-step t, of shape (n_x, m)\n da_prev -- Gradient w.r.t. the previous hidden state, numpy array of shape (n_a, m)\n dc_prev -- Gradient w.r.t. the previous memory state, of shape (n_a, m, T_x)\n dWf -- Gradient w.r.t. the weight matrix of the forget gate, numpy array of shape (n_a, n_a + n_x)\n dWi -- Gradient w.r.t. the weight matrix of the update gate, numpy array of shape (n_a, n_a + n_x)\n dWc -- Gradient w.r.t. the weight matrix of the memory gate, numpy array of shape (n_a, n_a + n_x)\n dWo -- Gradient w.r.t. the weight matrix of the output gate, numpy array of shape (n_a, n_a + n_x)\n dbf -- Gradient w.r.t. biases of the forget gate, of shape (n_a, 1)\n dbi -- Gradient w.r.t. biases of the update gate, of shape (n_a, 1)\n dbc -- Gradient w.r.t. biases of the memory gate, of shape (n_a, 1)\n dbo -- Gradient w.r.t. biases of the output gate, of shape (n_a, 1)\n \"\"\"\n\n # Retrieve information from \"cache\"\n (a_next, c_next, a_prev, c_prev, ft, it, cct, ot, xt, parameters) = cache\n \n ### START CODE HERE ###\n # Retrieve dimensions from xt's and a_next's shape (≈2 lines)\n n_x, m = xt.shape\n n_a, m = a_next.shape\n \n # Compute gates related derivatives, you can find their values can be found by looking carefully at equations (7) to (10) (≈4 lines)\n dot = da_next * np.tanh(c_next) * ot * (1 - ot)\n dcct = (dc_next * it + ot * (1 - np.square(np.tanh(c_next))) * it * da_next) * (1 - np.square(cct))\n dit = (dc_next * cct + ot * (1 - np.square(np.tanh(c_next))) * cct * da_next) * it * (1 - it)\n dft = (dc_next * c_prev + ot *(1 - np.square(np.tanh(c_next))) * c_prev * da_next) * ft * (1 - ft)\n \n # Code equations (7) to (10) (≈4 lines)\n #dit = None\n #dft = None\n #dot = None\n #dcct = None\n concat = np.concatenate((a_prev, xt), axis=0)\n \n # Compute parameters related derivatives. Use equations (11)-(14) (≈8 lines)\n dWf = np.dot(dft, concat.T)\n dWi = np.dot(dit, concat.T)\n dWc = np.dot(dcct, concat.T)\n dWo = np.dot(dot, concat.T)\n dbf = np.sum(dft, axis=1 ,keepdims = True)\n dbi = np.sum(dit, axis=1, keepdims = True)\n dbc = np.sum(dcct, axis=1, keepdims = True)\n dbo = np.sum(dot, axis=1, keepdims = True)\n\n # Compute derivatives w.r.t previous hidden state, previous memory state and input. Use equations (15)-(17). (≈3 lines)\n da_prev = np.dot(parameters['Wf'][:, :n_a].T, dft) + np.dot(parameters['Wi'][:, :n_a].T, dit) + np.dot(parameters['Wc'][:, :n_a].T, dcct) + np.dot(parameters['Wo'][:, :n_a].T, dot)\n dc_prev = dc_next * ft + ot * (1 - np.square(np.tanh(c_next))) * ft * da_next\n dxt = np.dot(parameters['Wf'][:, n_a:].T, dft) + np.dot(parameters['Wi'][:, n_a:].T, dit) + np.dot(parameters['Wc'][:, n_a:].T, dcct) + np.dot(parameters['Wo'][:, n_a:].T, dot)\n ### END CODE HERE ###\n \n # Save gradients in dictionary\n gradients = {\"dxt\": dxt, \"da_prev\": da_prev, \"dc_prev\": dc_prev, \"dWf\": dWf,\"dbf\": dbf, \"dWi\": dWi,\"dbi\": dbi,\n \"dWc\": dWc,\"dbc\": dbc, \"dWo\": dWo,\"dbo\": dbo}\n\n return gradients",
"_____no_output_____"
],
[
"np.random.seed(1)\nxt = np.random.randn(3,10)\na_prev = np.random.randn(5,10)\nc_prev = np.random.randn(5,10)\nWf = np.random.randn(5, 5+3)\nbf = np.random.randn(5,1)\nWi = np.random.randn(5, 5+3)\nbi = np.random.randn(5,1)\nWo = np.random.randn(5, 5+3)\nbo = np.random.randn(5,1)\nWc = np.random.randn(5, 5+3)\nbc = np.random.randn(5,1)\nWy = np.random.randn(2,5)\nby = np.random.randn(2,1)\n\nparameters = {\"Wf\": Wf, \"Wi\": Wi, \"Wo\": Wo, \"Wc\": Wc, \"Wy\": Wy, \"bf\": bf, \"bi\": bi, \"bo\": bo, \"bc\": bc, \"by\": by}\n\na_next, c_next, yt, cache = lstm_cell_forward(xt, a_prev, c_prev, parameters)\n\nda_next = np.random.randn(5,10)\ndc_next = np.random.randn(5,10)\ngradients = lstm_cell_backward(da_next, dc_next, cache)\nprint(\"gradients[\\\"dxt\\\"][1][2] =\", gradients[\"dxt\"][1][2])\nprint(\"gradients[\\\"dxt\\\"].shape =\", gradients[\"dxt\"].shape)\nprint(\"gradients[\\\"da_prev\\\"][2][3] =\", gradients[\"da_prev\"][2][3])\nprint(\"gradients[\\\"da_prev\\\"].shape =\", gradients[\"da_prev\"].shape)\nprint(\"gradients[\\\"dc_prev\\\"][2][3] =\", gradients[\"dc_prev\"][2][3])\nprint(\"gradients[\\\"dc_prev\\\"].shape =\", gradients[\"dc_prev\"].shape)\nprint(\"gradients[\\\"dWf\\\"][3][1] =\", gradients[\"dWf\"][3][1])\nprint(\"gradients[\\\"dWf\\\"].shape =\", gradients[\"dWf\"].shape)\nprint(\"gradients[\\\"dWi\\\"][1][2] =\", gradients[\"dWi\"][1][2])\nprint(\"gradients[\\\"dWi\\\"].shape =\", gradients[\"dWi\"].shape)\nprint(\"gradients[\\\"dWc\\\"][3][1] =\", gradients[\"dWc\"][3][1])\nprint(\"gradients[\\\"dWc\\\"].shape =\", gradients[\"dWc\"].shape)\nprint(\"gradients[\\\"dWo\\\"][1][2] =\", gradients[\"dWo\"][1][2])\nprint(\"gradients[\\\"dWo\\\"].shape =\", gradients[\"dWo\"].shape)\nprint(\"gradients[\\\"dbf\\\"][4] =\", gradients[\"dbf\"][4])\nprint(\"gradients[\\\"dbf\\\"].shape =\", gradients[\"dbf\"].shape)\nprint(\"gradients[\\\"dbi\\\"][4] =\", gradients[\"dbi\"][4])\nprint(\"gradients[\\\"dbi\\\"].shape =\", gradients[\"dbi\"].shape)\nprint(\"gradients[\\\"dbc\\\"][4] =\", gradients[\"dbc\"][4])\nprint(\"gradients[\\\"dbc\\\"].shape =\", gradients[\"dbc\"].shape)\nprint(\"gradients[\\\"dbo\\\"][4] =\", gradients[\"dbo\"][4])\nprint(\"gradients[\\\"dbo\\\"].shape =\", gradients[\"dbo\"].shape)",
"gradients[\"dxt\"][1][2] = 3.23055911511\ngradients[\"dxt\"].shape = (3, 10)\ngradients[\"da_prev\"][2][3] = -0.0639621419711\ngradients[\"da_prev\"].shape = (5, 10)\ngradients[\"dc_prev\"][2][3] = 0.797522038797\ngradients[\"dc_prev\"].shape = (5, 10)\ngradients[\"dWf\"][3][1] = -0.147954838164\ngradients[\"dWf\"].shape = (5, 8)\ngradients[\"dWi\"][1][2] = 1.05749805523\ngradients[\"dWi\"].shape = (5, 8)\ngradients[\"dWc\"][3][1] = 2.30456216369\ngradients[\"dWc\"].shape = (5, 8)\ngradients[\"dWo\"][1][2] = 0.331311595289\ngradients[\"dWo\"].shape = (5, 8)\ngradients[\"dbf\"][4] = [ 0.18864637]\ngradients[\"dbf\"].shape = (5, 1)\ngradients[\"dbi\"][4] = [-0.40142491]\ngradients[\"dbi\"].shape = (5, 1)\ngradients[\"dbc\"][4] = [ 0.25587763]\ngradients[\"dbc\"].shape = (5, 1)\ngradients[\"dbo\"][4] = [ 0.13893342]\ngradients[\"dbo\"].shape = (5, 1)\n"
]
],
[
[
"**Expected Output**:\n\n<table>\n <tr>\n <td>\n **gradients[\"dxt\"][1][2]** =\n </td>\n <td>\n 3.23055911511\n </td>\n </tr>\n <tr>\n <td>\n **gradients[\"dxt\"].shape** =\n </td>\n <td>\n (3, 10)\n </td>\n </tr>\n <tr>\n <td>\n **gradients[\"da_prev\"][2][3]** =\n </td>\n <td>\n -0.0639621419711\n </td>\n </tr>\n <tr>\n <td>\n **gradients[\"da_prev\"].shape** =\n </td>\n <td>\n (5, 10)\n </td>\n </tr>\n <tr>\n <td>\n **gradients[\"dc_prev\"][2][3]** =\n </td>\n <td>\n 0.797522038797\n </td>\n </tr>\n <tr>\n <td>\n **gradients[\"dc_prev\"].shape** =\n </td>\n <td>\n (5, 10)\n </td>\n </tr>\n <tr>\n <td>\n **gradients[\"dWf\"][3][1]** = \n </td>\n <td>\n -0.147954838164\n </td>\n </tr>\n <tr>\n <td>\n **gradients[\"dWf\"].shape** =\n </td>\n <td>\n (5, 8)\n </td>\n </tr>\n <tr>\n <td>\n **gradients[\"dWi\"][1][2]** = \n </td>\n <td>\n 1.05749805523\n </td>\n </tr>\n <tr>\n <td>\n **gradients[\"dWi\"].shape** = \n </td>\n <td>\n (5, 8)\n </td>\n </tr>\n <tr>\n <td>\n **gradients[\"dWc\"][3][1]** = \n </td>\n <td>\n 2.30456216369\n </td>\n </tr>\n <tr>\n <td>\n **gradients[\"dWc\"].shape** = \n </td>\n <td>\n (5, 8)\n </td>\n </tr>\n <tr>\n <td>\n **gradients[\"dWo\"][1][2]** = \n </td>\n <td>\n 0.331311595289\n </td>\n </tr>\n <tr>\n <td>\n **gradients[\"dWo\"].shape** = \n </td>\n <td>\n (5, 8)\n </td>\n </tr>\n <tr>\n <td>\n **gradients[\"dbf\"][4]** = \n </td>\n <td>\n [ 0.18864637]\n </td>\n </tr>\n <tr>\n <td>\n **gradients[\"dbf\"].shape** = \n </td>\n <td>\n (5, 1)\n </td>\n </tr>\n <tr>\n <td>\n **gradients[\"dbi\"][4]** = \n </td>\n <td>\n [-0.40142491]\n </td>\n </tr>\n <tr>\n <td>\n **gradients[\"dbi\"].shape** = \n </td>\n <td>\n (5, 1)\n </td>\n </tr>\n <tr>\n <td>\n **gradients[\"dbc\"][4]** = \n </td>\n <td>\n [ 0.25587763]\n </td>\n </tr>\n <tr>\n <td>\n **gradients[\"dbc\"].shape** = \n </td>\n <td>\n (5, 1)\n </td>\n </tr>\n <tr>\n <td>\n **gradients[\"dbo\"][4]** = \n </td>\n <td>\n [ 0.13893342]\n </td>\n </tr>\n <tr>\n <td>\n **gradients[\"dbo\"].shape** = \n </td>\n <td>\n (5, 1)\n </td>\n </tr>\n</table>",
"_____no_output_____"
],
[
"### 3.3 Backward pass through the LSTM RNN\n\nThis part is very similar to the `rnn_backward` function you implemented above. You will first create variables of the same dimension as your return variables. You will then iterate over all the time steps starting from the end and call the one step function you implemented for LSTM at each iteration. You will then update the parameters by summing them individually. Finally return a dictionary with the new gradients. \n\n**Instructions**: Implement the `lstm_backward` function. Create a for loop starting from $T_x$ and going backward. For each step call `lstm_cell_backward` and update the your old gradients by adding the new gradients to them. Note that `dxt` is not updated but is stored.",
"_____no_output_____"
]
],
[
[
"def lstm_backward(da, caches):\n \n \"\"\"\n Implement the backward pass for the RNN with LSTM-cell (over a whole sequence).\n\n Arguments:\n da -- Gradients w.r.t the hidden states, numpy-array of shape (n_a, m, T_x)\n dc -- Gradients w.r.t the memory states, numpy-array of shape (n_a, m, T_x)\n caches -- cache storing information from the forward pass (lstm_forward)\n\n Returns:\n gradients -- python dictionary containing:\n dx -- Gradient of inputs, of shape (n_x, m, T_x)\n da0 -- Gradient w.r.t. the previous hidden state, numpy array of shape (n_a, m)\n dWf -- Gradient w.r.t. the weight matrix of the forget gate, numpy array of shape (n_a, n_a + n_x)\n dWi -- Gradient w.r.t. the weight matrix of the update gate, numpy array of shape (n_a, n_a + n_x)\n dWc -- Gradient w.r.t. the weight matrix of the memory gate, numpy array of shape (n_a, n_a + n_x)\n dWo -- Gradient w.r.t. the weight matrix of the save gate, numpy array of shape (n_a, n_a + n_x)\n dbf -- Gradient w.r.t. biases of the forget gate, of shape (n_a, 1)\n dbi -- Gradient w.r.t. biases of the update gate, of shape (n_a, 1)\n dbc -- Gradient w.r.t. biases of the memory gate, of shape (n_a, 1)\n dbo -- Gradient w.r.t. biases of the save gate, of shape (n_a, 1)\n \"\"\"\n\n # Retrieve values from the first cache (t=1) of caches.\n (caches, x) = caches\n (a1, c1, a0, c0, f1, i1, cc1, o1, x1, parameters) = caches[0]\n \n ### START CODE HERE ###\n # Retrieve dimensions from da's and x1's shapes (≈2 lines)\n n_a, m, T_x = da.shape\n n_x, m = x1.shape\n \n # initialize the gradients with the right sizes (≈12 lines)\n dx = np.zeros((n_x, m, T_x))\n da0 = np.zeros((n_a, m))\n da_prevt = np.zeros(da0.shape)\n dc_prevt = np.zeros(da0.shape)\n dWf = np.zeros((n_a, n_a + n_x))\n dWi = np.zeros(dWf.shape)\n dWc = np.zeros(dWf.shape)\n dWo = np.zeros(dWf.shape)\n dbf = np.zeros((n_a, 1))\n dbi = np.zeros(dbf.shape)\n dbc = np.zeros(dbf.shape)\n dbo = np.zeros(dbf.shape)\n \n # loop back over the whole sequence\n for t in reversed(range(T_x)):\n # Compute all gradients using lstm_cell_backward\n gradients = lstm_cell_backward(da[:, :, t], dc_prevt, caches[t])\n # Store or add the gradient to the parameters' previous step's gradient\n dx[:,:,t] = gradients[\"dxt\"]\n dWf += gradients[\"dWf\"]\n dWi += gradients[\"dWi\"]\n dWc += gradients[\"dWc\"]\n dWo += gradients[\"dWo\"]\n dbf += gradients[\"dbf\"]\n dbi += gradients[\"dbi\"]\n dbc += gradients[\"dbc\"]\n dbo += gradients[\"dbo\"]\n # Set the first activation's gradient to the backpropagated gradient da_prev.\n da0 = gradients[\"da_prev\"]\n \n ### END CODE HERE ###\n\n # Store the gradients in a python dictionary\n gradients = {\"dx\": dx, \"da0\": da0, \"dWf\": dWf,\"dbf\": dbf, \"dWi\": dWi,\"dbi\": dbi,\n \"dWc\": dWc,\"dbc\": dbc, \"dWo\": dWo,\"dbo\": dbo}\n \n return gradients",
"_____no_output_____"
],
[
"np.random.seed(1)\nx = np.random.randn(3,10,7)\na0 = np.random.randn(5,10)\nWf = np.random.randn(5, 5+3)\nbf = np.random.randn(5,1)\nWi = np.random.randn(5, 5+3)\nbi = np.random.randn(5,1)\nWo = np.random.randn(5, 5+3)\nbo = np.random.randn(5,1)\nWc = np.random.randn(5, 5+3)\nbc = np.random.randn(5,1)\n\nparameters = {\"Wf\": Wf, \"Wi\": Wi, \"Wo\": Wo, \"Wc\": Wc, \"Wy\": Wy, \"bf\": bf, \"bi\": bi, \"bo\": bo, \"bc\": bc, \"by\": by}\n\na, y, c, caches = lstm_forward(x, a0, parameters)\n\nda = np.random.randn(5, 10, 4)\ngradients = lstm_backward(da, caches)\n\nprint(\"gradients[\\\"dx\\\"][1][2] =\", gradients[\"dx\"][1][2])\nprint(\"gradients[\\\"dx\\\"].shape =\", gradients[\"dx\"].shape)\nprint(\"gradients[\\\"da0\\\"][2][3] =\", gradients[\"da0\"][2][3])\nprint(\"gradients[\\\"da0\\\"].shape =\", gradients[\"da0\"].shape)\nprint(\"gradients[\\\"dWf\\\"][3][1] =\", gradients[\"dWf\"][3][1])\nprint(\"gradients[\\\"dWf\\\"].shape =\", gradients[\"dWf\"].shape)\nprint(\"gradients[\\\"dWi\\\"][1][2] =\", gradients[\"dWi\"][1][2])\nprint(\"gradients[\\\"dWi\\\"].shape =\", gradients[\"dWi\"].shape)\nprint(\"gradients[\\\"dWc\\\"][3][1] =\", gradients[\"dWc\"][3][1])\nprint(\"gradients[\\\"dWc\\\"].shape =\", gradients[\"dWc\"].shape)\nprint(\"gradients[\\\"dWo\\\"][1][2] =\", gradients[\"dWo\"][1][2])\nprint(\"gradients[\\\"dWo\\\"].shape =\", gradients[\"dWo\"].shape)\nprint(\"gradients[\\\"dbf\\\"][4] =\", gradients[\"dbf\"][4])\nprint(\"gradients[\\\"dbf\\\"].shape =\", gradients[\"dbf\"].shape)\nprint(\"gradients[\\\"dbi\\\"][4] =\", gradients[\"dbi\"][4])\nprint(\"gradients[\\\"dbi\\\"].shape =\", gradients[\"dbi\"].shape)\nprint(\"gradients[\\\"dbc\\\"][4] =\", gradients[\"dbc\"][4])\nprint(\"gradients[\\\"dbc\\\"].shape =\", gradients[\"dbc\"].shape)\nprint(\"gradients[\\\"dbo\\\"][4] =\", gradients[\"dbo\"][4])\nprint(\"gradients[\\\"dbo\\\"].shape =\", gradients[\"dbo\"].shape)",
"gradients[\"dx\"][1][2] = [-0.00173313 0.08287442 -0.30545663 -0.43281115]\ngradients[\"dx\"].shape = (3, 10, 4)\ngradients[\"da0\"][2][3] = -0.095911501954\ngradients[\"da0\"].shape = (5, 10)\ngradients[\"dWf\"][3][1] = -0.0698198561274\ngradients[\"dWf\"].shape = (5, 8)\ngradients[\"dWi\"][1][2] = 0.102371820249\ngradients[\"dWi\"].shape = (5, 8)\ngradients[\"dWc\"][3][1] = -0.0624983794927\ngradients[\"dWc\"].shape = (5, 8)\ngradients[\"dWo\"][1][2] = 0.0484389131444\ngradients[\"dWo\"].shape = (5, 8)\ngradients[\"dbf\"][4] = [-0.0565788]\ngradients[\"dbf\"].shape = (5, 1)\ngradients[\"dbi\"][4] = [-0.15399065]\ngradients[\"dbi\"].shape = (5, 1)\ngradients[\"dbc\"][4] = [-0.29691142]\ngradients[\"dbc\"].shape = (5, 1)\ngradients[\"dbo\"][4] = [-0.29798344]\ngradients[\"dbo\"].shape = (5, 1)\n"
]
],
[
[
"**Expected Output**:\n\n<table>\n <tr>\n <td>\n **gradients[\"dx\"][1][2]** =\n </td>\n <td>\n [-0.00173313 0.08287442 -0.30545663 -0.43281115]\n </td>\n </tr>\n <tr>\n <td>\n **gradients[\"dx\"].shape** =\n </td>\n <td>\n (3, 10, 4)\n </td>\n </tr>\n <tr>\n <td>\n **gradients[\"da0\"][2][3]** =\n </td>\n <td>\n -0.095911501954\n </td>\n </tr>\n <tr>\n <td>\n **gradients[\"da0\"].shape** =\n </td>\n <td>\n (5, 10)\n </td>\n </tr>\n <tr>\n <td>\n **gradients[\"dWf\"][3][1]** = \n </td>\n <td>\n -0.0698198561274\n </td>\n </tr>\n <tr>\n <td>\n **gradients[\"dWf\"].shape** =\n </td>\n <td>\n (5, 8)\n </td>\n </tr>\n <tr>\n <td>\n **gradients[\"dWi\"][1][2]** = \n </td>\n <td>\n 0.102371820249\n </td>\n </tr>\n <tr>\n <td>\n **gradients[\"dWi\"].shape** = \n </td>\n <td>\n (5, 8)\n </td>\n </tr>\n <tr>\n <td>\n **gradients[\"dWc\"][3][1]** = \n </td>\n <td>\n -0.0624983794927\n </td>\n </tr>\n <tr>\n <td>\n **gradients[\"dWc\"].shape** = \n </td>\n <td>\n (5, 8)\n </td>\n </tr>\n <tr>\n <td>\n **gradients[\"dWo\"][1][2]** = \n </td>\n <td>\n 0.0484389131444\n </td>\n </tr>\n <tr>\n <td>\n **gradients[\"dWo\"].shape** = \n </td>\n <td>\n (5, 8)\n </td>\n </tr>\n <tr>\n <td>\n **gradients[\"dbf\"][4]** = \n </td>\n <td>\n [-0.0565788]\n </td>\n </tr>\n <tr>\n <td>\n **gradients[\"dbf\"].shape** = \n </td>\n <td>\n (5, 1)\n </td>\n </tr>\n <tr>\n <td>\n **gradients[\"dbi\"][4]** = \n </td>\n <td>\n [-0.06997391]\n </td>\n </tr>\n <tr>\n <td>\n **gradients[\"dbi\"].shape** = \n </td>\n <td>\n (5, 1)\n </td>\n </tr>\n <tr>\n <td>\n **gradients[\"dbc\"][4]** = \n </td>\n <td>\n [-0.27441821]\n </td>\n </tr>\n <tr>\n <td>\n **gradients[\"dbc\"].shape** = \n </td>\n <td>\n (5, 1)\n </td>\n </tr>\n <tr>\n <td>\n **gradients[\"dbo\"][4]** = \n </td>\n <td>\n [ 0.16532821]\n </td>\n </tr>\n <tr>\n <td>\n **gradients[\"dbo\"].shape** = \n </td>\n <td>\n (5, 1)\n </td>\n </tr>\n</table>",
"_____no_output_____"
],
[
"### Congratulations !\n\nCongratulations on completing this assignment. You now understand how recurrent neural networks work! \n\nLets go on to the next exercise, where you'll use an RNN to build a character-level language model.\n",
"_____no_output_____"
]
]
] | [
"markdown",
"code",
"markdown",
"code",
"markdown",
"code",
"markdown",
"code",
"markdown",
"code",
"markdown",
"code",
"markdown",
"code",
"markdown",
"code",
"markdown",
"code",
"markdown"
] | [
[
"markdown",
"markdown"
],
[
"code"
],
[
"markdown",
"markdown",
"markdown"
],
[
"code",
"code"
],
[
"markdown",
"markdown"
],
[
"code",
"code"
],
[
"markdown",
"markdown",
"markdown",
"markdown"
],
[
"code",
"code"
],
[
"markdown",
"markdown"
],
[
"code",
"code"
],
[
"markdown",
"markdown",
"markdown",
"markdown",
"markdown"
],
[
"code",
"code"
],
[
"markdown",
"markdown"
],
[
"code",
"code"
],
[
"markdown",
"markdown",
"markdown"
],
[
"code",
"code"
],
[
"markdown",
"markdown"
],
[
"code",
"code"
],
[
"markdown",
"markdown"
]
] |
e768b3bdb557880b0a256f9097fd7442ed971b2f | 1,004,843 | ipynb | Jupyter Notebook | use-cases/computer_vision/1-metastases-detection-train-model.ipynb | sureindia-in/sagemaker-examples-good | be72218d4fd75bdb7f0df136026366fcd726fbe0 | [
"Apache-2.0"
] | 1 | 2021-05-31T17:19:10.000Z | 2021-05-31T17:19:10.000Z | use-cases/computer_vision/1-metastases-detection-train-model.ipynb | sureindia-in/sagemaker-examples-good | be72218d4fd75bdb7f0df136026366fcd726fbe0 | [
"Apache-2.0"
] | 1 | 2021-05-26T00:01:10.000Z | 2021-05-26T00:01:10.000Z | use-cases/computer_vision/1-metastases-detection-train-model.ipynb | sureindia-in/sagemaker-examples-good | be72218d4fd75bdb7f0df136026366fcd726fbe0 | [
"Apache-2.0"
] | null | null | null | 1,413.281294 | 983,700 | 0.957373 | [
[
[
"# Computer Vision for Medical Imaging: Part 1. Train Model with Hyperparameter Tuning Job\nThis notebook is part 1 of a 4-part series of techniques and services offer by SageMaker to build a model which predicts if an image of cells contains cancer. This notebook shows how to build a model using hyperparameter tuning.",
"_____no_output_____"
],
[
"## Dataset\nThe dataset for this demo comes from the [Camelyon16 Challenge](https://camelyon16.grand-challenge.org/) made available under the CC0 licencse. The raw data provided by the challenge has been processed into 96x96 pixel tiles by [Bas Veeling](https://github.com/basveeling/pcam) and also made available under the CC0 license. For detailed information on each dataset please see the papers below:\n* Ehteshami Bejnordi et al. Diagnostic Assessment of Deep Learning Algorithms for Detection of Lymph Node Metastases in Women With Breast Cancer. JAMA: The Journal of the American Medical Association, 318(22), 2199–2210. [doi:jama.2017.14585](https://doi.org/10.1001/jama.2017.14585)\n* B. S. Veeling, J. Linmans, J. Winkens, T. Cohen, M. Welling. \"Rotation Equivariant CNNs for Digital Pathology\". [arXiv:1806.03962](http://arxiv.org/abs/1806.03962)\n\nThe tiled dataset from Bas Veeling is over 6GB of data. In order to easily run this demo, the dataset has been pruned to the first 14,000 images of the tiled dataset and comes included in the repo with this notebook for convenience.",
"_____no_output_____"
],
[
"## Update Sagemaker SDK and Boto3\n\n<div class=\"alert alert-warning\">\n<b>NOTE</b> You may get an error from pip's dependency resolver; you can ignore this error.\n</div>",
"_____no_output_____"
]
],
[
[
"import pip\n\n\ndef import_or_install(package):\n try:\n __import__(package)\n except ImportError:\n pip.main([\"install\", package])\n\n\nrequired_packages = [\"sagemaker\", \"boto3\", \"mxnet\", \"h5py\", \"tqdm\", \"matplotlib\"]\n\nfor package in required_packages:\n import_or_install(package)",
"_____no_output_____"
],
[
"%store -r\n%store",
"_____no_output_____"
]
],
[
[
"## Import Libraries",
"_____no_output_____"
]
],
[
[
"import io\nimport os\nimport h5py\nimport zipfile\nimport boto3\nimport sagemaker\nimport mxnet as mx\nimport numpy as np\nfrom tqdm import tqdm\nimport matplotlib.pyplot as plt\nimport cv2",
"_____no_output_____"
]
],
[
[
"## Configure Boto3 Clients and Sessions",
"_____no_output_____"
]
],
[
[
"region = \"us-west-2\" # Change region as needed\nboto3.setup_default_session(region_name=region)\nboto_session = boto3.Session(region_name=region)\n\ns3_client = boto3.client(\"s3\", region_name=region)\n\nsagemaker_boto_client = boto_session.client(\"sagemaker\")\nsagemaker_session = sagemaker.session.Session(\n boto_session=boto_session, sagemaker_client=sagemaker_boto_client\n)\nsagemaker_role = sagemaker.get_execution_role()\n\nbucket = sagemaker.Session().default_bucket()",
"_____no_output_____"
]
],
[
[
"## Load Dataset",
"_____no_output_____"
]
],
[
[
"# check if directory exists\nif not os.path.isdir(\"data\"):\n os.mkdir(\"data\")\n\n# download zip file from public s3 bucket\n!wget -P data https://sagemaker-sample-files.s3.amazonaws.com/datasets/image/pcam/medical_images.zip",
"_____no_output_____"
],
[
"with zipfile.ZipFile(\"data/medical_images.zip\") as zf:\n zf.extractall()\nwith open(\"data/camelyon16_tiles.h5\", \"rb\") as hf:\n f = h5py.File(hf, \"r\")\n\n X = f[\"x\"][()]\n y = f[\"y\"][()]\n\nprint(\"Shape of X:\", X.shape)\nprint(\"Shape of y:\", y.shape)",
"Shape of X: (14000, 96, 96, 3)\nShape of y: (14000,)\n"
],
[
"# write to session s3 bucket\ns3_client.upload_file(\"data/medical_images.zip\", bucket, f\"data/medical_images.zip\")",
"_____no_output_____"
],
[
"# delete local copy\nimport os\n\nif os.path.exists(\"data/medical_images.zip\"):\n os.remove(\"data/medical_images.zip\")\nelse:\n print(\"The file does not exist\")",
"_____no_output_____"
]
],
[
[
"## View Sample Images from Dataset",
"_____no_output_____"
]
],
[
[
"def preview_images(X, y, n, cols):\n sample_images = X[:n]\n sample_labels = y[:n]\n\n rows = int(np.ceil(n / cols))\n fig, axs = plt.subplots(rows, cols, figsize=(11.5, 7))\n\n for i, ax in enumerate(axs.flatten()):\n image = sample_images[i]\n label = sample_labels[i]\n ax.imshow(image)\n ax.axis(\"off\")\n ax.set_title(f\"Label: {label}\")\n\n plt.tight_layout()\n\n\npreview_images(X, y, 15, 5)",
"_____no_output_____"
]
],
[
[
"## Shuffle and Split Dataset",
"_____no_output_____"
]
],
[
[
"from sklearn.model_selection import train_test_split\n\nX_numpy = X[:]\ny_numpy = y[:]\n\nX_train, X_test, y_train, y_test = train_test_split(\n X_numpy, y_numpy, test_size=1000, random_state=0\n)\nX_train, X_val, y_train, y_val = train_test_split(X_train, y_train, test_size=2000, random_state=1)\n\nprint(X_train.shape)\nprint(X_val.shape)\nprint(X_test.shape)",
"(11000, 96, 96, 3)\n(2000, 96, 96, 3)\n(1000, 96, 96, 3)\n"
]
],
[
[
"## Convert Splits to RecordIO Format",
"_____no_output_____"
]
],
[
[
"def write_to_recordio(X: np.ndarray, y: np.ndarray, prefix: str):\n record = mx.recordio.MXIndexedRecordIO(idx_path=f\"{prefix}.idx\", uri=f\"{prefix}.rec\", flag=\"w\")\n for idx, arr in enumerate(tqdm(X)):\n header = mx.recordio.IRHeader(0, y[idx], idx, 0)\n s = mx.recordio.pack_img(\n header,\n arr,\n quality=95,\n img_fmt=\".jpg\",\n )\n record.write_idx(idx, s)\n record.close()",
"_____no_output_____"
],
[
"write_to_recordio(X_train, y_train, prefix=\"data/train\")\nwrite_to_recordio(X_val, y_val, prefix=\"data/val\")\nwrite_to_recordio(X_test, y_test, prefix=\"data/test\")",
"_____no_output_____"
]
],
[
[
"## Upload Data Splits to S3",
"_____no_output_____"
]
],
[
[
"prefix = \"cv-metastasis\"\n\ntry:\n s3_client.create_bucket(\n Bucket=bucket, ACL=\"private\", CreateBucketConfiguration={\"LocationConstraint\": region}\n )\n print(f\"Created S3 bucket: {bucket}\")\n\nexcept Exception as e:\n if e.response[\"Error\"][\"Code\"] == \"BucketAlreadyOwnedByYou\":\n print(f\"Using existing bucket: {bucket}\")\n else:\n raise (e)",
"_____no_output_____"
],
[
"%store prefix",
"_____no_output_____"
],
[
"s3_client.upload_file(\"data/train.rec\", bucket, f\"{prefix}/data/train/train.rec\")\ns3_client.upload_file(\"data/val.rec\", bucket, f\"{prefix}/data/val/val.rec\")\ns3_client.upload_file(\"data/test.rec\", bucket, f\"{prefix}/data/test/test.rec\")",
"_____no_output_____"
]
],
[
[
"## Configure the Estimator",
"_____no_output_____"
]
],
[
[
"training_image = sagemaker.image_uris.retrieve(\"image-classification\", region)\nnum_training_samples = X_train.shape[0]\nnum_classes = len(np.unique(y_train))\n\nhyperparameters = {\n \"num_layers\": 18,\n \"use_pretrained_model\": 1,\n \"augmentation_type\": \"crop_color_transform\",\n \"image_shape\": \"3,96,96\",\n \"num_classes\": num_classes,\n \"num_training_samples\": num_training_samples,\n \"mini_batch_size\": 64,\n \"epochs\": 5,\n \"learning_rate\": 0.01,\n \"precision_dtype\": \"float32\",\n}\n\nestimator_config = {\n \"hyperparameters\": hyperparameters,\n \"image_uri\": training_image,\n \"role\": sagemaker.get_execution_role(),\n \"instance_count\": 1,\n \"instance_type\": \"ml.p3.2xlarge\",\n \"volume_size\": 100,\n \"max_run\": 360000,\n \"output_path\": f\"s3://{bucket}/{prefix}/training_jobs\",\n}\n\nimage_classifier = sagemaker.estimator.Estimator(**estimator_config)",
"_____no_output_____"
],
[
"%store num_training_samples",
"_____no_output_____"
]
],
[
[
"## Configure the Hyperparameter Tuner\n\nAlthough we would prefer to tune for recall, the current HyperparameterTuner implementation for Image Classification only supports validation accuracy.",
"_____no_output_____"
]
],
[
[
"hyperparameter_ranges = {\n \"mini_batch_size\": sagemaker.parameter.CategoricalParameter([16, 32, 64]),\n \"learning_rate\": sagemaker.parameter.CategoricalParameter([0.001, 0.01]),\n}\n\nhyperparameter_tuner = sagemaker.tuner.HyperparameterTuner(\n estimator=image_classifier,\n objective_metric_name=\"validation:accuracy\",\n hyperparameter_ranges=hyperparameter_ranges,\n max_jobs=6,\n max_parallel_jobs=2,\n base_tuning_job_name=prefix,\n)",
"_____no_output_____"
]
],
[
[
"## Define the Data Channels",
"_____no_output_____"
]
],
[
[
"train_input = sagemaker.inputs.TrainingInput(\n s3_data=f\"s3://{bucket}/{prefix}/data/train\",\n content_type=\"application/x-recordio\",\n s3_data_type=\"S3Prefix\",\n input_mode=\"Pipe\",\n)\n\nval_input = sagemaker.inputs.TrainingInput(\n s3_data=f\"s3://{bucket}/{prefix}/data/val\",\n content_type=\"application/x-recordio\",\n s3_data_type=\"S3Prefix\",\n input_mode=\"Pipe\",\n)\n\ndata_channels = {\"train\": train_input, \"validation\": val_input}",
"_____no_output_____"
]
],
[
[
"## Run Hyperparameter Tuning Jobs",
"_____no_output_____"
]
],
[
[
"if 'tuning_job_name' not in locals():\n hyperparameter_tuner.fit(inputs=data_channels)\n tuning_job_name = hyperparameter_tuner.describe().get('HyperParameterTuningJobName')\n %store tuning_job_name\nelse:\n print(f'Using previous tuning job: {tuning_job_name}')",
"_____no_output_____"
],
[
"%store tuning_job_name",
"_____no_output_____"
]
],
[
[
"## Examine Results\n\n<div class=\"alert alert-warning\">\n<b>NOTE:</b> If your kernel has restarted after running the hyperparameter tuning job, everyting you need has been persisted to SageMaker. You can continue on without having to run the tuning job again.\n</div>",
"_____no_output_____"
]
],
[
[
"results = sagemaker.analytics.HyperparameterTuningJobAnalytics(tuning_job_name)\nresults_df = results.dataframe()\nresults_df",
"_____no_output_____"
],
[
"best_training_job_summary = results.description()[\"BestTrainingJob\"]\nbest_training_job_name = best_training_job_summary[\"TrainingJobName\"]\n\n%store best_training_job_name",
"_____no_output_____"
]
]
] | [
"markdown",
"code",
"markdown",
"code",
"markdown",
"code",
"markdown",
"code",
"markdown",
"code",
"markdown",
"code",
"markdown",
"code",
"markdown",
"code",
"markdown",
"code",
"markdown",
"code",
"markdown",
"code",
"markdown",
"code",
"markdown",
"code"
] | [
[
"markdown",
"markdown",
"markdown"
],
[
"code",
"code"
],
[
"markdown"
],
[
"code"
],
[
"markdown"
],
[
"code"
],
[
"markdown"
],
[
"code",
"code",
"code",
"code"
],
[
"markdown"
],
[
"code"
],
[
"markdown"
],
[
"code"
],
[
"markdown"
],
[
"code",
"code"
],
[
"markdown"
],
[
"code",
"code",
"code"
],
[
"markdown"
],
[
"code",
"code"
],
[
"markdown"
],
[
"code"
],
[
"markdown"
],
[
"code"
],
[
"markdown"
],
[
"code",
"code"
],
[
"markdown"
],
[
"code",
"code"
]
] |
Subsets and Splits
No community queries yet
The top public SQL queries from the community will appear here once available.