hexsha
stringlengths 40
40
| size
int64 6
14.9M
| ext
stringclasses 1
value | lang
stringclasses 1
value | max_stars_repo_path
stringlengths 6
260
| max_stars_repo_name
stringlengths 6
119
| max_stars_repo_head_hexsha
stringlengths 40
41
| max_stars_repo_licenses
sequence | max_stars_count
int64 1
191k
โ | max_stars_repo_stars_event_min_datetime
stringlengths 24
24
โ | max_stars_repo_stars_event_max_datetime
stringlengths 24
24
โ | max_issues_repo_path
stringlengths 6
260
| max_issues_repo_name
stringlengths 6
119
| max_issues_repo_head_hexsha
stringlengths 40
41
| max_issues_repo_licenses
sequence | max_issues_count
int64 1
67k
โ | max_issues_repo_issues_event_min_datetime
stringlengths 24
24
โ | max_issues_repo_issues_event_max_datetime
stringlengths 24
24
โ | max_forks_repo_path
stringlengths 6
260
| max_forks_repo_name
stringlengths 6
119
| max_forks_repo_head_hexsha
stringlengths 40
41
| max_forks_repo_licenses
sequence | max_forks_count
int64 1
105k
โ | max_forks_repo_forks_event_min_datetime
stringlengths 24
24
โ | max_forks_repo_forks_event_max_datetime
stringlengths 24
24
โ | avg_line_length
float64 2
1.04M
| max_line_length
int64 2
11.2M
| alphanum_fraction
float64 0
1
| cells
sequence | cell_types
sequence | cell_type_groups
sequence |
---|---|---|---|---|---|---|---|---|---|---|---|---|---|---|---|---|---|---|---|---|---|---|---|---|---|---|---|---|---|---|
e768bf5245c9b9f0015a2456fe7f1d43d2bb0088 | 71,188 | ipynb | Jupyter Notebook | K-MEANS CLUSTERING.ipynb | PrasannaDataBus/CLUSTERING | d91ca8b1e9a75c65fa7137599da23b9c938d7eb5 | [
"Apache-2.0"
] | null | null | null | K-MEANS CLUSTERING.ipynb | PrasannaDataBus/CLUSTERING | d91ca8b1e9a75c65fa7137599da23b9c938d7eb5 | [
"Apache-2.0"
] | null | null | null | K-MEANS CLUSTERING.ipynb | PrasannaDataBus/CLUSTERING | d91ca8b1e9a75c65fa7137599da23b9c938d7eb5 | [
"Apache-2.0"
] | null | null | null | 133.310861 | 15,344 | 0.882396 | [
[
[
"# K-Means Clustering",
"_____no_output_____"
]
],
[
[
"# Import required packages\nimport pandas as pd\nfrom sklearn.preprocessing import MinMaxScaler\nfrom sklearn.cluster import KMeans\nimport matplotlib.pyplot as plt",
"_____no_output_____"
],
[
"from pandas import DataFrame\n\nData = {'x': [25,34,22,27,33,33,31,22,35,34,67,54,57,43,50,57,59,52,65,47,49,48,35,33,44,45,38,43,51,46],\n 'y': [79,51,53,78,59,74,73,57,69,75,51,32,40,47,53,36,35,58,59,50,25,20,14,12,20,5,29,27,8,7]\n }\n \ndf = DataFrame(Data,columns=['x','y'])\ndf.head()\n",
"_____no_output_____"
]
],
[
[
"Next youโll see how to use sklearn to find the centroids for 3 clusters, and then for 4 clusters.\nK-Means Clustering in Python โ 3 clusters\n\nOnce you created the DataFrame based on the above data, youโll need to import 2 additional Python modules:\n\n matplotlib โ for creating charts in Python\n sklearn โ for applying the K-Means Clustering in Python\n\nIn the code below, you can specify the number of clusters. For this example, assign 3 clusters as follows:\n\nKMeans(n_clusters=3).fit(df)",
"_____no_output_____"
]
],
[
[
"import matplotlib.pyplot as plt\nfrom sklearn.cluster import KMeans",
"_____no_output_____"
],
[
"mms = MinMaxScaler()\nmms.fit(df)\ndata_transformed = mms.transform(df)",
"_____no_output_____"
],
[
"Sum_of_squared_distances = []\nK = range(1,15)\nfor k in K:\n km = KMeans(n_clusters=k)\n km = km.fit(data_transformed)\n Sum_of_squared_distances.append(km.inertia_)",
"_____no_output_____"
],
[
"plt.plot(K, Sum_of_squared_distances, 'bx-')\nplt.xlabel('k')\nplt.ylabel('Sum_of_squared_distances')\nplt.title('Elbow Method For Optimal k')\nplt.show()",
"_____no_output_____"
],
[
" \nkmeans = KMeans(n_clusters=5).fit(df)\ncentroids = kmeans.cluster_centers_\nprint(centroids)\n",
"[[27.75 55. ]\n [56.75 35.75 ]\n [43.2 16.7 ]\n [54. 53. ]\n [30.83333333 74.66666667]]\n"
],
[
"plt.scatter(df['x'], df['y'], c= kmeans.labels_.astype(float), s=50, alpha=0.9)\nplt.scatter(centroids[:, 0], centroids[:, 1], c='red', s=100)",
"_____no_output_____"
],
[
"\nimport matplotlib.pyplot as plt\nfrom sklearn.cluster import KMeans\n\n \ndf = DataFrame(Data,columns=['x','y'])\n \nkmeans = KMeans(n_clusters=3).fit(df)\ncentroids = kmeans.cluster_centers_\nprint(centroids)โimport matplotlib.pyplot as plt\nfrom sklearn.cluster import KMeans\n\n \ndf = DataFrame(Data,columns=['x','y'])\n \nkmeans = KMeans(n_clusters=3).fit(df)\ncentroids = kmeans.cluster_centers_\nprint(centroids)\n\nplt.scatter(df['x'], df['y'], c= kmeans.labels_.astype(float), s=50, alpha=0.5)\nplt.scatter(centroids[:, 0], centroids[:, 1], c='red', s=50)\n\n\nplt.scatter(df['x'], df['y'], c= kmeans.labels_.astype(float), s=50, alpha=0.5)\nplt.scatter(centroids[:, 0], centroids[:, 1], c='red', s=50)\n",
"[[55.1 46.1]\n [29.6 66.8]\n [43.2 16.7]]\n"
],
[
"Note that the center of each cluster (in red) represents the mean of all the observations that belong to that cluster.\n\nAs you may also see, the observations that belong to a given cluster are closer to the center of that cluster, in comparison to the centers of other clusters.\nK-Means Clustering in Python โ 4 clusters\n\nLetโs now see what would happen if you use 4 clusters instead. In that case, the only thing youโll need to do is to change the n_clusters from 3 to 4:\n\nKMeans(n_clusters=4).fit(df)\n\nAnd so, your full Python code for 4 clusters would look like this:",
"_____no_output_____"
],
[
" \nkmeans = KMeans(n_clusters= 6).fit(df)\ncentroids = kmeans.cluster_centers_\nprint(centroids)\n\nplt.scatter(df['x'], df['y'], c= kmeans.labels_.astype(float), s=50, alpha=0.5)\nplt.scatter(centroids[:, 0], centroids[:, 1], c='red', s=50)\n\n",
"[[44.4 24.2 ]\n [30.83333333 74.66666667]\n [27.75 55. ]\n [56.75 35.75 ]\n [42. 9.2 ]\n [54. 53. ]]\n"
],
[
"Tkinter GUI to Display the Results\n\nYou can use the tkinter module in Python to display the clusters on a simple graphical user interface.\n\nThis is the code that you can use (for 3 clusters):",
"_____no_output_____"
]
]
] | [
"markdown",
"code",
"raw",
"code"
] | [
[
"markdown"
],
[
"code",
"code"
],
[
"raw"
],
[
"code",
"code",
"code",
"code",
"code",
"code",
"code",
"code",
"code",
"code"
]
] |
e768ca3c0368a377a17a6f56cfc54e325ca81777 | 112,404 | ipynb | Jupyter Notebook | notebooks/spark/AerospikeSparkPython.ipynb | dotyjim-work/aerospike-interactive-notebooks | 1ac22f59e679ef38210085273a2d0a2d51b2c80e | [
"MIT"
] | 1 | 2021-06-23T22:27:50.000Z | 2021-06-23T22:27:50.000Z | notebooks/spark/AerospikeSparkPython.ipynb | dotyjim-work/aerospike-interactive-notebooks | 1ac22f59e679ef38210085273a2d0a2d51b2c80e | [
"MIT"
] | 1 | 2021-11-09T21:04:53.000Z | 2021-11-09T23:53:51.000Z | notebooks/spark/AerospikeSparkPython.ipynb | dotyjim-work/aerospike-interactive-notebooks | 1ac22f59e679ef38210085273a2d0a2d51b2c80e | [
"MIT"
] | 2 | 2021-02-24T17:13:47.000Z | 2021-11-05T18:20:16.000Z | 64.488812 | 19,404 | 0.707599 | [
[
[
"# Aerospike Connect for Spark Tutorial for Python\n#### Tested with Spark connector 3.2.0, ASDB EE 5.7.0.7, Java 8, Apache Spark 3.0.2, Python 3.7 and Scala 2.12.11 and [Spylon]( https://pypi.org/project/spylon-kernel/)",
"_____no_output_____"
],
[
"## Setup",
"_____no_output_____"
],
[
"#### Ensure Database Is Running\nThis notebook requires that Aerospike datbase is running.",
"_____no_output_____"
]
],
[
[
"!asd >& /dev/null\n!pgrep -x asd >/dev/null && echo \"Aerospike database is running!\" || echo \"**Aerospike database is not running!**\"",
"Aerospike database is running!\n"
]
],
[
[
"#### Set Aerospike, Spark, and Spark Connector Paths and Parameters",
"_____no_output_____"
]
],
[
[
"# Directorie where spark related components are installed\nSPARK_NB_DIR = '/opt/spark-nb'\nSPARK_HOME = SPARK_NB_DIR + '/spark-3.0.3-bin-hadoop3.2'",
"_____no_output_____"
],
[
"# IP Address or DNS name for one host in your Aerospike cluster\nAS_HOST =\"localhost\"\n# Name of one of your namespaces. Type 'show namespaces' at the aql prompt if you are not sure\nAS_NAMESPACE = \"test\" \nAEROSPIKE_SPARK_JAR_VERSION=\"3.2.0\"\nAS_PORT = 3000 # Usually 3000, but change here if not\nAS_CONNECTION_STRING = AS_HOST + \":\"+ str(AS_PORT)",
"_____no_output_____"
],
[
"# Aerospike Spark Connector settings\nimport os \nAEROSPIKE_JAR_PATH = SPARK_NB_DIR + '/' + \"aerospike-spark-assembly-\" + AEROSPIKE_SPARK_JAR_VERSION + \".jar\"\nos.environ[\"PYSPARK_SUBMIT_ARGS\"] = '--jars ' + AEROSPIKE_JAR_PATH + ' pyspark-shell'",
"_____no_output_____"
]
],
[
[
"#### Alternative Setup for Running Notebook in Different Environment\nPlease follow the instructions below **instead of the setup above** if you are running this notebook in a different environment from the one provided by the Aerospike Intro-Notebooks container.\n```\n# IP Address or DNS name for one host in your Aerospike cluster\nAS_HOST = \"<seed-host-ip>\"\n# Name of one of your namespaces. Type 'show namespaces' at the aql prompt if you are not sure\nAS_NAMESPACE = \"<namespace>\" \nAEROSPIKE_SPARK_JAR_VERSION=\"<spark-connector-version>\"\nAS_PORT = 3000 # Usually 3000, but change here if not\nAS_CONNECTION_STRING = AS_HOST + \":\"+ str(AS_PORT)\n\n# Set SPARK_HOME path.\nSPARK_HOME = '<spark-home-dir>'\n\n# Please download the appropriate Aeropsike Connect for Spark from the [download page](https://enterprise.aerospike.com/enterprise/download/connectors/aerospike-spark/notes.html) \n# Set `AEROSPIKE_JAR_PATH` with path to the downloaded binary\nimport os \nAEROSPIKE_JAR_PATH= \"<aerospike-jar-dir>/aerospike-spark-assembly-\"+AEROSPIKE_SPARK_JAR_VERSION+\".jar\"\nos.environ[\"PYSPARK_SUBMIT_ARGS\"] = '--jars ' + AEROSPIKE_JAR_PATH + ' pyspark-shell'\n```",
"_____no_output_____"
],
[
"## Spark Initialization",
"_____no_output_____"
]
],
[
[
"# Next we locate the Spark installation - this will be found using the SPARK_HOME environment variable that you will have set \n\nimport findspark\nfindspark.init(SPARK_HOME)",
"_____no_output_____"
],
[
"import pyspark\nfrom pyspark.sql.types import *",
"_____no_output_____"
]
],
[
[
"#### Configure Aerospike properties in the Spark Session object. Please visit [Configuring Aerospike Connect for Spark](https://docs.aerospike.com/docs/connect/processing/spark/configuration.html) for more information about the properties used on this page.",
"_____no_output_____"
]
],
[
[
"from pyspark.sql import SparkSession\nfrom pyspark import SparkContext\nsc = SparkContext.getOrCreate()\nconf=sc._conf.setAll([(\"aerospike.namespace\",AS_NAMESPACE),(\"aerospike.seedhost\",AS_CONNECTION_STRING), (\"aerospike.log.level\",\"info\")])\nsc.stop()\nsc = pyspark.SparkContext(conf=conf)\nspark = SparkSession(sc)\n# sqlContext = SQLContext(sc)",
"_____no_output_____"
]
],
[
[
"## Schema in the Spark Connector\n\n- Aerospike is schemaless, however Spark adher to schema. After the schema is decided upon (either through inference or given), data within the bins must honor the types. \n\n- To infer schema, the connector samples a set of records (configurable through `aerospike.schema.scan`) to decide the name of bins/columns and their types. This implies that the derived schema depends entirely upon sampled records. \n\n- **Note that `__key` was not part of provided schema. So how can one query using `__key`? We can just add `__key` in provided schema with appropriate type. Similarly we can add `__gen` or `__ttl` etc.** \n \n schemaWithPK = StructType([\n StructField(\"__key\",IntegerType(), False), \n StructField(\"id\", IntegerType(), False),\n StructField(\"name\", StringType(), False),\n StructField(\"age\", IntegerType(), False),\n StructField(\"salary\",IntegerType(), False)])\n \n- **We recommend that you provide schema for queries that involve [collection data types](https://docs.aerospike.com/docs/guide/cdt.html) such as lists, maps, and mixed types. Using schema inference for CDT may cause unexpected issues.** ",
"_____no_output_____"
],
[
"### Flexible schema inference \nSpark assumes that the underlying data store (Aerospike in this case) follows a strict schema for all the records within a table. However, Aerospike is a No-SQL DB and is schemaless. For further information on the Spark connector reconciles those differences, visit [Flexible schema](https://docs.aerospike.com/docs/connect/processing/spark/configuration.html#flexible-schemas) page\n - aerospike.schema.flexible = true (default)\n - aerospike.schema.flexible = false",
"_____no_output_____"
]
],
[
[
"import random\nnum_records=200\n\nschema = StructType( \n [\n StructField(\"id\", IntegerType(), True),\n StructField(\"name\", StringType(), True)\n ]\n)\n\ninputBuf = []\nfor i in range(1, num_records) :\n name = \"name\" + str(i)\n id_ = i \n inputBuf.append((id_, name))\n \ninputRDD = spark.sparkContext.parallelize(inputBuf)\ninputDF=spark.createDataFrame(inputRDD,schema)\n\n#Write the Sample Data to Aerospike\ninputDF \\\n.write \\\n.mode('overwrite') \\\n.format(\"aerospike\") \\\n.option(\"aerospike.writeset\", \"py_input_data\")\\\n.option(\"aerospike.updateByKey\", \"id\") \\\n.save()",
"_____no_output_____"
]
],
[
[
"#### aerospike.schema.flexible = true (default) \n \n If none of the column types in the user-specified schema match the bin types of a record in Aerospike, a record with NULLs is returned in the result set. \n\nPlease use the filter() in Spark to filter out NULL records. For e.g. df.filter(\"gender == NULL\").show(false), where df is a dataframe and gender is a field that was not specified in the user-specified schema. \n\nIf the above mismatch is limited to fewer columns in the user-specified schema then NULL would be returned for those columns in the result set. **Note: there is no way to tell apart a NULL due to missing value in the original data set and the NULL due to mismatch, at this point. Hence, the user would have to treat all NULLs as missing values.** The columns that are not a part of the schema will be automatically filtered out in the result set by the connector.\n\nPlease note that if any field is set to NOT nullable i.e. nullable = false, your query will error out if thereโs a type mismatch between an Aerospike bin and the column type specified in the user-specified schema.\n \n ",
"_____no_output_____"
]
],
[
[
"schemaIncorrect = StructType( \n [\n StructField(\"id\", IntegerType(), True),\n StructField(\"name\", IntegerType(), True) ##Note incorrect type of name bin\n ]\n)\n\nflexSchemaInference=spark \\\n.read \\\n.format(\"aerospike\") \\\n.schema(schemaIncorrect) \\\n.option(\"aerospike.set\", \"py_input_data\").load()\n\nflexSchemaInference.show(5)\n\n##notice all the contents of name column is null due to schema mismatch and aerospike.schema.flexible = true (by default)",
"+---+----+\n| id|name|\n+---+----+\n| 10|null|\n| 50|null|\n|185|null|\n|117|null|\n| 88|null|\n+---+----+\nonly showing top 5 rows\n\n"
]
],
[
[
"#### aerospike.schema.flexible = false \n\nIf a mismatch between the user-specified schema and the schema of a record in Aerospike is detected at the bin/column level, your query will error out.\n",
"_____no_output_____"
]
],
[
[
"#When strict matching is set, we will get an exception due to type mismatch with schema provided.\n\ntry:\n errorDFStrictSchemaInference=spark \\\n .read \\\n .format(\"aerospike\") \\\n .schema(schemaIncorrect) \\\n .option(\"aerospike.schema.flexible\" ,\"false\") \\\n .option(\"aerospike.set\", \"py_input_data\").load()\n errorDFStrictSchemaInference.show(5)\nexcept Exception as e: \n pass\n \n#This will throw error due to type mismatch ",
"_____no_output_____"
]
],
[
[
"## Create sample data",
"_____no_output_____"
]
],
[
[
"# We create age vs salary data, using three different Gaussian distributions\nimport numpy as np\nimport matplotlib.pyplot as plt\nimport pandas as pd\nimport math\n\n# Make sure we get the same results every time this workbook is run\n# Otherwise we are occasionally exposed to results not working out as expected\nnp.random.seed(12345)\n\n# Create covariance matrix from std devs + correlation\ndef covariance_matrix(std_dev_1,std_dev_2,correlation):\n return [[std_dev_1 ** 2, correlation * std_dev_1 * std_dev_2], \n [correlation * std_dev_1 * std_dev_2, std_dev_2 ** 2]]\n\n# Return a bivariate sample given means/std dev/correlation\ndef age_salary_sample(distribution_params,sample_size):\n mean = [distribution_params[\"age_mean\"], distribution_params[\"salary_mean\"]]\n cov = covariance_matrix(distribution_params[\"age_std_dev\"],distribution_params[\"salary_std_dev\"],\n distribution_params[\"age_salary_correlation\"])\n return np.random.multivariate_normal(mean, cov, sample_size).T\n\n# Define the characteristics of our age/salary distribution\nage_salary_distribution_1 = {\"age_mean\":25,\"salary_mean\":50000,\n \"age_std_dev\":1,\"salary_std_dev\":5000,\"age_salary_correlation\":0.3}\n\nage_salary_distribution_2 = {\"age_mean\":45,\"salary_mean\":80000,\n \"age_std_dev\":4,\"salary_std_dev\":8000,\"age_salary_correlation\":0.7}\n\nage_salary_distribution_3 = {\"age_mean\":35,\"salary_mean\":70000,\n \"age_std_dev\":2,\"salary_std_dev\":9000,\"age_salary_correlation\":0.1}\n\ndistribution_data = [age_salary_distribution_1,age_salary_distribution_2,age_salary_distribution_3]\n\n# Sample age/salary data for each distributions\nsample_size_1 = 100;\nsample_size_2 = 120;\nsample_size_3 = 80;\nsample_sizes = [sample_size_1,sample_size_2,sample_size_3]\ngroup_1_ages,group_1_salaries = age_salary_sample(age_salary_distribution_1,sample_size=sample_size_1)\ngroup_2_ages,group_2_salaries = age_salary_sample(age_salary_distribution_2,sample_size=sample_size_2)\ngroup_3_ages,group_3_salaries = age_salary_sample(age_salary_distribution_3,sample_size=sample_size_3)\n\nages=np.concatenate([group_1_ages,group_2_ages,group_3_ages])\nsalaries=np.concatenate([group_1_salaries,group_2_salaries,group_3_salaries])\n\nprint(\"Data created\")",
"Data created\n"
]
],
[
[
"### Display simulated age/salary data",
"_____no_output_____"
]
],
[
[
"# Plot the sample data\ngroup_1_colour, group_2_colour, group_3_colour ='red','blue', 'pink'\nplt.xlabel('Age',fontsize=10)\nplt.ylabel(\"Salary\",fontsize=10) \n\nplt.scatter(group_1_ages,group_1_salaries,c=group_1_colour,label=\"Group 1\")\nplt.scatter(group_2_ages,group_2_salaries,c=group_2_colour,label=\"Group 2\")\nplt.scatter(group_3_ages,group_3_salaries,c=group_3_colour,label=\"Group 3\")\n\nplt.legend(loc='upper left')\nplt.show()",
"_____no_output_____"
]
],
[
[
"### Save data to Aerospike",
"_____no_output_____"
]
],
[
[
"# Turn the above records into a Data Frame\n# First of all, create an array of arrays\ninputBuf = []\n\nfor i in range(0, len(ages)) :\n id = i + 1 # Avoid counting from zero\n name = \"Individual: {:03d}\".format(id)\n # Note we need to make sure values are typed correctly\n # salary will have type numpy.float64 - if it is not cast as below, an error will be thrown\n age = float(ages[i])\n salary = int(salaries[i])\n inputBuf.append((id, name,age,salary))\n\n# Convert to an RDD \ninputRDD = spark.sparkContext.parallelize(inputBuf)\n \n# Convert to a data frame using a schema\nschema = StructType([\n StructField(\"id\", IntegerType(), True),\n StructField(\"name\", StringType(), True),\n StructField(\"age\", DoubleType(), True),\n StructField(\"salary\",IntegerType(), True)\n])\n\ninputDF=spark.createDataFrame(inputRDD,schema)\n\n#Write the data frame to Aerospike, the id field is used as the primary key\ninputDF \\\n.write \\\n.mode('overwrite') \\\n.format(\"aerospike\") \\\n.option(\"aerospike.set\", \"salary_data\")\\\n.option(\"aerospike.updateByKey\", \"id\") \\\n.save()",
"_____no_output_____"
]
],
[
[
"### Using Spark SQL syntax to insert data",
"_____no_output_____"
]
],
[
[
"#Aerospike DB needs a Primary key for record insertion. Hence, you must identify the primary key column \n#using for example .option(โaerospike.updateByKeyโ, โidโ), where โidโ is the name of the column that youโd \n#like to be the Primary key, while loading data from the DB. \n\ninsertDFWithSchema=spark \\\n.read \\\n.format(\"aerospike\") \\\n.schema(schema) \\\n.option(\"aerospike.set\", \"salary_data\") \\\n.option(\"aerospike.updateByKey\", \"id\") \\\n.load()\n\nsqlView=\"inserttable\"\n\n\n#\n# V2 datasource doesn't allow insert into a view. \n#\ninsertDFWithSchema.createTempView(sqlView)\nspark.sql(\"select * from inserttable\").show()",
"+---+---------------+------------------+------+\n| id| name| age|salary|\n+---+---------------+------------------+------+\n|239|Individual: 239|34.652141285212814| 61747|\n|101|Individual: 101| 46.53337694047585| 89019|\n|194|Individual: 194| 45.57430980213645| 94548|\n| 31|Individual: 031| 25.24920420954561| 54312|\n|139|Individual: 139| 38.84745269824981| 69645|\n| 14|Individual: 014|25.590430778495463| 51513|\n|142|Individual: 142| 42.5606479932568| 80357|\n|272|Individual: 272| 33.97918907293991| 66496|\n| 76|Individual: 076|25.457857266022874| 46214|\n|147|Individual: 147|43.186823515795496| 70158|\n| 79|Individual: 079|25.887490702675912| 48162|\n| 96|Individual: 096|24.084761701659602| 46328|\n|132|Individual: 132| 50.3039623703105| 78746|\n| 10|Individual: 010|25.082338749020728| 58345|\n|141|Individual: 141| 43.67491677796685| 79076|\n|140|Individual: 140|43.065120467057845| 78500|\n|160|Individual: 160| 54.98712625322743| 97029|\n|112|Individual: 112| 37.09568187885065| 72307|\n|120|Individual: 120|45.189080979167926| 80007|\n| 34|Individual: 034|22.794852985231497| 49882|\n+---+---------------+------------------+------+\nonly showing top 20 rows\n\n"
]
],
[
[
"## Load data into a DataFrame without specifying any Schema (uses schema inference)",
"_____no_output_____"
]
],
[
[
"# Create a Spark DataFrame by using the Connector Schema inference mechanism\n# The fields preceded with __ are metadata fields - key/digest/expiry/generation/ttl\n# By default you just get everything, with no column ordering, which is why it looks untidy\n# Note we don't get anything in the 'key' field as we have not chosen to save as a bin.\n# Use .option(\"aerospike.sendKey\", True) to do this\n\nloadedDFWithoutSchema = (\n spark.read.format(\"aerospike\") \\\n .option(\"aerospike.set\", \"salary_data\") \\\n .load()\n)\n\nloadedDFWithoutSchema.show(10)",
"+-----+--------------------+---------+------------+-------+------------------+---------------+------+---+\n|__key| __digest| __expiry|__generation| __ttl| age| name|salary| id|\n+-----+--------------------+---------+------------+-------+------------------+---------------+------+---+\n| null|[03 50 2E 7F 70 9...|378341961| 2|2591999|34.652141285212814|Individual: 239| 61747|239|\n| null|[0F 10 1A 93 B1 E...|378341961| 2|2591999| 45.57430980213645|Individual: 194| 94548|194|\n| null|[04 C0 5E 9A 68 5...|378341961| 2|2591999| 46.53337694047585|Individual: 101| 89019|101|\n| null|[1A E0 A8 A0 F2 3...|378341961| 2|2591999| 25.24920420954561|Individual: 031| 54312| 31|\n| null|[23 20 78 35 5D 7...|378341961| 2|2591998| 38.84745269824981|Individual: 139| 69645|139|\n| null|[35 00 8C 78 43 F...|378341961| 2|2591998|25.590430778495463|Individual: 014| 51513| 14|\n| null|[37 00 6D 21 08 9...|378341961| 2|2591998| 42.5606479932568|Individual: 142| 80357|142|\n| null|[59 00 4B C7 6D 9...|378341961| 2|2591998| 33.97918907293991|Individual: 272| 66496|272|\n| null|[61 50 89 B1 EC 0...|378341961| 2|2591998|25.457857266022874|Individual: 076| 46214| 76|\n| null|[6C 50 7F 9B FD C...|378341961| 2|2591998|43.186823515795496|Individual: 147| 70158|147|\n+-----+--------------------+---------+------------+-------+------------------+---------------+------+---+\nonly showing top 10 rows\n\n"
]
],
[
[
"## Load data into a DataFrame using user specified schema ",
"_____no_output_____"
]
],
[
[
"# If we explicitly set the schema, using the previously created schema object\n# we effectively type the rows in the Data Frame\n\nloadedDFWithSchema=spark \\\n.read \\\n.format(\"aerospike\") \\\n.schema(schema) \\\n.option(\"aerospike.set\", \"salary_data\").load()\n\nloadedDFWithSchema.show(5)",
"+---+---------------+------------------+------+\n| id| name| age|salary|\n+---+---------------+------------------+------+\n|239|Individual: 239|34.652141285212814| 61747|\n|101|Individual: 101| 46.53337694047585| 89019|\n|194|Individual: 194| 45.57430980213645| 94548|\n| 31|Individual: 031| 25.24920420954561| 54312|\n|139|Individual: 139| 38.84745269824981| 69645|\n+---+---------------+------------------+------+\nonly showing top 5 rows\n\n"
]
],
[
[
"## Sampling from Aerospike DB\n\n- Sample specified number of records from Aerospike to considerably reduce data movement between Aerospike and the Spark clusters. Depending on the aerospike.partition.factor setting, you may get more records than desired. Please use this property in conjunction with Spark `limit()` function to get the specified number of records. The sample read is not randomized, so sample more than you need and use the Spark `sample()` function to randomize if you see fit. You can use it in conjunction with `aerospike.recordspersecond` to control the load on the Aerospike server while sampling.\n\n- For more information, please see [documentation](https://docs.aerospike.com/docs/connect/processing/spark/configuration.html) page.",
"_____no_output_____"
]
],
[
[
"#number_of_spark_partitions (num_sp)=2^{aerospike.partition.factor}\n#total number of records = Math.ceil((float)aerospike.sample.size/num_sp) * (num_sp) \n#use lower partition factor for more accurate sampling\nsetname=\"py_input_data\"\nsample_size=101\n\ndf3=spark.read.format(\"aerospike\") \\\n.option(\"aerospike.partition.factor\",\"2\") \\\n.option(\"aerospike.set\",setname) \\\n.option(\"aerospike.sample.size\",\"101\") \\\n.load()\n\ndf4=spark.read.format(\"aerospike\") \\\n.option(\"aerospike.partition.factor\",\"6\") \\\n.option(\"aerospike.set\",setname) \\\n.option(\"aerospike.sample.size\",\"101\") \\\n.load()\n\n#Notice that more records were read than requested due to the underlying partitioning logic related to the partition factor as described earlier, hence we use Spark limit() function additionally to return the desired number of records.\ncount3=df3.count()\ncount4=df4.count()\n\n\n#Note how limit got only 101 records from df4.\ndfWithLimit=df4.limit(101)\nlimitCount=dfWithLimit.count()\n\nprint(\"count3= \", count3, \" count4= \", count4, \" limitCount=\", limitCount)",
"count3= 104 count4= 113 limitCount= 101\n"
]
],
[
[
"## Working with Collection Data Types (CDT) in Aerospike\n\n### Save JSON into Aerospike using a schema",
"_____no_output_____"
]
],
[
[
"# Schema specification\naliases_type = StructType([\n StructField(\"first_name\",StringType(),False),\n StructField(\"last_name\",StringType(),False)\n])\n\nid_type = StructType([\n StructField(\"first_name\",StringType(),False), \n StructField(\"last_name\",StringType(),False), \n StructField(\"aliases\",ArrayType(aliases_type),False)\n])\n\nstreet_adress_type = StructType([\n StructField(\"street_name\",StringType(),False), \n StructField(\"apt_number\",IntegerType(),False)\n])\n\naddress_type = StructType([\n StructField(\"zip\",LongType(),False), \n StructField(\"street\",street_adress_type,False), \n StructField(\"city\",StringType(),False)\n])\n\nworkHistory_type = StructType([\n StructField (\"company_name\",StringType(),False),\n StructField( \"company_address\",address_type,False),\n StructField(\"worked_from\",StringType(),False)\n])\n\nperson_type = StructType([\n StructField(\"name\",id_type,False),\n StructField(\"SSN\",StringType(),False),\n StructField(\"home_address\",ArrayType(address_type),False),\n StructField(\"work_history\",ArrayType(workHistory_type),False)\n])\n\n# JSON data location\ncomplex_data_json=\"resources/nested_data.json\"\n\n# Read data in using prepared schema\ncmplx_data_with_schema=spark.read.schema(person_type).json(complex_data_json)\n\n# Save data to Aerospike\ncmplx_data_with_schema \\\n.write \\\n.mode('overwrite') \\\n.format(\"aerospike\") \\\n.option(\"aerospike.writeset\", \"complex_input_data\") \\\n.option(\"aerospike.updateByKey\", \"SSN\") \\\n.save()",
"_____no_output_____"
]
],
[
[
"### Retrieve CDT from Aerospike into a DataFrame using schema ",
"_____no_output_____"
]
],
[
[
"loadedComplexDFWithSchema=spark \\\n.read \\\n.format(\"aerospike\") \\\n.option(\"aerospike.set\", \"complex_input_data\") \\\n.schema(person_type) \\\n.load() \nloadedComplexDFWithSchema.show(5)",
"+--------------------+-----------+--------------------+--------------------+\n| name| SSN| home_address| work_history|\n+--------------------+-----------+--------------------+--------------------+\n|[Carrie, Collier,...|611-70-8032|[[14908, [Frankli...|[[Russell Group, ...|\n|[Ashley, Davis, [...|708-19-4933|[[44679, [Duarte ...|[[Gaines LLC, [57...|\n|[Anthony, Dalton,...|466-55-4994|[[48032, [Mark Es...|[[Mora, Sherman a...|\n|[Jennifer, Willia...|438-70-6995|[[6917, [Gates Vi...|[[Cox, Olsen and ...|\n|[Robert, Robinson...|561-49-6700|[[29209, [Hernand...|[[Frye, Mckee and...|\n+--------------------+-----------+--------------------+--------------------+\nonly showing top 5 rows\n\n"
]
],
[
[
"## Data Exploration with Aerospike ",
"_____no_output_____"
]
],
[
[
"import pandas\nimport matplotlib\nimport matplotlib.pyplot as plt\n\n#convert Spark df to pandas df\npdf = loadedDFWithSchema.toPandas()\n\n# Describe the data\n\npdf.describe()",
"_____no_output_____"
],
[
"#Histogram - Age\nage_min, age_max = int(np.amin(pdf['age'])), math.ceil(np.amax(pdf['age']))\nage_bucket_size = 5\nprint(age_min,age_max)\npdf[['age']].plot(kind='hist',bins=range(age_min,age_max,age_bucket_size),rwidth=0.8)\nplt.xlabel('Age',fontsize=10)\nplt.legend(loc=None)\nplt.show()\n\n#Histogram - Salary\nsalary_min, salary_max = int(np.amin(pdf['salary'])), math.ceil(np.amax(pdf['salary']))\nsalary_bucket_size = 5000\npdf[['salary']].plot(kind='hist',bins=range(salary_min,salary_max,salary_bucket_size),rwidth=0.8)\nplt.xlabel('Salary',fontsize=10)\nplt.legend(loc=None)\nplt.show()\n\n# Heatmap\nage_bucket_count = math.ceil((age_max - age_min)/age_bucket_size)\nsalary_bucket_count = math.ceil((salary_max - salary_min)/salary_bucket_size)\n\nx = [[0 for i in range(salary_bucket_count)] for j in range(age_bucket_count)]\nfor i in range(len(pdf['age'])):\n age_bucket = math.floor((pdf['age'][i] - age_min)/age_bucket_size)\n salary_bucket = math.floor((pdf['salary'][i] - salary_min)/salary_bucket_size)\n x[age_bucket][salary_bucket] += 1\n\nplt.title(\"Salary/Age distribution heatmap\")\nplt.xlabel(\"Salary in '000s\")\nplt.ylabel(\"Age\")\n\nplt.imshow(x, cmap='YlOrRd', interpolation='nearest',extent=[salary_min/1000,salary_max/1000,age_min,age_max],\n origin=\"lower\")\nplt.colorbar(orientation=\"horizontal\")\nplt.show()",
"22 57\n"
]
],
[
[
" # Quering Aerospike Data using SparkSQL\n\n### Note:\n 1. Queries that involve Primary Key or Digest in the predicate trigger aerospike_batch_get()( https://www.aerospike.com/docs/client/c/usage/kvs/batch.html) and run extremely fast. For e.g. a query containing `__key` or `__digest` with, with no `OR` between two bins.\n 2. All other queries may entail a full scan of the Aerospike DB if they canโt be converted to Aerospike batchget. ",
"_____no_output_____"
],
[
"## Queries that include Primary Key in the Predicate\n\nWith batch get queries we can apply filters on metadata columns such as `__gen` or `__ttl`. To do this, these columns should be exposed through the schema.\n",
"_____no_output_____"
]
],
[
[
"# Basic PKey query\nbatchGet1= spark \\\n.read \\\n.format(\"aerospike\") \\\n.option(\"aerospike.set\", \"salary_data\") \\\n.option(\"aerospike.keyType\", \"int\") \\\n.load().where(\"__key = 100\") \\\n\nbatchGet1.show()\n#Note ASDB only supports equality test with PKs in primary key query. \n#So, a where clause with \"__key >10\", would result in scan query!",
"+-----+--------------------+---------+------------+-------+-----------------+---------------+------+---+\n|__key| __digest| __expiry|__generation| __ttl| age| name|salary| id|\n+-----+--------------------+---------+------------+-------+-----------------+---------------+------+---+\n| 100|[82 46 D4 AF BB 7...|378341961| 2|2591987|25.62963757719123|Individual: 100| 56483|100|\n+-----+--------------------+---------+------------+-------+-----------------+---------------+------+---+\n\n"
],
[
"# Batch get, primary key based query\nfrom pyspark.sql.functions import col\nsomePrimaryKeys= list(range(1,10))\nsomeMoreKeys= list(range(12,14))\nbatchGet2= spark \\\n.read \\\n.format(\"aerospike\") \\\n.option(\"aerospike.set\", \"salary_data\") \\\n.option(\"aerospike.keyType\", \"int\") \\\n.load().where((col(\"__key\").isin(somePrimaryKeys)) | ( col(\"__key\").isin(someMoreKeys))) \n\nbatchGet2.show(5)",
"+-----+--------------------+---------+------------+-------+------------------+---------------+------+---+\n|__key| __digest| __expiry|__generation| __ttl| age| name|salary| id|\n+-----+--------------------+---------+------------+-------+------------------+---------------+------+---+\n| 13|[27 B2 50 19 5B 5...|378341961| 2|2591985|24.945277952954463|Individual: 013| 47114| 13|\n| 5|[CC 73 E2 C2 23 2...|378341961| 2|2591985| 26.41972973144744|Individual: 005| 53845| 5|\n| 1|[85 36 18 55 4C B...|378341961| 2|2591985|25.395470523704972|Individual: 001| 48976| 1|\n| 9|[EB 86 7C 94 AA 4...|378341961| 2|2591985| 24.04479361358856|Individual: 009| 39991| 9|\n| 3|[B1 E9 BC 33 C7 9...|378341961| 2|2591985|26.918958635987867|Individual: 003| 59828| 3|\n+-----+--------------------+---------+------------+-------+------------------+---------------+------+---+\nonly showing top 5 rows\n\n"
]
],
[
[
"### batchget query using `__digest`\n - `__digest` can have only two types `BinaryType`(default type) or `StringType`.\n - If schema is not provided and `__digest` is `StringType`, then set `aerospike.digestType` to `string`.\n - Records retrieved with `__digest` batchget call will have null primary key (i.e.`__key` is `null`).",
"_____no_output_____"
]
],
[
[
"#convert digests to a list of byte[]\ndigest_list=batchGet2.select(\"__digest\").rdd.flatMap(lambda x: x).collect()\n\n#convert digest to hex string for querying. Only digests of type hex string and byte[] array are allowed.\nstring_digest=[ ''.join(format(x, '02x') for x in m) for m in digest_list]\n\n#option(\"aerospike.digestType\", \"string\") hints to assume that __digest type is string in schema inference.\n#please note that __key retrieved in this case is null. So be careful to use retrieved keys in downstream query!\nbatchGetWithDigest= spark \\\n.read \\\n.format(\"aerospike\") \\\n.option(\"aerospike.set\", \"salary_data\") \\\n.option(\"aerospike.digestType\", \"string\") \\\n.load().where(col(\"__digest\").isin(string_digest)) \nbatchGetWithDigest.show() \n\n\n#digests can be mixed with primary keys as well\nbatchGetWithDigestAndKey= spark \\\n.read \\\n.format(\"aerospike\") \\\n.option(\"aerospike.set\", \"salary_data\") \\\n.option(\"aerospike.digestType\", \"string\") \\\n.option(\"aerospike.keyType\", \"int\") \\\n.load().where(col(\"__digest\").isin(string_digest[0:1]) | ( col(\"__key\").isin(someMoreKeys))) \nbatchGetWithDigestAndKey.show()\n#please note to the null in key columns in both dataframe",
"+-----+--------------------+---------+------------+-------+------------------+---------------+------+---+\n|__key| __digest| __expiry|__generation| __ttl| age| name|salary| id|\n+-----+--------------------+---------+------------+-------+------------------+---------------+------+---+\n| null|27b250195b5a5ba13...|378341961| 2|2591977|24.945277952954463|Individual: 013| 47114| 13|\n| null|cc73e2c2232b35c49...|378341961| 2|2591977| 26.41972973144744|Individual: 005| 53845| 5|\n| null|853618554cb05c351...|378341961| 2|2591977|25.395470523704972|Individual: 001| 48976| 1|\n| null|eb867c94aa487a039...|378341961| 2|2591977| 24.04479361358856|Individual: 009| 39991| 9|\n| null|b1e9bc33c79b69e5c...|378341961| 2|2591977|26.918958635987867|Individual: 003| 59828| 3|\n| null|5a4a6223f73814afe...|378341961| 2|2591977|24.065640693038556|Individual: 006| 55035| 6|\n| null|db4ab2ffe4642f01c...|378341961| 2|2591976| 25.30086646117202|Individual: 007| 51374| 7|\n| null|86bbb52ef3b7d61eb...|378341961| 2|2591976| 24.31403545898676|Individual: 002| 47402| 2|\n| null|f84bdce243c7f1305...|378341961| 2|2591976|26.251474759555798|Individual: 008| 56764| 8|\n| null|849cbbf34c5ca14ab...|378341961| 2|2591976|25.000494245766017|Individual: 012| 66244| 12|\n| null|91dc5e91d4b9060f6...|378341961| 2|2591976|25.296641063103234|Individual: 004| 50464| 4|\n+-----+--------------------+---------+------------+-------+------------------+---------------+------+---+\n\n+-----+--------------------+---------+------------+-------+------------------+---------------+------+---+\n|__key| __digest| __expiry|__generation| __ttl| age| name|salary| id|\n+-----+--------------------+---------+------------+-------+------------------+---------------+------+---+\n| null|27b250195b5a5ba13...|378341961| 2|2591976|24.945277952954463|Individual: 013| 47114| 13|\n| 12|849cbbf34c5ca14ab...|378341961| 2|2591975|25.000494245766017|Individual: 012| 66244| 12|\n+-----+--------------------+---------+------------+-------+------------------+---------------+------+---+\n\n"
]
],
[
[
"## Queries including non-primary key conditions",
"_____no_output_____"
]
],
[
[
"# This query will run as a scan, which will be slower\nsomePrimaryKeys= list(range(1,10))\nscanQuery1= spark \\\n.read \\\n.format(\"aerospike\") \\\n.option(\"aerospike.set\", \"salary_data\") \\\n.option(\"aerospike.keyType\", \"int\") \\\n.load().where((col(\"__key\").isin(somePrimaryKeys)) | ( col(\"age\") >50 ))\n\nscanQuery1.show()",
"+-----+--------------------+---------+------------+-------+------------------+---------------+------+---+\n|__key| __digest| __expiry|__generation| __ttl| age| name|salary| id|\n+-----+--------------------+---------+------------+-------+------------------+---------------+------+---+\n| null|[9A 80 6A A1 FC C...|378341961| 2|2591974| 50.3039623703105|Individual: 132| 78746|132|\n| null|[EF A0 76 41 51 B...|378341961| 2|2591974| 54.98712625322743|Individual: 160| 97029|160|\n| null|[6E 92 74 77 95 D...|378341961| 2|2591974| 56.51623471593584|Individual: 196| 80848|196|\n| null|[71 65 79 9E 25 9...|378341961| 2|2591974| 50.4687163424899|Individual: 162| 96742|162|\n| null|[7C 66 F5 9E 99 6...|378341961| 2|2591974| 50.57144124293668|Individual: 156| 88377|156|\n| null|[7E A6 1C 30 4F 9...|378341961| 2|2591974| 50.58123004549132|Individual: 203| 91326|203|\n| null|[AB AA F1 86 BF C...|378341961| 2|2591973| 50.82155356588119|Individual: 106| 91658|106|\n| null|[BC 6A 1B 19 1A 9...|378341961| 2|2591973| 50.83291154818823|Individual: 187| 92796|187|\n| null|[0E 7B 68 E5 9C 9...|378341961| 2|2591973|52.636460763338036|Individual: 149| 90797|149|\n| null|[9E 5B 71 28 56 3...|378341961| 2|2591973|51.040523493441206|Individual: 214| 90306|214|\n| null|[28 CC 1A A7 5E 2...|378341961| 2|2591973| 56.14454565605453|Individual: 220| 94943|220|\n| null|[DF 6D 03 6F 18 2...|378341961| 2|2591973|51.405636565306544|Individual: 193| 97698|193|\n| null|[4B AF 54 1F E5 2...|378341961| 2|2591973| 51.28350713525771|Individual: 178| 90077|178|\n| null|[FD DF 68 1A 00 E...|378341961| 2|2591972|56.636218720385074|Individual: 206|105414|206|\n+-----+--------------------+---------+------------+-------+------------------+---------------+------+---+\n\n"
]
],
[
[
"## Pushdown [Aerospike Expressions](https://docs.aerospike.com/docs/guide/expressions/) from within a Spark API.\n\n - Make sure that you do not use no the WHERE clause or spark filters while querying\n - See [Aerospike Expressions](https://docs.aerospike.com/docs/guide/expressions/) for more information on how to construct expressions.\n - Contstructed expressions must be converted to Base64 before using them in the Spark API\n",
"_____no_output_____"
]
],
[
[
"scala_predexp= sc._jvm.com.aerospike.spark.utility.AerospikePushdownExpressions\n\n#id % 5 == 0 => get rows where mod(col(\"id\")) ==0\n#Equvalent java Exp: Exp.eq(Exp.mod(Exp.intBin(\"a\"), Exp.`val`(5)), Exp.`val`(0))\nexpIntBin=scala_predexp.intBin(\"id\") # id is the name of column\nexpMODIntBinEqualToZero=scala_predexp.eq(scala_predexp.mod(expIntBin, scala_predexp.val(5)),scala_predexp.val(0))\nexpMODIntBinToBase64= scala_predexp.build(expMODIntBinEqualToZero).getBase64()\n#expMODIntBinToBase64= \"kwGTGpNRAqJpZAUA\"\npushdownset = \"py_input_data\"\n\n\npushDownDF =spark\\\n .read \\\n .format(\"aerospike\") \\\n .schema(schema) \\\n .option(\"aerospike.set\", pushdownset) \\\n .option(\"aerospike.pushdown.expressions\", expMODIntBinToBase64) \\\n .load()\n\npushDownDF.count() #should get 39 records, we have 199/5 records whose id bin is divisble by 5",
"_____no_output_____"
],
[
"pushDownDF.show(2)",
"+---+------+----+------+\n| id| name| age|salary|\n+---+------+----+------+\n| 10|name10|null| null|\n| 50|name50|null| null|\n+---+------+----+------+\nonly showing top 2 rows\n\n"
]
],
[
[
"## Parameters for tuning Aerospike / Spark performance\n\n - aerospike.partition.factor: number of logical aerospike partitions [0-15]\n - aerospike.maxthreadcount : maximum number of threads to use for writing data into Aerospike\n - aerospike.compression : compression of java client-server communication\n - aerospike.batchMax : maximum number of records per read request (default 5000)\n - aerospike.recordspersecond : same as java client\n\n## Other useful parameters\n - aerospike.keyType : Primary key type hint for schema inference. Always set it properly if primary key type is not string \n\nSee https://www.aerospike.com/docs/connect/processing/spark/reference.html for detailed description of the above properties\n",
"_____no_output_____"
],
[
"# Machine Learning using Aerospike / Spark\n\nIn this section we use the data we took from Aerospike and apply a clustering algorithm to it.\n\nWe assume the data is composed of multiple data sets having a Gaussian multi-variate distribution\n\nWe don't know how many clusters there are, so we try clustering based on the assumption there are 1 through 20.\n\nWe compare the quality of the results using the Bayesian Information Criterion - https://en.wikipedia.org/wiki/Bayesian_information_criterion and pick the best.\n \n## Find Optimal Cluster Count ",
"_____no_output_____"
]
],
[
[
"from sklearn.mixture import GaussianMixture\n\n# We take the data we previously \nages=pdf['age']\nsalaries=pdf['salary']\n#age_salary_matrix=np.matrix([ages,salaries]).T\nage_salary_matrix=np.asarray([ages,salaries]).T\n\n# Find the optimal number of clusters\noptimal_cluster_count = 1\nbest_bic_score = GaussianMixture(1).fit(age_salary_matrix).bic(age_salary_matrix)\n\nfor count in range(1,20):\n gm=GaussianMixture(count)\n gm.fit(age_salary_matrix)\n if gm.bic(age_salary_matrix) < best_bic_score:\n best_bic_score = gm.bic(age_salary_matrix)\n optimal_cluster_count = count\n\nprint(\"Optimal cluster count found to be \"+str(optimal_cluster_count))",
"Optimal cluster count found to be 4\n"
]
],
[
[
"## Estimate cluster distribution parameters\nNext we fit our cluster using the optimal cluster count, and print out the discovered means and covariance matrix",
"_____no_output_____"
]
],
[
[
"gm = GaussianMixture(optimal_cluster_count)\ngm.fit(age_salary_matrix)\n\nestimates = []\n# Index\nfor index in range(0,optimal_cluster_count):\n estimated_mean_age = round(gm.means_[index][0],2)\n estimated_mean_salary = round(gm.means_[index][1],0)\n estimated_age_std_dev = round(math.sqrt(gm.covariances_[index][0][0]),2)\n estimated_salary_std_dev = round(math.sqrt(gm.covariances_[index][1][1]),0)\n estimated_correlation = round(gm.covariances_[index][0][1] / ( estimated_age_std_dev * estimated_salary_std_dev ),3)\n row = [estimated_mean_age,estimated_mean_salary,estimated_age_std_dev,estimated_salary_std_dev,estimated_correlation]\n estimates.append(row)\n \npd.DataFrame(estimates,columns = [\"Est Mean Age\",\"Est Mean Salary\",\"Est Age Std Dev\",\"Est Salary Std Dev\",\"Est Correlation\"]) \n",
"_____no_output_____"
]
],
[
[
"## Original Distribution Parameters",
"_____no_output_____"
]
],
[
[
"distribution_data_as_rows = []\nfor distribution in distribution_data:\n row = [distribution['age_mean'],distribution['salary_mean'],distribution['age_std_dev'],\n distribution['salary_std_dev'],distribution['age_salary_correlation']]\n distribution_data_as_rows.append(row)\n\npd.DataFrame(distribution_data_as_rows,columns = [\"Mean Age\",\"Mean Salary\",\"Age Std Dev\",\"Salary Std Dev\",\"Correlation\"])",
"_____no_output_____"
]
],
[
[
"You can see that the algorithm provides good estimates of the original parameters",
"_____no_output_____"
],
[
"## Prediction\n\nWe generate new age/salary pairs for each of the distributions and look at how accurate the prediction is",
"_____no_output_____"
]
],
[
[
"def prediction_accuracy(model,age_salary_distribution,sample_size):\n # Generate new values\n new_ages,new_salaries = age_salary_sample(age_salary_distribution,sample_size)\n #new_age_salary_matrix=np.matrix([new_ages,new_salaries]).T\n new_age_salary_matrix=np.asarray([new_ages,new_salaries]).T\n # Find which cluster the mean would be classified into\n #mean = np.matrix([age_salary_distribution['age_mean'],age_salary_distribution['salary_mean']])\n #mean = np.asarray([age_salary_distribution['age_mean'],age_salary_distribution['salary_mean']])\n mean = np.asarray(np.matrix([age_salary_distribution['age_mean'],age_salary_distribution['salary_mean']]))\n mean_cluster_index = model.predict(mean)[0]\n # How would new samples be classified\n classification = model.predict(new_age_salary_matrix)\n # How many were classified correctly\n correctly_classified = len([ 1 for x in classification if x == mean_cluster_index])\n return correctly_classified / sample_size\n\nprediction_accuracy_results = [None for x in range(3)]\nfor index, age_salary_distribution in enumerate(distribution_data):\n prediction_accuracy_results[index] = prediction_accuracy(gm,age_salary_distribution,1000)\n\noverall_accuracy = sum(prediction_accuracy_results)/ len(prediction_accuracy_results)\nprint(\"Accuracies for each distribution : \",\" ,\".join(map('{:.2%}'.format,prediction_accuracy_results)))\nprint(\"Overall accuracy : \",'{:.2%}'.format(overall_accuracy))\n",
"Accuracies for each distribution : 100.00% ,60.20% ,98.00%\nOverall accuracy : 86.07%\n"
]
],
[
[
"## aerolookup\n aerolookup allows you to look up records corresponding to a set of keys stored in a Spark DF, streaming or otherwise. It supports:\n \n - [Aerospike CDT](https://docs.aerospike.com/docs/guide/cdt.htmlarbitrary)\n - Quota and retry (these configurations are extracted from sparkconf) \n - [Flexible schema](https://docs.aerospike.com/docs/connect/processing/spark/configuration.html#flexible-schemas). To enable, set `aerospike.schema.flexible` to true in the SparkConf object.\n - Aerospike Expressions Pushdown (Note: This must be specified through SparkConf object.)\n",
"_____no_output_____"
]
],
[
[
"alias = StructType([StructField(\"first_name\", StringType(), False),\n StructField(\"last_name\", StringType(), False)])\nname = StructType([StructField(\"first_name\", StringType(), False),\n StructField(\"aliases\", ArrayType(alias), False)])\nstreet_adress = StructType([StructField(\"street_name\", StringType(), False),\n StructField(\"apt_number\", IntegerType(), False)])\naddress = StructType([StructField(\"zip\", LongType(), False),\n StructField(\"street\", street_adress, False),\n StructField(\"city\", StringType(), False)])\nwork_history = StructType([StructField(\"company_name\", StringType(), False),\n StructField(\"company_address\", address, False),\n StructField(\"worked_from\", StringType(), False)])\n\noutput_schema = StructType([StructField(\"name\", name, False),\n StructField(\"SSN\", StringType(), False),\n StructField(\"home_address\", ArrayType(address), False)])\n\nssns = [[\"825-55-3247\"], [\"289-18-1554\"], [\"756-46-4088\"], \n [\"525-31-0299\"], [\"456-45-2200\"], [\"200-71-7765\"]]\n\n#Create a set of PKs whose records you'd like to look up in the Aerospike database\ncustomerIdsDF=spark.createDataFrame(ssns,[\"SSN\"])\n\nfrom pyspark.sql import SQLContext\n\nscala2_object= sc._jvm.com.aerospike.spark.PythonUtil #Import the scala object\ngateway_df=scala2_object.aerolookup(customerIdsDF._jdf, #Please note ._jdf\n 'SSN',\n 'complex_input_data', #complex_input_data is the set in Aerospike database that you are using to look up the keys stored in SSN DF\n output_schema.json(),\n 'test')\naerolookup_df=pyspark.sql.DataFrame(gateway_df,spark._wrapped) \n#Note the wrapping of java object into python.sql.DataFrame \n",
"_____no_output_____"
],
[
"aerolookup_df.show()",
"+--------------------+-----------+--------------------+\n| name| SSN| home_address|\n+--------------------+-----------+--------------------+\n|[Gary, [[Cameron,...|825-55-3247|[[66428, [Kim Mil...|\n|[Megan, [[Robert,...|289-18-1554|[[81551, [Archer ...|\n|[Melanie, [[Justi...|756-46-4088|[[61327, [Jeanett...|\n|[Lisa, [[William,...|525-31-0299|[[98337, [Brittne...|\n|[Ryan, [[Jonathon...|456-45-2200|[[97077, [Davis D...|\n|[Lauren, [[Shaun,...|200-71-7765|[[6813, [Johnson ...|\n+--------------------+-----------+--------------------+\n\n"
]
]
] | [
"markdown",
"code",
"markdown",
"code",
"markdown",
"code",
"markdown",
"code",
"markdown",
"code",
"markdown",
"code",
"markdown",
"code",
"markdown",
"code",
"markdown",
"code",
"markdown",
"code",
"markdown",
"code",
"markdown",
"code",
"markdown",
"code",
"markdown",
"code",
"markdown",
"code",
"markdown",
"code",
"markdown",
"code",
"markdown",
"code",
"markdown",
"code",
"markdown",
"code",
"markdown",
"code",
"markdown",
"code",
"markdown",
"code",
"markdown",
"code",
"markdown",
"code",
"markdown",
"code"
] | [
[
"markdown",
"markdown",
"markdown"
],
[
"code"
],
[
"markdown"
],
[
"code",
"code",
"code"
],
[
"markdown",
"markdown"
],
[
"code",
"code"
],
[
"markdown"
],
[
"code"
],
[
"markdown",
"markdown"
],
[
"code"
],
[
"markdown"
],
[
"code"
],
[
"markdown"
],
[
"code"
],
[
"markdown"
],
[
"code"
],
[
"markdown"
],
[
"code"
],
[
"markdown"
],
[
"code"
],
[
"markdown"
],
[
"code"
],
[
"markdown"
],
[
"code"
],
[
"markdown"
],
[
"code"
],
[
"markdown"
],
[
"code"
],
[
"markdown"
],
[
"code"
],
[
"markdown"
],
[
"code"
],
[
"markdown"
],
[
"code",
"code"
],
[
"markdown",
"markdown"
],
[
"code",
"code"
],
[
"markdown"
],
[
"code"
],
[
"markdown"
],
[
"code"
],
[
"markdown"
],
[
"code",
"code"
],
[
"markdown",
"markdown"
],
[
"code"
],
[
"markdown"
],
[
"code"
],
[
"markdown"
],
[
"code"
],
[
"markdown",
"markdown"
],
[
"code"
],
[
"markdown"
],
[
"code",
"code"
]
] |
e768da290e65817975575f2fff7ecc110d881d75 | 158,520 | ipynb | Jupyter Notebook | Moon_classification_Exercise/Moon_Classification_Exercise.ipynb | NwekeChidi/Udacity_ML_with_SageMaker | fb707639cf622f8f3b104eecddc52aa09fea709b | [
"MIT"
] | null | null | null | Moon_classification_Exercise/Moon_Classification_Exercise.ipynb | NwekeChidi/Udacity_ML_with_SageMaker | fb707639cf622f8f3b104eecddc52aa09fea709b | [
"MIT"
] | null | null | null | Moon_classification_Exercise/Moon_Classification_Exercise.ipynb | NwekeChidi/Udacity_ML_with_SageMaker | fb707639cf622f8f3b104eecddc52aa09fea709b | [
"MIT"
] | null | null | null | 135.371477 | 95,880 | 0.830223 | [
[
[
"# Moon Data Classification\n\nIn this notebook, you'll be tasked with building and deploying a **custom model** in SageMaker. Specifically, you'll define and train a custom, PyTorch neural network to create a binary classifier for data that is separated into two classes; the data looks like two moon shapes when it is displayed, and is often referred to as **moon data**.\n\nThe notebook will be broken down into a few steps:\n* Generating the moon data\n* Loading it into an S3 bucket\n* Defining a PyTorch binary classifier\n* Completing a training script\n* Training and deploying the custom model\n* Evaluating its performance\n\nBeing able to train and deploy custom models is a really useful skill to have. Especially in applications that may not be easily solved by traditional algorithms like a LinearLearner.\n\n---",
"_____no_output_____"
],
[
"Load in required libraries, below.",
"_____no_output_____"
]
],
[
[
"# data \nimport pandas as pd \nimport numpy as np\nfrom sklearn.datasets import make_moons\nfrom sklearn.model_selection import train_test_split\nimport matplotlib.pyplot as plt\n\n%matplotlib inline",
"_____no_output_____"
]
],
[
[
"## Generating Moon Data\n\nBelow, I have written code to generate some moon data, using sklearn's [make_moons](https://scikit-learn.org/stable/modules/generated/sklearn.datasets.make_moons.html) and [train_test_split](https://scikit-learn.org/stable/modules/generated/sklearn.model_selection.train_test_split.html).\n\nI'm specifying the number of data points and a noise parameter to use for generation. Then, displaying the resulting data.",
"_____no_output_____"
]
],
[
[
"# set data params\nnp.random.seed(0)\nnum_pts = 1000\nnoise_val = 0.25\n\n# generate data\n# X = 2D points, Y = class labels (0 or 1)\nX, Y = make_moons(num_pts, noise=noise_val)\n\n# Split into test and training data\nX_train, X_test, Y_train, Y_test = train_test_split(X, Y,\n test_size=0.25, random_state=1)",
"_____no_output_____"
],
[
"# plot\n# points are colored by class, Y_train\n# 0 labels = purple, 1 = yellow\nplt.figure(figsize=(8,5))\nplt.scatter(X_train[:,0], X_train[:,1], c=Y_train)\nplt.title('Moon Data')\nplt.show()",
"_____no_output_____"
]
],
[
[
"## SageMaker Resources\n\nThe below cell stores the SageMaker session and role (for creating estimators and models), and creates a default S3 bucket. After creating this bucket, you can upload any locally stored data to S3.",
"_____no_output_____"
]
],
[
[
"# sagemaker\nimport boto3\nimport sagemaker\nfrom sagemaker import get_execution_role",
"_____no_output_____"
],
[
"# SageMaker session and role\nsagemaker_session = sagemaker.Session()\nrole = sagemaker.get_execution_role()\n\n# default S3 bucket\nbucket = sagemaker_session.default_bucket()",
"_____no_output_____"
]
],
[
[
"### EXERCISE: Create csv files\n\nDefine a function that takes in x (features) and y (labels) and saves them to one `.csv` file at the path `data_dir/filename`. SageMaker expects `.csv` files to be in a certain format, according to the [documentation](https://docs.aws.amazon.com/sagemaker/latest/dg/cdf-training.html):\n> Amazon SageMaker requires that a CSV file doesn't have a header record and that the target variable is in the first column.\n\nIt may be useful to use pandas to merge your features and labels into one DataFrame and then convert that into a `.csv` file. When you create a `.csv` file, make sure to set `header=False`, and `index=False` so you don't include anything extraneous, like column names, in the `.csv` file.",
"_____no_output_____"
]
],
[
[
"import os\n\ndef make_csv(x, y, filename, data_dir):\n '''Merges features and labels and converts them into one csv file with labels in the first column.\n :param x: Data features\n :param y: Data labels\n :param file_name: Name of csv file, ex. 'train.csv'\n :param data_dir: The directory where files will be saved\n '''\n # make data dir, if it does not exist\n if not os.path.exists(data_dir):\n os.makedirs(data_dir)\n \n # your code here\n pd.concat([pd.DataFrame(y), pd.DataFrame(x)], axis=1).to_csv(os.path.join(data_dir, filename), header=False, index=False)\n \n # nothing is returned, but a print statement indicates that the function has run\n print('Path created: '+str(data_dir)+'/'+str(filename))",
"_____no_output_____"
]
],
[
[
"The next cell runs the above function to create a `train.csv` file in a specified directory.",
"_____no_output_____"
]
],
[
[
"data_dir = 'data_moon' # the folder we will use for storing data\nname = 'train.csv'\n\n# create 'train.csv'\nmake_csv(X_train, Y_train, name, data_dir)",
"Path created: data_moon/train.csv\n"
]
],
[
[
"### Upload Data to S3\n\nUpload locally-stored `train.csv` file to S3 by using `sagemaker_session.upload_data`. This function needs to know: where the data is saved locally, and where to upload in S3 (a bucket and prefix).",
"_____no_output_____"
]
],
[
[
"# specify where to upload in S3\nprefix = 'sagemaker/moon-data'\n\n# upload to S3\ninput_data = sagemaker_session.upload_data(path=data_dir, bucket=bucket, key_prefix=prefix)\nprint(input_data)",
"s3://sagemaker-us-east-1-633655289115/sagemaker/moon-data\n"
]
],
[
[
"Check that you've uploaded the data, by printing the contents of the default bucket.",
"_____no_output_____"
]
],
[
[
"# iterate through S3 objects and print contents\nfor obj in boto3.resource('s3').Bucket(bucket).objects.all():\n print(obj.key)",
"fraud_detection/linear-learner-2020-10-24-06-45-32-777/output/model.tar.gz\nfraud_detection/linear-learner-2020-10-24-07-25-35-056/output/model.tar.gz\nfraud_detection/linear-learner-2020-10-24-07-48-22-409/output/model.tar.gz\nfraud_detection/linear-learner-2020-10-24-08-12-30-994/output/model.tar.gz\nfraud_detection/linear-learner-2020-10-24-08-35-11-726/output/model.tar.gz\npytorch-inference-2020-10-24-18-31-29-473/model.tar.gz\npytorch-inference-2020-10-24-19-11-32-308/model.tar.gz\npytorch-training-2020-10-24-18-19-07-459/source/sourcedir.tar.gz\npytorch-training-2020-10-24-18-25-03-445/source/sourcedir.tar.gz\nsagemaker-pytorch-2020-10-24-18-53-06-891/sourcedir.tar.gz\nsagemaker-record-sets/LinearLearner-2020-10-24-06-36-33-183/.amazon.manifest\nsagemaker-record-sets/LinearLearner-2020-10-24-06-36-33-183/matrix_0.pbr\nsagemaker-record-sets/LinearLearner-2020-10-24-06-45-22-344/.amazon.manifest\nsagemaker-record-sets/LinearLearner-2020-10-24-06-45-22-344/matrix_0.pbr\nsagemaker-record-sets/LinearLearner-2020-10-24-07-02-18-578/.amazon.manifest\nsagemaker-record-sets/LinearLearner-2020-10-24-07-02-18-578/matrix_0.pbr\nsagemaker-record-sets/LinearLearner-2020-10-24-07-14-28-034/.amazon.manifest\nsagemaker-record-sets/LinearLearner-2020-10-24-07-14-28-034/matrix_0.pbr\nsagemaker-record-sets/LinearLearner-2020-10-24-08-10-34-997/.amazon.manifest\nsagemaker-record-sets/LinearLearner-2020-10-24-08-10-34-997/matrix_0.pbr\nsagemaker/moon-data/pytorch-training-2020-10-24-18-25-03-445/debug-output/claim.smd\nsagemaker/moon-data/pytorch-training-2020-10-24-18-25-03-445/debug-output/collections/000000000/worker_0_collections.json\nsagemaker/moon-data/pytorch-training-2020-10-24-18-25-03-445/debug-output/events/000000000000/000000000000_worker_0.tfevents\nsagemaker/moon-data/pytorch-training-2020-10-24-18-25-03-445/debug-output/events/000000000500/000000000500_worker_0.tfevents\nsagemaker/moon-data/pytorch-training-2020-10-24-18-25-03-445/debug-output/index/000000000/000000000000_worker_0.json\nsagemaker/moon-data/pytorch-training-2020-10-24-18-25-03-445/debug-output/index/000000000/000000000500_worker_0.json\nsagemaker/moon-data/pytorch-training-2020-10-24-18-25-03-445/debug-output/training_job_end.ts\nsagemaker/moon-data/pytorch-training-2020-10-24-18-25-03-445/output/model.tar.gz\nsagemaker/moon-data/train.csv\n"
]
],
[
[
"---\n# Modeling\n\nNow that you've uploaded your training data, it's time to define and train a model!\n\nIn this notebook, you'll define and train a **custom PyTorch model**; a neural network that performs binary classification. \n\n### EXERCISE: Define a model in `model.py`\n\nTo implement a custom classifier, the first thing you'll do is define a neural network. You've been give some starting code in the directory `source`, where you can find the file, `model.py`. You'll need to complete the class `SimpleNet`; specifying the layers of the neural network and its feedforward behavior. It may be helpful to review the [code for a 3-layer MLP](https://github.com/udacity/deep-learning-v2-pytorch/blob/master/convolutional-neural-networks/mnist-mlp/mnist_mlp_solution.ipynb).\n\nThis model should be designed to: \n* Accept a number of `input_dim` features\n* Create some linear, hidden layers of a desired size\n* Return **a single output value** that indicates the class score\n\nThe returned output value should be a [sigmoid-activated](https://pytorch.org/docs/stable/nn.html#sigmoid) class score; a value between 0-1 that can be rounded to get a predicted, class label.\n\nBelow, you can use !pygmentize to display the code in the `model.py` file. Read through the code; all of your tasks are marked with TODO comments. You should navigate to the file, and complete the tasks to define a `SimpleNet`.",
"_____no_output_____"
]
],
[
[
"!pygmentize source/model.py",
"\u001b[34mimport\u001b[39;49;00m \u001b[04m\u001b[36mtorch\u001b[39;49;00m\n\u001b[34mimport\u001b[39;49;00m \u001b[04m\u001b[36mtorch\u001b[39;49;00m\u001b[04m\u001b[36m.\u001b[39;49;00m\u001b[04m\u001b[36mnn\u001b[39;49;00m \u001b[34mas\u001b[39;49;00m \u001b[04m\u001b[36mnn\u001b[39;49;00m\n\u001b[34mimport\u001b[39;49;00m \u001b[04m\u001b[36mtorch\u001b[39;49;00m\u001b[04m\u001b[36m.\u001b[39;49;00m\u001b[04m\u001b[36mnn\u001b[39;49;00m\u001b[04m\u001b[36m.\u001b[39;49;00m\u001b[04m\u001b[36mfunctional\u001b[39;49;00m \u001b[34mas\u001b[39;49;00m \u001b[04m\u001b[36mF\u001b[39;49;00m\n\n\u001b[37m## TODO: Complete this classifier\u001b[39;49;00m\n\u001b[34mclass\u001b[39;49;00m \u001b[04m\u001b[32mSimpleNet\u001b[39;49;00m(nn.Module):\n \n \u001b[37m## TODO: Define the init function\u001b[39;49;00m\n \u001b[34mdef\u001b[39;49;00m \u001b[32m__init__\u001b[39;49;00m(\u001b[36mself\u001b[39;49;00m, input_dim, hidden_dim, output_dim):\n \u001b[33m'''Defines layers of a neural network.\u001b[39;49;00m\n\u001b[33m :param input_dim: Number of input features\u001b[39;49;00m\n\u001b[33m :param hidden_dim: Size of hidden layer(s)\u001b[39;49;00m\n\u001b[33m :param output_dim: Number of outputs\u001b[39;49;00m\n\u001b[33m '''\u001b[39;49;00m\n \u001b[36msuper\u001b[39;49;00m(SimpleNet, \u001b[36mself\u001b[39;49;00m).\u001b[32m__init__\u001b[39;49;00m()\n \n \u001b[37m# define all layers, here\u001b[39;49;00m\n \u001b[37m# first layer\u001b[39;49;00m\n hidden_1 = hidden_dim\n \u001b[36mself\u001b[39;49;00m.fc1 = nn.Linear(input_dim, hidden_1)\n \u001b[37m# second layer\u001b[39;49;00m\n hidden_2 = \u001b[36mint\u001b[39;49;00m(hidden_dim/\u001b[34m2\u001b[39;49;00m)\n \u001b[36mself\u001b[39;49;00m.fc2 = nn.Linear(hidden_1, hidden_2)\n \u001b[37m# final layer\u001b[39;49;00m\n \u001b[36mself\u001b[39;49;00m.fc3 = nn.Linear(hidden_2, output_dim)\n \u001b[37m# dropout\u001b[39;49;00m\n \u001b[36mself\u001b[39;49;00m.dropout = nn.Dropout(\u001b[34m0.2\u001b[39;49;00m)\n \u001b[37m# sigmoid layer\u001b[39;49;00m\n \u001b[36mself\u001b[39;49;00m.sig = nn.Sigmoid()\n \n \n \n \u001b[37m## TODO: Define the feedforward behavior of the network\u001b[39;49;00m\n \u001b[34mdef\u001b[39;49;00m \u001b[32mforward\u001b[39;49;00m(\u001b[36mself\u001b[39;49;00m, x):\n \u001b[33m'''Feedforward behavior of the net.\u001b[39;49;00m\n\u001b[33m :param x: A batch of input features\u001b[39;49;00m\n\u001b[33m :return: A single, sigmoid activated value\u001b[39;49;00m\n\u001b[33m '''\u001b[39;49;00m\n \u001b[37m# your code, here\u001b[39;49;00m\n \u001b[37m# Computing layers with activation functions and dropout\u001b[39;49;00m\n \u001b[37m# add first layer\u001b[39;49;00m\n x = F.relu(\u001b[36mself\u001b[39;49;00m.fc1(x))\n x = \u001b[36mself\u001b[39;49;00m.dropout(x)\n \u001b[37m# add second layer\u001b[39;49;00m\n x = F.relu(\u001b[36mself\u001b[39;49;00m.fc2(x))\n x = \u001b[36mself\u001b[39;49;00m.dropout(x)\n \u001b[37m# add final layer\u001b[39;49;00m\n x = \u001b[36mself\u001b[39;49;00m.fc3(x)\n \u001b[37m# add sigmoid layer\u001b[39;49;00m\n x = \u001b[36mself\u001b[39;49;00m.sig(x)\n \u001b[34mreturn\u001b[39;49;00m x\n"
]
],
[
[
"## Training Script\n\nTo implement a custom classifier, you'll also need to complete a `train.py` script. You can find this in the `source` directory.\n\nA typical training script:\n\n* Loads training data from a specified directory\n* Parses any training & model hyperparameters (ex. nodes in a neural network, training epochs, etc.)\n* Instantiates a model of your design, with any specified hyperparams\n* Trains that model\n* Finally, saves the model so that it can be hosted/deployed, later\n\n### EXERCISE: Complete the `train.py` script\n\nMuch of the training script code is provided for you. Almost all of your work will be done in the if __name__ == '__main__': section. To complete the `train.py` file, you will:\n\n* Define any additional model training hyperparameters using `parser.add_argument`\n* Define a model in the if __name__ == '__main__': section\n* Train the model in that same section\n\nBelow, you can use !pygmentize to display an existing train.py file. Read through the code; all of your tasks are marked with TODO comments.",
"_____no_output_____"
]
],
[
[
"!pygmentize source/train.py",
"\u001b[34mfrom\u001b[39;49;00m \u001b[04m\u001b[36m__future__\u001b[39;49;00m \u001b[34mimport\u001b[39;49;00m print_function \u001b[37m# future proof\u001b[39;49;00m\n\u001b[34mimport\u001b[39;49;00m \u001b[04m\u001b[36margparse\u001b[39;49;00m\n\u001b[34mimport\u001b[39;49;00m \u001b[04m\u001b[36msys\u001b[39;49;00m\n\u001b[34mimport\u001b[39;49;00m \u001b[04m\u001b[36mos\u001b[39;49;00m\n\u001b[34mimport\u001b[39;49;00m \u001b[04m\u001b[36mjson\u001b[39;49;00m\n\n\u001b[34mimport\u001b[39;49;00m \u001b[04m\u001b[36mpandas\u001b[39;49;00m \u001b[34mas\u001b[39;49;00m \u001b[04m\u001b[36mpd\u001b[39;49;00m\n\n\u001b[37m# pytorch\u001b[39;49;00m\n\u001b[34mimport\u001b[39;49;00m \u001b[04m\u001b[36mtorch\u001b[39;49;00m\n\u001b[34mimport\u001b[39;49;00m \u001b[04m\u001b[36mtorch\u001b[39;49;00m\u001b[04m\u001b[36m.\u001b[39;49;00m\u001b[04m\u001b[36mnn\u001b[39;49;00m \u001b[34mas\u001b[39;49;00m \u001b[04m\u001b[36mnn\u001b[39;49;00m\n\u001b[34mimport\u001b[39;49;00m \u001b[04m\u001b[36mtorch\u001b[39;49;00m\u001b[04m\u001b[36m.\u001b[39;49;00m\u001b[04m\u001b[36moptim\u001b[39;49;00m \u001b[34mas\u001b[39;49;00m \u001b[04m\u001b[36moptim\u001b[39;49;00m\n\u001b[34mimport\u001b[39;49;00m \u001b[04m\u001b[36mtorch\u001b[39;49;00m\u001b[04m\u001b[36m.\u001b[39;49;00m\u001b[04m\u001b[36mutils\u001b[39;49;00m\u001b[04m\u001b[36m.\u001b[39;49;00m\u001b[04m\u001b[36mdata\u001b[39;49;00m\n\n\u001b[37m# import model\u001b[39;49;00m\n\u001b[34mfrom\u001b[39;49;00m \u001b[04m\u001b[36mmodel\u001b[39;49;00m \u001b[34mimport\u001b[39;49;00m SimpleNet\n\n\n\u001b[34mdef\u001b[39;49;00m \u001b[32mmodel_fn\u001b[39;49;00m(model_dir):\n \u001b[36mprint\u001b[39;49;00m(\u001b[33m\"\u001b[39;49;00m\u001b[33mLoading model.\u001b[39;49;00m\u001b[33m\"\u001b[39;49;00m)\n\n \u001b[37m# First, load the parameters used to create the model.\u001b[39;49;00m\n model_info = {}\n model_info_path = os.path.join(model_dir, \u001b[33m'\u001b[39;49;00m\u001b[33mmodel_info.pth\u001b[39;49;00m\u001b[33m'\u001b[39;49;00m)\n \u001b[34mwith\u001b[39;49;00m \u001b[36mopen\u001b[39;49;00m(model_info_path, \u001b[33m'\u001b[39;49;00m\u001b[33mrb\u001b[39;49;00m\u001b[33m'\u001b[39;49;00m) \u001b[34mas\u001b[39;49;00m f:\n model_info = torch.load(f)\n\n \u001b[36mprint\u001b[39;49;00m(\u001b[33m\"\u001b[39;49;00m\u001b[33mmodel_info: \u001b[39;49;00m\u001b[33m{}\u001b[39;49;00m\u001b[33m\"\u001b[39;49;00m.format(model_info))\n\n \u001b[37m# Determine the device and construct the model.\u001b[39;49;00m\n device = torch.device(\u001b[33m\"\u001b[39;49;00m\u001b[33mcuda\u001b[39;49;00m\u001b[33m\"\u001b[39;49;00m \u001b[34mif\u001b[39;49;00m torch.cuda.is_available() \u001b[34melse\u001b[39;49;00m \u001b[33m\"\u001b[39;49;00m\u001b[33mcpu\u001b[39;49;00m\u001b[33m\"\u001b[39;49;00m)\n model = SimpleNet(model_info[\u001b[33m'\u001b[39;49;00m\u001b[33minput_dim\u001b[39;49;00m\u001b[33m'\u001b[39;49;00m], \n model_info[\u001b[33m'\u001b[39;49;00m\u001b[33mhidden_dim\u001b[39;49;00m\u001b[33m'\u001b[39;49;00m], \n model_info[\u001b[33m'\u001b[39;49;00m\u001b[33moutput_dim\u001b[39;49;00m\u001b[33m'\u001b[39;49;00m])\n\n \u001b[37m# Load the stored model parameters.\u001b[39;49;00m\n model_path = os.path.join(model_dir, \u001b[33m'\u001b[39;49;00m\u001b[33mmodel.pth\u001b[39;49;00m\u001b[33m'\u001b[39;49;00m)\n \u001b[34mwith\u001b[39;49;00m \u001b[36mopen\u001b[39;49;00m(model_path, \u001b[33m'\u001b[39;49;00m\u001b[33mrb\u001b[39;49;00m\u001b[33m'\u001b[39;49;00m) \u001b[34mas\u001b[39;49;00m f:\n model.load_state_dict(torch.load(f))\n \n \u001b[34mreturn\u001b[39;49;00m model.to(device)\n\n\n\u001b[37m# Load the training data from a csv file\u001b[39;49;00m\n\u001b[34mdef\u001b[39;49;00m \u001b[32m_get_train_loader\u001b[39;49;00m(batch_size, data_dir):\n \u001b[36mprint\u001b[39;49;00m(\u001b[33m\"\u001b[39;49;00m\u001b[33mGet data loader.\u001b[39;49;00m\u001b[33m\"\u001b[39;49;00m)\n\n \u001b[37m# read in csv file\u001b[39;49;00m\n train_data = pd.read_csv(os.path.join(data_dir, \u001b[33m\"\u001b[39;49;00m\u001b[33mtrain.csv\u001b[39;49;00m\u001b[33m\"\u001b[39;49;00m), header=\u001b[34mNone\u001b[39;49;00m, names=\u001b[34mNone\u001b[39;49;00m)\n\n \u001b[37m# labels are first column\u001b[39;49;00m\n train_y = torch.from_numpy(train_data[[\u001b[34m0\u001b[39;49;00m]].values).float().squeeze()\n \u001b[37m# features are the rest\u001b[39;49;00m\n train_x = torch.from_numpy(train_data.drop([\u001b[34m0\u001b[39;49;00m], axis=\u001b[34m1\u001b[39;49;00m).values).float()\n\n \u001b[37m# create dataset\u001b[39;49;00m\n train_ds = torch.utils.data.TensorDataset(train_x, train_y)\n\n \u001b[34mreturn\u001b[39;49;00m torch.utils.data.DataLoader(train_ds, batch_size=batch_size)\n\n\n\u001b[37m# Provided train function\u001b[39;49;00m\n\u001b[34mdef\u001b[39;49;00m \u001b[32mtrain\u001b[39;49;00m(model, train_loader, epochs, optimizer, criterion, device):\n \u001b[33m\"\"\"\u001b[39;49;00m\n\u001b[33m This is the training method that is called by the PyTorch training script. The parameters\u001b[39;49;00m\n\u001b[33m passed are as follows:\u001b[39;49;00m\n\u001b[33m model - The PyTorch model that we wish to train.\u001b[39;49;00m\n\u001b[33m train_loader - The PyTorch DataLoader that should be used during training.\u001b[39;49;00m\n\u001b[33m epochs - The total number of epochs to train for.\u001b[39;49;00m\n\u001b[33m optimizer - The optimizer to use during training.\u001b[39;49;00m\n\u001b[33m criterion - The loss function used for training. \u001b[39;49;00m\n\u001b[33m device - Where the model and data should be loaded (gpu or cpu).\u001b[39;49;00m\n\u001b[33m \"\"\"\u001b[39;49;00m\n \n \u001b[34mfor\u001b[39;49;00m epoch \u001b[35min\u001b[39;49;00m \u001b[36mrange\u001b[39;49;00m(\u001b[34m1\u001b[39;49;00m, epochs + \u001b[34m1\u001b[39;49;00m):\n model.train()\n total_loss = \u001b[34m0\u001b[39;49;00m\n \u001b[34mfor\u001b[39;49;00m batch_idx, (data, target) \u001b[35min\u001b[39;49;00m \u001b[36menumerate\u001b[39;49;00m(train_loader, \u001b[34m1\u001b[39;49;00m):\n \u001b[37m# prep data\u001b[39;49;00m\n data, target = data.to(device), target.to(device)\n optimizer.zero_grad() \u001b[37m# zero accumulated gradients\u001b[39;49;00m\n \u001b[37m# get output of SimpleNet\u001b[39;49;00m\n output = model(data)\n \u001b[37m# calculate loss and perform backprop\u001b[39;49;00m\n loss = criterion(output, target)\n loss.backward()\n optimizer.step()\n \n total_loss += loss.item()\n \n \u001b[37m# print loss stats\u001b[39;49;00m\n \u001b[36mprint\u001b[39;49;00m(\u001b[33m\"\u001b[39;49;00m\u001b[33mEpoch: \u001b[39;49;00m\u001b[33m{}\u001b[39;49;00m\u001b[33m, Loss: \u001b[39;49;00m\u001b[33m{}\u001b[39;49;00m\u001b[33m\"\u001b[39;49;00m.format(epoch, total_loss / \u001b[36mlen\u001b[39;49;00m(train_loader)))\n\n \u001b[37m# save after all epochs\u001b[39;49;00m\n save_model(model, args.model_dir)\n\n\n\u001b[37m# Provided model saving functions\u001b[39;49;00m\n\u001b[34mdef\u001b[39;49;00m \u001b[32msave_model\u001b[39;49;00m(model, model_dir):\n \u001b[36mprint\u001b[39;49;00m(\u001b[33m\"\u001b[39;49;00m\u001b[33mSaving the model.\u001b[39;49;00m\u001b[33m\"\u001b[39;49;00m)\n path = os.path.join(model_dir, \u001b[33m'\u001b[39;49;00m\u001b[33mmodel.pth\u001b[39;49;00m\u001b[33m'\u001b[39;49;00m)\n \u001b[37m# save state dictionary\u001b[39;49;00m\n torch.save(model.cpu().state_dict(), path)\n \n\u001b[34mdef\u001b[39;49;00m \u001b[32msave_model_params\u001b[39;49;00m(model, model_dir):\n model_info_path = os.path.join(args.model_dir, \u001b[33m'\u001b[39;49;00m\u001b[33mmodel_info.pth\u001b[39;49;00m\u001b[33m'\u001b[39;49;00m)\n \u001b[34mwith\u001b[39;49;00m \u001b[36mopen\u001b[39;49;00m(model_info_path, \u001b[33m'\u001b[39;49;00m\u001b[33mwb\u001b[39;49;00m\u001b[33m'\u001b[39;49;00m) \u001b[34mas\u001b[39;49;00m f:\n model_info = {\n \u001b[33m'\u001b[39;49;00m\u001b[33minput_dim\u001b[39;49;00m\u001b[33m'\u001b[39;49;00m: args.input_dim,\n \u001b[33m'\u001b[39;49;00m\u001b[33mhidden_dim\u001b[39;49;00m\u001b[33m'\u001b[39;49;00m: args.hidden_dim,\n \u001b[33m'\u001b[39;49;00m\u001b[33moutput_dim\u001b[39;49;00m\u001b[33m'\u001b[39;49;00m: args.output_dim\n }\n torch.save(model_info, f)\n\n\n\u001b[37m## TODO: Complete the main code\u001b[39;49;00m\n\u001b[34mif\u001b[39;49;00m \u001b[31m__name__\u001b[39;49;00m == \u001b[33m'\u001b[39;49;00m\u001b[33m__main__\u001b[39;49;00m\u001b[33m'\u001b[39;49;00m:\n \u001b[37m# All of the model parameters and training parameters are sent as arguments\u001b[39;49;00m\n \u001b[37m# when this script is executed, during a training job\u001b[39;49;00m\n \n \u001b[37m# Here we set up an argument parser to easily access the parameters\u001b[39;49;00m\n parser = argparse.ArgumentParser()\n\n \u001b[37m# SageMaker parameters, like the directories for training data and saving models; set automatically\u001b[39;49;00m\n \u001b[37m# Do not need to change\u001b[39;49;00m\n parser.add_argument(\u001b[33m'\u001b[39;49;00m\u001b[33m--hosts\u001b[39;49;00m\u001b[33m'\u001b[39;49;00m, \u001b[36mtype\u001b[39;49;00m=\u001b[36mlist\u001b[39;49;00m, default=json.loads(os.environ[\u001b[33m'\u001b[39;49;00m\u001b[33mSM_HOSTS\u001b[39;49;00m\u001b[33m'\u001b[39;49;00m]))\n parser.add_argument(\u001b[33m'\u001b[39;49;00m\u001b[33m--current-host\u001b[39;49;00m\u001b[33m'\u001b[39;49;00m, \u001b[36mtype\u001b[39;49;00m=\u001b[36mstr\u001b[39;49;00m, default=os.environ[\u001b[33m'\u001b[39;49;00m\u001b[33mSM_CURRENT_HOST\u001b[39;49;00m\u001b[33m'\u001b[39;49;00m])\n parser.add_argument(\u001b[33m'\u001b[39;49;00m\u001b[33m--model-dir\u001b[39;49;00m\u001b[33m'\u001b[39;49;00m, \u001b[36mtype\u001b[39;49;00m=\u001b[36mstr\u001b[39;49;00m, default=os.environ[\u001b[33m'\u001b[39;49;00m\u001b[33mSM_MODEL_DIR\u001b[39;49;00m\u001b[33m'\u001b[39;49;00m])\n parser.add_argument(\u001b[33m'\u001b[39;49;00m\u001b[33m--data-dir\u001b[39;49;00m\u001b[33m'\u001b[39;49;00m, \u001b[36mtype\u001b[39;49;00m=\u001b[36mstr\u001b[39;49;00m, default=os.environ[\u001b[33m'\u001b[39;49;00m\u001b[33mSM_CHANNEL_TRAIN\u001b[39;49;00m\u001b[33m'\u001b[39;49;00m])\n \n \u001b[37m# Training Parameters, given\u001b[39;49;00m\n parser.add_argument(\u001b[33m'\u001b[39;49;00m\u001b[33m--batch-size\u001b[39;49;00m\u001b[33m'\u001b[39;49;00m, \u001b[36mtype\u001b[39;49;00m=\u001b[36mint\u001b[39;49;00m, default=\u001b[34m64\u001b[39;49;00m, metavar=\u001b[33m'\u001b[39;49;00m\u001b[33mN\u001b[39;49;00m\u001b[33m'\u001b[39;49;00m,\n help=\u001b[33m'\u001b[39;49;00m\u001b[33minput batch size for training (default: 64)\u001b[39;49;00m\u001b[33m'\u001b[39;49;00m)\n parser.add_argument(\u001b[33m'\u001b[39;49;00m\u001b[33m--epochs\u001b[39;49;00m\u001b[33m'\u001b[39;49;00m, \u001b[36mtype\u001b[39;49;00m=\u001b[36mint\u001b[39;49;00m, default=\u001b[34m10\u001b[39;49;00m, metavar=\u001b[33m'\u001b[39;49;00m\u001b[33mN\u001b[39;49;00m\u001b[33m'\u001b[39;49;00m,\n help=\u001b[33m'\u001b[39;49;00m\u001b[33mnumber of epochs to train (default: 10)\u001b[39;49;00m\u001b[33m'\u001b[39;49;00m)\n parser.add_argument(\u001b[33m'\u001b[39;49;00m\u001b[33m--lr\u001b[39;49;00m\u001b[33m'\u001b[39;49;00m, \u001b[36mtype\u001b[39;49;00m=\u001b[36mfloat\u001b[39;49;00m, default=\u001b[34m0.001\u001b[39;49;00m, metavar=\u001b[33m'\u001b[39;49;00m\u001b[33mLR\u001b[39;49;00m\u001b[33m'\u001b[39;49;00m,\n help=\u001b[33m'\u001b[39;49;00m\u001b[33mlearning rate (default: 0.001)\u001b[39;49;00m\u001b[33m'\u001b[39;49;00m)\n parser.add_argument(\u001b[33m'\u001b[39;49;00m\u001b[33m--seed\u001b[39;49;00m\u001b[33m'\u001b[39;49;00m, \u001b[36mtype\u001b[39;49;00m=\u001b[36mint\u001b[39;49;00m, default=\u001b[34m1\u001b[39;49;00m, metavar=\u001b[33m'\u001b[39;49;00m\u001b[33mS\u001b[39;49;00m\u001b[33m'\u001b[39;49;00m,\n help=\u001b[33m'\u001b[39;49;00m\u001b[33mrandom seed (default: 1)\u001b[39;49;00m\u001b[33m'\u001b[39;49;00m)\n \n \u001b[37m## TODO: Add args for the three model parameters: input_dim, hidden_dim, output_dim\u001b[39;49;00m\n \u001b[37m# Model parameters\u001b[39;49;00m\n parser.add_argument(\u001b[33m'\u001b[39;49;00m\u001b[33m--input_dim\u001b[39;49;00m\u001b[33m'\u001b[39;49;00m, \u001b[36mtype\u001b[39;49;00m=\u001b[36mint\u001b[39;49;00m, default=\u001b[34m2\u001b[39;49;00m, metavar=\u001b[33m'\u001b[39;49;00m\u001b[33mN\u001b[39;49;00m\u001b[33m'\u001b[39;49;00m,\n help=\u001b[33m'\u001b[39;49;00m\u001b[33minput dimension for training (default: 2)\u001b[39;49;00m\u001b[33m'\u001b[39;49;00m)\n parser.add_argument(\u001b[33m'\u001b[39;49;00m\u001b[33m--hidden_dim\u001b[39;49;00m\u001b[33m'\u001b[39;49;00m, \u001b[36mtype\u001b[39;49;00m=\u001b[36mint\u001b[39;49;00m, default=\u001b[34m20\u001b[39;49;00m, metavar=\u001b[33m'\u001b[39;49;00m\u001b[33mN\u001b[39;49;00m\u001b[33m'\u001b[39;49;00m,\n help=\u001b[33m'\u001b[39;49;00m\u001b[33mhidden dimension for training (default: 20)\u001b[39;49;00m\u001b[33m'\u001b[39;49;00m)\n parser.add_argument(\u001b[33m'\u001b[39;49;00m\u001b[33m--output_dim\u001b[39;49;00m\u001b[33m'\u001b[39;49;00m, \u001b[36mtype\u001b[39;49;00m=\u001b[36mint\u001b[39;49;00m, default=\u001b[34m1\u001b[39;49;00m, metavar=\u001b[33m'\u001b[39;49;00m\u001b[33mN\u001b[39;49;00m\u001b[33m'\u001b[39;49;00m,\n help=\u001b[33m'\u001b[39;49;00m\u001b[33mnumber of classes (default: 1)\u001b[39;49;00m\u001b[33m'\u001b[39;49;00m)\n\n \n args = parser.parse_args()\n\n device = torch.device(\u001b[33m\"\u001b[39;49;00m\u001b[33mcuda\u001b[39;49;00m\u001b[33m\"\u001b[39;49;00m \u001b[34mif\u001b[39;49;00m torch.cuda.is_available() \u001b[34melse\u001b[39;49;00m \u001b[33m\"\u001b[39;49;00m\u001b[33mcpu\u001b[39;49;00m\u001b[33m\"\u001b[39;49;00m)\n \n \u001b[37m# set the seed for generating random numbers\u001b[39;49;00m\n torch.manual_seed(args.seed)\n \u001b[34mif\u001b[39;49;00m torch.cuda.is_available():\n torch.cuda.manual_seed(args.seed)\n \n \u001b[37m# get train loader\u001b[39;49;00m\n train_loader = _get_train_loader(args.batch_size, args.data_dir) \u001b[37m# data_dir from above..\u001b[39;49;00m\n \n \n \u001b[37m## TODO: Build the model by passing in the input params\u001b[39;49;00m\n \u001b[37m# To get params from the parser, call args.argument_name, ex. args.epochs or ards.hidden_dim\u001b[39;49;00m\n \u001b[37m# Don't forget to move your model .to(device) to move to GPU , if appropriate\u001b[39;49;00m\n model = SimpleNet(args.input_dim, args.hidden_dim, args.output_dim).to(device)\n \n \u001b[37m# Given: save the parameters used to construct the model\u001b[39;49;00m\n save_model_params(model, args.model_dir)\n\n \u001b[37m## TODO: Define an optimizer and loss function for training\u001b[39;49;00m\n optimizer = optim.Adam(model.parameters(), lr=args.lr)\n criterion = nn.BCELoss()\n\n \n \u001b[37m# Trains the model (given line of code, which calls the above training function)\u001b[39;49;00m\n \u001b[37m# This function *also* saves the model state dictionary\u001b[39;49;00m\n train(model, train_loader, args.epochs, optimizer, criterion, device)\n \n"
]
],
[
[
"### EXERCISE: Create a PyTorch Estimator\n\nYou've had some practice instantiating built-in models in SageMaker. All estimators require some constructor arguments to be passed in. When a custom model is constructed in SageMaker, an **entry point** must be specified. The entry_point is the training script that will be executed when the model is trained; the `train.py` function you specified above! \n\nSee if you can complete this task, instantiating a PyTorch estimator, using only the [PyTorch estimator documentation](https://sagemaker.readthedocs.io/en/stable/sagemaker.pytorch.html) as a resource. It is suggested that you use the **latest version** of PyTorch as the optional `framework_version` parameter.\n\n#### Instance Types\n\nIt is suggested that you use instances that are available in the free tier of usage: `'ml.c4.xlarge'` for training and `'ml.t2.medium'` for deployment.",
"_____no_output_____"
]
],
[
[
"# import a PyTorch wrapper\nfrom sagemaker.pytorch import PyTorch\n\n# specify an output path\noutput_path = 's3://{}/{}'.format(bucket, prefix)\n\n# instantiate a pytorch estimator\nestimator = PyTorch(entry_point='train.py',\n source_dir='source',\n framework_version='1.0',\n role=role,\n train_instance_count=1,\n train_instance_type='ml.c4.xlarge',\n output_path=output_path,\n sagemaker_session=sagemaker_session,\n hyperparameters={\n 'epoch':80,\n 'input_dim':2,\n 'hidden_dim':20,\n 'output_dim':1\n })\n",
"_____no_output_____"
]
],
[
[
"## Train the Estimator\n\nAfter instantiating your estimator, train it with a call to `.fit()`. The `train.py` file explicitly loads in `.csv` data, so you do not need to convert the input data to any other format.",
"_____no_output_____"
]
],
[
[
"%%time \n# train the estimator on S3 training data\nestimator.fit({'train': input_data})",
"'create_image_uri' will be deprecated in favor of 'ImageURIProvider' class in SageMaker Python SDK v2.\n's3_input' class will be renamed to 'TrainingInput' in SageMaker Python SDK v2.\n'create_image_uri' will be deprecated in favor of 'ImageURIProvider' class in SageMaker Python SDK v2.\n"
]
],
[
[
"## Create a Trained Model\n\nPyTorch models do not automatically come with `.predict()` functions attached (as many Scikit-learn models do, for example) and you may have noticed that you've been give a `predict.py` file. This file is responsible for loading a trained model and applying it to passed in, numpy data. When you created a PyTorch estimator, you specified where the training script, `train.py` was located. \n\n> How can we tell a PyTorch model where the `predict.py` file is?\n\nBefore you can deploy this custom PyTorch model, you have to take one more step: creating a `PyTorchModel`. In earlier exercises you could see that a call to `.deploy()` created both a **model** and an **endpoint**, but for PyTorch models, these steps have to be separate.\n\n### EXERCISE: Instantiate a `PyTorchModel`\n\nYou can create a `PyTorchModel` (different that a PyTorch estimator) from your trained, estimator attributes. This model is responsible for knowing how to execute a specific `predict.py` script. And this model is what you'll deploy to create an endpoint.\n\n#### Model Parameters\n\nTo instantiate a `PyTorchModel`, ([documentation, here](https://sagemaker.readthedocs.io/en/stable/sagemaker.pytorch.html#sagemaker.pytorch.model.PyTorchModel)) you pass in the same arguments as your PyTorch estimator, with a few additions/modifications:\n* **model_data**: The trained `model.tar.gz` file created by your estimator, which can be accessed as `estimator.model_data`.\n* **entry_point**: This time, this is the path to the Python script SageMaker runs for **prediction** rather than training, `predict.py`.\n",
"_____no_output_____"
]
],
[
[
"%%time\n# importing PyTorchModel\nfrom sagemaker.pytorch import PyTorchModel\n\n# Create a model from the trained estimator data\n# And point to the prediction script\nmodel = PyTorchModel(model_data=estimator.model_data,\n role=role,\n framework_version='1.0',\n entry_point='predict.py',\n source_dir='source',\n )\n",
"Parameter image will be renamed to image_uri in SageMaker Python SDK v2.\n"
]
],
[
[
"### EXERCISE: Deploy the trained model\n\nDeploy your model to create a predictor. We'll use this to make predictions on our test data and evaluate the model.",
"_____no_output_____"
]
],
[
[
"%%time\n# deploy and create a predictor\npredictor = model.deploy(initial_instance_count=1, instance_type='ml.t2.medium')",
"'create_image_uri' will be deprecated in favor of 'ImageURIProvider' class in SageMaker Python SDK v2.\n"
]
],
[
[
"---\n## Evaluating Your Model\n\nOnce your model is deployed, you can see how it performs when applied to the test data.\n\nThe provided function below, takes in a deployed predictor, some test features and labels, and returns a dictionary of metrics; calculating false negatives and positives as well as recall, precision, and accuracy.",
"_____no_output_____"
]
],
[
[
"# code to evaluate the endpoint on test data\n# returns a variety of model metrics\ndef evaluate(predictor, test_features, test_labels, verbose=True):\n \"\"\"\n Evaluate a model on a test set given the prediction endpoint. \n Return binary classification metrics.\n :param predictor: A prediction endpoint\n :param test_features: Test features\n :param test_labels: Class labels for test data\n :param verbose: If True, prints a table of all performance metrics\n :return: A dictionary of performance metrics.\n \"\"\"\n \n # rounding and squeezing array\n test_preds = np.squeeze(np.round(predictor.predict(test_features)))\n \n # calculate true positives, false positives, true negatives, false negatives\n tp = np.logical_and(test_labels, test_preds).sum()\n fp = np.logical_and(1-test_labels, test_preds).sum()\n tn = np.logical_and(1-test_labels, 1-test_preds).sum()\n fn = np.logical_and(test_labels, 1-test_preds).sum()\n \n # calculate binary classification metrics\n recall = tp / (tp + fn)\n precision = tp / (tp + fp)\n accuracy = (tp + tn) / (tp + fp + tn + fn)\n \n # print metrics\n if verbose:\n print(pd.crosstab(test_labels, test_preds, rownames=['actuals'], colnames=['predictions']))\n print(\"\\n{:<11} {:.3f}\".format('Recall:', recall))\n print(\"{:<11} {:.3f}\".format('Precision:', precision))\n print(\"{:<11} {:.3f}\".format('Accuracy:', accuracy))\n print()\n \n return {'TP': tp, 'FP': fp, 'FN': fn, 'TN': tn, \n 'Precision': precision, 'Recall': recall, 'Accuracy': accuracy}\n\n",
"_____no_output_____"
]
],
[
[
"### Test Results\n\nThe cell below runs the `evaluate` function. \n\nThe code assumes that you have a defined `predictor` and `X_test` and `Y_test` from previously-run cells.",
"_____no_output_____"
]
],
[
[
"# get metrics for custom predictor\nmetrics = evaluate(predictor, X_test, Y_test, True)",
"predictions 0.0 1.0\nactuals \n0 107 11\n1 15 117\n\nRecall: 0.886\nPrecision: 0.914\nAccuracy: 0.896\n\n"
]
],
[
[
"## Delete the Endpoint\n\nFinally, I've add a convenience function to delete prediction endpoints after we're done with them. And if you're done evaluating the model, you should delete your model endpoint!",
"_____no_output_____"
]
],
[
[
"# Accepts a predictor endpoint as input\n# And deletes the endpoint by name\ndef delete_endpoint(predictor):\n try:\n boto3.client('sagemaker').delete_endpoint(EndpointName=predictor.endpoint)\n print('Deleted {}'.format(predictor.endpoint))\n except:\n print('Already deleted: {}'.format(predictor.endpoint))",
"_____no_output_____"
],
[
"# delete the predictor endpoint \ndelete_endpoint(predictor)",
"Deleted sagemaker-pytorch-2020-10-24-19-46-20-459\n"
]
],
[
[
"## Final Cleanup!\n\n* Double check that you have deleted all your endpoints.\n* I'd also suggest manually deleting your S3 bucket, models, and endpoint configurations directly from your AWS console.\n\nYou can find thorough cleanup instructions, [in the documentation](https://docs.aws.amazon.com/sagemaker/latest/dg/ex1-cleanup.html).",
"_____no_output_____"
],
[
"---\n# Conclusion\n\nIn this notebook, you saw how to train and deploy a custom, PyTorch model in SageMaker. SageMaker has many built-in models that are useful for common clustering and classification tasks, but it is useful to know how to create custom, deep learning models that are flexible enough to learn from a variety of data.",
"_____no_output_____"
]
]
] | [
"markdown",
"code",
"markdown",
"code",
"markdown",
"code",
"markdown",
"code",
"markdown",
"code",
"markdown",
"code",
"markdown",
"code",
"markdown",
"code",
"markdown",
"code",
"markdown",
"code",
"markdown",
"code",
"markdown",
"code",
"markdown",
"code",
"markdown",
"code",
"markdown",
"code",
"markdown",
"code",
"markdown"
] | [
[
"markdown",
"markdown"
],
[
"code"
],
[
"markdown"
],
[
"code",
"code"
],
[
"markdown"
],
[
"code",
"code"
],
[
"markdown"
],
[
"code"
],
[
"markdown"
],
[
"code"
],
[
"markdown"
],
[
"code"
],
[
"markdown"
],
[
"code"
],
[
"markdown"
],
[
"code"
],
[
"markdown"
],
[
"code"
],
[
"markdown"
],
[
"code"
],
[
"markdown"
],
[
"code"
],
[
"markdown"
],
[
"code"
],
[
"markdown"
],
[
"code"
],
[
"markdown"
],
[
"code"
],
[
"markdown"
],
[
"code"
],
[
"markdown"
],
[
"code",
"code"
],
[
"markdown",
"markdown"
]
] |
e768dc07ff496b3b09fcffe5c0fc43a44181fe9a | 199,043 | ipynb | Jupyter Notebook | C5-Applied Social Network Analysis with Python/Notebooks/Week1/Loading+Graphs+in+NetworkX.ipynb | nishamathi/coursera-data-science-python | 23d4a4af763c39dbe0e1a7e503b9d001766a65e9 | [
"MIT"
] | 1 | 2022-02-06T15:47:18.000Z | 2022-02-06T15:47:18.000Z | C5-Applied Social Network Analysis with Python/Notebooks/Week1/Loading+Graphs+in+NetworkX.ipynb | nishamathi/coursera-data-science-python | 23d4a4af763c39dbe0e1a7e503b9d001766a65e9 | [
"MIT"
] | null | null | null | C5-Applied Social Network Analysis with Python/Notebooks/Week1/Loading+Graphs+in+NetworkX.ipynb | nishamathi/coursera-data-science-python | 23d4a4af763c39dbe0e1a7e503b9d001766a65e9 | [
"MIT"
] | null | null | null | 52.050994 | 42,067 | 0.543631 | [
[
[
"# Loading Graphs in NetworkX",
"_____no_output_____"
]
],
[
[
"import networkx as nx\nimport numpy as np\nimport pandas as pd\n%matplotlib notebook\n\n# Instantiate the graph\nG1 = nx.Graph()\n# add node/edge pairs\nG1.add_edges_from([(0, 1),\n (0, 2),\n (0, 3),\n (0, 5),\n (1, 3),\n (1, 6),\n (3, 4),\n (4, 5),\n (4, 7),\n (5, 8),\n (8, 9)])\n\n# draw the network G1\nnx.draw_networkx(G1)",
"_____no_output_____"
],
[
"import networkx as nx\n\nG=nx.MultiGraph()\nG.add_node('A',role='manager')\nG.add_edge('A','B',relation = 'friend')\nG.add_edge('A','C', relation = 'business partner')\nG.add_edge('A','B', relation = 'classmate')\nG.node['A']['role'] = 'team member'\nG.node['B']['role'] = 'engineer'\n\nG.node['A']['role']",
"_____no_output_____"
]
],
[
[
"### Adjacency List",
"_____no_output_____"
],
[
"`G_adjlist.txt` is the adjaceny list representation of G1.\n\nIt can be read as follows:\n* `0 1 2 3 5` $\\rightarrow$ node `0` is adjacent to nodes `1, 2, 3, 5`\n* `1 3 6` $\\rightarrow$ node `1` is (also) adjacent to nodes `3, 6`\n* `2` $\\rightarrow$ node `2` is (also) adjacent to no new nodes\n* `3 4` $\\rightarrow$ node `3` is (also) adjacent to node `4` \n\nand so on. Note that adjacencies are only accounted for once (e.g. node `2` is adjacent to node `0`, but node `0` is not listed in node `2`'s row, because that edge has already been accounted for in node `0`'s row).",
"_____no_output_____"
]
],
[
[
"!cat G_adjlist.txt",
"0 1 2 3 5\r\n1 3 6\r\n2\r\n3 4\r\n4 5 7\r\n5 8\r\n6\r\n7\r\n8 9\r\n9\r\n"
]
],
[
[
"If we read in the adjacency list using `nx.read_adjlist`, we can see that it matches `G1`.",
"_____no_output_____"
]
],
[
[
"G2 = nx.read_adjlist('G_adjlist.txt', nodetype=int)\nG2.edges()",
"_____no_output_____"
]
],
[
[
"### Adjacency Matrix\n\nThe elements in an adjacency matrix indicate whether pairs of vertices are adjacent or not in the graph. Each node has a corresponding row and column. For example, row `0`, column `1` corresponds to the edge between node `0` and node `1`. \n\nReading across row `0`, there is a '`1`' in columns `1`, `2`, `3`, and `5`, which indicates that node `0` is adjacent to nodes 1, 2, 3, and 5",
"_____no_output_____"
]
],
[
[
"G_mat = np.array([[0, 1, 1, 1, 0, 1, 0, 0, 0, 0],\n [1, 0, 0, 1, 0, 0, 1, 0, 0, 0],\n [1, 0, 0, 0, 0, 0, 0, 0, 0, 0],\n [1, 1, 0, 0, 1, 0, 0, 0, 0, 0],\n [0, 0, 0, 1, 0, 1, 0, 1, 0, 0],\n [1, 0, 0, 0, 1, 0, 0, 0, 1, 0],\n [0, 1, 0, 0, 0, 0, 0, 0, 0, 0],\n [0, 0, 0, 0, 1, 0, 0, 0, 0, 0],\n [0, 0, 0, 0, 0, 1, 0, 0, 0, 1],\n [0, 0, 0, 0, 0, 0, 0, 0, 1, 0]])\nG_mat",
"_____no_output_____"
]
],
[
[
"If we convert the adjacency matrix to a networkx graph using `nx.Graph`, we can see that it matches G1.",
"_____no_output_____"
]
],
[
[
"G3 = nx.Graph(G_mat)\nG3.edges()",
"_____no_output_____"
]
],
[
[
"### Edgelist",
"_____no_output_____"
],
[
"The edge list format represents edge pairings in the first two columns. Additional edge attributes can be added in subsequent columns. Looking at `G_edgelist.txt` this is the same as the original graph `G1`, but now each edge has a weight. \n\nFor example, from the first row, we can see the edge between nodes `0` and `1`, has a weight of `4`.",
"_____no_output_____"
]
],
[
[
"!cat G_edgelist.txt",
"0 1 4\r\n0 2 3\r\n0 3 2\r\n0 5 6\r\n1 3 2\r\n1 6 5\r\n3 4 3\r\n4 5 1\r\n4 7 2\r\n5 8 6\r\n8 9 1\r\n"
]
],
[
[
"Using `read_edgelist` and passing in a list of tuples with the name and type of each edge attribute will create a graph with our desired edge attributes.",
"_____no_output_____"
]
],
[
[
"G4 = nx.read_edgelist('G_edgelist.txt', data=[('Weight', int)])\n\nG4.edges(data=True)",
"_____no_output_____"
]
],
[
[
"### Pandas DataFrame",
"_____no_output_____"
],
[
"Graphs can also be created from pandas dataframes if they are in edge list format.",
"_____no_output_____"
]
],
[
[
"G_df = pd.read_csv('G_edgelist.txt', delim_whitespace=True, \n header=None, names=['n1', 'n2', 'weight'])\nG_df",
"_____no_output_____"
],
[
"G5 = nx.from_pandas_dataframe(G_df, 'n1', 'n2', edge_attr='weight')\nG5.edges(data=True)",
"_____no_output_____"
]
],
[
[
"### Chess Example",
"_____no_output_____"
],
[
"Now let's load in a more complex graph and perform some basic analysis on it.\n\nWe will be looking at chess_graph.txt, which is a directed graph of chess games in edge list format.",
"_____no_output_____"
]
],
[
[
"!head -5 chess_graph.txt",
"1 2 0\t885635999.999997\r\n1 3 0\t885635999.999997\r\n1 4 0\t885635999.999997\r\n1 5 1\t885635999.999997\r\n1 6 0\t885635999.999997\r\n"
]
],
[
[
"Each node is a chess player, and each edge represents a game. The first column with an outgoing edge corresponds to the white player, the second column with an incoming edge corresponds to the black player.\n\nThe third column, the weight of the edge, corresponds to the outcome of the game. A weight of 1 indicates white won, a 0 indicates a draw, and a -1 indicates black won.\n\nThe fourth column corresponds to approximate timestamps of when the game was played.\n\nWe can read in the chess graph using `read_edgelist`, and tell it to create the graph using a `nx.MultiDiGraph`.",
"_____no_output_____"
]
],
[
[
"chess = nx.read_edgelist('chess_graph.txt', data=[('outcome', int), ('timestamp', float)], \n create_using=nx.MultiDiGraph())",
"_____no_output_____"
],
[
"chess.is_directed(), chess.is_multigraph()\nchess",
"_____no_output_____"
],
[
"chess.edges(data=True)",
"_____no_output_____"
]
],
[
[
"Looking at the degree of each node, we can see how many games each person played. A dictionary is returned where each key is the player, and each value is the number of games played.",
"_____no_output_____"
]
],
[
[
"games_played = chess.degree()\ngames_played",
"_____no_output_____"
]
],
[
[
"Using list comprehension, we can find which player played the most games.",
"_____no_output_____"
]
],
[
[
"max_value = max(games_played.values())\nmax_key, = [i for i in games_played.keys() if games_played[i] == max_value]\n\nprint('player {}\\n{} games'.format(max_key, max_value))",
"player 461\n280 games\n"
]
],
[
[
"Let's use pandas to find out which players won the most games. First let's convert our graph to a DataFrame.",
"_____no_output_____"
]
],
[
[
"df = pd.DataFrame(chess.edges(data=True), columns=['white', 'black', 'outcome'])\ndf.head()",
"_____no_output_____"
]
],
[
[
"Next we can use a lambda to pull out the outcome from the attributes dictionary.",
"_____no_output_____"
]
],
[
[
"df['outcome'] = df['outcome'].map(lambda x: x['outcome'])\ndf.head()",
"_____no_output_____"
]
],
[
[
"To count the number of times a player won as white, we find the rows where the outcome was '1', group by the white player, and sum.\n\nTo count the number of times a player won as back, we find the rows where the outcome was '-1', group by the black player, sum, and multiply by -1.\n\nThe we can add these together with a fill value of 0 for those players that only played as either black or white.",
"_____no_output_____"
]
],
[
[
"won_as_white = df[df['outcome']==1].groupby('white').sum()\nwon_as_black = -df[df['outcome']==-1].groupby('black').sum()\nwin_count = won_as_white.add(won_as_black, fill_value=0)\nwin_count.head()",
"_____no_output_____"
]
],
[
[
"Using `nlargest` we find that player 330 won the most games at 109.",
"_____no_output_____"
]
],
[
[
"win_count.nlargest(5, 'outcome')",
"_____no_output_____"
]
]
] | [
"markdown",
"code",
"markdown",
"code",
"markdown",
"code",
"markdown",
"code",
"markdown",
"code",
"markdown",
"code",
"markdown",
"code",
"markdown",
"code",
"markdown",
"code",
"markdown",
"code",
"markdown",
"code",
"markdown",
"code",
"markdown",
"code",
"markdown",
"code",
"markdown",
"code",
"markdown",
"code"
] | [
[
"markdown"
],
[
"code",
"code"
],
[
"markdown",
"markdown"
],
[
"code"
],
[
"markdown"
],
[
"code"
],
[
"markdown"
],
[
"code"
],
[
"markdown"
],
[
"code"
],
[
"markdown",
"markdown"
],
[
"code"
],
[
"markdown"
],
[
"code"
],
[
"markdown",
"markdown"
],
[
"code",
"code"
],
[
"markdown",
"markdown"
],
[
"code"
],
[
"markdown"
],
[
"code",
"code",
"code"
],
[
"markdown"
],
[
"code"
],
[
"markdown"
],
[
"code"
],
[
"markdown"
],
[
"code"
],
[
"markdown"
],
[
"code"
],
[
"markdown"
],
[
"code"
],
[
"markdown"
],
[
"code"
]
] |
e768dee51a87b833f1b2f49f4c614b0218d354c9 | 5,726 | ipynb | Jupyter Notebook | 4 - Sequences, Time Series and Prediction/Week 2/Course 4 Week 2.ipynb | chen-xin-94/DeepLearning.AI-TensorFlow-Developer-Course | 7d84b2ae362fa57a8c1541ca3007414ea9f8023b | [
"Apache-2.0"
] | 79 | 2020-11-05T07:50:41.000Z | 2022-03-31T04:49:58.000Z | Coursera/DeepLearning.AI TensorFlow Developer Professional Certificate/4 - Sequences, Time Series and Prediction/Week 2/Course 4 Week 2.ipynb | MaheshBabu11/Python | f708cd5f9c561b64a73c63d228c8039ae35e91df | [
"MIT"
] | null | null | null | Coursera/DeepLearning.AI TensorFlow Developer Professional Certificate/4 - Sequences, Time Series and Prediction/Week 2/Course 4 Week 2.ipynb | MaheshBabu11/Python | f708cd5f9c561b64a73c63d228c8039ae35e91df | [
"MIT"
] | 72 | 2020-12-16T03:42:34.000Z | 2022-03-31T08:24:05.000Z | 30.620321 | 104 | 0.48533 | [
[
[
"!pip install tf-nightly-2.0-preview\n",
"_____no_output_____"
],
[
"import tensorflow as tf\nimport numpy as np\nimport matplotlib.pyplot as plt\nprint(tf.__version__)",
"_____no_output_____"
],
[
"def plot_series(time, series, format=\"-\", start=0, end=None):\n plt.plot(time[start:end], series[start:end], format)\n plt.xlabel(\"Time\")\n plt.ylabel(\"Value\")\n plt.grid(False)\n\ndef trend(time, slope=0):\n return slope * time\n\ndef seasonal_pattern(season_time):\n \"\"\"Just an arbitrary pattern, you can change it if you wish\"\"\"\n return np.where(season_time < 0.1,\n np.cos(season_time * 6 * np.pi),\n 2 / np.exp(9 * season_time))\n\ndef seasonality(time, period, amplitude=1, phase=0):\n \"\"\"Repeats the same pattern at each period\"\"\"\n season_time = ((time + phase) % period) / period\n return amplitude * seasonal_pattern(season_time)\n\ndef noise(time, noise_level=1, seed=None):\n rnd = np.random.RandomState(seed)\n return rnd.randn(len(time)) * noise_level\n\ntime = np.arange(10 * 365 + 1, dtype=\"float32\")\nbaseline = 10\nseries = trend(time, 0.1) \nbaseline = 10\namplitude = 40\nslope = 0.005\nnoise_level = 3\n\n# Create the series\nseries = baseline + trend(time, slope) + seasonality(time, period=365, amplitude=amplitude)\n# Update with noise\nseries += noise(time, noise_level, seed=51)\n\nsplit_time = 3000\ntime_train = time[:split_time]\nx_train = series[:split_time]\ntime_valid = time[split_time:]\nx_valid = series[split_time:]\n\nwindow_size = 20\nbatch_size = 32\nshuffle_buffer_size = 1000\n\nplot_series(time, series)",
"_____no_output_____"
],
[
"def windowed_dataset(series, window_size, batch_size, shuffle_buffer):\n dataset = tf.data.Dataset.from_tensor_slices(series)\n dataset = dataset.window(window_size + 1, shift=1, drop_remainder=True)\n dataset = dataset.flat_map(lambda window: window.batch(window_size + 1))\n dataset = dataset.shuffle(shuffle_buffer).map(lambda window: (window[:-1], window[-1]))\n dataset = dataset.batch(batch_size).prefetch(1)\n return dataset",
"_____no_output_____"
],
[
"dataset = windowed_dataset(x_train, window_size, batch_size, shuffle_buffer_size)\n\n\nmodel = tf.keras.models.Sequential([\n tf.keras.layers.Dense(100, input_shape=[window_size], activation=\"relu\"), \n tf.keras.layers.Dense(10, activation=\"relu\"), \n tf.keras.layers.Dense(1)\n])\n\nmodel.compile(loss=\"mse\", optimizer=tf.keras.optimizers.SGD(lr=1e-6, momentum=0.9))\nmodel.fit(dataset,epochs=100,verbose=0)\n\n",
"_____no_output_____"
],
[
"forecast = []\nfor time in range(len(series) - window_size):\n forecast.append(model.predict(series[time:time + window_size][np.newaxis]))\n\nforecast = forecast[split_time-window_size:]\nresults = np.array(forecast)[:, 0, 0]\n\n\nplt.figure(figsize=(10, 6))\n\nplot_series(time_valid, x_valid)\nplot_series(time_valid, results)",
"_____no_output_____"
],
[
"tf.keras.metrics.mean_absolute_error(x_valid, results).numpy()",
"_____no_output_____"
]
]
] | [
"code"
] | [
[
"code",
"code",
"code",
"code",
"code",
"code",
"code"
]
] |
e768e1a9b10e4030c6f571928a238b41d96d6b9e | 419,784 | ipynb | Jupyter Notebook | jupyter_notebooks/classification.ipynb | lightning485/osterai | d1eb83ce56101ec226abe5d35d410381878e5d6e | [
"MIT"
] | null | null | null | jupyter_notebooks/classification.ipynb | lightning485/osterai | d1eb83ce56101ec226abe5d35d410381878e5d6e | [
"MIT"
] | null | null | null | jupyter_notebooks/classification.ipynb | lightning485/osterai | d1eb83ce56101ec226abe5d35d410381878e5d6e | [
"MIT"
] | null | null | null | 756.367568 | 74,673 | 0.806277 | [
[
[
"# Counting Easter eggs\nOur experiment compares the classification approach and the regression approach. The selection is done with the `class_mode` option in Keras' ImageDataGenerator flow_from_directory. `categorical` is used for the one-hot encoding and `sparse` for integers as classes.\n\nCareful: While this is convention there, in other contexts, 'sparse' might mean a vector representation with more-than-one-hot entries, and rather the term 'binary' would be used for integers, generalizing a binary 0/1 problem to several possible classes.\n\nIn the notebook, the class_mode is used as a switch for the different Net variants and evaluation scripting.",
"_____no_output_____"
]
],
[
[
"class_mode = \"categorical\"",
"_____no_output_____"
]
],
[
[
"## Imports and version numbers",
"_____no_output_____"
]
],
[
[
"import tensorflow as tf\nfrom tensorflow.keras.preprocessing import image_dataset_from_directory\nfrom tensorflow.keras.preprocessing.image import ImageDataGenerator\nfrom matplotlib import pyplot as plt\nimport os\nimport re\nimport numpy as np\nfrom tensorflow.keras.preprocessing.image import load_img",
"_____no_output_____"
],
[
"# Python version: 3.8\nprint(tf.__version__)",
"2.3.1\n"
],
[
"# CUDA version:\n!nvcc --version",
"nvcc: NVIDIA (R) Cuda compiler driver\nCopyright (c) 2005-2019 NVIDIA Corporation\nBuilt on Sun_Jul_28_19:12:52_Pacific_Daylight_Time_2019\nCuda compilation tools, release 10.1, V10.1.243\n"
]
],
[
[
"## Prepare data for the training\nIf you redo this notebook on your own, you'll need the images with 0..7 (without 5) eggs in the folders `./images/0` ... `./images/7` (`./images/5` must exist for the classification training, but be empty)",
"_____no_output_____"
]
],
[
[
"data_directory = \"./images\"\n\ninput_shape = [64,64,3] # 256\nbatch_size = 16\n\nseed = 123 # for val split\n\ntrain_datagen = ImageDataGenerator(\n validation_split=0.2,\n rescale=1.0/255.0\n )\n\ntrain_generator = train_datagen.flow_from_directory(\n data_directory,\n seed=seed,\n target_size=(input_shape[0],input_shape[1]),\n color_mode=\"rgb\",\n class_mode=class_mode,\n batch_size=batch_size,\n subset='training'\n)\n\nval_datagen = ImageDataGenerator(\n validation_split=0.2,\n rescale=1.0/255.0\n )\n \nval_generator = val_datagen.flow_from_directory(\n data_directory,\n seed=seed,\n target_size=(input_shape[0],input_shape[1]),\n color_mode=\"rgb\",\n class_mode=class_mode,\n batch_size=batch_size,\n subset='validation'\n)\n",
"Found 11200 images belonging to 8 classes.\nFound 2800 images belonging to 8 classes.\n"
]
],
[
[
"## Prepare the model",
"_____no_output_____"
]
],
[
[
"\nnum_classes = 8 # because 0..7 eggs\nif class_mode == \"categorical\":\n num_output_dimensions = num_classes\nif class_mode == \"sparse\":\n num_output_dimensions = 1\n\nmodel = tf.keras.Sequential()\n\nmodel.add( tf.keras.layers.Conv2D(\n filters = 4,\n kernel_size = 5,\n strides = 1,\n padding = 'same',\n activation = 'relu',\n input_shape = input_shape\n ))\nmodel.add( tf.keras.layers.MaxPooling2D(\n pool_size = 2, strides = 2\n ))\nmodel.add( tf.keras.layers.Conv2D(\n filters = 8,\n kernel_size = 5,\n strides = 1,\n padding = 'same',\n activation = 'relu'\n ))\nmodel.add( tf.keras.layers.MaxPooling2D(\n pool_size = 2, strides = 2\n ))\nmodel.add( tf.keras.layers.Flatten())\nmodel.add(tf.keras.layers.Dense(\n units = 16, activation = 'relu'\n ))\nif class_mode == \"categorical\":\n last_activation = 'softmax'\nif class_mode == \"sparse\":\n last_activation = None\nmodel.add(tf.keras.layers.Dense(\n units = num_output_dimensions, activation = last_activation\n ))\n\nif class_mode == \"categorical\":\n loss = 'categorical_crossentropy'\nif class_mode == \"sparse\":\n loss = 'mse'\nmodel.compile(\n optimizer = 'adam',\n loss = loss,\n metrics = ['accuracy']\n )\n\nmodel.summary()",
"Model: \"sequential\"\n_________________________________________________________________\nLayer (type) Output Shape Param # \n=================================================================\nconv2d (Conv2D) (None, 64, 64, 4) 304 \n_________________________________________________________________\nmax_pooling2d (MaxPooling2D) (None, 32, 32, 4) 0 \n_________________________________________________________________\nconv2d_1 (Conv2D) (None, 32, 32, 8) 808 \n_________________________________________________________________\nmax_pooling2d_1 (MaxPooling2 (None, 16, 16, 8) 0 \n_________________________________________________________________\nflatten (Flatten) (None, 2048) 0 \n_________________________________________________________________\ndense (Dense) (None, 16) 32784 \n_________________________________________________________________\ndense_1 (Dense) (None, 8) 136 \n=================================================================\nTotal params: 34,032\nTrainable params: 34,032\nNon-trainable params: 0\n_________________________________________________________________\n"
]
],
[
[
"## Train the model\n(on 0,1,2,3,4,6,7, but not 5 eggs)",
"_____no_output_____"
]
],
[
[
"epochs = 5\n\nmodel.fit(\n train_generator,\n epochs=epochs,\n validation_data=val_generator,\n )\n",
"Epoch 1/5\n700/700 [==============================] - 29s 41ms/step - loss: 0.8835 - accuracy: 0.6392 - val_loss: 0.1543 - val_accuracy: 0.9654\nEpoch 2/5\n700/700 [==============================] - 29s 41ms/step - loss: 0.1501 - accuracy: 0.9516 - val_loss: 0.0663 - val_accuracy: 0.9804\nEpoch 3/5\n700/700 [==============================] - 29s 41ms/step - loss: 0.0836 - accuracy: 0.9729 - val_loss: 0.1199 - val_accuracy: 0.9543\nEpoch 4/5\n700/700 [==============================] - 29s 41ms/step - loss: 0.0766 - accuracy: 0.9739 - val_loss: 0.0389 - val_accuracy: 0.9907\nEpoch 5/5\n700/700 [==============================] - 29s 41ms/step - loss: 0.0638 - accuracy: 0.9797 - val_loss: 0.0628 - val_accuracy: 0.9754\n"
],
[
"plt.figure()\n\nif class_mode == \"categorical\":\n plt.plot(model.history.history['accuracy'])\n plt.plot(model.history.history['val_accuracy'])\n plt.title('History')\n plt.ylabel('Value')\n plt.xlabel('Epoch')\n plt.legend(['accuracy','val_accuracy'], loc='best')\n plt.show()\n\nif class_mode == \"sparse\":\n plt.plot(model.history.history['loss'])\n plt.plot(model.history.history['val_loss'])\n plt.title('History')\n plt.ylabel('Value')\n plt.xlabel('Epoch')\n plt.legend(['loss','val_loss'], loc='best')\n plt.show()",
"_____no_output_____"
]
],
[
[
"## Illustrate performance on unknown and completely unknown input\n(5 eggs are completly unknown; all other numbers trained but at least the test image with 4 eggs was not used during training)\n\nIf you are running this notebook on you own, you might have to adjust the filepaths, and you'll have to put the images with 5 eggs in the folder `./images_other/5`",
"_____no_output_____"
]
],
[
[
"filepath_known = './images_known_unknown/4/0.png'\nfilepath_unknown = './images_unknown/5/0.png'\n\n# helper function to make the notebook more tidy\ndef make_prediction(filepath):\n img = load_img(\n filepath,\n target_size=(input_shape[0],input_shape[1])\n )\n img = np.array(img)\n img = img / 255.0\n data = np.expand_dims(img, axis=0)\n prediction = model.predict(data)[0]\n predicted_class = np.argmax(prediction)\n return img, prediction, predicted_class\n\nif class_mode == \"categorical\":\n img, prediction, predicted_class = make_prediction(filepath_known)\n\n plt.figure()\n plt.subplot(2,1,0+1)\n plt.imshow(img)\n plt.subplot(2,1,0+2)\n plt.plot(prediction,'og')\n plt.xlabel('Predicted \"class\"')\n plt.ylabel('Score')\n\n img, prediction, predicted_class = make_prediction(filepath_unknown)\n\n plt.figure()\n plt.subplot(2,1,0+1)\n plt.imshow(img)\n plt.subplot(2,1,0+2)\n plt.plot(prediction,'og')\n plt.xlabel('Predicted \"class\"')\n plt.ylabel('Score')\n\nif class_mode == \"sparse\":\n img, prediction, predicted_class = make_prediction(filepath_known)\n\n plt.figure()\n plt.imshow(img)\n _ = plt.title('Prediction: '+str(prediction[0])+' - '+str(round(prediction[0])))\n\n img, prediction, predicted_class = make_prediction(filepath_unknown)\n\n plt.figure()\n plt.imshow(img)\n _ = plt.title('Prediction: '+str(prediction[0])+' - '+str(round(prediction[0])))",
"_____no_output_____"
],
[
"data_test_directory = \"./images_unknown\"\n\ntest_datagen = ImageDataGenerator(\n rescale=1.0/255.0\n )\ntest_generator = test_datagen.flow_from_directory(\n data_test_directory,\n target_size=(input_shape[0],input_shape[1]),\n color_mode=\"rgb\",\n class_mode=class_mode,\n batch_size=1,\n subset=None,\n shuffle=False\n )\n\nall_predictions = model.predict(test_generator, verbose=1)\n\nplt.figure()\n\nif class_mode == 'categorical':\n _ = plt.imshow(all_predictions, cmap='summer', aspect='auto', interpolation='none')\n _ = plt.colorbar()\n _ = plt.xlabel('Predicted class')\n _ = plt.ylabel('Image number')\n _ = plt.title('Score heatmap for true class 5')\n\nif class_mode == 'sparse':\n num_bins = 70\n _ = plt.hist(all_predictions, num_bins, color='g')\n _ = plt.xlabel('Regression value')\n _ = plt.ylabel('Counts')\n _ = plt.title('Histogram of output values for true number 5')",
"Found 2000 images belonging to 8 classes.\n2000/2000 [==============================] - 5s 3ms/step\n"
]
],
[
[
"## Illustrate performance on completely known data\nFor the sake of completeness, we repeat the last plots again for the known data used in the training phase.",
"_____no_output_____"
]
],
[
[
"data_test_directory = \"./images\"\n\ntest_datagen = ImageDataGenerator(\n rescale=1.0/255.0\n )\ntest_generator = test_datagen.flow_from_directory(\n data_test_directory,\n target_size=(input_shape[0],input_shape[1]),\n color_mode=\"rgb\",\n class_mode=class_mode,\n batch_size=1,\n subset=None,\n shuffle=False\n )\n\ntest_labels = (test_generator.class_indices)\ntest_filenames = test_generator.filenames\n\nall_predictions = model.predict(test_generator, verbose=1)\n\nif class_mode == \"categorical\":\n _ = plt.imshow(all_predictions, cmap='summer', aspect='auto', interpolation='none')\n _ = plt.colorbar()\n _ = plt.xlabel('Class')\n _ = plt.ylabel('Image number')\n _ = plt.title('Score heatmap')\n\nif class_mode == \"sparse\":\n plt.figure()\n num_bins = 70\n _ = plt.hist(all_predictions, num_bins, color='g')\n _ = plt.xlabel('Regression value')\n _ = plt.ylabel('Counts')\n _ = plt.title('Histogram of output values')",
"Found 14000 images belonging to 8 classes.\n14000/14000 [==============================] - 37s 3ms/step\n"
]
]
] | [
"markdown",
"code",
"markdown",
"code",
"markdown",
"code",
"markdown",
"code",
"markdown",
"code",
"markdown",
"code",
"markdown",
"code"
] | [
[
"markdown"
],
[
"code"
],
[
"markdown"
],
[
"code",
"code",
"code"
],
[
"markdown"
],
[
"code"
],
[
"markdown"
],
[
"code"
],
[
"markdown"
],
[
"code",
"code"
],
[
"markdown"
],
[
"code",
"code"
],
[
"markdown"
],
[
"code"
]
] |
e768f08ed059c18ed2fc7aead29ee67e1c779ef1 | 7,316 | ipynb | Jupyter Notebook | 101-150/110.balanced-binary-tree.ipynb | Sorosliu1029/LeetCode | 4aefc25a2095b6c06004d06dc9e45fa3db26dc12 | [
"MIT"
] | 1 | 2022-01-14T07:59:49.000Z | 2022-01-14T07:59:49.000Z | 101-150/110.balanced-binary-tree.ipynb | Sorosliu1029/LeetCode | 4aefc25a2095b6c06004d06dc9e45fa3db26dc12 | [
"MIT"
] | null | null | null | 101-150/110.balanced-binary-tree.ipynb | Sorosliu1029/LeetCode | 4aefc25a2095b6c06004d06dc9e45fa3db26dc12 | [
"MIT"
] | null | null | null | 24.065789 | 286 | 0.492482 | [
[
[
"### 110. Balanced Binary Tree",
"_____no_output_____"
],
[
"#### Content\n<p>Given a binary tree, determine if it is height-balanced.</p>\n\n<p>For this problem, a height-balanced binary tree is defined as:</p>\n\n<blockquote>\n<p>a binary tree in which the left and right subtrees of <em>every</em> node differ in height by no more than 1.</p>\n</blockquote>\n\n<p> </p>\n<p><strong>Example 1:</strong></p>\n<img alt=\"\" src=\"https://assets.leetcode.com/uploads/2020/10/06/balance_1.jpg\" style=\"width: 342px; height: 221px;\" />\n<pre>\n<strong>Input:</strong> root = [3,9,20,null,null,15,7]\n<strong>Output:</strong> true\n</pre>\n\n<p><strong>Example 2:</strong></p>\n<img alt=\"\" src=\"https://assets.leetcode.com/uploads/2020/10/06/balance_2.jpg\" style=\"width: 452px; height: 301px;\" />\n<pre>\n<strong>Input:</strong> root = [1,2,2,3,3,null,null,4,4]\n<strong>Output:</strong> false\n</pre>\n\n<p><strong>Example 3:</strong></p>\n\n<pre>\n<strong>Input:</strong> root = []\n<strong>Output:</strong> true\n</pre>\n\n<p> </p>\n<p><strong>Constraints:</strong></p>\n\n<ul>\n\t<li>The number of nodes in the tree is in the range <code>[0, 5000]</code>.</li>\n\t<li><code>-10<sup>4</sup> <= Node.val <= 10<sup>4</sup></code></li>\n</ul>\n",
"_____no_output_____"
],
[
"#### Difficulty: Easy, AC rate: 45.4%\n\n#### Question Tags:\n- Tree\n- Depth-First Search\n- Binary Tree\n\n#### Links:\n ๐ [Question Detail](https://leetcode.com/problems/balanced-binary-tree/description/) | ๐ [Question Solution](https://leetcode.com/problems/balanced-binary-tree/solution/) | ๐ฌ [Question Discussion](https://leetcode.com/problems/balanced-binary-tree/discuss/?orderBy=most_votes)\n\n#### Hints:\n",
"_____no_output_____"
],
[
"#### Sample Test Case\n[3,9,20,null,null,15,7]",
"_____no_output_____"
],
[
"---\nWhat's your idea?\n\nDFS\n\nไธๆฆๆฃๆตๅฐๅทฆๅญๆ ๆ่
ๅณๅญๆ ๅทฒ็ปไธๅนณ่กกไบ๏ผๅ็ดๆฅ่ฟๅ๏ผไธๅๆฃๆฅๅฆไธๆฃตๅญๆ \n\nๅฆๅ๏ผๆ็
งๅนณ่กกๆ ็ๅฎไน๏ผๆฃๆฅๅทฆๅณๅญๆ ้ซๅบฆๅทฎ\n\n---",
"_____no_output_____"
]
],
[
[
"from typing import Tuple\n\n# Definition for a binary tree node.\nclass TreeNode:\n def __init__(self, val=0, left=None, right=None):\n self.val = val\n self.left = left\n self.right = right\n\nclass Solution:\n def isBalanced(self, root: TreeNode) -> bool:\n balanced, _ = self.visitSubTree(root)\n return balanced\n \n def visitSubTree(self, sub_root: TreeNode) -> Tuple[bool, int]:\n if not sub_root:\n return [True, 0]\n \n is_left_balanced, left_height = self.visitSubTree(sub_root.left)\n if not is_left_balanced:\n return [False, left_height + 1]\n \n is_right_balanced, right_height = self.visitSubTree(sub_root.right)\n if not is_right_balanced:\n return [False, right_height + 1]\n \n return [abs(left_height - right_height) <= 1, max(left_height, right_height)+1]",
"_____no_output_____"
],
[
"s = Solution()\n\nn7 = TreeNode(7)\nn15 = TreeNode(15)\nn20 = TreeNode(20, n15, n7)\nn9 = TreeNode(9)\nn3 = TreeNode(3, n9, n20)\ns.isBalanced(n3)",
"_____no_output_____"
],
[
"s.isBalanced(None)",
"_____no_output_____"
],
[
"n4l = TreeNode(4)\nn4r = TreeNode(4)\nn3l = TreeNode(3, n4l, n4r)\nn3r = TreeNode(3)\nn2l = TreeNode(2, n3l, n3r)\nn2r = TreeNode(2)\nn1 = TreeNode(1, n2l, n2r)\ns.isBalanced(n1)",
"_____no_output_____"
],
[
"n4l = TreeNode(4)\nn4r = TreeNode(4)\nn3l = TreeNode(3, n4l, n4r)\nn3r = TreeNode(3)\nn3rr = TreeNode(3)\nn2l = TreeNode(2, n3l, n3r)\nn2r = TreeNode(2, n3rr)\nn1 = TreeNode(1, n2l, n2r)\ns.isBalanced(n1)",
"_____no_output_____"
],
[
"import sys, os; sys.path.append(os.path.abspath('..'))\nfrom submitter import submit\nsubmit(110)",
"_____no_output_____"
]
]
] | [
"markdown",
"code"
] | [
[
"markdown",
"markdown",
"markdown",
"markdown",
"markdown"
],
[
"code",
"code",
"code",
"code",
"code",
"code"
]
] |
e768f28ecd37b184d9b99692f83e81c746f3a78a | 97,883 | ipynb | Jupyter Notebook | DCGAN-nb.ipynb | MJ10/DCGAN | 3985dededb884313571051c7abff61c6cb3d0c6b | [
"MIT"
] | null | null | null | DCGAN-nb.ipynb | MJ10/DCGAN | 3985dededb884313571051c7abff61c6cb3d0c6b | [
"MIT"
] | null | null | null | DCGAN-nb.ipynb | MJ10/DCGAN | 3985dededb884313571051c7abff61c6cb3d0c6b | [
"MIT"
] | null | null | null | 87.007111 | 1,657 | 0.607562 | [
[
[
"import tensorflow as tf\nfrom DCGAN import DCGAN",
"_____no_output_____"
],
[
"sess = tf.InteractiveSession()",
"_____no_output_____"
],
[
"dcgan = DCGAN(sess, input_height=32, input_width=32,\n output_height=32, output_width=32,\n dataset='cifar')",
"_____no_output_____"
],
[
"dcgan.train()",
"Reading checkpoints...\nFailed to find a checkpoint\nFailed to load checkpoint\nEpoch: [ 0] [ 0/ 937] time: 6.0490, d_loss: 2.59173012, g_loss: 1.18601978\nEpoch: [ 0] [ 1/ 937] time: 11.1601, d_loss: 1.90442324, g_loss: 0.89997661\nEpoch: [ 0] [ 2/ 937] time: 16.1806, d_loss: 1.58417368, g_loss: 0.75836051\nEpoch: [ 0] [ 3/ 937] time: 21.2193, d_loss: 1.55620551, g_loss: 0.73450166\nEpoch: [ 0] [ 4/ 937] time: 26.5115, d_loss: 1.45740724, g_loss: 0.69896019\nEpoch: [ 0] [ 5/ 937] time: 31.5558, d_loss: 1.50869536, g_loss: 0.73043072\nEpoch: [ 0] [ 6/ 937] time: 36.5812, d_loss: 1.45730567, g_loss: 0.69903827\nEpoch: [ 0] [ 7/ 937] time: 41.6219, d_loss: 1.41693139, g_loss: 0.67956841\nEpoch: [ 0] [ 8/ 937] time: 46.6552, d_loss: 1.44928205, g_loss: 0.68525624\nEpoch: [ 0] [ 9/ 937] time: 51.6940, d_loss: 1.45065784, g_loss: 0.69497186\nEpoch: [ 0] [ 10/ 937] time: 56.7280, d_loss: 1.50137901, g_loss: 0.72820050\nEpoch: [ 0] [ 11/ 937] time: 61.7560, d_loss: 1.49324143, g_loss: 0.74603361\nEpoch: [ 0] [ 12/ 937] time: 66.8258, d_loss: 1.46530938, g_loss: 0.71432257\nEpoch: [ 0] [ 13/ 937] time: 71.8529, d_loss: 1.43292451, g_loss: 0.69837409\nEpoch: [ 0] [ 14/ 937] time: 77.0762, d_loss: 1.41472137, g_loss: 0.69337851\nEpoch: [ 0] [ 15/ 937] time: 82.1434, d_loss: 1.42331147, g_loss: 0.70254797\nEpoch: [ 0] [ 16/ 937] time: 87.2552, d_loss: 1.44305038, g_loss: 0.70029283\nEpoch: [ 0] [ 17/ 937] time: 92.2964, d_loss: 1.43858027, g_loss: 0.71507376\nEpoch: [ 0] [ 18/ 937] time: 97.3276, d_loss: 1.44326389, g_loss: 0.72191072\nEpoch: [ 0] [ 19/ 937] time: 102.3867, d_loss: 1.48783875, g_loss: 0.72693336\nEpoch: [ 0] [ 20/ 937] time: 107.4240, d_loss: 1.49477708, g_loss: 0.74717087\nEpoch: [ 0] [ 21/ 937] time: 112.6652, d_loss: 1.47432077, g_loss: 0.73020190\nEpoch: [ 0] [ 22/ 937] time: 117.6987, d_loss: 1.49631453, g_loss: 0.73180121\nEpoch: [ 0] [ 23/ 937] time: 122.7362, d_loss: 1.44933319, g_loss: 0.73234898\nEpoch: [ 0] [ 24/ 937] time: 127.7642, d_loss: 1.41479754, g_loss: 0.71818578\nEpoch: [ 0] [ 25/ 937] time: 132.7915, d_loss: 1.40140045, g_loss: 0.72005343\nEpoch: [ 0] [ 26/ 937] time: 137.8336, d_loss: 1.40638852, g_loss: 0.70124120\nEpoch: [ 0] [ 27/ 937] time: 142.9652, d_loss: 1.40978432, g_loss: 0.71706247\nEpoch: [ 0] [ 28/ 937] time: 148.2093, d_loss: 1.40061271, g_loss: 0.71853358\nEpoch: [ 0] [ 29/ 937] time: 153.2568, d_loss: 1.37819576, g_loss: 0.70659864\nEpoch: [ 0] [ 30/ 937] time: 158.2994, d_loss: 1.40119290, g_loss: 0.70012355\nEpoch: [ 0] [ 31/ 937] time: 163.3346, d_loss: 1.44171679, g_loss: 0.65862226\nEpoch: [ 0] [ 32/ 937] time: 168.3663, d_loss: 1.46935034, g_loss: 0.64977467\nEpoch: [ 0] [ 33/ 937] time: 173.5501, d_loss: 1.46558285, g_loss: 0.65169674\nEpoch: [ 0] [ 34/ 937] time: 178.5836, d_loss: 1.48324931, g_loss: 0.66875708\nEpoch: [ 0] [ 35/ 937] time: 183.6162, d_loss: 1.46516228, g_loss: 0.64522517\nEpoch: [ 0] [ 36/ 937] time: 188.6527, d_loss: 1.44611502, g_loss: 0.65484929\nEpoch: [ 0] [ 37/ 937] time: 193.6802, d_loss: 1.42673695, g_loss: 0.65040290\nEpoch: [ 0] [ 38/ 937] time: 198.8256, d_loss: 1.42223585, g_loss: 0.65188771\nEpoch: [ 0] [ 39/ 937] time: 203.8599, d_loss: 1.43771005, g_loss: 0.64088213\nEpoch: [ 0] [ 40/ 937] time: 208.9135, d_loss: 1.43711901, g_loss: 0.65288460\nEpoch: [ 0] [ 41/ 937] time: 213.9568, d_loss: 1.45505738, g_loss: 0.64688981\nEpoch: [ 0] [ 42/ 937] time: 219.1775, d_loss: 1.44192815, g_loss: 0.66558123\nEpoch: [ 0] [ 43/ 937] time: 224.2312, d_loss: 1.43283713, g_loss: 0.69327235\nEpoch: [ 0] [ 44/ 937] time: 229.9868, d_loss: 1.43462038, g_loss: 0.71106315\nEpoch: [ 0] [ 45/ 937] time: 235.0203, d_loss: 1.52839041, g_loss: 0.76073879\nEpoch: [ 0] [ 46/ 937] time: 240.1449, d_loss: 1.46915960, g_loss: 0.72295779\nEpoch: [ 0] [ 47/ 937] time: 245.1604, d_loss: 1.40911472, g_loss: 0.69644666\nEpoch: [ 0] [ 48/ 937] time: 250.4431, d_loss: 1.44297767, g_loss: 0.68657351\nEpoch: [ 0] [ 49/ 937] time: 255.7939, d_loss: 1.43607056, g_loss: 0.67764741\n[Sample] d_loss: 1.41539836, g_loss: 0.70671487\nEpoch: [ 0] [ 50/ 937] time: 262.5147, d_loss: 1.43079257, g_loss: 0.67453402\nEpoch: [ 0] [ 51/ 937] time: 268.0494, d_loss: 1.42963302, g_loss: 0.65400088\nEpoch: [ 0] [ 52/ 937] time: 273.8062, d_loss: 1.43462992, g_loss: 0.63938296\nEpoch: [ 0] [ 53/ 937] time: 278.8458, d_loss: 1.43406773, g_loss: 0.66370511\nEpoch: [ 0] [ 54/ 937] time: 283.8852, d_loss: 1.44408774, g_loss: 0.67007232\nEpoch: [ 0] [ 55/ 937] time: 288.9126, d_loss: 1.46001506, g_loss: 0.67833257\nEpoch: [ 0] [ 56/ 937] time: 294.2839, d_loss: 1.44804454, g_loss: 0.67318153\nEpoch: [ 0] [ 57/ 937] time: 299.3566, d_loss: 1.54158795, g_loss: 0.73598719\nEpoch: [ 0] [ 58/ 937] time: 306.2094, d_loss: 1.47991490, g_loss: 0.71210855\nEpoch: [ 0] [ 59/ 937] time: 315.2801, d_loss: 1.43797565, g_loss: 0.69173729\nEpoch: [ 0] [ 60/ 937] time: 322.2859, d_loss: 1.43783641, g_loss: 0.69269550\nEpoch: [ 0] [ 61/ 937] time: 328.5991, d_loss: 1.42355847, g_loss: 0.66487408\nEpoch: [ 0] [ 62/ 937] time: 335.6898, d_loss: 1.42659938, g_loss: 0.67652994\nEpoch: [ 0] [ 63/ 937] time: 342.3009, d_loss: 1.45127273, g_loss: 0.71104729\nEpoch: [ 0] [ 64/ 937] time: 348.7355, d_loss: 1.45282292, g_loss: 0.72033286\nEpoch: [ 0] [ 65/ 937] time: 355.6712, d_loss: 1.44202673, g_loss: 0.66939586\nEpoch: [ 0] [ 66/ 937] time: 361.8670, d_loss: 1.44037843, g_loss: 0.66667873\nEpoch: [ 0] [ 67/ 937] time: 369.0172, d_loss: 1.42888761, g_loss: 0.67633748\nEpoch: [ 0] [ 68/ 937] time: 377.3477, d_loss: 1.42907608, g_loss: 0.67590606\nEpoch: [ 0] [ 69/ 937] time: 384.2622, d_loss: 1.41119361, g_loss: 0.67857325\nEpoch: [ 0] [ 70/ 937] time: 390.7584, d_loss: 1.42081499, g_loss: 0.66896480\nEpoch: [ 0] [ 71/ 937] time: 395.9085, d_loss: 1.42902780, g_loss: 0.67160779\nEpoch: [ 0] [ 72/ 937] time: 401.0078, d_loss: 1.47877002, g_loss: 0.70087510\nEpoch: [ 0] [ 73/ 937] time: 407.0248, d_loss: 1.46801949, g_loss: 0.71497977\nEpoch: [ 0] [ 74/ 937] time: 413.4784, d_loss: 1.43304241, g_loss: 0.68723845\nEpoch: [ 0] [ 75/ 937] time: 420.3856, d_loss: 1.40981674, g_loss: 0.67402101\nEpoch: [ 0] [ 76/ 937] time: 428.9129, d_loss: 1.43163919, g_loss: 0.66841280\nEpoch: [ 0] [ 77/ 937] time: 435.9046, d_loss: 1.45918131, g_loss: 0.70717907\nEpoch: [ 0] [ 78/ 937] time: 441.8583, d_loss: 1.45587587, g_loss: 0.67927438\nEpoch: [ 0] [ 79/ 937] time: 447.2098, d_loss: 1.43078995, g_loss: 0.69228077\nEpoch: [ 0] [ 80/ 937] time: 452.4900, d_loss: 1.40492070, g_loss: 0.69040143\nEpoch: [ 0] [ 81/ 937] time: 458.0961, d_loss: 1.39871240, g_loss: 0.69678247\nEpoch: [ 0] [ 82/ 937] time: 464.2524, d_loss: 1.41154504, g_loss: 0.69083619\nEpoch: [ 0] [ 83/ 937] time: 471.4646, d_loss: 1.42679608, g_loss: 0.67257833\nEpoch: [ 0] [ 84/ 937] time: 476.6869, d_loss: 1.42552495, g_loss: 0.67418766\nEpoch: [ 0] [ 85/ 937] time: 481.9385, d_loss: 1.42744994, g_loss: 0.68012822\nEpoch: [ 0] [ 86/ 937] time: 487.2227, d_loss: 1.43269515, g_loss: 0.67501462\nEpoch: [ 0] [ 87/ 937] time: 492.4398, d_loss: 1.41543150, g_loss: 0.67581111\nEpoch: [ 0] [ 88/ 937] time: 497.5949, d_loss: 1.41490972, g_loss: 0.67915374\nEpoch: [ 0] [ 89/ 937] time: 502.8161, d_loss: 1.42191005, g_loss: 0.67816079\nEpoch: [ 0] [ 90/ 937] time: 508.1942, d_loss: 1.41603422, g_loss: 0.67608774\nEpoch: [ 0] [ 91/ 937] time: 513.3684, d_loss: 1.42709506, g_loss: 0.68447745\nEpoch: [ 0] [ 92/ 937] time: 518.5175, d_loss: 1.41916621, g_loss: 0.67515683\nEpoch: [ 0] [ 93/ 937] time: 523.6782, d_loss: 1.40906763, g_loss: 0.68163878\nEpoch: [ 0] [ 94/ 937] time: 528.8403, d_loss: 1.40837300, g_loss: 0.68722904\nEpoch: [ 0] [ 95/ 937] time: 534.0037, d_loss: 1.40401471, g_loss: 0.68644756\nEpoch: [ 0] [ 96/ 937] time: 539.1586, d_loss: 1.40996814, g_loss: 0.67724109\nEpoch: [ 0] [ 97/ 937] time: 544.3025, d_loss: 1.40533197, g_loss: 0.68097639\nEpoch: [ 0] [ 98/ 937] time: 549.4949, d_loss: 1.41158473, g_loss: 0.67954963\nEpoch: [ 0] [ 99/ 937] time: 554.9463, d_loss: 1.42082906, g_loss: 0.68351531\n[Sample] d_loss: 1.41172981, g_loss: 0.70321292\nEpoch: [ 0] [ 100/ 937] time: 561.7062, d_loss: 1.40612757, g_loss: 0.68343288\nEpoch: [ 0] [ 101/ 937] time: 566.8245, d_loss: 1.41630709, g_loss: 0.68304616\n"
]
]
] | [
"code"
] | [
[
"code",
"code",
"code",
"code"
]
] |
e768f6626cbe7bae46c6c9c0479253b7b7fc24cd | 26,411 | ipynb | Jupyter Notebook | EmoDJ Music Player/EmoDJ_Music_Player_Code.ipynb | peggypytang/EmoDJ | 0897ea65fb5b651e1949e2bb6ebb5b91cd516664 | [
"MIT"
] | 10 | 2021-01-13T08:14:49.000Z | 2022-01-29T15:47:39.000Z | EmoDJ Music Player/EmoDJ_Music_Player_Code.ipynb | peggypytang/EmoDJ | 0897ea65fb5b651e1949e2bb6ebb5b91cd516664 | [
"MIT"
] | null | null | null | EmoDJ Music Player/EmoDJ_Music_Player_Code.ipynb | peggypytang/EmoDJ | 0897ea65fb5b651e1949e2bb6ebb5b91cd516664 | [
"MIT"
] | null | null | null | 40.632308 | 197 | 0.580667 | [
[
[
"# EmoDJ Music Player\n\nEmoDJ is a brand-new AI-powered offline music player desktop application that focuses on improving listeners' emotional wellness.\n\nThis application is designed based on psychology theories. It is powered by machine learning to automatically identify music emotion of your songs.\n\nTo start EmoDJ at first time, click Cell>Run All<br>\nTo restart EmoDJ after quit, click Kernel>Restart and Run All\n\nSupported music file format: .wav.\nSample music files in /musics folder are downloaded from Free Music Archive.",
"_____no_output_____"
],
[
"### Music Emotion Recognition Engine\nLoad trained models and predict arousal and valence value of the music <br>",
"_____no_output_____"
]
],
[
[
"import os\nimport librosa\nimport sklearn\n \ndef preprocess_feature(file_name):\n n_mfcc = 12\n mfcc_all = []\n #MFCC per time period (500ms)\n x, sr = librosa.load(MUSIC_FOLDER + file_name)\n for i in range(0, len(x), int(sr*500/1000)):\n x_cont = x[i:i+int(sr*500/1000)]\n mfccs = librosa.feature.mfcc(x_cont,sr=sr,n_mfcc=n_mfcc)\n #append feature value for music interval shorter than 500ms\n mfccs = np.hstack((mfccs, np.zeros((12,22 - mfccs.shape[1]))))\n mfccs = mfccs.flatten()\n mfcc_all.append(mfccs)\n return np.vstack(mfcc_all)\n\ndef normalise(input_data, feature_matrix_mean, feature_matrix_std):\n return (input_data - feature_matrix_mean) / feature_matrix_std\n\ndef emotion_predict(file_name):\n #Load trained models\n with open(MODEL_FOLDER + 'arousal_model.pkl', 'rb') as f:\n arousal_model = pickle.load(f)\n with open(MODEL_FOLDER + 'valence_model.pkl', 'rb') as f:\n valence_model = pickle.load(f)\n with open(MODEL_FOLDER + 'feature_matrix_mean.pkl', 'rb') as f:\n feature_matrix_mean = pickle.load(f)\n with open(MODEL_FOLDER + 'feature_matrix_std.pkl', 'rb') as f:\n feature_matrix_std = pickle.load(f)\n mfcc = preprocess_feature(file_name) \n mfcc_norm = normalise(mfcc, feature_matrix_mean, feature_matrix_std)\n #Predict arousal value per 5 second interval\n music_aro = arousal_model.predict(mfcc_norm).flatten()\n #Predict valence value per 5 second interval\n music_val = valence_model.predict(mfcc_norm).flatten()\n return music_aro, music_val\n ",
"_____no_output_____"
]
],
[
[
"### Music Emotion Retrieval Panel\nDisplay music by their valence and arousal value. Colour of marker represents colour association to average music emotion of that music piece.\n\nListeners can see annotation (song name, valence value, arousal value) of particular music piece by hovering its marker.\n\nListeners can retrieve and play the music piece by clicking on its marker.\n\nThe music currently playing is shown in yellow colour, the music played is shown in grey colour. This would be reset if the listener select new music piece to play (reconstruct the playlist).",
"_____no_output_____"
]
],
[
[
"HAPPY_COLOUR = 'lawngreen'\nSAD_COLOUR = 'darkblue'\nTENSE_COLOUR = 'red'\nCALM_COLOUR = 'darkcyan'\nBASE_COLOUR = 'darkgrey'\nPLAYING_COLOUR = 'gold'\nFILE_FORMAT = '.wav'\n\n#Start playing music when user pick the marker on scatter plot\ndef pick_music(event):\n ind = event.ind\n song_id = emotion_df.iloc[ind , :][ID_FIELD].values[0]\n start_music(song_id)\n\n#Show annotation when user hover the marker on scatter plot\n#(song name, valence value, arousal value)\ndef update_annot(ind):\n pos = scatter_panel.get_offsets()[ind[\"ind\"][0]]\n music_annot.xy = pos\n (x,y) = pos\n song_id = emotion_df[(emotion_df[VAL_FIELD]==x) & (emotion_df[ARO_FIELD]==y)][ID_FIELD].values[0]\n music_annot.set_text(get_song_name(song_id) + \\\n '\\nValence: '+ str(x.round(2)) + \\\n '\\nArousal: '+ str(y.round(2))) \n\ndef hover_music(event):\n vis = music_annot.get_visible()\n if event.inaxes == ax_panel:\n cont, ind = scatter_panel.contains(event)\n if cont:\n update_annot(ind)\n music_annot.set_visible(True)\n canvas_panel.draw_idle()\n else:\n if vis:\n music_annot.set_visible(False)\n canvas_panel.draw_idle()\n\n#List marker colour for marks on scatter plot \n#based on corresponding emotion of average arousal valence value of that music\ndef list_colour_panel(x_list, y_list):\n colour_list = []\n for x, y in zip(x_list,y_list):\n if x >= 0 and y > 0:\n colour_list.append(HAPPY_COLOUR)\n elif x <= 0 and y < 0:\n colour_list.append(SAD_COLOUR)\n elif x < 0 and y >= 0:\n colour_list.append(TENSE_COLOUR)\n else:\n colour_list.append(CALM_COLOUR)\n return colour_list",
"_____no_output_____"
]
],
[
[
"### Music Visualisation Engine\nWhile playing the music, Fast Fourier Transform was performed on each 1024 frames to show amplitude (converted to dB) and frequency.<br>\nColour of line represents colour association to time vary music emotion.",
"_____no_output_____"
]
],
[
[
"#Initialise visualition\ndef init_vis(): \n line.set_ydata([0] * len(vis_x))\n return line,\n\n#Update the visualisation\n#Line plot value based on real FFT (converted to dB)\n#Line colour based on emotion of arousal valence value at that time period\ndef animate_vis(i): \n global num_CHUNK\n #Show visualisation when\n #-music file is loaded\n #-is playing (not paused)\n #-the music file has not finished playing\n if wf is not None and isplay and wf.getnframes()-CHUNK*num_CHUNK>0:\n num_CHUNK += 1\n data = wf.readframes(CHUNK)\n audio_data = np.frombuffer(data, np.int16)\n dfft = 10.*np.log10(abs(np.fft.rfft(audio_data)+1)) # +1 to avoid log0\n line.set_xdata(np.arange(len(dfft))*10.)\n line.set_ydata(dfft)\n line.set_color(colour_vis.pop(0))\n else: \n line.set_xdata(vis_x)\n line.set_ydata([0] * len(vis_x))\n return line,\n\n#List colour for marks on scatter plot \n#Based on corresponding emotion of arousal valence value across time period\ndef list_colour_vis(song_id):\n global colour_vis\n colour_vis = []\n valence_list = valence_df[valence_df[ID_FIELD]==song_id][VAL_FIELD].values[0]\n arousal_list = arousal_df[arousal_df[ID_FIELD]==song_id][ARO_FIELD].values[0]\n for x, y in zip(valence_list,arousal_list):\n if x >= 0 and y > 0:\n colour_vis.extend([HAPPY_COLOUR]*int(TIME_PERIOD*(RATE/CHUNK)))\n elif x <= 0 and y < 0:\n colour_vis.extend([SAD_COLOUR]*int(TIME_PERIOD*(RATE/CHUNK)))\n elif x < 0 and y >= 0:\n colour_vis.extend([TENSE_COLOUR]*int(TIME_PERIOD*(RATE/CHUNK)))\n else:\n colour_vis.extend([CALM_COLOUR]*int(TIME_PERIOD*(RATE/CHUNK)))\n\ncolour_vis = []\nnum_CHUNK = 0\nTIME_PERIOD = 5 #5second",
"_____no_output_____"
]
],
[
[
"### Music Recommendation and Player Engine\nIn addition to standard functions (such as next, pause, resume), it provides recommended playlist based on similarity of music emotion with the music selection.\n\nIt would play the next music piece in playlist automatically, starting from the most similar one, until it reaches the end of playlist.",
"_____no_output_____"
]
],
[
[
"from tkinter import messagebox\nimport pygame\n\ndef get_song_name(song_id):\n return processed_music[processed_music[ID_FIELD]==song_id][NAME_FIELD].values[0]\n\ndef get_song_file_path(song_id):\n return MUSIC_FOLDER + get_song_name(song_id)\n\n#Construct playlist based on similarity with song selected\n#Euclidean distance by valence and arousal value (square root is ignored)\ndef construct_playlist(song_id):\n global playlist\n playlist = []\n playlist_dict = {}\n curr_val = emotion_df[emotion_df[ID_FIELD]==song_id][VAL_FIELD].values[0]\n curr_aro = emotion_df[emotion_df[ID_FIELD]==song_id][ARO_FIELD].values[0]\n song_list = list(emotion_df[ID_FIELD].values)\n song_list.remove(song_id)\n for compare_song_id in song_list:\n compare_val = emotion_df[emotion_df[ID_FIELD]==compare_song_id][VAL_FIELD].values[0]\n compare_aro = emotion_df[emotion_df[ID_FIELD]==compare_song_id][ARO_FIELD].values[0]\n playlist_dict[compare_song_id] = (curr_val-compare_val)**2 + (curr_aro-compare_aro)**2\n playlist_dict = sorted(playlist_dict.items(), key = lambda kv:(kv[1], kv[0]))\n playlist = [i[0] for i in playlist_dict]\n\n#Update setting to play song \ndef update_music_setting(song_id):\n global wf, num_CHUNK\n mixer.music.load(get_song_file_path(song_id))\n mixer.music.play()\n wf = wave.open(get_song_file_path(song_id), 'rb')\n songLabel.set(get_song_name(song_id))\n list_colour_vis(song_id)\n ax_panel.scatter(emotion_df[VAL_FIELD],emotion_df[ARO_FIELD], s=15,c=scatter_colour,picker=False)\n #Played songs are displayed as BASE_COLOUR\n ax_panel.scatter(emotion_df[emotion_df[ID_FIELD].isin(played_songs)][VAL_FIELD], \\\n emotion_df[emotion_df[ID_FIELD].isin(played_songs)][ARO_FIELD], s=16,c=BASE_COLOUR,picker=False)\n #Playing songs are displayed as PLAYING_COLOUR\n ax_panel.scatter(emotion_df[emotion_df[ID_FIELD]==song_id][VAL_FIELD], \\\n emotion_df[emotion_df[ID_FIELD]==song_id][ARO_FIELD], s=17,c=PLAYING_COLOUR,picker=False)\n canvas_panel.draw()\n played_songs.append(song_id)\n num_CHUNK = 0\n\n#User selected in panel to start song and construct new playlist\ndef start_music(song_id):\n global isplay, played_songs\n mixer.music.stop()\n #Construct playlist\n construct_playlist(song_id)\n played_songs = []\n #Load and play song selected\n isplay = True\n update_music_setting(song_id)\n\n#User clicked next button to play next song in playlist\ndef next_music():\n global wf, isplay\n mixer.music.stop()\n if playlist:\n isplay = True\n song_id = playlist.pop(0)\n update_music_setting(song_id)\n else:\n wf = None\n isplay = False\n messagebox.showinfo('EmoDJ', 'Reach the end of playlist.')\n \n#User clicked pause/resume button \ndef pause_music():\n global isplay\n if wf is None and isplay:\n messagebox.showinfo('EmoDJ', 'Please select music in Emotion Panel.')\n elif wf:\n if isplay:\n isplay = False\n mixer.music.pause()\n else:\n isplay = True\n mixer.music.unpause()\n\n#Auto play next music in playlist\ndef auto_next_music():\n global isplay, wf\n #Check if the song completes\n if isplay:\n if wf is not None:\n if wf.getnframes()-CHUNK*num_CHUNK<=0:\n #End playing if playlist exhaust\n if playlist == [] :\n isplay = False\n wf = None\n songLabel.set('')\n messagebox.showinfo('EmoDJ', 'Reach the end of playlist.')\n #Play next song in playlist\n elif isplay: \n wf = None\n song_id = playlist.pop(0)\n update_music_setting(song_id)\n window.after(2000, auto_next_music)\n \nwf = None\nplayed_songs = []\nplaylist = []\nisnext = False \nisplay = False",
"pygame 1.9.6\nHello from the pygame community. https://www.pygame.org/contribute.html\n"
]
],
[
[
"### Create Index\nCreate below search index files\n- Average emotion of musics \n- Time varying valence values of musics \n- Time varying arousal values of musics \n- Processed music (Music pieces with music emotion recognised)",
"_____no_output_____"
]
],
[
[
"import os\nimport pickle\nimport time, sys\nfrom IPython.display import clear_output\n\ndef update_progress(processed, total):\n bar_length = 20\n progress = processed/total\n \n if isinstance(progress, int):\n progress = float(progress)\n if not isinstance(progress, float):\n progress = 0\n if progress < 0:\n progress = 0\n if progress >= 1:\n progress = 1\n\n block = int(round(bar_length * progress))\n\n clear_output(wait = True)\n text = \"Music Emotion Recognition Progress: [{0}] {1} / {2} songs processed\".format( \"#\" * block + \"-\" * (bar_length - block), processed, total )\n print(text)\n \n \ndef create_index(emotion_df, arousal_df, valence_df, processed_music, music_files):\n #Remove music from processed music if it is not in the folder anymore\n musics_remove = set(processed_music[NAME_FIELD].values) - set(music_files)\n for music_name in musics_remove:\n song_id = processed_music[processed_music[NAME_FIELD]==music_name][ID_FIELD].values[0]\n processed_music = processed_music[processed_music.song_id != song_id]\n emotion_df = emotion_df[emotion_df.song_id != song_id]\n arousal_df = arousal_df[arousal_df.song_id != song_id]\n valence_df = valence_df[valence_df.song_id != song_id]\n \n #Process unprocessed musics in folder\n #Only process .wav files\n musics_new = set(music_files) - set(processed_music[NAME_FIELD].values)\n num_proceeded = 0\n \n for music_name in musics_new: \n update_progress(num_proceeded, len(musics_new))\n\n if music_name.find(FILE_FORMAT)>-1:\n music_aro, music_val = emotion_predict(music_name)\n new_song_id = int(np.nanmax([processed_music[ID_FIELD].max(),0])) +1\n processed_music = processed_music.append({ID_FIELD:new_song_id,NAME_FIELD:music_name}, ignore_index=True)\n arousal_df = arousal_df.append({ID_FIELD:new_song_id,ARO_FIELD:music_aro}, ignore_index=True)\n valence_df = valence_df.append({ID_FIELD:new_song_id,VAL_FIELD:music_val}, ignore_index=True)\n emotion_df = emotion_df.append({ID_FIELD:new_song_id,VAL_FIELD:music_val.mean(),ARO_FIELD:music_aro.mean()}, ignore_index=True)\n num_proceeded += 1\n \n #Save index\n with open(INDEX_FOLDER+'average_emotion.pkl', 'wb') as f:\n pickle.dump(emotion_df,f)\n with open(INDEX_FOLDER+'arousal.pkl', 'wb') as f:\n pickle.dump(arousal_df,f)\n with open(INDEX_FOLDER+'valence.pkl', 'wb') as f:\n pickle.dump(valence_df,f)\n with open(INDEX_FOLDER+'processed_music.pkl', 'wb') as f:\n pickle.dump(processed_music,f)",
"_____no_output_____"
]
],
[
[
"### Load Index\nLoad indexes if any. Otherwise, create index folder and empty indexes.<br>\nFolder structure:\n- musics/ (Music files)\n- index/ (Index files)\n- model/ (Music emotion recognition model)",
"_____no_output_____"
]
],
[
[
"import pandas as pd\nimport numpy as np\nMUSIC_FOLDER = 'musics/'\nINDEX_FOLDER = 'index/'\nMODEL_FOLDER = 'model/'\nVAL_FIELD = 'valence'\nARO_FIELD = 'arousal'\nNAME_FIELD = 'song_name'\nID_FIELD = 'song_id'\n\ndef load_index():\n #For first time using this program\n #Create initial index\n if not os.path.exists(INDEX_FOLDER):\n os.makedirs(INDEX_FOLDER)\n #Average emotion of the music\n try:\n with open(INDEX_FOLDER+'average_emotion.pkl', 'rb') as f:\n emotion_df = pickle.load(f)\n except:\n emotion_df = pd.DataFrame(columns=[ID_FIELD, VAL_FIELD, ARO_FIELD])\n #Dynamic arousal values of the music\n try:\n with open(INDEX_FOLDER+'arousal.pkl', 'rb') as f:\n arousal_df = pickle.load(f)\n except:\n arousal_df = pd.DataFrame(columns=[ID_FIELD, ARO_FIELD])\n #Dynamic valence values of the music\n try:\n with open(INDEX_FOLDER+'valence.pkl','rb') as f:\n valence_df = pickle.load(f)\n except:\n valence_df = pd.DataFrame(columns=[ID_FIELD, VAL_FIELD])\n #Processed music\n try:\n with open(INDEX_FOLDER+'processed_music.pkl','rb') as f:\n processed_music = pickle.load(f)\n except:\n processed_music = pd.DataFrame(columns=[ID_FIELD, NAME_FIELD])\n \n emotion_df = emotion_df.astype({ID_FIELD: int})\n arousal_df = arousal_df.astype({ID_FIELD: int})\n valence_df = valence_df.astype({ID_FIELD: int})\n processed_music = processed_music.astype({ID_FIELD: int})\n \n music_files = os.listdir(MUSIC_FOLDER)\n if '.DS_Store' in music_files:\n music_files.remove('.DS_Store')\n \n return emotion_df, arousal_df, valence_df, processed_music, music_files",
"_____no_output_____"
]
],
[
[
"### GUI Engine\nGraphical user interface to interact with listener.\n\nBefore launching GUI, it will check if there are unprocessed music. If so, process to get music emotion values of the unprocessed music and re-create of index.",
"_____no_output_____"
]
],
[
[
"#Due to system specification difference\n#Parameter to ensure synchronisation of visualisation and sound \nSYNC = 3.5 \n\nimport tkinter as tk\nimport wave \nfrom pygame import mixer\nimport matplotlib.animation as animation\nfrom matplotlib import style\nstyle.use('ggplot')\nimport matplotlib.pyplot as plt\nimport numpy as np\nimport math\nimport time\nfrom PIL import ImageTk, Image\nfrom matplotlib.figure import Figure\nfrom matplotlib.backends.backend_tkagg import FigureCanvasTkAgg\nfrom matplotlib.animation import FuncAnimation\n\ndef quit_window():\n mixer.music.stop()\n window.withdraw()\n window.update()\n window.destroy()\n \ndef cmdNext(): next_music() \ndef cmdPause(): pause_music()\ndef cmdQuit(): quit_window()\n\nemotion_df, arousal_df, valence_df, processed_music, music_files = load_index()\n\n#Check if there are unprocessed music\nunprocessed_music = set(music_files).symmetric_difference(set(processed_music[NAME_FIELD].values))\nif unprocessed_music:\n print('Processing musics...')\n create_index(emotion_df, arousal_df, valence_df, processed_music, music_files)\n \nemotion_df, arousal_df, valence_df, processed_music, _ = load_index()\n \nwindow = tk.Tk()\nwindow.title(\"EmoDJ\")\nwindow.config(background='white')\nwindow.geometry('1300x700')\n\n#EmoDJ logo\nlogo_image = ImageTk.PhotoImage(Image.open(MODEL_FOLDER + 'logo.jpeg').resize((150, 80), Image.ANTIALIAS))\ntk.Label(window, image = logo_image,background='white').grid(row = 0,column=0,sticky='W')\n\n#Song name\nsongLabel = tk.StringVar()\nsongLabel.set('')\ntk.Label(window, textvariable=songLabel,background='white').grid(row = 1, column=0)\n\n#music visualisation\nfig_vis, ax_vis = plt.subplots(figsize=(8,5))\nRATE = 44100\nCHUNK = 1024\nmax_x = CHUNK+1\nmax_y = 100\n\nvis_x = np.arange(0, max_x)\nax_vis.set_xlim(0, max_x)\nax_vis.set_ylim(0, max_y)\nax_vis.set_axis_off()\nline, = ax_vis.plot(vis_x, [0] * len(vis_x))\ncanvas_vis = FigureCanvasTkAgg(fig_vis, master=window)\ncanvas_vis.get_tk_widget().grid(row=2,column=0,rowspan=2,sticky='W'+'E'+'N'+'S')\nani = animation.FuncAnimation(fig_vis, animate_vis, init_func=init_vis, interval=int(math.ceil(1000/(RATE/CHUNK)))+SYNC, blit=True)\n\n#music player\ntk.Button(window, text=\"Quit\", command=cmdQuit).grid(row = 0, column=2,sticky='E'+'N')\nmixer.pre_init(frequency=RATE, size=-16, channels=2)\nmixer.init()\ntk.Button(window, text=\"Next\", command=cmdNext).grid(row = 1, column=1,sticky='W'+'E'+'N'+'S')\ntk.Button(window, text=\"Resume/Pause\", command=cmdPause).grid(row = 1, column=2,sticky='W'+'E'+'N'+'S')\n\n#music emotion panel\nfig_panel, ax_panel = plt.subplots(figsize=(5,5))\nscatter_colour = list_colour_panel(emotion_df[VAL_FIELD], emotion_df[ARO_FIELD])\nscatter_panel = ax_panel.scatter(emotion_df[VAL_FIELD],emotion_df[ARO_FIELD], s=15,c=scatter_colour, picker=True)\nax_panel.axvline(x=0,c=BASE_COLOUR)\nax_panel.axhline(y=0,c=BASE_COLOUR)\nax_panel.set_xlim(-1, 1)\nax_panel.set_ylim(-1, 1)\nax_panel.text(-1,0.05,s='-1 Valence',fontsize=9,color=BASE_COLOUR)\nax_panel.text(0.75,0.05,s='+1 Valence',fontsize=9,color=BASE_COLOUR)\nax_panel.text(0.05,-1,s='-1 Arousal',fontsize=9,color=BASE_COLOUR)\nax_panel.text(0.05,1,s='+1 Arousal',fontsize=9,color=BASE_COLOUR)\nax_panel.set_axis_off()\nmusic_annot = ax_panel.annotate(\"\", xy=(0,0), xytext=(20,20),textcoords=\"offset points\",bbox=dict(boxstyle=\"round\", fc=\"w\"))\nmusic_annot.set_visible(False)\ncanvas_panel = FigureCanvasTkAgg(fig_panel, master=window)\ncanvas_panel.get_tk_widget().grid(row=2,column=1,columnspan=2,sticky='W'+'E'+'N'+'S')\ncanvas_panel.mpl_connect('pick_event', pick_music)\ncanvas_panel.mpl_connect(\"motion_notify_event\", hover_music)\ntk.Label(window, text=\"Start playing music by clicking on the marker!\",background='white').grid(row = 3,column=1,columnspan=2,sticky='W'+'E'+'N'+'S')\nwindow.after(0, auto_next_music)\nwindow.mainloop()\nprint('Enjoy music! See you next time.')",
"Enjoy music! See you next time.\n"
]
]
] | [
"markdown",
"code",
"markdown",
"code",
"markdown",
"code",
"markdown",
"code",
"markdown",
"code",
"markdown",
"code",
"markdown",
"code"
] | [
[
"markdown",
"markdown"
],
[
"code"
],
[
"markdown"
],
[
"code"
],
[
"markdown"
],
[
"code"
],
[
"markdown"
],
[
"code"
],
[
"markdown"
],
[
"code"
],
[
"markdown"
],
[
"code"
],
[
"markdown"
],
[
"code"
]
] |
e768f9743567427ca96075ac0b60f269cea18993 | 10,998 | ipynb | Jupyter Notebook | doc/source/ray-core/examples/modin_xgboost/modin_xgboost.ipynb | richardsliu/ray | 0c27d925886e1fcfa0a22cb50715ac921091ea83 | [
"Apache-2.0"
] | 22 | 2018-05-08T05:52:34.000Z | 2020-04-01T10:09:55.000Z | doc/source/ray-core/examples/modin_xgboost/modin_xgboost.ipynb | richardsliu/ray | 0c27d925886e1fcfa0a22cb50715ac921091ea83 | [
"Apache-2.0"
] | 53 | 2021-10-06T20:08:04.000Z | 2022-03-21T20:17:25.000Z | doc/source/ray-core/examples/modin_xgboost/modin_xgboost.ipynb | richardsliu/ray | 0c27d925886e1fcfa0a22cb50715ac921091ea83 | [
"Apache-2.0"
] | 10 | 2018-04-27T10:50:59.000Z | 2020-02-24T02:41:43.000Z | 29.644205 | 100 | 0.569194 | [
[
[
"# XGBoost-Ray with Modin\n\nThis notebook includes an example workflow using\n[XGBoost-Ray](https://docs.ray.io/en/latest/xgboost-ray.html) and\n[Modin](https://modin.readthedocs.io/en/latest/) for distributed model\ntraining and prediction.\n\n## Cluster Setup\n\nFirst, we'll set up our Ray Cluster. The provided ``modin_xgboost.yaml``\ncluster config can be used to set up an AWS cluster with 64 CPUs.\n\nThe following steps assume you are in a directory with both\n``modin_xgboost.yaml`` and this file saved as ``modin_xgboost.ipynb``.\n\n**Step 1:** Bring up the Ray cluster.\n\n```bash\npip install ray boto3\nray up modin_xgboost.yaml\n```\n\n**Step 2:** Move ``modin_xgboost.ipynb`` to the cluster and start Jupyter.\n\n```bash\nray rsync_up modin_xgboost.yaml \"./modin_xgboost.ipynb\" \\\n \"~/modin_xgboost.ipynb\"\nray exec modin_xgboost.yaml --port-forward=9999 \"jupyter notebook \\\n --port=9999\"\n```\n\nYou can then access this notebook at the URL that is output:\n``http://localhost:9999/?token=<token>``\n\n## Python Setup\n\nFirst, we'll import all the libraries we'll be using. This step also helps us\nverify that the environment is configured correctly. If any of the imports\nare missing, an exception will be raised.",
"_____no_output_____"
]
],
[
[
"import argparse\nimport time\n\nimport modin.pandas as pd\nfrom modin.experimental.sklearn.model_selection import train_test_split\nfrom xgboost_ray import RayDMatrix, RayParams, train, predict\n\nimport ray",
"_____no_output_____"
]
],
[
[
"Next, let's parse some arguments. This will be used for executing the ``.py``\nfile, but not for the ``.ipynb``. If you are using the interactive notebook,\nyou can directly override the arguments manually.",
"_____no_output_____"
]
],
[
[
"parser = argparse.ArgumentParser()\nparser.add_argument(\n \"--address\", type=str, default=\"auto\", help=\"The address to use for Ray.\"\n)\nparser.add_argument(\n \"--smoke-test\",\n action=\"store_true\",\n help=\"Read a smaller dataset for quick testing purposes.\",\n)\nparser.add_argument(\n \"--num-actors\", type=int, default=4, help=\"Sets number of actors for training.\"\n)\nparser.add_argument(\n \"--cpus-per-actor\",\n type=int,\n default=8,\n help=\"The number of CPUs per actor for training.\",\n)\nparser.add_argument(\n \"--num-actors-inference\",\n type=int,\n default=16,\n help=\"Sets number of actors for inference.\",\n)\nparser.add_argument(\n \"--cpus-per-actor-inference\",\n type=int,\n default=2,\n help=\"The number of CPUs per actor for inference.\",\n)\n# Ignore -f from ipykernel_launcher\nargs, _ = parser.parse_known_args()",
"_____no_output_____"
]
],
[
[
" Override these arguments as needed:",
"_____no_output_____"
]
],
[
[
"address = args.address\nsmoke_test = args.smoke_test\nnum_actors = args.num_actors\ncpus_per_actor = args.cpus_per_actor\nnum_actors_inference = args.num_actors_inference\ncpus_per_actor_inference = args.cpus_per_actor_inference",
"_____no_output_____"
]
],
[
[
"## Connecting to the Ray cluster\n\nNow, let's connect our Python script to this newly deployed Ray cluster!",
"_____no_output_____"
]
],
[
[
"if not ray.is_initialized():\n ray.init(address=address)",
"_____no_output_____"
]
],
[
[
"## Data Preparation\n\nWe will use the [HIGGS dataset from the UCI Machine Learning dataset\nrepository](https://archive.ics.uci.edu/ml/datasets/HIGGS). The HIGGS\ndataset consists of 11,000,000 samples and 28 attributes, which is large\nenough size to show the benefits of distributed computation.",
"_____no_output_____"
]
],
[
[
"LABEL_COLUMN = \"label\"\nif smoke_test:\n # Test dataset with only 10,000 records.\n FILE_URL = \"https://ray-ci-higgs.s3.us-west-2.amazonaws.com/simpleHIGGS\" \".csv\"\nelse:\n # Full dataset. This may take a couple of minutes to load.\n FILE_URL = (\n \"https://archive.ics.uci.edu/ml/machine-learning-databases\"\n \"/00280/HIGGS.csv.gz\"\n )\n\ncolnames = [LABEL_COLUMN] + [\"feature-%02d\" % i for i in range(1, 29)]",
"_____no_output_____"
],
[
"load_data_start_time = time.time()\n\ndf = pd.read_csv(FILE_URL, names=colnames)\n\nload_data_end_time = time.time()\nload_data_duration = load_data_end_time - load_data_start_time\nprint(f\"Dataset loaded in {load_data_duration} seconds.\")",
"_____no_output_____"
]
],
[
[
"Split data into training and validation.",
"_____no_output_____"
]
],
[
[
"df_train, df_validation = train_test_split(df)\nprint(df_train, df_validation)",
"_____no_output_____"
]
],
[
[
"## Distributed Training\n\nThe ``train_xgboost`` function contains all the logic necessary for\ntraining using XGBoost-Ray.\n\nDistributed training can not only speed up the process, but also allow you\nto use datasets that are too large to fit in memory of a single node. With\ndistributed training, the dataset is sharded across different actors\nrunning on separate nodes. Those actors communicate with each other to\ncreate the final model.\n\nFirst, the dataframes are wrapped in ``RayDMatrix`` objects, which handle\ndata sharding across the cluster. Then, the ``train`` function is called.\nThe evaluation scores will be saved to ``evals_result`` dictionary. The\nfunction returns a tuple of the trained model (booster) and the evaluation\nscores.\n\nThe ``ray_params`` variable expects a ``RayParams`` object that contains\nRay-specific settings, such as the number of workers.",
"_____no_output_____"
]
],
[
[
"def train_xgboost(config, train_df, test_df, target_column, ray_params):\n train_set = RayDMatrix(train_df, target_column)\n test_set = RayDMatrix(test_df, target_column)\n\n evals_result = {}\n\n train_start_time = time.time()\n\n # Train the classifier\n bst = train(\n params=config,\n dtrain=train_set,\n evals=[(test_set, \"eval\")],\n evals_result=evals_result,\n verbose_eval=False,\n num_boost_round=100,\n ray_params=ray_params,\n )\n\n train_end_time = time.time()\n train_duration = train_end_time - train_start_time\n print(f\"Total time taken: {train_duration} seconds.\")\n\n model_path = \"model.xgb\"\n bst.save_model(model_path)\n print(\"Final validation error: {:.4f}\".format(evals_result[\"eval\"][\"error\"][-1]))\n\n return bst, evals_result",
"_____no_output_____"
]
],
[
[
"We can now pass our Modin dataframes and run the function. We will use\n``RayParams`` to specify that the number of actors and CPUs to train with.",
"_____no_output_____"
]
],
[
[
"# standard XGBoost config for classification\nconfig = {\n \"tree_method\": \"approx\",\n \"objective\": \"binary:logistic\",\n \"eval_metric\": [\"logloss\", \"error\"],\n}\n\nbst, evals_result = train_xgboost(\n config,\n df_train,\n df_validation,\n LABEL_COLUMN,\n RayParams(cpus_per_actor=cpus_per_actor, num_actors=num_actors),\n)\nprint(f\"Results: {evals_result}\")",
"_____no_output_____"
]
],
[
[
"## Prediction\n\nWith the model trained, we can now predict on unseen data. For the\npurposes of this example, we will use the same dataset for prediction as\nfor training.\n\nSince prediction is naively parallelizable, distributing it over multiple\nactors can measurably reduce the amount of time needed.",
"_____no_output_____"
]
],
[
[
"inference_df = RayDMatrix(df, ignore=[LABEL_COLUMN, \"partition\"])\nresults = predict(\n bst,\n inference_df,\n ray_params=RayParams(\n cpus_per_actor=cpus_per_actor_inference, num_actors=num_actors_inference\n ),\n)\n\nprint(results)",
"_____no_output_____"
]
]
] | [
"markdown",
"code",
"markdown",
"code",
"markdown",
"code",
"markdown",
"code",
"markdown",
"code",
"markdown",
"code",
"markdown",
"code",
"markdown",
"code",
"markdown",
"code"
] | [
[
"markdown"
],
[
"code"
],
[
"markdown"
],
[
"code"
],
[
"markdown"
],
[
"code"
],
[
"markdown"
],
[
"code"
],
[
"markdown"
],
[
"code",
"code"
],
[
"markdown"
],
[
"code"
],
[
"markdown"
],
[
"code"
],
[
"markdown"
],
[
"code"
],
[
"markdown"
],
[
"code"
]
] |
e7690074472ca0e2ad1e85e28050680c39c153a4 | 5,149 | ipynb | Jupyter Notebook | module_001_python/lesson_004_function/student_tasks/HomeWork.ipynb | VanyaTihonov/ML | baa1b2d7b16fe304f6ea5f513d44bbdedb65d8a5 | [
"Apache-2.0"
] | null | null | null | module_001_python/lesson_004_function/student_tasks/HomeWork.ipynb | VanyaTihonov/ML | baa1b2d7b16fe304f6ea5f513d44bbdedb65d8a5 | [
"Apache-2.0"
] | null | null | null | module_001_python/lesson_004_function/student_tasks/HomeWork.ipynb | VanyaTihonov/ML | baa1b2d7b16fe304f6ea5f513d44bbdedb65d8a5 | [
"Apache-2.0"
] | null | null | null | 28.447514 | 250 | 0.512332 | [
[
[
"# ะะฐะดะฐัะฐ 1\nะัะพะตะบัะธัะพะฒะฐะฝะธะต ััะฝะบัะธะน ะดะปั ะฟะพัััะพะตะฝะธั ะพะฑััะฐััะธั
ะผะพะดะตะปะตะน ะฟะพ ะดะฐะฝะฝัะผ. ะ ะดะฐะฝะฝะพะน ะทะฐะดะฐัะฐ ะฒะฐะผ ะฝัะถะฝะพ ัะฐะทัะฐะฑะพัะฐัั ะฟัะพัะพัะธะฟั ััะฝะบัะธะน(ะพะฑััะฒะปะตะฝะธะต ััะฝะบัะธะน ะฑะตะท ัะตะฐะปะธะทะฐัะธะน) ะดะปั ะทะฐะดะฐัะธ ะฐะฝะฐะปะธะทะฐ ะดะฐะฝะฝัั
ะธะท ะผะฐัะธะฝะฝะพะณะพ ะพะฑััะตะฝะธั, ะดะพะปะถะฝั ะฑััั ัััะตะฝั ัะปะตะดัััะธะต ัะฐะณะธ:\n* ะะฐะณััะทะบะฐ ะดะฐะฝะฝัั
ะธะท ะฒะฝะตัะฝะธั
ะธััะพัะฝะธะบะพะฒ\n* ะะฑัะฐะฑะพัะบะฐ ะฝะต ะทะฐะดะฐะฝะฝัั
ะทะฝะฐัะตะฝะธะน - ะฟัะพะฟััะบะพะฒ\n* ะฃะดะฐะปะตะฝะธะต ะฝะต ะธะฝัะพัะผะฐัะธะฒะฝัั
ะฟัะธะทะฝะฐะบะพะฒ ะธ ะพะฑัะตะบัะพะฒ\n* ะะพะปััะตะฝะธะต ะผะพะดะตะปะธ ะดะปั ะพะฑััะตะฝะธั\n* ะัะตะฝะบะฐ ะบะฐัะตััะฒะฐ ะผะพะดะตะปะธ\n* ะกะพั
ัะฐะฝะตะฝะธะต ะผะพะดะตะปะธ ะฒ ัะฐะนะป",
"_____no_output_____"
]
],
[
[
"def loading_dataframe(path,source=\"file\",type='csv'):\n \"\"\"\n ะคัะฝะบัะธั ะทะฐะณััะถะฐะตั ัะฐะนะป ะธะท ะฒะฝะตัะฝะธั
ะธััะพัะฝะธะบะพะฒ.\n ะะฐัะฐะผะตััั:\n path โ ะฟััั, ะธะท ะบะพัะพัะพะณะพ ะทะฐะณััะถะฐะตััั ะดะพะบัะผะตะฝั,\n source โ ัะธะฟ ะดะพะบัะผะตะฝัะฐ (file (ะฟะพ ัะผะพะปัะฐะฝะธั), http, https, ftp),\n type โ ัะฐััะธัะตะฝะธะต ะดะพะบัะผะตะฝัะฐ (txt,csv,xls).\n ะ ะตะทัะปััะฐั:\n load_data โ ัะฐะนะป.\n \"\"\"\n pass\n\ndef preparing_nones(dataframe,*columns,mode):\n \"\"\"\n ะคัะฝะบัะธั ะพะฑัะฐะฑะฐััะฒะฐะตั ะฝะตะทะฐะดะฐะฝะฝัะต ะทะฝะฐัะตะฝะธั (ะฟัะพะฟััะบะธ).\n ะะฐัะฐะผะตััั:\n dataframe โ ัะฐะนะป,\n columns โ ัะพ, ััะพ ะฝะฐะดะพ ะพะฑัะฐะฑะพัะฐัั,\n mode โ ัะพ, ััะพ ะฝัะถะฝะพ ัะดะตะปะฐัั ั ะฟัะพะฟััะบะฐะผะธ.\n ะ ะตะทัะปััะฐั:\n preparing_nones โ ัะฐะนะป ั ะพะฑัะฐะฑะพัะฐะฝะฝัะผะธ ะฟัะพะฟััะบะฐะผะธ.\n \"\"\"\n pass\ndef moving_dataframe(dataframe):\n \"\"\"ะคัะฝะบัะธั ะดะปั ัะดะฐะปะตะฝะธั ะฝะตะธะฝัะพัะผะฐัะธะฒะฝัั
ะฟัะธะทะฝะฐะบะพะฒ.\n ะะฐัะฐะผะตัั:\n dataframe โ ะพะฑัะตะบั, ะบะพัะพััะน ะฝัะถะฝะพ ัะดะฐะปะธัั.\n ะ ะตะทัะปััะฐั:\n moving_nones โ ัะดะฐะปะตะฝะธะต ัะฐะนะปะฐ\"\"\"\n pass\ndef constructing_model(dataframe, model_name, **params):\n \"\"\"\n ะคัะฝะบัะธั ะดะปั ะฟะพัััะพะตะฝะธั ะผะพะดะตะปะธ ะดะปั ะพะฑััะตะฝะธั.\n ะะฐัะฐะผะตััั:\n dataframe(dataframe) - ะธัั
ะพะดะฝัะน dataframe,\n model_name(str) - ะฝะฐะทะฒะฐะฝะธะต ะผะพะดะตะปะธ: xgboost, random_forest, sequential,\n params(dictionary) - ะฟะฐัะฐะผะตััั ะผะพะดะตะปะธ.\n ะ ะตะทัะปััะฐั:\n model ะฝะฐะด ะดะฐะฝะฝัะผะธ.\n \"\"\"\n pass\ndef scoring_model(model):\n \"\"\"\n ะคัะฝะบัะธั ะดะปั ะพัะตะฝะบะธ ะบะฐัะตััะฒะฐ ะผะพะดะตะปะธ.\n ะะฐัะฐะผะตัั:\n model.\n ะ ะตะทัะปััะฐั:\n ะัะตะฝะบะฐ ะผะพะดะตะปะธ\n \"\"\"\n pass\ndef saving_model(model,file):\n \"\"\"\n ะคัะฝะบัะธั ะดะปั ัะพั
ัะฐะฝะตะฝะธั ะผะพะดะตะปะธ ะฒ ัะฐะนะป.\n ะะฐัะฐะผะตััั:\n model โ ะฝะฐะทะฒะฐะฝะธะต ะผะพะดะตะปะธ,\n file โ ะฝะฐะทะฒะฐะฝะธะต ัะฐะนะปะฐ\n \"\"\"\n pass",
"_____no_output_____"
]
],
[
[
"# ะะฐะดะฐัะฐ 2\nะะฐะดะฐัะฐ ะฟะพะฒััะตะฝะฝะพะน ัะปะพะถะฝะพััะธ. ะ ะตะฐะปะธะทะพะฒะฐัั ะฒัะฒะพะด ััะตัะณะพะปัะฝะธะบะฐ ะฟะฐัะบะฐะปั, ัะตัะตะท ััะฝะบัะธั. ะัะธะผะตั ััะตัะณะพะปัะฝะธะบะฐ:\n\nะะปัะฑะธะฝะฐ 10 ะฟะพ ัะผะพะปัะฐะฝะธั",
"_____no_output_____"
]
],
[
[
"def print_pascal(primary,deep=10):\n for i in range(1,deep+1):\n print(pascal(primary,i))\ndef pascal(primary,deep):\n if deep == 1:\n new_list = [primary]\n elif deep == 2:\n new_list = []\n for i in range (deep):\n new_list.extend(pascal(primary,1))\n else:\n new_list = []\n for i in range(0,deep):\n if i == 0 or i == deep-1:\n new_list.append(primary)\n else:\n new_list.append(pascal(primary,deep-1)[i-1]+pascal(primary,deep-1)[i])\n return new_list\nprint_pascal(1)",
"[1]\n[1, 1]\n[1, 2, 1]\n[1, 3, 3, 1]\n[1, 4, 6, 4, 1]\n[1, 5, 10, 10, 5, 1]\n[1, 6, 15, 20, 15, 6, 1]\n[1, 7, 21, 35, 35, 21, 7, 1]\n[1, 8, 28, 56, 70, 56, 28, 8, 1]\n[1, 9, 36, 84, 126, 126, 84, 36, 9, 1]\n"
]
]
] | [
"markdown",
"code",
"markdown",
"code"
] | [
[
"markdown"
],
[
"code"
],
[
"markdown"
],
[
"code"
]
] |
e7690c5de18e88c49bfd2730a405aeac57e475a8 | 441,605 | ipynb | Jupyter Notebook | vietocr_gettingstart.ipynb | uMetalooper/vietocr | da2a2445c666f885c034a6983675974eff0b7ff4 | [
"Apache-2.0"
] | null | null | null | vietocr_gettingstart.ipynb | uMetalooper/vietocr | da2a2445c666f885c034a6983675974eff0b7ff4 | [
"Apache-2.0"
] | null | null | null | vietocr_gettingstart.ipynb | uMetalooper/vietocr | da2a2445c666f885c034a6983675974eff0b7ff4 | [
"Apache-2.0"
] | 1 | 2022-03-14T10:48:04.000Z | 2022-03-14T10:48:04.000Z | 362.565681 | 48,946 | 0.923583 | [
[
[
"<a href=\"https://colab.research.google.com/github/pbcquoc/vietocr/blob/master/vietocr_gettingstart.ipynb\" target=\"_parent\"><img src=\"https://colab.research.google.com/assets/colab-badge.svg\" alt=\"Open In Colab\"/></a>",
"_____no_output_____"
],
[
"\n# Introduction\n<p align=\"center\">\n<img src=\"https://raw.githubusercontent.com/pbcquoc/vietocr/master/image/vietocr.jpg\" width=\"512\" height=\"512\">\n</p>\nThis notebook describe how you can use VietOcr to train OCR model\n\n\n",
"_____no_output_____"
]
],
[
[
"! pip install --quiet vietocr",
"\u001b[?25l\r\u001b[K |โโโโโโ | 10kB 26.4MB/s eta 0:00:01\r\u001b[K |โโโโโโโโโโโ | 20kB 1.7MB/s eta 0:00:01\r\u001b[K |โโโโโโโโโโโโโโโโโ | 30kB 2.3MB/s eta 0:00:01\r\u001b[K |โโโโโโโโโโโโโโโโโโโโโโโ | 40kB 2.5MB/s eta 0:00:01\r\u001b[K |โโโโโโโโโโโโโโโโโโโโโโโโโโโโ | 51kB 2.0MB/s eta 0:00:01\r\u001b[K |โโโโโโโโโโโโโโโโโโโโโโโโโโโโโโโโ| 61kB 1.8MB/s \n\u001b[?25h Installing build dependencies ... \u001b[?25l\u001b[?25hdone\n Getting requirements to build wheel ... \u001b[?25l\u001b[?25hdone\n Preparing wheel metadata ... \u001b[?25l\u001b[?25hdone\n\u001b[K |โโโโโโโโโโโโโโโโโโโโโโโโโโโโโโโโ| 880kB 7.2MB/s \n\u001b[K |โโโโโโโโโโโโโโโโโโโโโโโโโโโโโโโโ| 952kB 17.0MB/s \n\u001b[?25h Building wheel for gdown (PEP 517) ... \u001b[?25l\u001b[?25hdone\n Building wheel for lmdb (setup.py) ... \u001b[?25l\u001b[?25hdone\n\u001b[31mERROR: albumentations 0.1.12 has requirement imgaug<0.2.7,>=0.2.5, but you'll have imgaug 0.4.0 which is incompatible.\u001b[0m\n"
]
],
[
[
"# Inference",
"_____no_output_____"
]
],
[
[
"import matplotlib.pyplot as plt\nfrom PIL import Image\n\nfrom vietocr.tool.predictor import Predictor\nfrom vietocr.tool.config import Cfg",
"_____no_output_____"
],
[
"config = Cfg.load_config_from_name('vgg_transformer')",
"_____no_output_____"
]
],
[
[
"Change weights to your weights or using default weights from our pretrained model. Path can be url or local file",
"_____no_output_____"
]
],
[
[
"# config['weights'] = './weights/transformerocr.pth'\nconfig['weights'] = 'https://drive.google.com/uc?id=13327Y1tz1ohsm5YZMyXVMPIOjoOA0OaA'\nconfig['cnn']['pretrained']=False\nconfig['device'] = 'cuda:0'\nconfig['predictor']['beamsearch']=False",
"_____no_output_____"
],
[
"detector = Predictor(config)",
"Cached Downloading: /root/.cache/gdown/https-COLON--SLASH--SLASH-drive.google.com-SLASH-uc-QUESTION-id-EQUAL-13327Y1tz1ohsm5YZMyXVMPIOjoOA0OaA\nDownloading...\nFrom: https://drive.google.com/uc?id=13327Y1tz1ohsm5YZMyXVMPIOjoOA0OaA\nTo: /root/.cache/gdown/tmpdw3empzl/dl\n152MB [00:00, 187MB/s]\n"
],
[
"! gdown --id 1uMVd6EBjY4Q0G2IkU5iMOQ34X0bysm0b\n! unzip -qq -o sample.zip",
"Downloading...\nFrom: https://drive.google.com/uc?id=1uMVd6EBjY4Q0G2IkU5iMOQ34X0bysm0b\nTo: /content/sample.zip\n\r 0% 0.00/306k [00:00<?, ?B/s]\r100% 306k/306k [00:00<00:00, 85.7MB/s]\n"
],
[
"! ls sample | shuf |head -n 5",
"97652.jpg\n037188000873.jpeg\n30036.jpg\n461_PIGTAIL_57575.jpg\n030068003051.jpeg\n"
],
[
"img = './sample/031189003299.jpeg'\nimg = Image.open(img)\nplt.imshow(img)\ns = detector.predict(img)\ns",
"_____no_output_____"
]
],
[
[
"# Download sample dataset",
"_____no_output_____"
]
],
[
[
"! gdown https://drive.google.com/uc?id=19QU4VnKtgm3gf0Uw_N2QKSquW1SQ5JiE",
"Downloading...\nFrom: https://drive.google.com/uc?id=19QU4VnKtgm3gf0Uw_N2QKSquW1SQ5JiE\nTo: /content/data_line.zip\n61.2MB [00:00, 67.2MB/s]\n"
],
[
"! unzip -qq -o ./data_line.zip",
"_____no_output_____"
]
],
[
[
"# Train model",
"_____no_output_____"
],
[
"\n\n1. Load your config\n2. Train model using your dataset above\n\n",
"_____no_output_____"
],
[
"Load the default config, we adopt VGG for image feature extraction",
"_____no_output_____"
]
],
[
[
"from vietocr.tool.config import Cfg\nfrom vietocr.model.trainer import Trainer",
"_____no_output_____"
]
],
[
[
"# Change the config \n\n* *data_root*: the folder save your all images\n* *train_annotation*: path to train annotation\n* *valid_annotation*: path to valid annotation\n* *print_every*: show train loss at every n steps\n* *valid_every*: show validation loss at every n steps\n* *iters*: number of iteration to train your model\n* *export*: export weights to folder that you can use for inference\n* *metrics*: number of sample in validation annotation you use for computing full_sequence_accuracy, for large dataset it will take too long, then you can reuduce this number\n",
"_____no_output_____"
]
],
[
[
"config = Cfg.load_config_from_name('vgg_transformer')",
"_____no_output_____"
],
[
"#config['vocab'] = 'aAร รแบฃแบขรฃรรกรแบกแบ ฤฤแบฑแบฐแบณแบฒแบตแบดแบฏแบฎแบทแบถรขรแบงแบฆแบฉแบจแบซแบชแบฅแบคแบญแบฌbBcCdDฤฤeEรจรแบปแบบแบฝแบผรฉรแบนแบธรชรแปแปแปแปแป
แปแบฟแบพแปแปfFgGhHiIรฌรแปแปฤฉฤจรญรแปแปjJkKlLmMnNoOรฒรแปแปรตรรณรแปแปรดรแปแปแปแปแปแปแปแปแปแปฦกฦ แปแปแปแปแปกแป แปแปแปฃแปขpPqQrRsStTuUรนรแปงแปฆลฉลจรบรแปฅแปคฦฐฦฏแปซแปชแปญแปฌแปฏแปฎแปฉแปจแปฑแปฐvVwWxXyYแปณแปฒแปทแปถแปนแปธรฝรแปตแปดzZ0123456789!\"#$%&\\'()*+,-./:;<=>?@[\\\\]^_`{|}~ '\n\ndataset_params = {\n 'name':'hw',\n 'data_root':'./data_line/',\n 'train_annotation':'train_line_annotation.txt',\n 'valid_annotation':'test_line_annotation.txt'\n}\n\nparams = {\n 'print_every':200,\n 'valid_every':15*200,\n 'iters':20000,\n 'checkpoint':'./checkpoint/transformerocr_checkpoint.pth', \n 'export':'./weights/transformerocr.pth',\n 'metrics': 10000\n }\n\nconfig['trainer'].update(params)\nconfig['dataset'].update(dataset_params)\nconfig['device'] = 'cuda:0'",
"_____no_output_____"
]
],
[
[
"you can change any of these params in this full list below",
"_____no_output_____"
]
],
[
[
"config",
"_____no_output_____"
]
],
[
[
"You should train model from our pretrained ",
"_____no_output_____"
]
],
[
[
"trainer = Trainer(config, pretrained=True)",
"Downloading: \"https://download.pytorch.org/models/vgg19_bn-c79401a0.pth\" to /root/.cache/torch/hub/checkpoints/vgg19_bn-c79401a0.pth\n"
]
],
[
[
"Save model configuration for inference, load_config_from_file",
"_____no_output_____"
]
],
[
[
"trainer.config.save('config.yml')",
"_____no_output_____"
]
],
[
[
"Visualize your dataset to check data augmentation is appropriate",
"_____no_output_____"
]
],
[
[
"trainer.visualize_dataset()",
"_____no_output_____"
]
],
[
[
"Train now",
"_____no_output_____"
]
],
[
[
"trainer.train()",
"iter: 000200 - train loss: 1.657 - lr: 1.91e-05 - load time: 1.08 - gpu time: 158.33\niter: 000400 - train loss: 1.429 - lr: 3.95e-05 - load time: 0.76 - gpu time: 158.76\niter: 000600 - train loss: 1.331 - lr: 7.14e-05 - load time: 0.73 - gpu time: 158.38\niter: 000800 - train loss: 1.252 - lr: 1.12e-04 - load time: 1.29 - gpu time: 158.43\niter: 001000 - train loss: 1.218 - lr: 1.56e-04 - load time: 0.84 - gpu time: 158.86\niter: 001200 - train loss: 1.192 - lr: 2.01e-04 - load time: 0.78 - gpu time: 160.20\niter: 001400 - train loss: 1.140 - lr: 2.41e-04 - load time: 1.54 - gpu time: 158.48\niter: 001600 - train loss: 1.129 - lr: 2.73e-04 - load time: 0.70 - gpu time: 159.42\niter: 001800 - train loss: 1.095 - lr: 2.93e-04 - load time: 0.74 - gpu time: 158.03\niter: 002000 - train loss: 1.098 - lr: 3.00e-04 - load time: 0.66 - gpu time: 159.21\niter: 002200 - train loss: 1.060 - lr: 3.00e-04 - load time: 1.52 - gpu time: 157.63\niter: 002400 - train loss: 1.055 - lr: 3.00e-04 - load time: 0.80 - gpu time: 159.34\niter: 002600 - train loss: 1.032 - lr: 2.99e-04 - load time: 0.74 - gpu time: 159.13\niter: 002800 - train loss: 1.019 - lr: 2.99e-04 - load time: 1.42 - gpu time: 158.27\n"
]
],
[
[
"Visualize prediction from our trained model\n",
"_____no_output_____"
]
],
[
[
"trainer.visualize_prediction()",
"_____no_output_____"
]
],
[
[
"Compute full seq accuracy for full valid dataset",
"_____no_output_____"
]
],
[
[
"trainer.precision()",
"_____no_output_____"
]
]
] | [
"markdown",
"code",
"markdown",
"code",
"markdown",
"code",
"markdown",
"code",
"markdown",
"code",
"markdown",
"code",
"markdown",
"code",
"markdown",
"code",
"markdown",
"code",
"markdown",
"code",
"markdown",
"code",
"markdown",
"code",
"markdown",
"code"
] | [
[
"markdown",
"markdown"
],
[
"code"
],
[
"markdown"
],
[
"code",
"code"
],
[
"markdown"
],
[
"code",
"code",
"code",
"code",
"code"
],
[
"markdown"
],
[
"code",
"code"
],
[
"markdown",
"markdown",
"markdown"
],
[
"code"
],
[
"markdown"
],
[
"code",
"code"
],
[
"markdown"
],
[
"code"
],
[
"markdown"
],
[
"code"
],
[
"markdown"
],
[
"code"
],
[
"markdown"
],
[
"code"
],
[
"markdown"
],
[
"code"
],
[
"markdown"
],
[
"code"
],
[
"markdown"
],
[
"code"
]
] |
e769181a78b4e6820e0520a085738386b3ae5473 | 26,611 | ipynb | Jupyter Notebook | HeroesOfPymoli/.ipynb_checkpoints/HeroesOfPymoli_Example-checkpoint.ipynb | dimpalsuthar91/RePanda | c10ccc5f3a704f017dd4452183fbfabcdc90356b | [
"ADSL"
] | null | null | null | HeroesOfPymoli/.ipynb_checkpoints/HeroesOfPymoli_Example-checkpoint.ipynb | dimpalsuthar91/RePanda | c10ccc5f3a704f017dd4452183fbfabcdc90356b | [
"ADSL"
] | null | null | null | HeroesOfPymoli/.ipynb_checkpoints/HeroesOfPymoli_Example-checkpoint.ipynb | dimpalsuthar91/RePanda | c10ccc5f3a704f017dd4452183fbfabcdc90356b | [
"ADSL"
] | null | null | null | 28.675647 | 191 | 0.327083 | [
[
[
"# Heroes Of Pymoli Data Analysis\n* Of the 1163 active players, the vast majority are male (82%). There also exists, a smaller, but notable proportion of female players (16%).\n\n* Our peak age demographic falls between 20-24 (42%) with secondary groups falling between 15-19 (17.80%) and 25-29 (15.48%).\n\n* Our players are putting in significant cash during the lifetime of their gameplay. Across all major age and gender demographics, the average purchase for a user is roughly $491. \n-----",
"_____no_output_____"
]
],
[
[
"import pandas as pd\nimport numpy as np",
"_____no_output_____"
]
],
[
[
"## Player Count",
"_____no_output_____"
],
[
"## Purchasing Analysis (Total)",
"_____no_output_____"
],
[
"## Gender Demographics",
"_____no_output_____"
],
[
"\n## Purchasing Analysis (Gender)",
"_____no_output_____"
],
[
"## Age Demographics",
"_____no_output_____"
],
[
"## Purchasing Analysis (Age)",
"_____no_output_____"
],
[
"## Top Spenders",
"_____no_output_____"
],
[
"## Most Popular Items",
"_____no_output_____"
],
[
"## Most Profitable Items",
"_____no_output_____"
]
]
] | [
"markdown",
"code",
"markdown"
] | [
[
"markdown"
],
[
"code"
],
[
"markdown",
"markdown",
"markdown",
"markdown",
"markdown",
"markdown",
"markdown",
"markdown",
"markdown"
]
] |
e769255bc7d8921f44323739104c8e6967bed19f | 21,749 | ipynb | Jupyter Notebook | tutorial/tutorial05_metadata_preprocessing.ipynb | milidris/melusine | 6f976bf44cf895b3de6e3a4b581d97544c1b281f | [
"Apache-2.0"
] | 1 | 2020-02-11T23:00:35.000Z | 2020-02-11T23:00:35.000Z | tutorial/tutorial05_metadata_preprocessing.ipynb | milidris/melusine | 6f976bf44cf895b3de6e3a4b581d97544c1b281f | [
"Apache-2.0"
] | null | null | null | tutorial/tutorial05_metadata_preprocessing.ipynb | milidris/melusine | 6f976bf44cf895b3de6e3a4b581d97544c1b281f | [
"Apache-2.0"
] | null | null | null | 28.998667 | 160 | 0.422364 | [
[
[
"# Metadata preprocessing tutorial",
"_____no_output_____"
],
[
"Melusine **prepare_data.metadata_engineering subpackage** provides classes to preprocess the metadata :\n- **MetaExtension :** a transformer which creates an 'extension' feature extracted from regex in metadata. It extracts the extensions of mail adresses.\n- **MetaDate :** a transformer which creates new features from dates such as: hour, minute, dayofweek.\n- **Dummifier :** a transformer to dummifies categorial features.\n\nAll the classes have **fit_transform** methods.",
"_____no_output_____"
],
[
"### Input dataframe",
"_____no_output_____"
],
[
"- To use a **MetaExtension** transformer : the dataframe requires a **from** column\n- To use a **MetaDate** transformer : the dataframe requires a **date** column",
"_____no_output_____"
]
],
[
[
"from melusine.data.data_loader import load_email_data\n\ndf_emails = load_email_data()\ndf_emails = df_emails[['from','date']]",
"_____no_output_____"
],
[
"df_emails['from']",
"_____no_output_____"
],
[
"df_emails['date']",
"_____no_output_____"
]
],
[
[
"### MetaExtension transformer",
"_____no_output_____"
],
[
"A **MetaExtension transformer** creates an *extension* feature extracted from regex in metadata. It extracts the extensions of mail adresses.",
"_____no_output_____"
]
],
[
[
"from melusine.prepare_email.metadata_engineering import MetaExtension\n\nmeta_extension = MetaExtension()",
"_____no_output_____"
],
[
"df_emails = meta_extension.fit_transform(df_emails)",
"_____no_output_____"
],
[
"df_emails.extension",
"_____no_output_____"
]
],
[
[
"### MetaExtension transformer",
"_____no_output_____"
],
[
"A **MetaDate transformer** creates new features from dates : **hour**, **minute** and **dayofweek**.",
"_____no_output_____"
]
],
[
[
"from melusine.prepare_email.metadata_engineering import MetaDate\n\nmeta_date = MetaDate()",
"_____no_output_____"
],
[
"df_emails = meta_date.fit_transform(df_emails)",
"_____no_output_____"
],
[
"df_emails.date[0]",
"_____no_output_____"
],
[
"df_emails.hour[0]",
"_____no_output_____"
],
[
"df_emails.loc[0,'min']",
"_____no_output_____"
],
[
"df_emails.dayofweek[0]",
"_____no_output_____"
]
],
[
[
"### Dummifier transformer",
"_____no_output_____"
],
[
"A **Dummifier transformer** dummifies categorial features.\n\nIts arguments are :\n- **columns_to_dummify** : a list of the metadata columns to dummify.",
"_____no_output_____"
]
],
[
[
"from melusine.prepare_email.metadata_engineering import Dummifier\n\ndummifier = Dummifier(columns_to_dummify=['extension', 'dayofweek', 'hour', 'min'])",
"_____no_output_____"
],
[
"df_meta = dummifier.fit_transform(df_emails)",
"_____no_output_____"
],
[
"df_meta.columns",
"_____no_output_____"
],
[
"df_meta.head()",
"_____no_output_____"
]
],
[
[
"### Custom metadata transformer",
"_____no_output_____"
],
[
"A custom transformer can be implemented to extract metadata from a column :",
"_____no_output_____"
],
[
"```python\nfrom sklearn.base import BaseEstimator, TransformerMixin\n\nclass MetaDataCustom(BaseEstimator, TransformerMixin):\n \"\"\"Transformer which creates custom matadata\n\n Compatible with scikit-learn API.\n \"\"\"\n\n def __init__(self):\n \"\"\"\n arguments\n \"\"\"\n\n def fit(self, X, y=None):\n \"\"\" Fit method\"\"\"\n return self\n\n def transform(self, X):\n \"\"\"Transform method\"\"\"\n X['custom_metadata'] = X['column'].apply(self.get_metadata)\n return X\n```",
"_____no_output_____"
],
[
"The name of the output column can then be given as argument to a Dummifier transformer :",
"_____no_output_____"
],
[
"```python\ndummifier = Dummifier(columns_to_dummify=['custom_metadata'])\n```",
"_____no_output_____"
]
]
] | [
"markdown",
"code",
"markdown",
"code",
"markdown",
"code",
"markdown",
"code",
"markdown"
] | [
[
"markdown",
"markdown",
"markdown",
"markdown"
],
[
"code",
"code",
"code"
],
[
"markdown",
"markdown"
],
[
"code",
"code",
"code"
],
[
"markdown",
"markdown"
],
[
"code",
"code",
"code",
"code",
"code",
"code"
],
[
"markdown",
"markdown"
],
[
"code",
"code",
"code",
"code"
],
[
"markdown",
"markdown",
"markdown",
"markdown",
"markdown"
]
] |
e7693351428bd7de423462393b7a6ada308005fd | 6,746 | ipynb | Jupyter Notebook | Haumea Data/Read Data from Haumea Data.ipynb | dallinspencer/MultiMoon_Files | af9b24eb190985665221d58fd055f715b215767e | [
"MIT"
] | null | null | null | Haumea Data/Read Data from Haumea Data.ipynb | dallinspencer/MultiMoon_Files | af9b24eb190985665221d58fd055f715b215767e | [
"MIT"
] | null | null | null | Haumea Data/Read Data from Haumea Data.ipynb | dallinspencer/MultiMoon_Files | af9b24eb190985665221d58fd055f715b215767e | [
"MIT"
] | null | null | null | 43.24359 | 79 | 0.572487 | [
[
[
"import pandas as pd\nimport numpy as np\nfrom astropy.time import Time\nfrom astroquery.jplhorizons import Horizons\nfrom astropy import units as u\n\nHiaka2016 = pd.read_csv('haumea_s1hst_2016.txt')\nNamaka2016 = pd.read_csv('haumea_s2hst_2016.txt')\n\nparamsHiaka = pd.DataFrame()\nparamsNamaka = pd.DataFrame()\n\ndateH = Hiaka2016['time']\ndateN = Namaka2016['time']\ndateListH = []\ndateListN = []\nfor i in dateH:\n jd = Time(i,format='jd')\n dateListH.append(jd)\n\nfor i in dateN:\n jd = Time(i,format='jd')\n dateListN.append(jd)\n\nHaumea1 = Horizons(id='Haumea',location=None,epochs = dateListH)\nHaumea2 = Horizons(id='Haumea',location=None,epochs = dateListN)\nhRA1 = Haumea1.ephemerides()['RA']\nhDEC1 = Haumea1.ephemerides()['DEC']\nhRA2 = Haumea2.ephemerides()['RA']\nhDEC2 = Haumea2.ephemerides()['DEC']\n\ndeltaRAH = Hiaka2016['x']\ndeltaDECH = Hiaka2016['y']\ndeltaRAN = Namaka2016['x']\ndeltaDECN = Namaka2016['y']\n\nparamsHiaka['Date'] = Hiaka2016['time']\nparamsHiaka['RA-Primary'] = hRA1\nparamsHiaka['DEC-Primary'] = hDEC1\n\nparamsNamaka['Date'] = Namaka2016['time']\nparamsNamaka['RA-Primary'] = hRA2\nparamsNamaka['DEC-Primary'] = hDEC2\n\nparamsHiaka['RA-Hiaka'] = deltaRAH/np.cos(hDEC1*np.pi/180)/3600+hRA1\nparamsHiaka['DEC-Hiaka'] = deltaDECH/3600+hDEC1\n\nparamsNamaka['RA-Namaka'] = deltaRAN/np.cos(hDEC2*np.pi/180)/3600+hRA2\nparamsNamaka['DEC-Namaka'] = deltaDECN/3600+hDEC2\n\n\nprint(paramsHiaka,'\\n')\nprint(paramsNamaka)",
" Date RA-Primary DEC-Primary RA-Hiaka DEC-Hiaka\n0 2453746.525 203.05282 19.39354 203.052759 19.393623\n1 2453746.554 203.05294 19.39378 203.052879 19.393865\n2 2454138.287 203.95697 19.40732 203.956908 19.407381\n3 2454138.304 203.95689 19.40750 203.956828 19.407562\n4 2454138.351 203.95668 19.40800 203.956617 19.408064\n5 2454138.368 203.95660 19.40818 203.956537 19.408245\n6 2454138.418 203.95637 19.40871 203.956306 19.408777\n7 2454138.435 203.95629 19.40889 203.956227 19.408958\n8 2454138.484 203.95607 19.40941 203.956006 19.409480\n9 2454138.501 203.95599 19.40959 203.955926 19.409661\n10 2454138.551 203.95576 19.41012 203.955695 19.410193\n11 2454138.567 203.95569 19.41029 203.955625 19.410364\n12 2454469.653 204.82324 18.85057 204.823310 18.850216\n13 2454593.726 203.66619 19.88401 203.666136 19.884313\n14 2454600.192 203.56909 19.89088 203.569122 19.890927\n15 2454601.990 203.54321 19.89134 203.543264 19.891304\n16 2454603.788 203.51787 19.89118 203.517944 19.891058\n17 2454605.788 203.49034 19.89027 203.490428 19.890061\n18 2455375.661 204.95350 19.23793 204.953421 19.238270\n19 2455375.727 204.95322 19.23744 204.953142 19.237780\n20 2455375.793 204.95295 19.23695 204.952872 19.237289\n21 2455375.859 204.95267 19.23647 204.952593 19.236808\n22 2455375.928 204.95239 19.23596 204.952314 19.236298\n23 2455375.993 204.95212 19.23548 204.952044 19.235818\n24 2455376.058 204.95185 19.23499 204.951775 19.235327\n25 2457155.338 210.01677 18.06191 210.016900 18.061716\n26 2457203.995 209.47326 17.91575 209.473383 17.915574 \n\n Date RA-Primary DEC-Primary RA-Namaka DEC-Namaka\n0 2453746.525 203.05282 19.39354 203.052832 19.393488\n1 2453746.554 203.05294 19.39378 203.052951 19.393727\n2 2454138.287 203.95697 19.40732 203.956962 19.407162\n3 2454138.304 203.95689 19.40750 203.956881 19.407343\n4 2454138.351 203.95668 19.40800 203.956671 19.407845\n5 2454138.368 203.95660 19.40818 203.956591 19.408024\n6 2454138.418 203.95637 19.40871 203.956361 19.408558\n7 2454138.435 203.95629 19.40889 203.956282 19.408738\n8 2454138.484 203.95607 19.40941 203.956061 19.409262\n9 2454138.501 203.95599 19.40959 203.955980 19.409441\n10 2454138.551 203.95576 19.41012 203.955750 19.409973\n11 2454138.567 203.95569 19.41029 203.955679 19.410144\n12 2454469.653 204.82324 18.85057 204.823233 18.850491\n13 2454593.726 203.66619 19.88401 203.666191 19.883799\n14 2454600.192 203.56909 19.89088 203.569083 19.890935\n15 2454601.990 203.54321 19.89134 203.543203 19.891479\n16 2454603.788 203.51787 19.89118 203.517867 19.891346\n17 2454605.788 203.49034 19.89027 203.490340 19.890353\n18 2455375.655 204.95352 19.23797 204.953518 19.238025\n19 2455375.673 204.95345 19.23784 204.953448 19.237892\n20 2455375.719 204.95325 19.23750 204.953248 19.237551\n21 2455375.737 204.95318 19.23737 204.953178 19.237420\n22 2455375.786 204.95297 19.23701 204.952967 19.237055\n23 2457155.338 210.01677 18.06191 210.016799 18.061783\n24 2457203.995 209.47326 17.91575 209.473216 17.915943\n"
]
]
] | [
"code"
] | [
[
"code"
]
] |
e76936ae5012580b29cb5d1542ab625489d294fa | 64,902 | ipynb | Jupyter Notebook | deep_learning/seq2seq/2_torch_seq2seq_attention.ipynb | certara-ShengnanHuang/machine-learning | d21dfbeabf2876ffe49fcef444ca4516c4d36df0 | [
"MIT"
] | 2,104 | 2016-04-15T13:35:55.000Z | 2022-03-28T10:39:51.000Z | deep_learning/seq2seq/2_torch_seq2seq_attention.ipynb | certara-ShengnanHuang/machine-learning | d21dfbeabf2876ffe49fcef444ca4516c4d36df0 | [
"MIT"
] | 10 | 2017-04-07T14:25:23.000Z | 2021-05-18T03:16:15.000Z | deep_learning/seq2seq/2_torch_seq2seq_attention.ipynb | certara-ShengnanHuang/machine-learning | d21dfbeabf2876ffe49fcef444ca4516c4d36df0 | [
"MIT"
] | 539 | 2015-12-10T04:23:44.000Z | 2022-03-31T07:15:28.000Z | 32.778788 | 1,868 | 0.53607 | [
[
[
"<h1>Table of Contents<span class=\"tocSkip\"></span></h1>\n<div class=\"toc\"><ul class=\"toc-item\"><li><span><a href=\"#Seq2Seq-With-Attention\" data-toc-modified-id=\"Seq2Seq-With-Attention-1\"><span class=\"toc-item-num\">1 </span>Seq2Seq With Attention</a></span><ul class=\"toc-item\"><li><span><a href=\"#Data-Preparation\" data-toc-modified-id=\"Data-Preparation-1.1\"><span class=\"toc-item-num\">1.1 </span>Data Preparation</a></span></li><li><span><a href=\"#Model-Implementation\" data-toc-modified-id=\"Model-Implementation-1.2\"><span class=\"toc-item-num\">1.2 </span>Model Implementation</a></span><ul class=\"toc-item\"><li><span><a href=\"#Encoder\" data-toc-modified-id=\"Encoder-1.2.1\"><span class=\"toc-item-num\">1.2.1 </span>Encoder</a></span></li><li><span><a href=\"#Attention\" data-toc-modified-id=\"Attention-1.2.2\"><span class=\"toc-item-num\">1.2.2 </span>Attention</a></span></li><li><span><a href=\"#Decoder\" data-toc-modified-id=\"Decoder-1.2.3\"><span class=\"toc-item-num\">1.2.3 </span>Decoder</a></span></li><li><span><a href=\"#Seq2Seq\" data-toc-modified-id=\"Seq2Seq-1.2.4\"><span class=\"toc-item-num\">1.2.4 </span>Seq2Seq</a></span></li></ul></li><li><span><a href=\"#Training-Seq2Seq\" data-toc-modified-id=\"Training-Seq2Seq-1.3\"><span class=\"toc-item-num\">1.3 </span>Training Seq2Seq</a></span></li><li><span><a href=\"#Evaluating-Seq2Seq\" data-toc-modified-id=\"Evaluating-Seq2Seq-1.4\"><span class=\"toc-item-num\">1.4 </span>Evaluating Seq2Seq</a></span></li><li><span><a href=\"#Summary\" data-toc-modified-id=\"Summary-1.5\"><span class=\"toc-item-num\">1.5 </span>Summary</a></span></li></ul></li><li><span><a href=\"#Reference\" data-toc-modified-id=\"Reference-2\"><span class=\"toc-item-num\">2 </span>Reference</a></span></li></ul></div>",
"_____no_output_____"
]
],
[
[
"# code for loading the format for the notebook\nimport os\n\n# path : store the current path to convert back to it later\npath = os.getcwd()\nos.chdir(os.path.join('..', '..', 'notebook_format'))\n\nfrom formats import load_style\nload_style(css_style='custom2.css', plot_style=False)",
"_____no_output_____"
],
[
"os.chdir(path)\n\n# 1. magic for inline plot\n# 2. magic to print version\n# 3. magic so that the notebook will reload external python modules\n# 4. magic to enable retina (high resolution) plots\n# https://gist.github.com/minrk/3301035\n%matplotlib inline\n%load_ext watermark\n%load_ext autoreload\n%autoreload 2\n%config InlineBackend.figure_format='retina'\n\nimport os\nimport math\nimport time\nimport spacy\nimport random\nimport torch\nimport numpy as np\nimport torch.nn as nn\nimport torch.optim as optim\nfrom torchtext.datasets import Multi30k\nfrom torchtext.data import Field, BucketIterator\n\n%watermark -a 'Ethen' -d -t -v -p numpy,torch,torchtext,spacy",
"Ethen 2019-10-09 13:46:01 \n\nCPython 3.6.4\nIPython 7.7.0\n\nnumpy 1.16.5\ntorch 1.1.0.post2\ntorchtext 0.3.1\nspacy 2.1.6\n"
]
],
[
[
"# Seq2Seq With Attention",
"_____no_output_____"
],
[
"Seq2Seq framework involves a family of encoders and decoders, where the encoder encodes a source sequence into a fixed length vector from which the decoder picks up and aims to correctly generates the target sequence. The vanilla version of this type of architecture looks something along the lines of:\n\n<img src=\"img/2_seq2seq.png\" width=\"70%\" height=\"70%\">\n\nThe RNN encoder has an input sequence $x_1, x_2, x_3, x_4$. We denote the encoder states by $c_1, c_2, c_3$. The encoder outputs a single output vector $c$ which is passed as input to the decoder. Like the encoder, the decoder is also a single-layered RNN, we denote the decoder states by $s_1, s_2, s_3$ and the network's output by $y_1, y_2, y_3, y_4$. A problem with this vanilla architecture lies in the fact that the decoder needs to represent the entire input sequence $x_1, x_2, x_3, x_4$ as a single vector $c$, which can cause information loss. In other words, the fixed-length context vector is hypothesized to be the bottleneck in this framework.\n\nThe attention mechanism that we'll be introducing here extends this approach by allowing the model to soft search for parts of the source sequence that are relevant to predicting the target sequence, which looks like the following:\n\n<img src=\"img/2_seq2seq_attention.png\" width=\"70%\" height=\"70%\">\n\nThe attention mechanism is located between the encoder and the decoder, its input is composed of the encoder's output vectors $h_1, h_2, h_3, h_4$ and the states of the decoder $s_0, s_1, s_2, s_3$, the attention's output is a sequence of vectors called context vectors denoted by $c_1, c_2, c_3, c_4$.",
"_____no_output_____"
],
[
"These context vectors enable the decoder to focus on certain parts of the input when predicting its output. Each context vector is a weighted sum of the encoder's output vectors $h_1, h_2, h_3, h_4$, where each vector $h_i$ contains information about the whole input sequence with a strong focus on the parts surrounding the i-th vector of the input sequence. The vectors $h_1, h_2, h_3, h_4$ are scaled by weights $\\alpha_{ij}$ capturing the degree of relevance of input $x_j$ to output at time $i$, $y_i$. The context vectors $c_1, c_2, c_3, c_4$ are calculated by:\n\n\\begin{align}\nc_i = \\sum_{j=1}^4 a_{ij} h_j\n\\end{align}\n\nThe attention weights $a_{ij}$ are learned using an additional fully-connected network, denoted by $fc$, whose input consists of the decoder's hidden state $s_0, s_1, s_2, s_3$ and the encoder's output $h_1, h_2, h_3, h_4$. It's computation can be more formally defined by:\n\n\\begin{align}\na_{ij} = \\frac{exp(e_{ij})}{\\sum_{k=1}^4exp(e_{ik})}\n\\end{align}\n\nWhere:\n\n\\begin{align}\ne_{ij} = fc(s_{i-1}, h_j)\n\\end{align}\n\n<img src=\"img/2_attention_cell.png\" width=\"70%\" height=\"70%\">\n\nAs can be seen in the above image, the fully-connected network receives the concatenation of vectors $[s_{i-1}, h_i]$ as input at time step $i$. The network has a single fully-connected layer, the outputs of the layer, denoted by $e_{ij}$, are passed through a softmax function computing the attention weights, which lie in $[0,1]$.\n\nNote that we are using the same fully-connected network for all the concatenated pairs $[s_{i-1},h_1], [s_{i-1},h_2], [s_{i-1},h_3], [s_{i-1},h_4]$, meaning there is a single network learning the attention weights.\n\n\n<img src=\"img/2_fully_connect.png\" width=\"70%\" height=\"70%\">\n\n\nTo re-emphasize the attention weights $\\alpha_{ij}$ reflects the importance of $h_j$ with respect to the previous hidden state $s_{iโ1}$ in deciding the next state $s_i$ and generating $y_i$. A large $\\alpha_{ij}$ attention weight causes the RNN to focus on input $x_j$ (represented by the encoder's output $h_j$), when predicting the output $y_i$.",
"_____no_output_____"
],
[
"We can talk through an iteration of the algorithm to see how it all ties together.\n\n<img src=\"img/2_seq2seq_attention1.png\" width=\"70%\" height=\"70%\">\n\nThe first computation performed is the computation of vectors $h_1, h_2, h_3, h_4$ by the encoder. These are then used as inputs to the attention mechanism. This is where the decoder is first involved by inputting its initial state vector $s_0$ (note that for this initial state of the decoder, we often times use the hidden state from the encoder) and we have the first attention input sequence $[s_0, h_1], [s_0, h_2], [s_0, h_3], [s_0, h_4]$.\n\n<img src=\"img/2_seq2seq_attention2.png\" width=\"70%\" height=\"70%\">\n\nThe attention mechanism picks up the inputs and computes the first set of attention weights $\\alpha_{11}, \\alpha_{12}, \\alpha_{13}, \\alpha_{14}$ enabling the computation of the first context vector $c_1$. The decoder now uses $[s_0,c_1]$ to generate the first output $y_1$. This process then repeats itself, until we've generated all the outputs.",
"_____no_output_____"
],
[
"## Data Preparation",
"_____no_output_____"
],
[
"This part is pretty much identical to that of the vanilla seq2seq, hence explanation is omitted.",
"_____no_output_____"
]
],
[
[
"# !python -m spacy download de\n# !python -m spacy download en",
"_____no_output_____"
],
[
"SEED = 2222\nrandom.seed(SEED)\ntorch.manual_seed(SEED)",
"_____no_output_____"
],
[
"# tokenize sentences into individual tokens\n# https://spacy.io/usage/spacy-101#annotations-token\nspacy_de = spacy.load('de_core_news_sm')\nspacy_en = spacy.load('en_core_web_sm')\n\ndef tokenize_de(text):\n return [tok.text for tok in spacy_de.tokenizer(text)][::-1]\n\ndef tokenize_en(text):\n return [tok.text for tok in spacy_en.tokenizer(text)]",
"_____no_output_____"
],
[
"source = Field(tokenize=tokenize_de, init_token='<sos>', eos_token='<eos>', lower=True)\ntarget = Field(tokenize=tokenize_en, init_token='<sos>', eos_token='<eos>', lower=True)",
"_____no_output_____"
],
[
"train_data, valid_data, test_data = Multi30k.splits(exts=('.de', '.en'), fields=(source, target))\nprint(f\"Number of training examples: {len(train_data.examples)}\")\nprint(f\"Number of validation examples: {len(valid_data.examples)}\")\nprint(f\"Number of testing examples: {len(test_data.examples)}\")",
"Number of training examples: 29000\nNumber of validation examples: 1014\nNumber of testing examples: 1000\n"
],
[
"train_data.examples[0].src",
"_____no_output_____"
],
[
"train_data.examples[0].trg",
"_____no_output_____"
],
[
"source.build_vocab(train_data, min_freq=2)\ntarget.build_vocab(train_data, min_freq=2)\nprint(f\"Unique tokens in source (de) vocabulary: {len(source.vocab)}\")\nprint(f\"Unique tokens in target (en) vocabulary: {len(target.vocab)}\")",
"Unique tokens in source (de) vocabulary: 7855\nUnique tokens in target (en) vocabulary: 5893\n"
],
[
"device = torch.device('cuda' if torch.cuda.is_available() else 'cpu')",
"_____no_output_____"
],
[
"BATCH_SIZE = 128\n\n# create batches out of the dataset and sends them to the appropriate device\ntrain_iterator, valid_iterator, test_iterator = BucketIterator.splits(\n (train_data, valid_data, test_data), batch_size=BATCH_SIZE, device=device)",
"_____no_output_____"
],
[
"test_batch = next(iter(test_iterator))\ntest_batch",
"_____no_output_____"
]
],
[
[
"## Model Implementation",
"_____no_output_____"
]
],
[
[
"# adjustable parameters\nINPUT_DIM = len(source.vocab)\nOUTPUT_DIM = len(target.vocab)\nENC_EMB_DIM = 256\nDEC_EMB_DIM = 256\nENC_HID_DIM = 512\nDEC_HID_DIM = 512\nN_LAYERS = 1\nENC_DROPOUT = 0.5\nDEC_DROPOUT = 0.5",
"_____no_output_____"
]
],
[
[
"The following sections are heavily \"borrowed\" from the wonderful tutorial on this topic listed below.\n\n- [Jupyter Notebook: Neural Machine Translation by Jointly Learning to Align and Translate](https://nbviewer.jupyter.org/github/bentrevett/pytorch-seq2seq/blob/master/3%20-%20Neural%20Machine%20Translation%20by%20Jointly%20Learning%20to%20Align%20and%20Translate.ipynb)\n\nSome personal preference modifications have been made.",
"_____no_output_____"
],
[
"### Encoder",
"_____no_output_____"
],
[
"Like other seq2seq-like architectures, we first need to specify an encoder. Here we'll be using a bidirectional GRU layer. With a bidirectional layer, we have a forward layer scanning the sentence from left to right (shown below in green), and a backward layer scanning the sentence from right to left (yellow). From the coding perspective, we need to set the `bidirectional=True` for the GRU layer's argument.\n\n<img src=\"img/2_bidirectional.png\" width=\"80%\" height=\"80%\">\n\nMore formally, we now have:\n\n$$\n\\begin{align}\nh_t^\\rightarrow &= \\text{EncoderGRU}^\\rightarrow(x_t^\\rightarrow,h_{t-1}^\\rightarrow)\\\\\nh_t^\\leftarrow &= \\text{EncoderGRU}^\\leftarrow(x_t^\\leftarrow,h_{t-1}^\\leftarrow)\n\\end{align}\n$$\n\nWhere $x_0^\\rightarrow = \\text{<sos>}, x_1^\\rightarrow = \\text{guten}$ and $x_0^\\leftarrow = \\text{<eos>}, x_1^\\leftarrow = \\text{morgen}$.\n\nAs before, we only pass an embedded input to our GRU layer. We'll get two context vectors, one from the forward layer after it has seen the final word in the sentence, $z^\\rightarrow=h_T^\\rightarrow$, and one from the backward layer after it has seen the first word in the sentence, $z^\\leftarrow=h_T^\\leftarrow$.\n\nAs we'll be using bidirectional layer, the next section is devoted to help us understand how the output looks like before we implement the actual encoder that we'll be using. The shape of the output is explicitly printed out to make it easier to comprehend. Here, we're using GRU layer, which can be replaced with a LSTM layer, which is similar, but return an additional cell state variable that has the same size as the hidden state.",
"_____no_output_____"
]
],
[
[
"class Encoder(nn.Module):\n def __init__(self, input_dim, emb_dim, hid_dim, n_layers, dropout):\n super().__init__()\n self.emb_dim = emb_dim\n self.hid_dim = hid_dim\n self.input_dim = input_dim\n self.n_layers = n_layers\n self.dropout = dropout\n\n self.embedding = nn.Embedding(input_dim, emb_dim)\n self.rnn = nn.GRU(emb_dim, hid_dim, n_layers, dropout=dropout,\n bidirectional=True)\n\n def forward(self, src_batch):\n # src [sent len, batch size]\n embedded = self.embedding(src_batch) # [sent len, batch size, emb dim]\n outputs, hidden = self.rnn(embedded) # [sent len, batch size, hidden dim]\n # outputs -> [sent len, batch size, hidden dim * n directions]\n # hidden -> [n layers * n directions, batch size, hidden dim]\n return outputs, hidden",
"_____no_output_____"
],
[
"# first experiment with n_layers = 1\nn_layers = 1\nencoder = Encoder(INPUT_DIM, ENC_EMB_DIM, ENC_HID_DIM, n_layers, ENC_DROPOUT).to(device)\noutputs, hidden = encoder(test_batch.src)\noutputs.shape, hidden.shape",
"_____no_output_____"
]
],
[
[
"Notice that output's last dimension is 1024, which is the hidden dimension (512) multiplied by the number of directions (2). Whereas the hidden's first dimension is 2, representing the number of directions (2).\n\n- The returned outputs of bidirectional RNN at timestep $t$ is the output after feeding input to both the reverse and normal RNN unit at timestep $t$, where normal RNN has seen inputs $1...t$ and reverse RNN has seen inputs $t...n$, with $n$ being the length of the sequence).\n- The returned hidden state of bidirectional RNN is the hidden state after the whole sequence is consume. For normal RNN it's after timestep $n$; for reverse RNN it's after timestep 1.\n\nThe following diagram can also come in handy when visualizing the difference between output and hidden.\n\n<img src=\"img/2_rnn_output_hidden.png\" width=\"60%\" height=\"60%\">\n\nIn the diagram $n$ notes each timestep, and $w$ denotes the number of layer.\n\n- output comprises all the hidden states in the last layer (\"last\" depth-wise, not time-wise).\n- ($h_n$, $c_n$) comprise of the hidden states after the last timestep, $t = n$, so we could potentially feed them into another LSTM layer.",
"_____no_output_____"
]
],
[
[
"# the outputs are concatenated at the last dimension\nassert (outputs[-1, :, :ENC_HID_DIM] == hidden[0]).all()\nassert (outputs[0, :, ENC_HID_DIM:] == hidden[1]).all()",
"_____no_output_____"
],
[
"# experiment with n_layers = 2\nn_layers = 2\nencoder = Encoder(INPUT_DIM, ENC_EMB_DIM, ENC_HID_DIM, n_layers, ENC_DROPOUT).to(device)\noutputs, hidden = encoder(test_batch.src)\noutputs.shape, hidden.shape",
"_____no_output_____"
]
],
[
[
"Notice now the first dimension of the hidden cell becomes 4, which represents the number of layers (2) multiplied by the number of directions (2). The order of the hidden state is stacked by [forward_1, backward_1, forward_2, backward_2, ...]",
"_____no_output_____"
]
],
[
[
"assert (outputs[-1, :, :ENC_HID_DIM] == hidden[2]).all()\nassert (outputs[0, :, ENC_HID_DIM:] == hidden[3]).all()",
"_____no_output_____"
]
],
[
[
"We'll need some final touches for our actual encoder. As our encoder's hidden state will be used as the decoder's initial hidden state, we need to make sure we make them the same shape. In our example, the decoder is not bidirectional, and only needs a single context vector, $z$, to use as its initial hidden state, $s_0$, and we currently have two, a forward and a backward one ($z^\\rightarrow=h_T^\\rightarrow$ and $z^\\leftarrow=h_T^\\leftarrow$, respectively). We solve this by concatenating the two context vectors together, passing them through a linear layer, $g$, and applying the $\\tanh$ activation function. \n\n$$\n\\begin{align}\nz=\\tanh(g(h_T^\\rightarrow, h_T^\\leftarrow)) = \\tanh(g(z^\\rightarrow, z^\\leftarrow)) = s_0\n\\end{align}\n$$",
"_____no_output_____"
]
],
[
[
"class Encoder(nn.Module):\n def __init__(self, input_dim, emb_dim, enc_hid_dim, dec_hid_dim, n_layers, dropout):\n super().__init__()\n self.emb_dim = emb_dim\n self.enc_hid_dim = enc_hid_dim\n self.dec_hid_dim = dec_hid_dim\n self.input_dim = input_dim\n self.n_layers = n_layers\n self.dropout = dropout\n\n self.embedding = nn.Embedding(input_dim, emb_dim)\n self.rnn = nn.GRU(emb_dim, enc_hid_dim, n_layers, dropout=dropout,\n bidirectional=True)\n self.fc = nn.Linear(enc_hid_dim * 2, dec_hid_dim)\n\n def forward(self, src_batch):\n # src [sent len, batch size]\n\n # [sent len, batch size, emb dim]\n embedded = self.embedding(src_batch)\n outputs, hidden = self.rnn(embedded)\n # outputs -> [sent len, batch size, hidden dim * n directions]\n # hidden -> [n layers * n directions, batch size, hidden dim]\n\n # initial decoder hidden is final hidden state of the forwards and\n # backwards encoder RNNs fed through a linear layer\n concated = torch.cat((hidden[-2, :, :], hidden[-1, :, :]), dim=1)\n hidden = torch.tanh(self.fc(concated))\n return outputs, hidden",
"_____no_output_____"
],
[
"encoder = Encoder(INPUT_DIM, ENC_EMB_DIM, ENC_HID_DIM, DEC_HID_DIM, N_LAYERS, ENC_DROPOUT).to(device)\noutputs, hidden = encoder(test_batch.src)\noutputs.shape, hidden.shape",
"_____no_output_____"
]
],
[
[
"### Attention",
"_____no_output_____"
],
[
"The next part is the hightlight. The attention layer will take in the previous hidden state of the decoder $s_{t-1}$, and all of the stacked forward and backward hidden state from the encoder $H$. The output will be an attention vector $a_t$, that is the length of the source sentece, each element of this vector will be a floating number between 0 and 1, and the entire vector sums up to 1.\n\nIntuitively, this layer takes in what we've decoded so far $s_{t-1}$, and all of what have encoded $H$, to produce a vector $a_t$, that represents which word in the source sentence should we pay the most attention to in order to correctly predict the next thing in the target sequence $y_{t+1}$.\n\nGraphically, this looks something like below. For the very first attention vector, where we use the encoder's hidden state as the initial hidden state from the decoder. The green/yellow blocks represent the hidden states from both the forward and backward RNNs, and the attention computation is all done within the pink block.\n\n<img src=\"img/2_attention.png\" weight=\"80%\" height=\"80%\">",
"_____no_output_____"
]
],
[
[
"class Attention(nn.Module):\n\n def __init__(self, enc_hid_dim, dec_hid_dim):\n super().__init__()\n self.enc_hid_dim = enc_hid_dim\n self.dec_hid_dim = dec_hid_dim\n\n # enc_hid_dim multiply by 2 due to bidirectional\n self.fc1 = nn.Linear(enc_hid_dim * 2 + dec_hid_dim, dec_hid_dim)\n self.fc2 = nn.Linear(dec_hid_dim, 1, bias=False)\n\n def forward(self, encoder_outputs, hidden):\n src_len = encoder_outputs.shape[0]\n batch_size = encoder_outputs.shape[1]\n \n # repeat encoder hidden state src_len times [batch size, sent len, dec hid dim]\n hidden = hidden.unsqueeze(1).repeat(1, src_len, 1)\n # reshape/permute the encoder output, so that the batch size comes first\n # [batch size, sent len, enc hid dim * 2], times 2 because of bidirectional\n outputs = encoder_outputs.permute(1, 0, 2)\n\n # the attention mechanism receives a concatenation of the hidden state\n # and the encoder output\n concat = torch.cat((hidden, outputs), dim=2)\n \n # fully connected layer and softmax layer to compute the attention weight\n # [batch size, sent len, dec hid dim]\n energy = torch.tanh(self.fc1(concat))\n\n # attention weight should be of [batch size, sent len]\n attention = self.fc2(energy).squeeze(dim=2) \n attention_weight = torch.softmax(attention, dim=1)\n return attention_weight",
"_____no_output_____"
],
[
"attention = Attention(ENC_HID_DIM, DEC_HID_DIM).to(device)\nattention_weight = attention(outputs, hidden)\nattention_weight.shape",
"_____no_output_____"
]
],
[
[
"### Decoder",
"_____no_output_____"
],
[
"Now comes the decoder, within the decoder, we first use the attention layer that we've created in the previous section to compute the attention weight, this gives us the weight for each source sentence that the model should pay attention to when generating the current target output in the sequence. Along with the output from the encoder, this gives us the context vector. Finally, the decoder takes the embedded input along with the context to generate the target output in the sequence.",
"_____no_output_____"
]
],
[
[
"class Decoder(nn.Module):\n\n def __init__(self, output_dim, emb_dim, enc_hid_dim, dec_hid_dim, n_layers,\n dropout, attention):\n super().__init__()\n self.emb_dim = emb_dim\n self.enc_hid_dim = enc_hid_dim\n self.dec_hid_dim = dec_hid_dim\n self.output_dim = output_dim\n self.n_layers = n_layers\n self.dropout = dropout\n self.attention = attention\n\n self.embedding = nn.Embedding(output_dim, emb_dim)\n self.rnn = nn.GRU(enc_hid_dim * 2 + emb_dim, dec_hid_dim, n_layers, dropout=dropout)\n self.linear = nn.Linear(dec_hid_dim, output_dim)\n\n def forward(self, trg, encoder_outputs, hidden):\n # trg [batch size]\n # outputs [src sen len, batch size, enc hid dim * 2], times 2 due to bidirectional\n # hidden [batch size, dec hid dim]\n\n # [batch size, 1, sent len] \n attention = self.attention(encoder_outputs, hidden).unsqueeze(1)\n\n # [batch size, sent len, enc hid dim * 2]\n outputs = encoder_outputs.permute(1, 0, 2)\n\n # [1, batch size, enc hid dim * 2]\n context = torch.bmm(attention, outputs).permute(1, 0, 2)\n\n # input sentence -> embedding\n # [1, batch size, emb dim]\n embedded = self.embedding(trg.unsqueeze(0))\n rnn_input = torch.cat((embedded, context), dim=2)\n\n outputs, hidden = self.rnn(rnn_input, hidden.unsqueeze(0))\n prediction = self.linear(outputs.squeeze(0))\n return prediction, hidden.squeeze(0)",
"_____no_output_____"
],
[
"decoder = Decoder(OUTPUT_DIM, DEC_EMB_DIM, ENC_HID_DIM, DEC_HID_DIM, N_LAYERS, DEC_DROPOUT, attention).to(device)\nprediction, decoder_hidden = decoder(test_batch.trg[0], outputs, hidden)\n\n# notice the decoder_hidden's shape should match the shape that's generated by\n# the encoder\nprediction.shape, decoder_hidden.shape",
"_____no_output_____"
]
],
[
[
"### Seq2Seq",
"_____no_output_____"
],
[
"This part is about putting the encoder and decoder together and is very much identical to the vanilla seq2seq framework, hence the explanation is omitted.",
"_____no_output_____"
]
],
[
[
"class Seq2Seq(nn.Module):\n def __init__(self, encoder, decoder, device):\n super().__init__()\n self.encoder = encoder\n self.decoder = decoder\n self.device = device\n\n def forward(self, src_batch, trg_batch, teacher_forcing_ratio=0.5):\n max_len, batch_size = trg_batch.shape\n trg_vocab_size = self.decoder.output_dim\n\n # tensor to store decoder's output\n outputs = torch.zeros(max_len, batch_size, trg_vocab_size).to(self.device)\n\n # encoder_outputs : all hidden states of the input sequence (forward and backward)\n # hidden : final forward and backward hidden states, passed through a linear layer\n encoder_outputs, hidden = self.encoder(src_batch)\n\n trg = trg_batch[0]\n for i in range(1, max_len):\n prediction, hidden = self.decoder(trg, encoder_outputs, hidden)\n outputs[i] = prediction\n\n if random.random() < teacher_forcing_ratio:\n trg = trg_batch[i]\n else:\n trg = prediction.argmax(1)\n\n return outputs",
"_____no_output_____"
],
[
"attention = Attention(ENC_HID_DIM, DEC_HID_DIM)\nencoder = Encoder(INPUT_DIM, ENC_EMB_DIM, ENC_HID_DIM, DEC_HID_DIM, N_LAYERS, ENC_DROPOUT)\ndecoder = Decoder(OUTPUT_DIM, DEC_EMB_DIM, ENC_HID_DIM, DEC_HID_DIM, N_LAYERS, DEC_DROPOUT, attention)\nseq2seq = Seq2Seq(encoder, decoder, device).to(device)\nseq2seq",
"_____no_output_____"
],
[
"outputs = seq2seq(test_batch.src, test_batch.trg)\noutputs.shape",
"_____no_output_____"
],
[
"def count_parameters(model):\n return sum(p.numel() for p in model.parameters() if p.requires_grad)\n\nprint(f'The model has {count_parameters(seq2seq):,} trainable parameters')",
"The model has 12,975,877 trainable parameters\n"
]
],
[
[
"## Training Seq2Seq",
"_____no_output_____"
],
[
"We've done the hard work of defining our seq2seq module. The final touch is to specify the training/evaluation loop.",
"_____no_output_____"
]
],
[
[
"optimizer = optim.Adam(seq2seq.parameters())\n\n# ignore the padding index when calculating the loss\nPAD_IDX = target.vocab.stoi['<pad>']\ncriterion = nn.CrossEntropyLoss(ignore_index=PAD_IDX)",
"_____no_output_____"
],
[
"def train(seq2seq, iterator, optimizer, criterion):\n seq2seq.train()\n \n epoch_loss = 0\n for batch in iterator:\n optimizer.zero_grad()\n outputs = seq2seq(batch.src, batch.trg)\n\n # the loss function only works on 2d inputs\n # and 1d targets we need to flatten each of them\n outputs_flatten = outputs[1:].view(-1, outputs.shape[-1])\n trg_flatten = batch.trg[1:].view(-1)\n loss = criterion(outputs_flatten, trg_flatten)\n \n loss.backward()\n optimizer.step()\n \n epoch_loss += loss.item()\n\n return epoch_loss / len(iterator)",
"_____no_output_____"
],
[
"def evaluate(seq2seq, iterator, criterion):\n seq2seq.eval()\n\n epoch_loss = 0\n with torch.no_grad():\n for batch in iterator:\n # turn off teacher forcing\n outputs = seq2seq(batch.src, batch.trg, teacher_forcing_ratio=0) \n\n # trg = [trg sent len, batch size]\n # output = [trg sent len, batch size, output dim]\n outputs_flatten = outputs[1:].view(-1, outputs.shape[-1])\n trg_flatten = batch.trg[1:].view(-1)\n loss = criterion(outputs_flatten, trg_flatten)\n epoch_loss += loss.item()\n \n return epoch_loss / len(iterator)",
"_____no_output_____"
],
[
"def epoch_time(start_time, end_time):\n elapsed_time = end_time - start_time\n elapsed_mins = int(elapsed_time / 60)\n elapsed_secs = int(elapsed_time - (elapsed_mins * 60))\n return elapsed_mins, elapsed_secs",
"_____no_output_____"
],
[
"N_EPOCHS = 10\nbest_valid_loss = float('inf')\n\nfor epoch in range(N_EPOCHS):\n start_time = time.time()\n train_loss = train(seq2seq, train_iterator, optimizer, criterion)\n valid_loss = evaluate(seq2seq, valid_iterator, criterion)\n end_time = time.time()\n\n epoch_mins, epoch_secs = epoch_time(start_time, end_time)\n\n if valid_loss < best_valid_loss:\n best_valid_loss = valid_loss\n torch.save(seq2seq.state_dict(), 'tut2-model.pt')\n\n print(f'Epoch: {epoch+1:02} | Time: {epoch_mins}m {epoch_secs}s')\n print(f'\\tTrain Loss: {train_loss:.3f} | Train PPL: {math.exp(train_loss):7.3f}')\n print(f'\\t Val. Loss: {valid_loss:.3f} | Val. PPL: {math.exp(valid_loss):7.3f}')",
"Epoch: 01 | Time: 2m 30s\n\tTrain Loss: 4.844 | Train PPL: 126.976\n\t Val. Loss: 4.691 | Val. PPL: 108.948\nEpoch: 02 | Time: 2m 30s\n\tTrain Loss: 3.948 | Train PPL: 51.808\n\t Val. Loss: 4.004 | Val. PPL: 54.793\nEpoch: 03 | Time: 2m 31s\n\tTrain Loss: 3.230 | Train PPL: 25.281\n\t Val. Loss: 3.498 | Val. PPL: 33.059\nEpoch: 04 | Time: 2m 29s\n\tTrain Loss: 2.733 | Train PPL: 15.379\n\t Val. Loss: 3.413 | Val. PPL: 30.360\nEpoch: 05 | Time: 2m 28s\n\tTrain Loss: 2.379 | Train PPL: 10.793\n\t Val. Loss: 3.269 | Val. PPL: 26.285\nEpoch: 06 | Time: 2m 32s\n\tTrain Loss: 2.089 | Train PPL: 8.079\n\t Val. Loss: 3.228 | Val. PPL: 25.229\nEpoch: 07 | Time: 2m 29s\n\tTrain Loss: 1.862 | Train PPL: 6.438\n\t Val. Loss: 3.201 | Val. PPL: 24.561\nEpoch: 08 | Time: 2m 30s\n\tTrain Loss: 1.626 | Train PPL: 5.084\n\t Val. Loss: 3.297 | Val. PPL: 27.044\nEpoch: 09 | Time: 2m 30s\n\tTrain Loss: 1.406 | Train PPL: 4.078\n\t Val. Loss: 3.312 | Val. PPL: 27.451\nEpoch: 10 | Time: 2m 31s\n\tTrain Loss: 1.239 | Train PPL: 3.453\n\t Val. Loss: 3.467 | Val. PPL: 32.050\n"
]
],
[
[
"## Evaluating Seq2Seq",
"_____no_output_____"
]
],
[
[
"seq2seq.load_state_dict(torch.load('tut2-model.pt'))\n\ntest_loss = evaluate(seq2seq, test_iterator, criterion)\nprint(f'| Test Loss: {test_loss:.3f} | Test PPL: {math.exp(test_loss):7.3f} |')",
"| Test Loss: 3.237 | Test PPL: 25.467 |\n"
]
],
[
[
"Here, we pick a random example in our dataset, print out the original source and target sentence. Then takes a look at whether the \"predicted\" target sentence generated by the model.",
"_____no_output_____"
]
],
[
[
"example_idx = 0\nexample = train_data.examples[example_idx]\nprint('source sentence: ', ' '.join(example.src))\nprint('target sentence: ', ' '.join(example.trg))",
"source sentence: . bรผsche vieler nรคhe der in freien im sind mรคnner weiรe junge zwei\ntarget sentence: two young , white males are outside near many bushes .\n"
],
[
"src_tensor = source.process([example.src]).to(device)\ntrg_tensor = target.process([example.trg]).to(device)\nprint(trg_tensor.shape)\n\nseq2seq.eval()\nwith torch.no_grad():\n outputs = seq2seq(src_tensor, trg_tensor, teacher_forcing_ratio=0)\n\noutputs.shape",
"torch.Size([13, 1])\n"
],
[
"output_idx = outputs[1:].squeeze(1).argmax(1)\n' '.join([target.vocab.itos[idx] for idx in output_idx])",
"_____no_output_____"
]
],
[
[
"## Summary",
"_____no_output_____"
],
[
"- Upon implementing the attention mechanism, we were able to achieve a better evaluation score on the test set, while even using less parameters. As mentioned in the original paper:\n\n> We extended the basic encoderโdecoder by letting a model (soft)search for a set of input words. This frees the model from having to encode the whole source sentence into a fixed-length vector, and also lets the model focus only on information relevant to the generation of the next target word. This has a major positive impact on the ability of the neural machine translation system to yield good results on longer sentences.\n\n- Note that another interesting thing that we're capable of doing but wasn't done here is to visualize the attention weight to see for a given translation, where the model is focusing on.",
"_____no_output_____"
],
[
"# Reference",
"_____no_output_____"
],
[
"- [Blog: Attention in RNNs](https://medium.com/datadriveninvestor/attention-in-rnns-321fbcd64f05)\n- [Stackoverflow: What's the difference between โhiddenโ and โoutputโ in PyTorch LSTM?](https://stackoverflow.com/questions/48302810/whats-the-difference-between-hidden-and-output-in-pytorch-lstm)\n- [Jupyter Notebook: Neural Machine Translation by Jointly Learning to Align and Translate](https://nbviewer.jupyter.org/github/bentrevett/pytorch-seq2seq/blob/master/3%20-%20Neural%20Machine%20Translation%20by%20Jointly%20Learning%20to%20Align%20and%20Translate.ipynb)\n- [Paper: D. Bahdanau, K. Cho, Y. Bengio (2014) - Neural Machine Translation by Jointly Learning to Align and Translate](https://arxiv.org/abs/1409.0473)",
"_____no_output_____"
]
]
] | [
"markdown",
"code",
"markdown",
"code",
"markdown",
"code",
"markdown",
"code",
"markdown",
"code",
"markdown",
"code",
"markdown",
"code",
"markdown",
"code",
"markdown",
"code",
"markdown",
"code",
"markdown",
"code",
"markdown",
"code",
"markdown",
"code",
"markdown"
] | [
[
"markdown"
],
[
"code",
"code"
],
[
"markdown",
"markdown",
"markdown",
"markdown",
"markdown",
"markdown"
],
[
"code",
"code",
"code",
"code",
"code",
"code",
"code",
"code",
"code",
"code",
"code"
],
[
"markdown"
],
[
"code"
],
[
"markdown",
"markdown",
"markdown"
],
[
"code",
"code"
],
[
"markdown"
],
[
"code",
"code"
],
[
"markdown"
],
[
"code"
],
[
"markdown"
],
[
"code",
"code"
],
[
"markdown",
"markdown"
],
[
"code",
"code"
],
[
"markdown",
"markdown"
],
[
"code",
"code"
],
[
"markdown",
"markdown"
],
[
"code",
"code",
"code",
"code"
],
[
"markdown",
"markdown"
],
[
"code",
"code",
"code",
"code",
"code"
],
[
"markdown"
],
[
"code"
],
[
"markdown"
],
[
"code",
"code",
"code"
],
[
"markdown",
"markdown",
"markdown",
"markdown"
]
] |
e7693a8e7624977033caed96fba2f679eb51467e | 3,418 | ipynb | Jupyter Notebook | notebooks/MD_incl_azi_to_XYZ_minCurv.ipynb | Zabamund/fracture-SFA | a62e0be3b8117666d174f054673d36c53750a57f | [
"MIT"
] | 3 | 2019-10-20T16:34:00.000Z | 2019-11-02T21:54:14.000Z | notebooks/MD_incl_azi_to_XYZ_minCurv.ipynb | Zabamund/fracture-SFA | a62e0be3b8117666d174f054673d36c53750a57f | [
"MIT"
] | 1 | 2018-09-12T14:18:46.000Z | 2018-09-12T14:18:46.000Z | notebooks/MD_incl_azi_to_XYZ_minCurv.ipynb | Zabamund/fracture-SFA | a62e0be3b8117666d174f054673d36c53750a57f | [
"MIT"
] | 3 | 2018-09-10T17:46:39.000Z | 2020-12-19T03:17:06.000Z | 34.18 | 122 | 0.548566 | [
[
[
"# these warning filters are needed for a numpy error\nimport warnings\nwarnings.filterwarnings(\"ignore\", message=\"numpy.dtype size changed\") # due a RuntimeWarning with numpy.dtype\nimport pandas as pd\nimport numpy as np\nimport matplotlib.pyplot as plt\n%matplotlib inline\nimport math",
"_____no_output_____"
],
[
"def mdia_to_xyz_minCurve(deviation):\n \"\"\"\n A function to convert a well deviation (path of csv file) given in MD[m], incl[deg], azi[deg]\n into an xyz array with x[m], y[m], z[m] using the minimum curvature method\n according to: [drillingformulas.com](http://bit.ly/2MNp7U0)\n \"\"\"\n # read data\n data = pd.read_csv(deviation, sep=',', header='infer')\n # clean data\n data.drop(columns=['Unnamed: 0'], inplace=True)\n #data['Dogleg [deg/30m]'].replace(np.nan, 0, inplace=True)\n # add columns needed for calculations\n data['Dogleg_rad [rad/30m]'] = np.radians(data['Dogleg [deg/30m]'])\n data['RatioFactor'] = (2 / data['Dogleg_rad [rad/30m]']) * np.tan(data['Dogleg_rad [rad/30m]'] / 2)\n # calculate intervals\n delta_MD = np.array(data['MD[m]'][1:]) - np.array(data['MD[m]'][:-1])\n # get uppers/lowers\n RF_lower = np.array(data['RatioFactor'][1:])\n incl_upper = np.array(data['Inc[deg]'][:-1])\n incl_lower = np.array(data['Inc[deg]'][1:])\n azi_upper = np.array(data['Azi[deg]'][:-1])\n azi_lower = np.array(data['Azi[deg]'][1:])\n # calculate xyz\n east_x = delta_MD / 2 * (np.sin(np.radians(incl_upper)) * np.sin(np.radians(azi_upper)) \n + np.sin(np.radians(incl_lower)) * np.sin(np.radians(azi_lower))) * RF_lower\n \n north_y = delta_MD / 2 * (np.sin(np.radians(incl_upper)) * np.cos(np.radians(azi_upper)) \n + np.sin(np.radians(incl_lower)) * np.cos(np.radians(azi_lower))) * RF_lower\n \n TVD_z = delta_MD / 2 * (np.cos(np.radians(incl_upper)) + np.cos(np.radians(incl_lower))) * RF_lower\n \n return east_x, north_y, TVD_z",
"_____no_output_____"
],
[
"x = mdia_to_xyz_minCurve('../data/cleanedData/survey_edt.csv')[0]\ny = mdia_to_xyz_minCurve('../data/cleanedData/survey_edt.csv')[1]\nz = mdia_to_xyz_minCurve('../data/cleanedData/survey_edt.csv')[2]",
"_____no_output_____"
]
]
] | [
"code"
] | [
[
"code",
"code",
"code"
]
] |
e7694a0fd15f9582e4b5f5c8c99037d0f1a612c8 | 2,938 | ipynb | Jupyter Notebook | pos_tagging/Bigram tagging of Greek and Latin with the CLTK.ipynb | kylepjohnson/ipython_notebooks | 7f77ec06a70169cc479a6f912b4888789bf28ac4 | [
"MIT"
] | 9 | 2016-08-10T09:03:09.000Z | 2021-01-06T21:34:20.000Z | pos_tagging/Bigram tagging of Greek and Latin with the CLTK.ipynb | kylepjohnson/ipython_notebooks | 7f77ec06a70169cc479a6f912b4888789bf28ac4 | [
"MIT"
] | null | null | null | pos_tagging/Bigram tagging of Greek and Latin with the CLTK.ipynb | kylepjohnson/ipython_notebooks | 7f77ec06a70169cc479a6f912b4888789bf28ac4 | [
"MIT"
] | 3 | 2018-10-07T01:56:22.000Z | 2021-01-06T21:33:28.000Z | 22.427481 | 164 | 0.515997 | [
[
[
"empty"
]
]
] | [
"empty"
] | [
[
"empty"
]
] |
e769506b0a15be832c9f322bd4204ca6b299a2d4 | 202,382 | ipynb | Jupyter Notebook | reasoning_engine/categorical reasoning/Categorical_deduction_generic_all_inferences.ipynb | rts1988/IntelligentTutoringSystem_Experiments | b2f797a5bfff18fb37c7a779a19a72a75db7eeef | [
"MIT"
] | 1 | 2020-05-30T17:10:30.000Z | 2020-05-30T17:10:30.000Z | reasoning_engine/categorical reasoning/Categorical_deduction_generic_all_inferences.ipynb | rts1988/IntelligentTutoringSystem_Experiments | b2f797a5bfff18fb37c7a779a19a72a75db7eeef | [
"MIT"
] | null | null | null | reasoning_engine/categorical reasoning/Categorical_deduction_generic_all_inferences.ipynb | rts1988/IntelligentTutoringSystem_Experiments | b2f797a5bfff18fb37c7a779a19a72a75db7eeef | [
"MIT"
] | 1 | 2019-05-02T05:11:15.000Z | 2019-05-02T05:11:15.000Z | 165.075041 | 19,240 | 0.858846 | [
[
[
"# Categorical deduction (generic and all inferences)\n\n1. Take a mix of generic and specific statements\n2. Create powerset of combinations of specific statements\n3. create a inference graph for each combination of specific statements.\n4. Make all possible inferences for each graph (chain)\n5. present the union of possible conclusions for each node",
"_____no_output_____"
]
],
[
[
"# Syllogism specific statements \n# First statement A __ B. \n# Second statement B __ C.\n# Third statement A ___ C -> look up tables to check if true, possible, or false.\n\nspecific_statement_options = {'disjoint from','overlaps with','subset of','superset of','identical to'}\n\n# make a dictionary. key is a tuple with first statement type, second statement type and third statement type and value is True, Possible, False\nTruth_Table = dict()\nTruth_Table[( 'subset of', 'subset of', 'subset of')] = 'True'\nTruth_Table[( 'identical to', 'subset of', 'subset of')] = 'True'\nTruth_Table[( 'overlaps with', 'subset of', 'subset of')] = 'Possible'\nTruth_Table[( 'disjoint from', 'subset of', 'subset of')] = 'Possible'\nTruth_Table[( 'superset of', 'subset of', 'subset of')] = 'Possible'\nTruth_Table[( 'subset of', 'identical to', 'subset of')] = 'True'\nTruth_Table[( 'identical to', 'identical to', 'subset of')] = 'False'\nTruth_Table[( 'overlaps with', 'identical to', 'subset of')] = 'False'\nTruth_Table[( 'disjoint from', 'identical to', 'subset of')] = 'False'\nTruth_Table[( 'superset of', 'identical to', 'subset of')] = 'False'\nTruth_Table[( 'subset of', 'overlaps with', 'subset of')] = 'Possible'\nTruth_Table[( 'identical to', 'overlaps with', 'subset of')] = 'False'\nTruth_Table[( 'overlaps with', 'overlaps with', 'subset of')] = 'Possible'\nTruth_Table[( 'disjoint from', 'overlaps with', 'subset of')] = 'Possible'\nTruth_Table[( 'superset of', 'overlaps with', 'subset of')] = 'False'\nTruth_Table[( 'subset of', 'disjoint from', 'subset of')] = 'False'\nTruth_Table[( 'identical to', 'disjoint from', 'subset of')] = 'False'\nTruth_Table[( 'overlaps with', 'disjoint from', 'subset of')] = 'False'\nTruth_Table[( 'disjoint from', 'disjoint from', 'subset of')] = 'Possible'\nTruth_Table[( 'superset of', 'disjoint from', 'subset of')] = 'False'\nTruth_Table[( 'subset of', 'superset of', 'subset of')] = 'Possible'\nTruth_Table[( 'identical to', 'superset of', 'subset of')] = 'False'\nTruth_Table[( 'overlaps with', 'superset of', 'subset of')] = 'False'\nTruth_Table[( 'disjoint from', 'superset of', 'subset of')] = 'False'\nTruth_Table[( 'superset of', 'superset of', 'subset of')] = 'False'\nTruth_Table[( 'subset of', 'subset of', 'identical to')] = 'False'\nTruth_Table[( 'identical to', 'subset of', 'identical to')] = 'False'\nTruth_Table[( 'overlaps with', 'subset of', 'identical to')] = 'False'\nTruth_Table[( 'disjoint from', 'subset of', 'identical to')] = 'False'\nTruth_Table[( 'superset of', 'subset of', 'identical to')] = 'Possible'\nTruth_Table[( 'subset of', 'identical to', 'identical to')] = 'False'\nTruth_Table[( 'identical to', 'identical to', 'identical to')] = 'True'\nTruth_Table[( 'overlaps with', 'identical to', 'identical to')] = 'False'\nTruth_Table[( 'disjoint from', 'identical to', 'identical to')] = 'False'\nTruth_Table[( 'superset of', 'identical to', 'identical to')] = 'False'\nTruth_Table[( 'subset of', 'overlaps with', 'identical to')] = 'False'\nTruth_Table[( 'identical to', 'overlaps with', 'identical to')] = 'False'\nTruth_Table[( 'overlaps with', 'overlaps with', 'identical to')] = 'Possible'\nTruth_Table[( 'disjoint from', 'overlaps with', 'identical to')] = 'False'\nTruth_Table[( 'superset of', 'overlaps with', 'identical to')] = 'False'\nTruth_Table[( 'subset of', 'disjoint from', 'identical to')] = 'False'\nTruth_Table[( 'identical to', 'disjoint from', 'identical to')] = 'False'\nTruth_Table[( 'overlaps with', 'disjoint from', 'identical to')] = 'False'\nTruth_Table[( 'disjoint from', 'disjoint from', 'identical to')] = 'Possible'\nTruth_Table[( 'superset of', 'disjoint from', 'identical to')] = 'False'\nTruth_Table[( 'subset of', 'superset of', 'identical to')] = 'Possible'\nTruth_Table[( 'identical to', 'superset of', 'identical to')] = 'False'\nTruth_Table[( 'overlaps with', 'superset of', 'identical to')] = 'False'\nTruth_Table[( 'disjoint from', 'superset of', 'identical to')] = 'False'\nTruth_Table[( 'superset of', 'superset of', 'identical to')] = 'False'\nTruth_Table[( 'subset of', 'subset of', 'overlaps with')] = 'False'\nTruth_Table[( 'identical to', 'subset of', 'overlaps with')] = 'False'\nTruth_Table[( 'overlaps with', 'subset of', 'overlaps with')] = 'Possible'\nTruth_Table[( 'disjoint from', 'subset of', 'overlaps with')] = 'Possible'\nTruth_Table[( 'superset of', 'subset of', 'overlaps with')] = 'Possible'\nTruth_Table[( 'subset of', 'identical to', 'overlaps with')] = 'False'\nTruth_Table[( 'identical to', 'identical to', 'overlaps with')] = 'False'\nTruth_Table[( 'overlaps with', 'identical to', 'overlaps with')] = 'True'\nTruth_Table[( 'disjoint from', 'identical to', 'overlaps with')] = 'False'\nTruth_Table[( 'superset of', 'identical to', 'overlaps with')] = 'False'\nTruth_Table[( 'subset of', 'overlaps with', 'overlaps with')] = 'Possible'\nTruth_Table[( 'identical to', 'overlaps with', 'overlaps with')] = 'True'\nTruth_Table[( 'overlaps with', 'overlaps with', 'overlaps with')] = 'Possible'\nTruth_Table[( 'disjoint from', 'overlaps with', 'overlaps with')] = 'Possible'\nTruth_Table[( 'superset of', 'overlaps with', 'overlaps with')] = 'Possible'\nTruth_Table[( 'subset of', 'disjoint from', 'overlaps with')] = 'False'\nTruth_Table[( 'identical to', 'disjoint from', 'overlaps with')] = 'False'\nTruth_Table[( 'overlaps with', 'disjoint from', 'overlaps with')] = 'Possible'\nTruth_Table[( 'disjoint from', 'disjoint from', 'overlaps with')] = 'Possible'\nTruth_Table[( 'superset of', 'disjoint from', 'overlaps with')] = 'Possible'\nTruth_Table[( 'subset of', 'superset of', 'overlaps with')] = 'Possible'\nTruth_Table[( 'identical to', 'superset of', 'overlaps with')] = 'False'\nTruth_Table[( 'overlaps with', 'superset of', 'overlaps with')] = 'Possible'\nTruth_Table[( 'disjoint from', 'superset of', 'overlaps with')] = 'False'\nTruth_Table[( 'superset of', 'superset of', 'overlaps with')] = 'False'\nTruth_Table[( 'subset of', 'subset of', 'disjoint from')] = 'False'\nTruth_Table[( 'identical to', 'subset of', 'disjoint from')] = 'False'\nTruth_Table[( 'overlaps with', 'subset of', 'disjoint from')] = 'False'\nTruth_Table[( 'disjoint from', 'subset of', 'disjoint from')] = 'Possible'\nTruth_Table[( 'superset of', 'subset of', 'disjoint from')] = 'False'\nTruth_Table[( 'subset of', 'identical to', 'disjoint from')] = 'False'\nTruth_Table[( 'identical to', 'identical to', 'disjoint from')] = 'False'\nTruth_Table[( 'overlaps with', 'identical to', 'disjoint from')] = 'False'\nTruth_Table[( 'disjoint from', 'identical to', 'disjoint from')] = 'True'\nTruth_Table[( 'superset of', 'identical to', 'disjoint from')] = 'False'\nTruth_Table[( 'subset of', 'overlaps with', 'disjoint from')] = 'Possible'\nTruth_Table[( 'identical to', 'overlaps with', 'disjoint from')] = 'False'\nTruth_Table[( 'overlaps with', 'overlaps with', 'disjoint from')] = 'Possible'\nTruth_Table[( 'disjoint from', 'overlaps with', 'disjoint from')] = 'Possible'\nTruth_Table[( 'superset of', 'overlaps with', 'disjoint from')] = 'False'\nTruth_Table[( 'subset of', 'disjoint from', 'disjoint from')] = 'True'\nTruth_Table[( 'identical to', 'disjoint from', 'disjoint from')] = 'True'\nTruth_Table[( 'overlaps with', 'disjoint from', 'disjoint from')] = 'Possible'\nTruth_Table[( 'disjoint from', 'disjoint from', 'disjoint from')] = 'Possible'\nTruth_Table[( 'superset of', 'disjoint from', 'disjoint from')] = 'Possible'\nTruth_Table[( 'subset of', 'superset of', 'disjoint from')] = 'Possible'\nTruth_Table[( 'identical to', 'superset of', 'disjoint from')] = 'False'\nTruth_Table[( 'overlaps with', 'superset of', 'disjoint from')] = 'Possible'\nTruth_Table[( 'disjoint from', 'superset of', 'disjoint from')] = 'True'\nTruth_Table[( 'superset of', 'superset of', 'disjoint from')] = 'False'\nTruth_Table[( 'subset of', 'subset of', 'superset of')] = 'False'\nTruth_Table[( 'identical to', 'subset of', 'superset of')] = 'False'\nTruth_Table[( 'overlaps with', 'subset of', 'superset of')] = 'Possible'\nTruth_Table[( 'disjoint from', 'subset of', 'superset of')] = 'False'\nTruth_Table[( 'superset of', 'subset of', 'superset of')] = 'Possible'\nTruth_Table[( 'subset of', 'identical to', 'superset of')] = 'False'\nTruth_Table[( 'identical to', 'identical to', 'superset of')] = 'False'\nTruth_Table[( 'overlaps with', 'identical to', 'superset of')] = 'False'\nTruth_Table[( 'disjoint from', 'identical to', 'superset of')] = 'False'\nTruth_Table[( 'superset of', 'identical to', 'superset of')] = 'True'\nTruth_Table[( 'subset of', 'overlaps with', 'superset of')] = 'False'\nTruth_Table[( 'identical to', 'overlaps with', 'superset of')] = 'False'\nTruth_Table[( 'overlaps with', 'overlaps with', 'superset of')] = 'Possible'\nTruth_Table[( 'disjoint from', 'overlaps with', 'superset of')] = 'False'\nTruth_Table[( 'superset of', 'overlaps with', 'superset of')] = 'Possible'\nTruth_Table[( 'subset of', 'disjoint from', 'superset of')] = 'False'\nTruth_Table[( 'identical to', 'disjoint from', 'superset of')] = 'False'\nTruth_Table[( 'overlaps with', 'disjoint from', 'superset of')] = 'Possible'\nTruth_Table[( 'disjoint from', 'disjoint from', 'superset of')] = 'Possible'\nTruth_Table[( 'superset of', 'disjoint from', 'superset of')] = 'Possible'\nTruth_Table[( 'subset of', 'superset of', 'superset of')] = 'Possible'\nTruth_Table[( 'identical to', 'superset of', 'superset of')] = 'True'\nTruth_Table[( 'overlaps with', 'superset of', 'superset of')] = 'Possible'\nTruth_Table[( 'disjoint from', 'superset of', 'superset of')] = 'False'\nTruth_Table[( 'superset of', 'superset of', 'superset of')] = 'True'\n\n",
"_____no_output_____"
],
[
"major_premise = 'subset of'\nminor_premise = 'subset of'\nconclusion = 'subset of'\ntruth_value = Truth_Table[(major_premise,minor_premise,conclusion)]\nprint(truth_value)\n\ndef truth_value_additive(major_premise,minor_premise,conclusion):\n return Truth_Table[(major_premise,minor_premise,conclusion)]\n\ndef all_true_specific(major_premise,minor_premise):\n return [x for x in specific_statement_options if Truth_Table[(major_premise,minor_premise,x)]=='True']\n\ndef all_possible_specific(major_premise,minor_premise):\n return [x for x in specific_statement_options if Truth_Table[(major_premise,minor_premise,x)]=='Possible']\n\ndef all_false_specific(major_premise,minor_premise):\n return [x for x in specific_statement_options if Truth_Table[(major_premise,minor_premise,x)]=='False']\n",
"True\n"
],
[
"truth_value_additive('subset of','superset of','overlaps with')",
"_____no_output_____"
],
[
"all_true_specific('subset of','overlaps with')",
"_____no_output_____"
],
[
"reverse_implications = dict()\nreverse_implications['subset of']='superset of'\nreverse_implications['identical to']='identical to'\nreverse_implications['overlaps with']='overlaps with'\nreverse_implications['disjoint from']='disjoint from'\nreverse_implications['superset of']='subset of'\n",
"_____no_output_____"
],
[
"generic_statement_options = {'All','Some','No','Some_not'} # universal affirmative, particular affirmative, universal negative, particular negative\ngeneric_to_specific = dict()\ngeneric_to_specific['All'] = {'subset of','identical to'} \ngeneric_to_specific['No'] = {'disjoint from'}\ngeneric_to_specific['Some'] = {'overlaps with','subset of','identical to','superset of'} # generic_to_specific['All'].union({'superset of','overlaps with'})\ngeneric_to_specific['Some_not'] = {'overlaps with','disjoint from','superset of'} # generic_to_specific['No'].union({'superset of','overlaps with'})\n",
"_____no_output_____"
],
[
"# generic premises and conclusion: tautology, fallacy, or possible if\n# take in generic premises, make powersets of major and minor premise possibilities,\n# get the truth value for each, and get the joint conclusion: \n# always true (tautology), sometimes true or possible, and always false\nimport itertools\n\ngeneric_major_premise = 'All'\ngeneric_minor_premise = 'No'\ngeneric_conclusion = 'No'\n\npossibilities = list(itertools.product(generic_to_specific[generic_major_premise],generic_to_specific[generic_minor_premise],generic_to_specific[generic_conclusion]))\n\ntruth_value_list = []\nfor p in possibilities:\n truth_value_list.append(truth_value_additive(p[0],p[1],p[2]))\nprint(possibilities,truth_value_list)",
"[('identical to', 'disjoint from', 'disjoint from'), ('subset of', 'disjoint from', 'disjoint from')] ['True', 'True']\n"
],
[
"def generic_truth_value_additive(generic_major_premise,generic_minor_premise,generic_conclusion):\n possibilities = list(itertools.product(generic_to_specific[generic_major_premise],generic_to_specific[generic_minor_premise],generic_to_specific[generic_conclusion]))\n truth_value_list = []\n for p in possibilities:\n truth_value_list.append(truth_value_additive(p[0],p[1],p[2]))\n print(possibilities,truth_value_list)\n if ('True' in truth_value_list) and ('False' not in truth_value_list) and ('Possible' not in truth_value_list):\n return 'True'\n elif ('False' in truth_value_list) and ('True' in truth_value_list):\n return 'Possible'\n elif ('Possible' in truth_value_list):\n return 'Possible'\n elif ('False' in truth_value_list) and ('Possible' not in truth_value_list) and ('True' not in truth_value_list):\n return 'False'\n else:\n return 'Not valid truth values'\n ",
"_____no_output_____"
],
[
"generic_truth_value_additive('Some','No','No')",
"[('identical to', 'disjoint from', 'disjoint from'), ('subset of', 'disjoint from', 'disjoint from'), ('overlaps with', 'disjoint from', 'disjoint from'), ('superset of', 'disjoint from', 'disjoint from')] ['True', 'True', 'Possible', 'Possible']\n"
],
[
"# reverse implications, additive only (A,B) - (B,C) - (A,C)\n# define sets\nsets = ['A','B','C']\n\nfirst_statement = ['B','subset of','A']\nsecond_statement = ['C','overlaps with','B']\nthird_statement = ['C','disjoint from','A']\n\nadditive_set_order_check = dict()\nadditive_set_order_check['first'] = (0,1)\nadditive_set_order_check['second'] = (1,2)\nadditive_set_order_check['third'] = (0,2)\n# check if a statement needs to be reversed\ndef check_reverse_specific(statement,stype,sets):\n if (statement[0]==sets[additive_set_order_check[stype][0]]) and (statement[2]==sets[additive_set_order_check[stype][1]]):\n print('straight')\n return statement\n else:\n print('reverse')\n return [statement[2],reverse_implications[statement[1]],statement[0]]\n \n# Ideally, should auto calculate order or sets. or alternatively, calculate the reverse of each statement as an inference.\n\n\n",
"_____no_output_____"
],
[
"# Given a set of statements in the form ['A', 'disjoint from','B'], make all inferences, find all contradictions. \nimport networkx as nx\ndel(infG)\nstatement_set = [['A','subset of','B'],['B','subset of','C'],['D','identical to','C']]\n# make a graph? \ninfG = nx.DiGraph()\n# get list of nodes from elt 0 and 2 from each statement\nsetnodes = set()\nfromnodes = set()\ntonodes = set()\nfor statement in statement_set:\n fromnodes.add(statement[0])\n tonodes.add(statement[2])\n infG.add_edge(statement[0],statement[2],rel = statement[1])\nsetnodes = fromnodes.union(tonodes)\nprint(fromnodes,tonodes, setnodes)\nroots = fromnodes-tonodes\nends = tonodes - fromnodes\nprint(roots,ends)",
"_____no_output_____"
],
[
"import matplotlib.pyplot as plt\n#nx.draw_spectral(infG,with_labels=True,edge_labels = 'rel' font_size=18,node_size=1200)",
"_____no_output_____"
],
[
"pos = nx.spectral_layout(infG)\nnx.draw(infG, pos, with_labels=True)\nedge_labels = nx.get_edge_attributes(infG,'rel')\nnx.draw_networkx_edge_labels(infG, pos, labels = edge_labels)\n#plt.savefig('this.png')\nplt.show()\n#nx.get_edge_attributes(infG,'rel')",
"_____no_output_____"
],
[
"# getting reverse implications and redrawing graph\ninfGr = nx.DiGraph()\nfor statement in statement_set:\n infGr.add_edge(statement[2],statement[0],rel = reverse_implications[statement[1]])\npos = nx.spectral_layout(infGr)\nnx.draw(infGr, pos, with_labels=True)\nedge_labels = nx.get_edge_attributes(infGr,'rel')\nnx.draw_networkx_edge_labels(infGr, pos, labels = edge_labels)\n#plt.savefig('this.png')\nplt.show()\n\n# note this rewrites the latest edges, and doesn't show multiple edges between nodes, which is annoying. ",
"_____no_output_____"
],
[
"def make_all_inferences(infGc):\n roots = {n for n in infG.nodes if list(infG.predecessors(n))==[]}\n ends = {n for n in infG.nodes if list(infG.successors(n))==[]}\n \n infG1 = infGc.copy()\n \n prev_paths = ['']\n no_more_inf_flag = 0\n contradiction_found = 0\n while no_more_inf_flag==0:\n # calculating paths between roots and ends\n paths = dict()\n num_infs= 0\n for r in roots:\n paths[r] = dict()\n for e in ends:\n paths[r][e] = list(nx.all_simple_paths(infG1,r,e))\n\n paths[r][e]= [p for p in paths[r][e] if p not in prev_paths]\n prev_paths = prev_paths + paths[r][e]\n \n \n \n for r in roots:\n for e in ends:\n for p in paths[r][e]:\n print(p)\n for i in range(len(p)-2):\n print(p[i],p[i+1],p[i+2])\n inf = all_true_specific(infG1.edges[(p[i],p[i+1])]['rel'],infG1.edges[(p[i+1],p[i+2])]['rel'])\n if len(inf)>0:\n # catch contradictions\n if (p[i],p[i+2]) in infG1.edges():\n if infG1.edges[(p[i],p[i+2])]['rel'] not in inf:\n print('Contradicting relationship between ',p[i],' and ',p[i+2],' already exists as ',infG1.edges[(p[i],p[i+2])]['rel'])\n contradiction_found = 1\n else:\n print('Since ',p[i],infG1.edges[(p[i],p[i+1])]['rel'],p[i+1],', and ',p[i+1],infG1.edges[(p[i+1],p[i+2])]['rel'],p[i+2],', this means')\n print(p[i],inf[0],p[i+2])\n infG1.add_edge(p[i],p[i+2],rel=inf[0])\n fromnodes.add(p[i])\n tonodes.add(p[i+2])\n num_infs= num_infs+1\n if (num_infs==0) or (contradiction_found==1):\n no_more_inf_flag = 1\n\n if contradiction_found==1:\n print('not updating graph since contradiction found')\n del(infG1)\n else: \n infGc = infG1.copy()\n del(infG1)\n\n edges = list(infGc.edges())\n for edge in edges:\n print(edge,infGc.edges[edge]['rel'])\n return (infGc,contradiction_found)",
"_____no_output_____"
],
[
"(infG,cd_found) = make_all_inferences(infG)\nif cd_found==1:\n print('not changing graph until contradiction resolved')\nelse:\n print('Updated graph')",
"_____no_output_____"
],
[
"del(infG2)",
"_____no_output_____"
],
[
"infG2 = nx.MultiDiGraph()\nfor (u,v) in infG.edges():\n infG2.add_edge(u,v,0,rel=infG.edges[(u,v)]['rel'])\n infG2.add_edge(v,u,1,rel=reverse_implications[infG.edges[(u,v)]['rel']])",
"_____no_output_____"
],
[
"list(nx.all_simple_paths(infG2,'D','A'))",
"_____no_output_____"
],
[
"def get_s_or_r_multi(infG3,u,v):\n if infG3.edges.get((u,v,0),'')=='':\n return 1\n else:\n return 0\n\ndef get_rel_multidigraph(infG3,u,v):\n return infG3.edges[(u,v,get_s_or_r_multi(infG3,u,v))]['rel']\n ",
"_____no_output_____"
],
[
"roots = {n for n in infG.nodes if list(infG.predecessors(n))==[]}\nends = {n for n in infG.nodes if list(infG.successors(n))==[]}\nprint(roots,ends)\n\nprev_paths = ['']\npaths = dict()\nfor r in roots:\n paths[r] = dict()\n for e in ends:\n paths[r][e] = list(nx.all_simple_paths(infG2,r,e))\nprint(paths)",
"_____no_output_____"
],
[
"all_true_specific(get_rel_multidigraph(infG2,'D','C'),get_rel_multidigraph(infG2,'C','B'))",
"_____no_output_____"
],
[
"def make_all_inferences_multi(infGc):\n # make multidigraph\n infG2 = nx.MultiDiGraph()\n roots = list(infG.nodes())\n ends = list(infG.nodes())\n if str(type(infGc))==\"<class 'networkx.classes.multidigraph.MultiDiGraph'>\": \n for (u,v) in infGc.edges():\n if get_s_or_r_multi(infGc,u,v)==0:\n infG2.add_edge(u,v,0,rel=get_rel_multidigraph(infGc,u,v))\n infG2.add_edge(v,u,1,rel=reverse_implications[get_rel_multidigraph(infGc,u,v)])\n else:\n infG2.add_edge(u,v,1,rel=get_rel_multidigraph(infGc,u,v))\n infG2.add_edge(v,u,0,rel=reverse_implications[get_rel_multidigraph(infGc,u,v)])\n elif str(type(infGc))==\"<class 'networkx.classes.digraph.DiGraph'>\":\n #roots = {n for n in infG.nodes if list(infG.predecessors(n))==[]}\n #ends = {n for n in infG.nodes if list(infG.successors(n))==[]}\n \n for (u,v) in infGc.edges():\n infG2.add_edge(u,v,0,rel=infGc.edges[(u,v)]['rel'])\n infG2.add_edge(v,u,1,rel=reverse_implications[infGc.edges[(u,v)]['rel']])\n else:\n print('Only directed graphs or multidirected graphs accepted')\n return ('','')\n\n prev_paths = []\n no_more_inf_flag = 0\n contradiction_found = 0\n while no_more_inf_flag==0:\n # calculating paths between roots and ends\n paths = dict()\n num_infs= 0\n for r in roots:\n paths[r] = dict()\n for e in ends:\n paths[r][e] = list(nx.all_simple_paths(infG2,r,e))\n\n paths[r][e]= [p for p in paths[r][e] if p not in prev_paths]\n prev_paths = prev_paths + paths[r][e]\n #print(prev_paths)\n \n \n for r in roots:\n for e in ends:\n for p in paths[r][e]:\n #print(p)\n for i in range(len(p)-2):\n #print(p[i],p[i+1],p[i+2])\n #print(get_rel_multidigraph(infG2,p[i],p[i+1]))\n #print(get_rel_multidigraph(infG2,p[i+1],p[i+2]))\n inf = all_true_specific(get_rel_multidigraph(infG2,p[i],p[i+1]),get_rel_multidigraph(infG2,p[i+1],p[i+2]))\n if len(inf)>0:\n # catch contradictions\n if (p[i],p[i+2]) in infG2.edges():\n if get_rel_multidigraph(infG2,p[i],p[i+2]) not in inf:\n print('Contradicting relationship between ',p[i],' and ',p[i+2],' already exists as ',get_rel_multidigraph(infG2,p[i],p[i+2]))\n contradiction_found = 1\n else:\n print('Since ',p[i],get_rel_multidigraph(infG2,p[i],p[i+1]),p[i+1],', and ',p[i+1],get_rel_multidigraph(infG2,p[i+1],p[i+2]),p[i+2],', this means')\n print(p[i],inf[0],p[i+2])\n infG2.add_edge(p[i],p[i+2],0,rel=inf[0])\n fromnodes.add(p[i])\n tonodes.add(p[i+2])\n num_infs= num_infs+1\n if (num_infs==0) or (contradiction_found==1):\n no_more_inf_flag = 1\n\n if contradiction_found==1:\n print('not updating graph since contradiction found')\n del(infG2)\n else: \n infGc = infG2.copy()\n del(infG2)\n\n edges = list(infGc.edges())\n for (u,v) in edges:\n print('(',u,',',v,')',get_rel_multidigraph(infGc,u,v))\n return (infGc,contradiction_found)",
"_____no_output_____"
],
[
"(infG,cd) = make_all_inferences_multi(infG)",
"_____no_output_____"
],
[
"roots = list(infG.nodes())\nends = list(infG.nodes())",
"_____no_output_____"
],
[
"for r in roots:\n for e in ends:\n print(list(nx.all_simple_paths(infG,r,e)))",
"_____no_output_____"
],
[
"print(generic_to_specific['All'].intersection(generic_to_specific['Some']))\nprint(generic_to_specific['Some'].intersection(generic_to_specific['Some_not']))\nprint(generic_to_specific['Some_not'].intersection(generic_to_specific['No']))\nprint(generic_to_specific['No'].intersection(generic_to_specific['All']))\nprint(generic_to_specific['All'].intersection(generic_to_specific['Some_not']))\nprint(generic_to_specific['Some'].intersection(generic_to_specific['No']))",
"_____no_output_____"
],
[
"def validate_statement(statement_set,new_statement):\n # validating each new statement against existing statement set: assuming that the existing statement is already done with chain inferencing. \n # statement set for each inference graph in possible ones should be considered, and if we can find the ones that satisfy. display the ones that don't and reduce possibilities. \n # if a statement is encountered (specific or generic) that is completely new nodes, add (mark citation)\n # if a statement is encountered (specific or generic) that uses one new node and one existing, add (mark citation)\n # if a statement is encountered that uses the same two nodes in same order: \n # if new statement and any old statement with same nodes are specific and different it is a contradiction and needs to be resolved.\n # consider saving for each edge which statements it is inferred from so a chain can be established and displayed\n # if new statement is specific and the combination of old ones is generic-specific combination, specific statement should be in generic_to_specific[dict] intersection of previous statements\n # if new statement is generic and the old ones are a combination, the intersection of the new with the old should be displayed and verified. if intersection is nullset, throw up contradiction to resolve. \n # if a statement with reverse nodes is encountered, reverse and follow above instructions. ",
"_____no_output_____"
],
[
"# Generic statements sets \nimport itertools\n#def powerset_generic_to_specific(generic_statement_set):\ngeneric_statement_set = [['A','No','B'],['B','All','C'],['C','Some','D']]\n\n# add one more step here for multiple generic statements between two nodes - compatible (All,Some), (Some, Some_not), (Some_not, no). incompatible (no,all), (all, some_not), (some, no)\n# the incompatible types should be filtered out during entry\n\n\n\n# set of converted generic to specific sets\npossibilities_set = [list(generic_to_specific[statement[1]]) if statement[1] in generic_statement_options else [statement[1]] for statement in generic_statement_set]\nnode_set = [[statement[0],statement[2]] for statement in generic_statement_set]\nprint(possibilities_set,'\\n\\n\\n')\n\ndef flattentup(tup):\n flatlist = []\n for elt in tup:\n #print(elt,type(elt))\n if type(elt) is not tuple:\n #print('elt appended')\n flatlist.append(elt)\n else:\n #print('calling recursive')\n flatlist = flatlist + flattentup(elt)\n return flatlist\n\n#combinations = list(itertools.product([ps for ps in possibilities_set]))\n#print(combinations)\n\ncombinations = possibilities_set[0]\nfor i in possibilities_set[1:len(possibilities_set)]:\n combinations = list(itertools.product(combinations,i))\n#print(combinations)\n\ncombinationslist = [flattentup(elt) for elt in combinations]\nprint(combinationslist)\n\ninfgraphdict =dict()\nfor i in range(len(combinationslist)):\n infgraphdict[i] = []\n for j in range(len(node_set)):\n infgraphdict[i].append([node_set[j][0],combinationslist[i][j],node_set[j][1]])\nprint(infgraphdict)\n\nimport networkx as nx\nimport matplotlib.pyplot as plt\n\ninfdict = dict()\nfor i in infgraphdict.keys():\n\n statement_set = infgraphdict[i]\n # make a graph? \n infG = nx.DiGraph()\n # get list of nodes from elt 0 and 2 from each statement\n setnodes = set()\n fromnodes = set()\n tonodes = set()\n for statement in statement_set:\n fromnodes.add(statement[0])\n tonodes.add(statement[2])\n infG.add_edge(statement[0],statement[2],rel = statement[1])\n setnodes = fromnodes.union(tonodes)\n print(fromnodes,tonodes, setnodes)\n roots = fromnodes-tonodes\n ends = tonodes - fromnodes\n print(roots,ends)\n pos = nx.spectral_layout(infG)\n nx.draw(infG, pos, with_labels=True)\n edge_labels = nx.get_edge_attributes(infG,'rel')\n nx.draw_networkx_edge_labels(infG, pos, labels = edge_labels)\n #plt.savefig('this.png')\n plt.show()\n \n (infdict[i],contradiction_found) = make_all_inferences_multi(infG)\n del(infG)",
"[['disjoint from'], ['identical to', 'subset of'], ['identical to', 'subset of', 'overlaps with', 'superset of']] \n\n\n\n[['disjoint from', 'identical to', 'identical to'], ['disjoint from', 'identical to', 'subset of'], ['disjoint from', 'identical to', 'overlaps with'], ['disjoint from', 'identical to', 'superset of'], ['disjoint from', 'subset of', 'identical to'], ['disjoint from', 'subset of', 'subset of'], ['disjoint from', 'subset of', 'overlaps with'], ['disjoint from', 'subset of', 'superset of']]\n{0: [['A', 'disjoint from', 'B'], ['B', 'identical to', 'C'], ['C', 'identical to', 'D']], 1: [['A', 'disjoint from', 'B'], ['B', 'identical to', 'C'], ['C', 'subset of', 'D']], 2: [['A', 'disjoint from', 'B'], ['B', 'identical to', 'C'], ['C', 'overlaps with', 'D']], 3: [['A', 'disjoint from', 'B'], ['B', 'identical to', 'C'], ['C', 'superset of', 'D']], 4: [['A', 'disjoint from', 'B'], ['B', 'subset of', 'C'], ['C', 'identical to', 'D']], 5: [['A', 'disjoint from', 'B'], ['B', 'subset of', 'C'], ['C', 'subset of', 'D']], 6: [['A', 'disjoint from', 'B'], ['B', 'subset of', 'C'], ['C', 'overlaps with', 'D']], 7: [['A', 'disjoint from', 'B'], ['B', 'subset of', 'C'], ['C', 'superset of', 'D']]}\n{'C', 'B', 'A'} {'D', 'C', 'B'} {'A', 'D', 'C', 'B'}\n{'A'} {'D'}\n"
],
[
"# get possible relationships for each edge\n\nedge_poss_dict = dict()\n\nfor i in infdict.keys():\n #print(i)\n for edge in infdict[i].edges():\n #print(edge)\n if edge not in edge_poss_dict.keys():\n #print('adding new key')\n edge_poss_dict[edge] = list()\n #print(edge_poss_dict[edge])\n #print(type(edge_poss_dict[edge]))\n t = [edge[0],get_rel_multidigraph(infdict[i],edge[0],edge[1]),edge[1]]\n if t not in edge_poss_dict[edge]:\n edge_poss_dict[edge].append(t)\n #print(edge_poss_dict[edge])\n\n\nprint(edge_poss_dict)\n\n# consider converting possibles to smaller generic statement for readability. ",
"{('A', 'B'): [['A', 'disjoint from', 'B']], ('A', 'C'): [['A', 'disjoint from', 'C']], ('A', 'D'): [['A', 'disjoint from', 'D']], ('B', 'A'): [['B', 'disjoint from', 'A']], ('B', 'C'): [['B', 'identical to', 'C'], ['B', 'subset of', 'C']], ('B', 'D'): [['B', 'identical to', 'D'], ['B', 'subset of', 'D'], ['B', 'overlaps with', 'D'], ['B', 'superset of', 'D']], ('C', 'B'): [['C', 'identical to', 'B'], ['C', 'superset of', 'B']], ('C', 'D'): [['C', 'identical to', 'D'], ['C', 'subset of', 'D'], ['C', 'overlaps with', 'D'], ['C', 'superset of', 'D']], ('C', 'A'): [['C', 'disjoint from', 'A']], ('D', 'C'): [['D', 'identical to', 'C'], ['D', 'superset of', 'C'], ['D', 'overlaps with', 'C'], ['D', 'subset of', 'C']], ('D', 'B'): [['D', 'identical to', 'B'], ['D', 'superset of', 'B'], ['D', 'overlaps with', 'B'], ['D', 'subset of', 'B']], ('D', 'A'): [['D', 'disjoint from', 'A']]}\n"
],
[
"infdict",
"_____no_output_____"
],
[
"del(edge_poss_dict)",
"_____no_output_____"
]
]
] | [
"markdown",
"code"
] | [
[
"markdown"
],
[
"code",
"code",
"code",
"code",
"code",
"code",
"code",
"code",
"code",
"code",
"code",
"code",
"code",
"code",
"code",
"code",
"code",
"code",
"code",
"code",
"code",
"code",
"code",
"code",
"code",
"code",
"code",
"code",
"code",
"code",
"code",
"code"
]
] |
e76950abc0adb9b781c2c867d2be22dd46f7809d | 6,032 | ipynb | Jupyter Notebook | notebooks/ROS_Tutorials/.ipynb_checkpoints/ROS Nodes-checkpoint.ipynb | GimpelZhang/git_test | 78dddbdc71209c3cfba58d831cfde1588989f8ab | [
"MIT"
] | 1 | 2020-11-30T03:23:22.000Z | 2020-11-30T03:23:22.000Z | notebooks/ROS_Tutorials/.ipynb_checkpoints/ROS Nodes-checkpoint.ipynb | GimpelZhang/git_test | 78dddbdc71209c3cfba58d831cfde1588989f8ab | [
"MIT"
] | null | null | null | notebooks/ROS_Tutorials/.ipynb_checkpoints/ROS Nodes-checkpoint.ipynb | GimpelZhang/git_test | 78dddbdc71209c3cfba58d831cfde1588989f8ab | [
"MIT"
] | null | null | null | 22.762264 | 213 | 0.528183 | [
[
[
"## Understanding ROS Nodes\n\nThis tutorial introduces ROS graph concepts and discusses the use of `roscore`, `rosnode`, and `rosrun` commandline tools.\n\nSource: [ROS Wiki](http://wiki.ros.org/ROS/Tutorials/UnderstandingNodes)",
"_____no_output_____"
],
[
"### Quick Overview of Graph Concepts\n* Nodes: A node is an executable that uses ROS to communicate with other nodes.\n* Messages: ROS data type used when subscribing or publishing to a topic.\n* Topics: Nodes can publish messages to a topic as well as subscribe to a topic to receive messages.\n* Master: Name service for ROS (i.e. helps nodes find each other)\n* rosout: ROS equivalent of stdout/stderr\n* roscore: Master + rosout + parameter server (parameter server will be introduced later)",
"_____no_output_____"
],
[
"### roscore\n\n`roscore` is the first thing you should run when using ROS.",
"_____no_output_____"
]
],
[
[
"%%bash --bg\nroscore",
"Starting job # 0 in a separate thread.\n"
]
],
[
[
"### Using `rosnode`\n\n`rosnode` displays information about the ROS nodes that are currently running. The `rosnode list` command lists these active nodes:",
"_____no_output_____"
]
],
[
[
"%%bash\nrosnode list",
"/rosout\n"
],
[
"%%bash\nrosnode info rosout",
"--------------------------------------------------------------------------------\nNode [/rosout]\nPublications: \n * /rosout_agg [rosgraph_msgs/Log]\n\nSubscriptions: \n * /rosout [unknown type]\n\nServices: \n * /rosout/get_loggers\n * /rosout/set_logger_level\n\n\ncontacting node http://localhost:43395/ ...\nPid: 18703\n\n"
]
],
[
[
"### Using `rosrun`\n\n`rosrun` allows you to use the package name to directly run a node within a package (without having to know the package path).",
"_____no_output_____"
]
],
[
[
"%%bash --bg\nrosrun turtlesim turtlesim_node",
"Starting job # 2 in a separate thread.\n"
]
],
[
[
"NOTE: The turtle may look different in your turtlesim window. Don't worry about it - there are [many types of turtle](http://wiki.ros.org/Distributions#Current_Distribution_Releases) and yours is a surprise!",
"_____no_output_____"
]
],
[
[
"%%bash\nrosnode list",
"/rosout\n/turtlesim\n"
]
],
[
[
"One powerful feature of ROS is that you can reassign Names from the command-line.\n\nClose the turtlesim window to stop the node. Now let's re-run it, but this time use a [Remapping Argument](http://wiki.ros.org/Remapping%20Arguments) to change the node's name:",
"_____no_output_____"
]
],
[
[
"%%bash --bg\nrosrun turtlesim turtlesim_node __name:=my_turtle",
"Starting job # 3 in a separate thread.\n"
]
],
[
[
"Now, if we go back and use `rosnode list`:",
"_____no_output_____"
]
],
[
[
"%%bash\nrosnode list",
"/my_turtle\n/rosout\n/turtlesim\n"
]
],
[
[
"### Review\nWhat was covered:\n\n* roscore = ros+core : master (provides name service for ROS) + rosout (stdout/stderr) + parameter server (parameter server will be introduced later)\n* rosnode = ros+node : ROS tool to get information about a node.\n* rosrun = ros+run : runs a node from a given package.\n\nNow that you understand how ROS nodes work, let's look at how [ROS topics](ROS%20Topics.ipynb) work.",
"_____no_output_____"
]
]
] | [
"markdown",
"code",
"markdown",
"code",
"markdown",
"code",
"markdown",
"code",
"markdown",
"code",
"markdown",
"code",
"markdown"
] | [
[
"markdown",
"markdown",
"markdown"
],
[
"code"
],
[
"markdown"
],
[
"code",
"code"
],
[
"markdown"
],
[
"code"
],
[
"markdown"
],
[
"code"
],
[
"markdown"
],
[
"code"
],
[
"markdown"
],
[
"code"
],
[
"markdown"
]
] |
e76955508fa3cdd41c073ea045c71001c4ab2a88 | 19,205 | ipynb | Jupyter Notebook | Quiz.ipynb | sandeepkumarpradhan71/sandeepkumar | d1d827dd6a16cedc5bf5f2e8311c5d633c46a149 | [
"Apache-2.0"
] | null | null | null | Quiz.ipynb | sandeepkumarpradhan71/sandeepkumar | d1d827dd6a16cedc5bf5f2e8311c5d633c46a149 | [
"Apache-2.0"
] | null | null | null | Quiz.ipynb | sandeepkumarpradhan71/sandeepkumar | d1d827dd6a16cedc5bf5f2e8311c5d633c46a149 | [
"Apache-2.0"
] | null | null | null | 41.932314 | 235 | 0.365894 | [
[
[
"<a href=\"https://colab.research.google.com/github/sandeepkumarpradhan71/sandeepkumar/blob/main/Quiz.ipynb\" target=\"_parent\"><img src=\"https://colab.research.google.com/assets/colab-badge.svg\" alt=\"Open In Colab\"/></a>",
"_____no_output_____"
]
],
[
[
"print('Welcome to Techno Quiz: ')\n\n\nans = input('''Ready to begin (yes/no): ''')\nscore=0\ntotal_Q=15\n\n\n\nif ans.lower() =='yes' :\n \n ans = input(''' 1.How to check your current python version ?\n A. python version\n B. python -V\n Ans:''')\n \n \n if ans.lower () == 'b' :\n score+= 1\n print('correct')\n else:\n print('Incorrect')\n\n ans = input( '''2.What is used to define a block of code in python ?\n A.Parenthesis\n B.Curly braces\n C.Indentation\n Ans:''')\n \n if ans.lower () == 'c':\n score+= 1\n print('correct')\n else:\n print('Incorrect')\n print('''Ans : C\n Explanation: Python uses indentation to define block of code.\n Indentations are simply Blank spaces or Tabs which is used as an indicator that indented code is the child part.\n As curlybraces are used in C/C++/Java.. So, Option B is correct.''')\n\n\n ans = input( '''3.All keyword in python are in\n A. Lowercase\n B. Uppercase\n C. Both uppercase & Lowercase\n Ans:''')\n \n if ans.lower () == 'c' :\n score+= 1\n print('correct')\n else:\n print('Incorrect')\n print('''Ans : C\n Explanation: All keywords in python are in lowercase except True, False and None.\n So, Option C is correct.''')\n\n\n ans = input( '''4. Dictionary keys must be immutable \n {true/false}\n Ans:''')\n \n if ans.lower () == 'true' :\n score+= 1\n print('correct')\n else:\n print('Incorrect')\n print(''' Explanation: Dictionary keys must be immutable.which means you can use strings,numbers or tuples\n as dictionary keys and you can't use any mutable object as the key such as list.''')\n\n\n ans = input('''5.Which of the following function convert a string to a float in python?\n A. int(x [,base])\n B. float(x)\n C. str(x)\n Ans:''') \n \n if ans.lower () == 'b':\n score+= 1\n print('correct')\n else:\n print('Incorrect')\n print('Explanation: float(x) โ Converts x to a floating-point number')\n\n \n ans = input( '''6. In Python, how are arguments passed?\n A. pass by value\n B. pass by reference\n C. It gives options to user to choose\n Ans:''')\n \n if ans.lower () =='b' :\n score+= 1\n print('correct')\n else:\n print('Incorrect')\n print('''Ans : B\n Explanation: All parameters (arguments) in the Python language are passed by reference.\n It means if you change what a parameter refers to within a function, the change also reflects back in the calling function''')\n\n ans = input('''7.Which function can be used on the file to display a dialog for saving a file ?\n A. Filename = savefilename()\n B. Filename = asksaveasfilename()\n C. No such option in python\n Ans:''')\n \n if ans.lower () == 'b' :\n score+= 1\n print('correct')\n else:\n print('Incorrect')\n\n ans = input( ''' 8.What command is used to shuffle a list 'L' ?\n A. L.shuffle()\n B. shuffle(L)\n C. random.Shuffle(L)\n Ans:''')\n \n if ans.lower () =='c' :\n score+= 1\n print('correct')\n else:\n print('Incorrect')\n print('''Ans : C\n Explanation: To shuffle the list we use random.shuffle(List-name)function''')\n\n ans = input( '''9.What is it called when a function is defined inside a class?\n A. method\n B. class\n C. module\n Ans:''')\n\n \n \n if ans.lower () == 'a' :\n score+= 1\n print('correct')\n else:\n print('Incorrect')\n print('''Ans : A\n Explanation: method is called when a function is defined inside a class. So, option C is correct.''')\n\n\n\n ans = input( '''10.Syntax error in python is detected by ______ at _____\n A. compiler/compile time\n B. interpreter/run time\n C. compiler/run time\n Ans:''')\n \n if ans.lower () == 'b':\n score+= 1\n print('correct')\n else:\n print('Incorrect')\n print('''Ans : B\n Explanation: Syntax error in python is detected by interpreter at run time.''')\n \n \n ans = input( '''11.Which among the following are mutable objects in Python\n (i) List\n (ii) Integer\n (iii) String \n (iv) Tuple\n\n A. i only\n B. i and ii only\n C. iii and iv only\n D. iv only\n Ans:''')\n \n if ans.lower () == 'a' :\n score+= 1\n print('correct')\n else:\n print('Incorrect') \n print('''Ans : A\n Explanation: List are mutable objects in Python.''')\n\n\n ans = input( '''12. In python what is method inside class ?\n A.attribute\n B.object\n C.function\n Ans:''')\n \n if ans.lower () =='c' :\n score+= 1\n print('correct')\n else:\n print('Incorrect')\n print('''Ans : C\n Explanation: In OOP of Python, function is known by \"method\".''')\n\n\n ans = input( '''13.The elements of a list are arranged in descending order. Which of the following two will give same outputs?\n i. print(list_name.sort())\n ii. print(max(list_name))\n iii. print(list_name.reverse())\n\n A. i, ii\n B. i, iii\n C. ii, iii\n Ans:''')\n \n if ans.lower () == 'b' :\n score+= 1\n print('correct')\n else:\n print('Incorrect')\n print('''Ans : B\n Explanation: print(list_name.sort()) and print(list_name.reverse()) will give same outputs''')\n\n\n ans = input( '''14.Which of the following is correct?\n {class A:\n def __init__(self,name):\n self.name=name\n a1=A(\"john\")\n a2=A(\"john\") }\n\n A. id(a1) and id(a2) will have same value.\n B. id(a1) and id(a2) will have different values.\n C. Two objects with same value of attribute cannot be created\n Ans:''')\n \n if ans.lower () =='b' :\n score+= 1\n print('correct')\n else:\n print('Incorrect')\n print('''Ans : B\n Explanation: Although both a1 and a2 have same value of attributes,\n but these two point to two different object.\n Hence, their id will be different.''')\n\n ans = input( '''15.Python was developed by\n A. Guido van Rossum\n B. James Gosling\n C. Dennis Ritchie\n Ans:''')\n \n if ans.lower () == 'a' :\n score+= 1\n print('correct')\n else:\n print('Incorrect')\n print('''Ans : A\n Explanation: A Dutch Programmer Guido van Rossum developed python\n at Centrum Wiskunde & Informatica (CWI) in the Netherlands as a successor to the ABC language.\n\n\n''')\n \nprint('Result :', score, \"questions correct.\")\nmarks = (score/total_Q) * 100\n\nprint (\"Marks Accqired [%] : \",marks)\n\n\nprint('''Thankyou for taking part in Techno Quiz \n Have a Nice Day!!! ''')\n",
"Welcome to Techno Quiz: \nReady to begin (yes/no): yes\n 1.How to check your current python version ?\n A. python version\n B. python -V\n Ans:b\ncorrect\n2.What is used to define a block of code in python ?\n A.Parenthesis\n B.Curly braces\n C.Indentation\n Ans:c\ncorrect\n3.All keyword in python are in\n A. Lowercase\n B. Uppercase\n C. Both uppercase & Lowercase\n Ans:c\ncorrect\n4. Dictionary keys must be immutable \n {true/false}\n Ans:true\ncorrect\n5.Which of the following function convert a string to a float in python?\n A. int(x [,base])\n B. float(x)\n C. str(x)\n Ans:float(x)\nIncorrect\nExplanation: float(x) โ Converts x to a floating-point number\n6. In Python, how are arguments passed?\n A . pass by value\n B. pass by reference\n C. It gives options to user to choose\n Ans:b\ncorrect\n7.Which function can be used on the file to display a dialog for saving a file ?\n A. Filename = savefilename()\n B. Filename = asksaveasfilename()\n C. No such option in python\n Ans:b\ncorrect\n 8.What command is used to shuffle a list 'L' ?\n A. L.shuffle()\n B. shuffle(L)\n C. random.Shuffle(L)\n Ans:a\nIncorrect\nAns : C\n Explanation: To shuffle the list we use random.shuffle(List-name)function\n9.What is it called when a function is defined inside a class?\n A.method\n B. class\n C.module\n Ans:b\nIncorrect\nAns : A\n Explanation: method is called when a function is defined inside a class. So, option C is correct.\n10.Syntax error in python is detected by ______ at _____\n A. compiler/compile time\n B.interpreter/run time\n C.compiler/run time\n Ans:b\ncorrect\n11.Which among the following are mutable objects in Python\n (i) List\n (ii) Integer\n (iii) String \n (iv) Tuple\n\n A. i only\n B. i and ii only\n C. iii and iv only\n D. iv only\n Ans:c\nIncorrect\nAns : A\n Explanation: List are mutable objects in Python.\n12. In python what is method inside class ?\n A.attribute\n B.object\n C.function\n Ans:c\ncorrect\n13.The elements of a list are arranged in descending order. Which of the following two will give same outputs?\n i. print(list_name.sort())\n ii. print(max(list_name))\n iii. print(list_name.reverse())\n\n A. i, ii\n B. i, iii\n C. ii, iii\n Ans:c\nIncorrect\nAns : B\n Explanation: print(list_name.sort()) and print(list_name.reverse()) will give same outputs\n14.Which of the following is correct?\n {class A:\n def __init__(self,name):\n self.name=name\n a1=A(\"john\")\n a2=A(\"john\") }\n\n A. id(a1) and id(a2) will have same value.\n B. id(a1) and id(a2) will have different values.\n C. Two objects with same value of attribute cannot be created\n Ans:b\ncorrect\n15.Python was developed by\n A. Guido van Rossum\n B. James Gosling\n C. Dennis Ritchie\n Ans:b\nIncorrect\nAns : A\n Explanation: A Dutch Programmer Guido van Rossum developed python\n at Centrum Wiskunde & Informatica (CWI) in the Netherlands as a successor to the ABC language.\n\n\n\nResult : 9 questions correct.\nMarks Accqired [%] : 60.0\nThankyou for taking part in Techno Quiz \n Have a Nice Day!!! \n"
],
[
"",
"_____no_output_____"
],
[
"",
"_____no_output_____"
]
]
] | [
"markdown",
"code"
] | [
[
"markdown"
],
[
"code",
"code",
"code"
]
] |
e76958bd3b226c39b22bf45b39f0701514f7b7fd | 671,304 | ipynb | Jupyter Notebook | nbs_probabilistic/07.2 - Difference of Input.ipynb | sagar-garg/WeatherBench | fdf208d9af6a896ccb012146dfef268722380a0d | [
"MIT"
] | null | null | null | nbs_probabilistic/07.2 - Difference of Input.ipynb | sagar-garg/WeatherBench | fdf208d9af6a896ccb012146dfef268722380a0d | [
"MIT"
] | 8 | 2020-04-28T08:21:21.000Z | 2020-12-08T06:07:52.000Z | nbs_probabilistic/07.2 - Difference of Input.ipynb | sagar-garg/WeatherBench | fdf208d9af6a896ccb012146dfef268722380a0d | [
"MIT"
] | null | null | null | 49.814782 | 7,460 | 0.513055 | [
[
[
"#input x, #truth y, #predict (y-x) in bins.\n\nmajor changes:\n- in Datagenerator(), add y=y-X[output_idxs]\n- in create_predictions(): when unnormalizing, only multiply with std, dont add mean\n- included adaptive bins\n\n#Observations\n- DOI takes much longer to train to same loss than normal categorical.\n- not much better performance with adaptive bins",
"_____no_output_____"
],
[
"ToDo:\n- change create_prediction() function for ensemble (not done) and binned (done) prediction. Currently x is added after predictions are made.\n- better method to get adaptive bins. Currently bins are made on 1 year data\n- unable to run compute_bin_crps() for full data. kernel dies. may load in chunks.",
"_____no_output_____"
]
],
[
[
"%load_ext autoreload\n%autoreload 2",
"_____no_output_____"
],
[
"import xarray as xr\nimport numpy as np\nimport matplotlib.pyplot as plt\nfrom src.data_generator import *\nfrom src.train import *\nfrom src.utils import *\nfrom src.networks import *",
"_____no_output_____"
],
[
"tf.__version__",
"_____no_output_____"
],
[
"import os\nimport tensorflow as tf\nos.environ[\"CUDA_VISIBLE_DEVICES\"]=str(0)\nprint(\"Num GPUs Available: \", len(tf.config.experimental.list_physical_devices('GPU')))",
"Num GPUs Available: 1\n"
],
[
"policy = mixed_precision.Policy('mixed_float16')\nmixed_precision.set_policy(policy)",
"_____no_output_____"
],
[
"args = load_args('../nn_configs/B/81.1-resnet_d3_dr_0.1.yml')\nargs['train_years']=['2017', '2017']\nargs['valid_years']=['2018-01-01','2018-03-31']\nargs['test_years']=['2018-04-01','2018-12-31']\nargs['model_save_dir'] ='/home/garg/data/WeatherBench/predictions/saved_models'\nargs['datadir']='/home/garg/data/WeatherBench/5.625deg'\nargs['is_categorical']=True",
"_____no_output_____"
],
[
"args['is_doi']=True\nargs['bin_min']=-2; args['bin_max']=2 #checked min, max of (x-y) in train.\nargs['adaptive_bins']=None\nargs['num_bins'], args['bin_min'], args['bin_max']",
"_____no_output_____"
],
[
"args['filters'] = [128, 128, 128, 128, 128, 128, 128, 128, \n 128, 128, 128, 128, 128, 128, 128, 128, \n 128, 128, 128, 128, 2*args['num_bins']]\n#args['loss'] = 'lat_categorical_loss'",
"_____no_output_____"
],
[
"dg_train, dg_valid, dg_test = load_data(**args)",
"_____no_output_____"
],
[
"x,y=dg_train[0]; print(x.shape, y.shape)\nx,y=dg_valid[0]; print(x.shape, y.shape) \nx,y=dg_test[0]; print(x.shape, y.shape)\n#changing valid shape too. maybe not a good idea/not needed.",
"(32, 32, 64, 114) (32, 32, 64, 2, 50)\n(32, 32, 64, 114) (32, 32, 64, 2, 50)\n(32, 32, 64, 114) (32, 32, 64, 2, 50)\n"
],
[
"y.min(), y.max(), y[0,0,0,0,:]",
"_____no_output_____"
]
],
[
[
"# Training",
"_____no_output_____"
]
],
[
[
"# model = build_resnet_categorical(\n# **args, input_shape=dg_train.shape,\n# )\n# # model.summary()\n\n# categorical_loss = create_lat_categorical_loss(dg_train.data.lat, 2)\n# model.compile(keras.optimizers.Adam(1e-3), loss=categorical_loss)\n# model_history=model.fit(dg_train, epochs=50)\n",
"_____no_output_____"
],
[
"#training is slower compared to normal categorical without DOI",
"_____no_output_____"
],
[
"# #exp_id=args['exp_id']\n# exp_id='categorical_doi_v1'\n# model_save_dir=args['model_save_dir']\n\n# model.save(f'{model_save_dir}/{exp_id}.h5')\n# model.save_weights(f'{model_save_dir}/{exp_id}_weights.h5')\n\n# #to_pickle(model_history.history, f'{model_save_dir}/{exp_id}_history.pkl')",
"_____no_output_____"
],
[
"# checking training",
"_____no_output_____"
],
[
"# # list all data in history\n# print(history.history.keys())\n# # summarize history for accuracy\n# plt.plot(history.history['accuracy'])\n# plt.plot(history.history['val_accuracy'])\n# plt.title('model accuracy')\n# plt.ylabel('accuracy')\n# plt.xlabel('epoch')\n# plt.legend(['train', 'test'], loc='upper left')\n# plt.show()\n# # summarize history for loss\n# plt.plot(history.history['loss'])\n# plt.plot(history.history['val_loss'])\n# plt.title('model loss')\n# plt.ylabel('loss')\n# plt.xlabel('epoch')\n# plt.legend(['train', 'test'], loc='upper left')\n# plt.show()",
"_____no_output_____"
]
],
[
[
"# Predictions",
"_____no_output_____"
]
],
[
[
"exp_id='categorical_doi_v1'\nmodel_save_dir=args['model_save_dir']",
"_____no_output_____"
],
[
"#args['ext_mean'] = xr.open_dataarray(f'{args[\"model_save_dir\"]}/{args[\"exp_id\"]}_mean.nc')\n#args['ext_std'] = xr.open_dataarray(f'{args[\"model_save_dir\"]}/{args[\"exp_id\"]}_std.nc')\n#dg_test = load_data(**args, only_test=True)",
"_____no_output_____"
],
[
"model = keras.models.load_model(\n f'{model_save_dir}/{exp_id}.h5',\n custom_objects={'PeriodicConv2D': PeriodicConv2D, 'categorical_loss': keras.losses.mse}\n)",
"_____no_output_____"
],
[
"# #small test\n# xtrue ,ytrue=dg_valid[0]\n# ypred=model.predict(xtrue)\n# print(ytrue.shape, ytrue.max(),ytrue.min(), ytrue.mean())\n# print(ypred.shape, ypred.max(),ypred.min(), ypred.mean())\n# print(xtrue[...,12].min(), xtrue[...,12].max(), xtrue[...,12].mean(), xtrue.shape)",
"_____no_output_____"
],
[
"#full-data (apr-dec 2018)\npreds = create_predictions(model, dg_test, is_categorical=True, \n is_doi=True, adaptive_bins=None,\n bin_min=args['bin_min'], bin_max=args['bin_max'])\npreds\n#maybe add bin_min, bin_max, is_doi, to **kwargs in function.",
"_____no_output_____"
],
[
"#extremely small values may increase numerical error? (x-y)\npreds.t.min(), preds.t.max(), preds.t.mean()",
"_____no_output_____"
],
[
"preds.t.bin_edges",
"_____no_output_____"
],
[
"#attempt 1: add actual x values to prediction\n#attempt 2: add unnormalized x (using mean, std of dg_test) -no need.",
"_____no_output_____"
],
[
"#attempt 1\ndatadir=args['datadir']\nz500_valid = load_test_data(f'{datadir}/geopotential_500', 'z').drop('level')\nt850_valid = load_test_data(f'{datadir}/temperature_850', 't').drop('level')\nvalid = xr.merge([z500_valid, t850_valid])\nvalid",
"_____no_output_____"
],
[
"dg_test.lead_time",
"_____no_output_____"
],
[
"# valid_x=valid.sel(time=preds.time-np.timedelta64(3,'D'))\n# valid_y=valid.sel(time=preds.time)",
"_____no_output_____"
],
[
"#Shifting left by 72 hours. Now this value can be added to preds.\nvalid_x_new=valid.shift(time=-dg_test.lead_time)\nvalid_x_new",
"_____no_output_____"
],
[
"print(\nvalid.t.isel(lat=0,lon=0).sel(time='2017-01-01T00:00:00').values,\nvalid.t.isel(lat=0,lon=0).sel(time='2017-01-04T00:00:00').values,\nvalid_x_new.t.isel(lat=0,lon=0).sel(time='2017-01-01T00:00:00').values)\n#valid.t.isel(lat=0,lon=0,time=72).values,valid_x_new.t.isel(lat=0,lon=0,time=0).values",
"257.84134 258.57373 258.57373\n"
]
],
[
[
"# Most likely class",
"_____no_output_____"
]
],
[
[
"# Using bin_mid_points of prediction with highest probability\ndas = []\nfor var in ['z', 't']:\n idxs = np.argmax(preds[var], -1)\n most_likely = preds[var].mid_points[idxs]\n das.append(xr.DataArray(\n most_likely, dims=['time', 'lat', 'lon'],\n coords = [preds.time, preds.lat, preds.lon],\n name=var\n ))\npreds_ml = xr.merge(das)\n\npreds_ml",
"_____no_output_____"
],
[
"preds_ml_new=preds_ml+valid.shift(time=-dg_test.lead_time)",
"_____no_output_____"
],
[
"#be careful of last points (2018-12-28) to 2018-12-31. \n#they must contain nan values\npreds_ml_new.t.isel(time=-36).values\n#preds_new.t.sel(time='2018-12-28T22:00:00').values",
"_____no_output_____"
],
[
"#removing last 3 days (naive approach)\npreds_ml_new=preds_ml_new.sel(time=slice(None,'2018-12-28T22:00:00'))",
"_____no_output_____"
],
[
"preds_ml_new.t.max().values, preds_ml_new.t.min().values, preds_ml_new.t.mean().values",
"_____no_output_____"
],
[
"valid.t.max().values, valid.t.min().values, valid.t.mean().values",
"_____no_output_____"
]
],
[
[
"# RMSE",
"_____no_output_____"
]
],
[
[
"compute_weighted_rmse(preds_ml_new, valid).load()\n#still very bad. for comparison, training on the same data for same epochs (loss=1.7) without difference to input method had rmse of 685 ",
"_____no_output_____"
]
],
[
[
"# Binned CRPS",
"_____no_output_____"
]
],
[
[
"preds['t'].mid_points",
"_____no_output_____"
],
[
"#changing Observation directly instead of predictions for binned crps\nobs=valid-valid.shift(time=-dg_test.lead_time)\nobs=obs.sel(time=preds.time) #reducing to preds size\nobs=obs.sel(time=slice(None,'2018-12-28T22:00:00'))#removing nan values\n",
"_____no_output_____"
],
[
"print(\nvalid.t.isel(lat=0,lon=0).sel(time='2018-05-05T22:00:00').values,\nvalid_x_new.t.isel(lat=0,lon=0).sel(time='2018-05-05T22:00:00').values,\nobs.t.isel(lat=0,lon=0).sel(time='2018-05-05T22:00:00').values\n)",
"240.97852 246.35167 -5.3731537\n"
],
[
"obs #reduced set. 2018-01-04 to 2018-12-28",
"_____no_output_____"
],
[
"def compute_bin_crps(obs, preds, bin_edges):\n \"\"\"\n Last axis must be bin axis\n obs: [...]\n preds: [..., n_bins]\n \"\"\"\n obs = obs.values\n preds = preds.values\n # Convert observation\n a = np.minimum(bin_edges[1:], obs[..., None])\n b = bin_edges[:-1] * (bin_edges[0:-1] > obs[..., None])\n y = np.maximum(a, b)\n # Convert predictions to cumulative predictions with a zero at the beginning\n cum_preds = np.cumsum(preds, -1)\n cum_preds_zero = np.concatenate([np.zeros((*cum_preds.shape[:-1], 1)), cum_preds], -1)\n xmin = bin_edges[..., :-1]\n xmax = bin_edges[..., 1:]\n lmass = cum_preds_zero[..., :-1]\n umass = 1 - cum_preds_zero[..., 1:]\n# y = np.atleast_1d(y)\n# xmin, xmax = np.atleast_1d(xmin), np.atleast_1d(xmax)\n# lmass, lmass = np.atleast_1d(lmass), np.atleast_1d(lmass)\n scale = xmax - xmin\n# print('scale =', scale)\n y_scale = (y - xmin) / scale\n# print('y_scale = ', y_scale)\n \n z = y_scale.copy()\n z[z < 0] = 0\n z[z > 1] = 1\n# print('z =', z)\n a = 1 - (lmass + umass)\n# print('a =', a)\n crps = (\n np.abs(y_scale - z) + z**2 * a - z * (1 - 2*lmass) + \n a**2 / 3 + (1 - lmass) * umass\n )\n return np.sum(scale * crps, -1)",
"_____no_output_____"
],
[
"def compute_weighted_bin_crps(da_fc, da_true, mean_dims=xr.ALL_DIMS):\n \"\"\"\n \"\"\"\n t = np.intersect1d(da_fc.time, da_true.time)\n da_fc, da_true = da_fc.sel(time=t), da_true.sel(time=t)\n weights_lat = np.cos(np.deg2rad(da_true.lat))\n weights_lat /= weights_lat.mean()\n dims = ['time', 'lat', 'lon']\n if type(da_true) is xr.Dataset:\n das = []\n for var in da_true:\n result = compute_bin_crps(da_true[var], da_fc[var], da_fc[var].bin_edges)\n das.append(xr.DataArray(\n result, dims=dims, coords=dict(da_true.coords), name=var\n ))\n crps = xr.merge(das)\n else:\n result = compute_bin_crps(da_true, da_fc, da_fc.bin_edges)\n crps = xr.DataArray(\n result, dims=dims, coords=dict(da_true.coords), name=da_fc.name\n )\n crps = (crps * weights_lat).mean(mean_dims)\n return crps",
"_____no_output_____"
],
[
"obs1 = obs.sel(time='2018-05-05')\npreds1 = preds.sel(time='2018-05-05')",
"_____no_output_____"
],
[
"compute_weighted_bin_crps(preds1, obs1).load()",
"_____no_output_____"
],
[
"#pretty bad again.",
"_____no_output_____"
]
],
[
[
"# compare to - Adaptive binning",
"_____no_output_____"
],
[
"# Adaptive binning",
"_____no_output_____"
]
],
[
[
"#Finding bin edges on full 1 year training data (Not possible for 40 years)\nargs['is_categorical']=False\ndg_train, dg_valid, dg_test = load_data(**args)\nargs['is_categorical']=True\n\nx,y=dg_train[0]; print(x.shape, y.shape)\ndiff=y-x[...,dg_train.output_idxs]\nprint(diff.min(), diff.max(), diff.mean())\nplt.hist(diff.reshape(-1))",
"(32, 32, 64, 114) (32, 32, 64, 2)\n-1.9876502 1.9121759 0.001743055\n"
],
[
"diff=[]\nfor x,y in dg_train:\n diff.append(y-x[...,dg_train.output_idxs])\ndiff = np.array([ elem for singleList in diff for elem in singleList])\ndiff.shape",
"_____no_output_____"
],
[
"diff_shape=diff.shape\ndiff2, bins=pd.qcut(diff.reshape(-1), args['num_bins'], \n labels=False, retbins=True)",
"_____no_output_____"
],
[
"bins",
"_____no_output_____"
],
[
"args['is_doi']=True\nargs['bin_min']=bins[0]; args['bin_max']=bins[-1]\nargs['adaptive_bins']=bins\nargs['num_bins'], args['bin_min'], args['bin_max']",
"_____no_output_____"
],
[
"#args",
"_____no_output_____"
],
[
"dg_train, dg_valid, dg_test = load_data(**args)",
"_____no_output_____"
],
[
"x,y=dg_train[0]; print(x.shape, y.shape)\nx,y=dg_valid[0]; print(x.shape, y.shape) \nx,y=dg_test[0]; print(x.shape, y.shape)\n#changing valid shape too. maybe not a good idea.",
"(32, 32, 64, 114) (32, 32, 64, 2, 50)\n(32, 32, 64, 114) (32, 32, 64, 2, 50)\n(32, 32, 64, 114) (32, 32, 64, 2, 50)\n"
],
[
"y.min(), y.max(), y[0,0,0,0,:]",
"_____no_output_____"
],
[
"x[0,0,0,0]",
"_____no_output_____"
],
[
"#checking if data generator worked\nidxs = np.argmax(y, -1)\nplt.hist(idxs[...,0].reshape(-1))",
"_____no_output_____"
],
[
"# #compare distribution to non-adaptive.\n# args['bins']=None; args['bin_min']=-2; args['bin_max']=2\n# dg_train, dg_valid, dg_test = load_data(**args)\n# x,y=dg_test[0]; print(x.shape, y.shape)\n# #remember y is not same bcoz of shuffle in train. so use test.",
"(32, 32, 64, 114) (32, 32, 64, 2, 50)\n"
],
[
"x[0,0,0,0]",
"_____no_output_____"
],
[
"idxs = np.argmax(y, -1)\nplt.hist(idxs[...,0].reshape(-1))",
"_____no_output_____"
],
[
"#so different distributions with adaptive/non-adaptive.",
"_____no_output_____"
]
],
[
[
"# Training for adaptive bins",
"_____no_output_____"
]
],
[
[
"# model2 = build_resnet_categorical(\n# **args, input_shape=dg_train.shape,\n# )\n# # model.summary()\n\n# categorical_loss = create_lat_categorical_loss(dg_train.data.lat, 2)\n# model2.compile(keras.optimizers.Adam(1e-3), loss=categorical_loss)\n\n\n# model_history=model2.fit(dg_train, epochs=50)",
"Epoch 1/50\n136/136 [==============================] - 49s 361ms/step - loss: 6.5560\nEpoch 2/50\n136/136 [==============================] - 50s 367ms/step - loss: 4.6104\nEpoch 3/50\n136/136 [==============================] - 51s 376ms/step - loss: 4.3396\nEpoch 4/50\n136/136 [==============================] - 51s 374ms/step - loss: 4.1535\nEpoch 5/50\n136/136 [==============================] - 51s 373ms/step - loss: 4.1815\nEpoch 6/50\n136/136 [==============================] - 51s 374ms/step - loss: 4.0734\nEpoch 7/50\n136/136 [==============================] - 51s 372ms/step - loss: 3.8996\nEpoch 8/50\n136/136 [==============================] - 51s 373ms/step - loss: 3.7947\nEpoch 9/50\n136/136 [==============================] - 51s 376ms/step - loss: 3.7118\nEpoch 10/50\n136/136 [==============================] - 51s 376ms/step - loss: 3.5961\nEpoch 11/50\n136/136 [==============================] - 50s 371ms/step - loss: 3.5001\nEpoch 12/50\n136/136 [==============================] - 51s 378ms/step - loss: 3.4141\nEpoch 13/50\n136/136 [==============================] - 52s 381ms/step - loss: 3.3114\nEpoch 14/50\n136/136 [==============================] - 51s 374ms/step - loss: 3.2153\nEpoch 15/50\n136/136 [==============================] - 51s 376ms/step - loss: 3.1381\nEpoch 16/50\n136/136 [==============================] - 51s 378ms/step - loss: 3.0896\nEpoch 17/50\n136/136 [==============================] - 51s 375ms/step - loss: 3.0259\nEpoch 18/50\n136/136 [==============================] - 51s 374ms/step - loss: 2.9567\nEpoch 19/50\n136/136 [==============================] - 51s 376ms/step - loss: 2.8986\nEpoch 20/50\n136/136 [==============================] - 51s 375ms/step - loss: 2.8602\nEpoch 21/50\n136/136 [==============================] - 51s 377ms/step - loss: 2.8153\nEpoch 22/50\n136/136 [==============================] - 51s 377ms/step - loss: 2.7757\nEpoch 23/50\n136/136 [==============================] - 51s 377ms/step - loss: 2.7431\nEpoch 24/50\n136/136 [==============================] - 51s 375ms/step - loss: 2.7130\nEpoch 25/50\n136/136 [==============================] - 51s 376ms/step - loss: 2.6858\nEpoch 26/50\n136/136 [==============================] - 51s 376ms/step - loss: 2.6713\nEpoch 27/50\n136/136 [==============================] - 51s 375ms/step - loss: 2.6361\nEpoch 28/50\n136/136 [==============================] - 51s 376ms/step - loss: 2.6132\nEpoch 29/50\n136/136 [==============================] - 51s 378ms/step - loss: 2.5988\nEpoch 30/50\n136/136 [==============================] - 51s 376ms/step - loss: 2.5775\nEpoch 31/50\n136/136 [==============================] - 51s 376ms/step - loss: 2.5693\nEpoch 32/50\n136/136 [==============================] - 51s 375ms/step - loss: 2.5469\nEpoch 33/50\n136/136 [==============================] - 51s 374ms/step - loss: 2.5441\nEpoch 34/50\n136/136 [==============================] - 51s 374ms/step - loss: 2.5184\nEpoch 35/50\n136/136 [==============================] - 51s 373ms/step - loss: 2.5093\nEpoch 36/50\n136/136 [==============================] - 51s 374ms/step - loss: 2.4929\nEpoch 37/50\n136/136 [==============================] - 51s 372ms/step - loss: 2.4852\nEpoch 38/50\n136/136 [==============================] - 51s 375ms/step - loss: 2.4829\nEpoch 39/50\n136/136 [==============================] - 51s 374ms/step - loss: 2.4620\nEpoch 40/50\n136/136 [==============================] - 51s 375ms/step - loss: 2.4501\nEpoch 41/50\n136/136 [==============================] - 51s 375ms/step - loss: 2.4444\nEpoch 42/50\n136/136 [==============================] - 51s 375ms/step - loss: 2.4382\nEpoch 43/50\n136/136 [==============================] - 51s 376ms/step - loss: 2.4270\nEpoch 44/50\n136/136 [==============================] - 51s 376ms/step - loss: 2.4236\nEpoch 45/50\n136/136 [==============================] - 51s 372ms/step - loss: 2.4164\nEpoch 46/50\n136/136 [==============================] - 51s 372ms/step - loss: 2.4103\nEpoch 47/50\n136/136 [==============================] - 51s 376ms/step - loss: 2.4036\nEpoch 48/50\n136/136 [==============================] - 51s 378ms/step - loss: 2.3950\nEpoch 49/50\n136/136 [==============================] - 51s 374ms/step - loss: 2.3972\nEpoch 50/50\n136/136 [==============================] - 51s 374ms/step - loss: 2.3860\n"
],
[
"# exp_id='categorical_doi_adaptive_bins_v1'\n# model_save_dir=args['model_save_dir']\n\n# model2.save(f'{model_save_dir}/{exp_id}.h5')\n# model2.save_weights(f'{model_save_dir}/{exp_id}_weights.h5')\n\n# to_pickle(model_history.history, f'{model_save_dir}/{exp_id}_history.pkl')",
"_____no_output_____"
],
[
"# checking training",
"_____no_output_____"
],
[
"# # list all data in history\n# print(history.history.keys())\n# # summarize history for accuracy\n# plt.plot(history.history['accuracy'])\n# plt.plot(history.history['val_accuracy'])\n# plt.title('model accuracy')\n# plt.ylabel('accuracy')\n# plt.xlabel('epoch')\n# plt.legend(['train', 'test'], loc='upper left')\n# plt.show()\n# # summarize history for loss\n# plt.plot(history.history['loss'])\n# plt.plot(history.history['val_loss'])\n# plt.title('model loss')\n# plt.ylabel('loss')\n# plt.xlabel('epoch')\n# plt.legend(['train', 'test'], loc='upper left')\n# plt.show()",
"_____no_output_____"
]
],
[
[
"# Predictions for Adaptive bins",
"_____no_output_____"
]
],
[
[
"exp_id='categorical_doi_adaptive_bins_v1'\nmodel_save_dir=args['model_save_dir']\nmodel2 = keras.models.load_model(\n f'{model_save_dir}/{exp_id}.h5',\n custom_objects={'PeriodicConv2D': PeriodicConv2D, 'categorical_loss': keras.losses.mse}\n)",
"_____no_output_____"
],
[
"#args",
"_____no_output_____"
],
[
"#full-data (apr-dec 2018)\npreds = create_predictions(model2, dg_test, is_categorical=True, is_doi=True,\n bin_min=args['bin_min'], bin_max=args['bin_max'],\n adaptive_bins=bins)\npreds",
"_____no_output_____"
],
[
"#extremely small values may increase numerical error? (x-y)\npreds.t.min(), preds.t.max(), preds.t.mean()",
"_____no_output_____"
],
[
"preds.t.bin_edges\n#surprisingly end points are much larger than with non-adaptive. will Check!!",
"_____no_output_____"
]
],
[
[
"# Most likely class",
"_____no_output_____"
]
],
[
[
"# Using bin_mid_points of prediction with highest probability\ndas = []\nfor var in ['z', 't']:\n idxs = np.argmax(preds[var], -1)\n most_likely = preds[var].mid_points[idxs]\n das.append(xr.DataArray(\n most_likely, dims=['time', 'lat', 'lon'],\n coords = [preds.time, preds.lat, preds.lon],\n name=var\n ))\npreds_ml = xr.merge(das)\n\npreds_ml",
"_____no_output_____"
],
[
"datadir=args['datadir']\nz500_valid = load_test_data(f'{datadir}/geopotential_500', 'z').drop('level')\nt850_valid = load_test_data(f'{datadir}/temperature_850', 't').drop('level')\nvalid = xr.merge([z500_valid, t850_valid])\nvalid",
"_____no_output_____"
],
[
"preds_ml_new=preds_ml+valid.shift(time=-dg_test.lead_time)",
"_____no_output_____"
],
[
"#be careful of last points (2018-12-28) to 2018-12-31. \n#they must contain nan values\npreds_ml_new.t.isel(time=-36).values\n#preds_new.t.sel(time='2018-12-28T22:00:00').values",
"_____no_output_____"
],
[
"#removing last 3 days (naive approach)\npreds_ml_new=preds_ml_new.sel(time=slice(None,'2018-12-28T22:00:00'))",
"_____no_output_____"
],
[
"preds_ml_new.t.max().values, preds_ml_new.t.min().values, preds_ml_new.t.mean().values\n#edges are more extreme!",
"_____no_output_____"
],
[
"valid.t.max().values, valid.t.min().values, valid.t.mean().values",
"_____no_output_____"
]
],
[
[
"# RMSE",
"_____no_output_____"
]
],
[
[
"compute_weighted_rmse(preds_ml_new, valid).load()",
"_____no_output_____"
],
[
"#almost same as non-adaptive. loss comparable (~2.9 for no-adaptive. ~2.3 for adaptive)",
"_____no_output_____"
]
],
[
[
"# Binned CRPS",
"_____no_output_____"
]
],
[
[
"preds['t'].mid_points",
"_____no_output_____"
],
[
"#changing Observation directly instead of predictions for binned crps\nobs=valid-valid.shift(time=-dg_test.lead_time)\nobs=obs.sel(time=preds.time) #reducing to preds size\nobs=obs.sel(time=slice(None,'2018-12-28T22:00:00'))#removing nan values\n",
"_____no_output_____"
],
[
"print(\nvalid.t.isel(lat=0,lon=0).sel(time='2018-05-05T22:00:00').values,\nvalid_x_new.t.isel(lat=0,lon=0).sel(time='2018-05-05T22:00:00').values,\nobs.t.isel(lat=0,lon=0).sel(time='2018-05-05T22:00:00').values\n)",
"240.97852 246.35167 -5.3731537\n"
],
[
"obs #reduced set. 2018-01-04 to 2018-12-28",
"_____no_output_____"
],
[
"obs1 = obs.sel(time='2018-05-05')\npreds1 = preds.sel(time='2018-05-05')",
"_____no_output_____"
],
[
"compute_weighted_bin_crps(preds1, obs1).load()",
"_____no_output_____"
],
[
"#pretty bad again.",
"_____no_output_____"
]
],
[
[
"# comparing to - without input difference",
"_____no_output_____"
]
],
[
[
"from src.data_generator import *",
"_____no_output_____"
],
[
"args['bin_min']=-5; args['bin_max']=5 #checked min, max of (x-y) in train.\nargs['num_bins'], args['bin_min'], args['bin_max']\ndg_train, dg_valid, dg_test = load_data(**args)",
"_____no_output_____"
],
[
"x,y=dg_train[0]; print(x.shape, y.shape)\nx,y=dg_valid[0]; print(x.shape, y.shape) \nx,y=dg_test[0]; print(x.shape, y.shape)",
"(32, 32, 64, 114) (32, 32, 64, 2, 50)\n(32, 32, 64, 114) (32, 32, 64, 2, 50)\n(32, 32, 64, 114) (32, 32, 64, 2, 50)\n"
],
[
"y[0,0,0,0,:]",
"_____no_output_____"
],
[
"args['bin_min']",
"_____no_output_____"
],
[
"model = build_resnet_categorical(\n **args, input_shape=dg_train.shape,\n)\n# model.summary()",
"_____no_output_____"
],
[
"categorical_loss = create_lat_categorical_loss(dg_train.data.lat, 2)\nmodel.compile(keras.optimizers.Adam(1e-3), loss=categorical_loss)\n",
"_____no_output_____"
],
[
"model.fit(dg_train, epochs=30)",
"Epoch 1/30\n136/136 [==============================] - 48s 355ms/step - loss: 5.3799\nEpoch 2/30\n136/136 [==============================] - 50s 369ms/step - loss: 3.4690\nEpoch 3/30\n136/136 [==============================] - 51s 377ms/step - loss: 3.2071\nEpoch 4/30\n136/136 [==============================] - 51s 373ms/step - loss: 3.1679\nEpoch 5/30\n136/136 [==============================] - 51s 377ms/step - loss: 3.0492\nEpoch 6/30\n136/136 [==============================] - 51s 378ms/step - loss: 2.9614\nEpoch 7/30\n136/136 [==============================] - 51s 377ms/step - loss: 2.9988\nEpoch 8/30\n136/136 [==============================] - 51s 373ms/step - loss: 2.8479\nEpoch 9/30\n136/136 [==============================] - 51s 376ms/step - loss: 2.7417\nEpoch 10/30\n136/136 [==============================] - 51s 373ms/step - loss: 2.6448\nEpoch 11/30\n136/136 [==============================] - 51s 377ms/step - loss: 2.6447\nEpoch 12/30\n136/136 [==============================] - 51s 376ms/step - loss: 2.5350\nEpoch 13/30\n136/136 [==============================] - 51s 375ms/step - loss: 2.4273\nEpoch 14/30\n136/136 [==============================] - 51s 375ms/step - loss: 2.3338\nEpoch 15/30\n136/136 [==============================] - 51s 373ms/step - loss: 2.2495\nEpoch 16/30\n136/136 [==============================] - 51s 374ms/step - loss: 2.1838\nEpoch 17/30\n136/136 [==============================] - 51s 377ms/step - loss: 2.1101\nEpoch 18/30\n136/136 [==============================] - 51s 374ms/step - loss: 2.0579\nEpoch 19/30\n136/136 [==============================] - 51s 376ms/step - loss: 2.0042\nEpoch 20/30\n136/136 [==============================] - 51s 375ms/step - loss: 1.9572\nEpoch 21/30\n136/136 [==============================] - 51s 377ms/step - loss: 1.9107\nEpoch 22/30\n136/136 [==============================] - 51s 374ms/step - loss: 1.8809\nEpoch 23/30\n136/136 [==============================] - 50s 371ms/step - loss: 1.8459\nEpoch 24/30\n136/136 [==============================] - 51s 373ms/step - loss: 1.8228\nEpoch 25/30\n136/136 [==============================] - 51s 377ms/step - loss: 1.8053\nEpoch 26/30\n136/136 [==============================] - 51s 373ms/step - loss: 1.7731\nEpoch 27/30\n136/136 [==============================] - 51s 374ms/step - loss: 1.7553\nEpoch 28/30\n136/136 [==============================] - 51s 374ms/step - loss: 1.7345\nEpoch 29/30\n136/136 [==============================] - 51s 377ms/step - loss: 1.7127\nEpoch 30/30\n136/136 [==============================] - 51s 376ms/step - loss: 1.7002\n"
],
[
"#Much faster training.",
"_____no_output_____"
],
[
"#small test",
"_____no_output_____"
],
[
"xtrue ,ytrue=dg_valid[0]\nypred=model.predict(xtrue)",
"_____no_output_____"
],
[
"ytrue.shape, ytrue.max(),ytrue.min(), ytrue.mean()",
"_____no_output_____"
],
[
"ypred.shape, ypred.max(),ypred.min(), ypred.mean()",
"_____no_output_____"
],
[
"#apr-dec",
"_____no_output_____"
],
[
"preds = create_predictions(model, dg_test, is_categorical=True)",
"_____no_output_____"
],
[
"preds",
"_____no_output_____"
],
[
"preds.t.min(), preds.t.max(), preds.t.mean()",
"_____no_output_____"
],
[
"idxs = np.argmax(preds.t.isel(time=0), -1)",
"_____no_output_____"
],
[
"mp = preds.t.mid_points",
"_____no_output_____"
],
[
"# Most likely bin\nplt.matshow(mp[idxs])\nplt.colorbar();",
"_____no_output_____"
],
[
"# Let's do this for all times and compute the RMSE\ndas = []\nfor var in ['z', 't']:\n idxs = np.argmax(preds[var], -1)\n most_likely = preds[var].mid_points[idxs]\n das.append(xr.DataArray(\n most_likely, dims=['time', 'lat', 'lon'],\n coords = [preds.time, preds.lat, preds.lon],\n name=var\n ))\npreds_ml = xr.merge(das)",
"_____no_output_____"
],
[
"preds_ml",
"_____no_output_____"
],
[
"datadir=args['datadir']\nz500_valid = load_test_data(f'{datadir}/geopotential_500', 'z').drop('level')\nt850_valid = load_test_data(f'{datadir}/temperature_850', 't').drop('level')\nvalid = xr.merge([z500_valid, t850_valid])",
"_____no_output_____"
],
[
"valid=valid.sel(time=preds_ml.time)",
"_____no_output_____"
],
[
"compute_weighted_rmse(preds_ml, valid).load()",
"_____no_output_____"
],
[
"preds.t.bin_width / 2",
"_____no_output_____"
],
[
"preds.t.isel(time=0).max('bin').plot()",
"_____no_output_____"
],
[
"plt.bar(preds.t.mid_points, preds.t.isel(time=0).sel(lat=50, lon=300, method='nearest'), preds.t.bin_width)\nplt.bar(preds.t.mid_points, preds.t.isel(time=0).sel(lat=0, lon=150, method='nearest'), preds.t.bin_width)",
"_____no_output_____"
],
[
"plt.bar(preds.t.mid_points, preds.t.isel(time=0).sel(lat=50, lon=20, method='nearest'), preds.t.bin_width)\nplt.bar(preds.t.mid_points, preds.t.isel(time=0).sel(lat=0, lon=0, method='nearest'), preds.t.bin_width)",
"_____no_output_____"
]
],
[
[
"## Binned CRPS",
"_____no_output_____"
]
],
[
[
"def compute_bin_crps(obs, preds, bin_edges):\n \"\"\"\n Last axis must be bin axis\n obs: [...]\n preds: [..., n_bins]\n \"\"\"\n obs = obs.values\n preds = preds.values\n # Convert observation\n a = np.minimum(bin_edges[1:], obs[..., None])\n b = bin_edges[:-1] * (bin_edges[0:-1] > obs[..., None])\n y = np.maximum(a, b)\n # Convert predictions to cumulative predictions with a zero at the beginning\n cum_preds = np.cumsum(preds, -1)\n cum_preds_zero = np.concatenate([np.zeros((*cum_preds.shape[:-1], 1)), cum_preds], -1)\n xmin = bin_edges[..., :-1]\n xmax = bin_edges[..., 1:]\n lmass = cum_preds_zero[..., :-1]\n umass = 1 - cum_preds_zero[..., 1:]\n# y = np.atleast_1d(y)\n# xmin, xmax = np.atleast_1d(xmin), np.atleast_1d(xmax)\n# lmass, lmass = np.atleast_1d(lmass), np.atleast_1d(lmass)\n scale = xmax - xmin\n# print('scale =', scale)\n y_scale = (y - xmin) / scale\n# print('y_scale = ', y_scale)\n \n z = y_scale.copy()\n z[z < 0] = 0\n z[z > 1] = 1\n# print('z =', z)\n a = 1 - (lmass + umass)\n# print('a =', a)\n crps = (\n np.abs(y_scale - z) + z**2 * a - z * (1 - 2*lmass) + \n a**2 / 3 + (1 - lmass) * umass\n )\n return np.sum(scale * crps, -1)",
"_____no_output_____"
],
[
"def compute_weighted_bin_crps(da_fc, da_true, mean_dims=xr.ALL_DIMS):\n \"\"\"\n \"\"\"\n t = np.intersect1d(da_fc.time, da_true.time)\n da_fc, da_true = da_fc.sel(time=t), da_true.sel(time=t)\n weights_lat = np.cos(np.deg2rad(da_true.lat))\n weights_lat /= weights_lat.mean()\n dims = ['time', 'lat', 'lon']\n if type(da_true) is xr.Dataset:\n das = []\n for var in da_true:\n result = compute_bin_crps(da_true[var], da_fc[var], da_fc[var].bin_edges)\n das.append(xr.DataArray(\n result, dims=dims, coords=dict(da_true.coords), name=var\n ))\n crps = xr.merge(das)\n else:\n result = compute_bin_crps(da_true, da_fc, da_fc.bin_edges)\n crps = xr.DataArray(\n result, dims=dims, coords=dict(da_true.coords), name=da_fc.name\n )\n crps = (crps * weights_lat).mean(mean_dims)\n return crps",
"_____no_output_____"
],
[
"valid",
"_____no_output_____"
],
[
"compute_weighted_bin_crps(preds, valid)",
"_____no_output_____"
],
[
"# Ignore below",
"_____no_output_____"
]
],
[
[
"# Adaptive binning",
"_____no_output_____"
]
],
[
[
"args['is_categorical']=False\ndg_train, dg_valid, dg_test = load_data(**args)\nargs['is_categorical']=True\nx,y=dg_train[0]; print(x.shape, y.shape)\n\ndiff=y-x[...,dg_train.output_idxs]\n\nprint(diff.min(), diff.max(), diff.mean())\n\nplt.hist(diff.reshape(-1))",
"_____no_output_____"
],
[
"diff=[]\nfor x,y in dg_train:\n diff.append(y-x[...,dg_train.output_idxs])",
"_____no_output_____"
],
[
"diff = np.array([ elem for singleList in diff for elem in singleList])",
"_____no_output_____"
],
[
"diff.shape",
"_____no_output_____"
],
[
"diff_shape=diff.shape\ndiff2, bins=pd.qcut(diff.reshape(-1), args['num_bins'], \n labels=False, retbins=True)",
"_____no_output_____"
],
[
"diff2=diff2.reshape(diff_shape)",
"_____no_output_____"
],
[
"diff2.shape, diff2.max(), diff2.min(), diff2.mean()",
"_____no_output_____"
],
[
"bins",
"_____no_output_____"
],
[
"diff2=np_utils.to_categorical(diff2, num_classes=args['num_bins'])",
"_____no_output_____"
],
[
"diff2.shape, diff2.max(), diff2.min(), diff2.mean()",
"_____no_output_____"
],
[
"diff3=diff\ndiff3_shape=diff3.shape\ndiff3.shape",
"_____no_output_____"
],
[
"diff3=pd.cut(diff3.reshape(-1), bins, labels=False).reshape(diff3_shape)",
"_____no_output_____"
],
[
"diff3.shape",
"_____no_output_____"
],
[
"diff3",
"_____no_output_____"
],
[
"diff3.shape, diff3.max(), diff3.min(), diff3.mean()",
"_____no_output_____"
],
[
"diff3[:,:,:,1].min()",
"_____no_output_____"
],
[
"np.argwhere(np.isnan(diff3))",
"_____no_output_____"
],
[
"diff[1743,25,49,1]",
"_____no_output_____"
]
],
[
[
"# Unnormalized Data",
"_____no_output_____"
]
],
[
[
"a1=np.arange(100)\nmean=np.mean(a1); std=np.std(a1)",
"_____no_output_____"
],
[
"a1_norm=(a1-mean)/std",
"_____no_output_____"
],
[
"a1_norm",
"_____no_output_____"
],
[
"a1[4]-a1[2]",
"_____no_output_____"
],
[
"diff=a1_norm[4]-a1_norm[2]\ndiff",
"_____no_output_____"
],
[
"diff*std",
"_____no_output_____"
]
]
] | [
"markdown",
"code",
"markdown",
"code",
"markdown",
"code",
"markdown",
"code",
"markdown",
"code",
"markdown",
"code",
"markdown",
"code",
"markdown",
"code",
"markdown",
"code",
"markdown",
"code",
"markdown",
"code",
"markdown",
"code",
"markdown",
"code",
"markdown",
"code",
"markdown",
"code",
"markdown",
"code"
] | [
[
"markdown",
"markdown"
],
[
"code",
"code",
"code",
"code",
"code",
"code",
"code",
"code",
"code",
"code",
"code"
],
[
"markdown"
],
[
"code",
"code",
"code",
"code",
"code"
],
[
"markdown"
],
[
"code",
"code",
"code",
"code",
"code",
"code",
"code",
"code",
"code",
"code",
"code",
"code",
"code"
],
[
"markdown"
],
[
"code",
"code",
"code",
"code",
"code",
"code"
],
[
"markdown"
],
[
"code"
],
[
"markdown"
],
[
"code",
"code",
"code",
"code",
"code",
"code",
"code",
"code",
"code"
],
[
"markdown",
"markdown"
],
[
"code",
"code",
"code",
"code",
"code",
"code",
"code",
"code",
"code",
"code",
"code",
"code",
"code",
"code",
"code"
],
[
"markdown"
],
[
"code",
"code",
"code",
"code"
],
[
"markdown"
],
[
"code",
"code",
"code",
"code",
"code"
],
[
"markdown"
],
[
"code",
"code",
"code",
"code",
"code",
"code",
"code"
],
[
"markdown"
],
[
"code",
"code"
],
[
"markdown"
],
[
"code",
"code",
"code",
"code",
"code",
"code",
"code"
],
[
"markdown"
],
[
"code",
"code",
"code",
"code",
"code",
"code",
"code",
"code",
"code",
"code",
"code",
"code",
"code",
"code",
"code",
"code",
"code",
"code",
"code",
"code",
"code",
"code",
"code",
"code",
"code",
"code",
"code",
"code",
"code"
],
[
"markdown"
],
[
"code",
"code",
"code",
"code",
"code"
],
[
"markdown"
],
[
"code",
"code",
"code",
"code",
"code",
"code",
"code",
"code",
"code",
"code",
"code",
"code",
"code",
"code",
"code",
"code",
"code",
"code"
],
[
"markdown"
],
[
"code",
"code",
"code",
"code",
"code",
"code"
]
] |
e7695cf49cc4639d0a03e796c942ca8025b6e398 | 2,904 | ipynb | Jupyter Notebook | sec5exercise02a.ipynb | teshenglin/computational_mathematics | 8aae728eb3239a72f18e24efc0c272830156795d | [
"MIT"
] | null | null | null | sec5exercise02a.ipynb | teshenglin/computational_mathematics | 8aae728eb3239a72f18e24efc0c272830156795d | [
"MIT"
] | null | null | null | sec5exercise02a.ipynb | teshenglin/computational_mathematics | 8aae728eb3239a72f18e24efc0c272830156795d | [
"MIT"
] | null | null | null | 20.892086 | 66 | 0.375689 | [
[
[
"$f(x)=exp(\\sin(\\pi x))$ integrate from $-1$ to $1$.\n\n---\n\n\n",
"_____no_output_____"
]
],
[
[
"import math\nimport numpy as np\n\ndef f(x):\n return math.exp(np.sin(np.pi*x))\n\nn=10\nk=-1\nresult=0\nfor i in range(n):\n result+=f(k)/n\n result+=f(k+2/n)/n\n k=k+2/n\nprint(result)",
"2.532131754402837\n"
],
[
"n=20\nk=-1\nresult=0\nfor i in range(n):\n result+=f(k)/n\n result+=f(k+2/n)/n\n k=k+2/n\nprint(result)",
"2.532131755504016\n"
],
[
"n=40\nk=-1\nresult=0\nfor i in range(n):\n result+=f(k)/n\n result+=f(k+2/n)/n\n k=k+2/n\nprint(result)",
"2.532131755504017\n"
],
[
"",
"_____no_output_____"
]
]
] | [
"markdown",
"code"
] | [
[
"markdown"
],
[
"code",
"code",
"code",
"code"
]
] |
e7696774af914eede65291607cb9d801d55b6657 | 147,337 | ipynb | Jupyter Notebook | Intro-To-Computer-Vision-1/1_1_Image_Representation/6_1. Visualizing the Data.ipynb | prakhargurawa/PyTorch-ML | 8959af849a2791e1409db99d682ed980299053a9 | [
"MIT"
] | null | null | null | Intro-To-Computer-Vision-1/1_1_Image_Representation/6_1. Visualizing the Data.ipynb | prakhargurawa/PyTorch-ML | 8959af849a2791e1409db99d682ed980299053a9 | [
"MIT"
] | null | null | null | Intro-To-Computer-Vision-1/1_1_Image_Representation/6_1. Visualizing the Data.ipynb | prakhargurawa/PyTorch-ML | 8959af849a2791e1409db99d682ed980299053a9 | [
"MIT"
] | null | null | null | 715.228155 | 141,952 | 0.952151 | [
[
[
"# Day and Night Image Classifier\n---\n\nThe day/night image dataset consists of 200 RGB color images in two categories: day and night. There are equal numbers of each example: 100 day images and 100 night images.\n\nWe'd like to build a classifier that can accurately label these images as day or night, and that relies on finding distinguishing features between the two types of images!\n\n*Note: All images come from the [AMOS dataset](http://cs.uky.edu/~jacobs/datasets/amos/) (Archive of Many Outdoor Scenes).*\n",
"_____no_output_____"
],
[
"### Import resources\n\nBefore you get started on the project code, import the libraries and resources that you'll need.",
"_____no_output_____"
]
],
[
[
"import cv2 # computer vision library\nimport helpers\n\nimport numpy as np\nimport matplotlib.pyplot as plt\nimport matplotlib.image as mpimg\n\n%matplotlib inline",
"_____no_output_____"
]
],
[
[
"## Training and Testing Data\nThe 200 day/night images are separated into training and testing datasets. \n\n* 60% of these images are training images, for you to use as you create a classifier.\n* 40% are test images, which will be used to test the accuracy of your classifier.\n\nFirst, we set some variables to keep track of some where our images are stored:\n\n image_dir_training: the directory where our training image data is stored\n image_dir_test: the directory where our test image data is stored",
"_____no_output_____"
]
],
[
[
"# Image data directories\nimage_dir_training = \"day_night_images/training/\"\nimage_dir_test = \"day_night_images/test/\"",
"_____no_output_____"
]
],
[
[
"## Load the datasets\n\nThese first few lines of code will load the training day/night images and store all of them in a variable, `IMAGE_LIST`. This list contains the images and their associated label (\"day\" or \"night\"). \n\nFor example, the first image-label pair in `IMAGE_LIST` can be accessed by index: \n``` IMAGE_LIST[0][:]```.\n",
"_____no_output_____"
]
],
[
[
"# Using the load_dataset function in helpers.py\n# Load training data\nIMAGE_LIST = helpers.load_dataset(image_dir_training)\nlen(IMAGE_LIST)",
"_____no_output_____"
]
],
[
[
"---\n# 1. Visualize the input images\n",
"_____no_output_____"
]
],
[
[
"# Select an image and its label by list index\nimage_index = 0\nselected_image = IMAGE_LIST[image_index][0]\nselected_label = IMAGE_LIST[image_index][1]\n\n## TODO: Print out 1. The shape of the image and 2. The image's label `selected_label`\nprint(selected_image.shape)\nprint(selected_label)\n## TODO: Display a night image\n# Note the differences between the day and night images\n# Any measurable differences can be used to classify these images\n\nimage_index = 200\nselected_image = IMAGE_LIST[image_index][0]\nselected_label = IMAGE_LIST[image_index][1]\n\n## TODO: Print out 1. The shape of the image and 2. The image's label `selected_label`\nprint(selected_image.shape)\nprint(selected_label)\nplt.imshow(selected_image)",
"(458, 800, 3)\nday\n(700, 1280, 3)\nnight\n"
]
]
] | [
"markdown",
"code",
"markdown",
"code",
"markdown",
"code",
"markdown",
"code"
] | [
[
"markdown",
"markdown"
],
[
"code"
],
[
"markdown"
],
[
"code"
],
[
"markdown"
],
[
"code"
],
[
"markdown"
],
[
"code"
]
] |
e7696e9644f42e946fe526f92a13e39cebdb2f27 | 4,960 | ipynb | Jupyter Notebook | tushare/datayes1.ipynb | phosphoric/databook | 9317bd835892f0198168ed760526f4670e67d03f | [
"Apache-2.0"
] | 20 | 2018-07-27T15:14:44.000Z | 2022-03-10T06:44:46.000Z | tushare/datayes1.ipynb | openthings/databook-old | 3b728f444f6c46e5c5d7f219cdf4d6041895b910 | [
"Apache-2.0"
] | 1 | 2020-11-18T22:15:54.000Z | 2020-11-18T22:15:54.000Z | tushare/datayes1.ipynb | openthings/databook-old | 3b728f444f6c46e5c5d7f219cdf4d6041895b910 | [
"Apache-2.0"
] | 19 | 2018-07-27T07:42:22.000Z | 2021-05-12T01:36:10.000Z | 32 | 117 | 0.429839 | [
[
[
"# -*- coding: utf-8 -*-\nimport http.client\nimport traceback\nimport urllib\n\nimport gzip\nfrom io import BytesIO\n\nHTTP_OK = 200\nHTTP_AUTHORIZATION_ERROR = 401\nclass Client:\n domain = 'api.wmcloud.com'\n port = 443\n token = ''\n #่ฎพ็ฝฎๅ ็ฝ็ป่ฟๆฅ๏ผ้่ฟ็ๆฌกๆฐ\n reconnectTimes=2\n httpClient = None\n def __init__( self ):\n self.httpClient = http.client.HTTPSConnection(self.domain, self.port, timeout=60)\n def __del__( self ):\n if self.httpClient is not None:\n self.httpClient.close()\n def encodepath(self, path):\n #่ฝฌๆขๅๆฐ็็ผ็ \n start=0\n n=len(path)\n re=''\n i=path.find('=',start)\n while i!=-1 :\n re+=path[start:i+1]\n start=i+1\n i=path.find('&',start)\n if(i>=0):\n for j in range(start,i):\n if(path[j]>'~'):\n re+=urllib.quote(path[j])\n else:\n re+=path[j] \n re+='&'\n start=i+1\n else:\n for j in range(start,n):\n if(path[j]>'~'):\n re+=urllib.quote(path[j])\n else:\n re+=path[j] \n start=n\n i=path.find('=',start)\n return re\n def init(self, token):\n self.token=token\n def getData(self, path):\n result = None\n path='/data/v1' + path\n print (path)\n path=self.encodepath(path)\n for i in range(self.reconnectTimes):\n try:\n #set http header here\n self.httpClient.request('GET', path, headers = {\"Authorization\": \"Bearer \" + self.token,\n \"Accept-Encoding\": \"gzip, deflate\"})\n #make request\n response = self.httpClient.getresponse()\n result = response.read()\n compressedstream = BytesIO(result) \n gziper = gzip.GzipFile(fileobj=compressedstream)\n try:\n result = gziper.read()\n except:\n pass\n return response.status, result\n except Exception as e:\n if i == self.reconnectTimes-1:\n raise e\n if self.httpClient is not None:\n self.httpClient.close()\n self.httpClient = http.client.HTTPSConnection(self.domain, self.port, timeout=60)\n return -1, result",
"_____no_output_____"
],
[
"# -*- coding: utf-8 -*-\nif __name__ == \"__main__\":\n try:\n client = Client()\n client.init('f8f7e9783547b0e0f1898a67bad529c82685094bc5e946fca2b74704ee8d78b2')\n url1='/api/HKequity/getequSHHKQuota.json?field=&exchangeCD=&tradeDate=20161027'\n code, result = client.getData(url1)\n if code==200:\n print(result.decode('utf-8'))\n else:\n print (code)\n print (result)\n except Exception as e:\n #traceback.print_exc()\n raise e",
"/data/v1/api/HKequity/getequSHHKQuota.json?field=&exchangeCD=&tradeDate=20161027\n{\"retCode\":-403,\"retMsg\":\"Need Privilege\"}\n"
]
]
] | [
"code"
] | [
[
"code",
"code"
]
] |
e7698369c1c837310c76c228770c2f3f001a683e | 45,111 | ipynb | Jupyter Notebook | Kaggle_Challenge_Assignment7.ipynb | JimKing100/DS-Unit-2-Kaggle-Challenge | d1a705987c5a4df8b3ab74daab453754b77045cc | [
"MIT"
] | null | null | null | Kaggle_Challenge_Assignment7.ipynb | JimKing100/DS-Unit-2-Kaggle-Challenge | d1a705987c5a4df8b3ab74daab453754b77045cc | [
"MIT"
] | null | null | null | Kaggle_Challenge_Assignment7.ipynb | JimKing100/DS-Unit-2-Kaggle-Challenge | d1a705987c5a4df8b3ab74daab453754b77045cc | [
"MIT"
] | null | null | null | 56.672111 | 280 | 0.541509 | [
[
[
"<a href=\"https://colab.research.google.com/github/JimKing100/DS-Unit-2-Kaggle-Challenge/blob/master/Kaggle_Challenge_Assignment7.ipynb\" target=\"_parent\"><img src=\"https://colab.research.google.com/assets/colab-badge.svg\" alt=\"Open In Colab\"/></a>",
"_____no_output_____"
]
],
[
[
"# Installs\n%%capture\n!pip install --upgrade category_encoders plotly",
"_____no_output_____"
],
[
"# Imports\nimport os, sys\n\nos.chdir('/content')\n!git init .\n!git remote add origin https://github.com/LambdaSchool/DS-Unit-2-Kaggle-Challenge.git\n!git pull origin master\n\n!pip install -r requirements.txt\n\nos.chdir('module1')",
"Reinitialized existing Git repository in /content/.git/\nfatal: remote origin already exists.\nFrom https://github.com/LambdaSchool/DS-Unit-2-Kaggle-Challenge\n * branch master -> FETCH_HEAD\nAlready up to date.\nRequirement already satisfied: category_encoders==2.0.0 in /usr/local/lib/python3.6/dist-packages (from -r requirements.txt (line 1)) (2.0.0)\nRequirement already satisfied: eli5==0.10.1 in /usr/local/lib/python3.6/dist-packages (from -r requirements.txt (line 2)) (0.10.1)\nRequirement already satisfied: matplotlib!=3.1.1 in /usr/local/lib/python3.6/dist-packages (from -r requirements.txt (line 3)) (3.0.3)\nRequirement already satisfied: pandas-profiling==2.3.0 in /usr/local/lib/python3.6/dist-packages (from -r requirements.txt (line 4)) (2.3.0)\nRequirement already satisfied: pdpbox==0.2.0 in /usr/local/lib/python3.6/dist-packages (from -r requirements.txt (line 5)) (0.2.0)\nRequirement already satisfied: plotly==4.1.1 in /usr/local/lib/python3.6/dist-packages (from -r requirements.txt (line 6)) (4.1.1)\nRequirement already satisfied: seaborn==0.9.0 in /usr/local/lib/python3.6/dist-packages (from -r requirements.txt (line 7)) (0.9.0)\nRequirement already satisfied: scikit-learn==0.21.3 in /usr/local/lib/python3.6/dist-packages (from -r requirements.txt (line 8)) (0.21.3)\nRequirement already satisfied: shap==0.30.0 in /usr/local/lib/python3.6/dist-packages (from -r requirements.txt (line 9)) (0.30.0)\nRequirement already satisfied: xgboost==0.90 in /usr/local/lib/python3.6/dist-packages (from -r requirements.txt (line 10)) (0.90)\nRequirement already satisfied: pandas>=0.21.1 in /usr/local/lib/python3.6/dist-packages (from category_encoders==2.0.0->-r requirements.txt (line 1)) (0.24.2)\nRequirement already satisfied: statsmodels>=0.6.1 in /usr/local/lib/python3.6/dist-packages (from category_encoders==2.0.0->-r requirements.txt (line 1)) (0.10.1)\nRequirement already satisfied: patsy>=0.4.1 in /usr/local/lib/python3.6/dist-packages (from category_encoders==2.0.0->-r requirements.txt (line 1)) (0.5.1)\nRequirement already satisfied: scipy>=0.19.0 in /usr/local/lib/python3.6/dist-packages (from category_encoders==2.0.0->-r requirements.txt (line 1)) (1.3.1)\nRequirement already satisfied: numpy>=1.11.3 in /usr/local/lib/python3.6/dist-packages (from category_encoders==2.0.0->-r requirements.txt (line 1)) (1.16.5)\nRequirement already satisfied: tabulate>=0.7.7 in /usr/local/lib/python3.6/dist-packages (from eli5==0.10.1->-r requirements.txt (line 2)) (0.8.3)\nRequirement already satisfied: graphviz in /usr/local/lib/python3.6/dist-packages (from eli5==0.10.1->-r requirements.txt (line 2)) (0.10.1)\nRequirement already satisfied: six in /usr/local/lib/python3.6/dist-packages (from eli5==0.10.1->-r requirements.txt (line 2)) (1.12.0)\nRequirement already satisfied: jinja2 in /usr/local/lib/python3.6/dist-packages (from eli5==0.10.1->-r requirements.txt (line 2)) (2.10.1)\nRequirement already satisfied: attrs>16.0.0 in /usr/local/lib/python3.6/dist-packages (from eli5==0.10.1->-r requirements.txt (line 2)) (19.1.0)\nRequirement already satisfied: kiwisolver>=1.0.1 in /usr/local/lib/python3.6/dist-packages (from matplotlib!=3.1.1->-r requirements.txt (line 3)) (1.1.0)\nRequirement already satisfied: cycler>=0.10 in /usr/local/lib/python3.6/dist-packages (from matplotlib!=3.1.1->-r requirements.txt (line 3)) (0.10.0)\nRequirement already satisfied: python-dateutil>=2.1 in /usr/local/lib/python3.6/dist-packages (from matplotlib!=3.1.1->-r requirements.txt (line 3)) (2.5.3)\nRequirement already satisfied: pyparsing!=2.0.4,!=2.1.2,!=2.1.6,>=2.0.1 in /usr/local/lib/python3.6/dist-packages (from matplotlib!=3.1.1->-r requirements.txt (line 3)) (2.4.2)\nRequirement already satisfied: missingno>=0.4.2 in /usr/local/lib/python3.6/dist-packages (from pandas-profiling==2.3.0->-r requirements.txt (line 4)) (0.4.2)\nRequirement already satisfied: htmlmin>=0.1.12 in /usr/local/lib/python3.6/dist-packages (from pandas-profiling==2.3.0->-r requirements.txt (line 4)) (0.1.12)\nRequirement already satisfied: confuse>=1.0.0 in /usr/local/lib/python3.6/dist-packages (from pandas-profiling==2.3.0->-r requirements.txt (line 4)) (1.0.0)\nRequirement already satisfied: astropy in /usr/local/lib/python3.6/dist-packages (from pandas-profiling==2.3.0->-r requirements.txt (line 4)) (3.0.5)\nRequirement already satisfied: phik>=0.9.8 in /usr/local/lib/python3.6/dist-packages (from pandas-profiling==2.3.0->-r requirements.txt (line 4)) (0.9.8)\nRequirement already satisfied: joblib in /usr/local/lib/python3.6/dist-packages (from pdpbox==0.2.0->-r requirements.txt (line 5)) (0.13.2)\nRequirement already satisfied: psutil in /usr/local/lib/python3.6/dist-packages (from pdpbox==0.2.0->-r requirements.txt (line 5)) (5.4.8)\nRequirement already satisfied: retrying>=1.3.3 in /usr/local/lib/python3.6/dist-packages (from plotly==4.1.1->-r requirements.txt (line 6)) (1.3.3)\nRequirement already satisfied: tqdm>4.25.0 in /usr/local/lib/python3.6/dist-packages (from shap==0.30.0->-r requirements.txt (line 9)) (4.28.1)\nRequirement already satisfied: ipython in /usr/local/lib/python3.6/dist-packages (from shap==0.30.0->-r requirements.txt (line 9)) (5.5.0)\nRequirement already satisfied: scikit-image in /usr/local/lib/python3.6/dist-packages (from shap==0.30.0->-r requirements.txt (line 9)) (0.15.0)\nRequirement already satisfied: pytz>=2011k in /usr/local/lib/python3.6/dist-packages (from pandas>=0.21.1->category_encoders==2.0.0->-r requirements.txt (line 1)) (2018.9)\nRequirement already satisfied: MarkupSafe>=0.23 in /usr/local/lib/python3.6/dist-packages (from jinja2->eli5==0.10.1->-r requirements.txt (line 2)) (1.1.1)\nRequirement already satisfied: setuptools in /usr/local/lib/python3.6/dist-packages (from kiwisolver>=1.0.1->matplotlib!=3.1.1->-r requirements.txt (line 3)) (41.2.0)\nRequirement already satisfied: pyyaml in /usr/local/lib/python3.6/dist-packages (from confuse>=1.0.0->pandas-profiling==2.3.0->-r requirements.txt (line 4)) (3.13)\nRequirement already satisfied: jupyter-client>=5.2.3 in /usr/local/lib/python3.6/dist-packages (from phik>=0.9.8->pandas-profiling==2.3.0->-r requirements.txt (line 4)) (5.3.1)\nRequirement already satisfied: pytest-pylint>=0.13.0 in /usr/local/lib/python3.6/dist-packages (from phik>=0.9.8->pandas-profiling==2.3.0->-r requirements.txt (line 4)) (0.14.1)\nRequirement already satisfied: nbconvert>=5.3.1 in /usr/local/lib/python3.6/dist-packages (from phik>=0.9.8->pandas-profiling==2.3.0->-r requirements.txt (line 4)) (5.6.0)\nRequirement already satisfied: pytest>=4.0.2 in /usr/local/lib/python3.6/dist-packages (from phik>=0.9.8->pandas-profiling==2.3.0->-r requirements.txt (line 4)) (5.1.2)\nRequirement already satisfied: numba>=0.38.1 in /usr/local/lib/python3.6/dist-packages (from phik>=0.9.8->pandas-profiling==2.3.0->-r requirements.txt (line 4)) (0.40.1)\nRequirement already satisfied: decorator in /usr/local/lib/python3.6/dist-packages (from ipython->shap==0.30.0->-r requirements.txt (line 9)) (4.4.0)\nRequirement already satisfied: simplegeneric>0.8 in /usr/local/lib/python3.6/dist-packages (from ipython->shap==0.30.0->-r requirements.txt (line 9)) (0.8.1)\nRequirement already satisfied: pygments in /usr/local/lib/python3.6/dist-packages (from ipython->shap==0.30.0->-r requirements.txt (line 9)) (2.1.3)\nRequirement already satisfied: pickleshare in /usr/local/lib/python3.6/dist-packages (from ipython->shap==0.30.0->-r requirements.txt (line 9)) (0.7.5)\nRequirement already satisfied: prompt-toolkit<2.0.0,>=1.0.4 in /usr/local/lib/python3.6/dist-packages (from ipython->shap==0.30.0->-r requirements.txt (line 9)) (1.0.16)\nRequirement already satisfied: pexpect; sys_platform != \"win32\" in /usr/local/lib/python3.6/dist-packages (from ipython->shap==0.30.0->-r requirements.txt (line 9)) (4.7.0)\nRequirement already satisfied: traitlets>=4.2 in /usr/local/lib/python3.6/dist-packages (from ipython->shap==0.30.0->-r requirements.txt (line 9)) (4.3.2)\nRequirement already satisfied: pillow>=4.3.0 in /usr/local/lib/python3.6/dist-packages (from scikit-image->shap==0.30.0->-r requirements.txt (line 9)) (4.3.0)\nRequirement already satisfied: networkx>=2.0 in /usr/local/lib/python3.6/dist-packages (from scikit-image->shap==0.30.0->-r requirements.txt (line 9)) (2.3)\nRequirement already satisfied: PyWavelets>=0.4.0 in /usr/local/lib/python3.6/dist-packages (from scikit-image->shap==0.30.0->-r requirements.txt (line 9)) (1.0.3)\nRequirement already satisfied: imageio>=2.0.1 in /usr/local/lib/python3.6/dist-packages (from scikit-image->shap==0.30.0->-r requirements.txt (line 9)) (2.4.1)\nRequirement already satisfied: jupyter-core in /usr/local/lib/python3.6/dist-packages (from jupyter-client>=5.2.3->phik>=0.9.8->pandas-profiling==2.3.0->-r requirements.txt (line 4)) (4.5.0)\nRequirement already satisfied: pyzmq>=13 in /usr/local/lib/python3.6/dist-packages (from jupyter-client>=5.2.3->phik>=0.9.8->pandas-profiling==2.3.0->-r requirements.txt (line 4)) (17.0.0)\nRequirement already satisfied: tornado>=4.1 in /usr/local/lib/python3.6/dist-packages (from jupyter-client>=5.2.3->phik>=0.9.8->pandas-profiling==2.3.0->-r requirements.txt (line 4)) (4.5.3)\nRequirement already satisfied: pylint>=1.4.5 in /usr/local/lib/python3.6/dist-packages (from pytest-pylint>=0.13.0->phik>=0.9.8->pandas-profiling==2.3.0->-r requirements.txt (line 4)) (2.3.1)\nRequirement already satisfied: nbformat>=4.4 in /usr/local/lib/python3.6/dist-packages (from nbconvert>=5.3.1->phik>=0.9.8->pandas-profiling==2.3.0->-r requirements.txt (line 4)) (4.4.0)\nRequirement already satisfied: entrypoints>=0.2.2 in /usr/local/lib/python3.6/dist-packages (from nbconvert>=5.3.1->phik>=0.9.8->pandas-profiling==2.3.0->-r requirements.txt (line 4)) (0.3)\nRequirement already satisfied: mistune<2,>=0.8.1 in /usr/local/lib/python3.6/dist-packages (from nbconvert>=5.3.1->phik>=0.9.8->pandas-profiling==2.3.0->-r requirements.txt (line 4)) (0.8.4)\nRequirement already satisfied: testpath in /usr/local/lib/python3.6/dist-packages (from nbconvert>=5.3.1->phik>=0.9.8->pandas-profiling==2.3.0->-r requirements.txt (line 4)) (0.4.2)\nRequirement already satisfied: pandocfilters>=1.4.1 in /usr/local/lib/python3.6/dist-packages (from nbconvert>=5.3.1->phik>=0.9.8->pandas-profiling==2.3.0->-r requirements.txt (line 4)) (1.4.2)\nRequirement already satisfied: defusedxml in /usr/local/lib/python3.6/dist-packages (from nbconvert>=5.3.1->phik>=0.9.8->pandas-profiling==2.3.0->-r requirements.txt (line 4)) (0.6.0)\nRequirement already satisfied: bleach in /usr/local/lib/python3.6/dist-packages (from nbconvert>=5.3.1->phik>=0.9.8->pandas-profiling==2.3.0->-r requirements.txt (line 4)) (3.1.0)\nRequirement already satisfied: atomicwrites>=1.0 in /usr/local/lib/python3.6/dist-packages (from pytest>=4.0.2->phik>=0.9.8->pandas-profiling==2.3.0->-r requirements.txt (line 4)) (1.3.0)\nRequirement already satisfied: pluggy<1.0,>=0.12 in /usr/local/lib/python3.6/dist-packages (from pytest>=4.0.2->phik>=0.9.8->pandas-profiling==2.3.0->-r requirements.txt (line 4)) (0.13.0)\nRequirement already satisfied: importlib-metadata>=0.12; python_version < \"3.8\" in /usr/local/lib/python3.6/dist-packages (from pytest>=4.0.2->phik>=0.9.8->pandas-profiling==2.3.0->-r requirements.txt (line 4)) (0.23)\nRequirement already satisfied: py>=1.5.0 in /usr/local/lib/python3.6/dist-packages (from pytest>=4.0.2->phik>=0.9.8->pandas-profiling==2.3.0->-r requirements.txt (line 4)) (1.8.0)\nRequirement already satisfied: wcwidth in /usr/local/lib/python3.6/dist-packages (from pytest>=4.0.2->phik>=0.9.8->pandas-profiling==2.3.0->-r requirements.txt (line 4)) (0.1.7)\nRequirement already satisfied: packaging in /usr/local/lib/python3.6/dist-packages (from pytest>=4.0.2->phik>=0.9.8->pandas-profiling==2.3.0->-r requirements.txt (line 4)) (19.1)\nRequirement already satisfied: more-itertools>=4.0.0 in /usr/local/lib/python3.6/dist-packages (from pytest>=4.0.2->phik>=0.9.8->pandas-profiling==2.3.0->-r requirements.txt (line 4)) (7.2.0)\nRequirement already satisfied: llvmlite>=0.25.0dev0 in /usr/local/lib/python3.6/dist-packages (from numba>=0.38.1->phik>=0.9.8->pandas-profiling==2.3.0->-r requirements.txt (line 4)) (0.29.0)\nRequirement already satisfied: ptyprocess>=0.5 in /usr/local/lib/python3.6/dist-packages (from pexpect; sys_platform != \"win32\"->ipython->shap==0.30.0->-r requirements.txt (line 9)) (0.6.0)\nRequirement already satisfied: ipython-genutils in /usr/local/lib/python3.6/dist-packages (from traitlets>=4.2->ipython->shap==0.30.0->-r requirements.txt (line 9)) (0.2.0)\nRequirement already satisfied: olefile in /usr/local/lib/python3.6/dist-packages (from pillow>=4.3.0->scikit-image->shap==0.30.0->-r requirements.txt (line 9)) (0.46)\nRequirement already satisfied: mccabe<0.7,>=0.6 in /usr/local/lib/python3.6/dist-packages (from pylint>=1.4.5->pytest-pylint>=0.13.0->phik>=0.9.8->pandas-profiling==2.3.0->-r requirements.txt (line 4)) (0.6.1)\nRequirement already satisfied: astroid<3,>=2.2.0 in /usr/local/lib/python3.6/dist-packages (from pylint>=1.4.5->pytest-pylint>=0.13.0->phik>=0.9.8->pandas-profiling==2.3.0->-r requirements.txt (line 4)) (2.2.5)\nRequirement already satisfied: isort<5,>=4.2.5 in /usr/local/lib/python3.6/dist-packages (from pylint>=1.4.5->pytest-pylint>=0.13.0->phik>=0.9.8->pandas-profiling==2.3.0->-r requirements.txt (line 4)) (4.3.21)\nRequirement already satisfied: jsonschema!=2.5.0,>=2.4 in /usr/local/lib/python3.6/dist-packages (from nbformat>=4.4->nbconvert>=5.3.1->phik>=0.9.8->pandas-profiling==2.3.0->-r requirements.txt (line 4)) (2.6.0)\nRequirement already satisfied: webencodings in /usr/local/lib/python3.6/dist-packages (from bleach->nbconvert>=5.3.1->phik>=0.9.8->pandas-profiling==2.3.0->-r requirements.txt (line 4)) (0.5.1)\nRequirement already satisfied: zipp>=0.5 in /usr/local/lib/python3.6/dist-packages (from importlib-metadata>=0.12; python_version < \"3.8\"->pytest>=4.0.2->phik>=0.9.8->pandas-profiling==2.3.0->-r requirements.txt (line 4)) (0.6.0)\nRequirement already satisfied: wrapt in /usr/local/lib/python3.6/dist-packages (from astroid<3,>=2.2.0->pylint>=1.4.5->pytest-pylint>=0.13.0->phik>=0.9.8->pandas-profiling==2.3.0->-r requirements.txt (line 4)) (1.11.2)\nRequirement already satisfied: lazy-object-proxy in /usr/local/lib/python3.6/dist-packages (from astroid<3,>=2.2.0->pylint>=1.4.5->pytest-pylint>=0.13.0->phik>=0.9.8->pandas-profiling==2.3.0->-r requirements.txt (line 4)) (1.4.2)\nRequirement already satisfied: typed-ast>=1.3.0; implementation_name == \"cpython\" in /usr/local/lib/python3.6/dist-packages (from astroid<3,>=2.2.0->pylint>=1.4.5->pytest-pylint>=0.13.0->phik>=0.9.8->pandas-profiling==2.3.0->-r requirements.txt (line 4)) (1.4.0)\n"
],
[
"# Disable warning\nimport warnings\nwarnings.filterwarnings(action='ignore', category=FutureWarning, module='numpy')",
"_____no_output_____"
],
[
"# Imports\nimport pandas as pd\nimport numpy as np\nimport math\n\nimport sklearn\nsklearn.__version__\n\n# Import the models\nfrom sklearn.linear_model import LogisticRegressionCV\nfrom sklearn.pipeline import make_pipeline\n\n# Import encoder and scaler and imputer\nimport category_encoders as ce\nfrom sklearn.preprocessing import StandardScaler\nfrom sklearn.impute import SimpleImputer\n\n# Import random forest classifier\nfrom sklearn.ensemble import RandomForestClassifier",
"_____no_output_____"
],
[
"# Import, load data and split data into train, validate and test\ntrain_features = pd.read_csv('../data/tanzania/train_features.csv')\ntrain_labels = pd.read_csv('../data/tanzania/train_labels.csv')\ntest_features = pd.read_csv('../data/tanzania/test_features.csv')\nsample_submission = pd.read_csv('../data/tanzania/sample_submission.csv')\n\nassert train_features.shape == (59400, 40)\nassert train_labels.shape == (59400, 2)\nassert test_features.shape == (14358, 40)\nassert sample_submission.shape == (14358, 2)\n\n# Load initial train features and labels\nfrom sklearn.model_selection import train_test_split\nX_train = train_features\ny_train = train_labels['status_group']\n\n# Split the initial train features and labels 80% into new train and new validation\nX_train, X_val, y_train, y_val = train_test_split(\n X_train, y_train, train_size = 0.80, test_size = 0.20,\n stratify = y_train, random_state=42\n)\n\nX_train.shape, X_val.shape, y_train.shape, y_val.shape",
"_____no_output_____"
],
[
"# Wrangle train, validate, and test sets\ndef wrangle(X):\n \n # Set bins value\n bins=20\n \n # Prevent SettingWithCopyWarning\n X = X.copy()\n \n # Clean installer\n X['installer'] = X['installer'].str.lower()\n X['installer'] = X['installer'].str.replace('danid', 'danida')\n X['installer'] = X['installer'].str.replace('disti', 'district council')\n X['installer'] = X['installer'].str.replace('commu', 'community')\n X['installer'] = X['installer'].str.replace('central government', 'government')\n X['installer'] = X['installer'].str.replace('kkkt _ konde and dwe', 'kkkt')\n X['installer'].value_counts(normalize=True)\n tops = X['installer'].value_counts()[:5].index\n X.loc[~X['installer'].isin(tops), 'installer'] = 'Other'\n \n # Clean funder and bin\n X['funder'] = X['funder'].str.lower()\n X['funder'] = X['funder'].str[:3]\n X['funder'].value_counts(normalize=True)\n tops = X['funder'].value_counts()[:20].index\n X.loc[~X['funder'].isin(tops), 'funder'] = 'Other'\n\n # Use mean for gps_height missing values\n X.loc[X['gps_height'] == 0, 'gps_height'] = X['gps_height'].mean()\n \n # Bin lga\n #tops = X['lga'].value_counts()[:10].index\n #X.loc[~X['lga'].isin(tops), 'lga'] = 'Other'\n\n # Bin ward \n #tops = X['ward'].value_counts()[:bins].index\n #X.loc[~X['ward'].isin(tops), 'ward'] = 'Other'\n \n # Bin subvillage\n tops = X_train['subvillage'].value_counts()[:10].index\n X_train.loc[~X_train['subvillage'].isin(tops), 'subvillage'] = 'Other'\n\n # Clean latitude and longitude\n average_lat = X.groupby('region').latitude.mean().reset_index()\n average_long = X.groupby('region').longitude.mean().reset_index()\n\n shinyanga_lat = average_lat.loc[average_lat['region'] == 'Shinyanga', 'latitude']\n shinyanga_long = average_long.loc[average_lat['region'] == 'Shinyanga', 'longitude']\n\n X.loc[(X['region'] == 'Shinyanga') & (X['latitude'] > -1), ['latitude']] = shinyanga_lat[17]\n X.loc[(X['region'] == 'Shinyanga') & (X['longitude'] == 0), ['longitude']] = shinyanga_long[17]\n\n mwanza_lat = average_lat.loc[average_lat['region'] == 'Mwanza', 'latitude']\n mwanza_long = average_long.loc[average_lat['region'] == 'Mwanza', 'longitude']\n\n X.loc[(X['region'] == 'Mwanza') & (X['latitude'] > -1), ['latitude']] = mwanza_lat[13]\n X.loc[(X['region'] == 'Mwanza') & (X['longitude'] == 0) , ['longitude']] = mwanza_long[13]\n \n # Impute mean for tsh based on mean of source_class/basin/waterpoint_type_group\n def tsh_calc(tsh, source, base, waterpoint):\n if tsh == 0:\n if (source, base, waterpoint) in tsh_dict:\n new_tsh = tsh_dict[source, base, waterpoint]\n return new_tsh\n else:\n return tsh\n return tsh\n \n temp = X[X['amount_tsh'] != 0].groupby(['source_class',\n 'basin',\n 'waterpoint_type_group'])['amount_tsh'].mean()\n\n tsh_dict = dict(temp)\n X['amount_tsh'] = X.apply(lambda x: tsh_calc(x['amount_tsh'], x['source_class'], x['basin'], x['waterpoint_type_group']), axis=1)\n\n # Impute mean for the feature based on latitude and longitude\n def latlong_conversion(feature, pop, long, lat):\n \n radius = 0.1\n radius_increment = 0.3\n \n if pop <= 1:\n pop_temp = pop\n while pop_temp <= 1 and radius <= 2:\n lat_from = lat - radius\n lat_to = lat + radius\n long_from = long - radius\n long_to = long + radius\n \n df = X[(X['latitude'] >= lat_from) & \n (X['latitude'] <= lat_to) &\n (X['longitude'] >= long_from) &\n (X['longitude'] <= long_to)]\n \n pop_temp = df[feature].mean()\n if math.isnan(pop_temp):\n pop_temp = pop\n radius = radius + radius_increment\n else:\n pop_temp = pop\n \n if pop_temp <= 1:\n new_pop = X_train[feature].mean()\n else:\n new_pop = pop_temp\n \n return new_pop\n \n # Impute gps_height based on location\n #X['population'] = X.apply(lambda x: latlong_conversion('population', x['population'], x['longitude'], x['latitude']), axis=1)\n \n # Impute gps_height based on location\n #X['gps_height'] = X.apply(lambda x: latlong_conversion('gps_height', x['gps_height'], x['longitude'], x['latitude']), axis=1)\n \n # quantity & quantity_group are duplicates, so drop quantity_group\n X = X.drop(columns='quantity_group')\n X = X.drop(columns='num_private')\n \n # return the wrangled dataframe\n return X\n",
"_____no_output_____"
],
[
"# Wrangle the data\nX_train = wrangle(X_train)\nX_val = wrangle(X_val)",
"/usr/local/lib/python3.6/dist-packages/pandas/core/indexing.py:543: SettingWithCopyWarning: \nA value is trying to be set on a copy of a slice from a DataFrame.\nTry using .loc[row_indexer,col_indexer] = value instead\n\nSee the caveats in the documentation: http://pandas.pydata.org/pandas-docs/stable/indexing.html#indexing-view-versus-copy\n self.obj[item] = s\n"
],
[
"# Feature engineering\ndef feature_engineer(X):\n \n # Create new feature pump_age\n X['pump_age'] = 2013 - X['construction_year']\n X.loc[X['pump_age'] == 2013, 'pump_age'] = 0\n X.loc[X['pump_age'] == 0, 'pump_age'] = 10\n \n # Create new feature region_district\n X['region_district'] = X['region_code'].astype(str) + X['district_code'].astype(str)\n \n #X['tsh_pop'] = X['amount_tsh']/X['population']\n\n return X",
"_____no_output_____"
],
[
"# Feature engineer the data\nX_train = feature_engineer(X_train)\nX_val = feature_engineer(X_val)",
"_____no_output_____"
],
[
"# Encode a feature\ndef encode_feature(X, y, str):\n X['status_group'] = y\n X.groupby(str)['status_group'].value_counts(normalize=True)\n X['functional']= (X['status_group'] == 'functional').astype(int)\n X[['status_group', 'functional']]\n return X",
"_____no_output_____"
],
[
"# Encode all the categorical features\ntrain = X_train.copy()\ntrain = encode_feature(train, y_train, 'quantity')\ntrain = encode_feature(train, y_train, 'waterpoint_type')\ntrain = encode_feature(train, y_train, 'extraction_type')\ntrain = encode_feature(train, y_train, 'installer')\ntrain = encode_feature(train, y_train, 'funder')\ntrain = encode_feature(train, y_train, 'water_quality')\ntrain = encode_feature(train, y_train, 'basin')\ntrain = encode_feature(train, y_train, 'region')\ntrain = encode_feature(train, y_train, 'payment')\ntrain = encode_feature(train, y_train, 'source')\n#train = encode_feature(train, y_train, 'lga')\n#train = encode_feature(train, y_train, 'ward')\n#train = encode_feature(train, y_train, 'scheme_management')\ntrain = encode_feature(train, y_train, 'management')\ntrain = encode_feature(train, y_train, 'region_district')\ntrain = encode_feature(train, y_train, 'subvillage')",
"_____no_output_____"
],
[
"# use quantity feature and the numerical features but drop id\ncategorical_features = ['quantity', 'waterpoint_type', 'extraction_type', 'installer',\n 'funder', 'water_quality', 'basin', 'region', 'payment', \n 'source', 'management', 'region_district', 'subvillage']\n \nnumeric_features = X_train.select_dtypes('number').columns.drop('id').tolist()\nfeatures = categorical_features + numeric_features\n\n# make subsets using the quantity feature all numeric features except id\nX_train = X_train[features]\nX_val = X_val[features]\n\n# Create the logistic regression pipeline\npipeline = make_pipeline (\n ce.OneHotEncoder(use_cat_names=True),\n StandardScaler(),\n LogisticRegressionCV(random_state=42, n_jobs=-1)\n)\n\npipeline.fit(X_train, y_train)\n\nprint('Validation Accuracy', pipeline.score(X_val, y_val)) ",
"/usr/local/lib/python3.6/dist-packages/sklearn/linear_model/logistic.py:469: FutureWarning: Default multi_class will be changed to 'auto' in 0.22. Specify the multi_class option to silence this warning.\n \"this warning.\", FutureWarning)\n/usr/local/lib/python3.6/dist-packages/sklearn/model_selection/_split.py:1978: FutureWarning: The default value of cv will change from 3 to 5 in version 0.22. Specify it explicitly to silence this warning.\n warnings.warn(CV_WARNING, FutureWarning)\n/usr/local/lib/python3.6/dist-packages/sklearn/linear_model/logistic.py:947: ConvergenceWarning: lbfgs failed to converge. Increase the number of iterations.\n \"of iterations.\", ConvergenceWarning)\n"
],
[
"# Create the random forest pipeline\npipeline = make_pipeline (\n ce.OneHotEncoder(use_cat_names=True),\n StandardScaler(),\n RandomForestClassifier(n_estimators=1000, \n random_state=42,\n min_samples_leaf=1,\n max_features = 'auto',\n n_jobs=-1,\n verbose = 1)\n)\n\npipeline.fit(X_train, y_train)\nprint('Validation Accuracy', pipeline.score(X_val, y_val)) ",
"[Parallel(n_jobs=-1)]: Using backend ThreadingBackend with 4 concurrent workers.\n[Parallel(n_jobs=-1)]: Done 42 tasks | elapsed: 3.0s\n[Parallel(n_jobs=-1)]: Done 192 tasks | elapsed: 13.1s\n[Parallel(n_jobs=-1)]: Done 442 tasks | elapsed: 30.1s\n[Parallel(n_jobs=-1)]: Done 792 tasks | elapsed: 53.9s\n[Parallel(n_jobs=-1)]: Done 1000 out of 1000 | elapsed: 1.1min finished\n[Parallel(n_jobs=4)]: Using backend ThreadingBackend with 4 concurrent workers.\n[Parallel(n_jobs=4)]: Done 42 tasks | elapsed: 0.1s\n[Parallel(n_jobs=4)]: Done 192 tasks | elapsed: 0.3s\n[Parallel(n_jobs=4)]: Done 442 tasks | elapsed: 0.7s\n[Parallel(n_jobs=4)]: Done 792 tasks | elapsed: 1.3s\n"
],
[
"pd.set_option('display.max_columns', 100)\nmodel = pipeline.named_steps['randomforestclassifier']\nencoder = pipeline.named_steps['onehotencoder']\nencoded_columns = encoder.transform(X_train).columns \nimportances = pd.Series(model.feature_importances_, encoded_columns)\nimportances.sort_values(ascending=False)",
"_____no_output_____"
],
[
"test_features['pump_age'] = 2013 - test_features['construction_year']\ntest_features.loc[test_features['pump_age'] == 2013, 'pump_age'] = 0\ntest_features.loc[test_features['pump_age'] == 0, 'pump_age'] = 10\n \ntest_features['region_district'] = test_features['region_code'].astype(str) + test_features['district_code'].astype(str)\n\ntest_features['tsh_pop'] = test_features['amount_tsh']/test_features['population']\n\ntest_features.drop(columns=['num_private'])\n\nX_test = test_features[features]\n\nassert all(X_test.columns == X_train.columns)\n\ny_pred = pipeline.predict(X_test)",
"[Parallel(n_jobs=4)]: Using backend ThreadingBackend with 4 concurrent workers.\n[Parallel(n_jobs=4)]: Done 42 tasks | elapsed: 0.1s\n[Parallel(n_jobs=4)]: Done 192 tasks | elapsed: 0.3s\n[Parallel(n_jobs=4)]: Done 442 tasks | elapsed: 0.7s\n[Parallel(n_jobs=4)]: Done 792 tasks | elapsed: 1.2s\n[Parallel(n_jobs=4)]: Done 1000 out of 1000 | elapsed: 1.6s finished\n"
],
[
"#submission = sample_submission.copy()\n#submission['status_group'] = y_pred\n#submission.to_csv('/content/submission-01.csv', index=False)",
"_____no_output_____"
]
]
] | [
"markdown",
"code"
] | [
[
"markdown"
],
[
"code",
"code",
"code",
"code",
"code",
"code",
"code",
"code",
"code",
"code",
"code",
"code",
"code",
"code",
"code",
"code"
]
] |
e76986d809314a2364b0a3bc339b9d6e3c431574 | 388,472 | ipynb | Jupyter Notebook | tutorials/Certification_Trainings/Healthcare/16.Adverse_Drug_Event_ADE_NER_and_Classifier.ipynb | gkovaig/spark-nlp-workshop | 2bd5c3f2bd5dc8bebca6af70b74efcfd282a1f51 | [
"Apache-2.0"
] | null | null | null | tutorials/Certification_Trainings/Healthcare/16.Adverse_Drug_Event_ADE_NER_and_Classifier.ipynb | gkovaig/spark-nlp-workshop | 2bd5c3f2bd5dc8bebca6af70b74efcfd282a1f51 | [
"Apache-2.0"
] | null | null | null | tutorials/Certification_Trainings/Healthcare/16.Adverse_Drug_Event_ADE_NER_and_Classifier.ipynb | gkovaig/spark-nlp-workshop | 2bd5c3f2bd5dc8bebca6af70b74efcfd282a1f51 | [
"Apache-2.0"
] | null | null | null | 388,472 | 388,472 | 0.877456 | [
[
[
"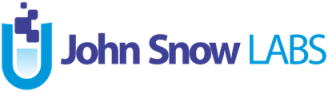",
"_____no_output_____"
],
[
"[](https://colab.research.google.com/github/JohnSnowLabs/spark-nlp-workshop/blob/master/tutorials/Certification_Trainings/Healthcare/16.Adverse_Drug_Event_ADE_NER_and_Classifier.ipynb)",
"_____no_output_____"
],
[
"# Adverse Drug Event (ADE) Pretrained NER and Classifier Models",
"_____no_output_____"
],
[
"`ADE NER`: Extracts ADE and DRUG entities from clinical texts.\n\n`ADE Classifier`: CLassify if a sentence is ADE-related (`True`) or not (`False`)\n\nWe use several datasets to train these models:\n\n- Twitter dataset, which is used in paper \"`Deep learning for pharmacovigilance: recurrent neural network architectures for labeling adverse drug reactions in Twitter posts`\" (https://pubmed.ncbi.nlm.nih.gov/28339747/)\n- ADE-Corpus-V2, which is used in paper \"`An Attentive Sequence Model for Adverse Drug Event Extraction from Biomedical Text`\" (https://arxiv.org/abs/1801.00625) and available online: https://sites.google.com/site/adecorpus/home/document.\n- CADEC dataset, which is used in paper `Cadec: A corpus of adverse drug event annotations` (https://pubmed.ncbi.nlm.nih.gov/25817970)",
"_____no_output_____"
]
],
[
[
"import json\n\nfrom google.colab import files\n\nlicense_keys = files.upload()\n\nwith open(list(license_keys.keys())[0]) as f:\n license_keys = json.load(f)",
"_____no_output_____"
],
[
"%%capture\nfor k,v in license_keys.items(): \n %set_env $k=$v\n\n!wget https://raw.githubusercontent.com/JohnSnowLabs/spark-nlp-workshop/master/jsl_colab_setup.sh\n!bash jsl_colab_setup.sh",
"_____no_output_____"
],
[
"import json\nimport os\nfrom pyspark.ml import Pipeline,PipelineModel\nfrom pyspark.sql import SparkSession\n\nfrom sparknlp.annotator import *\nfrom sparknlp_jsl.annotator import *\nfrom sparknlp.base import *\nimport sparknlp_jsl\nimport sparknlp\n\nparams = {\"spark.driver.memory\":\"16G\",\n\"spark.kryoserializer.buffer.max\":\"2000M\",\n\"spark.driver.maxResultSize\":\"2000M\"}\n\nspark = sparknlp_jsl.start(license_keys['SECRET'],params=params)\n\nprint (sparknlp.version())\nprint (sparknlp_jsl.version())",
"3.0.1\n3.0.0\n"
]
],
[
[
"## ADE Classifier Pipeline (with a pretrained model)\n\n`True` : The sentence is talking about a possible ADE\n\n`False` : The sentences doesn't have any information about an ADE.\n\n",
"_____no_output_____"
],
[
"### ADE Classifier with BioBert",
"_____no_output_____"
],
[
"",
"_____no_output_____"
]
],
[
[
"# Annotator that transforms a text column from dataframe into an Annotation ready for NLP\ndocumentAssembler = DocumentAssembler()\\\n .setInputCol(\"text\")\\\n .setOutputCol(\"sentence\")\n\n# Tokenizer splits words in a relevant format for NLP\ntokenizer = Tokenizer()\\\n .setInputCols([\"sentence\"])\\\n .setOutputCol(\"token\")\n\nbert_embeddings = BertEmbeddings.pretrained(\"biobert_pubmed_base_cased\")\\\n .setInputCols([\"sentence\", \"token\"])\\\n .setOutputCol(\"embeddings\")\\\n .setMaxSentenceLength(512)\n\nembeddingsSentence = SentenceEmbeddings() \\\n .setInputCols([\"sentence\", \"embeddings\"]) \\\n .setOutputCol(\"sentence_embeddings\") \\\n .setPoolingStrategy(\"AVERAGE\")\\\n .setStorageRef('biobert_pubmed_base_cased')\n\nclasssifierdl = ClassifierDLModel.pretrained(\"classifierdl_ade_biobert\", \"en\", \"clinical/models\")\\\n .setInputCols([\"sentence\", \"sentence_embeddings\"]) \\\n .setOutputCol(\"class\")\n\nade_clf_pipeline = Pipeline(\n stages=[documentAssembler, \n tokenizer,\n bert_embeddings,\n embeddingsSentence,\n classsifierdl])\n\n\nempty_data = spark.createDataFrame([[\"\"]]).toDF(\"text\")\n\nade_clf_model = ade_clf_pipeline.fit(empty_data)\n\nade_lp_pipeline = LightPipeline(ade_clf_model)",
"biobert_pubmed_base_cased download started this may take some time.\nApproximate size to download 386.4 MB\n[OK!]\nclassifierdl_ade_biobert download started this may take some time.\nApproximate size to download 21.8 MB\n[OK!]\n"
],
[
"text = \"I feel a bit drowsy & have a little blurred vision after taking an insulin\"\n\nade_lp_pipeline.annotate(text)['class'][0]",
"_____no_output_____"
],
[
"text=\"I just took an Advil and have no gastric problems so far.\"\n\nade_lp_pipeline.annotate(text)['class'][0]",
"_____no_output_____"
]
],
[
[
"As you can see `gastric problems` is not detected as `ADE` as it is in a negative context. So, classifier did a good job detecting that.",
"_____no_output_____"
]
],
[
[
"text=\"I just took a Metformin and started to feel dizzy.\"\n\nade_lp_pipeline.annotate(text)['class'][0]",
"_____no_output_____"
],
[
"t='''\nAlways tired, and possible blood clots. I was on Voltaren for about 4 years and all of the sudden had a minor stroke and had blood clots that traveled to my eye. I had every test in the book done at the hospital, and they couldn't find anything. I was completley healthy! I am thinking it was from the voltaren. I have been off of the drug for 8 months now, and have never felt better. I started eating healthy and working out and that has help alot. I can now sleep all thru the night. I wont take this again. If I have the back pain, I will pop a tylonol instead.\n'''\n\nade_lp_pipeline.annotate(t)['class'][0]\n",
"_____no_output_____"
],
[
"texts = [\"I feel a bit drowsy & have a little blurred vision, after taking a pill.\",\n\"I've been on Arthrotec 50 for over 10 years on and off, only taking it when I needed it.\",\n\"Due to my arthritis getting progressively worse, to the point where I am in tears with the agony, gp's started me on 75 twice a day and I have to take it every day for the next month to see how I get on, here goes.\",\n\"So far its been very good, pains almost gone, but I feel a bit weird, didn't have that when on 50.\"]\n\nfor text in texts:\n\n result = ade_lp_pipeline.annotate(text)\n\n print (result['class'][0])\n",
"True\nFalse\nTrue\nFalse\n"
]
],
[
[
"### ADE Classifier trained with conversational (short) sentences",
"_____no_output_____"
],
[
"This model is trained on short, conversational sentences related to ADE and is supposed to do better on the text that is short and used in a daily context.",
"_____no_output_____"
],
[
"",
"_____no_output_____"
]
],
[
[
"conv_classsifierdl = ClassifierDLModel.pretrained(\"classifierdl_ade_conversational_biobert\", \"en\", \"clinical/models\")\\\n .setInputCols([\"sentence\", \"sentence_embeddings\"]) \\\n .setOutputCol(\"class\")\n\nconv_ade_clf_pipeline = Pipeline(\n stages=[documentAssembler, \n tokenizer,\n bert_embeddings,\n embeddingsSentence,\n conv_classsifierdl])\n\nempty_data = spark.createDataFrame([[\"\"]]).toDF(\"text\")\n\nconv_ade_clf_model = conv_ade_clf_pipeline.fit(empty_data)\n\nconv_ade_lp_pipeline = LightPipeline(conv_ade_clf_model)",
"classifierdl_ade_conversational_biobert download started this may take some time.\nApproximate size to download 21.8 MB\n[OK!]\n"
],
[
"text = \"after taking a pill, he denies any pain\"\n\nconv_ade_lp_pipeline.annotate(text)['class'][0]",
"_____no_output_____"
]
],
[
[
"## ADE NER\n\nExtracts `ADE` and `DRUG` entities from text.",
"_____no_output_____"
],
[
"",
"_____no_output_____"
]
],
[
[
"documentAssembler = DocumentAssembler()\\\n .setInputCol(\"text\")\\\n .setOutputCol(\"document\")\n\nsentenceDetector = SentenceDetector()\\\n .setInputCols([\"document\"])\\\n .setOutputCol(\"sentence\")\n\ntokenizer = Tokenizer()\\\n .setInputCols([\"sentence\"])\\\n .setOutputCol(\"token\")\n\nword_embeddings = WordEmbeddingsModel.pretrained(\"embeddings_clinical\", \"en\", \"clinical/models\")\\\n .setInputCols([\"sentence\", \"token\"])\\\n .setOutputCol(\"embeddings\")\n\nade_ner = MedicalNerModel.pretrained(\"ner_ade_clinical\", \"en\", \"clinical/models\") \\\n .setInputCols([\"sentence\", \"token\", \"embeddings\"]) \\\n .setOutputCol(\"ner\")\n\nner_converter = NerConverter() \\\n .setInputCols([\"sentence\", \"token\", \"ner\"]) \\\n .setOutputCol(\"ner_chunk\")\n\nner_pipeline = Pipeline(stages=[\n documentAssembler, \n sentenceDetector,\n tokenizer,\n word_embeddings,\n ade_ner,\n ner_converter])\n\nempty_data = spark.createDataFrame([[\"\"]]).toDF(\"text\")\n\nade_ner_model = ner_pipeline.fit(empty_data)\n\nade_ner_lp = LightPipeline(ade_ner_model)",
"embeddings_clinical download started this may take some time.\nApproximate size to download 1.6 GB\n[OK!]\nner_ade_clinical download started this may take some time.\nApproximate size to download 13.9 MB\n[OK!]\n"
],
[
"light_result = ade_ner_lp.fullAnnotate(\"I feel a bit drowsy & have a little blurred vision, so far no gastric problems. I have been on Arthrotec 50 for over 10 years on and off, only taking it when I needed it. Due to my arthritis getting progressively worse, to the point where I am in tears with the agony, gp's started me on 75 twice a day and I have to take it every day for the next month to see how I get on, here goes. So far its been very good, pains almost gone, but I feel a bit weird, didn't have that when on 50.\")\n\nchunks = []\nentities = []\nbegin =[]\nend = []\n\nfor n in light_result[0]['ner_chunk']:\n\n begin.append(n.begin)\n end.append(n.end)\n chunks.append(n.result)\n entities.append(n.metadata['entity']) \n\nimport pandas as pd\n\ndf = pd.DataFrame({'chunks':chunks, 'entities':entities,\n 'begin': begin, 'end': end})\n\ndf",
"_____no_output_____"
]
],
[
[
"As you can see `gastric problems` is not detected as `ADE` as it is in a negative context. So, NER did a good job ignoring that.",
"_____no_output_____"
],
[
"#### ADE NER with Bert embeddings",
"_____no_output_____"
],
[
"",
"_____no_output_____"
]
],
[
[
"documentAssembler = DocumentAssembler()\\\n .setInputCol(\"text\")\\\n .setOutputCol(\"document\")\n\nsentenceDetector = SentenceDetector()\\\n .setInputCols([\"document\"])\\\n .setOutputCol(\"sentence\")\n\ntokenizer = Tokenizer()\\\n .setInputCols([\"sentence\"])\\\n .setOutputCol(\"token\")\n\nbert_embeddings = BertEmbeddings.pretrained(\"biobert_pubmed_base_cased\")\\\n .setInputCols([\"sentence\", \"token\"])\\\n .setOutputCol(\"embeddings\")\n \nade_ner_bert = MedicalNerModel.pretrained(\"ner_ade_biobert\", \"en\", \"clinical/models\") \\\n .setInputCols([\"sentence\", \"token\", \"embeddings\"]) \\\n .setOutputCol(\"ner\")\n\nner_converter = NerConverter() \\\n .setInputCols([\"sentence\", \"token\", \"ner\"]) \\\n .setOutputCol(\"ner_chunk\")\n\nner_pipeline = Pipeline(stages=[\n documentAssembler, \n sentenceDetector,\n tokenizer,\n bert_embeddings,\n ade_ner_bert,\n ner_converter])\n\nempty_data = spark.createDataFrame([[\"\"]]).toDF(\"text\")\n\nade_ner_model_bert = ner_pipeline.fit(empty_data)\n\nade_ner_lp_bert = LightPipeline(ade_ner_model_bert)",
"biobert_pubmed_base_cased download started this may take some time.\nApproximate size to download 386.4 MB\n[OK!]\nner_ade_biobert download started this may take some time.\nApproximate size to download 15.3 MB\n[OK!]\n"
],
[
"light_result = ade_ner_lp_bert.fullAnnotate(\"I feel a bit drowsy & have a little blurred vision, so far no gastric problems. I have been on Arthrotec 50 for over 10 years on and off, only taking it when I needed it. Due to my arthritis getting progressively worse, to the point where I am in tears with the agony, gp's started me on 75 twice a day and I have to take it every day for the next month to see how I get on, here goes. So far its been very good, pains almost gone, but I feel a bit weird, didn't have that when on 50.\")\n\nchunks = []\nentities = []\nbegin =[]\nend = []\n\nfor n in light_result[0]['ner_chunk']:\n\n begin.append(n.begin)\n end.append(n.end)\n chunks.append(n.result)\n entities.append(n.metadata['entity']) \n\nimport pandas as pd\n\ndf = pd.DataFrame({'chunks':chunks, 'entities':entities,\n 'begin': begin, 'end': end})\n\ndf",
"_____no_output_____"
]
],
[
[
"Looks like Bert version of NER returns more entities than clinical embeddings version.",
"_____no_output_____"
],
[
"## NER and Classifier combined with AssertionDL Model",
"_____no_output_____"
]
],
[
[
"assertion_ner_converter = NerConverter() \\\n .setInputCols([\"sentence\", \"token\", \"ner\"]) \\\n .setOutputCol(\"ass_ner_chunk\")\\\n .setWhiteList(['ADE'])\n\nbiobert_assertion = AssertionDLModel.pretrained(\"assertion_dl_biobert\", \"en\", \"clinical/models\") \\\n .setInputCols([\"sentence\", \"ass_ner_chunk\", \"embeddings\"]) \\\n .setOutputCol(\"assertion\")\n\nassertion_ner_pipeline = Pipeline(stages=[\n documentAssembler, \n sentenceDetector,\n tokenizer,\n bert_embeddings,\n ade_ner_bert,\n ner_converter,\n assertion_ner_converter,\n biobert_assertion])\n\nempty_data = spark.createDataFrame([[\"\"]]).toDF(\"text\")\n\nade_ass_ner_model_bert = assertion_ner_pipeline.fit(empty_data)\n\nade_ass_ner_model_lp_bert = LightPipeline(ade_ass_ner_model_bert)",
"assertion_dl_biobert download started this may take some time.\nApproximate size to download 3 MB\n[OK!]\n"
],
[
"import pandas as pd\ntext = \"I feel a bit drowsy & have a little blurred vision, so far no gastric problems. I have been on Arthrotec 50 for over 10 years on and off, only taking it when I needed it. Due to my arthritis getting progressively worse, to the point where I am in tears with the agony, gp's started me on 75 twice a day and I have to take it every day for the next month to see how I get on, here goes. So far its been very good, pains almost gone, but I feel a bit weird, didn't have that when on 50.\"\n\nprint (text)\n\nlight_result = ade_ass_ner_model_lp_bert.fullAnnotate(text)[0]\n\nchunks=[]\nentities=[]\nstatus=[]\n\nfor n,m in zip(light_result['ass_ner_chunk'],light_result['assertion']):\n \n chunks.append(n.result)\n entities.append(n.metadata['entity']) \n status.append(m.result)\n \ndf = pd.DataFrame({'chunks':chunks, 'entities':entities, 'assertion':status})\n\ndf",
"I feel a bit drowsy & have a little blurred vision, so far no gastric problems. I have been on Arthrotec 50 for over 10 years on and off, only taking it when I needed it. Due to my arthritis getting progressively worse, to the point where I am in tears with the agony, gp's started me on 75 twice a day and I have to take it every day for the next month to see how I get on, here goes. So far its been very good, pains almost gone, but I feel a bit weird, didn't have that when on 50.\n"
]
],
[
[
"Looks great ! `gastric problems` is detected as `ADE` and `absent`",
"_____no_output_____"
],
[
"## ADE models applied to Spark Dataframes",
"_____no_output_____"
]
],
[
[
"import pyspark.sql.functions as F\n\n! wget -q\thttps://raw.githubusercontent.com/JohnSnowLabs/spark-nlp-workshop/master/tutorials/Certification_Trainings/Healthcare/data/sample_ADE_dataset.csv\n\nade_DF = spark.read\\\n .option(\"header\", \"true\")\\\n .csv(\"./sample_ADE_dataset.csv\")\\\n .filter(F.col(\"label\").isin(['True','False']))\n\nade_DF.show(truncate=50)",
"+--------------------------------------------------+-----+\n| text|label|\n+--------------------------------------------------+-----+\n|Do U know what Meds are R for bipolar depressio...|False|\n|# hypercholesterol: Because of elevated CKs (pe...| True|\n|Her weight, respirtory status and I/O should be...|False|\n|* DM - Pt had several episodes of hypoglycemia ...| True|\n|We report the case of a female acromegalic pati...| True|\n|2 . Calcipotriene 0.005% Cream Sig: One (1) App...|False|\n|Always tired, and possible blood clots. I was o...| True|\n|A difference in chemical structure between thes...|False|\n|10 . She was left on prednisone 20mg qd due to ...|False|\n|The authors suggest that risperidone may increa...| True|\n|- Per oral maxillofacial surgery there is no ev...|False|\n|@marionjross Cipro is just as bad! Stay away fr...|False|\n|A young woman with epilepsy had tonic-clonic se...| True|\n|Intravenous methotrexate is an effective adjunc...|False|\n|PURPOSE: To report new indocyanine green angiog...|False|\n|2 . Docusate Sodium 50 mg/5 mL Liquid [**Hospit...|False|\n| consider neupogen.|False|\n|He was treated allopurinol and Rasburicase for ...|False|\n|Toxicity, pharmacokinetics, and in vitro hemodi...| True|\n|# thrombocytopenia: Secondary to chemotherapy a...| True|\n+--------------------------------------------------+-----+\nonly showing top 20 rows\n\n"
]
],
[
[
"**With BioBert version of NER** (will be slower but more accurate)",
"_____no_output_____"
]
],
[
[
"import pyspark.sql.functions as F\n\nner_converter = NerConverter() \\\n .setInputCols([\"sentence\", \"token\", \"ner\"]) \\\n .setOutputCol(\"ner_chunk\")\\\n .setWhiteList(['ADE'])\n\nner_pipeline = Pipeline(stages=[\n documentAssembler, \n sentenceDetector,\n tokenizer,\n bert_embeddings,\n ade_ner_bert,\n ner_converter])\n\n\nempty_data = spark.createDataFrame([[\"\"]]).toDF(\"text\")\n\nade_ner_model = ner_pipeline.fit(empty_data)\n\nresult = ade_ner_model.transform(ade_DF)\n\nsample_df = result.select('text','ner_chunk.result')\\\n.toDF('text','ADE_phrases').filter(F.size('ADE_phrases')>0).toPandas()",
"_____no_output_____"
],
[
"import pandas as pd\npd.set_option('display.max_colwidth', 0)",
"_____no_output_____"
],
[
"sample_df.sample(20)",
"_____no_output_____"
]
],
[
[
"**Doing the same with clinical embeddings version** (faster results)",
"_____no_output_____"
]
],
[
[
"import pyspark.sql.functions as F\n\nner_converter = NerConverter() \\\n .setInputCols([\"sentence\", \"token\", \"ner\"]) \\\n .setOutputCol(\"ner_chunk\")\\\n .setWhiteList(['ADE'])\n\nner_pipeline = Pipeline(stages=[\n documentAssembler, \n sentenceDetector,\n tokenizer,\n word_embeddings,\n ade_ner,\n ner_converter])\n\nempty_data = spark.createDataFrame([[\"\"]]).toDF(\"text\")\n\nade_ner_model = ner_pipeline.fit(empty_data)\n\nresult = ade_ner_model.transform(ade_DF)\n\nresult.select('text','ner_chunk.result')\\\n.toDF('text','ADE_phrases').filter(F.size('ADE_phrases')>0)\\\n.show(truncate=70)\n",
"+----------------------------------------------------------------------+----------------------------------------------------------------------+\n| text| ADE_phrases|\n+----------------------------------------------------------------------+----------------------------------------------------------------------+\n|# hypercholesterol: Because of elevated CKs (peaked at 819) the pat...| [elevated CKs]|\n|We report the case of a female acromegalic patient in whom multiple...| [multiple hepatic adenomas]|\n|Always tired, and possible blood clots. I was on Voltaren for about...| [blood clots that traveled to my eye, back pain]|\n|The authors suggest that risperidone may increase affect in patient...| [increase affect]|\n|A young woman with epilepsy had tonic-clonic seizures during antine...| [tonic-clonic seizures]|\n|Intravenous methotrexate is an effective adjunct to steroid therapy...| [dermatomyositis-polyositis]|\n|PURPOSE: To report new indocyanine green angiographic (ICGA) findin...| [indocyanine green angiographic (ICGA) findings]|\n|Toxicity, pharmacokinetics, and in vitro hemodialysis clearance of ...| [Toxicity]|\n| # thrombocytopenia: Secondary to chemotherapy and MDS/AML concerns.| [thrombocytopenia]|\n|A fatal massive pulmonary embolus developed in a patient treated wi...| [fatal massive pulmonary embolus]|\n|# Maculopapular rash: over extremities, chest and back, thought [**...| [Maculopapular rash]|\n| Hypokalemia after normal doses of neubulized albuterol (salbutamol).| [Hypokalemia]|\n|A transient tonic pupillary response, denervation supersensitivity,...|[transient tonic pupillary response, denervation supersensitivity, ...|\n|As per above, ID added Atovaquone for PCP [**Name9 (PRE) *] given t...| [BM suppression, liver damage]|\n|Electrocardiographic findings and laboratory data indicated a diagn...| [acute myocardial infarction]|\n| Hepatic reactions to cyclofenil.| [Hepatic reactions]|\n|Therefore, parenteral amiodarone was implicated as the cause of acu...| [acute hepatitis]|\n|Vincristine levels were also assayed and showed a dramatic decline ...| [dramatic decline in postexchange levels]|\n|Eight days after the end of interferon treatment, he showed signs o...| [inability to sit]|\n|2 years with no problems, then toe neuropathy for two years now and...|[toe neuropathy, toe neuropathy, stomach problems, pain, heart woul...|\n+----------------------------------------------------------------------+----------------------------------------------------------------------+\nonly showing top 20 rows\n\n"
]
],
[
[
"### Creating sentence dataframe (one sentence per row) and getting ADE entities and categories",
"_____no_output_____"
]
],
[
[
"documentAssembler = DocumentAssembler()\\\n .setInputCol(\"text\")\\\n .setOutputCol(\"document\")\n\nsentenceDetector = SentenceDetector()\\\n .setInputCols([\"document\"])\\\n .setOutputCol(\"sentence\")\\\n .setExplodeSentences(True)\n\ntokenizer = Tokenizer()\\\n .setInputCols([\"sentence\"])\\\n .setOutputCol(\"token\")\n\nbert_embeddings = BertEmbeddings.pretrained(\"biobert_pubmed_base_cased\")\\\n .setInputCols([\"sentence\", \"token\"])\\\n .setOutputCol(\"embeddings\")\n\nembeddingsSentence = SentenceEmbeddings() \\\n .setInputCols([\"sentence\", \"embeddings\"]) \\\n .setOutputCol(\"sentence_embeddings\") \\\n .setPoolingStrategy(\"AVERAGE\")\\\n .setStorageRef('biobert_pubmed_base_cased')\n\nclasssifierdl = ClassifierDLModel.pretrained(\"classifierdl_ade_biobert\", \"en\", \"clinical/models\")\\\n .setInputCols([\"sentence\", \"sentence_embeddings\"]) \\\n .setOutputCol(\"class\")\\\n .setStorageRef('biobert_pubmed_base_cased')\n\nade_ner = MedicalNerModel.pretrained(\"ner_ade_biobert\", \"en\", \"clinical/models\") \\\n .setInputCols([\"sentence\", \"token\", \"embeddings\"]) \\\n .setOutputCol(\"ner\")\n \nner_converter = NerConverter() \\\n .setInputCols([\"sentence\", \"token\", \"ner\"]) \\\n .setOutputCol(\"ner_chunk\")\\\n .setWhiteList(['ADE'])\n\nner_clf_pipeline = Pipeline(\n stages=[documentAssembler, \n sentenceDetector,\n tokenizer,\n bert_embeddings,\n embeddingsSentence,\n classsifierdl,\n ade_ner,\n ner_converter])\n\nade_Sentences = ner_clf_pipeline.fit(ade_DF)",
"biobert_pubmed_base_cased download started this may take some time.\nApproximate size to download 386.4 MB\n[OK!]\nclassifierdl_ade_biobert download started this may take some time.\nApproximate size to download 21.8 MB\n[OK!]\nner_ade_biobert download started this may take some time.\nApproximate size to download 15.3 MB\n[OK!]\n"
],
[
"import pyspark.sql.functions as F\n\nade_Sentences.transform(ade_DF).select('sentence.result','ner_chunk.result','class.result')\\\n.toDF('sentence','ADE_phrases','is_ADE').show(truncate=60)",
"+------------------------------------------------------------+---------------------------------------------+-------+\n| sentence| ADE_phrases| is_ADE|\n+------------------------------------------------------------+---------------------------------------------+-------+\n| [Do U know what Meds are R for bipolar depression?]| []|[False]|\n| [Currently #FDA approved #quetiapine AKA #Seroquel]| []|[False]|\n|[# hypercholesterol: Because of elevated CKs (peaked at 8...| [elevated CKs]|[False]|\n|[Her weight, respirtory status and I/O should be monitore...| []|[False]|\n|[* DM - Pt had several episodes of hypoglycemia on lantus...| [hypoglycemia]| [True]|\n|[We report the case of a female acromegalic patient in wh...| [hepatic adenomas]| [True]|\n| [2 .]| []|[False]|\n|[Calcipotriene 0.005% Cream Sig: One (1) Appl Topical [**...| []|[False]|\n| [Always tired, and possible blood clots.]| [tired, blood clots]|[False]|\n|[I was on Voltaren for about 4 years and all of the sudde...|[stroke, blood clots that traveled to my eye]|[False]|\n|[I had every test in the book done at the hospital, and t...| []|[False]|\n| [I was completley healthy!]| [completley healthy]|[False]|\n| [I am thinking it was from the voltaren.]| []|[False]|\n|[I have been off of the drug for 8 months now, and have n...| []|[False]|\n|[I started eating healthy and working out and that has he...| []|[False]|\n| [I can now sleep all thru the night.]| []|[False]|\n| [I wont take this again.]| []|[False]|\n| [If I have the back pain, I will pop a tylonol instead.]| []|[False]|\n|[A difference in chemical structure between these two dru...| []|[False]|\n| [10 .]| []|[False]|\n+------------------------------------------------------------+---------------------------------------------+-------+\nonly showing top 20 rows\n\n"
]
],
[
[
"## Creating a pretrained pipeline with ADE NER, Assertion and Classifer",
"_____no_output_____"
]
],
[
[
"# Annotator that transforms a text column from dataframe into an Annotation ready for NLP\ndocumentAssembler = DocumentAssembler()\\\n .setInputCol(\"text\")\\\n .setOutputCol(\"sentence\")\n\n# Tokenizer splits words in a relevant format for NLP\ntokenizer = Tokenizer()\\\n .setInputCols([\"sentence\"])\\\n .setOutputCol(\"token\")\n\nbert_embeddings = BertEmbeddings.pretrained(\"biobert_pubmed_base_cased\")\\\n .setInputCols([\"sentence\", \"token\"])\\\n .setOutputCol(\"embeddings\")\n\nade_ner = MedicalNerModel.pretrained(\"ner_ade_biobert\", \"en\", \"clinical/models\") \\\n .setInputCols([\"sentence\", \"token\", \"embeddings\"]) \\\n .setOutputCol(\"ner\")\\\n .setStorageRef('biobert_pubmed_base_cased')\n\nner_converter = NerConverter() \\\n .setInputCols([\"sentence\", \"token\", \"ner\"]) \\\n .setOutputCol(\"ner_chunk\")\n\nassertion_ner_converter = NerConverter() \\\n .setInputCols([\"sentence\", \"token\", \"ner\"]) \\\n .setOutputCol(\"ass_ner_chunk\")\\\n .setWhiteList(['ADE'])\n\nbiobert_assertion = AssertionDLModel.pretrained(\"assertion_dl_biobert\", \"en\", \"clinical/models\") \\\n .setInputCols([\"sentence\", \"ass_ner_chunk\", \"embeddings\"]) \\\n .setOutputCol(\"assertion\")\n\nembeddingsSentence = SentenceEmbeddings() \\\n .setInputCols([\"sentence\", \"embeddings\"]) \\\n .setOutputCol(\"sentence_embeddings\") \\\n .setPoolingStrategy(\"AVERAGE\")\\\n .setStorageRef('biobert_pubmed_base_cased')\n\nclasssifierdl = ClassifierDLModel.pretrained(\"classifierdl_ade_conversational_biobert\", \"en\", \"clinical/models\")\\\n .setInputCols([\"sentence\", \"sentence_embeddings\"]) \\\n .setOutputCol(\"class\")\n\nade_clf_pipeline = Pipeline(\n stages=[documentAssembler, \n tokenizer,\n bert_embeddings,\n ade_ner,\n ner_converter,\n assertion_ner_converter,\n biobert_assertion,\n embeddingsSentence,\n classsifierdl])\n\nempty_data = spark.createDataFrame([[\"\"]]).toDF(\"text\")\n\nade_ner_clf_model = ade_clf_pipeline.fit(empty_data)\n\nade_ner_clf_pipeline = LightPipeline(ade_ner_clf_model)",
"biobert_pubmed_base_cased download started this may take some time.\nApproximate size to download 386.4 MB\n[OK!]\nner_ade_biobert download started this may take some time.\nApproximate size to download 15.3 MB\n[OK!]\nassertion_dl_biobert download started this may take some time.\nApproximate size to download 3 MB\n[OK!]\nclassifierdl_ade_conversational_biobert download started this may take some time.\nApproximate size to download 21.8 MB\n[OK!]\n"
],
[
"classsifierdl.getStorageRef()",
"_____no_output_____"
],
[
"text = 'Always tired, and possible blood clots. I was on Voltaren for about 4 years and all of the sudden had a minor stroke and had blood clots that traveled to my eye. I had every test in the book done at the hospital, and they couldnt find anything. I was completley healthy! I am thinking it was from the voltaren. I have been off of the drug for 8 months now, and have never felt better. I started eating healthy and working out and that has help alot. I can now sleep all thru the night. I wont take this again. If I have the back pain, I will pop a tylonol instead.'\n\nlight_result = ade_ner_clf_pipeline.fullAnnotate(text)\n\nprint (light_result[0]['class'][0].metadata)\n\nchunks = []\nentities = []\nbegin =[]\nend = []\n\nfor n in light_result[0]['ner_chunk']:\n\n begin.append(n.begin)\n end.append(n.end)\n chunks.append(n.result)\n entities.append(n.metadata['entity']) \n\nimport pandas as pd\n\ndf = pd.DataFrame({'chunks':chunks, 'entities':entities,\n 'begin': begin, 'end': end})\n\ndf",
"{'sentence': '0', 'False': '0.018514052', 'True': '0.9814859'}\n"
],
[
"import pandas as pd\n\ntext = 'I have always felt tired, but no blood clots. I was on Voltaren for about 4 years and all of the sudden had a minor stroke and had blood clots that traveled to my eye. I had every test in the book done at the hospital, and they couldnt find anything. I was completley healthy! I am thinking it was from the voltaren. I have been off of the drug for 8 months now, and have never felt better. I started eating healthy and working out and that has help alot. I can now sleep all thru the night. I wont take this again. If I have the back pain, I will pop a tylonol instead.'\n\nprint (text)\n\nlight_result = ade_ass_ner_model_lp_bert.fullAnnotate(text)[0]\n\nchunks=[]\nentities=[]\nstatus=[]\n\nfor n,m in zip(light_result['ass_ner_chunk'],light_result['assertion']):\n \n chunks.append(n.result)\n entities.append(n.metadata['entity']) \n status.append(m.result)\n \ndf = pd.DataFrame({'chunks':chunks, 'entities':entities, 'assertion':status})\n\ndf",
"I have always felt tired, but no blood clots. I was on Voltaren for about 4 years and all of the sudden had a minor stroke and had blood clots that traveled to my eye. I had every test in the book done at the hospital, and they couldnt find anything. I was completley healthy! I am thinking it was from the voltaren. I have been off of the drug for 8 months now, and have never felt better. I started eating healthy and working out and that has help alot. I can now sleep all thru the night. I wont take this again. If I have the back pain, I will pop a tylonol instead.\n"
],
[
"result = ade_ner_clf_pipeline.annotate('I just took an Advil 100 mg and it made me drowsy')\n\nprint (result['class'])\nprint(list(zip(result['token'],result['ner'])))",
"['False']\n[('I', 'O'), ('just', 'O'), ('took', 'O'), ('an', 'O'), ('Advil', 'B-DRUG'), ('100', 'O'), ('mg', 'O'), ('and', 'O'), ('it', 'O'), ('made', 'O'), ('me', 'O'), ('drowsy', 'B-ADE')]\n"
],
[
"ade_ner_clf_model.save('ade_pretrained_pipeline')",
"_____no_output_____"
],
[
"from sparknlp.pretrained import PretrainedPipeline\n\nade_pipeline = PretrainedPipeline.from_disk('ade_pretrained_pipeline')\n\nade_pipeline.annotate('I just took an Advil 100 mg then it made me drowsy')",
"_____no_output_____"
],
[
"ade_pipeline.model.stages",
"_____no_output_____"
]
]
] | [
"markdown",
"code",
"markdown",
"code",
"markdown",
"code",
"markdown",
"code",
"markdown",
"code",
"markdown",
"code",
"markdown",
"code",
"markdown",
"code",
"markdown",
"code",
"markdown",
"code",
"markdown",
"code",
"markdown",
"code"
] | [
[
"markdown",
"markdown",
"markdown",
"markdown"
],
[
"code",
"code",
"code"
],
[
"markdown",
"markdown",
"markdown"
],
[
"code",
"code",
"code"
],
[
"markdown"
],
[
"code",
"code",
"code"
],
[
"markdown",
"markdown",
"markdown"
],
[
"code",
"code"
],
[
"markdown",
"markdown"
],
[
"code",
"code"
],
[
"markdown",
"markdown",
"markdown"
],
[
"code",
"code"
],
[
"markdown",
"markdown"
],
[
"code",
"code"
],
[
"markdown",
"markdown"
],
[
"code"
],
[
"markdown"
],
[
"code",
"code",
"code"
],
[
"markdown"
],
[
"code"
],
[
"markdown"
],
[
"code",
"code"
],
[
"markdown"
],
[
"code",
"code",
"code",
"code",
"code",
"code",
"code",
"code"
]
] |
e76997a58f273b7b49282021ed63f06364e9fd36 | 249,959 | ipynb | Jupyter Notebook | model.ipynb | abhi134/Brain_Tumor_Segmentation | c0b8a94f101137a506c5a1938c4c5c679e979018 | [
"MIT"
] | 101 | 2018-07-18T07:06:35.000Z | 2022-03-20T05:53:49.000Z | model.ipynb | Rda99/Brain-Tumor-Segmentation-using-Deep-Neural-networks | b9b8db4fef09942c0d38406834a90fa2e74dd6b8 | [
"MIT"
] | 3 | 2019-02-18T16:09:57.000Z | 2020-06-24T17:01:31.000Z | model.ipynb | Rda99/Brain-Tumor-Segmentation-using-Deep-Neural-networks | b9b8db4fef09942c0d38406834a90fa2e74dd6b8 | [
"MIT"
] | 68 | 2018-10-23T16:01:12.000Z | 2022-01-30T11:35:05.000Z | 249,959 | 249,959 | 0.815618 | [
[
[
"!apt-get install -y -qq software-properties-common python-software-properties module-init-tools\n!add-apt-repository -y ppa:alessandro-strada/ppa 2>&1 > /dev/null\n!apt-get update -qq 2>&1 > /dev/null\n!apt-get -y install -qq google-drive-ocamlfuse fuse\nfrom google.colab import auth\nauth.authenticate_user()\nfrom oauth2client.client import GoogleCredentials\ncreds = GoogleCredentials.get_application_default()\nimport getpass\n!google-drive-ocamlfuse -headless -id={creds.client_id} -secret={creds.client_secret} < /dev/null 2>&1 | grep URL\nvcode = getpass.getpass()\n!echo {vcode} | google-drive-ocamlfuse -headless -id={creds.client_id} -secret={creds.client_secret}",
"Please, open the following URL in a web browser: https://accounts.google.com/o/oauth2/auth?client_id=32555940559.apps.googleusercontent.com&redirect_uri=urn%3Aietf%3Awg%3Aoauth%3A2.0%3Aoob&scope=https%3A%2F%2Fwww.googleapis.com%2Fauth%2Fdrive&response_type=code&access_type=offline&approval_prompt=force\r\nยทยทยทยทยทยทยทยทยทยท\nPlease, open the following URL in a web browser: https://accounts.google.com/o/oauth2/auth?client_id=32555940559.apps.googleusercontent.com&redirect_uri=urn%3Aietf%3Awg%3Aoauth%3A2.0%3Aoob&scope=https%3A%2F%2Fwww.googleapis.com%2Fauth%2Fdrive&response_type=code&access_type=offline&approval_prompt=force\nPlease enter the verification code: Access token retrieved correctly.\n"
],
[
" !ls",
"datalab drive\r\n"
],
[
"!mkdir -p drive\n!google-drive-ocamlfuse drive",
"_____no_output_____"
],
[
"from keras import layers\nfrom keras.layers import Input, Dense, Activation, ZeroPadding2D, BatchNormalization, Flatten, Conv2D, Lambda,Concatenate\nfrom keras.layers import AveragePooling2D, MaxPooling2D, Dropout, GlobalMaxPooling2D, GlobalAveragePooling2D, Add\nfrom keras.models import Model\nfrom keras import regularizers\nfrom keras.preprocessing import image\nfrom keras.utils import layer_utils\nfrom keras.utils.data_utils import get_file\nfrom keras.applications.imagenet_utils import preprocess_input\nfrom keras.initializers import glorot_normal\n#import pydot\nfrom IPython.display import SVG\nfrom keras.utils.vis_utils import model_to_dot\nfrom keras.utils import plot_model",
"Using TensorFlow backend.\n"
],
[
"import tensorflow as tf",
"_____no_output_____"
],
[
"def two_path(X_input):\n \n X = Conv2D(64,(7,7),strides=(1,1),padding='valid')(X_input)\n X = BatchNormalization()(X)\n X1 = Conv2D(64,(7,7),strides=(1,1),padding='valid')(X_input)\n X1 = BatchNormalization()(X1)\n X = layers.Maximum()([X,X1])\n X = Conv2D(64,(4,4),strides=(1,1),padding='valid',activation='relu')(X)\n \n X2 = Conv2D(160,(13,13),strides=(1,1),padding='valid')(X_input)\n X2 = BatchNormalization()(X2)\n X21 = Conv2D(160,(13,13),strides=(1,1),padding='valid')(X_input)\n X21 = BatchNormalization()(X21)\n X2 = layers.Maximum()([X2,X21])\n \n X3 = Conv2D(64,(3,3),strides=(1,1),padding='valid')(X)\n X3 = BatchNormalization()(X3)\n X31 = Conv2D(64,(3,3),strides=(1,1),padding='valid')(X)\n X31 = BatchNormalization()(X31)\n X = layers.Maximum()([X3,X31])\n X = Conv2D(64,(2,2),strides=(1,1),padding='valid',activation='relu')(X)\n \n X = Concatenate()([X2,X])\n #X = Conv2D(5,(21,21),strides=(1,1))(X)\n #X = Activation('softmax')(X)\n \n #model = Model(inputs = X_input, outputs = X)\n return X",
"_____no_output_____"
],
[
"def input_cascade(input_shape1,input_shape2):\n \n X1_input = Input(input_shape1)\n X1 = two_path(X1_input)\n X1 = Conv2D(5,(21,21),strides=(1,1),padding='valid',activation='relu')(X1)\n X1 = BatchNormalization()(X1)\n \n X2_input = Input(input_shape2)\n X2_input1 = Concatenate()([X1,X2_input])\n #X2_input1 = Input(tensor = X2_input1)\n X2 = two_path(X2_input1)\n X2 = Conv2D(5,(21,21),strides=(1,1),padding='valid')(X2)\n X2 = BatchNormalization()(X2)\n X2 = Activation('softmax')(X2)\n \n model = Model(inputs=[X1_input,X2_input],outputs=X2)\n return model\n ",
"_____no_output_____"
],
[
"def MFCcascade(input_shape1,input_shape2):\n \n X1_input = Input(input_shape1)\n X1 = two_path(X1_input)\n X1 = Conv2D(5,(21,21),strides=(1,1),padding='valid',activation='relu')(X1)\n X1 = BatchNormalization()(X1)\n #X1 = MaxPooling2D((2,2))(X1)\n \n X2_input = Input(input_shape2)\n X2 = two_path(X2_input)\n \n X2 = Concatenate()([X1,X2])\n X2 = Conv2D(5,(21,21),strides=(1,1),padding='valid',activation='relu')(X2)\n X2 = BatchNormalization()(X2)\n X2 = Activation('softmax')(X2)\n \n model = Model(inputs=[X1_input,X2_input],outputs=X2)\n return model\n ",
"_____no_output_____"
],
[
"m = MFCcascade((53,53,4),(33,33,4))\nm.summary()",
"__________________________________________________________________________________________________\nLayer (type) Output Shape Param # Connected to \n==================================================================================================\ninput_1 (InputLayer) (None, 53, 53, 4) 0 \n__________________________________________________________________________________________________\nconv2d_1 (Conv2D) (None, 47, 47, 64) 12608 input_1[0][0] \n__________________________________________________________________________________________________\nconv2d_2 (Conv2D) (None, 47, 47, 64) 12608 input_1[0][0] \n__________________________________________________________________________________________________\nbatch_normalization_1 (BatchNor (None, 47, 47, 64) 256 conv2d_1[0][0] \n__________________________________________________________________________________________________\nbatch_normalization_2 (BatchNor (None, 47, 47, 64) 256 conv2d_2[0][0] \n__________________________________________________________________________________________________\ninput_2 (InputLayer) (None, 33, 33, 4) 0 \n__________________________________________________________________________________________________\nmaximum_1 (Maximum) (None, 47, 47, 64) 0 batch_normalization_1[0][0] \n batch_normalization_2[0][0] \n__________________________________________________________________________________________________\nconv2d_10 (Conv2D) (None, 27, 27, 64) 12608 input_2[0][0] \n__________________________________________________________________________________________________\nconv2d_11 (Conv2D) (None, 27, 27, 64) 12608 input_2[0][0] \n__________________________________________________________________________________________________\nconv2d_3 (Conv2D) (None, 44, 44, 64) 65600 maximum_1[0][0] \n__________________________________________________________________________________________________\nbatch_normalization_8 (BatchNor (None, 27, 27, 64) 256 conv2d_10[0][0] \n__________________________________________________________________________________________________\nbatch_normalization_9 (BatchNor (None, 27, 27, 64) 256 conv2d_11[0][0] \n__________________________________________________________________________________________________\nconv2d_6 (Conv2D) (None, 42, 42, 64) 36928 conv2d_3[0][0] \n__________________________________________________________________________________________________\nconv2d_7 (Conv2D) (None, 42, 42, 64) 36928 conv2d_3[0][0] \n__________________________________________________________________________________________________\nmaximum_4 (Maximum) (None, 27, 27, 64) 0 batch_normalization_8[0][0] \n batch_normalization_9[0][0] \n__________________________________________________________________________________________________\nconv2d_4 (Conv2D) (None, 41, 41, 160) 108320 input_1[0][0] \n__________________________________________________________________________________________________\nconv2d_5 (Conv2D) (None, 41, 41, 160) 108320 input_1[0][0] \n__________________________________________________________________________________________________\nbatch_normalization_5 (BatchNor (None, 42, 42, 64) 256 conv2d_6[0][0] \n__________________________________________________________________________________________________\nbatch_normalization_6 (BatchNor (None, 42, 42, 64) 256 conv2d_7[0][0] \n__________________________________________________________________________________________________\nconv2d_12 (Conv2D) (None, 24, 24, 64) 65600 maximum_4[0][0] \n__________________________________________________________________________________________________\nbatch_normalization_3 (BatchNor (None, 41, 41, 160) 640 conv2d_4[0][0] \n__________________________________________________________________________________________________\nbatch_normalization_4 (BatchNor (None, 41, 41, 160) 640 conv2d_5[0][0] \n__________________________________________________________________________________________________\nmaximum_3 (Maximum) (None, 42, 42, 64) 0 batch_normalization_5[0][0] \n batch_normalization_6[0][0] \n__________________________________________________________________________________________________\nconv2d_15 (Conv2D) (None, 22, 22, 64) 36928 conv2d_12[0][0] \n__________________________________________________________________________________________________\nconv2d_16 (Conv2D) (None, 22, 22, 64) 36928 conv2d_12[0][0] \n__________________________________________________________________________________________________\nmaximum_2 (Maximum) (None, 41, 41, 160) 0 batch_normalization_3[0][0] \n batch_normalization_4[0][0] \n__________________________________________________________________________________________________\nconv2d_8 (Conv2D) (None, 41, 41, 64) 16448 maximum_3[0][0] \n__________________________________________________________________________________________________\nconv2d_13 (Conv2D) (None, 21, 21, 160) 108320 input_2[0][0] \n__________________________________________________________________________________________________\nconv2d_14 (Conv2D) (None, 21, 21, 160) 108320 input_2[0][0] \n__________________________________________________________________________________________________\nbatch_normalization_12 (BatchNo (None, 22, 22, 64) 256 conv2d_15[0][0] \n__________________________________________________________________________________________________\nbatch_normalization_13 (BatchNo (None, 22, 22, 64) 256 conv2d_16[0][0] \n__________________________________________________________________________________________________\nconcatenate_1 (Concatenate) (None, 41, 41, 224) 0 maximum_2[0][0] \n conv2d_8[0][0] \n__________________________________________________________________________________________________\nbatch_normalization_10 (BatchNo (None, 21, 21, 160) 640 conv2d_13[0][0] \n__________________________________________________________________________________________________\nbatch_normalization_11 (BatchNo (None, 21, 21, 160) 640 conv2d_14[0][0] \n__________________________________________________________________________________________________\nmaximum_6 (Maximum) (None, 22, 22, 64) 0 batch_normalization_12[0][0] \n batch_normalization_13[0][0] \n__________________________________________________________________________________________________\nconv2d_9 (Conv2D) (None, 21, 21, 5) 493925 concatenate_1[0][0] \n__________________________________________________________________________________________________\nmaximum_5 (Maximum) (None, 21, 21, 160) 0 batch_normalization_10[0][0] \n batch_normalization_11[0][0] \n__________________________________________________________________________________________________\nconv2d_17 (Conv2D) (None, 21, 21, 64) 16448 maximum_6[0][0] \n__________________________________________________________________________________________________\nbatch_normalization_7 (BatchNor (None, 21, 21, 5) 20 conv2d_9[0][0] \n__________________________________________________________________________________________________\nconcatenate_2 (Concatenate) (None, 21, 21, 224) 0 maximum_5[0][0] \n conv2d_17[0][0] \n__________________________________________________________________________________________________\nconcatenate_3 (Concatenate) (None, 21, 21, 229) 0 batch_normalization_7[0][0] \n concatenate_2[0][0] \n__________________________________________________________________________________________________\nconv2d_18 (Conv2D) (None, 1, 1, 5) 504950 concatenate_3[0][0] \n__________________________________________________________________________________________________\nbatch_normalization_14 (BatchNo (None, 1, 1, 5) 20 conv2d_18[0][0] \n__________________________________________________________________________________________________\nactivation_1 (Activation) (None, 1, 1, 5) 0 batch_normalization_14[0][0] \n==================================================================================================\nTotal params: 1,799,043\nTrainable params: 1,796,719\nNon-trainable params: 2,324\n__________________________________________________________________________________________________\n"
],
[
"m.compile(optimizer='adam',loss='categorical_crossentropy',metrics=['accuracy'])",
"_____no_output_____"
],
[
"m.save('trial_0001_MFCcascade_acc.h5')",
"_____no_output_____"
],
[
"m1 = input_cascade((65,65,4),(33,33,4))\nm1.summary()",
"_____no_output_____"
],
[
"import os\nos.chdir('drive/brat')",
"_____no_output_____"
],
[
"def model_gen(input_dim,x,y,slice_no):\n X1 = []\n X2 = []\n Y = []\n print(int((input_dim)/2))\n for i in range(int((input_dim)/2),175-int((input_dim)/2)):\n for j in range(int((input_dim)/2),195-int((input_dim)/2)):\n X2.append(x[i-16:i+17,j-16:j+17,:])\n X1.append(x[i-int((input_dim)/2):i+int((input_dim)/2)+1,j-int((input_dim)/2):j+int((input_dim)/2)+1,:])\n Y.append(y[i,slice_no,j])\n \n X1 = np.asarray(X1)\n X2 = np.asarray(X2)\n Y = np.asarray(Y)\n d = [X1,X2,Y]\n return d",
"_____no_output_____"
],
[
"def data_gen(path,slice_no,model_no):\n p = os.listdir(path)\n p.sort(key=str.lower)\n arr = []\n for i in range(len(p)):\n if(i != 4):\n p1 = os.listdir(path+'/'+p[i])\n p1.sort()\n img = sitk.ReadImage(path+'/'+p[i]+'/'+p1[-1])\n arr.append(sitk.GetArrayFromImage(img))\n else:\n p1 = os.listdir(path+'/'+p[i])\n img = sitk.ReadImage(path+'/'+p[i]+'/'+p1[0])\n y = sitk.GetArrayFromImage(img) \n data = np.zeros((196,176,216,4))\n for i in range(196):\n data[i,:,:,0] = arr[0][:,i,:]\n data[i,:,:,1] = arr[1][:,i,:]\n data[i,:,:,2] = arr[2][:,i,:]\n data[i,:,:,3] = arr[3][:,i,:]\n x = data[slice_no]\n \n if(model_no == 0):\n X1 = []\n for i in range(16,159):\n for j in range(16,199):\n X1.append(x[i-16:i+17,j-16:j+17,:])\n Y1 = []\n for i in range(16,159):\n for j in range(16,199):\n Y1.append(y[i,slice_no,j]) \n X1 = np.asarray(X1)\n Y1 = np.asarray(Y1)\n d = [X1,Y1]\n elif(model_no == 1):\n d = model_gen(65,x,y,slice_no)\n elif(model_no == 2):\n d = model_gen(56,x,y,slice_no)\n elif(model_no == 3):\n d = model_gen(53,x,y,slice_no) \n \n return d ",
"_____no_output_____"
],
[
"d = data_gen('LG/0001',100,3)",
"26\n"
],
[
"d[2].all == 0",
"_____no_output_____"
],
[
"len(d[0])",
"_____no_output_____"
],
[
"!pip3 install SimpleITK",
"Collecting SimpleITK\n\u001b[?25l Downloading https://files.pythonhosted.org/packages/88/08/9be802a363c1259d1c9d8d684ee23d3cffa7f35be62ab8a2d8f7890cfa7c/SimpleITK-1.1.0-cp36-cp36m-manylinux1_x86_64.whl (41.0MB)\n\u001b[K 100% |โโโโโโโโโโโโโโโโโโโโโโโโโโโโโโโโ| 41.0MB 1.4MB/s \n\u001b[?25hInstalling collected packages: SimpleITK\nSuccessfully installed SimpleITK-1.1.0\n"
],
[
"import SimpleITK as sitk\nimport numpy as np",
"_____no_output_____"
],
[
"y = np.zeros((17589,1,1,5))",
"_____no_output_____"
],
[
"for i in range(y.shape[0]):\n y[i,:,:,d[2][i]] = 1",
"_____no_output_____"
],
[
"sample = np.zeros((5,1))\nfor i in range(5):\n sample[i] = np.sum(y[:,:,:,i])\nprint(sample/np.sum(sample)) ",
"[[9.62476548e-01]\n [2.06378987e-02]\n [4.20717494e-03]\n [1.21098414e-02]\n [5.68537154e-04]]\n"
],
[
"X1 = np.asarray(d[0])",
"_____no_output_____"
],
[
"X1.shape",
"_____no_output_____"
],
[
"X2 = np.asarray(d[1])",
"_____no_output_____"
],
[
"X2.shape",
"_____no_output_____"
],
[
"m1.inputs",
"_____no_output_____"
],
[
"m.compile(optimizer='adam',loss='categorical_crossentropy',metrics=[f1_score])",
"_____no_output_____"
],
[
"m_info = m.fit([X1,X2],y,epochs=20,batch_size=256)",
"_____no_output_____"
],
[
"m.compile(optimizer='adam',loss='categorical_crossentropy',metrics=['accuracy'])",
"_____no_output_____"
]
],
[
[
"Slice 136 patient 0002",
"_____no_output_____"
]
],
[
[
"from sklearn.utils import class_weight\nclass_weights = class_weight.compute_class_weight('balanced',\n np.unique(d[2]),\n d[2])",
"_____no_output_____"
],
[
"class_weights",
"_____no_output_____"
],
[
"import keras\nmodel = keras.models.load_model('trial_0001_MFCcas_dim2_128_acc.h5')",
"_____no_output_____"
],
[
"m_info = m.fit([X1,X2],y,epochs= 20,batch_size = 256,class_weight = class_weights)",
"Epoch 1/20\n17589/17589 [==============================] - 91s 5ms/step - loss: 1.1423 - acc: 0.9462\nEpoch 2/20\n17589/17589 [==============================] - 81s 5ms/step - loss: 1.0267 - acc: 0.9763\nEpoch 3/20\n17589/17589 [==============================] - 81s 5ms/step - loss: 0.9321 - acc: 0.9802\nEpoch 4/20\n17589/17589 [==============================] - 81s 5ms/step - loss: 0.8436 - acc: 0.9850\nEpoch 5/20\n16128/17589 [==========================>...] - ETA: 6s - loss: 0.7692 - acc: 0.9859"
],
[
"import matplotlib.pyplot as plt\nplt.plot(m_info.history['acc'])\n#plt.plot(m_info.history['val_acc'])\nplt.title('model accuracy')\nplt.ylabel('accuracy')\nplt.xlabel('epoch')\nplt.legend(['train', 'test'], loc='upper left')\nplt.show()",
"_____no_output_____"
],
[
"m.save('trial_MFCcascade_acc.h5')",
"_____no_output_____"
]
],
[
[
"eval on 128th slice 0002",
"_____no_output_____"
]
],
[
[
"model.evaluate([X1,X2],y,batch_size = 1024)",
"17589/17589 [==============================] - 77s 4ms/step\n"
],
[
"model_info = model.fit([X1,X2],y,epochs=30,batch_size=256,class_weight= class_weights)",
"Epoch 1/30\n17589/17589 [==============================] - 96s 5ms/step - loss: 0.1438 - acc: 0.9482\nEpoch 2/30\n17589/17589 [==============================] - 82s 5ms/step - loss: 0.0838 - acc: 0.9741\nEpoch 3/30\n17589/17589 [==============================] - 82s 5ms/step - loss: 0.0770 - acc: 0.9762\nEpoch 4/30\n17589/17589 [==============================] - 82s 5ms/step - loss: 0.0738 - acc: 0.9766\nEpoch 5/30\n16128/17589 [==========================>...] - ETA: 6s - loss: 0.0668 - acc: 0.9791"
],
[
"import matplotlib.pyplot as plt\nplt.plot(model_info.history['acc'])\n#plt.plot(m_info.history['val_acc'])\nplt.title('model accuracy')\nplt.ylabel('accuracy')\nplt.xlabel('epoch')\nplt.legend(['train', 'test'], loc='upper left')\nplt.show()",
"_____no_output_____"
],
[
"model.save('trial_0001_MFCcas_dim2_128_acc.h5')",
"_____no_output_____"
]
],
[
[
"eval on 100th slice 0001",
"_____no_output_____"
]
],
[
[
"model.evaluate([X1,X2],y,batch_size = 1024)",
"17589/17589 [==============================] - 76s 4ms/step\n"
],
[
"pred = model.predict([X1,X2],batch_size = 1024)\npred = np.around(pred)\npred1 = np.dot(pred.reshape(17589,5),np.array([0,1,2,3,4]))\ny1 = np.dot(y.reshape(17589,5),np.array([0,1,2,3,4]))",
"_____no_output_____"
],
[
"y2 = np.argmax(y.reshape(17589,5),axis = 1)\ny2.all() == 0",
"_____no_output_____"
],
[
"y1.all()==0",
"_____no_output_____"
],
[
"from sklearn import metrics",
"_____no_output_____"
],
[
"f1 = metrics.f1_score(y1,pred1,average='micro')\nf1",
"_____no_output_____"
],
[
"p1 = metrics.precision_score(y1,pred1,average='micro')\np1",
"_____no_output_____"
],
[
"r1 = metrics.recall_score(y1,pred1,average='micro')\nr1",
"_____no_output_____"
],
[
"p2 = metrics.precision_score(y1,pred2,average='micro')\np2",
"_____no_output_____"
],
[
"pred2 = np.zeros((17589))\nf2 = metrics.f1_score(y1,pred2,average='micro')\nf2",
"_____no_output_____"
]
],
[
[
"Slice 128 patient 0001",
"_____no_output_____"
]
],
[
[
"from sklearn.utils import class_weight",
"_____no_output_____"
],
[
"class_weights = class_weight.compute_class_weight('balanced',\n np.unique(d[2]),\n d[2])",
"_____no_output_____"
],
[
"class_weights",
"_____no_output_____"
],
[
"m1.compile(optimizer='adam',loss='categorical_crossentropy',metrics=['accuracy'])",
"_____no_output_____"
],
[
"m1_info = m1.fit([X1,X2],y,epochs=20,batch_size=256,class_weight= class_weights)",
"Epoch 1/20\n14541/14541 [==============================] - 127s 9ms/step - loss: 1.3402 - acc: 0.8345\nEpoch 2/20\n14541/14541 [==============================] - 123s 8ms/step - loss: 1.1816 - acc: 0.9560\nEpoch 3/20\n14541/14541 [==============================] - 123s 8ms/step - loss: 1.0906 - acc: 0.9647\nEpoch 4/20\n14541/14541 [==============================] - 123s 8ms/step - loss: 1.0021 - acc: 0.9735\nEpoch 5/20\n14541/14541 [==============================] - 123s 8ms/step - loss: 0.9231 - acc: 0.9801\nEpoch 6/20\n 5632/14541 [==========>...................] - ETA: 1:15 - loss: 0.8693 - acc: 0.9826"
]
],
[
[
"plot of inputcascade",
"_____no_output_____"
]
],
[
[
"import matplotlib.pyplot as plt",
"_____no_output_____"
],
[
"plt.plot(m1_info.history['acc'])\n#plt.plot(m_info.history['val_acc'])\nplt.title('model accuracy')\nplt.ylabel('accuracy')\nplt.xlabel('epoch')\nplt.legend(['train', 'test'], loc='upper left')\nplt.show()",
"_____no_output_____"
],
[
"m1.save('trial_0001_input_cascade_acc.h5')",
"_____no_output_____"
],
[
"plt.plot(m_info.history['acc'])\n#plt.plot(m_info.history['val_acc'])\nplt.title('model accuracy')\nplt.ylabel('accuracy')\nplt.xlabel('epoch')\nplt.legend(['train', 'test'], loc='upper left')\nplt.show()",
"_____no_output_____"
]
],
[
[
"Training on slice 128, evaluating on 136",
"_____no_output_____"
]
],
[
[
"m.evaluate([X1,X2],y,batch_size = 1024)",
"17589/17589 [==============================] - 74s 4ms/step\n"
],
[
"m.save('trial_0001_MFCcas_dim2_128_acc.h5')",
"_____no_output_____"
],
[
"pred = m.predict([X1,X2],batch_size = 1024)",
"_____no_output_____"
],
[
"print(((pred != 0.) & (pred != 1.)).any())",
"False\n"
],
[
"pred = np.around(pred)",
"_____no_output_____"
],
[
"type(y)",
"_____no_output_____"
],
[
"pred1 = np.dot(pred.reshape(17589,5),np.array([0,1,2,3,4]))",
"_____no_output_____"
],
[
"pred1.shape",
"_____no_output_____"
],
[
"y1 = np.dot(y.reshape(17589,5),np.array([0,1,2,3,4]))",
"_____no_output_____"
],
[
"from sklearn import metrics",
"_____no_output_____"
],
[
"f1 = metrics.f1_score(y1,pred1,average='micro')\nf1",
"_____no_output_____"
],
[
"pred2 = np.zeros((17589,1))\nf1 = f1 = metrics.f1_score(y1,pred2,average='micro')\nf1",
"_____no_output_____"
],
[
"f1 = metrics.f1_score(y1,pred1,average='weighted')\nf1",
"/usr/local/lib/python3.6/dist-packages/sklearn/metrics/classification.py:1137: UndefinedMetricWarning: F-score is ill-defined and being set to 0.0 in labels with no true samples.\n 'recall', 'true', average, warn_for)\n"
],
[
"f1 = metrics.f1_score(y1,pred1,average='macro')\nf1",
"/usr/local/lib/python3.6/dist-packages/sklearn/metrics/classification.py:1137: UndefinedMetricWarning: F-score is ill-defined and being set to 0.0 in labels with no true samples.\n 'recall', 'true', average, warn_for)\n"
],
[
"plt.plot(m_info.history['acc'])\n#plt.plot(m_info.history['val_acc'])\nplt.title('model accuracy')\nplt.ylabel('accuracy')\nplt.xlabel('epoch')\nplt.legend(['train', 'test'], loc='upper left')\nplt.show()",
"_____no_output_____"
],
[
"m_info = m.fit([X1,X2],y,epochs=20,batch_size=256,class_weight= 10*class_weights)",
"Epoch 1/20\n17589/17589 [==============================] - 80s 5ms/step - loss: 0.3351 - acc: 0.9581\nEpoch 2/20\n17589/17589 [==============================] - 80s 5ms/step - loss: 0.3181 - acc: 0.9580\nEpoch 3/20\n17589/17589 [==============================] - 81s 5ms/step - loss: 0.3040 - acc: 0.9581\nEpoch 4/20\n17589/17589 [==============================] - 80s 5ms/step - loss: 0.2909 - acc: 0.9583\nEpoch 5/20\n16128/17589 [==========================>...] - ETA: 6s - loss: 0.2797 - acc: 0.9583"
],
[
"plt.plot(m_info.history['acc'])\n#plt.plot(m_info.history['val_acc'])\nplt.title('model accuracy')\nplt.ylabel('accuracy')\nplt.xlabel('epoch')\nplt.legend(['train', 'test'], loc='upper left')\nplt.show()",
"_____no_output_____"
],
[
"import matplotlib.pyplot as plt",
"_____no_output_____"
],
[
"m.save('trial_0001_MFCcas_dim2_128_acc.h5')",
"_____no_output_____"
],
[
"import keras",
"_____no_output_____"
],
[
"def two_pathcnn(input_shape):\n \n X_input = Input(input_shape)\n \n X = Conv2D(64,(7,7),strides=(1,1),padding='valid')(X_input)\n X = BatchNormalization()(X)\n X1 = Conv2D(64,(7,7),strides=(1,1),padding='valid')(X_input)\n X1 = BatchNormalization()(X1)\n X = layers.Maximum()([X,X1])\n X = Conv2D(64,(4,4),strides=(1,1),padding='valid',activation='relu')(X)\n \n X2 = Conv2D(160,(13,13),strides=(1,1),padding='valid')(X_input)\n X2 = BatchNormalization()(X2)\n X21 = Conv2D(160,(13,13),strides=(1,1),padding='valid')(X_input)\n X21 = BatchNormalization()(X21)\n X2 = layers.Maximum()([X2,X21])\n \n X3 = Conv2D(64,(3,3),strides=(1,1),padding='valid')(X)\n X3 = BatchNormalization()(X3)\n X31 = Conv2D(64,(3,3),strides=(1,1),padding='valid')(X)\n X31 = BatchNormalization()(X31)\n X = layers.Maximum()([X3,X31])\n X = Conv2D(64,(2,2),strides=(1,1),padding='valid',activation='relu')(X)\n \n X = Concatenate()([X2,X])\n X = Conv2D(5,(21,21),strides=(1,1),padding='valid')(X)\n X = Activation('softmax')(X)\n \n model = Model(inputs = X_input, outputs = X)\n return model ",
"_____no_output_____"
],
[
"import os",
"_____no_output_____"
],
[
"m0 = two_pathcnn((33,33,4))\nm0.summary()",
"__________________________________________________________________________________________________\nLayer (type) Output Shape Param # Connected to \n==================================================================================================\ninput_1 (InputLayer) (None, 33, 33, 4) 0 \n__________________________________________________________________________________________________\nconv2d_1 (Conv2D) (None, 27, 27, 64) 12608 input_1[0][0] \n__________________________________________________________________________________________________\nconv2d_2 (Conv2D) (None, 27, 27, 64) 12608 input_1[0][0] \n__________________________________________________________________________________________________\nbatch_normalization_1 (BatchNor (None, 27, 27, 64) 256 conv2d_1[0][0] \n__________________________________________________________________________________________________\nbatch_normalization_2 (BatchNor (None, 27, 27, 64) 256 conv2d_2[0][0] \n__________________________________________________________________________________________________\nmaximum_1 (Maximum) (None, 27, 27, 64) 0 batch_normalization_1[0][0] \n batch_normalization_2[0][0] \n__________________________________________________________________________________________________\nconv2d_3 (Conv2D) (None, 24, 24, 64) 65600 maximum_1[0][0] \n__________________________________________________________________________________________________\nconv2d_6 (Conv2D) (None, 22, 22, 64) 36928 conv2d_3[0][0] \n__________________________________________________________________________________________________\nconv2d_7 (Conv2D) (None, 22, 22, 64) 36928 conv2d_3[0][0] \n__________________________________________________________________________________________________\nconv2d_4 (Conv2D) (None, 21, 21, 160) 108320 input_1[0][0] \n__________________________________________________________________________________________________\nconv2d_5 (Conv2D) (None, 21, 21, 160) 108320 input_1[0][0] \n__________________________________________________________________________________________________\nbatch_normalization_5 (BatchNor (None, 22, 22, 64) 256 conv2d_6[0][0] \n__________________________________________________________________________________________________\nbatch_normalization_6 (BatchNor (None, 22, 22, 64) 256 conv2d_7[0][0] \n__________________________________________________________________________________________________\nbatch_normalization_3 (BatchNor (None, 21, 21, 160) 640 conv2d_4[0][0] \n__________________________________________________________________________________________________\nbatch_normalization_4 (BatchNor (None, 21, 21, 160) 640 conv2d_5[0][0] \n__________________________________________________________________________________________________\nmaximum_3 (Maximum) (None, 22, 22, 64) 0 batch_normalization_5[0][0] \n batch_normalization_6[0][0] \n__________________________________________________________________________________________________\nmaximum_2 (Maximum) (None, 21, 21, 160) 0 batch_normalization_3[0][0] \n batch_normalization_4[0][0] \n__________________________________________________________________________________________________\nconv2d_8 (Conv2D) (None, 21, 21, 64) 16448 maximum_3[0][0] \n__________________________________________________________________________________________________\nconcatenate_1 (Concatenate) (None, 21, 21, 224) 0 maximum_2[0][0] \n conv2d_8[0][0] \n__________________________________________________________________________________________________\nconv2d_9 (Conv2D) (None, 1, 1, 5) 493925 concatenate_1[0][0] \n__________________________________________________________________________________________________\nactivation_1 (Activation) (None, 1, 1, 5) 0 conv2d_9[0][0] \n==================================================================================================\nTotal params: 893,989\nTrainable params: 892,837\nNon-trainable params: 1,152\n__________________________________________________________________________________________________\n"
],
[
"os.chdir('drive/brat')",
"_____no_output_____"
],
[
"!ls",
"data.ipynb\t\tmodel.ipynb\r\ndata_scan_0001.pickle\ttraining.ipynb\r\ndata_trial_81.h5\ttrial_0001_81_accuracy.h5\r\ndata_trial_dim2_128.h5\ttrial_0001_81_f1.h5\r\ndata_trial.h5\t\ttrial_0001_accuracy.h5\r\ndata_trial_X.pickle\ttrial_0001_f1.h5\r\ndata_trial_Y.pickle\ttrial_0001_input_cascade_acc.h5\r\ndata_Y_0001.pickle\ttrial_0001_input_cascasde_acc.h5\r\nHG\t\t\ttrial_0001_MFCcas_dim2_128_acc.h5\r\nLG\r\n"
]
],
[
[
"for training over entire image, create batch of patches for one image, batch of labels in Y",
"_____no_output_____"
]
],
[
[
"import h5py\nimport numpy as np",
"_____no_output_____"
],
[
"hf = h5py.File('data_trial_dim2_128.h5', 'r')\nX = hf.get('dataset_1')\nY = hf.get('dataset_2')",
"_____no_output_____"
],
[
"y = np.zeros((26169,1,1,5))",
"_____no_output_____"
],
[
"for i in range(y.shape[0]):\n y[i,:,:,Y[i]] = 1",
"_____no_output_____"
],
[
"X = np.asarray(X)",
"_____no_output_____"
],
[
"X.shape",
"_____no_output_____"
],
[
"keras.__version__",
"_____no_output_____"
],
[
"import keras.backend as K\n\ndef f1_score(y_true, y_pred):\n\n # Count positive samples.\n c1 = K.sum(K.round(K.clip(y_true * y_pred, 0, 1)))\n c2 = K.sum(K.round(K.clip(y_true, 0, 1)))\n c3 = K.sum(K.round(K.clip(y_pred, 0, 1)))\n\n # If there are no true samples, fix the F1 score at 0.\n if c3 == 0:\n return 0\n\n # How many selected items are relevant?\n precision = c1 / c2\n\n # How many relevant items are selected?\n recall = c1 / c3\n\n # Calculate f1_score\n f1_score = 2 * (precision * recall) / (precision + recall)\n return f1_score",
"_____no_output_____"
],
[
"from sklearn.utils import class_weight",
"_____no_output_____"
],
[
"class_weights = class_weight.compute_class_weight('balanced',\n np.unique(Y),\n Y)",
"_____no_output_____"
],
[
"",
"_____no_output_____"
],
[
"m0.compile(optimizer='adam',loss='categorical_hinge',metrics=[f1_score])",
"_____no_output_____"
],
[
"m0_info = m0.fit(X,y,epochs=20,batch_size=1024,class_weight = class_weights)",
"Epoch 1/20\n26169/26169 [==============================] - 24s 901us/step - loss: 0.0967 - f1_score: 0.9517\nEpoch 2/20\n26169/26169 [==============================] - 23s 878us/step - loss: 0.0967 - f1_score: 0.9517\nEpoch 3/20\n26169/26169 [==============================] - 23s 881us/step - loss: 0.0967 - f1_score: 0.9517\nEpoch 4/20\n26169/26169 [==============================] - 23s 879us/step - loss: 0.0967 - f1_score: 0.9517\nEpoch 5/20\n26169/26169 [==============================] - 23s 878us/step - loss: 0.0967 - f1_score: 0.9517\nEpoch 6/20\n26169/26169 [==============================] - 23s 882us/step - loss: 0.0967 - f1_score: 0.9517\nEpoch 7/20\n26169/26169 [==============================] - 23s 881us/step - loss: 0.0967 - f1_score: 0.9517\nEpoch 8/20\n23552/26169 [=========================>....] - ETA: 2s - loss: 0.0959 - f1_score: 0.9521"
],
[
"m0.save('trial_0001_dim2_128_f1.h5')",
"_____no_output_____"
],
[
"!ls",
"data.ipynb\t\tHG\r\ndata_scan_0001.pickle\tLG\r\ndata_trial_81.h5\tmodel.ipynb\r\ndata_trial_dim2_128.h5\ttrial_0001_81_accuracy.h5\r\ndata_trial.h5\t\ttrial_0001_81_f1.h5\r\ndata_trial_X.pickle\ttrial_0001_accuracy.h5\r\ndata_trial_Y.pickle\ttrial_0001_f1.h5\r\ndata_Y_0001.pickle\ttrial_0001_MFCcas_dim2_128_acc.h5\r\n"
],
[
"m0.compile(optimizer='adam',loss='categorical_crossentropy',metrics=['accuracy'])",
"_____no_output_____"
],
[
"m0_info = m0.fit(X,y,epochs=20,batch_size=4096,class_weight = class_weights)",
"Epoch 1/20\n26169/26169 [==============================] - 41s 2ms/step - loss: 0.7791 - acc: 0.9517\nEpoch 2/20\n26169/26169 [==============================] - 25s 939us/step - loss: 0.7791 - acc: 0.9517\nEpoch 3/20\n26169/26169 [==============================] - 25s 939us/step - loss: 0.7791 - acc: 0.9517\nEpoch 4/20\n26169/26169 [==============================] - 25s 941us/step - loss: 0.7791 - acc: 0.9517\nEpoch 5/20\n26169/26169 [==============================] - 25s 941us/step - loss: 0.7791 - acc: 0.9517\nEpoch 6/20\n26169/26169 [==============================] - 25s 940us/step - loss: 0.7791 - acc: 0.9517\nEpoch 7/20\n26169/26169 [==============================] - 25s 941us/step - loss: 0.7791 - acc: 0.9517\nEpoch 8/20\n26169/26169 [==============================] - 25s 942us/step - loss: 0.7791 - acc: 0.9517\nEpoch 9/20\n26169/26169 [==============================] - 25s 940us/step - loss: 0.7791 - acc: 0.9517\nEpoch 10/20\n26169/26169 [==============================] - 25s 940us/step - loss: 0.7791 - acc: 0.9517\nEpoch 11/20\n26169/26169 [==============================] - 25s 941us/step - loss: 0.7791 - acc: 0.9517\nEpoch 12/20\n26169/26169 [==============================] - 25s 942us/step - loss: 0.7791 - acc: 0.9517\nEpoch 13/20\n26169/26169 [==============================] - 25s 940us/step - loss: 0.7791 - acc: 0.9517\nEpoch 14/20\n12288/26169 [=============>................] - ETA: 13s - loss: 0.7634 - acc: 0.9526"
],
[
"m0.save('trial_0001_dim2_128_accuracy.h5')",
"_____no_output_____"
],
[
"!ls",
"_____no_output_____"
],
[
"mod = keras.models.load_model('trial_0001_81_accuracy.h5')",
"_____no_output_____"
],
[
"mod.evaluate(X,y,batch_size = 1024)",
"_____no_output_____"
],
[
"pred = m0.predict(X,batch_size = 1024)",
"_____no_output_____"
],
[
"pred.shape",
"_____no_output_____"
],
[
"pred = np.floor(pred)",
"_____no_output_____"
],
[
"y.reshape(26169,5)",
"_____no_output_____"
],
[
"pred.astype(int)",
"_____no_output_____"
],
[
"pred = pred.reshape(26169,5)\ny_pred = np.floor(np.dot(pred,np.array([0,1,2,3,4])))\ny_pred.reshape(26169,1)",
"_____no_output_____"
],
[
"y_pred.shape",
"_____no_output_____"
],
[
"print(((y_pred != 0.) & (y_pred != 1.)).any())",
"False\n"
],
[
"from matplotlib import pyplot as plt\nplt.imshow(np.uint8(y_pred*32))\nplt.show()",
"_____no_output_____"
],
[
"from sklearn import metrics",
"_____no_output_____"
],
[
"f1 = metrics.f1_score(y,pred)",
"_____no_output_____"
],
[
"!pip3 install SimpleITK",
"Requirement already satisfied: SimpleITK in /usr/local/lib/python3.6/dist-packages (1.1.0)\r\n"
],
[
"import SimpleITK as sitk\nimport numpy as np",
"_____no_output_____"
],
[
"path = 'LG/0001'\np = os.listdir(path)\np.sort(key=str.lower)\narr = []\nfor i in range(len(p)):\n if(i != 4):\n p1 = os.listdir(path+'/'+p[i])\n p1.sort()\n img = sitk.ReadImage(path+'/'+p[i]+'/'+p1[-1])\n arr.append(sitk.GetArrayFromImage(img))\n else:\n p1 = os.listdir(path+'/'+p[i])\n img = sitk.ReadImage(path+'/'+p[i]+'/'+p1[0])\n Y_labels = sitk.GetArrayFromImage(img) \ndata = np.zeros((Y_labels.shape[1],Y_labels.shape[0],Y_labels.shape[2],4))\nfor i in range(196):\n data[i,:,:,0] = arr[0][:,i,:]\n data[i,:,:,1] = arr[1][:,i,:]\n data[i,:,:,2] = arr[2][:,i,:]\n data[i,:,:,3] = arr[3][:,i,:]\n ",
"_____no_output_____"
],
[
"def model_gen(input_dim,x,y,slice_no):\n X1 = []\n X2 = []\n Y = []\n \n for i in range(int((input_dim)/2),175-int((input_dim)/2)):\n for j in range(int((input_dim)/2),195-int((input_dim)/2)):\n if(x[i-16:i+17,j-16:j+17,:].any != 0):\n X2.append(x[i-16:i+17,j-16:j+17,:])\n X1.append(x[i-int((input_dim)/2):i+int((input_dim)/2)+1,j-int((input_dim)/2):j+int((input_dim)/2)+1,:])\n Y.append(y[i,slice_no,j])\n \n \n X1 = np.asarray(X1)\n X2 = np.asarray(X2)\n Y = np.asarray(Y)\n d = [X1,X2,Y]\n return d",
"_____no_output_____"
],
[
"def data_gen(data,y,slice_no,model_no):\n d = []\n x = data[slice_no]\n if(x.any() != 0 and y.any() != 0):\n if(model_no == 0):\n X1 = []\n for i in range(16,159):\n for j in range(16,199):\n if(x[i-16:i+17,j-16:j+17,:].all != 0):\n X1.append(x[i-16:i+17,j-16:j+17,:])\n Y1 = []\n for i in range(16,159):\n for j in range(16,199):\n if(x[i-16:i+17,j-16:j+17,:].all != 0):\n Y1.append(y[i,slice_no,j]) \n X1 = np.asarray(X1)\n Y1 = np.asarray(Y1)\n d = [X1,Y1]\n elif(model_no == 1):\n d = model_gen(65,x,y,slice_no)\n elif(model_no == 2):\n d = model_gen(56,x,y,slice_no)\n elif(model_no == 3):\n d = model_gen(53,x,y,slice_no) \n \n return d ",
"_____no_output_____"
],
[
"from sklearn.utils import class_weight",
"_____no_output_____"
],
[
"m0.compile(optimizer='adam',loss='categorical_crossentropy',metrics=['accuracy'])",
"_____no_output_____"
],
[
"info = []\nfor i in range(90,data.shape[0],2):\n d = data_gen(data,Y_labels,i,0)\n if(len(d) != 0):\n y = np.zeros((d[-1].shape[0],1,1,5))\n for j in range(y.shape[0]):\n y[j,:,:,d[-1][j]] = 1\n X1 = d[0]\n class_weights = class_weight.compute_class_weight('balanced',\n np.unique(d[-1]),\n d[-1])\n print('slice no:'+str(i))\n info.append(m0.fit(X1,y,epochs=2,batch_size=32,class_weight= class_weights))\n m0.save('trial_0001_2path_acc.h5')",
"slice no:90\nEpoch 1/2\n24992/26169 [===========================>..] - ETA: 5s - loss: 1.1921e-07 - acc: 1.0000"
],
[
"",
"_____no_output_____"
]
]
] | [
"code",
"markdown",
"code",
"markdown",
"code",
"markdown",
"code",
"markdown",
"code",
"markdown",
"code",
"markdown",
"code",
"markdown",
"code"
] | [
[
"code",
"code",
"code",
"code",
"code",
"code",
"code",
"code",
"code",
"code",
"code",
"code",
"code",
"code",
"code",
"code",
"code",
"code",
"code",
"code",
"code",
"code",
"code",
"code",
"code",
"code",
"code",
"code",
"code",
"code",
"code"
],
[
"markdown"
],
[
"code",
"code",
"code",
"code",
"code",
"code"
],
[
"markdown"
],
[
"code",
"code",
"code",
"code"
],
[
"markdown"
],
[
"code",
"code",
"code",
"code",
"code",
"code",
"code",
"code",
"code",
"code"
],
[
"markdown"
],
[
"code",
"code",
"code",
"code",
"code"
],
[
"markdown"
],
[
"code",
"code",
"code",
"code"
],
[
"markdown"
],
[
"code",
"code",
"code",
"code",
"code",
"code",
"code",
"code",
"code",
"code",
"code",
"code",
"code",
"code",
"code",
"code",
"code",
"code",
"code",
"code",
"code",
"code",
"code",
"code",
"code"
],
[
"markdown"
],
[
"code",
"code",
"code",
"code",
"code",
"code",
"code",
"code",
"code",
"code",
"code",
"code",
"code",
"code",
"code",
"code",
"code",
"code",
"code",
"code",
"code",
"code",
"code",
"code",
"code",
"code",
"code",
"code",
"code",
"code",
"code",
"code",
"code",
"code",
"code",
"code",
"code",
"code",
"code",
"code",
"code"
]
] |
e769a23ab748aaf19419c956e8391bff185e287b | 93,782 | ipynb | Jupyter Notebook | .ipynb_checkpoints/Project 3-checkpoint.ipynb | junemore/traffic-accidents-analysis | 0d67e0dae0de1ec7650017292751c590b3dd0b22 | [
"MIT"
] | null | null | null | .ipynb_checkpoints/Project 3-checkpoint.ipynb | junemore/traffic-accidents-analysis | 0d67e0dae0de1ec7650017292751c590b3dd0b22 | [
"MIT"
] | null | null | null | .ipynb_checkpoints/Project 3-checkpoint.ipynb | junemore/traffic-accidents-analysis | 0d67e0dae0de1ec7650017292751c590b3dd0b22 | [
"MIT"
] | null | null | null | 63.53794 | 40,978 | 0.638833 | [
[
[
"%matplotlib inline\nimport matplotlib.pyplot as plt\nfrom dbfread import DBF\nimport pandas as pd\nimport numpy as np\nfrom pandas import DataFrame\nimport shelve\n\nplt.style.use('seaborn-dark')\nplt.rcParams['figure.figsize'] = (10, 6)",
"_____no_output_____"
]
],
[
[
"## Load 10 years of accident data, from 2007 to 2016",
"_____no_output_____"
]
],
[
[
"#load accidents data from 2007 to 2016 \ndbf07= DBF('accident/accident2007.dbf')\ndbf08= DBF('accident/accident2008.dbf')\ndbf09= DBF('accident/accident2009.dbf')\ndbf10= DBF('accident/accident2010.dbf')\ndbf11 = DBF('accident/accident2011.dbf')\ndbf12 = DBF('accident/accident2012.dbf')\ndbf13 = DBF('accident/accident2013.dbf')\ndbf14 = DBF('accident/accident2014.dbf')\ndbf15 = DBF('accident/accident2015.dbf')\ndbf16 = DBF('accident/accident2016.dbf')\naccidents07 = DataFrame(iter(dbf07))\naccidents08 = DataFrame(iter(dbf08))\naccidents09 = DataFrame(iter(dbf09))\naccidents10 = DataFrame(iter(dbf10))\naccidents11 = DataFrame(iter(dbf11))\naccidents12 = DataFrame(iter(dbf12))\naccidents13 = DataFrame(iter(dbf13))\naccidents14 = DataFrame(iter(dbf14))\naccidents15 = DataFrame(iter(dbf15))\naccidents16 = DataFrame(iter(dbf16))",
"_____no_output_____"
]
],
[
[
"First, we want to combine accidents10 ~ accidents16 to one dataframe. Since not all of the accident data downloaded from the U.S. Department of Transportation have the same features, by using the `jion:inner` option in `pd.concat` function, we can get the intersection of features.",
"_____no_output_____"
]
],
[
[
"# rename column name in frame07 so that columns names are the same with other frames\naccidents07.rename(columns={'latitude': 'LATITUDE', 'longitud': 'LONGITUD'}, inplace=True)",
"_____no_output_____"
],
[
"# take a look inside how the accident data file looks like\n#combine all accidents file\nallaccidents = pd.concat([accidents07,accidents08,accidents09,accidents10,accidents11,accidents12,accidents13,accidents14,accidents15,accidents16], axis=0,join='inner')\npd.set_option('display.max_columns', 100)\nallaccidents.head()",
"_____no_output_____"
]
],
[
[
"The allaccidents table recorded 320874 accidents from 2010-2016, and it has 42 features. Here are the meaning of some of the features according to the `FARS Analytical Userโs Manual`.\n\n### Explaination of variables\n*VE_TOTAL*: Number of Vehicle in crash <br/>\n*VE_FORMS*: Number of Motor Vehicles in Transport (MVIT) <br/>\n*PED*: Number of Persons Not in Motor Vehicles <br/>\n*NHS*: National Highway System<br/>\n*ROUTE*: Route Signing <br/>\n*SP_JUR*: Special Jurisdiction <br/>\n*HARM_EV*: First Harmful Event<br/>\n*TWAY_ID , TWAY_ID2* : Trafficway Identifier <br/>\n*MILEPT*: Milepoint <br/>\n*SP_JUR*: Special Jurisdiction<br/>\n*HARM_EV*: injury or damage producing First Harmful Event <br/> \n*MAN_COLL*:Manner of Collision <br/> \n*RELJCT1, RELJCT2*: Relation to Junction- Within Interchange Area, Specific Location. <br/>\n*TYP_INT*: Type of Intersection <br/>\n*REL_ROAD*: Relation to Trafficway <br/>\n*LGT_COND*: Light Condition<br/> \n*NOT_HOUR,MIN*: Min, Hour of Notification <br/>\n*ARR_HOUR,MIN*: Hour, Min arrival at scene <br/>\n*HOSP_HR,MIN*: Hour, Min arrival at hospital <br/>\n*CF1, CF2, CF3*:Related Factors- Crash Level, factors related to the crash <br/>\n*FATALS*: Fatalities<br/>\n*DRUNK_DR*: Number of Drinking Drivers<br/> \n*RAIL*: Rail Grade Crossing Identifier<br/>\n\nFor more detailed information, please refer to `FARS Analytical Userโs Manual`.",
"_____no_output_____"
],
[
"## Select variables and rename variables\nObserved from the table above, some of the variables in the table are not very readable. Therefore, in order to make it easier to understand the variables,we renamed some of the variables according to `FARS Analytical Userโs Manual` downloaded from the `U.S. Department of Transportation` website. In order to make all column values informative, we selected important column variables from allaccidents, replace numerical number to meaningful character description according to `FARS Analytical Userโs Manual`",
"_____no_output_____"
]
],
[
[
"import warnings\nwarnings.filterwarnings('ignore')\naccidents = allaccidents[['YEAR','ST_CASE','STATE','VE_TOTAL','PERSONS','FATALS','MONTH','DAY_WEEK','HOUR','NHS','LATITUDE','LONGITUD','MAN_COLL','LGT_COND','WEATHER','ARR_HOUR','ARR_MIN','CF1','DRUNK_DR']]\naccidents.rename(columns={'ST_CASE':'CASE_NUM','VE_TOTAL':'NUM_VEHICLE','NHS': 'HIGHWAY', 'MAN_COLL': 'COLLISION_TYPE','LGT_COND':'LIGHT_CONDITION','CF1':'CRASH_FACTOR','DRUNK_DR':'DRUNK_DRIVE'}, inplace=True)\naccidents['MONTH'] = accidents['MONTH'].map({1.0:'January', 2.0:'February', 3.0: 'March', 4.0:'April', 5.0:'May', 6.0:'June', 7.0:'July', 8.0:'August',9.0: 'September', 10.0:'October', 11.0:'November', 12.0:'December'})\naccidents['DAY_WEEK']= accidents['DAY_WEEK'].map({1.0:'Sunday',2.0:'Monday', 3.0:'Tuesday', 4.0: 'Wednesday', 5.0:'Thursday', 6.0:'Friday', 7.0:'Saturday'})\naccidents['HIGHWAY'] = accidents['HIGHWAY'].map({1.0:'On',0.0:'Off',9.0:'Unknow'})\naccidents['COLLISION_TYPE'] = accidents['COLLISION_TYPE'].map({0.0:'Not Collision',1.0:'Rear-End',2.0:'Head-On',3.0:'Rear-to-Rear',4.0:'Angle',5.0:'Sideswipe, Same Direction',6.0:'Sideswipe, Opposite Direction',7.0:'Sideswipe, Unknown Direction',9.0:'Unknown'})\naccidents['LIGHT_CONDITION'] = accidents['LIGHT_CONDITION'].map({1.0:'Daylight',2.0:'Dark' ,3.0:'Dark',5.0:'Dusk',6.0:'Dark',4.0:'Dawn', 7.0:'Other',8.0 :'Not Report', 9.0:'Not Report'})\n# accidents['WEATHER'] = accidents['WEATHER'].map({0.0:'Normal',1.0:'Clear',2.0:'Rain',3.0\naccidents.head()",
"_____no_output_____"
]
],
[
[
"combine \"year\" and \"case_num\" to reindex accidents dataframe.",
"_____no_output_____"
]
],
[
[
"accidents['STATE']=accidents['STATE'].astype(int)\naccidents['CASE_NUM']=accidents['CASE_NUM'].astype(int)\naccidents['YEAR']=accidents['YEAR'].astype(int)\naccidents.index = list(accidents['YEAR'].astype(str) + accidents['CASE_NUM'].astype(str))\naccidents.head()",
"_____no_output_____"
],
[
"accidents.shape",
"_____no_output_____"
]
],
[
[
"### Load vehicle data file which contains mortality rate",
"_____no_output_____"
],
[
"We also want to study the mortality rate of fatal accidents. The data element โFatalities in Vehicleโ in the Vehicle data file from the `U.S. Department of Transportation` website provides the number of deaths in a vehicle.",
"_____no_output_____"
]
],
[
[
"vdbf07= DBF('vehicle_deaths/vehicle2007.dbf')\nvdbf08= DBF('vehicle_deaths/vehicle2008.dbf')\nvdbf09= DBF('vehicle_deaths/vehicle2009.dbf')\nvdbf10= DBF('vehicle_deaths/vehicle2010.dbf')\nvdbf11= DBF('vehicle_deaths/vehicle2011.dbf')\nvdbf12= DBF('vehicle_deaths/vehicle2012.dbf')\nvdbf13= DBF('vehicle_deaths/vehicle2013.dbf')\nvdbf14= DBF('vehicle_deaths/vehicle2014.dbf')\n# vdbf15= DBF('vehicle_deaths/vehicle2015.csv')\nvdbf16= DBF('vehicle_deaths/vehicle2016.dbf')\nvehicle07 = DataFrame(iter(vdbf07))\nvehicle08 = DataFrame(iter(vdbf08))\nvehicle09 = DataFrame(iter(vdbf09))\nvehicle10 = DataFrame(iter(vdbf10))\nvehicle11 = DataFrame(iter(vdbf11))\nvehicle12 = DataFrame(iter(vdbf12))\nvehicle13 = DataFrame(iter(vdbf13))\nvehicle14 = DataFrame(iter(vdbf14))\n# vehicle15 = pd.read_csv('vehicle_deaths/vehicle2015.csv')\nvehicle16 = DataFrame(iter(vdbf16))",
"_____no_output_____"
],
[
"vehicle07['YEAR']=2007\nvehicle08['YEAR']=2008\nvehicle09['YEAR']=2009\nvehicle10['YEAR']=2010\nvehicle11['YEAR']=2011\nvehicle12['YEAR']=2012\nvehicle13['YEAR']=2013\nvehicle14['YEAR']=2014\n# vehicle15['YEAR']='2015.0'\nvehicle16['YEAR']=2016",
"_____no_output_____"
],
[
"allvehicles=pd.concat([vehicle07,vehicle08,vehicle09,vehicle10,vehicle11,vehicle12,vehicle13,vehicle14,vehicle16], axis=0,join='outer')\nvehicles = allvehicles[['STATE','YEAR','ST_CASE','HIT_RUN','TRAV_SP','ROLLOVER','FIRE_EXP','SPEEDREL','DEATHS']]\nvehicles.rename(columns={'ST_CASE':'CASE_NUM','TRAV_SP':'SPEED','FIRE_EXP': 'FIRE','SPEEDREL':'SPEEDING'}, inplace=True)\nvehicles['STATE']=vehicles['STATE'].astype(int)\nvehicles['CASE_NUM']=vehicles['CASE_NUM'].astype(int)\nvehicles['YEAR']=vehicles['YEAR'].astype(int)\nvehicles.index = list(vehicles['YEAR'].astype(str) + vehicles['CASE_NUM'].astype(str))\nvehicles.head()",
"_____no_output_____"
],
[
"all = pd.merge(vehicles, accidents, left_index=True, right_index=True, how='inner',on=('STATE', 'YEAR','CASE_NUM'))\nall.index=(all.index).astype(int)\nall.sort_index()\nall.head()",
"_____no_output_____"
]
],
[
[
"### plot ",
"_____no_output_____"
]
],
[
[
"#the total accidents number each year, analysis the difference between every year\nyear_acci=all[['YEAR','CASE_NUM']].groupby('YEAR').count()\nmonth_acci=all[['MONTH','CASE_NUM']].groupby('MONTH').count()\nday_acci=all[['DAY_WEEK','CASE_NUM']].groupby('DAY_WEEK').count()\nhour_acci=all[['HOUR','CASE_NUM']].groupby('HOUR').count()\nhour_acci=hour_acci.drop(hour_acci.index[-1])\nhour_acci.iloc[0]=hour_acci.iloc[0]+hour_acci.iloc[-1]\nhour_acci=hour_acci.drop(hour_acci.index[-1])\nday_acci.index = pd.CategoricalIndex(day_acci.index, \n categories=['Monday', 'Tuesday', 'Wednesday', 'Thursday','Friday','Saturday', 'Sunday'], \n sorted=True)\nday_acci = day_acci.sort_index()\nmonth_acci.index=pd.CategoricalIndex(month_acci.index, \n categories=['January', 'February', 'March', 'April','May','June', 'July','August','September','October','November','December'], \n sorted=True)\nmonth_acci=month_acci.sort_index()",
"_____no_output_____"
],
[
"import matplotlib.pyplot as plt\nf1,axarr = plt.subplots(2,2)\nf1.set_figwidth(15)\nf1.set_figheight(9)\n\naxarr[0,0].set_ylabel(\"count\")\naxarr[0,0].set_title(\"the total accidents number each year\")\naxarr[0,0].bar(year_acci.index,year_acci['CASE_NUM'])\n\n\nobjects1=np.array(month_acci.index)\nx1=np.arange(len(objects1))\naxarr[0,1].set_ylabel(\"count\")\naxarr[0,1].set_title(\"the total accidents number every month\")\naxarr[0,1].bar(x1,month_acci['CASE_NUM'])\naxarr[0,1].set_xticks(x1)\naxarr[0,1].set_xticklabels(objects1)\n\naxarr[1,0].set_ylabel(\"count\")\naxarr[1,0].set_title(\"the total accidents number every hour\")\naxarr[1,0].bar(hour_acci.index,hour_acci['CASE_NUM'])\naxarr[1,0].set_xticks(np.arange(0,24))\n\nobjects2=np.array(day_acci.index)\nx2=np.arange(len(objects2))\naxarr[1,1].set_ylabel(\"count\")\naxarr[1,1].set_title(\"the total accidents number every week\")\naxarr[1,1].bar(x2,day_acci['CASE_NUM'])\naxarr[1,1].set_xticks(x2)\naxarr[1,1].set_xticklabels(objects2)\nf1\n#f1.savefig(\"fig/time_relate_count.png\")",
"_____no_output_____"
],
[
"all.to_hdf('results/df1.h5', 'all')\n\n#with shelve.open('results/vars2') as db:\n #db['speech_words'] = speech_words\n #db['speeches_cleaned'] = speeches_cleaned",
"_____no_output_____"
]
]
] | [
"code",
"markdown",
"code",
"markdown",
"code",
"markdown",
"code",
"markdown",
"code",
"markdown",
"code",
"markdown",
"code"
] | [
[
"code"
],
[
"markdown"
],
[
"code"
],
[
"markdown"
],
[
"code",
"code"
],
[
"markdown",
"markdown"
],
[
"code"
],
[
"markdown"
],
[
"code",
"code"
],
[
"markdown",
"markdown"
],
[
"code",
"code",
"code",
"code"
],
[
"markdown"
],
[
"code",
"code",
"code"
]
] |
e769b1a96b0b59e11ba86786e211edacb3ab85ef | 20,043 | ipynb | Jupyter Notebook | Part09_Hither_to_Train_Thither_to_Test.ipynb | erikma/ColorMatching | 724937cf18334f93950a4cf84f2196111749e338 | [
"MIT"
] | null | null | null | Part09_Hither_to_Train_Thither_to_Test.ipynb | erikma/ColorMatching | 724937cf18334f93950a4cf84f2196111749e338 | [
"MIT"
] | null | null | null | Part09_Hither_to_Train_Thither_to_Test.ipynb | erikma/ColorMatching | 724937cf18334f93950a4cf84f2196111749e338 | [
"MIT"
] | null | null | null | 42.644681 | 416 | 0.578357 | [
[
[
"# Part 9: Hither to Train, Thither to Test\nOK, now we know a bit about perceptrons. We'll return to that subject again. But now let's do a couple of things with our 48 colors from lesson 7:\n\n* We're going to wiggle some more - perturb the color data - in order to generate even more data.\n* But now we're going to randomly split the data into two parts, 80% for training and 20% for testing.\n\nWhy split for training and testing?",
"_____no_output_____"
],
[
"## Repeating the Same Things Too Much Makes Jack a Dull Network\nIt's possible to *overtrain* your network, to provide it with so much similar data and so many epoch repetitions that it only learns the data you give it, so that if you give it something new it can't deal with it very well and its guesses come out wrong. A network that can make good predictions is a *generalized* network. \n\nSo if you have a lot of data - enough not to worry about *undertraining* by not providing enough examples - you can keep aside some of it as a test for after all your epochs are done, to see, if you give it data it was not trained on, it can still produce similar loss and provide accuracy.\n\nThis testing is called *scoring* the network against test data.",
"_____no_output_____"
],
[
"## But why weren't we splitting data before?\nIn the beginning we had no colors, then 3, then 11, then 24, then 48. Splitting with so little data does not do much good, as you'll keep important information about some colors out of training, and the network won't know what they are since it never saw them as an example. When we started perturbing - wiggling - the original colors to multiply how much data we have, splitting started to become possible.\n\nWhat we're going to do below is increase the amount of data even more, by adding more wiggle points. And then we're going to keep 20% of the data aside for testing the network to see whether it's becoming too focused - not generalized enough - and can't figure out what to do with the test data.",
"_____no_output_____"
],
[
"## Slightly Different Network\nThere are a bunch of differences in the network code below, based on what we learned in lesson 7:\n\n* We use only 4 perceptrons per color, used to be 8.\n* We use a batch size of 8 to avoid waiting too long for training. As we increase the data we can usually increase the batch size without making things much worse.\n* We split the data into training and test, then train on the training data.\n* Then after training we score the network against the test data which the network hasn't seen yet.",
"_____no_output_____"
]
],
[
[
"from keras.layers import Activation, Dense, Dropout\nfrom keras.models import Sequential\nimport keras.optimizers, keras.utils, numpy\nfrom sklearn.model_selection import train_test_split\nfrom sklearn.preprocessing import LabelBinarizer\n\ndef train(rgbValues, colorNames, epochs = 3, perceptronsPerColorName = 4, batchSize = 8):\n \"\"\"\n Trains a neural network to understand how to map color names to RGB triples.\n The provided lists of RGB triples must be floating point triples with each\n value in the range [0.0, 1.0], and the number of color names must be the same length.\n Different names are allowed to map to the same RGB triple.\n Returns a trained model that can be used for recognize().\n \"\"\"\n\n # Convert the Python map RGB values into a numpy array needed for training.\n rgbNumpyArray = numpy.array(rgbValues, numpy.float64)\n \n # Convert the color labels into a one-hot feature array.\n # Text labels for each array position are in the classes_ list on the binarizer.\n labelBinarizer = LabelBinarizer()\n oneHotLabels = labelBinarizer.fit_transform(colorNames)\n numColors = len(labelBinarizer.classes_)\n colorLabels = labelBinarizer.classes_\n \n # Partition the data into training and testing splits using 80% of\n # the data for training and the remaining 20% for testing.\n (trainingColors, testColors, trainingOneHotLabels, testOneHotLabels) = train_test_split(\n rgbNumpyArray, oneHotLabels, test_size=0.2)\n\n # Hyperparameters to define the network shape.\n numFullyConnectedPerceptrons = numColors * perceptronsPerColorName\n \n model = Sequential([\n # Layer 1: Fully connected layer with ReLU activation.\n Dense(numFullyConnectedPerceptrons, activation='relu', kernel_initializer='TruncatedNormal', input_shape=(3,)),\n\n # Outputs: SoftMax activation to get probabilities by color.\n Dense(numColors, activation='softmax')\n ])\n\n print(model.summary())\n\n # Compile for categorization.\n model.compile(\n optimizer = keras.optimizers.SGD(lr = 0.01, momentum = 0.9, decay = 1e-6, nesterov = True),\n loss = 'categorical_crossentropy',\n metrics = [ 'accuracy' ])\n\n history = model.fit(trainingColors, trainingOneHotLabels, epochs=epochs, batch_size=batchSize)\n\n print(\"\")\n print(\"Scoring result against test data after training with training data:\")\n score = model.evaluate(testColors, testOneHotLabels, batch_size=batchSize)\n \n print(\"\")\n print(\"Score: loss=%1.4f, accuracy=%1.4f\" % (score[0], score[1]))\n return (model, colorLabels)",
"Using TensorFlow backend.\n"
]
],
[
[
"Here's our createMoreTrainingData() function, mostly the same but we've doubled the number of perturbValues by adding points in between the previous ones.",
"_____no_output_____"
]
],
[
[
"def createMoreTrainingData(colorNameToRGBMap):\n # The incoming color map is not typically going to be oversubscribed with e.g.\n # extra 'red' samples pointing to slightly different colors. We generate a\n # training dataset by perturbing each color by a small amount positive and\n # negative. We do this for each color individually, by pairs, and for all three\n # at once, for each positive and negative value, resulting in dataset that is\n # many times as large.\n perturbValues = [ 0.0, 0.005, 0.01, 0.015, 0.02, 0.025, 0.03 ]\n rgbValues = []\n labels = []\n for colorName, rgb in colorNameToRGBMap.items():\n reds = []\n greens = []\n blues = []\n for perturb in perturbValues:\n if rgb[0] + perturb <= 1.0:\n reds.append(rgb[0] + perturb)\n if perturb != 0.0 and rgb[0] - perturb >= 0.0:\n reds.append(rgb[0] - perturb)\n if rgb[1] + perturb <= 1.0:\n greens.append(rgb[1] + perturb)\n if perturb != 0.0 and rgb[1] - perturb >= 0.0:\n greens.append(rgb[1] - perturb)\n if rgb[2] + perturb <= 1.0:\n blues.append(rgb[2] + perturb)\n if perturb != 0.0 and rgb[2] - perturb >= 0.0:\n blues.append(rgb[2] - perturb)\n for red in reds:\n for green in greens:\n for blue in blues:\n rgbValues.append((red, green, blue))\n labels.append(colorName)\n return (rgbValues, labels)",
"_____no_output_____"
]
],
[
[
"And our previous 48 crayon colors, and let's try training:",
"_____no_output_____"
]
],
[
[
"def rgbToFloat(r, g, b): # r, g, b in 0-255 range\n return (float(r) / 255.0, float(g) / 255.0, float(b) / 255.0)\n\n# http://www.jennyscrayoncollection.com/2017/10/complete-list-of-current-crayola-crayon.html\ncolorMap = {\n # 8-crayon box colors\n 'red': rgbToFloat(238, 32, 77),\n 'yellow': rgbToFloat(252, 232, 131),\n 'blue': rgbToFloat(31, 117, 254),\n 'brown': rgbToFloat(180, 103, 77),\n 'orange': rgbToFloat(255, 117, 56),\n 'green': rgbToFloat(28, 172, 20),\n 'violet': rgbToFloat(146, 110, 174),\n 'black': rgbToFloat(35, 35, 35),\n\n # Additional for 16-count box\n 'red-violet': rgbToFloat(192, 68, 143),\n 'red-orange': rgbToFloat(255, 117, 56),\n 'yellow-green': rgbToFloat(197, 227, 132),\n 'blue-violet': rgbToFloat(115, 102, 189),\n 'carnation-pink': rgbToFloat(255, 170, 204),\n 'yellow-orange': rgbToFloat(255, 182, 83),\n 'blue-green': rgbToFloat(25, 158, 189),\n 'white': rgbToFloat(237, 237, 237),\n\n # Additional for 24-count box\n 'violet-red': rgbToFloat(247, 83 ,148),\n 'apricot': rgbToFloat(253, 217, 181),\n 'cerulean': rgbToFloat(29, 172, 214),\n 'indigo': rgbToFloat(93, 118, 203),\n 'scarlet': rgbToFloat(242, 40, 71),\n 'green-yellow': rgbToFloat(240, 232, 145),\n 'bluetiful': rgbToFloat(46, 80, 144),\n 'gray': rgbToFloat(149, 145, 140),\n \n # Additional for 32-count box\n 'chestnut': rgbToFloat(188, 93, 88),\n 'peach': rgbToFloat(255, 207, 171),\n 'sky-blue': rgbToFloat(128, 215, 235),\n 'cadet-blue': rgbToFloat(176, 183, 198),\n 'melon': rgbToFloat(253, 188, 180),\n 'tan': rgbToFloat(250, 167, 108),\n 'wisteria': rgbToFloat(205, 164, 222),\n 'timberwolf': rgbToFloat(219, 215, 210),\n\n # Additional for 48-count box\n 'lavender': rgbToFloat(252, 180, 213),\n 'burnt-sienna': rgbToFloat(234, 126, 93),\n 'olive-green': rgbToFloat(186, 184, 108),\n 'purple-mountains-majesty': rgbToFloat(157, 129, 186),\n 'salmon': rgbToFloat(255, 155, 170),\n 'macaroni-and-cheese': rgbToFloat(255, 189, 136),\n 'granny-smith-apple': rgbToFloat(168, 228, 160),\n 'sepia': rgbToFloat(165, 105, 79),\n 'mauvelous': rgbToFloat(239, 152, 170),\n 'goldenrod': rgbToFloat(255, 217, 117),\n 'sea-green': rgbToFloat(159, 226, 191),\n 'raw-sienna': rgbToFloat(214, 138, 89),\n 'mahogany': rgbToFloat(205, 74, 74),\n 'spring-green': rgbToFloat(236, 234, 190),\n 'cornflower': rgbToFloat(154, 206, 235),\n 'tumbleweed': rgbToFloat(222, 170, 136),\n}\n\n(rgbValues, colorNames) = createMoreTrainingData(colorMap)\n(colorModel, colorLabels) = train(rgbValues, colorNames)",
"WARNING:tensorflow:From c:\\users\\erik\\appdata\\local\\programs\\python\\python36\\lib\\site-packages\\tensorflow\\python\\framework\\op_def_library.py:263: colocate_with (from tensorflow.python.framework.ops) is deprecated and will be removed in a future version.\nInstructions for updating:\nColocations handled automatically by placer.\n_________________________________________________________________\nLayer (type) Output Shape Param # \n=================================================================\ndense_1 (Dense) (None, 192) 768 \n_________________________________________________________________\ndense_2 (Dense) (None, 48) 9264 \n=================================================================\nTotal params: 10,032\nTrainable params: 10,032\nNon-trainable params: 0\n_________________________________________________________________\nNone\nWARNING:tensorflow:From c:\\users\\erik\\appdata\\local\\programs\\python\\python36\\lib\\site-packages\\tensorflow\\python\\ops\\math_ops.py:3066: to_int32 (from tensorflow.python.ops.math_ops) is deprecated and will be removed in a future version.\nInstructions for updating:\nUse tf.cast instead.\nEpoch 1/3\n74224/74224 [==============================] - 14s 185us/step - loss: 1.0514 - acc: 0.7338\nEpoch 2/3\n74224/74224 [==============================] - 12s 166us/step - loss: 0.2417 - acc: 0.9323\nEpoch 3/3\n74224/74224 [==============================] - 13s 169us/step - loss: 0.1757 - acc: 0.9456\n\nScoring result against test data after training with training data:\n18557/18557 [==============================] - 2s 86us/step\n\nScore: loss=0.1417, accuracy=0.9565\n"
]
],
[
[
"Not bad: We quickly got our loss down to 0.17 in only 3 epochs, but the larger batch size kept it from taking a really long time.\n\nBut let's examine our new addition, the test data scoring result. From my machine:\n\n `Score: loss=0.1681, accuracy=0.9464`\n\nNote that we trained with 74,000 data points, but we kept aside an additional 18,000 data points as test data the network was not allowed to train with. And when we ask the network to predict with the test data, the loss we get - 0.168 on my machine - is pretty close to the 0.172 loss I got on training.\n\nThis is good news! It means our network is well generalized: not overtrained, not too focused to deal with making predictions on new data.\n\nTry it out to make sure it still seems like a good result:",
"_____no_output_____"
]
],
[
[
"from ipywidgets import interact\nfrom IPython.core.display import display, HTML\n\ndef displayColor(r, g, b):\n rInt = min(255, max(0, int(r * 255.0)))\n gInt = min(255, max(0, int(g * 255.0)))\n bInt = min(255, max(0, int(b * 255.0)))\n hexColor = \"#%02X%02X%02X\" % (rInt, gInt, bInt)\n display(HTML('<div style=\"width: 50%; height: 50px; background: ' + hexColor + ';\"></div>'))\n\nnumPredictionsToShow = 5\n@interact(r = (0.0, 1.0, 0.01), g = (0.0, 1.0, 0.01), b = (0.0, 1.0, 0.01))\ndef getTopPredictionsFromModel(r, g, b):\n testColor = numpy.array([ (r, g, b) ])\n predictions = colorModel.predict(testColor, verbose=0) # Predictions shape (1, numColors)\n predictions *= 100.0\n predColorTuples = []\n for i in range(0, len(colorLabels)):\n predColorTuples.append((predictions[0][i], colorLabels[i]))\n predAndNames = numpy.array(predColorTuples, dtype=[('pred', float), ('colorName', 'U50')])\n sorted = numpy.sort(predAndNames, order=['pred', 'colorName'])\n sorted = sorted[::-1] # reverse rows to get highest on top\n for i in range(0, numPredictionsToShow):\n print(\"%2.1f\" % sorted[i][0] + \"%\", sorted[i][1])\n displayColor(r, g, b)",
"_____no_output_____"
]
],
[
[
"In my opinion the extra perturbation data made quite a bit of difference. It guesses over 70% for gray at (0.5, 0.5, 0.5), better than before.\n\nHere's the hyperparameter slider version so you can try out different epochs, batch sizes, and perceptrons:",
"_____no_output_____"
]
],
[
[
"@interact(epochs = (1, 10), perceptronsPerColorName = (1, 12), batchSize = (1, 50))\ndef trainModel(epochs=4, perceptronsPerColorName=3, batchSize=16):\n global colorModel\n global colorLabels\n (colorModel, colorLabels) = train(rgbValues, colorNames, epochs=epochs, perceptronsPerColorName=perceptronsPerColorName, batchSize=batchSize)",
"_____no_output_____"
],
[
"interact(getTopPredictionsFromModel, r = (0.0, 1.0, 0.01), g = (0.0, 1.0, 0.01), b = (0.0, 1.0, 0.01))",
"_____no_output_____"
]
],
[
[
"### Coming up...\nWe'll begin understanding why neurons and perceptrons by themselves are not enough, it's the connections that matter too. And we'll begin to learn how training works to create weights and biases that let ask the network for new predictions.",
"_____no_output_____"
]
]
] | [
"markdown",
"code",
"markdown",
"code",
"markdown",
"code",
"markdown",
"code",
"markdown",
"code",
"markdown"
] | [
[
"markdown",
"markdown",
"markdown",
"markdown"
],
[
"code"
],
[
"markdown"
],
[
"code"
],
[
"markdown"
],
[
"code"
],
[
"markdown"
],
[
"code"
],
[
"markdown"
],
[
"code",
"code"
],
[
"markdown"
]
] |
e769c051b8f6658e2df6a3f4a67b36a671418124 | 18,252 | ipynb | Jupyter Notebook | notebooks/lab-2/data-structures-part-2.ipynb | samuelcheang0419/python-labs | 6f32c141412a1af4d8a39b25a56cc211d0a9040f | [
"BSD-2-Clause-FreeBSD"
] | null | null | null | notebooks/lab-2/data-structures-part-2.ipynb | samuelcheang0419/python-labs | 6f32c141412a1af4d8a39b25a56cc211d0a9040f | [
"BSD-2-Clause-FreeBSD"
] | null | null | null | notebooks/lab-2/data-structures-part-2.ipynb | samuelcheang0419/python-labs | 6f32c141412a1af4d8a39b25a56cc211d0a9040f | [
"BSD-2-Clause-FreeBSD"
] | null | null | null | 31.964974 | 337 | 0.52712 | [
[
[
"# Lab 2: Data Structures ~ Advanced Applications\nWow, look at you! Congratulations on making it to the second part of the lab!\n\nThese assignments are *absolutely not required*! Even if you're here, you shouldn't try to solve all of the problems in this file. Our suggestion is that you should skim through these problems to find the ones that are most interesting to you.",
"_____no_output_____"
],
[
"## Pretty Pascal\nThis is a variation on the Pascal question from Part 1. Given a number `n`, print out the first `n` rows of Pascal's triangle, *centering* each line. You should use the `generate_pascal_row` function you wrote previously (copy it over from the other notebook). The Pascal's triangle with 1 row just contains the number `1`.\n\nTo center a string in Python, you can use use string format specifiers. `'{:^10}'.format(var)` will produce a string of length 10 or `len(var)` (whichever is longer), with `str(var)` centered.\n\n```python\n'{:^10}'.format('CS41') # => ' CS41 '\n```\n\nYou can even specify an optional `fillchar` to fill with characters other than spaces!\n\nFor example, for `n = 10`:\n```python\nprint_pascal_triangle(10)\n# 1 \n# 1 1 \n# 1 2 1 \n# 1 3 3 1 \n# 1 4 6 4 1 \n# 1 5 10 10 5 1 \n# 1 6 15 20 15 6 1 \n# 1 7 21 35 35 21 7 1 \n# 1 8 28 56 70 56 28 8 1 \n# 1 9 36 84 126 126 84 36 9 1\n```",
"_____no_output_____"
]
],
[
[
"def generate_pascal_row(row):\n \"\"\"Generate the next row of Pascal's triangle.\"\"\"\n if not row:\n return [1]\n row1, row2 = row + [0], [0] + row\n return list(map(sum, zip(row1, row2)))\n\ndef print_pascal_triangle(n):\n \"\"\"Print the first n rows of Pascal's triangle.\"\"\"\n total_spaces = n + n - 1\n prev_row = []\n for i in range(1, n + 1):\n prev_row = generate_pascal_row(prev_row)\n print_row = ' '.join(map(str, prev_row))\n space_either_side = (total_spaces - (i + i - 1)) // 2\n print_row = ' ' * space_either_side + print_row + ' ' * space_either_side\n print(print_row)\n\nprint_pascal_triangle(3)\nprint_pascal_triangle(10)",
" 1 \n 1 1 \n1 2 1\n 1 \n 1 1 \n 1 2 1 \n 1 3 3 1 \n 1 4 6 4 1 \n 1 5 10 10 5 1 \n 1 6 15 20 15 6 1 \n 1 7 21 35 35 21 7 1 \n 1 8 28 56 70 56 28 8 1 \n1 9 36 84 126 126 84 36 9 1\n"
]
],
[
[
"## Special Phrases\nFor the next few problems, just like cyclone phrases, we'll describe a criterion that makes a word or phrase special.\n\nLet's load up the dictionary file again. Remember, if you are using macOS or Linux, you should have a dictionary file available at `/usr/share/dict/words` and we've mirrored the file at `https://stanfordpython.com/res/misc/words`, so you can download the dictionary from there.\n\nCopy (or rewrite) `load_english` to load the words from this file.",
"_____no_output_____"
]
],
[
[
"# If you downloaded words from the course website,\n# change me to the path to the downloaded file.\nDICTIONARY_FILE = '/usr/share/dict/words'\n\ndef load_english():\n \"\"\"Load and return a collection of english words from a file.\"\"\"\n pass\n\nenglish = load_english()\nprint(len(english))",
"_____no_output_____"
]
],
[
[
"### Triad Phrases\n\nTriad words are English words for which the two smaller strings you make by extracting alternating letters both form valid words.\n\nFor example:\n\n\n\nWrite a function to determine whether an entire phrase passed into a function is made of triad words. You can assume that all words are made of only alphabetic characters, and are separated by whitespace. We will consider the empty string to be an invalid English word.\n\n```python\nis_triad_phrase(\"learned theorem\") # => True\nis_triad_phrase(\"studied theories\") # => False\nis_triad_phrase(\"wooded agrarians\") # => True\nis_triad_phrase(\"forrested farmers\") # => False\nis_triad_phrase(\"schooled oriole\") # => True\nis_triad_phrase(\"educated small bird\") # => False\nis_triad_phrase(\"a\") # => False\nis_triad_phrase(\"\") # => False\n```\n\nGenerate a list of all triad words. How many are there? We found 2770 distinct triad words (case-insensitive).",
"_____no_output_____"
]
],
[
[
"def is_triad_word(word, english):\n \"\"\"Return whether a word is a triad word.\"\"\"\n pass\n \ndef is_triad_phrase(phrase, english):\n \"\"\"Return whether a phrase is composed of only triad words.\"\"\"\n pass",
"_____no_output_____"
]
],
[
[
"### Surpassing Phrases (challenge)\n\nSurpassing words are English words for which the gap between each adjacent pair of letters strictly increases. These gaps are computed without \"wrapping around\" from Z to A.\n\nFor example:\n\n\n\nWrite a function to determine whether an entire phrase passed into a function is made of surpassing words. You can assume that all words are made of only alphabetic characters, and are separated by whitespace. We will consider the empty string and a 1-character string to be valid surpassing phrases.\n\n```python\nis_surpassing_phrase(\"superb subway\") # => True\nis_surpassing_phrase(\"excellent train\") # => False\nis_surpassing_phrase(\"porky hogs\") # => True\nis_surpassing_phrase(\"plump pigs\") # => False\nis_surpassing_phrase(\"turnip fields\") # => True\nis_surpassing_phrase(\"root vegetable lands\") # => False\nis_surpassing_phrase(\"a\") # => True\nis_surpassing_phrase(\"\") # => True\n```\n\nWe've provided a `character_gap` function that returns the gap between two characters. To understand how it works, you should first learn about the Python functions `ord` (one-character string to integer ordinal) and `chr` (integer ordinal to one-character string). For example:\n\n```python\nord('a') # => 97\nchr(97) # => 'a'\n```\n\nSo, in order to find the gap between `G` and `E`, we compute `abs(ord('G') - ord('E'))`, where `abs` returns the absolute value of its argument.\n\nGenerate a list of all surpassing words. How many are there? We found 1931 distinct surpassing words.",
"_____no_output_____"
]
],
[
[
"def character_gap(ch1, ch2):\n \"\"\"Return the absolute gap between two characters.\"\"\"\n return abs(ord(ch1) - ord(ch2))\n\ndef is_surpassing_word(word):\n \"\"\"Return whether a word is surpassing.\"\"\"\n pass\n\ndef is_surpassing_phrase(word):\n \"\"\"Return whether a word is surpassing.\"\"\"",
"_____no_output_____"
]
],
[
[
"### Triangle Words\nThe nth term of the sequence of triangle numbers is given by $1 + 2 + ... + n = \\frac{n(n+1)}{2}$. For example, the first ten triangle numbers are: `1, 3, 6, 10, 15, 21, 28, 36, 45, 55, ...`\n\nBy converting each letter in a word to a number corresponding to its alphabetical position (`A=1`, `B=2`, etc) and adding these values we form a word value. For example, the word value for SKY is `19 + 11 + 25 = 55` and 55 is a triangle number. If the word value is a triangle number then we shall call the word a triangle word.\n\nGenerate a list of all triangle words. How many are there? As a sanity check, we found 16303 distinct triangle words.\n\n*Hint: you can use `ord(ch)` to get the integer ASCII value of a character. You can also use a dictionary to accomplish this!*",
"_____no_output_____"
]
],
[
[
"def is_triangle_word(word):\n \"\"\"Return whether a word is a triangle word.\"\"\"\n pass\n\nprint(is_triangle_word(\"SKY\")) # => True",
"_____no_output_____"
]
],
[
[
"## Polygon Collision\n\nGiven two polygons in the form of lists of 2-tuples, determine whether the two polygons intersect.\n\nFormally, a polygon is represented by a list of (x, y) tuples, where each (x, y) tuple is a vertex of the polygon. Edges are assumed to be between adjacent vertices in the list, and the last vertex is connected to the first. For example, the unit square would be represented by\n\n```\nsquare = [(0,0), (0,1), (1,1), (1,0)]\n```\n\nYou can assume that the polygon described by the provided list of tuples is not self-intersecting, but do not assume that it is convex.\n\n**Note: this is a *hard* problem. Quite hard.**",
"_____no_output_____"
]
],
[
[
"# compare each edge of poly1 with each edge of poly2\n# how do two lines intersect? define line1 has (x1a, y1a) and (x1b, y1b), and line2 has (x2a, y2a) and (x2b, y2b). \n# they intersect when \ndef polygon_collision(poly1, poly2):\n pass\n\nunit_square = [(0,0), (0,1), (1,1), (1,0)]\ntriangle = [(0,0), (0.5,2), (1,0)]\n\nprint(polygon_collision(unit_square, triangle)) # => True",
"_____no_output_____"
]
],
[
[
"## Comprehensions\nWe haven't talked about data comprehensions yet, but if you're interested, you can read about them [here](https://docs.python.org/3/tutorial/datastructures.html#list-comprehensions) and then tackle the problems below.\n\n#### Read\n\nPredict the output of each of the following list comprehensions. After you have written down your hypothesis, run the code cell to see if you were correct. If you were incorrect, discuss with a partner why Python returns what it does.\n\n```Python\n[x for x in [1, 2, 3, 4]]\n[n - 2 for n in range(10)]\n[k % 10 for k in range(41) if k % 3 == 0]\n[s.lower() for s in ['PythOn', 'iS', 'cOoL'] if s[0] < s[-1]]\n\n# Something is fishy here. Can you spot it?\narr = [[3,2,1], ['a','b','c'], [('do',), ['re'], 'mi']]\nprint([el.append(el[0] * 4) for el in arr]) # What is printed?\nprint(arr) # What is the content of `arr` at this point?\n\n[letter for letter in \"pYthON\" if letter.isupper()]\n{len(w) for w in [\"its\", \"the\", \"remix\", \"to\", \"ignition\"]}\n```",
"_____no_output_____"
]
],
[
[
"# Predict the output of the following comprehensions. Does the output match what you expect?\nprint([x for x in [1, 2, 3, 4]])\n# [1, 2, 3, 4]",
"[1, 2, 3, 4]\n"
],
[
"print([n - 2 for n in range(10)])\n# -2, -1 ... 7",
"[-2, -1, 0, 1, 2, 3, 4, 5, 6, 7]\n"
],
[
"print([k % 10 for k in range(41) if k % 3 == 0])\n# 0, 3, ... , 9",
"[0, 3, 6, 9, 2, 5, 8, 1, 4, 7, 0, 3, 6, 9]\n"
],
[
"'P' < 'n'",
"_____no_output_____"
],
[
"print([s.lower() for s in ['PythOn', 'iS', 'cOoL'] if s[0] < s[-1]])\n# ['python']",
"['python']\n"
],
[
"# Something is fishy here. Can you spot it?\narr = [[3,2,1], ['a','b','c'], [('do',), ['re'], 'mi']]\nprint([el.append(el[0] * 4) for el in arr]) # What is printed?\n# None, None, None",
"[None, None, None]\n"
],
[
"print(arr) # What is the content of `arr` at this point?\n# [[3, 2, 1, 12], ['a', 'b', 'c', 'aaaa'], [not sure..]]",
"[[3, 2, 1, 12], ['a', 'b', 'c', 'aaaa'], [('do',), ['re'], 'mi', ('do', 'do', 'do', 'do')]]\n"
],
[
"print([letter for letter in \"pYthON\" if letter.isupper()])\n# ['Y', 'O', 'N']",
"['Y', 'O', 'N']\n"
],
[
"print({len(w) for w in [\"its\", \"the\", \"remix\", \"to\", \"ignition\"]})\n# {3, 5, 2, 8}",
"{8, 2, 3, 5}\n"
]
],
[
[
"#### Write\n\nWrite comprehensions to transform the input data structure into the output data structure:\n\n```python\n[0, 1, 2, 3] -> [1, 3, 5, 7] # Double and add one\n['apple', 'orange', 'pear'] -> ['A', 'O', 'P'] # Capitalize first letter\n['apple', 'orange', 'pear'] -> ['apple', 'pear'] # Contains a 'p'\n\n[\"TA_parth\", \"student_poohbear\", \"TA_michael\", \"TA_guido\", \"student_htiek\"] -> [\"parth\", \"michael\", \"guido\"]\n['apple', 'orange', 'pear'] -> [('apple', 5), ('orange', 6), ('pear', 4)]\n\n['apple', 'orange', 'pear'] -> {'apple': 5, 'orange': 6, 'pear': 4}\n```",
"_____no_output_____"
]
],
[
[
"nums = [0, 1, 2, 3]\nfruits = ['apple', 'orange', 'pear']\npeople = [\"TA_parth\", \"student_poohbear\", \"TA_michael\", \"TA_guido\", \"student_htiek\"]\n\n# Add your comprehensions here!\nprint([2 * n + 1 for n in nums])\nprint([c[0].upper() for c in fruits])\nprint([w for w in fruits if 'p' in w])\nprint('-'*20)\nprint([name[3:] for name in people if name[:3] == 'TA_'])\nprint([(fruit, len(fruit)) for fruit in fruits])\nprint('-'*20)\nprint({fruit: len(fruit) for fruit in fruits})",
"[1, 3, 5, 7]\n['A', 'O', 'P']\n['apple', 'pear']\n--------------------\n['parth', 'michael', 'guido']\n[('apple', 5), ('orange', 6), ('pear', 4)]\n--------------------\n{'apple': 5, 'orange': 6, 'pear': 4}\n"
]
],
[
[
"*Credit to Sam Redmond, Puzzling.SE (specifically [JLee](https://puzzling.stackexchange.com/users/463/jlee)), ProjectEuler and InterviewCake for several problem ideas*",
"_____no_output_____"
],
[
"> With ๐ฆ by @psarin and @coopermj",
"_____no_output_____"
]
]
] | [
"markdown",
"code",
"markdown",
"code",
"markdown",
"code",
"markdown",
"code",
"markdown",
"code",
"markdown",
"code",
"markdown",
"code",
"markdown",
"code",
"markdown"
] | [
[
"markdown",
"markdown"
],
[
"code"
],
[
"markdown"
],
[
"code"
],
[
"markdown"
],
[
"code"
],
[
"markdown"
],
[
"code"
],
[
"markdown"
],
[
"code"
],
[
"markdown"
],
[
"code"
],
[
"markdown"
],
[
"code",
"code",
"code",
"code",
"code",
"code",
"code",
"code",
"code"
],
[
"markdown"
],
[
"code"
],
[
"markdown",
"markdown"
]
] |
e769c5c7eaed9793196b401e7a7ce2987c8092bf | 8,028 | ipynb | Jupyter Notebook | ImageAlign.ipynb | emilswan/stockstats | 842d7d59cba81fba4af9259b3ec14f9b298bdbaa | [
"BSD-3-Clause"
] | null | null | null | ImageAlign.ipynb | emilswan/stockstats | 842d7d59cba81fba4af9259b3ec14f9b298bdbaa | [
"BSD-3-Clause"
] | null | null | null | ImageAlign.ipynb | emilswan/stockstats | 842d7d59cba81fba4af9259b3ec14f9b298bdbaa | [
"BSD-3-Clause"
] | null | null | null | 48.071856 | 906 | 0.563901 | [
[
[
"<a href=\"https://colab.research.google.com/github/emilswan/stockstats/blob/master/ImageAlign.ipynb\" target=\"_parent\"><img src=\"https://colab.research.google.com/assets/colab-badge.svg\" alt=\"Open In Colab\"/></a>",
"_____no_output_____"
]
],
[
[
"# import the necessary packages\nimport numpy as np\nimport imutils\nimport cv2\ndef align_images(image, template, maxFeatures=500, keepPercent=0.2,\n\tdebug=False):\n\t# convert both the input image and template to grayscale\n\timageGray = cv2.cvtColor(image, cv2.COLOR_BGR2GRAY)\n\ttemplateGray = cv2.cvtColor(template, cv2.COLOR_BGR2GRAY)\n \n \t# use ORB to detect keypoints and extract (binary) local\n\t# invariant features\n\torb = cv2.ORB_create(maxFeatures)\n\t(kpsA, descsA) = orb.detectAndCompute(imageGray, None)\n\t(kpsB, descsB) = orb.detectAndCompute(templateGray, None)\n\t# match the features\n\tmethod = cv2.DESCRIPTOR_MATCHER_BRUTEFORCE_HAMMING\n\tmatcher = cv2.DescriptorMatcher_create(method)\n\tmatches = matcher.match(descsA, descsB, None)\n \n # sort the matches by their distance (the smaller the distance,\n\t# the \"more similar\" the features are)\n\tmatches = sorted(matches, key=lambda x:x.distance)\n\t# keep only the top matches\n\tkeep = int(len(matches) * keepPercent)\n\tmatches = matches[:keep]\n\t# check to see if we should visualize the matched keypoints\n\tif debug:\n\t\tmatchedVis = cv2.drawMatches(image, kpsA, template, kpsB,\n\t\t\tmatches, None)\n\t\tmatchedVis = imutils.resize(matchedVis, width=1000)\n\t\tcv2.imshow(\"Matched Keypoints\", matchedVis)\n\t\tcv2.waitKey(0)\n \t# allocate memory for the keypoints (x, y)-coordinates from the\n\t# top matches -- we'll use these coordinates to compute our\n\t# homography matrix\n\tptsA = np.zeros((len(matches), 2), dtype=\"float\")\n\tptsB = np.zeros((len(matches), 2), dtype=\"float\")\n\t# loop over the top matches\n\tfor (i, m) in enumerate(matches):\n\t\t# indicate that the two keypoints in the respective images\n\t\t# map to each other\n\t\tptsA[i] = kpsA[m.queryIdx].pt\n\t\tptsB[i] = kpsB[m.trainIdx].pt\n\n \t# compute the homography matrix between the two sets of matched\n\t# points\n\t(H, mask) = cv2.findHomography(ptsA, ptsB, method=cv2.RANSAC)\n\t# use the homography matrix to align the images\n\t(h, w) = template.shape[:2]\n\taligned = cv2.warpPerspective(image, H, (w, h))\n\t# return the aligned image\n\treturn aligned\n\n# import the necessary packages\nfrom pyimagesearch.alignment import align_images\nimport numpy as np\nimport argparse\nimport imutils\nimport cv2\n# construct the argument parser and parse the arguments\nap = argparse.ArgumentParser()\nap.add_argument(\"-i\", \"--image\", required=True,\n\thelp=\"path to input image that we'll align to template\")\nap.add_argument(\"-t\", \"--template\", required=True,\n\thelp=\"path to input template image\")\nargs = vars(ap.parse_args())\n\n# load the input image and template from disk\nprint(\"[INFO] loading images...\")\nimage = cv2.imread(args[\"image\"])\ntemplate = cv2.imread(args[\"template\"])\n# align the images\nprint(\"[INFO] aligning images...\")\naligned = align_images(image, template, debug=True)\n\n# resize both the aligned and template images so we can easily\n# visualize them on our screen\naligned = imutils.resize(aligned, width=700)\ntemplate = imutils.resize(template, width=700)\n# our first output visualization of the image alignment will be a\n# side-by-side comparison of the output aligned image and the\n# template\nstacked = np.hstack([aligned, template])\n\n# our second image alignment visualization will be *overlaying* the\n# aligned image on the template, that way we can obtain an idea of\n# how good our image alignment is\noverlay = template.copy()\noutput = aligned.copy()\ncv2.addWeighted(overlay, 0.5, output, 0.5, 0, output)\n# show the two output image alignment visualizations\ncv2.imshow(\"Image Alignment Stacked\", stacked)\ncv2.imshow(\"Image Alignment Overlay\", output)\ncv2.waitKey(0)\n\n",
"_____no_output_____"
],
[
"",
"_____no_output_____"
]
]
] | [
"markdown",
"code"
] | [
[
"markdown"
],
[
"code",
"code"
]
] |
e769d3e990f44b0e2009d8654f69526c982f03fb | 13,467 | ipynb | Jupyter Notebook | Datasets/Vectors/us_census_tracts.ipynb | dmendelo/earthengine-py-notebooks | 515567fa2702b436daf449fff02f5c690003cf94 | [
"MIT"
] | 2 | 2020-02-05T02:36:18.000Z | 2021-03-23T11:02:39.000Z | Datasets/Vectors/us_census_tracts.ipynb | Fernigithub/earthengine-py-notebooks | 32689dc5da4a86e46ea30d8b22241866c1f7cf61 | [
"MIT"
] | null | null | null | Datasets/Vectors/us_census_tracts.ipynb | Fernigithub/earthengine-py-notebooks | 32689dc5da4a86e46ea30d8b22241866c1f7cf61 | [
"MIT"
] | 3 | 2021-01-06T17:33:08.000Z | 2022-02-18T02:14:18.000Z | 76.954286 | 8,024 | 0.832257 | [
[
[
"<table class=\"ee-notebook-buttons\" align=\"left\">\n <td><a target=\"_blank\" href=\"https://github.com/giswqs/earthengine-py-notebooks/tree/master/Datasets/Vectors/us_census_tracts.ipynb\"><img width=32px src=\"https://www.tensorflow.org/images/GitHub-Mark-32px.png\" /> View source on GitHub</a></td>\n <td><a target=\"_blank\" href=\"https://nbviewer.jupyter.org/github/giswqs/earthengine-py-notebooks/blob/master/Datasets/Vectors/us_census_tracts.ipynb\"><img width=26px src=\"https://upload.wikimedia.org/wikipedia/commons/thumb/3/38/Jupyter_logo.svg/883px-Jupyter_logo.svg.png\" />Notebook Viewer</a></td>\n <td><a target=\"_blank\" href=\"https://mybinder.org/v2/gh/giswqs/earthengine-py-notebooks/master?filepath=Datasets/Vectors/us_census_tracts.ipynb\"><img width=58px src=\"https://mybinder.org/static/images/logo_social.png\" />Run in binder</a></td>\n <td><a target=\"_blank\" href=\"https://colab.research.google.com/github/giswqs/earthengine-py-notebooks/blob/master/Datasets/Vectors/us_census_tracts.ipynb\"><img src=\"https://www.tensorflow.org/images/colab_logo_32px.png\" /> Run in Google Colab</a></td>\n</table>",
"_____no_output_____"
],
[
"## Install Earth Engine API\nInstall the [Earth Engine Python API](https://developers.google.com/earth-engine/python_install) and [geehydro](https://github.com/giswqs/geehydro). The **geehydro** Python package builds on the [folium](https://github.com/python-visualization/folium) package and implements several methods for displaying Earth Engine data layers, such as `Map.addLayer()`, `Map.setCenter()`, `Map.centerObject()`, and `Map.setOptions()`.\nThe magic command `%%capture` can be used to hide output from a specific cell. Uncomment these lines if you are running this notebook for the first time.",
"_____no_output_____"
]
],
[
[
"# %%capture\n# !pip install earthengine-api\n# !pip install geehydro",
"_____no_output_____"
]
],
[
[
"Import libraries",
"_____no_output_____"
]
],
[
[
"import ee\nimport folium\nimport geehydro",
"_____no_output_____"
]
],
[
[
"Authenticate and initialize Earth Engine API. You only need to authenticate the Earth Engine API once. Uncomment the line `ee.Authenticate()` \nif you are running this notebook for the first time or if you are getting an authentication error. ",
"_____no_output_____"
]
],
[
[
"# ee.Authenticate()\nee.Initialize()",
"_____no_output_____"
]
],
[
[
"## Create an interactive map \nThis step creates an interactive map using [folium](https://github.com/python-visualization/folium). The default basemap is the OpenStreetMap. Additional basemaps can be added using the `Map.setOptions()` function. \nThe optional basemaps can be `ROADMAP`, `SATELLITE`, `HYBRID`, `TERRAIN`, or `ESRI`.",
"_____no_output_____"
]
],
[
[
"Map = folium.Map(location=[40, -100], zoom_start=4)\nMap.setOptions('HYBRID')",
"_____no_output_____"
]
],
[
[
"## Add Earth Engine Python script ",
"_____no_output_____"
]
],
[
[
"dataset = ee.FeatureCollection('TIGER/2010/Tracts_DP1')\nvisParams = {\n 'min': 0,\n 'max': 4000,\n 'opacity': 0.8,\n}\n\n# Turn the strings into numbers\ndataset = dataset.map(lambda f: f.set('shape_area', ee.Number.parse(f.get('dp0010001'))))\n\n# Map.setCenter(-103.882, 43.036, 8)\nimage = ee.Image().float().paint(dataset, 'dp0010001')\n\nMap.addLayer(image, visParams, 'TIGER/2010/Tracts_DP1')\n# Map.addLayer(dataset, {}, 'for Inspector', False)",
"_____no_output_____"
]
],
[
[
"## Display Earth Engine data layers ",
"_____no_output_____"
]
],
[
[
"Map.setControlVisibility(layerControl=True, fullscreenControl=True, latLngPopup=True)\nMap",
"_____no_output_____"
]
]
] | [
"markdown",
"code",
"markdown",
"code",
"markdown",
"code",
"markdown",
"code",
"markdown",
"code",
"markdown",
"code"
] | [
[
"markdown",
"markdown"
],
[
"code"
],
[
"markdown"
],
[
"code"
],
[
"markdown"
],
[
"code"
],
[
"markdown"
],
[
"code"
],
[
"markdown"
],
[
"code"
],
[
"markdown"
],
[
"code"
]
] |
e769d93652ee62079654b36ab7b38791e2cbbb52 | 671,631 | ipynb | Jupyter Notebook | notebooks/old notebooks/dict to ASPA v2.ipynb | deKeijzer/SRON-DCGAN | 1ead827ebf549917435e6bc9ddd2d4d5951aa205 | [
"MIT"
] | null | null | null | notebooks/old notebooks/dict to ASPA v2.ipynb | deKeijzer/SRON-DCGAN | 1ead827ebf549917435e6bc9ddd2d4d5951aa205 | [
"MIT"
] | null | null | null | notebooks/old notebooks/dict to ASPA v2.ipynb | deKeijzer/SRON-DCGAN | 1ead827ebf549917435e6bc9ddd2d4d5951aa205 | [
"MIT"
] | null | null | null | 395.309594 | 202,552 | 0.932884 | [
[
[
"This notebook functionizes the 'Array to ASPA'. Goal is to convert any input dictionary to a usable ASPA for analysis.\n\nIMPORTANT:\nDuring the visualisation of the images. Each cmap per individual image is scaled depending on the contents. Therefor the images array has to be saved and used... Saving the PNG's will give faulty results. \n\nTODO:\nSplit up all features into e.g. 4 scales so they can be scaled and distuingished better?\nBut also reserve space for 'cloud' models. ",
"_____no_output_____"
],
[
"# Imports",
"_____no_output_____"
]
],
[
[
"import numpy as np\nimport seaborn as sns\nimport pandas as pd\n\n\nimport matplotlib.pyplot as plt\n\nfrom sklearn.preprocessing import MinMaxScaler\nfrom tqdm import tqdm\n\nfrom keijzer_exogan import *\n\n%matplotlib inline\n%config InlineBackend.print_figure_kwargs={'facecolor' : \"w\"} # Make sure the axis background of plots is white, this is usefull for the black theme in JupyterLab\nsns.set()",
"_____no_output_____"
]
],
[
[
"# Load chunk\nX[0] is a dict from regular chunk \nX[0][0] is a dict from .npy selection ",
"_____no_output_____"
]
],
[
[
"dir_ = '/datb/16011015/ExoGAN_data//'\n\nX = np.load(dir_+'selection/last_chunks_25_percent.npy')\nX = X.flatten()\n\nnp.random.seed(23) # Set seed for the np.random functions\n\n# Shuffle X along the first axis to make the order of simulations random\nnp.random.shuffle(X) # note that X = np.rand.... isn't required\n\nlen(X)",
"_____no_output_____"
],
[
"X[0].keys()",
"_____no_output_____"
],
[
"# scale the data\ndef scale_param(X, X_min, X_max):\n \"\"\"\n Formule source: \n https://scikit-learn.org/stable/modules/generated/sklearn.preprocessing.MinMaxScaler.html\n \n In this case 1 is max, 0 is min\n \"\"\"\n std = (X-X_min)/ (X_max - X_min)\n return std*(1 - 0)+0",
"_____no_output_____"
],
[
"x = X[0]",
"_____no_output_____"
],
[
"cmap = 'gray'\n\n\"\"\"\nTransforms the input dictionary (in the format of ExoGAN), to the ASPA format.\nTODO:\n\n- devide each parameter in bins and scale the data per bin (to hopefully increase the contrast in the data)\n- make sure to leave space for cloud model information (max2, min2 is currently double info from max1, min1)\n\"\"\"\n\nspectrum = x['data']['spectrum']\n\nif len(spectrum) != 515:\n print('Spectrum length != 515. breaking script')\n #break\n\n\"\"\"\nScale the spectrum\n\"\"\"\nspectrum = spectrum.reshape(-1, 1) # convert 1D array to 2D cause standardscaler requires it\n\nscaler = MinMaxScaler(feature_range=(0,1)).fit(spectrum)\nstd = np.std(spectrum)\nmin_ = spectrum.min()\nmax_ = spectrum.max()\n\nspectrum = scaler.transform(spectrum)\n\n# fill spectrum to have a size of 529, to then reshape to 23x23\nspectrum = np.append(spectrum, [0 for _ in range(14)]) # fill array to size 529 with zeroes\nspectrum = spectrum.reshape(23, 23) # building block one\n\n# Also scale min_ max_ from the spectrum\nmin_ = scale_param(min_, 6.5e-3, 2.6e-2)\nmax_ = scale_param(max_, 6.5e-3, 2.6e-2)\n\n\"\"\"\nAdd the different building blocks to each other\n\"\"\"\n\nmax1 = np.full((12,6), max_) # create array of shape 12,6 (height, width) with the max_ value\nmin1 = np.full((11,6), min_)\nmax1min1 = np.concatenate((max1, min1), axis=0) # Add min1 below max1 (axis=0) \n\nimage = np.concatenate((spectrum, max1min1), axis=1) # Add max1min1 to the right of spectrum (axis=1)\n\n\"\"\"\nGet all parameters and scale them\n\"\"\"\n# Get the param values\nch4 = x['param']['ch4_mixratio']\nco2 = x['param']['co2_mixratio']\nco = x['param']['co_mixratio']\nh2o = x['param']['h2o_mixratio']\nmass = x['param']['planet_mass']\nradius = x['param']['planet_radius']\ntemp = x['param']['temperature_profile']\n\n# Scale params (parm, min_value, max_value) where min/max should be the \nch4 = scale_param(ch4, 1e-8, 1e-1)\nco2 = scale_param(co2, 1e-8, 1e-1)\nco = scale_param(co, 1e-8, 1e-1)\nh2o = scale_param(h2o, 1e-8, 1e-1)\nmass = scale_param(mass, 1.5e27, 3.8e27)\nradius = scale_param(radius, 5.6e7, 1.0e8)\ntemp = scale_param(temp, 1e3, 2e3)\n\n# Create the building blocks\nco2 = np.full((23,1), co2)\nco = np.full((23,1), co)\nch4 = np.full((23,1), ch4)\n\n\nmass = np.full((1,23), mass)\nradius = np.full((1,23), radius)\ntemp = np.full((1,23), temp)\n\nh2o = np.full((9,9), h2o)\n\nmax2 = np.full((6,12), max_) # create array of shape 12,7 (height, width) with the max_ value\nmin2 = np.full((6,11), min_)\n\n\"\"\"\nPut building blocks together\n\"\"\"\nimage = np.concatenate((image, co2), axis=1)\nimage = np.concatenate((image, co), axis=1)\nimage = np.concatenate((image, ch4), axis=1)\n\nsub_image = np.concatenate((max2, min2), axis=1)\nsub_image = np.concatenate((sub_image, mass), axis=0)\nsub_image = np.concatenate((sub_image, radius), axis=0)\nsub_image = np.concatenate((sub_image, temp), axis=0)\nsub_image = np.concatenate((sub_image, h2o), axis=1)\n\nimage = np.concatenate((image, sub_image), axis=0)",
"_____no_output_____"
],
[
"plt.imshow(image, cmap='gray')",
"_____no_output_____"
]
],
[
[
"# New ASPA",
"_____no_output_____"
],
[
"## Load data, combine $(R_p/R_s)^2$ with the wavelength",
"_____no_output_____"
]
],
[
[
"i = np.random.randint(0,len(X))\nx = X[i] # select a dict from X\n\n\nwavelengths = pd.read_csv(dir_+'wnw_grid.txt', header=None).values\nspectrum = x['data']['spectrum']\nspectrum = np.expand_dims(spectrum, axis=1) # change shape from (515,) to (515,1)\nparams = x['param']\n\nfor param in params:\n if 'mixratio' in param: \n params[param] = np.log(np.abs(params[param])) # transform mixratio's because they are generated on logarithmic scale\n\nparams",
"_____no_output_____"
]
],
[
[
"# Normalize params",
"_____no_output_____"
]
],
[
[
"# Min max values from training set, in the same order as params above: planet mass, temp, .... co mixratio.\nmin_values = [1.518e26, 1e3, -18.42, 5.593e7, -18.42, -18.42, -18.42]\nmax_values = [3.796e27, 2e3, -2.303, 1.049e8, -2.306, -2.306, -2.306]\n\nfor i,param in enumerate(params):\n params[param] = scale_param(params[param], min_values[i], max_values[i])\n\nparams",
"_____no_output_____"
],
[
"wavelengths.shape, spectrum.shape",
"_____no_output_____"
],
[
"data = np.concatenate([wavelengths,spectrum], axis=1)\ndata = pd.DataFrame(data)\ndata.columns = ['x', 'y'] # x is wavelength, y is (R_p / R_s)^2",
"_____no_output_____"
]
],
[
[
"## Original ExoGAN simulation\nFrom 0.3 to 50 micron",
"_____no_output_____"
]
],
[
[
"plt.figure(figsize=(10,5))\n\nplt.plot(data.x, data.y, '.-', color='r')\nplt.xlabel(r'Wavelength [ยตm]')\nplt.ylabel(r'$(R_P / R_S)^2$')\n\nplt.xscale('log')\nlen(data)",
"_____no_output_____"
]
],
[
[
"## Select 0.3 to 16 micron",
"_____no_output_____"
]
],
[
[
"data = data[(data.x >= 0.3) & (data.x <= 16)] # select data between 0.3 and 16 micron\n\nplt.figure(figsize=(10,5))\n\nplt.plot(data.x, data.y, '.-', color='r')\nplt.xlabel(r'Wavelength [ยตm]')\nplt.ylabel(r'$(R_P / R_S)^2$')\n\n#plt.xscale('log')\nplt.xlim((2, 16))\nlen(data)",
"_____no_output_____"
]
],
[
[
"## Important!\nNotice how $(R_p/R_s)^2$ by index goes from a high to a low wavelength. \nApart from that, i'm assuming the spatial difference between peaks is due to plotting against the index instead of the wavelength. \nThe spectrum (below) will remain unchanged and is encoded this way into an ASPA, the wavelength values from above therefor have to be used to transform the ASPA back into $(R_p/R_s)^2$ with the wavelength values. ",
"_____no_output_____"
]
],
[
[
"#spectrum = np.flipud(data.y)\n\nplt.figure(figsize=(10,5))\n\nplt.plot(data.y, '.-', color='r')\nplt.xlabel(r'Index')\nplt.ylabel(r'$(R_P / R_S)^2$')",
"_____no_output_____"
]
],
[
[
"## Split the spectrum in bins ",
"_____no_output_____"
]
],
[
[
"# Could loop this, but right now this is more visual\nbin1 = data[data.x <= 0.8]\nbin2 = data[(data.x > 0.8) & (data.x <= 1.3)] # select data between 2 and 4 micron\nbin3 = data[(data.x > 1.3) & (data.x <= 2)]\nbin4 = data[(data.x > 2) & (data.x <= 4)]\nbin5 = data[(data.x > 4) & (data.x <= 6)]\nbin6 = data[(data.x > 6) & (data.x <= 10)]\nbin7 = data[(data.x > 10) & (data.x <= 14)]\nbin8 = data[data.x > 14]\n\nbin1.head()",
"_____no_output_____"
]
],
[
[
"## Bins against wavelength",
"_____no_output_____"
]
],
[
[
"\"\"\"\nVisualize the bins\n\"\"\"\n\nbins = [bin8, bin7, bin6, bin5, bin4, bin3, bin2, bin1]\n\nplt.figure(figsize=(10,5))\nfor b in bins:\n plt.plot(b.iloc[:,0], b.iloc[:,1], '.-')\n plt.xlabel(r'Wavelength [ยตm]')\n plt.ylabel(r'$(R_P / R_S)^2$')\n \n#plt.xlim((0.3, 9))",
"_____no_output_____"
]
],
[
[
"# Bins against index\nNotice how bin1 (0-2 micron) has way more datapoints than bin 8 (14-16 micron)",
"_____no_output_____"
]
],
[
[
"plt.figure(figsize=(10,5))\nfor b in bins:\n plt.plot(b.iloc[:,1], '.-')\n plt.xlabel(r'Index [-]')\n plt.ylabel(r'$(R_P / R_S)^2$')",
"_____no_output_____"
]
],
[
[
"## Normalize the spectrum in bins",
"_____no_output_____"
]
],
[
[
"scalers = [MinMaxScaler(feature_range=(0,1)).fit(b) for b in bins] # list of 8 scalers for the 8 bins\nmins = [ b.iloc[:,1].min() for b in bins] # .iloc[:,1] selects the R/R (y) only\nmaxs = [ b.iloc[:,1].max() for b in bins]\nstds = [ b.iloc[:,1].std() for b in bins]",
"_____no_output_____"
],
[
"bins_scaled = []\nfor i,b in enumerate(bins):\n bins_scaled.append(scalers[i].transform(b))",
"_____no_output_____"
],
[
"plt.figure(figsize=(10,5))\nfor i,b in enumerate(bins_scaled):\n plt.plot(b[:, 0], b[:,1], '.-', label=i)\nplt.legend()",
"_____no_output_____"
],
[
"np.concatenate(bins_scaled, axis=0).shape",
"_____no_output_____"
]
],
[
[
"## Scaled spectrum in bins",
"_____no_output_____"
]
],
[
[
"spectrum_scaled = np.concatenate(bins_scaled, axis=0)\nspectrum_scaled = spectrum_scaled[:,1]\n\nplt.plot(spectrum_scaled, '.-')\n\nlen(spectrum_scaled)",
"_____no_output_____"
]
],
[
[
"# Start creating the ASPA",
"_____no_output_____"
]
],
[
[
"import math",
"_____no_output_____"
],
[
"aspa = np.zeros((32,32))\n\nrow_length = 25 # amount of pixels used per row\nn_rows = math.ceil(len(spectrum_scaled) / row_length) # amount of rows the spectrum needs in the aspa, so for 415 data points, 415/32=12.96 -> 13 rows\nprint('Using %s rows' % n_rows)\n\nfor i in range(n_rows): # for i in \n\n start = i*row_length\n stop = start+row_length\n spec = spectrum_scaled[start:stop]\n \n if len(spec) != row_length:\n n_missing_points = row_length-len(spec)\n spec = np.append(spec, [0 for _ in range(n_missing_points)]) # for last row, if length != 32, fill remaining with 0's\n print('Filled row with %s points' % n_missing_points)\n \n aspa[i, :row_length] = spec\n\nplt.imshow(aspa, cmap='gray')",
"Using 16 rows\n"
]
],
[
[
"# Fill in the 7 ExoGAN params",
"_____no_output_____"
]
],
[
[
"params",
"_____no_output_____"
],
[
"for i,param in enumerate(params):\n aspa[:16, 25+i:32+i] = params[param]\n\nplt.imshow(aspa, cmap='gray')",
"_____no_output_____"
]
],
[
[
"# Fill in the min, max, std valued for the bins\nTODO: Normalize these properly",
"_____no_output_____"
]
],
[
[
"mins, maxs, stds",
"_____no_output_____"
],
[
"for i in range(len(mins)):\n min_ = scale_param(mins[i], 0.005, 0.03)\n max_ = scale_param(maxs[i], 0.005, 0.03)\n std_ = scale_param(stds[i], 1e-7, 1e-4)\n \n aspa[16:17, i*4:i*4+4] = min_\n aspa[17:18, i*4:i*4+4] = std_\n aspa[18:19, i*4:i*4+4] = max_\n \n \n print(min_, max_, std_)\n\nplt.imshow(aspa, cmap='gray')",
"0.18304209407699484 0.18501794207820269 0.12168214443077108\n0.1795876155164152 0.18350703182382339 0.3251502352023359\n0.17866701946658978 0.1806349121948885 0.1224142192152229\n0.17703549815458045 0.18609035470782206 0.6717569588276018\n0.17673002557975623 0.18317877235056546 0.4599526393679086\n0.17562721815244248 0.17967088755857144 0.27905825425832625\n0.17332491781668713 0.1769439181886055 0.27431291578560724\n0.17239153039711982 0.17512444145796277 0.1951118129395312\n"
]
],
[
[
"# Fill in unused space with noise",
"_____no_output_____"
]
],
[
[
"for i in range(13):\n noise = np.random.rand(32) # random noise betweem 0 and 1 for each row\n aspa[19+i:20+i*1, :] = noise\n\nplt.imshow(aspa, cmap='gray')",
"_____no_output_____"
]
],
[
[
"# Functionize ASPA v2",
"_____no_output_____"
]
],
[
[
"def ASPA_v2(x, wavelengths):\n spectrum = x['data']['spectrum']\n spectrum = np.expand_dims(spectrum, axis=1) # change shape from (515,) to (515,1)\n params = x['param']\n\n for param in params:\n if 'mixratio' in param: \n params[param] = np.log(np.abs(params[param])) # transform mixratio's because they are generated on logarithmic scale\n \n \"\"\"\n Normalize params\n \"\"\"\n # Min max values from training set, in the same order as params above: planet mass, temp, .... co mixratio.\n min_values = [1.518400e+27, \n 1.000000e+03, \n -1.842068e+01, \n 5.592880e+07, \n -1.842068e+01, \n -1.842068e+01, \n -1.842068e+01]\n \n max_values = [3.796000e+27, \n 2.000000e+03, \n -2.302585e+00, \n 1.048665e+08, \n -2.302585e+00, \n -2.302585e+00,\n -2.302585e+00]\n\n for i,param in enumerate(params):\n params[param] = scale_param(params[param], min_values[i], max_values[i])\n #print('%s: %s' % (param, params[param]))\n #print('-'*5)\n \"\"\"\n Select bins\n \"\"\"\n data = np.concatenate([wavelengths,spectrum], axis=1)\n data = pd.DataFrame(data)\n data.columns = ['x', 'y'] # x is wavelength, y is (R_p / R_s)^2\n \n # Could loop this, but right now this is more visual\n bin1 = data[data.x <= 0.8]\n bin2 = data[(data.x > 0.8) & (data.x <= 1.3)] # select data between 2 and 4 micron\n bin3 = data[(data.x > 1.3) & (data.x <= 2)]\n bin4 = data[(data.x > 2) & (data.x <= 4)]\n bin5 = data[(data.x > 4) & (data.x <= 6)]\n bin6 = data[(data.x > 6) & (data.x <= 10)]\n bin7 = data[(data.x > 10) & (data.x <= 14)]\n bin8 = data[data.x > 14]\n\n bins = [bin8, bin7, bin6, bin5, bin4, bin3, bin2, bin1]\n \n \"\"\"\n Normalize bins\n \"\"\"\n scalers = [MinMaxScaler(feature_range=(0,1)).fit(b) for b in bins] # list of 8 scalers for the 8 bins\n mins = [ b.iloc[:,1].min() for b in bins] # .iloc[:,1] selects the R/R (y) only\n maxs = [ b.iloc[:,1].max() for b in bins]\n stds = [ b.iloc[:,1].std() for b in bins]\n #print(min(mins), max(maxs))\n bins_scaled = []\n for i,b in enumerate(bins):\n bins_scaled.append(scalers[i].transform(b))\n \n spectrum_scaled = np.concatenate(bins_scaled, axis=0)\n spectrum_scaled = spectrum_scaled[:,1]\n \n \"\"\"\n Create the ASPA\n \"\"\"\n \n \"\"\"Spectrum\"\"\"\n aspa = np.zeros((32,32))\n\n row_length = 25 # amount of pixels used per row\n n_rows = math.ceil(len(spectrum_scaled) / row_length) # amount of rows the spectrum needs in the aspa, so for 415 data points, 415/32=12.96 -> 13 rows\n #print('Using %s rows' % n_rows)\n\n for i in range(n_rows): # for i in \n\n start = i*row_length\n stop = start+row_length\n spec = spectrum_scaled[start:stop]\n\n if len(spec) != row_length:\n n_missing_points = row_length-len(spec)\n spec = np.append(spec, [0 for _ in range(n_missing_points)]) # for last row, if length != 32, fill remaining with 0's\n #print('Filled row with %s points' % n_missing_points)\n\n aspa[i, :row_length] = spec\n \n \"\"\"ExoGAN params\"\"\"\n for i,param in enumerate(params):\n aspa[:16, 25+i:26+i] = params[param]\n \n \"\"\"min max std values for spectrum bins\"\"\"\n for i in range(len(mins)):\n min_ = scale_param(mins[i], 0.005, 0.03)\n max_ = scale_param(maxs[i], 0.005, 0.03)\n std_ = scale_param(stds[i], 9e-6, 2e-4)\n\n aspa[16:17, i*4:i*4+4] = min_\n aspa[17:18, i*4:i*4+4] = std_\n aspa[18:19, i*4:i*4+4] = max_\n \n \"\"\"Fill unused space with noice\"\"\"\n for i in range(13):\n noise = np.random.rand(32) # random noise betweem 0 and 1 for each row\n aspa[19+i:20+i*1, :] = noise\n \n return aspa",
"_____no_output_____"
]
],
[
[
"# Test ASPA v2 function",
"_____no_output_____"
]
],
[
[
"## Load data",
"_____no_output_____"
],
[
"i = np.random.randint(0,len(X))\ndict_ = X[i] # select a dict from X\n\nwavelengths = pd.read_csv(dir_+'wnw_grid.txt', header=None).values\n\ndict_['param']",
"_____no_output_____"
],
[
"aspa = ASPA_v2(dict_, wavelengths)",
"_____no_output_____"
],
[
"plt.imshow(aspa, cmap='gray')",
"_____no_output_____"
],
[
"np.random.shuffle(X)",
"_____no_output_____"
],
[
"plt.figure(figsize=(10,20))\n\nfor i in tqdm(range(8*4)):\n image = ASPA_v2(X[i], wavelengths)\n \n\n plt.subplot(8, 4, i+1)\n plt.imshow(image, cmap='gray', vmin=0, vmax=1.2)\n plt.tight_layout()",
"100%|โโโโโโโโโโ| 32/32 [00:06<00:00, 2.92it/s]\n"
]
],
[
[
"# Creating images from all simulations in the chunk",
"_____no_output_____"
]
],
[
[
"images = []\nfor i in tqdm(range(len(X))):\n image = ASPA_v2(X[i], wavelengths)\n image = image.reshape(1, 32, 32) # [images, channel, width, height]\n images.append(image)\n \nimages = np.array(images)\nimages.shape",
"_____no_output_____"
]
],
[
[
"# Saving this array to disk",
"_____no_output_____"
]
],
[
[
"%%time\nnp.save(dir_+'selection/last_chunks_25_percent_images.npy', images)",
"_____no_output_____"
]
],
[
[
"# Test loading and visualization",
"_____no_output_____"
]
],
[
[
"print('DONE')",
"_____no_output_____"
],
[
"print(\"DONE\")",
"_____no_output_____"
],
[
"print(\"DONE\")",
"DONE\n"
],
[
"images = np.load('/datb/16011015/ExoGAN_data/selection/first_chunks_25_percent_images.npy')",
"_____no_output_____"
],
[
"images.shape",
"_____no_output_____"
],
[
"plt.imshow(images[0,0,:,:])",
"_____no_output_____"
],
[
"plt.figure(figsize=(10,20))\n\nfor i in range(8*4):\n plt.subplot(8, 4, i+1)\n plt.imshow(images[i,0,:,:], cmap='gnuplot2')\n plt.tight_layout()",
"_____no_output_____"
]
],
[
[
"# Randomly mask pixels from the encoded spectrum",
"_____no_output_____"
]
],
[
[
"image = images[0, 0, :, :]\nplt.imshow(image)",
"_____no_output_____"
],
[
"# image[:23, :23] is the encoded spectrum.\nt = image.copy()\nprint(t.shape)\n#t[:23, :23] = 0\nplt.imshow(t)",
"_____no_output_____"
]
],
[
[
"# Random uniform dropout",
"_____no_output_____"
]
],
[
[
"t = image.copy()\ndropout = 0.9\n\nfor i in range(24): # loop over rows\n for j in range(24): # loop over cols\n a = np.random.random() # random uniform dist 0 - 1\n if a < dropout:\n t[i-1:i, j-1:j] = 0\n else:\n pass",
"_____no_output_____"
],
[
"plt.figure(figsize=(10,10))\nplt.imshow(t)",
"_____no_output_____"
],
[
"# image[:23, :23] is the encoded spectrum.\nt = image.copy()\n\n#t[:23, :23] = 0\nplt.imshow(t)\nt.shape",
"_____no_output_____"
]
],
[
[
"# Range dropout",
"_____no_output_____"
]
],
[
[
"# TODO: Mask everything but the visible spectrum\n\ndef mask_image(image, visible_length, random_visible_spectrum=True):\n \"\"\"\n Masks everything in an input image, apart from the start to visible_length. \n \n start = start wavelength/index value of the visible (non masked) spectrum\n visible_length = length of the visible spectrum (in pixels)\n output: masked_image\n \"\"\"\n\n image_masked = image.copy()\n \n spectrum_length = 23*23 # length of spectrum in ASPA\n start_max = spectrum_length - visible_length # maximum value start can have to still be able to show spectrum of length visible_length\n start = np.random.randint(0, start_max)\n\n # start stop index to mask before the visible (not masked) spectrum / sequence\n\n stop = start + visible_length # stop index of unmasked sequence\n\n spectrum = image_masked[:23, :23].flatten() # flatten the spectrum\n spectrum[:start] = 0\n spectrum[stop:] = 0\n spectrum = spectrum.reshape(23, 23)\n\n #t[:, :] = 0\n\n image_masked[:23, :23] = spectrum\n\n image_masked[:, 29:] = 0 # right side params\n image_masked[29:, :] = 0 # bottom params\n image_masked[23:, 23:] = 0 # h2o\n\n image_masked = image_masked.reshape(1, 32, 32) # add the channel dimension back \n \n return image_masked\n\n\nimage = images[0, 0, :, :].copy()\nvisible_length = 46 # length of the visible (not to mask) spectrum\n\nimage_masked = mask_image(image, visible_length)\nplt.imshow(image_masked[0, :, :])",
"_____no_output_____"
]
],
[
[
"## Also mask params and h2o\nLeaving min max values for now (they will get updated anyway)",
"_____no_output_____"
]
]
] | [
"markdown",
"code",
"markdown",
"code",
"markdown",
"code",
"markdown",
"code",
"markdown",
"code",
"markdown",
"code",
"markdown",
"code",
"markdown",
"code",
"markdown",
"code",
"markdown",
"code",
"markdown",
"code",
"markdown",
"code",
"markdown",
"code",
"markdown",
"code",
"markdown",
"code",
"markdown",
"code",
"markdown",
"code",
"markdown",
"code",
"markdown",
"raw",
"markdown",
"raw",
"markdown",
"code",
"markdown",
"code",
"markdown",
"code",
"markdown",
"code",
"markdown"
] | [
[
"markdown",
"markdown"
],
[
"code"
],
[
"markdown"
],
[
"code",
"code",
"code",
"code",
"code",
"code"
],
[
"markdown",
"markdown"
],
[
"code"
],
[
"markdown"
],
[
"code",
"code",
"code"
],
[
"markdown"
],
[
"code"
],
[
"markdown"
],
[
"code"
],
[
"markdown"
],
[
"code"
],
[
"markdown"
],
[
"code"
],
[
"markdown"
],
[
"code"
],
[
"markdown"
],
[
"code"
],
[
"markdown"
],
[
"code",
"code",
"code",
"code"
],
[
"markdown"
],
[
"code"
],
[
"markdown"
],
[
"code",
"code"
],
[
"markdown"
],
[
"code",
"code"
],
[
"markdown"
],
[
"code",
"code"
],
[
"markdown"
],
[
"code"
],
[
"markdown"
],
[
"code"
],
[
"markdown"
],
[
"code",
"code",
"code",
"code",
"code",
"code"
],
[
"markdown"
],
[
"raw"
],
[
"markdown"
],
[
"raw"
],
[
"markdown"
],
[
"code",
"code",
"code",
"code",
"code",
"code",
"code"
],
[
"markdown"
],
[
"code",
"code"
],
[
"markdown"
],
[
"code",
"code",
"code"
],
[
"markdown"
],
[
"code"
],
[
"markdown"
]
] |
e769e75382903899f1fd5ccf906e6914bfd94066 | 26,999 | ipynb | Jupyter Notebook | code/MobileNetv2-cifar.ipynb | TheShadow29/pyt-mobilenet | 2cdd6a271ad0691f3242834e7fe5205924d83ac1 | [
"MIT"
] | 8 | 2018-06-19T21:55:17.000Z | 2020-04-03T14:23:28.000Z | code/MobileNetv2-cifar.ipynb | TheShadow29/pyt-mobilenet | 2cdd6a271ad0691f3242834e7fe5205924d83ac1 | [
"MIT"
] | null | null | null | code/MobileNetv2-cifar.ipynb | TheShadow29/pyt-mobilenet | 2cdd6a271ad0691f3242834e7fe5205924d83ac1 | [
"MIT"
] | null | null | null | 71.805851 | 2,005 | 0.611467 | [
[
[
"from all_imports import *",
"_____no_output_____"
],
[
"%matplotlib inline\n%reload_ext autoreload\n%autoreload 2",
"_____no_output_____"
],
[
"from cifar10 import *",
"_____no_output_____"
],
[
"from mobile_net import *",
"_____no_output_____"
],
[
"bs=64\nsz=32",
"_____no_output_____"
],
[
"data = get_data(sz, bs)",
"_____no_output_____"
],
[
"class exp_dw_block(nn.Module):\n ## Thanks to https://github.com/kuangliu/pytorch-cifar/blob/master/models/mobilenetv2.py\n def __init__(self, in_c, out_c, expansion, stride):\n super().__init__()\n self.stride = stride\n exp_out_c = in_c * expansion\n \n self.ptwise_conv = nn.Conv2d(in_c, exp_out_c, kernel_size=1, bias=False)\n self.bn1 = nn.BatchNorm2d(exp_out_c)\n self.dwise_conv = nn.Conv2d(exp_out_c, exp_out_c, kernel_size=3, \n groups=exp_out_c, stride=self.stride, padding=1, bias=False)\n self.bn2 = nn.BatchNorm2d(exp_out_c)\n self.lin_conv = nn.Conv2d(exp_out_c, out_c, kernel_size=1, bias=False)\n self.bn3 = nn.BatchNorm2d(out_c)\n \n self.res = nn.Sequential()\n if self.stride == 1 and in_c != out_c:\n self.res = nn.Sequential(nn.Conv2d(in_c, out_c, kernel_size=1, bias=False), \n nn.BatchNorm2d(out_c))\n \n def forward(self, inp):\n out = F.relu6(self.bn1(self.ptwise_conv(inp)))\n out = F.relu6(self.bn2(self.dwise_conv(out)))\n out = self.bn3(self.lin_conv(out))\n if self.stride == 1:\n out = out + self.res(inp)\n return out\n \n",
"_____no_output_____"
],
[
"class mblnetv2(nn.Module):\n def __init__(self, block, inc_scale, inc_start, tuple_list, num_classes):\n super().__init__()\n # assuming tuple list of form:\n # expansion, out_planes, num_blocks, stride \n self.num_blocks = len(tuple_list)\n self.in_planes = inc_start // inc_scale\n self.conv1 = nn.Conv2d(3, self.in_planes, kernel_size=3, stride=1, padding=1, bias=False)\n self.bn1 = nn.BatchNorm2d(self.in_planes)\n lyrs = []\n for expf, inc, nb, strl in tuple_list:\n lyrs.append(self._make_layer(block, expf, inc, nb, strl))\n \n self.lyrs = nn.Sequential(*lyrs)\n self.linear = nn.Linear(tuple_list[-1][1], num_classes)\n \n \n def _make_layer(self, block, expf, planes, num_blocks, stride):\n strides = [stride] + [1]*(num_blocks-1)\n layers = []\n for stride in strides:\n layers.append(block(self.in_planes, planes, expf, stride))\n self.in_planes = planes\n return nn.Sequential(*layers)\n \n def forward(self, inp):\n out = F.relu(self.bn1(self.conv1(inp)))\n out = self.lyrs(out)\n out = F.adaptive_avg_pool2d(out, 1)\n out = out.view(out.size(0), -1)\n out = self.linear(out)\n return F.log_softmax(out, dim=-1)\n",
"_____no_output_____"
],
[
"tpl = [(1, 16, 1, 1),\n (6, 24, 2, 1), \n (6, 32, 3, 2),\n (6, 64, 4, 2),\n (6, 96, 3, 1),\n (6, 160, 3, 2),\n (6, 320, 1, 1)]\nmd_mbl1 = mblnetv2(exp_dw_block, 1, 32,\n tpl,\n num_classes=10)",
"_____no_output_____"
],
[
"data = get_data(sz, bs)",
"_____no_output_____"
],
[
"learn = ConvLearner.from_model_data(md_mbl1, data)\n\ntotal_model_params(learn.summary())",
"Total parameters in the model :1875162\n"
],
[
"learn.fit(1, 1, cycle_len=30, use_clr_beta=(20, 20, 0.95, 0.85), best_save_name='best_mblnv2_new_xp1',\n metrics=[accuracy])",
"_____no_output_____"
],
[
"# learn.fit(5e-2, 1, cycle_len=50, use_clr_beta=(20, 13.68, 0.95, 0.85), best_save_name='best_mblnetv2_xp_1', metrics=[accuracy])",
"_____no_output_____"
],
[
"learn.load('best_mblnetv2_xp_1')",
"_____no_output_____"
],
[
"learn.fit(0, 1)",
"_____no_output_____"
]
]
] | [
"code"
] | [
[
"code",
"code",
"code",
"code",
"code",
"code",
"code",
"code",
"code",
"code",
"code",
"code",
"code",
"code",
"code"
]
] |
e769e8eaed69ddebccb6d23cf81ee46148062362 | 63,167 | ipynb | Jupyter Notebook | 1.1-Types.ipynb | mohamedsuhaib/Python_Study | e0e306f6c3665d8dad9b9446a0bde18f58a1cf7c | [
"MIT"
] | null | null | null | 1.1-Types.ipynb | mohamedsuhaib/Python_Study | e0e306f6c3665d8dad9b9446a0bde18f58a1cf7c | [
"MIT"
] | null | null | null | 1.1-Types.ipynb | mohamedsuhaib/Python_Study | e0e306f6c3665d8dad9b9446a0bde18f58a1cf7c | [
"MIT"
] | null | null | null | 42.79607 | 1,710 | 0.443428 | [
[
[
"<div class=\"alert alert-block alert-info\" style=\"margin-top: 20px\">\n <a href=\"https://cocl.us/NotebooksPython101\">\n <img src=\"https://s3-api.us-geo.objectstorage.softlayer.net/cf-courses-data/CognitiveClass/PY0101EN/Ad/TopAd.png\" width=\"750\" align=\"center\">\n </a>\n</div>",
"_____no_output_____"
],
[
"<a href=\"https://cognitiveclass.ai/\">\n <img src=\"https://s3-api.us-geo.objectstorage.softlayer.net/cf-courses-data/CognitiveClass/PY0101EN/Ad/CCLog.png\" width=\"200\" align=\"center\">\n</a>",
"_____no_output_____"
],
[
"<h1>Python - Writing Your First Python Code!</h1>",
"_____no_output_____"
],
[
"<p><strong>Welcome!</strong> This notebook will teach you the basics of the Python programming language. Although the information presented here is quite basic, it is an important foundation that will help you read and write Python code. By the end of this notebook, you'll know the basics of Python, including how to write basic commands, understand some basic types, and how to perform simple operations on them.</p> ",
"_____no_output_____"
],
[
"<h2>Table of Contents</h2>\n<div class=\"alert alert-block alert-info\" style=\"margin-top: 20px\">\n <ul>\n <li>\n <a href=\"#hello\">Say \"Hello\" to the world in Python</a>\n <ul>\n <li><a href=\"version\">What version of Python are we using?</a></li>\n <li><a href=\"comments\">Writing comments in Python</a></li>\n <li><a href=\"errors\">Errors in Python</a></li>\n <li><a href=\"python_error\">Does Python know about your error before it runs your code?</a></li>\n <li><a href=\"exercise\">Exercise: Your First Program</a></li>\n </ul>\n </li>\n <li>\n <a href=\"#types_objects\">Types of objects in Python</a>\n <ul>\n <li><a href=\"int\">Integers</a></li>\n <li><a href=\"float\">Floats</a></li>\n <li><a href=\"convert\">Converting from one object type to a different object type</a></li>\n <li><a href=\"bool\">Boolean data type</a></li>\n <li><a href=\"exer_type\">Exercise: Types</a></li>\n </ul>\n </li>\n <li>\n <a href=\"#expressions\">Expressions and Variables</a>\n <ul>\n <li><a href=\"exp\">Expressions</a></li>\n <li><a href=\"exer_exp\">Exercise: Expressions</a></li>\n <li><a href=\"var\">Variables</a></li>\n <li><a href=\"exer_exp_var\">Exercise: Expression and Variables in Python</a></li>\n </ul>\n </li>\n </ul>\n <p>\n Estimated time needed: <strong>25 min</strong>\n </p>\n</div>\n\n<hr>",
"_____no_output_____"
],
[
"<h2 id=\"hello\">Say \"Hello\" to the world in Python</h2>",
"_____no_output_____"
],
[
"When learning a new programming language, it is customary to start with an \"hello world\" example. As simple as it is, this one line of code will ensure that we know how to print a string in output and how to execute code within cells in a notebook.",
"_____no_output_____"
],
[
"<hr/>\n<div class=\"alert alert-success alertsuccess\" style=\"margin-top: 20px\">\n[Tip]: To execute the Python code in the code cell below, click on the cell to select it and press <kbd>Shift</kbd> + <kbd>Enter</kbd>.\n</div>\n<hr/>",
"_____no_output_____"
]
],
[
[
"# Try your first Python output\n\nprint(\"Hello, Python!\")",
"Hello, Python!\n"
]
],
[
[
"\nAfter executing the cell above, you should see that Python prints <code>Hello, Python!</code>. Congratulations on running your first Python code!",
"_____no_output_____"
],
[
"<hr/>\n<div class=\"alert alert-success alertsuccess\" style=\"margin-top: 20px\">\n [Tip:] <code>print()</code> is a function. You passed the string <code>'Hello, Python!'</code> as an argument to instruct Python on what to print.\n</div>\n<hr/>",
"_____no_output_____"
],
[
"<h3 id=\"version\">What version of Python are we using?</h3>",
"_____no_output_____"
],
[
"<p>\n There are two popular versions of the Python programming language in use today: Python 2 and Python 3. The Python community has decided to move on from Python 2 to Python 3, and many popular libraries have announced that they will no longer support Python 2.\n</p>\n<p>\n Since Python 3 is the future, in this course we will be using it exclusively. How do we know that our notebook is executed by a Python 3 runtime? We can look in the top-right hand corner of this notebook and see \"Python 3\".\n</p>\n<p>\n We can also ask directly Python and obtain a detailed answer. Try executing the following code:\n</p>",
"_____no_output_____"
]
],
[
[
"# Check version runing on Jupyter notebook\nfrom platform import python_version\nprint(python_version())\n\n# Check version inside your Python program\nimport sys\nprint(sys.version)",
"3.7.10\n3.7.10 (default, Feb 26 2021, 18:47:35) \n[GCC 7.3.0]\n"
]
],
[
[
"<hr/>\n<div class=\"alert alert-success alertsuccess\" style=\"margin-top: 20px\">\n [Tip:] <code>sys</code> is a built-in module that contains many system-specific parameters and functions, including the Python version in use. Before using it, we must explictly <code>import</code> it.\n</div>\n<hr/>",
"_____no_output_____"
],
[
"<h3 id=\"comments\">Writing comments in Python</h3>",
"_____no_output_____"
],
[
"<p>\n In addition to writing code, note that it's always a good idea to add comments to your code. It will help others understand what you were trying to accomplish (the reason why you wrote a given snippet of code). Not only does this help <strong>other people</strong> understand your code, it can also serve as a reminder <strong>to you</strong> when you come back to it weeks or months later.</p>\n\n<p>\n To write comments in Python, use the number symbol <code>#</code> before writing your comment. When you run your code, Python will ignore everything past the <code>#</code> on a given line.\n</p>",
"_____no_output_____"
]
],
[
[
"# Practice on writing comments\n\nprint('Hello, Python!') # This line prints a string\n# print('Hi')",
"Hello, Python!\n"
]
],
[
[
"<p>\n After executing the cell above, you should notice that <code>This line prints a string</code> did not appear in the output, because it was a comment (and thus ignored by Python).\n</p>\n<p>\n The second line was also not executed because <code>print('Hi')</code> was preceded by the number sign (<code>#</code>) as well! Since this isn't an explanatory comment from the programmer, but an actual line of code, we might say that the programmer <em>commented out</em> that second line of code.\n</p>",
"_____no_output_____"
],
[
"<h3 id=\"errors\">Errors in Python</h3>",
"_____no_output_____"
],
[
"<p>Everyone makes mistakes. For many types of mistakes, Python will tell you that you have made a mistake by giving you an error message. It is important to read error messages carefully to really understand where you made a mistake and how you may go about correcting it.</p>\n<p>For example, if you spell <code>print</code> as <code>frint</code>, Python will display an error message. Give it a try:</p>",
"_____no_output_____"
]
],
[
[
"# Print string as error message\n\nfrint(\"Hello, Python!\")",
"_____no_output_____"
]
],
[
[
"<p>The error message tells you: \n<ol>\n <li>where the error occurred (more useful in large notebook cells or scripts), and</li> \n <li>what kind of error it was (NameError)</li> \n</ol>\n<p>Here, Python attempted to run the function <code>frint</code>, but could not determine what <code>frint</code> is since it's not a built-in function and it has not been previously defined by us either.</p>",
"_____no_output_____"
],
[
"<p>\n You'll notice that if we make a different type of mistake, by forgetting to close the string, we'll obtain a different error (i.e., a <code>SyntaxError</code>). Try it below:\n</p>",
"_____no_output_____"
]
],
[
[
"# Try to see build in error message\n\nprint(\"Hello, Python!)",
"_____no_output_____"
]
],
[
[
"<h3 id=\"python_error\">Does Python know about your error before it runs your code?</h3>",
"_____no_output_____"
],
[
"Python is what is called an <em>interpreted language</em>. Compiled languages examine your entire program at compile time, and are able to warn you about a whole class of errors prior to execution. In contrast, Python interprets your script line by line as it executes it. Python will stop executing the entire program when it encounters an error (unless the error is expected and handled by the programmer, a more advanced subject that we'll cover later on in this course).",
"_____no_output_____"
],
[
"Try to run the code in the cell below and see what happens:",
"_____no_output_____"
]
],
[
[
"# Print string and error to see the running order\n\nprint(\"This will be printed\")\nfrint(\"This will cause an error\")\nprint(\"This will NOT be printed\")",
"This will be printed\n"
]
],
[
[
"<h3 id=\"exercise\">Exercise: Your First Program</h3>",
"_____no_output_____"
],
[
"<p>Generations of programmers have started their coding careers by simply printing \"Hello, world!\". You will be following in their footsteps.</p>\n<p>In the code cell below, use the <code>print()</code> function to print out the phrase: <code>Hello, world!</code></p>",
"_____no_output_____"
]
],
[
[
"# Write your code below and press Shift+Enter to execute \nprint(\"Hello World!\")",
"Hello World!\n"
]
],
[
[
"Double-click __here__ for the solution.\n\n<!-- Your answer is below:\n\nprint(\"Hello, world!\")\n\n-->",
"_____no_output_____"
],
[
"<p>Now, let's enhance your code with a comment. In the code cell below, print out the phrase: <code>Hello, world!</code> and comment it with the phrase <code>Print the traditional hello world</code> all in one line of code.</p>",
"_____no_output_____"
]
],
[
[
"# Write your code below and press Shift+Enter to execute \n#print the traditional Hello World\nprint(\"Hello World!\")",
"Hello World!\n"
]
],
[
[
"Double-click __here__ for the solution.\n\n<!-- Your answer is below:\n\nprint(\"Hello, world!\") # Print the traditional hello world\n\n-->\n",
"_____no_output_____"
],
[
"<hr>",
"_____no_output_____"
],
[
"<h2 id=\"types_objects\" align=\"center\">Types of objects in Python</h2>",
"_____no_output_____"
],
[
"<p>Python is an object-oriented language. There are many different types of objects in Python. Let's start with the most common object types: <i>strings</i>, <i>integers</i> and <i>floats</i>. Anytime you write words (text) in Python, you're using <i>character strings</i> (strings for short). The most common numbers, on the other hand, are <i>integers</i> (e.g. -1, 0, 100) and <i>floats</i>, which represent real numbers (e.g. 3.14, -42.0).</p>",
"_____no_output_____"
],
[
"<a align=\"center\">\n <img src=\"https://s3-api.us-geo.objectstorage.softlayer.net/cf-courses-data/CognitiveClass/PY0101EN/Chapter%201/Images/TypesObjects.png\" width=\"600\">\n</a>",
"_____no_output_____"
],
[
"<p>The following code cells contain some examples.</p>",
"_____no_output_____"
]
],
[
[
"# Integer\n\n11",
"_____no_output_____"
],
[
"# Float\n\n2.14",
"_____no_output_____"
],
[
"# String\n\n\"Hello, Python 101!\"",
"_____no_output_____"
]
],
[
[
"<p>You can get Python to tell you the type of an expression by using the built-in <code>type()</code> function. You'll notice that Python refers to integers as <code>int</code>, floats as <code>float</code>, and character strings as <code>str</code>.</p>",
"_____no_output_____"
]
],
[
[
"# Type of 12\n\ntype(12)",
"_____no_output_____"
],
[
"# Type of 2.14\n\ntype(2.14)",
"_____no_output_____"
],
[
"# Type of \"Hello, Python 101!\"\n\ntype(\"Hello, Python 101!\")",
"_____no_output_____"
]
],
[
[
"<p>In the code cell below, use the <code>type()</code> function to check the object type of <code>12.0</code>.",
"_____no_output_____"
]
],
[
[
"# Write your code below. Don't forget to press Shift+Enter to execute the cell\ntype(12.0)",
"_____no_output_____"
]
],
[
[
"Double-click __here__ for the solution.\n\n<!-- Your answer is below:\n\ntype(12.0)\n\n-->",
"_____no_output_____"
],
[
"<h3 id=\"int\">Integers</h3>",
"_____no_output_____"
],
[
"<p>Here are some examples of integers. Integers can be negative or positive numbers:</p>",
"_____no_output_____"
],
[
"<a align=\"center\">\n <img src=\"https://s3-api.us-geo.objectstorage.softlayer.net/cf-courses-data/CognitiveClass/PY0101EN/Chapter%201/Images/TypesInt.png\" width=\"600\">\n</a>",
"_____no_output_____"
],
[
"<p>We can verify this is the case by using, you guessed it, the <code>type()</code> function:",
"_____no_output_____"
]
],
[
[
"# Print the type of -1\n\ntype(-1)",
"_____no_output_____"
],
[
"# Print the type of 4\n\ntype(4)",
"_____no_output_____"
],
[
"# Print the type of 0\n\ntype(0)",
"_____no_output_____"
]
],
[
[
"<h3 id=\"float\">Floats</h3> ",
"_____no_output_____"
],
[
"<p>Floats represent real numbers; they are a superset of integer numbers but also include \"numbers with decimals\". There are some limitations when it comes to machines representing real numbers, but floating point numbers are a good representation in most cases. You can learn more about the specifics of floats for your runtime environment, by checking the value of <code>sys.float_info</code>. This will also tell you what's the largest and smallest number that can be represented with them.</p>\n\n<p>Once again, can test some examples with the <code>type()</code> function:",
"_____no_output_____"
]
],
[
[
"# Print the type of 1.0\n\ntype(1.0) # Notice that 1 is an int, and 1.0 is a float",
"_____no_output_____"
],
[
"# Print the type of 0.5\n\ntype(0.5)",
"_____no_output_____"
],
[
"# Print the type of 0.56\n\ntype(0.56)",
"_____no_output_____"
],
[
"# System settings about float type\n\nsys.float_info",
"_____no_output_____"
]
],
[
[
"<h3 id=\"convert\">Converting from one object type to a different object type</h3>",
"_____no_output_____"
],
[
"<p>You can change the type of the object in Python; this is called typecasting. For example, you can convert an <i>integer</i> into a <i>float</i> (e.g. 2 to 2.0).</p>\n<p>Let's try it:</p>",
"_____no_output_____"
]
],
[
[
"# Verify that this is an integer\n\ntype(2)",
"_____no_output_____"
]
],
[
[
"<h4>Converting integers to floats</h4>\n<p>Let's cast integer 2 to float:</p>",
"_____no_output_____"
]
],
[
[
"# Convert 2 to a float\n\nfloat(2)",
"_____no_output_____"
],
[
"# Convert integer 2 to a float and check its type\n\ntype(float(2))",
"_____no_output_____"
]
],
[
[
"<p>When we convert an integer into a float, we don't really change the value (i.e., the significand) of the number. However, if we cast a float into an integer, we could potentially lose some information. For example, if we cast the float 1.1 to integer we will get 1 and lose the decimal information (i.e., 0.1):</p>",
"_____no_output_____"
]
],
[
[
"# Casting 1.1 to integer will result in loss of information\n\nint(1.1)",
"_____no_output_____"
]
],
[
[
"<h4>Converting from strings to integers or floats</h4>",
"_____no_output_____"
],
[
"<p>Sometimes, we can have a string that contains a number within it. If this is the case, we can cast that string that represents a number into an integer using <code>int()</code>:</p>",
"_____no_output_____"
]
],
[
[
"# Convert a string into an integer\n\nint('1')",
"_____no_output_____"
]
],
[
[
"<p>But if you try to do so with a string that is not a perfect match for a number, you'll get an error. Try the following:</p>",
"_____no_output_____"
]
],
[
[
"# Convert a string into an integer with error\n\nint('1 or 2 people')",
"_____no_output_____"
]
],
[
[
"<p>You can also convert strings containing floating point numbers into <i>float</i> objects:</p>",
"_____no_output_____"
]
],
[
[
"# Convert the string \"1.2\" into a float\n\nfloat('1.2')",
"_____no_output_____"
]
],
[
[
"<hr/>\n<div class=\"alert alert-success alertsuccess\" style=\"margin-top: 20px\">\n [Tip:] Note that strings can be represented with single quotes (<code>'1.2'</code>) or double quotes (<code>\"1.2\"</code>), but you can't mix both (e.g., <code>\"1.2'</code>).\n</div>\n<hr/>",
"_____no_output_____"
],
[
"<h4>Converting numbers to strings</h4>",
"_____no_output_____"
],
[
"<p>If we can convert strings to numbers, it is only natural to assume that we can convert numbers to strings, right?</p>",
"_____no_output_____"
]
],
[
[
"# Convert an integer to a string\n\nstr(1)",
"_____no_output_____"
]
],
[
[
"<p>And there is no reason why we shouldn't be able to make floats into strings as well:</p> ",
"_____no_output_____"
]
],
[
[
"# Convert a float to a string\n\nstr(1.2)",
"_____no_output_____"
]
],
[
[
"<h3 id=\"bool\">Boolean data type</h3>",
"_____no_output_____"
],
[
"<p><i>Boolean</i> is another important type in Python. An object of type <i>Boolean</i> can take on one of two values: <code>True</code> or <code>False</code>:</p>",
"_____no_output_____"
]
],
[
[
"# Value true\n\nTrue",
"_____no_output_____"
]
],
[
[
"<p>Notice that the value <code>True</code> has an uppercase \"T\". The same is true for <code>False</code> (i.e. you must use the uppercase \"F\").</p>",
"_____no_output_____"
]
],
[
[
"# Value false\n\nFalse",
"_____no_output_____"
]
],
[
[
"<p>When you ask Python to display the type of a boolean object it will show <code>bool</code> which stands for <i>boolean</i>:</p> ",
"_____no_output_____"
]
],
[
[
"# Type of True\n\ntype(True)",
"_____no_output_____"
],
[
"# Type of False\n\ntype(False)",
"_____no_output_____"
]
],
[
[
"<p>We can cast boolean objects to other data types. If we cast a boolean with a value of <code>True</code> to an integer or float we will get a one. If we cast a boolean with a value of <code>False</code> to an integer or float we will get a zero. Similarly, if we cast a 1 to a Boolean, you get a <code>True</code>. And if we cast a 0 to a Boolean we will get a <code>False</code>. Let's give it a try:</p> ",
"_____no_output_____"
]
],
[
[
"# Convert True to int\n\nint(True)",
"_____no_output_____"
],
[
"# Convert 1 to boolean\n\nbool(1)",
"_____no_output_____"
],
[
"# Convert 0 to boolean\n\nbool(0)",
"_____no_output_____"
],
[
"# Convert True to float\n\nfloat(True)",
"_____no_output_____"
]
],
[
[
"<h3 id=\"exer_type\">Exercise: Types</h3>",
"_____no_output_____"
],
[
"<p>What is the data type of the result of: <code>6 / 2</code>?</p>",
"_____no_output_____"
]
],
[
[
"# Write your code below. Don't forget to press Shift+Enter to execute the cell\ntype (6/2)",
"_____no_output_____"
]
],
[
[
"Double-click __here__ for the solution.\n\n<!-- Your answer is below:\ntype(6/2) # float\n-->",
"_____no_output_____"
],
[
"<p>What is the type of the result of: <code>6 // 2</code>? (Note the double slash <code>//</code>.)</p>",
"_____no_output_____"
]
],
[
[
"# Write your code below. Don't forget to press Shift+Enter to execute the cell\ntype (6//2)",
"_____no_output_____"
]
],
[
[
"Double-click __here__ for the solution.\n\n<!-- Your answer is below:\ntype(6//2) # int, as the double slashes stand for integer division \n-->",
"_____no_output_____"
],
[
"<hr>",
"_____no_output_____"
],
[
"<h2 id=\"expressions\">Expression and Variables</h2>",
"_____no_output_____"
],
[
"<h3 id=\"exp\">Expressions</h3>",
"_____no_output_____"
],
[
"<p>Expressions in Python can include operations among compatible types (e.g., integers and floats). For example, basic arithmetic operations like adding multiple numbers:</p>",
"_____no_output_____"
]
],
[
[
"# Addition operation expression\n\n43 + 60 + 16 + 41",
"_____no_output_____"
]
],
[
[
"<p>We can perform subtraction operations using the minus operator. In this case the result is a negative number:</p>",
"_____no_output_____"
]
],
[
[
"# Subtraction operation expression\n\n50 - 60",
"_____no_output_____"
]
],
[
[
"<p>We can do multiplication using an asterisk:</p>",
"_____no_output_____"
]
],
[
[
"# Multiplication operation expression\n\n5 * 5",
"_____no_output_____"
]
],
[
[
"<p>We can also perform division with the forward slash:",
"_____no_output_____"
]
],
[
[
"# Division operation expression\n\n25 / 5",
"_____no_output_____"
],
[
"# Division operation expression\n25 / 6",
"_____no_output_____"
]
],
[
[
"<p>As seen in the quiz above, we can use the double slash for integer division, where the result is rounded to the nearest integer:",
"_____no_output_____"
]
],
[
[
"# Integer division operation expression\n\n25 // 5",
"_____no_output_____"
],
[
"# Integer division operation expression\n\n25 // 6",
"_____no_output_____"
]
],
[
[
"<h3 id=\"exer_exp\">Exercise: Expression</h3>",
"_____no_output_____"
],
[
"<p>Let's write an expression that calculates how many hours there are in 160 minutes:",
"_____no_output_____"
]
],
[
[
"# Write your code below. Don't forget to press Shift+Enter to execute the cell\n160 / 60",
"_____no_output_____"
]
],
[
[
"Double-click __here__ for the solution.\n\n<!-- Your answer is below:\n160/60 \n# Or \n160//60\n-->",
"_____no_output_____"
],
[
"<p>Python follows well accepted mathematical conventions when evaluating mathematical expressions. In the following example, Python adds 30 to the result of the multiplication (i.e., 120).",
"_____no_output_____"
]
],
[
[
"# Mathematical expression\n\n30 + 2 * 60",
"_____no_output_____"
]
],
[
[
"<p>And just like mathematics, expressions enclosed in parentheses have priority. So the following multiplies 32 by 60.",
"_____no_output_____"
]
],
[
[
"# Mathematical expression\n\n(30 + 2) * 60",
"_____no_output_____"
]
],
[
[
"<h3 id=\"var\">Variables</h3>",
"_____no_output_____"
],
[
"<p>Just like with most programming languages, we can store values in <i>variables</i>, so we can use them later on. For example:</p>",
"_____no_output_____"
]
],
[
[
"# Store value into variable\n\nx = 43 + 60 + 16 + 41",
"_____no_output_____"
]
],
[
[
"<p>To see the value of <code>x</code> in a Notebook, we can simply place it on the last line of a cell:</p>",
"_____no_output_____"
]
],
[
[
"# Print out the value in variable\n\nx",
"_____no_output_____"
]
],
[
[
"<p>We can also perform operations on <code>x</code> and save the result to a new variable:</p>",
"_____no_output_____"
]
],
[
[
"# Use another variable to store the result of the operation between variable and value\n\ny = x / 60\ny",
"_____no_output_____"
]
],
[
[
"<p>If we save a value to an existing variable, the new value will overwrite the previous value:</p>",
"_____no_output_____"
]
],
[
[
"# Overwrite variable with new value\n\nx = x / 60\nx",
"_____no_output_____"
]
],
[
[
"<p>It's a good practice to use meaningful variable names, so you and others can read the code and understand it more easily:</p>",
"_____no_output_____"
]
],
[
[
"# Name the variables meaningfully\n\ntotal_min = 43 + 42 + 57 # Total length of albums in minutes\ntotal_min",
"_____no_output_____"
],
[
"# Name the variables meaningfully\n\ntotal_hours = total_min / 60 # Total length of albums in hours \ntotal_hours",
"_____no_output_____"
]
],
[
[
"<p>In the cells above we added the length of three albums in minutes and stored it in <code>total_min</code>. We then divided it by 60 to calculate total length <code>total_hours</code> in hours. You can also do it all at once in a single expression, as long as you use parenthesis to add the albums length before you divide, as shown below.</p>",
"_____no_output_____"
]
],
[
[
"# Complicate expression\n\ntotal_hours = (43 + 42 + 57) / 60 # Total hours in a single expression\ntotal_hours",
"_____no_output_____"
]
],
[
[
"<p>If you'd rather have total hours as an integer, you can of course replace the floating point division with integer division (i.e., <code>//</code>).</p>",
"_____no_output_____"
],
[
"<h3 id=\"exer_exp_var\">Exercise: Expression and Variables in Python</h3>",
"_____no_output_____"
],
[
"<p>What is the value of <code>x</code> where <code>x = 3 + 2 * 2</code></p>",
"_____no_output_____"
]
],
[
[
"# Write your code below. Don't forget to press Shift+Enter to execute the cell\nx = 3 + 2 * 2\nx",
"_____no_output_____"
]
],
[
[
"Double-click __here__ for the solution.\n\n<!-- Your answer is below:\n7\n-->\n",
"_____no_output_____"
],
[
"<p>What is the value of <code>y</code> where <code>y = (3 + 2) * 2</code>?</p>",
"_____no_output_____"
]
],
[
[
"# Write your code below. Don't forget to press Shift+Enter to execute the cell\ny = (3+2)*2\ny",
"_____no_output_____"
]
],
[
[
"Double-click __here__ for the solution.\n\n<!-- Your answer is below:\n10\n-->",
"_____no_output_____"
],
[
"<p>What is the value of <code>z</code> where <code>z = x + y</code>?</p>",
"_____no_output_____"
]
],
[
[
"# Write your code below. Don't forget to press Shift+Enter to execute the cell\nz= x+y\nz",
"_____no_output_____"
]
],
[
[
"Double-click __here__ for the solution.\n\n<!-- Your answer is below:\n17\n-->",
"_____no_output_____"
],
[
"<hr>\n<h2>The last exercise!</h2>\n<p>Congratulations, you have completed your first lesson and hands-on lab in Python. However, there is one more thing you need to do. The Data Science community encourages sharing work. The best way to share and showcase your work is to share it on GitHub. By sharing your notebook on GitHub you are not only building your reputation with fellow data scientists, but you can also show it off when applying for a job. Even though this was your first piece of work, it is never too early to start building good habits. So, please read and follow <a href=\"https://cognitiveclass.ai/blog/data-scientists-stand-out-by-sharing-your-notebooks/\" target=\"_blank\">this article</a> to learn how to share your work.\n<hr>",
"_____no_output_____"
],
[
"<div class=\"alert alert-block alert-info\" style=\"margin-top: 20px\">\n<h2>Get IBM Watson Studio free of charge!</h2>\n <p><a href=\"https://cocl.us/NotebooksPython101bottom\"><img src=\"https://s3-api.us-geo.objectstorage.softlayer.net/cf-courses-data/CognitiveClass/PY0101EN/Ad/BottomAd.png\" width=\"750\" align=\"center\"></a></p>\n</div>",
"_____no_output_____"
],
[
"<h3>About the Authors:</h3> \n<p><a href=\"https://www.linkedin.com/in/joseph-s-50398b136/\" target=\"_blank\">Joseph Santarcangelo</a> is a Data Scientist at IBM, and holds a PhD in Electrical Engineering. His research focused on using Machine Learning, Signal Processing, and Computer Vision to determine how videos impact human cognition. Joseph has been working for IBM since he completed his PhD.</p>",
"_____no_output_____"
],
[
"Other contributors: <a href=\"www.linkedin.com/in/jiahui-mavis-zhou-a4537814a\">Mavis Zhou</a>",
"_____no_output_____"
],
[
"<hr>\n<p>Copyright © 2018 IBM Developer Skills Network. This notebook and its source code are released under the terms of the <a href=\"https://cognitiveclass.ai/mit-license/\">MIT License</a>.</p>",
"_____no_output_____"
]
]
] | [
"markdown",
"code",
"markdown",
"code",
"markdown",
"code",
"markdown",
"code",
"markdown",
"code",
"markdown",
"code",
"markdown",
"code",
"markdown",
"code",
"markdown",
"code",
"markdown",
"code",
"markdown",
"code",
"markdown",
"code",
"markdown",
"code",
"markdown",
"code",
"markdown",
"code",
"markdown",
"code",
"markdown",
"code",
"markdown",
"code",
"markdown",
"code",
"markdown",
"code",
"markdown",
"code",
"markdown",
"code",
"markdown",
"code",
"markdown",
"code",
"markdown",
"code",
"markdown",
"code",
"markdown",
"code",
"markdown",
"code",
"markdown",
"code",
"markdown",
"code",
"markdown",
"code",
"markdown",
"code",
"markdown",
"code",
"markdown",
"code",
"markdown",
"code",
"markdown",
"code",
"markdown",
"code",
"markdown",
"code",
"markdown",
"code",
"markdown",
"code",
"markdown",
"code",
"markdown",
"code",
"markdown",
"code",
"markdown",
"code",
"markdown"
] | [
[
"markdown",
"markdown",
"markdown",
"markdown",
"markdown",
"markdown",
"markdown",
"markdown"
],
[
"code"
],
[
"markdown",
"markdown",
"markdown",
"markdown"
],
[
"code"
],
[
"markdown",
"markdown",
"markdown"
],
[
"code"
],
[
"markdown",
"markdown",
"markdown"
],
[
"code"
],
[
"markdown",
"markdown"
],
[
"code"
],
[
"markdown",
"markdown",
"markdown"
],
[
"code"
],
[
"markdown",
"markdown"
],
[
"code"
],
[
"markdown",
"markdown"
],
[
"code"
],
[
"markdown",
"markdown",
"markdown",
"markdown",
"markdown",
"markdown"
],
[
"code",
"code",
"code"
],
[
"markdown"
],
[
"code",
"code",
"code"
],
[
"markdown"
],
[
"code"
],
[
"markdown",
"markdown",
"markdown",
"markdown",
"markdown"
],
[
"code",
"code",
"code"
],
[
"markdown",
"markdown"
],
[
"code",
"code",
"code",
"code"
],
[
"markdown",
"markdown"
],
[
"code"
],
[
"markdown"
],
[
"code",
"code"
],
[
"markdown"
],
[
"code"
],
[
"markdown",
"markdown"
],
[
"code"
],
[
"markdown"
],
[
"code"
],
[
"markdown"
],
[
"code"
],
[
"markdown",
"markdown",
"markdown"
],
[
"code"
],
[
"markdown"
],
[
"code"
],
[
"markdown",
"markdown"
],
[
"code"
],
[
"markdown"
],
[
"code"
],
[
"markdown"
],
[
"code",
"code"
],
[
"markdown"
],
[
"code",
"code",
"code",
"code"
],
[
"markdown",
"markdown"
],
[
"code"
],
[
"markdown",
"markdown"
],
[
"code"
],
[
"markdown",
"markdown",
"markdown",
"markdown",
"markdown"
],
[
"code"
],
[
"markdown"
],
[
"code"
],
[
"markdown"
],
[
"code"
],
[
"markdown"
],
[
"code",
"code"
],
[
"markdown"
],
[
"code",
"code"
],
[
"markdown",
"markdown"
],
[
"code"
],
[
"markdown",
"markdown"
],
[
"code"
],
[
"markdown"
],
[
"code"
],
[
"markdown",
"markdown"
],
[
"code"
],
[
"markdown"
],
[
"code"
],
[
"markdown"
],
[
"code"
],
[
"markdown"
],
[
"code"
],
[
"markdown"
],
[
"code",
"code"
],
[
"markdown"
],
[
"code"
],
[
"markdown",
"markdown",
"markdown"
],
[
"code"
],
[
"markdown",
"markdown"
],
[
"code"
],
[
"markdown",
"markdown"
],
[
"code"
],
[
"markdown",
"markdown",
"markdown",
"markdown",
"markdown",
"markdown"
]
] |
e769f188a6e94308c25ea2b88c06fb6f36e91ead | 144,514 | ipynb | Jupyter Notebook | check_correlation.ipynb | heros-lab/colaboratory | ad8312473422228ede8cb206de346f5a064b0e99 | [
"MIT"
] | null | null | null | check_correlation.ipynb | heros-lab/colaboratory | ad8312473422228ede8cb206de346f5a064b0e99 | [
"MIT"
] | null | null | null | check_correlation.ipynb | heros-lab/colaboratory | ad8312473422228ede8cb206de346f5a064b0e99 | [
"MIT"
] | null | null | null | 850.082353 | 139,226 | 0.947514 | [
[
[
"<a href=\"https://colab.research.google.com/github/heros-lab/colaboratory/blob/master/check_correlation.ipynb\" target=\"_parent\"><img src=\"https://colab.research.google.com/assets/colab-badge.svg\" alt=\"Open In Colab\"/></a>",
"_____no_output_____"
]
],
[
[
"from google.colab import drive\ndrive.mount('/content/drive')\n\nimport numpy as np\nimport pandas as pd\nimport matplotlib.pyplot as plt\n\nwork_path = \"/content/drive/My Drive/Colab Notebooks\"\n",
"_____no_output_____"
],
[
"free_x = pd.read_csv(f\"{work_path}/data/free_x.csv\")\nfree_y = pd.read_csv(f\"{work_path}/data/free_y.csv\")\n\nstep_x = pd.read_csv(f\"{work_path}/data/step_x.csv\")\nstep_y = pd.read_csv(f\"{work_path}/data/step_y.csv\")\n\nms1a_x = pd.read_csv(f\"{work_path}/data/ms1a_x.csv\")\nms1a_y = pd.read_csv(f\"{work_path}/data/ms1a_y.csv\")\n\nms2a_x = pd.read_csv(f\"{work_path}/data/ms2a_x.csv\")\nms2a_y = pd.read_csv(f\"{work_path}/data/ms2a_y.csv\")\n\nms3a_x = pd.read_csv(f\"{work_path}/data/ms3a_x.csv\")\nms3a_y = pd.read_csv(f\"{work_path}/data/ms3a_y.csv\")",
"_____no_output_____"
],
[
"label_x = list(free_x.columns)\nlabel_y = list(free_y.columns)\n\nlabel_corr_x = label_x[1:7]\nlabel_corr_y = label_y[1:]",
"_____no_output_____"
],
[
"df_free = free_x[label_corr_x].join(free_y[label_corr_y])\ndf_step = step_x[label_corr_x].join(step_y[label_corr_y])\ndf_ms1a = ms1a_x[label_corr_x].join(ms1a_y[label_corr_y])\ndf_ms2a = ms2a_x[label_corr_x].join(ms2a_y[label_corr_y])\ndf_ms3a = ms3a_x[label_corr_x].join(ms3a_y[label_corr_y])\n\ncorr_free = df_free.corr()[label_corr_x][6:]\ncorr_step = df_step.corr()[label_corr_x][6:]\ncorr_ms1a = df_ms1a.corr()[label_corr_x][6:]\ncorr_ms2a = df_ms2a.corr()[label_corr_x][6:]\ncorr_ms3a = df_ms3a.corr()[label_corr_x][6:]",
"_____no_output_____"
],
[
"fig, ax = plt.subplots(3, 2, figsize=(12,8), dpi=200)\nfig.subplots_adjust(hspace=0.35, wspace=0.12)\n\ncorr_free.abs().plot.bar(ax=ax[0,0], rot=0, legend=False, title=\"Free Response\")\ncorr_step.abs().plot.bar(ax=ax[0,1], rot=0, legend=False, title=\"Step Response\")\ncorr_ms1a.abs().plot.bar(ax=ax[1,0], rot=0, legend=False, title=\"M-Series1 Response\")\ncorr_ms2a.abs().plot.bar(ax=ax[1,1], rot=0, legend=False, title=\"M-Series2 Response\")\ncorr_ms3a.abs().plot.bar(ax=ax[2,0], rot=0, legend=False, title=\"M-Series3 Response\")\nax[1,1].legend(bbox_to_anchor=(1.2, 1.2), loc='center', fontsize=14)\n\nfor i in range(3):\n for j in range(2):\n ax[i,j].hlines([0.7], -1, 5, color=\"r\", linestyle=\":\")",
"_____no_output_____"
],
[
"",
"_____no_output_____"
]
]
] | [
"markdown",
"code"
] | [
[
"markdown"
],
[
"code",
"code",
"code",
"code",
"code",
"code"
]
] |
e769f7662e135477b38ab44157edfbabe78a76cd | 504,166 | ipynb | Jupyter Notebook | 07-happy-hotel/python/happy_hotel.ipynb | leslem/insight-data-challenges | 14c56d30663d7fef178b820d2128dbf4782c1200 | [
"MIT"
] | null | null | null | 07-happy-hotel/python/happy_hotel.ipynb | leslem/insight-data-challenges | 14c56d30663d7fef178b820d2128dbf4782c1200 | [
"MIT"
] | 1 | 2021-06-08T02:43:08.000Z | 2021-06-08T03:05:21.000Z | 07-happy-hotel/python/happy_hotel.ipynb | leslem/insight-data-challenges | 14c56d30663d7fef178b820d2128dbf4782c1200 | [
"MIT"
] | null | null | null | 42.299354 | 60,728 | 0.626318 | [
[
[
"# Planning",
"_____no_output_____"
],
[
"## Challenge",
"_____no_output_____"
],
[
"As a data scientist at a hotel chain, I'm trying to find out what customers are happy and unhappy with, based on reviews. I'd like to know the topics in each review and a score for the topic.",
"_____no_output_____"
],
[
"## Approach",
"_____no_output_____"
],
[
"- Use standard NLP techniques (tokenization, TF-IDF, etc.) to process the reviews\n- Use LDA to identify topics in the reviews for each hotel\n - Learn the topics from whole reviews\n - For each hotel, combine all of the reviews into a metareview\n - Use the fit LDA model to score the appropriateness of each topic for this hotel\n - Also across all hotels\n- Look at topics coming up in happy vs. unhappy reviews for each hotel",
"_____no_output_____"
],
[
"## Results\n",
"_____no_output_____"
],
[
"## Takeaways\n",
"_____no_output_____"
]
],
[
[
"import logging\nimport matplotlib.pyplot as plt\nimport numpy as np\nimport os\nimport pandas as pd\nimport plotly.express as px\nimport plotly.io as pio\nimport pyLDAvis # Has a warning on import\nimport pyLDAvis.sklearn\nimport pyLDAvis.gensim\nimport seaborn as sns\n\nfrom gensim.corpora.dictionary import Dictionary\nfrom gensim.models import LdaMulticore, Phrases, TfidfModel # Has a warning on import\nfrom gensim.parsing.preprocessing import STOPWORDS\nfrom IPython.display import display\nfrom nltk.corpus import stopwords # Has a warning on import\nfrom nltk.stem import WordNetLemmatizer, SnowballStemmer\nfrom nltk.tokenize import RegexpTokenizer\nfrom pprint import pprint\nfrom vaderSentiment.vaderSentiment import SentimentIntensityAnalyzer\n\n\nlemmatizer = WordNetLemmatizer()\nstemmer = SnowballStemmer(\"english\")\nregex_tokenizer = RegexpTokenizer(r'\\w+')\nvader_analyzer = SentimentIntensityAnalyzer()",
"unable to import 'smart_open.gcs', disabling that module\n"
],
[
"# Plot settings\nsns.set(style=\"whitegrid\", font_scale=1.10)\npio.templates.default = \"plotly_white\"",
"_____no_output_____"
],
[
"# Set random number seed for reproducibility\nnp.random.seed(48)",
"_____no_output_____"
],
[
"# Set logging level for gensim\nlogging.basicConfig(format='%(asctime)s : %(levelname)s : %(message)s', level=logging.DEBUG)",
"_____no_output_____"
],
[
"data_dir = '~/devel/insight-data-challenges/07-happy-hotel/data'\noutput_dir = '~/devel/insight-data-challenges/07-happy-hotel/output'",
"_____no_output_____"
]
],
[
[
"## Read in and clean the data",
"_____no_output_____"
],
[
"Before reading in all of the files I downloaded from the GDrive, I used `diff` to compare the files because they looked like they might be duplicates. \n\n```\ndiff hotel_happy_reviews\\ -\\ hotel_happy_reviews.csv hotel_happy_reviews\\ -\\ hotel_happy_reviews.csv.csv\ndiff hotel_happy_reviews\\ -\\ hotel_happy_reviews.csv hotel_happy_reviews(1)\\ -\\ hotel_happy_reviews.csv\n```\n\nThis indicated that three of the files were exact duplicates, leaving me with one file of happy reviews and one file of not happy reviews.\n```\nhotel_happy_reviews - hotel_happy_reviews.csv\nhotel_not_happy_reviews - hotel_not_happy_reviews.csv.csv\n```",
"_____no_output_____"
]
],
[
[
"happy_reviews = pd.read_csv(\n os.path.join(os.path.expanduser(data_dir), 'hotel_happy_reviews - hotel_happy_reviews.csv'),\n)\ndisplay(happy_reviews.info())\ndisplay(happy_reviews)\n\n# Name this bad_reviews so it's easier to distinguish\nbad_reviews = pd.read_csv(\n os.path.join(os.path.expanduser(data_dir), 'hotel_not_happy_reviews - hotel_not_happy_reviews.csv.csv'),\n)\ndisplay(bad_reviews.info())\ndisplay(bad_reviews)",
"<class 'pandas.core.frame.DataFrame'>\nRangeIndex: 26521 entries, 0 to 26520\nData columns (total 4 columns):\n # Column Non-Null Count Dtype \n--- ------ -------------- ----- \n 0 User_ID 26521 non-null object\n 1 Description 26521 non-null object\n 2 Is_Response 26521 non-null object\n 3 hotel_ID 26521 non-null int64 \ndtypes: int64(1), object(3)\nmemory usage: 828.9+ KB\n"
]
],
[
[
"### Check that the two dfs are formatted the same",
"_____no_output_____"
]
],
[
[
"assert happy_reviews.columns.to_list() == bad_reviews.columns.to_list()\nassert happy_reviews.dtypes.to_list() == bad_reviews.dtypes.to_list()",
"_____no_output_____"
]
],
[
[
"## Look at the data in detail",
"_____no_output_____"
]
],
[
[
"display(happy_reviews['hotel_ID'].value_counts())\ndisplay(happy_reviews['User_ID'].describe())\n\ndisplay(bad_reviews['hotel_ID'].value_counts())\ndisplay(bad_reviews['User_ID'].describe())",
"_____no_output_____"
]
],
[
[
"## Process review text",
"_____no_output_____"
],
[
"### Tokenize",
"_____no_output_____"
],
[
"Split the reviews up into individual words",
"_____no_output_____"
]
],
[
[
"def tokenize(review):\n '''Split review string into tokens; remove stop words.\n\n Returns: list of strings, one for each word in the review\n '''\n s = review.lower() # Make lowercase\n s = regex_tokenizer.tokenize(s) # Split into words and remove punctuation.\n s = [t for t in s if not t.isnumeric()] # Remove numbers but not words containing numbers.\n s = [t for t in s if len(t) > 2] # Remove 1- and 2-character tokens.\n # I found that the lemmatizer didn't work very well here - it needs a little more tuning to be useful.\n # For example, \"was\" and \"has\" were lemmatized to \"wa\" and \"ha\", which was counterproductive.\n s = [stemmer.stem(lemmatizer.lemmatize(t, pos='v')) for t in s] # Stem and lemmatize verbs\n s = [t for t in s if t not in STOPWORDS] # Remove stop words\n return s\n\n\nhappy_tokens = happy_reviews['Description'].apply(tokenize)\nbad_tokens = bad_reviews['Description'].apply(tokenize)\n\ndisplay(happy_tokens.head())\ndisplay(bad_tokens.head())\n\nall_tokens = happy_tokens.append(bad_tokens, ignore_index=True)",
"_____no_output_____"
]
],
[
[
"### Find bigrams and trigrams",
"_____no_output_____"
],
[
"Identify word pairs and triplets that are above a given count threshold across all reviews.",
"_____no_output_____"
]
],
[
[
"# Add bigrams to single tokens\nbigrammer = Phrases(all_tokens, min_count=20)\ntrigrammer = Phrases(bigrammer[all_tokens], min_count=20)\n\n# For bigrams and trigrams meeting the min and threshold, add them to the token lists.\nfor idx in range(len(all_tokens)):\n all_tokens.iloc[idx].extend([token for token in trigrammer[all_tokens.iloc[idx]]\n if '_' in token]) # Bigrams and trigrams are joined by underscores",
"2020-04-09 15:31:27,829 : INFO : collecting all words and their counts\n"
]
],
[
[
"### Remove rare and common tokens, and limit vocabulary",
"_____no_output_____"
]
],
[
[
"dictionary = Dictionary(all_tokens)\ndictionary.filter_extremes(no_below=30, no_above=0.5, keep_n=20000)\n\n# Look at the top 100 and bottom 100 tokens\n\ntemp = dictionary[0] # Initialize the dict\n\ntoken_counts = pd.DataFrame(np.array(\n [[token_id, dictionary.id2token[token_id], dictionary.cfs[token_id]]\n for token_id in dictionary.keys()\n if token_id in dictionary.cfs.keys() and token_id in dictionary.id2token.keys()\n ]\n), columns=['id', 'token', 'count'])\n\ntoken_counts['count'] = token_counts['count'].astype('int')\ntoken_counts['count'].describe()\ntoken_counts = token_counts.sort_values('count')\n\nplt.rcParams.update({'figure.figsize': (5, 3.5), 'figure.dpi': 200})\ntoken_counts['count'].head(5000).hist(bins=100)\nplt.suptitle(\"Counts for 5,000 least frequent included words\")\nplt.show()\ndisplay(token_counts.head(50))\n\nplt.rcParams.update({'figure.figsize': (5, 3.5), 'figure.dpi': 200})\ntoken_counts['count'].tail(1000).hist(bins=100)\nplt.suptitle(\"Counts for 1,000 most frequent included words\")\nplt.show()\ndisplay(token_counts.tail(50))",
"2020-04-09 15:31:55,317 : INFO : adding document #0 to Dictionary(0 unique tokens: [])\n"
],
[
"# Replace the split data with the data updated with phrases\ndisplay(happy_tokens.shape, bad_tokens.shape)\nhappy_tokens = all_tokens.iloc[:len(happy_tokens)].copy().reset_index(drop=True)\nbad_tokens = all_tokens.iloc[len(happy_tokens):].copy().reset_index(drop=True)\ndisplay(happy_tokens.shape, bad_tokens.shape)",
"_____no_output_____"
]
],
[
[
"### Look at two examples before and after preprocessing",
"_____no_output_____"
]
],
[
[
"happy_idx = np.random.randint(1, len(happy_tokens))\nbad_idx = np.random.randint(1, len(bad_tokens))\n\nprint('HAPPY before:')\ndisplay(happy_reviews['Description'].iloc[happy_idx])\nprint('HAPPY after:')\ndisplay(happy_tokens.iloc[happy_idx])\n\nprint('NOT HAPPY before:')\ndisplay(bad_reviews['Description'].iloc[bad_idx])\nprint('NOT HAPPY after:')\ndisplay(bad_tokens.iloc[bad_idx])",
"HAPPY before:\n"
]
],
[
[
"### Vectorize with Bag of Words and TF-IDF",
"_____no_output_____"
]
],
[
[
"bow_corpus = [dictionary.doc2bow(review) for review in all_tokens]\ntfidf_model = TfidfModel(bow_corpus)\ntfidf_corpus = tfidf_model[bow_corpus]\nprint('Number of unique tokens: {}'.format(len(dictionary)))\nprint('Number of documents: {}'.format(len(bow_corpus)))\nlen(tfidf_corpus)",
"2020-04-09 15:32:02,623 : INFO : collecting document frequencies\n"
]
],
[
[
"## LDA topic modeling",
"_____no_output_____"
]
],
[
[
"# Fit a single version of the LDA model.\nnum_topics = 10\nchunksize = 5000\npasses = 4\niterations = 200\neval_every = 1 # Evaluate convergence at the end\n\nid2word = dictionary.id2token\n\nlda_model = LdaMulticore(\n corpus=tfidf_corpus,\n id2word=id2word,\n chunksize=chunksize,\n alpha='symmetric',\n eta='auto',\n iterations=iterations,\n num_topics=num_topics,\n passes=passes,\n eval_every=eval_every,\n workers=4 # Use all four cores\n)\n\ntop_topics = lda_model.top_topics(tfidf_corpus)\npprint(top_topics)",
"2020-04-09 15:32:02,990 : INFO : using symmetric alpha at 0.1\n"
]
],
[
[
"Gensim calculates the [intrinsic coherence score](http://qpleple.com/topic-coherence-to-evaluate-topic-models/) for\neach topic. By averaging across all of the topics in the model you can get an average coherence score. Coherence\nis a measure of the strength of the association between words in a topic cluster. It is supposed to be an objective\nway to evaluate the quailty of the topic clusters. Higher scores are better.",
"_____no_output_____"
]
],
[
[
"# Average topic coherence is the sum of topic coherences of all topics, divided by the number of topics.\navg_topic_coherence = sum([t[1] for t in top_topics]) / num_topics\nprint('Average topic coherence: %.4f.' % avg_topic_coherence)",
"Average topic coherence: -1.2838.\n"
]
],
[
[
"References:\n- https://radimrehurek.com/gensim/auto_examples/tutorials/run_lda.html#sphx-glr-auto-examples-tutorials-run-lda-py\n- https://towardsdatascience.com/topic-modeling-and-latent-dirichlet-allocation-in-python-9bf156893c24",
"_____no_output_____"
]
],
[
[
"# This code is used to run the .py script from beginning to end in the python interpreter\n# with open('python/happy_hotel.py', 'r') as f:\n# exec(f.read())\n\n# plt.close('all')",
"_____no_output_____"
]
]
] | [
"markdown",
"code",
"markdown",
"code",
"markdown",
"code",
"markdown",
"code",
"markdown",
"code",
"markdown",
"code",
"markdown",
"code",
"markdown",
"code",
"markdown",
"code",
"markdown",
"code",
"markdown",
"code",
"markdown",
"code"
] | [
[
"markdown",
"markdown",
"markdown",
"markdown",
"markdown",
"markdown",
"markdown"
],
[
"code",
"code",
"code",
"code",
"code"
],
[
"markdown",
"markdown"
],
[
"code"
],
[
"markdown"
],
[
"code"
],
[
"markdown"
],
[
"code"
],
[
"markdown",
"markdown",
"markdown"
],
[
"code"
],
[
"markdown",
"markdown"
],
[
"code"
],
[
"markdown"
],
[
"code",
"code"
],
[
"markdown"
],
[
"code"
],
[
"markdown"
],
[
"code"
],
[
"markdown"
],
[
"code"
],
[
"markdown"
],
[
"code"
],
[
"markdown"
],
[
"code"
]
] |
e769f90bae8956859ee266d50c5e600d501f7e34 | 15,647 | ipynb | Jupyter Notebook | notebooks/19-content-recommender.ipynb | sujitpal/content-engineering-tutorial | 7acb6ca846753426638bc3c29d90ce450a9e27bb | [
"Apache-2.0"
] | 6 | 2018-09-20T15:43:12.000Z | 2020-08-09T10:22:23.000Z | notebooks/19-content-recommender.ipynb | sujitpal/content-engineering-tutorial | 7acb6ca846753426638bc3c29d90ce450a9e27bb | [
"Apache-2.0"
] | null | null | null | notebooks/19-content-recommender.ipynb | sujitpal/content-engineering-tutorial | 7acb6ca846753426638bc3c29d90ce450a9e27bb | [
"Apache-2.0"
] | 1 | 2019-02-15T15:41:32.000Z | 2019-02-15T15:41:32.000Z | 35.400452 | 370 | 0.571356 | [
[
[
"# Recommendations via Dimensionality Reduction\n\nAll the content discovery approaches we have explored in previous notebooks can be used to do content recommendations. Here we explore yet another approach to do that, but instead of considering a single article as input, we will look at situations where we know that a user has read a set of articles and he is looking for recommendations on what to read next.\n\nSince we have already extracted the authors, orgs and keywords for each article, we can now construct a bipartite graph between author and article, orgs and article and keywords and article, which gives us the basis for a recommender.",
"_____no_output_____"
]
],
[
[
"from sklearn.decomposition import NMF\nimport joblib\nimport json\nimport numpy as np\nimport os\nimport requests\nimport urllib",
"_____no_output_____"
],
[
"DATA_DIR = \"../data\"\nMODEL_DIR = \"../models\"\n\nSOLR_URL = \"http://localhost:8983/solr/nips2index\"\nFEATURES_DUMP_FILE = os.path.join(DATA_DIR, \"comb-features.tsv\")\n\nNMF_MODEL_FILE = os.path.join(MODEL_DIR, \"recommender-nmf.pkl\")\n\nPAPERS_METADATA = os.path.join(DATA_DIR, \"papers_metadata.tsv\")",
"_____no_output_____"
]
],
[
[
"## Extract features from index",
"_____no_output_____"
]
],
[
[
"query_string = \"*:*\"\nfield_list = \"id,keywords,authors,orgs\"\ncursor_mark = \"*\"\nnum_docs, num_keywords = 0, 0\ndoc_keyword_pairs = []\n\nfdump = open(FEATURES_DUMP_FILE, \"w\")\nall_keywords, all_authors, all_orgs = set(), set(), set()\n\nwhile True:\n if num_docs % 1000 == 0:\n print(\"{:d} documents ({:d} keywords, {:d} authors, {:d} orgs) retrieved\"\n .format(num_docs, len(all_keywords), len(all_authors), len(all_orgs)))\n payload = {\n \"q\": query_string,\n \"fl\": field_list,\n \"sort\": \"id asc\",\n \"rows\": 100,\n \"cursorMark\": cursor_mark\n }\n params = urllib.parse.urlencode(payload, quote_via=urllib.parse.quote_plus)\n search_url = SOLR_URL + \"/select?\" + params\n resp = requests.get(search_url)\n resp_json = json.loads(resp.text)\n docs = resp_json[\"response\"][\"docs\"]\n \n docs_retrieved = 0\n for doc in docs:\n doc_id = int(doc[\"id\"])\n keywords, authors, orgs = [\"NA\"], [\"NA\"], [\"NA\"]\n if \"keywords\" in doc.keys():\n keywords = doc[\"keywords\"]\n all_keywords.update(keywords)\n if \"authors\" in doc.keys():\n authors = doc[\"authors\"]\n all_authors.update(authors)\n if \"orgs\" in doc.keys():\n orgs = doc[\"orgs\"]\n all_orgs.update(orgs)\n fdump.write(\"{:d}\\t{:s}\\t{:s}\\t{:s}\\n\"\n .format(doc_id, \"|\".join(keywords), \"|\".join(authors), \n \"|\".join(orgs)))\n num_docs += 1\n docs_retrieved += 1\n if docs_retrieved == 0:\n break\n\n # for next batch of ${rows} rows\n cursor_mark = resp_json[\"nextCursorMark\"]\n\nprint(\"{:d} documents ({:d} keywords, {:d} authors, {:d} orgs) retrieved, COMPLETE\"\n .format(num_docs, len(all_keywords), len(all_authors), len(all_orgs)))\nfdump.close()",
"0 documents (0 keywords, 0 authors, 0 orgs) retrieved\n1000 documents (1628 keywords, 1347 authors, 159 orgs) retrieved\n2000 documents (1756 keywords, 2601 authors, 214 orgs) retrieved\n3000 documents (1814 keywords, 3948 authors, 269 orgs) retrieved\n4000 documents (1833 keywords, 5210 authors, 311 orgs) retrieved\n5000 documents (1842 keywords, 6537 authors, 350 orgs) retrieved\n6000 documents (1847 keywords, 7983 authors, 385 orgs) retrieved\n7000 documents (1847 keywords, 9517 authors, 420 orgs) retrieved\n7238 documents (1847 keywords, 9719 authors, 426 orgs) retrieved, COMPLETE\n"
]
],
[
[
"## Build sparse feature vector for documents\n\nThe feature vector for each document will consist of a sparse vector of size 11992 (1847+9719+426). An entry is 1 if the item occurs in the document, 0 otherwise.",
"_____no_output_____"
]
],
[
[
"def build_lookup_table(item_set):\n item2idx = {}\n for idx, item in enumerate(item_set):\n item2idx[item] = idx\n return item2idx\n\nkeyword2idx = build_lookup_table(all_keywords)\nauthor2idx = build_lookup_table(all_authors)\norg2idx = build_lookup_table(all_orgs)\nprint(len(keyword2idx), len(author2idx), len(org2idx))",
"1847 9719 426\n"
],
[
"def build_feature_vector(items, item2idx):\n vec = np.zeros((len(item2idx)))\n if items == \"NA\":\n return vec\n for item in items.split(\"|\"):\n idx = item2idx[item]\n vec[idx] = 1\n return vec\n\n\nXk = np.zeros((num_docs, len(keyword2idx)))\nXa = np.zeros((num_docs, len(author2idx)))\nXo = np.zeros((num_docs, len(org2idx)))\n\nfdump = open(FEATURES_DUMP_FILE, \"r\")\nfor line in fdump:\n doc_id, keywords, authors, orgs = line.strip().split(\"\\t\")\n doc_id = int(doc_id)\n Xk[doc_id] = build_feature_vector(keywords, keyword2idx)\n Xa[doc_id] = build_feature_vector(authors, author2idx)\n Xo[doc_id] = build_feature_vector(orgs, org2idx)\nfdump.close() \n\nX = np.concatenate((Xk, Xa, Xo), axis=1)\nprint(X.shape)\nprint(X)",
"(7238, 11992)\n[[0. 0. 0. ... 0. 0. 0.]\n [0. 0. 0. ... 0. 0. 0.]\n [0. 0. 0. ... 0. 0. 0.]\n ...\n [0. 0. 0. ... 0. 0. 0.]\n [0. 0. 0. ... 0. 0. 0.]\n [0. 0. 0. ... 0. 0. 0.]]\n"
]
],
[
[
"## Reduce dimensionality\n\nWe reduce the sparse feature vector to a lower dimensional dense vector which effectively maps the original vector to a new \"taste\" vector space. Topic modeling has the same effect. We will use non-negative matrix factorization.\n\nIdea here is to factorize the input matrix X into two smaller matrices W and H, which can be multiplied back together with minimal reconstruction error. The training phase will try to minimize the reconstruction error.\n\n$$X = WH \\approx X$$\n\nThe W matrix can be used as a reduced denser proxy for X.",
"_____no_output_____"
]
],
[
[
"if os.path.exists(NMF_MODEL_FILE):\n print(\"model already generated, loading\")\n model = joblib.load(NMF_MODEL_FILE)\n W = model.transform(X)\n H = model.components_\nelse: \n model = NMF(n_components=150, init='random', solver=\"cd\", \n verbose=True, random_state=42)\n W = model.fit_transform(X)\n H = model.components_\n joblib.dump(model, NMF_MODEL_FILE)\n \nprint(W.shape, H.shape)",
"model already generated, loading\nviolation: 1.0\nviolation: 0.2411207712867099\nviolation: 0.0225518954481444\nviolation: 0.00395945567371017\nviolation: 0.0004979448419219516\nviolation: 8.176770536033433e-05\nConverged at iteration 6\n(7238, 150) (150, 11992)\n"
]
],
[
[
"## Similar Documents",
"_____no_output_____"
]
],
[
[
"sim = np.matmul(W, np.transpose(W))\nprint(sim.shape)",
"(7238, 7238)\n"
],
[
"def similar_docs(filename, sim, topn):\n doc_id = int(filename.split(\".\")[0])\n row = sim[doc_id, :]\n target_docs = np.argsort(-row)[0:topn].tolist()\n scores = row[target_docs].tolist()\n target_filenames = [\"{:d}.txt\".format(x) for x in target_docs]\n return target_filenames, scores\n \n\nfilename2title = {}\nwith open(PAPERS_METADATA, \"r\") as f:\n for line in f:\n if line.startswith(\"#\"):\n continue\n cols = line.strip().split(\"\\t\")\n filename2title[\"{:s}.txt\".format(cols[0])] = cols[2]\n\nsource_filename = \"1032.txt\"\ntop_n = 10\ntarget_filenames, scores = similar_docs(source_filename, sim, top_n)\nprint(\"Source: {:s}\".format(filename2title[source_filename]))\nprint(\"--- top {:d} similar docs ---\".format(top_n))\nfor target_filename, score in zip(target_filenames, scores):\n if target_filename == source_filename:\n continue\n print(\"({:.5f}) {:s}\".format(score, filename2title[target_filename]))",
"Source: Forward-backward retraining of recurrent neural networks\n--- top 10 similar docs ---\n(0.05010) Context-Dependent Multiple Distribution Phonetic Modeling with MLPs\n(0.04715) Is Learning The n-th Thing Any Easier Than Learning The First?\n(0.04123) Learning Statistically Neutral Tasks without Expert Guidance\n(0.04110) Combining Visual and Acoustic Speech Signals with a Neural Network Improves Intelligibility\n(0.04087) The Ni1000: High Speed Parallel VLSI for Implementing Multilayer Perceptrons\n(0.04038) Subset Selection and Summarization in Sequential Data\n(0.04003) Back Propagation is Sensitive to Initial Conditions\n(0.03939) Semi-Supervised Multitask Learning\n(0.03862) SoundNet: Learning Sound Representations from Unlabeled Video\n"
]
],
[
[
"## Suggesting Documents based on Read Collection\n\nWe consider an arbitary set of documents that we know a user has read or liked or marked somehow, and we want to recommend other documents that he may like.\n\nTo do this, we compute the average feature among these documents (starting from the sparse features) convert it to a average dense feature vector, then find the most similar compared to that one.",
"_____no_output_____"
]
],
[
[
"collection_size = np.random.randint(3, high=10, size=1)[0]\ncollection_ids = np.random.randint(0, high=num_docs+1, size=collection_size)\n\nfeat_vec = np.zeros((1, 11992))\nfor collection_id in collection_ids:\n feat_vec += X[collection_id, :]\nfeat_vec /= collection_size\ny = model.transform(feat_vec)\ndoc_sims = np.matmul(W, np.transpose(y)).squeeze(axis=1)\ntarget_ids = np.argsort(-doc_sims)[0:top_n]\nscores = doc_sims[target_ids]\n\nprint(\"--- Source collection ---\")\nfor collection_id in collection_ids:\n print(\"{:s}\".format(filename2title[\"{:d}.txt\".format(collection_id)]))\nprint(\"--- Recommendations ---\")\nfor target_id, score in zip(target_ids, scores):\n print(\"({:.5f}) {:s}\".format(score, filename2title[\"{:d}.txt\".format(target_id)]))",
"violation: 1.0\nviolation: 0.23129634545431624\nviolation: 0.03209572604136983\nviolation: 0.007400997221153011\nviolation: 0.0012999049199094925\nviolation: 0.0001959522250959198\nviolation: 4.179248920879007e-05\nConverged at iteration 7\n--- Source collection ---\nA Generic Approach for Identification of Event Related Brain Potentials via a Competitive Neural Network Structure\nImplicit Surfaces with Globally Regularised and Compactly Supported Basis Functions\nLearning Trajectory Preferences for Manipulators via Iterative Improvement\nStatistical Modeling of Cell Assemblies Activities in Associative Cortex of Behaving Monkeys\nLearning to Traverse Image Manifolds\n--- Recommendations ---\n(0.06628) Fast Second Order Stochastic Backpropagation for Variational Inference\n(0.06128) Scalable Model Selection for Belief Networks\n(0.05793) Large Margin Discriminant Dimensionality Reduction in Prediction Space\n(0.05643) Efficient Globally Convergent Stochastic Optimization for Canonical Correlation Analysis\n(0.05629) Recognizing Activities by Attribute Dynamics\n(0.05622) Efficient Match Kernel between Sets of Features for Visual Recognition\n(0.05565) Learning Wake-Sleep Recurrent Attention Models\n(0.05466) Boosting Density Estimation\n(0.05422) Sparse deep belief net model for visual area V2\n(0.05350) Cluster Kernels for Semi-Supervised Learning\n"
]
]
] | [
"markdown",
"code",
"markdown",
"code",
"markdown",
"code",
"markdown",
"code",
"markdown",
"code",
"markdown",
"code"
] | [
[
"markdown"
],
[
"code",
"code"
],
[
"markdown"
],
[
"code"
],
[
"markdown"
],
[
"code",
"code"
],
[
"markdown"
],
[
"code"
],
[
"markdown"
],
[
"code",
"code"
],
[
"markdown"
],
[
"code"
]
] |
e76a0bf634118b3156f662fad445baa74f42b0c4 | 38,333 | ipynb | Jupyter Notebook | VapourSynthColab.ipynb | 03stevensmi/VapourSynthColab | 4bddbf9f2c920cef280a5167da5ae6da7a2afa2b | [
"MIT"
] | 1 | 2021-07-19T16:36:11.000Z | 2021-07-19T16:36:11.000Z | VapourSynthColab.ipynb | 03stevensmi/VapourSynthColab | 4bddbf9f2c920cef280a5167da5ae6da7a2afa2b | [
"MIT"
] | null | null | null | VapourSynthColab.ipynb | 03stevensmi/VapourSynthColab | 4bddbf9f2c920cef280a5167da5ae6da7a2afa2b | [
"MIT"
] | null | null | null | 43.363122 | 246 | 0.539353 | [
[
[
"<a href=\"https://colab.research.google.com/github/03stevensmi/VapourSynthColab/blob/master/VapourSynthColab.ipynb\" target=\"_parent\"><img src=\"https://colab.research.google.com/assets/colab-badge.svg\" alt=\"Open In Colab\"/></a>",
"_____no_output_____"
],
[
"Welcome to VapourSynth in Colab!\n\nBasic usage instructions: run the setup script, and run all the tabs in the \"processing\" script for example output.\n\nFor links to instructions, tutorials, and help, see https://github.com/AlphaAtlas/VapourSynthColab",
"_____no_output_____"
],
[
"# Init\n",
"_____no_output_____"
]
],
[
[
"#@title Check GPU\n#@markdown Run this to connect to a Colab Instance, and see what GPU Google gave you.\n\ngpu = !nvidia-smi --query-gpu=gpu_name --format=csv\nprint(gpu[1])\nprint(\"The Tesla T4 and P100 are fast and support hardware encoding. The K80 and P4 are slower.\")\nprint(\"Sometimes resetting the instance in the 'runtime' tab will give you a different GPU.\")",
"_____no_output_____"
],
[
"!apt-get install python3-distutils\n!apt-get install python3-apt\n\n!wget https://www.python.org/ftp/python/3.6.3/Python-3.6.3.tgz\n!tar -xvf Python-3.6.3.tgz\n%cd Python-3.6.3\n!sudo ./configure --enable-optimizations\n%cd /content/\n!rm -rfv \"./Python-3.6.3\"\n!rm -rfv \"./Python-3.6.3.tgz\"\n\n!wget https://bootstrap.pypa.io/get-pip.py\n!sudo python3.6 get-pip.py\n!!rm -rfv \"./get-pip.py\"\n",
"_____no_output_____"
],
[
"!pip install torch\n!pip install cupy-cuda101",
"_____no_output_____"
],
[
"%cd /content/\n!wget http://lliurex.net/bionic/pool/universe/f/ffmpeg/ffmpeg_3.4.2-2_amd64.deb\n!sudo apt install ./ffmpeg_3.4.2-2_amd64.deb\n!rm ./ffmpeg_3.4.2-2_amd64.deb",
"_____no_output_____"
],
[
"#@title Setup {display-mode: \"form\"}\n#@markdown Run this to install VapourSynth, VapourSynth plugins and scripts, as well as some example upscaling models.\n#NOTE: running this more than once may or may not work. \n#The buggy console output is due to the threaded installing\n#Currently TPU support is broken and incomplete, but it isn't particularly useful since it doesn't support opencl anyway \n\n#Init\nimport os, sys, shutil, tempfile\nimport collections\nfrom datetime import datetime, timedelta\nimport requests\nimport threading\nimport ipywidgets as widgets\nfrom IPython import display\nimport PIL\nfrom google.colab import files\nimport time\n%cd /\n\n\n#Function defs\n#---------------------------------------------------------\n\n#Like shutil.copytree(), but doesn't complain about existing directories\n#Note this is fixed in newer version of Python 3\ndef copytree(src, dst, symlinks=False, ignore=None):\n for item in os.listdir(src):\n s = os.path.join(src, item)\n d = os.path.join(dst, item)\n if os.path.isdir(s):\n shutil.copytree(s, d, symlinks, ignore)\n else:\n shutil.copy2(s, d)\n\n#Download and extract the .py scripts from the VapourSynth fatpack\ndef download_fatpack_scripts():\n %cd /\n print(\"Downloading VS FatPack Scripts...\")\n dlurl = r\"https://github.com/theChaosCoder/vapoursynth-portable-FATPACK/releases/download/r3/VapourSynth64Portable_2019_11_02.7z\"\n with tempfile.TemporaryDirectory() as t:\n dpath = os.path.join(t, \"VapourSynth64Portable_2019_11_02.7z\")\n os.chdir(t)\n !wget {dlurl}\n %cd /\n !7z x -o{t} {dpath}\n scriptsd = os.path.abspath(os.path.join(t, \"VapourSynth64Portable\", \"Scripts\"))\n s = os.path.normpath(\"VapourSynthImports\")\n os.makedirs(s, exist_ok = True)\n copytree(scriptsd, s)\n sys.path.append(s)\n \n #Get some additional scripts.\n !wget -O /VapourSynthImports/muvsfunc_numpy.py https://raw.githubusercontent.com/WolframRhodium/muvsfunc/master/Collections/muvsfunc_numpy.py\n !wget -O /VapourSynthImports/edi_rpow2.py https://gist.githubusercontent.com/YamashitaRen/020c497524e794779d9c/raw/2a20385e50804f8b24f2a2479e2c0f3c335d4853/edi_rpow2.py\n !wget -O /VapourSynthImports/BMToolkit.py https://raw.githubusercontent.com/IFeelBloated/BlockMatchingToolkit/master/BMToolkit.py\n if accelerator == \"CUDA\":\n !wget -O /VapourSynthImports/Alpha_CuPy.py https://raw.githubusercontent.com/AlphaAtlas/VapourSynth-Super-Resolution-Helper/master/Scripts/Alpha_CuPy.py\n !wget -O /VapourSynthImports/dpid.cu https://raw.githubusercontent.com/WolframRhodium/muvsfunc/master/Collections/examples/Dpid_cupy/dpid.cu\n !wget -O /VapourSynthImports/bilateral.cu https://raw.githubusercontent.com/WolframRhodium/muvsfunc/master/Collections/examples/BilateralGPU_cupy/bilateral.cu\n\n #Get an example model:\n import gdown\n gdown.download(r\"https://drive.google.com/uc?id=1KToK9mOz05wgxeMaWj9XFLOE4cnvo40D\", \"/content/4X_Box.pth\", quiet=False)\n\ndef getdep1():\n %cd /\n #Install apt-fast, for faster installing\n !/bin/bash -c \"$(curl -sL https://git.io/vokNn)\"\n #Get some basic dependancies\n !apt-fast install -y -q -q subversion davfs2 p7zip-full p7zip-rar ninja-build \n\n#Get VapourSynth and ImageMagick built just for a colab environment\ndef getvs():\n %cd /\n #%cd var/cache/apt/archives\n #Artifacts hosted on bintray. If they fail to install, they can be built from source. \n !curl -L \"https://github.com/03stevensmi/VapourSynthColab/raw/master/imagemagick_7.0.9-8-1_amd64.deb\" -o /var/cache/apt/archives/imagemagick.deb\n !dpkg -i /var/cache/apt/archives/imagemagick.deb\n !ldconfig /usr/local/lib\n !curl -L \"https://github.com/03stevensmi/VapourSynthColab/raw/master/vapoursynth_48-1_amd64.deb\" -o /var/cache/apt/archives/vapoursynth.deb\n !dpkg -i /var/cache/apt/archives/vapoursynth.deb\n !ldconfig /usr/local/lib\n #%cd /\n\ndef getvsplugins():\n %cd /\n #Allow unauthenticated sources\n if not os.path.isfile(\"/etc/apt/apt.conf.d/99myown\"):\n with open(\"/etc/apt/apt.conf.d/99myown\", \"w+\") as f:\n f.write(r'APT::Get::AllowUnauthenticated \"true\";')\n sources = \"/etc/apt/sources.list\"\n #Backup original apt sources file, just in case\n with tempfile.TemporaryDirectory() as t:\n tsources = os.path.join(t, os.path.basename(sources))\n shutil.copy(sources, tsources)\n #Add deb-multimedia repo\n #Because building dozens of VS plugins is not fun, and takes forever\n with open(sources, \"a+\") as f:\n deb = \"deb https://www.deb-multimedia.org sid main non-free\\n\"\n if not \"deb-multimedia\" in f.read():\n f.write(deb)\n\n with open(sources, \"a+\") as f:\n #Temporarily use Debian unstable for some required dependencies \n if not \"ftp.us.debian.org\" in f.read():\n f.write(\"deb http://ftp.us.debian.org/debian/ sid main\\n\")\n !add-apt-repository -y ppa:deadsnakes/ppa\n !apt-fast update -oAcquire::AllowInsecureRepositories=true\n !apt-fast install -y --allow-unauthenticated deb-multimedia-keyring\n !apt-fast update \n\n #Parse plugins to install\n out = !apt-cache search vapoursynth\n vspackages = \"\"\n #exclude packages with these strings in the name\n exclude = [\"waifu\", \"wobbly\", \"editor\", \"dctfilter\", \"vapoursynth-dev\", \"vapoursynth-doc\"]\n for line in out:\n p = line.split(\" - \")[0].strip()\n if not any(x in p for x in exclude) and \"vapoursynth\" in p and p != \"vapoursynth\":\n vspackages = vspackages + p + \" \"\n print(vspackages)\n #Install VS plugins and a newer ffmpeg build\n !apt-fast install -y --allow-unauthenticated --no-install-recommends ffmpeg youtube-dl libzimg-dev {vspackages} libfftw3-3 libfftw3-double3 libfftw3-dev libfftw3-bin libfftw3-double3 libfftw3-single3 checkinstall\n #Get a tiny example video\n !youtube-dl -o /content/enhance.webm -f 278 https://www.youtube.com/watch?v=I_8ZH1Ggjk0\n #Restore original sources\n os.remove(sources)\n shutil.copy(tsources, sources)\n #Congrats! Apt may or may not be borked.\n copytree(\"/usr/lib/x86_64-linux-gnu/vapoursynth\", \"/usr/local/lib/vapoursynth\")\n !ldconfig /usr/local/lib/vapoursynth\n\n#Install vapoursynth python modules\ndef getpythonstuff():\n %cd /\n !python3.6 -m pip install vapoursynth meson opencv-python\n\ndef cudastuff():\n %cd /\n out = !nvcc --version\n cudaver = (str(out).split(\"Cuda compilation tools, release \")[1].split(\", \")[0].replace(\".\", \"\"))\n #Note this download sometimes times out\n !python3.6 -m pip install mxnet-cu{cudaver} #cupy-cuda{cudaver}\n !pip install git+https://github.com/AlphaAtlas/VSGAN.git\n\n #Mxnet stuff\n \n modelurl = \"https://github.com/WolframRhodium/Super-Resolution-Zoo/trunk\"\n if os.path.isdir(\"/NeuralNetworks\"):\n !svn update --set-depth immediates /NeuralNetworks\n !svn update --set-depth infinity /NeuralNetworks/ARAN\n else:\n !svn checkout --depth immediates {modelurl} /NeuralNetworks\n\ndef makesrcd(name):\n %cd /\n srpath = os.path.abspath(os.path.join(\"/src\", name))\n os.makedirs(srpath, exist_ok = False)\n %cd {srpath}\n\ndef mesongit(giturl):\n p = os.path.basename(giturl)[:-4]\n makesrcd(p)\n !git clone {giturl}\n %cd {p}\n !meson build\n !ninja -C build\n !ninja -C build install\n\n#Taken from https://stackoverflow.com/a/31614591\n#Allows exceptions to be caught from threads\nfrom threading import Thread\n\nclass PropagatingThread(Thread):\n def run(self):\n self.exc = None\n try:\n if hasattr(self, '_Thread__target'):\n # Thread uses name mangling prior to Python 3.\n self.ret = self._Thread__target(*self._Thread__args, **self._Thread__kwargs)\n else:\n self.ret = self._target(*self._args, **self._kwargs)\n except BaseException as e:\n self.exc = e\n\n def join(self):\n super(PropagatingThread, self).join()\n if self.exc:\n raise self.exc\n return self.ret\n\n\n#Interpolation experiment\n#%cd /\n#os.makedirs(\"/videotools\")\n#%cd /videotools\n#!git clone https://github.com/sniklaus/pytorch-sepconv.git\n#%cd /\n\n#Function for testing vapoursynth scripts\n#Takes the path of the script, and a boolean for generating a test frame.\n\n#-----------------------------------------------------------\n\n#Init functions are threaded for speed\n#\"PropagatingThread\" class is used to return exceptions from threads, otherwise they fail silently\n\nt1 = PropagatingThread(target = getdep1)\nt1.start()\nprint(\"apt init thread started\")\n\nt2 = PropagatingThread(target = download_fatpack_scripts)\nt2.start()\nprint(\"VS script downloader thread started.\")\n\n#Get rid of memory usage log spam from MXnet\nos.environ[\"TCMALLOC_LARGE_ALLOC_REPORT_THRESHOLD\"] = \"107374182400\"\n\n#Check for an accelerator\naccelerator = None\ngpu = None\nif 'COLAB_TPU_ADDR' in os.environ:\n #WIP\n raise Exception(\"TPUs are (currently) not supported! Please use a GPU or CPU instance.\")\nelse:\n #Check for Nvidia GPU, and identify it \n out = !command -v nvidia-smi\n if out != []:\n out = !nvidia-smi\n for l in out:\n if \"Driver Version\" in l:\n accelerator = \"CUDA\"\n print(\"Nvidia GPU detected:\")\n gpu = !nvidia-smi --query-gpu=gpu_name --format=csv\n gpu = gpu[1]\n #print(\"Tesla K80 < Tesla T4 < Tesla P100\")\n break\nif accelerator == None:\n print(\"Warning: No Accelerator Detected!\")\n\nt1.join()\nprint(\"Apt init thread done.\")\n\nt1 = PropagatingThread(target = getvs)\nt1.start()\nprint(\"Vapoursynth/Imagemagick downloader thread started.\")\nt1.join()\nprint(\"Vapoursynth/Imagemagick installed\")\n\nt3 = PropagatingThread(target = getpythonstuff)\nt3.start()\nprint(\"Pip thread started\")\n\nt1 = PropagatingThread(target = getvsplugins)\nt1.start()\nprint(\"VS plugin downloader thread started.\")\n\nt3.join()\nprint(\"pip thread done\")\n\nif accelerator == \"TPU\":\n #WIP!\n pass\n\nelif accelerator == \"CUDA\":\n t3 = PropagatingThread(target = cudastuff)\n t3.start()\n print(\"CUDA pip thread started.\")\nelse:\n pass\n\nt2.join()\nprint(\"VS script downloader thread done.\")\n\nt3.join()\nprint(\"CUDA pip thread done.\")\n\nt1.join()\nprint(\"VS plugin thread done.\")\n\n\n\n#Build some more plugins(s)\n#TODO: Build without changing working directory, or try the multiprocessing module, so building can run asynchronously \nprint(\"Building additional plugins\")\nmesongit(r\"https://github.com/HomeOfVapourSynthEvolution/VapourSynth-DCTFilter.git\")\nmesongit(r\"https://github.com/HomeOfVapourSynthEvolution/VapourSynth-TTempSmooth.git\")\n\ngoogpath = None\n%cd /\n\nClear_Console_Output_When_Done = True #@param {type:\"boolean\"}\nif Clear_Console_Output_When_Done:\n display.clear_output()\n#if gpu is not None:\n# print(gpu[1])\n# print(\"A Tesla T4 or P100 is significantly faster than a K80\")\n# print(\"And the K80 doesn't support hardware encoding.\")\n",
"_____no_output_____"
],
[
"#@title Mount Google Drive\n#@markdown Highly recommended!\n\nimport os\n%cd /\n\n#Check if Google Drive is mounted, and mount if its not.\ngoogpath = os.path.abspath(os.path.join(\"gdrive\", \"MyDrive\"))\nif not os.path.isdir(googpath):\n from google.colab import drive\n drive.mount('/gdrive', force_remount=True)",
"_____no_output_____"
],
[
"#@title Mount a Nextcloud Drive\n\nimport os\nnextcloud = \"/nextcloud\"\nos.makedirs(nextcloud, exist_ok=True)\nNextcloud_URL = \"https://us.hostiso.cloud/remote.php/webdav/\" #@param {type:\"string\"}\n\n%cd /\nif os.path.isfile(\"/etc/fstab\"):\n os.remove(\"/etc/fstab\")\nwith open(\"/etc/fstab\" , \"a\") as f:\n f.write(Nextcloud_URL + \" \" + nextcloud + \" davfs user,rw,auto 0 0\")\n!mount {nextcloud}",
"_____no_output_____"
]
],
[
[
"# Processing",
"_____no_output_____"
],
[
"",
"_____no_output_____"
]
],
[
[
"%%writefile /content/autogenerated.vpy\n\n#This is the Vapoursynth Script!\n#Running this cell will write the code in this cell to disk, for VSPipe to read.\n#Later cells will check to see if it executes.\n#Edit it just like a regular python VS script.\n#Search for functions and function reference in http://vsdb.top/, or browse the \"VapourSynthImports\" folder. \n\n#Import functions\nimport sys, os, cv2\nsys.path.append('/VapourSynthImports')\nimport vapoursynth as vs\nimport vsgan as VSGAN\nimport mvsfunc as mvf\n#import muvsfunc as muf\n#import fvsfunc as fvf\nimport havsfunc as haf\nimport Alpha_CuPy as ape\nimport muvsfunc_numpy as mufnp\n#import BMToolkit as bm\nimport G41Fun as G41\n#import vsutil as util\n#import edi_rpow2 as edi\n#import kagefunc as kage\n#import lostfunc as lost\n#import vsTAAmbk as taa\n#import xvs as xvs\nfrom vapoursynth import core\n\n#Set RAM cache size, in MB\ncore.max_cache_size = 10500\n\n#Get Video(s) or Image(s). ffms2 (ffmpeg) or imwri (imagemagick) will read just about anything.\n#Lsmash sometimes works if ffms2 failes, d2v reads mpeg2 files\nclip = core.ffms2.Source(r\"/content/enhance.webm\")\n#clip = core.lsmas.LWLibavSource(\"/tmp/%d.png\")\n#clip = core.imwri.Read(\"testimage.tiff\")\n\n#Store source for previewing\nsrc = clip\n\n#Convert to 16 bit YUV for preprocessing\n#clip = core.resize.Spline36(clip, format = vs.YUV444P16)\n\n#Deinterlace\n#clip = G41.QTGMC(clip, Preset='Medium')\n\n#Mild deblocking\n#clip = fvf.AutoDeblock(clip)\n\n#Convert to floating point RGB\nclip = mvf.ToRGB(clip, depth = 32)\n\n#Spatio-temportal GPU denoiser. https://github.com/Khanattila/KNLMeansCL/wiki/Filter-description\nclip = core.knlm.KNLMeansCL(clip, a = 8, d = 4, h = 1.4)\n\n\npreupscale = clip\n#Run ESRGAN model. See https://upscale.wiki/wiki/Model_Database\nvsgan_device = VSGAN.VSGAN()\nvsgan_device.load_model(model=r\"/content/4X_Box.pth\", scale=4)\nclip = vsgan_device.run(clip=clip, chunk = False, pad = 16)\n\nclip = core.knlm.KNLMeansCL(clip, a = 7, d = 3, h = 1.4)\n\n\n\n#Run MXNet model. See the \"MXNet\" cell.\n#Tensorflow models are also supported!\n#sr_args = dict(model_filename=r'/NeuralNetworks/ARAN/aran_c0_s1_x4', up_scale=4, device_id=0, block_w=256, block_h=128, is_rgb_model=True, pad=None, crop=None, pre_upscale=False)\n#clip = mufnp.super_resolution(clip, **sr_args)\n\n#HQ downscale on the GPU with dpid\n#clip = ape.GPU_Downscale(clip, width = 3840, height = 2160)\n\n#Convert back to YUV 444 format/Rec 709 colorspace\nclip = core.resize.Spline36(clip, format = vs.YUV444P16, matrix_s = \"709\")\n\n#Strong temporal denoiser and stabilizer with the LR as a motion reference clip, for stabilizing.\nprefilter = core.resize.Spline36(preupscale, format = clip.format, width = clip.width, height = clip.height, matrix_s = \"709\")\nclip = G41.SMDegrain(clip, tr=3, RefineMotion=True, pel = 1, prefilter = prefilter)\n\n#Another CPU denoiser/stabilizer. \"very high\" is very slow.\n#clip = haf.MCTemporalDenoise(clip, settings = \"very high\", useTTmpSm = True, maxr=4, stabilize = True)\n\n#Stabilized Anti Aliasing, with some GPU acceleration\n#clip = taa.TAAmbk(clip, opencl=True, stabilize = 3)\n\n#Example sharpeners that work well on high-res images\n#Masks or mvf.limitfilter are good ways to keep artifacts in check\n#clip = core.warp.AWarpSharp2(clip)\n#clip = G41.NonlinUSM(clip, z=3, sstr=0.28, rad=9, power=1)\n\n#High quality, strong debanding\n#clip = fvf.GradFun3(clip, smode = 2)\n\n#Convert back to 8 bit YUV420 for output. \nclip = core.resize.Spline36(clip, format = vs.YUV420P8, matrix_s = \"709\", dither_type = \"error_diffusion\")\n\n#Interpolate to double the source framerate\n#super = core.mv.Super(inter)\n#backward_vectors = core.mv.Analyse(super, isb = True, overlap=4, search = 3)\n#forward_vectors = core.mv.Analyse(super, isb = False, overlap=4, search = 3)\n#inter = core.mv.FlowFPS(inter, super, backward_vectors, forward_vectors, num=0, den=0)\n\n#Stack the source on top of the processed clip for comparison\nsrc = core.resize.Point(src, width = clip.width, height = clip.height, format = clip.format)\n#clip = core.std.StackVertical([clip, src])\n#Alternatively, interleave the source and slow down the framerate for easy comparison.\nclip = core.std.Interleave([clip, src])\nclip = core.std.AssumeFPS(clip, fpsnum = 2)\n\n#clip = core.std.SelectEvery(clip=clip, cycle=48, offsets=[0,1])\n\nclip.set_output()",
"_____no_output_____"
],
[
"#@title Preview Options\n#@markdown Run this cell to check the .vpy script, and set preview options. \n#@markdown * Software encoding is relatively slow on colab's single CPU core, but returns a smaller video.\n#@markdown * Hardware encoding doesn't work on older GPUs or a TPU, but can be faster.\n#@markdown * Sometimes video previews don't work. Chrome seems more reliable than Firefox, but its video player doesn't support scrubbing. Alternatively, you can download the preview in the \"/content\" folder with the Colab UI.\n#@markdown * HEVC support in browsers is iffy.\n#@markdown * PNG previews are more reliable, but less optimal. \n#@markdown * In video previews, you can interleave the source and processed clips and change the framerate for easy comparisons. \n#@markdown ***\n\n#TODO: Make vpy file path editable\nvpyscript = \"/content/autogenerated.vpy\"\n#@markdown Use hardware encoding.\nHardware_Encoding = True #@param {type:\"boolean\"}\n#@markdown Encode preview as lossless or high quality lossy video\nLossless = False #@param {type:\"boolean\"}\n#@markdown Use HEVC instead of AVC for preview. Experimental.\nHEVC = False #@param {type:\"boolean\"}\n#@markdown Generate a single PNG instead of a video.\nWrite_PNG = False #@param {type:\"boolean\"}\n#@markdown Don't display any video preview, just write it to /content\nDisplay_Video = False #@param {type:\"boolean\"}\n#@markdown Number of preview frames to generate\npreview_frames = 120 #@param {type:\"integer\"}\n#Check script with test frame (for debugging)\nTest_Frame = False \n\nfrom IPython.display import clear_output\nimport ipywidgets as widgets\nfrom pprint import pprint\n\n\n\ndef checkscript(vpyfile, checkoutput):\n \n #Clear the preview cache folder, as the script could have changed\n \n quotepath = r'\"' + vpyfile + r'\"'\n print(\"Testing script...\")\n if checkoutput:\n #See if the script will really output a frame\n test = !vspipe -y -s 0 -e 0 {quotepath} .\n #Parse the script, and return information about it. \n rawinfo = !vspipe -i {quotepath} -\n #Store clip properties as a dict\n #I really need to learn regex...\n clipinfo = eval(r\"{\" + str(rawinfo)[1:].replace(r\"\\n\", r\"','\").replace(r\": \", r\"': '\")[:-1] + r\"}\")\n !clear\n if not isinstance(clipinfo, dict):\n print(rawinfo)\n raise Exception(\"Error parsing VapourSynth script!\")\n #print(\"Script output properties: \")\n #!echo {clipinfo}\n return clipinfo, rawinfo, quotepath\n\n#Make a preview button, and a frame slider\n#Note that the slider won't appear with single frame scripts\n%cd /\n#display.clear_output()\n!clear\nclipinfo, rawinfo, quotepath = checkscript(vpyscript, Test_Frame)\nframeslider = None\ndrawslider = int(clipinfo[\"Frames\"]) > 1\nif drawslider:\n frameslider = widgets.IntSlider(value=0, max=(int(clipinfo[\"Frames\"]) - 1), layout=widgets.Layout(width='100%', height='150%'))\nelse:\n preview_frames = 1\nfv = None\n\n\nif not(preview_frames > 0 and preview_frames <= int(clipinfo[\"Frames\"])):\n raise Exception(\"preview_frames must be a valid integer\")\nif drawslider:\n fv = int(frameslider.value)\nelse:\n fv = 0\n\nencstr = \"\"\npreviewfile = r\"/usr/local/share/jupyter/nbextensions/preview.mp4\"\nif os.path.isfile(previewfile):\n os.remove(previewfile)\nev = min((int(fv + preview_frames - 1), int(clipinfo[\"Frames\"])- 1))\nenctup = (Hardware_Encoding, HEVC, Lossless) \nif enctup == (True, True, True):\n encstr = r\"-c:v hevc_nvenc -profile main10 -preset lossless -spatial_aq:v 1 -aq-strength 15 \"\nelif enctup == (True, True, False):\n encstr = r\"-c:v hevc_nvenc -pix_fmt yuv420p10le -preset:v medium -profile:v main10 -spatial_aq:v 1 -aq-strength 15 -rc:v constqp -qp:v 9\"\nelif enctup == (True, False, True):\n encstr = r\"-c:v h264_nvenc -preset lossless -profile high444p -spatial-aq 1 -aq-strength 15\"\nelif enctup == (False, True, True):\n encstr = r\"-c:v libx265 -pix_fmt yuv420p10le -preset slow -x265-params lossless=1\"\nelif enctup == (True, False, False):\n encstr = r\"-c:v h264_nvenc -pix_fmt yuv420p -preset:v medium -rc:v constqp -qp:v 10 -spatial-aq 1 -aq-strength 15\"\nelif enctup == (False, False, True):\n encstr = r\"-c:v libx264 -preset veryslow -crf 0\"\nelif enctup == (False, True, False):\n encstr = r\"-c:v libx265 -pix_fmt yuv420p10le -preset slow -crf 9\"\nelif enctup == (False, False, False):\n encstr = r\"-c:v libx264 -pix_fmt yuv420p -preset veryslow -crf 9\"\nelse:\n raise Exception(\"Invalid parameters!\")\nclear_output()\nprint(*rawinfo, sep = ' ')\nprint(\"Select the frame(s) you want to preview with the slider and 'preview frames', then run the next cell.\")\ndisplay.display(frameslider)\n\n",
"_____no_output_____"
],
[
"#@title Generate Preview\n\nimport os, time\n\n\npreviewdisplay = r\"\"\"\n<video controls autoplay>\n <source src=\"/nbextensions/preview.mp4\" type='video/mp4;\"'>\n Your browser does not support the video tag.\n</video>\n\"\"\"\npreviewpng = \"/content/preview\" + str(frameslider.value) + \".png\"\nif os.path.isfile(previewfile):\n os.remove(previewfile)\nif os.path.isfile(previewpng):\n os.remove(previewpng)\nframes = str(clipinfo[\"Frames\"])\nend = min(frameslider.value + preview_frames - 1, int(clipinfo[\"Frames\"]) - 1)\nif Write_PNG:\n !vspipe -y -s {frameslider.value} -e {frameslider.value} /content/autogenerated.vpy - | ffmpeg -y -hide_banner -loglevel warning -i pipe: {previewpng} \n if os.path.isfile(previewpng):\n import PIL\n display.display(PIL.Image.open(previewpng, mode='r'))\n else:\n raise Exception(\"Error generating preview!\")\nelse:\n out = !vspipe --progress -y -s {frameslider.value} -e {end} /content/autogenerated.vpy - | ffmpeg -y -hide_banner -progress pipe:1 -loglevel warning -i pipe: {encstr} {previewfile} | grep \"fps\"\n if os.path.isfile(previewfile):\n if os.path.isfile(\"/content/preview.mp4\"):\n os.remove(\"/content/preview.mp4\")\n !ln {previewfile} \"/content/preview.mp4\"\n clear_output()\n for temp in out:\n if \"Output\" in temp:\n print(temp)\n if Display_Video:\n display.display(display.HTML(previewdisplay))\n else:\n raise Exception(\"Error generating preview!\")",
"_____no_output_____"
]
],
[
[
"# Scratch Space\n\n\n---\n\n",
"_____no_output_____"
]
],
[
[
"#Do stuff here\n\n#Example ffmpeg script:\n\n!vspipe -y /content/autogenerated.vpy - | ffmpeg -i pipe: -c:v hevc_nvenc -profile:v main10 -preset lossless -spatial_aq:v 1 -aq-strength 15 \"/gdrive/MyDrive/upscale.mkv\"\n\n#TODO: Figure out why vspipe's progress isn't showing up in colab.",
"_____no_output_____"
]
],
[
[
"# Extra Functions\n",
"_____no_output_____"
]
],
[
[
"#@title Build ImageMagick and VapourSynth for Colab\n#@markdown VapourSynth needs to be built for Python 3.6, and Imagemagick needs to be built for the VapourSynth imwri plugin. The setup script pulls from bintray, but this cell will rebuild and reinstall them if those debs dont work. \n#@markdown The built debs can be found in the \"src\" folder.\n\n#Get some requirements for building\ndef getbuildstuff():\n !apt-fast install software-properties-common autoconf automake libtool build-essential cython3 coreutils pkg-config\n !python3.6 -m pip install tesseract cython\n\n#Build imagemagick, for imwri and local image manipulation, and create a deb\ndef buildmagick():\n makesrcd(\"imagemagick\")\n !wget https://imagemagick.org/download/ImageMagick-7.0.9-8.tar.gz\n !tar xvzf ImageMagick-7.0.9-8.tar.gz\n %cd ImageMagick-7.0.9-8\n !./configure --enable-hdri=yes --with-quantum-depth=32\n !make -j 4 --quiet\n !sudo checkinstall -D --fstrans=no --install=yes --default --pakdir=/src --pkgname=imagemagick --pkgversion=\"8:7.0.9-8\"\n !ldconfig /usr/local/lib\n\n#Build vapoursynth for colab (python 3.6, Broadwell SIMD, etc.), and create a deb\ndef buildvs():\n makesrcd(\"vapoursynth\")\n !wget https://github.com/vapoursynth/vapoursynth/archive/R48.tar.gz\n !tar -xf R48.tar.gz\n %cd vapoursynth-R48\n !./autogen.sh\n !./configure --enable-imwri\n !make -j 4 --quiet\n !sudo checkinstall -D --fstrans=no --install=yes --default --pakdir=/src --pkgname=vapoursynth --pkgversion=48\n !ldconfig /usr/local/lib\n \ngetbuildstuff()\nbuildmagick()\nbuildvs()",
"_____no_output_____"
],
[
"#@title MXnet\n#@markdown This cell will pull pretrained models from https://github.com/WolframRhodium/Super-Resolution-Zoo\n#@markdown For usage examples, see [this](https://github.com/WolframRhodium/muvsfunc/blob/master/Collections/examples/super_resolution_mxnet.vpy)\n#@markdown and [this](https://github.com/WolframRhodium/Super-Resolution-Zoo/wiki/Explanation-of-configurations-in-info.md)\n#Note that there's no release for the mxnet C++ plugin, and I can't get it to build in colab, but the header pulls and installs mxnet and the numpy super resolution function\nn = \"ESRGAN\" #@param {type:\"string\"}\n!svn update --set-depth infinity NeuralNetworks/{n}",
"_____no_output_____"
]
]
] | [
"markdown",
"code",
"markdown",
"code",
"markdown",
"code",
"markdown",
"code"
] | [
[
"markdown",
"markdown",
"markdown"
],
[
"code",
"code",
"code",
"code",
"code",
"code",
"code"
],
[
"markdown",
"markdown"
],
[
"code",
"code",
"code"
],
[
"markdown"
],
[
"code"
],
[
"markdown"
],
[
"code",
"code"
]
] |
e76a17634005214b1598539729a8c34c2acb8c39 | 18,715 | ipynb | Jupyter Notebook | Metodo de Runge-Kutta_Actividad.ipynb | ebucheli/F1014B | 1599ed81cfc736ab41705c672ed6e26fe928f8cf | [
"MIT"
] | 3 | 2020-05-17T20:36:38.000Z | 2021-04-08T21:34:29.000Z | Metodo de Runge-Kutta_Actividad.ipynb | ebucheli/F1014B | 1599ed81cfc736ab41705c672ed6e26fe928f8cf | [
"MIT"
] | null | null | null | Metodo de Runge-Kutta_Actividad.ipynb | ebucheli/F1014B | 1599ed81cfc736ab41705c672ed6e26fe928f8cf | [
"MIT"
] | 9 | 2020-05-18T14:14:15.000Z | 2021-06-10T21:37:15.000Z | 32.046233 | 290 | 0.549826 | [
[
[
"# Mรฉtodo de Runge-Kutta de 4to orden\n\nClase: F1014B Modelaciรณn Computacional de Sistemas Electromagnรฉticos\n\nAutor: Edoardo Bucheli\n\nProfesor de Cรกtedra, Tec de Monterrey Campus Santa Fe",
"_____no_output_____"
],
[
"## Introducciรณn\nEn esta sesiรณn aprenderemos un mรฉtodo numรฉrico para la soluciรณn de problemas de valor inicial con la siguiente forma,\n\n$$\\frac{dy}{dx} = f(x,y)\\qquad y(x_0) = y_0$$\n\nEl mรฉtodo que estudiaremos se conoce como el mรฉtodo de Runge-Kutta desarrollado por los matemรกticos alemanes Carl Runge y Wilhem Kutta.\n\nEste mรฉtodo es a su vez una versiรณn mรกs precisa del **Mรฉtodo de Euler** el cual estudiaremos rรกpidamente antes de pasar a Runge-Kutta.",
"_____no_output_____"
],
[
"## Mรฉtodo de Euler\n\nEl mรฉtodo de Euler se basa en un principio muy sencillo. Dado un campo de pendientes, creamos una trayectoria que se mueve conforme a la pendiente en un punto $(x,y)$ dado un movimiento horizontal $\\Delta x = h$. Este procedimiento se visualiza en la siguiente imรกgen.\n\n<img src=\"imgs/euler_method.png\">\n\n\nEn la imรกgen se aprecia de manera muy clara el error que ocurre al llevar a cabo la aproximaciรณn. Para mejorar la aproximaciรณn, podemos reducir el tamaรฑo de $h$ como se muestra en la siguiente figura,\n\n<img src=\"imgs/euler_2.png\">\n\nEn la imagen anterior se muestran aproximaciones con $h = 1$, $h = 0.2$ y $h = 0.05$.\n\nAunque un valor para $h$ puede ser lo suficientemente chico para obtener un resultado, si el valor final de $x$ que estamos buscando es muy grande entonces nuestro algoritmo puede ser muy costoso computacionalmente. Es por ello que resulta necesario encontrar un mรฉtodo mรกs eficiente.",
"_____no_output_____"
],
[
"## Ejercicio: Implementa el mรฉtodo de Euler\n\nAhora si empezaremos a programar, en esta secciรณn necesitas implementar el Mรฉtodo de Euler.\n\nPara hacer eso definamos el mรฉtodo formalmente:\n\n\n### Definiciรณn: Mรฉtodo de Euler\n\nPara el problema de valor inicial,\n\n$$\\frac{dy}{dx} = f(x,y)\\qquad y(x_0)=y_0$$\n\nEl mรฉtodo de Euler con salto de tamaรฑo $h$ consiste en aplicar la fรณrmula iterativa,\n\n$$y_{n+1} = y_n + h\\cdot f(x_n,y_n)$$\n\ny\n\n$$x_{n+1} = x_n + h$$\n\npara calcular las aproximaciones $y_1,y_2,y_3,\\dots$ de los valores reales $y(x_1),y(x_2),y(x_3),\\dots$ que emanan de la soluciรณn exacta $y(x)$",
"_____no_output_____"
],
[
"### Soluciรณn Guiada\n\nEmpecemos por importar las librerรญas necesarias.",
"_____no_output_____"
]
],
[
[
"import numpy as np\nimport matplotlib.pyplot as plt",
"_____no_output_____"
]
],
[
[
"Para la soluciรณn del problema, intentemos hacer una implementaciรณn similar a lo que harรญa un/a programador/a con mรกs experiencia.\n\nPor lo tanto separaremos la soluciรณn en dos funciones. Una se encargarรก de calcular un paso del mรฉtodo de euler y la segunda utilizarรก esta funciรณn para repetir el proceso tantas veces sea necesario.\n\nEmpecemos entonces por implementar la funciรณn `euler_step()` que se encargarรก de llevar a cabo un paso del mรฉtodo de Euler.",
"_____no_output_____"
]
],
[
[
"def euler_step(x_n,y_n,f,h):\n \"\"\"\n Calcula un paso del mรฉtodo de euler\n Entradas:\n x_n: int,float\n El valor inicial de x en este paso\n y_n: int, float\n El valor inicial de y en este paso\n f: funciรณn\n Una funciรณn que represente f(x,y) en el problema de valor inicial.\n h: int, float\n El tamaรฑo del salto de un paso al siguiente\n \n Salida:\n x_n_plus_1: float\n El valor de x actualizado de acuerdo al mรฉtodo de euler\n y_n_plus_1: float\n El valor de y actualizado de acuerdo al mรฉtodo de euler\n \"\"\"\n \n # Empieza tu cรณdigo aquรญ (alrededor de 2 lรญneas)\n \n \n return x_n_plus_1, y_n_plus_1\n ",
"_____no_output_____"
]
],
[
[
"Probemos tu funciรณn con el siguiente problema,\n\n$$\\frac{dy}{dx} = x + \\frac{1}{5}y \\qquad y(0) = -3 $$\n\nUtilizando $h = 1$",
"_____no_output_____"
]
],
[
[
"x_0 = 0\ny_0 = -3\n\ndef f(x,y):\n return x + (1/5)*y\n\nh = 1\n\nprint(euler_step(x_0,y_0,f,h))",
"_____no_output_____"
]
],
[
[
"Tu resultado deberรญa ser `(1,-3.6)`",
"_____no_output_____"
],
[
"Ahora que tenemos una funciรณn que calcula un paso del mรฉtodo, implementemos la funciรณn `euler_method()` que use la funciรณn `euler_step()` para generar una lista de valores hasta una nueva variable `x_goal`",
"_____no_output_____"
]
],
[
[
"def euler_method(x_0,y_0,x_goal,f,h):\n \"\"\"\n Regresa una lista para aproximaciones de y con el mรฉtodo de euler hasta un cierto valor x_goal\n Entradas:\n x_n: int,float\n El valor inicial de x\n y_n: int, float\n El valor inicial de y\n x_goal: int,float\n El valor hasta donde queremos calcular las aproximaciones del mรฉtodo de euler\n f: funciรณn\n Una funciรณn que represente f(x,y) en el problema de valor inicial.\n h: int, float\n El tamaรฑo del salto de un paso al siguiente\n \n Salida:\n x_n_list: list\n Una lista con los valores de 'x' desde x_0 hasta el valor x_{n+1} mรกs cercano a x_goal que sea tambiรฉn menor\n y_n_list: list\n Una lista con las aproximaciones 'y' evaluadas con los valores de x desde x_0 hasta el valor x_n+1 mรกs cercano a x_goal que sea tambiรฉn menor\n \"\"\"\n \n # Definimos x_n y y_n como los valores iniciales\n x_n = x_0\n y_n = y_0\n \n # Crea las listas x_n_list y y_n_list por ahora solo contienen los valores iniciales\n x_n_list = [x_0]\n y_n_list = [y_0]\n \n # Crea aquรญ un ciclo donde lleves a cabo el procedimiento tantas veces sea necesario,\n # no olvides guardar cada valor que calcules en las listas 'x_n_list' y 'y_n_list'\n # Aprox 4 lรญneas\n \n return x_n_list,y_n_list",
"_____no_output_____"
],
[
"x_0 = 0\ny_0 = -3\n\ndef f(x,y):\n return x + (1/5)*y\n\nh = 1\nx_goal = 5\n\nprint(euler_method(x_0,y_0,x_goal,f,h))",
"_____no_output_____"
]
],
[
[
"La salida de la celda anterior deberรญa ser:\n\n`([0, 1, 2, 3, 4, 5], [-3, -3.6, -3.3200000000000003, -1.9840000000000004, 0.6191999999999993, 4.743039999999999])`",
"_____no_output_____"
],
[
"## Un Mรฉtodo de Euler Mejorado\n\nUna manera sencilla de mejorar el mรฉtodo de Euler serรก obteniendo mรกs de una pendiente y tomando el promedio de las pendientes para mejorar la predicciรณn como se muestra en la siguiente figura.\n\n<img src=\"imgs/euler_3.png\">\n\nVemos que una vez que generamos un nuevo punto $y(x_{n+1})$ podemos obtener la pendiente en este punto, tomar el promedio entre esta pendiente y la encontrada en $y(x_n)$ para encontrar una nueva predicciรณn un poco mejor.",
"_____no_output_____"
],
[
"## Mรฉtodo de Runge-Kutta\n\nUtilizando el **Teorema Fundamental del Cรกlculo** podemos derivar la siguiente expresiรณn,\n\n$$y(x_{n+1})-y(x_n) = \\int_{x_n}^{x_{n+1}}y'(x)dx$$\n\nY a su vez, por la **Ley de Simpson** de integraciรณn podemos aproximar esto como,\n\n$$y(x_{n+1})-y(x_n)\\approx \\frac{h}{6}\\bigg[y'\\big(x_n\\big)+4y'\\bigg(x_n+\\frac{h}{2}\\bigg)+y'\\big(x_{n+1}\\big)\\bigg]$$\n\nY por lo tanto podemos despejar $y_{n+1}$ de la siguiente forma,\n\n$$y(x_{n+1})\\approx y(x_n) + \\frac{h}{6}\\bigg[y'\\big(x_n\\big)+2y'\\bigg(x_n+\\frac{h}{2}\\bigg)+2y'\\bigg(x_n+\\frac{h}{2}\\bigg)+y'\\big(x_{n+1}\\big)\\bigg]$$\n\nDefiniremos los tรฉrminos dentro del parรฉntesis $y'\\big(x_n\\big)$, $y'\\big(x_n+\\frac{h}{2}\\big)$, $y'\\big(x_n+\\frac{h}{2}\\big)$ y $y'\\big(x_{n+1}\\big)$ como $k_1,k_2,k_3,k_4$ respectivamente de la siguiente forma,\n\n### $k_1 = f(x_n,y_n)$\n\nEsto es la pendiente en $(x_n,y_n)$ misma pendiente que utilizarรญamos en el Mรฉtodo de Euler original.\n\n### $k_2 = f(x_n+\\frac{1}{2}h,y_n+\\frac{1}{2}hk_1)$\n\nEsto es la pendiente en el punto medio de $x_n$ y $x_{n+1}$ de acuerdo a la pendiente $k_1$\n \n### $k_3 = f(x_n+\\frac{1}{2}h,y_n+\\frac{1}{2}hk_2)$\n\nEsto es una correciรณn de la pendiente del punto medio de $x_n$ y $x_{n+1}$ como se hace en el mรฉtodo de Euler corregido.\n\n### $k_4 = f(x_{n+1},y_n+hk_3)$\n\nEsto es la pendiente del punto $(x_{n+1},y_{n+1})$ basado en la pendiente corregida $k_3$.\n\nLo que finalmente nos lleva a la forma,\n\n$$y_{n+1}=y_n+\\frac{h}{6}(k_1+2k_2+2k_3+k_4)$$\n\nNota que se usan las cuatro pendientes calculadas de manera ponderada. Es decir que no es exactamente un promedio, sino que le damos un poco mรกs de peso a ciertas pendientes. Especรญficamente a las de los puntos medios.\n\nDefinamos entonces de manera formal el algoritmo.",
"_____no_output_____"
],
[
"### Definiciรณn: Mรฉtodo de Runge-Kutta\n\nPara el problema de valor inicial,\n\n$$\\frac{dy}{dx} = f(x,y)\\qquad y(x_0)=y_0$$\n\nEl mรฉtodo de Runge-Kutta con salto de tamaรฑo $h$ consiste en aplicar la fรณrmula iterativa,\n\n$$y_{n+1}=y_n+\\frac{h}{6}(k_1+2k_2+2k_3+k_4)$$\n\nDรณnde,\n\n* $k_1 = f(x_n,y_n)$\n* $k_2 = f(x_n+\\frac{1}{2}h,y_n+\\frac{1}{2}hk_1)$\n* $k_3 = f(x_n+\\frac{1}{2}h,y_n+\\frac{1}{2}hk_2)$\n* $k_4 = f(x_{n+1},y_n+hk_3)$\n\npara calcular las aproximaciones $y_1,y_2,y_3,\\dots$ de los valores reales $y(x_1),y(x_2),y(x_3),\\dots$ que emanan de la soluciรณn exacta $y(x)$",
"_____no_output_____"
],
[
"## Ejercicio: Implementa el Mรฉtodo de Runge-Kutta\n\nAhora implementaremos el mรฉtodo de Runge-Kutta. Al igual que Euler convendrรก hacerlo como una funciรณn que podamos llamar para implementar un paso combinada de una funciรณn que implemente un cierto nรบmero de iteraciones.",
"_____no_output_____"
],
[
"Empecemos por definir la funciรณn `runge_kutta_step()` que llevarรก a cabo una iteraciรณn del mรฉtodo.",
"_____no_output_____"
]
],
[
[
"def runge_kutta_step(x_n,y_n,f,h):\n \"\"\"\n Calcula una iteraciรณn del mรฉtodo de Runge-Kutta de cuarto orden\n Entradas:\n x_n: int,float\n El valor inicial de x en este paso\n y_n: int, float\n El valor inicial de y en este paso\n f: funciรณn\n Una funciรณn que represente f(x,y) en el problema de valor inicial.\n h: int, float\n El tamaรฑo del salto de un paso al siguiente\n \n Salida:\n x_n_plus_1: float\n El valor de x actualizado de acuerdo al mรฉtodo de euler\n y_n_plus_1: float\n El valor de y actualizado de acuerdo al mรฉtodo de euler\n \"\"\"\n \n # Calcula cada una de las pendientes k de acuerdo al mรฉtodo de Runge-Kutta\n k_1 = #\n k_2 = #\n k_3 = #\n k_4 = #\n \n # Y ahora calcula y_n_plus_1 y x_n_plus_1\n # Aprox 2 lรญneas\n \n return x_n_plus_1, y_n_plus_1",
"_____no_output_____"
],
[
"def f(x,y):\n return x + y\n\nx_0 = 0\ny_0 = 1\nh = 0.5",
"_____no_output_____"
],
[
"x_1_test, y_1_test = runge_kutta_step(x_0,y_0,f,h)\nprint(f\"x_1 = {x_1_test}\\ny_1 = {y_1_test}\")",
"_____no_output_____"
]
],
[
[
"El resultado anterior deberรญa ser:\n```\nx_1 = 0.5\ny_1 = 1.796875\n```\n\nPara terminar, implementemos la funciรณn `runge_kutta()`",
"_____no_output_____"
]
],
[
[
"def runge_kutta(x_0,y_0,x_goal,f,h):\n \"\"\"\n Regresa una lista para aproximaciones de y con el mรฉtodo de Runge-Kutta hasta un cierto valor x_goal\n Entradas:\n x_n: int,float\n El valor inicial de x\n y_n: int, float\n El valor inicial de y\n x_goal: int,float\n El valor hasta donde queremos calcular las aproximaciones del mรฉtodo de euler\n f: funciรณn\n Una funciรณn que represente f(x,y) en el problema de valor inicial.\n h: int, float\n El tamaรฑo del salto de un paso al siguiente\n \n Salida:\n x_n_list: list\n Una lista con los valores de 'x' desde x_0 hasta el valor x_{n+1} mรกs cercano a x_goal que sea tambiรฉn menor\n y_n_list: list\n Una lista con las aproximaciones 'y' evaluadas con los valores de x desde x_0 hasta el valor x_n+1 mรกs cercano a x_goal que sea tambiรฉn menor\n \"\"\"\n \n # Definimos x_n y y_n como los valores iniciales\n x_n = x_0\n y_n = y_0\n \n # Crea las listas x_n_list y y_n_list por ahora solo contienen los valores iniciales\n x_n_list = [x_0]\n y_n_list = [y_0]\n \n # Crea aquรญ un ciclo donde lleves a cabo el procedimiento tantas veces sea necesario,\n # no olvides guardar cada valor que calcules en las listas 'x_n_list' y 'y_n_list'\n # Aprox 4 lรญneas\n \n return x_n_list,y_n_list",
"_____no_output_____"
],
[
"def f(x,y):\n return x + y\n\nx_0 = 0\ny_0 = 1\nh = 0.1\nx_goal = 1\n\n\nx_list, y_list = runge_kutta(x_0,y_0,x_goal,f,h)",
"_____no_output_____"
]
],
[
[
"Usemos una librerรญa llamada `prettytable` para imprimir nuestro resultado. Si no la tienes instalada entonces la siguiente linea arrojarรญa un error.",
"_____no_output_____"
]
],
[
[
"from prettytable import PrettyTable",
"_____no_output_____"
]
],
[
[
"En caso de que no tengas la librerรญa la puedes instalar corriendo el siguiente comando en una celda,\n\n`!pip install PrettyTable`\n\nO puedes simplemente correr el comando `pip install PrettyTable` en una ventana de la terminal (mac y linux) o el prompt de anaconda (windows).",
"_____no_output_____"
]
],
[
[
"mytable = PrettyTable()\n\nfor x,y in zip(x_list,y_list):\n mytable.add_row([\"{:0.2f}\".format(x),\"{:0.6f}\".format(y)])\nprint(mytable)",
"_____no_output_____"
]
],
[
[
"La salida de la celda anterior deberรญa ser:\n```\n+---------+----------+\n| Field 1 | Field 2 |\n+---------+----------+\n| 0.00 | 1.000000 |\n| 0.10 | 1.110342 |\n| 0.20 | 1.242805 |\n| 0.30 | 1.399717 |\n| 0.40 | 1.583648 |\n| 0.50 | 1.797441 |\n| 0.60 | 2.044236 |\n| 0.70 | 2.327503 |\n| 0.80 | 2.651079 |\n| 0.90 | 3.019203 |\n| 1.00 | 3.436559 |\n| 1.10 | 3.908327 |\n+---------+----------+\n```",
"_____no_output_____"
]
]
] | [
"markdown",
"code",
"markdown",
"code",
"markdown",
"code",
"markdown",
"code",
"markdown",
"code",
"markdown",
"code",
"markdown",
"code",
"markdown",
"code",
"markdown"
] | [
[
"markdown",
"markdown",
"markdown",
"markdown",
"markdown"
],
[
"code"
],
[
"markdown"
],
[
"code"
],
[
"markdown"
],
[
"code"
],
[
"markdown",
"markdown"
],
[
"code",
"code"
],
[
"markdown",
"markdown",
"markdown",
"markdown",
"markdown",
"markdown"
],
[
"code",
"code",
"code"
],
[
"markdown"
],
[
"code",
"code"
],
[
"markdown"
],
[
"code"
],
[
"markdown"
],
[
"code"
],
[
"markdown"
]
] |
e76a2bbce12f364388fc04ff4117d69311a13595 | 7,595 | ipynb | Jupyter Notebook | notebooks/collimator_with_DAC.ipynb | Fahima-Islam/c3dp | f8eb9235dd4fba7edcc0642ed68e325346ff577e | [
"MIT"
] | null | null | null | notebooks/collimator_with_DAC.ipynb | Fahima-Islam/c3dp | f8eb9235dd4fba7edcc0642ed68e325346ff577e | [
"MIT"
] | 1 | 2019-05-03T20:16:49.000Z | 2019-05-03T20:16:49.000Z | notebooks/collimator_with_DAC.ipynb | Fahima-Islam/c3dp | f8eb9235dd4fba7edcc0642ed68e325346ff577e | [
"MIT"
] | null | null | null | 25.833333 | 207 | 0.572219 | [
[
[
"import os, sys\n# import mcvine modules\nfrom instrument.geometry.pml import weave\nfrom instrument.geometry import operations",
"_____no_output_____"
],
[
"thisdir = os.path.abspath(os.path.dirname(\"__file__\"))\nlibpath = os.path.join(thisdir, '../c3dp_source')\nif not libpath in sys.path:\n sys.path.insert(0, libpath)",
"_____no_output_____"
],
[
"import collimator_support as colli_original",
"_____no_output_____"
],
[
"import SCADGen.Parser\nfrom create_collimator_geometry import Collimator_geom\nfrom clampcell_geo import Clampcell\nfrom DAC_geo import DAC",
"_____no_output_____"
],
[
"clampcell=Clampcell(total_height=True)\nouter_body=clampcell.outer_body()\ninner_sleeve=clampcell.inner_sleeve()\nsample=clampcell.sample()\n\ncell_sample_assembly=operations.unite(operations.unite(outer_body, sample), inner_sleeve)",
"_____no_output_____"
],
[
"dac=DAC()\nanvil=dac.anvil()\n\n# gasket=dac.gasket()\n# gasket_holder=dac.gasket_holder()\nsorrounding_gasket=dac.sorrounding_gasket()\n\nseat=dac.seat()\npiston=dac.piston()\nseat_pistion=dac.seat_piston()\nbar=dac.body_bar()\nbar=dac.body_bar_rotated()\n\ndac_cell=operations.unite(operations.unite(operations.unite(anvil,sorrounding_gasket),\n seat_pistion), bar)\n# dac_cell_beam= operations.rotate (dac_cell, transversal=1, angle='%s *degree' %(90))",
"_____no_output_____"
],
[
"collimator_fr_center=dac.gasket_diameter/2.\nprint (collimator_fr_center)",
"3.0\n"
],
[
"scad_flag = True ########CHANGE CAD FLAG HERE\n\nif scad_flag is True:\n samplepath = os.path.join(thisdir, '../figures')\nelse:\n samplepath = os.path.join(thisdir, '../sample')",
"_____no_output_____"
],
[
"colli_height=dac.pavilion_total_triangle_height()+dac.girdle_height+dac.seat_skirt_height+dac.seat_shaft_height+dac.piston_shaft_height+20\ncollimator_height=2*colli_height\n\nprint (collimator_height)",
"119.56781044332605\n"
],
[
"for coll_length in [collimator_height]: #100, 230,380\n\n\n channel_length=coll_length-15. #32, 17\n\n min_channel_wall_thickness=1.\n\n coll = colli_original.Collimator_geom()\n# coll = Collimator_geom()\n coll.set_constraints(max_coll_height_detector=collimator_height, max_coll_width_detector=collimator_height,\n min_channel_wall_thickness=min_channel_wall_thickness,\n max_coll_length=coll_length, min_channel_size=3.,\n truss_base_thickness=10., trass_final_height_factor=0.34,\n truss_blade_length=10,touch_to_halfcircle=3,\n SNAP_acceptance_angle=False,\n collimator_front_end_from_center=collimator_fr_center)\n\n\n coll.set_parameters(number_channels=5.,channel_length =channel_length)\n\n filename = 'coll_geometry_{coll_length}_{coll_height}_{coll_width}_{channel_length}_{wall_thickness}.xml'.\\\n format(coll_length=coll_length, coll_height=coll.max_coll_height_detector, coll_width=coll.max_coll_height_detector, channel_length=channel_length, wall_thickness=min_channel_wall_thickness)\n\n outputfile = os.path.join(samplepath, filename)\n\n\n supports=coll.support()\n\n truss=coll.support_design()\n\n coli = coll.gen_one_col(collimator_Nosupport=True)\n \n coli_transversal = coll.gen_collimators(detector_angles=[90], \n multiple_collimator=False,collimator_Nosupport=False)\n\n# collimator=coll.gen_collimators_xml(multiple_collimator=False, scad_flag=scad_flag,detector_angles=[0], collimator_Nosupport=False, coll_file=outputfile)",
"_____no_output_____"
],
[
"# both=operations.unite(dac_cell_beam, coli_transversal)\n# both= truss\n# both=supports\n# both=operations.unite(anvil, sorrounding_gasket)\nboth=operations.unite(dac_cell, coli)\nfile='dac_cell_coli'\nfilename='%s.xml'%(file)\noutputfile=os.path.join(samplepath, filename)\nwith open (outputfile,'wt') as file_h:\n weave(both,file_h, print_docs = False)",
"_____no_output_____"
],
[
"p = SCADGen.Parser.Parser(outputfile)\np.createSCAD()\ntest = p.rootelems[0]",
"_____no_output_____"
],
[
"cadFile_name='%s.scad'%(file)\ncad_file_path=os.path.abspath(os.path.join(samplepath, cadFile_name))",
"_____no_output_____"
],
[
"cad_file_path",
"_____no_output_____"
],
[
"!vglrun openscad {cad_file_path}",
"_____no_output_____"
]
]
] | [
"code"
] | [
[
"code",
"code",
"code",
"code",
"code",
"code",
"code",
"code",
"code",
"code",
"code",
"code",
"code",
"code",
"code"
]
] |
e76a2d637243fdba58ba2eb0289c65b27f086af0 | 166,664 | ipynb | Jupyter Notebook | notebooks/Prototype_data_prep.ipynb | nateGeorge/udacity_dend_capstone | 9d3eff1ed4cd38a4698ade0d4aca75b187fb0d54 | [
"Apache-2.0"
] | null | null | null | notebooks/Prototype_data_prep.ipynb | nateGeorge/udacity_dend_capstone | 9d3eff1ed4cd38a4698ade0d4aca75b187fb0d54 | [
"Apache-2.0"
] | null | null | null | notebooks/Prototype_data_prep.ipynb | nateGeorge/udacity_dend_capstone | 9d3eff1ed4cd38a4698ade0d4aca75b187fb0d54 | [
"Apache-2.0"
] | null | null | null | 34.830512 | 216 | 0.351006 | [
[
[
"This notebook is for prototyping data preparation for insertion into the database.\n\n# Data for installer table.\n\nNeed:\n- installer name\n- installer primary module manufacurer (e.g. mode of manufacturer name for all installers)",
"_____no_output_____"
]
],
[
[
"import pandas as pd\nimport numpy as np",
"_____no_output_____"
],
[
"def load_lbnl_data(replace_nans=True):\n df1 = pd.read_csv('../data/TTS_LBNL_public_file_10-Dec-2019_p1.csv', encoding='latin-1', low_memory=False)\n df2 = pd.read_csv('../data/TTS_LBNL_public_file_10-Dec-2019_p2.csv', encoding='latin-1', low_memory=False)\n lbnl_df = pd.concat([df1, df2], axis=0)\n if replace_nans:\n lbnl_df.replace(-9999, np.nan, inplace=True)\n lbnl_df.replace('-9999', np.nan, inplace=True)\n \n return lbnl_df",
"_____no_output_____"
],
[
"lbnl_df = load_lbnl_data(replace_nans=False)\nlbnl_df_nonan = load_lbnl_data()",
"_____no_output_____"
],
[
"lbnl_df.head()",
"_____no_output_____"
],
[
"lbnl_df.info()",
"<class 'pandas.core.frame.DataFrame'>\nInt64Index: 1543831 entries, 0 to 843830\nData columns (total 60 columns):\n # Column Non-Null Count Dtype \n--- ------ -------------- ----- \n 0 Data Provider 1543831 non-null object \n 1 System ID (from first Data Provider) 1543831 non-null object \n 2 System ID (from second Data Provider, if applicable) 1543831 non-null object \n 3 System ID (Tracking the Sun) 1543831 non-null object \n 4 Installation Date 1543831 non-null object \n 5 System Size 1543831 non-null float64\n 6 Total Installed Price 1543831 non-null float64\n 7 Appraised Value Flag 1543831 non-null bool \n 8 Sales Tax Cost 1543831 non-null float64\n 9 Rebate or Grant 1543831 non-null float64\n 10 Performance-Based Incentive (Annual Payment) 1543831 non-null float64\n 11 Performance-Based Incentives (Duration) 1543831 non-null int64 \n 12 Feed-in Tariff (Annual Payment) 1543831 non-null float64\n 13 Feed-in Tariff (Duration) 1543831 non-null int64 \n 14 Customer Segment 1543831 non-null object \n 15 New Construction 1543831 non-null int64 \n 16 Tracking 1543831 non-null int64 \n 17 Ground Mounted 1543831 non-null int64 \n 18 Battery System 1543831 non-null int64 \n 19 Zip Code 1543831 non-null object \n 20 City 1543831 non-null object \n 21 State 1543831 non-null object \n 22 Utility Service Territory 1543831 non-null object \n 23 Third-Party Owned 1543831 non-null int64 \n 24 Installer Name 1543831 non-null object \n 25 Self-Installed 1543831 non-null int64 \n 26 Azimuth #1 1543831 non-null float64\n 27 Azimuth #2 1543831 non-null float64\n 28 Azimuth #3 1543831 non-null float64\n 29 Tilt #1 1543831 non-null float64\n 30 Tilt #2 1543831 non-null int64 \n 31 Tilt #3 1543831 non-null int64 \n 32 Module Manufacturer #1 1543831 non-null object \n 33 Module Model #1 1543831 non-null object \n 34 Module Manufacturer #2 1543831 non-null object \n 35 Module Model #2 1543831 non-null object \n 36 Module Manufacturer #3 1543831 non-null object \n 37 Module Model #3 1543831 non-null object \n 38 Additional module model 1543831 non-null int64 \n 39 Module Technology #1 1543831 non-null object \n 40 Module Technology #2 1543831 non-null object \n 41 Module Technology #3 1543831 non-null object \n 42 BIPV Module #1 1543831 non-null int64 \n 43 BIPV Module #2 1543831 non-null int64 \n 44 BIPV Module #3 1543831 non-null int64 \n 45 Module Efficiency #1 1543831 non-null float64\n 46 Module Efficiency #2 1543831 non-null float64\n 47 Module Efficiency #3 1543831 non-null float64\n 48 Inverter Manufacturer #1 1543831 non-null object \n 49 Inverter Manufacturer #2 1543831 non-null object \n 50 Inverter Manufacturer #3 1543831 non-null object \n 51 Inverter Model #1 1543831 non-null object \n 52 Inverter Model #2 1543831 non-null object \n 53 Inverter Model #3 1543831 non-null object \n 54 Microinverter #1 1543831 non-null int64 \n 55 Microinverter #2 1543831 non-null int64 \n 56 Microinverter #3 1543831 non-null int64 \n 57 System Inverter Capacity 1543831 non-null float64\n 58 DC Optimizer 1543831 non-null int64 \n 59 Inverter Loading Ratio 1543831 non-null float64\ndtypes: bool(1), float64(15), int64(18), object(26)\nmemory usage: 708.2+ MB\n"
],
[
"# get mode of module manufacturer #1 for each install company\n# doesn't seem to work when -9999 values are replaced with NaNs\nmanufacturer_modes = lbnl_df[['Installer Name', 'Module Manufacturer #1']].groupby('Installer Name').agg(lambda x: x.value_counts().index[0])",
"_____no_output_____"
],
[
"manufacturer_modes.head()",
"_____no_output_____"
],
[
"lbnl_zip_data = lbnl_df[['Battery System', 'Feed-in Tariff (Annual Payment)', 'Zip Code']].copy()",
"_____no_output_____"
]
],
[
[
"Relpace missing values with 0 so it doesn't screw up the average calculation.",
"_____no_output_____"
]
],
[
[
"lbnl_zip_data.replace(-9999, 0, inplace=True)\nlbnl_zip_groups = lbnl_zip_data.groupby('Zip Code').mean()",
"_____no_output_____"
],
[
"lbnl_zip_groups.head()",
"_____no_output_____"
],
[
"lbnl_zip_groups.info()",
"<class 'pandas.core.frame.DataFrame'>\nIndex: 36744 entries, 85351 to 99403\nData columns (total 2 columns):\n # Column Non-Null Count Dtype \n--- ------ -------------- ----- \n 0 Battery System 36744 non-null float64\n 1 Feed-in Tariff (Annual Payment) 36744 non-null float64\ndtypes: float64(2)\nmemory usage: 861.2+ KB\n"
]
],
[
[
"Drop missing zip codes.",
"_____no_output_____"
]
],
[
[
"lbnl_zip_groups = lbnl_zip_groups[~(lbnl_zip_groups.index == '-9999')]",
"_____no_output_____"
],
[
"lbnl_zip_groups.reset_index(inplace=True)",
"_____no_output_____"
],
[
"lbnl_zip_groups.head()",
"_____no_output_____"
]
],
[
[
"# Data for the Utility table.\n\nNeed:\n- zipcode\n- utility name\n- ownership\n- service type\n\nJoin EIA-861 report data with EIA IOU rates by zipcode",
"_____no_output_____"
]
],
[
[
"eia861_df = pd.read_excel('../data/Sales_Ult_Cust_2018.xlsx', header=[0, 1, 2])",
"_____no_output_____"
],
[
"def load_eia_iou_data():\n iou_df = pd.read_csv('../data/iouzipcodes2017.csv')\n noniou_df = pd.read_csv('../data/noniouzipcodes2017.csv')\n eia_zipcode_df = pd.concat([iou_df, noniou_df], axis=0)\n \n # zip codes are ints without zero padding\n eia_zipcode_df['zip'] = eia_zipcode_df['zip'].astype('str')\n eia_zipcode_df['zip'] = eia_zipcode_df['zip'].apply(lambda x: x.zfill(5))\n \n return eia_zipcode_df",
"_____no_output_____"
],
[
"eia_zip_df = load_eia_iou_data()",
"_____no_output_____"
],
[
"eia_zip_df.info()",
"<class 'pandas.core.frame.DataFrame'>\nInt64Index: 86672 entries, 0 to 34073\nData columns (total 9 columns):\n # Column Non-Null Count Dtype \n--- ------ -------------- ----- \n 0 zip 86672 non-null object \n 1 eiaid 86672 non-null int64 \n 2 utility_name 86672 non-null object \n 3 state 86672 non-null object \n 4 service_type 86672 non-null object \n 5 ownership 86672 non-null object \n 6 comm_rate 86672 non-null float64\n 7 ind_rate 86672 non-null float64\n 8 res_rate 86672 non-null float64\ndtypes: float64(3), int64(1), object(5)\nmemory usage: 6.6+ MB\n"
],
[
"# util number here is eiaia in the IOU data\nutility_number = eia861_df['Utility Characteristics', 'Unnamed: 1_level_1', 'Utility Number']\nutility_name = eia861_df['Utility Characteristics', 'Unnamed: 2_level_1', 'Utility Name']\nservice_type = eia861_df['Utility Characteristics', 'Unnamed: 4_level_1', 'Service Type']\nownership = eia861_df['Utility Characteristics', 'Unnamed: 7_level_1', 'Ownership']\n\neia_utility_data = pd.concat([utility_number, utility_name, service_type, ownership], axis=1)\neia_utility_data.columns = eia_utility_data.columns.droplevel(0).droplevel(0)\neia_utility_data.head()",
"_____no_output_____"
],
[
"res_data = eia861_df['RESIDENTIAL'].copy()",
"_____no_output_____"
],
[
"res_data.head()",
"_____no_output_____"
],
[
"res_data[res_data['Revenues', 'Thousand Dollars'] == '.']",
"_____no_output_____"
]
],
[
[
"Missing data seems to be a period.",
"_____no_output_____"
]
],
[
[
"res_data.replace('.', np.nan, inplace=True)",
"_____no_output_____"
],
[
"for c in res_data.columns:\n print(c)\n res_data[c] = res_data[c].astype('float')",
"('Revenues', 'Thousand Dollars')\n('Sales', 'Megawatthours')\n('Customers', 'Count')\n"
],
[
"res_data['average_yearly_bill'] = res_data['Revenues', 'Thousand Dollars'] * 1000 / res_data['Customers', 'Count']",
"_____no_output_____"
],
[
"res_data.head()",
"_____no_output_____"
],
[
"res_data['average_yearly_kwh'] = (res_data['Sales', 'Megawatthours'] * 1000) / res_data['Customers', 'Count']",
"_____no_output_____"
],
[
"res_data.head()",
"_____no_output_____"
]
],
[
[
"Get average bill and kWh used by zip code.",
"_____no_output_____"
]
],
[
[
"res_columns = ['average_yearly_bill', 'average_yearly_kwh']",
"_____no_output_____"
],
[
"res_data.columns = res_data.columns.droplevel(1)",
"_____no_output_____"
],
[
"res_data[res_columns].head()",
"_____no_output_____"
],
[
"eia_861_data = pd.concat([res_data[res_columns], eia_utility_data], axis=1)\neia_861_data.head()",
"_____no_output_____"
],
[
"eia_861_data_zipcode = eia_861_data.merge(eia_zip_df, left_on='Utility Number', right_on='eiaid')",
"_____no_output_____"
],
[
"eia_861_data_zipcode.head()",
"_____no_output_____"
]
],
[
[
"Double-check res_rate",
"_____no_output_____"
]
],
[
[
"eia_861_data_zipcode['res_rate_recalc'] = eia_861_data_zipcode['average_yearly_bill'] / eia_861_data_zipcode['average_yearly_kwh']",
"_____no_output_____"
],
[
"eia_861_data_zipcode.head()",
"_____no_output_____"
],
[
"eia_861_data_zipcode.drop_duplicates(inplace=True)",
"_____no_output_____"
],
[
"eia_861_data_zipcode.tail()",
"_____no_output_____"
],
[
"eia_861_data_zipcode.info()",
"<class 'pandas.core.frame.DataFrame'>\nInt64Index: 152322 entries, 0 to 159485\nData columns (total 16 columns):\n # Column Non-Null Count Dtype \n--- ------ -------------- ----- \n 0 average_yearly_bill 143449 non-null float64\n 1 average_yearly_kwh 143449 non-null float64\n 2 Utility Number 152322 non-null float64\n 3 Utility Name 152322 non-null object \n 4 Service Type 152322 non-null object \n 5 Ownership 152322 non-null object \n 6 zip 152322 non-null object \n 7 eiaid 152322 non-null int64 \n 8 utility_name 152322 non-null object \n 9 state 152322 non-null object \n 10 service_type 152322 non-null object \n 11 ownership 152322 non-null object \n 12 comm_rate 152322 non-null float64\n 13 ind_rate 152322 non-null float64\n 14 res_rate 152322 non-null float64\n 15 res_rate_recalc 143449 non-null float64\ndtypes: float64(7), int64(1), object(8)\nmemory usage: 19.8+ MB\n"
]
],
[
[
"# Join project solar, ACS, EIA, and LBNL data to get main table\n\nTry and save all of required data from bigquery.",
"_____no_output_____"
]
],
[
[
"# Set up GCP API\nfrom google.cloud import bigquery\n# Construct a BigQuery client object.\nclient = bigquery.Client()",
"_____no_output_____"
],
[
"# ACS US census data\nACS_DB = '`bigquery-public-data`.census_bureau_acs'\nACS_TABLE = 'zip_codes_2017_5yr'\n\n# project sunroof\nPSR_DB = '`bigquery-public-data`.sunroof_solar'\nPSR_TABLE = 'solar_potential_by_postal_code'",
"_____no_output_____"
],
[
"# columns to keep from ACS data\nACS_COLS = ['geo_id', # zipcode\n 'median_age',\n 'housing_units',\n 'median_income',\n 'owner_occupied_housing_units',\n 'occupied_housing_units',\n # housing units which will be used to calculate total single-family homes\n 'dwellings_1_units_detached',\n 'dwellings_1_units_attached',\n 'dwellings_2_units',\n 'dwellings_3_to_4_units',\n 'bachelors_degree_2',\n 'different_house_year_ago_different_city',\n 'different_house_year_ago_same_city']",
"_____no_output_____"
],
[
"query = \"\"\"SELECT {} FROM {}.{} LIMIT 20;\"\"\".format(', '.join(ACS_COLS), ACS_DB, ACS_TABLE)\nacs_df = pd.read_gbq(query)\nacs_df",
"Downloading: 100%|โโโโโโโโโโ| 20/20 [00:00<00:00, 120.87rows/s]\n"
],
[
"query = f\"\"\"SELECT geo_id,\n median_age,\n housing_units,\n median_income,\n owner_occupied_housing_units,\n occupied_housing_units,\n dwellings_1_units_detached + dwellings_1_units_attached + dwellings_2_units + dwellings_3_to_4_units AS family_homes,\n bachelors_degree_2,\n different_house_year_ago_different_city + different_house_year_ago_same_city AS moved_recently\n FROM {ACS_DB}.{ACS_TABLE}\n LIMIT 10;\"\"\"\n\ntest_df = pd.read_gbq(query)\ntest_df",
"Downloading: 100%|โโโโโโโโโโ| 10/10 [00:00<00:00, 55.20rows/s]\n"
],
[
"acs_data_query = f\"\"\"SELECT geo_id,\n median_age,\n housing_units,\n median_income,\n owner_occupied_housing_units,\n occupied_housing_units,\n dwellings_1_units_detached + dwellings_1_units_attached + dwellings_2_units + dwellings_3_to_4_units AS family_homes,\n bachelors_degree_2,\n different_house_year_ago_different_city + different_house_year_ago_same_city AS moved_recently\n FROM {ACS_DB}.{ACS_TABLE}\"\"\"\n\nacs_data = pd.read_gbq(acs_data_query)",
"\nDownloading: 0%| | 0/33120 [00:00<?, ?rows/s]\u001b[A\nDownloading: 100%|โโโโโโโโโโ| 33120/33120 [00:02<00:00, 11087.15rows/s]\u001b[A\n"
],
[
"acs_data.to_csv('../data/acs_data.csv', index=False)",
"_____no_output_____"
],
[
"acs_data.shape",
"_____no_output_____"
],
[
"acs_data.head()",
"_____no_output_____"
]
],
[
[
"Project sunroof data",
"_____no_output_____"
]
],
[
[
"psr_cols = ['region_name',\n 'percent_covered',\n 'percent_qualified',\n 'number_of_panels_total',\n 'kw_median',\n 'count_qualified',\n 'existing_installs_count']",
"_____no_output_____"
],
[
"psr_query = f\"\"\"SELECT region_name,\n percent_covered,\n percent_qualified,\n number_of_panels_total,\n kw_median,\n (count_qualified - existing_installs_count) AS potential_installs\n FROM {PSR_DB}.{PSR_TABLE}\n LIMIT 10;\n \"\"\"\n\ntest_df = pd.read_gbq(psr_query)\ntest_df",
"Downloading: 100%|โโโโโโโโโโ| 10/10 [00:00<00:00, 70.47rows/s]\n"
],
[
"psr_query = f\"\"\"SELECT region_name,\n percent_covered,\n percent_qualified,\n number_of_panels_total,\n kw_median,\n (count_qualified - existing_installs_count) AS potential_installs\n FROM {PSR_DB}.{PSR_TABLE};\n \"\"\"\n\npsr_df = pd.read_gbq(psr_query)",
"Downloading: 100%|โโโโโโโโโโ| 11516/11516 [00:00<00:00, 12087.98rows/s]\n"
],
[
"psr_df.to_csv('../data/psr_data.csv')",
"_____no_output_____"
],
[
"psr_df.head()",
"_____no_output_____"
]
],
[
[
"# Join data for main data table",
"_____no_output_____"
]
],
[
[
"psr_acs = psr_df.merge(acs_data, left_on='region_name', right_on='geo_id', how='outer')",
"_____no_output_____"
],
[
"psr_acs.head()",
"_____no_output_____"
],
[
"psr_acs_lbnl = psr_acs.merge(lbnl_zip_groups, left_on='region_name', right_on='Zip Code', how='outer')",
"_____no_output_____"
],
[
"psr_acs_lbnl_eia = psr_acs_lbnl.merge(eia_861_data_zipcode, left_on='region_name', right_on='zip', how='outer')",
"_____no_output_____"
],
[
"psr_acs_lbnl_eia.head()",
"_____no_output_____"
],
[
"psr_acs_lbnl_eia.columns",
"_____no_output_____"
],
[
"psr_acs_lbnl_eia.info()",
"<class 'pandas.core.frame.DataFrame'>\nInt64Index: 206079 entries, 0 to 206078\nData columns (total 34 columns):\n # Column Non-Null Count Dtype \n--- ------ -------------- ----- \n 0 region_name 42105 non-null object \n 1 percent_covered 42105 non-null float64\n 2 percent_qualified 42105 non-null float64\n 3 number_of_panels_total 42020 non-null float64\n 4 kw_median 42020 non-null float64\n 5 potential_installs 42105 non-null float64\n 6 geo_id 62530 non-null object \n 7 median_age 61729 non-null float64\n 8 housing_units 62530 non-null float64\n 9 median_income 59662 non-null float64\n 10 owner_occupied_housing_units 62530 non-null float64\n 11 occupied_housing_units 62530 non-null float64\n 12 family_homes 62530 non-null float64\n 13 bachelors_degree_2 62399 non-null float64\n 14 moved_recently 62399 non-null float64\n 15 Zip Code 54245 non-null object \n 16 Battery System 54245 non-null float64\n 17 Feed-in Tariff (Annual Payment) 54245 non-null float64\n 18 average_yearly_bill 143459 non-null float64\n 19 average_yearly_kwh 143459 non-null float64\n 20 Utility Number 152332 non-null float64\n 21 Utility Name 152332 non-null object \n 22 Service Type 152332 non-null object \n 23 Ownership 152332 non-null object \n 24 zip 152332 non-null object \n 25 eiaid 152332 non-null float64\n 26 utility_name 152332 non-null object \n 27 state 152332 non-null object \n 28 service_type 152332 non-null object \n 29 ownership 152332 non-null object \n 30 comm_rate 152332 non-null float64\n 31 ind_rate 152332 non-null float64\n 32 res_rate 152332 non-null float64\n 33 res_rate_recalc 143459 non-null float64\ndtypes: float64(23), object(11)\nmemory usage: 55.0+ MB\n"
]
],
[
[
"Looks like we have a lot of missing data. Combine the zip code columns to have one zip column with no missing data.",
"_____no_output_____"
]
],
[
[
"def fill_zips(x):\n if not pd.isna(x['zip']):\n return x['zip']\n elif not pd.isna(x['Zip Code']):\n return x['Zip Code']\n elif not pd.isna(x['geo_id']):\n return x['geo_id']\n elif not pd.isna(x['region_name']):\n return x['region_name']\n else:\n return np.nan",
"_____no_output_____"
],
[
"psr_acs_lbnl_eia['full_zip'] = psr_acs_lbnl_eia.apply(fill_zips, axis=1)",
"_____no_output_____"
],
[
"# columns we'll use in the same order as the DB table\ncols_to_use = ['full_zip',\n 'percent_qualified',\n 'number_of_panels_total',\n 'kw_median',\n 'potential_installs',\n 'median_income',\n 'median_age',\n 'occupied_housing_units',\n 'owner_occupied_housing_units',\n 'family_homes',\n 'bachelors_degree_2',\n 'moved_recently',\n 'average_yearly_bill',\n 'average_yearly_kwh',\n # note: installer ID has to be gotten from the installer table\n 'Battery System',\n 'Feed-in Tariff (Annual Payment)']",
"_____no_output_____"
],
[
"df_to_write = psr_acs_lbnl_eia[cols_to_use]\ndf_to_write.head()",
"_____no_output_____"
],
[
"df_to_write.describe()",
"_____no_output_____"
],
[
"df_to_write.info()",
"<class 'pandas.core.frame.DataFrame'>\nInt64Index: 206079 entries, 0 to 206078\nData columns (total 16 columns):\n # Column Non-Null Count Dtype \n--- ------ -------------- ----- \n 0 full_zip 206079 non-null object \n 1 percent_qualified 42105 non-null float64\n 2 number_of_panels_total 42020 non-null float64\n 3 kw_median 42020 non-null float64\n 4 potential_installs 42105 non-null float64\n 5 median_income 59662 non-null float64\n 6 median_age 61729 non-null float64\n 7 occupied_housing_units 62530 non-null float64\n 8 owner_occupied_housing_units 62530 non-null float64\n 9 family_homes 62530 non-null float64\n 10 bachelors_degree_2 62399 non-null float64\n 11 moved_recently 62399 non-null float64\n 12 average_yearly_bill 143459 non-null float64\n 13 average_yearly_kwh 143459 non-null float64\n 14 Battery System 54245 non-null float64\n 15 Feed-in Tariff (Annual Payment) 54245 non-null float64\ndtypes: float64(15), object(1)\nmemory usage: 26.7+ MB\n"
]
],
[
[
"That's a lot of missing data. Something is wrong though, since there should only be ~41k zip codes, and this is showing 206k.",
"_____no_output_____"
]
],
[
[
"df_to_write.to_csv('../data/solar_metrics_data.csv', index=False)",
"_____no_output_____"
],
[
"import pandas as pd",
"_____no_output_____"
],
[
"df = pd.read_csv('../data/solar_metrics_data.csv')",
"/home/nate/anaconda3/envs/dend_capstone/lib/python3.7/site-packages/IPython/core/interactiveshell.py:3063: DtypeWarning: Columns (0) have mixed types.Specify dtype option on import or set low_memory=False.\n interactivity=interactivity, compiler=compiler, result=result)\n"
],
[
"df.drop_duplicates().shape",
"_____no_output_____"
],
[
"df['full_zip'].drop_duplicates().shape",
"_____no_output_____"
],
[
"df['full_zip'].head()",
"_____no_output_____"
]
]
] | [
"markdown",
"code",
"markdown",
"code",
"markdown",
"code",
"markdown",
"code",
"markdown",
"code",
"markdown",
"code",
"markdown",
"code",
"markdown",
"code",
"markdown",
"code",
"markdown",
"code",
"markdown",
"code",
"markdown",
"code"
] | [
[
"markdown"
],
[
"code",
"code",
"code",
"code",
"code",
"code",
"code",
"code"
],
[
"markdown"
],
[
"code",
"code",
"code"
],
[
"markdown"
],
[
"code",
"code",
"code"
],
[
"markdown"
],
[
"code",
"code",
"code",
"code",
"code",
"code",
"code",
"code"
],
[
"markdown"
],
[
"code",
"code",
"code",
"code",
"code",
"code"
],
[
"markdown"
],
[
"code",
"code",
"code",
"code",
"code",
"code"
],
[
"markdown"
],
[
"code",
"code",
"code",
"code",
"code"
],
[
"markdown"
],
[
"code",
"code",
"code",
"code",
"code",
"code",
"code",
"code",
"code"
],
[
"markdown"
],
[
"code",
"code",
"code",
"code",
"code"
],
[
"markdown"
],
[
"code",
"code",
"code",
"code",
"code",
"code",
"code"
],
[
"markdown"
],
[
"code",
"code",
"code",
"code",
"code",
"code"
],
[
"markdown"
],
[
"code",
"code",
"code",
"code",
"code",
"code"
]
] |
e76a4d6b41cb128cacccc7af444bb019b534c80f | 183,666 | ipynb | Jupyter Notebook | titanic/nb/0 - Getting to know the data.ipynb | mapa17/Kaggle | 0c75a2b750858524d8871249420fa510404f2d2b | [
"MIT"
] | null | null | null | titanic/nb/0 - Getting to know the data.ipynb | mapa17/Kaggle | 0c75a2b750858524d8871249420fa510404f2d2b | [
"MIT"
] | null | null | null | titanic/nb/0 - Getting to know the data.ipynb | mapa17/Kaggle | 0c75a2b750858524d8871249420fa510404f2d2b | [
"MIT"
] | null | null | null | 129.524683 | 44,204 | 0.825526 | [
[
[
"import numpy as np\nimport pandas as pd\nfrom matplotlib import pyplot as plt\nimport seaborn as sns\n%matplotlib inline\nplt.rcParams['figure.figsize'] = [15, 5]",
"_____no_output_____"
]
],
[
[
"# Loading the data",
"_____no_output_____"
]
],
[
[
"!ls ../input_data/",
"gender_submission.csv test.csv train.csv\r\n"
],
[
"train = pd.read_csv('../input_data/train.csv')\ntest = pd.read_csv('../input_data/train.csv')",
"_____no_output_____"
],
[
"train.head(10)",
"_____no_output_____"
]
],
[
[
"# Features\nFrom the competetion documentation\n\n|Feature | Explanation | Values |\n|--------|-------------|--------|\n|survival|\tSurvival\t|0 = No, 1 = Yes|\n|pclass|\tTicket class\t|1 = 1st, 2 = 2nd, 3 = 3rd|\n|sex|\tSex| male/female|\n|Age|\tAge| in years\t|\n|sibsp|\t# of siblings / spouses aboard the Titanic| numeric|\t\n|parch|\t# of parents / children aboard the Titanic|\tnumeric|\n|ticket|\tTicket number\t| string|\n|fare|\tPassenger fare\t| numeric |\n|cabin|\tCabin number\t| string|\n|embarked|\tPort of Embarkation|\tC = Cherbourg, Q = Queenstown, S = Southampton|\n\n**Notes**\npclass: A proxy for socio-economic status (SES)\n\n1st = Upper\n\n2nd = Middle\n\n3rd = Lower\n\n\nage: Age is fractional if less than 1. If the age is estimated, is it in the form of xx.5\n\n\nsibsp: The dataset defines family relations in this way...\n\nSibling = brother, sister, stepbrother, stepsister\n\nSpouse = husband, wife (mistresses and fiancรฉs were ignored)\n\nparch: The dataset defines family relations in this way...\n\nParent = mother, father\n\nChild = daughter, son, stepdaughter, stepson\n\nSome children travelled only with a nanny, therefore parch=0 for them.",
"_____no_output_____"
],
[
"# Data Exploration",
"_____no_output_____"
]
],
[
[
"print('DataSet Size')\ntrain.shape",
"DataSet Size\n"
],
[
"print(\"Number of missing values\")\npd.DataFrame(train.isna().sum(axis=0)).T",
"Number of missing values\n"
],
[
"train['Pclass'].hist(grid=False)",
"_____no_output_____"
],
[
"train.describe()",
"_____no_output_____"
],
[
"print('Numaber of missing Cabin strings per class')\ntrain[['Pclass', 'Cabin']].groupby('Pclass').agg(lambda x: x.isna().sum())",
"Numaber of missing Cabin strings per class\n"
],
[
"print('Numaber of missing Age per class')\ntrain[['Pclass', 'Age']].groupby('Pclass').agg(lambda x: x.isna().sum())",
"Numaber of missing Age per class\n"
],
[
"train['Embarked'].value_counts()",
"_____no_output_____"
],
[
"print('Whats the influence of the port?')\ntrain[['Embarked', 'Survived']].groupby('Embarked').agg(lambda x: x.sum())",
"Whats the influence of the port?\n"
],
[
"print('Relative surival rate per port')\ntrain[['Embarked', 'Survived']].groupby('Embarked').agg(lambda x: x.sum())['Survived'] / train['Embarked'].value_counts()",
"Relative surival rate per port\n"
],
[
"print('Number of Pclass per port')\ntrain[['Pclass', 'Embarked']].groupby('Embarked').apply(lambda x: x['Pclass'].value_counts(sort=False))",
"Number of Pclass per port\n"
],
[
"# Mark if a cabin is known or not\ntrain['UnknownCabin'] = train['Cabin'].isna()\ntrain['UnknownAge'] = train['Age'].isna()\ntrain['Sp-Pa'] = train['SibSp'] - train['Parch']",
"_____no_output_____"
],
[
"train.corr()",
"_____no_output_____"
]
],
[
[
"## Correlation Interpretation\n* Pclass: the higher the pclass (worse class) decreases the chance of survival significantly (the riches first)\n* Age: higher age decreases survival slightly (the children first)\n* SipSp: more siblings has a light negative effect on survival (bigger families have it more difficult?)\n* Parch: having more parent figures increases the chance of survival\n* Fare: a higher fare increases the chance of survival significantly",
"_____no_output_____"
]
],
[
[
"fig, ax = plt.subplots()\nclasses = []\nfor pclass, df in train[['Pclass', 'Fare']].groupby('Pclass', as_index=False):\n df['Fare'].plot(kind='kde', ax=ax)\n classes.append(pclass)\nax.legend(classes)\nax.set_xlim(-10, 200)",
"_____no_output_____"
],
[
"fig, ax = plt.subplots()\nclasses = []\nfor pclass, df in train[['Pclass', 'Age']].groupby('Pclass', as_index=False):\n df['Age'].plot(kind='kde', ax=ax)\n classes.append(pclass)\nax.legend(classes)",
"_____no_output_____"
],
[
"g = sns.FacetGrid(train, col=\"Sex\", row=\"Pclass\")\ng = g.map(plt.hist, \"Survived\", density=True, bins=[0, 1, 2], rwidth=0.8)",
"_____no_output_____"
],
[
"g = sns.FacetGrid(train, col=\"Survived\", row=\"Pclass\", hue='Sex')\ng = g.map(lambda S, **kwargs: S.plot('hist', **kwargs, alpha=0.5), \"Age\")\ng.add_legend()",
"_____no_output_____"
],
[
"g = sns.FacetGrid(train, col=\"Survived\", row=\"Pclass\")\ng = g.map(lambda S, **kwargs: S.plot('hist', **kwargs), \"Fare\")",
"_____no_output_____"
]
]
] | [
"code",
"markdown",
"code",
"markdown",
"code",
"markdown",
"code"
] | [
[
"code"
],
[
"markdown"
],
[
"code",
"code",
"code"
],
[
"markdown",
"markdown"
],
[
"code",
"code",
"code",
"code",
"code",
"code",
"code",
"code",
"code",
"code",
"code",
"code"
],
[
"markdown"
],
[
"code",
"code",
"code",
"code",
"code"
]
] |
e76a4fc24d7bcd0892cc81815cb835d63bf4313a | 165,792 | ipynb | Jupyter Notebook | book/_build/html/_sources/descriptive/m3-demo-05-SkewnessAndKurtosisUsingPandas.ipynb | hossainlab/statswithpy | 981ecfe4937f18e4c8a8420f7c362cb187d6cbeb | [
"MIT"
] | null | null | null | book/_build/html/_sources/descriptive/m3-demo-05-SkewnessAndKurtosisUsingPandas.ipynb | hossainlab/statswithpy | 981ecfe4937f18e4c8a8420f7c362cb187d6cbeb | [
"MIT"
] | null | null | null | book/_build/html/_sources/descriptive/m3-demo-05-SkewnessAndKurtosisUsingPandas.ipynb | hossainlab/statswithpy | 981ecfe4937f18e4c8a8420f7c362cb187d6cbeb | [
"MIT"
] | null | null | null | 225.874659 | 29,600 | 0.917451 | [
[
[
"import pandas as pd\nimport matplotlib.pyplot as plt",
"_____no_output_____"
],
[
"height_weight_data = pd.read_csv('datasets/500_Person_Gender_Height_Weight_Index.csv')\n\nheight_weight_data.head()",
"_____no_output_____"
],
[
"height_weight_data.drop('Index', inplace=True, axis=1)\n\nheight_weight_data.shape",
"_____no_output_____"
],
[
"height_weight_data[['Height']].plot(kind = 'hist',\n title = 'Height', figsize=(12, 8))",
"_____no_output_____"
],
[
"height_weight_data[['Weight']].plot(kind = 'hist',\n title = 'weight', figsize=(12, 8))",
"_____no_output_____"
],
[
"height_weight_data[['Height']].plot(kind = 'kde',\n title = 'Height', figsize=(12, 8))",
"_____no_output_____"
],
[
"height_weight_data[['Weight']].plot(kind = 'kde',\n title = 'Weight', figsize=(12, 8))",
"_____no_output_____"
]
],
[
[
"### Skewness\n\nIt is the degree of distortion from the normal distribution. It measures the lack of symmetry in data distribution.\n\n-- Positive Skewness means when the tail on the right side of the distribution is longer or fatter. The mean and median will be greater than the mode. \n\n-- Negative Skewness is when the tail of the left side of the distribution is longer or fatter than the tail on the right side. The mean and median will be less than the mode. ",
"_____no_output_____"
]
],
[
[
"height_weight_data['Height'].skew()",
"_____no_output_____"
],
[
"height_weight_data['Weight'].skew()",
"_____no_output_____"
],
[
"listOfSeries = [pd.Series(['Male', 400, 300], index=height_weight_data.columns ), \n pd.Series(['Female', 660, 370], index=height_weight_data.columns ), \n pd.Series(['Female', 199, 410], index=height_weight_data.columns ),\n pd.Series(['Male', 202, 390], index=height_weight_data.columns ), \n pd.Series(['Female', 770, 210], index=height_weight_data.columns ),\n pd.Series(['Male', 880, 203], index=height_weight_data.columns )]",
"_____no_output_____"
],
[
"height_weight_updated = height_weight_data.append(listOfSeries , ignore_index=True)\n\nheight_weight_updated.tail()",
"_____no_output_____"
],
[
"height_weight_updated[['Height']].plot(kind = 'hist', bins=100,\n title = 'Height', figsize=(12, 8))",
"_____no_output_____"
],
[
"height_weight_updated[['Weight']].plot(kind = 'hist', bins=100,\n title = 'weight', figsize=(12, 8))",
"_____no_output_____"
],
[
"height_weight_updated[['Height']].plot(kind = 'kde',\n title = 'Height', figsize=(12, 8))",
"_____no_output_____"
],
[
"height_weight_updated[['Weight']].plot(kind = 'kde',\n title = 'weight', figsize=(12, 8))",
"_____no_output_____"
],
[
"height_weight_updated['Height'].skew()",
"_____no_output_____"
],
[
"height_weight_updated['Weight'].skew()",
"_____no_output_____"
]
],
[
[
"### Kurtosis\n\nIt is actually the measure of outliers present in the distribution.\n\n-- High kurtosis in a data set is an indicator that data has heavy tails or outliers. \n-- Low kurtosis in a data set is an indicator that data has light tails or lack of outliers.",
"_____no_output_____"
]
],
[
[
"height_weight_data['Height'].kurtosis()",
"_____no_output_____"
],
[
"height_weight_data['Weight'].kurtosis()",
"_____no_output_____"
],
[
"height_weight_updated['Height'].kurtosis()",
"_____no_output_____"
],
[
"height_weight_updated['Weight'].kurtosis()",
"_____no_output_____"
]
]
] | [
"code",
"markdown",
"code",
"markdown",
"code"
] | [
[
"code",
"code",
"code",
"code",
"code",
"code",
"code"
],
[
"markdown"
],
[
"code",
"code",
"code",
"code",
"code",
"code",
"code",
"code",
"code",
"code"
],
[
"markdown"
],
[
"code",
"code",
"code",
"code"
]
] |
e76a588ed194e963bfc50c3b256f72b994dd97dc | 42,298 | ipynb | Jupyter Notebook | Training and Output.ipynb | iamshamikb/Walmart_M5_Accuracy | 9d155e620e3d8552402e4856292630cf63c50f62 | [
"MIT"
] | null | null | null | Training and Output.ipynb | iamshamikb/Walmart_M5_Accuracy | 9d155e620e3d8552402e4856292630cf63c50f62 | [
"MIT"
] | null | null | null | Training and Output.ipynb | iamshamikb/Walmart_M5_Accuracy | 9d155e620e3d8552402e4856292630cf63c50f62 | [
"MIT"
] | null | null | null | 33.730463 | 132 | 0.370278 | [
[
[
"# Imports",
"_____no_output_____"
]
],
[
[
"# ! pip install pandas\n# ! pip install calender\n# ! pip install numpy\n# ! pip install datetime\n# ! pip install matplotlib\n# ! pip install collections\n# ! pip install random\n# ! pip install tqdm\n# ! pip install sklearn\n# ! pip install lightgbm\n# ! pip install xgboost",
"_____no_output_____"
],
[
"import pandas as pd\nimport calendar\nfrom datetime import datetime\nimport numpy as np\nfrom sklearn.model_selection import train_test_split\nfrom sklearn.preprocessing import LabelEncoder\nfrom IPython.display import clear_output as cclear\nfrom lightgbm import LGBMRegressor\nimport joblib",
"_____no_output_____"
]
],
[
[
"# Load data",
"_____no_output_____"
]
],
[
[
"def get_csv(X):\n return pd.read_csv(path+X)\npath = ''\ncalender, sales_train_ev, sales_train_val, sell_prices = get_csv('calendar.csv'), get_csv('sales_train_evaluation.csv'), \\\n get_csv('sales_train_validation.csv'), get_csv('sell_prices.csv')",
"_____no_output_____"
],
[
"non_numeric_col_list = ['id','item_id','dept_id','cat_id','store_id','state_id','d', 'date']\nstore_dict = {'CA_1':0, 'CA_2':0, 'CA_3':0, 'CA_4':0, 'WI_1':0, 'WI_2':0, 'WI_3':0, 'TX_1':0, 'TX_2':0, 'TX_3':0}",
"_____no_output_____"
],
[
"# Encoding Categorical Columns\ndef encode_cat_cols(new_df):\n le = [0]*len(non_numeric_col_list) \n for i in range(len(non_numeric_col_list)):\n print(\"Encoding col: \", non_numeric_col_list[i])\n le[i] = LabelEncoder()\n new_df[non_numeric_col_list[i]] = le[i].fit_transform( new_df[non_numeric_col_list[i]] )\n return le, new_df\n\n\n# Function for reversing the long form\ndef reverse_long_form(le, X_test, train_out):\n for i in range(len(non_numeric_col_list)):\n X_test[non_numeric_col_list[i]] = le[i].inverse_transform(X_test[non_numeric_col_list[i]])\n\n X_test['unit_sale'] = train_out\n kk = X_test.pivot(index='id', columns='d')['unit_sale']\n kk['id'] = kk.index\n kk.reset_index(drop=True, inplace=True)\n\n cols = list(kk)\n cols = [cols[-1]] + cols[:-1]\n kk = kk[cols]\n\n return kk\n\n\n# This function does feature engineering on sales_train_ev or sales_train_val\n# There is another feature engineering function for adding columns to dataframe containing rows of only onw store\ndef feature_engineer(df):\n day_columns = list(df.columns[6:])\n other_var = list(df.columns[:6])\n \n print('Melting out...')\n df = pd.melt(df, id_vars = other_var, value_vars = day_columns)\n df = df.rename(columns = {\"variable\": \"d\", \"value\": \"unit_sale\"})\n # print(df.shape)\n \n print('Adding Feature \\'date\\'...')\n cal_dict = dict(zip(calender.d,calender.date))\n df[\"date\"] = df[\"d\"].map(cal_dict)\n # df.head()\n \n print('Adding Feature \\'day_of_week\\'...')\n day_of_week_dict = dict(zip(calender.d,calender.wday))\n df['day_of_week'] = df[\"d\"].map(day_of_week_dict)\n\n print('Adding Feature \\'month_no\\'...')\n month_no_dict = dict(zip(calender.d,calender.month))\n df['month_no'] = df[\"d\"].map(month_no_dict)\n \n print('Adding Feature \\'day_of_month\\'...')\n l = [i[-2:] for i in list(calender.date)]\n calender['day_of_month'] = l\n \n day_of_month_dict = dict(zip(calender.d,calender.day_of_month))\n df['day_of_month'] = df[\"d\"].map(day_of_month_dict)\n \n print('Done.')\n print('Here is how featurised data looks like...')\n print(df.head(3))\n return df\n\n\ndef reorder_data(df, csv_name):\n df['sp_index'] = (df.index)\n index_dict = dict(zip(df.id, df.sp_index))\n df = df.drop('sp_index', axis=1)\n\n kk = pd.read_csv(str(csv_name)+'.csv')\n # kk = kk.drop(kk.columns[0], axis=1)\n\n kk['sp_index'] = kk[\"id\"].map(index_dict)\n kk = kk.sort_values(by='sp_index', axis=0)\n kk = kk.drop('sp_index', axis=1)\n kk.to_csv(str(csv_name)+'.csv')",
"_____no_output_____"
]
],
[
[
"# Train Function",
"_____no_output_____"
]
],
[
[
"def startegy7dot1(new_df, dept):\n print('Using strategy ', strategy)\n evaluation, validation = new_df.id.iloc[0].find('evaluation'), new_df.id.iloc[0].find('validation')\n \n new_df = new_df[new_df.dept_id == dept]\n print('Total rows: ', len(new_df))\n \n rows_per_day = len(new_df[new_df.d == 'd_1'])\n print('Rows per day: ', rows_per_day)\n \n new_df['day_of_month'] = new_df['day_of_month'].fillna(0)\n new_df = new_df.astype({'day_of_month': 'int32'}) # Making day_of_month column as int\n new_df['date'] = new_df['date'].astype(str)\n \n y = new_df.unit_sale # getting the label\n new_df = new_df.drop('unit_sale', axis=1)\n\n print('Encoding categorical features...')\n le, new_df = encode_cat_cols(new_df) # Encoding Categorical Columns\n\n X = new_df\n \n ev_train_start, ev_train_end, val_train_start, val_train_end = rows_per_day*(0), rows_per_day*1941,\\\n rows_per_day*(0), rows_per_day*1913\n \n model = LGBMRegressor(boosting_type = 'gbdt', \n objective = 'tweedie',\n tweedie_variance_power = 1.3, \n metric = 'rmse',\n subsample = 0.5,\n subsample_freq = 1,\n learning_rate = 0.03,\n num_leaves = 3000, \n min_data_in_leaf = 5000, \n feature_fraction = 0.5,\n max_bin = 300, \n n_estimators = 500,\n boost_from_average = False,\n verbose = -1,\n n_jobs = -1)\n \n if evaluation != -1: # if evaluation data\n print('Getting X_train, y_train...')\n X_train, y_train = X.iloc[ev_train_start:ev_train_end], y[ev_train_start:ev_train_end] \n X_test, y_test = X.iloc[ev_train_end:], y[ev_train_end:] \n model_name = 'Eval_'+str(dept)+'.pkl'\n joblib.dump(le, 'le_Eval_'+str(dept)+'.pkl') \n \n if validation != -1: # if validation data\n print('Getting X_train, y_train...')\n X_train, y_train = X.iloc[val_train_start:val_train_end], y[val_train_start:val_train_end]\n X_test, y_test = X.iloc[val_train_end:], y[val_train_end:]\n model_name = 'Val_'+str(dept)+'.pkl'\n joblib.dump(le, 'le_Val_'+str(dept)+'.pkl')\n \n print('X_train len', len(X_train), 'y_train len', len(y_train), 'X_test len', len(X_test))\n \n print('Fitting model...')\n model.fit(X_train, y_train)\n \n print('Fitting done. Saving model...')\n joblib.dump(model, model_name)\n \n joblib_model = joblib.load(model_name)\n \n print('Making predictions...')\n train_out = joblib_model.predict(X_test)\n\n print('Done.')\n return le, X_test, train_out\n\ndef get_output_of_eval_or_val(df):\n main_out_df = pd.DataFrame()\n \n list_dept = list(set(df.dept_id))\n for i in list_dept:\n print('Sequence of depts processing: ', list_dept)\n print('Working on Dept: ', i)\n \n le, X_test, train_out = startegy7dot1(df, i)\n \n print('Reversing the long form...')\n out_df = reverse_long_form(le, X_test, train_out)\n main_out_df = pd.concat([main_out_df, out_df], ignore_index=False)\n cclear()\n\n l = [] # In this part we rename the columns to F_1, F_2 ....\n for i in range(1,29):\n l.append('F'+str(i))\n l = ['id']+l\n\n main_out_df.columns = l\n \n return main_out_df",
"_____no_output_____"
]
],
[
[
"# Run",
"_____no_output_____"
]
],
[
[
"strategy = 7.1",
"_____no_output_____"
],
[
"############## Eval data",
"_____no_output_____"
],
[
"%%time\ndf = sales_train_ev.copy()\nempty_list = [0]*30490\nfor i in range(1942, 1970):\n df['d_'+str(i)] = empty_list\ndf = feature_engineer(df)",
"Melting out...\nAdding Feature 'date'...\nAdding Feature 'day_of_week'...\nAdding Feature 'month_no'...\nAdding Feature 'day_of_month'...\nDone.\nHere is how featurised data looks like...\n id item_id dept_id cat_id store_id \\\n0 HOBBIES_1_001_CA_1_evaluation HOBBIES_1_001 HOBBIES_1 HOBBIES CA_1 \n1 HOBBIES_1_002_CA_1_evaluation HOBBIES_1_002 HOBBIES_1 HOBBIES CA_1 \n2 HOBBIES_1_003_CA_1_evaluation HOBBIES_1_003 HOBBIES_1 HOBBIES CA_1 \n\n state_id d unit_sale date day_of_week month_no day_of_month \n0 CA d_1 0 2011-01-29 1 1 29 \n1 CA d_1 0 2011-01-29 1 1 29 \n2 CA d_1 0 2011-01-29 1 1 29 \nCPU times: user 32.6 s, sys: 8.82 s, total: 41.4 s\nWall time: 41.3 s\n"
],
[
"%%time\nmain_out_df_ev = get_output_of_eval_or_val(df)\nmain_out_df_ev.to_csv('main_out_ev.csv', index=False)",
"CPU times: user 4h 35min 40s, sys: 1min 14s, total: 4h 36min 54s\nWall time: 51min 14s\n"
],
[
"############# Val Data",
"_____no_output_____"
],
[
"%%time\ndf = sales_train_val.copy()\nempty_list = [0]*30490\nfor i in range(1914, 1942):\n df['d_'+str(i)] = empty_list\ndf = feature_engineer(df)",
"Melting out...\nAdding Feature 'date'...\nAdding Feature 'day_of_week'...\nAdding Feature 'month_no'...\nAdding Feature 'day_of_month'...\nDone.\nHere is how featurised data looks like...\n id item_id dept_id cat_id store_id \\\n0 HOBBIES_1_001_CA_1_validation HOBBIES_1_001 HOBBIES_1 HOBBIES CA_1 \n1 HOBBIES_1_002_CA_1_validation HOBBIES_1_002 HOBBIES_1 HOBBIES CA_1 \n2 HOBBIES_1_003_CA_1_validation HOBBIES_1_003 HOBBIES_1 HOBBIES CA_1 \n\n state_id d unit_sale date day_of_week month_no day_of_month \n0 CA d_1 0 2011-01-29 1 1 29 \n1 CA d_1 0 2011-01-29 1 1 29 \n2 CA d_1 0 2011-01-29 1 1 29 \nCPU times: user 38 s, sys: 9.85 s, total: 47.9 s\nWall time: 47.7 s\n"
],
[
"%%time\nmain_out_df_val = get_output_of_eval_or_val(df)\nmain_out_df_val.to_csv('main_out_val.csv', index=False)",
"CPU times: user 4h 15min 3s, sys: 1min 8s, total: 4h 16min 12s\nWall time: 47min 47s\n"
],
[
"############# Reorder and Write the output",
"_____no_output_____"
],
[
"sales_train_val",
"_____no_output_____"
],
[
"reorder_data(sales_train_val, 'main_out_val')\nreorder_data(sales_train_ev, 'main_out_ev')",
"_____no_output_____"
],
[
"main_out_ev = pd.read_csv('main_out_ev.csv')\nmain_out_val = pd.read_csv('main_out_val.csv')\n\nsub_df = pd.concat([main_out_ev, main_out_val], ignore_index=True)\nsub_df = sub_df.round(2)",
"_____no_output_____"
],
[
"sub_df.drop([sub_df.columns[0], sub_df.columns[-1]], axis=1, inplace=True)",
"_____no_output_____"
],
[
"sub_df",
"_____no_output_____"
],
[
"sub_df.to_csv('submission.csv', index=False)",
"_____no_output_____"
]
]
] | [
"markdown",
"code",
"markdown",
"code",
"markdown",
"code",
"markdown",
"code"
] | [
[
"markdown"
],
[
"code",
"code"
],
[
"markdown"
],
[
"code",
"code",
"code"
],
[
"markdown"
],
[
"code"
],
[
"markdown"
],
[
"code",
"code",
"code",
"code",
"code",
"code",
"code",
"code",
"code",
"code",
"code",
"code",
"code",
"code"
]
] |
e76a5d14f289660fb59af87dea1f4a38cd0995af | 16,357 | ipynb | Jupyter Notebook | analyze_vocab.ipynb | quentin-auge/blogger | 1a9ff596e7b4402acf926dd69ba423d2dce1a9f2 | [
"BSD-3-Clause"
] | null | null | null | analyze_vocab.ipynb | quentin-auge/blogger | 1a9ff596e7b4402acf926dd69ba423d2dce1a9f2 | [
"BSD-3-Clause"
] | null | null | null | analyze_vocab.ipynb | quentin-auge/blogger | 1a9ff596e7b4402acf926dd69ba423d2dce1a9f2 | [
"BSD-3-Clause"
] | null | null | null | 33.044444 | 237 | 0.506817 | [
[
[
"import operator\n\nfrom collections import Counter",
"_____no_output_____"
]
],
[
[
"# Get text",
"_____no_output_____"
]
],
[
[
"with open('data/one_txt/blogger.txt') as f:\n blogger = f.read()",
"_____no_output_____"
],
[
"with open('data/one_txt/wordpress.txt') as f:\n wordpress = f.read()",
"_____no_output_____"
],
[
"txt = wordpress + blogger",
"_____no_output_____"
]
],
[
[
"# Explore vocabulary",
"_____no_output_____"
]
],
[
[
"vocab_count = dict(Counter(txt))",
"_____no_output_____"
],
[
"vocab_freq = {char: count / len(txt) for char, count in vocab_count.items()}",
"_____no_output_____"
],
[
"sorted(zip(vocab_count.keys(), vocab_count.values(), vocab_freq.values()), key=operator.itemgetter(1))",
"_____no_output_____"
],
[
"full_vocab = sorted(vocab_count.keys(), key=vocab_count.get, reverse=True)\nfull_vocab = ''.join(full_vocab)\nfull_vocab",
"_____no_output_____"
]
],
[
[
"## Normalize some of the text characters",
"_____no_output_____"
]
],
[
[
"def normalize_txt(txt):\n\n # Non-breaking spaces -> regular spaces\n txt = txt.replace('\\xa0', ' ')\n\n # Double quotes\n double_quotes_chars = 'โโยปยซ'\n for double_quotes_char in double_quotes_chars:\n txt = txt.replace(double_quotes_char, '\"')\n\n # Single quotes\n single_quote_chars = 'โ`ยดโ'\n for single_quote_char in single_quote_chars:\n txt = txt.replace(single_quote_char, \"'\")\n\n # Triple dots\n txt = txt.replace('โฆ', '...')\n\n # Hyphens\n hyphen_chars = 'โโ'\n for hyphen_char in hyphen_chars:\n txt = txt.replace(hyphen_char, '-')\n\n return txt",
"_____no_output_____"
],
[
"txt = normalize_txt(txt)",
"_____no_output_____"
],
[
"vocab_count = dict(Counter(txt))\nfull_vocab = sorted(vocab_count.keys(), key=vocab_count.get, reverse=True)\nfull_vocab = ''.join(full_vocab)\nfull_vocab",
"_____no_output_____"
]
],
[
[
"## Restrict text to a sensible vocabulary",
"_____no_output_____"
]
],
[
[
"vocab = ' !\"$%\\'()+,-./0123456789:;=>?ABCDEFGHIJKLMNOPQRSTUVWXYZ_abcdefghijklmnopqrstuvwxyz~ยฐร รขรงรจรฉรชรซรฎรฏรดรนรปละพโฌ'",
"_____no_output_____"
],
[
"# Restrict text to vocabulary\ndef restrict_to_vocab(txt, vocab):\n txt = ''.join(char for char in txt if char in vocab)\n return txt",
"_____no_output_____"
],
[
"txt = restrict_to_vocab(txt, vocab)",
"_____no_output_____"
],
[
"# Double check new vocabulary\nassert ''.join(sorted(set(txt))) == vocab",
"_____no_output_____"
]
]
] | [
"code",
"markdown",
"code",
"markdown",
"code",
"markdown",
"code",
"markdown",
"code"
] | [
[
"code"
],
[
"markdown"
],
[
"code",
"code",
"code"
],
[
"markdown"
],
[
"code",
"code",
"code",
"code"
],
[
"markdown"
],
[
"code",
"code",
"code"
],
[
"markdown"
],
[
"code",
"code",
"code",
"code"
]
] |
e76a6ea68a1538f224fc89d8a86ac0cf51a80d24 | 449,991 | ipynb | Jupyter Notebook | notebooks/lagrange_interpolation.ipynb | jomorlier/FEM-Notes | 3b81053aee79dc59965c3622bc0d0eb6cfc7e8ae | [
"MIT"
] | 1 | 2020-04-15T01:53:14.000Z | 2020-04-15T01:53:14.000Z | notebooks/lagrange_interpolation.ipynb | jomorlier/FEM-Notes | 3b81053aee79dc59965c3622bc0d0eb6cfc7e8ae | [
"MIT"
] | null | null | null | notebooks/lagrange_interpolation.ipynb | jomorlier/FEM-Notes | 3b81053aee79dc59965c3622bc0d0eb6cfc7e8ae | [
"MIT"
] | 1 | 2020-05-25T17:19:53.000Z | 2020-05-25T17:19:53.000Z | 157.33951 | 257,427 | 0.838199 | [
[
[
"# One-dimensional Lagrange Interpolation",
"_____no_output_____"
],
[
"The problem of interpolation or finding the value of a function at an arbitrary point $X$ inside a given domain, provided we have discrete known values of the function inside the same domain is at the heart of the finite element method. In this notebooke we use Lagrange interpolation where the approximation $\\hat f(x)$ to the function $f(x)$ is built like:\n\n\\begin{equation}\n\\hat f(x)={L^I}(x)f^I\n\\end{equation}\n\nIn the expression above $L^I$ represents the $I$ Lagrange Polynomial of order $n-1$ and $f^1, f^2,,...,f^n$ are the $n$ known values of the function. Here we are using the summation convention over the repeated superscripts.\n\nThe $I$ Lagrange polynomial is given by the recursive expression:\n\n\\begin{equation}\n{L^I}(x)=\\prod_{J=1, J \\ne I}^{n}{\\frac{{\\left( {x - {x^J}} \\right)}}{{\\left( {{x^I} - {x^J}} \\right)}}} \n\\end{equation}\n\nin the domain $x\\in[-1.0,1.0]$.\n\nWe wish to interpolate the function $ f(x)=x^3+4x^2-10 $ assuming we know its value at points $x=-1.0$, $x=1.0$ and $x=0.0$.",
"_____no_output_____"
]
],
[
[
"from __future__ import division\nimport numpy as np\nfrom scipy import interpolate\nimport sympy as sym\nimport matplotlib.pyplot as plt\nfrom mpl_toolkits.mplot3d import Axes3D",
"_____no_output_____"
],
[
"%matplotlib notebook\n",
"_____no_output_____"
]
],
[
[
"First we use a function to generate the Lagrage polynomial of order $order$ at point $i$",
"_____no_output_____"
]
],
[
[
"def basis_lagrange(x_data, var, cont):\n \"\"\"Find the basis for the Lagrange interpolant\"\"\"\n prod = sym.prod((var - x_data[i])/(x_data[cont] - x_data[i])\n for i in range(len(x_data)) if i != cont)\n return sym.simplify(prod)",
"_____no_output_____"
]
],
[
[
"we now define the function $ f(x)=x^3+4x^2-10 $:",
"_____no_output_____"
]
],
[
[
"fun = lambda x: x**3 + 4*x**2 - 10",
"_____no_output_____"
],
[
"x = sym.symbols('x')\nx_data = np.array([-1, 1, 0])\nf_data = fun(x_data)",
"_____no_output_____"
]
],
[
[
"And obtain the Lagrange polynomials using:\n",
"_____no_output_____"
]
],
[
[
"basis = []\nfor cont in range(len(x_data)):\n basis.append(basis_lagrange(x_data, x, cont))\n sym.pprint(basis[cont])\n",
"xโ
(x - 1)\nโโโโโโโโโ\n 2 \nxโ
(x + 1)\nโโโโโโโโโ\n 2 \n 2 \n- x + 1\n"
]
],
[
[
"which are shown in the following plots/",
"_____no_output_____"
]
],
[
[
"npts = 101\nx_eval = np.linspace(-1, 1, npts)\nbasis_num = sym.lambdify((x), basis, \"numpy\") # Create a lambda function for the polynomials",
"_____no_output_____"
],
[
"plt.figure(figsize=(6, 4))\nfor k in range(3): \n y_eval = basis_num(x_eval)[k]\n plt.plot(x_eval, y_eval)",
"_____no_output_____"
],
[
"y_interp = sym.simplify(sum(f_data[k]*basis[k] for k in range(3)))\ny_interp",
"_____no_output_____"
]
],
[
[
"Now we plot the complete approximating polynomial, the actual function and the points where the function was known.",
"_____no_output_____"
]
],
[
[
"y_interp = sum(f_data[k]*basis_num(x_eval)[k] for k in range(3))\ny_original = fun(x_eval)\n\nplt.figure(figsize=(6, 4))\nplt.plot(x_eval, y_original)\nplt.plot(x_eval, y_interp)\nplt.plot([-1, 1, 0], f_data, 'ko')\nplt.show()",
"_____no_output_____"
]
],
[
[
"## Interpolation in 2 dimensions\n\nWe can extend the concept of Lagrange interpolation to 2 or more dimensions.\nIn the case of bilinear interpolation (2ร2, 4 vertices) in $[-1, 1]^2$,\nthe base functions are given by (**prove it**):\n\n\\begin{align}\nN_0 = \\frac{1}{4}(1 - x)(1 - y)\\\\\nN_1 = \\frac{1}{4}(1 + x)(1 - y)\\\\\nN_2 = \\frac{1}{4}(1 + x)(1 + y)\\\\\nN_3 = \\frac{1}{4}(1 - x)(1 + y)\n\\end{align}\n\nLet's see an example using piecewise bilinear interpolation.",
"_____no_output_____"
]
],
[
[
"def rect_grid(Lx, Ly, nx, ny):\n u\"\"\"Create a rectilinear grid for a rectangle\n \n The rectangle has dimensiones Lx by Ly. nx are \n the number of nodes in x, and ny are the number of nodes\n in y\n \"\"\"\n y, x = np.mgrid[-Ly/2:Ly/2:ny*1j, -Lx/2:Lx/2:nx*1j]\n els = np.zeros(((nx - 1)*(ny - 1), 4), dtype=int)\n for row in range(ny - 1):\n for col in range(nx - 1):\n cont = row*(nx - 1) + col\n els[cont, :] = [cont + row, cont + row + 1,\n cont + row + nx + 1, cont + row + nx]\n return x.flatten(), y.flatten(), els",
"_____no_output_____"
],
[
"def interp_bilinear(coords, f_vals, grid=(10, 10)):\n \"\"\"Piecewise bilinear interpolation for rectangular domains\"\"\"\n x_min, y_min = np.min(coords, axis=0)\n x_max, y_max = np.max(coords, axis=0)\n x, y = np.mgrid[-1:1:grid[0]*1j,-1:1:grid[1]*1j]\n N0 = (1 - x) * (1 - y)\n N1 = (1 + x) * (1 - y)\n N2 = (1 + x) * (1 + y)\n N3 = (1 - x) * (1 + y)\n interp_fun = N0 * f_vals[0] + N1 * f_vals[1] + N2 * f_vals[2] + N3 * f_vals[3]\n interp_fun = 0.25*interp_fun\n x, y = np.mgrid[x_min:x_max:grid[0]*1j, y_min:y_max:grid[1]*1j]\n return x, y, interp_fun",
"_____no_output_____"
],
[
"def fun(x, y):\n \"\"\"Monkey saddle function\"\"\"\n return y**3 + 3*y*x**2",
"_____no_output_____"
],
[
"x_coords, y_coords, els = rect_grid(2, 2, 4, 4)\nnels = els.shape[0]\nz_coords = fun(x_coords, y_coords)\nz_min = np.min(z_coords)\nz_max = np.max(z_coords)",
"_____no_output_____"
],
[
"fig = plt.figure(figsize=(6, 6))\nax = fig.add_subplot(111, projection='3d')\nx, y = np.mgrid[-1:1:51j,-1:1:51j]\nz = fun(x, y)\nsurf = ax.plot_surface(x, y, z, rstride=1, cstride=1, linewidth=0, alpha=0.6,\n cmap=\"viridis\")\nplt.colorbar(surf, shrink=0.5, aspect=10)\nax.plot(x_coords, y_coords, z_coords, 'ok')\nfor k in range(nels):\n x_vals = x_coords[els[k, :]]\n y_vals = y_coords[els[k, :]]\n coords = np.column_stack([x_vals, y_vals])\n f_vals = fun(x_vals, y_vals)\n x, y, z = interp_bilinear(coords, f_vals, grid=[4, 4])\n inter = ax.plot_wireframe(x, y, z, color=\"black\", cstride=3, rstride=3)\nplt.xlabel(r\"$x$\", fontsize=18)\nplt.ylabel(r\"$y$\", fontsize=18)\nax.legend([inter], [u\"Interpolation\"])\nplt.show();",
"_____no_output_____"
]
],
[
[
"<sub>The next cell change the format of the Notebook.</sub>",
"_____no_output_____"
]
],
[
[
"from IPython.core.display import HTML\ndef css_styling():\n styles = open('../styles/custom_barba.css', 'r').read()\n return HTML(styles)\ncss_styling()",
"_____no_output_____"
]
]
] | [
"markdown",
"code",
"markdown",
"code",
"markdown",
"code",
"markdown",
"code",
"markdown",
"code",
"markdown",
"code",
"markdown",
"code",
"markdown",
"code"
] | [
[
"markdown",
"markdown"
],
[
"code",
"code"
],
[
"markdown"
],
[
"code"
],
[
"markdown"
],
[
"code",
"code"
],
[
"markdown"
],
[
"code"
],
[
"markdown"
],
[
"code",
"code",
"code"
],
[
"markdown"
],
[
"code"
],
[
"markdown"
],
[
"code",
"code",
"code",
"code",
"code"
],
[
"markdown"
],
[
"code"
]
] |
e76a7f35ab84ebce637687ef330d887a8dc2cebc | 30,797 | ipynb | Jupyter Notebook | exercise-missing-values.ipynb | Kartik-Bhardwaj192/Machine-Learning-Intro-And-Intermediate | 107905e2e04085d12dec25a4c8fd07c9fce3c0a1 | [
"MIT"
] | null | null | null | exercise-missing-values.ipynb | Kartik-Bhardwaj192/Machine-Learning-Intro-And-Intermediate | 107905e2e04085d12dec25a4c8fd07c9fce3c0a1 | [
"MIT"
] | null | null | null | exercise-missing-values.ipynb | Kartik-Bhardwaj192/Machine-Learning-Intro-And-Intermediate | 107905e2e04085d12dec25a4c8fd07c9fce3c0a1 | [
"MIT"
] | null | null | null | 30,797 | 30,797 | 0.662662 | [
[
[
"**This notebook is an exercise in the [Intermediate Machine Learning](https://www.kaggle.com/learn/intermediate-machine-learning) course. You can reference the tutorial at [this link](https://www.kaggle.com/alexisbcook/missing-values).**\n\n---\n",
"_____no_output_____"
],
[
"Now it's your turn to test your new knowledge of **missing values** handling. You'll probably find it makes a big difference.\n\n# Setup\n\nThe questions will give you feedback on your work. Run the following cell to set up the feedback system.",
"_____no_output_____"
]
],
[
[
"# Set up code checking\nimport os\nif not os.path.exists(\"../input/train.csv\"):\n os.symlink(\"../input/home-data-for-ml-course/train.csv\", \"../input/train.csv\") \n os.symlink(\"../input/home-data-for-ml-course/test.csv\", \"../input/test.csv\") \nfrom learntools.core import binder\nbinder.bind(globals())\nfrom learntools.ml_intermediate.ex2 import *\nprint(\"Setup Complete\")",
"Setup Complete\n"
]
],
[
[
"In this exercise, you will work with data from the [Housing Prices Competition for Kaggle Learn Users](https://www.kaggle.com/c/home-data-for-ml-course). \n\n\n\nRun the next code cell without changes to load the training and validation sets in `X_train`, `X_valid`, `y_train`, and `y_valid`. The test set is loaded in `X_test`.",
"_____no_output_____"
]
],
[
[
"import pandas as pd\nfrom sklearn.model_selection import train_test_split\n\n# Read the data\nX_full = pd.read_csv('../input/train.csv', index_col='Id')\nX_test_full = pd.read_csv('../input/test.csv', index_col='Id')\n\n# Remove rows with missing target, separate target from predictors\nX_full.dropna(axis=0, subset=['SalePrice'], inplace=True)\ny = X_full.SalePrice\nX_full.drop(['SalePrice'], axis=1, inplace=True)\n\n# To keep things simple, we'll use only numerical predictors\nX = X_full.select_dtypes(exclude=['object'])\nX_test = X_test_full.select_dtypes(exclude=['object'])\n\n# Break off validation set from training data\nX_train, X_valid, y_train, y_valid = train_test_split(X, y, train_size=0.8, test_size=0.2,\n random_state=0)",
"_____no_output_____"
]
],
[
[
"Use the next code cell to print the first five rows of the data.",
"_____no_output_____"
]
],
[
[
"X_train.head()",
"_____no_output_____"
]
],
[
[
"You can already see a few missing values in the first several rows. In the next step, you'll obtain a more comprehensive understanding of the missing values in the dataset.\n\n# Step 1: Preliminary investigation\n\nRun the code cell below without changes.",
"_____no_output_____"
]
],
[
[
"# Shape of training data (num_rows, num_columns)\nprint(X_train.shape)\n\n# Number of missing values in each column of training data\nmissing_val_count_by_column = (X_train.isnull().sum())\nprint(missing_val_count_by_column[missing_val_count_by_column > 0])\n#print(X_train.isnull().sum(axis=0))",
"(1168, 36)\nLotFrontage 212\nMasVnrArea 6\nGarageYrBlt 58\ndtype: int64\n"
]
],
[
[
"### Part A\n\nUse the above output to answer the questions below.",
"_____no_output_____"
]
],
[
[
"# Fill in the line below: How many rows are in the training data?\nnum_rows = 1168\n\n# Fill in the line below: How many columns in the training data\n# have missing values?\nnum_cols_with_missing = 3\n\n# Fill in the line below: How many missing entries are contained in \n# all of the training data?\ntot_missing = 276\n\n# Check your answers\nstep_1.a.check()",
"_____no_output_____"
],
[
"# Lines below will give you a hint or solution code\n#step_1.a.hint()\n#step_1.a.solution()",
"_____no_output_____"
]
],
[
[
"### Part B\nConsidering your answers above, what do you think is likely the best approach to dealing with the missing values?",
"_____no_output_____"
]
],
[
[
"# Check your answer (Run this code cell to receive credit!)\nstep_1.b.check()",
"_____no_output_____"
],
[
"step_1.b.hint()",
"_____no_output_____"
]
],
[
[
"To compare different approaches to dealing with missing values, you'll use the same `score_dataset()` function from the tutorial. This function reports the [mean absolute error](https://en.wikipedia.org/wiki/Mean_absolute_error) (MAE) from a random forest model.",
"_____no_output_____"
]
],
[
[
"from sklearn.ensemble import RandomForestRegressor\nfrom sklearn.metrics import mean_absolute_error\n\n# Function for comparing different approaches\ndef score_dataset(X_train, X_valid, y_train, y_valid):\n model = RandomForestRegressor(n_estimators=100, random_state=0)\n model.fit(X_train, y_train)\n preds = model.predict(X_valid)\n return mean_absolute_error(y_valid, preds)",
"_____no_output_____"
]
],
[
[
"# Step 2: Drop columns with missing values\n\nIn this step, you'll preprocess the data in `X_train` and `X_valid` to remove columns with missing values. Set the preprocessed DataFrames to `reduced_X_train` and `reduced_X_valid`, respectively. ",
"_____no_output_____"
]
],
[
[
"# Fill in the line below: get names of columns with missing values\nmissing_col_names = ['LotFrontage','MasVnrArea','GarageYrBlt'] # Your code here\ninclude_column_names = [cols for cols in X_train.columns \n if cols not in missing_col_names]\n# Fill in the lines below: drop columns in training and validation data\nreduced_X_train = X_train[include_column_names]\nreduced_X_valid = X_valid[include_column_names]\n#print(reduced_X_train)\n# Check your answers\nstep_2.check()",
"_____no_output_____"
],
[
"# Lines below will give you a hint or solution code\n#step_2.hint()\nstep_2.solution()",
"_____no_output_____"
]
],
[
[
"Run the next code cell without changes to obtain the MAE for this approach.",
"_____no_output_____"
]
],
[
[
"print(\"MAE (Drop columns with missing values):\")\nprint(score_dataset(reduced_X_train, reduced_X_valid, y_train, y_valid))",
"MAE (Drop columns with missing values):\n17837.82570776256\n"
]
],
[
[
"# Step 3: Imputation\n\n### Part A\n\nUse the next code cell to impute missing values with the mean value along each column. Set the preprocessed DataFrames to `imputed_X_train` and `imputed_X_valid`. Make sure that the column names match those in `X_train` and `X_valid`.",
"_____no_output_____"
]
],
[
[
"from sklearn.impute import SimpleImputer\n\n# Fill in the lines below: imputation\n # Your code here \nmyimputer = SimpleImputer()\nimputed_X_train = pd.DataFrame(myimputer.fit_transform(X_train))\nimputed_X_valid = pd.DataFrame(myimputer.transform(X_valid))\n\n# Fill in the lines below: imputation removed column names; put them back\nimputed_X_train.columns = X_train.columns\nimputed_X_valid.columns = X_valid.columns\n\n# Check your answers\nstep_3.a.check()",
"_____no_output_____"
],
[
"# Lines below will give you a hint or solution code\n#step_3.a.hint()\n#step_3.a.solution()",
"_____no_output_____"
]
],
[
[
"Run the next code cell without changes to obtain the MAE for this approach.",
"_____no_output_____"
]
],
[
[
"print(\"MAE (Imputation):\")\nprint(score_dataset(imputed_X_train, imputed_X_valid, y_train, y_valid))",
"MAE (Imputation):\n18062.894611872147\n"
]
],
[
[
"### Part B\n\nCompare the MAE from each approach. Does anything surprise you about the results? Why do you think one approach performed better than the other?",
"_____no_output_____"
]
],
[
[
"# Check your answer (Run this code cell to receive credit!)\nstep_3.b.check()",
"_____no_output_____"
],
[
"#step_3.b.hint()",
"_____no_output_____"
]
],
[
[
"# Step 4: Generate test predictions\n\nIn this final step, you'll use any approach of your choosing to deal with missing values. Once you've preprocessed the training and validation features, you'll train and evaluate a random forest model. Then, you'll preprocess the test data before generating predictions that can be submitted to the competition!\n\n### Part A\n\nUse the next code cell to preprocess the training and validation data. Set the preprocessed DataFrames to `final_X_train` and `final_X_valid`. **You can use any approach of your choosing here!** in order for this step to be marked as correct, you need only ensure:\n- the preprocessed DataFrames have the same number of columns,\n- the preprocessed DataFrames have no missing values, \n- `final_X_train` and `y_train` have the same number of rows, and\n- `final_X_valid` and `y_valid` have the same number of rows.",
"_____no_output_____"
]
],
[
[
"# Preprocessed training and validation features\nfinal_X_train = reduced_X_train\nfinal_X_valid = reduced_X_valid\n\n# Check your answers\nstep_4.a.check()",
"_____no_output_____"
],
[
"# Lines below will give you a hint or solution code\n#step_4.a.hint()\n#step_4.a.solution()",
"_____no_output_____"
]
],
[
[
"Run the next code cell to train and evaluate a random forest model. (*Note that we don't use the `score_dataset()` function above, because we will soon use the trained model to generate test predictions!*)",
"_____no_output_____"
]
],
[
[
"# Define and fit model\nmodel = RandomForestRegressor(n_estimators=100, random_state=0)\nmodel.fit(final_X_train, y_train)\n\n# Get validation predictions and MAE\npreds_valid = model.predict(final_X_valid)\nprint(\"MAE (Your approach):\")\nprint(mean_absolute_error(y_valid, preds_valid))",
"MAE (Your approach):\n17837.82570776256\n"
]
],
[
[
"### Part B\n\nUse the next code cell to preprocess your test data. Make sure that you use a method that agrees with how you preprocessed the training and validation data, and set the preprocessed test features to `final_X_test`.\n\nThen, use the preprocessed test features and the trained model to generate test predictions in `preds_test`.\n\nIn order for this step to be marked correct, you need only ensure:\n- the preprocessed test DataFrame has no missing values, and\n- `final_X_test` has the same number of rows as `X_test`.",
"_____no_output_____"
]
],
[
[
"# Fill in the line below: preprocess test data\nfinal_X_test = X_test[include_column_names]\n\n# Fill in the line below: get test predictions\nimputed_final_X_test = pd.DataFrame(myimputer.fit_transform(final_X_test))\nimputed_final_X_test.columns = final_X_test.columns\nfinal_X_test = imputed_final_X_test\npreds_test = model.predict(final_X_test)\n\n# Check your answers\nstep_4.b.check()",
"_____no_output_____"
],
[
"# Lines below will give you a hint or solution code\n#step_4.b.hint()\n#step_4.b.solution()",
"_____no_output_____"
]
],
[
[
"Run the next code cell without changes to save your results to a CSV file that can be submitted directly to the competition.",
"_____no_output_____"
]
],
[
[
"# Save test predictions to file\noutput = pd.DataFrame({'Id': X_test.index,\n 'SalePrice': preds_test})\noutput.to_csv('submission.csv', index=False)",
"_____no_output_____"
]
],
[
[
"# Submit your results\n\nOnce you have successfully completed Step 4, you're ready to submit your results to the leaderboard! (_You also learned how to do this in the previous exercise. If you need a reminder of how to do this, please use the instructions below._) \n\nFirst, you'll need to join the competition if you haven't already. So open a new window by clicking on [this link](https://www.kaggle.com/c/home-data-for-ml-course). Then click on the **Join Competition** button.\n\n\n\nNext, follow the instructions below:\n1. Begin by clicking on the blue **Save Version** button in the top right corner of the window. This will generate a pop-up window. \n2. Ensure that the **Save and Run All** option is selected, and then click on the blue **Save** button.\n3. This generates a window in the bottom left corner of the notebook. After it has finished running, click on the number to the right of the **Save Version** button. This pulls up a list of versions on the right of the screen. Click on the ellipsis **(...)** to the right of the most recent version, and select **Open in Viewer**. This brings you into view mode of the same page. You will need to scroll down to get back to these instructions.\n4. Click on the **Output** tab on the right of the screen. Then, click on the file you would like to submit, and click on the blue **Submit** button to submit your results to the leaderboard.\n\nYou have now successfully submitted to the competition!\n\nIf you want to keep working to improve your performance, select the blue **Edit** button in the top right of the screen. Then you can change your code and repeat the process. There's a lot of room to improve, and you will climb up the leaderboard as you work.\n\n\n# Keep going\n\nMove on to learn what **[categorical variables](https://www.kaggle.com/alexisbcook/categorical-variables)** are, along with how to incorporate them into your machine learning models. Categorical variables are very common in real-world data, but you'll get an error if you try to plug them into your models without processing them first!",
"_____no_output_____"
],
[
"---\n\n\n\n\n*Have questions or comments? Visit the [Learn Discussion forum](https://www.kaggle.com/learn-forum/161289) to chat with other Learners.*",
"_____no_output_____"
]
]
] | [
"markdown",
"code",
"markdown",
"code",
"markdown",
"code",
"markdown",
"code",
"markdown",
"code",
"markdown",
"code",
"markdown",
"code",
"markdown",
"code",
"markdown",
"code",
"markdown",
"code",
"markdown",
"code",
"markdown",
"code",
"markdown",
"code",
"markdown",
"code",
"markdown",
"code",
"markdown",
"code",
"markdown"
] | [
[
"markdown",
"markdown"
],
[
"code"
],
[
"markdown"
],
[
"code"
],
[
"markdown"
],
[
"code"
],
[
"markdown"
],
[
"code"
],
[
"markdown"
],
[
"code",
"code"
],
[
"markdown"
],
[
"code",
"code"
],
[
"markdown"
],
[
"code"
],
[
"markdown"
],
[
"code",
"code"
],
[
"markdown"
],
[
"code"
],
[
"markdown"
],
[
"code",
"code"
],
[
"markdown"
],
[
"code"
],
[
"markdown"
],
[
"code",
"code"
],
[
"markdown"
],
[
"code",
"code"
],
[
"markdown"
],
[
"code"
],
[
"markdown"
],
[
"code",
"code"
],
[
"markdown"
],
[
"code"
],
[
"markdown",
"markdown"
]
] |
e76a8166e34404fb671b28c011c25a12683c2a40 | 4,227 | ipynb | Jupyter Notebook | isye_6501_sim_hw/aarti_solution/Question 13.2.ipynb | oskrgab/isye-6644_project | acc3c6a3f2b4c80f834851243c8671e5da6c7f67 | [
"Apache-2.0"
] | null | null | null | isye_6501_sim_hw/aarti_solution/Question 13.2.ipynb | oskrgab/isye-6644_project | acc3c6a3f2b4c80f834851243c8671e5da6c7f67 | [
"Apache-2.0"
] | null | null | null | isye_6501_sim_hw/aarti_solution/Question 13.2.ipynb | oskrgab/isye-6644_project | acc3c6a3f2b4c80f834851243c8671e5da6c7f67 | [
"Apache-2.0"
] | 1 | 2021-06-14T14:06:15.000Z | 2021-06-14T14:06:15.000Z | 29.767606 | 98 | 0.518098 | [
[
[
"**Installing the packages**",
"_____no_output_____"
]
],
[
[
"#!pip install simpy\nimport random\nimport simpy as sy",
"_____no_output_____"
]
],
[
[
"**Intializing the variable and creating the simulation**",
"_____no_output_____"
]
],
[
[
"#Declaring the variables\n\nnum_checkers = 10 # Number of Checkers \nnum_scanners = 5 #Number of scanners \nwait_time = 0 #Initial Waiting Time set to 0\ntotal_pax = 1 #Total number of passengers initialized to 1\nnum_pax = 100 #Overall Passengers set to 100\nruntime = 500 #End simulation when runtime crosses 500 mins\n\narrival_rate = 50 #To simulate a busy airport\ncheck_rate = 0.75 #As mentioned in the \n\nclass Airport(object):\n def __init__(self, env, num_checkers, num_scanners):\n self.env = env\n self.checker = sy.Resource(env, num_checkers) #Number of boarding pass checkers \n self.scanners = []\n for i in range(0, num_scanners): #Number of scanners \n self.scanners.append(sy.Resource(env))\n \n def BP_check(self, pax):\n service_time = random.expovariate(1/check_rate)\n yield self.env.timeout(service_time)\n \n def scan(self, pax):\n scan_time = random.uniform(0.5, 1)\n yield self.env.timeout(scan_time)\n \n def Passenger(self, env, number):\n global wait_time #global average wait time\n global total_pax \n arrival_time = env.now\n scan_queue = [] #Every scanner has its own queue\n \n with self.checker.request() as request:\n yield request\n yield env.process(self.BP_check(number))\n \n for scanner in self.scanners:\n scan_queue.append(len(scanner.queue)) #getting the length of each scanner \n \n #Find the shortest scanner queue\n min_index = min(scan_queue)\n short_queue_index = scan_queue.index(min_index)\n \n with self.scanners[short_queue_index].request() as request:\n yield request\n yield env.process(self.scan(number))\n \n exit_time = env.now\n wait_time += (exit_time - arrival_time)\n total_pax += 1\n\n def setup(self, env, num_pax):\n yield env.timeout(random.expovariate(arrival_rate)) \n env.process(self.Passenger(env, num_pax))\n \n \n#Running the simulation\nenv = sy.Environment()\napi = Airport(env, num_checkers, num_scanners)\n\nfor i in range(0,num_pax):\n env.process(api.setup(env, i))\n\nenv.run(until = runtime)\navg_wait_time = wait_time / total_pax\n\nprint(\"Avg waiting time is %f\" %avg_wait_time)\n",
"Avg waiting time is 8.104722\n"
]
]
] | [
"markdown",
"code",
"markdown",
"code"
] | [
[
"markdown"
],
[
"code"
],
[
"markdown"
],
[
"code"
]
] |
e76a8bba95f06b9355848084078c720e520a85dc | 58,591 | ipynb | Jupyter Notebook | doc/example/conversion_reaction.ipynb | LarsFroehling/pyPESTO | f9f4a526c0f34cda2c2670b7d61d4f9872a8e368 | [
"BSD-3-Clause"
] | null | null | null | doc/example/conversion_reaction.ipynb | LarsFroehling/pyPESTO | f9f4a526c0f34cda2c2670b7d61d4f9872a8e368 | [
"BSD-3-Clause"
] | null | null | null | doc/example/conversion_reaction.ipynb | LarsFroehling/pyPESTO | f9f4a526c0f34cda2c2670b7d61d4f9872a8e368 | [
"BSD-3-Clause"
] | null | null | null | 194.009934 | 22,460 | 0.913092 | [
[
[
"Conversion reaction\n===================",
"_____no_output_____"
]
],
[
[
"import importlib\nimport os\nimport sys\nimport numpy as np\nimport amici\nimport amici.plotting\nimport pypesto\n\n# sbml file we want to import\nsbml_file = 'conversion_reaction/model_conversion_reaction.xml'\n# name of the model that will also be the name of the python module\nmodel_name = 'model_conversion_reaction'\n# directory to which the generated model code is written\nmodel_output_dir = 'tmp/' + model_name",
"_____no_output_____"
]
],
[
[
"## Compile AMICI model",
"_____no_output_____"
]
],
[
[
"# import sbml model, compile and generate amici module\nsbml_importer = amici.SbmlImporter(sbml_file)\nsbml_importer.sbml2amici(model_name,\n model_output_dir,\n verbose=False)",
"_____no_output_____"
]
],
[
[
"## Load AMICI model",
"_____no_output_____"
]
],
[
[
"# load amici module (the usual starting point later for the analysis)\nsys.path.insert(0, os.path.abspath(model_output_dir))\nmodel_module = importlib.import_module(model_name)\nmodel = model_module.getModel()\nmodel.requireSensitivitiesForAllParameters()\nmodel.setTimepoints(amici.DoubleVector(np.linspace(0, 10, 11)))\nmodel.setParameterScale(amici.ParameterScaling_log10)\nmodel.setParameters(amici.DoubleVector([-0.3,-0.7]))\nsolver = model.getSolver()\nsolver.setSensitivityMethod(amici.SensitivityMethod_forward)\nsolver.setSensitivityOrder(amici.SensitivityOrder_first)\n\n# how to run amici now:\nrdata = amici.runAmiciSimulation(model, solver, None)\namici.plotting.plotStateTrajectories(rdata)\nedata = amici.ExpData(rdata, 0.2, 0.0)",
"_____no_output_____"
]
],
[
[
"## Optimize",
"_____no_output_____"
]
],
[
[
"# create objective function from amici model\n# pesto.AmiciObjective is derived from pesto.Objective, \n# the general pesto objective function class\nobjective = pypesto.AmiciObjective(model, solver, [edata], 1)\n\n# create optimizer object which contains all information for doing the optimization\noptimizer = pypesto.ScipyOptimizer(method='ls_trf')\n\n#optimizer.solver = 'bfgs|meigo'\n# if select meigo -> also set default values in solver_options\n#optimizer.options = {'maxiter': 1000, 'disp': True} # = pesto.default_options_meigo()\n#optimizer.startpoints = []\n#optimizer.startpoint_method = 'lhs|uniform|something|function'\n#optimizer.n_starts = 100\n\n# see PestoOptions.m for more required options here\n# returns OptimizationResult, see parameters.MS for what to return\n# list of final optim results foreach multistart, times, hess, grad, \n# flags, meta information (which optimizer -> optimizer.get_repr())\n\n# create problem object containing all information on the problem to be solved\nproblem = pypesto.Problem(objective=objective, \n lb=[-2,-2], ub=[2,2])\n\n# maybe lb, ub = inf\n# other constraints: kwargs, class pesto.Constraints\n# constraints on pams, states, esp. pesto.AmiciConstraints (e.g. pam1 + pam2<= const)\n# if optimizer cannot handle -> error\n# maybe also scaling / transformation of parameters encoded here\n\n# do the optimization\nresult = pypesto.minimize(problem=problem, \n optimizer=optimizer, \n n_starts=10)\n# optimize is a function since it does not need an internal memory,\n# just takes input and returns output in the form of a Result object\n# 'result' parameter: e.g. some results from somewhere -> pick best start points",
"_____no_output_____"
]
],
[
[
"## Visualize",
"_____no_output_____"
]
],
[
[
"# waterfall, parameter space, scatter plots, fits to data\n# different functions for different plotting types\nimport pypesto.visualize\n\npypesto.visualize.waterfall(result)\npypesto.visualize.parameters(result)",
"_____no_output_____"
]
],
[
[
"## Data storage",
"_____no_output_____"
]
],
[
[
"# result = pypesto.storage.load('db_file.db')",
"_____no_output_____"
]
],
[
[
"## Profiles",
"_____no_output_____"
]
],
[
[
"# there are three main parts: optimize, profile, sample. the overall structure of profiles and sampling\n# will be similar to optimizer like above.\n# we intend to only have just one result object which can be reused everywhere, but the problem of how to \n# not have one huge class but\n# maybe simplified views on it for optimization, profiles and sampling is still to be solved\n\n# profiler = pypesto.Profiler()\n\n# result = pypesto.profile(problem, profiler, result=None)\n# possibly pass result object from optimization to get good parameter guesses",
"_____no_output_____"
]
],
[
[
"## Sampling",
"_____no_output_____"
]
],
[
[
"# sampler = pypesto.Sampler()\n\n# result = pypesto.sample(problem, sampler, result=None)",
"_____no_output_____"
],
[
"# open: how to parallelize. the idea is to use methods similar to those in pyabc for working on clusters.\n# one way would be to specify an additional 'engine' object passed to optimize(), profile(), sample(),\n# which in the default setting just does a for loop, but can also be customized.",
"_____no_output_____"
]
]
] | [
"markdown",
"code",
"markdown",
"code",
"markdown",
"code",
"markdown",
"code",
"markdown",
"code",
"markdown",
"code",
"markdown",
"code",
"markdown",
"code"
] | [
[
"markdown"
],
[
"code"
],
[
"markdown"
],
[
"code"
],
[
"markdown"
],
[
"code"
],
[
"markdown"
],
[
"code"
],
[
"markdown"
],
[
"code"
],
[
"markdown"
],
[
"code"
],
[
"markdown"
],
[
"code"
],
[
"markdown"
],
[
"code",
"code"
]
] |
e76a999b4ec95dfe7c0772d0a118eb7036b1287b | 26,363 | ipynb | Jupyter Notebook | cmp_cmp/ModelPredict_plot.ipynb | 3upperm2n/block_trace_analyzer | 2e786d316679fc329310785722e140d7ee8d7f58 | [
"MIT"
] | null | null | null | cmp_cmp/ModelPredict_plot.ipynb | 3upperm2n/block_trace_analyzer | 2e786d316679fc329310785722e140d7ee8d7f58 | [
"MIT"
] | null | null | null | cmp_cmp/ModelPredict_plot.ipynb | 3upperm2n/block_trace_analyzer | 2e786d316679fc329310785722e140d7ee8d7f58 | [
"MIT"
] | null | null | null | 48.640221 | 13,452 | 0.684406 | [
[
[
"import warnings\nimport pandas as pd\nimport numpy as np\nimport matplotlib.pyplot as plt\n\nwarnings.filterwarnings(\"ignore\", category=np.VisibleDeprecationWarning)",
"_____no_output_____"
],
[
"df_trace = pd.read_csv('model_results.csv')",
"_____no_output_____"
],
[
"df_trace.head()",
"_____no_output_____"
],
[
"df_sorted = df_trace.sort_values(['datasize'], ascending=True)",
"_____no_output_____"
],
[
"df_sorted.head()",
"_____no_output_____"
],
[
"datasize = []\nreal_ls = []\npred_ls = []\n\n# select the 1st 10 samples\ncount = 0\nfor index,row in df_sorted.iterrows():\n datasize.append(float(row['datasize']))\n real_ls.append(float(row['real']))\n pred_ls.append(float(row['model']))\n \n count += 1\n if count == 20:\n break",
"_____no_output_____"
],
[
"print datasize[0]\nprint real_ls[0]\nprint pred_ls[0]",
"40000.0\n0.100801\n0.0924000000001\n"
],
[
"n_groups = len(datasize)\n\n# create plot\nfig, ax = plt.subplots()\nindex = np.arange(n_groups)\nbar_width = 0.3\nopacity = 0.8\n\nbar1 = plt.bar(index, real_ls, bar_width, alpha=opacity, color='b', label='Real')\nbar2 = plt.bar(index + bar_width, pred_ls, bar_width, alpha=opacity, color='g', label='Pred')\n\nplt.tick_params(\n axis='x', # changes apply to the x-axis\n which='both', # both major and minor ticks are affected\n bottom='off', # ticks along the bottom edge are off\n top='off', # ticks along the top edge are off\n labelbottom='off') # labels along the bottom edge are off\n\n\nplt.xlabel('Test Cases')\nplt.ylabel('Runtime (ms)')\nplt.title('Compare Model Predicted Runtime with Real Runtime for Two CKE')\n\nplt.legend(prop={'size':9})\n \nplt.tight_layout()\nplt.show()",
"_____no_output_____"
]
],
[
[
"### compute the error rate",
"_____no_output_____"
]
],
[
[
"df_sorted.head()",
"_____no_output_____"
],
[
"# df_sorted['diff'] = df_sorted['real'] - df_sorted['model']\ndf_sorted['error'] = abs(df_sorted['real'] - df_sorted['model'] )/ df_sorted['real'] ",
"_____no_output_____"
],
[
"df_sorted.head()",
"_____no_output_____"
],
[
"df_sorted['error'].mean()",
"_____no_output_____"
],
[
"df_sorted['error'].std()",
"_____no_output_____"
],
[
"df_sorted.shape",
"_____no_output_____"
]
]
] | [
"code",
"markdown",
"code"
] | [
[
"code",
"code",
"code",
"code",
"code",
"code",
"code",
"code"
],
[
"markdown"
],
[
"code",
"code",
"code",
"code",
"code",
"code"
]
] |
e76ab79bf50e5c9a1be146edb08010e2db07125d | 867,116 | ipynb | Jupyter Notebook | Lectures/Lecture_5/Pokemon.ipynb | lev1khachatryan/DataVisualization | 80f13ee56e6808bf076b7fc7fa5f0d61d80a42bc | [
"MIT"
] | 1 | 2020-05-19T01:41:20.000Z | 2020-05-19T01:41:20.000Z | Lectures/Lecture_5/Pokemon.ipynb | lev1khachatryan/DataVisualization | 80f13ee56e6808bf076b7fc7fa5f0d61d80a42bc | [
"MIT"
] | null | null | null | Lectures/Lecture_5/Pokemon.ipynb | lev1khachatryan/DataVisualization | 80f13ee56e6808bf076b7fc7fa5f0d61d80a42bc | [
"MIT"
] | null | null | null | 32.624102 | 30,885 | 0.42535 | [
[
[
"# Plot types by function\n\n1. Comparison\n2. Proportion\n3. Relationship\n4. Part to a whole\n5. Distribution\n6. Change over time",
"_____no_output_____"
]
],
[
[
"import numpy as np \nimport pandas as pd \nimport seaborn as sns\nfrom plotly import tools\nimport plotly.plotly as py\nfrom plotly.offline import init_notebook_mode,iplot\ninit_notebook_mode(connected=True)\nimport plotly.graph_objs as go\nimport plotly.figure_factory as ff\nimport matplotlib.pyplot as plt",
"_____no_output_____"
],
[
"data = pd.read_csv(\"Pokemon.csv\")",
"_____no_output_____"
],
[
"print(data.shape)",
"(800, 13)\n"
],
[
"data.head()",
"_____no_output_____"
],
[
"data.isna().sum()",
"_____no_output_____"
],
[
"fig = ff.create_distplot([data.HP],['HP'],bin_size=5)\niplot(fig, filename='Basic Distplot')",
"_____no_output_____"
],
[
"hist_data = [data['Attack'],data['Defense']]\ngroup_labels = ['Attack','Defense']\n\nfig = ff.create_distplot(hist_data, group_labels, bin_size=5, show_hist=False, show_rug=False)\niplot(fig, filename='Distplot of attack and defense')",
"_____no_output_____"
],
[
"trace0 = go.Box(y=data[\"HP\"],name=\"HP\", boxmean=True)\ntrace1 = go.Box(y=data[\"Attack\"],name=\"Attack\", boxmean=True)\ntrace2 = go.Box(y=data[\"Defense\"],name=\"Defense\", boxmean=True)\ntrace3 = go.Box(y=data[\"Sp. Atk\"],name=\"Sp. Atk\", boxmean=True)\ntrace4 = go.Box(y=data[\"Sp. Def\"],name=\"Sp. Def\", boxmean=True)\ntrace5 = go.Box(y=data[\"Speed\"],name=\"Speed\", boxmean=True)\ndat = [trace0, trace1, trace2,trace3, trace4, trace5]\niplot(dat)",
"_____no_output_____"
],
[
"feats = ['HP','Attack','Defense','Sp. Atk','Sp. Def','Speed']",
"_____no_output_____"
],
[
"x = data[data[\"Name\"] == \"Charizard\"]\nt1 = go.Scatterpolar(theta = feats,\n r = [x[i].values[0] for i in feats], fill = 'toself', name = x.Name.values[0])\n\nlayout = go.Layout(\n polar = dict(\n radialaxis = dict(\n visible = True,\n range = [0, 255]\n )\n ),\n showlegend = True,\n title = \"Stats of {}\".format(x.Name.values[0])\n)\ndat = [t1]\nfig = go.Figure(data=dat, layout=layout)\niplot(fig)",
"_____no_output_____"
],
[
"x = data[data[\"Name\"] == \"Charizard\"]\nt1 = go.Scatterpolar(theta = feats,\n r = [x[i].values[0] for i in feats], fill = 'toself', name = x.Name.values[0])\n\ny = data[data[\"Name\"] == \"Pikachu\"]\nt2 = go.Scatterpolar(theta = feats,\n r = [y[i].values[0] for i in feats], fill = 'toself', name = y.Name.values[0])\n\nlayout = go.Layout(\n polar = dict(\n radialaxis = dict(\n visible = True,\n range = [0, 255]\n )\n ),\n showlegend = True,\n title = \"{} vs {}\".format(x.Name.values[0],y.Name.values[0])\n)\ndat = [t1, t2]\nfig = go.Figure(data=dat, layout=layout)\niplot(fig)",
"_____no_output_____"
],
[
"t1 = go.Scatter(\n x = data[\"Defense\"],\n y = data[\"Attack\"],\n mode='markers',\n marker=dict(\n size=10\n ),\n text=data[\"Name\"]\n)\ndat = [t1]\nlayout = go.Layout(\n showlegend = True,\n font=dict(family='Courier New, monospace', size=10, color='#ffffff'),\n title=\"Scatter plot of Defense vs Attack with Speed as colorscale\",\n xaxis = dict(showgrid = True),yaxis = dict(showgrid = True)\n)\nfig = go.Figure(data=dat, layout=layout)\niplot(fig, filename = \"Scatterplot\")",
"_____no_output_____"
],
[
"t1 = go.Scatter(\n x = data[\"Defense\"],\n y = data[\"Attack\"],\n mode='markers',\n marker=dict(\n size = data[\"Speed\"]/10,\n ),\n text=data[\"Name\"]\n)\ndat = [t1]\nlayout = go.Layout(\n showlegend = True,\n font=dict(family='Courier New, monospace', size=10, color='#ffffff'),\n title=\"Scatter plot of Defense vs Attack with Speed as colorscale\",\n xaxis = dict(showgrid = True),yaxis = dict(showgrid = True)\n)\nfig = go.Figure(data=dat, layout=layout)\niplot(fig, filename = \"Scatterplot\")",
"_____no_output_____"
],
[
"t1 = go.Scatter(\n x = data[\"Defense\"],\n y = data[\"Attack\"],\n mode='markers',\n marker=dict(\n size=10,\n color = data[\"Speed\"],\n showscale=True\n ),\n text=data[\"Name\"]\n)\ndat = [t1]\nlayout = go.Layout(\n showlegend = True,\n font=dict(family='Courier New, monospace', size=10, color='#ffffff'),\n title=\"Scatter plot of Defense vs Attack with Speed as colorscale\",\n xaxis = dict(showgrid = True),yaxis = dict(showgrid = True)\n)\nfig = go.Figure(data=dat, layout=layout)\niplot(fig, filename = \"Scatterplot\")",
"_____no_output_____"
],
[
"t = go.Scatter3d(\n x=data[\"Speed\"],\n y=data[\"Attack\"],\n z=data[\"Defense\"],\n mode='markers',\n marker=dict(\n size=3,\n line=dict(\n color='rgba(217, 217, 217, 0.14)',\n width=0.5\n ),\n opacity=1\n )\n)\ndat = [t]\nlayout = go.Layout(\n margin=dict(\n l=0,\n r=0,\n b=0,\n t=0\n ),\n xaxis=dict(title=\"Speed\"),\n yaxis=dict(title=\"Attack\"),\n title = \"Speed vs Attack vs Defense\"\n)\nfig = go.Figure(data=dat, layout=layout)\niplot(fig, filename='3d-scatter')",
"_____no_output_____"
],
[
"legendary_grouped = data.groupby(['Legendary', 'Generation']).mean()[['Attack', 'Defense', \"Sp. Atk\",\n \"Sp. Def\", \"Speed\"]]",
"_____no_output_____"
],
[
"names = [\"False\" + \"_\" + str(i) for i in range(1,7)]",
"_____no_output_____"
],
[
"names.extend([\"True\" + \"_\" + str(i) for i in range(1,7)])",
"_____no_output_____"
],
[
"t1 = go.Bar(x=names, y=legendary_grouped.Attack, name=\"Attack\")\nt2 = go.Bar(x=names, y=legendary_grouped.Defense, name=\"Defense\")\n\n#layout = dict(barmode = 'group')\nlayout = dict(barmode = 'stack')\n\ndat = [t1, t2]\nfigure = dict(data=dat,layout=layout)\niplot(figure)",
"_____no_output_____"
],
[
"t1 = go.Bar(x=names, y=legendary_grouped.Attack, name=\"Attack\")\nt2 = go.Bar(x=names, y=legendary_grouped.Defense, name=\"Defense\")\n\nlayout = dict(barmode = 'group')\n#layout = dict(barmode = 'stack')\n\ndat = [t1, t2]\nfigure = dict(data=dat,layout=layout)\niplot(figure)",
"_____no_output_____"
],
[
"t1 = go.Bar(x=names, y=legendary_grouped.Attack, name=\"Attack\")\nt2 = go.Bar(x=names, y=legendary_grouped.Defense, name=\"Defense\")\n\ndat = [t1, t2]\nfigure = tools.make_subplots(rows=2, cols=1, subplot_titles=('Plot 1', 'Plot 2'))\n\nfigure.append_trace(t1, 1, 1)\nfigure.append_trace(t2, 2, 1)\niplot(figure)",
"This is the format of your plot grid:\n[ (1,1) x1,y1 ]\n[ (2,1) x2,y2 ]\n\n"
],
[
"t1 = go.Bar(x=names, y=legendary_grouped.Attack, name=\"Attack\")\nt2 = go.Bar(x=names, y=legendary_grouped.Defense, name=\"Defense\")\n\ndat = [t1, t2]\nfigure = tools.make_subplots(rows=1, cols=2, subplot_titles=('Attack', 'Defence'))\n\nfigure.append_trace(t1, 1, 1)\nfigure.append_trace(t2, 1, 2)\niplot(figure)",
"This is the format of your plot grid:\n[ (1,1) x1,y1 ] [ (1,2) x2,y2 ]\n\n"
],
[
"legendary_grouped = data.groupby(['Legendary', 'Generation']).mean()[['HP', 'Attack', 'Defense', \"Sp. Atk\",\n \"Sp. Def\", \"Speed\"]]",
"_____no_output_____"
],
[
"data.head()",
"_____no_output_____"
],
[
"type_grouped = data.groupby(\"Type 1\").mean()[['HP','Attack', 'Defense', \"Sp. Atk\", \"Sp. Def\", \"Speed\"]]",
"_____no_output_____"
],
[
"dat = [go.Bar(x=type_grouped.index, y=type_grouped[i], name=i) for i in type_grouped.columns]\n\nfigure = tools.make_subplots(rows=6, cols=1, subplot_titles=('Attack', 'Defence'))\n\nfor i in range(1,7):\n figure.append_trace(dat[i-1], i, 1)\n\niplot(figure)",
"This is the format of your plot grid:\n[ (1,1) x1,y1 ]\n[ (2,1) x2,y2 ]\n[ (3,1) x3,y3 ]\n[ (4,1) x4,y4 ]\n[ (5,1) x5,y5 ]\n[ (6,1) x6,y6 ]\n\n"
],
[
"type_grouped",
"_____no_output_____"
],
[
"dat = [go.Bar(x=type_grouped.index, y=type_grouped[i], name=i) for i in type_grouped.columns]\n\n#layout = dict(barmode = 'group')\nlayout = dict(barmode = 'stack')\n\nfigure = dict(data=dat,layout=layout)\niplot(figure)",
"_____no_output_____"
],
[
"dat = [go.Box(x=data[data[\"Type 1\"] == i][\"HP\"], name=\"HP\" + \"_\" + i, boxmean=True, orientation = \"h\",) \n for i in list(set(data[\"Type 1\"].tolist()))]\niplot(dat)",
"_____no_output_____"
],
[
"dat = [go.Box(y=data[data[\"Type 1\"] == i][\"HP\"], name=\"HP\" + \"_\" + i, boxmean=True, orientation = \"v\",)\n for i in list(set(data[\"Type 1\"].tolist()))]\niplot(dat)",
"_____no_output_____"
],
[
"corr_matrix_list = data[[\"HP\", \"Attack\", \"Defense\", \"Sp. Atk\", \"Sp. Def\", \"Speed\",\n \"Generation\", \"Legendary\"]].corr().values.tolist()\nx_axis = data.corr().columns\ny_axis = data.corr().index.values\n\ntrace = go.Heatmap(x=x_axis ,y=y_axis, z=corr_matrix_list, colorscale='Blues')\n\ndat = [trace]\nfigure = dict(data=dat)\niplot(figure)",
"_____no_output_____"
]
]
] | [
"markdown",
"code"
] | [
[
"markdown"
],
[
"code",
"code",
"code",
"code",
"code",
"code",
"code",
"code",
"code",
"code",
"code",
"code",
"code",
"code",
"code",
"code",
"code",
"code",
"code",
"code",
"code",
"code",
"code",
"code",
"code",
"code",
"code",
"code",
"code",
"code",
"code"
]
] |
e76ac86e9b788b18ef463178eb146b5158f0b9eb | 215,705 | ipynb | Jupyter Notebook | python/.ipynb_checkpoints/SRL_Data-checkpoint.ipynb | sanjaymeena/SemanticRoleLabeler | f31fce09905a5c0d13354e7b4614a2d995c9d880 | [
"MIT"
] | null | null | null | python/.ipynb_checkpoints/SRL_Data-checkpoint.ipynb | sanjaymeena/SemanticRoleLabeler | f31fce09905a5c0d13354e7b4614a2d995c9d880 | [
"MIT"
] | null | null | null | python/.ipynb_checkpoints/SRL_Data-checkpoint.ipynb | sanjaymeena/SemanticRoleLabeler | f31fce09905a5c0d13354e7b4614a2d995c9d880 | [
"MIT"
] | 1 | 2019-02-14T07:07:46.000Z | 2019-02-14T07:07:46.000Z | 314.89781 | 95,202 | 0.837217 | [
[
[
"# Load libraries\nimport pandas as pd\nimport json\n\n\nimport matplotlib.pyplot as plt\n%matplotlib inline\n\n\n#Plot the data:\nmy_colors = 'rgbkymc' #red, green, blue, black, etc.\nfrom IPython.display import set_matplotlib_formats\n# set_matplotlib_formats('pdf', 'png')\n# plt.rcParams['savefig.dpi'] = 75\n\n# plt.rcParams['figure.autolayout'] = False\n# plt.rcParams['figure.figsize'] = 10, 6\n# plt.rcParams['axes.labelsize'] = 18\n# plt.rcParams['axes.titlesize'] = 20\n# plt.rcParams['font.size'] = 16\n# plt.rcParams['lines.linewidth'] = 2.0\n# plt.rcParams['lines.markersize'] = 8\n# plt.rcParams['legend.fontsize'] = 14\n\n# plt.rcParams['text.usetex'] = True\n# plt.rcParams['font.family'] = \"serif\"\n# plt.rcParams['font.serif'] = \"cm\"\n# plt.rcParams['text.latex.preamble'] = \"\\\\usepackage{subdepth}, \\\\usepackage{type1cm}\"",
"_____no_output_____"
],
[
"\ndata_path=\"data/srl_test.json\"\n\nwith open(data_path) as json_data:\n data = json.load(json_data)\n\ndata=data['sentences'] \ndf=pd.DataFrame(data)\n",
"_____no_output_____"
],
[
"df.head()",
"_____no_output_____"
],
[
"# preds=df['predicates'];\n# preds.columns=['predicates']\n# preds.head()\ndf['predicates'] = df['predicates'].apply(tuple)\ndf.head()\ndf_tokens_temp=df['predicates'].value_counts(dropna=True)",
"_____no_output_____"
],
[
"f = plt.figure(figsize=(45, 75)) # Change the size as necessary\n#Plot the data:\ndf_tokens_temp.plot.barh(ax=f.gca(),color=my_colors) # figure.gca means \"get current axis\"",
"_____no_output_____"
],
[
"df1 = df['src']\ndf1.value_counts(dropna=True)",
"_____no_output_____"
],
[
"df1 = df['tokenLength']\ndf1.value_counts(dropna=True,ascending=False)",
"_____no_output_____"
],
[
"#print (df.iloc[0]['A1'])",
"_____no_output_____"
],
[
"col_list= list(df)\ncol_list.remove('sentence')\ncol_list.remove('predicates')\ncol_list.remove('multiplePredicates')\ncol_list.remove('tokenLength')\ncol_list.remove('src')\n\n# Create subset of dataframe\ndf1 = df[col_list]",
"_____no_output_____"
],
[
"sentences_with_multiple_preds=df['multiplePredicates'].sum(axis=0)",
"_____no_output_____"
],
[
"print (\"total sentences : \", len(df))\nprint (\"sentences with multiple predicates : \", sentences_with_multiple_preds)\nprint (\"statistics on argument relations : \", \"\\n\")\nprint (\"Rel\" , \"\\t\", \"Count\")\nprint (df1.sum(axis=0))",
"total sentences : 12271\nsentences with multiple predicates : 6269\nstatistics on argument relations : \n\nRel \t Count\n0 1\nA0 5426\nA1 4050\nA2 977\nA3 42\nA4 77\nADV 4768\nAFT 477\nAM-REC 23\nAT 6092\nBNF 149\nC- 50\nC-ARG 48\nCAU 64\nCND 122\nCON 13\nCST 3\nCTS 22\nDIR 249\nDIS 827\nEXT 248\nFRQ 8\nLOC 508\nMNR 37\nMOD 3044\nMOD0 1\nNEG 1106\nPRP 72\nRAG 58\nREC 101\nTGT 9\nTMP 909\ndtype: int64\n"
],
[
"plt.style.use('ggplot')",
"_____no_output_____"
],
[
"df_frame=df1.sum(axis=0).to_frame()\n\nf = plt.figure(figsize=(15, 15)) # Change the size as necessary\n#Plot the data:\nmy_colors = 'rgbkymc' #red, green, blue, black, etc.\n\n# 2\ndf_frame.plot.barh(ax=f.gca(),color=my_colors) # figure.gca means \"get current axis\"\nplt.title('SRL Relations Vs Counts', color='black')\n\n\n",
"_____no_output_____"
]
]
] | [
"code"
] | [
[
"code",
"code",
"code",
"code",
"code",
"code",
"code",
"code",
"code",
"code",
"code",
"code",
"code"
]
] |
e76ac913a6aa5c0edb67b737d5473bc1a7b57d27 | 2,923 | ipynb | Jupyter Notebook | _build/jupyter_execute/Module3/m3_07.ipynb | liuzhengqi1996/math452_Spring2022 | b01d1d9bee4778b3069e314c775a54f16dd44053 | [
"MIT"
] | null | null | null | _build/jupyter_execute/Module3/m3_07.ipynb | liuzhengqi1996/math452_Spring2022 | b01d1d9bee4778b3069e314c775a54f16dd44053 | [
"MIT"
] | null | null | null | _build/jupyter_execute/Module3/m3_07.ipynb | liuzhengqi1996/math452_Spring2022 | b01d1d9bee4778b3069e314c775a54f16dd44053 | [
"MIT"
] | null | null | null | 39.5 | 813 | 0.677386 | [
[
[
"# DNN for image classification",
"_____no_output_____"
]
],
[
[
"from IPython.display import IFrame\n\nIFrame(src= \"https://cdnapisec.kaltura.com/p/2356971/sp/235697100/embedIframeJs/uiconf_id/41416911/partner_id/2356971?iframeembed=true&playerId=kaltura_player&entry_id=1_zltbjpto&flashvars[streamerType]=auto&flashvars[localizationCode]=en&flashvars[leadWithHTML5]=true&flashvars[sideBarContainer.plugin]=true&flashvars[sideBarContainer.position]=left&flashvars[sideBarContainer.clickToClose]=true&flashvars[chapters.plugin]=true&flashvars[chapters.layout]=vertical&flashvars[chapters.thumbnailRotator]=false&flashvars[streamSelector.plugin]=true&flashvars[EmbedPlayer.SpinnerTarget]=videoHolder&flashvars[dualScreen.plugin]=true&flashvars[hotspots.plugin]=1&flashvars[Kaltura.addCrossoriginToIframe]=true&&wid=1_gjz238z7\" ,width='800', height='500')",
"_____no_output_____"
]
]
] | [
"markdown",
"code"
] | [
[
"markdown"
],
[
"code"
]
] |
e76ace9fa53f20c36727e9fdaf7fd41be74ea7c1 | 14,672 | ipynb | Jupyter Notebook | Metodo_de_Bayes_Censo.ipynb | VictorCalebeIFG/MachineLearning_Python | 1a0fc0d1c77d00446f2358322127e51d4a148db8 | [
"MIT"
] | null | null | null | Metodo_de_Bayes_Censo.ipynb | VictorCalebeIFG/MachineLearning_Python | 1a0fc0d1c77d00446f2358322127e51d4a148db8 | [
"MIT"
] | null | null | null | Metodo_de_Bayes_Censo.ipynb | VictorCalebeIFG/MachineLearning_Python | 1a0fc0d1c77d00446f2358322127e51d4a148db8 | [
"MIT"
] | null | null | null | 62.434043 | 8,966 | 0.764722 | [
[
[
"<a href=\"https://colab.research.google.com/github/VictorCalebeIFG/MachineLearning_Python/blob/main/Metodo_de_Bayes_Censo.ipynb\" target=\"_parent\"><img src=\"https://colab.research.google.com/assets/colab-badge.svg\" alt=\"Open In Colab\"/></a>",
"_____no_output_____"
],
[
"# **Mรฉtodos de Bayes - Dados do Censo**",
"_____no_output_____"
],
[
"### Importaรงรฃo dos *dados*",
"_____no_output_____"
]
],
[
[
"import pickle\n\nwith open(\"/content/censo.pkl\",\"rb\") as f:\n x_censo_treino,y_censo_treino,x_censo_teste,y_censo_teste = pickle.load(f)\n",
"_____no_output_____"
]
],
[
[
"### Treinar o modelo preditivo:",
"_____no_output_____"
]
],
[
[
"from sklearn.naive_bayes import GaussianNB\n\nnaive = GaussianNB()\nnaive.fit(x_censo_treino,y_censo_treino)",
"_____no_output_____"
]
],
[
[
"### Previsรตes",
"_____no_output_____"
]
],
[
[
"previsoes = naive.predict(x_censo_teste)\nprevisoes",
"_____no_output_____"
]
],
[
[
"### Verificando a acurracia do modelo",
"_____no_output_____"
]
],
[
[
"from sklearn.metrics import accuracy_score\n\naccuracy_score(y_censo_teste,previsoes)",
"_____no_output_____"
]
],
[
[
"### Como pode ser visto, a acuracia do modelo รฉ bem baixa. As vezes serรก necessรกrio modificar o preprocessamento (\"de acordo com o professor, ao retirar a padronizaรงรฃo do preprocessamento, neste algorรญtmo e para essa base de dados em especรญfico, a acurรกcia aumentou para 75%\")",
"_____no_output_____"
]
],
[
[
"from yellowbrick.classifier import ConfusionMatrix",
"_____no_output_____"
],
[
"cm = ConfusionMatrix(naive)\ncm.fit(x_censo_treino,y_censo_treino)\ncm.score(x_censo_teste,y_censo_teste)",
"_____no_output_____"
]
]
] | [
"markdown",
"code",
"markdown",
"code",
"markdown",
"code",
"markdown",
"code",
"markdown",
"code"
] | [
[
"markdown",
"markdown",
"markdown"
],
[
"code"
],
[
"markdown"
],
[
"code"
],
[
"markdown"
],
[
"code"
],
[
"markdown"
],
[
"code"
],
[
"markdown"
],
[
"code",
"code"
]
] |
e76aea71813ccd42e318d89b6df44121758f91d9 | 190,988 | ipynb | Jupyter Notebook | Lightcurve/Lightcurve tutorial.ipynb | jdswinbank/notebooks | 900c32c6358862af6a948259edfc8c17ed8f8e43 | [
"MIT"
] | 12 | 2017-03-10T05:57:19.000Z | 2021-11-21T07:38:37.000Z | Lightcurve/Lightcurve tutorial.ipynb | jdswinbank/notebooks | 900c32c6358862af6a948259edfc8c17ed8f8e43 | [
"MIT"
] | 34 | 2016-05-27T05:48:45.000Z | 2021-05-10T14:55:21.000Z | Lightcurve/Lightcurve tutorial.ipynb | jdswinbank/notebooks | 900c32c6358862af6a948259edfc8c17ed8f8e43 | [
"MIT"
] | 28 | 2016-04-06T18:40:34.000Z | 2021-06-28T06:15:50.000Z | 89.121792 | 49,472 | 0.841969 | [
[
[
"Start here to begin with Stingray.",
"_____no_output_____"
]
],
[
[
"import numpy as np\n%matplotlib inline\nimport warnings\nwarnings.filterwarnings('ignore')",
"_____no_output_____"
]
],
[
[
"# Creating a light curve",
"_____no_output_____"
]
],
[
[
"from stingray import Lightcurve",
"_____no_output_____"
]
],
[
[
"A `Lightcurve` object can be created in two ways :\n\n1. From an array of time stamps and an array of counts.\n2. From photon arrival times.",
"_____no_output_____"
],
[
"## 1. Array of time stamps and counts",
"_____no_output_____"
],
[
"Create 1000 time stamps",
"_____no_output_____"
]
],
[
[
"times = np.arange(1000)\ntimes[:10]",
"_____no_output_____"
]
],
[
[
"Create 1000 random Poisson-distributed counts:",
"_____no_output_____"
]
],
[
[
"counts = np.random.poisson(100, size=len(times))\ncounts[:10]",
"_____no_output_____"
]
],
[
[
"Create a Lightcurve object with the times and counts array.",
"_____no_output_____"
]
],
[
[
"lc = Lightcurve(times, counts)",
"WARNING:root:Checking if light curve is well behaved. This can take time, so if you are sure it is already sorted, specify skip_checks=True at light curve creation.\nWARNING:root:Checking if light curve is sorted.\nWARNING:root:Computing the bin time ``dt``. This can take time. If you know the bin time, please specify it at light curve creation\n"
]
],
[
[
"The number of data points can be counted with the `len` function.",
"_____no_output_____"
]
],
[
[
"len(lc)",
"_____no_output_____"
]
],
[
[
"Note the warnings thrown by the syntax above. By default, `stingray` does a number of checks on the data that is put into the `Lightcurve` class. For example, it checks whether it's evenly sampled. It also computes the time resolution `dt`. All of these checks take time. If you know the time resolution, it's a good idea to put it in manually. If you know that your light curve is well-behaved (for example, because you know the data really well, or because you've generated it yourself, as we've done above), you can skip those checks and save a bit of time:",
"_____no_output_____"
]
],
[
[
"dt = 1 \nlc = Lightcurve(times, counts, dt=dt, skip_checks=True)",
"_____no_output_____"
]
],
[
[
"## 2. Photon Arrival Times\n\nOften, you might have unbinned photon arrival times, rather than a light curve with time stamps and associated measurements. If this is the case, you can use the `make_lightcurve` method to turn these photon arrival times into a regularly binned light curve.",
"_____no_output_____"
]
],
[
[
"arrivals = np.loadtxt(\"photon_arrivals.txt\")\narrivals[:10]",
"_____no_output_____"
],
[
"lc_new = Lightcurve.make_lightcurve(arrivals, dt=1)",
"_____no_output_____"
]
],
[
[
"The time bins and respective counts can be seen with `lc.counts` and `lc.time`",
"_____no_output_____"
]
],
[
[
"lc_new.counts",
"_____no_output_____"
],
[
"lc_new.time",
"_____no_output_____"
]
],
[
[
"One useful feature is that you can explicitly pass in the start time and the duration of the observation. This can be helpful because the chance that a photon will arrive exactly at the start of the observation and the end of the observation is very small. In practice, when making multiple light curves from the same observation (e.g. individual light curves of multiple detectors, of for different energy ranges) this can lead to the creation of light curves with time bins that are *slightly* offset from one another. Here, passing in the total duration of the observation and the start time can be helpful.",
"_____no_output_____"
]
],
[
[
"lc_new = Lightcurve.make_lightcurve(arrivals, dt=1.0, tstart=1.0, tseg=9.0)",
"_____no_output_____"
]
],
[
[
"# Properties",
"_____no_output_____"
],
[
"A Lightcurve object has the following properties :\n\n1. `time` : numpy array of time values\n2. `counts` : numpy array of counts per bin values\n3. `counts_err`: numpy array with the uncertainties on the values in `counts`\n4. `countrate` : numpy array of counts per second\n5. `countrate_err`: numpy array of the uncertainties on the values in `countrate`\n4. `n` : Number of data points in the lightcurve\n5. `dt` : Time resolution of the light curve\n6. `tseg` : Total duration of the light curve\n7. `tstart` : Start time of the light curve\n8. `meancounts`: The mean counts of the light curve\n9. `meanrate`: The mean count rate of the light curve\n10. `mjdref`: MJD reference date (``tstart`` / 86400 gives the date in MJD at the start of the observation)\n11. `gti`:Good Time Intervals. They indicate the \"safe\" time intervals to be used during the analysis of the light curve. \n12. `err_dist`: Statistic of the Lightcurve, it is used to calculate the uncertainties and other statistical values appropriately. It propagates to Spectrum classes\n",
"_____no_output_____"
]
],
[
[
"lc.n == len(lc)",
"_____no_output_____"
]
],
[
[
"Note that by default, `stingray` assumes that the user is passing a light curve in **counts per bin**. That is, the counts in bin $i$ will be the number of photons that arrived in the interval $t_i - 0.5\\Delta t$ and $t_i + 0.5\\Delta t$. Sometimes, data is given in **count rate**, i.e. the number of events that arrive within an interval of a *second*. The two will only be the same if the time resolution of the light curve is exactly 1 second.\n\nWhether the input data is in counts per bin or in count rate can be toggled via the boolean `input_counts` keyword argument. By default, this argument is set to `True`, and the code assumes the light curve passed into the object is in counts/bin. By setting it to `False`, the user can pass in count rates:",
"_____no_output_____"
]
],
[
[
"# times with a resolution of 0.1\ndt = 0.1\ntimes = np.arange(0, 100, dt)\ntimes[:10]",
"_____no_output_____"
],
[
"mean_countrate = 100.0\ncountrate = np.random.poisson(mean_countrate, size=len(times))",
"_____no_output_____"
],
[
"lc = Lightcurve(times, counts=countrate, dt=dt, skip_checks=True, input_counts=False)",
"_____no_output_____"
]
],
[
[
"Internally, both `counts` and `countrate` attribute will be defined no matter what the user passes in, since they're trivially converted between each other through a multiplication/division with `dt:",
"_____no_output_____"
]
],
[
[
"print(mean_countrate)\nprint(lc.countrate[:10])",
"100.0\n[113 92 110 97 101 102 103 101 124 89]\n"
],
[
"mean_counts = mean_countrate * dt\nprint(mean_counts)\nprint(lc.counts[:10])",
"10.0\n[11.3 9.2 11. 9.7 10.1 10.2 10.3 10.1 12.4 8.9]\n"
]
],
[
[
"## Error Distributions in `stingray.Lightcurve`\n\nThe instruments that record our data impose measurement noise on our measurements. Depending on the type of instrument, the statistical distribution of that noise can be different. `stingray` was originally developed with X-ray data in mind, where most data comes in the form of _photon arrival times_, which generate measurements distributed according to a Poisson distribution. By default, `err_dist` is assumed to Poisson, and this is the only statistical distribution currently fully supported. But you *can* put in your own errors (via `counts_err` or `countrate_err`). It'll produce a warning, and be aware that some of the statistical assumptions made about downstream products (e.g. the normalization of periodograms) may not be correct:",
"_____no_output_____"
]
],
[
[
"times = np.arange(1000)\n\nmean_flux = 100.0 # mean flux\nstd_flux = 2.0 # standard deviation on the flux\n\n# generate fluxes with a Gaussian distribution and \n# an array of associated uncertainties\nflux = np.random.normal(loc=mean_flux, scale=std_flux, size=len(times)) \nflux_err = np.ones_like(flux) * std_flux",
"_____no_output_____"
],
[
"lc = Lightcurve(times, flux, err=flux_err, err_dist=\"gauss\", dt=1.0, skip_checks=True)",
"_____no_output_____"
]
],
[
[
"## Good Time Intervals\n\n`Lightcurve` (and most other core `stingray` classes) support the use of *Good Time Intervals* (or GTIs), which denote the parts of an observation that are reliable for scientific purposes. Often, GTIs introduce gaps (e.g. where the instrument was off, or affected by solar flares). By default. GTIs are passed and don't apply to the data within a `Lightcurve` object, but become relevant in a number of circumstances, such as when generating `Powerspectrum` objects. \n\nIf no GTIs are given at instantiation of the `Lightcurve` class, an artificial GTI will be created spanning the entire length of the data set being passed in:",
"_____no_output_____"
]
],
[
[
"times = np.arange(1000)\ncounts = np.random.poisson(100, size=len(times))\n\nlc = Lightcurve(times, counts, dt=1, skip_checks=True)",
"_____no_output_____"
],
[
"lc.gti",
"_____no_output_____"
],
[
"print(times[0]) # first time stamp in the light curve\nprint(times[-1]) # last time stamp in the light curve\nprint(lc.gti) # the GTIs generated within Lightcurve",
"0\n999\n[[-5.000e-01 9.995e+02]]\n"
]
],
[
[
"GTIs are defined as a list of tuples:",
"_____no_output_____"
]
],
[
[
"gti = [(0, 500), (600, 1000)]",
"_____no_output_____"
],
[
"lc = Lightcurve(times, counts, dt=1, skip_checks=True, gti=gti)",
"_____no_output_____"
],
[
"print(lc.gti)",
"[[ 0 500]\n [ 600 1000]]\n"
]
],
[
[
"We'll get back to these when we talk more about some of the methods that apply GTIs to the data.\n\n# Operations",
"_____no_output_____"
],
[
"## Addition/Subtraction",
"_____no_output_____"
],
[
"Two light curves can be summed up or subtracted from each other if they have same time arrays.",
"_____no_output_____"
]
],
[
[
"lc = Lightcurve(times, counts, dt=1, skip_checks=True)\nlc_rand = Lightcurve(np.arange(1000), [500]*1000, dt=1, skip_checks=True)",
"_____no_output_____"
],
[
"lc_sum = lc + lc_rand",
"_____no_output_____"
],
[
"print(\"Counts in light curve 1: \" + str(lc.counts[:5]))\nprint(\"Counts in light curve 2: \" + str(lc_rand.counts[:5]))\nprint(\"Counts in summed light curve: \" + str(lc_sum.counts[:5]))",
"Counts in light curve 1: [103 99 102 109 104]\nCounts in light curve 2: [500 500 500 500 500]\nCounts in summed light curve: [603 599 602 609 604]\n"
]
],
[
[
"## Negation",
"_____no_output_____"
],
[
"A negation operation on the lightcurve object inverts the count array from positive to negative values.",
"_____no_output_____"
]
],
[
[
"lc_neg = -lc",
"_____no_output_____"
],
[
"lc_sum = lc + lc_neg",
"_____no_output_____"
],
[
"np.all(lc_sum.counts == 0) # All the points on lc and lc_neg cancel each other",
"_____no_output_____"
]
],
[
[
"## Indexing",
"_____no_output_____"
],
[
"Count value at a particular time can be obtained using indexing.",
"_____no_output_____"
]
],
[
[
"lc[120]",
"_____no_output_____"
]
],
[
[
"A Lightcurve can also be sliced to generate a new object.",
"_____no_output_____"
]
],
[
[
"lc_sliced = lc[100:200]",
"_____no_output_____"
],
[
"len(lc_sliced.counts)",
"_____no_output_____"
]
],
[
[
"# Methods",
"_____no_output_____"
],
[
"## Concatenation",
"_____no_output_____"
],
[
"Two light curves can be combined into a single object using the `join` method. Note that both of them must not have overlapping time arrays.",
"_____no_output_____"
]
],
[
[
"lc_1 = lc",
"_____no_output_____"
],
[
"lc_2 = Lightcurve(np.arange(1000, 2000), np.random.rand(1000)*1000, dt=1, skip_checks=True)",
"_____no_output_____"
],
[
"lc_long = lc_1.join(lc_2, skip_checks=True) # Or vice-versa",
"_____no_output_____"
],
[
"print(len(lc_long))",
"2000\n"
]
],
[
[
"## Truncation",
"_____no_output_____"
],
[
"A light curve can also be truncated.",
"_____no_output_____"
]
],
[
[
"lc_cut = lc_long.truncate(start=0, stop=1000)",
"_____no_output_____"
],
[
"len(lc_cut)",
"_____no_output_____"
]
],
[
[
"**Note** : By default, the `start` and `stop` parameters are assumed to be given as **indices** of the time array. However, the `start` and `stop` values can also be given as time values in the same value as the time array.",
"_____no_output_____"
]
],
[
[
"lc_cut = lc_long.truncate(start=500, stop=1500, method='time')",
"_____no_output_____"
],
[
"lc_cut.time[0], lc_cut.time[-1]",
"_____no_output_____"
]
],
[
[
"## Re-binning",
"_____no_output_____"
],
[
"The time resolution (`dt`) can also be changed to a larger value.\n\n**Note** : While the new resolution need not be an integer multiple of the previous time resolution, be aware that if it is not, the last bin will be cut off by the fraction left over by the integer division.",
"_____no_output_____"
]
],
[
[
"lc_rebinned = lc_long.rebin(2)",
"_____no_output_____"
],
[
"print(\"Old time resolution = \" + str(lc_long.dt))\nprint(\"Number of data points = \" + str(lc_long.n))\nprint(\"New time resolution = \" + str(lc_rebinned.dt))\nprint(\"Number of data points = \" + str(lc_rebinned.n))",
"Old time resolution = 1\nNumber of data points = 2000\nNew time resolution = 2\nNumber of data points = 1000\n"
]
],
[
[
"## Sorting",
"_____no_output_____"
],
[
"A lightcurve can be sorted using the `sort` method. This function sorts `time` array and the `counts` array is changed accordingly.",
"_____no_output_____"
]
],
[
[
"new_lc_long = lc_long[:] # Copying into a new object",
"_____no_output_____"
],
[
"new_lc_long = new_lc_long.sort(reverse=True)",
"_____no_output_____"
],
[
"new_lc_long.time[0] == max(lc_long.time)",
"_____no_output_____"
]
],
[
[
"You can sort by the `counts` array using `sort_counts` method which changes `time` array accordingly:",
"_____no_output_____"
]
],
[
[
"new_lc = lc_long[:]\nnew_lc = new_lc.sort_counts()\nnew_lc.counts[-1] == max(lc_long.counts)",
"_____no_output_____"
]
],
[
[
"## Plotting",
"_____no_output_____"
],
[
"A curve can be plotted with the `plot` method.",
"_____no_output_____"
]
],
[
[
"lc.plot()",
"_____no_output_____"
]
],
[
[
"A plot can also be customized using several keyword arguments.",
"_____no_output_____"
]
],
[
[
"lc.plot(labels=('Time', \"Counts\"), # (xlabel, ylabel)\n axis=(0, 1000, -50, 150), # (xmin, xmax, ymin, ymax)\n title=\"Random generated lightcurve\",\n marker='c:') # c is for cyan and : is the marker style",
"_____no_output_____"
]
],
[
[
"The figure drawn can also be saved in a file using keywords arguments in the plot method itself.",
"_____no_output_____"
]
],
[
[
"lc.plot(marker = 'k', save=True, filename=\"lightcurve.png\")",
"_____no_output_____"
]
],
[
[
"**Note** : See `utils.savefig` function for more options on saving a file.",
"_____no_output_____"
],
[
"# Sample Data",
"_____no_output_____"
],
[
"Stingray also has a sample `Lightcurve` data which can be imported from within the library.",
"_____no_output_____"
]
],
[
[
"from stingray import sampledata",
"_____no_output_____"
],
[
"lc = sampledata.sample_data()",
"WARNING:root:Checking if light curve is well behaved. This can take time, so if you are sure it is already sorted, specify skip_checks=True at light curve creation.\nWARNING:root:Checking if light curve is sorted.\nWARNING:root:Computing the bin time ``dt``. This can take time. If you know the bin time, please specify it at light curve creation\n"
],
[
"lc.plot()",
"_____no_output_____"
]
],
[
[
"## Checking the Light Curve for Irregularities\n\nYou can perform checks on the behaviour of the light curve, similar to what's done when instantiating a `Lightcurve` object when `skip_checks=False`, by calling the relevant method:",
"_____no_output_____"
]
],
[
[
"time = np.hstack([np.arange(0, 10, 0.1), np.arange(10, 20, 0.3)]) # uneven time resolution\ncounts = np.random.poisson(100, size=len(time))\n\nlc = Lightcurve(time, counts, dt=1.0, skip_checks=True)",
"_____no_output_____"
],
[
"lc.check_lightcurve()",
"_____no_output_____"
]
],
[
[
"Let's add some badly formatted GTIs:",
"_____no_output_____"
]
],
[
[
"gti = [(10, 100), (20, 30, 40), ((1, 2), (3, 4, (5, 6)))] # not a well-behaved GTI\nlc = Lightcurve(time, counts, dt=0.1, skip_checks=True, gti=gti)",
"_____no_output_____"
],
[
"lc.check_lightcurve()",
"_____no_output_____"
]
],
[
[
"## MJDREF and Shifting Times\n\nThe `mjdref` keyword argument defines a reference time in Modified Julian Date. Often, X-ray missions count their internal time in seconds from a given reference date and time (so that numbers don't become arbitrarily large). The data is then in the format of Mission Elapsed Time (MET), or seconds since that reference time. \n\n`mjdref` is generally passed into the `Lightcurve` object at instantiation, but it can be changed later:",
"_____no_output_____"
]
],
[
[
"mjdref = 91254\ntime = np.arange(1000)\ncounts = np.random.poisson(100, size=len(time))\n\nlc = Lightcurve(time, counts, dt=1, skip_checks=True, mjdref=mjdref)\nprint(lc.mjdref)",
"91254\n"
],
[
"mjdref_new = 91254 + 20\nlc_new = lc.change_mjdref(mjdref_new)\nprint(lc_new.mjdref)",
"91274\n"
]
],
[
[
"This change only affects the *reference time*, not the values given in the `time` attribute. However, it is also possible to shift the *entire light curve*, along with its GTIs:",
"_____no_output_____"
]
],
[
[
"gti = [(0,500), (600, 1000)]\nlc.gti = gti",
"_____no_output_____"
],
[
"print(\"first three time bins: \" + str(lc.time[:3]))\nprint(\"GTIs: \" + str(lc.gti))",
"first three time bins: [0 1 2]\nGTIs: [[ 0 500]\n [ 600 1000]]\n"
],
[
"time_shift = 10.0\nlc_shifted = lc.shift(time_shift)",
"_____no_output_____"
],
[
"print(\"Shifted first three time bins: \" + str(lc_shifted.time[:3]))\nprint(\"Shifted GTIs: \" + str(lc_shifted.gti))",
"Shifted first three time bins: [10. 11. 12.]\nShifted GTIs: [[ 10. 510.]\n [ 610. 1010.]]\n"
]
],
[
[
"## Calculating a baseline\n\n**TODO**: Need to document this method",
"_____no_output_____"
],
[
"## Working with GTIs and Splitting Light Curves\n\nIt is possible to split light curves into multiple segments. In particular, it can be useful to split light curves with large gaps into individual contiguous segments without gaps. ",
"_____no_output_____"
]
],
[
[
"# make a time array with a big gap and a small gap\ntime = np.array([1, 2, 3, 10, 11, 12, 13, 14, 17, 18, 19, 20])\ncounts = np.random.poisson(100, size=len(time))\n\nlc = Lightcurve(time, counts, skip_checks=True)",
"WARNING:root:Computing the bin time ``dt``. This can take time. If you know the bin time, please specify it at light curve creation\n"
],
[
"lc.gti",
"_____no_output_____"
]
],
[
[
"This light curve has uneven bins. It has a large gap between 3 and 10, and a smaller gap between 14 and 17. We can use the `split` method to split it into three contiguous segments:",
"_____no_output_____"
]
],
[
[
"lc_split = lc.split(min_gap=2*lc.dt)",
"_____no_output_____"
],
[
"for lc_tmp in lc_split:\n print(lc_tmp.time)",
"[1 2 3]\n[10 11 12 13 14]\n[17 18 19 20]\n"
]
],
[
[
"This has split the light curve into three contiguous segments. You can adjust the tolerance for the size of gap that's acceptable via the `min_gap` attribute. You can also require a minimum number of data points in the output light curves. This is helpful when you're only interested in contiguous segments of a certain length:",
"_____no_output_____"
]
],
[
[
"lc_split = lc.split(min_gap=6.0)",
"_____no_output_____"
],
[
"for lc_tmp in lc_split:\n print(lc_tmp.time)",
"[1 2 3]\n[10 11 12 13 14 17 18 19 20]\n"
]
],
[
[
"What if we only want the long segment?",
"_____no_output_____"
]
],
[
[
"lc_split = lc.split(min_gap=6.0, min_points=4)",
"_____no_output_____"
],
[
"for lc_tmp in lc_split:\n print(lc_tmp.time)",
"[10 11 12 13 14 17 18 19 20]\n"
]
],
[
[
"A special case of splitting your light curve object is to split by GTIs. This can be helpful if you want to look at individual contiguous segments separately:",
"_____no_output_____"
]
],
[
[
"# make a time array with a big gap and a small gap\ntime = np.arange(20)\ncounts = np.random.poisson(100, size=len(time))\ngti = [(0,8), (12,20)]\n\n\nlc = Lightcurve(time, counts, dt=1, skip_checks=True, gti=gti)",
"_____no_output_____"
],
[
"lc_split = lc.split_by_gti()",
"_____no_output_____"
],
[
"for lc_tmp in lc_split:\n print(lc_tmp.time)",
"[1 2 3 4 5 6 7]\n[13 14 15 16 17 18 19]\n"
]
],
[
[
"Because I'd passed in GTIs that define the range from 0-8 and from 12-20 as good time intervals, the light curve will be split into two individual ones containing all data points falling within these ranges.\n\nYou can also apply the GTIs *directly* to the original light curve, which will filter `time`, `counts`, `countrate`, `counts_err` and `countrate_err` to only fall within the bounds of the GTIs:",
"_____no_output_____"
]
],
[
[
"# make a time array with a big gap and a small gap\ntime = np.arange(20)\ncounts = np.random.poisson(100, size=len(time))\ngti = [(0,8), (12,20)]\n\n\nlc = Lightcurve(time, counts, dt=1, skip_checks=True, gti=gti)",
"_____no_output_____"
]
],
[
[
"**Caution**: This is one of the few methods that change the original state of the object, rather than returning a new copy of it with the changes applied! So any events falling outside of the range of the GTIs will be lost:",
"_____no_output_____"
]
],
[
[
"# time array before applying GTIs:\nlc.time",
"_____no_output_____"
],
[
"lc.apply_gtis()",
"_____no_output_____"
],
[
"# time array after applying GTIs\nlc.time",
"_____no_output_____"
]
],
[
[
"As you can see, the time bins 8-12 have been dropped, since they fall outside of the GTIs. \n\n## Analyzing Light Curve Segments\n\nThere's some functionality in `stingray` aimed at making analysis of individual light curve segments (or chunks, as they're called throughout the code) efficient. \n\nOne helpful function tells you the length that segments should have to satisfy two conditions: (1) the minimum number of time bins in the segment, and (2) the minimum total number of counts (or flux) in each segment.\n\nLet's give this a try with an example:",
"_____no_output_____"
]
],
[
[
"dt=1.0\ntime = np.arange(0, 100, dt)\ncounts = np.random.poisson(100, size=len(time))\n\nlc = Lightcurve(time, counts, dt=dt, skip_checks=True)\n",
"_____no_output_____"
],
[
"min_total_counts = 300\nmin_total_bins = 2\nestimated_chunk_length = lc.estimate_chunk_length(min_total_counts, min_total_bins)\n\nprint(\"The estimated length of each segment in seconds to satisfy both conditions is: \" + str(estimated_chunk_length))",
"The estimated length of each segment in seconds to satisfy both conditions is: 4.0\n"
]
],
[
[
"So we have time bins of 1 second time resolution, each with an average of 100 counts/bin. We require at least 2 time bins in each segment, and also a minimum number of total counts in the segment of 300. In theory, you'd expect to need 3 time bins (so 3-second segments) to satisfy the condition above. However, the Poisson distribution is quite variable, so we cannot guarantee that all bins will have a total number of counts above 300. Hence, our segments need to be 4 seconds long. \n\nWe can now use these segments to do some analysis, using the `analyze_by_chunks` method. In the simplest, case we can use a standard `numpy` operation to learn something about the properties of each segment:",
"_____no_output_____"
]
],
[
[
"start_times, stop_times, lc_sums = lc.analyze_lc_chunks(chunk_length = 10.0, func=np.median)",
"_____no_output_____"
],
[
"lc_sums",
"_____no_output_____"
]
],
[
[
"This splits the light curve into 10-second segments, and then finds the median number of counts/bin in each segment. For a flat light curve like the one we generated above, this isn't super interesting, but this method can be helpful for more complex analyses. Instead of `np.median`, you can also pass in your own function:",
"_____no_output_____"
]
],
[
[
"def myfunc(lc):\n \"\"\"\n Not a very interesting function\n \"\"\"\n return np.sum(lc.counts) * 10.0",
"_____no_output_____"
],
[
"start_times, stop_times, lc_result = lc.analyze_lc_chunks(chunk_length=10.0, func=myfunc)",
"_____no_output_____"
],
[
"lc_result",
"_____no_output_____"
]
],
[
[
"## Compatibility with `Lightkurve`\n\nThe [`Lightkurve` package](https://docs.lightkurve.org) provides a large amount of complementary functionality to stingray, in particular for data observed with Kepler and TESS, stars and exoplanets, and unevenly sampled data. We have implemented a conversion method that converts to/from `stingray`'s native `Lightcurve` object and `Lightkurve`'s native `LightCurve` object. Equivalent functionality exists in `Lightkurve`, too. ",
"_____no_output_____"
]
],
[
[
"import lightkurve",
"_____no_output_____"
],
[
"lc_new = lc.to_lightkurve()",
"_____no_output_____"
],
[
"type(lc_new)",
"_____no_output_____"
],
[
"lc_new.time",
"_____no_output_____"
],
[
"lc_new.flux",
"_____no_output_____"
]
],
[
[
"Let's do the rountrip to stingray:",
"_____no_output_____"
]
],
[
[
"lc_back = lc_new.to_stingray()",
"WARNING:root:Checking if light curve is well behaved. This can take time, so if you are sure it is already sorted, specify skip_checks=True at light curve creation.\nWARNING:root:Checking if light curve is sorted.\nWARNING:root:Computing the bin time ``dt``. This can take time. If you know the bin time, please specify it at light curve creation\n"
],
[
"lc_back.time",
"_____no_output_____"
],
[
"lc_back.counts",
"_____no_output_____"
]
],
[
[
"Similarly, we can transform `Lightcurve` objects to and from `astropy.TimeSeries` objects:",
"_____no_output_____"
]
],
[
[
"dt=1.0\ntime = np.arange(0, 100, dt)\ncounts = np.random.poisson(100, size=len(time))\n\nlc = Lightcurve(time, counts, dt=dt, skip_checks=True)\n\n# convet to astropy.TimeSeries object\nts = lc.to_astropy_timeseries()",
"_____no_output_____"
],
[
"type(ts)",
"_____no_output_____"
],
[
"ts[:10]",
"_____no_output_____"
]
],
[
[
"lc_back = Lightcurve.from_astropy_timeseries(ts)",
"_____no_output_____"
],
[
"## Reading/Writing Lightcurves to/from files\n\nThe `Lightcurve` class has some rudimentary reading/writing capabilities via the `read` and `write` methods. For more information `stingray` inputs and outputs, please refer to the I/O tutorial.",
"_____no_output_____"
]
]
] | [
"markdown",
"code",
"markdown",
"code",
"markdown",
"code",
"markdown",
"code",
"markdown",
"code",
"markdown",
"code",
"markdown",
"code",
"markdown",
"code",
"markdown",
"code",
"markdown",
"code",
"markdown",
"code",
"markdown",
"code",
"markdown",
"code",
"markdown",
"code",
"markdown",
"code",
"raw",
"code",
"markdown",
"code",
"markdown",
"code",
"markdown",
"code",
"markdown",
"code",
"markdown",
"code",
"markdown",
"code",
"markdown",
"code",
"markdown",
"code",
"markdown",
"code",
"markdown",
"code",
"markdown",
"code",
"markdown",
"code",
"markdown",
"code",
"markdown",
"code",
"markdown",
"code",
"markdown",
"code",
"markdown",
"code",
"markdown",
"code",
"markdown",
"code",
"markdown",
"code",
"markdown",
"code",
"markdown",
"code",
"markdown",
"code",
"markdown",
"code",
"markdown",
"code",
"markdown",
"code",
"markdown",
"code",
"markdown",
"code",
"markdown",
"code",
"markdown",
"code",
"markdown",
"code",
"markdown"
] | [
[
"markdown"
],
[
"code"
],
[
"markdown"
],
[
"code"
],
[
"markdown",
"markdown",
"markdown"
],
[
"code"
],
[
"markdown"
],
[
"code"
],
[
"markdown"
],
[
"code"
],
[
"markdown"
],
[
"code"
],
[
"markdown"
],
[
"code"
],
[
"markdown"
],
[
"code",
"code"
],
[
"markdown"
],
[
"code",
"code"
],
[
"markdown"
],
[
"code"
],
[
"markdown",
"markdown"
],
[
"code"
],
[
"markdown"
],
[
"code",
"code",
"code"
],
[
"markdown"
],
[
"code",
"code"
],
[
"markdown"
],
[
"code",
"code"
],
[
"markdown"
],
[
"code",
"code",
"code"
],
[
"raw"
],
[
"code",
"code",
"code"
],
[
"markdown",
"markdown",
"markdown"
],
[
"code",
"code",
"code"
],
[
"markdown",
"markdown"
],
[
"code",
"code",
"code"
],
[
"markdown",
"markdown"
],
[
"code"
],
[
"markdown"
],
[
"code",
"code"
],
[
"markdown",
"markdown",
"markdown"
],
[
"code",
"code",
"code",
"code"
],
[
"markdown",
"markdown"
],
[
"code",
"code"
],
[
"markdown"
],
[
"code",
"code"
],
[
"markdown",
"markdown"
],
[
"code",
"code"
],
[
"markdown",
"markdown"
],
[
"code",
"code",
"code"
],
[
"markdown"
],
[
"code"
],
[
"markdown",
"markdown"
],
[
"code"
],
[
"markdown"
],
[
"code"
],
[
"markdown"
],
[
"code"
],
[
"markdown",
"markdown",
"markdown"
],
[
"code",
"code",
"code"
],
[
"markdown"
],
[
"code",
"code"
],
[
"markdown"
],
[
"code",
"code"
],
[
"markdown"
],
[
"code",
"code"
],
[
"markdown"
],
[
"code",
"code",
"code",
"code"
],
[
"markdown",
"markdown"
],
[
"code",
"code"
],
[
"markdown"
],
[
"code",
"code"
],
[
"markdown"
],
[
"code",
"code"
],
[
"markdown"
],
[
"code",
"code"
],
[
"markdown"
],
[
"code",
"code",
"code"
],
[
"markdown"
],
[
"code"
],
[
"markdown"
],
[
"code",
"code",
"code"
],
[
"markdown"
],
[
"code",
"code"
],
[
"markdown"
],
[
"code",
"code"
],
[
"markdown"
],
[
"code",
"code",
"code"
],
[
"markdown"
],
[
"code",
"code",
"code",
"code",
"code"
],
[
"markdown"
],
[
"code",
"code",
"code"
],
[
"markdown"
],
[
"code",
"code",
"code"
],
[
"markdown",
"markdown"
]
] |
e76b0949340372e9c73eab47e0df1175b01e69d0 | 153,336 | ipynb | Jupyter Notebook | Ro6.ipynb | Roland236/ADS-Assignment-6 | 778fac0915d3c68f48d0c8c293e3faaff57c6cb8 | [
"MIT"
] | null | null | null | Ro6.ipynb | Roland236/ADS-Assignment-6 | 778fac0915d3c68f48d0c8c293e3faaff57c6cb8 | [
"MIT"
] | null | null | null | Ro6.ipynb | Roland236/ADS-Assignment-6 | 778fac0915d3c68f48d0c8c293e3faaff57c6cb8 | [
"MIT"
] | null | null | null | 29.329763 | 33,842 | 0.367259 | [
[
[
"import pandas as pd\nimport numpy as np\nimport plotly.graph_objects as go\nimport plotly.express as px\nimport json",
"_____no_output_____"
],
[
"Df=px.data.gapminder()\nDf.head()",
"_____no_output_____"
],
[
"y=np.mean(Df['lifeExp'].values.tolist())\n",
"_____no_output_____"
],
[
"df1=px.data.gapminder().query(\"continent=='Asia'\")\nfig = px.choropleth(df1, locations = 'iso_alpha', hover_data = ['lifeExp'],\n hover_name = 'country', color = 'country',scope = 'asia')\nfig.show()",
"_____no_output_____"
],
[
"#Deviation in GDP of each country in Europe and South America.\n#Df.groupby('continent').count()\nDf1=px.data.gapminder().query(\"continent=='Americas'\")\nfig=px.choropleth(Df1, locations = 'gdpPerca',\n hover_name = 'country', color = 'country',scope ='africa')\nfig.show()",
"_____no_output_____"
],
[
"#The change in population of each African country in the last 3 decades\nDf2=df1=px.data.gapminder().query(\"continent=='africa'\")\nfig=px.choropleth(Df2, locations = 'iso_alpha', hover_data = ['pop'],\n hover_name = 'country', color = 'country',scope ='africa')\nfig.show()",
"_____no_output_____"
]
]
] | [
"code"
] | [
[
"code",
"code",
"code",
"code",
"code",
"code"
]
] |
e76b0f4a0fb7b2035d6b72d3b6ee02526ae5d1e0 | 3,844 | ipynb | Jupyter Notebook | Reading Gas detector/testing_value.ipynb | dongwon18/MyProject | 12383b500324af3214bba8ba40f15c7857599fa5 | [
"MIT"
] | null | null | null | Reading Gas detector/testing_value.ipynb | dongwon18/MyProject | 12383b500324af3214bba8ba40f15c7857599fa5 | [
"MIT"
] | null | null | null | Reading Gas detector/testing_value.ipynb | dongwon18/MyProject | 12383b500324af3214bba8ba40f15c7857599fa5 | [
"MIT"
] | null | null | null | 26.328767 | 90 | 0.561134 | [
[
[
"from pymodbus.client.sync import ModbusTcpClient\r\nfrom pymodbus.constants import Endian\r\nfrom pymodbus.payload import BinaryPayloadBuilder\r\nimport socket\r\nimport time",
"_____no_output_____"
],
[
"# get local IP address\r\nhostname = socket.gethostname()\r\nserver_ip_addr = socket.gethostbyname(hostname)\r\nserver_port = 502",
"_____no_output_____"
],
[
"# connect to server\r\nclient = ModbusTcpClient(server_ip_addr, server_port)",
"_____no_output_____"
],
[
"print(\"[+]Info : Connection\" + str(client.connect()))\r\n",
"[+]Info : ConnectionTrue\n"
],
[
"\"\"\"\r\nWrite values to registers in server \r\n according to GASTRON GTD-5000 Address Map\r\n\r\n40001: flow correction 1, fault active 0 alarm 01\r\n 1000 0001 0000 0000 = 0x8100 = 33024\r\n40002: gas ID, catridge ID\r\n 0000 0001 0001 1001 = 0x0119 = 281\r\n40003: gas value word 1 of 2\r\n 0000 0000 0000 0001 = 0x0001 = 1\r\n40004: gas value word 2 of 2\r\n 1010 0000 0000 0100 = 0xA004 = 40964\r\n40006: error code\r\n 0000 0101 0000 0000 = 0x0500 = 1280\r\n40007 : units PPB\r\n 0000 0000 0010 0000 = 0x0020 = 32\r\n40013 : 1st alarm limit word 1 of 2\r\n 0000 0000 0000 0001 = 0x0001 = 1\r\n40014 : 1st alarm limit word 2 of 2\r\n 1010 0000 0000 1000 = 0xA008 = 40968\r\n40015 : 2nd alarm limit word 1 of 2\r\n 0000 0000 0000 0010 = 0x0002 = 2 \r\n40016 : 2nd alarm limit word 1 of 2\r\n 0000 0000 0000 0000 = 0x0000 = 0\r\n\r\n1st alarm on, 2nd alarm off\r\n\"\"\"\r\n# when reading values from registers, values are decimal\r\n# no matter how it was written(hex or decimal) \r\nclient.write_registers(0, 0x8100) \r\nclient.write_registers(1, 0x0119)\r\nclient.write_registers(2, 1) \r\nclient.write_registers(3, 40964) \r\nclient.write_registers(5, 1280) \r\nclient.write_registers(6, 32) \r\nclient.write_registers(12, 1) \r\nclient.write_registers(13, 40968) \r\nclient.write_registers(14, 2) \r\nclient.write_registers(15, 0) ",
"_____no_output_____"
]
]
] | [
"code"
] | [
[
"code",
"code",
"code",
"code",
"code"
]
] |
e76b10c5d4ad004de086907f7bf1ca08cbf7f426 | 82,010 | ipynb | Jupyter Notebook | 02_SQL_query_Data_merge.ipynb | Pamaland1/Flip | 9eb601aaddf23d0eeed22e888f5ce5b157d342af | [
"MIT"
] | null | null | null | 02_SQL_query_Data_merge.ipynb | Pamaland1/Flip | 9eb601aaddf23d0eeed22e888f5ce5b157d342af | [
"MIT"
] | null | null | null | 02_SQL_query_Data_merge.ipynb | Pamaland1/Flip | 9eb601aaddf23d0eeed22e888f5ce5b157d342af | [
"MIT"
] | null | null | null | 33.015298 | 184 | 0.357603 | [
[
[
"import pandas as pd\n\n#get pandas and sql to work together\nimport psycopg2 as pg\nimport pandas.io.sql as pd_sql\n\nfrom psycopg2 import connect\nfrom psycopg2.extensions import ISOLATION_LEVEL_AUTOCOMMIT\nimport matplotlib.pyplot as plt",
"_____no_output_____"
],
[
"params = {\n 'host': 'localhost', # We are connecting to our _local_ version of psql\n 'user': 'agar',\n 'dbname': 'votes', # DB that we are connecting to\n 'port': 5432 # port we opened on AWS\n}\n\n# We will talk about this magic Python trick!\nconnection = pg.connect(**params)",
"_____no_output_____"
],
[
"sql_query_demographics = \"\"\"\nselect \n\"Code\" as district_id, \ncast(\"2010 Census Adult Population by Race\" as float) as white_adult_percent, \ncast(\"Unnamed: 33\" as float) as black_adult_percent, \ncast(\"Unnamed: 34\" as float) as latino_adult_percent,\ncast(\"Unnamed: 35\" as float) as asian_adult_percent,\ncast(\"Unnamed: 36\" as float) as native_adult_percent,\ncast(\"Unnamed: 37\" as float) as other_adult_percent,\ncast(\"2016 American Community Survey Income and Education\" as float) as bachelors,\ncast(\"Unnamed: 40\" as float) as white_bachelors,\ncast(\"Non-College White Share\" as float) as white_no_college,\ncast (REPLACE(replace(\"Unnamed: 42\", ',', ''), '$', '') as integer) as income_median,\ncast(replace(\"Census Voting Age Population_Includes Latinos of Any Race\", ',', '') as integer) as total_vote,\ncast(replace(\"Unnamed: 94\", ',', '') as integer) as white_vote,\ncast(replace(\"Unnamed: 95\", ',', '') as integer) as black_vote,\ncast(replace(\"Unnamed: 96\", ',', '') as integer) as latino_vote,\ncast(replace(\"Unnamed: 97\", ',', '') as integer) as asian_vote,\ncast(replace(\"Unnamed: 98\", ',', '') as integer) as native_vote,\ncast(replace(\"Unnamed: 99\", ',', '') as integer) as other_vote,\ncast(replace(\"Census Total Population_Includes Latinos of Any Race\", ',', '') as integer) as total_pop,\ncast(replace(\"Unnamed: 87\", ',', '') as integer) as white_pop,\ncast(replace(\"Unnamed: 88\", ',', '') as integer) as black_pop,\ncast(replace(\"Unnamed: 89\", ',', '') as integer) as latino_pop,\ncast(replace(\"Unnamed: 90\", ',', '') as integer) as asian_pop,\ncast(replace(\"Unnamed: 91\", ',', '') as integer) as native_pop,\ncast(replace(\"Unnamed: 92\", ',', '') as integer) as other_pop\n\nfrom \"demographic_115_con\" \n\"\"\"",
"_____no_output_____"
],
[
"sql_query_largest_geo = \"\"\"\nselect \n\"District\" as district_id,\n\"p_of CD\" as larg1_percent_pop,\n\"p_of CD.1\" as larg2_percent_pop,\n\"p_of CD.2\" as larg3_percent_pop\nfrom \"geography_116+\" g \n\"\"\"",
"_____no_output_____"
],
[
"sql_query_metro = \"\"\"\nselect \n\"District\" as district_id,\n\"p_of CD\" as metro1_percent_pop,\n\"p_of CD.1\" as metro2_percent_pop,\n\"p_of CD.2\" as metro3_percent_pop,\n\"p_no metro\" as metro_none_percent_pop\nfrom \"geography_116_metro\" \n\"\"\"",
"_____no_output_____"
],
[
"sql_query_votes = \"\"\"\nselect year, \ncase \n\twhen district<10 \n\t\tthen concat(state_po, '-0', district)\n\twhen district>9\t\n\t\tthen concat(state_po, '-', district)\n\t\tend as district_id,\nstate_fips, party,\ncast(replace(candidatevotes, ',', '') as integer) as candidate_votes, \ntotalvotes\n\nfrom \"H_of_Rep\" hor \n\nwhere year>2009 and year<2020\norder by year, district_id, party\n\"\"\"",
"_____no_output_____"
],
[
"df_demographics = pd.read_sql_query(sql_query_demographics, connection, index_col=None, coerce_float=True, params=None, parse_dates=None, chunksize=None)",
"_____no_output_____"
],
[
"df_demographics.head(440)",
"_____no_output_____"
],
[
"df_largest_geo = pd.read_sql_query(sql_query_largest_geo, connection, index_col=None, coerce_float=True, params=None, parse_dates=None, chunksize=None)",
"_____no_output_____"
],
[
"df_largest_geo.head()",
"_____no_output_____"
],
[
"df_metro = pd.read_sql_query(sql_query_metro, connection, index_col=None, coerce_float=True, params=None, parse_dates=None, chunksize=None)",
"_____no_output_____"
],
[
"df_metro.head()",
"_____no_output_____"
],
[
"df_metro[\"district_id\"][0] = \"AK-00\"",
"/Users/agar/opt/anaconda3/envs/metis/lib/python3.7/site-packages/ipykernel_launcher.py:1: SettingWithCopyWarning: \nA value is trying to be set on a copy of a slice from a DataFrame\n\nSee the caveats in the documentation: http://pandas.pydata.org/pandas-docs/stable/user_guide/indexing.html#returning-a-view-versus-a-copy\n \"\"\"Entry point for launching an IPython kernel.\n"
],
[
"district_merge_1 = pd.merge(df_metro, df_largest_geo, how='inner', on=\"district_id\")",
"_____no_output_____"
],
[
"district_merge_1b = pd.merge(district_merge_1, df_demographics, how='inner', on=\"district_id\")",
"_____no_output_____"
],
[
"district_merge_1b.head()",
"_____no_output_____"
],
[
"new = district_merge_1[\"district_id\"].str.split(\"-\", n = 1, expand = True) ",
"_____no_output_____"
],
[
"new.head()",
"_____no_output_____"
],
[
"new_num = new[1].replace(\"AL\", '00')",
"_____no_output_____"
],
[
"new_num.head()",
"_____no_output_____"
],
[
"news = pd.concat([new[0], new_num], axis=1, sort=False)",
"_____no_output_____"
],
[
"news_district_fix = news[0] + \"-\" + news[1]",
"_____no_output_____"
],
[
"news_district_fix",
"_____no_output_____"
],
[
"district_merge_2 = pd.concat([news_district_fix, district_merge_1b], axis=1, sort=False)",
"_____no_output_____"
],
[
"district_merge_2 = district_merge_2.drop(columns = \"district_id\")",
"_____no_output_____"
],
[
"district_merge_2.columns",
"_____no_output_____"
],
[
"district_merge_2 = district_merge_2.rename(columns={0: \"district_id\"})",
"_____no_output_____"
],
[
"district_merge_2.head()",
"_____no_output_____"
],
[
"df_votes = pd.read_sql_query(sql_query_votes, connection, index_col=None, coerce_float=True, params=None, parse_dates=None, chunksize=None)",
"_____no_output_____"
],
[
"df_votes.head()",
"_____no_output_____"
],
[
"df_votes.tail()",
"_____no_output_____"
],
[
"sql_query_winners_ten = \"\"\"\nselect year, party, district_id\nfrom \"Winners+\" w \nwhere year>2009 and year<2020\norder by year, district_id, party\n\"\"\"",
"_____no_output_____"
],
[
"df_winners_ten = pd.read_sql_query(sql_query_winners_ten, connection, index_col=None, coerce_float=True, params=None, parse_dates=None, chunksize=None)",
"_____no_output_____"
],
[
"df_winners_ten",
"_____no_output_____"
],
[
"winner_votes = pd.merge(df_winners_ten, df_votes, how='left', on=[\"year\", \"district_id\", \"party\"])",
"_____no_output_____"
],
[
"sql_query_flips_agg = \"\"\"\nselect * from\n(\nselect *, \nsum(party_change_simple) over (partition by district_id order by year asc) as sum_flips_total\nfrom \"Flips\" \n) as total_flips\nwhere year>2009 and year<2019 \n\"\"\"",
"_____no_output_____"
],
[
"df_flips_agg = pd.read_sql_query(sql_query_flips_agg, connection, index_col=None, coerce_float=True, params=None, parse_dates=None, chunksize=None)",
"_____no_output_____"
],
[
"df_flips_agg.head()",
"_____no_output_____"
],
[
"winner_votes_agg = pd.merge(df_flips_agg, winner_votes, how='left', on=[\"year\", \"district_id\"])",
"_____no_output_____"
],
[
"winner_votes_agg.head()",
"_____no_output_____"
],
[
"complete_df = pd.merge(winner_votes_agg, district_merge_2, how='left', on=[\"district_id\"])",
"_____no_output_____"
],
[
"complete_df.head()",
"_____no_output_____"
],
[
"complete_df.info()",
"<class 'pandas.core.frame.DataFrame'>\nInt64Index: 2229 entries, 0 to 2228\nData columns (total 39 columns):\nyear 2229 non-null int64\ndistrict_id 2229 non-null object\nparty_change_simple 2229 non-null float64\nsum_flips_total 2229 non-null float64\nparty 2229 non-null object\nstate_fips 2229 non-null int64\ncandidate_votes 2229 non-null int64\ntotalvotes 2229 non-null float64\nmetro1_percent_pop 2229 non-null float64\nmetro2_percent_pop 2229 non-null float64\nmetro3_percent_pop 2229 non-null float64\nmetro_none_percent_pop 2229 non-null float64\nlarg1_percent_pop 2229 non-null float64\nlarg2_percent_pop 2229 non-null float64\nlarg3_percent_pop 2229 non-null float64\nwhite_adult_percent 2229 non-null float64\nblack_adult_percent 2229 non-null float64\nlatino_adult_percent 2229 non-null float64\nasian_adult_percent 2229 non-null float64\nnative_adult_percent 2229 non-null float64\nother_adult_percent 2229 non-null float64\nbachelors 2229 non-null float64\nwhite_bachelors 2229 non-null float64\nwhite_no_college 2229 non-null float64\nincome_median 2229 non-null float64\ntotal_vote 2229 non-null float64\nwhite_vote 2229 non-null float64\nblack_vote 2229 non-null float64\nlatino_vote 2229 non-null float64\nasian_vote 2229 non-null float64\nnative_vote 2229 non-null float64\nother_vote 2229 non-null float64\ntotal_pop 2229 non-null float64\nwhite_pop 2229 non-null float64\nblack_pop 2229 non-null float64\nlatino_pop 2229 non-null float64\nasian_pop 2229 non-null float64\nnative_pop 2229 non-null float64\nother_pop 2229 non-null float64\ndtypes: float64(34), int64(3), object(2)\nmemory usage: 696.6+ KB\n"
],
[
"complete_df = complete_df.fillna(0)",
"_____no_output_____"
],
[
"complete_df.to_csv('/Users/agar/_METIS/exercises/Project_3/data_source/complete_df') ",
"_____no_output_____"
],
[
"from sqlalchemy import create_engine\nconnection_string = f'postgres://agar:{params[\"host\"]}@{params[\"host\"]}:{params[\"port\"]}/votes'\nengine = create_engine(connection_string, pool_pre_ping=True)",
"_____no_output_____"
],
[
"complete_df.iloc[:0].to_sql(\"Complete\", engine, index=False)\ncomplete_df.iloc[:].to_sql(\"Complete\", engine, index=False, if_exists = 'append', chunksize = 1000)",
"_____no_output_____"
],
[
" sql_query_finance = \"\"\"\nselect * from \"financials\"\n\"\"\"",
"_____no_output_____"
],
[
"df_financials = pd.read_sql_query(sql_query_finance, connection, index_col=None, coerce_float=True, params=None, parse_dates=None, chunksize=None)",
"_____no_output_____"
],
[
"#get only House of Representatives\nmask = df_financials['Cand_Office'] == \"H\"\nhouse_df = df_financials[mask]\n\n#select financial columns \ndf_in = house_df[['Cand_State', 'Cand_Office_Dist','year', 'Total_Receipt', 'Total_Disbursement', 'Individual_Itemized_Contribution',\n 'Individual_Unitemized_Contribution', 'Individual_Contribution',\n 'Other_Committee_Contribution', 'Party_Committee_Contribution',\n 'Cand_Contribution', 'Total_Contribution', 'Operating_Expenditure']]\n\n#sum expenditures by district and year\ndf_financial_sum = df_in.groupby(['Cand_State', 'Cand_Office_Dist','year']).agg('sum')\ndf_financial_sum_r = df_financial_sum.reset_index()\n\n#set columns for joins\ndf_financial_sum_r['Cand_Office_Dist'] = df_financial_sum_r.Cand_Office_Dist.map(\"{:02}\".format)\ndf_financial_sum_r['ID_DIST'] = df_financial_sum_r['year'].astype(str)+ \"-\" + df_financial_sum_r['Cand_State'] + \"-\" + df_financial_sum_r['Cand_Office_Dist'].astype(str) \ndf_financial_sum_r['district_id'] = df_financial_sum_r['Cand_State']+ \"-\" + df_financial_sum_r['Cand_Office_Dist'].astype(str) \ndf_r.to_csv(\"financials_df.csv\")\n\ndf_r.to_csv(\"financials_df.csv\")",
"_____no_output_____"
]
]
] | [
"code"
] | [
[
"code",
"code",
"code",
"code",
"code",
"code",
"code",
"code",
"code",
"code",
"code",
"code",
"code",
"code",
"code",
"code",
"code",
"code",
"code",
"code",
"code",
"code",
"code",
"code",
"code",
"code",
"code",
"code",
"code",
"code",
"code",
"code",
"code",
"code",
"code",
"code",
"code",
"code",
"code",
"code",
"code",
"code",
"code",
"code",
"code",
"code",
"code",
"code",
"code",
"code"
]
] |
e76b298c54f206a83a3d659e22f5c9392227f642 | 93,506 | ipynb | Jupyter Notebook | summary worksheet/M6 Function Fundamentals and Best Practices.ipynb | Lavendulaa/programming-in-python-for-data-science | bc41da8afacf4c180ae0ff9c6dc26a7e6292252f | [
"MIT"
] | null | null | null | summary worksheet/M6 Function Fundamentals and Best Practices.ipynb | Lavendulaa/programming-in-python-for-data-science | bc41da8afacf4c180ae0ff9c6dc26a7e6292252f | [
"MIT"
] | null | null | null | summary worksheet/M6 Function Fundamentals and Best Practices.ipynb | Lavendulaa/programming-in-python-for-data-science | bc41da8afacf4c180ae0ff9c6dc26a7e6292252f | [
"MIT"
] | null | null | null | 44.484301 | 2,905 | 0.596058 | [
[
[
"SUMMARY\nEvaluate the readability, complexity and performance of a function.\nWrite docstrings for functions following the NumPy/SciPy format.\nWrite comments within a function to improve readability.\nWrite and design functions with default arguments.\nExplain the importance of scoping and environments in Python as they relate to functions.\nFormulate test cases to prove a function design specification.\nUse assert statements to formulate a test case to prove a function design specification.\nUse test-driven development principles to define a function that accepts parameters, returns values and passes all tests.\nHandle errors gracefully via exception handling.",
"_____no_output_____"
],
[
"In the last module, we were introduced to the DRY principle and how creating functions helps comply with it.\n\nLetโs do a little bit of a recap.\n\nDRY stands for Donโt Repeat Yourself.\n\nWe can avoid writing repetitive code by creating a function that takes in arguments, performs some operations, and returns the results.\n\nThe example in Module 5 converted code that creates a list of squared elements from an existing list of numbers into a function.",
"_____no_output_____"
]
],
[
[
"#example loop\nnumbers = [2, 3, 5]\nsquared = list()\nfor number in numbers: \n squared.append(number ** 2)\nsquared\n",
"_____no_output_____"
],
[
"#ex1 loop as function\ndef squares_a_list(numerical_list):#function name and agruement\n new_squared_list = list() #initialize output list\n for number in numerical_list:\n new_squared_list.append(number ** 2)\n return new_squared_list\nsquares_a_list(numbers) #function call",
"_____no_output_____"
]
],
[
[
"This function gave us the ability to do the same operation for multiple lists without having to rewrite any code and just calling the function.",
"_____no_output_____"
]
],
[
[
"larger_numbers = [5, 44, 55, 23, 11]\npromoted_numbers = [73, 84, 95]\nexecutive_numbers = [100, 121, 250, 103, 183, 222, 214]\n\n",
"_____no_output_____"
],
[
"squares_a_list(larger_numbers)",
"_____no_output_____"
],
[
"squares_a_list(promoted_numbers)",
"_____no_output_____"
],
[
"squares_a_list(executive_numbers)",
"_____no_output_____"
]
],
[
[
"Itโs important to know what exactly is going on inside and outside of a function.\n\nIn our function squares_a_list() we saw that we created a variable named new_squared_list.\n\nWe can print this variable and watch all the elements be appended to it as we loop through the input list.\n\nBut what happens if we try and print this variable outside of the function?\n\nYikes! Where did new_squared_list go?\n\nIt doesnโt seem to exist! Thatโs not entirely true.\n\nIn Python, new_squared_list is something we call a local variable.\n\nLocal variables are any objects that have been created within a function and only exist inside the function where they are made.\n\nCode within a function is described as a local environment.\n\nSince we called new_squared_list outside of the functionโs body, Python fails to recognize it.",
"_____no_output_____"
]
],
[
[
"def squares_a_list(numerical_list):\n new_squared_list = list()\n for number in numerical_list:\n new_squared_list.append(number ** 2)\n print(new_squared_list)\n return new_squared_list",
"_____no_output_____"
],
[
"squares_a_list(numbers)",
"[4]\n[4, 9]\n[4, 9, 25]\n"
],
[
"new_squared_list",
"_____no_output_____"
]
],
[
[
"Letโs talk more about function arguments.\n\nArguments play a paramount role when it comes to adhering to the DRY principle as well as adding flexibility to your code.\n\nLetโs bring back the function we made named squares_a_list().\n\nThe reason we made this function in the first place was to DRY out our code and avoid repeating the same for loop for any additional list we wished to operate on.\n\nWhat happens now if we no longer wanted to square a number but calculate a specified exponential of each element, perhaps (n^3), or (n^4)?\n\nWould we need a new function?\n\nWe could make a similar new function for cubing the numbers.\n\nBut this feels repetitive.\n\n\nA better solution that adheres to the DRY principle is to tweak our original function but add an additional argument.\n\nTake a look at exponent_a_list() which now takes 2 arguments; the original numerical_list, and now a new argument named exponent.\n\nThis gives us a choice of the exponent. We could use the same function now for any exponent we want instead of making a new function for each.\n\nThis makes sense to do if we foresee needing this versatility, else the additional argument isnโt necessary.",
"_____no_output_____"
]
],
[
[
"def exponent_a_list(numerical_list, exponent):\n new_exponent_list = list()\n\n for number in numerical_list:\n new_exponent_list.append(number ** exponent)\n\n return new_exponent_list",
"_____no_output_____"
],
[
"numbers = [2, 3, 5]\nexponent_a_list(numbers, 3) #the 2nd arguement allows us to specify an exponent value",
"_____no_output_____"
],
[
"exponent_a_list(numbers, 5)",
"_____no_output_____"
]
],
[
[
"Functions can have any number of arguments and any number of optional arguments, but we must be careful with the order of the arguments.\n\nWhen we define our arguments in a function, all arguments with default values (aka optional arguments) need to be placed after required arguments.\n\nIf any required arguments follow any arguments with default values, an error will occur.\n\nLetโs take our original function exponent_a_list() and re-order it so the optional exponent argument is defined first.\n\nWe will see Python throw an error.",
"_____no_output_____"
]
],
[
[
"def exponent_a_list(exponent=2, numerical_list):\n new_exponent_list = list()\n\n for number in numerical_list:\n new_exponent_list.append(number ** exponent)\n\n return new_exponent_list",
"_____no_output_____"
]
],
[
[
"Up to this point, we have been calling functions with multiple arguments in a single way.\n\nWhen we call our function, we have been ordering the arguments in the order the function defined them in.\n\nSo, in exponent_a_list(), the argument numerical_list is defined first, followed by the argument exponent.\n\nNaturally, we have been calling our function with the arguments in this order as well.",
"_____no_output_____"
]
],
[
[
"def exponent_a_list(numerical_list, exponent=2):\n new_exponent_list = list()\n\n for number in numerical_list:\n new_exponent_list.append(number ** exponent)\n\n return new_exponent_list\nexponent_a_list([2, 3, 5], 5)",
"_____no_output_____"
]
],
[
[
"We showed earlier that we could also call the function by specifying exponent=5.\n\nAnother way of calling this would be to also specify any of the argument names that do not have default values, in this case, numerical_list.\n\nWhat happens if we switch up the order of the arguments and put exponent=5 followed by numerical_list=numbers?\n\nIt still works!",
"_____no_output_____"
]
],
[
[
"exponent_a_list(numerical_list=[2, 3, 5], exponent=5)",
"_____no_output_____"
],
[
"exponent_a_list(exponent=5, numerical_list=[2, 3, 5])",
"_____no_output_____"
]
],
[
[
"What about if we switch up the ordering of the arguments without specifying any of the argument names.\n\nOur function doesnโt recognize the input arguments, and an error occurs because the two arguments are being swapped - it thinks 5 is the list, and [2, 3, 5] is the exponent.\n\nItโs important to take care when ordering and calling a function.\n\nThe rule of thumb to remember is if you are going to call a function where the arguments are in a different order from how they were defined, you need to assign the argument name to the value when you call the function.",
"_____no_output_____"
]
],
[
[
"exponent_a_list(5, [2, 3, 5]) #this wont work because it thinkg that 5 is the list",
"_____no_output_____"
]
],
[
[
"Functions can get very complicated, so it is not always obvious what they do just from looking at the name, arguments, or code.\n\nTherefore, people like to explain what the function does.\n\nThe standard format for doing this is called a docstring.\n\nA docstring is a literal string that comes directly after the function def and documents the functionโs purpose and usage.\n\nWriting a docstring documents what your code does so that collaborators (and you in 6 monthsโ time!) are not struggling to decipher and reuse your code.\n\nIn the last section we had our function squares_a_list().\n\nAlthough our function name is quite descriptive, it could mean various things.\n\nHow do we know what data type it takes in and returns?\n\nHaving documentation for it can be useful in answering these questions.",
"_____no_output_____"
],
[
"Here is the code for a function from the pandas package called truncate().\n\nYou can view the complete code here. https://github.com/pandas-dev/pandas/blob/v1.1.0/pandas/core/generic.py#L9258\n\nI think we can all agree that it would take a bit of time to figure out what the function is doing, the expected input variable types, and what the function is returning.\n\nLuckily pandas provides detailed documentation to explain the functionโs code.",
"_____no_output_____"
],
[
"Ah. This documentation gives us a much clearer idea of what the function is doing and how to use it.\n\nWe can see what it requires as input arguments and what it returns.\n\nIt also explains the expectations of the function.\n\nReading this instead of the code saved us some time and definitely potential confusion.\n\nThere are several styles of docstrings; this one and the one weโll be using is called the NumPy style.",
"_____no_output_____"
],
[
"All docstrings, not just the Numpy formatted ones, are contained within 3 sets of quotations\"\"\". We discussed in module 4 that this was one of the ways to implement string values.\n\nAdding this additional string to our function has no effect on our code, and the sole purpose of the docstring is for human consumption.\n\nThe NumPy format includes 4 main sections:\n- A brief description of the function\n- Explaining the input Parameters\n- What the function Returns\n- Examplesgit a",
"_____no_output_____"
]
],
[
[
"string1 = \"\"\"This is a string\"\"\"\ntype(string1)",
"_____no_output_____"
]
],
[
[
"Writing documentation for squares_a_list() using the NumPy style takes the following format.\n\nWe can identify the brief description of the function at the top, the parameters that it takes in, and what object type they should be, as well as what to expect as an output.\n\nHere we can even see examples of how to run it and what is returned.",
"_____no_output_____"
]
],
[
[
"def squares_a_list(numerical_list):\n \"\"\"\n Squared every element in a list.\n\n Parameters\n ----------\n numerical_list : list\n The list from which to calculate squared values \n\n Returns\n -------\n list\n A new list containing the squared value of each of the elements from the input list \n\n Examples\n --------\n >>> squares_a_list([1, 2, 3, 4])\n [1, 4, 9, 16]\n \"\"\"\n new_squared_list = list()\n for number in numerical_list:\n new_squared_list.append(number ** 2)\n return new_squared_list",
"_____no_output_____"
]
],
[
[
"Using exponent_a_list(), a function from the previous section as an example, we include an optional note in the parameter definition and an explanation of the default value in the parameter description.\n",
"_____no_output_____"
]
],
[
[
"def exponent_a_list(numerical_list, exponent=2):\n \"\"\"\n Creates a new list containing specified exponential values of the input list. \n\n Parameters\n ----------\n numerical_list : list\n The list from which to calculate exponential values from\n exponent: int or float, optional\n The exponent value (the default is 2, which implies the square).\n\n Returns\n -------\n new_exponent_list : list\n A new list containing the exponential value specified of each \n of the elements from the input list \n\n Examples\n --------\n >>> exponent_a_list([1, 2, 3, 4])\n [1, 4, 9, 16]\n \"\"\"\n\n new_exponent_list = list()\n\n for number in numerical_list:\n new_exponent_list.append(number ** exponent)",
"_____no_output_____"
]
],
[
[
"Ah, remember how we talked about side effects back at the beginning of this module?\n\nAlthough we recommend avoiding side effects in your functions, there may be occasions where theyโre unavoidable or required.\n\nIn these cases, we must make it clear in the documentation so that the user of the function knows that their objects are going to be modified. (As an analogy: If someone wants you to babysit their cat, you would probably tell them first if you were going to paint it red while you had it!)\n\nSo how we include side effects in our docstrings?\n\nItโs best to include your function side effects in the first sentence of the docstring.",
"_____no_output_____"
]
],
[
[
"def function_name(param1, param2):\n \"\"\"The first line is a short description of the function. \n\n If your function includes side effects, explain it clearly here.\n\n\n Parameters\n ----------\n param1 : datatype\n A description of param1.\n\n .\n .\n .\n Etc.\n \"\"\"",
"_____no_output_____"
]
],
[
[
"Ok great! Now that weโve written and explained our functions with a standardized format, we can read it in our file easily, but what if our function is located in a different file?\n\nHow can we learn what it does when reading our code?\n\nWe learned in the first assignment that we can read more about built-in functions using the question mark before the function name.\n\nThis returns the docstring of the function.\n?function_name",
"_____no_output_____"
]
],
[
[
"?len # For example, if we want the docstring for the function len():",
"Object `len # For example, if we want the docstring for the function len():` not found.\n"
]
],
[
[
"We all know that mistakes are a regular part of life.\n\nIn coding, every line of code is at risk for potential errors, so naturally, we want a way of defending our functions against potential issues.\n\nDefensive programming is code written in such a way that, if errors do occur, they are handled in a graceful, fast and informative manner.\n\nIf something goes wrong, we donโt want the code to crash on its own terms - we want it to fail gracefully, in a way we pre-determined.\n\nTo help soften the landing, we write code that throws our own Exceptions.\n\nExceptions are used in Defensive programming to disrupt the normal flow of instructions. When Python encounters code that it cannot execute, it will throw an exception.\n\nBefore we dive into exceptions, letโs revisit our function exponent_a_list().\n\nIt works somewhat well, but what happens if we try to use it with an input string instead of a list.\n\nWe get an error that explains a little bit of whatโs causing the issue but not directly.\n\nThis error, called a TypeError here, is itself a Python exception. But the error message, which is a default Python message, is not super clear.\n\nThis is where raising our own Exception steps in to help.",
"_____no_output_____"
]
],
[
[
"def exponent_a_list(numerical_list, exponent=2):\n new_exponent_list = list()\n for number in numerical_list:\n new_exponent_list.append(number ** exponent)\n return new_exponent_list\nnumerical_string = \"123\"\nexponent_a_list(numerical_string)",
"_____no_output_____"
],
[
"def exponent_a_list(numerical_list, exponent=2):\n\n if type(numerical_list) is not list:\n raise Exception(\"You are not using a list for the numerical_list input.\")\n\n new_exponent_list = list()\n for number in numerical_list:\n new_exponent_list.append(number ** exponent)\n return new_exponent_list",
"_____no_output_____"
]
],
[
[
"Exceptions disrupt the regular execution of our code. When we raise an Exception, we are forcing our own error with our own message.\n\nIf we wanted to raise an exception to solve the problem on the last slide, we could do the following.",
"_____no_output_____"
]
],
[
[
"numerical_string = \"123\"\nexponent_a_list(numerical_string)",
"_____no_output_____"
]
],
[
[
"Letโs take a closer look.\n\nThe first line of code is an if statement - what needs to occur to trigger this new code weโve written.\n\nThis code translates to โIf numerical_list is not of the type listโฆโ.\n\nThe second line does the complaining.\n\nWe tell it to raise an Exception (throw an error) with this message.\n\nNow we get an error message that is straightforward on why our code is failing.\n\nException: You are not using a list for the numerical_list input.\n\nI hope we can agree that this message is easier to decipher than the original.\n\nThe new message made the cause of the error much clearer to the user, making our function more usable.",
"_____no_output_____"
],
[
"Letโs now learn more about the possible different types of Exceptions.\n\nThe exception type called Exception is a generic, catch-all exception type.\n\nThere are also many other exception types; for example, you may have encountered ValueError or a TypeError at some point.\n\nException, which is used in our previous examples, may not be the best option for the raises we made.\n\nLetโs take a look now at the exception we wrote that checks if the input value for numerical_list was the correct type.\n\nSince this is a type error, a better-raised exception over Exception would be TypeError.\n\nLetโs make our correction here and change Exception in our function to TypeError.",
"_____no_output_____"
]
],
[
[
"if type(numerical_list) is not list:\n raise Exception(\"You are not using a list for the numerical_list input.\")",
"_____no_output_____"
],
[
"def exponent_a_list(numerical_list, exponent=2):\n\n if type(numerical_list) is not list:\n raise TypeError(\"You are not using a list for the numerical_list input.\")\n\n new_exponent_list = list()\n for number in numerical_list:\n new_exponent_list.append(number ** exponent)\n return new_exponent_list",
"_____no_output_____"
],
[
"numerical_string = \"123\"\nexponent_a_list(numerical_string)",
"_____no_output_____"
]
],
[
[
"Now that we can write exceptions, itโs important to document them.\n\nItโs a good idea to include details of any included exceptions in our functionโs docstring.\n\nUnder the NumPy docstring format, we explain our raised exception after the โReturnsโ section.\n\nWe first specify the exception type and then an explanation of what causes the exception to be raised.\n\nFor example, weโve added a โRaisesโ section in our exponent_a_list docstring here.",
"_____no_output_____"
]
],
[
[
"def exponent_a_list(numerical_list, exponent=2):\n \"\"\"\n Creates a new list containing specified exponential values of the input list. \n\n Parameters\n ----------\n numerical_list : list\n The list from which to calculate exponential values from\n exponent : int or float, optional\n The exponent value (the default is 2, which implies the square).\n\n Returns\n -------\n new_exponent_list : list\n A new list containing the exponential value specified of each \n of the elements from the input list \n\n Raises\n ------\n TypeError\n If the input argument numerical_list is not of type list\n\n Examples\n --------\n >>> exponent_a_list([1, 2, 3, 4])\n [1, 4, 9, 16]\n \"\"\"",
"_____no_output_____"
]
],
[
[
"In the last section, we learned about raising exceptions, which, in a lot of cases, helps the function user identify if they are using it correctly.\n\nBut there are still some questions remaining:\n\nHow can we be so sure that the code we wrote is doing what we want it to?\n\nDoes our code work 100% of the time?\n\nThese questions can be answered by using something called units tests.\n\nWeโll be implementing unit tests in Python using assert statements.\" assert statements are just one way of implementing unit tests.\n\nLetโs first discuss the syntax of an assert statement and then how they can be applied to the bigger concept, which is unit tests.",
"_____no_output_____"
],
[
"assert statements can be used as sanity checks for our program.\n\nWe implement them as a โdebuggingโ tactic to make sure our code runs as we expect it to.\n\nWhen Python reaches an assert statement, it evaluates the condition to a Boolean value.\n\nIf the statement is True, Python will continue to run. However, if the Boolean is False, the code stops running, and an error message is printed.\n\nLetโs take a look at one.\n\nHere we have the keyword assert that checks if 1==2. Since this is False, an error is thrown, and the message beside the condition \"1 is not equal to 2.\" is outputted.",
"_____no_output_____"
]
],
[
[
"assert 1 == 2 , \"1 is not equal to 2.\"",
"_____no_output_____"
]
],
[
[
"https://prog-learn.mds.ubc.ca/module6/assert2.png",
"_____no_output_____"
],
[
"Letโs take a look at an example where the Boolean is True.\n\nHere, since the assert statement results in a True values, Python continues to run, and the next line of code is executed.\n\nWhen an assert is thrown due to a Boolean evaluating to False, the next line of code does not get an opportunity to be executed.",
"_____no_output_____"
]
],
[
[
"assert 1 == 1 , \"1 is not equal to 1.\"\nprint('Will this line execute?')",
"Will this line execute?\n"
],
[
"assert 1 == 2 , \"1 is not equal to 2.\"\nprint('Will this line execute?')",
"_____no_output_____"
],
[
"Not all assert statements need to have a message.\n\nWe can re-write the statement from before without one.\n\nThis time youโll notice that the error doesnโt contain the particular message beside AssertionError like we had before.",
"_____no_output_____"
],
[
"assert 1 == 2 ",
"_____no_output_____"
]
],
[
[
"Where do assert statements come in handy?\n\nUp to this point, we have been creating functions, and only after we have written them, weโve tested if they work.\n\nSome programmers use a different approach: writing tests before the actual function. This is called Test-Driven Development.\n\nThis may seem a little counter-intuitive, but weโre creating the expectations of our function before the actual function code.\n\nOften we have an idea of what our function should be able to do and what output is expected.\n\nIf we write our tests before the function, it helps understand exactly what code we need to write and it avoids encountering large time-consuming bugs down the line.\n\nOnce we have a serious of tests for the function, we can put them into assert statements as an easy way of checking that all the tests pass.",
"_____no_output_____"
],
[
"https://prog-learn.mds.ubc.ca/module6/why.png",
"_____no_output_____"
],
[
"So, what kind of tests do we want?\n\nWe want to keep these tests simple - things that we know are true or could be easily calculated by hand.\n\nFor example, letโs look at our exponent_a_list() function.\n\nEasy cases for this function would be lists containing numbers that we can easily square or cube.\n\nFor example, we expect the square output of [1, 2, 4, 7] to be [1, 4, 16, 49].\n\nThe test for this would look like the one shown here.\n\nIt is recommended to write multiple tests.\n\nLetโs write another test for a differently sized list as well as different values for both input arguments numerical_list and exponent.\n\nLetโs make another test for exponent = 3. Again, we use numbers that we know the cube of.\n\nWe can also test that the type of the returned object is correct.",
"_____no_output_____"
]
],
[
[
"def exponent_a_list(numerical_list, exponent=2):\n new_exponent_list = list()\n\n for number in numerical_list:\n new_exponent_list.append(number ** exponent)\n\n return new_exponent_list",
"_____no_output_____"
],
[
"assert exponent_a_list([1, 2, 4, 7], 2) == [1, 4, 16, 49], \"incorrect output for exponent = 2\"",
"_____no_output_____"
],
[
"assert exponent_a_list([1, 2, 3], 3) == [1, 8, 27], \"incorrect output for exponent = 3\"",
"_____no_output_____"
],
[
"assert type(exponent_a_list([1,2,4], 2)) == list, \"output type not a list\"",
"_____no_output_____"
]
],
[
[
"Just because all our tests pass, this does not mean our program is necessarily correct.\n\nItโs common that our tests can pass, but our code contains errors.\n\nLetโs take a look at the function bad_function(). Itโs very similar to exponent_a_list except that it separately computes the first entry before doing the rest in the loop.\n\nThis function looks like it would work perfectly fine, but what happens if we get an input argument for numerical_list that cannot be sliced?\n\nLetโs write some unit tests using assert statements and see what happens.\n\nHere, it looks like our tests pass at first.\n\nBut what happens if we try our function with an empty list?\n\nWe get an unexpected error! How do we avoid this?\n\nWrite a lot of tests and donโt be overconfident, even after writing a lot of tests!\n\nChecking an empty list in our bad_function() function is an example of checking a corner case.\n\nA corner case is an input that is reasonable but a bit unusual and may trip up our code.",
"_____no_output_____"
]
],
[
[
"def bad_function(numerical_list, exponent=2):\n new_exponent_list = [numerical_list[0] ** exponent] # seed list with first element\n for number in numerical_list[1:]:\n new_exponent_list.append(number ** exponent)\n return new_exponent_list",
"_____no_output_____"
],
[
"assert bad_function([1, 2, 4, 7], 2) == [1, 4, 16, 49], \"incorrect output for exponent = 2\"\nassert bad_function([2, 1, 3], 3) == [8, 1, 27], \"incorrect output for exponent = 3\"",
"_____no_output_____"
],
[
"bad_function([], 2)",
"_____no_output_____"
]
],
[
[
"Often, we will be making functions that work on data.\n\nFor example, perhaps we want to write a function called column_stats that returns some summary statistics in the form of a dictionary.\n\nThe function here is something we might have envisioned. (Note that if weโre using test-driven development, this function will just be an idea, not completed code.)\n\nIn these situations, we need to invent some sort of data so that we can easily calculate the max, min, range, and mean and write unit tests to check that our function does the correct operations.\n\nThe data can be made from scratch using functions such as pd.DataFrame() or pd.DataFrame.from_dict() which we learned about in module 4.\n\nYou can also upload a very small slice of an existing dataframe.",
"_____no_output_____"
]
],
[
[
"def column_stats(df, column):\n stats_dict = {'max': df[column].max(),\n 'min': df[column].min(),\n 'mean': round(df[column].mean()),\n 'range': df[column].max() - df[column].min()}\n return stats_dict",
"_____no_output_____"
]
],
[
[
"The values we chose in our columns should be simple enough to easily calculate the expected output of our function.\n\nJust like how we made unit tests using calculations we know to be true, we do the same using a simple dataset we call helper data.\n\nThe dataframe must have a small dimension to keep the calculations simple.\n\nThe tests we write for the function column_stats() are now easy to calculate since the values we are using are few and simple.\n\nWe wrote tests that check different columns in our forest dataframe.",
"_____no_output_____"
]
],
[
[
"import pandas as pd\ndata = {'name': ['Cherry', 'Oak', 'Willow', 'Fir', 'Oak'], \n 'height': [15, 20, 10, 5, 10], \n 'diameter': [2, 5, 3, 10, 5], \n 'age': [0, 0, 0, 0, 0], \n 'flowering': [True, False, True, False, False]}\n\nforest = pd.DataFrame.from_dict(data)\nforest",
"_____no_output_____"
],
[
"assert column_stats(forest, 'height') == {'max': 20, 'min': 5, 'mean': 12.0, 'range': 15}\nassert column_stats(forest, 'diameter') == {'max': 10, 'min': 2, 'mean': 5.0, 'range': 8}\nassert column_stats(forest, 'age') == {'max': 0, 'min': 0, 'mean': 0, 'range': 0}\n",
"_____no_output_____"
]
],
[
[
"We use a systematic approach to design our function using a general set of steps to follow when writing programs.\n\nThe approach we recommend includes 5 steps:\n\n1. Write the function stub: a function that does nothing but accepts all input parameters and returns the correct datatype.\n\nThis means we are writing the skeleton of a function.\n\nWe include the line that defines the function with the input arguments and the return statement returning the object with the desired data type.\n\nUsing our exponent_a_list() function as an example, we include the functionโs first line and the return statement.",
"_____no_output_____"
]
],
[
[
"def exponent_a_list(numerical_list, exponent=2):\n return list()",
"_____no_output_____"
]
],
[
[
"2. Write tests to satisfy the design specifications.\n\nThis is where our assert statements come in.\n\nWe write tests that we want our function to pass.\n\nIn our exponent_a_list() example, we expect that our function will take in a list and an optional argument named exponent and then returns a list with the exponential value of each element of the input list.\n\nHere we can see our code fails since we have no function code yet!",
"_____no_output_____"
]
],
[
[
"def exponent_a_list(numerical_list, exponent=2):\n return list()\n\nassert type(exponent_a_list([1,2,4], 2)) == list, \"output type not a list\"\nassert exponent_a_list([1, 2, 4, 7], 2) == [1, 4, 16, 49], \"incorrect output for exponent = 2\"\nassert exponent_a_list([1, 2, 3], 3) == [1, 8, 27], \"incorrect output for exponent = 3\"",
"_____no_output_____"
]
],
[
[
"3. Outline the program with pseudo-code.\n\nPseudo-code is an informal but high-level description of the code and operations that we wish to implement.\n\nIn this step, we are essentially writing the steps that we anticipate needing to complete our function as comments within the function.\n\nSo for our function pseudo-code includes:",
"_____no_output_____"
]
],
[
[
"def exponent_a_list(numerical_list, exponent=2):\n\n # create a new empty list\n # loop through all the elements in numerical_list\n # for each element calculate element ** exponent\n # append it to the new list \n\n return list()\n\nassert type(exponent_a_list([1,2,4], 2)) == list, \"output type not a list\"\nassert exponent_a_list([1, 2, 4, 7], 2) == [1, 4, 16, 49], \"incorrect output for exponent = 2\"\nassert exponent_a_list([1, 2, 3], 3) == [1, 8, 27], \"incorrect output for exponent = 3\"",
"_____no_output_____"
]
],
[
[
"4. Write code and test frequently.\n\nHere is where we fill in our function.\n\nAs you work on the code, more and more tests of the tests that you wrote will pass until finally, all your assert statements no longer produce any error messages.",
"_____no_output_____"
]
],
[
[
"def exponent_a_list(numerical_list, exponent=2):\n new_exponent_list = list()\n\n for number in numerical_list:\n new_exponent_list.append(number ** exponent)\n\n return new_exponent_list\n\nassert type(exponent_a_list([1,2,4], 2)) == list, \"output type not a list\"\nassert exponent_a_list([1, 2, 4, 7], 2) == [1, 4, 16, 49], \"incorrect output for exponent = 2\"\nassert exponent_a_list([1, 2, 3], 3) == [1, 8, 27], \"incorrect output for exponent = 3\"\n",
"_____no_output_____"
]
],
[
[
"5. Write documentation.\n\nFinally, we finish writing our function with a docstring.",
"_____no_output_____"
]
],
[
[
"def exponent_a_list(numerical_list, exponent=2):\n \"\"\" Creates a new list containing specified exponential values of the input list. \n\n Parameters\n ----------\n numerical_list : list\n The list from which to calculate exponential values from\n exponent : int or float, optional\n The exponent value (the default is 2, which implies the square).\n\n Returns\n -------\n new_exponent_list : list\n A new list containing the exponential value specified of each of\n the elements from the input list \n\n Examples\n --------\n >>> exponent_a_list([1, 2, 3, 4])\n [1, 4, 9, 16]\n \"\"\"\n new_exponent_list = list()\n for number in numerical_list:\n new_exponent_list.append(number ** exponent)\n return new_exponent_list\n",
"_____no_output_____"
]
],
[
[
"This has been quite a full module!\n\nWeโve learned how to make functions, how to handle errors gracefully, how to test our functions, and write the necessary documentation to keep our code comprehensible.\n\nThese skills will all contribute to writing effective code.\n\nOne thing we have not discussed yet is the actual code within a function.\n\nWhat makes a function useful?\n\nIs a function more useful when it performs more operations?\n\nDoes adding parameters make your functions more or less useful?\n\nThese are all questions we need to think about when writing functions.\n\nWe are going to list some habits to adopt when writing and designing your functions.",
"_____no_output_____"
],
[
"Hard coding is the process of embedding values directly into your code without saving them in variables\n\nWhen we hardcode values into our code, it decreases flexibility.\n\nBeing inflexible can cause you to end up writing more functions and/or violating the DRY principle.\n\nThis, in turn, can decrease the readability and makes code problematic to maintain. In short, hard coding is a breeding ground for bugs.\n\nRemember our function squares_a_list()?\n\nIn this function, we โhard-codedโ in 2 when we calculated number ** 2.\n\nThere are a couple of approaches to improving the situation. One is to assign 2 to a variable in the function before doing this calculation. That way, if you need to reuse that number, later on, you can just refer to the variable; and if you need to change the 2 to a 3, you only need to change it in one place. Another benefit is that youโre giving it a variable name, which acts as a little bit of documentation.\n\nThe other approach is to turn the value into an argument like we did when we made exponent_a_list().\n\nThis new function now gives us more flexibility with our code.\n\nIf we now encounter a situation where we need to calculate each element to a different exponent like 4 or 0, we can do so without writing new code and potentially making a new error in doing so.\n\nWe reduce our long term workload.\n\nThis version is more maintainable code, but it doesnโt give the function caller any flexibility. What you decide depends on how you expect your function to be used.",
"_____no_output_____"
]
],
[
[
"def squares_a_list(numerical_list):\n new_squared_list = list()\n\n for number in numerical_list:\n new_squared_list.append(number ** 2)\n\n return new_squared_list",
"_____no_output_____"
],
[
"def exponent_a_list(numerical_list, exponent):\n new_exponent_list = list()\n\n for number in numerical_list:\n new_exponent_list.append(number ** exponent)\n\n return new_exponent_list",
"_____no_output_____"
]
],
[
[
"Although it may seem useful when a function acts as a one-stop-shop that does everything you want in a single function, this also limits your ability to reuse code that lies within it.\n\nIdeally, functions should serve a single purpose.\n\nFor example, letโs say we have a function that reads in a csv, finds the mean of each group in a column, and plots a specified variable.\n\nAlthough this may seem nice, we may want to break this up into multiple smaller functions. For example, what if we donโt want the plot? Perhaps the plot is just something we wanted a single time, and now we are committed to it for each time we use the function.\n\nAnother problem with this function is that the means are only printed and not returned. Thus, we have no way of accessing the statistics to use further in our code (we would have to repeat ourselves and rewrite ",
"_____no_output_____"
]
],
[
[
"import altair as alt\n\ndef load_filter_and_average(file, grouping_column, ploting_column):\n df = pd.read_csv(file)\n source = df.groupby(grouping_column).mean().reset_index()\n chart = alt.Chart(source, width = 500, height = 300).mark_bar().encode(\n x=alt.X(grouping_column),\n y=alt.Y(ploting_column)\n )\n return chart\nbad_idea = load_filter_and_average('cereal.csv', 'mfr', 'rating')\nbad_idea",
"_____no_output_____"
]
],
[
[
"In this case, you want to simplify the function.\n\nHaving a function that only calculates the mean values of the groups in the specified column is much more usable.\n\nA preferred function would look something like this, where the input is a dataframe we have already read in, and the output is the dataframe of mean values for all the columns.",
"_____no_output_____"
]
],
[
[
"def grouped_means(df, grouping_column):\n grouped_mean = df.groupby(grouping_column).mean().reset_index()\n return grouped_mean\n\ncereal_mfr = grouped_means(cereal, 'mfr')\ncereal_mfr",
"_____no_output_____"
],
[
"If we wanted, we could then make a second function that creates the desired plot part of the previous function.",
"_____no_output_____"
],
[
"def plot_mean(df, grouping_column, ploting_column):\n chart = alt.Chart(df, width = 500, height = 300).mark_bar().encode(\n x=alt.X(grouping_column),\n y=alt.Y(ploting_column)\n )\n return chart\n\nplot1 = plot_mean(cereal_mfr, 'mfr', 'rating')\nplot1",
"_____no_output_____"
]
],
[
[
"3. Return a single object\n\nFor the most part, we have only lightly touched on the fact that functions can return multiple objects, and itโs with good reason.\n\nAlthough functions are capable of returning multiple objects, that doesnโt mean that itโs the best option.\n\nFor instance, what if we converted our function load_filter_and_average() so that it returns a dataframe and a plot.",
"_____no_output_____"
]
],
[
[
"def load_filter_and_average(file, grouping_column, ploting_column):\n df = pd.read_csv(file)\n source = df.groupby(grouping_column).mean().reset_index()\n chart = alt.Chart(source, width = 500, height = 300).mark_bar().encode(\n x=alt.X(grouping_column),\n y=alt.Y(ploting_column)\n )\n return chart, source\n\nanother_bad_idea = load_filter_and_average('cereal.csv', 'mfr', 'rating')\nanother_bad_idea",
"_____no_output_____"
]
],
[
[
"Since our function returns a tuple, we can obtain the plot by selecting the first element of the output.\n\nThis can be quite confusing. We would recommend separating the code into two functions and can have each one return a single object.\n\nItโs best to think of programming functions in the same way as mathematical functions where most times, mathematical functions return a single value.",
"_____no_output_____"
]
],
[
[
"another_bad_idea[0]",
"_____no_output_____"
]
],
[
[
"Itโs generally bad form to include objects in a function that were created outside of it.\n\nTake our grouped_means() function.\n\nWhat if instead of including df as an input argument, we just used cereal that we loaded earlier?\n\nThe number one problem with doing this is now our function only works on the cereal data - itโs not usable on other data.",
"_____no_output_____"
]
],
[
[
"def grouped_means(df, grouping_column):\n grouped_mean = df.groupby(grouping_column).mean().reset_index()\n return grouped_mean",
"_____no_output_____"
],
[
"cereal = pd.read_csv('cereal.csv')\n\ndef bad_grouped_means(grouping_column):\n grouped_mean = cereal.groupby(grouping_column).mean().reset_index()\n return grouped_mean",
"_____no_output_____"
]
],
[
[
"Ok, letโs say we still use it, then what happens?\n\nAlthough it does work, global variables have the opportunity to be altered in the global environment.\n\nWhen we change the global variable outside the function and try to use the function again, it will refer to the new global variable and potentially no longer work.\n\nOf course, like in any case, these habits are suggestions and not strict rules.\n\nThere will be times where adhering to one of these may not be possible or will hinder your code instead of enhancing it.\n\nThe rule of thumb is to ask yourself how helpful is your function if you or someone else wishes to reuse it.",
"_____no_output_____"
]
]
] | [
"markdown",
"code",
"markdown",
"code",
"markdown",
"code",
"markdown",
"code",
"markdown",
"code",
"markdown",
"code",
"markdown",
"code",
"markdown",
"code",
"markdown",
"code",
"markdown",
"code",
"markdown",
"code",
"markdown",
"code",
"markdown",
"code",
"markdown",
"code",
"markdown",
"code",
"markdown",
"code",
"markdown",
"code",
"markdown",
"code",
"markdown",
"code",
"markdown",
"code",
"markdown",
"code",
"markdown",
"code",
"markdown",
"code",
"markdown",
"code",
"markdown",
"code",
"markdown",
"code",
"markdown",
"code",
"markdown",
"code",
"markdown",
"code",
"markdown",
"code",
"markdown",
"code",
"markdown",
"code",
"markdown",
"code",
"markdown",
"code",
"markdown"
] | [
[
"markdown",
"markdown"
],
[
"code",
"code"
],
[
"markdown"
],
[
"code",
"code",
"code",
"code"
],
[
"markdown"
],
[
"code",
"code",
"code"
],
[
"markdown"
],
[
"code",
"code",
"code"
],
[
"markdown"
],
[
"code"
],
[
"markdown"
],
[
"code"
],
[
"markdown"
],
[
"code",
"code"
],
[
"markdown"
],
[
"code"
],
[
"markdown",
"markdown",
"markdown",
"markdown"
],
[
"code"
],
[
"markdown"
],
[
"code"
],
[
"markdown"
],
[
"code"
],
[
"markdown"
],
[
"code"
],
[
"markdown"
],
[
"code"
],
[
"markdown"
],
[
"code",
"code"
],
[
"markdown"
],
[
"code"
],
[
"markdown",
"markdown"
],
[
"code",
"code",
"code"
],
[
"markdown"
],
[
"code"
],
[
"markdown",
"markdown"
],
[
"code"
],
[
"markdown",
"markdown"
],
[
"code",
"code",
"code",
"code"
],
[
"markdown",
"markdown",
"markdown"
],
[
"code",
"code",
"code",
"code"
],
[
"markdown"
],
[
"code",
"code",
"code"
],
[
"markdown"
],
[
"code"
],
[
"markdown"
],
[
"code",
"code"
],
[
"markdown"
],
[
"code"
],
[
"markdown"
],
[
"code"
],
[
"markdown"
],
[
"code"
],
[
"markdown"
],
[
"code"
],
[
"markdown"
],
[
"code"
],
[
"markdown",
"markdown"
],
[
"code",
"code"
],
[
"markdown"
],
[
"code"
],
[
"markdown"
],
[
"code",
"code",
"code"
],
[
"markdown"
],
[
"code"
],
[
"markdown"
],
[
"code"
],
[
"markdown"
],
[
"code",
"code"
],
[
"markdown"
]
] |
e76b5a71ab713ecd14ba45dfcad64e8084c7c648 | 128 | ipynb | Jupyter Notebook | notebooks/dsp_image/010_segmentation_2.ipynb | bilha-analytics/school | d3491eb3f88386dcef35abe13ff8d494790a607d | [
"MIT"
] | null | null | null | notebooks/dsp_image/010_segmentation_2.ipynb | bilha-analytics/school | d3491eb3f88386dcef35abe13ff8d494790a607d | [
"MIT"
] | null | null | null | notebooks/dsp_image/010_segmentation_2.ipynb | bilha-analytics/school | d3491eb3f88386dcef35abe13ff8d494790a607d | [
"MIT"
] | 1 | 2021-04-08T07:12:48.000Z | 2021-04-08T07:12:48.000Z | 32 | 75 | 0.882813 | [
[
[
"empty"
]
]
] | [
"empty"
] | [
[
"empty"
]
] |
e76b6b1bdd469d2b7aba08a7bd0b3a5f393d14ce | 18,838 | ipynb | Jupyter Notebook | dft_workflow/run_slabs/run_oh_covered/setup_dft_oh.ipynb | raulf2012/PROJ_IrOx_OER | 56883d6f5b62e67703fe40899e2e68b3f5de143b | [
"MIT"
] | 1 | 2022-03-21T04:43:47.000Z | 2022-03-21T04:43:47.000Z | dft_workflow/run_slabs/run_oh_covered/setup_dft_oh.ipynb | raulf2012/PROJ_IrOx_OER | 56883d6f5b62e67703fe40899e2e68b3f5de143b | [
"MIT"
] | null | null | null | dft_workflow/run_slabs/run_oh_covered/setup_dft_oh.ipynb | raulf2012/PROJ_IrOx_OER | 56883d6f5b62e67703fe40899e2e68b3f5de143b | [
"MIT"
] | 1 | 2021-02-13T12:55:02.000Z | 2021-02-13T12:55:02.000Z | 33.579323 | 113 | 0.415649 | [
[
[
"# Setup *OH OER jobs\n---",
"_____no_output_____"
],
[
"### Import Modules",
"_____no_output_____"
]
],
[
[
"import os\nprint(os.getcwd())\nimport sys\nimport time; ti = time.time()\n\nsys.path.insert(0, \"..\")\n\nimport copy\nimport json\nimport pickle\nfrom shutil import copyfile\n\nimport numpy as np\nimport pandas as pd\nfrom pandas import MultiIndex\n\nfrom ase import io\n\n# #########################################################\nfrom methods import (\n# get_df_slab,\n get_df_slabs_to_run,\n get_df_jobs,\n get_df_jobs_anal,\n get_df_jobs_data,\n get_df_jobs_paths,\n get_df_active_sites,\n get_df_slabs_oh,\n )\n\n# #########################################################\nfrom dft_workflow_methods import get_job_spec_dft_params",
"/home/raulf2012/Dropbox/01_norskov/00_git_repos/PROJ_IrOx_OER/dft_workflow/run_slabs/run_oh_covered\n"
],
[
"from methods import isnotebook \nisnotebook_i = isnotebook()\nif isnotebook_i:\n from tqdm.notebook import tqdm\n verbose = True\nelse:\n from tqdm import tqdm\n verbose = False",
"_____no_output_____"
]
],
[
[
"### Script Inputs",
"_____no_output_____"
]
],
[
[
"# Slac queue to submit to\nslac_sub_queue_i = \"suncat3\" # 'suncat', 'suncat2', 'suncat3'",
"_____no_output_____"
]
],
[
[
"### Read Data",
"_____no_output_____"
]
],
[
[
"# #########################################################\ndf_jobs_data = get_df_jobs_data()\n\n# #########################################################\ndf_jobs = get_df_jobs()\n\n# #########################################################\ndf_jobs_anal = get_df_jobs_anal()\n\n# #########################################################\ndf_active_sites = get_df_active_sites()\n\n# #########################################################\ndf_slabs_to_run = get_df_slabs_to_run()\ndf_slabs_to_run = df_slabs_to_run.set_index([\"compenv\", \"slab_id\", \"att_num\"], drop=False)\n\n# #########################################################\ndf_slabs_oh = get_df_slabs_oh()",
"_____no_output_____"
]
],
[
[
"### Filtering down to `oer_adsorbate` jobs",
"_____no_output_____"
]
],
[
[
"df_ind = df_jobs_anal.index.to_frame()\ndf_jobs_anal = df_jobs_anal.loc[\n df_ind[df_ind.job_type == \"oer_adsorbate\"].index\n ]\ndf_jobs_anal = df_jobs_anal.droplevel(level=0)",
"_____no_output_____"
]
],
[
[
"### Setup",
"_____no_output_____"
]
],
[
[
"directory = os.path.join(\n os.environ[\"PROJ_irox_oer\"],\n \"dft_workflow/run_slabs/run_oh_covered\",\n \"out_data/dft_jobs\")\nif not os.path.exists(directory):\n os.makedirs(directory)\n\ndirectory = os.path.join(\n os.environ[\"PROJ_irox_oer\"],\n \"dft_workflow/run_slabs/run_oh_covered\",\n \"out_data/__temp__\")\nif not os.path.exists(directory):\n os.makedirs(directory)\n\ncompenv = os.environ[\"COMPENV\"]",
"_____no_output_____"
]
],
[
[
"### Filtering `df_jobs_anal`",
"_____no_output_____"
]
],
[
[
"from methods_run_slabs import get_systems_to_run_bare_and_oh\n\nindices_to_process = get_systems_to_run_bare_and_oh(df_jobs_anal)\ndf_jobs_anal_i = df_jobs_anal.loc[indices_to_process]\n\n\n# #########################################################\n# Removing systems that were marked to be ignored\nfrom methods import get_systems_to_stop_run_indices\nindices_to_stop_running = get_systems_to_stop_run_indices(df_jobs_anal=df_jobs_anal)\n\nindices_to_drop = []\nfor index_i in df_jobs_anal_i.index:\n if index_i in indices_to_stop_running:\n indices_to_drop.append(index_i)\n\ndf_jobs_anal_i = df_jobs_anal_i.drop(index=indices_to_drop)\n\n\n# #########################################################\n# Drop redundent indices (adsorbate and active site)\ndf_jobs_anal_i = df_jobs_anal_i.set_index(\n df_jobs_anal_i.index.droplevel(level=[2, 3, ])\n )\n\n\n# #########################################################\nidx = np.intersect1d(\n df_jobs_anal_i.index,\n df_slabs_to_run.index,\n )\nshared_indices = idx\n\ndf_i = pd.concat([\n df_slabs_to_run.loc[shared_indices].status,\n df_jobs_anal_i.loc[shared_indices],\n ], axis=1)\ndf_i = df_i[df_i.status == \"ok\"]\n\n# df_i.head()",
"_____no_output_____"
],
[
"indices_not_in = []\nfor i in df_jobs_anal_i.index:\n if i not in df_slabs_to_run.index:\n indices_not_in.append(i)\n\nprint(\n \"Number of systems that have not been manually approved:\",\n len(indices_not_in),\n )",
"Number of systems that have not been manually approved: 0\n"
],
[
"# #########################################################\ndata_dict_list = []\n# #########################################################\nfor name_i, row_i in df_i.iterrows():\n # #####################################################\n compenv_i = name_i[0]\n slab_id_i = name_i[1]\n att_num_i = name_i[2]\n # #####################################################\n\n # if verbose:\n # print(40 * \"=\")\n # print(\"compenv:\", compenv_i, \"|\", \"slab_id:\", slab_id_i, \"|\", \"att_num:\", att_num_i)\n\n # #####################################################\n job_id_max_i = row_i.job_id_max\n # #####################################################\n\n # #####################################################\n df_jobs_i = df_jobs[df_jobs.compenv == compenv_i]\n row_jobs_i = df_jobs_i[df_jobs_i.job_id == job_id_max_i]\n row_jobs_i = row_jobs_i.iloc[0]\n # #####################################################\n bulk_id_i = row_jobs_i.bulk_id\n facet_i = row_jobs_i.facet\n # #####################################################\n\n # #####################################################\n df_jobs_data_i = df_jobs_data[df_jobs_data.compenv == compenv_i]\n row_data_i = df_jobs_data_i[df_jobs_data_i.job_id == job_id_max_i]\n row_data_i = row_data_i.iloc[0]\n # #####################################################\n slab_i = row_data_i.final_atoms\n # #####################################################\n\n # #####################################################\n row_active_site_i = df_active_sites[df_active_sites.slab_id == slab_id_i]\n row_active_site_i = row_active_site_i.iloc[0]\n # #####################################################\n active_sites_unique_i = row_active_site_i.active_sites_unique\n num_active_sites_unique_i = row_active_site_i.num_active_sites_unique\n # #####################################################\n\n # print(len(active_sites_unique_i))\n # print(\"TEMP\")\n # active_sites_unique_i = [24, ]\n\n for active_site_j in active_sites_unique_i:\n df_slabs_oh_i = df_slabs_oh.loc[(compenv_i, slab_id_i, \"o\", active_site_j, att_num_i)]\n for att_num_oh_k, row_k in df_slabs_oh_i.iterrows():\n data_dict_i = dict()\n\n slab_oh_k = row_k.slab_oh\n num_atoms_k = slab_oh_k.get_global_number_of_atoms()\n\n # #############################################\n # attempt = 1\n rev = 1\n\n path_i = os.path.join(\n\n \"out_data/dft_jobs\",\n compenv_i, bulk_id_i, facet_i,\n \"oh\",\n \"active_site__\" + str(active_site_j).zfill(2),\n str(att_num_oh_k).zfill(2) + \"_attempt\", # Attempt\n \"_\" + str(rev).zfill(2), # Revision\n )\n\n path_full_i = os.path.join(\n os.environ[\"PROJ_irox_oer_gdrive\"],\n \"dft_workflow/run_slabs/run_oh_covered\",\n path_i)\n\n # if verbose:\n # print(path_full_i )\n\n path_exists = False\n if os.path.exists(path_full_i):\n path_exists = True\n\n\n # #############################################\n data_dict_i = dict()\n # #############################################\n data_dict_i[\"compenv\"] = compenv_i\n data_dict_i[\"slab_id\"] = slab_id_i\n data_dict_i[\"att_num\"] = att_num_oh_k\n data_dict_i[\"active_site\"] = active_site_j\n data_dict_i[\"path_short\"] = path_i\n data_dict_i[\"path_full\"] = path_full_i\n data_dict_i[\"path_exists\"] = path_exists\n data_dict_i[\"slab_oh\"] = slab_oh_k\n # #############################################\n data_dict_list.append(data_dict_i)\n # #############################################\n\n# #########################################################\ndf_to_setup = pd.DataFrame(data_dict_list)\ndf_to_setup = df_to_setup.set_index(\n [\"compenv\", \"slab_id\", \"att_num\", \"active_site\", ], drop=False)\n\ndf_to_setup_i = df_to_setup[df_to_setup.path_exists == False]",
"_____no_output_____"
],
[
"# #########################################################\ndata_dict_list = []\n# #########################################################\nfor name_i, row_i in df_to_setup_i.iterrows():\n # #####################################################\n data_dict_i = dict()\n # #####################################################\n compenv_i = name_i[0]\n slab_id_i = name_i[1]\n att_num_i = name_i[2]\n active_site_i = name_i[3]\n # #####################################################\n path_full_i = row_i.path_full\n path_short_i = row_i.path_short\n slab_oh_i = row_i.slab_oh\n # #####################################################\n\n # print(name_i, \"|\", active_site_i, \"|\", path_full_i)\n if verbose:\n print(name_i, \"|\", active_site_i)\n print(path_full_i)\n\n os.makedirs(path_full_i)\n\n # #####################################################\n # Copy dft script to job folder\n copyfile(\n os.path.join(os.environ[\"PROJ_irox_oer\"], \"dft_workflow/dft_scripts/slab_dft.py\"),\n os.path.join(path_full_i, \"model.py\"),\n )\n\n # #####################################################\n # Copy atoms object to job folder\n slab_oh_i.write(\n os.path.join(path_full_i, \"init.traj\")\n )\n slab_oh_i.write(\n os.path.join(path_full_i, \"init.cif\")\n )\n num_atoms_i = slab_oh_i.get_global_number_of_atoms()\n\n # #####################################################\n data_dict_i[\"compenv\"] = compenv_i\n data_dict_i[\"slab_id\"] = slab_id_i\n data_dict_i[\"bulk_id\"] = bulk_id_i\n data_dict_i[\"att_num\"] = att_num_i\n data_dict_i[\"rev_num\"] = rev\n data_dict_i[\"active_site\"] = active_site_j\n data_dict_i[\"facet\"] = facet_i\n data_dict_i[\"slab_oh\"] = slab_oh_i\n data_dict_i[\"num_atoms\"] = num_atoms_i\n data_dict_i[\"path_short\"] = path_short_i\n data_dict_i[\"path_full\"] = path_full_i\n # #####################################################\n data_dict_list.append(data_dict_i)\n # #####################################################\n\n\n# #########################################################\ndf_jobs_new = pd.DataFrame(data_dict_list)\n\n# Create empty dataframe with columns if dataframe is empty\nif df_jobs_new.shape[0] == 0:\n df_jobs_new = pd.DataFrame(\n columns=[\"compenv\", \"slab_id\", \"att_num\", \"active_site\", ])",
"_____no_output_____"
],
[
"# #########################################################\ndata_dict_list = []\n# #########################################################\nfor i_cnt, row_i in df_jobs_new.iterrows():\n # #####################################################\n compenv_i = row_i.compenv\n num_atoms = row_i.num_atoms\n path_full_i = row_i.path_full\n # ####################################################\n dft_params_i = get_job_spec_dft_params(\n compenv=compenv_i,\n slac_sub_queue=slac_sub_queue_i,\n )\n dft_params_i[\"ispin\"] = 2\n\n # print(path_full_i)\n\n # #####################################################\n with open(os.path.join(path_full_i, \"dft-params.json\"), \"w+\") as fle:\n json.dump(dft_params_i, fle, indent=2, skipkeys=True)\n\n # #####################################################\n data_dict_i = dict()\n # #####################################################\n data_dict_i[\"compenv\"] = compenv_i\n data_dict_i[\"slab_id\"] = slab_id_i\n data_dict_i[\"att_num\"] = att_num_i\n data_dict_i[\"active_site\"] = active_site_i\n data_dict_i[\"dft_params\"] = dft_params_i\n # #####################################################\n data_dict_list.append(data_dict_i)\n # #####################################################\n\n\n# #########################################################\ndf_dft_params = pd.DataFrame(data_dict_list)\n\n\n# Create empty dataframe with columns if dataframe is empty\nif df_dft_params.shape[0] == 0:\n tmp = 42\n # df_jobs_new = pd.DataFrame(\n # columns=[\"compenv\", \"slab_id\", \"att_num\", \"active_site\", ])\nelse:\n keys = [\"compenv\", \"slab_id\", \"att_num\", \"active_site\"]\n df_dft_params = df_dft_params.set_index(keys, drop=False)",
"_____no_output_____"
],
[
"# #########################################################\nprint(20 * \"# # \")\nprint(\"All done!\")\nprint(\"Run time:\", np.round((time.time() - ti) / 60, 3), \"min\")\nprint(\"setup_dft_oh.ipynb\")\nprint(20 * \"# # \")\n# #########################################################",
"# # # # # # # # # # # # # # # # # # # # # # # # # # # # # # # # # # # # # # # # \nAll done!\nRun time: 0.091 min\nsetup_dft_oh.ipynb\n# # # # # # # # # # # # # # # # # # # # # # # # # # # # # # # # # # # # # # # # \n"
]
],
[
[
"\n\n",
"_____no_output_____"
]
]
] | [
"markdown",
"code",
"markdown",
"code",
"markdown",
"code",
"markdown",
"code",
"markdown",
"code",
"markdown",
"code",
"raw"
] | [
[
"markdown",
"markdown"
],
[
"code",
"code"
],
[
"markdown"
],
[
"code"
],
[
"markdown"
],
[
"code"
],
[
"markdown"
],
[
"code"
],
[
"markdown"
],
[
"code"
],
[
"markdown"
],
[
"code",
"code",
"code",
"code",
"code",
"code"
],
[
"raw"
]
] |
e76b6f392039b486baa7bf2c08fb486105cac043 | 25,830 | ipynb | Jupyter Notebook | molecules/PILS_spectrum.ipynb | CambridgeUniversityPress/IntroductionInterstellarMedium | fbfe64c7d50d15da93ebf2fbc7d86d83cbf8941a | [
"CC0-1.0"
] | 3 | 2021-04-26T15:37:13.000Z | 2021-05-13T04:42:15.000Z | molecules/PILS_spectrum.ipynb | interstellarmedium/interstellarmedium.github.io | 0440a5bd80052ab87575e70fc39acd4bf8e225b3 | [
"CC0-1.0"
] | null | null | null | molecules/PILS_spectrum.ipynb | interstellarmedium/interstellarmedium.github.io | 0440a5bd80052ab87575e70fc39acd4bf8e225b3 | [
"CC0-1.0"
] | null | null | null | 253.235294 | 23,608 | 0.917615 | [
[
[
"## Introduction to the Interstellar Medium\n### Jonathan Williams",
"_____no_output_____"
],
[
"### Figure 7.4: molecular rich spectrum",
"_____no_output_____"
],
[
"#### this is a small portion (centered around CO 3-2) from the published spectrum in https://www.aanda.org/articles/aa/abs/2016/11/aa28648-16/aa28648-16.html\n#### the ascii file was provided by Jes Jorgensen",
"_____no_output_____"
]
],
[
[
"import numpy as np\nimport matplotlib.pyplot as plt\nfrom matplotlib.ticker import FormatStrFormatter\n%matplotlib inline",
"_____no_output_____"
],
[
"nu, flux = np.loadtxt('PILS_spectrum.txt', unpack=True)\n\nfig = plt.figure(figsize=(6,4))\nax = fig.add_subplot(1,1,1)\nax.set_xlabel(r\"$\\nu$ [GHz]\", fontsize=16)\nax.set_ylabel(r\"Flux [Jy]\", fontsize=16)\n\nax.plot(nu, flux, color='k', lw=1)\n\nax.xaxis.set_major_formatter(FormatStrFormatter('%5.1f'))\nax.set_xlim(344.55, 347.44)\nax.set_ylim(-0.07, 1.3)\n\nfig.tight_layout() \nplt.savefig('PILS_spectrum.pdf')",
"_____no_output_____"
]
]
] | [
"markdown",
"code"
] | [
[
"markdown",
"markdown",
"markdown"
],
[
"code",
"code"
]
] |
e76b7256ea8c030764be7349c122dffcef9d3f82 | 21,031 | ipynb | Jupyter Notebook | demo/mAP_evaluate.ipynb | alanwanga/mmdetection3d | a9031dc357f37c961a9d225c9ededec912e50ede | [
"Apache-2.0"
] | null | null | null | demo/mAP_evaluate.ipynb | alanwanga/mmdetection3d | a9031dc357f37c961a9d225c9ededec912e50ede | [
"Apache-2.0"
] | null | null | null | demo/mAP_evaluate.ipynb | alanwanga/mmdetection3d | a9031dc357f37c961a9d225c9ededec912e50ede | [
"Apache-2.0"
] | null | null | null | 41.399606 | 132 | 0.531549 | [
[
[
"'''\nThe intput files expected to have the format:\n\nExpected fields:\n\ngt = [{\n 'sample_token': '0f0e3ce89d2324d8b45aa55a7b4f8207fbb039a550991a5149214f98cec136ac',\n 'translation': [974.2811881299899, 1714.6815014457964, -23.689857123368846],\n 'size': [1.796, 4.488, 1.664],\n 'rotation': [0.14882026466054782, 0, 0, 0.9888642620837121],\n 'name': 'car'\n}]\n\nprediction_result = {\n 'sample_token': '0f0e3ce89d2324d8b45aa55a7b4f8207fbb039a550991a5149214f98cec136ac',\n 'translation': [971.8343488872263, 1713.6816097857359, -25.82534357061308],\n 'size': [2.519726579986132, 7.810161372666739, 3.483438286096803],\n 'rotation': [0.10913582721095375, 0.04099572636992043, 0.01927712319721745, 1.029328402625659],\n 'name': 'car',\n 'score': 0.3077029437237213\n}\n\ninput arguments:\n\n--pred_file: file with predictions\n--gt_file: ground truth file\n--iou_threshold: IOU threshold\n\nIn general we would be interested in average of mAP at thresholds [0.5, 0.55, 0.6, 0.65,...0.95], similar to the\nstandard COCO => one needs to run this file N times for every IOU threshold independently.\n'''\n\nfrom scipy.spatial.transform import Rotation as R\nimport itertools\n\nimport argparse\nimport json\nfrom collections import defaultdict\nfrom pathlib import Path\n\nimport math\nimport numpy as np\nfrom pyquaternion import Quaternion\nfrom shapely.geometry import Polygon\n\nclass Box3D:\n \"\"\"Data class used during detection evaluation. Can be a prediction or ground truth.\"\"\"\n\n def __init__(self, **kwargs):\n sample_token = kwargs[\"sample_token\"]\n translation = kwargs[\"translation\"]\n size = kwargs[\"size\"]\n rotation = kwargs[\"rotation\"]\n name = kwargs[\"name\"]\n score = kwargs.get(\"score\", -1)\n\n if not isinstance(sample_token, str):\n raise TypeError(\"Sample_token must be a string!\")\n\n if not len(translation) == 3:\n raise ValueError(\"Translation must have 3 elements!\")\n\n if np.any(np.isnan(translation)):\n raise ValueError(\"Translation may not be NaN!\")\n\n if not len(size) == 3:\n raise ValueError(\"Size must have 3 elements!\")\n\n if np.any(np.isnan(size)):\n raise ValueError(\"Size may not be NaN!\")\n\n if not len(rotation) == 4:\n raise ValueError(\"Rotation must have 4 elements!\")\n\n if np.any(np.isnan(rotation)):\n raise ValueError(\"Rotation may not be NaN!\")\n\n if name is None:\n raise ValueError(\"Name cannot be empty!\")\n\n # Assign.\n self.sample_token = sample_token\n self.translation = translation\n self.size = size\n self.volume = np.prod(self.size)\n self.score = score\n\n assert np.all([x > 0 for x in size])\n self.rotation = rotation\n self.name = name\n self.quaternion = Quaternion(self.rotation)\n\n self.width, self.length, self.height = size\n\n self.center_x, self.center_y, self.center_z = self.translation\n\n self.min_z = self.center_z - self.height / 2\n self.max_z = self.center_z + self.height / 2\n\n self.ground_bbox_coords = None\n self.ground_bbox_coords = self.get_ground_bbox_coords()\n\n @staticmethod\n def check_orthogonal(a, b, c):\n \"\"\"Check that vector (b - a) is orthogonal to the vector (c - a).\"\"\"\n return np.isclose((b[0] - a[0]) * (c[0] - a[0]) + (b[1] - a[1]) * (c[1] - a[1]), 0)\n\n def get_ground_bbox_coords(self):\n if self.ground_bbox_coords is not None:\n return self.ground_bbox_coords\n return self.calculate_ground_bbox_coords()\n\n def calculate_ground_bbox_coords(self):\n \"\"\"We assume that the 3D box has lower plane parallel to the ground.\n Returns: Polygon with 4 points describing the base.\n \"\"\"\n if self.ground_bbox_coords is not None:\n return self.ground_bbox_coords\n\n rotation_matrix = self.quaternion.rotation_matrix\n\n cos_angle = rotation_matrix[0, 0]\n sin_angle = rotation_matrix[1, 0]\n\n point_0_x = self.center_x + self.length / 2 * cos_angle + self.width / 2 * sin_angle\n point_0_y = self.center_y + self.length / 2 * sin_angle - self.width / 2 * cos_angle\n\n point_1_x = self.center_x + self.length / 2 * cos_angle - self.width / 2 * sin_angle\n point_1_y = self.center_y + self.length / 2 * sin_angle + self.width / 2 * cos_angle\n\n point_2_x = self.center_x - self.length / 2 * cos_angle - self.width / 2 * sin_angle\n point_2_y = self.center_y - self.length / 2 * sin_angle + self.width / 2 * cos_angle\n\n point_3_x = self.center_x - self.length / 2 * cos_angle + self.width / 2 * sin_angle\n point_3_y = self.center_y - self.length / 2 * sin_angle - self.width / 2 * cos_angle\n\n point_0 = point_0_x, point_0_y\n point_1 = point_1_x, point_1_y\n point_2 = point_2_x, point_2_y\n point_3 = point_3_x, point_3_y\n\n assert self.check_orthogonal(point_0, point_1, point_3)\n assert self.check_orthogonal(point_1, point_0, point_2)\n assert self.check_orthogonal(point_2, point_1, point_3)\n assert self.check_orthogonal(point_3, point_0, point_2)\n\n self.ground_bbox_coords = Polygon(\n [\n (point_0_x, point_0_y),\n (point_1_x, point_1_y),\n (point_2_x, point_2_y),\n (point_3_x, point_3_y),\n (point_0_x, point_0_y),\n ]\n )\n\n return self.ground_bbox_coords\n\n def get_height_intersection(self, other):\n min_z = max(other.min_z, self.min_z)\n max_z = min(other.max_z, self.max_z)\n\n return max(0, max_z - min_z)\n\n def get_area_intersection(self, other) -> float:\n result = self.ground_bbox_coords.intersection(other.ground_bbox_coords).area\n\n assert result <= self.width * self.length\n\n return result\n\n def get_intersection(self, other) -> float:\n height_intersection = self.get_height_intersection(other)\n\n area_intersection = self.ground_bbox_coords.intersection(other.ground_bbox_coords).area\n\n return height_intersection * area_intersection\n\n def get_iou(self, other):\n intersection = self.get_intersection(other)\n union = self.volume + other.volume - intersection\n\n iou = np.clip(intersection / union, 0, 1)\n\n return iou\n\n def __repr__(self):\n return str(self.serialize())\n\n def serialize(self) -> dict:\n \"\"\"Returns: Serialized instance as dict.\"\"\"\n\n return {\n \"sample_token\": self.sample_token,\n \"translation\": self.translation,\n \"size\": self.size,\n \"rotation\": self.rotation,\n \"name\": self.name,\n \"volume\": self.volume,\n \"score\": self.score,\n }\n\n\ndef group_by_key(detections, key):\n groups = defaultdict(list)\n for detection in detections:\n groups[detection[key]].append(detection)\n return groups\n\n\ndef wrap_in_box(input):\n result = {}\n for key, value in input.items():\n result[key] = [Box3D(**x) for x in value]\n\n return result\n\n\ndef get_envelope(precisions):\n \"\"\"Compute the precision envelope.\n Args:\n precisions:\n Returns:\n \"\"\"\n for i in range(precisions.size - 1, 0, -1):\n precisions[i - 1] = np.maximum(precisions[i - 1], precisions[i])\n return precisions\n\n\ndef get_ap(recalls, precisions):\n \"\"\"Calculate average precision.\n Args:\n recalls:\n precisions: Returns (float): average precision.\n Returns:\n \"\"\"\n # correct AP calculation\n # first append sentinel values at the end\n recalls = np.concatenate(([0.0], recalls, [1.0]))\n precisions = np.concatenate(([0.0], precisions, [0.0]))\n\n precisions = get_envelope(precisions)\n\n # to calculate area under PR curve, look for points where X axis (recall) changes value\n i = np.where(recalls[1:] != recalls[:-1])[0]\n\n # and sum (\\Delta recall) * prec\n ap = np.sum((recalls[i + 1] - recalls[i]) * precisions[i + 1])\n return ap\n\n\ndef get_ious(gt_boxes, predicted_box):\n return [predicted_box.get_iou(x) for x in gt_boxes]\n\n\ndef recall_precision(gt, predictions, iou_threshold):\n num_gts = len(gt)\n image_gts = group_by_key(gt, \"sample_token\")\n image_gts = wrap_in_box(image_gts)\n\n sample_gt_checked = {sample_token: np.zeros(len(boxes)) for sample_token, boxes in image_gts.items()}\n\n predictions = sorted(predictions, key=lambda x: x[\"score\"], reverse=True)\n\n # go down dets and mark TPs and FPs\n num_predictions = len(predictions)\n tp = np.zeros(num_predictions)\n fp = np.zeros(num_predictions)\n\n for prediction_index, prediction in enumerate(predictions):\n predicted_box = Box3D(**prediction)\n\n sample_token = prediction[\"sample_token\"]\n\n max_overlap = -np.inf\n jmax = -1\n\n try:\n gt_boxes = image_gts[sample_token] # gt_boxes per sample\n gt_checked = sample_gt_checked[sample_token] # gt flags per sample\n except KeyError:\n gt_boxes = []\n gt_checked = None\n\n if len(gt_boxes) > 0:\n overlaps = get_ious(gt_boxes, predicted_box)\n\n max_overlap = np.max(overlaps)\n\n jmax = np.argmax(overlaps)\n\n if max_overlap > iou_threshold:\n if gt_checked[jmax] == 0:\n tp[prediction_index] = 1.0\n gt_checked[jmax] = 1\n else:\n fp[prediction_index] = 1.0\n else:\n fp[prediction_index] = 1.0\n\n # compute precision recall\n fp = np.cumsum(fp, axis=0)\n tp = np.cumsum(tp, axis=0)\n\n recalls = tp / float(num_gts)\n\n assert np.all(0 <= recalls) & np.all(recalls <= 1)\n\n # avoid divide by zero in case the first detection matches a difficult ground truth\n precisions = tp / np.maximum(tp + fp, np.finfo(np.float64).eps)\n\n assert np.all(0 <= precisions) & np.all(precisions <= 1)\n\n ap = get_ap(recalls, precisions)\n\n return recalls, precisions, ap\n\n\ndef get_average_precisions(gt: list, predictions: list, class_names: list, iou_threshold: float) -> np.array:\n \"\"\"Returns an array with an average precision per class.\n Args:\n gt: list of dictionaries in the format described below.\n predictions: list of dictionaries in the format described below.\n class_names: list of the class names.\n iou_threshold: IOU threshold used to calculate TP / FN\n Returns an array with an average precision per class.\n Ground truth and predictions should have schema:\n gt = [{\n 'sample_token': '0f0e3ce89d2324d8b45aa55a7b4f8207fbb039a550991a5149214f98cec136ac',\n 'translation': [974.2811881299899, 1714.6815014457964, -23.689857123368846],\n 'size': [1.796, 4.488, 1.664],\n 'rotation': [0.14882026466054782, 0, 0, 0.9888642620837121],\n 'name': 'car'\n }]\n predictions = [{\n 'sample_token': '0f0e3ce89d2324d8b45aa55a7b4f8207fbb039a550991a5149214f98cec136ac',\n 'translation': [971.8343488872263, 1713.6816097857359, -25.82534357061308],\n 'size': [2.519726579986132, 7.810161372666739, 3.483438286096803],\n 'rotation': [0.10913582721095375, 0.04099572636992043, 0.01927712319721745, 1.029328402625659],\n 'name': 'car',\n 'score': 0.3077029437237213\n }]\n \"\"\"\n assert 0 <= iou_threshold <= 1\n\n gt_by_class_name = group_by_key(gt, \"name\")\n pred_by_class_name = group_by_key(predictions, \"name\")\n\n average_precisions = np.zeros(len(class_names))\n\n for class_id, class_name in enumerate(class_names):\n if class_name in pred_by_class_name:\n recalls, precisions, average_precision = recall_precision(\n gt_by_class_name[class_name], pred_by_class_name[class_name], iou_threshold\n )\n average_precisions[class_id] = average_precision\n\n return average_precisions\n\n\ndef get_class_names(gt: dict) -> list:\n \"\"\"Get sorted list of class names.\n Args:\n gt:\n Returns: Sorted list of class names.\n \"\"\"\n return sorted(list(set([x[\"name\"] for x in gt])))\n\n\n# if __name__ == \"__main__\":\n# parser = argparse.ArgumentParser()\n# arg = parser.add_argument\n# arg(\"-p\", \"--pred_file\", type=str, help=\"Path to the predictions file.\", required=True)\n# arg(\"-g\", \"--gt_file\", type=str, help=\"Path to the ground truth file.\", required=True)\n# arg(\"-t\", \"--iou_threshold\", type=float, help=\"iou threshold\", default=0.5)\n\n# args = parser.parse_args()\n\n# gt_path = Path(args.gt_file)\n# pred_path = Path(args.pred_file)\n\n# with open(args.pred_file) as f:\n# predictions = json.load(f)\n\n# with open(args.gt_file) as f:\n# gt = json.load(f)\n\n# class_names = get_class_names(gt)\n# print(\"Class_names = \", class_names)\n\n# average_precisions = get_average_precisions(gt, predictions, class_names, args.iou_threshold)\n\n# mAP = np.mean(average_precisions)\n# print(\"Average per class mean average precision = \", mAP)\n\n# for class_id in sorted(list(zip(class_names, average_precisions.flatten().tolist()))):\n# print(class_id)",
"_____no_output_____"
],
[
"gt_file = './review.json'\npred_file = './pred.json'\n\ndef build_scene(file: str) -> list:\n scene = []\n with open(file) as f:\n data = json.load(f)\n for frame in data['frames']:\n items = []\n for o in frame['items']:\n# if math.sqrt(o['position']['x'] ** 2 + o['dimension']['y'] ** 2 + (o['position']['z'] + 1.84) ** 2) > 50:\n# continue\n item = {}\n item['sample_token'] = str(frame['frameId'])\n item['translation'] = [o['position']['x'], o['position']['y'], o['position']['z']]\n item['size'] = [o['dimension']['x'], o['dimension']['y'], o['dimension']['z']]\n euler = R.from_euler('xyz',[o['rotation']['x'],o['rotation']['y'],o['rotation']['z']])\n quat = euler.as_quat()\n item['rotation'] = quat\n item['name'] = o['category']\n item['score'] = 1 if 'score' not in o else o['score']\n items.append(item)\n scene.append(items)\n return list(itertools.chain.from_iterable(scene))\n\ngt = build_scene(gt_file)\npred = build_scene(pred_file)\n\nfor iou_threshold in np.arange(0.01, 0.92, 0.05):\n iou_threshold = round(iou_threshold, 2)\n recalls, precisions, ap = recall_precision(gt, pred, iou_threshold)\n print(\"IoU threshold: {:.2f} Precsion: {:.12f}, Recall: {:.12f}\"\n .format(iou_threshold, precisions[-1], recalls[-1]))",
"IoU threshold: 0.01 Precsion: 0.075000000000, Recall: 0.187500000000\nIoU threshold: 0.06 Precsion: 0.075000000000, Recall: 0.187500000000\nIoU threshold: 0.11 Precsion: 0.075000000000, Recall: 0.187500000000\nIoU threshold: 0.16 Precsion: 0.050000000000, Recall: 0.125000000000\nIoU threshold: 0.21 Precsion: 0.050000000000, Recall: 0.125000000000\nIoU threshold: 0.26 Precsion: 0.050000000000, Recall: 0.125000000000\nIoU threshold: 0.31 Precsion: 0.050000000000, Recall: 0.125000000000\nIoU threshold: 0.36 Precsion: 0.050000000000, Recall: 0.125000000000\nIoU threshold: 0.41 Precsion: 0.050000000000, Recall: 0.125000000000\nIoU threshold: 0.46 Precsion: 0.050000000000, Recall: 0.125000000000\nIoU threshold: 0.51 Precsion: 0.025000000000, Recall: 0.062500000000\nIoU threshold: 0.56 Precsion: 0.025000000000, Recall: 0.062500000000\nIoU threshold: 0.61 Precsion: 0.000000000000, Recall: 0.000000000000\nIoU threshold: 0.66 Precsion: 0.000000000000, Recall: 0.000000000000\nIoU threshold: 0.71 Precsion: 0.000000000000, Recall: 0.000000000000\nIoU threshold: 0.76 Precsion: 0.000000000000, Recall: 0.000000000000\nIoU threshold: 0.81 Precsion: 0.000000000000, Recall: 0.000000000000\nIoU threshold: 0.86 Precsion: 0.000000000000, Recall: 0.000000000000\nIoU threshold: 0.91 Precsion: 0.000000000000, Recall: 0.000000000000\n"
]
]
] | [
"code"
] | [
[
"code",
"code"
]
] |
e76b7d2af0850f751e396eadce77d6479b61b9a0 | 59,900 | ipynb | Jupyter Notebook | 2016/tutorial_final/175/tutorial.ipynb | zeromtmu/practicaldatascience.github.io | 62950a3a3e7833552b0f2269cc3ee5c34a1d6d7b | [
"MIT"
] | 1 | 2021-07-06T17:36:24.000Z | 2021-07-06T17:36:24.000Z | 2016/tutorial_final/175/tutorial.ipynb | zeromtmu/practicaldatascience.github.io | 62950a3a3e7833552b0f2269cc3ee5c34a1d6d7b | [
"MIT"
] | null | null | null | 2016/tutorial_final/175/tutorial.ipynb | zeromtmu/practicaldatascience.github.io | 62950a3a3e7833552b0f2269cc3ee5c34a1d6d7b | [
"MIT"
] | 1 | 2021-07-06T17:36:34.000Z | 2021-07-06T17:36:34.000Z | 102.568493 | 20,660 | 0.819032 | [
[
[
"# K-Nearest Neighbors Algorithm and Its Application\n\n## Introduction\n\nAs we have learnt, NaiveBayes and decision tree are all eager learning algorithm which constructs a classification model before receiving new data to do queries. In contrast, lazy learning algorithm stores all training data until a query is made. K-nearest neighbors (a.k.a k-NN) algorithm is one of the most famous lazy learning algorithms, as well as among the simplest of all machine learning algorithms.\n\nThe idea of k-NN algorithm is quite simple. Whenever we have a new point to classify, we find its K nearest neighbors from the training data. Let's say we have two class of data labeled separately as green square and red circle. The new data which is a blue triangle is waiting for classification. Right now we don't know class the triangle belongs to and our goal is to find a proper family for it.",
"_____no_output_____"
],
[
"",
"_____no_output_____"
],
[
"Usually, the easiest way for us to decide whether a person is good or bad is through searching his or her friends. Here, we should study all the friends of the blue triangle. However, how we define friendship for data? We know that each data is actually a spot in space and it has a distance to all other data spots. In k-NN algorithm, k decides the friendly distance. Given the classification criteria above, if k = 3 (solid line circle), the blue triangle is assigned to the class of red circles because there are 2 red circles and only 1 green square inside the inner circle; if k = 5 (dashed line circle), the blue triangle is assigned to the class of green square because there are 3 green squares but 2 red circles inside the outer circle. This seems a statistical way to help us find the right class for new data. **We assign a class label only considering the number of friends near by and their classes.**\n\nThe distance here can be calculated using one of the following measures:\n- Euclidean Distance\n- Minkowski Distance\n- Mahalanobis Distance",
"_____no_output_____"
],
[
"## Weighted K-NN Algorithm\n\nAs we discussed in last session, we begin with a naive k-NN algorithm which only take the number of appearance of data in each class into consideration. We may get a wrong classification result in a situation like below:",
"_____no_output_____"
],
[
"",
"_____no_output_____"
],
[
"Within a proper distance, there are more green squares than red circles. However, the blue triangle is closely surrounded by red circles and all green squares locate farther from it. The blue triangle is most likely to be one of the class of red circles even though there are more green squares show up in the friendly circle.\n\n> One way to overcome this problem is to weight the classification, taking into account the distance from the test point to each of its k nearest neighbors.\n\nThere are several approaches to apply weight to the class of each of the k nearest points. Here I introduce one approach called **attribute/feature weighted k-NN**.\n\nThere are two assumptions:\n- All the attribute values are numerical or real\n- Class attribute values are discrete integer values\n\nDetailed steps:\n\n- Read the training data\n- Set K to some value\n- Calculate Euclidean distances to all training data points\n- Find the K nearest neighbors based on the distances\n- Assign weight to each feature\n- Return the class that represents the maximum of the k instances\n- Calculate the accuracy",
"_____no_output_____"
],
[
"## Other Improvements\n\n### Density based k-NN\n\nIn Density based K-NN the distance between test and training instances is increased in sparse area and reduced in dense areas because it not only considers the density of test instance but also the densities of its K neighbors.\n\n### Variable k-NN\n\nIt has been observed that the values in K nearest neighbor classification results heavily depends upon the number of neighbors and each data has different K value that is suitable for it. This approach finds the optimum K value for each classification and generate an array which contains the best K value for each training set instances.\n\n### Class based k-NN\n\nSometimes the size of each class can also cause problem. When one class have too many instances\nwhile others have too few instances, the class with smallest size won't be selected by our algorithm. We should take this factor into consideration as well.",
"_____no_output_____"
],
[
"## Application\n\nIn this tutorial, we are going to apply k-NN algorithm on sentences from the abstract and introduction of 30 scientific articles. Firstly, we call KNeighborsClassifier from sklearn library, train it with training data set and calculate prediction accuracy on testing data. Then we implement our own K-NN algorithm. We will compare the accuracy of our algorithm with the one of sklearn library. Finally, we implement an improved k-NN algorithm based on naive k-NN.\n\n### Data Set\n\n[Sentence Classification Data Set from UCI](http://archive.ics.uci.edu/ml/datasets/Sentence+Classification#)\n\n### Training Data Format\n\nA snippet of training data may like:\n> MISC although the internet as level topology has been extensively studied over the past few years little is known about the details of the as taxonomy\n\n> MISC an as node can represent a wide variety of organizations e g large isp or small private business university with vastly different network characteristics externs\n\n> AIMX in this paper we introduce a radically new approach based on machine learning techniques to map all the ases in the internet into a natural as taxonomy\n\n> OWNX we successfully classify NUMBER NUMBER percent of ases with expected accuracy of NUMBER NUMBER percent\n\nThe first attribute is a label of:\n1. AIM: \"A specific research goal of the current paper\"\n2. OWNX: \"(Neutral) description of own work presented in current paper\"\n3. CONT: \"Statements of comparison with or contrast to other work; weaknesses of other work\"\n4. BASIS: \"Statements of agreement with other work or continuation of other work\"\n5. MISC: \"(Neutral) description of other researchers' work\"\nThe second part is a sentence in the paper.\n\nOur goal here is to use K-NN to guess the category of a given sentense.",
"_____no_output_____"
],
[
"Here are libraries we need:",
"_____no_output_____"
]
],
[
[
"import numpy as np\nimport pandas as pd\nfrom nltk import word_tokenize\nfrom sklearn.neighbors import KNeighborsClassifier\nfrom collections import Counter\nimport matplotlib\nmatplotlib.use('svg')\n%matplotlib inline\nimport matplotlib.pyplot as plt\nplt.style.use('ggplot')",
"_____no_output_____"
]
],
[
[
"### Feature Scoring\nWe are provided with an extra key words list for each category. We can use those words to score the likelihood of a senctence's category. For example, sentences contain words like \"we\", \"introduce\" and \"design\" are more likely to describe the research goal of the current paper, which should belongs to category AIM. If a sentence contain `N` words in AIM word list, we give it's AIM feature score `N`.",
"_____no_output_____"
]
],
[
[
"# Read in key words for each category\ndef build_word_set(filename):\n with open(filename) as f:\n lines = f.readlines()\n return {word.strip() for word in lines if word}\n\naim_set = build_word_set(\"./word_lists/aim.txt\")\nbase_set = build_word_set(\"./word_lists/base.txt\")\nown_set = build_word_set(\"./word_lists/own.txt\")\nconstract_set = build_word_set(\"./word_lists/contrast.txt\")\nstopwords_set = build_word_set(\"./word_lists/stopwords.txt\")",
"_____no_output_____"
]
],
[
[
"Train data on classifier. Return feature matrix and label vector. If `train` is `false`, the output label vector will be empty.",
"_____no_output_____"
]
],
[
[
"def load_data(filename, train=True):\n \"\"\"\n Training data format like:\n \n 'AIMX In this paper we derive the equations for Loop Corrected Belief Propagation on a continuous variable Gaussian model'\n \n For each single line of raw data, caculate the feature score of it and put it into feature matrix (and label matrix if it's training data) \n \"\"\"\n feature_m = np.empty((0,4), dtype=int)\n label_m = np.empty((0,1))\n def caculate_score(line):\n aim_score, base_score, own_score, cons_score = 0, 0, 0, 0\n word_list = [w for w in word_tokenize(line.lower()) if w not in stopwords_set]\n for word in word_list:\n if word in aim_set:\n aim_score += 1\n if word in base_set:\n base_score += 1\n if word in own_set:\n own_score += 1\n if word in constract_set:\n cons_score += 1\n return aim_score, base_score, own_score, cons_score\n \n with open(filename) as f:\n for line in f:\n if line.startswith(\"###\"):\n continue\n else:\n if train:\n label, sentence = line.split(' ', 1)\n a, b, o, c = caculate_score(sentence)\n feature_m = np.append(feature_m, np.array([[a, b, o, c]]), axis=0)\n label_m = np.append(label_m, np.array([[label]]), axis=0)\n else:\n a, b, o, c = caculate_score(line)\n feature_m = np.append(feature_m, np.array([[a, b, o, c]]), axis=0)\n\n return feature_m, label_m",
"_____no_output_____"
]
],
[
[
"Let's try out one training data and see the accuracy of our classifier!",
"_____no_output_____"
]
],
[
[
"f, l = load_data('./arxiv_annotate10_7_3.txt') \nsklearn_knn = KNeighborsClassifier(n_neighbors=3)\nsklearn_knn.fit(f, l.ravel())\n\ncorrect = 0.0\nfor i in range(len(f)):\n if sklearn_knn.predict(f[i].reshape(1,-1)) == l[i]:\n correct += 1\nprint correct/len(f)",
"0.716417910448\n"
]
],
[
[
"## Performance Analysis\n\nIn this part, we are going to evaluate the performance of our algorithm in contrast to the sklearn library.\n\nThere are several perspectives for us to analysis an algorithm, time complexity, space complexity, error rate, etc.\nBasic kNN algorithm stores all samples, so the space complexity depends on the volume of samples. The total time complexity is `O(nk+nd)` with n examples each of dimension d, including time to compute distance to all samples and find k closest examples. Here, let's take a deep look at how k influence the error rate.\n\n### How to Choose k?\n\nIn theory, if infinite number of samples available, the larger is k, the better is classification. The lower bound of k is 1. In this case, the k-nn model may be too sensitive to โnoiseโ. The upper bound is n, the number of samples. If k is that big, then we will take all samples into consideration. This means that the class of the testing data only depends on the majority of the class of all the samples and it's nothing to do with distance.\n\nThe program we implemented below is for generating error rates with different k. We choose k from 1 to 50. Then draw a figure to show the relationship between k and accuracy rate.",
"_____no_output_____"
],
[
"## Visualized Result",
"_____no_output_____"
],
[
"### Load Data\n\nFirstly, we load data for use. `data.txt` is a much bigger data set which runs much slower.",
"_____no_output_____"
]
],
[
[
"f, l = load_data('./data.txt')",
"_____no_output_____"
]
],
[
[
"### Test Sklearn Library\n\nOn one hand, we test sklearn library with different k.",
"_____no_output_____"
]
],
[
[
"x_points = [x for x in range(1,10)] + [x for x in range(10, 50, 3)]\ny_points = []\n\nfor k in x_points:\n correct = 0.0\n sklearn_knn = KNeighborsClassifier(n_neighbors=k)\n sklearn_knn.fit(f, l.ravel())\n for i in range(len(f)):\n if sklearn_knn.predict(f[i].reshape(1,-1)) == l[i]:\n correct += 1\n y_points.append(correct/len(f))\n \nplt.plot(x_points, y_points, 'b-',lw=1.5)",
"_____no_output_____"
]
],
[
[
"### Test Our K-NN\n\nOn the other hand, we implement our own simplest K-NN algorithm first and test its accuracy rate.",
"_____no_output_____"
]
],
[
[
"class KNN:\n def __init__(self, k):\n self.k = k\n\n def _euclidean_distance(self, data1, data2):\n diff = np.power(data1 - data2, 2)\n diff_sum = np.sum(diff, axis=0)\n return np.sqrt(diff_sum)\n\n def majority_vote(self, neighbors):\n clusters = [neighbour[1][0] for neighbour in neighbors]\n counter = Counter(clusters)\n return counter.most_common()[0][0]\n\n def predict(self, training_data, test_entry):\n def add_distance_attr(training_entry, test_entry):\n return (training_entry, self._euclidean_distance(test_entry, training_entry[0]))\n\n distances = [add_distance_attr(training_entry, test_entry) for training_entry in training_data]\n distances.sort(key=lambda x: x[1])\n sorted_training = [entry[0] for entry in distances]\n # Replace last line with next line when call majority_vote2 method for weighted KNN\n # sorted_training = [entry for entry in distances]\n neighbors = sorted_training[:self.k]\n\n return self.majority_vote(neighbors)",
"_____no_output_____"
],
[
"training = [(f[i], l[i]) for i in range(f.shape[0])]\n\nx_points = [x for x in range(1,10)] + [x for x in range(10, 40, 3)]\ny_points = []\n\nfor k in x_points:\n knn = KNN(k)\n correct = 0\n for i in training:\n if knn.predict(training, i[0]) == i[1][0]:\n correct += 1\n y_points.append(correct*1.0/len(training))\nplt.plot(x_points, y_points, 'r-',lw=1.5)",
"_____no_output_____"
]
],
[
[
"### Conclusion\n\nFrom the figures above, we find that both method have a peak accuracy rate of 77 near 6. Congratulations! Our simplest k-NN algorithm works very well on this data set compared with sklearn library. \n\nWhen k is small than 6, there is a wave due to the sensitivity of the noise. At the same time, we can infer that larger k gives smoother boundaries, better for generalization, but could have worse performance when boundaries become too blurry.",
"_____no_output_____"
],
[
"## Improved K-NN\n\nAs we discussed before, assign a weight to each feature is a way to improve K-NN. In original K-NN, we assign a class label based on the majority of each feature in the range of k neighbors. Now we multiply the value of each feature with a weight. The weight comes from inverse distance and the distance is the mean distance test point and all points of that feature.\n\nThe following method is one way we implement the idea above. We can insert into our K-NN implementation to see the difference.",
"_____no_output_____"
]
],
[
[
"def majority_vote2(neighbors):\n\n \"\"\"\n neighbors[0] like: ([[1,2,3,4], ['MISC']], dist)\n list of (label, weight), weight = # of same label * 1.0 / (avg dist / # of same )\n of them\n \"\"\"\n AIM_label_cnt = 0\n AIM_label_total_dist = 0\n OWNX_label_cnt = 0\n OWNX_label_total_dist = 0\n BASIS_label_cnt = 0\n BASIS_label_total_dist = 0\n MISC_label_cnt = 0\n MISC_label_total_dist = 0\n CONT_label_cnt = 0\n CONT_label_total_dist = 0\n\n for row in neighbors:\n label, dist = row[0][1][0], row[1]\n if label == \"AIM\":\n AIM_label_cnt += 1\n AIM_label_total_dist += dist\n elif label == \"OWNX\":\n OWNX_label_cnt += 1\n OWNX_label_total_dist += dist\n elif label == \"BASIS\":\n BASIS_label_cnt += 1\n BASIS_label_total_dist += dist\n elif label == \"MISC\":\n MISC_label_cnt += 1\n MISC_label_total_dist += dist\n elif label == \"CONT\":\n CONT_label_cnt += 1\n CONT_label_total_dist += dist\n\n if AIM_label_total_dist == 0:\n AIM_label_total_dist = float('inf')\n if OWNX_label_total_dist == 0:\n OWNX_label_total_dist = float('inf')\n if BASIS_label_total_dist == 0:\n BASIS_label_total_dist = float('inf')\n if MISC_label_total_dist == 0:\n MISC_label_total_dist = float('inf')\n if CONT_label_total_dist == 0:\n CONT_label_total_dist = float('inf')\n\n label_list = [(\"AIM\", AIM_label_cnt**2*1.0/AIM_label_total_dist),\n (\"OWNX\", OWNX_label_cnt**2*1.0/OWNX_label_total_dist),\n (\"BASIS\", BASIS_label_cnt**2*1.0/BASIS_label_total_dist),\n (\"MISC\", MISC_label_cnt**2*1.0/MISC_label_total_dist),\n (\"CONT\", CONT_label_cnt**2*1.0/CONT_label_total_dist)]\n label_list.sort(key = lambda x: x[1], reverse=True)\n return label_list[0][0]",
"_____no_output_____"
]
],
[
[
"## Reference\n\n- [Wiki for K-NN](https://www.wikiwand.com/en/K-nearest_neighbors_algorithm)\n- [Weighted K-NN](http://www.csee.umbc.edu/~tinoosh/cmpe650/slides/K_Nearest_Neighbor_Algorithm.pdf)\n- [Attribute weighting in K-nearest neighbor classification](https://tampub.uta.fi/bitstream/handle/10024/96376/GRADU-1417607625.pdf?sequence=1)\n- [From Cambridge KNN](https://blog.cambridgecoding.com/2016/01/16/machine-learning-under-the-hood-writing-your-own-k-nearest-neighbour-algorithm/)\n",
"_____no_output_____"
]
]
] | [
"markdown",
"code",
"markdown",
"code",
"markdown",
"code",
"markdown",
"code",
"markdown",
"code",
"markdown",
"code",
"markdown",
"code",
"markdown",
"code",
"markdown"
] | [
[
"markdown",
"markdown",
"markdown",
"markdown",
"markdown",
"markdown",
"markdown",
"markdown",
"markdown"
],
[
"code"
],
[
"markdown"
],
[
"code"
],
[
"markdown"
],
[
"code"
],
[
"markdown"
],
[
"code"
],
[
"markdown",
"markdown",
"markdown"
],
[
"code"
],
[
"markdown"
],
[
"code"
],
[
"markdown"
],
[
"code",
"code"
],
[
"markdown",
"markdown"
],
[
"code"
],
[
"markdown"
]
] |
e76b805889cad33e7578d908c515377e3ad542f4 | 169,099 | ipynb | Jupyter Notebook | Lesson04/Exercise12.ipynb | TrainingByPackt/Applied-Data-Learning-with-Keras-eLearning | 3fff15883acb52e441264fd7a996cfbb7c2cb140 | [
"MIT"
] | 2 | 2019-11-23T16:22:45.000Z | 2020-04-27T13:02:31.000Z | Lesson04/Exercise12.ipynb | TrainingByPackt/Applied-Data-Learning-with-Keras-eLearning | 3fff15883acb52e441264fd7a996cfbb7c2cb140 | [
"MIT"
] | null | null | null | Lesson04/Exercise12.ipynb | TrainingByPackt/Applied-Data-Learning-with-Keras-eLearning | 3fff15883acb52e441264fd7a996cfbb7c2cb140 | [
"MIT"
] | 5 | 2019-11-25T10:35:32.000Z | 2020-11-11T16:54:27.000Z | 52.046476 | 161 | 0.370984 | [
[
[
"# step 1\n# create some simulated regression data\nimport numpy\nfrom sklearn.datasets import make_regression\nX, y = make_regression(n_samples=500, n_features=2, n_informative=2, noise=5, random_state=0)\n\n# Print the sizes of the dataset\nprint(\"Number of Examples in the Dataset = \", X.shape[0])\nprint(\"Number of Features for each example = \", X.shape[1])\n# print output range\nprint(\"Output Range = [%f, %f]\" %(min(y), max(y)))",
"Number of Examples in the Dataset = 500\nNumber of Features for each example = 2\nOutput Range = [-288.225754, 271.270135]\n"
],
[
"# step 2\nfrom keras.models import Sequential\nfrom keras.layers import Dense, Activation\n# Create the function that returns the keras model\ndef build_model():\n # build the Keras model\n model = Sequential()\n model.add(Dense(16, input_dim=2, activation='relu'))\n model.add(Dense(8, activation='relu'))\n model.add(Dense(1))\n # Compile the model\n model.compile(loss='mean_squared_error', optimizer='adam')\n # return the model\n return model",
"Using TensorFlow backend.\n"
],
[
"# step 3\n# build the scikit-Learn interface for the keras model\nfrom keras.wrappers.scikit_learn import KerasRegressor\nYourModel = KerasRegressor(build_fn= build_model, epochs=300, batch_size=10, verbose=1)",
"_____no_output_____"
],
[
"# step 4\n# define the iterator to perform 5-fold cross validation\nfrom sklearn.model_selection import KFold\nkf = KFold(n_splits=5)",
"_____no_output_____"
],
[
"# step 5\n# perform cross validation on X, y\nfrom sklearn.model_selection import cross_val_score\nresults = cross_val_score(YourModel, X, y, cv=kf)",
"Epoch 1/300\n400/400 [==============================] - 2s 5ms/step - loss: 10787.5895\nEpoch 2/300\n400/400 [==============================] - 0s 300us/step - loss: 10721.5124\nEpoch 3/300\n400/400 [==============================] - 0s 300us/step - loss: 10635.4636\nEpoch 4/300\n400/400 [==============================] - 0s 300us/step - loss: 10523.7216\nEpoch 5/300\n400/400 [==============================] - 0s 300us/step - loss: 10377.7988\nEpoch 6/300\n400/400 [==============================] - 0s 300us/step - loss: 10151.4446\nEpoch 7/300\n400/400 [==============================] - 0s 300us/step - loss: 9818.6851\nEpoch 8/300\n400/400 [==============================] - 0s 300us/step - loss: 9412.7773\nEpoch 9/300\n400/400 [==============================] - 0s 275us/step - loss: 8911.5203\nEpoch 10/300\n400/400 [==============================] - 0s 275us/step - loss: 8333.2434\nEpoch 11/300\n400/400 [==============================] - 0s 300us/step - loss: 7663.1001\nEpoch 12/300\n400/400 [==============================] - 0s 325us/step - loss: 6942.4922\nEpoch 13/300\n400/400 [==============================] - 0s 300us/step - loss: 6134.9481\nEpoch 14/300\n400/400 [==============================] - 0s 300us/step - loss: 5294.6609\nEpoch 15/300\n400/400 [==============================] - 0s 300us/step - loss: 4449.6244\nEpoch 16/300\n400/400 [==============================] - 0s 300us/step - loss: 3651.5002\nEpoch 17/300\n400/400 [==============================] - 0s 300us/step - loss: 2949.0617\nEpoch 18/300\n400/400 [==============================] - 0s 300us/step - loss: 2342.6644\nEpoch 19/300\n400/400 [==============================] - 0s 325us/step - loss: 1843.6199\nEpoch 20/300\n400/400 [==============================] - 0s 275us/step - loss: 1451.4539\nEpoch 21/300\n400/400 [==============================] - 0s 325us/step - loss: 1148.7504\nEpoch 22/300\n400/400 [==============================] - 0s 300us/step - loss: 902.9738\nEpoch 23/300\n400/400 [==============================] - 0s 300us/step - loss: 723.2597\nEpoch 24/300\n400/400 [==============================] - 0s 300us/step - loss: 592.7049\nEpoch 25/300\n400/400 [==============================] - 0s 300us/step - loss: 498.9209\nEpoch 26/300\n400/400 [==============================] - 0s 300us/step - loss: 431.2456\nEpoch 27/300\n400/400 [==============================] - 0s 300us/step - loss: 384.6888\nEpoch 28/300\n400/400 [==============================] - 0s 300us/step - loss: 347.2039\nEpoch 29/300\n400/400 [==============================] - 0s 300us/step - loss: 319.5052\nEpoch 30/300\n400/400 [==============================] - 0s 275us/step - loss: 298.3060\nEpoch 31/300\n400/400 [==============================] - 0s 300us/step - loss: 279.3497\nEpoch 32/300\n400/400 [==============================] - 0s 325us/step - loss: 264.4646\nEpoch 33/300\n400/400 [==============================] - 0s 300us/step - loss: 251.8541\nEpoch 34/300\n400/400 [==============================] - 0s 300us/step - loss: 243.1705\nEpoch 35/300\n400/400 [==============================] - 0s 300us/step - loss: 234.3055\nEpoch 36/300\n400/400 [==============================] - 0s 300us/step - loss: 226.6559\nEpoch 37/300\n400/400 [==============================] - 0s 300us/step - loss: 224.7824\nEpoch 38/300\n400/400 [==============================] - 0s 300us/step - loss: 221.5335\nEpoch 39/300\n400/400 [==============================] - 0s 300us/step - loss: 219.7768\nEpoch 40/300\n400/400 [==============================] - 0s 300us/step - loss: 218.4055\nEpoch 41/300\n400/400 [==============================] - 0s 300us/step - loss: 216.3338\nEpoch 42/300\n400/400 [==============================] - 0s 300us/step - loss: 214.4206\nEpoch 43/300\n400/400 [==============================] - 0s 300us/step - loss: 212.7957\nEpoch 44/300\n400/400 [==============================] - 0s 300us/step - loss: 210.9274\nEpoch 45/300\n400/400 [==============================] - 0s 325us/step - loss: 208.9156\nEpoch 46/300\n400/400 [==============================] - 0s 275us/step - loss: 208.6731\nEpoch 47/300\n400/400 [==============================] - 0s 275us/step - loss: 206.4077\nEpoch 48/300\n400/400 [==============================] - 0s 300us/step - loss: 204.4343\nEpoch 49/300\n400/400 [==============================] - 0s 300us/step - loss: 203.2349\nEpoch 50/300\n400/400 [==============================] - 0s 300us/step - loss: 200.6065\nEpoch 51/300\n400/400 [==============================] - 0s 300us/step - loss: 199.0318\nEpoch 52/300\n400/400 [==============================] - 0s 300us/step - loss: 196.7662\nEpoch 53/300\n400/400 [==============================] - 0s 300us/step - loss: 195.4331\nEpoch 54/300\n400/400 [==============================] - 0s 300us/step - loss: 193.6124\nEpoch 55/300\n400/400 [==============================] - 0s 300us/step - loss: 190.5613\nEpoch 56/300\n400/400 [==============================] - 0s 300us/step - loss: 189.2990\nEpoch 57/300\n400/400 [==============================] - 0s 275us/step - loss: 188.1379\nEpoch 58/300\n400/400 [==============================] - 0s 275us/step - loss: 184.7554\nEpoch 59/300\n400/400 [==============================] - 0s 300us/step - loss: 183.4578\nEpoch 60/300\n400/400 [==============================] - 0s 300us/step - loss: 180.3344\nEpoch 61/300\n400/400 [==============================] - 0s 300us/step - loss: 178.4621\nEpoch 62/300\n400/400 [==============================] - 0s 300us/step - loss: 174.4733\nEpoch 63/300\n400/400 [==============================] - 0s 300us/step - loss: 172.0028\nEpoch 64/300\n400/400 [==============================] - 0s 300us/step - loss: 169.6489\nEpoch 65/300\n400/400 [==============================] - 0s 300us/step - loss: 166.9882\nEpoch 66/300\n400/400 [==============================] - 0s 300us/step - loss: 163.6969\nEpoch 67/300\n400/400 [==============================] - 0s 300us/step - loss: 161.3114\nEpoch 68/300\n400/400 [==============================] - 0s 300us/step - loss: 159.3438\nEpoch 69/300\n400/400 [==============================] - 0s 300us/step - loss: 155.3219\nEpoch 70/300\n400/400 [==============================] - 0s 300us/step - loss: 152.7521\nEpoch 71/300\n400/400 [==============================] - 0s 300us/step - loss: 150.2530\nEpoch 72/300\n400/400 [==============================] - 0s 275us/step - loss: 147.1582\nEpoch 73/300\n400/400 [==============================] - 0s 275us/step - loss: 143.5714\nEpoch 74/300\n400/400 [==============================] - 0s 300us/step - loss: 140.2247\nEpoch 75/300\n400/400 [==============================] - 0s 325us/step - loss: 137.1724\nEpoch 76/300\n400/400 [==============================] - 0s 300us/step - loss: 134.0049\nEpoch 77/300\n400/400 [==============================] - 0s 325us/step - loss: 130.8275\nEpoch 78/300\n400/400 [==============================] - 0s 300us/step - loss: 127.8174\nEpoch 79/300\n400/400 [==============================] - 0s 300us/step - loss: 125.5010\nEpoch 80/300\n400/400 [==============================] - 0s 300us/step - loss: 122.3663\nEpoch 81/300\n400/400 [==============================] - 0s 300us/step - loss: 118.5379\nEpoch 82/300\n400/400 [==============================] - 0s 300us/step - loss: 116.1261\nEpoch 83/300\n400/400 [==============================] - 0s 300us/step - loss: 113.4813\nEpoch 84/300\n400/400 [==============================] - 0s 300us/step - loss: 110.1145\nEpoch 85/300\n400/400 [==============================] - 0s 300us/step - loss: 107.1337\nEpoch 86/300\n400/400 [==============================] - 0s 300us/step - loss: 104.5438\nEpoch 87/300\n400/400 [==============================] - 0s 300us/step - loss: 101.6203\nEpoch 88/300\n400/400 [==============================] - 0s 300us/step - loss: 99.6564\nEpoch 89/300\n400/400 [==============================] - 0s 300us/step - loss: 96.8610\nEpoch 90/300\n400/400 [==============================] - 0s 300us/step - loss: 93.4498\nEpoch 91/300\n400/400 [==============================] - 0s 300us/step - loss: 91.3700\nEpoch 92/300\n400/400 [==============================] - 0s 300us/step - loss: 88.5714\nEpoch 93/300\n400/400 [==============================] - 0s 275us/step - loss: 85.7000\nEpoch 94/300\n400/400 [==============================] - 0s 275us/step - loss: 83.7117\nEpoch 95/300\n"
],
[
"# step 6\n# print the result\nprint(\"Final Cross Validation Loss =\", abs(results.mean()))",
"Final Cross Validation Loss = 25.39843524932861\n"
]
]
] | [
"code"
] | [
[
"code",
"code",
"code",
"code",
"code",
"code"
]
] |
e76b82973c2b153c16ab05e0733b0c259d2fc6cd | 10,183 | ipynb | Jupyter Notebook | tutorials/compiler/20newsgroup/sample.ipynb | SebastianWolf-SAP/contextual-ai | 1d38ef7b3b4e12aa8e966146eb9d53f809c54204 | [
"Apache-2.0"
] | 83 | 2020-06-17T04:07:29.000Z | 2022-03-12T13:45:24.000Z | tutorials/compiler/20newsgroup/sample.ipynb | SebastianWolf-SAP/contextual-ai | 1d38ef7b3b4e12aa8e966146eb9d53f809c54204 | [
"Apache-2.0"
] | 15 | 2020-06-30T09:22:19.000Z | 2021-11-11T10:52:40.000Z | tutorials/compiler/20newsgroup/sample.ipynb | SebastianWolf-SAP/contextual-ai | 1d38ef7b3b4e12aa8e966146eb9d53f809c54204 | [
"Apache-2.0"
] | 11 | 2020-06-17T17:01:24.000Z | 2022-02-27T18:53:03.000Z | 32.224684 | 590 | 0.591967 | [
[
[
"# LIME Text Explainer via XAI\n\nThis tutorial demonstrates how to generate explanations using LIME's text explainer implemented by the Contextual AI library. Much of the tutorial overlaps with what is covered in the [LIME tabular tutorial](lime_tabular_explainer.ipynb). To recap, the main steps for generating explanations are:\n\n1. Get an explainer via the `ExplainerFactory` class\n2. Build the text explainer\n3. Call `explain_instance`\n\n\n## Credits\n1. Pramodh, Manduri <[email protected]>",
"_____no_output_____"
],
[
"### Step 1: Import libraries",
"_____no_output_____"
]
],
[
[
"# Some auxiliary imports for the tutorial\nimport pprint\nimport sys\nimport random\nimport numpy as np\nfrom pprint import pprint\nfrom sklearn import datasets\nfrom sklearn.model_selection import train_test_split\nfrom sklearn.naive_bayes import MultinomialNB\nfrom sklearn.feature_extraction.text import TfidfVectorizer\n\n# Set seed for reproducibility\nnp.random.seed(123456)\n\n# Set the path so that we can import ExplainerFactory\nsys.path.append('../../../')\n\n# Main Contextual AI imports\nimport xai\nfrom xai.explainer import ExplainerFactory",
"_____no_output_____"
]
],
[
[
"### Step 2: Load dataset and train a model\n\nIn this tutorial, we rely on the 20newsgroups text dataset, which can be loaded via sklearn's dataset utility. Documentation on the dataset itself can be found [here](https://scikit-learn.org/0.19/datasets/twenty_newsgroups.html). To keep things simple, we will extract data for 3 topics - baseball, Christianity, and medicine.\n\nOur target model is a multinomial Naive Bayes classifier, which we train using TF-IDF vectors.",
"_____no_output_____"
]
],
[
[
"# Train on a subset of categories\n\ncategories = [\n 'rec.sport.baseball',\n 'soc.religion.christian',\n 'sci.med'\n]\n\nraw_train = datasets.fetch_20newsgroups(subset='train', categories=categories)\nprint(list(raw_train.keys()))\nprint(raw_train.target_names)\nprint(raw_train.target[:10])\nraw_test = datasets.fetch_20newsgroups(subset='test', categories=categories)\n\nX_train = raw_train.data\nvectorizer = TfidfVectorizer()\nX_train_vec = vectorizer.fit_transform(X_train)\ny_train = raw_train.target\n\nX_test_vec = vectorizer.transform(raw_test.data)\ny_test = raw_test.target\n\nclf = MultinomialNB(alpha=0.1)\nclf.fit(X_train_vec, y_train)\n\nlimit_size=200\npprint('Subsetting training sample to %s to speed up.' % limit_size)\nX_train = X_train[:limit_size]\npprint('Classifier score: %s' % clf.score(X_test_vec, y_test))\npprint('Classifier predict func %s:' % clf.predict_proba)",
"['data', 'filenames', 'target_names', 'target', 'DESCR']\n['rec.sport.baseball', 'sci.med', 'soc.religion.christian']\n[1 0 2 2 0 2 0 0 0 1]\n'Subsetting training sample to 200 to speed up.'\n'Classifier score: 0.9689336691855583'\n('Classifier predict func <bound method _BaseNB.predict_proba of '\n 'MultinomialNB(alpha=0.1, class_prior=None, fit_prior=True)>:')\n"
]
],
[
[
"### Step 3: Instantiate the explainer\n\nHere, we will use the LIME Text Explainer.",
"_____no_output_____"
]
],
[
[
"explainer = ExplainerFactory.get_explainer(domain=xai.DOMAIN.TEXT)\nclf.predict_proba",
"_____no_output_____"
]
],
[
[
"### Step 4: Build the explainer\n\nThis initializes the underlying explainer object. We provide the `explain_instance` method below with the raw text - LIME's text explainer algorithm will conduct its own preprocessing in order to generate interpretable representations of the data. Hence we must define a custom `predict_fn` which takes a raw piece of text, vectorizes it via a pre-trained TF-IDF vectorizer, and passes the vector into the trained Naive Bayes model to generate class probabilities. LIME uses `predict_fn` to query our Naive Bayes model in order to learn its behavior around the provided data instance.",
"_____no_output_____"
]
],
[
[
"def predict_fn(instance):\n vec = vectorizer.transform(instance)\n return clf.predict_proba(vec)\n\nexplainer.build_explainer(predict_fn)",
"_____no_output_____"
],
[
"clf = clf\nfeature_names = []\nclf_fn = predict_fn\ntarget_names_list = []\n\nimport os\nimport json\nimport sys\nsys.path.append('../../../')\nfrom xai.compiler.base import Configuration, Controller\njson_config = 'lime-text-classification-model-interpreter.json'\nwith open(json_config) as file:\n config = json.load(file)\nconfig",
"The sklearn.metrics.classification module is deprecated in version 0.22 and will be removed in version 0.24. The corresponding classes / functions should instead be imported from sklearn.metrics. Anything that cannot be imported from sklearn.metrics is now part of the private API.\n"
],
[
"controller = Controller(config=Configuration(config, locals()))\ncontroller.render()",
"Interpret 100/200 samples\nInterpret 200/200 samples\nWarning: figure will exceed the page bottom, adding a new page.\n"
]
],
[
[
"### Results",
"_____no_output_____"
]
],
[
[
"pprint(\"report generated : %s/20newsgroup-clsssification-model-interpreter-report.pdf\" % os.getcwd())\n('report generated : '\n '/Users/i062308/Development/Explainable_AI/tutorials/compiler/20newsgroup/20newsgroup-clsssification-model-interpreter-report.pdf')",
"('report generated : '\n '/Users/i062308/Development/Explainable_AI/tutorials/compiler/20newsgroup/20newsgroup-clsssification-model-interpreter-report.pdf')\n"
]
]
] | [
"markdown",
"code",
"markdown",
"code",
"markdown",
"code",
"markdown",
"code",
"markdown",
"code"
] | [
[
"markdown",
"markdown"
],
[
"code"
],
[
"markdown"
],
[
"code"
],
[
"markdown"
],
[
"code"
],
[
"markdown"
],
[
"code",
"code",
"code"
],
[
"markdown"
],
[
"code"
]
] |
e76b8b0c4b5b84025cf6a64006186b7a93a096b4 | 82,512 | ipynb | Jupyter Notebook | src/original/MJT_UoEdinburghUK/demo/demo_fit_dce.ipynb | JonathanArvidsson/DCE-DSC-MRI_CodeCollection | 7ddddbe302a23f51bcec5444e2a3cab31f5a9bb4 | [
"Apache-2.0"
] | null | null | null | src/original/MJT_UoEdinburghUK/demo/demo_fit_dce.ipynb | JonathanArvidsson/DCE-DSC-MRI_CodeCollection | 7ddddbe302a23f51bcec5444e2a3cab31f5a9bb4 | [
"Apache-2.0"
] | null | null | null | src/original/MJT_UoEdinburghUK/demo/demo_fit_dce.ipynb | JonathanArvidsson/DCE-DSC-MRI_CodeCollection | 7ddddbe302a23f51bcec5444e2a3cab31f5a9bb4 | [
"Apache-2.0"
] | null | null | null | 263.616613 | 17,432 | 0.920242 | [
[
[
"## Fit DCE data",
"_____no_output_____"
]
],
[
[
"import sys\nimport matplotlib.pyplot as plt\nimport numpy as np\nsys.path.append('..')\nimport dce_fit, relaxivity, signal_models, water_ex_models, aifs, pk_models\n%load_ext autoreload\n%autoreload 2",
"_____no_output_____"
]
],
[
[
"---\n### First get the signal data",
"_____no_output_____"
]
],
[
[
"# Input time and signal values (subject 4)\nt = np.array([19.810000,59.430000,99.050000,138.670000,178.290000,217.910000,257.530000,297.150000,336.770000,376.390000,416.010000,455.630000,495.250000,534.870000,574.490000,614.110000,653.730000,693.350000,732.970000,772.590000,812.210000,851.830000,891.450000,931.070000,970.690000,1010.310000,1049.930000,1089.550000,1129.170000,1168.790000,1208.410000,1248.030000])\ns_vif = np.array([411.400000,420.200000,419.600000,399.000000,1650.400000,3229.200000,3716.200000,3375.600000,3022.000000,2801.200000,2669.800000,2413.800000,2321.400000,2231.400000,2152.800000,2138.200000,2059.200000,2037.600000,2008.200000,1998.800000,1936.800000,1939.400000,1887.000000,1872.800000,1840.200000,1820.400000,1796.200000,1773.000000,1775.600000,1762.000000,1693.400000,1675.800000])\ns_tissue = np.array([378.774277,380.712810,378.789773,382.467975,407.950413,443.482955,446.239153,433.392045,425.428202,426.274793,420.676653,417.144112,410.072831,422.042355,414.013430,410.885847,405.251033,415.864669,418.615186,406.327479,408.692149,406.797004,418.646694,408.176136,404.993285,405.098140,417.022211,408.189050,409.819731,401.988636,405.866219,406.299587])\n\n# Calculate the enhancement\nbaseline_idx = [0, 1, 2]\nenh_vif = dce_fit.sig_to_enh(s_vif, baseline_idx)\nenh_tissue = dce_fit.sig_to_enh(s_tissue, baseline_idx)\n\nfig, ax = plt.subplots(2,2)\nax[0,0].plot(t, s_tissue, '.', label='tissue signal')\nax[1,0].plot(t, s_vif, '.', label='VIF signal')\nax[1,0].set_xlabel('time (s)');\nax[0,1].plot(t, enh_tissue, '.', label='tissue enh (%)')\nax[1,1].plot(t, enh_vif, '.', label='VIF enh (%)')\nax[1,1].set_xlabel('time (s)');\n[a.legend() for a in ax.flatten()];\n",
"_____no_output_____"
]
],
[
[
"### Convert enhancement to concentration",
"_____no_output_____"
]
],
[
[
"# First define some relevant parameters\nR10_tissue, R10_vif = 1/1.3651, 1/1.7206\nk_vif, k_tissue = 0.9946, 1.2037 # flip angle correction factor\nhct = 0.46\n\n# Specify relaxivity model, i.e. concentration --> relaxation rate relationship\nc_to_r_model = relaxivity.c_to_r_linear(r1=5.0, r2=7.1)\n\n# Specify signal model, i.e. relaxation rate --> signal relationship\nsignal_model = signal_models.spgr(tr=3.4e-3, fa_rad=15.*(np.pi/180.), te=1.7e-3)\n\n# Calculate concentrations\nC_t = dce_fit.enh_to_conc(enh_tissue, k_tissue, R10_tissue, c_to_r_model, signal_model)\nc_p_vif = dce_fit.enh_to_conc(enh_vif, k_vif, R10_vif, c_to_r_model, signal_model) / (1-hct)\n\nfig, ax = plt.subplots(2,1)\nax[0].plot(t, C_t, '.', label='tissue conc (mM)')\nax[0].set_xlabel('time (s)');\nax[1].plot(t, c_p_vif, '.', label='VIF plasma conc (mM)')\nax[1].set_xlabel('time (s)');\n[a.legend() for a in ax.flatten()];",
"_____no_output_____"
]
],
[
[
"### Fit the concentration data using a pharmacokinetic model",
"_____no_output_____"
]
],
[
[
"# First create an AIF object\naif = aifs.patient_specific(t, c_p_vif)\n\n# Now create a pharmacokinetic model object\npk_model = pk_models.patlak(t, aif)\n\n# Set some initial parameters and fit the concentration data\nweights = np.concatenate([np.zeros(7), np.ones(25)]) # (exclude first few points from fit)\npk_pars_0 = [{'vp': 0.2, 'ps': 1e-4}] # (just use 1 set of starting parameters here)\n\n%time pk_pars, C_t_fit = dce_fit.conc_to_pkp(C_t, pk_model, pk_pars_0, weights)\n\nplt.plot(t, C_t, '.', label='tissue conc (mM)')\nplt.plot(t, C_t_fit, '-', label='model fit (mM)')\nplt.legend();\n\nprint(f\"Fitted parameters: {pk_pars}\")\nprint(f\"Expected: vp = 0.0081, ps = 2.00e-4\")",
"Wall time: 30.9 ms\nFitted parameters: {'vp': 0.008097170500283217, 'ps': 0.00019992401629213917}\nExpected: vp = 0.0081, ps = 2.00e-4\n"
]
],
[
[
"### Alternative approach: fit the tissue signal directly\nTo do this, we also need to create a water_ex_model object, which determines the relationship between R1 in each tissue compartment and the exponential R1 components. \nWe start by assuming the fast water exchange limit (as implicitly assumed above when estimating tissue concentration).\nThe result should be very similar to fitting the concentration curve.",
"_____no_output_____"
]
],
[
[
"# Create a water exchange model object.\nwater_ex_model = water_ex_models.fxl()\n\n# Now fit the enhancement curve\n%time pk_pars_enh, enh_fit = dce_fit.enh_to_pkp(enh_tissue, hct, k_tissue, R10_tissue, R10_vif, pk_model, c_to_r_model, water_ex_model, signal_model, pk_pars_0, weights)\n\nplt.plot(t, enh_tissue, '.', label='tissue enh (%)')\nplt.plot(t, enh_fit, '-', label='model fit (%)')\nplt.legend();\n\nprint(f\"Fitted parameters: {pk_pars_enh}\")\nprint(f\"Expected: vp = 0.0081, ps = 2.00e-4\")",
"Wall time: 159 ms\nFitted parameters: {'vp': 0.008081262743467564, 'ps': 0.00019935657535213955}\nExpected: vp = 0.0081, ps = 2.00e-4\n"
]
],
[
[
"### Repeat the fit assuming slow water exchange...\nThis time, we assume slow water exchange across the vessel wall. The result will be very different compared with fitting the concentration curve.",
"_____no_output_____"
]
],
[
[
"# Create a water exchange model object.\nwater_ex_model = water_ex_models.ntexl() # slow exchange across vessel wall, fast exchange across cell wall\n\n# Now fit the enhancement curve\n%time pk_pars_enh_ntexl, enh_fit_ntexl = dce_fit.enh_to_pkp(enh_tissue, hct, k_tissue, R10_tissue, R10_vif, pk_model, c_to_r_model, water_ex_model, signal_model, pk_pars_0, weights)\n\nplt.plot(t, enh_tissue, '.', label='tissue enh (%)')\nplt.plot(t, enh_fit_ntexl, '-', label='model fit (%)')\nplt.legend();\n\nprint(f\"Fitted parameters: {pk_pars_enh_ntexl}\")\nprint(f\"Expected: vp = 0.0113, ps = 1.12e-4\")",
"Wall time: 166 ms\nFitted parameters: {'vp': 0.011282424728448814, 'ps': 0.00011163566464040331}\nExpected: vp = 0.0113, ps = 1.12e-4\n"
]
]
] | [
"markdown",
"code",
"markdown",
"code",
"markdown",
"code",
"markdown",
"code",
"markdown",
"code",
"markdown",
"code"
] | [
[
"markdown"
],
[
"code"
],
[
"markdown"
],
[
"code"
],
[
"markdown"
],
[
"code"
],
[
"markdown"
],
[
"code"
],
[
"markdown"
],
[
"code"
],
[
"markdown"
],
[
"code"
]
] |
e76b934427fc03dd5ac83a3efa79f302674340e9 | 319,889 | ipynb | Jupyter Notebook | cvnd/CVND_Exercises/1_2_Convolutional_Filters_Edge_Detection/5. Canny Edge Detection.ipynb | sijoonlee/deep_learning | 437ee14b478688d671257310c33071f75764e3ed | [
"MIT"
] | 20 | 2019-09-29T13:32:00.000Z | 2022-03-28T09:57:51.000Z | cvnd/CVND_Exercises/1_2_Convolutional_Filters_Edge_Detection/5. Canny Edge Detection.ipynb | sijoonlee/deep_learning | 437ee14b478688d671257310c33071f75764e3ed | [
"MIT"
] | 11 | 2021-06-08T20:32:58.000Z | 2022-03-12T00:05:43.000Z | cvnd/CVND_Exercises/1_2_Convolutional_Filters_Edge_Detection/5. Canny Edge Detection.ipynb | sijoonlee/deep_learning | 437ee14b478688d671257310c33071f75764e3ed | [
"MIT"
] | null | null | null | 1,249.566406 | 112,460 | 0.960396 | [
[
[
"import numpy as np\nimport matplotlib.pyplot as plt\nimport cv2\n\n%matplotlib inline\n\n# Read in the image\nimage = cv2.imread('images/brain_MR.jpg')\n\n# Change color to RGB (from BGR)\nimage = cv2.cvtColor(image, cv2.COLOR_BGR2RGB)\n\nplt.imshow(image)",
"_____no_output_____"
],
[
"# Convert the image to grayscale for processing\ngray = cv2.cvtColor(image, cv2.COLOR_RGB2GRAY)\n\nplt.imshow(gray, cmap='gray')",
"_____no_output_____"
]
],
[
[
"### Implement Canny edge detection",
"_____no_output_____"
]
],
[
[
"# Try Canny using \"wide\" and \"tight\" thresholds\n\nwide = cv2.Canny(gray, 30, 100)\ntight = cv2.Canny(gray, 200, 240)\n \n \n# Display the images\nf, (ax1, ax2) = plt.subplots(1, 2, figsize=(20,10))\n\nax1.set_title('wide')\nax1.imshow(wide, cmap='gray')\n\nax2.set_title('tight')\nax2.imshow(tight, cmap='gray')",
"_____no_output_____"
]
],
[
[
"### TODO: Try to find the edges of this flower\n\nSet a small enough threshold to isolate the boundary of the flower.",
"_____no_output_____"
]
],
[
[
"# Read in the image\nimage = cv2.imread('images/sunflower.jpg')\n\n# Change color to RGB (from BGR)\nimage = cv2.cvtColor(image, cv2.COLOR_BGR2RGB)\n\nplt.imshow(image)",
"_____no_output_____"
],
[
"# Convert the image to grayscale\ngray = cv2.cvtColor(image, cv2.COLOR_RGB2GRAY)\n\n## TODO: Define lower and upper thresholds for hysteresis\n# right now the threshold is so small and low that it will pick up a lot of noise\nlower = 150\nupper = 200\n\nedges = cv2.Canny(gray, lower, upper)\n\nplt.figure(figsize=(20,10))\nplt.imshow(edges, cmap='gray')",
"_____no_output_____"
]
]
] | [
"code",
"markdown",
"code",
"markdown",
"code"
] | [
[
"code",
"code"
],
[
"markdown"
],
[
"code"
],
[
"markdown"
],
[
"code",
"code"
]
] |
e76b95b00e456ef0e4af2d0ccf9aa5d1722245e6 | 5,344 | ipynb | Jupyter Notebook | part2/lab4/LAB_Sarcasm_Detector.ipynb | yasheshshroff/ODSC2021_NLP_PyTorch | 359a9df1a97dff00eaeaa354f07df82e70ac23a2 | [
"MIT"
] | 4 | 2020-10-28T22:54:10.000Z | 2020-11-06T21:17:18.000Z | part2/lab4/LAB_Sarcasm_Detector.ipynb | yasheshshroff/ODSC2021_NLP_PyTorch | 359a9df1a97dff00eaeaa354f07df82e70ac23a2 | [
"MIT"
] | null | null | null | part2/lab4/LAB_Sarcasm_Detector.ipynb | yasheshshroff/ODSC2021_NLP_PyTorch | 359a9df1a97dff00eaeaa354f07df82e70ac23a2 | [
"MIT"
] | 15 | 2021-03-12T19:57:47.000Z | 2021-11-18T19:45:29.000Z | 22.266667 | 129 | 0.553331 | [
[
[
"## LAB - Sarcasm Detector",
"_____no_output_____"
],
[
"## LAB\n\n* Analyze input data, determine the sequence length (max)\n* Train BERT Sequence Classifier to detect sarcasm in the given dataset\n* Save the best model in './bert_sarcasm_detection_state_dict.pth'\n* Predict the sacasm for some headlines\n",
"_____no_output_____"
],
[
"### Download data and import packages",
"_____no_output_____"
]
],
[
[
"!wget https://github.com/ravi-ilango/acm-dec-2020-nlp/blob/main/lab4/sarcasm_data.zip?raw=true -O sarcasm_data.zip\n\n!unzip sarcasm_data.zip",
"_____no_output_____"
],
[
"!pip install transformers",
"_____no_output_____"
],
[
"# imports\nimport torch\nfrom torch.utils.data import TensorDataset, DataLoader, RandomSampler, SequentialSampler\nfrom sklearn.model_selection import train_test_split\nfrom transformers import BertForSequenceClassification, BertTokenizer, BertConfig, AdamW\n\nfrom tqdm import trange\n\nimport json\nimport numpy as np\nimport pandas as pd\nimport os\n\nimport matplotlib.pyplot as plt\n%matplotlib inline",
"_____no_output_____"
],
[
"model_path = './bert_sarcasm_detection_state_dict.pth'",
"_____no_output_____"
]
],
[
[
"### Load data and explore",
"_____no_output_____"
]
],
[
[
"def read_json(json_file):\n json_data = []\n file = open(json_file)\n for line in file:\n json_line = json.loads(line)\n json_data.append(json_line)\n return json_data\n\njson_data = []\nfor json_file in ['./sarcasm_data/Sarcasm_Headlines_Dataset.json', './sarcasm_data/Sarcasm_Headlines_Dataset_v2.json']:\n json_data = json_data + read_json(json_file)",
"_____no_output_____"
],
[
"df = pd.DataFrame(json_data)\n\nheadline_data_train = df.headline.values\nis_sarcastic_label_train = df.is_sarcastic.values\n\nprint(headline_data_train.shape)",
"_____no_output_____"
],
[
"for _, row in df[df.is_sarcastic==1].head().iterrows():\n print (f\"\\n{row.headline}\")",
"_____no_output_____"
],
[
"labels = is_sarcastic_label_train\nplt.hist(labels)\nplt.xlabel('is_sarcastic')\nplt.ylabel('nb samples')\nplt.title('is_sarcastic distribution')\nplt.xticks(np.arange(len(np.unique(labels))));",
"_____no_output_____"
]
],
[
[
"### YOUR CODE STARTS HERE",
"_____no_output_____"
]
]
] | [
"markdown",
"code",
"markdown",
"code",
"markdown"
] | [
[
"markdown",
"markdown",
"markdown"
],
[
"code",
"code",
"code",
"code"
],
[
"markdown"
],
[
"code",
"code",
"code",
"code"
],
[
"markdown"
]
] |
e76b9c65508492c54ecff40d467ae6e7f7493517 | 555,517 | ipynb | Jupyter Notebook | licensed_sponsors_uk/.ipynb_checkpoints/Untitled-checkpoint.ipynb | for-hk/Schindler | e676fb57248a18fd9102eee9c667bd93f8fbdb18 | [
"MIT"
] | null | null | null | licensed_sponsors_uk/.ipynb_checkpoints/Untitled-checkpoint.ipynb | for-hk/Schindler | e676fb57248a18fd9102eee9c667bd93f8fbdb18 | [
"MIT"
] | 19 | 2020-06-11T19:05:57.000Z | 2020-07-16T10:04:10.000Z | licensed_sponsors_uk/.ipynb_checkpoints/Untitled-checkpoint.ipynb | for-hk/Schindler | e676fb57248a18fd9102eee9c667bd93f8fbdb18 | [
"MIT"
] | null | null | null | 215.233243 | 472,047 | 0.598671 | [
[
[
"from lxml import html\nimport requests",
"_____no_output_____"
],
[
"page = requests.get('https://www.gov.uk/government/publications/register-of-licensed-sponsors-workers')\ntree = html.fromstring(page.content)",
"_____no_output_____"
],
[
"download_path = tree.xpath('//*[@id=\"attachment_4271537\"]/div[2]/h2/a')[0].get(\"href\")",
"_____no_output_____"
],
[
"print(download_path)",
"https://assets.publishing.service.gov.uk/government/uploads/system/uploads/attachment_data/file/891847/2020-06-12_Tier_2_5_Register_of_Sponsors.pdf\n"
],
[
"response = requests.get(download_path)",
"_____no_output_____"
],
[
"with open('/tmp/metadata.pdf', 'wb') as f:\n f.write(response.content)",
"_____no_output_____"
],
[
"from PyPDF2.pdf import PdfFileReader, PdfFileWriter, ContentStream, TextStringObject",
"_____no_output_____"
],
[
"pdfFileObj = open('/tmp/metadata.pdf','rb') ",
"_____no_output_____"
],
[
"pdfReader = PdfFileReader(pdfFileObj)",
"_____no_output_____"
],
[
"pdfReader.numPages",
"_____no_output_____"
],
[
"pageObj = pdfReader.getPage(0)",
"_____no_output_____"
],
[
"print(pageObj)\n",
"{'/Type': '/Page', '/Parent': IndirectObject(2, 0), '/Resources': IndirectObject(6, 0), '/Contents': [IndirectObject(5, 0)], '/MediaBox': [0, 0, 841, 595], '/CropBox': [0, 0, 841, 595]}\n"
],
[
"content = pageObj[\"/Contents\"].getObject()",
"_____no_output_____"
],
[
"print(content)",
"[IndirectObject(5, 0)]\n"
],
[
"import sys\nif sys.version_info[0] < 3:\n def b_(s):\n return s\nelse:\n B_CACHE = {}\n\n def b_(s):\n bc = B_CACHE\n if s in bc:\n return bc[s]\n if type(s) == bytes:\n return s\n else:\n r = s.encode('latin-1')\n if len(s) < 2:\n bc[s] = r\n return r",
"_____no_output_____"
],
[
"def get_text(content):\n if not isinstance(content, ContentStream):\n content = ContentStream(content, pageObj.pdf)\n\n text = \"\"\n for operands, operator in content.operations:\n if operator == b_(\"Tj\"):\n _text = operands[0]\n # print(operands)\n # print(operator)\n if isinstance(_text, TextStringObject):\n text += _text\n text += \"\\n\"\n elif operator == b_(\"T*\"):\n text += \"\\n\"\n elif operator == b_(\"'\"):\n text += \"\\n\"\n _text = operands[0]\n if isinstance(_text, TextStringObject):\n text += operands[0]\n elif operator == b_('\"'):\n _text = operands[2]\n if isinstance(_text, TextStringObject):\n text += \"\\n\"\n text += _text\n elif operator == b_(\"TJ\"):\n for i in operands[0]:\n if isinstance(i, TextStringObject):\n text += i\n text += \"\\n\"\n return text",
"_____no_output_____"
],
[
"all_text = \"\"\nfor i in range(pdfReader.numPages): \n pageObj = pdfReader.getPage(i)\n content = pageObj[\"/Contents\"].getObject()\n all_text += get_text(content)\n print(\"processing page : \", i)\n# print(text)",
"processing page : 0\nprocessing page : 1\nprocessing page : 2\nprocessing page : 3\nprocessing page : 4\nprocessing page : 5\nprocessing page : 6\nprocessing page : 7\nprocessing page : 8\nprocessing page : 9\nprocessing page : 10\nprocessing page : 11\nprocessing page : 12\nprocessing page : 13\nprocessing page : 14\nprocessing page : 15\nprocessing page : 16\nprocessing page : 17\nprocessing page : 18\nprocessing page : 19\nprocessing page : 20\nprocessing page : 21\nprocessing page : 22\nprocessing page : 23\nprocessing page : 24\nprocessing page : 25\nprocessing page : 26\nprocessing page : 27\nprocessing page : 28\nprocessing page : 29\nprocessing page : 30\nprocessing page : 31\nprocessing page : 32\nprocessing page : 33\nprocessing page : 34\nprocessing page : 35\nprocessing page : 36\nprocessing page : 37\nprocessing page : 38\nprocessing page : 39\nprocessing page : 40\nprocessing page : 41\nprocessing page : 42\nprocessing page : 43\nprocessing page : 44\nprocessing page : 45\nprocessing page : 46\nprocessing page : 47\nprocessing page : 48\nprocessing page : 49\nprocessing page : 50\nprocessing page : 51\nprocessing page : 52\nprocessing page : 53\nprocessing page : 54\nprocessing page : 55\nprocessing page : 56\nprocessing page : 57\nprocessing page : 58\nprocessing page : 59\nprocessing page : 60\nprocessing page : 61\nprocessing page : 62\nprocessing page : 63\nprocessing page : 64\nprocessing page : 65\nprocessing page : 66\nprocessing page : 67\nprocessing page : 68\nprocessing page : 69\nprocessing page : 70\nprocessing page : 71\nprocessing page : 72\nprocessing page : 73\nprocessing page : 74\nprocessing page : 75\nprocessing page : 76\nprocessing page : 77\nprocessing page : 78\nprocessing page : 79\nprocessing page : 80\nprocessing page : 81\nprocessing page : 82\nprocessing page : 83\nprocessing page : 84\nprocessing page : 85\nprocessing page : 86\nprocessing page : 87\nprocessing page : 88\nprocessing page : 89\nprocessing page : 90\nprocessing page : 91\nprocessing page : 92\nprocessing page : 93\nprocessing page : 94\nprocessing page : 95\nprocessing page : 96\nprocessing page : 97\nprocessing page : 98\nprocessing page : 99\nprocessing page : 100\nprocessing page : 101\nprocessing page : 102\nprocessing page : 103\nprocessing page : 104\nprocessing page : 105\nprocessing page : 106\nprocessing page : 107\nprocessing page : 108\nprocessing page : 109\nprocessing page : 110\nprocessing page : 111\nprocessing page : 112\nprocessing page : 113\nprocessing page : 114\nprocessing page : 115\nprocessing page : 116\nprocessing page : 117\nprocessing page : 118\nprocessing page : 119\nprocessing page : 120\nprocessing page : 121\nprocessing page : 122\nprocessing page : 123\nprocessing page : 124\nprocessing page : 125\nprocessing page : 126\nprocessing page : 127\nprocessing page : 128\nprocessing page : 129\nprocessing page : 130\nprocessing page : 131\nprocessing page : 132\nprocessing page : 133\nprocessing page : 134\nprocessing page : 135\nprocessing page : 136\nprocessing page : 137\nprocessing page : 138\nprocessing page : 139\nprocessing page : 140\nprocessing page : 141\nprocessing page : 142\nprocessing page : 143\nprocessing page : 144\nprocessing page : 145\nprocessing page : 146\nprocessing page : 147\nprocessing page : 148\nprocessing page : 149\nprocessing page : 150\nprocessing page : 151\nprocessing page : 152\nprocessing page : 153\nprocessing page : 154\nprocessing page : 155\nprocessing page : 156\nprocessing page : 157\nprocessing page : 158\nprocessing page : 159\nprocessing page : 160\nprocessing page : 161\nprocessing page : 162\nprocessing page : 163\nprocessing page : 164\nprocessing page : 165\nprocessing page : 166\nprocessing page : 167\nprocessing page : 168\nprocessing page : 169\nprocessing page : 170\nprocessing page : 171\nprocessing page : 172\nprocessing page : 173\nprocessing page : 174\nprocessing page : 175\nprocessing page : 176\nprocessing page : 177\nprocessing page : 178\nprocessing page : 179\nprocessing page : 180\nprocessing page : 181\nprocessing page : 182\nprocessing page : 183\nprocessing page : 184\nprocessing page : 185\nprocessing page : 186\nprocessing page : 187\nprocessing page : 188\nprocessing page : 189\nprocessing page : 190\nprocessing page : 191\nprocessing page : 192\nprocessing page : 193\nprocessing page : 194\nprocessing page : 195\nprocessing page : 196\nprocessing page : 197\nprocessing page : 198\nprocessing page : 199\nprocessing page : 200\nprocessing page : 201\nprocessing page : 202\nprocessing page : 203\nprocessing page : 204\nprocessing page : 205\nprocessing page : 206\nprocessing page : 207\nprocessing page : 208\nprocessing page : 209\nprocessing page : 210\nprocessing page : 211\nprocessing page : 212\nprocessing page : 213\nprocessing page : 214\nprocessing page : 215\nprocessing page : 216\nprocessing page : 217\nprocessing page : 218\nprocessing page : 219\nprocessing page : 220\nprocessing page : 221\nprocessing page : 222\nprocessing page : 223\nprocessing page : 224\nprocessing page : 225\nprocessing page : 226\nprocessing page : 227\nprocessing page : 228\nprocessing page : 229\nprocessing page : 230\nprocessing page : 231\nprocessing page : 232\nprocessing page : 233\nprocessing page : 234\nprocessing page : 235\nprocessing page : 236\nprocessing page : 237\nprocessing page : 238\nprocessing page : 239\nprocessing page : 240\nprocessing page : 241\nprocessing page : 242\nprocessing page : 243\nprocessing page : 244\nprocessing page : 245\nprocessing page : 246\nprocessing page : 247\nprocessing page : 248\nprocessing page : 249\nprocessing page : 250\nprocessing page : 251\nprocessing page : 252\nprocessing page : 253\nprocessing page : 254\nprocessing page : 255\nprocessing page : 256\nprocessing page : 257\nprocessing page : 258\nprocessing page : 259\nprocessing page : 260\nprocessing page : 261\nprocessing page : 262\nprocessing page : 263\nprocessing page : 264\nprocessing page : 265\nprocessing page : 266\nprocessing page : 267\nprocessing page : 268\nprocessing page : 269\nprocessing page : 270\nprocessing page : 271\nprocessing page : 272\nprocessing page : 273\nprocessing page : 274\nprocessing page : 275\nprocessing page : 276\nprocessing page : 277\nprocessing page : 278\nprocessing page : 279\nprocessing page : 280\nprocessing page : 281\nprocessing page : 282\nprocessing page : 283\nprocessing page : 284\nprocessing page : 285\nprocessing page : 286\nprocessing page : 287\nprocessing page : 288\nprocessing page : 289\nprocessing page : 290\nprocessing page : 291\nprocessing page : 292\nprocessing page : 293\nprocessing page : 294\nprocessing page : 295\nprocessing page : 296\nprocessing page : 297\nprocessing page : 298\nprocessing page : 299\nprocessing page : 300\nprocessing page : 301\nprocessing page : 302\nprocessing page : 303\nprocessing page : 304\nprocessing page : 305\nprocessing page : 306\nprocessing page : 307\nprocessing page : 308\nprocessing page : 309\nprocessing page : 310\nprocessing page : 311\nprocessing page : 312\nprocessing page : 313\nprocessing page : 314\nprocessing page : 315\nprocessing page : 316\nprocessing page : 317\nprocessing page : 318\nprocessing page : 319\nprocessing page : 320\nprocessing page : 321\nprocessing page : 322\nprocessing page : 323\nprocessing page : 324\nprocessing page : 325\nprocessing page : 326\nprocessing page : 327\nprocessing page : 328\nprocessing page : 329\nprocessing page : 330\nprocessing page : 331\nprocessing page : 332\nprocessing page : 333\nprocessing page : 334\nprocessing page : 335\nprocessing page : 336\nprocessing page : 337\nprocessing page : 338\nprocessing page : 339\nprocessing page : 340\nprocessing page : 341\nprocessing page : 342\nprocessing page : 343\nprocessing page : 344\nprocessing page : 345\nprocessing page : 346\nprocessing page : 347\nprocessing page : 348\nprocessing page : 349\nprocessing page : 350\nprocessing page : 351\nprocessing page : 352\nprocessing page : 353\nprocessing page : 354\nprocessing page : 355\nprocessing page : 356\nprocessing page : 357\nprocessing page : 358\nprocessing page : 359\nprocessing page : 360\nprocessing page : 361\nprocessing page : 362\nprocessing page : 363\nprocessing page : 364\nprocessing page : 365\nprocessing page : 366\nprocessing page : 367\nprocessing page : 368\nprocessing page : 369\n"
],
[
"# for content in contents:\n# text_object = content.getObject()\n# print(text_object)",
"_____no_output_____"
],
[
"with open(\"raw_text.csv\", \"w\") as text_file:\n text_file.write(all_text)",
"_____no_output_____"
],
[
"# pdftotext -layout /tmp/metadata.pdf",
"_____no_output_____"
],
[
"pageObj.extractText()",
"_____no_output_____"
],
[
"contents = pageObj.getContents()",
"_____no_output_____"
],
[
"contents[0]",
"_____no_output_____"
],
[
"def findInDict(needle,haystack):\n for key in haystack.keys():\n try:\n value = haystack[key]\n except:\n continue\n if key == needle:\n return value\n if type(value) == types.DictType or isinstance(value,pyPdf.generic.DictionaryObject): \n x = findInDict(needle,value)\n if x is not None:\n return x",
"_____no_output_____"
],
[
"print(pdfReader.resolvedObjects)",
"{(0, 1): {'/Type': '/Catalog', '/Pages': IndirectObject(2, 0), '/PageMode': '/UseNone', '/ViewerPreferences': {'/FitWindow': <PyPDF2.generic.BooleanObject object at 0x109eb87f0>, '/PageLayout': '/SinglePage', '/NonFullScreenPageMode': '/UseNone'}}, (0, 2): {'/Type': '/Pages', '/Kids': [IndirectObject(8, 0), IndirectObject(17, 0), IndirectObject(21, 0), IndirectObject(25, 0), IndirectObject(29, 0), IndirectObject(33, 0), IndirectObject(37, 0), IndirectObject(41, 0), IndirectObject(45, 0), IndirectObject(49, 0), IndirectObject(53, 0), IndirectObject(57, 0), IndirectObject(61, 0), IndirectObject(65, 0), IndirectObject(69, 0), IndirectObject(73, 0), IndirectObject(77, 0), IndirectObject(81, 0), IndirectObject(85, 0), IndirectObject(89, 0), IndirectObject(93, 0), IndirectObject(97, 0), IndirectObject(101, 0), IndirectObject(105, 0), IndirectObject(109, 0), IndirectObject(113, 0), IndirectObject(117, 0), IndirectObject(121, 0), IndirectObject(125, 0), IndirectObject(129, 0), IndirectObject(133, 0), IndirectObject(137, 0), IndirectObject(141, 0), IndirectObject(145, 0), IndirectObject(149, 0), IndirectObject(153, 0), IndirectObject(157, 0), IndirectObject(161, 0), IndirectObject(165, 0), IndirectObject(169, 0), IndirectObject(173, 0), IndirectObject(177, 0), IndirectObject(181, 0), IndirectObject(185, 0), IndirectObject(189, 0), IndirectObject(193, 0), IndirectObject(197, 0), IndirectObject(201, 0), IndirectObject(205, 0), IndirectObject(209, 0), IndirectObject(213, 0), IndirectObject(217, 0), IndirectObject(221, 0), IndirectObject(225, 0), IndirectObject(229, 0), IndirectObject(233, 0), IndirectObject(237, 0), IndirectObject(241, 0), IndirectObject(245, 0), IndirectObject(249, 0), IndirectObject(253, 0), IndirectObject(257, 0), IndirectObject(261, 0), IndirectObject(265, 0), IndirectObject(269, 0), IndirectObject(273, 0), IndirectObject(277, 0), IndirectObject(281, 0), IndirectObject(285, 0), IndirectObject(289, 0), IndirectObject(293, 0), IndirectObject(297, 0), IndirectObject(301, 0), IndirectObject(305, 0), IndirectObject(309, 0), IndirectObject(313, 0), IndirectObject(317, 0), IndirectObject(321, 0), IndirectObject(325, 0), IndirectObject(329, 0), IndirectObject(333, 0), IndirectObject(337, 0), IndirectObject(341, 0), IndirectObject(345, 0), IndirectObject(349, 0), IndirectObject(353, 0), IndirectObject(357, 0), IndirectObject(361, 0), IndirectObject(365, 0), IndirectObject(369, 0), IndirectObject(373, 0), IndirectObject(377, 0), IndirectObject(381, 0), IndirectObject(385, 0), IndirectObject(389, 0), IndirectObject(393, 0), IndirectObject(397, 0), IndirectObject(401, 0), IndirectObject(405, 0), IndirectObject(409, 0), IndirectObject(413, 0), IndirectObject(417, 0), IndirectObject(421, 0), IndirectObject(425, 0), IndirectObject(429, 0), IndirectObject(433, 0), IndirectObject(437, 0), IndirectObject(441, 0), IndirectObject(445, 0), IndirectObject(449, 0), IndirectObject(453, 0), IndirectObject(457, 0), IndirectObject(461, 0), IndirectObject(465, 0), IndirectObject(469, 0), IndirectObject(473, 0), IndirectObject(477, 0), IndirectObject(481, 0), IndirectObject(485, 0), IndirectObject(489, 0), IndirectObject(493, 0), IndirectObject(497, 0), IndirectObject(501, 0), IndirectObject(505, 0), IndirectObject(509, 0), IndirectObject(513, 0), IndirectObject(517, 0), IndirectObject(521, 0), IndirectObject(525, 0), IndirectObject(529, 0), IndirectObject(533, 0), IndirectObject(537, 0), IndirectObject(541, 0), IndirectObject(545, 0), IndirectObject(549, 0), IndirectObject(553, 0), IndirectObject(557, 0), IndirectObject(561, 0), IndirectObject(565, 0), IndirectObject(569, 0), IndirectObject(573, 0), IndirectObject(577, 0), IndirectObject(581, 0), IndirectObject(585, 0), IndirectObject(589, 0), IndirectObject(593, 0), IndirectObject(597, 0), IndirectObject(601, 0), IndirectObject(605, 0), IndirectObject(609, 0), IndirectObject(613, 0), IndirectObject(617, 0), IndirectObject(621, 0), IndirectObject(625, 0), IndirectObject(629, 0), IndirectObject(633, 0), IndirectObject(637, 0), IndirectObject(641, 0), IndirectObject(645, 0), IndirectObject(649, 0), IndirectObject(653, 0), IndirectObject(657, 0), IndirectObject(661, 0), IndirectObject(665, 0), IndirectObject(669, 0), IndirectObject(673, 0), IndirectObject(677, 0), IndirectObject(681, 0), IndirectObject(685, 0), IndirectObject(689, 0), IndirectObject(693, 0), IndirectObject(697, 0), IndirectObject(701, 0), IndirectObject(705, 0), IndirectObject(709, 0), IndirectObject(713, 0), IndirectObject(717, 0), IndirectObject(721, 0), IndirectObject(725, 0), IndirectObject(729, 0), IndirectObject(733, 0), IndirectObject(737, 0), IndirectObject(741, 0), IndirectObject(745, 0), IndirectObject(749, 0), IndirectObject(753, 0), IndirectObject(757, 0), IndirectObject(761, 0), IndirectObject(765, 0), IndirectObject(769, 0), IndirectObject(773, 0), IndirectObject(777, 0), IndirectObject(781, 0), IndirectObject(785, 0), IndirectObject(789, 0), IndirectObject(793, 0), IndirectObject(797, 0), IndirectObject(801, 0), IndirectObject(805, 0), IndirectObject(809, 0), IndirectObject(813, 0), IndirectObject(817, 0), IndirectObject(821, 0), IndirectObject(825, 0), IndirectObject(829, 0), IndirectObject(833, 0), IndirectObject(837, 0), IndirectObject(841, 0), IndirectObject(845, 0), IndirectObject(849, 0), IndirectObject(853, 0), IndirectObject(857, 0), IndirectObject(861, 0), IndirectObject(865, 0), IndirectObject(869, 0), IndirectObject(873, 0), IndirectObject(877, 0), IndirectObject(881, 0), IndirectObject(885, 0), IndirectObject(889, 0), IndirectObject(893, 0), IndirectObject(897, 0), IndirectObject(901, 0), IndirectObject(905, 0), IndirectObject(909, 0), IndirectObject(913, 0), IndirectObject(917, 0), IndirectObject(921, 0), IndirectObject(925, 0), IndirectObject(929, 0), IndirectObject(933, 0), IndirectObject(937, 0), IndirectObject(941, 0), IndirectObject(945, 0), IndirectObject(949, 0), IndirectObject(953, 0), IndirectObject(957, 0), IndirectObject(961, 0), IndirectObject(965, 0), IndirectObject(969, 0), IndirectObject(973, 0), IndirectObject(977, 0), IndirectObject(981, 0), IndirectObject(985, 0), IndirectObject(989, 0), IndirectObject(993, 0), IndirectObject(997, 0), IndirectObject(1001, 0), IndirectObject(1005, 0), IndirectObject(1009, 0), IndirectObject(1013, 0), IndirectObject(1017, 0), IndirectObject(1021, 0), IndirectObject(1025, 0), IndirectObject(1029, 0), IndirectObject(1033, 0), IndirectObject(1037, 0), IndirectObject(1041, 0), IndirectObject(1045, 0), IndirectObject(1049, 0), IndirectObject(1053, 0), IndirectObject(1057, 0), IndirectObject(1061, 0), IndirectObject(1065, 0), IndirectObject(1069, 0), IndirectObject(1073, 0), IndirectObject(1077, 0), IndirectObject(1081, 0), IndirectObject(1085, 0), IndirectObject(1089, 0), IndirectObject(1093, 0), IndirectObject(1097, 0), IndirectObject(1101, 0), IndirectObject(1105, 0), IndirectObject(1109, 0), IndirectObject(1113, 0), IndirectObject(1117, 0), IndirectObject(1121, 0), IndirectObject(1125, 0), IndirectObject(1129, 0), IndirectObject(1133, 0), IndirectObject(1137, 0), IndirectObject(1141, 0), IndirectObject(1145, 0), IndirectObject(1149, 0), IndirectObject(1153, 0), IndirectObject(1157, 0), IndirectObject(1161, 0), IndirectObject(1165, 0), IndirectObject(1169, 0), IndirectObject(1173, 0), IndirectObject(1177, 0), IndirectObject(1181, 0), IndirectObject(1185, 0), IndirectObject(1189, 0), IndirectObject(1193, 0), IndirectObject(1197, 0), IndirectObject(1201, 0), IndirectObject(1205, 0), IndirectObject(1209, 0), IndirectObject(1213, 0), IndirectObject(1217, 0), IndirectObject(1221, 0), IndirectObject(1225, 0), IndirectObject(1229, 0), IndirectObject(1233, 0), IndirectObject(1237, 0), IndirectObject(1241, 0), IndirectObject(1245, 0), IndirectObject(1249, 0), IndirectObject(1253, 0), IndirectObject(1257, 0), IndirectObject(1261, 0), IndirectObject(1265, 0), IndirectObject(1269, 0), IndirectObject(1273, 0), IndirectObject(1277, 0), IndirectObject(1281, 0), IndirectObject(1285, 0), IndirectObject(1289, 0), IndirectObject(1293, 0), IndirectObject(1297, 0), IndirectObject(1301, 0), IndirectObject(1305, 0), IndirectObject(1309, 0), IndirectObject(1313, 0), IndirectObject(1317, 0), IndirectObject(1321, 0), IndirectObject(1325, 0), IndirectObject(1329, 0), IndirectObject(1333, 0), IndirectObject(1337, 0), IndirectObject(1341, 0), IndirectObject(1345, 0), IndirectObject(1349, 0), IndirectObject(1353, 0), IndirectObject(1357, 0), IndirectObject(1361, 0), IndirectObject(1365, 0), IndirectObject(1369, 0), IndirectObject(1373, 0), IndirectObject(1377, 0), IndirectObject(1381, 0), IndirectObject(1385, 0), IndirectObject(1389, 0), IndirectObject(1393, 0), IndirectObject(1397, 0), IndirectObject(1401, 0), IndirectObject(1405, 0), IndirectObject(1409, 0), IndirectObject(1413, 0), IndirectObject(1417, 0), IndirectObject(1421, 0), IndirectObject(1425, 0), IndirectObject(1429, 0), IndirectObject(1433, 0), IndirectObject(1437, 0), IndirectObject(1441, 0), IndirectObject(1445, 0), IndirectObject(1449, 0), IndirectObject(1453, 0), IndirectObject(1457, 0), IndirectObject(1461, 0), IndirectObject(1465, 0), IndirectObject(1469, 0), IndirectObject(1473, 0), IndirectObject(1477, 0), IndirectObject(1481, 0), IndirectObject(1485, 0), IndirectObject(1489, 0), IndirectObject(1493, 0), IndirectObject(1497, 0), IndirectObject(1501, 0), IndirectObject(1505, 0), IndirectObject(1509, 0), IndirectObject(1513, 0), IndirectObject(1517, 0), IndirectObject(1521, 0), IndirectObject(1525, 0), IndirectObject(1529, 0), IndirectObject(1533, 0), IndirectObject(1537, 0), IndirectObject(1541, 0), IndirectObject(1545, 0), IndirectObject(1549, 0), IndirectObject(1553, 0), IndirectObject(1557, 0), IndirectObject(1561, 0), IndirectObject(1565, 0), IndirectObject(1569, 0), IndirectObject(1573, 0), IndirectObject(1577, 0), IndirectObject(1581, 0), IndirectObject(1585, 0), IndirectObject(1589, 0), IndirectObject(1593, 0), IndirectObject(1597, 0), IndirectObject(1601, 0), IndirectObject(1605, 0), IndirectObject(1609, 0), IndirectObject(1613, 0), IndirectObject(1617, 0), IndirectObject(1621, 0), IndirectObject(1625, 0), IndirectObject(1629, 0), IndirectObject(1633, 0), IndirectObject(1637, 0), IndirectObject(1641, 0), IndirectObject(1645, 0), IndirectObject(1649, 0), IndirectObject(1653, 0), IndirectObject(1657, 0), IndirectObject(1661, 0), IndirectObject(1665, 0), IndirectObject(1669, 0), IndirectObject(1673, 0), IndirectObject(1677, 0), IndirectObject(1681, 0), IndirectObject(1685, 0), IndirectObject(1689, 0), IndirectObject(1693, 0), IndirectObject(1697, 0), IndirectObject(1701, 0), IndirectObject(1705, 0), IndirectObject(1709, 0), IndirectObject(1713, 0), IndirectObject(1717, 0), IndirectObject(1721, 0), IndirectObject(1725, 0), IndirectObject(1729, 0), IndirectObject(1733, 0), IndirectObject(1737, 0), IndirectObject(1741, 0), IndirectObject(1745, 0), IndirectObject(1749, 0), IndirectObject(1753, 0), IndirectObject(1757, 0), IndirectObject(1761, 0), IndirectObject(1765, 0), IndirectObject(1769, 0), IndirectObject(1773, 0), IndirectObject(1777, 0), IndirectObject(1781, 0), IndirectObject(1785, 0), IndirectObject(1789, 0), IndirectObject(1793, 0), IndirectObject(1797, 0), IndirectObject(1801, 0), IndirectObject(1805, 0), IndirectObject(1809, 0), IndirectObject(1813, 0), IndirectObject(1817, 0), IndirectObject(1821, 0), IndirectObject(1825, 0), IndirectObject(1829, 0), IndirectObject(1833, 0), IndirectObject(1837, 0), IndirectObject(1841, 0), IndirectObject(1845, 0), IndirectObject(1849, 0), IndirectObject(1853, 0), IndirectObject(1857, 0), IndirectObject(1861, 0), IndirectObject(1865, 0), IndirectObject(1869, 0), IndirectObject(1873, 0), IndirectObject(1877, 0), IndirectObject(1881, 0), IndirectObject(1885, 0), IndirectObject(1889, 0), IndirectObject(1893, 0), IndirectObject(1897, 0), IndirectObject(1901, 0), IndirectObject(1905, 0), IndirectObject(1909, 0), IndirectObject(1913, 0), IndirectObject(1917, 0), IndirectObject(1921, 0), IndirectObject(1925, 0), IndirectObject(1929, 0), IndirectObject(1933, 0), IndirectObject(1937, 0), IndirectObject(1941, 0), IndirectObject(1945, 0), IndirectObject(1949, 0), IndirectObject(1953, 0), IndirectObject(1957, 0), IndirectObject(1961, 0), IndirectObject(1965, 0), IndirectObject(1969, 0), IndirectObject(1973, 0), IndirectObject(1977, 0), IndirectObject(1981, 0), IndirectObject(1985, 0), IndirectObject(1989, 0), IndirectObject(1993, 0), IndirectObject(1997, 0), IndirectObject(2001, 0), IndirectObject(2005, 0), IndirectObject(2009, 0), IndirectObject(2013, 0), IndirectObject(2017, 0), IndirectObject(2021, 0), IndirectObject(2025, 0), IndirectObject(2029, 0), IndirectObject(2033, 0), IndirectObject(2037, 0), IndirectObject(2041, 0), IndirectObject(2045, 0), IndirectObject(2049, 0), IndirectObject(2053, 0), IndirectObject(2057, 0), IndirectObject(2061, 0), IndirectObject(2065, 0), IndirectObject(2069, 0), IndirectObject(2073, 0), IndirectObject(2077, 0), IndirectObject(2081, 0), IndirectObject(2085, 0), IndirectObject(2089, 0), IndirectObject(2093, 0), IndirectObject(2097, 0), IndirectObject(2101, 0), IndirectObject(2105, 0), IndirectObject(2109, 0), IndirectObject(2113, 0), IndirectObject(2117, 0), IndirectObject(2121, 0), IndirectObject(2125, 0), IndirectObject(2129, 0), IndirectObject(2133, 0), IndirectObject(2137, 0), IndirectObject(2141, 0), IndirectObject(2145, 0), IndirectObject(2149, 0), IndirectObject(2153, 0), IndirectObject(2157, 0), IndirectObject(2161, 0), IndirectObject(2165, 0), IndirectObject(2169, 0), IndirectObject(2173, 0), IndirectObject(2177, 0), IndirectObject(2181, 0), IndirectObject(2185, 0), IndirectObject(2189, 0), IndirectObject(2193, 0), IndirectObject(2197, 0), IndirectObject(2201, 0), IndirectObject(2205, 0), IndirectObject(2209, 0), IndirectObject(2213, 0), IndirectObject(2217, 0), IndirectObject(2221, 0), IndirectObject(2225, 0), IndirectObject(2229, 0), IndirectObject(2233, 0), IndirectObject(2237, 0), IndirectObject(2241, 0), IndirectObject(2245, 0), IndirectObject(2249, 0), IndirectObject(2253, 0), IndirectObject(2257, 0), IndirectObject(2261, 0), IndirectObject(2265, 0), IndirectObject(2269, 0), IndirectObject(2273, 0), IndirectObject(2277, 0), IndirectObject(2281, 0), IndirectObject(2285, 0), IndirectObject(2289, 0), IndirectObject(2293, 0), IndirectObject(2297, 0), IndirectObject(2301, 0), IndirectObject(2305, 0), IndirectObject(2309, 0), IndirectObject(2313, 0), IndirectObject(2317, 0), IndirectObject(2321, 0), IndirectObject(2325, 0), IndirectObject(2329, 0), IndirectObject(2333, 0), IndirectObject(2337, 0), IndirectObject(2341, 0), IndirectObject(2345, 0), IndirectObject(2349, 0), IndirectObject(2353, 0), IndirectObject(2357, 0), IndirectObject(2361, 0), IndirectObject(2365, 0), IndirectObject(2369, 0), IndirectObject(2373, 0), IndirectObject(2377, 0), IndirectObject(2381, 0), IndirectObject(2385, 0), IndirectObject(2389, 0), IndirectObject(2393, 0), IndirectObject(2397, 0), IndirectObject(2401, 0), IndirectObject(2405, 0), IndirectObject(2409, 0), IndirectObject(2413, 0), IndirectObject(2417, 0), IndirectObject(2421, 0), IndirectObject(2425, 0), IndirectObject(2429, 0), IndirectObject(2433, 0), IndirectObject(2437, 0), IndirectObject(2441, 0), IndirectObject(2445, 0), IndirectObject(2449, 0), IndirectObject(2453, 0), IndirectObject(2457, 0), IndirectObject(2461, 0), IndirectObject(2465, 0), IndirectObject(2469, 0), IndirectObject(2473, 0), IndirectObject(2477, 0), IndirectObject(2481, 0), IndirectObject(2485, 0), IndirectObject(2489, 0), IndirectObject(2493, 0), IndirectObject(2497, 0), IndirectObject(2501, 0), IndirectObject(2505, 0), IndirectObject(2509, 0), IndirectObject(2513, 0), IndirectObject(2517, 0), IndirectObject(2521, 0), IndirectObject(2525, 0), IndirectObject(2529, 0), IndirectObject(2533, 0), IndirectObject(2537, 0), IndirectObject(2541, 0), IndirectObject(2545, 0), IndirectObject(2549, 0), IndirectObject(2553, 0), IndirectObject(2557, 0), IndirectObject(2561, 0), IndirectObject(2565, 0), IndirectObject(2569, 0), IndirectObject(2573, 0), IndirectObject(2577, 0), IndirectObject(2581, 0), IndirectObject(2585, 0), IndirectObject(2589, 0), IndirectObject(2593, 0), IndirectObject(2597, 0), IndirectObject(2601, 0), IndirectObject(2605, 0), IndirectObject(2609, 0), IndirectObject(2613, 0), IndirectObject(2617, 0), IndirectObject(2621, 0), IndirectObject(2625, 0), IndirectObject(2629, 0), IndirectObject(2633, 0), IndirectObject(2637, 0), IndirectObject(2641, 0), IndirectObject(2645, 0), IndirectObject(2649, 0), IndirectObject(2653, 0), IndirectObject(2657, 0), IndirectObject(2661, 0), IndirectObject(2665, 0), IndirectObject(2669, 0), IndirectObject(2673, 0), IndirectObject(2677, 0), IndirectObject(2681, 0), IndirectObject(2685, 0), IndirectObject(2689, 0), IndirectObject(2693, 0), IndirectObject(2697, 0), IndirectObject(2701, 0), IndirectObject(2705, 0), IndirectObject(2709, 0), IndirectObject(2713, 0), IndirectObject(2717, 0), IndirectObject(2721, 0), IndirectObject(2725, 0), IndirectObject(2729, 0), IndirectObject(2733, 0), IndirectObject(2737, 0), IndirectObject(2741, 0), IndirectObject(2745, 0), IndirectObject(2749, 0), IndirectObject(2753, 0), IndirectObject(2757, 0), IndirectObject(2761, 0), IndirectObject(2765, 0), IndirectObject(2769, 0), IndirectObject(2773, 0), IndirectObject(2777, 0), IndirectObject(2781, 0), IndirectObject(2785, 0), IndirectObject(2789, 0), IndirectObject(2793, 0), IndirectObject(2797, 0), IndirectObject(2801, 0), IndirectObject(2805, 0), IndirectObject(2809, 0), IndirectObject(2813, 0), IndirectObject(2817, 0), IndirectObject(2821, 0), IndirectObject(2825, 0), IndirectObject(2829, 0), IndirectObject(2833, 0), IndirectObject(2837, 0), IndirectObject(2841, 0), IndirectObject(2845, 0), IndirectObject(2849, 0), IndirectObject(2853, 0), IndirectObject(2857, 0), IndirectObject(2861, 0), IndirectObject(2865, 0), IndirectObject(2869, 0), IndirectObject(2873, 0), IndirectObject(2877, 0), IndirectObject(2881, 0), IndirectObject(2885, 0), IndirectObject(2889, 0), IndirectObject(2893, 0), IndirectObject(2897, 0), IndirectObject(2901, 0), IndirectObject(2905, 0), IndirectObject(2909, 0), IndirectObject(2913, 0), IndirectObject(2917, 0), IndirectObject(2921, 0), IndirectObject(2925, 0), IndirectObject(2929, 0), IndirectObject(2933, 0), IndirectObject(2937, 0), IndirectObject(2941, 0), IndirectObject(2945, 0), IndirectObject(2949, 0), IndirectObject(2953, 0), IndirectObject(2957, 0), IndirectObject(2961, 0), IndirectObject(2965, 0), IndirectObject(2969, 0), IndirectObject(2973, 0), IndirectObject(2977, 0), IndirectObject(2981, 0), IndirectObject(2985, 0), IndirectObject(2989, 0), IndirectObject(2993, 0), IndirectObject(2997, 0), IndirectObject(3001, 0), IndirectObject(3005, 0), IndirectObject(3009, 0), IndirectObject(3013, 0), IndirectObject(3017, 0), IndirectObject(3021, 0), IndirectObject(3025, 0), IndirectObject(3029, 0), IndirectObject(3033, 0), IndirectObject(3037, 0), IndirectObject(3041, 0), IndirectObject(3045, 0), IndirectObject(3049, 0), IndirectObject(3053, 0), IndirectObject(3057, 0), IndirectObject(3061, 0), IndirectObject(3065, 0), IndirectObject(3069, 0), IndirectObject(3073, 0), IndirectObject(3077, 0), IndirectObject(3081, 0), IndirectObject(3085, 0), IndirectObject(3089, 0), IndirectObject(3093, 0), IndirectObject(3097, 0), IndirectObject(3101, 0), IndirectObject(3105, 0), IndirectObject(3109, 0), IndirectObject(3113, 0), IndirectObject(3117, 0), IndirectObject(3121, 0), IndirectObject(3125, 0), IndirectObject(3129, 0), IndirectObject(3133, 0), IndirectObject(3137, 0), IndirectObject(3141, 0), IndirectObject(3145, 0), IndirectObject(3149, 0), IndirectObject(3153, 0), IndirectObject(3157, 0), IndirectObject(3161, 0), IndirectObject(3165, 0), IndirectObject(3169, 0), IndirectObject(3173, 0), IndirectObject(3177, 0), IndirectObject(3181, 0), IndirectObject(3185, 0), IndirectObject(3189, 0), IndirectObject(3193, 0), IndirectObject(3197, 0), IndirectObject(3201, 0), IndirectObject(3205, 0), IndirectObject(3209, 0), IndirectObject(3213, 0), IndirectObject(3217, 0), IndirectObject(3221, 0), IndirectObject(3225, 0), IndirectObject(3229, 0), IndirectObject(3233, 0), IndirectObject(3237, 0), IndirectObject(3241, 0), IndirectObject(3245, 0), IndirectObject(3249, 0), IndirectObject(3253, 0), IndirectObject(3257, 0), IndirectObject(3261, 0), IndirectObject(3265, 0), IndirectObject(3269, 0), IndirectObject(3273, 0), IndirectObject(3277, 0), IndirectObject(3281, 0), IndirectObject(3285, 0), IndirectObject(3289, 0), IndirectObject(3293, 0), IndirectObject(3297, 0), IndirectObject(3301, 0), IndirectObject(3305, 0), IndirectObject(3309, 0), IndirectObject(3313, 0), IndirectObject(3317, 0), IndirectObject(3321, 0), IndirectObject(3325, 0), IndirectObject(3329, 0), IndirectObject(3333, 0), IndirectObject(3337, 0), IndirectObject(3341, 0), IndirectObject(3345, 0), IndirectObject(3349, 0), IndirectObject(3353, 0), IndirectObject(3357, 0), IndirectObject(3361, 0), IndirectObject(3365, 0), IndirectObject(3369, 0), IndirectObject(3373, 0), IndirectObject(3377, 0), IndirectObject(3381, 0), IndirectObject(3385, 0), IndirectObject(3389, 0), IndirectObject(3393, 0), IndirectObject(3397, 0), IndirectObject(3401, 0), IndirectObject(3405, 0), IndirectObject(3409, 0), IndirectObject(3413, 0), IndirectObject(3417, 0), IndirectObject(3421, 0), IndirectObject(3425, 0), IndirectObject(3429, 0), IndirectObject(3433, 0), IndirectObject(3437, 0), IndirectObject(3441, 0), IndirectObject(3445, 0), IndirectObject(3449, 0), IndirectObject(3453, 0), IndirectObject(3457, 0), IndirectObject(3461, 0), IndirectObject(3465, 0), IndirectObject(3469, 0), IndirectObject(3473, 0), IndirectObject(3477, 0), IndirectObject(3481, 0), IndirectObject(3485, 0), IndirectObject(3489, 0), IndirectObject(3493, 0), IndirectObject(3497, 0), IndirectObject(3501, 0), IndirectObject(3505, 0), IndirectObject(3509, 0), IndirectObject(3513, 0), IndirectObject(3517, 0), IndirectObject(3521, 0), IndirectObject(3525, 0), IndirectObject(3529, 0), IndirectObject(3533, 0), IndirectObject(3537, 0), IndirectObject(3541, 0), IndirectObject(3545, 0), IndirectObject(3549, 0), IndirectObject(3553, 0), IndirectObject(3557, 0), IndirectObject(3561, 0), IndirectObject(3565, 0), IndirectObject(3569, 0), IndirectObject(3573, 0), IndirectObject(3577, 0), IndirectObject(3581, 0), IndirectObject(3585, 0), IndirectObject(3589, 0), IndirectObject(3593, 0), IndirectObject(3597, 0), IndirectObject(3601, 0), IndirectObject(3605, 0), IndirectObject(3609, 0), IndirectObject(3613, 0), IndirectObject(3617, 0), IndirectObject(3621, 0), IndirectObject(3625, 0), IndirectObject(3629, 0), IndirectObject(3633, 0), IndirectObject(3637, 0), IndirectObject(3641, 0), IndirectObject(3645, 0), IndirectObject(3649, 0), IndirectObject(3653, 0), IndirectObject(3657, 0), IndirectObject(3661, 0), IndirectObject(3665, 0), IndirectObject(3669, 0), IndirectObject(3673, 0), IndirectObject(3677, 0), IndirectObject(3681, 0), IndirectObject(3685, 0), IndirectObject(3689, 0), IndirectObject(3693, 0), IndirectObject(3697, 0), IndirectObject(3701, 0), IndirectObject(3705, 0), IndirectObject(3709, 0), IndirectObject(3713, 0), IndirectObject(3717, 0), IndirectObject(3721, 0), IndirectObject(3725, 0), IndirectObject(3729, 0), IndirectObject(3733, 0), IndirectObject(3737, 0), IndirectObject(3741, 0), IndirectObject(3745, 0), IndirectObject(3749, 0), IndirectObject(3753, 0), IndirectObject(3757, 0), IndirectObject(3761, 0), IndirectObject(3765, 0), IndirectObject(3769, 0), IndirectObject(3773, 0), IndirectObject(3777, 0), IndirectObject(3781, 0), IndirectObject(3785, 0), IndirectObject(3789, 0), IndirectObject(3793, 0), IndirectObject(3797, 0), IndirectObject(3801, 0), IndirectObject(3805, 0), IndirectObject(3809, 0), IndirectObject(3813, 0), IndirectObject(3817, 0), IndirectObject(3821, 0), IndirectObject(3825, 0), IndirectObject(3829, 0), IndirectObject(3833, 0), IndirectObject(3837, 0), IndirectObject(3841, 0), IndirectObject(3845, 0), IndirectObject(3849, 0), IndirectObject(3853, 0), IndirectObject(3857, 0), IndirectObject(3861, 0), IndirectObject(3865, 0), IndirectObject(3869, 0), IndirectObject(3873, 0), IndirectObject(3877, 0), IndirectObject(3881, 0), IndirectObject(3885, 0), IndirectObject(3889, 0), IndirectObject(3893, 0), IndirectObject(3897, 0), IndirectObject(3901, 0), IndirectObject(3905, 0), IndirectObject(3909, 0), IndirectObject(3913, 0), IndirectObject(3917, 0), IndirectObject(3921, 0), IndirectObject(3925, 0), IndirectObject(3929, 0), IndirectObject(3933, 0), IndirectObject(3937, 0), IndirectObject(3941, 0), IndirectObject(3945, 0), IndirectObject(3949, 0), IndirectObject(3953, 0), IndirectObject(3957, 0), IndirectObject(3961, 0), IndirectObject(3965, 0), IndirectObject(3969, 0), IndirectObject(3973, 0), IndirectObject(3977, 0), IndirectObject(3981, 0), IndirectObject(3985, 0), IndirectObject(3989, 0), IndirectObject(3993, 0), IndirectObject(3997, 0), IndirectObject(4001, 0), IndirectObject(4005, 0), IndirectObject(4009, 0), IndirectObject(4013, 0), IndirectObject(4017, 0), IndirectObject(4021, 0), IndirectObject(4025, 0), IndirectObject(4029, 0), IndirectObject(4033, 0), IndirectObject(4037, 0), IndirectObject(4041, 0), IndirectObject(4045, 0), IndirectObject(4049, 0), IndirectObject(4053, 0), IndirectObject(4057, 0), IndirectObject(4061, 0), IndirectObject(4065, 0), IndirectObject(4069, 0), IndirectObject(4073, 0), IndirectObject(4077, 0), IndirectObject(4081, 0), IndirectObject(4085, 0), IndirectObject(4089, 0), IndirectObject(4093, 0), IndirectObject(4097, 0), IndirectObject(4101, 0), IndirectObject(4105, 0), IndirectObject(4109, 0), IndirectObject(4113, 0), IndirectObject(4117, 0), IndirectObject(4121, 0), IndirectObject(4125, 0), IndirectObject(4129, 0), IndirectObject(4133, 0), IndirectObject(4137, 0), IndirectObject(4141, 0), IndirectObject(4145, 0), IndirectObject(4149, 0), IndirectObject(4153, 0), IndirectObject(4157, 0), IndirectObject(4161, 0), IndirectObject(4165, 0), IndirectObject(4169, 0), IndirectObject(4173, 0), IndirectObject(4177, 0), IndirectObject(4181, 0), IndirectObject(4185, 0), IndirectObject(4189, 0), IndirectObject(4193, 0), IndirectObject(4197, 0), IndirectObject(4201, 0), IndirectObject(4205, 0), IndirectObject(4209, 0), IndirectObject(4213, 0), IndirectObject(4217, 0), IndirectObject(4221, 0), IndirectObject(4225, 0), IndirectObject(4229, 0), IndirectObject(4233, 0), IndirectObject(4237, 0), IndirectObject(4241, 0), IndirectObject(4245, 0), IndirectObject(4249, 0), IndirectObject(4253, 0), IndirectObject(4257, 0), IndirectObject(4261, 0), IndirectObject(4265, 0), IndirectObject(4269, 0), IndirectObject(4273, 0), IndirectObject(4277, 0), IndirectObject(4281, 0), IndirectObject(4285, 0), IndirectObject(4289, 0), IndirectObject(4293, 0), IndirectObject(4297, 0), IndirectObject(4301, 0), IndirectObject(4305, 0), IndirectObject(4309, 0), IndirectObject(4313, 0), IndirectObject(4317, 0), IndirectObject(4321, 0), IndirectObject(4325, 0), IndirectObject(4329, 0), IndirectObject(4333, 0), IndirectObject(4337, 0), IndirectObject(4341, 0), IndirectObject(4345, 0), IndirectObject(4349, 0), IndirectObject(4353, 0), IndirectObject(4357, 0), IndirectObject(4361, 0), IndirectObject(4365, 0), IndirectObject(4369, 0), IndirectObject(4373, 0), IndirectObject(4377, 0), IndirectObject(4381, 0), IndirectObject(4385, 0), IndirectObject(4389, 0), IndirectObject(4393, 0), IndirectObject(4397, 0), IndirectObject(4401, 0), IndirectObject(4405, 0), IndirectObject(4409, 0), IndirectObject(4413, 0), IndirectObject(4417, 0), IndirectObject(4421, 0), IndirectObject(4425, 0), IndirectObject(4429, 0), IndirectObject(4433, 0), IndirectObject(4437, 0), IndirectObject(4441, 0), IndirectObject(4445, 0), IndirectObject(4449, 0), IndirectObject(4453, 0), IndirectObject(4457, 0), IndirectObject(4461, 0), IndirectObject(4465, 0), IndirectObject(4469, 0), IndirectObject(4473, 0), IndirectObject(4477, 0), IndirectObject(4481, 0), IndirectObject(4485, 0), IndirectObject(4489, 0), IndirectObject(4493, 0), IndirectObject(4497, 0), IndirectObject(4501, 0), IndirectObject(4505, 0), IndirectObject(4509, 0), IndirectObject(4513, 0), IndirectObject(4517, 0), IndirectObject(4521, 0), IndirectObject(4525, 0), IndirectObject(4529, 0), IndirectObject(4533, 0), IndirectObject(4537, 0), IndirectObject(4541, 0), IndirectObject(4545, 0), IndirectObject(4549, 0), IndirectObject(4553, 0), IndirectObject(4557, 0), IndirectObject(4561, 0), IndirectObject(4565, 0), IndirectObject(4569, 0), IndirectObject(4573, 0), IndirectObject(4577, 0), IndirectObject(4581, 0), IndirectObject(4585, 0), IndirectObject(4589, 0), IndirectObject(4593, 0), IndirectObject(4597, 0), IndirectObject(4601, 0), IndirectObject(4605, 0), IndirectObject(4609, 0), IndirectObject(4613, 0), IndirectObject(4617, 0), IndirectObject(4621, 0), IndirectObject(4625, 0), IndirectObject(4629, 0), IndirectObject(4633, 0), IndirectObject(4637, 0), IndirectObject(4641, 0), IndirectObject(4645, 0), IndirectObject(4649, 0), IndirectObject(4653, 0), IndirectObject(4657, 0), IndirectObject(4661, 0), IndirectObject(4665, 0), IndirectObject(4669, 0), IndirectObject(4673, 0), IndirectObject(4677, 0), IndirectObject(4681, 0), IndirectObject(4685, 0), IndirectObject(4689, 0), IndirectObject(4693, 0), IndirectObject(4697, 0), IndirectObject(4701, 0), IndirectObject(4705, 0), IndirectObject(4709, 0), IndirectObject(4713, 0), IndirectObject(4717, 0), IndirectObject(4721, 0), IndirectObject(4725, 0), IndirectObject(4729, 0), IndirectObject(4733, 0), IndirectObject(4737, 0), IndirectObject(4741, 0), IndirectObject(4745, 0), IndirectObject(4749, 0), IndirectObject(4753, 0), IndirectObject(4757, 0), IndirectObject(4761, 0), IndirectObject(4765, 0), IndirectObject(4769, 0), IndirectObject(4773, 0), IndirectObject(4777, 0), IndirectObject(4781, 0), IndirectObject(4785, 0), IndirectObject(4789, 0), IndirectObject(4793, 0), IndirectObject(4797, 0), IndirectObject(4801, 0), IndirectObject(4805, 0), IndirectObject(4809, 0), IndirectObject(4813, 0), IndirectObject(4817, 0), IndirectObject(4821, 0), IndirectObject(4825, 0), IndirectObject(4829, 0), IndirectObject(4833, 0), IndirectObject(4837, 0), IndirectObject(4841, 0), IndirectObject(4845, 0), IndirectObject(4849, 0), IndirectObject(4853, 0), IndirectObject(4857, 0), IndirectObject(4861, 0), IndirectObject(4865, 0), IndirectObject(4869, 0), IndirectObject(4873, 0), IndirectObject(4877, 0), IndirectObject(4881, 0), IndirectObject(4885, 0), IndirectObject(4889, 0), IndirectObject(4893, 0), IndirectObject(4897, 0), IndirectObject(4901, 0), IndirectObject(4905, 0), IndirectObject(4909, 0), IndirectObject(4913, 0), IndirectObject(4917, 0), IndirectObject(4921, 0), IndirectObject(4925, 0), IndirectObject(4929, 0), IndirectObject(4933, 0), IndirectObject(4937, 0), IndirectObject(4941, 0), IndirectObject(4945, 0), IndirectObject(4949, 0), IndirectObject(4953, 0), IndirectObject(4957, 0), IndirectObject(4961, 0), IndirectObject(4965, 0), IndirectObject(4969, 0), IndirectObject(4973, 0), IndirectObject(4977, 0), IndirectObject(4981, 0), IndirectObject(4985, 0), IndirectObject(4989, 0), IndirectObject(4993, 0), IndirectObject(4997, 0), IndirectObject(5001, 0), IndirectObject(5005, 0), IndirectObject(5009, 0), IndirectObject(5013, 0), IndirectObject(5017, 0), IndirectObject(5021, 0), IndirectObject(5025, 0), IndirectObject(5029, 0), IndirectObject(5033, 0), IndirectObject(5037, 0), IndirectObject(5041, 0), IndirectObject(5045, 0), IndirectObject(5049, 0), IndirectObject(5053, 0), IndirectObject(5057, 0), IndirectObject(5061, 0), IndirectObject(5065, 0), IndirectObject(5069, 0), IndirectObject(5073, 0), IndirectObject(5077, 0), IndirectObject(5081, 0), IndirectObject(5085, 0), IndirectObject(5089, 0), IndirectObject(5093, 0), IndirectObject(5097, 0), IndirectObject(5101, 0), IndirectObject(5105, 0), IndirectObject(5109, 0), IndirectObject(5113, 0), IndirectObject(5117, 0), IndirectObject(5121, 0), IndirectObject(5125, 0), IndirectObject(5129, 0), IndirectObject(5133, 0), IndirectObject(5137, 0), IndirectObject(5141, 0), IndirectObject(5145, 0), IndirectObject(5149, 0), IndirectObject(5153, 0), IndirectObject(5157, 0), IndirectObject(5161, 0), IndirectObject(5165, 0), IndirectObject(5169, 0), IndirectObject(5173, 0), IndirectObject(5177, 0), IndirectObject(5181, 0), IndirectObject(5185, 0), IndirectObject(5189, 0), IndirectObject(5193, 0), IndirectObject(5197, 0), IndirectObject(5201, 0), IndirectObject(5205, 0), IndirectObject(5209, 0), IndirectObject(5213, 0), IndirectObject(5217, 0), IndirectObject(5221, 0), IndirectObject(5225, 0), IndirectObject(5229, 0), IndirectObject(5233, 0), IndirectObject(5237, 0), IndirectObject(5241, 0), IndirectObject(5245, 0), IndirectObject(5249, 0), IndirectObject(5253, 0), IndirectObject(5257, 0), IndirectObject(5261, 0), IndirectObject(5265, 0), IndirectObject(5269, 0), IndirectObject(5273, 0), IndirectObject(5277, 0), IndirectObject(5281, 0), IndirectObject(5285, 0), IndirectObject(5289, 0), IndirectObject(5293, 0), IndirectObject(5297, 0), IndirectObject(5301, 0), IndirectObject(5305, 0), IndirectObject(5309, 0), IndirectObject(5313, 0), IndirectObject(5317, 0), IndirectObject(5321, 0), IndirectObject(5325, 0), IndirectObject(5329, 0), IndirectObject(5333, 0), IndirectObject(5337, 0), IndirectObject(5341, 0), IndirectObject(5345, 0), IndirectObject(5349, 0), IndirectObject(5353, 0), IndirectObject(5357, 0), IndirectObject(5361, 0), IndirectObject(5365, 0), IndirectObject(5369, 0), IndirectObject(5373, 0), IndirectObject(5377, 0), IndirectObject(5381, 0), IndirectObject(5385, 0), IndirectObject(5389, 0), IndirectObject(5393, 0), IndirectObject(5397, 0), IndirectObject(5401, 0), IndirectObject(5405, 0), IndirectObject(5409, 0), IndirectObject(5413, 0), IndirectObject(5417, 0), IndirectObject(5421, 0), IndirectObject(5425, 0), IndirectObject(5429, 0), IndirectObject(5433, 0), IndirectObject(5437, 0), IndirectObject(5441, 0), IndirectObject(5445, 0), IndirectObject(5449, 0), IndirectObject(5453, 0), IndirectObject(5457, 0), IndirectObject(5461, 0), IndirectObject(5465, 0), IndirectObject(5469, 0), IndirectObject(5473, 0), IndirectObject(5477, 0), IndirectObject(5481, 0), IndirectObject(5485, 0), IndirectObject(5489, 0), IndirectObject(5493, 0), IndirectObject(5497, 0), IndirectObject(5501, 0), IndirectObject(5505, 0), IndirectObject(5509, 0), IndirectObject(5513, 0), IndirectObject(5517, 0), IndirectObject(5521, 0), IndirectObject(5525, 0), IndirectObject(5529, 0), IndirectObject(5533, 0), IndirectObject(5537, 0), IndirectObject(5541, 0), IndirectObject(5545, 0), IndirectObject(5549, 0), IndirectObject(5553, 0), IndirectObject(5557, 0), IndirectObject(5561, 0), IndirectObject(5565, 0), IndirectObject(5569, 0), IndirectObject(5573, 0), IndirectObject(5577, 0), IndirectObject(5581, 0), IndirectObject(5585, 0), IndirectObject(5589, 0), IndirectObject(5593, 0), IndirectObject(5597, 0), IndirectObject(5601, 0), IndirectObject(5605, 0), IndirectObject(5609, 0), IndirectObject(5613, 0), IndirectObject(5617, 0), IndirectObject(5621, 0), IndirectObject(5625, 0), IndirectObject(5629, 0), IndirectObject(5633, 0), IndirectObject(5637, 0), IndirectObject(5641, 0), IndirectObject(5645, 0), IndirectObject(5649, 0), IndirectObject(5653, 0), IndirectObject(5657, 0), IndirectObject(5661, 0), IndirectObject(5665, 0), IndirectObject(5669, 0), IndirectObject(5673, 0), IndirectObject(5677, 0), IndirectObject(5681, 0), IndirectObject(5685, 0), IndirectObject(5689, 0), IndirectObject(5693, 0), IndirectObject(5697, 0), IndirectObject(5701, 0), IndirectObject(5705, 0), IndirectObject(5709, 0), IndirectObject(5713, 0), IndirectObject(5717, 0), IndirectObject(5721, 0), IndirectObject(5725, 0), IndirectObject(5729, 0), IndirectObject(5733, 0), IndirectObject(5737, 0), IndirectObject(5741, 0), IndirectObject(5745, 0), IndirectObject(5749, 0), IndirectObject(5753, 0), IndirectObject(5757, 0), IndirectObject(5761, 0), IndirectObject(5765, 0), IndirectObject(5769, 0), IndirectObject(5773, 0), IndirectObject(5777, 0), IndirectObject(5781, 0), IndirectObject(5785, 0), IndirectObject(5789, 0), IndirectObject(5793, 0), IndirectObject(5797, 0), IndirectObject(5801, 0), IndirectObject(5805, 0), IndirectObject(5809, 0), IndirectObject(5813, 0), IndirectObject(5817, 0), IndirectObject(5821, 0), IndirectObject(5825, 0), IndirectObject(5829, 0), IndirectObject(5833, 0), IndirectObject(5837, 0), IndirectObject(5841, 0), IndirectObject(5845, 0), IndirectObject(5849, 0), IndirectObject(5853, 0), IndirectObject(5857, 0), IndirectObject(5861, 0), IndirectObject(5865, 0), IndirectObject(5869, 0), IndirectObject(5873, 0), IndirectObject(5877, 0), IndirectObject(5881, 0), IndirectObject(5885, 0), IndirectObject(5889, 0), IndirectObject(5893, 0), IndirectObject(5897, 0), IndirectObject(5901, 0), IndirectObject(5905, 0), IndirectObject(5909, 0), IndirectObject(5913, 0), IndirectObject(5917, 0), IndirectObject(5921, 0), IndirectObject(5925, 0), IndirectObject(5929, 0), IndirectObject(5933, 0), IndirectObject(5937, 0), IndirectObject(5941, 0), IndirectObject(5945, 0), IndirectObject(5949, 0), IndirectObject(5953, 0), IndirectObject(5957, 0), IndirectObject(5961, 0), IndirectObject(5965, 0), IndirectObject(5969, 0), IndirectObject(5973, 0), IndirectObject(5977, 0), IndirectObject(5981, 0), IndirectObject(5985, 0), IndirectObject(5989, 0), IndirectObject(5993, 0), IndirectObject(5997, 0), IndirectObject(6001, 0), IndirectObject(6005, 0), IndirectObject(6009, 0), IndirectObject(6013, 0), IndirectObject(6017, 0), IndirectObject(6021, 0), IndirectObject(6025, 0), IndirectObject(6029, 0), IndirectObject(6033, 0), IndirectObject(6037, 0), IndirectObject(6041, 0), IndirectObject(6045, 0), IndirectObject(6049, 0), IndirectObject(6053, 0), IndirectObject(6057, 0), IndirectObject(6061, 0), IndirectObject(6065, 0), IndirectObject(6069, 0), IndirectObject(6073, 0), IndirectObject(6077, 0), IndirectObject(6081, 0), IndirectObject(6085, 0), IndirectObject(6089, 0), IndirectObject(6093, 0), IndirectObject(6097, 0), IndirectObject(6101, 0), IndirectObject(6105, 0), IndirectObject(6109, 0), IndirectObject(6113, 0), IndirectObject(6117, 0), IndirectObject(6121, 0), IndirectObject(6125, 0), IndirectObject(6129, 0), IndirectObject(6133, 0), IndirectObject(6137, 0), IndirectObject(6141, 0), IndirectObject(6145, 0), IndirectObject(6149, 0), IndirectObject(6153, 0), IndirectObject(6157, 0), IndirectObject(6161, 0), IndirectObject(6165, 0), IndirectObject(6169, 0), IndirectObject(6173, 0), IndirectObject(6177, 0), IndirectObject(6181, 0), IndirectObject(6185, 0), IndirectObject(6189, 0), IndirectObject(6193, 0), IndirectObject(6197, 0), IndirectObject(6201, 0), IndirectObject(6205, 0), IndirectObject(6209, 0), IndirectObject(6213, 0), IndirectObject(6217, 0), IndirectObject(6221, 0), IndirectObject(6225, 0), IndirectObject(6229, 0), IndirectObject(6233, 0), IndirectObject(6237, 0), IndirectObject(6241, 0), IndirectObject(6245, 0), IndirectObject(6249, 0), IndirectObject(6253, 0), IndirectObject(6257, 0), IndirectObject(6261, 0), IndirectObject(6265, 0), IndirectObject(6269, 0), IndirectObject(6273, 0), IndirectObject(6277, 0), IndirectObject(6281, 0), IndirectObject(6285, 0), IndirectObject(6289, 0), IndirectObject(6293, 0), IndirectObject(6297, 0), IndirectObject(6301, 0), IndirectObject(6305, 0), IndirectObject(6309, 0), IndirectObject(6313, 0), IndirectObject(6317, 0), IndirectObject(6321, 0), IndirectObject(6325, 0), IndirectObject(6329, 0), IndirectObject(6333, 0), IndirectObject(6337, 0), IndirectObject(6341, 0), IndirectObject(6345, 0), IndirectObject(6349, 0), IndirectObject(6353, 0), IndirectObject(6357, 0), IndirectObject(6361, 0), IndirectObject(6365, 0), IndirectObject(6369, 0), IndirectObject(6373, 0), IndirectObject(6377, 0), IndirectObject(6381, 0), IndirectObject(6385, 0), IndirectObject(6389, 0), IndirectObject(6393, 0), IndirectObject(6397, 0), IndirectObject(6401, 0), IndirectObject(6405, 0), IndirectObject(6409, 0), IndirectObject(6413, 0), IndirectObject(6417, 0), IndirectObject(6421, 0), IndirectObject(6425, 0), IndirectObject(6429, 0), IndirectObject(6433, 0), IndirectObject(6437, 0), IndirectObject(6441, 0), IndirectObject(6445, 0), IndirectObject(6449, 0), IndirectObject(6453, 0), IndirectObject(6457, 0), IndirectObject(6461, 0), IndirectObject(6465, 0), IndirectObject(6469, 0), IndirectObject(6473, 0), IndirectObject(6477, 0), IndirectObject(6481, 0), IndirectObject(6485, 0), IndirectObject(6489, 0), IndirectObject(6493, 0), IndirectObject(6497, 0), IndirectObject(6501, 0), IndirectObject(6505, 0), IndirectObject(6509, 0), IndirectObject(6513, 0), IndirectObject(6517, 0), IndirectObject(6521, 0), IndirectObject(6525, 0), IndirectObject(6529, 0), IndirectObject(6533, 0), IndirectObject(6537, 0), IndirectObject(6541, 0), IndirectObject(6545, 0), IndirectObject(6549, 0), IndirectObject(6553, 0), IndirectObject(6557, 0), IndirectObject(6561, 0), IndirectObject(6565, 0), IndirectObject(6569, 0), IndirectObject(6573, 0), IndirectObject(6577, 0), IndirectObject(6581, 0), IndirectObject(6585, 0), IndirectObject(6589, 0), IndirectObject(6593, 0), IndirectObject(6597, 0), IndirectObject(6601, 0), IndirectObject(6605, 0), IndirectObject(6609, 0), IndirectObject(6613, 0), IndirectObject(6617, 0), IndirectObject(6621, 0), IndirectObject(6625, 0), IndirectObject(6629, 0), IndirectObject(6633, 0), IndirectObject(6637, 0), IndirectObject(6641, 0), IndirectObject(6645, 0), IndirectObject(6649, 0), IndirectObject(6653, 0), IndirectObject(6657, 0), IndirectObject(6661, 0), IndirectObject(6665, 0), IndirectObject(6669, 0), IndirectObject(6673, 0), IndirectObject(6677, 0), IndirectObject(6681, 0), IndirectObject(6685, 0), IndirectObject(6689, 0), IndirectObject(6693, 0), IndirectObject(6697, 0), IndirectObject(6701, 0), IndirectObject(6705, 0), IndirectObject(6709, 0), IndirectObject(6713, 0), IndirectObject(6717, 0), IndirectObject(6721, 0), IndirectObject(6725, 0), IndirectObject(6729, 0), IndirectObject(6733, 0), IndirectObject(6737, 0), IndirectObject(6741, 0), IndirectObject(6745, 0), IndirectObject(6749, 0), IndirectObject(6753, 0), IndirectObject(6757, 0), IndirectObject(6761, 0), IndirectObject(6765, 0), IndirectObject(6769, 0), IndirectObject(6773, 0), IndirectObject(6777, 0), IndirectObject(6781, 0), IndirectObject(6785, 0), IndirectObject(6789, 0), IndirectObject(6793, 0), IndirectObject(6797, 0), IndirectObject(6801, 0), IndirectObject(6805, 0), IndirectObject(6809, 0), IndirectObject(6813, 0), IndirectObject(6817, 0), IndirectObject(6821, 0), IndirectObject(6825, 0), IndirectObject(6829, 0), IndirectObject(6833, 0), IndirectObject(6837, 0), IndirectObject(6841, 0), IndirectObject(6845, 0), IndirectObject(6849, 0), IndirectObject(6853, 0), IndirectObject(6857, 0), IndirectObject(6861, 0), IndirectObject(6865, 0), IndirectObject(6869, 0), IndirectObject(6873, 0), IndirectObject(6877, 0), IndirectObject(6881, 0), IndirectObject(6885, 0), IndirectObject(6889, 0), IndirectObject(6893, 0), IndirectObject(6897, 0), IndirectObject(6901, 0), IndirectObject(6905, 0), IndirectObject(6909, 0), IndirectObject(6913, 0), IndirectObject(6917, 0), IndirectObject(6921, 0), IndirectObject(6925, 0), IndirectObject(6929, 0), IndirectObject(6933, 0), IndirectObject(6937, 0), IndirectObject(6941, 0), IndirectObject(6945, 0), IndirectObject(6949, 0), IndirectObject(6953, 0), IndirectObject(6957, 0), IndirectObject(6961, 0), IndirectObject(6965, 0), IndirectObject(6969, 0), IndirectObject(6973, 0), IndirectObject(6977, 0), IndirectObject(6981, 0), IndirectObject(6985, 0), IndirectObject(6989, 0), IndirectObject(6993, 0), IndirectObject(6997, 0), IndirectObject(7001, 0), IndirectObject(7005, 0), IndirectObject(7009, 0), IndirectObject(7013, 0), IndirectObject(7017, 0), IndirectObject(7021, 0), IndirectObject(7025, 0), IndirectObject(7029, 0), IndirectObject(7033, 0), IndirectObject(7037, 0), IndirectObject(7041, 0), IndirectObject(7045, 0), IndirectObject(7049, 0), IndirectObject(7053, 0), IndirectObject(7057, 0), IndirectObject(7061, 0), IndirectObject(7065, 0), IndirectObject(7069, 0), IndirectObject(7073, 0), IndirectObject(7077, 0), IndirectObject(7081, 0), IndirectObject(7085, 0), IndirectObject(7089, 0), IndirectObject(7093, 0), IndirectObject(7097, 0), IndirectObject(7101, 0), IndirectObject(7105, 0), IndirectObject(7109, 0), IndirectObject(7113, 0), IndirectObject(7117, 0), IndirectObject(7121, 0), IndirectObject(7125, 0), IndirectObject(7129, 0), IndirectObject(7133, 0), IndirectObject(7137, 0), IndirectObject(7141, 0), IndirectObject(7145, 0), IndirectObject(7149, 0), IndirectObject(7153, 0), IndirectObject(7157, 0), IndirectObject(7161, 0), IndirectObject(7165, 0), IndirectObject(7169, 0), IndirectObject(7173, 0), IndirectObject(7177, 0), IndirectObject(7181, 0), IndirectObject(7185, 0), IndirectObject(7189, 0), IndirectObject(7193, 0), IndirectObject(7197, 0), IndirectObject(7201, 0), IndirectObject(7205, 0), IndirectObject(7209, 0), IndirectObject(7213, 0), IndirectObject(7217, 0), IndirectObject(7221, 0), IndirectObject(7225, 0), IndirectObject(7229, 0), IndirectObject(7233, 0), IndirectObject(7237, 0), IndirectObject(7241, 0), IndirectObject(7245, 0), IndirectObject(7249, 0), IndirectObject(7253, 0), IndirectObject(7257, 0), IndirectObject(7261, 0), IndirectObject(7265, 0), IndirectObject(7269, 0), IndirectObject(7273, 0), IndirectObject(7277, 0), IndirectObject(7281, 0), IndirectObject(7285, 0), IndirectObject(7289, 0), IndirectObject(7293, 0), IndirectObject(7297, 0), IndirectObject(7301, 0), IndirectObject(7305, 0), IndirectObject(7309, 0), IndirectObject(7313, 0), IndirectObject(7317, 0), IndirectObject(7321, 0), IndirectObject(7325, 0), IndirectObject(7329, 0), IndirectObject(7333, 0), IndirectObject(7337, 0), IndirectObject(7341, 0), IndirectObject(7345, 0), IndirectObject(7349, 0), IndirectObject(7353, 0), IndirectObject(7357, 0), IndirectObject(7361, 0), IndirectObject(7365, 0), IndirectObject(7369, 0), IndirectObject(7373, 0), IndirectObject(7377, 0), IndirectObject(7381, 0), IndirectObject(7385, 0), IndirectObject(7389, 0), IndirectObject(7393, 0), IndirectObject(7397, 0), IndirectObject(7401, 0), IndirectObject(7405, 0), IndirectObject(7409, 0), IndirectObject(7413, 0), IndirectObject(7417, 0), IndirectObject(7421, 0), IndirectObject(7425, 0), IndirectObject(7429, 0), IndirectObject(7433, 0), IndirectObject(7437, 0), IndirectObject(7441, 0), IndirectObject(7445, 0), IndirectObject(7449, 0), IndirectObject(7453, 0), IndirectObject(7457, 0), IndirectObject(7461, 0), IndirectObject(7465, 0), IndirectObject(7469, 0), IndirectObject(7473, 0), IndirectObject(7477, 0), IndirectObject(7481, 0), IndirectObject(7485, 0), IndirectObject(7489, 0), IndirectObject(7493, 0), IndirectObject(7497, 0), IndirectObject(7501, 0), IndirectObject(7505, 0), IndirectObject(7509, 0), IndirectObject(7513, 0), IndirectObject(7517, 0), IndirectObject(7521, 0), IndirectObject(7525, 0), IndirectObject(7529, 0), IndirectObject(7533, 0), IndirectObject(7537, 0), IndirectObject(7541, 0), IndirectObject(7545, 0), IndirectObject(7549, 0), IndirectObject(7553, 0), IndirectObject(7557, 0), IndirectObject(7561, 0), IndirectObject(7565, 0), IndirectObject(7569, 0), IndirectObject(7573, 0), IndirectObject(7577, 0), IndirectObject(7581, 0), IndirectObject(7585, 0), IndirectObject(7589, 0), IndirectObject(7593, 0), IndirectObject(7597, 0), IndirectObject(7601, 0), IndirectObject(7605, 0), IndirectObject(7609, 0), IndirectObject(7613, 0), IndirectObject(7617, 0), IndirectObject(7621, 0), IndirectObject(7625, 0), IndirectObject(7629, 0), IndirectObject(7633, 0), IndirectObject(7637, 0), IndirectObject(7641, 0), IndirectObject(7645, 0), IndirectObject(7649, 0), IndirectObject(7653, 0), IndirectObject(7657, 0), IndirectObject(7661, 0), IndirectObject(7665, 0), IndirectObject(7669, 0), IndirectObject(7673, 0), IndirectObject(7677, 0), IndirectObject(7681, 0), IndirectObject(7685, 0), IndirectObject(7689, 0), IndirectObject(7693, 0), IndirectObject(7697, 0), IndirectObject(7701, 0), IndirectObject(7705, 0), IndirectObject(7709, 0), IndirectObject(7713, 0), IndirectObject(7717, 0), IndirectObject(7721, 0), IndirectObject(7725, 0), IndirectObject(7729, 0), IndirectObject(7733, 0), IndirectObject(7737, 0), IndirectObject(7741, 0), IndirectObject(7745, 0), IndirectObject(7749, 0), IndirectObject(7753, 0), IndirectObject(7757, 0), IndirectObject(7761, 0), IndirectObject(7765, 0), IndirectObject(7769, 0), IndirectObject(7773, 0), IndirectObject(7777, 0), IndirectObject(7781, 0), IndirectObject(7785, 0), IndirectObject(7789, 0), IndirectObject(7793, 0), IndirectObject(7797, 0), IndirectObject(7801, 0), IndirectObject(7805, 0), IndirectObject(7809, 0), IndirectObject(7813, 0), IndirectObject(7817, 0), IndirectObject(7821, 0), IndirectObject(7825, 0), IndirectObject(7829, 0), IndirectObject(7833, 0), IndirectObject(7837, 0), IndirectObject(7841, 0), IndirectObject(7845, 0), IndirectObject(7849, 0), IndirectObject(7853, 0), IndirectObject(7857, 0), IndirectObject(7861, 0), IndirectObject(7865, 0), IndirectObject(7869, 0), IndirectObject(7873, 0), IndirectObject(7877, 0), IndirectObject(7881, 0), IndirectObject(7885, 0), IndirectObject(7889, 0), IndirectObject(7893, 0), IndirectObject(7897, 0), IndirectObject(7901, 0), IndirectObject(7905, 0), IndirectObject(7909, 0), IndirectObject(7913, 0), IndirectObject(7917, 0), IndirectObject(7921, 0), IndirectObject(7925, 0), IndirectObject(7929, 0), IndirectObject(7933, 0), IndirectObject(7937, 0), IndirectObject(7941, 0), IndirectObject(7945, 0), IndirectObject(7949, 0), IndirectObject(7953, 0), IndirectObject(7957, 0), IndirectObject(7961, 0), IndirectObject(7965, 0), IndirectObject(7969, 0), IndirectObject(7973, 0), IndirectObject(7977, 0), IndirectObject(7981, 0), IndirectObject(7985, 0), IndirectObject(7989, 0), IndirectObject(7993, 0), IndirectObject(7997, 0), IndirectObject(8001, 0), IndirectObject(8005, 0), IndirectObject(8009, 0), IndirectObject(8013, 0), IndirectObject(8017, 0), IndirectObject(8021, 0), IndirectObject(8025, 0), IndirectObject(8029, 0), IndirectObject(8033, 0), IndirectObject(8037, 0), IndirectObject(8041, 0), IndirectObject(8045, 0), IndirectObject(8049, 0), IndirectObject(8053, 0), IndirectObject(8057, 0), IndirectObject(8061, 0), IndirectObject(8065, 0), IndirectObject(8069, 0), IndirectObject(8073, 0), IndirectObject(8077, 0), IndirectObject(8081, 0), IndirectObject(8085, 0), IndirectObject(8089, 0), IndirectObject(8093, 0), IndirectObject(8097, 0), IndirectObject(8101, 0), IndirectObject(8105, 0), IndirectObject(8109, 0), IndirectObject(8113, 0), IndirectObject(8117, 0), IndirectObject(8121, 0), IndirectObject(8125, 0), IndirectObject(8129, 0), IndirectObject(8133, 0), IndirectObject(8137, 0), IndirectObject(8141, 0), IndirectObject(8145, 0), IndirectObject(8149, 0), IndirectObject(8153, 0), IndirectObject(8157, 0), IndirectObject(8161, 0), IndirectObject(8165, 0), IndirectObject(8169, 0), IndirectObject(8173, 0), IndirectObject(8177, 0), IndirectObject(8181, 0), IndirectObject(8185, 0), IndirectObject(8189, 0), IndirectObject(8193, 0), IndirectObject(8197, 0), IndirectObject(8201, 0), IndirectObject(8205, 0), IndirectObject(8209, 0), IndirectObject(8213, 0), IndirectObject(8217, 0), IndirectObject(8221, 0), IndirectObject(8225, 0), IndirectObject(8229, 0), IndirectObject(8233, 0), IndirectObject(8237, 0), IndirectObject(8241, 0), IndirectObject(8245, 0), IndirectObject(8249, 0), IndirectObject(8253, 0), IndirectObject(8257, 0), IndirectObject(8261, 0), IndirectObject(8265, 0)], '/Count': 2064, '/MediaBox': IndirectObject(3, 0), '/CropBox': IndirectObject(4, 0)}, (0, 3): [0, 0, 841, 595], (0, 4): [0, 0, 841, 595], (0, 8): {'/Type': '/Page', '/Parent': IndirectObject(2, 0), '/Resources': IndirectObject(6, 0), '/Contents': [IndirectObject(5, 0)], '/MediaBox': [0, 0, 841, 595], '/CropBox': [0, 0, 841, 595]}, (0, 17): {'/Type': '/Page', '/Parent': IndirectObject(2, 0), '/Resources': IndirectObject(15, 0), '/Contents': [IndirectObject(14, 0)], '/MediaBox': [0, 0, 841, 595], '/CropBox': [0, 0, 841, 595]}, (0, 21): {'/Type': '/Page', '/Parent': IndirectObject(2, 0), '/Resources': IndirectObject(19, 0), '/Contents': [IndirectObject(18, 0)], '/MediaBox': [0, 0, 841, 595], '/CropBox': [0, 0, 841, 595]}, (0, 25): {'/Type': '/Page', '/Parent': IndirectObject(2, 0), '/Resources': IndirectObject(23, 0), '/Contents': [IndirectObject(22, 0)], '/MediaBox': [0, 0, 841, 595], '/CropBox': [0, 0, 841, 595]}, (0, 29): {'/Type': '/Page', '/Parent': IndirectObject(2, 0), '/Resources': IndirectObject(27, 0), '/Contents': [IndirectObject(26, 0)], '/MediaBox': [0, 0, 841, 595], '/CropBox': [0, 0, 841, 595]}, (0, 33): {'/Type': '/Page', '/Parent': IndirectObject(2, 0), '/Resources': IndirectObject(31, 0), '/Contents': [IndirectObject(30, 0)], '/MediaBox': [0, 0, 841, 595], '/CropBox': [0, 0, 841, 595]}, (0, 37): {'/Type': '/Page', '/Parent': IndirectObject(2, 0), '/Resources': IndirectObject(35, 0), '/Contents': [IndirectObject(34, 0)], '/MediaBox': [0, 0, 841, 595], '/CropBox': [0, 0, 841, 595]}, (0, 41): {'/Type': '/Page', '/Parent': IndirectObject(2, 0), '/Resources': IndirectObject(39, 0), '/Contents': [IndirectObject(38, 0)], '/MediaBox': [0, 0, 841, 595], '/CropBox': [0, 0, 841, 595]}, (0, 45): {'/Type': '/Page', '/Parent': IndirectObject(2, 0), '/Resources': IndirectObject(43, 0), '/Contents': [IndirectObject(42, 0)], '/MediaBox': [0, 0, 841, 595], '/CropBox': [0, 0, 841, 595]}, (0, 49): {'/Type': '/Page', '/Parent': IndirectObject(2, 0), '/Resources': IndirectObject(47, 0), '/Contents': [IndirectObject(46, 0)], '/MediaBox': [0, 0, 841, 595], '/CropBox': [0, 0, 841, 595]}, (0, 53): {'/Type': '/Page', '/Parent': IndirectObject(2, 0), '/Resources': IndirectObject(51, 0), '/Contents': [IndirectObject(50, 0)], '/MediaBox': [0, 0, 841, 595], '/CropBox': [0, 0, 841, 595]}, (0, 57): {'/Type': '/Page', '/Parent': IndirectObject(2, 0), '/Resources': IndirectObject(55, 0), '/Contents': [IndirectObject(54, 0)], '/MediaBox': [0, 0, 841, 595], '/CropBox': [0, 0, 841, 595]}, (0, 61): {'/Type': '/Page', '/Parent': IndirectObject(2, 0), '/Resources': IndirectObject(59, 0), '/Contents': [IndirectObject(58, 0)], '/MediaBox': [0, 0, 841, 595], '/CropBox': [0, 0, 841, 595]}, (0, 65): {'/Type': '/Page', '/Parent': IndirectObject(2, 0), '/Resources': IndirectObject(63, 0), '/Contents': [IndirectObject(62, 0)], '/MediaBox': [0, 0, 841, 595], '/CropBox': [0, 0, 841, 595]}, (0, 69): {'/Type': '/Page', '/Parent': IndirectObject(2, 0), '/Resources': IndirectObject(67, 0), '/Contents': [IndirectObject(66, 0)], '/MediaBox': [0, 0, 841, 595], '/CropBox': [0, 0, 841, 595]}, (0, 73): {'/Type': '/Page', '/Parent': IndirectObject(2, 0), '/Resources': IndirectObject(71, 0), '/Contents': [IndirectObject(70, 0)], '/MediaBox': [0, 0, 841, 595], '/CropBox': [0, 0, 841, 595]}, (0, 77): {'/Type': '/Page', '/Parent': IndirectObject(2, 0), '/Resources': IndirectObject(75, 0), '/Contents': [IndirectObject(74, 0)], '/MediaBox': [0, 0, 841, 595], '/CropBox': [0, 0, 841, 595]}, (0, 81): {'/Type': '/Page', '/Parent': IndirectObject(2, 0), '/Resources': IndirectObject(79, 0), '/Contents': [IndirectObject(78, 0)], '/MediaBox': [0, 0, 841, 595], '/CropBox': [0, 0, 841, 595]}, (0, 85): {'/Type': '/Page', '/Parent': IndirectObject(2, 0), '/Resources': IndirectObject(83, 0), '/Contents': [IndirectObject(82, 0)], '/MediaBox': [0, 0, 841, 595], '/CropBox': [0, 0, 841, 595]}, (0, 89): {'/Type': '/Page', '/Parent': IndirectObject(2, 0), '/Resources': IndirectObject(87, 0), '/Contents': [IndirectObject(86, 0)], '/MediaBox': [0, 0, 841, 595], '/CropBox': [0, 0, 841, 595]}, (0, 93): {'/Type': '/Page', '/Parent': IndirectObject(2, 0), '/Resources': IndirectObject(91, 0), '/Contents': [IndirectObject(90, 0)], '/MediaBox': [0, 0, 841, 595], '/CropBox': [0, 0, 841, 595]}, (0, 97): {'/Type': '/Page', '/Parent': IndirectObject(2, 0), '/Resources': IndirectObject(95, 0), '/Contents': [IndirectObject(94, 0)], '/MediaBox': [0, 0, 841, 595], '/CropBox': [0, 0, 841, 595]}, (0, 101): {'/Type': '/Page', '/Parent': IndirectObject(2, 0), '/Resources': IndirectObject(99, 0), '/Contents': [IndirectObject(98, 0)], '/MediaBox': [0, 0, 841, 595], '/CropBox': [0, 0, 841, 595]}, (0, 105): {'/Type': '/Page', '/Parent': IndirectObject(2, 0), '/Resources': IndirectObject(103, 0), '/Contents': [IndirectObject(102, 0)], '/MediaBox': [0, 0, 841, 595], '/CropBox': [0, 0, 841, 595]}, (0, 109): {'/Type': '/Page', '/Parent': IndirectObject(2, 0), '/Resources': IndirectObject(107, 0), '/Contents': [IndirectObject(106, 0)], '/MediaBox': [0, 0, 841, 595], '/CropBox': [0, 0, 841, 595]}, (0, 113): {'/Type': '/Page', '/Parent': IndirectObject(2, 0), '/Resources': IndirectObject(111, 0), '/Contents': [IndirectObject(110, 0)], '/MediaBox': [0, 0, 841, 595], '/CropBox': [0, 0, 841, 595]}, (0, 117): {'/Type': '/Page', '/Parent': IndirectObject(2, 0), '/Resources': IndirectObject(115, 0), '/Contents': [IndirectObject(114, 0)], '/MediaBox': [0, 0, 841, 595], '/CropBox': [0, 0, 841, 595]}, (0, 121): {'/Type': '/Page', '/Parent': IndirectObject(2, 0), '/Resources': IndirectObject(119, 0), '/Contents': [IndirectObject(118, 0)], '/MediaBox': [0, 0, 841, 595], '/CropBox': [0, 0, 841, 595]}, (0, 125): {'/Type': '/Page', '/Parent': IndirectObject(2, 0), '/Resources': IndirectObject(123, 0), '/Contents': [IndirectObject(122, 0)], '/MediaBox': [0, 0, 841, 595], '/CropBox': [0, 0, 841, 595]}, (0, 129): {'/Type': '/Page', '/Parent': IndirectObject(2, 0), '/Resources': IndirectObject(127, 0), '/Contents': [IndirectObject(126, 0)], '/MediaBox': [0, 0, 841, 595], '/CropBox': [0, 0, 841, 595]}, (0, 133): {'/Type': '/Page', '/Parent': IndirectObject(2, 0), '/Resources': IndirectObject(131, 0), '/Contents': [IndirectObject(130, 0)], '/MediaBox': [0, 0, 841, 595], '/CropBox': [0, 0, 841, 595]}, (0, 137): {'/Type': '/Page', '/Parent': IndirectObject(2, 0), '/Resources': IndirectObject(135, 0), '/Contents': [IndirectObject(134, 0)], '/MediaBox': [0, 0, 841, 595], '/CropBox': [0, 0, 841, 595]}, (0, 141): {'/Type': '/Page', '/Parent': IndirectObject(2, 0), '/Resources': IndirectObject(139, 0), '/Contents': [IndirectObject(138, 0)], '/MediaBox': [0, 0, 841, 595], '/CropBox': [0, 0, 841, 595]}, (0, 145): {'/Type': '/Page', '/Parent': IndirectObject(2, 0), '/Resources': IndirectObject(143, 0), '/Contents': [IndirectObject(142, 0)], '/MediaBox': [0, 0, 841, 595], '/CropBox': [0, 0, 841, 595]}, (0, 149): {'/Type': '/Page', '/Parent': IndirectObject(2, 0), '/Resources': IndirectObject(147, 0), '/Contents': [IndirectObject(146, 0)], '/MediaBox': [0, 0, 841, 595], '/CropBox': [0, 0, 841, 595]}, (0, 153): {'/Type': '/Page', '/Parent': IndirectObject(2, 0), '/Resources': IndirectObject(151, 0), '/Contents': [IndirectObject(150, 0)], '/MediaBox': [0, 0, 841, 595], '/CropBox': [0, 0, 841, 595]}, (0, 157): {'/Type': '/Page', '/Parent': IndirectObject(2, 0), '/Resources': IndirectObject(155, 0), '/Contents': [IndirectObject(154, 0)], '/MediaBox': [0, 0, 841, 595], '/CropBox': [0, 0, 841, 595]}, (0, 161): {'/Type': '/Page', '/Parent': IndirectObject(2, 0), '/Resources': IndirectObject(159, 0), '/Contents': [IndirectObject(158, 0)], '/MediaBox': [0, 0, 841, 595], '/CropBox': [0, 0, 841, 595]}, (0, 165): {'/Type': '/Page', '/Parent': IndirectObject(2, 0), '/Resources': IndirectObject(163, 0), '/Contents': [IndirectObject(162, 0)], '/MediaBox': [0, 0, 841, 595], '/CropBox': [0, 0, 841, 595]}, (0, 169): {'/Type': '/Page', '/Parent': IndirectObject(2, 0), '/Resources': IndirectObject(167, 0), '/Contents': [IndirectObject(166, 0)], '/MediaBox': [0, 0, 841, 595], '/CropBox': [0, 0, 841, 595]}, (0, 173): {'/Type': '/Page', '/Parent': IndirectObject(2, 0), '/Resources': IndirectObject(171, 0), '/Contents': [IndirectObject(170, 0)], '/MediaBox': [0, 0, 841, 595], '/CropBox': [0, 0, 841, 595]}, (0, 177): {'/Type': '/Page', '/Parent': IndirectObject(2, 0), '/Resources': IndirectObject(175, 0), '/Contents': [IndirectObject(174, 0)], '/MediaBox': [0, 0, 841, 595], '/CropBox': [0, 0, 841, 595]}, (0, 181): {'/Type': '/Page', '/Parent': IndirectObject(2, 0), '/Resources': IndirectObject(179, 0), '/Contents': [IndirectObject(178, 0)], '/MediaBox': [0, 0, 841, 595], '/CropBox': [0, 0, 841, 595]}, (0, 185): {'/Type': '/Page', '/Parent': IndirectObject(2, 0), '/Resources': IndirectObject(183, 0), '/Contents': [IndirectObject(182, 0)], '/MediaBox': [0, 0, 841, 595], '/CropBox': [0, 0, 841, 595]}, (0, 189): {'/Type': '/Page', '/Parent': IndirectObject(2, 0), '/Resources': IndirectObject(187, 0), '/Contents': [IndirectObject(186, 0)], '/MediaBox': [0, 0, 841, 595], '/CropBox': [0, 0, 841, 595]}, (0, 193): {'/Type': '/Page', '/Parent': IndirectObject(2, 0), '/Resources': IndirectObject(191, 0), '/Contents': [IndirectObject(190, 0)], '/MediaBox': [0, 0, 841, 595], '/CropBox': [0, 0, 841, 595]}, (0, 197): {'/Type': '/Page', '/Parent': IndirectObject(2, 0), '/Resources': IndirectObject(195, 0), '/Contents': [IndirectObject(194, 0)], '/MediaBox': [0, 0, 841, 595], '/CropBox': [0, 0, 841, 595]}, (0, 201): {'/Type': '/Page', '/Parent': IndirectObject(2, 0), '/Resources': IndirectObject(199, 0), '/Contents': [IndirectObject(198, 0)], '/MediaBox': [0, 0, 841, 595], '/CropBox': [0, 0, 841, 595]}, (0, 205): {'/Type': '/Page', '/Parent': IndirectObject(2, 0), '/Resources': IndirectObject(203, 0), '/Contents': [IndirectObject(202, 0)], '/MediaBox': [0, 0, 841, 595], '/CropBox': [0, 0, 841, 595]}, (0, 209): {'/Type': '/Page', '/Parent': IndirectObject(2, 0), '/Resources': IndirectObject(207, 0), '/Contents': [IndirectObject(206, 0)], '/MediaBox': [0, 0, 841, 595], '/CropBox': [0, 0, 841, 595]}, (0, 213): {'/Type': '/Page', '/Parent': IndirectObject(2, 0), '/Resources': IndirectObject(211, 0), '/Contents': [IndirectObject(210, 0)], '/MediaBox': [0, 0, 841, 595], '/CropBox': [0, 0, 841, 595]}, (0, 217): {'/Type': '/Page', '/Parent': IndirectObject(2, 0), '/Resources': IndirectObject(215, 0), '/Contents': [IndirectObject(214, 0)], '/MediaBox': [0, 0, 841, 595], '/CropBox': [0, 0, 841, 595]}, (0, 221): {'/Type': '/Page', '/Parent': IndirectObject(2, 0), '/Resources': IndirectObject(219, 0), '/Contents': [IndirectObject(218, 0)], '/MediaBox': [0, 0, 841, 595], '/CropBox': [0, 0, 841, 595]}, (0, 225): {'/Type': '/Page', '/Parent': IndirectObject(2, 0), '/Resources': IndirectObject(223, 0), '/Contents': [IndirectObject(222, 0)], '/MediaBox': [0, 0, 841, 595], '/CropBox': [0, 0, 841, 595]}, (0, 229): {'/Type': '/Page', '/Parent': IndirectObject(2, 0), '/Resources': IndirectObject(227, 0), '/Contents': [IndirectObject(226, 0)], '/MediaBox': [0, 0, 841, 595], '/CropBox': [0, 0, 841, 595]}, (0, 233): {'/Type': '/Page', '/Parent': IndirectObject(2, 0), '/Resources': IndirectObject(231, 0), '/Contents': [IndirectObject(230, 0)], '/MediaBox': [0, 0, 841, 595], '/CropBox': [0, 0, 841, 595]}, (0, 237): {'/Type': '/Page', '/Parent': IndirectObject(2, 0), '/Resources': IndirectObject(235, 0), '/Contents': [IndirectObject(234, 0)], '/MediaBox': [0, 0, 841, 595], '/CropBox': [0, 0, 841, 595]}, (0, 241): {'/Type': '/Page', '/Parent': IndirectObject(2, 0), '/Resources': IndirectObject(239, 0), '/Contents': [IndirectObject(238, 0)], '/MediaBox': [0, 0, 841, 595], '/CropBox': [0, 0, 841, 595]}, (0, 245): {'/Type': '/Page', '/Parent': IndirectObject(2, 0), '/Resources': IndirectObject(243, 0), '/Contents': [IndirectObject(242, 0)], '/MediaBox': [0, 0, 841, 595], '/CropBox': [0, 0, 841, 595]}, (0, 249): {'/Type': '/Page', '/Parent': IndirectObject(2, 0), '/Resources': IndirectObject(247, 0), '/Contents': [IndirectObject(246, 0)], '/MediaBox': [0, 0, 841, 595], '/CropBox': [0, 0, 841, 595]}, (0, 253): {'/Type': '/Page', '/Parent': IndirectObject(2, 0), '/Resources': IndirectObject(251, 0), '/Contents': [IndirectObject(250, 0)], '/MediaBox': [0, 0, 841, 595], '/CropBox': [0, 0, 841, 595]}, (0, 257): {'/Type': '/Page', '/Parent': IndirectObject(2, 0), '/Resources': IndirectObject(255, 0), '/Contents': [IndirectObject(254, 0)], '/MediaBox': [0, 0, 841, 595], '/CropBox': [0, 0, 841, 595]}, (0, 261): {'/Type': '/Page', '/Parent': IndirectObject(2, 0), '/Resources': IndirectObject(259, 0), '/Contents': [IndirectObject(258, 0)], '/MediaBox': [0, 0, 841, 595], '/CropBox': [0, 0, 841, 595]}, (0, 265): {'/Type': '/Page', '/Parent': IndirectObject(2, 0), '/Resources': IndirectObject(263, 0), '/Contents': [IndirectObject(262, 0)], '/MediaBox': [0, 0, 841, 595], '/CropBox': [0, 0, 841, 595]}, (0, 269): {'/Type': '/Page', '/Parent': IndirectObject(2, 0), '/Resources': IndirectObject(267, 0), '/Contents': [IndirectObject(266, 0)], '/MediaBox': [0, 0, 841, 595], '/CropBox': [0, 0, 841, 595]}, (0, 273): {'/Type': '/Page', '/Parent': IndirectObject(2, 0), '/Resources': IndirectObject(271, 0), '/Contents': [IndirectObject(270, 0)], '/MediaBox': [0, 0, 841, 595], '/CropBox': [0, 0, 841, 595]}, (0, 277): {'/Type': '/Page', '/Parent': IndirectObject(2, 0), '/Resources': IndirectObject(275, 0), '/Contents': [IndirectObject(274, 0)], '/MediaBox': [0, 0, 841, 595], '/CropBox': [0, 0, 841, 595]}, (0, 281): {'/Type': '/Page', '/Parent': IndirectObject(2, 0), '/Resources': IndirectObject(279, 0), '/Contents': [IndirectObject(278, 0)], '/MediaBox': [0, 0, 841, 595], '/CropBox': [0, 0, 841, 595]}, (0, 285): {'/Type': '/Page', '/Parent': IndirectObject(2, 0), '/Resources': IndirectObject(283, 0), '/Contents': [IndirectObject(282, 0)], '/MediaBox': [0, 0, 841, 595], '/CropBox': [0, 0, 841, 595]}, (0, 289): {'/Type': '/Page', '/Parent': IndirectObject(2, 0), '/Resources': IndirectObject(287, 0), '/Contents': [IndirectObject(286, 0)], '/MediaBox': [0, 0, 841, 595], '/CropBox': [0, 0, 841, 595]}, (0, 293): {'/Type': '/Page', '/Parent': IndirectObject(2, 0), '/Resources': IndirectObject(291, 0), '/Contents': [IndirectObject(290, 0)], '/MediaBox': [0, 0, 841, 595], '/CropBox': [0, 0, 841, 595]}, (0, 297): {'/Type': '/Page', '/Parent': IndirectObject(2, 0), '/Resources': IndirectObject(295, 0), '/Contents': [IndirectObject(294, 0)], '/MediaBox': [0, 0, 841, 595], '/CropBox': [0, 0, 841, 595]}, (0, 301): {'/Type': '/Page', '/Parent': IndirectObject(2, 0), '/Resources': IndirectObject(299, 0), '/Contents': [IndirectObject(298, 0)], '/MediaBox': [0, 0, 841, 595], '/CropBox': [0, 0, 841, 595]}, (0, 305): {'/Type': '/Page', '/Parent': IndirectObject(2, 0), '/Resources': IndirectObject(303, 0), '/Contents': [IndirectObject(302, 0)], '/MediaBox': [0, 0, 841, 595], '/CropBox': [0, 0, 841, 595]}, (0, 309): {'/Type': '/Page', '/Parent': IndirectObject(2, 0), '/Resources': IndirectObject(307, 0), '/Contents': [IndirectObject(306, 0)], '/MediaBox': [0, 0, 841, 595], '/CropBox': [0, 0, 841, 595]}, (0, 313): {'/Type': '/Page', '/Parent': IndirectObject(2, 0), '/Resources': IndirectObject(311, 0), '/Contents': [IndirectObject(310, 0)], '/MediaBox': [0, 0, 841, 595], '/CropBox': [0, 0, 841, 595]}, (0, 317): {'/Type': '/Page', '/Parent': IndirectObject(2, 0), '/Resources': IndirectObject(315, 0), '/Contents': [IndirectObject(314, 0)], '/MediaBox': [0, 0, 841, 595], '/CropBox': [0, 0, 841, 595]}, (0, 321): {'/Type': '/Page', '/Parent': IndirectObject(2, 0), '/Resources': IndirectObject(319, 0), '/Contents': [IndirectObject(318, 0)], '/MediaBox': [0, 0, 841, 595], '/CropBox': [0, 0, 841, 595]}, (0, 325): {'/Type': '/Page', '/Parent': IndirectObject(2, 0), '/Resources': IndirectObject(323, 0), '/Contents': [IndirectObject(322, 0)], '/MediaBox': [0, 0, 841, 595], '/CropBox': [0, 0, 841, 595]}, (0, 329): {'/Type': '/Page', '/Parent': IndirectObject(2, 0), '/Resources': IndirectObject(327, 0), '/Contents': [IndirectObject(326, 0)], '/MediaBox': [0, 0, 841, 595], '/CropBox': [0, 0, 841, 595]}, (0, 333): {'/Type': '/Page', '/Parent': IndirectObject(2, 0), '/Resources': IndirectObject(331, 0), '/Contents': [IndirectObject(330, 0)], '/MediaBox': [0, 0, 841, 595], '/CropBox': [0, 0, 841, 595]}, (0, 337): {'/Type': '/Page', '/Parent': IndirectObject(2, 0), '/Resources': IndirectObject(335, 0), '/Contents': [IndirectObject(334, 0)], '/MediaBox': [0, 0, 841, 595], '/CropBox': [0, 0, 841, 595]}, (0, 341): {'/Type': '/Page', '/Parent': IndirectObject(2, 0), '/Resources': IndirectObject(339, 0), '/Contents': [IndirectObject(338, 0)], '/MediaBox': [0, 0, 841, 595], '/CropBox': [0, 0, 841, 595]}, (0, 345): {'/Type': '/Page', '/Parent': IndirectObject(2, 0), '/Resources': IndirectObject(343, 0), '/Contents': [IndirectObject(342, 0)], '/MediaBox': [0, 0, 841, 595], '/CropBox': [0, 0, 841, 595]}, (0, 349): {'/Type': '/Page', '/Parent': IndirectObject(2, 0), '/Resources': IndirectObject(347, 0), '/Contents': [IndirectObject(346, 0)], '/MediaBox': [0, 0, 841, 595], '/CropBox': [0, 0, 841, 595]}, (0, 353): {'/Type': '/Page', '/Parent': IndirectObject(2, 0), '/Resources': IndirectObject(351, 0), '/Contents': [IndirectObject(350, 0)], '/MediaBox': [0, 0, 841, 595], '/CropBox': [0, 0, 841, 595]}, (0, 357): {'/Type': '/Page', '/Parent': IndirectObject(2, 0), '/Resources': IndirectObject(355, 0), '/Contents': [IndirectObject(354, 0)], '/MediaBox': [0, 0, 841, 595], '/CropBox': [0, 0, 841, 595]}, (0, 361): {'/Type': '/Page', '/Parent': IndirectObject(2, 0), '/Resources': IndirectObject(359, 0), '/Contents': [IndirectObject(358, 0)], '/MediaBox': [0, 0, 841, 595], '/CropBox': [0, 0, 841, 595]}, (0, 365): {'/Type': '/Page', '/Parent': IndirectObject(2, 0), '/Resources': IndirectObject(363, 0), '/Contents': [IndirectObject(362, 0)], '/MediaBox': [0, 0, 841, 595], '/CropBox': [0, 0, 841, 595]}, (0, 369): {'/Type': '/Page', '/Parent': IndirectObject(2, 0), '/Resources': IndirectObject(367, 0), '/Contents': [IndirectObject(366, 0)], '/MediaBox': [0, 0, 841, 595], '/CropBox': [0, 0, 841, 595]}, (0, 373): {'/Type': '/Page', '/Parent': IndirectObject(2, 0), '/Resources': IndirectObject(371, 0), '/Contents': [IndirectObject(370, 0)], '/MediaBox': [0, 0, 841, 595], '/CropBox': [0, 0, 841, 595]}, (0, 377): {'/Type': '/Page', '/Parent': IndirectObject(2, 0), '/Resources': IndirectObject(375, 0), '/Contents': [IndirectObject(374, 0)], '/MediaBox': [0, 0, 841, 595], '/CropBox': [0, 0, 841, 595]}, (0, 381): {'/Type': '/Page', '/Parent': IndirectObject(2, 0), '/Resources': IndirectObject(379, 0), '/Contents': [IndirectObject(378, 0)], '/MediaBox': [0, 0, 841, 595], '/CropBox': [0, 0, 841, 595]}, (0, 385): {'/Type': '/Page', '/Parent': IndirectObject(2, 0), '/Resources': IndirectObject(383, 0), '/Contents': [IndirectObject(382, 0)], '/MediaBox': [0, 0, 841, 595], '/CropBox': [0, 0, 841, 595]}, (0, 389): {'/Type': '/Page', '/Parent': IndirectObject(2, 0), '/Resources': IndirectObject(387, 0), '/Contents': [IndirectObject(386, 0)], '/MediaBox': [0, 0, 841, 595], '/CropBox': [0, 0, 841, 595]}, (0, 393): {'/Type': '/Page', '/Parent': IndirectObject(2, 0), '/Resources': IndirectObject(391, 0), '/Contents': [IndirectObject(390, 0)], '/MediaBox': [0, 0, 841, 595], '/CropBox': [0, 0, 841, 595]}, (0, 397): {'/Type': '/Page', '/Parent': IndirectObject(2, 0), '/Resources': IndirectObject(395, 0), '/Contents': [IndirectObject(394, 0)], '/MediaBox': [0, 0, 841, 595], '/CropBox': [0, 0, 841, 595]}, (0, 401): {'/Type': '/Page', '/Parent': IndirectObject(2, 0), '/Resources': IndirectObject(399, 0), '/Contents': [IndirectObject(398, 0)], '/MediaBox': [0, 0, 841, 595], '/CropBox': [0, 0, 841, 595]}, (0, 405): {'/Type': '/Page', '/Parent': IndirectObject(2, 0), '/Resources': IndirectObject(403, 0), '/Contents': [IndirectObject(402, 0)], '/MediaBox': [0, 0, 841, 595], '/CropBox': [0, 0, 841, 595]}, (0, 409): {'/Type': '/Page', '/Parent': IndirectObject(2, 0), '/Resources': IndirectObject(407, 0), '/Contents': [IndirectObject(406, 0)], '/MediaBox': [0, 0, 841, 595], '/CropBox': [0, 0, 841, 595]}, (0, 413): {'/Type': '/Page', '/Parent': IndirectObject(2, 0), '/Resources': IndirectObject(411, 0), '/Contents': [IndirectObject(410, 0)], '/MediaBox': [0, 0, 841, 595], '/CropBox': [0, 0, 841, 595]}, (0, 417): {'/Type': '/Page', '/Parent': IndirectObject(2, 0), '/Resources': IndirectObject(415, 0), '/Contents': [IndirectObject(414, 0)], '/MediaBox': [0, 0, 841, 595], '/CropBox': [0, 0, 841, 595]}, (0, 421): {'/Type': '/Page', '/Parent': IndirectObject(2, 0), '/Resources': IndirectObject(419, 0), '/Contents': [IndirectObject(418, 0)], '/MediaBox': [0, 0, 841, 595], '/CropBox': [0, 0, 841, 595]}, (0, 425): {'/Type': '/Page', '/Parent': IndirectObject(2, 0), '/Resources': IndirectObject(423, 0), '/Contents': [IndirectObject(422, 0)], '/MediaBox': [0, 0, 841, 595], '/CropBox': [0, 0, 841, 595]}, (0, 429): {'/Type': '/Page', '/Parent': IndirectObject(2, 0), '/Resources': IndirectObject(427, 0), '/Contents': [IndirectObject(426, 0)], '/MediaBox': [0, 0, 841, 595], '/CropBox': [0, 0, 841, 595]}, (0, 433): {'/Type': '/Page', '/Parent': IndirectObject(2, 0), '/Resources': IndirectObject(431, 0), '/Contents': [IndirectObject(430, 0)], '/MediaBox': [0, 0, 841, 595], '/CropBox': [0, 0, 841, 595]}, (0, 437): {'/Type': '/Page', '/Parent': IndirectObject(2, 0), '/Resources': IndirectObject(435, 0), '/Contents': [IndirectObject(434, 0)], '/MediaBox': [0, 0, 841, 595], '/CropBox': [0, 0, 841, 595]}, (0, 441): {'/Type': '/Page', '/Parent': IndirectObject(2, 0), '/Resources': IndirectObject(439, 0), '/Contents': [IndirectObject(438, 0)], '/MediaBox': [0, 0, 841, 595], '/CropBox': [0, 0, 841, 595]}, (0, 445): {'/Type': '/Page', '/Parent': IndirectObject(2, 0), '/Resources': IndirectObject(443, 0), '/Contents': [IndirectObject(442, 0)], '/MediaBox': [0, 0, 841, 595], '/CropBox': [0, 0, 841, 595]}, (0, 449): {'/Type': '/Page', '/Parent': IndirectObject(2, 0), '/Resources': IndirectObject(447, 0), '/Contents': [IndirectObject(446, 0)], '/MediaBox': [0, 0, 841, 595], '/CropBox': [0, 0, 841, 595]}, (0, 453): {'/Type': '/Page', '/Parent': IndirectObject(2, 0), '/Resources': IndirectObject(451, 0), '/Contents': [IndirectObject(450, 0)], '/MediaBox': [0, 0, 841, 595], '/CropBox': [0, 0, 841, 595]}, (0, 457): {'/Type': '/Page', '/Parent': IndirectObject(2, 0), '/Resources': IndirectObject(455, 0), '/Contents': [IndirectObject(454, 0)], '/MediaBox': [0, 0, 841, 595], '/CropBox': [0, 0, 841, 595]}, (0, 461): {'/Type': '/Page', '/Parent': IndirectObject(2, 0), '/Resources': IndirectObject(459, 0), '/Contents': [IndirectObject(458, 0)], '/MediaBox': [0, 0, 841, 595], '/CropBox': [0, 0, 841, 595]}, (0, 465): {'/Type': '/Page', '/Parent': IndirectObject(2, 0), '/Resources': IndirectObject(463, 0), '/Contents': [IndirectObject(462, 0)], '/MediaBox': [0, 0, 841, 595], '/CropBox': [0, 0, 841, 595]}, (0, 469): {'/Type': '/Page', '/Parent': IndirectObject(2, 0), '/Resources': IndirectObject(467, 0), '/Contents': [IndirectObject(466, 0)], '/MediaBox': [0, 0, 841, 595], '/CropBox': [0, 0, 841, 595]}, (0, 473): {'/Type': '/Page', '/Parent': IndirectObject(2, 0), '/Resources': IndirectObject(471, 0), '/Contents': [IndirectObject(470, 0)], '/MediaBox': [0, 0, 841, 595], '/CropBox': [0, 0, 841, 595]}, (0, 477): {'/Type': '/Page', '/Parent': IndirectObject(2, 0), '/Resources': IndirectObject(475, 0), '/Contents': [IndirectObject(474, 0)], '/MediaBox': [0, 0, 841, 595], '/CropBox': [0, 0, 841, 595]}, (0, 481): {'/Type': '/Page', '/Parent': IndirectObject(2, 0), '/Resources': IndirectObject(479, 0), '/Contents': [IndirectObject(478, 0)], '/MediaBox': [0, 0, 841, 595], '/CropBox': [0, 0, 841, 595]}, (0, 485): {'/Type': '/Page', '/Parent': IndirectObject(2, 0), '/Resources': IndirectObject(483, 0), '/Contents': [IndirectObject(482, 0)], '/MediaBox': [0, 0, 841, 595], '/CropBox': [0, 0, 841, 595]}, (0, 489): {'/Type': '/Page', '/Parent': IndirectObject(2, 0), '/Resources': IndirectObject(487, 0), '/Contents': [IndirectObject(486, 0)], '/MediaBox': [0, 0, 841, 595], '/CropBox': [0, 0, 841, 595]}, (0, 493): {'/Type': '/Page', '/Parent': IndirectObject(2, 0), '/Resources': IndirectObject(491, 0), '/Contents': [IndirectObject(490, 0)], '/MediaBox': [0, 0, 841, 595], '/CropBox': [0, 0, 841, 595]}, (0, 497): {'/Type': '/Page', '/Parent': IndirectObject(2, 0), '/Resources': IndirectObject(495, 0), '/Contents': [IndirectObject(494, 0)], '/MediaBox': [0, 0, 841, 595], '/CropBox': [0, 0, 841, 595]}, (0, 501): {'/Type': '/Page', '/Parent': IndirectObject(2, 0), '/Resources': IndirectObject(499, 0), '/Contents': [IndirectObject(498, 0)], '/MediaBox': [0, 0, 841, 595], '/CropBox': [0, 0, 841, 595]}, (0, 505): {'/Type': '/Page', '/Parent': IndirectObject(2, 0), '/Resources': IndirectObject(503, 0), '/Contents': [IndirectObject(502, 0)], '/MediaBox': [0, 0, 841, 595], '/CropBox': [0, 0, 841, 595]}, (0, 509): {'/Type': '/Page', '/Parent': IndirectObject(2, 0), '/Resources': IndirectObject(507, 0), '/Contents': [IndirectObject(506, 0)], '/MediaBox': [0, 0, 841, 595], '/CropBox': [0, 0, 841, 595]}, (0, 513): {'/Type': '/Page', '/Parent': IndirectObject(2, 0), '/Resources': IndirectObject(511, 0), '/Contents': [IndirectObject(510, 0)], '/MediaBox': [0, 0, 841, 595], '/CropBox': [0, 0, 841, 595]}, (0, 517): {'/Type': '/Page', '/Parent': IndirectObject(2, 0), '/Resources': IndirectObject(515, 0), '/Contents': [IndirectObject(514, 0)], '/MediaBox': [0, 0, 841, 595], '/CropBox': [0, 0, 841, 595]}, (0, 521): {'/Type': '/Page', '/Parent': IndirectObject(2, 0), '/Resources': IndirectObject(519, 0), '/Contents': [IndirectObject(518, 0)], '/MediaBox': [0, 0, 841, 595], '/CropBox': [0, 0, 841, 595]}, (0, 525): {'/Type': '/Page', '/Parent': IndirectObject(2, 0), '/Resources': IndirectObject(523, 0), '/Contents': [IndirectObject(522, 0)], '/MediaBox': [0, 0, 841, 595], '/CropBox': [0, 0, 841, 595]}, (0, 529): {'/Type': '/Page', '/Parent': IndirectObject(2, 0), '/Resources': IndirectObject(527, 0), '/Contents': [IndirectObject(526, 0)], '/MediaBox': [0, 0, 841, 595], '/CropBox': [0, 0, 841, 595]}, (0, 533): {'/Type': '/Page', '/Parent': IndirectObject(2, 0), '/Resources': IndirectObject(531, 0), '/Contents': [IndirectObject(530, 0)], '/MediaBox': [0, 0, 841, 595], '/CropBox': [0, 0, 841, 595]}, (0, 537): {'/Type': '/Page', '/Parent': IndirectObject(2, 0), '/Resources': IndirectObject(535, 0), '/Contents': [IndirectObject(534, 0)], '/MediaBox': [0, 0, 841, 595], '/CropBox': [0, 0, 841, 595]}, (0, 541): {'/Type': '/Page', '/Parent': IndirectObject(2, 0), '/Resources': IndirectObject(539, 0), '/Contents': [IndirectObject(538, 0)], '/MediaBox': [0, 0, 841, 595], '/CropBox': [0, 0, 841, 595]}, (0, 545): {'/Type': '/Page', '/Parent': IndirectObject(2, 0), '/Resources': IndirectObject(543, 0), '/Contents': [IndirectObject(542, 0)], '/MediaBox': [0, 0, 841, 595], '/CropBox': [0, 0, 841, 595]}, (0, 549): {'/Type': '/Page', '/Parent': IndirectObject(2, 0), '/Resources': IndirectObject(547, 0), '/Contents': [IndirectObject(546, 0)], '/MediaBox': [0, 0, 841, 595], '/CropBox': [0, 0, 841, 595]}, (0, 553): {'/Type': '/Page', '/Parent': IndirectObject(2, 0), '/Resources': IndirectObject(551, 0), '/Contents': [IndirectObject(550, 0)], '/MediaBox': [0, 0, 841, 595], '/CropBox': [0, 0, 841, 595]}, (0, 557): {'/Type': '/Page', '/Parent': IndirectObject(2, 0), '/Resources': IndirectObject(555, 0), '/Contents': [IndirectObject(554, 0)], '/MediaBox': [0, 0, 841, 595], '/CropBox': [0, 0, 841, 595]}, (0, 561): {'/Type': '/Page', '/Parent': IndirectObject(2, 0), '/Resources': IndirectObject(559, 0), '/Contents': [IndirectObject(558, 0)], '/MediaBox': [0, 0, 841, 595], '/CropBox': [0, 0, 841, 595]}, (0, 565): {'/Type': '/Page', '/Parent': IndirectObject(2, 0), '/Resources': IndirectObject(563, 0), '/Contents': [IndirectObject(562, 0)], '/MediaBox': [0, 0, 841, 595], '/CropBox': [0, 0, 841, 595]}, (0, 569): {'/Type': '/Page', '/Parent': IndirectObject(2, 0), '/Resources': IndirectObject(567, 0), '/Contents': [IndirectObject(566, 0)], '/MediaBox': [0, 0, 841, 595], '/CropBox': [0, 0, 841, 595]}, (0, 573): {'/Type': '/Page', '/Parent': IndirectObject(2, 0), '/Resources': IndirectObject(571, 0), '/Contents': [IndirectObject(570, 0)], '/MediaBox': [0, 0, 841, 595], '/CropBox': [0, 0, 841, 595]}, (0, 577): {'/Type': '/Page', '/Parent': IndirectObject(2, 0), '/Resources': IndirectObject(575, 0), '/Contents': [IndirectObject(574, 0)], '/MediaBox': [0, 0, 841, 595], '/CropBox': [0, 0, 841, 595]}, (0, 581): {'/Type': '/Page', '/Parent': IndirectObject(2, 0), '/Resources': IndirectObject(579, 0), '/Contents': [IndirectObject(578, 0)], '/MediaBox': [0, 0, 841, 595], '/CropBox': [0, 0, 841, 595]}, (0, 585): {'/Type': '/Page', '/Parent': IndirectObject(2, 0), '/Resources': IndirectObject(583, 0), '/Contents': [IndirectObject(582, 0)], '/MediaBox': [0, 0, 841, 595], '/CropBox': [0, 0, 841, 595]}, (0, 589): {'/Type': '/Page', '/Parent': IndirectObject(2, 0), '/Resources': IndirectObject(587, 0), '/Contents': [IndirectObject(586, 0)], '/MediaBox': [0, 0, 841, 595], '/CropBox': [0, 0, 841, 595]}, (0, 593): {'/Type': '/Page', '/Parent': IndirectObject(2, 0), '/Resources': IndirectObject(591, 0), '/Contents': [IndirectObject(590, 0)], '/MediaBox': [0, 0, 841, 595], '/CropBox': [0, 0, 841, 595]}, (0, 597): {'/Type': '/Page', '/Parent': IndirectObject(2, 0), '/Resources': IndirectObject(595, 0), '/Contents': [IndirectObject(594, 0)], '/MediaBox': [0, 0, 841, 595], '/CropBox': [0, 0, 841, 595]}, (0, 601): {'/Type': '/Page', '/Parent': IndirectObject(2, 0), '/Resources': IndirectObject(599, 0), '/Contents': [IndirectObject(598, 0)], '/MediaBox': [0, 0, 841, 595], '/CropBox': [0, 0, 841, 595]}, (0, 605): {'/Type': '/Page', '/Parent': IndirectObject(2, 0), '/Resources': IndirectObject(603, 0), '/Contents': [IndirectObject(602, 0)], '/MediaBox': [0, 0, 841, 595], '/CropBox': [0, 0, 841, 595]}, (0, 609): {'/Type': '/Page', '/Parent': IndirectObject(2, 0), '/Resources': IndirectObject(607, 0), '/Contents': [IndirectObject(606, 0)], '/MediaBox': [0, 0, 841, 595], '/CropBox': [0, 0, 841, 595]}, (0, 613): {'/Type': '/Page', '/Parent': IndirectObject(2, 0), '/Resources': IndirectObject(611, 0), '/Contents': [IndirectObject(610, 0)], '/MediaBox': [0, 0, 841, 595], '/CropBox': [0, 0, 841, 595]}, (0, 617): {'/Type': '/Page', '/Parent': IndirectObject(2, 0), '/Resources': IndirectObject(615, 0), '/Contents': [IndirectObject(614, 0)], '/MediaBox': [0, 0, 841, 595], '/CropBox': [0, 0, 841, 595]}, (0, 621): {'/Type': '/Page', '/Parent': IndirectObject(2, 0), '/Resources': IndirectObject(619, 0), '/Contents': [IndirectObject(618, 0)], '/MediaBox': [0, 0, 841, 595], '/CropBox': [0, 0, 841, 595]}, (0, 625): {'/Type': '/Page', '/Parent': IndirectObject(2, 0), '/Resources': IndirectObject(623, 0), '/Contents': [IndirectObject(622, 0)], '/MediaBox': [0, 0, 841, 595], '/CropBox': [0, 0, 841, 595]}, (0, 629): {'/Type': '/Page', '/Parent': IndirectObject(2, 0), '/Resources': IndirectObject(627, 0), '/Contents': [IndirectObject(626, 0)], '/MediaBox': [0, 0, 841, 595], '/CropBox': [0, 0, 841, 595]}, (0, 633): {'/Type': '/Page', '/Parent': IndirectObject(2, 0), '/Resources': IndirectObject(631, 0), '/Contents': [IndirectObject(630, 0)], '/MediaBox': [0, 0, 841, 595], '/CropBox': [0, 0, 841, 595]}, (0, 637): {'/Type': '/Page', '/Parent': IndirectObject(2, 0), '/Resources': IndirectObject(635, 0), '/Contents': [IndirectObject(634, 0)], '/MediaBox': [0, 0, 841, 595], '/CropBox': [0, 0, 841, 595]}, (0, 641): {'/Type': '/Page', '/Parent': IndirectObject(2, 0), '/Resources': IndirectObject(639, 0), '/Contents': [IndirectObject(638, 0)], '/MediaBox': [0, 0, 841, 595], '/CropBox': [0, 0, 841, 595]}, (0, 645): {'/Type': '/Page', '/Parent': IndirectObject(2, 0), '/Resources': IndirectObject(643, 0), '/Contents': [IndirectObject(642, 0)], '/MediaBox': [0, 0, 841, 595], '/CropBox': [0, 0, 841, 595]}, (0, 649): {'/Type': '/Page', '/Parent': IndirectObject(2, 0), '/Resources': IndirectObject(647, 0), '/Contents': [IndirectObject(646, 0)], '/MediaBox': [0, 0, 841, 595], '/CropBox': [0, 0, 841, 595]}, (0, 653): {'/Type': '/Page', '/Parent': IndirectObject(2, 0), '/Resources': IndirectObject(651, 0), '/Contents': [IndirectObject(650, 0)], '/MediaBox': [0, 0, 841, 595], '/CropBox': [0, 0, 841, 595]}, (0, 657): {'/Type': '/Page', '/Parent': IndirectObject(2, 0), '/Resources': IndirectObject(655, 0), '/Contents': [IndirectObject(654, 0)], '/MediaBox': [0, 0, 841, 595], '/CropBox': [0, 0, 841, 595]}, (0, 661): {'/Type': '/Page', '/Parent': IndirectObject(2, 0), '/Resources': IndirectObject(659, 0), '/Contents': [IndirectObject(658, 0)], '/MediaBox': [0, 0, 841, 595], '/CropBox': [0, 0, 841, 595]}, (0, 665): {'/Type': '/Page', '/Parent': IndirectObject(2, 0), '/Resources': IndirectObject(663, 0), '/Contents': [IndirectObject(662, 0)], '/MediaBox': [0, 0, 841, 595], '/CropBox': [0, 0, 841, 595]}, (0, 669): {'/Type': '/Page', '/Parent': IndirectObject(2, 0), '/Resources': IndirectObject(667, 0), '/Contents': [IndirectObject(666, 0)], '/MediaBox': [0, 0, 841, 595], '/CropBox': [0, 0, 841, 595]}, (0, 673): {'/Type': '/Page', '/Parent': IndirectObject(2, 0), '/Resources': IndirectObject(671, 0), '/Contents': [IndirectObject(670, 0)], '/MediaBox': [0, 0, 841, 595], '/CropBox': [0, 0, 841, 595]}, (0, 677): {'/Type': '/Page', '/Parent': IndirectObject(2, 0), '/Resources': IndirectObject(675, 0), '/Contents': [IndirectObject(674, 0)], '/MediaBox': [0, 0, 841, 595], '/CropBox': [0, 0, 841, 595]}, (0, 681): {'/Type': '/Page', '/Parent': IndirectObject(2, 0), '/Resources': IndirectObject(679, 0), '/Contents': [IndirectObject(678, 0)], '/MediaBox': [0, 0, 841, 595], '/CropBox': [0, 0, 841, 595]}, (0, 685): {'/Type': '/Page', '/Parent': IndirectObject(2, 0), '/Resources': IndirectObject(683, 0), '/Contents': [IndirectObject(682, 0)], '/MediaBox': [0, 0, 841, 595], '/CropBox': [0, 0, 841, 595]}, (0, 689): {'/Type': '/Page', '/Parent': IndirectObject(2, 0), '/Resources': IndirectObject(687, 0), '/Contents': [IndirectObject(686, 0)], '/MediaBox': [0, 0, 841, 595], '/CropBox': [0, 0, 841, 595]}, (0, 693): {'/Type': '/Page', '/Parent': IndirectObject(2, 0), '/Resources': IndirectObject(691, 0), '/Contents': [IndirectObject(690, 0)], '/MediaBox': [0, 0, 841, 595], '/CropBox': [0, 0, 841, 595]}, (0, 697): {'/Type': '/Page', '/Parent': IndirectObject(2, 0), '/Resources': IndirectObject(695, 0), '/Contents': [IndirectObject(694, 0)], '/MediaBox': [0, 0, 841, 595], '/CropBox': [0, 0, 841, 595]}, (0, 701): {'/Type': '/Page', '/Parent': IndirectObject(2, 0), '/Resources': IndirectObject(699, 0), '/Contents': [IndirectObject(698, 0)], '/MediaBox': [0, 0, 841, 595], '/CropBox': [0, 0, 841, 595]}, (0, 705): {'/Type': '/Page', '/Parent': IndirectObject(2, 0), '/Resources': IndirectObject(703, 0), '/Contents': [IndirectObject(702, 0)], '/MediaBox': [0, 0, 841, 595], '/CropBox': [0, 0, 841, 595]}, (0, 709): {'/Type': '/Page', '/Parent': IndirectObject(2, 0), '/Resources': IndirectObject(707, 0), '/Contents': [IndirectObject(706, 0)], '/MediaBox': [0, 0, 841, 595], '/CropBox': [0, 0, 841, 595]}, (0, 713): {'/Type': '/Page', '/Parent': IndirectObject(2, 0), '/Resources': IndirectObject(711, 0), '/Contents': [IndirectObject(710, 0)], '/MediaBox': [0, 0, 841, 595], '/CropBox': [0, 0, 841, 595]}, (0, 717): {'/Type': '/Page', '/Parent': IndirectObject(2, 0), '/Resources': IndirectObject(715, 0), '/Contents': [IndirectObject(714, 0)], '/MediaBox': [0, 0, 841, 595], '/CropBox': [0, 0, 841, 595]}, (0, 721): {'/Type': '/Page', '/Parent': IndirectObject(2, 0), '/Resources': IndirectObject(719, 0), '/Contents': [IndirectObject(718, 0)], '/MediaBox': [0, 0, 841, 595], '/CropBox': [0, 0, 841, 595]}, (0, 725): {'/Type': '/Page', '/Parent': IndirectObject(2, 0), '/Resources': IndirectObject(723, 0), '/Contents': [IndirectObject(722, 0)], '/MediaBox': [0, 0, 841, 595], '/CropBox': [0, 0, 841, 595]}, (0, 729): {'/Type': '/Page', '/Parent': IndirectObject(2, 0), '/Resources': IndirectObject(727, 0), '/Contents': [IndirectObject(726, 0)], '/MediaBox': [0, 0, 841, 595], '/CropBox': [0, 0, 841, 595]}, (0, 733): {'/Type': '/Page', '/Parent': IndirectObject(2, 0), '/Resources': IndirectObject(731, 0), '/Contents': [IndirectObject(730, 0)], '/MediaBox': [0, 0, 841, 595], '/CropBox': [0, 0, 841, 595]}, (0, 737): {'/Type': '/Page', '/Parent': IndirectObject(2, 0), '/Resources': IndirectObject(735, 0), '/Contents': [IndirectObject(734, 0)], '/MediaBox': [0, 0, 841, 595], '/CropBox': [0, 0, 841, 595]}, (0, 741): {'/Type': '/Page', '/Parent': IndirectObject(2, 0), '/Resources': IndirectObject(739, 0), '/Contents': [IndirectObject(738, 0)], '/MediaBox': [0, 0, 841, 595], '/CropBox': [0, 0, 841, 595]}, (0, 745): {'/Type': '/Page', '/Parent': IndirectObject(2, 0), '/Resources': IndirectObject(743, 0), '/Contents': [IndirectObject(742, 0)], '/MediaBox': [0, 0, 841, 595], '/CropBox': [0, 0, 841, 595]}, (0, 749): {'/Type': '/Page', '/Parent': IndirectObject(2, 0), '/Resources': IndirectObject(747, 0), '/Contents': [IndirectObject(746, 0)], '/MediaBox': [0, 0, 841, 595], '/CropBox': [0, 0, 841, 595]}, (0, 753): {'/Type': '/Page', '/Parent': IndirectObject(2, 0), '/Resources': IndirectObject(751, 0), '/Contents': [IndirectObject(750, 0)], '/MediaBox': [0, 0, 841, 595], '/CropBox': [0, 0, 841, 595]}, (0, 757): {'/Type': '/Page', '/Parent': IndirectObject(2, 0), '/Resources': IndirectObject(755, 0), '/Contents': [IndirectObject(754, 0)], '/MediaBox': [0, 0, 841, 595], '/CropBox': [0, 0, 841, 595]}, (0, 761): {'/Type': '/Page', '/Parent': IndirectObject(2, 0), '/Resources': IndirectObject(759, 0), '/Contents': [IndirectObject(758, 0)], '/MediaBox': [0, 0, 841, 595], '/CropBox': [0, 0, 841, 595]}, (0, 765): {'/Type': '/Page', '/Parent': IndirectObject(2, 0), '/Resources': IndirectObject(763, 0), '/Contents': [IndirectObject(762, 0)], '/MediaBox': [0, 0, 841, 595], '/CropBox': [0, 0, 841, 595]}, (0, 769): {'/Type': '/Page', '/Parent': IndirectObject(2, 0), '/Resources': IndirectObject(767, 0), '/Contents': [IndirectObject(766, 0)], '/MediaBox': [0, 0, 841, 595], '/CropBox': [0, 0, 841, 595]}, (0, 773): {'/Type': '/Page', '/Parent': IndirectObject(2, 0), '/Resources': IndirectObject(771, 0), '/Contents': [IndirectObject(770, 0)], '/MediaBox': [0, 0, 841, 595], '/CropBox': [0, 0, 841, 595]}, (0, 777): {'/Type': '/Page', '/Parent': IndirectObject(2, 0), '/Resources': IndirectObject(775, 0), '/Contents': [IndirectObject(774, 0)], '/MediaBox': [0, 0, 841, 595], '/CropBox': [0, 0, 841, 595]}, (0, 781): {'/Type': '/Page', '/Parent': IndirectObject(2, 0), '/Resources': IndirectObject(779, 0), '/Contents': [IndirectObject(778, 0)], '/MediaBox': [0, 0, 841, 595], '/CropBox': [0, 0, 841, 595]}, (0, 785): {'/Type': '/Page', '/Parent': IndirectObject(2, 0), '/Resources': IndirectObject(783, 0), '/Contents': [IndirectObject(782, 0)], '/MediaBox': [0, 0, 841, 595], '/CropBox': [0, 0, 841, 595]}, (0, 789): {'/Type': '/Page', '/Parent': IndirectObject(2, 0), '/Resources': IndirectObject(787, 0), '/Contents': [IndirectObject(786, 0)], '/MediaBox': [0, 0, 841, 595], '/CropBox': [0, 0, 841, 595]}, (0, 793): {'/Type': '/Page', '/Parent': IndirectObject(2, 0), '/Resources': IndirectObject(791, 0), '/Contents': [IndirectObject(790, 0)], '/MediaBox': [0, 0, 841, 595], '/CropBox': [0, 0, 841, 595]}, (0, 797): {'/Type': '/Page', '/Parent': IndirectObject(2, 0), '/Resources': IndirectObject(795, 0), '/Contents': [IndirectObject(794, 0)], '/MediaBox': [0, 0, 841, 595], '/CropBox': [0, 0, 841, 595]}, (0, 801): {'/Type': '/Page', '/Parent': IndirectObject(2, 0), '/Resources': IndirectObject(799, 0), '/Contents': [IndirectObject(798, 0)], '/MediaBox': [0, 0, 841, 595], '/CropBox': [0, 0, 841, 595]}, (0, 805): {'/Type': '/Page', '/Parent': IndirectObject(2, 0), '/Resources': IndirectObject(803, 0), '/Contents': [IndirectObject(802, 0)], '/MediaBox': [0, 0, 841, 595], '/CropBox': [0, 0, 841, 595]}, (0, 809): {'/Type': '/Page', '/Parent': IndirectObject(2, 0), '/Resources': IndirectObject(807, 0), '/Contents': [IndirectObject(806, 0)], '/MediaBox': [0, 0, 841, 595], '/CropBox': [0, 0, 841, 595]}, (0, 813): {'/Type': '/Page', '/Parent': IndirectObject(2, 0), '/Resources': IndirectObject(811, 0), '/Contents': [IndirectObject(810, 0)], '/MediaBox': [0, 0, 841, 595], '/CropBox': [0, 0, 841, 595]}, (0, 817): {'/Type': '/Page', '/Parent': IndirectObject(2, 0), '/Resources': IndirectObject(815, 0), '/Contents': [IndirectObject(814, 0)], '/MediaBox': [0, 0, 841, 595], '/CropBox': [0, 0, 841, 595]}, (0, 821): {'/Type': '/Page', '/Parent': IndirectObject(2, 0), '/Resources': IndirectObject(819, 0), '/Contents': [IndirectObject(818, 0)], '/MediaBox': [0, 0, 841, 595], '/CropBox': [0, 0, 841, 595]}, (0, 825): {'/Type': '/Page', '/Parent': IndirectObject(2, 0), '/Resources': IndirectObject(823, 0), '/Contents': [IndirectObject(822, 0)], '/MediaBox': [0, 0, 841, 595], '/CropBox': [0, 0, 841, 595]}, (0, 829): {'/Type': '/Page', '/Parent': IndirectObject(2, 0), '/Resources': IndirectObject(827, 0), '/Contents': [IndirectObject(826, 0)], '/MediaBox': [0, 0, 841, 595], '/CropBox': [0, 0, 841, 595]}, (0, 833): {'/Type': '/Page', '/Parent': IndirectObject(2, 0), '/Resources': IndirectObject(831, 0), '/Contents': [IndirectObject(830, 0)], '/MediaBox': [0, 0, 841, 595], '/CropBox': [0, 0, 841, 595]}, (0, 837): {'/Type': '/Page', '/Parent': IndirectObject(2, 0), '/Resources': IndirectObject(835, 0), '/Contents': [IndirectObject(834, 0)], '/MediaBox': [0, 0, 841, 595], '/CropBox': [0, 0, 841, 595]}, (0, 841): {'/Type': '/Page', '/Parent': IndirectObject(2, 0), '/Resources': IndirectObject(839, 0), '/Contents': [IndirectObject(838, 0)], '/MediaBox': [0, 0, 841, 595], '/CropBox': [0, 0, 841, 595]}, (0, 845): {'/Type': '/Page', '/Parent': IndirectObject(2, 0), '/Resources': IndirectObject(843, 0), '/Contents': [IndirectObject(842, 0)], '/MediaBox': [0, 0, 841, 595], '/CropBox': [0, 0, 841, 595]}, (0, 849): {'/Type': '/Page', '/Parent': IndirectObject(2, 0), '/Resources': IndirectObject(847, 0), '/Contents': [IndirectObject(846, 0)], '/MediaBox': [0, 0, 841, 595], '/CropBox': [0, 0, 841, 595]}, (0, 853): {'/Type': '/Page', '/Parent': IndirectObject(2, 0), '/Resources': IndirectObject(851, 0), '/Contents': [IndirectObject(850, 0)], '/MediaBox': [0, 0, 841, 595], '/CropBox': [0, 0, 841, 595]}, (0, 857): {'/Type': '/Page', '/Parent': IndirectObject(2, 0), '/Resources': IndirectObject(855, 0), '/Contents': [IndirectObject(854, 0)], '/MediaBox': [0, 0, 841, 595], '/CropBox': [0, 0, 841, 595]}, (0, 861): {'/Type': '/Page', '/Parent': IndirectObject(2, 0), '/Resources': IndirectObject(859, 0), '/Contents': [IndirectObject(858, 0)], '/MediaBox': [0, 0, 841, 595], '/CropBox': [0, 0, 841, 595]}, (0, 865): {'/Type': '/Page', '/Parent': IndirectObject(2, 0), '/Resources': IndirectObject(863, 0), '/Contents': [IndirectObject(862, 0)], '/MediaBox': [0, 0, 841, 595], '/CropBox': [0, 0, 841, 595]}, (0, 869): {'/Type': '/Page', '/Parent': IndirectObject(2, 0), '/Resources': IndirectObject(867, 0), '/Contents': [IndirectObject(866, 0)], '/MediaBox': [0, 0, 841, 595], '/CropBox': [0, 0, 841, 595]}, (0, 873): {'/Type': '/Page', '/Parent': IndirectObject(2, 0), '/Resources': IndirectObject(871, 0), '/Contents': [IndirectObject(870, 0)], '/MediaBox': [0, 0, 841, 595], '/CropBox': [0, 0, 841, 595]}, (0, 877): {'/Type': '/Page', '/Parent': IndirectObject(2, 0), '/Resources': IndirectObject(875, 0), '/Contents': [IndirectObject(874, 0)], '/MediaBox': [0, 0, 841, 595], '/CropBox': [0, 0, 841, 595]}, (0, 881): {'/Type': '/Page', '/Parent': IndirectObject(2, 0), '/Resources': IndirectObject(879, 0), '/Contents': [IndirectObject(878, 0)], '/MediaBox': [0, 0, 841, 595], '/CropBox': [0, 0, 841, 595]}, (0, 885): {'/Type': '/Page', '/Parent': IndirectObject(2, 0), '/Resources': IndirectObject(883, 0), '/Contents': [IndirectObject(882, 0)], '/MediaBox': [0, 0, 841, 595], '/CropBox': [0, 0, 841, 595]}, (0, 889): {'/Type': '/Page', '/Parent': IndirectObject(2, 0), '/Resources': IndirectObject(887, 0), '/Contents': [IndirectObject(886, 0)], '/MediaBox': [0, 0, 841, 595], '/CropBox': [0, 0, 841, 595]}, (0, 893): {'/Type': '/Page', '/Parent': IndirectObject(2, 0), '/Resources': IndirectObject(891, 0), '/Contents': [IndirectObject(890, 0)], '/MediaBox': [0, 0, 841, 595], '/CropBox': [0, 0, 841, 595]}, (0, 897): {'/Type': '/Page', '/Parent': IndirectObject(2, 0), '/Resources': IndirectObject(895, 0), '/Contents': [IndirectObject(894, 0)], '/MediaBox': [0, 0, 841, 595], '/CropBox': [0, 0, 841, 595]}, (0, 901): {'/Type': '/Page', '/Parent': IndirectObject(2, 0), '/Resources': IndirectObject(899, 0), '/Contents': [IndirectObject(898, 0)], '/MediaBox': [0, 0, 841, 595], '/CropBox': [0, 0, 841, 595]}, (0, 905): {'/Type': '/Page', '/Parent': IndirectObject(2, 0), '/Resources': IndirectObject(903, 0), '/Contents': [IndirectObject(902, 0)], '/MediaBox': [0, 0, 841, 595], '/CropBox': [0, 0, 841, 595]}, (0, 909): {'/Type': '/Page', '/Parent': IndirectObject(2, 0), '/Resources': IndirectObject(907, 0), '/Contents': [IndirectObject(906, 0)], '/MediaBox': [0, 0, 841, 595], '/CropBox': [0, 0, 841, 595]}, (0, 913): {'/Type': '/Page', '/Parent': IndirectObject(2, 0), '/Resources': IndirectObject(911, 0), '/Contents': [IndirectObject(910, 0)], '/MediaBox': [0, 0, 841, 595], '/CropBox': [0, 0, 841, 595]}, (0, 917): {'/Type': '/Page', '/Parent': IndirectObject(2, 0), '/Resources': IndirectObject(915, 0), '/Contents': [IndirectObject(914, 0)], '/MediaBox': [0, 0, 841, 595], '/CropBox': [0, 0, 841, 595]}, (0, 921): {'/Type': '/Page', '/Parent': IndirectObject(2, 0), '/Resources': IndirectObject(919, 0), '/Contents': [IndirectObject(918, 0)], '/MediaBox': [0, 0, 841, 595], '/CropBox': [0, 0, 841, 595]}, (0, 925): {'/Type': '/Page', '/Parent': IndirectObject(2, 0), '/Resources': IndirectObject(923, 0), '/Contents': [IndirectObject(922, 0)], '/MediaBox': [0, 0, 841, 595], '/CropBox': [0, 0, 841, 595]}, (0, 929): {'/Type': '/Page', '/Parent': IndirectObject(2, 0), '/Resources': IndirectObject(927, 0), '/Contents': [IndirectObject(926, 0)], '/MediaBox': [0, 0, 841, 595], '/CropBox': [0, 0, 841, 595]}, (0, 933): {'/Type': '/Page', '/Parent': IndirectObject(2, 0), '/Resources': IndirectObject(931, 0), '/Contents': [IndirectObject(930, 0)], '/MediaBox': [0, 0, 841, 595], '/CropBox': [0, 0, 841, 595]}, (0, 937): {'/Type': '/Page', '/Parent': IndirectObject(2, 0), '/Resources': IndirectObject(935, 0), '/Contents': [IndirectObject(934, 0)], '/MediaBox': [0, 0, 841, 595], '/CropBox': [0, 0, 841, 595]}, (0, 941): {'/Type': '/Page', '/Parent': IndirectObject(2, 0), '/Resources': IndirectObject(939, 0), '/Contents': [IndirectObject(938, 0)], '/MediaBox': [0, 0, 841, 595], '/CropBox': [0, 0, 841, 595]}, (0, 945): {'/Type': '/Page', '/Parent': IndirectObject(2, 0), '/Resources': IndirectObject(943, 0), '/Contents': [IndirectObject(942, 0)], '/MediaBox': [0, 0, 841, 595], '/CropBox': [0, 0, 841, 595]}, (0, 949): {'/Type': '/Page', '/Parent': IndirectObject(2, 0), '/Resources': IndirectObject(947, 0), '/Contents': [IndirectObject(946, 0)], '/MediaBox': [0, 0, 841, 595], '/CropBox': [0, 0, 841, 595]}, (0, 953): {'/Type': '/Page', '/Parent': IndirectObject(2, 0), '/Resources': IndirectObject(951, 0), '/Contents': [IndirectObject(950, 0)], '/MediaBox': [0, 0, 841, 595], '/CropBox': [0, 0, 841, 595]}, (0, 957): {'/Type': '/Page', '/Parent': IndirectObject(2, 0), '/Resources': IndirectObject(955, 0), '/Contents': [IndirectObject(954, 0)], '/MediaBox': [0, 0, 841, 595], '/CropBox': [0, 0, 841, 595]}, (0, 961): {'/Type': '/Page', '/Parent': IndirectObject(2, 0), '/Resources': IndirectObject(959, 0), '/Contents': [IndirectObject(958, 0)], '/MediaBox': [0, 0, 841, 595], '/CropBox': [0, 0, 841, 595]}, (0, 965): {'/Type': '/Page', '/Parent': IndirectObject(2, 0), '/Resources': IndirectObject(963, 0), '/Contents': [IndirectObject(962, 0)], '/MediaBox': [0, 0, 841, 595], '/CropBox': [0, 0, 841, 595]}, (0, 969): {'/Type': '/Page', '/Parent': IndirectObject(2, 0), '/Resources': IndirectObject(967, 0), '/Contents': [IndirectObject(966, 0)], '/MediaBox': [0, 0, 841, 595], '/CropBox': [0, 0, 841, 595]}, (0, 973): {'/Type': '/Page', '/Parent': IndirectObject(2, 0), '/Resources': IndirectObject(971, 0), '/Contents': [IndirectObject(970, 0)], '/MediaBox': [0, 0, 841, 595], '/CropBox': [0, 0, 841, 595]}, (0, 977): {'/Type': '/Page', '/Parent': IndirectObject(2, 0), '/Resources': IndirectObject(975, 0), '/Contents': [IndirectObject(974, 0)], '/MediaBox': [0, 0, 841, 595], '/CropBox': [0, 0, 841, 595]}, (0, 981): {'/Type': '/Page', '/Parent': IndirectObject(2, 0), '/Resources': IndirectObject(979, 0), '/Contents': [IndirectObject(978, 0)], '/MediaBox': [0, 0, 841, 595], '/CropBox': [0, 0, 841, 595]}, (0, 985): {'/Type': '/Page', '/Parent': IndirectObject(2, 0), '/Resources': IndirectObject(983, 0), '/Contents': [IndirectObject(982, 0)], '/MediaBox': [0, 0, 841, 595], '/CropBox': [0, 0, 841, 595]}, (0, 989): {'/Type': '/Page', '/Parent': IndirectObject(2, 0), '/Resources': IndirectObject(987, 0), '/Contents': [IndirectObject(986, 0)], '/MediaBox': [0, 0, 841, 595], '/CropBox': [0, 0, 841, 595]}, (0, 993): {'/Type': '/Page', '/Parent': IndirectObject(2, 0), '/Resources': IndirectObject(991, 0), '/Contents': [IndirectObject(990, 0)], '/MediaBox': [0, 0, 841, 595], '/CropBox': [0, 0, 841, 595]}, (0, 997): {'/Type': '/Page', '/Parent': IndirectObject(2, 0), '/Resources': IndirectObject(995, 0), '/Contents': [IndirectObject(994, 0)], '/MediaBox': [0, 0, 841, 595], '/CropBox': [0, 0, 841, 595]}, (0, 1001): {'/Type': '/Page', '/Parent': IndirectObject(2, 0), '/Resources': IndirectObject(999, 0), '/Contents': [IndirectObject(998, 0)], '/MediaBox': [0, 0, 841, 595], '/CropBox': [0, 0, 841, 595]}, (0, 1005): {'/Type': '/Page', '/Parent': IndirectObject(2, 0), '/Resources': IndirectObject(1003, 0), '/Contents': [IndirectObject(1002, 0)], '/MediaBox': [0, 0, 841, 595], '/CropBox': [0, 0, 841, 595]}, (0, 1009): {'/Type': '/Page', '/Parent': IndirectObject(2, 0), '/Resources': IndirectObject(1007, 0), '/Contents': [IndirectObject(1006, 0)], '/MediaBox': [0, 0, 841, 595], '/CropBox': [0, 0, 841, 595]}, (0, 1013): {'/Type': '/Page', '/Parent': IndirectObject(2, 0), '/Resources': IndirectObject(1011, 0), '/Contents': [IndirectObject(1010, 0)], '/MediaBox': [0, 0, 841, 595], '/CropBox': [0, 0, 841, 595]}, (0, 1017): {'/Type': '/Page', '/Parent': IndirectObject(2, 0), '/Resources': IndirectObject(1015, 0), '/Contents': [IndirectObject(1014, 0)], '/MediaBox': [0, 0, 841, 595], '/CropBox': [0, 0, 841, 595]}, (0, 1021): {'/Type': '/Page', '/Parent': IndirectObject(2, 0), '/Resources': IndirectObject(1019, 0), '/Contents': [IndirectObject(1018, 0)], '/MediaBox': [0, 0, 841, 595], '/CropBox': [0, 0, 841, 595]}, (0, 1025): {'/Type': '/Page', '/Parent': IndirectObject(2, 0), '/Resources': IndirectObject(1023, 0), '/Contents': [IndirectObject(1022, 0)], '/MediaBox': [0, 0, 841, 595], '/CropBox': [0, 0, 841, 595]}, (0, 1029): {'/Type': '/Page', '/Parent': IndirectObject(2, 0), '/Resources': IndirectObject(1027, 0), '/Contents': [IndirectObject(1026, 0)], '/MediaBox': [0, 0, 841, 595], '/CropBox': [0, 0, 841, 595]}, (0, 1033): {'/Type': '/Page', '/Parent': IndirectObject(2, 0), '/Resources': IndirectObject(1031, 0), '/Contents': [IndirectObject(1030, 0)], '/MediaBox': [0, 0, 841, 595], '/CropBox': [0, 0, 841, 595]}, (0, 1037): {'/Type': '/Page', '/Parent': IndirectObject(2, 0), '/Resources': IndirectObject(1035, 0), '/Contents': [IndirectObject(1034, 0)], '/MediaBox': [0, 0, 841, 595], '/CropBox': [0, 0, 841, 595]}, (0, 1041): {'/Type': '/Page', '/Parent': IndirectObject(2, 0), '/Resources': IndirectObject(1039, 0), '/Contents': [IndirectObject(1038, 0)], '/MediaBox': [0, 0, 841, 595], '/CropBox': [0, 0, 841, 595]}, (0, 1045): {'/Type': '/Page', '/Parent': IndirectObject(2, 0), '/Resources': IndirectObject(1043, 0), '/Contents': [IndirectObject(1042, 0)], '/MediaBox': [0, 0, 841, 595], '/CropBox': [0, 0, 841, 595]}, (0, 1049): {'/Type': '/Page', '/Parent': IndirectObject(2, 0), '/Resources': IndirectObject(1047, 0), '/Contents': [IndirectObject(1046, 0)], '/MediaBox': [0, 0, 841, 595], '/CropBox': [0, 0, 841, 595]}, (0, 1053): {'/Type': '/Page', '/Parent': IndirectObject(2, 0), '/Resources': IndirectObject(1051, 0), '/Contents': [IndirectObject(1050, 0)], '/MediaBox': [0, 0, 841, 595], '/CropBox': [0, 0, 841, 595]}, (0, 1057): {'/Type': '/Page', '/Parent': IndirectObject(2, 0), '/Resources': IndirectObject(1055, 0), '/Contents': [IndirectObject(1054, 0)], '/MediaBox': [0, 0, 841, 595], '/CropBox': [0, 0, 841, 595]}, (0, 1061): {'/Type': '/Page', '/Parent': IndirectObject(2, 0), '/Resources': IndirectObject(1059, 0), '/Contents': [IndirectObject(1058, 0)], '/MediaBox': [0, 0, 841, 595], '/CropBox': [0, 0, 841, 595]}, (0, 1065): {'/Type': '/Page', '/Parent': IndirectObject(2, 0), '/Resources': IndirectObject(1063, 0), '/Contents': [IndirectObject(1062, 0)], '/MediaBox': [0, 0, 841, 595], '/CropBox': [0, 0, 841, 595]}, (0, 1069): {'/Type': '/Page', '/Parent': IndirectObject(2, 0), '/Resources': IndirectObject(1067, 0), '/Contents': [IndirectObject(1066, 0)], '/MediaBox': [0, 0, 841, 595], '/CropBox': [0, 0, 841, 595]}, (0, 1073): {'/Type': '/Page', '/Parent': IndirectObject(2, 0), '/Resources': IndirectObject(1071, 0), '/Contents': [IndirectObject(1070, 0)], '/MediaBox': [0, 0, 841, 595], '/CropBox': [0, 0, 841, 595]}, (0, 1077): {'/Type': '/Page', '/Parent': IndirectObject(2, 0), '/Resources': IndirectObject(1075, 0), '/Contents': [IndirectObject(1074, 0)], '/MediaBox': [0, 0, 841, 595], '/CropBox': [0, 0, 841, 595]}, (0, 1081): {'/Type': '/Page', '/Parent': IndirectObject(2, 0), '/Resources': IndirectObject(1079, 0), '/Contents': [IndirectObject(1078, 0)], '/MediaBox': [0, 0, 841, 595], '/CropBox': [0, 0, 841, 595]}, (0, 1085): {'/Type': '/Page', '/Parent': IndirectObject(2, 0), '/Resources': IndirectObject(1083, 0), '/Contents': [IndirectObject(1082, 0)], '/MediaBox': [0, 0, 841, 595], '/CropBox': [0, 0, 841, 595]}, (0, 1089): {'/Type': '/Page', '/Parent': IndirectObject(2, 0), '/Resources': IndirectObject(1087, 0), '/Contents': [IndirectObject(1086, 0)], '/MediaBox': [0, 0, 841, 595], '/CropBox': [0, 0, 841, 595]}, (0, 1093): {'/Type': '/Page', '/Parent': IndirectObject(2, 0), '/Resources': IndirectObject(1091, 0), '/Contents': [IndirectObject(1090, 0)], '/MediaBox': [0, 0, 841, 595], '/CropBox': [0, 0, 841, 595]}, (0, 1097): {'/Type': '/Page', '/Parent': IndirectObject(2, 0), '/Resources': IndirectObject(1095, 0), '/Contents': [IndirectObject(1094, 0)], '/MediaBox': [0, 0, 841, 595], '/CropBox': [0, 0, 841, 595]}, (0, 1101): {'/Type': '/Page', '/Parent': IndirectObject(2, 0), '/Resources': IndirectObject(1099, 0), '/Contents': [IndirectObject(1098, 0)], '/MediaBox': [0, 0, 841, 595], '/CropBox': [0, 0, 841, 595]}, (0, 1105): {'/Type': '/Page', '/Parent': IndirectObject(2, 0), '/Resources': IndirectObject(1103, 0), '/Contents': [IndirectObject(1102, 0)], '/MediaBox': [0, 0, 841, 595], '/CropBox': [0, 0, 841, 595]}, (0, 1109): {'/Type': '/Page', '/Parent': IndirectObject(2, 0), '/Resources': IndirectObject(1107, 0), '/Contents': [IndirectObject(1106, 0)], '/MediaBox': [0, 0, 841, 595], '/CropBox': [0, 0, 841, 595]}, (0, 1113): {'/Type': '/Page', '/Parent': IndirectObject(2, 0), '/Resources': IndirectObject(1111, 0), '/Contents': [IndirectObject(1110, 0)], '/MediaBox': [0, 0, 841, 595], '/CropBox': [0, 0, 841, 595]}, (0, 1117): {'/Type': '/Page', '/Parent': IndirectObject(2, 0), '/Resources': IndirectObject(1115, 0), '/Contents': [IndirectObject(1114, 0)], '/MediaBox': [0, 0, 841, 595], '/CropBox': [0, 0, 841, 595]}, (0, 1121): {'/Type': '/Page', '/Parent': IndirectObject(2, 0), '/Resources': IndirectObject(1119, 0), '/Contents': [IndirectObject(1118, 0)], '/MediaBox': [0, 0, 841, 595], '/CropBox': [0, 0, 841, 595]}, (0, 1125): {'/Type': '/Page', '/Parent': IndirectObject(2, 0), '/Resources': IndirectObject(1123, 0), '/Contents': [IndirectObject(1122, 0)], '/MediaBox': [0, 0, 841, 595], '/CropBox': [0, 0, 841, 595]}, (0, 1129): {'/Type': '/Page', '/Parent': IndirectObject(2, 0), '/Resources': IndirectObject(1127, 0), '/Contents': [IndirectObject(1126, 0)], '/MediaBox': [0, 0, 841, 595], '/CropBox': [0, 0, 841, 595]}, (0, 1133): {'/Type': '/Page', '/Parent': IndirectObject(2, 0), '/Resources': IndirectObject(1131, 0), '/Contents': [IndirectObject(1130, 0)], '/MediaBox': [0, 0, 841, 595], '/CropBox': [0, 0, 841, 595]}, (0, 1137): {'/Type': '/Page', '/Parent': IndirectObject(2, 0), '/Resources': IndirectObject(1135, 0), '/Contents': [IndirectObject(1134, 0)], '/MediaBox': [0, 0, 841, 595], '/CropBox': [0, 0, 841, 595]}, (0, 1141): {'/Type': '/Page', '/Parent': IndirectObject(2, 0), '/Resources': IndirectObject(1139, 0), '/Contents': [IndirectObject(1138, 0)], '/MediaBox': [0, 0, 841, 595], '/CropBox': [0, 0, 841, 595]}, (0, 1145): {'/Type': '/Page', '/Parent': IndirectObject(2, 0), '/Resources': IndirectObject(1143, 0), '/Contents': [IndirectObject(1142, 0)], '/MediaBox': [0, 0, 841, 595], '/CropBox': [0, 0, 841, 595]}, (0, 1149): {'/Type': '/Page', '/Parent': IndirectObject(2, 0), '/Resources': IndirectObject(1147, 0), '/Contents': [IndirectObject(1146, 0)], '/MediaBox': [0, 0, 841, 595], '/CropBox': [0, 0, 841, 595]}, (0, 1153): {'/Type': '/Page', '/Parent': IndirectObject(2, 0), '/Resources': IndirectObject(1151, 0), '/Contents': [IndirectObject(1150, 0)], '/MediaBox': [0, 0, 841, 595], '/CropBox': [0, 0, 841, 595]}, (0, 1157): {'/Type': '/Page', '/Parent': IndirectObject(2, 0), '/Resources': IndirectObject(1155, 0), '/Contents': [IndirectObject(1154, 0)], '/MediaBox': [0, 0, 841, 595], '/CropBox': [0, 0, 841, 595]}, (0, 1161): {'/Type': '/Page', '/Parent': IndirectObject(2, 0), '/Resources': IndirectObject(1159, 0), '/Contents': [IndirectObject(1158, 0)], '/MediaBox': [0, 0, 841, 595], '/CropBox': [0, 0, 841, 595]}, (0, 1165): {'/Type': '/Page', '/Parent': IndirectObject(2, 0), '/Resources': IndirectObject(1163, 0), '/Contents': [IndirectObject(1162, 0)], '/MediaBox': [0, 0, 841, 595], '/CropBox': [0, 0, 841, 595]}, (0, 1169): {'/Type': '/Page', '/Parent': IndirectObject(2, 0), '/Resources': IndirectObject(1167, 0), '/Contents': [IndirectObject(1166, 0)], '/MediaBox': [0, 0, 841, 595], '/CropBox': [0, 0, 841, 595]}, (0, 1173): {'/Type': '/Page', '/Parent': IndirectObject(2, 0), '/Resources': IndirectObject(1171, 0), '/Contents': [IndirectObject(1170, 0)], '/MediaBox': [0, 0, 841, 595], '/CropBox': [0, 0, 841, 595]}, (0, 1177): {'/Type': '/Page', '/Parent': IndirectObject(2, 0), '/Resources': IndirectObject(1175, 0), '/Contents': [IndirectObject(1174, 0)], '/MediaBox': [0, 0, 841, 595], '/CropBox': [0, 0, 841, 595]}, (0, 1181): {'/Type': '/Page', '/Parent': IndirectObject(2, 0), '/Resources': IndirectObject(1179, 0), '/Contents': [IndirectObject(1178, 0)], '/MediaBox': [0, 0, 841, 595], '/CropBox': [0, 0, 841, 595]}, (0, 1185): {'/Type': '/Page', '/Parent': IndirectObject(2, 0), '/Resources': IndirectObject(1183, 0), '/Contents': [IndirectObject(1182, 0)], '/MediaBox': [0, 0, 841, 595], '/CropBox': [0, 0, 841, 595]}, (0, 1189): {'/Type': '/Page', '/Parent': IndirectObject(2, 0), '/Resources': IndirectObject(1187, 0), '/Contents': [IndirectObject(1186, 0)], '/MediaBox': [0, 0, 841, 595], '/CropBox': [0, 0, 841, 595]}, (0, 1193): {'/Type': '/Page', '/Parent': IndirectObject(2, 0), '/Resources': IndirectObject(1191, 0), '/Contents': [IndirectObject(1190, 0)], '/MediaBox': [0, 0, 841, 595], '/CropBox': [0, 0, 841, 595]}, (0, 1197): {'/Type': '/Page', '/Parent': IndirectObject(2, 0), '/Resources': IndirectObject(1195, 0), '/Contents': [IndirectObject(1194, 0)], '/MediaBox': [0, 0, 841, 595], '/CropBox': [0, 0, 841, 595]}, (0, 1201): {'/Type': '/Page', '/Parent': IndirectObject(2, 0), '/Resources': IndirectObject(1199, 0), '/Contents': [IndirectObject(1198, 0)], '/MediaBox': [0, 0, 841, 595], '/CropBox': [0, 0, 841, 595]}, (0, 1205): {'/Type': '/Page', '/Parent': IndirectObject(2, 0), '/Resources': IndirectObject(1203, 0), '/Contents': [IndirectObject(1202, 0)], '/MediaBox': [0, 0, 841, 595], '/CropBox': [0, 0, 841, 595]}, (0, 1209): {'/Type': '/Page', '/Parent': IndirectObject(2, 0), '/Resources': IndirectObject(1207, 0), '/Contents': [IndirectObject(1206, 0)], '/MediaBox': [0, 0, 841, 595], '/CropBox': [0, 0, 841, 595]}, (0, 1213): {'/Type': '/Page', '/Parent': IndirectObject(2, 0), '/Resources': IndirectObject(1211, 0), '/Contents': [IndirectObject(1210, 0)], '/MediaBox': [0, 0, 841, 595], '/CropBox': [0, 0, 841, 595]}, (0, 1217): {'/Type': '/Page', '/Parent': IndirectObject(2, 0), '/Resources': IndirectObject(1215, 0), '/Contents': [IndirectObject(1214, 0)], '/MediaBox': [0, 0, 841, 595], '/CropBox': [0, 0, 841, 595]}, (0, 1221): {'/Type': '/Page', '/Parent': IndirectObject(2, 0), '/Resources': IndirectObject(1219, 0), '/Contents': [IndirectObject(1218, 0)], '/MediaBox': [0, 0, 841, 595], '/CropBox': [0, 0, 841, 595]}, (0, 1225): {'/Type': '/Page', '/Parent': IndirectObject(2, 0), '/Resources': IndirectObject(1223, 0), '/Contents': [IndirectObject(1222, 0)], '/MediaBox': [0, 0, 841, 595], '/CropBox': [0, 0, 841, 595]}, (0, 1229): {'/Type': '/Page', '/Parent': IndirectObject(2, 0), '/Resources': IndirectObject(1227, 0), '/Contents': [IndirectObject(1226, 0)], '/MediaBox': [0, 0, 841, 595], '/CropBox': [0, 0, 841, 595]}, (0, 1233): {'/Type': '/Page', '/Parent': IndirectObject(2, 0), '/Resources': IndirectObject(1231, 0), '/Contents': [IndirectObject(1230, 0)], '/MediaBox': [0, 0, 841, 595], '/CropBox': [0, 0, 841, 595]}, (0, 1237): {'/Type': '/Page', '/Parent': IndirectObject(2, 0), '/Resources': IndirectObject(1235, 0), '/Contents': [IndirectObject(1234, 0)], '/MediaBox': [0, 0, 841, 595], '/CropBox': [0, 0, 841, 595]}, (0, 1241): {'/Type': '/Page', '/Parent': IndirectObject(2, 0), '/Resources': IndirectObject(1239, 0), '/Contents': [IndirectObject(1238, 0)], '/MediaBox': [0, 0, 841, 595], '/CropBox': [0, 0, 841, 595]}, (0, 1245): {'/Type': '/Page', '/Parent': IndirectObject(2, 0), '/Resources': IndirectObject(1243, 0), '/Contents': [IndirectObject(1242, 0)], '/MediaBox': [0, 0, 841, 595], '/CropBox': [0, 0, 841, 595]}, (0, 1249): {'/Type': '/Page', '/Parent': IndirectObject(2, 0), '/Resources': IndirectObject(1247, 0), '/Contents': [IndirectObject(1246, 0)], '/MediaBox': [0, 0, 841, 595], '/CropBox': [0, 0, 841, 595]}, (0, 1253): {'/Type': '/Page', '/Parent': IndirectObject(2, 0), '/Resources': IndirectObject(1251, 0), '/Contents': [IndirectObject(1250, 0)], '/MediaBox': [0, 0, 841, 595], '/CropBox': [0, 0, 841, 595]}, (0, 1257): {'/Type': '/Page', '/Parent': IndirectObject(2, 0), '/Resources': IndirectObject(1255, 0), '/Contents': [IndirectObject(1254, 0)], '/MediaBox': [0, 0, 841, 595], '/CropBox': [0, 0, 841, 595]}, (0, 1261): {'/Type': '/Page', '/Parent': IndirectObject(2, 0), '/Resources': IndirectObject(1259, 0), '/Contents': [IndirectObject(1258, 0)], '/MediaBox': [0, 0, 841, 595], '/CropBox': [0, 0, 841, 595]}, (0, 1265): {'/Type': '/Page', '/Parent': IndirectObject(2, 0), '/Resources': IndirectObject(1263, 0), '/Contents': [IndirectObject(1262, 0)], '/MediaBox': [0, 0, 841, 595], '/CropBox': [0, 0, 841, 595]}, (0, 1269): {'/Type': '/Page', '/Parent': IndirectObject(2, 0), '/Resources': IndirectObject(1267, 0), '/Contents': [IndirectObject(1266, 0)], '/MediaBox': [0, 0, 841, 595], '/CropBox': [0, 0, 841, 595]}, (0, 1273): {'/Type': '/Page', '/Parent': IndirectObject(2, 0), '/Resources': IndirectObject(1271, 0), '/Contents': [IndirectObject(1270, 0)], '/MediaBox': [0, 0, 841, 595], '/CropBox': [0, 0, 841, 595]}, (0, 1277): {'/Type': '/Page', '/Parent': IndirectObject(2, 0), '/Resources': IndirectObject(1275, 0), '/Contents': [IndirectObject(1274, 0)], '/MediaBox': [0, 0, 841, 595], '/CropBox': [0, 0, 841, 595]}, (0, 1281): {'/Type': '/Page', '/Parent': IndirectObject(2, 0), '/Resources': IndirectObject(1279, 0), '/Contents': [IndirectObject(1278, 0)], '/MediaBox': [0, 0, 841, 595], '/CropBox': [0, 0, 841, 595]}, (0, 1285): {'/Type': '/Page', '/Parent': IndirectObject(2, 0), '/Resources': IndirectObject(1283, 0), '/Contents': [IndirectObject(1282, 0)], '/MediaBox': [0, 0, 841, 595], '/CropBox': [0, 0, 841, 595]}, (0, 1289): {'/Type': '/Page', '/Parent': IndirectObject(2, 0), '/Resources': IndirectObject(1287, 0), '/Contents': [IndirectObject(1286, 0)], '/MediaBox': [0, 0, 841, 595], '/CropBox': [0, 0, 841, 595]}, (0, 1293): {'/Type': '/Page', '/Parent': IndirectObject(2, 0), '/Resources': IndirectObject(1291, 0), '/Contents': [IndirectObject(1290, 0)], '/MediaBox': [0, 0, 841, 595], '/CropBox': [0, 0, 841, 595]}, (0, 1297): {'/Type': '/Page', '/Parent': IndirectObject(2, 0), '/Resources': IndirectObject(1295, 0), '/Contents': [IndirectObject(1294, 0)], '/MediaBox': [0, 0, 841, 595], '/CropBox': [0, 0, 841, 595]}, (0, 1301): {'/Type': '/Page', '/Parent': IndirectObject(2, 0), '/Resources': IndirectObject(1299, 0), '/Contents': [IndirectObject(1298, 0)], '/MediaBox': [0, 0, 841, 595], '/CropBox': [0, 0, 841, 595]}, (0, 1305): {'/Type': '/Page', '/Parent': IndirectObject(2, 0), '/Resources': IndirectObject(1303, 0), '/Contents': [IndirectObject(1302, 0)], '/MediaBox': [0, 0, 841, 595], '/CropBox': [0, 0, 841, 595]}, (0, 1309): {'/Type': '/Page', '/Parent': IndirectObject(2, 0), '/Resources': IndirectObject(1307, 0), '/Contents': [IndirectObject(1306, 0)], '/MediaBox': [0, 0, 841, 595], '/CropBox': [0, 0, 841, 595]}, (0, 1313): {'/Type': '/Page', '/Parent': IndirectObject(2, 0), '/Resources': IndirectObject(1311, 0), '/Contents': [IndirectObject(1310, 0)], '/MediaBox': [0, 0, 841, 595], '/CropBox': [0, 0, 841, 595]}, (0, 1317): {'/Type': '/Page', '/Parent': IndirectObject(2, 0), '/Resources': IndirectObject(1315, 0), '/Contents': [IndirectObject(1314, 0)], '/MediaBox': [0, 0, 841, 595], '/CropBox': [0, 0, 841, 595]}, (0, 1321): {'/Type': '/Page', '/Parent': IndirectObject(2, 0), '/Resources': IndirectObject(1319, 0), '/Contents': [IndirectObject(1318, 0)], '/MediaBox': [0, 0, 841, 595], '/CropBox': [0, 0, 841, 595]}, (0, 1325): {'/Type': '/Page', '/Parent': IndirectObject(2, 0), '/Resources': IndirectObject(1323, 0), '/Contents': [IndirectObject(1322, 0)], '/MediaBox': [0, 0, 841, 595], '/CropBox': [0, 0, 841, 595]}, (0, 1329): {'/Type': '/Page', '/Parent': IndirectObject(2, 0), '/Resources': IndirectObject(1327, 0), '/Contents': [IndirectObject(1326, 0)], '/MediaBox': [0, 0, 841, 595], '/CropBox': [0, 0, 841, 595]}, (0, 1333): {'/Type': '/Page', '/Parent': IndirectObject(2, 0), '/Resources': IndirectObject(1331, 0), '/Contents': [IndirectObject(1330, 0)], '/MediaBox': [0, 0, 841, 595], '/CropBox': [0, 0, 841, 595]}, (0, 1337): {'/Type': '/Page', '/Parent': IndirectObject(2, 0), '/Resources': IndirectObject(1335, 0), '/Contents': [IndirectObject(1334, 0)], '/MediaBox': [0, 0, 841, 595], '/CropBox': [0, 0, 841, 595]}, (0, 1341): {'/Type': '/Page', '/Parent': IndirectObject(2, 0), '/Resources': IndirectObject(1339, 0), '/Contents': [IndirectObject(1338, 0)], '/MediaBox': [0, 0, 841, 595], '/CropBox': [0, 0, 841, 595]}, (0, 1345): {'/Type': '/Page', '/Parent': IndirectObject(2, 0), '/Resources': IndirectObject(1343, 0), '/Contents': [IndirectObject(1342, 0)], '/MediaBox': [0, 0, 841, 595], '/CropBox': [0, 0, 841, 595]}, (0, 1349): {'/Type': '/Page', '/Parent': IndirectObject(2, 0), '/Resources': IndirectObject(1347, 0), '/Contents': [IndirectObject(1346, 0)], '/MediaBox': [0, 0, 841, 595], '/CropBox': [0, 0, 841, 595]}, (0, 1353): {'/Type': '/Page', '/Parent': IndirectObject(2, 0), '/Resources': IndirectObject(1351, 0), '/Contents': [IndirectObject(1350, 0)], '/MediaBox': [0, 0, 841, 595], '/CropBox': [0, 0, 841, 595]}, (0, 1357): {'/Type': '/Page', '/Parent': IndirectObject(2, 0), '/Resources': IndirectObject(1355, 0), '/Contents': [IndirectObject(1354, 0)], '/MediaBox': [0, 0, 841, 595], '/CropBox': [0, 0, 841, 595]}, (0, 1361): {'/Type': '/Page', '/Parent': IndirectObject(2, 0), '/Resources': IndirectObject(1359, 0), '/Contents': [IndirectObject(1358, 0)], '/MediaBox': [0, 0, 841, 595], '/CropBox': [0, 0, 841, 595]}, (0, 1365): {'/Type': '/Page', '/Parent': IndirectObject(2, 0), '/Resources': IndirectObject(1363, 0), '/Contents': [IndirectObject(1362, 0)], '/MediaBox': [0, 0, 841, 595], '/CropBox': [0, 0, 841, 595]}, (0, 1369): {'/Type': '/Page', '/Parent': IndirectObject(2, 0), '/Resources': IndirectObject(1367, 0), '/Contents': [IndirectObject(1366, 0)], '/MediaBox': [0, 0, 841, 595], '/CropBox': [0, 0, 841, 595]}, (0, 1373): {'/Type': '/Page', '/Parent': IndirectObject(2, 0), '/Resources': IndirectObject(1371, 0), '/Contents': [IndirectObject(1370, 0)], '/MediaBox': [0, 0, 841, 595], '/CropBox': [0, 0, 841, 595]}, (0, 1377): {'/Type': '/Page', '/Parent': IndirectObject(2, 0), '/Resources': IndirectObject(1375, 0), '/Contents': [IndirectObject(1374, 0)], '/MediaBox': [0, 0, 841, 595], '/CropBox': [0, 0, 841, 595]}, (0, 1381): {'/Type': '/Page', '/Parent': IndirectObject(2, 0), '/Resources': IndirectObject(1379, 0), '/Contents': [IndirectObject(1378, 0)], '/MediaBox': [0, 0, 841, 595], '/CropBox': [0, 0, 841, 595]}, (0, 1385): {'/Type': '/Page', '/Parent': IndirectObject(2, 0), '/Resources': IndirectObject(1383, 0), '/Contents': [IndirectObject(1382, 0)], '/MediaBox': [0, 0, 841, 595], '/CropBox': [0, 0, 841, 595]}, (0, 1389): {'/Type': '/Page', '/Parent': IndirectObject(2, 0), '/Resources': IndirectObject(1387, 0), '/Contents': [IndirectObject(1386, 0)], '/MediaBox': [0, 0, 841, 595], '/CropBox': [0, 0, 841, 595]}, (0, 1393): {'/Type': '/Page', '/Parent': IndirectObject(2, 0), '/Resources': IndirectObject(1391, 0), '/Contents': [IndirectObject(1390, 0)], '/MediaBox': [0, 0, 841, 595], '/CropBox': [0, 0, 841, 595]}, (0, 1397): {'/Type': '/Page', '/Parent': IndirectObject(2, 0), '/Resources': IndirectObject(1395, 0), '/Contents': [IndirectObject(1394, 0)], '/MediaBox': [0, 0, 841, 595], '/CropBox': [0, 0, 841, 595]}, (0, 1401): {'/Type': '/Page', '/Parent': IndirectObject(2, 0), '/Resources': IndirectObject(1399, 0), '/Contents': [IndirectObject(1398, 0)], '/MediaBox': [0, 0, 841, 595], '/CropBox': [0, 0, 841, 595]}, (0, 1405): {'/Type': '/Page', '/Parent': IndirectObject(2, 0), '/Resources': IndirectObject(1403, 0), '/Contents': [IndirectObject(1402, 0)], '/MediaBox': [0, 0, 841, 595], '/CropBox': [0, 0, 841, 595]}, (0, 1409): {'/Type': '/Page', '/Parent': IndirectObject(2, 0), '/Resources': IndirectObject(1407, 0), '/Contents': [IndirectObject(1406, 0)], '/MediaBox': [0, 0, 841, 595], '/CropBox': [0, 0, 841, 595]}, (0, 1413): {'/Type': '/Page', '/Parent': IndirectObject(2, 0), '/Resources': IndirectObject(1411, 0), '/Contents': [IndirectObject(1410, 0)], '/MediaBox': [0, 0, 841, 595], '/CropBox': [0, 0, 841, 595]}, (0, 1417): {'/Type': '/Page', '/Parent': IndirectObject(2, 0), '/Resources': IndirectObject(1415, 0), '/Contents': [IndirectObject(1414, 0)], '/MediaBox': [0, 0, 841, 595], '/CropBox': [0, 0, 841, 595]}, (0, 1421): {'/Type': '/Page', '/Parent': IndirectObject(2, 0), '/Resources': IndirectObject(1419, 0), '/Contents': [IndirectObject(1418, 0)], '/MediaBox': [0, 0, 841, 595], '/CropBox': [0, 0, 841, 595]}, (0, 1425): {'/Type': '/Page', '/Parent': IndirectObject(2, 0), '/Resources': IndirectObject(1423, 0), '/Contents': [IndirectObject(1422, 0)], '/MediaBox': [0, 0, 841, 595], '/CropBox': [0, 0, 841, 595]}, (0, 1429): {'/Type': '/Page', '/Parent': IndirectObject(2, 0), '/Resources': IndirectObject(1427, 0), '/Contents': [IndirectObject(1426, 0)], '/MediaBox': [0, 0, 841, 595], '/CropBox': [0, 0, 841, 595]}, (0, 1433): {'/Type': '/Page', '/Parent': IndirectObject(2, 0), '/Resources': IndirectObject(1431, 0), '/Contents': [IndirectObject(1430, 0)], '/MediaBox': [0, 0, 841, 595], '/CropBox': [0, 0, 841, 595]}, (0, 1437): {'/Type': '/Page', '/Parent': IndirectObject(2, 0), '/Resources': IndirectObject(1435, 0), '/Contents': [IndirectObject(1434, 0)], '/MediaBox': [0, 0, 841, 595], '/CropBox': [0, 0, 841, 595]}, (0, 1441): {'/Type': '/Page', '/Parent': IndirectObject(2, 0), '/Resources': IndirectObject(1439, 0), '/Contents': [IndirectObject(1438, 0)], '/MediaBox': [0, 0, 841, 595], '/CropBox': [0, 0, 841, 595]}, (0, 1445): {'/Type': '/Page', '/Parent': IndirectObject(2, 0), '/Resources': IndirectObject(1443, 0), '/Contents': [IndirectObject(1442, 0)], '/MediaBox': [0, 0, 841, 595], '/CropBox': [0, 0, 841, 595]}, (0, 1449): {'/Type': '/Page', '/Parent': IndirectObject(2, 0), '/Resources': IndirectObject(1447, 0), '/Contents': [IndirectObject(1446, 0)], '/MediaBox': [0, 0, 841, 595], '/CropBox': [0, 0, 841, 595]}, (0, 1453): {'/Type': '/Page', '/Parent': IndirectObject(2, 0), '/Resources': IndirectObject(1451, 0), '/Contents': [IndirectObject(1450, 0)], '/MediaBox': [0, 0, 841, 595], '/CropBox': [0, 0, 841, 595]}, (0, 1457): {'/Type': '/Page', '/Parent': IndirectObject(2, 0), '/Resources': IndirectObject(1455, 0), '/Contents': [IndirectObject(1454, 0)], '/MediaBox': [0, 0, 841, 595], '/CropBox': [0, 0, 841, 595]}, (0, 1461): {'/Type': '/Page', '/Parent': IndirectObject(2, 0), '/Resources': IndirectObject(1459, 0), '/Contents': [IndirectObject(1458, 0)], '/MediaBox': [0, 0, 841, 595], '/CropBox': [0, 0, 841, 595]}, (0, 1465): {'/Type': '/Page', '/Parent': IndirectObject(2, 0), '/Resources': IndirectObject(1463, 0), '/Contents': [IndirectObject(1462, 0)], '/MediaBox': [0, 0, 841, 595], '/CropBox': [0, 0, 841, 595]}, (0, 1469): {'/Type': '/Page', '/Parent': IndirectObject(2, 0), '/Resources': IndirectObject(1467, 0), '/Contents': [IndirectObject(1466, 0)], '/MediaBox': [0, 0, 841, 595], '/CropBox': [0, 0, 841, 595]}, (0, 1473): {'/Type': '/Page', '/Parent': IndirectObject(2, 0), '/Resources': IndirectObject(1471, 0), '/Contents': [IndirectObject(1470, 0)], '/MediaBox': [0, 0, 841, 595], '/CropBox': [0, 0, 841, 595]}, (0, 1477): {'/Type': '/Page', '/Parent': IndirectObject(2, 0), '/Resources': IndirectObject(1475, 0), '/Contents': [IndirectObject(1474, 0)], '/MediaBox': [0, 0, 841, 595], '/CropBox': [0, 0, 841, 595]}, (0, 1481): {'/Type': '/Page', '/Parent': IndirectObject(2, 0), '/Resources': IndirectObject(1479, 0), '/Contents': [IndirectObject(1478, 0)], '/MediaBox': [0, 0, 841, 595], '/CropBox': [0, 0, 841, 595]}, (0, 1485): {'/Type': '/Page', '/Parent': IndirectObject(2, 0), '/Resources': IndirectObject(1483, 0), '/Contents': [IndirectObject(1482, 0)], '/MediaBox': [0, 0, 841, 595], '/CropBox': [0, 0, 841, 595]}, (0, 1489): {'/Type': '/Page', '/Parent': IndirectObject(2, 0), '/Resources': IndirectObject(1487, 0), '/Contents': [IndirectObject(1486, 0)], '/MediaBox': [0, 0, 841, 595], '/CropBox': [0, 0, 841, 595]}, (0, 1493): {'/Type': '/Page', '/Parent': IndirectObject(2, 0), '/Resources': IndirectObject(1491, 0), '/Contents': [IndirectObject(1490, 0)], '/MediaBox': [0, 0, 841, 595], '/CropBox': [0, 0, 841, 595]}, (0, 1497): {'/Type': '/Page', '/Parent': IndirectObject(2, 0), '/Resources': IndirectObject(1495, 0), '/Contents': [IndirectObject(1494, 0)], '/MediaBox': [0, 0, 841, 595], '/CropBox': [0, 0, 841, 595]}, (0, 1501): {'/Type': '/Page', '/Parent': IndirectObject(2, 0), '/Resources': IndirectObject(1499, 0), '/Contents': [IndirectObject(1498, 0)], '/MediaBox': [0, 0, 841, 595], '/CropBox': [0, 0, 841, 595]}, (0, 1505): {'/Type': '/Page', '/Parent': IndirectObject(2, 0), '/Resources': IndirectObject(1503, 0), '/Contents': [IndirectObject(1502, 0)], '/MediaBox': [0, 0, 841, 595], '/CropBox': [0, 0, 841, 595]}, (0, 1509): {'/Type': '/Page', '/Parent': IndirectObject(2, 0), '/Resources': IndirectObject(1507, 0), '/Contents': [IndirectObject(1506, 0)], '/MediaBox': [0, 0, 841, 595], '/CropBox': [0, 0, 841, 595]}, (0, 1513): {'/Type': '/Page', '/Parent': IndirectObject(2, 0), '/Resources': IndirectObject(1511, 0), '/Contents': [IndirectObject(1510, 0)], '/MediaBox': [0, 0, 841, 595], '/CropBox': [0, 0, 841, 595]}, (0, 1517): {'/Type': '/Page', '/Parent': IndirectObject(2, 0), '/Resources': IndirectObject(1515, 0), '/Contents': [IndirectObject(1514, 0)], '/MediaBox': [0, 0, 841, 595], '/CropBox': [0, 0, 841, 595]}, (0, 1521): {'/Type': '/Page', '/Parent': IndirectObject(2, 0), '/Resources': IndirectObject(1519, 0), '/Contents': [IndirectObject(1518, 0)], '/MediaBox': [0, 0, 841, 595], '/CropBox': [0, 0, 841, 595]}, (0, 1525): {'/Type': '/Page', '/Parent': IndirectObject(2, 0), '/Resources': IndirectObject(1523, 0), '/Contents': [IndirectObject(1522, 0)], '/MediaBox': [0, 0, 841, 595], '/CropBox': [0, 0, 841, 595]}, (0, 1529): {'/Type': '/Page', '/Parent': IndirectObject(2, 0), '/Resources': IndirectObject(1527, 0), '/Contents': [IndirectObject(1526, 0)], '/MediaBox': [0, 0, 841, 595], '/CropBox': [0, 0, 841, 595]}, (0, 1533): {'/Type': '/Page', '/Parent': IndirectObject(2, 0), '/Resources': IndirectObject(1531, 0), '/Contents': [IndirectObject(1530, 0)], '/MediaBox': [0, 0, 841, 595], '/CropBox': [0, 0, 841, 595]}, (0, 1537): {'/Type': '/Page', '/Parent': IndirectObject(2, 0), '/Resources': IndirectObject(1535, 0), '/Contents': [IndirectObject(1534, 0)], '/MediaBox': [0, 0, 841, 595], '/CropBox': [0, 0, 841, 595]}, (0, 1541): {'/Type': '/Page', '/Parent': IndirectObject(2, 0), '/Resources': IndirectObject(1539, 0), '/Contents': [IndirectObject(1538, 0)], '/MediaBox': [0, 0, 841, 595], '/CropBox': [0, 0, 841, 595]}, (0, 1545): {'/Type': '/Page', '/Parent': IndirectObject(2, 0), '/Resources': IndirectObject(1543, 0), '/Contents': [IndirectObject(1542, 0)], '/MediaBox': [0, 0, 841, 595], '/CropBox': [0, 0, 841, 595]}, (0, 1549): {'/Type': '/Page', '/Parent': IndirectObject(2, 0), '/Resources': IndirectObject(1547, 0), '/Contents': [IndirectObject(1546, 0)], '/MediaBox': [0, 0, 841, 595], '/CropBox': [0, 0, 841, 595]}, (0, 1553): {'/Type': '/Page', '/Parent': IndirectObject(2, 0), '/Resources': IndirectObject(1551, 0), '/Contents': [IndirectObject(1550, 0)], '/MediaBox': [0, 0, 841, 595], '/CropBox': [0, 0, 841, 595]}, (0, 1557): {'/Type': '/Page', '/Parent': IndirectObject(2, 0), '/Resources': IndirectObject(1555, 0), '/Contents': [IndirectObject(1554, 0)], '/MediaBox': [0, 0, 841, 595], '/CropBox': [0, 0, 841, 595]}, (0, 1561): {'/Type': '/Page', '/Parent': IndirectObject(2, 0), '/Resources': IndirectObject(1559, 0), '/Contents': [IndirectObject(1558, 0)], '/MediaBox': [0, 0, 841, 595], '/CropBox': [0, 0, 841, 595]}, (0, 1565): {'/Type': '/Page', '/Parent': IndirectObject(2, 0), '/Resources': IndirectObject(1563, 0), '/Contents': [IndirectObject(1562, 0)], '/MediaBox': [0, 0, 841, 595], '/CropBox': [0, 0, 841, 595]}, (0, 1569): {'/Type': '/Page', '/Parent': IndirectObject(2, 0), '/Resources': IndirectObject(1567, 0), '/Contents': [IndirectObject(1566, 0)], '/MediaBox': [0, 0, 841, 595], '/CropBox': [0, 0, 841, 595]}, (0, 1573): {'/Type': '/Page', '/Parent': IndirectObject(2, 0), '/Resources': IndirectObject(1571, 0), '/Contents': [IndirectObject(1570, 0)], '/MediaBox': [0, 0, 841, 595], '/CropBox': [0, 0, 841, 595]}, (0, 1577): {'/Type': '/Page', '/Parent': IndirectObject(2, 0), '/Resources': IndirectObject(1575, 0), '/Contents': [IndirectObject(1574, 0)], '/MediaBox': [0, 0, 841, 595], '/CropBox': [0, 0, 841, 595]}, (0, 1581): {'/Type': '/Page', '/Parent': IndirectObject(2, 0), '/Resources': IndirectObject(1579, 0), '/Contents': [IndirectObject(1578, 0)], '/MediaBox': [0, 0, 841, 595], '/CropBox': [0, 0, 841, 595]}, (0, 1585): {'/Type': '/Page', '/Parent': IndirectObject(2, 0), '/Resources': IndirectObject(1583, 0), '/Contents': [IndirectObject(1582, 0)], '/MediaBox': [0, 0, 841, 595], '/CropBox': [0, 0, 841, 595]}, (0, 1589): {'/Type': '/Page', '/Parent': IndirectObject(2, 0), '/Resources': IndirectObject(1587, 0), '/Contents': [IndirectObject(1586, 0)], '/MediaBox': [0, 0, 841, 595], '/CropBox': [0, 0, 841, 595]}, (0, 1593): {'/Type': '/Page', '/Parent': IndirectObject(2, 0), '/Resources': IndirectObject(1591, 0), '/Contents': [IndirectObject(1590, 0)], '/MediaBox': [0, 0, 841, 595], '/CropBox': [0, 0, 841, 595]}, (0, 1597): {'/Type': '/Page', '/Parent': IndirectObject(2, 0), '/Resources': IndirectObject(1595, 0), '/Contents': [IndirectObject(1594, 0)], '/MediaBox': [0, 0, 841, 595], '/CropBox': [0, 0, 841, 595]}, (0, 1601): {'/Type': '/Page', '/Parent': IndirectObject(2, 0), '/Resources': IndirectObject(1599, 0), '/Contents': [IndirectObject(1598, 0)], '/MediaBox': [0, 0, 841, 595], '/CropBox': [0, 0, 841, 595]}, (0, 1605): {'/Type': '/Page', '/Parent': IndirectObject(2, 0), '/Resources': IndirectObject(1603, 0), '/Contents': [IndirectObject(1602, 0)], '/MediaBox': [0, 0, 841, 595], '/CropBox': [0, 0, 841, 595]}, (0, 1609): {'/Type': '/Page', '/Parent': IndirectObject(2, 0), '/Resources': IndirectObject(1607, 0), '/Contents': [IndirectObject(1606, 0)], '/MediaBox': [0, 0, 841, 595], '/CropBox': [0, 0, 841, 595]}, (0, 1613): {'/Type': '/Page', '/Parent': IndirectObject(2, 0), '/Resources': IndirectObject(1611, 0), '/Contents': [IndirectObject(1610, 0)], '/MediaBox': [0, 0, 841, 595], '/CropBox': [0, 0, 841, 595]}, (0, 1617): {'/Type': '/Page', '/Parent': IndirectObject(2, 0), '/Resources': IndirectObject(1615, 0), '/Contents': [IndirectObject(1614, 0)], '/MediaBox': [0, 0, 841, 595], '/CropBox': [0, 0, 841, 595]}, (0, 1621): {'/Type': '/Page', '/Parent': IndirectObject(2, 0), '/Resources': IndirectObject(1619, 0), '/Contents': [IndirectObject(1618, 0)], '/MediaBox': [0, 0, 841, 595], '/CropBox': [0, 0, 841, 595]}, (0, 1625): {'/Type': '/Page', '/Parent': IndirectObject(2, 0), '/Resources': IndirectObject(1623, 0), '/Contents': [IndirectObject(1622, 0)], '/MediaBox': [0, 0, 841, 595], '/CropBox': [0, 0, 841, 595]}, (0, 1629): {'/Type': '/Page', '/Parent': IndirectObject(2, 0), '/Resources': IndirectObject(1627, 0), '/Contents': [IndirectObject(1626, 0)], '/MediaBox': [0, 0, 841, 595], '/CropBox': [0, 0, 841, 595]}, (0, 1633): {'/Type': '/Page', '/Parent': IndirectObject(2, 0), '/Resources': IndirectObject(1631, 0), '/Contents': [IndirectObject(1630, 0)], '/MediaBox': [0, 0, 841, 595], '/CropBox': [0, 0, 841, 595]}, (0, 1637): {'/Type': '/Page', '/Parent': IndirectObject(2, 0), '/Resources': IndirectObject(1635, 0), '/Contents': [IndirectObject(1634, 0)], '/MediaBox': [0, 0, 841, 595], '/CropBox': [0, 0, 841, 595]}, (0, 1641): {'/Type': '/Page', '/Parent': IndirectObject(2, 0), '/Resources': IndirectObject(1639, 0), '/Contents': [IndirectObject(1638, 0)], '/MediaBox': [0, 0, 841, 595], '/CropBox': [0, 0, 841, 595]}, (0, 1645): {'/Type': '/Page', '/Parent': IndirectObject(2, 0), '/Resources': IndirectObject(1643, 0), '/Contents': [IndirectObject(1642, 0)], '/MediaBox': [0, 0, 841, 595], '/CropBox': [0, 0, 841, 595]}, (0, 1649): {'/Type': '/Page', '/Parent': IndirectObject(2, 0), '/Resources': IndirectObject(1647, 0), '/Contents': [IndirectObject(1646, 0)], '/MediaBox': [0, 0, 841, 595], '/CropBox': [0, 0, 841, 595]}, (0, 1653): {'/Type': '/Page', '/Parent': IndirectObject(2, 0), '/Resources': IndirectObject(1651, 0), '/Contents': [IndirectObject(1650, 0)], '/MediaBox': [0, 0, 841, 595], '/CropBox': [0, 0, 841, 595]}, (0, 1657): {'/Type': '/Page', '/Parent': IndirectObject(2, 0), '/Resources': IndirectObject(1655, 0), '/Contents': [IndirectObject(1654, 0)], '/MediaBox': [0, 0, 841, 595], '/CropBox': [0, 0, 841, 595]}, (0, 1661): {'/Type': '/Page', '/Parent': IndirectObject(2, 0), '/Resources': IndirectObject(1659, 0), '/Contents': [IndirectObject(1658, 0)], '/MediaBox': [0, 0, 841, 595], '/CropBox': [0, 0, 841, 595]}, (0, 1665): {'/Type': '/Page', '/Parent': IndirectObject(2, 0), '/Resources': IndirectObject(1663, 0), '/Contents': [IndirectObject(1662, 0)], '/MediaBox': [0, 0, 841, 595], '/CropBox': [0, 0, 841, 595]}, (0, 1669): {'/Type': '/Page', '/Parent': IndirectObject(2, 0), '/Resources': IndirectObject(1667, 0), '/Contents': [IndirectObject(1666, 0)], '/MediaBox': [0, 0, 841, 595], '/CropBox': [0, 0, 841, 595]}, (0, 1673): {'/Type': '/Page', '/Parent': IndirectObject(2, 0), '/Resources': IndirectObject(1671, 0), '/Contents': [IndirectObject(1670, 0)], '/MediaBox': [0, 0, 841, 595], '/CropBox': [0, 0, 841, 595]}, (0, 1677): {'/Type': '/Page', '/Parent': IndirectObject(2, 0), '/Resources': IndirectObject(1675, 0), '/Contents': [IndirectObject(1674, 0)], '/MediaBox': [0, 0, 841, 595], '/CropBox': [0, 0, 841, 595]}, (0, 1681): {'/Type': '/Page', '/Parent': IndirectObject(2, 0), '/Resources': IndirectObject(1679, 0), '/Contents': [IndirectObject(1678, 0)], '/MediaBox': [0, 0, 841, 595], '/CropBox': [0, 0, 841, 595]}, (0, 1685): {'/Type': '/Page', '/Parent': IndirectObject(2, 0), '/Resources': IndirectObject(1683, 0), '/Contents': [IndirectObject(1682, 0)], '/MediaBox': [0, 0, 841, 595], '/CropBox': [0, 0, 841, 595]}, (0, 1689): {'/Type': '/Page', '/Parent': IndirectObject(2, 0), '/Resources': IndirectObject(1687, 0), '/Contents': [IndirectObject(1686, 0)], '/MediaBox': [0, 0, 841, 595], '/CropBox': [0, 0, 841, 595]}, (0, 1693): {'/Type': '/Page', '/Parent': IndirectObject(2, 0), '/Resources': IndirectObject(1691, 0), '/Contents': [IndirectObject(1690, 0)], '/MediaBox': [0, 0, 841, 595], '/CropBox': [0, 0, 841, 595]}, (0, 1697): {'/Type': '/Page', '/Parent': IndirectObject(2, 0), '/Resources': IndirectObject(1695, 0), '/Contents': [IndirectObject(1694, 0)], '/MediaBox': [0, 0, 841, 595], '/CropBox': [0, 0, 841, 595]}, (0, 1701): {'/Type': '/Page', '/Parent': IndirectObject(2, 0), '/Resources': IndirectObject(1699, 0), '/Contents': [IndirectObject(1698, 0)], '/MediaBox': [0, 0, 841, 595], '/CropBox': [0, 0, 841, 595]}, (0, 1705): {'/Type': '/Page', '/Parent': IndirectObject(2, 0), '/Resources': IndirectObject(1703, 0), '/Contents': [IndirectObject(1702, 0)], '/MediaBox': [0, 0, 841, 595], '/CropBox': [0, 0, 841, 595]}, (0, 1709): {'/Type': '/Page', '/Parent': IndirectObject(2, 0), '/Resources': IndirectObject(1707, 0), '/Contents': [IndirectObject(1706, 0)], '/MediaBox': [0, 0, 841, 595], '/CropBox': [0, 0, 841, 595]}, (0, 1713): {'/Type': '/Page', '/Parent': IndirectObject(2, 0), '/Resources': IndirectObject(1711, 0), '/Contents': [IndirectObject(1710, 0)], '/MediaBox': [0, 0, 841, 595], '/CropBox': [0, 0, 841, 595]}, (0, 1717): {'/Type': '/Page', '/Parent': IndirectObject(2, 0), '/Resources': IndirectObject(1715, 0), '/Contents': [IndirectObject(1714, 0)], '/MediaBox': [0, 0, 841, 595], '/CropBox': [0, 0, 841, 595]}, (0, 1721): {'/Type': '/Page', '/Parent': IndirectObject(2, 0), '/Resources': IndirectObject(1719, 0), '/Contents': [IndirectObject(1718, 0)], '/MediaBox': [0, 0, 841, 595], '/CropBox': [0, 0, 841, 595]}, (0, 1725): {'/Type': '/Page', '/Parent': IndirectObject(2, 0), '/Resources': IndirectObject(1723, 0), '/Contents': [IndirectObject(1722, 0)], '/MediaBox': [0, 0, 841, 595], '/CropBox': [0, 0, 841, 595]}, (0, 1729): {'/Type': '/Page', '/Parent': IndirectObject(2, 0), '/Resources': IndirectObject(1727, 0), '/Contents': [IndirectObject(1726, 0)], '/MediaBox': [0, 0, 841, 595], '/CropBox': [0, 0, 841, 595]}, (0, 1733): {'/Type': '/Page', '/Parent': IndirectObject(2, 0), '/Resources': IndirectObject(1731, 0), '/Contents': [IndirectObject(1730, 0)], '/MediaBox': [0, 0, 841, 595], '/CropBox': [0, 0, 841, 595]}, (0, 1737): {'/Type': '/Page', '/Parent': IndirectObject(2, 0), '/Resources': IndirectObject(1735, 0), '/Contents': [IndirectObject(1734, 0)], '/MediaBox': [0, 0, 841, 595], '/CropBox': [0, 0, 841, 595]}, (0, 1741): {'/Type': '/Page', '/Parent': IndirectObject(2, 0), '/Resources': IndirectObject(1739, 0), '/Contents': [IndirectObject(1738, 0)], '/MediaBox': [0, 0, 841, 595], '/CropBox': [0, 0, 841, 595]}, (0, 1745): {'/Type': '/Page', '/Parent': IndirectObject(2, 0), '/Resources': IndirectObject(1743, 0), '/Contents': [IndirectObject(1742, 0)], '/MediaBox': [0, 0, 841, 595], '/CropBox': [0, 0, 841, 595]}, (0, 1749): {'/Type': '/Page', '/Parent': IndirectObject(2, 0), '/Resources': IndirectObject(1747, 0), '/Contents': [IndirectObject(1746, 0)], '/MediaBox': [0, 0, 841, 595], '/CropBox': [0, 0, 841, 595]}, (0, 1753): {'/Type': '/Page', '/Parent': IndirectObject(2, 0), '/Resources': IndirectObject(1751, 0), '/Contents': [IndirectObject(1750, 0)], '/MediaBox': [0, 0, 841, 595], '/CropBox': [0, 0, 841, 595]}, (0, 1757): {'/Type': '/Page', '/Parent': IndirectObject(2, 0), '/Resources': IndirectObject(1755, 0), '/Contents': [IndirectObject(1754, 0)], '/MediaBox': [0, 0, 841, 595], '/CropBox': [0, 0, 841, 595]}, (0, 1761): {'/Type': '/Page', '/Parent': IndirectObject(2, 0), '/Resources': IndirectObject(1759, 0), '/Contents': [IndirectObject(1758, 0)], '/MediaBox': [0, 0, 841, 595], '/CropBox': [0, 0, 841, 595]}, (0, 1765): {'/Type': '/Page', '/Parent': IndirectObject(2, 0), '/Resources': IndirectObject(1763, 0), '/Contents': [IndirectObject(1762, 0)], '/MediaBox': [0, 0, 841, 595], '/CropBox': [0, 0, 841, 595]}, (0, 1769): {'/Type': '/Page', '/Parent': IndirectObject(2, 0), '/Resources': IndirectObject(1767, 0), '/Contents': [IndirectObject(1766, 0)], '/MediaBox': [0, 0, 841, 595], '/CropBox': [0, 0, 841, 595]}, (0, 1773): {'/Type': '/Page', '/Parent': IndirectObject(2, 0), '/Resources': IndirectObject(1771, 0), '/Contents': [IndirectObject(1770, 0)], '/MediaBox': [0, 0, 841, 595], '/CropBox': [0, 0, 841, 595]}, (0, 1777): {'/Type': '/Page', '/Parent': IndirectObject(2, 0), '/Resources': IndirectObject(1775, 0), '/Contents': [IndirectObject(1774, 0)], '/MediaBox': [0, 0, 841, 595], '/CropBox': [0, 0, 841, 595]}, (0, 1781): {'/Type': '/Page', '/Parent': IndirectObject(2, 0), '/Resources': IndirectObject(1779, 0), '/Contents': [IndirectObject(1778, 0)], '/MediaBox': [0, 0, 841, 595], '/CropBox': [0, 0, 841, 595]}, (0, 1785): {'/Type': '/Page', '/Parent': IndirectObject(2, 0), '/Resources': IndirectObject(1783, 0), '/Contents': [IndirectObject(1782, 0)], '/MediaBox': [0, 0, 841, 595], '/CropBox': [0, 0, 841, 595]}, (0, 1789): {'/Type': '/Page', '/Parent': IndirectObject(2, 0), '/Resources': IndirectObject(1787, 0), '/Contents': [IndirectObject(1786, 0)], '/MediaBox': [0, 0, 841, 595], '/CropBox': [0, 0, 841, 595]}, (0, 1793): {'/Type': '/Page', '/Parent': IndirectObject(2, 0), '/Resources': IndirectObject(1791, 0), '/Contents': [IndirectObject(1790, 0)], '/MediaBox': [0, 0, 841, 595], '/CropBox': [0, 0, 841, 595]}, (0, 1797): {'/Type': '/Page', '/Parent': IndirectObject(2, 0), '/Resources': IndirectObject(1795, 0), '/Contents': [IndirectObject(1794, 0)], '/MediaBox': [0, 0, 841, 595], '/CropBox': [0, 0, 841, 595]}, (0, 1801): {'/Type': '/Page', '/Parent': IndirectObject(2, 0), '/Resources': IndirectObject(1799, 0), '/Contents': [IndirectObject(1798, 0)], '/MediaBox': [0, 0, 841, 595], '/CropBox': [0, 0, 841, 595]}, (0, 1805): {'/Type': '/Page', '/Parent': IndirectObject(2, 0), '/Resources': IndirectObject(1803, 0), '/Contents': [IndirectObject(1802, 0)], '/MediaBox': [0, 0, 841, 595], '/CropBox': [0, 0, 841, 595]}, (0, 1809): {'/Type': '/Page', '/Parent': IndirectObject(2, 0), '/Resources': IndirectObject(1807, 0), '/Contents': [IndirectObject(1806, 0)], '/MediaBox': [0, 0, 841, 595], '/CropBox': [0, 0, 841, 595]}, (0, 1813): {'/Type': '/Page', '/Parent': IndirectObject(2, 0), '/Resources': IndirectObject(1811, 0), '/Contents': [IndirectObject(1810, 0)], '/MediaBox': [0, 0, 841, 595], '/CropBox': [0, 0, 841, 595]}, (0, 1817): {'/Type': '/Page', '/Parent': IndirectObject(2, 0), '/Resources': IndirectObject(1815, 0), '/Contents': [IndirectObject(1814, 0)], '/MediaBox': [0, 0, 841, 595], '/CropBox': [0, 0, 841, 595]}, (0, 1821): {'/Type': '/Page', '/Parent': IndirectObject(2, 0), '/Resources': IndirectObject(1819, 0), '/Contents': [IndirectObject(1818, 0)], '/MediaBox': [0, 0, 841, 595], '/CropBox': [0, 0, 841, 595]}, (0, 1825): {'/Type': '/Page', '/Parent': IndirectObject(2, 0), '/Resources': IndirectObject(1823, 0), '/Contents': [IndirectObject(1822, 0)], '/MediaBox': [0, 0, 841, 595], '/CropBox': [0, 0, 841, 595]}, (0, 1829): {'/Type': '/Page', '/Parent': IndirectObject(2, 0), '/Resources': IndirectObject(1827, 0), '/Contents': [IndirectObject(1826, 0)], '/MediaBox': [0, 0, 841, 595], '/CropBox': [0, 0, 841, 595]}, (0, 1833): {'/Type': '/Page', '/Parent': IndirectObject(2, 0), '/Resources': IndirectObject(1831, 0), '/Contents': [IndirectObject(1830, 0)], '/MediaBox': [0, 0, 841, 595], '/CropBox': [0, 0, 841, 595]}, (0, 1837): {'/Type': '/Page', '/Parent': IndirectObject(2, 0), '/Resources': IndirectObject(1835, 0), '/Contents': [IndirectObject(1834, 0)], '/MediaBox': [0, 0, 841, 595], '/CropBox': [0, 0, 841, 595]}, (0, 1841): {'/Type': '/Page', '/Parent': IndirectObject(2, 0), '/Resources': IndirectObject(1839, 0), '/Contents': [IndirectObject(1838, 0)], '/MediaBox': [0, 0, 841, 595], '/CropBox': [0, 0, 841, 595]}, (0, 1845): {'/Type': '/Page', '/Parent': IndirectObject(2, 0), '/Resources': IndirectObject(1843, 0), '/Contents': [IndirectObject(1842, 0)], '/MediaBox': [0, 0, 841, 595], '/CropBox': [0, 0, 841, 595]}, (0, 1849): {'/Type': '/Page', '/Parent': IndirectObject(2, 0), '/Resources': IndirectObject(1847, 0), '/Contents': [IndirectObject(1846, 0)], '/MediaBox': [0, 0, 841, 595], '/CropBox': [0, 0, 841, 595]}, (0, 1853): {'/Type': '/Page', '/Parent': IndirectObject(2, 0), '/Resources': IndirectObject(1851, 0), '/Contents': [IndirectObject(1850, 0)], '/MediaBox': [0, 0, 841, 595], '/CropBox': [0, 0, 841, 595]}, (0, 1857): {'/Type': '/Page', '/Parent': IndirectObject(2, 0), '/Resources': IndirectObject(1855, 0), '/Contents': [IndirectObject(1854, 0)], '/MediaBox': [0, 0, 841, 595], '/CropBox': [0, 0, 841, 595]}, (0, 1861): {'/Type': '/Page', '/Parent': IndirectObject(2, 0), '/Resources': IndirectObject(1859, 0), '/Contents': [IndirectObject(1858, 0)], '/MediaBox': [0, 0, 841, 595], '/CropBox': [0, 0, 841, 595]}, (0, 1865): {'/Type': '/Page', '/Parent': IndirectObject(2, 0), '/Resources': IndirectObject(1863, 0), '/Contents': [IndirectObject(1862, 0)], '/MediaBox': [0, 0, 841, 595], '/CropBox': [0, 0, 841, 595]}, (0, 1869): {'/Type': '/Page', '/Parent': IndirectObject(2, 0), '/Resources': IndirectObject(1867, 0), '/Contents': [IndirectObject(1866, 0)], '/MediaBox': [0, 0, 841, 595], '/CropBox': [0, 0, 841, 595]}, (0, 1873): {'/Type': '/Page', '/Parent': IndirectObject(2, 0), '/Resources': IndirectObject(1871, 0), '/Contents': [IndirectObject(1870, 0)], '/MediaBox': [0, 0, 841, 595], '/CropBox': [0, 0, 841, 595]}, (0, 1877): {'/Type': '/Page', '/Parent': IndirectObject(2, 0), '/Resources': IndirectObject(1875, 0), '/Contents': [IndirectObject(1874, 0)], '/MediaBox': [0, 0, 841, 595], '/CropBox': [0, 0, 841, 595]}, (0, 1881): {'/Type': '/Page', '/Parent': IndirectObject(2, 0), '/Resources': IndirectObject(1879, 0), '/Contents': [IndirectObject(1878, 0)], '/MediaBox': [0, 0, 841, 595], '/CropBox': [0, 0, 841, 595]}, (0, 1885): {'/Type': '/Page', '/Parent': IndirectObject(2, 0), '/Resources': IndirectObject(1883, 0), '/Contents': [IndirectObject(1882, 0)], '/MediaBox': [0, 0, 841, 595], '/CropBox': [0, 0, 841, 595]}, (0, 1889): {'/Type': '/Page', '/Parent': IndirectObject(2, 0), '/Resources': IndirectObject(1887, 0), '/Contents': [IndirectObject(1886, 0)], '/MediaBox': [0, 0, 841, 595], '/CropBox': [0, 0, 841, 595]}, (0, 1893): {'/Type': '/Page', '/Parent': IndirectObject(2, 0), '/Resources': IndirectObject(1891, 0), '/Contents': [IndirectObject(1890, 0)], '/MediaBox': [0, 0, 841, 595], '/CropBox': [0, 0, 841, 595]}, (0, 1897): {'/Type': '/Page', '/Parent': IndirectObject(2, 0), '/Resources': IndirectObject(1895, 0), '/Contents': [IndirectObject(1894, 0)], '/MediaBox': [0, 0, 841, 595], '/CropBox': [0, 0, 841, 595]}, (0, 1901): {'/Type': '/Page', '/Parent': IndirectObject(2, 0), '/Resources': IndirectObject(1899, 0), '/Contents': [IndirectObject(1898, 0)], '/MediaBox': [0, 0, 841, 595], '/CropBox': [0, 0, 841, 595]}, (0, 1905): {'/Type': '/Page', '/Parent': IndirectObject(2, 0), '/Resources': IndirectObject(1903, 0), '/Contents': [IndirectObject(1902, 0)], '/MediaBox': [0, 0, 841, 595], '/CropBox': [0, 0, 841, 595]}, (0, 1909): {'/Type': '/Page', '/Parent': IndirectObject(2, 0), '/Resources': IndirectObject(1907, 0), '/Contents': [IndirectObject(1906, 0)], '/MediaBox': [0, 0, 841, 595], '/CropBox': [0, 0, 841, 595]}, (0, 1913): {'/Type': '/Page', '/Parent': IndirectObject(2, 0), '/Resources': IndirectObject(1911, 0), '/Contents': [IndirectObject(1910, 0)], '/MediaBox': [0, 0, 841, 595], '/CropBox': [0, 0, 841, 595]}, (0, 1917): {'/Type': '/Page', '/Parent': IndirectObject(2, 0), '/Resources': IndirectObject(1915, 0), '/Contents': [IndirectObject(1914, 0)], '/MediaBox': [0, 0, 841, 595], '/CropBox': [0, 0, 841, 595]}, (0, 1921): {'/Type': '/Page', '/Parent': IndirectObject(2, 0), '/Resources': IndirectObject(1919, 0), '/Contents': [IndirectObject(1918, 0)], '/MediaBox': [0, 0, 841, 595], '/CropBox': [0, 0, 841, 595]}, (0, 1925): {'/Type': '/Page', '/Parent': IndirectObject(2, 0), '/Resources': IndirectObject(1923, 0), '/Contents': [IndirectObject(1922, 0)], '/MediaBox': [0, 0, 841, 595], '/CropBox': [0, 0, 841, 595]}, (0, 1929): {'/Type': '/Page', '/Parent': IndirectObject(2, 0), '/Resources': IndirectObject(1927, 0), '/Contents': [IndirectObject(1926, 0)], '/MediaBox': [0, 0, 841, 595], '/CropBox': [0, 0, 841, 595]}, (0, 1933): {'/Type': '/Page', '/Parent': IndirectObject(2, 0), '/Resources': IndirectObject(1931, 0), '/Contents': [IndirectObject(1930, 0)], '/MediaBox': [0, 0, 841, 595], '/CropBox': [0, 0, 841, 595]}, (0, 1937): {'/Type': '/Page', '/Parent': IndirectObject(2, 0), '/Resources': IndirectObject(1935, 0), '/Contents': [IndirectObject(1934, 0)], '/MediaBox': [0, 0, 841, 595], '/CropBox': [0, 0, 841, 595]}, (0, 1941): {'/Type': '/Page', '/Parent': IndirectObject(2, 0), '/Resources': IndirectObject(1939, 0), '/Contents': [IndirectObject(1938, 0)], '/MediaBox': [0, 0, 841, 595], '/CropBox': [0, 0, 841, 595]}, (0, 1945): {'/Type': '/Page', '/Parent': IndirectObject(2, 0), '/Resources': IndirectObject(1943, 0), '/Contents': [IndirectObject(1942, 0)], '/MediaBox': [0, 0, 841, 595], '/CropBox': [0, 0, 841, 595]}, (0, 1949): {'/Type': '/Page', '/Parent': IndirectObject(2, 0), '/Resources': IndirectObject(1947, 0), '/Contents': [IndirectObject(1946, 0)], '/MediaBox': [0, 0, 841, 595], '/CropBox': [0, 0, 841, 595]}, (0, 1953): {'/Type': '/Page', '/Parent': IndirectObject(2, 0), '/Resources': IndirectObject(1951, 0), '/Contents': [IndirectObject(1950, 0)], '/MediaBox': [0, 0, 841, 595], '/CropBox': [0, 0, 841, 595]}, (0, 1957): {'/Type': '/Page', '/Parent': IndirectObject(2, 0), '/Resources': IndirectObject(1955, 0), '/Contents': [IndirectObject(1954, 0)], '/MediaBox': [0, 0, 841, 595], '/CropBox': [0, 0, 841, 595]}, (0, 1961): {'/Type': '/Page', '/Parent': IndirectObject(2, 0), '/Resources': IndirectObject(1959, 0), '/Contents': [IndirectObject(1958, 0)], '/MediaBox': [0, 0, 841, 595], '/CropBox': [0, 0, 841, 595]}, (0, 1965): {'/Type': '/Page', '/Parent': IndirectObject(2, 0), '/Resources': IndirectObject(1963, 0), '/Contents': [IndirectObject(1962, 0)], '/MediaBox': [0, 0, 841, 595], '/CropBox': [0, 0, 841, 595]}, (0, 1969): {'/Type': '/Page', '/Parent': IndirectObject(2, 0), '/Resources': IndirectObject(1967, 0), '/Contents': [IndirectObject(1966, 0)], '/MediaBox': [0, 0, 841, 595], '/CropBox': [0, 0, 841, 595]}, (0, 1973): {'/Type': '/Page', '/Parent': IndirectObject(2, 0), '/Resources': IndirectObject(1971, 0), '/Contents': [IndirectObject(1970, 0)], '/MediaBox': [0, 0, 841, 595], '/CropBox': [0, 0, 841, 595]}, (0, 1977): {'/Type': '/Page', '/Parent': IndirectObject(2, 0), '/Resources': IndirectObject(1975, 0), '/Contents': [IndirectObject(1974, 0)], '/MediaBox': [0, 0, 841, 595], '/CropBox': [0, 0, 841, 595]}, (0, 1981): {'/Type': '/Page', '/Parent': IndirectObject(2, 0), '/Resources': IndirectObject(1979, 0), '/Contents': [IndirectObject(1978, 0)], '/MediaBox': [0, 0, 841, 595], '/CropBox': [0, 0, 841, 595]}, (0, 1985): {'/Type': '/Page', '/Parent': IndirectObject(2, 0), '/Resources': IndirectObject(1983, 0), '/Contents': [IndirectObject(1982, 0)], '/MediaBox': [0, 0, 841, 595], '/CropBox': [0, 0, 841, 595]}, (0, 1989): {'/Type': '/Page', '/Parent': IndirectObject(2, 0), '/Resources': IndirectObject(1987, 0), '/Contents': [IndirectObject(1986, 0)], '/MediaBox': [0, 0, 841, 595], '/CropBox': [0, 0, 841, 595]}, (0, 1993): {'/Type': '/Page', '/Parent': IndirectObject(2, 0), '/Resources': IndirectObject(1991, 0), '/Contents': [IndirectObject(1990, 0)], '/MediaBox': [0, 0, 841, 595], '/CropBox': [0, 0, 841, 595]}, (0, 1997): {'/Type': '/Page', '/Parent': IndirectObject(2, 0), '/Resources': IndirectObject(1995, 0), '/Contents': [IndirectObject(1994, 0)], '/MediaBox': [0, 0, 841, 595], '/CropBox': [0, 0, 841, 595]}, (0, 2001): {'/Type': '/Page', '/Parent': IndirectObject(2, 0), '/Resources': IndirectObject(1999, 0), '/Contents': [IndirectObject(1998, 0)], '/MediaBox': [0, 0, 841, 595], '/CropBox': [0, 0, 841, 595]}, (0, 2005): {'/Type': '/Page', '/Parent': IndirectObject(2, 0), '/Resources': IndirectObject(2003, 0), '/Contents': [IndirectObject(2002, 0)], '/MediaBox': [0, 0, 841, 595], '/CropBox': [0, 0, 841, 595]}, (0, 2009): {'/Type': '/Page', '/Parent': IndirectObject(2, 0), '/Resources': IndirectObject(2007, 0), '/Contents': [IndirectObject(2006, 0)], '/MediaBox': [0, 0, 841, 595], '/CropBox': [0, 0, 841, 595]}, (0, 2013): {'/Type': '/Page', '/Parent': IndirectObject(2, 0), '/Resources': IndirectObject(2011, 0), '/Contents': [IndirectObject(2010, 0)], '/MediaBox': [0, 0, 841, 595], '/CropBox': [0, 0, 841, 595]}, (0, 2017): {'/Type': '/Page', '/Parent': IndirectObject(2, 0), '/Resources': IndirectObject(2015, 0), '/Contents': [IndirectObject(2014, 0)], '/MediaBox': [0, 0, 841, 595], '/CropBox': [0, 0, 841, 595]}, (0, 2021): {'/Type': '/Page', '/Parent': IndirectObject(2, 0), '/Resources': IndirectObject(2019, 0), '/Contents': [IndirectObject(2018, 0)], '/MediaBox': [0, 0, 841, 595], '/CropBox': [0, 0, 841, 595]}, (0, 2025): {'/Type': '/Page', '/Parent': IndirectObject(2, 0), '/Resources': IndirectObject(2023, 0), '/Contents': [IndirectObject(2022, 0)], '/MediaBox': [0, 0, 841, 595], '/CropBox': [0, 0, 841, 595]}, (0, 2029): {'/Type': '/Page', '/Parent': IndirectObject(2, 0), '/Resources': IndirectObject(2027, 0), '/Contents': [IndirectObject(2026, 0)], '/MediaBox': [0, 0, 841, 595], '/CropBox': [0, 0, 841, 595]}, (0, 2033): {'/Type': '/Page', '/Parent': IndirectObject(2, 0), '/Resources': IndirectObject(2031, 0), '/Contents': [IndirectObject(2030, 0)], '/MediaBox': [0, 0, 841, 595], '/CropBox': [0, 0, 841, 595]}, (0, 2037): {'/Type': '/Page', '/Parent': IndirectObject(2, 0), '/Resources': IndirectObject(2035, 0), '/Contents': [IndirectObject(2034, 0)], '/MediaBox': [0, 0, 841, 595], '/CropBox': [0, 0, 841, 595]}, (0, 2041): {'/Type': '/Page', '/Parent': IndirectObject(2, 0), '/Resources': IndirectObject(2039, 0), '/Contents': [IndirectObject(2038, 0)], '/MediaBox': [0, 0, 841, 595], '/CropBox': [0, 0, 841, 595]}, (0, 2045): {'/Type': '/Page', '/Parent': IndirectObject(2, 0), '/Resources': IndirectObject(2043, 0), '/Contents': [IndirectObject(2042, 0)], '/MediaBox': [0, 0, 841, 595], '/CropBox': [0, 0, 841, 595]}, (0, 2049): {'/Type': '/Page', '/Parent': IndirectObject(2, 0), '/Resources': IndirectObject(2047, 0), '/Contents': [IndirectObject(2046, 0)], '/MediaBox': [0, 0, 841, 595], '/CropBox': [0, 0, 841, 595]}, (0, 2053): {'/Type': '/Page', '/Parent': IndirectObject(2, 0), '/Resources': IndirectObject(2051, 0), '/Contents': [IndirectObject(2050, 0)], '/MediaBox': [0, 0, 841, 595], '/CropBox': [0, 0, 841, 595]}, (0, 2057): {'/Type': '/Page', '/Parent': IndirectObject(2, 0), '/Resources': IndirectObject(2055, 0), '/Contents': [IndirectObject(2054, 0)], '/MediaBox': [0, 0, 841, 595], '/CropBox': [0, 0, 841, 595]}, (0, 2061): {'/Type': '/Page', '/Parent': IndirectObject(2, 0), '/Resources': IndirectObject(2059, 0), '/Contents': [IndirectObject(2058, 0)], '/MediaBox': [0, 0, 841, 595], '/CropBox': [0, 0, 841, 595]}, (0, 2065): {'/Type': '/Page', '/Parent': IndirectObject(2, 0), '/Resources': IndirectObject(2063, 0), '/Contents': [IndirectObject(2062, 0)], '/MediaBox': [0, 0, 841, 595], '/CropBox': [0, 0, 841, 595]}, (0, 2069): {'/Type': '/Page', '/Parent': IndirectObject(2, 0), '/Resources': IndirectObject(2067, 0), '/Contents': [IndirectObject(2066, 0)], '/MediaBox': [0, 0, 841, 595], '/CropBox': [0, 0, 841, 595]}, (0, 2073): {'/Type': '/Page', '/Parent': IndirectObject(2, 0), '/Resources': IndirectObject(2071, 0), '/Contents': [IndirectObject(2070, 0)], '/MediaBox': [0, 0, 841, 595], '/CropBox': [0, 0, 841, 595]}, (0, 2077): {'/Type': '/Page', '/Parent': IndirectObject(2, 0), '/Resources': IndirectObject(2075, 0), '/Contents': [IndirectObject(2074, 0)], '/MediaBox': [0, 0, 841, 595], '/CropBox': [0, 0, 841, 595]}, (0, 2081): {'/Type': '/Page', '/Parent': IndirectObject(2, 0), '/Resources': IndirectObject(2079, 0), '/Contents': [IndirectObject(2078, 0)], '/MediaBox': [0, 0, 841, 595], '/CropBox': [0, 0, 841, 595]}, (0, 2085): {'/Type': '/Page', '/Parent': IndirectObject(2, 0), '/Resources': IndirectObject(2083, 0), '/Contents': [IndirectObject(2082, 0)], '/MediaBox': [0, 0, 841, 595], '/CropBox': [0, 0, 841, 595]}, (0, 2089): {'/Type': '/Page', '/Parent': IndirectObject(2, 0), '/Resources': IndirectObject(2087, 0), '/Contents': [IndirectObject(2086, 0)], '/MediaBox': [0, 0, 841, 595], '/CropBox': [0, 0, 841, 595]}, (0, 2093): {'/Type': '/Page', '/Parent': IndirectObject(2, 0), '/Resources': IndirectObject(2091, 0), '/Contents': [IndirectObject(2090, 0)], '/MediaBox': [0, 0, 841, 595], '/CropBox': [0, 0, 841, 595]}, (0, 2097): {'/Type': '/Page', '/Parent': IndirectObject(2, 0), '/Resources': IndirectObject(2095, 0), '/Contents': [IndirectObject(2094, 0)], '/MediaBox': [0, 0, 841, 595], '/CropBox': [0, 0, 841, 595]}, (0, 2101): {'/Type': '/Page', '/Parent': IndirectObject(2, 0), '/Resources': IndirectObject(2099, 0), '/Contents': [IndirectObject(2098, 0)], '/MediaBox': [0, 0, 841, 595], '/CropBox': [0, 0, 841, 595]}, (0, 2105): {'/Type': '/Page', '/Parent': IndirectObject(2, 0), '/Resources': IndirectObject(2103, 0), '/Contents': [IndirectObject(2102, 0)], '/MediaBox': [0, 0, 841, 595], '/CropBox': [0, 0, 841, 595]}, (0, 2109): {'/Type': '/Page', '/Parent': IndirectObject(2, 0), '/Resources': IndirectObject(2107, 0), '/Contents': [IndirectObject(2106, 0)], '/MediaBox': [0, 0, 841, 595], '/CropBox': [0, 0, 841, 595]}, (0, 2113): {'/Type': '/Page', '/Parent': IndirectObject(2, 0), '/Resources': IndirectObject(2111, 0), '/Contents': [IndirectObject(2110, 0)], '/MediaBox': [0, 0, 841, 595], '/CropBox': [0, 0, 841, 595]}, (0, 2117): {'/Type': '/Page', '/Parent': IndirectObject(2, 0), '/Resources': IndirectObject(2115, 0), '/Contents': [IndirectObject(2114, 0)], '/MediaBox': [0, 0, 841, 595], '/CropBox': [0, 0, 841, 595]}, (0, 2121): {'/Type': '/Page', '/Parent': IndirectObject(2, 0), '/Resources': IndirectObject(2119, 0), '/Contents': [IndirectObject(2118, 0)], '/MediaBox': [0, 0, 841, 595], '/CropBox': [0, 0, 841, 595]}, (0, 2125): {'/Type': '/Page', '/Parent': IndirectObject(2, 0), '/Resources': IndirectObject(2123, 0), '/Contents': [IndirectObject(2122, 0)], '/MediaBox': [0, 0, 841, 595], '/CropBox': [0, 0, 841, 595]}, (0, 2129): {'/Type': '/Page', '/Parent': IndirectObject(2, 0), '/Resources': IndirectObject(2127, 0), '/Contents': [IndirectObject(2126, 0)], '/MediaBox': [0, 0, 841, 595], '/CropBox': [0, 0, 841, 595]}, (0, 2133): {'/Type': '/Page', '/Parent': IndirectObject(2, 0), '/Resources': IndirectObject(2131, 0), '/Contents': [IndirectObject(2130, 0)], '/MediaBox': [0, 0, 841, 595], '/CropBox': [0, 0, 841, 595]}, (0, 2137): {'/Type': '/Page', '/Parent': IndirectObject(2, 0), '/Resources': IndirectObject(2135, 0), '/Contents': [IndirectObject(2134, 0)], '/MediaBox': [0, 0, 841, 595], '/CropBox': [0, 0, 841, 595]}, (0, 2141): {'/Type': '/Page', '/Parent': IndirectObject(2, 0), '/Resources': IndirectObject(2139, 0), '/Contents': [IndirectObject(2138, 0)], '/MediaBox': [0, 0, 841, 595], '/CropBox': [0, 0, 841, 595]}, (0, 2145): {'/Type': '/Page', '/Parent': IndirectObject(2, 0), '/Resources': IndirectObject(2143, 0), '/Contents': [IndirectObject(2142, 0)], '/MediaBox': [0, 0, 841, 595], '/CropBox': [0, 0, 841, 595]}, (0, 2149): {'/Type': '/Page', '/Parent': IndirectObject(2, 0), '/Resources': IndirectObject(2147, 0), '/Contents': [IndirectObject(2146, 0)], '/MediaBox': [0, 0, 841, 595], '/CropBox': [0, 0, 841, 595]}, (0, 2153): {'/Type': '/Page', '/Parent': IndirectObject(2, 0), '/Resources': IndirectObject(2151, 0), '/Contents': [IndirectObject(2150, 0)], '/MediaBox': [0, 0, 841, 595], '/CropBox': [0, 0, 841, 595]}, (0, 2157): {'/Type': '/Page', '/Parent': IndirectObject(2, 0), '/Resources': IndirectObject(2155, 0), '/Contents': [IndirectObject(2154, 0)], '/MediaBox': [0, 0, 841, 595], '/CropBox': [0, 0, 841, 595]}, (0, 2161): {'/Type': '/Page', '/Parent': IndirectObject(2, 0), '/Resources': IndirectObject(2159, 0), '/Contents': [IndirectObject(2158, 0)], '/MediaBox': [0, 0, 841, 595], '/CropBox': [0, 0, 841, 595]}, (0, 2165): {'/Type': '/Page', '/Parent': IndirectObject(2, 0), '/Resources': IndirectObject(2163, 0), '/Contents': [IndirectObject(2162, 0)], '/MediaBox': [0, 0, 841, 595], '/CropBox': [0, 0, 841, 595]}, (0, 2169): {'/Type': '/Page', '/Parent': IndirectObject(2, 0), '/Resources': IndirectObject(2167, 0), '/Contents': [IndirectObject(2166, 0)], '/MediaBox': [0, 0, 841, 595], '/CropBox': [0, 0, 841, 595]}, (0, 2173): {'/Type': '/Page', '/Parent': IndirectObject(2, 0), '/Resources': IndirectObject(2171, 0), '/Contents': [IndirectObject(2170, 0)], '/MediaBox': [0, 0, 841, 595], '/CropBox': [0, 0, 841, 595]}, (0, 2177): {'/Type': '/Page', '/Parent': IndirectObject(2, 0), '/Resources': IndirectObject(2175, 0), '/Contents': [IndirectObject(2174, 0)], '/MediaBox': [0, 0, 841, 595], '/CropBox': [0, 0, 841, 595]}, (0, 2181): {'/Type': '/Page', '/Parent': IndirectObject(2, 0), '/Resources': IndirectObject(2179, 0), '/Contents': [IndirectObject(2178, 0)], '/MediaBox': [0, 0, 841, 595], '/CropBox': [0, 0, 841, 595]}, (0, 2185): {'/Type': '/Page', '/Parent': IndirectObject(2, 0), '/Resources': IndirectObject(2183, 0), '/Contents': [IndirectObject(2182, 0)], '/MediaBox': [0, 0, 841, 595], '/CropBox': [0, 0, 841, 595]}, (0, 2189): {'/Type': '/Page', '/Parent': IndirectObject(2, 0), '/Resources': IndirectObject(2187, 0), '/Contents': [IndirectObject(2186, 0)], '/MediaBox': [0, 0, 841, 595], '/CropBox': [0, 0, 841, 595]}, (0, 2193): {'/Type': '/Page', '/Parent': IndirectObject(2, 0), '/Resources': IndirectObject(2191, 0), '/Contents': [IndirectObject(2190, 0)], '/MediaBox': [0, 0, 841, 595], '/CropBox': [0, 0, 841, 595]}, (0, 2197): {'/Type': '/Page', '/Parent': IndirectObject(2, 0), '/Resources': IndirectObject(2195, 0), '/Contents': [IndirectObject(2194, 0)], '/MediaBox': [0, 0, 841, 595], '/CropBox': [0, 0, 841, 595]}, (0, 2201): {'/Type': '/Page', '/Parent': IndirectObject(2, 0), '/Resources': IndirectObject(2199, 0), '/Contents': [IndirectObject(2198, 0)], '/MediaBox': [0, 0, 841, 595], '/CropBox': [0, 0, 841, 595]}, (0, 2205): {'/Type': '/Page', '/Parent': IndirectObject(2, 0), '/Resources': IndirectObject(2203, 0), '/Contents': [IndirectObject(2202, 0)], '/MediaBox': [0, 0, 841, 595], '/CropBox': [0, 0, 841, 595]}, (0, 2209): {'/Type': '/Page', '/Parent': IndirectObject(2, 0), '/Resources': IndirectObject(2207, 0), '/Contents': [IndirectObject(2206, 0)], '/MediaBox': [0, 0, 841, 595], '/CropBox': [0, 0, 841, 595]}, (0, 2213): {'/Type': '/Page', '/Parent': IndirectObject(2, 0), '/Resources': IndirectObject(2211, 0), '/Contents': [IndirectObject(2210, 0)], '/MediaBox': [0, 0, 841, 595], '/CropBox': [0, 0, 841, 595]}, (0, 2217): {'/Type': '/Page', '/Parent': IndirectObject(2, 0), '/Resources': IndirectObject(2215, 0), '/Contents': [IndirectObject(2214, 0)], '/MediaBox': [0, 0, 841, 595], '/CropBox': [0, 0, 841, 595]}, (0, 2221): {'/Type': '/Page', '/Parent': IndirectObject(2, 0), '/Resources': IndirectObject(2219, 0), '/Contents': [IndirectObject(2218, 0)], '/MediaBox': [0, 0, 841, 595], '/CropBox': [0, 0, 841, 595]}, (0, 2225): {'/Type': '/Page', '/Parent': IndirectObject(2, 0), '/Resources': IndirectObject(2223, 0), '/Contents': [IndirectObject(2222, 0)], '/MediaBox': [0, 0, 841, 595], '/CropBox': [0, 0, 841, 595]}, (0, 2229): {'/Type': '/Page', '/Parent': IndirectObject(2, 0), '/Resources': IndirectObject(2227, 0), '/Contents': [IndirectObject(2226, 0)], '/MediaBox': [0, 0, 841, 595], '/CropBox': [0, 0, 841, 595]}, (0, 2233): {'/Type': '/Page', '/Parent': IndirectObject(2, 0), '/Resources': IndirectObject(2231, 0), '/Contents': [IndirectObject(2230, 0)], '/MediaBox': [0, 0, 841, 595], '/CropBox': [0, 0, 841, 595]}, (0, 2237): {'/Type': '/Page', '/Parent': IndirectObject(2, 0), '/Resources': IndirectObject(2235, 0), '/Contents': [IndirectObject(2234, 0)], '/MediaBox': [0, 0, 841, 595], '/CropBox': [0, 0, 841, 595]}, (0, 2241): {'/Type': '/Page', '/Parent': IndirectObject(2, 0), '/Resources': IndirectObject(2239, 0), '/Contents': [IndirectObject(2238, 0)], '/MediaBox': [0, 0, 841, 595], '/CropBox': [0, 0, 841, 595]}, (0, 2245): {'/Type': '/Page', '/Parent': IndirectObject(2, 0), '/Resources': IndirectObject(2243, 0), '/Contents': [IndirectObject(2242, 0)], '/MediaBox': [0, 0, 841, 595], '/CropBox': [0, 0, 841, 595]}, (0, 2249): {'/Type': '/Page', '/Parent': IndirectObject(2, 0), '/Resources': IndirectObject(2247, 0), '/Contents': [IndirectObject(2246, 0)], '/MediaBox': [0, 0, 841, 595], '/CropBox': [0, 0, 841, 595]}, (0, 2253): {'/Type': '/Page', '/Parent': IndirectObject(2, 0), '/Resources': IndirectObject(2251, 0), '/Contents': [IndirectObject(2250, 0)], '/MediaBox': [0, 0, 841, 595], '/CropBox': [0, 0, 841, 595]}, (0, 2257): {'/Type': '/Page', '/Parent': IndirectObject(2, 0), '/Resources': IndirectObject(2255, 0), '/Contents': [IndirectObject(2254, 0)], '/MediaBox': [0, 0, 841, 595], '/CropBox': [0, 0, 841, 595]}, (0, 2261): {'/Type': '/Page', '/Parent': IndirectObject(2, 0), '/Resources': IndirectObject(2259, 0), '/Contents': [IndirectObject(2258, 0)], '/MediaBox': [0, 0, 841, 595], '/CropBox': [0, 0, 841, 595]}, (0, 2265): {'/Type': '/Page', '/Parent': IndirectObject(2, 0), '/Resources': IndirectObject(2263, 0), '/Contents': [IndirectObject(2262, 0)], '/MediaBox': [0, 0, 841, 595], '/CropBox': [0, 0, 841, 595]}, (0, 2269): {'/Type': '/Page', '/Parent': IndirectObject(2, 0), '/Resources': IndirectObject(2267, 0), '/Contents': [IndirectObject(2266, 0)], '/MediaBox': [0, 0, 841, 595], '/CropBox': [0, 0, 841, 595]}, (0, 2273): {'/Type': '/Page', '/Parent': IndirectObject(2, 0), '/Resources': IndirectObject(2271, 0), '/Contents': [IndirectObject(2270, 0)], '/MediaBox': [0, 0, 841, 595], '/CropBox': [0, 0, 841, 595]}, (0, 2277): {'/Type': '/Page', '/Parent': IndirectObject(2, 0), '/Resources': IndirectObject(2275, 0), '/Contents': [IndirectObject(2274, 0)], '/MediaBox': [0, 0, 841, 595], '/CropBox': [0, 0, 841, 595]}, (0, 2281): {'/Type': '/Page', '/Parent': IndirectObject(2, 0), '/Resources': IndirectObject(2279, 0), '/Contents': [IndirectObject(2278, 0)], '/MediaBox': [0, 0, 841, 595], '/CropBox': [0, 0, 841, 595]}, (0, 2285): {'/Type': '/Page', '/Parent': IndirectObject(2, 0), '/Resources': IndirectObject(2283, 0), '/Contents': [IndirectObject(2282, 0)], '/MediaBox': [0, 0, 841, 595], '/CropBox': [0, 0, 841, 595]}, (0, 2289): {'/Type': '/Page', '/Parent': IndirectObject(2, 0), '/Resources': IndirectObject(2287, 0), '/Contents': [IndirectObject(2286, 0)], '/MediaBox': [0, 0, 841, 595], '/CropBox': [0, 0, 841, 595]}, (0, 2293): {'/Type': '/Page', '/Parent': IndirectObject(2, 0), '/Resources': IndirectObject(2291, 0), '/Contents': [IndirectObject(2290, 0)], '/MediaBox': [0, 0, 841, 595], '/CropBox': [0, 0, 841, 595]}, (0, 2297): {'/Type': '/Page', '/Parent': IndirectObject(2, 0), '/Resources': IndirectObject(2295, 0), '/Contents': [IndirectObject(2294, 0)], '/MediaBox': [0, 0, 841, 595], '/CropBox': [0, 0, 841, 595]}, (0, 2301): {'/Type': '/Page', '/Parent': IndirectObject(2, 0), '/Resources': IndirectObject(2299, 0), '/Contents': [IndirectObject(2298, 0)], '/MediaBox': [0, 0, 841, 595], '/CropBox': [0, 0, 841, 595]}, (0, 2305): {'/Type': '/Page', '/Parent': IndirectObject(2, 0), '/Resources': IndirectObject(2303, 0), '/Contents': [IndirectObject(2302, 0)], '/MediaBox': [0, 0, 841, 595], '/CropBox': [0, 0, 841, 595]}, (0, 2309): {'/Type': '/Page', '/Parent': IndirectObject(2, 0), '/Resources': IndirectObject(2307, 0), '/Contents': [IndirectObject(2306, 0)], '/MediaBox': [0, 0, 841, 595], '/CropBox': [0, 0, 841, 595]}, (0, 2313): {'/Type': '/Page', '/Parent': IndirectObject(2, 0), '/Resources': IndirectObject(2311, 0), '/Contents': [IndirectObject(2310, 0)], '/MediaBox': [0, 0, 841, 595], '/CropBox': [0, 0, 841, 595]}, (0, 2317): {'/Type': '/Page', '/Parent': IndirectObject(2, 0), '/Resources': IndirectObject(2315, 0), '/Contents': [IndirectObject(2314, 0)], '/MediaBox': [0, 0, 841, 595], '/CropBox': [0, 0, 841, 595]}, (0, 2321): {'/Type': '/Page', '/Parent': IndirectObject(2, 0), '/Resources': IndirectObject(2319, 0), '/Contents': [IndirectObject(2318, 0)], '/MediaBox': [0, 0, 841, 595], '/CropBox': [0, 0, 841, 595]}, (0, 2325): {'/Type': '/Page', '/Parent': IndirectObject(2, 0), '/Resources': IndirectObject(2323, 0), '/Contents': [IndirectObject(2322, 0)], '/MediaBox': [0, 0, 841, 595], '/CropBox': [0, 0, 841, 595]}, (0, 2329): {'/Type': '/Page', '/Parent': IndirectObject(2, 0), '/Resources': IndirectObject(2327, 0), '/Contents': [IndirectObject(2326, 0)], '/MediaBox': [0, 0, 841, 595], '/CropBox': [0, 0, 841, 595]}, (0, 2333): {'/Type': '/Page', '/Parent': IndirectObject(2, 0), '/Resources': IndirectObject(2331, 0), '/Contents': [IndirectObject(2330, 0)], '/MediaBox': [0, 0, 841, 595], '/CropBox': [0, 0, 841, 595]}, (0, 2337): {'/Type': '/Page', '/Parent': IndirectObject(2, 0), '/Resources': IndirectObject(2335, 0), '/Contents': [IndirectObject(2334, 0)], '/MediaBox': [0, 0, 841, 595], '/CropBox': [0, 0, 841, 595]}, (0, 2341): {'/Type': '/Page', '/Parent': IndirectObject(2, 0), '/Resources': IndirectObject(2339, 0), '/Contents': [IndirectObject(2338, 0)], '/MediaBox': [0, 0, 841, 595], '/CropBox': [0, 0, 841, 595]}, (0, 2345): {'/Type': '/Page', '/Parent': IndirectObject(2, 0), '/Resources': IndirectObject(2343, 0), '/Contents': [IndirectObject(2342, 0)], '/MediaBox': [0, 0, 841, 595], '/CropBox': [0, 0, 841, 595]}, (0, 2349): {'/Type': '/Page', '/Parent': IndirectObject(2, 0), '/Resources': IndirectObject(2347, 0), '/Contents': [IndirectObject(2346, 0)], '/MediaBox': [0, 0, 841, 595], '/CropBox': [0, 0, 841, 595]}, (0, 2353): {'/Type': '/Page', '/Parent': IndirectObject(2, 0), '/Resources': IndirectObject(2351, 0), '/Contents': [IndirectObject(2350, 0)], '/MediaBox': [0, 0, 841, 595], '/CropBox': [0, 0, 841, 595]}, (0, 2357): {'/Type': '/Page', '/Parent': IndirectObject(2, 0), '/Resources': IndirectObject(2355, 0), '/Contents': [IndirectObject(2354, 0)], '/MediaBox': [0, 0, 841, 595], '/CropBox': [0, 0, 841, 595]}, (0, 2361): {'/Type': '/Page', '/Parent': IndirectObject(2, 0), '/Resources': IndirectObject(2359, 0), '/Contents': [IndirectObject(2358, 0)], '/MediaBox': [0, 0, 841, 595], '/CropBox': [0, 0, 841, 595]}, (0, 2365): {'/Type': '/Page', '/Parent': IndirectObject(2, 0), '/Resources': IndirectObject(2363, 0), '/Contents': [IndirectObject(2362, 0)], '/MediaBox': [0, 0, 841, 595], '/CropBox': [0, 0, 841, 595]}, (0, 2369): {'/Type': '/Page', '/Parent': IndirectObject(2, 0), '/Resources': IndirectObject(2367, 0), '/Contents': [IndirectObject(2366, 0)], '/MediaBox': [0, 0, 841, 595], '/CropBox': [0, 0, 841, 595]}, (0, 2373): {'/Type': '/Page', '/Parent': IndirectObject(2, 0), '/Resources': IndirectObject(2371, 0), '/Contents': [IndirectObject(2370, 0)], '/MediaBox': [0, 0, 841, 595], '/CropBox': [0, 0, 841, 595]}, (0, 2377): {'/Type': '/Page', '/Parent': IndirectObject(2, 0), '/Resources': IndirectObject(2375, 0), '/Contents': [IndirectObject(2374, 0)], '/MediaBox': [0, 0, 841, 595], '/CropBox': [0, 0, 841, 595]}, (0, 2381): {'/Type': '/Page', '/Parent': IndirectObject(2, 0), '/Resources': IndirectObject(2379, 0), '/Contents': [IndirectObject(2378, 0)], '/MediaBox': [0, 0, 841, 595], '/CropBox': [0, 0, 841, 595]}, (0, 2385): {'/Type': '/Page', '/Parent': IndirectObject(2, 0), '/Resources': IndirectObject(2383, 0), '/Contents': [IndirectObject(2382, 0)], '/MediaBox': [0, 0, 841, 595], '/CropBox': [0, 0, 841, 595]}, (0, 2389): {'/Type': '/Page', '/Parent': IndirectObject(2, 0), '/Resources': IndirectObject(2387, 0), '/Contents': [IndirectObject(2386, 0)], '/MediaBox': [0, 0, 841, 595], '/CropBox': [0, 0, 841, 595]}, (0, 2393): {'/Type': '/Page', '/Parent': IndirectObject(2, 0), '/Resources': IndirectObject(2391, 0), '/Contents': [IndirectObject(2390, 0)], '/MediaBox': [0, 0, 841, 595], '/CropBox': [0, 0, 841, 595]}, (0, 2397): {'/Type': '/Page', '/Parent': IndirectObject(2, 0), '/Resources': IndirectObject(2395, 0), '/Contents': [IndirectObject(2394, 0)], '/MediaBox': [0, 0, 841, 595], '/CropBox': [0, 0, 841, 595]}, (0, 2401): {'/Type': '/Page', '/Parent': IndirectObject(2, 0), '/Resources': IndirectObject(2399, 0), '/Contents': [IndirectObject(2398, 0)], '/MediaBox': [0, 0, 841, 595], '/CropBox': [0, 0, 841, 595]}, (0, 2405): {'/Type': '/Page', '/Parent': IndirectObject(2, 0), '/Resources': IndirectObject(2403, 0), '/Contents': [IndirectObject(2402, 0)], '/MediaBox': [0, 0, 841, 595], '/CropBox': [0, 0, 841, 595]}, (0, 2409): {'/Type': '/Page', '/Parent': IndirectObject(2, 0), '/Resources': IndirectObject(2407, 0), '/Contents': [IndirectObject(2406, 0)], '/MediaBox': [0, 0, 841, 595], '/CropBox': [0, 0, 841, 595]}, (0, 2413): {'/Type': '/Page', '/Parent': IndirectObject(2, 0), '/Resources': IndirectObject(2411, 0), '/Contents': [IndirectObject(2410, 0)], '/MediaBox': [0, 0, 841, 595], '/CropBox': [0, 0, 841, 595]}, (0, 2417): {'/Type': '/Page', '/Parent': IndirectObject(2, 0), '/Resources': IndirectObject(2415, 0), '/Contents': [IndirectObject(2414, 0)], '/MediaBox': [0, 0, 841, 595], '/CropBox': [0, 0, 841, 595]}, (0, 2421): {'/Type': '/Page', '/Parent': IndirectObject(2, 0), '/Resources': IndirectObject(2419, 0), '/Contents': [IndirectObject(2418, 0)], '/MediaBox': [0, 0, 841, 595], '/CropBox': [0, 0, 841, 595]}, (0, 2425): {'/Type': '/Page', '/Parent': IndirectObject(2, 0), '/Resources': IndirectObject(2423, 0), '/Contents': [IndirectObject(2422, 0)], '/MediaBox': [0, 0, 841, 595], '/CropBox': [0, 0, 841, 595]}, (0, 2429): {'/Type': '/Page', '/Parent': IndirectObject(2, 0), '/Resources': IndirectObject(2427, 0), '/Contents': [IndirectObject(2426, 0)], '/MediaBox': [0, 0, 841, 595], '/CropBox': [0, 0, 841, 595]}, (0, 2433): {'/Type': '/Page', '/Parent': IndirectObject(2, 0), '/Resources': IndirectObject(2431, 0), '/Contents': [IndirectObject(2430, 0)], '/MediaBox': [0, 0, 841, 595], '/CropBox': [0, 0, 841, 595]}, (0, 2437): {'/Type': '/Page', '/Parent': IndirectObject(2, 0), '/Resources': IndirectObject(2435, 0), '/Contents': [IndirectObject(2434, 0)], '/MediaBox': [0, 0, 841, 595], '/CropBox': [0, 0, 841, 595]}, (0, 2441): {'/Type': '/Page', '/Parent': IndirectObject(2, 0), '/Resources': IndirectObject(2439, 0), '/Contents': [IndirectObject(2438, 0)], '/MediaBox': [0, 0, 841, 595], '/CropBox': [0, 0, 841, 595]}, (0, 2445): {'/Type': '/Page', '/Parent': IndirectObject(2, 0), '/Resources': IndirectObject(2443, 0), '/Contents': [IndirectObject(2442, 0)], '/MediaBox': [0, 0, 841, 595], '/CropBox': [0, 0, 841, 595]}, (0, 2449): {'/Type': '/Page', '/Parent': IndirectObject(2, 0), '/Resources': IndirectObject(2447, 0), '/Contents': [IndirectObject(2446, 0)], '/MediaBox': [0, 0, 841, 595], '/CropBox': [0, 0, 841, 595]}, (0, 2453): {'/Type': '/Page', '/Parent': IndirectObject(2, 0), '/Resources': IndirectObject(2451, 0), '/Contents': [IndirectObject(2450, 0)], '/MediaBox': [0, 0, 841, 595], '/CropBox': [0, 0, 841, 595]}, (0, 2457): {'/Type': '/Page', '/Parent': IndirectObject(2, 0), '/Resources': IndirectObject(2455, 0), '/Contents': [IndirectObject(2454, 0)], '/MediaBox': [0, 0, 841, 595], '/CropBox': [0, 0, 841, 595]}, (0, 2461): {'/Type': '/Page', '/Parent': IndirectObject(2, 0), '/Resources': IndirectObject(2459, 0), '/Contents': [IndirectObject(2458, 0)], '/MediaBox': [0, 0, 841, 595], '/CropBox': [0, 0, 841, 595]}, (0, 2465): {'/Type': '/Page', '/Parent': IndirectObject(2, 0), '/Resources': IndirectObject(2463, 0), '/Contents': [IndirectObject(2462, 0)], '/MediaBox': [0, 0, 841, 595], '/CropBox': [0, 0, 841, 595]}, (0, 2469): {'/Type': '/Page', '/Parent': IndirectObject(2, 0), '/Resources': IndirectObject(2467, 0), '/Contents': [IndirectObject(2466, 0)], '/MediaBox': [0, 0, 841, 595], '/CropBox': [0, 0, 841, 595]}, (0, 2473): {'/Type': '/Page', '/Parent': IndirectObject(2, 0), '/Resources': IndirectObject(2471, 0), '/Contents': [IndirectObject(2470, 0)], '/MediaBox': [0, 0, 841, 595], '/CropBox': [0, 0, 841, 595]}, (0, 2477): {'/Type': '/Page', '/Parent': IndirectObject(2, 0), '/Resources': IndirectObject(2475, 0), '/Contents': [IndirectObject(2474, 0)], '/MediaBox': [0, 0, 841, 595], '/CropBox': [0, 0, 841, 595]}, (0, 2481): {'/Type': '/Page', '/Parent': IndirectObject(2, 0), '/Resources': IndirectObject(2479, 0), '/Contents': [IndirectObject(2478, 0)], '/MediaBox': [0, 0, 841, 595], '/CropBox': [0, 0, 841, 595]}, (0, 2485): {'/Type': '/Page', '/Parent': IndirectObject(2, 0), '/Resources': IndirectObject(2483, 0), '/Contents': [IndirectObject(2482, 0)], '/MediaBox': [0, 0, 841, 595], '/CropBox': [0, 0, 841, 595]}, (0, 2489): {'/Type': '/Page', '/Parent': IndirectObject(2, 0), '/Resources': IndirectObject(2487, 0), '/Contents': [IndirectObject(2486, 0)], '/MediaBox': [0, 0, 841, 595], '/CropBox': [0, 0, 841, 595]}, (0, 2493): {'/Type': '/Page', '/Parent': IndirectObject(2, 0), '/Resources': IndirectObject(2491, 0), '/Contents': [IndirectObject(2490, 0)], '/MediaBox': [0, 0, 841, 595], '/CropBox': [0, 0, 841, 595]}, (0, 2497): {'/Type': '/Page', '/Parent': IndirectObject(2, 0), '/Resources': IndirectObject(2495, 0), '/Contents': [IndirectObject(2494, 0)], '/MediaBox': [0, 0, 841, 595], '/CropBox': [0, 0, 841, 595]}, (0, 2501): {'/Type': '/Page', '/Parent': IndirectObject(2, 0), '/Resources': IndirectObject(2499, 0), '/Contents': [IndirectObject(2498, 0)], '/MediaBox': [0, 0, 841, 595], '/CropBox': [0, 0, 841, 595]}, (0, 2505): {'/Type': '/Page', '/Parent': IndirectObject(2, 0), '/Resources': IndirectObject(2503, 0), '/Contents': [IndirectObject(2502, 0)], '/MediaBox': [0, 0, 841, 595], '/CropBox': [0, 0, 841, 595]}, (0, 2509): {'/Type': '/Page', '/Parent': IndirectObject(2, 0), '/Resources': IndirectObject(2507, 0), '/Contents': [IndirectObject(2506, 0)], '/MediaBox': [0, 0, 841, 595], '/CropBox': [0, 0, 841, 595]}, (0, 2513): {'/Type': '/Page', '/Parent': IndirectObject(2, 0), '/Resources': IndirectObject(2511, 0), '/Contents': [IndirectObject(2510, 0)], '/MediaBox': [0, 0, 841, 595], '/CropBox': [0, 0, 841, 595]}, (0, 2517): {'/Type': '/Page', '/Parent': IndirectObject(2, 0), '/Resources': IndirectObject(2515, 0), '/Contents': [IndirectObject(2514, 0)], '/MediaBox': [0, 0, 841, 595], '/CropBox': [0, 0, 841, 595]}, (0, 2521): {'/Type': '/Page', '/Parent': IndirectObject(2, 0), '/Resources': IndirectObject(2519, 0), '/Contents': [IndirectObject(2518, 0)], '/MediaBox': [0, 0, 841, 595], '/CropBox': [0, 0, 841, 595]}, (0, 2525): {'/Type': '/Page', '/Parent': IndirectObject(2, 0), '/Resources': IndirectObject(2523, 0), '/Contents': [IndirectObject(2522, 0)], '/MediaBox': [0, 0, 841, 595], '/CropBox': [0, 0, 841, 595]}, (0, 2529): {'/Type': '/Page', '/Parent': IndirectObject(2, 0), '/Resources': IndirectObject(2527, 0), '/Contents': [IndirectObject(2526, 0)], '/MediaBox': [0, 0, 841, 595], '/CropBox': [0, 0, 841, 595]}, (0, 2533): {'/Type': '/Page', '/Parent': IndirectObject(2, 0), '/Resources': IndirectObject(2531, 0), '/Contents': [IndirectObject(2530, 0)], '/MediaBox': [0, 0, 841, 595], '/CropBox': [0, 0, 841, 595]}, (0, 2537): {'/Type': '/Page', '/Parent': IndirectObject(2, 0), '/Resources': IndirectObject(2535, 0), '/Contents': [IndirectObject(2534, 0)], '/MediaBox': [0, 0, 841, 595], '/CropBox': [0, 0, 841, 595]}, (0, 2541): {'/Type': '/Page', '/Parent': IndirectObject(2, 0), '/Resources': IndirectObject(2539, 0), '/Contents': [IndirectObject(2538, 0)], '/MediaBox': [0, 0, 841, 595], '/CropBox': [0, 0, 841, 595]}, (0, 2545): {'/Type': '/Page', '/Parent': IndirectObject(2, 0), '/Resources': IndirectObject(2543, 0), '/Contents': [IndirectObject(2542, 0)], '/MediaBox': [0, 0, 841, 595], '/CropBox': [0, 0, 841, 595]}, (0, 2549): {'/Type': '/Page', '/Parent': IndirectObject(2, 0), '/Resources': IndirectObject(2547, 0), '/Contents': [IndirectObject(2546, 0)], '/MediaBox': [0, 0, 841, 595], '/CropBox': [0, 0, 841, 595]}, (0, 2553): {'/Type': '/Page', '/Parent': IndirectObject(2, 0), '/Resources': IndirectObject(2551, 0), '/Contents': [IndirectObject(2550, 0)], '/MediaBox': [0, 0, 841, 595], '/CropBox': [0, 0, 841, 595]}, (0, 2557): {'/Type': '/Page', '/Parent': IndirectObject(2, 0), '/Resources': IndirectObject(2555, 0), '/Contents': [IndirectObject(2554, 0)], '/MediaBox': [0, 0, 841, 595], '/CropBox': [0, 0, 841, 595]}, (0, 2561): {'/Type': '/Page', '/Parent': IndirectObject(2, 0), '/Resources': IndirectObject(2559, 0), '/Contents': [IndirectObject(2558, 0)], '/MediaBox': [0, 0, 841, 595], '/CropBox': [0, 0, 841, 595]}, (0, 2565): {'/Type': '/Page', '/Parent': IndirectObject(2, 0), '/Resources': IndirectObject(2563, 0), '/Contents': [IndirectObject(2562, 0)], '/MediaBox': [0, 0, 841, 595], '/CropBox': [0, 0, 841, 595]}, (0, 2569): {'/Type': '/Page', '/Parent': IndirectObject(2, 0), '/Resources': IndirectObject(2567, 0), '/Contents': [IndirectObject(2566, 0)], '/MediaBox': [0, 0, 841, 595], '/CropBox': [0, 0, 841, 595]}, (0, 2573): {'/Type': '/Page', '/Parent': IndirectObject(2, 0), '/Resources': IndirectObject(2571, 0), '/Contents': [IndirectObject(2570, 0)], '/MediaBox': [0, 0, 841, 595], '/CropBox': [0, 0, 841, 595]}, (0, 2577): {'/Type': '/Page', '/Parent': IndirectObject(2, 0), '/Resources': IndirectObject(2575, 0), '/Contents': [IndirectObject(2574, 0)], '/MediaBox': [0, 0, 841, 595], '/CropBox': [0, 0, 841, 595]}, (0, 2581): {'/Type': '/Page', '/Parent': IndirectObject(2, 0), '/Resources': IndirectObject(2579, 0), '/Contents': [IndirectObject(2578, 0)], '/MediaBox': [0, 0, 841, 595], '/CropBox': [0, 0, 841, 595]}, (0, 2585): {'/Type': '/Page', '/Parent': IndirectObject(2, 0), '/Resources': IndirectObject(2583, 0), '/Contents': [IndirectObject(2582, 0)], '/MediaBox': [0, 0, 841, 595], '/CropBox': [0, 0, 841, 595]}, (0, 2589): {'/Type': '/Page', '/Parent': IndirectObject(2, 0), '/Resources': IndirectObject(2587, 0), '/Contents': [IndirectObject(2586, 0)], '/MediaBox': [0, 0, 841, 595], '/CropBox': [0, 0, 841, 595]}, (0, 2593): {'/Type': '/Page', '/Parent': IndirectObject(2, 0), '/Resources': IndirectObject(2591, 0), '/Contents': [IndirectObject(2590, 0)], '/MediaBox': [0, 0, 841, 595], '/CropBox': [0, 0, 841, 595]}, (0, 2597): {'/Type': '/Page', '/Parent': IndirectObject(2, 0), '/Resources': IndirectObject(2595, 0), '/Contents': [IndirectObject(2594, 0)], '/MediaBox': [0, 0, 841, 595], '/CropBox': [0, 0, 841, 595]}, (0, 2601): {'/Type': '/Page', '/Parent': IndirectObject(2, 0), '/Resources': IndirectObject(2599, 0), '/Contents': [IndirectObject(2598, 0)], '/MediaBox': [0, 0, 841, 595], '/CropBox': [0, 0, 841, 595]}, (0, 2605): {'/Type': '/Page', '/Parent': IndirectObject(2, 0), '/Resources': IndirectObject(2603, 0), '/Contents': [IndirectObject(2602, 0)], '/MediaBox': [0, 0, 841, 595], '/CropBox': [0, 0, 841, 595]}, (0, 2609): {'/Type': '/Page', '/Parent': IndirectObject(2, 0), '/Resources': IndirectObject(2607, 0), '/Contents': [IndirectObject(2606, 0)], '/MediaBox': [0, 0, 841, 595], '/CropBox': [0, 0, 841, 595]}, (0, 2613): {'/Type': '/Page', '/Parent': IndirectObject(2, 0), '/Resources': IndirectObject(2611, 0), '/Contents': [IndirectObject(2610, 0)], '/MediaBox': [0, 0, 841, 595], '/CropBox': [0, 0, 841, 595]}, (0, 2617): {'/Type': '/Page', '/Parent': IndirectObject(2, 0), '/Resources': IndirectObject(2615, 0), '/Contents': [IndirectObject(2614, 0)], '/MediaBox': [0, 0, 841, 595], '/CropBox': [0, 0, 841, 595]}, (0, 2621): {'/Type': '/Page', '/Parent': IndirectObject(2, 0), '/Resources': IndirectObject(2619, 0), '/Contents': [IndirectObject(2618, 0)], '/MediaBox': [0, 0, 841, 595], '/CropBox': [0, 0, 841, 595]}, (0, 2625): {'/Type': '/Page', '/Parent': IndirectObject(2, 0), '/Resources': IndirectObject(2623, 0), '/Contents': [IndirectObject(2622, 0)], '/MediaBox': [0, 0, 841, 595], '/CropBox': [0, 0, 841, 595]}, (0, 2629): {'/Type': '/Page', '/Parent': IndirectObject(2, 0), '/Resources': IndirectObject(2627, 0), '/Contents': [IndirectObject(2626, 0)], '/MediaBox': [0, 0, 841, 595], '/CropBox': [0, 0, 841, 595]}, (0, 2633): {'/Type': '/Page', '/Parent': IndirectObject(2, 0), '/Resources': IndirectObject(2631, 0), '/Contents': [IndirectObject(2630, 0)], '/MediaBox': [0, 0, 841, 595], '/CropBox': [0, 0, 841, 595]}, (0, 2637): {'/Type': '/Page', '/Parent': IndirectObject(2, 0), '/Resources': IndirectObject(2635, 0), '/Contents': [IndirectObject(2634, 0)], '/MediaBox': [0, 0, 841, 595], '/CropBox': [0, 0, 841, 595]}, (0, 2641): {'/Type': '/Page', '/Parent': IndirectObject(2, 0), '/Resources': IndirectObject(2639, 0), '/Contents': [IndirectObject(2638, 0)], '/MediaBox': [0, 0, 841, 595], '/CropBox': [0, 0, 841, 595]}, (0, 2645): {'/Type': '/Page', '/Parent': IndirectObject(2, 0), '/Resources': IndirectObject(2643, 0), '/Contents': [IndirectObject(2642, 0)], '/MediaBox': [0, 0, 841, 595], '/CropBox': [0, 0, 841, 595]}, (0, 2649): {'/Type': '/Page', '/Parent': IndirectObject(2, 0), '/Resources': IndirectObject(2647, 0), '/Contents': [IndirectObject(2646, 0)], '/MediaBox': [0, 0, 841, 595], '/CropBox': [0, 0, 841, 595]}, (0, 2653): {'/Type': '/Page', '/Parent': IndirectObject(2, 0), '/Resources': IndirectObject(2651, 0), '/Contents': [IndirectObject(2650, 0)], '/MediaBox': [0, 0, 841, 595], '/CropBox': [0, 0, 841, 595]}, (0, 2657): {'/Type': '/Page', '/Parent': IndirectObject(2, 0), '/Resources': IndirectObject(2655, 0), '/Contents': [IndirectObject(2654, 0)], '/MediaBox': [0, 0, 841, 595], '/CropBox': [0, 0, 841, 595]}, (0, 2661): {'/Type': '/Page', '/Parent': IndirectObject(2, 0), '/Resources': IndirectObject(2659, 0), '/Contents': [IndirectObject(2658, 0)], '/MediaBox': [0, 0, 841, 595], '/CropBox': [0, 0, 841, 595]}, (0, 2665): {'/Type': '/Page', '/Parent': IndirectObject(2, 0), '/Resources': IndirectObject(2663, 0), '/Contents': [IndirectObject(2662, 0)], '/MediaBox': [0, 0, 841, 595], '/CropBox': [0, 0, 841, 595]}, (0, 2669): {'/Type': '/Page', '/Parent': IndirectObject(2, 0), '/Resources': IndirectObject(2667, 0), '/Contents': [IndirectObject(2666, 0)], '/MediaBox': [0, 0, 841, 595], '/CropBox': [0, 0, 841, 595]}, (0, 2673): {'/Type': '/Page', '/Parent': IndirectObject(2, 0), '/Resources': IndirectObject(2671, 0), '/Contents': [IndirectObject(2670, 0)], '/MediaBox': [0, 0, 841, 595], '/CropBox': [0, 0, 841, 595]}, (0, 2677): {'/Type': '/Page', '/Parent': IndirectObject(2, 0), '/Resources': IndirectObject(2675, 0), '/Contents': [IndirectObject(2674, 0)], '/MediaBox': [0, 0, 841, 595], '/CropBox': [0, 0, 841, 595]}, (0, 2681): {'/Type': '/Page', '/Parent': IndirectObject(2, 0), '/Resources': IndirectObject(2679, 0), '/Contents': [IndirectObject(2678, 0)], '/MediaBox': [0, 0, 841, 595], '/CropBox': [0, 0, 841, 595]}, (0, 2685): {'/Type': '/Page', '/Parent': IndirectObject(2, 0), '/Resources': IndirectObject(2683, 0), '/Contents': [IndirectObject(2682, 0)], '/MediaBox': [0, 0, 841, 595], '/CropBox': [0, 0, 841, 595]}, (0, 2689): {'/Type': '/Page', '/Parent': IndirectObject(2, 0), '/Resources': IndirectObject(2687, 0), '/Contents': [IndirectObject(2686, 0)], '/MediaBox': [0, 0, 841, 595], '/CropBox': [0, 0, 841, 595]}, (0, 2693): {'/Type': '/Page', '/Parent': IndirectObject(2, 0), '/Resources': IndirectObject(2691, 0), '/Contents': [IndirectObject(2690, 0)], '/MediaBox': [0, 0, 841, 595], '/CropBox': [0, 0, 841, 595]}, (0, 2697): {'/Type': '/Page', '/Parent': IndirectObject(2, 0), '/Resources': IndirectObject(2695, 0), '/Contents': [IndirectObject(2694, 0)], '/MediaBox': [0, 0, 841, 595], '/CropBox': [0, 0, 841, 595]}, (0, 2701): {'/Type': '/Page', '/Parent': IndirectObject(2, 0), '/Resources': IndirectObject(2699, 0), '/Contents': [IndirectObject(2698, 0)], '/MediaBox': [0, 0, 841, 595], '/CropBox': [0, 0, 841, 595]}, (0, 2705): {'/Type': '/Page', '/Parent': IndirectObject(2, 0), '/Resources': IndirectObject(2703, 0), '/Contents': [IndirectObject(2702, 0)], '/MediaBox': [0, 0, 841, 595], '/CropBox': [0, 0, 841, 595]}, (0, 2709): {'/Type': '/Page', '/Parent': IndirectObject(2, 0), '/Resources': IndirectObject(2707, 0), '/Contents': [IndirectObject(2706, 0)], '/MediaBox': [0, 0, 841, 595], '/CropBox': [0, 0, 841, 595]}, (0, 2713): {'/Type': '/Page', '/Parent': IndirectObject(2, 0), '/Resources': IndirectObject(2711, 0), '/Contents': [IndirectObject(2710, 0)], '/MediaBox': [0, 0, 841, 595], '/CropBox': [0, 0, 841, 595]}, (0, 2717): {'/Type': '/Page', '/Parent': IndirectObject(2, 0), '/Resources': IndirectObject(2715, 0), '/Contents': [IndirectObject(2714, 0)], '/MediaBox': [0, 0, 841, 595], '/CropBox': [0, 0, 841, 595]}, (0, 2721): {'/Type': '/Page', '/Parent': IndirectObject(2, 0), '/Resources': IndirectObject(2719, 0), '/Contents': [IndirectObject(2718, 0)], '/MediaBox': [0, 0, 841, 595], '/CropBox': [0, 0, 841, 595]}, (0, 2725): {'/Type': '/Page', '/Parent': IndirectObject(2, 0), '/Resources': IndirectObject(2723, 0), '/Contents': [IndirectObject(2722, 0)], '/MediaBox': [0, 0, 841, 595], '/CropBox': [0, 0, 841, 595]}, (0, 2729): {'/Type': '/Page', '/Parent': IndirectObject(2, 0), '/Resources': IndirectObject(2727, 0), '/Contents': [IndirectObject(2726, 0)], '/MediaBox': [0, 0, 841, 595], '/CropBox': [0, 0, 841, 595]}, (0, 2733): {'/Type': '/Page', '/Parent': IndirectObject(2, 0), '/Resources': IndirectObject(2731, 0), '/Contents': [IndirectObject(2730, 0)], '/MediaBox': [0, 0, 841, 595], '/CropBox': [0, 0, 841, 595]}, (0, 2737): {'/Type': '/Page', '/Parent': IndirectObject(2, 0), '/Resources': IndirectObject(2735, 0), '/Contents': [IndirectObject(2734, 0)], '/MediaBox': [0, 0, 841, 595], '/CropBox': [0, 0, 841, 595]}, (0, 2741): {'/Type': '/Page', '/Parent': IndirectObject(2, 0), '/Resources': IndirectObject(2739, 0), '/Contents': [IndirectObject(2738, 0)], '/MediaBox': [0, 0, 841, 595], '/CropBox': [0, 0, 841, 595]}, (0, 2745): {'/Type': '/Page', '/Parent': IndirectObject(2, 0), '/Resources': IndirectObject(2743, 0), '/Contents': [IndirectObject(2742, 0)], '/MediaBox': [0, 0, 841, 595], '/CropBox': [0, 0, 841, 595]}, (0, 2749): {'/Type': '/Page', '/Parent': IndirectObject(2, 0), '/Resources': IndirectObject(2747, 0), '/Contents': [IndirectObject(2746, 0)], '/MediaBox': [0, 0, 841, 595], '/CropBox': [0, 0, 841, 595]}, (0, 2753): {'/Type': '/Page', '/Parent': IndirectObject(2, 0), '/Resources': IndirectObject(2751, 0), '/Contents': [IndirectObject(2750, 0)], '/MediaBox': [0, 0, 841, 595], '/CropBox': [0, 0, 841, 595]}, (0, 2757): {'/Type': '/Page', '/Parent': IndirectObject(2, 0), '/Resources': IndirectObject(2755, 0), '/Contents': [IndirectObject(2754, 0)], '/MediaBox': [0, 0, 841, 595], '/CropBox': [0, 0, 841, 595]}, (0, 2761): {'/Type': '/Page', '/Parent': IndirectObject(2, 0), '/Resources': IndirectObject(2759, 0), '/Contents': [IndirectObject(2758, 0)], '/MediaBox': [0, 0, 841, 595], '/CropBox': [0, 0, 841, 595]}, (0, 2765): {'/Type': '/Page', '/Parent': IndirectObject(2, 0), '/Resources': IndirectObject(2763, 0), '/Contents': [IndirectObject(2762, 0)], '/MediaBox': [0, 0, 841, 595], '/CropBox': [0, 0, 841, 595]}, (0, 2769): {'/Type': '/Page', '/Parent': IndirectObject(2, 0), '/Resources': IndirectObject(2767, 0), '/Contents': [IndirectObject(2766, 0)], '/MediaBox': [0, 0, 841, 595], '/CropBox': [0, 0, 841, 595]}, (0, 2773): {'/Type': '/Page', '/Parent': IndirectObject(2, 0), '/Resources': IndirectObject(2771, 0), '/Contents': [IndirectObject(2770, 0)], '/MediaBox': [0, 0, 841, 595], '/CropBox': [0, 0, 841, 595]}, (0, 2777): {'/Type': '/Page', '/Parent': IndirectObject(2, 0), '/Resources': IndirectObject(2775, 0), '/Contents': [IndirectObject(2774, 0)], '/MediaBox': [0, 0, 841, 595], '/CropBox': [0, 0, 841, 595]}, (0, 2781): {'/Type': '/Page', '/Parent': IndirectObject(2, 0), '/Resources': IndirectObject(2779, 0), '/Contents': [IndirectObject(2778, 0)], '/MediaBox': [0, 0, 841, 595], '/CropBox': [0, 0, 841, 595]}, (0, 2785): {'/Type': '/Page', '/Parent': IndirectObject(2, 0), '/Resources': IndirectObject(2783, 0), '/Contents': [IndirectObject(2782, 0)], '/MediaBox': [0, 0, 841, 595], '/CropBox': [0, 0, 841, 595]}, (0, 2789): {'/Type': '/Page', '/Parent': IndirectObject(2, 0), '/Resources': IndirectObject(2787, 0), '/Contents': [IndirectObject(2786, 0)], '/MediaBox': [0, 0, 841, 595], '/CropBox': [0, 0, 841, 595]}, (0, 2793): {'/Type': '/Page', '/Parent': IndirectObject(2, 0), '/Resources': IndirectObject(2791, 0), '/Contents': [IndirectObject(2790, 0)], '/MediaBox': [0, 0, 841, 595], '/CropBox': [0, 0, 841, 595]}, (0, 2797): {'/Type': '/Page', '/Parent': IndirectObject(2, 0), '/Resources': IndirectObject(2795, 0), '/Contents': [IndirectObject(2794, 0)], '/MediaBox': [0, 0, 841, 595], '/CropBox': [0, 0, 841, 595]}, (0, 2801): {'/Type': '/Page', '/Parent': IndirectObject(2, 0), '/Resources': IndirectObject(2799, 0), '/Contents': [IndirectObject(2798, 0)], '/MediaBox': [0, 0, 841, 595], '/CropBox': [0, 0, 841, 595]}, (0, 2805): {'/Type': '/Page', '/Parent': IndirectObject(2, 0), '/Resources': IndirectObject(2803, 0), '/Contents': [IndirectObject(2802, 0)], '/MediaBox': [0, 0, 841, 595], '/CropBox': [0, 0, 841, 595]}, (0, 2809): {'/Type': '/Page', '/Parent': IndirectObject(2, 0), '/Resources': IndirectObject(2807, 0), '/Contents': [IndirectObject(2806, 0)], '/MediaBox': [0, 0, 841, 595], '/CropBox': [0, 0, 841, 595]}, (0, 2813): {'/Type': '/Page', '/Parent': IndirectObject(2, 0), '/Resources': IndirectObject(2811, 0), '/Contents': [IndirectObject(2810, 0)], '/MediaBox': [0, 0, 841, 595], '/CropBox': [0, 0, 841, 595]}, (0, 2817): {'/Type': '/Page', '/Parent': IndirectObject(2, 0), '/Resources': IndirectObject(2815, 0), '/Contents': [IndirectObject(2814, 0)], '/MediaBox': [0, 0, 841, 595], '/CropBox': [0, 0, 841, 595]}, (0, 2821): {'/Type': '/Page', '/Parent': IndirectObject(2, 0), '/Resources': IndirectObject(2819, 0), '/Contents': [IndirectObject(2818, 0)], '/MediaBox': [0, 0, 841, 595], '/CropBox': [0, 0, 841, 595]}, (0, 2825): {'/Type': '/Page', '/Parent': IndirectObject(2, 0), '/Resources': IndirectObject(2823, 0), '/Contents': [IndirectObject(2822, 0)], '/MediaBox': [0, 0, 841, 595], '/CropBox': [0, 0, 841, 595]}, (0, 2829): {'/Type': '/Page', '/Parent': IndirectObject(2, 0), '/Resources': IndirectObject(2827, 0), '/Contents': [IndirectObject(2826, 0)], '/MediaBox': [0, 0, 841, 595], '/CropBox': [0, 0, 841, 595]}, (0, 2833): {'/Type': '/Page', '/Parent': IndirectObject(2, 0), '/Resources': IndirectObject(2831, 0), '/Contents': [IndirectObject(2830, 0)], '/MediaBox': [0, 0, 841, 595], '/CropBox': [0, 0, 841, 595]}, (0, 2837): {'/Type': '/Page', '/Parent': IndirectObject(2, 0), '/Resources': IndirectObject(2835, 0), '/Contents': [IndirectObject(2834, 0)], '/MediaBox': [0, 0, 841, 595], '/CropBox': [0, 0, 841, 595]}, (0, 2841): {'/Type': '/Page', '/Parent': IndirectObject(2, 0), '/Resources': IndirectObject(2839, 0), '/Contents': [IndirectObject(2838, 0)], '/MediaBox': [0, 0, 841, 595], '/CropBox': [0, 0, 841, 595]}, (0, 2845): {'/Type': '/Page', '/Parent': IndirectObject(2, 0), '/Resources': IndirectObject(2843, 0), '/Contents': [IndirectObject(2842, 0)], '/MediaBox': [0, 0, 841, 595], '/CropBox': [0, 0, 841, 595]}, (0, 2849): {'/Type': '/Page', '/Parent': IndirectObject(2, 0), '/Resources': IndirectObject(2847, 0), '/Contents': [IndirectObject(2846, 0)], '/MediaBox': [0, 0, 841, 595], '/CropBox': [0, 0, 841, 595]}, (0, 2853): {'/Type': '/Page', '/Parent': IndirectObject(2, 0), '/Resources': IndirectObject(2851, 0), '/Contents': [IndirectObject(2850, 0)], '/MediaBox': [0, 0, 841, 595], '/CropBox': [0, 0, 841, 595]}, (0, 2857): {'/Type': '/Page', '/Parent': IndirectObject(2, 0), '/Resources': IndirectObject(2855, 0), '/Contents': [IndirectObject(2854, 0)], '/MediaBox': [0, 0, 841, 595], '/CropBox': [0, 0, 841, 595]}, (0, 2861): {'/Type': '/Page', '/Parent': IndirectObject(2, 0), '/Resources': IndirectObject(2859, 0), '/Contents': [IndirectObject(2858, 0)], '/MediaBox': [0, 0, 841, 595], '/CropBox': [0, 0, 841, 595]}, (0, 2865): {'/Type': '/Page', '/Parent': IndirectObject(2, 0), '/Resources': IndirectObject(2863, 0), '/Contents': [IndirectObject(2862, 0)], '/MediaBox': [0, 0, 841, 595], '/CropBox': [0, 0, 841, 595]}, (0, 2869): {'/Type': '/Page', '/Parent': IndirectObject(2, 0), '/Resources': IndirectObject(2867, 0), '/Contents': [IndirectObject(2866, 0)], '/MediaBox': [0, 0, 841, 595], '/CropBox': [0, 0, 841, 595]}, (0, 2873): {'/Type': '/Page', '/Parent': IndirectObject(2, 0), '/Resources': IndirectObject(2871, 0), '/Contents': [IndirectObject(2870, 0)], '/MediaBox': [0, 0, 841, 595], '/CropBox': [0, 0, 841, 595]}, (0, 2877): {'/Type': '/Page', '/Parent': IndirectObject(2, 0), '/Resources': IndirectObject(2875, 0), '/Contents': [IndirectObject(2874, 0)], '/MediaBox': [0, 0, 841, 595], '/CropBox': [0, 0, 841, 595]}, (0, 2881): {'/Type': '/Page', '/Parent': IndirectObject(2, 0), '/Resources': IndirectObject(2879, 0), '/Contents': [IndirectObject(2878, 0)], '/MediaBox': [0, 0, 841, 595], '/CropBox': [0, 0, 841, 595]}, (0, 2885): {'/Type': '/Page', '/Parent': IndirectObject(2, 0), '/Resources': IndirectObject(2883, 0), '/Contents': [IndirectObject(2882, 0)], '/MediaBox': [0, 0, 841, 595], '/CropBox': [0, 0, 841, 595]}, (0, 2889): {'/Type': '/Page', '/Parent': IndirectObject(2, 0), '/Resources': IndirectObject(2887, 0), '/Contents': [IndirectObject(2886, 0)], '/MediaBox': [0, 0, 841, 595], '/CropBox': [0, 0, 841, 595]}, (0, 2893): {'/Type': '/Page', '/Parent': IndirectObject(2, 0), '/Resources': IndirectObject(2891, 0), '/Contents': [IndirectObject(2890, 0)], '/MediaBox': [0, 0, 841, 595], '/CropBox': [0, 0, 841, 595]}, (0, 2897): {'/Type': '/Page', '/Parent': IndirectObject(2, 0), '/Resources': IndirectObject(2895, 0), '/Contents': [IndirectObject(2894, 0)], '/MediaBox': [0, 0, 841, 595], '/CropBox': [0, 0, 841, 595]}, (0, 2901): {'/Type': '/Page', '/Parent': IndirectObject(2, 0), '/Resources': IndirectObject(2899, 0), '/Contents': [IndirectObject(2898, 0)], '/MediaBox': [0, 0, 841, 595], '/CropBox': [0, 0, 841, 595]}, (0, 2905): {'/Type': '/Page', '/Parent': IndirectObject(2, 0), '/Resources': IndirectObject(2903, 0), '/Contents': [IndirectObject(2902, 0)], '/MediaBox': [0, 0, 841, 595], '/CropBox': [0, 0, 841, 595]}, (0, 2909): {'/Type': '/Page', '/Parent': IndirectObject(2, 0), '/Resources': IndirectObject(2907, 0), '/Contents': [IndirectObject(2906, 0)], '/MediaBox': [0, 0, 841, 595], '/CropBox': [0, 0, 841, 595]}, (0, 2913): {'/Type': '/Page', '/Parent': IndirectObject(2, 0), '/Resources': IndirectObject(2911, 0), '/Contents': [IndirectObject(2910, 0)], '/MediaBox': [0, 0, 841, 595], '/CropBox': [0, 0, 841, 595]}, (0, 2917): {'/Type': '/Page', '/Parent': IndirectObject(2, 0), '/Resources': IndirectObject(2915, 0), '/Contents': [IndirectObject(2914, 0)], '/MediaBox': [0, 0, 841, 595], '/CropBox': [0, 0, 841, 595]}, (0, 2921): {'/Type': '/Page', '/Parent': IndirectObject(2, 0), '/Resources': IndirectObject(2919, 0), '/Contents': [IndirectObject(2918, 0)], '/MediaBox': [0, 0, 841, 595], '/CropBox': [0, 0, 841, 595]}, (0, 2925): {'/Type': '/Page', '/Parent': IndirectObject(2, 0), '/Resources': IndirectObject(2923, 0), '/Contents': [IndirectObject(2922, 0)], '/MediaBox': [0, 0, 841, 595], '/CropBox': [0, 0, 841, 595]}, (0, 2929): {'/Type': '/Page', '/Parent': IndirectObject(2, 0), '/Resources': IndirectObject(2927, 0), '/Contents': [IndirectObject(2926, 0)], '/MediaBox': [0, 0, 841, 595], '/CropBox': [0, 0, 841, 595]}, (0, 2933): {'/Type': '/Page', '/Parent': IndirectObject(2, 0), '/Resources': IndirectObject(2931, 0), '/Contents': [IndirectObject(2930, 0)], '/MediaBox': [0, 0, 841, 595], '/CropBox': [0, 0, 841, 595]}, (0, 2937): {'/Type': '/Page', '/Parent': IndirectObject(2, 0), '/Resources': IndirectObject(2935, 0), '/Contents': [IndirectObject(2934, 0)], '/MediaBox': [0, 0, 841, 595], '/CropBox': [0, 0, 841, 595]}, (0, 2941): {'/Type': '/Page', '/Parent': IndirectObject(2, 0), '/Resources': IndirectObject(2939, 0), '/Contents': [IndirectObject(2938, 0)], '/MediaBox': [0, 0, 841, 595], '/CropBox': [0, 0, 841, 595]}, (0, 2945): {'/Type': '/Page', '/Parent': IndirectObject(2, 0), '/Resources': IndirectObject(2943, 0), '/Contents': [IndirectObject(2942, 0)], '/MediaBox': [0, 0, 841, 595], '/CropBox': [0, 0, 841, 595]}, (0, 2949): {'/Type': '/Page', '/Parent': IndirectObject(2, 0), '/Resources': IndirectObject(2947, 0), '/Contents': [IndirectObject(2946, 0)], '/MediaBox': [0, 0, 841, 595], '/CropBox': [0, 0, 841, 595]}, (0, 2953): {'/Type': '/Page', '/Parent': IndirectObject(2, 0), '/Resources': IndirectObject(2951, 0), '/Contents': [IndirectObject(2950, 0)], '/MediaBox': [0, 0, 841, 595], '/CropBox': [0, 0, 841, 595]}, (0, 2957): {'/Type': '/Page', '/Parent': IndirectObject(2, 0), '/Resources': IndirectObject(2955, 0), '/Contents': [IndirectObject(2954, 0)], '/MediaBox': [0, 0, 841, 595], '/CropBox': [0, 0, 841, 595]}, (0, 2961): {'/Type': '/Page', '/Parent': IndirectObject(2, 0), '/Resources': IndirectObject(2959, 0), '/Contents': [IndirectObject(2958, 0)], '/MediaBox': [0, 0, 841, 595], '/CropBox': [0, 0, 841, 595]}, (0, 2965): {'/Type': '/Page', '/Parent': IndirectObject(2, 0), '/Resources': IndirectObject(2963, 0), '/Contents': [IndirectObject(2962, 0)], '/MediaBox': [0, 0, 841, 595], '/CropBox': [0, 0, 841, 595]}, (0, 2969): {'/Type': '/Page', '/Parent': IndirectObject(2, 0), '/Resources': IndirectObject(2967, 0), '/Contents': [IndirectObject(2966, 0)], '/MediaBox': [0, 0, 841, 595], '/CropBox': [0, 0, 841, 595]}, (0, 2973): {'/Type': '/Page', '/Parent': IndirectObject(2, 0), '/Resources': IndirectObject(2971, 0), '/Contents': [IndirectObject(2970, 0)], '/MediaBox': [0, 0, 841, 595], '/CropBox': [0, 0, 841, 595]}, (0, 2977): {'/Type': '/Page', '/Parent': IndirectObject(2, 0), '/Resources': IndirectObject(2975, 0), '/Contents': [IndirectObject(2974, 0)], '/MediaBox': [0, 0, 841, 595], '/CropBox': [0, 0, 841, 595]}, (0, 2981): {'/Type': '/Page', '/Parent': IndirectObject(2, 0), '/Resources': IndirectObject(2979, 0), '/Contents': [IndirectObject(2978, 0)], '/MediaBox': [0, 0, 841, 595], '/CropBox': [0, 0, 841, 595]}, (0, 2985): {'/Type': '/Page', '/Parent': IndirectObject(2, 0), '/Resources': IndirectObject(2983, 0), '/Contents': [IndirectObject(2982, 0)], '/MediaBox': [0, 0, 841, 595], '/CropBox': [0, 0, 841, 595]}, (0, 2989): {'/Type': '/Page', '/Parent': IndirectObject(2, 0), '/Resources': IndirectObject(2987, 0), '/Contents': [IndirectObject(2986, 0)], '/MediaBox': [0, 0, 841, 595], '/CropBox': [0, 0, 841, 595]}, (0, 2993): {'/Type': '/Page', '/Parent': IndirectObject(2, 0), '/Resources': IndirectObject(2991, 0), '/Contents': [IndirectObject(2990, 0)], '/MediaBox': [0, 0, 841, 595], '/CropBox': [0, 0, 841, 595]}, (0, 2997): {'/Type': '/Page', '/Parent': IndirectObject(2, 0), '/Resources': IndirectObject(2995, 0), '/Contents': [IndirectObject(2994, 0)], '/MediaBox': [0, 0, 841, 595], '/CropBox': [0, 0, 841, 595]}, (0, 3001): {'/Type': '/Page', '/Parent': IndirectObject(2, 0), '/Resources': IndirectObject(2999, 0), '/Contents': [IndirectObject(2998, 0)], '/MediaBox': [0, 0, 841, 595], '/CropBox': [0, 0, 841, 595]}, (0, 3005): {'/Type': '/Page', '/Parent': IndirectObject(2, 0), '/Resources': IndirectObject(3003, 0), '/Contents': [IndirectObject(3002, 0)], '/MediaBox': [0, 0, 841, 595], '/CropBox': [0, 0, 841, 595]}, (0, 3009): {'/Type': '/Page', '/Parent': IndirectObject(2, 0), '/Resources': IndirectObject(3007, 0), '/Contents': [IndirectObject(3006, 0)], '/MediaBox': [0, 0, 841, 595], '/CropBox': [0, 0, 841, 595]}, (0, 3013): {'/Type': '/Page', '/Parent': IndirectObject(2, 0), '/Resources': IndirectObject(3011, 0), '/Contents': [IndirectObject(3010, 0)], '/MediaBox': [0, 0, 841, 595], '/CropBox': [0, 0, 841, 595]}, (0, 3017): {'/Type': '/Page', '/Parent': IndirectObject(2, 0), '/Resources': IndirectObject(3015, 0), '/Contents': [IndirectObject(3014, 0)], '/MediaBox': [0, 0, 841, 595], '/CropBox': [0, 0, 841, 595]}, (0, 3021): {'/Type': '/Page', '/Parent': IndirectObject(2, 0), '/Resources': IndirectObject(3019, 0), '/Contents': [IndirectObject(3018, 0)], '/MediaBox': [0, 0, 841, 595], '/CropBox': [0, 0, 841, 595]}, (0, 3025): {'/Type': '/Page', '/Parent': IndirectObject(2, 0), '/Resources': IndirectObject(3023, 0), '/Contents': [IndirectObject(3022, 0)], '/MediaBox': [0, 0, 841, 595], '/CropBox': [0, 0, 841, 595]}, (0, 3029): {'/Type': '/Page', '/Parent': IndirectObject(2, 0), '/Resources': IndirectObject(3027, 0), '/Contents': [IndirectObject(3026, 0)], '/MediaBox': [0, 0, 841, 595], '/CropBox': [0, 0, 841, 595]}, (0, 3033): {'/Type': '/Page', '/Parent': IndirectObject(2, 0), '/Resources': IndirectObject(3031, 0), '/Contents': [IndirectObject(3030, 0)], '/MediaBox': [0, 0, 841, 595], '/CropBox': [0, 0, 841, 595]}, (0, 3037): {'/Type': '/Page', '/Parent': IndirectObject(2, 0), '/Resources': IndirectObject(3035, 0), '/Contents': [IndirectObject(3034, 0)], '/MediaBox': [0, 0, 841, 595], '/CropBox': [0, 0, 841, 595]}, (0, 3041): {'/Type': '/Page', '/Parent': IndirectObject(2, 0), '/Resources': IndirectObject(3039, 0), '/Contents': [IndirectObject(3038, 0)], '/MediaBox': [0, 0, 841, 595], '/CropBox': [0, 0, 841, 595]}, (0, 3045): {'/Type': '/Page', '/Parent': IndirectObject(2, 0), '/Resources': IndirectObject(3043, 0), '/Contents': [IndirectObject(3042, 0)], '/MediaBox': [0, 0, 841, 595], '/CropBox': [0, 0, 841, 595]}, (0, 3049): {'/Type': '/Page', '/Parent': IndirectObject(2, 0), '/Resources': IndirectObject(3047, 0), '/Contents': [IndirectObject(3046, 0)], '/MediaBox': [0, 0, 841, 595], '/CropBox': [0, 0, 841, 595]}, (0, 3053): {'/Type': '/Page', '/Parent': IndirectObject(2, 0), '/Resources': IndirectObject(3051, 0), '/Contents': [IndirectObject(3050, 0)], '/MediaBox': [0, 0, 841, 595], '/CropBox': [0, 0, 841, 595]}, (0, 3057): {'/Type': '/Page', '/Parent': IndirectObject(2, 0), '/Resources': IndirectObject(3055, 0), '/Contents': [IndirectObject(3054, 0)], '/MediaBox': [0, 0, 841, 595], '/CropBox': [0, 0, 841, 595]}, (0, 3061): {'/Type': '/Page', '/Parent': IndirectObject(2, 0), '/Resources': IndirectObject(3059, 0), '/Contents': [IndirectObject(3058, 0)], '/MediaBox': [0, 0, 841, 595], '/CropBox': [0, 0, 841, 595]}, (0, 3065): {'/Type': '/Page', '/Parent': IndirectObject(2, 0), '/Resources': IndirectObject(3063, 0), '/Contents': [IndirectObject(3062, 0)], '/MediaBox': [0, 0, 841, 595], '/CropBox': [0, 0, 841, 595]}, (0, 3069): {'/Type': '/Page', '/Parent': IndirectObject(2, 0), '/Resources': IndirectObject(3067, 0), '/Contents': [IndirectObject(3066, 0)], '/MediaBox': [0, 0, 841, 595], '/CropBox': [0, 0, 841, 595]}, (0, 3073): {'/Type': '/Page', '/Parent': IndirectObject(2, 0), '/Resources': IndirectObject(3071, 0), '/Contents': [IndirectObject(3070, 0)], '/MediaBox': [0, 0, 841, 595], '/CropBox': [0, 0, 841, 595]}, (0, 3077): {'/Type': '/Page', '/Parent': IndirectObject(2, 0), '/Resources': IndirectObject(3075, 0), '/Contents': [IndirectObject(3074, 0)], '/MediaBox': [0, 0, 841, 595], '/CropBox': [0, 0, 841, 595]}, (0, 3081): {'/Type': '/Page', '/Parent': IndirectObject(2, 0), '/Resources': IndirectObject(3079, 0), '/Contents': [IndirectObject(3078, 0)], '/MediaBox': [0, 0, 841, 595], '/CropBox': [0, 0, 841, 595]}, (0, 3085): {'/Type': '/Page', '/Parent': IndirectObject(2, 0), '/Resources': IndirectObject(3083, 0), '/Contents': [IndirectObject(3082, 0)], '/MediaBox': [0, 0, 841, 595], '/CropBox': [0, 0, 841, 595]}, (0, 3089): {'/Type': '/Page', '/Parent': IndirectObject(2, 0), '/Resources': IndirectObject(3087, 0), '/Contents': [IndirectObject(3086, 0)], '/MediaBox': [0, 0, 841, 595], '/CropBox': [0, 0, 841, 595]}, (0, 3093): {'/Type': '/Page', '/Parent': IndirectObject(2, 0), '/Resources': IndirectObject(3091, 0), '/Contents': [IndirectObject(3090, 0)], '/MediaBox': [0, 0, 841, 595], '/CropBox': [0, 0, 841, 595]}, (0, 3097): {'/Type': '/Page', '/Parent': IndirectObject(2, 0), '/Resources': IndirectObject(3095, 0), '/Contents': [IndirectObject(3094, 0)], '/MediaBox': [0, 0, 841, 595], '/CropBox': [0, 0, 841, 595]}, (0, 3101): {'/Type': '/Page', '/Parent': IndirectObject(2, 0), '/Resources': IndirectObject(3099, 0), '/Contents': [IndirectObject(3098, 0)], '/MediaBox': [0, 0, 841, 595], '/CropBox': [0, 0, 841, 595]}, (0, 3105): {'/Type': '/Page', '/Parent': IndirectObject(2, 0), '/Resources': IndirectObject(3103, 0), '/Contents': [IndirectObject(3102, 0)], '/MediaBox': [0, 0, 841, 595], '/CropBox': [0, 0, 841, 595]}, (0, 3109): {'/Type': '/Page', '/Parent': IndirectObject(2, 0), '/Resources': IndirectObject(3107, 0), '/Contents': [IndirectObject(3106, 0)], '/MediaBox': [0, 0, 841, 595], '/CropBox': [0, 0, 841, 595]}, (0, 3113): {'/Type': '/Page', '/Parent': IndirectObject(2, 0), '/Resources': IndirectObject(3111, 0), '/Contents': [IndirectObject(3110, 0)], '/MediaBox': [0, 0, 841, 595], '/CropBox': [0, 0, 841, 595]}, (0, 3117): {'/Type': '/Page', '/Parent': IndirectObject(2, 0), '/Resources': IndirectObject(3115, 0), '/Contents': [IndirectObject(3114, 0)], '/MediaBox': [0, 0, 841, 595], '/CropBox': [0, 0, 841, 595]}, (0, 3121): {'/Type': '/Page', '/Parent': IndirectObject(2, 0), '/Resources': IndirectObject(3119, 0), '/Contents': [IndirectObject(3118, 0)], '/MediaBox': [0, 0, 841, 595], '/CropBox': [0, 0, 841, 595]}, (0, 3125): {'/Type': '/Page', '/Parent': IndirectObject(2, 0), '/Resources': IndirectObject(3123, 0), '/Contents': [IndirectObject(3122, 0)], '/MediaBox': [0, 0, 841, 595], '/CropBox': [0, 0, 841, 595]}, (0, 3129): {'/Type': '/Page', '/Parent': IndirectObject(2, 0), '/Resources': IndirectObject(3127, 0), '/Contents': [IndirectObject(3126, 0)], '/MediaBox': [0, 0, 841, 595], '/CropBox': [0, 0, 841, 595]}, (0, 3133): {'/Type': '/Page', '/Parent': IndirectObject(2, 0), '/Resources': IndirectObject(3131, 0), '/Contents': [IndirectObject(3130, 0)], '/MediaBox': [0, 0, 841, 595], '/CropBox': [0, 0, 841, 595]}, (0, 3137): {'/Type': '/Page', '/Parent': IndirectObject(2, 0), '/Resources': IndirectObject(3135, 0), '/Contents': [IndirectObject(3134, 0)], '/MediaBox': [0, 0, 841, 595], '/CropBox': [0, 0, 841, 595]}, (0, 3141): {'/Type': '/Page', '/Parent': IndirectObject(2, 0), '/Resources': IndirectObject(3139, 0), '/Contents': [IndirectObject(3138, 0)], '/MediaBox': [0, 0, 841, 595], '/CropBox': [0, 0, 841, 595]}, (0, 3145): {'/Type': '/Page', '/Parent': IndirectObject(2, 0), '/Resources': IndirectObject(3143, 0), '/Contents': [IndirectObject(3142, 0)], '/MediaBox': [0, 0, 841, 595], '/CropBox': [0, 0, 841, 595]}, (0, 3149): {'/Type': '/Page', '/Parent': IndirectObject(2, 0), '/Resources': IndirectObject(3147, 0), '/Contents': [IndirectObject(3146, 0)], '/MediaBox': [0, 0, 841, 595], '/CropBox': [0, 0, 841, 595]}, (0, 3153): {'/Type': '/Page', '/Parent': IndirectObject(2, 0), '/Resources': IndirectObject(3151, 0), '/Contents': [IndirectObject(3150, 0)], '/MediaBox': [0, 0, 841, 595], '/CropBox': [0, 0, 841, 595]}, (0, 3157): {'/Type': '/Page', '/Parent': IndirectObject(2, 0), '/Resources': IndirectObject(3155, 0), '/Contents': [IndirectObject(3154, 0)], '/MediaBox': [0, 0, 841, 595], '/CropBox': [0, 0, 841, 595]}, (0, 3161): {'/Type': '/Page', '/Parent': IndirectObject(2, 0), '/Resources': IndirectObject(3159, 0), '/Contents': [IndirectObject(3158, 0)], '/MediaBox': [0, 0, 841, 595], '/CropBox': [0, 0, 841, 595]}, (0, 3165): {'/Type': '/Page', '/Parent': IndirectObject(2, 0), '/Resources': IndirectObject(3163, 0), '/Contents': [IndirectObject(3162, 0)], '/MediaBox': [0, 0, 841, 595], '/CropBox': [0, 0, 841, 595]}, (0, 3169): {'/Type': '/Page', '/Parent': IndirectObject(2, 0), '/Resources': IndirectObject(3167, 0), '/Contents': [IndirectObject(3166, 0)], '/MediaBox': [0, 0, 841, 595], '/CropBox': [0, 0, 841, 595]}, (0, 3173): {'/Type': '/Page', '/Parent': IndirectObject(2, 0), '/Resources': IndirectObject(3171, 0), '/Contents': [IndirectObject(3170, 0)], '/MediaBox': [0, 0, 841, 595], '/CropBox': [0, 0, 841, 595]}, (0, 3177): {'/Type': '/Page', '/Parent': IndirectObject(2, 0), '/Resources': IndirectObject(3175, 0), '/Contents': [IndirectObject(3174, 0)], '/MediaBox': [0, 0, 841, 595], '/CropBox': [0, 0, 841, 595]}, (0, 3181): {'/Type': '/Page', '/Parent': IndirectObject(2, 0), '/Resources': IndirectObject(3179, 0), '/Contents': [IndirectObject(3178, 0)], '/MediaBox': [0, 0, 841, 595], '/CropBox': [0, 0, 841, 595]}, (0, 3185): {'/Type': '/Page', '/Parent': IndirectObject(2, 0), '/Resources': IndirectObject(3183, 0), '/Contents': [IndirectObject(3182, 0)], '/MediaBox': [0, 0, 841, 595], '/CropBox': [0, 0, 841, 595]}, (0, 3189): {'/Type': '/Page', '/Parent': IndirectObject(2, 0), '/Resources': IndirectObject(3187, 0), '/Contents': [IndirectObject(3186, 0)], '/MediaBox': [0, 0, 841, 595], '/CropBox': [0, 0, 841, 595]}, (0, 3193): {'/Type': '/Page', '/Parent': IndirectObject(2, 0), '/Resources': IndirectObject(3191, 0), '/Contents': [IndirectObject(3190, 0)], '/MediaBox': [0, 0, 841, 595], '/CropBox': [0, 0, 841, 595]}, (0, 3197): {'/Type': '/Page', '/Parent': IndirectObject(2, 0), '/Resources': IndirectObject(3195, 0), '/Contents': [IndirectObject(3194, 0)], '/MediaBox': [0, 0, 841, 595], '/CropBox': [0, 0, 841, 595]}, (0, 3201): {'/Type': '/Page', '/Parent': IndirectObject(2, 0), '/Resources': IndirectObject(3199, 0), '/Contents': [IndirectObject(3198, 0)], '/MediaBox': [0, 0, 841, 595], '/CropBox': [0, 0, 841, 595]}, (0, 3205): {'/Type': '/Page', '/Parent': IndirectObject(2, 0), '/Resources': IndirectObject(3203, 0), '/Contents': [IndirectObject(3202, 0)], '/MediaBox': [0, 0, 841, 595], '/CropBox': [0, 0, 841, 595]}, (0, 3209): {'/Type': '/Page', '/Parent': IndirectObject(2, 0), '/Resources': IndirectObject(3207, 0), '/Contents': [IndirectObject(3206, 0)], '/MediaBox': [0, 0, 841, 595], '/CropBox': [0, 0, 841, 595]}, (0, 3213): {'/Type': '/Page', '/Parent': IndirectObject(2, 0), '/Resources': IndirectObject(3211, 0), '/Contents': [IndirectObject(3210, 0)], '/MediaBox': [0, 0, 841, 595], '/CropBox': [0, 0, 841, 595]}, (0, 3217): {'/Type': '/Page', '/Parent': IndirectObject(2, 0), '/Resources': IndirectObject(3215, 0), '/Contents': [IndirectObject(3214, 0)], '/MediaBox': [0, 0, 841, 595], '/CropBox': [0, 0, 841, 595]}, (0, 3221): {'/Type': '/Page', '/Parent': IndirectObject(2, 0), '/Resources': IndirectObject(3219, 0), '/Contents': [IndirectObject(3218, 0)], '/MediaBox': [0, 0, 841, 595], '/CropBox': [0, 0, 841, 595]}, (0, 3225): {'/Type': '/Page', '/Parent': IndirectObject(2, 0), '/Resources': IndirectObject(3223, 0), '/Contents': [IndirectObject(3222, 0)], '/MediaBox': [0, 0, 841, 595], '/CropBox': [0, 0, 841, 595]}, (0, 3229): {'/Type': '/Page', '/Parent': IndirectObject(2, 0), '/Resources': IndirectObject(3227, 0), '/Contents': [IndirectObject(3226, 0)], '/MediaBox': [0, 0, 841, 595], '/CropBox': [0, 0, 841, 595]}, (0, 3233): {'/Type': '/Page', '/Parent': IndirectObject(2, 0), '/Resources': IndirectObject(3231, 0), '/Contents': [IndirectObject(3230, 0)], '/MediaBox': [0, 0, 841, 595], '/CropBox': [0, 0, 841, 595]}, (0, 3237): {'/Type': '/Page', '/Parent': IndirectObject(2, 0), '/Resources': IndirectObject(3235, 0), '/Contents': [IndirectObject(3234, 0)], '/MediaBox': [0, 0, 841, 595], '/CropBox': [0, 0, 841, 595]}, (0, 3241): {'/Type': '/Page', '/Parent': IndirectObject(2, 0), '/Resources': IndirectObject(3239, 0), '/Contents': [IndirectObject(3238, 0)], '/MediaBox': [0, 0, 841, 595], '/CropBox': [0, 0, 841, 595]}, (0, 3245): {'/Type': '/Page', '/Parent': IndirectObject(2, 0), '/Resources': IndirectObject(3243, 0), '/Contents': [IndirectObject(3242, 0)], '/MediaBox': [0, 0, 841, 595], '/CropBox': [0, 0, 841, 595]}, (0, 3249): {'/Type': '/Page', '/Parent': IndirectObject(2, 0), '/Resources': IndirectObject(3247, 0), '/Contents': [IndirectObject(3246, 0)], '/MediaBox': [0, 0, 841, 595], '/CropBox': [0, 0, 841, 595]}, (0, 3253): {'/Type': '/Page', '/Parent': IndirectObject(2, 0), '/Resources': IndirectObject(3251, 0), '/Contents': [IndirectObject(3250, 0)], '/MediaBox': [0, 0, 841, 595], '/CropBox': [0, 0, 841, 595]}, (0, 3257): {'/Type': '/Page', '/Parent': IndirectObject(2, 0), '/Resources': IndirectObject(3255, 0), '/Contents': [IndirectObject(3254, 0)], '/MediaBox': [0, 0, 841, 595], '/CropBox': [0, 0, 841, 595]}, (0, 3261): {'/Type': '/Page', '/Parent': IndirectObject(2, 0), '/Resources': IndirectObject(3259, 0), '/Contents': [IndirectObject(3258, 0)], '/MediaBox': [0, 0, 841, 595], '/CropBox': [0, 0, 841, 595]}, (0, 3265): {'/Type': '/Page', '/Parent': IndirectObject(2, 0), '/Resources': IndirectObject(3263, 0), '/Contents': [IndirectObject(3262, 0)], '/MediaBox': [0, 0, 841, 595], '/CropBox': [0, 0, 841, 595]}, (0, 3269): {'/Type': '/Page', '/Parent': IndirectObject(2, 0), '/Resources': IndirectObject(3267, 0), '/Contents': [IndirectObject(3266, 0)], '/MediaBox': [0, 0, 841, 595], '/CropBox': [0, 0, 841, 595]}, (0, 3273): {'/Type': '/Page', '/Parent': IndirectObject(2, 0), '/Resources': IndirectObject(3271, 0), '/Contents': [IndirectObject(3270, 0)], '/MediaBox': [0, 0, 841, 595], '/CropBox': [0, 0, 841, 595]}, (0, 3277): {'/Type': '/Page', '/Parent': IndirectObject(2, 0), '/Resources': IndirectObject(3275, 0), '/Contents': [IndirectObject(3274, 0)], '/MediaBox': [0, 0, 841, 595], '/CropBox': [0, 0, 841, 595]}, (0, 3281): {'/Type': '/Page', '/Parent': IndirectObject(2, 0), '/Resources': IndirectObject(3279, 0), '/Contents': [IndirectObject(3278, 0)], '/MediaBox': [0, 0, 841, 595], '/CropBox': [0, 0, 841, 595]}, (0, 3285): {'/Type': '/Page', '/Parent': IndirectObject(2, 0), '/Resources': IndirectObject(3283, 0), '/Contents': [IndirectObject(3282, 0)], '/MediaBox': [0, 0, 841, 595], '/CropBox': [0, 0, 841, 595]}, (0, 3289): {'/Type': '/Page', '/Parent': IndirectObject(2, 0), '/Resources': IndirectObject(3287, 0), '/Contents': [IndirectObject(3286, 0)], '/MediaBox': [0, 0, 841, 595], '/CropBox': [0, 0, 841, 595]}, (0, 3293): {'/Type': '/Page', '/Parent': IndirectObject(2, 0), '/Resources': IndirectObject(3291, 0), '/Contents': [IndirectObject(3290, 0)], '/MediaBox': [0, 0, 841, 595], '/CropBox': [0, 0, 841, 595]}, (0, 3297): {'/Type': '/Page', '/Parent': IndirectObject(2, 0), '/Resources': IndirectObject(3295, 0), '/Contents': [IndirectObject(3294, 0)], '/MediaBox': [0, 0, 841, 595], '/CropBox': [0, 0, 841, 595]}, (0, 3301): {'/Type': '/Page', '/Parent': IndirectObject(2, 0), '/Resources': IndirectObject(3299, 0), '/Contents': [IndirectObject(3298, 0)], '/MediaBox': [0, 0, 841, 595], '/CropBox': [0, 0, 841, 595]}, (0, 3305): {'/Type': '/Page', '/Parent': IndirectObject(2, 0), '/Resources': IndirectObject(3303, 0), '/Contents': [IndirectObject(3302, 0)], '/MediaBox': [0, 0, 841, 595], '/CropBox': [0, 0, 841, 595]}, (0, 3309): {'/Type': '/Page', '/Parent': IndirectObject(2, 0), '/Resources': IndirectObject(3307, 0), '/Contents': [IndirectObject(3306, 0)], '/MediaBox': [0, 0, 841, 595], '/CropBox': [0, 0, 841, 595]}, (0, 3313): {'/Type': '/Page', '/Parent': IndirectObject(2, 0), '/Resources': IndirectObject(3311, 0), '/Contents': [IndirectObject(3310, 0)], '/MediaBox': [0, 0, 841, 595], '/CropBox': [0, 0, 841, 595]}, (0, 3317): {'/Type': '/Page', '/Parent': IndirectObject(2, 0), '/Resources': IndirectObject(3315, 0), '/Contents': [IndirectObject(3314, 0)], '/MediaBox': [0, 0, 841, 595], '/CropBox': [0, 0, 841, 595]}, (0, 3321): {'/Type': '/Page', '/Parent': IndirectObject(2, 0), '/Resources': IndirectObject(3319, 0), '/Contents': [IndirectObject(3318, 0)], '/MediaBox': [0, 0, 841, 595], '/CropBox': [0, 0, 841, 595]}, (0, 3325): {'/Type': '/Page', '/Parent': IndirectObject(2, 0), '/Resources': IndirectObject(3323, 0), '/Contents': [IndirectObject(3322, 0)], '/MediaBox': [0, 0, 841, 595], '/CropBox': [0, 0, 841, 595]}, (0, 3329): {'/Type': '/Page', '/Parent': IndirectObject(2, 0), '/Resources': IndirectObject(3327, 0), '/Contents': [IndirectObject(3326, 0)], '/MediaBox': [0, 0, 841, 595], '/CropBox': [0, 0, 841, 595]}, (0, 3333): {'/Type': '/Page', '/Parent': IndirectObject(2, 0), '/Resources': IndirectObject(3331, 0), '/Contents': [IndirectObject(3330, 0)], '/MediaBox': [0, 0, 841, 595], '/CropBox': [0, 0, 841, 595]}, (0, 3337): {'/Type': '/Page', '/Parent': IndirectObject(2, 0), '/Resources': IndirectObject(3335, 0), '/Contents': [IndirectObject(3334, 0)], '/MediaBox': [0, 0, 841, 595], '/CropBox': [0, 0, 841, 595]}, (0, 3341): {'/Type': '/Page', '/Parent': IndirectObject(2, 0), '/Resources': IndirectObject(3339, 0), '/Contents': [IndirectObject(3338, 0)], '/MediaBox': [0, 0, 841, 595], '/CropBox': [0, 0, 841, 595]}, (0, 3345): {'/Type': '/Page', '/Parent': IndirectObject(2, 0), '/Resources': IndirectObject(3343, 0), '/Contents': [IndirectObject(3342, 0)], '/MediaBox': [0, 0, 841, 595], '/CropBox': [0, 0, 841, 595]}, (0, 3349): {'/Type': '/Page', '/Parent': IndirectObject(2, 0), '/Resources': IndirectObject(3347, 0), '/Contents': [IndirectObject(3346, 0)], '/MediaBox': [0, 0, 841, 595], '/CropBox': [0, 0, 841, 595]}, (0, 3353): {'/Type': '/Page', '/Parent': IndirectObject(2, 0), '/Resources': IndirectObject(3351, 0), '/Contents': [IndirectObject(3350, 0)], '/MediaBox': [0, 0, 841, 595], '/CropBox': [0, 0, 841, 595]}, (0, 3357): {'/Type': '/Page', '/Parent': IndirectObject(2, 0), '/Resources': IndirectObject(3355, 0), '/Contents': [IndirectObject(3354, 0)], '/MediaBox': [0, 0, 841, 595], '/CropBox': [0, 0, 841, 595]}, (0, 3361): {'/Type': '/Page', '/Parent': IndirectObject(2, 0), '/Resources': IndirectObject(3359, 0), '/Contents': [IndirectObject(3358, 0)], '/MediaBox': [0, 0, 841, 595], '/CropBox': [0, 0, 841, 595]}, (0, 3365): {'/Type': '/Page', '/Parent': IndirectObject(2, 0), '/Resources': IndirectObject(3363, 0), '/Contents': [IndirectObject(3362, 0)], '/MediaBox': [0, 0, 841, 595], '/CropBox': [0, 0, 841, 595]}, (0, 3369): {'/Type': '/Page', '/Parent': IndirectObject(2, 0), '/Resources': IndirectObject(3367, 0), '/Contents': [IndirectObject(3366, 0)], '/MediaBox': [0, 0, 841, 595], '/CropBox': [0, 0, 841, 595]}, (0, 3373): {'/Type': '/Page', '/Parent': IndirectObject(2, 0), '/Resources': IndirectObject(3371, 0), '/Contents': [IndirectObject(3370, 0)], '/MediaBox': [0, 0, 841, 595], '/CropBox': [0, 0, 841, 595]}, (0, 3377): {'/Type': '/Page', '/Parent': IndirectObject(2, 0), '/Resources': IndirectObject(3375, 0), '/Contents': [IndirectObject(3374, 0)], '/MediaBox': [0, 0, 841, 595], '/CropBox': [0, 0, 841, 595]}, (0, 3381): {'/Type': '/Page', '/Parent': IndirectObject(2, 0), '/Resources': IndirectObject(3379, 0), '/Contents': [IndirectObject(3378, 0)], '/MediaBox': [0, 0, 841, 595], '/CropBox': [0, 0, 841, 595]}, (0, 3385): {'/Type': '/Page', '/Parent': IndirectObject(2, 0), '/Resources': IndirectObject(3383, 0), '/Contents': [IndirectObject(3382, 0)], '/MediaBox': [0, 0, 841, 595], '/CropBox': [0, 0, 841, 595]}, (0, 3389): {'/Type': '/Page', '/Parent': IndirectObject(2, 0), '/Resources': IndirectObject(3387, 0), '/Contents': [IndirectObject(3386, 0)], '/MediaBox': [0, 0, 841, 595], '/CropBox': [0, 0, 841, 595]}, (0, 3393): {'/Type': '/Page', '/Parent': IndirectObject(2, 0), '/Resources': IndirectObject(3391, 0), '/Contents': [IndirectObject(3390, 0)], '/MediaBox': [0, 0, 841, 595], '/CropBox': [0, 0, 841, 595]}, (0, 3397): {'/Type': '/Page', '/Parent': IndirectObject(2, 0), '/Resources': IndirectObject(3395, 0), '/Contents': [IndirectObject(3394, 0)], '/MediaBox': [0, 0, 841, 595], '/CropBox': [0, 0, 841, 595]}, (0, 3401): {'/Type': '/Page', '/Parent': IndirectObject(2, 0), '/Resources': IndirectObject(3399, 0), '/Contents': [IndirectObject(3398, 0)], '/MediaBox': [0, 0, 841, 595], '/CropBox': [0, 0, 841, 595]}, (0, 3405): {'/Type': '/Page', '/Parent': IndirectObject(2, 0), '/Resources': IndirectObject(3403, 0), '/Contents': [IndirectObject(3402, 0)], '/MediaBox': [0, 0, 841, 595], '/CropBox': [0, 0, 841, 595]}, (0, 3409): {'/Type': '/Page', '/Parent': IndirectObject(2, 0), '/Resources': IndirectObject(3407, 0), '/Contents': [IndirectObject(3406, 0)], '/MediaBox': [0, 0, 841, 595], '/CropBox': [0, 0, 841, 595]}, (0, 3413): {'/Type': '/Page', '/Parent': IndirectObject(2, 0), '/Resources': IndirectObject(3411, 0), '/Contents': [IndirectObject(3410, 0)], '/MediaBox': [0, 0, 841, 595], '/CropBox': [0, 0, 841, 595]}, (0, 3417): {'/Type': '/Page', '/Parent': IndirectObject(2, 0), '/Resources': IndirectObject(3415, 0), '/Contents': [IndirectObject(3414, 0)], '/MediaBox': [0, 0, 841, 595], '/CropBox': [0, 0, 841, 595]}, (0, 3421): {'/Type': '/Page', '/Parent': IndirectObject(2, 0), '/Resources': IndirectObject(3419, 0), '/Contents': [IndirectObject(3418, 0)], '/MediaBox': [0, 0, 841, 595], '/CropBox': [0, 0, 841, 595]}, (0, 3425): {'/Type': '/Page', '/Parent': IndirectObject(2, 0), '/Resources': IndirectObject(3423, 0), '/Contents': [IndirectObject(3422, 0)], '/MediaBox': [0, 0, 841, 595], '/CropBox': [0, 0, 841, 595]}, (0, 3429): {'/Type': '/Page', '/Parent': IndirectObject(2, 0), '/Resources': IndirectObject(3427, 0), '/Contents': [IndirectObject(3426, 0)], '/MediaBox': [0, 0, 841, 595], '/CropBox': [0, 0, 841, 595]}, (0, 3433): {'/Type': '/Page', '/Parent': IndirectObject(2, 0), '/Resources': IndirectObject(3431, 0), '/Contents': [IndirectObject(3430, 0)], '/MediaBox': [0, 0, 841, 595], '/CropBox': [0, 0, 841, 595]}, (0, 3437): {'/Type': '/Page', '/Parent': IndirectObject(2, 0), '/Resources': IndirectObject(3435, 0), '/Contents': [IndirectObject(3434, 0)], '/MediaBox': [0, 0, 841, 595], '/CropBox': [0, 0, 841, 595]}, (0, 3441): {'/Type': '/Page', '/Parent': IndirectObject(2, 0), '/Resources': IndirectObject(3439, 0), '/Contents': [IndirectObject(3438, 0)], '/MediaBox': [0, 0, 841, 595], '/CropBox': [0, 0, 841, 595]}, (0, 3445): {'/Type': '/Page', '/Parent': IndirectObject(2, 0), '/Resources': IndirectObject(3443, 0), '/Contents': [IndirectObject(3442, 0)], '/MediaBox': [0, 0, 841, 595], '/CropBox': [0, 0, 841, 595]}, (0, 3449): {'/Type': '/Page', '/Parent': IndirectObject(2, 0), '/Resources': IndirectObject(3447, 0), '/Contents': [IndirectObject(3446, 0)], '/MediaBox': [0, 0, 841, 595], '/CropBox': [0, 0, 841, 595]}, (0, 3453): {'/Type': '/Page', '/Parent': IndirectObject(2, 0), '/Resources': IndirectObject(3451, 0), '/Contents': [IndirectObject(3450, 0)], '/MediaBox': [0, 0, 841, 595], '/CropBox': [0, 0, 841, 595]}, (0, 3457): {'/Type': '/Page', '/Parent': IndirectObject(2, 0), '/Resources': IndirectObject(3455, 0), '/Contents': [IndirectObject(3454, 0)], '/MediaBox': [0, 0, 841, 595], '/CropBox': [0, 0, 841, 595]}, (0, 3461): {'/Type': '/Page', '/Parent': IndirectObject(2, 0), '/Resources': IndirectObject(3459, 0), '/Contents': [IndirectObject(3458, 0)], '/MediaBox': [0, 0, 841, 595], '/CropBox': [0, 0, 841, 595]}, (0, 3465): {'/Type': '/Page', '/Parent': IndirectObject(2, 0), '/Resources': IndirectObject(3463, 0), '/Contents': [IndirectObject(3462, 0)], '/MediaBox': [0, 0, 841, 595], '/CropBox': [0, 0, 841, 595]}, (0, 3469): {'/Type': '/Page', '/Parent': IndirectObject(2, 0), '/Resources': IndirectObject(3467, 0), '/Contents': [IndirectObject(3466, 0)], '/MediaBox': [0, 0, 841, 595], '/CropBox': [0, 0, 841, 595]}, (0, 3473): {'/Type': '/Page', '/Parent': IndirectObject(2, 0), '/Resources': IndirectObject(3471, 0), '/Contents': [IndirectObject(3470, 0)], '/MediaBox': [0, 0, 841, 595], '/CropBox': [0, 0, 841, 595]}, (0, 3477): {'/Type': '/Page', '/Parent': IndirectObject(2, 0), '/Resources': IndirectObject(3475, 0), '/Contents': [IndirectObject(3474, 0)], '/MediaBox': [0, 0, 841, 595], '/CropBox': [0, 0, 841, 595]}, (0, 3481): {'/Type': '/Page', '/Parent': IndirectObject(2, 0), '/Resources': IndirectObject(3479, 0), '/Contents': [IndirectObject(3478, 0)], '/MediaBox': [0, 0, 841, 595], '/CropBox': [0, 0, 841, 595]}, (0, 3485): {'/Type': '/Page', '/Parent': IndirectObject(2, 0), '/Resources': IndirectObject(3483, 0), '/Contents': [IndirectObject(3482, 0)], '/MediaBox': [0, 0, 841, 595], '/CropBox': [0, 0, 841, 595]}, (0, 3489): {'/Type': '/Page', '/Parent': IndirectObject(2, 0), '/Resources': IndirectObject(3487, 0), '/Contents': [IndirectObject(3486, 0)], '/MediaBox': [0, 0, 841, 595], '/CropBox': [0, 0, 841, 595]}, (0, 3493): {'/Type': '/Page', '/Parent': IndirectObject(2, 0), '/Resources': IndirectObject(3491, 0), '/Contents': [IndirectObject(3490, 0)], '/MediaBox': [0, 0, 841, 595], '/CropBox': [0, 0, 841, 595]}, (0, 3497): {'/Type': '/Page', '/Parent': IndirectObject(2, 0), '/Resources': IndirectObject(3495, 0), '/Contents': [IndirectObject(3494, 0)], '/MediaBox': [0, 0, 841, 595], '/CropBox': [0, 0, 841, 595]}, (0, 3501): {'/Type': '/Page', '/Parent': IndirectObject(2, 0), '/Resources': IndirectObject(3499, 0), '/Contents': [IndirectObject(3498, 0)], '/MediaBox': [0, 0, 841, 595], '/CropBox': [0, 0, 841, 595]}, (0, 3505): {'/Type': '/Page', '/Parent': IndirectObject(2, 0), '/Resources': IndirectObject(3503, 0), '/Contents': [IndirectObject(3502, 0)], '/MediaBox': [0, 0, 841, 595], '/CropBox': [0, 0, 841, 595]}, (0, 3509): {'/Type': '/Page', '/Parent': IndirectObject(2, 0), '/Resources': IndirectObject(3507, 0), '/Contents': [IndirectObject(3506, 0)], '/MediaBox': [0, 0, 841, 595], '/CropBox': [0, 0, 841, 595]}, (0, 3513): {'/Type': '/Page', '/Parent': IndirectObject(2, 0), '/Resources': IndirectObject(3511, 0), '/Contents': [IndirectObject(3510, 0)], '/MediaBox': [0, 0, 841, 595], '/CropBox': [0, 0, 841, 595]}, (0, 3517): {'/Type': '/Page', '/Parent': IndirectObject(2, 0), '/Resources': IndirectObject(3515, 0), '/Contents': [IndirectObject(3514, 0)], '/MediaBox': [0, 0, 841, 595], '/CropBox': [0, 0, 841, 595]}, (0, 3521): {'/Type': '/Page', '/Parent': IndirectObject(2, 0), '/Resources': IndirectObject(3519, 0), '/Contents': [IndirectObject(3518, 0)], '/MediaBox': [0, 0, 841, 595], '/CropBox': [0, 0, 841, 595]}, (0, 3525): {'/Type': '/Page', '/Parent': IndirectObject(2, 0), '/Resources': IndirectObject(3523, 0), '/Contents': [IndirectObject(3522, 0)], '/MediaBox': [0, 0, 841, 595], '/CropBox': [0, 0, 841, 595]}, (0, 3529): {'/Type': '/Page', '/Parent': IndirectObject(2, 0), '/Resources': IndirectObject(3527, 0), '/Contents': [IndirectObject(3526, 0)], '/MediaBox': [0, 0, 841, 595], '/CropBox': [0, 0, 841, 595]}, (0, 3533): {'/Type': '/Page', '/Parent': IndirectObject(2, 0), '/Resources': IndirectObject(3531, 0), '/Contents': [IndirectObject(3530, 0)], '/MediaBox': [0, 0, 841, 595], '/CropBox': [0, 0, 841, 595]}, (0, 3537): {'/Type': '/Page', '/Parent': IndirectObject(2, 0), '/Resources': IndirectObject(3535, 0), '/Contents': [IndirectObject(3534, 0)], '/MediaBox': [0, 0, 841, 595], '/CropBox': [0, 0, 841, 595]}, (0, 3541): {'/Type': '/Page', '/Parent': IndirectObject(2, 0), '/Resources': IndirectObject(3539, 0), '/Contents': [IndirectObject(3538, 0)], '/MediaBox': [0, 0, 841, 595], '/CropBox': [0, 0, 841, 595]}, (0, 3545): {'/Type': '/Page', '/Parent': IndirectObject(2, 0), '/Resources': IndirectObject(3543, 0), '/Contents': [IndirectObject(3542, 0)], '/MediaBox': [0, 0, 841, 595], '/CropBox': [0, 0, 841, 595]}, (0, 3549): {'/Type': '/Page', '/Parent': IndirectObject(2, 0), '/Resources': IndirectObject(3547, 0), '/Contents': [IndirectObject(3546, 0)], '/MediaBox': [0, 0, 841, 595], '/CropBox': [0, 0, 841, 595]}, (0, 3553): {'/Type': '/Page', '/Parent': IndirectObject(2, 0), '/Resources': IndirectObject(3551, 0), '/Contents': [IndirectObject(3550, 0)], '/MediaBox': [0, 0, 841, 595], '/CropBox': [0, 0, 841, 595]}, (0, 3557): {'/Type': '/Page', '/Parent': IndirectObject(2, 0), '/Resources': IndirectObject(3555, 0), '/Contents': [IndirectObject(3554, 0)], '/MediaBox': [0, 0, 841, 595], '/CropBox': [0, 0, 841, 595]}, (0, 3561): {'/Type': '/Page', '/Parent': IndirectObject(2, 0), '/Resources': IndirectObject(3559, 0), '/Contents': [IndirectObject(3558, 0)], '/MediaBox': [0, 0, 841, 595], '/CropBox': [0, 0, 841, 595]}, (0, 3565): {'/Type': '/Page', '/Parent': IndirectObject(2, 0), '/Resources': IndirectObject(3563, 0), '/Contents': [IndirectObject(3562, 0)], '/MediaBox': [0, 0, 841, 595], '/CropBox': [0, 0, 841, 595]}, (0, 3569): {'/Type': '/Page', '/Parent': IndirectObject(2, 0), '/Resources': IndirectObject(3567, 0), '/Contents': [IndirectObject(3566, 0)], '/MediaBox': [0, 0, 841, 595], '/CropBox': [0, 0, 841, 595]}, (0, 3573): {'/Type': '/Page', '/Parent': IndirectObject(2, 0), '/Resources': IndirectObject(3571, 0), '/Contents': [IndirectObject(3570, 0)], '/MediaBox': [0, 0, 841, 595], '/CropBox': [0, 0, 841, 595]}, (0, 3577): {'/Type': '/Page', '/Parent': IndirectObject(2, 0), '/Resources': IndirectObject(3575, 0), '/Contents': [IndirectObject(3574, 0)], '/MediaBox': [0, 0, 841, 595], '/CropBox': [0, 0, 841, 595]}, (0, 3581): {'/Type': '/Page', '/Parent': IndirectObject(2, 0), '/Resources': IndirectObject(3579, 0), '/Contents': [IndirectObject(3578, 0)], '/MediaBox': [0, 0, 841, 595], '/CropBox': [0, 0, 841, 595]}, (0, 3585): {'/Type': '/Page', '/Parent': IndirectObject(2, 0), '/Resources': IndirectObject(3583, 0), '/Contents': [IndirectObject(3582, 0)], '/MediaBox': [0, 0, 841, 595], '/CropBox': [0, 0, 841, 595]}, (0, 3589): {'/Type': '/Page', '/Parent': IndirectObject(2, 0), '/Resources': IndirectObject(3587, 0), '/Contents': [IndirectObject(3586, 0)], '/MediaBox': [0, 0, 841, 595], '/CropBox': [0, 0, 841, 595]}, (0, 3593): {'/Type': '/Page', '/Parent': IndirectObject(2, 0), '/Resources': IndirectObject(3591, 0), '/Contents': [IndirectObject(3590, 0)], '/MediaBox': [0, 0, 841, 595], '/CropBox': [0, 0, 841, 595]}, (0, 3597): {'/Type': '/Page', '/Parent': IndirectObject(2, 0), '/Resources': IndirectObject(3595, 0), '/Contents': [IndirectObject(3594, 0)], '/MediaBox': [0, 0, 841, 595], '/CropBox': [0, 0, 841, 595]}, (0, 3601): {'/Type': '/Page', '/Parent': IndirectObject(2, 0), '/Resources': IndirectObject(3599, 0), '/Contents': [IndirectObject(3598, 0)], '/MediaBox': [0, 0, 841, 595], '/CropBox': [0, 0, 841, 595]}, (0, 3605): {'/Type': '/Page', '/Parent': IndirectObject(2, 0), '/Resources': IndirectObject(3603, 0), '/Contents': [IndirectObject(3602, 0)], '/MediaBox': [0, 0, 841, 595], '/CropBox': [0, 0, 841, 595]}, (0, 3609): {'/Type': '/Page', '/Parent': IndirectObject(2, 0), '/Resources': IndirectObject(3607, 0), '/Contents': [IndirectObject(3606, 0)], '/MediaBox': [0, 0, 841, 595], '/CropBox': [0, 0, 841, 595]}, (0, 3613): {'/Type': '/Page', '/Parent': IndirectObject(2, 0), '/Resources': IndirectObject(3611, 0), '/Contents': [IndirectObject(3610, 0)], '/MediaBox': [0, 0, 841, 595], '/CropBox': [0, 0, 841, 595]}, (0, 3617): {'/Type': '/Page', '/Parent': IndirectObject(2, 0), '/Resources': IndirectObject(3615, 0), '/Contents': [IndirectObject(3614, 0)], '/MediaBox': [0, 0, 841, 595], '/CropBox': [0, 0, 841, 595]}, (0, 3621): {'/Type': '/Page', '/Parent': IndirectObject(2, 0), '/Resources': IndirectObject(3619, 0), '/Contents': [IndirectObject(3618, 0)], '/MediaBox': [0, 0, 841, 595], '/CropBox': [0, 0, 841, 595]}, (0, 3625): {'/Type': '/Page', '/Parent': IndirectObject(2, 0), '/Resources': IndirectObject(3623, 0), '/Contents': [IndirectObject(3622, 0)], '/MediaBox': [0, 0, 841, 595], '/CropBox': [0, 0, 841, 595]}, (0, 3629): {'/Type': '/Page', '/Parent': IndirectObject(2, 0), '/Resources': IndirectObject(3627, 0), '/Contents': [IndirectObject(3626, 0)], '/MediaBox': [0, 0, 841, 595], '/CropBox': [0, 0, 841, 595]}, (0, 3633): {'/Type': '/Page', '/Parent': IndirectObject(2, 0), '/Resources': IndirectObject(3631, 0), '/Contents': [IndirectObject(3630, 0)], '/MediaBox': [0, 0, 841, 595], '/CropBox': [0, 0, 841, 595]}, (0, 3637): {'/Type': '/Page', '/Parent': IndirectObject(2, 0), '/Resources': IndirectObject(3635, 0), '/Contents': [IndirectObject(3634, 0)], '/MediaBox': [0, 0, 841, 595], '/CropBox': [0, 0, 841, 595]}, (0, 3641): {'/Type': '/Page', '/Parent': IndirectObject(2, 0), '/Resources': IndirectObject(3639, 0), '/Contents': [IndirectObject(3638, 0)], '/MediaBox': [0, 0, 841, 595], '/CropBox': [0, 0, 841, 595]}, (0, 3645): {'/Type': '/Page', '/Parent': IndirectObject(2, 0), '/Resources': IndirectObject(3643, 0), '/Contents': [IndirectObject(3642, 0)], '/MediaBox': [0, 0, 841, 595], '/CropBox': [0, 0, 841, 595]}, (0, 3649): {'/Type': '/Page', '/Parent': IndirectObject(2, 0), '/Resources': IndirectObject(3647, 0), '/Contents': [IndirectObject(3646, 0)], '/MediaBox': [0, 0, 841, 595], '/CropBox': [0, 0, 841, 595]}, (0, 3653): {'/Type': '/Page', '/Parent': IndirectObject(2, 0), '/Resources': IndirectObject(3651, 0), '/Contents': [IndirectObject(3650, 0)], '/MediaBox': [0, 0, 841, 595], '/CropBox': [0, 0, 841, 595]}, (0, 3657): {'/Type': '/Page', '/Parent': IndirectObject(2, 0), '/Resources': IndirectObject(3655, 0), '/Contents': [IndirectObject(3654, 0)], '/MediaBox': [0, 0, 841, 595], '/CropBox': [0, 0, 841, 595]}, (0, 3661): {'/Type': '/Page', '/Parent': IndirectObject(2, 0), '/Resources': IndirectObject(3659, 0), '/Contents': [IndirectObject(3658, 0)], '/MediaBox': [0, 0, 841, 595], '/CropBox': [0, 0, 841, 595]}, (0, 3665): {'/Type': '/Page', '/Parent': IndirectObject(2, 0), '/Resources': IndirectObject(3663, 0), '/Contents': [IndirectObject(3662, 0)], '/MediaBox': [0, 0, 841, 595], '/CropBox': [0, 0, 841, 595]}, (0, 3669): {'/Type': '/Page', '/Parent': IndirectObject(2, 0), '/Resources': IndirectObject(3667, 0), '/Contents': [IndirectObject(3666, 0)], '/MediaBox': [0, 0, 841, 595], '/CropBox': [0, 0, 841, 595]}, (0, 3673): {'/Type': '/Page', '/Parent': IndirectObject(2, 0), '/Resources': IndirectObject(3671, 0), '/Contents': [IndirectObject(3670, 0)], '/MediaBox': [0, 0, 841, 595], '/CropBox': [0, 0, 841, 595]}, (0, 3677): {'/Type': '/Page', '/Parent': IndirectObject(2, 0), '/Resources': IndirectObject(3675, 0), '/Contents': [IndirectObject(3674, 0)], '/MediaBox': [0, 0, 841, 595], '/CropBox': [0, 0, 841, 595]}, (0, 3681): {'/Type': '/Page', '/Parent': IndirectObject(2, 0), '/Resources': IndirectObject(3679, 0), '/Contents': [IndirectObject(3678, 0)], '/MediaBox': [0, 0, 841, 595], '/CropBox': [0, 0, 841, 595]}, (0, 3685): {'/Type': '/Page', '/Parent': IndirectObject(2, 0), '/Resources': IndirectObject(3683, 0), '/Contents': [IndirectObject(3682, 0)], '/MediaBox': [0, 0, 841, 595], '/CropBox': [0, 0, 841, 595]}, (0, 3689): {'/Type': '/Page', '/Parent': IndirectObject(2, 0), '/Resources': IndirectObject(3687, 0), '/Contents': [IndirectObject(3686, 0)], '/MediaBox': [0, 0, 841, 595], '/CropBox': [0, 0, 841, 595]}, (0, 3693): {'/Type': '/Page', '/Parent': IndirectObject(2, 0), '/Resources': IndirectObject(3691, 0), '/Contents': [IndirectObject(3690, 0)], '/MediaBox': [0, 0, 841, 595], '/CropBox': [0, 0, 841, 595]}, (0, 3697): {'/Type': '/Page', '/Parent': IndirectObject(2, 0), '/Resources': IndirectObject(3695, 0), '/Contents': [IndirectObject(3694, 0)], '/MediaBox': [0, 0, 841, 595], '/CropBox': [0, 0, 841, 595]}, (0, 3701): {'/Type': '/Page', '/Parent': IndirectObject(2, 0), '/Resources': IndirectObject(3699, 0), '/Contents': [IndirectObject(3698, 0)], '/MediaBox': [0, 0, 841, 595], '/CropBox': [0, 0, 841, 595]}, (0, 3705): {'/Type': '/Page', '/Parent': IndirectObject(2, 0), '/Resources': IndirectObject(3703, 0), '/Contents': [IndirectObject(3702, 0)], '/MediaBox': [0, 0, 841, 595], '/CropBox': [0, 0, 841, 595]}, (0, 3709): {'/Type': '/Page', '/Parent': IndirectObject(2, 0), '/Resources': IndirectObject(3707, 0), '/Contents': [IndirectObject(3706, 0)], '/MediaBox': [0, 0, 841, 595], '/CropBox': [0, 0, 841, 595]}, (0, 3713): {'/Type': '/Page', '/Parent': IndirectObject(2, 0), '/Resources': IndirectObject(3711, 0), '/Contents': [IndirectObject(3710, 0)], '/MediaBox': [0, 0, 841, 595], '/CropBox': [0, 0, 841, 595]}, (0, 3717): {'/Type': '/Page', '/Parent': IndirectObject(2, 0), '/Resources': IndirectObject(3715, 0), '/Contents': [IndirectObject(3714, 0)], '/MediaBox': [0, 0, 841, 595], '/CropBox': [0, 0, 841, 595]}, (0, 3721): {'/Type': '/Page', '/Parent': IndirectObject(2, 0), '/Resources': IndirectObject(3719, 0), '/Contents': [IndirectObject(3718, 0)], '/MediaBox': [0, 0, 841, 595], '/CropBox': [0, 0, 841, 595]}, (0, 3725): {'/Type': '/Page', '/Parent': IndirectObject(2, 0), '/Resources': IndirectObject(3723, 0), '/Contents': [IndirectObject(3722, 0)], '/MediaBox': [0, 0, 841, 595], '/CropBox': [0, 0, 841, 595]}, (0, 3729): {'/Type': '/Page', '/Parent': IndirectObject(2, 0), '/Resources': IndirectObject(3727, 0), '/Contents': [IndirectObject(3726, 0)], '/MediaBox': [0, 0, 841, 595], '/CropBox': [0, 0, 841, 595]}, (0, 3733): {'/Type': '/Page', '/Parent': IndirectObject(2, 0), '/Resources': IndirectObject(3731, 0), '/Contents': [IndirectObject(3730, 0)], '/MediaBox': [0, 0, 841, 595], '/CropBox': [0, 0, 841, 595]}, (0, 3737): {'/Type': '/Page', '/Parent': IndirectObject(2, 0), '/Resources': IndirectObject(3735, 0), '/Contents': [IndirectObject(3734, 0)], '/MediaBox': [0, 0, 841, 595], '/CropBox': [0, 0, 841, 595]}, (0, 3741): {'/Type': '/Page', '/Parent': IndirectObject(2, 0), '/Resources': IndirectObject(3739, 0), '/Contents': [IndirectObject(3738, 0)], '/MediaBox': [0, 0, 841, 595], '/CropBox': [0, 0, 841, 595]}, (0, 3745): {'/Type': '/Page', '/Parent': IndirectObject(2, 0), '/Resources': IndirectObject(3743, 0), '/Contents': [IndirectObject(3742, 0)], '/MediaBox': [0, 0, 841, 595], '/CropBox': [0, 0, 841, 595]}, (0, 3749): {'/Type': '/Page', '/Parent': IndirectObject(2, 0), '/Resources': IndirectObject(3747, 0), '/Contents': [IndirectObject(3746, 0)], '/MediaBox': [0, 0, 841, 595], '/CropBox': [0, 0, 841, 595]}, (0, 3753): {'/Type': '/Page', '/Parent': IndirectObject(2, 0), '/Resources': IndirectObject(3751, 0), '/Contents': [IndirectObject(3750, 0)], '/MediaBox': [0, 0, 841, 595], '/CropBox': [0, 0, 841, 595]}, (0, 3757): {'/Type': '/Page', '/Parent': IndirectObject(2, 0), '/Resources': IndirectObject(3755, 0), '/Contents': [IndirectObject(3754, 0)], '/MediaBox': [0, 0, 841, 595], '/CropBox': [0, 0, 841, 595]}, (0, 3761): {'/Type': '/Page', '/Parent': IndirectObject(2, 0), '/Resources': IndirectObject(3759, 0), '/Contents': [IndirectObject(3758, 0)], '/MediaBox': [0, 0, 841, 595], '/CropBox': [0, 0, 841, 595]}, (0, 3765): {'/Type': '/Page', '/Parent': IndirectObject(2, 0), '/Resources': IndirectObject(3763, 0), '/Contents': [IndirectObject(3762, 0)], '/MediaBox': [0, 0, 841, 595], '/CropBox': [0, 0, 841, 595]}, (0, 3769): {'/Type': '/Page', '/Parent': IndirectObject(2, 0), '/Resources': IndirectObject(3767, 0), '/Contents': [IndirectObject(3766, 0)], '/MediaBox': [0, 0, 841, 595], '/CropBox': [0, 0, 841, 595]}, (0, 3773): {'/Type': '/Page', '/Parent': IndirectObject(2, 0), '/Resources': IndirectObject(3771, 0), '/Contents': [IndirectObject(3770, 0)], '/MediaBox': [0, 0, 841, 595], '/CropBox': [0, 0, 841, 595]}, (0, 3777): {'/Type': '/Page', '/Parent': IndirectObject(2, 0), '/Resources': IndirectObject(3775, 0), '/Contents': [IndirectObject(3774, 0)], '/MediaBox': [0, 0, 841, 595], '/CropBox': [0, 0, 841, 595]}, (0, 3781): {'/Type': '/Page', '/Parent': IndirectObject(2, 0), '/Resources': IndirectObject(3779, 0), '/Contents': [IndirectObject(3778, 0)], '/MediaBox': [0, 0, 841, 595], '/CropBox': [0, 0, 841, 595]}, (0, 3785): {'/Type': '/Page', '/Parent': IndirectObject(2, 0), '/Resources': IndirectObject(3783, 0), '/Contents': [IndirectObject(3782, 0)], '/MediaBox': [0, 0, 841, 595], '/CropBox': [0, 0, 841, 595]}, (0, 3789): {'/Type': '/Page', '/Parent': IndirectObject(2, 0), '/Resources': IndirectObject(3787, 0), '/Contents': [IndirectObject(3786, 0)], '/MediaBox': [0, 0, 841, 595], '/CropBox': [0, 0, 841, 595]}, (0, 3793): {'/Type': '/Page', '/Parent': IndirectObject(2, 0), '/Resources': IndirectObject(3791, 0), '/Contents': [IndirectObject(3790, 0)], '/MediaBox': [0, 0, 841, 595], '/CropBox': [0, 0, 841, 595]}, (0, 3797): {'/Type': '/Page', '/Parent': IndirectObject(2, 0), '/Resources': IndirectObject(3795, 0), '/Contents': [IndirectObject(3794, 0)], '/MediaBox': [0, 0, 841, 595], '/CropBox': [0, 0, 841, 595]}, (0, 3801): {'/Type': '/Page', '/Parent': IndirectObject(2, 0), '/Resources': IndirectObject(3799, 0), '/Contents': [IndirectObject(3798, 0)], '/MediaBox': [0, 0, 841, 595], '/CropBox': [0, 0, 841, 595]}, (0, 3805): {'/Type': '/Page', '/Parent': IndirectObject(2, 0), '/Resources': IndirectObject(3803, 0), '/Contents': [IndirectObject(3802, 0)], '/MediaBox': [0, 0, 841, 595], '/CropBox': [0, 0, 841, 595]}, (0, 3809): {'/Type': '/Page', '/Parent': IndirectObject(2, 0), '/Resources': IndirectObject(3807, 0), '/Contents': [IndirectObject(3806, 0)], '/MediaBox': [0, 0, 841, 595], '/CropBox': [0, 0, 841, 595]}, (0, 3813): {'/Type': '/Page', '/Parent': IndirectObject(2, 0), '/Resources': IndirectObject(3811, 0), '/Contents': [IndirectObject(3810, 0)], '/MediaBox': [0, 0, 841, 595], '/CropBox': [0, 0, 841, 595]}, (0, 3817): {'/Type': '/Page', '/Parent': IndirectObject(2, 0), '/Resources': IndirectObject(3815, 0), '/Contents': [IndirectObject(3814, 0)], '/MediaBox': [0, 0, 841, 595], '/CropBox': [0, 0, 841, 595]}, (0, 3821): {'/Type': '/Page', '/Parent': IndirectObject(2, 0), '/Resources': IndirectObject(3819, 0), '/Contents': [IndirectObject(3818, 0)], '/MediaBox': [0, 0, 841, 595], '/CropBox': [0, 0, 841, 595]}, (0, 3825): {'/Type': '/Page', '/Parent': IndirectObject(2, 0), '/Resources': IndirectObject(3823, 0), '/Contents': [IndirectObject(3822, 0)], '/MediaBox': [0, 0, 841, 595], '/CropBox': [0, 0, 841, 595]}, (0, 3829): {'/Type': '/Page', '/Parent': IndirectObject(2, 0), '/Resources': IndirectObject(3827, 0), '/Contents': [IndirectObject(3826, 0)], '/MediaBox': [0, 0, 841, 595], '/CropBox': [0, 0, 841, 595]}, (0, 3833): {'/Type': '/Page', '/Parent': IndirectObject(2, 0), '/Resources': IndirectObject(3831, 0), '/Contents': [IndirectObject(3830, 0)], '/MediaBox': [0, 0, 841, 595], '/CropBox': [0, 0, 841, 595]}, (0, 3837): {'/Type': '/Page', '/Parent': IndirectObject(2, 0), '/Resources': IndirectObject(3835, 0), '/Contents': [IndirectObject(3834, 0)], '/MediaBox': [0, 0, 841, 595], '/CropBox': [0, 0, 841, 595]}, (0, 3841): {'/Type': '/Page', '/Parent': IndirectObject(2, 0), '/Resources': IndirectObject(3839, 0), '/Contents': [IndirectObject(3838, 0)], '/MediaBox': [0, 0, 841, 595], '/CropBox': [0, 0, 841, 595]}, (0, 3845): {'/Type': '/Page', '/Parent': IndirectObject(2, 0), '/Resources': IndirectObject(3843, 0), '/Contents': [IndirectObject(3842, 0)], '/MediaBox': [0, 0, 841, 595], '/CropBox': [0, 0, 841, 595]}, (0, 3849): {'/Type': '/Page', '/Parent': IndirectObject(2, 0), '/Resources': IndirectObject(3847, 0), '/Contents': [IndirectObject(3846, 0)], '/MediaBox': [0, 0, 841, 595], '/CropBox': [0, 0, 841, 595]}, (0, 3853): {'/Type': '/Page', '/Parent': IndirectObject(2, 0), '/Resources': IndirectObject(3851, 0), '/Contents': [IndirectObject(3850, 0)], '/MediaBox': [0, 0, 841, 595], '/CropBox': [0, 0, 841, 595]}, (0, 3857): {'/Type': '/Page', '/Parent': IndirectObject(2, 0), '/Resources': IndirectObject(3855, 0), '/Contents': [IndirectObject(3854, 0)], '/MediaBox': [0, 0, 841, 595], '/CropBox': [0, 0, 841, 595]}, (0, 3861): {'/Type': '/Page', '/Parent': IndirectObject(2, 0), '/Resources': IndirectObject(3859, 0), '/Contents': [IndirectObject(3858, 0)], '/MediaBox': [0, 0, 841, 595], '/CropBox': [0, 0, 841, 595]}, (0, 3865): {'/Type': '/Page', '/Parent': IndirectObject(2, 0), '/Resources': IndirectObject(3863, 0), '/Contents': [IndirectObject(3862, 0)], '/MediaBox': [0, 0, 841, 595], '/CropBox': [0, 0, 841, 595]}, (0, 3869): {'/Type': '/Page', '/Parent': IndirectObject(2, 0), '/Resources': IndirectObject(3867, 0), '/Contents': [IndirectObject(3866, 0)], '/MediaBox': [0, 0, 841, 595], '/CropBox': [0, 0, 841, 595]}, (0, 3873): {'/Type': '/Page', '/Parent': IndirectObject(2, 0), '/Resources': IndirectObject(3871, 0), '/Contents': [IndirectObject(3870, 0)], '/MediaBox': [0, 0, 841, 595], '/CropBox': [0, 0, 841, 595]}, (0, 3877): {'/Type': '/Page', '/Parent': IndirectObject(2, 0), '/Resources': IndirectObject(3875, 0), '/Contents': [IndirectObject(3874, 0)], '/MediaBox': [0, 0, 841, 595], '/CropBox': [0, 0, 841, 595]}, (0, 3881): {'/Type': '/Page', '/Parent': IndirectObject(2, 0), '/Resources': IndirectObject(3879, 0), '/Contents': [IndirectObject(3878, 0)], '/MediaBox': [0, 0, 841, 595], '/CropBox': [0, 0, 841, 595]}, (0, 3885): {'/Type': '/Page', '/Parent': IndirectObject(2, 0), '/Resources': IndirectObject(3883, 0), '/Contents': [IndirectObject(3882, 0)], '/MediaBox': [0, 0, 841, 595], '/CropBox': [0, 0, 841, 595]}, (0, 3889): {'/Type': '/Page', '/Parent': IndirectObject(2, 0), '/Resources': IndirectObject(3887, 0), '/Contents': [IndirectObject(3886, 0)], '/MediaBox': [0, 0, 841, 595], '/CropBox': [0, 0, 841, 595]}, (0, 3893): {'/Type': '/Page', '/Parent': IndirectObject(2, 0), '/Resources': IndirectObject(3891, 0), '/Contents': [IndirectObject(3890, 0)], '/MediaBox': [0, 0, 841, 595], '/CropBox': [0, 0, 841, 595]}, (0, 3897): {'/Type': '/Page', '/Parent': IndirectObject(2, 0), '/Resources': IndirectObject(3895, 0), '/Contents': [IndirectObject(3894, 0)], '/MediaBox': [0, 0, 841, 595], '/CropBox': [0, 0, 841, 595]}, (0, 3901): {'/Type': '/Page', '/Parent': IndirectObject(2, 0), '/Resources': IndirectObject(3899, 0), '/Contents': [IndirectObject(3898, 0)], '/MediaBox': [0, 0, 841, 595], '/CropBox': [0, 0, 841, 595]}, (0, 3905): {'/Type': '/Page', '/Parent': IndirectObject(2, 0), '/Resources': IndirectObject(3903, 0), '/Contents': [IndirectObject(3902, 0)], '/MediaBox': [0, 0, 841, 595], '/CropBox': [0, 0, 841, 595]}, (0, 3909): {'/Type': '/Page', '/Parent': IndirectObject(2, 0), '/Resources': IndirectObject(3907, 0), '/Contents': [IndirectObject(3906, 0)], '/MediaBox': [0, 0, 841, 595], '/CropBox': [0, 0, 841, 595]}, (0, 3913): {'/Type': '/Page', '/Parent': IndirectObject(2, 0), '/Resources': IndirectObject(3911, 0), '/Contents': [IndirectObject(3910, 0)], '/MediaBox': [0, 0, 841, 595], '/CropBox': [0, 0, 841, 595]}, (0, 3917): {'/Type': '/Page', '/Parent': IndirectObject(2, 0), '/Resources': IndirectObject(3915, 0), '/Contents': [IndirectObject(3914, 0)], '/MediaBox': [0, 0, 841, 595], '/CropBox': [0, 0, 841, 595]}, (0, 3921): {'/Type': '/Page', '/Parent': IndirectObject(2, 0), '/Resources': IndirectObject(3919, 0), '/Contents': [IndirectObject(3918, 0)], '/MediaBox': [0, 0, 841, 595], '/CropBox': [0, 0, 841, 595]}, (0, 3925): {'/Type': '/Page', '/Parent': IndirectObject(2, 0), '/Resources': IndirectObject(3923, 0), '/Contents': [IndirectObject(3922, 0)], '/MediaBox': [0, 0, 841, 595], '/CropBox': [0, 0, 841, 595]}, (0, 3929): {'/Type': '/Page', '/Parent': IndirectObject(2, 0), '/Resources': IndirectObject(3927, 0), '/Contents': [IndirectObject(3926, 0)], '/MediaBox': [0, 0, 841, 595], '/CropBox': [0, 0, 841, 595]}, (0, 3933): {'/Type': '/Page', '/Parent': IndirectObject(2, 0), '/Resources': IndirectObject(3931, 0), '/Contents': [IndirectObject(3930, 0)], '/MediaBox': [0, 0, 841, 595], '/CropBox': [0, 0, 841, 595]}, (0, 3937): {'/Type': '/Page', '/Parent': IndirectObject(2, 0), '/Resources': IndirectObject(3935, 0), '/Contents': [IndirectObject(3934, 0)], '/MediaBox': [0, 0, 841, 595], '/CropBox': [0, 0, 841, 595]}, (0, 3941): {'/Type': '/Page', '/Parent': IndirectObject(2, 0), '/Resources': IndirectObject(3939, 0), '/Contents': [IndirectObject(3938, 0)], '/MediaBox': [0, 0, 841, 595], '/CropBox': [0, 0, 841, 595]}, (0, 3945): {'/Type': '/Page', '/Parent': IndirectObject(2, 0), '/Resources': IndirectObject(3943, 0), '/Contents': [IndirectObject(3942, 0)], '/MediaBox': [0, 0, 841, 595], '/CropBox': [0, 0, 841, 595]}, (0, 3949): {'/Type': '/Page', '/Parent': IndirectObject(2, 0), '/Resources': IndirectObject(3947, 0), '/Contents': [IndirectObject(3946, 0)], '/MediaBox': [0, 0, 841, 595], '/CropBox': [0, 0, 841, 595]}, (0, 3953): {'/Type': '/Page', '/Parent': IndirectObject(2, 0), '/Resources': IndirectObject(3951, 0), '/Contents': [IndirectObject(3950, 0)], '/MediaBox': [0, 0, 841, 595], '/CropBox': [0, 0, 841, 595]}, (0, 3957): {'/Type': '/Page', '/Parent': IndirectObject(2, 0), '/Resources': IndirectObject(3955, 0), '/Contents': [IndirectObject(3954, 0)], '/MediaBox': [0, 0, 841, 595], '/CropBox': [0, 0, 841, 595]}, (0, 3961): {'/Type': '/Page', '/Parent': IndirectObject(2, 0), '/Resources': IndirectObject(3959, 0), '/Contents': [IndirectObject(3958, 0)], '/MediaBox': [0, 0, 841, 595], '/CropBox': [0, 0, 841, 595]}, (0, 3965): {'/Type': '/Page', '/Parent': IndirectObject(2, 0), '/Resources': IndirectObject(3963, 0), '/Contents': [IndirectObject(3962, 0)], '/MediaBox': [0, 0, 841, 595], '/CropBox': [0, 0, 841, 595]}, (0, 3969): {'/Type': '/Page', '/Parent': IndirectObject(2, 0), '/Resources': IndirectObject(3967, 0), '/Contents': [IndirectObject(3966, 0)], '/MediaBox': [0, 0, 841, 595], '/CropBox': [0, 0, 841, 595]}, (0, 3973): {'/Type': '/Page', '/Parent': IndirectObject(2, 0), '/Resources': IndirectObject(3971, 0), '/Contents': [IndirectObject(3970, 0)], '/MediaBox': [0, 0, 841, 595], '/CropBox': [0, 0, 841, 595]}, (0, 3977): {'/Type': '/Page', '/Parent': IndirectObject(2, 0), '/Resources': IndirectObject(3975, 0), '/Contents': [IndirectObject(3974, 0)], '/MediaBox': [0, 0, 841, 595], '/CropBox': [0, 0, 841, 595]}, (0, 3981): {'/Type': '/Page', '/Parent': IndirectObject(2, 0), '/Resources': IndirectObject(3979, 0), '/Contents': [IndirectObject(3978, 0)], '/MediaBox': [0, 0, 841, 595], '/CropBox': [0, 0, 841, 595]}, (0, 3985): {'/Type': '/Page', '/Parent': IndirectObject(2, 0), '/Resources': IndirectObject(3983, 0), '/Contents': [IndirectObject(3982, 0)], '/MediaBox': [0, 0, 841, 595], '/CropBox': [0, 0, 841, 595]}, (0, 3989): {'/Type': '/Page', '/Parent': IndirectObject(2, 0), '/Resources': IndirectObject(3987, 0), '/Contents': [IndirectObject(3986, 0)], '/MediaBox': [0, 0, 841, 595], '/CropBox': [0, 0, 841, 595]}, (0, 3993): {'/Type': '/Page', '/Parent': IndirectObject(2, 0), '/Resources': IndirectObject(3991, 0), '/Contents': [IndirectObject(3990, 0)], '/MediaBox': [0, 0, 841, 595], '/CropBox': [0, 0, 841, 595]}, (0, 3997): {'/Type': '/Page', '/Parent': IndirectObject(2, 0), '/Resources': IndirectObject(3995, 0), '/Contents': [IndirectObject(3994, 0)], '/MediaBox': [0, 0, 841, 595], '/CropBox': [0, 0, 841, 595]}, (0, 4001): {'/Type': '/Page', '/Parent': IndirectObject(2, 0), '/Resources': IndirectObject(3999, 0), '/Contents': [IndirectObject(3998, 0)], '/MediaBox': [0, 0, 841, 595], '/CropBox': [0, 0, 841, 595]}, (0, 4005): {'/Type': '/Page', '/Parent': IndirectObject(2, 0), '/Resources': IndirectObject(4003, 0), '/Contents': [IndirectObject(4002, 0)], '/MediaBox': [0, 0, 841, 595], '/CropBox': [0, 0, 841, 595]}, (0, 4009): {'/Type': '/Page', '/Parent': IndirectObject(2, 0), '/Resources': IndirectObject(4007, 0), '/Contents': [IndirectObject(4006, 0)], '/MediaBox': [0, 0, 841, 595], '/CropBox': [0, 0, 841, 595]}, (0, 4013): {'/Type': '/Page', '/Parent': IndirectObject(2, 0), '/Resources': IndirectObject(4011, 0), '/Contents': [IndirectObject(4010, 0)], '/MediaBox': [0, 0, 841, 595], '/CropBox': [0, 0, 841, 595]}, (0, 4017): {'/Type': '/Page', '/Parent': IndirectObject(2, 0), '/Resources': IndirectObject(4015, 0), '/Contents': [IndirectObject(4014, 0)], '/MediaBox': [0, 0, 841, 595], '/CropBox': [0, 0, 841, 595]}, (0, 4021): {'/Type': '/Page', '/Parent': IndirectObject(2, 0), '/Resources': IndirectObject(4019, 0), '/Contents': [IndirectObject(4018, 0)], '/MediaBox': [0, 0, 841, 595], '/CropBox': [0, 0, 841, 595]}, (0, 4025): {'/Type': '/Page', '/Parent': IndirectObject(2, 0), '/Resources': IndirectObject(4023, 0), '/Contents': [IndirectObject(4022, 0)], '/MediaBox': [0, 0, 841, 595], '/CropBox': [0, 0, 841, 595]}, (0, 4029): {'/Type': '/Page', '/Parent': IndirectObject(2, 0), '/Resources': IndirectObject(4027, 0), '/Contents': [IndirectObject(4026, 0)], '/MediaBox': [0, 0, 841, 595], '/CropBox': [0, 0, 841, 595]}, (0, 4033): {'/Type': '/Page', '/Parent': IndirectObject(2, 0), '/Resources': IndirectObject(4031, 0), '/Contents': [IndirectObject(4030, 0)], '/MediaBox': [0, 0, 841, 595], '/CropBox': [0, 0, 841, 595]}, (0, 4037): {'/Type': '/Page', '/Parent': IndirectObject(2, 0), '/Resources': IndirectObject(4035, 0), '/Contents': [IndirectObject(4034, 0)], '/MediaBox': [0, 0, 841, 595], '/CropBox': [0, 0, 841, 595]}, (0, 4041): {'/Type': '/Page', '/Parent': IndirectObject(2, 0), '/Resources': IndirectObject(4039, 0), '/Contents': [IndirectObject(4038, 0)], '/MediaBox': [0, 0, 841, 595], '/CropBox': [0, 0, 841, 595]}, (0, 4045): {'/Type': '/Page', '/Parent': IndirectObject(2, 0), '/Resources': IndirectObject(4043, 0), '/Contents': [IndirectObject(4042, 0)], '/MediaBox': [0, 0, 841, 595], '/CropBox': [0, 0, 841, 595]}, (0, 4049): {'/Type': '/Page', '/Parent': IndirectObject(2, 0), '/Resources': IndirectObject(4047, 0), '/Contents': [IndirectObject(4046, 0)], '/MediaBox': [0, 0, 841, 595], '/CropBox': [0, 0, 841, 595]}, (0, 4053): {'/Type': '/Page', '/Parent': IndirectObject(2, 0), '/Resources': IndirectObject(4051, 0), '/Contents': [IndirectObject(4050, 0)], '/MediaBox': [0, 0, 841, 595], '/CropBox': [0, 0, 841, 595]}, (0, 4057): {'/Type': '/Page', '/Parent': IndirectObject(2, 0), '/Resources': IndirectObject(4055, 0), '/Contents': [IndirectObject(4054, 0)], '/MediaBox': [0, 0, 841, 595], '/CropBox': [0, 0, 841, 595]}, (0, 4061): {'/Type': '/Page', '/Parent': IndirectObject(2, 0), '/Resources': IndirectObject(4059, 0), '/Contents': [IndirectObject(4058, 0)], '/MediaBox': [0, 0, 841, 595], '/CropBox': [0, 0, 841, 595]}, (0, 4065): {'/Type': '/Page', '/Parent': IndirectObject(2, 0), '/Resources': IndirectObject(4063, 0), '/Contents': [IndirectObject(4062, 0)], '/MediaBox': [0, 0, 841, 595], '/CropBox': [0, 0, 841, 595]}, (0, 4069): {'/Type': '/Page', '/Parent': IndirectObject(2, 0), '/Resources': IndirectObject(4067, 0), '/Contents': [IndirectObject(4066, 0)], '/MediaBox': [0, 0, 841, 595], '/CropBox': [0, 0, 841, 595]}, (0, 4073): {'/Type': '/Page', '/Parent': IndirectObject(2, 0), '/Resources': IndirectObject(4071, 0), '/Contents': [IndirectObject(4070, 0)], '/MediaBox': [0, 0, 841, 595], '/CropBox': [0, 0, 841, 595]}, (0, 4077): {'/Type': '/Page', '/Parent': IndirectObject(2, 0), '/Resources': IndirectObject(4075, 0), '/Contents': [IndirectObject(4074, 0)], '/MediaBox': [0, 0, 841, 595], '/CropBox': [0, 0, 841, 595]}, (0, 4081): {'/Type': '/Page', '/Parent': IndirectObject(2, 0), '/Resources': IndirectObject(4079, 0), '/Contents': [IndirectObject(4078, 0)], '/MediaBox': [0, 0, 841, 595], '/CropBox': [0, 0, 841, 595]}, (0, 4085): {'/Type': '/Page', '/Parent': IndirectObject(2, 0), '/Resources': IndirectObject(4083, 0), '/Contents': [IndirectObject(4082, 0)], '/MediaBox': [0, 0, 841, 595], '/CropBox': [0, 0, 841, 595]}, (0, 4089): {'/Type': '/Page', '/Parent': IndirectObject(2, 0), '/Resources': IndirectObject(4087, 0), '/Contents': [IndirectObject(4086, 0)], '/MediaBox': [0, 0, 841, 595], '/CropBox': [0, 0, 841, 595]}, (0, 4093): {'/Type': '/Page', '/Parent': IndirectObject(2, 0), '/Resources': IndirectObject(4091, 0), '/Contents': [IndirectObject(4090, 0)], '/MediaBox': [0, 0, 841, 595], '/CropBox': [0, 0, 841, 595]}, (0, 4097): {'/Type': '/Page', '/Parent': IndirectObject(2, 0), '/Resources': IndirectObject(4095, 0), '/Contents': [IndirectObject(4094, 0)], '/MediaBox': [0, 0, 841, 595], '/CropBox': [0, 0, 841, 595]}, (0, 4101): {'/Type': '/Page', '/Parent': IndirectObject(2, 0), '/Resources': IndirectObject(4099, 0), '/Contents': [IndirectObject(4098, 0)], '/MediaBox': [0, 0, 841, 595], '/CropBox': [0, 0, 841, 595]}, (0, 4105): {'/Type': '/Page', '/Parent': IndirectObject(2, 0), '/Resources': IndirectObject(4103, 0), '/Contents': [IndirectObject(4102, 0)], '/MediaBox': [0, 0, 841, 595], '/CropBox': [0, 0, 841, 595]}, (0, 4109): {'/Type': '/Page', '/Parent': IndirectObject(2, 0), '/Resources': IndirectObject(4107, 0), '/Contents': [IndirectObject(4106, 0)], '/MediaBox': [0, 0, 841, 595], '/CropBox': [0, 0, 841, 595]}, (0, 4113): {'/Type': '/Page', '/Parent': IndirectObject(2, 0), '/Resources': IndirectObject(4111, 0), '/Contents': [IndirectObject(4110, 0)], '/MediaBox': [0, 0, 841, 595], '/CropBox': [0, 0, 841, 595]}, (0, 4117): {'/Type': '/Page', '/Parent': IndirectObject(2, 0), '/Resources': IndirectObject(4115, 0), '/Contents': [IndirectObject(4114, 0)], '/MediaBox': [0, 0, 841, 595], '/CropBox': [0, 0, 841, 595]}, (0, 4121): {'/Type': '/Page', '/Parent': IndirectObject(2, 0), '/Resources': IndirectObject(4119, 0), '/Contents': [IndirectObject(4118, 0)], '/MediaBox': [0, 0, 841, 595], '/CropBox': [0, 0, 841, 595]}, (0, 4125): {'/Type': '/Page', '/Parent': IndirectObject(2, 0), '/Resources': IndirectObject(4123, 0), '/Contents': [IndirectObject(4122, 0)], '/MediaBox': [0, 0, 841, 595], '/CropBox': [0, 0, 841, 595]}, (0, 4129): {'/Type': '/Page', '/Parent': IndirectObject(2, 0), '/Resources': IndirectObject(4127, 0), '/Contents': [IndirectObject(4126, 0)], '/MediaBox': [0, 0, 841, 595], '/CropBox': [0, 0, 841, 595]}, (0, 4133): {'/Type': '/Page', '/Parent': IndirectObject(2, 0), '/Resources': IndirectObject(4131, 0), '/Contents': [IndirectObject(4130, 0)], '/MediaBox': [0, 0, 841, 595], '/CropBox': [0, 0, 841, 595]}, (0, 4137): {'/Type': '/Page', '/Parent': IndirectObject(2, 0), '/Resources': IndirectObject(4135, 0), '/Contents': [IndirectObject(4134, 0)], '/MediaBox': [0, 0, 841, 595], '/CropBox': [0, 0, 841, 595]}, (0, 4141): {'/Type': '/Page', '/Parent': IndirectObject(2, 0), '/Resources': IndirectObject(4139, 0), '/Contents': [IndirectObject(4138, 0)], '/MediaBox': [0, 0, 841, 595], '/CropBox': [0, 0, 841, 595]}, (0, 4145): {'/Type': '/Page', '/Parent': IndirectObject(2, 0), '/Resources': IndirectObject(4143, 0), '/Contents': [IndirectObject(4142, 0)], '/MediaBox': [0, 0, 841, 595], '/CropBox': [0, 0, 841, 595]}, (0, 4149): {'/Type': '/Page', '/Parent': IndirectObject(2, 0), '/Resources': IndirectObject(4147, 0), '/Contents': [IndirectObject(4146, 0)], '/MediaBox': [0, 0, 841, 595], '/CropBox': [0, 0, 841, 595]}, (0, 4153): {'/Type': '/Page', '/Parent': IndirectObject(2, 0), '/Resources': IndirectObject(4151, 0), '/Contents': [IndirectObject(4150, 0)], '/MediaBox': [0, 0, 841, 595], '/CropBox': [0, 0, 841, 595]}, (0, 4157): {'/Type': '/Page', '/Parent': IndirectObject(2, 0), '/Resources': IndirectObject(4155, 0), '/Contents': [IndirectObject(4154, 0)], '/MediaBox': [0, 0, 841, 595], '/CropBox': [0, 0, 841, 595]}, (0, 4161): {'/Type': '/Page', '/Parent': IndirectObject(2, 0), '/Resources': IndirectObject(4159, 0), '/Contents': [IndirectObject(4158, 0)], '/MediaBox': [0, 0, 841, 595], '/CropBox': [0, 0, 841, 595]}, (0, 4165): {'/Type': '/Page', '/Parent': IndirectObject(2, 0), '/Resources': IndirectObject(4163, 0), '/Contents': [IndirectObject(4162, 0)], '/MediaBox': [0, 0, 841, 595], '/CropBox': [0, 0, 841, 595]}, (0, 4169): {'/Type': '/Page', '/Parent': IndirectObject(2, 0), '/Resources': IndirectObject(4167, 0), '/Contents': [IndirectObject(4166, 0)], '/MediaBox': [0, 0, 841, 595], '/CropBox': [0, 0, 841, 595]}, (0, 4173): {'/Type': '/Page', '/Parent': IndirectObject(2, 0), '/Resources': IndirectObject(4171, 0), '/Contents': [IndirectObject(4170, 0)], '/MediaBox': [0, 0, 841, 595], '/CropBox': [0, 0, 841, 595]}, (0, 4177): {'/Type': '/Page', '/Parent': IndirectObject(2, 0), '/Resources': IndirectObject(4175, 0), '/Contents': [IndirectObject(4174, 0)], '/MediaBox': [0, 0, 841, 595], '/CropBox': [0, 0, 841, 595]}, (0, 4181): {'/Type': '/Page', '/Parent': IndirectObject(2, 0), '/Resources': IndirectObject(4179, 0), '/Contents': [IndirectObject(4178, 0)], '/MediaBox': [0, 0, 841, 595], '/CropBox': [0, 0, 841, 595]}, (0, 4185): {'/Type': '/Page', '/Parent': IndirectObject(2, 0), '/Resources': IndirectObject(4183, 0), '/Contents': [IndirectObject(4182, 0)], '/MediaBox': [0, 0, 841, 595], '/CropBox': [0, 0, 841, 595]}, (0, 4189): {'/Type': '/Page', '/Parent': IndirectObject(2, 0), '/Resources': IndirectObject(4187, 0), '/Contents': [IndirectObject(4186, 0)], '/MediaBox': [0, 0, 841, 595], '/CropBox': [0, 0, 841, 595]}, (0, 4193): {'/Type': '/Page', '/Parent': IndirectObject(2, 0), '/Resources': IndirectObject(4191, 0), '/Contents': [IndirectObject(4190, 0)], '/MediaBox': [0, 0, 841, 595], '/CropBox': [0, 0, 841, 595]}, (0, 4197): {'/Type': '/Page', '/Parent': IndirectObject(2, 0), '/Resources': IndirectObject(4195, 0), '/Contents': [IndirectObject(4194, 0)], '/MediaBox': [0, 0, 841, 595], '/CropBox': [0, 0, 841, 595]}, (0, 4201): {'/Type': '/Page', '/Parent': IndirectObject(2, 0), '/Resources': IndirectObject(4199, 0), '/Contents': [IndirectObject(4198, 0)], '/MediaBox': [0, 0, 841, 595], '/CropBox': [0, 0, 841, 595]}, (0, 4205): {'/Type': '/Page', '/Parent': IndirectObject(2, 0), '/Resources': IndirectObject(4203, 0), '/Contents': [IndirectObject(4202, 0)], '/MediaBox': [0, 0, 841, 595], '/CropBox': [0, 0, 841, 595]}, (0, 4209): {'/Type': '/Page', '/Parent': IndirectObject(2, 0), '/Resources': IndirectObject(4207, 0), '/Contents': [IndirectObject(4206, 0)], '/MediaBox': [0, 0, 841, 595], '/CropBox': [0, 0, 841, 595]}, (0, 4213): {'/Type': '/Page', '/Parent': IndirectObject(2, 0), '/Resources': IndirectObject(4211, 0), '/Contents': [IndirectObject(4210, 0)], '/MediaBox': [0, 0, 841, 595], '/CropBox': [0, 0, 841, 595]}, (0, 4217): {'/Type': '/Page', '/Parent': IndirectObject(2, 0), '/Resources': IndirectObject(4215, 0), '/Contents': [IndirectObject(4214, 0)], '/MediaBox': [0, 0, 841, 595], '/CropBox': [0, 0, 841, 595]}, (0, 4221): {'/Type': '/Page', '/Parent': IndirectObject(2, 0), '/Resources': IndirectObject(4219, 0), '/Contents': [IndirectObject(4218, 0)], '/MediaBox': [0, 0, 841, 595], '/CropBox': [0, 0, 841, 595]}, (0, 4225): {'/Type': '/Page', '/Parent': IndirectObject(2, 0), '/Resources': IndirectObject(4223, 0), '/Contents': [IndirectObject(4222, 0)], '/MediaBox': [0, 0, 841, 595], '/CropBox': [0, 0, 841, 595]}, (0, 4229): {'/Type': '/Page', '/Parent': IndirectObject(2, 0), '/Resources': IndirectObject(4227, 0), '/Contents': [IndirectObject(4226, 0)], '/MediaBox': [0, 0, 841, 595], '/CropBox': [0, 0, 841, 595]}, (0, 4233): {'/Type': '/Page', '/Parent': IndirectObject(2, 0), '/Resources': IndirectObject(4231, 0), '/Contents': [IndirectObject(4230, 0)], '/MediaBox': [0, 0, 841, 595], '/CropBox': [0, 0, 841, 595]}, (0, 4237): {'/Type': '/Page', '/Parent': IndirectObject(2, 0), '/Resources': IndirectObject(4235, 0), '/Contents': [IndirectObject(4234, 0)], '/MediaBox': [0, 0, 841, 595], '/CropBox': [0, 0, 841, 595]}, (0, 4241): {'/Type': '/Page', '/Parent': IndirectObject(2, 0), '/Resources': IndirectObject(4239, 0), '/Contents': [IndirectObject(4238, 0)], '/MediaBox': [0, 0, 841, 595], '/CropBox': [0, 0, 841, 595]}, (0, 4245): {'/Type': '/Page', '/Parent': IndirectObject(2, 0), '/Resources': IndirectObject(4243, 0), '/Contents': [IndirectObject(4242, 0)], '/MediaBox': [0, 0, 841, 595], '/CropBox': [0, 0, 841, 595]}, (0, 4249): {'/Type': '/Page', '/Parent': IndirectObject(2, 0), '/Resources': IndirectObject(4247, 0), '/Contents': [IndirectObject(4246, 0)], '/MediaBox': [0, 0, 841, 595], '/CropBox': [0, 0, 841, 595]}, (0, 4253): {'/Type': '/Page', '/Parent': IndirectObject(2, 0), '/Resources': IndirectObject(4251, 0), '/Contents': [IndirectObject(4250, 0)], '/MediaBox': [0, 0, 841, 595], '/CropBox': [0, 0, 841, 595]}, (0, 4257): {'/Type': '/Page', '/Parent': IndirectObject(2, 0), '/Resources': IndirectObject(4255, 0), '/Contents': [IndirectObject(4254, 0)], '/MediaBox': [0, 0, 841, 595], '/CropBox': [0, 0, 841, 595]}, (0, 4261): {'/Type': '/Page', '/Parent': IndirectObject(2, 0), '/Resources': IndirectObject(4259, 0), '/Contents': [IndirectObject(4258, 0)], '/MediaBox': [0, 0, 841, 595], '/CropBox': [0, 0, 841, 595]}, (0, 4265): {'/Type': '/Page', '/Parent': IndirectObject(2, 0), '/Resources': IndirectObject(4263, 0), '/Contents': [IndirectObject(4262, 0)], '/MediaBox': [0, 0, 841, 595], '/CropBox': [0, 0, 841, 595]}, (0, 4269): {'/Type': '/Page', '/Parent': IndirectObject(2, 0), '/Resources': IndirectObject(4267, 0), '/Contents': [IndirectObject(4266, 0)], '/MediaBox': [0, 0, 841, 595], '/CropBox': [0, 0, 841, 595]}, (0, 4273): {'/Type': '/Page', '/Parent': IndirectObject(2, 0), '/Resources': IndirectObject(4271, 0), '/Contents': [IndirectObject(4270, 0)], '/MediaBox': [0, 0, 841, 595], '/CropBox': [0, 0, 841, 595]}, (0, 4277): {'/Type': '/Page', '/Parent': IndirectObject(2, 0), '/Resources': IndirectObject(4275, 0), '/Contents': [IndirectObject(4274, 0)], '/MediaBox': [0, 0, 841, 595], '/CropBox': [0, 0, 841, 595]}, (0, 4281): {'/Type': '/Page', '/Parent': IndirectObject(2, 0), '/Resources': IndirectObject(4279, 0), '/Contents': [IndirectObject(4278, 0)], '/MediaBox': [0, 0, 841, 595], '/CropBox': [0, 0, 841, 595]}, (0, 4285): {'/Type': '/Page', '/Parent': IndirectObject(2, 0), '/Resources': IndirectObject(4283, 0), '/Contents': [IndirectObject(4282, 0)], '/MediaBox': [0, 0, 841, 595], '/CropBox': [0, 0, 841, 595]}, (0, 4289): {'/Type': '/Page', '/Parent': IndirectObject(2, 0), '/Resources': IndirectObject(4287, 0), '/Contents': [IndirectObject(4286, 0)], '/MediaBox': [0, 0, 841, 595], '/CropBox': [0, 0, 841, 595]}, (0, 4293): {'/Type': '/Page', '/Parent': IndirectObject(2, 0), '/Resources': IndirectObject(4291, 0), '/Contents': [IndirectObject(4290, 0)], '/MediaBox': [0, 0, 841, 595], '/CropBox': [0, 0, 841, 595]}, (0, 4297): {'/Type': '/Page', '/Parent': IndirectObject(2, 0), '/Resources': IndirectObject(4295, 0), '/Contents': [IndirectObject(4294, 0)], '/MediaBox': [0, 0, 841, 595], '/CropBox': [0, 0, 841, 595]}, (0, 4301): {'/Type': '/Page', '/Parent': IndirectObject(2, 0), '/Resources': IndirectObject(4299, 0), '/Contents': [IndirectObject(4298, 0)], '/MediaBox': [0, 0, 841, 595], '/CropBox': [0, 0, 841, 595]}, (0, 4305): {'/Type': '/Page', '/Parent': IndirectObject(2, 0), '/Resources': IndirectObject(4303, 0), '/Contents': [IndirectObject(4302, 0)], '/MediaBox': [0, 0, 841, 595], '/CropBox': [0, 0, 841, 595]}, (0, 4309): {'/Type': '/Page', '/Parent': IndirectObject(2, 0), '/Resources': IndirectObject(4307, 0), '/Contents': [IndirectObject(4306, 0)], '/MediaBox': [0, 0, 841, 595], '/CropBox': [0, 0, 841, 595]}, (0, 4313): {'/Type': '/Page', '/Parent': IndirectObject(2, 0), '/Resources': IndirectObject(4311, 0), '/Contents': [IndirectObject(4310, 0)], '/MediaBox': [0, 0, 841, 595], '/CropBox': [0, 0, 841, 595]}, (0, 4317): {'/Type': '/Page', '/Parent': IndirectObject(2, 0), '/Resources': IndirectObject(4315, 0), '/Contents': [IndirectObject(4314, 0)], '/MediaBox': [0, 0, 841, 595], '/CropBox': [0, 0, 841, 595]}, (0, 4321): {'/Type': '/Page', '/Parent': IndirectObject(2, 0), '/Resources': IndirectObject(4319, 0), '/Contents': [IndirectObject(4318, 0)], '/MediaBox': [0, 0, 841, 595], '/CropBox': [0, 0, 841, 595]}, (0, 4325): {'/Type': '/Page', '/Parent': IndirectObject(2, 0), '/Resources': IndirectObject(4323, 0), '/Contents': [IndirectObject(4322, 0)], '/MediaBox': [0, 0, 841, 595], '/CropBox': [0, 0, 841, 595]}, (0, 4329): {'/Type': '/Page', '/Parent': IndirectObject(2, 0), '/Resources': IndirectObject(4327, 0), '/Contents': [IndirectObject(4326, 0)], '/MediaBox': [0, 0, 841, 595], '/CropBox': [0, 0, 841, 595]}, (0, 4333): {'/Type': '/Page', '/Parent': IndirectObject(2, 0), '/Resources': IndirectObject(4331, 0), '/Contents': [IndirectObject(4330, 0)], '/MediaBox': [0, 0, 841, 595], '/CropBox': [0, 0, 841, 595]}, (0, 4337): {'/Type': '/Page', '/Parent': IndirectObject(2, 0), '/Resources': IndirectObject(4335, 0), '/Contents': [IndirectObject(4334, 0)], '/MediaBox': [0, 0, 841, 595], '/CropBox': [0, 0, 841, 595]}, (0, 4341): {'/Type': '/Page', '/Parent': IndirectObject(2, 0), '/Resources': IndirectObject(4339, 0), '/Contents': [IndirectObject(4338, 0)], '/MediaBox': [0, 0, 841, 595], '/CropBox': [0, 0, 841, 595]}, (0, 4345): {'/Type': '/Page', '/Parent': IndirectObject(2, 0), '/Resources': IndirectObject(4343, 0), '/Contents': [IndirectObject(4342, 0)], '/MediaBox': [0, 0, 841, 595], '/CropBox': [0, 0, 841, 595]}, (0, 4349): {'/Type': '/Page', '/Parent': IndirectObject(2, 0), '/Resources': IndirectObject(4347, 0), '/Contents': [IndirectObject(4346, 0)], '/MediaBox': [0, 0, 841, 595], '/CropBox': [0, 0, 841, 595]}, (0, 4353): {'/Type': '/Page', '/Parent': IndirectObject(2, 0), '/Resources': IndirectObject(4351, 0), '/Contents': [IndirectObject(4350, 0)], '/MediaBox': [0, 0, 841, 595], '/CropBox': [0, 0, 841, 595]}, (0, 4357): {'/Type': '/Page', '/Parent': IndirectObject(2, 0), '/Resources': IndirectObject(4355, 0), '/Contents': [IndirectObject(4354, 0)], '/MediaBox': [0, 0, 841, 595], '/CropBox': [0, 0, 841, 595]}, (0, 4361): {'/Type': '/Page', '/Parent': IndirectObject(2, 0), '/Resources': IndirectObject(4359, 0), '/Contents': [IndirectObject(4358, 0)], '/MediaBox': [0, 0, 841, 595], '/CropBox': [0, 0, 841, 595]}, (0, 4365): {'/Type': '/Page', '/Parent': IndirectObject(2, 0), '/Resources': IndirectObject(4363, 0), '/Contents': [IndirectObject(4362, 0)], '/MediaBox': [0, 0, 841, 595], '/CropBox': [0, 0, 841, 595]}, (0, 4369): {'/Type': '/Page', '/Parent': IndirectObject(2, 0), '/Resources': IndirectObject(4367, 0), '/Contents': [IndirectObject(4366, 0)], '/MediaBox': [0, 0, 841, 595], '/CropBox': [0, 0, 841, 595]}, (0, 4373): {'/Type': '/Page', '/Parent': IndirectObject(2, 0), '/Resources': IndirectObject(4371, 0), '/Contents': [IndirectObject(4370, 0)], '/MediaBox': [0, 0, 841, 595], '/CropBox': [0, 0, 841, 595]}, (0, 4377): {'/Type': '/Page', '/Parent': IndirectObject(2, 0), '/Resources': IndirectObject(4375, 0), '/Contents': [IndirectObject(4374, 0)], '/MediaBox': [0, 0, 841, 595], '/CropBox': [0, 0, 841, 595]}, (0, 4381): {'/Type': '/Page', '/Parent': IndirectObject(2, 0), '/Resources': IndirectObject(4379, 0), '/Contents': [IndirectObject(4378, 0)], '/MediaBox': [0, 0, 841, 595], '/CropBox': [0, 0, 841, 595]}, (0, 4385): {'/Type': '/Page', '/Parent': IndirectObject(2, 0), '/Resources': IndirectObject(4383, 0), '/Contents': [IndirectObject(4382, 0)], '/MediaBox': [0, 0, 841, 595], '/CropBox': [0, 0, 841, 595]}, (0, 4389): {'/Type': '/Page', '/Parent': IndirectObject(2, 0), '/Resources': IndirectObject(4387, 0), '/Contents': [IndirectObject(4386, 0)], '/MediaBox': [0, 0, 841, 595], '/CropBox': [0, 0, 841, 595]}, (0, 4393): {'/Type': '/Page', '/Parent': IndirectObject(2, 0), '/Resources': IndirectObject(4391, 0), '/Contents': [IndirectObject(4390, 0)], '/MediaBox': [0, 0, 841, 595], '/CropBox': [0, 0, 841, 595]}, (0, 4397): {'/Type': '/Page', '/Parent': IndirectObject(2, 0), '/Resources': IndirectObject(4395, 0), '/Contents': [IndirectObject(4394, 0)], '/MediaBox': [0, 0, 841, 595], '/CropBox': [0, 0, 841, 595]}, (0, 4401): {'/Type': '/Page', '/Parent': IndirectObject(2, 0), '/Resources': IndirectObject(4399, 0), '/Contents': [IndirectObject(4398, 0)], '/MediaBox': [0, 0, 841, 595], '/CropBox': [0, 0, 841, 595]}, (0, 4405): {'/Type': '/Page', '/Parent': IndirectObject(2, 0), '/Resources': IndirectObject(4403, 0), '/Contents': [IndirectObject(4402, 0)], '/MediaBox': [0, 0, 841, 595], '/CropBox': [0, 0, 841, 595]}, (0, 4409): {'/Type': '/Page', '/Parent': IndirectObject(2, 0), '/Resources': IndirectObject(4407, 0), '/Contents': [IndirectObject(4406, 0)], '/MediaBox': [0, 0, 841, 595], '/CropBox': [0, 0, 841, 595]}, (0, 4413): {'/Type': '/Page', '/Parent': IndirectObject(2, 0), '/Resources': IndirectObject(4411, 0), '/Contents': [IndirectObject(4410, 0)], '/MediaBox': [0, 0, 841, 595], '/CropBox': [0, 0, 841, 595]}, (0, 4417): {'/Type': '/Page', '/Parent': IndirectObject(2, 0), '/Resources': IndirectObject(4415, 0), '/Contents': [IndirectObject(4414, 0)], '/MediaBox': [0, 0, 841, 595], '/CropBox': [0, 0, 841, 595]}, (0, 4421): {'/Type': '/Page', '/Parent': IndirectObject(2, 0), '/Resources': IndirectObject(4419, 0), '/Contents': [IndirectObject(4418, 0)], '/MediaBox': [0, 0, 841, 595], '/CropBox': [0, 0, 841, 595]}, (0, 4425): {'/Type': '/Page', '/Parent': IndirectObject(2, 0), '/Resources': IndirectObject(4423, 0), '/Contents': [IndirectObject(4422, 0)], '/MediaBox': [0, 0, 841, 595], '/CropBox': [0, 0, 841, 595]}, (0, 4429): {'/Type': '/Page', '/Parent': IndirectObject(2, 0), '/Resources': IndirectObject(4427, 0), '/Contents': [IndirectObject(4426, 0)], '/MediaBox': [0, 0, 841, 595], '/CropBox': [0, 0, 841, 595]}, (0, 4433): {'/Type': '/Page', '/Parent': IndirectObject(2, 0), '/Resources': IndirectObject(4431, 0), '/Contents': [IndirectObject(4430, 0)], '/MediaBox': [0, 0, 841, 595], '/CropBox': [0, 0, 841, 595]}, (0, 4437): {'/Type': '/Page', '/Parent': IndirectObject(2, 0), '/Resources': IndirectObject(4435, 0), '/Contents': [IndirectObject(4434, 0)], '/MediaBox': [0, 0, 841, 595], '/CropBox': [0, 0, 841, 595]}, (0, 4441): {'/Type': '/Page', '/Parent': IndirectObject(2, 0), '/Resources': IndirectObject(4439, 0), '/Contents': [IndirectObject(4438, 0)], '/MediaBox': [0, 0, 841, 595], '/CropBox': [0, 0, 841, 595]}, (0, 4445): {'/Type': '/Page', '/Parent': IndirectObject(2, 0), '/Resources': IndirectObject(4443, 0), '/Contents': [IndirectObject(4442, 0)], '/MediaBox': [0, 0, 841, 595], '/CropBox': [0, 0, 841, 595]}, (0, 4449): {'/Type': '/Page', '/Parent': IndirectObject(2, 0), '/Resources': IndirectObject(4447, 0), '/Contents': [IndirectObject(4446, 0)], '/MediaBox': [0, 0, 841, 595], '/CropBox': [0, 0, 841, 595]}, (0, 4453): {'/Type': '/Page', '/Parent': IndirectObject(2, 0), '/Resources': IndirectObject(4451, 0), '/Contents': [IndirectObject(4450, 0)], '/MediaBox': [0, 0, 841, 595], '/CropBox': [0, 0, 841, 595]}, (0, 4457): {'/Type': '/Page', '/Parent': IndirectObject(2, 0), '/Resources': IndirectObject(4455, 0), '/Contents': [IndirectObject(4454, 0)], '/MediaBox': [0, 0, 841, 595], '/CropBox': [0, 0, 841, 595]}, (0, 4461): {'/Type': '/Page', '/Parent': IndirectObject(2, 0), '/Resources': IndirectObject(4459, 0), '/Contents': [IndirectObject(4458, 0)], '/MediaBox': [0, 0, 841, 595], '/CropBox': [0, 0, 841, 595]}, (0, 4465): {'/Type': '/Page', '/Parent': IndirectObject(2, 0), '/Resources': IndirectObject(4463, 0), '/Contents': [IndirectObject(4462, 0)], '/MediaBox': [0, 0, 841, 595], '/CropBox': [0, 0, 841, 595]}, (0, 4469): {'/Type': '/Page', '/Parent': IndirectObject(2, 0), '/Resources': IndirectObject(4467, 0), '/Contents': [IndirectObject(4466, 0)], '/MediaBox': [0, 0, 841, 595], '/CropBox': [0, 0, 841, 595]}, (0, 4473): {'/Type': '/Page', '/Parent': IndirectObject(2, 0), '/Resources': IndirectObject(4471, 0), '/Contents': [IndirectObject(4470, 0)], '/MediaBox': [0, 0, 841, 595], '/CropBox': [0, 0, 841, 595]}, (0, 4477): {'/Type': '/Page', '/Parent': IndirectObject(2, 0), '/Resources': IndirectObject(4475, 0), '/Contents': [IndirectObject(4474, 0)], '/MediaBox': [0, 0, 841, 595], '/CropBox': [0, 0, 841, 595]}, (0, 4481): {'/Type': '/Page', '/Parent': IndirectObject(2, 0), '/Resources': IndirectObject(4479, 0), '/Contents': [IndirectObject(4478, 0)], '/MediaBox': [0, 0, 841, 595], '/CropBox': [0, 0, 841, 595]}, (0, 4485): {'/Type': '/Page', '/Parent': IndirectObject(2, 0), '/Resources': IndirectObject(4483, 0), '/Contents': [IndirectObject(4482, 0)], '/MediaBox': [0, 0, 841, 595], '/CropBox': [0, 0, 841, 595]}, (0, 4489): {'/Type': '/Page', '/Parent': IndirectObject(2, 0), '/Resources': IndirectObject(4487, 0), '/Contents': [IndirectObject(4486, 0)], '/MediaBox': [0, 0, 841, 595], '/CropBox': [0, 0, 841, 595]}, (0, 4493): {'/Type': '/Page', '/Parent': IndirectObject(2, 0), '/Resources': IndirectObject(4491, 0), '/Contents': [IndirectObject(4490, 0)], '/MediaBox': [0, 0, 841, 595], '/CropBox': [0, 0, 841, 595]}, (0, 4497): {'/Type': '/Page', '/Parent': IndirectObject(2, 0), '/Resources': IndirectObject(4495, 0), '/Contents': [IndirectObject(4494, 0)], '/MediaBox': [0, 0, 841, 595], '/CropBox': [0, 0, 841, 595]}, (0, 4501): {'/Type': '/Page', '/Parent': IndirectObject(2, 0), '/Resources': IndirectObject(4499, 0), '/Contents': [IndirectObject(4498, 0)], '/MediaBox': [0, 0, 841, 595], '/CropBox': [0, 0, 841, 595]}, (0, 4505): {'/Type': '/Page', '/Parent': IndirectObject(2, 0), '/Resources': IndirectObject(4503, 0), '/Contents': [IndirectObject(4502, 0)], '/MediaBox': [0, 0, 841, 595], '/CropBox': [0, 0, 841, 595]}, (0, 4509): {'/Type': '/Page', '/Parent': IndirectObject(2, 0), '/Resources': IndirectObject(4507, 0), '/Contents': [IndirectObject(4506, 0)], '/MediaBox': [0, 0, 841, 595], '/CropBox': [0, 0, 841, 595]}, (0, 4513): {'/Type': '/Page', '/Parent': IndirectObject(2, 0), '/Resources': IndirectObject(4511, 0), '/Contents': [IndirectObject(4510, 0)], '/MediaBox': [0, 0, 841, 595], '/CropBox': [0, 0, 841, 595]}, (0, 4517): {'/Type': '/Page', '/Parent': IndirectObject(2, 0), '/Resources': IndirectObject(4515, 0), '/Contents': [IndirectObject(4514, 0)], '/MediaBox': [0, 0, 841, 595], '/CropBox': [0, 0, 841, 595]}, (0, 4521): {'/Type': '/Page', '/Parent': IndirectObject(2, 0), '/Resources': IndirectObject(4519, 0), '/Contents': [IndirectObject(4518, 0)], '/MediaBox': [0, 0, 841, 595], '/CropBox': [0, 0, 841, 595]}, (0, 4525): {'/Type': '/Page', '/Parent': IndirectObject(2, 0), '/Resources': IndirectObject(4523, 0), '/Contents': [IndirectObject(4522, 0)], '/MediaBox': [0, 0, 841, 595], '/CropBox': [0, 0, 841, 595]}, (0, 4529): {'/Type': '/Page', '/Parent': IndirectObject(2, 0), '/Resources': IndirectObject(4527, 0), '/Contents': [IndirectObject(4526, 0)], '/MediaBox': [0, 0, 841, 595], '/CropBox': [0, 0, 841, 595]}, (0, 4533): {'/Type': '/Page', '/Parent': IndirectObject(2, 0), '/Resources': IndirectObject(4531, 0), '/Contents': [IndirectObject(4530, 0)], '/MediaBox': [0, 0, 841, 595], '/CropBox': [0, 0, 841, 595]}, (0, 4537): {'/Type': '/Page', '/Parent': IndirectObject(2, 0), '/Resources': IndirectObject(4535, 0), '/Contents': [IndirectObject(4534, 0)], '/MediaBox': [0, 0, 841, 595], '/CropBox': [0, 0, 841, 595]}, (0, 4541): {'/Type': '/Page', '/Parent': IndirectObject(2, 0), '/Resources': IndirectObject(4539, 0), '/Contents': [IndirectObject(4538, 0)], '/MediaBox': [0, 0, 841, 595], '/CropBox': [0, 0, 841, 595]}, (0, 4545): {'/Type': '/Page', '/Parent': IndirectObject(2, 0), '/Resources': IndirectObject(4543, 0), '/Contents': [IndirectObject(4542, 0)], '/MediaBox': [0, 0, 841, 595], '/CropBox': [0, 0, 841, 595]}, (0, 4549): {'/Type': '/Page', '/Parent': IndirectObject(2, 0), '/Resources': IndirectObject(4547, 0), '/Contents': [IndirectObject(4546, 0)], '/MediaBox': [0, 0, 841, 595], '/CropBox': [0, 0, 841, 595]}, (0, 4553): {'/Type': '/Page', '/Parent': IndirectObject(2, 0), '/Resources': IndirectObject(4551, 0), '/Contents': [IndirectObject(4550, 0)], '/MediaBox': [0, 0, 841, 595], '/CropBox': [0, 0, 841, 595]}, (0, 4557): {'/Type': '/Page', '/Parent': IndirectObject(2, 0), '/Resources': IndirectObject(4555, 0), '/Contents': [IndirectObject(4554, 0)], '/MediaBox': [0, 0, 841, 595], '/CropBox': [0, 0, 841, 595]}, (0, 4561): {'/Type': '/Page', '/Parent': IndirectObject(2, 0), '/Resources': IndirectObject(4559, 0), '/Contents': [IndirectObject(4558, 0)], '/MediaBox': [0, 0, 841, 595], '/CropBox': [0, 0, 841, 595]}, (0, 4565): {'/Type': '/Page', '/Parent': IndirectObject(2, 0), '/Resources': IndirectObject(4563, 0), '/Contents': [IndirectObject(4562, 0)], '/MediaBox': [0, 0, 841, 595], '/CropBox': [0, 0, 841, 595]}, (0, 4569): {'/Type': '/Page', '/Parent': IndirectObject(2, 0), '/Resources': IndirectObject(4567, 0), '/Contents': [IndirectObject(4566, 0)], '/MediaBox': [0, 0, 841, 595], '/CropBox': [0, 0, 841, 595]}, (0, 4573): {'/Type': '/Page', '/Parent': IndirectObject(2, 0), '/Resources': IndirectObject(4571, 0), '/Contents': [IndirectObject(4570, 0)], '/MediaBox': [0, 0, 841, 595], '/CropBox': [0, 0, 841, 595]}, (0, 4577): {'/Type': '/Page', '/Parent': IndirectObject(2, 0), '/Resources': IndirectObject(4575, 0), '/Contents': [IndirectObject(4574, 0)], '/MediaBox': [0, 0, 841, 595], '/CropBox': [0, 0, 841, 595]}, (0, 4581): {'/Type': '/Page', '/Parent': IndirectObject(2, 0), '/Resources': IndirectObject(4579, 0), '/Contents': [IndirectObject(4578, 0)], '/MediaBox': [0, 0, 841, 595], '/CropBox': [0, 0, 841, 595]}, (0, 4585): {'/Type': '/Page', '/Parent': IndirectObject(2, 0), '/Resources': IndirectObject(4583, 0), '/Contents': [IndirectObject(4582, 0)], '/MediaBox': [0, 0, 841, 595], '/CropBox': [0, 0, 841, 595]}, (0, 4589): {'/Type': '/Page', '/Parent': IndirectObject(2, 0), '/Resources': IndirectObject(4587, 0), '/Contents': [IndirectObject(4586, 0)], '/MediaBox': [0, 0, 841, 595], '/CropBox': [0, 0, 841, 595]}, (0, 4593): {'/Type': '/Page', '/Parent': IndirectObject(2, 0), '/Resources': IndirectObject(4591, 0), '/Contents': [IndirectObject(4590, 0)], '/MediaBox': [0, 0, 841, 595], '/CropBox': [0, 0, 841, 595]}, (0, 4597): {'/Type': '/Page', '/Parent': IndirectObject(2, 0), '/Resources': IndirectObject(4595, 0), '/Contents': [IndirectObject(4594, 0)], '/MediaBox': [0, 0, 841, 595], '/CropBox': [0, 0, 841, 595]}, (0, 4601): {'/Type': '/Page', '/Parent': IndirectObject(2, 0), '/Resources': IndirectObject(4599, 0), '/Contents': [IndirectObject(4598, 0)], '/MediaBox': [0, 0, 841, 595], '/CropBox': [0, 0, 841, 595]}, (0, 4605): {'/Type': '/Page', '/Parent': IndirectObject(2, 0), '/Resources': IndirectObject(4603, 0), '/Contents': [IndirectObject(4602, 0)], '/MediaBox': [0, 0, 841, 595], '/CropBox': [0, 0, 841, 595]}, (0, 4609): {'/Type': '/Page', '/Parent': IndirectObject(2, 0), '/Resources': IndirectObject(4607, 0), '/Contents': [IndirectObject(4606, 0)], '/MediaBox': [0, 0, 841, 595], '/CropBox': [0, 0, 841, 595]}, (0, 4613): {'/Type': '/Page', '/Parent': IndirectObject(2, 0), '/Resources': IndirectObject(4611, 0), '/Contents': [IndirectObject(4610, 0)], '/MediaBox': [0, 0, 841, 595], '/CropBox': [0, 0, 841, 595]}, (0, 4617): {'/Type': '/Page', '/Parent': IndirectObject(2, 0), '/Resources': IndirectObject(4615, 0), '/Contents': [IndirectObject(4614, 0)], '/MediaBox': [0, 0, 841, 595], '/CropBox': [0, 0, 841, 595]}, (0, 4621): {'/Type': '/Page', '/Parent': IndirectObject(2, 0), '/Resources': IndirectObject(4619, 0), '/Contents': [IndirectObject(4618, 0)], '/MediaBox': [0, 0, 841, 595], '/CropBox': [0, 0, 841, 595]}, (0, 4625): {'/Type': '/Page', '/Parent': IndirectObject(2, 0), '/Resources': IndirectObject(4623, 0), '/Contents': [IndirectObject(4622, 0)], '/MediaBox': [0, 0, 841, 595], '/CropBox': [0, 0, 841, 595]}, (0, 4629): {'/Type': '/Page', '/Parent': IndirectObject(2, 0), '/Resources': IndirectObject(4627, 0), '/Contents': [IndirectObject(4626, 0)], '/MediaBox': [0, 0, 841, 595], '/CropBox': [0, 0, 841, 595]}, (0, 4633): {'/Type': '/Page', '/Parent': IndirectObject(2, 0), '/Resources': IndirectObject(4631, 0), '/Contents': [IndirectObject(4630, 0)], '/MediaBox': [0, 0, 841, 595], '/CropBox': [0, 0, 841, 595]}, (0, 4637): {'/Type': '/Page', '/Parent': IndirectObject(2, 0), '/Resources': IndirectObject(4635, 0), '/Contents': [IndirectObject(4634, 0)], '/MediaBox': [0, 0, 841, 595], '/CropBox': [0, 0, 841, 595]}, (0, 4641): {'/Type': '/Page', '/Parent': IndirectObject(2, 0), '/Resources': IndirectObject(4639, 0), '/Contents': [IndirectObject(4638, 0)], '/MediaBox': [0, 0, 841, 595], '/CropBox': [0, 0, 841, 595]}, (0, 4645): {'/Type': '/Page', '/Parent': IndirectObject(2, 0), '/Resources': IndirectObject(4643, 0), '/Contents': [IndirectObject(4642, 0)], '/MediaBox': [0, 0, 841, 595], '/CropBox': [0, 0, 841, 595]}, (0, 4649): {'/Type': '/Page', '/Parent': IndirectObject(2, 0), '/Resources': IndirectObject(4647, 0), '/Contents': [IndirectObject(4646, 0)], '/MediaBox': [0, 0, 841, 595], '/CropBox': [0, 0, 841, 595]}, (0, 4653): {'/Type': '/Page', '/Parent': IndirectObject(2, 0), '/Resources': IndirectObject(4651, 0), '/Contents': [IndirectObject(4650, 0)], '/MediaBox': [0, 0, 841, 595], '/CropBox': [0, 0, 841, 595]}, (0, 4657): {'/Type': '/Page', '/Parent': IndirectObject(2, 0), '/Resources': IndirectObject(4655, 0), '/Contents': [IndirectObject(4654, 0)], '/MediaBox': [0, 0, 841, 595], '/CropBox': [0, 0, 841, 595]}, (0, 4661): {'/Type': '/Page', '/Parent': IndirectObject(2, 0), '/Resources': IndirectObject(4659, 0), '/Contents': [IndirectObject(4658, 0)], '/MediaBox': [0, 0, 841, 595], '/CropBox': [0, 0, 841, 595]}, (0, 4665): {'/Type': '/Page', '/Parent': IndirectObject(2, 0), '/Resources': IndirectObject(4663, 0), '/Contents': [IndirectObject(4662, 0)], '/MediaBox': [0, 0, 841, 595], '/CropBox': [0, 0, 841, 595]}, (0, 4669): {'/Type': '/Page', '/Parent': IndirectObject(2, 0), '/Resources': IndirectObject(4667, 0), '/Contents': [IndirectObject(4666, 0)], '/MediaBox': [0, 0, 841, 595], '/CropBox': [0, 0, 841, 595]}, (0, 4673): {'/Type': '/Page', '/Parent': IndirectObject(2, 0), '/Resources': IndirectObject(4671, 0), '/Contents': [IndirectObject(4670, 0)], '/MediaBox': [0, 0, 841, 595], '/CropBox': [0, 0, 841, 595]}, (0, 4677): {'/Type': '/Page', '/Parent': IndirectObject(2, 0), '/Resources': IndirectObject(4675, 0), '/Contents': [IndirectObject(4674, 0)], '/MediaBox': [0, 0, 841, 595], '/CropBox': [0, 0, 841, 595]}, (0, 4681): {'/Type': '/Page', '/Parent': IndirectObject(2, 0), '/Resources': IndirectObject(4679, 0), '/Contents': [IndirectObject(4678, 0)], '/MediaBox': [0, 0, 841, 595], '/CropBox': [0, 0, 841, 595]}, (0, 4685): {'/Type': '/Page', '/Parent': IndirectObject(2, 0), '/Resources': IndirectObject(4683, 0), '/Contents': [IndirectObject(4682, 0)], '/MediaBox': [0, 0, 841, 595], '/CropBox': [0, 0, 841, 595]}, (0, 4689): {'/Type': '/Page', '/Parent': IndirectObject(2, 0), '/Resources': IndirectObject(4687, 0), '/Contents': [IndirectObject(4686, 0)], '/MediaBox': [0, 0, 841, 595], '/CropBox': [0, 0, 841, 595]}, (0, 4693): {'/Type': '/Page', '/Parent': IndirectObject(2, 0), '/Resources': IndirectObject(4691, 0), '/Contents': [IndirectObject(4690, 0)], '/MediaBox': [0, 0, 841, 595], '/CropBox': [0, 0, 841, 595]}, (0, 4697): {'/Type': '/Page', '/Parent': IndirectObject(2, 0), '/Resources': IndirectObject(4695, 0), '/Contents': [IndirectObject(4694, 0)], '/MediaBox': [0, 0, 841, 595], '/CropBox': [0, 0, 841, 595]}, (0, 4701): {'/Type': '/Page', '/Parent': IndirectObject(2, 0), '/Resources': IndirectObject(4699, 0), '/Contents': [IndirectObject(4698, 0)], '/MediaBox': [0, 0, 841, 595], '/CropBox': [0, 0, 841, 595]}, (0, 4705): {'/Type': '/Page', '/Parent': IndirectObject(2, 0), '/Resources': IndirectObject(4703, 0), '/Contents': [IndirectObject(4702, 0)], '/MediaBox': [0, 0, 841, 595], '/CropBox': [0, 0, 841, 595]}, (0, 4709): {'/Type': '/Page', '/Parent': IndirectObject(2, 0), '/Resources': IndirectObject(4707, 0), '/Contents': [IndirectObject(4706, 0)], '/MediaBox': [0, 0, 841, 595], '/CropBox': [0, 0, 841, 595]}, (0, 4713): {'/Type': '/Page', '/Parent': IndirectObject(2, 0), '/Resources': IndirectObject(4711, 0), '/Contents': [IndirectObject(4710, 0)], '/MediaBox': [0, 0, 841, 595], '/CropBox': [0, 0, 841, 595]}, (0, 4717): {'/Type': '/Page', '/Parent': IndirectObject(2, 0), '/Resources': IndirectObject(4715, 0), '/Contents': [IndirectObject(4714, 0)], '/MediaBox': [0, 0, 841, 595], '/CropBox': [0, 0, 841, 595]}, (0, 4721): {'/Type': '/Page', '/Parent': IndirectObject(2, 0), '/Resources': IndirectObject(4719, 0), '/Contents': [IndirectObject(4718, 0)], '/MediaBox': [0, 0, 841, 595], '/CropBox': [0, 0, 841, 595]}, (0, 4725): {'/Type': '/Page', '/Parent': IndirectObject(2, 0), '/Resources': IndirectObject(4723, 0), '/Contents': [IndirectObject(4722, 0)], '/MediaBox': [0, 0, 841, 595], '/CropBox': [0, 0, 841, 595]}, (0, 4729): {'/Type': '/Page', '/Parent': IndirectObject(2, 0), '/Resources': IndirectObject(4727, 0), '/Contents': [IndirectObject(4726, 0)], '/MediaBox': [0, 0, 841, 595], '/CropBox': [0, 0, 841, 595]}, (0, 4733): {'/Type': '/Page', '/Parent': IndirectObject(2, 0), '/Resources': IndirectObject(4731, 0), '/Contents': [IndirectObject(4730, 0)], '/MediaBox': [0, 0, 841, 595], '/CropBox': [0, 0, 841, 595]}, (0, 4737): {'/Type': '/Page', '/Parent': IndirectObject(2, 0), '/Resources': IndirectObject(4735, 0), '/Contents': [IndirectObject(4734, 0)], '/MediaBox': [0, 0, 841, 595], '/CropBox': [0, 0, 841, 595]}, (0, 4741): {'/Type': '/Page', '/Parent': IndirectObject(2, 0), '/Resources': IndirectObject(4739, 0), '/Contents': [IndirectObject(4738, 0)], '/MediaBox': [0, 0, 841, 595], '/CropBox': [0, 0, 841, 595]}, (0, 4745): {'/Type': '/Page', '/Parent': IndirectObject(2, 0), '/Resources': IndirectObject(4743, 0), '/Contents': [IndirectObject(4742, 0)], '/MediaBox': [0, 0, 841, 595], '/CropBox': [0, 0, 841, 595]}, (0, 4749): {'/Type': '/Page', '/Parent': IndirectObject(2, 0), '/Resources': IndirectObject(4747, 0), '/Contents': [IndirectObject(4746, 0)], '/MediaBox': [0, 0, 841, 595], '/CropBox': [0, 0, 841, 595]}, (0, 4753): {'/Type': '/Page', '/Parent': IndirectObject(2, 0), '/Resources': IndirectObject(4751, 0), '/Contents': [IndirectObject(4750, 0)], '/MediaBox': [0, 0, 841, 595], '/CropBox': [0, 0, 841, 595]}, (0, 4757): {'/Type': '/Page', '/Parent': IndirectObject(2, 0), '/Resources': IndirectObject(4755, 0), '/Contents': [IndirectObject(4754, 0)], '/MediaBox': [0, 0, 841, 595], '/CropBox': [0, 0, 841, 595]}, (0, 4761): {'/Type': '/Page', '/Parent': IndirectObject(2, 0), '/Resources': IndirectObject(4759, 0), '/Contents': [IndirectObject(4758, 0)], '/MediaBox': [0, 0, 841, 595], '/CropBox': [0, 0, 841, 595]}, (0, 4765): {'/Type': '/Page', '/Parent': IndirectObject(2, 0), '/Resources': IndirectObject(4763, 0), '/Contents': [IndirectObject(4762, 0)], '/MediaBox': [0, 0, 841, 595], '/CropBox': [0, 0, 841, 595]}, (0, 4769): {'/Type': '/Page', '/Parent': IndirectObject(2, 0), '/Resources': IndirectObject(4767, 0), '/Contents': [IndirectObject(4766, 0)], '/MediaBox': [0, 0, 841, 595], '/CropBox': [0, 0, 841, 595]}, (0, 4773): {'/Type': '/Page', '/Parent': IndirectObject(2, 0), '/Resources': IndirectObject(4771, 0), '/Contents': [IndirectObject(4770, 0)], '/MediaBox': [0, 0, 841, 595], '/CropBox': [0, 0, 841, 595]}, (0, 4777): {'/Type': '/Page', '/Parent': IndirectObject(2, 0), '/Resources': IndirectObject(4775, 0), '/Contents': [IndirectObject(4774, 0)], '/MediaBox': [0, 0, 841, 595], '/CropBox': [0, 0, 841, 595]}, (0, 4781): {'/Type': '/Page', '/Parent': IndirectObject(2, 0), '/Resources': IndirectObject(4779, 0), '/Contents': [IndirectObject(4778, 0)], '/MediaBox': [0, 0, 841, 595], '/CropBox': [0, 0, 841, 595]}, (0, 4785): {'/Type': '/Page', '/Parent': IndirectObject(2, 0), '/Resources': IndirectObject(4783, 0), '/Contents': [IndirectObject(4782, 0)], '/MediaBox': [0, 0, 841, 595], '/CropBox': [0, 0, 841, 595]}, (0, 4789): {'/Type': '/Page', '/Parent': IndirectObject(2, 0), '/Resources': IndirectObject(4787, 0), '/Contents': [IndirectObject(4786, 0)], '/MediaBox': [0, 0, 841, 595], '/CropBox': [0, 0, 841, 595]}, (0, 4793): {'/Type': '/Page', '/Parent': IndirectObject(2, 0), '/Resources': IndirectObject(4791, 0), '/Contents': [IndirectObject(4790, 0)], '/MediaBox': [0, 0, 841, 595], '/CropBox': [0, 0, 841, 595]}, (0, 4797): {'/Type': '/Page', '/Parent': IndirectObject(2, 0), '/Resources': IndirectObject(4795, 0), '/Contents': [IndirectObject(4794, 0)], '/MediaBox': [0, 0, 841, 595], '/CropBox': [0, 0, 841, 595]}, (0, 4801): {'/Type': '/Page', '/Parent': IndirectObject(2, 0), '/Resources': IndirectObject(4799, 0), '/Contents': [IndirectObject(4798, 0)], '/MediaBox': [0, 0, 841, 595], '/CropBox': [0, 0, 841, 595]}, (0, 4805): {'/Type': '/Page', '/Parent': IndirectObject(2, 0), '/Resources': IndirectObject(4803, 0), '/Contents': [IndirectObject(4802, 0)], '/MediaBox': [0, 0, 841, 595], '/CropBox': [0, 0, 841, 595]}, (0, 4809): {'/Type': '/Page', '/Parent': IndirectObject(2, 0), '/Resources': IndirectObject(4807, 0), '/Contents': [IndirectObject(4806, 0)], '/MediaBox': [0, 0, 841, 595], '/CropBox': [0, 0, 841, 595]}, (0, 4813): {'/Type': '/Page', '/Parent': IndirectObject(2, 0), '/Resources': IndirectObject(4811, 0), '/Contents': [IndirectObject(4810, 0)], '/MediaBox': [0, 0, 841, 595], '/CropBox': [0, 0, 841, 595]}, (0, 4817): {'/Type': '/Page', '/Parent': IndirectObject(2, 0), '/Resources': IndirectObject(4815, 0), '/Contents': [IndirectObject(4814, 0)], '/MediaBox': [0, 0, 841, 595], '/CropBox': [0, 0, 841, 595]}, (0, 4821): {'/Type': '/Page', '/Parent': IndirectObject(2, 0), '/Resources': IndirectObject(4819, 0), '/Contents': [IndirectObject(4818, 0)], '/MediaBox': [0, 0, 841, 595], '/CropBox': [0, 0, 841, 595]}, (0, 4825): {'/Type': '/Page', '/Parent': IndirectObject(2, 0), '/Resources': IndirectObject(4823, 0), '/Contents': [IndirectObject(4822, 0)], '/MediaBox': [0, 0, 841, 595], '/CropBox': [0, 0, 841, 595]}, (0, 4829): {'/Type': '/Page', '/Parent': IndirectObject(2, 0), '/Resources': IndirectObject(4827, 0), '/Contents': [IndirectObject(4826, 0)], '/MediaBox': [0, 0, 841, 595], '/CropBox': [0, 0, 841, 595]}, (0, 4833): {'/Type': '/Page', '/Parent': IndirectObject(2, 0), '/Resources': IndirectObject(4831, 0), '/Contents': [IndirectObject(4830, 0)], '/MediaBox': [0, 0, 841, 595], '/CropBox': [0, 0, 841, 595]}, (0, 4837): {'/Type': '/Page', '/Parent': IndirectObject(2, 0), '/Resources': IndirectObject(4835, 0), '/Contents': [IndirectObject(4834, 0)], '/MediaBox': [0, 0, 841, 595], '/CropBox': [0, 0, 841, 595]}, (0, 4841): {'/Type': '/Page', '/Parent': IndirectObject(2, 0), '/Resources': IndirectObject(4839, 0), '/Contents': [IndirectObject(4838, 0)], '/MediaBox': [0, 0, 841, 595], '/CropBox': [0, 0, 841, 595]}, (0, 4845): {'/Type': '/Page', '/Parent': IndirectObject(2, 0), '/Resources': IndirectObject(4843, 0), '/Contents': [IndirectObject(4842, 0)], '/MediaBox': [0, 0, 841, 595], '/CropBox': [0, 0, 841, 595]}, (0, 4849): {'/Type': '/Page', '/Parent': IndirectObject(2, 0), '/Resources': IndirectObject(4847, 0), '/Contents': [IndirectObject(4846, 0)], '/MediaBox': [0, 0, 841, 595], '/CropBox': [0, 0, 841, 595]}, (0, 4853): {'/Type': '/Page', '/Parent': IndirectObject(2, 0), '/Resources': IndirectObject(4851, 0), '/Contents': [IndirectObject(4850, 0)], '/MediaBox': [0, 0, 841, 595], '/CropBox': [0, 0, 841, 595]}, (0, 4857): {'/Type': '/Page', '/Parent': IndirectObject(2, 0), '/Resources': IndirectObject(4855, 0), '/Contents': [IndirectObject(4854, 0)], '/MediaBox': [0, 0, 841, 595], '/CropBox': [0, 0, 841, 595]}, (0, 4861): {'/Type': '/Page', '/Parent': IndirectObject(2, 0), '/Resources': IndirectObject(4859, 0), '/Contents': [IndirectObject(4858, 0)], '/MediaBox': [0, 0, 841, 595], '/CropBox': [0, 0, 841, 595]}, (0, 4865): {'/Type': '/Page', '/Parent': IndirectObject(2, 0), '/Resources': IndirectObject(4863, 0), '/Contents': [IndirectObject(4862, 0)], '/MediaBox': [0, 0, 841, 595], '/CropBox': [0, 0, 841, 595]}, (0, 4869): {'/Type': '/Page', '/Parent': IndirectObject(2, 0), '/Resources': IndirectObject(4867, 0), '/Contents': [IndirectObject(4866, 0)], '/MediaBox': [0, 0, 841, 595], '/CropBox': [0, 0, 841, 595]}, (0, 4873): {'/Type': '/Page', '/Parent': IndirectObject(2, 0), '/Resources': IndirectObject(4871, 0), '/Contents': [IndirectObject(4870, 0)], '/MediaBox': [0, 0, 841, 595], '/CropBox': [0, 0, 841, 595]}, (0, 4877): {'/Type': '/Page', '/Parent': IndirectObject(2, 0), '/Resources': IndirectObject(4875, 0), '/Contents': [IndirectObject(4874, 0)], '/MediaBox': [0, 0, 841, 595], '/CropBox': [0, 0, 841, 595]}, (0, 4881): {'/Type': '/Page', '/Parent': IndirectObject(2, 0), '/Resources': IndirectObject(4879, 0), '/Contents': [IndirectObject(4878, 0)], '/MediaBox': [0, 0, 841, 595], '/CropBox': [0, 0, 841, 595]}, (0, 4885): {'/Type': '/Page', '/Parent': IndirectObject(2, 0), '/Resources': IndirectObject(4883, 0), '/Contents': [IndirectObject(4882, 0)], '/MediaBox': [0, 0, 841, 595], '/CropBox': [0, 0, 841, 595]}, (0, 4889): {'/Type': '/Page', '/Parent': IndirectObject(2, 0), '/Resources': IndirectObject(4887, 0), '/Contents': [IndirectObject(4886, 0)], '/MediaBox': [0, 0, 841, 595], '/CropBox': [0, 0, 841, 595]}, (0, 4893): {'/Type': '/Page', '/Parent': IndirectObject(2, 0), '/Resources': IndirectObject(4891, 0), '/Contents': [IndirectObject(4890, 0)], '/MediaBox': [0, 0, 841, 595], '/CropBox': [0, 0, 841, 595]}, (0, 4897): {'/Type': '/Page', '/Parent': IndirectObject(2, 0), '/Resources': IndirectObject(4895, 0), '/Contents': [IndirectObject(4894, 0)], '/MediaBox': [0, 0, 841, 595], '/CropBox': [0, 0, 841, 595]}, (0, 4901): {'/Type': '/Page', '/Parent': IndirectObject(2, 0), '/Resources': IndirectObject(4899, 0), '/Contents': [IndirectObject(4898, 0)], '/MediaBox': [0, 0, 841, 595], '/CropBox': [0, 0, 841, 595]}, (0, 4905): {'/Type': '/Page', '/Parent': IndirectObject(2, 0), '/Resources': IndirectObject(4903, 0), '/Contents': [IndirectObject(4902, 0)], '/MediaBox': [0, 0, 841, 595], '/CropBox': [0, 0, 841, 595]}, (0, 4909): {'/Type': '/Page', '/Parent': IndirectObject(2, 0), '/Resources': IndirectObject(4907, 0), '/Contents': [IndirectObject(4906, 0)], '/MediaBox': [0, 0, 841, 595], '/CropBox': [0, 0, 841, 595]}, (0, 4913): {'/Type': '/Page', '/Parent': IndirectObject(2, 0), '/Resources': IndirectObject(4911, 0), '/Contents': [IndirectObject(4910, 0)], '/MediaBox': [0, 0, 841, 595], '/CropBox': [0, 0, 841, 595]}, (0, 4917): {'/Type': '/Page', '/Parent': IndirectObject(2, 0), '/Resources': IndirectObject(4915, 0), '/Contents': [IndirectObject(4914, 0)], '/MediaBox': [0, 0, 841, 595], '/CropBox': [0, 0, 841, 595]}, (0, 4921): {'/Type': '/Page', '/Parent': IndirectObject(2, 0), '/Resources': IndirectObject(4919, 0), '/Contents': [IndirectObject(4918, 0)], '/MediaBox': [0, 0, 841, 595], '/CropBox': [0, 0, 841, 595]}, (0, 4925): {'/Type': '/Page', '/Parent': IndirectObject(2, 0), '/Resources': IndirectObject(4923, 0), '/Contents': [IndirectObject(4922, 0)], '/MediaBox': [0, 0, 841, 595], '/CropBox': [0, 0, 841, 595]}, (0, 4929): {'/Type': '/Page', '/Parent': IndirectObject(2, 0), '/Resources': IndirectObject(4927, 0), '/Contents': [IndirectObject(4926, 0)], '/MediaBox': [0, 0, 841, 595], '/CropBox': [0, 0, 841, 595]}, (0, 4933): {'/Type': '/Page', '/Parent': IndirectObject(2, 0), '/Resources': IndirectObject(4931, 0), '/Contents': [IndirectObject(4930, 0)], '/MediaBox': [0, 0, 841, 595], '/CropBox': [0, 0, 841, 595]}, (0, 4937): {'/Type': '/Page', '/Parent': IndirectObject(2, 0), '/Resources': IndirectObject(4935, 0), '/Contents': [IndirectObject(4934, 0)], '/MediaBox': [0, 0, 841, 595], '/CropBox': [0, 0, 841, 595]}, (0, 4941): {'/Type': '/Page', '/Parent': IndirectObject(2, 0), '/Resources': IndirectObject(4939, 0), '/Contents': [IndirectObject(4938, 0)], '/MediaBox': [0, 0, 841, 595], '/CropBox': [0, 0, 841, 595]}, (0, 4945): {'/Type': '/Page', '/Parent': IndirectObject(2, 0), '/Resources': IndirectObject(4943, 0), '/Contents': [IndirectObject(4942, 0)], '/MediaBox': [0, 0, 841, 595], '/CropBox': [0, 0, 841, 595]}, (0, 4949): {'/Type': '/Page', '/Parent': IndirectObject(2, 0), '/Resources': IndirectObject(4947, 0), '/Contents': [IndirectObject(4946, 0)], '/MediaBox': [0, 0, 841, 595], '/CropBox': [0, 0, 841, 595]}, (0, 4953): {'/Type': '/Page', '/Parent': IndirectObject(2, 0), '/Resources': IndirectObject(4951, 0), '/Contents': [IndirectObject(4950, 0)], '/MediaBox': [0, 0, 841, 595], '/CropBox': [0, 0, 841, 595]}, (0, 4957): {'/Type': '/Page', '/Parent': IndirectObject(2, 0), '/Resources': IndirectObject(4955, 0), '/Contents': [IndirectObject(4954, 0)], '/MediaBox': [0, 0, 841, 595], '/CropBox': [0, 0, 841, 595]}, (0, 4961): {'/Type': '/Page', '/Parent': IndirectObject(2, 0), '/Resources': IndirectObject(4959, 0), '/Contents': [IndirectObject(4958, 0)], '/MediaBox': [0, 0, 841, 595], '/CropBox': [0, 0, 841, 595]}, (0, 4965): {'/Type': '/Page', '/Parent': IndirectObject(2, 0), '/Resources': IndirectObject(4963, 0), '/Contents': [IndirectObject(4962, 0)], '/MediaBox': [0, 0, 841, 595], '/CropBox': [0, 0, 841, 595]}, (0, 4969): {'/Type': '/Page', '/Parent': IndirectObject(2, 0), '/Resources': IndirectObject(4967, 0), '/Contents': [IndirectObject(4966, 0)], '/MediaBox': [0, 0, 841, 595], '/CropBox': [0, 0, 841, 595]}, (0, 4973): {'/Type': '/Page', '/Parent': IndirectObject(2, 0), '/Resources': IndirectObject(4971, 0), '/Contents': [IndirectObject(4970, 0)], '/MediaBox': [0, 0, 841, 595], '/CropBox': [0, 0, 841, 595]}, (0, 4977): {'/Type': '/Page', '/Parent': IndirectObject(2, 0), '/Resources': IndirectObject(4975, 0), '/Contents': [IndirectObject(4974, 0)], '/MediaBox': [0, 0, 841, 595], '/CropBox': [0, 0, 841, 595]}, (0, 4981): {'/Type': '/Page', '/Parent': IndirectObject(2, 0), '/Resources': IndirectObject(4979, 0), '/Contents': [IndirectObject(4978, 0)], '/MediaBox': [0, 0, 841, 595], '/CropBox': [0, 0, 841, 595]}, (0, 4985): {'/Type': '/Page', '/Parent': IndirectObject(2, 0), '/Resources': IndirectObject(4983, 0), '/Contents': [IndirectObject(4982, 0)], '/MediaBox': [0, 0, 841, 595], '/CropBox': [0, 0, 841, 595]}, (0, 4989): {'/Type': '/Page', '/Parent': IndirectObject(2, 0), '/Resources': IndirectObject(4987, 0), '/Contents': [IndirectObject(4986, 0)], '/MediaBox': [0, 0, 841, 595], '/CropBox': [0, 0, 841, 595]}, (0, 4993): {'/Type': '/Page', '/Parent': IndirectObject(2, 0), '/Resources': IndirectObject(4991, 0), '/Contents': [IndirectObject(4990, 0)], '/MediaBox': [0, 0, 841, 595], '/CropBox': [0, 0, 841, 595]}, (0, 4997): {'/Type': '/Page', '/Parent': IndirectObject(2, 0), '/Resources': IndirectObject(4995, 0), '/Contents': [IndirectObject(4994, 0)], '/MediaBox': [0, 0, 841, 595], '/CropBox': [0, 0, 841, 595]}, (0, 5001): {'/Type': '/Page', '/Parent': IndirectObject(2, 0), '/Resources': IndirectObject(4999, 0), '/Contents': [IndirectObject(4998, 0)], '/MediaBox': [0, 0, 841, 595], '/CropBox': [0, 0, 841, 595]}, (0, 5005): {'/Type': '/Page', '/Parent': IndirectObject(2, 0), '/Resources': IndirectObject(5003, 0), '/Contents': [IndirectObject(5002, 0)], '/MediaBox': [0, 0, 841, 595], '/CropBox': [0, 0, 841, 595]}, (0, 5009): {'/Type': '/Page', '/Parent': IndirectObject(2, 0), '/Resources': IndirectObject(5007, 0), '/Contents': [IndirectObject(5006, 0)], '/MediaBox': [0, 0, 841, 595], '/CropBox': [0, 0, 841, 595]}, (0, 5013): {'/Type': '/Page', '/Parent': IndirectObject(2, 0), '/Resources': IndirectObject(5011, 0), '/Contents': [IndirectObject(5010, 0)], '/MediaBox': [0, 0, 841, 595], '/CropBox': [0, 0, 841, 595]}, (0, 5017): {'/Type': '/Page', '/Parent': IndirectObject(2, 0), '/Resources': IndirectObject(5015, 0), '/Contents': [IndirectObject(5014, 0)], '/MediaBox': [0, 0, 841, 595], '/CropBox': [0, 0, 841, 595]}, (0, 5021): {'/Type': '/Page', '/Parent': IndirectObject(2, 0), '/Resources': IndirectObject(5019, 0), '/Contents': [IndirectObject(5018, 0)], '/MediaBox': [0, 0, 841, 595], '/CropBox': [0, 0, 841, 595]}, (0, 5025): {'/Type': '/Page', '/Parent': IndirectObject(2, 0), '/Resources': IndirectObject(5023, 0), '/Contents': [IndirectObject(5022, 0)], '/MediaBox': [0, 0, 841, 595], '/CropBox': [0, 0, 841, 595]}, (0, 5029): {'/Type': '/Page', '/Parent': IndirectObject(2, 0), '/Resources': IndirectObject(5027, 0), '/Contents': [IndirectObject(5026, 0)], '/MediaBox': [0, 0, 841, 595], '/CropBox': [0, 0, 841, 595]}, (0, 5033): {'/Type': '/Page', '/Parent': IndirectObject(2, 0), '/Resources': IndirectObject(5031, 0), '/Contents': [IndirectObject(5030, 0)], '/MediaBox': [0, 0, 841, 595], '/CropBox': [0, 0, 841, 595]}, (0, 5037): {'/Type': '/Page', '/Parent': IndirectObject(2, 0), '/Resources': IndirectObject(5035, 0), '/Contents': [IndirectObject(5034, 0)], '/MediaBox': [0, 0, 841, 595], '/CropBox': [0, 0, 841, 595]}, (0, 5041): {'/Type': '/Page', '/Parent': IndirectObject(2, 0), '/Resources': IndirectObject(5039, 0), '/Contents': [IndirectObject(5038, 0)], '/MediaBox': [0, 0, 841, 595], '/CropBox': [0, 0, 841, 595]}, (0, 5045): {'/Type': '/Page', '/Parent': IndirectObject(2, 0), '/Resources': IndirectObject(5043, 0), '/Contents': [IndirectObject(5042, 0)], '/MediaBox': [0, 0, 841, 595], '/CropBox': [0, 0, 841, 595]}, (0, 5049): {'/Type': '/Page', '/Parent': IndirectObject(2, 0), '/Resources': IndirectObject(5047, 0), '/Contents': [IndirectObject(5046, 0)], '/MediaBox': [0, 0, 841, 595], '/CropBox': [0, 0, 841, 595]}, (0, 5053): {'/Type': '/Page', '/Parent': IndirectObject(2, 0), '/Resources': IndirectObject(5051, 0), '/Contents': [IndirectObject(5050, 0)], '/MediaBox': [0, 0, 841, 595], '/CropBox': [0, 0, 841, 595]}, (0, 5057): {'/Type': '/Page', '/Parent': IndirectObject(2, 0), '/Resources': IndirectObject(5055, 0), '/Contents': [IndirectObject(5054, 0)], '/MediaBox': [0, 0, 841, 595], '/CropBox': [0, 0, 841, 595]}, (0, 5061): {'/Type': '/Page', '/Parent': IndirectObject(2, 0), '/Resources': IndirectObject(5059, 0), '/Contents': [IndirectObject(5058, 0)], '/MediaBox': [0, 0, 841, 595], '/CropBox': [0, 0, 841, 595]}, (0, 5065): {'/Type': '/Page', '/Parent': IndirectObject(2, 0), '/Resources': IndirectObject(5063, 0), '/Contents': [IndirectObject(5062, 0)], '/MediaBox': [0, 0, 841, 595], '/CropBox': [0, 0, 841, 595]}, (0, 5069): {'/Type': '/Page', '/Parent': IndirectObject(2, 0), '/Resources': IndirectObject(5067, 0), '/Contents': [IndirectObject(5066, 0)], '/MediaBox': [0, 0, 841, 595], '/CropBox': [0, 0, 841, 595]}, (0, 5073): {'/Type': '/Page', '/Parent': IndirectObject(2, 0), '/Resources': IndirectObject(5071, 0), '/Contents': [IndirectObject(5070, 0)], '/MediaBox': [0, 0, 841, 595], '/CropBox': [0, 0, 841, 595]}, (0, 5077): {'/Type': '/Page', '/Parent': IndirectObject(2, 0), '/Resources': IndirectObject(5075, 0), '/Contents': [IndirectObject(5074, 0)], '/MediaBox': [0, 0, 841, 595], '/CropBox': [0, 0, 841, 595]}, (0, 5081): {'/Type': '/Page', '/Parent': IndirectObject(2, 0), '/Resources': IndirectObject(5079, 0), '/Contents': [IndirectObject(5078, 0)], '/MediaBox': [0, 0, 841, 595], '/CropBox': [0, 0, 841, 595]}, (0, 5085): {'/Type': '/Page', '/Parent': IndirectObject(2, 0), '/Resources': IndirectObject(5083, 0), '/Contents': [IndirectObject(5082, 0)], '/MediaBox': [0, 0, 841, 595], '/CropBox': [0, 0, 841, 595]}, (0, 5089): {'/Type': '/Page', '/Parent': IndirectObject(2, 0), '/Resources': IndirectObject(5087, 0), '/Contents': [IndirectObject(5086, 0)], '/MediaBox': [0, 0, 841, 595], '/CropBox': [0, 0, 841, 595]}, (0, 5093): {'/Type': '/Page', '/Parent': IndirectObject(2, 0), '/Resources': IndirectObject(5091, 0), '/Contents': [IndirectObject(5090, 0)], '/MediaBox': [0, 0, 841, 595], '/CropBox': [0, 0, 841, 595]}, (0, 5097): {'/Type': '/Page', '/Parent': IndirectObject(2, 0), '/Resources': IndirectObject(5095, 0), '/Contents': [IndirectObject(5094, 0)], '/MediaBox': [0, 0, 841, 595], '/CropBox': [0, 0, 841, 595]}, (0, 5101): {'/Type': '/Page', '/Parent': IndirectObject(2, 0), '/Resources': IndirectObject(5099, 0), '/Contents': [IndirectObject(5098, 0)], '/MediaBox': [0, 0, 841, 595], '/CropBox': [0, 0, 841, 595]}, (0, 5105): {'/Type': '/Page', '/Parent': IndirectObject(2, 0), '/Resources': IndirectObject(5103, 0), '/Contents': [IndirectObject(5102, 0)], '/MediaBox': [0, 0, 841, 595], '/CropBox': [0, 0, 841, 595]}, (0, 5109): {'/Type': '/Page', '/Parent': IndirectObject(2, 0), '/Resources': IndirectObject(5107, 0), '/Contents': [IndirectObject(5106, 0)], '/MediaBox': [0, 0, 841, 595], '/CropBox': [0, 0, 841, 595]}, (0, 5113): {'/Type': '/Page', '/Parent': IndirectObject(2, 0), '/Resources': IndirectObject(5111, 0), '/Contents': [IndirectObject(5110, 0)], '/MediaBox': [0, 0, 841, 595], '/CropBox': [0, 0, 841, 595]}, (0, 5117): {'/Type': '/Page', '/Parent': IndirectObject(2, 0), '/Resources': IndirectObject(5115, 0), '/Contents': [IndirectObject(5114, 0)], '/MediaBox': [0, 0, 841, 595], '/CropBox': [0, 0, 841, 595]}, (0, 5121): {'/Type': '/Page', '/Parent': IndirectObject(2, 0), '/Resources': IndirectObject(5119, 0), '/Contents': [IndirectObject(5118, 0)], '/MediaBox': [0, 0, 841, 595], '/CropBox': [0, 0, 841, 595]}, (0, 5125): {'/Type': '/Page', '/Parent': IndirectObject(2, 0), '/Resources': IndirectObject(5123, 0), '/Contents': [IndirectObject(5122, 0)], '/MediaBox': [0, 0, 841, 595], '/CropBox': [0, 0, 841, 595]}, (0, 5129): {'/Type': '/Page', '/Parent': IndirectObject(2, 0), '/Resources': IndirectObject(5127, 0), '/Contents': [IndirectObject(5126, 0)], '/MediaBox': [0, 0, 841, 595], '/CropBox': [0, 0, 841, 595]}, (0, 5133): {'/Type': '/Page', '/Parent': IndirectObject(2, 0), '/Resources': IndirectObject(5131, 0), '/Contents': [IndirectObject(5130, 0)], '/MediaBox': [0, 0, 841, 595], '/CropBox': [0, 0, 841, 595]}, (0, 5137): {'/Type': '/Page', '/Parent': IndirectObject(2, 0), '/Resources': IndirectObject(5135, 0), '/Contents': [IndirectObject(5134, 0)], '/MediaBox': [0, 0, 841, 595], '/CropBox': [0, 0, 841, 595]}, (0, 5141): {'/Type': '/Page', '/Parent': IndirectObject(2, 0), '/Resources': IndirectObject(5139, 0), '/Contents': [IndirectObject(5138, 0)], '/MediaBox': [0, 0, 841, 595], '/CropBox': [0, 0, 841, 595]}, (0, 5145): {'/Type': '/Page', '/Parent': IndirectObject(2, 0), '/Resources': IndirectObject(5143, 0), '/Contents': [IndirectObject(5142, 0)], '/MediaBox': [0, 0, 841, 595], '/CropBox': [0, 0, 841, 595]}, (0, 5149): {'/Type': '/Page', '/Parent': IndirectObject(2, 0), '/Resources': IndirectObject(5147, 0), '/Contents': [IndirectObject(5146, 0)], '/MediaBox': [0, 0, 841, 595], '/CropBox': [0, 0, 841, 595]}, (0, 5153): {'/Type': '/Page', '/Parent': IndirectObject(2, 0), '/Resources': IndirectObject(5151, 0), '/Contents': [IndirectObject(5150, 0)], '/MediaBox': [0, 0, 841, 595], '/CropBox': [0, 0, 841, 595]}, (0, 5157): {'/Type': '/Page', '/Parent': IndirectObject(2, 0), '/Resources': IndirectObject(5155, 0), '/Contents': [IndirectObject(5154, 0)], '/MediaBox': [0, 0, 841, 595], '/CropBox': [0, 0, 841, 595]}, (0, 5161): {'/Type': '/Page', '/Parent': IndirectObject(2, 0), '/Resources': IndirectObject(5159, 0), '/Contents': [IndirectObject(5158, 0)], '/MediaBox': [0, 0, 841, 595], '/CropBox': [0, 0, 841, 595]}, (0, 5165): {'/Type': '/Page', '/Parent': IndirectObject(2, 0), '/Resources': IndirectObject(5163, 0), '/Contents': [IndirectObject(5162, 0)], '/MediaBox': [0, 0, 841, 595], '/CropBox': [0, 0, 841, 595]}, (0, 5169): {'/Type': '/Page', '/Parent': IndirectObject(2, 0), '/Resources': IndirectObject(5167, 0), '/Contents': [IndirectObject(5166, 0)], '/MediaBox': [0, 0, 841, 595], '/CropBox': [0, 0, 841, 595]}, (0, 5173): {'/Type': '/Page', '/Parent': IndirectObject(2, 0), '/Resources': IndirectObject(5171, 0), '/Contents': [IndirectObject(5170, 0)], '/MediaBox': [0, 0, 841, 595], '/CropBox': [0, 0, 841, 595]}, (0, 5177): {'/Type': '/Page', '/Parent': IndirectObject(2, 0), '/Resources': IndirectObject(5175, 0), '/Contents': [IndirectObject(5174, 0)], '/MediaBox': [0, 0, 841, 595], '/CropBox': [0, 0, 841, 595]}, (0, 5181): {'/Type': '/Page', '/Parent': IndirectObject(2, 0), '/Resources': IndirectObject(5179, 0), '/Contents': [IndirectObject(5178, 0)], '/MediaBox': [0, 0, 841, 595], '/CropBox': [0, 0, 841, 595]}, (0, 5185): {'/Type': '/Page', '/Parent': IndirectObject(2, 0), '/Resources': IndirectObject(5183, 0), '/Contents': [IndirectObject(5182, 0)], '/MediaBox': [0, 0, 841, 595], '/CropBox': [0, 0, 841, 595]}, (0, 5189): {'/Type': '/Page', '/Parent': IndirectObject(2, 0), '/Resources': IndirectObject(5187, 0), '/Contents': [IndirectObject(5186, 0)], '/MediaBox': [0, 0, 841, 595], '/CropBox': [0, 0, 841, 595]}, (0, 5193): {'/Type': '/Page', '/Parent': IndirectObject(2, 0), '/Resources': IndirectObject(5191, 0), '/Contents': [IndirectObject(5190, 0)], '/MediaBox': [0, 0, 841, 595], '/CropBox': [0, 0, 841, 595]}, (0, 5197): {'/Type': '/Page', '/Parent': IndirectObject(2, 0), '/Resources': IndirectObject(5195, 0), '/Contents': [IndirectObject(5194, 0)], '/MediaBox': [0, 0, 841, 595], '/CropBox': [0, 0, 841, 595]}, (0, 5201): {'/Type': '/Page', '/Parent': IndirectObject(2, 0), '/Resources': IndirectObject(5199, 0), '/Contents': [IndirectObject(5198, 0)], '/MediaBox': [0, 0, 841, 595], '/CropBox': [0, 0, 841, 595]}, (0, 5205): {'/Type': '/Page', '/Parent': IndirectObject(2, 0), '/Resources': IndirectObject(5203, 0), '/Contents': [IndirectObject(5202, 0)], '/MediaBox': [0, 0, 841, 595], '/CropBox': [0, 0, 841, 595]}, (0, 5209): {'/Type': '/Page', '/Parent': IndirectObject(2, 0), '/Resources': IndirectObject(5207, 0), '/Contents': [IndirectObject(5206, 0)], '/MediaBox': [0, 0, 841, 595], '/CropBox': [0, 0, 841, 595]}, (0, 5213): {'/Type': '/Page', '/Parent': IndirectObject(2, 0), '/Resources': IndirectObject(5211, 0), '/Contents': [IndirectObject(5210, 0)], '/MediaBox': [0, 0, 841, 595], '/CropBox': [0, 0, 841, 595]}, (0, 5217): {'/Type': '/Page', '/Parent': IndirectObject(2, 0), '/Resources': IndirectObject(5215, 0), '/Contents': [IndirectObject(5214, 0)], '/MediaBox': [0, 0, 841, 595], '/CropBox': [0, 0, 841, 595]}, (0, 5221): {'/Type': '/Page', '/Parent': IndirectObject(2, 0), '/Resources': IndirectObject(5219, 0), '/Contents': [IndirectObject(5218, 0)], '/MediaBox': [0, 0, 841, 595], '/CropBox': [0, 0, 841, 595]}, (0, 5225): {'/Type': '/Page', '/Parent': IndirectObject(2, 0), '/Resources': IndirectObject(5223, 0), '/Contents': [IndirectObject(5222, 0)], '/MediaBox': [0, 0, 841, 595], '/CropBox': [0, 0, 841, 595]}, (0, 5229): {'/Type': '/Page', '/Parent': IndirectObject(2, 0), '/Resources': IndirectObject(5227, 0), '/Contents': [IndirectObject(5226, 0)], '/MediaBox': [0, 0, 841, 595], '/CropBox': [0, 0, 841, 595]}, (0, 5233): {'/Type': '/Page', '/Parent': IndirectObject(2, 0), '/Resources': IndirectObject(5231, 0), '/Contents': [IndirectObject(5230, 0)], '/MediaBox': [0, 0, 841, 595], '/CropBox': [0, 0, 841, 595]}, (0, 5237): {'/Type': '/Page', '/Parent': IndirectObject(2, 0), '/Resources': IndirectObject(5235, 0), '/Contents': [IndirectObject(5234, 0)], '/MediaBox': [0, 0, 841, 595], '/CropBox': [0, 0, 841, 595]}, (0, 5241): {'/Type': '/Page', '/Parent': IndirectObject(2, 0), '/Resources': IndirectObject(5239, 0), '/Contents': [IndirectObject(5238, 0)], '/MediaBox': [0, 0, 841, 595], '/CropBox': [0, 0, 841, 595]}, (0, 5245): {'/Type': '/Page', '/Parent': IndirectObject(2, 0), '/Resources': IndirectObject(5243, 0), '/Contents': [IndirectObject(5242, 0)], '/MediaBox': [0, 0, 841, 595], '/CropBox': [0, 0, 841, 595]}, (0, 5249): {'/Type': '/Page', '/Parent': IndirectObject(2, 0), '/Resources': IndirectObject(5247, 0), '/Contents': [IndirectObject(5246, 0)], '/MediaBox': [0, 0, 841, 595], '/CropBox': [0, 0, 841, 595]}, (0, 5253): {'/Type': '/Page', '/Parent': IndirectObject(2, 0), '/Resources': IndirectObject(5251, 0), '/Contents': [IndirectObject(5250, 0)], '/MediaBox': [0, 0, 841, 595], '/CropBox': [0, 0, 841, 595]}, (0, 5257): {'/Type': '/Page', '/Parent': IndirectObject(2, 0), '/Resources': IndirectObject(5255, 0), '/Contents': [IndirectObject(5254, 0)], '/MediaBox': [0, 0, 841, 595], '/CropBox': [0, 0, 841, 595]}, (0, 5261): {'/Type': '/Page', '/Parent': IndirectObject(2, 0), '/Resources': IndirectObject(5259, 0), '/Contents': [IndirectObject(5258, 0)], '/MediaBox': [0, 0, 841, 595], '/CropBox': [0, 0, 841, 595]}, (0, 5265): {'/Type': '/Page', '/Parent': IndirectObject(2, 0), '/Resources': IndirectObject(5263, 0), '/Contents': [IndirectObject(5262, 0)], '/MediaBox': [0, 0, 841, 595], '/CropBox': [0, 0, 841, 595]}, (0, 5269): {'/Type': '/Page', '/Parent': IndirectObject(2, 0), '/Resources': IndirectObject(5267, 0), '/Contents': [IndirectObject(5266, 0)], '/MediaBox': [0, 0, 841, 595], '/CropBox': [0, 0, 841, 595]}, (0, 5273): {'/Type': '/Page', '/Parent': IndirectObject(2, 0), '/Resources': IndirectObject(5271, 0), '/Contents': [IndirectObject(5270, 0)], '/MediaBox': [0, 0, 841, 595], '/CropBox': [0, 0, 841, 595]}, (0, 5277): {'/Type': '/Page', '/Parent': IndirectObject(2, 0), '/Resources': IndirectObject(5275, 0), '/Contents': [IndirectObject(5274, 0)], '/MediaBox': [0, 0, 841, 595], '/CropBox': [0, 0, 841, 595]}, (0, 5281): {'/Type': '/Page', '/Parent': IndirectObject(2, 0), '/Resources': IndirectObject(5279, 0), '/Contents': [IndirectObject(5278, 0)], '/MediaBox': [0, 0, 841, 595], '/CropBox': [0, 0, 841, 595]}, (0, 5285): {'/Type': '/Page', '/Parent': IndirectObject(2, 0), '/Resources': IndirectObject(5283, 0), '/Contents': [IndirectObject(5282, 0)], '/MediaBox': [0, 0, 841, 595], '/CropBox': [0, 0, 841, 595]}, (0, 5289): {'/Type': '/Page', '/Parent': IndirectObject(2, 0), '/Resources': IndirectObject(5287, 0), '/Contents': [IndirectObject(5286, 0)], '/MediaBox': [0, 0, 841, 595], '/CropBox': [0, 0, 841, 595]}, (0, 5293): {'/Type': '/Page', '/Parent': IndirectObject(2, 0), '/Resources': IndirectObject(5291, 0), '/Contents': [IndirectObject(5290, 0)], '/MediaBox': [0, 0, 841, 595], '/CropBox': [0, 0, 841, 595]}, (0, 5297): {'/Type': '/Page', '/Parent': IndirectObject(2, 0), '/Resources': IndirectObject(5295, 0), '/Contents': [IndirectObject(5294, 0)], '/MediaBox': [0, 0, 841, 595], '/CropBox': [0, 0, 841, 595]}, (0, 5301): {'/Type': '/Page', '/Parent': IndirectObject(2, 0), '/Resources': IndirectObject(5299, 0), '/Contents': [IndirectObject(5298, 0)], '/MediaBox': [0, 0, 841, 595], '/CropBox': [0, 0, 841, 595]}, (0, 5305): {'/Type': '/Page', '/Parent': IndirectObject(2, 0), '/Resources': IndirectObject(5303, 0), '/Contents': [IndirectObject(5302, 0)], '/MediaBox': [0, 0, 841, 595], '/CropBox': [0, 0, 841, 595]}, (0, 5309): {'/Type': '/Page', '/Parent': IndirectObject(2, 0), '/Resources': IndirectObject(5307, 0), '/Contents': [IndirectObject(5306, 0)], '/MediaBox': [0, 0, 841, 595], '/CropBox': [0, 0, 841, 595]}, (0, 5313): {'/Type': '/Page', '/Parent': IndirectObject(2, 0), '/Resources': IndirectObject(5311, 0), '/Contents': [IndirectObject(5310, 0)], '/MediaBox': [0, 0, 841, 595], '/CropBox': [0, 0, 841, 595]}, (0, 5317): {'/Type': '/Page', '/Parent': IndirectObject(2, 0), '/Resources': IndirectObject(5315, 0), '/Contents': [IndirectObject(5314, 0)], '/MediaBox': [0, 0, 841, 595], '/CropBox': [0, 0, 841, 595]}, (0, 5321): {'/Type': '/Page', '/Parent': IndirectObject(2, 0), '/Resources': IndirectObject(5319, 0), '/Contents': [IndirectObject(5318, 0)], '/MediaBox': [0, 0, 841, 595], '/CropBox': [0, 0, 841, 595]}, (0, 5325): {'/Type': '/Page', '/Parent': IndirectObject(2, 0), '/Resources': IndirectObject(5323, 0), '/Contents': [IndirectObject(5322, 0)], '/MediaBox': [0, 0, 841, 595], '/CropBox': [0, 0, 841, 595]}, (0, 5329): {'/Type': '/Page', '/Parent': IndirectObject(2, 0), '/Resources': IndirectObject(5327, 0), '/Contents': [IndirectObject(5326, 0)], '/MediaBox': [0, 0, 841, 595], '/CropBox': [0, 0, 841, 595]}, (0, 5333): {'/Type': '/Page', '/Parent': IndirectObject(2, 0), '/Resources': IndirectObject(5331, 0), '/Contents': [IndirectObject(5330, 0)], '/MediaBox': [0, 0, 841, 595], '/CropBox': [0, 0, 841, 595]}, (0, 5337): {'/Type': '/Page', '/Parent': IndirectObject(2, 0), '/Resources': IndirectObject(5335, 0), '/Contents': [IndirectObject(5334, 0)], '/MediaBox': [0, 0, 841, 595], '/CropBox': [0, 0, 841, 595]}, (0, 5341): {'/Type': '/Page', '/Parent': IndirectObject(2, 0), '/Resources': IndirectObject(5339, 0), '/Contents': [IndirectObject(5338, 0)], '/MediaBox': [0, 0, 841, 595], '/CropBox': [0, 0, 841, 595]}, (0, 5345): {'/Type': '/Page', '/Parent': IndirectObject(2, 0), '/Resources': IndirectObject(5343, 0), '/Contents': [IndirectObject(5342, 0)], '/MediaBox': [0, 0, 841, 595], '/CropBox': [0, 0, 841, 595]}, (0, 5349): {'/Type': '/Page', '/Parent': IndirectObject(2, 0), '/Resources': IndirectObject(5347, 0), '/Contents': [IndirectObject(5346, 0)], '/MediaBox': [0, 0, 841, 595], '/CropBox': [0, 0, 841, 595]}, (0, 5353): {'/Type': '/Page', '/Parent': IndirectObject(2, 0), '/Resources': IndirectObject(5351, 0), '/Contents': [IndirectObject(5350, 0)], '/MediaBox': [0, 0, 841, 595], '/CropBox': [0, 0, 841, 595]}, (0, 5357): {'/Type': '/Page', '/Parent': IndirectObject(2, 0), '/Resources': IndirectObject(5355, 0), '/Contents': [IndirectObject(5354, 0)], '/MediaBox': [0, 0, 841, 595], '/CropBox': [0, 0, 841, 595]}, (0, 5361): {'/Type': '/Page', '/Parent': IndirectObject(2, 0), '/Resources': IndirectObject(5359, 0), '/Contents': [IndirectObject(5358, 0)], '/MediaBox': [0, 0, 841, 595], '/CropBox': [0, 0, 841, 595]}, (0, 5365): {'/Type': '/Page', '/Parent': IndirectObject(2, 0), '/Resources': IndirectObject(5363, 0), '/Contents': [IndirectObject(5362, 0)], '/MediaBox': [0, 0, 841, 595], '/CropBox': [0, 0, 841, 595]}, (0, 5369): {'/Type': '/Page', '/Parent': IndirectObject(2, 0), '/Resources': IndirectObject(5367, 0), '/Contents': [IndirectObject(5366, 0)], '/MediaBox': [0, 0, 841, 595], '/CropBox': [0, 0, 841, 595]}, (0, 5373): {'/Type': '/Page', '/Parent': IndirectObject(2, 0), '/Resources': IndirectObject(5371, 0), '/Contents': [IndirectObject(5370, 0)], '/MediaBox': [0, 0, 841, 595], '/CropBox': [0, 0, 841, 595]}, (0, 5377): {'/Type': '/Page', '/Parent': IndirectObject(2, 0), '/Resources': IndirectObject(5375, 0), '/Contents': [IndirectObject(5374, 0)], '/MediaBox': [0, 0, 841, 595], '/CropBox': [0, 0, 841, 595]}, (0, 5381): {'/Type': '/Page', '/Parent': IndirectObject(2, 0), '/Resources': IndirectObject(5379, 0), '/Contents': [IndirectObject(5378, 0)], '/MediaBox': [0, 0, 841, 595], '/CropBox': [0, 0, 841, 595]}, (0, 5385): {'/Type': '/Page', '/Parent': IndirectObject(2, 0), '/Resources': IndirectObject(5383, 0), '/Contents': [IndirectObject(5382, 0)], '/MediaBox': [0, 0, 841, 595], '/CropBox': [0, 0, 841, 595]}, (0, 5389): {'/Type': '/Page', '/Parent': IndirectObject(2, 0), '/Resources': IndirectObject(5387, 0), '/Contents': [IndirectObject(5386, 0)], '/MediaBox': [0, 0, 841, 595], '/CropBox': [0, 0, 841, 595]}, (0, 5393): {'/Type': '/Page', '/Parent': IndirectObject(2, 0), '/Resources': IndirectObject(5391, 0), '/Contents': [IndirectObject(5390, 0)], '/MediaBox': [0, 0, 841, 595], '/CropBox': [0, 0, 841, 595]}, (0, 5397): {'/Type': '/Page', '/Parent': IndirectObject(2, 0), '/Resources': IndirectObject(5395, 0), '/Contents': [IndirectObject(5394, 0)], '/MediaBox': [0, 0, 841, 595], '/CropBox': [0, 0, 841, 595]}, (0, 5401): {'/Type': '/Page', '/Parent': IndirectObject(2, 0), '/Resources': IndirectObject(5399, 0), '/Contents': [IndirectObject(5398, 0)], '/MediaBox': [0, 0, 841, 595], '/CropBox': [0, 0, 841, 595]}, (0, 5405): {'/Type': '/Page', '/Parent': IndirectObject(2, 0), '/Resources': IndirectObject(5403, 0), '/Contents': [IndirectObject(5402, 0)], '/MediaBox': [0, 0, 841, 595], '/CropBox': [0, 0, 841, 595]}, (0, 5409): {'/Type': '/Page', '/Parent': IndirectObject(2, 0), '/Resources': IndirectObject(5407, 0), '/Contents': [IndirectObject(5406, 0)], '/MediaBox': [0, 0, 841, 595], '/CropBox': [0, 0, 841, 595]}, (0, 5413): {'/Type': '/Page', '/Parent': IndirectObject(2, 0), '/Resources': IndirectObject(5411, 0), '/Contents': [IndirectObject(5410, 0)], '/MediaBox': [0, 0, 841, 595], '/CropBox': [0, 0, 841, 595]}, (0, 5417): {'/Type': '/Page', '/Parent': IndirectObject(2, 0), '/Resources': IndirectObject(5415, 0), '/Contents': [IndirectObject(5414, 0)], '/MediaBox': [0, 0, 841, 595], '/CropBox': [0, 0, 841, 595]}, (0, 5421): {'/Type': '/Page', '/Parent': IndirectObject(2, 0), '/Resources': IndirectObject(5419, 0), '/Contents': [IndirectObject(5418, 0)], '/MediaBox': [0, 0, 841, 595], '/CropBox': [0, 0, 841, 595]}, (0, 5425): {'/Type': '/Page', '/Parent': IndirectObject(2, 0), '/Resources': IndirectObject(5423, 0), '/Contents': [IndirectObject(5422, 0)], '/MediaBox': [0, 0, 841, 595], '/CropBox': [0, 0, 841, 595]}, (0, 5429): {'/Type': '/Page', '/Parent': IndirectObject(2, 0), '/Resources': IndirectObject(5427, 0), '/Contents': [IndirectObject(5426, 0)], '/MediaBox': [0, 0, 841, 595], '/CropBox': [0, 0, 841, 595]}, (0, 5433): {'/Type': '/Page', '/Parent': IndirectObject(2, 0), '/Resources': IndirectObject(5431, 0), '/Contents': [IndirectObject(5430, 0)], '/MediaBox': [0, 0, 841, 595], '/CropBox': [0, 0, 841, 595]}, (0, 5437): {'/Type': '/Page', '/Parent': IndirectObject(2, 0), '/Resources': IndirectObject(5435, 0), '/Contents': [IndirectObject(5434, 0)], '/MediaBox': [0, 0, 841, 595], '/CropBox': [0, 0, 841, 595]}, (0, 5441): {'/Type': '/Page', '/Parent': IndirectObject(2, 0), '/Resources': IndirectObject(5439, 0), '/Contents': [IndirectObject(5438, 0)], '/MediaBox': [0, 0, 841, 595], '/CropBox': [0, 0, 841, 595]}, (0, 5445): {'/Type': '/Page', '/Parent': IndirectObject(2, 0), '/Resources': IndirectObject(5443, 0), '/Contents': [IndirectObject(5442, 0)], '/MediaBox': [0, 0, 841, 595], '/CropBox': [0, 0, 841, 595]}, (0, 5449): {'/Type': '/Page', '/Parent': IndirectObject(2, 0), '/Resources': IndirectObject(5447, 0), '/Contents': [IndirectObject(5446, 0)], '/MediaBox': [0, 0, 841, 595], '/CropBox': [0, 0, 841, 595]}, (0, 5453): {'/Type': '/Page', '/Parent': IndirectObject(2, 0), '/Resources': IndirectObject(5451, 0), '/Contents': [IndirectObject(5450, 0)], '/MediaBox': [0, 0, 841, 595], '/CropBox': [0, 0, 841, 595]}, (0, 5457): {'/Type': '/Page', '/Parent': IndirectObject(2, 0), '/Resources': IndirectObject(5455, 0), '/Contents': [IndirectObject(5454, 0)], '/MediaBox': [0, 0, 841, 595], '/CropBox': [0, 0, 841, 595]}, (0, 5461): {'/Type': '/Page', '/Parent': IndirectObject(2, 0), '/Resources': IndirectObject(5459, 0), '/Contents': [IndirectObject(5458, 0)], '/MediaBox': [0, 0, 841, 595], '/CropBox': [0, 0, 841, 595]}, (0, 5465): {'/Type': '/Page', '/Parent': IndirectObject(2, 0), '/Resources': IndirectObject(5463, 0), '/Contents': [IndirectObject(5462, 0)], '/MediaBox': [0, 0, 841, 595], '/CropBox': [0, 0, 841, 595]}, (0, 5469): {'/Type': '/Page', '/Parent': IndirectObject(2, 0), '/Resources': IndirectObject(5467, 0), '/Contents': [IndirectObject(5466, 0)], '/MediaBox': [0, 0, 841, 595], '/CropBox': [0, 0, 841, 595]}, (0, 5473): {'/Type': '/Page', '/Parent': IndirectObject(2, 0), '/Resources': IndirectObject(5471, 0), '/Contents': [IndirectObject(5470, 0)], '/MediaBox': [0, 0, 841, 595], '/CropBox': [0, 0, 841, 595]}, (0, 5477): {'/Type': '/Page', '/Parent': IndirectObject(2, 0), '/Resources': IndirectObject(5475, 0), '/Contents': [IndirectObject(5474, 0)], '/MediaBox': [0, 0, 841, 595], '/CropBox': [0, 0, 841, 595]}, (0, 5481): {'/Type': '/Page', '/Parent': IndirectObject(2, 0), '/Resources': IndirectObject(5479, 0), '/Contents': [IndirectObject(5478, 0)], '/MediaBox': [0, 0, 841, 595], '/CropBox': [0, 0, 841, 595]}, (0, 5485): {'/Type': '/Page', '/Parent': IndirectObject(2, 0), '/Resources': IndirectObject(5483, 0), '/Contents': [IndirectObject(5482, 0)], '/MediaBox': [0, 0, 841, 595], '/CropBox': [0, 0, 841, 595]}, (0, 5489): {'/Type': '/Page', '/Parent': IndirectObject(2, 0), '/Resources': IndirectObject(5487, 0), '/Contents': [IndirectObject(5486, 0)], '/MediaBox': [0, 0, 841, 595], '/CropBox': [0, 0, 841, 595]}, (0, 5493): {'/Type': '/Page', '/Parent': IndirectObject(2, 0), '/Resources': IndirectObject(5491, 0), '/Contents': [IndirectObject(5490, 0)], '/MediaBox': [0, 0, 841, 595], '/CropBox': [0, 0, 841, 595]}, (0, 5497): {'/Type': '/Page', '/Parent': IndirectObject(2, 0), '/Resources': IndirectObject(5495, 0), '/Contents': [IndirectObject(5494, 0)], '/MediaBox': [0, 0, 841, 595], '/CropBox': [0, 0, 841, 595]}, (0, 5501): {'/Type': '/Page', '/Parent': IndirectObject(2, 0), '/Resources': IndirectObject(5499, 0), '/Contents': [IndirectObject(5498, 0)], '/MediaBox': [0, 0, 841, 595], '/CropBox': [0, 0, 841, 595]}, (0, 5505): {'/Type': '/Page', '/Parent': IndirectObject(2, 0), '/Resources': IndirectObject(5503, 0), '/Contents': [IndirectObject(5502, 0)], '/MediaBox': [0, 0, 841, 595], '/CropBox': [0, 0, 841, 595]}, (0, 5509): {'/Type': '/Page', '/Parent': IndirectObject(2, 0), '/Resources': IndirectObject(5507, 0), '/Contents': [IndirectObject(5506, 0)], '/MediaBox': [0, 0, 841, 595], '/CropBox': [0, 0, 841, 595]}, (0, 5513): {'/Type': '/Page', '/Parent': IndirectObject(2, 0), '/Resources': IndirectObject(5511, 0), '/Contents': [IndirectObject(5510, 0)], '/MediaBox': [0, 0, 841, 595], '/CropBox': [0, 0, 841, 595]}, (0, 5517): {'/Type': '/Page', '/Parent': IndirectObject(2, 0), '/Resources': IndirectObject(5515, 0), '/Contents': [IndirectObject(5514, 0)], '/MediaBox': [0, 0, 841, 595], '/CropBox': [0, 0, 841, 595]}, (0, 5521): {'/Type': '/Page', '/Parent': IndirectObject(2, 0), '/Resources': IndirectObject(5519, 0), '/Contents': [IndirectObject(5518, 0)], '/MediaBox': [0, 0, 841, 595], '/CropBox': [0, 0, 841, 595]}, (0, 5525): {'/Type': '/Page', '/Parent': IndirectObject(2, 0), '/Resources': IndirectObject(5523, 0), '/Contents': [IndirectObject(5522, 0)], '/MediaBox': [0, 0, 841, 595], '/CropBox': [0, 0, 841, 595]}, (0, 5529): {'/Type': '/Page', '/Parent': IndirectObject(2, 0), '/Resources': IndirectObject(5527, 0), '/Contents': [IndirectObject(5526, 0)], '/MediaBox': [0, 0, 841, 595], '/CropBox': [0, 0, 841, 595]}, (0, 5533): {'/Type': '/Page', '/Parent': IndirectObject(2, 0), '/Resources': IndirectObject(5531, 0), '/Contents': [IndirectObject(5530, 0)], '/MediaBox': [0, 0, 841, 595], '/CropBox': [0, 0, 841, 595]}, (0, 5537): {'/Type': '/Page', '/Parent': IndirectObject(2, 0), '/Resources': IndirectObject(5535, 0), '/Contents': [IndirectObject(5534, 0)], '/MediaBox': [0, 0, 841, 595], '/CropBox': [0, 0, 841, 595]}, (0, 5541): {'/Type': '/Page', '/Parent': IndirectObject(2, 0), '/Resources': IndirectObject(5539, 0), '/Contents': [IndirectObject(5538, 0)], '/MediaBox': [0, 0, 841, 595], '/CropBox': [0, 0, 841, 595]}, (0, 5545): {'/Type': '/Page', '/Parent': IndirectObject(2, 0), '/Resources': IndirectObject(5543, 0), '/Contents': [IndirectObject(5542, 0)], '/MediaBox': [0, 0, 841, 595], '/CropBox': [0, 0, 841, 595]}, (0, 5549): {'/Type': '/Page', '/Parent': IndirectObject(2, 0), '/Resources': IndirectObject(5547, 0), '/Contents': [IndirectObject(5546, 0)], '/MediaBox': [0, 0, 841, 595], '/CropBox': [0, 0, 841, 595]}, (0, 5553): {'/Type': '/Page', '/Parent': IndirectObject(2, 0), '/Resources': IndirectObject(5551, 0), '/Contents': [IndirectObject(5550, 0)], '/MediaBox': [0, 0, 841, 595], '/CropBox': [0, 0, 841, 595]}, (0, 5557): {'/Type': '/Page', '/Parent': IndirectObject(2, 0), '/Resources': IndirectObject(5555, 0), '/Contents': [IndirectObject(5554, 0)], '/MediaBox': [0, 0, 841, 595], '/CropBox': [0, 0, 841, 595]}, (0, 5561): {'/Type': '/Page', '/Parent': IndirectObject(2, 0), '/Resources': IndirectObject(5559, 0), '/Contents': [IndirectObject(5558, 0)], '/MediaBox': [0, 0, 841, 595], '/CropBox': [0, 0, 841, 595]}, (0, 5565): {'/Type': '/Page', '/Parent': IndirectObject(2, 0), '/Resources': IndirectObject(5563, 0), '/Contents': [IndirectObject(5562, 0)], '/MediaBox': [0, 0, 841, 595], '/CropBox': [0, 0, 841, 595]}, (0, 5569): {'/Type': '/Page', '/Parent': IndirectObject(2, 0), '/Resources': IndirectObject(5567, 0), '/Contents': [IndirectObject(5566, 0)], '/MediaBox': [0, 0, 841, 595], '/CropBox': [0, 0, 841, 595]}, (0, 5573): {'/Type': '/Page', '/Parent': IndirectObject(2, 0), '/Resources': IndirectObject(5571, 0), '/Contents': [IndirectObject(5570, 0)], '/MediaBox': [0, 0, 841, 595], '/CropBox': [0, 0, 841, 595]}, (0, 5577): {'/Type': '/Page', '/Parent': IndirectObject(2, 0), '/Resources': IndirectObject(5575, 0), '/Contents': [IndirectObject(5574, 0)], '/MediaBox': [0, 0, 841, 595], '/CropBox': [0, 0, 841, 595]}, (0, 5581): {'/Type': '/Page', '/Parent': IndirectObject(2, 0), '/Resources': IndirectObject(5579, 0), '/Contents': [IndirectObject(5578, 0)], '/MediaBox': [0, 0, 841, 595], '/CropBox': [0, 0, 841, 595]}, (0, 5585): {'/Type': '/Page', '/Parent': IndirectObject(2, 0), '/Resources': IndirectObject(5583, 0), '/Contents': [IndirectObject(5582, 0)], '/MediaBox': [0, 0, 841, 595], '/CropBox': [0, 0, 841, 595]}, (0, 5589): {'/Type': '/Page', '/Parent': IndirectObject(2, 0), '/Resources': IndirectObject(5587, 0), '/Contents': [IndirectObject(5586, 0)], '/MediaBox': [0, 0, 841, 595], '/CropBox': [0, 0, 841, 595]}, (0, 5593): {'/Type': '/Page', '/Parent': IndirectObject(2, 0), '/Resources': IndirectObject(5591, 0), '/Contents': [IndirectObject(5590, 0)], '/MediaBox': [0, 0, 841, 595], '/CropBox': [0, 0, 841, 595]}, (0, 5597): {'/Type': '/Page', '/Parent': IndirectObject(2, 0), '/Resources': IndirectObject(5595, 0), '/Contents': [IndirectObject(5594, 0)], '/MediaBox': [0, 0, 841, 595], '/CropBox': [0, 0, 841, 595]}, (0, 5601): {'/Type': '/Page', '/Parent': IndirectObject(2, 0), '/Resources': IndirectObject(5599, 0), '/Contents': [IndirectObject(5598, 0)], '/MediaBox': [0, 0, 841, 595], '/CropBox': [0, 0, 841, 595]}, (0, 5605): {'/Type': '/Page', '/Parent': IndirectObject(2, 0), '/Resources': IndirectObject(5603, 0), '/Contents': [IndirectObject(5602, 0)], '/MediaBox': [0, 0, 841, 595], '/CropBox': [0, 0, 841, 595]}, (0, 5609): {'/Type': '/Page', '/Parent': IndirectObject(2, 0), '/Resources': IndirectObject(5607, 0), '/Contents': [IndirectObject(5606, 0)], '/MediaBox': [0, 0, 841, 595], '/CropBox': [0, 0, 841, 595]}, (0, 5613): {'/Type': '/Page', '/Parent': IndirectObject(2, 0), '/Resources': IndirectObject(5611, 0), '/Contents': [IndirectObject(5610, 0)], '/MediaBox': [0, 0, 841, 595], '/CropBox': [0, 0, 841, 595]}, (0, 5617): {'/Type': '/Page', '/Parent': IndirectObject(2, 0), '/Resources': IndirectObject(5615, 0), '/Contents': [IndirectObject(5614, 0)], '/MediaBox': [0, 0, 841, 595], '/CropBox': [0, 0, 841, 595]}, (0, 5621): {'/Type': '/Page', '/Parent': IndirectObject(2, 0), '/Resources': IndirectObject(5619, 0), '/Contents': [IndirectObject(5618, 0)], '/MediaBox': [0, 0, 841, 595], '/CropBox': [0, 0, 841, 595]}, (0, 5625): {'/Type': '/Page', '/Parent': IndirectObject(2, 0), '/Resources': IndirectObject(5623, 0), '/Contents': [IndirectObject(5622, 0)], '/MediaBox': [0, 0, 841, 595], '/CropBox': [0, 0, 841, 595]}, (0, 5629): {'/Type': '/Page', '/Parent': IndirectObject(2, 0), '/Resources': IndirectObject(5627, 0), '/Contents': [IndirectObject(5626, 0)], '/MediaBox': [0, 0, 841, 595], '/CropBox': [0, 0, 841, 595]}, (0, 5633): {'/Type': '/Page', '/Parent': IndirectObject(2, 0), '/Resources': IndirectObject(5631, 0), '/Contents': [IndirectObject(5630, 0)], '/MediaBox': [0, 0, 841, 595], '/CropBox': [0, 0, 841, 595]}, (0, 5637): {'/Type': '/Page', '/Parent': IndirectObject(2, 0), '/Resources': IndirectObject(5635, 0), '/Contents': [IndirectObject(5634, 0)], '/MediaBox': [0, 0, 841, 595], '/CropBox': [0, 0, 841, 595]}, (0, 5641): {'/Type': '/Page', '/Parent': IndirectObject(2, 0), '/Resources': IndirectObject(5639, 0), '/Contents': [IndirectObject(5638, 0)], '/MediaBox': [0, 0, 841, 595], '/CropBox': [0, 0, 841, 595]}, (0, 5645): {'/Type': '/Page', '/Parent': IndirectObject(2, 0), '/Resources': IndirectObject(5643, 0), '/Contents': [IndirectObject(5642, 0)], '/MediaBox': [0, 0, 841, 595], '/CropBox': [0, 0, 841, 595]}, (0, 5649): {'/Type': '/Page', '/Parent': IndirectObject(2, 0), '/Resources': IndirectObject(5647, 0), '/Contents': [IndirectObject(5646, 0)], '/MediaBox': [0, 0, 841, 595], '/CropBox': [0, 0, 841, 595]}, (0, 5653): {'/Type': '/Page', '/Parent': IndirectObject(2, 0), '/Resources': IndirectObject(5651, 0), '/Contents': [IndirectObject(5650, 0)], '/MediaBox': [0, 0, 841, 595], '/CropBox': [0, 0, 841, 595]}, (0, 5657): {'/Type': '/Page', '/Parent': IndirectObject(2, 0), '/Resources': IndirectObject(5655, 0), '/Contents': [IndirectObject(5654, 0)], '/MediaBox': [0, 0, 841, 595], '/CropBox': [0, 0, 841, 595]}, (0, 5661): {'/Type': '/Page', '/Parent': IndirectObject(2, 0), '/Resources': IndirectObject(5659, 0), '/Contents': [IndirectObject(5658, 0)], '/MediaBox': [0, 0, 841, 595], '/CropBox': [0, 0, 841, 595]}, (0, 5665): {'/Type': '/Page', '/Parent': IndirectObject(2, 0), '/Resources': IndirectObject(5663, 0), '/Contents': [IndirectObject(5662, 0)], '/MediaBox': [0, 0, 841, 595], '/CropBox': [0, 0, 841, 595]}, (0, 5669): {'/Type': '/Page', '/Parent': IndirectObject(2, 0), '/Resources': IndirectObject(5667, 0), '/Contents': [IndirectObject(5666, 0)], '/MediaBox': [0, 0, 841, 595], '/CropBox': [0, 0, 841, 595]}, (0, 5673): {'/Type': '/Page', '/Parent': IndirectObject(2, 0), '/Resources': IndirectObject(5671, 0), '/Contents': [IndirectObject(5670, 0)], '/MediaBox': [0, 0, 841, 595], '/CropBox': [0, 0, 841, 595]}, (0, 5677): {'/Type': '/Page', '/Parent': IndirectObject(2, 0), '/Resources': IndirectObject(5675, 0), '/Contents': [IndirectObject(5674, 0)], '/MediaBox': [0, 0, 841, 595], '/CropBox': [0, 0, 841, 595]}, (0, 5681): {'/Type': '/Page', '/Parent': IndirectObject(2, 0), '/Resources': IndirectObject(5679, 0), '/Contents': [IndirectObject(5678, 0)], '/MediaBox': [0, 0, 841, 595], '/CropBox': [0, 0, 841, 595]}, (0, 5685): {'/Type': '/Page', '/Parent': IndirectObject(2, 0), '/Resources': IndirectObject(5683, 0), '/Contents': [IndirectObject(5682, 0)], '/MediaBox': [0, 0, 841, 595], '/CropBox': [0, 0, 841, 595]}, (0, 5689): {'/Type': '/Page', '/Parent': IndirectObject(2, 0), '/Resources': IndirectObject(5687, 0), '/Contents': [IndirectObject(5686, 0)], '/MediaBox': [0, 0, 841, 595], '/CropBox': [0, 0, 841, 595]}, (0, 5693): {'/Type': '/Page', '/Parent': IndirectObject(2, 0), '/Resources': IndirectObject(5691, 0), '/Contents': [IndirectObject(5690, 0)], '/MediaBox': [0, 0, 841, 595], '/CropBox': [0, 0, 841, 595]}, (0, 5697): {'/Type': '/Page', '/Parent': IndirectObject(2, 0), '/Resources': IndirectObject(5695, 0), '/Contents': [IndirectObject(5694, 0)], '/MediaBox': [0, 0, 841, 595], '/CropBox': [0, 0, 841, 595]}, (0, 5701): {'/Type': '/Page', '/Parent': IndirectObject(2, 0), '/Resources': IndirectObject(5699, 0), '/Contents': [IndirectObject(5698, 0)], '/MediaBox': [0, 0, 841, 595], '/CropBox': [0, 0, 841, 595]}, (0, 5705): {'/Type': '/Page', '/Parent': IndirectObject(2, 0), '/Resources': IndirectObject(5703, 0), '/Contents': [IndirectObject(5702, 0)], '/MediaBox': [0, 0, 841, 595], '/CropBox': [0, 0, 841, 595]}, (0, 5709): {'/Type': '/Page', '/Parent': IndirectObject(2, 0), '/Resources': IndirectObject(5707, 0), '/Contents': [IndirectObject(5706, 0)], '/MediaBox': [0, 0, 841, 595], '/CropBox': [0, 0, 841, 595]}, (0, 5713): {'/Type': '/Page', '/Parent': IndirectObject(2, 0), '/Resources': IndirectObject(5711, 0), '/Contents': [IndirectObject(5710, 0)], '/MediaBox': [0, 0, 841, 595], '/CropBox': [0, 0, 841, 595]}, (0, 5717): {'/Type': '/Page', '/Parent': IndirectObject(2, 0), '/Resources': IndirectObject(5715, 0), '/Contents': [IndirectObject(5714, 0)], '/MediaBox': [0, 0, 841, 595], '/CropBox': [0, 0, 841, 595]}, (0, 5721): {'/Type': '/Page', '/Parent': IndirectObject(2, 0), '/Resources': IndirectObject(5719, 0), '/Contents': [IndirectObject(5718, 0)], '/MediaBox': [0, 0, 841, 595], '/CropBox': [0, 0, 841, 595]}, (0, 5725): {'/Type': '/Page', '/Parent': IndirectObject(2, 0), '/Resources': IndirectObject(5723, 0), '/Contents': [IndirectObject(5722, 0)], '/MediaBox': [0, 0, 841, 595], '/CropBox': [0, 0, 841, 595]}, (0, 5729): {'/Type': '/Page', '/Parent': IndirectObject(2, 0), '/Resources': IndirectObject(5727, 0), '/Contents': [IndirectObject(5726, 0)], '/MediaBox': [0, 0, 841, 595], '/CropBox': [0, 0, 841, 595]}, (0, 5733): {'/Type': '/Page', '/Parent': IndirectObject(2, 0), '/Resources': IndirectObject(5731, 0), '/Contents': [IndirectObject(5730, 0)], '/MediaBox': [0, 0, 841, 595], '/CropBox': [0, 0, 841, 595]}, (0, 5737): {'/Type': '/Page', '/Parent': IndirectObject(2, 0), '/Resources': IndirectObject(5735, 0), '/Contents': [IndirectObject(5734, 0)], '/MediaBox': [0, 0, 841, 595], '/CropBox': [0, 0, 841, 595]}, (0, 5741): {'/Type': '/Page', '/Parent': IndirectObject(2, 0), '/Resources': IndirectObject(5739, 0), '/Contents': [IndirectObject(5738, 0)], '/MediaBox': [0, 0, 841, 595], '/CropBox': [0, 0, 841, 595]}, (0, 5745): {'/Type': '/Page', '/Parent': IndirectObject(2, 0), '/Resources': IndirectObject(5743, 0), '/Contents': [IndirectObject(5742, 0)], '/MediaBox': [0, 0, 841, 595], '/CropBox': [0, 0, 841, 595]}, (0, 5749): {'/Type': '/Page', '/Parent': IndirectObject(2, 0), '/Resources': IndirectObject(5747, 0), '/Contents': [IndirectObject(5746, 0)], '/MediaBox': [0, 0, 841, 595], '/CropBox': [0, 0, 841, 595]}, (0, 5753): {'/Type': '/Page', '/Parent': IndirectObject(2, 0), '/Resources': IndirectObject(5751, 0), '/Contents': [IndirectObject(5750, 0)], '/MediaBox': [0, 0, 841, 595], '/CropBox': [0, 0, 841, 595]}, (0, 5757): {'/Type': '/Page', '/Parent': IndirectObject(2, 0), '/Resources': IndirectObject(5755, 0), '/Contents': [IndirectObject(5754, 0)], '/MediaBox': [0, 0, 841, 595], '/CropBox': [0, 0, 841, 595]}, (0, 5761): {'/Type': '/Page', '/Parent': IndirectObject(2, 0), '/Resources': IndirectObject(5759, 0), '/Contents': [IndirectObject(5758, 0)], '/MediaBox': [0, 0, 841, 595], '/CropBox': [0, 0, 841, 595]}, (0, 5765): {'/Type': '/Page', '/Parent': IndirectObject(2, 0), '/Resources': IndirectObject(5763, 0), '/Contents': [IndirectObject(5762, 0)], '/MediaBox': [0, 0, 841, 595], '/CropBox': [0, 0, 841, 595]}, (0, 5769): {'/Type': '/Page', '/Parent': IndirectObject(2, 0), '/Resources': IndirectObject(5767, 0), '/Contents': [IndirectObject(5766, 0)], '/MediaBox': [0, 0, 841, 595], '/CropBox': [0, 0, 841, 595]}, (0, 5773): {'/Type': '/Page', '/Parent': IndirectObject(2, 0), '/Resources': IndirectObject(5771, 0), '/Contents': [IndirectObject(5770, 0)], '/MediaBox': [0, 0, 841, 595], '/CropBox': [0, 0, 841, 595]}, (0, 5777): {'/Type': '/Page', '/Parent': IndirectObject(2, 0), '/Resources': IndirectObject(5775, 0), '/Contents': [IndirectObject(5774, 0)], '/MediaBox': [0, 0, 841, 595], '/CropBox': [0, 0, 841, 595]}, (0, 5781): {'/Type': '/Page', '/Parent': IndirectObject(2, 0), '/Resources': IndirectObject(5779, 0), '/Contents': [IndirectObject(5778, 0)], '/MediaBox': [0, 0, 841, 595], '/CropBox': [0, 0, 841, 595]}, (0, 5785): {'/Type': '/Page', '/Parent': IndirectObject(2, 0), '/Resources': IndirectObject(5783, 0), '/Contents': [IndirectObject(5782, 0)], '/MediaBox': [0, 0, 841, 595], '/CropBox': [0, 0, 841, 595]}, (0, 5789): {'/Type': '/Page', '/Parent': IndirectObject(2, 0), '/Resources': IndirectObject(5787, 0), '/Contents': [IndirectObject(5786, 0)], '/MediaBox': [0, 0, 841, 595], '/CropBox': [0, 0, 841, 595]}, (0, 5793): {'/Type': '/Page', '/Parent': IndirectObject(2, 0), '/Resources': IndirectObject(5791, 0), '/Contents': [IndirectObject(5790, 0)], '/MediaBox': [0, 0, 841, 595], '/CropBox': [0, 0, 841, 595]}, (0, 5797): {'/Type': '/Page', '/Parent': IndirectObject(2, 0), '/Resources': IndirectObject(5795, 0), '/Contents': [IndirectObject(5794, 0)], '/MediaBox': [0, 0, 841, 595], '/CropBox': [0, 0, 841, 595]}, (0, 5801): {'/Type': '/Page', '/Parent': IndirectObject(2, 0), '/Resources': IndirectObject(5799, 0), '/Contents': [IndirectObject(5798, 0)], '/MediaBox': [0, 0, 841, 595], '/CropBox': [0, 0, 841, 595]}, (0, 5805): {'/Type': '/Page', '/Parent': IndirectObject(2, 0), '/Resources': IndirectObject(5803, 0), '/Contents': [IndirectObject(5802, 0)], '/MediaBox': [0, 0, 841, 595], '/CropBox': [0, 0, 841, 595]}, (0, 5809): {'/Type': '/Page', '/Parent': IndirectObject(2, 0), '/Resources': IndirectObject(5807, 0), '/Contents': [IndirectObject(5806, 0)], '/MediaBox': [0, 0, 841, 595], '/CropBox': [0, 0, 841, 595]}, (0, 5813): {'/Type': '/Page', '/Parent': IndirectObject(2, 0), '/Resources': IndirectObject(5811, 0), '/Contents': [IndirectObject(5810, 0)], '/MediaBox': [0, 0, 841, 595], '/CropBox': [0, 0, 841, 595]}, (0, 5817): {'/Type': '/Page', '/Parent': IndirectObject(2, 0), '/Resources': IndirectObject(5815, 0), '/Contents': [IndirectObject(5814, 0)], '/MediaBox': [0, 0, 841, 595], '/CropBox': [0, 0, 841, 595]}, (0, 5821): {'/Type': '/Page', '/Parent': IndirectObject(2, 0), '/Resources': IndirectObject(5819, 0), '/Contents': [IndirectObject(5818, 0)], '/MediaBox': [0, 0, 841, 595], '/CropBox': [0, 0, 841, 595]}, (0, 5825): {'/Type': '/Page', '/Parent': IndirectObject(2, 0), '/Resources': IndirectObject(5823, 0), '/Contents': [IndirectObject(5822, 0)], '/MediaBox': [0, 0, 841, 595], '/CropBox': [0, 0, 841, 595]}, (0, 5829): {'/Type': '/Page', '/Parent': IndirectObject(2, 0), '/Resources': IndirectObject(5827, 0), '/Contents': [IndirectObject(5826, 0)], '/MediaBox': [0, 0, 841, 595], '/CropBox': [0, 0, 841, 595]}, (0, 5833): {'/Type': '/Page', '/Parent': IndirectObject(2, 0), '/Resources': IndirectObject(5831, 0), '/Contents': [IndirectObject(5830, 0)], '/MediaBox': [0, 0, 841, 595], '/CropBox': [0, 0, 841, 595]}, (0, 5837): {'/Type': '/Page', '/Parent': IndirectObject(2, 0), '/Resources': IndirectObject(5835, 0), '/Contents': [IndirectObject(5834, 0)], '/MediaBox': [0, 0, 841, 595], '/CropBox': [0, 0, 841, 595]}, (0, 5841): {'/Type': '/Page', '/Parent': IndirectObject(2, 0), '/Resources': IndirectObject(5839, 0), '/Contents': [IndirectObject(5838, 0)], '/MediaBox': [0, 0, 841, 595], '/CropBox': [0, 0, 841, 595]}, (0, 5845): {'/Type': '/Page', '/Parent': IndirectObject(2, 0), '/Resources': IndirectObject(5843, 0), '/Contents': [IndirectObject(5842, 0)], '/MediaBox': [0, 0, 841, 595], '/CropBox': [0, 0, 841, 595]}, (0, 5849): {'/Type': '/Page', '/Parent': IndirectObject(2, 0), '/Resources': IndirectObject(5847, 0), '/Contents': [IndirectObject(5846, 0)], '/MediaBox': [0, 0, 841, 595], '/CropBox': [0, 0, 841, 595]}, (0, 5853): {'/Type': '/Page', '/Parent': IndirectObject(2, 0), '/Resources': IndirectObject(5851, 0), '/Contents': [IndirectObject(5850, 0)], '/MediaBox': [0, 0, 841, 595], '/CropBox': [0, 0, 841, 595]}, (0, 5857): {'/Type': '/Page', '/Parent': IndirectObject(2, 0), '/Resources': IndirectObject(5855, 0), '/Contents': [IndirectObject(5854, 0)], '/MediaBox': [0, 0, 841, 595], '/CropBox': [0, 0, 841, 595]}, (0, 5861): {'/Type': '/Page', '/Parent': IndirectObject(2, 0), '/Resources': IndirectObject(5859, 0), '/Contents': [IndirectObject(5858, 0)], '/MediaBox': [0, 0, 841, 595], '/CropBox': [0, 0, 841, 595]}, (0, 5865): {'/Type': '/Page', '/Parent': IndirectObject(2, 0), '/Resources': IndirectObject(5863, 0), '/Contents': [IndirectObject(5862, 0)], '/MediaBox': [0, 0, 841, 595], '/CropBox': [0, 0, 841, 595]}, (0, 5869): {'/Type': '/Page', '/Parent': IndirectObject(2, 0), '/Resources': IndirectObject(5867, 0), '/Contents': [IndirectObject(5866, 0)], '/MediaBox': [0, 0, 841, 595], '/CropBox': [0, 0, 841, 595]}, (0, 5873): {'/Type': '/Page', '/Parent': IndirectObject(2, 0), '/Resources': IndirectObject(5871, 0), '/Contents': [IndirectObject(5870, 0)], '/MediaBox': [0, 0, 841, 595], '/CropBox': [0, 0, 841, 595]}, (0, 5877): {'/Type': '/Page', '/Parent': IndirectObject(2, 0), '/Resources': IndirectObject(5875, 0), '/Contents': [IndirectObject(5874, 0)], '/MediaBox': [0, 0, 841, 595], '/CropBox': [0, 0, 841, 595]}, (0, 5881): {'/Type': '/Page', '/Parent': IndirectObject(2, 0), '/Resources': IndirectObject(5879, 0), '/Contents': [IndirectObject(5878, 0)], '/MediaBox': [0, 0, 841, 595], '/CropBox': [0, 0, 841, 595]}, (0, 5885): {'/Type': '/Page', '/Parent': IndirectObject(2, 0), '/Resources': IndirectObject(5883, 0), '/Contents': [IndirectObject(5882, 0)], '/MediaBox': [0, 0, 841, 595], '/CropBox': [0, 0, 841, 595]}, (0, 5889): {'/Type': '/Page', '/Parent': IndirectObject(2, 0), '/Resources': IndirectObject(5887, 0), '/Contents': [IndirectObject(5886, 0)], '/MediaBox': [0, 0, 841, 595], '/CropBox': [0, 0, 841, 595]}, (0, 5893): {'/Type': '/Page', '/Parent': IndirectObject(2, 0), '/Resources': IndirectObject(5891, 0), '/Contents': [IndirectObject(5890, 0)], '/MediaBox': [0, 0, 841, 595], '/CropBox': [0, 0, 841, 595]}, (0, 5897): {'/Type': '/Page', '/Parent': IndirectObject(2, 0), '/Resources': IndirectObject(5895, 0), '/Contents': [IndirectObject(5894, 0)], '/MediaBox': [0, 0, 841, 595], '/CropBox': [0, 0, 841, 595]}, (0, 5901): {'/Type': '/Page', '/Parent': IndirectObject(2, 0), '/Resources': IndirectObject(5899, 0), '/Contents': [IndirectObject(5898, 0)], '/MediaBox': [0, 0, 841, 595], '/CropBox': [0, 0, 841, 595]}, (0, 5905): {'/Type': '/Page', '/Parent': IndirectObject(2, 0), '/Resources': IndirectObject(5903, 0), '/Contents': [IndirectObject(5902, 0)], '/MediaBox': [0, 0, 841, 595], '/CropBox': [0, 0, 841, 595]}, (0, 5909): {'/Type': '/Page', '/Parent': IndirectObject(2, 0), '/Resources': IndirectObject(5907, 0), '/Contents': [IndirectObject(5906, 0)], '/MediaBox': [0, 0, 841, 595], '/CropBox': [0, 0, 841, 595]}, (0, 5913): {'/Type': '/Page', '/Parent': IndirectObject(2, 0), '/Resources': IndirectObject(5911, 0), '/Contents': [IndirectObject(5910, 0)], '/MediaBox': [0, 0, 841, 595], '/CropBox': [0, 0, 841, 595]}, (0, 5917): {'/Type': '/Page', '/Parent': IndirectObject(2, 0), '/Resources': IndirectObject(5915, 0), '/Contents': [IndirectObject(5914, 0)], '/MediaBox': [0, 0, 841, 595], '/CropBox': [0, 0, 841, 595]}, (0, 5921): {'/Type': '/Page', '/Parent': IndirectObject(2, 0), '/Resources': IndirectObject(5919, 0), '/Contents': [IndirectObject(5918, 0)], '/MediaBox': [0, 0, 841, 595], '/CropBox': [0, 0, 841, 595]}, (0, 5925): {'/Type': '/Page', '/Parent': IndirectObject(2, 0), '/Resources': IndirectObject(5923, 0), '/Contents': [IndirectObject(5922, 0)], '/MediaBox': [0, 0, 841, 595], '/CropBox': [0, 0, 841, 595]}, (0, 5929): {'/Type': '/Page', '/Parent': IndirectObject(2, 0), '/Resources': IndirectObject(5927, 0), '/Contents': [IndirectObject(5926, 0)], '/MediaBox': [0, 0, 841, 595], '/CropBox': [0, 0, 841, 595]}, (0, 5933): {'/Type': '/Page', '/Parent': IndirectObject(2, 0), '/Resources': IndirectObject(5931, 0), '/Contents': [IndirectObject(5930, 0)], '/MediaBox': [0, 0, 841, 595], '/CropBox': [0, 0, 841, 595]}, (0, 5937): {'/Type': '/Page', '/Parent': IndirectObject(2, 0), '/Resources': IndirectObject(5935, 0), '/Contents': [IndirectObject(5934, 0)], '/MediaBox': [0, 0, 841, 595], '/CropBox': [0, 0, 841, 595]}, (0, 5941): {'/Type': '/Page', '/Parent': IndirectObject(2, 0), '/Resources': IndirectObject(5939, 0), '/Contents': [IndirectObject(5938, 0)], '/MediaBox': [0, 0, 841, 595], '/CropBox': [0, 0, 841, 595]}, (0, 5945): {'/Type': '/Page', '/Parent': IndirectObject(2, 0), '/Resources': IndirectObject(5943, 0), '/Contents': [IndirectObject(5942, 0)], '/MediaBox': [0, 0, 841, 595], '/CropBox': [0, 0, 841, 595]}, (0, 5949): {'/Type': '/Page', '/Parent': IndirectObject(2, 0), '/Resources': IndirectObject(5947, 0), '/Contents': [IndirectObject(5946, 0)], '/MediaBox': [0, 0, 841, 595], '/CropBox': [0, 0, 841, 595]}, (0, 5953): {'/Type': '/Page', '/Parent': IndirectObject(2, 0), '/Resources': IndirectObject(5951, 0), '/Contents': [IndirectObject(5950, 0)], '/MediaBox': [0, 0, 841, 595], '/CropBox': [0, 0, 841, 595]}, (0, 5957): {'/Type': '/Page', '/Parent': IndirectObject(2, 0), '/Resources': IndirectObject(5955, 0), '/Contents': [IndirectObject(5954, 0)], '/MediaBox': [0, 0, 841, 595], '/CropBox': [0, 0, 841, 595]}, (0, 5961): {'/Type': '/Page', '/Parent': IndirectObject(2, 0), '/Resources': IndirectObject(5959, 0), '/Contents': [IndirectObject(5958, 0)], '/MediaBox': [0, 0, 841, 595], '/CropBox': [0, 0, 841, 595]}, (0, 5965): {'/Type': '/Page', '/Parent': IndirectObject(2, 0), '/Resources': IndirectObject(5963, 0), '/Contents': [IndirectObject(5962, 0)], '/MediaBox': [0, 0, 841, 595], '/CropBox': [0, 0, 841, 595]}, (0, 5969): {'/Type': '/Page', '/Parent': IndirectObject(2, 0), '/Resources': IndirectObject(5967, 0), '/Contents': [IndirectObject(5966, 0)], '/MediaBox': [0, 0, 841, 595], '/CropBox': [0, 0, 841, 595]}, (0, 5973): {'/Type': '/Page', '/Parent': IndirectObject(2, 0), '/Resources': IndirectObject(5971, 0), '/Contents': [IndirectObject(5970, 0)], '/MediaBox': [0, 0, 841, 595], '/CropBox': [0, 0, 841, 595]}, (0, 5977): {'/Type': '/Page', '/Parent': IndirectObject(2, 0), '/Resources': IndirectObject(5975, 0), '/Contents': [IndirectObject(5974, 0)], '/MediaBox': [0, 0, 841, 595], '/CropBox': [0, 0, 841, 595]}, (0, 5981): {'/Type': '/Page', '/Parent': IndirectObject(2, 0), '/Resources': IndirectObject(5979, 0), '/Contents': [IndirectObject(5978, 0)], '/MediaBox': [0, 0, 841, 595], '/CropBox': [0, 0, 841, 595]}, (0, 5985): {'/Type': '/Page', '/Parent': IndirectObject(2, 0), '/Resources': IndirectObject(5983, 0), '/Contents': [IndirectObject(5982, 0)], '/MediaBox': [0, 0, 841, 595], '/CropBox': [0, 0, 841, 595]}, (0, 5989): {'/Type': '/Page', '/Parent': IndirectObject(2, 0), '/Resources': IndirectObject(5987, 0), '/Contents': [IndirectObject(5986, 0)], '/MediaBox': [0, 0, 841, 595], '/CropBox': [0, 0, 841, 595]}, (0, 5993): {'/Type': '/Page', '/Parent': IndirectObject(2, 0), '/Resources': IndirectObject(5991, 0), '/Contents': [IndirectObject(5990, 0)], '/MediaBox': [0, 0, 841, 595], '/CropBox': [0, 0, 841, 595]}, (0, 5997): {'/Type': '/Page', '/Parent': IndirectObject(2, 0), '/Resources': IndirectObject(5995, 0), '/Contents': [IndirectObject(5994, 0)], '/MediaBox': [0, 0, 841, 595], '/CropBox': [0, 0, 841, 595]}, (0, 6001): {'/Type': '/Page', '/Parent': IndirectObject(2, 0), '/Resources': IndirectObject(5999, 0), '/Contents': [IndirectObject(5998, 0)], '/MediaBox': [0, 0, 841, 595], '/CropBox': [0, 0, 841, 595]}, (0, 6005): {'/Type': '/Page', '/Parent': IndirectObject(2, 0), '/Resources': IndirectObject(6003, 0), '/Contents': [IndirectObject(6002, 0)], '/MediaBox': [0, 0, 841, 595], '/CropBox': [0, 0, 841, 595]}, (0, 6009): {'/Type': '/Page', '/Parent': IndirectObject(2, 0), '/Resources': IndirectObject(6007, 0), '/Contents': [IndirectObject(6006, 0)], '/MediaBox': [0, 0, 841, 595], '/CropBox': [0, 0, 841, 595]}, (0, 6013): {'/Type': '/Page', '/Parent': IndirectObject(2, 0), '/Resources': IndirectObject(6011, 0), '/Contents': [IndirectObject(6010, 0)], '/MediaBox': [0, 0, 841, 595], '/CropBox': [0, 0, 841, 595]}, (0, 6017): {'/Type': '/Page', '/Parent': IndirectObject(2, 0), '/Resources': IndirectObject(6015, 0), '/Contents': [IndirectObject(6014, 0)], '/MediaBox': [0, 0, 841, 595], '/CropBox': [0, 0, 841, 595]}, (0, 6021): {'/Type': '/Page', '/Parent': IndirectObject(2, 0), '/Resources': IndirectObject(6019, 0), '/Contents': [IndirectObject(6018, 0)], '/MediaBox': [0, 0, 841, 595], '/CropBox': [0, 0, 841, 595]}, (0, 6025): {'/Type': '/Page', '/Parent': IndirectObject(2, 0), '/Resources': IndirectObject(6023, 0), '/Contents': [IndirectObject(6022, 0)], '/MediaBox': [0, 0, 841, 595], '/CropBox': [0, 0, 841, 595]}, (0, 6029): {'/Type': '/Page', '/Parent': IndirectObject(2, 0), '/Resources': IndirectObject(6027, 0), '/Contents': [IndirectObject(6026, 0)], '/MediaBox': [0, 0, 841, 595], '/CropBox': [0, 0, 841, 595]}, (0, 6033): {'/Type': '/Page', '/Parent': IndirectObject(2, 0), '/Resources': IndirectObject(6031, 0), '/Contents': [IndirectObject(6030, 0)], '/MediaBox': [0, 0, 841, 595], '/CropBox': [0, 0, 841, 595]}, (0, 6037): {'/Type': '/Page', '/Parent': IndirectObject(2, 0), '/Resources': IndirectObject(6035, 0), '/Contents': [IndirectObject(6034, 0)], '/MediaBox': [0, 0, 841, 595], '/CropBox': [0, 0, 841, 595]}, (0, 6041): {'/Type': '/Page', '/Parent': IndirectObject(2, 0), '/Resources': IndirectObject(6039, 0), '/Contents': [IndirectObject(6038, 0)], '/MediaBox': [0, 0, 841, 595], '/CropBox': [0, 0, 841, 595]}, (0, 6045): {'/Type': '/Page', '/Parent': IndirectObject(2, 0), '/Resources': IndirectObject(6043, 0), '/Contents': [IndirectObject(6042, 0)], '/MediaBox': [0, 0, 841, 595], '/CropBox': [0, 0, 841, 595]}, (0, 6049): {'/Type': '/Page', '/Parent': IndirectObject(2, 0), '/Resources': IndirectObject(6047, 0), '/Contents': [IndirectObject(6046, 0)], '/MediaBox': [0, 0, 841, 595], '/CropBox': [0, 0, 841, 595]}, (0, 6053): {'/Type': '/Page', '/Parent': IndirectObject(2, 0), '/Resources': IndirectObject(6051, 0), '/Contents': [IndirectObject(6050, 0)], '/MediaBox': [0, 0, 841, 595], '/CropBox': [0, 0, 841, 595]}, (0, 6057): {'/Type': '/Page', '/Parent': IndirectObject(2, 0), '/Resources': IndirectObject(6055, 0), '/Contents': [IndirectObject(6054, 0)], '/MediaBox': [0, 0, 841, 595], '/CropBox': [0, 0, 841, 595]}, (0, 6061): {'/Type': '/Page', '/Parent': IndirectObject(2, 0), '/Resources': IndirectObject(6059, 0), '/Contents': [IndirectObject(6058, 0)], '/MediaBox': [0, 0, 841, 595], '/CropBox': [0, 0, 841, 595]}, (0, 6065): {'/Type': '/Page', '/Parent': IndirectObject(2, 0), '/Resources': IndirectObject(6063, 0), '/Contents': [IndirectObject(6062, 0)], '/MediaBox': [0, 0, 841, 595], '/CropBox': [0, 0, 841, 595]}, (0, 6069): {'/Type': '/Page', '/Parent': IndirectObject(2, 0), '/Resources': IndirectObject(6067, 0), '/Contents': [IndirectObject(6066, 0)], '/MediaBox': [0, 0, 841, 595], '/CropBox': [0, 0, 841, 595]}, (0, 6073): {'/Type': '/Page', '/Parent': IndirectObject(2, 0), '/Resources': IndirectObject(6071, 0), '/Contents': [IndirectObject(6070, 0)], '/MediaBox': [0, 0, 841, 595], '/CropBox': [0, 0, 841, 595]}, (0, 6077): {'/Type': '/Page', '/Parent': IndirectObject(2, 0), '/Resources': IndirectObject(6075, 0), '/Contents': [IndirectObject(6074, 0)], '/MediaBox': [0, 0, 841, 595], '/CropBox': [0, 0, 841, 595]}, (0, 6081): {'/Type': '/Page', '/Parent': IndirectObject(2, 0), '/Resources': IndirectObject(6079, 0), '/Contents': [IndirectObject(6078, 0)], '/MediaBox': [0, 0, 841, 595], '/CropBox': [0, 0, 841, 595]}, (0, 6085): {'/Type': '/Page', '/Parent': IndirectObject(2, 0), '/Resources': IndirectObject(6083, 0), '/Contents': [IndirectObject(6082, 0)], '/MediaBox': [0, 0, 841, 595], '/CropBox': [0, 0, 841, 595]}, (0, 6089): {'/Type': '/Page', '/Parent': IndirectObject(2, 0), '/Resources': IndirectObject(6087, 0), '/Contents': [IndirectObject(6086, 0)], '/MediaBox': [0, 0, 841, 595], '/CropBox': [0, 0, 841, 595]}, (0, 6093): {'/Type': '/Page', '/Parent': IndirectObject(2, 0), '/Resources': IndirectObject(6091, 0), '/Contents': [IndirectObject(6090, 0)], '/MediaBox': [0, 0, 841, 595], '/CropBox': [0, 0, 841, 595]}, (0, 6097): {'/Type': '/Page', '/Parent': IndirectObject(2, 0), '/Resources': IndirectObject(6095, 0), '/Contents': [IndirectObject(6094, 0)], '/MediaBox': [0, 0, 841, 595], '/CropBox': [0, 0, 841, 595]}, (0, 6101): {'/Type': '/Page', '/Parent': IndirectObject(2, 0), '/Resources': IndirectObject(6099, 0), '/Contents': [IndirectObject(6098, 0)], '/MediaBox': [0, 0, 841, 595], '/CropBox': [0, 0, 841, 595]}, (0, 6105): {'/Type': '/Page', '/Parent': IndirectObject(2, 0), '/Resources': IndirectObject(6103, 0), '/Contents': [IndirectObject(6102, 0)], '/MediaBox': [0, 0, 841, 595], '/CropBox': [0, 0, 841, 595]}, (0, 6109): {'/Type': '/Page', '/Parent': IndirectObject(2, 0), '/Resources': IndirectObject(6107, 0), '/Contents': [IndirectObject(6106, 0)], '/MediaBox': [0, 0, 841, 595], '/CropBox': [0, 0, 841, 595]}, (0, 6113): {'/Type': '/Page', '/Parent': IndirectObject(2, 0), '/Resources': IndirectObject(6111, 0), '/Contents': [IndirectObject(6110, 0)], '/MediaBox': [0, 0, 841, 595], '/CropBox': [0, 0, 841, 595]}, (0, 6117): {'/Type': '/Page', '/Parent': IndirectObject(2, 0), '/Resources': IndirectObject(6115, 0), '/Contents': [IndirectObject(6114, 0)], '/MediaBox': [0, 0, 841, 595], '/CropBox': [0, 0, 841, 595]}, (0, 6121): {'/Type': '/Page', '/Parent': IndirectObject(2, 0), '/Resources': IndirectObject(6119, 0), '/Contents': [IndirectObject(6118, 0)], '/MediaBox': [0, 0, 841, 595], '/CropBox': [0, 0, 841, 595]}, (0, 6125): {'/Type': '/Page', '/Parent': IndirectObject(2, 0), '/Resources': IndirectObject(6123, 0), '/Contents': [IndirectObject(6122, 0)], '/MediaBox': [0, 0, 841, 595], '/CropBox': [0, 0, 841, 595]}, (0, 6129): {'/Type': '/Page', '/Parent': IndirectObject(2, 0), '/Resources': IndirectObject(6127, 0), '/Contents': [IndirectObject(6126, 0)], '/MediaBox': [0, 0, 841, 595], '/CropBox': [0, 0, 841, 595]}, (0, 6133): {'/Type': '/Page', '/Parent': IndirectObject(2, 0), '/Resources': IndirectObject(6131, 0), '/Contents': [IndirectObject(6130, 0)], '/MediaBox': [0, 0, 841, 595], '/CropBox': [0, 0, 841, 595]}, (0, 6137): {'/Type': '/Page', '/Parent': IndirectObject(2, 0), '/Resources': IndirectObject(6135, 0), '/Contents': [IndirectObject(6134, 0)], '/MediaBox': [0, 0, 841, 595], '/CropBox': [0, 0, 841, 595]}, (0, 6141): {'/Type': '/Page', '/Parent': IndirectObject(2, 0), '/Resources': IndirectObject(6139, 0), '/Contents': [IndirectObject(6138, 0)], '/MediaBox': [0, 0, 841, 595], '/CropBox': [0, 0, 841, 595]}, (0, 6145): {'/Type': '/Page', '/Parent': IndirectObject(2, 0), '/Resources': IndirectObject(6143, 0), '/Contents': [IndirectObject(6142, 0)], '/MediaBox': [0, 0, 841, 595], '/CropBox': [0, 0, 841, 595]}, (0, 6149): {'/Type': '/Page', '/Parent': IndirectObject(2, 0), '/Resources': IndirectObject(6147, 0), '/Contents': [IndirectObject(6146, 0)], '/MediaBox': [0, 0, 841, 595], '/CropBox': [0, 0, 841, 595]}, (0, 6153): {'/Type': '/Page', '/Parent': IndirectObject(2, 0), '/Resources': IndirectObject(6151, 0), '/Contents': [IndirectObject(6150, 0)], '/MediaBox': [0, 0, 841, 595], '/CropBox': [0, 0, 841, 595]}, (0, 6157): {'/Type': '/Page', '/Parent': IndirectObject(2, 0), '/Resources': IndirectObject(6155, 0), '/Contents': [IndirectObject(6154, 0)], '/MediaBox': [0, 0, 841, 595], '/CropBox': [0, 0, 841, 595]}, (0, 6161): {'/Type': '/Page', '/Parent': IndirectObject(2, 0), '/Resources': IndirectObject(6159, 0), '/Contents': [IndirectObject(6158, 0)], '/MediaBox': [0, 0, 841, 595], '/CropBox': [0, 0, 841, 595]}, (0, 6165): {'/Type': '/Page', '/Parent': IndirectObject(2, 0), '/Resources': IndirectObject(6163, 0), '/Contents': [IndirectObject(6162, 0)], '/MediaBox': [0, 0, 841, 595], '/CropBox': [0, 0, 841, 595]}, (0, 6169): {'/Type': '/Page', '/Parent': IndirectObject(2, 0), '/Resources': IndirectObject(6167, 0), '/Contents': [IndirectObject(6166, 0)], '/MediaBox': [0, 0, 841, 595], '/CropBox': [0, 0, 841, 595]}, (0, 6173): {'/Type': '/Page', '/Parent': IndirectObject(2, 0), '/Resources': IndirectObject(6171, 0), '/Contents': [IndirectObject(6170, 0)], '/MediaBox': [0, 0, 841, 595], '/CropBox': [0, 0, 841, 595]}, (0, 6177): {'/Type': '/Page', '/Parent': IndirectObject(2, 0), '/Resources': IndirectObject(6175, 0), '/Contents': [IndirectObject(6174, 0)], '/MediaBox': [0, 0, 841, 595], '/CropBox': [0, 0, 841, 595]}, (0, 6181): {'/Type': '/Page', '/Parent': IndirectObject(2, 0), '/Resources': IndirectObject(6179, 0), '/Contents': [IndirectObject(6178, 0)], '/MediaBox': [0, 0, 841, 595], '/CropBox': [0, 0, 841, 595]}, (0, 6185): {'/Type': '/Page', '/Parent': IndirectObject(2, 0), '/Resources': IndirectObject(6183, 0), '/Contents': [IndirectObject(6182, 0)], '/MediaBox': [0, 0, 841, 595], '/CropBox': [0, 0, 841, 595]}, (0, 6189): {'/Type': '/Page', '/Parent': IndirectObject(2, 0), '/Resources': IndirectObject(6187, 0), '/Contents': [IndirectObject(6186, 0)], '/MediaBox': [0, 0, 841, 595], '/CropBox': [0, 0, 841, 595]}, (0, 6193): {'/Type': '/Page', '/Parent': IndirectObject(2, 0), '/Resources': IndirectObject(6191, 0), '/Contents': [IndirectObject(6190, 0)], '/MediaBox': [0, 0, 841, 595], '/CropBox': [0, 0, 841, 595]}, (0, 6197): {'/Type': '/Page', '/Parent': IndirectObject(2, 0), '/Resources': IndirectObject(6195, 0), '/Contents': [IndirectObject(6194, 0)], '/MediaBox': [0, 0, 841, 595], '/CropBox': [0, 0, 841, 595]}, (0, 6201): {'/Type': '/Page', '/Parent': IndirectObject(2, 0), '/Resources': IndirectObject(6199, 0), '/Contents': [IndirectObject(6198, 0)], '/MediaBox': [0, 0, 841, 595], '/CropBox': [0, 0, 841, 595]}, (0, 6205): {'/Type': '/Page', '/Parent': IndirectObject(2, 0), '/Resources': IndirectObject(6203, 0), '/Contents': [IndirectObject(6202, 0)], '/MediaBox': [0, 0, 841, 595], '/CropBox': [0, 0, 841, 595]}, (0, 6209): {'/Type': '/Page', '/Parent': IndirectObject(2, 0), '/Resources': IndirectObject(6207, 0), '/Contents': [IndirectObject(6206, 0)], '/MediaBox': [0, 0, 841, 595], '/CropBox': [0, 0, 841, 595]}, (0, 6213): {'/Type': '/Page', '/Parent': IndirectObject(2, 0), '/Resources': IndirectObject(6211, 0), '/Contents': [IndirectObject(6210, 0)], '/MediaBox': [0, 0, 841, 595], '/CropBox': [0, 0, 841, 595]}, (0, 6217): {'/Type': '/Page', '/Parent': IndirectObject(2, 0), '/Resources': IndirectObject(6215, 0), '/Contents': [IndirectObject(6214, 0)], '/MediaBox': [0, 0, 841, 595], '/CropBox': [0, 0, 841, 595]}, (0, 6221): {'/Type': '/Page', '/Parent': IndirectObject(2, 0), '/Resources': IndirectObject(6219, 0), '/Contents': [IndirectObject(6218, 0)], '/MediaBox': [0, 0, 841, 595], '/CropBox': [0, 0, 841, 595]}, (0, 6225): {'/Type': '/Page', '/Parent': IndirectObject(2, 0), '/Resources': IndirectObject(6223, 0), '/Contents': [IndirectObject(6222, 0)], '/MediaBox': [0, 0, 841, 595], '/CropBox': [0, 0, 841, 595]}, (0, 6229): {'/Type': '/Page', '/Parent': IndirectObject(2, 0), '/Resources': IndirectObject(6227, 0), '/Contents': [IndirectObject(6226, 0)], '/MediaBox': [0, 0, 841, 595], '/CropBox': [0, 0, 841, 595]}, (0, 6233): {'/Type': '/Page', '/Parent': IndirectObject(2, 0), '/Resources': IndirectObject(6231, 0), '/Contents': [IndirectObject(6230, 0)], '/MediaBox': [0, 0, 841, 595], '/CropBox': [0, 0, 841, 595]}, (0, 6237): {'/Type': '/Page', '/Parent': IndirectObject(2, 0), '/Resources': IndirectObject(6235, 0), '/Contents': [IndirectObject(6234, 0)], '/MediaBox': [0, 0, 841, 595], '/CropBox': [0, 0, 841, 595]}, (0, 6241): {'/Type': '/Page', '/Parent': IndirectObject(2, 0), '/Resources': IndirectObject(6239, 0), '/Contents': [IndirectObject(6238, 0)], '/MediaBox': [0, 0, 841, 595], '/CropBox': [0, 0, 841, 595]}, (0, 6245): {'/Type': '/Page', '/Parent': IndirectObject(2, 0), '/Resources': IndirectObject(6243, 0), '/Contents': [IndirectObject(6242, 0)], '/MediaBox': [0, 0, 841, 595], '/CropBox': [0, 0, 841, 595]}, (0, 6249): {'/Type': '/Page', '/Parent': IndirectObject(2, 0), '/Resources': IndirectObject(6247, 0), '/Contents': [IndirectObject(6246, 0)], '/MediaBox': [0, 0, 841, 595], '/CropBox': [0, 0, 841, 595]}, (0, 6253): {'/Type': '/Page', '/Parent': IndirectObject(2, 0), '/Resources': IndirectObject(6251, 0), '/Contents': [IndirectObject(6250, 0)], '/MediaBox': [0, 0, 841, 595], '/CropBox': [0, 0, 841, 595]}, (0, 6257): {'/Type': '/Page', '/Parent': IndirectObject(2, 0), '/Resources': IndirectObject(6255, 0), '/Contents': [IndirectObject(6254, 0)], '/MediaBox': [0, 0, 841, 595], '/CropBox': [0, 0, 841, 595]}, (0, 6261): {'/Type': '/Page', '/Parent': IndirectObject(2, 0), '/Resources': IndirectObject(6259, 0), '/Contents': [IndirectObject(6258, 0)], '/MediaBox': [0, 0, 841, 595], '/CropBox': [0, 0, 841, 595]}, (0, 6265): {'/Type': '/Page', '/Parent': IndirectObject(2, 0), '/Resources': IndirectObject(6263, 0), '/Contents': [IndirectObject(6262, 0)], '/MediaBox': [0, 0, 841, 595], '/CropBox': [0, 0, 841, 595]}, (0, 6269): {'/Type': '/Page', '/Parent': IndirectObject(2, 0), '/Resources': IndirectObject(6267, 0), '/Contents': [IndirectObject(6266, 0)], '/MediaBox': [0, 0, 841, 595], '/CropBox': [0, 0, 841, 595]}, (0, 6273): {'/Type': '/Page', '/Parent': IndirectObject(2, 0), '/Resources': IndirectObject(6271, 0), '/Contents': [IndirectObject(6270, 0)], '/MediaBox': [0, 0, 841, 595], '/CropBox': [0, 0, 841, 595]}, (0, 6277): {'/Type': '/Page', '/Parent': IndirectObject(2, 0), '/Resources': IndirectObject(6275, 0), '/Contents': [IndirectObject(6274, 0)], '/MediaBox': [0, 0, 841, 595], '/CropBox': [0, 0, 841, 595]}, (0, 6281): {'/Type': '/Page', '/Parent': IndirectObject(2, 0), '/Resources': IndirectObject(6279, 0), '/Contents': [IndirectObject(6278, 0)], '/MediaBox': [0, 0, 841, 595], '/CropBox': [0, 0, 841, 595]}, (0, 6285): {'/Type': '/Page', '/Parent': IndirectObject(2, 0), '/Resources': IndirectObject(6283, 0), '/Contents': [IndirectObject(6282, 0)], '/MediaBox': [0, 0, 841, 595], '/CropBox': [0, 0, 841, 595]}, (0, 6289): {'/Type': '/Page', '/Parent': IndirectObject(2, 0), '/Resources': IndirectObject(6287, 0), '/Contents': [IndirectObject(6286, 0)], '/MediaBox': [0, 0, 841, 595], '/CropBox': [0, 0, 841, 595]}, (0, 6293): {'/Type': '/Page', '/Parent': IndirectObject(2, 0), '/Resources': IndirectObject(6291, 0), '/Contents': [IndirectObject(6290, 0)], '/MediaBox': [0, 0, 841, 595], '/CropBox': [0, 0, 841, 595]}, (0, 6297): {'/Type': '/Page', '/Parent': IndirectObject(2, 0), '/Resources': IndirectObject(6295, 0), '/Contents': [IndirectObject(6294, 0)], '/MediaBox': [0, 0, 841, 595], '/CropBox': [0, 0, 841, 595]}, (0, 6301): {'/Type': '/Page', '/Parent': IndirectObject(2, 0), '/Resources': IndirectObject(6299, 0), '/Contents': [IndirectObject(6298, 0)], '/MediaBox': [0, 0, 841, 595], '/CropBox': [0, 0, 841, 595]}, (0, 6305): {'/Type': '/Page', '/Parent': IndirectObject(2, 0), '/Resources': IndirectObject(6303, 0), '/Contents': [IndirectObject(6302, 0)], '/MediaBox': [0, 0, 841, 595], '/CropBox': [0, 0, 841, 595]}, (0, 6309): {'/Type': '/Page', '/Parent': IndirectObject(2, 0), '/Resources': IndirectObject(6307, 0), '/Contents': [IndirectObject(6306, 0)], '/MediaBox': [0, 0, 841, 595], '/CropBox': [0, 0, 841, 595]}, (0, 6313): {'/Type': '/Page', '/Parent': IndirectObject(2, 0), '/Resources': IndirectObject(6311, 0), '/Contents': [IndirectObject(6310, 0)], '/MediaBox': [0, 0, 841, 595], '/CropBox': [0, 0, 841, 595]}, (0, 6317): {'/Type': '/Page', '/Parent': IndirectObject(2, 0), '/Resources': IndirectObject(6315, 0), '/Contents': [IndirectObject(6314, 0)], '/MediaBox': [0, 0, 841, 595], '/CropBox': [0, 0, 841, 595]}, (0, 6321): {'/Type': '/Page', '/Parent': IndirectObject(2, 0), '/Resources': IndirectObject(6319, 0), '/Contents': [IndirectObject(6318, 0)], '/MediaBox': [0, 0, 841, 595], '/CropBox': [0, 0, 841, 595]}, (0, 6325): {'/Type': '/Page', '/Parent': IndirectObject(2, 0), '/Resources': IndirectObject(6323, 0), '/Contents': [IndirectObject(6322, 0)], '/MediaBox': [0, 0, 841, 595], '/CropBox': [0, 0, 841, 595]}, (0, 6329): {'/Type': '/Page', '/Parent': IndirectObject(2, 0), '/Resources': IndirectObject(6327, 0), '/Contents': [IndirectObject(6326, 0)], '/MediaBox': [0, 0, 841, 595], '/CropBox': [0, 0, 841, 595]}, (0, 6333): {'/Type': '/Page', '/Parent': IndirectObject(2, 0), '/Resources': IndirectObject(6331, 0), '/Contents': [IndirectObject(6330, 0)], '/MediaBox': [0, 0, 841, 595], '/CropBox': [0, 0, 841, 595]}, (0, 6337): {'/Type': '/Page', '/Parent': IndirectObject(2, 0), '/Resources': IndirectObject(6335, 0), '/Contents': [IndirectObject(6334, 0)], '/MediaBox': [0, 0, 841, 595], '/CropBox': [0, 0, 841, 595]}, (0, 6341): {'/Type': '/Page', '/Parent': IndirectObject(2, 0), '/Resources': IndirectObject(6339, 0), '/Contents': [IndirectObject(6338, 0)], '/MediaBox': [0, 0, 841, 595], '/CropBox': [0, 0, 841, 595]}, (0, 6345): {'/Type': '/Page', '/Parent': IndirectObject(2, 0), '/Resources': IndirectObject(6343, 0), '/Contents': [IndirectObject(6342, 0)], '/MediaBox': [0, 0, 841, 595], '/CropBox': [0, 0, 841, 595]}, (0, 6349): {'/Type': '/Page', '/Parent': IndirectObject(2, 0), '/Resources': IndirectObject(6347, 0), '/Contents': [IndirectObject(6346, 0)], '/MediaBox': [0, 0, 841, 595], '/CropBox': [0, 0, 841, 595]}, (0, 6353): {'/Type': '/Page', '/Parent': IndirectObject(2, 0), '/Resources': IndirectObject(6351, 0), '/Contents': [IndirectObject(6350, 0)], '/MediaBox': [0, 0, 841, 595], '/CropBox': [0, 0, 841, 595]}, (0, 6357): {'/Type': '/Page', '/Parent': IndirectObject(2, 0), '/Resources': IndirectObject(6355, 0), '/Contents': [IndirectObject(6354, 0)], '/MediaBox': [0, 0, 841, 595], '/CropBox': [0, 0, 841, 595]}, (0, 6361): {'/Type': '/Page', '/Parent': IndirectObject(2, 0), '/Resources': IndirectObject(6359, 0), '/Contents': [IndirectObject(6358, 0)], '/MediaBox': [0, 0, 841, 595], '/CropBox': [0, 0, 841, 595]}, (0, 6365): {'/Type': '/Page', '/Parent': IndirectObject(2, 0), '/Resources': IndirectObject(6363, 0), '/Contents': [IndirectObject(6362, 0)], '/MediaBox': [0, 0, 841, 595], '/CropBox': [0, 0, 841, 595]}, (0, 6369): {'/Type': '/Page', '/Parent': IndirectObject(2, 0), '/Resources': IndirectObject(6367, 0), '/Contents': [IndirectObject(6366, 0)], '/MediaBox': [0, 0, 841, 595], '/CropBox': [0, 0, 841, 595]}, (0, 6373): {'/Type': '/Page', '/Parent': IndirectObject(2, 0), '/Resources': IndirectObject(6371, 0), '/Contents': [IndirectObject(6370, 0)], '/MediaBox': [0, 0, 841, 595], '/CropBox': [0, 0, 841, 595]}, (0, 6377): {'/Type': '/Page', '/Parent': IndirectObject(2, 0), '/Resources': IndirectObject(6375, 0), '/Contents': [IndirectObject(6374, 0)], '/MediaBox': [0, 0, 841, 595], '/CropBox': [0, 0, 841, 595]}, (0, 6381): {'/Type': '/Page', '/Parent': IndirectObject(2, 0), '/Resources': IndirectObject(6379, 0), '/Contents': [IndirectObject(6378, 0)], '/MediaBox': [0, 0, 841, 595], '/CropBox': [0, 0, 841, 595]}, (0, 6385): {'/Type': '/Page', '/Parent': IndirectObject(2, 0), '/Resources': IndirectObject(6383, 0), '/Contents': [IndirectObject(6382, 0)], '/MediaBox': [0, 0, 841, 595], '/CropBox': [0, 0, 841, 595]}, (0, 6389): {'/Type': '/Page', '/Parent': IndirectObject(2, 0), '/Resources': IndirectObject(6387, 0), '/Contents': [IndirectObject(6386, 0)], '/MediaBox': [0, 0, 841, 595], '/CropBox': [0, 0, 841, 595]}, (0, 6393): {'/Type': '/Page', '/Parent': IndirectObject(2, 0), '/Resources': IndirectObject(6391, 0), '/Contents': [IndirectObject(6390, 0)], '/MediaBox': [0, 0, 841, 595], '/CropBox': [0, 0, 841, 595]}, (0, 6397): {'/Type': '/Page', '/Parent': IndirectObject(2, 0), '/Resources': IndirectObject(6395, 0), '/Contents': [IndirectObject(6394, 0)], '/MediaBox': [0, 0, 841, 595], '/CropBox': [0, 0, 841, 595]}, (0, 6401): {'/Type': '/Page', '/Parent': IndirectObject(2, 0), '/Resources': IndirectObject(6399, 0), '/Contents': [IndirectObject(6398, 0)], '/MediaBox': [0, 0, 841, 595], '/CropBox': [0, 0, 841, 595]}, (0, 6405): {'/Type': '/Page', '/Parent': IndirectObject(2, 0), '/Resources': IndirectObject(6403, 0), '/Contents': [IndirectObject(6402, 0)], '/MediaBox': [0, 0, 841, 595], '/CropBox': [0, 0, 841, 595]}, (0, 6409): {'/Type': '/Page', '/Parent': IndirectObject(2, 0), '/Resources': IndirectObject(6407, 0), '/Contents': [IndirectObject(6406, 0)], '/MediaBox': [0, 0, 841, 595], '/CropBox': [0, 0, 841, 595]}, (0, 6413): {'/Type': '/Page', '/Parent': IndirectObject(2, 0), '/Resources': IndirectObject(6411, 0), '/Contents': [IndirectObject(6410, 0)], '/MediaBox': [0, 0, 841, 595], '/CropBox': [0, 0, 841, 595]}, (0, 6417): {'/Type': '/Page', '/Parent': IndirectObject(2, 0), '/Resources': IndirectObject(6415, 0), '/Contents': [IndirectObject(6414, 0)], '/MediaBox': [0, 0, 841, 595], '/CropBox': [0, 0, 841, 595]}, (0, 6421): {'/Type': '/Page', '/Parent': IndirectObject(2, 0), '/Resources': IndirectObject(6419, 0), '/Contents': [IndirectObject(6418, 0)], '/MediaBox': [0, 0, 841, 595], '/CropBox': [0, 0, 841, 595]}, (0, 6425): {'/Type': '/Page', '/Parent': IndirectObject(2, 0), '/Resources': IndirectObject(6423, 0), '/Contents': [IndirectObject(6422, 0)], '/MediaBox': [0, 0, 841, 595], '/CropBox': [0, 0, 841, 595]}, (0, 6429): {'/Type': '/Page', '/Parent': IndirectObject(2, 0), '/Resources': IndirectObject(6427, 0), '/Contents': [IndirectObject(6426, 0)], '/MediaBox': [0, 0, 841, 595], '/CropBox': [0, 0, 841, 595]}, (0, 6433): {'/Type': '/Page', '/Parent': IndirectObject(2, 0), '/Resources': IndirectObject(6431, 0), '/Contents': [IndirectObject(6430, 0)], '/MediaBox': [0, 0, 841, 595], '/CropBox': [0, 0, 841, 595]}, (0, 6437): {'/Type': '/Page', '/Parent': IndirectObject(2, 0), '/Resources': IndirectObject(6435, 0), '/Contents': [IndirectObject(6434, 0)], '/MediaBox': [0, 0, 841, 595], '/CropBox': [0, 0, 841, 595]}, (0, 6441): {'/Type': '/Page', '/Parent': IndirectObject(2, 0), '/Resources': IndirectObject(6439, 0), '/Contents': [IndirectObject(6438, 0)], '/MediaBox': [0, 0, 841, 595], '/CropBox': [0, 0, 841, 595]}, (0, 6445): {'/Type': '/Page', '/Parent': IndirectObject(2, 0), '/Resources': IndirectObject(6443, 0), '/Contents': [IndirectObject(6442, 0)], '/MediaBox': [0, 0, 841, 595], '/CropBox': [0, 0, 841, 595]}, (0, 6449): {'/Type': '/Page', '/Parent': IndirectObject(2, 0), '/Resources': IndirectObject(6447, 0), '/Contents': [IndirectObject(6446, 0)], '/MediaBox': [0, 0, 841, 595], '/CropBox': [0, 0, 841, 595]}, (0, 6453): {'/Type': '/Page', '/Parent': IndirectObject(2, 0), '/Resources': IndirectObject(6451, 0), '/Contents': [IndirectObject(6450, 0)], '/MediaBox': [0, 0, 841, 595], '/CropBox': [0, 0, 841, 595]}, (0, 6457): {'/Type': '/Page', '/Parent': IndirectObject(2, 0), '/Resources': IndirectObject(6455, 0), '/Contents': [IndirectObject(6454, 0)], '/MediaBox': [0, 0, 841, 595], '/CropBox': [0, 0, 841, 595]}, (0, 6461): {'/Type': '/Page', '/Parent': IndirectObject(2, 0), '/Resources': IndirectObject(6459, 0), '/Contents': [IndirectObject(6458, 0)], '/MediaBox': [0, 0, 841, 595], '/CropBox': [0, 0, 841, 595]}, (0, 6465): {'/Type': '/Page', '/Parent': IndirectObject(2, 0), '/Resources': IndirectObject(6463, 0), '/Contents': [IndirectObject(6462, 0)], '/MediaBox': [0, 0, 841, 595], '/CropBox': [0, 0, 841, 595]}, (0, 6469): {'/Type': '/Page', '/Parent': IndirectObject(2, 0), '/Resources': IndirectObject(6467, 0), '/Contents': [IndirectObject(6466, 0)], '/MediaBox': [0, 0, 841, 595], '/CropBox': [0, 0, 841, 595]}, (0, 6473): {'/Type': '/Page', '/Parent': IndirectObject(2, 0), '/Resources': IndirectObject(6471, 0), '/Contents': [IndirectObject(6470, 0)], '/MediaBox': [0, 0, 841, 595], '/CropBox': [0, 0, 841, 595]}, (0, 6477): {'/Type': '/Page', '/Parent': IndirectObject(2, 0), '/Resources': IndirectObject(6475, 0), '/Contents': [IndirectObject(6474, 0)], '/MediaBox': [0, 0, 841, 595], '/CropBox': [0, 0, 841, 595]}, (0, 6481): {'/Type': '/Page', '/Parent': IndirectObject(2, 0), '/Resources': IndirectObject(6479, 0), '/Contents': [IndirectObject(6478, 0)], '/MediaBox': [0, 0, 841, 595], '/CropBox': [0, 0, 841, 595]}, (0, 6485): {'/Type': '/Page', '/Parent': IndirectObject(2, 0), '/Resources': IndirectObject(6483, 0), '/Contents': [IndirectObject(6482, 0)], '/MediaBox': [0, 0, 841, 595], '/CropBox': [0, 0, 841, 595]}, (0, 6489): {'/Type': '/Page', '/Parent': IndirectObject(2, 0), '/Resources': IndirectObject(6487, 0), '/Contents': [IndirectObject(6486, 0)], '/MediaBox': [0, 0, 841, 595], '/CropBox': [0, 0, 841, 595]}, (0, 6493): {'/Type': '/Page', '/Parent': IndirectObject(2, 0), '/Resources': IndirectObject(6491, 0), '/Contents': [IndirectObject(6490, 0)], '/MediaBox': [0, 0, 841, 595], '/CropBox': [0, 0, 841, 595]}, (0, 6497): {'/Type': '/Page', '/Parent': IndirectObject(2, 0), '/Resources': IndirectObject(6495, 0), '/Contents': [IndirectObject(6494, 0)], '/MediaBox': [0, 0, 841, 595], '/CropBox': [0, 0, 841, 595]}, (0, 6501): {'/Type': '/Page', '/Parent': IndirectObject(2, 0), '/Resources': IndirectObject(6499, 0), '/Contents': [IndirectObject(6498, 0)], '/MediaBox': [0, 0, 841, 595], '/CropBox': [0, 0, 841, 595]}, (0, 6505): {'/Type': '/Page', '/Parent': IndirectObject(2, 0), '/Resources': IndirectObject(6503, 0), '/Contents': [IndirectObject(6502, 0)], '/MediaBox': [0, 0, 841, 595], '/CropBox': [0, 0, 841, 595]}, (0, 6509): {'/Type': '/Page', '/Parent': IndirectObject(2, 0), '/Resources': IndirectObject(6507, 0), '/Contents': [IndirectObject(6506, 0)], '/MediaBox': [0, 0, 841, 595], '/CropBox': [0, 0, 841, 595]}, (0, 6513): {'/Type': '/Page', '/Parent': IndirectObject(2, 0), '/Resources': IndirectObject(6511, 0), '/Contents': [IndirectObject(6510, 0)], '/MediaBox': [0, 0, 841, 595], '/CropBox': [0, 0, 841, 595]}, (0, 6517): {'/Type': '/Page', '/Parent': IndirectObject(2, 0), '/Resources': IndirectObject(6515, 0), '/Contents': [IndirectObject(6514, 0)], '/MediaBox': [0, 0, 841, 595], '/CropBox': [0, 0, 841, 595]}, (0, 6521): {'/Type': '/Page', '/Parent': IndirectObject(2, 0), '/Resources': IndirectObject(6519, 0), '/Contents': [IndirectObject(6518, 0)], '/MediaBox': [0, 0, 841, 595], '/CropBox': [0, 0, 841, 595]}, (0, 6525): {'/Type': '/Page', '/Parent': IndirectObject(2, 0), '/Resources': IndirectObject(6523, 0), '/Contents': [IndirectObject(6522, 0)], '/MediaBox': [0, 0, 841, 595], '/CropBox': [0, 0, 841, 595]}, (0, 6529): {'/Type': '/Page', '/Parent': IndirectObject(2, 0), '/Resources': IndirectObject(6527, 0), '/Contents': [IndirectObject(6526, 0)], '/MediaBox': [0, 0, 841, 595], '/CropBox': [0, 0, 841, 595]}, (0, 6533): {'/Type': '/Page', '/Parent': IndirectObject(2, 0), '/Resources': IndirectObject(6531, 0), '/Contents': [IndirectObject(6530, 0)], '/MediaBox': [0, 0, 841, 595], '/CropBox': [0, 0, 841, 595]}, (0, 6537): {'/Type': '/Page', '/Parent': IndirectObject(2, 0), '/Resources': IndirectObject(6535, 0), '/Contents': [IndirectObject(6534, 0)], '/MediaBox': [0, 0, 841, 595], '/CropBox': [0, 0, 841, 595]}, (0, 6541): {'/Type': '/Page', '/Parent': IndirectObject(2, 0), '/Resources': IndirectObject(6539, 0), '/Contents': [IndirectObject(6538, 0)], '/MediaBox': [0, 0, 841, 595], '/CropBox': [0, 0, 841, 595]}, (0, 6545): {'/Type': '/Page', '/Parent': IndirectObject(2, 0), '/Resources': IndirectObject(6543, 0), '/Contents': [IndirectObject(6542, 0)], '/MediaBox': [0, 0, 841, 595], '/CropBox': [0, 0, 841, 595]}, (0, 6549): {'/Type': '/Page', '/Parent': IndirectObject(2, 0), '/Resources': IndirectObject(6547, 0), '/Contents': [IndirectObject(6546, 0)], '/MediaBox': [0, 0, 841, 595], '/CropBox': [0, 0, 841, 595]}, (0, 6553): {'/Type': '/Page', '/Parent': IndirectObject(2, 0), '/Resources': IndirectObject(6551, 0), '/Contents': [IndirectObject(6550, 0)], '/MediaBox': [0, 0, 841, 595], '/CropBox': [0, 0, 841, 595]}, (0, 6557): {'/Type': '/Page', '/Parent': IndirectObject(2, 0), '/Resources': IndirectObject(6555, 0), '/Contents': [IndirectObject(6554, 0)], '/MediaBox': [0, 0, 841, 595], '/CropBox': [0, 0, 841, 595]}, (0, 6561): {'/Type': '/Page', '/Parent': IndirectObject(2, 0), '/Resources': IndirectObject(6559, 0), '/Contents': [IndirectObject(6558, 0)], '/MediaBox': [0, 0, 841, 595], '/CropBox': [0, 0, 841, 595]}, (0, 6565): {'/Type': '/Page', '/Parent': IndirectObject(2, 0), '/Resources': IndirectObject(6563, 0), '/Contents': [IndirectObject(6562, 0)], '/MediaBox': [0, 0, 841, 595], '/CropBox': [0, 0, 841, 595]}, (0, 6569): {'/Type': '/Page', '/Parent': IndirectObject(2, 0), '/Resources': IndirectObject(6567, 0), '/Contents': [IndirectObject(6566, 0)], '/MediaBox': [0, 0, 841, 595], '/CropBox': [0, 0, 841, 595]}, (0, 6573): {'/Type': '/Page', '/Parent': IndirectObject(2, 0), '/Resources': IndirectObject(6571, 0), '/Contents': [IndirectObject(6570, 0)], '/MediaBox': [0, 0, 841, 595], '/CropBox': [0, 0, 841, 595]}, (0, 6577): {'/Type': '/Page', '/Parent': IndirectObject(2, 0), '/Resources': IndirectObject(6575, 0), '/Contents': [IndirectObject(6574, 0)], '/MediaBox': [0, 0, 841, 595], '/CropBox': [0, 0, 841, 595]}, (0, 6581): {'/Type': '/Page', '/Parent': IndirectObject(2, 0), '/Resources': IndirectObject(6579, 0), '/Contents': [IndirectObject(6578, 0)], '/MediaBox': [0, 0, 841, 595], '/CropBox': [0, 0, 841, 595]}, (0, 6585): {'/Type': '/Page', '/Parent': IndirectObject(2, 0), '/Resources': IndirectObject(6583, 0), '/Contents': [IndirectObject(6582, 0)], '/MediaBox': [0, 0, 841, 595], '/CropBox': [0, 0, 841, 595]}, (0, 6589): {'/Type': '/Page', '/Parent': IndirectObject(2, 0), '/Resources': IndirectObject(6587, 0), '/Contents': [IndirectObject(6586, 0)], '/MediaBox': [0, 0, 841, 595], '/CropBox': [0, 0, 841, 595]}, (0, 6593): {'/Type': '/Page', '/Parent': IndirectObject(2, 0), '/Resources': IndirectObject(6591, 0), '/Contents': [IndirectObject(6590, 0)], '/MediaBox': [0, 0, 841, 595], '/CropBox': [0, 0, 841, 595]}, (0, 6597): {'/Type': '/Page', '/Parent': IndirectObject(2, 0), '/Resources': IndirectObject(6595, 0), '/Contents': [IndirectObject(6594, 0)], '/MediaBox': [0, 0, 841, 595], '/CropBox': [0, 0, 841, 595]}, (0, 6601): {'/Type': '/Page', '/Parent': IndirectObject(2, 0), '/Resources': IndirectObject(6599, 0), '/Contents': [IndirectObject(6598, 0)], '/MediaBox': [0, 0, 841, 595], '/CropBox': [0, 0, 841, 595]}, (0, 6605): {'/Type': '/Page', '/Parent': IndirectObject(2, 0), '/Resources': IndirectObject(6603, 0), '/Contents': [IndirectObject(6602, 0)], '/MediaBox': [0, 0, 841, 595], '/CropBox': [0, 0, 841, 595]}, (0, 6609): {'/Type': '/Page', '/Parent': IndirectObject(2, 0), '/Resources': IndirectObject(6607, 0), '/Contents': [IndirectObject(6606, 0)], '/MediaBox': [0, 0, 841, 595], '/CropBox': [0, 0, 841, 595]}, (0, 6613): {'/Type': '/Page', '/Parent': IndirectObject(2, 0), '/Resources': IndirectObject(6611, 0), '/Contents': [IndirectObject(6610, 0)], '/MediaBox': [0, 0, 841, 595], '/CropBox': [0, 0, 841, 595]}, (0, 6617): {'/Type': '/Page', '/Parent': IndirectObject(2, 0), '/Resources': IndirectObject(6615, 0), '/Contents': [IndirectObject(6614, 0)], '/MediaBox': [0, 0, 841, 595], '/CropBox': [0, 0, 841, 595]}, (0, 6621): {'/Type': '/Page', '/Parent': IndirectObject(2, 0), '/Resources': IndirectObject(6619, 0), '/Contents': [IndirectObject(6618, 0)], '/MediaBox': [0, 0, 841, 595], '/CropBox': [0, 0, 841, 595]}, (0, 6625): {'/Type': '/Page', '/Parent': IndirectObject(2, 0), '/Resources': IndirectObject(6623, 0), '/Contents': [IndirectObject(6622, 0)], '/MediaBox': [0, 0, 841, 595], '/CropBox': [0, 0, 841, 595]}, (0, 6629): {'/Type': '/Page', '/Parent': IndirectObject(2, 0), '/Resources': IndirectObject(6627, 0), '/Contents': [IndirectObject(6626, 0)], '/MediaBox': [0, 0, 841, 595], '/CropBox': [0, 0, 841, 595]}, (0, 6633): {'/Type': '/Page', '/Parent': IndirectObject(2, 0), '/Resources': IndirectObject(6631, 0), '/Contents': [IndirectObject(6630, 0)], '/MediaBox': [0, 0, 841, 595], '/CropBox': [0, 0, 841, 595]}, (0, 6637): {'/Type': '/Page', '/Parent': IndirectObject(2, 0), '/Resources': IndirectObject(6635, 0), '/Contents': [IndirectObject(6634, 0)], '/MediaBox': [0, 0, 841, 595], '/CropBox': [0, 0, 841, 595]}, (0, 6641): {'/Type': '/Page', '/Parent': IndirectObject(2, 0), '/Resources': IndirectObject(6639, 0), '/Contents': [IndirectObject(6638, 0)], '/MediaBox': [0, 0, 841, 595], '/CropBox': [0, 0, 841, 595]}, (0, 6645): {'/Type': '/Page', '/Parent': IndirectObject(2, 0), '/Resources': IndirectObject(6643, 0), '/Contents': [IndirectObject(6642, 0)], '/MediaBox': [0, 0, 841, 595], '/CropBox': [0, 0, 841, 595]}, (0, 6649): {'/Type': '/Page', '/Parent': IndirectObject(2, 0), '/Resources': IndirectObject(6647, 0), '/Contents': [IndirectObject(6646, 0)], '/MediaBox': [0, 0, 841, 595], '/CropBox': [0, 0, 841, 595]}, (0, 6653): {'/Type': '/Page', '/Parent': IndirectObject(2, 0), '/Resources': IndirectObject(6651, 0), '/Contents': [IndirectObject(6650, 0)], '/MediaBox': [0, 0, 841, 595], '/CropBox': [0, 0, 841, 595]}, (0, 6657): {'/Type': '/Page', '/Parent': IndirectObject(2, 0), '/Resources': IndirectObject(6655, 0), '/Contents': [IndirectObject(6654, 0)], '/MediaBox': [0, 0, 841, 595], '/CropBox': [0, 0, 841, 595]}, (0, 6661): {'/Type': '/Page', '/Parent': IndirectObject(2, 0), '/Resources': IndirectObject(6659, 0), '/Contents': [IndirectObject(6658, 0)], '/MediaBox': [0, 0, 841, 595], '/CropBox': [0, 0, 841, 595]}, (0, 6665): {'/Type': '/Page', '/Parent': IndirectObject(2, 0), '/Resources': IndirectObject(6663, 0), '/Contents': [IndirectObject(6662, 0)], '/MediaBox': [0, 0, 841, 595], '/CropBox': [0, 0, 841, 595]}, (0, 6669): {'/Type': '/Page', '/Parent': IndirectObject(2, 0), '/Resources': IndirectObject(6667, 0), '/Contents': [IndirectObject(6666, 0)], '/MediaBox': [0, 0, 841, 595], '/CropBox': [0, 0, 841, 595]}, (0, 6673): {'/Type': '/Page', '/Parent': IndirectObject(2, 0), '/Resources': IndirectObject(6671, 0), '/Contents': [IndirectObject(6670, 0)], '/MediaBox': [0, 0, 841, 595], '/CropBox': [0, 0, 841, 595]}, (0, 6677): {'/Type': '/Page', '/Parent': IndirectObject(2, 0), '/Resources': IndirectObject(6675, 0), '/Contents': [IndirectObject(6674, 0)], '/MediaBox': [0, 0, 841, 595], '/CropBox': [0, 0, 841, 595]}, (0, 6681): {'/Type': '/Page', '/Parent': IndirectObject(2, 0), '/Resources': IndirectObject(6679, 0), '/Contents': [IndirectObject(6678, 0)], '/MediaBox': [0, 0, 841, 595], '/CropBox': [0, 0, 841, 595]}, (0, 6685): {'/Type': '/Page', '/Parent': IndirectObject(2, 0), '/Resources': IndirectObject(6683, 0), '/Contents': [IndirectObject(6682, 0)], '/MediaBox': [0, 0, 841, 595], '/CropBox': [0, 0, 841, 595]}, (0, 6689): {'/Type': '/Page', '/Parent': IndirectObject(2, 0), '/Resources': IndirectObject(6687, 0), '/Contents': [IndirectObject(6686, 0)], '/MediaBox': [0, 0, 841, 595], '/CropBox': [0, 0, 841, 595]}, (0, 6693): {'/Type': '/Page', '/Parent': IndirectObject(2, 0), '/Resources': IndirectObject(6691, 0), '/Contents': [IndirectObject(6690, 0)], '/MediaBox': [0, 0, 841, 595], '/CropBox': [0, 0, 841, 595]}, (0, 6697): {'/Type': '/Page', '/Parent': IndirectObject(2, 0), '/Resources': IndirectObject(6695, 0), '/Contents': [IndirectObject(6694, 0)], '/MediaBox': [0, 0, 841, 595], '/CropBox': [0, 0, 841, 595]}, (0, 6701): {'/Type': '/Page', '/Parent': IndirectObject(2, 0), '/Resources': IndirectObject(6699, 0), '/Contents': [IndirectObject(6698, 0)], '/MediaBox': [0, 0, 841, 595], '/CropBox': [0, 0, 841, 595]}, (0, 6705): {'/Type': '/Page', '/Parent': IndirectObject(2, 0), '/Resources': IndirectObject(6703, 0), '/Contents': [IndirectObject(6702, 0)], '/MediaBox': [0, 0, 841, 595], '/CropBox': [0, 0, 841, 595]}, (0, 6709): {'/Type': '/Page', '/Parent': IndirectObject(2, 0), '/Resources': IndirectObject(6707, 0), '/Contents': [IndirectObject(6706, 0)], '/MediaBox': [0, 0, 841, 595], '/CropBox': [0, 0, 841, 595]}, (0, 6713): {'/Type': '/Page', '/Parent': IndirectObject(2, 0), '/Resources': IndirectObject(6711, 0), '/Contents': [IndirectObject(6710, 0)], '/MediaBox': [0, 0, 841, 595], '/CropBox': [0, 0, 841, 595]}, (0, 6717): {'/Type': '/Page', '/Parent': IndirectObject(2, 0), '/Resources': IndirectObject(6715, 0), '/Contents': [IndirectObject(6714, 0)], '/MediaBox': [0, 0, 841, 595], '/CropBox': [0, 0, 841, 595]}, (0, 6721): {'/Type': '/Page', '/Parent': IndirectObject(2, 0), '/Resources': IndirectObject(6719, 0), '/Contents': [IndirectObject(6718, 0)], '/MediaBox': [0, 0, 841, 595], '/CropBox': [0, 0, 841, 595]}, (0, 6725): {'/Type': '/Page', '/Parent': IndirectObject(2, 0), '/Resources': IndirectObject(6723, 0), '/Contents': [IndirectObject(6722, 0)], '/MediaBox': [0, 0, 841, 595], '/CropBox': [0, 0, 841, 595]}, (0, 6729): {'/Type': '/Page', '/Parent': IndirectObject(2, 0), '/Resources': IndirectObject(6727, 0), '/Contents': [IndirectObject(6726, 0)], '/MediaBox': [0, 0, 841, 595], '/CropBox': [0, 0, 841, 595]}, (0, 6733): {'/Type': '/Page', '/Parent': IndirectObject(2, 0), '/Resources': IndirectObject(6731, 0), '/Contents': [IndirectObject(6730, 0)], '/MediaBox': [0, 0, 841, 595], '/CropBox': [0, 0, 841, 595]}, (0, 6737): {'/Type': '/Page', '/Parent': IndirectObject(2, 0), '/Resources': IndirectObject(6735, 0), '/Contents': [IndirectObject(6734, 0)], '/MediaBox': [0, 0, 841, 595], '/CropBox': [0, 0, 841, 595]}, (0, 6741): {'/Type': '/Page', '/Parent': IndirectObject(2, 0), '/Resources': IndirectObject(6739, 0), '/Contents': [IndirectObject(6738, 0)], '/MediaBox': [0, 0, 841, 595], '/CropBox': [0, 0, 841, 595]}, (0, 6745): {'/Type': '/Page', '/Parent': IndirectObject(2, 0), '/Resources': IndirectObject(6743, 0), '/Contents': [IndirectObject(6742, 0)], '/MediaBox': [0, 0, 841, 595], '/CropBox': [0, 0, 841, 595]}, (0, 6749): {'/Type': '/Page', '/Parent': IndirectObject(2, 0), '/Resources': IndirectObject(6747, 0), '/Contents': [IndirectObject(6746, 0)], '/MediaBox': [0, 0, 841, 595], '/CropBox': [0, 0, 841, 595]}, (0, 6753): {'/Type': '/Page', '/Parent': IndirectObject(2, 0), '/Resources': IndirectObject(6751, 0), '/Contents': [IndirectObject(6750, 0)], '/MediaBox': [0, 0, 841, 595], '/CropBox': [0, 0, 841, 595]}, (0, 6757): {'/Type': '/Page', '/Parent': IndirectObject(2, 0), '/Resources': IndirectObject(6755, 0), '/Contents': [IndirectObject(6754, 0)], '/MediaBox': [0, 0, 841, 595], '/CropBox': [0, 0, 841, 595]}, (0, 6761): {'/Type': '/Page', '/Parent': IndirectObject(2, 0), '/Resources': IndirectObject(6759, 0), '/Contents': [IndirectObject(6758, 0)], '/MediaBox': [0, 0, 841, 595], '/CropBox': [0, 0, 841, 595]}, (0, 6765): {'/Type': '/Page', '/Parent': IndirectObject(2, 0), '/Resources': IndirectObject(6763, 0), '/Contents': [IndirectObject(6762, 0)], '/MediaBox': [0, 0, 841, 595], '/CropBox': [0, 0, 841, 595]}, (0, 6769): {'/Type': '/Page', '/Parent': IndirectObject(2, 0), '/Resources': IndirectObject(6767, 0), '/Contents': [IndirectObject(6766, 0)], '/MediaBox': [0, 0, 841, 595], '/CropBox': [0, 0, 841, 595]}, (0, 6773): {'/Type': '/Page', '/Parent': IndirectObject(2, 0), '/Resources': IndirectObject(6771, 0), '/Contents': [IndirectObject(6770, 0)], '/MediaBox': [0, 0, 841, 595], '/CropBox': [0, 0, 841, 595]}, (0, 6777): {'/Type': '/Page', '/Parent': IndirectObject(2, 0), '/Resources': IndirectObject(6775, 0), '/Contents': [IndirectObject(6774, 0)], '/MediaBox': [0, 0, 841, 595], '/CropBox': [0, 0, 841, 595]}, (0, 6781): {'/Type': '/Page', '/Parent': IndirectObject(2, 0), '/Resources': IndirectObject(6779, 0), '/Contents': [IndirectObject(6778, 0)], '/MediaBox': [0, 0, 841, 595], '/CropBox': [0, 0, 841, 595]}, (0, 6785): {'/Type': '/Page', '/Parent': IndirectObject(2, 0), '/Resources': IndirectObject(6783, 0), '/Contents': [IndirectObject(6782, 0)], '/MediaBox': [0, 0, 841, 595], '/CropBox': [0, 0, 841, 595]}, (0, 6789): {'/Type': '/Page', '/Parent': IndirectObject(2, 0), '/Resources': IndirectObject(6787, 0), '/Contents': [IndirectObject(6786, 0)], '/MediaBox': [0, 0, 841, 595], '/CropBox': [0, 0, 841, 595]}, (0, 6793): {'/Type': '/Page', '/Parent': IndirectObject(2, 0), '/Resources': IndirectObject(6791, 0), '/Contents': [IndirectObject(6790, 0)], '/MediaBox': [0, 0, 841, 595], '/CropBox': [0, 0, 841, 595]}, (0, 6797): {'/Type': '/Page', '/Parent': IndirectObject(2, 0), '/Resources': IndirectObject(6795, 0), '/Contents': [IndirectObject(6794, 0)], '/MediaBox': [0, 0, 841, 595], '/CropBox': [0, 0, 841, 595]}, (0, 6801): {'/Type': '/Page', '/Parent': IndirectObject(2, 0), '/Resources': IndirectObject(6799, 0), '/Contents': [IndirectObject(6798, 0)], '/MediaBox': [0, 0, 841, 595], '/CropBox': [0, 0, 841, 595]}, (0, 6805): {'/Type': '/Page', '/Parent': IndirectObject(2, 0), '/Resources': IndirectObject(6803, 0), '/Contents': [IndirectObject(6802, 0)], '/MediaBox': [0, 0, 841, 595], '/CropBox': [0, 0, 841, 595]}, (0, 6809): {'/Type': '/Page', '/Parent': IndirectObject(2, 0), '/Resources': IndirectObject(6807, 0), '/Contents': [IndirectObject(6806, 0)], '/MediaBox': [0, 0, 841, 595], '/CropBox': [0, 0, 841, 595]}, (0, 6813): {'/Type': '/Page', '/Parent': IndirectObject(2, 0), '/Resources': IndirectObject(6811, 0), '/Contents': [IndirectObject(6810, 0)], '/MediaBox': [0, 0, 841, 595], '/CropBox': [0, 0, 841, 595]}, (0, 6817): {'/Type': '/Page', '/Parent': IndirectObject(2, 0), '/Resources': IndirectObject(6815, 0), '/Contents': [IndirectObject(6814, 0)], '/MediaBox': [0, 0, 841, 595], '/CropBox': [0, 0, 841, 595]}, (0, 6821): {'/Type': '/Page', '/Parent': IndirectObject(2, 0), '/Resources': IndirectObject(6819, 0), '/Contents': [IndirectObject(6818, 0)], '/MediaBox': [0, 0, 841, 595], '/CropBox': [0, 0, 841, 595]}, (0, 6825): {'/Type': '/Page', '/Parent': IndirectObject(2, 0), '/Resources': IndirectObject(6823, 0), '/Contents': [IndirectObject(6822, 0)], '/MediaBox': [0, 0, 841, 595], '/CropBox': [0, 0, 841, 595]}, (0, 6829): {'/Type': '/Page', '/Parent': IndirectObject(2, 0), '/Resources': IndirectObject(6827, 0), '/Contents': [IndirectObject(6826, 0)], '/MediaBox': [0, 0, 841, 595], '/CropBox': [0, 0, 841, 595]}, (0, 6833): {'/Type': '/Page', '/Parent': IndirectObject(2, 0), '/Resources': IndirectObject(6831, 0), '/Contents': [IndirectObject(6830, 0)], '/MediaBox': [0, 0, 841, 595], '/CropBox': [0, 0, 841, 595]}, (0, 6837): {'/Type': '/Page', '/Parent': IndirectObject(2, 0), '/Resources': IndirectObject(6835, 0), '/Contents': [IndirectObject(6834, 0)], '/MediaBox': [0, 0, 841, 595], '/CropBox': [0, 0, 841, 595]}, (0, 6841): {'/Type': '/Page', '/Parent': IndirectObject(2, 0), '/Resources': IndirectObject(6839, 0), '/Contents': [IndirectObject(6838, 0)], '/MediaBox': [0, 0, 841, 595], '/CropBox': [0, 0, 841, 595]}, (0, 6845): {'/Type': '/Page', '/Parent': IndirectObject(2, 0), '/Resources': IndirectObject(6843, 0), '/Contents': [IndirectObject(6842, 0)], '/MediaBox': [0, 0, 841, 595], '/CropBox': [0, 0, 841, 595]}, (0, 6849): {'/Type': '/Page', '/Parent': IndirectObject(2, 0), '/Resources': IndirectObject(6847, 0), '/Contents': [IndirectObject(6846, 0)], '/MediaBox': [0, 0, 841, 595], '/CropBox': [0, 0, 841, 595]}, (0, 6853): {'/Type': '/Page', '/Parent': IndirectObject(2, 0), '/Resources': IndirectObject(6851, 0), '/Contents': [IndirectObject(6850, 0)], '/MediaBox': [0, 0, 841, 595], '/CropBox': [0, 0, 841, 595]}, (0, 6857): {'/Type': '/Page', '/Parent': IndirectObject(2, 0), '/Resources': IndirectObject(6855, 0), '/Contents': [IndirectObject(6854, 0)], '/MediaBox': [0, 0, 841, 595], '/CropBox': [0, 0, 841, 595]}, (0, 6861): {'/Type': '/Page', '/Parent': IndirectObject(2, 0), '/Resources': IndirectObject(6859, 0), '/Contents': [IndirectObject(6858, 0)], '/MediaBox': [0, 0, 841, 595], '/CropBox': [0, 0, 841, 595]}, (0, 6865): {'/Type': '/Page', '/Parent': IndirectObject(2, 0), '/Resources': IndirectObject(6863, 0), '/Contents': [IndirectObject(6862, 0)], '/MediaBox': [0, 0, 841, 595], '/CropBox': [0, 0, 841, 595]}, (0, 6869): {'/Type': '/Page', '/Parent': IndirectObject(2, 0), '/Resources': IndirectObject(6867, 0), '/Contents': [IndirectObject(6866, 0)], '/MediaBox': [0, 0, 841, 595], '/CropBox': [0, 0, 841, 595]}, (0, 6873): {'/Type': '/Page', '/Parent': IndirectObject(2, 0), '/Resources': IndirectObject(6871, 0), '/Contents': [IndirectObject(6870, 0)], '/MediaBox': [0, 0, 841, 595], '/CropBox': [0, 0, 841, 595]}, (0, 6877): {'/Type': '/Page', '/Parent': IndirectObject(2, 0), '/Resources': IndirectObject(6875, 0), '/Contents': [IndirectObject(6874, 0)], '/MediaBox': [0, 0, 841, 595], '/CropBox': [0, 0, 841, 595]}, (0, 6881): {'/Type': '/Page', '/Parent': IndirectObject(2, 0), '/Resources': IndirectObject(6879, 0), '/Contents': [IndirectObject(6878, 0)], '/MediaBox': [0, 0, 841, 595], '/CropBox': [0, 0, 841, 595]}, (0, 6885): {'/Type': '/Page', '/Parent': IndirectObject(2, 0), '/Resources': IndirectObject(6883, 0), '/Contents': [IndirectObject(6882, 0)], '/MediaBox': [0, 0, 841, 595], '/CropBox': [0, 0, 841, 595]}, (0, 6889): {'/Type': '/Page', '/Parent': IndirectObject(2, 0), '/Resources': IndirectObject(6887, 0), '/Contents': [IndirectObject(6886, 0)], '/MediaBox': [0, 0, 841, 595], '/CropBox': [0, 0, 841, 595]}, (0, 6893): {'/Type': '/Page', '/Parent': IndirectObject(2, 0), '/Resources': IndirectObject(6891, 0), '/Contents': [IndirectObject(6890, 0)], '/MediaBox': [0, 0, 841, 595], '/CropBox': [0, 0, 841, 595]}, (0, 6897): {'/Type': '/Page', '/Parent': IndirectObject(2, 0), '/Resources': IndirectObject(6895, 0), '/Contents': [IndirectObject(6894, 0)], '/MediaBox': [0, 0, 841, 595], '/CropBox': [0, 0, 841, 595]}, (0, 6901): {'/Type': '/Page', '/Parent': IndirectObject(2, 0), '/Resources': IndirectObject(6899, 0), '/Contents': [IndirectObject(6898, 0)], '/MediaBox': [0, 0, 841, 595], '/CropBox': [0, 0, 841, 595]}, (0, 6905): {'/Type': '/Page', '/Parent': IndirectObject(2, 0), '/Resources': IndirectObject(6903, 0), '/Contents': [IndirectObject(6902, 0)], '/MediaBox': [0, 0, 841, 595], '/CropBox': [0, 0, 841, 595]}, (0, 6909): {'/Type': '/Page', '/Parent': IndirectObject(2, 0), '/Resources': IndirectObject(6907, 0), '/Contents': [IndirectObject(6906, 0)], '/MediaBox': [0, 0, 841, 595], '/CropBox': [0, 0, 841, 595]}, (0, 6913): {'/Type': '/Page', '/Parent': IndirectObject(2, 0), '/Resources': IndirectObject(6911, 0), '/Contents': [IndirectObject(6910, 0)], '/MediaBox': [0, 0, 841, 595], '/CropBox': [0, 0, 841, 595]}, (0, 6917): {'/Type': '/Page', '/Parent': IndirectObject(2, 0), '/Resources': IndirectObject(6915, 0), '/Contents': [IndirectObject(6914, 0)], '/MediaBox': [0, 0, 841, 595], '/CropBox': [0, 0, 841, 595]}, (0, 6921): {'/Type': '/Page', '/Parent': IndirectObject(2, 0), '/Resources': IndirectObject(6919, 0), '/Contents': [IndirectObject(6918, 0)], '/MediaBox': [0, 0, 841, 595], '/CropBox': [0, 0, 841, 595]}, (0, 6925): {'/Type': '/Page', '/Parent': IndirectObject(2, 0), '/Resources': IndirectObject(6923, 0), '/Contents': [IndirectObject(6922, 0)], '/MediaBox': [0, 0, 841, 595], '/CropBox': [0, 0, 841, 595]}, (0, 6929): {'/Type': '/Page', '/Parent': IndirectObject(2, 0), '/Resources': IndirectObject(6927, 0), '/Contents': [IndirectObject(6926, 0)], '/MediaBox': [0, 0, 841, 595], '/CropBox': [0, 0, 841, 595]}, (0, 6933): {'/Type': '/Page', '/Parent': IndirectObject(2, 0), '/Resources': IndirectObject(6931, 0), '/Contents': [IndirectObject(6930, 0)], '/MediaBox': [0, 0, 841, 595], '/CropBox': [0, 0, 841, 595]}, (0, 6937): {'/Type': '/Page', '/Parent': IndirectObject(2, 0), '/Resources': IndirectObject(6935, 0), '/Contents': [IndirectObject(6934, 0)], '/MediaBox': [0, 0, 841, 595], '/CropBox': [0, 0, 841, 595]}, (0, 6941): {'/Type': '/Page', '/Parent': IndirectObject(2, 0), '/Resources': IndirectObject(6939, 0), '/Contents': [IndirectObject(6938, 0)], '/MediaBox': [0, 0, 841, 595], '/CropBox': [0, 0, 841, 595]}, (0, 6945): {'/Type': '/Page', '/Parent': IndirectObject(2, 0), '/Resources': IndirectObject(6943, 0), '/Contents': [IndirectObject(6942, 0)], '/MediaBox': [0, 0, 841, 595], '/CropBox': [0, 0, 841, 595]}, (0, 6949): {'/Type': '/Page', '/Parent': IndirectObject(2, 0), '/Resources': IndirectObject(6947, 0), '/Contents': [IndirectObject(6946, 0)], '/MediaBox': [0, 0, 841, 595], '/CropBox': [0, 0, 841, 595]}, (0, 6953): {'/Type': '/Page', '/Parent': IndirectObject(2, 0), '/Resources': IndirectObject(6951, 0), '/Contents': [IndirectObject(6950, 0)], '/MediaBox': [0, 0, 841, 595], '/CropBox': [0, 0, 841, 595]}, (0, 6957): {'/Type': '/Page', '/Parent': IndirectObject(2, 0), '/Resources': IndirectObject(6955, 0), '/Contents': [IndirectObject(6954, 0)], '/MediaBox': [0, 0, 841, 595], '/CropBox': [0, 0, 841, 595]}, (0, 6961): {'/Type': '/Page', '/Parent': IndirectObject(2, 0), '/Resources': IndirectObject(6959, 0), '/Contents': [IndirectObject(6958, 0)], '/MediaBox': [0, 0, 841, 595], '/CropBox': [0, 0, 841, 595]}, (0, 6965): {'/Type': '/Page', '/Parent': IndirectObject(2, 0), '/Resources': IndirectObject(6963, 0), '/Contents': [IndirectObject(6962, 0)], '/MediaBox': [0, 0, 841, 595], '/CropBox': [0, 0, 841, 595]}, (0, 6969): {'/Type': '/Page', '/Parent': IndirectObject(2, 0), '/Resources': IndirectObject(6967, 0), '/Contents': [IndirectObject(6966, 0)], '/MediaBox': [0, 0, 841, 595], '/CropBox': [0, 0, 841, 595]}, (0, 6973): {'/Type': '/Page', '/Parent': IndirectObject(2, 0), '/Resources': IndirectObject(6971, 0), '/Contents': [IndirectObject(6970, 0)], '/MediaBox': [0, 0, 841, 595], '/CropBox': [0, 0, 841, 595]}, (0, 6977): {'/Type': '/Page', '/Parent': IndirectObject(2, 0), '/Resources': IndirectObject(6975, 0), '/Contents': [IndirectObject(6974, 0)], '/MediaBox': [0, 0, 841, 595], '/CropBox': [0, 0, 841, 595]}, (0, 6981): {'/Type': '/Page', '/Parent': IndirectObject(2, 0), '/Resources': IndirectObject(6979, 0), '/Contents': [IndirectObject(6978, 0)], '/MediaBox': [0, 0, 841, 595], '/CropBox': [0, 0, 841, 595]}, (0, 6985): {'/Type': '/Page', '/Parent': IndirectObject(2, 0), '/Resources': IndirectObject(6983, 0), '/Contents': [IndirectObject(6982, 0)], '/MediaBox': [0, 0, 841, 595], '/CropBox': [0, 0, 841, 595]}, (0, 6989): {'/Type': '/Page', '/Parent': IndirectObject(2, 0), '/Resources': IndirectObject(6987, 0), '/Contents': [IndirectObject(6986, 0)], '/MediaBox': [0, 0, 841, 595], '/CropBox': [0, 0, 841, 595]}, (0, 6993): {'/Type': '/Page', '/Parent': IndirectObject(2, 0), '/Resources': IndirectObject(6991, 0), '/Contents': [IndirectObject(6990, 0)], '/MediaBox': [0, 0, 841, 595], '/CropBox': [0, 0, 841, 595]}, (0, 6997): {'/Type': '/Page', '/Parent': IndirectObject(2, 0), '/Resources': IndirectObject(6995, 0), '/Contents': [IndirectObject(6994, 0)], '/MediaBox': [0, 0, 841, 595], '/CropBox': [0, 0, 841, 595]}, (0, 7001): {'/Type': '/Page', '/Parent': IndirectObject(2, 0), '/Resources': IndirectObject(6999, 0), '/Contents': [IndirectObject(6998, 0)], '/MediaBox': [0, 0, 841, 595], '/CropBox': [0, 0, 841, 595]}, (0, 7005): {'/Type': '/Page', '/Parent': IndirectObject(2, 0), '/Resources': IndirectObject(7003, 0), '/Contents': [IndirectObject(7002, 0)], '/MediaBox': [0, 0, 841, 595], '/CropBox': [0, 0, 841, 595]}, (0, 7009): {'/Type': '/Page', '/Parent': IndirectObject(2, 0), '/Resources': IndirectObject(7007, 0), '/Contents': [IndirectObject(7006, 0)], '/MediaBox': [0, 0, 841, 595], '/CropBox': [0, 0, 841, 595]}, (0, 7013): {'/Type': '/Page', '/Parent': IndirectObject(2, 0), '/Resources': IndirectObject(7011, 0), '/Contents': [IndirectObject(7010, 0)], '/MediaBox': [0, 0, 841, 595], '/CropBox': [0, 0, 841, 595]}, (0, 7017): {'/Type': '/Page', '/Parent': IndirectObject(2, 0), '/Resources': IndirectObject(7015, 0), '/Contents': [IndirectObject(7014, 0)], '/MediaBox': [0, 0, 841, 595], '/CropBox': [0, 0, 841, 595]}, (0, 7021): {'/Type': '/Page', '/Parent': IndirectObject(2, 0), '/Resources': IndirectObject(7019, 0), '/Contents': [IndirectObject(7018, 0)], '/MediaBox': [0, 0, 841, 595], '/CropBox': [0, 0, 841, 595]}, (0, 7025): {'/Type': '/Page', '/Parent': IndirectObject(2, 0), '/Resources': IndirectObject(7023, 0), '/Contents': [IndirectObject(7022, 0)], '/MediaBox': [0, 0, 841, 595], '/CropBox': [0, 0, 841, 595]}, (0, 7029): {'/Type': '/Page', '/Parent': IndirectObject(2, 0), '/Resources': IndirectObject(7027, 0), '/Contents': [IndirectObject(7026, 0)], '/MediaBox': [0, 0, 841, 595], '/CropBox': [0, 0, 841, 595]}, (0, 7033): {'/Type': '/Page', '/Parent': IndirectObject(2, 0), '/Resources': IndirectObject(7031, 0), '/Contents': [IndirectObject(7030, 0)], '/MediaBox': [0, 0, 841, 595], '/CropBox': [0, 0, 841, 595]}, (0, 7037): {'/Type': '/Page', '/Parent': IndirectObject(2, 0), '/Resources': IndirectObject(7035, 0), '/Contents': [IndirectObject(7034, 0)], '/MediaBox': [0, 0, 841, 595], '/CropBox': [0, 0, 841, 595]}, (0, 7041): {'/Type': '/Page', '/Parent': IndirectObject(2, 0), '/Resources': IndirectObject(7039, 0), '/Contents': [IndirectObject(7038, 0)], '/MediaBox': [0, 0, 841, 595], '/CropBox': [0, 0, 841, 595]}, (0, 7045): {'/Type': '/Page', '/Parent': IndirectObject(2, 0), '/Resources': IndirectObject(7043, 0), '/Contents': [IndirectObject(7042, 0)], '/MediaBox': [0, 0, 841, 595], '/CropBox': [0, 0, 841, 595]}, (0, 7049): {'/Type': '/Page', '/Parent': IndirectObject(2, 0), '/Resources': IndirectObject(7047, 0), '/Contents': [IndirectObject(7046, 0)], '/MediaBox': [0, 0, 841, 595], '/CropBox': [0, 0, 841, 595]}, (0, 7053): {'/Type': '/Page', '/Parent': IndirectObject(2, 0), '/Resources': IndirectObject(7051, 0), '/Contents': [IndirectObject(7050, 0)], '/MediaBox': [0, 0, 841, 595], '/CropBox': [0, 0, 841, 595]}, (0, 7057): {'/Type': '/Page', '/Parent': IndirectObject(2, 0), '/Resources': IndirectObject(7055, 0), '/Contents': [IndirectObject(7054, 0)], '/MediaBox': [0, 0, 841, 595], '/CropBox': [0, 0, 841, 595]}, (0, 7061): {'/Type': '/Page', '/Parent': IndirectObject(2, 0), '/Resources': IndirectObject(7059, 0), '/Contents': [IndirectObject(7058, 0)], '/MediaBox': [0, 0, 841, 595], '/CropBox': [0, 0, 841, 595]}, (0, 7065): {'/Type': '/Page', '/Parent': IndirectObject(2, 0), '/Resources': IndirectObject(7063, 0), '/Contents': [IndirectObject(7062, 0)], '/MediaBox': [0, 0, 841, 595], '/CropBox': [0, 0, 841, 595]}, (0, 7069): {'/Type': '/Page', '/Parent': IndirectObject(2, 0), '/Resources': IndirectObject(7067, 0), '/Contents': [IndirectObject(7066, 0)], '/MediaBox': [0, 0, 841, 595], '/CropBox': [0, 0, 841, 595]}, (0, 7073): {'/Type': '/Page', '/Parent': IndirectObject(2, 0), '/Resources': IndirectObject(7071, 0), '/Contents': [IndirectObject(7070, 0)], '/MediaBox': [0, 0, 841, 595], '/CropBox': [0, 0, 841, 595]}, (0, 7077): {'/Type': '/Page', '/Parent': IndirectObject(2, 0), '/Resources': IndirectObject(7075, 0), '/Contents': [IndirectObject(7074, 0)], '/MediaBox': [0, 0, 841, 595], '/CropBox': [0, 0, 841, 595]}, (0, 7081): {'/Type': '/Page', '/Parent': IndirectObject(2, 0), '/Resources': IndirectObject(7079, 0), '/Contents': [IndirectObject(7078, 0)], '/MediaBox': [0, 0, 841, 595], '/CropBox': [0, 0, 841, 595]}, (0, 7085): {'/Type': '/Page', '/Parent': IndirectObject(2, 0), '/Resources': IndirectObject(7083, 0), '/Contents': [IndirectObject(7082, 0)], '/MediaBox': [0, 0, 841, 595], '/CropBox': [0, 0, 841, 595]}, (0, 7089): {'/Type': '/Page', '/Parent': IndirectObject(2, 0), '/Resources': IndirectObject(7087, 0), '/Contents': [IndirectObject(7086, 0)], '/MediaBox': [0, 0, 841, 595], '/CropBox': [0, 0, 841, 595]}, (0, 7093): {'/Type': '/Page', '/Parent': IndirectObject(2, 0), '/Resources': IndirectObject(7091, 0), '/Contents': [IndirectObject(7090, 0)], '/MediaBox': [0, 0, 841, 595], '/CropBox': [0, 0, 841, 595]}, (0, 7097): {'/Type': '/Page', '/Parent': IndirectObject(2, 0), '/Resources': IndirectObject(7095, 0), '/Contents': [IndirectObject(7094, 0)], '/MediaBox': [0, 0, 841, 595], '/CropBox': [0, 0, 841, 595]}, (0, 7101): {'/Type': '/Page', '/Parent': IndirectObject(2, 0), '/Resources': IndirectObject(7099, 0), '/Contents': [IndirectObject(7098, 0)], '/MediaBox': [0, 0, 841, 595], '/CropBox': [0, 0, 841, 595]}, (0, 7105): {'/Type': '/Page', '/Parent': IndirectObject(2, 0), '/Resources': IndirectObject(7103, 0), '/Contents': [IndirectObject(7102, 0)], '/MediaBox': [0, 0, 841, 595], '/CropBox': [0, 0, 841, 595]}, (0, 7109): {'/Type': '/Page', '/Parent': IndirectObject(2, 0), '/Resources': IndirectObject(7107, 0), '/Contents': [IndirectObject(7106, 0)], '/MediaBox': [0, 0, 841, 595], '/CropBox': [0, 0, 841, 595]}, (0, 7113): {'/Type': '/Page', '/Parent': IndirectObject(2, 0), '/Resources': IndirectObject(7111, 0), '/Contents': [IndirectObject(7110, 0)], '/MediaBox': [0, 0, 841, 595], '/CropBox': [0, 0, 841, 595]}, (0, 7117): {'/Type': '/Page', '/Parent': IndirectObject(2, 0), '/Resources': IndirectObject(7115, 0), '/Contents': [IndirectObject(7114, 0)], '/MediaBox': [0, 0, 841, 595], '/CropBox': [0, 0, 841, 595]}, (0, 7121): {'/Type': '/Page', '/Parent': IndirectObject(2, 0), '/Resources': IndirectObject(7119, 0), '/Contents': [IndirectObject(7118, 0)], '/MediaBox': [0, 0, 841, 595], '/CropBox': [0, 0, 841, 595]}, (0, 7125): {'/Type': '/Page', '/Parent': IndirectObject(2, 0), '/Resources': IndirectObject(7123, 0), '/Contents': [IndirectObject(7122, 0)], '/MediaBox': [0, 0, 841, 595], '/CropBox': [0, 0, 841, 595]}, (0, 7129): {'/Type': '/Page', '/Parent': IndirectObject(2, 0), '/Resources': IndirectObject(7127, 0), '/Contents': [IndirectObject(7126, 0)], '/MediaBox': [0, 0, 841, 595], '/CropBox': [0, 0, 841, 595]}, (0, 7133): {'/Type': '/Page', '/Parent': IndirectObject(2, 0), '/Resources': IndirectObject(7131, 0), '/Contents': [IndirectObject(7130, 0)], '/MediaBox': [0, 0, 841, 595], '/CropBox': [0, 0, 841, 595]}, (0, 7137): {'/Type': '/Page', '/Parent': IndirectObject(2, 0), '/Resources': IndirectObject(7135, 0), '/Contents': [IndirectObject(7134, 0)], '/MediaBox': [0, 0, 841, 595], '/CropBox': [0, 0, 841, 595]}, (0, 7141): {'/Type': '/Page', '/Parent': IndirectObject(2, 0), '/Resources': IndirectObject(7139, 0), '/Contents': [IndirectObject(7138, 0)], '/MediaBox': [0, 0, 841, 595], '/CropBox': [0, 0, 841, 595]}, (0, 7145): {'/Type': '/Page', '/Parent': IndirectObject(2, 0), '/Resources': IndirectObject(7143, 0), '/Contents': [IndirectObject(7142, 0)], '/MediaBox': [0, 0, 841, 595], '/CropBox': [0, 0, 841, 595]}, (0, 7149): {'/Type': '/Page', '/Parent': IndirectObject(2, 0), '/Resources': IndirectObject(7147, 0), '/Contents': [IndirectObject(7146, 0)], '/MediaBox': [0, 0, 841, 595], '/CropBox': [0, 0, 841, 595]}, (0, 7153): {'/Type': '/Page', '/Parent': IndirectObject(2, 0), '/Resources': IndirectObject(7151, 0), '/Contents': [IndirectObject(7150, 0)], '/MediaBox': [0, 0, 841, 595], '/CropBox': [0, 0, 841, 595]}, (0, 7157): {'/Type': '/Page', '/Parent': IndirectObject(2, 0), '/Resources': IndirectObject(7155, 0), '/Contents': [IndirectObject(7154, 0)], '/MediaBox': [0, 0, 841, 595], '/CropBox': [0, 0, 841, 595]}, (0, 7161): {'/Type': '/Page', '/Parent': IndirectObject(2, 0), '/Resources': IndirectObject(7159, 0), '/Contents': [IndirectObject(7158, 0)], '/MediaBox': [0, 0, 841, 595], '/CropBox': [0, 0, 841, 595]}, (0, 7165): {'/Type': '/Page', '/Parent': IndirectObject(2, 0), '/Resources': IndirectObject(7163, 0), '/Contents': [IndirectObject(7162, 0)], '/MediaBox': [0, 0, 841, 595], '/CropBox': [0, 0, 841, 595]}, (0, 7169): {'/Type': '/Page', '/Parent': IndirectObject(2, 0), '/Resources': IndirectObject(7167, 0), '/Contents': [IndirectObject(7166, 0)], '/MediaBox': [0, 0, 841, 595], '/CropBox': [0, 0, 841, 595]}, (0, 7173): {'/Type': '/Page', '/Parent': IndirectObject(2, 0), '/Resources': IndirectObject(7171, 0), '/Contents': [IndirectObject(7170, 0)], '/MediaBox': [0, 0, 841, 595], '/CropBox': [0, 0, 841, 595]}, (0, 7177): {'/Type': '/Page', '/Parent': IndirectObject(2, 0), '/Resources': IndirectObject(7175, 0), '/Contents': [IndirectObject(7174, 0)], '/MediaBox': [0, 0, 841, 595], '/CropBox': [0, 0, 841, 595]}, (0, 7181): {'/Type': '/Page', '/Parent': IndirectObject(2, 0), '/Resources': IndirectObject(7179, 0), '/Contents': [IndirectObject(7178, 0)], '/MediaBox': [0, 0, 841, 595], '/CropBox': [0, 0, 841, 595]}, (0, 7185): {'/Type': '/Page', '/Parent': IndirectObject(2, 0), '/Resources': IndirectObject(7183, 0), '/Contents': [IndirectObject(7182, 0)], '/MediaBox': [0, 0, 841, 595], '/CropBox': [0, 0, 841, 595]}, (0, 7189): {'/Type': '/Page', '/Parent': IndirectObject(2, 0), '/Resources': IndirectObject(7187, 0), '/Contents': [IndirectObject(7186, 0)], '/MediaBox': [0, 0, 841, 595], '/CropBox': [0, 0, 841, 595]}, (0, 7193): {'/Type': '/Page', '/Parent': IndirectObject(2, 0), '/Resources': IndirectObject(7191, 0), '/Contents': [IndirectObject(7190, 0)], '/MediaBox': [0, 0, 841, 595], '/CropBox': [0, 0, 841, 595]}, (0, 7197): {'/Type': '/Page', '/Parent': IndirectObject(2, 0), '/Resources': IndirectObject(7195, 0), '/Contents': [IndirectObject(7194, 0)], '/MediaBox': [0, 0, 841, 595], '/CropBox': [0, 0, 841, 595]}, (0, 7201): {'/Type': '/Page', '/Parent': IndirectObject(2, 0), '/Resources': IndirectObject(7199, 0), '/Contents': [IndirectObject(7198, 0)], '/MediaBox': [0, 0, 841, 595], '/CropBox': [0, 0, 841, 595]}, (0, 7205): {'/Type': '/Page', '/Parent': IndirectObject(2, 0), '/Resources': IndirectObject(7203, 0), '/Contents': [IndirectObject(7202, 0)], '/MediaBox': [0, 0, 841, 595], '/CropBox': [0, 0, 841, 595]}, (0, 7209): {'/Type': '/Page', '/Parent': IndirectObject(2, 0), '/Resources': IndirectObject(7207, 0), '/Contents': [IndirectObject(7206, 0)], '/MediaBox': [0, 0, 841, 595], '/CropBox': [0, 0, 841, 595]}, (0, 7213): {'/Type': '/Page', '/Parent': IndirectObject(2, 0), '/Resources': IndirectObject(7211, 0), '/Contents': [IndirectObject(7210, 0)], '/MediaBox': [0, 0, 841, 595], '/CropBox': [0, 0, 841, 595]}, (0, 7217): {'/Type': '/Page', '/Parent': IndirectObject(2, 0), '/Resources': IndirectObject(7215, 0), '/Contents': [IndirectObject(7214, 0)], '/MediaBox': [0, 0, 841, 595], '/CropBox': [0, 0, 841, 595]}, (0, 7221): {'/Type': '/Page', '/Parent': IndirectObject(2, 0), '/Resources': IndirectObject(7219, 0), '/Contents': [IndirectObject(7218, 0)], '/MediaBox': [0, 0, 841, 595], '/CropBox': [0, 0, 841, 595]}, (0, 7225): {'/Type': '/Page', '/Parent': IndirectObject(2, 0), '/Resources': IndirectObject(7223, 0), '/Contents': [IndirectObject(7222, 0)], '/MediaBox': [0, 0, 841, 595], '/CropBox': [0, 0, 841, 595]}, (0, 7229): {'/Type': '/Page', '/Parent': IndirectObject(2, 0), '/Resources': IndirectObject(7227, 0), '/Contents': [IndirectObject(7226, 0)], '/MediaBox': [0, 0, 841, 595], '/CropBox': [0, 0, 841, 595]}, (0, 7233): {'/Type': '/Page', '/Parent': IndirectObject(2, 0), '/Resources': IndirectObject(7231, 0), '/Contents': [IndirectObject(7230, 0)], '/MediaBox': [0, 0, 841, 595], '/CropBox': [0, 0, 841, 595]}, (0, 7237): {'/Type': '/Page', '/Parent': IndirectObject(2, 0), '/Resources': IndirectObject(7235, 0), '/Contents': [IndirectObject(7234, 0)], '/MediaBox': [0, 0, 841, 595], '/CropBox': [0, 0, 841, 595]}, (0, 7241): {'/Type': '/Page', '/Parent': IndirectObject(2, 0), '/Resources': IndirectObject(7239, 0), '/Contents': [IndirectObject(7238, 0)], '/MediaBox': [0, 0, 841, 595], '/CropBox': [0, 0, 841, 595]}, (0, 7245): {'/Type': '/Page', '/Parent': IndirectObject(2, 0), '/Resources': IndirectObject(7243, 0), '/Contents': [IndirectObject(7242, 0)], '/MediaBox': [0, 0, 841, 595], '/CropBox': [0, 0, 841, 595]}, (0, 7249): {'/Type': '/Page', '/Parent': IndirectObject(2, 0), '/Resources': IndirectObject(7247, 0), '/Contents': [IndirectObject(7246, 0)], '/MediaBox': [0, 0, 841, 595], '/CropBox': [0, 0, 841, 595]}, (0, 7253): {'/Type': '/Page', '/Parent': IndirectObject(2, 0), '/Resources': IndirectObject(7251, 0), '/Contents': [IndirectObject(7250, 0)], '/MediaBox': [0, 0, 841, 595], '/CropBox': [0, 0, 841, 595]}, (0, 7257): {'/Type': '/Page', '/Parent': IndirectObject(2, 0), '/Resources': IndirectObject(7255, 0), '/Contents': [IndirectObject(7254, 0)], '/MediaBox': [0, 0, 841, 595], '/CropBox': [0, 0, 841, 595]}, (0, 7261): {'/Type': '/Page', '/Parent': IndirectObject(2, 0), '/Resources': IndirectObject(7259, 0), '/Contents': [IndirectObject(7258, 0)], '/MediaBox': [0, 0, 841, 595], '/CropBox': [0, 0, 841, 595]}, (0, 7265): {'/Type': '/Page', '/Parent': IndirectObject(2, 0), '/Resources': IndirectObject(7263, 0), '/Contents': [IndirectObject(7262, 0)], '/MediaBox': [0, 0, 841, 595], '/CropBox': [0, 0, 841, 595]}, (0, 7269): {'/Type': '/Page', '/Parent': IndirectObject(2, 0), '/Resources': IndirectObject(7267, 0), '/Contents': [IndirectObject(7266, 0)], '/MediaBox': [0, 0, 841, 595], '/CropBox': [0, 0, 841, 595]}, (0, 7273): {'/Type': '/Page', '/Parent': IndirectObject(2, 0), '/Resources': IndirectObject(7271, 0), '/Contents': [IndirectObject(7270, 0)], '/MediaBox': [0, 0, 841, 595], '/CropBox': [0, 0, 841, 595]}, (0, 7277): {'/Type': '/Page', '/Parent': IndirectObject(2, 0), '/Resources': IndirectObject(7275, 0), '/Contents': [IndirectObject(7274, 0)], '/MediaBox': [0, 0, 841, 595], '/CropBox': [0, 0, 841, 595]}, (0, 7281): {'/Type': '/Page', '/Parent': IndirectObject(2, 0), '/Resources': IndirectObject(7279, 0), '/Contents': [IndirectObject(7278, 0)], '/MediaBox': [0, 0, 841, 595], '/CropBox': [0, 0, 841, 595]}, (0, 7285): {'/Type': '/Page', '/Parent': IndirectObject(2, 0), '/Resources': IndirectObject(7283, 0), '/Contents': [IndirectObject(7282, 0)], '/MediaBox': [0, 0, 841, 595], '/CropBox': [0, 0, 841, 595]}, (0, 7289): {'/Type': '/Page', '/Parent': IndirectObject(2, 0), '/Resources': IndirectObject(7287, 0), '/Contents': [IndirectObject(7286, 0)], '/MediaBox': [0, 0, 841, 595], '/CropBox': [0, 0, 841, 595]}, (0, 7293): {'/Type': '/Page', '/Parent': IndirectObject(2, 0), '/Resources': IndirectObject(7291, 0), '/Contents': [IndirectObject(7290, 0)], '/MediaBox': [0, 0, 841, 595], '/CropBox': [0, 0, 841, 595]}, (0, 7297): {'/Type': '/Page', '/Parent': IndirectObject(2, 0), '/Resources': IndirectObject(7295, 0), '/Contents': [IndirectObject(7294, 0)], '/MediaBox': [0, 0, 841, 595], '/CropBox': [0, 0, 841, 595]}, (0, 7301): {'/Type': '/Page', '/Parent': IndirectObject(2, 0), '/Resources': IndirectObject(7299, 0), '/Contents': [IndirectObject(7298, 0)], '/MediaBox': [0, 0, 841, 595], '/CropBox': [0, 0, 841, 595]}, (0, 7305): {'/Type': '/Page', '/Parent': IndirectObject(2, 0), '/Resources': IndirectObject(7303, 0), '/Contents': [IndirectObject(7302, 0)], '/MediaBox': [0, 0, 841, 595], '/CropBox': [0, 0, 841, 595]}, (0, 7309): {'/Type': '/Page', '/Parent': IndirectObject(2, 0), '/Resources': IndirectObject(7307, 0), '/Contents': [IndirectObject(7306, 0)], '/MediaBox': [0, 0, 841, 595], '/CropBox': [0, 0, 841, 595]}, (0, 7313): {'/Type': '/Page', '/Parent': IndirectObject(2, 0), '/Resources': IndirectObject(7311, 0), '/Contents': [IndirectObject(7310, 0)], '/MediaBox': [0, 0, 841, 595], '/CropBox': [0, 0, 841, 595]}, (0, 7317): {'/Type': '/Page', '/Parent': IndirectObject(2, 0), '/Resources': IndirectObject(7315, 0), '/Contents': [IndirectObject(7314, 0)], '/MediaBox': [0, 0, 841, 595], '/CropBox': [0, 0, 841, 595]}, (0, 7321): {'/Type': '/Page', '/Parent': IndirectObject(2, 0), '/Resources': IndirectObject(7319, 0), '/Contents': [IndirectObject(7318, 0)], '/MediaBox': [0, 0, 841, 595], '/CropBox': [0, 0, 841, 595]}, (0, 7325): {'/Type': '/Page', '/Parent': IndirectObject(2, 0), '/Resources': IndirectObject(7323, 0), '/Contents': [IndirectObject(7322, 0)], '/MediaBox': [0, 0, 841, 595], '/CropBox': [0, 0, 841, 595]}, (0, 7329): {'/Type': '/Page', '/Parent': IndirectObject(2, 0), '/Resources': IndirectObject(7327, 0), '/Contents': [IndirectObject(7326, 0)], '/MediaBox': [0, 0, 841, 595], '/CropBox': [0, 0, 841, 595]}, (0, 7333): {'/Type': '/Page', '/Parent': IndirectObject(2, 0), '/Resources': IndirectObject(7331, 0), '/Contents': [IndirectObject(7330, 0)], '/MediaBox': [0, 0, 841, 595], '/CropBox': [0, 0, 841, 595]}, (0, 7337): {'/Type': '/Page', '/Parent': IndirectObject(2, 0), '/Resources': IndirectObject(7335, 0), '/Contents': [IndirectObject(7334, 0)], '/MediaBox': [0, 0, 841, 595], '/CropBox': [0, 0, 841, 595]}, (0, 7341): {'/Type': '/Page', '/Parent': IndirectObject(2, 0), '/Resources': IndirectObject(7339, 0), '/Contents': [IndirectObject(7338, 0)], '/MediaBox': [0, 0, 841, 595], '/CropBox': [0, 0, 841, 595]}, (0, 7345): {'/Type': '/Page', '/Parent': IndirectObject(2, 0), '/Resources': IndirectObject(7343, 0), '/Contents': [IndirectObject(7342, 0)], '/MediaBox': [0, 0, 841, 595], '/CropBox': [0, 0, 841, 595]}, (0, 7349): {'/Type': '/Page', '/Parent': IndirectObject(2, 0), '/Resources': IndirectObject(7347, 0), '/Contents': [IndirectObject(7346, 0)], '/MediaBox': [0, 0, 841, 595], '/CropBox': [0, 0, 841, 595]}, (0, 7353): {'/Type': '/Page', '/Parent': IndirectObject(2, 0), '/Resources': IndirectObject(7351, 0), '/Contents': [IndirectObject(7350, 0)], '/MediaBox': [0, 0, 841, 595], '/CropBox': [0, 0, 841, 595]}, (0, 7357): {'/Type': '/Page', '/Parent': IndirectObject(2, 0), '/Resources': IndirectObject(7355, 0), '/Contents': [IndirectObject(7354, 0)], '/MediaBox': [0, 0, 841, 595], '/CropBox': [0, 0, 841, 595]}, (0, 7361): {'/Type': '/Page', '/Parent': IndirectObject(2, 0), '/Resources': IndirectObject(7359, 0), '/Contents': [IndirectObject(7358, 0)], '/MediaBox': [0, 0, 841, 595], '/CropBox': [0, 0, 841, 595]}, (0, 7365): {'/Type': '/Page', '/Parent': IndirectObject(2, 0), '/Resources': IndirectObject(7363, 0), '/Contents': [IndirectObject(7362, 0)], '/MediaBox': [0, 0, 841, 595], '/CropBox': [0, 0, 841, 595]}, (0, 7369): {'/Type': '/Page', '/Parent': IndirectObject(2, 0), '/Resources': IndirectObject(7367, 0), '/Contents': [IndirectObject(7366, 0)], '/MediaBox': [0, 0, 841, 595], '/CropBox': [0, 0, 841, 595]}, (0, 7373): {'/Type': '/Page', '/Parent': IndirectObject(2, 0), '/Resources': IndirectObject(7371, 0), '/Contents': [IndirectObject(7370, 0)], '/MediaBox': [0, 0, 841, 595], '/CropBox': [0, 0, 841, 595]}, (0, 7377): {'/Type': '/Page', '/Parent': IndirectObject(2, 0), '/Resources': IndirectObject(7375, 0), '/Contents': [IndirectObject(7374, 0)], '/MediaBox': [0, 0, 841, 595], '/CropBox': [0, 0, 841, 595]}, (0, 7381): {'/Type': '/Page', '/Parent': IndirectObject(2, 0), '/Resources': IndirectObject(7379, 0), '/Contents': [IndirectObject(7378, 0)], '/MediaBox': [0, 0, 841, 595], '/CropBox': [0, 0, 841, 595]}, (0, 7385): {'/Type': '/Page', '/Parent': IndirectObject(2, 0), '/Resources': IndirectObject(7383, 0), '/Contents': [IndirectObject(7382, 0)], '/MediaBox': [0, 0, 841, 595], '/CropBox': [0, 0, 841, 595]}, (0, 7389): {'/Type': '/Page', '/Parent': IndirectObject(2, 0), '/Resources': IndirectObject(7387, 0), '/Contents': [IndirectObject(7386, 0)], '/MediaBox': [0, 0, 841, 595], '/CropBox': [0, 0, 841, 595]}, (0, 7393): {'/Type': '/Page', '/Parent': IndirectObject(2, 0), '/Resources': IndirectObject(7391, 0), '/Contents': [IndirectObject(7390, 0)], '/MediaBox': [0, 0, 841, 595], '/CropBox': [0, 0, 841, 595]}, (0, 7397): {'/Type': '/Page', '/Parent': IndirectObject(2, 0), '/Resources': IndirectObject(7395, 0), '/Contents': [IndirectObject(7394, 0)], '/MediaBox': [0, 0, 841, 595], '/CropBox': [0, 0, 841, 595]}, (0, 7401): {'/Type': '/Page', '/Parent': IndirectObject(2, 0), '/Resources': IndirectObject(7399, 0), '/Contents': [IndirectObject(7398, 0)], '/MediaBox': [0, 0, 841, 595], '/CropBox': [0, 0, 841, 595]}, (0, 7405): {'/Type': '/Page', '/Parent': IndirectObject(2, 0), '/Resources': IndirectObject(7403, 0), '/Contents': [IndirectObject(7402, 0)], '/MediaBox': [0, 0, 841, 595], '/CropBox': [0, 0, 841, 595]}, (0, 7409): {'/Type': '/Page', '/Parent': IndirectObject(2, 0), '/Resources': IndirectObject(7407, 0), '/Contents': [IndirectObject(7406, 0)], '/MediaBox': [0, 0, 841, 595], '/CropBox': [0, 0, 841, 595]}, (0, 7413): {'/Type': '/Page', '/Parent': IndirectObject(2, 0), '/Resources': IndirectObject(7411, 0), '/Contents': [IndirectObject(7410, 0)], '/MediaBox': [0, 0, 841, 595], '/CropBox': [0, 0, 841, 595]}, (0, 7417): {'/Type': '/Page', '/Parent': IndirectObject(2, 0), '/Resources': IndirectObject(7415, 0), '/Contents': [IndirectObject(7414, 0)], '/MediaBox': [0, 0, 841, 595], '/CropBox': [0, 0, 841, 595]}, (0, 7421): {'/Type': '/Page', '/Parent': IndirectObject(2, 0), '/Resources': IndirectObject(7419, 0), '/Contents': [IndirectObject(7418, 0)], '/MediaBox': [0, 0, 841, 595], '/CropBox': [0, 0, 841, 595]}, (0, 7425): {'/Type': '/Page', '/Parent': IndirectObject(2, 0), '/Resources': IndirectObject(7423, 0), '/Contents': [IndirectObject(7422, 0)], '/MediaBox': [0, 0, 841, 595], '/CropBox': [0, 0, 841, 595]}, (0, 7429): {'/Type': '/Page', '/Parent': IndirectObject(2, 0), '/Resources': IndirectObject(7427, 0), '/Contents': [IndirectObject(7426, 0)], '/MediaBox': [0, 0, 841, 595], '/CropBox': [0, 0, 841, 595]}, (0, 7433): {'/Type': '/Page', '/Parent': IndirectObject(2, 0), '/Resources': IndirectObject(7431, 0), '/Contents': [IndirectObject(7430, 0)], '/MediaBox': [0, 0, 841, 595], '/CropBox': [0, 0, 841, 595]}, (0, 7437): {'/Type': '/Page', '/Parent': IndirectObject(2, 0), '/Resources': IndirectObject(7435, 0), '/Contents': [IndirectObject(7434, 0)], '/MediaBox': [0, 0, 841, 595], '/CropBox': [0, 0, 841, 595]}, (0, 7441): {'/Type': '/Page', '/Parent': IndirectObject(2, 0), '/Resources': IndirectObject(7439, 0), '/Contents': [IndirectObject(7438, 0)], '/MediaBox': [0, 0, 841, 595], '/CropBox': [0, 0, 841, 595]}, (0, 7445): {'/Type': '/Page', '/Parent': IndirectObject(2, 0), '/Resources': IndirectObject(7443, 0), '/Contents': [IndirectObject(7442, 0)], '/MediaBox': [0, 0, 841, 595], '/CropBox': [0, 0, 841, 595]}, (0, 7449): {'/Type': '/Page', '/Parent': IndirectObject(2, 0), '/Resources': IndirectObject(7447, 0), '/Contents': [IndirectObject(7446, 0)], '/MediaBox': [0, 0, 841, 595], '/CropBox': [0, 0, 841, 595]}, (0, 7453): {'/Type': '/Page', '/Parent': IndirectObject(2, 0), '/Resources': IndirectObject(7451, 0), '/Contents': [IndirectObject(7450, 0)], '/MediaBox': [0, 0, 841, 595], '/CropBox': [0, 0, 841, 595]}, (0, 7457): {'/Type': '/Page', '/Parent': IndirectObject(2, 0), '/Resources': IndirectObject(7455, 0), '/Contents': [IndirectObject(7454, 0)], '/MediaBox': [0, 0, 841, 595], '/CropBox': [0, 0, 841, 595]}, (0, 7461): {'/Type': '/Page', '/Parent': IndirectObject(2, 0), '/Resources': IndirectObject(7459, 0), '/Contents': [IndirectObject(7458, 0)], '/MediaBox': [0, 0, 841, 595], '/CropBox': [0, 0, 841, 595]}, (0, 7465): {'/Type': '/Page', '/Parent': IndirectObject(2, 0), '/Resources': IndirectObject(7463, 0), '/Contents': [IndirectObject(7462, 0)], '/MediaBox': [0, 0, 841, 595], '/CropBox': [0, 0, 841, 595]}, (0, 7469): {'/Type': '/Page', '/Parent': IndirectObject(2, 0), '/Resources': IndirectObject(7467, 0), '/Contents': [IndirectObject(7466, 0)], '/MediaBox': [0, 0, 841, 595], '/CropBox': [0, 0, 841, 595]}, (0, 7473): {'/Type': '/Page', '/Parent': IndirectObject(2, 0), '/Resources': IndirectObject(7471, 0), '/Contents': [IndirectObject(7470, 0)], '/MediaBox': [0, 0, 841, 595], '/CropBox': [0, 0, 841, 595]}, (0, 7477): {'/Type': '/Page', '/Parent': IndirectObject(2, 0), '/Resources': IndirectObject(7475, 0), '/Contents': [IndirectObject(7474, 0)], '/MediaBox': [0, 0, 841, 595], '/CropBox': [0, 0, 841, 595]}, (0, 7481): {'/Type': '/Page', '/Parent': IndirectObject(2, 0), '/Resources': IndirectObject(7479, 0), '/Contents': [IndirectObject(7478, 0)], '/MediaBox': [0, 0, 841, 595], '/CropBox': [0, 0, 841, 595]}, (0, 7485): {'/Type': '/Page', '/Parent': IndirectObject(2, 0), '/Resources': IndirectObject(7483, 0), '/Contents': [IndirectObject(7482, 0)], '/MediaBox': [0, 0, 841, 595], '/CropBox': [0, 0, 841, 595]}, (0, 7489): {'/Type': '/Page', '/Parent': IndirectObject(2, 0), '/Resources': IndirectObject(7487, 0), '/Contents': [IndirectObject(7486, 0)], '/MediaBox': [0, 0, 841, 595], '/CropBox': [0, 0, 841, 595]}, (0, 7493): {'/Type': '/Page', '/Parent': IndirectObject(2, 0), '/Resources': IndirectObject(7491, 0), '/Contents': [IndirectObject(7490, 0)], '/MediaBox': [0, 0, 841, 595], '/CropBox': [0, 0, 841, 595]}, (0, 7497): {'/Type': '/Page', '/Parent': IndirectObject(2, 0), '/Resources': IndirectObject(7495, 0), '/Contents': [IndirectObject(7494, 0)], '/MediaBox': [0, 0, 841, 595], '/CropBox': [0, 0, 841, 595]}, (0, 7501): {'/Type': '/Page', '/Parent': IndirectObject(2, 0), '/Resources': IndirectObject(7499, 0), '/Contents': [IndirectObject(7498, 0)], '/MediaBox': [0, 0, 841, 595], '/CropBox': [0, 0, 841, 595]}, (0, 7505): {'/Type': '/Page', '/Parent': IndirectObject(2, 0), '/Resources': IndirectObject(7503, 0), '/Contents': [IndirectObject(7502, 0)], '/MediaBox': [0, 0, 841, 595], '/CropBox': [0, 0, 841, 595]}, (0, 7509): {'/Type': '/Page', '/Parent': IndirectObject(2, 0), '/Resources': IndirectObject(7507, 0), '/Contents': [IndirectObject(7506, 0)], '/MediaBox': [0, 0, 841, 595], '/CropBox': [0, 0, 841, 595]}, (0, 7513): {'/Type': '/Page', '/Parent': IndirectObject(2, 0), '/Resources': IndirectObject(7511, 0), '/Contents': [IndirectObject(7510, 0)], '/MediaBox': [0, 0, 841, 595], '/CropBox': [0, 0, 841, 595]}, (0, 7517): {'/Type': '/Page', '/Parent': IndirectObject(2, 0), '/Resources': IndirectObject(7515, 0), '/Contents': [IndirectObject(7514, 0)], '/MediaBox': [0, 0, 841, 595], '/CropBox': [0, 0, 841, 595]}, (0, 7521): {'/Type': '/Page', '/Parent': IndirectObject(2, 0), '/Resources': IndirectObject(7519, 0), '/Contents': [IndirectObject(7518, 0)], '/MediaBox': [0, 0, 841, 595], '/CropBox': [0, 0, 841, 595]}, (0, 7525): {'/Type': '/Page', '/Parent': IndirectObject(2, 0), '/Resources': IndirectObject(7523, 0), '/Contents': [IndirectObject(7522, 0)], '/MediaBox': [0, 0, 841, 595], '/CropBox': [0, 0, 841, 595]}, (0, 7529): {'/Type': '/Page', '/Parent': IndirectObject(2, 0), '/Resources': IndirectObject(7527, 0), '/Contents': [IndirectObject(7526, 0)], '/MediaBox': [0, 0, 841, 595], '/CropBox': [0, 0, 841, 595]}, (0, 7533): {'/Type': '/Page', '/Parent': IndirectObject(2, 0), '/Resources': IndirectObject(7531, 0), '/Contents': [IndirectObject(7530, 0)], '/MediaBox': [0, 0, 841, 595], '/CropBox': [0, 0, 841, 595]}, (0, 7537): {'/Type': '/Page', '/Parent': IndirectObject(2, 0), '/Resources': IndirectObject(7535, 0), '/Contents': [IndirectObject(7534, 0)], '/MediaBox': [0, 0, 841, 595], '/CropBox': [0, 0, 841, 595]}, (0, 7541): {'/Type': '/Page', '/Parent': IndirectObject(2, 0), '/Resources': IndirectObject(7539, 0), '/Contents': [IndirectObject(7538, 0)], '/MediaBox': [0, 0, 841, 595], '/CropBox': [0, 0, 841, 595]}, (0, 7545): {'/Type': '/Page', '/Parent': IndirectObject(2, 0), '/Resources': IndirectObject(7543, 0), '/Contents': [IndirectObject(7542, 0)], '/MediaBox': [0, 0, 841, 595], '/CropBox': [0, 0, 841, 595]}, (0, 7549): {'/Type': '/Page', '/Parent': IndirectObject(2, 0), '/Resources': IndirectObject(7547, 0), '/Contents': [IndirectObject(7546, 0)], '/MediaBox': [0, 0, 841, 595], '/CropBox': [0, 0, 841, 595]}, (0, 7553): {'/Type': '/Page', '/Parent': IndirectObject(2, 0), '/Resources': IndirectObject(7551, 0), '/Contents': [IndirectObject(7550, 0)], '/MediaBox': [0, 0, 841, 595], '/CropBox': [0, 0, 841, 595]}, (0, 7557): {'/Type': '/Page', '/Parent': IndirectObject(2, 0), '/Resources': IndirectObject(7555, 0), '/Contents': [IndirectObject(7554, 0)], '/MediaBox': [0, 0, 841, 595], '/CropBox': [0, 0, 841, 595]}, (0, 7561): {'/Type': '/Page', '/Parent': IndirectObject(2, 0), '/Resources': IndirectObject(7559, 0), '/Contents': [IndirectObject(7558, 0)], '/MediaBox': [0, 0, 841, 595], '/CropBox': [0, 0, 841, 595]}, (0, 7565): {'/Type': '/Page', '/Parent': IndirectObject(2, 0), '/Resources': IndirectObject(7563, 0), '/Contents': [IndirectObject(7562, 0)], '/MediaBox': [0, 0, 841, 595], '/CropBox': [0, 0, 841, 595]}, (0, 7569): {'/Type': '/Page', '/Parent': IndirectObject(2, 0), '/Resources': IndirectObject(7567, 0), '/Contents': [IndirectObject(7566, 0)], '/MediaBox': [0, 0, 841, 595], '/CropBox': [0, 0, 841, 595]}, (0, 7573): {'/Type': '/Page', '/Parent': IndirectObject(2, 0), '/Resources': IndirectObject(7571, 0), '/Contents': [IndirectObject(7570, 0)], '/MediaBox': [0, 0, 841, 595], '/CropBox': [0, 0, 841, 595]}, (0, 7577): {'/Type': '/Page', '/Parent': IndirectObject(2, 0), '/Resources': IndirectObject(7575, 0), '/Contents': [IndirectObject(7574, 0)], '/MediaBox': [0, 0, 841, 595], '/CropBox': [0, 0, 841, 595]}, (0, 7581): {'/Type': '/Page', '/Parent': IndirectObject(2, 0), '/Resources': IndirectObject(7579, 0), '/Contents': [IndirectObject(7578, 0)], '/MediaBox': [0, 0, 841, 595], '/CropBox': [0, 0, 841, 595]}, (0, 7585): {'/Type': '/Page', '/Parent': IndirectObject(2, 0), '/Resources': IndirectObject(7583, 0), '/Contents': [IndirectObject(7582, 0)], '/MediaBox': [0, 0, 841, 595], '/CropBox': [0, 0, 841, 595]}, (0, 7589): {'/Type': '/Page', '/Parent': IndirectObject(2, 0), '/Resources': IndirectObject(7587, 0), '/Contents': [IndirectObject(7586, 0)], '/MediaBox': [0, 0, 841, 595], '/CropBox': [0, 0, 841, 595]}, (0, 7593): {'/Type': '/Page', '/Parent': IndirectObject(2, 0), '/Resources': IndirectObject(7591, 0), '/Contents': [IndirectObject(7590, 0)], '/MediaBox': [0, 0, 841, 595], '/CropBox': [0, 0, 841, 595]}, (0, 7597): {'/Type': '/Page', '/Parent': IndirectObject(2, 0), '/Resources': IndirectObject(7595, 0), '/Contents': [IndirectObject(7594, 0)], '/MediaBox': [0, 0, 841, 595], '/CropBox': [0, 0, 841, 595]}, (0, 7601): {'/Type': '/Page', '/Parent': IndirectObject(2, 0), '/Resources': IndirectObject(7599, 0), '/Contents': [IndirectObject(7598, 0)], '/MediaBox': [0, 0, 841, 595], '/CropBox': [0, 0, 841, 595]}, (0, 7605): {'/Type': '/Page', '/Parent': IndirectObject(2, 0), '/Resources': IndirectObject(7603, 0), '/Contents': [IndirectObject(7602, 0)], '/MediaBox': [0, 0, 841, 595], '/CropBox': [0, 0, 841, 595]}, (0, 7609): {'/Type': '/Page', '/Parent': IndirectObject(2, 0), '/Resources': IndirectObject(7607, 0), '/Contents': [IndirectObject(7606, 0)], '/MediaBox': [0, 0, 841, 595], '/CropBox': [0, 0, 841, 595]}, (0, 7613): {'/Type': '/Page', '/Parent': IndirectObject(2, 0), '/Resources': IndirectObject(7611, 0), '/Contents': [IndirectObject(7610, 0)], '/MediaBox': [0, 0, 841, 595], '/CropBox': [0, 0, 841, 595]}, (0, 7617): {'/Type': '/Page', '/Parent': IndirectObject(2, 0), '/Resources': IndirectObject(7615, 0), '/Contents': [IndirectObject(7614, 0)], '/MediaBox': [0, 0, 841, 595], '/CropBox': [0, 0, 841, 595]}, (0, 7621): {'/Type': '/Page', '/Parent': IndirectObject(2, 0), '/Resources': IndirectObject(7619, 0), '/Contents': [IndirectObject(7618, 0)], '/MediaBox': [0, 0, 841, 595], '/CropBox': [0, 0, 841, 595]}, (0, 7625): {'/Type': '/Page', '/Parent': IndirectObject(2, 0), '/Resources': IndirectObject(7623, 0), '/Contents': [IndirectObject(7622, 0)], '/MediaBox': [0, 0, 841, 595], '/CropBox': [0, 0, 841, 595]}, (0, 7629): {'/Type': '/Page', '/Parent': IndirectObject(2, 0), '/Resources': IndirectObject(7627, 0), '/Contents': [IndirectObject(7626, 0)], '/MediaBox': [0, 0, 841, 595], '/CropBox': [0, 0, 841, 595]}, (0, 7633): {'/Type': '/Page', '/Parent': IndirectObject(2, 0), '/Resources': IndirectObject(7631, 0), '/Contents': [IndirectObject(7630, 0)], '/MediaBox': [0, 0, 841, 595], '/CropBox': [0, 0, 841, 595]}, (0, 7637): {'/Type': '/Page', '/Parent': IndirectObject(2, 0), '/Resources': IndirectObject(7635, 0), '/Contents': [IndirectObject(7634, 0)], '/MediaBox': [0, 0, 841, 595], '/CropBox': [0, 0, 841, 595]}, (0, 7641): {'/Type': '/Page', '/Parent': IndirectObject(2, 0), '/Resources': IndirectObject(7639, 0), '/Contents': [IndirectObject(7638, 0)], '/MediaBox': [0, 0, 841, 595], '/CropBox': [0, 0, 841, 595]}, (0, 7645): {'/Type': '/Page', '/Parent': IndirectObject(2, 0), '/Resources': IndirectObject(7643, 0), '/Contents': [IndirectObject(7642, 0)], '/MediaBox': [0, 0, 841, 595], '/CropBox': [0, 0, 841, 595]}, (0, 7649): {'/Type': '/Page', '/Parent': IndirectObject(2, 0), '/Resources': IndirectObject(7647, 0), '/Contents': [IndirectObject(7646, 0)], '/MediaBox': [0, 0, 841, 595], '/CropBox': [0, 0, 841, 595]}, (0, 7653): {'/Type': '/Page', '/Parent': IndirectObject(2, 0), '/Resources': IndirectObject(7651, 0), '/Contents': [IndirectObject(7650, 0)], '/MediaBox': [0, 0, 841, 595], '/CropBox': [0, 0, 841, 595]}, (0, 7657): {'/Type': '/Page', '/Parent': IndirectObject(2, 0), '/Resources': IndirectObject(7655, 0), '/Contents': [IndirectObject(7654, 0)], '/MediaBox': [0, 0, 841, 595], '/CropBox': [0, 0, 841, 595]}, (0, 7661): {'/Type': '/Page', '/Parent': IndirectObject(2, 0), '/Resources': IndirectObject(7659, 0), '/Contents': [IndirectObject(7658, 0)], '/MediaBox': [0, 0, 841, 595], '/CropBox': [0, 0, 841, 595]}, (0, 7665): {'/Type': '/Page', '/Parent': IndirectObject(2, 0), '/Resources': IndirectObject(7663, 0), '/Contents': [IndirectObject(7662, 0)], '/MediaBox': [0, 0, 841, 595], '/CropBox': [0, 0, 841, 595]}, (0, 7669): {'/Type': '/Page', '/Parent': IndirectObject(2, 0), '/Resources': IndirectObject(7667, 0), '/Contents': [IndirectObject(7666, 0)], '/MediaBox': [0, 0, 841, 595], '/CropBox': [0, 0, 841, 595]}, (0, 7673): {'/Type': '/Page', '/Parent': IndirectObject(2, 0), '/Resources': IndirectObject(7671, 0), '/Contents': [IndirectObject(7670, 0)], '/MediaBox': [0, 0, 841, 595], '/CropBox': [0, 0, 841, 595]}, (0, 7677): {'/Type': '/Page', '/Parent': IndirectObject(2, 0), '/Resources': IndirectObject(7675, 0), '/Contents': [IndirectObject(7674, 0)], '/MediaBox': [0, 0, 841, 595], '/CropBox': [0, 0, 841, 595]}, (0, 7681): {'/Type': '/Page', '/Parent': IndirectObject(2, 0), '/Resources': IndirectObject(7679, 0), '/Contents': [IndirectObject(7678, 0)], '/MediaBox': [0, 0, 841, 595], '/CropBox': [0, 0, 841, 595]}, (0, 7685): {'/Type': '/Page', '/Parent': IndirectObject(2, 0), '/Resources': IndirectObject(7683, 0), '/Contents': [IndirectObject(7682, 0)], '/MediaBox': [0, 0, 841, 595], '/CropBox': [0, 0, 841, 595]}, (0, 7689): {'/Type': '/Page', '/Parent': IndirectObject(2, 0), '/Resources': IndirectObject(7687, 0), '/Contents': [IndirectObject(7686, 0)], '/MediaBox': [0, 0, 841, 595], '/CropBox': [0, 0, 841, 595]}, (0, 7693): {'/Type': '/Page', '/Parent': IndirectObject(2, 0), '/Resources': IndirectObject(7691, 0), '/Contents': [IndirectObject(7690, 0)], '/MediaBox': [0, 0, 841, 595], '/CropBox': [0, 0, 841, 595]}, (0, 7697): {'/Type': '/Page', '/Parent': IndirectObject(2, 0), '/Resources': IndirectObject(7695, 0), '/Contents': [IndirectObject(7694, 0)], '/MediaBox': [0, 0, 841, 595], '/CropBox': [0, 0, 841, 595]}, (0, 7701): {'/Type': '/Page', '/Parent': IndirectObject(2, 0), '/Resources': IndirectObject(7699, 0), '/Contents': [IndirectObject(7698, 0)], '/MediaBox': [0, 0, 841, 595], '/CropBox': [0, 0, 841, 595]}, (0, 7705): {'/Type': '/Page', '/Parent': IndirectObject(2, 0), '/Resources': IndirectObject(7703, 0), '/Contents': [IndirectObject(7702, 0)], '/MediaBox': [0, 0, 841, 595], '/CropBox': [0, 0, 841, 595]}, (0, 7709): {'/Type': '/Page', '/Parent': IndirectObject(2, 0), '/Resources': IndirectObject(7707, 0), '/Contents': [IndirectObject(7706, 0)], '/MediaBox': [0, 0, 841, 595], '/CropBox': [0, 0, 841, 595]}, (0, 7713): {'/Type': '/Page', '/Parent': IndirectObject(2, 0), '/Resources': IndirectObject(7711, 0), '/Contents': [IndirectObject(7710, 0)], '/MediaBox': [0, 0, 841, 595], '/CropBox': [0, 0, 841, 595]}, (0, 7717): {'/Type': '/Page', '/Parent': IndirectObject(2, 0), '/Resources': IndirectObject(7715, 0), '/Contents': [IndirectObject(7714, 0)], '/MediaBox': [0, 0, 841, 595], '/CropBox': [0, 0, 841, 595]}, (0, 7721): {'/Type': '/Page', '/Parent': IndirectObject(2, 0), '/Resources': IndirectObject(7719, 0), '/Contents': [IndirectObject(7718, 0)], '/MediaBox': [0, 0, 841, 595], '/CropBox': [0, 0, 841, 595]}, (0, 7725): {'/Type': '/Page', '/Parent': IndirectObject(2, 0), '/Resources': IndirectObject(7723, 0), '/Contents': [IndirectObject(7722, 0)], '/MediaBox': [0, 0, 841, 595], '/CropBox': [0, 0, 841, 595]}, (0, 7729): {'/Type': '/Page', '/Parent': IndirectObject(2, 0), '/Resources': IndirectObject(7727, 0), '/Contents': [IndirectObject(7726, 0)], '/MediaBox': [0, 0, 841, 595], '/CropBox': [0, 0, 841, 595]}, (0, 7733): {'/Type': '/Page', '/Parent': IndirectObject(2, 0), '/Resources': IndirectObject(7731, 0), '/Contents': [IndirectObject(7730, 0)], '/MediaBox': [0, 0, 841, 595], '/CropBox': [0, 0, 841, 595]}, (0, 7737): {'/Type': '/Page', '/Parent': IndirectObject(2, 0), '/Resources': IndirectObject(7735, 0), '/Contents': [IndirectObject(7734, 0)], '/MediaBox': [0, 0, 841, 595], '/CropBox': [0, 0, 841, 595]}, (0, 7741): {'/Type': '/Page', '/Parent': IndirectObject(2, 0), '/Resources': IndirectObject(7739, 0), '/Contents': [IndirectObject(7738, 0)], '/MediaBox': [0, 0, 841, 595], '/CropBox': [0, 0, 841, 595]}, (0, 7745): {'/Type': '/Page', '/Parent': IndirectObject(2, 0), '/Resources': IndirectObject(7743, 0), '/Contents': [IndirectObject(7742, 0)], '/MediaBox': [0, 0, 841, 595], '/CropBox': [0, 0, 841, 595]}, (0, 7749): {'/Type': '/Page', '/Parent': IndirectObject(2, 0), '/Resources': IndirectObject(7747, 0), '/Contents': [IndirectObject(7746, 0)], '/MediaBox': [0, 0, 841, 595], '/CropBox': [0, 0, 841, 595]}, (0, 7753): {'/Type': '/Page', '/Parent': IndirectObject(2, 0), '/Resources': IndirectObject(7751, 0), '/Contents': [IndirectObject(7750, 0)], '/MediaBox': [0, 0, 841, 595], '/CropBox': [0, 0, 841, 595]}, (0, 7757): {'/Type': '/Page', '/Parent': IndirectObject(2, 0), '/Resources': IndirectObject(7755, 0), '/Contents': [IndirectObject(7754, 0)], '/MediaBox': [0, 0, 841, 595], '/CropBox': [0, 0, 841, 595]}, (0, 7761): {'/Type': '/Page', '/Parent': IndirectObject(2, 0), '/Resources': IndirectObject(7759, 0), '/Contents': [IndirectObject(7758, 0)], '/MediaBox': [0, 0, 841, 595], '/CropBox': [0, 0, 841, 595]}, (0, 7765): {'/Type': '/Page', '/Parent': IndirectObject(2, 0), '/Resources': IndirectObject(7763, 0), '/Contents': [IndirectObject(7762, 0)], '/MediaBox': [0, 0, 841, 595], '/CropBox': [0, 0, 841, 595]}, (0, 7769): {'/Type': '/Page', '/Parent': IndirectObject(2, 0), '/Resources': IndirectObject(7767, 0), '/Contents': [IndirectObject(7766, 0)], '/MediaBox': [0, 0, 841, 595], '/CropBox': [0, 0, 841, 595]}, (0, 7773): {'/Type': '/Page', '/Parent': IndirectObject(2, 0), '/Resources': IndirectObject(7771, 0), '/Contents': [IndirectObject(7770, 0)], '/MediaBox': [0, 0, 841, 595], '/CropBox': [0, 0, 841, 595]}, (0, 7777): {'/Type': '/Page', '/Parent': IndirectObject(2, 0), '/Resources': IndirectObject(7775, 0), '/Contents': [IndirectObject(7774, 0)], '/MediaBox': [0, 0, 841, 595], '/CropBox': [0, 0, 841, 595]}, (0, 7781): {'/Type': '/Page', '/Parent': IndirectObject(2, 0), '/Resources': IndirectObject(7779, 0), '/Contents': [IndirectObject(7778, 0)], '/MediaBox': [0, 0, 841, 595], '/CropBox': [0, 0, 841, 595]}, (0, 7785): {'/Type': '/Page', '/Parent': IndirectObject(2, 0), '/Resources': IndirectObject(7783, 0), '/Contents': [IndirectObject(7782, 0)], '/MediaBox': [0, 0, 841, 595], '/CropBox': [0, 0, 841, 595]}, (0, 7789): {'/Type': '/Page', '/Parent': IndirectObject(2, 0), '/Resources': IndirectObject(7787, 0), '/Contents': [IndirectObject(7786, 0)], '/MediaBox': [0, 0, 841, 595], '/CropBox': [0, 0, 841, 595]}, (0, 7793): {'/Type': '/Page', '/Parent': IndirectObject(2, 0), '/Resources': IndirectObject(7791, 0), '/Contents': [IndirectObject(7790, 0)], '/MediaBox': [0, 0, 841, 595], '/CropBox': [0, 0, 841, 595]}, (0, 7797): {'/Type': '/Page', '/Parent': IndirectObject(2, 0), '/Resources': IndirectObject(7795, 0), '/Contents': [IndirectObject(7794, 0)], '/MediaBox': [0, 0, 841, 595], '/CropBox': [0, 0, 841, 595]}, (0, 7801): {'/Type': '/Page', '/Parent': IndirectObject(2, 0), '/Resources': IndirectObject(7799, 0), '/Contents': [IndirectObject(7798, 0)], '/MediaBox': [0, 0, 841, 595], '/CropBox': [0, 0, 841, 595]}, (0, 7805): {'/Type': '/Page', '/Parent': IndirectObject(2, 0), '/Resources': IndirectObject(7803, 0), '/Contents': [IndirectObject(7802, 0)], '/MediaBox': [0, 0, 841, 595], '/CropBox': [0, 0, 841, 595]}, (0, 7809): {'/Type': '/Page', '/Parent': IndirectObject(2, 0), '/Resources': IndirectObject(7807, 0), '/Contents': [IndirectObject(7806, 0)], '/MediaBox': [0, 0, 841, 595], '/CropBox': [0, 0, 841, 595]}, (0, 7813): {'/Type': '/Page', '/Parent': IndirectObject(2, 0), '/Resources': IndirectObject(7811, 0), '/Contents': [IndirectObject(7810, 0)], '/MediaBox': [0, 0, 841, 595], '/CropBox': [0, 0, 841, 595]}, (0, 7817): {'/Type': '/Page', '/Parent': IndirectObject(2, 0), '/Resources': IndirectObject(7815, 0), '/Contents': [IndirectObject(7814, 0)], '/MediaBox': [0, 0, 841, 595], '/CropBox': [0, 0, 841, 595]}, (0, 7821): {'/Type': '/Page', '/Parent': IndirectObject(2, 0), '/Resources': IndirectObject(7819, 0), '/Contents': [IndirectObject(7818, 0)], '/MediaBox': [0, 0, 841, 595], '/CropBox': [0, 0, 841, 595]}, (0, 7825): {'/Type': '/Page', '/Parent': IndirectObject(2, 0), '/Resources': IndirectObject(7823, 0), '/Contents': [IndirectObject(7822, 0)], '/MediaBox': [0, 0, 841, 595], '/CropBox': [0, 0, 841, 595]}, (0, 7829): {'/Type': '/Page', '/Parent': IndirectObject(2, 0), '/Resources': IndirectObject(7827, 0), '/Contents': [IndirectObject(7826, 0)], '/MediaBox': [0, 0, 841, 595], '/CropBox': [0, 0, 841, 595]}, (0, 7833): {'/Type': '/Page', '/Parent': IndirectObject(2, 0), '/Resources': IndirectObject(7831, 0), '/Contents': [IndirectObject(7830, 0)], '/MediaBox': [0, 0, 841, 595], '/CropBox': [0, 0, 841, 595]}, (0, 7837): {'/Type': '/Page', '/Parent': IndirectObject(2, 0), '/Resources': IndirectObject(7835, 0), '/Contents': [IndirectObject(7834, 0)], '/MediaBox': [0, 0, 841, 595], '/CropBox': [0, 0, 841, 595]}, (0, 7841): {'/Type': '/Page', '/Parent': IndirectObject(2, 0), '/Resources': IndirectObject(7839, 0), '/Contents': [IndirectObject(7838, 0)], '/MediaBox': [0, 0, 841, 595], '/CropBox': [0, 0, 841, 595]}, (0, 7845): {'/Type': '/Page', '/Parent': IndirectObject(2, 0), '/Resources': IndirectObject(7843, 0), '/Contents': [IndirectObject(7842, 0)], '/MediaBox': [0, 0, 841, 595], '/CropBox': [0, 0, 841, 595]}, (0, 7849): {'/Type': '/Page', '/Parent': IndirectObject(2, 0), '/Resources': IndirectObject(7847, 0), '/Contents': [IndirectObject(7846, 0)], '/MediaBox': [0, 0, 841, 595], '/CropBox': [0, 0, 841, 595]}, (0, 7853): {'/Type': '/Page', '/Parent': IndirectObject(2, 0), '/Resources': IndirectObject(7851, 0), '/Contents': [IndirectObject(7850, 0)], '/MediaBox': [0, 0, 841, 595], '/CropBox': [0, 0, 841, 595]}, (0, 7857): {'/Type': '/Page', '/Parent': IndirectObject(2, 0), '/Resources': IndirectObject(7855, 0), '/Contents': [IndirectObject(7854, 0)], '/MediaBox': [0, 0, 841, 595], '/CropBox': [0, 0, 841, 595]}, (0, 7861): {'/Type': '/Page', '/Parent': IndirectObject(2, 0), '/Resources': IndirectObject(7859, 0), '/Contents': [IndirectObject(7858, 0)], '/MediaBox': [0, 0, 841, 595], '/CropBox': [0, 0, 841, 595]}, (0, 7865): {'/Type': '/Page', '/Parent': IndirectObject(2, 0), '/Resources': IndirectObject(7863, 0), '/Contents': [IndirectObject(7862, 0)], '/MediaBox': [0, 0, 841, 595], '/CropBox': [0, 0, 841, 595]}, (0, 7869): {'/Type': '/Page', '/Parent': IndirectObject(2, 0), '/Resources': IndirectObject(7867, 0), '/Contents': [IndirectObject(7866, 0)], '/MediaBox': [0, 0, 841, 595], '/CropBox': [0, 0, 841, 595]}, (0, 7873): {'/Type': '/Page', '/Parent': IndirectObject(2, 0), '/Resources': IndirectObject(7871, 0), '/Contents': [IndirectObject(7870, 0)], '/MediaBox': [0, 0, 841, 595], '/CropBox': [0, 0, 841, 595]}, (0, 7877): {'/Type': '/Page', '/Parent': IndirectObject(2, 0), '/Resources': IndirectObject(7875, 0), '/Contents': [IndirectObject(7874, 0)], '/MediaBox': [0, 0, 841, 595], '/CropBox': [0, 0, 841, 595]}, (0, 7881): {'/Type': '/Page', '/Parent': IndirectObject(2, 0), '/Resources': IndirectObject(7879, 0), '/Contents': [IndirectObject(7878, 0)], '/MediaBox': [0, 0, 841, 595], '/CropBox': [0, 0, 841, 595]}, (0, 7885): {'/Type': '/Page', '/Parent': IndirectObject(2, 0), '/Resources': IndirectObject(7883, 0), '/Contents': [IndirectObject(7882, 0)], '/MediaBox': [0, 0, 841, 595], '/CropBox': [0, 0, 841, 595]}, (0, 7889): {'/Type': '/Page', '/Parent': IndirectObject(2, 0), '/Resources': IndirectObject(7887, 0), '/Contents': [IndirectObject(7886, 0)], '/MediaBox': [0, 0, 841, 595], '/CropBox': [0, 0, 841, 595]}, (0, 7893): {'/Type': '/Page', '/Parent': IndirectObject(2, 0), '/Resources': IndirectObject(7891, 0), '/Contents': [IndirectObject(7890, 0)], '/MediaBox': [0, 0, 841, 595], '/CropBox': [0, 0, 841, 595]}, (0, 7897): {'/Type': '/Page', '/Parent': IndirectObject(2, 0), '/Resources': IndirectObject(7895, 0), '/Contents': [IndirectObject(7894, 0)], '/MediaBox': [0, 0, 841, 595], '/CropBox': [0, 0, 841, 595]}, (0, 7901): {'/Type': '/Page', '/Parent': IndirectObject(2, 0), '/Resources': IndirectObject(7899, 0), '/Contents': [IndirectObject(7898, 0)], '/MediaBox': [0, 0, 841, 595], '/CropBox': [0, 0, 841, 595]}, (0, 7905): {'/Type': '/Page', '/Parent': IndirectObject(2, 0), '/Resources': IndirectObject(7903, 0), '/Contents': [IndirectObject(7902, 0)], '/MediaBox': [0, 0, 841, 595], '/CropBox': [0, 0, 841, 595]}, (0, 7909): {'/Type': '/Page', '/Parent': IndirectObject(2, 0), '/Resources': IndirectObject(7907, 0), '/Contents': [IndirectObject(7906, 0)], '/MediaBox': [0, 0, 841, 595], '/CropBox': [0, 0, 841, 595]}, (0, 7913): {'/Type': '/Page', '/Parent': IndirectObject(2, 0), '/Resources': IndirectObject(7911, 0), '/Contents': [IndirectObject(7910, 0)], '/MediaBox': [0, 0, 841, 595], '/CropBox': [0, 0, 841, 595]}, (0, 7917): {'/Type': '/Page', '/Parent': IndirectObject(2, 0), '/Resources': IndirectObject(7915, 0), '/Contents': [IndirectObject(7914, 0)], '/MediaBox': [0, 0, 841, 595], '/CropBox': [0, 0, 841, 595]}, (0, 7921): {'/Type': '/Page', '/Parent': IndirectObject(2, 0), '/Resources': IndirectObject(7919, 0), '/Contents': [IndirectObject(7918, 0)], '/MediaBox': [0, 0, 841, 595], '/CropBox': [0, 0, 841, 595]}, (0, 7925): {'/Type': '/Page', '/Parent': IndirectObject(2, 0), '/Resources': IndirectObject(7923, 0), '/Contents': [IndirectObject(7922, 0)], '/MediaBox': [0, 0, 841, 595], '/CropBox': [0, 0, 841, 595]}, (0, 7929): {'/Type': '/Page', '/Parent': IndirectObject(2, 0), '/Resources': IndirectObject(7927, 0), '/Contents': [IndirectObject(7926, 0)], '/MediaBox': [0, 0, 841, 595], '/CropBox': [0, 0, 841, 595]}, (0, 7933): {'/Type': '/Page', '/Parent': IndirectObject(2, 0), '/Resources': IndirectObject(7931, 0), '/Contents': [IndirectObject(7930, 0)], '/MediaBox': [0, 0, 841, 595], '/CropBox': [0, 0, 841, 595]}, (0, 7937): {'/Type': '/Page', '/Parent': IndirectObject(2, 0), '/Resources': IndirectObject(7935, 0), '/Contents': [IndirectObject(7934, 0)], '/MediaBox': [0, 0, 841, 595], '/CropBox': [0, 0, 841, 595]}, (0, 7941): {'/Type': '/Page', '/Parent': IndirectObject(2, 0), '/Resources': IndirectObject(7939, 0), '/Contents': [IndirectObject(7938, 0)], '/MediaBox': [0, 0, 841, 595], '/CropBox': [0, 0, 841, 595]}, (0, 7945): {'/Type': '/Page', '/Parent': IndirectObject(2, 0), '/Resources': IndirectObject(7943, 0), '/Contents': [IndirectObject(7942, 0)], '/MediaBox': [0, 0, 841, 595], '/CropBox': [0, 0, 841, 595]}, (0, 7949): {'/Type': '/Page', '/Parent': IndirectObject(2, 0), '/Resources': IndirectObject(7947, 0), '/Contents': [IndirectObject(7946, 0)], '/MediaBox': [0, 0, 841, 595], '/CropBox': [0, 0, 841, 595]}, (0, 7953): {'/Type': '/Page', '/Parent': IndirectObject(2, 0), '/Resources': IndirectObject(7951, 0), '/Contents': [IndirectObject(7950, 0)], '/MediaBox': [0, 0, 841, 595], '/CropBox': [0, 0, 841, 595]}, (0, 7957): {'/Type': '/Page', '/Parent': IndirectObject(2, 0), '/Resources': IndirectObject(7955, 0), '/Contents': [IndirectObject(7954, 0)], '/MediaBox': [0, 0, 841, 595], '/CropBox': [0, 0, 841, 595]}, (0, 7961): {'/Type': '/Page', '/Parent': IndirectObject(2, 0), '/Resources': IndirectObject(7959, 0), '/Contents': [IndirectObject(7958, 0)], '/MediaBox': [0, 0, 841, 595], '/CropBox': [0, 0, 841, 595]}, (0, 7965): {'/Type': '/Page', '/Parent': IndirectObject(2, 0), '/Resources': IndirectObject(7963, 0), '/Contents': [IndirectObject(7962, 0)], '/MediaBox': [0, 0, 841, 595], '/CropBox': [0, 0, 841, 595]}, (0, 7969): {'/Type': '/Page', '/Parent': IndirectObject(2, 0), '/Resources': IndirectObject(7967, 0), '/Contents': [IndirectObject(7966, 0)], '/MediaBox': [0, 0, 841, 595], '/CropBox': [0, 0, 841, 595]}, (0, 7973): {'/Type': '/Page', '/Parent': IndirectObject(2, 0), '/Resources': IndirectObject(7971, 0), '/Contents': [IndirectObject(7970, 0)], '/MediaBox': [0, 0, 841, 595], '/CropBox': [0, 0, 841, 595]}, (0, 7977): {'/Type': '/Page', '/Parent': IndirectObject(2, 0), '/Resources': IndirectObject(7975, 0), '/Contents': [IndirectObject(7974, 0)], '/MediaBox': [0, 0, 841, 595], '/CropBox': [0, 0, 841, 595]}, (0, 7981): {'/Type': '/Page', '/Parent': IndirectObject(2, 0), '/Resources': IndirectObject(7979, 0), '/Contents': [IndirectObject(7978, 0)], '/MediaBox': [0, 0, 841, 595], '/CropBox': [0, 0, 841, 595]}, (0, 7985): {'/Type': '/Page', '/Parent': IndirectObject(2, 0), '/Resources': IndirectObject(7983, 0), '/Contents': [IndirectObject(7982, 0)], '/MediaBox': [0, 0, 841, 595], '/CropBox': [0, 0, 841, 595]}, (0, 7989): {'/Type': '/Page', '/Parent': IndirectObject(2, 0), '/Resources': IndirectObject(7987, 0), '/Contents': [IndirectObject(7986, 0)], '/MediaBox': [0, 0, 841, 595], '/CropBox': [0, 0, 841, 595]}, (0, 7993): {'/Type': '/Page', '/Parent': IndirectObject(2, 0), '/Resources': IndirectObject(7991, 0), '/Contents': [IndirectObject(7990, 0)], '/MediaBox': [0, 0, 841, 595], '/CropBox': [0, 0, 841, 595]}, (0, 7997): {'/Type': '/Page', '/Parent': IndirectObject(2, 0), '/Resources': IndirectObject(7995, 0), '/Contents': [IndirectObject(7994, 0)], '/MediaBox': [0, 0, 841, 595], '/CropBox': [0, 0, 841, 595]}, (0, 8001): {'/Type': '/Page', '/Parent': IndirectObject(2, 0), '/Resources': IndirectObject(7999, 0), '/Contents': [IndirectObject(7998, 0)], '/MediaBox': [0, 0, 841, 595], '/CropBox': [0, 0, 841, 595]}, (0, 8005): {'/Type': '/Page', '/Parent': IndirectObject(2, 0), '/Resources': IndirectObject(8003, 0), '/Contents': [IndirectObject(8002, 0)], '/MediaBox': [0, 0, 841, 595], '/CropBox': [0, 0, 841, 595]}, (0, 8009): {'/Type': '/Page', '/Parent': IndirectObject(2, 0), '/Resources': IndirectObject(8007, 0), '/Contents': [IndirectObject(8006, 0)], '/MediaBox': [0, 0, 841, 595], '/CropBox': [0, 0, 841, 595]}, (0, 8013): {'/Type': '/Page', '/Parent': IndirectObject(2, 0), '/Resources': IndirectObject(8011, 0), '/Contents': [IndirectObject(8010, 0)], '/MediaBox': [0, 0, 841, 595], '/CropBox': [0, 0, 841, 595]}, (0, 8017): {'/Type': '/Page', '/Parent': IndirectObject(2, 0), '/Resources': IndirectObject(8015, 0), '/Contents': [IndirectObject(8014, 0)], '/MediaBox': [0, 0, 841, 595], '/CropBox': [0, 0, 841, 595]}, (0, 8021): {'/Type': '/Page', '/Parent': IndirectObject(2, 0), '/Resources': IndirectObject(8019, 0), '/Contents': [IndirectObject(8018, 0)], '/MediaBox': [0, 0, 841, 595], '/CropBox': [0, 0, 841, 595]}, (0, 8025): {'/Type': '/Page', '/Parent': IndirectObject(2, 0), '/Resources': IndirectObject(8023, 0), '/Contents': [IndirectObject(8022, 0)], '/MediaBox': [0, 0, 841, 595], '/CropBox': [0, 0, 841, 595]}, (0, 8029): {'/Type': '/Page', '/Parent': IndirectObject(2, 0), '/Resources': IndirectObject(8027, 0), '/Contents': [IndirectObject(8026, 0)], '/MediaBox': [0, 0, 841, 595], '/CropBox': [0, 0, 841, 595]}, (0, 8033): {'/Type': '/Page', '/Parent': IndirectObject(2, 0), '/Resources': IndirectObject(8031, 0), '/Contents': [IndirectObject(8030, 0)], '/MediaBox': [0, 0, 841, 595], '/CropBox': [0, 0, 841, 595]}, (0, 8037): {'/Type': '/Page', '/Parent': IndirectObject(2, 0), '/Resources': IndirectObject(8035, 0), '/Contents': [IndirectObject(8034, 0)], '/MediaBox': [0, 0, 841, 595], '/CropBox': [0, 0, 841, 595]}, (0, 8041): {'/Type': '/Page', '/Parent': IndirectObject(2, 0), '/Resources': IndirectObject(8039, 0), '/Contents': [IndirectObject(8038, 0)], '/MediaBox': [0, 0, 841, 595], '/CropBox': [0, 0, 841, 595]}, (0, 8045): {'/Type': '/Page', '/Parent': IndirectObject(2, 0), '/Resources': IndirectObject(8043, 0), '/Contents': [IndirectObject(8042, 0)], '/MediaBox': [0, 0, 841, 595], '/CropBox': [0, 0, 841, 595]}, (0, 8049): {'/Type': '/Page', '/Parent': IndirectObject(2, 0), '/Resources': IndirectObject(8047, 0), '/Contents': [IndirectObject(8046, 0)], '/MediaBox': [0, 0, 841, 595], '/CropBox': [0, 0, 841, 595]}, (0, 8053): {'/Type': '/Page', '/Parent': IndirectObject(2, 0), '/Resources': IndirectObject(8051, 0), '/Contents': [IndirectObject(8050, 0)], '/MediaBox': [0, 0, 841, 595], '/CropBox': [0, 0, 841, 595]}, (0, 8057): {'/Type': '/Page', '/Parent': IndirectObject(2, 0), '/Resources': IndirectObject(8055, 0), '/Contents': [IndirectObject(8054, 0)], '/MediaBox': [0, 0, 841, 595], '/CropBox': [0, 0, 841, 595]}, (0, 8061): {'/Type': '/Page', '/Parent': IndirectObject(2, 0), '/Resources': IndirectObject(8059, 0), '/Contents': [IndirectObject(8058, 0)], '/MediaBox': [0, 0, 841, 595], '/CropBox': [0, 0, 841, 595]}, (0, 8065): {'/Type': '/Page', '/Parent': IndirectObject(2, 0), '/Resources': IndirectObject(8063, 0), '/Contents': [IndirectObject(8062, 0)], '/MediaBox': [0, 0, 841, 595], '/CropBox': [0, 0, 841, 595]}, (0, 8069): {'/Type': '/Page', '/Parent': IndirectObject(2, 0), '/Resources': IndirectObject(8067, 0), '/Contents': [IndirectObject(8066, 0)], '/MediaBox': [0, 0, 841, 595], '/CropBox': [0, 0, 841, 595]}, (0, 8073): {'/Type': '/Page', '/Parent': IndirectObject(2, 0), '/Resources': IndirectObject(8071, 0), '/Contents': [IndirectObject(8070, 0)], '/MediaBox': [0, 0, 841, 595], '/CropBox': [0, 0, 841, 595]}, (0, 8077): {'/Type': '/Page', '/Parent': IndirectObject(2, 0), '/Resources': IndirectObject(8075, 0), '/Contents': [IndirectObject(8074, 0)], '/MediaBox': [0, 0, 841, 595], '/CropBox': [0, 0, 841, 595]}, (0, 8081): {'/Type': '/Page', '/Parent': IndirectObject(2, 0), '/Resources': IndirectObject(8079, 0), '/Contents': [IndirectObject(8078, 0)], '/MediaBox': [0, 0, 841, 595], '/CropBox': [0, 0, 841, 595]}, (0, 8085): {'/Type': '/Page', '/Parent': IndirectObject(2, 0), '/Resources': IndirectObject(8083, 0), '/Contents': [IndirectObject(8082, 0)], '/MediaBox': [0, 0, 841, 595], '/CropBox': [0, 0, 841, 595]}, (0, 8089): {'/Type': '/Page', '/Parent': IndirectObject(2, 0), '/Resources': IndirectObject(8087, 0), '/Contents': [IndirectObject(8086, 0)], '/MediaBox': [0, 0, 841, 595], '/CropBox': [0, 0, 841, 595]}, (0, 8093): {'/Type': '/Page', '/Parent': IndirectObject(2, 0), '/Resources': IndirectObject(8091, 0), '/Contents': [IndirectObject(8090, 0)], '/MediaBox': [0, 0, 841, 595], '/CropBox': [0, 0, 841, 595]}, (0, 8097): {'/Type': '/Page', '/Parent': IndirectObject(2, 0), '/Resources': IndirectObject(8095, 0), '/Contents': [IndirectObject(8094, 0)], '/MediaBox': [0, 0, 841, 595], '/CropBox': [0, 0, 841, 595]}, (0, 8101): {'/Type': '/Page', '/Parent': IndirectObject(2, 0), '/Resources': IndirectObject(8099, 0), '/Contents': [IndirectObject(8098, 0)], '/MediaBox': [0, 0, 841, 595], '/CropBox': [0, 0, 841, 595]}, (0, 8105): {'/Type': '/Page', '/Parent': IndirectObject(2, 0), '/Resources': IndirectObject(8103, 0), '/Contents': [IndirectObject(8102, 0)], '/MediaBox': [0, 0, 841, 595], '/CropBox': [0, 0, 841, 595]}, (0, 8109): {'/Type': '/Page', '/Parent': IndirectObject(2, 0), '/Resources': IndirectObject(8107, 0), '/Contents': [IndirectObject(8106, 0)], '/MediaBox': [0, 0, 841, 595], '/CropBox': [0, 0, 841, 595]}, (0, 8113): {'/Type': '/Page', '/Parent': IndirectObject(2, 0), '/Resources': IndirectObject(8111, 0), '/Contents': [IndirectObject(8110, 0)], '/MediaBox': [0, 0, 841, 595], '/CropBox': [0, 0, 841, 595]}, (0, 8117): {'/Type': '/Page', '/Parent': IndirectObject(2, 0), '/Resources': IndirectObject(8115, 0), '/Contents': [IndirectObject(8114, 0)], '/MediaBox': [0, 0, 841, 595], '/CropBox': [0, 0, 841, 595]}, (0, 8121): {'/Type': '/Page', '/Parent': IndirectObject(2, 0), '/Resources': IndirectObject(8119, 0), '/Contents': [IndirectObject(8118, 0)], '/MediaBox': [0, 0, 841, 595], '/CropBox': [0, 0, 841, 595]}, (0, 8125): {'/Type': '/Page', '/Parent': IndirectObject(2, 0), '/Resources': IndirectObject(8123, 0), '/Contents': [IndirectObject(8122, 0)], '/MediaBox': [0, 0, 841, 595], '/CropBox': [0, 0, 841, 595]}, (0, 8129): {'/Type': '/Page', '/Parent': IndirectObject(2, 0), '/Resources': IndirectObject(8127, 0), '/Contents': [IndirectObject(8126, 0)], '/MediaBox': [0, 0, 841, 595], '/CropBox': [0, 0, 841, 595]}, (0, 8133): {'/Type': '/Page', '/Parent': IndirectObject(2, 0), '/Resources': IndirectObject(8131, 0), '/Contents': [IndirectObject(8130, 0)], '/MediaBox': [0, 0, 841, 595], '/CropBox': [0, 0, 841, 595]}, (0, 8137): {'/Type': '/Page', '/Parent': IndirectObject(2, 0), '/Resources': IndirectObject(8135, 0), '/Contents': [IndirectObject(8134, 0)], '/MediaBox': [0, 0, 841, 595], '/CropBox': [0, 0, 841, 595]}, (0, 8141): {'/Type': '/Page', '/Parent': IndirectObject(2, 0), '/Resources': IndirectObject(8139, 0), '/Contents': [IndirectObject(8138, 0)], '/MediaBox': [0, 0, 841, 595], '/CropBox': [0, 0, 841, 595]}, (0, 8145): {'/Type': '/Page', '/Parent': IndirectObject(2, 0), '/Resources': IndirectObject(8143, 0), '/Contents': [IndirectObject(8142, 0)], '/MediaBox': [0, 0, 841, 595], '/CropBox': [0, 0, 841, 595]}, (0, 8149): {'/Type': '/Page', '/Parent': IndirectObject(2, 0), '/Resources': IndirectObject(8147, 0), '/Contents': [IndirectObject(8146, 0)], '/MediaBox': [0, 0, 841, 595], '/CropBox': [0, 0, 841, 595]}, (0, 8153): {'/Type': '/Page', '/Parent': IndirectObject(2, 0), '/Resources': IndirectObject(8151, 0), '/Contents': [IndirectObject(8150, 0)], '/MediaBox': [0, 0, 841, 595], '/CropBox': [0, 0, 841, 595]}, (0, 8157): {'/Type': '/Page', '/Parent': IndirectObject(2, 0), '/Resources': IndirectObject(8155, 0), '/Contents': [IndirectObject(8154, 0)], '/MediaBox': [0, 0, 841, 595], '/CropBox': [0, 0, 841, 595]}, (0, 8161): {'/Type': '/Page', '/Parent': IndirectObject(2, 0), '/Resources': IndirectObject(8159, 0), '/Contents': [IndirectObject(8158, 0)], '/MediaBox': [0, 0, 841, 595], '/CropBox': [0, 0, 841, 595]}, (0, 8165): {'/Type': '/Page', '/Parent': IndirectObject(2, 0), '/Resources': IndirectObject(8163, 0), '/Contents': [IndirectObject(8162, 0)], '/MediaBox': [0, 0, 841, 595], '/CropBox': [0, 0, 841, 595]}, (0, 8169): {'/Type': '/Page', '/Parent': IndirectObject(2, 0), '/Resources': IndirectObject(8167, 0), '/Contents': [IndirectObject(8166, 0)], '/MediaBox': [0, 0, 841, 595], '/CropBox': [0, 0, 841, 595]}, (0, 8173): {'/Type': '/Page', '/Parent': IndirectObject(2, 0), '/Resources': IndirectObject(8171, 0), '/Contents': [IndirectObject(8170, 0)], '/MediaBox': [0, 0, 841, 595], '/CropBox': [0, 0, 841, 595]}, (0, 8177): {'/Type': '/Page', '/Parent': IndirectObject(2, 0), '/Resources': IndirectObject(8175, 0), '/Contents': [IndirectObject(8174, 0)], '/MediaBox': [0, 0, 841, 595], '/CropBox': [0, 0, 841, 595]}, (0, 8181): {'/Type': '/Page', '/Parent': IndirectObject(2, 0), '/Resources': IndirectObject(8179, 0), '/Contents': [IndirectObject(8178, 0)], '/MediaBox': [0, 0, 841, 595], '/CropBox': [0, 0, 841, 595]}, (0, 8185): {'/Type': '/Page', '/Parent': IndirectObject(2, 0), '/Resources': IndirectObject(8183, 0), '/Contents': [IndirectObject(8182, 0)], '/MediaBox': [0, 0, 841, 595], '/CropBox': [0, 0, 841, 595]}, (0, 8189): {'/Type': '/Page', '/Parent': IndirectObject(2, 0), '/Resources': IndirectObject(8187, 0), '/Contents': [IndirectObject(8186, 0)], '/MediaBox': [0, 0, 841, 595], '/CropBox': [0, 0, 841, 595]}, (0, 8193): {'/Type': '/Page', '/Parent': IndirectObject(2, 0), '/Resources': IndirectObject(8191, 0), '/Contents': [IndirectObject(8190, 0)], '/MediaBox': [0, 0, 841, 595], '/CropBox': [0, 0, 841, 595]}, (0, 8197): {'/Type': '/Page', '/Parent': IndirectObject(2, 0), '/Resources': IndirectObject(8195, 0), '/Contents': [IndirectObject(8194, 0)], '/MediaBox': [0, 0, 841, 595], '/CropBox': [0, 0, 841, 595]}, (0, 8201): {'/Type': '/Page', '/Parent': IndirectObject(2, 0), '/Resources': IndirectObject(8199, 0), '/Contents': [IndirectObject(8198, 0)], '/MediaBox': [0, 0, 841, 595], '/CropBox': [0, 0, 841, 595]}, (0, 8205): {'/Type': '/Page', '/Parent': IndirectObject(2, 0), '/Resources': IndirectObject(8203, 0), '/Contents': [IndirectObject(8202, 0)], '/MediaBox': [0, 0, 841, 595], '/CropBox': [0, 0, 841, 595]}, (0, 8209): {'/Type': '/Page', '/Parent': IndirectObject(2, 0), '/Resources': IndirectObject(8207, 0), '/Contents': [IndirectObject(8206, 0)], '/MediaBox': [0, 0, 841, 595], '/CropBox': [0, 0, 841, 595]}, (0, 8213): {'/Type': '/Page', '/Parent': IndirectObject(2, 0), '/Resources': IndirectObject(8211, 0), '/Contents': [IndirectObject(8210, 0)], '/MediaBox': [0, 0, 841, 595], '/CropBox': [0, 0, 841, 595]}, (0, 8217): {'/Type': '/Page', '/Parent': IndirectObject(2, 0), '/Resources': IndirectObject(8215, 0), '/Contents': [IndirectObject(8214, 0)], '/MediaBox': [0, 0, 841, 595], '/CropBox': [0, 0, 841, 595]}, (0, 8221): {'/Type': '/Page', '/Parent': IndirectObject(2, 0), '/Resources': IndirectObject(8219, 0), '/Contents': [IndirectObject(8218, 0)], '/MediaBox': [0, 0, 841, 595], '/CropBox': [0, 0, 841, 595]}, (0, 8225): {'/Type': '/Page', '/Parent': IndirectObject(2, 0), '/Resources': IndirectObject(8223, 0), '/Contents': [IndirectObject(8222, 0)], '/MediaBox': [0, 0, 841, 595], '/CropBox': [0, 0, 841, 595]}, (0, 8229): {'/Type': '/Page', '/Parent': IndirectObject(2, 0), '/Resources': IndirectObject(8227, 0), '/Contents': [IndirectObject(8226, 0)], '/MediaBox': [0, 0, 841, 595], '/CropBox': [0, 0, 841, 595]}, (0, 8233): {'/Type': '/Page', '/Parent': IndirectObject(2, 0), '/Resources': IndirectObject(8231, 0), '/Contents': [IndirectObject(8230, 0)], '/MediaBox': [0, 0, 841, 595], '/CropBox': [0, 0, 841, 595]}, (0, 8237): {'/Type': '/Page', '/Parent': IndirectObject(2, 0), '/Resources': IndirectObject(8235, 0), '/Contents': [IndirectObject(8234, 0)], '/MediaBox': [0, 0, 841, 595], '/CropBox': [0, 0, 841, 595]}, (0, 8241): {'/Type': '/Page', '/Parent': IndirectObject(2, 0), '/Resources': IndirectObject(8239, 0), '/Contents': [IndirectObject(8238, 0)], '/MediaBox': [0, 0, 841, 595], '/CropBox': [0, 0, 841, 595]}, (0, 8245): {'/Type': '/Page', '/Parent': IndirectObject(2, 0), '/Resources': IndirectObject(8243, 0), '/Contents': [IndirectObject(8242, 0)], '/MediaBox': [0, 0, 841, 595], '/CropBox': [0, 0, 841, 595]}, (0, 8249): {'/Type': '/Page', '/Parent': IndirectObject(2, 0), '/Resources': IndirectObject(8247, 0), '/Contents': [IndirectObject(8246, 0)], '/MediaBox': [0, 0, 841, 595], '/CropBox': [0, 0, 841, 595]}, (0, 8253): {'/Type': '/Page', '/Parent': IndirectObject(2, 0), '/Resources': IndirectObject(8251, 0), '/Contents': [IndirectObject(8250, 0)], '/MediaBox': [0, 0, 841, 595], '/CropBox': [0, 0, 841, 595]}, (0, 8257): {'/Type': '/Page', '/Parent': IndirectObject(2, 0), '/Resources': IndirectObject(8255, 0), '/Contents': [IndirectObject(8254, 0)], '/MediaBox': [0, 0, 841, 595], '/CropBox': [0, 0, 841, 595]}, (0, 8261): {'/Type': '/Page', '/Parent': IndirectObject(2, 0), '/Resources': IndirectObject(8259, 0), '/Contents': [IndirectObject(8258, 0)], '/MediaBox': [0, 0, 841, 595], '/CropBox': [0, 0, 841, 595]}, (0, 8265): {'/Type': '/Page', '/Parent': IndirectObject(2, 0), '/Resources': IndirectObject(8263, 0), '/Contents': [IndirectObject(8262, 0)], '/MediaBox': [0, 0, 841, 595], '/CropBox': [0, 0, 841, 595]}, (0, 46): {'/Filter': ['/FlateDecode']}}\n"
],
[
"from tabula import read_pdf",
"_____no_output_____"
],
[
"df = read_pdf('/tmp/metadata.pdf')",
"'pages' argument isn't specified.Will extract only from page 1 by default.\nGot stderr: Jun 13, 2020 2:58:35 AM org.apache.pdfbox.rendering.PDFRenderer suggestKCMS\nINFO: Your current java version is: 1.8.0_121\nJun 13, 2020 2:58:35 AM org.apache.pdfbox.rendering.PDFRenderer suggestKCMS\nINFO: To get higher rendering speed on old java 1.8 or 9 versions,\nJun 13, 2020 2:58:35 AM org.apache.pdfbox.rendering.PDFRenderer suggestKCMS\nINFO: update to the latest 1.8 or 9 version (>= 1.8.0_191 or >= 9.0.4),\nJun 13, 2020 2:58:35 AM org.apache.pdfbox.rendering.PDFRenderer suggestKCMS\nINFO: or\nJun 13, 2020 2:58:35 AM org.apache.pdfbox.rendering.PDFRenderer suggestKCMS\nINFO: use the option -Dsun.java2d.cmm=sun.java2d.cmm.kcms.KcmsServiceProvider\nJun 13, 2020 2:58:35 AM org.apache.pdfbox.rendering.PDFRenderer suggestKCMS\nINFO: or call System.setProperty(\"sun.java2d.cmm\", \"sun.java2d.cmm.kcms.KcmsServiceProvider\")\n\n"
],
[
"df",
"_____no_output_____"
]
]
] | [
"code"
] | [
[
"code",
"code",
"code",
"code",
"code",
"code",
"code",
"code",
"code",
"code",
"code",
"code",
"code",
"code",
"code",
"code",
"code",
"code",
"code",
"code",
"code",
"code",
"code",
"code",
"code",
"code",
"code",
"code"
]
] |
e76b9cd901f216ab74850181e36638c2ce82a955 | 81,730 | ipynb | Jupyter Notebook | notebooks/data-notebooks/cmip6/cmip6-zarr-jasmin.ipynb | RuthPetrie/ceda-notebooks | b1fb0a4106e19dab8bcf9c802bf34483a992a33f | [
"BSD-2-Clause"
] | 4 | 2020-06-16T12:17:19.000Z | 2021-05-12T15:35:41.000Z | notebooks/data-notebooks/cmip6/cmip6-zarr-jasmin.ipynb | RuthPetrie/ceda-notebooks | b1fb0a4106e19dab8bcf9c802bf34483a992a33f | [
"BSD-2-Clause"
] | 16 | 2020-05-26T14:49:57.000Z | 2022-02-21T13:50:47.000Z | notebooks/data-notebooks/cmip6/cmip6-zarr-jasmin.ipynb | RuthPetrie/ceda-notebooks | b1fb0a4106e19dab8bcf9c802bf34483a992a33f | [
"BSD-2-Clause"
] | 10 | 2020-05-19T15:02:18.000Z | 2022-03-24T10:55:26.000Z | 180.022026 | 60,576 | 0.884755 | [
[
[
"# Working with CMIP6 data in the JASMIN Object Store\nThis Notebook describes how to set up a virtual environment and then work with CMIP6 data in the JASMIN Object Store (stored in Zarr format).",
"_____no_output_____"
],
[
"## Start by creating a virtual environment and getting the packages installed...",
"_____no_output_____"
]
],
[
[
"# Import the required packages\nimport virtualenv\nimport pip\nimport os\n\n# Define and create the base directory install virtual environments\nvenvs_dir = os.path.join(os.path.expanduser(\"~\"), \"nb-venvs\")\n\nif not os.path.isdir(venvs_dir):\n os.makedirs(venvs_dir)\n \n# Define the venv directory\nvenv_dir = os.path.join(venvs_dir, 'venv-cmip6-zarr')",
"_____no_output_____"
],
[
"# Create the virtual environment\nvirtualenv.create_environment(venv_dir)",
"copying /opt/jaspy/bin/python => /home/users/astephen/nb-venvs/venv-cmip6-zarr/bin/python\n"
],
[
"# Activate the venv\nactivate_file = os.path.join(venv_dir, \"bin\", \"activate_this.py\")\nexec(open(activate_file).read(), dict(__file__=activate_file))",
"_____no_output_____"
],
[
"# Install a set of required packages via `pip`\nrequirements = ['fsspec', 'intake', 'intake_esm', 'aiohttp']\n\nfor pkg in requirements:\n pip.main([\"install\", \"--prefix\", venv_dir, pkg])",
"WARNING: pip is being invoked by an old script wrapper. This will fail in a future version of pip.\nPlease see https://github.com/pypa/pip/issues/5599 for advice on fixing the underlying issue.\nTo avoid this problem you can invoke Python with '-m pip' instead of running pip directly.\n"
]
],
[
[
"# Accessing CMIP6 Data from the JASMIN (Zarr) Object Store",
"_____no_output_____"
],
[
"**Pre-requisites**\n1. Required packages: `['xarray', 'zarr', 'fsspec', 'intake', 'intake_esm', 'aiohttp']`\n2. Data access: must be able to see JASMIN Object Store for CMIP6 (currently inside JASMIN firewall)",
"_____no_output_____"
],
[
"## Step 1: Import required packages",
"_____no_output_____"
]
],
[
[
"import xarray as xr\nimport intake\nimport intake_esm\nimport fsspec",
"_____no_output_____"
]
],
[
[
"## Step 2: read the CMIP6 Intake (ESM) catalog from github\nWe define a collection (\"col\") that can be searched/filtered for required datasets.",
"_____no_output_____"
]
],
[
[
"col_url = \"https://raw.githubusercontent.com/cedadev/\" \\\n \"cmip6-object-store/master/catalogs/ceda-zarr-cmip6.json\"\ncol = intake.open_esm_datastore(col_url)",
"_____no_output_____"
]
],
[
[
"How many datasets are currently stored?",
"_____no_output_____"
]
],
[
[
"f'There are {len(col.df)} datasets'",
"_____no_output_____"
]
],
[
[
"## Step 3: Filter the catalog for historical and future data\nIn this example, we want to compare the surface temperature (\"tas\") from the\nUKESM1-0-LL model, for a historical and future (\"ssp585-bgc\") scenario.",
"_____no_output_____"
]
],
[
[
"cat = col.search(source_id=\"UKESM1-0-LL\",\n experiment_id=[\"historical\", \"ssp585-bgc\"], \n member_id=[\"r4i1p1f2\", \"r12i1p1f2\"],\n table_id=\"Amon\",\n variable_id=\"tas\")\n\n# Extract the single record subsets for historical and future experiments\nhist_cat = cat.search(experiment_id='historical')\nssp_cat = cat.search(experiment_id='ssp585-bgc')",
"_____no_output_____"
]
],
[
[
"## Step 4: Convert to xarray datasets",
"_____no_output_____"
],
[
"Define a quick function to convert a catalog to an xarray `Dataset`.",
"_____no_output_____"
]
],
[
[
"def cat_to_ds(cat):\n zarr_path = cat.df['zarr_path'][0]\n fsmap = fsspec.get_mapper(zarr_path)\n return xr.open_zarr(fsmap, consolidated=True, use_cftime=True)",
"_____no_output_____"
]
],
[
[
"Extract the `tas` (surface air temperture) variable for the historical and future experiments.",
"_____no_output_____"
]
],
[
[
"hist_tas = cat_to_ds(hist_cat)['tas']\nssp_tas = cat_to_ds(ssp_cat)['tas']",
"_____no_output_____"
]
],
[
[
"## Step 5: Subtract the historical from the future average\nGenerate time-series means of historical and future data. Subtract the historical from the future scenario and plot the difference.",
"_____no_output_____"
]
],
[
[
"# Calculate time means\ndiff = ssp_tas.mean(axis=0) - hist_tas.mean(axis=0)\n\n# Plot a map of the time-series means\ndiff.plot()",
"_____no_output_____"
]
],
[
[
"## References\n- CMIP6 Object Store code (github): https://github.com/cedadev/cmip6-object-store\n- This notebook: https://github.com/cedadev/cmip6-object-store/blob/master/notebooks/cmip6-zarr-jasmin.ipynb",
"_____no_output_____"
]
]
] | [
"markdown",
"code",
"markdown",
"code",
"markdown",
"code",
"markdown",
"code",
"markdown",
"code",
"markdown",
"code",
"markdown",
"code",
"markdown",
"code",
"markdown"
] | [
[
"markdown",
"markdown"
],
[
"code",
"code",
"code",
"code"
],
[
"markdown",
"markdown",
"markdown"
],
[
"code"
],
[
"markdown"
],
[
"code"
],
[
"markdown"
],
[
"code"
],
[
"markdown"
],
[
"code"
],
[
"markdown",
"markdown"
],
[
"code"
],
[
"markdown"
],
[
"code"
],
[
"markdown"
],
[
"code"
],
[
"markdown"
]
] |
e76b9e33ceab9a2aaa73a7826495c82a31ad0aa7 | 92,625 | ipynb | Jupyter Notebook | basic codes/training_deep_neuralnet.ipynb | MachineLearningWithHuman/ComputerVision | 9929a3115241067da2dd4bcbdd628d4c78fa8072 | [
"Apache-2.0"
] | 3 | 2019-07-10T15:29:59.000Z | 2020-06-15T17:10:15.000Z | basic codes/training_deep_neuralnet.ipynb | MachineLearningWithHuman/ComputerVision | 9929a3115241067da2dd4bcbdd628d4c78fa8072 | [
"Apache-2.0"
] | null | null | null | basic codes/training_deep_neuralnet.ipynb | MachineLearningWithHuman/ComputerVision | 9929a3115241067da2dd4bcbdd628d4c78fa8072 | [
"Apache-2.0"
] | 1 | 2020-06-15T16:27:44.000Z | 2020-06-15T16:27:44.000Z | 148.914791 | 31,354 | 0.783136 | [
[
[
"!wget --no-check-certificate \\\n https://storage.googleapis.com/laurencemoroney-blog.appspot.com/horse-or-human.zip \\\n -O /tmp/horse-or-human.zip\n\n!wget --no-check-certificate \\\n https://storage.googleapis.com/laurencemoroney-blog.appspot.com/validation-horse-or-human.zip \\\n -O /tmp/validation-horse-or-human.zip\n \nimport os\nimport zipfile\n\nlocal_zip = '/tmp/horse-or-human.zip'\nzip_ref = zipfile.ZipFile(local_zip, 'r')\nzip_ref.extractall('/tmp/horse-or-human')\nlocal_zip = '/tmp/validation-horse-or-human.zip'\nzip_ref = zipfile.ZipFile(local_zip, 'r')\nzip_ref.extractall('/tmp/validation-horse-or-human')\nzip_ref.close()\n# Directory with our training horse pictures\ntrain_horse_dir = os.path.join('/tmp/horse-or-human/horses')\n\n# Directory with our training human pictures\ntrain_human_dir = os.path.join('/tmp/horse-or-human/humans')\n\n# Directory with our training horse pictures\nvalidation_horse_dir = os.path.join('/tmp/validation-horse-or-human/horses')\n\n# Directory with our training human pictures\nvalidation_human_dir = os.path.join('/tmp/validation-horse-or-human/humans')",
"--2020-06-14 21:48:48-- https://storage.googleapis.com/laurencemoroney-blog.appspot.com/horse-or-human.zip\nResolving storage.googleapis.com (storage.googleapis.com)... 74.125.135.128, 2607:f8b0:400e:c01::80\nConnecting to storage.googleapis.com (storage.googleapis.com)|74.125.135.128|:443... connected.\nHTTP request sent, awaiting response... 200 OK\nLength: 149574867 (143M) [application/zip]\nSaving to: โ/tmp/horse-or-human.zipโ\n\n\r/tmp/horse-or-human 0%[ ] 0 --.-KB/s \r/tmp/horse-or-human 4%[ ] 7.04M 35.2MB/s \r/tmp/horse-or-human 20%[===> ] 28.70M 71.7MB/s \r/tmp/horse-or-human 44%[=======> ] 63.11M 105MB/s \r/tmp/horse-or-human 50%[=========> ] 72.01M 81.4MB/s \r/tmp/horse-or-human 74%[=============> ] 106.13M 97.9MB/s \r/tmp/horse-or-human 100%[===================>] 142.65M 112MB/s in 1.3s \n\n2020-06-14 21:48:49 (112 MB/s) - โ/tmp/horse-or-human.zipโ saved [149574867/149574867]\n\n--2020-06-14 21:48:53-- https://storage.googleapis.com/laurencemoroney-blog.appspot.com/validation-horse-or-human.zip\nResolving storage.googleapis.com (storage.googleapis.com)... 74.125.142.128, 2607:f8b0:400e:c07::80\nConnecting to storage.googleapis.com (storage.googleapis.com)|74.125.142.128|:443... connected.\nHTTP request sent, awaiting response... 200 OK\nLength: 11480187 (11M) [application/zip]\nSaving to: โ/tmp/validation-horse-or-human.zipโ\n\n/tmp/validation-hor 100%[===================>] 10.95M 44.6MB/s in 0.2s \n\n2020-06-14 21:48:54 (44.6 MB/s) - โ/tmp/validation-horse-or-human.zipโ saved [11480187/11480187]\n\n"
]
],
[
[
"## Building a Small Model from Scratch\n\nBut before we continue, let's start defining the model:\n\nStep 1 will be to import tensorflow.",
"_____no_output_____"
]
],
[
[
"import tensorflow as tf",
"_____no_output_____"
]
],
[
[
"We then add convolutional layers as in the previous example, and flatten the final result to feed into the densely connected layers.",
"_____no_output_____"
],
[
"Finally we add the densely connected layers. \n\nNote that because we are facing a two-class classification problem, i.e. a *binary classification problem*, we will end our network with a [*sigmoid* activation](https://wikipedia.org/wiki/Sigmoid_function), so that the output of our network will be a single scalar between 0 and 1, encoding the probability that the current image is class 1 (as opposed to class 0).",
"_____no_output_____"
]
],
[
[
"model = tf.keras.models.Sequential([\n # Note the input shape is the desired size of the image 300x300 with 3 bytes color\n # This is the first convolution\n tf.keras.layers.Conv2D(16, (3,3), activation='relu', input_shape=(300, 300, 3)),\n tf.keras.layers.MaxPooling2D(2, 2),\n # The second convolution\n tf.keras.layers.Conv2D(32, (3,3), activation='relu'),\n tf.keras.layers.MaxPooling2D(2,2),\n # The third convolution\n tf.keras.layers.Conv2D(64, (3,3), activation='relu'),\n tf.keras.layers.MaxPooling2D(2,2),\n # The fourth convolution\n tf.keras.layers.Conv2D(64, (3,3), activation='relu'),\n tf.keras.layers.MaxPooling2D(2,2),\n # The fifth convolution\n tf.keras.layers.Conv2D(64, (3,3), activation='relu'),\n tf.keras.layers.MaxPooling2D(2,2),\n # Flatten the results to feed into a DNN\n tf.keras.layers.Flatten(),\n # 512 neuron hidden layer\n tf.keras.layers.Dense(512, activation='relu'),\n # Only 1 output neuron. It will contain a value from 0-1 where 0 for 1 class ('horses') and 1 for the other ('humans')\n tf.keras.layers.Dense(1, activation='sigmoid')\n])",
"_____no_output_____"
],
[
"from tensorflow.keras.optimizers import RMSprop\n\nmodel.compile(loss='binary_crossentropy',\n optimizer=RMSprop(lr=1e-4),\n metrics=['accuracy'])",
"_____no_output_____"
],
[
"model.summary()",
"Model: \"sequential\"\n_________________________________________________________________\nLayer (type) Output Shape Param # \n=================================================================\nconv2d (Conv2D) (None, 298, 298, 16) 448 \n_________________________________________________________________\nmax_pooling2d (MaxPooling2D) (None, 149, 149, 16) 0 \n_________________________________________________________________\nconv2d_1 (Conv2D) (None, 147, 147, 32) 4640 \n_________________________________________________________________\nmax_pooling2d_1 (MaxPooling2 (None, 73, 73, 32) 0 \n_________________________________________________________________\nconv2d_2 (Conv2D) (None, 71, 71, 64) 18496 \n_________________________________________________________________\nmax_pooling2d_2 (MaxPooling2 (None, 35, 35, 64) 0 \n_________________________________________________________________\nconv2d_3 (Conv2D) (None, 33, 33, 64) 36928 \n_________________________________________________________________\nmax_pooling2d_3 (MaxPooling2 (None, 16, 16, 64) 0 \n_________________________________________________________________\nconv2d_4 (Conv2D) (None, 14, 14, 64) 36928 \n_________________________________________________________________\nmax_pooling2d_4 (MaxPooling2 (None, 7, 7, 64) 0 \n_________________________________________________________________\nflatten (Flatten) (None, 3136) 0 \n_________________________________________________________________\ndense (Dense) (None, 512) 1606144 \n_________________________________________________________________\ndense_1 (Dense) (None, 1) 513 \n=================================================================\nTotal params: 1,704,097\nTrainable params: 1,704,097\nNon-trainable params: 0\n_________________________________________________________________\n"
],
[
"from tensorflow.keras.preprocessing.image import ImageDataGenerator\n\n# All images will be rescaled by 1./255\ntrain_datagen = ImageDataGenerator(\n rescale=1./255,\n rotation_range=40,\n width_shift_range=0.2,\n height_shift_range=0.2,\n shear_range=0.2,\n zoom_range=0.2,\n horizontal_flip=True,\n fill_mode='nearest')\n\nvalidation_datagen = ImageDataGenerator(rescale=1/255)\n\n# Flow training images in batches of 128 using train_datagen generator\ntrain_generator = train_datagen.flow_from_directory(\n '/tmp/horse-or-human/', # This is the source directory for training images\n target_size=(300, 300), # All images will be resized to 150x150\n batch_size=128,\n # Since we use binary_crossentropy loss, we need binary labels\n class_mode='binary')\n\n# Flow training images in batches of 128 using train_datagen generator\nvalidation_generator = validation_datagen.flow_from_directory(\n '/tmp/validation-horse-or-human/', # This is the source directory for training images\n target_size=(300, 300), # All images will be resized to 150x150\n batch_size=32,\n # Since we use binary_crossentropy loss, we need binary labels\n class_mode='binary')",
"Found 1027 images belonging to 2 classes.\nFound 256 images belonging to 2 classes.\n"
],
[
"history = model.fit(\n train_generator,\n steps_per_epoch=8, \n epochs=100,\n verbose=1,\n validation_data = validation_generator,\n validation_steps=8)",
"Epoch 1/100\n8/8 [==============================] - 16s 2s/step - loss: 0.6850 - accuracy: 0.5195 - val_loss: 0.6723 - val_accuracy: 0.5078\nEpoch 2/100\n8/8 [==============================] - 18s 2s/step - loss: 0.6714 - accuracy: 0.6107 - val_loss: 0.6565 - val_accuracy: 0.5156\nEpoch 3/100\n8/8 [==============================] - 18s 2s/step - loss: 0.6411 - accuracy: 0.6997 - val_loss: 0.5843 - val_accuracy: 0.7500\nEpoch 4/100\n8/8 [==============================] - 18s 2s/step - loss: 0.6114 - accuracy: 0.6830 - val_loss: 0.9304 - val_accuracy: 0.5000\nEpoch 5/100\n8/8 [==============================] - 18s 2s/step - loss: 0.6033 - accuracy: 0.6763 - val_loss: 0.4969 - val_accuracy: 0.7773\nEpoch 6/100\n8/8 [==============================] - 18s 2s/step - loss: 0.5549 - accuracy: 0.7308 - val_loss: 0.6154 - val_accuracy: 0.6797\nEpoch 7/100\n8/8 [==============================] - 20s 2s/step - loss: 0.5323 - accuracy: 0.7402 - val_loss: 0.5816 - val_accuracy: 0.7422\nEpoch 8/100\n8/8 [==============================] - 18s 2s/step - loss: 0.5727 - accuracy: 0.7419 - val_loss: 0.8476 - val_accuracy: 0.6094\nEpoch 9/100\n8/8 [==============================] - 18s 2s/step - loss: 0.4732 - accuracy: 0.7875 - val_loss: 0.8447 - val_accuracy: 0.6758\nEpoch 10/100\n8/8 [==============================] - 18s 2s/step - loss: 0.4938 - accuracy: 0.7664 - val_loss: 0.8145 - val_accuracy: 0.7070\nEpoch 11/100\n8/8 [==============================] - 18s 2s/step - loss: 0.6010 - accuracy: 0.7475 - val_loss: 0.9323 - val_accuracy: 0.6875\nEpoch 12/100\n8/8 [==============================] - 20s 3s/step - loss: 0.4725 - accuracy: 0.7720 - val_loss: 1.0448 - val_accuracy: 0.6602\nEpoch 13/100\n8/8 [==============================] - 20s 2s/step - loss: 0.4456 - accuracy: 0.7939 - val_loss: 1.0014 - val_accuracy: 0.6914\nEpoch 14/100\n8/8 [==============================] - 18s 2s/step - loss: 0.4696 - accuracy: 0.7675 - val_loss: 0.9309 - val_accuracy: 0.7031\nEpoch 15/100\n8/8 [==============================] - 18s 2s/step - loss: 0.4436 - accuracy: 0.7931 - val_loss: 1.3374 - val_accuracy: 0.6016\nEpoch 16/100\n8/8 [==============================] - 18s 2s/step - loss: 0.4536 - accuracy: 0.7753 - val_loss: 1.5529 - val_accuracy: 0.5781\nEpoch 17/100\n8/8 [==============================] - 18s 2s/step - loss: 0.4360 - accuracy: 0.7909 - val_loss: 0.9800 - val_accuracy: 0.6992\nEpoch 18/100\n8/8 [==============================] - 18s 2s/step - loss: 0.4015 - accuracy: 0.8320 - val_loss: 0.9342 - val_accuracy: 0.7109\nEpoch 19/100\n8/8 [==============================] - 18s 2s/step - loss: 0.4028 - accuracy: 0.8209 - val_loss: 0.6104 - val_accuracy: 0.8086\nEpoch 20/100\n8/8 [==============================] - 18s 2s/step - loss: 0.4738 - accuracy: 0.7820 - val_loss: 1.3574 - val_accuracy: 0.6641\nEpoch 21/100\n8/8 [==============================] - 20s 2s/step - loss: 0.3849 - accuracy: 0.8350 - val_loss: 1.0417 - val_accuracy: 0.7109\nEpoch 22/100\n8/8 [==============================] - 18s 2s/step - loss: 0.4200 - accuracy: 0.8087 - val_loss: 1.3183 - val_accuracy: 0.6719\nEpoch 23/100\n8/8 [==============================] - 18s 2s/step - loss: 0.3451 - accuracy: 0.8576 - val_loss: 0.4415 - val_accuracy: 0.8633\nEpoch 24/100\n8/8 [==============================] - 20s 3s/step - loss: 0.3420 - accuracy: 0.8532 - val_loss: 1.3908 - val_accuracy: 0.6875\nEpoch 25/100\n8/8 [==============================] - 18s 2s/step - loss: 0.3571 - accuracy: 0.8509 - val_loss: 2.6670 - val_accuracy: 0.5586\nEpoch 26/100\n8/8 [==============================] - 18s 2s/step - loss: 0.4049 - accuracy: 0.8131 - val_loss: 1.5005 - val_accuracy: 0.6758\nEpoch 27/100\n8/8 [==============================] - 18s 2s/step - loss: 0.3602 - accuracy: 0.8409 - val_loss: 1.8096 - val_accuracy: 0.6445\nEpoch 28/100\n8/8 [==============================] - 18s 2s/step - loss: 0.3194 - accuracy: 0.8710 - val_loss: 1.6951 - val_accuracy: 0.6680\nEpoch 29/100\n8/8 [==============================] - 21s 3s/step - loss: 0.3483 - accuracy: 0.8509 - val_loss: 1.6056 - val_accuracy: 0.6758\nEpoch 30/100\n8/8 [==============================] - 18s 2s/step - loss: 0.2935 - accuracy: 0.8843 - val_loss: 2.3601 - val_accuracy: 0.5859\nEpoch 31/100\n8/8 [==============================] - 18s 2s/step - loss: 0.3262 - accuracy: 0.8532 - val_loss: 2.1762 - val_accuracy: 0.6289\nEpoch 32/100\n8/8 [==============================] - 20s 3s/step - loss: 0.3651 - accuracy: 0.8365 - val_loss: 2.0033 - val_accuracy: 0.6211\nEpoch 33/100\n8/8 [==============================] - 18s 2s/step - loss: 0.3573 - accuracy: 0.8487 - val_loss: 1.8806 - val_accuracy: 0.6406\nEpoch 34/100\n8/8 [==============================] - 20s 2s/step - loss: 0.2932 - accuracy: 0.8779 - val_loss: 2.0488 - val_accuracy: 0.6133\nEpoch 35/100\n8/8 [==============================] - 18s 2s/step - loss: 0.2915 - accuracy: 0.8810 - val_loss: 1.5599 - val_accuracy: 0.6641\nEpoch 36/100\n8/8 [==============================] - 20s 3s/step - loss: 0.2844 - accuracy: 0.8936 - val_loss: 1.6776 - val_accuracy: 0.6875\nEpoch 37/100\n8/8 [==============================] - 18s 2s/step - loss: 0.2876 - accuracy: 0.8699 - val_loss: 1.5051 - val_accuracy: 0.6953\nEpoch 38/100\n8/8 [==============================] - 18s 2s/step - loss: 0.3352 - accuracy: 0.8699 - val_loss: 1.8497 - val_accuracy: 0.6484\nEpoch 39/100\n8/8 [==============================] - 20s 3s/step - loss: 0.2391 - accuracy: 0.9150 - val_loss: 2.2378 - val_accuracy: 0.6289\nEpoch 40/100\n8/8 [==============================] - 18s 2s/step - loss: 0.2856 - accuracy: 0.8776 - val_loss: 1.5164 - val_accuracy: 0.6992\nEpoch 41/100\n8/8 [==============================] - 18s 2s/step - loss: 0.2760 - accuracy: 0.8799 - val_loss: 2.1761 - val_accuracy: 0.6367\nEpoch 42/100\n8/8 [==============================] - 18s 2s/step - loss: 0.2208 - accuracy: 0.9088 - val_loss: 2.6893 - val_accuracy: 0.6055\nEpoch 43/100\n8/8 [==============================] - 18s 2s/step - loss: 0.2667 - accuracy: 0.8821 - val_loss: 1.8392 - val_accuracy: 0.6836\nEpoch 44/100\n8/8 [==============================] - 18s 2s/step - loss: 0.2285 - accuracy: 0.9143 - val_loss: 1.8571 - val_accuracy: 0.6914\nEpoch 45/100\n8/8 [==============================] - 18s 2s/step - loss: 0.3035 - accuracy: 0.8699 - val_loss: 2.4545 - val_accuracy: 0.6172\nEpoch 46/100\n8/8 [==============================] - 20s 3s/step - loss: 0.2251 - accuracy: 0.9010 - val_loss: 2.4441 - val_accuracy: 0.6484\nEpoch 47/100\n8/8 [==============================] - 18s 2s/step - loss: 0.3360 - accuracy: 0.8598 - val_loss: 2.7625 - val_accuracy: 0.6328\nEpoch 48/100\n8/8 [==============================] - 18s 2s/step - loss: 0.2022 - accuracy: 0.9199 - val_loss: 2.4598 - val_accuracy: 0.6484\nEpoch 49/100\n8/8 [==============================] - 20s 2s/step - loss: 0.1929 - accuracy: 0.9131 - val_loss: 2.2525 - val_accuracy: 0.6562\nEpoch 50/100\n8/8 [==============================] - 18s 2s/step - loss: 0.2752 - accuracy: 0.8821 - val_loss: 2.1146 - val_accuracy: 0.6680\nEpoch 51/100\n8/8 [==============================] - 18s 2s/step - loss: 0.2255 - accuracy: 0.8988 - val_loss: 1.9542 - val_accuracy: 0.6953\nEpoch 52/100\n8/8 [==============================] - 18s 2s/step - loss: 0.2108 - accuracy: 0.9210 - val_loss: 3.4228 - val_accuracy: 0.5781\nEpoch 53/100\n8/8 [==============================] - 18s 2s/step - loss: 0.1980 - accuracy: 0.9210 - val_loss: 2.4943 - val_accuracy: 0.6562\nEpoch 54/100\n8/8 [==============================] - 18s 2s/step - loss: 0.2021 - accuracy: 0.9199 - val_loss: 1.7572 - val_accuracy: 0.7109\nEpoch 55/100\n8/8 [==============================] - 20s 3s/step - loss: 0.2130 - accuracy: 0.9155 - val_loss: 2.5870 - val_accuracy: 0.6484\nEpoch 56/100\n8/8 [==============================] - 18s 2s/step - loss: 0.1931 - accuracy: 0.9188 - val_loss: 3.2605 - val_accuracy: 0.6172\nEpoch 57/100\n8/8 [==============================] - 20s 2s/step - loss: 0.1945 - accuracy: 0.9131 - val_loss: 2.2119 - val_accuracy: 0.6914\nEpoch 58/100\n8/8 [==============================] - 21s 3s/step - loss: 0.1416 - accuracy: 0.9511 - val_loss: 2.6175 - val_accuracy: 0.6680\nEpoch 59/100\n8/8 [==============================] - 18s 2s/step - loss: 0.2204 - accuracy: 0.9188 - val_loss: 2.4795 - val_accuracy: 0.6680\nEpoch 60/100\n8/8 [==============================] - 18s 2s/step - loss: 0.2644 - accuracy: 0.8966 - val_loss: 3.0486 - val_accuracy: 0.6445\nEpoch 61/100\n8/8 [==============================] - 18s 2s/step - loss: 0.1537 - accuracy: 0.9388 - val_loss: 2.9839 - val_accuracy: 0.6562\nEpoch 62/100\n8/8 [==============================] - 20s 2s/step - loss: 0.1723 - accuracy: 0.9307 - val_loss: 1.5448 - val_accuracy: 0.7383\nEpoch 63/100\n8/8 [==============================] - 18s 2s/step - loss: 0.2173 - accuracy: 0.9132 - val_loss: 2.5974 - val_accuracy: 0.6641\nEpoch 64/100\n8/8 [==============================] - 18s 2s/step - loss: 0.1546 - accuracy: 0.9455 - val_loss: 2.8638 - val_accuracy: 0.6562\nEpoch 65/100\n8/8 [==============================] - 18s 2s/step - loss: 0.1736 - accuracy: 0.9232 - val_loss: 4.7983 - val_accuracy: 0.5547\nEpoch 66/100\n8/8 [==============================] - 18s 2s/step - loss: 0.2429 - accuracy: 0.9066 - val_loss: 2.5306 - val_accuracy: 0.6641\nEpoch 67/100\n8/8 [==============================] - 20s 2s/step - loss: 0.1566 - accuracy: 0.9453 - val_loss: 2.4748 - val_accuracy: 0.6875\nEpoch 68/100\n8/8 [==============================] - 18s 2s/step - loss: 0.1462 - accuracy: 0.9444 - val_loss: 3.0807 - val_accuracy: 0.6562\nEpoch 69/100\n8/8 [==============================] - 20s 2s/step - loss: 0.1743 - accuracy: 0.9326 - val_loss: 1.6064 - val_accuracy: 0.7422\nEpoch 70/100\n8/8 [==============================] - 18s 2s/step - loss: 0.1594 - accuracy: 0.9399 - val_loss: 1.6763 - val_accuracy: 0.7422\nEpoch 71/100\n8/8 [==============================] - 18s 2s/step - loss: 0.3120 - accuracy: 0.8843 - val_loss: 2.3008 - val_accuracy: 0.6797\nEpoch 72/100\n8/8 [==============================] - 18s 2s/step - loss: 0.1494 - accuracy: 0.9477 - val_loss: 2.8251 - val_accuracy: 0.6562\nEpoch 73/100\n8/8 [==============================] - 18s 2s/step - loss: 0.1376 - accuracy: 0.9433 - val_loss: 3.7275 - val_accuracy: 0.6289\nEpoch 74/100\n8/8 [==============================] - 18s 2s/step - loss: 0.1485 - accuracy: 0.9377 - val_loss: 4.3073 - val_accuracy: 0.6016\nEpoch 75/100\n8/8 [==============================] - 18s 2s/step - loss: 0.2358 - accuracy: 0.8921 - val_loss: 3.1323 - val_accuracy: 0.6484\nEpoch 76/100\n8/8 [==============================] - 20s 3s/step - loss: 0.1771 - accuracy: 0.9266 - val_loss: 3.6355 - val_accuracy: 0.6484\nEpoch 77/100\n8/8 [==============================] - 18s 2s/step - loss: 0.1238 - accuracy: 0.9533 - val_loss: 3.6633 - val_accuracy: 0.6562\nEpoch 78/100\n8/8 [==============================] - 18s 2s/step - loss: 0.1981 - accuracy: 0.9155 - val_loss: 1.3377 - val_accuracy: 0.7500\nEpoch 79/100\n8/8 [==============================] - 18s 2s/step - loss: 0.1512 - accuracy: 0.9466 - val_loss: 2.6734 - val_accuracy: 0.6758\nEpoch 80/100\n8/8 [==============================] - 18s 2s/step - loss: 0.1289 - accuracy: 0.9511 - val_loss: 2.5404 - val_accuracy: 0.6914\nEpoch 81/100\n8/8 [==============================] - 18s 2s/step - loss: 0.1487 - accuracy: 0.9444 - val_loss: 2.4587 - val_accuracy: 0.6875\nEpoch 82/100\n8/8 [==============================] - 18s 2s/step - loss: 0.1104 - accuracy: 0.9588 - val_loss: 2.2757 - val_accuracy: 0.7266\nEpoch 83/100\n8/8 [==============================] - 18s 2s/step - loss: 0.2143 - accuracy: 0.9166 - val_loss: 2.7385 - val_accuracy: 0.6797\nEpoch 84/100\n8/8 [==============================] - 18s 2s/step - loss: 0.1224 - accuracy: 0.9511 - val_loss: 2.8706 - val_accuracy: 0.6836\nEpoch 85/100\n8/8 [==============================] - 18s 2s/step - loss: 0.1633 - accuracy: 0.9366 - val_loss: 3.9889 - val_accuracy: 0.6328\nEpoch 86/100\n8/8 [==============================] - 18s 2s/step - loss: 0.1328 - accuracy: 0.9511 - val_loss: 3.1196 - val_accuracy: 0.6719\nEpoch 87/100\n8/8 [==============================] - 18s 2s/step - loss: 0.1318 - accuracy: 0.9477 - val_loss: 2.7268 - val_accuracy: 0.7070\nEpoch 88/100\n8/8 [==============================] - 18s 2s/step - loss: 0.1296 - accuracy: 0.9488 - val_loss: 4.3136 - val_accuracy: 0.6445\nEpoch 89/100\n8/8 [==============================] - 18s 2s/step - loss: 0.1905 - accuracy: 0.9188 - val_loss: 2.7030 - val_accuracy: 0.6836\nEpoch 90/100\n8/8 [==============================] - 18s 2s/step - loss: 0.1243 - accuracy: 0.9533 - val_loss: 2.3421 - val_accuracy: 0.7344\nEpoch 91/100\n8/8 [==============================] - 18s 2s/step - loss: 0.1282 - accuracy: 0.9433 - val_loss: 4.2297 - val_accuracy: 0.6445\nEpoch 92/100\n8/8 [==============================] - 18s 2s/step - loss: 0.1192 - accuracy: 0.9511 - val_loss: 2.0731 - val_accuracy: 0.7422\nEpoch 93/100\n8/8 [==============================] - 18s 2s/step - loss: 0.3976 - accuracy: 0.8932 - val_loss: 4.0525 - val_accuracy: 0.6367\nEpoch 94/100\n8/8 [==============================] - 18s 2s/step - loss: 0.1021 - accuracy: 0.9577 - val_loss: 3.3658 - val_accuracy: 0.6602\nEpoch 95/100\n8/8 [==============================] - 18s 2s/step - loss: 0.1499 - accuracy: 0.9366 - val_loss: 2.2549 - val_accuracy: 0.7148\nEpoch 96/100\n8/8 [==============================] - 18s 2s/step - loss: 0.1106 - accuracy: 0.9644 - val_loss: 2.9970 - val_accuracy: 0.6758\nEpoch 97/100\n8/8 [==============================] - 18s 2s/step - loss: 0.1820 - accuracy: 0.9388 - val_loss: 4.2064 - val_accuracy: 0.6406\nEpoch 98/100\n8/8 [==============================] - 18s 2s/step - loss: 0.1164 - accuracy: 0.9499 - val_loss: 2.8604 - val_accuracy: 0.6992\nEpoch 99/100\n8/8 [==============================] - 20s 3s/step - loss: 0.1121 - accuracy: 0.9522 - val_loss: 3.4937 - val_accuracy: 0.6602\nEpoch 100/100\n8/8 [==============================] - 18s 2s/step - loss: 0.1067 - accuracy: 0.9566 - val_loss: 3.2441 - val_accuracy: 0.6758\n"
],
[
"import matplotlib.pyplot as plt\nacc = history.history['accuracy']\nval_acc = history.history['val_accuracy']\nloss = history.history['loss']\nval_loss = history.history['val_loss']\n\nepochs = range(len(acc))\n\nplt.plot(epochs, acc, 'r', label='Training accuracy')\nplt.plot(epochs, val_acc, 'b', label='Validation accuracy')\nplt.title('Training and validation accuracy')\n\nplt.figure()\n\nplt.plot(epochs, loss, 'r', label='Training Loss')\nplt.plot(epochs, val_loss, 'b', label='Validation Loss')\nplt.title('Training and validation loss')\nplt.legend()\n\nplt.show()",
"_____no_output_____"
]
],
[
[
"around 40+ min ",
"_____no_output_____"
]
],
[
[
"",
"_____no_output_____"
]
]
] | [
"code",
"markdown",
"code",
"markdown",
"code",
"markdown",
"code"
] | [
[
"code"
],
[
"markdown"
],
[
"code"
],
[
"markdown",
"markdown"
],
[
"code",
"code",
"code",
"code",
"code",
"code"
],
[
"markdown"
],
[
"code"
]
] |
e76ba2399ce5d3655e8fa26cadebdab70eb9ff75 | 347,393 | ipynb | Jupyter Notebook | tdu1s3.ipynb | cardstud/DS-Unit-1-Sprint-2-Data-Wrangling-and-Storytelling | 36ead9c43b9613bdecdea8899efaf24c42776c6a | [
"MIT"
] | null | null | null | tdu1s3.ipynb | cardstud/DS-Unit-1-Sprint-2-Data-Wrangling-and-Storytelling | 36ead9c43b9613bdecdea8899efaf24c42776c6a | [
"MIT"
] | null | null | null | tdu1s3.ipynb | cardstud/DS-Unit-1-Sprint-2-Data-Wrangling-and-Storytelling | 36ead9c43b9613bdecdea8899efaf24c42776c6a | [
"MIT"
] | null | null | null | 101.964485 | 55,002 | 0.750179 | [
[
[
"<a href=\"https://colab.research.google.com/github/cardstud/DS-Unit-1-Sprint-2-Data-Wrangling-and-Storytelling/blob/master/tdu1s3.ipynb\" target=\"_parent\"><img src=\"https://colab.research.google.com/assets/colab-badge.svg\" alt=\"Open In Colab\"/></a>",
"_____no_output_____"
],
[
"# Data Science Unit 1 Sprint Challenge 2\n\n## Data Wrangling and Storytelling\n\nTaming data from its raw form into informative insights and stories.",
"_____no_output_____"
],
[
"## Data Wrangling\n\nIn this Sprint Challenge you will first \"wrangle\" some data from [Gapminder](https://www.gapminder.org/about-gapminder/), a Swedish non-profit co-founded by Hans Rosling. \"Gapminder produces free teaching resources making the world understandable based on reliable statistics.\"\n- [Cell phones (total), by country and year](https://raw.githubusercontent.com/open-numbers/ddf--gapminder--systema_globalis/master/ddf--datapoints--cell_phones_total--by--geo--time.csv)\n- [Population (total), by country and year](https://raw.githubusercontent.com/open-numbers/ddf--gapminder--systema_globalis/master/ddf--datapoints--population_total--by--geo--time.csv)\n- [Geo country codes](https://github.com/open-numbers/ddf--gapminder--systema_globalis/blob/master/ddf--entities--geo--country.csv)\n\nThese two links have everything you need to successfully complete the first part of this sprint challenge.\n- [Pandas documentation: Working with Text Data](https://pandas.pydata.org/pandas-docs/stable/text.html) (one question)\n- [Pandas Cheat Sheet](https://github.com/pandas-dev/pandas/blob/master/doc/cheatsheet/Pandas_Cheat_Sheet.pdf) (everything else)",
"_____no_output_____"
],
[
"### Part 0. Load data\n\nYou don't need to add or change anything here. Just run this cell and it loads the data for you, into three dataframes.",
"_____no_output_____"
]
],
[
[
"import pandas as pd\n\ncell_phones = pd.read_csv('https://raw.githubusercontent.com/open-numbers/ddf--gapminder--systema_globalis/master/ddf--datapoints--cell_phones_total--by--geo--time.csv')\n\npopulation = pd.read_csv('https://raw.githubusercontent.com/open-numbers/ddf--gapminder--systema_globalis/master/ddf--datapoints--population_total--by--geo--time.csv')\n\ngeo_country_codes = (pd.read_csv('https://raw.githubusercontent.com/open-numbers/ddf--gapminder--systema_globalis/master/ddf--entities--geo--country.csv')\n .rename(columns={'country': 'geo', 'name': 'country'}))",
"_____no_output_____"
]
],
[
[
"### Part 1. Join data",
"_____no_output_____"
],
[
"First, join the `cell_phones` and `population` dataframes (with an inner join on `geo` and `time`).\n\nThe resulting dataframe's shape should be: (8590, 4)",
"_____no_output_____"
]
],
[
[
"df = pd.merge(cell_phones, population, how='inner')",
"_____no_output_____"
],
[
"df.shape",
"_____no_output_____"
]
],
[
[
"Then, select the `geo` and `country` columns from the `geo_country_codes` dataframe, and join with your population and cell phone data.\n\nThe resulting dataframe's shape should be: (8590, 5)",
"_____no_output_____"
]
],
[
[
"df = pd.merge(geo_country_codes[['geo', 'country']], df)",
"_____no_output_____"
],
[
"df.shape",
"_____no_output_____"
]
],
[
[
"### Part 2. Make features",
"_____no_output_____"
],
[
"Calculate the number of cell phones per person, and add this column onto your dataframe.\n\n(You've calculated correctly if you get 1.220 cell phones per person in the United States in 2017.)",
"_____no_output_____"
]
],
[
[
"df.head()",
"_____no_output_____"
],
[
"df[df['country'] == 'United States']",
"_____no_output_____"
],
[
"df.dtypes",
"_____no_output_____"
],
[
"df['cell_phones_total'].value_counts().sum()",
"_____no_output_____"
],
[
"condition = (df['country'] == 'United States') & (df['time'] == 2017)\ncolumns = ['country', 'time', 'cell_phones_total', 'population_total']\nsubset = df[condition][columns]",
"_____no_output_____"
],
[
"subset.shape",
"_____no_output_____"
],
[
"subset.head()",
"_____no_output_____"
],
[
"#value = subset['cell_phones_total'] / subset['population_total']\ndf['cell_phones_per_person'] = df['cell_phones_total'] / df['population_total'] # better way to do this\ndf[(df.country=='United States') & (df.time==2017)]",
"_____no_output_____"
],
[
"value",
"_____no_output_____"
]
],
[
[
"Modify the `geo` column to make the geo codes uppercase instead of lowercase.",
"_____no_output_____"
]
],
[
[
"df.head(1)",
"_____no_output_____"
],
[
"df['geo'] = df['geo'].str.upper()",
"_____no_output_____"
],
[
"df.head()",
"_____no_output_____"
]
],
[
[
"### Part 3. Process data",
"_____no_output_____"
],
[
"Use the describe function, to describe your dataframe's numeric columns, and then its non-numeric columns.\n\n(You'll see the time period ranges from 1960 to 2017, and there are 195 unique countries represented.)",
"_____no_output_____"
]
],
[
[
"# numeric columns\ndf.describe()",
"_____no_output_____"
],
[
"# non-numeric columns\ndf.describe(exclude='number')",
"_____no_output_____"
]
],
[
[
"In 2017, what were the top 5 countries with the most cell phones total?\n\nYour list of countries should have these totals:\n\n| country | cell phones total |\n|:-------:|:-----------------:|\n| ? | 1,474,097,000 |\n| ? | 1,168,902,277 |\n| ? | 458,923,202 |\n| ? | 395,881,000 |\n| ? | 236,488,548 |\n\n",
"_____no_output_____"
]
],
[
[
"# This optional code formats float numbers with comma separators\npd.options.display.float_format = '{:,}'.format",
"_____no_output_____"
],
[
"condition = (df['time'] == 2017)\ncolumns = ['country', 'cell_phones_total']\nsubset = df[condition][columns]",
"_____no_output_____"
],
[
"subset.head()",
"_____no_output_____"
],
[
"subset = subset.sort_values(by=['cell_phones_total'], ascending=False)",
"_____no_output_____"
],
[
"subset.head(5)",
"_____no_output_____"
]
],
[
[
"2017 was the first year that China had more cell phones than people.\n\nWhat was the first year that the USA had more cell phones than people?",
"_____no_output_____"
]
],
[
[
"condition = (df['country'] == 'United States') \ncolumns = ['time', 'country', 'cell_phones_total', 'population_total']\nsubset1 = df[condition][columns]",
"_____no_output_____"
],
[
"subset1.sort_values(by='time', ascending=False).head(5)",
"_____no_output_____"
],
[
"df[(df.geo=='USA') & (df.cell_phones_per_person > 1)].time.min() # the way to get the answer via coding",
"_____no_output_____"
],
[
"# The year that USA had more cell phones than people is in 2014 when cell_phones_total = 355,500,000 vs population_total = 317,718,779",
"_____no_output_____"
]
],
[
[
"### Part 4. Reshape data",
"_____no_output_____"
],
[
"*This part is not needed to pass the sprint challenge, only to get a 3! Only work on this after completing the other sections.*\n\nCreate a pivot table:\n- Columns: Years 2007โ2017\n- Rows: China, India, United States, Indonesia, Brazil (order doesn't matter)\n- Values: Cell Phones Total\n\nThe table's shape should be: (5, 11)",
"_____no_output_____"
]
],
[
[
"condition = subset1['country'] == ('China', 'United States', 'Indonesia', 'Brazil')\ncolumns = ['time', 'country', 'cell_phones_total', 'population_total']\nsubset2 = subset1[condition][columns]\nsubset2",
"_____no_output_____"
],
[
"subset1.pivot_table(index='columns', columns='time', values='cell_phones_total')\n# ran out of time...oh well",
"_____no_output_____"
]
],
[
[
"Sort these 5 countries, by biggest increase in cell phones from 2007 to 2017.\n\nWhich country had 935,282,277 more cell phones in 2017 versus 2007?",
"_____no_output_____"
]
],
[
[
"",
"_____no_output_____"
]
],
[
[
"If you have the time and curiosity, what other questions can you ask and answer with this data?",
"_____no_output_____"
],
[
"## Data Storytelling\n\nIn this part of the sprint challenge you'll work with a dataset from **FiveThirtyEight's article, [Every Guest Jon Stewart Ever Had On โThe Daily Showโ](https://fivethirtyeight.com/features/every-guest-jon-stewart-ever-had-on-the-daily-show/)**!",
"_____no_output_____"
],
[
"### Part 0 โ Run this starter code\n\nYou don't need to add or change anything here. Just run this cell and it loads the data for you, into a dataframe named `df`.\n\n(You can explore the data if you want, but it's not required to pass the Sprint Challenge.)",
"_____no_output_____"
]
],
[
[
"%matplotlib inline\nimport matplotlib.pyplot as plt\nimport numpy as np\nimport pandas as pd\n\nurl = 'https://raw.githubusercontent.com/fivethirtyeight/data/master/daily-show-guests/daily_show_guests.csv'\ndf = pd.read_csv(url).rename(columns={'YEAR': 'Year', 'Raw_Guest_List': 'Guest'})\n\ndef get_occupation(group):\n if group in ['Acting', 'Comedy', 'Musician']:\n return 'Acting, Comedy & Music'\n elif group in ['Media', 'media']:\n return 'Media'\n elif group in ['Government', 'Politician', 'Political Aide']:\n return 'Government and Politics'\n else:\n return 'Other'\n \ndf['Occupation'] = df['Group'].apply(get_occupation)",
"_____no_output_____"
]
],
[
[
"### Part 1 โ What's the breakdown of guestsโ occupations per year?\n\nFor example, in 1999, what percentage of guests were actors, comedians, or musicians? What percentage were in the media? What percentage were in politics? What percentage were from another occupation?\n\nThen, what about in 2000? In 2001? And so on, up through 2015.\n\nSo, **for each year of _The Daily Show_, calculate the percentage of guests from each occupation:**\n- Acting, Comedy & Music\n- Government and Politics\n- Media\n- Other\n\n#### Hints:\nYou can make a crosstab. (See pandas documentation for examples, explanation, and parameters.)\n\nYou'll know you've calculated correctly when the percentage of \"Acting, Comedy & Music\" guests is 90.36% in 1999, and 45% in 2015.",
"_____no_output_____"
]
],
[
[
"df.head()",
"_____no_output_____"
],
[
"df.describe()",
"_____no_output_____"
],
[
"df.describe(exclude='number')",
"_____no_output_____"
],
[
"df1 = pd.crosstab(df['Year'], df['Occupation'], normalize='index')\ndf1",
"_____no_output_____"
]
],
[
[
"### Part 2 โ Recreate this explanatory visualization:",
"_____no_output_____"
]
],
[
[
"from IPython.display import display, Image\npng = 'https://fivethirtyeight.com/wp-content/uploads/2015/08/hickey-datalab-dailyshow.png'\nexample = Image(png, width=500)\ndisplay(example)",
"_____no_output_____"
]
],
[
[
"**Hints:**\n- You can choose any Python visualization library you want. I've verified the plot can be reproduced with matplotlib, pandas plot, or seaborn. I assume other libraries like altair or plotly would work too.\n- If you choose to use seaborn, you may want to upgrade the version to 0.9.0.\n\n**Expectations:** Your plot should include:\n- 3 lines visualizing \"occupation of guests, by year.\" The shapes of the lines should look roughly identical to 538's example. Each line should be a different color. (But you don't need to use the _same_ colors as 538.)\n- Legend or labels for the lines. (But you don't need each label positioned next to its line or colored like 538.)\n- Title in the upper left: _\"Who Got To Be On 'The Daily Show'?\"_ with more visual emphasis than the subtitle. (Bolder and/or larger font.)\n- Subtitle underneath the title: _\"Occupation of guests, by year\"_\n\n**Optional Bonus Challenge:**\n- Give your plot polished aesthetics, with improved resemblance to the 538 example.\n- Any visual element not specifically mentioned in the expectations is an optional bonus.",
"_____no_output_____"
]
],
[
[
"display(example)",
"_____no_output_____"
],
[
"df2 = df1.drop(['Other'], axis=1)\ndf2",
"_____no_output_____"
],
[
"display(example)",
"_____no_output_____"
],
[
"plt.style.use('fivethirtyeight')\nyax = ['0', '25', '50', '75', '100%']\nax = df2.plot()\nax.patch.set_alpha(0.1)\nplt.title(\"Who Got To Be On 'The Daily Show'?\",\n fontsize=18,\n x=-0.1,\n y=1.1,loc='left',\n fontweight='bold');\nsubtitle_string = 'Occupation of guests, by year'\nplt.suptitle(subtitle_string, fontsize=14, x= 0.24, y=0.94)\nplt.xlabel(' ')\nplt.legend(bbox_to_anchor = [0.6, 0.75]);",
"_____no_output_____"
]
],
[
[
"###Example by Alex",
"_____no_output_____"
]
],
[
[
"import matplotlib.pyplot as plt\nimport seaborn as sns",
"_____no_output_____"
],
[
"import numpy as np",
"_____no_output_____"
],
[
"x = np.arange(0,10)",
"_____no_output_____"
],
[
"x",
"_____no_output_____"
],
[
"y = x**2",
"_____no_output_____"
],
[
"y",
"_____no_output_____"
],
[
"plt.plot(x,y)",
"_____no_output_____"
],
[
"y_labels = [f'{i}%' for i in y]",
"_____no_output_____"
],
[
"plt.yticks(y, y_labels);",
"_____no_output_____"
],
[
"y_labels = [f'{i}' if i!= 100 else f'{i}%' for i in range(0, 101, 10)]\n# y_labels = [f'{i}' if i= 100 else f'{i}%' for i in range(0, 101, 10)]",
"_____no_output_____"
],
[
"plt.plot(x,y)\nplt.yticks(range(0,101,10), y_labels);\nplt.title('My plot')\nplt.text(x=4, y=50, s='My text')",
"_____no_output_____"
],
[
"import pandas as pd\ndf = pd.DataFrame({'x':X, 'y':y})",
"_____no_output_____"
],
[
"import seaborn as sns\nsns.replot()\nplt.yticks(range(0,101,10), y_labels);\nplt.title('My plot')\nplt.text(x=4, y=50, s='My text')",
"_____no_output_____"
],
[
"",
"_____no_output_____"
]
]
] | [
"markdown",
"code",
"markdown",
"code",
"markdown",
"code",
"markdown",
"code",
"markdown",
"code",
"markdown",
"code",
"markdown",
"code",
"markdown",
"code",
"markdown",
"code",
"markdown",
"code",
"markdown",
"code",
"markdown",
"code",
"markdown",
"code",
"markdown",
"code",
"markdown",
"code"
] | [
[
"markdown",
"markdown",
"markdown",
"markdown"
],
[
"code"
],
[
"markdown",
"markdown"
],
[
"code",
"code"
],
[
"markdown"
],
[
"code",
"code"
],
[
"markdown",
"markdown"
],
[
"code",
"code",
"code",
"code",
"code",
"code",
"code",
"code",
"code"
],
[
"markdown"
],
[
"code",
"code",
"code"
],
[
"markdown",
"markdown"
],
[
"code",
"code"
],
[
"markdown"
],
[
"code",
"code",
"code",
"code",
"code"
],
[
"markdown"
],
[
"code",
"code",
"code",
"code"
],
[
"markdown",
"markdown"
],
[
"code",
"code"
],
[
"markdown"
],
[
"code"
],
[
"markdown",
"markdown",
"markdown"
],
[
"code"
],
[
"markdown"
],
[
"code",
"code",
"code",
"code"
],
[
"markdown"
],
[
"code"
],
[
"markdown"
],
[
"code",
"code",
"code",
"code"
],
[
"markdown"
],
[
"code",
"code",
"code",
"code",
"code",
"code",
"code",
"code",
"code",
"code",
"code",
"code",
"code",
"code"
]
] |
e76ba54dab2dd9c9a685f686c20e85ee5f2c5c39 | 4,353 | ipynb | Jupyter Notebook | IFTTT/IFTTT_Trigger_workflow.ipynb | vivard/awesome-notebooks | 899558bcc2165bb2155f5ab69ac922c6458e1799 | [
"BSD-3-Clause"
] | null | null | null | IFTTT/IFTTT_Trigger_workflow.ipynb | vivard/awesome-notebooks | 899558bcc2165bb2155f5ab69ac922c6458e1799 | [
"BSD-3-Clause"
] | null | null | null | IFTTT/IFTTT_Trigger_workflow.ipynb | vivard/awesome-notebooks | 899558bcc2165bb2155f5ab69ac922c6458e1799 | [
"BSD-3-Clause"
] | null | null | null | 20.728571 | 282 | 0.518034 | [
[
[
"<img width=\"10%\" alt=\"Naas\" src=\"https://landen.imgix.net/jtci2pxwjczr/assets/5ice39g4.png?w=160\"/>",
"_____no_output_____"
],
[
"# IFTTT - Trigger workflow\n<a href=\"https://app.naas.ai/user-redirect/naas/downloader?url=https://raw.githubusercontent.com/jupyter-naas/awesome-notebooks/master/IFTTT/IFTTT_Trigger_workflow.ipynb\" target=\"_parent\"><img src=\"https://naasai-public.s3.eu-west-3.amazonaws.com/open_in_naas.svg\"/></a>",
"_____no_output_____"
],
[
"**Tags:** #ifttt #automation #nocode",
"_____no_output_____"
],
[
"## Input",
"_____no_output_____"
],
[
"### Import library",
"_____no_output_____"
]
],
[
[
"from naas_drivers import ifttt",
"_____no_output_____"
]
],
[
[
"### Variables",
"_____no_output_____"
]
],
[
[
"event = \"myevent\"\nkey = \"cl9U-VaeBu1**********\"\ndata = { \"value1\": \"Bryan\", \"value2\": \"Helmig\", \"value3\": 27 }",
"_____no_output_____"
]
],
[
[
"## Model",
"_____no_output_____"
],
[
"### Connect to IFTTT",
"_____no_output_____"
]
],
[
[
"result = ifttt.connect(key)",
"_____no_output_____"
]
],
[
[
"## Output",
"_____no_output_____"
],
[
"### Display result",
"_____no_output_____"
]
],
[
[
"result = ifttt.send(event, data)",
"_____no_output_____"
]
]
] | [
"markdown",
"code",
"markdown",
"code",
"markdown",
"code",
"markdown",
"code"
] | [
[
"markdown",
"markdown",
"markdown",
"markdown",
"markdown"
],
[
"code"
],
[
"markdown"
],
[
"code"
],
[
"markdown",
"markdown"
],
[
"code"
],
[
"markdown",
"markdown"
],
[
"code"
]
] |
e76bb7206f327704b5dff86c34af6cbebf16b7c6 | 17,471 | ipynb | Jupyter Notebook | It-can-see-you.ipynb | Ryukijano/it-can-see-you | fae8dc0e30986c4a81712a9f1b0f28acc49b36a7 | [
"MIT"
] | null | null | null | It-can-see-you.ipynb | Ryukijano/it-can-see-you | fae8dc0e30986c4a81712a9f1b0f28acc49b36a7 | [
"MIT"
] | null | null | null | It-can-see-you.ipynb | Ryukijano/it-can-see-you | fae8dc0e30986c4a81712a9f1b0f28acc49b36a7 | [
"MIT"
] | null | null | null | 31.310036 | 191 | 0.501631 | [
[
[
"# Project - Face Mask Detection\n",
"_____no_output_____"
],
[
"import numpy as np\nimport pandas as pd\n%matplotlib inline\nimport matplotlib as mpl\nimport matplotlib.pyplot as plt\nimport os",
"_____no_output_____"
],
[
"import tensorflow as tf\nfrom tensorflow import keras",
"_____no_output_____"
]
],
[
[
"### Please enter the correct file path",
"_____no_output_____"
]
],
[
[
"train_dir = r'C:\\Users\\Ryukijano\\Python_notebooks\\Face_ Mask_ Dataset\\Train'\nvalidation_dir = r'C:\\Users\\Ryukijano\\Python_notebooks\\Face_ Mask_ Dataset\\Validation'\ntest_dir =r'C:\\Users\\Ryukijano\\Python_notebooks\\Face_ Mask_ Dataset\\Test'",
"_____no_output_____"
],
[
"from tensorflow.keras.preprocessing.image import ImageDataGenerator",
"_____no_output_____"
],
[
"train_datagen = ImageDataGenerator(rescale=1./255)\ntest_datagen = ImageDataGenerator(rescale=1./255)\n\ntrain_generator = train_datagen.flow_from_directory(\n train_dir,\n target_size=(128, 128),\n batch_size=20,\n class_mode='binary')\n\nvalidation_generator = test_datagen.flow_from_directory(\n validation_dir,\n target_size=(128, 128),\n batch_size=20,\n class_mode='binary')",
"Found 10000 images belonging to 2 classes.\nFound 800 images belonging to 2 classes.\n"
],
[
"from tensorflow.keras import layers\nfrom tensorflow.keras import models",
"_____no_output_____"
],
[
"model = models.Sequential()\n\nmodel.add(layers.Conv2D(32, (3, 3), activation='relu',\n input_shape=(128, 128, 3)))\nmodel.add(layers.MaxPooling2D((2, 2)))\n\nmodel.add(layers.Conv2D(64, (3, 3), activation='relu'))\nmodel.add(layers.MaxPooling2D((2, 2)))\n\nmodel.add(layers.Conv2D(128, (3, 3), activation='relu'))\nmodel.add(layers.MaxPooling2D((2, 2)))\n\nmodel.add(layers.Conv2D(128, (3, 3), activation='relu'))\nmodel.add(layers.MaxPooling2D((2, 2)))\n\nmodel.add(layers.Flatten())\n\nmodel.add(layers.Dense(512, activation='relu'))\n\nmodel.add(layers.Dense(1, activation='sigmoid'))",
"_____no_output_____"
],
[
"from tensorflow.keras import optimizers\n\nmodel.compile(loss='binary_crossentropy',\n optimizer=optimizers.RMSprop(lr=1e-4),\n metrics=['acc'])",
"_____no_output_____"
],
[
"history = model.fit_generator(\n train_generator,\n steps_per_epoch=500,\n epochs=20,\n validation_data=validation_generator,\n validation_steps=40)",
"WARNING:tensorflow:From <ipython-input-16-0f3e0e957b18>:6: Model.fit_generator (from tensorflow.python.keras.engine.training) is deprecated and will be removed in a future version.\nInstructions for updating:\nPlease use Model.fit, which supports generators.\nEpoch 1/20\n500/500 [==============================] - 99s 199ms/step - loss: 0.1468 - acc: 0.9429 - val_loss: 0.0382 - val_acc: 0.9887\nEpoch 2/20\n500/500 [==============================] - 98s 196ms/step - loss: 0.0448 - acc: 0.9843 - val_loss: 0.0151 - val_acc: 0.9950\nEpoch 3/20\n500/500 [==============================] - 101s 202ms/step - loss: 0.0297 - acc: 0.9888 - val_loss: 0.0133 - val_acc: 0.9937\nEpoch 4/20\n500/500 [==============================] - 99s 198ms/step - loss: 0.0227 - acc: 0.9923 - val_loss: 0.0122 - val_acc: 0.9950\nEpoch 5/20\n500/500 [==============================] - 99s 198ms/step - loss: 0.0200 - acc: 0.9927 - val_loss: 0.0156 - val_acc: 0.9962\nEpoch 6/20\n500/500 [==============================] - 101s 202ms/step - loss: 0.0166 - acc: 0.9940 - val_loss: 0.0081 - val_acc: 0.9962\nEpoch 7/20\n500/500 [==============================] - 100s 200ms/step - loss: 0.0155 - acc: 0.9947 - val_loss: 0.0144 - val_acc: 0.9950\nEpoch 8/20\n500/500 [==============================] - 99s 197ms/step - loss: 0.0145 - acc: 0.9946 - val_loss: 0.0105 - val_acc: 0.9950\nEpoch 9/20\n500/500 [==============================] - 98s 195ms/step - loss: 0.0126 - acc: 0.9958 - val_loss: 0.0115 - val_acc: 0.9962\nEpoch 10/20\n500/500 [==============================] - 98s 196ms/step - loss: 0.0108 - acc: 0.9963 - val_loss: 0.0148 - val_acc: 0.9962\nEpoch 11/20\n500/500 [==============================] - 100s 199ms/step - loss: 0.0109 - acc: 0.9962 - val_loss: 0.0180 - val_acc: 0.9962\nEpoch 12/20\n500/500 [==============================] - 100s 199ms/step - loss: 0.0071 - acc: 0.9970 - val_loss: 0.0131 - val_acc: 0.9962\nEpoch 13/20\n500/500 [==============================] - 99s 198ms/step - loss: 0.0075 - acc: 0.9975 - val_loss: 0.0165 - val_acc: 0.9962\nEpoch 14/20\n500/500 [==============================] - 99s 198ms/step - loss: 0.0064 - acc: 0.9976 - val_loss: 0.0162 - val_acc: 0.9962\nEpoch 15/20\n500/500 [==============================] - 99s 198ms/step - loss: 0.0062 - acc: 0.9976 - val_loss: 0.0098 - val_acc: 0.9975\nEpoch 16/20\n500/500 [==============================] - 100s 199ms/step - loss: 0.0060 - acc: 0.9982 - val_loss: 0.0112 - val_acc: 0.9962\nEpoch 17/20\n500/500 [==============================] - 101s 203ms/step - loss: 0.0058 - acc: 0.9981 - val_loss: 0.0164 - val_acc: 0.9975\nEpoch 18/20\n500/500 [==============================] - 100s 199ms/step - loss: 0.0050 - acc: 0.9984 - val_loss: 0.0359 - val_acc: 0.9950\nEpoch 19/20\n500/500 [==============================] - 100s 199ms/step - loss: 0.0056 - acc: 0.9986 - val_loss: 0.0130 - val_acc: 0.9962\nEpoch 20/20\n500/500 [==============================] - 100s 200ms/step - loss: 0.0027 - acc: 0.9991 - val_loss: 0.0122 - val_acc: 0.9975\n"
],
[
"model.save(\"model_cnn_project_P1.h5\")",
"_____no_output_____"
],
[
"from tensorflow.keras import backend as K \n\nK.clear_session()\ndel model",
"_____no_output_____"
],
[
"train_datagen = ImageDataGenerator(\n rescale=1./255,\n rotation_range=40,\n width_shift_range=0.2,\n height_shift_range=0.2,\n shear_range=0.2,\n zoom_range=0.2,\n horizontal_flip=True,)\n\ntest_datagen = ImageDataGenerator(rescale=1./255)\n\ntrain_generator = train_datagen.flow_from_directory(\n train_dir,\n target_size=(128, 128),\n batch_size=32,\n class_mode='binary')\n\nvalidation_generator = test_datagen.flow_from_directory(\n validation_dir,\n target_size=(128, 128),\n batch_size=32,\n class_mode='binary')",
"Found 10000 images belonging to 2 classes.\nFound 800 images belonging to 2 classes.\n"
],
[
"model = models.Sequential()\nmodel.add(layers.Conv2D(32, (3, 3), activation='relu',\n input_shape=(128, 128, 3)))\nmodel.add(layers.MaxPooling2D((2, 2)))\nmodel.add(layers.Conv2D(64, (3, 3), activation='relu'))\nmodel.add(layers.MaxPooling2D((2, 2)))\nmodel.add(layers.Conv2D(128, (3, 3), activation='relu'))\nmodel.add(layers.MaxPooling2D((2, 2)))\nmodel.add(layers.Conv2D(128, (3, 3), activation='relu'))\nmodel.add(layers.MaxPooling2D((2, 2)))\nmodel.add(layers.Flatten())\nmodel.add(layers.Dropout(0.5))\nmodel.add(layers.Dense(512, activation='relu'))\nmodel.add(layers.Dense(1, activation='sigmoid'))\n\nmodel.compile(loss='binary_crossentropy',\n optimizer=optimizers.RMSprop(lr=1e-4),\n metrics=['acc'])",
"_____no_output_____"
],
[
"history = model.fit_generator(\n train_generator,\n steps_per_epoch=300,\n epochs=10,\n validation_data=validation_generator,\n validation_steps=25)",
"Epoch 1/10\n300/300 [==============================] - 99s 331ms/step - loss: 0.3014 - acc: 0.8710 - val_loss: 0.1483 - val_acc: 0.9463\nEpoch 2/10\n300/300 [==============================] - 97s 322ms/step - loss: 0.1984 - acc: 0.9222 - val_loss: 0.0983 - val_acc: 0.9675\nEpoch 3/10\n300/300 [==============================] - 96s 319ms/step - loss: 0.1727 - acc: 0.9360 - val_loss: 0.1028 - val_acc: 0.9688\nEpoch 4/10\n300/300 [==============================] - 96s 319ms/step - loss: 0.1640 - acc: 0.9379 - val_loss: 0.0906 - val_acc: 0.9762\nEpoch 5/10\n300/300 [==============================] - 96s 320ms/step - loss: 0.1596 - acc: 0.9426 - val_loss: 0.0756 - val_acc: 0.9787\nEpoch 6/10\n300/300 [==============================] - 98s 327ms/step - loss: 0.1517 - acc: 0.9466 - val_loss: 0.0654 - val_acc: 0.9775\nEpoch 7/10\n300/300 [==============================] - 98s 327ms/step - loss: 0.1395 - acc: 0.9482 - val_loss: 0.0625 - val_acc: 0.9837\nEpoch 8/10\n300/300 [==============================] - 96s 321ms/step - loss: 0.1348 - acc: 0.9508 - val_loss: 0.0684 - val_acc: 0.9850\nEpoch 9/10\n300/300 [==============================] - 93s 311ms/step - loss: 0.1216 - acc: 0.9558 - val_loss: 0.0388 - val_acc: 0.9875\nEpoch 10/10\n300/300 [==============================] - 94s 315ms/step - loss: 0.1196 - acc: 0.9581 - val_loss: 0.0356 - val_acc: 0.9850\n"
],
[
"from tensorflow.keras.applications import VGG19\n\nconv_base = VGG19(weights='imagenet',\n include_top=False,\n input_shape=(128, 128, 3))",
"Downloading data from https://storage.googleapis.com/tensorflow/keras-applications/vgg19/vgg19_weights_tf_dim_ordering_tf_kernels_notop.h5\n80142336/80134624 [==============================] - 5s 0us/step\n"
],
[
"from tensorflow.keras import models\nfrom tensorflow.keras import layers\n\nmodel = models.Sequential()\nmodel.add(conv_base)\nmodel.add(layers.Flatten())\nmodel.add(layers.Dense(256, activation='relu'))\nmodel.add(layers.Dense(1, activation='sigmoid'))",
"_____no_output_____"
],
[
"from tensorflow.keras import optimizers\n\nmodel.compile(loss='binary_crossentropy',\n optimizer=optimizers.RMSprop(lr=2e-5),\n metrics=['acc'])",
"_____no_output_____"
],
[
"checkpoint_cb = keras.callbacks.ModelCheckpoint(\"CNN_Final_Project_Model-{epoch:02d}.h5\")",
"_____no_output_____"
],
[
"history = model.fit_generator(\n train_generator,\n steps_per_epoch=300,\n epochs=10,\n validation_data=validation_generator,\n validation_steps=25,\n callbacks=[checkpoint_cb])",
"Epoch 1/10\n300/300 [==============================] - 1267s 4s/step - loss: 0.0816 - acc: 0.9660 - val_loss: 0.0050 - val_acc: 0.9987\nEpoch 2/10\n300/300 [==============================] - 1303s 4s/step - loss: 0.0268 - acc: 0.9908 - val_loss: 0.0028 - val_acc: 0.9987\nEpoch 3/10\n300/300 [==============================] - 1357s 5s/step - loss: 0.0203 - acc: 0.9941 - val_loss: 4.2187e-04 - val_acc: 1.0000\nEpoch 4/10\n300/300 [==============================] - 1293s 4s/step - loss: 0.0156 - acc: 0.9957 - val_loss: 0.0172 - val_acc: 0.9975\nEpoch 5/10\n300/300 [==============================] - 1249s 4s/step - loss: 0.0123 - acc: 0.9966 - val_loss: 0.0050 - val_acc: 0.9987\nEpoch 6/10\n300/300 [==============================] - 1244s 4s/step - loss: 0.0135 - acc: 0.9966 - val_loss: 0.0031 - val_acc: 0.9987\nEpoch 7/10\n300/300 [==============================] - 1222s 4s/step - loss: 0.0115 - acc: 0.9968 - val_loss: 8.7311e-05 - val_acc: 1.0000\nEpoch 8/10\n109/300 [=========>....................] - ETA: 12:55 - loss: 0.0036 - acc: 0.9986"
],
[
"test_generator = test_datagen.flow_from_directory(\n test_dir,\n target_size=(128, 128),\n batch_size=32,\n class_mode='binary')",
"_____no_output_____"
],
[
"model.evaluate_generator(test_generator, steps=31)",
"_____no_output_____"
]
]
] | [
"code",
"markdown",
"code"
] | [
[
"code",
"code",
"code"
],
[
"markdown"
],
[
"code",
"code",
"code",
"code",
"code",
"code",
"code",
"code",
"code",
"code",
"code",
"code",
"code",
"code",
"code",
"code",
"code",
"code",
"code"
]
] |
e76bbdd871f3f514d15f829fc9f344821dc9a686 | 10,360 | ipynb | Jupyter Notebook | L15_model evaluation 2/code/L15_confidence intervals holdout.ipynb | pedrogomes-dev/MA28CP-Intro-to-Machine-Learning | fd24017b8195a0d9ec9511071d4f8842dd596861 | [
"MIT"
] | 21 | 2020-08-24T20:23:24.000Z | 2022-03-17T13:47:59.000Z | L15_model evaluation 2/code/L15_confidence intervals holdout.ipynb | pedrogomes-dev/MA28CP-Intro-to-Machine-Learning | fd24017b8195a0d9ec9511071d4f8842dd596861 | [
"MIT"
] | null | null | null | L15_model evaluation 2/code/L15_confidence intervals holdout.ipynb | pedrogomes-dev/MA28CP-Intro-to-Machine-Learning | fd24017b8195a0d9ec9511071d4f8842dd596861 | [
"MIT"
] | 22 | 2020-08-29T14:30:40.000Z | 2022-03-24T13:42:17.000Z | 10,360 | 10,360 | 0.716216 | [
[
[
"# L15 - Model evaluation 2 (confidence intervals)\n\n---\n\n\n- Instructor: Dalcimar Casanova ([email protected])\n- Course website: https://www.dalcimar.com/disciplinas/aprendizado-de-maquina\n- Bibliography: based on lectures of Dr. Sebastian Raschka",
"_____no_output_____"
]
],
[
[
"import numpy as np\nimport matplotlib.pyplot as plt",
"_____no_output_____"
]
],
[
[
"<img src=\"https://sebastianraschka.com/images/blog/2016/model-evaluation-selection-part3/holdout-validation_01.png\" width=\"600\">",
"_____no_output_____"
]
],
[
[
"from mlxtend.data import iris_data\nfrom sklearn.neighbors import KNeighborsClassifier\nfrom sklearn.model_selection import train_test_split\n\nX, y = iris_data()\n\nprint(np.shape(y))\n\nX_train_valid, X_test, y_train_valid, y_test = train_test_split(X, y, test_size=0.3, random_state=1, stratify=y)\nX_train, X_valid, y_train, y_valid = train_test_split(X_train_valid, y_train_valid, test_size=0.5, random_state=1)\n\nprint(np.shape(y_train))\nprint(np.shape(y_valid))\nprint(np.shape(y_test))",
"(150,)\n(52,)\n(53,)\n(45,)\n"
],
[
"pip install hypopt",
"Requirement already satisfied: hypopt in /usr/local/lib/python3.6/dist-packages (1.0.9)\nRequirement already satisfied: numpy>=1.11.3 in /usr/local/lib/python3.6/dist-packages (from hypopt) (1.19.5)\nRequirement already satisfied: scikit-learn>=0.18 in /usr/local/lib/python3.6/dist-packages (from hypopt) (0.22.2.post1)\nRequirement already satisfied: joblib>=0.11 in /usr/local/lib/python3.6/dist-packages (from scikit-learn>=0.18->hypopt) (1.0.0)\nRequirement already satisfied: scipy>=0.17.0 in /usr/local/lib/python3.6/dist-packages (from scikit-learn>=0.18->hypopt) (1.4.1)\n"
]
],
[
[
"<img src=\"https://sebastianraschka.com/images/blog/2016/model-evaluation-selection-part3/holdout-validation_02.png\" width=\"600\">",
"_____no_output_____"
]
],
[
[
"from hypopt import GridSearch\n#from sklearn.model_selection import GridSearchCV\n\nknn = KNeighborsClassifier()\n\nparam_grid = {\n 'n_neighbors': [2, 3, 4, 5]\n}\n\ngrid = GridSearch(knn, param_grid=param_grid)\ngrid.fit(X_train, y_train, X_valid, y_valid)",
"100%|โโโโโโโโโโ| 4/4 [00:00<00:00, 265.79it/s]\n"
]
],
[
[
"<img src=\"https://sebastianraschka.com/images/blog/2016/model-evaluation-selection-part3/holdout-validation_03.png\" width=\"600\">",
"_____no_output_____"
]
],
[
[
"print(grid.param_scores)\n\nprint(grid.best_params)\nprint(grid.best_score)\nprint(grid.best_estimator_)\n\nclf = grid.best_estimator_",
"[({'n_neighbors': 5}, 0.8867924528301887), ({'n_neighbors': 4}, 0.8867924528301887), ({'n_neighbors': 3}, 0.8679245283018868), ({'n_neighbors': 2}, 0.8679245283018868)]\n{'n_neighbors': 5}\n0.8867924528301887\nKNeighborsClassifier(algorithm='auto', leaf_size=30, metric='minkowski',\n metric_params=None, n_jobs=None, n_neighbors=4, p=2,\n weights='uniform')\n"
],
[
"from sklearn.metrics import accuracy_score\n\ny_test_pred = clf.predict(X_test)\nacc_test = accuracy_score(y_test, y_test_pred)\n\nprint(acc_test)",
"0.9333333333333333\n"
]
],
[
[
"<img src=\"https://sebastianraschka.com/images/blog/2016/model-evaluation-selection-part3/holdout-validation_04.png\" width=\"600\">",
"_____no_output_____"
]
],
[
[
"clf.fit(X_train_valid, y_train_valid)",
"_____no_output_____"
]
],
[
[
"<img src=\"https://sebastianraschka.com/images/blog/2016/model-evaluation-selection-part3/holdout-validation_05.png\" width=\"600\">",
"_____no_output_____"
]
],
[
[
"y_test_pred = clf.predict(X_test)\nacc_test = accuracy_score(y_test, y_test_pred)\n\nprint(acc_test)",
"0.9777777777777777\n"
]
],
[
[
"## Confidence interval (via normal approximation)\n\n",
"_____no_output_____"
]
],
[
[
"ci_test = 1.96 * np.sqrt((acc_test*(1-acc_test)) / y_test.shape[0])\n\ntest_lower = acc_test-ci_test\ntest_upper = acc_test+ci_test\n\nprint(test_lower, test_upper)",
"0.9347088917490147 1.0208466638065408\n"
],
[
"ci_test = 2.58 * np.sqrt((acc_test*(1-acc_test)) / y_test.shape[0])\n\ntest_lower = acc_test-ci_test\ntest_upper = acc_test+ci_test\n\nprint(test_lower, test_upper)",
"0.921085060454202 1.0344704951013535\n"
]
]
] | [
"markdown",
"code",
"markdown",
"code",
"markdown",
"code",
"markdown",
"code",
"markdown",
"code",
"markdown",
"code",
"markdown",
"code"
] | [
[
"markdown"
],
[
"code"
],
[
"markdown"
],
[
"code",
"code"
],
[
"markdown"
],
[
"code"
],
[
"markdown"
],
[
"code",
"code"
],
[
"markdown"
],
[
"code"
],
[
"markdown"
],
[
"code"
],
[
"markdown"
],
[
"code",
"code"
]
] |
e76bbe2aba1232d10a97f606febe0689855d8738 | 84,742 | ipynb | Jupyter Notebook | docs/tutorial/inventory.ipynb | brigade-automation/brigade | 290ecbf785be313e82d05e9f187865f2fd65e998 | [
"Apache-2.0"
] | 63 | 2018-04-05T02:46:24.000Z | 2018-05-15T13:16:15.000Z | docs/tutorial/inventory.ipynb | napalm-automation/brigade | 4622a754d46b49fe5866e8bfb6fb84f3e7bb46f6 | [
"Apache-2.0"
] | 65 | 2016-12-17T11:55:43.000Z | 2018-03-28T09:41:11.000Z | docs/tutorial/inventory.ipynb | brigade-automation/brigade | 290ecbf785be313e82d05e9f187865f2fd65e998 | [
"Apache-2.0"
] | 7 | 2018-04-03T08:18:54.000Z | 2018-05-15T13:11:47.000Z | 59.551651 | 432 | 0.551887 | [
[
[
"# ignore this cell, this is just a helper cell to provide the magic %highlight_file\n%run ../highlighter.py",
"_____no_output_____"
]
],
[
[
"## Inventory\n\nThe Inventory is arguably the most important piece of nornir. Let's see how it works. To begin with the [inventory](../api/nornir/core/inventory.html#module-nornir.core.inventory) is comprised of [hosts](../api/nornir/core/inventory.html#nornir.core.inventory.Hosts), [groups](../api/nornir/core/inventory.html#nornir.core.inventory.Groups) and [defaults](../api/nornir/core/inventory.html#nornir.core.inventory.Defaults).\n\nIn this tutorial we are using the [SimpleInventory](../api/nornir/plugins/inventory/simple.html#nornir.plugins.inventory.simple.SimpleInventory) plugin. This inventory plugin stores all the relevant data in three files. Letโs start by checking them:",
"_____no_output_____"
]
],
[
[
"# hosts file\n%highlight_file inventory/hosts.yaml",
"_____no_output_____"
]
],
[
[
"The hosts file is basically a map where the outermost key is the name of the host and then a `Host` object. You can see the schema of the object by executing:",
"_____no_output_____"
]
],
[
[
"from nornir.core.inventory import Host\nimport json\nprint(json.dumps(Host.schema(), indent=4))",
"{\n \"name\": \"str\",\n \"connection_options\": {\n \"$connection_type\": {\n \"extras\": {\n \"$key\": \"$value\"\n },\n \"hostname\": \"str\",\n \"port\": \"int\",\n \"username\": \"str\",\n \"password\": \"str\",\n \"platform\": \"str\"\n }\n },\n \"groups\": [\n \"$group_name\"\n ],\n \"data\": {\n \"$key\": \"$value\"\n },\n \"hostname\": \"str\",\n \"port\": \"int\",\n \"username\": \"str\",\n \"password\": \"str\",\n \"platform\": \"str\"\n}\n"
]
],
[
[
"The `groups_file` follows the same rules as the `hosts_file`.",
"_____no_output_____"
]
],
[
[
"# groups file\n%highlight_file inventory/groups.yaml",
"_____no_output_____"
]
],
[
[
"Finally, the defaults file has the same schema as the `Host` we described before but without outer keys to denote individual elements. We will see how the data in the groups and defaults file is used later on in this tutorial.",
"_____no_output_____"
]
],
[
[
"# defaults file\n%highlight_file inventory/defaults.yaml",
"_____no_output_____"
]
],
[
[
"### Accessing the inventory\n\nYou can access the [inventory](../api/nornir/core/inventory.html#module-nornir.core.inventory) with the `inventory` attribute:",
"_____no_output_____"
]
],
[
[
"from nornir import InitNornir\nnr = InitNornir(config_file=\"config.yaml\")\n\nprint(nr.inventory.hosts)",
"{'host1.cmh': Host: host1.cmh, 'host2.cmh': Host: host2.cmh, 'spine00.cmh': Host: spine00.cmh, 'spine01.cmh': Host: spine01.cmh, 'leaf00.cmh': Host: leaf00.cmh, 'leaf01.cmh': Host: leaf01.cmh, 'host1.bma': Host: host1.bma, 'host2.bma': Host: host2.bma, 'spine00.bma': Host: spine00.bma, 'spine01.bma': Host: spine01.bma, 'leaf00.bma': Host: leaf00.bma, 'leaf01.bma': Host: leaf01.bma}\n"
]
],
[
[
"The inventory has two dict-like attributes `hosts` and `groups` that you can use to access the hosts and groups respectively:",
"_____no_output_____"
]
],
[
[
"nr.inventory.hosts",
"_____no_output_____"
],
[
"nr.inventory.groups",
"_____no_output_____"
],
[
"nr.inventory.hosts[\"leaf01.bma\"]",
"_____no_output_____"
]
],
[
[
"Hosts and groups are also dict-like objects:",
"_____no_output_____"
]
],
[
[
"host = nr.inventory.hosts[\"leaf01.bma\"]\nhost.keys()",
"_____no_output_____"
],
[
"host[\"site\"]",
"_____no_output_____"
]
],
[
[
"### Inheritance model\n\nLet's see how the inheritance models works by example. Let's start by looking again at the groups file:",
"_____no_output_____"
]
],
[
[
"# groups file\n%highlight_file inventory/groups.yaml",
"_____no_output_____"
]
],
[
[
"The host `leaf01.bma` belongs to the group `bma` which in turn belongs to the groups `eu` and `global`. The host `spine00.cmh` belongs to the group `cmh` which doesn't belong to any other group.\n\nData resolution works by iterating recursively over all the parent groups and trying to see if that parent group (or any of it's parents) contains the data. For instance:",
"_____no_output_____"
]
],
[
[
"leaf01_bma = nr.inventory.hosts[\"leaf01.bma\"]\nleaf01_bma[\"domain\"] # comes from the group `global`",
"_____no_output_____"
],
[
"leaf01_bma[\"asn\"] # comes from group `eu`",
"_____no_output_____"
]
],
[
[
"Values in `defaults` will be returned if neither the host nor the parents have a specific value for it.",
"_____no_output_____"
]
],
[
[
"leaf01_cmh = nr.inventory.hosts[\"leaf01.cmh\"]\nleaf01_cmh[\"domain\"] # comes from defaults",
"_____no_output_____"
]
],
[
[
"If nornir can't resolve the data you should get a KeyError as usual:",
"_____no_output_____"
]
],
[
[
"try:\n leaf01_cmh[\"non_existent\"]\nexcept KeyError as e:\n print(f\"Couldn't find key: {e}\")",
"Couldn't find key: 'non_existent'\n"
]
],
[
[
"You can also try to access data without recursive resolution by using the `data` attribute. For example, if we try to access `leaf01_cmh.data[\"domain\"]` we should get an error as the host itself doesn't have that data:",
"_____no_output_____"
]
],
[
[
"try:\n leaf01_cmh.data[\"domain\"]\nexcept KeyError as e:\n print(f\"Couldn't find key: {e}\")",
"Couldn't find key: 'domain'\n"
]
],
[
[
"### Filtering the inventory\n\nSo far we have seen that `nr.inventory.hosts` and `nr.inventory.groups` are dict-like objects that we can use to iterate over all the hosts and groups or to access any particular one directly. Now we are going to see how we can do some fancy filtering that will enable us to operate on groups of hosts based on their properties.\n\nThe simpler way of filtering hosts is by `<key, value>` pairs. For instance:",
"_____no_output_____"
]
],
[
[
"nr.filter(site=\"cmh\").inventory.hosts.keys()",
"_____no_output_____"
]
],
[
[
"You can also filter using multiple `<key, value>` pairs:",
"_____no_output_____"
]
],
[
[
"nr.filter(site=\"cmh\", role=\"spine\").inventory.hosts.keys()",
"_____no_output_____"
]
],
[
[
"Filter is cumulative:",
"_____no_output_____"
]
],
[
[
"nr.filter(site=\"cmh\").filter(role=\"spine\").inventory.hosts.keys()",
"_____no_output_____"
]
],
[
[
"Or:",
"_____no_output_____"
]
],
[
[
"cmh = nr.filter(site=\"cmh\")\ncmh.filter(role=\"spine\").inventory.hosts.keys()",
"_____no_output_____"
],
[
"cmh.filter(role=\"leaf\").inventory.hosts.keys()",
"_____no_output_____"
]
],
[
[
"You can also grab the children of a group:",
"_____no_output_____"
]
],
[
[
"nr.inventory.children_of_group(\"eu\")",
"_____no_output_____"
]
],
[
[
"#### Advanced filtering\n\nSometimes you need more fancy filtering. For those cases you have two options:\n\n1. Use a filter function.\n2. Use a filter object.\n\n##### Filter functions\n\nThe ``filter_func`` parameter let's you run your own code to filter the hosts. The function signature is as simple as ``my_func(host)`` where host is an object of type [Host](../api/nornir/core/inventory.html#nornir.core.inventory.Host) and it has to return either ``True`` or ``False`` to indicate if you want to host or not.",
"_____no_output_____"
]
],
[
[
"def has_long_name(host):\n return len(host.name) == 11\n\nnr.filter(filter_func=has_long_name).inventory.hosts.keys()",
"_____no_output_____"
],
[
"# Or a lambda function\nnr.filter(filter_func=lambda h: len(h.name) == 9).inventory.hosts.keys()",
"_____no_output_____"
]
],
[
[
"##### Filter Object\n\nYou can also use a filter objects to incrementally create a complex query objects. Let's see how it works by example:",
"_____no_output_____"
]
],
[
[
"# first you need to import the F object\nfrom nornir.core.filter import F",
"_____no_output_____"
],
[
"# hosts in group cmh\ncmh = nr.filter(F(groups__contains=\"cmh\"))\nprint(cmh.inventory.hosts.keys())",
"dict_keys(['host1.cmh', 'host2.cmh', 'spine00.cmh', 'spine01.cmh', 'leaf00.cmh', 'leaf01.cmh'])\n"
],
[
"# devices running either linux or eos\nlinux_or_eos = nr.filter(F(platform=\"linux\") | F(platform=\"eos\"))\nprint(linux_or_eos.inventory.hosts.keys())",
"dict_keys(['host1.cmh', 'host2.cmh', 'spine00.cmh', 'leaf00.cmh', 'host1.bma', 'host2.bma', 'spine00.bma', 'leaf00.bma'])\n"
],
[
"# spines in cmh\ncmh_and_spine = nr.filter(F(groups__contains=\"cmh\") & F(role=\"spine\"))\nprint(cmh_and_spine.inventory.hosts.keys())",
"dict_keys(['spine00.cmh', 'spine01.cmh'])\n"
],
[
"# cmh devices that are not spines\ncmh_and_not_spine = nr.filter(F(groups__contains=\"cmh\") & ~F(role=\"spine\"))\nprint(cmh_and_not_spine.inventory.hosts.keys())",
"dict_keys(['host1.cmh', 'host2.cmh', 'leaf00.cmh', 'leaf01.cmh'])\n"
]
],
[
[
"You can also access nested data and even check if dicts/lists/strings contains elements. Again, let's see by example:",
"_____no_output_____"
]
],
[
[
"nested_string_asd = nr.filter(F(nested_data__a_string__contains=\"asd\"))\nprint(nested_string_asd.inventory.hosts.keys())",
"dict_keys(['host1.cmh'])\n"
],
[
"a_dict_element_equals = nr.filter(F(nested_data__a_dict__c=3))\nprint(a_dict_element_equals.inventory.hosts.keys())",
"dict_keys(['host2.cmh'])\n"
],
[
"a_list_contains = nr.filter(F(nested_data__a_list__contains=2))\nprint(a_list_contains.inventory.hosts.keys())",
"dict_keys(['host1.cmh', 'host2.cmh'])\n"
]
],
[
[
"You can basically access any nested data by separating the elements in the path with two underscores `__`. Then you can use `__contains` to check if an element exists or if a string has a particular substring.",
"_____no_output_____"
]
]
] | [
"code",
"markdown",
"code",
"markdown",
"code",
"markdown",
"code",
"markdown",
"code",
"markdown",
"code",
"markdown",
"code",
"markdown",
"code",
"markdown",
"code",
"markdown",
"code",
"markdown",
"code",
"markdown",
"code",
"markdown",
"code",
"markdown",
"code",
"markdown",
"code",
"markdown",
"code",
"markdown",
"code",
"markdown",
"code",
"markdown",
"code",
"markdown",
"code",
"markdown",
"code",
"markdown"
] | [
[
"code"
],
[
"markdown"
],
[
"code"
],
[
"markdown"
],
[
"code"
],
[
"markdown"
],
[
"code"
],
[
"markdown"
],
[
"code"
],
[
"markdown"
],
[
"code"
],
[
"markdown"
],
[
"code",
"code",
"code"
],
[
"markdown"
],
[
"code",
"code"
],
[
"markdown"
],
[
"code"
],
[
"markdown"
],
[
"code",
"code"
],
[
"markdown"
],
[
"code"
],
[
"markdown"
],
[
"code"
],
[
"markdown"
],
[
"code"
],
[
"markdown"
],
[
"code"
],
[
"markdown"
],
[
"code"
],
[
"markdown"
],
[
"code"
],
[
"markdown"
],
[
"code",
"code"
],
[
"markdown"
],
[
"code"
],
[
"markdown"
],
[
"code",
"code"
],
[
"markdown"
],
[
"code",
"code",
"code",
"code",
"code"
],
[
"markdown"
],
[
"code",
"code",
"code"
],
[
"markdown"
]
] |
e76bcabb1fd4ebd68247e87e5f40dd00c6840c81 | 47,497 | ipynb | Jupyter Notebook | Sentiment_Analysis_of_Twitter_using_Naive_Bayes.ipynb | shreenath2001/Sentiment_Analysis_of_Twitter | 800b9159d26abcb33a8974a2fdf40a68d81023ce | [
"MIT"
] | 1 | 2021-01-25T14:14:03.000Z | 2021-01-25T14:14:03.000Z | Sentiment_Analysis_of_Twitter_using_Naive_Bayes.ipynb | shreenath2001/Sentiment_Analysis_of_Twitter | 800b9159d26abcb33a8974a2fdf40a68d81023ce | [
"MIT"
] | null | null | null | Sentiment_Analysis_of_Twitter_using_Naive_Bayes.ipynb | shreenath2001/Sentiment_Analysis_of_Twitter | 800b9159d26abcb33a8974a2fdf40a68d81023ce | [
"MIT"
] | null | null | null | 37.517378 | 7,048 | 0.631514 | [
[
[
"# Importing Libraries",
"_____no_output_____"
]
],
[
[
"# important packages\n\nimport pandas as pd\t\t\t\t\t# data manipulation using dataframes\nimport numpy as np\t\t\t\t\t# data statistical analysis\nimport seaborn as sns\t\t\t\t# Statistical data visualization\nimport cv2\t\t\t\t\t\t\t# Image and Video processing library\n\nimport matplotlib.pyplot as plt\t\t# data visualisation\n%matplotlib inline\n\npd.set_option('display.max_colwidth',1000)",
"/usr/local/lib/python3.6/dist-packages/statsmodels/tools/_testing.py:19: FutureWarning: pandas.util.testing is deprecated. Use the functions in the public API at pandas.testing instead.\n import pandas.util.testing as tm\n"
],
[
"import re # for regular expressions\n\nimport nltk # for text manipulation\nnltk.download('punkt') # Punkt Sentence Tokenizer\n\nimport warnings \nwarnings.filterwarnings(\"ignore\", category=DeprecationWarning)",
"[nltk_data] Downloading package punkt to /root/nltk_data...\n[nltk_data] Package punkt is already up-to-date!\n"
],
[
"from google.colab import drive\ndrive.mount('/content/drive')",
"Drive already mounted at /content/drive; to attempt to forcibly remount, call drive.mount(\"/content/drive\", force_remount=True).\n"
],
[
"!pwd",
"/content\n"
]
],
[
[
"# Importing Dataset",
"_____no_output_____"
]
],
[
[
"df = pd.read_csv(\"/content/drive/My Drive/P1: Twitter Sentiment Analysis/train.txt\")\ndf_test = pd.read_csv(\"/content/drive/My Drive/P1: Twitter Sentiment Analysis/test_samples.txt\")\ndf.head()",
"_____no_output_____"
],
[
"df.shape",
"_____no_output_____"
],
[
"df_test.head()",
"_____no_output_____"
],
[
"df.info()",
"<class 'pandas.core.frame.DataFrame'>\nRangeIndex: 21465 entries, 0 to 21464\nData columns (total 3 columns):\n # Column Non-Null Count Dtype \n--- ------ -------------- ----- \n 0 tweet_id 21465 non-null int64 \n 1 sentiment 21465 non-null object\n 2 tweet_text 21465 non-null object\ndtypes: int64(1), object(2)\nmemory usage: 503.2+ KB\n"
],
[
"df[\"sentiment\"].unique()",
"_____no_output_____"
]
],
[
[
"# Data Visualization",
"_____no_output_____"
]
],
[
[
"sns.countplot(df['sentiment'], label = \"Count\")",
"_____no_output_____"
],
[
"positive = df[df[\"sentiment\"] == 'positive']\nnegative = df[df[\"sentiment\"] == 'negative']\nneutral = df[df[\"sentiment\"] == 'neutral']",
"_____no_output_____"
],
[
"positive_percentage = (positive.shape[0]/df.shape[0])*100\nnegative_percentage = (negative.shape[0]/df.shape[0])*100\nneutral_percentage = (neutral.shape[0]/df.shape[0])*100\nprint(f\"Positve Tweets = {positive_percentage:.2f}%\\nNegative Tweets = {negative_percentage:.2f}%\\nNeutral Tweets = {neutral_percentage:.2f}%\")",
"Positve Tweets = 42.23%\nNegative Tweets = 15.78%\nNeutral Tweets = 41.99%\n"
]
],
[
[
"# Data Cleaning",
"_____no_output_____"
]
],
[
[
"df_test[\"sentiment\"] = \"NA\"\n\ndf_total = pd.concat((df, df_test), ignore_index=True)\ndf_total.head()",
"_____no_output_____"
],
[
"df_total.shape",
"_____no_output_____"
],
[
"#### Removing Twitter Handles (@user)\n\ndef remove_pattern(input_txt, pattern):\n r = re.findall(pattern, input_txt)\n for i in r:\n input_txt = re.sub(i, '', input_txt)\n \n return input_txt",
"_____no_output_____"
],
[
"import re\nimport nltk\nnltk.download('wordnet')\nnltk.download('stopwords')\nfrom nltk.corpus import stopwords\nfrom nltk.stem.porter import PorterStemmer\nfrom nltk.stem.wordnet import WordNetLemmatizer",
"[nltk_data] Downloading package wordnet to /root/nltk_data...\n[nltk_data] Package wordnet is already up-to-date!\n[nltk_data] Downloading package stopwords to /root/nltk_data...\n[nltk_data] Package stopwords is already up-to-date!\n"
],
[
"corpus = []\nfor i in range(df_total.shape[0]):\n text = np.vectorize(remove_pattern)(df_total['tweet_text'][i], \"@[\\w]*\") # Removing Twitter Handles (@user)\n text = str(text) \n text = re.sub('[^a-zA-Z]', ' ', text) # Removing Punctuations, Numbers, and Special Characters\n text = re.sub(r'\\s+', ' ', text).strip() # Remove_trailing_spaces(input_txt)\n text = text.lower() # Convert to lower case\n text = text.split() # Split-data\n lemmatizer = WordNetLemmatizer() #WordNet Lemmatization\n #ps = PorterStemmer() #porter's stemmer\n all_stopwords = stopwords.words('english') # List of Stopwords\n all_stopwords.remove('not')\n text = [lemmatizer.lemmatize(word) for word in text if not word in set(all_stopwords)]\n #text = [ps.stem(word) for word in text if not word in set(all_stopwords)]\n text = ' '.join(text)\n corpus.append(text)",
"_____no_output_____"
],
[
"len(corpus)",
"_____no_output_____"
],
[
"### to find no. of sentences with words >500\n\nnum = 0\nfor i in range(len(corpus)):\n if (len(corpus[i]) >= 500):\n num = num + 1\nnum",
"_____no_output_____"
]
],
[
[
"# Data Visualization",
"_____no_output_____"
]
],
[
[
"sns.countplot(df['sentiment'], label = \"Count\")",
"_____no_output_____"
],
[
"positive = df[df[\"sentiment\"] == 'positive']\nnegative = df[df[\"sentiment\"] == 'negative']\nneutral = df[df[\"sentiment\"] == 'neutral']",
"_____no_output_____"
],
[
"positive_percentage = (positive.shape[0]/df.shape[0])*100\nnegative_percentage = (negative.shape[0]/df.shape[0])*100\nneutral_percentage = (neutral.shape[0]/df.shape[0])*100\nprint(f\"Positve Tweets = {positive_percentage:.2f}%\\nNegative Tweets = {negative_percentage:.2f}%\\nNeutral Tweets = {neutral_percentage:.2f}%\")",
"Positve Tweets = 42.23%\nNegative Tweets = 15.78%\nNeutral Tweets = 41.99%\n"
]
],
[
[
"# Bag of Words Model",
"_____no_output_____"
],
[
"#### CountVectorizer",
"_____no_output_____"
]
],
[
[
"from sklearn.feature_extraction.text import CountVectorizer, TfidfVectorizer\ncv = CountVectorizer()\nX= cv.fit_transform(corpus).toarray()",
"_____no_output_____"
],
[
"len(X[0])",
"_____no_output_____"
]
],
[
[
"### TfidfVectorizer",
"_____no_output_____"
]
],
[
[
"#from sklearn.feature_extraction.text import CountVectorizer, TfidfVectorizer\n#tv = TfidfVectorizer()\n#X = tv.fit_transform(corpus).toarray()",
"_____no_output_____"
],
[
"len(X[0])",
"_____no_output_____"
]
],
[
[
"# Data Splitting",
"_____no_output_____"
]
],
[
[
"X_train = X[:21465]\nX_test = X[21465:]\ny_train = df.iloc[:, 1].values",
"_____no_output_____"
],
[
"X_train.shape",
"_____no_output_____"
],
[
"y_train.shape",
"_____no_output_____"
],
[
"from sklearn.naive_bayes import MultinomialNB\n\nclassifier = MultinomialNB()\nclassifier.fit(X_train, y_train)",
"_____no_output_____"
]
],
[
[
"# Model Prediction",
"_____no_output_____"
]
],
[
[
"y_pred = classifier.predict(X_test)",
"_____no_output_____"
],
[
"print(y_pred)\nprint(y_pred.shape)",
"['neutral' 'positive' 'neutral' ... 'positive' 'neutral' 'positive']\n(5398,)\n"
],
[
"list1 = []\nheading = ['tweet_id', 'sentiment']\n\nlist1.append(heading)\n\nfor i in range(len(y_pred)):\n sub = []\n sub.append(df_test[\"tweet_id\"][i])\n sub.append(y_pred[i])\n list1.append(sub)",
"_____no_output_____"
]
],
[
[
"# Generate Submission File",
"_____no_output_____"
]
],
[
[
"import csv\nwith open('/content/drive/My Drive/P1: Twitter Sentiment Analysis/Models/NB_TV.csv', 'w', newline='') as fp:\n a = csv.writer(fp, delimiter = \",\")\n data = list1\n a.writerows(data)",
"_____no_output_____"
]
],
[
[
"# Test Accuracy (Count_Vectorizer )= 61.13%\n\n# Test Accuracy (Tf-idf_Vectorizer )= 60.10%\n",
"_____no_output_____"
]
]
] | [
"markdown",
"code",
"markdown",
"code",
"markdown",
"code",
"markdown",
"code",
"markdown",
"code",
"markdown",
"code",
"markdown",
"code",
"markdown",
"code",
"markdown",
"code",
"markdown",
"code",
"markdown"
] | [
[
"markdown"
],
[
"code",
"code",
"code",
"code"
],
[
"markdown"
],
[
"code",
"code",
"code",
"code",
"code"
],
[
"markdown"
],
[
"code",
"code",
"code"
],
[
"markdown"
],
[
"code",
"code",
"code",
"code",
"code",
"code",
"code"
],
[
"markdown"
],
[
"code",
"code",
"code"
],
[
"markdown",
"markdown"
],
[
"code",
"code"
],
[
"markdown"
],
[
"code",
"code"
],
[
"markdown"
],
[
"code",
"code",
"code",
"code"
],
[
"markdown"
],
[
"code",
"code",
"code"
],
[
"markdown"
],
[
"code"
],
[
"markdown"
]
] |
e76bcdf7050dcc17068593ef3feaa327565e6a59 | 3,080 | ipynb | Jupyter Notebook | analysis/optical flow.ipynb | hitennirmal/goucher | aef7810b282a2249aae4e8a22470f55b2ea33485 | [
"MIT"
] | 1 | 2018-04-06T14:24:55.000Z | 2018-04-06T14:24:55.000Z | analysis/optical flow.ipynb | hitennirmal/goucher | aef7810b282a2249aae4e8a22470f55b2ea33485 | [
"MIT"
] | null | null | null | analysis/optical flow.ipynb | hitennirmal/goucher | aef7810b282a2249aae4e8a22470f55b2ea33485 | [
"MIT"
] | 3 | 2018-04-06T14:24:57.000Z | 2019-03-04T00:30:44.000Z | 23.875969 | 155 | 0.523052 | [
[
[
"# Optical Flow test \n\nThis notebook will try to follow optical flow \n\n\ninitial code comes from : \nhttps://docs.opencv.org/3.3.1/d7/d8b/tutorial_py_lucas_kanade.html",
"_____no_output_____"
]
],
[
[
"%matplotlib inline\nimport cv2 \nimport os\nimport sys\nimport numpy as np \nimport glob\nimport matplotlib.pyplot as plt",
"_____no_output_____"
],
[
"samplehash = '10a278dc5ebd2b93e1572a136578f9dbe84d10157cc6cca178c339d9ca762c52' #'7fafc640d446cab1872e4376b5c2649f8c67e658b3fc89d2bced3b47c929e608'#",
"_____no_output_____"
],
[
"files = sorted(glob.glob( \"../data/train/data/\" + samplehash + \"/frame*.png\" ))",
"_____no_output_____"
],
[
"images = [ cv2.imread(x,1) for x in files ]",
"_____no_output_____"
],
[
"cap = cv2.VideoCapture(\"vtest.avi\")\nframe1 = images[0]\nprvs = cv2.cvtColor(frame1,cv2.COLOR_BGR2GRAY)\nhsv = np.zeros_like(frame1)\nhsv[...,1] = 255\nindex =0 \nwhile(1):\n index += 1\n if index == 100: break\n frame2 = images[index]\n next = cv2.cvtColor(frame2,cv2.COLOR_BGR2GRAY)\n flow = cv2.calcOpticalFlowFarneback(prvs,next, None, 0.5, 3, 15, 3, 5, 1.2, 0)\n mag, ang = cv2.cartToPolar(flow[...,0], flow[...,1])\n hsv[...,0] = ang*180/np.pi/2\n hsv[...,2] = cv2.normalize(mag,None,0,255,cv2.NORM_MINMAX)\n bgr = cv2.cvtColor(hsv,cv2.COLOR_HSV2BGR)\n cv2.imshow('frame2',bgr)\n k = cv2.waitKey(30) & 0xff\n if k == 27:\n break\n elif k == ord('s'):\n cv2.imwrite('opticalfb.png',frame2)\n cv2.imwrite('opticalhsv.png',bgr)\n prvs = next\ncap.release()\ncv2.destroyAllWindows()",
"_____no_output_____"
]
]
] | [
"markdown",
"code"
] | [
[
"markdown"
],
[
"code",
"code",
"code",
"code",
"code"
]
] |
e76bd73462b22a4952d3c8385eb34ec8fd747a2a | 2,418 | ipynb | Jupyter Notebook | if-else/comparacoes-contraintuitivas.ipynb | amarelopiupiu/python | bbd07a4b5e52d011c77f20622e17126f78fa3051 | [
"MIT"
] | null | null | null | if-else/comparacoes-contraintuitivas.ipynb | amarelopiupiu/python | bbd07a4b5e52d011c77f20622e17126f78fa3051 | [
"MIT"
] | null | null | null | if-else/comparacoes-contraintuitivas.ipynb | amarelopiupiu/python | bbd07a4b5e52d011c77f20622e17126f78fa3051 | [
"MIT"
] | 1 | 2021-06-09T01:05:59.000Z | 2021-06-09T01:05:59.000Z | 29.487805 | 251 | 0.609595 | [
[
[
"# Comparaรงรตes Contraintuitivas\n\nExistem algumas comparaรงรตes no Python que nรฃo sรฃo tรฃo intuitivas quando vemos pela primeira vez, mas que sรฃo muito usadas, principalmente por programadores mais experientes.\n\nร bom sabermos alguns exemplos e buscar sempre entender o que aquela comparaรงรฃo estรก buscando verificar.\n\n### Exemplo 1:\n\nDigamos que vocรช estรก construindo um sistema de controle de vendas e precisa de algumas informaรงรตes para fazer o cรกlculo do resultado da loja no fim de um mรชs.",
"_____no_output_____"
]
],
[
[
"faturamento = input('Qual foi o faturamento da loja nesse mรชs?')\ncusto = input('Qual foi o custo da loja nesse mรชs?')\n\nif faturamento and custo: # serve para o usuรกrio nรฃo deixar os valores vazios\n lucro = int(faturamento) - int(custo)\n print(\"O lucro da loja foi de {} reais\".format(lucro)) \nelse: # se os valores forem vazios vai aparecer essa mensagem\n print('Preencha o faturamento e o lucro corretamente')",
"Qual foi o faturamento da loja nesse mรชs?500\nQual foi o custo da loja nesse mรชs?\nPreencha o faturamento e o lucro corretamente\n"
]
],
[
[
"## Resumo\n\nAlgumas comparaรงรตes contraintuitivas muito usadas:\n\nIf 0:\n\nIf '':\n\nTemos outras tambรฉm, mas que sรฃo usadas para verificar listas vazias, dicionรกrios vazios, objetos vazios e assim vai. Quando chegarmos nesses mรณdulos vamos relembrar esse conceito, mas o importante รฉ saber dessa possibilidade e entender seu uso.",
"_____no_output_____"
]
]
] | [
"markdown",
"code",
"markdown"
] | [
[
"markdown"
],
[
"code"
],
[
"markdown"
]
] |
e76bf7e4fd5c0b4b2fe4d5daa4fb355263fd15f4 | 15,064 | ipynb | Jupyter Notebook | first-neural-network/Untitled.ipynb | scollins83/deep-learning | 5b1353fe1dea90065cdf7b0a61cc5f5b68f72094 | [
"MIT"
] | 2 | 2018-05-06T13:11:53.000Z | 2019-01-31T14:10:11.000Z | first-neural-network/Untitled.ipynb | scollins83/deep-learning | 5b1353fe1dea90065cdf7b0a61cc5f5b68f72094 | [
"MIT"
] | null | null | null | first-neural-network/Untitled.ipynb | scollins83/deep-learning | 5b1353fe1dea90065cdf7b0a61cc5f5b68f72094 | [
"MIT"
] | 2 | 2017-06-14T13:34:30.000Z | 2019-11-02T08:26:55.000Z | 38.824742 | 127 | 0.49303 | [
[
[
"import numpy as np\nfrom data_prep import features, targets, features_test, targets_test\n\nnp.random.seed(21)\n\ndef sigmoid(x):\n \"\"\"\n Calculate sigmoid\n \"\"\"\n return 1 / (1 + np.exp(-x))\n\n\n# Hyperparameters\nn_hidden = 2 # number of hidden units\nepochs = 900\nlearnrate = 0.005\n\nn_records, n_features = features.shape\nlast_loss = None\n# Initialize weights\nweights_input_hidden = np.random.normal(scale=1 / n_features ** .5,\n size=(n_features, n_hidden))\nweights_hidden_output = np.random.normal(scale=1 / n_features ** .5,\n size=n_hidden)\n\nfor e in range(epochs):\n del_w_input_hidden = np.zeros(weights_input_hidden.shape)\n del_w_hidden_output = np.zeros(weights_hidden_output.shape)\n for x, y in zip(features.values, targets):\n ## Forward pass ##\n # TODO: Calculate the output\n hidden_input = np.dot(x, weights_input_hidden)\n hidden_output = sigmoid(hidden_input)\n\n output = sigmoid(np.dot(hidden_output,\n weights_hidden_output))\n\n ## Backward pass ##\n # TODO: Calculate the network's prediction error\n error = y - output\n\n # TODO: Calculate error term for the output unit\n output_error_term = error * output * (1 - output)\n\n ## propagate errors to hidden layer\n\n # TODO: Calculate the hidden layer's contribution to the error\n hidden_error = np.dot(output_error_term, weights_hidden_output)\n\n # TODO: Calculate the error term for the hidden layer\n hidden_error_term = hidden_error * hidden_output * (1 - hidden_output)\n\n # TODO: Update the change in weights\n del_w_hidden_output += output_error_term * hidden_output\n del_w_input_hidden += hidden_error_term * x[:, None]\n\n # TODO: Update weights\n weights_input_hidden += learnrate * del_w_input_hidden / n_records\n weights_hidden_output += learnrate * del_w_hidden_output / n_records\n\n # Printing out the mean square error on the training set\n if e % (epochs / 10) == 0:\n hidden_output = sigmoid(np.dot(x, weights_input_hidden))\n out = sigmoid(np.dot(hidden_output,\n weights_hidden_output))\n loss = np.mean((out - targets) ** 2)\n\n if last_loss and last_loss < loss:\n print(\"Train loss: \", loss, \" WARNING - Loss Increasing\")\n else:\n print(\"Train loss: \", loss)\n last_loss = loss\n\n# Calculate accuracy on test data\nhidden = sigmoid(np.dot(features_test, weights_input_hidden))\nout = sigmoid(np.dot(hidden, weights_hidden_output))\npredictions = out > 0.5\naccuracy = np.mean(predictions == targets_test)\nprint(\"Prediction accuracy: {:.3f}\".format(accuracy))",
"_____no_output_____"
],
[
"import numpy as np\n\nclass Node:\n \"\"\"\n Base class for nodes in the network.\n\n Arguments:\n\n `inbound_nodes`: A list of nodes with edges into this node.\n \"\"\"\n def __init__(self, inbound_nodes=[]):\n \"\"\"\n Node's constructor (runs when the object is instantiated). Sets\n properties that all nodes need.\n \"\"\"\n # A list of nodes with edges into this node.\n self.inbound_nodes = inbound_nodes\n # The eventual value of this node. Set by running\n # the forward() method.\n self.value = None\n # A list of nodes that this node outputs to.\n self.outbound_nodes = []\n # New property! Keys are the inputs to this node and\n # their values are the partials of this node with\n # respect to that input.\n self.gradients = {}\n # Sets this node as an outbound node for all of\n # this node's inputs.\n for node in inbound_nodes:\n node.outbound_nodes.append(self)\n\n def forward(self):\n \"\"\"\n Every node that uses this class as a base class will\n need to define its own `forward` method.\n \"\"\"\n raise NotImplementedError\n\n def backward(self):\n \"\"\"\n Every node that uses this class as a base class will\n need to define its own `backward` method.\n \"\"\"\n raise NotImplementedError\n\n\nclass Input(Node):\n \"\"\"\n A generic input into the network.\n \"\"\"\n def __init__(self):\n # The base class constructor has to run to set all\n # the properties here.\n #\n # The most important property on an Input is value.\n # self.value is set during `topological_sort` later.\n Node.__init__(self)\n\n def forward(self):\n # Do nothing because nothing is calculated.\n pass\n\n def backward(self):\n # An Input node has no inputs so the gradient (derivative)\n # is zero.\n # The key, `self`, is reference to this object.\n self.gradients = {self: 0}\n # Weights and bias may be inputs, so you need to sum\n # the gradient from output gradients.\n for n in self.outbound_nodes:\n self.gradients[self] += n.gradients[self]\n\nclass Linear(Node):\n \"\"\"\n Represents a node that performs a linear transform.\n \"\"\"\n def __init__(self, X, W, b):\n # The base class (Node) constructor. Weights and bias\n # are treated like inbound nodes.\n Node.__init__(self, [X, W, b])\n\n def forward(self):\n \"\"\"\n Performs the math behind a linear transform.\n \"\"\"\n X = self.inbound_nodes[0].value\n W = self.inbound_nodes[1].value\n b = self.inbound_nodes[2].value\n self.value = np.dot(X, W) + b\n\n def backward(self):\n \"\"\"\n Calculates the gradient based on the output values.\n \"\"\"\n # Initialize a partial for each of the inbound_nodes.\n self.gradients = {n: np.zeros_like(n.value) for n in self.inbound_nodes}\n # Cycle through the outputs. The gradient will change depending\n # on each output, so the gradients are summed over all outputs.\n for n in self.outbound_nodes:\n # Get the partial of the cost with respect to this node.\n grad_cost = n.gradients[self]\n # Set the partial of the loss with respect to this node's inputs.\n self.gradients[self.inbound_nodes[0]] += np.dot(grad_cost, self.inbound_nodes[1].value.T)\n # Set the partial of the loss with respect to this node's weights.\n self.gradients[self.inbound_nodes[1]] += np.dot(self.inbound_nodes[0].value.T, grad_cost)\n # Set the partial of the loss with respect to this node's bias.\n self.gradients[self.inbound_nodes[2]] += np.sum(grad_cost, axis=0, keepdims=False)\n\n\nclass Sigmoid(Node):\n \"\"\"\n Represents a node that performs the sigmoid activation function.\n \"\"\"\n def __init__(self, node):\n # The base class constructor.\n Node.__init__(self, [node])\n\n def _sigmoid(self, x):\n \"\"\"\n This method is separate from `forward` because it\n will be used with `backward` as well.\n\n `x`: A numpy array-like object.\n \"\"\"\n return 1. / (1. + np.exp(-x))\n\n def forward(self):\n \"\"\"\n Perform the sigmoid function and set the value.\n \"\"\"\n input_value = self.inbound_nodes[0].value\n self.value = self._sigmoid(input_value)\n\n def backward(self):\n \"\"\"\n Calculates the gradient using the derivative of\n the sigmoid function.\n \"\"\"\n # Initialize the gradients to 0.\n self.gradients = {n: np.zeros_like(n.value) for n in self.inbound_nodes}\n # Sum the partial with respect to the input over all the outputs.\n for n in self.outbound_nodes:\n grad_cost = n.gradients[self]\n sigmoid = self.value\n self.gradients[self.inbound_nodes[0]] += sigmoid * (1 - sigmoid) * grad_cost\n\n\nclass MSE(Node):\n def __init__(self, y, a):\n \"\"\"\n The mean squared error cost function.\n Should be used as the last node for a network.\n \"\"\"\n # Call the base class' constructor.\n Node.__init__(self, [y, a])\n\n def forward(self):\n \"\"\"\n Calculates the mean squared error.\n \"\"\"\n # NOTE: We reshape these to avoid possible matrix/vector broadcast\n # errors.\n #\n # For example, if we subtract an array of shape (3,) from an array of shape\n # (3,1) we get an array of shape(3,3) as the result when we want\n # an array of shape (3,1) instead.\n #\n # Making both arrays (3,1) insures the result is (3,1) and does\n # an elementwise subtraction as expected.\n y = self.inbound_nodes[0].value.reshape(-1, 1)\n a = self.inbound_nodes[1].value.reshape(-1, 1)\n\n self.m = self.inbound_nodes[0].value.shape[0]\n # Save the computed output for backward.\n self.diff = y - a\n self.value = np.mean(self.diff**2)\n\n def backward(self):\n \"\"\"\n Calculates the gradient of the cost.\n \"\"\"\n self.gradients[self.inbound_nodes[0]] = (2 / self.m) * self.diff\n self.gradients[self.inbound_nodes[1]] = (-2 / self.m) * self.diff\n\n\ndef topological_sort(feed_dict):\n \"\"\"\n Sort the nodes in topological order using Kahn's Algorithm.\n\n `feed_dict`: A dictionary where the key is a `Input` Node and the value is the respective value feed to that Node.\n\n Returns a list of sorted nodes.\n \"\"\"\n\n input_nodes = [n for n in feed_dict.keys()]\n\n G = {}\n nodes = [n for n in input_nodes]\n while len(nodes) > 0:\n n = nodes.pop(0)\n if n not in G:\n G[n] = {'in': set(), 'out': set()}\n for m in n.outbound_nodes:\n if m not in G:\n G[m] = {'in': set(), 'out': set()}\n G[n]['out'].add(m)\n G[m]['in'].add(n)\n nodes.append(m)\n\n L = []\n S = set(input_nodes)\n while len(S) > 0:\n n = S.pop()\n\n if isinstance(n, Input):\n n.value = feed_dict[n]\n\n L.append(n)\n for m in n.outbound_nodes:\n G[n]['out'].remove(m)\n G[m]['in'].remove(n)\n # if no other incoming edges add to S\n if len(G[m]['in']) == 0:\n S.add(m)\n return L\n\n\ndef forward_and_backward(graph):\n \"\"\"\n Performs a forward pass and a backward pass through a list of sorted Nodes.\n\n Arguments:\n\n `graph`: The result of calling `topological_sort`.\n \"\"\"\n # Forward pass\n for n in graph:\n n.forward()\n\n # Backward pass\n # see: https://docs.python.org/2.3/whatsnew/section-slices.html\n for n in graph[::-1]:\n n.backward()\n\n\ndef sgd_update(trainables, learning_rate=1e-2):\n \"\"\"\n Updates the value of each trainable with SGD.\n\n Arguments:\n\n `trainables`: A list of `Input` Nodes representing weights/biases.\n `learning_rate`: The learning rate.\n \"\"\"\n # Performs SGD\n #\n # Loop over the trainables\n for t in trainables:\n # Change the trainable's value by subtracting the learning rate\n # multiplied by the partial of the cost with respect to this\n # trainable.\n partial = t.gradients[t]\n t.value -= learning_rate * partial\n",
"_____no_output_____"
]
]
] | [
"code"
] | [
[
"code",
"code"
]
] |
e76bfb498625ec6a305769a2bae2f4dff5afaba8 | 14,222 | ipynb | Jupyter Notebook | 02-Basic Python/07-Cond Stat.ipynb | Goliath-Research/Introduction-to-Data-Science | f2c2fdd426684758eb1d918bd9affbeaa154ed91 | [
"MIT"
] | null | null | null | 02-Basic Python/07-Cond Stat.ipynb | Goliath-Research/Introduction-to-Data-Science | f2c2fdd426684758eb1d918bd9affbeaa154ed91 | [
"MIT"
] | null | null | null | 02-Basic Python/07-Cond Stat.ipynb | Goliath-Research/Introduction-to-Data-Science | f2c2fdd426684758eb1d918bd9affbeaa154ed91 | [
"MIT"
] | null | null | null | 18.688568 | 292 | 0.456757 | [
[
[
"# Conditional Statements in Python",
"_____no_output_____"
],
[
"Conditional statement controls the flow of execution depending on some condition.",
"_____no_output_____"
],
[
"## Python conditions\n\nPython supports the usual logical conditions from mathematics:",
"_____no_output_____"
],
[
"| **Condition** | **Expression** | \n|----:|:----:|\n| Equal |a == b|\n| Not Equal |a != b|\n| Less than |a < b|\n| Less than or equal to |a <= b|\n| Greater than |a > b|\n| Greater than or equal to |a >= b|",
"_____no_output_____"
]
],
[
[
"a = 2\nb = 5",
"_____no_output_____"
],
[
"# Equal\na == b",
"_____no_output_____"
],
[
"# Not equal\na != b",
"_____no_output_____"
],
[
"# Less than\na < b",
"_____no_output_____"
],
[
"# Less than or equal to\na <= b",
"_____no_output_____"
],
[
"# Greater than\na > b",
"_____no_output_____"
],
[
"# Greater than or equal to\na >= b",
"_____no_output_____"
]
],
[
[
"Python Logical Operators:\n\n- `and`: Returns True if both statements are true\n- `or`: Returns True if one of the statements is true\n- `not`: Reverse the result. Returns False if the result is true, and True is the result is False\n",
"_____no_output_____"
]
],
[
[
"a = 1\nb = 2\nc = 10",
"_____no_output_____"
],
[
"# True and True\na < c and b < c",
"_____no_output_____"
],
[
"# True and False\na < c and b > c",
"_____no_output_____"
],
[
"# True or False\na < c or b > c",
"_____no_output_____"
],
[
"# False or True\na > c or b < c",
"_____no_output_____"
],
[
"# True or True\na < c or b < c",
"_____no_output_____"
],
[
"# False or False\na > c or b > c",
"_____no_output_____"
]
],
[
[
"Using `not` before a boolean expression inverts it:",
"_____no_output_____"
]
],
[
[
"print(not False)",
"True\n"
],
[
"not(a < c)",
"_____no_output_____"
],
[
"not(a > c)",
"_____no_output_____"
]
],
[
[
"## If statements",
"_____no_output_____"
]
],
[
[
"a = 10\nb = 20\nif b > a:\n print(\"The condition is True\")\n print('All these sentences are executed!')",
"The condition is True\nAll these sentences are executed!\n"
]
],
[
[
"Remember Python relies on indentation (whitespace at the beginning of a line) to define scope in the code. \n\nThe same sentence, without indentation, raises an error.",
"_____no_output_____"
]
],
[
[
"if b > a: # This will raise an error\nprint(\"The condition is True\")\nprint('All these sentences are executed')",
"_____no_output_____"
]
],
[
[
"When the condition is False, the sentence is not executed. ",
"_____no_output_____"
]
],
[
[
"a = 10\nb = 20\nif b < a:\n print(\"The condition is False\")\n print('These sentences are NOT executed!')",
"_____no_output_____"
]
],
[
[
"The else keyword catches anything which isn't caught by the preceding conditions.",
"_____no_output_____"
]
],
[
[
"a = 5\nb = 10\nif b < a:\n print(\"The condition is True.\")\nelse:\n print(\"The condition is False.\") ",
"The condition is False.\n"
]
],
[
[
"The elif keyword is pythons way of saying \"if the previous conditions were not true, then try this condition\".",
"_____no_output_____"
]
],
[
[
"# using elif\na = 3\nb = 3\nif b > a:\n print(\"b is greater than a\")\nelif a == b:\n print(\"a and b are equal\")",
"a and b are equal\n"
],
[
"# using else\na = 6\nb = 4\nif b > a:\n print(\"b is greater than a\")\nelif a == b:\n print(\"a and b are equal\")\nelse:\n print(\"a is greater than b\")",
"a is greater than b\n"
]
],
[
[
"An arbitrary number of `elif` clauses can be specified. The `else` clause is optional. If it is present, there can be only one, and it must be specified last.",
"_____no_output_____"
]
],
[
[
"name = 'Anna'\nif name == 'Maria':\n print('Hello Maria!')\nelif name == 'Sarah':\n print('Hello Sarah!')\nelif name == 'Anna':\n print('Hello Anna!')\nelif name == 'Sofia':\n print('Hello Sofia!')\nelse:\n print(\"I do not know who you are!\")",
"Hello Anna!\n"
],
[
"name = 'Julia'\nif name == 'Maria':\n print('Hello Maria!')\nelif name == 'Sarah':\n print('Hello Sarah!')\nelif name == 'Anna':\n print('Hello Anna!')\nelif name == 'Sofia':\n print('Hello Sofia!')\nelse:\n print(\"I do not know who you are!\")",
"I do not know who you are!\n"
],
[
"# Processing user input\nusername = input('Enter username:')\nprint('Your name is', username)",
"Your name is Ana\n"
],
[
"age = input('Enter your age')\nif int(age) < 18:\n print('You are a child!')\nelse:\n print('You are an adult!')",
"You are a child!\n"
],
[
"# Nested if\nx = 14\n\nif x > 10:\n print('Above 10,')\n if x > 20:\n print('and also above 20.')\n else:\n print('but not above 20.') ",
"Above 10,\nbut not above 20.\n"
],
[
"x = 35\n\nif x > 10:\n print('Above 10,')\n if x > 20:\n print('and also above 20.')\n else:\n print('but not above 20.') ",
"Above 10,\nand also above 20.\n"
]
],
[
[
"The `pass` Statement: if statements cannot be empty, but if you for some reason have an if statement with no content, put in the `pass` statement to avoid getting an error.",
"_____no_output_____"
]
],
[
[
"a = 33\nb = 200\nif b > a:\n pass\nelse:\n print('b <= a')",
"_____no_output_____"
]
]
] | [
"markdown",
"code",
"markdown",
"code",
"markdown",
"code",
"markdown",
"code",
"markdown",
"code",
"markdown",
"code",
"markdown",
"code",
"markdown",
"code",
"markdown",
"code",
"markdown",
"code"
] | [
[
"markdown",
"markdown",
"markdown",
"markdown"
],
[
"code",
"code",
"code",
"code",
"code",
"code",
"code"
],
[
"markdown"
],
[
"code",
"code",
"code",
"code",
"code",
"code",
"code"
],
[
"markdown"
],
[
"code",
"code",
"code"
],
[
"markdown"
],
[
"code"
],
[
"markdown"
],
[
"code"
],
[
"markdown"
],
[
"code"
],
[
"markdown"
],
[
"code"
],
[
"markdown"
],
[
"code",
"code"
],
[
"markdown"
],
[
"code",
"code",
"code",
"code",
"code",
"code"
],
[
"markdown"
],
[
"code"
]
] |
e76bfd3c4c830161466f68ed74d9e185f5e2c17d | 7,421 | ipynb | Jupyter Notebook | algoExpert/numbers_in_pi/solution.ipynb | maple1eaf/learning_algorithm | a9296c083ba9b79af1be10f56365ee8c95d3aac6 | [
"MIT"
] | null | null | null | algoExpert/numbers_in_pi/solution.ipynb | maple1eaf/learning_algorithm | a9296c083ba9b79af1be10f56365ee8c95d3aac6 | [
"MIT"
] | null | null | null | algoExpert/numbers_in_pi/solution.ipynb | maple1eaf/learning_algorithm | a9296c083ba9b79af1be10f56365ee8c95d3aac6 | [
"MIT"
] | null | null | null | 28.109848 | 211 | 0.509231 | [
[
[
"# Numbers In Pi\n[link](https://www.algoexpert.io/questions/Numbers%20In%20Pi)",
"_____no_output_____"
],
[
"## My Solution",
"_____no_output_____"
]
],
[
[
"def numbersInPi(pi, numbers):\n # Write your code here.\n # brute force\n d1 = {number: True for number in numbers}\n minSpaces = [float('inf')]\n numbersInPiHelper(pi, d1, 0, minSpaces, 0)\n return minSpaces[0] if minSpaces[0] != float('inf') else -1\n \ndef numbersInPiHelper(pi, d1, startIdx, minSpaces, numberOfSpaces):\n for endIdx in range(startIdx, len(pi)):\n cur = pi[startIdx:endIdx + 1]\n \n if cur in d1:\n if endIdx == len(pi) - 1 and numberOfSpaces < minSpaces[0]:\n minSpaces[0] = numberOfSpaces\n continue\n numbersInPiHelper(pi, d1, endIdx + 1, minSpaces, numberOfSpaces + 1)",
"_____no_output_____"
],
[
"def numbersInPi(pi, numbers):\n # Write your code here.\n # dp: O(n^3 + m) time | O(n + m)\n # n - the number of digits in pi\n # m - length of the number list\n d1 = {number: True for number in numbers}\n opt = [-1 for i in range(len(pi))]\n \n for i in range(len(pi)):\n if pi[:i + 1] in d1:\n opt[i] = 0\n else:\n minValue = float('inf')\n for j in range(i):\n if opt[j] != -1 and pi[j + 1:i + 1] in d1:\n minValue = min(opt[j], minValue)\n if minValue != float('inf'):\n opt[i] = minValue + 1\n \n return opt[-1]",
"_____no_output_____"
]
],
[
[
"## Expert Solution",
"_____no_output_____"
]
],
[
[
"# O(n^3 + m) time | O(n + m) space, where n is the number of digits in Pi and m is the number of favorite numbers\n# recursive solution\ndef numbersInPi(pi, numbers):\n\tnumbersTable = {number: True for number in numbers}\n\tminSpaces = getMinSpaces(pi, numbersTable, {}, 0)\n\treturn -1 if minSpaces == float(\"inf\") else minSpaces\n\ndef getMinSpaces(pi, numbersTable, cache, idx):\n\tif idx == len(pi):\n\t\treturn -1\n\tif idx in cache:\n\t\treturn cache[idx]\n\tminSpaces = float(\"inf\")\n\tfor i in range(idx, len(pi)):\n\t\tprefix = pi[idx: i + 1]\n\t\tif prefix in numbersTable:\n\t\t\tminSpacesInSuffix = getMinSpaces(pi, numbersTable, cache, i + 1)\n\t\t\tminSpaces = min(minSpaces, minSpacesInSuffix + 1)\n\tcache[idx] = minSpaces\n\treturn cache[idx]",
"_____no_output_____"
],
[
"# O(n^3 + m) time | O(n + m) space, where n is the number of digits in Pi and m is the number of favorite numbers\n# dp\ndef numbersInPi(pi, numbers):\n\tnumbersTable = {number: True for number in numbers}\n cache = {}\n for i in reversed(range(len(pi))):\n getMinSpaces(pi, numbersTable, cache, i)\n return -1 if cache[0] == float(\"inf\") else cache[0]\n\ndef getMinSpaces(pi, numbersTable, cache, idx):\n if idx == len(pi):\n return -1\n if idx in cache:\n return cache[idx]\n minSpaces = float(\"inf\")\n for i in range(idx, len(pi)):\n prefix = pi[idx : i + 1]\n if prefix in numbersTable:\n minSpacesInSuffix = getMinSpaces(pi, numbersTable, cache, i + 1)\n minSpaces = min(minSpaces, minSpacesInSuffix)\n cache[idx] = minSpaces\n return cache[idx]",
"_____no_output_____"
]
],
[
[
"## Thoughts\n### my solution 1\n- this is a brute force solution\n- there are many duplicate calculations because we may deploy the recursive function many times at same string inputs. for example, let `pi = \"31415926\"`, recursive function is `fun(piece of string)`.\n - one moment, we may have `\"3\" + \"14\" + fun(\"15926\")`\n - another moment, we may have `\"314\" + fun(\"15926\")`\n - `fun(\"15926\")` is computed more than once\n - an idea is to store the value of `fun(\"15926\")` when we get it at first time.\n\n### expert solution 1\n- `cache[i]` stores the min number of spaces add of `pi[i:end]`\n- `cache[i]` is computed in the order from i = end to i = 0\n- this is basically the memo version of dp\n\n### expert solution 2\n- why can we solve the question with the same direction of generate `cache[i]`? this will turns to be a dp solution.\n\n### my solution 2\n- actually we can solve in an order of start to end.\n- `opt[i]` means the the min number of spaces added to string `pi[:i + 1]` (i + 1 due to the exclusive)",
"_____no_output_____"
]
]
] | [
"markdown",
"code",
"markdown",
"code",
"markdown"
] | [
[
"markdown",
"markdown"
],
[
"code",
"code"
],
[
"markdown"
],
[
"code",
"code"
],
[
"markdown"
]
] |
e76c063c72167167c7a8ef770db063c797ad76b0 | 184,090 | ipynb | Jupyter Notebook | notebooks/sddp.ipynb | leclere/DPnotebooks | c432dd1d9897516debc20f2e03948793e8a6e7be | [
"MIT"
] | 1 | 2019-08-09T15:31:28.000Z | 2019-08-09T15:31:28.000Z | notebooks/sddp.ipynb | leclere/DPnotebooks | c432dd1d9897516debc20f2e03948793e8a6e7be | [
"MIT"
] | null | null | null | notebooks/sddp.ipynb | leclere/DPnotebooks | c432dd1d9897516debc20f2e03948793e8a6e7be | [
"MIT"
] | 1 | 2020-06-15T21:42:52.000Z | 2020-06-15T21:42:52.000Z | 260.014124 | 35,018 | 0.922054 | [
[
[
"empty"
]
]
] | [
"empty"
] | [
[
"empty"
]
] |
e76c0adcbaa8aec10357676075866badd770fbba | 403,106 | ipynb | Jupyter Notebook | harmonic_oscillator.ipynb | sju-chem264-2019/10-24-19-introduction-to-harmonic-oscillator-daliahassan98 | 2f753a1159ed2fc6942fea9223414aa815ac2ad8 | [
"MIT"
] | null | null | null | harmonic_oscillator.ipynb | sju-chem264-2019/10-24-19-introduction-to-harmonic-oscillator-daliahassan98 | 2f753a1159ed2fc6942fea9223414aa815ac2ad8 | [
"MIT"
] | null | null | null | harmonic_oscillator.ipynb | sju-chem264-2019/10-24-19-introduction-to-harmonic-oscillator-daliahassan98 | 2f753a1159ed2fc6942fea9223414aa815ac2ad8 | [
"MIT"
] | null | null | null | 249.601238 | 23,760 | 0.922861 | [
[
[
"# Introduction to the Harmonic Oscillator",
"_____no_output_____"
],
[
"*Note:* Much of this is adapted/copied from https://flothesof.github.io/harmonic-oscillator-three-methods-solution.html",
"_____no_output_____"
],
[
"This week week we are going to begin studying molecular dynamics, which uses classical mechanics to study molecular systems. Our \"hydrogen atom\" in this section will be the 1D harmomic oscillator. \n\n ",
"_____no_output_____"
],
[
"The harmonic oscillator is a system that, when displaced from its equilibrium position, experiences a restoring force F proportional to the displacement x:\n\n$$F=-kx$$\n\nThe potential energy of this system is \n\n$$V = {1 \\over 2}k{x^2}$$",
"_____no_output_____"
],
[
"These are sometime rewritten as\n\n$$ F=- \\omega_0^2 m x, \\text{ } V(x) = {1 \\over 2} \\omega_0^2 m {x^2}$$\n\nWhere $\\omega_0 = \\sqrt {{k \\over m}} $",
"_____no_output_____"
],
[
"In classical mechanics, our goal is to determine the equations of motion, $x(t),y(t)$, that describe our system. \n\nIn this notebook we will use sympy to solve an second order, ordinary differential equation.",
"_____no_output_____"
],
[
"## 1. Solving differential equations with sympy",
"_____no_output_____"
],
[
"Soliving differential equations can be tough, and there is not always a set plan on how to proceed. Luckily for us, the harmonic osscillator is the classic second order diffferential eqations.",
"_____no_output_____"
],
[
"Consider the following second order differential equation\n\n$$ay(t)''+by(t)'=c$$\n\nwhere $y(t)'' = {{{d^2}y} \\over {dt^2}}$, and $y(t)' = {{{d}y} \\over {dt}}$",
"_____no_output_____"
],
[
"We can rewrite this as a homogeneous linear differential equations\n\n$$ay(t)''+by(t)'-c=0$$",
"_____no_output_____"
],
[
"The goal here is to find $y(t)$, similar to our classical mechanics problems. Lets use sympy to solve this equation",
"_____no_output_____"
],
[
"### Second order ordinary differential equation",
"_____no_output_____"
],
[
"First we import the sympy library",
"_____no_output_____"
]
],
[
[
"import sympy as sym",
"_____no_output_____"
]
],
[
[
"Next we initialize pretty printing",
"_____no_output_____"
]
],
[
[
"sym.init_printing()",
"_____no_output_____"
]
],
[
[
"Next we will set our symbols",
"_____no_output_____"
]
],
[
[
"t,a,b,c=sym.symbols(\"t,a,b,c\")",
"_____no_output_____"
]
],
[
[
"Now for somehting new. We can define functions using `sym.Function(\"f\")`",
"_____no_output_____"
]
],
[
[
"y=sym.Function(\"y\")\ny(t)",
"_____no_output_____"
]
],
[
[
"Now, If I want to define a first or second derivative, I can use `sym.diff`",
"_____no_output_____"
]
],
[
[
"sym.diff(y(t),(t,1)),sym.diff(y(t),(t,2))",
"_____no_output_____"
]
],
[
[
"My differential equation can be written as follows",
"_____no_output_____"
]
],
[
[
"dfeq=a*sym.diff(y(t),(t,2))+b*sym.diff(y(t),(t,1))-c\ndfeq",
"_____no_output_____"
],
[
"sol = sym.dsolve(dfeq)\nsol",
"_____no_output_____"
]
],
[
[
"The two constants $C_1$ and $C_2$ can be determined by setting boundry conditions.\nFirst, we can set the condition $y(t=0)=y_0$\n\nThe next intial condition we will set is $y'(t=0)=v_0$\n\nTo setup the equality we want to solve, we are using `sym.Eq`. This function sets up an equaility between a lhs aand rhs of an equation",
"_____no_output_____"
]
],
[
[
"# sym.Eq example\nalpha,beta=sym.symbols(\"alpha,beta\")\nsym.Eq(alpha+2,beta)",
"_____no_output_____"
]
],
[
[
"Back to the actual problem",
"_____no_output_____"
]
],
[
[
"y0,v0=sym.symbols(\"y_0,v_0\")\nics=[sym.Eq(sol.args[1].subs(t, 0), y0),\n sym.Eq(sol.args[1].diff(t).subs(t, 0), v0)]\nics",
"_____no_output_____"
]
],
[
[
"We can use this result to first solve for $C_2$ and then solve for $C_1$.\nOr we can use sympy to solve this for us.",
"_____no_output_____"
]
],
[
[
"solved_ics=sym.solve(ics)\nsolved_ics",
"_____no_output_____"
]
],
[
[
"Substitute the result back into $y(t)$",
"_____no_output_____"
]
],
[
[
"full_sol = sol.subs(solved_ics[0])\nfull_sol",
"_____no_output_____"
]
],
[
[
"We can plot this result too. Assume that $a,b,c=1$ and that the starting conditions are $y_0=0,v_0=0$\n\n\nWe will use two sample problems:\n\n* case 1 : initial position is nonzero and initial velocity is zero\n* case 2 : initial position is zero and initialvelocity is nonzero\n",
"_____no_output_____"
]
],
[
[
"# Print plots\n%matplotlib inline",
"_____no_output_____"
]
],
[
[
"#### Initial velocity set to zero",
"_____no_output_____"
]
],
[
[
"case1 = sym.simplify(full_sol.subs({y0:0, v0:0, a:1, b:1, c:1}))\ncase1",
"_____no_output_____"
],
[
"sym.plot(case1.rhs)\nsym.plot(case1.rhs,(t,-2,2))",
"_____no_output_____"
]
],
[
[
"#### Initial velocity set to one",
"_____no_output_____"
]
],
[
[
"case2 = sym.simplify(full_sol.subs({y0:0, v0:1, a:1, b:1, c:1}))\ncase2",
"_____no_output_____"
],
[
"sym.plot(case2.lhs,(t,-2,2))",
"_____no_output_____"
]
],
[
[
"## Calculate the phase space",
"_____no_output_____"
],
[
"As we will see in lecture, the state of our classical systems are defined as points in phase space, a hyperspace defined by ${{\\bf{r}}^N},{{\\bf{p}}^N}$. We will convert our sympy expression into a numerical function so that we can plot the path of $y(t)$ in phase space $y,y'$.",
"_____no_output_____"
]
],
[
[
"case1",
"_____no_output_____"
],
[
"# Import numpy library\nimport numpy as np\n\n# Make numerical functions out of symbolic expressions\nyfunc=sym.lambdify(t,case1.rhs,'numpy')\nvfunc=sym.lambdify(t,case1.rhs.diff(t),'numpy')\n\n# Make list of numbers\ntlst=np.linspace(-2,2,100)\n\n# Import pyplot\nimport matplotlib\nimport matplotlib.pyplot as plt\n# Make plot\nplt.plot(yfunc(tlst),vfunc(tlst))\nplt.xlabel('$y$')\nplt.ylabel(\"$y'$\")\nplt.show()",
"_____no_output_____"
]
],
[
[
"### Exercise 1.1 \n\nChange the initial starting conditions and see how that changes the plots. Make three different plots with different starting conditions",
"_____no_output_____"
]
],
[
[
"#Making initial velocity equal 10 and change initial positions to 5,5,5\ncase3 = sym.simplify(full_sol.subs({y0:1, v0:10, a:5, b:5, c:5}))\ncase3",
"_____no_output_____"
],
[
"sym.plot(case3.rhs)\nsym.plot(case3.rhs,(t,-2,2))",
"_____no_output_____"
],
[
"case4 = sym.simplify(full_sol.subs({y0:5, v0:2, a:3, b:4, c:5}))\ncase4",
"_____no_output_____"
],
[
"sym.plot(case4.rhs)\nsym.plot(case4.rhs,(t,-2,2))",
"_____no_output_____"
],
[
"case5 = sym.simplify(full_sol.subs({y0:10, v0:0, a:0, b:1, c:1}))\ncase5",
"_____no_output_____"
],
[
"sym.plot(case5.rhs)\nsym.plot(case5.rhs,(t,-2,2))",
"_____no_output_____"
]
],
[
[
"## 2. Harmonic oscillator ",
"_____no_output_____"
],
[
"Applying the harmonic oscillator force to Newton's second law leads to the following second order differential equation\n\n$$ F = m a $$\n\n$$ F= - \\omega_0^2 m x $$\n\n$$ a = - \\omega_0^2 x $$\n\n$$ x(t)'' = - \\omega_0^2 x $$",
"_____no_output_____"
],
[
"The final expression can be rearranged into a second order homogenous differential equation, and can be solved using the methods we used above",
"_____no_output_____"
],
[
"Your goal is determine and plot the equations of motion of a 1D harmomnic oscillator",
"_____no_output_____"
],
[
"### Exercise 2.1 ",
"_____no_output_____"
],
[
"1. Use the methodology above to determine the equations of motion $x(t), v(t)$ for a harmonic ocillator\n1. Solve for any constants by using the following initial conditions: $x(0)=x_0, v(0)=v_0$\n1. Show expressions for and plot the equations of motion for the following cases:\n 1. $x(0)=0, v(0)=0$\n 1. $x(0)=0, v(0)>0$\n 1. $x(0)>0, v(0)=0$\n 1. $x(0)<0, v(0)=0$\n1. Plot the phasespace diagram for the harmonic oscillator",
"_____no_output_____"
]
],
[
[
"# Your code here\nm,t,omega=sym.symbols(\"m,t,omega\")\nx=sym.Function(\"x\")\nx(t)",
"_____no_output_____"
],
[
"sym.diff(x(t),(t,1)),sym.diff(x(t),(t,2))",
"_____no_output_____"
],
[
"dfeq1=sym.diff(x(t),(t,2))+omega**2*x(t)\ndfeq1",
"_____no_output_____"
],
[
"sol1 = sym.dsolve(dfeq1)\nsol1",
"_____no_output_____"
],
[
"x0,v0=sym.symbols(\"x_0,v_0\")\nics1=[sym.Eq(sol1.args[1].subs(t, 0), x0),\n sym.Eq(sol1.args[1].diff(t).subs(t, 0), v0)]\nics1",
"_____no_output_____"
],
[
"solved_ics1=sym.solve(ics1)\nsolved_ics1",
"_____no_output_____"
],
[
"full_sol1 = sol.subs(solved_ics1[0])\nfull_sol1",
"_____no_output_____"
],
[
"# Print plots\n%matplotlib inline",
"_____no_output_____"
],
[
"case_100 = sym.simplify(full_sol1.subs({x0:0, v0:0, omega:1}))\ncase_100",
"_____no_output_____"
],
[
"sym.plot(case_100.rhs)\nsym.plot(case_100.rhs,(t,-2,2))",
"_____no_output_____"
],
[
"case_101 = sym.simplify(full_sol1.subs({x0:0, v0:5, omega:1}))\ncase_101",
"_____no_output_____"
],
[
"sym.plot(case_101.rhs)\nsym.plot(case_101.rhs,(t,-2,2))",
"_____no_output_____"
],
[
"case_102 = sym.simplify(full_sol1.subs({x0:5, v0:0, omega:1}))\ncase_102",
"_____no_output_____"
],
[
"sym.plot(case_102.rhs)\nsym.plot(case_102.rhs,(t,-2,2))",
"_____no_output_____"
],
[
"case_103 = sym.simplify(full_sol1.subs({x0:-5, v0:0, omega:1}))\ncase_103",
"_____no_output_____"
],
[
"sym.plot(case_103.rhs)\nsym.plot(case_103.rhs,(t,-2,2))",
"_____no_output_____"
],
[
"case_100",
"_____no_output_____"
],
[
"# Import numpy library\nimport numpy as np\n\n# Make numerical functions out of symbolic expressions\nxfunc=sym.lambdify(t,case_100.rhs,'numpy')\nvfunc=sym.lambdify(t,case_100.rhs.diff(t),'numpy')\n\n# Make list of numbers\ntlst=np.linspace(-2,2,100)\n\n# Import pyplot\nimport matplotlib\nimport matplotlib.pyplot as plt\n# Make plot\nplt.plot(xfunc(tlst),vfunc(tlst))\nplt.xlabel('$y$')\nplt.ylabel(\"$y'$\")\nplt.show()",
"_____no_output_____"
],
[
"# Import numpy library\nimport numpy as np\n\n# Make numerical functions out of symbolic expressions\nxfunc=sym.lambdify(t,case_101.rhs,'numpy')\nvfunc=sym.lambdify(t,case_101.rhs.diff(t),'numpy')\n\n# Make list of numbers\ntlst=np.linspace(-5,5,100)\n\n# Import pyplot\nimport matplotlib\nimport matplotlib.pyplot as plt\n# Make plot\nplt.plot(xfunc(tlst),vfunc(tlst))\nplt.xlabel('$y$')\nplt.ylabel(\"$y'$\")\nplt.show()",
"_____no_output_____"
],
[
"# Import numpy library\nimport numpy as np\n\n# Make numerical functions out of symbolic expressions\nxfunc=sym.lambdify(t,case_102.rhs,'numpy')\nvfunc=sym.lambdify(t,case_102.rhs.diff(t),'numpy')\n\n# Make list of numbers\ntlst=np.linspace(-5,5,100)\n\n# Import pyplot\nimport matplotlib\nimport matplotlib.pyplot as plt\n# Make plot\nplt.plot(xfunc(tlst),vfunc(tlst))\nplt.xlabel('$y$')\nplt.ylabel(\"$y'$\")\nplt.show()",
"_____no_output_____"
]
]
] | [
"markdown",
"code",
"markdown",
"code",
"markdown",
"code",
"markdown",
"code",
"markdown",
"code",
"markdown",
"code",
"markdown",
"code",
"markdown",
"code",
"markdown",
"code",
"markdown",
"code",
"markdown",
"code",
"markdown",
"code",
"markdown",
"code",
"markdown",
"code",
"markdown",
"code",
"markdown",
"code"
] | [
[
"markdown",
"markdown",
"markdown",
"markdown",
"markdown",
"markdown",
"markdown",
"markdown",
"markdown",
"markdown",
"markdown",
"markdown",
"markdown"
],
[
"code"
],
[
"markdown"
],
[
"code"
],
[
"markdown"
],
[
"code"
],
[
"markdown"
],
[
"code"
],
[
"markdown"
],
[
"code"
],
[
"markdown"
],
[
"code",
"code"
],
[
"markdown"
],
[
"code"
],
[
"markdown"
],
[
"code"
],
[
"markdown"
],
[
"code"
],
[
"markdown"
],
[
"code"
],
[
"markdown"
],
[
"code"
],
[
"markdown"
],
[
"code",
"code"
],
[
"markdown"
],
[
"code",
"code"
],
[
"markdown",
"markdown"
],
[
"code",
"code"
],
[
"markdown"
],
[
"code",
"code",
"code",
"code",
"code",
"code"
],
[
"markdown",
"markdown",
"markdown",
"markdown",
"markdown",
"markdown"
],
[
"code",
"code",
"code",
"code",
"code",
"code",
"code",
"code",
"code",
"code",
"code",
"code",
"code",
"code",
"code",
"code",
"code",
"code",
"code",
"code"
]
] |
e76c20ddc822d5339c26f977d95effd12bfc5d72 | 79,928 | ipynb | Jupyter Notebook | binder/DM_workshop_082721/Interactive_Measured_Data_Plotting.ipynb | villano-lab/galactic-spin | 16b67b319008a4bb91ef3b2e80828e98cf13173b | [
"MIT"
] | 2 | 2020-04-04T23:05:15.000Z | 2021-10-01T15:42:53.000Z | binder/DM_workshop_082721/Interactive_Measured_Data_Plotting.ipynb | villano-lab/galactic-spin | 16b67b319008a4bb91ef3b2e80828e98cf13173b | [
"MIT"
] | 3 | 2021-11-12T18:51:21.000Z | 2022-01-14T19:39:59.000Z | binder/DM_workshop_082721/Interactive_Measured_Data_Plotting.ipynb | villano-lab/galactic-spin | 16b67b319008a4bb91ef3b2e80828e98cf13173b | [
"MIT"
] | null | null | null | 302.757576 | 66,540 | 0.911896 | [
[
[
"import sys\nsys.path.append('python/')\n\nimport time\nstartTime = time.time() # Calculate time for running this notebook\n\nimport numpy as np\nimport matplotlib.pyplot as plt \nimport load_galaxies as lg # Load load_galaxies.py library",
"_____no_output_____"
]
],
[
[
"_Python help: Running the notebook the first time, make sure to run all cells to be able to make changes in the notebook. Hit Shift+Enter to run the cell or click on the top menu: Kernel > Restart & Run All > Restart and Run All Cells to rerun the whole notebook. If you make any changes in a cell, rerun that cell._",
"_____no_output_____"
],
[
"# Measured data plotting",
"_____no_output_____"
],
[
"In this notebook you can load radial velocity measurements of multiple galaxies from a prepared Python library. Plot them all in a single graph so you can compare them with each other. <br><br>\nPlotting these measurements is the first step of producing a rotation curve for a galaxy and an indication of how much mass the galaxy contains. Setting Newton's law of gravitation and the circular motion equation equal to each other, you can derive the equation for circular velocity in terms of enclosed mass and radius: \n\n\\begin{equation}\nv(r) = \\sqrt{\\frac{G M_{enc}(r)}{r}}\n\\end{equation}\n<br>\n\n>where:<br>\n $G$ = gravitational constant<br>\n $M_{enc}(r)$ = enclosed mass as a function of radius<br>\n $r$ = radius or distance from the center of the galaxy\n <br>\n\nKnowing the radial velocity of stars at different radii, you can estimate the mass that is enclosed in that radius. By measuring the brightnesses (photometric profile) of stars and the amount of gas, you can approximate the mass of \"visible\" matter. Compare it with the actual mass calculated from the radial velocities to get an idea of how much mass is \"missing\". The result is a ratio (mass-to-light ratio or M/L) that has been useful to describe the amount of dark matter in galaxies. <br>\n\n### Vocabulary\n__Rotation curve__: velocity of stars and gas at distances from the center of the galaxy and plotted as a curve<br>\n__Radial velocity__: how fast stars and gas are moving at different distances from the center of the galaxy<br>\n__NGC__: New General Catalogue of galaxies<br>\n__UGC__: Uppsala General Catalogue of galaxies<br>\n__kpc__: kiloparsec: 1 kpc = 3262 light years = 3.086e+19 meters = 1.917e+16 miles",
"_____no_output_____"
],
[
"## Load data of multiple galaxies",
"_____no_output_____"
],
[
"Load the radii, velocities, and errors in velocities of multiple galaxies from a Python library. ",
"_____no_output_____"
]
],
[
[
"# NGC 5533\nr_NGC5533, v_NGC5533, v_err_NGC5533 = lg.NGC5533['m_radii'],lg.NGC5533['m_velocities'],lg.NGC5533['m_v_errors']\n\n# NGC 891\nr_NGC0891, v_NGC0891, v_err_NGC0891 = lg.NGC0891['m_radii'],lg.NGC0891['m_velocities'],lg.NGC0891['m_v_errors']\n\n# NGC 7814\nr_NGC7814, v_NGC7814, v_err_NGC7814 = lg.NGC7814['m_radii'],lg.NGC7814['m_velocities'],lg.NGC7814['m_v_errors']\n\n# NGC 5005\nr_NGC5005, v_NGC5005, v_err_NGC5005 = lg.NGC5005['m_radii'],lg.NGC5005['m_velocities'],lg.NGC5005['m_v_errors']\n\n# NGC 3198\nr_NGC3198, v_NGC3198, v_err_NGC3198 = lg.NGC3198['m_radii'],lg.NGC3198['m_velocities'],lg.NGC3198['m_v_errors']\n\n# UGC 89\n#r_UGC89, v_UGC89, v_err_UGC89 = lg.UGC89['m_radii'],lg.UGC89['m_velocities'],lg.UGC89['m_v_errors']\n\n# UGC 477\nr_UGC477, v_UGC477, v_err_UGC477 = lg.UGC477['m_radii'],lg.UGC477['m_velocities'],lg.UGC477['m_v_errors']\n\n# UGC 1281\nr_UGC1281, v_UGC1281, v_err_UGC1281 = lg.UGC1281['m_radii'],lg.UGC1281['m_velocities'],lg.UGC1281['m_v_errors']\n\n# UGC 1437\nr_UGC1437, v_UGC1437, v_err_UGC1437 = lg.UGC1437['m_radii'],lg.UGC1437['m_velocities'],lg.UGC1437['m_v_errors']\n\n# UGC 2953\nr_UGC2953, v_UGC2953, v_err_UGC2953 = lg.UGC2953['m_radii'],lg.UGC2953['m_velocities'],lg.UGC2953['m_v_errors']\n\n# UGC 4325\nr_UGC4325, v_UGC4325, v_err_UGC4325 = lg.UGC4325['m_radii'],lg.UGC4325['m_velocities'],lg.UGC4325['m_v_errors']\n\n# UGC 5253\nr_UGC5253, v_UGC5253, v_err_UGC5253 = lg.UGC5253['m_radii'],lg.UGC5253['m_velocities'],lg.UGC5253['m_v_errors']\n\n# UGC 6787\nr_UGC6787, v_UGC6787, v_err_UGC6787 = lg.UGC6787['m_radii'],lg.UGC6787['m_velocities'],lg.UGC6787['m_v_errors']\n\n# UGC 10075\nr_UGC10075, v_UGC10075, v_err_UGC10075 = lg.UGC10075['m_radii'],lg.UGC10075['m_velocities'],lg.UGC10075['m_v_errors']",
"_____no_output_____"
]
],
[
[
"## Plot measured data with errorbars",
"_____no_output_____"
],
[
"Measured data points of 13 galaxies are plotted below.<br>\n\n1. __Change the limits of the x-axis to zoom in and out of the graph.__ <br>\n_Python help: change the limits of the x-axis by modifying the two numbers (left and right) of the line: plt.xlim then rerun the notebook or the cell._ <br><br>\n\n2. __Finding supermassive black holes:__ A high velocity at a radius close to zero (close to the center of the galaxy) indicates that there is a supermassive black hole is present at the center of that galaxy, changing the velocities of the close-by stars only. The reason the black hole does not have that much effect on the motion of stars at larger distances is because it acts as a point mass with negligible radius and the velocity drops off as $1 / \\sqrt r$. <br>\n__Can you find the galaxies with a possible central supermassive black hole and hide the curves of the rest of the galaxies?__ <br>\n_Python help: Turn off the display of all lines and go through them one by one. You can \"turn off\" the display of each galaxy by typing a # sign in front of the line \"plt.errorbar\"._<br>\n_Sneak peak: In the Interactive_\\__Rotation_\\__Curve_\\__Plotting notebook you will be able to find out which stars are affected by the central supermassive black hole, or in other words, what we mean by \"close-by\" stars._ <br><br> \n\n3. __What do you notice about the size of the error bars at radii close to the center and far from the center? What could be the reason?__",
"_____no_output_____"
]
],
[
[
"# Define radius for plotting\nr = np.linspace(0,100,100)\n\n# Plot\nplt.figure(figsize=(10.0,7.0)) # size of the plot\nplt.title('Measured data of multiple galaxies', fontsize=14) # giving the plot a title\nplt.xlabel('Radius (kpc)', fontsize=12) # labeling the x-axis\nplt.ylabel('Velocity (km/s)', fontsize=12) # labeling the y-axis\nplt.xlim(0,20) # limits of the x-axis (default from 0 to 20 kpc)\nplt.ylim(0,420) # limits of the y-axis (default from 0 to 420 km/s)\n\n# Plotting the measured data\nplt.errorbar(r_NGC5533,v_NGC5533,yerr=v_err_NGC5533, label='NGC 5533', marker='o', markersize=6, linestyle='none', color='royalblue')\nplt.errorbar(r_NGC0891,v_NGC0891,yerr=v_err_NGC0891, label='NGC 891', marker='o', markersize=6, linestyle='none', color='seagreen')\nplt.errorbar(r_NGC7814,v_NGC7814,yerr=v_err_NGC7814, label='NGC 7814', marker='o', markersize=6, linestyle='none', color='m')\nplt.errorbar(r_NGC5005,v_NGC5005,yerr=v_err_NGC5005, label='NGC 5005', marker='o', markersize=6, linestyle='none', color='red')\nplt.errorbar(r_NGC3198,v_NGC3198,yerr=v_err_NGC3198, label='NGC 3198', marker='o', markersize=6, linestyle='none', color='gold')\nplt.errorbar(r_UGC477,v_UGC477,yerr=v_err_UGC477, label='UGC 477', marker='o', markersize=6, linestyle='none', color='lightpink')\nplt.errorbar(r_UGC1281,v_UGC1281,yerr=v_err_UGC1281, label='UGC 1281', marker='o', markersize=6, linestyle='none', color='aquamarine')\nplt.errorbar(r_UGC1437,v_UGC1437,yerr=v_err_UGC1437, label='UGC 1437', marker='o', markersize=6, linestyle='none', color='peru')\nplt.errorbar(r_UGC2953,v_UGC2953,yerr=v_err_UGC2953, label='UGC 2953', marker='o', markersize=6, linestyle='none', color='lightslategrey')\nplt.errorbar(r_UGC4325,v_UGC4325,yerr=v_err_UGC4325, label='UGC 4325', marker='o', markersize=6, linestyle='none', color='darkorange')\nplt.errorbar(r_UGC5253,v_UGC5253,yerr=v_err_UGC5253, label='UGC 5253', marker='o', markersize=6, linestyle='none', color='maroon')\nplt.errorbar(r_UGC6787,v_UGC6787,yerr=v_err_UGC6787, label='UGC 6787', marker='o', markersize=6, linestyle='none', color='midnightblue')\nplt.errorbar(r_UGC10075,v_UGC10075,yerr=v_err_UGC10075, label='UGC 10075', marker='o', markersize=6, linestyle='none', color='y')\n\nplt.legend(loc='upper right')\nplt.show()",
"_____no_output_____"
],
[
"# Time\nexecutionTime = (time.time() - startTime)\nttt=executionTime/60\nprint(f'Execution time: {ttt:.2f} minutes')",
"Execution time: 8.77 minutes\n"
]
],
[
[
"# References\n>De Naray, Rachel Kuzio, Stacy S. McGaugh, W. J. G. De Blok, and A. Bosma. __\"High-resolution optical velocity fields of 11 low surface brightness galaxies.\"__ The Astrophysical Journal Supplement Series 165, no. 2 (2006): 461. https://doi.org/10.1086/505345.<br><br>\n>De Naray, Rachel Kuzio, Stacy S. McGaugh, and W. J. G. De Blok. __\"Mass models for low surface brightness galaxies with high-resolution optical velocity fields.\"__ The Astrophysical Journal 676, no. 2 (2008): 920. https://doi.org/10.1086/527543.<br><br>\n>Epinat, B., P. Amram, M. Marcelin, C. Balkowski, O. Daigle, O. Hernandez, L. Chemin, C. Carignan, J.-L. Gach, and P. Balard. __โGHASP: An Hฮฑ Kinematic Survey of Spiral and Irregular GALAXIES โ Vi. New Hฮ Data Cubes for 108 Galaxies.โ__ Monthly Notices of the Royal Astronomical Society 388, no. 2 (July 19, 2008): 500โ550. https://doi.org/10.1111/j.1365-2966.2008.13422.x. <br><br>\n>Fraternali, F., R. Sancisi, and P. Kamphuis. __โA Tale of Two Galaxies: Light and Mass in NGC 891 and NGC 7814.โ__ Astronomy & Astrophysics 531 (June 13, 2011). https://doi.org/10.1051/0004-6361/201116634.<br><br> \n>Karukes, E. V., P. Salucci, and Gianfranco Gentile. __\"The dark matter distribution in the spiral NGC 3198 out to 0.22 $R_{vir}$.\"__ _Astronomy & Astrophysics_ 578 (2015): A13. https://doi.org/10.1051/0004-6361/201425339. <br><br>\n>Lelli, Federico, ed. __โSpitzer Photometry and Accurate Rotation Curves.โ__ SPARC, May 29, 2020. http://astroweb.cwru.edu/SPARC/. <br><br> \n>Noordermeer, Edo. __\"The rotation curves of flattened Sรฉrsic bulges.\"__ _Monthly Notices of the Royal Astronomical Society_ 385, no. 3 (2007): 1359-1364. https://doi.org/10.1111/j.1365-2966.2008.12837.x<br><br>\n>Richards, Emily E., L. van Zee, K. L. Barnes, S. Staudaher, D. A. Dale, T. T. Braun, D. C. Wavle, et al. __โBaryonic Distributions in the Dark Matter Halo of NGC 5005.โ__ Monthly Notices of the Royal Astronomical Society 449, no. 4 (June 1, 2015): 3981โ96. https://doi.org/10.1093/mnras/stv568. \n***",
"_____no_output_____"
]
]
] | [
"code",
"markdown",
"code",
"markdown",
"code",
"markdown"
] | [
[
"code"
],
[
"markdown",
"markdown",
"markdown",
"markdown",
"markdown"
],
[
"code"
],
[
"markdown",
"markdown"
],
[
"code",
"code"
],
[
"markdown"
]
] |
e76c286aa83d3f330f800610e3bf310296be51c2 | 30,720 | ipynb | Jupyter Notebook | notebooks/Merging_Annotations.ipynb | jackson-waschura/marine-acoustics-2021 | 300a5856de402d52342523c6138751a5ca7e07a8 | [
"MIT"
] | null | null | null | notebooks/Merging_Annotations.ipynb | jackson-waschura/marine-acoustics-2021 | 300a5856de402d52342523c6138751a5ca7e07a8 | [
"MIT"
] | null | null | null | notebooks/Merging_Annotations.ipynb | jackson-waschura/marine-acoustics-2021 | 300a5856de402d52342523c6138751a5ca7e07a8 | [
"MIT"
] | 2 | 2022-01-13T16:16:28.000Z | 2022-01-20T17:39:51.000Z | 34.672686 | 136 | 0.468001 | [
[
[
"import math\nimport pandas as pd\nimport numpy as np\nimport librosa\nimport librosa.display\nimport matplotlib.pyplot as plt\nfrom matplotlib.patches import Rectangle\nfrom scipy.io import wavfile\nfrom collections import OrderedDict\nfrom tqdm import tqdm",
"_____no_output_____"
],
[
"recording_name = \"671658014.180929033558\"\nwav_name = \"../data/{}_norm_8k-resample.wav\".format(recording_name)\ninitials = [\"AW\",\"MS\"]\nselection_tables = [\"../data/{}-{}.txt\".format(recording_name, ins) for ins in initials]\nselection_tables = [pd.read_csv(p, sep=\"\\t\").drop([\"Selection\", \"View\", \"Channel\"], axis=1) for p in selection_tables]\nfor i in range(len(selection_tables)):\n selection_tables[i][\"Source\"] = initials[i]\n \nall_annotations = pd.concat(selection_tables, ignore_index=True)\nall_annotations[\"Species Confidence\"] = all_annotations[\"Species\"].str.strip().str[-1]\nall_annotations.loc[all_annotations[\"Species Confidence\"].isna(), \"Species Confidence\"] = \"5\"\nhas_conf = all_annotations[\"Species Confidence\"].apply(lambda x: x.isdigit())\nall_annotations.loc[has_conf, \"Species\"] = all_annotations.loc[has_conf, \"Species\"].str[:-1].str.strip().str.lower()\nall_annotations.loc[~has_conf, \"Species Confidence\"] = \"5\"\nall_annotations[\"Species Confidence\"] = all_annotations[\"Species Confidence\"].astype(int)\nall_annotations",
"_____no_output_____"
],
[
"all_annotations = all_annotations.loc[all_annotations[\"species\"].isna()]\nall_annotations = all_annotations.drop(\"species\", axis=1)",
"_____no_output_____"
],
[
"all_annotations = all_annotations.loc[all_annotations[\"Uncertainty\"].isna()]\nall_annotations = all_annotations.drop(\"Uncertainty\", axis=1)",
"_____no_output_____"
],
[
"all_annotations.loc[all_annotations[\"Species\"].isna(), \"Species\"] = \"hb whale\"",
"_____no_output_____"
],
[
"all_annotations[\"Species\"].value_counts(dropna=False)",
"_____no_output_____"
],
[
"species_cleaning_dict = {\n\"hb whale\": \"Humpback Whale\",\n\"hb\" : \"Humpback Whale\",\n\"mechanical\": \"Mechanical\",\n\"??\": \"Unknown\",\n\"?\": \"Unknown\",\n\"sea lion\": \"Sea Lion\",\n\"unknown\": \"Unknown\",\n\"mech.\": \"Mechanical\",\n\"hb whale\": \"Humpback Whale\",\n\"hb whale 5\": \"Humpback Whale\",\n\"unkown\": \"Unknown\",\n\"mechanic\": \"Mechanical\",\n\"?hb whale\": \"Humpback Whale\",\n\"helicopter/plane\": \"Mechanical\",\n\"mech\": \"Mechanical\",\n\"hb whale5\": \"Humpback Whale\",\n\"hb whale 3\": \"Humpback Whale\",\n\"hb\": \"Humpback Whale\",\n\"hbwhale\": \"Humpback Whale\",\n\" hb whale\": \"Humpback Whale\",\n\"hb whael\": \"Humpback Whale\",\n\"boat\": \"Mechanical\",\n}\nall_annotations[\"Species\"] = all_annotations[\"Species\"].map(species_cleaning_dict)\nall_annotations[\"Species\"].value_counts(dropna=False)",
"_____no_output_____"
],
[
"# TODO: measure speed of different fns for opening wav files\ndef read_wavfile(wav_name, normalize=True, verbose=False):\n if verbose:\n print(\"Reading {}\".format(wav_name))\n sr, data = wavfile.read(wav_name)\n if verbose:\n print(\"{} samples at {} samples/sec --> {} seconds\".format(data.shape[0], sr, data.shape[0]/sr))\n\n if normalize:\n data = data.astype(float)\n data = data - data.min()\n data = data / data.max()\n data = data - 0.5\n \n return sr, data\n\nsamplerate, data = read_wavfile(wav_name, verbose=True)",
"_____no_output_____"
],
[
"def plot_annotated_mel_spec(data, samplerate, annotations, cls_col=None, n_fft=4096, hop_length=64,\n n_mels=512, fmax=1600, adjust_fmax=True, figsize=(15, 5), buffer_s=0.125,\n title=None):\n # Extract annotation bounds\n start_s, end_s = annotations[\"Begin Time (s)\"].min() - buffer_s, annotations[\"End Time (s)\"].max() + buffer_s\n start_s, end_s = max(start_s, 0.0), min(end_s, len(data)/samplerate)\n observed_max = annotations[\"High Freq (Hz)\"].max()\n if adjust_fmax and observed_max > fmax:\n new_fmax = observed_max*1.1\n print(\"Annotations extend above frequency max of {} Hz, increasing to {:g} Hz.\".format(fmax, new_fmax))\n fmax = new_fmax\n start_i, end_i = int(math.floor(start_s*samplerate) - n_fft/2), int(math.ceil(end_s*samplerate) + n_fft/2)\n if start_i < 0:\n print(\"Start Index < 0! Setting to 0 instead.\")\n start_i = 0\n start_s = (start_i + n_fft/2) / samplerate\n if end_i >= len(data):\n print(\"End Index > length of sequence. Setting to end of sequence instead.\")\n end_i = len(data)-1\n end_s = (end_i - n_fft/2) / samplerate\n \n # Compute & Draw Mel Spectrogram\n mel_spec = librosa.feature.melspectrogram(y=data[start_i:end_i],\n sr=samplerate,\n n_fft=n_fft,\n hop_length=hop_length,\n n_mels=n_mels,\n fmax=fmax,\n center=False)\n S_dB = librosa.power_to_db(mel_spec, ref=np.max)\n plt.figure(figsize=figsize)\n librosa.display.specshow(S_dB,\n x_axis='time',\n y_axis='mel',\n sr=samplerate,\n hop_length=hop_length,\n fmax=fmax)\n \n # Draw Annotations\n ax = plt.gca()\n if cls_col is not None:\n classes = annotations[cls_col].unique()\n else:\n classes = [\"NA\"]\n colors = plt.cm.get_cmap(\"hsv\")\n class_colors = {classes[c]: colors(c / (len(classes)+1)) for c in range(len(classes))}\n for b_i in annotations.index:\n box = annotations.loc[b_i]\n left, right, top, bot = box[\"Begin Time (s)\"], box[\"End Time (s)\"], \\\n box[\"High Freq (Hz)\"], max(box[\"Low Freq (Hz)\"], 5)\n if cls_col is not None:\n cls = box[cls_col]\n else:\n cls = \"NA\"\n \n rect = Rectangle((left - start_s, bot), # X,Y of bottom left\n right-left, # Width\n top-bot, # Height\n linewidth=2,\n edgecolor=class_colors[cls],\n facecolor='none',\n label=cls)\n ax.add_patch(rect)\n \n # Decorate Plot\n x_ticks = np.linspace(0.0, end_s - start_s, num=5)\n x_tick_labels = [\"{:.3f}\".format(t) for t in (x_ticks+start_s)]\n plt.xticks(x_ticks, x_tick_labels)\n plt.xlabel(\"Time (Seconds)\")\n plt.ylabel(\"Frequency (Hz)\")\n if title is None:\n plt.title(\"Mel Spectrogram\")\n else:\n plt.title(\"Mel Spectrogram ({})\".format(title))\n if cls_col is not None:\n handles, labels = plt.gca().get_legend_handles_labels()\n by_label = OrderedDict(zip(labels, handles))\n plt.legend(by_label.values(), by_label.keys(), loc='upper right')\n plt.show()\n plt.close()",
"_____no_output_____"
],
[
"for start in [15*s for s in range(4)]:\n mask = (all_annotations[\"End Time (s)\"] > start) & (all_annotations[\"Begin Time (s)\"] < start+30)\n n_boxes = mask.sum()\n if n_boxes > 0:\n print(\"Found {} annotations between {} and {} seconds.\".format(mask.sum(), start, start+30))\n plot_annotated_mel_spec(data, samplerate,\n all_annotations.loc[mask],\n figsize=(15,5),\n buffer_s=3.0,\n cls_col=\"Source\")",
"_____no_output_____"
],
[
"# Also called \"Jaccard Index\"\ndef IOU(box1, box2):\n # (left, right, top, bottom) is the box order\n l1, r1, t1, b1 = box1\n l2, r2, t2, b2 = box2\n \n # Quick check if boxes do not overlap\n # Time dimension (r/l) checked first since it is more likely to filter\n if r1 < l2 or r2 < l1 or t1 < b2 or t2 < b1:\n return 0.0\n \n # IOU Calculation\n intersection_area = (min(r1, r2) - max(l1, l2)) * (min(t1, t2) - max(b1, b2))\n union_area = (r1 - l1) * (t1 - b1) + (r2 - l2) * (t2 - b2) - intersection_area\n \n return intersection_area / union_area",
"_____no_output_____"
],
[
"def calculate_agreements(annotations, verbose=True):\n agreements = np.zeros(shape=(len(annotations), len(annotations)))\n iter1 = range(len(annotations))\n if verbose:\n iter1 = tqdm(iter1, desc='Calculating Agreements')\n for i1 in iter1:\n for i2 in range(i1+1, len(annotations)):\n a1, a2 = annotations.iloc[i1], annotations.iloc[i2]\n # Left, Right, Top, Bottom\n agreements[i1, i2] = IOU((a1[\"Begin Time (s)\"],\n a1[\"End Time (s)\"],\n a1[\"High Freq (Hz)\"],\n a1[\"Low Freq (Hz)\"]),\n (a2[\"Begin Time (s)\"],\n a2[\"End Time (s)\"],\n a2[\"High Freq (Hz)\"],\n a2[\"Low Freq (Hz)\"]))\n agreements[i2, i1] = agreements[i1, i2]\n return agreements\n\n\ndef extract_trivial_annotations(annotations, agreements=None, thresh=0.02, verbose=True):\n if agreements is None:\n agreements = calculate_agreements(annotations)\n \n trivials = (agreements <= thresh).all(axis=1)\n \n if verbose:\n print(\"N Trivial: \", trivials.sum())\n print(\"% Trivial :\", (trivials.sum())/agreements.shape[0])\n \n done_annotations = annotations.loc[trivials]\n remaining_agreements = agreements[~trivials,:][:,~trivials]\n remaining_annotations = annotations.loc[~trivials]\n \n if verbose:\n print(\"Remaining Annotations: \", len(remaining_annotations))\n \n return done_annotations, remaining_annotations, remaining_agreements\n\n\ndef extract_merged_annotations(annotations, class_col, agreements=None, thresh=0.55, verbose=True):\n if agreements is None:\n agreements = calculate_agreements(annotations)\n \n classes = annotations[class_col].to_numpy()\n pairings = ((agreements == agreements.max(axis=1))\n & (agreements > thresh)\n & (classes.reshape((1,-1)) == classes.reshape((-1,1))))\n matches = np.triu(pairings & pairings.T, 0)\n \n if verbose:\n print(\"N Pairings: \", pairings.sum()//2, \"N Matches: \", matches.sum())\n print(\"% Matched :\", (matches.sum())/agreements.shape[0])\n \n matched_boxes = []\n for b_i, b_j in zip(*np.nonzero(matches)):\n box_i, box_j = annotations.iloc[b_i], annotations.iloc[b_j]\n new_box = box_i.copy()\n for c in [\"Begin Time (s)\", \"End Time (s)\", \"High Freq (Hz)\", \"Low Freq (Hz)\", \"Species Confidence\"]:\n new_box[c] = (box_i[c] + box_j[c]) / 2.0\n new_box[\"Source\"] = \"Merged\"\n matched_boxes.append(new_box)\n done_annotations = pd.DataFrame(matched_boxes)\n matched_mask = (matches | matches.T).any(axis=1)\n remaining_annotations = annotations[~matched_mask]\n \n if verbose:\n print(\"Remaining Annotations: \", len(remaining_annotations))\n \n return done_annotations, remaining_annotations, None\n\n\ndef extract_aggregate_annotations(annotations, agreements=None, ratio_thresh=2.0, verbose=True):\n if agreements is None:\n agreements = calculate_agreements(annotations)\n \n areas = np.zeros(shape=(len(annotations)))\n for i1 in tqdm(range(len(annotations))):\n a1 = annotations.iloc[i1]\n areas[i1] = (a1[\"End Time (s)\"]-a1[\"Begin Time (s)\"])*(a1[\"High Freq (Hz)\"]-a1[\"Low Freq (Hz)\"])\n \n # area_ratios[i,j] = areas[i] / areas[j]\n area_ratios = areas.reshape((-1, 1)) / areas.reshape((1, -1))\n \n # Aggregates (i big)\n aggregate_boxes = ((area_ratios > ratio_thresh) & (agreements > 0.0)).sum(axis=1) > 2\n \n if verbose:\n print(\"N Aggregators: \", aggregate_boxes.sum())\n total = aggregate_boxes.sum()\n print(\"N Total: \", total)\n print(\"% Total: \", total/agreements.shape[0])\n \n remaining_agreements = agreements[~aggregate_boxes,:][:,~aggregate_boxes]\n remaining_annotations = annotations[~aggregate_boxes]\n \n if verbose:\n print(\"Remaining Annotations: \", len(remaining_annotations))\n \n return None, remaining_annotations, remaining_agreements",
"_____no_output_____"
],
[
"done_boxes = []\n\n# 1. Pass along trivial boxes so that future steps don't need to consider them\ndone, rem_annotations, rem_agreements = extract_trivial_annotations(annotations_to_merge)\ndone_boxes.append(done)",
"_____no_output_____"
],
[
"# 2. Merge matched boxed (Union vs Mean strategies) -- currently using mean strat\ndone, rem_annotations, _ = extract_merged_annotations(rem_annotations, \"Species\", agreements=rem_agreements)\ndone_boxes.append(done)",
"_____no_output_____"
],
[
"# 3. Detect aggregate boxes and remove them.\n_, rem_annotations, rem_agreements = extract_aggregate_annotations(rem_annotations)",
"_____no_output_____"
],
[
"# 4. Finally check whether new trivials have been revealed\ndone, rem_annotations, rem_agreements = extract_trivial_annotations(rem_annotations,\n agreements=rem_agreements,\n thresh=0.04)\ndone_boxes.append(done)",
"_____no_output_____"
],
[
"done_boxes = pd.concat(done_boxes)",
"_____no_output_____"
],
[
"print(\"Produced {} done annotations\".format(len(done_boxes)))\nprint(\"Handled {} of {} initial annotations ({:.2f}%)\".format(\n len(annotations_to_merge)-len(rem_annotations),\n len(annotations_to_merge),\n 100*(len(annotations_to_merge)-len(rem_annotations)) / len(annotations_to_merge)))",
"_____no_output_____"
],
[
"# Visualize all of the remaining boxes and manually merge\nrem_annotations[\"Index+Class\"] = rem_annotations[\"Species\"].str.cat(rem_annotations.index.astype(str))\nfor i in range(len(rem_annotations)):\n tmp = rem_annotations.iloc[i]\n l_edge, r_edge = tmp[\"Begin Time (s)\"], tmp[\"End Time (s)\"]\n buffer = 2.0\n mask = (rem_annotations[\"End Time (s)\"] > (l_edge-buffer)) & (rem_annotations[\"Begin Time (s)\"] < (r_edge+buffer))\n plot_annotated_mel_spec(data, samplerate,\n rem_annotations.loc[mask],\n figsize=(10,5),\n buffer_s=1.5,\n cls_col=\"Index+Class\",\n adjust_fmax=False,\n title=tmp.name)",
"_____no_output_____"
],
[
"# Instructions\n# INSTR, ID_1[, ID_2, ..., ID_N]\n# r --> remove\n# i --> intersection\n# u --> union\n# m --> mean\n# c -->create new box\n\ndef validate_instructions(instructions):\n all_nums = []\n for i in instructions:\n words = i.split(\",\")\n if words[0] == \"c\":\n continue\n nums = words[1:]\n all_nums.extend([int(n) for n in nums])\n n_occurences = pd.Series(all_nums).value_counts()\n return n_occurences.loc[(n_occurences > 1)]\n\ninstructions = [\n\"r,1159\",\n\"i,10,1163\",\n\"r,14\",\n\"r,1196\",\n\"r,39\",\n\"r,879\",\n\"r,46\",\n\"r,48\",\n\"r,1100\",\n\"r,49\",\n\"r,1102\",\n\"m,55,1249\",\n\"r,1264\",\n\"r,1265\",\n\"r,74\",\n\"r,1268\",\n\"r,1269\",\n\"m,82,1275\",\n\"r,1280\",\n\"r,88\",\n\"m,96,1289\",\n\"r,1292\",\n\"m,105,1296\",\n\"r,1298\",\n\"u,111,1300\",\n\"m,134,1318\",\n\"m,140,1324\",\n\"r,1330\",\n\"r,1336\",\n\"r,1341\",\n\"r,1342\",\n\"m,185,1363\",\n\"u,187,1365\",\n\"u,188,1366\",\n\"r,189\",\n\"m,194,1369\",\n\"u,196,1370\",\n\"r,1371\",\n\"r,1372\",\n\"r,1373\",\n\"m,924,1374\",\n\"r,1375\",\n\"m,204,1376\",\n\"u,206,1380\",\n\"m,207,1381\",\n\"r,214\",\n\"r,1399\",\n\"m,223,1403\",\n\"r,1404\",\n\"r,1405\",\n\"m,228,1409\",\n\"r,233\",\n\"m,239,1419\",\n\"r,245\",\n\"r,259\",\n\"r,260\",\n\"r,1445\",\n\"u,283,1454\",\n\"m,289,1464\",\n\"r,1474\",\n\"m,299,1475\",\n\"r,301\",\n\"m,322,1497\",\n\"u,324,1498\",\n\"u,329,1504\",\n\"u,331,1506\",\n\"u,332,1507\",\n\"r,333\",\n\"r,336\",\n\"r,337\",\n\"u,340,341,1524\",\n\"m,344,1528\",\n\"u,345,1529\",\n\"u,346,1530\",\n\"u,351,1536\",\n\"u,359,1541\",\n\"m,372,1544\",\n\"u,373,1545\",\n\"r,1548\",\n\"u,378,1551\",\n\"u,1038,1550\",\n\"r,1552\",\n\"u,383,1556\",\n\"u,1040,1557\",\n\"u,384,1558\",\n\"m,386,1561\",\n\"u,390,1564\",\n\"u,392,1565\",\n\"u,393,1566\",\n\"r,395\",\n\"r,401\",\n\"r,1573\",\n\"u,409,1579\",\n\"r,1589\",\n\"r,1590\",\n\"r,1595\",\n\"r,1598\",\n\"r,1614\",\n\"m,429,1617\",\n\"r,1618\",\n\"u,431,1619\", # Consider lowering bottom to 0Hz\n\"r,1623\",\n\"r,1627\",\n\"r,1635\",\n\"r,1639\",\n\"r,1640\",\n\"r,1643\",\n\"u,460,1674\",\n\"u,464,1677\",\n\"m,473,1686\",\n\"r,1689\",\n\"u,477,1690\", # Consider dropping bottom to 0Hz\n\"r,1692\",\n\"r,1694\",\n\"m,483,1696\",\n\"u,485,1698\",\n\"u,486,1699\",\n\"r,1704\",\n\"m,492,1706\",\n\"r,1707\",\n\"u,496,1073,1710\",\n\"r,1713\",\n\"r,1716\",\n\"r,506\",\n\"r,1722\",\n\"u,511,1723\",\n\"u,520,1727\",\n\"r,1729\",\n\"r,1732\",\n\"r,1733\",\n\"u,531,1735\",\n\"r,1736\",\n\"r,1737\",\n\"r,1738\",\n\"u,544,1747\", # Consider dropping bottom to 0Hz\n\"u,546,1749\",\n\"u,548,1751\", # Consider dropping bottom to 0Hz\n\"r,549\",\n\"r,553\",\n\"m,556,1765\",\n\"u,558,1779\",\n\"u,559,1783\",\n\"r,1788\",\n\"r,563\",\n\"c,6778.01,6780.9,256,0.0\",# l,r,t,b\n\"r,1791\",\n\"u,570,1796\",\n\"u,571,1797\",\n\"m,573,1799\",\n\"u,575,1800\",\n\"r,576\",\n\"r,1801\",\n\"c,6939.5,6942.7,460,0.0\", # l,r,t,b\n\"r,1802\",\n\"r,1803\",\n\"r,1814\",\n\"r,1815\",\n\"u,610,1817\",\n\"u,620,1827\",\n\"u,625,1830\",\n\"u,626,1833\",\n\"u,629,1836\",\n\"r,631\",\n\"u,634,1842\",\n\"r,1847\",\n\"r,1849\",\n\"r,1857\",\n\"u,658,1859\",\n\"u,660,1861\",\n\"r,1863\",\n\"r,1864\",\n\"r,1865\",\n\"m,666,1866\",\n\"u,670,1869\",\n\"u,677,1873\",\n\"u,678,1874\",\n\"u,679,1875\",\n\"m,681,1877\",\n\"r,682\",\n\"u,689,1882\",\n\"u,690,1883\",\n\"r,1890\",\n\"r,1893\",\n\"r,1894\",\n\"u,701,1897,1898\",\n\"u,703,1900\",\n\"u,705,1888\",\n\"u,712,1903\",\n\"r,1904\",\n\"r,1905\",\n\"u,722,1906\",\n\"r,1911\",\n\"u,757,1913\",\n\"u,769,1917\", # Consider dropping bottom to 0Hz\n\"r,1918\",\n\"m,777,1922\",\n\"r,1923\",\n\"r,779\",\n\"r,780\",\n\"m,781,1928\",\n\"r,1929\",\n\"r,1930\",\n\"r,1931\",\n\"r,1932\",\n\"r,1934\",\n\"u,797,1938\",\n\"u,799,1939\",\n\"u,800,1940\",\n\"r,802\",\n\"u,803,1946\",\n\"u,805,1948\",\n\"u,807,1950\",\n\"u,810,1953\",\n\"u,824,1960\",\n\"u,825,1961\",\n\"m,827,1964\",\n\"r,1965\",\n\"u,834,1967\",\n\"u,847,1980\",\n\"u,848,1981\",\n\"r,1982\",\n\"u,851,1984\",\n\"m,863,1150\",\n\"r,865\",\n\"u,866,1172\",\n\"u,938,1448\",\n\"u,963,1502\",\n\"u,994,1509\",\n\"u,995,1511\",\n\"u,997,1513\",\n\"u,1056,1601\",\n\"u,1057,1602\",\n\"r,1603\",\n\"u,1067,1615\",\n\"u,1070,1662\",\n\"u,1075,1715\",\n\"u,1076,1726\",\n\"m,1089,1205\",\n\"r,1090\",\n\"r,1222\",\n\"r,1223\",\n\"r,1227\",\n\"r,1235\",\n\"u,1116,1637\",\n\"u,1117,1638\",\n\"r,1121\",\n\"m,1122,1645\",\n\"u,1124,1657\",\n\"r,1128\",\n\"m,1134,1767\",\n\"m,1138,1772\",\n\"r,1792\"\n]\n\nvalidate_instructions(instructions)",
"_____no_output_____"
],
[
"def execute_instructions(instructions, rem_annotations):\n to_drop = np.zeros(len(rem_annotations), dtype=bool)\n new_boxes = []\n for i in instructions:\n words = i.split(\",\")\n code = words[0]\n parameters = words[1:]\n if code == \"r\":\n for n in parameters:\n to_drop[rem_annotations.index.to_numpy()==int(n)] = True\n elif code == \"i\":\n # Intersection\n boxes = rem_annotations.loc[[int(n) for n in parameters]]\n new_box = boxes.iloc[0].copy()\n for c in [\"Begin Time (s)\", \"Low Freq (Hz)\", \"Species Confidence\"]:\n new_box[c] = boxes[c].max()\n for c in [\"End Time (s)\", \"High Freq (Hz)\"]:\n new_box[c] = boxes[c].min()\n new_box[\"Source\"] = \"Merged\"\n new_boxes.append(new_box)\n for n in parameters:\n to_drop[rem_annotations.index.to_numpy()==int(n)] = True\n elif code == \"u\":\n # Union\n boxes = rem_annotations.loc[[int(n) for n in parameters]]\n new_box = boxes.iloc[0].copy()\n for c in [\"End Time (s)\", \"High Freq (Hz)\", \"Species Confidence\"]:\n new_box[c] = boxes[c].max()\n for c in [\"Begin Time (s)\", \"Low Freq (Hz)\"]:\n new_box[c] = boxes[c].min()\n new_box[\"Source\"] = \"Merged\"\n new_boxes.append(new_box)\n for n in parameters:\n to_drop[rem_annotations.index.to_numpy()==int(n)] = True\n elif code == \"m\":\n # Mean\n boxes = rem_annotations.loc[[int(n) for n in parameters]]\n new_box = boxes.iloc[0].copy()\n for c in [\"Begin Time (s)\", \"End Time (s)\", \"High Freq (Hz)\", \"Low Freq (Hz)\"]:\n new_box[c] = boxes[c].mean()\n new_box[\"Species Confidence\"] = boxes[\"Species Confidence\"].max()\n new_box[\"Source\"] = \"Merged\"\n new_boxes.append(new_box)\n for n in parameters:\n to_drop[rem_annotations.index.to_numpy()==int(n)] = True\n elif code == \"c\":\n # Create new box\n parameters = [float(p) for p in parameters]\n new_box = pd.Series({\n \"Begin Time (s)\": parameters[0],\n \"End Time (s)\": parameters[1],\n \"High Freq (Hz)\": parameters[2],\n \"Low Freq (Hz)\": parameters[3]})\n new_boxes.append(new_box)\n else:\n raise ValueError(\"Instruction not recognized\")\n return ~to_drop, pd.DataFrame(new_boxes)",
"_____no_output_____"
],
[
"to_keep, new_boxes = execute_instructions(instructions, rem_annotations)",
"_____no_output_____"
],
[
"final_annotations = pd.concat([done_boxes, rem_annotations.loc[to_keep], new_boxes], ignore_index=True)\nfinal_annotations = final_annotations.drop([\"Source\"], axis=1)",
"_____no_output_____"
],
[
"final_annotations.to_csv(\"../data/{}-final.txt\".format(recording_name), index=False)",
"_____no_output_____"
]
]
] | [
"code"
] | [
[
"code",
"code",
"code",
"code",
"code",
"code",
"code",
"code",
"code",
"code",
"code",
"code",
"code",
"code",
"code",
"code",
"code",
"code",
"code",
"code",
"code",
"code",
"code",
"code"
]
] |
e76c31f840dd3c2c79cb59cf87acf2de75517485 | 4,164 | ipynb | Jupyter Notebook | test_nrt.ipynb | sepal-contrib/alert_module | fa1b45308bb04da5269177e5a05f37f4cf6add1e | [
"MIT"
] | null | null | null | test_nrt.ipynb | sepal-contrib/alert_module | fa1b45308bb04da5269177e5a05f37f4cf6add1e | [
"MIT"
] | 3 | 2020-09-21T11:42:26.000Z | 2020-10-07T16:19:20.000Z | test_nrt.ipynb | sepal-contrib/alert_module | fa1b45308bb04da5269177e5a05f37f4cf6add1e | [
"MIT"
] | null | null | null | 27.576159 | 104 | 0.564121 | [
[
[
"# create a map to display everything\nfrom sepal_ui import mapping\n\nMap = mapping.SepalMap()",
"_____no_output_____"
],
[
"# create the model for testing purposes\nfrom component import model\nfrom datetime import datetime\n\nalert_model = model.AlertModel()\nalert_model.alert_type = \"RECENT\"\nalert_model.alert_collection = \"GLAD-S\"\nalert_model.start = datetime.strptime(\"2022-04-25\", \"%Y-%m-%d\")\nalert_model.end = datetime.strptime(\"2022-03-25\", \"%Y-%m-%d\")\nalert_model.min_size = 1",
"_____no_output_____"
],
[
"# create the aoi_model\nfrom sepal_ui import aoi\nfrom sepal_ui import color as sc\nfrom sepal_ui import sepalwidgets as sw\nimport ee\n\nee.Initialize()\n\naoi_model = aoi.AoiModel(\n asset=\"users/bornToBeAlive/cambodia_alert_aoi\", alert=sw.Alert()\n)\nMap.zoom_ee_object(aoi_model.feature_collection.geometry())\nempty = ee.Image().byte()\noutline = empty.paint(featureCollection=aoi_model.feature_collection, color=1, width=4)\nMap.addLayer(outline, {\"palette\": sc.primary}, \"aoi\")",
"_____no_output_____"
],
[
"# generate the grid\nfrom component import scripts as cs\nfrom ipyleaflet import GeoJSON\nimport json\n\nx = 74\ngrid = cs.set_grid(aoi_model.gdf)\ndata = json.loads(grid[grid.index.isin([x])].to_json())\nstyle = {\"stroke\": True, \"color\": \"grey\", \"weight\": 2, \"opacity\": 1, \"fill\": False}\nipygeojson = GeoJSON(data=data, style=style, name=\"grid\")\nMap.add_layer(ipygeojson)\n\nlen(grid)",
"_____no_output_____"
],
[
"# display all alerts\na = alert_model\ngeom = ee.FeatureCollection(data)\nalerts = cs.get_alerts(a.alert_collection, a.start, a.end, geom, a.asset)\nMap.addLayer(\n alerts.select(\"alert\").clip(geom),\n {\"min\": 1, \"max\": 2, \"palette\": [\"red\", \"yellow\"]},\n \"alerts\",\n)\nMap.zoom_ee_object(geom.geometry());",
"_____no_output_____"
],
[
"# extract eh alerts\nimport geopandas as gpd\n\nalert_clump = cs.get_alerts_clump(alerts=alerts, aoi=geom)\njson = alert_clump.getInfo()\ngdf = gpd.GeoDataFrame.from_features(json)\nstyle = {\"stroke\": True, \"color\": \"BLUE\", \"weight\": 2, \"opacity\": 1, \"fill\": True}\nipygeojson = GeoJSON(data=json, style=style, name=\"alerts\")\nMap.add_layer(ipygeojson);",
"_____no_output_____"
],
[
"Map",
"_____no_output_____"
]
]
] | [
"code"
] | [
[
"code",
"code",
"code",
"code",
"code",
"code",
"code"
]
] |
e76c5212d0eadf1a8dbd80077f3285179bb4dac6 | 112,627 | ipynb | Jupyter Notebook | Chapter6.ipynb | VandyChris/HandsOnMachineLearning | 2c3d9b5869d82eca97cdfe047f56d3fea65e27bb | [
"MIT"
] | null | null | null | Chapter6.ipynb | VandyChris/HandsOnMachineLearning | 2c3d9b5869d82eca97cdfe047f56d3fea65e27bb | [
"MIT"
] | null | null | null | Chapter6.ipynb | VandyChris/HandsOnMachineLearning | 2c3d9b5869d82eca97cdfe047f56d3fea65e27bb | [
"MIT"
] | null | null | null | 236.115304 | 63,020 | 0.920516 | [
[
[
"Import packages",
"_____no_output_____"
]
],
[
[
"#\nimport sklearn\nimport numpy as np\nimport matplotlib.pyplot as plt\n%matplotlib inline",
"_____no_output_____"
]
],
[
[
"define two functions for visualization",
"_____no_output_____"
]
],
[
[
"#\nfrom matplotlib.colors import ListedColormap\n\ndef plot_decision_boundary(clf, X, y, axes=[0, 7.5, 0, 3], iris=True, legend=False, plot_training=True):\n x1s = np.linspace(axes[0], axes[1], 100)\n x2s = np.linspace(axes[2], axes[3], 100)\n x1, x2 = np.meshgrid(x1s, x2s)\n X_new = np.c_[x1.ravel(), x2.ravel()]\n y_pred = clf.predict(X_new).reshape(x1.shape)\n custom_cmap = ListedColormap(['#fafab0','#9898ff','#a0faa0'])\n plt.contourf(x1, x2, y_pred, alpha=0.3, cmap=custom_cmap)\n if not iris:\n custom_cmap2 = ListedColormap(['#7d7d58','#4c4c7f','#507d50'])\n plt.contour(x1, x2, y_pred, cmap=custom_cmap2, alpha=0.8)\n if plot_training:\n plt.plot(X[:, 0][y==0], X[:, 1][y==0], \"yo\", label=\"Iris-Setosa\")\n plt.plot(X[:, 0][y==1], X[:, 1][y==1], \"bs\", label=\"Iris-Versicolor\")\n plt.plot(X[:, 0][y==2], X[:, 1][y==2], \"g^\", label=\"Iris-Virginica\")\n plt.axis(axes)\n if iris:\n plt.xlabel(\"Petal length\", fontsize=14)\n plt.ylabel(\"Petal width\", fontsize=14)\n else:\n plt.xlabel(r\"$x_1$\", fontsize=18)\n plt.ylabel(r\"$x_2$\", fontsize=18, rotation=0)\n if legend:\n plt.legend(loc=\"lower right\", fontsize=14)",
"_____no_output_____"
],
[
"#\ndef plot_regression_predictions(tree_reg, X, y, axes=[0, 1, -0.2, 1], ylabel=\"$y$\"):\n x1 = np.linspace(axes[0], axes[1], 500).reshape(-1, 1)\n y_pred = tree_reg.predict(x1)\n plt.axis(axes)\n plt.xlabel(\"$x_1$\", fontsize=18)\n if ylabel:\n plt.ylabel(ylabel, fontsize=18, rotation=0)\n plt.plot(X, y, \"b.\")\n plt.plot(x1, y_pred, \"r.-\", linewidth=2, label=r\"$\\hat{y}$\")",
"_____no_output_____"
]
],
[
[
"Load the Iris data from sklearn. Use petal length and width as the training input. ",
"_____no_output_____"
]
],
[
[
"from sklearn.datasets import load_iris",
"_____no_output_____"
],
[
"iris = load_iris()",
"_____no_output_____"
],
[
"iris.feature_names",
"_____no_output_____"
],
[
"X = iris.data[:, 2:]\ny = iris.target",
"_____no_output_____"
]
],
[
[
"Now fit a decision tree classifier. Set max depth at 2.",
"_____no_output_____"
]
],
[
[
"from sklearn.tree import DecisionTreeClassifier",
"_____no_output_____"
],
[
"clf_tree = DecisionTreeClassifier(max_depth=2)\nclf_tree.fit(X, y)",
"_____no_output_____"
]
],
[
[
"Now visualize the model by the plot_decision_boundary function.",
"_____no_output_____"
]
],
[
[
"plt.figure(figsize=(10, 6))\nplot_decision_boundary(clf_tree, X, y, axes=[0, 7.5, 0, 3], iris=True, legend=False, plot_training=True)",
"_____no_output_____"
]
],
[
[
"Predict the probability of each class for [5, 1.5]",
"_____no_output_____"
]
],
[
[
"clf_tree.predict_proba([[5, 1.5]])",
"_____no_output_____"
]
],
[
[
"Run next cell to generate 100 moon data at noise=0.25 and random_state=53",
"_____no_output_____"
]
],
[
[
"from sklearn.datasets import make_moons\nX, y = make_moons(n_samples=100, noise=0.25, random_state=53)",
"_____no_output_____"
]
],
[
[
"Now fit two decision tree model. One has no restriction, and another has min_samples_leaf = 4",
"_____no_output_____"
]
],
[
[
"clf_tree = DecisionTreeClassifier()\nclf_tree_4 = DecisionTreeClassifier(min_samples_leaf=4)\nclf_tree.fit(X, y)\nclf_tree_4.fit(X, y)",
"_____no_output_____"
]
],
[
[
"Now use function plot_decision_boundary to visualize and compare these two models. Check for overfitting.",
"_____no_output_____"
]
],
[
[
"limit = [X[:, 0].min(), X[:, 0].max(), X[:, 1].min(), X[:, 1].max()]\nplt.figure(figsize=(12, 6))\nplt.subplot(121)\nplot_decision_boundary(clf_tree, X, y, axes=limit, iris=False)\nplt.title('no restriction')\nplt.subplot(122)\nplot_decision_boundary(clf_tree_4, X, y, axes=limit, iris=False)\nplt.title('min_samples_leaf=4')",
"_____no_output_____"
]
],
[
[
"# Regression",
"_____no_output_____"
],
[
"Run next cell to generate synthetic data",
"_____no_output_____"
]
],
[
[
"#\nnp.random.seed(42)\nm = 200\nX = np.random.rand(m, 1)\ny = 4 * (X - 0.5) ** 2\ny = y + np.random.randn(m, 1) / 10\ny = y.ravel()",
"_____no_output_____"
]
],
[
[
"Fit two regression trees. The first three have max_depth of 2, 3, 5; and the last one has no restriction.",
"_____no_output_____"
]
],
[
[
"from sklearn.tree import DecisionTreeRegressor",
"_____no_output_____"
],
[
"reg_tree_2 = DecisionTreeRegressor(max_depth=2)\nreg_tree_3 = DecisionTreeRegressor(max_depth=3)\nreg_tree_5 = DecisionTreeRegressor(max_depth=5)\nreg_tree_none = DecisionTreeRegressor()",
"_____no_output_____"
],
[
"reg_tree_2.fit(X, y)\nreg_tree_3.fit(X, y)\nreg_tree_5.fit(X, y)\nreg_tree_none.fit(X, y)",
"_____no_output_____"
]
],
[
[
"Now visualize these four trees by the function of plot_regression_predictions",
"_____no_output_____"
]
],
[
[
"limit = [X.min(), X.max(), y.min(), y.max()]\nplt.figure(figsize=(10, 10))\nplt.subplot(221)\nplot_regression_predictions(reg_tree_2, X, y, axes=limit)\nplt.subplot(222)\nplot_regression_predictions(reg_tree_3, X, y, axes=limit)\nplt.subplot(223)\nplot_regression_predictions(reg_tree_5, X, y, axes=limit)\nplt.subplot(224)\nplot_regression_predictions(reg_tree_none, X, y, axes=limit)",
"_____no_output_____"
]
]
] | [
"markdown",
"code",
"markdown",
"code",
"markdown",
"code",
"markdown",
"code",
"markdown",
"code",
"markdown",
"code",
"markdown",
"code",
"markdown",
"code",
"markdown",
"code",
"markdown",
"code",
"markdown",
"code",
"markdown",
"code"
] | [
[
"markdown"
],
[
"code"
],
[
"markdown"
],
[
"code",
"code"
],
[
"markdown"
],
[
"code",
"code",
"code",
"code"
],
[
"markdown"
],
[
"code",
"code"
],
[
"markdown"
],
[
"code"
],
[
"markdown"
],
[
"code"
],
[
"markdown"
],
[
"code"
],
[
"markdown"
],
[
"code"
],
[
"markdown"
],
[
"code"
],
[
"markdown",
"markdown"
],
[
"code"
],
[
"markdown"
],
[
"code",
"code",
"code"
],
[
"markdown"
],
[
"code"
]
] |
e76c687c36c02a2a6f9436498435adcb8c096d91 | 31,431 | ipynb | Jupyter Notebook | birds/bird_csv_to_czml.ipynb | rsignell-usgs/CZML | 11d1f6684eff89818308cc0db32e3e0f65cc223d | [
"CC0-1.0"
] | 1 | 2019-05-06T00:29:01.000Z | 2019-05-06T00:29:01.000Z | birds/bird_csv_to_czml.ipynb | ChBeil/CZML | 11d1f6684eff89818308cc0db32e3e0f65cc223d | [
"CC0-1.0"
] | null | null | null | birds/bird_csv_to_czml.ipynb | ChBeil/CZML | 11d1f6684eff89818308cc0db32e3e0f65cc223d | [
"CC0-1.0"
] | 2 | 2021-05-16T18:39:04.000Z | 2021-05-18T15:09:17.000Z | 103.052459 | 25,048 | 0.659954 | [
[
[
"from czml import czml\n\n# Initialize a document\ndoc = czml.CZML()",
"_____no_output_____"
],
[
"clock = {\n \"step\": \"SYSTEM_CLOCK_MULTIPLIER\",\n \"range\": \"LOOP_STOP\",\n \"multiplier\": 2160000,\n \"interval\": \"2015-01-01/2015-12-31\",\n \"currentTime\": \"2015-01-01\"\n }",
"_____no_output_____"
],
[
"# Create and append the document packet\npacket1 = czml.CZMLPacket(id='document',version='1.0',clock=clock)",
"_____no_output_____"
],
[
"packet1.dumps()",
"_____no_output_____"
],
[
"doc.packets.append(packet1)",
"_____no_output_____"
],
[
"packet2 = czml.CZMLPacket(id='flycatcher', availability=\"2015-01-01/2015-12-31\")",
"_____no_output_____"
],
[
"packet2.dumps()",
"_____no_output_____"
],
[
"point={\n \"color\": {\n \"rgba\": [\n 255, 255, 0, 255\n ]\n },\n \"outlineWidth\": 0,\n \"pixelSize\": 10,\n \"show\": True\n }",
"_____no_output_____"
],
[
"packet2.point = point",
"_____no_output_____"
]
],
[
[
"## Read the actual Bird Data CSV",
"_____no_output_____"
],
[
"# Convert Cornell Bird Migration CSV files to CZML\nTrying out the CZML python package. Installed from PIP until we can build our own conda package",
"_____no_output_____"
]
],
[
[
"import pandas as pd\nimport datetime as dt\nimport numpy as np",
"_____no_output_____"
],
[
"# parser to convert integer yeardays to datetimes in 2015\ndef parse(day):\n date = dt.datetime(2015,1,1,0,0) + dt.timedelta(days=(day.astype(np.int32)-1))\n return date",
"_____no_output_____"
],
[
"def csv_to_position(file='Acadian_Flycatcher.csv'):\n df = pd.read_csv(file, parse_dates=True, date_parser=parse, index_col=0, na_values='NA')\n df.dropna(how=\"all\", inplace=True) \n df['z']=0.0\n df['str']= df.index.strftime('%Y-%m-%d')\n df2 = df.ix[:,[3,0,1,2]]\n a = df2.values.tolist()\n return {'cartographicDegrees':[val for sublist in a for val in sublist]}",
"_____no_output_____"
],
[
"import glob",
"_____no_output_____"
],
[
"csv_files = glob.glob('*.csv')",
"_____no_output_____"
],
[
"for csv_file in csv_files:\n bird = csv_file.split('.')[0]\n packet = czml.CZMLPacket(id=bird, availability=\"2015-01-01/2015-12-31\")\n packet.point = point\n pos = csv_to_position(file=csv_file)\n packet.position = pos\n desc = czml.Description(string=bird)\n packet.description = desc\n doc.packets.append(packet)\n\n ",
"_____no_output_____"
],
[
"# inspect the last packet\npacket.dumps()",
"_____no_output_____"
],
[
"# Write the CZML document to a file\nfilename = \"all_birds.czml\"\ndoc.write(filename)",
"_____no_output_____"
]
]
] | [
"code",
"markdown",
"code"
] | [
[
"code",
"code",
"code",
"code",
"code",
"code",
"code",
"code",
"code"
],
[
"markdown",
"markdown"
],
[
"code",
"code",
"code",
"code",
"code",
"code",
"code",
"code"
]
] |
e76c78ecf2d369b9169e112e6b27622754cc9d5e | 48,458 | ipynb | Jupyter Notebook | Colab NoteBooks/BertSum_and_PreSumm_preprocessing.ipynb | gagan94/PreSumm | 65b5c46d0352d0c1e818efa0e6628efb132a63c4 | [
"MIT"
] | null | null | null | Colab NoteBooks/BertSum_and_PreSumm_preprocessing.ipynb | gagan94/PreSumm | 65b5c46d0352d0c1e818efa0e6628efb132a63c4 | [
"MIT"
] | null | null | null | Colab NoteBooks/BertSum_and_PreSumm_preprocessing.ipynb | gagan94/PreSumm | 65b5c46d0352d0c1e818efa0e6628efb132a63c4 | [
"MIT"
] | null | null | null | 54.325112 | 8,140 | 0.537228 | [
[
[
"import pandas as pd\n!pip install stanfordnlp\nimport stanfordnlp\n!pip install pytorch_pretrained_bert\nimport torch,gc",
"Requirement already satisfied: stanfordnlp in /usr/local/lib/python3.6/dist-packages (0.2.0)\nRequirement already satisfied: tqdm in /usr/local/lib/python3.6/dist-packages (from stanfordnlp) (4.28.1)\nRequirement already satisfied: torch>=1.0.0 in /usr/local/lib/python3.6/dist-packages (from stanfordnlp) (1.3.1)\nRequirement already satisfied: requests in /usr/local/lib/python3.6/dist-packages (from stanfordnlp) (2.21.0)\nRequirement already satisfied: protobuf in /usr/local/lib/python3.6/dist-packages (from stanfordnlp) (3.10.0)\nRequirement already satisfied: numpy in /usr/local/lib/python3.6/dist-packages (from stanfordnlp) (1.17.5)\nRequirement already satisfied: chardet<3.1.0,>=3.0.2 in /usr/local/lib/python3.6/dist-packages (from requests->stanfordnlp) (3.0.4)\nRequirement already satisfied: certifi>=2017.4.17 in /usr/local/lib/python3.6/dist-packages (from requests->stanfordnlp) (2019.11.28)\nRequirement already satisfied: urllib3<1.25,>=1.21.1 in /usr/local/lib/python3.6/dist-packages (from requests->stanfordnlp) (1.24.3)\nRequirement already satisfied: idna<2.9,>=2.5 in /usr/local/lib/python3.6/dist-packages (from requests->stanfordnlp) (2.8)\nRequirement already satisfied: six>=1.9 in /usr/local/lib/python3.6/dist-packages (from protobuf->stanfordnlp) (1.12.0)\nRequirement already satisfied: setuptools in /usr/local/lib/python3.6/dist-packages (from protobuf->stanfordnlp) (42.0.2)\nRequirement already satisfied: pytorch_pretrained_bert in /usr/local/lib/python3.6/dist-packages (0.6.2)\nRequirement already satisfied: tqdm in /usr/local/lib/python3.6/dist-packages (from pytorch_pretrained_bert) (4.28.1)\nRequirement already satisfied: numpy in /usr/local/lib/python3.6/dist-packages (from pytorch_pretrained_bert) (1.17.5)\nRequirement already satisfied: regex in /usr/local/lib/python3.6/dist-packages (from pytorch_pretrained_bert) (2019.12.20)\nRequirement already satisfied: boto3 in /usr/local/lib/python3.6/dist-packages (from pytorch_pretrained_bert) (1.10.47)\nRequirement already satisfied: torch>=0.4.1 in /usr/local/lib/python3.6/dist-packages (from pytorch_pretrained_bert) (1.3.1)\nRequirement already satisfied: requests in /usr/local/lib/python3.6/dist-packages (from pytorch_pretrained_bert) (2.21.0)\nRequirement already satisfied: jmespath<1.0.0,>=0.7.1 in /usr/local/lib/python3.6/dist-packages (from boto3->pytorch_pretrained_bert) (0.9.4)\nRequirement already satisfied: s3transfer<0.3.0,>=0.2.0 in /usr/local/lib/python3.6/dist-packages (from boto3->pytorch_pretrained_bert) (0.2.1)\nRequirement already satisfied: botocore<1.14.0,>=1.13.47 in /usr/local/lib/python3.6/dist-packages (from boto3->pytorch_pretrained_bert) (1.13.47)\nRequirement already satisfied: idna<2.9,>=2.5 in /usr/local/lib/python3.6/dist-packages (from requests->pytorch_pretrained_bert) (2.8)\nRequirement already satisfied: urllib3<1.25,>=1.21.1 in /usr/local/lib/python3.6/dist-packages (from requests->pytorch_pretrained_bert) (1.24.3)\nRequirement already satisfied: certifi>=2017.4.17 in /usr/local/lib/python3.6/dist-packages (from requests->pytorch_pretrained_bert) (2019.11.28)\nRequirement already satisfied: chardet<3.1.0,>=3.0.2 in /usr/local/lib/python3.6/dist-packages (from requests->pytorch_pretrained_bert) (3.0.4)\nRequirement already satisfied: python-dateutil<3.0.0,>=2.1; python_version >= \"2.7\" in /usr/local/lib/python3.6/dist-packages (from botocore<1.14.0,>=1.13.47->boto3->pytorch_pretrained_bert) (2.6.1)\nRequirement already satisfied: docutils<0.16,>=0.10 in /usr/local/lib/python3.6/dist-packages (from botocore<1.14.0,>=1.13.47->boto3->pytorch_pretrained_bert) (0.15.2)\nRequirement already satisfied: six>=1.5 in /usr/local/lib/python3.6/dist-packages (from python-dateutil<3.0.0,>=2.1; python_version >= \"2.7\"->botocore<1.14.0,>=1.13.47->boto3->pytorch_pretrained_bert) (1.12.0)\n"
]
],
[
[
"CoreNLP running on Python Server",
"_____no_output_____"
]
],
[
[
"!echo \"Downloading CoreNLP...\"\n!wget \"http://nlp.stanford.edu/software/stanford-corenlp-full-2018-10-05.zip\" -O corenlp.zip\n!unzip corenlp.zip\n!mv ./stanford-corenlp-full-2018-10-05 ./corenlp\n\n# Set the CORENLP_HOME environment variable to point to the installation location\nimport os\nos.environ[\"CORENLP_HOME\"] = \"./corenlp\"\n\n# Import client module\nfrom stanfordnlp.server import CoreNLPClient",
"Downloading CoreNLP...\n--2020-01-23 06:13:53-- http://nlp.stanford.edu/software/stanford-corenlp-full-2018-10-05.zip\nResolving nlp.stanford.edu (nlp.stanford.edu)... 171.64.67.140\nConnecting to nlp.stanford.edu (nlp.stanford.edu)|171.64.67.140|:80... connected.\nHTTP request sent, awaiting response... 302 Found\nLocation: https://nlp.stanford.edu/software/stanford-corenlp-full-2018-10-05.zip [following]\n--2020-01-23 06:13:54-- https://nlp.stanford.edu/software/stanford-corenlp-full-2018-10-05.zip\nConnecting to nlp.stanford.edu (nlp.stanford.edu)|171.64.67.140|:443... connected.\nHTTP request sent, awaiting response... 200 OK\nLength: 393239982 (375M) [application/zip]\nSaving to: โcorenlp.zipโ\n\ncorenlp.zip 100%[===================>] 375.02M 7.33MB/s in 2m 2s \n\n2020-01-23 06:15:56 (3.07 MB/s) - โcorenlp.zipโ saved [393239982/393239982]\n\nArchive: corenlp.zip\n creating: stanford-corenlp-full-2018-10-05/\n inflating: stanford-corenlp-full-2018-10-05/jaxb-core-2.3.0.1-sources.jar \n inflating: stanford-corenlp-full-2018-10-05/xom-1.2.10-src.jar \n inflating: stanford-corenlp-full-2018-10-05/CoreNLP-to-HTML.xsl \n inflating: stanford-corenlp-full-2018-10-05/README.txt \n inflating: stanford-corenlp-full-2018-10-05/jollyday-0.4.9-sources.jar \n inflating: stanford-corenlp-full-2018-10-05/LIBRARY-LICENSES \n creating: stanford-corenlp-full-2018-10-05/sutime/\n inflating: stanford-corenlp-full-2018-10-05/sutime/british.sutime.txt \n inflating: stanford-corenlp-full-2018-10-05/sutime/defs.sutime.txt \n inflating: stanford-corenlp-full-2018-10-05/sutime/spanish.sutime.txt \n inflating: stanford-corenlp-full-2018-10-05/sutime/english.sutime.txt \n inflating: stanford-corenlp-full-2018-10-05/sutime/english.holidays.sutime.txt \n extracting: stanford-corenlp-full-2018-10-05/ejml-0.23-src.zip \n inflating: stanford-corenlp-full-2018-10-05/input.txt.xml \n inflating: stanford-corenlp-full-2018-10-05/build.xml \n inflating: stanford-corenlp-full-2018-10-05/pom.xml \n inflating: stanford-corenlp-full-2018-10-05/stanford-corenlp-3.9.2-javadoc.jar \n creating: stanford-corenlp-full-2018-10-05/tokensregex/\n inflating: stanford-corenlp-full-2018-10-05/tokensregex/color.input.txt \n inflating: stanford-corenlp-full-2018-10-05/tokensregex/retokenize.txt \n inflating: stanford-corenlp-full-2018-10-05/tokensregex/color.properties \n inflating: stanford-corenlp-full-2018-10-05/tokensregex/color.rules.txt \n inflating: stanford-corenlp-full-2018-10-05/javax.json-api-1.0-sources.jar \n inflating: stanford-corenlp-full-2018-10-05/jaxb-api-2.4.0-b180830.0359-sources.jar \n inflating: stanford-corenlp-full-2018-10-05/stanford-corenlp-3.9.2-models.jar \n inflating: stanford-corenlp-full-2018-10-05/protobuf.jar \n inflating: stanford-corenlp-full-2018-10-05/javax.activation-api-1.2.0.jar \n inflating: stanford-corenlp-full-2018-10-05/StanfordDependenciesManual.pdf \n creating: stanford-corenlp-full-2018-10-05/patterns/\n inflating: stanford-corenlp-full-2018-10-05/patterns/example.properties \n extracting: stanford-corenlp-full-2018-10-05/patterns/otherpeople.txt \n extracting: stanford-corenlp-full-2018-10-05/patterns/goldplaces.txt \n inflating: stanford-corenlp-full-2018-10-05/patterns/stopwords.txt \n inflating: stanford-corenlp-full-2018-10-05/patterns/presidents.txt \n inflating: stanford-corenlp-full-2018-10-05/patterns/names.txt \n extracting: stanford-corenlp-full-2018-10-05/patterns/places.txt \n inflating: stanford-corenlp-full-2018-10-05/patterns/goldnames.txt \n inflating: stanford-corenlp-full-2018-10-05/slf4j-simple.jar \n inflating: stanford-corenlp-full-2018-10-05/stanford-corenlp-3.9.2-sources.jar \n inflating: stanford-corenlp-full-2018-10-05/input.txt \n inflating: stanford-corenlp-full-2018-10-05/joda-time.jar \n inflating: stanford-corenlp-full-2018-10-05/xom.jar \n inflating: stanford-corenlp-full-2018-10-05/jaxb-impl-2.4.0-b180830.0438-sources.jar \n inflating: stanford-corenlp-full-2018-10-05/StanfordCoreNlpDemo.java \n inflating: stanford-corenlp-full-2018-10-05/jaxb-core-2.3.0.1.jar \n inflating: stanford-corenlp-full-2018-10-05/RESOURCE-LICENSES \n inflating: stanford-corenlp-full-2018-10-05/javax.activation-api-1.2.0-sources.jar \n inflating: stanford-corenlp-full-2018-10-05/slf4j-api.jar \n inflating: stanford-corenlp-full-2018-10-05/pom-java-11.xml \n inflating: stanford-corenlp-full-2018-10-05/ejml-0.23.jar \n inflating: stanford-corenlp-full-2018-10-05/javax.json.jar \n inflating: stanford-corenlp-full-2018-10-05/Makefile \n inflating: stanford-corenlp-full-2018-10-05/corenlp.sh \n inflating: stanford-corenlp-full-2018-10-05/joda-time-2.9-sources.jar \n inflating: stanford-corenlp-full-2018-10-05/jaxb-api-2.4.0-b180830.0359.jar \n inflating: stanford-corenlp-full-2018-10-05/jollyday.jar \n inflating: stanford-corenlp-full-2018-10-05/ShiftReduceDemo.java \n inflating: stanford-corenlp-full-2018-10-05/jaxb-impl-2.4.0-b180830.0438.jar \n inflating: stanford-corenlp-full-2018-10-05/stanford-corenlp-3.9.2.jar \n inflating: stanford-corenlp-full-2018-10-05/SemgrexDemo.java \n inflating: stanford-corenlp-full-2018-10-05/LICENSE.txt \n"
],
[
"# Construct a CoreNLPClient with some basic annotators, a memory allocation of 4GB, and port number 9001\nclient = CoreNLPClient(annotators=['tokenize','ssplit', 'pos', 'lemma', 'ner'], memory='4G', endpoint='http://localhost:9001')\nprint(client)\n\n# Start the background server and wait for some time\n# Note that in practice this is totally optional, as by default the server will be started when the first annotation is performed\nclient.start()\nimport time; time.sleep(10)",
"<stanfordnlp.server.client.CoreNLPClient object at 0x7f6ed0cf68d0>\nStarting server with command: java -Xmx4G -cp ./corenlp/* edu.stanford.nlp.pipeline.StanfordCoreNLPServer -port 9001 -timeout 60000 -threads 5 -maxCharLength 100000 -quiet True -serverProperties corenlp_server-5f4e4d7044944e52.props -preload tokenize,ssplit,pos,lemma,ner\n"
]
],
[
[
"Add the Number of Rows to be considered as training data, test data and validation data in the input CSV which contains the training data",
"_____no_output_____"
]
],
[
[
"Trainrow = 1\ntestrow = 45\nvalrow = 49",
"_____no_output_____"
]
],
[
[
"Reading the input text from CSV file mentioned here. Change according to requirement.",
"_____no_output_____"
]
],
[
[
"import json\ndef format_to_lines():\n p_ct = 0\n i = 0\n for i in range(Trainrow):\n df_csv = pd.read_csv('./fincident.csv',encoding='cp1252')\n dataset = []\n d = _format_to_lines_new(df_csv,i)\n dataset.append(d)\n i += 1\n pt_file = \"{:s}.{:s}.{:d}.json\".format('bert_data', \"train\", p_ct)\n with open(pt_file, 'w') as save:\n # save.write('\\n'.join(dataset))\n save.write(json.dumps(dataset))\n p_ct += 1\n print(p_ct)\n dataset = []\n",
"_____no_output_____"
],
[
"REMAP = {\"-lrb-\": \"(\", \"-rrb-\": \")\", \"-lcb-\": \"{\", \"-rcb-\": \"}\",\n \"-lsb-\": \"[\", \"-rsb-\": \"]\", \"``\": '\"', \"''\": '\"'}\n\nimport re\ndef clean(x):\n return re.sub(\n r\"-lrb-|-rrb-|-lcb-|-rcb-|-lsb-|-rsb-|``|''\",\n lambda m: REMAP.get(m.group()), x)\n \nlower = True\n\ndef _format_to_lines_new(df_csv,i):\n source = []\n tgt = []\n flag = False\n print(df_csv['Texts'][i])\n ann = client.annotate(str(df_csv['Texts'][i]),annotators='tokenize,ssplit', output_format='json' )\n for sent in ann['sentences']:\n tokens = [t['word'] for t in sent['tokens']]\n if (lower):\n tokens = [t.lower() for t in tokens]\n if (tokens[0] == '@highlight'):\n flag = True\n continue\n if (flag):\n tgt.append(tokens)\n flag = False\n else:\n source.append(tokens)\n\n\n ann = client.annotate(str(df_csv['Analysis'][i]),annotators='tokenize,ssplit', output_format='json' )\n for sent in ann['sentences']:\n tokens = [t['word'] for t in sent['tokens']]\n if (lower):\n tokens = [t.lower() for t in tokens]\n if (tokens[0] == '@highlight'):\n flag = True\n continue\n if (flag):\n tgt.append(tokens)\n flag = False\n else:\n tgt.append(tokens)\n \n\n source = [clean(' '.join(sent)).split() for sent in source]\n tgt = [clean(' '.join(sent)).split() for sent in tgt]\n\n return {'src': source, 'tgt': tgt}",
"_____no_output_____"
],
[
"from multiprocess import Pool\ndef format_to_bert(args):\n for i in range(Trainrow):\n a_lst = []\n for json_f in glob.glob(pjoin(args.raw_path, '*' + corpus_type + '.*.json')):\n real_name = json_f.split('/')[-1]\n a_lst.append((json_f, args, pjoin(args.save_path, real_name.replace('json', 'bert.pt'))))\n print(a_lst)\n pool = Pool(args.n_cpus)\n for d in pool.imap(_format_to_bert, a_lst):\n pass\n\n pool.close()\n pool.join()\n",
"_____no_output_____"
]
],
[
[
"Genereate JSON file of the trianing data from th CSV",
"_____no_output_____"
]
],
[
[
"format_to_lines()",
" Hello Colleagues The solution creation is failing in QTC 906 with all the users with generic error message saying authorisation is missing. but with the same users the solutions were created previously also. NOTE : Even after the error message is shown the solution can be seen in solution explorer window but when clicked on it to sync then it says solution does not exist in the system. can you please check this ? This is blocking us for creating solution to test our automates. Regards Vishwanath A. Telsang \n\n Hi Colleagues The issue is coming up because .myproj is not getting created in XREP. Please take over and check what can be done. Thanks Regards Sasi. \n\n Hi I debugged the SDk and found out thar we call the PDI_1o_product_create to create product and after the product creation is done we make a separate call to generate solution file (.myproj .sln and .suo ) The load in system QTC 906 is too heavy. thera are in total 4700 solution present in the tenant. Since the system load is too high the solution creation is being timedout and we can make a backend call for creating these solution realted files. To resolve the issue we need to make system faster by removing the unwanted solution. To workaround: 1. Create a dummy solution in any tenant 2. Do a download as copy and deploy to QTC 906. 3. Create patch in 906 . 4. Use this solution for automate related task. Regards Nadeem \n\n Hi I debugged the SDk and found out thar we call the PDI_1o_product_create to create product and after the product creation is done we make a separate call to generate solution file (.myproj .sln and .suo ) The load in system QTC 906 is too heavy. thera are in total 4700 solution present in the tenant. Since the system load is too high the solution creation is being timedout and we can make a backend call for creating these solution realted files. To resolve the issue we need to make system faster by removing the unwanted solution. To workaround: 1. Create a dummy solution in any tenant 2. Do a download as copy and deploy to QTC 906. 3. Create patch in 906 . 4. Use this solution for automate related task. Regards Nadeem \n\n\n1\n"
]
],
[
[
"Different Modules which are required below for Preprocessing",
"_____no_output_____"
]
],
[
[
"def _get_word_ngrams(n, sentences):\n \"\"\"Calculates word n-grams for multiple sentences.\n \"\"\"\n assert len(sentences) > 0\n assert n > 0\n\n # words = _split_into_words(sentences)\n\n words = sum(sentences, [])\n # words = [w for w in words if w not in stopwords]\n return _get_ngrams(n, words)",
"_____no_output_____"
],
[
"def _get_ngrams(n, text):\n \"\"\"Calcualtes n-grams.\n Args:\n n: which n-grams to calculate\n text: An array of tokens\n Returns:\n A set of n-grams\n \"\"\"\n ngram_set = set()\n text_length = len(text)\n max_index_ngram_start = text_length - n\n for i in range(max_index_ngram_start + 1):\n ngram_set.add(tuple(text[i:i + n]))\n return ngram_set",
"_____no_output_____"
],
[
"def greedy_selection(doc_sent_list, abstract_sent_list, summary_size):\n def _rouge_clean(s):\n return re.sub(r'[^a-zA-Z0-9 ]', '', s)\n\n max_rouge = 0.0\n abstract = sum(abstract_sent_list, [])\n abstract = _rouge_clean(' '.join(abstract)).split()\n sents = [_rouge_clean(' '.join(s)).split() for s in doc_sent_list]\n evaluated_1grams = [_get_word_ngrams(1, [sent]) for sent in sents]\n reference_1grams = _get_word_ngrams(1, [abstract])\n evaluated_2grams = [_get_word_ngrams(2, [sent]) for sent in sents]\n reference_2grams = _get_word_ngrams(2, [abstract])\n\n selected = []\n for s in range(summary_size):\n cur_max_rouge = max_rouge\n cur_id = -1\n for i in range(len(sents)):\n if (i in selected):\n continue\n c = selected + [i]\n candidates_1 = [evaluated_1grams[idx] for idx in c]\n candidates_1 = set.union(*map(set, candidates_1))\n candidates_2 = [evaluated_2grams[idx] for idx in c]\n candidates_2 = set.union(*map(set, candidates_2))\n rouge_1 = cal_rouge(candidates_1, reference_1grams)['f']\n rouge_2 = cal_rouge(candidates_2, reference_2grams)['f']\n rouge_score = rouge_1 + rouge_2\n if rouge_score > cur_max_rouge:\n cur_max_rouge = rouge_score\n cur_id = i\n if (cur_id == -1):\n return selected\n selected.append(cur_id)\n max_rouge = cur_max_rouge\n\n return sorted(selected)\n",
"_____no_output_____"
],
[
"def cal_rouge(evaluated_ngrams, reference_ngrams):\n reference_count = len(reference_ngrams)\n evaluated_count = len(evaluated_ngrams)\n\n overlapping_ngrams = evaluated_ngrams.intersection(reference_ngrams)\n overlapping_count = len(overlapping_ngrams)\n\n if evaluated_count == 0:\n precision = 0.0\n else:\n precision = overlapping_count / evaluated_count\n\n if reference_count == 0:\n recall = 0.0\n else:\n recall = overlapping_count / reference_count\n\n f1_score = 2.0 * ((precision * recall) / (precision + recall + 1e-8))\n return {\"f\": f1_score, \"p\": precision, \"r\": recall}",
"_____no_output_____"
],
[
"from pytorch_pretrained_bert import BertTokenizer",
"_____no_output_____"
]
],
[
[
"Execute the below code for Preprocessing for PreSumm Only",
"_____no_output_____"
]
],
[
[
"# For PreSumm\n\nclass BertData():\n def __init__(self):\n self.tokenizer = BertTokenizer.from_pretrained('bert-base-uncased', do_lower_case=True)\n\n self.sep_token = '[SEP]'\n self.cls_token = '[CLS]'\n self.pad_token = '[PAD]'\n self.tgt_bos = '[unused0]'\n self.tgt_eos = '[unused1]'\n self.tgt_sent_split = '[unused2]'\n self.sep_vid = self.tokenizer.vocab[self.sep_token]\n self.cls_vid = self.tokenizer.vocab[self.cls_token]\n self.pad_vid = self.tokenizer.vocab[self.pad_token]\n\n def preprocess(self, src, tgt, sent_labels, use_bert_basic_tokenizer=False, is_test=False):\n\n if ((not is_test) and len(src) == 0):\n print(\"returned none\")\n return None\n\n original_src_txt = [' '.join(s) for s in src]\n\n idxs = [i for i, s in enumerate(src) if (len(s) > 5)]\n\n _sent_labels = [0] * len(src)\n for l in sent_labels:\n _sent_labels[l] = 1\n\n src = [src[i][:200] for i in idxs]\n sent_labels = [_sent_labels[i] for i in idxs]\n src = src[:200]\n sent_labels = sent_labels[:200]\n\n if ((not is_test) and len(src) < 3):\n print(\"returned none\")\n return None\n\n src_txt = [' '.join(sent) for sent in src]\n text = ' {} {} '.format(self.sep_token, self.cls_token).join(src_txt)\n\n src_subtokens = self.tokenizer.tokenize(text)\n\n src_subtokens = [self.cls_token] + src_subtokens + [self.sep_token]\n src_subtoken_idxs = self.tokenizer.convert_tokens_to_ids(src_subtokens)\n _segs = [-1] + [i for i, t in enumerate(src_subtoken_idxs) if t == self.sep_vid]\n segs = [_segs[i] - _segs[i - 1] for i in range(1, len(_segs))]\n segments_ids = []\n for i, s in enumerate(segs):\n if (i % 2 == 0):\n segments_ids += s * [0]\n else:\n segments_ids += s * [1]\n cls_ids = [i for i, t in enumerate(src_subtoken_idxs) if t == self.cls_vid]\n sent_labels = sent_labels[:len(cls_ids)]\n\n tgt_subtokens_str = '[unused0] ' + ' [unused2] '.join(\n [' '.join(self.tokenizer.tokenize(' '.join(tt))) for tt in tgt]) + ' [unused1]'\n tgt_subtoken = tgt_subtokens_str.split()[:200]\n if ((not is_test) and len(tgt_subtoken) < 20):\n print(\"returned none <20\")\n print(len(tgt_subtoken))\n return None\n\n tgt_subtoken_idxs = self.tokenizer.convert_tokens_to_ids(tgt_subtoken)\n\n tgt_txt = '<q>'.join([' '.join(tt) for tt in tgt])\n src_txt = [original_src_txt[i] for i in idxs]\n\n return src_subtoken_idxs, sent_labels, tgt_subtoken_idxs, segments_ids, cls_ids, src_txt, tgt_txt",
"_____no_output_____"
]
],
[
[
"Execute the below code for Preprocessing for BertSumm Only",
"_____no_output_____"
]
],
[
[
"# For BertSum\nclass BertData():\n def __init__(self):\n self.tokenizer = BertTokenizer.from_pretrained('bert-base-uncased', do_lower_case=True)\n self.sep_vid = self.tokenizer.vocab['[SEP]']\n self.cls_vid = self.tokenizer.vocab['[CLS]']\n self.pad_vid = self.tokenizer.vocab['[PAD]']\n\n def preprocess(self, src, tgt, oracle_ids):\n\n if (len(src) == 0):\n return None\n\n original_src_txt = [' '.join(s) for s in src]\n\n labels = [0] * len(src)\n for l in oracle_ids:\n labels[l] = 1\n\n idxs = [i for i, s in enumerate(src) if (len(s) > 5)]\n\n src = [src[i][:200] for i in idxs]\n labels = [labels[i] for i in idxs]\n src = src[:200]\n labels = labels[:200]\n\n print(\"length of label\")\n print(len(labels))\n if (len(src) < 10):\n return None\n if (len(labels) == 0):\n return None\n\n src_txt = [' '.join(sent) for sent in src]\n # text = [' '.join(ex['src_txt'][i].split()[:self.args.max_src_ntokens]) for i in idxs]\n # text = [_clean(t) for t in text]\n text = ' [SEP] [CLS] '.join(src_txt)\n src_subtokens = self.tokenizer.tokenize(text)\n src_subtokens = src_subtokens[:510]\n src_subtokens = ['[CLS]'] + src_subtokens + ['[SEP]']\n\n src_subtoken_idxs = self.tokenizer.convert_tokens_to_ids(src_subtokens)\n _segs = [-1] + [i for i, t in enumerate(src_subtoken_idxs) if t == self.sep_vid]\n segs = [_segs[i] - _segs[i - 1] for i in range(1, len(_segs))]\n segments_ids = []\n for i, s in enumerate(segs):\n if (i % 2 == 0):\n segments_ids += s * [0]\n else:\n segments_ids += s * [1]\n cls_ids = [i for i, t in enumerate(src_subtoken_idxs) if t == self.cls_vid]\n labels = labels[:len(cls_ids)]\n\n tgt_txt = '<q>'.join([' '.join(tt) for tt in tgt])\n src_txt = [original_src_txt[i] for i in idxs]\n return src_subtoken_idxs, labels, segments_ids, cls_ids, src_txt, tgt_txt\n",
"_____no_output_____"
]
],
[
[
"Execute the below code for Preprocessing for PreSumm Only",
"_____no_output_____"
]
],
[
[
"## For PreSumm Only\n\ndef _format_to_bert():\n p_ct = 0\n for i in range(Trainrow):\n pt_file = \"{:s}.{:s}.{:d}.json\".format('bert_data', \"train\", p_ct)\n json_file = pt_file\n save_file = pt_file.replace('.json','.pt')\n p_ct += 1\n bert = BertData()\n\n jobs = json.load(open(json_file))\n datasets = []\n for d in jobs:\n source, tgt = d['src'], d['tgt']\n print(\"inside\")\n oracle_ids = greedy_selection(source, tgt, 3)\n #elif (args.oracle_mode == 'combination'):\n # oracle_ids = combination_selection(source, tgt, 3)\n print(oracle_ids)\n b_data = bert.preprocess(source, tgt, oracle_ids)\n if (b_data is None):\n print(\"None\")\n continue\n src_subtoken_idxs, sent_labels, tgt_subtoken_idxs, segments_ids, cls_ids, src_txt, tgt_txt = b_data\n #for BertSumm\n #indexed_tokens, labels, segments_ids, cls_ids, src_txt, tgt_txt = b_data\n # for BertSumm\n #b_data_dict = {\"src\": indexed_tokens, \"labels\": labels, \"segs\": segments_ids, 'clss': cls_ids,\n # 'src_txt': src_txt, \"tgt_txt\": tgt_txt}\n b_data_dict = {\"src\": src_subtoken_idxs, \"tgt\": tgt_subtoken_idxs,\n \"src_sent_labels\": sent_labels, \"segs\": segments_ids, 'clss': cls_ids,\n 'src_txt': src_txt, \"tgt_txt\": tgt_txt}\n print(b_data_dict)\n datasets.append(b_data_dict)\n torch.save(datasets, save_file)\n datasets = []\n gc.collect()",
"_____no_output_____"
]
],
[
[
"Execute the below code for Preprocessing for PreSumm Only",
"_____no_output_____"
]
],
[
[
"## For BertSumm Only\n\ndef _format_to_bert():\n p_ct = 0\n for i in range(Trainrow):\n pt_file = \"{:s}.{:s}.{:d}.json\".format('bert_data', \"train\", p_ct)\n json_file = pt_file\n save_file = pt_file.replace('.json','.pt')\n p_ct += 1\n bert = BertData()\n\n jobs = json.load(open(json_file))\n datasets = []\n for d in jobs:\n source, tgt = d['src'], d['tgt']\n print(\"inside\")\n oracle_ids = greedy_selection(source, tgt, 3)\n #elif (args.oracle_mode == 'combination'):\n # oracle_ids = combination_selection(source, tgt, 3)\n print(oracle_ids)\n b_data = bert.preprocess(source, tgt, oracle_ids)\n if (b_data is None):\n print(\"None\")\n continue\n #for BertSumm\n indexed_tokens, labels, segments_ids, cls_ids, src_txt, tgt_txt = b_data\n # for BertSumm\n b_data_dict = {\"src\": indexed_tokens, \"labels\": labels, \"segs\": segments_ids, 'clss': cls_ids,\n 'src_txt': src_txt, \"tgt_txt\": tgt_txt}\n print(b_data_dict)\n datasets.append(b_data_dict)\n torch.save(datasets, save_file)\n datasets = []\n gc.collect()",
"_____no_output_____"
],
[
"_format_to_bert()",
"inside\n[0, 18, 22]\n{'src': [101, 7592, 8628, 1996, 5576, 4325, 2003, 7989, 1999, 1053, 13535, 3938, 2575, 2007, 2035, 1996, 5198, 2007, 12391, 7561, 4471, 3038, 3166, 6648, 2003, 4394, 1012, 102, 101, 2021, 2007, 1996, 2168, 5198, 1996, 7300, 2020, 2580, 3130, 2036, 1012, 102, 101, 3602, 1024, 2130, 2044, 1996, 7561, 4471, 2003, 3491, 1996, 5576, 2064, 2022, 2464, 1999, 5576, 10566, 3332, 2021, 2043, 13886, 2006, 2009, 2000, 26351, 2059, 2009, 2758, 5576, 2515, 2025, 4839, 1999, 1996, 2291, 1012, 102, 101, 2064, 2017, 3531, 4638, 2023, 1029, 102, 101, 2023, 2003, 10851, 2149, 2005, 4526, 5576, 2000, 3231, 2256, 8285, 15416, 1012, 102, 101, 12362, 25292, 18663, 16207, 1037, 1012, 10093, 8791, 2290, 7632, 8628, 1996, 3277, 2003, 2746, 2039, 2138, 1012, 102, 101, 2026, 21572, 3501, 2003, 2025, 2893, 2580, 1999, 1060, 2890, 2361, 1012, 102, 101, 3531, 2202, 2058, 1998, 4638, 2054, 2064, 2022, 2589, 1012, 102, 101, 7632, 1045, 2139, 8569, 15567, 1996, 17371, 2243, 1998, 2179, 2041, 22794, 2099, 2057, 2655, 1996, 22851, 2072, 1035, 1015, 2080, 1035, 4031, 1035, 3443, 2000, 3443, 4031, 1998, 2044, 1996, 4031, 4325, 2003, 2589, 2057, 2191, 1037, 3584, 2655, 2000, 9699, 5576, 5371, 1006, 1012, 102, 101, 10514, 2080, 1007, 1996, 7170, 1999, 2291, 1053, 13535, 3938, 2575, 2003, 2205, 3082, 1012, 102, 101, 1996, 2527, 2024, 1999, 2561, 21064, 2692, 5576, 2556, 1999, 1996, 16713, 1012, 102, 101, 2144, 1996, 2291, 7170, 2003, 2205, 2152, 1996, 5576, 4325, 2003, 2108, 22313, 5833, 1998, 2057, 2064, 2191, 1037, 2067, 10497, 2655, 2005, 4526, 2122, 5576, 2613, 3064, 6764, 1012, 102, 101, 2000, 10663, 1996, 3277, 2057, 2342, 2000, 2191, 2291, 5514, 2011, 9268, 1996, 18162, 5576, 1012, 102, 101, 3443, 1037, 24369, 5576, 1999, 2151, 16713, 1016, 1012, 102, 101, 2079, 1037, 8816, 2004, 6100, 1998, 21296, 2000, 1053, 13535, 3938, 2575, 1012, 102, 101, 2224, 2023, 5576, 2005, 8285, 8585, 3141, 4708, 1012, 102, 101, 12362, 23233, 21564, 7632, 1045, 2139, 8569, 15567, 1996, 17371, 2243, 1998, 2179, 2041, 22794, 2099, 2057, 2655, 1996, 22851, 2072, 1035, 1015, 2080, 1035, 4031, 1035, 3443, 2000, 3443, 4031, 1998, 2044, 1996, 4031, 4325, 2003, 2589, 2057, 2191, 1037, 3584, 2655, 2000, 9699, 5576, 5371, 1006, 1012, 102, 101, 10514, 2080, 1007, 1996, 7170, 1999, 2291, 1053, 13535, 3938, 2575, 2003, 2205, 3082, 1012, 102, 101, 1996, 2527, 2024, 1999, 2561, 21064, 2692, 5576, 2556, 1999, 1996, 16713, 1012, 102, 101, 2144, 1996, 2291, 7170, 2003, 2205, 2152, 1996, 5576, 4325, 2003, 2108, 22313, 5833, 1998, 2057, 2064, 2191, 1037, 2067, 10497, 2655, 2005, 4526, 2122, 5576, 2613, 3064, 6764, 1012, 102, 101, 2000, 10663, 1996, 3277, 2057, 2342, 2000, 2191, 2291, 5514, 2011, 9268, 1996, 18162, 5576, 1012, 102, 101, 3443, 1037, 24369, 5576, 1999, 2151, 16713, 1016, 1012, 102, 101, 2079, 1037, 8816, 2004, 6100, 1998, 21296, 2000, 1053, 13535, 3938, 2575, 1012, 102, 101, 2224, 2023, 5576, 2005, 8285, 8585, 3141, 4708, 1012, 102], 'tgt': [1, 1996, 5576, 4325, 2003, 7989, 1999, 1053, 13535, 3938, 2575, 2007, 2035, 1996, 5198, 2007, 12391, 7561, 4471, 3038, 3166, 6648, 2003, 4394, 1012, 3, 1996, 3277, 2003, 2746, 2039, 2138, 1012, 3, 2026, 21572, 3501, 2003, 2025, 2893, 2580, 1999, 1060, 2890, 2361, 1012, 3, 1996, 7170, 1999, 2291, 1053, 13535, 3938, 2575, 2003, 2205, 3082, 1012, 3, 2045, 2024, 1999, 2561, 21064, 2692, 5576, 2556, 1999, 1996, 16713, 1012, 3, 2144, 1996, 2291, 7170, 2003, 2205, 2152, 1996, 5576, 4325, 2003, 2108, 22313, 5833, 1012, 3, 2000, 2147, 24490, 1024, 1015, 1012, 3, 3443, 1037, 24369, 5576, 1999, 2151, 16713, 1016, 1012, 3, 2079, 1037, 8816, 2004, 6100, 1998, 21296, 2000, 1053, 13535, 3938, 2575, 1012, 3, 1017, 1012, 3, 3443, 8983, 1999, 3938, 2575, 1012, 3, 1018, 1012, 3, 2224, 2023, 5576, 2005, 8285, 8585, 3141, 4708, 1012, 2], 'src_sent_labels': [1, 0, 0, 0, 0, 0, 0, 0, 0, 0, 0, 0, 0, 0, 1, 1, 0, 0, 0, 0, 0, 0, 0, 0], 'segs': [0, 0, 0, 0, 0, 0, 0, 0, 0, 0, 0, 0, 0, 0, 0, 0, 0, 0, 0, 0, 0, 0, 0, 0, 0, 0, 0, 0, 1, 1, 1, 1, 1, 1, 1, 1, 1, 1, 1, 1, 1, 1, 0, 0, 0, 0, 0, 0, 0, 0, 0, 0, 0, 0, 0, 0, 0, 0, 0, 0, 0, 0, 0, 0, 0, 0, 0, 0, 0, 0, 0, 0, 0, 0, 0, 0, 0, 0, 0, 0, 1, 1, 1, 1, 1, 1, 1, 1, 0, 0, 0, 0, 0, 0, 0, 0, 0, 0, 0, 0, 0, 0, 0, 1, 1, 1, 1, 1, 1, 1, 1, 1, 1, 1, 1, 1, 1, 1, 1, 1, 1, 1, 1, 0, 0, 0, 0, 0, 0, 0, 0, 0, 0, 0, 0, 0, 0, 1, 1, 1, 1, 1, 1, 1, 1, 1, 1, 1, 1, 0, 0, 0, 0, 0, 0, 0, 0, 0, 0, 0, 0, 0, 0, 0, 0, 0, 0, 0, 0, 0, 0, 0, 0, 0, 0, 0, 0, 0, 0, 0, 0, 0, 0, 0, 0, 0, 0, 0, 0, 0, 0, 0, 0, 0, 0, 0, 0, 1, 1, 1, 1, 1, 1, 1, 1, 1, 1, 1, 1, 1, 1, 1, 1, 1, 0, 0, 0, 0, 0, 0, 0, 0, 0, 0, 0, 0, 0, 0, 0, 1, 1, 1, 1, 1, 1, 1, 1, 1, 1, 1, 1, 1, 1, 1, 1, 1, 1, 1, 1, 1, 1, 1, 1, 1, 1, 1, 1, 1, 1, 1, 1, 0, 0, 0, 0, 0, 0, 0, 0, 0, 0, 0, 0, 0, 0, 0, 0, 0, 0, 1, 1, 1, 1, 1, 1, 1, 1, 1, 1, 1, 0, 0, 0, 0, 0, 0, 0, 0, 0, 0, 0, 0, 0, 0, 0, 1, 1, 1, 1, 1, 1, 1, 1, 1, 1, 1, 0, 0, 0, 0, 0, 0, 0, 0, 0, 0, 0, 0, 0, 0, 0, 0, 0, 0, 0, 0, 0, 0, 0, 0, 0, 0, 0, 0, 0, 0, 0, 0, 0, 0, 0, 0, 0, 0, 0, 0, 0, 0, 0, 0, 0, 0, 0, 0, 0, 0, 0, 1, 1, 1, 1, 1, 1, 1, 1, 1, 1, 1, 1, 1, 1, 1, 1, 1, 0, 0, 0, 0, 0, 0, 0, 0, 0, 0, 0, 0, 0, 0, 0, 1, 1, 1, 1, 1, 1, 1, 1, 1, 1, 1, 1, 1, 1, 1, 1, 1, 1, 1, 1, 1, 1, 1, 1, 1, 1, 1, 1, 1, 1, 1, 1, 0, 0, 0, 0, 0, 0, 0, 0, 0, 0, 0, 0, 0, 0, 0, 0, 0, 0, 1, 1, 1, 1, 1, 1, 1, 1, 1, 1, 1, 0, 0, 0, 0, 0, 0, 0, 0, 0, 0, 0, 0, 0, 0, 0, 1, 1, 1, 1, 1, 1, 1, 1, 1, 1, 1], 'clss': [0, 28, 42, 80, 88, 103, 123, 137, 149, 197, 214, 229, 261, 279, 290, 305, 316, 367, 384, 399, 431, 449, 460, 475], 'src_txt': ['hello colleagues the solution creation is failing in qtc 906 with all the users with generic error message saying authorisation is missing .', 'but with the same users the solutions were created previously also .', 'note : even after the error message is shown the solution can be seen in solution explorer window but when clicked on it to sync then it says solution does not exist in the system .', 'can you please check this ?', 'this is blocking us for creating solution to test our automates .', 'regards vishwanath a. telsang hi colleagues the issue is coming up because .', 'myproj is not getting created in xrep .', 'please take over and check what can be done .', 'hi i debugged the sdk and found out thar we call the pdi _ 1o _ product _ create to create product and after the product creation is done we make a separate call to generate solution file ( .', 'suo ) the load in system qtc 906 is too heavy .', 'thera are in total 4700 solution present in the tenant .', 'since the system load is too high the solution creation is being timedout and we can make a backend call for creating these solution realted files .', 'to resolve the issue we need to make system faster by removing the unwanted solution .', 'create a dummy solution in any tenant 2 .', 'do a download as copy and deploy to qtc 906 .', 'use this solution for automate related task .', 'regards nadeem hi i debugged the sdk and found out thar we call the pdi _ 1o _ product _ create to create product and after the product creation is done we make a separate call to generate solution file ( .', 'suo ) the load in system qtc 906 is too heavy .', 'thera are in total 4700 solution present in the tenant .', 'since the system load is too high the solution creation is being timedout and we can make a backend call for creating these solution realted files .', 'to resolve the issue we need to make system faster by removing the unwanted solution .', 'create a dummy solution in any tenant 2 .', 'do a download as copy and deploy to qtc 906 .', 'use this solution for automate related task .'], 'tgt_txt': 'the solution creation is failing in qtc 906 with all the users with generic error message saying authorisation is missing .<q>the issue is coming up because .<q>myproj is not getting created in xrep .<q>the load in system qtc 906 is too heavy .<q>there are in total 4700 solution present in the tenant .<q>since the system load is too high the solution creation is being timedout .<q>to workaround : 1 .<q>create a dummy solution in any tenant 2 .<q>do a download as copy and deploy to qtc 906 .<q>3 .<q>create patch in 906 .<q>4 .<q>use this solution for automate related task .'}\n"
]
]
] | [
"code",
"markdown",
"code",
"markdown",
"code",
"markdown",
"code",
"markdown",
"code",
"markdown",
"code",
"markdown",
"code",
"markdown",
"code",
"markdown",
"code",
"markdown",
"code"
] | [
[
"code"
],
[
"markdown"
],
[
"code",
"code"
],
[
"markdown"
],
[
"code"
],
[
"markdown"
],
[
"code",
"code",
"code"
],
[
"markdown"
],
[
"code"
],
[
"markdown"
],
[
"code",
"code",
"code",
"code",
"code"
],
[
"markdown"
],
[
"code"
],
[
"markdown"
],
[
"code"
],
[
"markdown"
],
[
"code"
],
[
"markdown"
],
[
"code",
"code"
]
] |
e76c7cfcf3c63baa1db7b84cac5b46da5926364a | 4,939 | ipynb | Jupyter Notebook | workshops/2019-scipy/index.ipynb | whocaresustc/skimage-tutorials | 2e4c8ba9f09b377ebe363b04562aa33c8df3b0f5 | [
"CC-BY-4.0"
] | null | null | null | workshops/2019-scipy/index.ipynb | whocaresustc/skimage-tutorials | 2e4c8ba9f09b377ebe363b04562aa33c8df3b0f5 | [
"CC-BY-4.0"
] | null | null | null | workshops/2019-scipy/index.ipynb | whocaresustc/skimage-tutorials | 2e4c8ba9f09b377ebe363b04562aa33c8df3b0f5 | [
"CC-BY-4.0"
] | null | null | null | 36.316176 | 552 | 0.604778 | [
[
[
"# Image analysis in Python with SciPy and scikit-image\n\n<div style=\"border: solid 1px; background: #abcfef; font-size: 150%; padding: 1em; margin: 1em; width: 75%;\">\n\n<p>To participate, please follow the preparation instructions at</p>\n<p>https://github.com/scikit-image/skimage-tutorials/</p>\n<p>(click on **preparation.md**).</p>\n\n</div>\n\n<hr/>\nTL;DR: Install Python 3.6, scikit-image, and the Jupyter notebook. Then clone this repo:\n\n```python\ngit clone --depth=1 https://github.com/scikit-image/skimage-tutorials\n```\n<hr/>\n\nIf you cloned it before today, use `git pull origin` to get the latest changes.",
"_____no_output_____"
]
],
[
[
"%run ../../check_setup.py",
"[โ] scikit-image 0.15.0\n[โ] numpy 1.16.2\n[โ] scipy 1.2.1\n[โ] matplotlib 3.0.3\n[โ] notebook 5.7.6\n[โ] scikit-learn 0.20.3\n"
]
],
[
[
"scikit-image is a collection of image processing algorithms for the\nSciPy ecosystem. It aims to have a Pythonic API (read: does what you'd expect), \nis well documented, and provides researchers and practitioners with well-tested,\nfundamental building blocks for rapidly constructing sophisticated image\nprocessing pipelines.\n\nIn this tutorial, we provide an interactive overview of the library,\nwhere participants have the opportunity to try their hand at various\nimage processing challenges.\n\nAttendees are expected to have a working knowledge of NumPy, SciPy, and Matplotlib.\n\nAcross domains, modalities, and scales of exploration, images form an integral subset of scientific measurements. Despite a deep appeal to human intuition, gaining understanding of image content remains challenging, and often relies on heuristics. Even so, the wealth of knowledge contained inside of images cannot be understated, and <a href=\"http://scikit-image.org\">scikit-image</a>, along with <a href=\"http://scipy.org\">SciPy</a>, provides a strong foundation upon which to build algorithms and applications for exploring this domain.\n\n\n# Prerequisites\n\nPlease see the [preparation instructions](https://github.com/scikit-image/skimage-tutorials/blob/master/preparation.md).\n\n# Schedule\n\n- 1:30โ2:20: Introduction & [images as NumPy arrays](../../lectures/00_images_are_arrays.ipynb)\n- 2:30โ3:20: [Filters](../../lectures/1_image_filters.ipynb)\n- 3:30โ4:20: [Segmentation](../../lectures/4_segmentation.ipynb)\n- 4:30โ5:00: [Advanced workflow example](../../lectures/adv5-pores.ipynb)\n- 5:00โ5:20: [Tour of scikit-image](../../lectures/tour_of_skimage.ipynb)\n- 5:20โ5:30: Q&A\n\n**Note:** Snacks are available 2:15-4:00; coffee & tea until 5.\n\n# For later\n\n- Check out the other [lectures](../../lectures)\n- Check out a [3D segmentation workflow](../../lectures/three_dimensional_image_processing.ipynb)\n- Some [real world use cases](http://bit.ly/skimage_real_world)\n\n\n# After the tutorial\n\nStay in touch!\n\n- Come to the [sprint](https://www.scipy2019.scipy.org/sprints-schedule)!\n- Follow the project's progress [on GitHub](https://github.com/scikit-image/scikit-image).\n- Ask the team questions on the [mailing list](https://mail.python.org/mailman/listinfo/scikit-image)\n- [Contribute!](http://scikit-image.org/docs/dev/contribute.html)\n- Read [our paper](https://peerj.com/articles/453/) (or [this other paper, for skimage in microtomography](https://ascimaging.springeropen.com/articles/10.1186/s40679-016-0031-0))",
"_____no_output_____"
]
]
] | [
"markdown",
"code",
"markdown"
] | [
[
"markdown"
],
[
"code"
],
[
"markdown"
]
] |
e76c80a4baddc04f3a44466b1d5ee26a31146cf5 | 3,187 | ipynb | Jupyter Notebook | judges_in_ties.ipynb | daldunin/judges_in_ties | ee81d63534ac2fb5f3af69fc5d5510e43b2b6e27 | [
"MIT"
] | null | null | null | judges_in_ties.ipynb | daldunin/judges_in_ties | ee81d63534ac2fb5f3af69fc5d5510e43b2b6e27 | [
"MIT"
] | null | null | null | judges_in_ties.ipynb | daldunin/judges_in_ties | ee81d63534ac2fb5f3af69fc5d5510e43b2b6e27 | [
"MIT"
] | null | null | null | 27.95614 | 101 | 0.490116 | [
[
[
"import random\n\ndef repetitive_testing(\n teams_qty: int, judges_qty: int, points_qty: int,\n experiments_qty: int):\n \n ties_qty = 0\n \n for experiment in range(experiments_qty):\n results = []\n for r in range(0, teams_qty):\n results.append([r,0])\n #print('results: ',results)\n for judge in range(judges_qty):\n teams = random.sample(range(teams_qty), points_qty)\n #print('teams: ',teams)\n points = points_qty\n for team in teams:\n team_points = random.randint(1, points)\n results[team][1] += team_points\n\n points -= team_points\n if points == 0:\n #print('results: ',results)\n break\n \n #print('results again: ',results)\n results.sort(key=lambda x:x[1])\n #print('sorted_results: ',results)\n \n if results[teams_qty - 1][1] == results[teams_qty - 2][1]:\n ties_qty += 1\n \n #print('ties_qty: ',ties_qty)\n \n return ties_qty\n",
"_____no_output_____"
],
[
"teams_qty = 17 #teams quantity\njudges_qty = 4 #jugdes quantity\nmax_points = 6 #maximum number of points that judge can spread over teams\nexperiments_qty = 10000 #number of experiments for each points quantity from 1 to max_points\n\nfor points_qty in range(1, max_points + 1):\n ties_qty = repetitive_testing(teams_qty, judges_qty, points_qty, experiments_qty)\n print('points_qty: ', points_qty)\n print('ties_pct: ', (ties_qty / experiments_qty) * 100)\n ",
"points_qty: 1\nties_pct: 69.11\npoints_qty: 2\nties_pct: 53.290000000000006\npoints_qty: 3\nties_pct: 39.15\npoints_qty: 4\nties_pct: 31.52\npoints_qty: 5\nties_pct: 26.169999999999998\npoints_qty: 6\nties_pct: 22.78\n"
]
]
] | [
"code"
] | [
[
"code",
"code"
]
] |
e76c965e31be58694bcd2db57bff7fcb4d6d680e | 31,032 | ipynb | Jupyter Notebook | Thinkful Program/Natural Language Processing/Thinkful 36.3 Challenge 0.ipynb | rleary90/sturdy-octo-happiness | 0b11c8575fe984f632f52f4e326defab5a28fd2e | [
"MIT"
] | null | null | null | Thinkful Program/Natural Language Processing/Thinkful 36.3 Challenge 0.ipynb | rleary90/sturdy-octo-happiness | 0b11c8575fe984f632f52f4e326defab5a28fd2e | [
"MIT"
] | null | null | null | Thinkful Program/Natural Language Processing/Thinkful 36.3 Challenge 0.ipynb | rleary90/sturdy-octo-happiness | 0b11c8575fe984f632f52f4e326defab5a28fd2e | [
"MIT"
] | null | null | null | 39.231353 | 290 | 0.480601 | [
[
[
"%matplotlib inline\nimport numpy as np\nimport pandas as pd\nimport scipy\nimport sklearn\nimport spacy\nimport matplotlib.pyplot as plt\nimport seaborn as sns\nimport re\nfrom nltk.corpus import gutenberg, stopwords\nfrom collections import Counter\nimport nltk\n\nimport warnings\n\nnltk.download('gutenberg')\n!python -m spacy download en",
"[nltk_data] Downloading package gutenberg to\n[nltk_data] /Users/rodrickleary/nltk_data...\n[nltk_data] Unzipping corpora/gutenberg.zip.\n"
],
[
"# supress future warnings\nwarnings.simplefilter(action='ignore', category=FutureWarning)",
"_____no_output_____"
],
[
"# Utility function for standard text cleaning.\ndef text_cleaner(text):\n # Visual inspection identifies a form of punctuation spaCy does not\n # recognize: the double dash '--'. Better get rid of it now!\n text = re.sub(r'--',' ',text)\n text = re.sub(\"[\\[].*?[\\]]\", \"\", text)\n text = ' '.join(text.split())\n return text\n \n# Load and clean the data.\npersuasion = gutenberg.raw('austen-persuasion.txt')\nalice = gutenberg.raw('carroll-alice.txt')\n\n# The Chapter indicator is idiosyncratic\npersuasion = re.sub(r'Chapter \\d+', '', persuasion)\nalice = re.sub(r'CHAPTER .*', '', alice)\n \nalice = text_cleaner(alice[:int(len(alice)/10)])\npersuasion = text_cleaner(persuasion[:int(len(persuasion)/10)])",
"_____no_output_____"
],
[
"# Parse the cleaned novels. This can take a bit.\nnlp = spacy.load('en')\nalice_doc = nlp(alice)\npersuasion_doc = nlp(persuasion)",
"_____no_output_____"
],
[
"# Utility function to create a list of the 2000 most common words.\ndef bag_of_words(text):\n \n # Filter out punctuation and stop words.\n allwords = [token.lemma_\n for token in text\n if not token.is_punct\n and not token.is_stop]\n \n # Return the most common words.\n return [item[0] for item in Counter(allwords).most_common(2000)]",
"_____no_output_____"
],
[
"import collections\n# define a new bag of words approach that should get us some additional information\n# we want to look at number of words in sentence, how many times puncutation is used, how many nouns, verbs, adj, etc. length of previous sentence vs. length of next sentence\ndef bow_feature_generator(sentences, common_words, include_extra_counts=True):\n # we want to go through each sentence and count the number of occurances of verbs, nouns, adj, etc.\n # we also want to count how many words are in each text\n rows = []\n for index, row in enumerate(sentences.itertuples()):\n \n sentence = row.text_sentence\n source = row.text_source\n \n info_row = collections.defaultdict(int)\n \n for token in sentence:\n if include_extra_counts:\n part_of_speech = token.pos_\n\n if part_of_speech == \"VERB\":\n # if it is a verb, add 1 for the sentence\n info_row[\"n_verbs\"] += 1\n elif part_of_speech == \"NOUN\":\n # if it is a noun, add 1 for the noun to the sentence\n info_row[\"n_nouns\"] += 1\n\n elif part_of_speech == \"ADJ\":\n # if it is an adjective, add 1 for the adjective count for this sentence\n info_row[\"n_adjectives\"] += 1\n\n info_row[\"n_words\"] += 1\n \n \n if token.is_punct:\n info_row[\"n_puncutated_words\"] += 1\n \n if token.is_right_punct:\n info_row[\"n_right_puncutated_words\"] += 1\n \n if token.is_left_punct:\n info_row[\"n_left_puncutated_words\"] += 1\n \n # named entities\n if token.ent_type_ == \"PERSON\":\n info_row[\"n_people_mentioned\"] += 1\n \n if token.ent_type_ == \"LOC\":\n info_row[\"n_locations_metioned\"] += 1\n \n if token.ent_type_ == \"PRODUCT\":\n info_row[\"n_products_mentioned\"] += 1\n \n if not token.is_punct and not token.is_stop and token.lemma_ in common_words:\n info_row[token.lemma_] += 1\n\n \n info_row = dict(info_row)\n info_row[\"text_sentence\"] = sentence\n info_row[\"text_source\"] = source\n rows.append(info_row)\n \n if include_extra_counts:\n extra_columns = [\"n_verbs\", \"n_nouns\",\n \"n_adjectives\", \"n_words\",\n \"n_puncutated_words\", \"n_right_puncutated_words\",\n \"n_left_puncutated_words\", \"n_people_mentioned\",\n \"n_locations_metioned\", \"n_products_mentioned\"\n ]\n else:\n extra_columns = []\n \n df = pd.DataFrame(data=rows, columns=[\"text_sentence\"] + extra_columns + list(common_words) + [\"text_source\"])\n \n df = df.fillna(0)\n \n return df",
"_____no_output_____"
],
[
"# Group into sentences.\nalice_sents = [[sent, \"Carroll\"] for sent in alice_doc.sents]\npersuasion_sents = [[sent, \"Austen\"] for sent in persuasion_doc.sents]\n\n# Combine the sentences from the two novels into one data frame.\nsentences = pd.DataFrame(alice_sents + persuasion_sents)\nsentences[\"text_sentence\"] = sentences[0]\nsentences[\"text_source\"] = sentences[1]\nsentences = sentences[[\"text_sentence\", \"text_source\"]]\nsentences.head()",
"_____no_output_____"
],
[
"from sklearn.svm import SVC\nfrom sklearn.linear_model import LogisticRegression\nfrom sklearn import ensemble\nfrom sklearn.model_selection import GridSearchCV\nfrom sklearn.model_selection import train_test_split",
"_____no_output_____"
],
[
"def find_best_model(models, X_train, X_test, y_train, y_test):\n \n for model, grid_parameters in models.items():\n print(\"Running for {}\".format(str(model.__name__)))\n print(\"--------\")\n grid = GridSearchCV(model(), grid_parameters, refit=True, verbose=0)\n \n # fit\n grid.fit(X_train, y_train)\n \n # print the score, and the best parameters\n print(\"Best Score: {}\".format(grid.best_score_))\n print(\"Train Score: {}\".format(grid.score(X_train, y_train)))\n print(\"Test Score: {}\".format(grid.score(X_test, y_test)))\n print(\"Best Params: {}\".format(grid.best_params_))\n print(\"\")",
"_____no_output_____"
],
[
"# create some grid searching tools for Logistic Regression, Gradient Boosting, Random Forrest, and Support Vector Classifier\n\n# logistic regression parameters\nlr_params = {\"C\": [0.1, 1.0, 10., 100.], \"penalty\": [\"l1\", \"l2\"], \"solver\": [\"liblinear\"]}\n\n# gradient boosting possible parameters\ngb_params = {\"loss\": [\"deviance\", \"exponential\"], \"n_estimators\": [5, 10, 50, 100, 1000], \"subsample\": [0.1, 0.3, 0.6, 0.9, 1]}\n\n# random forrest parameters\nrdf_params = {\"n_estimators\": [1, 5, 10, 50, 100, 1000], \"criterion\": [\"gini\", \"entropy\"]}\n\n# support vector classifier parameters\nsvc_params = grid_parameters = {'C': [1, 10, 100, 1000], 'gamma': [1, 0.1, 0.001, 0.0001, \"scale\", \"auto\"], 'kernel': ['linear','rbf', 'sigmoid']}",
"_____no_output_____"
],
[
"models_dict = {\n LogisticRegression: lr_params,\n ensemble.GradientBoostingClassifier: gb_params,\n ensemble.RandomForestClassifier: rdf_params,\n SVC: svc_params\n}",
"_____no_output_____"
],
[
"# Set up the bags.\nalicewords = bag_of_words(alice_doc)\npersuasionwords = bag_of_words(persuasion_doc)\n\n# Combine bags to create a set of unique words.\ncommon_words = set(alicewords + persuasionwords)",
"_____no_output_____"
],
[
"data1 = bow_feature_generator(sentences, common_words, include_extra_counts=False)\ndata2 = bow_feature_generator(sentences, common_words, include_extra_counts=True)",
"_____no_output_____"
],
[
"data2.head()",
"_____no_output_____"
],
[
"features1 = data1.drop(columns=[\"text_source\", \"text_sentence\"])\ntarget1 = data1[\"text_source\"]\n\nfeatures2 = data2.drop(columns=[\"text_source\", \"text_sentence\"])\ntarget2 = data2[\"text_source\"]",
"_____no_output_____"
],
[
"X_train1, X_test1, y_train1, y_test1 = train_test_split(features1, target1, test_size=0.2, random_state=42)\nX_train2, X_test2, y_train2, y_test2 = train_test_split(features2, target2, test_size=0.2, random_state=42)",
"_____no_output_____"
],
[
"find_best_model(models_dict, X_train2, X_test2, y_train2, y_test2)",
"Running for LogisticRegression\n--------\n"
]
]
] | [
"code"
] | [
[
"code",
"code",
"code",
"code",
"code",
"code",
"code",
"code",
"code",
"code",
"code",
"code",
"code",
"code",
"code",
"code",
"code"
]
] |
e76c9b49f6be5840f008d02cedcf7434b7fdef3c | 23,483 | ipynb | Jupyter Notebook | pattern-classification/machine_learning/scikit-learn/tfidf_scikit-learn.ipynb | gopala-kr/ds-notebooks | bc35430ecdd851f2ceab8f2437eec4d77cb59423 | [
"MIT"
] | 1 | 2021-12-13T15:41:48.000Z | 2021-12-13T15:41:48.000Z | pattern-classification/machine_learning/scikit-learn/tfidf_scikit-learn.ipynb | gopala-kr/ds-notebooks | bc35430ecdd851f2ceab8f2437eec4d77cb59423 | [
"MIT"
] | 15 | 2021-09-12T15:06:13.000Z | 2022-03-31T19:02:08.000Z | pattern-classification/machine_learning/scikit-learn/tfidf_scikit-learn.ipynb | gopala-kr/ds-notebooks | bc35430ecdd851f2ceab8f2437eec4d77cb59423 | [
"MIT"
] | 1 | 2022-01-29T00:37:52.000Z | 2022-01-29T00:37:52.000Z | 22.64513 | 543 | 0.522889 | [
[
[
"%load_ext watermark\n%watermark -a 'Sebastian Raschka' -p scikit-learn,numpy,scipy -v -d",
"Sebastian Raschka 23/05/2015 \n\nCPython 3.4.3\nIPython 3.1.0\n\nscikit-learn 0.16.1\nnumpy 1.9.2\nscipy 0.15.1\n"
]
],
[
[
"<br>\n<br>",
"_____no_output_____"
],
[
"#Tf-idf Walkthrough for scikit-learn",
"_____no_output_____"
],
[
"When I was using scikit-learn extremely handy `TfidfVectorizer` I had some trouble interpreting the results since they seem to be a little bit different from the standard convention of how the *term frequency-inverse document frequency* (tf-idf) is calculated. Here, I just put together a brief overview of how the `TfidfVectorizer` works -- mainly as personal reference sheet, but maybe it is useful to one or the other.",
"_____no_output_____"
],
[
"<hr>\n### Sections\n- [What are Tf-idfs all about?](What-are-Tf-idfs-all-about?)\n- [Example data](#Example-data)\n- [Raw term frequency](#Raw-term-frequency)\n- [Normalized term frequency](#Normalized-term-frequency)\n- [Term frequency-inverse document frequency -- tf-idf](#Term-frequency-inverse-document-frequency----tf-idf)\n- [Inverse document frequency](#Inverse-document-frequency)\n- [Normalized tf-idf](#Normalized-tf-idf)\n- [Smooth idf](#Smooth-idf)\n- [Tf-idf in scikit-learn](#Tf-idf-in-scikit-learn)\n- [TfidfVectorizer defaults](#TfidfVectorizer-defaults)\n\n<hr>",
"_____no_output_____"
],
[
"<br>\n<br>",
"_____no_output_____"
],
[
"## What are Tf-idfs all about?",
"_____no_output_____"
],
[
"[[back to top](#Sections)]",
"_____no_output_____"
],
[
"Tf-idfs are a way to represent documents as feature vectors. Tf-idfs can be understood as a modification of the *raw term frequencies* (tf); the tf is the count of how often a particular word occurs in a given document. The concept behind the tf-idf is to downweight terms proportionally to the number of documents in which they occur. Here, the idea is that terms that occur in many different documents are likely unimportant or don't contain any useful information for Natural Language Processing tasks such as document classification.",
"_____no_output_____"
],
[
"<br>\n<br>",
"_____no_output_____"
],
[
"## Example data",
"_____no_output_____"
],
[
"[[back to top](#Sections)]",
"_____no_output_____"
],
[
"For the following sections, let us consider the following dataset that consists of 3 documents:",
"_____no_output_____"
]
],
[
[
"import numpy as np\ndocs = np.array([\n 'The sun is shining',\n 'The weather is sweet',\n 'The sun is shining and the weather is sweet'])",
"_____no_output_____"
]
],
[
[
"<br>\n<br>",
"_____no_output_____"
],
[
"## Raw term frequency",
"_____no_output_____"
],
[
"[[back to top](#Sections)]",
"_____no_output_____"
],
[
"First, we will start with the *raw term frequency* tf(t, d), which is defined by the number of times a term *t* occurs in a document *t*\n\n<hr>\nAlternative term frequency definitions include the binary term frequency, log-scaled term frequency, and augmented term frequency [[1](#References)].\n<hr>",
"_____no_output_____"
],
[
"Using the [`CountVectorizer`](http://scikit-learn.org/stable/modules/generated/sklearn.feature_extraction.text.CountVectorizer.html) from scikit-learn, we can construct a bag-of-words model with the term frequencies as follows:",
"_____no_output_____"
]
],
[
[
"from sklearn.feature_extraction.text import CountVectorizer\ncv = CountVectorizer()\ntf = cv.fit_transform(docs).toarray()\ntf",
"_____no_output_____"
],
[
"cv.vocabulary_",
"_____no_output_____"
]
],
[
[
"Based on the vocabulary, the word \"and\" would be the 1st feature in each document vector in `tf`, the word \"is\" the 2nd, the word \"shining\" the 3rd, etc.",
"_____no_output_____"
],
[
"<br>\n<br>",
"_____no_output_____"
],
[
"## Normalized term frequency",
"_____no_output_____"
],
[
"[[back to top](#Sections)]",
"_____no_output_____"
],
[
"In this section, we will take a brief look at how the tf-feature vector can be normalized, which will be useful later.",
"_____no_output_____"
],
[
"The most common way to normalize the raw term frequency is l2-normalization, i.e., dividing the raw term frequency vector $v$ by its length $||v||_2$ (L2- or Euclidean norm).\n\n$$v_{norm} = \\frac{v}{||v||_2} = \\frac{v}{\\sqrt{v{_1}^2 + v{_2}^2 + \\dots + v{_n}^2}} = \\frac{v}{\\big(\\sum_{i=1}^n v_i \\big)^{\\frac{1}{2}}}$$",
"_____no_output_____"
],
[
"For example, we would normalize our 3rd document `'The sun is shining and the weather is sweet'` as follows:",
"_____no_output_____"
],
[
"$\\frac{[1, 2, 1, 1, 1, 2, 1]}{2} = [ 0.2773501, 0.5547002, 0.2773501, 0.2773501, 0.2773501,\n 0.5547002, 0.2773501]$",
"_____no_output_____"
]
],
[
[
"tf_norm = tf[2] / np.sqrt(np.sum(tf[2]**2))\ntf_norm",
"_____no_output_____"
]
],
[
[
"In scikit-learn, we can use the [`TfidfTransformer`](http://scikit-learn.org/stable/modules/generated/sklearn.feature_extraction.text.TfidfTransformer.html) to normalize the term frequencies if we disable the inverse document frequency calculation (`use_idf: False` and `smooth_idf=False`):",
"_____no_output_____"
]
],
[
[
"from sklearn.feature_extraction.text import TfidfTransformer\ntfidf = TfidfTransformer(use_idf=False, norm='l2', smooth_idf=False)\ntf_norm = tfidf.fit_transform(tf).toarray()\nprint('Normalized term frequencies of document 3:\\n %s' % tf_norm[-1])",
"Normalized term frequencies of document 3:\n [ 0.2773501 0.5547002 0.2773501 0.2773501 0.2773501 0.5547002\n 0.2773501]\n"
]
],
[
[
"<br>\n<br>",
"_____no_output_____"
],
[
"## Term frequency-inverse document frequency -- tf-idf",
"_____no_output_____"
],
[
"[[back to top](#Sections)]",
"_____no_output_____"
],
[
"Most commonly, the term frequency-inverse document frequency (tf-idf) is calculated as follows [[1](#References)]:",
"_____no_output_____"
],
[
"$$\\text{tf-idf}(t, d) = \\text{tf}(t, d) \\times \\text{idf}(t),$$\nwhere idf is the inverse document frequency, which we will introduce in the next section.",
"_____no_output_____"
],
[
"<br>\n<br>",
"_____no_output_____"
],
[
"## Inverse document frequency",
"_____no_output_____"
],
[
"[[back to top](#Sections)]",
"_____no_output_____"
],
[
"In order to understand the *inverse document frequency* idf, let us first introduce the term *document frequency* $\\text{df}(d,t)$, which simply the number of documents $d$ that contain the term $t$. We can then define the idf as follows:",
"_____no_output_____"
],
[
"$$\\text{idf}(t) = log{\\frac{n_d}{1+\\text{df}(d,t)}},$$ \nwhere \n$n_d$: The total number of documents \n$\\text{df}(d,t)$: The number of documents that contain term $t$.\n\nNote that the constant 1 is added to the denominator to avoid a zero-division error if a term is not contained in any document in the test dataset.",
"_____no_output_____"
],
[
"Now, Let us calculate the idfs of the words \"and\", \"is,\" and \"shining:\"",
"_____no_output_____"
]
],
[
[
"n_docs = len(docs)\n\ndf_and = 1\nidf_and = np.log(n_docs / (1 + df_and))\nprint('idf \"and\": %s' % idf_and)\n\ndf_is = 3\nidf_is = np.log(n_docs / (1 + df_is))\nprint('idf \"is\": %s' % idf_is)\n\ndf_shining = 2\nidf_shining = np.log(n_docs / (1 + df_shining))\nprint('idf \"shining\": %s' % idf_shining)",
"idf \"and\": 0.405465108108\nidf \"is\": -0.287682072452\nidf \"shining\": 0.0\n"
]
],
[
[
"Using those idfs, we can eventually calculate the tf-idfs for the 3rd document:",
"_____no_output_____"
]
],
[
[
"print('Tf-idfs in document 3:\\n')\nprint('tf-idf \"and\": %s' % (1 * idf_and))\nprint('tf-idf \"is\": %s' % (2 * idf_is))\nprint('tf-idf \"shining\": %s' % (1 * idf_shining))",
"Tf-idfs in document 3:\n\ntf-idf \"and\": 0.405465108108\ntf-idf \"is\": -0.575364144904\ntf-idf \"shining\": 0.0\n"
]
],
[
[
"<br>\n<br>",
"_____no_output_____"
],
[
"## Tf-idf in scikit-learn",
"_____no_output_____"
],
[
"[[back to top](#Sections)]",
"_____no_output_____"
],
[
"The tf-idfs in scikit-learn are calculated a little bit differently. Here, the `+1` count is added to the idf, whereas instead of the denominator if the df:",
"_____no_output_____"
],
[
"$$\\text{idf}(t) = log{\\frac{n_d}{\\text{df}(d,t)}} + 1$$ ",
"_____no_output_____"
],
[
"We can demonstrate this by calculating the idfs manually using the equation above and comparing the results to the TfidfTransformer output using the settings `use_idf=True, smooth_idf=False, norm=None`.\nIn the following examples, we will be again using the words \"and,\" \"is,\" and \"shining:\" from document 3.",
"_____no_output_____"
]
],
[
[
"tf_and = 1 \ndf_and = 1 \ntf_and * (np.log(n_docs / df_and) + 1)",
"_____no_output_____"
],
[
"tf_shining = 2 \ndf_shining = 3 \ntf_shining * (np.log(n_docs / df_shining) + 1)",
"_____no_output_____"
],
[
"tf_is = 1 \ndf_is = 2 \ntf_is * (np.log(n_docs / df_is) + 1)",
"_____no_output_____"
],
[
"tfidf = TfidfTransformer(use_idf=True, smooth_idf=False, norm=None)\ntfidf.fit_transform(tf).toarray()[-1][0:3]",
"_____no_output_____"
]
],
[
[
"<br>\n<br>",
"_____no_output_____"
],
[
"## Normalized tf-idf",
"_____no_output_____"
],
[
"[[back to top](#Sections)]",
"_____no_output_____"
],
[
"Now, let us calculate the normalized tf-idfs. Our feature vector of un-normalized tf-idfs for document 3 would look as follows if we'd applied the equation from the previous section to all words in the document:",
"_____no_output_____"
]
],
[
[
"tf_idfs_d3 = np.array([[ 2.09861229, 2.0, 1.40546511, 1.40546511, 1.40546511, 2.0, 1.40546511]])",
"_____no_output_____"
]
],
[
[
"Using the l2-norm, we then normalize the tf-idfs as follows:",
"_____no_output_____"
]
],
[
[
"tf_idfs_d3_norm = tf_idfs_d3[-1] / np.sqrt(np.sum(tf_idfs_d3[-1]**2))\ntf_idfs_d3_norm",
"_____no_output_____"
]
],
[
[
"And finally, we compare the results to the results that the `TfidfTransformer` returns.",
"_____no_output_____"
]
],
[
[
"tfidf = TfidfTransformer(use_idf=True, smooth_idf=False, norm='l2')\ntfidf.fit_transform(tf).toarray()[-1]",
"_____no_output_____"
]
],
[
[
"<br>\n<br>",
"_____no_output_____"
],
[
"## Smooth idf",
"_____no_output_____"
],
[
"[[back to top](#Sections)]",
"_____no_output_____"
],
[
"Another parameter in the `TfidfTransformer` is the `smooth_idf`, which is described as\n> smooth_idf : boolean, default=True \nSmooth idf weights by adding one to document frequencies, as if an extra document was seen containing every term in the collection exactly once. Prevents zero divisions.",
"_____no_output_____"
],
[
"So, our idf would then be defined as follows:",
"_____no_output_____"
],
[
"$$\\text{idf}(t) = log{\\frac{1 + n_d}{1+\\text{df}(d,t)}} + 1$$ ",
"_____no_output_____"
],
[
"To confirm that we understand the `smooth_idf` parameter correctly, let us walk through the 3-word example again:",
"_____no_output_____"
]
],
[
[
"tfidf = TfidfTransformer(use_idf=True, smooth_idf=True, norm=None)\ntfidf.fit_transform(tf).toarray()[-1][:3]",
"_____no_output_____"
],
[
"tf_and = 1 \ndf_and = 1 \ntf_and * (np.log((n_docs+1) / (df_and+1)) + 1) ",
"_____no_output_____"
],
[
"tf_is = 2\ndf_is = 3 \ntf_is * (np.log((n_docs+1) / (df_is+1)) + 1) ",
"_____no_output_____"
],
[
"tf_shining = 1 \ndf_shining = 2\ntf_shining * (np.log((n_docs+1) / (df_shining+1)) + 1) ",
"_____no_output_____"
]
],
[
[
"<br>\n<br>",
"_____no_output_____"
],
[
"#TfidfVectorizer defaults",
"_____no_output_____"
],
[
"[[back to top](#Sections)]",
"_____no_output_____"
],
[
"Finally, we now understand the default settings in the `TfidfTransformer`, which are:",
"_____no_output_____"
],
[
"- `use_idf=True`\n- `smooth_idf=True`\n- `norm='l2'`",
"_____no_output_____"
],
[
"And the equation can be summarized as \n$\\text{tf-idf} = \\text{tf}(t) \\times (\\text{idf}(t, d) + 1),$ \nwhere \n$\\text{idf}(t) = log{\\frac{1 + n_d}{1+\\text{df}(d,t)}}.$",
"_____no_output_____"
]
],
[
[
"tfidf = TfidfTransformer()\ntfidf.fit_transform(tf).toarray()[-1]",
"_____no_output_____"
],
[
"smooth_tfidfs_d3 = np.array([[ 1.69314718, 2.0, 1.28768207, 1.28768207, 1.28768207, 2.0, 1.28768207]])\nsmooth_tfidfs_d3_norm = smooth_tfidfs_d3[-1] / np.sqrt(np.sum(smooth_tfidfs_d3[-1]**2))\nsmooth_tfidfs_d3_norm",
"_____no_output_____"
]
],
[
[
"<br>\n<br>",
"_____no_output_____"
],
[
"### References",
"_____no_output_____"
],
[
"[[back to top](#Sections)]",
"_____no_output_____"
],
[
"[1] G. Salton and M. J. McGill. Introduction to modern information retrieval. 1983.",
"_____no_output_____"
]
]
] | [
"code",
"markdown",
"code",
"markdown",
"code",
"markdown",
"code",
"markdown",
"code",
"markdown",
"code",
"markdown",
"code",
"markdown",
"code",
"markdown",
"code",
"markdown",
"code",
"markdown",
"code",
"markdown",
"code",
"markdown",
"code",
"markdown"
] | [
[
"code"
],
[
"markdown",
"markdown",
"markdown",
"markdown",
"markdown",
"markdown",
"markdown",
"markdown",
"markdown",
"markdown",
"markdown",
"markdown"
],
[
"code"
],
[
"markdown",
"markdown",
"markdown",
"markdown",
"markdown"
],
[
"code",
"code"
],
[
"markdown",
"markdown",
"markdown",
"markdown",
"markdown",
"markdown",
"markdown",
"markdown"
],
[
"code"
],
[
"markdown"
],
[
"code"
],
[
"markdown",
"markdown",
"markdown",
"markdown",
"markdown",
"markdown",
"markdown",
"markdown",
"markdown",
"markdown",
"markdown"
],
[
"code"
],
[
"markdown"
],
[
"code"
],
[
"markdown",
"markdown",
"markdown",
"markdown",
"markdown",
"markdown"
],
[
"code",
"code",
"code",
"code"
],
[
"markdown",
"markdown",
"markdown",
"markdown"
],
[
"code"
],
[
"markdown"
],
[
"code"
],
[
"markdown"
],
[
"code"
],
[
"markdown",
"markdown",
"markdown",
"markdown",
"markdown",
"markdown",
"markdown"
],
[
"code",
"code",
"code",
"code"
],
[
"markdown",
"markdown",
"markdown",
"markdown",
"markdown",
"markdown"
],
[
"code",
"code"
],
[
"markdown",
"markdown",
"markdown",
"markdown"
]
] |
e76ca072e162f92a8652ae37c0ae609c9147473d | 443,546 | ipynb | Jupyter Notebook | examples/benchmark.ipynb | bogdan-kulynych/trials | 1611f4572e7be341c52a4c34e1c785e51b7070b3 | [
"MIT"
] | 29 | 2015-02-02T21:44:37.000Z | 2021-09-08T04:49:44.000Z | examples/benchmark.ipynb | bogdan-kulynych/trials | 1611f4572e7be341c52a4c34e1c785e51b7070b3 | [
"MIT"
] | null | null | null | examples/benchmark.ipynb | bogdan-kulynych/trials | 1611f4572e7be341c52a4c34e1c785e51b7070b3 | [
"MIT"
] | 18 | 2015-02-07T16:50:27.000Z | 2019-09-15T16:42:01.000Z | 1,692.923664 | 213,955 | 0.94489 | [
[
[
"empty"
]
]
] | [
"empty"
] | [
[
"empty"
]
] |
e76ca658592a04b071c43ea95a7845cdeeb0ccb8 | 17,281 | ipynb | Jupyter Notebook | notebooks/beamblockage/wradlib_beamblock.ipynb | kmuehlbauer/wradlib-notebooks | 11f46c3f2130582fa5c46908bac3820a7f48d294 | [
"MIT"
] | null | null | null | notebooks/beamblockage/wradlib_beamblock.ipynb | kmuehlbauer/wradlib-notebooks | 11f46c3f2130582fa5c46908bac3820a7f48d294 | [
"MIT"
] | null | null | null | notebooks/beamblockage/wradlib_beamblock.ipynb | kmuehlbauer/wradlib-notebooks | 11f46c3f2130582fa5c46908bac3820a7f48d294 | [
"MIT"
] | 1 | 2020-06-07T21:25:47.000Z | 2020-06-07T21:25:47.000Z | 28.70598 | 335 | 0.54152 | [
[
[
"This notebook is part of the $\\omega radlib$ documentation: http://wradlib.org/wradlib-docs.\n\nCopyright (c) 2018, $\\omega radlib$ developers.\nDistributed under the MIT License. See LICENSE.txt for more info.",
"_____no_output_____"
],
[
"# Beam Blockage Calculation using a DEM",
"_____no_output_____"
],
[
"Here, we derive (**p**artial) **b**eam-**b**lockage (**PBB**) from a **D**igital **E**levation **M**odel (**DEM**). ",
"_____no_output_____"
],
[
"We require\n- the local radar setup (sitecoords, number of rays, number of bins, antenna elevation, beamwidth, and the range resolution);\n- a **DEM** with a adequate resolution. \n\nHere we use pre-processed data from the [GTOPO30](https://lta.cr.usgs.gov/GTOPO30) and [SRTM](http://www2.jpl.nasa.gov/srtm) missions.",
"_____no_output_____"
]
],
[
[
"import wradlib as wrl\nimport matplotlib.pyplot as pl\nimport matplotlib as mpl\nimport warnings\nwarnings.filterwarnings('ignore')\ntry:\n get_ipython().magic(\"matplotlib inline\")\nexcept:\n pl.ion()\nimport numpy as np",
"_____no_output_____"
]
],
[
[
"## Setup for Bonn radar",
"_____no_output_____"
],
[
"First, we need to define some radar specifications (here: *University of Bonn*).",
"_____no_output_____"
]
],
[
[
"sitecoords = (7.071663, 50.73052, 99.5)\nnrays = 360 # number of rays\nnbins = 1000 # number of range bins\nel = 1.0 # vertical antenna pointing angle (deg)\nbw = 1.0 # half power beam width (deg)\nrange_res = 100. # range resolution (meters)",
"_____no_output_____"
]
],
[
[
"Create the range, azimuth, and beamradius arrays.",
"_____no_output_____"
]
],
[
[
"r = np.arange(nbins) * range_res\nbeamradius = wrl.util.half_power_radius(r, bw)",
"_____no_output_____"
]
],
[
[
"We use \n\n- [wradlib.georef.sweep_centroids](http://wradlib.org/wradlib-docs/latest/generated/wradlib.georef.sweep_centroids.html) and \n- [wradlib.georef.spherical_to_proj](http://wradlib.org/wradlib-docs/latest/generated/wradlib.georef.spherical_to_proj.html) \n\nto calculate the spherical coordinates of the bin centroids and their longitude, latitude and altitude.",
"_____no_output_____"
]
],
[
[
"coord = wrl.georef.sweep_centroids(nrays, range_res, nbins, el)\ncoords = wrl.georef.spherical_to_proj(coord[..., 0], \n np.degrees(coord[..., 1]),\n coord[..., 2], sitecoords)\nlon = coords[..., 0]\nlat = coords[..., 1]\nalt = coords[..., 2]",
"_____no_output_____"
],
[
"polcoords = coords[..., :2]\nprint(\"lon,lat,alt:\", coords.shape)",
"_____no_output_____"
],
[
"rlimits = (lon.min(), lat.min(), lon.max(), lat.max())\nprint(\"Radar bounding box:\\n\\t%.2f\\n%.2f %.2f\\n\\t%.2f\" % \n (lat.max(), lon.min(), lon.max(), lat.min()))",
"_____no_output_____"
]
],
[
[
"## Preprocessing the digitial elevation model",
"_____no_output_____"
],
[
"- Read the DEM from a ``geotiff`` file (in `WRADLIB_DATA`);\n- clip the region inside the bounding box;\n- map the DEM values to the polar grid points. \n\n*Note*: You can choose between the coarser resolution `bonn_gtopo.tif` (from GTOPO30) and the finer resolution `bonn_new.tif` (from the SRTM mission).\n\nThe DEM raster data is opened via [wradlib.io.open_raster](http://wradlib.org/wradlib-docs/latest/generated/wradlib.io.open_raster.html) and extracted via [wradlib.georef.extract_raster_dataset](http://wradlib.org/wradlib-docs/latest/generated/wradlib.georef.extract_raster_dataset.html).",
"_____no_output_____"
]
],
[
[
"#rasterfile = wrl.util.get_wradlib_data_file('geo/bonn_gtopo.tif')\nrasterfile = wrl.util.get_wradlib_data_file('geo/bonn_new.tif')\n\nds = wrl.io.open_raster(rasterfile)\nrastervalues, rastercoords, proj = wrl.georef.extract_raster_dataset(ds, nodata=-32768.)\n\n# Clip the region inside our bounding box \nind = wrl.util.find_bbox_indices(rastercoords, rlimits)\nrastercoords = rastercoords[ind[1]:ind[3], ind[0]:ind[2], ...]\nrastervalues = rastervalues[ind[1]:ind[3], ind[0]:ind[2]]\n\n# Map rastervalues to polar grid points\npolarvalues = wrl.ipol.cart2irregular_spline(rastercoords, rastervalues,\n polcoords, order=3,\n prefilter=False)",
"_____no_output_____"
]
],
[
[
"## Calculate Beam-Blockage",
"_____no_output_____"
],
[
"Now we can finally apply the [wradlib.qual.beam_block_frac](http://wradlib.org/wradlib-docs/latest/generated/wradlib.qual.beam_block_frac.html) function to calculate the PBB.",
"_____no_output_____"
]
],
[
[
"PBB = wrl.qual.beam_block_frac(polarvalues, alt, beamradius)\nPBB = np.ma.masked_invalid(PBB)\nprint(PBB.shape)",
"_____no_output_____"
]
],
[
[
"So far, we calculated the fraction of beam blockage for each bin.\n\nBut we need to into account that the radar signal travels along a beam. Cumulative beam blockage (CBB) in one bin along a beam will always be at least as high as the maximum PBB of the preceeding bins (see [wradlib.qual.cum_beam_block_frac](http://wradlib.org/wradlib-docs/latest/generated/wradlib.qual.cum_beam_block_frac.html))",
"_____no_output_____"
]
],
[
[
"CBB = wrl.qual.cum_beam_block_frac(PBB)\nprint(CBB.shape)",
"_____no_output_____"
]
],
[
[
"## Visualize Beamblockage",
"_____no_output_____"
],
[
"Now we visualize\n- the average terrain altitude per radar bin\n- a beam blockage map\n- interaction with terrain along a single beam",
"_____no_output_____"
]
],
[
[
"# just a little helper function to style x and y axes of our maps\ndef annotate_map(ax, cm=None, title=\"\"):\n ticks = (ax.get_xticks()/1000).astype(np.int)\n ax.set_xticklabels(ticks)\n ticks = (ax.get_yticks()/1000).astype(np.int)\n ax.set_yticklabels(ticks)\n ax.set_xlabel(\"Kilometers\")\n ax.set_ylabel(\"Kilometers\")\n if not cm is None:\n pl.colorbar(cm, ax=ax)\n if not title==\"\":\n ax.set_title(title)\n ax.grid()",
"_____no_output_____"
],
[
"fig = pl.figure(figsize=(10, 8))\n\n# create subplots\nax1 = pl.subplot2grid((2, 2), (0, 0))\nax2 = pl.subplot2grid((2, 2), (0, 1))\nax3 = pl.subplot2grid((2, 2), (1, 0), colspan=2, rowspan=1)\n\n# azimuth angle\nangle = 225\n\n# Plot terrain (on ax1)\nax1, dem = wrl.vis.plot_ppi(polarvalues, \n ax=ax1, r=r, \n az=np.degrees(coord[:,0,1]), \n cmap=mpl.cm.terrain, vmin=0.)\nax1.plot([0,np.sin(np.radians(angle))*1e5],\n [0,np.cos(np.radians(angle))*1e5],\"r-\")\nax1.plot(sitecoords[0], sitecoords[1], 'ro')\nannotate_map(ax1, dem, 'Terrain within {0} km range'.format(np.max(r / 1000.) + 0.1))\n\n# Plot CBB (on ax2)\nax2, cbb = wrl.vis.plot_ppi(CBB, ax=ax2, r=r, \n az=np.degrees(coord[:,0,1]),\n cmap=mpl.cm.PuRd, vmin=0, vmax=1)\nannotate_map(ax2, cbb, 'Beam-Blockage Fraction')\n\n# Plot single ray terrain profile on ax3\nbc, = ax3.plot(r / 1000., alt[angle, :], '-b',\n linewidth=3, label='Beam Center')\nb3db, = ax3.plot(r / 1000., (alt[angle, :] + beamradius), ':b',\n linewidth=1.5, label='3 dB Beam width')\nax3.plot(r / 1000., (alt[angle, :] - beamradius), ':b')\nax3.fill_between(r / 1000., 0.,\n polarvalues[angle, :],\n color='0.75')\nax3.set_xlim(0., np.max(r / 1000.) + 0.1)\nax3.set_ylim(0., 3000)\nax3.set_xlabel('Range (km)')\nax3.set_ylabel('Altitude (m)')\nax3.grid()\n\naxb = ax3.twinx()\nbbf, = axb.plot(r / 1000., CBB[angle, :], '-k',\n label='BBF')\naxb.set_ylabel('Beam-blockage fraction')\naxb.set_ylim(0., 1.)\naxb.set_xlim(0., np.max(r / 1000.) + 0.1)\n\n\nlegend = ax3.legend((bc, b3db, bbf), \n ('Beam Center', '3 dB Beam width', 'BBF'),\n loc='upper left', fontsize=10)",
"_____no_output_____"
]
],
[
[
"## Visualize Beam Propagation showing earth curvature",
"_____no_output_____"
],
[
"Now we visualize\n- interaction with terrain along a single beam\n\nIn this representation the earth curvature is shown. For this we assume the earth a sphere with exactly 6370000 m radius. This is needed to get the height ticks at nice position.",
"_____no_output_____"
]
],
[
[
"def height_formatter(x, pos):\n x = (x - 6370000) / 1000\n fmt_str = '{:g}'.format(x)\n return fmt_str\n \ndef range_formatter(x, pos):\n x = x / 1000.\n fmt_str = '{:g}'.format(x)\n return fmt_str",
"_____no_output_____"
]
],
[
[
"- The [wradlib.vis.create_cg](http://wradlib.org/wradlib-docs/latest/generated/wradlib.vis.create_cg.html)-function is facilitated to create the curved geometries. \n- The actual data is plottet as (theta, range) on the parasite axis. \n- Some tweaking is needed to get the final plot look nice.",
"_____no_output_____"
]
],
[
[
"fig = pl.figure(figsize=(10, 6))\n\ncgax, caax, paax = wrl.vis.create_cg('RHI', fig, 111)\n\n# azimuth angle\nangle = 225\n\n# fix grid_helper\ner = 6370000\ngh = cgax.get_grid_helper()\ngh.grid_finder.grid_locator2._nbins=80\ngh.grid_finder.grid_locator2._steps=[1,2,4,5,10]\n\n# calculate beam_height and arc_distance for ke=1\n# means line of sight\nbhe = wrl.georef.bin_altitude(r, 0, sitecoords[2], re=er, ke=1.)\nade = wrl.georef.bin_distance(r, 0, sitecoords[2], re=er, ke=1.)\nnn0 = np.zeros_like(r)\n# for nice plotting we assume earth_radius = 6370000 m\necp = nn0 + er\n# theta (arc_distance sector angle)\nthetap = - np.degrees(ade/er) + 90.0\n\n# zero degree elevation with standard refraction\nbh0 = wrl.georef.bin_altitude(r, 0, sitecoords[2], re=er)\n\n# plot (ecp is earth surface normal null)\nbes, = paax.plot(thetap, ecp, '-k', linewidth=3, label='Earth Surface NN')\nbc, = paax.plot(thetap, ecp + alt[angle, :], '-b', linewidth=3, label='Beam Center')\nbc0r, = paax.plot(thetap, ecp + bh0 + alt[angle, 0] , '-g', label='0 deg Refraction')\nbc0n, = paax.plot(thetap, ecp + bhe + alt[angle, 0], '-r', label='0 deg line of sight')\nb3db, = paax.plot(thetap, ecp + alt[angle, :] + beamradius, ':b', label='+3 dB Beam width')\npaax.plot(thetap, ecp + alt[angle, :] - beamradius, ':b', label='-3 dB Beam width')\n\n# orography\npaax.fill_between(thetap, ecp,\n ecp + polarvalues[angle, :],\n color='0.75')\n\n# shape axes\ncgax.set_xlim(0, np.max(ade))\ncgax.set_ylim([ecp.min()-1000, ecp.max()+2500])\ncaax.grid(True, axis='x')\ncgax.grid(True, axis='y')\ncgax.axis['top'].toggle(all=False)\ncaax.yaxis.set_major_locator(mpl.ticker.MaxNLocator(steps=[1,2,4,5,10], nbins=20, prune='both'))\ncaax.xaxis.set_major_locator(mpl.ticker.MaxNLocator())\ncaax.yaxis.set_major_formatter(mpl.ticker.FuncFormatter(height_formatter))\ncaax.xaxis.set_major_formatter(mpl.ticker.FuncFormatter(range_formatter))\n\ncaax.set_xlabel('Range (km)')\ncaax.set_ylabel('Altitude (km)')\n \nlegend = paax.legend((bes, bc0n, bc0r, bc, b3db), \n ('Earth Surface NN', '0 deg line of sight', '0 deg std refraction', 'Beam Center', '3 dB Beam width'),\n loc='upper left', fontsize=10)",
"_____no_output_____"
]
],
[
[
"Go back to [Read DEM Raster Data](#Read-DEM-Raster-Data), change the rasterfile to use the other resolution DEM and process again.",
"_____no_output_____"
]
]
] | [
"markdown",
"code",
"markdown",
"code",
"markdown",
"code",
"markdown",
"code",
"markdown",
"code",
"markdown",
"code",
"markdown",
"code",
"markdown",
"code",
"markdown",
"code",
"markdown",
"code",
"markdown"
] | [
[
"markdown",
"markdown",
"markdown",
"markdown"
],
[
"code"
],
[
"markdown",
"markdown"
],
[
"code"
],
[
"markdown"
],
[
"code"
],
[
"markdown"
],
[
"code",
"code",
"code"
],
[
"markdown",
"markdown"
],
[
"code"
],
[
"markdown",
"markdown"
],
[
"code"
],
[
"markdown"
],
[
"code"
],
[
"markdown",
"markdown"
],
[
"code",
"code"
],
[
"markdown",
"markdown"
],
[
"code"
],
[
"markdown"
],
[
"code"
],
[
"markdown"
]
] |
e76ca912dfb11b6adb3c0cdde6d345458e785619 | 12,052 | ipynb | Jupyter Notebook | key_features/ptn_4.2_serverless-inference/serverless_endpoint_kornlp_nsmc.ipynb | aws-samples/sm-model-serving-patterns | b2c086a1ad512c05ed4195e9a9cde3e7a595bd39 | [
"MIT"
] | 7 | 2022-01-28T04:52:30.000Z | 2022-03-30T01:44:22.000Z | key_features/ptn_4.2_serverless-inference/serverless_endpoint_kornlp_nsmc.ipynb | aws-samples/sm-model-serving-patterns | b2c086a1ad512c05ed4195e9a9cde3e7a595bd39 | [
"MIT"
] | null | null | null | key_features/ptn_4.2_serverless-inference/serverless_endpoint_kornlp_nsmc.ipynb | aws-samples/sm-model-serving-patterns | b2c086a1ad512c05ed4195e9a9cde3e7a595bd39 | [
"MIT"
] | null | null | null | 29.611794 | 320 | 0.568204 | [
[
[
"# Deploy SageMaker Serverless Endpoint\n\n## Sentiment Binary Classification (fine-tuning with KoELECTRA-Small-v3 model and Naver Sentiment Movie Corpus dataset)\n\n- KoELECTRA: https://github.com/monologg/KoELECTRA\n- Naver Sentiment Movie Corpus Dataset: https://github.com/e9t/nsmc\n\n---\n\n## Overview\n\nAmazon SageMaker Serverless Inference๋ re:Invent 2021์ ๋ฐ์นญ๋ ์ ๊ท ์ถ๋ก ์ต์
์ผ๋ก ํธ์คํ
์ธํ๋ผ ๊ด๋ฆฌ์ ๋ํ ๋ถ๋ด ์์ด ๋จธ์ ๋ฌ๋์ ๋ชจ๋ธ์ ์ฝ๊ฒ ๋ฐฐํฌํ๊ณ ํ์ฅํ ์ ์๋๋ก ์ ์๋ ์ ๊ท ์ถ๋ก ์ต์
์
๋๋ค. SageMaker Serverless Inference๋ ์ปดํจํ
๋ฆฌ์์ค๋ฅผ ์๋์ผ๋ก ์์ํ๊ณ ํธ๋ํฝ์ ๋ฐ๋ผ ์๋์ผ๋ก ์ค์ผ์ผ ์ธ/์์์ ์ํํ๋ฏ๋ก ์ธ์คํด์ค ์ ํ์ ์ ํํ๊ฑฐ๋ ์ค์ผ์ผ๋ง ์ ์ฑ
์ ๊ด๋ฆฌํ ํ์๊ฐ ์์ต๋๋ค. ๋ฐ๋ผ์, ํธ๋ํฝ ๊ธ์ฆ ์ฌ์ด์ ์ ํด ๊ธฐ๊ฐ์ด ์๊ณ ์ฝ๋ ์คํํธ๋ฅผ ํ์ฉํ ์ ์๋ ์ํฌ๋ก๋์ ์ด์์ ์
๋๋ค.\n\n## Difference from Lambda Serverless Inference\n\n\n### Lambda Serverless Inference\n\n- Lambda ์ปจํ
์ด๋์ฉ ๋์ปค ์ด๋ฏธ์ง ๋น๋/๋๋ฒ๊ทธ ํ Amazon ECR(Amazon Elastic Container Registry)์ ํธ์\n- Option 1: Lambda ํจ์๋ฅผ ์์ฑํ์ฌ ์ง์ ๋ชจ๋ธ ๋ฐฐํฌ ์ํ\n- Option 2: SageMaker API๋ก SageMaker์์ ๋ชจ๋ธ ๋ฐฐํฌ ์ํ (`LambdaModel` ๋ฐ `LambdaPredictor` ๋ฆฌ์์ค๋ฅผ ์์ฐจ์ ์ผ๋ก ์์ฑ) ๋จ, Option 2๋ฅผ ์ฌ์ฉํ๋ ๊ฒฝ์ฐ ์ ์ ํ ๊ถํ์ ์ง์ ์ค์ ํด ์ค์ผ ํฉ๋๋ค.\n - SageMaker๊ณผ ์ฐ๊ฒฐ๋ role ๋ํด ECR ์ต์ธ์ค๋ฅผ ํ์ฉํ๋ policy ์์ฑ ๋ฐ ์ฐ๊ฒฐ\n - SageMaker ๋
ธํธ๋ถ์์ lambda๋ฅผ ์คํํ ์ ์๋ role ์์ฑ\n - Lambda ํจ์๊ฐ ECR private ๋ฆฌํฌ์งํ ๋ฆฌ์ ์ฐ๊ฒฐํ๋ ์ต์ธ์ค๋ฅผ ํ์ฉํ๋ policy ์์ฑ ๋ฐ ์ฐ๊ฒฐ \n\n\n### SageMaker Serverless Inference\n\n๊ธฐ์กด Endpoint ๋ฐฐํฌ ์ฝ๋์์ Endpoint ์ค์ ๋ง ๋ณ๊ฒฝํด ์ฃผ์๋ฉด ๋๋ฉฐ, ๋ณ๋์ ๋์ปค ์ด๋ฏธ์ง ๋น๋๊ฐ ํ์ ์๊ธฐ์ ์ฝ๊ณ ๋น ๋ฅด๊ฒ ์๋ฒ๋ฆฌ์ค ์ถ๋ก ์ ์ํํ ์ ์์ต๋๋ค.\n\n**์ฃผ์**\n- ํ์ฌ ์์ธ ๋ฆฌ์ ์ ์ง์ํ์ง ์๊ธฐ ๋๋ฌธ์ ์๋ ๋ฆฌ์ ์ค ํ๋๋ฅผ ์ ํํด์ ์ํํ์
์ผ ํฉ๋๋ค.\n - ํ์ฌ ์ง์ํ๋ ๋ฆฌ์ : US East (Northern Virginia), US East (Ohio), US West (Oregon), EU (Ireland), Asia Pacific (Tokyo) and Asia Pacific (Sydney)\n- boto3, botocore, sagemaker, awscli๋ 2021๋
12์ ๋ฒ์ ์ดํ๋ฅผ ์ฌ์ฉํ์
์ผ ํฉ๋๋ค.",
"_____no_output_____"
],
[
"<br>\n\n## 1. Upload Model Artifacts\n---\n\n๋ชจ๋ธ์ ์์นด์ด๋นํ์ฌ S3๋ก ์
๋ก๋ํฉ๋๋ค.",
"_____no_output_____"
]
],
[
[
"!pip install -qU sagemaker botocore boto3 awscli\n!pip install --ignore-installed PyYAML\n!pip install transformers==4.12.5",
"_____no_output_____"
],
[
"import torch\nimport torchvision\nimport torchvision.models as models\nimport sagemaker\nfrom sagemaker import get_execution_role\nfrom sagemaker.utils import name_from_base\nfrom sagemaker.pytorch import PyTorchModel\nimport boto3\nimport datetime\nimport time\nfrom time import strftime,gmtime\nimport json\nimport os\nimport io\nimport torchvision.transforms as transforms\nfrom src.utils import print_outputs, upload_model_artifact_to_s3, NLPPredictor \n\nrole = get_execution_role()\nboto_session = boto3.session.Session()\nsm_session = sagemaker.session.Session()\nsm_client = boto_session.client(\"sagemaker\")\nsm_runtime = boto_session.client(\"sagemaker-runtime\")\nregion = boto_session.region_name\nbucket = sm_session.default_bucket()\nprefix = 'serverless-inference-kornlp-nsmc'\n\nprint(f'region = {region}')\nprint(f'role = {role}')\nprint(f'bucket = {bucket}')\nprint(f'prefix = {prefix}')",
"_____no_output_____"
],
[
"model_variant = 'modelA'\nnlp_task = 'nsmc'\nmodel_path = f'model-{nlp_task}'\nmodel_s3_uri = upload_model_artifact_to_s3(model_variant, model_path, bucket, prefix)",
"_____no_output_____"
]
],
[
[
"<br>\n\n## 2. Create SageMaker Serverless Endpoint\n---\n\nSageMaker Serverless Endpoint๋ ๊ธฐ์กด SageMaker ๋ฆฌ์ผํ์ ์๋ํฌ์ธํธ ๋ฐฐํฌ์ 99% ์ ์ฌํฉ๋๋ค. 1%์ ์ฐจ์ด๊ฐ ๋ฌด์์ผ๊น์? Endpoint ๊ตฌ์ฑ ์ค์ ์, ServerlessConfig์์ ๋ฉ๋ชจ๋ฆฌ ํฌ๊ธฐ(`MemorySizeInMB`), ์ต๋ ๋์ ์ ์(`MaxConcurrency`)์ ๋ํ ํ๋ผ๋ฉํฐ๋ง ์ถ๊ฐํ์๋ฉด ๋ฉ๋๋ค.\n\n```python\nsm_client.create_endpoint_config(\n ...\n \"ServerlessConfig\": {\n \"MemorySizeInMB\": 2048,\n \"MaxConcurrency\": 20\n }\n)\n```\n\n์์ธํ ๋ด์ฉ์ ์๋ ๋งํฌ๋ฅผ ์ฐธ์กฐํด ์ฃผ์ธ์.\n- Amazon SageMaker Developer Guide - Serverless Inference: https://docs.aws.amazon.com/sagemaker/latest/dg/serverless-endpoints.html",
"_____no_output_____"
],
[
"### Create Inference containter definition for Model",
"_____no_output_____"
]
],
[
[
"from sagemaker.image_uris import retrieve\n\ndeploy_instance_type = 'ml.m5.xlarge'\npt_ecr_image_uri = retrieve(\n framework='pytorch',\n region=region,\n version='1.7.1',\n py_version='py3',\n instance_type = deploy_instance_type,\n accelerator_type=None,\n image_scope='inference'\n)",
"_____no_output_____"
]
],
[
[
"### Create a SageMaker Model\n\n`create_model` API๋ฅผ ํธ์ถํ์ฌ ์ ์ฝ๋ ์
์์ ์์ฑํ ์ปจํ
์ด๋์ ์ ์๋ฅผ ํฌํจํ๋ ๋ชจ๋ธ์ ์์ฑํฉ๋๋ค.",
"_____no_output_____"
]
],
[
[
"model_name = f\"KorNLPServerless-{nlp_task}-{strftime('%Y-%m-%d-%H-%M-%S', gmtime())}\"\n\ncreate_model_response = sm_client.create_model(\n ModelName=model_name,\n Containers=[\n {\n \"Image\": pt_ecr_image_uri,\n \"Mode\": \"SingleModel\",\n \"ModelDataUrl\": model_s3_uri,\n \"Environment\": {\n \"SAGEMAKER_CONTAINER_LOG_LEVEL\": \"20\",\n \"SAGEMAKER_PROGRAM\": \"inference_nsmc.py\",\n \"SAGEMAKER_SUBMIT_DIRECTORY\": model_s3_uri,\n }, \n } \n \n ],\n ExecutionRoleArn=role,\n)\nprint(f\"Created Model: {create_model_response['ModelArn']}\")",
"_____no_output_____"
]
],
[
[
"### Create Endpoint Configuration\n\n`ServerlessConfig`์ผ๋ก ์๋ํฌ์ธํธ์ ๋ํ ์๋ฒ๋ฆฌ์ค ์ค์ ์ ์กฐ์ ํ ์ ์์ต๋๋ค. ์ต๋ ๋์ ํธ์ถ(`MaxConcurrency`; max concurrent invocations)์ 1์์ 50 ์ฌ์ด์ด๋ฉฐ, `MemorySize`๋ 1024MB, 2048MB, 3072MB, 4096MB, 5120MB ๋๋ 6144MB๋ฅผ ์ ํํ ์ ์์ต๋๋ค.",
"_____no_output_____"
]
],
[
[
"endpoint_config_name = f\"KorNLPServerlessEndpointConfig-{nlp_task}-{strftime('%Y-%m-%d-%H-%M-%S', gmtime())}\"\nendpoint_config_response = sm_client.create_endpoint_config(\n EndpointConfigName=endpoint_config_name,\n ProductionVariants=[\n {\n \"VariantName\": \"AllTraffic\",\n \"ModelName\": model_name,\n \"ServerlessConfig\": {\n \"MemorySizeInMB\": 4096,\n \"MaxConcurrency\": 20,\n }, \n },\n ],\n)\nprint(f\"Created EndpointConfig: {endpoint_config_response['EndpointConfigArn']}\")",
"_____no_output_____"
]
],
[
[
"### Create a SageMaker Multi-container endpoint\n\ncreate_endpoint API๋ก ๋ฉํฐ ์ปจํ
์ด๋ ์๋ํฌ์ธํธ๋ฅผ ์์ฑํฉ๋๋ค. ๊ธฐ์กด์ ์๋ํฌ์ธํธ ์์ฑ ๋ฐฉ๋ฒ๊ณผ ๋์ผํฉ๋๋ค.",
"_____no_output_____"
]
],
[
[
"endpoint_name = f\"KorNLPServerlessEndpoint-{nlp_task}-{strftime('%Y-%m-%d-%H-%M-%S', gmtime())}\"\nendpoint_response = sm_client.create_endpoint(\n EndpointName=endpoint_name, \n EndpointConfigName=endpoint_config_name\n)\nprint(f\"Creating Endpoint: {endpoint_response['EndpointArn']}\")",
"_____no_output_____"
]
],
[
[
"`describe_endpoint` API๋ฅผ ์ฌ์ฉํ์ฌ ์๋ํฌ์ธํธ ์์ฑ ์ํ๋ฅผ ํ์ธํ ์ ์์ต๋๋ค. SageMaker ์๋ฒ๋ฆฌ์ค ์๋ํฌ์ธํธ๋ ์ผ๋ฐ์ ์ธ ์๋ํฌ์ธํธ ์์ฑ๋ณด๋ค ๋น ๋ฅด๊ฒ ์์ฑ๋ฉ๋๋ค. (์ฝ 2-3๋ถ)",
"_____no_output_____"
]
],
[
[
"%%time\nwaiter = boto3.client('sagemaker').get_waiter('endpoint_in_service')\nprint(\"Waiting for endpoint to create...\")\nwaiter.wait(EndpointName=endpoint_name)\nresp = sm_client.describe_endpoint(EndpointName=endpoint_name)\nprint(f\"Endpoint Status: {resp['EndpointStatus']}\")",
"_____no_output_____"
]
],
[
[
"### Direct Invocation for Model \n\n์ต์ด ํธ์ถ ์ Cold start๋ก ์ง์ฐ ์๊ฐ์ด ๋ฐ์ํ์ง๋ง, ์ต์ด ํธ์ถ ์ดํ์๋ warm ์ํ๋ฅผ ์ ์งํ๊ธฐ ๋๋ฌธ์ ๋น ๋ฅด๊ฒ ์๋ตํฉ๋๋ค. ๋ฌผ๋ก ์ ๋ถ ๋์ ํธ์ถ์ด ๋์ง ์๊ฑฐ๋ ์์ฒญ์ด ๋ง์์ง๋ฉด cold ์ํ๋ก ๋ฐ๋๋ค๋ ์ ์ ์ ์ํด ์ฃผ์ธ์.",
"_____no_output_____"
]
],
[
[
"model_sample_path = 'samples/nsmc.txt'\n!cat $model_sample_path\nwith open(model_sample_path, mode='rb') as file:\n model_input_data = file.read() \n\nmodel_response = sm_runtime.invoke_endpoint(\n EndpointName=endpoint_name,\n ContentType=\"application/jsonlines\",\n Accept=\"application/jsonlines\",\n Body=model_input_data\n)\n\nmodel_outputs = model_response['Body'].read().decode()\nprint()\nprint_outputs(model_outputs)",
"_____no_output_____"
]
],
[
[
"### Check Model Latency",
"_____no_output_____"
]
],
[
[
"import time\nstart = time.time()\nfor _ in range(10):\n model_response = sm_runtime.invoke_endpoint(\n EndpointName=endpoint_name,\n ContentType=\"application/jsonlines\",\n Accept=\"application/jsonlines\",\n Body=model_input_data\n)\ninference_time = (time.time()-start)\nprint(f'Inference time is {inference_time:.4f} ms.')",
"_____no_output_____"
]
],
[
[
"<br>\n\n## Clean Up\n---",
"_____no_output_____"
]
],
[
[
"sm_client.delete_endpoint(EndpointName=endpoint_name)\nsm_client.delete_endpoint_config(EndpointConfigName=endpoint_config_name)\nsm_client.delete_model(ModelName=model_name)",
"_____no_output_____"
]
]
] | [
"markdown",
"code",
"markdown",
"code",
"markdown",
"code",
"markdown",
"code",
"markdown",
"code",
"markdown",
"code",
"markdown",
"code",
"markdown",
"code",
"markdown",
"code"
] | [
[
"markdown",
"markdown"
],
[
"code",
"code",
"code"
],
[
"markdown",
"markdown"
],
[
"code"
],
[
"markdown"
],
[
"code"
],
[
"markdown"
],
[
"code"
],
[
"markdown"
],
[
"code"
],
[
"markdown"
],
[
"code"
],
[
"markdown"
],
[
"code"
],
[
"markdown"
],
[
"code"
],
[
"markdown"
],
[
"code"
]
] |
Subsets and Splits
No saved queries yet
Save your SQL queries to embed, download, and access them later. Queries will appear here once saved.