hexsha
stringlengths 40
40
| size
int64 6
14.9M
| ext
stringclasses 1
value | lang
stringclasses 1
value | max_stars_repo_path
stringlengths 6
260
| max_stars_repo_name
stringlengths 6
119
| max_stars_repo_head_hexsha
stringlengths 40
41
| max_stars_repo_licenses
sequence | max_stars_count
int64 1
191k
⌀ | max_stars_repo_stars_event_min_datetime
stringlengths 24
24
⌀ | max_stars_repo_stars_event_max_datetime
stringlengths 24
24
⌀ | max_issues_repo_path
stringlengths 6
260
| max_issues_repo_name
stringlengths 6
119
| max_issues_repo_head_hexsha
stringlengths 40
41
| max_issues_repo_licenses
sequence | max_issues_count
int64 1
67k
⌀ | max_issues_repo_issues_event_min_datetime
stringlengths 24
24
⌀ | max_issues_repo_issues_event_max_datetime
stringlengths 24
24
⌀ | max_forks_repo_path
stringlengths 6
260
| max_forks_repo_name
stringlengths 6
119
| max_forks_repo_head_hexsha
stringlengths 40
41
| max_forks_repo_licenses
sequence | max_forks_count
int64 1
105k
⌀ | max_forks_repo_forks_event_min_datetime
stringlengths 24
24
⌀ | max_forks_repo_forks_event_max_datetime
stringlengths 24
24
⌀ | avg_line_length
float64 2
1.04M
| max_line_length
int64 2
11.2M
| alphanum_fraction
float64 0
1
| cells
sequence | cell_types
sequence | cell_type_groups
sequence |
---|---|---|---|---|---|---|---|---|---|---|---|---|---|---|---|---|---|---|---|---|---|---|---|---|---|---|---|---|---|---|
e770ab14f4db42b0a17bafa4fd72fa8b9c4b311f | 40,656 | ipynb | Jupyter Notebook | database/pymongo.ipynb | Junhojuno/TIL | c252b62b94dc519ccd528c2cd8b638e85adee89c | [
"MIT"
] | null | null | null | database/pymongo.ipynb | Junhojuno/TIL | c252b62b94dc519ccd528c2cd8b638e85adee89c | [
"MIT"
] | null | null | null | database/pymongo.ipynb | Junhojuno/TIL | c252b62b94dc519ccd528c2cd8b638e85adee89c | [
"MIT"
] | 3 | 2018-05-23T03:33:41.000Z | 2018-07-09T14:34:15.000Z | 30.846737 | 611 | 0.452086 | [
[
[
"### install pymongo package\n- mac\n - pip(3) install pymongo\n- window\n - conda install -c anaconda pymongo",
"_____no_output_____"
]
],
[
[
"import pymongo, requests",
"_____no_output_____"
]
],
[
[
"##### 1. server에 연결(client생성)",
"_____no_output_____"
]
],
[
[
"client = pymongo.MongoClient('mongodb://13.125.237.246:27017')\nclient",
"_____no_output_____"
]
],
[
[
"##### 2. db선택",
"_____no_output_____"
]
],
[
[
"db = client.dss\ndb",
"_____no_output_____"
]
],
[
[
"##### 3. db의 collection 리스트를 확인",
"_____no_output_____"
]
],
[
[
"db.collection_names()",
"_____no_output_____"
]
],
[
[
"##### 4. collection 선택",
"_____no_output_____"
]
],
[
[
"collection = db.info\ncollection",
"_____no_output_____"
]
],
[
[
"##### 5. find",
"_____no_output_____"
]
],
[
[
"# find_one : 한 개의 document를 가져옵니다.\ndocument = collection.find_one({\"subject\" : \"java\"})\ntype(document), document",
"_____no_output_____"
],
[
"# find : 여러 개의 documents를 가져옵니다\ndocuments = collection.find({\"subject\": \"java\"})\ndocuments",
"_____no_output_____"
],
[
"datas = list(documents)\nlen(datas)",
"_____no_output_____"
],
[
"datas",
"_____no_output_____"
],
[
"list(documents)",
"_____no_output_____"
]
],
[
[
"다 사라짐.",
"_____no_output_____"
]
],
[
[
"# count - documents의 갯수를 가져오는 함수\ndocuments = collection.find()\ndocuments.count()",
"_____no_output_____"
],
[
"# sort - 정렬\ndocuments = collection.find({\"level\":{\"$lte\":3}}).sort(\"level\", pymongo.DESCENDING)\nlist(documents)",
"_____no_output_____"
]
],
[
[
"##### 6. insert",
"_____no_output_____"
]
],
[
[
"# insert_one\ndata = {\"subject\":\"css\", \"level\":1, \"comments\":[{\"name\":\"peter\", \"msg\":\"easy\"}]}\nresult = collection.insert_one(data)\nresult",
"_____no_output_____"
],
[
"result.inserted_id",
"_____no_output_____"
],
[
"# insert_many\ndatas = [\n {\"subject\":\"webpack\", \"level\":2, \"comments\":[{\"name\":\"peter\", \"msg\":\"easy\"}]},\n {\"subject\":\"gulp\", \"level\":3, \"comments\":[{\"name\":\"peter\", \"msg\":\"easy\"}]},\n {\"subject\":\"bower\", \"level\":4, \"comments\":[{\"name\":\"peter\", \"msg\":\"easy\"}]}\n]\nresult = collection.insert_many(datas)\nresult",
"_____no_output_____"
],
[
"result.inserted_ids",
"_____no_output_____"
]
],
[
[
"### 직방 데이터 크롤링 후 저장",
"_____no_output_____"
]
],
[
[
"url = \"https://api.zigbang.com/v3/items?detail=true&item_ids=[12258942,12217921,12251354,12042761,12270198,12263778,12149733,12263079,12046500,12227516,12245261,12258364,11741210,11947081,12081429,12248641,12039772,12148952,12271001,12201879,12269163,12268373,12268568,12204018,12247416,12241201,12174611,12254380,12233724,12139836,11869595,12178704,12262681,12261598,12106912,12248115,12154374,12240537,12245412,12155533,12198385,12203883,12251810,12239779,12013638,12218505,12249844,12184761,12258707,12096937,12191641,12256520,12163720,12241556,12245758,12272387,12256200,12260120,12195600,12263256]\"",
"_____no_output_____"
],
[
"response = requests.get(url)\nresponse",
"_____no_output_____"
],
[
"# parsing - [{},{},{},{},{},..........]\nzigbang_dict_list = response.json().get(\"items\") # 최상단 items를 벗겨냄\nlen(zigbang_dict_list)",
"_____no_output_____"
],
[
"items = [item[\"item\"] for item in zigbang_dict_list]\nlen(items)",
"_____no_output_____"
],
[
"items[:2]",
"_____no_output_____"
],
[
"collection = client.crawling.zigbang\nresult_zigbang = collection.insert_many(items)\nresult_zigbang",
"_____no_output_____"
]
],
[
[
"##### 렌트비용이 50이하인 데이터 추출",
"_____no_output_____"
]
],
[
[
"query = {\"rent\":{\"$lte\":50}}\n\ndocuments = collection.find(query)\ndocuments",
"_____no_output_____"
],
[
"datas = list(documents)\nlen(datas)",
"_____no_output_____"
],
[
"# pandas로 만들어보자\ndf = pd.DataFrame(datas)\ndf.tail()",
"_____no_output_____"
],
[
"filtered_df = df[['rent','options','size','deposit']]\nfiltered_df.tail()",
"_____no_output_____"
],
[
"query = {\"rent\":{\"$lte\":50}}\n\ndocuments = collection.find(query, {\"_id\":False,\"deposit\":True, \"rent\":True, \"options\":True,\"size\":True})\ndocuments",
"_____no_output_____"
],
[
"df = pd.DataFrame(list(documents))\ndf.tail()",
"_____no_output_____"
]
],
[
[
"##### delete - database",
"_____no_output_____"
]
],
[
[
"client.drop_database(\"crawling\")",
"_____no_output_____"
]
],
[
[
"##### delete - collection",
"_____no_output_____"
]
],
[
[
"client.crawling.drop_collection(\"zigbang\")",
"_____no_output_____"
]
]
] | [
"markdown",
"code",
"markdown",
"code",
"markdown",
"code",
"markdown",
"code",
"markdown",
"code",
"markdown",
"code",
"markdown",
"code",
"markdown",
"code",
"markdown",
"code",
"markdown",
"code",
"markdown",
"code",
"markdown",
"code"
] | [
[
"markdown"
],
[
"code"
],
[
"markdown"
],
[
"code"
],
[
"markdown"
],
[
"code"
],
[
"markdown"
],
[
"code"
],
[
"markdown"
],
[
"code"
],
[
"markdown"
],
[
"code",
"code",
"code",
"code",
"code"
],
[
"markdown"
],
[
"code",
"code"
],
[
"markdown"
],
[
"code",
"code",
"code",
"code"
],
[
"markdown"
],
[
"code",
"code",
"code",
"code",
"code",
"code"
],
[
"markdown"
],
[
"code",
"code",
"code",
"code",
"code",
"code"
],
[
"markdown"
],
[
"code"
],
[
"markdown"
],
[
"code"
]
] |
e770adac60d59454deb9dfcf4ce5e312b25d16a7 | 58,258 | ipynb | Jupyter Notebook | .ipynb_checkpoints/category-checkpoint.ipynb | danhtaihoang/expectation-reflection | ae89c77da1e47ffc0ea09fb2e919d29308998b95 | [
"MIT"
] | null | null | null | .ipynb_checkpoints/category-checkpoint.ipynb | danhtaihoang/expectation-reflection | ae89c77da1e47ffc0ea09fb2e919d29308998b95 | [
"MIT"
] | null | null | null | .ipynb_checkpoints/category-checkpoint.ipynb | danhtaihoang/expectation-reflection | ae89c77da1e47ffc0ea09fb2e919d29308998b95 | [
"MIT"
] | null | null | null | 144.920398 | 26,476 | 0.869408 | [
[
[
"## Synthetic data: Catogorical variables",
"_____no_output_____"
]
],
[
[
"import numpy as np\nimport pandas as pd\nfrom sklearn.model_selection import train_test_split\nfrom sklearn.model_selection import KFold\nfrom sklearn.utils import shuffle\nfrom sklearn.metrics import accuracy_score\n\nfrom synthesize_data import synthesize_data\nimport expectation_reflection as ER\nfrom sklearn.linear_model import LogisticRegression\nfrom sklearn.naive_bayes import GaussianNB\nfrom sklearn.tree import DecisionTreeClassifier\nfrom sklearn.ensemble import RandomForestClassifier\n\nimport matplotlib.pyplot as plt\n%matplotlib inline",
"_____no_output_____"
],
[
"np.random.seed(1)",
"_____no_output_____"
],
[
"def inference(X_train,y_train,X_test,y_test,method='expectation_reflection'):\n if method == 'expectation_reflection':\n h0,w = ER.fit(X_train,y_train,niter_max=100,regu=0.001)\n y_pred = ER.predict(X_test,h0,w)\n\n else:\n if method == 'logistic_regression':\n model = LogisticRegression(solver='liblinear')\n\n if method == 'naive_bayes': \n model = GaussianNB()\n\n if method == 'random_forest':\n model = RandomForestClassifier(criterion = \"gini\", random_state = 1,\n max_depth=3, min_samples_leaf=5,n_estimators=100) \n \n if method == 'decision_tree':\n model = DecisionTreeClassifier() \n\n model.fit(X_train, y_train)\n y_pred = model.predict(X_test)\n \n accuracy = accuracy_score(y_test,y_pred) \n \n return accuracy",
"_____no_output_____"
],
[
"def compare_inference(X,y,train_size):\n npred = 100\n accuracy = np.zeros((len(list_methods),npred))\n for ipred in range(npred):\n X, y = shuffle(X, y)\n X_train0,X_test,y_train0,y_test = train_test_split(X,y,test_size=0.2,random_state = ipred)\n idx_train = np.random.choice(len(y_train0),size=int(train_size*len(y)),replace=False)\n X_train,y_train = X_train0[idx_train],y_train0[idx_train]\n\n for i,method in enumerate(list_methods):\n accuracy[i,ipred] = inference(X_train,y_train,X_test,y_test,method)\n \n return accuracy.mean(axis=1),accuracy.std(axis=1)",
"_____no_output_____"
],
[
"l = 10000 ; n = 40 ; g = 4.",
"_____no_output_____"
],
[
"X,y = synthesize_data(l,n,g,data_type='categorical')",
"_____no_output_____"
],
[
"np.unique(y,return_counts=True)",
"_____no_output_____"
],
[
"list_train_size = [0.8,0.6,0.4,0.2,0.1]\nlist_methods=['logistic_regression','naive_bayes','random_forest','decision_tree','expectation_reflection']\nacc = np.zeros((len(list_train_size),len(list_methods)))\nacc_std = np.zeros((len(list_train_size),len(list_methods)))\nfor i,train_size in enumerate(list_train_size):\n acc[i,:],acc_std[i,:] = compare_inference(X,y,train_size)\n print(train_size,acc[i,:])",
"0.8 [0.98133 0.94237 0.68139 0.666445 0.979465]\n0.6 [0.97942 0.93552 0.679885 0.66155 0.977335]\n0.4 [0.97332 0.922375 0.679445 0.659095 0.97189 ]\n0.2 [0.95689 0.896125 0.680675 0.648245 0.957745]\n0.1 [0.925955 0.860555 0.68053 0.63509 0.92841 ]\n"
],
[
"acc_std",
"_____no_output_____"
],
[
"df = pd.DataFrame(acc,columns = list_methods)\ndf.insert(0, \"train_size\",list_train_size, True)\ndf",
"_____no_output_____"
],
[
"plt.figure(figsize=(4,3)) \nplt.plot(list_train_size,acc[:,0],'k--',marker='o',mfc='none',label='Logistic Regression')\nplt.plot(list_train_size,acc[:,1],'b--',marker='s',mfc='none',label='Naive Bayes')\nplt.plot(list_train_size,acc[:,2],'r--',marker='^',mfc='none',label='Random Forest')\nplt.plot(list_train_size,acc[:,4],'k-',marker='o',label='Expectation Reflection')\nplt.xlabel('train size')\nplt.ylabel('accuracy mean')\nplt.legend()",
"_____no_output_____"
],
[
"plt.figure(figsize=(4,3)) \nplt.plot(list_train_size,acc_std[:,0],'k--',marker='o',mfc='none',label='Logistic Regression')\nplt.plot(list_train_size,acc_std[:,1],'b--',marker='s',mfc='none',label='Naive Bayes')\nplt.plot(list_train_size,acc_std[:,2],'r--',marker='^',mfc='none',label='Random Forest')\nplt.plot(list_train_size,acc_std[:,4],'k-',marker='o',label='Expectation Reflection')\nplt.xlabel('train size')\nplt.ylabel('accuracy standard deviation')\nplt.legend()",
"_____no_output_____"
]
]
] | [
"markdown",
"code"
] | [
[
"markdown"
],
[
"code",
"code",
"code",
"code",
"code",
"code",
"code",
"code",
"code",
"code",
"code",
"code"
]
] |
e770bea263af23f89f47ea2f29a104c57b090c8b | 27,632 | ipynb | Jupyter Notebook | ActorCritic/.ipynb_checkpoints/DDPG-Copy1-checkpoint.ipynb | bluemapleman/Maple-Reinforcement-Learning | 16ec0d377e2be0375401fb7bd576eef5f4c14de8 | [
"MIT"
] | 9 | 2018-11-15T05:04:14.000Z | 2021-12-15T22:01:01.000Z | ActorCritic/.ipynb_checkpoints/DDPG-Copy1-checkpoint.ipynb | bluemapleman/Maple-Reinforcement-Learning | 16ec0d377e2be0375401fb7bd576eef5f4c14de8 | [
"MIT"
] | null | null | null | ActorCritic/.ipynb_checkpoints/DDPG-Copy1-checkpoint.ipynb | bluemapleman/Maple-Reinforcement-Learning | 16ec0d377e2be0375401fb7bd576eef5f4c14de8 | [
"MIT"
] | 1 | 2019-12-19T07:01:54.000Z | 2019-12-19T07:01:54.000Z | 46.207358 | 636 | 0.533657 | [
[
[
"# DDPG - BipedalWalker-v2\n\n- Xinyao Qian\n- Tianhao Liu",
"_____no_output_____"
],
[
"- Get familiar with the BipedalWalker-v2 environment first\n\nFind that BipedalWalker behaves embarrasingly bad if taking random walking strategy.",
"_____no_output_____"
]
],
[
[
"import tensorflow as tf\nimport numpy as np\nimport gym\n\n# Load Environment\nENV_NAME = 'BipedalWalker-v2'\nenv = gym.make(ENV_NAME)\n# Repeoducible environment parameters\nenv.seed(1)\n\ns=env.reset()\nepisode=100\nsteps=5000\nwhile i in range(episode):\n for j in range(steps):\n env.render()\n a=env.action_space.sample()\n s_,r,d,_=env.step(a)\n\n if d:\n s=env.reset()",
"\u001b[33mWARN: gym.spaces.Box autodetected dtype as <class 'numpy.float32'>. Please provide explicit dtype.\u001b[0m\n\u001b[33mWARN: gym.spaces.Box autodetected dtype as <class 'numpy.float32'>. Please provide explicit dtype.\u001b[0m\n"
]
],
[
[
"# Our solution",
"_____no_output_____"
],
[
"\n**Since the action space of BipedalWalker is consecutive, that means value based models such as Q-Learning or DQN, are not applicable**, because value based models generally try to fit a better value function that tells us how good it is to be at a certain state s (V(s)) or to take action a at the state s (Q(s,a)), and then we still need to choose specific action based on our exploring strategy (e.g. $\\epsilon$-greedy). Obviously, it can't work when our actions are consecutive/countless.\n\nSo then we consider using **policy based models**, for example, REINFORCE. However, there is another problem that REINFORCE can only update parameters/learn everytime an episode ends, which slowed the convergence process. \n\nThen we get to know that there is another series of models that called **Actor Critic which combines the advantages of both the value based model and the policy based model and make it possible for policy based models to update itself at every step**. \n\nSpecifically, we simultaneously train a policy gradients network and a Q-Learning network. The policy network behaves as the actor which takes in observations and outputs best actions to be taken, while the value network will behave as a critic to take in observations and tell the actor how 'good' to be at the current state, so that the actor can know how good its last action that brought it here was, and update its parameters according to this feedback, while the critic can also update its own parameters in the way Q-Learning does. **In a sense, actor and critic are supervising each other to become better and better**.\n\n<center>\n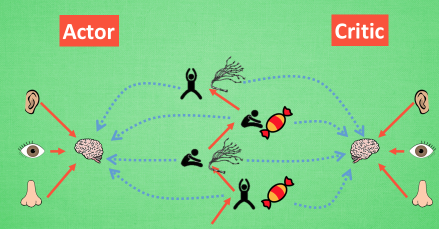\n</center>\n\n\n> https://morvanzhou.github.io/static/results/ML-intro/AC3.png\n",
"_____no_output_____"
],
[
"# Environment preparation & Definition of Classes: Actor, Critic, Memory",
"_____no_output_____"
]
],
[
[
"import gym\nimport os\nimport tensorflow as tf\nimport numpy as np\nimport shutil\n\nnp.random.seed(1)\ntf.set_random_seed(1)\n\n# Load Environment\nENV_NAME = 'BipedalWalker-v2'\nenv = gym.make(ENV_NAME)\n# Repeoducible environment parameters\nenv.seed(1)\n\n\nSTATE_DIM = env.observation_space.shape[0] # 24 environment variables\nACTION_DIM = env.action_space.shape[0] # 4 consecutive actions\nACTION_BOUND = env.action_space.high # [1, 1, 1, 1]\n\n# all placeholder for tf\nwith tf.name_scope('S'):\n S = tf.placeholder(tf.float32, shape=[None, STATE_DIM], name='s')\nwith tf.name_scope('R'):\n R = tf.placeholder(tf.float32, [None, 1], name='r')\nwith tf.name_scope('S_'):\n S_ = tf.placeholder(tf.float32, shape=[None, STATE_DIM], name='s_')\n\n############################### Actor ####################################\n\nclass Actor(object):\n def __init__(self, sess, action_dim, action_bound, learning_rate, t_replace_iter):\n self.sess = sess\n self.a_dim = action_dim\n self.action_bound = action_bound\n self.lr = learning_rate\n self.t_replace_iter = t_replace_iter\n self.t_replace_counter = 0\n\n with tf.variable_scope('Actor'):\n # input s, output a\n self.a = self._build_net(S, scope='eval_net', trainable=True)\n\n # input s_, output a, get a_ for critic\n self.a_ = self._build_net(S_, scope='target_net', trainable=False)\n\n self.e_params = tf.get_collection(tf.GraphKeys.GLOBAL_VARIABLES, scope='Actor/eval_net')\n self.t_params = tf.get_collection(tf.GraphKeys.GLOBAL_VARIABLES, scope='Actor/target_net')\n\n def _build_net(self, s, scope, trainable):\n with tf.variable_scope(scope):\n init_w = tf.random_normal_initializer(0., 0.01)\n init_b = tf.constant_initializer(0.01)\n net = tf.layers.dense(s, 500, activation=tf.nn.relu,\n kernel_initializer=init_w, bias_initializer=init_b, name='l1', trainable=trainable)\n net = tf.layers.dense(net, 200, activation=tf.nn.relu,\n kernel_initializer=init_w, bias_initializer=init_b, name='l2', trainable=trainable)\n\n with tf.variable_scope('a'):\n actions = tf.layers.dense(net, self.a_dim, activation=tf.nn.tanh, kernel_initializer=init_w,\n bias_initializer=init_b, name='a', trainable=trainable)\n scaled_a = tf.multiply(actions, self.action_bound, name='scaled_a') # Scale output to -action_bound to action_bound\n return scaled_a\n\n def learn(self, s): # batch update\n self.sess.run(self.train_op, feed_dict={S: s})\n if self.t_replace_counter % self.t_replace_iter == 0:\n self.sess.run([tf.assign(t, e) for t, e in zip(self.t_params, self.e_params)])\n self.t_replace_counter += 1\n\n def choose_action(self, s):\n s = s[np.newaxis, :] # single state\n return self.sess.run(self.a, feed_dict={S: s})[0] # single action\n\n def add_grad_to_graph(self, a_grads):\n with tf.variable_scope('policy_grads'):\n # ys = policy;\n # xs = policy's parameters;\n # self.a_grads = the gradients of the policy to get more Q\n # tf.gradients will calculate dys/dxs with a initial gradients for ys, so this is dq/da * da/dparams\n self.policy_grads_and_vars = tf.gradients(ys=self.a, xs=self.e_params, grad_ys=a_grads)\n\n with tf.variable_scope('A_train'):\n opt = tf.train.RMSPropOptimizer(-self.lr) # (- learning rate) for ascent policy\n self.train_op = opt.apply_gradients(zip(self.policy_grads_and_vars, self.e_params), global_step=GLOBAL_STEP)\n\n\n######################################## Critic #########################################\n\nclass Critic(object):\n def __init__(self, sess, state_dim, action_dim, learning_rate, gamma, t_replace_iter, a, a_):\n self.sess = sess\n self.s_dim = state_dim\n self.a_dim = action_dim\n self.lr = learning_rate\n self.gamma = gamma\n self.t_replace_iter = t_replace_iter\n self.t_replace_counter = 0\n\n with tf.variable_scope('Critic'):\n # Input (s, a), output q\n self.a = a\n self.q = self._build_net(S, self.a, 'eval_net', trainable=True)\n\n # Input (s_, a_), output q_ for q_target\n self.q_ = self._build_net(S_, a_, 'target_net', trainable=False) # target_q is based on a_ from Actor's target_net\n\n self.e_params = tf.get_collection(tf.GraphKeys.GLOBAL_VARIABLES, scope='Critic/eval_net')\n self.t_params = tf.get_collection(tf.GraphKeys.GLOBAL_VARIABLES, scope='Critic/target_net')\n\n with tf.variable_scope('target_q'):\n self.target_q = R + self.gamma * self.q_\n\n with tf.variable_scope('abs_TD'):\n self.abs_td = tf.abs(self.target_q - self.q)\n self.ISWeights = tf.placeholder(tf.float32, [None, 1], name='IS_weights')\n with tf.variable_scope('TD_error'):\n self.loss = tf.reduce_mean(self.ISWeights * tf.squared_difference(self.target_q, self.q))\n\n with tf.variable_scope('C_train'):\n self.train_op = tf.train.AdamOptimizer(self.lr).minimize(self.loss, global_step=GLOBAL_STEP)\n\n with tf.variable_scope('a_grad'):\n self.a_grads = tf.gradients(self.q, a)[0] # tensor of gradients of each sample (None, a_dim)\n\n def _build_net(self, s, a, scope, trainable):\n with tf.variable_scope(scope):\n init_w = tf.random_normal_initializer(0., 0.01)\n init_b = tf.constant_initializer(0.01)\n\n with tf.variable_scope('l1'):\n n_l1 = 700\n # combine the action and states together in this way\n w1_s = tf.get_variable('w1_s', [self.s_dim, n_l1], initializer=init_w, trainable=trainable)\n w1_a = tf.get_variable('w1_a', [self.a_dim, n_l1], initializer=init_w, trainable=trainable)\n b1 = tf.get_variable('b1', [1, n_l1], initializer=init_b, trainable=trainable)\n net = tf.nn.relu(tf.matmul(s, w1_s) + tf.matmul(a, w1_a) + b1)\n with tf.variable_scope('l2'):\n net = tf.layers.dense(net, 20, activation=tf.nn.relu, kernel_initializer=init_w,\n bias_initializer=init_b, name='l2', trainable=trainable)\n with tf.variable_scope('q'):\n q = tf.layers.dense(net, 1, kernel_initializer=init_w, bias_initializer=init_b, trainable=trainable) # Q(s,a)\n return q\n\n def learn(self, s, a, r, s_, ISW):\n _, abs_td = self.sess.run([self.train_op, self.abs_td], feed_dict={S: s, self.a: a, R: r, S_: s_, self.ISWeights: ISW})\n if self.t_replace_counter % self.t_replace_iter == 0:\n self.sess.run([tf.assign(t, e) for t, e in zip(self.t_params, self.e_params)])\n self.t_replace_counter += 1\n return abs_td\n\n######################################## Assistanting Class: SumTree and Memory #########################################\n\nclass SumTree(object):\n \"\"\"\n This SumTree code is modified version and the original code is from:\n https://github.com/jaara/AI-blog/blob/master/SumTree.py\n Story the data with it priority in tree and data frameworks.\n \"\"\"\n data_pointer = 0\n\n def __init__(self, capacity):\n self.capacity = capacity # for all priority values\n self.tree = np.zeros(2 * capacity - 1)+1e-5\n # [--------------Parent nodes-------------][-------leaves to recode priority-------]\n # size: capacity - 1 size: capacity\n self.data = np.zeros(capacity, dtype=object) # for all transitions\n # [--------------data frame-------------]\n # size: capacity\n\n def add_new_priority(self, p, data):\n leaf_idx = self.data_pointer + self.capacity - 1\n\n self.data[self.data_pointer] = data # update data_frame\n self.update(leaf_idx, p) # update tree_frame\n self.data_pointer += 1\n if self.data_pointer >= self.capacity: # replace when exceed the capacity\n self.data_pointer = 0\n\n def update(self, tree_idx, p):\n change = p - self.tree[tree_idx]\n\n self.tree[tree_idx] = p\n self._propagate_change(tree_idx, change)\n\n def _propagate_change(self, tree_idx, change):\n \"\"\"change the sum of priority value in all parent nodes\"\"\"\n parent_idx = (tree_idx - 1) // 2\n self.tree[parent_idx] += change\n if parent_idx != 0:\n self._propagate_change(parent_idx, change)\n\n def get_leaf(self, lower_bound):\n leaf_idx = self._retrieve(lower_bound) # search the max leaf priority based on the lower_bound\n data_idx = leaf_idx - self.capacity + 1\n return [leaf_idx, self.tree[leaf_idx], self.data[data_idx]]\n\n def _retrieve(self, lower_bound, parent_idx=0):\n \"\"\"\n Tree structure and array storage:\n Tree index:\n 0 -> storing priority sum\n / \\\n 1 2\n / \\ / \\\n 3 4 5 6 -> storing priority for transitions\n Array type for storing:\n [0,1,2,3,4,5,6]\n \"\"\"\n left_child_idx = 2 * parent_idx + 1\n right_child_idx = left_child_idx + 1\n\n if left_child_idx >= len(self.tree): # end search when no more child\n return parent_idx\n\n if self.tree[left_child_idx] == self.tree[right_child_idx]:\n return self._retrieve(lower_bound, np.random.choice([left_child_idx, right_child_idx]))\n if lower_bound <= self.tree[left_child_idx]: # downward search, always search for a higher priority node\n return self._retrieve(lower_bound, left_child_idx)\n else:\n return self._retrieve(lower_bound - self.tree[left_child_idx], right_child_idx)\n\n @property\n def root_priority(self):\n return self.tree[0] # the root\n\n\nclass Memory(object): # stored as ( s, a, r, s_ ) in SumTree\n \"\"\"\n This SumTree code is modified version and the original code is from:\n https://github.com/jaara/AI-blog/blob/master/Seaquest-DDQN-PER.py\n \"\"\"\n epsilon = 0.001 # small amount to avoid zero priority\n alpha = 0.6 # [0~1] convert the importance of TD error to priority\n beta = 0.4 # importance-sampling, from initial value increasing to 1\n beta_increment_per_sampling = 1e-5 # annealing the bias\n abs_err_upper = 1 # for stability refer to paper\n\n def __init__(self, capacity):\n self.tree = SumTree(capacity)\n\n def store(self, error, transition):\n p = self._get_priority(error)\n self.tree.add_new_priority(p, transition)\n\n def prio_sample(self, n):\n batch_idx, batch_memory, ISWeights = [], [], []\n segment = self.tree.root_priority / n\n self.beta = np.min([1, self.beta + self.beta_increment_per_sampling]) # max = 1\n\n min_prob = np.min(self.tree.tree[-self.tree.capacity:]) / self.tree.root_priority\n maxiwi = np.power(self.tree.capacity * min_prob, -self.beta) # for later normalizing ISWeights\n for i in range(n):\n a = segment * i\n b = segment * (i + 1)\n lower_bound = np.random.uniform(a, b)\n while True:\n idx, p, data = self.tree.get_leaf(lower_bound)\n if type(data) is int:\n i -= 1\n lower_bound = np.random.uniform(segment * i, segment * (i+1))\n else:\n break\n prob = p / self.tree.root_priority\n ISWeights.append(self.tree.capacity * prob)\n batch_idx.append(idx)\n batch_memory.append(data)\n\n ISWeights = np.vstack(ISWeights)\n ISWeights = np.power(ISWeights, -self.beta) / maxiwi # normalize\n return batch_idx, np.vstack(batch_memory), ISWeights\n\n def random_sample(self, n):\n idx = np.random.randint(0, self.tree.capacity, size=n, dtype=np.int)\n return np.vstack(self.tree.data[idx])\n\n def update(self, idx, error):\n p = self._get_priority(error)\n self.tree.update(idx, p)\n\n def _get_priority(self, error):\n error += self.epsilon # avoid 0\n clipped_error = np.clip(error, 0, self.abs_err_upper)\n return np.power(clipped_error, self.alpha)\n\nprint('Finished!')",
"\u001b[33mWARN: gym.spaces.Box autodetected dtype as <class 'numpy.float32'>. Please provide explicit dtype.\u001b[0m\n\u001b[33mWARN: gym.spaces.Box autodetected dtype as <class 'numpy.float32'>. Please provide explicit dtype.\u001b[0m\nFinished!\n"
]
],
[
[
"# Main loop for trainning",
"_____no_output_____"
]
],
[
[
"######################################## Hyperparameters ########################################\n\nMAX_EPISODES = 500\nLR_A = 0.000005 # learning rate for actor\nLR_C = 0.000005 # learning rate for mcritic\nGAMMA = 0.999 # reward discount\nREPLACE_ITER_A = 1700\nREPLACE_ITER_C = 1500\nMEMORY_CAPACITY = 200000\nBATCH_SIZE = 32\nDISPLAY_THRESHOLD = 100 # display until the running reward > 100\nDATA_PATH = './data'\nSAVE_MODEL_ITER = 100000\nRENDER = False\nOUTPUT_GRAPH = False\n\nGLOBAL_STEP = tf.Variable(0, trainable=False)\nINCREASE_GS = GLOBAL_STEP.assign(tf.add(GLOBAL_STEP, 1))\nLR_A = tf.train.exponential_decay(LR_A, GLOBAL_STEP, 10000, .97, staircase=True)\nLR_C = tf.train.exponential_decay(LR_C, GLOBAL_STEP, 10000, .97, staircase=True)\nEND_POINT = (200 - 10) * (14/30) # from game\n\n##################################################\nLOAD_MODEL = True # Whether to load trained model#\n##################################################\n\nsess = tf.Session()\n\n# Create actor and critic.\nactor = Actor(sess, ACTION_DIM, ACTION_BOUND, LR_A, REPLACE_ITER_A)\ncritic = Critic(sess, STATE_DIM, ACTION_DIM, LR_C, GAMMA, REPLACE_ITER_C, actor.a, actor.a_)\nactor.add_grad_to_graph(critic.a_grads)\n\nM = Memory(MEMORY_CAPACITY)\n\nsaver = tf.train.Saver(max_to_keep=100) # Maximum number of recent checkpoints to keep. Defaults to 5.\n\n\n################################# Determine whether it's a new training or going-on training ###############3\nif LOAD_MODEL: # Returns CheckpointState proto from the \"checkpoint\" file.\n all_ckpt = tf.train.get_checkpoint_state('./data', 'checkpoint').all_model_checkpoint_paths\n saver.restore(sess, all_ckpt[-1]) # reload trained parameters into the tf session\nelse:\n if os.path.isdir(DATA_PATH): shutil.rmtree(DATA_PATH) # recursively remove all files under directory\n os.mkdir(DATA_PATH)\n sess.run(tf.global_variables_initializer())\n\nif OUTPUT_GRAPH:\n tf.summary.FileWriter('logs', graph=sess.graph)\n\nvar = 0.0000001 # control exploration\nvar_min = 0.000001\n\n\n################################# Main loop for training #################################\nfor i_episode in range(MAX_EPISODES):\n \n s = env.reset()\n ep_r = 0 # the episode reward\n \n while True:\n if RENDER:\n env.render()\n \n a = actor.choose_action(s)\n a = np.clip(np.random.normal(a, var), -1, 1) # explore using randomness\n s_, r, done, _ = env.step(a) # r = total 300+ points up to the far end. If the robot falls, it gets -100.\n \n # when r=-100, that means BipedalWalker has falled to the groud\n if r == -100: r = -2\n ep_r += r\n\n transition = np.hstack((s, a, [r], s_))\n max_p = np.max(M.tree.tree[-M.tree.capacity:])\n M.store(max_p, transition)\n \n \n # when the training reaches certain stage, we lessen the probability of exploration\n if GLOBAL_STEP.eval(sess) > MEMORY_CAPACITY/20:\n var = max([var*0.9999, var_min]) # decay the action randomness\n tree_idx, b_M, ISWeights = M.prio_sample(BATCH_SIZE) # for critic update\n b_s = b_M[:, :STATE_DIM]\n b_a = b_M[:, STATE_DIM: STATE_DIM + ACTION_DIM]\n b_r = b_M[:, -STATE_DIM - 1: -STATE_DIM]\n b_s_ = b_M[:, -STATE_DIM:]\n \n # Critic updates its parameters\n abs_td = critic.learn(b_s, b_a, b_r, b_s_, ISWeights)\n \n # Actor updates its parameters\n actor.learn(b_s)\n \n for i in range(len(tree_idx)): # update priority\n idx = tree_idx[i]\n M.update(idx, abs_td[i])\n \n if GLOBAL_STEP.eval(sess) % SAVE_MODEL_ITER == 0:\n ckpt_path = os.path.join(DATA_PATH, 'DDPG.ckpt')\n save_path = saver.save(sess, ckpt_path, global_step=GLOBAL_STEP, write_meta_graph=False)\n print(\"\\nSave Model %s\\n\" % save_path)\n\n if done:\n if \"running_r\" not in globals():\n running_r = ep_r\n else:\n running_r = 0.95*running_r + 0.05*ep_r\n if running_r > DISPLAY_THRESHOLD: RENDER = True\n else: RENDER = False\n\n done = '| Achieve ' if env.unwrapped.hull.position[0] >= END_POINT else '| -----'\n \n print('Episode:', i_episode,\n done,\n '| Running_r: %i' % int(running_r),\n '| Epi_r: %.2f' % ep_r,\n '| Exploration: %.3f' % var,\n '| Pos: %.i' % int(env.unwrapped.hull.position[0]),\n '| LR_A: %.6f' % sess.run(LR_A),\n '| LR_C: %.6f' % sess.run(LR_C),\n )\n break\n\n s = s_\n sess.run(INCREASE_GS)",
"INFO:tensorflow:Restoring parameters from ./data/DDPG.ckpt-1200000\nEpisode: 0 | Achieve | Running_r: 271 | Epi_r: 271.74 | Exploration: 0.000 | Pos: 88 | LR_A: 0.000000 | LR_C: 0.000000\nEpisode: 1 | Achieve | Running_r: 271 | Epi_r: 269.24 | Exploration: 0.000 | Pos: 88 | LR_A: 0.000000 | LR_C: 0.000000\nEpisode: 2 | Achieve | Running_r: 271 | Epi_r: 273.15 | Exploration: 0.000 | Pos: 88 | LR_A: 0.000000 | LR_C: 0.000000\nEpisode: 3 | Achieve | Running_r: 271 | Epi_r: 271.24 | Exploration: 0.000 | Pos: 88 | LR_A: 0.000000 | LR_C: 0.000000\nEpisode: 4 | Achieve | Running_r: 271 | Epi_r: 269.90 | Exploration: 0.000 | Pos: 88 | LR_A: 0.000000 | LR_C: 0.000000\nEpisode: 5 | Achieve | Running_r: 271 | Epi_r: 268.49 | Exploration: 0.000 | Pos: 88 | LR_A: 0.000000 | LR_C: 0.000000\nEpisode: 6 | Achieve | Running_r: 271 | Epi_r: 271.28 | Exploration: 0.000 | Pos: 88 | LR_A: 0.000000 | LR_C: 0.000000\nEpisode: 7 | Achieve | Running_r: 271 | Epi_r: 269.52 | Exploration: 0.000 | Pos: 88 | LR_A: 0.000000 | LR_C: 0.000000\nEpisode: 8 | Achieve | Running_r: 271 | Epi_r: 270.98 | Exploration: 0.000 | Pos: 88 | LR_A: 0.000000 | LR_C: 0.000000\nEpisode: 9 | Achieve | Running_r: 271 | Epi_r: 270.82 | Exploration: 0.000 | Pos: 88 | LR_A: 0.000000 | LR_C: 0.000000\nEpisode: 10 | Achieve | Running_r: 271 | Epi_r: 268.31 | Exploration: 0.000 | Pos: 88 | LR_A: 0.000000 | LR_C: 0.000000\n"
]
]
] | [
"markdown",
"code",
"markdown",
"code",
"markdown",
"code"
] | [
[
"markdown",
"markdown"
],
[
"code"
],
[
"markdown",
"markdown",
"markdown"
],
[
"code"
],
[
"markdown"
],
[
"code"
]
] |
e770d22e07d5d1ce06f0fe13fbeaffb0c31b4462 | 182,110 | ipynb | Jupyter Notebook | Practice/Practice1.ipynb | Mentors4EDU/Python-Notebooks | 73fbf6b4eaca09f4ec94d555bb0acad18684bc3a | [
"MIT"
] | null | null | null | Practice/Practice1.ipynb | Mentors4EDU/Python-Notebooks | 73fbf6b4eaca09f4ec94d555bb0acad18684bc3a | [
"MIT"
] | null | null | null | Practice/Practice1.ipynb | Mentors4EDU/Python-Notebooks | 73fbf6b4eaca09f4ec94d555bb0acad18684bc3a | [
"MIT"
] | null | null | null | 329.90942 | 68,476 | 0.905826 | [
[
[
"import pandas as pd\ndf = pd.read_csv(\"Downloads/LaptopSales.csv\")\nprint(df)",
" Date Configuration Customer Postcode Store Postcode \\\n0 1/1/2008 0:01 163 EC4V 5BH SE1 2BN \n1 1/1/2008 0:02 320 SW4 0JL SW12 9HD \n2 1/1/2008 0:04 23 EC3V 1LR E2 0RY \n3 1/1/2008 0:04 169 SW1P 3AU SE1 2BN \n4 1/1/2008 0:06 365 EC4V 4EG SW1V 4QQ \n... ... ... ... ... \n297567 12/30/2008 23:55 703 SE1 2UP SW1P 3AU \n297568 12/30/2008 23:55 731 N13 4JD N3 1DH \n297569 12/30/2008 23:57 375 SE25 6EF CR7 8LE \n297570 12/30/2008 23:58 101 SW8 1LA SW1P 3AU \n297571 12/30/2008 23:58 343 SE16 4QZ SE8 3JD \n\n Retail Price Screen Size (Inches) Battery Life (Hours) RAM (GB) \\\n0 455.0 15 5 1 \n1 545.0 15 6 1 \n2 515.0 15 4 1 \n3 395.0 15 5 1 \n4 585.0 15 6 2 \n... ... ... ... ... \n297567 NaN 17 5 4 \n297568 392.0 17 6 1 \n297569 441.0 15 6 2 \n297570 406.0 15 4 4 \n297571 530.0 15 6 2 \n\n Processor Speeds (GHz) Integrated Wireless? HD Size (GB) \\\n0 2.0 Yes 80 \n1 2.0 No 300 \n2 2.0 Yes 300 \n3 2.0 No 40 \n4 2.0 No 120 \n... ... ... ... \n297567 2.0 No 300 \n297568 1.5 No 80 \n297569 2.4 Yes 300 \n297570 1.5 Yes 120 \n297571 1.5 Yes 300 \n\n Bundled Applications? customer X customer Y store X store Y \n0 Yes 532041 180995 534057.0 179682.0 \n1 No 529240 175537 528739.0 173080.0 \n2 Yes 533095 181047 535652.0 182961.0 \n3 Yes 529902 179641 534057.0 179682.0 \n4 Yes 531684 180948 528924.0 178440.0 \n... ... ... ... ... ... \n297567 Yes 533595 180025 529902.0 179641.0 \n297568 Yes 531165 192973 525109.0 190628.0 \n297569 Yes 534086 168521 532714.0 168302.0 \n297570 Yes 530716 177488 529902.0 179641.0 \n297571 Yes 534348 179162 537175.0 177885.0 \n\n[297572 rows x 16 columns]\n"
],
[
"df = pd.read_csv('Downloads/LaptopSales.csv', dtype=str)",
"_____no_output_____"
],
[
"missing_values = [\"n/a\", \"na\", \"--\"]\ndf = pd.read_csv(\"Downloads/LaptopSales.csv\", na_values = missing_values)\nprint(df['Retail Price'])",
"0 455.0\n1 545.0\n2 515.0\n3 395.0\n4 585.0\n ... \n297567 NaN\n297568 392.0\n297569 441.0\n297570 406.0\n297571 530.0\nName: Retail Price, Length: 297572, dtype: float64\n"
],
[
"missing_values = [\"n/a\", \"na\", \"--\"]\ndf = pd.read_csv(\"Downloads/LaptopSales.csv\", na_values = missing_values)\nprint(df['Bundled Applications?'])",
"0 Yes\n1 No\n2 Yes\n3 Yes\n4 Yes\n ... \n297567 Yes\n297568 Yes\n297569 Yes\n297570 Yes\n297571 Yes\nName: Bundled Applications?, Length: 297572, dtype: object\n"
],
[
"d = {'col1': [1, 2], 'col2': [3, 4]}\ndf = pd.DataFrame(data=d)\ndf.dtypes\ndtype: object",
"_____no_output_____"
],
[
"df = pd.read_csv(\"Downloads/LaptopSales.csv\")\ndef calculate_mean(n):\n s = sum(\"Retail Price\")\n N = len(n)\n mean = s / N\n return mean\nprint(mean)",
"<function mean at 0x7f862fe5fdd0>\n"
],
[
"import numpy as np\nimport matplotlib.pyplot as plt\nfrom scipy.stats import norm",
"_____no_output_____"
],
[
"import csv\nimport nltk",
"_____no_output_____"
],
[
"import pandas as pd\ndf = pd.read_csv (r'Downloads/LaptopSales.csv')\nmean1 = df['Retail Price'].mean()\nsum1 = df['Retail Price'].sum()\nmax1 = df['Retail Price'].max()\nmin1 = df['Retail Price'].min()\ncount1 = df['Retail Price'].count()\nmedian1 = df['Retail Price'].median() \nstd1 = df['Retail Price'].std() \nvar1 = df['Retail Price'].var() \nprint ('Mean price ' + str(mean1))\nprint ('Sum of price: ' + str(sum1))\nprint ('Max price: ' + str(max1))\nprint ('Min price: ' + str(min1))\nprint ('Count of prices: ' + str(count1))\nprint ('Median price: ' + str(median1))\nprint ('Std of prices: ' + str(std1))\nprint ('Var of sprice: ' + str(var1))\n# Source: https://datatofish.com/use-pandas-to-calculate-stats-from-an-imported-csv-file/\ngroupby_mean1 = df.groupby(['Store Postcode']).mean() \ngroupby_count1 = df.groupby(['Store Postcode']).count()\ngroupby_mean2 = df.groupby(['Integrated Wireless?']).mean() \ngroupby_count2 = df.groupby(['Integrated Wireless?']).count()\nprint ('Sum of values, grouped by store: ' + str(groupby_mean1))\nprint ('Sum of values, grouped by wireless?: ' + str(groupby_mean2))",
"Mean price 508.12593575453405\nSum of price: 144373314.0\nMax price: 890.0\nMin price: 168.0\nCount of prices: 284129\nMedian price: 500.0\nStd of prices: 104.61178904404392\nVar of sprice: 10943.626406995547\nSum of values, grouped by store: Configuration Retail Price Screen Size (Inches) \\\nStore Postcode \nCR7 8LE 379.596019 471.828844 15.801072 \nE2 0RY 379.262774 520.186446 15.807829 \nE7 8NW 374.561380 466.946338 15.799262 \nKT2 5AU 382.545769 521.514872 15.820844 \nN17 6QA 381.658022 522.943285 15.817626 \nN3 1DH 386.736632 471.305644 15.840917 \nNW5 2QH 382.514822 521.081869 15.822544 \nS1P 3AU 394.189189 512.084507 15.878378 \nSE1 2BN 378.857376 520.028106 15.809611 \nSE8 3JD 381.562097 520.706581 15.814703 \nSW12 9HD 381.230448 521.222406 15.819773 \nSW18 1NN 378.419224 520.333191 15.809179 \nSW1P 3AU 379.833595 469.919544 15.814413 \nSW1V 4QQ 380.267252 520.359460 15.812339 \nW10 6HQ 382.010057 520.046876 15.815478 \nW4 3PH 379.367222 469.218710 15.806299 \n\n Battery Life (Hours) RAM (GB) Processor Speeds (GHz) \\\nStore Postcode \nCR7 8LE 4.983540 2.108332 1.935549 \nE2 0RY 4.973198 2.096688 1.932751 \nE7 8NW 4.958904 2.080084 1.925685 \nKT2 5AU 4.978095 2.112520 1.918446 \nN17 6QA 4.972170 2.118701 1.934177 \nN3 1DH 4.975423 2.109930 1.928263 \nNW5 2QH 4.975577 2.095749 1.928874 \nS1P 3AU 4.979730 2.094595 1.910811 \nSE1 2BN 4.968157 2.098173 1.932721 \nSE8 3JD 4.979090 2.108466 1.926725 \nSW12 9HD 4.966809 2.115421 1.928910 \nSW18 1NN 4.966255 2.093919 1.934953 \nSW1P 3AU 4.968317 2.097492 1.930566 \nSW1V 4QQ 4.973615 2.097534 1.932347 \nW10 6HQ 4.981931 2.096991 1.931544 \nW4 3PH 4.979055 2.085614 1.935117 \n\n HD Size (GB) customer X customer Y store X store Y \nStore Postcode \nCR7 8LE 133.548552 533456.899834 169430.070946 532714.0 168302.0 \nE2 0RY 133.161168 534659.607581 182051.479370 535652.0 182961.0 \nE7 8NW 130.089568 540738.618546 184929.938883 541428.0 184515.0 \nKT2 5AU 131.367305 518835.566059 171278.788564 517917.0 170243.0 \nN17 6QA 131.537002 532585.718954 187615.972591 533788.0 189994.0 \nN3 1DH 127.120558 526529.338758 188172.724344 525109.0 190628.0 \nNW5 2QH 132.085337 529777.960420 182985.656806 529248.0 185213.0 \nS1P 3AU 147.162162 531746.371622 179742.858108 NaN NaN \nSE1 2BN 132.013615 532787.513548 180657.067225 534057.0 179682.0 \nSE8 3JD 130.906900 536600.161012 177738.370111 537175.0 177885.0 \nSW12 9HD 130.766320 531385.559760 171352.760249 528739.0 173080.0 \nSW18 1NN 133.407218 524827.332481 173245.441319 525155.0 175180.0 \nSW1P 3AU 132.231405 530518.215943 181080.108489 529902.0 179641.0 \nSW1V 4QQ 131.757913 529612.209261 180288.726353 528924.0 178440.0 \nW10 6HQ 130.946902 525155.015597 181342.246570 524190.0 181567.0 \nW4 3PH 132.517964 519234.183152 174915.857973 519585.0 177640.0 \nSum of values, grouped by wireless?: Configuration Retail Price Screen Size (Inches) \\\nIntegrated Wireless? \nNo 390.695215 502.398237 15.831000 \nYes 369.632329 513.912599 15.795498 \n\n Battery Life (Hours) RAM (GB) Processor Speeds (GHz) \\\nIntegrated Wireless? \nNo 4.983835 2.124953 1.935290 \nYes 4.960281 2.072821 1.927022 \n\n HD Size (GB) customer X customer Y \\\nIntegrated Wireless? \nNo 132.902660 530753.257494 179892.870183 \nYes 131.138297 530754.337897 179867.651786 \n\n store X store Y \nIntegrated Wireless? \nNo 530647.522622 179765.153983 \nYes 530645.860008 179731.724218 \n"
],
[
"d = pd.read_csv('Downloads/LaptopSales.csv')\ndata= d['Configuration']\nprices = d['Retail Price']\nplt.scatter(data, prices, edgecolors='r')\nplt.xlabel('Configuration')\nplt.ylabel('Prices')\nplt.title('Rise in Prices')\nplt.show()\n# Source: https://medium.com/python-pandemonium/data-visualization-in-python-scatter-plots-in-matplotlib-da90ac4c99f9",
"_____no_output_____"
],
[
"d = pd.read_csv('Downloads/LaptopSales.csv')\ndata= d['Screen Size (Inches)']\nprices = d['Retail Price']\nplt.scatter(data, prices, edgecolors='r')\nplt.xlabel('Screen Size (Inches)')\nplt.ylabel('Prices')\nplt.title('Rise in Prices')\nplt.show()",
"_____no_output_____"
],
[
"d = pd.read_csv('Downloads/LaptopSales.csv')\ndata= d['Battery Life (Hours)']\nprices = d['Retail Price']\nplt.scatter(data, prices, edgecolors='r')\nplt.xlabel('Battery Life (Hours)')\nplt.ylabel('Prices')\nplt.title('Rise in Prices')\nplt.show()",
"_____no_output_____"
],
[
"d = pd.read_csv('Downloads/LaptopSales.csv')\ndata= d['RAM (GB)']\nprices = d['Retail Price']\nplt.scatter(data, prices, edgecolors='r')\nplt.xlabel('RAM (GB)')\nplt.ylabel('Prices')\nplt.title('Rise in Prices')\nplt.show()",
"_____no_output_____"
],
[
"d = pd.read_csv('Downloads/LaptopSales.csv')\ndata= d['Processor Speeds (GHz)']\nprices = d['Retail Price']\nplt.scatter(data, prices, edgecolors='r')\nplt.xlabel('Processor Speeds (GHz)')\nplt.ylabel('Prices')\nplt.title('Rise in Prices')\nplt.show()",
"_____no_output_____"
],
[
"d = pd.read_csv('Downloads/LaptopSales.csv')\ndata= d['HD Size (GB)']\nprices = d['Retail Price']\nplt.scatter(data, prices, edgecolors='r')\nplt.xlabel('HD Size (GB)')\nplt.ylabel('Prices')\nplt.title('Rise in Prices')\nplt.show()",
"_____no_output_____"
],
[
"# Regarding Questions #\nprint('Data types are str, float64, and object')\nprint('Some of the data has missing values and it shows w n/a, such as 297567 NaN')\nprint('The average price of the laptops sold is 508.12593575453405 which is rounded to 508, the median price is 500.0')\nprint('Prices do vary between stores, and I used store postal code to sort them out. The cheapest store is postal code E7 8NW, while more expensive seems to be N17 6QA')\nprint('The price varies slightly w/ the integrated wireless feature')\nprint('The scatter plots were helpful to an extent, and for sake of ease seperated them by category')\nprint('The most expesive features seem to be screen size and RAM. Configurations seem to be incremental as well as others')",
"Data types are str, float64, and object\nSome of the data has missing values and it shows w n/a, such as 297567 NaN\nThe average price of the laptops sold is 508.12593575453405 which is rounded to 508, the median price is 500.0\nPrices do vary between stores, and I used store postal code to sort them out. The cheapest store is postal code E7 8NW, while more expensive seems to be N17 6QA\nThe price varies slightly w/ the integrated wireless feature\nThe scatter plots were helpful to an extent, and for sake of ease seperated them by category\nThe most expesive features seem to be screen size and RAM. Configurations seem to be incremental as well as others\n"
]
]
] | [
"code"
] | [
[
"code",
"code",
"code",
"code",
"code",
"code",
"code",
"code",
"code",
"code",
"code",
"code",
"code",
"code",
"code",
"code"
]
] |
e770d4cedd80e932634c4ee2794fc0fc838264c8 | 41,383 | ipynb | Jupyter Notebook | ES Module 3 Soln.ipynb | ds-modules/ETHSTD-21AC- | ef1362ed19c537beb241e8f1545f3a49ffb3a5a4 | [
"MIT"
] | 4 | 2017-09-13T18:07:57.000Z | 2017-11-06T18:28:17.000Z | ES Module 3 Soln.ipynb | ds-modules/ETHSTD-21AC- | ef1362ed19c537beb241e8f1545f3a49ffb3a5a4 | [
"MIT"
] | null | null | null | ES Module 3 Soln.ipynb | ds-modules/ETHSTD-21AC- | ef1362ed19c537beb241e8f1545f3a49ffb3a5a4 | [
"MIT"
] | null | null | null | 31.857583 | 496 | 0.478385 | [
[
[
"# ES Module 3",
"_____no_output_____"
],
[
"Welcome to Module 3!\n\nLast time, we went over:\n 1. Strings and Intergers\n 2. Arrays\n 3. Tables\n \nToday we will continue working with tables, and introduce a new procedure called filtering. Before you start, run the following cell.",
"_____no_output_____"
]
],
[
[
"# Loading our libraries, i.e. tool box for our module\nimport numpy as np\nfrom datascience import *",
"_____no_output_____"
]
],
[
[
"### Paired Programming \nToday we want to introduce a new system of work called paired programming. Wikipedia defines paired programming in the following way:\n\nPair programming is an agile software development technique in which two programmers work together at one workstation. One, the driver, writes code while the other, the observer or navigator, reviews each line of code as it is typed in. The two programmers switch roles frequently.\n\nThis methodolgy is quite known in the computer science realm, and we want to try and see how well it would work in our little class room. Hopefully we would all benefit from this, by closing the gap between more experienced coders and less so we could move forward to more advanced topics! Additionally, there is always the benefit of having a friend when all hell breaks loose (or the code just would not work..)\n\nSo after this brief introduction, please team up with a class-mate, hopefully someone you did not know from before that is at a slightly different level of programming experience.",
"_____no_output_____"
],
[
"Please start now, as one takes the controls and the other is reviewing the code.",
"_____no_output_____"
],
[
"## 0. Comments",
"_____no_output_____"
],
[
"Comments are ways of making your code more human readable. It's good practice to add comments to your code so someone else reading your code can get an idea of what's going on. \n\nYou can add a comment to your code by preceeding it with a `#` symbol. When the computer sees any line preceeded by a `#` symbol, it'll ignore it. Here's an example below: ",
"_____no_output_____"
]
],
[
[
"# Calculating the total number of pets in my house.\nnum_cats = 4\nnum_dogs = 10\ntotal = num_cats + num_dogs\ntotal",
"_____no_output_____"
]
],
[
[
"Now, write a comment in the cell below explaining what it is doing, then run the cell to see if you're correct.",
"_____no_output_____"
]
],
[
[
"animals = make_array('Cat', 'Dog', 'Bird', 'Spider')\nnum_legs = make_array(4, 4, 2, 8)\nmy_table = Table().with_columns('Animal', animals,\n 'Number of Legs', num_legs)\nmy_table",
"_____no_output_____"
]
],
[
[
"## 1. Tables (Continued) ",
"_____no_output_____"
],
[
"It is time to practice tables again. We want to load the table files you have uploaded last module. This time, you do it by yourself. Load the table \"inmates_by_year.csv\" and \"correctional_population.csv\" and assign it to a variable. Remember, to load a table we use `Table.read_table()` and pass the name of the table as an argument to the function.",
"_____no_output_____"
]
],
[
[
"inmates_by_year = Table.read_table('inmates_by_year.csv')\ncorrectional_population = Table.read_table('correctional_population.csv')\n",
"_____no_output_____"
]
],
[
[
"Good job! Now we have all the tables loaded. \n\nIt is time to extract some information from these tables!\nIn the next several cells, we would guide you through a quick manipulation that will allow us to extract information about the entire correctional population using both tables we have loaded above.",
"_____no_output_____"
],
[
"In the correctional_population table, we are given data about the number of supervised per 100,000 U.S. adult residents. That means that if we want to have the approximated number of the entire population under supervision we need to multiply by 100,000.",
"_____no_output_____"
]
],
[
[
"# First, extract the column name \"Number supervised per 100,000 U.S. adult residents/c\" from \n# the correctional_population table and assign it to the variable provided.\nc_p = correctional_population.column('Number supervised per 100,000 U.S. adult residents/c')\nc_p",
"_____no_output_____"
]
],
[
[
"#### filtering\nWhen you run the cell above, you may notice that the values in our array are actually strings (you can tell because each value has quotation marks around it). However, we can't do mathematical operations on strings, so we'll have to convert this array first so it has integers instead of strings. This is called filtering, or cleaning the data, so we can actually do some work on it. In the following cells, when you see the `# filtering` sign, know that we have yet to cover this topic.\n\nRun the following cell to do clean the table. We'll go over how to do this in a later section of this module. If you have any questions about how it works, feel free to ask any of us!",
"_____no_output_____"
]
],
[
[
"# filtering\ndef string_to_int(val):\n return int(val.replace(',', ''))\n\nc_p = correctional_population.apply(string_to_int, 'Number supervised per 100,000 U.S. adult residents/c')",
"_____no_output_____"
]
],
[
[
"Now, let's continue finding the real value of c_p. ",
"_____no_output_____"
]
],
[
[
"# In this cell, multiply the correctional population column name \"Number supervised per 100,000 U.S. adult residents/c\" \n# by 100000 and assign it to a new variable (c_p stands for correctional population)\nreal_c_p = c_p * 100000\nreal_c_p",
"_____no_output_____"
]
],
[
[
"Next we want to assign the Total column from inmates_by_year to a variable in order to be able to operate on it.",
"_____no_output_____"
]
],
[
[
"total_inmates = inmates_by_year.column('Total')\ntotal_inmates",
"_____no_output_____"
]
],
[
[
"Again, run the following line to convert the values in `total_inmates` to ints.",
"_____no_output_____"
]
],
[
[
"# filtering \ntotal_inmates = inmates_by_year.apply(string_to_int, 'Total')\ntotal_inmates",
"_____no_output_____"
]
],
[
[
"#### Switch position, the navigator now takes the wheel.",
"_____no_output_____"
],
[
"Now that we have the variables holding all the information we want to manipulate, we can start digging into it.\n\nWe want to come up with a scheme that will allow us to see the precentage of people that are incarcerated, from the total supervised population, by year.\n\nBefore we do that, though, examine your two variables, `total_inmates` and `real_c_p` and their corresponding tables. Do you foresee any issues with directly comparing these two tables? \n\nThe `correctional_population` table has a row corresponding to 2000, which `inmates_by_year` does not have. This not only means that the data from our two tables doesn't match up, but also that our arrays are two different lengths. Recall that we cannot do operations on arrays with different lengths. \n\nTo fix this, run the following cell, in which we get rid of the value corresponding to the year 2000 from `real_c_p`. Again, if you have questions about how this works, feel free to ask us! ",
"_____no_output_____"
]
],
[
[
"# filtering\nreal_c_p = real_c_p.take(np.arange(1, real_c_p.size))\nreal_c_p",
"_____no_output_____"
]
],
[
[
"Now our arrays both correspond to data from the same years and we can do operations with both of them!",
"_____no_output_____"
]
],
[
[
"# Write a short code that stores the precentage of people incarcerated from the supervised population \n# (rel stands for relative, c_p stands from correctional population)\ninmates_rel_c_p = (total_inmates / real_c_p) * 100\ninmates_rel_c_p",
"_____no_output_____"
]
],
[
[
"Now, this actually gives us useful information!\nWhy not write it down? Please write down what this information tells you about the judicial infrastructure - we are looking for more mathy/dry explanation (rather than observation of how poorly it is). ",
"_____no_output_____"
]
],
[
[
"# A simple sentence will suffice, we want to see intuitive understanding. Please call a teacher when done to check!\nextract_information_shows = \"The percentage of people, supervisied by US adult correctional system, who are incarcerated\"",
"_____no_output_____"
]
],
[
[
"For a final touch, please sort inmates_rel_c_p by descending order in the next cell. We won't tell you how to sort, this time please check the last lab module on how to sort a table. It is an important quality of a programmer to be able to reuse code you already have. \n\nHint: Remember that you can only use `sort` on tables. How might you manipulate your array so that you can sort it?",
"_____no_output_____"
]
],
[
[
"# Please sort inmates_rel_c_p in descending order and print it out\ninmates_rel_c_p = Table().with_columns('Inmate_percentage', inmates_rel_c_p)\ninmates_rel_c_p.sort('Inmate_percentage',descending = True)\ninmates_rel_c_p",
"_____no_output_____"
]
],
[
[
"#### Before starting, please switch positions",
"_____no_output_____"
],
[
"## Filtering",
"_____no_output_____"
],
[
"Right now, we can't really get much extra information from our tables other than by sorting them. In this section, we'll learn how to filter our data so we can get more useful insights from it. This is especially useful when dealing with larger data sets!\n\nFor example, say we wanted insights about the total number of inmates after 2012. We can find this out using the `where` function. Check out the cell below for an example of how to use this. ",
"_____no_output_____"
]
],
[
[
"inmates_by_year.where('Year', are.above(2012))",
"_____no_output_____"
]
],
[
[
"Notice that `where` takes in two arguments: the name of the column, and the condition we are filtering by. \n\nNow, try it for yourself! In the cell below, filter `correctional_population` so it only includes years after 2008. ",
"_____no_output_____"
],
[
"If you run the following cell, you'll find a complete description of all such conditions (which we'll call predicates) that you can pass into where. This information can also be found [here](https://www.inferentialthinking.com/chapters/05/2/selecting-rows.html).",
"_____no_output_____"
]
],
[
[
"functions = make_array('are.equal_to(Z)', 'are.above(x)', 'are.above_or_equal_to(x)', 'are.below(x)', \n 'are.below_or_equal_to(x)', 'are.between(x, y)', 'are.strictly_between(x, y)',\n 'are.between_or_equal_to(x, y)', 'are.containing(S)')\ndescriptions = make_array('Equal to Z', 'Greater than x', 'Greater than or equal to x', 'Below x', \n 'Less than or equal to x', 'Greater than or equal to x, and less than y',\n 'Greater than x and less than y', 'Greater than or equal to x, and less than or equal to y',\n 'Contains the string S')\npredicates = Table().with_columns('Predicate', functions,\n 'Description', descriptions)\npredicates",
"_____no_output_____"
]
],
[
[
"Now, we'll be using filtering to gain more insights about our two tables. Before we start, be sure to run the following cell so we can ensure every column we're working with is numerical.",
"_____no_output_____"
]
],
[
[
"inmates_by_year = inmates_by_year.drop('Total').with_column('Total', total_inmates).select('Year', 'Total', 'Standard error/a')\ncorrectional_population = correctional_population.drop('Number supervised per 100,000 U.S. adult residents/c').with_column('Number supervised per 100,000 U.S. adult residents/c', c_p).select('Year', 'Number supervised per 100,000 U.S. adult residents/c', 'U.S. adult residents under correctional supervision ').relabel('U.S. adult residents under correctional supervision ', 'U.S. adult residents under correctional supervision')\n",
"_____no_output_____"
]
],
[
[
"First, find the mean of the total number of inmates. Hint: You can use the `np.mean()` function on arrays to calculate this.",
"_____no_output_____"
]
],
[
[
"avg_inmates = np.mean(inmates_by_year.column('Total'))\navg_inmates",
"_____no_output_____"
]
],
[
[
"Now, filter `inmates_by_year` to find data for the years in which the number of total inmates was under the average.",
"_____no_output_____"
]
],
[
[
"filtered_inmates = inmates_by_year.where('Total', are.below(avg_inmates))\nfiltered_inmates",
"_____no_output_____"
]
],
[
[
"What does this tell you about the total inmate population? Write your answer in the cell below.",
"_____no_output_____"
]
],
[
[
"answer = \"YOUR TEXT HERE\"",
"_____no_output_____"
]
],
[
[
"#### Before continuing, please switch positions.",
"_____no_output_____"
],
[
"Now, similarly, find the average number of adults under correctional supervision, and filter the table to find the years in which the number of adults under correctional supervision was under the average.",
"_____no_output_____"
]
],
[
[
"avg = np.mean(correctional_population.column('Number supervised per 100,000 U.S. adult residents/c'))\nfiltered_c_p = correctional_population.where('Number supervised per 100,000 U.S. adult residents/c', are.below(avg))\nfiltered_c_p",
"_____no_output_____"
]
],
[
[
"Do the years match up? Does this make sense based on the proportions you calculated above in `inmates_rel_c_p`?",
"_____no_output_____"
]
],
[
[
"answer = \"YOUR TEXT HERE\"",
"_____no_output_____"
]
],
[
[
"Now, from `correctional_population`, filter the table so the value of U.S. adult residents under correctional supervision is 1 in 31. Remember, the values in this column are strings.",
"_____no_output_____"
]
],
[
[
"c_p_1_in_34 = correctional_population.where('U.S. adult residents under correctional supervision', are.containing('1 in 31'))\nc_p_1_in_34",
"_____no_output_____"
]
],
[
[
"Now, we have one last challenge exercise. Before doing this, finish the challenge exercises from last module. We highly encourage you to work with your partner on this one.\n\nIn the following cell, find the year with the max number of supervised adults for which the proportion of US adult residents under correctional supervision was 1 in 32. ",
"_____no_output_____"
]
],
[
[
"one_in_32 = correctional_population.where('U.S. adult residents under correctional supervision', are.containing('1 in 32'))\none_in_32_sorted = one_in_32.sort('Number supervised per 100,000 U.S. adult residents/c', descending = True)\nyear = one_in_32_sorted.column('Year').item(0)\nyear",
"_____no_output_____"
]
],
[
[
"Congratulations, you're done with this module! Before you leave, please fill out this [link](https://docs.google.com/a/berkeley.edu/forms/d/1KQHzw-rh_E--lnQ7ItLrOcH7WJUTexDiKMLcuPwClzo/edit?usp=drive_web) to give us feedback on how we can make these modules more useful for you.",
"_____no_output_____"
]
]
] | [
"markdown",
"code",
"markdown",
"code",
"markdown",
"code",
"markdown",
"code",
"markdown",
"code",
"markdown",
"code",
"markdown",
"code",
"markdown",
"code",
"markdown",
"code",
"markdown",
"code",
"markdown",
"code",
"markdown",
"code",
"markdown",
"code",
"markdown",
"code",
"markdown",
"code",
"markdown",
"code",
"markdown",
"code",
"markdown",
"code",
"markdown",
"code",
"markdown",
"code",
"markdown",
"code",
"markdown",
"code",
"markdown",
"code",
"markdown"
] | [
[
"markdown",
"markdown"
],
[
"code"
],
[
"markdown",
"markdown",
"markdown",
"markdown"
],
[
"code"
],
[
"markdown"
],
[
"code"
],
[
"markdown",
"markdown"
],
[
"code"
],
[
"markdown",
"markdown"
],
[
"code"
],
[
"markdown"
],
[
"code"
],
[
"markdown"
],
[
"code"
],
[
"markdown"
],
[
"code"
],
[
"markdown"
],
[
"code"
],
[
"markdown",
"markdown"
],
[
"code"
],
[
"markdown"
],
[
"code"
],
[
"markdown"
],
[
"code"
],
[
"markdown"
],
[
"code"
],
[
"markdown",
"markdown",
"markdown"
],
[
"code"
],
[
"markdown",
"markdown"
],
[
"code"
],
[
"markdown"
],
[
"code"
],
[
"markdown"
],
[
"code"
],
[
"markdown"
],
[
"code"
],
[
"markdown"
],
[
"code"
],
[
"markdown",
"markdown"
],
[
"code"
],
[
"markdown"
],
[
"code"
],
[
"markdown"
],
[
"code"
],
[
"markdown"
],
[
"code"
],
[
"markdown"
]
] |
e770dd34a791c6f7985610b824e50abfce3851d5 | 162,497 | ipynb | Jupyter Notebook | climate_starter.ipynb | RShailza/sqlalchemy-challenge | 46d0772c247889d8e6759d945e82b3b76cc4201b | [
"MIT"
] | null | null | null | climate_starter.ipynb | RShailza/sqlalchemy-challenge | 46d0772c247889d8e6759d945e82b3b76cc4201b | [
"MIT"
] | null | null | null | climate_starter.ipynb | RShailza/sqlalchemy-challenge | 46d0772c247889d8e6759d945e82b3b76cc4201b | [
"MIT"
] | null | null | null | 63.450605 | 53,712 | 0.689342 | [
[
[
"%matplotlib inline\nfrom matplotlib import style\nstyle.use('fivethirtyeight')\nimport matplotlib.pyplot as plt",
"_____no_output_____"
],
[
"import numpy as np\nimport pandas as pd",
"_____no_output_____"
],
[
"import datetime as dt",
"_____no_output_____"
]
],
[
[
"# Reflect Tables into SQLAlchemy ORM",
"_____no_output_____"
]
],
[
[
"# Python SQL toolkit and Object Relational Mapper\nimport sqlalchemy\nfrom sqlalchemy.ext.automap import automap_base\nfrom sqlalchemy.orm import Session\nfrom sqlalchemy import create_engine, func\nfrom sqlalchemy import create_engine, inspect",
"_____no_output_____"
],
[
"engine = create_engine(\"sqlite:///Resources/hawaii.sqlite\")",
"_____no_output_____"
],
[
"# reflect an existing database into a new model\n\nBase = automap_base()\n\n# reflect the tables\n\nBase.prepare(engine, reflect=True)",
"_____no_output_____"
],
[
"# We can view all of the classes that automap found\n\nBase.classes.keys()",
"_____no_output_____"
],
[
"# Save references to each table\nMeasurement = Base.classes.measurement\n\nStation = Base.classes.station",
"_____no_output_____"
],
[
"# Create our session (link) from Python to the DB\n\nsession = Session(engine)",
"_____no_output_____"
],
[
"m_table = session.query(Measurement).first()\nm_table.__dict__",
"_____no_output_____"
],
[
"#measurements table rows\n\nfor row in session.query(Measurement.id, Measurement.date, Measurement.tobs, Measurement.prcp, Measurement.station).limit(10).all():\n print(row)",
"(1, '2010-01-01', 65.0, 0.08, 'USC00519397')\n(2, '2010-01-02', 63.0, 0.0, 'USC00519397')\n(3, '2010-01-03', 74.0, 0.0, 'USC00519397')\n(4, '2010-01-04', 76.0, 0.0, 'USC00519397')\n(5, '2010-01-06', 73.0, None, 'USC00519397')\n(6, '2010-01-07', 70.0, 0.06, 'USC00519397')\n(7, '2010-01-08', 64.0, 0.0, 'USC00519397')\n(8, '2010-01-09', 68.0, 0.0, 'USC00519397')\n(9, '2010-01-10', 73.0, 0.0, 'USC00519397')\n(10, '2010-01-11', 64.0, 0.01, 'USC00519397')\n"
],
[
"s_table = session.query(Station).first()\ns_table.__dict__",
"_____no_output_____"
],
[
"for row in session.query(Station.id, Station.name, Station.station, Station.longitude, Station.latitude, Station.elevation).all():\n print(row)",
"(1, 'WAIKIKI 717.2, HI US', 'USC00519397', -157.8168, 21.2716, 3.0)\n(2, 'KANEOHE 838.1, HI US', 'USC00513117', -157.8015, 21.4234, 14.6)\n(3, 'KUALOA RANCH HEADQUARTERS 886.9, HI US', 'USC00514830', -157.8374, 21.5213, 7.0)\n(4, 'PEARL CITY, HI US', 'USC00517948', -157.9751, 21.3934, 11.9)\n(5, 'UPPER WAHIAWA 874.3, HI US', 'USC00518838', -158.0111, 21.4992, 306.6)\n(6, 'WAIMANALO EXPERIMENTAL FARM, HI US', 'USC00519523', -157.71139, 21.33556, 19.5)\n(7, 'WAIHEE 837.5, HI US', 'USC00519281', -157.84888999999998, 21.45167, 32.9)\n(8, 'HONOLULU OBSERVATORY 702.2, HI US', 'USC00511918', -157.9992, 21.3152, 0.9)\n(9, 'MANOA LYON ARBO 785.2, HI US', 'USC00516128', -157.8025, 21.3331, 152.4)\n"
]
],
[
[
"# OR",
"_____no_output_____"
]
],
[
[
"# Create the inspector and connect it to the engine\ninspector = inspect(engine)\n\n# Collect the names of tables within the database\ninspector.get_table_names()\n",
"_____no_output_____"
],
[
"# Using the inspector to print the column names within the 'measuremnts' table and its types\ncolumns1 = inspector.get_columns('measurements')\n\nfor column in columns1:\n print(column[\"name\"], column[\"type\"])",
"_____no_output_____"
],
[
"# Using the inspector to print the column names within the 'station' table and its types\ncolumns2 = inspector.get_columns('station')\n\nfor column in columns2:\n print(column[\"name\"], column[\"type\"])",
"id INTEGER\nstation TEXT\nname TEXT\nlatitude FLOAT\nlongitude FLOAT\nelevation FLOAT\n"
]
],
[
[
"# Exploratory Climate Analysis",
"_____no_output_____"
],
[
"--------------------------------------------------------------------------------------------------------------------------",
"_____no_output_____"
],
[
"********************* Precipitation Analysis ********************* ",
"_____no_output_____"
],
[
"--------------------------------------------------------------------------------------------------------------------------",
"_____no_output_____"
]
],
[
[
"# Design a query to retrieve the last 12 months of precipitation data and plot the results\n\n#calulation the last date.\nsession.query(Measurement.date).order_by(Measurement.date.desc()).first()",
"_____no_output_____"
],
[
"# Calculate the date 1 year ago from the last data point in the database\nyear_ago_date= dt.date(2017, 8, 23) - dt.timedelta(days=366)\nprint('Query Date:', year_ago_date)\n",
"Query Date: 2016-08-22\n"
],
[
"# Perform a query to retrieve the data and precipitation scores\n\nprcp_date = session.query(Measurement.date, Measurement.prcp).\\\n filter(func.strftime('%Y-%m-%d',Measurement.date) > year_ago_date).order_by(Measurement.date).all()\nprcp_date\n ",
"_____no_output_____"
],
[
"# Save the query results as a Pandas DataFrame and set the index to the date column\n\nprcp_df = pd.DataFrame(prcp_date, columns=['date', 'prcp'])\nprcp_df.set_index('date', inplace = True)",
"_____no_output_____"
],
[
"# Sort the dataframe by date\n\nsort_df = prcp_df.sort_values('date')\nsort_df\n",
"_____no_output_____"
],
[
"prcp_df.plot(title=\"Precipitation Analysis\", figsize=(12,8))\nplt.legend(loc='upper center')\n#plt.savefig(\"Images/precipitation.png\")\nplt.tight_layout()\n\nplt.show()\n",
"_____no_output_____"
],
[
"# Use Pandas to calcualte the summary statistics for the precipitation data\nprcp_df.describe()",
"_____no_output_____"
]
],
[
[
"--------------------------------------------------------------------------------------------------------------------------",
"_____no_output_____"
],
[
"********************* Station Analysis ********************* ",
"_____no_output_____"
],
[
"--------------------------------------------------------------------------------------------------------------------------",
"_____no_output_____"
]
],
[
[
"# Design a query to show how many stations are available in this dataset?\nnumber_of_stations = session.query(Station).count()\nnumber_of_stations",
"_____no_output_____"
],
[
"# What are the most active stations? (i.e. what stations have the most rows)?\n# List the stations and the counts in descending order.\nactive_stations = (session.query(Measurement.station, func.count(Measurement.station))\n .group_by(Measurement.station)\n .order_by(func.count(Measurement.station).desc()).all())\nactive_stations",
"_____no_output_____"
],
[
"# Using the station id from the previous query, calculate the lowest temperature recorded, \n# highest temperature recorded, and average temperature of the most active station?\n\ntobs = [Measurement.station, func.min(Measurement.tobs),\n func.max(Measurement.tobs),func.avg(Measurement.tobs)]\n\nactiveStation = session.query(*tobs).filter(Measurement.station=='USC00519281').all()\nactiveStation",
"_____no_output_____"
],
[
"pd.DataFrame(activeStation, columns=['station', 'min_temp', 'max_temp', 'avg_temp']).set_index('station')",
"_____no_output_____"
],
[
"# Choose the station with the highest number of temperature observations.\n# Query the last 12 months of temperature observation data for this station and plot the results as a histogram\n#year_high",
"_____no_output_____"
],
[
"# Choose the station with the highest number of temperature observations.\n# Query the last 12 months of temperature observation data for this station and plot the results as a histogram\nyear_high_temp =(session.query(Measurement.date,(Measurement.tobs))\n .filter(func.strftime(Measurement.date) > year_ago_date)\n .filter(Measurement.station=='USC00519281')\n .all())\nyear_high_temp",
"_____no_output_____"
],
[
"tobs_df = pd.DataFrame(year_high_temp, columns=['date', 'temp']) \ntobs_df.set_index('date', inplace = True)\n",
"_____no_output_____"
],
[
"plt.rcParams['figure.figsize']=(10,7)\n\nplt.hist(tobs_df['temp'], bins=12, alpha=0.6 )\n\nplt.title('Temperature Observation Aug 2016 - Aug 2017\\nHonolulu, Hawaii',fontsize=20)\nplt.xlabel('Temperature (F)',fontsize=16)\nplt.ylabel('Frequency',fontsize=16)\nplt.xticks(fontsize=12)\nplt.yticks(fontsize=12)\nplt.ylim(0,70)\n\nplt.show()",
"_____no_output_____"
]
],
[
[
"## Bonus Challenge Assignment",
"_____no_output_____"
]
],
[
[
"# This function called `calc_temps` will accept start date and end date in the format '%Y-%m-%d' \n# and return the minimum, average, and maximum temperatures for that range of dates\ndef calc_temps(start_date, end_date):\n \"\"\"TMIN, TAVG, and TMAX for a list of dates.\n \n Args:\n start_date (string): A date string in the format %Y-%m-%d\n end_date (string): A date string in the format %Y-%m-%d\n \n Returns:\n TMIN, TAVE, and TMAX\n \"\"\"\n \n return session.query(func.min(Measurement.tobs), func.avg(Measurement.tobs), func.max(Measurement.tobs)).\\\n filter(Measurement.date >= start_date).filter(Measurement.date <= end_date).all()\n\n# function usage example\nprint(calc_temps('2012-02-28', '2012-03-05'))",
"[(62.0, 69.57142857142857, 74.0)]\n"
],
[
"# Use your previous function `calc_temps` to calculate the tmin, tavg, and tmax \n# for your trip using the previous year's data for those same dates.\n",
"_____no_output_____"
],
[
"# Plot the results from your previous query as a bar chart. \n# Use \"Trip Avg Temp\" as your Title\n# Use the average temperature for the y value\n# Use the peak-to-peak (tmax-tmin) value as the y error bar (yerr)\n",
"_____no_output_____"
],
[
"# Calculate the total amount of rainfall per weather station for your trip dates using the previous year's matching dates.\n# Sort this in descending order by precipitation amount and list the station, name, latitude, longitude, and elevation\n\n",
"_____no_output_____"
],
[
"# Create a query that will calculate the daily normals \n# (i.e. the averages for tmin, tmax, and tavg for all historic data matching a specific month and day)\n\ndef daily_normals(date):\n \"\"\"Daily Normals.\n \n Args:\n date (str): A date string in the format '%m-%d'\n \n Returns:\n A list of tuples containing the daily normals, tmin, tavg, and tmax\n \n \"\"\"\n \n sel = [func.min(Measurement.tobs), func.avg(Measurement.tobs), func.max(Measurement.tobs)]\n return session.query(*sel).filter(func.strftime(\"%m-%d\", Measurement.date) == date).all()\n \ndaily_normals(\"01-01\")",
"_____no_output_____"
],
[
"# calculate the daily normals for your trip\n# push each tuple of calculations into a list called `normals`\n\n# Set the start and end date of the trip\n\n# Use the start and end date to create a range of dates\n\n# Stip off the year and save a list of %m-%d strings\n\n# Loop through the list of %m-%d strings and calculate the normals for each date\n",
"_____no_output_____"
],
[
"# Load the previous query results into a Pandas DataFrame and add the `trip_dates` range as the `date` index\n",
"_____no_output_____"
],
[
"# Plot the daily normals as an area plot with `stacked=False`\n",
"_____no_output_____"
]
],
[
[
"# ROUGH WORK FOR APP.PY \n",
"_____no_output_____"
]
],
[
[
"# from flask import Flask, jsonify\n",
"_____no_output_____"
],
[
"# def precipitation():\n# # Create session (link) from Python to the DB\n# session = Session(engine)\n\n# # Query Measurement\n# results = (session.query(Measurement.date, Measurement.prcp)\n# .order_by(Measurement.date))\n \n# # Create a dictionary\n# precipitation_date = []\n# for each_row in results:\n# dt_dict = {}\n# dt_dict[\"date\"] = each_row.date\n# dt_dict[\"prcp\"] = each_row.prcp\n# precipitation_date.append(dt_dict)\n\n# # return jsonify(precipitation_date)\n# return(precipitation_date)",
"_____no_output_____"
],
[
"# precipitation()",
"_____no_output_____"
],
[
"# #def tobs():\n \n# #create a session\n# session3 = Session(engine)\n\n# # Query measurement for latest datre \n# last_date = session3.query(Measurement.date).order_by(Measurement.date.desc()).first()\n\n# print(last_date)\n# last_12mnth = (dt.datetime.strptime(last_date[0],'%Y-%m-%d') -dt.timedelta(days=365)).date()\n# print(last_12mnth)\n\n# # year_ago_date= dt.date(2017, 8, 23) - dt.timedelta(days=366)\n# # # print('Query Date:', year_ago_date)\n\n# tobs_results = session3.query(Measurement.date, Measurement.tobs).\\\n# filter(Measurement.date >= last_12mnth).order_by(Measurement.date).all()\n\n# # Create a list of dicts with `date` and `tobs` as the keys and values\n# tobs_totals = []\n# for result in tobs_results:\n# row = {}\n# row[\"date\"] = result[0]\n# row[\"tobs\"] = result[1]\n# tobs_totals.append(row)\n\n# tobs_totals",
"_____no_output_____"
],
[
"# def start_date(start):\n# # print(\"start_date status:OK\")\n# #convert the tsring from user to date\n# start_date = dt.datetime.strptime(start, '%Y-%m-%d').date()\n# last_date_dd = (dt.datetime.strptime(last_date[0][0], '%Y-%m-%d')).date() \n# first_date_dd = (dt.datetime.strptime(first_date[0][0], '%Y-%m-%d')).date()\n# #if fgiven start_date greater than last or lesser than first available date in dataset, print the following \n# if start_date > last_date_dd or start_date < first_date_dd:\n# return(f\"Select date range between {first_date[0][0]} and {last_date[0][0]}\")\n# else:\n# #Return a JSON list of the minimum temperature, the average temperature, \n# #and the max temperature for a given start range.\n# start_min_max_temp = session.query(func.min(Measurement.tobs), func.avg(Measurement.tobs),\\\n# func.max(Measurement.tobs)).filter(Measurement.date >= start_date).all()\n# start_date_data = list(np.ravel(start_min_max_temp))\n# #return jsonify(start_date_data)\n \n \n",
"_____no_output_____"
]
]
] | [
"code",
"markdown",
"code",
"markdown",
"code",
"markdown",
"code",
"markdown",
"code",
"markdown",
"code",
"markdown",
"code"
] | [
[
"code",
"code",
"code"
],
[
"markdown"
],
[
"code",
"code",
"code",
"code",
"code",
"code",
"code",
"code",
"code",
"code"
],
[
"markdown"
],
[
"code",
"code",
"code"
],
[
"markdown",
"markdown",
"markdown",
"markdown"
],
[
"code",
"code",
"code",
"code",
"code",
"code",
"code"
],
[
"markdown",
"markdown",
"markdown"
],
[
"code",
"code",
"code",
"code",
"code",
"code",
"code",
"code"
],
[
"markdown"
],
[
"code",
"code",
"code",
"code",
"code",
"code",
"code",
"code"
],
[
"markdown"
],
[
"code",
"code",
"code",
"code",
"code"
]
] |
e770e479ebe922a50a36bdce0300d0da79c4d607 | 13,499 | ipynb | Jupyter Notebook | test/notebooks/Interact Manual Tests.ipynb | JobJob/Interact.jl | a35440f45b429ed7a9b19c98b55e174380a75524 | [
"MIT"
] | null | null | null | test/notebooks/Interact Manual Tests.ipynb | JobJob/Interact.jl | a35440f45b429ed7a9b19c98b55e174380a75524 | [
"MIT"
] | null | null | null | test/notebooks/Interact Manual Tests.ipynb | JobJob/Interact.jl | a35440f45b429ed7a9b19c98b55e174380a75524 | [
"MIT"
] | null | null | null | 27.492872 | 128 | 0.56019 | [
[
[
"empty"
]
]
] | [
"empty"
] | [
[
"empty"
]
] |
e770e75ae1f1668a7748672b28dde6d5fb131e19 | 33,991 | ipynb | Jupyter Notebook | 03-NLP/introNLTK.ipynb | holabayor/datascience | 50cdd9c328ac50754bfeab15494af900a3027443 | [
"Apache-2.0"
] | 37 | 2016-09-25T12:35:31.000Z | 2021-11-25T16:57:01.000Z | 03-NLP/introNLTK.ipynb | saini12/datascience | dad8cbf80cf3cdd38bee32e6b1e10d87c9c2f1b0 | [
"Apache-2.0"
] | 1 | 2018-03-29T00:21:23.000Z | 2018-03-31T10:10:47.000Z | 03-NLP/introNLTK.ipynb | saini12/datascience | dad8cbf80cf3cdd38bee32e6b1e10d87c9c2f1b0 | [
"Apache-2.0"
] | 35 | 2016-01-23T12:48:19.000Z | 2021-03-03T23:03:45.000Z | 24.471562 | 331 | 0.512606 | [
[
[
"# Introduction to NLTK\n\nWe have seen how to do [some basic text processing in Python](https://github.com/Mashimo/datascience/blob/master/03-NLP/helloworld-nlp.ipynb), now we introduce an open source framework for natural language processing that can further help to work with human languages: [NLTK (Natural Language ToolKit)](http://www.nltk.org/).",
"_____no_output_____"
],
[
"## Tokenise a text",
"_____no_output_____"
],
[
"Let's start with a simple text in a Python string:",
"_____no_output_____"
]
],
[
[
"sampleText1 = \"The Elephant's 4 legs: THE Pub! You can't believe it or can you, the believer?\"\nsampleText2 = \"Pierre Vinken, 61 years old, will join the board as a nonexecutive director Nov. 29.\"",
"_____no_output_____"
]
],
[
[
"### Tokens",
"_____no_output_____"
],
[
"The basic atomic part of each text are the tokens. A token is the NLP name for a sequence of characters that we want to treat as a group.\nWe have seen how we can extract tokens by splitting the text at the blank spaces. \nNTLK has a function word_tokenize() for it:",
"_____no_output_____"
]
],
[
[
"import nltk",
"_____no_output_____"
],
[
"s1Tokens = nltk.word_tokenize(sampleText1)\ns1Tokens",
"_____no_output_____"
],
[
"len(s1Tokens)",
"_____no_output_____"
]
],
[
[
"21 tokens extracted, which include words and punctuation. \nNote that the tokens are different than what a split by blank spaces would obtained, e.g. \"can't\" is by NTLK considered TWO tokens: \"can\" and \"n't\" (= \"not\") while a tokeniser that splits text by spaces would consider it a single token: \"can't\". \nLet's see another example: ",
"_____no_output_____"
]
],
[
[
"s2Tokens = nltk.word_tokenize(sampleText2)\ns2Tokens",
"_____no_output_____"
]
],
[
[
"And we can apply it to an entire book, \"The Prince\" by Machiavelli that we used last time:",
"_____no_output_____"
]
],
[
[
"# If you would like to work with the raw text you can use 'bookRaw'\nwith open('../datasets/ThePrince.txt', 'r') as f:\n bookRaw = f.read()",
"_____no_output_____"
],
[
"bookTokens = nltk.word_tokenize(bookRaw)\nbookText = nltk.Text(bookTokens) # special format\nnBookTokens= len(bookTokens) # or alternatively len(bookText)",
"_____no_output_____"
],
[
"print (\"*** Analysing book ***\") \nprint (\"The book is {} chars long\".format (len(bookRaw)))\nprint (\"The book has {} tokens\".format (nBookTokens))",
"*** Analysing book ***\nThe book is 300814 chars long\nThe book has 59792 tokens\n"
]
],
[
[
"As mentioned above, the NTLK tokeniser works in a more sophisticated way than just splitting by spaces, therefore we got this time more tokens.",
"_____no_output_____"
],
[
"## Sentences\n\nNTLK has a function to tokenise a text not in words but in sentences. ",
"_____no_output_____"
]
],
[
[
"text1 = \"This is the first sentence. A liter of milk in the U.S. costs $0.99. Is this the third sentence? Yes, it is!\"\nsentences = nltk.sent_tokenize(text1)\nlen(sentences)",
"_____no_output_____"
],
[
"sentences",
"_____no_output_____"
]
],
[
[
"As you see, it is not splitting just after each full stop but check if it's part of an acronym (U.S.) or a number (0.99). \nIt also splits correctly sentences after question or exclamation marks but not after commas.",
"_____no_output_____"
]
],
[
[
"sentences = nltk.sent_tokenize(bookRaw) # extract sentences\nnSent = len(sentences)\nprint (\"The book has {} sentences\".format (nSent))\nprint (\"and each sentence has in average {} tokens\".format (nBookTokens / nSent))",
"The book has 1416 sentences\nand each sentence has in average 42.22598870056497 tokens\n"
]
],
[
[
"### Most common tokens\n\nWhat are the 20 most frequently occurring (unique) tokens in the text? What is their frequency?\n\nThe NTLK FreqDist class is used to encode “frequency distributions”, which count the number of times that something occurs, for example a token.\n\nIts `most_common()` method then returns a list of tuples where each tuple is of the form `(token, frequency)`. The list is sorted in descending order of frequency.",
"_____no_output_____"
]
],
[
[
"def get_top_words(tokens):\n # Calculate frequency distribution\n fdist = nltk.FreqDist(tokens)\n return fdist.most_common()",
"_____no_output_____"
],
[
"topBook = get_top_words(bookTokens)\n # Output top 20 words\ntopBook[:20]",
"_____no_output_____"
]
],
[
[
"Comma is the most common: we need to remove the punctuation.",
"_____no_output_____"
],
[
"### Most common alphanumeric tokens\n\nWe can use `isalpha()` to check if the token is a word and not punctuation.",
"_____no_output_____"
]
],
[
[
"topWords = [(freq, word) for (word,freq) in topBook if word.isalpha() and freq > 400]\ntopWords",
"_____no_output_____"
]
],
[
[
"We can also remove any capital letters before tokenising:",
"_____no_output_____"
]
],
[
[
"def preprocessText(text, lowercase=True):\n if lowercase:\n tokens = nltk.word_tokenize(text.lower())\n else:\n tokens = nltk.word_tokenize(text)\n\n return [word for word in tokens if word.isalpha()]",
"_____no_output_____"
],
[
"bookWords = preprocessText(bookRaw)",
"_____no_output_____"
],
[
"topBook = get_top_words(bookWords)\n# Output top 20 words\ntopBook[:20]",
"_____no_output_____"
],
[
"print (\"*** Analysing book ***\") \nprint (\"The text has now {} words (tokens)\".format (len(bookWords)))",
"*** Analysing book ***\nThe text has now 52202 words (tokens)\n"
]
],
[
[
"Now we removed the punctuation and the capital letters but the most common token is \"the\", not a significative word ... \nAs we have seen last time, these are so-called **stop words** that are very common and are normally stripped from a text when doing these kind of analysis.",
"_____no_output_____"
],
[
"### Meaningful most common tokens\n\nA simple approach could be to filter the tokens that have a length greater than 5 and frequency of more than 150.",
"_____no_output_____"
]
],
[
[
"meaningfulWords = [word for (word,freq) in topBook if len(word) > 5 and freq > 80]\nsorted(meaningfulWords)",
"_____no_output_____"
]
],
[
[
"This would work but would leave out also tokens such as `I` and `you` which are actually significative. \nThe better approach - that we have seen earlier how - is to remove stopwords using external files containing the stop words. \nNLTK has a corpus of stop words in several languages:",
"_____no_output_____"
]
],
[
[
"from nltk.corpus import stopwords",
"_____no_output_____"
],
[
"stopwordsEN = set(stopwords.words('english')) # english language",
"_____no_output_____"
],
[
"betterWords = [w for w in bookWords if w not in stopwordsEN]",
"_____no_output_____"
],
[
"topBook = get_top_words(betterWords)\n# Output top 20 words\ntopBook[:20]",
"_____no_output_____"
]
],
[
[
"Now we excluded words such as `the` but we can improve further the list by looking at semantically similar words, such as plural and singular versions.",
"_____no_output_____"
]
],
[
[
"'princes' in betterWords",
"_____no_output_____"
],
[
"betterWords.count(\"prince\") + betterWords.count(\"princes\") ",
"_____no_output_____"
]
],
[
[
"## Stemming",
"_____no_output_____"
],
[
"Above, in the list of words we have both `prince` and `princes` which are respectively the singular and plural version of the same word (the **stem**). The same would happen with verb conjugation (`love` and `loving` are considered different words but are actually *inflections* of the same verb). \n**Stemmer** is the tool that reduces such inflectional forms into their stem, base or root form and NLTK has several of them (each with a different heuristic algorithm).",
"_____no_output_____"
]
],
[
[
"input1 = \"List listed lists listing listings\"\nwords1 = input1.lower().split(' ')\nwords1",
"_____no_output_____"
]
],
[
[
"And now we apply one of the NLTK stemmer, the Porter stemmer:",
"_____no_output_____"
]
],
[
[
"porter = nltk.PorterStemmer()\n[porter.stem(t) for t in words1]",
"_____no_output_____"
]
],
[
[
"As you see, all 5 different words have been reduced to the same stem and would be now the same lexical token.",
"_____no_output_____"
]
],
[
[
"stemmedWords = [porter.stem(w) for w in betterWords]\ntopBook = get_top_words(stemmedWords)\ntopBook[:20] # Output top 20 words",
"_____no_output_____"
]
],
[
[
"Now the word `princ` is counted 281 times, exactly like the sum of prince and princes. \n\nA note here: Stemming usually refers to a crude heuristic process that chops off the ends of words in the hope of achieving this goal correctly most of the time, and often includes the removal of derivational affixes. \n`Prince` and `princes` become `princ`. \nA different flavour is the **lemmatisation** that we will see in one second, but first a note about stemming in other languages than English.",
"_____no_output_____"
],
[
"### Stemming in other languages",
"_____no_output_____"
],
[
"**`Snowball`** is an improvement created by Porter: a language to create stemmers and have rules for many more languages than English. \nFor example Italian:",
"_____no_output_____"
]
],
[
[
"from nltk.stem.snowball import SnowballStemmer\nstemmerIT = SnowballStemmer(\"italian\")",
"_____no_output_____"
],
[
"inputIT = \"Io ho tre mele gialle, tu hai una mela gialla e due pere verdi\"\nwordsIT = inputIT.split(' ')",
"_____no_output_____"
],
[
"[stemmerIT.stem(w) for w in wordsIT]",
"_____no_output_____"
]
],
[
[
"## Lemma\nLemmatization usually refers to doing things properly with the use of a vocabulary and morphological analysis of words, normally aiming to remove inflectional endings only and to return the **base or dictionary form of a word, which is known as the lemma**. \nWhile a stemmer operates on a single word without knowledge of the context, a lemmatiser can take the context in consideration. ",
"_____no_output_____"
],
[
"NLTK has also a built-in lemmatiser, so let's see it in action:",
"_____no_output_____"
]
],
[
[
"from nltk.stem import WordNetLemmatizer\n\nlemmatizer = WordNetLemmatizer()",
"_____no_output_____"
],
[
"words1",
"_____no_output_____"
],
[
"[lemmatizer.lemmatize(w, 'n') for w in words1] # n = nouns",
"_____no_output_____"
]
],
[
[
"We tell the lemmatise that the words are nouns. In this case it considers the same lemma words such as list (singular noun) and lists (plural noun) but leave as they are the other words.",
"_____no_output_____"
]
],
[
[
"[lemmatizer.lemmatize(w, 'v') for w in words1] # v = verbs",
"_____no_output_____"
]
],
[
[
"We get a different result if we say that the words are verbs. \nThey have all the same lemma, in fact they could be all different inflections or conjugation of a verb.",
"_____no_output_____"
],
[
"The type of words that can be used are: \n'n' = noun, 'v'=verb, 'a'=adjective, 'r'=adverb",
"_____no_output_____"
]
],
[
[
"words2 = ['good', 'better']",
"_____no_output_____"
],
[
"[porter.stem(w) for w in words2]",
"_____no_output_____"
],
[
"[lemmatizer.lemmatize(w, 'a') for w in words2] ",
"_____no_output_____"
]
],
[
[
"It works with different adjectives, it doesn't look only at prefixes and suffixes. \nYou would wonder why stemmers are used, instead of always using lemmatisers: stemmers are much simpler, smaller and faster and for many applications good enough.",
"_____no_output_____"
],
[
"Now we lemmatise the book:",
"_____no_output_____"
]
],
[
[
"lemmatisedWords = [lemmatizer.lemmatize(w, 'n') for w in betterWords]\ntopBook = get_top_words(lemmatisedWords)\ntopBook[:20] # Output top 20 words",
"_____no_output_____"
]
],
[
[
"Yes, the lemma now is `prince`. \nBut note that we consider all words in the book as nouns, while actually a proper way would be to apply the correct type to each single word.",
"_____no_output_____"
],
[
"## Part of speech (PoS)\n\nIn traditional grammar, a part of speech (abbreviated form: PoS or POS) is a category of words which have similar grammatical properties. \n\nFor example, an adjective (red, big, quiet, ...) describe properties while a verb (throw, walk, have) describe actions or states.\n\nCommonly listed parts of speech are noun, verb, adjective, adverb, pronoun, preposition, conjunction, interjection.",
"_____no_output_____"
]
],
[
[
"text1 = \"Children shouldn't drink a sugary drink before bed.\"\ntokensT1 = nltk.word_tokenize(text1)\nnltk.pos_tag(tokensT1)",
"_____no_output_____"
]
],
[
[
"The NLTK function `pos_tag()` will tag each token with the estimated PoS. \nNLTK has 13 categories of PoS. You can check the acronym using the NLTK help function: ",
"_____no_output_____"
]
],
[
[
"nltk.help.upenn_tagset('RB')",
"RB: adverb\n occasionally unabatingly maddeningly adventurously professedly\n stirringly prominently technologically magisterially predominately\n swiftly fiscally pitilessly ...\n"
]
],
[
[
"Which are the most common PoS in The Prince book?",
"_____no_output_____"
]
],
[
[
"tokensAndPos = nltk.pos_tag(bookTokens)\nposList = [thePOS for (word, thePOS) in tokensAndPos]\nfdistPos = nltk.FreqDist(posList)\nfdistPos.most_common(5)",
"_____no_output_____"
],
[
"nltk.help.upenn_tagset('IN')",
"IN: preposition or conjunction, subordinating\n astride among uppon whether out inside pro despite on by throughout\n below within for towards near behind atop around if like until below\n next into if beside ...\n"
]
],
[
[
"It's not nouns (NN) but interections (IN) such as preposition or conjunction.",
"_____no_output_____"
],
[
"## Extra note: Parsing the grammar structure",
"_____no_output_____"
],
[
"Words can be ambiguous and sometimes is not easy to understand which kind of POS is a word, for example in the sentence \"visiting aunts can be a nuisance\", is visiting a verb or an adjective? \nTagging a PoS depends on the context, which can be ambiguous.",
"_____no_output_____"
],
[
"Making sense of a sentence is easier if it follows a well-defined grammatical structure, such as : subject + verb + object \nNLTK allows to define a formal grammar which can then be used to parse a text. The NLTK ChartParser is a procedure for finding one or more trees (sentences have internal organisation that can be represented using a tree) corresponding to a grammatically well-formed sentence.",
"_____no_output_____"
]
],
[
[
"# Parsing sentence structure\ntext2 = nltk.word_tokenize(\"Alice loves Bob\")\ngrammar = nltk.CFG.fromstring(\"\"\"\nS -> NP VP\nVP -> V NP\nNP -> 'Alice' | 'Bob'\nV -> 'loves'\n\"\"\")\n\nparser = nltk.ChartParser(grammar)\ntrees = parser.parse_all(text2)\nfor tree in trees:\n print(tree)",
"(S (NP Alice) (VP (V loves) (NP Bob)))\n"
]
],
[
[
"This is a \"toy grammar,\" a small grammar that illustrate the key aspects of parsing. But there is an obvious question as to whether the approach can be scaled up to cover large corpora of natural languages. How hard would it be to construct such a set of productions by hand? In general, the answer is: very hard. \nNevertheless, there are efforts to develop broad-coverage grammars, such as weighted and probabilistic grammars.",
"_____no_output_____"
],
[
"## The world outside NLTK",
"_____no_output_____"
],
[
"As a final note, NLTK was used here for educational purpose but you should be aware that has its own limitations. \nNLTK is a solid library but it's old and slow. Especially the NLTK's lemmatisation functionality is slow enough that it will become the bottleneck in almost any application that will use it.\n\nFor industrial NLP application a very performance-minded Python library is [SpaCy.io](https://spacy.io/) instead. \nAnd for robust multi-lingual support there is [polyglot](http://polyglot.readthedocs.io/en/latest/) that has a much wider language support of all the above.",
"_____no_output_____"
],
[
"Other tools exist in other computer languages such as Stanford CoreNLP and Apache OpenNLP, both in Java.",
"_____no_output_____"
]
]
] | [
"markdown",
"code",
"markdown",
"code",
"markdown",
"code",
"markdown",
"code",
"markdown",
"code",
"markdown",
"code",
"markdown",
"code",
"markdown",
"code",
"markdown",
"code",
"markdown",
"code",
"markdown",
"code",
"markdown",
"code",
"markdown",
"code",
"markdown",
"code",
"markdown",
"code",
"markdown",
"code",
"markdown",
"code",
"markdown",
"code",
"markdown",
"code",
"markdown",
"code",
"markdown",
"code",
"markdown",
"code",
"markdown",
"code",
"markdown",
"code",
"markdown"
] | [
[
"markdown",
"markdown",
"markdown"
],
[
"code"
],
[
"markdown",
"markdown"
],
[
"code",
"code",
"code"
],
[
"markdown"
],
[
"code"
],
[
"markdown"
],
[
"code",
"code",
"code"
],
[
"markdown",
"markdown"
],
[
"code",
"code"
],
[
"markdown"
],
[
"code"
],
[
"markdown"
],
[
"code",
"code"
],
[
"markdown",
"markdown"
],
[
"code"
],
[
"markdown"
],
[
"code",
"code",
"code",
"code"
],
[
"markdown",
"markdown"
],
[
"code"
],
[
"markdown"
],
[
"code",
"code",
"code",
"code"
],
[
"markdown"
],
[
"code",
"code"
],
[
"markdown",
"markdown"
],
[
"code"
],
[
"markdown"
],
[
"code"
],
[
"markdown"
],
[
"code"
],
[
"markdown",
"markdown",
"markdown"
],
[
"code",
"code",
"code"
],
[
"markdown",
"markdown"
],
[
"code",
"code",
"code"
],
[
"markdown"
],
[
"code"
],
[
"markdown",
"markdown"
],
[
"code",
"code",
"code"
],
[
"markdown",
"markdown"
],
[
"code"
],
[
"markdown",
"markdown"
],
[
"code"
],
[
"markdown"
],
[
"code"
],
[
"markdown"
],
[
"code",
"code"
],
[
"markdown",
"markdown",
"markdown",
"markdown"
],
[
"code"
],
[
"markdown",
"markdown",
"markdown",
"markdown"
]
] |
e77102194d4a0a97b05f38e269cc9b00225f5f66 | 3,370 | ipynb | Jupyter Notebook | udacity_ml/software_engineering/holiday_gifts/optimizing_code_holiday_gifts.ipynb | issagaliyeva/machine_learning | 63f4d39a95147cdac4ef760cb47dffc318793a99 | [
"MIT"
] | null | null | null | udacity_ml/software_engineering/holiday_gifts/optimizing_code_holiday_gifts.ipynb | issagaliyeva/machine_learning | 63f4d39a95147cdac4ef760cb47dffc318793a99 | [
"MIT"
] | null | null | null | udacity_ml/software_engineering/holiday_gifts/optimizing_code_holiday_gifts.ipynb | issagaliyeva/machine_learning | 63f4d39a95147cdac4ef760cb47dffc318793a99 | [
"MIT"
] | null | null | null | 27.622951 | 428 | 0.581306 | [
[
[
"# Optimizing Code: Holiday Gifts\nIn the last example, you learned that using vectorized operations and more efficient data structures can optimize your code. Let's use these tips for one more example.\n\nSay your online gift store has one million users that each listed a gift on a wish list. You have the prices for each of these gifts stored in `gift_costs.txt`. For the holidays, you're going to give each customer their wish list gift for free if it is under 25 dollars. Now, you want to calculate the total cost of all gifts under 25 dollars to see how much you'd spend on free gifts. Here's one way you could've done it.",
"_____no_output_____"
]
],
[
[
"import time\nimport numpy as np",
"_____no_output_____"
],
[
"with open('gift_costs.txt') as f:\n gift_costs = f.read().split('\\n')\n \ngift_costs = np.array(gift_costs).astype(int) # convert string to int",
"_____no_output_____"
],
[
"start = time.time()\n\ntotal_price = 0\nfor cost in gift_costs:\n if cost < 25:\n total_price += cost * 1.08 # add cost after tax\n\nprint(total_price)\nprint('Duration: {} seconds'.format(time.time() - start))",
"32765421.24\nDuration: 6.560739994049072 seconds\n"
]
],
[
[
"Here you iterate through each cost in the list, and check if it's less than 25. If so, you add the cost to the total price after tax. This works, but there is a much faster way to do this. Can you refactor this to run under half a second?",
"_____no_output_____"
],
[
"## Refactor Code\n**Hint:** Using numpy makes it very easy to select all the elements in an array that meet a certain condition, and then perform operations on them together all at once. You can them find the sum of what those values end up being.",
"_____no_output_____"
]
],
[
[
"start = time.time()\n\ntotal_price = np.sum(gift_costs[gift_costs < 25] * 1.08) # compute the total price\n\nprint(total_price)\nprint('Duration: {} seconds'.format(time.time() - start))",
"32765421.24\nDuration: 0.09631609916687012 seconds\n"
]
]
] | [
"markdown",
"code",
"markdown",
"code"
] | [
[
"markdown"
],
[
"code",
"code",
"code"
],
[
"markdown",
"markdown"
],
[
"code"
]
] |
e771060a85f911cd95eb9ca1cd0a7b809e225f39 | 111,166 | ipynb | Jupyter Notebook | 1st_DNN.ipynb | joyjeni/-Learn-Artificial-Intelligence-with-TensorFlow | 8ae05456241a3ead3dcb83dd315797380d7acacf | [
"MIT"
] | null | null | null | 1st_DNN.ipynb | joyjeni/-Learn-Artificial-Intelligence-with-TensorFlow | 8ae05456241a3ead3dcb83dd315797380d7acacf | [
"MIT"
] | null | null | null | 1st_DNN.ipynb | joyjeni/-Learn-Artificial-Intelligence-with-TensorFlow | 8ae05456241a3ead3dcb83dd315797380d7acacf | [
"MIT"
] | null | null | null | 159.035765 | 83,538 | 0.846401 | [
[
[
"<a href=\"https://colab.research.google.com/github/joyjeni/-Learn-Artificial-Intelligence-with-TensorFlow/blob/master/1st_DNN.ipynb\" target=\"_parent\"><img src=\"https://colab.research.google.com/assets/colab-badge.svg\" alt=\"Open In Colab\"/></a>",
"_____no_output_____"
],
[
"# **Import Libraries and modules**",
"_____no_output_____"
]
],
[
[
"# https://keras.io/\n!pip install -q keras\nimport keras",
"_____no_output_____"
],
[
"import numpy as np\n\nfrom keras.models import Sequential\nfrom keras.layers import Dense, Dropout, Activation, Flatten, Add\nfrom keras.layers import Convolution2D, MaxPooling2D\nfrom keras.utils import np_utils\n\nfrom keras.datasets import mnist",
"Using TensorFlow backend.\n"
]
],
[
[
"### Load pre-shuffled MNIST data into train and test sets",
"_____no_output_____"
]
],
[
[
"(X_train, y_train), (X_test, y_test) = mnist.load_data()",
"Downloading data from https://s3.amazonaws.com/img-datasets/mnist.npz\n11493376/11490434 [==============================] - 0s 0us/step\n"
],
[
"print (X_train.shape)\nfrom matplotlib import pyplot as plt\n%matplotlib inline\nplt.imshow(X_train[0])",
"(60000, 28, 28)\n"
],
[
"X_train = X_train.reshape(X_train.shape[0], 28, 28,1)\nX_test = X_test.reshape(X_test.shape[0], 28, 28,1)",
"_____no_output_____"
],
[
"X_train = X_train.astype('float32')\nX_test = X_test.astype('float32')\nX_train /= 255\nX_test /= 255",
"_____no_output_____"
],
[
"y_train[:10]",
"_____no_output_____"
],
[
"# Convert 1-dimensional class arrays to 10-dimensional class matrices\nY_train = np_utils.to_categorical(y_train, 10)\nY_test = np_utils.to_categorical(y_test, 10)",
"_____no_output_____"
],
[
"Y_train[:10]\n",
"_____no_output_____"
],
[
"from keras.layers import Activation\nmodel = Sequential()\n\n \nmodel.add(Convolution2D(32, 3, 3, activation='relu', input_shape=(28,28,1)))\nmodel.add(Convolution2D(10, 1, activation='relu'))\nmodel.add(Convolution2D(10, 26))\nmodel.add(Flatten())\nmodel.add(Activation('softmax'))",
"/usr/local/lib/python3.6/dist-packages/ipykernel_launcher.py:5: UserWarning: Update your `Conv2D` call to the Keras 2 API: `Conv2D(32, (3, 3), activation=\"relu\", input_shape=(28, 28, 1...)`\n \"\"\"\n"
],
[
"model.summary()",
"_________________________________________________________________\nLayer (type) Output Shape Param # \n=================================================================\nconv2d_1 (Conv2D) (None, 26, 26, 32) 320 \n_________________________________________________________________\nconv2d_2 (Conv2D) (None, 26, 26, 10) 330 \n_________________________________________________________________\nconv2d_3 (Conv2D) (None, 1, 1, 10) 67610 \n_________________________________________________________________\nflatten_1 (Flatten) (None, 10) 0 \n_________________________________________________________________\nactivation_1 (Activation) (None, 10) 0 \n=================================================================\nTotal params: 68,260\nTrainable params: 68,260\nNon-trainable params: 0\n_________________________________________________________________\n"
],
[
"model.compile(loss='categorical_crossentropy',\n optimizer='adam',\n metrics=['accuracy'])",
"_____no_output_____"
],
[
"model.fit(X_train, Y_train, batch_size=32, nb_epoch=10, verbose=1)",
"/usr/local/lib/python3.6/dist-packages/keras/models.py:942: UserWarning: The `nb_epoch` argument in `fit` has been renamed `epochs`.\n warnings.warn('The `nb_epoch` argument in `fit` '\n"
],
[
"score = model.evaluate(X_test, Y_test, verbose=0)",
"_____no_output_____"
],
[
"print(score)",
"[0.07641638516254279, 0.9829]\n"
],
[
"y_pred = model.predict(X_test)",
"_____no_output_____"
],
[
"print(y_pred[:9])\nprint(y_test[:9])",
"[[2.0593689e-17 6.3046323e-17 8.9005447e-13 1.8406407e-10 8.0754371e-19\n 8.0671096e-15 1.1510667e-24 1.0000000e+00 2.1262439e-10 2.7205176e-11]\n [2.5421015e-15 5.1460576e-12 1.0000000e+00 1.7606363e-12 6.3726551e-23\n 3.3248325e-16 4.4326671e-09 1.8222993e-27 1.8873889e-12 3.6800765e-21]\n [3.7568029e-08 9.9987435e-01 6.9531761e-06 6.3134886e-10 9.9738187e-05\n 5.2485571e-08 1.0483473e-07 1.0138228e-06 1.7757657e-05 1.9014761e-10]\n [1.0000000e+00 2.2739057e-15 6.6718070e-10 5.6225624e-15 4.5025926e-16\n 2.7407175e-13 1.3530634e-09 3.9892802e-13 5.8574483e-14 4.0855683e-12]\n [1.0115652e-12 6.9521903e-14 1.3467061e-13 1.9833676e-13 9.9999976e-01\n 4.2785103e-16 1.6022580e-12 5.5436229e-11 6.7551126e-10 2.2112354e-07]\n [2.3979660e-10 9.9982810e-01 3.5553690e-08 3.5910691e-11 1.0332796e-05\n 1.4169725e-10 2.0029900e-11 1.5314015e-04 8.3647601e-06 3.8377021e-10]\n [1.4182455e-19 9.8074493e-11 5.7774190e-09 2.3251079e-13 9.9966061e-01\n 6.0978769e-09 2.3871165e-16 2.2344653e-09 3.3876873e-04 5.9758122e-07]\n [7.2593749e-24 1.0864400e-09 7.5089712e-11 6.4676060e-12 5.3071453e-06\n 3.0447264e-08 5.8216405e-18 1.4018439e-14 9.3028554e-09 9.9999464e-01]\n [5.8572938e-11 5.5615773e-23 4.0553398e-19 1.0435163e-14 7.1205892e-15\n 3.1688964e-01 6.8311024e-01 4.5070697e-21 8.7986400e-08 1.6301742e-10]]\n[7 2 1 0 4 1 4 9 5]\n"
],
[
"layer_dict = dict([(layer.name, layer) for layer in model.layers])",
"_____no_output_____"
],
[
"import numpy as np\nfrom matplotlib import pyplot as plt\nfrom keras import backend as K\n%matplotlib inline\n# util function to convert a tensor into a valid image\ndef deprocess_image(x):\n # normalize tensor: center on 0., ensure std is 0.1\n x -= x.mean()\n x /= (x.std() + 1e-5)\n x *= 0.1\n\n # clip to [0, 1]\n x += 0.5\n x = np.clip(x, 0, 1)\n\n # convert to RGB array\n x *= 255\n #x = x.transpose((1, 2, 0))\n x = np.clip(x, 0, 255).astype('uint8')\n return x\n\ndef vis_img_in_filter(img = np.array(X_train[2]).reshape((1, 28, 28, 1)).astype(np.float64), \n layer_name = 'conv2d_14'):\n layer_output = layer_dict[layer_name].output\n img_ascs = list()\n for filter_index in range(layer_output.shape[3]):\n # build a loss function that maximizes the activation\n # of the nth filter of the layer considered\n loss = K.mean(layer_output[:, :, :, filter_index])\n\n # compute the gradient of the input picture wrt this loss\n grads = K.gradients(loss, model.input)[0]\n\n # normalization trick: we normalize the gradient\n grads /= (K.sqrt(K.mean(K.square(grads))) + 1e-5)\n\n # this function returns the loss and grads given the input picture\n iterate = K.function([model.input], [loss, grads])\n\n # step size for gradient ascent\n step = 5.\n\n img_asc = np.array(img)\n # run gradient ascent for 20 steps\n for i in range(20):\n loss_value, grads_value = iterate([img_asc])\n img_asc += grads_value * step\n\n img_asc = img_asc[0]\n img_ascs.append(deprocess_image(img_asc).reshape((28, 28)))\n \n if layer_output.shape[3] >= 35:\n plot_x, plot_y = 6, 6\n elif layer_output.shape[3] >= 23:\n plot_x, plot_y = 4, 6\n elif layer_output.shape[3] >= 11:\n plot_x, plot_y = 2, 6\n else:\n plot_x, plot_y = 1, 2\n fig, ax = plt.subplots(plot_x, plot_y, figsize = (12, 12))\n ax[0, 0].imshow(img.reshape((28, 28)), cmap = 'gray')\n ax[0, 0].set_title('Input image')\n fig.suptitle('Input image and %s filters' % (layer_name,))\n fig.tight_layout(pad = 0.3, rect = [0, 0, 0.9, 0.9])\n for (x, y) in [(i, j) for i in range(plot_x) for j in range(plot_y)]:\n if x == 0 and y == 0:\n continue\n ax[x, y].imshow(img_ascs[x * plot_y + y - 1], cmap = 'gray')\n ax[x, y].set_title('filter %d' % (x * plot_y + y - 1))\n\nvis_img_in_filter()",
"_____no_output_____"
],
[
"",
"_____no_output_____"
]
]
] | [
"markdown",
"code",
"markdown",
"code"
] | [
[
"markdown",
"markdown"
],
[
"code",
"code"
],
[
"markdown"
],
[
"code",
"code",
"code",
"code",
"code",
"code",
"code",
"code",
"code",
"code",
"code",
"code",
"code",
"code",
"code",
"code",
"code",
"code"
]
] |
e7710cc1e216abcd849f0dbacc400346f42a2592 | 126,110 | ipynb | Jupyter Notebook | project.ipynb | gcaracas/ds_project | 3304614dd7aa80ad5979a5b6db80ae0cdccc4463 | [
"MIT"
] | null | null | null | project.ipynb | gcaracas/ds_project | 3304614dd7aa80ad5979a5b6db80ae0cdccc4463 | [
"MIT"
] | null | null | null | project.ipynb | gcaracas/ds_project | 3304614dd7aa80ad5979a5b6db80ae0cdccc4463 | [
"MIT"
] | null | null | null | 125.732802 | 20,012 | 0.853945 | [
[
[
"import pandas as pd\nimport numpy as np\nimport matplotlib, matplotlib.pyplot as plt\nfrom matplotlib.ticker import MaxNLocator\nimport seaborn as sns",
"_____no_output_____"
],
[
"df=pd.read_csv(\"./PreK_project.csv\")\ndf.dropna(subset=['Student_ID'], how='all', inplace = True)",
"_____no_output_____"
],
[
"# We need to arrange our StudentID from float to int, and then to string\ndef convertToBetterIndex(df_t):\n strTbl=[]\n for a in df_t:\n strTbl.append(str(int(a)))\n return strTbl\n ",
"_____no_output_____"
],
[
"df['Student_ID']=convertToBetterIndex(df['Student_ID'].values)\ndf.set_index('Student_ID', inplace=True)",
"_____no_output_____"
],
[
"# As you can see, here we have a duplicate, and this is preventing us to replicate the brochure plot.\n# We will deal with this by removing this entry.\ndf.loc['164658334']",
"_____no_output_____"
],
[
"# Removing duplicate entries in index\ndf = df[~df.index.duplicated(keep='first')]",
"_____no_output_____"
],
[
"students=['945765668', '577523557','142728168','325383322','514537372','532834342','275522143','236438768',\n '912457324','492575653','286764562','982494857','2358183654','273972562','127648521',\n '834171656','852221239','643655377','872582144','666683785','855745397','989538648','183837445',\n '983366665','164658334','854644586','923951547','853248442','945653567','386148753','672424336',\n '227388697','296549775','618975655','733624274','867823644','823135663']\n# The following table contains all students that were part of the program prek\nprekst=df.loc[students]",
"_____no_output_____"
],
[
"noprk = df.index.isin(students)\n# The followint table contains students that were not in the prek program\nnonprek=df[~noprk]",
"_____no_output_____"
],
[
"def getMean(dataset, indexStr):\n # Remove all Nan in row/index\n dataset.dropna(subset=[indexStr], how='all', inplace = True)\n col=dataset[indexStr]\n return col.mean()",
"_____no_output_____"
]
],
[
[
"<body>\n <section style=\"background-color:White; font-family:Georgia;text-align:center\">\n <h2 style=\"font-family:Garamond; color:solid #229954\">Result 1</h2>\n <h3 style=\"font-family:Garamond;\">Replicating results from Boise Pre-K Program Evaluation 2017</h3>\n <h4 style=\"font-family:Garamond;\">Page 7</h4>\n <hr/>\n </section>\n</body>",
"_____no_output_____"
]
],
[
[
"print(\"Fall LSF No Vista Pre-k = \", getMean(nonprek.copy(), 'Fall_LSF'))\nprint(\"Fall LSF Vista Pre-k = \", getMean(prekst.copy(), 'Fall_LSF'))\nprint(\"Fall LNF No Vista Pre-k = \", getMean(nonprek.copy(), 'Fall_LNF'))\nprint(\"Fall LNF Vista Pre-k = \", getMean(prekst.copy(), 'Fall_LNF'))",
"Fall LSF No Vista Pre-k = 6.3375\nFall LSF Vista Pre-k = 13.409090909090908\nFall LNF No Vista Pre-k = 16.2\nFall LNF Vista Pre-k = 26.363636363636363\n"
],
[
"print(\"Winter LSF No Vista Pre-k = \", getMean(nonprek.copy(), 'Winter_LSF'))\nprint(\"Winter LSF Vista Pre-k = \", getMean(prekst.copy(), 'Winter_LSF'))\nprint(\"Winter LNF No Vista Pre-k = \", getMean(nonprek.copy(), 'Winter_LNF'))\nprint(\"Winter LNF Vista Pre-k = \", getMean(prekst.copy(), 'Winter_LNF'))",
"Winter LSF No Vista Pre-k = 25.22093023255814\nWinter LSF Vista Pre-k = 31.347826086956523\nWinter LNF No Vista Pre-k = 32.86046511627907\nWinter LNF Vista Pre-k = 36.52173913043478\n"
],
[
"print(\"Spring LSF No Vista Pre-k = \", getMean(nonprek.copy(), 'Spring_LSF'))\nprint(\"Spring LSF Vista Pre-k = \", getMean(prekst.copy(), 'Spring_LSF'))\nprint(\"Spring LNF No Vista Pre-k = \", getMean(nonprek.copy(), 'Spring_LNF'))\nprint(\"Spring LNF Vista Pre-k = \", getMean(prekst.copy(), 'Spring_LNF'))",
"Spring LSF No Vista Pre-k = 40.38297872340426\nSpring LSF Vista Pre-k = 45.0\nSpring LNF No Vista Pre-k = 41.07446808510638\nSpring LNF Vista Pre-k = 46.26086956521739\n"
]
],
[
[
"<body>\n <section style=\"background-color:White; font-family:Georgia;text-align:center\">\n <h2 style=\"font-family:Garamond; color:solid #229954\">Result 1</h2>\n <h3 style=\"font-family:Garamond;\">Replicating results from Boise Pre-K Program Evaluation 2017</h3>\n <h4 style=\"font-family:Garamond;\">Page 9</h4>\n <hr/>\n </section>\n</body>",
"_____no_output_____"
]
],
[
[
"# We need to arrange our StudentID from float to int, and then to string\ndef convertToInt(df_t):\n strTbl=[]\n for a in df_t:\n strTbl.append(int(a))\n return strTbl\n ",
"_____no_output_____"
],
[
"def getListValues(dataset, firstSelector, secondSelector):\n tbl = dataset.reset_index()\n data = tbl.groupby([firstSelector])[[secondSelector]].count()\n data = data.reset_index()\n data['firstSelector']=convertToInt(data[firstSelector].values)\n return list(data[secondSelector])",
"_____no_output_____"
],
[
"def getBelowAverages(dataset):\n tbl = dataset.reset_index()\n data = tbl.groupby(['Fall_GRTR_Level'])[['Student_ID']].count()\n data = data.reset_index()\n data['Fall_GRTR_Level']=convertToInt(data['Fall_GRTR_Level'].values)\n fall = (list(data['Student_ID']))[0]\n \n data = tbl.groupby(['Winter_GRTR_Level'])[['Student_ID']].count()\n data = data.reset_index()\n data['Winter_GRTR_Level']=convertToInt(data['Winter_GRTR_Level'].values)\n winter = (list(data['Student_ID']))[0]\n \n data = tbl.groupby(['Spring_GRTR_Level'])[['Student_ID']].count()\n data = data.reset_index()\n data['Spring_GRTR_Level']=convertToInt(data['Spring_GRTR_Level'].values)\n spring = (list(data['Student_ID']))[0]\n \n return [fall, winter, spring]\ndef getAverages(dataset):\n tbl = dataset.reset_index()\n data = tbl.groupby(['Fall_GRTR_Level'])[['Student_ID']].count()\n data = data.reset_index()\n data['Fall_GRTR_Level']=convertToInt(data['Fall_GRTR_Level'].values)\n fall = (list(data['Student_ID']))[1]\n \n data = tbl.groupby(['Winter_GRTR_Level'])[['Student_ID']].count()\n data = data.reset_index()\n data['Winter_GRTR_Level']=convertToInt(data['Winter_GRTR_Level'].values)\n winter = (list(data['Student_ID']))[1]\n \n data = tbl.groupby(['Spring_GRTR_Level'])[['Student_ID']].count()\n data = data.reset_index()\n data['Spring_GRTR_Level']=convertToInt(data['Spring_GRTR_Level'].values)\n spring = (list(data['Student_ID']))[1]\n \n return [fall, winter, spring]\ndef getAboveAverages(dataset):\n tbl = dataset.reset_index()\n data = tbl.groupby(['Fall_GRTR_Level'])[['Student_ID']].count()\n data = data.reset_index()\n data['Fall_GRTR_Level']=convertToInt(data['Fall_GRTR_Level'].values)\n fall = (list(data['Student_ID']))[2]\n \n data = tbl.groupby(['Winter_GRTR_Level'])[['Student_ID']].count()\n data = data.reset_index()\n data['Winter_GRTR_Level']=convertToInt(data['Winter_GRTR_Level'].values)\n winter = (list(data['Student_ID']))[2]\n \n data = tbl.groupby(['Spring_GRTR_Level'])[['Student_ID']].count()\n data = data.reset_index()\n data['Spring_GRTR_Level']=convertToInt(data['Spring_GRTR_Level'].values)\n spring = (list(data['Student_ID']))[2]\n \n return [fall, winter, spring]",
"_____no_output_____"
],
[
"fig, ax = plt.subplots(figsize=(12, 7))\nN = 3 #Number of groups\nwidth = 0.40 # the width of the bars\nind = np.arange(N) # the x locations for the groups\n\nx=[0, 2, 4]\nfall = getListValues(prekst.copy(), 'Fall_GRTR_Level','Student_ID')\n\nba = getBelowAverages(prekst.copy())\nav = getAverages(prekst.copy())\naa = getAboveAverages(prekst.copy())\n\n#ax = plt.figure().gca()\nax.yaxis.set_major_locator(MaxNLocator(integer=True))\n\np1 = ax.bar(x,ba, width, color='deepskyblue', bottom=0)\np2 = ax.bar(ind*2 + width , av, width,color='orangered')\np3 = ax.bar(ind*2 + width*2, aa, width,color='royalblue')\n\nax.set_title('Cohort 1 Get Ready To Read Levels (2015-2016)')\nax.set_xticks((ind*2) + width/2)\nax.set_xticklabels(('Fall', 'Winter', 'Spring'))\nax.set_ylabel('number of students')\n\nax.grid(True)\nax.legend((p1[0], p2[0], p3[0]), ('below average', 'average', 'above average'),loc='upper left')\nax.autoscale_view()\nplt.show()",
"_____no_output_____"
],
[
"prekst.columns",
"_____no_output_____"
]
],
[
[
"<body>\n <section style=\"background-color:White; font-family:Georgia;text-align:center\">\n <h2 style=\"font-family:Garamond; color:solid #229954\">Trending improvement in Get Ready To Read score</h2>\n <h3 style=\"font-family:Garamond;\">Question: Are students who start the pre-k program, show improvement from fall to spring on their Get Ready To Read scores?</h3>\n <hr/>\n </section>\n</body>",
"_____no_output_____"
]
],
[
[
"sns.pairplot(prekst, x_vars=\"Fall_GRTR_Score\", y_vars=\"Spring_GRTR_Score\",kind=\"reg\")",
"_____no_output_____"
]
],
[
[
"<body>\n <section style=\"background-color:White; font-family:Georgia;text-align:center\">\n <h2 style=\"font-family:Garamond; color:solid #229954\">Rate of improvement on pre-k and no pre-k students together</h2>\n <h3 style=\"font-family:Garamond;\">Question: On Kindergardent, do we see any difference on improvement rate between kids with and without pre-k?</h3>\n <h4>Preliminary observation: Here we will use the slope of our regression to measure that and we do see that kids with pre-k have a higher rate of imporovement (higher slope) on both, LNF and LSF</h4>\n <hr/>\n </section>\n</body>",
"_____no_output_____"
]
],
[
[
"print(\"LNF Scores for pre-k Students\")\np1=sns.pairplot(prekst, x_vars=[\"Fall_LNF\"],y_vars=\"Spring_LNF\", kind='reg')\naxes = p1.axes\naxes[0,0].set_ylim(0,100)\nprint(\"LNF Scores for pre-k Students\")\np2=sns.pairplot(prekst, x_vars=[\"Fall_LSF\"],y_vars=\"Spring_LSF\", kind='reg')\naxes = p2.axes\naxes[0,0].set_ylim(0,100)",
"LNF Scores for pre-k Students\nLNF Scores for pre-k Students\n"
],
[
"print(\"LNF Scores for no pre-k Students\")\np1=sns.pairplot(nonprek, x_vars=[\"Fall_LNF\"],y_vars=\"Spring_LNF\", kind='reg')\naxes = p1.axes\naxes[0,0].set_ylim(0,100)\nprint(\"LSF Scores for no pre-k Students\")\np2=sns.pairplot(nonprek, x_vars=[\"Fall_LSF\"],y_vars=\"Spring_LSF\", kind='reg')\naxes = p2.axes\naxes[0,0].set_ylim(0,100)",
"LNF Scores for no pre-k Students\nLSF Scores for no pre-k Students\n"
]
],
[
[
"Now let's get the real numbers on rate of learning (our m slope from the regression)",
"_____no_output_____"
]
],
[
[
"# Import SK Learn train test split\nfrom sklearn.linear_model import LinearRegression\nfrom sklearn.cross_validation import train_test_split\ndef getSlope(X, y):\n # Assign variables to capture train test split output\n #X_train, X_test, y_train, y_test = train_test_split(X, y)\n # Instantiate\n linreg = LinearRegression()\n linreg.fit(X, y)\n return linreg.coef_[0]",
"_____no_output_____"
]
],
[
[
"<body>\n <section style=\"background-color:White; font-family:Georgia;text-align:center\">\n <h3 style=\"font-family:Garamond;\">Question: What is the quantitative learning rate in LNF from students with and without Pre-k</h3>\n <hr/>\n </section>\n</body>",
"_____no_output_____"
]
],
[
[
"toEval = nonprek.copy()\nX = toEval['Fall_LNF']\ny = toEval['Winter_LNF']\nX[0]=0 # Fix an issue because the first sample is Nan thus ffill is ineffective for first sample\nX=X.fillna(method='ffill')\ny=y.fillna(method='ffill')\nX=X.values.reshape(-1,1)\ny=y.values.reshape(-1,1)\nprint(\"LNF Learning rate for studenst non pre-K From Fall to Winter =\",getSlope(X,y))\n",
"LNF Learning rate for studenst non pre-K From Fall to Winter = [0.69921721]\n"
],
[
"toEval = prekst.copy()\nX = toEval['Fall_LNF']\ny = toEval['Winter_LNF']\nX[0]=0 # Fix an issue because the first sample is Nan thus ffill is ineffective for first sample\nX=X.fillna(method='ffill')\ny=y.fillna(method='ffill')\nX=X.values.reshape(-1,1)\ny=y.values.reshape(-1,1)\nprint(\"LNF Learning rate for studenst pre-K From Fall to Winter =\",getSlope(X,y))",
"LNF Learning rate for studenst pre-K From Fall to Winter = [1.01872332]\n"
]
],
[
[
"<body>\n <section style=\"background-color:White; font-family:Georgia;text-align:center\">\n <h3 style=\"font-family:Garamond;\">Question: What is the quantitative learning rate in LSF from students with and without Pre-k</h3>\n <hr/>\n </section>\n</body>",
"_____no_output_____"
]
],
[
[
"toEval = nonprek.copy()\nX = toEval['Fall_LSF']\ny = toEval['Winter_LSF']\nX[0]=0 # Fix an issue because the first sample is Nan thus ffill is ineffective for first sample\nX=X.fillna(method='ffill')\ny=y.fillna(method='ffill')\nX=X.values.reshape(-1,1)\ny=y.values.reshape(-1,1)\nprint(\"LSF Learning rate for studenst non pre-K From Fall to Winter =\",getSlope(X,y))\n",
"LSF Learning rate for studenst non pre-K From Fall to Winter = [1.1361879]\n"
],
[
"toEval = prekst.copy()\nX = toEval['Fall_LSF']\ny = toEval['Winter_LSF']\nX[0]=0 # Fix an issue because the first sample is Nan thus ffill is ineffective for first sample\nX=X.fillna(method='ffill')\ny=y.fillna(method='ffill')\nX=X.values.reshape(-1,1)\ny=y.values.reshape(-1,1)\nprint(\"LSF Learning rate for studenst pre-K From Fall to Winter =\",getSlope(X,y))",
"LSF Learning rate for studenst pre-K From Fall to Winter = [1.194067]\n"
]
],
[
[
"<body>\n <section style=\"background-color:White; font-family:Georgia;text-align:center\">\n <h3 style=\"font-family:Garamond;\">Question: Is there a difference in learning rate between high performers from both groups?</h3>\n <h3 style=\"font-family:Garamond;\">Observation: The following plots have the same scale</h3>\n <hr/>\n </section>\n</body>",
"_____no_output_____"
]
],
[
[
"pkhp = prekst[prekst['Fall_Level'] == 3]\nnpkhp = nonprek[nonprek['Fall_Level'] == 3]",
"_____no_output_____"
],
[
"print(\"LNF Scores for pre-k Students\")\np1=sns.pairplot(pkhp, x_vars=[\"Fall_LNF\"],y_vars=\"Spring_LNF\", kind='reg')\naxes = p1.axes\naxes[0,0].set_ylim(0,100)\ntype(p1)",
"LNF Scores for pre-k Students\n"
],
[
"print(\"LSF Scores for no pre-k Students\")\np2=sns.pairplot(npkhp, x_vars=[\"Fall_LNF\"],y_vars=\"Spring_LNF\", kind='reg')\naxes = p2.axes\naxes[0,0].set_ylim(0,100)",
"LSF Scores for no pre-k Students\n"
]
],
[
[
"Observations so far that we can formulate questions/answers.",
"_____no_output_____"
],
[
"We did this for mid and low performers and alghouth they scored higher from Fall to Spring, the learning rate was flat or decreased a bit. This might explain why in ",
"_____no_output_____"
]
]
] | [
"code",
"markdown",
"code",
"markdown",
"code",
"markdown",
"code",
"markdown",
"code",
"markdown",
"code",
"markdown",
"code",
"markdown",
"code",
"markdown",
"code",
"markdown"
] | [
[
"code",
"code",
"code",
"code",
"code",
"code",
"code",
"code",
"code"
],
[
"markdown"
],
[
"code",
"code",
"code"
],
[
"markdown"
],
[
"code",
"code",
"code",
"code",
"code"
],
[
"markdown"
],
[
"code"
],
[
"markdown"
],
[
"code",
"code"
],
[
"markdown"
],
[
"code"
],
[
"markdown"
],
[
"code",
"code"
],
[
"markdown"
],
[
"code",
"code"
],
[
"markdown"
],
[
"code",
"code",
"code"
],
[
"markdown",
"markdown"
]
] |
e7711ac031f7a768897111e73705cdf4f692ee98 | 16,089 | ipynb | Jupyter Notebook | think_python/.ipynb_checkpoints/ch_2_variables-checkpoint.ipynb | Lawrence-Krukrubo/Effective_Python | 1b4c513f5aab37657b171c0e1b373ea67814a498 | [
"MIT"
] | 2 | 2021-02-06T05:12:18.000Z | 2021-02-09T12:25:33.000Z | think_python/ch_2_variables.ipynb | Blackman9t/Effective_Python | 1b4c513f5aab37657b171c0e1b373ea67814a498 | [
"MIT"
] | null | null | null | think_python/ch_2_variables.ipynb | Blackman9t/Effective_Python | 1b4c513f5aab37657b171c0e1b373ea67814a498 | [
"MIT"
] | 2 | 2020-06-14T21:40:34.000Z | 2020-11-21T13:57:32.000Z | 24.563359 | 192 | 0.557151 | [
[
[
"<h2>2.5 Expressions and statements</h2>",
"_____no_output_____"
],
[
"**An expression** is a combination of values, variables, and operators. A value all by itself\nis considered an expression, and so is a variable, so the following are all legal expressions\n(assuming that the variable x has been assigned a value):<br>\n17<br>\nx<br>\nx + 17<br>\n**A statement** is a unit of code that the Python interpreter can execute. We have seen two\nkinds of statement: print and assignment.\nTechnically an expression is also a statement, but it is probably simpler to think of them\nas different things. The important difference is that an expression has a value; a statement\ndoes not.",
"_____no_output_____"
],
[
"A value is an expression so it gets printed out in interpreter mode",
"_____no_output_____"
]
],
[
[
"5 ",
"_____no_output_____"
]
],
[
[
"An assignment is a statement and so does not get printed out technically by the python shell in interpreter mode",
"_____no_output_____"
]
],
[
[
"x = 5 ",
"_____no_output_____"
]
],
[
[
"The value of an expression gets printed out in the python shell interpreter mode",
"_____no_output_____"
]
],
[
[
"x + 1",
"_____no_output_____"
]
],
[
[
"<h2>2.7 Order of operations</h2>\n\nWhen more than one operator appears in an expression, the order of evaluation depends\non the rules of precedence. For mathematical operators, Python follows mathematical\nconvention. The acronym **PEMDAS** is a useful way to remember the rules:",
"_____no_output_____"
],
[
"• **Parentheses** have the highest precedence and can be used to force an expression to\nevaluate in the order you want. Since expressions in parentheses are evaluated first,\n2 * (3-1) is 4, and (1+1)^(5-2) is 8. You can also use parentheses to make an\nexpression easier to read, as in (minute * 100) / 60, even if it doesn’t change the\nresult.\n\n• **Exponentiation** has the next highest precedence, so 2^1+1 is 3, not 4, and 3*1^3 is\n3, not 27.\n\n• **Multiplication and Division** have the same precedence, which is higher than\n**Addition and Subtraction**, which also have the same precedence. So 2*3-1 is 5, not\n4, and 6+4/2 is 8, not 5.\n\n• Operators with the same precedence are evaluated from left to right (except exponentiation). So in the expression degrees / 2 * pi, the division happens first and the\nresult is multiplied by pi. To divide by 2π, you can use parentheses or write degrees\n/ 2 / pi.\n\nI don’t work very hard to remember rules of precedence for other operators. If I can’t tell\nby looking at the expression, I use parentheses to make it obvious.",
"_____no_output_____"
],
[
"<h2>2.9 Comments</h2>\n\nAs programs get bigger and more complicated, they get more difficult to read. Formal\nlanguages are dense, and it is often difficult to look at a piece of code and figure out what\nit is doing, or why.\n",
"_____no_output_____"
],
[
"Comments are most useful when they document non-obvious features of the code. It is\nreasonable to assume that the reader can figure out what the code does; it is much more\nuseful to explain why.<br>\nThis comment is redundant with the code and useless:<br>\n`v = 5` # assign 5 to v<br>\nThis comment contains useful information that is not in the code:<br>\n`v = 5` # velocity in meters/second.<br>\nGood variable names can reduce the need for comments, but long names can make complex expressions hard to read, so there is a tradeoff.",
"_____no_output_____"
],
[
"<h2>2.11 Glossary</h2>\n\n1. **value:** One of the basic units of data, like a number or string, that a program manipulates.\n2. **type:** A category of values. The types we have seen so far are integers (type int), floatingpoint numbers (type float), and strings (type str).\n3. **integer:** A type that represents whole numbers.\n4. **floating-point:** A type that represents numbers with fractional parts.\n5. **string:** A type that represents sequences of characters.\n6. **variable:** A name that refers to a value.\n7. **statement:** A section of code that represents a command or action. So far, the statements\nwe have seen are assignments and print statements.\n8. **assignment:** A statement that assigns a value to a variable.\n9. **state diagram:** A graphical representation of a set of variables and the values they refer to.\n10. **keyword:** A reserved word that is used by the compiler to parse a program; you cannot\nuse keywords like if, def, and while as variable names.",
"_____no_output_____"
],
[
"11. **operator:** A special symbol that represents a simple computation like addition, multiplication, or string concatenation.\n12. **operand:** One of the values on which an operator operates.\n13. **floor division:** The operation that divides two numbers and chops off the fraction part.\n14. **expression:** A combination of variables, operators, and values that represents a single result value.\n15. **evaluate:** To simplify an expression by performing the operations in order to yield a single value.\n16. **rules of precedence:** The set of rules governing the order in which expressions involving multiple operators and operands are evaluated.\n17. **concatenate:** To join two operands end-to-end.\n18. **comment:** Information in a program that is meant for other programmers (or anyone reading the source code) and has no effect on the execution of the program.\n",
"_____no_output_____"
],
[
"<h2>2.12 Exercises</h2>",
"_____no_output_____"
],
[
"**Exercise 2.2.** Assume that we execute the following assignment statements:",
"_____no_output_____"
]
],
[
[
"width = 17\nheight = 12.0\ndelimiter = '.'",
"_____no_output_____"
]
],
[
[
"For each of the following expressions, write the value of the expression and the type (of the value of\nthe expression).\n\n1. width/2\n2. width/2.0\n3. height/3\n4. 1 + 2 * 5\n5. delimiter * 5",
"_____no_output_____"
]
],
[
[
"width / 2 # Type of value of expression in float",
"_____no_output_____"
],
[
"width / 2.0 # Type of value of expression in float",
"_____no_output_____"
],
[
"height / 3 # Type of value of expression in float",
"_____no_output_____"
],
[
"1+2 * 5 # Type of value of expression is int, value is 11",
"_____no_output_____"
],
[
"delimiter * 5 # Type of value of expression is string",
"_____no_output_____"
]
],
[
[
"**Exercise 2.3.** Practice using the Python interpreter as a calculator:",
"_____no_output_____"
],
[
"1. The volume of a sphere with radius r is ${4\\over3}\\pi r^3$ What is the volume of a sphere with radius 5? Hint: 392.7 is wrong!\n2. Suppose the cover price of a book is $$24.95, but bookstores get a 40% discount. Shipping costs\n$3 for the first copy and 75 cents for each additional copy. What is the total wholesale cost for\n60 copies?\n3. If I leave my house at 6:52 am and run 1 mile at an easy pace (8:15 per mile), then 3 miles at\ntempo (7:12 per mile) and 1 mile at easy pace again, what time do I get home for breakfast?",
"_____no_output_____"
]
],
[
[
"import math",
"_____no_output_____"
]
],
[
[
"**Quest 1.**",
"_____no_output_____"
]
],
[
[
"radius = 5\nvolume = (4/3*math.pi)*radius**3\nvolume",
"_____no_output_____"
]
],
[
[
"**Quest 2.**",
"_____no_output_____"
]
],
[
[
"cover_price = 24.95\nbook_stores_discount = 0.4",
"_____no_output_____"
]
],
[
[
"Total wholesale cost for each book will be the cover_price cost less discounts plus shipping cost. The fisrst copy has shipping of $$3 and the rest 0.75 cents. So add it up for 60 copies",
"_____no_output_____"
]
],
[
[
"net_cover_price = cover_price - (cover_price * book_stores_discount)\nnet_cover_price",
"_____no_output_____"
],
[
"First_shipping_cost = 3\nsubsequent_shipping_cost = 0.75",
"_____no_output_____"
],
[
"first_book_cost = net_cover_price + First_shipping_cost\nfifty_nine_books_cost = (net_cover_price + subsequent_shipping_cost) * 59",
"_____no_output_____"
],
[
"total_wholesale_cost = first_book_cost + fifty_nine_books_cost\ntotal_wholesale_cost",
"_____no_output_____"
]
],
[
[
"**Quest 3.**",
"_____no_output_____"
]
],
[
[
"min_sec = 60\nhours_sec = 3600",
"_____no_output_____"
],
[
"start_time_secs = 6 * hours_sec + 52 * min_sec\nstart_time_secs",
"_____no_output_____"
],
[
"easy_pace_per_mile = 8 * min_sec + 15\ntempo_space_per_mile = 7 * min_sec + 12",
"_____no_output_____"
]
],
[
[
"Now add 2 * easy-pace + 3 * tempo-pace to start-time ",
"_____no_output_____"
]
],
[
[
"finish_time_secs = start_time_secs + (2 * easy_pace_per_mile) + (3 * tempo_space_per_mile)\nfinish_time_secs",
"_____no_output_____"
]
],
[
[
"Now convert finish-time-secs to hours and minutes",
"_____no_output_____"
]
],
[
[
"import time \n \ndef convert(seconds): \n return time.strftime(\"%H:%M:%S\", time.gmtime(seconds)) ",
"_____no_output_____"
],
[
"# Now call it on the start_time to check, start-time is 06.52\nconvert(start_time_secs)",
"_____no_output_____"
],
[
"# Now call it on the end_time to get the answer\nconvert(finish_time_secs)",
"_____no_output_____"
]
],
[
[
"Therefore, I get home for breakfast by 07:30:06 a.m",
"_____no_output_____"
]
]
] | [
"markdown",
"code",
"markdown",
"code",
"markdown",
"code",
"markdown",
"code",
"markdown",
"code",
"markdown",
"code",
"markdown",
"code",
"markdown",
"code",
"markdown",
"code",
"markdown",
"code",
"markdown",
"code",
"markdown",
"code",
"markdown"
] | [
[
"markdown",
"markdown",
"markdown"
],
[
"code"
],
[
"markdown"
],
[
"code"
],
[
"markdown"
],
[
"code"
],
[
"markdown",
"markdown",
"markdown",
"markdown",
"markdown",
"markdown",
"markdown",
"markdown"
],
[
"code"
],
[
"markdown"
],
[
"code",
"code",
"code",
"code",
"code"
],
[
"markdown",
"markdown"
],
[
"code"
],
[
"markdown"
],
[
"code"
],
[
"markdown"
],
[
"code"
],
[
"markdown"
],
[
"code",
"code",
"code",
"code"
],
[
"markdown"
],
[
"code",
"code",
"code"
],
[
"markdown"
],
[
"code"
],
[
"markdown"
],
[
"code",
"code",
"code"
],
[
"markdown"
]
] |
e7712b66fd6a9aa32cd74e382b3c0ab1e9eef2f2 | 359,991 | ipynb | Jupyter Notebook | Starbucks_Capstone_notebook.ipynb | amit-singh-rathore/Starbucks-Capstone | 5e6a1701a4985cf4a001f7dd0d7a80e2991b9613 | [
"CNRI-Python"
] | null | null | null | Starbucks_Capstone_notebook.ipynb | amit-singh-rathore/Starbucks-Capstone | 5e6a1701a4985cf4a001f7dd0d7a80e2991b9613 | [
"CNRI-Python"
] | null | null | null | Starbucks_Capstone_notebook.ipynb | amit-singh-rathore/Starbucks-Capstone | 5e6a1701a4985cf4a001f7dd0d7a80e2991b9613 | [
"CNRI-Python"
] | null | null | null | 95.185352 | 45,694 | 0.767322 | [
[
[
"# Starbucks Capstone Challenge\n\n### Introduction\n\nThis data set contains simulated data that mimics customer behavior on the Starbucks rewards mobile app. Once every few days, Starbucks sends out an offer to users of the mobile app. An offer can be merely an advertisement for a drink or an actual offer such as a discount or BOGO (buy one get one free). Some users might not receive any offer during certain weeks. \n\nNot all users receive the same offer, and that is the challenge to solve with this data set.\n\nYour task is to combine transaction, demographic and offer data to determine which demographic groups respond best to which offer type. This data set is a simplified version of the real Starbucks app because the underlying simulator only has one product whereas Starbucks actually sells dozens of products.\n\nEvery offer has a validity period before the offer expires. As an example, a BOGO offer might be valid for only 5 days. You'll see in the data set that informational offers have a validity period even though these ads are merely providing information about a product; for example, if an informational offer has 7 days of validity, you can assume the customer is feeling the influence of the offer for 7 days after receiving the advertisement.\n\nYou'll be given transactional data showing user purchases made on the app including the timestamp of purchase and the amount of money spent on a purchase. This transactional data also has a record for each offer that a user receives as well as a record for when a user actually views the offer. There are also records for when a user completes an offer. \n\nKeep in mind as well that someone using the app might make a purchase through the app without having received an offer or seen an offer.\n\n### Example\n\nTo give an example, a user could receive a discount offer buy 10 dollars get 2 off on Monday. The offer is valid for 10 days from receipt. If the customer accumulates at least 10 dollars in purchases during the validity period, the customer completes the offer.\n\nHowever, there are a few things to watch out for in this data set. Customers do not opt into the offers that they receive; in other words, a user can receive an offer, never actually view the offer, and still complete the offer. For example, a user might receive the \"buy 10 dollars get 2 dollars off offer\", but the user never opens the offer during the 10 day validity period. The customer spends 15 dollars during those ten days. There will be an offer completion record in the data set; however, the customer was not influenced by the offer because the customer never viewed the offer.\n\n### Cleaning\n\nThis makes data cleaning especially important and tricky.\n\nYou'll also want to take into account that some demographic groups will make purchases even if they don't receive an offer. From a business perspective, if a customer is going to make a 10 dollar purchase without an offer anyway, you wouldn't want to send a buy 10 dollars get 2 dollars off offer. You'll want to try to assess what a certain demographic group will buy when not receiving any offers.\n\n### Final Advice\n\nBecause this is a capstone project, you are free to analyze the data any way you see fit. For example, you could build a machine learning model that predicts how much someone will spend based on demographics and offer type. Or you could build a model that predicts whether or not someone will respond to an offer. Or, you don't need to build a machine learning model at all. You could develop a set of heuristics that determine what offer you should send to each customer (i.e., 75 percent of women customers who were 35 years old responded to offer A vs 40 percent from the same demographic to offer B, so send offer A).",
"_____no_output_____"
],
[
"# Data Sets\n\nThe data is contained in three files:\n\n* portfolio.json - containing offer ids and meta data about each offer (duration, type, etc.)\n* profile.json - demographic data for each customer\n* transcript.json - records for transactions, offers received, offers viewed, and offers completed\n\nHere is the schema and explanation of each variable in the files:\n\n**portfolio.json**\n* id (string) - offer id\n* offer_type (string) - type of offer ie BOGO, discount, informational\n* difficulty (int) - minimum required spend to complete an offer\n* reward (int) - reward given for completing an offer\n* duration (int) - time for offer to be open, in days\n* channels (list of strings)\n\n**profile.json**\n* age (int) - age of the customer \n* became_member_on (int) - date when customer created an app account\n* gender (str) - gender of the customer (note some entries contain 'O' for other rather than M or F)\n* id (str) - customer id\n* income (float) - customer's income\n\n**transcript.json**\n* event (str) - record description (ie transaction, offer received, offer viewed, etc.)\n* person (str) - customer id\n* time (int) - time in hours since start of test. The data begins at time t=0\n* value - (dict of strings) - either an offer id or transaction amount depending on the record",
"_____no_output_____"
]
],
[
[
"# Import required libraries\nfrom datetime import datetime\nimport json\nimport math\nimport matplotlib.pyplot as plt\nimport numpy as np\nimport pandas as pd\nimport seaborn as sns\n\nfrom sklearn.ensemble import RandomForestClassifier\nfrom sklearn.linear_model import LogisticRegression \nfrom sklearn.metrics import fbeta_score, accuracy_score\nfrom sklearn.model_selection import cross_val_score\nfrom sklearn.model_selection import train_test_split\nfrom sklearn.neighbors import KNeighborsClassifier\nfrom sklearn.preprocessing import MinMaxScaler\nfrom sklearn.tree import DecisionTreeClassifier\n\nimport warnings\nwarnings.filterwarnings('ignore')\n% matplotlib inline",
"_____no_output_____"
]
],
[
[
"##Data Loading",
"_____no_output_____"
]
],
[
[
"# Load the json files for processing\nportfolio = pd.read_json('data/portfolio.json', orient='records', lines=True)\nprofile = pd.read_json('data/profile.json', orient='records', lines=True)\ntranscript = pd.read_json('data/transcript.json', orient='records', lines=True)",
"_____no_output_____"
]
],
[
[
"# Data Exploration",
"_____no_output_____"
],
[
"## Portfolio",
"_____no_output_____"
]
],
[
[
"portfolio.head()",
"_____no_output_____"
],
[
"items, attributes = portfolio.shape\nprint(\"Portfolio dataset has {} records and {} attributes\".format(items, attributes))",
"Portfolio dataset has 10 records and 6 attributes\n"
],
[
"portfolio.info()",
"<class 'pandas.core.frame.DataFrame'>\nRangeIndex: 10 entries, 0 to 9\nData columns (total 6 columns):\n # Column Non-Null Count Dtype \n--- ------ -------------- ----- \n 0 reward 10 non-null int64 \n 1 channels 10 non-null object\n 2 difficulty 10 non-null int64 \n 3 duration 10 non-null int64 \n 4 offer_type 10 non-null object\n 5 id 10 non-null object\ndtypes: int64(3), object(3)\nmemory usage: 608.0+ bytes\n"
],
[
"portfolio.describe(include='all')",
"_____no_output_____"
],
[
"plt.figure(figsize=[5,5])\nfig, ax = plt.subplots() \ncategory_count = portfolio.offer_type.value_counts()\ncategory_count.plot(kind='barh') \nfor i, count in enumerate(category_count):\n ax.text(count, i, str(count))\nplt.title(\"Offer distribution per offer Type\")",
"_____no_output_____"
],
[
"#Get all possible channels\nimport itertools\nset(itertools.chain.from_iterable(portfolio.channels))",
"_____no_output_____"
]
],
[
[
"## Profile",
"_____no_output_____"
]
],
[
[
"profile.head(5)",
"_____no_output_____"
],
[
"items, attributes = profile.shape\nprint(\"Portfolio dataset has {} records and {} attributes\".format(items, attributes))",
"Portfolio dataset has 17000 records and 5 attributes\n"
],
[
"profile.info()",
"<class 'pandas.core.frame.DataFrame'>\nRangeIndex: 17000 entries, 0 to 16999\nData columns (total 5 columns):\n # Column Non-Null Count Dtype \n--- ------ -------------- ----- \n 0 gender 14825 non-null object \n 1 age 17000 non-null int64 \n 2 id 17000 non-null object \n 3 became_member_on 17000 non-null int64 \n 4 income 14825 non-null float64\ndtypes: float64(1), int64(2), object(2)\nmemory usage: 664.2+ KB\n"
],
[
"profile.describe(include=\"all\")",
"_____no_output_____"
],
[
"#check for null values \nprofile.isnull().sum()",
"_____no_output_____"
],
[
"profile.duplicated().sum()",
"_____no_output_____"
],
[
"# age distribution\nprofile.age.hist();",
"_____no_output_____"
],
[
"sns.boxplot(profile['age'], width=0.5);",
"_____no_output_____"
]
],
[
[
"Age 118 seems outlier. Lets explore it further.",
"_____no_output_____"
]
],
[
[
"profile[profile['age']== 118].age.count()",
"_____no_output_____"
],
[
"profile[profile.age == 118][['gender','income']]",
"_____no_output_____"
]
],
[
[
"As per above analysis we see that wherever age is 118, the values in Gender and income is null. And also 2175 is count of such of rows. Also we saw that 2175 instances had gender and income was null. So we will drop all instances where age equals 118 as these are errorneous record.",
"_____no_output_____"
]
],
[
[
"## Gender-wise age distribution\nsns.distplot(profile[profile.gender=='M'].age,label='Male')\nsns.distplot(profile[profile.gender=='F'].age,label='Female')\nsns.distplot(profile[profile.gender=='O'].age,label='Other')\nplt.legend()\nplt.show()",
"_____no_output_____"
],
[
"# distribution of income\nprofile.income.hist();",
"_____no_output_____"
],
[
"profile['income'].mean()",
"_____no_output_____"
],
[
"# Gender wise data distribution\nprofile.gender.value_counts()",
"_____no_output_____"
],
[
"## Gender-wise Income Distribution\nsns.distplot(profile[profile.gender=='M'].income,label='Male')\nsns.distplot(profile[profile.gender=='F'].income,label='Female')\nsns.distplot(profile[profile.gender=='O'].income,label='Other')\nplt.legend()\nplt.show()",
"_____no_output_____"
]
],
[
[
"## Transcript",
"_____no_output_____"
]
],
[
[
"transcript.head()",
"_____no_output_____"
],
[
"items, attributes = transcript.shape\nprint(\"Transcript dataset has {} records and {} attributes\".format(items, attributes))",
"Transcript dataset has 306534 records and 4 attributes\n"
],
[
"transcript.info()",
"<class 'pandas.core.frame.DataFrame'>\nRangeIndex: 306534 entries, 0 to 306533\nData columns (total 4 columns):\n # Column Non-Null Count Dtype \n--- ------ -------------- ----- \n 0 person 306534 non-null object\n 1 event 306534 non-null object\n 2 value 306534 non-null object\n 3 time 306534 non-null int64 \ndtypes: int64(1), object(3)\nmemory usage: 9.4+ MB\n"
],
[
"#check for null values\ntranscript.isnull().sum()",
"_____no_output_____"
],
[
"transcript['event'].value_counts()",
"_____no_output_____"
],
[
"keys = transcript['value'].apply(lambda x: list(x.keys()))\npossible_keys = set()\nfor key in keys:\n for item in key:\n possible_keys.add(item)\nprint(possible_keys)",
"{'offer id', 'amount', 'offer_id', 'reward'}\n"
]
],
[
[
"For the **value** attribute have 3 possible value.\n1. offer id/ offer_id\n2. amount\n3. reward",
"_____no_output_____"
],
[
"# Data cleaning & Transformation",
"_____no_output_____"
],
[
"## Portfolio",
"_____no_output_____"
],
[
"Renaming columns for better understanding and meaningfulness",
"_____no_output_____"
]
],
[
[
"#Rename columns\nnew_cols_name = {'difficulty':'offer_difficulty' , 'id':'offer_id', 'duration':'offer_duration', 'reward': 'offer_reward'}\nportfolio = portfolio.rename(columns=new_cols_name )",
"_____no_output_____"
]
],
[
[
"Exploding the channel attribute into four separate attribute - (email, mobile, social, web)",
"_____no_output_____"
]
],
[
[
"dummy = pd.get_dummies(portfolio.channels.apply(pd.Series).stack()).sum(level=0)\nportfolio = pd.concat([portfolio, dummy], axis=1)\nportfolio.drop(columns='channels', inplace=True)",
"_____no_output_____"
],
[
"portfolio.head()",
"_____no_output_____"
]
],
[
[
"## Profile",
"_____no_output_____"
],
[
"Renaming columns for better understaning & meaningfulness",
"_____no_output_____"
]
],
[
[
"#Rename columns\ncols_profile = {'id':'customer_id' , 'income':'customer_income'}\nprofile = profile.rename(columns=cols_profile)",
"_____no_output_____"
]
],
[
[
"Removing rows with missing values. We saw above that all nulls belong to age 118 which are outliers.",
"_____no_output_____"
]
],
[
[
"#drop all rows which has null value \nprofile = profile.loc[profile['gender'].isnull() == False]",
"_____no_output_____"
]
],
[
[
"Classifying ages into groups for better understanding in Exploratory Data Analysis later:\n* Under 20\n* 21 - 35\n* 35 - 50\n* 50 - 65\n* Above 65",
"_____no_output_____"
]
],
[
[
"#Convert ages into age group\nprofile.loc[(profile.age <= 20) , 'Age_group'] = 'Under 20'\nprofile.loc[(profile.age >= 21) & (profile.age <= 35) , 'Age_group'] = '21-35'\nprofile.loc[(profile.age >= 36) & (profile.age <= 50) , 'Age_group'] = '36-50'\nprofile.loc[(profile.age >= 51) & (profile.age <= 65) , 'Age_group'] = '51-65'\nprofile.loc[(profile.age >= 66) , 'Age_group'] = 'Above 65'\nprofile.drop('age',axis=1,inplace=True)",
"_____no_output_____"
]
],
[
[
"Classifying income into income_groups for better understanding in Exploratory Data Analysis later:\n* 30-50K\n* 50-80K\n* 80-110K\n* Above 110K",
"_____no_output_____"
]
],
[
[
"#Convert income into income group\nprofile.loc[(profile.customer_income >= 30000) & (profile.customer_income <= 50000) , 'Income_group'] = '30-50K'\nprofile.loc[(profile.customer_income >= 50001) & (profile.customer_income <= 80000) , 'Income_group'] = '50-80K'\nprofile.loc[(profile.customer_income >= 80001) & (profile.customer_income <= 110000) , 'Income_group'] = '80-110K'\nprofile.loc[(profile.customer_income >= 110001) , 'Income_group'] = 'Above 110K'\nprofile.drop('customer_income',axis=1,inplace=True)",
"_____no_output_____"
]
],
[
[
"Converting became_member_on to a more quantitative term member_since_days. This will depict how long the customer has been member of the program.",
"_____no_output_____"
]
],
[
[
"#Convert joining date to duration in days for which the customer is member\nprofile['became_member_on'] = pd.to_datetime(profile['became_member_on'], format='%Y%m%d')\nbaseline_date = max(profile['became_member_on'])\nprofile['member_since_days'] = profile['became_member_on'].apply(lambda x: (baseline_date - x).days)\nprofile.drop('became_member_on',axis=1,inplace=True)",
"_____no_output_____"
],
[
"profile.head()",
"_____no_output_____"
]
],
[
[
"## Transcript",
"_____no_output_____"
],
[
"Renaming columns for better understaning & meaningfulness",
"_____no_output_____"
]
],
[
[
"#Rename columns\ntranscript_cols = {'person':'customer_id'}\ntranscript = transcript.rename(columns=transcript_cols)",
"_____no_output_____"
]
],
[
[
"Removing space in event as when we explode, its easier to maintain columns name without space.",
"_____no_output_____"
]
],
[
[
"transcript['event'] = transcript['event'].str.replace(' ', '-')",
"_____no_output_____"
]
],
[
[
"Split the value column into three columns as the keys of the dictionary which represents offer_id, reward, amount. Also we will merge offer_id and \"offer id\" into single attribute offer_id.",
"_____no_output_____"
]
],
[
[
"transcript['offer_id'] = transcript['value'].apply(lambda x: x.get('offer_id'))\ntranscript['offer id'] = transcript['value'].apply(lambda x: x.get('offer id'))\ntranscript['reward'] = transcript['value'].apply(lambda x: x.get('reward'))\ntranscript['amount'] = transcript['value'].apply(lambda x: x.get('amount'))\n\ntranscript['offer_id'] = transcript.apply(lambda x : x['offer id'] if x['offer_id'] == None else x['offer_id'], axis=1)\ntranscript.drop(['offer id' , 'value'] , axis=1, inplace=True)\ntranscript.fillna(0 , inplace=True)\n",
"_____no_output_____"
],
[
"transcript.head()",
"_____no_output_____"
]
],
[
[
"# Preparing data for Analysis",
"_____no_output_____"
],
[
"## Merging the three tables",
"_____no_output_____"
]
],
[
[
"merged_df = pd.merge(portfolio, transcript, on='offer_id')\nmerged_df = pd.merge(merged_df, profile, on='customer_id')",
"_____no_output_____"
],
[
"merged_df.head()",
"_____no_output_____"
],
[
"merged_df.groupby(['event','offer_type'])['offer_type'].count()",
"_____no_output_____"
],
[
"merged_df['event'] = merged_df['event'].map({'offer-received':1, 'offer-viewed':2, 'offer-completed':3})",
"_____no_output_____"
]
],
[
[
"## Generating the target variable",
"_____no_output_____"
],
[
"When a customer completes the offer against an offer_id we will label that as a success. If the status is not in Offer-completed then the cust_id, order_id detail we be considerd as unsuccessful ad targeting.",
"_____no_output_____"
]
],
[
[
"#Create a target variable from event\nmerged_df['Offer_Encashed'] = 0\nfor row in range(merged_df.shape[0]):\n current_event = merged_df.at[row,'event']\n if current_event == 3:\n merged_df.at[row,'Offer_Encashed'] = 1",
"_____no_output_____"
],
[
"merged_df.Offer_Encashed.value_counts()",
"_____no_output_____"
],
[
"merged_df['offer_type'].value_counts().plot.barh(title='Offer Type distribution')",
"_____no_output_____"
]
],
[
[
"Buy One Get One & discount Offer type have similar distribution.",
"_____no_output_____"
]
],
[
[
"merged_df['Age_group'].value_counts().plot.barh(title=' Distribution of age groups')",
"_____no_output_____"
]
],
[
[
"It is quite surprising to see that customers Above 60 use Starbucks application the most, those with age 40-60 are on the second. One would usually think that customers between age 20-45 use app the most, but this is not the case here.",
"_____no_output_____"
]
],
[
[
"merged_df['event'].value_counts().plot.barh(title=' Event distribution')",
"_____no_output_____"
]
],
[
[
"From distribution it follows the sales funnel. \nOffer received > Offer Viewed > Offer completed.",
"_____no_output_____"
]
],
[
[
"plt.figure(figsize=(15, 5))\nsns.countplot(x=\"Age_group\", hue=\"gender\", data=merged_df)\nsns.set(style=\"whitegrid\")\nplt.title('Gender distribution in different age groups')\nplt.ylabel('No of instances')\nplt.xlabel('Age Group')\nplt.legend(title='Gender')",
"_____no_output_____"
]
],
[
[
"The male customers are more than the female ones in each age group. Buut in above 60 range the distribution is almost 50-50",
"_____no_output_____"
]
],
[
[
"plt.figure(figsize=(15, 5))\nsns.countplot(x=\"event\", hue=\"gender\", data=merged_df)\nplt.title('Distribution of Event Type by Gender ')\nplt.ylabel('No of instances')\nplt.xlabel('Event Type')\nplt.legend(title='Gender')",
"_____no_output_____"
],
[
"plt.figure(figsize=(15, 5))\nsns.countplot(x=\"event\", hue=\"offer_type\", data=merged_df)\nplt.title('Distribution of offer types in events')\nplt.ylabel('No of instances')\nplt.xlabel('Event Type')\nplt.legend(title='Offer Type')",
"_____no_output_____"
]
],
[
[
"From the graph we can infer that the discount offer type once viewed are very likely to be completed. ",
"_____no_output_____"
]
],
[
[
"plt.figure(figsize=(15, 5))\nsns.countplot(x=\"Age_group\", hue=\"event\", data=merged_df)\nplt.title('Event type distribution by age group')\nplt.ylabel('No of instances')\nplt.xlabel('Age Group')\nplt.legend(title='Event Type')",
"_____no_output_____"
]
],
[
[
"# iv) Build a Machine Learning model to predict response of a customer to an offer",
"_____no_output_____"
],
[
"## 1. Data Preparation and Cleaning II",
"_____no_output_____"
],
[
"#### Tasks\n1. Encode categorical data such as gender, offer type and age groups.\n2. Encode the 'event' data to numerical values:\n * offer received ---> 1\n * offer viewed ---> 2\n * offer completed ---> 3\n3. Encode offer id.\n4. Scale and normalize numerical data.",
"_____no_output_____"
]
],
[
[
"dummy = pd.get_dummies(merged_df.offer_type.apply(pd.Series).stack()).sum(level=0)\nmerged_df = pd.concat([merged_df, dummy], axis=1)\nmerged_df.drop(columns='offer_type', inplace=True)",
"_____no_output_____"
],
[
"dummy = pd.get_dummies(merged_df.gender.apply(pd.Series).stack()).sum(level=0)\nmerged_df = pd.concat([merged_df, dummy], axis=1)\nmerged_df.drop(columns='gender', inplace=True)",
"_____no_output_____"
],
[
"dummy = pd.get_dummies(merged_df.Age_group.apply(pd.Series).stack()).sum(level=0)\nmerged_df = pd.concat([merged_df, dummy], axis=1)\nmerged_df.drop(columns='Age_group', inplace=True)",
"_____no_output_____"
],
[
"dummy = pd.get_dummies(merged_df.Income_group.apply(pd.Series).stack()).sum(level=0)\nmerged_df = pd.concat([merged_df, dummy], axis=1)\nmerged_df.drop(columns='Income_group', inplace=True)",
"_____no_output_____"
],
[
"offerids = merged_df['offer_id'].unique().tolist()\no_mapping = dict( zip(offerids,range(len(offerids))) )\nmerged_df.replace({'offer_id': o_mapping},inplace=True)",
"_____no_output_____"
]
],
[
[
"Distribution of encashemnt of offer by Age group and gender.",
"_____no_output_____"
]
],
[
[
"sns.set_style('whitegrid')\nbar_color= ['r', 'g', 'y', 'c', 'm']\nfig,ax= plt.subplots(1,3,figsize=(15,5))\nfig.tight_layout()\n\nmerged_df[merged_df['Offer_Encashed']==1][['F','M','O']].sum().plot.bar(ax=ax[0], fontsize=10,color=bar_color) \nax[0].set_title(\" Offer Encashed - Gender Wise\")\nax[0].set_xlabel(\"Gender\")\nax[0].set_ylabel(\"No of Encashment\")\n\nage_cols=['Under 20','21-35', '36-50', '51-65', 'Above 65']\nmerged_df[merged_df['Offer_Encashed']==1][age_cols].sum().plot.bar(ax=ax[1], fontsize=10,color=bar_color) \nax[1].set_title(\"Offer Encashed - Age Wise\")\nax[1].set_xlabel(\"Age Group\")\nax[1].set_ylabel(\"No of Encashment\")\n\nincome_cols=['30-50K', '50-80K', '80-110K', 'Above 110K']\nmerged_df[merged_df['Offer_Encashed']==1][income_cols].sum().plot.bar(ax=ax[2], fontsize=10, color=bar_color) \nax[2].set_title(\"Offer Encashed - Income Wise\")\nax[2].set_xlabel(\"Income\")\nax[2].set_ylabel(\"No of Encashment\")\nplt.show()",
"_____no_output_____"
],
[
"#drop customer_id, time, amount, event\nmerged_df.drop(['customer_id', 'time', 'amount', 'event', 'reward'], axis=1, inplace=True)",
"_____no_output_____"
],
[
"from sklearn.preprocessing import MinMaxScaler\nscaler = MinMaxScaler()\nnumerical = ['offer_difficulty', 'offer_duration', 'offer_reward', 'member_since_days']\nmerged_df[numerical] = scaler.fit_transform(merged_df[numerical])",
"_____no_output_____"
],
[
"merged_df.drop_duplicates(inplace=True)",
"_____no_output_____"
],
[
"merged_df.head()",
"_____no_output_____"
]
],
[
[
"## 2. Split train and test data",
"_____no_output_____"
],
[
"Final data is ready after tasks 1-5. We will now split the data (both features and their labels) into training and test sets, taking 60% of data for training and 40% for testing.",
"_____no_output_____"
]
],
[
[
"data = merged_df.drop('Offer_Encashed', axis=1)\nlabel = merged_df['Offer_Encashed']",
"_____no_output_____"
],
[
"X_train, X_test, y_train, y_test = train_test_split(data, label, test_size = 0.3, random_state = 4756)\nprint(\"Train: {} Test {}\".format(X_train.shape[0], X_test.shape[0]))",
"Train: 52300 Test 22415\n"
]
],
[
[
"## Model training and testing",
"_____no_output_____"
],
[
"### Metrics",
"_____no_output_____"
],
[
"We will consider the F1 score as the model metric to assess the quality of the approach and determine which model gives the best results. It can be interpreted as the weighted average of the precision and recall. The traditional or balanced F-score (F1 score) is the harmonic mean of precision and recall, where an F1 score reaches its best value at 100 and worst at 0.",
"_____no_output_____"
]
],
[
[
"def get_model_scores(classifier):\n train_prediction = (classifier.fit(X_train, y_train)).predict(X_train)\n test_predictions = (classifier.fit(X_train, y_train)).predict(X_test)\n f1_train = accuracy_score(y_train, train_prediction)*100\n f1_test = fbeta_score(y_test, test_predictions, beta = 0.5, average='micro' )*100\n clf_name = classifier.__class__.__name__\n \n return f1_train, f1_test, clf_name",
"_____no_output_____"
]
],
[
[
"### LogisticRegression (Benchmark)",
"_____no_output_____"
],
[
"I am using LogisticRegression classifier to build the benchmark, and evaluate the model result by the F1 score metric.",
"_____no_output_____"
]
],
[
[
"lr_clf = LogisticRegression(random_state = 10)\nlr_f1_train, lr_f1_test, lr_model = get_model_scores(lr_clf)",
"_____no_output_____"
],
[
"linear = {'Benchmark Model': [ lr_model], 'F1-Score(Training)':[lr_f1_train], 'F1-Score(Test)': [lr_f1_test]}\nbenchmark = pd.DataFrame(linear)",
"_____no_output_____"
],
[
"benchmark",
"_____no_output_____"
]
],
[
[
"### RandomForestClassifier",
"_____no_output_____"
]
],
[
[
"rf_clf = RandomForestClassifier(random_state = 10, criterion='gini', min_samples_leaf=10, min_samples_split=2, n_estimators=100)\nrf_f1_train, rf_f1_test, rf_model = get_model_scores(rf_clf)",
"_____no_output_____"
]
],
[
[
"### DecisionTreeClassifier",
"_____no_output_____"
]
],
[
[
"dt_clf = DecisionTreeClassifier(random_state = 10)\ndt_f1_train, dt_f1_test, dt_model = get_model_scores(dt_clf)",
"_____no_output_____"
]
],
[
[
"### K Nearest Neighbors",
"_____no_output_____"
]
],
[
[
"knn_clf = KNeighborsClassifier(n_neighbors = 5)\nknn_f1_train, knn_f1_test, knn_model = get_model_scores(knn_clf)",
"_____no_output_____"
]
],
[
[
"## Classifier Evaluation Summary",
"_____no_output_____"
]
],
[
[
"performance_summary = {'Classifier': [lr_model, rf_model, dt_model, knn_model], \n 'F1-Score':[lr_f1_train, rf_f1_train, dt_f1_train, knn_f1_train] }\n \nperformance_summary = pd.DataFrame(performance_summary)",
"_____no_output_____"
],
[
"performance_summary",
"_____no_output_____"
]
]
] | [
"markdown",
"code",
"markdown",
"code",
"markdown",
"code",
"markdown",
"code",
"markdown",
"code",
"markdown",
"code",
"markdown",
"code",
"markdown",
"code",
"markdown",
"code",
"markdown",
"code",
"markdown",
"code",
"markdown",
"code",
"markdown",
"code",
"markdown",
"code",
"markdown",
"code",
"markdown",
"code",
"markdown",
"code",
"markdown",
"code",
"markdown",
"code",
"markdown",
"code",
"markdown",
"code",
"markdown",
"code",
"markdown",
"code",
"markdown",
"code",
"markdown",
"code",
"markdown",
"code",
"markdown",
"code",
"markdown",
"code",
"markdown",
"code",
"markdown",
"code",
"markdown",
"code",
"markdown",
"code",
"markdown",
"code"
] | [
[
"markdown",
"markdown"
],
[
"code"
],
[
"markdown"
],
[
"code"
],
[
"markdown",
"markdown"
],
[
"code",
"code",
"code",
"code",
"code",
"code"
],
[
"markdown"
],
[
"code",
"code",
"code",
"code",
"code",
"code",
"code",
"code"
],
[
"markdown"
],
[
"code",
"code"
],
[
"markdown"
],
[
"code",
"code",
"code",
"code",
"code"
],
[
"markdown"
],
[
"code",
"code",
"code",
"code",
"code",
"code"
],
[
"markdown",
"markdown",
"markdown",
"markdown"
],
[
"code"
],
[
"markdown"
],
[
"code",
"code"
],
[
"markdown",
"markdown"
],
[
"code"
],
[
"markdown"
],
[
"code"
],
[
"markdown"
],
[
"code"
],
[
"markdown"
],
[
"code"
],
[
"markdown"
],
[
"code",
"code"
],
[
"markdown",
"markdown"
],
[
"code"
],
[
"markdown"
],
[
"code"
],
[
"markdown"
],
[
"code",
"code"
],
[
"markdown",
"markdown"
],
[
"code",
"code",
"code",
"code"
],
[
"markdown",
"markdown"
],
[
"code",
"code",
"code"
],
[
"markdown"
],
[
"code"
],
[
"markdown"
],
[
"code"
],
[
"markdown"
],
[
"code"
],
[
"markdown"
],
[
"code",
"code"
],
[
"markdown"
],
[
"code"
],
[
"markdown",
"markdown",
"markdown"
],
[
"code",
"code",
"code",
"code",
"code"
],
[
"markdown"
],
[
"code",
"code",
"code",
"code",
"code"
],
[
"markdown",
"markdown"
],
[
"code",
"code"
],
[
"markdown",
"markdown",
"markdown"
],
[
"code"
],
[
"markdown",
"markdown"
],
[
"code",
"code",
"code"
],
[
"markdown"
],
[
"code"
],
[
"markdown"
],
[
"code"
],
[
"markdown"
],
[
"code"
],
[
"markdown"
],
[
"code",
"code"
]
] |
e7714383197c30b2cfa27a5a4f4c19ad3b01a452 | 11,200 | ipynb | Jupyter Notebook | Recommendations/recommendation_kmeans/recommendation_project_part2.ipynb | ankit-kothari/data_science_journey | 350c9df0848545250a60b6e870dbfdc870e97c2e | [
"MIT"
] | null | null | null | Recommendations/recommendation_kmeans/recommendation_project_part2.ipynb | ankit-kothari/data_science_journey | 350c9df0848545250a60b6e870dbfdc870e97c2e | [
"MIT"
] | null | null | null | Recommendations/recommendation_kmeans/recommendation_project_part2.ipynb | ankit-kothari/data_science_journey | 350c9df0848545250a60b6e870dbfdc870e97c2e | [
"MIT"
] | null | null | null | 28.35443 | 248 | 0.505268 | [
[
[
"import pandas as pd\nimport numpy as np\nimport time\nimport ast\nimport datetime\nimport matplotlib.pyplot as plt\nfrom sklearn.preprocessing import StandardScaler\nfrom sklearn.feature_selection import RFECV\nimport numpy as np\nimport seaborn as sns\nfrom sklearn.model_selection import cross_val_score\nfrom sklearn import metrics\nimport matplotlib.pyplot as plt\nimport warnings\nfrom sklearn.preprocessing import StandardScaler\nfrom sklearn import preprocessing\nfrom sklearn.manifold import TSNE\nwarnings.filterwarnings(\"ignore\")\nfrom scipy import stats\nfrom scipy.stats import shapiro\nfrom scipy.stats import anderson\nfrom sklearn.model_selection import train_test_split\nfrom sklearn.decomposition import PCA\nfrom sklearn import preprocessing",
"_____no_output_____"
]
],
[
[
"# About the Dataset",
"_____no_output_____"
]
],
[
[
"\n#nextcell\nratings = pd.read_csv('/Users/ankitkothari/Documents/gdrivre/UMD/MSML-602-DS/final_project/ratings_small.csv')\nmovies = pd.read_csv('/Users/ankitkothari/Documents/gdrivre/UMD/MSML-602-DS/final_project/movies_metadata_features.csv')\n\n",
"_____no_output_____"
]
],
[
[
"# Data Cleaning\n\n## Dropping Columns",
"_____no_output_____"
]
],
[
[
"movies.drop(columns=['Unnamed: 0'],inplace=True)\nratings = pd.merge(movies,ratings).drop(['genres','timestamp','imdb_id','overview','popularity','production_companies','production_countries','release_date','revenue','runtime','vote_average','year','vote_count','original_language'],axis=1)\n",
"_____no_output_____"
],
[
"usri = int(input()) #587 #15 #468\nselect_user = ratings.loc[ratings['userId'] == usri]\n\n",
"15\n"
]
],
[
[
"## Finding Similarity Matrix\n### Creating a Pivot Table of Title against userId for ratings",
"_____no_output_____"
]
],
[
[
"userRatings = ratings.pivot_table(index=['title'],columns=['userId'],values='rating')\nuserRatings = userRatings.dropna(thresh=10, axis=1).fillna(0,axis=1)\n\n",
"_____no_output_____"
],
[
"corrMatrix = userRatings.corr(method='pearson')\n#corrMatrix = userRatings.corr(method='spearman')\n#corrMatrix = userRatings.corr(method='kendall')\n",
"_____no_output_____"
]
],
[
[
"### Creating Similarity Matrix using Pearson Correlation method",
"_____no_output_____"
]
],
[
[
"def get_similar(usrid):\n similar_ratings = corrMatrix[usrid]\n similar_ratings = similar_ratings.sort_values(ascending=False)\n return similar_ratings\n\n",
"_____no_output_____"
]
],
[
[
"# Recommendation",
"_____no_output_____"
]
],
[
[
"moidofotus = [0,0,0,0]\ns_m = pd.DataFrame()\ns_m = s_m.append(get_similar(usri), ignore_index=True)\nfor c in range(0,4):\n moidofotus[c]=s_m.columns[c]\n\nif moidofotus[0] == usri:\n moidofotus.pop(0)\nprint(moidofotus)\n\nmovie_match=[]\nfor i in moidofotus:\n select_user = ratings.loc[ratings['userId'] == i]\n #print(select_user)\n print(\"For user\", i)\n final_use = select_user.loc[select_user['rating'] >= 4.0].sort_values(by=['rating'],ascending=False).iloc[0:10,:]\n print(final_use['title'])\n movie_match.append(final_use['title'].to_list())",
"[388, 461, 509]\nFor user 388\n14337 The Chronicles of Riddick: Dark Fury\n6683 Mr. & Mrs. Smith\n4016 Love Actually\n11089 The Golden Compass\n9419 Notes on a Scandal\n4792 Dawn of the Dead\n5204 Zatoichi\n10750 Postal\n10478 Across the Universe\n7090 Cockles and Muscles\nName: title, dtype: object\nFor user 461\n818 The Million Dollar Hotel\n2030 Monsoon Wedding\n14347 The Chronicles of Riddick: Dark Fury\n1126 Under the Sand\n5399 Two Brothers\n13730 Windows on Monday\n13107 Shriek If You Know What I Did Last Friday the ...\n12576 The Garden of Eden\n12046 Reclaim Your Brain\n1012 One Night at McCool's\nName: title, dtype: object\nFor user 509\n12882 The Red Elvis\n13114 Shriek If You Know What I Did Last Friday the ...\n3037 The Hours\n2330 Enough\n4460 The Butterfly Effect\n1825 The Shipping News\n4381 Cold Mountain\n14195 Totally Blonde\n7271 Saw II\n4614 Monsieur Ibrahim\nName: title, dtype: object\n"
],
[
"select_user['title']",
"_____no_output_____"
]
],
[
[
"## Performance Evaluation",
"_____no_output_____"
]
],
[
[
"movies_suggested_and_he_watched=0\ntotal_suggest_movies = 0\nfor movies in movie_match:\n total_suggest_movies=total_suggest_movies+len(movies)\n for movie in movies:\n if movie in select_user['title'].to_list():\n movies_suggested_and_he_watched=movies_suggested_and_he_watched+1\nprint(movies_suggested_and_he_watched)\nprint(total_suggest_movies)",
"27\n30\n"
]
]
] | [
"code",
"markdown",
"code",
"markdown",
"code",
"markdown",
"code",
"markdown",
"code",
"markdown",
"code",
"markdown",
"code"
] | [
[
"code"
],
[
"markdown"
],
[
"code"
],
[
"markdown"
],
[
"code",
"code"
],
[
"markdown"
],
[
"code",
"code"
],
[
"markdown"
],
[
"code"
],
[
"markdown"
],
[
"code",
"code"
],
[
"markdown"
],
[
"code"
]
] |
e7714c4c036b5c2a124279ed8459d3be41d769be | 503,699 | ipynb | Jupyter Notebook | src/survey/results/interpretation.ipynb | maybe-hello-world/team_blue_291A | 2da35ffae79742f968d95f1be83bdc8910f90b81 | [
"MIT"
] | null | null | null | src/survey/results/interpretation.ipynb | maybe-hello-world/team_blue_291A | 2da35ffae79742f968d95f1be83bdc8910f90b81 | [
"MIT"
] | 1 | 2021-12-04T04:00:57.000Z | 2021-12-04T04:00:57.000Z | src/survey/results/interpretation.ipynb | maybe-hello-world/team_blue_291A | 2da35ffae79742f968d95f1be83bdc8910f90b81 | [
"MIT"
] | null | null | null | 1,119.331111 | 450,436 | 0.952583 | [
[
[
"import pandas as pd\nimport numpy as np\nimport seaborn as sns\nimport matplotlib.pyplot as plt\n\nsns.set(font_scale=1.3)",
"_____no_output_____"
],
[
"import sys\nsys.version",
"_____no_output_____"
],
[
"df = pd.read_csv(\"raw_data.csv\")\nprint(df.head())\nprint(len(df))",
" key value user\n0 yuaku.png 2 -5785923211323718779\n1 wbyzz.png 3 -5785923211323718779\n2 diukc.png 2 -5785923211323718779\n3 gnoij.png 1 -5785923211323718779\n4 nohcv.png 5 -5785923211323718779\n1914\n"
],
[
"df['predicted'] = pd.to_numeric(df.value)",
"_____no_output_____"
],
[
"mapping = {'img0_blue_8.png': 'sfrxq.png', 'img0_original_8.png': 'jgaxu.png', 'img10_blue_9.png': 'ybbjw.png', 'img10_original_9.png': 'vgfwg.png', 'img11_blue_12.png': 'strdd.png', 'img11_original_12.png': 'qzubf.png', 'img12_blue_6.png': 'gnoij.png', 'img12_original_6.png': 'xwtuu.png', 'img13_blue_10.png': 'nohcv.png', 'img13_original_10.png': 'diukc.png', 'img14_blue_5.png': 'hkshk.png', 'img14_original_5.png': 'yuaku.png', 'img1_blue_3.png': 'rftjn.png', 'img1_original_3.png': 'wwjkt.png', 'img2_blue_7.png': 'dnocu.png', 'img2_original_7.png': 'wbyzz.png', 'img3_blue_8.png': 'mepqp.png', 'img3_original_8.png': 'mregu.png', 'img4_blue_7.png': 'pofzm.png', 'img4_original_7.png': 'crelm.png', 'img5_blue_12.png': 'frofx.png', 'img5_original_12.png': 'tuliw.png', 'img6_blue_13.png': 'fsgtc.png', 'img6_original_13.png': 'zlltm.png', 'img7_blue_8.png': 'mcbvs.png', 'img7_original_8.png': 'nkebh.png', 'img8_blue_4.png': 'wdfpk.png', 'img8_original_4.png': 'msqxw.png', 'img9_blue_5.png': 'sbstp.png', 'img9_original_5.png': 'vxlcq.png'}",
"_____no_output_____"
],
[
"reverse_mapping = dict(((value, key) for key, value in mapping.items()))",
"_____no_output_____"
],
[
"df['picture'] = df.apply(lambda x: reverse_mapping[x['key']], axis=1)",
"_____no_output_____"
],
[
"df = df.filter(['user', 'picture', 'predicted'])",
"_____no_output_____"
],
[
"def parse_name(name: pd.Series) -> (str, bool, int):\n name = name['picture'].removesuffix('.png').split(\"_\")\n return name[0], name[1] == 'blue', int(name[2])",
"_____no_output_____"
],
[
"df[['picture', 'our_solution', 'true_objects']] = df.apply(parse_name, axis=1, result_type='expand')",
"_____no_output_____"
],
[
"df = df[df.predicted < 20]",
"_____no_output_____"
],
[
"df['absolute_error'] = abs(df['true_objects'] - df['predicted'])",
"_____no_output_____"
],
[
"print(len(df[df.our_solution == True]), len(df[df.our_solution != True]))",
"985 923\n"
],
[
"df.head()",
"_____no_output_____"
],
[
"plt.figure(figsize=(10, 5))\nsns.histplot(data=df, x='absolute_error', hue='our_solution', kde=True, multiple='dodge')",
"_____no_output_____"
],
[
"fig, axarr = plt.subplots(5, 3, figsize=(20, 25))\nfor i, picture in enumerate(f\"img{i}\" for i in range(15)):\n i, j = i // 3, i % 3\n data = df[df.picture == picture]\n title = f\"{picture}, total answers: {len(data)}\"\n sns.histplot(data=data, x='absolute_error', hue='our_solution', kde=True, multiple='dodge', ax=axarr[i, j]).set_title(title)\nplt.tight_layout()",
"_____no_output_____"
],
[
"original_df = df[df.our_solution == False]\nour_df = df[df.our_solution == True]\n\nprint(f\"Original images: mean={original_df.absolute_error.mean():.3f}, std={original_df.absolute_error.std():.3f}\")\nprint(f\"Our images: mean={our_df.absolute_error.mean():.3f}, std={our_df.absolute_error.std():.3f}\")",
"Original images: mean=6.113, std=2.834\nOur images: mean=5.298, std=2.784\n"
],
[
"for i, picture in enumerate(f\"img{i}\" for i in range(15)):\n data = df[df.picture == picture]\n original_data = data[data.our_solution == False]\n our_data = data[data.our_solution == True]\n print(f\"{picture} & {original_data.absolute_error.mean():.3f} & {original_data.absolute_error.std():.3f} & {our_data.absolute_error.mean():.3f} & {our_data.absolute_error.std():.3f} \\\\\\\\\")",
"img0 & 6.685 & 1.039 & 5.218 & 1.166 \\\\\nimg1 & 1.939 & 0.345 & 0.929 & 0.759 \\\\\nimg2 & 5.491 & 0.663 & 5.397 & 0.493 \\\\\nimg3 & 4.723 & 1.218 & 4.737 & 1.717 \\\\\nimg4 & 5.115 & 0.858 & 3.628 & 0.723 \\\\\nimg5 & 10.407 & 0.922 & 10.174 & 0.985 \\\\\nimg6 & 10.647 & 1.219 & 8.937 & 1.693 \\\\\nimg7 & 6.163 & 0.943 & 5.457 & 0.973 \\\\\nimg8 & 2.746 & 0.659 & 1.906 & 0.904 \\\\\nimg9 & 3.712 & 0.651 & 3.383 & 0.555 \\\\\nimg10 & 7.029 & 0.170 & 5.676 & 0.692 \\\\\nimg11 & 10.983 & 0.296 & 9.500 & 0.985 \\\\\nimg12 & 4.966 & 0.417 & 3.972 & 0.985 \\\\\nimg13 & 7.726 & 0.813 & 6.325 & 1.586 \\\\\nimg14 & 3.738 & 0.603 & 3.000 & 1.008 \\\\\n"
],
[
"len(set(df.user))",
"_____no_output_____"
]
]
] | [
"code"
] | [
[
"code",
"code",
"code",
"code",
"code",
"code",
"code",
"code",
"code",
"code",
"code",
"code",
"code",
"code",
"code",
"code",
"code",
"code",
"code"
]
] |
e77151d9a694ca5de838d0ed48c5a80a5e597617 | 40,966 | ipynb | Jupyter Notebook | busca v0.5.ipynb | carvalhoandre/interpretacao_dados | 64e094e820d7dd09eb44207077c3c733418aff03 | [
"MIT"
] | null | null | null | busca v0.5.ipynb | carvalhoandre/interpretacao_dados | 64e094e820d7dd09eb44207077c3c733418aff03 | [
"MIT"
] | null | null | null | busca v0.5.ipynb | carvalhoandre/interpretacao_dados | 64e094e820d7dd09eb44207077c3c733418aff03 | [
"MIT"
] | null | null | null | 129.230284 | 1,946 | 0.675048 | [
[
[
"### Uninove \n\nData: 17/02/2022\n\nProfessor: Leandro Romualdo da Silva\n\nDisciplina: Inteligência Artificial\n\nMatéria: Algoritmos de Busca \n\nResumo: O código abaixo cria o ambiente do labirinto usando a biblioteca turtle e o agente precisa encontrar o caminho de saida do labirinto, a busca com objetivo de encontrar a saida utiliza algumas funções que fazer o agente testar caminhos e após encontrar o caminho de volta retorna a posição inicial. \n\nReferências: https://panda.ime.usp.br/panda/static/pythonds_pt/04-Recursao/10-labirinto.html\nhttps://docs.python.org/3/library/turtle.html\n\n\nOutro material de referência muito interessante é este trabalho que usa algoritmos de busca no pacman. http://www.ic.uff.br/~bianca/ia-pos/t1.html",
"_____no_output_____"
]
],
[
[
"import turtle\n\n'''\n\nParâmetros que delimitam o labirinto, indicam os obstaculos, caminhos livres para seguir, saida do labirinto e caminho correto identificado.\n\nPART_OF_PART - O caminho correto é sinalizado retornando ao ponto de partida.\nTRIED - Caminho percorrido pelo agente. Sinaliza o caminho que ele esta buscando pela saida. \nOBSTACLE - O caminho contém obstaculos que delimitam o labirinto e são representados pelo simbolo +.\nDEAD_END - Sinaliza caminhos que o agente já percorreu e estão errados. \n\n'''\nPART_OF_PATH = 'O'\nTRIED = '.'\nOBSTACLE = '+'\nDEAD_END = '-'\n\nclass Maze:\n\n '''\n A função __init__ lê o arquivo com a matriz que representa o labirinto, lê a quantidade de linhas e colunas, bem como linha a coluna de inicio\n Instância o Turtle para gerar a interface gráfica e utiliza como coordenadas as linhas e colunas da nossa matriz\n A posição inicial do agente é lida através do loop na função. \n Instanciamos o turtle, definimos um formato do agente que pode ser turtle, arrow, circle, square, triangle, classic.\n\n '''\n def __init__(self,mazeFileName):\n rowsInMaze = 0\n columnsInMaze = 0\n self.mazelist = []\n mazeFile = open(mazeFileName,'r')\n rowsInMaze = 0\n for line in mazeFile:\n rowList = []\n col = 0\n for ch in line[:-1]:\n rowList.append(ch)\n if ch == 'S':\n self.startRow = rowsInMaze\n self.startCol = col\n col = col + 1\n rowsInMaze = rowsInMaze + 1\n self.mazelist.append(rowList)\n columnsInMaze = len(rowList)\n\n self.rowsInMaze = rowsInMaze\n self.columnsInMaze = columnsInMaze\n self.xTranslate = -columnsInMaze/2\n self.yTranslate = rowsInMaze/2\n self.t = turtle.Turtle()\n self.t.shape('turtle')\n turtle.title('Desafio saida de labirinto')\n self.wn = turtle.Screen()\n self.wn.setworldcoordinates(-(columnsInMaze-1)/2-.5,-(rowsInMaze-1)/2-.5,(columnsInMaze-1)/2+.5,(rowsInMaze-1)/2+.5)\n\n def drawMaze(self):\n '''\n Função que cria a interação do gráfico do labirinto, temos a velocidade, o tracer, criamos uma lista com a linha e coluna\n checamos se é um obstáculo e pintamos de laranja para gerar o mapa do labirinto.\n O rastro do agente é da cor cinza e o agente da cor vermelho e pode ser alterado nas configurações abaixo.\n '''\n self.t.speed(10)\n self.wn.tracer(0)\n for y in range(self.rowsInMaze):\n for x in range(self.columnsInMaze):\n if self.mazelist[y][x] == OBSTACLE:\n self.drawCenteredBox(x+self.xTranslate,-y+self.yTranslate,'orange')\n self.t.color('gray')\n self.t.fillcolor('red')\n self.wn.update()\n self.wn.tracer(1)\n\n def drawCenteredBox(self,x,y,color):\n\n '''\n Esta função recebe coluna, linha e cor que será aplicada para o centro do box. \n '''\n self.t.up()\n self.t.goto(x-.5,y-.5)\n self.t.color(color)\n self.t.fillcolor(color)\n self.t.setheading(90)\n self.t.down()\n self.t.begin_fill()\n for i in range(4):\n self.t.forward(1)\n self.t.right(90)\n self.t.end_fill()\n\n def moveAgent(self,x,y):\n\n '''\n Função que move o agente, a chamada \"goto\" faz o movimento do agente.\n '''\n self.t.up()\n self.t.setheading(self.t.towards(x+self.xTranslate,-y+self.yTranslate))\n self.t.goto(x+self.xTranslate,-y+self.yTranslate)\n\n def dropBreadcrumb(self,color): \n self.t.dot(10,color)\n\n def updatePosition(self,row,col,val=None):\n\n '''\n Checa se a posição indicada é valida e movimenta o agente para nova posição, Se a posição é valida a cor azul é aplicada ao rastro,\n Se for um caminho já explorado a cor vermelha é aplicada, caso tenha finalizado a saida o percurso de volta é salvo em verde. \n '''\n \n if val:\n self.mazelist[row][col] = val\n self.moveAgent(col,row)\n\n if val == PART_OF_PATH:\n color = 'green'\n elif val == OBSTACLE:\n color = 'red'\n elif val == TRIED:\n color = 'blue'\n elif val == DEAD_END:\n color = 'red'\n else:\n color = None\n\n if color:\n self.dropBreadcrumb(color)\n\n def isExit(self,row,col):\n '''\n Função de saida de acordo com as regras da matriz 0 ou rowsInMaze-1 determinam a saida.\n '''\n\n return (row == 0 or\n row == self.rowsInMaze-1 or\n col == 0 or\n col == self.columnsInMaze-1 )\n\n def __getitem__(self,idx):\n return self.mazelist[idx]\n\ndef searchFrom(maze, startRow, startColumn):\n\n '''\n Função de busca em si, recebe a matriz (maze) linha e coluna de inicio. Aqui aplicamos os testes de direção e vamos explorando o caminho\n usando as demais funções. \n '''\n # Tente cada uma das posições até encontrar a saida\n # Valores de retorno na saida da base\n # 1. Se encontrar um obstaculo retornar false\n maze.updatePosition(startRow, startColumn)\n if maze[startRow][startColumn] == OBSTACLE :\n return False\n # 2. Encontrou uma área que já foi explorada\n if maze[startRow][startColumn] == TRIED or maze[startRow][startColumn] == DEAD_END:\n return False\n # 3. Encontrou uma borda não ocupada por um obstáculo\n if maze.isExit(startRow,startColumn):\n maze.updatePosition(startRow, startColumn, PART_OF_PATH)\n return True\n maze.updatePosition(startRow, startColumn, TRIED)\n print(startColumn, startRow)\n # Caso contrário teste cada direção novamente\n found = searchFrom(maze, startRow-1, startColumn) or \\\n searchFrom(maze, startRow+1, startColumn) or \\\n searchFrom(maze, startRow, startColumn-1) or \\\n searchFrom(maze, startRow, startColumn+1)\n if found:\n maze.updatePosition(startRow, startColumn, PART_OF_PATH)\n else:\n maze.updatePosition(startRow, startColumn, DEAD_END)\n return found",
"_____no_output_____"
],
[
"myMaze = Maze('D:\\Users\\andre\\Documents\\Faculdade\\inteligencia artificial\\maze2.txt')\nmyMaze.drawMaze()\nmyMaze.updatePosition(myMaze.startRow,myMaze.startCol)",
"_____no_output_____"
],
[
"searchFrom(myMaze, myMaze.startRow, myMaze.startCol)",
"15 8\n15 7\n14 7\n14 6\n14 5\n14 4\n13 4\n13 5\n13 6\n12 6\n12 7\n12 8\n12 9\n"
]
]
] | [
"markdown",
"code"
] | [
[
"markdown"
],
[
"code",
"code",
"code"
]
] |
e7715e72b57e1ce785b5c9fe91223a4d0f369ed1 | 6,075 | ipynb | Jupyter Notebook | Chapter04/Exercise 4.06/Exercise 4.06.ipynb | abhishekr128/The-Natural-Language-Processing-Workshop | fcf1e529a5d8e2da1e5b51570339b8337ba4774b | [
"MIT"
] | 23 | 2020-05-04T18:36:41.000Z | 2022-03-16T09:22:34.000Z | Chapter04/Exercise 4.06/Exercise 4.06.ipynb | nipunsadvilkar/The-Natural-Language-Processing-Workshop | c4b815cb69235c7140908ddd61c0e61afdd9b877 | [
"MIT"
] | 9 | 2020-03-31T18:28:50.000Z | 2022-03-12T00:40:50.000Z | Chapter04/Exercise 4.06/Exercise 4.06.ipynb | nipunsadvilkar/The-Natural-Language-Processing-Workshop | c4b815cb69235c7140908ddd61c0e61afdd9b877 | [
"MIT"
] | 37 | 2020-04-11T19:08:55.000Z | 2022-03-16T09:22:40.000Z | 28.125 | 108 | 0.371523 | [
[
[
"# Exercise 6: Collect data using APIs\nUse Exchange Rates API to get USD to other currency rate for today: https://www.exchangerate-api.com/",
"_____no_output_____"
]
],
[
[
"import json\nimport pprint\nimport requests\nimport pandas as pd",
"_____no_output_____"
],
[
"r = requests.get(\"https://api.exchangerate-api.com/v4/latest/USD\")\ndata = r.json()\npprint.pprint(data)",
"{'base': 'USD',\n 'date': '2020-01-26',\n 'rates': {'AED': 3.672058,\n 'ARS': 60.073152,\n 'AUD': 1.462619,\n 'BGN': 1.772324,\n 'BRL': 4.175311,\n 'BSD': 1,\n 'CAD': 1.313949,\n 'CHF': 0.970542,\n 'CLP': 775.032232,\n 'CNY': 6.937035,\n 'COP': 3356.26087,\n 'CZK': 22.774105,\n 'DKK': 6.769282,\n 'DOP': 53.200551,\n 'EGP': 15.731404,\n 'EUR': 0.906153,\n 'FJD': 2.173377,\n 'GBP': 0.763843,\n 'GTQ': 7.686349,\n 'HKD': 7.772394,\n 'HRK': 6.743727,\n 'HUF': 304.680441,\n 'IDR': 13481.299912,\n 'ILS': 3.454776,\n 'INR': 71.322065,\n 'ISK': 124.593825,\n 'JPY': 109.43859,\n 'KRW': 1168.893381,\n 'KZT': 378.401961,\n 'MXN': 18.789109,\n 'MYR': 4.06712,\n 'NOK': 9.020184,\n 'NZD': 1.513791,\n 'PAB': 1,\n 'PEN': 3.322153,\n 'PHP': 50.850544,\n 'PKR': 154.697395,\n 'PLN': 3.850458,\n 'PYG': 6432.833333,\n 'RON': 4.330082,\n 'RUB': 61.891661,\n 'SAR': 3.750937,\n 'SEK': 9.552551,\n 'SGD': 1.351475,\n 'THB': 30.561937,\n 'TRY': 5.940419,\n 'TWD': 30.040088,\n 'UAH': 24.306582,\n 'USD': 1,\n 'UYU': 37.255792,\n 'ZAR': 14.392269},\n 'time_last_updated': 1579997437}\n"
],
[
"df = pd.DataFrame(data)\ndf.head()",
"_____no_output_____"
]
]
] | [
"markdown",
"code"
] | [
[
"markdown"
],
[
"code",
"code",
"code"
]
] |
e77164297ce8346c0d06cf1280187dda85c8a37c | 13,560 | ipynb | Jupyter Notebook | white paper.ipynb | shubham0704/parallelGSO | 46e8b6f194d339b4f4512b463009e0671a16de81 | [
"MIT"
] | 16 | 2018-07-28T15:06:07.000Z | 2021-02-13T13:42:54.000Z | white paper.ipynb | shubham0704/parallelGSO | 46e8b6f194d339b4f4512b463009e0671a16de81 | [
"MIT"
] | null | null | null | white paper.ipynb | shubham0704/parallelGSO | 46e8b6f194d339b4f4512b463009e0671a16de81 | [
"MIT"
] | null | null | null | 67.128713 | 1,026 | 0.684145 | [
[
[
"# Parallel Galactic Swarm Optimization",
"_____no_output_____"
],
[
"## Project Description\n\nDeep Learning is a family of machine learning algorithms which involve a deeper cascade of smaller learning models than traditional machine learning models have. These algorithms are used extensively for automate tasks which were previously thought to be only possible for humans to do. Today the go-to algorithm for optimizing the deep learning algorithms is Gradient Descent. Although, it works very well in practice. It gets stuck when there are multiple optimal solutions available for a given function. i.e A multimodal function. In practice a different version of it called Stochastic Gradient Descent introduces stochasticity to help navigate out of tough spots but the requirement of finding gradients limits its use case to just that.\n\nFortunately, We have a family of algorithms that are very good at dealing with the functions which are highly non-convex, the kind of ones for which we cannot find gradients to, called the metaheuristic algorithms. A research work was recently published by our university which proposed an algorithm called Galactic Swarm Optimization (GSO) which is currently the state-of-the-art. GSO doesn't need any gradient finding. So even for non-convex functions (the kind of ones which have the min/max) are also suitable. What it lacked was that it could not scale in with hardware. \n\n*Our primary contribution is that we have come up with a solution which modifies the GSO algorithm to scale in with increase in hardware. We have also created a library which is open-sourced on github along with provisions to rerun the benchmarks.*\n\n----",
"_____no_output_____"
],
[
"## Related Work\n**Particle Swarm Optimization (PSO)**\n\nPSO is inspired from how birds search for food or how a school of fishes navigate. Where a bird is termed as a particle and the search for food/best global optima is guided by each particls position, velocity and intertia.Each particle's movement is influenced by its local best known position, but is also guided toward the best known positions in the search-space, which are updated as better positions are found by other particles. This is expected to move the swarm toward the best solutions. The equation for this is given by -\n\n$V_{(t+1)}^{i} = Current motion + Particle Memory Influence + Swarm Influence$\n\nor,\n\n$V_{(t+1)}^{i} = wv_{t}^{i} + C_1r_1(p_t^i - x_{(t)}^i) + C_2r_2(G - x_{(t)}^i)$\n\nwhere $C_1$ and $C_2$ are cognition and social learning factors respectively and $r_1$, $r_2$ are randomly generated numbers in the range [0,1], G is the global best, $p_t^i$ is the local best and $v_{t}^{i}$ is the velocity of the particle at time t. \n\nThe next position of the particle is determines as follows - \n\n$X_{(t+1)}^{i} = x_{(t)}^i + v_{(t+1)}^{i}$\n\n**Simulation of PSO**\n\n\nCredits - Ephramac",
"_____no_output_____"
],
[
"## Implementation details\n\n**The sequential GSO algorithm**\n\nThe GSO algorithm is a modification of the PSO algorithm which eliminates the pain points of the PSO algorithm. Most variants of PSO first have a full exploration phase which gradually becomes a full exploitation by using the learning rate decay to strike the balance. GSO has multiple cycles of exploration and exploitation by dividing search in terms of epochs. This allows us to explore the global minima more accurately.\nConsider each galaxy as a subswarm which have a centre of mass. These galaxies are part of a larger supercluster. Where they look like point masses revolving inside. Now using PSO we find the best solution a.k.a the centre of mass of galaxy which represents the galaxy in the supercluster. Now these representative points are used to find centre of mass of this large supercluster. This heirarchy can go on even more but we currently restrict it to 2 levels. We use PSO to find the centre of mass of a galaxy/supercluster. The algorithm looks as follows-\n```\ndef GSO(M, bounds, num_particles, max_iter):\n subswarm_bests = []\n dims = len(bounds)\n lb, ub = bounds \n \n for i in range(M):\n swarm_init = list of randomly initialized num_particles in the range (lb, ub)\n subswarm_best,_ = PSO(error,bounds,maxiter, swarm_init=swarm_init)\n subswarm_bests.append(subswarm_best)\n best_position, best_error = PSO(error, bounds, maxiter, swarm_init=subswarm_bests)\n return best_position, best_error\n```\n\n\n\n**Bottleneck identification:**\n\nWe can see that there is a for-loop above where we are calling PSO function M times and collecting the output sequentially can be identified as a clear case where we can apply **SIMD (Single Instruction Multiple Data)** based parallelism. So we fork out M threads which have their own stack. We tried not to use any shared datastructure for exchanging information in this fork procedure since we do not want any latency introduced due to synchronization. But in practice we have found that doing information exchange midway on where is the best solution greatly speeds up our exploitation phase, the global_best variable is present in the shared_list internally present inside a heap visible to all threads. We have introduced a lock region which gets activate midway once, where each PSO thread updates on where is the global best. This tradeoff is done in order to encourage exploitation which ultimately helps us reach the goal faster. Below is a diagram representing the proposed and implemented fork-join model -\n\n**The parallel GSO diagram**\n\n\n\n---\n\n## Software specifications\n\n**Libraries**\n+ **Numba** - Used for speeding up math-intensive computations in python and maximizing CPU utilization\n+ **multiprocessing** - Used for spawning threads with PSO function calls\n+ **numpy** - Used some standard factory functions from numpy which have C like performance and are implicitly highly parallel\n+ **matplotlib, seaborn** - plotting graphs of CPU utilization and functions\n\n**Profiling tools**\n+ **line_profiler** - For getting line by line execution time and number of hits\n+ **timeit** - For timing the whole function and taking the best average among top N executions\n+ **psutil** - For checking individual CPU utilization when our algorithm runs (reading taken at 1ms interval).\n---\n## Code Optimizations\n\nGenerally whenever we prototype an algorithm the general strategies that were followed by famous libraries like scikit-learn, numpy are to profile the expensive parts and write them in C and then use the Cython API to call the C code into your python program. But, that is no longer the case. Why? - Because we have Numba. Numba lets you write C like performant Python Code. All you have to do is to know where and how to gain maximum performance. Our implementation is **fully vectorized and multi-threaded**. Changes we have made in our code to gain performance are as follows -\n1. Defined a custom numpy datatype for creating particle objects instead of creating class Particle. The benefit we got from this was that Numba recognizes numpy datatype because it then knows what size it can take and therefore the intermediate bytecode generated by the LLVM compiler can assign type easily to the numpy object instead of it being a standard python object with no type definitiion. In short, **this helped us with save Memory** and also made it easier for Numba to recognize and generate efficient intermediate bytecode.\n2. Inherently, numpy code is faster than Numba code (by a small factor) and therfore we have used Numpy methods in our code where possible because, numpy is heavyily optimized and scales in smoothly with increase in number of cores.\n3. Used Numba's just in time compilation for each method making sure code written in each function is easily recognized by numba (see examples on how we do it for a sample function) which **helped gain maximum performance**.\n4. After a one time run, code is automatically cached and compilation is not needed again even for the JIT compiler.\n5. Vectorized IO - Input/Output for all functions are N-dimensional numpy arrays. All transformations performed on them do not have any excess overhead and helped us with speed gains.\n6. MultiThreading - Spawning PSO functions as threads using the multiprocessing library. Threading allows multiple PSO functions to run parallely.\n\n**Simple Numba Demo to see which part of code numba speeds up**\n\n\nThe green part shows which part is sped up in the whole code.\n\n----\n## Performance Numbers\n\nWe measure our performance as follows -\n\nWe are not interested in how much time we get a solution rather in the same time if a we are able to explore the search space more agressively then we are at profit. So our parameters of algorithms worthiness is -\n\n+ Per CPU utilization\n+ Closeness of the output of GSO function to actual Global minima\n\n**Per CPU utilisation on ROSENS function**\n\n(a) 2 CPUs\n\n\n(b) 4 CPUs\n\n\n(b) 8 CPUs\n\n\n\n**Closeness to actual Global Minimas**\n\n|Function|Actual minima| After PGSO | Error |\n|-------------|: ------------- |:-------------:| -----:|\n|sphere| [0,0]| [-1.15653952e-19 2.48066460e-19] | 0.0 | \n|rosen| [1,1] | [0.99495864 1.05122347] | 0.9949590570932898 | \n|rastrigin| [0,0]| [-1.35843176e-10 -8.35954051e-10] | 0.0 | \n|griewank| [0,0]| [ 1.56490468e-09 -1.08337460e-08] | 0.0 | \n|zakharov| [0,0] | [ 2.95537177e-24 -1.80678038e-25] | 0.0 | \n|nonContinuousRastrigin| [0,0] | [-4.52851948e-10 2.70235658e-09] | 0.0 | \n\n\nWe can clearly see all are errors are less than almost 0 which shows the success of our algorithm. We can also clearly see that as we increase the number of processors the per-cpu utilization increases. The detailed reports on all the variants of the benchmarks are included in our notebooks also avaiable on github. The other experiments are more interesting and can be found in the experiments/tests directory.\n\n----\n",
"_____no_output_____"
],
[
"## Benefits to the community\n\nWe all have seen the boon of genetic programming - from Neural Architecture Search [[ 1 ]](https://arxiv.org/pdf/1704.00764) (AutoML) to automatically design better chassis for cars that humans could not possibly think of[[ 2 ]](http://boxcar2d.com/) is truly amazing. The Genetic algorithms family comes under the meta-heuristic algorithms.\n\nA comparison was made by [(R.Hassan et. al 2004)](https://bee22.com/resources/Hassan%202004.pdf) where they found PSO to be performing way better than GAs in strong settings. Our approach greatly improves the PSO and makes the search more sufficient utilizing hardware at full capacity helping narrow down the search space quickly and therfore argue that our algorithm be put to use in the above areas. We tend to offer this algorithm to the community to bolster the following areas along with the ones mentioned above. As per survey done by [(R Poli 2008)](https://www.hindawi.com/journals/jaea/2008/685175/) the areas where our algorithm will benefit are:\n\n+ Combinatorial Optimization\n+ Communication Networks\n+ Controllers\n+ Clustering and Classification\n+ Design\n+ Finance\n+ Faults\n+ Images and Videos\n\nand much more...\n\nWe can't wait enough to see what can be done further on with our work and warmly welcome constructive feedback from both the developer and the research community",
"_____no_output_____"
]
]
] | [
"markdown"
] | [
[
"markdown",
"markdown",
"markdown",
"markdown",
"markdown"
]
] |
e7717388e7c5367ec6e2c996b9a2f7c4eb54fe94 | 98,815 | ipynb | Jupyter Notebook | Section 5/Bivariate Analysis - Titanic.ipynb | kamaleshreddy/Exploratory-Data-Analysis-with-Pandas-and-Python-3.x | 54fa94962e35e1d3d83752bca99be38b646c37a3 | [
"MIT"
] | 39 | 2019-05-01T06:56:36.000Z | 2021-11-08T13:12:57.000Z | Section 5/Bivariate Analysis - Titanic.ipynb | kamaleshreddy/Exploratory-Data-Analysis-with-Pandas-and-Python-3.x | 54fa94962e35e1d3d83752bca99be38b646c37a3 | [
"MIT"
] | null | null | null | Section 5/Bivariate Analysis - Titanic.ipynb | kamaleshreddy/Exploratory-Data-Analysis-with-Pandas-and-Python-3.x | 54fa94962e35e1d3d83752bca99be38b646c37a3 | [
"MIT"
] | 29 | 2019-04-30T17:12:28.000Z | 2022-01-28T00:59:55.000Z | 99.411469 | 10,068 | 0.790993 | [
[
[
"#### Dataset Used : Titanic ( https://www.kaggle.com/c/titanic )\n\nThis dataset basically includes information regarding all the passengers on Titanic . Various attributes of passengers like age , sex , class ,etc. is recorded and final label 'survived' determines whether or the passenger survived or not .",
"_____no_output_____"
]
],
[
[
"import numpy as np\nimport pandas as pd\nimport matplotlib.pyplot as plt\nimport seaborn as sns\n%matplotlib inline\n",
"_____no_output_____"
],
[
"titanic_data_df = pd.read_csv('titanic-data.csv')",
"_____no_output_____"
]
],
[
[
"1. **Survived:** Outcome of survival (0 = No; 1 = Yes)\n2. **Pclass:** Socio-economic class (1 = Upper class; 2 = Middle class; 3 = Lower class)\n3. **Name:** Name of passenger\n4. **Sex:** Sex of the passenger\n5. **Age:** Age of the passenger (Some entries contain NaN)\n6. **SibSp:** Number of siblings and spouses of the passenger aboard\n7. **Parch:** Number of parents and children of the passenger aboard\n8. **Ticket:** Ticket number of the passenger\n9. **Fare: **Fare paid by the passenger\n10. **Cabin** Cabin number of the passenger (Some entries contain NaN)\n11. **Embarked:** Port of embarkation of the passenger (C = Cherbourg; Q = Queenstown; S = Southampton)",
"_____no_output_____"
]
],
[
[
"g = sns.countplot(x='Sex', hue='Survived', data=titanic_data_df)",
"_____no_output_____"
],
[
"g = sns.catplot(x=\"Embarked\", col=\"Survived\",\n data=titanic_data_df, kind=\"count\",\n height=4, aspect=.7);",
"_____no_output_____"
],
[
"g = sns.countplot(x='Embarked', hue='Survived', data=titanic_data_df)",
"_____no_output_____"
],
[
"g = sns.countplot(x='Embarked', hue='Pclass', data=titanic_data_df)",
"_____no_output_____"
],
[
"g = sns.countplot(x='Pclass', hue='Survived', data=titanic_data_df)",
"_____no_output_____"
]
],
[
[
"## Add a new column - Family size \nI will be adding a new column 'Family Size' which will be the SibSp and Parch + 1",
"_____no_output_____"
]
],
[
[
"#Function to add new column 'FamilySize'\ndef add_family(df):\n df['FamilySize'] = df['SibSp'] + df['Parch'] + 1 \n return df\n\ntitanic_data_df = add_family(titanic_data_df)\ntitanic_data_df.head(10)",
"_____no_output_____"
],
[
"g = sns.countplot(x=\"FamilySize\", hue=\"Survived\",\n data=titanic_data_df);",
"_____no_output_____"
],
[
"g = sns.countplot(x=\"FamilySize\", hue=\"Sex\",\n data=titanic_data_df);",
"_____no_output_____"
]
],
[
[
"## Add a new column - Age Group \n",
"_____no_output_____"
]
],
[
[
"age_df = titanic_data_df[~titanic_data_df['Age'].isnull()]\n#Make bins and group all passengers into these bins and store those values in a new column 'ageGroup'\nage_bins = ['0-9', '10-19', '20-29', '30-39', '40-49', '50-59', '60-69', '70-79']\nage_df['ageGroup'] = pd.cut(titanic_data_df.Age, range(0, 81, 10), right=False, labels=age_bins)",
"/home/kashif/anaconda3/lib/python3.7/site-packages/ipykernel_launcher.py:4: SettingWithCopyWarning: \nA value is trying to be set on a copy of a slice from a DataFrame.\nTry using .loc[row_indexer,col_indexer] = value instead\n\nSee the caveats in the documentation: http://pandas.pydata.org/pandas-docs/stable/indexing.html#indexing-view-versus-copy\n after removing the cwd from sys.path.\n"
],
[
"age_df[['Age', 'ageGroup']]",
"_____no_output_____"
],
[
"sns.countplot(x='ageGroup', hue='Survived', data=age_df)",
"_____no_output_____"
]
]
] | [
"markdown",
"code",
"markdown",
"code",
"markdown",
"code",
"markdown",
"code"
] | [
[
"markdown"
],
[
"code",
"code"
],
[
"markdown"
],
[
"code",
"code",
"code",
"code",
"code"
],
[
"markdown"
],
[
"code",
"code",
"code"
],
[
"markdown"
],
[
"code",
"code",
"code"
]
] |
e771747d27faba95da1c879d424438fd3b24dcaf | 1,560 | ipynb | Jupyter Notebook | insert 20 random letters in the range a through 'f' into a list.ipynb | AntonioRobG/programming-fundamentals-for-data-science | ae943238dd064d884f808ba182be4f6845f7ad20 | [
"MIT"
] | null | null | null | insert 20 random letters in the range a through 'f' into a list.ipynb | AntonioRobG/programming-fundamentals-for-data-science | ae943238dd064d884f808ba182be4f6845f7ad20 | [
"MIT"
] | null | null | null | insert 20 random letters in the range a through 'f' into a list.ipynb | AntonioRobG/programming-fundamentals-for-data-science | ae943238dd064d884f808ba182be4f6845f7ad20 | [
"MIT"
] | null | null | null | 22.608696 | 77 | 0.550641 | [
[
[
"\"\"\"\nInsert 20 random letters in the range \"a\" through 'f' into a list.\na)\tSort the list in ascending order.\nb)\tSort the list in descending order.\nc)\tGet the unique values and sort them in ascending order.\n\"\"\" ",
"_____no_output_____"
],
[
"import random\nmylist = list(\"abcdef\")\nnewlist = []\nfor i in range(21):\n newlist.append(random.choice(mylist))\nnewlist.sort()\nprint(newlist)\nnewlist.sort(reverse=True)\nprint(newlist)\nnewlist = list(dict.fromkeys(newlist))\nnewlist.sort()\nprint(newlist)",
"_____no_output_____"
]
]
] | [
"code"
] | [
[
"code",
"code"
]
] |
e77176c3c8f56d3c9acfd425d6e2b87623258acb | 9,813 | ipynb | Jupyter Notebook | examples/notebooks/formulas.ipynb | diego-mazon/statsmodels | af8b5b5dc78acb600ffd08cda6bd9b1ca5200e10 | [
"BSD-3-Clause"
] | null | null | null | examples/notebooks/formulas.ipynb | diego-mazon/statsmodels | af8b5b5dc78acb600ffd08cda6bd9b1ca5200e10 | [
"BSD-3-Clause"
] | 1 | 2019-07-29T08:35:08.000Z | 2019-07-29T08:35:08.000Z | examples/notebooks/formulas.ipynb | ozeno/statsmodels | 9271ced806b807a4dd325238df38b60f1aa363e2 | [
"BSD-3-Clause"
] | null | null | null | 25.033163 | 435 | 0.573525 | [
[
[
"# Formulas: Fitting models using R-style formulas",
"_____no_output_____"
],
[
"Since version 0.5.0, ``statsmodels`` allows users to fit statistical models using R-style formulas. Internally, ``statsmodels`` uses the [patsy](http://patsy.readthedocs.org/) package to convert formulas and data to the matrices that are used in model fitting. The formula framework is quite powerful; this tutorial only scratches the surface. A full description of the formula language can be found in the ``patsy`` docs: \n\n* [Patsy formula language description](http://patsy.readthedocs.org/)\n\n## Loading modules and functions",
"_____no_output_____"
]
],
[
[
"import numpy as np # noqa:F401 needed in namespace for patsy\nimport statsmodels.api as sm",
"_____no_output_____"
]
],
[
[
"#### Import convention",
"_____no_output_____"
],
[
"You can import explicitly from statsmodels.formula.api",
"_____no_output_____"
]
],
[
[
"from statsmodels.formula.api import ols",
"_____no_output_____"
]
],
[
[
"Alternatively, you can just use the `formula` namespace of the main `statsmodels.api`.",
"_____no_output_____"
]
],
[
[
"sm.formula.ols",
"_____no_output_____"
]
],
[
[
"Or you can use the following conventioin",
"_____no_output_____"
]
],
[
[
"import statsmodels.formula.api as smf",
"_____no_output_____"
]
],
[
[
"These names are just a convenient way to get access to each model's `from_formula` classmethod. See, for instance",
"_____no_output_____"
]
],
[
[
"sm.OLS.from_formula",
"_____no_output_____"
]
],
[
[
"All of the lower case models accept ``formula`` and ``data`` arguments, whereas upper case ones take ``endog`` and ``exog`` design matrices. ``formula`` accepts a string which describes the model in terms of a ``patsy`` formula. ``data`` takes a [pandas](https://pandas.pydata.org/) data frame or any other data structure that defines a ``__getitem__`` for variable names like a structured array or a dictionary of variables. \n\n``dir(sm.formula)`` will print a list of available models. \n\nFormula-compatible models have the following generic call signature: ``(formula, data, subset=None, *args, **kwargs)``",
"_____no_output_____"
],
[
"\n## OLS regression using formulas\n\nTo begin, we fit the linear model described on the [Getting Started](gettingstarted.html) page. Download the data, subset columns, and list-wise delete to remove missing observations:",
"_____no_output_____"
]
],
[
[
"dta = sm.datasets.get_rdataset(\"Guerry\", \"HistData\", cache=True)",
"_____no_output_____"
],
[
"df = dta.data[['Lottery', 'Literacy', 'Wealth', 'Region']].dropna()\ndf.head()",
"_____no_output_____"
]
],
[
[
"Fit the model:",
"_____no_output_____"
]
],
[
[
"mod = ols(formula='Lottery ~ Literacy + Wealth + Region', data=df)\nres = mod.fit()\nprint(res.summary())",
"_____no_output_____"
]
],
[
[
"## Categorical variables\n\nLooking at the summary printed above, notice that ``patsy`` determined that elements of *Region* were text strings, so it treated *Region* as a categorical variable. `patsy`'s default is also to include an intercept, so we automatically dropped one of the *Region* categories.\n\nIf *Region* had been an integer variable that we wanted to treat explicitly as categorical, we could have done so by using the ``C()`` operator: ",
"_____no_output_____"
]
],
[
[
"res = ols(formula='Lottery ~ Literacy + Wealth + C(Region)', data=df).fit()\nprint(res.params)",
"_____no_output_____"
]
],
[
[
"Patsy's mode advanced features for categorical variables are discussed in: [Patsy: Contrast Coding Systems for categorical variables](contrasts.html)",
"_____no_output_____"
],
[
"## Operators\n\nWe have already seen that \"~\" separates the left-hand side of the model from the right-hand side, and that \"+\" adds new columns to the design matrix. \n\n### Removing variables\n\nThe \"-\" sign can be used to remove columns/variables. For instance, we can remove the intercept from a model by: ",
"_____no_output_____"
]
],
[
[
"res = ols(formula='Lottery ~ Literacy + Wealth + C(Region) -1 ', data=df).fit()\nprint(res.params)",
"_____no_output_____"
]
],
[
[
"### Multiplicative interactions\n\n\":\" adds a new column to the design matrix with the interaction of the other two columns. \"*\" will also include the individual columns that were multiplied together:",
"_____no_output_____"
]
],
[
[
"res1 = ols(formula='Lottery ~ Literacy : Wealth - 1', data=df).fit()\nres2 = ols(formula='Lottery ~ Literacy * Wealth - 1', data=df).fit()\nprint(res1.params, '\\n')\nprint(res2.params)",
"_____no_output_____"
]
],
[
[
"Many other things are possible with operators. Please consult the [patsy docs](https://patsy.readthedocs.org/en/latest/formulas.html) to learn more.",
"_____no_output_____"
],
[
"## Functions\n\nYou can apply vectorized functions to the variables in your model: ",
"_____no_output_____"
]
],
[
[
"res = smf.ols(formula='Lottery ~ np.log(Literacy)', data=df).fit()\nprint(res.params)",
"_____no_output_____"
]
],
[
[
"Define a custom function:",
"_____no_output_____"
]
],
[
[
"def log_plus_1(x):\n return np.log(x) + 1.\nres = smf.ols(formula='Lottery ~ log_plus_1(Literacy)', data=df).fit()\nprint(res.params)",
"_____no_output_____"
]
],
[
[
"Any function that is in the calling namespace is available to the formula.",
"_____no_output_____"
],
[
"## Using formulas with models that do not (yet) support them\n\nEven if a given `statsmodels` function does not support formulas, you can still use `patsy`'s formula language to produce design matrices. Those matrices \ncan then be fed to the fitting function as `endog` and `exog` arguments. \n\nTo generate ``numpy`` arrays: ",
"_____no_output_____"
]
],
[
[
"import patsy\nf = 'Lottery ~ Literacy * Wealth'\ny,X = patsy.dmatrices(f, df, return_type='matrix')\nprint(y[:5])\nprint(X[:5])",
"_____no_output_____"
]
],
[
[
"To generate pandas data frames: ",
"_____no_output_____"
]
],
[
[
"f = 'Lottery ~ Literacy * Wealth'\ny,X = patsy.dmatrices(f, df, return_type='dataframe')\nprint(y[:5])\nprint(X[:5])",
"_____no_output_____"
],
[
"print(sm.OLS(y, X).fit().summary())",
"_____no_output_____"
]
]
] | [
"markdown",
"code",
"markdown",
"code",
"markdown",
"code",
"markdown",
"code",
"markdown",
"code",
"markdown",
"code",
"markdown",
"code",
"markdown",
"code",
"markdown",
"code",
"markdown",
"code",
"markdown",
"code",
"markdown",
"code",
"markdown",
"code",
"markdown",
"code"
] | [
[
"markdown",
"markdown"
],
[
"code"
],
[
"markdown",
"markdown"
],
[
"code"
],
[
"markdown"
],
[
"code"
],
[
"markdown"
],
[
"code"
],
[
"markdown"
],
[
"code"
],
[
"markdown",
"markdown"
],
[
"code",
"code"
],
[
"markdown"
],
[
"code"
],
[
"markdown"
],
[
"code"
],
[
"markdown",
"markdown"
],
[
"code"
],
[
"markdown"
],
[
"code"
],
[
"markdown",
"markdown"
],
[
"code"
],
[
"markdown"
],
[
"code"
],
[
"markdown",
"markdown"
],
[
"code"
],
[
"markdown"
],
[
"code",
"code"
]
] |
e7717d4e87e61324d3b07f9dbaebb47c8043ad06 | 9,085 | ipynb | Jupyter Notebook | Codes/run.ipynb | Reself-C/COMAP-MCM-ICM-2022 | 30fe1de5b58de99878bc1358662f3ae7d7689b20 | [
"MIT"
] | 1 | 2022-03-13T20:15:41.000Z | 2022-03-13T20:15:41.000Z | Codes/run.ipynb | Reself-C/COMAP-MCM-ICM-2022 | 30fe1de5b58de99878bc1358662f3ae7d7689b20 | [
"MIT"
] | null | null | null | Codes/run.ipynb | Reself-C/COMAP-MCM-ICM-2022 | 30fe1de5b58de99878bc1358662f3ae7d7689b20 | [
"MIT"
] | 1 | 2022-03-04T16:07:51.000Z | 2022-03-04T16:07:51.000Z | 30.589226 | 85 | 0.409906 | [
[
[
"# This file is to plot our final results",
"_____no_output_____"
],
[
"import numpy as np\nimport seaborn as sns\nimport pandas as pd\nimport matplotlib.pyplot as plt\n\nplt.figure(figsize=(20, 5)) \nsns.set(style='whitegrid')\n\ndf = pd.read_csv('DATA.csv')\ndf.head()",
"_____no_output_____"
],
[
"def value_computation(name, alpha_b=0.02, alpha_g=0.01 ,data=df):\n # enter your col name, alpha_b = 0.02, alpha_g = 0.01 for example\n # n_b n_g is the number of bitcoin and gol\n n_b = np.array(df[name+'b'])\n n_g = np.array(df[name+'g'])\n \n GP = np.array(df['Gold_Prediction'])\n GG = np.array(df['Gold_GroundTruth'])\n BP = np.array(df['BITCOIN_Prediction'])\n BG = np.array(df['BITCOIN_GroundTruth'])\n n = GG.shape[0]\n \n # Weight for Prediction\n value_P = np.zeros((n, 1))\n value_P[0] = 1000\n cash_P = np.zeros((n,1))\n cash_P[0] = 1000\n for day in range(1,n):\n del_B = n_b[day] - n_b[day-1]\n del_G = n_g[day] - n_g[day-1]\n if del_B > 0:\n change_B = - del_B*BP[day]*(1+alpha_b)\n else:\n change_B = - del_B*BP[day]*(1-alpha_b)\n if del_G > 0:\n change_G = - del_B*BP[day]*(1+alpha_g)\n else:\n change_G = - del_B*BP[day]*(1-alpha_g)\n cash_P[day] = cash_P[day-1] + change_B + change_G\n value_P[day] = cash_P[day] + n_b[day]*BP[day] + n_g[day]*GP[day]\n\n # Weight for Ground Truth\n value_G = np.zeros((n, 1))\n value_G[0] = 1000\n cash_G = np.zeros((n,1))\n cash_G[0] = 1000\n for day in range(1,n):\n del_B = n_b[day] - n_b[day-1]\n del_G = n_g[day] - n_g[day-1]\n if del_B > 0:\n change_B = - del_B*BG[day]*(1+alpha_b)\n else:\n change_B = - del_B*BG[day]*(1-alpha_b)\n if del_G > 0:\n change_G = - del_B*BG[day]*(1+alpha_g)\n else:\n change_G = - del_B*BG[day]*(1-alpha_g)\n cash_P[day] = cash_P[day-1] + change_B + change_G\n value_P[day] = cash_P[day] + n_b[day]*BG[day] + n_g[day]*GG[day]\n \n return value_P, value_G",
"_____no_output_____"
],
[
"def weight_plot(name, P, data=df):\n n_b = np.array(df[name+'b'])\n n_g = np.array(df[name+'g'])\n GG = np.array(df['Gold_GroundTruth'])\n BG = np.array(df['BITCOIN_GroundTruth'])\n \n w_b = BG * n_b / P\n w_g = GG * n_g / P\n w_c = 1 - w_b - w_g\n \n sns.lineplot(data=[w_c.flatten(),w_b.flatten(),w_g.flatten()])\n plt.xlabel('Day')\n plt.ylabel('Weight')\n plt.show()\n plt.savefig('../img/'+name+'W.png')\n\ndef value_plot(name, P):\n sns.lineplot(data=P.flatten(),color='black')\n plt.xlabel('Day')\n plt.ylabel('Value [$]')\n plt.show()\n plt.savefig('../img/'+name+'P.png')\n \ndef ratio_plot(name, P, G):\n rP = P[1:]/P[:-1].flatten()\n rG = G[1:]/P[:-1].flatten()\n sns.lineplot(data=[rP,rG])\n plt.xlabel('Day')\n plt.ylabel('Ratio')\n plt.show()\n plt.savefig('../img/'+name+'R.png')",
"_____no_output_____"
],
[
"name = 'test1'\n\nP, G = value_computation(name)\nweight_plot(name, P)\nvalue_plot(name, P)\nratio_plot(name, P, G)",
"_____no_output_____"
]
]
] | [
"code"
] | [
[
"code",
"code",
"code",
"code",
"code"
]
] |
e77187842c5611c78db93b9af3924a2bda872586 | 61,327 | ipynb | Jupyter Notebook | 05-data-mining/labs/CH6EJ3-Descomposicion-en-valores-singulares.ipynb | quiquegv/NEOLAND-DS2020-datalabs | 192b394b5a4a1fee700324623d3d933ba234b8fd | [
"MIT"
] | null | null | null | 05-data-mining/labs/CH6EJ3-Descomposicion-en-valores-singulares.ipynb | quiquegv/NEOLAND-DS2020-datalabs | 192b394b5a4a1fee700324623d3d933ba234b8fd | [
"MIT"
] | null | null | null | 05-data-mining/labs/CH6EJ3-Descomposicion-en-valores-singulares.ipynb | quiquegv/NEOLAND-DS2020-datalabs | 192b394b5a4a1fee700324623d3d933ba234b8fd | [
"MIT"
] | null | null | null | 123.146586 | 13,834 | 0.850947 | [
[
[
"<div style='float:left'>\n<h1>\nCH6EJ3 Extracción Componentes Principales\n</h1>\n",
"_____no_output_____"
],
[
"## Procedimiento",
"_____no_output_____"
],
[
"### Cargamos y/o instalamos las librerias necesarios",
"_____no_output_____"
]
],
[
[
"if(!require(devtools)){\n install.packages('devtools',dependencies =c(\"Depends\", \"Imports\"),repos='http://cran.es.r-project.org')\n require(devtools)\n}\nif(!require(ggbiplot)){\n install.packages('ggbiplot',dependencies =c(\"Depends\", \"Imports\"),repos='http://cran.es.r-project.org')\n require(ggbiplot)\n}\nif(!require(scales)){\n install.packages('scales',dependencies =c(\"Depends\", \"Imports\"),repos='http://cran.es.r-project.org')\n require(scales)\n}\nif(!require(grid)){\n install.packages('grid',dependencies =c(\"Depends\", \"Imports\"),repos='http://cran.es.r-project.org')\n require(grid)\n}\nif(!require(plyr)){\n install.packages('plyr',dependencies =c(\"Depends\", \"Imports\"),repos='http://cran.es.r-project.org')\n require(plyr)\n}",
"Loading required package: devtools\nWarning message:\n\"package 'devtools' was built under R version 3.3.3\"Loading required package: ggbiplot\nWarning message:\n\"package 'ggbiplot' was built under R version 3.3.3\"Loading required package: ggplot2\nWarning message:\n\"package 'ggplot2' was built under R version 3.3.3\"Loading required package: plyr\nLoading required package: scales\nLoading required package: grid\n"
]
],
[
[
"### Cargamos los datos de un directorio local.",
"_____no_output_____"
]
],
[
[
"Alumnos_usos_sociales <- read.csv(\"B2.332_Students.csv\", comment.char=\"#\")\n# X contiene las variables que queremos trabajar\nR <- Alumnos_usos_sociales[,c(31:34)]\nhead(R)",
"_____no_output_____"
]
],
[
[
"### Cálculo de la Singular value decomposition y de los valores que lo caracterizan.",
"_____no_output_____"
]
],
[
[
"# Generamos SVD\nR.order <- R\nR.svd <-svd(R.order[,c(1:3)])\n\n# D, U y V\nR.svd$d\nhead(R.svd$u)\nR.svd$v",
"_____no_output_____"
]
],
[
[
"### Calculo de la varianza acumulada en el primer factor",
"_____no_output_____"
]
],
[
[
"sum(R.svd$d)\nvar=sum(R.svd$d[1])\nvar\nvar/sum(R.svd$d)",
"_____no_output_____"
]
],
[
[
"### Porcentaje de la varianza explicada por los svd generados",
"_____no_output_____"
]
],
[
[
"plot(R.svd$d^2/sum(R.svd$d^2),type=\"l\",xlab=\"Singular vector\",ylab=\"Varianza explicada\")",
"_____no_output_____"
]
],
[
[
"### Porcentaje de la varianza acumulada explicada ",
"_____no_output_____"
]
],
[
[
"plot(cumsum(R.svd$d^2/sum(R.svd$d^2)),type=\"l\",xlab=\"Singular vector\",ylab=\"Varianza explicada acumulada\")",
"_____no_output_____"
]
],
[
[
"### Creamos un gráfico con el primer y segundo vector asignando colores. Rojo no supera, verde supera",
"_____no_output_____"
]
],
[
[
"# Dibujamos primero todos los scores de comp2 y comp1\nY <- R.order[,4]\nplot(R.svd$u[,1],R.svd$u[,2])\n# Asignamos rojo a no supera y verde a si supera\npoints(R.svd$u[Y==\"No\",1],R.svd$u[Y==\"No\",2],col=\"red\")\npoints(R.svd$u[Y==\"Si\",1],R.svd$u[Y==\"Si\",2],col=\"green\")",
"_____no_output_____"
]
],
[
[
"### Reconstrucción de la imagen de los datos a partir de los SVD",
"_____no_output_____"
]
],
[
[
"R.recon1=R.svd$u[,1]%*%diag(R.svd$d[1],length(1),length(1))%*%t(R.svd$v[,1])\nR.recon2=R.svd$u[,2]%*%diag(R.svd$d[2],length(2),length(2))%*%t(R.svd$v[,2])\nR.recon3=R.svd$u[,3]%*%diag(R.svd$d[3],length(3),length(3))%*%t(R.svd$v[,3])\npar(mfrow=c(2,2))\nimage(as.matrix(R.order[,c(1:3)]),main=\"Matriz Original\")\nimage(R.recon1,main=\"Matriz Factor 1\")\nimage(R.recon2,main=\"Matriz Factor 2\")\nimage(R.recon3,main=\"Matriz Factor 3\")",
"_____no_output_____"
]
]
] | [
"markdown",
"code",
"markdown",
"code",
"markdown",
"code",
"markdown",
"code",
"markdown",
"code",
"markdown",
"code",
"markdown",
"code",
"markdown",
"code"
] | [
[
"markdown",
"markdown",
"markdown"
],
[
"code"
],
[
"markdown"
],
[
"code"
],
[
"markdown"
],
[
"code"
],
[
"markdown"
],
[
"code"
],
[
"markdown"
],
[
"code"
],
[
"markdown"
],
[
"code"
],
[
"markdown"
],
[
"code"
],
[
"markdown"
],
[
"code"
]
] |
e7719e0cc2e91162738804d69b5a8a263ccf24f0 | 1,191 | ipynb | Jupyter Notebook | examples/introduction.ipynb | vidartf/jupyter-scales | c826f7466e93f31946c535f12243267b02cf9af7 | [
"BSD-3-Clause"
] | 13 | 2017-08-31T13:51:17.000Z | 2021-08-10T08:52:10.000Z | examples/introduction.ipynb | vidartf/jupyter-scales | c826f7466e93f31946c535f12243267b02cf9af7 | [
"BSD-3-Clause"
] | 10 | 2018-09-19T00:52:40.000Z | 2021-05-13T11:08:57.000Z | examples/introduction.ipynb | vidartf/jupyter-scales | c826f7466e93f31946c535f12243267b02cf9af7 | [
"BSD-3-Clause"
] | 4 | 2017-09-18T08:14:45.000Z | 2019-11-14T00:47:23.000Z | 19.52459 | 118 | 0.518052 | [
[
[
"# Introduction",
"_____no_output_____"
]
],
[
[
"import ipyscales",
"_____no_output_____"
],
[
"# Make a default scale, and list its trait values:\nscale = ipyscales.LinearScale()\nprint(', '.join('%s: %s' % (key, getattr(scale, key)) for key in sorted(scale.keys) if not key.startswith('_')))",
"clamp: False, domain: (0.0, 1.0), interpolator: interpolate, range: (0.0, 1.0)\n"
]
]
] | [
"markdown",
"code"
] | [
[
"markdown"
],
[
"code",
"code"
]
] |
e7719eee47fd01dbe17bebf7cfb8721e07baa007 | 14,339 | ipynb | Jupyter Notebook | inference_finetuned_35000-step.ipynb | snoop2head/KoGPT-Joong-2 | 118d830231d3afc2f59e02ffd2439ab5cc1d10fd | [
"MIT"
] | 7 | 2021-11-18T06:58:54.000Z | 2022-02-05T10:59:33.000Z | inference_finetuned_35000-step.ipynb | snoop2head/KoGPT-Joong-2 | 118d830231d3afc2f59e02ffd2439ab5cc1d10fd | [
"MIT"
] | 1 | 2021-12-09T03:12:31.000Z | 2021-12-09T03:12:31.000Z | inference_finetuned_35000-step.ipynb | snoop2head/KoGPT-Joong-2 | 118d830231d3afc2f59e02ffd2439ab5cc1d10fd | [
"MIT"
] | 1 | 2021-12-02T09:23:22.000Z | 2021-12-02T09:23:22.000Z | 33.738824 | 741 | 0.573889 | [
[
[
"import os\n\n# root path\nROOT_PATH = os.path.abspath(\".\") # this makes compatible absolute path both for local and server\n\n# designate root path for the data\nDATA_ROOT_PATH = os.path.join(ROOT_PATH, 'data')\n\n# designate path for each dataset files\nLYRIC_PATH = os.path.join(DATA_ROOT_PATH, \"lyrics_kor.txt\")\nBILLBOARD_PATH = os.path.join(DATA_ROOT_PATH, \"rawdata_김지훈_201500844.tsv\")\nGEULSTAGRAM_PATH = os.path.join(DATA_ROOT_PATH, \"geulstagram.csv\")\n\nprint(ROOT_PATH)",
"/Users/noopy/Documents/BERT-PROJECTS/kogpt-ghost-writer\n"
],
[
"from datetime import datetime\nfrom easydict import EasyDict\n\n# Initialize configuration\nCFG = EasyDict()\n\n# Dataset Config as constants\nCFG.DEBUG = False\nCFG.num_workers = 4\nCFG.train_batch_size = 16\n\n# Train configuration\nCFG.user_name = \"snoop2head\"\ntoday = datetime.now().strftime(\"%m%d_%H:%M\")\nCFG.file_base_name = f\"{CFG.user_name}_{today}\"\nCFG.model_dir = \"skt/ko-gpt-trinity-1.2B-v0.5\" # designate the model's name registered on huggingface: https://huggingface.co/skt/ko-gpt-trinity-1.2B-v0.5\nCFG.max_token_length = 42\nCFG.learning_rate = 5e-5\nCFG.weight_decay = 1e-2 # https://paperswithcode.com/method/weight-decay\n\n# training steps configurations\nCFG.save_steps = 500\nCFG.early_stopping_patience = 5\nCFG.warmup_steps = 500\nCFG.logging_steps = 100\nCFG.evaluation_strategy = 'epoch'\nCFG.evaluation_steps = 500\n\n# Directory configuration\nCFG.result_dir = os.path.join(ROOT_PATH, \"results\")\nCFG.saved_model_dir = os.path.join(ROOT_PATH, \"best_models\")\nCFG.logging_dir = os.path.join(ROOT_PATH, \"logs\")\nCFG.baseline_dir = os.path.join(ROOT_PATH, 'baseline-code')\n\nprint(CFG)",
"{'DEBUG': False, 'num_workers': 4, 'train_batch_size': 16, 'user_name': 'snoop2head', 'file_base_name': 'snoop2head_1118_02:32', 'model_dir': 'skt/ko-gpt-trinity-1.2B-v0.5', 'max_token_length': 42, 'learning_rate': 5e-05, 'weight_decay': 0.01, 'save_steps': 500, 'early_stopping_patience': 5, 'warmup_steps': 500, 'logging_steps': 100, 'evaluation_strategy': 'epoch', 'evaluation_steps': 500, 'result_dir': '/Users/noopy/Documents/BERT-PROJECTS/kogpt-ghost-writer/results', 'saved_model_dir': '/Users/noopy/Documents/BERT-PROJECTS/kogpt-ghost-writer/best_models', 'logging_dir': '/Users/noopy/Documents/BERT-PROJECTS/kogpt-ghost-writer/logs', 'baseline_dir': '/Users/noopy/Documents/BERT-PROJECTS/kogpt-ghost-writer/baseline-code'}\n"
],
[
"import random\nimport torch\nimport pandas as pd\nimport numpy as np\n\nos.environ[\"TOKENIZERS_PARALLELISM\"] = \"true\"\nos.environ[\"CUDA_LAUNCH_BLOCKING\"] = \"1\"\n\ndef seed_everything(seed) :\n torch.manual_seed(seed)\n torch.cuda.manual_seed(seed)\n torch.cuda.manual_seed_all(seed) # if use multi-GPU\n torch.backends.cudnn.deterministic = True\n torch.backends.cudnn.benchmark = False\n np.random.seed(seed)\n random.seed(seed)\nseed_everything(42)",
"_____no_output_____"
],
[
"# read txt file from line by line\ndef read_txt(path):\n with open(path, 'r', encoding='utf-8') as f:\n lines = f.readlines()\n return lines\n\n# make sampling function from the list\ndef sampling(list_lines:list, n:int) -> list:\n # sampling\n list_lines = np.random.choice(list_lines, n)\n list_lines = list(list_lines)\n return list_lines",
"_____no_output_____"
],
[
"import torch\nfrom transformers import GPT2LMHeadModel, AutoModelWithLMHead\n\n# CFG.saved_model_dir = \"./results\"\nCFG.model_dir = \"snoop2head/KoGPT-Joong-2\"\n\n# Attach Language model Head to the pretrained GPT model\nmodel = AutoModelWithLMHead.from_pretrained(CFG.model_dir) # KoGPT3 shares the same structure as KoGPT2. \n\n\n# move the model to device\nif torch.cuda.is_available() and CFG.DEBUG == False:\n device = torch.device(\"cuda:0\")\nelif CFG.DEBUG == True or not torch.cuda.is_available():\n device = torch.device(\"cpu\")\n\nmodel.to(device)\nmodel.eval()\nprint(device)",
"/Users/noopy/.pyenv/versions/3.8.3/envs/korpoet/lib/python3.8/site-packages/transformers/models/auto/modeling_auto.py:694: FutureWarning: The class `AutoModelWithLMHead` is deprecated and will be removed in a future version. Please use `AutoModelForCausalLM` for causal language models, `AutoModelForMaskedLM` for masked language models and `AutoModelForSeq2SeqLM` for encoder-decoder models.\n warnings.warn(\n"
],
[
"if device == torch.device(\"cuda:0\"):\n os.system(\"nvidia-smi\")",
"_____no_output_____"
],
[
"from transformers import GPT2Tokenizer, PreTrainedTokenizerFast, AutoTokenizer\n\n# https://huggingface.co/transformers/preprocessing.html\n# Load the Tokenizer: \"Fast\" means that the tokenizer code is written in Rust Lang\ntokenizer = AutoTokenizer.from_pretrained(\n CFG.model_dir,\n max_len = CFG.max_token_length,\n padding='max_length',\n add_special_tokens = True,\n return_tensors=\"pt\",\n truncation = True,\n bos_token = \"<s>\",\n eos_token = \"</s>\",\n unk_token = \"<unk>\",\n pad_token = \"<pad>\",\n mask_token = \"<mask>\",\n)",
"_____no_output_____"
],
[
"def infer_sentence(input_sentence, k, output_token_length):\n\n # encode the sample sentence\n input_ids = tokenizer.encode(\n input_sentence, \n add_special_tokens=False, \n return_tensors=\"pt\"\n )\n\n # decode the output sequence and print its outcome\n list_decoded_sequences = []\n while len(list_decoded_sequences) < k:\n # generate output sequence from the given encoded input sequence\n output_sequences = model.generate(\n input_ids=input_ids.to(device), \n do_sample=True, \n max_length=output_token_length, \n num_return_sequences=k\n )\n\n for index, generated_sequence in enumerate(output_sequences):\n generated_sequence = generated_sequence.tolist()\n # remove padding from the generated sequence\n generated_sequence = generated_sequence[:generated_sequence.index(tokenizer.pad_token_id)]\n decoded_sequence = tokenizer.decode(generated_sequence, clean_up_tokenization_spaces=True)\n # print(f\"{index} : {decoded_sequence}\")\n list_decoded_sequences.append(decoded_sequence)\n list_decoded_sequences = list(set(list_decoded_sequences))\n \n return list_decoded_sequences\n\ninput_sentence = \"너는 나의\"\nprint(f\"Inferred sentences given '{input_sentence}'\")\ninferred_sentences = infer_sentence(input_sentence, k=10, output_token_length=CFG.max_token_length)\ninferred_sentences",
"Inferred sentences given '너는 나의'\n"
],
[
"def make_samhaengshi(input_letter, k, output_token_length):\n list_samhaengshi = []\n for one_letter in input_letter:\n list_decoded_sequences = infer_sentence(one_letter, k=k, output_token_length=output_token_length)\n list_samhaengshi.extend(list_decoded_sequences)\n return list_samhaengshi\n\nmake_samhaengshi(input_letter=\"자탄풍\", k=1, output_token_length=CFG.max_token_length)",
"_____no_output_____"
],
[
"def make_residual_samhaengshi(input_letter, k, output_token_length):\n # make letter string into \n list_samhaengshi = []\n \n # initializing text and index for iteration purpose\n index = 0\n\n # iterating over the input letter string\n for index, letter_item in enumerate(input_letter):\n # initializing the input_letter\n if index == 0:\n residual_text = letter_item\n else:\n pass\n \n # infer and add to the output\n list_sentences = infer_sentence(residual_text, 3, output_token_length)\n for sentence in list_sentences:\n if len(sentence) == 1:\n pass\n elif len(sentence) >= 2:\n inferred_sentence = sentence # first item of the inferred list\n if index != 0:\n # remove previous sentence from the output\n inferred_sentence = inferred_sentence.replace(list_samhaengshi[index-1], \"\").strip() \n else:\n pass\n list_samhaengshi.append(inferred_sentence)\n \n # until the end of the input_letter, give the previous residual_text to the next iteration\n if index < len(input_letter) - 1: \n residual_sentence = list_samhaengshi[index]\n next_letter = input_letter[index + 1]\n residual_text = f\"{residual_sentence} {next_letter}\" # previous sentence + next letter\n # print(residual_text)\n\n elif index == len(input_letter) - 1: # end of the input_letter\n # Concatenate strings in the list without intersection\n\n return list_samhaengshi",
"_____no_output_____"
],
[
"sample_item = \"가을잎\" \ninferred_samhaengshi = make_residual_samhaengshi(sample_item, k=1, output_token_length=CFG.max_token_length)\nfor item in inferred_samhaengshi:\n print(item)",
"가물어 메마른 땅에 단비를 내리시듯 성령의 단비를 부어 새 생명 주옵소서\n을씨년\n잎을 씨부렸다\n"
]
],
[
[
"### ToDo\n- probably make candidate 10 sentences per letter and pick sentences with sentence transformer trained with Next Sentence Prediction Task?\n- Filter out similar sentences based on levenstein distance or sentence bert\n- remove curse words, person words with pororo or other tools -> either from dataset or inference process",
"_____no_output_____"
]
],
[
[
"# https://github.com/lovit/levenshtein_finder",
"_____no_output_____"
]
]
] | [
"code",
"markdown",
"code"
] | [
[
"code",
"code",
"code",
"code",
"code",
"code",
"code",
"code",
"code",
"code",
"code"
],
[
"markdown"
],
[
"code"
]
] |
e771ad9e53901ea0c65aa066bb53ecd94f97b3a9 | 8,757 | ipynb | Jupyter Notebook | my_sheets/week7.ipynb | isomorphicdude/MATH50003NumericalAnalysis | bca66f71b6e1147fb8c1abe0c2a78c558c1ea73e | [
"MIT"
] | null | null | null | my_sheets/week7.ipynb | isomorphicdude/MATH50003NumericalAnalysis | bca66f71b6e1147fb8c1abe0c2a78c558c1ea73e | [
"MIT"
] | null | null | null | my_sheets/week7.ipynb | isomorphicdude/MATH50003NumericalAnalysis | bca66f71b6e1147fb8c1abe0c2a78c558c1ea73e | [
"MIT"
] | null | null | null | 30.092784 | 184 | 0.47836 | [
[
[
"empty"
]
]
] | [
"empty"
] | [
[
"empty"
]
] |
e771c49447d9b5c54c890af6657c188f175e5bb0 | 7,107 | ipynb | Jupyter Notebook | code/personal_practice_space/Untitled.ipynb | Adamthe1st/PROJECT_1_SAUDI-TRAFFIC-ACCIDENTS-STATISTICAL-SUMMARIES-AND-TRENDS | 093578156284ba89fb86b9be85e2a607bece2ad0 | [
"Xnet",
"X11"
] | null | null | null | code/personal_practice_space/Untitled.ipynb | Adamthe1st/PROJECT_1_SAUDI-TRAFFIC-ACCIDENTS-STATISTICAL-SUMMARIES-AND-TRENDS | 093578156284ba89fb86b9be85e2a607bece2ad0 | [
"Xnet",
"X11"
] | null | null | null | code/personal_practice_space/Untitled.ipynb | Adamthe1st/PROJECT_1_SAUDI-TRAFFIC-ACCIDENTS-STATISTICAL-SUMMARIES-AND-TRENDS | 093578156284ba89fb86b9be85e2a607bece2ad0 | [
"Xnet",
"X11"
] | null | null | null | 42.813253 | 932 | 0.549177 | [
[
[
"\n\n# importing created functions\nfrom basic_functions import *\n\n# tree is a decision classifier which takes in specific criteria (e.g a string)\n# and make a splitter decision.The strategy is to choose the split at each node.\n# i.e chooses the \"best\" split option and “random” to choose the best random split. \n\nfrom sklearn import tree\n\n# NumPy can be used as multi-dimensional container of generic data.\n# in which data-types can be defined. \n\nimport numpy as np\n\nfrom sklearn.externals.six import StringIO\n# pydot creates, modifies and process graphs dot language.\n#import pydot\n\n## ===================\n## 0- Loading the data\n## ===================\n# calling function to read all cells from data file \nramdt = read_file(\"../project_raw_data/ram.xlsx\",\"all\")\n\n# calling function to transform row to col\nrdtcol = transform_row_to_col(ramdt)\n\n\n## ===================\n## 1- Prepare the data\n## ===================\n# creating a variable to hold the target (cell type) data\ntrain_tgt = rdtcol['Type'][0:11] + rdtcol['Type'][15:18] + rdtcol['Type'][21:63] + rdtcol['Type'][75:112]\n\n# train_ds is variable to hold extracted dataset from row data (data we want to train)\n# the (extract_ds_from_row_data) function passes the follwing parameters:\n# ramdt,range of rows excluding headers,\n# range of columns based on index zero (headers) of ramdt.\n# (ie. exclude columns[0,1] and include rest of columns. \n# list range 1,12 corresponds to cell type 1\n# list range 16,19 corresponds to cell type 2\n# list range 22,64 corresponds to cell type 3\n# list range 76,113 corresponds to cell type 4\n\ntrain_ds = extract_ds_from_row_data(ramdt, list(range(1,12)) + list(range(16,19)) + list(range(22,64)) + list(range(76,113)),range(2,len(ramdt[0])))\n\n#loop to print the train data\nprint(\"======= Train data ============\")\nprint(\"Target [Features]\")\nfor i in range(0,len(train_tgt)):\n print(train_tgt[i], train_ds[i])\n\n\n# test_tgt is variable holding cell type range we want to test.\ntest_tgt = rdtcol['Type'][11:15] + rdtcol['Type'][19:21] + rdtcol['Type'][64:75] + rdtcol['Type'][113:123]\n\ntest_ds = extract_ds_from_row_data(ramdt,\nlist(range(12,16)) + list(range(19,22)) + list(range(64,76)) +\nlist(range(113,124)),range(2,len(ramdt[0])))\n\n#loop to print the test data\nprint(\"======= Test data ============\")\nprint(\"Target [Features]\")\nfor i in range(0,len(test_tgt)):\n print(test_tgt[i], test_ds[i])\n\n\n## ===========\n## 2- Learning\n## ===========\n# fitting the data to the decision tree classifier algorithm \nclf = tree.DecisionTreeClassifier()\nclf.fit(train_ds,train_tgt)\n\n## ============================\n## 3- test the learning process\n## ============================\n\npredicted_tgt = clf.predict(test_ds)\n\n## =======================================\n## 4- Compute the accuracy of perdiction\n## =======================================\n\nprint(\"======= Prediction ============\")\ncorrect = 0\nfor i in range(0,len(test_tgt)):\n if predicted_tgt[i] == test_tgt[i]: # when prediction matches with actual\n correct = correct + 1 # increment the correct counter\n print(\"Actual Cell Type:\", test_tgt[i], \"Predicted Cell Type:\", predicted_tgt[i])\n\naccuracy = correct / len(test_tgt) * 100\nprint(\"======= Statistics ============\")\nprint(\"Accuracy over one run\" , round( accuracy , 2), \"%\")\n\n\n\n########### RUNNING 100 TIMES ###################\n#this a loop to run the classifier 100 times\n\naccuracy = []\nfor run in range(0,100):\n ## learn\n clf = tree.DecisionTreeClassifier()\n clf.fit(train_ds,train_tgt)\n ## test\n predicted_tgt = clf.predict(test_ds)\n ## compute\n correct = 0\n for i in range(0,len(test_tgt)):\n if predicted_tgt[i] == test_tgt[i]: # when prediction matches with actual\n correct = correct + 1 # increment the correct counter\n \n # calculating accuracty of prediction (%)\n accuracy.append(correct / len(test_tgt) * 100)\n\n# rounding predicted output to the nearest decimal. \nprint(\"Avg. accuracy over 100 runs\",round(np.mean(accuracy) , 2) , \"%\")",
"_____no_output_____"
]
]
] | [
"code"
] | [
[
"code"
]
] |
e771d9d0bd30f47637defc04a5894aa7a94336b5 | 4,385 | ipynb | Jupyter Notebook | python-sdk/experimental/using-xgboost/2.distributed-cpu.ipynb | msftcoderdjw/azureml-examples | ec56311eb3c9c366d62fdd008ecc1022136c8dfc | [
"MIT"
] | 1 | 2021-04-28T11:26:59.000Z | 2021-04-28T11:26:59.000Z | python-sdk/experimental/using-xgboost/2.distributed-cpu.ipynb | msftcoderdjw/azureml-examples | ec56311eb3c9c366d62fdd008ecc1022136c8dfc | [
"MIT"
] | null | null | null | python-sdk/experimental/using-xgboost/2.distributed-cpu.ipynb | msftcoderdjw/azureml-examples | ec56311eb3c9c366d62fdd008ecc1022136c8dfc | [
"MIT"
] | 1 | 2021-06-26T00:41:47.000Z | 2021-06-26T00:41:47.000Z | 25.643275 | 278 | 0.567617 | [
[
[
"# Distributed XGBoost (CPU)\n\nScaling out on AmlCompute is simple! The code from the previous notebook has been modified and adapted in [src/run.py](src/run.py). In particular, changes include:\n\n- use ``dask_mpi`` to initialize Dask on MPI\n- use ``argparse`` to allow for command line argument inputs\n- use ``mlflow`` logging \n\nThe [environment.yml](environment.yml) contains the conda environment specification.",
"_____no_output_____"
],
[
"## Get Workspace",
"_____no_output_____"
]
],
[
[
"from azureml.core import Workspace\n\nws = Workspace.from_config()\nws",
"_____no_output_____"
]
],
[
[
"## Distributed Remotely\n\nSimply use ``MpiConfiguration`` with the desired node count.",
"_____no_output_____"
],
[
"**Important**: see the [``dask-mpi`` documentation](http://mpi.dask.org/en/latest/) for details on how the Dask workers and scheduler are started.\n\nBy default with the Azuer ML MPI configuration, two nodes are used for the scheduler and script process.\n\nThis means you should add two additional nodes to reach the desired number of worker nodes. Additionally, we need to pass in the number of vCPUs per node, which will be used to intiialize the same number of threads via ``dask_mpi.initialize(nthreads=args.cpus_per_node)``.",
"_____no_output_____"
]
],
[
[
"nodes = 8 + 2 # number of workers + 2 needed for scheduler and script process\ncpus_per_node = 4 # number of vCPUs per node; to initialize one thread per CPU\n\nprint(f\"Nodes: {nodes}\\nCPUs/node: {cpus_per_node}\")",
"_____no_output_____"
],
[
"arguments = [\n \"--cpus_per_node\",\n cpus_per_node,\n \"--num_boost_round\",\n 100,\n \"--learning_rate\",\n 0.2,\n \"--gamma\",\n 0,\n]\narguments",
"_____no_output_____"
],
[
"from azureml.core import ScriptRunConfig, Experiment, Environment\nfrom azureml.core.runconfig import MpiConfiguration\n\nenv = Environment.from_conda_specification(\"xgboost-cpu-tutorial\", \"environment.yml\")\nmpi_config = MpiConfiguration(node_count=nodes)\nsrc = ScriptRunConfig(\n source_directory=\"src\",\n script=\"run.py\",\n arguments=arguments,\n compute_target=\"cpu-cluster\",\n environment=env,\n distributed_job_config=mpi_config,\n max_run_duration_seconds=60 * 60,\n)\nrun = Experiment(ws, \"xgboost-cpu-tutorial\").submit(src)\nrun",
"_____no_output_____"
]
],
[
[
"## View Widget\n\nOptionally, view the output in the run widget.",
"_____no_output_____"
]
],
[
[
"from azureml.widgets import RunDetails\n\nRunDetails(run).show()",
"_____no_output_____"
]
],
[
[
"for testing, wait for the run to complete",
"_____no_output_____"
]
],
[
[
"run.wait_for_completion(show_output=True)",
"_____no_output_____"
]
]
] | [
"markdown",
"code",
"markdown",
"code",
"markdown",
"code",
"markdown",
"code"
] | [
[
"markdown",
"markdown"
],
[
"code"
],
[
"markdown",
"markdown"
],
[
"code",
"code",
"code"
],
[
"markdown"
],
[
"code"
],
[
"markdown"
],
[
"code"
]
] |
e771df371cbf24138c2ca57bac708f37f96c9600 | 48,339 | ipynb | Jupyter Notebook | tutorials/streamlit_notebooks/NER_BTC.ipynb | Laurasgmt/spark-nlp-workshop | d9f7984022179fbdd18918f795a0a27d2d07aafd | [
"Apache-2.0"
] | 1 | 2022-01-14T00:40:14.000Z | 2022-01-14T00:40:14.000Z | tutorials/streamlit_notebooks/NER_BTC.ipynb | Laurasgmt/spark-nlp-workshop | d9f7984022179fbdd18918f795a0a27d2d07aafd | [
"Apache-2.0"
] | null | null | null | tutorials/streamlit_notebooks/NER_BTC.ipynb | Laurasgmt/spark-nlp-workshop | d9f7984022179fbdd18918f795a0a27d2d07aafd | [
"Apache-2.0"
] | null | null | null | 44.104927 | 991 | 0.416889 | [
[
[
"\n\n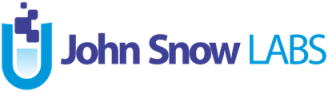\n\n[](https://colab.research.google.com/github/JohnSnowLabs/spark-nlp-workshop/blob/master/tutorials/streamlit_notebooks/NER_BTC.ipynb)\n\n\n\n\n",
"_____no_output_____"
],
[
"# **Detect Entities in Twitter texts**",
"_____no_output_____"
],
[
"## 1. Colab Setup",
"_____no_output_____"
]
],
[
[
"!wget http://setup.johnsnowlabs.com/colab.sh -O - | bash",
"--2021-11-17 05:37:04-- http://setup.johnsnowlabs.com/colab.sh\nResolving setup.johnsnowlabs.com (setup.johnsnowlabs.com)... 51.158.130.125\nConnecting to setup.johnsnowlabs.com (setup.johnsnowlabs.com)|51.158.130.125|:80... connected.\nHTTP request sent, awaiting response... 302 Found\nLocation: https://setup.johnsnowlabs.com/colab.sh [following]\n--2021-11-17 05:37:04-- https://setup.johnsnowlabs.com/colab.sh\nConnecting to setup.johnsnowlabs.com (setup.johnsnowlabs.com)|51.158.130.125|:443... connected.\nHTTP request sent, awaiting response... 302 Moved Temporarily\nLocation: https://raw.githubusercontent.com/JohnSnowLabs/spark-nlp/master/scripts/colab_setup.sh [following]\n--2021-11-17 05:37:05-- https://raw.githubusercontent.com/JohnSnowLabs/spark-nlp/master/scripts/colab_setup.sh\nResolving raw.githubusercontent.com (raw.githubusercontent.com)... 185.199.108.133, 185.199.111.133, 185.199.110.133, ...\nConnecting to raw.githubusercontent.com (raw.githubusercontent.com)|185.199.108.133|:443... connected.\nHTTP request sent, awaiting response... 200 OK\nLength: 1275 (1.2K) [text/plain]\nSaving to: ‘STDOUT’\n\n- 0%[ ] 0 --.-KB/s setup Colab for PySpark 3.0.3 and Spark NLP 3.3.2\nInstalling PySpark 3.0.3 and Spark NLP 3.3.2\n- 100%[===================>] 1.25K --.-KB/s in 0s \n\n2021-11-17 05:37:05 (50.9 MB/s) - written to stdout [1275/1275]\n\n\u001b[K |████████████████████████████████| 209.1 MB 12 kB/s \n\u001b[K |████████████████████████████████| 130 kB 38.1 MB/s \n\u001b[K |████████████████████████████████| 198 kB 41.9 MB/s \n\u001b[?25h Building wheel for pyspark (setup.py) ... \u001b[?25l\u001b[?25hdone\n"
],
[
"!pip install --ignore-installed spark-nlp-display",
"Collecting spark-nlp-display\n Downloading spark_nlp_display-1.8-py3-none-any.whl (95 kB)\n\u001b[K |████████████████████████████████| 95 kB 2.4 MB/s \n\u001b[?25hCollecting spark-nlp\n Using cached spark_nlp-3.3.2-py2.py3-none-any.whl (130 kB)\nCollecting ipython\n Downloading ipython-7.29.0-py3-none-any.whl (790 kB)\n\u001b[K |████████████████████████████████| 790 kB 18.0 MB/s \n\u001b[?25hCollecting pandas\n Downloading pandas-1.3.4-cp37-cp37m-manylinux_2_17_x86_64.manylinux2014_x86_64.whl (11.3 MB)\n\u001b[K |████████████████████████████████| 11.3 MB 16.9 MB/s \n\u001b[?25hCollecting svgwrite==1.4\n Downloading svgwrite-1.4-py3-none-any.whl (66 kB)\n\u001b[K |████████████████████████████████| 66 kB 4.6 MB/s \n\u001b[?25hCollecting numpy\n Downloading numpy-1.21.4-cp37-cp37m-manylinux_2_12_x86_64.manylinux2010_x86_64.whl (15.7 MB)\n\u001b[K |████████████████████████████████| 15.7 MB 63 kB/s \n\u001b[?25hCollecting pexpect>4.3\n Downloading pexpect-4.8.0-py2.py3-none-any.whl (59 kB)\n\u001b[K |████████████████████████████████| 59 kB 6.2 MB/s \n\u001b[?25hCollecting prompt-toolkit!=3.0.0,!=3.0.1,<3.1.0,>=2.0.0\n Downloading prompt_toolkit-3.0.22-py3-none-any.whl (374 kB)\n\u001b[K |████████████████████████████████| 374 kB 38.1 MB/s \n\u001b[?25hCollecting traitlets>=4.2\n Downloading traitlets-5.1.1-py3-none-any.whl (102 kB)\n\u001b[K |████████████████████████████████| 102 kB 10.0 MB/s \n\u001b[?25hCollecting setuptools>=18.5\n Downloading setuptools-59.1.1-py3-none-any.whl (951 kB)\n\u001b[K |████████████████████████████████| 951 kB 35.6 MB/s \n\u001b[?25hCollecting pickleshare\n Downloading pickleshare-0.7.5-py2.py3-none-any.whl (6.9 kB)\nCollecting jedi>=0.16\n Downloading jedi-0.18.1-py2.py3-none-any.whl (1.6 MB)\n\u001b[K |████████████████████████████████| 1.6 MB 39.9 MB/s \n\u001b[?25hCollecting backcall\n Downloading backcall-0.2.0-py2.py3-none-any.whl (11 kB)\nCollecting matplotlib-inline\n Downloading matplotlib_inline-0.1.3-py3-none-any.whl (8.2 kB)\nCollecting pygments\n Downloading Pygments-2.10.0-py3-none-any.whl (1.0 MB)\n\u001b[K |████████████████████████████████| 1.0 MB 33.3 MB/s \n\u001b[?25hCollecting decorator\n Downloading decorator-5.1.0-py3-none-any.whl (9.1 kB)\nCollecting parso<0.9.0,>=0.8.0\n Downloading parso-0.8.2-py2.py3-none-any.whl (94 kB)\n\u001b[K |████████████████████████████████| 94 kB 2.4 MB/s \n\u001b[?25hCollecting ptyprocess>=0.5\n Downloading ptyprocess-0.7.0-py2.py3-none-any.whl (13 kB)\nCollecting wcwidth\n Downloading wcwidth-0.2.5-py2.py3-none-any.whl (30 kB)\nCollecting pytz>=2017.3\n Downloading pytz-2021.3-py2.py3-none-any.whl (503 kB)\n\u001b[K |████████████████████████████████| 503 kB 39.2 MB/s \n\u001b[?25hCollecting python-dateutil>=2.7.3\n Downloading python_dateutil-2.8.2-py2.py3-none-any.whl (247 kB)\n\u001b[K |████████████████████████████████| 247 kB 40.4 MB/s \n\u001b[?25hCollecting six>=1.5\n Downloading six-1.16.0-py2.py3-none-any.whl (11 kB)\nInstalling collected packages: wcwidth, traitlets, six, ptyprocess, parso, setuptools, pytz, python-dateutil, pygments, prompt-toolkit, pickleshare, pexpect, numpy, matplotlib-inline, jedi, decorator, backcall, svgwrite, spark-nlp, pandas, ipython, spark-nlp-display\n\u001b[31mERROR: pip's dependency resolver does not currently take into account all the packages that are installed. This behaviour is the source of the following dependency conflicts.\nyellowbrick 1.3.post1 requires numpy<1.20,>=1.16.0, but you have numpy 1.21.4 which is incompatible.\nnbclient 0.5.8 requires jupyter-client>=6.1.5, but you have jupyter-client 5.3.5 which is incompatible.\nmoviepy 0.2.3.5 requires decorator<5.0,>=4.0.2, but you have decorator 5.1.0 which is incompatible.\njupyter-console 5.2.0 requires prompt-toolkit<2.0.0,>=1.0.0, but you have prompt-toolkit 3.0.22 which is incompatible.\ngoogle-colab 1.0.0 requires ipython~=5.5.0, but you have ipython 7.29.0 which is incompatible.\ngoogle-colab 1.0.0 requires pandas~=1.1.0; python_version >= \"3.0\", but you have pandas 1.3.4 which is incompatible.\ngoogle-colab 1.0.0 requires six~=1.15.0, but you have six 1.16.0 which is incompatible.\ndatascience 0.10.6 requires folium==0.2.1, but you have folium 0.8.3 which is incompatible.\nalbumentations 0.1.12 requires imgaug<0.2.7,>=0.2.5, but you have imgaug 0.2.9 which is incompatible.\u001b[0m\nSuccessfully installed backcall-0.2.0 decorator-5.1.0 ipython-7.29.0 jedi-0.18.1 matplotlib-inline-0.1.3 numpy-1.21.4 pandas-1.3.4 parso-0.8.2 pexpect-4.8.0 pickleshare-0.7.5 prompt-toolkit-3.0.22 ptyprocess-0.7.0 pygments-2.10.0 python-dateutil-2.8.2 pytz-2021.3 setuptools-59.1.1 six-1.16.0 spark-nlp-3.3.2 spark-nlp-display-1.8 svgwrite-1.4 traitlets-5.1.1 wcwidth-0.2.5\n"
],
[
"import pandas as pd\nimport numpy as np\nimport json\nfrom pyspark.ml import Pipeline\nfrom pyspark.sql import SparkSession\nimport pyspark.sql.functions as F\nfrom sparknlp.annotator import *\nfrom sparknlp.base import *\nimport sparknlp\nfrom sparknlp.pretrained import PretrainedPipeline",
"_____no_output_____"
]
],
[
[
"## 2. Start Spark Session",
"_____no_output_____"
]
],
[
[
"spark = sparknlp.start()",
"_____no_output_____"
]
],
[
[
"## 3. Some sample examples",
"_____no_output_____"
]
],
[
[
"text_list = test_sentences = [\"\"\"Wengers big mistakes is not being ruthless enough with bad players.\"\"\",\n \"\"\"Aguero goal . From being someone previously so reliable , he 's been terrible this year .\"\"\",\n \"\"\"Paul Scholes approached Alex Ferguson about making a comeback . Ferguson clearly only too happy to accommodate him .\"\"\",\n \"\"\"Wikipedia today , as soon as you load the website , hit ESC to prevent the 'blackout ' from loading.\"\"\",\n \"\"\"David Attenborough shows us a duck billed platypus.\"\"\",\n \"\"\"London GET UPDATES FROM Peter Hotez\"\"\",\n \"\"\"Pentagram's Dominic Lippa is working on a new identity for University of Arts London \"\"\"]",
"_____no_output_____"
]
],
[
[
"## 4. Define Spark NLP pipeline",
"_____no_output_____"
]
],
[
[
"document = DocumentAssembler()\\\n .setInputCol(\"text\")\\\n .setOutputCol(\"document\")\n\ntokenizer = Tokenizer()\\\n .setInputCols(\"document\")\\\n .setOutputCol(\"token\") \n\ntokenClassifier = BertForTokenClassification.pretrained(\"bert_token_classifier_ner_btc\", \"en\")\\\n .setInputCols(\"token\", \"document\")\\\n .setOutputCol(\"ner\")\\\n .setCaseSensitive(True)\n\nner_converter = NerConverter()\\\n .setInputCols([\"document\",\"token\",\"ner\"])\\\n .setOutputCol(\"ner_chunk\")\\\n \n\npipeline = Pipeline(stages=[document, tokenizer, tokenClassifier, ner_converter])\n\n",
"bert_token_classifier_ner_btc download started this may take some time.\nApproximate size to download 385.3 MB\n[OK!]\n"
]
],
[
[
"## 5. Run the pipeline",
"_____no_output_____"
]
],
[
[
"model = pipeline.fit(spark.createDataFrame(pd.DataFrame({'text': ['']})))\n\nresult = model.transform(spark.createDataFrame(pd.DataFrame({'text': text_list})))\n",
"_____no_output_____"
]
],
[
[
"## 6. Visualize results",
"_____no_output_____"
]
],
[
[
"\nresult.select(F.explode(F.arrays_zip('document.result', 'ner_chunk.result',\"ner_chunk.metadata\")).alias(\"cols\")) \\\n.select(\n F.expr(\"cols['1']\").alias(\"chunk\"),\n F.expr(\"cols['2'].entity\").alias('result')).show(truncate=False)",
"+-------------------------+------+\n|chunk |result|\n+-------------------------+------+\n|Wengers |PER |\n|Aguero |PER |\n|Paul Scholes |PER |\n|Alex Ferguson |PER |\n|Ferguson |PER |\n|Wikipedia |ORG |\n|David Attenborough |PER |\n|London |LOC |\n|Peter Hotez |PER |\n|Pentagram's |ORG |\n|Dominic Lippa |PER |\n|University of Arts London|ORG |\n+-------------------------+------+\n\n"
],
[
"from sparknlp_display import NerVisualizer\n\nfor i in range(len(text_list)):\n NerVisualizer().display(\n result = result.collect()[i],\n label_col = 'ner_chunk',\n document_col = 'document'\n )\n\n",
"_____no_output_____"
],
[
"",
"_____no_output_____"
]
]
] | [
"markdown",
"code",
"markdown",
"code",
"markdown",
"code",
"markdown",
"code",
"markdown",
"code",
"markdown",
"code"
] | [
[
"markdown",
"markdown",
"markdown"
],
[
"code",
"code",
"code"
],
[
"markdown"
],
[
"code"
],
[
"markdown"
],
[
"code"
],
[
"markdown"
],
[
"code"
],
[
"markdown"
],
[
"code"
],
[
"markdown"
],
[
"code",
"code",
"code"
]
] |
e771e8183b6aef22d2229437c8c6c8714a7f3dc1 | 298,518 | ipynb | Jupyter Notebook | Population_Segmentation/Pop_Segmentation_Exercise.ipynb | fradeleo/Sagemaker_Case_Studies | 29ed6ea423e4c4db5715435d6da9ac5fd053eb85 | [
"MIT"
] | null | null | null | Population_Segmentation/Pop_Segmentation_Exercise.ipynb | fradeleo/Sagemaker_Case_Studies | 29ed6ea423e4c4db5715435d6da9ac5fd053eb85 | [
"MIT"
] | null | null | null | Population_Segmentation/Pop_Segmentation_Exercise.ipynb | fradeleo/Sagemaker_Case_Studies | 29ed6ea423e4c4db5715435d6da9ac5fd053eb85 | [
"MIT"
] | null | null | null | 58.590383 | 17,096 | 0.692608 | [
[
[
"# Population Segmentation with SageMaker\n\nIn this notebook, you'll employ two, unsupervised learning algorithms to do **population segmentation**. Population segmentation aims to find natural groupings in population data that reveal some feature-level similarities between different regions in the US.\n\nUsing **principal component analysis** (PCA) you will reduce the dimensionality of the original census data. Then, you'll use **k-means clustering** to assign each US county to a particular cluster based on where a county lies in component space. How each cluster is arranged in component space can tell you which US counties are most similar and what demographic traits define that similarity; this information is most often used to inform targeted, marketing campaigns that want to appeal to a specific group of people. This cluster information is also useful for learning more about a population by revealing patterns between regions that you otherwise may not have noticed.\n\n### US Census Data\n\nYou'll be using data collected by the [US Census](https://en.wikipedia.org/wiki/United_States_Census), which aims to count the US population, recording demographic traits about labor, age, population, and so on, for each county in the US. The bulk of this notebook was taken from an existing SageMaker example notebook and [blog post](https://aws.amazon.com/blogs/machine-learning/analyze-us-census-data-for-population-segmentation-using-amazon-sagemaker/), and I've broken it down further into demonstrations and exercises for you to complete.\n\n### Machine Learning Workflow\n\nTo implement population segmentation, you'll go through a number of steps:\n* Data loading and exploration\n* Data cleaning and pre-processing \n* Dimensionality reduction with PCA\n* Feature engineering and data transformation\n* Clustering transformed data with k-means\n* Extracting trained model attributes and visualizing k clusters\n\nThese tasks make up a complete, machine learning workflow from data loading and cleaning to model deployment. Each exercise is designed to give you practice with part of the machine learning workflow, and to demonstrate how to use SageMaker tools, such as built-in data management with S3 and built-in algorithms.\n\n---",
"_____no_output_____"
],
[
"First, import the relevant libraries into this SageMaker notebook. ",
"_____no_output_____"
]
],
[
[
"# data managing and display libs\nimport pandas as pd\nimport numpy as np\nimport os\nimport io\n\nimport matplotlib.pyplot as plt\nimport matplotlib\n%matplotlib inline ",
"_____no_output_____"
],
[
"# sagemaker libraries\nimport boto3\nimport sagemaker",
"_____no_output_____"
]
],
[
[
"## Loading the Data from Amazon S3\n\nThis particular dataset is already in an Amazon S3 bucket; you can load the data by pointing to this bucket and getting a data file by name. \n\n> You can interact with S3 using a `boto3` client.",
"_____no_output_____"
]
],
[
[
"# boto3 client to get S3 data\ns3_client = boto3.client('s3')\nbucket_name='aws-ml-blog-sagemaker-census-segmentation'",
"_____no_output_____"
]
],
[
[
"Take a look at the contents of this bucket; get a list of objects that are contained within the bucket and print out the names of the objects. You should see that there is one file, 'Census_Data_for_SageMaker.csv'.",
"_____no_output_____"
]
],
[
[
"# get a list of objects in the bucket\nobj_list=s3_client.list_objects(Bucket=bucket_name)\n\n# print object(s)in S3 bucket\nfiles=[]\nfor contents in obj_list['Contents']:\n files.append(contents['Key'])\n \nprint(files)",
"['Census_Data_for_SageMaker.csv']\n"
],
[
"# there is one file --> one key\nfile_name=files[0]\n\nprint(file_name)",
"Census_Data_for_SageMaker.csv\n"
]
],
[
[
"Retrieve the data file from the bucket with a call to `client.get_object()`.",
"_____no_output_____"
]
],
[
[
"# get an S3 object by passing in the bucket and file name\ndata_object = s3_client.get_object(Bucket=bucket_name, Key=file_name)\n\n# what info does the object contain?\ndisplay(data_object)",
"_____no_output_____"
],
[
"# information is in the \"Body\" of the object\ndata_body = data_object[\"Body\"].read()\nprint('Data type: ', type(data_body))",
"Data type: <class 'bytes'>\n"
]
],
[
[
"This is a `bytes` datatype, which you can read it in using [io.BytesIO(file)](https://docs.python.org/3/library/io.html#binary-i-o).",
"_____no_output_____"
]
],
[
[
"# read in bytes data\ndata_stream = io.BytesIO(data_body)\n\n# create a dataframe\ncounties_df = pd.read_csv(data_stream, header=0, delimiter=\",\") \ncounties_df.head()",
"_____no_output_____"
]
],
[
[
"## Exploratory Data Analysis (EDA)\n\nNow that you've loaded in the data, it is time to clean it up, explore it, and pre-process it. Data exploration is one of the most important parts of the machine learning workflow because it allows you to notice any initial patterns in data distribution and features that may inform how you proceed with modeling and clustering the data.\n\n### EXERCISE: Explore data & drop any incomplete rows of data\n\nWhen you first explore the data, it is good to know what you are working with. How many data points and features are you starting with, and what kind of information can you get at a first glance? In this notebook, you're required to use complete data points to train a model. So, your first exercise will be to investigate the shape of this data and implement a simple, data cleaning step: dropping any incomplete rows of data.\n\nYou should be able to answer the **question**: How many data points and features are in the original, provided dataset? (And how many points are left after dropping any incomplete rows?)",
"_____no_output_____"
]
],
[
[
"counties_df.shape",
"_____no_output_____"
],
[
"# print out stats about data\ncounties_df.shape\n# drop any incomplete rows of data, and create a new df\nclean_counties_df = counties_df.dropna()\nclean_counties_df.shape",
"_____no_output_____"
]
],
[
[
"### EXERCISE: Create a new DataFrame, indexed by 'State-County'\n\nEventually, you'll want to feed these features into a machine learning model. Machine learning models need numerical data to learn from and not categorical data like strings (State, County). So, you'll reformat this data such that it is indexed by region and you'll also drop any features that are not useful for clustering.\n\nTo complete this task, perform the following steps, using your *clean* DataFrame, generated above:\n1. Combine the descriptive columns, 'State' and 'County', into one, new categorical column, 'State-County'. \n2. Index the data by this unique State-County name.\n3. After doing this, drop the old State and County columns and the CensusId column, which does not give us any meaningful demographic information.\n\nAfter completing this task, you should have a DataFrame with 'State-County' as the index, and 34 columns of numerical data for each county. You should get a resultant DataFrame that looks like the following (truncated for display purposes):\n```\n TotalPop\t Men\t Women\tHispanic\t...\n \nAlabama-Autauga\t55221\t 26745\t28476\t2.6 ...\nAlabama-Baldwin\t195121\t95314\t99807\t4.5 ...\nAlabama-Barbour\t26932\t 14497\t12435\t4.6 ...\n...\n\n```",
"_____no_output_____"
]
],
[
[
"# index data by 'State-County'\nclean_counties_df.index= clean_counties_df.State + '-' + clean_counties_df.County",
"_____no_output_____"
],
[
"clean_counties_df.head(1)",
"_____no_output_____"
],
[
"# drop the old State and County columns, and the CensusId column\n# clean df should be modified or created anew\ncolumns_to_drop = ['State', 'County','CensusId']\nclean_counties_df = clean_counties_df.drop(columns = columns_to_drop)\nclean_counties_df.head(1)",
"_____no_output_____"
]
],
[
[
"Now, what features do you have to work with?",
"_____no_output_____"
]
],
[
[
"# features\nfeatures_list = clean_counties_df.columns.values\nprint('Features: \\n', features_list)",
"Features: \n ['TotalPop' 'Men' 'Women' 'Hispanic' 'White' 'Black' 'Native' 'Asian'\n 'Pacific' 'Citizen' 'Income' 'IncomeErr' 'IncomePerCap' 'IncomePerCapErr'\n 'Poverty' 'ChildPoverty' 'Professional' 'Service' 'Office' 'Construction'\n 'Production' 'Drive' 'Carpool' 'Transit' 'Walk' 'OtherTransp'\n 'WorkAtHome' 'MeanCommute' 'Employed' 'PrivateWork' 'PublicWork'\n 'SelfEmployed' 'FamilyWork' 'Unemployment']\n"
]
],
[
[
"## Visualizing the Data\n\nIn general, you can see that features come in a variety of ranges, mostly percentages from 0-100, and counts that are integer values in a large range. Let's visualize the data in some of our feature columns and see what the distribution, over all counties, looks like.\n\nThe below cell displays **histograms**, which show the distribution of data points over discrete feature ranges. The x-axis represents the different bins; each bin is defined by a specific range of values that a feature can take, say between the values 0-5 and 5-10, and so on. The y-axis is the frequency of occurrence or the number of county data points that fall into each bin. I find it helpful to use the y-axis values for relative comparisons between different features.\n\nBelow, I'm plotting a histogram comparing methods of commuting to work over all of the counties. I just copied these feature names from the list of column names, printed above. I also know that all of these features are represented as percentages (%) in the original data, so the x-axes of these plots will be comparable.",
"_____no_output_____"
]
],
[
[
"# transportation (to work)\ntransport_list = ['Drive', 'Carpool', 'Transit', 'Walk', 'OtherTransp']\nn_bins = 30 # can decrease to get a wider bin (or vice versa)\n\nfor column_name in transport_list:\n ax=plt.subplots(figsize=(6,3))\n # get data by column_name and display a histogram\n ax = plt.hist(clean_counties_df[column_name], bins=n_bins)\n title=\"Histogram of \" + column_name\n plt.title(title, fontsize=12)\n plt.show()",
"_____no_output_____"
]
],
[
[
"### EXERCISE: Create histograms of your own\n\nCommute transportation method is just one category of features. If you take a look at the 34 features, you can see data on profession, race, income, and more. Display a set of histograms that interest you!\n",
"_____no_output_____"
]
],
[
[
"# create a list of features that you want to compare or examine\nmy_list = ['Hispanic', 'White', 'Black', 'Native', 'Asian', 'Pacific']\nn_bins = 50 # define n_bins\n\n# histogram creation code is similar to above\nfor column_name in my_list:\n ax=plt.subplots(figsize=(6,3))\n # get data by column_name and display a histogram\n ax = plt.hist(clean_counties_df[column_name], bins=n_bins)\n title=\"Histogram of \" + column_name\n plt.title(title, fontsize=12)\n plt.show()",
"_____no_output_____"
]
],
[
[
"### EXERCISE: Normalize the data\n\nYou need to standardize the scale of the numerical columns in order to consistently compare the values of different features. You can use a [MinMaxScaler](https://scikit-learn.org/stable/modules/generated/sklearn.preprocessing.MinMaxScaler.html) to transform the numerical values so that they all fall between 0 and 1.",
"_____no_output_____"
]
],
[
[
"# scale numerical features into a normalized range, 0-1\nfrom sklearn.preprocessing import MinMaxScaler\nscaler = MinMaxScaler()\n# store them in this dataframe\ncounties_scaled = pd.DataFrame(scaler.fit_transform(clean_counties_df.astype(float)))\n\ncounties_scaled.columns=clean_counties_df.columns\ncounties_scaled.index=clean_counties_df.index",
"_____no_output_____"
],
[
"counties_scaled.head()",
"_____no_output_____"
]
],
[
[
"---\n# Data Modeling\n\n\nNow, the data is ready to be fed into a machine learning model!\n\nEach data point has 34 features, which means the data is 34-dimensional. Clustering algorithms rely on finding clusters in n-dimensional feature space. For higher dimensions, an algorithm like k-means has a difficult time figuring out which features are most important, and the result is, often, noisier clusters.\n\nSome dimensions are not as important as others. For example, if every county in our dataset has the same rate of unemployment, then that particular feature doesn’t give us any distinguishing information; it will not help to separate counties into different groups because its value doesn’t *vary* between counties.\n\n> Instead, we really want to find the features that help to separate and group data. We want to find features that cause the **most variance** in the dataset!\n\nSo, before I cluster this data, I’ll want to take a dimensionality reduction step. My aim will be to form a smaller set of features that will better help to separate our data. The technique I’ll use is called PCA or **principal component analysis**\n\n## Dimensionality Reduction\n\nPCA attempts to reduce the number of features within a dataset while retaining the “principal components”, which are defined as *weighted*, linear combinations of existing features that are designed to be linearly independent and account for the largest possible variability in the data! You can think of this method as taking many features and combining similar or redundant features together to form a new, smaller feature set.\n\nWe can reduce dimensionality with the built-in SageMaker model for PCA.",
"_____no_output_____"
],
[
"### Roles and Buckets\n\n> To create a model, you'll first need to specify an IAM role, and to save the model attributes, you'll need to store them in an S3 bucket.\n\nThe `get_execution_role` function retrieves the IAM role you created at the time you created your notebook instance. Roles are essentially used to manage permissions and you can read more about that [in this documentation](https://docs.aws.amazon.com/sagemaker/latest/dg/sagemaker-roles.html). For now, know that we have a FullAccess notebook, which allowed us to access and download the census data stored in S3.\n\nYou must specify a bucket name for an S3 bucket in your account where you want SageMaker model parameters to be stored. Note that the bucket must be in the same region as this notebook. You can get a default S3 bucket, which automatically creates a bucket for you and in your region, by storing the current SageMaker session and calling `session.default_bucket()`.",
"_____no_output_____"
]
],
[
[
"from sagemaker import get_execution_role\n\nsession = sagemaker.Session() # store the current SageMaker session\n\n# get IAM role\nrole = get_execution_role()\nprint(role)",
"arn:aws:iam::730357687813:role/service-role/AmazonSageMaker-ExecutionRole-20200522T082389\n"
],
[
"# get default bucket\nbucket_name = session.default_bucket()\nprint(bucket_name)\nprint()",
"sagemaker-eu-central-1-730357687813\n\n"
]
],
[
[
"## Define a PCA Model\n\nTo create a PCA model, I'll use the built-in SageMaker resource. A SageMaker estimator requires a number of parameters to be specified; these define the type of training instance to use and the model hyperparameters. A PCA model requires the following constructor arguments:\n\n* role: The IAM role, which was specified, above.\n* train_instance_count: The number of training instances (typically, 1).\n* train_instance_type: The type of SageMaker instance for training.\n* num_components: An integer that defines the number of PCA components to produce.\n* sagemaker_session: The session used to train on SageMaker.\n\nDocumentation on the PCA model can be found [here](http://sagemaker.readthedocs.io/en/latest/pca.html).\n\nBelow, I first specify where to save the model training data, the `output_path`.",
"_____no_output_____"
]
],
[
[
"# define location to store model artifacts\nprefix = 'counties'\n\noutput_path='s3://{}/{}/'.format(bucket_name, prefix)\n\nprint('Training artifacts will be uploaded to: {}'.format(output_path))",
"Training artifacts will be uploaded to: s3://sagemaker-eu-central-1-730357687813/counties/\n"
],
[
"# define a PCA model\nfrom sagemaker import PCA\n\n# this is current features - 1\n# you'll select only a portion of these to use, later\nN_COMPONENTS=33\n\npca_SM = PCA(role=role,\n train_instance_count=1,\n train_instance_type='ml.c4.xlarge',\n output_path=output_path, # specified, above\n num_components=N_COMPONENTS, \n sagemaker_session=session)\n",
"_____no_output_____"
]
],
[
[
"### Convert data into a RecordSet format\n\nNext, prepare the data for a built-in model by converting the DataFrame to a numpy array of float values.\n\nThe *record_set* function in the SageMaker PCA model converts a numpy array into a **RecordSet** format that is the required format for the training input data. This is a requirement for _all_ of SageMaker's built-in models. The use of this data type is one of the reasons that allows training of models within Amazon SageMaker to perform faster, especially for large datasets.",
"_____no_output_____"
]
],
[
[
"# convert df to np array\ntrain_data_np = counties_scaled.values.astype('float32')\n\n# convert to RecordSet format\nformatted_train_data = pca_SM.record_set(train_data_np)",
"_____no_output_____"
]
],
[
[
"## Train the model\n\nCall the fit function on the PCA model, passing in our formatted, training data. This spins up a training instance to perform the training job.\n\nNote that it takes the longest to launch the specified training instance; the fitting itself doesn't take much time.",
"_____no_output_____"
]
],
[
[
"%%time\n\n# train the PCA mode on the formatted data\npca_SM.fit(formatted_train_data)",
"2020-05-23 05:40:14 Starting - Starting the training job...\n2020-05-23 05:40:16 Starting - Launching requested ML instances.........\n2020-05-23 05:41:46 Starting - Preparing the instances for training......\n2020-05-23 05:43:02 Downloading - Downloading input data\n2020-05-23 05:43:02 Training - Downloading the training image..\u001b[34mDocker entrypoint called with argument(s): train\u001b[0m\n\u001b[34mRunning default environment configuration script\u001b[0m\n\u001b[34m[05/23/2020 05:43:18 INFO 140677512759104] Reading default configuration from /opt/amazon/lib/python2.7/site-packages/algorithm/resources/default-conf.json: {u'_num_gpus': u'auto', u'_log_level': u'info', u'subtract_mean': u'true', u'force_dense': u'true', u'epochs': 1, u'algorithm_mode': u'regular', u'extra_components': u'-1', u'_kvstore': u'dist_sync', u'_num_kv_servers': u'auto'}\u001b[0m\n\u001b[34m[05/23/2020 05:43:18 INFO 140677512759104] Reading provided configuration from /opt/ml/input/config/hyperparameters.json: {u'feature_dim': u'34', u'mini_batch_size': u'500', u'num_components': u'33'}\u001b[0m\n\u001b[34m[05/23/2020 05:43:18 INFO 140677512759104] Final configuration: {u'num_components': u'33', u'_num_gpus': u'auto', u'_log_level': u'info', u'subtract_mean': u'true', u'force_dense': u'true', u'epochs': 1, u'algorithm_mode': u'regular', u'feature_dim': u'34', u'extra_components': u'-1', u'_kvstore': u'dist_sync', u'_num_kv_servers': u'auto', u'mini_batch_size': u'500'}\u001b[0m\n\u001b[34m[05/23/2020 05:43:18 WARNING 140677512759104] Loggers have already been setup.\u001b[0m\n\u001b[34m[05/23/2020 05:43:20 INFO 140677512759104] Launching parameter server for role scheduler\u001b[0m\n\u001b[34m[05/23/2020 05:43:20 INFO 140677512759104] {'ECS_CONTAINER_METADATA_URI': 'http://169.254.170.2/v3/76e3ea69-dccf-4e9b-aa4d-467320032ebb', 'PROTOCOL_BUFFERS_PYTHON_IMPLEMENTATION_VERSION': '2', 'PATH': '/opt/amazon/bin:/usr/local/nvidia/bin:/usr/local/sbin:/usr/local/bin:/usr/sbin:/usr/bin:/sbin:/bin:/opt/amazon/bin:/opt/amazon/bin', 'SAGEMAKER_HTTP_PORT': '8080', 'HOME': '/root', 'PYTHONUNBUFFERED': 'TRUE', 'CANONICAL_ENVROOT': '/opt/amazon', 'LD_LIBRARY_PATH': '/opt/amazon/lib/python2.7/site-packages/cv2/../../../../lib:/usr/local/nvidia/lib64:/opt/amazon/lib', 'LANG': 'en_US.utf8', 'DMLC_INTERFACE': 'eth0', 'SHLVL': '1', 'AWS_REGION': 'eu-central-1', 'NVIDIA_VISIBLE_DEVICES': 'void', 'TRAINING_JOB_NAME': 'pca-2020-05-23-05-40-13-839', 'PROTOCOL_BUFFERS_PYTHON_IMPLEMENTATION': 'cpp', 'ENVROOT': '/opt/amazon', 'SAGEMAKER_DATA_PATH': '/opt/ml', 'NVIDIA_DRIVER_CAPABILITIES': 'compute,utility', 'NVIDIA_REQUIRE_CUDA': 'cuda>=9.0', 'OMP_NUM_THREADS': '2', 'HOSTNAME': 'ip-10-0-133-43.eu-central-1.compute.internal', 'AWS_CONTAINER_CREDENTIALS_RELATIVE_URI': '/v2/credentials/d6ef282a-7c64-41e9-9d5a-34e911f2beb7', 'PWD': '/', 'TRAINING_JOB_ARN': 'arn:aws:sagemaker:eu-central-1:730357687813:training-job/pca-2020-05-23-05-40-13-839', 'AWS_EXECUTION_ENV': 'AWS_ECS_EC2'}\u001b[0m\n\u001b[34m[05/23/2020 05:43:20 INFO 140677512759104] envs={'ECS_CONTAINER_METADATA_URI': 'http://169.254.170.2/v3/76e3ea69-dccf-4e9b-aa4d-467320032ebb', 'PROTOCOL_BUFFERS_PYTHON_IMPLEMENTATION_VERSION': '2', 'DMLC_NUM_WORKER': '1', 'DMLC_PS_ROOT_PORT': '9000', 'PATH': '/opt/amazon/bin:/usr/local/nvidia/bin:/usr/local/sbin:/usr/local/bin:/usr/sbin:/usr/bin:/sbin:/bin:/opt/amazon/bin:/opt/amazon/bin', 'SAGEMAKER_HTTP_PORT': '8080', 'HOME': '/root', 'PYTHONUNBUFFERED': 'TRUE', 'CANONICAL_ENVROOT': '/opt/amazon', 'LD_LIBRARY_PATH': '/opt/amazon/lib/python2.7/site-packages/cv2/../../../../lib:/usr/local/nvidia/lib64:/opt/amazon/lib', 'LANG': 'en_US.utf8', 'DMLC_INTERFACE': 'eth0', 'SHLVL': '1', 'DMLC_PS_ROOT_URI': '10.0.133.43', 'AWS_REGION': 'eu-central-1', 'NVIDIA_VISIBLE_DEVICES': 'void', 'TRAINING_JOB_NAME': 'pca-2020-05-23-05-40-13-839', 'PROTOCOL_BUFFERS_PYTHON_IMPLEMENTATION': 'cpp', 'ENVROOT': '/opt/amazon', 'SAGEMAKER_DATA_PATH': '/opt/ml', 'NVIDIA_DRIVER_CAPABILITIES': 'compute,utility', 'NVIDIA_REQUIRE_CUDA': 'cuda>=9.0', 'OMP_NUM_THREADS': '2', 'HOSTNAME': 'ip-10-0-133-43.eu-central-1.compute.internal', 'AWS_CONTAINER_CREDENTIALS_RELATIVE_URI': '/v2/credentials/d6ef282a-7c64-41e9-9d5a-34e911f2beb7', 'DMLC_ROLE': 'scheduler', 'PWD': '/', 'DMLC_NUM_SERVER': '1', 'TRAINING_JOB_ARN': 'arn:aws:sagemaker:eu-central-1:730357687813:training-job/pca-2020-05-23-05-40-13-839', 'AWS_EXECUTION_ENV': 'AWS_ECS_EC2'}\u001b[0m\n\u001b[34m[05/23/2020 05:43:20 INFO 140677512759104] Launching parameter server for role server\u001b[0m\n\u001b[34m[05/23/2020 05:43:20 INFO 140677512759104] {'ECS_CONTAINER_METADATA_URI': 'http://169.254.170.2/v3/76e3ea69-dccf-4e9b-aa4d-467320032ebb', 'PROTOCOL_BUFFERS_PYTHON_IMPLEMENTATION_VERSION': '2', 'PATH': '/opt/amazon/bin:/usr/local/nvidia/bin:/usr/local/sbin:/usr/local/bin:/usr/sbin:/usr/bin:/sbin:/bin:/opt/amazon/bin:/opt/amazon/bin', 'SAGEMAKER_HTTP_PORT': '8080', 'HOME': '/root', 'PYTHONUNBUFFERED': 'TRUE', 'CANONICAL_ENVROOT': '/opt/amazon', 'LD_LIBRARY_PATH': '/opt/amazon/lib/python2.7/site-packages/cv2/../../../../lib:/usr/local/nvidia/lib64:/opt/amazon/lib', 'LANG': 'en_US.utf8', 'DMLC_INTERFACE': 'eth0', 'SHLVL': '1', 'AWS_REGION': 'eu-central-1', 'NVIDIA_VISIBLE_DEVICES': 'void', 'TRAINING_JOB_NAME': 'pca-2020-05-23-05-40-13-839', 'PROTOCOL_BUFFERS_PYTHON_IMPLEMENTATION': 'cpp', 'ENVROOT': '/opt/amazon', 'SAGEMAKER_DATA_PATH': '/opt/ml', 'NVIDIA_DRIVER_CAPABILITIES': 'compute,utility', 'NVIDIA_REQUIRE_CUDA': 'cuda>=9.0', 'OMP_NUM_THREADS': '2', 'HOSTNAME': 'ip-10-0-133-43.eu-central-1.compute.internal', 'AWS_CONTAINER_CREDENTIALS_RELATIVE_URI': '/v2/credentials/d6ef282a-7c64-41e9-9d5a-34e911f2beb7', 'PWD': '/', 'TRAINING_JOB_ARN': 'arn:aws:sagemaker:eu-central-1:730357687813:training-job/pca-2020-05-23-05-40-13-839', 'AWS_EXECUTION_ENV': 'AWS_ECS_EC2'}\u001b[0m\n\u001b[34m[05/23/2020 05:43:20 INFO 140677512759104] envs={'ECS_CONTAINER_METADATA_URI': 'http://169.254.170.2/v3/76e3ea69-dccf-4e9b-aa4d-467320032ebb', 'PROTOCOL_BUFFERS_PYTHON_IMPLEMENTATION_VERSION': '2', 'DMLC_NUM_WORKER': '1', 'DMLC_PS_ROOT_PORT': '9000', 'PATH': '/opt/amazon/bin:/usr/local/nvidia/bin:/usr/local/sbin:/usr/local/bin:/usr/sbin:/usr/bin:/sbin:/bin:/opt/amazon/bin:/opt/amazon/bin', 'SAGEMAKER_HTTP_PORT': '8080', 'HOME': '/root', 'PYTHONUNBUFFERED': 'TRUE', 'CANONICAL_ENVROOT': '/opt/amazon', 'LD_LIBRARY_PATH': '/opt/amazon/lib/python2.7/site-packages/cv2/../../../../lib:/usr/local/nvidia/lib64:/opt/amazon/lib', 'LANG': 'en_US.utf8', 'DMLC_INTERFACE': 'eth0', 'SHLVL': '1', 'DMLC_PS_ROOT_URI': '10.0.133.43', 'AWS_REGION': 'eu-central-1', 'NVIDIA_VISIBLE_DEVICES': 'void', 'TRAINING_JOB_NAME': 'pca-2020-05-23-05-40-13-839', 'PROTOCOL_BUFFERS_PYTHON_IMPLEMENTATION': 'cpp', 'ENVROOT': '/opt/amazon', 'SAGEMAKER_DATA_PATH': '/opt/ml', 'NVIDIA_DRIVER_CAPABILITIES': 'compute,utility', 'NVIDIA_REQUIRE_CUDA': 'cuda>=9.0', 'OMP_NUM_THREADS': '2', 'HOSTNAME': 'ip-10-0-133-43.eu-central-1.compute.internal', 'AWS_CONTAINER_CREDENTIALS_RELATIVE_URI': '/v2/credentials/d6ef282a-7c64-41e9-9d5a-34e911f2beb7', 'DMLC_ROLE': 'server', 'PWD': '/', 'DMLC_NUM_SERVER': '1', 'TRAINING_JOB_ARN': 'arn:aws:sagemaker:eu-central-1:730357687813:training-job/pca-2020-05-23-05-40-13-839', 'AWS_EXECUTION_ENV': 'AWS_ECS_EC2'}\u001b[0m\n\u001b[34m[05/23/2020 05:43:20 INFO 140677512759104] Environment: {'ECS_CONTAINER_METADATA_URI': 'http://169.254.170.2/v3/76e3ea69-dccf-4e9b-aa4d-467320032ebb', 'PROTOCOL_BUFFERS_PYTHON_IMPLEMENTATION_VERSION': '2', 'DMLC_PS_ROOT_PORT': '9000', 'DMLC_NUM_WORKER': '1', 'SAGEMAKER_HTTP_PORT': '8080', 'PATH': '/opt/amazon/bin:/usr/local/nvidia/bin:/usr/local/sbin:/usr/local/bin:/usr/sbin:/usr/bin:/sbin:/bin:/opt/amazon/bin:/opt/amazon/bin', 'PYTHONUNBUFFERED': 'TRUE', 'CANONICAL_ENVROOT': '/opt/amazon', 'LD_LIBRARY_PATH': '/opt/amazon/lib/python2.7/site-packages/cv2/../../../../lib:/usr/local/nvidia/lib64:/opt/amazon/lib', 'LANG': 'en_US.utf8', 'DMLC_INTERFACE': 'eth0', 'SHLVL': '1', 'DMLC_PS_ROOT_URI': '10.0.133.43', 'AWS_REGION': 'eu-central-1', 'NVIDIA_VISIBLE_DEVICES': 'void', 'TRAINING_JOB_NAME': 'pca-2020-05-23-05-40-13-839', 'HOME': '/root', 'PROTOCOL_BUFFERS_PYTHON_IMPLEMENTATION': 'cpp', 'ENVROOT': '/opt/amazon', 'SAGEMAKER_DATA_PATH': '/opt/ml', 'NVIDIA_DRIVER_CAPABILITIES': 'compute,utility', 'NVIDIA_REQUIRE_CUDA': 'cuda>=9.0', 'OMP_NUM_THREADS': '2', 'HOSTNAME': 'ip-10-0-133-43.eu-central-1.compute.internal', 'AWS_CONTAINER_CREDENTIALS_RELATIVE_URI': '/v2/credentials/d6ef282a-7c64-41e9-9d5a-34e911f2beb7', 'DMLC_ROLE': 'worker', 'PWD': '/', 'DMLC_NUM_SERVER': '1', 'TRAINING_JOB_ARN': 'arn:aws:sagemaker:eu-central-1:730357687813:training-job/pca-2020-05-23-05-40-13-839', 'AWS_EXECUTION_ENV': 'AWS_ECS_EC2'}\u001b[0m\n\u001b[34mProcess 60 is a shell:scheduler.\u001b[0m\n\u001b[34mProcess 69 is a shell:server.\u001b[0m\n\u001b[34mProcess 1 is a worker.\u001b[0m\n\u001b[34m[05/23/2020 05:43:20 INFO 140677512759104] Using default worker.\u001b[0m\n\u001b[34m[05/23/2020 05:43:20 INFO 140677512759104] Loaded iterator creator application/x-recordio-protobuf for content type ('application/x-recordio-protobuf', '1.0')\u001b[0m\n\u001b[34m[05/23/2020 05:43:20 INFO 140677512759104] Loaded iterator creator application/x-labeled-vector-protobuf for content type ('application/x-labeled-vector-protobuf', '1.0')\u001b[0m\n\u001b[34m[05/23/2020 05:43:20 INFO 140677512759104] Loaded iterator creator protobuf for content type ('protobuf', '1.0')\u001b[0m\n\u001b[34m[05/23/2020 05:43:20 INFO 140677512759104] Create Store: dist_sync\u001b[0m\n\u001b[34m[05/23/2020 05:43:21 INFO 140677512759104] nvidia-smi took: 0.0252349376678 secs to identify 0 gpus\u001b[0m\n\u001b[34m[05/23/2020 05:43:21 INFO 140677512759104] Number of GPUs being used: 0\u001b[0m\n\u001b[34m[05/23/2020 05:43:21 INFO 140677512759104] The default executor is <PCAExecutor on cpu(0)>.\u001b[0m\n\u001b[34m[05/23/2020 05:43:21 INFO 140677512759104] 34 feature(s) found in 'data'.\u001b[0m\n\u001b[34m[05/23/2020 05:43:21 INFO 140677512759104] <PCAExecutor on cpu(0)> is assigned to batch slice from 0 to 499.\u001b[0m\n\u001b[34m#metrics {\"Metrics\": {\"initialize.time\": {\"count\": 1, \"max\": 742.8948879241943, \"sum\": 742.8948879241943, \"min\": 742.8948879241943}}, \"EndTime\": 1590212601.11761, \"Dimensions\": {\"Host\": \"algo-1\", \"Operation\": \"training\", \"Algorithm\": \"PCA\"}, \"StartTime\": 1590212600.365208}\n\u001b[0m\n\u001b[34m#metrics {\"Metrics\": {\"Max Batches Seen Between Resets\": {\"count\": 1, \"max\": 0, \"sum\": 0.0, \"min\": 0}, \"Number of Batches Since Last Reset\": {\"count\": 1, \"max\": 0, \"sum\": 0.0, \"min\": 0}, \"Number of Records Since Last Reset\": {\"count\": 1, \"max\": 0, \"sum\": 0.0, \"min\": 0}, \"Total Batches Seen\": {\"count\": 1, \"max\": 0, \"sum\": 0.0, \"min\": 0}, \"Total Records Seen\": {\"count\": 1, \"max\": 0, \"sum\": 0.0, \"min\": 0}, \"Max Records Seen Between Resets\": {\"count\": 1, \"max\": 0, \"sum\": 0.0, \"min\": 0}, \"Reset Count\": {\"count\": 1, \"max\": 0, \"sum\": 0.0, \"min\": 0}}, \"EndTime\": 1590212601.117854, \"Dimensions\": {\"Host\": \"algo-1\", \"Meta\": \"init_train_data_iter\", \"Operation\": \"training\", \"Algorithm\": \"PCA\"}, \"StartTime\": 1590212601.117795}\n\u001b[0m\n\u001b[34m[2020-05-23 05:43:21.118] [tensorio] [info] epoch_stats={\"data_pipeline\": \"/opt/ml/input/data/train\", \"epoch\": 0, \"duration\": 752, \"num_examples\": 1, \"num_bytes\": 82000}\u001b[0m\n\u001b[34m[2020-05-23 05:43:21.159] [tensorio] [info] epoch_stats={\"data_pipeline\": \"/opt/ml/input/data/train\", \"epoch\": 1, \"duration\": 33, \"num_examples\": 7, \"num_bytes\": 527752}\u001b[0m\n\u001b[34m#metrics {\"Metrics\": {\"epochs\": {\"count\": 1, \"max\": 1, \"sum\": 1.0, \"min\": 1}, \"update.time\": {\"count\": 1, \"max\": 41.612863540649414, \"sum\": 41.612863540649414, \"min\": 41.612863540649414}}, \"EndTime\": 1590212601.159863, \"Dimensions\": {\"Host\": \"algo-1\", \"Operation\": \"training\", \"Algorithm\": \"PCA\"}, \"StartTime\": 1590212601.117719}\n\u001b[0m\n\u001b[34m[05/23/2020 05:43:21 INFO 140677512759104] #progress_metric: host=algo-1, completed 100 % of epochs\u001b[0m\n\u001b[34m#metrics {\"Metrics\": {\"Max Batches Seen Between Resets\": {\"count\": 1, \"max\": 7, \"sum\": 7.0, \"min\": 7}, \"Number of Batches Since Last Reset\": {\"count\": 1, \"max\": 7, \"sum\": 7.0, \"min\": 7}, \"Number of Records Since Last Reset\": {\"count\": 1, \"max\": 3218, \"sum\": 3218.0, \"min\": 3218}, \"Total Batches Seen\": {\"count\": 1, \"max\": 7, \"sum\": 7.0, \"min\": 7}, \"Total Records Seen\": {\"count\": 1, \"max\": 3218, \"sum\": 3218.0, \"min\": 3218}, \"Max Records Seen Between Resets\": {\"count\": 1, \"max\": 3218, \"sum\": 3218.0, \"min\": 3218}, \"Reset Count\": {\"count\": 1, \"max\": 1, \"sum\": 1.0, \"min\": 1}}, \"EndTime\": 1590212601.16041, \"Dimensions\": {\"Host\": \"algo-1\", \"Meta\": \"training_data_iter\", \"Operation\": \"training\", \"Algorithm\": \"PCA\", \"epoch\": 0}, \"StartTime\": 1590212601.118201}\n\u001b[0m\n\u001b[34m[05/23/2020 05:43:21 INFO 140677512759104] #throughput_metric: host=algo-1, train throughput=75987.4469923 records/second\u001b[0m\n\u001b[34m#metrics {\"Metrics\": {\"finalize.time\": {\"count\": 1, \"max\": 24.407148361206055, \"sum\": 24.407148361206055, \"min\": 24.407148361206055}}, \"EndTime\": 1590212601.185214, \"Dimensions\": {\"Host\": \"algo-1\", \"Operation\": \"training\", \"Algorithm\": \"PCA\"}, \"StartTime\": 1590212601.160081}\n\u001b[0m\n\u001b[34m[05/23/2020 05:43:21 INFO 140677512759104] Test data is not provided.\u001b[0m\n\u001b[34m#metrics {\"Metrics\": {\"totaltime\": {\"count\": 1, \"max\": 2453.641891479492, \"sum\": 2453.641891479492, \"min\": 2453.641891479492}, \"setuptime\": {\"count\": 1, \"max\": 1556.6868782043457, \"sum\": 1556.6868782043457, \"min\": 1556.6868782043457}}, \"EndTime\": 1590212601.188449, \"Dimensions\": {\"Host\": \"algo-1\", \"Operation\": \"training\", \"Algorithm\": \"PCA\"}, \"StartTime\": 1590212601.185625}\n\u001b[0m\n"
]
],
[
[
"## Accessing the PCA Model Attributes\n\nAfter the model is trained, we can access the underlying model parameters.\n\n### Unzip the Model Details\n\nNow that the training job is complete, you can find the job under **Jobs** in the **Training** subsection in the Amazon SageMaker console. You can find the job name listed in the training jobs. Use that job name in the following code to specify which model to examine.\n\nModel artifacts are stored in S3 as a TAR file; a compressed file in the output path we specified + 'output/model.tar.gz'. The artifacts stored here can be used to deploy a trained model.",
"_____no_output_____"
]
],
[
[
"# Get the name of the training job, it's suggested that you copy-paste\n# from the notebook or from a specific job in the AWS console\n\ntraining_job_name='pca-2020-05-22-09-14-18-586'\n\n# where the model is saved, by default\nmodel_key = os.path.join(prefix, training_job_name, 'output/model.tar.gz')\nprint(model_key)\n\n# download and unzip model\nboto3.resource('s3').Bucket(bucket_name).download_file(model_key, 'model.tar.gz')\n\n# unzipping as model_algo-1\nos.system('tar -zxvf model.tar.gz')\nos.system('unzip model_algo-1')",
"counties/pca-2020-05-22-09-14-18-586/output/model.tar.gz\n"
]
],
[
[
"### MXNet Array\n\nMany of the Amazon SageMaker algorithms use MXNet for computational speed, including PCA, and so the model artifacts are stored as an array. After the model is unzipped and decompressed, we can load the array using MXNet.\n\nYou can take a look at the MXNet [documentation, here](https://aws.amazon.com/mxnet/).",
"_____no_output_____"
]
],
[
[
"import mxnet as mx\n\n# loading the unzipped artifacts\npca_model_params = mx.ndarray.load('model_algo-1')\n\n# what are the params\nprint(pca_model_params)",
"{'s': \n[1.7896362e-02 3.0864021e-02 3.2130770e-02 3.5486195e-02 9.4831578e-02\n 1.2699370e-01 4.0288666e-01 1.4084760e+00 1.5100485e+00 1.5957943e+00\n 1.7783760e+00 2.1662524e+00 2.2966361e+00 2.3856051e+00 2.6954880e+00\n 2.8067985e+00 3.0175958e+00 3.3952675e+00 3.5731301e+00 3.6966958e+00\n 4.1890211e+00 4.3457499e+00 4.5410376e+00 5.0189657e+00 5.5786467e+00\n 5.9809699e+00 6.3925138e+00 7.6952214e+00 7.9913125e+00 1.0180052e+01\n 1.1718245e+01 1.3035975e+01 1.9592180e+01]\n<NDArray 33 @cpu(0)>, 'v': \n[[ 2.46869749e-03 2.56468095e-02 2.50773830e-03 ... -7.63925165e-02\n 1.59879066e-02 5.04589686e-03]\n [-2.80601848e-02 -6.86634064e-01 -1.96283013e-02 ... -7.59587288e-02\n 1.57304872e-02 4.95312130e-03]\n [ 3.25766727e-02 7.17300594e-01 2.40726061e-02 ... -7.68136829e-02\n 1.62378680e-02 5.13597298e-03]\n ...\n [ 1.12151138e-01 -1.17030945e-02 -2.88011521e-01 ... 1.39890045e-01\n -3.09406728e-01 -6.34506866e-02]\n [ 2.99992133e-02 -3.13433539e-03 -7.63589665e-02 ... 4.17341813e-02\n -7.06735924e-02 -1.42857227e-02]\n [ 7.33537527e-05 3.01008171e-04 -8.00925500e-06 ... 6.97060227e-02\n 1.20169498e-01 2.33626723e-01]]\n<NDArray 34x33 @cpu(0)>, 'mean': \n[[0.00988273 0.00986636 0.00989863 0.11017046 0.7560245 0.10094159\n 0.0186819 0.02940491 0.0064698 0.01154038 0.31539047 0.1222766\n 0.3030056 0.08220861 0.256217 0.2964254 0.28914267 0.40191284\n 0.57868284 0.2854676 0.28294644 0.82774544 0.34378946 0.01576072\n 0.04649627 0.04115358 0.12442778 0.47014 0.00980645 0.7608103\n 0.19442631 0.21674445 0.0294168 0.22177474]]\n<NDArray 1x34 @cpu(0)>}\n"
]
],
[
[
"## PCA Model Attributes\n\nThree types of model attributes are contained within the PCA model.\n\n* **mean**: The mean that was subtracted from a component in order to center it.\n* **v**: The makeup of the principal components; (same as ‘components_’ in an sklearn PCA model).\n* **s**: The singular values of the components for the PCA transformation. This does not exactly give the % variance from the original feature space, but can give the % variance from the projected feature space.\n \nWe are only interested in v and s. \n\nFrom s, we can get an approximation of the data variance that is covered in the first `n` principal components. The approximate explained variance is given by the formula: the sum of squared s values for all top n components over the sum over squared s values for _all_ components:\n\n\\begin{equation*}\n\\frac{\\sum_{n}^{ } s_n^2}{\\sum s^2}\n\\end{equation*}\n\nFrom v, we can learn more about the combinations of original features that make up each principal component.\n",
"_____no_output_____"
]
],
[
[
"# get selected params\ns=pd.DataFrame(pca_model_params['s'].asnumpy())\nv=pd.DataFrame(pca_model_params['v'].asnumpy())",
"_____no_output_____"
]
],
[
[
"## Data Variance\n\nOur current PCA model creates 33 principal components, but when we create new dimensionality-reduced training data, we'll only select a few, top n components to use. To decide how many top components to include, it's helpful to look at how much **data variance** the components capture. For our original, high-dimensional data, 34 features captured 100% of our data variance. If we discard some of these higher dimensions, we will lower the amount of variance we can capture.\n\n### Tradeoff: dimensionality vs. data variance\n\nAs an illustrative example, say we have original data in three dimensions. So, three dimensions capture 100% of our data variance; these dimensions cover the entire spread of our data. The below images are taken from the PhD thesis, [“Approaches to analyse and interpret biological profile data”](https://publishup.uni-potsdam.de/opus4-ubp/frontdoor/index/index/docId/696) by Matthias Scholz, (2006, University of Potsdam, Germany).\n\n<img src='notebook_ims/3d_original_data.png' width=35% />\n\nNow, you may also note that most of this data seems related; it falls close to a 2D plane, and just by looking at the spread of the data, we can visualize that the original, three dimensions have some correlation. So, we can instead choose to create two new dimensions, made up of linear combinations of the original, three dimensions. These dimensions are represented by the two axes/lines, centered in the data. \n\n<img src='notebook_ims/pca_2d_dim_reduction.png' width=70% />\n\nIf we project this in a new, 2D space, we can see that we still capture most of the original data variance using *just* two dimensions. There is a tradeoff between the amount of variance we can capture and the number of component-dimensions we use to represent our data.\n\nWhen we select the top n components to use in a new data model, we'll typically want to include enough components to capture about 80-90% of the original data variance. In this project, we are looking at generalizing over a lot of data and we'll aim for about 80% coverage.",
"_____no_output_____"
],
[
"**Note**: The _top_ principal components, with the largest s values, are actually at the end of the s DataFrame. Let's print out the s values for the top n, principal components.",
"_____no_output_____"
]
],
[
[
"# looking at top 5 components\nn_principal_components = 5\n\nstart_idx = N_COMPONENTS - n_principal_components # 33-n\n\n# print a selection of s\nprint(s.iloc[start_idx:, :])",
" 0\n28 7.991313\n29 10.180052\n30 11.718245\n31 13.035975\n32 19.592180\n"
]
],
[
[
"### EXERCISE: Calculate the explained variance\n\nIn creating new training data, you'll want to choose the top n principal components that account for at least 80% data variance. \n\nComplete a function, `explained_variance` that takes in the entire array `s` and a number of top principal components to consider. Then return the approximate, explained variance for those top n components. \n\nFor example, to calculate the explained variance for the top 5 components, calculate s squared for *each* of the top 5 components, add those up and normalize by the sum of *all* squared s values, according to this formula:\n\n\\begin{equation*}\n\\frac{\\sum_{5}^{ } s_n^2}{\\sum s^2}\n\\end{equation*}\n\n> Using this function, you should be able to answer the **question**: What is the smallest number of principal components that captures at least 80% of the total variance in the dataset?",
"_____no_output_____"
]
],
[
[
"# Calculate the explained variance for the top n principal components\n# you may assume you have access to the global var N_COMPONENTS\ndef explained_variance(s, n_top_components):\n '''Calculates the approx. data variance that n_top_components captures.\n :param s: A dataframe of singular values for top components; \n the top value is in the last row.\n :param n_top_components: An integer, the number of top components to use.\n :return: The expected data variance covered by the n_top_components.'''\n \n num = (s.iloc[-n_top_components:, :].values ** 2).sum()\n denom = (s.values ** 2).sum()\n exp_var = num/denom\n \n return exp_var\n",
"_____no_output_____"
]
],
[
[
"### Test Cell\n\nTest out your own code by seeing how it responds to different inputs; does it return a reasonable value for the single, top component? What about for the top 5 components?",
"_____no_output_____"
]
],
[
[
"# test cell\nn_top_components = 7 # select a value for the number of top components\n\n# calculate the explained variance\nexp_variance = explained_variance(s, n_top_components)\nprint('Explained variance: ', exp_variance)",
"Explained variance: 0.80167246\n"
]
],
[
[
"As an example, you should see that the top principal component accounts for about 32% of our data variance! Next, you may be wondering what makes up this (and other components); what linear combination of features make these components so influential in describing the spread of our data?\n\nBelow, let's take a look at our original features and use that as a reference.",
"_____no_output_____"
]
],
[
[
"# features\nfeatures_list = counties_scaled.columns.values\nprint('Features: \\n', features_list)",
"Features: \n ['TotalPop' 'Men' 'Women' 'Hispanic' 'White' 'Black' 'Native' 'Asian'\n 'Pacific' 'Citizen' 'Income' 'IncomeErr' 'IncomePerCap' 'IncomePerCapErr'\n 'Poverty' 'ChildPoverty' 'Professional' 'Service' 'Office' 'Construction'\n 'Production' 'Drive' 'Carpool' 'Transit' 'Walk' 'OtherTransp'\n 'WorkAtHome' 'MeanCommute' 'Employed' 'PrivateWork' 'PublicWork'\n 'SelfEmployed' 'FamilyWork' 'Unemployment']\n"
]
],
[
[
"## Component Makeup\n\nWe can now examine the makeup of each PCA component based on **the weightings of the original features that are included in the component**. The following code shows the feature-level makeup of the first component.\n\nNote that the components are again ordered from smallest to largest and so I am getting the correct rows by calling N_COMPONENTS-1 to get the top, 1, component.",
"_____no_output_____"
]
],
[
[
"import seaborn as sns\n\ndef display_component(v, features_list, component_num, n_weights=10):\n \n # get index of component (last row - component_num)\n row_idx = N_COMPONENTS-component_num\n\n # get the list of weights from a row in v, dataframe\n v_1_row = v.iloc[:, row_idx]\n v_1 = np.squeeze(v_1_row.values)\n\n # match weights to features in counties_scaled dataframe, using list comporehension\n comps = pd.DataFrame(list(zip(v_1, features_list)), \n columns=['weights', 'features'])\n\n # we'll want to sort by the largest n_weights\n # weights can be neg/pos and we'll sort by magnitude\n comps['abs_weights']=comps['weights'].apply(lambda x: np.abs(x))\n sorted_weight_data = comps.sort_values('abs_weights', ascending=False).head(n_weights)\n\n # display using seaborn\n ax=plt.subplots(figsize=(10,6))\n ax=sns.barplot(data=sorted_weight_data,\n x=\"weights\", \n y=\"features\",\n palette=\"Blues_d\")\n ax.set_title(\"PCA Component Makeup, Component #\" + str(component_num))\n plt.show()\n",
"_____no_output_____"
],
[
"# display makeup of first component\nnum=1\ndisplay_component(v, counties_scaled.columns.values, component_num=num, n_weights=10)",
"_____no_output_____"
]
],
[
[
"# Deploying the PCA Model\n\nWe can now deploy this model and use it to make \"predictions\". Instead of seeing what happens with some test data, we'll actually want to pass our training data into the deployed endpoint to create principal components for each data point. \n\nRun the cell below to deploy/host this model on an instance_type that we specify.",
"_____no_output_____"
]
],
[
[
"%%time\n# this takes a little while, around 7mins\npca_predictor = pca_SM.deploy(initial_instance_count=1, \n instance_type='ml.t2.medium')",
"-----------------!CPU times: user 319 ms, sys: 14 ms, total: 333 ms\nWall time: 8min 32s\n"
]
],
[
[
"We can pass the original, numpy dataset to the model and transform the data using the model we created. Then we can take the largest n components to reduce the dimensionality of our data.",
"_____no_output_____"
]
],
[
[
"# pass np train data to the PCA model\ntrain_pca = pca_predictor.predict(train_data_np)",
"_____no_output_____"
],
[
"# check out the first item in the produced training features\ndata_idx = 0\nprint(train_pca[data_idx])",
"label {\n key: \"projection\"\n value {\n float32_tensor {\n values: 0.0002009272575378418\n values: 0.0002455431967973709\n values: -0.0005782842636108398\n values: -0.0007815659046173096\n values: -0.00041911262087523937\n values: -0.0005133943632245064\n values: -0.0011316537857055664\n values: 0.0017268601804971695\n values: -0.005361668765544891\n values: -0.009066537022590637\n values: -0.008141040802001953\n values: -0.004735097289085388\n values: -0.00716288760304451\n values: 0.0003725700080394745\n values: -0.01208949089050293\n values: 0.02134685218334198\n values: 0.0009293854236602783\n values: 0.002417147159576416\n values: -0.0034637749195098877\n values: 0.01794189214706421\n values: -0.01639425754547119\n values: 0.06260128319263458\n values: 0.06637358665466309\n values: 0.002479255199432373\n values: 0.10011336207389832\n values: -0.1136140376329422\n values: 0.02589476853609085\n values: 0.04045158624649048\n values: -0.01082391943782568\n values: 0.1204797774553299\n values: -0.0883558839559555\n values: 0.16052711009979248\n values: -0.06027412414550781\n }\n }\n}\n\n"
]
],
[
[
"### EXERCISE: Create a transformed DataFrame\n\nFor each of our data points, get the top n component values from the list of component data points, returned by our predictor above, and put those into a new DataFrame.\n\nYou should end up with a DataFrame that looks something like the following:\n```\n c_1\t c_2\t c_3\t c_4\t c_5\t ...\nAlabama-Autauga\t-0.060274\t0.160527\t-0.088356\t 0.120480\t-0.010824\t...\nAlabama-Baldwin\t-0.149684\t0.185969\t-0.145743\t-0.023092\t-0.068677\t...\nAlabama-Barbour\t0.506202\t 0.296662\t 0.146258\t 0.297829\t0.093111\t...\n...\n```",
"_____no_output_____"
]
],
[
[
"# create dimensionality-reduced data\ndef create_transformed_df(train_pca, counties_scaled, n_top_components):\n ''' Return a dataframe of data points with component features. \n The dataframe should be indexed by State-County and contain component values.\n :param train_pca: A list of pca training data, returned by a PCA model.\n :param counties_scaled: A dataframe of normalized, original features.\n :param n_top_components: An integer, the number of top components to use.\n :return: A dataframe, indexed by State-County, with n_top_component values as columns. \n '''\n # create new dataframe to add data to\n counties_transformed=pd.DataFrame()\n\n # for each of our new, transformed data points\n # append the component values to the dataframe\n for data in train_pca:\n # get component values for each data point\n components=data.label['projection'].float32_tensor.values\n counties_transformed=counties_transformed.append([list(components)])\n\n # index by county, just like counties_scaled\n counties_transformed.index=counties_scaled.index\n\n # keep only the top n components\n start_idx = N_COMPONENTS - n_top_components\n counties_transformed = counties_transformed.iloc[:,start_idx:]\n \n # reverse columns, component order \n return counties_transformed.iloc[:, ::-1]\n",
"_____no_output_____"
]
],
[
[
"Now we can create a dataset where each county is described by the top n principle components that we analyzed earlier. Each of these components is a linear combination of the original feature space. We can interpret each of these components by analyzing the makeup of the component, shown previously.\n\n### Define the `top_n` components to use in this transformed data\n\nYour code should return data, indexed by 'State-County' and with as many columns as `top_n` components.\n\nYou can also choose to add descriptive column names for this data; names that correspond to the component number or feature-level makeup.",
"_____no_output_____"
]
],
[
[
"## Specify top n\ntop_n = 7\n\n# call your function and create a new dataframe\ncounties_transformed = create_transformed_df(train_pca, counties_scaled, n_top_components=top_n)\n\n## TODO: Add descriptive column names\nPCA_list=['c_1', 'c_2', 'c_3', 'c_4', 'c_5', 'c_6', 'c_7']\ncounties_transformed.columns=PCA_list \n\n# print result\ncounties_transformed.head()",
"_____no_output_____"
]
],
[
[
"### Delete the Endpoint!\n\nNow that we've deployed the mode and created our new, transformed training data, we no longer need the PCA endpoint.\n\nAs a clean up step, you should always delete your endpoints after you are done using them (and if you do not plan to deploy them to a website, for example).",
"_____no_output_____"
]
],
[
[
"# delete predictor endpoint\nsession.delete_endpoint(pca_predictor.endpoint)",
"_____no_output_____"
]
],
[
[
"---\n# Population Segmentation \n\nNow, you’ll use the unsupervised clustering algorithm, k-means, to segment counties using their PCA attributes, which are in the transformed DataFrame we just created. K-means is a clustering algorithm that identifies clusters of similar data points based on their component makeup. Since we have ~3000 counties and 34 attributes in the original dataset, the large feature space may have made it difficult to cluster the counties effectively. Instead, we have reduced the feature space to 7 PCA components, and we’ll cluster on this transformed dataset.",
"_____no_output_____"
],
[
"### EXERCISE: Define a k-means model\n\nYour task will be to instantiate a k-means model. A `KMeans` estimator requires a number of parameters to be instantiated, which allow us to specify the type of training instance to use, and the model hyperparameters. \n\nYou can read about the required parameters, in the [`KMeans` documentation](https://sagemaker.readthedocs.io/en/stable/kmeans.html); note that not all of the possible parameters are required.\n",
"_____no_output_____"
],
[
"### Choosing a \"Good\" K\n\nOne method for choosing a \"good\" k, is to choose based on empirical data. A bad k would be one so *high* that only one or two very close data points are near it, and another bad k would be one so *low* that data points are really far away from the centers.\n\nYou want to select a k such that data points in a single cluster are close together but that there are enough clusters to effectively separate the data. You can approximate this separation by measuring how close your data points are to each cluster center; the average centroid distance between cluster points and a centroid. After trying several values for k, the centroid distance typically reaches some \"elbow\"; it stops decreasing at a sharp rate and this indicates a good value of k. The graph below indicates the average centroid distance for value of k between 5 and 12.\n\n<img src='notebook_ims/elbow_graph.png' width=50% />\n\nA distance elbow can be seen around 8 when the distance starts to increase and then decrease at a slower rate. This indicates that there is enough separation to distinguish the data points in each cluster, but also that you included enough clusters so that the data points aren’t *extremely* far away from each cluster.",
"_____no_output_____"
]
],
[
[
"# define a KMeans estimator\nkmeans = sagemaker.KMeans(role = role,\n train_instance_count = 1,\n train_instance_type='ml.c4.xlarge',\n output_path = output_path,\n k = 8)\n \nprint('Training artifacts will be uploaded to: {}'.format(output_path))",
"Training artifacts will be uploaded to: s3://sagemaker-eu-central-1-730357687813/counties/\n"
]
],
[
[
"### EXERCISE: Create formatted, k-means training data\n\nJust as before, you should convert the `counties_transformed` df into a numpy array and then into a RecordSet. This is the required format for passing training data into a `KMeans` model.",
"_____no_output_____"
]
],
[
[
"# convert the transformed dataframe into record_set data\nkmeans_train_data_np = counties_transformed.values.astype('float32')\nkmeans_formatted_train_data = kmeans.record_set(kmeans_train_data_np)",
"_____no_output_____"
]
],
[
[
"### EXERCISE: Train the k-means model\n\nPass in the formatted training data and train the k-means model.",
"_____no_output_____"
]
],
[
[
"%%time\nkmeans.fit(kmeans_formatted_train_data)",
"2020-05-23 06:55:58 Starting - Starting the training job...\n2020-05-23 06:56:00 Starting - Launching requested ML instances......\n2020-05-23 06:57:03 Starting - Preparing the instances for training......\n2020-05-23 06:58:26 Downloading - Downloading input data\n2020-05-23 06:58:26 Training - Downloading the training image...\n2020-05-23 06:58:58 Uploading - Uploading generated training model\n2020-05-23 06:58:58 Completed - Training job completed\n\u001b[34mDocker entrypoint called with argument(s): train\u001b[0m\n\u001b[34mRunning default environment configuration script\u001b[0m\n\u001b[34m[05/23/2020 06:58:48 INFO 140047905527616] Reading default configuration from /opt/amazon/lib/python2.7/site-packages/algorithm/resources/default-input.json: {u'_enable_profiler': u'false', u'_tuning_objective_metric': u'', u'_num_gpus': u'auto', u'local_lloyd_num_trials': u'auto', u'_log_level': u'info', u'_kvstore': u'auto', u'local_lloyd_init_method': u'kmeans++', u'force_dense': u'true', u'epochs': u'1', u'init_method': u'random', u'local_lloyd_tol': u'0.0001', u'local_lloyd_max_iter': u'300', u'_disable_wait_to_read': u'false', u'extra_center_factor': u'auto', u'eval_metrics': u'[\"msd\"]', u'_num_kv_servers': u'1', u'mini_batch_size': u'5000', u'half_life_time_size': u'0', u'_num_slices': u'1'}\u001b[0m\n\u001b[34m[05/23/2020 06:58:48 INFO 140047905527616] Reading provided configuration from /opt/ml/input/config/hyperparameters.json: {u'feature_dim': u'7', u'k': u'8', u'force_dense': u'True'}\u001b[0m\n\u001b[34m[05/23/2020 06:58:48 INFO 140047905527616] Final configuration: {u'_tuning_objective_metric': u'', u'extra_center_factor': u'auto', u'local_lloyd_init_method': u'kmeans++', u'force_dense': u'True', u'epochs': u'1', u'feature_dim': u'7', u'local_lloyd_tol': u'0.0001', u'_disable_wait_to_read': u'false', u'eval_metrics': u'[\"msd\"]', u'_num_kv_servers': u'1', u'mini_batch_size': u'5000', u'_enable_profiler': u'false', u'_num_gpus': u'auto', u'local_lloyd_num_trials': u'auto', u'_log_level': u'info', u'init_method': u'random', u'half_life_time_size': u'0', u'local_lloyd_max_iter': u'300', u'_kvstore': u'auto', u'k': u'8', u'_num_slices': u'1'}\u001b[0m\n\u001b[34m[05/23/2020 06:58:48 WARNING 140047905527616] Loggers have already been setup.\u001b[0m\n\u001b[34mProcess 1 is a worker.\u001b[0m\n\u001b[34m[05/23/2020 06:58:48 INFO 140047905527616] Using default worker.\u001b[0m\n\u001b[34m[05/23/2020 06:58:48 INFO 140047905527616] Loaded iterator creator application/x-recordio-protobuf for content type ('application/x-recordio-protobuf', '1.0')\u001b[0m\n\u001b[34m[05/23/2020 06:58:48 INFO 140047905527616] Create Store: local\u001b[0m\n\u001b[34m[05/23/2020 06:58:48 INFO 140047905527616] nvidia-smi took: 0.0252118110657 secs to identify 0 gpus\u001b[0m\n\u001b[34m[05/23/2020 06:58:48 INFO 140047905527616] Number of GPUs being used: 0\u001b[0m\n\u001b[34m[05/23/2020 06:58:48 INFO 140047905527616] Setting up with params: {u'_tuning_objective_metric': u'', u'extra_center_factor': u'auto', u'local_lloyd_init_method': u'kmeans++', u'force_dense': u'True', u'epochs': u'1', u'feature_dim': u'7', u'local_lloyd_tol': u'0.0001', u'_disable_wait_to_read': u'false', u'eval_metrics': u'[\"msd\"]', u'_num_kv_servers': u'1', u'mini_batch_size': u'5000', u'_enable_profiler': u'false', u'_num_gpus': u'auto', u'local_lloyd_num_trials': u'auto', u'_log_level': u'info', u'init_method': u'random', u'half_life_time_size': u'0', u'local_lloyd_max_iter': u'300', u'_kvstore': u'auto', u'k': u'8', u'_num_slices': u'1'}\u001b[0m\n\u001b[34m[05/23/2020 06:58:48 INFO 140047905527616] 'extra_center_factor' was set to 'auto', evaluated to 10.\u001b[0m\n\u001b[34m[05/23/2020 06:58:48 INFO 140047905527616] Number of GPUs being used: 0\u001b[0m\n\u001b[34m[05/23/2020 06:58:48 INFO 140047905527616] number of center slices 1\u001b[0m\n\u001b[34m[05/23/2020 06:58:48 WARNING 140047905527616] Batch size 5000 is bigger than the first batch data. Effective batch size used to initialize is 3218\u001b[0m\n\u001b[34m#metrics {\"Metrics\": {\"Max Batches Seen Between Resets\": {\"count\": 1, \"max\": 1, \"sum\": 1.0, \"min\": 1}, \"Number of Batches Since Last Reset\": {\"count\": 1, \"max\": 1, \"sum\": 1.0, \"min\": 1}, \"Number of Records Since Last Reset\": {\"count\": 1, \"max\": 3218, \"sum\": 3218.0, \"min\": 3218}, \"Total Batches Seen\": {\"count\": 1, \"max\": 1, \"sum\": 1.0, \"min\": 1}, \"Total Records Seen\": {\"count\": 1, \"max\": 3218, \"sum\": 3218.0, \"min\": 3218}, \"Max Records Seen Between Resets\": {\"count\": 1, \"max\": 3218, \"sum\": 3218.0, \"min\": 3218}, \"Reset Count\": {\"count\": 1, \"max\": 0, \"sum\": 0.0, \"min\": 0}}, \"EndTime\": 1590217128.442506, \"Dimensions\": {\"Host\": \"algo-1\", \"Meta\": \"init_train_data_iter\", \"Operation\": \"training\", \"Algorithm\": \"AWS/KMeansWebscale\"}, \"StartTime\": 1590217128.442472}\n\u001b[0m\n\u001b[34m[2020-05-23 06:58:48.442] [tensorio] [info] epoch_stats={\"data_pipeline\": \"/opt/ml/input/data/train\", \"epoch\": 0, \"duration\": 33, \"num_examples\": 1, \"num_bytes\": 167336}\u001b[0m\n\u001b[34m[2020-05-23 06:58:48.489] [tensorio] [info] epoch_stats={\"data_pipeline\": \"/opt/ml/input/data/train\", \"epoch\": 1, \"duration\": 46, \"num_examples\": 1, \"num_bytes\": 167336}\u001b[0m\n\u001b[34m[05/23/2020 06:58:48 INFO 140047905527616] processed a total of 3218 examples\u001b[0m\n\u001b[34m[05/23/2020 06:58:48 INFO 140047905527616] #progress_metric: host=algo-1, completed 100 % of epochs\u001b[0m\n\u001b[34m#metrics {\"Metrics\": {\"Max Batches Seen Between Resets\": {\"count\": 1, \"max\": 1, \"sum\": 1.0, \"min\": 1}, \"Number of Batches Since Last Reset\": {\"count\": 1, \"max\": 1, \"sum\": 1.0, \"min\": 1}, \"Number of Records Since Last Reset\": {\"count\": 1, \"max\": 3218, \"sum\": 3218.0, \"min\": 3218}, \"Total Batches Seen\": {\"count\": 1, \"max\": 2, \"sum\": 2.0, \"min\": 2}, \"Total Records Seen\": {\"count\": 1, \"max\": 6436, \"sum\": 6436.0, \"min\": 6436}, \"Max Records Seen Between Resets\": {\"count\": 1, \"max\": 3218, \"sum\": 3218.0, \"min\": 3218}, \"Reset Count\": {\"count\": 1, \"max\": 1, \"sum\": 1.0, \"min\": 1}}, \"EndTime\": 1590217128.490535, \"Dimensions\": {\"Host\": \"algo-1\", \"Meta\": \"training_data_iter\", \"Operation\": \"training\", \"Algorithm\": \"AWS/KMeansWebscale\", \"epoch\": 0}, \"StartTime\": 1590217128.442763}\n\u001b[0m\n\u001b[34m[05/23/2020 06:58:48 INFO 140047905527616] #throughput_metric: host=algo-1, train throughput=67151.9347251 records/second\u001b[0m\n\u001b[34m[05/23/2020 06:58:48 WARNING 140047905527616] wait_for_all_workers will not sync workers since the kv store is not running distributed\u001b[0m\n\u001b[34m[05/23/2020 06:58:48 INFO 140047905527616] shrinking 80 centers into 8\u001b[0m\n\u001b[34m[05/23/2020 06:58:48 INFO 140047905527616] local kmeans attempt #0. Current mean square distance 0.062246\u001b[0m\n\u001b[34m[05/23/2020 06:58:48 INFO 140047905527616] local kmeans attempt #1. Current mean square distance 0.063014\u001b[0m\n\u001b[34m[05/23/2020 06:58:48 INFO 140047905527616] local kmeans attempt #2. Current mean square distance 0.059803\u001b[0m\n\u001b[34m[05/23/2020 06:58:48 INFO 140047905527616] local kmeans attempt #3. Current mean square distance 0.063063\u001b[0m\n\u001b[34m[05/23/2020 06:58:48 INFO 140047905527616] local kmeans attempt #4. Current mean square distance 0.064876\u001b[0m\n\u001b[34m[05/23/2020 06:58:48 INFO 140047905527616] local kmeans attempt #5. Current mean square distance 0.063535\u001b[0m\n\u001b[34m[05/23/2020 06:58:48 INFO 140047905527616] local kmeans attempt #6. Current mean square distance 0.063639\u001b[0m\n\u001b[34m[05/23/2020 06:58:48 INFO 140047905527616] local kmeans attempt #7. Current mean square distance 0.064357\u001b[0m\n\u001b[34m[05/23/2020 06:58:48 INFO 140047905527616] local kmeans attempt #8. Current mean square distance 0.061033\u001b[0m\n\u001b[34m[05/23/2020 06:58:48 INFO 140047905527616] local kmeans attempt #9. Current mean square distance 0.060658\u001b[0m\n\u001b[34m[05/23/2020 06:58:48 INFO 140047905527616] finished shrinking process. Mean Square Distance = 0\u001b[0m\n\u001b[34m[05/23/2020 06:58:48 INFO 140047905527616] #quality_metric: host=algo-1, train msd <loss>=0.0598029382527\u001b[0m\n\u001b[34m[05/23/2020 06:58:48 INFO 140047905527616] compute all data-center distances: inner product took: 30.7809%, (0.017753 secs)\u001b[0m\n\u001b[34m[05/23/2020 06:58:48 INFO 140047905527616] collect from kv store took: 18.8244%, (0.010857 secs)\u001b[0m\n\u001b[34m[05/23/2020 06:58:48 INFO 140047905527616] splitting centers key-value pair took: 18.6784%, (0.010773 secs)\u001b[0m\n\u001b[34m[05/23/2020 06:58:48 INFO 140047905527616] batch data loading with context took: 7.2903%, (0.004205 secs)\u001b[0m\n\u001b[34m[05/23/2020 06:58:48 INFO 140047905527616] compute all data-center distances: point norm took: 7.0377%, (0.004059 secs)\u001b[0m\n\u001b[34m[05/23/2020 06:58:48 INFO 140047905527616] predict compute msd took: 6.0613%, (0.003496 secs)\u001b[0m\n\u001b[34m[05/23/2020 06:58:48 INFO 140047905527616] gradient: one_hot took: 5.6905%, (0.003282 secs)\u001b[0m\n\u001b[34m[05/23/2020 06:58:48 INFO 140047905527616] gradient: cluster size took: 2.3579%, (0.001360 secs)\u001b[0m\n\u001b[34m[05/23/2020 06:58:48 INFO 140047905527616] gradient: cluster center took: 1.6853%, (0.000972 secs)\u001b[0m\n\u001b[34m[05/23/2020 06:58:48 INFO 140047905527616] update state and report convergance took: 0.8408%, (0.000485 secs)\u001b[0m\n\u001b[34m[05/23/2020 06:58:48 INFO 140047905527616] update set-up time took: 0.3795%, (0.000219 secs)\u001b[0m\n\u001b[34m[05/23/2020 06:58:48 INFO 140047905527616] compute all data-center distances: center norm took: 0.3278%, (0.000189 secs)\u001b[0m\n\u001b[34m[05/23/2020 06:58:48 INFO 140047905527616] predict minus dist took: 0.0451%, (0.000026 secs)\u001b[0m\n\u001b[34m[05/23/2020 06:58:48 INFO 140047905527616] TOTAL took: 0.0576758384705\u001b[0m\n\u001b[34m[05/23/2020 06:58:48 INFO 140047905527616] Number of GPUs being used: 0\u001b[0m\n\u001b[34m#metrics {\"Metrics\": {\"finalize.time\": {\"count\": 1, \"max\": 331.773042678833, \"sum\": 331.773042678833, \"min\": 331.773042678833}, \"initialize.time\": {\"count\": 1, \"max\": 28.280019760131836, \"sum\": 28.280019760131836, \"min\": 28.280019760131836}, \"model.serialize.time\": {\"count\": 1, \"max\": 0.14591217041015625, \"sum\": 0.14591217041015625, \"min\": 0.14591217041015625}, \"update.time\": {\"count\": 1, \"max\": 47.55997657775879, \"sum\": 47.55997657775879, \"min\": 47.55997657775879}, \"epochs\": {\"count\": 1, \"max\": 1, \"sum\": 1.0, \"min\": 1}, \"state.serialize.time\": {\"count\": 1, \"max\": 1.5878677368164062, \"sum\": 1.5878677368164062, \"min\": 1.5878677368164062}, \"_shrink.time\": {\"count\": 1, \"max\": 329.76484298706055, \"sum\": 329.76484298706055, \"min\": 329.76484298706055}}, \"EndTime\": 1590217128.824555, \"Dimensions\": {\"Host\": \"algo-1\", \"Operation\": \"training\", \"Algorithm\": \"AWS/KMeansWebscale\"}, \"StartTime\": 1590217128.408428}\n\u001b[0m\n\u001b[34m[05/23/2020 06:58:48 INFO 140047905527616] Test data is not provided.\u001b[0m\n\u001b[34m#metrics {\"Metrics\": {\"totaltime\": {\"count\": 1, \"max\": 479.54416275024414, \"sum\": 479.54416275024414, \"min\": 479.54416275024414}, \"setuptime\": {\"count\": 1, \"max\": 13.439178466796875, \"sum\": 13.439178466796875, \"min\": 13.439178466796875}}, \"EndTime\": 1590217128.824923, \"Dimensions\": {\"Host\": \"algo-1\", \"Operation\": \"training\", \"Algorithm\": \"AWS/KMeansWebscale\"}, \"StartTime\": 1590217128.824649}\n\u001b[0m\n"
]
],
[
[
"### EXERCISE: Deploy the k-means model\n\nDeploy the trained model to create a `kmeans_predictor`.\n",
"_____no_output_____"
]
],
[
[
"%%time\n# deploy the model to create a predictor\nkmeans_predictor = kmeans.deploy(initial_instance_count=1,\n instance_type='ml.t2.medium')",
"-----------------!CPU times: user 316 ms, sys: 14 ms, total: 330 ms\nWall time: 8min 32s\n"
]
],
[
[
"### EXERCISE: Pass in the training data and assign predicted cluster labels\n\nAfter deploying the model, you can pass in the k-means training data, as a numpy array, and get resultant, predicted cluster labels for each data point.",
"_____no_output_____"
]
],
[
[
"# get the predicted clusters for all the kmeans training data\ncluster_info= kmeans_predictor.predict(train_data_kmeans_np)",
"_____no_output_____"
]
],
[
[
"## Exploring the resultant clusters\n\nThe resulting predictions should give you information about the cluster that each data point belongs to.\n\nYou should be able to answer the **question**: which cluster does a given data point belong to?",
"_____no_output_____"
]
],
[
[
"# print cluster info for first data point\ndata_idx = 3\n\nprint('County is: ', counties_transformed.index[data_idx])\nprint()\nprint(cluster_info[data_idx])",
"County is: Alabama-Bibb\n\nlabel {\n key: \"closest_cluster\"\n value {\n float32_tensor {\n values: 3.0\n }\n }\n}\nlabel {\n key: \"distance_to_cluster\"\n value {\n float32_tensor {\n values: 0.3843974173069\n }\n }\n}\n\n"
]
],
[
[
"### Visualize the distribution of data over clusters\n\nGet the cluster labels for each of our data points (counties) and visualize the distribution of points over each cluster.",
"_____no_output_____"
]
],
[
[
"# get all cluster labels\ncluster_labels = [c.label['closest_cluster'].float32_tensor.values[0] for c in cluster_info]",
"_____no_output_____"
],
[
"# count up the points in each cluster\ncluster_df = pd.DataFrame(cluster_labels)[0].value_counts()\n\nprint(cluster_df)",
"3.0 907\n6.0 842\n0.0 386\n7.0 375\n1.0 368\n5.0 167\n2.0 87\n4.0 86\nName: 0, dtype: int64\n"
]
],
[
[
"Now, you may be wondering, what do each of these clusters tell us about these data points? To improve explainability, we need to access the underlying model to get the cluster centers. These centers will help describe which features characterize each cluster.",
"_____no_output_____"
],
[
"### Delete the Endpoint!\n\nNow that you've deployed the k-means model and extracted the cluster labels for each data point, you no longer need the k-means endpoint.",
"_____no_output_____"
]
],
[
[
"# delete kmeans endpoint\nsession.delete_endpoint(kmeans_predictor.endpoint)",
"_____no_output_____"
]
],
[
[
"---\n# Model Attributes & Explainability\n\nExplaining the result of the modeling is an important step in making use of our analysis. By combining PCA and k-means, and the information contained in the model attributes within a SageMaker trained model, you can learn about a population and remark on some patterns you've found, based on the data.",
"_____no_output_____"
],
[
"### EXERCISE: Access the k-means model attributes\n\nExtract the k-means model attributes from where they are saved as a TAR file in an S3 bucket.\n\nYou'll need to access the model by the k-means training job name, and then unzip the file into `model_algo-1`. Then you can load that file using MXNet, as before.",
"_____no_output_____"
]
],
[
[
"# download and unzip the kmeans model file\n# use the name model_algo-1\n\n# download and unzip the kmeans model file\nkmeans_job_name = 'kmeans-2020-05-23-06-55-58-261'\n\nmodel_key = os.path.join(prefix, kmeans_job_name, 'output/model.tar.gz')\n\n# download the model file\nboto3.resource('s3').Bucket(bucket_name).download_file(model_key, 'model.tar.gz')\nos.system('tar -zxvf model.tar.gz')\nos.system('unzip model_algo-1')\n",
"_____no_output_____"
],
[
"# get the trained kmeans params using mxnet\nkmeans_model_params = mx.ndarray.load('model_algo-1')\n\nprint(kmeans_model_params)",
"[\n[[ 0.35492653 0.23771921 0.07889839 0.2500726 0.09919675 -0.05618306\n 0.04399072]\n [-0.23379213 -0.3808242 0.07702101 0.08526881 0.0603863 -0.00519104\n 0.0597847 ]\n [ 1.3077838 -0.2294502 -0.17610097 -0.42974427 -0.11858643 0.11248738\n 0.15853602]\n [-0.02278126 0.07436099 0.12951738 -0.05602401 -0.04330579 0.05682565\n -0.03048567]\n [ 0.5819005 -0.45450625 -0.03150757 0.04155013 -0.09733208 -0.02300905\n -0.13401571]\n [ 0.25074974 -0.1768499 -0.10482205 -0.22392033 0.23187745 -0.19118813\n -0.10258509]\n [-0.24812227 0.04720467 -0.02500745 -0.06317183 -0.03199761 -0.04560736\n 0.00395537]\n [-0.04086831 0.03606306 -0.3563783 0.10303619 -0.01080673 0.07729725\n -0.01095549]]\n<NDArray 8x7 @cpu(0)>]\n"
]
],
[
[
"There is only 1 set of model parameters contained within the k-means model: the cluster centroid locations in PCA-transformed, component space.\n\n* **centroids**: The location of the centers of each cluster in component space, identified by the k-means algorithm. \n",
"_____no_output_____"
]
],
[
[
"# get all the centroids\ncluster_centroids=pd.DataFrame(kmeans_model_params[0].asnumpy())\ncluster_centroids.columns=counties_transformed.columns\n\ndisplay(cluster_centroids)",
"_____no_output_____"
]
],
[
[
"### Visualizing Centroids in Component Space\n\nYou can't visualize 7-dimensional centroids in space, but you can plot a heatmap of the centroids and their location in the transformed feature space. \n\nThis gives you insight into what characteristics define each cluster. Often with unsupervised learning, results are hard to interpret. This is one way to make use of the results of PCA + clustering techniques, together. Since you were able to examine the makeup of each PCA component, you can understand what each centroid represents in terms of the PCA components.",
"_____no_output_____"
]
],
[
[
"# generate a heatmap in component space, using the seaborn library\nplt.figure(figsize = (12,9))\nax = sns.heatmap(cluster_centroids.T, cmap = 'YlGnBu')\nax.set_xlabel(\"Cluster\")\nplt.yticks(fontsize = 16)\nplt.xticks(fontsize = 16)\nax.set_title(\"Attribute Value by Centroid\")\nplt.show()",
"_____no_output_____"
]
],
[
[
"If you've forgotten what each component corresponds to at an original-feature-level, that's okay! You can use the previously defined `display_component` function to see the feature-level makeup.",
"_____no_output_____"
]
],
[
[
"# what do each of these components mean again?\n# let's use the display function, from above\ncomponent_num=5\ndisplay_component(v, counties_scaled.columns.values, component_num=component_num)",
"_____no_output_____"
]
],
[
[
"### Natural Groupings\n\nYou can also map the cluster labels back to each individual county and examine which counties are naturally grouped together.",
"_____no_output_____"
]
],
[
[
"# add a 'labels' column to the dataframe\ncounties_transformed['labels']=list(map(int, cluster_labels))\n\n# sort by cluster label 0-6\nsorted_counties = counties_transformed.sort_values('labels', ascending=True)\n# view some pts in cluster 0\nsorted_counties.head(20)",
"_____no_output_____"
]
],
[
[
"You can also examine one of the clusters in more detail, like cluster 1, for example. A quick glance at the location of the centroid in component space (the heatmap) tells us that it has the highest value for the `comp_6` attribute. You can now see which counties fit that description.",
"_____no_output_____"
]
],
[
[
"# get all counties with label == 1\ncluster=counties_transformed[counties_transformed['labels']==1]\ncluster.head()",
"_____no_output_____"
]
],
[
[
"## Final Cleanup!\n\n* Double check that you have deleted all your endpoints.\n* I'd also suggest manually deleting your S3 bucket, models, and endpoint configurations directly from your AWS console.\n\nYou can find thorough cleanup instructions, [in the documentation](https://docs.aws.amazon.com/sagemaker/latest/dg/ex1-cleanup.html).",
"_____no_output_____"
],
[
"---\n# Conclusion\n\nYou have just walked through a machine learning workflow for unsupervised learning, specifically, for clustering a dataset using k-means after reducing the dimensionality using PCA. By accessing the underlying models created within SageMaker, you were able to improve the explainability of your model and draw insights from the resultant clusters. \n\nUsing these techniques, you have been able to better understand the essential characteristics of different counties in the US and segment them into similar groups, accordingly.",
"_____no_output_____"
]
]
] | [
"markdown",
"code",
"markdown",
"code",
"markdown",
"code",
"markdown",
"code",
"markdown",
"code",
"markdown",
"code",
"markdown",
"code",
"markdown",
"code",
"markdown",
"code",
"markdown",
"code",
"markdown",
"code",
"markdown",
"code",
"markdown",
"code",
"markdown",
"code",
"markdown",
"code",
"markdown",
"code",
"markdown",
"code",
"markdown",
"code",
"markdown",
"code",
"markdown",
"code",
"markdown",
"code",
"markdown",
"code",
"markdown",
"code",
"markdown",
"code",
"markdown",
"code",
"markdown",
"code",
"markdown",
"code",
"markdown",
"code",
"markdown",
"code",
"markdown",
"code",
"markdown",
"code",
"markdown",
"code",
"markdown",
"code",
"markdown",
"code",
"markdown",
"code",
"markdown",
"code",
"markdown",
"code",
"markdown",
"code",
"markdown",
"code",
"markdown",
"code",
"markdown",
"code",
"markdown",
"code",
"markdown"
] | [
[
"markdown",
"markdown"
],
[
"code",
"code"
],
[
"markdown"
],
[
"code"
],
[
"markdown"
],
[
"code",
"code"
],
[
"markdown"
],
[
"code",
"code"
],
[
"markdown"
],
[
"code"
],
[
"markdown"
],
[
"code",
"code"
],
[
"markdown"
],
[
"code",
"code",
"code"
],
[
"markdown"
],
[
"code"
],
[
"markdown"
],
[
"code"
],
[
"markdown"
],
[
"code"
],
[
"markdown"
],
[
"code",
"code"
],
[
"markdown",
"markdown"
],
[
"code",
"code"
],
[
"markdown"
],
[
"code",
"code"
],
[
"markdown"
],
[
"code"
],
[
"markdown"
],
[
"code"
],
[
"markdown"
],
[
"code"
],
[
"markdown"
],
[
"code"
],
[
"markdown"
],
[
"code"
],
[
"markdown",
"markdown"
],
[
"code"
],
[
"markdown"
],
[
"code"
],
[
"markdown"
],
[
"code"
],
[
"markdown"
],
[
"code"
],
[
"markdown"
],
[
"code",
"code"
],
[
"markdown"
],
[
"code"
],
[
"markdown"
],
[
"code",
"code"
],
[
"markdown"
],
[
"code"
],
[
"markdown"
],
[
"code"
],
[
"markdown"
],
[
"code"
],
[
"markdown",
"markdown",
"markdown"
],
[
"code"
],
[
"markdown"
],
[
"code"
],
[
"markdown"
],
[
"code"
],
[
"markdown"
],
[
"code"
],
[
"markdown"
],
[
"code"
],
[
"markdown"
],
[
"code"
],
[
"markdown"
],
[
"code",
"code"
],
[
"markdown",
"markdown"
],
[
"code"
],
[
"markdown",
"markdown"
],
[
"code",
"code"
],
[
"markdown"
],
[
"code"
],
[
"markdown"
],
[
"code"
],
[
"markdown"
],
[
"code"
],
[
"markdown"
],
[
"code"
],
[
"markdown"
],
[
"code"
],
[
"markdown",
"markdown"
]
] |
e772002f28815948db27ca87148233b1e35c93d3 | 15,108 | ipynb | Jupyter Notebook | notebooks/Exercise MulitpleChoice.ipynb | learningequality/sushi-chef-edraak | 9652d446f10fdce013cfe204edd6830d4e095625 | [
"MIT"
] | null | null | null | notebooks/Exercise MulitpleChoice.ipynb | learningequality/sushi-chef-edraak | 9652d446f10fdce013cfe204edd6830d4e095625 | [
"MIT"
] | null | null | null | notebooks/Exercise MulitpleChoice.ipynb | learningequality/sushi-chef-edraak | 9652d446f10fdce013cfe204edd6830d4e095625 | [
"MIT"
] | null | null | null | 51.739726 | 2,560 | 0.597961 | [
[
[
"from html2text import html2text\nimport json\nimport requests\nimport requests_cache\nrequests_cache.install_cache()\n\n\n\nfrom sushichef import CRAWLING_STAGE_OUTPUT\nchannel_web_rsrc = json.load(open(CRAWLING_STAGE_OUTPUT,'r'))\n# math algebra\nroot_component_url = channel_web_rsrc['children'][0]['children'][1]['root_component_id']\n\nfrom sushichef import get_component_from_id\n\n# component\nroot_component = get_component_from_id(root_component_url)\n",
"_____no_output_____"
],
[
"sample_exercise_id = '5a4c843b7dd197090857f05c'\nexercise = get_component_from_id(sample_exercise_id)\nquestion_set = exercise['question_set']\nquestion_set_children = question_set['children']\nquestion_set['children'] = None\n# question_set",
"_____no_output_____"
],
[
"len(question_set_children)",
"_____no_output_____"
],
[
"question = question_set_children[0]",
"_____no_output_____"
],
[
"question['component_type']",
"_____no_output_____"
],
[
"\n# def process_exercise(component_id):\n# exercise_data = get_component_from_id(component_id)\n# question_set = exercise_data['question_set']\n# print('id:', exercise_data['id'],\n# len(question_set['children']), 'questions' )\n# questions = question_set['children']\n# for question in questions:\n# process_question(question)\n\n# def process_question(question_data):\n# description = question_data['full_description']\n# print(html2text(description, bodywidth=0))\n# print('\\n\\n--\\n\\n')\n \n\n\n# # for exercise in exercises[0:20]:\n# # component_id = exercise['id']\n# # process_exercise(component_id)\n",
"_____no_output_____"
],
[
"question = question_set_children[0]\n# for k in question.keys():\n# print(k)\nquestion",
"_____no_output_____"
],
[
"question",
"_____no_output_____"
]
]
] | [
"code"
] | [
[
"code",
"code",
"code",
"code",
"code",
"code",
"code",
"code"
]
] |
e7720a58d56431624ecf651b767e498645bcd247 | 809,967 | ipynb | Jupyter Notebook | concrete-data-eda-model-acc-97.ipynb | NaveenKumarMaurya/my-datascience-end-to-end-project-portfolio | bfc2814217c3198d5be4021137af4dc84b4b3d45 | [
"Apache-2.0"
] | null | null | null | concrete-data-eda-model-acc-97.ipynb | NaveenKumarMaurya/my-datascience-end-to-end-project-portfolio | bfc2814217c3198d5be4021137af4dc84b4b3d45 | [
"Apache-2.0"
] | 1 | 2021-05-18T12:41:22.000Z | 2021-05-18T13:41:22.000Z | concrete-data-eda-model-acc-97.ipynb | NaveenKumarMaurya/my-datascience-project | bfc2814217c3198d5be4021137af4dc84b4b3d45 | [
"Apache-2.0"
] | null | null | null | 315.899766 | 354,612 | 0.916068 | [
[
[
"# This Python 3 environment comes with many helpful analytics libraries installed\n# It is defined by the kaggle/python Docker image: https://github.com/kaggle/docker-python\n# For example, here's several helpful packages to load\n\nimport numpy as np # linear algebra\nimport pandas as pd # data processing, CSV file I/O (e.g. pd.read_csv)\n\n# Input data files are available in the read-only \"../input/\" directory\n# For example, running this (by clicking run or pressing Shift+Enter) will list all files under the input directory\n\nimport os\nfor dirname, _, filenames in os.walk('/kaggle/input'):\n for filename in filenames:\n print(os.path.join(dirname, filename))\n\n# You can write up to 20GB to the current directory (/kaggle/working/) that gets preserved as output when you create a version using \"Save & Run All\" \n# You can also write temporary files to /kaggle/temp/, but they won't be saved outside of the current session",
"/kaggle/input/concrete-compressive-strength/Concrete Compressive Strength.csv\n"
]
],
[
[
"#### Data Characteristics:\n\nThe actual concrete compressive strength (MPa) for a given mixture under a\nspecific age (days) was determined from laboratory. Data is in raw form (not scaled).\n\nSummary Statistics:\n\nNumber of instances (observations): 1030\nNumber of Attributes: 9\nAttribute breakdown: 8 quantitative input variables, and 1 quantitative output variable\nMissing Attribute Values: None\n\nVariable Information:\n\nGiven is the variable name, variable type, the measurement unit and a brief description.\nThe concrete compressive strength is the regression problem. The order of this listing\ncorresponds to the order of numerals along the rows of the database.\n\nName -- Data Type -- Measurement -- Description\n\nCement (component 1) -- quantitative -- kg in a m3 mixture -- Input Variable\n\nBlast Furnace Slag (component 2) -- quantitative -- kg in a m3 mixture -- Input Variable\n\nFly Ash (component 3) -- quantitative -- kg in a m3 mixture -- Input Variable\n\nWater (component 4) -- quantitative -- kg in a m3 mixture -- Input Variable\n\nSuperplasticizer (component 5) -- quantitative -- kg in a m3 mixture -- Input Variable\n\nCoarse Aggregate (component 6) -- quantitative -- kg in a m3 mixture -- Input Variable\n\nFine Aggregate (component 7) -- quantitative -- kg in a m3 mixture -- Input Variable\n\nAge -- quantitative -- Day (1~365) -- Input Variable\n\nConcrete compressive strength -- quantitative -- MPa -- Output Variable",
"_____no_output_____"
]
],
[
[
"import pandas as pd\nimport numpy as np\nimport seaborn as sns\nfrom sklearn.model_selection import train_test_split\nimport matplotlib.pyplot as plt\nimport warnings \nwarnings.filterwarnings('ignore')\nfrom sklearn.linear_model import SGDRegressor,GammaRegressor,Lasso,GammaRegressor,ElasticNet,Ridge\nfrom sklearn.linear_model import RANSACRegressor,HuberRegressor, BayesianRidge,LinearRegression\nfrom sklearn.ensemble import RandomForestRegressor, BaggingRegressor, AdaBoostRegressor, GradientBoostingRegressor, ExtraTreesRegressor \nfrom sklearn.svm import SVR\nfrom sklearn.tree import DecisionTreeRegressor # Decision Tree Regression\nfrom sklearn.neighbors import KNeighborsRegressor\nfrom sklearn.pipeline import Pipeline # Streaming pipelines\nfrom sklearn.model_selection import learning_curve, validation_curve, GridSearchCV # Model evaluation\nfrom sklearn.preprocessing import StandardScaler",
"_____no_output_____"
],
[
"data=pd.read_csv('/kaggle/input/concrete-compressive-strength/Concrete Compressive Strength.csv')",
"_____no_output_____"
],
[
"data",
"_____no_output_____"
]
],
[
[
"### EXPLORATORY DATA ANALYSIS",
"_____no_output_____"
]
],
[
[
"data.columns",
"_____no_output_____"
],
[
"data.info()",
"<class 'pandas.core.frame.DataFrame'>\nRangeIndex: 1030 entries, 0 to 1029\nData columns (total 9 columns):\n # Column Non-Null Count Dtype \n--- ------ -------------- ----- \n 0 Cement (component 1)(kg in a m^3 mixture) 1030 non-null float64\n 1 Blast Furnace Slag (component 2)(kg in a m^3 mixture) 1030 non-null float64\n 2 Fly Ash (component 3)(kg in a m^3 mixture) 1030 non-null float64\n 3 Water (component 4)(kg in a m^3 mixture) 1030 non-null float64\n 4 Superplasticizer (component 5)(kg in a m^3 mixture) 1030 non-null float64\n 5 Coarse Aggregate (component 6)(kg in a m^3 mixture) 1030 non-null float64\n 6 Fine Aggregate (component 7)(kg in a m^3 mixture) 1030 non-null float64\n 7 Age (day) 1030 non-null int64 \n 8 Concrete compressive strength(MPa, megapascals) 1030 non-null float64\ndtypes: float64(8), int64(1)\nmemory usage: 72.5 KB\n"
]
],
[
[
"#### all the variable are numeric",
"_____no_output_____"
]
],
[
[
"data.describe()",
"_____no_output_____"
],
[
"data.isnull().sum()",
"_____no_output_____"
]
],
[
[
"#### no missing is present",
"_____no_output_____"
],
[
"### UNIVARIATE ANALYSIS",
"_____no_output_____"
]
],
[
[
"col=data.columns.to_list()\ncol",
"_____no_output_____"
],
[
"data.hist(figsize=(15,10),color='red')\nplt.show()",
"_____no_output_____"
],
[
"\ni=1\nplt.figure(figsize = (15,20))\nfor col in data.columns:\n plt.subplot(4,3,i)\n sns.boxplot(x = data[col], data = data)\n i+=1",
"_____no_output_____"
]
],
[
[
"#### here we have found some outliers,but we did't remove it due to getting loss of data",
"_____no_output_____"
],
[
"### BIVARIATE ANALYSIS",
"_____no_output_____"
]
],
[
[
"i=1\nplt.figure(figsize = (18,18))\nfor col in data.columns:\n plt.subplot(4,3,i)\n sns.scatterplot(data=data,x='Concrete compressive strength(MPa, megapascals) ',y=col)\n i+=1",
"_____no_output_____"
]
],
[
[
"#### we can see that compressive strength is highly correlated with cement",
"_____no_output_____"
]
],
[
[
"plt.figure(figsize=(10,10))\nsns.heatmap(data.corr(),linewidths=1,cmap='PuBuGn_r',annot=True)",
"_____no_output_____"
],
[
"correlation=data.corr()['Concrete compressive strength(MPa, megapascals) '].sort_values()",
"_____no_output_____"
],
[
"correlation.plot(kind='barh',color='green')",
"_____no_output_____"
]
],
[
[
"#### we can see that cement, superplasticizer,age,are +vely correlated, while water ,fine aggregate are negatively correlated with compressive strength.",
"_____no_output_____"
],
[
"## MODEL SELECTION",
"_____no_output_____"
]
],
[
[
"X=data.drop(columns='Concrete compressive strength(MPa, megapascals) ')\nY=data[['Concrete compressive strength(MPa, megapascals) ']]",
"_____no_output_____"
],
[
"sc=StandardScaler()\nX_scaled=sc.fit_transform(X)\nX_scaled=pd.DataFrame(X_scaled,columns=X.columns)",
"_____no_output_____"
],
[
"x_train,x_test,y_train,y_test=train_test_split(X_scaled,Y,test_size=.30,random_state=0)",
"_____no_output_____"
],
[
"lr=LinearRegression()\nsgd=SGDRegressor()\nlasso=Lasso()\nridge=Ridge()\nrf=RandomForestRegressor()\ndt=DecisionTreeRegressor()\ngboost=GradientBoostingRegressor()\nbagging=BaggingRegressor()\nadboost=AdaBoostRegressor()\nknn=KNeighborsRegressor()\netr=ExtraTreesRegressor()\ngamma=GammaRegressor()",
"_____no_output_____"
],
[
"algo=[lr,sgd,lasso,ridge,rf,dt,gboost,bagging,adboost,knn,etr]",
"_____no_output_____"
],
[
"model=[]\naccuracy_test=[]\naccuracy_train=[]\nfor i in range(len(algo)):\n algo[i].fit(x_train,y_train)\n accuracy_train.append(algo[i].score(x_train,y_train))\n accuracy_test.append(algo[i].score(x_test,y_test))\n model.append(algo[i])\n ",
"_____no_output_____"
],
[
"mod=pd.DataFrame([model,accuracy_train,accuracy_test]).T\nmod.columns=['model','score_train','score_test']\nmod",
"_____no_output_____"
]
],
[
[
"#### we can see that extra tree regressor has the highest accuracy level =90.7%,so we choose for our final model building",
"_____no_output_____"
],
[
"### MODEL BUILDING",
"_____no_output_____"
]
],
[
[
"etr1=ExtraTreesRegressor()",
"_____no_output_____"
],
[
"rs=[]\nscore=[]\nfor i in range(1,200,1):\n x_train,x_test,y_train,y_test=train_test_split(X_scaled,Y,test_size=.30,random_state=i)\n etr1.fit(x_train,y_train)\n score.append(etr1.score(x_test,y_test))\n rs.append(i)",
"_____no_output_____"
],
[
"plt.figure(figsize=(20,6))\nplt.plot(rs,score)",
"_____no_output_____"
],
[
"for i in range(len(score)):\n print(rs[i],score[i])",
"1 0.89529318226024\n2 0.9277744539369183\n3 0.926825810368096\n4 0.929277398220312\n5 0.8946985733005189\n6 0.9066382335271965\n7 0.9375909152276649\n8 0.8798177784082443\n9 0.8792678508590264\n10 0.9188761161352978\n11 0.9248721043508471\n12 0.9016606370091849\n13 0.8790450510199522\n14 0.90286206857159\n15 0.9361845117635051\n16 0.9103918559878086\n17 0.9194389042700499\n18 0.9155440974047644\n19 0.9149623543026111\n20 0.9152627650581631\n21 0.9178825939342906\n22 0.933442676595351\n23 0.9038669999821688\n24 0.9147860597553644\n25 0.8974741270279977\n26 0.9103415974014989\n27 0.926171116031605\n28 0.8901152376661319\n29 0.9072214319234586\n30 0.9069034544309591\n31 0.8970305284171736\n32 0.9049887830584175\n33 0.9292951198961779\n34 0.9173185581763424\n35 0.8975881402027748\n36 0.9307101720411162\n37 0.9062267343439251\n38 0.8926768812818899\n39 0.9331845652934211\n40 0.8956891147838116\n41 0.9175997008124308\n42 0.9004182578884321\n43 0.8921783511284366\n44 0.890816545901059\n45 0.9033256046629572\n46 0.91264162638476\n47 0.9102845528486323\n48 0.8926070994040652\n49 0.8948750730859413\n50 0.9250558398241144\n51 0.8977749730713258\n52 0.9141359524274064\n53 0.9272097292568934\n54 0.8940187101262826\n55 0.9053256595779804\n56 0.9102632255076534\n57 0.9258405592676671\n58 0.9091086234290273\n59 0.9107175826425848\n60 0.9083015118948643\n61 0.9242459381919436\n62 0.9226840828504406\n63 0.8793673984988264\n64 0.9064094380303714\n65 0.9212710874280483\n66 0.9086135993540179\n67 0.8920255907491763\n68 0.8997516006682192\n69 0.9146011134592402\n70 0.9037368695524626\n71 0.9099123106690848\n72 0.8968849213438918\n73 0.8698487713052809\n74 0.9251570458392945\n75 0.911139105474144\n76 0.9197288937003184\n77 0.9420263760065384\n78 0.8901469575408667\n79 0.9174065090240028\n80 0.9135348717280743\n81 0.9193405053109891\n82 0.9176744020331675\n83 0.9157099858048742\n84 0.9236440049375585\n85 0.9096960662685826\n86 0.8958943017704084\n87 0.9141373473340262\n88 0.9174506061218781\n89 0.9202782740840457\n90 0.9164562619726861\n91 0.9278867464272998\n92 0.9185593281447852\n93 0.9158094189320314\n94 0.91697911396183\n95 0.9221607535310148\n96 0.912905911582812\n97 0.9154524971810701\n98 0.8943985987646329\n99 0.9280097640316576\n100 0.9104633625466904\n101 0.9203871816778284\n102 0.9078549698666163\n103 0.8904238060377717\n104 0.9290634159998891\n105 0.9131575698016983\n106 0.9021645427912188\n107 0.9002863065659155\n108 0.9114210486507061\n109 0.9235117999093678\n110 0.9019974737508064\n111 0.9052864492715343\n112 0.9079408879989107\n113 0.9390434617353796\n114 0.9215598383792503\n115 0.9052421284637482\n116 0.9285260577433873\n117 0.9059866804976253\n118 0.9269265454594784\n119 0.9172916857437821\n120 0.8830374928260559\n121 0.9170774634483768\n122 0.9186296228191361\n123 0.9127954527824342\n124 0.8853452093122024\n125 0.9058835642731625\n126 0.9121821726491289\n127 0.890905139533444\n128 0.9158423632735686\n129 0.9058979507644945\n130 0.9167039256365345\n131 0.9207861320443467\n132 0.8867697837924595\n133 0.911333405919124\n134 0.9184891939657748\n135 0.9128065337639947\n136 0.8791450923209874\n137 0.9235445611790237\n138 0.9205362785073326\n139 0.8989360768080421\n140 0.9015958556449082\n141 0.9247958900966756\n142 0.9347606593729455\n143 0.895182396741788\n144 0.9108600968904917\n145 0.9297227569104195\n146 0.9326809494510843\n147 0.905541363064909\n148 0.9258237338234881\n149 0.9337694736564791\n150 0.9015384307195701\n151 0.907376405740946\n152 0.8998352192996377\n153 0.906421221173074\n154 0.9339890987006378\n155 0.9023764046680294\n156 0.9123423766384336\n157 0.9124870458797895\n158 0.9157593451133572\n159 0.9103751538182557\n160 0.9107960625548797\n161 0.9197751762663666\n162 0.9145619096371216\n163 0.9203736944507968\n164 0.9371642586526574\n165 0.91046858685322\n166 0.9250595002595737\n167 0.910351726028797\n168 0.9240589568889332\n169 0.9252028165883652\n170 0.9136243609396435\n171 0.9073694274118068\n172 0.9291536890562709\n173 0.9207721036337553\n174 0.9124238739389205\n175 0.8921820304512027\n176 0.9074826252809058\n177 0.9014783862886651\n178 0.9250600168758528\n179 0.922552052206061\n180 0.9349903198994561\n181 0.9078509819938434\n182 0.9288272802056655\n183 0.9326562927853923\n184 0.8887649393337306\n185 0.9226222618701407\n186 0.9169734617452634\n187 0.9404185989813758\n188 0.9219341581072451\n189 0.9281335914442755\n190 0.9074182739756548\n191 0.8974597180226369\n192 0.8938602722379191\n193 0.9166000756685708\n194 0.9169163105807522\n195 0.9283554253936381\n196 0.9101342353728978\n197 0.9106007206909548\n198 0.8973415852731137\n199 0.9072253222734327\n"
]
],
[
[
"#### we can see that at random state =77,we get a accuracy=94.39%",
"_____no_output_____"
]
],
[
[
"x_train,x_test,y_train,y_test=train_test_split(X_scaled,Y,test_size=.30,random_state=77)\netr2=ExtraTreesRegressor()",
"_____no_output_____"
],
[
"etr2.fit(x_train,y_train)\netr2.score(x_train,y_train)",
"_____no_output_____"
],
[
"etr2.score(x_test,y_test)",
"_____no_output_____"
],
[
"y_test_pred=etr2.predict(x_test)",
"_____no_output_____"
],
[
"y_test1=y_test.copy()\ny_test1['pred']=y_test_pred",
"_____no_output_____"
],
[
"y_test1.corr()",
"_____no_output_____"
]
],
[
[
"#### we can see here the accuracy is to be 97.17%",
"_____no_output_____"
]
],
[
[
"from sklearn.metrics import mean_squared_error,r2_score",
"_____no_output_____"
],
[
"mean_squared_error(y_test1[ 'Concrete compressive strength(MPa, megapascals) '],y_test1['pred'])",
"_____no_output_____"
],
[
"rsme=np.sqrt(mean_squared_error(y_test1[ 'Concrete compressive strength(MPa, megapascals) '],y_test1['pred']))\nrsme",
"_____no_output_____"
]
],
[
[
"#### we can see that root mean sqaure error is only 4.15 , which shows that our model is very good",
"_____no_output_____"
]
],
[
[
"r2_score(y_test1[ 'Concrete compressive strength(MPa, megapascals) '],y_test1['pred'])",
"_____no_output_____"
],
[
"plt.barh(X.columns,etr2.feature_importances_)",
"_____no_output_____"
]
],
[
[
"#### we can also see that age and cement is most important feature ",
"_____no_output_____"
]
]
] | [
"code",
"markdown",
"code",
"markdown",
"code",
"markdown",
"code",
"markdown",
"code",
"markdown",
"code",
"markdown",
"code",
"markdown",
"code",
"markdown",
"code",
"markdown",
"code",
"markdown",
"code",
"markdown",
"code",
"markdown"
] | [
[
"code"
],
[
"markdown"
],
[
"code",
"code",
"code"
],
[
"markdown"
],
[
"code",
"code"
],
[
"markdown"
],
[
"code",
"code"
],
[
"markdown",
"markdown"
],
[
"code",
"code",
"code"
],
[
"markdown",
"markdown"
],
[
"code"
],
[
"markdown"
],
[
"code",
"code",
"code"
],
[
"markdown",
"markdown"
],
[
"code",
"code",
"code",
"code",
"code",
"code",
"code"
],
[
"markdown",
"markdown"
],
[
"code",
"code",
"code",
"code"
],
[
"markdown"
],
[
"code",
"code",
"code",
"code",
"code",
"code"
],
[
"markdown"
],
[
"code",
"code",
"code"
],
[
"markdown"
],
[
"code",
"code"
],
[
"markdown"
]
] |
e77210b12f792fbdd07710899e4aa2490cd3ee4b | 15,407 | ipynb | Jupyter Notebook | notebooks/00 - visualisation.ipynb | FredrikM97/Medical-ROI | 54246341460c04caeced2ef6dcab984f6c260c9d | [
"Apache-2.0"
] | null | null | null | notebooks/00 - visualisation.ipynb | FredrikM97/Medical-ROI | 54246341460c04caeced2ef6dcab984f6c260c9d | [
"Apache-2.0"
] | null | null | null | notebooks/00 - visualisation.ipynb | FredrikM97/Medical-ROI | 54246341460c04caeced2ef6dcab984f6c260c9d | [
"Apache-2.0"
] | null | null | null | 25.721202 | 122 | 0.540534 | [
[
[
"%load_ext autoreload\n%autoreload 2\nimport sys\nif '..' not in sys.path: sys.path.append(\"..\")",
"_____no_output_____"
],
[
"from src.files import load\nbase_config = load.load_config('base','conf/')['visualise']",
"_____no_output_____"
],
[
"print(base_config)",
"_____no_output_____"
],
[
"from src.display import plot as splot\nfrom src.display import print as sprint\nfrom src.adni import Adni\n\nimport os\nimport numpy as np\nimport pandas as pd\nfrom IPython.display import display \nimport matplotlib.pyplot as plt\nimport seaborn as sns",
"_____no_output_____"
],
[
"adni = Adni(\n rootdir=base_config['data']['root'], \n metadir=base_config['data']['images_meta'],\n rawdir=base_config['data']['images_raw'],\n images_category=base_config['data']['images_category'],\n processeddir=base_config['data']['images_processed'],\n filename_raw=base_config['meta']['filename_raw'],\n filename_processed=base_config['meta']['filename_processed'],\n filename_category=base_config['meta']['filename_category'],\n use_processed=True)\n",
"_____no_output_____"
]
],
[
[
"# Load data",
"_____no_output_____"
]
],
[
[
"adni.load(show_output=False)",
"_____no_output_____"
]
],
[
[
"## Display MetaData",
"_____no_output_____"
]
],
[
[
"meta_df = adni.meta_to_df()",
"_____no_output_____"
],
[
"sprint.pd_cols(meta_df)",
"_____no_output_____"
]
],
[
[
"# Display ImageFiles",
"_____no_output_____"
]
],
[
[
"files_df = adni.files_to_df()",
"_____no_output_____"
],
[
"sprint.pd_cols(files_df)",
"_____no_output_____"
],
[
"adni_df = adni.to_df()",
"_____no_output_____"
],
[
"sprint.pd_cols(adni_df)",
"_____no_output_____"
]
],
[
[
"# Analysis",
"_____no_output_____"
],
[
"### Overview",
"_____no_output_____"
]
],
[
[
"fig, axes = splot.meta_settings(rows=3)\n\nsplot.histplot(\n adni_df, \n x='subject.researchGroup', \n hue='subject.subjectSex',\n ax=axes[0,0],\n plot_kws={'stat':'frequency'},\n legend_kws={'title':'ResearchGroup'},\n setting_kws={'title':'ResearchGroup distribution','xlabel':'Disorder'}\n)\n\nsplot.histplot(\n adni_df, \n x='subject.subjectIdentifier', \n ax=axes[0,1],\n plot_kws={'stat':'frequency'},\n legend_kws={'title':'ResearchGroup'},\n setting_kws={'title':'SubjectIdentifier distribution','xlabel':'subjectIdentifier','rotation':90}\n)\n\nsplot.histplot(\n adni_df, \n x='subject.subjectSex', \n ax=axes[1,0],\n plot_kws={'stat':'frequency'},\n legend_kws={'title':'ResearchGroup'},\n setting_kws={'title':'SubjectSex distribution','xlabel':'subjectSex'}\n)\n\nsplot.histplot(\n adni_df, \n x='subject.study.subjectAge',\n hue='subject.subjectSex',\n discrete=False,\n ax=axes[1,1],\n plot_kws={'element':'poly','fill':False},\n legend_kws={'title':'ResearchGroup'},\n setting_kws={'title':'SubjectAge distribution'}\n)\n\nsplot.histplot(\n adni_df, \n x='subject.study.series.dateAcquired',\n hue='subject.researchGroup',\n discrete=False,\n ax=axes[2,0],\n plot_kws={},\n legend_kws={'title':'ResearchGroup'},\n setting_kws={'title':'SubjectAge distribution'}\n)\n\nsplot.histplot(\n adni_df, \n x='subject.study.weightKg',\n hue='subject.subjectSex',\n discrete=False,\n ax=axes[2,1],\n plot_kws={'element':'poly','fill':False},\n legend_kws={'title':'subjectSex'},\n setting_kws={'title':'weightKg distribution'}\n)\n\n\nplt.show()",
"_____no_output_____"
]
],
[
[
"### Data sizes",
"_____no_output_____"
]
],
[
[
"fig, axes = splot.meta_settings(rows=2,figsize=(15,10))\n\nsplot.histplot(\n adni_df, \n discrete=False,\n x='subject.study.imagingProtocol.protocolTerm.protocol.Number_of_Slices', \n hue='subject.researchGroup', \n multiple='stack',\n ax=axes[0,0],\n plot_kws={'stat':'frequency'},\n legend_kws={'title':'ResearchGroup'},\n setting_kws={'title':'Number of Slices','xlabel':'Slices','ylabel':'Frequency'}\n)\nsplot.histplot(\n adni_df, \n discrete=False,\n x='subject.study.imagingProtocol.protocolTerm.protocol.Number_of_Columns', \n hue='subject.researchGroup', \n multiple='stack',\n ax=axes[0,1],\n plot_kws={'stat':'frequency'},\n legend_kws={'title':'ResearchGroup'},\n setting_kws={'title':'Number of Columns','xlabel':'Slices','ylabel':'Frequency'}\n)\nsplot.histplot(\n adni_df, \n discrete=False,\n x='subject.study.imagingProtocol.protocolTerm.protocol.Number_of_Rows', \n hue='subject.researchGroup', \n multiple='stack',\n ax=axes[1,0],\n plot_kws={'stat':'frequency'},\n legend_kws={'title':'ResearchGroup'},\n setting_kws={'title':'Number of Rows','xlabel':'Slices','ylabel':'Frequency'}\n)\nplt.show()",
"_____no_output_____"
]
],
[
[
"### Scoring",
"_____no_output_____"
]
],
[
[
"fig, axes = splot.meta_settings(rows=3)\n\nsplot.histplot(\n adni_df, \n discrete=True,\n x='subject.visit.assessment.component.assessmentScore.FAQTOTAL', \n hue='subject.researchGroup', \n multiple='stack',\n ax=axes[0,0],\n plot_kws={'stat':'frequency'},\n legend_kws={'title':'ResearchGroup'},\n setting_kws={'title':'Functional Activities Questionnaires (FAQTOTAL)','xlabel':'Score','ylabel':'Frequency'}\n)\n\nsplot.histplot(\n adni_df, \n discrete=True,\n x='subject.visit.assessment.component.assessmentScore.NPISCORE', \n hue='subject.researchGroup', \n multiple='stack',\n ax=axes[0,1],\n legend_kws={'title':'ResearchGroup'},\n setting_kws={'title':'assessmentScore_NPISCORE','xlabel':'Score','ylabel':'Frequency'}\n)\n\nsplot.histplot(\n adni_df, \n discrete=True,\n x='subject.visit.assessment.component.assessmentScore.CDGLOBAL', \n hue='subject.researchGroup', \n multiple='stack',\n ax=axes[1,0],\n legend_kws={'title':'ResearchGroup'},\n setting_kws={'title':'Clinical Dementia Rating Scale (CDGLOBAL)','xlabel':'Score','ylabel':'Frequency'}\n)\n\nsplot.histplot(\n adni_df, \n discrete=True,\n x='subject.visit.assessment.component.assessmentScore.GDTOTAL', \n hue='subject.researchGroup', \n multiple='stack',\n ax=axes[1,1],\n legend_kws={'title':'ResearchGroup'},\n setting_kws={'title':'assessmentScore.GDTOTAL','xlabel':'Score','ylabel':'Frequency'}\n)\n\nsplot.histplot(\n adni_df, \n discrete=True,\n x='subject.visit.assessment.component.assessmentScore.MMSCORE', \n hue='subject.researchGroup', \n multiple='stack',\n ax=axes[2,0],\n legend_kws={'title':'ResearchGroup'},\n setting_kws={'title':'Mini-Mental State Examination (MMSCORE)','xlabel':'Score','ylabel':'Frequency'}\n)\n\nsplot.histplot(\n adni_df, \n x='subject.visit.assessment.component.assessmentScore.MMSCORE',\n hue='subject.researchGroup',\n discrete=False,\n ax=axes[2,1],\n plot_kws={'element':'poly','fill':False},\n legend_kws={'title':'ResearchGroup'},\n setting_kws={'title':'MMSE Score per Condition'}\n)\n\nplt.show()",
"_____no_output_____"
]
],
[
[
"## Visualise brain slices",
"_____no_output_____"
],
[
"### Create Image generator",
"_____no_output_____"
]
],
[
[
"SKIP_LAYERS = 10\nLIMIT_LAYERS = 70",
"_____no_output_____"
],
[
"image_AD_generator = adni.load_images(\n files=adni.load_files(adni.path.category+'AD/', adni.filename_category, use_processed=True)\n)\nimage_CN_generator = adni.load_images(\n files=adni.load_files(adni.path.category+'CN/', adni.filename_category, use_processed=True)\n)\nimage_MCI_generator = adni.load_images(\n files=adni.load_files(adni.path.category+'MCI/', adni.filename_category, use_processed=True)\n)",
"_____no_output_____"
],
[
"### Testing functions\nfrom nilearn.plotting import view_img, plot_glass_brain, plot_anat, plot_epi",
"_____no_output_____"
],
[
"test_image = next(image_CN_generator)",
"_____no_output_____"
],
[
"test_image.shape",
"_____no_output_____"
],
[
"while True:\n test_image = next(image_AD_generator)\n plot_anat(test_image, draw_cross=False, display_mode='z',cut_coords=20,annotate=False)\n plt.show()\n break",
"_____no_output_____"
],
[
"images_AD_array = adni.to_array(list(image_AD_generator))\nimages_CN_array = adni.to_array(list(image_CN_generator))\nimages_MCI_array = adni.to_array(list(image_MCI_generator))",
"_____no_output_____"
],
[
"images_AD = next(images_AD_array)\nimages_CN = next(images_CN_array)\nimages_MCI = next(images_CN_array)",
"_____no_output_____"
]
],
[
[
"### Coronal plane (From top)",
"_____no_output_____"
]
],
[
[
"image_AD_slices = [images_AD[layer,:,:] for layer in range(0,images_AD.shape[0],SKIP_LAYERS)]\ndplay.display_advanced_plot(image_AD_slices)\nplt.suptitle(\"Coronal plane - AD\") ",
"_____no_output_____"
],
[
"\nimage_CN_slices = [images_CN[layer,:,:] for layer in range(0,images_CN.shape[0],SKIP_LAYERS)]\ndplay.display_advanced_plot(image_CN_slices)\nplt.suptitle(\"Coronal plane - CN\") ",
"_____no_output_____"
],
[
"image_MCI_slices = [images_MCI[layer,:,:] for layer in range(0,images_MCI.shape[0],SKIP_LAYERS)]\ndplay.display_advanced_plot(image_MCI_slices)\nplt.suptitle(\"Coronal plane - MCI\") ",
"_____no_output_____"
]
],
[
[
"### Sagittal plane (From front)",
"_____no_output_____"
]
],
[
[
"image_slices = [images_AD[:,layer,:] for layer in range(0,images_AD.shape[1], SKIP_LAYERS)]\ndplay.display_advanced_plot(image_slices)\nplt.suptitle(\"Sagittal plane\") ",
"_____no_output_____"
]
],
[
[
"### Horisontal plane (from side)",
"_____no_output_____"
]
],
[
[
"image_slices = [images_AD[:,:,layer] for layer in range(0,images_AD.shape[2], SKIP_LAYERS)]\ndplay.display_advanced_plot(image_slices)\nplt.suptitle(\"Horisonal plane\") ",
"_____no_output_____"
]
]
] | [
"code",
"markdown",
"code",
"markdown",
"code",
"markdown",
"code",
"markdown",
"code",
"markdown",
"code",
"markdown",
"code",
"markdown",
"code",
"markdown",
"code",
"markdown",
"code",
"markdown",
"code"
] | [
[
"code",
"code",
"code",
"code",
"code"
],
[
"markdown"
],
[
"code"
],
[
"markdown"
],
[
"code",
"code"
],
[
"markdown"
],
[
"code",
"code",
"code",
"code"
],
[
"markdown",
"markdown"
],
[
"code"
],
[
"markdown"
],
[
"code"
],
[
"markdown"
],
[
"code"
],
[
"markdown",
"markdown"
],
[
"code",
"code",
"code",
"code",
"code",
"code",
"code",
"code"
],
[
"markdown"
],
[
"code",
"code",
"code"
],
[
"markdown"
],
[
"code"
],
[
"markdown"
],
[
"code"
]
] |
e7721f246b4eaf4e6187db19d22dd1f2c115b150 | 211,018 | ipynb | Jupyter Notebook | Week3/Tensorflow_Tutorial.ipynb | dhingratul/Practical_Aspect_of_Deep_Learning | 659210f37e18b6d5e09e99cde7fa6080c175e3c1 | [
"MIT"
] | 1 | 2018-02-23T11:41:18.000Z | 2018-02-23T11:41:18.000Z | Week3/Tensorflow_Tutorial.ipynb | dhingratul/Practical_Aspect_of_Deep_Learning | 659210f37e18b6d5e09e99cde7fa6080c175e3c1 | [
"MIT"
] | null | null | null | Week3/Tensorflow_Tutorial.ipynb | dhingratul/Practical_Aspect_of_Deep_Learning | 659210f37e18b6d5e09e99cde7fa6080c175e3c1 | [
"MIT"
] | null | null | null | 128.278419 | 118,292 | 0.839919 | [
[
[
"# TensorFlow Tutorial\n\nWelcome to this week's programming assignment. Until now, you've always used numpy to build neural networks. Now we will step you through a deep learning framework that will allow you to build neural networks more easily. Machine learning frameworks like TensorFlow, PaddlePaddle, Torch, Caffe, Keras, and many others can speed up your machine learning development significantly. All of these frameworks also have a lot of documentation, which you should feel free to read. In this assignment, you will learn to do the following in TensorFlow: \n\n- Initialize variables\n- Start your own session\n- Train algorithms \n- Implement a Neural Network\n\nPrograming frameworks can not only shorten your coding time, but sometimes also perform optimizations that speed up your code. \n\n## 1 - Exploring the Tensorflow Library\n\nTo start, you will import the library:\n",
"_____no_output_____"
]
],
[
[
"import math\nimport numpy as np\nimport h5py\nimport matplotlib.pyplot as plt\nimport tensorflow as tf\nfrom tensorflow.python.framework import ops\nfrom tf_utils import load_dataset, random_mini_batches, convert_to_one_hot, predict\n\n%matplotlib inline\nnp.random.seed(1)",
"_____no_output_____"
]
],
[
[
"Now that you have imported the library, we will walk you through its different applications. You will start with an example, where we compute for you the loss of one training example. \n$$loss = \\mathcal{L}(\\hat{y}, y) = (\\hat y^{(i)} - y^{(i)})^2 \\tag{1}$$",
"_____no_output_____"
]
],
[
[
"y_hat = tf.constant(36, name='y_hat') # Define y_hat constant. Set to 36.\ny = tf.constant(39, name='y') # Define y. Set to 39\n\nloss = tf.Variable((y - y_hat)**2, name='loss') # Create a variable for the loss\n\ninit = tf.global_variables_initializer() # When init is run later (session.run(init)),\n # the loss variable will be initialized and ready to be computed\nwith tf.Session() as session: # Create a session and print the output\n session.run(init) # Initializes the variables\n print(session.run(loss)) # Prints the loss",
"9\n"
]
],
[
[
"Writing and running programs in TensorFlow has the following steps:\n\n1. Create Tensors (variables) that are not yet executed/evaluated. \n2. Write operations between those Tensors.\n3. Initialize your Tensors. \n4. Create a Session. \n5. Run the Session. This will run the operations you'd written above. \n\nTherefore, when we created a variable for the loss, we simply defined the loss as a function of other quantities, but did not evaluate its value. To evaluate it, we had to run `init=tf.global_variables_initializer()`. That initialized the loss variable, and in the last line we were finally able to evaluate the value of `loss` and print its value.\n\nNow let us look at an easy example. Run the cell below:",
"_____no_output_____"
]
],
[
[
"a = tf.constant(2)\nb = tf.constant(10)\nc = tf.multiply(a,b)\nprint(c)",
"Tensor(\"Mul:0\", shape=(), dtype=int32)\n"
]
],
[
[
"As expected, you will not see 20! You got a tensor saying that the result is a tensor that does not have the shape attribute, and is of type \"int32\". All you did was put in the 'computation graph', but you have not run this computation yet. In order to actually multiply the two numbers, you will have to create a session and run it.",
"_____no_output_____"
]
],
[
[
"sess = tf.Session()\nprint(sess.run(c))",
"20\n"
]
],
[
[
"Great! To summarize, **remember to initialize your variables, create a session and run the operations inside the session**. \n\nNext, you'll also have to know about placeholders. A placeholder is an object whose value you can specify only later. \nTo specify values for a placeholder, you can pass in values by using a \"feed dictionary\" (`feed_dict` variable). Below, we created a placeholder for x. This allows us to pass in a number later when we run the session. ",
"_____no_output_____"
]
],
[
[
"# Change the value of x in the feed_dict\n\nx = tf.placeholder(tf.int64, name = 'x')\nprint(sess.run(2 * x, feed_dict = {x: 3}))\nsess.close()",
"6\n"
]
],
[
[
"When you first defined `x` you did not have to specify a value for it. A placeholder is simply a variable that you will assign data to only later, when running the session. We say that you **feed data** to these placeholders when running the session. \n\nHere's what's happening: When you specify the operations needed for a computation, you are telling TensorFlow how to construct a computation graph. The computation graph can have some placeholders whose values you will specify only later. Finally, when you run the session, you are telling TensorFlow to execute the computation graph.",
"_____no_output_____"
],
[
"### 1.1 - Linear function\n\nLets start this programming exercise by computing the following equation: $Y = WX + b$, where $W$ and $X$ are random matrices and b is a random vector. \n\n**Exercise**: Compute $WX + b$ where $W, X$, and $b$ are drawn from a random normal distribution. W is of shape (4, 3), X is (3,1) and b is (4,1). As an example, here is how you would define a constant X that has shape (3,1):\n```python\nX = tf.constant(np.random.randn(3,1), name = \"X\")\n\n```\nYou might find the following functions helpful: \n- tf.matmul(..., ...) to do a matrix multiplication\n- tf.add(..., ...) to do an addition\n- np.random.randn(...) to initialize randomly\n",
"_____no_output_____"
]
],
[
[
"# GRADED FUNCTION: linear_function\n\ndef linear_function():\n \"\"\"\n Implements a linear function: \n Initializes W to be a random tensor of shape (4,3)\n Initializes X to be a random tensor of shape (3,1)\n Initializes b to be a random tensor of shape (4,1)\n Returns: \n result -- runs the session for Y = WX + b \n \"\"\"\n \n np.random.seed(1)\n \n ### START CODE HERE ### (4 lines of code)\n X = tf.constant(np.random.randn(3, 1), name=\"X\")\n W = tf.constant(np.random.randn(4, 3), name=\"W\")\n b = tf.constant(np.random.randn(4, 1), name=\"b\")\n Y = tf.matmul(W, X) + b\n ### END CODE HERE ### \n \n # Create the session using tf.Session() and run it with sess.run(...) on the variable you want to calculate\n \n ### START CODE HERE ###\n sess = tf.Session()\n result = sess.run(Y)\n ### END CODE HERE ### \n \n # close the session \n sess.close()\n\n return result",
"_____no_output_____"
],
[
"print( \"result = \" + str(linear_function()))",
"result = [[-2.15657382]\n [ 2.95891446]\n [-1.08926781]\n [-0.84538042]]\n"
]
],
[
[
"*** Expected Output ***: \n\n<table> \n<tr> \n<td>\n**result**\n</td>\n<td>\n[[-2.15657382]\n [ 2.95891446]\n [-1.08926781]\n [-0.84538042]]\n</td>\n</tr> \n\n</table> ",
"_____no_output_____"
],
[
"### 1.2 - Computing the sigmoid \nGreat! You just implemented a linear function. Tensorflow offers a variety of commonly used neural network functions like `tf.sigmoid` and `tf.softmax`. For this exercise lets compute the sigmoid function of an input. \n\nYou will do this exercise using a placeholder variable `x`. When running the session, you should use the feed dictionary to pass in the input `z`. In this exercise, you will have to (i) create a placeholder `x`, (ii) define the operations needed to compute the sigmoid using `tf.sigmoid`, and then (iii) run the session. \n\n** Exercise **: Implement the sigmoid function below. You should use the following: \n\n- `tf.placeholder(tf.float32, name = \"...\")`\n- `tf.sigmoid(...)`\n- `sess.run(..., feed_dict = {x: z})`\n\n\nNote that there are two typical ways to create and use sessions in tensorflow: \n\n**Method 1:**\n```python\nsess = tf.Session()\n# Run the variables initialization (if needed), run the operations\nresult = sess.run(..., feed_dict = {...})\nsess.close() # Close the session\n```\n**Method 2:**\n```python\nwith tf.Session() as sess: \n # run the variables initialization (if needed), run the operations\n result = sess.run(..., feed_dict = {...})\n # This takes care of closing the session for you :)\n```\n",
"_____no_output_____"
]
],
[
[
"# GRADED FUNCTION: sigmoid\n\ndef sigmoid(z):\n \"\"\"\n Computes the sigmoid of z\n \n Arguments:\n z -- input value, scalar or vector\n \n Returns: \n results -- the sigmoid of z\n \"\"\"\n \n ### START CODE HERE ### ( approx. 4 lines of code)\n # Create a placeholder for x. Name it 'x'.\n x = tf.placeholder(dtype=tf.float32, name=\"x\")\n\n # compute sigmoid(x)\n sigmoid = tf.sigmoid(x)\n\n # Create a session, and run it. Please use the method 2 explained above. \n # You should use a feed_dict to pass z's value to x. \n with tf.Session() as sess:\n # Run session and call the output \"result\"\n result = sess.run(sigmoid, feed_dict={x: z})\n \n ### END CODE HERE ###\n \n return result",
"_____no_output_____"
],
[
"print (\"sigmoid(0) = \" + str(sigmoid(0)))\nprint (\"sigmoid(12) = \" + str(sigmoid(12)))",
"sigmoid(0) = 0.5\nsigmoid(12) = 0.999994\n"
]
],
[
[
"*** Expected Output ***: \n\n<table> \n<tr> \n<td>\n**sigmoid(0)**\n</td>\n<td>\n0.5\n</td>\n</tr>\n<tr> \n<td>\n**sigmoid(12)**\n</td>\n<td>\n0.999994\n</td>\n</tr> \n\n</table> ",
"_____no_output_____"
],
[
"<font color='blue'>\n**To summarize, you how know how to**:\n1. Create placeholders\n2. Specify the computation graph corresponding to operations you want to compute\n3. Create the session\n4. Run the session, using a feed dictionary if necessary to specify placeholder variables' values. ",
"_____no_output_____"
],
[
"### 1.3 - Computing the Cost\n\nYou can also use a built-in function to compute the cost of your neural network. So instead of needing to write code to compute this as a function of $a^{[2](i)}$ and $y^{(i)}$ for i=1...m: \n$$ J = - \\frac{1}{m} \\sum_{i = 1}^m \\large ( \\small y^{(i)} \\log a^{ [2] (i)} + (1-y^{(i)})\\log (1-a^{ [2] (i)} )\\large )\\small\\tag{2}$$\n\nyou can do it in one line of code in tensorflow!\n\n**Exercise**: Implement the cross entropy loss. The function you will use is: \n\n\n- `tf.nn.sigmoid_cross_entropy_with_logits(logits = ..., labels = ...)`\n\nYour code should input `z`, compute the sigmoid (to get `a`) and then compute the cross entropy cost $J$. All this can be done using one call to `tf.nn.sigmoid_cross_entropy_with_logits`, which computes\n\n$$- \\frac{1}{m} \\sum_{i = 1}^m \\large ( \\small y^{(i)} \\log \\sigma(z^{[2](i)}) + (1-y^{(i)})\\log (1-\\sigma(z^{[2](i)})\\large )\\small\\tag{2}$$\n\n",
"_____no_output_____"
]
],
[
[
"# GRADED FUNCTION: cost\n\ndef cost(logits, labels):\n \"\"\"\n Computes the cost using the sigmoid cross entropy\n \n Arguments:\n logits -- vector containing z, output of the last linear unit (before the final sigmoid activation)\n labels -- vector of labels y (1 or 0) \n \n Note: What we've been calling \"z\" and \"y\" in this class are respectively called \"logits\" and \"labels\" \n in the TensorFlow documentation. So logits will feed into z, and labels into y. \n \n Returns:\n cost -- runs the session of the cost (formula (2))\n \"\"\"\n \n ### START CODE HERE ### \n \n # Create the placeholders for \"logits\" (z) and \"labels\" (y) (approx. 2 lines)\n z = tf.placeholder(dtype=tf.float32, name=\"logits\")\n y = tf.placeholder(dtype=tf.float32, name=\"labels\")\n \n # Use the loss function (approx. 1 line)\n cost = tf.nn.sigmoid_cross_entropy_with_logits(logits=z, labels=y)\n \n # Create a session (approx. 1 line). See method 1 above.\n sess = tf.Session()\n \n # Run the session (approx. 1 line).\n cost = sess.run(cost, feed_dict={z: logits, y: labels})\n \n # Close the session (approx. 1 line). See method 1 above.\n sess.close()\n \n ### END CODE HERE ###\n \n return cost",
"_____no_output_____"
],
[
"logits = sigmoid(np.array([0.2,0.4,0.7,0.9]))\ncost = cost(logits, np.array([0,0,1,1]))\nprint (\"cost = \" + str(cost))",
"cost = [ 1.00538719 1.03664088 0.41385433 0.39956614]\n"
]
],
[
[
"** Expected Output** : \n\n<table> \n <tr> \n <td>\n **cost**\n </td>\n <td>\n [ 1.00538719 1.03664088 0.41385433 0.39956614]\n </td>\n </tr>\n\n</table>",
"_____no_output_____"
],
[
"### 1.4 - Using One Hot encodings\n\nMany times in deep learning you will have a y vector with numbers ranging from 0 to C-1, where C is the number of classes. If C is for example 4, then you might have the following y vector which you will need to convert as follows:\n\n\n<img src=\"images/onehot.png\" style=\"width:600px;height:150px;\">\n\nThis is called a \"one hot\" encoding, because in the converted representation exactly one element of each column is \"hot\" (meaning set to 1). To do this conversion in numpy, you might have to write a few lines of code. In tensorflow, you can use one line of code: \n\n- tf.one_hot(labels, depth, axis) \n\n**Exercise:** Implement the function below to take one vector of labels and the total number of classes $C$, and return the one hot encoding. Use `tf.one_hot()` to do this. ",
"_____no_output_____"
]
],
[
[
"# GRADED FUNCTION: one_hot_matrix\n\ndef one_hot_matrix(labels, C):\n \"\"\"\n Creates a matrix where the i-th row corresponds to the ith class number and the jth column\n corresponds to the jth training example. So if example j had a label i. Then entry (i,j) \n will be 1. \n \n Arguments:\n labels -- vector containing the labels \n C -- number of classes, the depth of the one hot dimension\n \n Returns: \n one_hot -- one hot matrix\n \"\"\"\n \n ### START CODE HERE ###\n \n # Create a tf.constant equal to C (depth), name it 'C'. (approx. 1 line)\n C = tf.constant(C, dtype=tf.int32, name=\"C\")\n \n # Use tf.one_hot, be careful with the axis (approx. 1 line)\n one_hot_matrix = tf.one_hot(labels, C, axis=0)\n \n # Create the session (approx. 1 line)\n sess = tf.Session()\n \n # Run the session (approx. 1 line)\n one_hot = sess.run(one_hot_matrix)\n \n # Close the session (approx. 1 line). See method 1 above.\n sess.close()\n \n ### END CODE HERE ###\n \n return one_hot",
"_____no_output_____"
],
[
"labels = np.array([1,2,3,0,2,1])\none_hot = one_hot_matrix(labels, C = 4)\nprint (\"one_hot = \" + str(one_hot))",
"one_hot = [[ 0. 0. 0. 1. 0. 0.]\n [ 1. 0. 0. 0. 0. 1.]\n [ 0. 1. 0. 0. 1. 0.]\n [ 0. 0. 1. 0. 0. 0.]]\n"
]
],
[
[
"**Expected Output**: \n\n<table> \n <tr> \n <td>\n **one_hot**\n </td>\n <td>\n [[ 0. 0. 0. 1. 0. 0.]\n [ 1. 0. 0. 0. 0. 1.]\n [ 0. 1. 0. 0. 1. 0.]\n [ 0. 0. 1. 0. 0. 0.]]\n </td>\n </tr>\n\n</table>\n",
"_____no_output_____"
],
[
"### 1.5 - Initialize with zeros and ones\n\nNow you will learn how to initialize a vector of zeros and ones. The function you will be calling is `tf.ones()`. To initialize with zeros you could use tf.zeros() instead. These functions take in a shape and return an array of dimension shape full of zeros and ones respectively. \n\n**Exercise:** Implement the function below to take in a shape and to return an array (of the shape's dimension of ones). \n\n - tf.ones(shape)\n",
"_____no_output_____"
]
],
[
[
"# GRADED FUNCTION: ones\n\ndef ones(shape):\n \"\"\"\n Creates an array of ones of dimension shape\n \n Arguments:\n shape -- shape of the array you want to create\n \n Returns: \n ones -- array containing only ones\n \"\"\"\n \n ### START CODE HERE ###\n \n # Create \"ones\" tensor using tf.ones(...). (approx. 1 line)\n ones = tf.ones(shape)\n \n # Create the session (approx. 1 line)\n sess = tf.Session()\n \n # Run the session to compute 'ones' (approx. 1 line)\n ones = sess.run(ones)\n \n # Close the session (approx. 1 line). See method 1 above.\n sess.close()\n \n ### END CODE HERE ###\n return ones",
"_____no_output_____"
],
[
"print (\"ones = \" + str(ones([3])))",
"ones = [ 1. 1. 1.]\n"
]
],
[
[
"**Expected Output:**\n\n<table> \n <tr> \n <td>\n **ones**\n </td>\n <td>\n [ 1. 1. 1.]\n </td>\n </tr>\n\n</table>",
"_____no_output_____"
],
[
"# 2 - Building your first neural network in tensorflow\n\nIn this part of the assignment you will build a neural network using tensorflow. Remember that there are two parts to implement a tensorflow model:\n\n- Create the computation graph\n- Run the graph\n\nLet's delve into the problem you'd like to solve!\n\n### 2.0 - Problem statement: SIGNS Dataset\n\nOne afternoon, with some friends we decided to teach our computers to decipher sign language. We spent a few hours taking pictures in front of a white wall and came up with the following dataset. It's now your job to build an algorithm that would facilitate communications from a speech-impaired person to someone who doesn't understand sign language.\n\n- **Training set**: 1080 pictures (64 by 64 pixels) of signs representing numbers from 0 to 5 (180 pictures per number).\n- **Test set**: 120 pictures (64 by 64 pixels) of signs representing numbers from 0 to 5 (20 pictures per number).\n\nNote that this is a subset of the SIGNS dataset. The complete dataset contains many more signs.\n\nHere are examples for each number, and how an explanation of how we represent the labels. These are the original pictures, before we lowered the image resolutoion to 64 by 64 pixels.\n<img src=\"images/hands.png\" style=\"width:800px;height:350px;\"><caption><center> <u><font color='purple'> **Figure 1**</u><font color='purple'>: SIGNS dataset <br> <font color='black'> </center>\n\n\nRun the following code to load the dataset.",
"_____no_output_____"
]
],
[
[
"# Loading the dataset\nX_train_orig, Y_train_orig, X_test_orig, Y_test_orig, classes = load_dataset()",
"_____no_output_____"
]
],
[
[
"Change the index below and run the cell to visualize some examples in the dataset.",
"_____no_output_____"
]
],
[
[
"# Example of a picture\nindex = 0\nplt.imshow(X_train_orig[index])\nprint (\"y = \" + str(np.squeeze(Y_train_orig[:, index])))",
"y = 5\n"
]
],
[
[
"As usual you flatten the image dataset, then normalize it by dividing by 255. On top of that, you will convert each label to a one-hot vector as shown in Figure 1. Run the cell below to do so.",
"_____no_output_____"
]
],
[
[
"# Flatten the training and test images\nX_train_flatten = X_train_orig.reshape(X_train_orig.shape[0], -1).T\nX_test_flatten = X_test_orig.reshape(X_test_orig.shape[0], -1).T\n# Normalize image vectors\nX_train = X_train_flatten/255.\nX_test = X_test_flatten/255.\n# Convert training and test labels to one hot matrices\nY_train = convert_to_one_hot(Y_train_orig, 6)\nY_test = convert_to_one_hot(Y_test_orig, 6)\n\nprint (\"number of training examples = \" + str(X_train.shape[1]))\nprint (\"number of test examples = \" + str(X_test.shape[1]))\nprint (\"X_train shape: \" + str(X_train.shape))\nprint (\"Y_train shape: \" + str(Y_train.shape))\nprint (\"X_test shape: \" + str(X_test.shape))\nprint (\"Y_test shape: \" + str(Y_test.shape))",
"number of training examples = 1080\nnumber of test examples = 120\nX_train shape: (12288, 1080)\nY_train shape: (6, 1080)\nX_test shape: (12288, 120)\nY_test shape: (6, 120)\n"
]
],
[
[
"**Note** that 12288 comes from $64 \\times 64 \\times 3$. Each image is square, 64 by 64 pixels, and 3 is for the RGB colors. Please make sure all these shapes make sense to you before continuing.",
"_____no_output_____"
],
[
"**Your goal** is to build an algorithm capable of recognizing a sign with high accuracy. To do so, you are going to build a tensorflow model that is almost the same as one you have previously built in numpy for cat recognition (but now using a softmax output). It is a great occasion to compare your numpy implementation to the tensorflow one. \n\n**The model** is *LINEAR -> RELU -> LINEAR -> RELU -> LINEAR -> SOFTMAX*. The SIGMOID output layer has been converted to a SOFTMAX. A SOFTMAX layer generalizes SIGMOID to when there are more than two classes. ",
"_____no_output_____"
],
[
"### 2.1 - Create placeholders\n\nYour first task is to create placeholders for `X` and `Y`. This will allow you to later pass your training data in when you run your session. \n\n**Exercise:** Implement the function below to create the placeholders in tensorflow.",
"_____no_output_____"
]
],
[
[
"# GRADED FUNCTION: create_placeholders\n\ndef create_placeholders(n_x, n_y):\n \"\"\"\n Creates the placeholders for the tensorflow session.\n \n Arguments:\n n_x -- scalar, size of an image vector (num_px * num_px = 64 * 64 * 3 = 12288)\n n_y -- scalar, number of classes (from 0 to 5, so -> 6)\n \n Returns:\n X -- placeholder for the data input, of shape [n_x, None] and dtype \"float\"\n Y -- placeholder for the input labels, of shape [n_y, None] and dtype \"float\"\n \n Tips:\n - You will use None because it let's us be flexible on the number of examples you will for the placeholders.\n In fact, the number of examples during test/train is different.\n \"\"\"\n\n ### START CODE HERE ### (approx. 2 lines)\n X = tf.placeholder(dtype=tf.float32, shape=[n_x, None], name=\"X\")\n Y = tf.placeholder(dtype=tf.float32, shape=[n_y, None], name= \"Y\")\n ### END CODE HERE ###\n \n return X, Y",
"_____no_output_____"
],
[
"X, Y = create_placeholders(12288, 6)\nprint (\"X = \" + str(X))\nprint (\"Y = \" + str(Y))",
"X = Tensor(\"X_3:0\", shape=(12288, ?), dtype=float32)\nY = Tensor(\"Y_2:0\", shape=(6, ?), dtype=float32)\n"
]
],
[
[
"**Expected Output**: \n\n<table> \n <tr> \n <td>\n **X**\n </td>\n <td>\n Tensor(\"Placeholder_1:0\", shape=(12288, ?), dtype=float32) (not necessarily Placeholder_1)\n </td>\n </tr>\n <tr> \n <td>\n **Y**\n </td>\n <td>\n Tensor(\"Placeholder_2:0\", shape=(10, ?), dtype=float32) (not necessarily Placeholder_2)\n </td>\n </tr>\n\n</table>",
"_____no_output_____"
],
[
"### 2.2 - Initializing the parameters\n\nYour second task is to initialize the parameters in tensorflow.\n\n**Exercise:** Implement the function below to initialize the parameters in tensorflow. You are going use Xavier Initialization for weights and Zero Initialization for biases. The shapes are given below. As an example, to help you, for W1 and b1 you could use: \n\n```python\nW1 = tf.get_variable(\"W1\", [25,12288], initializer = tf.contrib.layers.xavier_initializer(seed = 1))\nb1 = tf.get_variable(\"b1\", [25,1], initializer = tf.zeros_initializer())\n```\nPlease use `seed = 1` to make sure your results match ours.",
"_____no_output_____"
]
],
[
[
"# GRADED FUNCTION: initialize_parameters\n\ndef initialize_parameters():\n \"\"\"\n Initializes parameters to build a neural network with tensorflow. The shapes are:\n W1 : [25, 12288]\n b1 : [25, 1]\n W2 : [12, 25]\n b2 : [12, 1]\n W3 : [6, 12]\n b3 : [6, 1]\n \n Returns:\n parameters -- a dictionary of tensors containing W1, b1, W2, b2, W3, b3\n \"\"\"\n \n tf.set_random_seed(1) # so that your \"random\" numbers match ours\n \n ### START CODE HERE ### (approx. 6 lines of code)\n W1 = tf.get_variable(\"W1\", [25,12288], initializer = tf.contrib.layers.xavier_initializer(seed = 1))\n b1 = tf.get_variable(\"b1\", [25,1], initializer = tf.zeros_initializer())\n W2 = tf.get_variable(\"W2\", [12,25], initializer = tf.contrib.layers.xavier_initializer(seed = 1))\n b2 = tf.get_variable(\"b2\", [12,1], initializer = tf.zeros_initializer())\n W3 = tf.get_variable(\"W3\", [6,12], initializer = tf.contrib.layers.xavier_initializer(seed = 1))\n b3 = tf.get_variable(\"b3\", [6,1], initializer = tf.zeros_initializer())\n ### END CODE HERE ###\n\n parameters = {\"W1\": W1,\n \"b1\": b1,\n \"W2\": W2,\n \"b2\": b2,\n \"W3\": W3,\n \"b3\": b3}\n \n return parameters",
"_____no_output_____"
],
[
"tf.reset_default_graph()\nwith tf.Session() as sess:\n parameters = initialize_parameters()\n print(\"W1 = \" + str(parameters[\"W1\"]))\n print(\"b1 = \" + str(parameters[\"b1\"]))\n print(\"W2 = \" + str(parameters[\"W2\"]))\n print(\"b2 = \" + str(parameters[\"b2\"]))",
"W1 = <tf.Variable 'W1:0' shape=(25, 12288) dtype=float32_ref>\nb1 = <tf.Variable 'b1:0' shape=(25, 1) dtype=float32_ref>\nW2 = <tf.Variable 'W2:0' shape=(12, 25) dtype=float32_ref>\nb2 = <tf.Variable 'b2:0' shape=(12, 1) dtype=float32_ref>\n"
]
],
[
[
"**Expected Output**: \n\n<table> \n <tr> \n <td>\n **W1**\n </td>\n <td>\n < tf.Variable 'W1:0' shape=(25, 12288) dtype=float32_ref >\n </td>\n </tr>\n <tr> \n <td>\n **b1**\n </td>\n <td>\n < tf.Variable 'b1:0' shape=(25, 1) dtype=float32_ref >\n </td>\n </tr>\n <tr> \n <td>\n **W2**\n </td>\n <td>\n < tf.Variable 'W2:0' shape=(12, 25) dtype=float32_ref >\n </td>\n </tr>\n <tr> \n <td>\n **b2**\n </td>\n <td>\n < tf.Variable 'b2:0' shape=(12, 1) dtype=float32_ref >\n </td>\n </tr>\n\n</table>",
"_____no_output_____"
],
[
"As expected, the parameters haven't been evaluated yet.",
"_____no_output_____"
],
[
"### 2.3 - Forward propagation in tensorflow \n\nYou will now implement the forward propagation module in tensorflow. The function will take in a dictionary of parameters and it will complete the forward pass. The functions you will be using are: \n\n- `tf.add(...,...)` to do an addition\n- `tf.matmul(...,...)` to do a matrix multiplication\n- `tf.nn.relu(...)` to apply the ReLU activation\n\n**Question:** Implement the forward pass of the neural network. We commented for you the numpy equivalents so that you can compare the tensorflow implementation to numpy. It is important to note that the forward propagation stops at `z3`. The reason is that in tensorflow the last linear layer output is given as input to the function computing the loss. Therefore, you don't need `a3`!\n\n",
"_____no_output_____"
]
],
[
[
"# GRADED FUNCTION: forward_propagation\n\ndef forward_propagation(X, parameters):\n \"\"\"\n Implements the forward propagation for the model: LINEAR -> RELU -> LINEAR -> RELU -> LINEAR -> SOFTMAX\n \n Arguments:\n X -- input dataset placeholder, of shape (input size, number of examples)\n parameters -- python dictionary containing your parameters \"W1\", \"b1\", \"W2\", \"b2\", \"W3\", \"b3\"\n the shapes are given in initialize_parameters\n\n Returns:\n Z3 -- the output of the last LINEAR unit\n \"\"\"\n \n # Retrieve the parameters from the dictionary \"parameters\" \n W1 = parameters['W1']\n b1 = parameters['b1']\n W2 = parameters['W2']\n b2 = parameters['b2']\n W3 = parameters['W3']\n b3 = parameters['b3']\n \n ### START CODE HERE ### (approx. 5 lines) # Numpy Equivalents:\n Z1 = tf.add(tf.matmul(W1, X), b1) # Z1 = np.dot(W1, X) + b1\n A1 = tf.nn.relu(Z1) # A1 = relu(Z1)\n Z2 = tf.add(tf.matmul(W2, A1), b2) # Z2 = np.dot(W2, a1) + b2\n A2 = tf.nn.relu(Z2) # A2 = relu(Z2)\n Z3 = tf.add(tf.matmul(W3, A2), b3) # Z3 = np.dot(W3,Z2) + b3\n ### END CODE HERE ###\n \n return Z3",
"_____no_output_____"
],
[
"tf.reset_default_graph()\n\nwith tf.Session() as sess:\n X, Y = create_placeholders(12288, 6)\n parameters = initialize_parameters()\n Z3 = forward_propagation(X, parameters)\n print(\"Z3 = \" + str(Z3))",
"Z3 = Tensor(\"Add_2:0\", shape=(6, ?), dtype=float32)\n"
]
],
[
[
"**Expected Output**: \n\n<table> \n <tr> \n <td>\n **Z3**\n </td>\n <td>\n Tensor(\"Add_2:0\", shape=(6, ?), dtype=float32)\n </td>\n </tr>\n\n</table>",
"_____no_output_____"
],
[
"You may have noticed that the forward propagation doesn't output any cache. You will understand why below, when we get to brackpropagation.",
"_____no_output_____"
],
[
"### 2.4 Compute cost\n\nAs seen before, it is very easy to compute the cost using:\n```python\ntf.reduce_mean(tf.nn.softmax_cross_entropy_with_logits(logits = ..., labels = ...))\n```\n**Question**: Implement the cost function below. \n- It is important to know that the \"`logits`\" and \"`labels`\" inputs of `tf.nn.softmax_cross_entropy_with_logits` are expected to be of shape (number of examples, num_classes). We have thus transposed Z3 and Y for you.\n- Besides, `tf.reduce_mean` basically does the summation over the examples.",
"_____no_output_____"
]
],
[
[
"# GRADED FUNCTION: compute_cost \n\ndef compute_cost(Z3, Y):\n \"\"\"\n Computes the cost\n \n Arguments:\n Z3 -- output of forward propagation (output of the last LINEAR unit), of shape (6, number of examples)\n Y -- \"true\" labels vector placeholder, same shape as Z3\n \n Returns:\n cost - Tensor of the cost function\n \"\"\"\n \n # to fit the tensorflow requirement for tf.nn.softmax_cross_entropy_with_logits(...,...)\n logits = tf.transpose(Z3)\n labels = tf.transpose(Y)\n \n ### START CODE HERE ### (1 line of code)\n cost = tf.reduce_mean(tf.nn.softmax_cross_entropy_with_logits(logits = logits, labels = labels))\n ### END CODE HERE ###\n \n return cost",
"_____no_output_____"
],
[
"tf.reset_default_graph()\n\nwith tf.Session() as sess:\n X, Y = create_placeholders(12288, 6)\n parameters = initialize_parameters()\n Z3 = forward_propagation(X, parameters)\n cost = compute_cost(Z3, Y)\n print(\"cost = \" + str(cost))",
"cost = Tensor(\"Mean:0\", shape=(), dtype=float32)\n"
]
],
[
[
"**Expected Output**: \n\n<table> \n <tr> \n <td>\n **cost**\n </td>\n <td>\n Tensor(\"Mean:0\", shape=(), dtype=float32)\n </td>\n </tr>\n\n</table>",
"_____no_output_____"
],
[
"### 2.5 - Backward propagation & parameter updates\n\nThis is where you become grateful to programming frameworks. All the backpropagation and the parameters update is taken care of in 1 line of code. It is very easy to incorporate this line in the model.\n\nAfter you compute the cost function. You will create an \"`optimizer`\" object. You have to call this object along with the cost when running the tf.session. When called, it will perform an optimization on the given cost with the chosen method and learning rate.\n\nFor instance, for gradient descent the optimizer would be:\n```python\noptimizer = tf.train.GradientDescentOptimizer(learning_rate = learning_rate).minimize(cost)\n```\n\nTo make the optimization you would do:\n```python\n_ , c = sess.run([optimizer, cost], feed_dict={X: minibatch_X, Y: minibatch_Y})\n```\n\nThis computes the backpropagation by passing through the tensorflow graph in the reverse order. From cost to inputs.\n\n**Note** When coding, we often use `_` as a \"throwaway\" variable to store values that we won't need to use later. Here, `_` takes on the evaluated value of `optimizer`, which we don't need (and `c` takes the value of the `cost` variable). ",
"_____no_output_____"
],
[
"### 2.6 - Building the model\n\nNow, you will bring it all together! \n\n**Exercise:** Implement the model. You will be calling the functions you had previously implemented.",
"_____no_output_____"
]
],
[
[
"def model(X_train, Y_train, X_test, Y_test, learning_rate = 0.0001,\n num_epochs = 1500, minibatch_size = 32, print_cost = True):\n \"\"\"\n Implements a three-layer tensorflow neural network: LINEAR->RELU->LINEAR->RELU->LINEAR->SOFTMAX.\n \n Arguments:\n X_train -- training set, of shape (input size = 12288, number of training examples = 1080)\n Y_train -- test set, of shape (output size = 6, number of training examples = 1080)\n X_test -- training set, of shape (input size = 12288, number of training examples = 120)\n Y_test -- test set, of shape (output size = 6, number of test examples = 120)\n learning_rate -- learning rate of the optimization\n num_epochs -- number of epochs of the optimization loop\n minibatch_size -- size of a minibatch\n print_cost -- True to print the cost every 100 epochs\n \n Returns:\n parameters -- parameters learnt by the model. They can then be used to predict.\n \"\"\"\n \n ops.reset_default_graph() # to be able to rerun the model without overwriting tf variables\n tf.set_random_seed(1) # to keep consistent results\n seed = 3 # to keep consistent results\n (n_x, m) = X_train.shape # (n_x: input size, m : number of examples in the train set)\n n_y = Y_train.shape[0] # n_y : output size\n costs = [] # To keep track of the cost\n \n # Create Placeholders of shape (n_x, n_y)\n ### START CODE HERE ### (1 line)\n X, Y = create_placeholders(n_x, n_y)\n ### END CODE HERE ###\n\n # Initialize parameters\n ### START CODE HERE ### (1 line)\n parameters = initialize_parameters()\n ### END CODE HERE ###\n \n # Forward propagation: Build the forward propagation in the tensorflow graph\n ### START CODE HERE ### (1 line)\n Z3 = forward_propagation(X, parameters)\n ### END CODE HERE ###\n \n # Cost function: Add cost function to tensorflow graph\n ### START CODE HERE ### (1 line)\n cost = compute_cost(Z3, Y)\n ### END CODE HERE ###\n \n # Backpropagation: Define the tensorflow optimizer. Use an AdamOptimizer.\n ### START CODE HERE ### (1 line)\n optimizer = tf.train.AdamOptimizer(learning_rate).minimize(cost)\n ### END CODE HERE ###\n \n # Initialize all the variables\n init = tf.global_variables_initializer()\n\n # Start the session to compute the tensorflow graph\n with tf.Session() as sess:\n \n # Run the initialization\n sess.run(init)\n \n # Do the training loop\n for epoch in range(num_epochs):\n\n epoch_cost = 0. # Defines a cost related to an epoch\n num_minibatches = int(m / minibatch_size) # number of minibatches of size minibatch_size in the train set\n seed = seed + 1\n minibatches = random_mini_batches(X_train, Y_train, minibatch_size, seed)\n\n for minibatch in minibatches:\n\n # Select a minibatch\n (minibatch_X, minibatch_Y) = minibatch\n \n # IMPORTANT: The line that runs the graph on a minibatch.\n # Run the session to execute the \"optimizer\" and the \"cost\", the feedict should contain a minibatch for (X,Y).\n ### START CODE HERE ### (1 line)\n _ , minibatch_cost = sess.run([optimizer, cost], feed_dict={X: minibatch_X, Y: minibatch_Y})\n ### END CODE HERE ###\n \n epoch_cost += minibatch_cost / num_minibatches\n\n # Print the cost every epoch\n if print_cost == True and epoch % 100 == 0:\n print (\"Cost after epoch %i: %f\" % (epoch, epoch_cost))\n if print_cost == True and epoch % 5 == 0:\n costs.append(epoch_cost)\n \n # plot the cost\n plt.plot(np.squeeze(costs))\n plt.ylabel('cost')\n plt.xlabel('iterations (per tens)')\n plt.title(\"Learning rate =\" + str(learning_rate))\n plt.show()\n\n # lets save the parameters in a variable\n parameters = sess.run(parameters)\n print (\"Parameters have been trained!\")\n\n # Calculate the correct predictions\n correct_prediction = tf.equal(tf.argmax(Z3), tf.argmax(Y))\n\n # Calculate accuracy on the test set\n accuracy = tf.reduce_mean(tf.cast(correct_prediction, \"float\"))\n\n print (\"Train Accuracy:\", accuracy.eval({X: X_train, Y: Y_train}))\n print (\"Test Accuracy:\", accuracy.eval({X: X_test, Y: Y_test}))\n \n return parameters",
"_____no_output_____"
]
],
[
[
"Run the following cell to train your model! On our machine it takes about 5 minutes. Your \"Cost after epoch 100\" should be 1.016458. If it's not, don't waste time; interrupt the training by clicking on the square (⬛) in the upper bar of the notebook, and try to correct your code. If it is the correct cost, take a break and come back in 5 minutes!",
"_____no_output_____"
]
],
[
[
"parameters = model(X_train, Y_train, X_test, Y_test)",
"Cost after epoch 0: 1.855702\nCost after epoch 100: 1.016458\nCost after epoch 200: 0.733102\nCost after epoch 300: 0.572940\nCost after epoch 400: 0.468774\nCost after epoch 500: 0.381021\nCost after epoch 600: 0.313822\nCost after epoch 700: 0.254158\nCost after epoch 800: 0.203829\nCost after epoch 900: 0.166421\nCost after epoch 1000: 0.141486\nCost after epoch 1100: 0.107580\nCost after epoch 1200: 0.086270\nCost after epoch 1300: 0.059371\nCost after epoch 1400: 0.052228\n"
]
],
[
[
"**Expected Output**:\n\n<table> \n <tr> \n <td>\n **Train Accuracy**\n </td>\n <td>\n 0.999074\n </td>\n </tr>\n <tr> \n <td>\n **Test Accuracy**\n </td>\n <td>\n 0.716667\n </td>\n </tr>\n\n</table>\n\nAmazing, your algorithm can recognize a sign representing a figure between 0 and 5 with 71.7% accuracy.\n\n**Insights**:\n- Your model seems big enough to fit the training set well. However, given the difference between train and test accuracy, you could try to add L2 or dropout regularization to reduce overfitting. \n- Think about the session as a block of code to train the model. Each time you run the session on a minibatch, it trains the parameters. In total you have run the session a large number of times (1500 epochs) until you obtained well trained parameters.",
"_____no_output_____"
],
[
"### 2.7 - Test with your own image (optional / ungraded exercise)\n\nCongratulations on finishing this assignment. You can now take a picture of your hand and see the output of your model. To do that:\n 1. Click on \"File\" in the upper bar of this notebook, then click \"Open\" to go on your Coursera Hub.\n 2. Add your image to this Jupyter Notebook's directory, in the \"images\" folder\n 3. Write your image's name in the following code\n 4. Run the code and check if the algorithm is right!",
"_____no_output_____"
]
],
[
[
"import scipy\nfrom PIL import Image\nfrom scipy import ndimage\n\n## START CODE HERE ## (PUT YOUR IMAGE NAME) \nmy_image = \"thumbs_up.jpg\"\n## END CODE HERE ##\n\n# We preprocess your image to fit your algorithm.\nfname = \"images/\" + my_image\nimage = np.array(ndimage.imread(fname, flatten=False))\nmy_image = scipy.misc.imresize(image, size=(64,64)).reshape((1, 64*64*3)).T\nmy_image_prediction = predict(my_image, parameters)\n\nplt.imshow(image)\nprint(\"Your algorithm predicts: y = \" + str(np.squeeze(my_image_prediction)))",
"Your algorithm predicts: y = 3\n"
]
],
[
[
"You indeed deserved a \"thumbs-up\" although as you can see the algorithm seems to classify it incorrectly. The reason is that the training set doesn't contain any \"thumbs-up\", so the model doesn't know how to deal with it! We call that a \"mismatched data distribution\" and it is one of the various of the next course on \"Structuring Machine Learning Projects\".",
"_____no_output_____"
],
[
"<font color='blue'>\n**What you should remember**:\n- Tensorflow is a programming framework used in deep learning\n- The two main object classes in tensorflow are Tensors and Operators. \n- When you code in tensorflow you have to take the following steps:\n - Create a graph containing Tensors (Variables, Placeholders ...) and Operations (tf.matmul, tf.add, ...)\n - Create a session\n - Initialize the session\n - Run the session to execute the graph\n- You can execute the graph multiple times as you've seen in model()\n- The backpropagation and optimization is automatically done when running the session on the \"optimizer\" object.",
"_____no_output_____"
]
]
] | [
"markdown",
"code",
"markdown",
"code",
"markdown",
"code",
"markdown",
"code",
"markdown",
"code",
"markdown",
"code",
"markdown",
"code",
"markdown",
"code",
"markdown",
"code",
"markdown",
"code",
"markdown",
"code",
"markdown",
"code",
"markdown",
"code",
"markdown",
"code",
"markdown",
"code",
"markdown",
"code",
"markdown",
"code",
"markdown",
"code",
"markdown",
"code",
"markdown",
"code",
"markdown"
] | [
[
"markdown"
],
[
"code"
],
[
"markdown"
],
[
"code"
],
[
"markdown"
],
[
"code"
],
[
"markdown"
],
[
"code"
],
[
"markdown"
],
[
"code"
],
[
"markdown",
"markdown"
],
[
"code",
"code"
],
[
"markdown",
"markdown"
],
[
"code",
"code"
],
[
"markdown",
"markdown",
"markdown"
],
[
"code",
"code"
],
[
"markdown",
"markdown"
],
[
"code",
"code"
],
[
"markdown",
"markdown"
],
[
"code",
"code"
],
[
"markdown",
"markdown"
],
[
"code"
],
[
"markdown"
],
[
"code"
],
[
"markdown"
],
[
"code"
],
[
"markdown",
"markdown",
"markdown"
],
[
"code",
"code"
],
[
"markdown",
"markdown"
],
[
"code",
"code"
],
[
"markdown",
"markdown",
"markdown"
],
[
"code",
"code"
],
[
"markdown",
"markdown",
"markdown"
],
[
"code",
"code"
],
[
"markdown",
"markdown",
"markdown"
],
[
"code"
],
[
"markdown"
],
[
"code"
],
[
"markdown",
"markdown"
],
[
"code"
],
[
"markdown",
"markdown"
]
] |
e77229a5e161a366d1456376cb911d0173649817 | 3,609 | ipynb | Jupyter Notebook | .ipynb_checkpoints/LCP 4-checkpoint.ipynb | thomaspingel/lcpy | 6bf2893010d1dad5ad783d7c4f0351166425a1a7 | [
"MIT"
] | null | null | null | .ipynb_checkpoints/LCP 4-checkpoint.ipynb | thomaspingel/lcpy | 6bf2893010d1dad5ad783d7c4f0351166425a1a7 | [
"MIT"
] | null | null | null | .ipynb_checkpoints/LCP 4-checkpoint.ipynb | thomaspingel/lcpy | 6bf2893010d1dad5ad783d7c4f0351166425a1a7 | [
"MIT"
] | null | null | null | 27.340909 | 114 | 0.560543 | [
[
[
"import numpy as np\nimport pandas as pd\nimport geopandas\nimport neilpy\n\ngeopandas.io.file.fiona.drvsupport.supported_drivers['KML'] = 'rw'",
"_____no_output_____"
],
[
"# Load data\n\n# nodes must have a unique ID in the first column, and then longitudes and latitudes in columns 2 and 3.\n# edges must have start and destination unique IDs that match those in nodes\n\nnodes = pd.read_csv('data/nodes.csv',index_col=0)\nedges = pd.read_csv('data/edges.csv')\n\nE, meta = neilpy.imread('data/cusco_elevation.tif')\nS = neilpy.slope(E,meta['cellsize'])",
"_____no_output_____"
],
[
"#%% DIRECT\n\ngdf = neilpy.direct_routes(nodes,edges)\ngdf.to_file('out/direct.shp')",
"_____no_output_____"
],
[
"#%% AREAL SLOPE EXAMPLE\n\nlabel = 'tobler'\nC = neilpy.cost_tobler_hiking_function(S)\ngdf = neilpy.get_areal_routes(nodes,edges,C,meta,label=label)\ngdf.to_file('out/' + label + '.shp')\ngdf.to_file('out/' + label + '.kml',driver='KML')\n\nlabel = 'rademaker'\nC = neilpy.cost_rademaker(S)\ngdf = neilpy.get_areal_routes(nodes,edges,C,meta,label=label)\ngdf.to_file('out/' + label + '.shp')\ngdf.to_file('out/' + label + '.kml',driver='KML')",
"Creating surface network for tobler\nDone creating surface network.\nCalculating costs and routes.\nDone calculating costs and routes.\nCalculating costs and routes.\nDone calculating costs and routes.\nCreating surface network for rademaker\nDone creating surface network.\nCalculating costs and routes.\nDone calculating costs and routes.\nCalculating costs and routes.\nDone calculating costs and routes.\n"
],
[
"#%% LINEAR SLOPE EXAMPLE\n\nlabel = 'pingel_linear_9.25'\nscale_factor = 9.25\n\ndf = neilpy.create_raster_network(E)\ndf['slope'] = np.abs(df['source_value'] - df['target_value']) / (meta['cellsize']*df['distance']) #dx/dz\ndf['slope'] = np.rad2deg(np.arctan(df['slope']))\ndf['slope'] = neilpy.cost_pingel_exponential(df['slope'],scale_factor)\ndf['weight'] = df['distance'] * df['slope']\n\ngdf = neilpy.get_linear_routes(nodes, edges, df, meta, label=label)\ngdf.to_file('out/' + label + '.shp')\ngdf.to_file('out/' + label + '.kml',driver='KML')",
"_____no_output_____"
]
]
] | [
"code"
] | [
[
"code",
"code",
"code",
"code",
"code"
]
] |
e7722cc6ef69995883eab8949277d11ae1756dab | 140,460 | ipynb | Jupyter Notebook | Week 01 - Introduction to Python/Python I.ipynb | TheAIDojo/Machine_Learning_Bootcamp | ebe9f64976acf57b7c33bfe9a9a99b91e6c39111 | [
"MIT"
] | 12 | 2022-01-12T11:42:43.000Z | 2022-03-01T20:02:25.000Z | Week 01 - Introduction to Python/Python I.ipynb | TheAIDojo/Machine_Learning_Bootcamp | ebe9f64976acf57b7c33bfe9a9a99b91e6c39111 | [
"MIT"
] | null | null | null | Week 01 - Introduction to Python/Python I.ipynb | TheAIDojo/Machine_Learning_Bootcamp | ebe9f64976acf57b7c33bfe9a9a99b91e6c39111 | [
"MIT"
] | 8 | 2022-01-12T11:40:52.000Z | 2022-02-18T13:32:23.000Z | 45.55952 | 45,134 | 0.624804 | [
[
[
"# Python I\n<!--<badge>--><a href=\"https://colab.research.google.com/github/TheAIDojo/Machine_Learning_Bootcamp/blob/main/Week 01 - Introduction to Python/Python I.ipynb\" target=\"_parent\"><img src=\"https://colab.research.google.com/assets/colab-badge.svg\" alt=\"Open In Colab\"/></a><!--</badge>-->\n\nPython is an interpreted high-level general-purpose programming language. Its design philosophy emphasizes code readability with its use of significant indentation. Its language constructs as well as its object-oriented approach aim to help programmers write clear, logical code for small and large-scale projects.\n\n* [Cheatsheet](https://perso.limsi.fr/pointal/_media/python:cours:mementopython3-english.pdf)",
"_____no_output_____"
],
[
"## The Python Interpreter\n<a id='interpreter'></a>\n\nExcecution of Python programs is often performed by an **interpreter**, meaning that program statements are converted to machine executable code at **runtime** (i.e., when the program is actually run) as opposed to **compiled** into executable code before it is run by the end user. This is one of the primary ways we'll interact with Python, especially at first. We'll type some Python code and then hit the `Enter` key. This causes the code to be translated and executed. \n\nInterpretation allows great flexibility (interpreted programs can modify their source code at run time), but it's often the case that interpreted programs run much more slowly than their compiled counterparts. It's also often more difficult to find errors in interpreted programs. \n\nWe can interact with the Python interpreter via a prompt, which looks something like the following:\n\n (base) C:\\Users\\nimda>python\n Python 3.6.4 |Anaconda, Inc.| (default, Jan 16 2018, 10:22:32) [MSC v.190064 bit (AMD64)] on win32\n Type \"help\", \"copyright\", \"credits\" or \"license\" for more information.\n >>> print(\"Hello World\") # note: this will print something to the screen. \n Hello World\n >>>\n\nAbove, the `print` function prints out a string representation of the argument. The `#` denotes a comment, and the interpreter skips anything on the line after it (that is, it won't try to interpret anything after the `#`). \n\nOf course, we can save Python code into a program file and execute it later, too. \n\n\n### Jupyter Notebooks\n\nWe'll also interact with Python using Jupyter Notebooks (like this one). When we hit `Run` in the menu bar, we are performing an action analogous to hitting enter from a command prompt. The code in the active cell will be executed. Users should be aware that though we are intereacting with a Web page, there is a Web server and Python enviroment running behind the scenes. This adds a later of complexity, but the ability to mix well-formatted documentation and code makes using Jupyter Notebook worthwhile.\n\n\n<a id='variables'></a>\n## Python Identifiers and Variables\n\n### Identifiers\n\nAn **identifier** in Python is a word (a string) used to identify a variable, function, class, etc. in a Python program. It can be thought of as a proper name. Identifiers start with a letter (A-Z) or an underscore `_`; this first character is followed by a sequence of letters numbers, and underscores.\n\nCertain identifiers, such as `class` or `if` are builtin keywords and cannot be redefined by users. \n\n### Variables\n\nAs in most programming languages, **variables** play a central role in Python. We need a way to store and refer to data in our programs, and variables are the primary way to do this. Specifically, we assign data values variables using the `=`. After the assignment has been made, we may use the variable to access the data as many times as we like. \n\nIn general, the righthand side of an assignment is evaluated first (e.g., 1+1 is evaluated to 2), and afterwards the result is stored in the variable specified on the left. That explains why the last line below results in a value of 6 being printed. On evaluation of the righthand side, the current value of `blue_fish` (3) is added to itself, and the resulting value is assigned to `blue_fish`, overwriting the 3.",
"_____no_output_____"
]
],
[
[
"one_fish = 1\ntwo_fish = one_fish + 1\nblue_fish = one_fish + two_fish\nprint(one_fish)\nprint(two_fish)\nprint(blue_fish)\nblue_fish = blue_fish + blue_fish\nprint(blue_fish)",
"1\n2\n3\n6\n"
]
],
[
[
"### Dynamic Typing\n\nNote that no data type (e.g., integer, string) is specified in an assignment, even the first time a variable is used. In general, variables and types are *not* declared in Python before a value is assigned. Python is said to be a **dynamically typed** language. \n\nThe below code is perfectly fine in Python, but assigning a number and then a string in another lanauge such as Java would cause an error.",
"_____no_output_____"
]
],
[
[
"a = 1\nprint(a)\na = \"hello\"\nprint(a)",
"1\nhello\n"
]
],
[
[
"## Data Types\n<a id='datatypes'></a>\n\nAs in most programming languages, each data value in a Python program has a **data type** (even though we typically don't specify it). We'll discuss some of the datatypes here. \n\nFor a given data value, we can get its type using the `type` function, which takes an argument. The below print expressions show several of the built-in data types (and how literal values are parsed by default). ",
"_____no_output_____"
]
],
[
[
"print(type(1)) # an integer\nprint(type(2.0)) # a float\nprint(type(\"hi!\")) # a string\nprint(type(True)) # a boolean value\nprint(type([1, 2, 3, 4, 5])) # a list (a mutable collection)\nprint(type((1, 2, 3, 4, 5))) # a tuple (an immutable collection)\nprint(type({\"fname\": \"john\", \"lname\": \"doe\"})) # a dictionary (a collection of key-value pairs)",
"<class 'int'>\n<class 'float'>\n<class 'str'>\n<class 'bool'>\n<class 'list'>\n<class 'tuple'>\n<class 'dict'>\n"
]
],
[
[
"<a id='numbers'></a>\n### Numbers\n\nThe basic numerical data types of python are:\n* `int` (integer values), \n* `float` (floating point numbers), and \n* `complex` (complex numbers). ",
"_____no_output_____"
]
],
[
[
"x = 1 # \ny = 1.0\nz = 1 + 2j\nw = 1e10\nv = 1.0\nu = 2j\nprint(type(x), \": \", x)\nprint(type(y), \": \", y)\nprint(type(z), \": \", z)\nprint(type(w), \": \", w)\nprint(type(u), \": \", v)\nprint(type(u), \": \", u)",
"<class 'int'> : 1\n<class 'float'> : 1.0\n<class 'complex'> : (1+2j)\n<class 'float'> : 10000000000.0\n<class 'complex'> : 1.0\n<class 'complex'> : 2j\n"
]
],
[
[
"In general, a number written as a simply integer will, unsurprisingly, be interpreted in Python as an `int`.\n\nNumbers written using a `.` or scientific notation are interpreted as floats. Numbers written using `j` are interpreted as complex numbers.\n\n**NOTE**: Unlike some other languages, Python 3 does not have minimum or maxium integer values (Python 2 does, however). \n\n<a id='arithmetic'></a>\n### Arithmetic\n\nThe arithmetic operations available in most languages are also present in Python (with a default precedence on operations). ",
"_____no_output_____"
]
],
[
[
"1 + 3 - (3 - 2) # simple addition and subtraction",
"_____no_output_____"
],
[
"4 * 2.0 # multiplication of an int and a float (yields a float)",
"_____no_output_____"
],
[
"5 / 2 # floating point division",
"_____no_output_____"
],
[
"print(5.6 // 2) # integer division\nprint(type(5.6 // 2))",
"2.0\n<class 'float'>\n"
],
[
"5 % 2 # modulo operator (straightforwardly, the integer remainder of 5/2)",
"_____no_output_____"
],
[
"2 % -5 # (not so intuitive if negative numbers are involved)",
"_____no_output_____"
],
[
"2**4 # exponentiation",
"_____no_output_____"
]
],
[
[
"### Data Type of results\n\nWhen two numbers of different types are used in an arithmetic operation, the data type is usually what one would expect, but there are some cases where it's different than either operand. For instance, though 5 and 2 are both integers, the result of `5/2` is a `float`, and the result of `5.2//2` (integer division) is a float. ",
"_____no_output_____"
],
[
"### Strings\n<a id='strings'></a>\nStrings in Python (datatype `str`) can be enclosed in single (`'`) or double (`\"`) quotes. It doesn't matter which is used, but the opening and closing marks must be of the same type. The backslash `\\` is used to escape quotes in a string as well as to indicate other escape characters (e.g., `\\n` indicates a new line). Upon printing, the string is formatted appropriately. ",
"_____no_output_____"
]
],
[
[
"print(\"This is a string\")\nprint('this is a string containing \"quotes\"')\nprint('this is another string containing \"quotes\"')\nprint(\"this is string\\nhas two lines\")",
"This is a string\nthis is a string containing \"quotes\"\nthis is another string containing \"quotes\"\nthis is string\nhas two lines\n"
]
],
[
[
"To prevent processing of escape characters, you can use indicate a *raw* string by putting an `r` before the string. ",
"_____no_output_____"
]
],
[
[
"print(r\"this is string \\n has only one line\")",
"this is string \\n has only one line\n"
]
],
[
[
"#### Multiline Strings\nMultiline strings can be delineated using 3 quotes. If you do not wish to include a line end in the output, you can end the line with `\\`.",
"_____no_output_____"
]
],
[
[
"print(\n \"\"\"Line 1\nLine 2\nLine 3\\\nLine 3 continued\"\"\"\n)",
"Line 1\nLine 2\nLine 3Line 3 continued\n"
]
],
[
[
"#### String Concatenation \nStrings can be concatenated. You must be careful when trying to concatenate other types to a string, however. They must be \nconverted to strings first using `str()`. ",
"_____no_output_____"
]
],
[
[
"print(\"This\" + \" line contains \" + str(4) + \" components\")\nprint(\n \"Here are some things converted to strings: \"\n + str(2.3)\n + \", \"\n + str(True)\n + \", \"\n + str((1, 2))\n)",
"This line contains 4 components\nHere are some things converted to strings: 2.3, True, (1, 2)\n"
]
],
[
[
"`print` can take an arbitrary number of arguments. Leveraging this eliminates the need to explicitly convert data values to strings (because we're no longer attempting to concatenate strings).",
"_____no_output_____"
]
],
[
[
"print(\"This\", \"line contains\", 4, \"components\")\nprint(\"Here are some things converted to strings:\", 2.3, \",\", True, \",\", (1, 2))",
"This line contains 4 components\nHere are some things converted to strings: 2.3 , True , (1, 2)\n"
]
],
[
[
"Note, however, that `print` will by default insert a space between elements. If you wish to change the separator between items (e.g. to `,`) , add `sep=\",\"` as an argument. ",
"_____no_output_____"
]
],
[
[
"print(\"This\", \"line contains\", 4, \"components\", sep=\"---\")\nprint(\n \"Here are some things converted to strings:\", 2.3, \",\", True, \",\", (1, 2), sep=\"---\"\n)",
"This---line contains---4---components\nHere are some things converted to strings:---2.3---,---True---,---(1, 2)\n"
]
],
[
[
"You can also create a string from another string by *multiplying* it with a number",
"_____no_output_____"
]
],
[
[
"word1 = \"abba\"\nword2 = 3 * word1\nprint(word2)",
"abbaabbaabba\n"
]
],
[
[
"Also, if multiple **string literals** (as opposed to variables or string expressions) appear consecutively, they will be combined into one string. ",
"_____no_output_____"
]
],
[
[
"a = \"this \" \"is \" \"the \" \"way \" \"the \" \"world \" \"ends.\"\nprint(a)\nprint(type(a))\na = \"this \", \"is \", \"the \", \"way \", \"the \", \"world \", \"ends.\"\nprint(a)\nprint(type(a))",
"this is the way the world ends.\n<class 'str'>\n('this ', 'is ', 'the ', 'way ', 'the ', 'world ', 'ends.')\n<class 'tuple'>\n"
]
],
[
[
"#### Substrings: Indexing and Slicing\n\nA character of a string can be extracted using an index (starting at 0), and a substring can be extracted using **slices**. Slices indicate a range of indexes. The notation is similar to that used for arrays in other languages.\n\nIt also happens that indexing from the right (staring at -1) is possible. ",
"_____no_output_____"
]
],
[
[
"string1 = \"this is the way the world ends.\"\nprint(string1[12]) # the substring at index 12 (1 character)\nprint(string1[0:4]) # from the start of the string to index 4 (but 4 is excluded)\nprint(string1[5:]) # from index 5 to the end of the string\nprint(string1[:4]) # from the start of the string to index 4 (4 is excluded)\nprint(string1[-1]) # The last character of the string\nprint(string1[-5:-1]) # from index -5 to -1 (but excluding -1)\nprint(string1[-5:]) # from index -5 to the end of the string",
"w\nthis\nis the way the world ends.\nthis\n.\nends\nends.\n"
]
],
[
[
"**NOTE**: Strings are **immutable**. We cannot reassign a character or sequence in a string as we might assign values to an array in some other programming languages. When the below code is executed, an exception (error) will be raised. ",
"_____no_output_____"
]
],
[
[
"a = \"abc\"",
"_____no_output_____"
],
[
"a[0] = \"b\" # this will raise an exception",
"_____no_output_____"
]
],
[
[
"#### Splitting and Joining Strings\n\nIt's often the case that we want to split strings into multiple substrings, e.g., when reading a comma-delimited list of values. The `split` method of a string does just that. It retuns a list object (lists are covered later). \n\nTo combine strings using a delimeter (e.g., to create a comma-delimited list), we can use `join`. ",
"_____no_output_____"
]
],
[
[
"text = \"The quick brown fox jumped over the lazy dog\"\nspl = text.split() # This returns a list of strings (lists are covered later)\nprint(spl)\njoined = \",\".join(spl)\nprint(joined) # and this re-joins them, separating words with commas\nspl = joined.split(\",\") # and this re-splits them, again based on commas\nprint(spl)\njoined = \"-\".join(spl) # and this re-joins them, separating words with dashes\nprint(joined)",
"['The', 'quick', 'brown', 'fox', 'jumped', 'over', 'the', 'lazy', 'dog']\nThe,quick,brown,fox,jumped,over,the,lazy,dog\n['The', 'quick', 'brown', 'fox', 'jumped', 'over', 'the', 'lazy', 'dog']\nThe-quick-brown-fox-jumped-over-the-lazy-dog\n"
]
],
[
[
"Similarly, to split a multiline string into a list of lines (each a string), we can use `splitlines`. ",
"_____no_output_____"
]
],
[
[
"lines = \"\"\"one\ntwo\nthree\"\"\"\nli = lines.splitlines() # Split the multiple line string \nprint(li)",
"['one', 'two', 'three']\n"
]
],
[
[
"To join strings into multiple lines, we can again use `join`. ",
"_____no_output_____"
]
],
[
[
"lines = [\"one\", \"two\", \"three\"]\ndata = \"\\n\".join(lines)# join list of strings to multiple line string \nprint(data)",
"one\ntwo\nthree\n"
]
],
[
[
"<a id='boolean'></a>\n### Boolean Values, and None\n\nPython has two Boolean values, `True` and `False`. The normal logical operations (`and`, `or`, `not`) are present. ",
"_____no_output_____"
]
],
[
[
"print(True and False)\nprint(True or False)\nprint(not True)",
"False\nTrue\nFalse\n"
]
],
[
[
"There is also the value `None` (the only value of the `NoneType` data type). `None` is used to stand for the absence of a value. However, it can be used in place of False, as can zero numerical values (of any numerical type), empty sequences/collections (`[]`,`()`, `{}`, etc.). \n\nOther values are treated as `True`. Note that Boolean expressions are short-circuited. As soon as the interpreter knows enough to compute the appropriate Boolean value of the expression, it stops further evaluation. Also, the retun value of the Boolean expression need not be a Boolean value, as indicated below. The value of the last item evaluated is returned. ",
"_____no_output_____"
]
],
[
[
"print(1 and True)\nprint(True and 66)\nprint(True and \"aa\")\nprint(False and \"aa\")\nprint(True or {})\nprint(not [])\nprint(True and ())",
"True\n66\naa\nFalse\nTrue\nTrue\n()\n"
]
],
[
[
"<a id='comparisons'></a>\n#### Boolean Comparisons",
"_____no_output_____"
],
[
"There are 8 basic comparison operations in Python.\n\n\n| Symbol | Note | \n| --- | --- |\n| `<` | less than | \n| `<=` | less than or equal to | \n| `>` | greater than | \n| `>=` | greater than or equal to | \n| `==` | equal to | \n| `!=` | not equal to | \n| `is` | identical to (for objects) | \n| `is not` | not identical to (for objects) | \n\n\nRegarding the first 6, these will work as expected for numerical values. Note, however, that they can be applied to other datatypes as well. Strings are compared on a character-by-character basis, based on a lexicographic ordering. Sequences such as lists are compared on an element by element basis.",
"_____no_output_____"
]
],
[
[
"print(\"abc\" > \"ac\")\nprint(\"a\" < \"1\")\nprint(\"A\" < \"a\")\nprint((1, 1, 2) < (1, 1, 3))",
"False\nFalse\nTrue\nTrue\n"
]
],
[
[
"Note that `is` is true only if the two items compared are the *same* object, whereas `==` only checks for eqaulity in a weaker sense. Below, the elements of the two lists `x` and `y` have elements that evaluate as being equal, but the two lists are nevertheless distinct in memory. As such, the first `print` statement should yield `True`, while the second should yield `False`.",
"_____no_output_____"
]
],
[
[
"x = (1, 1, 2)\ny = (1, 1, 2)\nprint(x == y)\nprint(x is y)",
"True\nFalse\n"
],
[
"x = \"hello\"\ny = x\na = \"hel\"\nb = \"lo\"\nz = a + b\nw = x[:]\nprint(x)\nprint(y)\nprint(z)\nprint(\"x==y: \", x == y)\nprint(\"x==z: \", x == z)\nprint(\"x is y: \", x is y)\nprint(\"x is z: \", x is z)\nprint(\"x is w: \", x is w)",
"hello\nhello\nhello\nx==y: True\nx==z: True\nx is y: True\nx is z: False\nx is w: True\n"
]
],
[
[
"<a id=\"conversions\"></a>\n### Converting between Types\n\nValues of certain data types can be converted to values of other datatypes (actually, a new value of the desired data type is produced). If the conversion cannot take place (becuase the datatypes are incompatible), an exception will be raised.",
"_____no_output_____"
]
],
[
[
"x = 1\ns = str(x) # convert x to a string\ns_int = int(s)\ns_float = float(s)\ns_comp = complex(s)\nx_float = float(x)\n\nprint(s)\nprint(s_int) # convert to an integer\nprint(s_float) # convert to a floating point number\nprint(s_comp) # convert to a complext number\nprint(x_float)\n\n# Let's check their IDs\nprint(id(x))\nprint(id(s))\nprint(id(s_int))\nprint(id(s_float))\nprint(id(x_float))\nprint(id(int(x_float)))",
"1\n1\n1.0\n(1+0j)\n1.0\n93926537898496\n140538951529264\n93926537898496\n140538952028656\n140538952028464\n93926537898496\n"
]
],
[
[
"## The `id()` function\n\nThe `id()` function can be used to identify an object in memory. It returns an integer value that is guaranteed to uniquely identify an object for the duration of its existence. ",
"_____no_output_____"
]
],
[
[
"print(\"id(x): \", id(x))\nprint(\"id(y): \", id(y))\nprint(\"id(z): \", id(z))\nprint(\"id(w): \", id(w))",
"id(x): 140539018862384\nid(y): 140539018862384\nid(z): 140538951488880\nid(w): 140539018862384\n"
]
],
[
[
"## Lists,Tuples, Sets, and Dictionaries\n",
"_____no_output_____"
],
[
"<a id='lists'></a>\n### Lists \n\nMany languages (e.g., Java) have what are often called **arrays**. In Python the object most like them are called **lists**. Like arrays in other languages, Python lists are represented syntactically using `[...]` blocks. Their elements can be referenced via indexes, and just like arrays in other languages, Python lists are **mutable** objects. That is, it is possible to change the value of an individual cell in a list. In this way, Python lists are unlike Python strings (which are immutable). ",
"_____no_output_____"
]
],
[
[
"a = [0, 1, 2, 3] # a list of integers\nprint(a)\na[0] = 3 # overwrite the first element of the list\nprint(a)\na[1:3] = [4, 5]\n# overwrite the last two elements of the list (using values from a new list)\nprint(a)",
"[0, 1, 2, 3]\n[3, 1, 2, 3]\n[3, 4, 5, 3]\n"
]
],
[
[
"Note that some operations on lists return other lists. ",
"_____no_output_____"
]
],
[
[
"a = [1, 2, 3]\nb = [4, 5, 6]\nc = a + b\nprint(a)\nprint(b)\nprint(c)\nprint(\"-\" * 25)\nc[0] = 10\nb[0] = 40\nprint(a)\nprint(b)\nprint(c)",
"[1, 2, 3]\n[4, 5, 6]\n[1, 2, 3, 4, 5, 6]\n-------------------------\n[1, 2, 3]\n[40, 5, 6]\n[10, 2, 3, 4, 5, 6]\n"
]
],
[
[
"Above, `c` is a new list containing elements copied from `a` and `b`. Subsequent changes to `a` or `b` do not affect `c`, and changes to `c` do not affect `a` or `b`. \n\nThe length of a list can be obtained using `len()`, and a single element can be added to a list using `append()`. Note the syntax used for each. ",
"_____no_output_____"
]
],
[
[
"a = []\na.append(1) # add an element to the end of the list\na.append(2)\na.append([3, 4])\nprint(a)\nprint(\"length of 'a': \", len(a))",
"[1, 2, [3, 4]]\nlength of 'a': 3\n"
]
],
[
[
"Some additional list operations are shown below. Pay careful attention to how `a` and `b` are related.",
"_____no_output_____"
]
],
[
[
"a = [10]\na.extend([11, 12]) # append elements of one list to the end of another one\nb = a\nc = a.copy() # copy the elements of a to a new list, and then assign it to c\nb[0] = 20\nc[0] = 30\nprint(\"a:\", a)\nprint(\"b:\", b)\nprint(\"c:\", c)",
"a: [20, 11, 12]\nb: [20, 11, 12]\nc: [30, 11, 12]\n"
],
[
"b.reverse() # reverse the elements of the list in place\nprint(\"a reversed:\", a)\nb.sort()\nprint(\"a sorted:\", a)\na.clear() # empty the list\nprint(\"b is \", b, \" having length \", len(b))",
"a reversed: [12, 11, 20]\na sorted: [11, 12, 20]\nb is [] having length 0\n"
],
[
"list1 = [\"a\", \"b\", \"d\", \"e\"]\nlist1.insert(2, \"c\") # insert element \"c\" at position 2, increasing the length by 1\nprint(list1)\ne = list1.pop() # remove the last element of the list\nprint(\"popped: \", e, list1)\nlist1 = [\"d\", \"b\", \"b\", \"c\", \"d\", \"d\", \"a\"]\nlist1.sort() # sort the list\nprint(\"new list, sorted:\", list1)\nprint(\"count of 'd': \", list1.count(\"d\")) # count the number of times \"d\" occurs\nprint(\"first index of 'd': \", list1.index(\"d\")) # return the index of the first occurrence of \"d\"\nprint(list1)\n\ndel list1[2] # remove the element at index 2\nprint(\"element at index 2 removed:\", list1)\n\ndel list1[2:4] # remove the elements from index 2 to 4\nprint(\"elements at index 2-4 removed:\", list1)",
"['a', 'b', 'c', 'd', 'e']\npopped: e ['a', 'b', 'c', 'd']\nnew list, sorted: ['a', 'b', 'b', 'c', 'd', 'd', 'd']\ncount of 'd': 3\nfirst index of 'd': 4\n['a', 'b', 'b', 'c', 'd', 'd', 'd']\nele at index 2 removed: ['a', 'b', 'c', 'd', 'd', 'd']\nelements at index 2-4 removed: ['a', 'b', 'd', 'd']\n"
]
],
[
[
"<a id='tuples'></a>\n### Tuples\n\nThere also exists an immutable counterpart to a list, the **tuple**. Elements can also be referenced by index, but (as with Python strings) new values cannot be assigned. Unlike a list, Tuples are created using either `(...)` or simply by using a comma-delimeted sequence of 1 or more elements. ",
"_____no_output_____"
]
],
[
[
"a = () # the empty tuple\nb = (1, 2) # a tuple of 2 elements\nc = 3, 4, 5 # another way of creating a tuple\nd = (6,) # a singleton tuple\ne = (7,) # another singleton tuple\nprint(a)\nprint(b)\nprint(c)\nprint(d)\nprint(len(d))\nprint(e)\nprint(b[1])",
"()\n(1, 2)\n(3, 4, 5)\n(6,)\n1\n(7,)\n2\n"
]
],
[
[
"As with lists, we can combine tuples to form new tuples",
"_____no_output_____"
]
],
[
[
"a = (1, 2, 3, 4) # Create python tuple\nb = \"x\", \"y\", \"z\" # Another way to create python tuple\nc = a[0:3] + b # Concatenate two python tuples\nprint(c)",
"(1, 2, 3, 'x', 'y', 'z')\n"
]
],
[
[
"<a id='sets'></a>\n### Sets\n\nSets, created using `{...}` or `set(...)` in Python, are unordered collections without duplicate elements. If the same element is added again, the set will not change. \n",
"_____no_output_____"
]
],
[
[
"a = {\"a\", \"b\", \"c\", \"d\"} # create a new set containing these elements\nb = set(\n \"hello world\"\n) # create a set containing the distinct characters of 'hello world'\nprint(a)\nprint(b)\nprint(a | b) # print the union of a and b\nprint(a & b) # print the intersection of a and b\nprint(a - b) # print elements of a not in b\nprint(b - a) # print elements of b not in a\nprint(b ^ a) # print elements in either but not both",
"{'a', 'd', 'c', 'b'}\n{'l', 'r', 'w', 'e', 'd', 'h', ' ', 'o'}\n{'l', 'b', 'c', 'r', 'w', 'e', 'd', 'h', ' ', 'a', 'o'}\n{'d'}\n{'a', 'c', 'b'}\n{'l', 'r', 'w', 'e', 'h', ' ', 'o'}\n{'l', 'b', 'c', 'r', 'w', 'e', 'h', ' ', 'a', 'o'}\n"
]
],
[
[
"Given the below, it appears that `==` is used to evaluate membership. ",
"_____no_output_____"
]
],
[
[
"a = \"hello\"\nb = \"hel\"\nc = \"lo\"\nd = b + c # Concatenate string \ns = {a, b, c, d}\nprint(\"id(a):\", a)\nprint(\"id(d):\", d)\nprint(s)",
"id(a): hello\nid(d): hello\n{'lo', 'hel', 'hello'}\n"
]
],
[
[
"<a id='dictionaries'></a>\n### Dictionaries\n\nDictionaries are collections of key-value pairs. A dictionary can be created using `d = {key1:value1, key2:value2, ...}` syntax, or else from 2-ary tuples using `dictionary()`. New key value pairs can be assigned, and old values referenced, using `d[key]`. ",
"_____no_output_____"
]
],
[
[
"employee = {\"last\": \"smth\", \"first\": \"joe\"} # Create dictionary \nemployee[\"middle\"] = \"william\" # Add new key and value to the dictionary \nemployee[\"last\"] = \"smith\"\naddr = {} # an empty dictionary\naddr[\"number\"] = 1234\naddr[\"street\"] = \"Elm St\" # Add new key and value to the dictionary \naddr[\"city\"] = \"Athens\" # Add new key and value to the dictionary \naddr[\"state\"] = \"GA\" # Add new key and value to the dictionary \naddr[\"zip\"] = \"30602\" # Add new key and value to the dictionary \nemployee[\"address\"] = addr\nprint(employee)\nkeys = list(employee.keys()) # list the keys of 'employee'\nprint(\"keys: \" + str(sorted(keys)))\nprint(\"last\" in keys) # Print whether 'last' is in keys or not (prints True or False)\nprint(\"lastt\" in keys) # Print whether 'lastt' is in keys or not (prints True or False)\n\nemployee2 = employee.copy() # create a shallow copy of the employee\nemployee2[\"last\"] = \"jones\"\nemployee2[\"address\"][\n \"street\"\n] = \"beech\" # reassign the street name of the employee's address\nprint(employee)\nprint(employee2)",
"{'last': 'smith', 'first': 'joe', 'middle': 'william', 'address': {'number': 1234, 'street': 'Elm St', 'city': 'Athens', 'state': 'GA', 'zip': '30602'}}\nkeys: ['address', 'first', 'last', 'middle']\nTrue\nFalse\n{'last': 'smith', 'first': 'joe', 'middle': 'william', 'address': {'number': 1234, 'street': 'beech', 'city': 'Athens', 'state': 'GA', 'zip': '30602'}}\n{'last': 'jones', 'first': 'joe', 'middle': 'william', 'address': {'number': 1234, 'street': 'beech', 'city': 'Athens', 'state': 'GA', 'zip': '30602'}}\n"
]
],
[
[
"<a id=\"conversions\"></a>\n### Conversion Between Types",
"_____no_output_____"
]
],
[
[
"y = (1, 2, 3, 1, 1) # Create tuple \nz = list(y) # convert tuple to a list\nprint(y)\nprint(z)\nprint(tuple(z)) # convert z to a tuple\nprint(set(z)) # convert z to a set\n\nw = ((\"one\", 1), (\"two\", 2), (\"three\", 3)) # Create special tuple to convert it to dictionary \nv = dict(w) # Convert the tuple to dictionary \nprint(v)\nprint(tuple(v)) # Convert the dictionary to tuple\nprint(tuple(v.keys())) # Get the keys of the dictionary \nprint(tuple(v.values())) # Get the values of the keys in the dictionary ",
"(1, 2, 3, 1, 1)\n[1, 2, 3, 1, 1]\n(1, 2, 3, 1, 1)\n{1, 2, 3}\n{'one': 1, 'two': 2, 'three': 3}\n('one', 'two', 'three')\n('one', 'two', 'three')\n(1, 2, 3)\n"
]
],
[
[
"## Controlling the Flow of Program Execution\n\nAs in most programing languages, Python allows program execution to branch when certain conditions are met, and it also allows arbitrary execution loops. Without such features, Python would not be very useful (or Turing complete). \n",
"_____no_output_____"
],
[
"### If Statements\n\nIn Python, *if-then-else* statements are specified using the keywords `if`, `elif` (else if), and `else` (else). The general form is given below: \n\n if condition1:\n do_something\n elif condition2:\n do_something_else\n ...\n elif condition_n:\n do_something_else\n else:\n if_all_else_fails_do_this\n\nThe `elif` and `else` clauses are optional. There can be many `elif` clauses, but there can be only 1 `else` clause in the `if`-`elif`-`else` sequence.",
"_____no_output_____"
]
],
[
[
"x = 3\n\n# Test the number if it bigger than 10\nif x > 10:\n print(\"value \" + str(x) + \" is greater than 10\")\n# Test the number if it bigger than or equal to 7 and less than 10\nelif x >= 7 and x < 10:\n print(\"value \" + str(x) + \" is in range [7,10)\")\n# Test the number if it bigger than or equal to 5 and less than 7\nelif x >= 5 and x < 7:\n print(\"value \" + str(x) + \" is in range [5,7)\")\n# Test the number if it's less than 5\nelse:\n print(\"value \" + str(x) + \" is less than 5\")\n",
"value 3 is less than 5\n"
]
],
[
[
"### While Loops\n\nPython provides both `while` loops and `for` loops. The former are arguably lower-level but not as natural-looking to a human eye. \n\nBelow is a simple `while` loop. So long as the condition specified evaluates to a value comparable to `True`, the code in the body of the loop will be executed. As such, without the statement incrementing `i`, the loop would halt. ",
"_____no_output_____"
],
[
"\n\n```\nwhile condition:\n do_something\n```\n\n",
"_____no_output_____"
]
],
[
[
"string = \"hello world\"\nlength = len(string)# get the length of the string\ni = 0\nwhile i < length:\n print(string[i])\n i = i + 1",
"h\ne\nl\nl\no\n \nw\no\nr\nl\nd\n"
]
],
[
[
"Loops, including while loops, can contain break statements (which aborts execution of the loop) and continue statements (which tell the loop to proceed to the next cycle).\n\n",
"_____no_output_____"
]
],
[
[
"num = 0\n\nwhile num < 5:\n num += 1 # num += 1 is same as num = num + 1\n print('num = ', num)\n if num == 3: # condition before exiting a loop\n break",
"num = 1\nnum = 2\nnum = 3\n"
],
[
"num = 0\n\nwhile num < 5:\n\tnum += 1 \n\tif num > 3: # condition before exiting a loop\n\t\tcontinue\n\tprint('num = ', num) # the statement after 'continue' statement is skipped",
"num = 1\nnum = 2\nnum = 3\n"
]
],
[
[
"While Loop with else Block\n",
"_____no_output_____"
]
],
[
[
"num = 0\n\nwhile num < 3:\n\tnum += 1 \n\tprint('num = ', num)\nelse:\n print('else block executed')",
"num = 1\nnum = 2\nnum = 3\nelse block executed\n"
],
[
"a = ['A', 'B', 'C', 'D']\ns = 'd'\n\ni = 0\nwhile i < len(a):\n if a[i] == s:\n # Processing for item found\n break\n i += 1\nelse:\n # Processing for item not found\n print(s, 'not found in list')",
"d not found in list\n"
]
],
[
[
"Below we sort a string, identifying its unique members. Then we use a `while` loop together with the previously defined `count` function to count the occurrences of each character. ",
"_____no_output_____"
]
],
[
[
"# unique characters\n\nraw_string = 'Hello'\n\nresult = set()\ni = 0\nlength = len(raw_string)\n\nwhile i < length:\n result.add(raw_string[i])\n i = i + 1\nprint(sorted(list(result)))\n\n",
"['H', 'e', 'l', 'o']\n"
]
],
[
[
"Here is the explanation for the code above: \n1. We create an empty set called result and assign it to an empty set. \n2. We create a variable called i and assign it to 0. \n3. We create a variable called length and assign it to the length of the raw_string. \n4. We create a while loop that will go on forever until i is equal to the length of the raw_string. \n5. We add the character at index i of the raw_string to the set result. 6. We increment i by 1. \n7. We print the sorted list of the set result.",
"_____no_output_____"
],
[
"It turns out that as far as loops and evaluating the truth of conditional statements are concerned, a great many values and not just `True` and `False` will suffice. E.g., nonzero numbers, or nonempty strings or lists will be taken as `True`, and 0 and empty lists, etc., will be taken as `False`. ",
"_____no_output_____"
]
],
[
[
"\nx = []\ns = \"a\"\n\ncount = 0\nprint(x, \":\", end=\"\")\nwhile x:\n print(s, end=\"\")\n count = count + 1\n if count > 3:\n break\nprint(\"\")\n",
"[] :\n"
]
],
[
[
"### For Loops and the Range function\n\nIn Python, `for` statements iterate over sequences and utilize the `in` keyword. Like `while` loops, `for` loops can contain `break` and `continue`. They can also contain `else` statements; these are executed when the loop ends via something other than `break`).",
"_____no_output_____"
]
],
[
[
"raw_string = \"hello world\"\ncharacters = set(raw_string)\nfor w in characters:\n print(w, \",\", raw_string.count(w))",
"l , 3\nr , 1\nw , 1\ne , 1\nd , 1\nh , 1\n , 1\no , 2\n"
]
],
[
[
"The `range()` function can be used to generate a sequence of numbers, which can then be used in a loop. ",
"_____no_output_____"
]
],
[
[
"x = range(11)\nfor i in x:\n print(i, i * i)",
"0 0\n1 1\n2 4\n3 9\n4 16\n5 25\n6 36\n7 49\n8 64\n9 81\n10 100\n"
]
],
[
[
"Minimum and maximum (exlcusive) values can also be specified, as can an integer step-size.",
"_____no_output_____"
]
],
[
[
"x = range(20, 31, 2)\nfor i in x:\n print(i)",
"20\n22\n24\n26\n28\n30\n"
]
]
] | [
"markdown",
"code",
"markdown",
"code",
"markdown",
"code",
"markdown",
"code",
"markdown",
"code",
"markdown",
"code",
"markdown",
"code",
"markdown",
"code",
"markdown",
"code",
"markdown",
"code",
"markdown",
"code",
"markdown",
"code",
"markdown",
"code",
"markdown",
"code",
"markdown",
"code",
"markdown",
"code",
"markdown",
"code",
"markdown",
"code",
"markdown",
"code",
"markdown",
"code",
"markdown",
"code",
"markdown",
"code",
"markdown",
"code",
"markdown",
"code",
"markdown",
"code",
"markdown",
"code",
"markdown",
"code",
"markdown",
"code",
"markdown",
"code",
"markdown",
"code",
"markdown",
"code",
"markdown",
"code",
"markdown",
"code",
"markdown",
"code",
"markdown",
"code",
"markdown",
"code",
"markdown",
"code",
"markdown",
"code",
"markdown",
"code",
"markdown",
"code",
"markdown",
"code",
"markdown",
"code",
"markdown",
"code"
] | [
[
"markdown",
"markdown"
],
[
"code"
],
[
"markdown"
],
[
"code"
],
[
"markdown"
],
[
"code"
],
[
"markdown"
],
[
"code"
],
[
"markdown"
],
[
"code",
"code",
"code",
"code",
"code",
"code",
"code"
],
[
"markdown",
"markdown"
],
[
"code"
],
[
"markdown"
],
[
"code"
],
[
"markdown"
],
[
"code"
],
[
"markdown"
],
[
"code"
],
[
"markdown"
],
[
"code"
],
[
"markdown"
],
[
"code"
],
[
"markdown"
],
[
"code"
],
[
"markdown"
],
[
"code"
],
[
"markdown"
],
[
"code"
],
[
"markdown"
],
[
"code",
"code"
],
[
"markdown"
],
[
"code"
],
[
"markdown"
],
[
"code"
],
[
"markdown"
],
[
"code"
],
[
"markdown"
],
[
"code"
],
[
"markdown"
],
[
"code"
],
[
"markdown",
"markdown"
],
[
"code"
],
[
"markdown"
],
[
"code",
"code"
],
[
"markdown"
],
[
"code"
],
[
"markdown"
],
[
"code"
],
[
"markdown",
"markdown"
],
[
"code"
],
[
"markdown"
],
[
"code"
],
[
"markdown"
],
[
"code"
],
[
"markdown"
],
[
"code",
"code",
"code"
],
[
"markdown"
],
[
"code"
],
[
"markdown"
],
[
"code"
],
[
"markdown"
],
[
"code"
],
[
"markdown"
],
[
"code"
],
[
"markdown"
],
[
"code"
],
[
"markdown"
],
[
"code"
],
[
"markdown",
"markdown"
],
[
"code"
],
[
"markdown",
"markdown"
],
[
"code"
],
[
"markdown"
],
[
"code",
"code"
],
[
"markdown"
],
[
"code",
"code"
],
[
"markdown"
],
[
"code"
],
[
"markdown",
"markdown"
],
[
"code"
],
[
"markdown"
],
[
"code"
],
[
"markdown"
],
[
"code"
],
[
"markdown"
],
[
"code"
]
] |
e7722de7d915c5456075c8ea6fec73747b37e433 | 125,902 | ipynb | Jupyter Notebook | docs/tutorial/Getting_started.ipynb | yoelcortes/biosteam | 8d90788f87efb3b23428387d79097817962cad97 | [
"MIT"
] | 29 | 2019-03-01T04:47:43.000Z | 2020-02-23T01:34:40.000Z | docs/tutorial/Getting_started.ipynb | yoelcortes/biosteam | 8d90788f87efb3b23428387d79097817962cad97 | [
"MIT"
] | 15 | 2019-11-01T13:57:50.000Z | 2020-02-28T06:40:46.000Z | docs/tutorial/Getting_started.ipynb | yoelcortes/biosteam | 8d90788f87efb3b23428387d79097817962cad97 | [
"MIT"
] | 4 | 2019-06-05T15:39:04.000Z | 2020-01-08T20:42:04.000Z | 102.526059 | 52,628 | 0.826405 | [
[
[
"# Getting started",
"_____no_output_____"
],
[
"### Initialize streams",
"_____no_output_____"
],
[
"[Stream](https://thermosteam.readthedocs.io/en/latest/Stream.html) objects define material flow rates along with its thermodynamic state. Before creating streams, a [Thermo](https://thermosteam.readthedocs.io/en/latest/Thermo.html) property package must be defined. Alternatively, we can just pass chemical names and BioSTEAM will automatically create a property package based on ideal mixing rules and UNIFAC activity coefficients for thermodynamic equilibrium. More complex packages can be defined through Thermosteam, BioSTEAM's premier thermodynamic engine. Please visit [Thermosteam's documentation](https://thermosteam.readthedocs.io/en/latest/index.html) for a complete tutorial on [Stream](https://thermosteam.readthedocs.io/en/latest/Stream.html) objects and how to create a property package. In this example, a simple feed stream with a few common chemicals will be initialized:",
"_____no_output_____"
]
],
[
[
"import biosteam as bst\nbst.settings.set_thermo(['Water', 'Methanol'])\nfeed = bst.Stream(Water=50, Methanol=20)\nfeed.show()",
"Stream: s1\n phase: 'l', T: 298.15 K, P: 101325 Pa\n flow (kmol/hr): Water 50\n Methanol 20\n"
]
],
[
[
"Set prices for performing techno-economic analysis later:",
"_____no_output_____"
]
],
[
[
"feed.price = 0.15 # USD/kg\nfeed.cost # USD/hr",
"_____no_output_____"
]
],
[
[
"### Process settings",
"_____no_output_____"
],
[
"Process settings include price of feeds and products, conditions of utilities, and the chemical engineering plant cost index. These should be set before simulating a system.",
"_____no_output_____"
],
[
"Set the chemical engineering plant cost index:",
"_____no_output_____"
]
],
[
[
"bst.CE # Default year is 2017",
"_____no_output_____"
],
[
"bst.CE = 603.1 # To year 2018",
"_____no_output_____"
]
],
[
[
"Set [PowerUtility](../PowerUtility.txt) options:",
"_____no_output_____"
]
],
[
[
"bst.PowerUtility.price # Default price (USD/kJ)",
"_____no_output_____"
],
[
"bst.PowerUtility.price = 0.065 # Adjust price",
"_____no_output_____"
]
],
[
[
"Set [HeatUtility](../HeatUtility.txt) options via [UtilityAgent](../UtilityAgent.txt) objects, which are [Stream](https://thermosteam.readthedocs.io/en/latest/Stream.html) objects with additional attributes to describe a utility agent:",
"_____no_output_____"
]
],
[
[
"bst.HeatUtility.cooling_agents # All available cooling agents",
"_____no_output_____"
],
[
"cooling_water = bst.HeatUtility.get_cooling_agent('cooling_water')\ncooling_water.show() # A UtilityAgent",
"UtilityAgent: cooling_water\n heat_transfer_efficiency: 1.000\n heat_transfer_price: 0 USD/kJ\n regeneration_price: 0.000488 USD/kmol\n T_limit: 325 K\n phase: 'l'\n T: 305.37 K\n P: 101325 Pa\n flow (kmol/hr): Water 1\n"
],
[
"# Price of regenerating the utility in USD/kmol\ncooling_water.regeneration_price",
"_____no_output_____"
],
[
"# Other utilities may be priced for amount of heat transfered in USD/kJ\nchilled_water = bst.HeatUtility.get_cooling_agent('chilled_water')\nchilled_water.heat_transfer_price",
"_____no_output_____"
],
[
"cooling_water.T = 302 # Change the temperature of cooling water (K)",
"_____no_output_____"
],
[
"bst.HeatUtility.heating_agents # All available heating agents",
"_____no_output_____"
],
[
"lps = bst.HeatUtility.get_heating_agent('low_pressure_steam') # A UtilityAgent\nlps.show() # Note that because utility changes phase, T_limit is None",
"UtilityAgent: low_pressure_steam\n heat_transfer_efficiency: 0.950\n heat_transfer_price: 0 USD/kJ\n regeneration_price: 0.238 USD/kmol\n T_limit: None\n phase: 'g'\n T: 412.19 K\n P: 344738 Pa\n flow (kmol/hr): Water 1\n"
],
[
"lps.regeneration_price = 0.20 # Adjust price (USD/kmol)",
"_____no_output_____"
]
],
[
[
"### Find design requirements and cost with Unit objects",
"_____no_output_____"
],
[
"[Creating a Unit](./Creating_a_Unit.ipynb) can be flexible. But in summary, a [Unit](../Unit.txt) object is initialized with an ID, and unit-specific arguments. BioSTEAM includes [essential unit operations](../units/units.txt) with rigorous modeling and design algorithms. Here we create a [Flash](../units/Flash.txt) object as an example:",
"_____no_output_____"
]
],
[
[
"from biosteam import units\n\n# Specify vapor fraction and isobaric conditions\nF1 = units.Flash('F1', V=0.5, P=101325)\nF1.show()",
"Flash: F1\nins...\n[0] missing stream\nouts...\n[0] s2\n phase: 'l', T: 298.15 K, P: 101325 Pa\n flow: 0\n[1] s3\n phase: 'l', T: 298.15 K, P: 101325 Pa\n flow: 0\n"
]
],
[
[
"Note that, by default, Missing Stream objects are given to inputs, `ins`, and empty streams to outputs, `outs`:",
"_____no_output_____"
]
],
[
[
"F1.ins",
"_____no_output_____"
],
[
"F1.outs",
"_____no_output_____"
]
],
[
[
"You can connect streams by setting the `ins` and `outs`:",
"_____no_output_____"
]
],
[
[
"F1.ins[0] = feed\nF1.show()",
"Flash: F1\nins...\n[0] s1\n phase: 'l', T: 298.15 K, P: 101325 Pa\n flow (kmol/hr): Water 50\n Methanol 20\nouts...\n[0] s2\n phase: 'l', T: 298.15 K, P: 101325 Pa\n flow: 0\n[1] s3\n phase: 'l', T: 298.15 K, P: 101325 Pa\n flow: 0\n"
]
],
[
[
"To simulate the flash, use the `simulate` method:",
"_____no_output_____"
]
],
[
[
"F1.simulate()\nF1.show()",
"Flash: F1\nins...\n[0] s1\n phase: 'l', T: 298.15 K, P: 101325 Pa\n flow (kmol/hr): Water 50\n Methanol 20\nouts...\n[0] s2\n phase: 'g', T: 359.6 K, P: 101325 Pa\n flow (kmol/hr): Water 19\n Methanol 16\n[1] s3\n phase: 'l', T: 359.6 K, P: 101325 Pa\n flow (kmol/hr): Water 31\n Methanol 4.01\n"
]
],
[
[
"Note that warnings notify you whether purchase cost correlations are out of range for the given design. This is ok for the example, but its important to make sure that the process is well designed and cost correlations are suitable for the domain.",
"_____no_output_____"
],
[
"The `results` method returns simulation results:",
"_____no_output_____"
]
],
[
[
"F1.results() # Default returns DataFrame object with units",
"_____no_output_____"
],
[
"F1.results(with_units=False) # Returns Series object without units",
"_____no_output_____"
]
],
[
[
"Although BioSTEAM includes a large set of essential unit operations, many process specific unit operations are not yet available. In this case, you can create new [Unit subclasses](./Inheriting_from_Unit.ipynb) to model unit operations not yet available in BioSTEAM.",
"_____no_output_____"
],
[
"### Solve recycle loops and process specifications with System objects",
"_____no_output_____"
],
[
"Designing a chemical process is no easy task. A simple recycle process consisting of a flash with a partial liquid recycle is presented here.",
"_____no_output_____"
],
[
"Create a [Mixer](../units/mixing.txt) object and a [Splitter](../units/splitting.txt) object:",
"_____no_output_____"
]
],
[
[
"M1 = units.Mixer('M1')\nS1 = units.Splitter('S1', outs=('liquid_recycle', 'liquid_product'),\n split=0.5) # Split to 0th output stream\nF1.outs[0].ID = 'vapor_product'\nF1.outs[1].ID = 'liquid'",
"_____no_output_____"
]
],
[
[
"You can [find unit operations and manage flowsheets](./Managing_flowsheets.ipynb) with the `main_flowsheet`:",
"_____no_output_____"
]
],
[
[
"bst.main_flowsheet.diagram()",
"_____no_output_____"
]
],
[
[
"Connect streams and make a recycle loop using [-pipe- notation](./-pipe-_notation.ipynb):",
"_____no_output_____"
]
],
[
[
"feed = bst.Stream('feed', Methanol=100, Water=450)\n\n# Broken down -pipe- notation\n[S1-0, feed]-M1 # M1.ins[:] = [S1.outs[0], feed]\nM1-F1 # F1.ins[:] = M1.outs\nF1-1-S1 # S1.ins[:] = [F1.outs[1]]\n\n# All together\n[S1-0, feed]-M1-F1-1-S1;",
"_____no_output_____"
]
],
[
[
"Now lets check the diagram again:",
"_____no_output_____"
]
],
[
[
"bst.main_flowsheet.diagram(format='png')",
"_____no_output_____"
]
],
[
[
"[System](../System.txt) objects take care of solving recycle loops and simulating all unit operations.\nAlthough there are many ways of [creating a system](./Creating_a_System.ipynb), the most recommended way is to use the flowsheet:",
"_____no_output_____"
]
],
[
[
"flowsheet_sys = bst.main_flowsheet.create_system('flowsheet_sys')\nflowsheet_sys.show()",
"System: flowsheet_sys\nHighest convergence error among components in recycle\nstream S1-0 after 0 loops:\n- flow rate 0.00e+00 kmol/hr (0%)\n- temperature 0.00e+00 K (0%)\nins...\n[0] feed\n phase: 'l', T: 298.15 K, P: 101325 Pa\n flow (kmol/hr): Water 450\n Methanol 100\nouts...\n[0] vapor_product\n phase: 'g', T: 359.6 K, P: 101325 Pa\n flow (kmol/hr): Water 19\n Methanol 16\n[1] liquid_product\n phase: 'l', T: 298.15 K, P: 101325 Pa\n flow: 0\n"
]
],
[
[
"Although not recommened due to the likelyhood of human error, a [System](../System.txt) object may also be created by specifying an ID, a `recycle` stream and a `path` of units to run element by element:",
"_____no_output_____"
]
],
[
[
"sys = bst.System('sys', path=(M1, F1, S1), recycle=S1-0) # recycle=S1.outs[0]\nsys.show()",
"System: sys\nHighest convergence error among components in recycle\nstream S1-0 after 0 loops:\n- flow rate 0.00e+00 kmol/hr (0%)\n- temperature 0.00e+00 K (0%)\nins...\n[0] feed\n phase: 'l', T: 298.15 K, P: 101325 Pa\n flow (kmol/hr): Water 450\n Methanol 100\nouts...\n[0] vapor_product\n phase: 'g', T: 359.6 K, P: 101325 Pa\n flow (kmol/hr): Water 19\n Methanol 16\n[1] liquid_product\n phase: 'l', T: 298.15 K, P: 101325 Pa\n flow: 0\n"
]
],
[
[
"Simulate the System object:",
"_____no_output_____"
]
],
[
[
"sys.simulate()\nsys.show()",
"System: sys\nHighest convergence error among components in recycle\nstream S1-0 after 4 loops:\n- flow rate 1.38e-01 kmol/hr (0.16%)\n- temperature 4.44e-03 K (0.0012%)\nins...\n[0] feed\n phase: 'l', T: 298.15 K, P: 101325 Pa\n flow (kmol/hr): Water 450\n Methanol 100\nouts...\n[0] vapor_product\n phase: 'g', T: 366.34 K, P: 101325 Pa\n flow (kmol/hr): Water 275\n Methanol 92.1\n[1] liquid_product\n phase: 'l', T: 366.34 K, P: 101325 Pa\n flow (kmol/hr): Water 175\n Methanol 7.91\n"
]
],
[
[
"Note how the recycle stream converged and all unit operations (including the flash vessel) were simulated:",
"_____no_output_____"
]
],
[
[
"F1.results()",
"_____no_output_____"
]
],
[
[
"You can retrieve summarized power and heat utilities from the system as well:",
"_____no_output_____"
]
],
[
[
"sys.power_utility.show()",
"PowerUtility:\n consumption: 0 kW\n production: 0 kW\n rate: 0 kW\n cost: 0 USD/hr\n"
],
[
"for i in sys.heat_utilities: i.show()",
"HeatUtility: low_pressure_steam\n duty: 1.82e+07 kJ/hr\n flow: 470 kmol/hr\n cost: 94 USD/hr\n"
]
],
[
[
"Once your system has been simulated, you can save a system report to view all results in an excel spreadsheet:",
"_____no_output_____"
]
],
[
[
"# Try this on your computer and open excel\n# sys.save_report('Example.xlsx') ",
"_____no_output_____"
]
],
[
[
"Note that the cash flow analysis did not appear in the report because it requires a [TEA](../TEA.txt) object with all the necessary parameters (e.g., depreciation schedule, plant lifetime, construction schedule) to perform the analysis. A [TEA](../TEA.txt) object may also solve for economic indicators such as internal rate of return, minimum product selling price (MPSP), and maximum feedstock purchase price (MFPP). [Techno-economic analysis](./Techno-economic_analysis.ipynb) is discussed in detail later in the tutorial due to the extensive nature of the cash flow analysis.",
"_____no_output_____"
]
]
] | [
"markdown",
"code",
"markdown",
"code",
"markdown",
"code",
"markdown",
"code",
"markdown",
"code",
"markdown",
"code",
"markdown",
"code",
"markdown",
"code",
"markdown",
"code",
"markdown",
"code",
"markdown",
"code",
"markdown",
"code",
"markdown",
"code",
"markdown",
"code",
"markdown",
"code",
"markdown",
"code",
"markdown",
"code",
"markdown",
"code",
"markdown",
"code",
"markdown",
"code",
"markdown"
] | [
[
"markdown",
"markdown",
"markdown"
],
[
"code"
],
[
"markdown"
],
[
"code"
],
[
"markdown",
"markdown",
"markdown"
],
[
"code",
"code"
],
[
"markdown"
],
[
"code",
"code"
],
[
"markdown"
],
[
"code",
"code",
"code",
"code",
"code",
"code",
"code",
"code"
],
[
"markdown",
"markdown"
],
[
"code"
],
[
"markdown"
],
[
"code",
"code"
],
[
"markdown"
],
[
"code"
],
[
"markdown"
],
[
"code"
],
[
"markdown",
"markdown"
],
[
"code",
"code"
],
[
"markdown",
"markdown",
"markdown",
"markdown"
],
[
"code"
],
[
"markdown"
],
[
"code"
],
[
"markdown"
],
[
"code"
],
[
"markdown"
],
[
"code"
],
[
"markdown"
],
[
"code"
],
[
"markdown"
],
[
"code"
],
[
"markdown"
],
[
"code"
],
[
"markdown"
],
[
"code"
],
[
"markdown"
],
[
"code",
"code"
],
[
"markdown"
],
[
"code"
],
[
"markdown"
]
] |
e772326bc6912d558b34cf41daa0041f06dd55e1 | 171,507 | ipynb | Jupyter Notebook | multivariate_BWAS_replicability_analysis_FC.ipynb | spisakt/BWAS_comment | 517ea47b04f1d77dd81ba96bd6b4fb497c0faedf | [
"MIT"
] | null | null | null | multivariate_BWAS_replicability_analysis_FC.ipynb | spisakt/BWAS_comment | 517ea47b04f1d77dd81ba96bd6b4fb497c0faedf | [
"MIT"
] | null | null | null | multivariate_BWAS_replicability_analysis_FC.ipynb | spisakt/BWAS_comment | 517ea47b04f1d77dd81ba96bd6b4fb497c0faedf | [
"MIT"
] | null | null | null | 60.135694 | 6,690 | 0.497263 | [
[
[
"# Analyzing replicability of functional connectivity-based multivariate BWAS on the Human Connectome Project dataset\n\n## Imports",
"_____no_output_____"
]
],
[
[
"import numpy as np\nimport pandas as pd\nimport seaborn as sns\nimport matplotlib.pyplot as plt\nfrom sklearn.linear_model import Ridge\nfrom sklearn.svm import SVR\nfrom sklearn.model_selection import KFold, train_test_split\nfrom sklearn.pipeline import Pipeline\nfrom sklearn.decomposition import PCA\nfrom joblib import Parallel, delayed\nfrom mlxtend.evaluate import permutation_test\nsns.set(rc={\"figure.figsize\":(4, 2)})\nsns.set_style(\"whitegrid\")",
"_____no_output_____"
]
],
[
[
"## Load HCP data\n\nWe load functional network matrices (netmats) from the HCP1200-release, as published on connectomeDB: https://db.humanconnectome.org/\nDue to licensoing issues, data is not supplied with the repository, but can be downloaded from the ConnectomeDB.\nSee [hcp_data/readme.md](hcp_data/readme.md) for more details.",
"_____no_output_____"
]
],
[
[
"# HCP data can be obtained from the connectomeDB\n# data is not part of this repository\nsubjectIDs = pd.read_csv('hcp_data/subjectIDs.txt', header=None)\n\nnetmats_pearson = pd.read_csv('hcp_data/netmats1_correlationZ.txt',\n sep=' ',\n header=None)\nnetmats_pearson['ID'] = subjectIDs[0]\nnetmats_pearson.set_index('ID', drop=True, inplace=True)\n\n\nnetmats_parcor = pd.read_csv('hcp_data/netmats2_partial-correlation.txt',\n sep=' ',\n header=None)\nnetmats_parcor['ID'] = subjectIDs[0]\nnetmats_parcor.set_index('ID', drop=True, inplace=True)\n\nbehavior = pd.read_csv('hcp_data/hcp1200_behavioral_data.csv')\nbehavior = behavior.set_index('Subject', drop=True)\n\n# convert age to numeric\nage = []\nfor s in behavior['Age']:\n if s == '36+':\n age.append(36)\n else:\n split = s.split(sep='-')\n age.append(np.mean((float(split[0]), float(split[1]))))\n\nbehavior['age'] = age\nbehavior",
"_____no_output_____"
]
],
[
[
"# Function to prepare target variable\n",
"_____no_output_____"
]
],
[
[
"def create_data(target='CogTotalComp_AgeAdj', feature_data=netmats_parcor):\n # it's a good practice to use pandas for merging, messing up subject order can be painful\n features = feature_data.columns\n df = behavior\n df = df.merge(feature_data, left_index=True, right_index=True, how='left')\n\n df = df.dropna(subset = [target] + features.values.tolist())\n y = df[target].values\n X = df[features].values\n return X, y",
"_____no_output_____"
]
],
[
[
"# Function implementing a single bootstrap iteration\n\nWe define a workhorse function which:\n- randomly samples the discovery and the replication datasets,\n- creates cross-validated estimates of predictive performance within the discovery sample\n- finalizes the model by fitting it to the whole discovery sample (overfits the discovery but not the replication sample)\n- use it to predict the replication sample",
"_____no_output_____"
]
],
[
[
"def bootstrap_workhorse(X, y, sample_size, model, random_state, shuffle_y=False):\n\n #create discovery and replication samples by random sampling from the whole dataset (without replacement)\n\n # if shuffle_y is true, a null model is created bz permuting y\n if shuffle_y:\n rng = np.random.default_rng(random_state)\n y = rng.permutation(y)\n\n # sample the discovery and replication sets *without replacement* (with replacement introduces spurious dependencies)\n X_discovery, X_replication, y_discovery, y_replication = train_test_split(X, y, train_size=sample_size, test_size=sample_size, shuffle=True, random_state=random_state)\n\n # standard 10-fold cross-validation\n cv = KFold(10)\n\n # below we obtain cross-validated predictions in the discovery sample\n predicted_discovery_cv = np.zeros_like(y_discovery) # here we collect the predictions for each fold\n cor_per_fold = np.zeros(cv.n_splits) # here we collect the predictive performance in each fold\n i = 0 # just a counter\n for train, test in cv.split(X=X_discovery, y=y_discovery): # loop to leave one fold out\n model.fit(X=X_discovery[train], y=y_discovery[train]) # fit model to the training set\n predicted_discovery_cv[test] = model.predict(X=X_discovery[test]) # use fitted model to predict teh test set\n cor_per_fold[i] = np.corrcoef(y_discovery[test], predicted_discovery_cv[test])[0,1] # calculate performance on tne test set\n i += 1\n # calculate mean test performance across all folds\n r_disc_cv = np.mean(cor_per_fold)\n # 'finalize' model by training it on the full discovery sample (without cross-validation)\n final_model = model.fit(X=X_discovery, y=y_discovery)\n # obtain predictions with the final model on the discovery sample, note that this model actually overfits this sample.\n # we do this only to demonstrate biased estimates\n predicted_discovery_overfit = final_model.predict(X=X_discovery)\n # here we obtain the biased effect size (r) estimates for demonstrational purposes\n r_disc_overfit = np.corrcoef(predicted_discovery_overfit, y_discovery)[0, 1]\n\n # We use the final model to predict the replication sample\n # This is correct (no overfitting here), the final model did not see this data during training\n predicted_replication = final_model.predict(X=X_replication)\n # we obtain the out-of-sample prediction performance estimates\n r_rep = np.corrcoef(predicted_replication, y_replication)[0, 1]\n\n # below we calculate permutation-based p-values for all three effect size estimates (in-sample unbiased, in-sample biased, out-of-sample)\n # (one sided tests, testing for positive correlation)\n p_disc_cv = permutation_test(predicted_discovery_cv, y_discovery, method='approximate', num_rounds=1000, func=lambda x, y: np.corrcoef(x, y)[1][0],seed=random_state)\n p_disc_overfit = permutation_test(predicted_discovery_overfit, y_discovery, method='approximate', num_rounds=1000, func=lambda x, y: np.corrcoef(x, y)[1][0],seed=random_state)\n p_rep = permutation_test(predicted_replication, y_replication, method='approximate', num_rounds=1000, func=lambda x, y: np.corrcoef(x, y)[1][0],seed=random_state)\n # return results\n return r_disc_cv, r_disc_overfit, r_rep, p_disc_cv, p_disc_overfit, p_rep",
"_____no_output_____"
]
],
[
[
"All set, now we start the analysis.",
"_____no_output_____"
],
[
"# Replicability with sample sizes n=50, 100, 200, 300 and max\nHere we train a few different models on 100 bootstrap samples.\n\nWe aggregate the results of our workhorse function in `n_bootstrap`=100 bootstrap cases (run in parallel).\n\nThe whole process is repeated for all sample sizes, fetaure_sets and target variables.\n\n## Here we test age and 5 cognitive variables, including 'cognitive ability' (the main target variable in the target paper)\n- age: age group of the participants\n- CogTotalComp_AgeAdj: total cognitive ability\n- PMAT24_A_CR, : Fluid Intelligence (Penn Progressive Matrices)\n- CardSort_AgeAdj: Executive Function/Cognitive Flexibility (Dimensional Change Card Sort)\n- Flanker_AgeAdj: Executive Function/Inhibition (Flanker Task)\n- PicSeq_AgeAdj: Episodic Memory (Picture Sequence Memory)",
"_____no_output_____"
],
[
"# Reproducing the PCA+SVR-based model from the target paper\n### Like in the target paper:\n- Both PCA and SVR are done inside the cross-validation\n- PCA reatains the firts k principal components that together explain 50% of the variance\n- scikit-learn makes sure that PCA is only fit for the training samples\n- both for the test sets (in the cross-validation) and the replication sample PCA is not re-fit, bt features are simply transformed with the already fit PCA",
"_____no_output_____"
]
],
[
[
"%%time\n\nrandom_state = 42\nn_bootstrap = 100\n\nfeatures = {\n 'netmats_parcor': netmats_parcor,\n 'netmats_pearson': netmats_pearson\n}\n\nmodels = {\n 'PCA_SVR': Pipeline([('pca', PCA(n_components=0.5)),\n ('svr', SVR())])\n\n}\n\n# We aggregate all results here:\ndf = pd.DataFrame(columns=['connectivity','model','target','n','r_discovery_cv','r_discovery_overfit','r_replication','p_discovery_cv','p_discovery_overfit','p_replication'])\n\nfor feature_set in features:\n for model in models:\n for target_var in ['age', 'CogTotalComp_AgeAdj', 'PMAT24_A_CR', 'Flanker_AgeAdj', 'CardSort_AgeAdj', 'PicSeq_AgeAdj']:\n for sample_size in [50, 100, 200, 300, 'max']:\n\n print('*****************************************************************')\n print(feature_set, model, target_var, sample_size)\n\n X, y = create_data(target=target_var, feature_data=features[feature_set])\n\n if sample_size=='max':\n sample_size = int(len(y)/2)\n\n # create random seeds for each bootstrap iteration for reproducibility\n rng = np.random.default_rng(random_state)\n random_sates = rng.integers(np.iinfo(np.int32).max, size=n_bootstrap)\n\n # run bootstrap iterations in parallel\n r_discovery_cv, r_discovery_overfit, r_replication, p_discovery_cv, p_discovery_overfit, p_replication = zip(\n *Parallel(n_jobs=-1)(\n delayed(bootstrap_workhorse)(X, y, sample_size, models[model], seed) for seed in random_sates))\n\n tmp_data_frame = pd.DataFrame({\n 'connectivity' : feature_set,\n 'model' : model,\n 'target' : target_var,\n 'n' : sample_size,\n 'r_discovery_cv': r_discovery_cv,\n 'r_discovery_overfit': r_discovery_overfit,\n 'r_replication': r_replication,\n 'p_discovery_cv': p_discovery_cv,\n 'p_discovery_overfit': p_discovery_overfit,\n 'p_replication': p_replication\n })\n #sns.scatterplot(x='r_replication', y='r_discovery_cv', data=tmp_data_frame)\n #plt.ylabel('in-sample (r)')\n #plt.xlabel('out-of-sample (r_pred)')\n #plt.show()\n print(tmp_data_frame.r_discovery_cv.mean(), tmp_data_frame.r_replication.mean())\n\n for alpha in [0.05, 0.01, 0.005, 0.001]:\n print('Replicability at alpha =', alpha, ':',\n (tmp_data_frame.loc[tmp_data_frame['p_discovery_cv']<alpha,'p_replication']<alpha).sum() / (tmp_data_frame['p_discovery_cv']<0.05).sum() * 100, '%')\n\n df = pd.concat((df, tmp_data_frame))\n df.reset_index(drop=True, inplace=True)\n df.to_csv('res/results_PCA_SVR.csv')\n\ndf",
"*****************************************************************\nnetmats_parcor PCA_SVR age 50\n0.18451221232892587 0.18901378266057708\nReplicability at alpha = 0.05 : 57.14285714285714 %\nReplicability at alpha = 0.01 : 14.285714285714285 %\nReplicability at alpha = 0.005 : 0.0 %\nReplicability at alpha = 0.001 : 0.0 %\n*****************************************************************\nnetmats_parcor PCA_SVR age 100\n0.24729849550421484 0.27347377929445293\nReplicability at alpha = 0.05 : 89.55223880597015 %\nReplicability at alpha = 0.01 : 40.298507462686565 %\nReplicability at alpha = 0.005 : 31.343283582089555 %\nReplicability at alpha = 0.001 : 11.940298507462686 %\n*****************************************************************\nnetmats_parcor PCA_SVR age 200\n0.34201730090591836 0.36175079133098437\nReplicability at alpha = 0.05 : 100.0 %\nReplicability at alpha = 0.01 : 98.9795918367347 %\nReplicability at alpha = 0.005 : 95.91836734693877 %\nReplicability at alpha = 0.001 : 80.61224489795919 %\n*****************************************************************\nnetmats_parcor PCA_SVR age 300\n0.3874316088394154 0.39438101148062005\nReplicability at alpha = 0.05 : 100.0 %\nReplicability at alpha = 0.01 : 100.0 %\nReplicability at alpha = 0.005 : 100.0 %\nReplicability at alpha = 0.001 : 100.0 %\n*****************************************************************\nnetmats_parcor PCA_SVR age max\n0.4261365660134988 0.44371738829107926\nReplicability at alpha = 0.05 : 100.0 %\nReplicability at alpha = 0.01 : 100.0 %\nReplicability at alpha = 0.005 : 100.0 %\nReplicability at alpha = 0.001 : 100.0 %\n*****************************************************************\nnetmats_parcor PCA_SVR CogTotalComp_AgeAdj 50\n0.1281291166293238 0.14032170544200848\nReplicability at alpha = 0.05 : nan %\nReplicability at alpha = 0.01 : nan %\nReplicability at alpha = 0.005 : nan %\nReplicability at alpha = 0.001 : nan %\n*****************************************************************\nnetmats_parcor PCA_SVR CogTotalComp_AgeAdj 100\n"
]
],
[
[
"# Now we fit a simple Ridge regression\n(no feature selection, no hyperparameter optimization)\nThis can be expected to perform better on low samples than SVR.",
"_____no_output_____"
]
],
[
[
"%%time\n\nrandom_state = 42\nn_bootstrap = 100\n\nfeatures = {\n 'netmats_parcor': netmats_parcor,\n 'netmats_pearson': netmats_pearson\n}\n\nmodels = {\n 'ridge': Ridge()\n}\n\n# We aggregate all results here:\ndf = pd.DataFrame(columns=['connectivity','model','target','n','r_discovery_cv','r_discovery_overfit','r_replication','p_discovery_cv','p_discovery_overfit','p_replication'])\n\nfor feature_set in features:\n for model in models:\n for target_var in ['age', 'CogTotalComp_AgeAdj', 'PMAT24_A_CR', 'Flanker_AgeAdj', 'CardSort_AgeAdj', 'PicSeq_AgeAdj']:\n for sample_size in [50, 100, 200, 300, 'max']:\n\n print('*****************************************************************')\n print(feature_set, model, target_var, sample_size)\n\n X, y = create_data(target=target_var, feature_data=features[feature_set])\n\n if sample_size=='max':\n sample_size = int(len(y)/2)\n\n # create random seeds for each bootstrap iteration for reproducibility\n rng = np.random.default_rng(random_state)\n random_sates = rng.integers(np.iinfo(np.int32).max, size=n_bootstrap)\n\n # run bootstrap iterations in parallel\n r_discovery_cv, r_discovery_overfit, r_replication, p_discovery_cv, p_discovery_overfit, p_replication = zip(\n *Parallel(n_jobs=-1)(\n delayed(bootstrap_workhorse)(X, y, sample_size, models[model], seed) for seed in random_sates))\n\n tmp_data_frame = pd.DataFrame({\n 'connectivity' : feature_set,\n 'model' : model,\n 'target' : target_var,\n 'n' : sample_size,\n 'r_discovery_cv': r_discovery_cv,\n 'r_discovery_overfit': r_discovery_overfit,\n 'r_replication': r_replication,\n 'p_discovery_cv': p_discovery_cv,\n 'p_discovery_overfit': p_discovery_overfit,\n 'p_replication': p_replication\n })\n #sns.scatterplot(x='r_replication', y='r_discovery_cv', data=tmp_data_frame)\n #plt.ylabel('in-sample (r)')\n #plt.xlabel('out-of-sample (r_pred)')\n #plt.show()\n print(tmp_data_frame.r_discovery_cv.mean(), tmp_data_frame.r_replication.mean())\n\n for alpha in [0.05, 0.01, 0.005, 0.001]:\n print('Replicability at alpha =', alpha, ':',\n (tmp_data_frame.loc[tmp_data_frame['p_discovery_cv']<alpha,'p_replication']<alpha).sum() / (tmp_data_frame['p_discovery_cv']<0.05).sum() * 100, '%')\n\n df = pd.concat((df, tmp_data_frame))\n df.reset_index(drop=True, inplace=True)\n df.to_csv('res/results_Ridge.csv')\n\ndf\n",
"*****************************************************************\nnetmats_parcor ridge age 50\n0.24233370132686197 0.2609198136325508\nReplicability at alpha = 0.05 : 58.536585365853654 %\nReplicability at alpha = 0.01 : 14.634146341463413 %\nReplicability at alpha = 0.005 : 12.195121951219512 %\nReplicability at alpha = 0.001 : 7.317073170731707 %\n*****************************************************************\nnetmats_parcor ridge age 100\n0.3323209164524509 0.34287580012326385\nReplicability at alpha = 0.05 : 97.75280898876404 %\nReplicability at alpha = 0.01 : 71.91011235955057 %\nReplicability at alpha = 0.005 : 58.42696629213483 %\nReplicability at alpha = 0.001 : 38.20224719101123 %\n*****************************************************************\nnetmats_parcor ridge age 200\n0.39528891792691084 0.4213171713707975\nReplicability at alpha = 0.05 : 100.0 %\nReplicability at alpha = 0.01 : 100.0 %\nReplicability at alpha = 0.005 : 99.0 %\nReplicability at alpha = 0.001 : 97.0 %\n*****************************************************************\nnetmats_parcor ridge age 300\n0.44147299146433366 0.4482536389663905\nReplicability at alpha = 0.05 : 100.0 %\nReplicability at alpha = 0.01 : 100.0 %\nReplicability at alpha = 0.005 : 100.0 %\nReplicability at alpha = 0.001 : 100.0 %\n*****************************************************************\nnetmats_parcor ridge age max\n0.48020846359551633 0.48967065593640496\nReplicability at alpha = 0.05 : 100.0 %\nReplicability at alpha = 0.01 : 100.0 %\nReplicability at alpha = 0.005 : 100.0 %\nReplicability at alpha = 0.001 : 100.0 %\n*****************************************************************\nnetmats_parcor ridge CogTotalComp_AgeAdj 50\n0.21283412855006123 0.25667317377477333\nReplicability at alpha = 0.05 : 64.86486486486487 %\nReplicability at alpha = 0.01 : 21.62162162162162 %\nReplicability at alpha = 0.005 : 10.81081081081081 %\nReplicability at alpha = 0.001 : 0.0 %\n*****************************************************************\nnetmats_parcor ridge CogTotalComp_AgeAdj 100\n0.30814190972607364 0.3244129647896814\nReplicability at alpha = 0.05 : 97.5 %\nReplicability at alpha = 0.01 : 68.75 %\nReplicability at alpha = 0.005 : 63.74999999999999 %\nReplicability at alpha = 0.001 : 33.75 %\n*****************************************************************\nnetmats_parcor ridge CogTotalComp_AgeAdj 200\n0.40452415449126483 0.4062055329971098\nReplicability at alpha = 0.05 : 100.0 %\nReplicability at alpha = 0.01 : 99.0 %\nReplicability at alpha = 0.005 : 99.0 %\nReplicability at alpha = 0.001 : 98.0 %\n*****************************************************************\nnetmats_parcor ridge CogTotalComp_AgeAdj 300\n0.4310543037446978 0.4438901556711544\nReplicability at alpha = 0.05 : 100.0 %\nReplicability at alpha = 0.01 : 100.0 %\nReplicability at alpha = 0.005 : 100.0 %\nReplicability at alpha = 0.001 : 100.0 %\n*****************************************************************\nnetmats_parcor ridge CogTotalComp_AgeAdj max\n0.4726518883300038 0.47903946590450225\nReplicability at alpha = 0.05 : 100.0 %\nReplicability at alpha = 0.01 : 100.0 %\nReplicability at alpha = 0.005 : 100.0 %\nReplicability at alpha = 0.001 : 100.0 %\n*****************************************************************\nnetmats_parcor ridge PMAT24_A_CR 50\n0.1823401535280456 0.2012726045326664\nReplicability at alpha = 0.05 : 45.83333333333333 %\nReplicability at alpha = 0.01 : 8.333333333333332 %\nReplicability at alpha = 0.005 : 4.166666666666666 %\nReplicability at alpha = 0.001 : 0.0 %\n*****************************************************************\nnetmats_parcor ridge PMAT24_A_CR 100\n0.2267392726585016 0.22748732178394315\nReplicability at alpha = 0.05 : 75.40983606557377 %\nReplicability at alpha = 0.01 : 32.78688524590164 %\nReplicability at alpha = 0.005 : 22.950819672131146 %\nReplicability at alpha = 0.001 : 6.557377049180328 %\n*****************************************************************\nnetmats_parcor ridge PMAT24_A_CR 200\n0.2721367270595466 0.27987993716710663\nReplicability at alpha = 0.05 : 97.89473684210527 %\nReplicability at alpha = 0.01 : 87.36842105263159 %\nReplicability at alpha = 0.005 : 80.0 %\nReplicability at alpha = 0.001 : 47.368421052631575 %\n*****************************************************************\nnetmats_parcor ridge PMAT24_A_CR 300\n0.2861113545492929 0.2902385092001479\nReplicability at alpha = 0.05 : 100.0 %\nReplicability at alpha = 0.01 : 98.9795918367347 %\nReplicability at alpha = 0.005 : 98.9795918367347 %\nReplicability at alpha = 0.001 : 86.73469387755102 %\n*****************************************************************\nnetmats_parcor ridge PMAT24_A_CR max\n0.2922426473940083 0.301492004778787\nReplicability at alpha = 0.05 : 100.0 %\nReplicability at alpha = 0.01 : 100.0 %\nReplicability at alpha = 0.005 : 100.0 %\nReplicability at alpha = 0.001 : 100.0 %\n*****************************************************************\nnetmats_parcor ridge Flanker_AgeAdj 50\n0.0443711981293818 0.06932189595874623\nReplicability at alpha = 0.05 : 10.0 %\nReplicability at alpha = 0.01 : 0.0 %\nReplicability at alpha = 0.005 : 0.0 %\nReplicability at alpha = 0.001 : 0.0 %\n*****************************************************************\nnetmats_parcor ridge Flanker_AgeAdj 100\n0.07383591946659811 0.08508903100867408\nReplicability at alpha = 0.05 : 21.052631578947366 %\nReplicability at alpha = 0.01 : 5.263157894736842 %\nReplicability at alpha = 0.005 : 0.0 %\nReplicability at alpha = 0.001 : 0.0 %\n*****************************************************************\nnetmats_parcor ridge Flanker_AgeAdj 200\n0.1043401785816992 0.10467365119965867\nReplicability at alpha = 0.05 : 44.44444444444444 %\nReplicability at alpha = 0.01 : 11.11111111111111 %\nReplicability at alpha = 0.005 : 2.7777777777777777 %\nReplicability at alpha = 0.001 : 0.0 %\n*****************************************************************\nnetmats_parcor ridge Flanker_AgeAdj 300\n0.11740601607603006 0.11951226654893972\nReplicability at alpha = 0.05 : 64.61538461538461 %\nReplicability at alpha = 0.01 : 16.923076923076923 %\nReplicability at alpha = 0.005 : 10.76923076923077 %\nReplicability at alpha = 0.001 : 0.0 %\n*****************************************************************\nnetmats_parcor ridge Flanker_AgeAdj max\n0.13226123494919054 0.14022631939127772\nReplicability at alpha = 0.05 : 98.87640449438202 %\nReplicability at alpha = 0.01 : 61.79775280898876 %\nReplicability at alpha = 0.005 : 42.69662921348314 %\nReplicability at alpha = 0.001 : 12.359550561797752 %\n*****************************************************************\nnetmats_parcor ridge CardSort_AgeAdj 50\n0.03322269613512982 0.09078700181744905\nReplicability at alpha = 0.05 : 25.0 %\nReplicability at alpha = 0.01 : 0.0 %\nReplicability at alpha = 0.005 : 0.0 %\nReplicability at alpha = 0.001 : 0.0 %\n*****************************************************************\nnetmats_parcor ridge CardSort_AgeAdj 100\n0.07355512218057152 0.10144797710118604\nReplicability at alpha = 0.05 : 36.36363636363637 %\nReplicability at alpha = 0.01 : 0.0 %\nReplicability at alpha = 0.005 : 0.0 %\nReplicability at alpha = 0.001 : 0.0 %\n*****************************************************************\nnetmats_parcor ridge CardSort_AgeAdj 200\n0.1191089234752379 0.14201690360065491\nReplicability at alpha = 0.05 : 53.48837209302325 %\nReplicability at alpha = 0.01 : 13.953488372093023 %\nReplicability at alpha = 0.005 : 11.627906976744185 %\nReplicability at alpha = 0.001 : 2.3255813953488373 %\n*****************************************************************\nnetmats_parcor ridge CardSort_AgeAdj 300\n0.16484711750220982 0.1671880335883637\nReplicability at alpha = 0.05 : 89.74358974358975 %\nReplicability at alpha = 0.01 : 46.15384615384615 %\nReplicability at alpha = 0.005 : 33.33333333333333 %\nReplicability at alpha = 0.001 : 5.128205128205128 %\n*****************************************************************\nnetmats_parcor ridge CardSort_AgeAdj max\n0.1789658621130238 0.1895769375836651\nReplicability at alpha = 0.05 : 100.0 %\nReplicability at alpha = 0.01 : 96.90721649484536 %\nReplicability at alpha = 0.005 : 91.75257731958763 %\nReplicability at alpha = 0.001 : 65.97938144329896 %\n*****************************************************************\nnetmats_parcor ridge PicSeq_AgeAdj 50\n0.10629000587633886 0.09860417658851763\nReplicability at alpha = 0.05 : 10.0 %\nReplicability at alpha = 0.01 : 0.0 %\nReplicability at alpha = 0.005 : 0.0 %\nReplicability at alpha = 0.001 : 0.0 %\n*****************************************************************\nnetmats_parcor ridge PicSeq_AgeAdj 100\n0.0949755744370731 0.1314332174937417\nReplicability at alpha = 0.05 : 47.05882352941176 %\nReplicability at alpha = 0.01 : 0.0 %\nReplicability at alpha = 0.005 : 0.0 %\nReplicability at alpha = 0.001 : 0.0 %\n*****************************************************************\nnetmats_parcor ridge PicSeq_AgeAdj 200\n0.146383528748086 0.1703337821724498\nReplicability at alpha = 0.05 : 82.25806451612904 %\nReplicability at alpha = 0.01 : 24.193548387096776 %\nReplicability at alpha = 0.005 : 22.58064516129032 %\nReplicability at alpha = 0.001 : 11.29032258064516 %\n*****************************************************************\nnetmats_parcor ridge PicSeq_AgeAdj 300\n0.1703237435255263 0.17241277384991086\nReplicability at alpha = 0.05 : 95.23809523809523 %\nReplicability at alpha = 0.01 : 60.71428571428571 %\nReplicability at alpha = 0.005 : 44.047619047619044 %\nReplicability at alpha = 0.001 : 16.666666666666664 %\n*****************************************************************\nnetmats_parcor ridge PicSeq_AgeAdj max\n0.18233069324505247 0.185930935195939\nReplicability at alpha = 0.05 : 100.0 %\nReplicability at alpha = 0.01 : 93.87755102040816 %\nReplicability at alpha = 0.005 : 90.81632653061224 %\nReplicability at alpha = 0.001 : 66.3265306122449 %\n*****************************************************************\nnetmats_pearson ridge age 50\n0.14436849357647855 0.18481463200614492\nReplicability at alpha = 0.05 : 43.333333333333336 %\nReplicability at alpha = 0.01 : 13.333333333333334 %\nReplicability at alpha = 0.005 : 3.3333333333333335 %\nReplicability at alpha = 0.001 : 0.0 %\n*****************************************************************\nnetmats_pearson ridge age 100\n0.25465817480605085 0.26862478264613243\nReplicability at alpha = 0.05 : 77.02702702702703 %\nReplicability at alpha = 0.01 : 37.83783783783784 %\nReplicability at alpha = 0.005 : 27.027027027027028 %\nReplicability at alpha = 0.001 : 13.513513513513514 %\n*****************************************************************\nnetmats_pearson ridge age 200\n0.3287743917063903 0.36869205100237123\nReplicability at alpha = 0.05 : 100.0 %\nReplicability at alpha = 0.01 : 96.96969696969697 %\nReplicability at alpha = 0.005 : 95.95959595959596 %\nReplicability at alpha = 0.001 : 82.82828282828282 %\n*****************************************************************\nnetmats_pearson ridge age 300\n0.39936921527356317 0.4055241903617708\nReplicability at alpha = 0.05 : 100.0 %\nReplicability at alpha = 0.01 : 100.0 %\nReplicability at alpha = 0.005 : 100.0 %\nReplicability at alpha = 0.001 : 100.0 %\n*****************************************************************\nnetmats_pearson ridge age max\n0.4362665842811234 0.4411349049881781\nReplicability at alpha = 0.05 : 100.0 %\nReplicability at alpha = 0.01 : 100.0 %\nReplicability at alpha = 0.005 : 100.0 %\nReplicability at alpha = 0.001 : 100.0 %\n*****************************************************************\nnetmats_pearson ridge CogTotalComp_AgeAdj 50\n0.1345837098547966 0.21503639335855204\nReplicability at alpha = 0.05 : 40.625 %\nReplicability at alpha = 0.01 : 9.375 %\nReplicability at alpha = 0.005 : 6.25 %\nReplicability at alpha = 0.001 : 0.0 %\n*****************************************************************\nnetmats_pearson ridge CogTotalComp_AgeAdj 100\n0.24669203314719976 0.2515114436083911\nReplicability at alpha = 0.05 : 78.66666666666666 %\nReplicability at alpha = 0.01 : 42.66666666666667 %\nReplicability at alpha = 0.005 : 26.666666666666668 %\nReplicability at alpha = 0.001 : 8.0 %\n*****************************************************************\nnetmats_pearson ridge CogTotalComp_AgeAdj 200\n0.30007058738420606 0.31597434384989187\nReplicability at alpha = 0.05 : 100.0 %\nReplicability at alpha = 0.01 : 92.85714285714286 %\nReplicability at alpha = 0.005 : 85.71428571428571 %\nReplicability at alpha = 0.001 : 67.3469387755102 %\n*****************************************************************\nnetmats_pearson ridge CogTotalComp_AgeAdj 300\n0.33590718006555087 0.34932970770652483\nReplicability at alpha = 0.05 : 100.0 %\nReplicability at alpha = 0.01 : 98.98989898989899 %\nReplicability at alpha = 0.005 : 98.98989898989899 %\nReplicability at alpha = 0.001 : 97.97979797979798 %\n*****************************************************************\nnetmats_pearson ridge CogTotalComp_AgeAdj max\n0.37141193149251367 0.38191979503949747\nReplicability at alpha = 0.05 : 100.0 %\nReplicability at alpha = 0.01 : 100.0 %\nReplicability at alpha = 0.005 : 100.0 %\nReplicability at alpha = 0.001 : 100.0 %\n*****************************************************************\nnetmats_pearson ridge PMAT24_A_CR 50\n0.14934222791452376 0.1388799182188397\nReplicability at alpha = 0.05 : 11.11111111111111 %\nReplicability at alpha = 0.01 : 0.0 %\nReplicability at alpha = 0.005 : 0.0 %\nReplicability at alpha = 0.001 : 0.0 %\n*****************************************************************\nnetmats_pearson ridge PMAT24_A_CR 100\n0.18566220036570222 0.1838033314380429\nReplicability at alpha = 0.05 : 70.0 %\nReplicability at alpha = 0.01 : 16.0 %\nReplicability at alpha = 0.005 : 10.0 %\nReplicability at alpha = 0.001 : 2.0 %\n*****************************************************************\nnetmats_pearson ridge PMAT24_A_CR 200\n0.2017473008751673 0.22291863490268424\nReplicability at alpha = 0.05 : 96.15384615384616 %\nReplicability at alpha = 0.01 : 67.94871794871796 %\nReplicability at alpha = 0.005 : 57.692307692307686 %\nReplicability at alpha = 0.001 : 23.076923076923077 %\n*****************************************************************\nnetmats_pearson ridge PMAT24_A_CR 300\n0.21904483791623303 0.23218168028471226\nReplicability at alpha = 0.05 : 100.0 %\nReplicability at alpha = 0.01 : 86.45833333333334 %\nReplicability at alpha = 0.005 : 76.04166666666666 %\nReplicability at alpha = 0.001 : 45.83333333333333 %\n*****************************************************************\nnetmats_pearson ridge PMAT24_A_CR max\n0.23273225340700723 0.24325742843831086\nReplicability at alpha = 0.05 : 100.0 %\nReplicability at alpha = 0.01 : 98.0 %\nReplicability at alpha = 0.005 : 98.0 %\nReplicability at alpha = 0.001 : 91.0 %\n*****************************************************************\nnetmats_pearson ridge Flanker_AgeAdj 50\n0.035976819031413136 0.05747397295177521\nReplicability at alpha = 0.05 : 6.666666666666667 %\nReplicability at alpha = 0.01 : 0.0 %\nReplicability at alpha = 0.005 : 0.0 %\nReplicability at alpha = 0.001 : 0.0 %\n*****************************************************************\nnetmats_pearson ridge Flanker_AgeAdj 100\n0.0731436100166337 0.07234018621938115\nReplicability at alpha = 0.05 : 16.0 %\nReplicability at alpha = 0.01 : 4.0 %\nReplicability at alpha = 0.005 : 4.0 %\nReplicability at alpha = 0.001 : 0.0 %\n*****************************************************************\nnetmats_pearson ridge Flanker_AgeAdj 200\n0.08157419252004719 0.08879863978328496\nReplicability at alpha = 0.05 : 25.806451612903224 %\nReplicability at alpha = 0.01 : 3.225806451612903 %\nReplicability at alpha = 0.005 : 0.0 %\nReplicability at alpha = 0.001 : 0.0 %\n*****************************************************************\nnetmats_pearson ridge Flanker_AgeAdj 300\n0.09695735940209599 0.09971177201685358\nReplicability at alpha = 0.05 : 51.92307692307693 %\nReplicability at alpha = 0.01 : 11.538461538461538 %\nReplicability at alpha = 0.005 : 9.615384615384617 %\nReplicability at alpha = 0.001 : 1.9230769230769231 %\n*****************************************************************\nnetmats_pearson ridge Flanker_AgeAdj max\n0.1250866282647015 0.11999535001191594\nReplicability at alpha = 0.05 : 87.95180722891565 %\nReplicability at alpha = 0.01 : 49.39759036144578 %\nReplicability at alpha = 0.005 : 33.734939759036145 %\nReplicability at alpha = 0.001 : 8.433734939759036 %\n*****************************************************************\nnetmats_pearson ridge CardSort_AgeAdj 50\n0.05282206047453426 0.07921422079367145\nReplicability at alpha = 0.05 : 6.666666666666667 %\nReplicability at alpha = 0.01 : 0.0 %\nReplicability at alpha = 0.005 : 0.0 %\nReplicability at alpha = 0.001 : 0.0 %\n*****************************************************************\nnetmats_pearson ridge CardSort_AgeAdj 100\n0.07708630087091589 0.11347807860036273\nReplicability at alpha = 0.05 : 37.03703703703704 %\nReplicability at alpha = 0.01 : 11.11111111111111 %\nReplicability at alpha = 0.005 : 3.7037037037037033 %\nReplicability at alpha = 0.001 : 0.0 %\n*****************************************************************\nnetmats_pearson ridge CardSort_AgeAdj 200\n0.11411395755490421 0.12452129430914514\nReplicability at alpha = 0.05 : 59.57446808510638 %\nReplicability at alpha = 0.01 : 19.148936170212767 %\nReplicability at alpha = 0.005 : 10.638297872340425 %\nReplicability at alpha = 0.001 : 0.0 %\n*****************************************************************\nnetmats_pearson ridge CardSort_AgeAdj 300\n0.1350543645885287 0.14640649744766188\nReplicability at alpha = 0.05 : 83.82352941176471 %\nReplicability at alpha = 0.01 : 39.705882352941174 %\nReplicability at alpha = 0.005 : 33.82352941176471 %\nReplicability at alpha = 0.001 : 7.352941176470589 %\n*****************************************************************\nnetmats_pearson ridge CardSort_AgeAdj max\n0.14317504409223422 0.15294162337000017\nReplicability at alpha = 0.05 : 98.91304347826086 %\nReplicability at alpha = 0.01 : 72.82608695652173 %\nReplicability at alpha = 0.005 : 64.13043478260869 %\nReplicability at alpha = 0.001 : 20.652173913043477 %\n*****************************************************************\nnetmats_pearson ridge PicSeq_AgeAdj 50\n0.0978742832036127 0.0940846981022576\nReplicability at alpha = 0.05 : 20.0 %\nReplicability at alpha = 0.01 : 0.0 %\nReplicability at alpha = 0.005 : 0.0 %\nReplicability at alpha = 0.001 : 0.0 %\n*****************************************************************\nnetmats_pearson ridge PicSeq_AgeAdj 100\n0.09582738513044785 0.12424545727649311\nReplicability at alpha = 0.05 : 25.0 %\nReplicability at alpha = 0.01 : 5.555555555555555 %\nReplicability at alpha = 0.005 : 2.7777777777777777 %\nReplicability at alpha = 0.001 : 0.0 %\n*****************************************************************\nnetmats_pearson ridge PicSeq_AgeAdj 200\n0.13141849959842392 0.13875213635359748\nReplicability at alpha = 0.05 : 69.0909090909091 %\nReplicability at alpha = 0.01 : 20.0 %\nReplicability at alpha = 0.005 : 9.090909090909092 %\nReplicability at alpha = 0.001 : 3.6363636363636362 %\n*****************************************************************\nnetmats_pearson ridge PicSeq_AgeAdj 300\n0.15438375099781623 0.15965154764724945\nReplicability at alpha = 0.05 : 84.14634146341463 %\nReplicability at alpha = 0.01 : 48.78048780487805 %\nReplicability at alpha = 0.005 : 39.02439024390244 %\nReplicability at alpha = 0.001 : 8.536585365853659 %\n*****************************************************************\nnetmats_pearson ridge PicSeq_AgeAdj max\n0.1768425004782625 0.18968608293893335\nReplicability at alpha = 0.05 : 100.0 %\nReplicability at alpha = 0.01 : 90.625 %\nReplicability at alpha = 0.005 : 86.45833333333334 %\nReplicability at alpha = 0.001 : 61.458333333333336 %\nCPU times: user 22.6 s, sys: 5.94 s, total: 28.5 s\nWall time: 16min 48s\n"
]
],
[
[
"# Null scenario with random target\nTo evaluate false positives with biased estimates",
"_____no_output_____"
]
],
[
[
"%%time\n\nrandom_state = 42\nn_bootstrap = 100\n\nfeatures = {\n 'netmats_parcor': netmats_parcor,\n 'netmats_pearson': netmats_pearson\n}\n\nmodels = {\n 'PCA_SVR': Pipeline([('pca', PCA(n_components=0.5)),\n ('svr', SVR())])\n\n}\n\n# We aggregate all results here:\ndf = pd.DataFrame(columns=['connectivity','model','target','n','r_discovery_cv','r_discovery_overfit','r_replication','p_discovery_cv','p_discovery_overfit','p_replication'])\n\nfor feature_set in features:\n for model in models:\n for target_var in ['age', 'CogTotalComp_AgeAdj', 'PMAT24_A_CR', 'Flanker_AgeAdj', 'CardSort_AgeAdj', 'PicSeq_AgeAdj']:\n for sample_size in [50, 100, 200, 300, 'max']:\n\n print('*****************************************************************')\n print(feature_set, model, target_var, sample_size)\n\n X, y = create_data(target=target_var, feature_data=features[feature_set]) # gives a random y when target is None\n\n if sample_size=='max':\n sample_size = int(len(y)/2)\n\n # create random seeds for each bootstrap iteration for reproducibility\n rng = np.random.default_rng(random_state)\n random_sates = rng.integers(np.iinfo(np.int32).max, size=n_bootstrap)\n\n # run bootstrap iterations in parallel, with shuffle_y=True\n r_discovery_cv, r_discovery_overfit, r_replication, p_discovery_cv, p_discovery_overfit, p_replication = zip(\n *Parallel(n_jobs=-1)(\n delayed(bootstrap_workhorse)(X, y, sample_size, models[model], seed, shuffle_y=True) for seed in random_sates))\n\n tmp_data_frame = pd.DataFrame({\n 'connectivity' : feature_set,\n 'model' : model,\n 'target' : target_var,\n 'n' : sample_size,\n 'r_discovery_cv': r_discovery_cv,\n 'r_discovery_overfit': r_discovery_overfit,\n 'r_replication': r_replication,\n 'p_discovery_cv': p_discovery_cv,\n 'p_discovery_overfit': p_discovery_overfit,\n 'p_replication': p_replication\n })\n\n #sns.scatterplot(x='r_replication', y='r_discovery_cv', data=tmp_data_frame)\n #plt.ylabel('in-sample (r)')\n #plt.xlabel('out-of-sample (r_pred)')\n #plt.show()\n print(tmp_data_frame.r_discovery_cv.mean(), tmp_data_frame.r_replication.mean())\n\n for alpha in [0.05, 0.01, 0.005, 0.001]:\n print('Replicability at alpha =', alpha, ':',\n (tmp_data_frame.loc[tmp_data_frame['p_discovery_cv']<alpha,'p_replication']<alpha).sum() / (tmp_data_frame['p_discovery_cv']<0.05).sum() * 100, '%')\n\n df = pd.concat((df, tmp_data_frame))\n df.reset_index(drop=True, inplace=True)\n df.to_csv('res/results_null_PCA_SVR.csv')\n\ndf",
"*****************************************************************\nnetmats_parcor PCA_SVR age 50\n0.015718268671406327 -0.009632305254739223\nReplicability at alpha = 0.05 : 0.0 %\nReplicability at alpha = 0.01 : 0.0 %\nReplicability at alpha = 0.005 : 0.0 %\nReplicability at alpha = 0.001 : 0.0 %\n*****************************************************************\nnetmats_parcor PCA_SVR age 100\n0.02118342357629429 0.0008314530617750957\nReplicability at alpha = 0.05 : 0.0 %\nReplicability at alpha = 0.01 : 0.0 %\nReplicability at alpha = 0.005 : 0.0 %\nReplicability at alpha = 0.001 : 0.0 %\n*****************************************************************\nnetmats_parcor PCA_SVR age 200\n0.0020095024919472414 -0.0020659580620204566\nReplicability at alpha = 0.05 : 12.5 %\nReplicability at alpha = 0.01 : 0.0 %\nReplicability at alpha = 0.005 : 0.0 %\nReplicability at alpha = 0.001 : 0.0 %\n*****************************************************************\nnetmats_parcor PCA_SVR age 300\n0.010896406643841883 -0.014502589400244913\nReplicability at alpha = 0.05 : 0.0 %\nReplicability at alpha = 0.01 : 0.0 %\nReplicability at alpha = 0.005 : 0.0 %\nReplicability at alpha = 0.001 : 0.0 %\n*****************************************************************\nnetmats_parcor PCA_SVR age max\n0.007978059019951798 -0.001729976147067709\nReplicability at alpha = 0.05 : 0.0 %\nReplicability at alpha = 0.01 : 0.0 %\nReplicability at alpha = 0.005 : 0.0 %\nReplicability at alpha = 0.001 : 0.0 %\n*****************************************************************\nnetmats_parcor PCA_SVR CogTotalComp_AgeAdj 50\n-0.0016014510611288468 0.004006549132410664\nReplicability at alpha = 0.05 : nan %\nReplicability at alpha = 0.01 : nan %\nReplicability at alpha = 0.005 : nan %\nReplicability at alpha = 0.001 : nan %\n*****************************************************************\nnetmats_parcor PCA_SVR CogTotalComp_AgeAdj 100\n"
],
[
"%%time\n\nrandom_state = 42\nn_bootstrap = 100\n\nfeatures = {\n 'netmats_parcor': netmats_parcor,\n 'netmats_pearson': netmats_pearson\n}\n\nmodels = {\n 'Ridge': Ridge()\n\n}\n\n# We aggregate all results here:\ndf = pd.DataFrame(columns=['connectivity','model','target','n','r_discovery_cv','r_discovery_overfit','r_replication','p_discovery_cv','p_discovery_overfit','p_replication'])\n\nfor feature_set in features:\n for model in models:\n for target_var in ['age', 'CogTotalComp_AgeAdj', 'PMAT24_A_CR', 'Flanker_AgeAdj', 'CardSort_AgeAdj', 'PicSeq_AgeAdj']:\n for sample_size in [50, 100, 200, 300, 'max']:\n\n print('*****************************************************************')\n print(feature_set, model, target_var, sample_size)\n\n X, y = create_data(target=target_var, feature_data=features[feature_set]) # gives a random y when target is None\n\n if sample_size=='max':\n sample_size = int(len(y)/2)\n\n # create random seeds for each bootstrap iteration for reproducibility\n rng = np.random.default_rng(random_state)\n random_sates = rng.integers(np.iinfo(np.int32).max, size=n_bootstrap)\n\n # run bootstrap iterations in parallel, with shuffle_y=True\n r_discovery_cv, r_discovery_overfit, r_replication, p_discovery_cv, p_discovery_overfit, p_replication = zip(\n *Parallel(n_jobs=-1)(\n delayed(bootstrap_workhorse)(X, y, sample_size, models[model], seed, shuffle_y=True) for seed in random_sates))\n\n tmp_data_frame = pd.DataFrame({\n 'connectivity' : feature_set,\n 'model' : model,\n 'target' : target_var,\n 'n' : sample_size,\n 'r_discovery_cv': r_discovery_cv,\n 'r_discovery_overfit': r_discovery_overfit,\n 'r_replication': r_replication,\n 'p_discovery_cv': p_discovery_cv,\n 'p_discovery_overfit': p_discovery_overfit,\n 'p_replication': p_replication\n })\n\n #sns.scatterplot(x='r_replication', y='r_discovery_cv', data=tmp_data_frame)\n #plt.ylabel('in-sample (r)')\n #plt.xlabel('out-of-sample (r_pred)')\n #plt.show()\n print(tmp_data_frame.r_discovery_cv.mean(), tmp_data_frame.r_replication.mean())\n\n for alpha in [0.05, 0.01, 0.005, 0.001]:\n print('Replicability at alpha =', alpha, ':',\n (tmp_data_frame.loc[tmp_data_frame['p_discovery_cv']<alpha,'p_replication']<alpha).sum() / (tmp_data_frame['p_discovery_cv']<0.05).sum() * 100, '%')\n\n df = pd.concat((df, tmp_data_frame))\n df.reset_index(drop=True, inplace=True)\n df.to_csv('res/results_null_Ridge.csv')\n\ndf",
"*****************************************************************\nnetmats_parcor Ridge age 50\n-0.014348756240858624 -0.019865509971863777\nReplicability at alpha = 0.05 : 0.0 %\nReplicability at alpha = 0.01 : 0.0 %\nReplicability at alpha = 0.005 : 0.0 %\nReplicability at alpha = 0.001 : 0.0 %\n*****************************************************************\nnetmats_parcor Ridge age 100\n0.011020054799004317 0.00818911468614934\nReplicability at alpha = 0.05 : 0.0 %\nReplicability at alpha = 0.01 : 0.0 %\nReplicability at alpha = 0.005 : 0.0 %\nReplicability at alpha = 0.001 : 0.0 %\n*****************************************************************\nnetmats_parcor Ridge age 200\n0.014167298702132609 -0.0043645513332393575\nReplicability at alpha = 0.05 : 11.11111111111111 %\nReplicability at alpha = 0.01 : 0.0 %\nReplicability at alpha = 0.005 : 0.0 %\nReplicability at alpha = 0.001 : 0.0 %\n*****************************************************************\nnetmats_parcor Ridge age 300\n-0.0021402405014510047 -0.0034273125837230166\nReplicability at alpha = 0.05 : 11.11111111111111 %\nReplicability at alpha = 0.01 : 0.0 %\nReplicability at alpha = 0.005 : 0.0 %\nReplicability at alpha = 0.001 : 0.0 %\n*****************************************************************\nnetmats_parcor Ridge age max\n-0.000933870201982066 0.007505519687752571\nReplicability at alpha = 0.05 : 8.333333333333332 %\nReplicability at alpha = 0.01 : 0.0 %\nReplicability at alpha = 0.005 : 0.0 %\nReplicability at alpha = 0.001 : 0.0 %\n*****************************************************************\nnetmats_parcor Ridge CogTotalComp_AgeAdj 50\n0.02293901689413578 -0.003553262241010552\nReplicability at alpha = 0.05 : 33.33333333333333 %\nReplicability at alpha = 0.01 : 0.0 %\nReplicability at alpha = 0.005 : 0.0 %\nReplicability at alpha = 0.001 : 0.0 %\n*****************************************************************\nnetmats_parcor Ridge CogTotalComp_AgeAdj 100\n-0.002251849386081027 -0.007802699331008118\nReplicability at alpha = 0.05 : 0.0 %\nReplicability at alpha = 0.01 : 0.0 %\nReplicability at alpha = 0.005 : 0.0 %\nReplicability at alpha = 0.001 : 0.0 %\n*****************************************************************\nnetmats_parcor Ridge CogTotalComp_AgeAdj 200\n-0.0174070410572923 0.008146498429030416\nReplicability at alpha = 0.05 : 0.0 %\nReplicability at alpha = 0.01 : 0.0 %\nReplicability at alpha = 0.005 : 0.0 %\nReplicability at alpha = 0.001 : 0.0 %\n*****************************************************************\nnetmats_parcor Ridge CogTotalComp_AgeAdj 300\n-0.009671674488352178 -0.0057145689425816115\nReplicability at alpha = 0.05 : 14.285714285714285 %\nReplicability at alpha = 0.01 : 0.0 %\nReplicability at alpha = 0.005 : 0.0 %\nReplicability at alpha = 0.001 : 0.0 %\n*****************************************************************\nnetmats_parcor Ridge CogTotalComp_AgeAdj max\n0.008419829728109098 -0.0014326956221230609\nReplicability at alpha = 0.05 : 0.0 %\nReplicability at alpha = 0.01 : 0.0 %\nReplicability at alpha = 0.005 : 0.0 %\nReplicability at alpha = 0.001 : 0.0 %\n*****************************************************************\nnetmats_parcor Ridge PMAT24_A_CR 50\n0.002179824425776267 -0.021615718801904202\nReplicability at alpha = 0.05 : 0.0 %\nReplicability at alpha = 0.01 : 0.0 %\nReplicability at alpha = 0.005 : 0.0 %\nReplicability at alpha = 0.001 : 0.0 %\n*****************************************************************\nnetmats_parcor Ridge PMAT24_A_CR 100\n-0.003001451650394738 -0.012717970196495827\nReplicability at alpha = 0.05 : 0.0 %\nReplicability at alpha = 0.01 : 0.0 %\nReplicability at alpha = 0.005 : 0.0 %\nReplicability at alpha = 0.001 : 0.0 %\n*****************************************************************\nnetmats_parcor Ridge PMAT24_A_CR 200\n0.00010517823509648986 0.003351224006509012\nReplicability at alpha = 0.05 : 0.0 %\nReplicability at alpha = 0.01 : 0.0 %\nReplicability at alpha = 0.005 : 0.0 %\nReplicability at alpha = 0.001 : 0.0 %\n*****************************************************************\nnetmats_parcor Ridge PMAT24_A_CR 300\n-0.012993875534405668 -0.005839465329505123\nReplicability at alpha = 0.05 : 11.11111111111111 %\nReplicability at alpha = 0.01 : 0.0 %\nReplicability at alpha = 0.005 : 0.0 %\nReplicability at alpha = 0.001 : 0.0 %\n*****************************************************************\nnetmats_parcor Ridge PMAT24_A_CR max\n-0.0025841296777914524 -0.002606715356799574\nReplicability at alpha = 0.05 : 0.0 %\nReplicability at alpha = 0.01 : 0.0 %\nReplicability at alpha = 0.005 : 0.0 %\nReplicability at alpha = 0.001 : 0.0 %\n*****************************************************************\nnetmats_parcor Ridge Flanker_AgeAdj 50\n-0.005863964548830961 0.007938764878329231\nReplicability at alpha = 0.05 : 0.0 %\nReplicability at alpha = 0.01 : 0.0 %\nReplicability at alpha = 0.005 : 0.0 %\nReplicability at alpha = 0.001 : 0.0 %\n*****************************************************************\nnetmats_parcor Ridge Flanker_AgeAdj 100\n0.005747604717167121 -0.0013614969701774996\nReplicability at alpha = 0.05 : 0.0 %\nReplicability at alpha = 0.01 : 0.0 %\nReplicability at alpha = 0.005 : 0.0 %\nReplicability at alpha = 0.001 : 0.0 %\n*****************************************************************\nnetmats_parcor Ridge Flanker_AgeAdj 200\n0.004707590693397557 0.00441893901695366\nReplicability at alpha = 0.05 : 0.0 %\nReplicability at alpha = 0.01 : 0.0 %\nReplicability at alpha = 0.005 : 0.0 %\nReplicability at alpha = 0.001 : 0.0 %\n*****************************************************************\nnetmats_parcor Ridge Flanker_AgeAdj 300\n-0.01615270249560154 0.00695399615510614\nReplicability at alpha = 0.05 : 20.0 %\nReplicability at alpha = 0.01 : 0.0 %\nReplicability at alpha = 0.005 : 0.0 %\nReplicability at alpha = 0.001 : 0.0 %\n*****************************************************************\nnetmats_parcor Ridge Flanker_AgeAdj max\n0.009222660248893805 0.003326531328318057\nReplicability at alpha = 0.05 : 6.25 %\nReplicability at alpha = 0.01 : 0.0 %\nReplicability at alpha = 0.005 : 0.0 %\nReplicability at alpha = 0.001 : 0.0 %\n*****************************************************************\nnetmats_parcor Ridge CardSort_AgeAdj 50\n0.03164431766422981 -0.00012087256372228794\nReplicability at alpha = 0.05 : 11.11111111111111 %\nReplicability at alpha = 0.01 : 11.11111111111111 %\nReplicability at alpha = 0.005 : 0.0 %\nReplicability at alpha = 0.001 : 0.0 %\n*****************************************************************\nnetmats_parcor Ridge CardSort_AgeAdj 100\n0.0074788574270472285 0.014062402717247841\nReplicability at alpha = 0.05 : 0.0 %\nReplicability at alpha = 0.01 : 0.0 %\nReplicability at alpha = 0.005 : 0.0 %\nReplicability at alpha = 0.001 : 0.0 %\n*****************************************************************\nnetmats_parcor Ridge CardSort_AgeAdj 200\n-0.02048848428661857 0.010242473789039303\nReplicability at alpha = 0.05 : 0.0 %\nReplicability at alpha = 0.01 : 0.0 %\nReplicability at alpha = 0.005 : 0.0 %\nReplicability at alpha = 0.001 : 0.0 %\n*****************************************************************\nnetmats_parcor Ridge CardSort_AgeAdj 300\n-0.002088869610819724 -0.0016562721816369152\nReplicability at alpha = 0.05 : 10.0 %\nReplicability at alpha = 0.01 : 0.0 %\nReplicability at alpha = 0.005 : 0.0 %\nReplicability at alpha = 0.001 : 0.0 %\n*****************************************************************\nnetmats_parcor Ridge CardSort_AgeAdj max\n-0.00011425447587010948 -0.0019204116368759024\nReplicability at alpha = 0.05 : 8.333333333333332 %\nReplicability at alpha = 0.01 : 0.0 %\nReplicability at alpha = 0.005 : 0.0 %\nReplicability at alpha = 0.001 : 0.0 %\n*****************************************************************\nnetmats_parcor Ridge PicSeq_AgeAdj 50\n-0.027364134838531836 -0.012612204598756137\nReplicability at alpha = 0.05 : 0.0 %\nReplicability at alpha = 0.01 : 0.0 %\nReplicability at alpha = 0.005 : 0.0 %\nReplicability at alpha = 0.001 : 0.0 %\n*****************************************************************\nnetmats_parcor Ridge PicSeq_AgeAdj 100\n0.02162427958430202 -0.0016765017965614526\nReplicability at alpha = 0.05 : 0.0 %\nReplicability at alpha = 0.01 : 0.0 %\nReplicability at alpha = 0.005 : 0.0 %\nReplicability at alpha = 0.001 : 0.0 %\n*****************************************************************\nnetmats_parcor Ridge PicSeq_AgeAdj 200\n0.011211144099184587 0.00199440458162263\nReplicability at alpha = 0.05 : 10.0 %\nReplicability at alpha = 0.01 : 0.0 %\nReplicability at alpha = 0.005 : 0.0 %\nReplicability at alpha = 0.001 : 0.0 %\n*****************************************************************\nnetmats_parcor Ridge PicSeq_AgeAdj 300\n0.0018836019547171668 -0.003350605418368111\nReplicability at alpha = 0.05 : 0.0 %\nReplicability at alpha = 0.01 : 0.0 %\nReplicability at alpha = 0.005 : 0.0 %\nReplicability at alpha = 0.001 : 0.0 %\n*****************************************************************\nnetmats_parcor Ridge PicSeq_AgeAdj max\n0.002580691922613251 -0.004660561329078452\nReplicability at alpha = 0.05 : 0.0 %\nReplicability at alpha = 0.01 : 0.0 %\nReplicability at alpha = 0.005 : 0.0 %\nReplicability at alpha = 0.001 : 0.0 %\n*****************************************************************\nnetmats_pearson Ridge age 50\n-0.006906498280608221 -0.02084510055673394\nReplicability at alpha = 0.05 : 0.0 %\nReplicability at alpha = 0.01 : 0.0 %\nReplicability at alpha = 0.005 : 0.0 %\nReplicability at alpha = 0.001 : 0.0 %\n*****************************************************************\nnetmats_pearson Ridge age 100\n0.0018870701419332263 0.009290129018297911\nReplicability at alpha = 0.05 : 0.0 %\nReplicability at alpha = 0.01 : 0.0 %\nReplicability at alpha = 0.005 : 0.0 %\nReplicability at alpha = 0.001 : 0.0 %\n*****************************************************************\nnetmats_pearson Ridge age 200\n-0.005733712797873304 0.0013692427758450934\nReplicability at alpha = 0.05 : 0.0 %\nReplicability at alpha = 0.01 : 0.0 %\nReplicability at alpha = 0.005 : 0.0 %\nReplicability at alpha = 0.001 : 0.0 %\n*****************************************************************\nnetmats_pearson Ridge age 300\n0.005958789216373247 -2.6707429672553214e-06\nReplicability at alpha = 0.05 : 0.0 %\nReplicability at alpha = 0.01 : 0.0 %\nReplicability at alpha = 0.005 : 0.0 %\nReplicability at alpha = 0.001 : 0.0 %\n*****************************************************************\nnetmats_pearson Ridge age max\n-0.004046570632425921 0.008780889471408157\nReplicability at alpha = 0.05 : 0.0 %\nReplicability at alpha = 0.01 : 0.0 %\nReplicability at alpha = 0.005 : 0.0 %\nReplicability at alpha = 0.001 : 0.0 %\n*****************************************************************\nnetmats_pearson Ridge CogTotalComp_AgeAdj 50\n0.04452033934625877 0.004645613395684581\nReplicability at alpha = 0.05 : 0.0 %\nReplicability at alpha = 0.01 : 0.0 %\nReplicability at alpha = 0.005 : 0.0 %\nReplicability at alpha = 0.001 : 0.0 %\n*****************************************************************\nnetmats_pearson Ridge CogTotalComp_AgeAdj 100\n-0.01334481086593422 0.0017249662835642403\nReplicability at alpha = 0.05 : 0.0 %\nReplicability at alpha = 0.01 : 0.0 %\nReplicability at alpha = 0.005 : 0.0 %\nReplicability at alpha = 0.001 : 0.0 %\n*****************************************************************\nnetmats_pearson Ridge CogTotalComp_AgeAdj 200\n-0.005665794302928501 0.010043349069329719\nReplicability at alpha = 0.05 : 0.0 %\nReplicability at alpha = 0.01 : 0.0 %\nReplicability at alpha = 0.005 : 0.0 %\nReplicability at alpha = 0.001 : 0.0 %\n*****************************************************************\nnetmats_pearson Ridge CogTotalComp_AgeAdj 300\n0.004127101455001631 -0.00022099001637482533\nReplicability at alpha = 0.05 : 0.0 %\nReplicability at alpha = 0.01 : 0.0 %\nReplicability at alpha = 0.005 : 0.0 %\nReplicability at alpha = 0.001 : 0.0 %\n*****************************************************************\nnetmats_pearson Ridge CogTotalComp_AgeAdj max\n0.0020425582931248593 -0.005491111765428025\nReplicability at alpha = 0.05 : 8.333333333333332 %\nReplicability at alpha = 0.01 : 0.0 %\nReplicability at alpha = 0.005 : 0.0 %\nReplicability at alpha = 0.001 : 0.0 %\n*****************************************************************\nnetmats_pearson Ridge PMAT24_A_CR 50\n-0.0024029493700688073 0.0037007006870455883\nReplicability at alpha = 0.05 : 0.0 %\nReplicability at alpha = 0.01 : 0.0 %\nReplicability at alpha = 0.005 : 0.0 %\nReplicability at alpha = 0.001 : 0.0 %\n*****************************************************************\nnetmats_pearson Ridge PMAT24_A_CR 100\n-0.012463739742387382 -0.014600647516890147\nReplicability at alpha = 0.05 : 0.0 %\nReplicability at alpha = 0.01 : 0.0 %\nReplicability at alpha = 0.005 : 0.0 %\nReplicability at alpha = 0.001 : 0.0 %\n*****************************************************************\nnetmats_pearson Ridge PMAT24_A_CR 200\n0.0005711164432832821 -0.0013372491637859774\nReplicability at alpha = 0.05 : 0.0 %\nReplicability at alpha = 0.01 : 0.0 %\nReplicability at alpha = 0.005 : 0.0 %\nReplicability at alpha = 0.001 : 0.0 %\n*****************************************************************\nnetmats_pearson Ridge PMAT24_A_CR 300\n0.0015506273996479592 -0.005956391028788252\nReplicability at alpha = 0.05 : 8.333333333333332 %\nReplicability at alpha = 0.01 : 0.0 %\nReplicability at alpha = 0.005 : 0.0 %\nReplicability at alpha = 0.001 : 0.0 %\n*****************************************************************\nnetmats_pearson Ridge PMAT24_A_CR max\n0.0024854592676686227 -0.005750926411234354\nReplicability at alpha = 0.05 : 0.0 %\nReplicability at alpha = 0.01 : 0.0 %\nReplicability at alpha = 0.005 : 0.0 %\nReplicability at alpha = 0.001 : 0.0 %\n*****************************************************************\nnetmats_pearson Ridge Flanker_AgeAdj 50\n-0.007542184869513156 0.0037214085113836098\nReplicability at alpha = 0.05 : 0.0 %\nReplicability at alpha = 0.01 : 0.0 %\nReplicability at alpha = 0.005 : 0.0 %\nReplicability at alpha = 0.001 : 0.0 %\n*****************************************************************\nnetmats_pearson Ridge Flanker_AgeAdj 100\n0.0017518303717718228 0.005815788042763308\nReplicability at alpha = 0.05 : 0.0 %\nReplicability at alpha = 0.01 : 0.0 %\nReplicability at alpha = 0.005 : 0.0 %\nReplicability at alpha = 0.001 : 0.0 %\n*****************************************************************\nnetmats_pearson Ridge Flanker_AgeAdj 200\n-0.0020655683810509627 0.013489449893209167\nReplicability at alpha = 0.05 : 11.11111111111111 %\nReplicability at alpha = 0.01 : 0.0 %\nReplicability at alpha = 0.005 : 0.0 %\nReplicability at alpha = 0.001 : 0.0 %\n*****************************************************************\nnetmats_pearson Ridge Flanker_AgeAdj 300\n-0.01964099882234682 0.006051075234929582\nReplicability at alpha = 0.05 : 10.0 %\nReplicability at alpha = 0.01 : 0.0 %\nReplicability at alpha = 0.005 : 0.0 %\nReplicability at alpha = 0.001 : 0.0 %\n*****************************************************************\nnetmats_pearson Ridge Flanker_AgeAdj max\n0.00392584113499522 -0.0021512790689787466\nReplicability at alpha = 0.05 : 0.0 %\nReplicability at alpha = 0.01 : 0.0 %\nReplicability at alpha = 0.005 : 0.0 %\nReplicability at alpha = 0.001 : 0.0 %\n*****************************************************************\nnetmats_pearson Ridge CardSort_AgeAdj 50\n0.03202175309491191 -0.035334855289345164\nReplicability at alpha = 0.05 : 18.181818181818183 %\nReplicability at alpha = 0.01 : 0.0 %\nReplicability at alpha = 0.005 : 0.0 %\nReplicability at alpha = 0.001 : 0.0 %\n*****************************************************************\nnetmats_pearson Ridge CardSort_AgeAdj 100\n-0.013892768388936544 -0.005578276451901454\nReplicability at alpha = 0.05 : 0.0 %\nReplicability at alpha = 0.01 : 0.0 %\nReplicability at alpha = 0.005 : 0.0 %\nReplicability at alpha = 0.001 : 0.0 %\n*****************************************************************\nnetmats_pearson Ridge CardSort_AgeAdj 200\n-0.003954998848861015 -0.0014015193666770496\nReplicability at alpha = 0.05 : 11.11111111111111 %\nReplicability at alpha = 0.01 : 0.0 %\nReplicability at alpha = 0.005 : 0.0 %\nReplicability at alpha = 0.001 : 0.0 %\n*****************************************************************\nnetmats_pearson Ridge CardSort_AgeAdj 300\n0.0007623050906288291 -0.002683838450646227\nReplicability at alpha = 0.05 : 14.285714285714285 %\nReplicability at alpha = 0.01 : 0.0 %\nReplicability at alpha = 0.005 : 0.0 %\nReplicability at alpha = 0.001 : 0.0 %\n*****************************************************************\nnetmats_pearson Ridge CardSort_AgeAdj max\n0.0010255118249216128 0.004723830633075918\nReplicability at alpha = 0.05 : 18.181818181818183 %\nReplicability at alpha = 0.01 : 0.0 %\nReplicability at alpha = 0.005 : 0.0 %\nReplicability at alpha = 0.001 : 0.0 %\n*****************************************************************\nnetmats_pearson Ridge PicSeq_AgeAdj 50\n0.005861404075807947 -0.012902137975928594\nReplicability at alpha = 0.05 : 0.0 %\nReplicability at alpha = 0.01 : 0.0 %\nReplicability at alpha = 0.005 : 0.0 %\nReplicability at alpha = 0.001 : 0.0 %\n*****************************************************************\nnetmats_pearson Ridge PicSeq_AgeAdj 100\n-0.005921935550682716 0.0013951601093495367\nReplicability at alpha = 0.05 : 0.0 %\nReplicability at alpha = 0.01 : 0.0 %\nReplicability at alpha = 0.005 : 0.0 %\nReplicability at alpha = 0.001 : 0.0 %\n*****************************************************************\nnetmats_pearson Ridge PicSeq_AgeAdj 200\n-0.014861398438188926 -0.0029650977727130423\nReplicability at alpha = 0.05 : 14.285714285714285 %\nReplicability at alpha = 0.01 : 0.0 %\nReplicability at alpha = 0.005 : 0.0 %\nReplicability at alpha = 0.001 : 0.0 %\n*****************************************************************\nnetmats_pearson Ridge PicSeq_AgeAdj 300\n-0.003700412480677308 -0.00564380546601192\nReplicability at alpha = 0.05 : 0.0 %\nReplicability at alpha = 0.01 : 0.0 %\nReplicability at alpha = 0.005 : 0.0 %\nReplicability at alpha = 0.001 : 0.0 %\n*****************************************************************\nnetmats_pearson Ridge PicSeq_AgeAdj max\n-0.0019113224353064999 -0.00041829586934283086\nReplicability at alpha = 0.05 : 12.5 %\nReplicability at alpha = 0.01 : 0.0 %\nReplicability at alpha = 0.005 : 0.0 %\nReplicability at alpha = 0.001 : 0.0 %\nCPU times: user 22.1 s, sys: 5.95 s, total: 28.1 s\nWall time: 16min 23s\n"
]
],
[
[
"*See the notebook called 'plot_results.ipynb' for the results.*",
"_____no_output_____"
]
],
[
[
"model = Pipeline([('pca', PCA(n_components=0.5)), ('svr', SVR())])\nrandom_state = 42\ncv = KFold(10, shuffle=True, random_state=random_state)\n\nbar_data_svr = []\n\nfor target_var in ['age', 'CogTotalComp_AgeAdj', 'PMAT24_A_CR', 'Flanker_AgeAdj', 'CardSort_AgeAdj', 'PicSeq_AgeAdj']:\n print(target_var)\n X, y = create_data(target=target_var, feature_data=netmats_pearson)\n\n predicted_discovery_cv = np.zeros_like(y)\n cor_per_fold = np.zeros(cv.n_splits)\n i = 0\n for train, test in cv.split(X=X, y=y):\n model.fit(X=X[train], y=y[train])\n predicted_discovery_cv[test] = model.predict(X=X[test])\n cor_per_fold[i] = np.corrcoef(y[test], predicted_discovery_cv[test])[0,1]\n i += 1\n # correlation between the cross-validated predictions and observations in the discovery sample\n # this is the correct, unbiased estimate!\n # calculated as mean test performance across all folds\n r_disc_cv = np.mean(cor_per_fold)\n # finalize model by training it on the full discovery sample (without cross-validation)\n final_model = model.fit(X=X, y=y)\n # obtain predictions with the final model on the discovery sample, note that this model actually overfits this sample.\n # we do this only to demonstrate biased estimates\n predicted_discovery_overfit = final_model.predict(X=X)\n # here we obtain the biased effect size (r) estimates for demonstrational purposes\n r_disc_overfit = np.corrcoef(predicted_discovery_overfit, y)[0, 1]\n\n # below we calculate permutation-based p-values for all three effect size estimates (in-sample unbiased, in-sample biased, out-of-sample)\n # (one sided tests, testing for positive correlation)\n p_disc_cv = permutation_test(predicted_discovery_cv, y, method='approximate', num_rounds=1000, func=lambda x, y: np.corrcoef(x, y)[1][0],seed=random_state)\n p_disc_overfit = permutation_test(predicted_discovery_overfit, y, method='approximate', num_rounds=1000, func=lambda x, y: np.corrcoef(x, y)[1][0],seed=random_state)\n\n bar_data_svr.append(r_disc_cv)\n\n print('r =', np.round(r_disc_cv, 2), '\\tp =', np.round(p_disc_cv, 3), '\\tR2 =', np.round(r_disc_cv**2 * 100, 1), '%')",
"age\nr = 0.21 \tp = 0.001 \tR2 = 4.2 %\nCogTotalComp_AgeAdj\nr = 0.2 \tp = 0.001 \tR2 = 3.9 %\nPMAT24_A_CR\nr = 0.21 \tp = 0.001 \tR2 = 4.4 %\nFlanker_AgeAdj\nr = 0.12 \tp = 0.001 \tR2 = 1.5 %\nCardSort_AgeAdj\nr = 0.15 \tp = 0.001 \tR2 = 2.1 %\nPicSeq_AgeAdj\nr = 0.15 \tp = 0.001 \tR2 = 2.2 %\n"
],
[
"model = Ridge()\nrandom_state = 42\ncv = KFold(10, shuffle=True, random_state=random_state)\n\nbar_data_ridge = []\n\nfor target_var in ['age', 'CogTotalComp_AgeAdj', 'PMAT24_A_CR', 'Flanker_AgeAdj', 'CardSort_AgeAdj', 'PicSeq_AgeAdj']:\n print(target_var)\n X, y = create_data(target=target_var, feature_data=netmats_parcor)\n\n predicted_discovery_cv = np.zeros_like(y)\n cor_per_fold = np.zeros(cv.n_splits)\n i = 0\n for train, test in cv.split(X=X, y=y):\n model.fit(X=X[train], y=y[train])\n predicted_discovery_cv[test] = model.predict(X=X[test])\n cor_per_fold[i] = np.corrcoef(y[test], predicted_discovery_cv[test])[0,1]\n i += 1\n # correlation between the cross-validated predictions and observations in the discovery sample\n # this is the correct, unbiased estimate!\n # calculated as mean test performance across all folds\n r_disc_cv = np.mean(cor_per_fold)\n # finalize model by training it on the full discovery sample (without cross-validation)\n final_model = model.fit(X=X, y=y)\n # obtain predictions with the final model on the discovery sample, note that this model actually overfits this sample.\n # we do this only to demonstrate biased estimates\n predicted_discovery_overfit = final_model.predict(X=X)\n # here we obtain the biased effect size (r) estimates for demonstrational purposes\n r_disc_overfit = np.corrcoef(predicted_discovery_overfit, y)[0, 1]\n\n # below we calculate permutation-based p-values for all three effect size estimates (in-sample unbiased, in-sample biased, out-of-sample)\n # (one sided tests, testing for positive correlation)\n p_disc_cv = permutation_test(predicted_discovery_cv, y, method='approximate', num_rounds=1000, func=lambda x, y: np.corrcoef(x, y)[1][0],seed=random_state)\n p_disc_overfit = permutation_test(predicted_discovery_overfit, y, method='approximate', num_rounds=1000, func=lambda x, y: np.corrcoef(x, y)[1][0],seed=random_state)\n\n bar_data_ridge.append(r_disc_cv)\n\n print('r =', np.round(r_disc_cv, 2), '\\tp =', np.round(p_disc_cv, 3), '\\tR2 =', np.round(r_disc_cv**2 * 100, 1), '%')",
"age\nr = 0.52 \tp = 0.001 \tR2 = 26.7 %\nCogTotalComp_AgeAdj\nr = 0.5 \tp = 0.001 \tR2 = 25.0 %\nPMAT24_A_CR\nr = 0.28 \tp = 0.001 \tR2 = 8.1 %\nFlanker_AgeAdj\nr = 0.15 \tp = 0.001 \tR2 = 2.1 %\nCardSort_AgeAdj\nr = 0.24 \tp = 0.001 \tR2 = 5.8 %\nPicSeq_AgeAdj\nr = 0.17 \tp = 0.001 \tR2 = 2.8 %\n"
],
[
"model = Ridge()\nrandom_state = 42\ncv = KFold(10, shuffle=True, random_state=random_state)\n\nfor target_var in ['age', 'CogTotalComp_AgeAdj', 'PMAT24_A_CR', 'Flanker_AgeAdj', 'CardSort_AgeAdj', 'PicSeq_AgeAdj']:\n print(target_var)\n X, y = create_data(target=target_var, feature_data=netmats_pearson)\n\n predicted_discovery_cv = np.zeros_like(y)\n cor_per_fold = np.zeros(cv.n_splits)\n i = 0\n for train, test in cv.split(X=X, y=y):\n model.fit(X=X[train], y=y[train])\n predicted_discovery_cv[test] = model.predict(X=X[test])\n cor_per_fold[i] = np.corrcoef(y[test], predicted_discovery_cv[test])[0,1]\n i += 1\n # correlation between the cross-validated predictions and observations in the discovery sample\n # this is the correct, unbiased estimate!\n # calculated as mean test performance across all folds\n r_disc_cv = np.mean(cor_per_fold)\n # finalize model by training it on the full discovery sample (without cross-validation)\n final_model = model.fit(X=X, y=y)\n # obtain predictions with the final model on the discovery sample, note that this model actually overfits this sample.\n # we do this only to demonstrate biased estimates\n predicted_discovery_overfit = final_model.predict(X=X)\n # here we obtain the biased effect size (r) estimates for demonstrational purposes\n r_disc_overfit = np.corrcoef(predicted_discovery_overfit, y)[0, 1]\n\n # below we calculate permutation-based p-values for all three effect size estimates (in-sample unbiased, in-sample biased, out-of-sample)\n # (one sided tests, testing for positive correlation)\n p_disc_cv = permutation_test(predicted_discovery_cv, y, method='approximate', num_rounds=1000, func=lambda x, y: np.corrcoef(x, y)[1][0],seed=random_state)\n p_disc_overfit = permutation_test(predicted_discovery_overfit, y, method='approximate', num_rounds=1000, func=lambda x, y: np.corrcoef(x, y)[1][0],seed=random_state)\n\n print('r =', np.round(r_disc_cv, 2), '\\tp =', np.round(p_disc_cv, 3), '\\tR2 =', np.round(r_disc_cv**2 * 100, 1), '%')",
"age\nr = 0.45 \tp = 0.001 \tR2 = 20.0 %\nCogTotalComp_AgeAdj\nr = 0.4 \tp = 0.001 \tR2 = 16.2 %\nPMAT24_A_CR\nr = 0.25 \tp = 0.001 \tR2 = 6.3 %\nFlanker_AgeAdj\nr = 0.16 \tp = 0.001 \tR2 = 2.6 %\nCardSort_AgeAdj\nr = 0.17 \tp = 0.001 \tR2 = 2.8 %\nPicSeq_AgeAdj\nr = 0.23 \tp = 0.001 \tR2 = 5.5 %\n"
]
],
[
[
"### *See the notebook called 'plot_results_FC.ipynb' for the results.*",
"_____no_output_____"
]
]
] | [
"markdown",
"code",
"markdown",
"code",
"markdown",
"code",
"markdown",
"code",
"markdown",
"code",
"markdown",
"code",
"markdown",
"code",
"markdown",
"code",
"markdown"
] | [
[
"markdown"
],
[
"code"
],
[
"markdown"
],
[
"code"
],
[
"markdown"
],
[
"code"
],
[
"markdown"
],
[
"code"
],
[
"markdown",
"markdown",
"markdown"
],
[
"code"
],
[
"markdown"
],
[
"code"
],
[
"markdown"
],
[
"code",
"code"
],
[
"markdown"
],
[
"code",
"code",
"code"
],
[
"markdown"
]
] |
e772543a71f1cbe2b67f5f1b3772b28a4ffa36c4 | 329,084 | ipynb | Jupyter Notebook | Charity.ipynb | robsonzagrejr/hobs_nico_charity | 7ca6885b854cd91cd4e86a4cdbede08c6af0bd46 | [
"MIT"
] | null | null | null | Charity.ipynb | robsonzagrejr/hobs_nico_charity | 7ca6885b854cd91cd4e86a4cdbede08c6af0bd46 | [
"MIT"
] | null | null | null | Charity.ipynb | robsonzagrejr/hobs_nico_charity | 7ca6885b854cd91cd4e86a4cdbede08c6af0bd46 | [
"MIT"
] | null | null | null | 60.783894 | 44,330 | 0.475724 | [
[
[
"#Setup\n",
"_____no_output_____"
]
],
[
[
"pip install -U plotly",
"Requirement already up-to-date: plotly in /usr/local/lib/python3.6/dist-packages (4.14.3)\nRequirement already satisfied, skipping upgrade: six in /usr/local/lib/python3.6/dist-packages (from plotly) (1.15.0)\nRequirement already satisfied, skipping upgrade: retrying>=1.3.3 in /usr/local/lib/python3.6/dist-packages (from plotly) (1.3.3)\n"
]
],
[
[
"Make sure that sklearn version is 0.24.1",
"_____no_output_____"
]
],
[
[
"!pip install --user --upgrade scikit-learn==0.24.1\nimport sklearn\nprint('The scikit-learn version is {}.'.format(sklearn.__version__))",
"Requirement already up-to-date: scikit-learn==0.24.1 in /root/.local/lib/python3.6/site-packages (0.24.1)\nRequirement already satisfied, skipping upgrade: numpy>=1.13.3 in /usr/local/lib/python3.6/dist-packages (from scikit-learn==0.24.1) (1.19.5)\nRequirement already satisfied, skipping upgrade: scipy>=0.19.1 in /usr/local/lib/python3.6/dist-packages (from scikit-learn==0.24.1) (1.4.1)\nRequirement already satisfied, skipping upgrade: joblib>=0.11 in /usr/local/lib/python3.6/dist-packages (from scikit-learn==0.24.1) (1.0.0)\nRequirement already satisfied, skipping upgrade: threadpoolctl>=2.0.0 in /root/.local/lib/python3.6/site-packages (from scikit-learn==0.24.1) (2.1.0)\nThe scikit-learn version is 0.24.1.\n"
],
[
"# To support both python 2 and python 3\nfrom __future__ import division, print_function, unicode_literals\n\n# Common imports\nimport numpy as np\nimport os\nimport pandas as pd\n\nimport plotly.io as pio\nimport seaborn as sb\n\n# to make plotly as default plot engine in pandas\npd.options.plotting.backend = \"plotly\" #plotly\n\n# to make this notebook's output stable across runs\nnp.random.seed(42)\n\n# To plot pretty figures\n#%matplotlib inline\n#import matplotlib as mpl\nimport matplotlib.pyplot as plt\n#mpl.rc('axes', labelsize=14)\n#mpl.rc('xtick', labelsize=12)\n#mpl.rc('ytick', labelsize=12)\n\n# Ignore useless warnings (see SciPy issue #5998)\n# import warnings\n# warnings.filterwarnings(action=\"ignore\", message=\"^internal gelsd\")",
"_____no_output_____"
]
],
[
[
"#Data Raw Description\n\n**Features**\n- `age`: Age\n- `workclass`: Working Class (Private, Self-emp-not-inc, Self-emp-inc, Federal-gov, Local-gov, State-gov, Without-pay, Never-worked)\n- `education_level`: Level of Education (Bachelors, Some-college, 11th, HS-grad, Prof-school, Assoc-acdm, Assoc-voc, 9th, 7th-8th, 12th, Masters, 1st-4th, 10th, Doctorate, 5th-6th, Preschool)\n- `education-num`: Number of educational years completed\n- `marital-status`: Marital status (Married-civ-spouse, Divorced, Never-married, Separated, Widowed, Married-spouse-absent, Married-AF-spouse)\n- `occupation`: Work Occupation (Tech-support, Craft-repair, Other-service, Sales, Exec-managerial, Prof-specialty, Handlers-cleaners, Machine-op-inspct, Adm-clerical, Farming-fishing, Transport-moving, Priv-house-serv, Protective-serv, Armed-Forces)\n- `relationship`: Relationship Status (Wife, Own-child, Husband, Not-in-family, Other-relative, Unmarried)\n- `race`: Race (White, Asian-Pac-Islander, Amer-Indian-Eskimo, Other, Black)\n- `sex`: Sex (Female, Male)\n- `capital-gain`: Monetary Capital Gains\n- `capital-loss`: Monetary Capital Losses\n- `hours-per-week`: Average Hours Per Week Worked\n- `native-country`: Native Country (United-States, Cambodia, England, Puerto-Rico, Canada, Germany, Outlying-US(Guam-USVI-etc), India, Japan, Greece, South, China, Cuba, Iran, Honduras, Philippines, Italy, Poland, Jamaica, Vietnam, Mexico, Portugal, Ireland, France, Dominican-Republic, Laos, Ecuador, Taiwan, Haiti, Columbia, Hungary, Guatemala, Nicaragua, Scotland, Thailand, Yugoslavia, El-Salvador, Trinadad&Tobago, Peru, Hong, Holand-Netherlands)\n\n**Target Variable**\n- `income`: Income Class (<=50K, >50K)\n\nSource: https://www.kaggle.com/c/udacity-mlcharity-competition/leaderboard\n\nselect=census.csv",
"_____no_output_____"
],
[
"#Get the data\n",
"_____no_output_____"
]
],
[
[
"import requests\nr = requests.get('https://raw.githubusercontent.com/ngoeldner/Machine-Learning-Project/main/finding_donors/census.csv')",
"_____no_output_____"
],
[
"file_path = '/content/census.csv'\nf = open(file_path,'wb')\nf.write(r.content)",
"_____no_output_____"
],
[
"df = pd.read_csv(file_path)",
"_____no_output_____"
],
[
"df.head()",
"_____no_output_____"
]
],
[
[
"lower_snake_case",
"_____no_output_____"
]
],
[
[
"df = df.rename(\n columns={\n 'education-num': 'education_num',\n 'marital-status': 'marital_status',\n 'capital-gain': 'capital_gain',\n 'capital-loss': 'capital_loss',\n 'hours-per-week': 'hours_per_week',\n 'native-country': 'native_country',\n 'income': 'y'\n }\n)",
"_____no_output_____"
],
[
"df.head()",
"_____no_output_____"
]
],
[
[
"#Data Analysis",
"_____no_output_____"
],
[
"In this part, we take a quick glance at the whole dataset, then split it and look more carefully at the training dataset. ",
"_____no_output_____"
]
],
[
[
"df.info()",
"<class 'pandas.core.frame.DataFrame'>\nRangeIndex: 45222 entries, 0 to 45221\nData columns (total 14 columns):\n # Column Non-Null Count Dtype \n--- ------ -------------- ----- \n 0 age 45222 non-null int64 \n 1 workclass 45222 non-null object \n 2 education_level 45222 non-null object \n 3 education_num 45222 non-null float64\n 4 marital_status 45222 non-null object \n 5 occupation 45222 non-null object \n 6 relationship 45222 non-null object \n 7 race 45222 non-null object \n 8 sex 45222 non-null object \n 9 capital_gain 45222 non-null float64\n 10 capital_loss 45222 non-null float64\n 11 hours_per_week 45222 non-null float64\n 12 native_country 45222 non-null object \n 13 y 45222 non-null object \ndtypes: float64(4), int64(1), object(9)\nmemory usage: 4.8+ MB\n"
]
],
[
[
"So we got more categorical columns than numeric columns, later we will analyse what is the more appropriate cat->num transformation and if we should do any kind of feature engineering besides the cat->num.",
"_____no_output_____"
]
],
[
[
"numeric_columns = ['age', 'education_num', 'capital_gain', 'capital_loss', 'hours_per_week']",
"_____no_output_____"
]
],
[
[
"##Split",
"_____no_output_____"
]
],
[
[
"df_used = df.copy()",
"_____no_output_____"
],
[
"df_used['y'] = df_used['y'] == '>50K'",
"_____no_output_____"
],
[
"df_used.head()",
"_____no_output_____"
],
[
"X = df_used.drop(['y'], axis=1)\ny = df_used['y']",
"_____no_output_____"
],
[
"from sklearn.model_selection import train_test_split\nfrom sklearn.preprocessing import StandardScaler\nX_train_npre, X_test_npre, y_train, y_test = train_test_split(X, y, test_size=0.2, random_state=42)",
"_____no_output_____"
],
[
"df_train_npre = pd.concat([pd.DataFrame(X_train_npre),pd.DataFrame(y_train)], axis=1)\ndf_train_npre",
"_____no_output_____"
]
],
[
[
"##Analysis",
"_____no_output_____"
]
],
[
[
"print(df_train_npre['y'].count())\nprint(df_train_npre['y'].value_counts(normalize=True))\ntrue_y_prop = df_train_npre['y'].value_counts(normalize=True)[True]\nprint(true_y_prop)",
"36177\nFalse 0.753766\nTrue 0.246234\nName: y, dtype: float64\n0.24623379495259418\n"
],
[
"df_train_npre.describe()",
"_____no_output_____"
]
],
[
[
"Here we got some weird values, for example, working 99 hours/week (14 hours by 7 days in week).\nThe almost 100K is a possible value in capital gain, but this is clearly an outsider.\nMaybe letting this values in the training dataset can have a good influence in the model, unless we know that it was generated from wrong data imputation or mistype kind of error. Lets take a more carefull look.",
"_____no_output_____"
]
],
[
[
"pd.options.plotting.backend = \"matplotlib\" #plotly\ndf_train_npre[numeric_columns].hist(bins=50, figsize=(20,15))\nplt.show()\npd.options.plotting.backend = \"plotly\" #plotly",
"_____no_output_____"
]
],
[
[
"In the next cells, we can notice the huge amount of intances with 0 in capital_gain and in capital_loss",
"_____no_output_____"
]
],
[
[
"print(df_train_npre['capital_gain'].value_counts())\nprint(df_train_npre['capital_gain'].value_counts()[0]/df_train_npre.count()['capital_gain'])",
"0.0 33182\n15024.0 391\n7688.0 300\n7298.0 282\n99999.0 185\n ... \n3432.0 1\n2961.0 1\n1731.0 1\n2387.0 1\n7262.0 1\nName: capital_gain, Length: 119, dtype: int64\n0.917212593636841\n"
],
[
"print(df_train_npre['capital_loss'].value_counts())\nprint(df_train_npre['capital_loss'].value_counts()[0]/df_train_npre.count()['capital_loss'])",
"0.0 34488\n1902.0 226\n1887.0 188\n1977.0 186\n1485.0 54\n ... \n1421.0 1\n2282.0 1\n2163.0 1\n1510.0 1\n4356.0 1\nName: capital_loss, Length: 92, dtype: int64\n0.9533128783481217\n"
],
[
"df_train_npre.head()",
"_____no_output_____"
]
],
[
[
"### Proportion of true values of y in each class",
"_____no_output_____"
]
],
[
[
"def plot_true_porcent(column):\n return (\n df_train_npre\n .groupby(\n [column]\n )\n ['y']\n .value_counts(normalize=True)\n .xs(True, level=1)\n .plot(kind='bar')\n )",
"_____no_output_____"
],
[
"df_train_npre.groupby(['workclass'])['y'].value_counts(normalize=True)",
"_____no_output_____"
],
[
"plot_true_porcent('workclass')",
"_____no_output_____"
],
[
"plot_true_porcent('education_level')",
"_____no_output_____"
],
[
"df_train_npre['education_level'].unique()",
"_____no_output_____"
],
[
"plot_true_porcent('marital_status')",
"_____no_output_____"
],
[
"plot_true_porcent('occupation')",
"_____no_output_____"
],
[
"plot_true_porcent('relationship')",
"_____no_output_____"
],
[
"plot_true_porcent('race')",
"_____no_output_____"
],
[
"plot_true_porcent('sex')",
"_____no_output_____"
],
[
"plot_true_porcent('native_country')",
"_____no_output_____"
]
],
[
[
"# Preprocessing and Feature Engineering",
"_____no_output_____"
],
[
"##Pipeline",
"_____no_output_____"
]
],
[
[
"from sklearn.pipeline import Pipeline\nfrom sklearn.compose import ColumnTransformer\nfrom sklearn.preprocessing import OneHotEncoder, StandardScaler, FunctionTransformer\nfrom sklearn.impute import SimpleImputer\nfrom sklearn.base import BaseEstimator, TransformerMixin",
"_____no_output_____"
],
[
"categorical_columns = ['workclass', 'marital_status', 'occupation', 'relationship', 'race', 'sex']",
"_____no_output_____"
],
[
"numeric_columns = ['age', 'education_num', 'capital_gain', 'capital_loss', 'hours_per_week']",
"_____no_output_____"
]
],
[
[
"###Partial pipeline to get the name of the new features",
"_____no_output_____"
]
],
[
[
"partial_pipeline = ColumnTransformer([\n # (\"num\", StandardScaler(), numeric_columns),\n (\"cat\", OneHotEncoder(sparse=False, handle_unknown='ignore'), categorical_columns),\n # ('edu_level', MyCat2Num('education_level'), ['education_level']),\n # ('nat_country', MyCat2Num('native_country'), ['native_country']),\n \n ], n_jobs = -1)",
"_____no_output_____"
],
[
"partial_pipeline.fit(X_train_npre)",
"_____no_output_____"
],
[
"all_cat_names = partial_pipeline.get_feature_names()",
"_____no_output_____"
],
[
"all_cat_names",
"_____no_output_____"
]
],
[
[
"###Full Pipeline",
"_____no_output_____"
]
],
[
[
"class MyCat2Num(BaseEstimator, TransformerMixin):\n def __init__(self, column): # no *args or **kwargs\n self.column = column\n\n def fit(self, X, y):\n self.df_Xy = pd.concat([X,y], axis=1)\n self.column_y_true = (1-self.df_Xy.groupby([self.column])['y'].value_counts(normalize=True).xs(False, level=1)).sort_values()\n return self\n\n def transform(self, X, y=None):\n def transform_helper(level):\n try:\n return self.column_y_true[level]\n except:\n return -1\n return X.applymap(transform_helper)",
"_____no_output_____"
],
[
"full_pipeline = ColumnTransformer([\n (\"num\", StandardScaler(), numeric_columns),\n (\"cat\", OneHotEncoder(sparse=False, handle_unknown='ignore'), categorical_columns),\n ('edu-level', MyCat2Num('education_level'), ['education_level']),\n ('nat-country', MyCat2Num('native_country'), ['native_country']),\n \n ], n_jobs = -1)\n\nX_train_pre = full_pipeline.fit_transform(X_train_npre, y)\npd.DataFrame(X_train_pre, columns=numeric_columns+all_cat_names+['education_level']+['native_country'], index=X_train_npre.index)",
"_____no_output_____"
]
],
[
[
"#Model Training",
"_____no_output_____"
],
[
"In this part, we train different models using the GridSearchCV to search for the best hyperparameters. Since the dataset is not very large, we can test many combinations of hyperparameters for each model.",
"_____no_output_____"
]
],
[
[
"from sklearn.linear_model import LogisticRegression, SGDClassifier\nfrom sklearn.ensemble import ExtraTreesClassifier, RandomForestClassifier, GradientBoostingClassifier, AdaBoostClassifier\nfrom sklearn.model_selection import GridSearchCV, StratifiedKFold\nfrom sklearn.svm import LinearSVC, SVC\nfrom sklearn.tree import DecisionTreeClassifier\nfrom xgboost import XGBClassifier",
"_____no_output_____"
],
[
"#we can't set random_state, for example StratifiedKFold(n_splits=5, shuffle=False, random_state=0), \n# because it raises an error since random_state may only be set if shuffle=True. This is why we do not give a seed to random_state \n# and we don't suffle the data because it has already been shuffled in train_test_split \nskf = StratifiedKFold(n_splits=5)",
"_____no_output_____"
]
],
[
[
"##SGDClassifier",
"_____no_output_____"
]
],
[
[
"parameters = {'estimator__l1_ratio':[0.025, 0.05, 0.1, 0.3, 0.9, 1], 'estimator__alpha':[0.00001, 0.0001, 0.001]}\nsgd_class = SGDClassifier(random_state=0, max_iter=200, penalty='elasticnet')\npreproc_sgd_class = Pipeline(steps= [('preproc', full_pipeline),('estimator', sgd_class)] , verbose=3)\nsgd_class_gscv = GridSearchCV(estimator=preproc_sgd_class, param_grid=parameters, n_jobs=-1, cv=skf, scoring='roc_auc',\n error_score='raise', return_train_score=True, verbose=3)",
"_____no_output_____"
],
[
"sgd_class_gscv.fit(X_train_npre, y_train)\r\nprint(sgd_class_gscv.best_params_)\r\nprint(sgd_class_gscv.best_score_)",
"Fitting 5 folds for each of 18 candidates, totalling 90 fits\n[Pipeline] ........... (step 1 of 2) Processing preproc, total= 0.6s\n[Pipeline] ......... (step 2 of 2) Processing estimator, total= 0.5s\n{'estimator__alpha': 0.0001, 'estimator__l1_ratio': 0.05}\n0.8999891506432934\n"
],
[
"pd.concat([pd.DataFrame(sgd_class_gscv.cv_results_[\"params\"]),\r\n pd.DataFrame(sgd_class_gscv.cv_results_[\"rank_test_score\"], columns=[\"rank_test_score\"]),\r\n pd.DataFrame(sgd_class_gscv.cv_results_[\"mean_test_score\"], columns=[\"mean_test_score\"]),\r\n pd.DataFrame(sgd_class_gscv.cv_results_[\"mean_train_score\"], columns=[\"mean_train_score\"]),\r\n pd.DataFrame(sgd_class_gscv.cv_results_[\"std_test_score\"], columns=[\"std_test_score\"]),\r\n pd.DataFrame(sgd_class_gscv.cv_results_[\"std_train_score\"], columns=[\"std_train_score\"])],axis=1)",
"_____no_output_____"
]
],
[
[
"##Logistc Regression",
"_____no_output_____"
]
],
[
[
"parameters = {'estimator__l1_ratio':[0.05, 0.1, 0.3,0.6, 0.9, 1], 'estimator__C':[0.1,1,10]}\nlog_reg = LogisticRegression(solver='saga',penalty='elasticnet', random_state=0, max_iter=200)\npreproc_logreg = Pipeline(steps= [('preproc', full_pipeline),('estimator', log_reg)] , verbose=3)\nlog_reg_gscv = GridSearchCV(estimator=preproc_logreg, param_grid=parameters, n_jobs=-1, cv=skf, scoring='roc_auc',\n error_score='raise', return_train_score=True, verbose=3)",
"_____no_output_____"
],
[
"log_reg_gscv.fit(X_train_npre, y_train)\r\nprint(log_reg_gscv.best_params_)\r\nprint(log_reg_gscv.best_score_)",
"Fitting 5 folds for each of 18 candidates, totalling 90 fits\n[Pipeline] ........... (step 1 of 2) Processing preproc, total= 0.6s\n[Pipeline] ......... (step 2 of 2) Processing estimator, total= 9.4s\n{'estimator__C': 1, 'estimator__l1_ratio': 0.05}\n0.9026324667393995\n"
],
[
"pd.concat([pd.DataFrame(log_reg_gscv.cv_results_[\"params\"]),\r\n pd.DataFrame(log_reg_gscv.cv_results_[\"rank_test_score\"], columns=[\"rank_test_score\"]),\r\n pd.DataFrame(log_reg_gscv.cv_results_[\"mean_test_score\"], columns=[\"mean_test_score\"]),\r\n pd.DataFrame(log_reg_gscv.cv_results_[\"mean_train_score\"], columns=[\"mean_train_score\"]),\r\n pd.DataFrame(log_reg_gscv.cv_results_[\"std_test_score\"], columns=[\"std_test_score\"]),\r\n pd.DataFrame(log_reg_gscv.cv_results_[\"std_train_score\"], columns=[\"std_train_score\"])],axis=1)",
"_____no_output_____"
]
],
[
[
"##SVM\n",
"_____no_output_____"
],
[
"###Linear",
"_____no_output_____"
]
],
[
[
"parameters = {'estimator__loss':['squared_epsilon_insensitive'], 'estimator__C':[0.00001,0.0001,0.001,0.01,0.1,1,10,100,1000]}\n# parameters = {'estimator__loss':['squared_epsilon_insensitive'], 'estimator__C':[1]}\nlin_svc = LinearSVC(dual=False, random_state=0)\npreproc_linsvc = Pipeline(steps= [('preproc', full_pipeline),('estimator', lin_svc)])\nlin_svc_gscv = GridSearchCV(estimator=preproc_linsvc, param_grid=parameters, n_jobs=-1, cv=skf, scoring='roc_auc',\n error_score='raise', return_train_score=True, verbose=3)",
"_____no_output_____"
],
[
"lin_svc_gscv.fit(X_train_npre, y_train)\r\nprint(lin_svc_gscv.best_params_)\r\nprint(lin_svc_gscv.best_score_)",
"Fitting 5 folds for each of 9 candidates, totalling 45 fits\n{'estimator__C': 0.01, 'estimator__loss': 'squared_epsilon_insensitive'}\n0.8929176507490396\n"
],
[
"pd.concat([pd.DataFrame(lin_svc_gscv.cv_results_[\"params\"]),\r\n pd.DataFrame(lin_svc_gscv.cv_results_[\"rank_test_score\"], columns=[\"rank_test_score\"]),\r\n pd.DataFrame(lin_svc_gscv.cv_results_[\"mean_test_score\"], columns=[\"mean_test_score\"]),\r\n pd.DataFrame(lin_svc_gscv.cv_results_[\"mean_train_score\"], columns=[\"mean_train_score\"]),\r\n pd.DataFrame(lin_svc_gscv.cv_results_[\"std_test_score\"], columns=[\"std_test_score\"]),\r\n pd.DataFrame(lin_svc_gscv.cv_results_[\"std_train_score\"], columns=[\"std_train_score\"])],axis=1)",
"_____no_output_____"
]
],
[
[
"###Nonlinear",
"_____no_output_____"
]
],
[
[
"# parameters = [{'kernel':['poly'], 'C':[0.001,0.01,0.1,1,10,100,300], 'degree':[2,3,4,5,6,7,8]},\n# {'kernel':['rbf'], 'C':[0.001,0.01,0.1,1,10,100,300]},\n# {'kernel':['sigmoid'], 'C':[0.001,0.01,0.1,1,10,100,300]}\n# ]\nparameters = [{'estimator__kernel':['poly'], 'estimator__C':[0.1,], 'estimator__degree':[3,]},\n {'estimator__kernel':['rbf'], 'estimator__C':[0.1,]},\n {'estimator__kernel':['sigmoid'], 'estimator__C':[0.1,]}\n ]\nnlin_svc = SVC()\npreproc_nlinsvc = Pipeline(steps= [('preproc', full_pipeline),('estimator', nlin_svc)] , verbose=3)\nnlin_svc_gscv = GridSearchCV(estimator=preproc_nlinsvc, param_grid=parameters, n_jobs=-1, cv=skf, scoring='roc_auc',\n error_score='raise', verbose=3)",
"_____no_output_____"
],
[
"nlin_svc_gscv.fit(X_train_npre, y_train)\r\nprint(nlin_svc_gscv.best_params_)\r\nprint(nlin_svc_gscv.best_score_)",
"Fitting 5 folds for each of 3 candidates, totalling 15 fits\n"
],
[
"pd.concat([pd.DataFrame(nlin_svc_gscv.cv_results_[\"params\"]),\r\n pd.DataFrame(nlin_svc_gscv.cv_results_[\"rank_test_score\"], columns=[\"rank_test_score\"]),\r\n pd.DataFrame(nlin_svc_gscv.cv_results_[\"mean_test_score\"], columns=[\"mean_test_score\"]),\r\n pd.DataFrame(nlin_svc_gscv.cv_results_[\"std_test_score\"], columns=[\"std_test_score\"])],axis=1)",
"_____no_output_____"
]
],
[
[
"##Random Forest",
"_____no_output_____"
]
],
[
[
"# parameters = {'max_leaf_nodes':[700, 800,850, 900,950, 1000]}\nparameters = {'estimator__n_estimators':[100, 200, 300, 400]}\nrf = RandomForestClassifier(random_state=0, max_leaf_nodes=950)\npreproc_rf = Pipeline(steps= [('preproc', full_pipeline),('estimator', rf)] , verbose=4)\nrf_gscv = GridSearchCV(estimator=preproc_rf, param_grid=parameters, n_jobs=-1, cv=skf, scoring='roc_auc',\n error_score='raise', verbose=4)",
"_____no_output_____"
],
[
"rf_gscv.fit(X_train_npre, y_train)\r\nprint(rf_gscv.best_params_)\r\nprint(rf_gscv.best_score_)",
"Fitting 5 folds for each of 4 candidates, totalling 20 fits\n[Pipeline] ........... (step 1 of 2) Processing preproc, total= 0.6s\n[Pipeline] ......... (step 2 of 2) Processing estimator, total= 8.8s\n{'estimator__n_estimators': 300}\n0.9153413929112661\n"
],
[
"pd.concat([pd.DataFrame(rf_gscv.cv_results_[\"params\"]),\r\n pd.DataFrame(rf_gscv.cv_results_[\"rank_test_score\"], columns=[\"rank_test_score\"]),\r\n pd.DataFrame(rf_gscv.cv_results_[\"mean_test_score\"], columns=[\"mean_test_score\"]),\r\n pd.DataFrame(rf_gscv.cv_results_[\"std_test_score\"], columns=[\"std_test_score\"])],axis=1)",
"_____no_output_____"
]
],
[
[
"##Extra-Trees",
"_____no_output_____"
]
],
[
[
"# parameters = {'max_leaf_nodes':[1500, 1750, 2000, 2250, 2500]}\nparameters = {'estimator__n_estimators':[100, 200, 300, 400]}\net = ExtraTreesClassifier(random_state=0, max_leaf_nodes=2000)\npreproc_et = Pipeline(steps= [('preproc', full_pipeline),('estimator', et)] , verbose=3)\net_gscv = GridSearchCV(estimator=preproc_et, param_grid=parameters, n_jobs=-1, cv=skf, scoring='roc_auc',\n error_score='raise', verbose=3)",
"_____no_output_____"
],
[
"et_gscv.fit(X_train_npre, y_train)\r\nprint(et_gscv.best_estimator_)\r\nprint(et_gscv.best_score_)",
"Fitting 5 folds for each of 4 candidates, totalling 20 fits\n[Pipeline] ........... (step 1 of 2) Processing preproc, total= 0.6s\n[Pipeline] ......... (step 2 of 2) Processing estimator, total= 15.8s\nPipeline(steps=[('preproc',\n ColumnTransformer(n_jobs=-1,\n transformers=[('num', StandardScaler(),\n ['age', 'education_num',\n 'capital_gain',\n 'capital_loss',\n 'hours_per_week']),\n ('cat',\n OneHotEncoder(handle_unknown='ignore',\n sparse=False),\n ['workclass',\n 'marital_status',\n 'occupation', 'relationship',\n 'race', 'sex']),\n ('edu_level',\n MyCat2Num(column='education_level'),\n ['education_level']),\n ('nat_country',\n MyCat2Num(column='native_country'),\n ['native_country'])])),\n ('estimator',\n ExtraTreesClassifier(max_leaf_nodes=2000, n_estimators=400,\n random_state=0))],\n verbose=3)\n0.9025559038171602\n"
],
[
"pd.concat([pd.DataFrame(et_gscv.cv_results_[\"params\"]),\r\n pd.DataFrame(et_gscv.cv_results_[\"rank_test_score\"], columns=[\"rank_test_score\"]),\r\n pd.DataFrame(et_gscv.cv_results_[\"mean_test_score\"], columns=[\"mean_test_score\"]),\r\n pd.DataFrame(et_gscv.cv_results_[\"std_test_score\"], columns=[\"std_test_score\"])],axis=1)",
"_____no_output_____"
]
],
[
[
"##AdaBoost",
"_____no_output_____"
]
],
[
[
"parameters = {'estimator__n_estimators':[100,300], 'estimator__learning_rate' : [1,2,3]}\ndt = DecisionTreeClassifier(random_state=0)\nada = AdaBoostClassifier(base_estimator=dt, random_state=0)\npreproc_ada = Pipeline(steps= [('preproc', full_pipeline),('estimator', ada)] , verbose=4)\nada_gscv = GridSearchCV(estimator=preproc_ada, param_grid=parameters, n_jobs=-1, cv=skf, scoring='roc_auc',\n error_score='raise', verbose=3)",
"_____no_output_____"
],
[
"ada_gscv.fit(X_train_npre, y_train)\r\nprint(ada_gscv.best_estimator_)\r\nprint(ada_gscv.best_score_)",
"Fitting 5 folds for each of 6 candidates, totalling 30 fits\n[Pipeline] ........... (step 1 of 2) Processing preproc, total= 0.6s\n[Pipeline] ......... (step 2 of 2) Processing estimator, total= 17.0s\nPipeline(steps=[('preproc',\n ColumnTransformer(n_jobs=-1,\n transformers=[('num', StandardScaler(),\n ['age', 'education_num',\n 'capital_gain',\n 'capital_loss',\n 'hours_per_week']),\n ('cat',\n OneHotEncoder(handle_unknown='ignore',\n sparse=False),\n ['workclass',\n 'marital_status',\n 'occupation', 'relationship',\n 'race', 'sex']),\n ('edu_level',\n MyCat2Num(column='education_level'),\n ['education_level']),\n ('nat_country',\n MyCat2Num(column='native_country'),\n ['native_country'])])),\n ('estimator',\n AdaBoostClassifier(base_estimator=DecisionTreeClassifier(random_state=0),\n learning_rate=1, n_estimators=100,\n random_state=0))],\n verbose=4)\n0.8767016482964076\n"
],
[
"pd.concat([pd.DataFrame(ada_gscv.cv_results_[\"params\"]),\r\n pd.DataFrame(ada_gscv.cv_results_[\"rank_test_score\"], columns=[\"rank_test_score\"]),\r\n pd.DataFrame(ada_gscv.cv_results_[\"mean_test_score\"], columns=[\"mean_test_score\"]),\r\n pd.DataFrame(ada_gscv.cv_results_[\"std_test_score\"], columns=[\"std_test_score\"])],axis=1)",
"_____no_output_____"
]
],
[
[
"##Gradient Boosting",
"_____no_output_____"
]
],
[
[
"parameters = {'estimator__n_estimators':[400, 500],'estimator__max_depth':[2,3], 'estimator__learning_rate' : [0.1, 1]}\ngb = GradientBoostingClassifier(random_state=0, loss='deviance', subsample=0.8) \npreproc_gb = Pipeline(steps= [('preproc', full_pipeline),('estimator', gb)] , verbose=4)\ngb_gscv = GridSearchCV(estimator=preproc_gb, param_grid=parameters, n_jobs=-1, cv=skf, scoring='roc_auc',\n error_score='raise', verbose=4)",
"_____no_output_____"
],
[
"gb_gscv.fit(X_train_npre, y_train)\r\nprint(gb_gscv.best_params_)\r\nprint(gb_gscv.best_score_)",
"Fitting 5 folds for each of 8 candidates, totalling 40 fits\n[Pipeline] ........... (step 1 of 2) Processing preproc, total= 0.6s\n[Pipeline] ......... (step 2 of 2) Processing estimator, total= 33.7s\n{'estimator__learning_rate': 0.1, 'estimator__max_depth': 3, 'estimator__n_estimators': 500}\n0.9249179325978168\n"
],
[
"pd.concat([pd.DataFrame(gb_gscv.cv_results_[\"params\"]),\r\n pd.DataFrame(gb_gscv.cv_results_[\"rank_test_score\"], columns=[\"rank_test_score\"]),\r\n pd.DataFrame(gb_gscv.cv_results_[\"mean_test_score\"], columns=[\"mean_test_score\"]),\r\n pd.DataFrame(gb_gscv.cv_results_[\"std_test_score\"], columns=[\"std_test_score\"])],axis=1)",
"_____no_output_____"
]
],
[
[
"##XGBoost",
"_____no_output_____"
]
],
[
[
"parameters = {\n 'estimator__n_estimators' : [500], #400],\n \"estimator__eta\" : [0.05],# 0.10, 1],#0.15, 0.20, 0.25, 0.30 ],\n \"estimator__max_depth\" : [4],#3, 5, 6, 8],#, 10, 12, 15],\n \"estimator__gamma\" : [0.2],# 0.0,0.2 , 0.3, 0.4 ],\n #\"colsample_bytree\" : [ 0.3]#, 0.4, 0.5 , 0.7 ]\n}\nxgb = XGBClassifier(random_state=0)\npreproc_xgb = Pipeline(steps= [('preproc', full_pipeline),('estimator', xgb)] , verbose=4)\nxgb_gscv = GridSearchCV(estimator=preproc_xgb, param_grid=parameters, n_jobs=-1, cv=skf, scoring='roc_auc',\n error_score='raise', return_train_score=True, verbose=4)",
"_____no_output_____"
],
[
"xgb_gscv.fit(X_train_npre, y_train)\r\nprint(xgb_gscv.best_params_)\r\nprint(xgb_gscv.best_score_)",
"Fitting 5 folds for each of 1 candidates, totalling 5 fits\n[Pipeline] ........... (step 1 of 2) Processing preproc, total= 0.6s\n[Pipeline] ......... (step 2 of 2) Processing estimator, total= 27.0s\n{'estimator__eta': 0.05, 'estimator__gamma': 0.2, 'estimator__max_depth': 4, 'estimator__n_estimators': 500}\n0.9270605153713147\n"
],
[
"pd.concat([pd.DataFrame(xgb_gscv.cv_results_[\"params\"]),\r\n pd.DataFrame(xgb_gscv.cv_results_[\"rank_test_score\"], columns=[\"rank_test_score\"]),\r\n pd.DataFrame(xgb_gscv.cv_results_[\"mean_test_score\"], columns=[\"mean_test_score\"]),\r\n pd.DataFrame(xgb_gscv.cv_results_[\"std_test_score\"], columns=[\"std_test_score\"])],axis=1)",
"_____no_output_____"
]
],
[
[
"#Final Evaluation, Final Training and Saving the Model ",
"_____no_output_____"
],
[
"We have chosen the SGDClassifier for the final model because it presented a good roc_auc score, which was not so different from the score of a much more complex model, the XGBoost. Also, the SGDClassifier allows online learning.\r\n\r\nHere, we evaluate the final model in the test dataset. Then, we train the SGDClassifier in the entire dataset and export it to a file.",
"_____no_output_____"
]
],
[
[
"from sklearn.metrics import roc_auc_score\nfrom sklearn.base import clone",
"_____no_output_____"
],
[
"best_model = sgd_class_gscv.best_estimator_\ny_pred = best_model.predict(X_test_npre)\nroc_auc_score(y_test, y_pred)",
"_____no_output_____"
],
[
"sgd_class_gscv.best_params_",
"_____no_output_____"
],
[
"final_model = clone(sgd_class_gscv.best_estimator_)",
"_____no_output_____"
],
[
"final_model.fit(X, y)",
"[Pipeline] ........... (step 1 of 2) Processing preproc, total= 0.7s\n[Pipeline] ......... (step 2 of 2) Processing estimator, total= 0.5s\n"
],
[
"import joblib\nfilename = 'sgd_model.sav'\njoblib.dump(final_model, filename)",
"_____no_output_____"
],
[
"edu_df_Xy = pd.concat([X['education_level'],y], axis=1)\nedu_y_true = (1-edu_df_Xy.groupby(['education_level'])['y'].value_counts(normalize=True).xs(False, level=1)).sort_values()\n\nnat_df_Xy = pd.concat([X['native_country'],y], axis=1)\nnat_y_true = (1-nat_df_Xy.groupby(['native_country'])['y'].value_counts(normalize=True).xs(False, level=1)).sort_values()\n\ndef func_edu_helper(level):\n try:\n return edu_y_true[level]\n except:\n return -1\n\ndef func_nat_helper(level):\n try:\n return nat_y_true[level]\n except:\n return -1",
"_____no_output_____"
],
[
"X_new_edu = X['education_level'].map(func_edu_helper)\nX_new = X.copy()\nX_new['education_level'] = X_new_edu\n\nX_new_nat = X['native_country'].map(func_nat_helper)\nX_new = X.copy()\nX_new['native_country'] = X_new_nat\n",
"_____no_output_____"
],
[
"deploy_pipeline = ColumnTransformer([\n (\"num\", StandardScaler(), numeric_columns),\n (\"cat\", OneHotEncoder(sparse=False, handle_unknown='ignore'), categorical_columns),\n ], n_jobs = -1)",
"_____no_output_____"
],
[
"deploy_sgd_class = SGDClassifier(random_state=0, max_iter=200, penalty='elasticnet',alpha= 0.0001, l1_ratio= 0.05)\ndeploy_preproc_sgd_class = Pipeline(steps= [('preproc', deploy_pipeline),('estimator', deploy_sgd_class)] , verbose=3)",
"_____no_output_____"
],
[
"deploy_preproc_sgd_class.fit(X_new, y)",
"[Pipeline] ........... (step 1 of 2) Processing preproc, total= 1.9s\n[Pipeline] ......... (step 2 of 2) Processing estimator, total= 0.6s\n"
],
[
"import pickle\nsgd_class_model = deploy_preproc_sgd_class\n# Save to file in the current working directory\npkl_filename = \"sgd_class_.pkl\"\nwith open(pkl_filename, 'wb') as file:\n pickle.dump(sgd_class_model, file)\n#load file\nwith open(pkl_filename,'rb') as io:\n loaded_model=pickle.load(io)",
"_____no_output_____"
],
[
"loaded_model.predict(X)",
"_____no_output_____"
],
[
"import json\nedu_y_true.to_json('edu.json')\nnat_y_true.to_json('nat.json')",
"_____no_output_____"
]
]
] | [
"markdown",
"code",
"markdown",
"code",
"markdown",
"code",
"markdown",
"code",
"markdown",
"code",
"markdown",
"code",
"markdown",
"code",
"markdown",
"code",
"markdown",
"code",
"markdown",
"code",
"markdown",
"code",
"markdown",
"code",
"markdown",
"code",
"markdown",
"code",
"markdown",
"code",
"markdown",
"code",
"markdown",
"code",
"markdown",
"code",
"markdown",
"code",
"markdown",
"code",
"markdown",
"code",
"markdown",
"code",
"markdown",
"code",
"markdown",
"code",
"markdown",
"code"
] | [
[
"markdown"
],
[
"code"
],
[
"markdown"
],
[
"code",
"code"
],
[
"markdown",
"markdown"
],
[
"code",
"code",
"code",
"code"
],
[
"markdown"
],
[
"code",
"code"
],
[
"markdown",
"markdown"
],
[
"code"
],
[
"markdown"
],
[
"code"
],
[
"markdown"
],
[
"code",
"code",
"code",
"code",
"code",
"code"
],
[
"markdown"
],
[
"code",
"code"
],
[
"markdown"
],
[
"code"
],
[
"markdown"
],
[
"code",
"code",
"code"
],
[
"markdown"
],
[
"code",
"code",
"code",
"code",
"code",
"code",
"code",
"code",
"code",
"code",
"code"
],
[
"markdown",
"markdown"
],
[
"code",
"code",
"code"
],
[
"markdown"
],
[
"code",
"code",
"code",
"code"
],
[
"markdown"
],
[
"code",
"code"
],
[
"markdown",
"markdown"
],
[
"code",
"code"
],
[
"markdown"
],
[
"code",
"code",
"code"
],
[
"markdown"
],
[
"code",
"code",
"code"
],
[
"markdown",
"markdown"
],
[
"code",
"code",
"code"
],
[
"markdown"
],
[
"code",
"code",
"code"
],
[
"markdown"
],
[
"code",
"code",
"code"
],
[
"markdown"
],
[
"code",
"code",
"code"
],
[
"markdown"
],
[
"code",
"code",
"code"
],
[
"markdown"
],
[
"code",
"code",
"code"
],
[
"markdown"
],
[
"code",
"code",
"code"
],
[
"markdown",
"markdown"
],
[
"code",
"code",
"code",
"code",
"code",
"code",
"code",
"code",
"code",
"code",
"code",
"code",
"code",
"code"
]
] |
e7725a8f3e35dd318542753eaba1e59d100763f5 | 155,516 | ipynb | Jupyter Notebook | 08 - Create a Pipeline.ipynb | MeteorF/MS-AZ-DP100-Labs | c71e538050cda18d64c13d9d86390029ec9e8571 | [
"MIT"
] | null | null | null | 08 - Create a Pipeline.ipynb | MeteorF/MS-AZ-DP100-Labs | c71e538050cda18d64c13d9d86390029ec9e8571 | [
"MIT"
] | null | null | null | 08 - Create a Pipeline.ipynb | MeteorF/MS-AZ-DP100-Labs | c71e538050cda18d64c13d9d86390029ec9e8571 | [
"MIT"
] | null | null | null | 66.57363 | 9,261 | 0.633626 | [
[
[
"# Create a Pipeline\n\nYou can perform the various steps required to ingest data, train a model, and register the model individually by using the Azure ML SDK to run script-based experiments. However, in an enterprise environment it is common to encapsulate the sequence of discrete steps required to build a machine learning solution into a *pipeline* that can be run on one or more compute targets, either on-demand by a user, from an automated build process, or on a schedule.\n\nIn this notebook, you'll bring together all of these elements to create a simple pipeline that pre-processes data and then trains and registers a model.",
"_____no_output_____"
],
[
"## Connect to your workspace\n\nTo get started, connect to your workspace.\n\n> **Note**: If you haven't already established an authenticated session with your Azure subscription, you'll be prompted to authenticate by clicking a link, entering an authentication code, and signing into Azure.",
"_____no_output_____"
]
],
[
[
"import azureml.core\nfrom azureml.core import Workspace\n\n# Load the workspace from the saved config file\nws = Workspace.from_config()\nprint('Ready to use Azure ML {} to work with {}'.format(azureml.core.VERSION, ws.name))",
"Ready to use Azure ML 1.26.0 to work with mls-dp100\n"
]
],
[
[
"## Prepare data\n\nIn your pipeline, you'll use a dataset containing details of diabetes patients. Run the cell below to create this dataset (if you created it in previously, the code will find the existing version)",
"_____no_output_____"
]
],
[
[
"from azureml.core import Dataset\n\ndefault_ds = ws.get_default_datastore()\n\nif 'diabetes dataset' not in ws.datasets:\n default_ds.upload_files(files=['./data/diabetes.csv', './data/diabetes2.csv'], # Upload the diabetes csv files in /data\n target_path='diabetes-data/', # Put it in a folder path in the datastore\n overwrite=True, # Replace existing files of the same name\n show_progress=True)\n\n #Create a tabular dataset from the path on the datastore (this may take a short while)\n tab_data_set = Dataset.Tabular.from_delimited_files(path=(default_ds, 'diabetes-data/*.csv'))\n\n # Register the tabular dataset\n try:\n tab_data_set = tab_data_set.register(workspace=ws, \n name='diabetes dataset',\n description='diabetes data',\n tags = {'format':'CSV'},\n create_new_version=True)\n print('Dataset registered.')\n except Exception as ex:\n print(ex)\nelse:\n print('Dataset already registered.')",
"Dataset already registered.\n"
]
],
[
[
"## Create scripts for pipeline steps\n\nPipelines consist of one or more *steps*, which can be Python scripts, or specialized steps like a data transfer step that copies data from one location to another. Each step can run in its own compute context. In this exercise, you'll build a simple pipeline that contains two Python script steps: one to pre-process some training data, and another to use the pre-processed data to train and register a model.\n\nFirst, let's create a folder for the script files we'll use in the pipeline steps.",
"_____no_output_____"
]
],
[
[
"import os\n# Create a folder for the pipeline step files\nexperiment_folder = 'diabetes_pipeline'\nos.makedirs(experiment_folder, exist_ok=True)\n\nprint(experiment_folder)",
"diabetes_pipeline\n"
]
],
[
[
"Now let's create the first script, which will read data from the diabetes dataset and apply some simple pre-processing to remove any rows with missing data and normalize the numeric features so they're on a similar scale.\n\nThe script includes a argument named **--prepped-data**, which references the folder where the resulting data should be saved.",
"_____no_output_____"
]
],
[
[
"%%writefile $experiment_folder/prep_diabetes.py\n# Import libraries\nimport os\nimport argparse\nimport pandas as pd\nfrom azureml.core import Run\nfrom sklearn.preprocessing import MinMaxScaler\n\n# Get parameters\nparser = argparse.ArgumentParser()\nparser.add_argument(\"--input-data\", type=str, dest='raw_dataset_id', help='raw dataset')\nparser.add_argument('--prepped-data', type=str, dest='prepped_data', default='prepped_data', help='Folder for results')\nargs = parser.parse_args()\nsave_folder = args.prepped_data\n\n# Get the experiment run context\nrun = Run.get_context()\n\n# load the data (passed as an input dataset)\nprint(\"Loading Data...\")\ndiabetes = run.input_datasets['raw_data'].to_pandas_dataframe()\n\n# Log raw row count\nrow_count = (len(diabetes))\nrun.log('raw_rows', row_count)\n\n# remove nulls\ndiabetes = diabetes.dropna()\n\n# Normalize the numeric columns\nscaler = MinMaxScaler()\nnum_cols = ['Pregnancies','PlasmaGlucose','DiastolicBloodPressure','TricepsThickness','SerumInsulin','BMI','DiabetesPedigree']\ndiabetes[num_cols] = scaler.fit_transform(diabetes[num_cols])\n\n# Log processed rows\nrow_count = (len(diabetes))\nrun.log('processed_rows', row_count)\n\n# Save the prepped data\nprint(\"Saving Data...\")\nos.makedirs(save_folder, exist_ok=True)\nsave_path = os.path.join(save_folder,'data.csv')\ndiabetes.to_csv(save_path, index=False, header=True)\n\n# End the run\nrun.complete()",
"Writing diabetes_pipeline/prep_diabetes.py\n"
]
],
[
[
"Now you can create the script for the second step, which will train a model. The script includes a argument named **--training-folder**, which references the folder where the prepared data was saved by the previous step.",
"_____no_output_____"
]
],
[
[
"%%writefile $experiment_folder/train_diabetes.py\n# Import libraries\nfrom azureml.core import Run, Model\nimport argparse\nimport pandas as pd\nimport numpy as np\nimport joblib\nimport os\nfrom sklearn.model_selection import train_test_split\nfrom sklearn.tree import DecisionTreeClassifier\nfrom sklearn.metrics import roc_auc_score\nfrom sklearn.metrics import roc_curve\nimport matplotlib.pyplot as plt\n\n# Get parameters\nparser = argparse.ArgumentParser()\nparser.add_argument(\"--training-folder\", type=str, dest='training_folder', help='training data folder')\nargs = parser.parse_args()\ntraining_folder = args.training_folder\n\n# Get the experiment run context\nrun = Run.get_context()\n\n# load the prepared data file in the training folder\nprint(\"Loading Data...\")\nfile_path = os.path.join(training_folder,'data.csv')\ndiabetes = pd.read_csv(file_path)\n\n# Separate features and labels\nX, y = diabetes[['Pregnancies','PlasmaGlucose','DiastolicBloodPressure','TricepsThickness','SerumInsulin','BMI','DiabetesPedigree','Age']].values, diabetes['Diabetic'].values\n\n# Split data into training set and test set\nX_train, X_test, y_train, y_test = train_test_split(X, y, test_size=0.30, random_state=0)\n\n# Train adecision tree model\nprint('Training a decision tree model...')\nmodel = DecisionTreeClassifier().fit(X_train, y_train)\n\n# calculate accuracy\ny_hat = model.predict(X_test)\nacc = np.average(y_hat == y_test)\nprint('Accuracy:', acc)\nrun.log('Accuracy', np.float(acc))\n\n# calculate AUC\ny_scores = model.predict_proba(X_test)\nauc = roc_auc_score(y_test,y_scores[:,1])\nprint('AUC: ' + str(auc))\nrun.log('AUC', np.float(auc))\n\n# plot ROC curve\nfpr, tpr, thresholds = roc_curve(y_test, y_scores[:,1])\nfig = plt.figure(figsize=(6, 4))\n# Plot the diagonal 50% line\nplt.plot([0, 1], [0, 1], 'k--')\n# Plot the FPR and TPR achieved by our model\nplt.plot(fpr, tpr)\nplt.xlabel('False Positive Rate')\nplt.ylabel('True Positive Rate')\nplt.title('ROC Curve')\nrun.log_image(name = \"ROC\", plot = fig)\nplt.show()\n\n# Save the trained model in the outputs folder\nprint(\"Saving model...\")\nos.makedirs('outputs', exist_ok=True)\nmodel_file = os.path.join('outputs', 'diabetes_model.pkl')\njoblib.dump(value=model, filename=model_file)\n\n# Register the model\nprint('Registering model...')\nModel.register(workspace=run.experiment.workspace,\n model_path = model_file,\n model_name = 'diabetes_model',\n tags={'Training context':'Pipeline'},\n properties={'AUC': np.float(auc), 'Accuracy': np.float(acc)})\n\n\nrun.complete()",
"Writing diabetes_pipeline/train_diabetes.py\n"
]
],
[
[
"## Prepare a compute environment for the pipeline steps\n\nIn this exercise, you'll use the same compute for both steps, but it's important to realize that each step is run independently; so you could specify different compute contexts for each step if appropriate.\n\nFirst, get the compute target you created in a previous lab (if it doesn't exist, it will be created).\n\n> **Important**: Change *your-compute-cluster* to the name of your compute cluster in the code below before running it! Cluster names must be globally unique names between 2 to 16 characters in length. Valid characters are letters, digits, and the - character.",
"_____no_output_____"
]
],
[
[
"from azureml.core.compute import ComputeTarget, AmlCompute\nfrom azureml.core.compute_target import ComputeTargetException\n\ncluster_name = \"alazureml-cc0408\"\n\ntry:\n # Check for existing compute target\n pipeline_cluster = ComputeTarget(workspace=ws, name=cluster_name)\n print('Found existing cluster, use it.')\nexcept ComputeTargetException:\n # If it doesn't already exist, create it\n try:\n compute_config = AmlCompute.provisioning_configuration(vm_size='STANDARD_DS11_V2', max_nodes=2)\n pipeline_cluster = ComputeTarget.create(ws, cluster_name, compute_config)\n pipeline_cluster.wait_for_completion(show_output=True)\n except Exception as ex:\n print(ex)\n ",
"Found existing cluster, use it.\n"
]
],
[
[
"The compute will require a Python environment with the necessary package dependencies installed, so you'll need to create a run configuration.",
"_____no_output_____"
]
],
[
[
"from azureml.core import Environment\nfrom azureml.core.conda_dependencies import CondaDependencies\nfrom azureml.core.runconfig import RunConfiguration\n\n# Create a Python environment for the experiment\ndiabetes_env = Environment(\"diabetes-pipeline-env\")\ndiabetes_env.python.user_managed_dependencies = False # Let Azure ML manage dependencies\ndiabetes_env.docker.enabled = True # Use a docker container\n\n# Create a set of package dependencies\ndiabetes_packages = CondaDependencies.create(conda_packages=['scikit-learn','ipykernel','matplotlib','pandas','pip'],\n pip_packages=['azureml-defaults','azureml-dataprep[pandas]','pyarrow'])\n\n# Add the dependencies to the environment\ndiabetes_env.python.conda_dependencies = diabetes_packages\n\n# Register the environment \ndiabetes_env.register(workspace=ws)\nregistered_env = Environment.get(ws, 'diabetes-pipeline-env')\n\n# Create a new runconfig object for the pipeline\npipeline_run_config = RunConfiguration()\n\n# Use the compute you created above. \npipeline_run_config.target = pipeline_cluster\n\n# Assign the environment to the run configuration\npipeline_run_config.environment = registered_env\n\nprint (\"Run configuration created.\")",
"'enabled' is deprecated. Please use the azureml.core.runconfig.DockerConfiguration object with the 'use_docker' param instead.\n"
]
],
[
[
"## Create and run a pipeline\n\nNow you're ready to create and run a pipeline.\n\nFirst you need to define the steps for the pipeline, and any data references that need to be passed between them. In this case, the first step must write the prepared data to a folder that can be read from by the second step. Since the steps will be run on remote compute (and in fact, could each be run on different compute), the folder path must be passed as a data reference to a location in a datastore within the workspace. The **PipelineData** object is a special kind of data reference that is used for interim storage locations that can be passed between pipeline steps, so you'll create one and use at as the output for the first step and the input for the second step. Note that you also need to pass it as a script argument so our code can access the datastore location referenced by the data reference.",
"_____no_output_____"
]
],
[
[
"from azureml.pipeline.core import PipelineData\nfrom azureml.pipeline.steps import PythonScriptStep\n\n# Get the training dataset\ndiabetes_ds = ws.datasets.get(\"diabetes dataset\")\n\n# Create a PipelineData (temporary Data Reference) for the model folder\nprepped_data_folder = PipelineData(\"prepped_data_folder\", datastore=ws.get_default_datastore())\n\n# Step 1, Run the data prep script\nprep_step = PythonScriptStep(name = \"Prepare Data\",\n source_directory = experiment_folder,\n script_name = \"prep_diabetes.py\",\n arguments = ['--input-data', diabetes_ds.as_named_input('raw_data'),\n '--prepped-data', prepped_data_folder],\n outputs=[prepped_data_folder],\n compute_target = pipeline_cluster,\n runconfig = pipeline_run_config,\n allow_reuse = True)\n\n# Step 2, run the training script\ntrain_step = PythonScriptStep(name = \"Train and Register Model\",\n source_directory = experiment_folder,\n script_name = \"train_diabetes.py\",\n arguments = ['--training-folder', prepped_data_folder],\n inputs=[prepped_data_folder],\n compute_target = pipeline_cluster,\n runconfig = pipeline_run_config,\n allow_reuse = True)\n\nprint(\"Pipeline steps defined\")",
"Pipeline steps defined\n"
]
],
[
[
"OK, you're ready build the pipeline from the steps you've defined and run it as an experiment.",
"_____no_output_____"
]
],
[
[
"from azureml.core import Experiment\nfrom azureml.pipeline.core import Pipeline\nfrom azureml.widgets import RunDetails\n\n# Construct the pipeline\npipeline_steps = [prep_step, train_step]\npipeline = Pipeline(workspace=ws, steps=pipeline_steps)\nprint(\"Pipeline is built.\")\n\n# Create an experiment and run the pipeline\nexperiment = Experiment(workspace=ws, name = 'mslearn-diabetes-pipeline')\npipeline_run = experiment.submit(pipeline, regenerate_outputs=True)\nprint(\"Pipeline submitted for execution.\")\nRunDetails(pipeline_run).show()\npipeline_run.wait_for_completion(show_output=True)",
"Pipeline is built.\nCreated step Prepare Data [50c39f8a][74934260-06aa-4c4d-a4cd-230f602c6eb9], (This step will run and generate new outputs)\nCreated step Train and Register Model [6b28353a][1873f725-ebd6-4cab-a043-38d79745045a], (This step will run and generate new outputs)\nSubmitted PipelineRun 5e79d151-67ed-40d1-9ebc-18f03c44bfda\nLink to Azure Machine Learning Portal: https://ml.azure.com/runs/5e79d151-67ed-40d1-9ebc-18f03c44bfda?wsid=/subscriptions/c0a4d868-4fa1-4023-b058-13dfc12ea9be/resourcegroups/rg-dp100/workspaces/mls-dp100&tid=ffb6df9b-a626-4119-8765-20cd966f4661\nPipeline submitted for execution.\n"
]
],
[
[
"A graphical representation of the pipeline experiment will be displayed in the widget as it runs. Keep an eye on the kernel indicator at the top right of the page, when it turns from **⚫** to **◯**, the code has finished running. You can also monitor pipeline runs in the **Experiments** page in [Azure Machine Learning studio](https://ml.azure.com).\n\nWhen the pipeline has finished, you can examine the metrics recorded by it's child runs.",
"_____no_output_____"
]
],
[
[
"for run in pipeline_run.get_children():\n print(run.name, ':')\n metrics = run.get_metrics()\n for metric_name in metrics:\n print('\\t',metric_name, \":\", metrics[metric_name])",
"Train and Register Model :\n\t Accuracy : 0.9004444444444445\n\t AUC : 0.8859105592722003\n\t ROC : aml://artifactId/ExperimentRun/dcid.1d216aff-706b-4b92-a858-5de6e744d173/ROC_1617931737.png\nPrepare Data :\n\t raw_rows : 15000\n\t processed_rows : 15000\n"
]
],
[
[
"Assuming the pipeline was successful, a new model should be registered with a *Training context* tag indicating it was trained in a pipeline. Run the following code to verify this.",
"_____no_output_____"
]
],
[
[
"from azureml.core import Model\n\nfor model in Model.list(ws):\n print(model.name, 'version:', model.version)\n for tag_name in model.tags:\n tag = model.tags[tag_name]\n print ('\\t',tag_name, ':', tag)\n for prop_name in model.properties:\n prop = model.properties[prop_name]\n print ('\\t',prop_name, ':', prop)\n print('\\n')",
"diabetes_model version: 8\n\t Training context : Pipeline\n\t AUC : 0.8859105592722003\n\t Accuracy : 0.9004444444444445\n\n\ndiabetes_model version: 7\n\t Training context : Inline Training\n\t AUC : 0.8760759241753321\n\t Accuracy : 0.8876666666666667\n\n\ndiabetes_model version: 6\n\t Training context : Inline Training\n\t AUC : 0.8751082143390218\n\t Accuracy : 0.888\n\n\ndiabetes_model version: 5\n\t Training context : Compute cluster\n\t AUC : 0.886087297746153\n\t Accuracy : 0.9011111111111111\n\n\ndiabetes_model version: 4\n\t Training context : File dataset\n\t AUC : 0.8468331741963582\n\t Accuracy : 0.7793333333333333\n\n\ndiabetes_model version: 3\n\t Training context : Tabular dataset\n\t AUC : 0.8568509052814499\n\t Accuracy : 0.7891111111111111\n\n\ndiabetes_model version: 2\n\t Training context : Parameterized script\n\t AUC : 0.8484357430717946\n\t Accuracy : 0.774\n\n\ndiabetes_model version: 1\n\t Training context : Script\n\t AUC : 0.8483203144435048\n\t Accuracy : 0.774\n\n\namlstudio-designer-predict-dia version: 1\n\t CreatedByAMLStudio : true\n\n\nAutoML29253f2ad0 version: 1\n\n\n"
]
],
[
[
"## Publish the pipeline\n\nAfter you've created and tested a pipeline, you can publish it as a REST service.",
"_____no_output_____"
]
],
[
[
"# Publish the pipeline from the run\npublished_pipeline = pipeline_run.publish_pipeline(\n name=\"diabetes-training-pipeline\", description=\"Trains diabetes model\", version=\"1.0\")\n\npublished_pipeline",
"_____no_output_____"
]
],
[
[
"Note that the published pipeline has an endpoint, which you can see in the **Endpoints** page (on the **Pipeline Endpoints** tab) in [Azure Machine Learning studio](https://ml.azure.com). You can also find its URI as a property of the published pipeline object:",
"_____no_output_____"
]
],
[
[
"rest_endpoint = published_pipeline.endpoint\nprint(rest_endpoint)",
"https://eastasia.api.azureml.ms/pipelines/v1.0/subscriptions/c0a4d868-4fa1-4023-b058-13dfc12ea9be/resourceGroups/rg-dp100/providers/Microsoft.MachineLearningServices/workspaces/mls-dp100/PipelineRuns/PipelineSubmit/27573b5e-5a7c-4296-9439-29b2d6a03b52\n"
]
],
[
[
"## Call the pipeline endpoint\n\nTo use the endpoint, client applications need to make a REST call over HTTP. This request must be authenticated, so an authorization header is required. A real application would require a service principal with which to be authenticated, but to test this out, we'll use the authorization header from your current connection to your Azure workspace, which you can get using the following code:",
"_____no_output_____"
]
],
[
[
"from azureml.core.authentication import InteractiveLoginAuthentication\n\ninteractive_auth = InteractiveLoginAuthentication()\nauth_header = interactive_auth.get_authentication_header()\nprint(\"Authentication header ready.\")",
"Authentication header ready.\n"
]
],
[
[
"Now we're ready to call the REST interface. The pipeline runs asynchronously, so we'll get an identifier back, which we can use to track the pipeline experiment as it runs:",
"_____no_output_____"
]
],
[
[
"import requests\n\nexperiment_name = 'mslearn-diabetes-pipeline'\n\nrest_endpoint = published_pipeline.endpoint\nresponse = requests.post(rest_endpoint, \n headers=auth_header, \n json={\"ExperimentName\": experiment_name})\nrun_id = response.json()[\"Id\"]\nrun_id",
"_____no_output_____"
]
],
[
[
"Since you have the run ID, you can use it to wait for the run to complete.\n\n> **Note**: The pipeline should complete quickly, because each step was configured to allow output reuse. This was done primarily for convenience and to save time in this course. In reality, you'd likely want the first step to run every time in case the data has changed, and trigger the subsequent steps only if the output from step one changes.",
"_____no_output_____"
]
],
[
[
"from azureml.pipeline.core.run import PipelineRun\n\npublished_pipeline_run = PipelineRun(ws.experiments[experiment_name], run_id)\npublished_pipeline_run.wait_for_completion(show_output=True)",
"PipelineRunId: 2285dce2-df5b-4c9f-86b7-7e51618aa387\nLink to Azure Machine Learning Portal: https://ml.azure.com/runs/2285dce2-df5b-4c9f-86b7-7e51618aa387?wsid=/subscriptions/c0a4d868-4fa1-4023-b058-13dfc12ea9be/resourcegroups/rg-dp100/workspaces/mls-dp100&tid=ffb6df9b-a626-4119-8765-20cd966f4661\nPipelineRun Status: Running\n\nPipelineRun Execution Summary\n==============================\nPipelineRun Status: Finished\n{'runId': '2285dce2-df5b-4c9f-86b7-7e51618aa387', 'status': 'Completed', 'startTimeUtc': '2021-04-09T01:29:36.401211Z', 'endTimeUtc': '2021-04-09T01:29:39.315667Z', 'properties': {'azureml.runsource': 'azureml.PipelineRun', 'runSource': 'Unavailable', 'runType': 'HTTP', 'azureml.parameters': '{}', 'azureml.pipelineid': '27573b5e-5a7c-4296-9439-29b2d6a03b52'}, 'inputDatasets': [], 'outputDatasets': [], 'logFiles': {'logs/azureml/executionlogs.txt': 'https://mlsdp1007826913596.blob.core.windows.net/azureml/ExperimentRun/dcid.2285dce2-df5b-4c9f-86b7-7e51618aa387/logs/azureml/executionlogs.txt?sv=2019-02-02&sr=b&sig=U%2FqenChKdQrxLwUWQbSV1X2o8q%2BAp%2Fz%2BD0%2BcLgFFXmk%3D&st=2021-04-09T01%3A19%3A39Z&se=2021-04-09T09%3A29%3A39Z&sp=r', 'logs/azureml/stderrlogs.txt': 'https://mlsdp1007826913596.blob.core.windows.net/azureml/ExperimentRun/dcid.2285dce2-df5b-4c9f-86b7-7e51618aa387/logs/azureml/stderrlogs.txt?sv=2019-02-02&sr=b&sig=wexpNKdEY0HSLDxGDoNg952ep6CUiBaCPprjqiVa9QQ%3D&st=2021-04-09T01%3A19%3A39Z&se=2021-04-09T09%3A29%3A39Z&sp=r', 'logs/azureml/stdoutlogs.txt': 'https://mlsdp1007826913596.blob.core.windows.net/azureml/ExperimentRun/dcid.2285dce2-df5b-4c9f-86b7-7e51618aa387/logs/azureml/stdoutlogs.txt?sv=2019-02-02&sr=b&sig=3dK%2FOIuqg7KtkrijAjAOjkwwCg4g8%2FkBg%2FC4xCyFMdY%3D&st=2021-04-09T01%3A19%3A39Z&se=2021-04-09T09%3A29%3A39Z&sp=r'}, 'submittedBy': 'MeteoR Figel'}\n\n"
]
],
[
[
"## Schedule the Pipeline\n\nSuppose the clinic for the diabetes patients collects new data each week, and adds it to the dataset. You could run the pipeline every week to retrain the model with the new data.",
"_____no_output_____"
]
],
[
[
"from azureml.pipeline.core import ScheduleRecurrence, Schedule\n\n# Submit the Pipeline every Monday at 00:00 UTC\nrecurrence = ScheduleRecurrence(frequency=\"Week\", interval=1, week_days=[\"Monday\"], time_of_day=\"00:00\")\nweekly_schedule = Schedule.create(ws, name=\"weekly-diabetes-training\", \n description=\"Based on time\",\n pipeline_id=published_pipeline.id, \n experiment_name='mslearn-diabetes-pipeline', \n recurrence=recurrence)\nprint('Pipeline scheduled.')",
"Pipeline scheduled.\n"
]
],
[
[
"You can retrieve the schedules that are defined in the workspace like this:",
"_____no_output_____"
]
],
[
[
"schedules = Schedule.list(ws)\nschedules",
"_____no_output_____"
]
],
[
[
"You can check the latest run like this:",
"_____no_output_____"
]
],
[
[
"pipeline_experiment = ws.experiments.get('mslearn-diabetes-pipeline')\nlatest_run = list(pipeline_experiment.get_runs())[0]\n\nlatest_run.get_details()",
"_____no_output_____"
]
],
[
[
"This is a simple example, designed to demonstrate the principle. In reality, you could build more sophisticated logic into the pipeline steps - for example, evaluating the model against some test data to calculate a performance metric like AUC or accuracy, comparing the metric to that of any previously registered versions of the model, and only registering the new model if it performs better.\n\nYou can use the [Azure Machine Learning extension for Azure DevOps](https://marketplace.visualstudio.com/items?itemName=ms-air-aiagility.vss-services-azureml) to combine Azure ML pipelines with Azure DevOps pipelines (yes, it *is* confusing that they have the same name!) and integrate model retraining into a *continuous integration/continuous deployment (CI/CD)* process. For example you could use an Azure DevOps *build* pipeline to trigger an Azure ML pipeline that trains and registers a model, and when the model is registered it could trigger an Azure Devops *release* pipeline that deploys the model as a web service, along with the application or service that consumes the model.",
"_____no_output_____"
]
]
] | [
"markdown",
"code",
"markdown",
"code",
"markdown",
"code",
"markdown",
"code",
"markdown",
"code",
"markdown",
"code",
"markdown",
"code",
"markdown",
"code",
"markdown",
"code",
"markdown",
"code",
"markdown",
"code",
"markdown",
"code",
"markdown",
"code",
"markdown",
"code",
"markdown",
"code",
"markdown",
"code",
"markdown",
"code",
"markdown",
"code",
"markdown",
"code",
"markdown"
] | [
[
"markdown",
"markdown"
],
[
"code"
],
[
"markdown"
],
[
"code"
],
[
"markdown"
],
[
"code"
],
[
"markdown"
],
[
"code"
],
[
"markdown"
],
[
"code"
],
[
"markdown"
],
[
"code"
],
[
"markdown"
],
[
"code"
],
[
"markdown"
],
[
"code"
],
[
"markdown"
],
[
"code"
],
[
"markdown"
],
[
"code"
],
[
"markdown"
],
[
"code"
],
[
"markdown"
],
[
"code"
],
[
"markdown"
],
[
"code"
],
[
"markdown"
],
[
"code"
],
[
"markdown"
],
[
"code"
],
[
"markdown"
],
[
"code"
],
[
"markdown"
],
[
"code"
],
[
"markdown"
],
[
"code"
],
[
"markdown"
],
[
"code"
],
[
"markdown"
]
] |
e77260a4f5a475196ab55fad10b0c63e00ee33f0 | 86,858 | ipynb | Jupyter Notebook | DataPreparation.ipynb | schoolofdata-ch/openfarming-Decision-Support | 43daa22289c151340f659e409886fae502a6bb0f | [
"MIT"
] | null | null | null | DataPreparation.ipynb | schoolofdata-ch/openfarming-Decision-Support | 43daa22289c151340f659e409886fae502a6bb0f | [
"MIT"
] | null | null | null | DataPreparation.ipynb | schoolofdata-ch/openfarming-Decision-Support | 43daa22289c151340f659e409886fae502a6bb0f | [
"MIT"
] | null | null | null | 39.679306 | 2,618 | 0.446522 | [
[
[
"# Prepare Data to be Analyzed with Modulos AutoML",
"_____no_output_____"
],
[
"Note: For all of these operations to work, we are relying on the data being sorted, as it's done in the notebook DataCleaning.ipynb.",
"_____no_output_____"
],
[
"## Imports",
"_____no_output_____"
]
],
[
[
"import pandas as pd\nimport matplotlib.pyplot as plt\nimport glob\nimport os\nfrom IPython.display import display\nimport tqdm\nfrom collections import Counter\nimport matplotlib\npd.options.display.max_columns = None\nfrom pandas.plotting import register_matplotlib_converters\nregister_matplotlib_converters()\nimport numpy as np",
"_____no_output_____"
]
],
[
[
"## Configure path variables and number of samples",
"_____no_output_____"
]
],
[
[
"# Path where the cleaned data is stored\nfpath_clean_data_dir = 'clean_data/'\n\n# Path where the data ready for the ML analysis is stored and filename of output file\nfpath_prepared_data_dir = 'ready_data/'\nfoldername_prepared_data = 'ai_basic_all/'\n\n# Number of unique Cow IDs to consider (the computation is very slow\n# Including all samples is only advised once one is happy with the sample)\nnsamples = None # Full length for nsamples = None\n# nsamples = 10000",
"_____no_output_____"
],
[
"!mkdir -p {fpath_prepared_data_dir}{foldername_prepared_data}",
"_____no_output_____"
],
[
"def select_cow_by_id(df, cow_id, id_label='idani_anon'):\n \"\"\"\n Function to return all the entries matching a specific cow ID.\n \n :param df: Pandas dataframe\n :param cow_id: Specific cow ID to select\n :return: Entries for the corresponding cow ID\n \"\"\"\n return df[df[id_label]==cow_id]",
"_____no_output_____"
]
],
[
[
"# Data Loading",
"_____no_output_____"
],
[
"Load all relevant tables into one dictionary. Note that we are not considering hm_BCS and hm_pregnancy in this first implementation.",
"_____no_output_____"
]
],
[
[
"# Columns with datetime entries & file names\ndatetime_cols = {#'hm_BCS': ['BCS_date'],\n 'hm_lactation': ['calving_date'],\n 'hm_NSAIET': ['nsaiet_date'],\n 'hm_animal': ['birth_date'], \n 'hm_milkrecording': ['mlksmpl_date', 'lab_date'],\n 'hm_ebv': False,\n# 'hm_pregnancy': ['pregnancy_detection_date'],\n 'hm_health': ['healthevent_date']\n }\n\nfnames = list(datetime_cols.keys())\nprint('File names all:', fnames)",
"File names all: ['hm_lactation', 'hm_NSAIET', 'hm_animal', 'hm_milkrecording', 'hm_ebv', 'hm_health']\n"
],
[
"data_frames = {}\nfor fname in fnames:\n print('----- Reading in {:}.csv -----'.format(fname))\n fpath = fpath_clean_data_dir+fname+'.csv'\n data_frames[fname] = pd.read_csv(fpath, parse_dates=datetime_cols[fname])\n print(data_frames[fname].head(10))\n print()",
"----- Reading in hm_lactation.csv -----\n parity calving_date calving_ease idani_anon\n0 1 2018-09-06 2 CHE000000000561\n1 2 2019-09-15 2 CHE000000000561\n2 1 2016-09-07 2 CHE000000000781\n3 2 2017-08-05 1 CHE000000000781\n4 3 2018-10-18 2.5 CHE000000000781\n5 4 2019-09-29 1 CHE000000000781\n6 1 2017-11-01 1 CHE000000001494\n7 2 2018-11-01 2 CHE000000001494\n8 3 2020-01-01 2 CHE000000001494\n9 1 2013-09-28 1 CHE000000002000\n\n----- Reading in hm_NSAIET.csv -----\n parity nsaiet_date nsaiet_type AI_technician idani_anon \\\n0 0 2017-11-05 Besamung 10 CHE000000000561 \n1 0 2017-11-28 Besamung 10 CHE000000000561 \n2 1 2018-11-16 Besamung 10 CHE000000000561 \n3 1 2018-12-05 Besamung 10 CHE000000000561 \n4 2 2019-12-09 Besamung 10 CHE000000000561 \n5 2 2019-12-10 Besamung 10 CHE000000000561 \n6 2 2020-01-31 Besamung 10 CHE000000000561 \n7 0 2015-11-19 Belegung 5 CHE000000000781 \n8 1 2016-10-22 Besamung 10 CHE000000000781 \n9 2 2017-11-05 Besamung 10 CHE000000000781 \n\n idani_anon_aisire \n0 CHE000020282537 \n1 CHE000020282537 \n2 CHE000002123235 \n3 CHE000002123235 \n4 CHE000001110094 \n5 CHE000001110094 \n6 CHE000090893216 \n7 CHE000018236237 \n8 CHE000064498506 \n9 CHE000076479306 \n\n----- Reading in hm_animal.csv -----\n birth_date brd_abbr_icar idani_anon\n0 2016-03-08 HOL CHE000000000559\n1 2016-02-27 HOL CHE000000000561\n2 2011-05-09 HOL CHE000000000620\n3 2014-06-23 HOL CHE000000000781\n4 2015-11-25 HOL CHE000000001494\n5 2018-04-09 HOL CHE000000001501\n6 2011-10-11 HOL CHE000000002000\n7 2013-04-21 HOL CHE000000002068\n8 2008-07-26 HOL CHE000000002251\n9 2010-09-14 HOL CHE000000002451\n\n----- Reading in hm_milkrecording.csv -----\n mlksmpl_date milking_time_morning milking_time_evening lab_date DIM \\\n0 2018-10-10 50000.0 163000.0 2018-10-11 34.0 \n1 2018-11-13 50000.0 163000.0 2018-11-15 68.0 \n2 2018-12-18 50000.0 163000.0 2018-12-19 103.0 \n3 2019-01-21 50000.0 163000.0 2019-01-23 137.0 \n4 2019-02-23 50000.0 163000.0 2019-02-26 170.0 \n5 2019-03-29 50000.0 163500.0 2019-04-02 204.0 \n6 2019-05-06 50000.0 163500.0 2019-05-07 242.0 \n7 2019-06-07 50000.0 163000.0 2019-06-12 274.0 \n8 2019-07-12 50000.0 163000.0 2019-07-15 309.0 \n9 2019-09-20 50000.0 163000.0 2019-09-23 5.0 \n\n pruefmethode melkmethode milk_yield_24h fat_24h protein_24h lactose_24h \\\n0 AT4 Normal 34.2 36.5 24.9 49.8 \n1 AT4 Normal 34.1 34.9 29.2 50.3 \n2 AT4 Normal 30.9 37.2 26.7 48.3 \n3 AT4 Normal 36.3 36.8 31.1 50.0 \n4 AT4 Normal 35.3 34.2 29.6 48.6 \n5 AT4 Normal 37.0 35.7 31.2 48.8 \n6 AT4 Normal 34.6 37.0 30.1 48.9 \n7 AT4 Normal 30.5 39.5 31.2 48.3 \n8 AT4 Normal 30.9 34.5 29.2 47.8 \n9 AT4 Normal 40.1 40.2 42.3 47.5 \n\n scc_24h urea_24h AR_PESEE_PESCODEALPAGE idani_anon \\\n0 21.0 2.4 0.0 CHE000000000561 \n1 80.0 2.2 0.0 CHE000000000561 \n2 132.0 1.6 0.0 CHE000000000561 \n3 106.0 2.1 0.0 CHE000000000561 \n4 133.0 1.5 0.0 CHE000000000561 \n5 103.0 2.1 0.0 CHE000000000561 \n6 67.0 1.4 0.0 CHE000000000561 \n7 129.0 2.4 0.0 CHE000000000561 \n8 22.0 2.0 0.0 CHE000000000561 \n9 34.0 1.8 0.0 CHE000000000561 \n\n idhrd_anon milk_yield_msrmt_type fat_protein_24h_ratio \n0 CHE000000095710 2 1.465863 \n1 CHE000000095710 3 1.195205 \n2 CHE000000095710 2 1.393258 \n3 CHE000000095710 3 1.183280 \n4 CHE000000095710 2 1.155405 \n5 CHE000000095710 3 1.144231 \n6 CHE000000095710 2 1.229236 \n7 CHE000000095710 3 1.266026 \n8 CHE000000095710 2 1.181507 \n9 CHE000000095710 2 0.950355 \n\n----- Reading in hm_ebv.csv -----\n base label idani_anon ekg epr fkg fpr mkg per scs\n0 HO20 A CHE000000000559 -23.0 -0.02 -31.0 -0.07 -636.0 93.0 102.0\n1 HO20 CH CHE000000000561 18.0 -0.23 26.0 -0.24 1173.0 93.0 89.0\n2 HO20 A CHE000000000620 5.0 0.11 -1.0 0.05 -147.0 99.0 94.0\n3 HO20 CH CHE000000000781 0.0 -0.07 -2.0 -0.11 179.0 95.0 106.0\n4 HO20 CH CHE000000001494 3.0 0.11 -4.0 0.04 -179.0 93.0 111.0\n5 HO20 A CHE000000001501 36.0 0.08 30.0 -0.07 887.0 87.0 99.0\n6 HO20 CH CHE000000002000 -14.0 0.04 -17.0 0.05 -514.0 92.0 101.0\n7 HO20 CH CHE000000002068 -23.0 -0.06 -23.0 -0.01 -546.0 96.0 110.0\n8 HO20 CH CHE000000002251 -2.0 -0.15 7.0 -0.07 328.0 98.0 100.0\n9 HO20 CH CHE000000002451 -11.0 -0.06 -30.0 -0.28 -177.0 95.0 94.0\n\n----- Reading in hm_health.csv -----\n hecode_ASR healthevent_date idani_anon idhrd_anon\n0 10.7.1. 2018-11-19 CHE000000005877 CHE000000079291\n1 2.1.1. 2018-12-20 CHE000000005877 CHE000000079291\n2 10.4. 2019-09-06 CHE000000005877 CHE000000079291\n3 3.5. 2020-03-14 CHE000000005877 CHE000000079291\n4 6.1. 2014-06-02 CHE000000006772 CHE000000055108\n5 1.2. 2015-11-30 CHE000000006772 CHE000000055108\n6 3.3.3. 2018-04-12 CHE000000006772 CHE000000094671\n7 10.8. 2019-01-22 CHE000000006772 CHE000000055108\n8 10.4. 2019-01-22 CHE000000006772 CHE000000055108\n9 2.2.3. 2016-12-29 CHE000000019899 CHE000000006820\n\n"
]
],
[
[
"## Data Manipulation & Enhancement",
"_____no_output_____"
],
[
"### Remove all parity = 0 entries (i.e. inseminations before the cow has even given birth and milk)",
"_____no_output_____"
]
],
[
[
"orig_rows = data_frames['hm_NSAIET'].shape[0]\nmask = np.argwhere(data_frames['hm_NSAIET']['parity'].values == 0).flatten()\ndata_frames['hm_NSAIET'] = data_frames['hm_NSAIET'].drop(mask, axis=0).reset_index(drop=True)\nprint('Removed {:} entries ({:.2f}%)'.format(orig_rows-data_frames['hm_NSAIET'].shape[0],\n (1-data_frames['hm_NSAIET'].shape[0]/orig_rows)*100))",
"Removed 329889 entries (24.15%)\n"
]
],
[
[
"### List of unique cow IDs by considering intersection of all the tables with necessary inputs for prediction",
"_____no_output_____"
]
],
[
[
"# Tables necessary for the prediction ('hm_health' doesn't contain many cows and\n# one would have to throw away much data)\nfnames_necessary = [fname for fname in fnames if fname != 'hm_health']\n\n# Select subset\nunique_cow_ids = [set(data_frames[fname]['idani_anon'].values) for fname in fnames_necessary]\nunique_cow_ids = list(set.intersection(*unique_cow_ids))\n\nprint('Number of individual cows in sample: {:}'.format(len(unique_cow_ids)))",
"Number of individual cows in sample: 180005\n"
]
],
[
[
"### Convert parity to labels (= column used for prediction)\nIf the same parity number occurs multiple times only the one with the most recent time stamp is considered a success. The other are considered failures. Parities that only appear once are considered success by default.",
"_____no_output_____"
]
],
[
[
"def parity_to_label_for_single_cow(df):\n \"\"\"\n Function to return a new column called 'parity_labels', which contains True/False depending on the\n outcome of the artificial insemination.\n \n :param df: Subset of a Pandas dataframe containing all the relevant entries for a single cow\n :return: Column with labels encoding a successful/unsuccessful insemination (1 or 0)\n \"\"\"\n\n parity_values = df['parity'].values\n\n parity_labels = []\n parity_values_seen = []\n\n for p in parity_values[::-1]:\n if not p in parity_values_seen:\n parity_labels.append(1)\n parity_values_seen.append(p)\n else:\n parity_labels.append(0)\n\n return parity_labels[::-1]",
"_____no_output_____"
]
],
[
[
"#### Convert labels for all cows (using unique_cow_ids from above)",
"_____no_output_____"
]
],
[
[
"ids_to_remove = 0\n\nparity_labels_all = np.zeros(data_frames['hm_NSAIET'].shape[0], dtype=np.int)\nfor cow_id in tqdm.tqdm(unique_cow_ids):\n left = data_frames['hm_NSAIET'][\"idani_anon\"].searchsorted(cow_id, 'left')\n right = data_frames['hm_NSAIET'][\"idani_anon\"].searchsorted(cow_id, 'right')\n \n single_cow = data_frames['hm_NSAIET'][left:right]\n \n parity_values = single_cow['parity'].values\n if (parity_values != sorted(parity_values)).all():\n unique_cow_ids.remove(cow_id)\n ids_to_remove += 1\n \n else:\n parity_labels_all[left:right] = parity_to_label_for_single_cow(single_cow)\n \ndata_frames['hm_NSAIET']['parity_labels'] = parity_labels_all\n\nprint('Samples removed due to inconsistencies between the parities and the NSAIET-date: {:}'.format(ids_to_remove))",
"100%|██████████| 180005/180005 [00:33<00:00, 5410.62it/s]"
]
],
[
[
"## Display all dataframes individually (sanity check)",
"_____no_output_____"
]
],
[
[
"data_frames['hm_lactation']",
"_____no_output_____"
],
[
"data_frames['hm_NSAIET']",
"_____no_output_____"
],
[
"data_frames['hm_animal']",
"_____no_output_____"
],
[
"data_frames['hm_milkrecording']",
"_____no_output_____"
],
[
"data_frames['hm_ebv']",
"_____no_output_____"
],
[
"data_frames['hm_health']",
"_____no_output_____"
]
],
[
[
"## Functions to contain hm_NSAIET with other datasets",
"_____no_output_____"
]
],
[
[
"def combine_nsaeit_with_milkrecording_single_cow(df_nsaiet, df_milkrec, columns_both='idani_anon'):\n \"\"\"\n Function combining the dataframes hm_NSAIET and hm_milkrecording for a single cow ID.\n The tables are combined such that for every insemination, the date of the previous milkrecording is chosen.\n \n :param df_nsaiet: Subset of the NSAEIT Pandas dataframe containing the relevant entries for a single cow\n :param df_milkrec: Subset of the milkrecording Pandas dataframe containing the relevant entries for a single cow\n :param columns_both: Identical columns in both dataframes\n :return: Merged dataframe\n \"\"\"\n \n combined_df = []\n for idx_parity, parity_date in enumerate(df_nsaiet['nsaiet_date'].values): \n # Milk recording dates before the insemination date\n indices = np.argwhere((df_milkrec['mlksmpl_date'].values < parity_date)==True).flatten()\n \n # Throw away values, where there is no milk recording date before the insemination date\n if indices.size == 0:\n continue\n \n idx_milkrec = np.argwhere((df_milkrec['mlksmpl_date'].values < parity_date)==True).flatten()[-1]\n \n # Throw away the value, if the difference between the last milk recording and\n # the artificial insemination is longer than 60 days\n delta = np.timedelta64(parity_date - df_milkrec['mlksmpl_date'].values[idx_milkrec], 'D') // np.timedelta64(1, 'D')\n if delta > 60:\n continue\n \n df = pd.merge(df_nsaiet.iloc[[idx_parity]],\n df_milkrec.iloc[[idx_milkrec]],\n \"inner\", on=columns_both)\n combined_df.append(df)\n \n # Return None for an emtpy dataframe\n if len(combined_df) == 0:\n return None\n\n return pd.concat(combined_df).reset_index(drop=True)\n\n\ndef combine_nsaeit_with_lactation_single_cow(df_nsaiet, df_lactation, columns_both='idani_anon'):\n \"\"\"\n Function combining the dataframes hm_NSAIET and hm_lactation for a single cow ID.\n The tables are combined such that for every insemination, the entry with the same parity is chosen.\n \n :param df_nsaiet: Subset of the NSAEIT Pandas dataframe containing the relevant entries for a single cow\n :param df_lactation: Subset of the lactation Pandas dataframe containing the relevant entries for a single cow\n :param columns_both: Identical columns in both dataframes\n :return: Merged dataframe\n \"\"\"\n \n combined_df = []\n for idx_parity, parity in enumerate(df_nsaiet['parity'].values):\n idx_lactation = np.argwhere((df_lactation['parity'].values == parity)).flatten()[0]\n \n df = pd.merge(df_nsaiet.iloc[[idx_parity]],\n df_lactation.iloc[[idx_lactation]],\n \"inner\", on=columns_both)\n combined_df.append(df)\n\n return pd.concat(combined_df).reset_index(drop=True)\n\n\ndef combine_with_health_single_cow(df_nsaiet, df_health, threshold_health_date=45):\n \"\"\"\n Add health-events related columns depending on whether there were any recorded health events XX days before the\n insemination date.\n \n :param df_nsaiet: Subset of the NSAEIT Pandas dataframe containing all the relevant entries for a single cow\n :param df_other: Subset of the health Pandas dataframe containing all the relevant entries for a single cow\n :param threshold_health_date: Number of days before the insemination that a health event is considered to be relevant \n :return: Column with number of health events XX days before the artificial insemination\n \"\"\"\n \n healthevents = np.zeros(df_nsaiet.shape[0], dtype=np.float)\n\n if df_health is not None: \n health_dates = df_health['healthevent_date'].values\n\n for idx_parity, parity_date in enumerate(df_nsaiet['nsaiet_date'].values):\n deltas = [np.timedelta64(parity_date-date_health, 'D') // np.timedelta64(1, 'D') for date_health in health_dates]\n deltas = np.array(deltas, dtype=np.float)\n healthevents[idx_parity] = np.sum((deltas <= threshold_health_date) & (deltas >= 0))\n\n return healthevents\n\n\ndef combine_with_other_datasets_single_cow(df_nsaiet, df_other, columns_both='idani_anon'):\n \"\"\"\n Function combining the dataframes hm_NSAIET and hm_milkrecording (already combined) with another dataframe\n for a single cow ID.\n \n :param df_nsaiet: Subset of the NSAEIT Pandas dataframe containing all the relevant entries for a single cow\n :param df_other: Subset of the other Pandas dataframe containing all the relevant entries for a single cow\n :param columns_both: Identical columns in both dataframes\n :return: Merged dataframe\n \"\"\"\n \n combined_df = []\n for idx_parity, parity_date in enumerate(df_nsaiet['nsaiet_date'].values):\n df = pd.merge(df_nsaiet.iloc[[idx_parity]],\n df_other.iloc[[0]],\n \"inner\", on=columns_both)\n combined_df.append(df)\n\n return pd.concat(combined_df).reset_index(drop=True)\n\n\ndef return_single_cow_subset(df, cow_id, identifier_col='idani_anon'):\n \"\"\"\n For a given dataframe, return the subset of the dataframe for a given cow_id.\n \n :param df: Pandas dataframe\n :param cow_id: ID of the cow, whose data is to be selected\n :param identifier_col: Name of the column containing the ID\n :return: Subset of Pandas dataframe\n \"\"\"\n \n left = df[identifier_col].searchsorted(cow_id, 'left')\n right = df[identifier_col].searchsorted(cow_id, 'right')\n return df[left:right]",
"_____no_output_____"
]
],
[
[
"## Merge all dataframes",
"_____no_output_____"
]
],
[
[
"datetime_cols = {#'hm_BCS': ['BCS_date'],\n 'hm_lactation': ['calving_date'],\n 'hm_NSAIET': ['nsaiet_date'],\n 'hm_animal': ['birth_date'], \n 'hm_milkrecording': ['mlksmpl_date', 'lab_date'],\n 'hm_ebv': False,\n# 'hm_pregnancy': ['pregnancy_detection_date'],\n 'hm_health': ['healthevent_date']\n }\n\nfnames = list(datetime_cols.keys())\n\nfnames_wo_nsaiet_milkrec = [fname for fname in fnames if (fname != 'hm_NSAIET') and (fname != 'hm_milkrecording')]",
"_____no_output_____"
],
[
"df_merged = []\n\ncounter = 0\nbunchsize = 500\n\nfor cow_id in tqdm.tqdm(unique_cow_ids[:nsamples]):\n # Merge NSAIET & milkrecording\n single_cow_nsaiet = return_single_cow_subset(data_frames['hm_NSAIET'], cow_id)\n single_cow_milkrecording = return_single_cow_subset(data_frames['hm_milkrecording'], cow_id)\n \n dfcomb = combine_nsaeit_with_milkrecording_single_cow(single_cow_nsaiet, single_cow_milkrecording)\n \n # Skip Cow ID in case of an emtpy dataframe\n if dfcomb is None:\n continue\n \n for fname in fnames_wo_nsaiet_milkrec:\n single_cow = return_single_cow_subset(data_frames[fname], cow_id)\n col_both = list(set.intersection(set(dfcomb.keys().values), set(data_frames[fname].keys().values)))\n\n # Combine with hm_lactation\n if fname == 'hm_lactation':\n dfcomb = combine_nsaeit_with_lactation_single_cow(dfcomb, single_cow, col_both)\n\n # Combine with hm_ebv\n if fname == 'hm_ebv':\n dfcomb = combine_with_other_datasets_single_cow(dfcomb, single_cow, col_both)\n\n # Combine with hm_animal\n if fname == 'hm_animal':\n dfcomb = combine_with_other_datasets_single_cow(dfcomb, single_cow, col_both)\n\n # Combine with hm_health\n if fname == 'hm_health':\n dfcomb['healthevents'] = combine_with_health_single_cow(dfcomb, single_cow, threshold_health_date=45)\n \n # Append dataframes\n df_merged.append(dfcomb)\n \n counter += 1\n\n # Concatenate all dataframe and reset list after N=bunchsize samples\n if counter == bunchsize:\n df_merged_all = pd.concat(df_merged).reset_index(drop=True)\n df_merged = []\n elif counter % bunchsize == 0:\n df_merged_all = df_merged_all.append(pd.concat(df_merged).reset_index(drop=True), ignore_index=True)\n df_merged = []\n\nif counter < bunchsize:\n df_merged_all = pd.concat(df_merged).reset_index(drop=True)\nelif len(df_merged) != 0:\n df_merged_all = df_merged_all.append(pd.concat(df_merged).reset_index(drop=True), ignore_index=True) ",
" 0%| | 341/180005 [00:52<7:37:04, 6.55it/s] \n"
]
],
[
[
"## Add columns with age and days since calving, drop datetime columns",
"_____no_output_____"
]
],
[
[
"# Add columns (deltas between dates)\ndf_merged_all['age'] = (df_merged_all['nsaiet_date'] - df_merged_all['birth_date']).values // np.timedelta64(1, 'D')\ndf_merged_all['days_since_calving'] = (df_merged_all['nsaiet_date'] - df_merged_all['calving_date']).values // np.timedelta64(1, 'D')\ndf_merged_all['days_since_mlksample'] = (df_merged_all['nsaiet_date'] - df_merged_all['mlksmpl_date']).values // np.timedelta64(1, 'D')\n\n# # Drop columns with datetimes, since only the deltas are relevant\n# columns_to_drop = ['nsaiet_date', 'birth_date', 'calving_date', 'mlksmpl_date', 'lab_date', 'birth_date']\n# df_merged_all = df_merged_all.drop(labels=columns_to_drop, axis=1)",
"_____no_output_____"
],
[
"df_merged_all",
"_____no_output_____"
]
],
[
[
"## Save data, create a dataset structure file for the AutoML platform, and tar the dataset",
"_____no_output_____"
],
[
"Save dataset",
"_____no_output_____"
]
],
[
[
"folderpath = fpath_prepared_data_dir + foldername_prepared_data\ndf_merged_all.to_csv(folderpath+'data.csv', index=False)",
"_____no_output_____"
]
],
[
[
"Save dataset structure file (DSSF), which is needed for the AutoML analysis",
"_____no_output_____"
]
],
[
[
"# Content of DSSF\ndssf_string = ['[',\n ' {',\n ' \\\"name\\\": \\\"{}\\\",'.format(foldername_prepared_data[:-1]),\n ' \\\"path\\\": \\\"data.csv\\\",',\n ' \\\"type\\\": \\\"table\\\"',\n ' },',\n ' {',\n ' \\\"_version\\\": \\\"0.1\\\"',\n ' }',\n ']'\n ]\n\nprint('\\n'.join(dssf_string))\n\n# Write DSSF\ntext_file = open(folderpath+'dataset_structure.json', 'w')\nn = text_file.write('\\n'.join(dssf_string))\ntext_file.close()",
"[\n {\n \"name\": \"ai_basic_all\",\n \"path\": \"data.csv\",\n \"type\": \"table\"\n },\n {\n \"_version\": \"0.1\"\n }\n]\n"
]
],
[
[
"Create a tarball of all the contents",
"_____no_output_____"
]
],
[
[
"!tar -cf {fpath_prepared_data_dir}{foldername_prepared_data[:-1]}.tar -C {fpath_prepared_data_dir} {foldername_prepared_data[:-1]}",
"_____no_output_____"
]
],
[
[
"## Prepare a file for a regression task (predict optimal date for insemination)",
"_____no_output_____"
]
],
[
[
"foldername_prepared_data = 'ai_basic_all_predict_date/'",
"_____no_output_____"
],
[
"!mkdir -p {fpath_prepared_data_dir}{foldername_prepared_data}",
"_____no_output_____"
],
[
"# Remove all non-successful inseminations\nmask = df_merged_all['parity_labels'].values == 0\ndf_merged_subset = df_merged_all.drop(np.arange(mask.size)[mask], axis=0).reset_index(drop=True)\n\nfolderpath = fpath_prepared_data_dir + foldername_prepared_data\ndf_merged_subset.to_csv(folderpath+'data.csv', index=False)",
"_____no_output_____"
],
[
"# Content of DSSF\ndssf_string = ['[',\n ' {',\n ' \\\"name\\\": \\\"{}\\\",'.format(foldername_prepared_data[:-1]),\n ' \\\"path\\\": \\\"data.csv\\\",',\n ' \\\"type\\\": \\\"table\\\"',\n ' },',\n ' {',\n ' \\\"_version\\\": \\\"0.1\\\"',\n ' }',\n ']'\n ]\n\nprint('\\n'.join(dssf_string))\n\n# Write DSSF\ntext_file = open(folderpath+'dataset_structure.json', 'w')\nn = text_file.write('\\n'.join(dssf_string))\ntext_file.close()",
"[\n {\n \"name\": \"ai_basic_all_predict_date\",\n \"path\": \"data.csv\",\n \"type\": \"table\"\n },\n {\n \"_version\": \"0.1\"\n }\n]\n"
],
[
"!tar -cf {fpath_prepared_data_dir}{foldername_prepared_data[:-1]}.tar -C {fpath_prepared_data_dir} {foldername_prepared_data[:-1]}",
"_____no_output_____"
]
]
] | [
"markdown",
"code",
"markdown",
"code",
"markdown",
"code",
"markdown",
"code",
"markdown",
"code",
"markdown",
"code",
"markdown",
"code",
"markdown",
"code",
"markdown",
"code",
"markdown",
"code",
"markdown",
"code",
"markdown",
"code",
"markdown",
"code",
"markdown",
"code",
"markdown",
"code"
] | [
[
"markdown",
"markdown",
"markdown"
],
[
"code"
],
[
"markdown"
],
[
"code",
"code",
"code"
],
[
"markdown",
"markdown"
],
[
"code",
"code"
],
[
"markdown",
"markdown"
],
[
"code"
],
[
"markdown"
],
[
"code"
],
[
"markdown"
],
[
"code"
],
[
"markdown"
],
[
"code"
],
[
"markdown"
],
[
"code",
"code",
"code",
"code",
"code",
"code"
],
[
"markdown"
],
[
"code"
],
[
"markdown"
],
[
"code",
"code"
],
[
"markdown"
],
[
"code",
"code"
],
[
"markdown",
"markdown"
],
[
"code"
],
[
"markdown"
],
[
"code"
],
[
"markdown"
],
[
"code"
],
[
"markdown"
],
[
"code",
"code",
"code",
"code",
"code"
]
] |
e7726596b3c4c282b3f322b3046346f4cd9ca8f7 | 19,848 | ipynb | Jupyter Notebook | _notebooks/2020-10-20-julia.ipynb | ChristopherTh/statistics-blog | cca58e1cb0cb62e8a434b08724fe4739a8a37fd3 | [
"Apache-2.0"
] | null | null | null | _notebooks/2020-10-20-julia.ipynb | ChristopherTh/statistics-blog | cca58e1cb0cb62e8a434b08724fe4739a8a37fd3 | [
"Apache-2.0"
] | 2 | 2021-09-28T05:31:10.000Z | 2022-02-26T09:50:55.000Z | _notebooks/2020-10-20-julia.ipynb | ChristopherTh/statistics-blog | cca58e1cb0cb62e8a434b08724fe4739a8a37fd3 | [
"Apache-2.0"
] | null | null | null | 21.003175 | 144 | 0.37495 | [
[
[
"# \"Julia\"\n> \"Basics of the julia language\"\n\n- author: Christopher Thiemann\n- toc: true\n- branch: master\n- badges: true\n- comments: true\n- categories: [julia ]\n- hide: true\n- search_exclude: true",
"_____no_output_____"
]
],
[
[
"answer = 43",
"_____no_output_____"
],
[
"x = 2",
"_____no_output_____"
],
[
"1 < x < 3",
"_____no_output_____"
],
[
"M = [1 0; 0 1]",
"_____no_output_____"
],
[
"typeof(size(M))",
"_____no_output_____"
]
],
[
[
"## Basics",
"_____no_output_____"
],
[
"### Assignement",
"_____no_output_____"
]
],
[
[
"answer = 42\nx, y, z = 1, [1:10; ], \"A string\" # just like in python !\nx, y = y, x # swap x and y",
"_____no_output_____"
]
],
[
[
"### Declaring Constants",
"_____no_output_____"
]
],
[
[
"const DATE_OF_BIRTH = 2012",
"_____no_output_____"
]
],
[
[
"### Commenting",
"_____no_output_____"
]
],
[
[
"1 + 1 # Hello, this is a comment!",
"_____no_output_____"
]
],
[
[
"### Delimited comment",
"_____no_output_____"
]
],
[
[
"1 + #= This comment is inside code! =# 1",
"_____no_output_____"
]
],
[
[
"### Chaining",
"_____no_output_____"
]
],
[
[
"x = y = z = 1 # right-to-left",
"_____no_output_____"
],
[
"0 < x < 3 #works",
"_____no_output_____"
],
[
"z = 10\nb = 2\nx < y < z < b # works too!",
"_____no_output_____"
]
],
[
[
"### Function definition\n",
"_____no_output_____"
]
],
[
[
"function add_one(i)\n return i + 1 # Just a bit different to python.\nend\n\nadd_one(2)",
"_____no_output_____"
]
],
[
[
"### Insert LaTeX symbols\nNow this is a cool feature...in a code cell type for example \\alpha + Tab ",
"_____no_output_____"
]
],
[
[
"β = 1",
"_____no_output_____"
]
],
[
[
"## Operators",
"_____no_output_____"
],
[
"### Basic Arithmetic \nworks as expected",
"_____no_output_____"
]
],
[
[
"println(1 + 1)\nprintln(1 - 3)\nprintln(3 * 3)\nprintln(4 / 2)",
"2\n-2\n9\n2.0\n"
]
],
[
[
"### Exponentiation",
"_____no_output_____"
]
],
[
[
"2^2 # note the difference to python 2 ** 2",
"_____no_output_____"
]
],
[
[
"### Remainder",
"_____no_output_____"
]
],
[
[
"4 % 3",
"_____no_output_____"
]
],
[
[
"### Negation",
"_____no_output_____"
]
],
[
[
"!true # note the difference to numpys ~",
"_____no_output_____"
]
],
[
[
"### Equality",
"_____no_output_____"
]
],
[
[
"true == true",
"_____no_output_____"
]
],
[
[
"### Inequality",
"_____no_output_____"
]
],
[
[
"true != true",
"_____no_output_____"
]
],
[
[
"### Elementwise operation",
"_____no_output_____"
]
],
[
[
"[1 2; 3 3] .* [9 9;9 9] # elementwise",
"_____no_output_____"
],
[
"[1 2; 3 3] * [9 9;9 9] #matrix materix product",
"_____no_output_____"
]
],
[
[
"### Check for nan",
"_____no_output_____"
]
],
[
[
"isnan(9)",
"_____no_output_____"
]
],
[
[
"## Ternary operator",
"_____no_output_____"
],
[
"The syntax is\n\ncond ? do_true : else",
"_____no_output_____"
]
],
[
[
"1 != 1 ? println(3) : println(999)",
"999\n"
]
],
[
[
"### And/or",
"_____no_output_____"
]
],
[
[
"true && true",
"_____no_output_____"
],
[
"false || true",
"_____no_output_____"
],
[
"",
"_____no_output_____"
]
],
[
[
"## Sources",
"_____no_output_____"
],
[
"- [ ] https://juliadocs.github.io/Julia-Cheat-Sheet/\n- [ ] https://github.com/JuliaLang/julia\n\n- [ ] https://arxiv.org/pdf/2003.10146.pdf\n\n- [ ] https://github.com/h-Klok/StatsWithJuliaBook\n\n- [ ] juliahub\n\n- [ ] juliaacademy\n\n- [ ] https://www.sas.upenn.edu/~jesusfv/Chapter_HPC_8_Julia.pdf\n\n- [ ] https://www.packtpub.com/product/hands-on-design-patterns-and-best-practices-with-julia/9781838648817\n\n- [ ] https://www.elsevier.com/books/introduction-to-quantitative-macroeconomics-using-julia/caraiani/978-0-12-812219-8\n\n- [ ] https://colab.research.google.com/github/ageron/julia_notebooks/blob/master/Julia_for_Pythonistas.ipynb#scrollTo=EEzvvzCl1i0F\n\n- [ ] https://cheatsheets.quantecon.org/",
"_____no_output_____"
]
],
[
[
"",
"_____no_output_____"
]
]
] | [
"markdown",
"code",
"markdown",
"code",
"markdown",
"code",
"markdown",
"code",
"markdown",
"code",
"markdown",
"code",
"markdown",
"code",
"markdown",
"code",
"markdown",
"code",
"markdown",
"code",
"markdown",
"code",
"markdown",
"code",
"markdown",
"code",
"markdown",
"code",
"markdown",
"code",
"markdown",
"code",
"markdown",
"code",
"markdown",
"code",
"markdown",
"code"
] | [
[
"markdown"
],
[
"code",
"code",
"code",
"code",
"code"
],
[
"markdown",
"markdown"
],
[
"code"
],
[
"markdown"
],
[
"code"
],
[
"markdown"
],
[
"code"
],
[
"markdown"
],
[
"code"
],
[
"markdown"
],
[
"code",
"code",
"code"
],
[
"markdown"
],
[
"code"
],
[
"markdown"
],
[
"code"
],
[
"markdown",
"markdown"
],
[
"code"
],
[
"markdown"
],
[
"code"
],
[
"markdown"
],
[
"code"
],
[
"markdown"
],
[
"code"
],
[
"markdown"
],
[
"code"
],
[
"markdown"
],
[
"code"
],
[
"markdown"
],
[
"code",
"code"
],
[
"markdown"
],
[
"code"
],
[
"markdown",
"markdown"
],
[
"code"
],
[
"markdown"
],
[
"code",
"code",
"code"
],
[
"markdown",
"markdown"
],
[
"code"
]
] |
e7727cefd3f176d2a3e3192d02dc307ec0830f3e | 50,255 | ipynb | Jupyter Notebook | herramientas/procesamiento_informes_EPI/COVID_Descarga_y_Preprocesamiento_Informes_EPI_MINSAL.ipynb | DiazSalinas/COVID-19 | c79bc5487363a76baa2b9eb282991077eaeddf14 | [
"MIT"
] | 24 | 2020-04-02T04:35:32.000Z | 2020-08-11T00:48:06.000Z | herramientas/procesamiento_informes_EPI/COVID_Descarga_y_Preprocesamiento_Informes_EPI_MINSAL.ipynb | DiazSalinas/COVID-19 | c79bc5487363a76baa2b9eb282991077eaeddf14 | [
"MIT"
] | 26 | 2020-04-03T15:07:15.000Z | 2020-09-01T08:12:08.000Z | herramientas/procesamiento_informes_EPI/COVID_Descarga_y_Preprocesamiento_Informes_EPI_MINSAL.ipynb | DiazSalinas/COVID-19 | c79bc5487363a76baa2b9eb282991077eaeddf14 | [
"MIT"
] | 21 | 2020-04-02T21:29:08.000Z | 2020-09-01T19:25:22.000Z | 30.110845 | 1,392 | 0.436733 | [
[
[
"#Documento creado por https://github.com/Stepp1\n#Modificado por esteban\n\nimport subprocess\nimport shlex\nimport requests",
"_____no_output_____"
],
[
"#!pip install tabula-py \n#Sólo una vez",
"Collecting tabula-py\n Downloading tabula_py-2.1.0-py3-none-any.whl (10.4 MB)\n\u001b[K |████████████████████████████████| 10.4 MB 787 kB/s eta 0:00:01 |████████████████▊ | 5.4 MB 734 kB/s eta 0:00:07 |█████████████████ | 5.6 MB 734 kB/s eta 0:00:07\n\u001b[?25hCollecting distro\n Downloading distro-1.5.0-py2.py3-none-any.whl (18 kB)\nRequirement already satisfied: numpy in /opt/anaconda3/lib/python3.7/site-packages (from tabula-py) (1.18.1)\nRequirement already satisfied: pandas>=0.25.3 in /opt/anaconda3/lib/python3.7/site-packages (from tabula-py) (1.0.1)\nRequirement already satisfied: pytz>=2017.2 in /opt/anaconda3/lib/python3.7/site-packages (from pandas>=0.25.3->tabula-py) (2019.3)\nRequirement already satisfied: python-dateutil>=2.6.1 in /opt/anaconda3/lib/python3.7/site-packages (from pandas>=0.25.3->tabula-py) (2.8.1)\nRequirement already satisfied: six>=1.5 in /opt/anaconda3/lib/python3.7/site-packages (from python-dateutil>=2.6.1->pandas>=0.25.3->tabula-py) (1.14.0)\nInstalling collected packages: distro, tabula-py\nSuccessfully installed distro-1.5.0 tabula-py-2.1.0\n"
]
],
[
[
"### Eliminamos descargas anteriores",
"_____no_output_____"
]
],
[
[
"!rm *pdf",
"rm: *pdf: No such file or directory\r\n"
]
],
[
[
"### Obtenemos las urls de los informes (EPI y Generales)",
"_____no_output_____"
]
],
[
[
"response = subprocess.check_output(shlex.split('curl --request GET https://www.gob.cl/coronavirus/cifrasoficiales/'))\n\n\nurl_reporte = []\nurl_informe_epi = []\nfor line in response.decode().splitlines():\n if \"Reporte_Covid19.pdf\" in line:\n url = line.strip().split('https://')[1].split(\"\\\"\")[0]\n \n url_reporte.append(url)\n \n #El informe a veces está en minúsculas \n elif \"INFORME_EPI\" in line:\n \n test = line.strip()\n test = test.split('https://')[1].split(\"\\\"\")[0]\n url_informe_epi.append(test)\n \n ",
"_____no_output_____"
],
[
"url_informe_epi",
"_____no_output_____"
]
],
[
[
"#### Double Check",
"_____no_output_____"
]
],
[
[
"url_reporte",
"_____no_output_____"
],
[
"url_informe_epi",
"_____no_output_____"
]
],
[
[
"# Descarga Informes",
"_____no_output_____"
]
],
[
[
"#for url in set(url_reporte):\n# subprocess.check_output(shlex.split(\"wget \"+ url))",
"_____no_output_____"
],
[
"for url in set(url_informe_epi):\n subprocess.check_output(shlex.split(\"wget \"+ url))",
"_____no_output_____"
],
[
"!ls",
"_____no_output_____"
]
],
[
[
"# Preprocesamiento\n\nUsamos tabula-py: wrapper de Tabula App (escrita en Java). A library for extracting tables from PDF files \nhttps://github.com/chezou/tabula-py",
"_____no_output_____"
]
],
[
[
"import tabula\n\ndfs_files = {}\nfor url in url_informe_epi:\n pdf_file = url.split('/')[-1]\n df = tabula.read_pdf(pdf_file, pages='all', multiple_tables=True)\n \n fecha = pdf_file.split('_')[-1].split('.')[0]\n print(fecha)\n dfs_files['tablas_' + fecha] = df\n \n ",
"_____no_output_____"
]
],
[
[
"Verificamos algunas tablas",
"_____no_output_____"
]
],
[
[
"tablas_20200401 = dfs_files['tablas_20200401v2']\ntablas_20200330 = dfs_files['tablas_20200330']",
"_____no_output_____"
],
[
"df_comunas_20200401 = {}\nunnamed_primeraCol = {}\nfor idx, df in enumerate(tablas_20200401):\n\n if 'Comuna' in df.columns:\n \n key= 'tabla_' + str(idx + 1)\n print(key)\n df_comunas_20200401[key] = df",
"tabla_6\ntabla_7\ntabla_8\ntabla_9\ntabla_10\ntabla_11\ntabla_14\ntabla_15\ntabla_16\ntabla_17\ntabla_18\ntabla_19\ntabla_20\ntabla_21\ntabla_22\n"
],
[
"df_comunas_20200401['tabla_6'].head()",
"_____no_output_____"
]
],
[
[
"Tabla empieza con un *Unnamed: 0*",
"_____no_output_____"
]
],
[
[
"df_comunas_20200401['tabla_22'].head()",
"_____no_output_____"
]
],
[
[
"Tabla **no** empieza con un *Unnamed: 0*",
"_____no_output_____"
]
],
[
[
"df_comunas_20200330 = {}\nunnamed_primeraCol = {}\nfor idx, df in enumerate(tablas_20200330):\n\n if 'Comuna' in df.columns:\n \n key= 'tabla_' + str(idx + 1)\n print(key)\n df_comunas_20200330[key] = df",
"tabla_7\ntabla_8\ntabla_9\ntabla_10\ntabla_11\ntabla_12\ntabla_13\ntabla_15\ntabla_16\ntabla_17\ntabla_18\ntabla_19\ntabla_20\ntabla_21\ntabla_22\ntabla_23\n"
],
[
"df_comunas_20200330['tabla_7'].head()",
"_____no_output_____"
]
],
[
[
"Misma tabla empieza con un *Unnamed: 0*",
"_____no_output_____"
]
],
[
[
"df_comunas_20200330['tabla_23'].head()",
"_____no_output_____"
]
],
[
[
"Misma tabla **no** empieza con un *Unnamed: 0*",
"_____no_output_____"
],
[
"# Separamos estas dos categorias:",
"_____no_output_____"
]
],
[
[
"df_comunas_20200401 = {}\nunnamed_primeraCol_20200401 = {}\n\nfor idx, df in enumerate(tablas_20200401):\n if 'Comuna' in df.columns:\n \n key = 'tabla_' + str(idx + 1)\n df_comunas_20200401[key] = df\n \n if 'Unnamed' in df.columns[0]: \n print(key)\n unnamed_primeraCol_20200401[key] = df",
"tabla_6\ntabla_17\ntabla_18\ntabla_21\n"
],
[
"df_comunas_20200330 = {}\nunnamed_primeraCol_20200330 = {}\n\nfor idx, df in enumerate(tablas_20200330):\n if 'Comuna' in df.columns:\n \n key= 'tabla_' + str(idx + 1)\n df_comunas_20200330[key] = df\n \n if 'Unnamed' in df.columns[0]:\n print(key) \n unnamed_primeraCol_20200330[key] = df",
"tabla_7\ntabla_13\ntabla_18\ntabla_19\ntabla_22\n"
]
],
[
[
"# Resumen",
"_____no_output_____"
],
[
"* El informe 20200330 tiene una tabla más al parecer (en realidad esto no es así y parecer que un cambio en el gráfico dejo la kgá).\n\n* La extracción de tablas parece tener los mismos errores en las mismas tablas.",
"_____no_output_____"
]
],
[
[
"%%capture\n\"\"\"\n\nfor tup_1, tup_2 in zip(df_comunas.items(), df_comunas_2.items()):\n key_1, df_1 = tup_1\n key_2, df_2 = tup_2\n \n if (key_1 or key_2) in unnamed_primeraCol:\n if (df_1.columns == df_2.columns).all: \n print(\"LAS COLUMNAS DE LAS TABLAS *diferentes* coinciden!\", key_1, key_2)\n \n\"\"\"",
"_____no_output_____"
]
],
[
[
"## Estandarizamos las tablas",
"_____no_output_____"
]
],
[
[
"for key in df_comunas_20200401.keys():\n df = df_comunas_20200401[key]\n \n if key in unnamed_primeraCol_20200401.keys():\n df['Comuna'] = df['Unnamed: 0']\n df['N°'] = df['Unnamed: 1']\n df['Tasa'] = df['Unnamed: 2']\n \n df_comunas_20200401[key] = df.drop(labels='Unnamed: 0', \n axis=1).drop(labels='Unnamed: 1',\n axis=1).drop(labels='Unnamed: 2', axis=1)\n \n else:\n if key == 'tabla_22': continue \n \n df_comunas_20200401[key] = df.drop(labels='Unnamed: 0', \n axis=1).drop(labels='Unnamed: 1', axis=1)",
"_____no_output_____"
],
[
"for key in df_comunas_20200330.keys():\n df = df_comunas_20200330[key]\n \n if key in unnamed_primeraCol_20200330.keys():\n df['Comuna'] = df['Unnamed: 0']\n df['N°'] = df['Unnamed: 1']\n df['Tasa'] = df['Unnamed: 2']\n \n df_comunas_20200330[key] = df.drop(labels='Unnamed: 0', \n axis=1).drop(labels='Unnamed: 1',\n axis=1).drop(labels='Unnamed: 2', axis=1)\n else:\n if key == 'tabla_22': continue \n \n df_comunas_20200330[key] = df.drop(labels='Unnamed: 0', \n axis=1).drop(labels='Unnamed: 1',axis=1)",
"_____no_output_____"
],
[
"for key, region in df_comunas_20200401.items():\n print(key, region.columns)",
"tabla_6 Index(['Comuna', 'N°', 'Población', 'Tasa'], dtype='object')\ntabla_7 Index(['Comuna', 'N°', 'Población', 'Tasa'], dtype='object')\ntabla_8 Index(['Comuna', 'N°', 'Población', 'Tasa'], dtype='object')\ntabla_9 Index(['Comuna', 'N°', 'Población', 'Tasa'], dtype='object')\ntabla_10 Index(['Comuna', 'N°', 'Población', 'Tasa'], dtype='object')\ntabla_11 Index(['Comuna', 'N°', 'Población', 'Tasa'], dtype='object')\ntabla_14 Index(['Comuna', 'N°', 'Población', 'Tasa'], dtype='object')\ntabla_15 Index(['Comuna', 'N°', 'Población', 'Tasa'], dtype='object')\ntabla_16 Index(['Comuna', 'N°', 'Población', 'Tasa'], dtype='object')\ntabla_17 Index(['Comuna', 'N°', 'Población', 'Tasa'], dtype='object')\ntabla_18 Index(['Comuna', 'N°', 'Población', 'Tasa'], dtype='object')\ntabla_19 Index(['Comuna', 'N°', 'Población', 'Tasa'], dtype='object')\ntabla_20 Index(['Comuna', 'N°', 'Población', 'Tasa'], dtype='object')\ntabla_21 Index(['Comuna', 'N°', 'Población', 'Tasa'], dtype='object')\ntabla_22 Index(['Comuna', 'N°', 'Unnamed: 0', 'Población', 'Unnamed: 1', 'Tasa',\n 'Unnamed: 2'],\n dtype='object')\n"
],
[
"for key, region in df_comunas_20200330.items():\n print(key, region.columns)",
"tabla_7 Index(['Comuna', 'N°', 'Población', 'Tasa'], dtype='object')\ntabla_8 Index(['Comuna', 'N°', 'Población', 'Tasa'], dtype='object')\ntabla_9 Index(['Comuna', 'N°', 'Población', 'Tasa'], dtype='object')\ntabla_10 Index(['Comuna', 'N°', 'Población', 'Tasa'], dtype='object')\ntabla_11 Index(['Comuna', 'N°', 'Población', 'Tasa'], dtype='object')\ntabla_12 Index(['Comuna', 'N°', 'Población', 'Tasa'], dtype='object')\ntabla_13 Index(['Comuna', 'N°', 'Población', 'Tasa'], dtype='object')\ntabla_15 Index(['Comuna', 'N°', 'Población', 'Tasa'], dtype='object')\ntabla_16 Index(['Comuna', 'N°', 'Población', 'Tasa'], dtype='object')\ntabla_17 Index(['Comuna', 'N°', 'Población', 'Tasa'], dtype='object')\ntabla_18 Index(['Comuna', 'N°', 'Población', 'Tasa'], dtype='object')\ntabla_19 Index(['Comuna', 'N°', 'Población', 'Tasa'], dtype='object')\ntabla_20 Index(['Comuna', 'N°', 'Población', 'Tasa'], dtype='object')\ntabla_21 Index(['Comuna', 'N°', 'Población', 'Tasa'], dtype='object')\ntabla_22 Index(['Comuna', 'N°', 'Población', 'Tasa'], dtype='object')\ntabla_23 Index(['Comuna', 'N°', 'Población', 'Tasa', 'Unnamed: 2'], dtype='object')\n"
]
],
[
[
"## Ultima tabla tiene *Unnamed: 2*",
"_____no_output_____"
]
],
[
[
"df_comunas_20200401['tabla_21']",
"_____no_output_____"
],
[
"df_comunas_20200330['tabla_23']",
"_____no_output_____"
]
]
] | [
"code",
"markdown",
"code",
"markdown",
"code",
"markdown",
"code",
"markdown",
"code",
"markdown",
"code",
"markdown",
"code",
"markdown",
"code",
"markdown",
"code",
"markdown",
"code",
"markdown",
"code",
"markdown",
"code",
"markdown",
"code",
"markdown",
"code"
] | [
[
"code",
"code"
],
[
"markdown"
],
[
"code"
],
[
"markdown"
],
[
"code",
"code"
],
[
"markdown"
],
[
"code",
"code"
],
[
"markdown"
],
[
"code",
"code",
"code"
],
[
"markdown"
],
[
"code"
],
[
"markdown"
],
[
"code",
"code",
"code"
],
[
"markdown"
],
[
"code"
],
[
"markdown"
],
[
"code",
"code"
],
[
"markdown"
],
[
"code"
],
[
"markdown",
"markdown"
],
[
"code",
"code"
],
[
"markdown",
"markdown"
],
[
"code"
],
[
"markdown"
],
[
"code",
"code",
"code",
"code"
],
[
"markdown"
],
[
"code",
"code"
]
] |
e7728c485f5a550262f01a71478f5eb9bb913f79 | 39,559 | ipynb | Jupyter Notebook | Neural_network_e_deep_learning/Deep learning II.ipynb | RafaelTarachuck/Pratica_curso_DS | 654bb999fd688a34189d9d6db95d56fd807527be | [
"MIT"
] | null | null | null | Neural_network_e_deep_learning/Deep learning II.ipynb | RafaelTarachuck/Pratica_curso_DS | 654bb999fd688a34189d9d6db95d56fd807527be | [
"MIT"
] | null | null | null | Neural_network_e_deep_learning/Deep learning II.ipynb | RafaelTarachuck/Pratica_curso_DS | 654bb999fd688a34189d9d6db95d56fd807527be | [
"MIT"
] | null | null | null | 38.669599 | 142 | 0.346015 | [
[
[
"import pandas as pd\nfrom keras.models import Sequential\nfrom keras.layers import Dense\nfrom sklearn.model_selection import train_test_split\nfrom sklearn.metrics import confusion_matrix\nfrom sklearn.preprocessing import LabelEncoder, OneHotEncoder\nfrom sklearn.preprocessing import StandardScaler\nfrom sklearn.compose import make_column_transformer",
"_____no_output_____"
],
[
"dataset = pd.read_csv('Credit2.csv', sep= ';')\ndataset\n",
"_____no_output_____"
],
[
"#separação de dados em suas variáveis\nx = dataset.iloc[:,1:10].values # coluna ID ignorada por não ter valores úteis\ny = dataset.iloc[:,10].values\n\nx\n",
"_____no_output_____"
],
[
"#transformar a coluna \"Checking_status\" em números de 0 a 3\nlabelencoder = LabelEncoder() # utilizado a função LabelEncoder por se tratar de classificação sequencial \nx[:,0] = labelencoder.fit_transform(x[:,0])\nx",
"_____no_output_____"
],
[
"# traformação dos dados classificatórios \"credit_history\" em números\n#utilizada a função OneHotEncoder por se tratar de de classificação categórica, então cada característica será colocada em uma coluna\nonehotencoder = make_column_transformer((OneHotEncoder(categories= 'auto', sparse= False), [1]), remainder= 'passthrough')\nx = onehotencoder.fit_transform(x)\nx",
"_____no_output_____"
],
[
"# exclusão de uma das colunas criadas pelo processo ONeHotEncoder para evitar erros gerados pela correlação delas\nx = x[:,1:]\nx",
"_____no_output_____"
],
[
"#tranformação dos das da classe em valoes numéricos\nlabelencoder_y = LabelEncoder()\ny = labelencoder_y.fit_transform(y)\ny ",
"_____no_output_____"
],
[
"x_train, x_test, y_train, y_teste = train_test_split(x, y, test_size= 0.2, random_state= 0)\nprint(len(x_train), len(x_test), len(y_train), len(y_teste)) # visualização do tamanho das amostras de treino e teste",
"800 200 800 200\n"
],
[
"# normalização do intervalo de variáveis transformando todos os dados em uma mesma escala\nsc = StandardScaler()\nx_train = sc.fit_transform(x_train)\nx_test = sc.fit_transform(x_test)\nx_test",
"_____no_output_____"
],
[
"classifier = Sequential()\nclassifier.add(Dense(units= 6, kernel_initializer= 'uniform', activation= 'relu', input_dim= 12))\nclassifier.add(Dense(units= 6, kernel_initializer= 'uniform', activation= 'relu'))\nclassifier.add(Dense(units= 1, kernel_initializer= 'uniform', activation= 'sigmoid'))\nclassifier.compile(optimizer= 'adam', loss= 'binary_crossentropy', metrics= ['accuracy'])\nclassifier.fit(x_train, y_train, batch_size= 10, epochs= 100)",
"Epoch 1/100\n80/80 [==============================] - 4s 6ms/step - loss: 0.6814 - accuracy: 0.6975\nEpoch 2/100\n80/80 [==============================] - 0s 5ms/step - loss: 0.6166 - accuracy: 0.6975\nEpoch 3/100\n80/80 [==============================] - 0s 5ms/step - loss: 0.5528 - accuracy: 0.6975\nEpoch 4/100\n80/80 [==============================] - 0s 5ms/step - loss: 0.5398 - accuracy: 0.6975\nEpoch 5/100\n80/80 [==============================] - 0s 6ms/step - loss: 0.5337 - accuracy: 0.6975\nEpoch 6/100\n80/80 [==============================] - 0s 5ms/step - loss: 0.5300 - accuracy: 0.6975\nEpoch 7/100\n80/80 [==============================] - 0s 4ms/step - loss: 0.5269 - accuracy: 0.6975\nEpoch 8/100\n80/80 [==============================] - 0s 4ms/step - loss: 0.5246 - accuracy: 0.6975\nEpoch 9/100\n80/80 [==============================] - 0s 4ms/step - loss: 0.5233 - accuracy: 0.6975\nEpoch 10/100\n80/80 [==============================] - 0s 4ms/step - loss: 0.5214 - accuracy: 0.6975\nEpoch 11/100\n80/80 [==============================] - 0s 4ms/step - loss: 0.5200 - accuracy: 0.6975\nEpoch 12/100\n80/80 [==============================] - 0s 4ms/step - loss: 0.5190 - accuracy: 0.6975\nEpoch 13/100\n80/80 [==============================] - 0s 4ms/step - loss: 0.5175 - accuracy: 0.7375\nEpoch 14/100\n80/80 [==============================] - 0s 3ms/step - loss: 0.5169 - accuracy: 0.7412\nEpoch 15/100\n80/80 [==============================] - 0s 1ms/step - loss: 0.5153 - accuracy: 0.7487\nEpoch 16/100\n80/80 [==============================] - 0s 2ms/step - loss: 0.5145 - accuracy: 0.7450\nEpoch 17/100\n80/80 [==============================] - 0s 2ms/step - loss: 0.5140 - accuracy: 0.7513\nEpoch 18/100\n80/80 [==============================] - 0s 1ms/step - loss: 0.5135 - accuracy: 0.7500\nEpoch 19/100\n80/80 [==============================] - 0s 2ms/step - loss: 0.5136 - accuracy: 0.7513\nEpoch 20/100\n80/80 [==============================] - 0s 2ms/step - loss: 0.5118 - accuracy: 0.7487\nEpoch 21/100\n80/80 [==============================] - 0s 1ms/step - loss: 0.5109 - accuracy: 0.7550\nEpoch 22/100\n80/80 [==============================] - 0s 1ms/step - loss: 0.5103 - accuracy: 0.7563\nEpoch 23/100\n80/80 [==============================] - 0s 1ms/step - loss: 0.5102 - accuracy: 0.7513\nEpoch 24/100\n80/80 [==============================] - 0s 1ms/step - loss: 0.5084 - accuracy: 0.7525\nEpoch 25/100\n80/80 [==============================] - 0s 1ms/step - loss: 0.5081 - accuracy: 0.7513\nEpoch 26/100\n80/80 [==============================] - 0s 1ms/step - loss: 0.5075 - accuracy: 0.7525\nEpoch 27/100\n80/80 [==============================] - 0s 1ms/step - loss: 0.5070 - accuracy: 0.7513\nEpoch 28/100\n80/80 [==============================] - 0s 1ms/step - loss: 0.5056 - accuracy: 0.7500\nEpoch 29/100\n80/80 [==============================] - 0s 2ms/step - loss: 0.5050 - accuracy: 0.7563\nEpoch 30/100\n80/80 [==============================] - 0s 2ms/step - loss: 0.5039 - accuracy: 0.7513\nEpoch 31/100\n80/80 [==============================] - 0s 1ms/step - loss: 0.5030 - accuracy: 0.7538\nEpoch 32/100\n80/80 [==============================] - 0s 1ms/step - loss: 0.5026 - accuracy: 0.7538\nEpoch 33/100\n80/80 [==============================] - 0s 1ms/step - loss: 0.5018 - accuracy: 0.7575\nEpoch 34/100\n80/80 [==============================] - 0s 1ms/step - loss: 0.5004 - accuracy: 0.7588\nEpoch 35/100\n80/80 [==============================] - 0s 1ms/step - loss: 0.5001 - accuracy: 0.7575\nEpoch 36/100\n80/80 [==============================] - 0s 1ms/step - loss: 0.4985 - accuracy: 0.7588\nEpoch 37/100\n80/80 [==============================] - 0s 1ms/step - loss: 0.4977 - accuracy: 0.7575\nEpoch 38/100\n80/80 [==============================] - 0s 1ms/step - loss: 0.4977 - accuracy: 0.7538\nEpoch 39/100\n80/80 [==============================] - 0s 1ms/step - loss: 0.4970 - accuracy: 0.7613\nEpoch 40/100\n80/80 [==============================] - 0s 1ms/step - loss: 0.4965 - accuracy: 0.7613\nEpoch 41/100\n80/80 [==============================] - 0s 1ms/step - loss: 0.4957 - accuracy: 0.7638\nEpoch 42/100\n80/80 [==============================] - 0s 1ms/step - loss: 0.4949 - accuracy: 0.7700\nEpoch 43/100\n80/80 [==============================] - 0s 1ms/step - loss: 0.4941 - accuracy: 0.7638\nEpoch 44/100\n80/80 [==============================] - 0s 1ms/step - loss: 0.4932 - accuracy: 0.7663\nEpoch 45/100\n80/80 [==============================] - 0s 1ms/step - loss: 0.4928 - accuracy: 0.7675\nEpoch 46/100\n80/80 [==============================] - 0s 1ms/step - loss: 0.4926 - accuracy: 0.7650\nEpoch 47/100\n80/80 [==============================] - 0s 1ms/step - loss: 0.4917 - accuracy: 0.7663\nEpoch 48/100\n80/80 [==============================] - 0s 1ms/step - loss: 0.4907 - accuracy: 0.7713\nEpoch 49/100\n80/80 [==============================] - 0s 1ms/step - loss: 0.4905 - accuracy: 0.7713\nEpoch 50/100\n80/80 [==============================] - 0s 2ms/step - loss: 0.4894 - accuracy: 0.7725\nEpoch 51/100\n80/80 [==============================] - 0s 1ms/step - loss: 0.4887 - accuracy: 0.7763\nEpoch 52/100\n80/80 [==============================] - 0s 1ms/step - loss: 0.4888 - accuracy: 0.7725\nEpoch 53/100\n80/80 [==============================] - 0s 1ms/step - loss: 0.4877 - accuracy: 0.7725\nEpoch 54/100\n80/80 [==============================] - 0s 1ms/step - loss: 0.4885 - accuracy: 0.7713\nEpoch 55/100\n80/80 [==============================] - 0s 1ms/step - loss: 0.4869 - accuracy: 0.7725\nEpoch 56/100\n80/80 [==============================] - 0s 2ms/step - loss: 0.4872 - accuracy: 0.7750\nEpoch 57/100\n80/80 [==============================] - 0s 2ms/step - loss: 0.4863 - accuracy: 0.7750\nEpoch 58/100\n80/80 [==============================] - 0s 2ms/step - loss: 0.4860 - accuracy: 0.7738\nEpoch 59/100\n80/80 [==============================] - 0s 2ms/step - loss: 0.4850 - accuracy: 0.7775\nEpoch 60/100\n80/80 [==============================] - 0s 2ms/step - loss: 0.4856 - accuracy: 0.7763\nEpoch 61/100\n80/80 [==============================] - 0s 2ms/step - loss: 0.4842 - accuracy: 0.7800\nEpoch 62/100\n80/80 [==============================] - 0s 2ms/step - loss: 0.4839 - accuracy: 0.7775\nEpoch 63/100\n80/80 [==============================] - 0s 2ms/step - loss: 0.4837 - accuracy: 0.7750\nEpoch 64/100\n80/80 [==============================] - 0s 3ms/step - loss: 0.4830 - accuracy: 0.7750\nEpoch 65/100\n80/80 [==============================] - 0s 2ms/step - loss: 0.4822 - accuracy: 0.7713\nEpoch 66/100\n80/80 [==============================] - 0s 3ms/step - loss: 0.4817 - accuracy: 0.7763\nEpoch 67/100\n80/80 [==============================] - 0s 3ms/step - loss: 0.4813 - accuracy: 0.7738\nEpoch 68/100\n80/80 [==============================] - 0s 4ms/step - loss: 0.4814 - accuracy: 0.7763\nEpoch 69/100\n80/80 [==============================] - 0s 4ms/step - loss: 0.4810 - accuracy: 0.7788\nEpoch 70/100\n80/80 [==============================] - 0s 2ms/step - loss: 0.4803 - accuracy: 0.7763\nEpoch 71/100\n80/80 [==============================] - 0s 2ms/step - loss: 0.4794 - accuracy: 0.7775\nEpoch 72/100\n80/80 [==============================] - 0s 2ms/step - loss: 0.4792 - accuracy: 0.7763\nEpoch 73/100\n80/80 [==============================] - 0s 2ms/step - loss: 0.4793 - accuracy: 0.7750\nEpoch 74/100\n80/80 [==============================] - 0s 2ms/step - loss: 0.4788 - accuracy: 0.7763\nEpoch 75/100\n80/80 [==============================] - 0s 2ms/step - loss: 0.4781 - accuracy: 0.7800\nEpoch 76/100\n80/80 [==============================] - 0s 2ms/step - loss: 0.4778 - accuracy: 0.7763\nEpoch 77/100\n80/80 [==============================] - 0s 2ms/step - loss: 0.4773 - accuracy: 0.7750\nEpoch 78/100\n80/80 [==============================] - 0s 1ms/step - loss: 0.4771 - accuracy: 0.7763\nEpoch 79/100\n80/80 [==============================] - 0s 1ms/step - loss: 0.4767 - accuracy: 0.7763\nEpoch 80/100\n80/80 [==============================] - 0s 1ms/step - loss: 0.4759 - accuracy: 0.7812\nEpoch 81/100\n80/80 [==============================] - 0s 1ms/step - loss: 0.4765 - accuracy: 0.7713\nEpoch 82/100\n80/80 [==============================] - 0s 1ms/step - loss: 0.4759 - accuracy: 0.7725\nEpoch 83/100\n80/80 [==============================] - 0s 1ms/step - loss: 0.4754 - accuracy: 0.7763\nEpoch 84/100\n80/80 [==============================] - 0s 1ms/step - loss: 0.4752 - accuracy: 0.7800\nEpoch 85/100\n80/80 [==============================] - 0s 1ms/step - loss: 0.4746 - accuracy: 0.7800\nEpoch 86/100\n80/80 [==============================] - 0s 1ms/step - loss: 0.4757 - accuracy: 0.7812\nEpoch 87/100\n80/80 [==============================] - 0s 1ms/step - loss: 0.4737 - accuracy: 0.7763\nEpoch 88/100\n80/80 [==============================] - 0s 1ms/step - loss: 0.4736 - accuracy: 0.7812\nEpoch 89/100\n80/80 [==============================] - 0s 1ms/step - loss: 0.4730 - accuracy: 0.7812\nEpoch 90/100\n80/80 [==============================] - 0s 1ms/step - loss: 0.4728 - accuracy: 0.7825\nEpoch 91/100\n80/80 [==============================] - 0s 1ms/step - loss: 0.4723 - accuracy: 0.7837\nEpoch 92/100\n80/80 [==============================] - 0s 1ms/step - loss: 0.4723 - accuracy: 0.7800\nEpoch 93/100\n80/80 [==============================] - 0s 1ms/step - loss: 0.4718 - accuracy: 0.7837\nEpoch 94/100\n80/80 [==============================] - 0s 1ms/step - loss: 0.4716 - accuracy: 0.7788\nEpoch 95/100\n80/80 [==============================] - 0s 1ms/step - loss: 0.4715 - accuracy: 0.7763\nEpoch 96/100\n80/80 [==============================] - 0s 1ms/step - loss: 0.4720 - accuracy: 0.7800\nEpoch 97/100\n80/80 [==============================] - 0s 1ms/step - loss: 0.4704 - accuracy: 0.7800\nEpoch 98/100\n80/80 [==============================] - 0s 1ms/step - loss: 0.4707 - accuracy: 0.7837\nEpoch 99/100\n80/80 [==============================] - 0s 1ms/step - loss: 0.4702 - accuracy: 0.7788\nEpoch 100/100\n80/80 [==============================] - 0s 2ms/step - loss: 0.4711 - accuracy: 0.7775\n"
],
[
"#previsão com os dados de teste\ny_pred = classifier.predict(x_test)\n#resultado é entregue em probabilidades, então será convertido para true ou false\ny_pred = (y_pred > 0.5)\ny_pred",
"_____no_output_____"
],
[
"confusao = confusion_matrix(y_teste, y_pred)\nconfusao",
"_____no_output_____"
]
],
[
[
"O modelo acertou 145 (24+121) previsões de 200",
"_____no_output_____"
]
]
] | [
"code",
"markdown"
] | [
[
"code",
"code",
"code",
"code",
"code",
"code",
"code",
"code",
"code",
"code",
"code",
"code"
],
[
"markdown"
]
] |
e772ab116f587753f7e2dd3876ee02252d9500e1 | 26,444 | ipynb | Jupyter Notebook | spotify/Spotify Create Good and Bad Playlists.ipynb | chemicoPy/spotify-ml | 596c572b20b4e585142dc9606109d59f185db94e | [
"Apache-2.0"
] | null | null | null | spotify/Spotify Create Good and Bad Playlists.ipynb | chemicoPy/spotify-ml | 596c572b20b4e585142dc9606109d59f185db94e | [
"Apache-2.0"
] | null | null | null | spotify/Spotify Create Good and Bad Playlists.ipynb | chemicoPy/spotify-ml | 596c572b20b4e585142dc9606109d59f185db94e | [
"Apache-2.0"
] | null | null | null | 86.986842 | 1,960 | 0.650507 | [
[
[
"import pandas as pd \nimport spotipy\nimport spotipy.util as util\nsp = spotipy.Spotify() \nfrom spotipy.oauth2 import SpotifyClientCredentials \nfrom random import randint\n\ncid =\"CLIENT-ID\" \nsecret = \"SECRET\" \nusername = \"USERNAME\"\nclient_credentials_manager = SpotifyClientCredentials(client_id=cid, client_secret=secret) \nsp = spotipy.Spotify(client_credentials_manager=client_credentials_manager) \nsp.trace=False ",
"_____no_output_____"
],
[
"username = \"USERNAME\"\nscope = 'playlist-modify-private playlist-read-private user-library-read'\ntoken = util.prompt_for_user_token(username, scope)\n#Good Playlist\nif token:\n sp = spotipy.Spotify(auth=token)\n# results = sp.current_user_saved_tracks()\n# while results['next']:\n# ids = []\n# results = sp.next(results)\n# for item in results['items']:\n# track = item['track']\n# ids.append(track['id'])\n# sp.user_playlist_add_tracks(\"1287242681\", \"5OdH7PmotfAO7qDGxKdw3J\", ids)\n# else:\n# print(\"Can't get token for\", username)\n ",
"_____no_output_____"
],
[
"#Good Playlist\nplaylist = sp.user_playlist(\"suicidesheepify\", \"5EFG2g2Csw020N69ax0ac7\")\ntracks = playlist[\"tracks\"]\nsongs = tracks[\"items\"] \nwhile tracks['next']:\n tracks = sp.next(tracks)\n for item in tracks[\"items\"]:\n songs.append(item)\nids = [] \nprint(len(songs))\nprint(songs[0]['track']['id'])\ni = 0\nfor i in range(len(songs)):\n sp.user_playlist_add_tracks(\"1287242681\", \"5OdH7PmotfAO7qDGxKdw3J\", [songs[i][\"track\"][\"id\"]])",
"1260\n1hLKGOpquBi12snIayBz3e\n"
],
[
"#Bad Playlist\n#sp.user_playlist_add_tracks(\"1287242681\", \"1UlFeD3AElsMTjOKmbinJx\", ids)\ndata = pd.read_csv('data/playlistDump.csv')\ndata.head()",
"_____no_output_____"
],
[
"bad_playlists = []\nfor playlist_id in data[data['playlist_title'].str.contains(\"Country\")]['playlistId']:\n bad_playlists.append(playlist_id)\nfor playlist_id in data[data['playlist_title'].str.contains(\"R&B\")]['playlistId']:\n bad_playlists.append(playlist_id)\nfor playlist_id in data[data['playlist_title'].str.contains(\"Jazz\")]['playlistId']:\n bad_playlists.append(playlist_id)\n \nprint(bad_playlists)\n\nfor playlist_id in bad_playlists:\n playlist = sp.user_playlist(\"thesoundsofspotify\", playlist_id)\n tracks = playlist[\"tracks\"]\n songs = tracks[\"items\"] \n while tracks['next']:\n tracks = sp.next(tracks)\n for item in tracks[\"items\"]:\n songs.append(item)\n ids = [] \n i = 0\n if(len(songs)> 50):\n for i in range(len(songs)):\n sp.user_playlist_add_tracks(\"1287242681\", \"3ySDAXYGUwRrp8C4ejIm9m\", [songs[i]['track']['id']])\n else:\n for i in range(len(songs)):\n ids.append(songs[i][\"track\"][\"id\"])\n sp.user_playlist_add_tracks(\"1287242681\", \"3ySDAXYGUwRrp8C4ejIm9m\", ids)",
"['5BmMjQp8OwPGdg7OOINCHm', '1hseduxVpn5p3ukaREgsSi', '72nKBOJxfLUr3iuUUMn2nV', '0VZfpqcbBUWC6kpP1vVrvA', '4mijVkpSXJziPiOrK7YX4M', '5LpV4RS7WgWaDyKxu0W60r', '1PzGsPdOacIEdFsZG92bOI', '2qk8D3ZyEBXDwR02alzjXq', '7DZDNqoxWp3uIgwTMHdBN6', '4fj8PNbbwGXBWHKodGQhfD', '2yykv7TMUr4jPHPAE8Q3vW', '5TqoG1t47RTBqODKy6xjdK', '208eKWeHXifDir7qV5DQYB', '6ZoVYWfvloSUP0sYwAk0Eg', '01b3JSy4UfA2iiKodhAXse', '42xcPVnKaE1gu7PScFIG9H', '5D1IoXQGtdt84Mx8QvvSSY', '4HbGCzaH6bTsF1FGAESEzG', '7yV5vLKdCyBDi4MW0It39c', '34X13zwfKF95I5PKO6GEKk', '0Hwb2a9DJdom4yoe5V41K9', '0RgxqbxFEgx3wnqabByQRg', '2BvxkVFgxGelSCrWV53I2o', '2xblssDewgd1AA5R7Lap6w', '7N35r1mrsCLe66LczBV7EQ', '1rLnwJimWCmjp3f0mEbnkY', '7K7eQm5eNmjvR1avm2Uikd', '7vyHBfRfPOT8Sxy87P5osJ', '72YNCrIywbtPaFgFSQNI6X', '68qffdP3LzsrDkrmrt0xKi', '0M2STcvX62sBK5YuLTE076', '3RtFvzIXD7ulUCXkWdIOWW', '2XFrsvPGbamN9w2C57a9hg', '6lMZE2UCxN6J7un54fIwAy', '4kG2qiyLY2O24LPEYMv6vf', '47quI8Re3ItWH8Rvn9HSvk', '5aYcWZehrK6hKtR3EiWdqb', '4tH5CLOMXHah6ngcr3Z2Gu', '5KudWqpwRKdH5CY6yQ1nwA', '62h3iZoEvjJrmy42ucMytJ', '4pkdnCP0Wo9Yg9Mn0XGmC8', '2Dsz5JxEeRz0nAC0plSEMF', '293CDKWmDYutJjtVGJaq3L', '6WxllqF4D8Gj8Veg1WBY0r', '3nxdNdIA45HbkTwzlqZjQ0', '6ye4xUHWNCBV1GNFQ4yNaj', '6LgnHN3mGCfSTnYy7Cd81C', '40C4RcHHLDxFUuBeMryCPw', '0Uq6sBasJecSFwXoQxRtbt', '5EyFMotmvSfDAZ4hSdKrbx', '6cgvY9wIuV29oh36jxytWC', '4ehHChcQBZTZ0kP9CkHdzc', '7m2b2nBy51rTT8kHKJ7HyK', '7HV8yRXe49LmIRGoQdt1j4', '3djem4UfHRQUBeOMxtpY3p', '3GomZqnwKeecLnI3sOkoEq', '0gRjtLM7ocp0jndjkncxOf', '5eKFlGWniCD4d70sY7tXGa', '6Pixe6bdAwQIkLv4q85U7E', '3bhnRBlvTikmxpW50jBaIZ', '4g8jY2NYAlj7sBdFHtrgdF', '7kQQGS5m9U07fe7CPWiql7', '0iMiZcvIy26MqHQln5kkrI', '0jB6UfkDXKDPt4Q5wYluty', '26PD3pjcSfPuKUDV1jgfX8', '7wx58jJTsUZs2EIzNfGHth', '4GMYsnPRt6GT3VARNJQeKt', '51YToRmnFC3U4iPj6LgHgF', '0XRhBXYCpp2CBuISbi8dgr', '066Ws83mBgawkpYWWvjmp6', '2lsqobg83L55zSnJ8z7MuV', '2XWuLY8J7ViTQ7Gl3ZV9KV', '4UUXB0Mz6E3VbmRdbi2o5P', '2ZazIXecBCVmTlbyKJHxOc', '0WRIEExyzsjtbPZsUOAURR']\n"
]
]
] | [
"code"
] | [
[
"code",
"code",
"code",
"code",
"code"
]
] |
e772b4afd3561b193adc88af83d82b5c4c6a3cf1 | 108,092 | ipynb | Jupyter Notebook | conditional/main_conditional_disentangle_cifar_bs900_sratio_0_5_drop_0_5_sn100_run1.ipynb | minhtannguyen/ffjord | f3418249eaa4647f4339aea8d814cf2ce33be141 | [
"MIT"
] | null | null | null | conditional/main_conditional_disentangle_cifar_bs900_sratio_0_5_drop_0_5_sn100_run1.ipynb | minhtannguyen/ffjord | f3418249eaa4647f4339aea8d814cf2ce33be141 | [
"MIT"
] | null | null | null | conditional/main_conditional_disentangle_cifar_bs900_sratio_0_5_drop_0_5_sn100_run1.ipynb | minhtannguyen/ffjord | f3418249eaa4647f4339aea8d814cf2ce33be141 | [
"MIT"
] | null | null | null | 71.631544 | 1,046 | 0.514774 | [
[
[
"import os\nos.environ['CUDA_VISIBLE_DEVICES']='0,1,2,3'",
"_____no_output_____"
],
[
"%run -p ../train_cnf_disentangle_cifar.py --data cifar10 --dims 64,64,64 --strides 1,1,1,1 --num_blocks 2 --layer_type concat --multiscale True --rademacher True --batch_size 900 --test_batch_size 500 --save ../experiments_published/cnf_conditional_disentangle_cifar10_bs900_sratio_0_5_drop_0_5_sn100_run1 --seed 1 --lr 0.001 --conditional True --controlled_tol False --train_mode semisup --log_freq 10 --weight_y 0.5 --condition_ratio 0.5 --dropout_rate 0.5 --spectral_norm True --spectral_norm_niter 100\n#",
"/tancode/repos/tan-ffjord/train_cnf_disentangle_cifar.py\nimport argparse\nimport os\nimport time\nimport numpy as np\n\nimport torch\nimport torch.optim as optim\nimport torchvision.datasets as dset\nimport torchvision.transforms as tforms\nfrom torchvision.utils import save_image\n\nimport lib.layers as layers\nimport lib.utils as utils\nimport lib.multiscale_parallel as multiscale_parallel\nimport lib.modules as modules\nimport lib.thops as thops\n\nfrom train_misc import standard_normal_logprob\nfrom train_misc import set_cnf_options, count_nfe, count_parameters, count_total_time\nfrom train_misc import add_spectral_norm, spectral_norm_power_iteration\nfrom train_misc import create_regularization_fns, get_regularization, append_regularization_to_log\n\nfrom tensorboardX import SummaryWriter\n\n# go fast boi!!\ntorch.backends.cudnn.benchmark = True\nSOLVERS = [\"dopri5\", \"bdf\", \"rk4\", \"midpoint\", 'adams', 'explicit_adams']\nparser = argparse.ArgumentParser(\"Continuous Normalizing Flow\")\nparser.add_argument(\"--data\", choices=[\"mnist\", \"svhn\", \"cifar10\", 'lsun_church'], type=str, default=\"mnist\")\nparser.add_argument(\"--dims\", type=str, default=\"8,32,32,8\")\nparser.add_argument(\"--strides\", type=str, default=\"2,2,1,-2,-2\")\nparser.add_argument(\"--num_blocks\", type=int, default=1, help='Number of stacked CNFs.')\n\nparser.add_argument(\"--conv\", type=eval, default=True, choices=[True, False])\nparser.add_argument(\n \"--layer_type\", type=str, default=\"ignore\",\n choices=[\"ignore\", \"concat\", \"concat_v2\", \"squash\", \"concatsquash\", \"concatcoord\", \"hyper\", \"blend\"]\n)\nparser.add_argument(\"--divergence_fn\", type=str, default=\"approximate\", choices=[\"brute_force\", \"approximate\"])\nparser.add_argument(\n \"--nonlinearity\", type=str, default=\"softplus\", choices=[\"tanh\", \"relu\", \"softplus\", \"elu\", \"swish\"]\n)\n\nparser.add_argument(\"--seed\", type=int, default=0)\n\nparser.add_argument('--solver', type=str, default='dopri5', choices=SOLVERS)\nparser.add_argument('--atol', type=float, default=1e-5)\nparser.add_argument('--rtol', type=float, default=1e-5)\nparser.add_argument(\"--step_size\", type=float, default=None, help=\"Optional fixed step size.\")\n\nparser.add_argument('--test_solver', type=str, default=None, choices=SOLVERS + [None])\nparser.add_argument('--test_atol', type=float, default=None)\nparser.add_argument('--test_rtol', type=float, default=None)\n\nparser.add_argument(\"--imagesize\", type=int, default=None)\nparser.add_argument(\"--alpha\", type=float, default=1e-6)\nparser.add_argument('--time_length', type=float, default=1.0)\nparser.add_argument('--train_T', type=eval, default=True)\n\nparser.add_argument(\"--num_epochs\", type=int, default=1000)\nparser.add_argument(\"--batch_size\", type=int, default=200)\nparser.add_argument(\n \"--batch_size_schedule\", type=str, default=\"\", help=\"Increases the batchsize at every given epoch, dash separated.\"\n)\nparser.add_argument(\"--test_batch_size\", type=int, default=200)\nparser.add_argument(\"--lr\", type=float, default=1e-3)\nparser.add_argument(\"--warmup_iters\", type=float, default=1000)\nparser.add_argument(\"--weight_decay\", type=float, default=0.0)\nparser.add_argument(\"--spectral_norm_niter\", type=int, default=10)\nparser.add_argument(\"--weight_y\", type=float, default=0.5)\n\nparser.add_argument(\"--add_noise\", type=eval, default=True, choices=[True, False])\nparser.add_argument(\"--batch_norm\", type=eval, default=False, choices=[True, False])\nparser.add_argument('--residual', type=eval, default=False, choices=[True, False])\nparser.add_argument('--autoencode', type=eval, default=False, choices=[True, False])\nparser.add_argument('--rademacher', type=eval, default=True, choices=[True, False])\nparser.add_argument('--spectral_norm', type=eval, default=False, choices=[True, False])\nparser.add_argument('--multiscale', type=eval, default=False, choices=[True, False])\nparser.add_argument('--parallel', type=eval, default=False, choices=[True, False])\nparser.add_argument('--conditional', type=eval, default=False, choices=[True, False])\nparser.add_argument('--controlled_tol', type=eval, default=False, choices=[True, False])\nparser.add_argument(\"--train_mode\", choices=[\"semisup\", \"sup\", \"unsup\"], type=str, default=\"semisup\")\nparser.add_argument(\"--condition_ratio\", type=float, default=0.5)\nparser.add_argument(\"--dropout_rate\", type=float, default=0.0)\n\n\n# Regularizations\nparser.add_argument('--l1int', type=float, default=None, help=\"int_t ||f||_1\")\nparser.add_argument('--l2int', type=float, default=None, help=\"int_t ||f||_2\")\nparser.add_argument('--dl2int', type=float, default=None, help=\"int_t ||f^T df/dt||_2\")\nparser.add_argument('--JFrobint', type=float, default=None, help=\"int_t ||df/dx||_F\")\nparser.add_argument('--JdiagFrobint', type=float, default=None, help=\"int_t ||df_i/dx_i||_F\")\nparser.add_argument('--JoffdiagFrobint', type=float, default=None, help=\"int_t ||df/dx - df_i/dx_i||_F\")\n\nparser.add_argument(\"--time_penalty\", type=float, default=0, help=\"Regularization on the end_time.\")\nparser.add_argument(\n \"--max_grad_norm\", type=float, default=1e10,\n help=\"Max norm of graidents (default is just stupidly high to avoid any clipping)\"\n)\n\nparser.add_argument(\"--begin_epoch\", type=int, default=1)\nparser.add_argument(\"--resume\", type=str, default=None)\nparser.add_argument(\"--save\", type=str, default=\"experiments/cnf\")\nparser.add_argument(\"--val_freq\", type=int, default=1)\nparser.add_argument(\"--log_freq\", type=int, default=1)\n\nargs = parser.parse_args()\n\nif args.controlled_tol:\n import lib.odenvp_conditional_tol as odenvp\nelse:\n import lib.odenvp_conditional as odenvp\n \n# set seed\ntorch.manual_seed(args.seed)\nnp.random.seed(args.seed)\n\n# logger\nutils.makedirs(args.save)\nlogger = utils.get_logger(logpath=os.path.join(args.save, 'logs'), filepath=os.path.abspath(__file__)) # write to log file\nwriter = SummaryWriter(os.path.join(args.save, 'tensorboard')) # write to tensorboard\n\nif args.layer_type == \"blend\":\n logger.info(\"!! Setting time_length from None to 1.0 due to use of Blend layers.\")\n args.time_length = 1.0\n\nlogger.info(args)\n\n\ndef add_noise(x):\n \"\"\"\n [0, 1] -> [0, 255] -> add noise -> [0, 1]\n \"\"\"\n if args.add_noise:\n noise = x.new().resize_as_(x).uniform_()\n x = x * 255 + noise\n x = x / 256\n return x\n\n\ndef update_lr(optimizer, itr):\n iter_frac = min(float(itr + 1) / max(args.warmup_iters, 1), 1.0)\n lr = args.lr * iter_frac\n for param_group in optimizer.param_groups:\n param_group[\"lr\"] = lr\n\n\ndef get_train_loader(train_set, epoch):\n if args.batch_size_schedule != \"\":\n epochs = [0] + list(map(int, args.batch_size_schedule.split(\"-\")))\n n_passed = sum(np.array(epochs) <= epoch)\n current_batch_size = int(args.batch_size * n_passed)\n else:\n current_batch_size = args.batch_size\n train_loader = torch.utils.data.DataLoader(\n dataset=train_set, batch_size=current_batch_size, shuffle=True, drop_last=True, pin_memory=True\n )\n logger.info(\"===> Using batch size {}. Total {} iterations/epoch.\".format(current_batch_size, len(train_loader)))\n return train_loader\n\n\ndef get_dataset(args):\n trans = lambda im_size: tforms.Compose([tforms.Resize(im_size), tforms.ToTensor(), add_noise])\n\n if args.data == \"mnist\":\n im_dim = 1\n im_size = 28 if args.imagesize is None else args.imagesize\n train_set = dset.MNIST(root=\"../data\", train=True, transform=trans(im_size), download=True)\n test_set = dset.MNIST(root=\"../data\", train=False, transform=trans(im_size), download=True)\n elif args.data == \"svhn\":\n im_dim = 3\n im_size = 32 if args.imagesize is None else args.imagesize\n train_set = dset.SVHN(root=\"../data\", split=\"train\", transform=trans(im_size), download=True)\n test_set = dset.SVHN(root=\"../data\", split=\"test\", transform=trans(im_size), download=True)\n elif args.data == \"cifar10\":\n im_dim = 3\n im_size = 32 if args.imagesize is None else args.imagesize\n train_set = dset.CIFAR10(\n root=\"../data\", train=True, transform=tforms.Compose([\n tforms.Resize(im_size),\n tforms.RandomHorizontalFlip(),\n tforms.ToTensor(),\n add_noise,\n ]), download=True\n )\n test_set = dset.CIFAR10(root=\"../data\", train=False, transform=trans(im_size), download=True)\n elif args.data == 'celeba':\n im_dim = 3\n im_size = 64 if args.imagesize is None else args.imagesize\n train_set = dset.CelebA(\n train=True, transform=tforms.Compose([\n tforms.ToPILImage(),\n tforms.Resize(im_size),\n tforms.RandomHorizontalFlip(),\n tforms.ToTensor(),\n add_noise,\n ])\n )\n test_set = dset.CelebA(\n train=False, transform=tforms.Compose([\n tforms.ToPILImage(),\n tforms.Resize(im_size),\n tforms.ToTensor(),\n add_noise,\n ])\n )\n elif args.data == 'lsun_church':\n im_dim = 3\n im_size = 64 if args.imagesize is None else args.imagesize\n train_set = dset.LSUN(\n '../data', ['church_outdoor_train'], transform=tforms.Compose([\n tforms.Resize(96),\n tforms.RandomCrop(64),\n tforms.Resize(im_size),\n tforms.ToTensor(),\n add_noise,\n ])\n )\n test_set = dset.LSUN(\n '../data', ['church_outdoor_val'], transform=tforms.Compose([\n tforms.Resize(96),\n tforms.RandomCrop(64),\n tforms.Resize(im_size),\n tforms.ToTensor(),\n add_noise,\n ])\n ) \n elif args.data == 'imagenet_64':\n im_dim = 3\n im_size = 64 if args.imagesize is None else args.imagesize\n train_set = dset.ImageFolder(\n train=True, transform=tforms.Compose([\n tforms.ToPILImage(),\n tforms.Resize(im_size),\n tforms.RandomHorizontalFlip(),\n tforms.ToTensor(),\n add_noise,\n ])\n )\n test_set = dset.ImageFolder(\n train=False, transform=tforms.Compose([\n tforms.ToPILImage(),\n tforms.Resize(im_size),\n tforms.ToTensor(),\n add_noise,\n ])\n )\n \n data_shape = (im_dim, im_size, im_size)\n if not args.conv:\n data_shape = (im_dim * im_size * im_size,)\n\n test_loader = torch.utils.data.DataLoader(\n dataset=test_set, batch_size=args.test_batch_size, shuffle=False, drop_last=True\n )\n return train_set, test_loader, data_shape\n\n\ndef compute_bits_per_dim(x, model):\n zero = torch.zeros(x.shape[0], 1).to(x)\n\n # Don't use data parallelize if batch size is small.\n # if x.shape[0] < 200:\n # model = model.module\n \n z, delta_logp = model(x, zero) # run model forward\n\n logpz = standard_normal_logprob(z).view(z.shape[0], -1).sum(1, keepdim=True) # logp(z)\n logpx = logpz - delta_logp\n\n logpx_per_dim = torch.sum(logpx) / x.nelement() # averaged over batches\n bits_per_dim = -(logpx_per_dim - np.log(256)) / np.log(2)\n\n return bits_per_dim\n\ndef compute_bits_per_dim_conditional(x, y, model):\n zero = torch.zeros(x.shape[0], 1).to(x)\n y_onehot = thops.onehot(y, num_classes=model.module.y_class).to(x)\n\n # Don't use data parallelize if batch size is small.\n # if x.shape[0] < 200:\n # model = model.module\n \n z, delta_logp = model(x, zero) # run model forward\n \n dim_sup = int(args.condition_ratio * np.prod(z.size()[1:]))\n \n # prior\n mean, logs = model.module._prior(y_onehot)\n\n logpz_sup = modules.GaussianDiag.logp(mean, logs, z[:, 0:dim_sup]).view(-1,1) # logp(z)_sup\n logpz_unsup = standard_normal_logprob(z[:, dim_sup:]).view(z.shape[0], -1).sum(1, keepdim=True)\n logpz = logpz_sup + logpz_unsup\n logpx = logpz - delta_logp\n\n logpx_per_dim = torch.sum(logpx) / x.nelement() # averaged over batches\n bits_per_dim = -(logpx_per_dim - np.log(256)) / np.log(2)\n \n # dropout\n if args.dropout_rate > 0:\n zsup = model.module.dropout(z[:, 0:dim_sup])\n else:\n zsup = z[:, 0:dim_sup]\n \n # compute xentropy loss\n y_logits = model.module.project_class(zsup)\n loss_xent = model.module.loss_class(y_logits, y.to(x.get_device()))\n y_predicted = np.argmax(y_logits.cpu().detach().numpy(), axis=1)\n\n return bits_per_dim, loss_xent, y_predicted\n\ndef create_model(args, data_shape, regularization_fns):\n hidden_dims = tuple(map(int, args.dims.split(\",\")))\n strides = tuple(map(int, args.strides.split(\",\")))\n\n if args.multiscale:\n model = odenvp.ODENVP(\n (args.batch_size, *data_shape),\n n_blocks=args.num_blocks,\n intermediate_dims=hidden_dims,\n nonlinearity=args.nonlinearity,\n alpha=args.alpha,\n cnf_kwargs={\"T\": args.time_length, \"train_T\": args.train_T, \"regularization_fns\": regularization_fns, \"solver\": args.solver, \"atol\": args.atol, \"rtol\": args.rtol},\n condition_ratio=args.condition_ratio,\n dropout_rate=args.dropout_rate,)\n elif args.parallel:\n model = multiscale_parallel.MultiscaleParallelCNF(\n (args.batch_size, *data_shape),\n n_blocks=args.num_blocks,\n intermediate_dims=hidden_dims,\n alpha=args.alpha,\n time_length=args.time_length,\n )\n else:\n if args.autoencode:\n\n def build_cnf():\n autoencoder_diffeq = layers.AutoencoderDiffEqNet(\n hidden_dims=hidden_dims,\n input_shape=data_shape,\n strides=strides,\n conv=args.conv,\n layer_type=args.layer_type,\n nonlinearity=args.nonlinearity,\n )\n odefunc = layers.AutoencoderODEfunc(\n autoencoder_diffeq=autoencoder_diffeq,\n divergence_fn=args.divergence_fn,\n residual=args.residual,\n rademacher=args.rademacher,\n )\n cnf = layers.CNF(\n odefunc=odefunc,\n T=args.time_length,\n regularization_fns=regularization_fns,\n solver=args.solver,\n )\n return cnf\n else:\n\n def build_cnf():\n diffeq = layers.ODEnet(\n hidden_dims=hidden_dims,\n input_shape=data_shape,\n strides=strides,\n conv=args.conv,\n layer_type=args.layer_type,\n nonlinearity=args.nonlinearity,\n )\n odefunc = layers.ODEfunc(\n diffeq=diffeq,\n divergence_fn=args.divergence_fn,\n residual=args.residual,\n rademacher=args.rademacher,\n )\n cnf = layers.CNF(\n odefunc=odefunc,\n T=args.time_length,\n train_T=args.train_T,\n regularization_fns=regularization_fns,\n solver=args.solver,\n )\n return cnf\n\n chain = [layers.LogitTransform(alpha=args.alpha)] if args.alpha > 0 else [layers.ZeroMeanTransform()]\n chain = chain + [build_cnf() for _ in range(args.num_blocks)]\n if args.batch_norm:\n chain.append(layers.MovingBatchNorm2d(data_shape[0]))\n model = layers.SequentialFlow(chain)\n return model\n\n\nif __name__ == \"__main__\":\n\n # get deivce\n device = torch.device(\"cuda:0\" if torch.cuda.is_available() else \"cpu\")\n cvt = lambda x: x.type(torch.float32).to(device, non_blocking=True)\n\n # load dataset\n train_set, test_loader, data_shape = get_dataset(args)\n\n # build model\n regularization_fns, regularization_coeffs = create_regularization_fns(args)\n model = create_model(args, data_shape, regularization_fns)\n\n if args.spectral_norm: add_spectral_norm(model, logger)\n set_cnf_options(args, model)\n\n logger.info(model)\n logger.info(\"Number of trainable parameters: {}\".format(count_parameters(model)))\n \n writer.add_text('info', \"Number of trainable parameters: {}\".format(count_parameters(model)))\n\n # optimizer\n optimizer = optim.Adam(model.parameters(), lr=args.lr, weight_decay=args.weight_decay)\n \n # set initial iter\n itr = 1\n \n # set the meters\n time_epoch_meter = utils.RunningAverageMeter(0.97)\n time_meter = utils.RunningAverageMeter(0.97)\n loss_meter = utils.RunningAverageMeter(0.97) # track total loss\n nll_meter = utils.RunningAverageMeter(0.97) # track negative log-likelihood\n xent_meter = utils.RunningAverageMeter(0.97) # track xentropy score\n error_meter = utils.RunningAverageMeter(0.97) # track error score\n steps_meter = utils.RunningAverageMeter(0.97)\n grad_meter = utils.RunningAverageMeter(0.97)\n tt_meter = utils.RunningAverageMeter(0.97)\n\n # restore parameters\n if args.resume is not None:\n checkpt = torch.load(args.resume, map_location=lambda storage, loc: storage)\n model.load_state_dict(checkpt[\"state_dict\"])\n if \"optim_state_dict\" in checkpt.keys():\n optimizer.load_state_dict(checkpt[\"optim_state_dict\"])\n # Manually move optimizer state to device.\n for state in optimizer.state.values():\n for k, v in state.items():\n if torch.is_tensor(v):\n state[k] = cvt(v)\n args.begin_epoch = checkpt['epoch'] + 1\n itr = checkpt['iter'] + 1\n time_epoch_meter.set(checkpt['epoch_time_avg'])\n time_meter.set(checkpt['time_train'])\n loss_meter.set(checkpt['loss_train'])\n nll_meter.set(checkpt['bits_per_dim_train'])\n xent_meter.set(checkpt['xent_train'])\n error_meter.set(checkpt['error_train'])\n steps_meter.set(checkpt['nfe_train'])\n grad_meter.set(checkpt['grad_train'])\n tt_meter.set(checkpt['total_time_train'])\n\n if torch.cuda.is_available():\n model = torch.nn.DataParallel(model).cuda()\n\n # For visualization.\n if args.conditional:\n dim_unsup = int((1.0 - args.condition_ratio) * np.prod(data_shape))\n fixed_y = torch.from_numpy(np.arange(model.module.y_class)).repeat(model.module.y_class).type(torch.long).to(device, non_blocking=True)\n fixed_y_onehot = thops.onehot(fixed_y, num_classes=model.module.y_class)\n with torch.no_grad():\n mean, logs = model.module._prior(fixed_y_onehot)\n fixed_z_sup = modules.GaussianDiag.sample(mean, logs)\n fixed_z_unsup = cvt(torch.randn(model.module.y_class**2, dim_unsup))\n fixed_z = torch.cat((fixed_z_sup, fixed_z_unsup),1)\n else:\n fixed_z = cvt(torch.randn(100, *data_shape))\n \n\n if args.spectral_norm and not args.resume: spectral_norm_power_iteration(model, 500)\n\n best_loss_nll = float(\"inf\")\n best_error_score = float(\"inf\")\n \n for epoch in range(args.begin_epoch, args.num_epochs + 1):\n start_epoch = time.time()\n model.train()\n train_loader = get_train_loader(train_set, epoch)\n for _, (x, y) in enumerate(train_loader):\n start = time.time()\n update_lr(optimizer, itr)\n optimizer.zero_grad()\n\n if not args.conv:\n x = x.view(x.shape[0], -1)\n\n # cast data and move to device\n x = cvt(x)\n \n # compute loss\n if args.conditional:\n loss_nll, loss_xent, y_predicted = compute_bits_per_dim_conditional(x, y, model)\n if args.train_mode == \"semisup\":\n loss = loss_nll + args.weight_y * loss_xent\n elif args.train_mode == \"sup\":\n loss = loss_xent\n elif args.train_mode == \"unsup\":\n loss = loss_nll\n else:\n raise ValueError('Choose supported train_mode: semisup, sup, unsup')\n error_score = 1. - np.mean(y_predicted.astype(int) == y.numpy()) \n \n else:\n loss = compute_bits_per_dim(x, model)\n loss_nll, loss_xent, error_score = loss, 0., 0.\n \n if regularization_coeffs:\n reg_states = get_regularization(model, regularization_coeffs)\n reg_loss = sum(\n reg_state * coeff for reg_state, coeff in zip(reg_states, regularization_coeffs) if coeff != 0\n )\n loss = loss + reg_loss\n total_time = count_total_time(model)\n loss = loss + total_time * args.time_penalty\n\n loss.backward()\n grad_norm = torch.nn.utils.clip_grad_norm_(model.parameters(), args.max_grad_norm)\n\n optimizer.step()\n\n if args.spectral_norm: spectral_norm_power_iteration(model, args.spectral_norm_niter)\n \n time_meter.update(time.time() - start)\n loss_meter.update(loss.item())\n nll_meter.update(loss_nll.item())\n if args.conditional:\n xent_meter.update(loss_xent.item())\n else:\n xent_meter.update(loss_xent)\n error_meter.update(error_score)\n steps_meter.update(count_nfe(model))\n grad_meter.update(grad_norm)\n tt_meter.update(total_time)\n \n # write to tensorboard\n writer.add_scalars('time', {'train_iter': time_meter.val}, itr)\n writer.add_scalars('loss', {'train_iter': loss_meter.val}, itr)\n writer.add_scalars('bits_per_dim', {'train_iter': nll_meter.val}, itr)\n writer.add_scalars('xent', {'train_iter': xent_meter.val}, itr)\n writer.add_scalars('error', {'train_iter': error_meter.val}, itr)\n writer.add_scalars('nfe', {'train_iter': steps_meter.val}, itr)\n writer.add_scalars('grad', {'train_iter': grad_meter.val}, itr)\n writer.add_scalars('total_time', {'train_iter': tt_meter.val}, itr)\n\n if itr % args.log_freq == 0:\n log_message = (\n \"Iter {:04d} | Time {:.4f}({:.4f}) | Bit/dim {:.4f}({:.4f}) | Xent {:.4f}({:.4f}) | Loss {:.4f}({:.4f}) | Error {:.4f}({:.4f}) \"\n \"Steps {:.0f}({:.2f}) | Grad Norm {:.4f}({:.4f}) | Total Time {:.2f}({:.2f})\".format(\n itr, time_meter.val, time_meter.avg, nll_meter.val, nll_meter.avg, xent_meter.val, xent_meter.avg, loss_meter.val, loss_meter.avg, error_meter.val, error_meter.avg, steps_meter.val, steps_meter.avg, grad_meter.val, grad_meter.avg, tt_meter.val, tt_meter.avg\n )\n )\n if regularization_coeffs:\n log_message = append_regularization_to_log(log_message, regularization_fns, reg_states)\n logger.info(log_message)\n writer.add_text('info', log_message, itr)\n\n itr += 1\n \n # compute test loss\n model.eval()\n if epoch % args.val_freq == 0:\n with torch.no_grad():\n # write to tensorboard\n writer.add_scalars('time', {'train_epoch': time_meter.avg}, epoch)\n writer.add_scalars('loss', {'train_epoch': loss_meter.avg}, epoch)\n writer.add_scalars('bits_per_dim', {'train_epoch': nll_meter.avg}, epoch)\n writer.add_scalars('xent', {'train_epoch': xent_meter.avg}, epoch)\n writer.add_scalars('error', {'train_epoch': error_meter.avg}, epoch)\n writer.add_scalars('nfe', {'train_epoch': steps_meter.avg}, epoch)\n writer.add_scalars('grad', {'train_epoch': grad_meter.avg}, epoch)\n writer.add_scalars('total_time', {'train_epoch': tt_meter.avg}, epoch)\n \n start = time.time()\n logger.info(\"validating...\")\n writer.add_text('info', \"validating...\", epoch)\n losses_nll = []; losses_xent = []; losses = []\n total_correct = 0\n \n for (x, y) in test_loader:\n if not args.conv:\n x = x.view(x.shape[0], -1)\n x = cvt(x)\n if args.conditional:\n loss_nll, loss_xent, y_predicted = compute_bits_per_dim_conditional(x, y, model)\n if args.train_mode == \"semisup\":\n loss = loss_nll + args.weight_y * loss_xent\n elif args.train_mode == \"sup\":\n loss = loss_xent\n elif args.train_mode == \"unsup\":\n loss = loss_nll\n else:\n raise ValueError('Choose supported train_mode: semisup, sup, unsup')\n total_correct += np.sum(y_predicted.astype(int) == y.numpy())\n else:\n loss = compute_bits_per_dim(x, model)\n loss_nll, loss_xent = loss, 0.\n losses_nll.append(loss_nll.cpu().numpy()); losses.append(loss.cpu().numpy())\n if args.conditional: \n losses_xent.append(loss_xent.cpu().numpy())\n else:\n losses_xent.append(loss_xent)\n \n loss_nll = np.mean(losses_nll); loss_xent = np.mean(losses_xent); loss = np.mean(losses)\n error_score = 1. - total_correct / len(test_loader.dataset)\n time_epoch_meter.update(time.time() - start_epoch)\n \n # write to tensorboard\n test_time_spent = time.time() - start\n writer.add_scalars('time', {'validation': test_time_spent}, epoch)\n writer.add_scalars('epoch_time', {'validation': time_epoch_meter.val}, epoch)\n writer.add_scalars('bits_per_dim', {'validation': loss_nll}, epoch)\n writer.add_scalars('xent', {'validation': loss_xent}, epoch)\n writer.add_scalars('loss', {'validation': loss}, epoch)\n writer.add_scalars('error', {'validation': error_score}, epoch)\n \n log_message = \"Epoch {:04d} | Time {:.4f}, Epoch Time {:.4f}({:.4f}), Bit/dim {:.4f}(best: {:.4f}), Xent {:.4f}, Loss {:.4f}, Error {:.4f}(best: {:.4f})\".format(epoch, time.time() - start, time_epoch_meter.val, time_epoch_meter.avg, loss_nll, best_loss_nll, loss_xent, loss, error_score, best_error_score)\n logger.info(log_message)\n writer.add_text('info', log_message, epoch)\n \n for name, param in model.named_parameters():\n writer.add_histogram(name, param.clone().cpu().data.numpy(), epoch)\n \n \n utils.makedirs(args.save)\n torch.save({\n \"args\": args,\n \"state_dict\": model.module.state_dict() if torch.cuda.is_available() else model.state_dict(),\n \"optim_state_dict\": optimizer.state_dict(),\n \"epoch\": epoch,\n \"iter\": itr-1,\n \"error\": error_score,\n \"loss\": loss,\n \"xent\": loss_xent,\n \"bits_per_dim\": loss_nll,\n \"best_bits_per_dim\": best_loss_nll,\n \"best_error_score\": best_error_score,\n \"epoch_time\": time_epoch_meter.val,\n \"epoch_time_avg\": time_epoch_meter.avg,\n \"time\": test_time_spent,\n \"error_train\": error_meter.avg,\n \"loss_train\": loss_meter.avg,\n \"xent_train\": xent_meter.avg,\n \"bits_per_dim_train\": nll_meter.avg,\n \"total_time_train\": tt_meter.avg,\n \"time_train\": time_meter.avg,\n \"nfe_train\": steps_meter.avg,\n \"grad_train\": grad_meter.avg,\n }, os.path.join(args.save, \"epoch_%i_checkpt.pth\"%epoch))\n \n torch.save({\n \"args\": args,\n \"state_dict\": model.module.state_dict() if torch.cuda.is_available() else model.state_dict(),\n \"optim_state_dict\": optimizer.state_dict(),\n \"epoch\": epoch,\n \"iter\": itr-1,\n \"error\": error_score,\n \"loss\": loss,\n \"xent\": loss_xent,\n \"bits_per_dim\": loss_nll,\n \"best_bits_per_dim\": best_loss_nll,\n \"best_error_score\": best_error_score,\n \"epoch_time\": time_epoch_meter.val,\n \"epoch_time_avg\": time_epoch_meter.avg,\n \"time\": test_time_spent,\n \"error_train\": error_meter.avg,\n \"loss_train\": loss_meter.avg,\n \"xent_train\": xent_meter.avg,\n \"bits_per_dim_train\": nll_meter.avg,\n \"total_time_train\": tt_meter.avg,\n \"time_train\": time_meter.avg,\n \"nfe_train\": steps_meter.avg,\n \"grad_train\": grad_meter.avg,\n }, os.path.join(args.save, \"current_checkpt.pth\"))\n \n if loss_nll < best_loss_nll:\n best_loss_nll = loss_nll\n utils.makedirs(args.save)\n torch.save({\n \"args\": args,\n \"state_dict\": model.module.state_dict() if torch.cuda.is_available() else model.state_dict(),\n \"optim_state_dict\": optimizer.state_dict(),\n \"epoch\": epoch,\n \"iter\": itr-1,\n \"error\": error_score,\n \"loss\": loss,\n \"xent\": loss_xent,\n \"bits_per_dim\": loss_nll,\n \"best_bits_per_dim\": best_loss_nll,\n \"best_error_score\": best_error_score,\n \"epoch_time\": time_epoch_meter.val,\n \"epoch_time_avg\": time_epoch_meter.avg,\n \"time\": test_time_spent,\n \"error_train\": error_meter.avg,\n \"loss_train\": loss_meter.avg,\n \"xent_train\": xent_meter.avg,\n \"bits_per_dim_train\": nll_meter.avg,\n \"total_time_train\": tt_meter.avg,\n \"time_train\": time_meter.avg,\n \"nfe_train\": steps_meter.avg,\n \"grad_train\": grad_meter.avg,\n }, os.path.join(args.save, \"best_nll_checkpt.pth\"))\n \n if args.conditional:\n if error_score < best_error_score:\n best_error_score = error_score\n utils.makedirs(args.save)\n torch.save({\n \"args\": args,\n \"state_dict\": model.module.state_dict() if torch.cuda.is_available() else model.state_dict(),\n \"optim_state_dict\": optimizer.state_dict(),\n \"epoch\": epoch,\n \"iter\": itr-1,\n \"error\": error_score,\n \"loss\": loss,\n \"xent\": loss_xent,\n \"bits_per_dim\": loss_nll,\n \"best_bits_per_dim\": best_loss_nll,\n \"best_error_score\": best_error_score,\n \"epoch_time\": time_epoch_meter.val,\n \"epoch_time_avg\": time_epoch_meter.avg,\n \"time\": test_time_spent,\n \"error_train\": error_meter.avg,\n \"loss_train\": loss_meter.avg,\n \"xent_train\": xent_meter.avg,\n \"bits_per_dim_train\": nll_meter.avg,\n \"total_time_train\": tt_meter.avg,\n \"time_train\": time_meter.avg,\n \"nfe_train\": steps_meter.avg,\n \"grad_train\": grad_meter.avg,\n }, os.path.join(args.save, \"best_error_checkpt.pth\"))\n \n\n # visualize samples and density\n with torch.no_grad():\n fig_filename = os.path.join(args.save, \"figs\", \"{:04d}.jpg\".format(epoch))\n utils.makedirs(os.path.dirname(fig_filename))\n generated_samples = model(fixed_z, reverse=True).view(-1, *data_shape)\n save_image(generated_samples, fig_filename, nrow=10)\n if args.data == \"mnist\":\n writer.add_images('generated_images', generated_samples.repeat(1,3,1,1), epoch)\n else:\n writer.add_images('generated_images', generated_samples.repeat(1,1,1,1), epoch)\n\nNamespace(JFrobint=None, JdiagFrobint=None, JoffdiagFrobint=None, add_noise=True, alpha=1e-06, atol=1e-05, autoencode=False, batch_norm=False, batch_size=900, batch_size_schedule='', begin_epoch=1, condition_ratio=0.5, conditional=True, controlled_tol=False, conv=True, data='cifar10', dims='64,64,64', divergence_fn='approximate', dl2int=None, dropout_rate=0.5, imagesize=None, l1int=None, l2int=None, layer_type='concat', log_freq=10, lr=0.001, max_grad_norm=10000000000.0, multiscale=True, nonlinearity='softplus', num_blocks=2, num_epochs=1000, parallel=False, rademacher=True, residual=False, resume=None, rtol=1e-05, save='../experiments_published/cnf_conditional_disentangle_cifar10_bs900_sratio_0_5_drop_0_5_sn100_run1', seed=1, solver='dopri5', spectral_norm=True, spectral_norm_niter=100, step_size=None, strides='1,1,1,1', test_atol=None, test_batch_size=500, test_rtol=None, test_solver=None, time_length=1.0, time_penalty=0, train_T=True, train_mode='semisup', val_freq=1, warmup_iters=1000, weight_decay=0.0, weight_y=0.5)\n"
]
]
] | [
"code"
] | [
[
"code",
"code"
]
] |
e772bb5abe2de4addd65a08a01bdcf0edafae8ca | 10,297 | ipynb | Jupyter Notebook | OOP_58001_OOP_Concepts_2.ipynb | AndreiBenavidez/OOP-58001 | a37fb4361c777419e7bc063aee456f4ad5b2b3ea | [
"Apache-2.0"
] | null | null | null | OOP_58001_OOP_Concepts_2.ipynb | AndreiBenavidez/OOP-58001 | a37fb4361c777419e7bc063aee456f4ad5b2b3ea | [
"Apache-2.0"
] | null | null | null | OOP_58001_OOP_Concepts_2.ipynb | AndreiBenavidez/OOP-58001 | a37fb4361c777419e7bc063aee456f4ad5b2b3ea | [
"Apache-2.0"
] | null | null | null | 27.458667 | 246 | 0.452073 | [
[
[
"<a href=\"https://colab.research.google.com/github/AndreiBenavidez/OOP-58001/blob/main/OOP_58001_OOP_Concepts_2.ipynb\" target=\"_parent\"><img src=\"https://colab.research.google.com/assets/colab-badge.svg\" alt=\"Open In Colab\"/></a>",
"_____no_output_____"
],
[
"Class with Multiple Objects",
"_____no_output_____"
]
],
[
[
"class Birds:\n def __init__(self,bird_name):\n self.bird_name = bird_name\n def flying_birds(self):\n print(f\"{self.bird_name} flies above clouds\")\n def non_flying_birds(self):\n print(f\"{self.bird_name} is the national bird of the Philippines\")\n \nvulture = Birds(\"Griffon Vulture\")\ncrane = Birds(\"Common Crane\")\nemu = Birds (\"Emu\")\n\nvulture.flying_birds()\ncrane.flying_birds()\nemu.non_flying_birds()",
"Griffon Vulture flies above clouds\nCommon Crane flies above clouds\nEmu is the national bird of the Philippines\n"
]
],
[
[
"Encapsulation with Private Attributes",
"_____no_output_____"
]
],
[
[
"class foo:\n def __init__(self,a,b):\n self.a = a\n self.b = b\n def add(self):\n return self.a + self.b\nfoo_object = foo(3,4)\nfoo_object.add()\n\nfoo_object.a = 6\nfoo_object.add()",
"_____no_output_____"
],
[
"class foo:\n def __init__(self,a,b):\n self._a = a\n self._b = b\n def add(self):\n return self._a + self._b\nfoo_object = foo(3,4)\nfoo_object.add()\n\nfoo_object.a = 6\nfoo_object.add()",
"_____no_output_____"
]
],
[
[
"Encapsulation by mangling with double underscores",
"_____no_output_____"
]
],
[
[
"class Counter:\n def __init__(self):\n self.current = 0\n \n def increment(self):\n self.current += 1 #current = current+1\n\n def value(self):\n return self.current\n\n def reset(self):\n self.current = 0\n\ncounter = Counter()\n\ncounter.increment()\ncounter.increment()\ncounter.increment()\n\nprint(counter.value())",
"3\n"
]
],
[
[
"Inheritance",
"_____no_output_____"
]
],
[
[
"class Person:\n def __init__(self,fname,sname):\n self.fname = fname\n self.sname = sname\n def printname(self):\n print(self.fname,self.sname)\n\nx = Person(\"Andrei\",\"Benavidez\")\nx.printname()\n\nclass Teacher(Person):\n pass\n\nx = Teacher(\"Drei\", \"Benavidez\")\nx.printname()",
"Andrei Benavidez\nDrei Benavidez\n"
]
],
[
[
"Polymorphism",
"_____no_output_____"
]
],
[
[
"class RegularPolygon:\n def __init__(self,side):\n self._side = side\nclass Square(RegularPolygon):\n def area(self):\n return self._side * self._side\nclass EquilateralTriangle(RegularPolygon):\n def area(self):\n return self._side * self._side * 0.433\n\nobj1 = Square(4)\nobj2 = EquilateralTriangle(3)\n\nobj1.area()\nobj2.area()",
"_____no_output_____"
]
],
[
[
"\n\n* Create a Python program that displays the name of 3 students (Student 1, Student 2, Student 3) and their grades\n* Create a class name \"Person\" and attributes - std1, std2, std3, pre, mid, fin\n* Compute for the average grade of each term using Grade() method\n* Information about student's grades must be hidden from others\n\n\n\n",
"_____no_output_____"
]
],
[
[
"import random\n\nclass Person:\n def __init__ (self, student, pre, mid, fin):\n self.student = student\n self.pre = pre *0.30\n self.mid = mid *0.30\n self.fin = fin *0.40\n\n def Grade (self):\n print (self.student, \"has an average grade of\", self.pre, \"in Prelims\")\n print (self.student, \"has an average grade of\", self.mid, \"in Midterms\")\n print (self.student, \"has an average grade of\", self.fin, \"in Finals\")\n\nstd1 = Person (\"Andrei\", random.randint(70,100), random.randint(70,100), random.randint(70,100))\nstd2 = Person (\"Ady\", random.randint(70,100), random.randint(70,100), random.randint(70,100))\nstd3 = Person (\"Drei\", random.randint(70,100), random.randint(70,100), random.randint(70,100))\n\nstd1.Grade()\nstd2.Grade()\nstd3.Grade()",
"Andrei has an average grade of 21.9 in Prelims\nAndrei has an average grade of 23.4 in Midterms\nAndrei has an average grade of 33.2 in Finals\nAdy has an average grade of 26.4 in Prelims\nAdy has an average grade of 27.599999999999998 in Midterms\nAdy has an average grade of 28.0 in Finals\nDrei has an average grade of 28.2 in Prelims\nDrei has an average grade of 29.099999999999998 in Midterms\nDrei has an average grade of 38.800000000000004 in Finals\n"
]
]
] | [
"markdown",
"code",
"markdown",
"code",
"markdown",
"code",
"markdown",
"code",
"markdown",
"code",
"markdown",
"code"
] | [
[
"markdown",
"markdown"
],
[
"code"
],
[
"markdown"
],
[
"code",
"code"
],
[
"markdown"
],
[
"code"
],
[
"markdown"
],
[
"code"
],
[
"markdown"
],
[
"code"
],
[
"markdown"
],
[
"code"
]
] |
e772be6a7cfe2954c6821cac8967add0525fe25d | 8,582 | ipynb | Jupyter Notebook | enumerate.ipynb | chmoe/NLPLearning-CNWordSegmentation | 356e96914e4185f0588fb8274c7a4490bd817109 | [
"Apache-2.0"
] | null | null | null | enumerate.ipynb | chmoe/NLPLearning-CNWordSegmentation | 356e96914e4185f0588fb8274c7a4490bd817109 | [
"Apache-2.0"
] | null | null | null | enumerate.ipynb | chmoe/NLPLearning-CNWordSegmentation | 356e96914e4185f0588fb8274c7a4490bd817109 | [
"Apache-2.0"
] | null | null | null | 30.21831 | 141 | 0.494873 | [
[
[
"### Part 1.1 基于枚举方法来搭建中文分词工具(新)\n\n此项目需要的数据:\n1. 综合类中文词库.xlsx: 包含了中文词,当做词典来用\n2. 以变量的方式提供了部分unigram概率 word_prob\n\n\n举个例子: 给定词典=[我们 学习 人工 智能 人工智能 未来 是], 另外我们给定unigram概率:p(我们)=0.25, p(学习)=0.15, p(人工)=0.05, p(智能)=0.1, p(人工智能)=0.2, p(未来)=0.1, p(是)=0.15\n\n#### Step 1: 对于给定字符串:”我们学习人工智能,人工智能是未来“, 找出所有可能的分割方式\n- [我们,学习,人工智能,人工智能,是,未来]\n- [我们,学习,人工,智能,人工智能,是,未来]\n- [我们,学习,人工,智能,人工,智能,是,未来]\n- [我们,学习,人工智能,人工,智能,是,未来]\n.......\n\n\n#### Step 2: 我们也可以计算出每一个切分之后句子的概率\n- p(我们,学习,人工智能,人工智能,是,未来)= -log p(我们)-log p(学习)-log p(人工智能)-log p(人工智能)-log p(是)-log p(未来)\n- p(我们,学习,人工,智能,人工智能,是,未来)=-log p(我们)-log p(学习)-log p(人工)-log p(智能)-log p(人工智能)-log p(是)-log p(未来)\n- p(我们,学习,人工,智能,人工,智能,是,未来)=-log p(我们)-log p(学习)-log p(人工)-log p(智能)-log p(人工)-log p(智能)-log p(是)-log p(未来)\n- p(我们,学习,人工智能,人工,智能,是,未来)=-log p(我们)-log p(学习)-log p(人工智能)-log p(人工)-log p(智能)-log(是)-log p(未来)\n.....\n\n#### Step 3: 返回第二步中概率最大的结果",
"_____no_output_____"
]
],
[
[
"import pandas as pd\nimport numpy as np",
"_____no_output_____"
],
[
"path = \"./data/综合类中文词库.xlsx\"\ndata_frame = pd.read_excel(path, header = None)",
"_____no_output_____"
],
[
"dic_word_list = data_frame[data_frame.columns[0]].tolist()",
"_____no_output_____"
],
[
"dic_words = dic_word_list # 保存词典库中读取的单词\n\n# 以下是每一个单词出现的概率。为了问题的简化,我们只列出了一小部分单词的概率。 在这里没有出现的的单词但是出现在词典里的,统一把概率设置成为0.00001\n# 比如 p(\"学院\")=p(\"概率\")=...0.00001\n\nword_prob = {\"北京\":0.03,\"的\":0.08,\"天\":0.005,\"气\":0.005,\"天气\":0.06,\"真\":0.04,\"好\":0.05,\"真好\":0.04,\"啊\":0.01,\"真好啊\":0.02, \n \"今\":0.01,\"今天\":0.07,\"课程\":0.06,\"内容\":0.06,\"有\":0.05,\"很\":0.03,\"很有\":0.04,\"意思\":0.06,\"有意思\":0.005,\"课\":0.01,\n \"程\":0.005,\"经常\":0.08,\"意见\":0.08,\"意\":0.01,\"见\":0.005,\"有意见\":0.02,\"分歧\":0.04,\"分\":0.02, \"歧\":0.005}",
"_____no_output_____"
],
[
"for item in dic_words:\n word_prob.setdefault(item, 0.00001)",
"_____no_output_____"
],
[
"def split_word_with_dic_front_max(dic=[], input_str=\"\"):\n '''前项最大分词'''\n input_str_tmp = input_str\n segments = []\n while(input_str_tmp!=\"\"):\n for i in range(len(input_str_tmp), -1, -1):\n word = input_str_tmp[:i]\n if word in dic:\n segments.append(word)\n input_str_tmp = input_str_tmp[len(word):]\n break\n return segments",
"_____no_output_____"
],
[
"def split_word_with_dic_front_min(dic=[], input_str=\"\"):\n '''前项最小分词'''\n input_str_tmp = input_str\n segments = []\n while(input_str_tmp!=\"\"):\n for i in range(len(input_str_tmp)):\n word = input_str_tmp[:i] if len(input_str_tmp) != 1 else input_str_tmp[0] # 防止为空时找不到索引而循环\n if word in dic:\n segments.append(word)\n input_str_tmp = input_str_tmp[len(word):]\n break\n return segments",
"_____no_output_____"
],
[
"def split_word_with_dic_back_max(dic=[], input_str=\"\"):\n '''后项最大分词'''\n input_str_tmp = input_str\n segments = []\n while(input_str_tmp!=\"\"):\n for i in range(len(input_str_tmp)):\n word = input_str_tmp[i:]\n if word in dic:\n segments.append(word)\n input_str_tmp = input_str_tmp[:-len(word)]\n break\n return segments[::-1]",
"_____no_output_____"
],
[
"def split_word_with_dic_back_min(dic=[], input_str=\"\"):\n '''后项最小分词'''\n input_str_tmp = input_str\n segments = []\n while(input_str_tmp!=\"\"):\n for i in range(len(input_str_tmp), -1, -1):\n word = input_str_tmp[i:]\n if word in dic:\n segments.append(word)\n input_str_tmp = input_str_tmp[:-len(word)]\n break\n return segments[::-1]",
"_____no_output_____"
],
[
"def split_word(dic=[], input=\"\"):\n tmp_result = []\n tmp_result.append(split_word_with_dic_front_max(dic, input))\n tmp_result.append(split_word_with_dic_back_max(dic, input)) \n tmp_result.append(split_word_with_dic_front_min(dic, input))\n tmp_result.append(split_word_with_dic_back_min(dic, input)) \n return tmp_result",
"_____no_output_____"
],
[
"def get_split_probability_use_segment(split_word_segment=[]):\n sum_result = 0\n for seg in split_word_segment:\n sum_result -= np.log(word_prob.get(seg))\n return sum_result\ndef get_split_probability(split_word_segments=[[], ]):\n '''\n 根据传入的分词结果计算出概率最高的分词结果并返回\n '''\n index = 0\n max_index = 0\n sum = get_split_probability_use_segment(split_word_segments[0])\n for segment in split_word_segments:\n tmp = get_split_probability_use_segment(segment)\n if(sum>tmp): \n sum = tmp\n max_index = index\n index += 1\n return split_word_segments[max_index], sum",
"_____no_output_____"
],
[
"# 分数(10)\n## TODO 请编写word_segment_naive函数来实现对输入字符串的分词\ndef word_segment_naive(input_str):\n \"\"\"\n 1. 对于输入字符串做分词,并返回所有可行的分词之后的结果。\n 2. 针对于每一个返回结果,计算句子的概率\n 3. 返回概率最高的最作为最后结果\n \n input_str: 输入字符串 输入格式:“今天天气好”\n best_segment: 最好的分词结果 输出格式:[\"今天\",\"天气\",\"好\"]\n \"\"\"\n\n # TODO: 第一步: 计算所有可能的分词结果,要保证每个分完的词存在于词典里,这个结果有可能会非常多。 \n segments = split_word(list(word_prob.keys()), input_str) # 存储所有分词的结果。如果次字符串不可能被完全切分,则返回空列表(list)\n # 格式为:segments = [[\"今天\",“天气”,“好”],[\"今天\",“天“,”气”,“好”],[\"今“,”天\",“天气”,“好”],...]\n \n # TODO: 第二步:循环所有的分词结果,并计算出概率最高的分词结果,并返回\n best_segment, best_score = get_split_probability(segments)\n \n return best_segment ",
"_____no_output_____"
],
[
"# 测试\nprint(word_segment_naive(\"北京的天气真好啊\"))\nprint(word_segment_naive(\"今天的课程内容很有意思\"))\nprint(word_segment_naive(\"经常有意见分歧\"))",
"['北京', '的', '天气', '真好啊']\n['今天', '的', '课程', '内容', '很有', '意思']\n['经常', '有意见', '分歧']\n"
]
]
] | [
"markdown",
"code"
] | [
[
"markdown"
],
[
"code",
"code",
"code",
"code",
"code",
"code",
"code",
"code",
"code",
"code",
"code",
"code",
"code"
]
] |
e772c8f50d116baf0c0cb8c5fb0da745348e7fed | 66,567 | ipynb | Jupyter Notebook | ex2/2_1_Logistic_regression.ipynb | surajsimon/Andrew-ng-machine-learning-course-python-implementation | 7596e636b0b75a0a09eb15a8a51c71dea3fc59fc | [
"MIT"
] | 20 | 2019-01-21T23:20:24.000Z | 2022-01-22T10:31:35.000Z | ex2/2_1_Logistic_regression.ipynb | ZER-0-NE/Ng_ML | 7596e636b0b75a0a09eb15a8a51c71dea3fc59fc | [
"MIT"
] | null | null | null | ex2/2_1_Logistic_regression.ipynb | ZER-0-NE/Ng_ML | 7596e636b0b75a0a09eb15a8a51c71dea3fc59fc | [
"MIT"
] | 17 | 2019-02-04T10:02:54.000Z | 2021-05-18T18:08:53.000Z | 113.789744 | 33,940 | 0.858428 | [
[
[
"# 1 Logistic Regression",
"_____no_output_____"
],
[
"## 1.1 Visualizing the data",
"_____no_output_____"
]
],
[
[
"import matplotlib.pyplot as plt\nimport numpy as np\nfrom numpy import genfromtxt",
"_____no_output_____"
],
[
"data = genfromtxt('data/ex2data1.txt', delimiter=',')\n# Print first five rows to see what it looks like\nprint(data[:5, :])",
"[[ 34.62365962 78.02469282 0. ]\n [ 30.28671077 43.89499752 0. ]\n [ 35.84740877 72.90219803 0. ]\n [ 60.18259939 86.3085521 1. ]\n [ 79.03273605 75.34437644 1. ]]\n"
],
[
"X = data[:, 0:2] # scores on test1, test2\nY = data[:, 2] # admitted yes/no\n\nprint(X[:5])",
"[[ 34.62365962 78.02469282]\n [ 30.28671077 43.89499752]\n [ 35.84740877 72.90219803]\n [ 60.18259939 86.3085521 ]\n [ 79.03273605 75.34437644]]\n"
],
[
"print(Y[:5])",
"[ 0. 0. 0. 1. 1.]\n"
],
[
"plt.figure(figsize=(10, 7))\nplt.scatter(X[Y==1, 0], X[Y==1, 1], c='g', marker='P')\nplt.scatter(X[Y==0, 0], X[Y==0, 1], c='r', marker='o')\nplt.xlabel('Exam 1 score')\nplt.ylabel('Exam 2 score')\nplt.legend(['Admitted','Not admitted'])\nplt.show()",
"_____no_output_____"
]
],
[
[
"## 1.2 Implementation",
"_____no_output_____"
],
[
"### 1.2.1 Warmup exercise: sigmoid function",
"_____no_output_____"
]
],
[
[
"import math\n\ndef sigmoid(z):\n g = 1. / (1. + math.exp(-z))\n return g\n\n# Vectorize sigmoid function so it works on all elements of a numpy array\nsigmoid = np.vectorize(sigmoid)",
"_____no_output_____"
],
[
"# Test sigmoid function\ntest = np.array([[0]])\nsigmoid(test)",
"_____no_output_____"
],
[
"sigmoid(0)",
"_____no_output_____"
],
[
"test = np.array([[-10,-1], [0,0], [1,10]])\nsigmoid(test)",
"_____no_output_____"
]
],
[
[
"### 1.2.2 Cost function and gradient",
"_____no_output_____"
]
],
[
[
"# Setup the data matrix appropriately, and add ones for the intercept term\n[m, n] = X.shape",
"_____no_output_____"
],
[
"# Add intercept term to X\nX = np.column_stack((np.ones(m), X))",
"_____no_output_____"
],
[
"# Initialize fitting parameters\ninitial_theta = np.zeros([n + 1, 1])",
"_____no_output_____"
],
[
"def costFunction(theta, X, y):\n \n # Cost\n J = 0\n m = len(y)\n \n for i in range(m):\n z = np.dot(theta.T, X[i])\n J += -y[i]*math.log(sigmoid(z)) - (1 - y[i])*math.log((1 - sigmoid(z)))\n \n J = J/m\n \n # Gradient\n grad = np.zeros(theta.shape)\n \n for j in range(X.shape[1]):\n for i in range(m):\n z = np.dot(theta.T, X[i])\n grad[j] += (sigmoid(z) - y[i]) * X[i,j]\n grad[j] = grad[j]/m\n \n return J, grad",
"_____no_output_____"
],
[
"# Compute and display initial cost and gradient\ncost, grad = costFunction(initial_theta, X, Y)\n\nprint('Cost at initial theta (zeros):\\n', cost)\nprint('Expected cost (approx):\\n 0.693\\n')\nprint('Gradient at initial theta (zeros):\\n', grad)\nprint('\\nExpected gradients (approx):\\n -0.1000\\n -12.0092\\n -11.2628\\n')",
"Cost at initial theta (zeros):\n 0.69314718056\nExpected cost (approx):\n 0.693\n\nGradient at initial theta (zeros):\n [[ -0.1 ]\n [-12.00921659]\n [-11.26284221]]\n\nExpected gradients (approx):\n -0.1000\n -12.0092\n -11.2628\n\n"
],
[
"# Compute and display cost and gradient with non-zero theta\ntest_theta = np.array([-24, 0.2, 0.2])\ncost, grad = costFunction(test_theta, X, Y)\n\nprint('Cost at test theta (zeros):\\n', cost)\nprint('Expected cost (approx):\\n 0.218\\n')\nprint('Gradient at test theta (zeros):\\n', grad)\nprint('\\nExpected gradients (approx):\\n 0.043\\t 2.566\\t 2.647')",
"Cost at test theta (zeros):\n 0.218330193827\nExpected cost (approx):\n 0.218\n\nGradient at test theta (zeros):\n [ 0.04290299 2.56623412 2.64679737]\n\nExpected gradients (approx):\n 0.043\t 2.566\t 2.647\n"
]
],
[
[
"### 1.2.3 Learning parameters using fminunc",
"_____no_output_____"
],
[
"We're supposed to use Octave's ```fminunc``` function for this. I can't find a python implementation of this, so let's use ```scipy.optimize.minimize(method='TNC')``` instead.",
"_____no_output_____"
]
],
[
[
"from scipy.optimize import minimize\nres = minimize(fun=costFunction, x0=initial_theta, args=(X, Y), method='TNC', jac=True, options={'maxiter':400})\nres",
"_____no_output_____"
],
[
"theta = res.x\n\nprint('Cost at theta found by fmin_tnc:\\n', res.fun)\nprint('Expected cost (approx):\\n 0.203\\n')\nprint('Theta:\\n', res.x)\nprint('Expected theta (approx):\\n -25.161\\t 0.206\\t 0.201')",
"Cost at theta found by fmin_tnc:\n 0.203497701589\nExpected cost (approx):\n 0.203\n\nTheta:\n [-25.16131869 0.20623159 0.20147149]\nExpected theta (approx):\n -25.161\t 0.206\t 0.201\n"
],
[
"def plotDecisionBoundary(theta, X, Y):\n \n plt.figure(figsize=(10, 7))\n plt.scatter(X[Y==1, 1], X[Y==1, 2], c='g', marker='P')\n plt.scatter(X[Y==0, 1], X[Y==0, 2], c='r', marker='o')\n plt.xlabel('Exam 1 score')\n plt.ylabel('Exam 2 score')\n \n plot_x = [min(X[:,1]-2), max(X[:,1])+2]\n plot_y = [(-1/theta[2])*(theta[1]*plot_x[0] + theta[0]), (-1/theta[2])*(theta[1]*plot_x[1] + theta[0])]\n plt.plot(plot_x, plot_y)\n \n plt.xlim(min(X[:,1]-2),max(X[:,1])+2)\n plt.ylim(min(X[:,2]-2),max(X[:,2])+2)\n plt.legend(['Decision boundary', 'Admitted', 'Not admitted'])\n plt.show()",
"_____no_output_____"
],
[
"plotDecisionBoundary(theta, X, Y)",
"_____no_output_____"
]
],
[
[
"### 1.2.4 Evaluating logistic regression",
"_____no_output_____"
]
],
[
[
"# Predict probability of admission for a student with score 45 on exam 1 and score 85 on exam 2\n\nprob = sigmoid(np.dot([1, 45, 85], theta))\nprint('For a student with scores 45 and 85, we predict an admission probability of:\\n', prob)\nprint('Expected value:\\n 0.775 +/- 0.002\\n\\n')",
"For a student with scores 45 and 85, we predict an admission probability of:\n 0.7762906253511527\nExpected value:\n 0.775 +/- 0.002\n\n\n"
],
[
"# Compute accuracy on our training set\n\ndef predict(theta, X):\n \n m = X.shape[0] # Number of training examples\n p = np.zeros(m)\n\n for i in range(m):\n prob = sigmoid(np.dot(X[i,:], theta))\n if prob >= 0.5:\n p[i] = 1 # Predict \"Admitted\" if prob >= 0.5\n \n return p",
"_____no_output_____"
],
[
"p = predict(theta, X)\naccuracy = sum(p==Y) / m\n\nprint('Training accuracy:\\n', accuracy * 100, '%')\nprint('Expected accuracy (approx):\\n 89.0 %\\n')",
"Training accuracy:\n 89.0 %\nExpected accuracy (approx):\n 89.0 %\n\n"
]
]
] | [
"markdown",
"code",
"markdown",
"code",
"markdown",
"code",
"markdown",
"code",
"markdown",
"code"
] | [
[
"markdown",
"markdown"
],
[
"code",
"code",
"code",
"code",
"code"
],
[
"markdown",
"markdown"
],
[
"code",
"code",
"code",
"code"
],
[
"markdown"
],
[
"code",
"code",
"code",
"code",
"code",
"code"
],
[
"markdown",
"markdown"
],
[
"code",
"code",
"code",
"code"
],
[
"markdown"
],
[
"code",
"code",
"code"
]
] |
e772cfd7c987d8a6c8602f2b98b9b915c811cfb7 | 742,356 | ipynb | Jupyter Notebook | notebook/distribution_qvals_dmmpmm.ipynb | isabelleberger/isabelle- | d1337814fac73e7e6d516d1fb5e118a5dd6aeced | [
"MIT"
] | null | null | null | notebook/distribution_qvals_dmmpmm.ipynb | isabelleberger/isabelle- | d1337814fac73e7e6d516d1fb5e118a5dd6aeced | [
"MIT"
] | null | null | null | notebook/distribution_qvals_dmmpmm.ipynb | isabelleberger/isabelle- | d1337814fac73e7e6d516d1fb5e118a5dd6aeced | [
"MIT"
] | null | null | null | 168.029878 | 249,696 | 0.842422 | [
[
[
"import re\nimport pandas as pd\nimport numpy as np\nimport seaborn as sb\nimport matplotlib.pyplot as plt\n%matplotlib notebook \n\n",
"_____no_output_____"
],
[
"TFtable = []\nhits_list = []\nwith open('/Users/bergeric/Projects/s2rnai/data/hitcount.txt') as g: \n for line in g:\n if not line.strip().startswith('1 ##gff-version'):\n hits = int(line.split()[0])\n hits_list.append(hits)\n TF = line.split()[1]\n if hits > 100:\n TFtable.append((TF))\n\nhitdf = pd.DataFrame(TFtable, columns=['TF'])\n\n\nhitdf.head()",
"_____no_output_____"
],
[
"table = []\nwith open('/Users/bergeric/Projects/s2rnai/data/motif_alignments_dmmpmm2009_dm6.gff') as f:\n for line in f: \n if not line.startswith('##'):\n broken = line.split('\\t')\n pattern = broken[8].split(';')[3]\n pval = float(broken[8].split(';')[2][7:])\n qval = float(pattern[7:].strip())\n TF = broken[8].split(';')[0][5:]\n gene = broken[0]\n stuff = (TF, gene, qval, pval)\n table.append(stuff)",
"_____no_output_____"
],
[
"df= pd.DataFrame(table, columns=['TF', 'gene', 'q-value', 'p-value'])\ndf.head()",
"_____no_output_____"
],
[
"grp1 = df.groupby('TF')\ngrp1.describe()",
"_____no_output_____"
],
[
"indexTF = df.set_index(['TF'])\ndf2 = df[[\"TF\",\"q-value\",\"p-value\"]]\n#sub = df2.iloc[:5000,:]\ngroups = df2.groupby(['TF'])\nprint(groups['q-value'])",
"<pandas.core.groupby.SeriesGroupBy object at 0x13d11cfd0>\n"
]
],
[
[
"###### zip(df2, axes.flatten())\n",
"_____no_output_____"
]
],
[
[
"fig, axes = plt.subplots(8,2)",
"_____no_output_____"
],
[
"x= groups['q-value']\n\nfig, axes = plt.subplots(6,6, figsize=(12,12), sharex=True)\n#axr = axes.ravel()\n\n#zip(groups, axes.flatten())\nfor ax, x in zip(axes.flat, x):\n sb.distplot(x[1], ax=ax)\n ax.set_title(x[0])\n ax.axvline(0.05, color='r', ls=':')\n #axes.flat[-1].set_visible(False)\nax.set_xlim(0,1)\nplt.tight_layout()",
"_____no_output_____"
],
[
"fig, ax = plt.subplots(1, 1)\nprint(x[1])\nsb.distplot(x[1]['q-value'], hist=False, ax=ax, )\nax.set_title(x[0])\nax.axvline(0.05, color='r', ls=':')",
"_____no_output_____"
],
[
"plt.gca()\nplt.show()",
"_____no_output_____"
],
[
"x= groups['p-value']\n\nfig, axes = plt.subplots(6,6, figsize=(12,12), sharex=True)\n#axr = axes.ravel()\n\n#zip(groups, axes.flatten())\nfor ax, x in zip(axes.flat, x):\n sb.distplot(x[1], ax=ax)\n ax.set_title(x[0])\n ax.axvline(0.05, color='r', ls=':')\n #axes.flat[-1].set_visible(False)\nax.set_xlim(0,0.001)\nplt.tight_layout()",
"_____no_output_____"
]
]
] | [
"code",
"markdown",
"code"
] | [
[
"code",
"code",
"code",
"code",
"code",
"code"
],
[
"markdown"
],
[
"code",
"code",
"code",
"code",
"code"
]
] |
e772de99b0ffa20969d5c521aec25bcdbb01bade | 95,513 | ipynb | Jupyter Notebook | xspec/example_xspec.ipynb | ianan/nsigh | f76d57ced900098061ec1b1bf23cfe403e55a35a | [
"MIT"
] | 1 | 2020-07-02T20:55:39.000Z | 2020-07-02T20:55:39.000Z | xspec/example_xspec.ipynb | ianan/nsigh | f76d57ced900098061ec1b1bf23cfe403e55a35a | [
"MIT"
] | null | null | null | xspec/example_xspec.ipynb | ianan/nsigh | f76d57ced900098061ec1b1bf23cfe403e55a35a | [
"MIT"
] | 1 | 2020-07-23T14:56:20.000Z | 2020-07-23T14:56:20.000Z | 305.153355 | 43,492 | 0.919697 | [
[
[
"Example notebook that does stuff with the output files from a xspec, namely:\n* the .txt from wdata that saves the data/model,\n* the *.fits from writefits that save out the fit parameters.\n\nIGH 14 Feb 2020 - Started \nIGH 20 Feb 2020 - Better latex font, and fancier error label",
"_____no_output_____"
]
],
[
[
"from astropy.io import fits\nimport numpy as np\nimport matplotlib.pyplot as plt\nimport matplotlib\nimport warnings\nwarnings.simplefilter('ignore')\n\n# Some useful parameters\n# norm = 1e-14/(4piD_A^2)*\\int n_e n_p dV\n# The norm factor from the XSPEC APEC model is defined here: https://heasarc.gsfc.nasa.gov/xanadu/xspec/manual/node134.html\nkev2mk=0.0861733\nemfact=3.5557e-42",
"_____no_output_____"
],
[
"# An example file produced from writefits using FPMA and FPMA and a model of const*apec\nhdumok = fits.open('mod_thf2prb.fits')\n# hdumok.info()\nmokprm=hdumok[1].data\nmokcol=hdumok[1].columns.names\nhdumok.close()\nprint(mokcol)",
"['DIRPATH', 'PHAFILE', 'BACKFILE', 'RESPFILE', 'ARFFILE', 'EXPOSURE', 'STATISTIC', 'kT2', 'EkT2', 'norm5', 'Enorm5', 'factor6', 'Efactor6', 'kT7', 'EkT7', 'Abundanc8', 'EAbundanc8', 'Redshift9', 'ERedshift9', 'norm10', 'Enorm10']\n"
],
[
"t1=mokprm['kt2'][0]/kev2mk\nt1_cr=mokprm['ekt2'][0]/kev2mk\nprint('T1: ',t1,'MK, Err Rng: ',t1_cr)\nem1=np.double(mokprm['norm5'][0])/emfact\nem1_cr=np.double(mokprm['enorm5'][0])/emfact\nprint('EM1: ',em1,'cm^-3, Err Rng: ',em1_cr)\nfac=mokprm['factor6'][0]\nfac_cr=mokprm['efactor6'][0]\nprint('Fac: ',fac,' Fac Rng: ',fac_cr)",
"T1: 4.057312357339133 MK, Err Rng: [4.0522065 4.0625744]\nEM1: 4.862277469977783e+46 cm^-3, Err Rng: [4.79379412e+46 4.93071116e+46]\nFac: 1.05385 Fac Rng: [1.0454307 1.0623616]\n"
],
[
"# An example file produced from wdata from an iplot ldata ufspec delchi\ndd=[]\nwith open('mod_thf2prb.txt', 'r') as f:\n lines = f.readlines()\n dd.append(lines)\n# Get's rid of the first 3 lines which are normally not the data\ndd=dd[0][3:]",
"_____no_output_____"
],
[
"# Different plots separated by 'NO NO NO NO NO\\n' so need to find where this occurs\nid_break=[i for i, value in enumerate(dd) if value == 'NO NO NO NO NO\\n']\n\ndd_ld=dd[:id_break[0]]\ndd_uf=dd[id_break[0]+1:id_break[1]]\ndd_dc=dd[id_break[1]+1:]\n\n# For this example just assign the ldata plot\neng_ld=[]\ndeng_ld=[]\ndata_ld=[]\nedata_ld=[]\nmod_ld=[]\nfor i in dd_ld:\n temp_ld=i.split()\n eng_ld.append(float(temp_ld[0]))\n deng_ld.append(float(temp_ld[1]))\n data_ld.append(float(temp_ld[2]))\n edata_ld.append(float(temp_ld[3]))\n mod_ld.append(float(temp_ld[4]))\n \neng_ld=np.array(eng_ld)\ndeng_ld=np.array(deng_ld)\ndata_ld=np.array(data_ld)\nedata_ld=np.array(edata_ld)\nmod_ld=np.array(mod_ld)\n",
"_____no_output_____"
],
[
"# # Setup the font used for plotting\nmatplotlib.rcParams['font.sans-serif'] = \"Arial\"\nmatplotlib.rcParams['font.family'] = \"sans-serif\"\nmatplotlib.rcParams['font.size'] = 18\nmatplotlib.rcParams['mathtext.default']=\"regular\"",
"_____no_output_____"
],
[
"# A lot of this is just to get the plot exactly how I want it \n\nfig,axs=plt.subplots(2,1,figsize=(7,10),gridspec_kw=dict( height_ratios=[4,1],hspace=0.05))\n\n# Plot the data and model fit on the top plot\naxs[0].semilogy(eng_ld,data_ld,'.',ms=0.5,color='k')\nfor i in np.arange(len(data_ld)):\n axs[0].plot([eng_ld[i],eng_ld[i]],[data_ld[i]-edata_ld[i],data_ld[i]+edata_ld[i]],color='k')\n axs[0].plot([eng_ld[i]-deng_ld[i],eng_ld[i]+deng_ld[i]],[data_ld[i],data_ld[i]],color='k')\naxs[0].plot(eng_ld,mod_ld,color='firebrick',drawstyle='steps-mid')\naxs[0].set_ylabel('NuSTAR count s$^{-1}$ keV$^{-1}$')\nylim=[2e-1,7e4]\nxlim=[2,9]\naxs[0].set_ylim(ylim)\nfor aa in axs:\n aa.set_xlim(xlim)\n aa.label_outer()\n \n# Put the actual fit values on the top plot\nparam_labelt=\"{0:5.3f} ({1:5.3f} - {2:5.3f}) MK \".format(t1,t1_cr[0],t1_cr[1])\nparam_labelem=\"{0:5.2e} ({1:5.2e} - {2:5.2e}) \".format(em1,em1_cr[0],em1_cr[1])+\"$cm^{-3}$\"\naxs[0].text(0.95,0.92,param_labelt,color='firebrick',ha='right',transform=axs[0].transAxes)\naxs[0].text(0.95,0.86,param_labelem,color='firebrick',ha='right',transform=axs[0].transAxes)\naxs[0].text(0.95,0.80,\"{0:4.2f}\".format(fac),color='k',ha='right',transform=axs[0].transAxes)\n\n# You need to specify this yourself\nfiter=[2.5,7.0]\naxs[0].plot([fiter[0],fiter[0]],[ylim[0],10**(0.8*np.log10(ylim[1]))],':',color='grey')\naxs[0].plot([fiter[1],fiter[1]],[ylim[0],10**(0.8*np.log10(ylim[1]))],':',color='grey')\n\n# Calculate and plot the residuals on the bottom plot\nresid=(data_ld-mod_ld)/edata_ld\naxs[1].plot(eng_ld,resid,'.',ms=0.5,color='k')\naxs[1].set_ylim([-4,4])\naxs[1].set_xlabel('Energy [keV]')\naxs[1].plot(xlim,[0,0],'--',color='grey')\nfor i in np.arange(len(data_ld)):\n axs[1].plot([eng_ld[i]-deng_ld[i],eng_ld[i]+deng_ld[i]],[resid[i],resid[i]],color='firebrick')\naxs[1].set_ylabel('Resid')\nplt.show()\n ",
"_____no_output_____"
],
[
"# Same as above but just a fancier way of plotting the error ranges \nfig,axs=plt.subplots(2,1,figsize=(7,10),gridspec_kw=dict( height_ratios=[4,1],hspace=0.05))\n\n# Plot the data and model fit on the top plot\naxs[0].semilogy(eng_ld,data_ld,'.',ms=0.5,color='k')\nfor i in np.arange(len(data_ld)):\n axs[0].plot([eng_ld[i],eng_ld[i]],[data_ld[i]-edata_ld[i],data_ld[i]+edata_ld[i]],color='k')\n axs[0].plot([eng_ld[i]-deng_ld[i],eng_ld[i]+deng_ld[i]],[data_ld[i],data_ld[i]],color='k')\naxs[0].plot(eng_ld,mod_ld,color='firebrick',drawstyle='steps-mid')\naxs[0].set_ylabel('NuSTAR count s$^{-1}$ keV$^{-1}$')\nylim=[2e-1,7e4]\nxlim=[2,9]\naxs[0].set_ylim(ylim)\nfor aa in axs:\n aa.set_xlim(xlim)\n aa.label_outer()\n \n# Put the actual fit values on the top plot\nlabt=\"{0:5.3f}\".format(t1)\nlabtup=\"{0:5.3f}\".format(t1_cr[1]-t1)\nlabtlw=\"{0:5.3f}\".format(t1-t1_cr[0])\n\npowem1=np.floor(np.log10(em1))\nlabpowem1=\"{0:2d}\".format(int(powem1))\n\nlabem=\"{0:5.2f}\".format(em1/10**powem1)\nlabemup=\"{0:5.2f}\".format((em1_cr[1]-em1)/10**powem1)\nlabemlw=\"{0:5.2f}\".format((em1-em1_cr[0])/10**powem1)\n\nlabf=\"{0:5.2f}\".format(fac)\nlabfup=\"{0:5.2f}\".format(fac_cr[1]-fac)\nlabflw=\"{0:5.2f}\".format(fac-fac_cr[0])\n\naxs[0].text(0.02,0.92,labt+\" $^{+\"+labtup+\"}_{-\"+labtlw+\"}\\;MK,$\",color='firebrick',ha='left',transform=axs[0].transAxes)\naxs[0].text(0.34,0.92,labem+\" $^{+\"+labemup+\"}_{-\"+labemlw+\"}\\,×\\,10^{\"+labpowem1+\"}\\;cm^{-3},$\",color='firebrick',ha='left',transform=axs[0].transAxes)\naxs[0].text(0.78,0.92,labf+\" $^{+\"+labfup+\"}_{-\"+labflw+\"}$\",color='k',ha='left',transform=axs[0].transAxes)\n\n# You need to specify this yourself\nfiter=[2.5,7.0]\naxs[0].plot([fiter[0],fiter[0]],[ylim[0],10**(0.8*np.log10(ylim[1]))],':',color='grey')\naxs[0].plot([fiter[1],fiter[1]],[ylim[0],10**(0.8*np.log10(ylim[1]))],':',color='grey')\n\n# Calculate and plot the residuals on the bottom plot\nresid=(data_ld-mod_ld)/edata_ld\naxs[1].plot(eng_ld,resid,'.',ms=0.5,color='k')\naxs[1].set_ylim([-4,4])\naxs[1].set_xlabel('Energy [keV]')\naxs[1].plot(xlim,[0,0],'--',color='grey')\nfor i in np.arange(len(data_ld)):\n axs[1].plot([eng_ld[i]-deng_ld[i],eng_ld[i]+deng_ld[i]],[resid[i],resid[i]],color='firebrick')\naxs[1].set_ylabel('Resid')\nplt.show()\n ",
"_____no_output_____"
]
]
] | [
"markdown",
"code"
] | [
[
"markdown"
],
[
"code",
"code",
"code",
"code",
"code",
"code",
"code",
"code"
]
] |
e772deb4e7a94cef96486e05d342bbf5ca745e60 | 37,171 | ipynb | Jupyter Notebook | project-I/backup/load.ipynb | geraldmc/DL4CV-2022 | d734290849244816e4861efc0578325684c58409 | [
"MIT"
] | null | null | null | project-I/backup/load.ipynb | geraldmc/DL4CV-2022 | d734290849244816e4861efc0578325684c58409 | [
"MIT"
] | null | null | null | project-I/backup/load.ipynb | geraldmc/DL4CV-2022 | d734290849244816e4861efc0578325684c58409 | [
"MIT"
] | null | null | null | 46.003713 | 492 | 0.545533 | [
[
[
"import os\nimport argparse\nimport numpy as np\nimport torch\nimport torch.nn as nn\nimport torch.nn.functional as F\nimport torch.optim as optim\nfrom torch.autograd import Variable\nimport torch.utils.data as data\nimport torchvision\nfrom torchvision import datasets, transforms\nfrom torchsummary import summary",
"_____no_output_____"
],
[
"# Automatic reloading and inline plotting\n%reload_ext autoreload\n%autoreload 2\n%matplotlib inline",
"_____no_output_____"
],
[
"import sys\n\nCOLAB = 'google.colab' in str(get_ipython())\nAUGMENTED = False\nHAS_GPU = torch.cuda.is_available()\nis_local = not COLAB\n\nif COLAB: # running on Colab\n \n from google.colab import drive\n drive.flush_and_unmount()\n \n drive.mount('/content/drive', force_remount=True)\n gdrive_path = '/content/drive//MyDrive/DL4CV-2022/project-I/'\n sys.path.append(gdrive_path)\n\n zip_path_train = gdrive_path + 'data/Final_Training.zip'\n zip_path_val = gdrive_path + 'data/Final_Validation.zip'\n zip_path_test = gdrive_path + 'data/Final_Test.zip'\n !unzip -q \"{zip_path_train}\"\n !unzip -q \"{zip_path_val}\"\n !unzip -q \"{zip_path_test}\"\n \n if HAS_GPU:\n print(\"Using GPU\")\nelse:\n HAS_GPU = False\n print(\"Using CPU\")\n \nimport utils.helpers as utils\nimport loader.gtsrb_data as dataset\n\n# If we run cuda then accomodate these datatypes.\nFloatTensor = torch.cuda.FloatTensor if HAS_GPU else torch.FloatTensor\nLongTensor = torch.cuda.LongTensor if HAS_GPU else torch.LongTensor\nByteTensor = torch.cuda.ByteTensor if HAS_GPU else torch.ByteTensor\nTensor = FloatTensor",
"Using CPU\n"
],
[
"if COLAB:\n !ls /content/Final_Training/Images\nelse:\n !ls data/Final_Training/Images",
"\u001b[1m\u001b[36m00000\u001b[m\u001b[m \u001b[1m\u001b[36m00004\u001b[m\u001b[m \u001b[1m\u001b[36m00008\u001b[m\u001b[m \u001b[1m\u001b[36m00012\u001b[m\u001b[m \u001b[1m\u001b[36m00016\u001b[m\u001b[m \u001b[1m\u001b[36m00020\u001b[m\u001b[m \u001b[1m\u001b[36m00024\u001b[m\u001b[m \u001b[1m\u001b[36m00028\u001b[m\u001b[m \u001b[1m\u001b[36m00032\u001b[m\u001b[m \u001b[1m\u001b[36m00036\u001b[m\u001b[m \u001b[1m\u001b[36m00040\u001b[m\u001b[m\n\u001b[1m\u001b[36m00001\u001b[m\u001b[m \u001b[1m\u001b[36m00005\u001b[m\u001b[m \u001b[1m\u001b[36m00009\u001b[m\u001b[m \u001b[1m\u001b[36m00013\u001b[m\u001b[m \u001b[1m\u001b[36m00017\u001b[m\u001b[m \u001b[1m\u001b[36m00021\u001b[m\u001b[m \u001b[1m\u001b[36m00025\u001b[m\u001b[m \u001b[1m\u001b[36m00029\u001b[m\u001b[m \u001b[1m\u001b[36m00033\u001b[m\u001b[m \u001b[1m\u001b[36m00037\u001b[m\u001b[m \u001b[1m\u001b[36m00041\u001b[m\u001b[m\n\u001b[1m\u001b[36m00002\u001b[m\u001b[m \u001b[1m\u001b[36m00006\u001b[m\u001b[m \u001b[1m\u001b[36m00010\u001b[m\u001b[m \u001b[1m\u001b[36m00014\u001b[m\u001b[m \u001b[1m\u001b[36m00018\u001b[m\u001b[m \u001b[1m\u001b[36m00022\u001b[m\u001b[m \u001b[1m\u001b[36m00026\u001b[m\u001b[m \u001b[1m\u001b[36m00030\u001b[m\u001b[m \u001b[1m\u001b[36m00034\u001b[m\u001b[m \u001b[1m\u001b[36m00038\u001b[m\u001b[m \u001b[1m\u001b[36m00042\u001b[m\u001b[m\n\u001b[1m\u001b[36m00003\u001b[m\u001b[m \u001b[1m\u001b[36m00007\u001b[m\u001b[m \u001b[1m\u001b[36m00011\u001b[m\u001b[m \u001b[1m\u001b[36m00015\u001b[m\u001b[m \u001b[1m\u001b[36m00019\u001b[m\u001b[m \u001b[1m\u001b[36m00023\u001b[m\u001b[m \u001b[1m\u001b[36m00027\u001b[m\u001b[m \u001b[1m\u001b[36m00031\u001b[m\u001b[m \u001b[1m\u001b[36m00035\u001b[m\u001b[m \u001b[1m\u001b[36m00039\u001b[m\u001b[m\n"
],
[
"import loader.args as load_args\nargs = load_args.get_args(is_local)\n\nTRAIN_DATA_PATH = args.data_dir_train\nVAL_DATA_PATH = args.data_dir_val\nTEST_DATA_PATH = args.data_dir_test\nBATCH_SIZE = args.batch_size\nEPOCHS = 10\nBATCH_SIZE = args.batch_size\nLEARNING_RATE = args.learning_rate",
"_____no_output_____"
],
[
"from loader import transforms as tfs\n\nif AUGMENTED:\n\n train_loader = torch.utils.data.DataLoader(\n torch.utils.data.ConcatDataset([datasets.ImageFolder(\n TRAIN_DATA_PATH, \n transform=tfs.base_transform),\n datasets.ImageFolder(\n TRAIN_DATA_PATH,\n transform=tfs.data_translate),\n datasets.ImageFolder(\n TRAIN_DATA_PATH,\n transform=tfs.data_grayscale),\n datasets.ImageFolder(\n TRAIN_DATA_PATH,\n transform=tfs.data_center),\n datasets.ImageFolder(\n TRAIN_DATA_PATH,\n transform=tfs.data_rotate),\n datasets.ImageFolder(\n TRAIN_DATA_PATH,\n transform=tfs.data_jitter_hue)]), \n batch_size=args.batch_size, \n shuffle=True, num_workers=2, \n pin_memory=HAS_GPU)\n\n val_loader = torch.utils.data.DataLoader(\n torch.utils.data.ConcatDataset([datasets.ImageFolder(\n VAL_DATA_PATH, \n transform=tfs.base_transform),\n datasets.ImageFolder(\n VAL_DATA_PATH,\n transform=tfs.data_translate),\n datasets.ImageFolder(\n VAL_DATA_PATH,\n transform=tfs.data_grayscale),\n datasets.ImageFolder(\n VAL_DATA_PATH,\n transform=tfs.data_center),\n datasets.ImageFolder(\n VAL_DATA_PATH,\n transform=tfs.data_rotate),\n datasets.ImageFolder(\n VAL_DATA_PATH,\n transform=tfs.data_jitter_hue)]), \n batch_size=args.batch_size, \n shuffle=False, num_workers=2, \n pin_memory=HAS_GPU)\nelse:\n # NO augmentation--> train=39209, test=12630, val=3870.\n\n train_data = datasets.ImageFolder(\n root=TRAIN_DATA_PATH, \n transform=tfs.base_transform)\n train_loader = data.DataLoader(train_data, \n batch_size=BATCH_SIZE, shuffle=True, \n num_workers=2)\n \n test_data = datasets.ImageFolder(\n root=TEST_DATA_PATH, \n transform=tfs.base_transform)\n test_loader = data.DataLoader(test_data, \n batch_size=BATCH_SIZE, shuffle=True, \n num_workers=2)\n \n val_data = datasets.ImageFolder(\n root=VAL_DATA_PATH, \n transform=tfs.base_transform)\n val_loader = data.DataLoader(test_data, \n batch_size=BATCH_SIZE, shuffle=False, \n num_workers=2)",
"_____no_output_____"
],
[
"import matplotlib.pyplot as plt\n\nif AUGMENTED:\n print('Augmented dataset: %d training samples & %d validation samples\\n' % (\n len(train_loader.dataset), len(val_loader.dataset)))\n\n # Get labels from concatenated datasets.\n train_dataset_list = train_loader.dataset.datasets\n train_concat_labels = []\n for ds in train_dataset_list:\n train_concat_labels.extend(ds.targets)\n\n val_dataset_list = val_loader.dataset.datasets\n val_concat_labels = []\n for ds in val_dataset_list:\n val_concat_labels.extend(ds.targets)\n\n class_labels = range(43)\n\n print('Distribution of classes in augmented train dataset:')\n fig, ax = plt.subplots()\n _, counts = np.unique(train_concat_labels, return_counts=True)\n ax.bar(class_labels, counts)\n ax.set_xticks(class_labels, minor=True)\n plt.show()\nelse:\n print('Unaugmented dataset: %d training samples & %d validation samples\\n' % (\n len(train_loader)*args.batch_size, len(val_loader)*args.batch_size))",
"Unaugmented dataset: 39250 training samples & 12650 validation samples\n\n"
],
[
"from model.models import Net1\n\nmodel = Net1()\nif HAS_GPU:\n model.cuda()\n\n# Optimizer is updated to *not* include non-gradient weights. \noptimizer = optim.Adam(filter(lambda p: p.requires_grad, model.parameters()),\n lr=args.learning_rate)\n# Reduce learning rate when a metric has stopped improving. \nscheduler = optim.lr_scheduler.ReduceLROnPlateau(optimizer, 'min',\n patience=5,\n factor=0.5,\n verbose=True)",
"_____no_output_____"
],
[
"summary(model,(3,32,32))",
"----------------------------------------------------------------\n Layer (type) Output Shape Param #\n================================================================\n Conv2d-1 [-1, 8, 26, 26] 1,184\n MaxPool2d-2 [-1, 8, 13, 13] 0\n ReLU-3 [-1, 8, 13, 13] 0\n Conv2d-4 [-1, 10, 9, 9] 2,010\n MaxPool2d-5 [-1, 10, 4, 4] 0\n ReLU-6 [-1, 10, 4, 4] 0\n Linear-7 [-1, 32] 5,152\n ReLU-8 [-1, 32] 0\n Linear-9 [-1, 6] 198\n Conv2d-10 [-1, 100, 28, 28] 7,600\n BatchNorm2d-11 [-1, 100, 14, 14] 200\n Dropout2d-12 [-1, 100, 14, 14] 0\n Conv2d-13 [-1, 150, 12, 12] 135,150\n BatchNorm2d-14 [-1, 150, 6, 6] 300\n Dropout2d-15 [-1, 150, 6, 6] 0\n Conv2d-16 [-1, 250, 4, 4] 337,750\n BatchNorm2d-17 [-1, 250, 2, 2] 500\n Dropout2d-18 [-1, 250, 2, 2] 0\n Linear-19 [-1, 350] 350,350\n Linear-20 [-1, 43] 15,093\n================================================================\nTotal params: 855,487\nTrainable params: 855,487\nNon-trainable params: 0\n----------------------------------------------------------------\nInput size (MB): 0.01\nForward/backward pass size (MB): 1.26\nParams size (MB): 3.26\nEstimated Total Size (MB): 4.54\n----------------------------------------------------------------\n"
],
[
"from utils.meter import AvgMeter, calc_accuracy\n\ntrain_losses = []\ntrain_accuracies = []\n\ndef train(epoch):\n print('Epoch {} -------------------------------------------------------->'.format(epoch) )\n print()\n losses = AvgMeter()\n accuracies = AvgMeter()\n model.train()\n correct = 0\n training_loss = 0\n \n for batch_idx, (data, target) in enumerate(train_loader):\n data, target = Variable(data), Variable(target)\n if HAS_GPU:\n data = data.cuda()\n target = target.cuda()\n optimizer.zero_grad()\n output = model(data)\n loss = F.nll_loss(output, target)\n \n losses.update(loss.item(), data.size(0))\n accuracy = calc_accuracy(output, target)[0]\n train_accuracies.append(int(accuracy))\n accuracies.update(accuracy, data.size(0))\n \n loss.backward()\n optimizer.step()\n max_index = output.max(dim = 1)[1] # _, predicted\n correct += (max_index == target).sum()\n training_loss += loss\n \n if batch_idx % args.log_interval == 0:\n print('Training set: Average loss: {:.4f}, Accuracy: {}/{} ({:.3f}%)\\n'.format(\n losses.avg, int(accuracies.sum/100), accuracies.count, accuracies.avg))\n \n return train_accuracies",
"_____no_output_____"
],
[
"val_losses = []\nval_accuracies = []\n\ndef validate(epoch):\n losses = AvgMeter()\n accuracies = AvgMeter()\n error_cases = []\n \n model.eval() # changes the forward() behaviour of the module for val/test.\n \n with torch.no_grad(): # disable tracking of gradients in autograd.\n for data, target in val_loader:\n if HAS_GPU:\n data = data.cuda()\n target = target.cuda()\n output = model(data)\n loss = F.nll_loss(output, target)\n\n losses.update(loss.item(), data.size(0))\n accuracy = calc_accuracy(output, target)[0]\n val_accuracies.append(accuracy)\n accuracies.update(accuracy, data.size(0))\n\n _, pred = output.topk(1, 1, True, True)\n pred = pred.t()\n\n print('\\nValidation set: Average loss: {:.4f}, Accuracy: {}/{} ({:.2f}%)\\n'.format(\n losses.avg, int(accuracies.sum/100), accuracies.count, accuracies.avg))\n\n return val_accuracies",
"_____no_output_____"
],
[
"training_accuracy = []\nvalidation_accuracy = []\n\nfor epoch in range(1, args.num_epochs + 1):\n training_accuracy.append(train(epoch))\n validation_accuracy.append(validate(epoch))",
"Epoch 1 -------------------------------------------------------->\n\nTraining set: Average loss: 3.9154, Accuracy: 2/50 (4.000%)\n\nTraining set: Average loss: 3.5809, Accuracy: 397/5050 (7.861%)\n\nTraining set: Average loss: 3.3679, Accuracy: 1314/10050 (13.075%)\n\nTraining set: Average loss: 3.1832, Accuracy: 2603/15050 (17.296%)\n\nTraining set: Average loss: 3.0023, Accuracy: 4285/20050 (21.372%)\n\nTraining set: Average loss: 2.8333, Accuracy: 6302/25050 (25.158%)\n\nTraining set: Average loss: 2.6767, Accuracy: 8642/30050 (28.759%)\n\nTraining set: Average loss: 2.5248, Accuracy: 11318/35050 (32.291%)\n\n"
],
[
"#plot accuracy\n\nplt.plot(training_accuracy[4],'-o')\nplt.plot(validation_accuracy[4],'-o')\nplt.xlabel('Sample # per Epoch')\nplt.ylabel('Accuracy')\nplt.legend(['Train','Valid'])\nplt.title('Train vs Valid Accuracy per Epoch (Best)')\nNone",
"_____no_output_____"
],
[
"'''\n train_data = torchvision.datasets.ImageFolder(root=TRAIN_DATA_PATH, \n transform=tfs.data_transforms)\n train_loader = data.DataLoader(train_data, batch_size=BATCH_SIZE, shuffle=True, num_workers=2)\n test_data = torchvision.datasets.ImageFolder(root=TEST_DATA_PATH, \n transform=tfs.data_transforms)\n test_loader = data.DataLoader(test_data, batch_size=BATCH_SIZE, shuffle=True, num_workers=2)\n val_data = torchvision.datasets.ImageFolder(root=VAL_DATA_PATH, \n transform=tfs.data_transforms)\n val_loader = data.DataLoader(val_data, batch_size=BATCH_SIZE, shuffle=True, num_workers=2)\n \n \nds = datasets.ImageFolder(VAL_DATA_PATH, transform=None)\n#dir(ds)\n#ds.samples[0:5] # the first five samples\n#ds.imgs[33] # img 33\n#ds.samples[33] # sample 33, equivalent of previous line\n#ds.samples[33][0] # a sample path\n#ds.samples[33][1] # a sample class label\n#ds.find_classes(VAL_DATA_PATH)\n#ds.targets\n\n\ndef validation():\n model.eval()\n validation_loss = 0\n correct = 0\n for data, target in val_loader:\n with torch.no_grad():\n data, target = Variable(data), Variable(target)\n if HAS_GPU:\n data = data.cuda()\n target = target.cuda()\n output = model(data)\n validation_loss += F.nll_loss(output, target, size_average=False).data.item() # sum up batch loss\n pred = output.data.max(1, keepdim=True)[1] # get the index of the max log-probability\n correct += pred.eq(target.data.view_as(pred)).cpu().sum()\n\n validation_loss /= len(val_loader.dataset)\n scheduler.step(np.around(validation_loss,2))\n \n print('\\nValidation set: Average loss: {:.4f}, Accuracy: {}/{} ({:.0f}%)\\n'.format(\n validation_loss, correct, len(val_loader.dataset),\n 100. * correct / len(val_loader.dataset))) \n \n \ndef train(epoch):\n losses = AvgMeter()\n accuracies = AvgMeter()\n \n model.train()\n correct = 0\n training_loss = 0\n \n for batch_idx, (data, target) in enumerate(train_loader):\n data, target = Variable(data), Variable(target)\n if HAS_GPU:\n data = data.cuda()\n target = target.cuda()\n optimizer.zero_grad()\n output = model(data)\n loss = F.nll_loss(output, target)\n \n #losses.update(loss.item(), data.size(0))\n #accuracy = calc_accuracy(output, target)[0]\n #accuracies.update(accuracy, data.size(0))\n \n loss.backward()\n optimizer.step()\n max_index = output.max(dim = 1)[1] # _, predicted\n correct += (max_index == target).sum()\n training_loss += loss\n \n if batch_idx % args.log_interval == 0:\n train_loss=training_loss / len(train_loader.dataset)\n accu = 100. * correct / len(train_loader.dataset)\n print('Train Loss: %.3f | Accuracy: %.3f'%(train_loss,accu))\n print('---------------------------------------------------')\n print('Epoch: {} [{}/{} ({:.0f}%)]\\tLoss per example: {:.6f}\\tLoss: {:.6f}'.format(\n epoch, \n batch_idx * len(data), # this batch number, times total length\n len(train_loader.dataset), # length of the entire dataset.\n 100. * batch_idx / len(train_loader), \n loss.data.item()/(args.batch_size * args.log_interval),\n loss.data.item()) )\n \n train_accu.append(accu.detach().cpu().numpy())\n train_losses.append(train_loss.detach().cpu().numpy())\n \n \ndef validation():\n eval_losses=[]\n eval_accu_ls=[]\n\n model.eval()\n validation_loss = 0\n correct = 0\n for data, target in val_loader:\n with torch.no_grad():\n data, target = Variable(data), Variable(target)\n if HAS_GPU:\n data = data.cuda()\n target = target.cuda()\n output = model(data)\n # sum up batch loss\n validation_loss += F.nll_loss(output, \n target, \n size_average=False).data.item()\n # get the index of the max log-probability\n pred = output.data.max(1, keepdim=True)[1]\n correct += pred.eq(target.data.view_as(pred)).cpu().sum()\n\n validation_loss /= len(val_loader.dataset)\n scheduler.step(np.around(validation_loss,2))\n\n eval_loss=validation_loss / len(val_loader.dataset)\n eval_accu = 100. * correct / len(val_loader.dataset)\n\n eval_losses.append(eval_loss.detach().cpu().numpy())\n eval_accu_ls.append(eval_accu.detach().cpu().numpy())\n\n print('Validation Loss: %.3f | Accuracy: %.3f'%(test_loss,accu)) \n\n print('\\nValidation set: Average loss: {:.4f}, Accuracy: {}/{} ({:.0f}%)\\n'.format(\n validation_loss, \n correct, \n len(val_loader.dataset),\n 100. * correct / len(val_loader.dataset))\n\n'''",
"_____no_output_____"
]
]
] | [
"code"
] | [
[
"code",
"code",
"code",
"code",
"code",
"code",
"code",
"code",
"code",
"code",
"code",
"code",
"code",
"code"
]
] |
e772e4780f1200f9a5bb2ed4a0d1ff314f8c263d | 18,829 | ipynb | Jupyter Notebook | tutorials/3-IK-optimization.ipynb | Zwoelf12/rai-python | 6f86b9b45e897e81a0c61d0f33e267fa1164c92e | [
"MIT"
] | null | null | null | tutorials/3-IK-optimization.ipynb | Zwoelf12/rai-python | 6f86b9b45e897e81a0c61d0f33e267fa1164c92e | [
"MIT"
] | null | null | null | tutorials/3-IK-optimization.ipynb | Zwoelf12/rai-python | 6f86b9b45e897e81a0c61d0f33e267fa1164c92e | [
"MIT"
] | null | null | null | 34.93321 | 537 | 0.599979 | [
[
[
"# Inverse Kinematics Optimization\n\nThe previous doc explained features and how they define objectives of a constrained optimization problem. Here we show how to use this to solve IK optimization problems.\n\nAt the bottom there is more general text explaining the basic concepts.",
"_____no_output_____"
],
[
"## Demo of features in Inverse Kinematics\n\nLet's setup a standard configuration. (Lock the window with \"Always on Top\".)",
"_____no_output_____"
]
],
[
[
"import sys\nsys.path.append('../build') #rai/lib')\nimport numpy as np\nimport libry as ry",
"**ry-c++-log** /home/student/Desktop/KOMO/rai-python/rai/rai/ry/ry.cpp:init_LogToPythonConsole:34(0) initializing ry log callback** INFO:/home/student/Desktop/KOMO/rai-python/rai/rai/Core/util.cpp:initCmdLine:602(1) ** cmd line arguments: 'rai-pybind -python'\n** INFO:/home/student/Desktop/KOMO/rai-python/rai/rai/Core/util.cpp:initCmdLine:606(1) ** run path: '/home/student/Desktop/KOMO/rai-python/tutorials'\n** INFO:/home/student/Desktop/KOMO/rai-python/rai/rai/Core/graph.cpp:initParameters:1363(1) ** parsed parameters:\n{python,\nLGP/cameraFocus:[1, 0.5, 1],\nLGP/collisions:1,\nLGP/stopTime:300,\nLGP/stopSol:6,\nopt/maxStep:0.1}\n\n\n\n** INFO:/home/student/Desktop/KOMO/rai-python/rai/rai/ry/ry.cpp:init_LogToPythonConsole:34(0) initializing ry log callback\n"
],
[
"C = ry.Config()\nC.addFile('../rai-robotModels/pr2/pr2.g')\nC.addFile('../rai-robotModels/objects/kitchen.g')\nC.view()",
"_____no_output_____"
]
],
[
[
"For simplicity, let's add a frame that represents goals",
"_____no_output_____"
]
],
[
[
"goal = C.addFrame(\"goal\")\ngoal.setShape(ry.ST.sphere, [.05])\ngoal.setColor([.5,1,1])\ngoal.setPosition([1,.5,1])\nX0 = C.getFrameState() #store the initial configuration",
"_____no_output_____"
]
],
[
[
"We create an IK engine. The only objective is that the `positionDiff` (position difference in world coordinates) between `pr2L` (the yellow blob in the left hand) and `goal` is equal to zero:",
"_____no_output_____"
]
],
[
[
"IK = C.komo_IK(False)\nIK.addObjective(type=ry.OT.eq, times = [1,2], feature=ry.FS.positionDiff, frames=['pr2L', 'goal'])",
"_____no_output_____"
]
],
[
[
"We now call the optimizer (True means with random initialization/restart).",
"_____no_output_____"
]
],
[
[
"IK.optimize()\nIK.getReport()",
"** KOMO::run solver:dense collisions:0 x-dim:25 T:1 k:1 phases:1 stepsPerPhase:1 tau:1 #timeSlices:2 #totalDOFs:25 #frames:358\n** optimization time:0.00914103 (kin:0.000131 coll:0.000132 feat:0 newton: 0.00105) setJointStateCount:35\n sos:0.0808073 ineq:0 eq:0.238354\n"
]
],
[
[
"The best way to retrieve the result is to copy the optimized IK configuration back into your working configuration C, which is now also displayed",
"_____no_output_____"
]
],
[
[
"#IK.getFrameState(1)\nC.setFrameState(IK.getFrameState(0))",
"_____no_output_____"
]
],
[
[
"We can redo the optimization, but for a different configuration, namely a configuration where the goal is in another location.\nFor this we move goal in our working configuration C, then copy C back into the IK engine's configurations:",
"_____no_output_____"
]
],
[
[
"## (iterate executing this cell for different goal locations!)\n\n# move goal\ngoal.setPosition([.8,.2,.5])\n\n# copy C into the IK's internal configuration(s)\nIK.setConfigurations(C)\n\n# reoptimize\nIK.optimize(0.) # 0: no adding of noise for a random restart\n#print(IK.getReport())\nprint(np.shape(IK.getFrameState(0)))\nprint(np.shape(IK.getFrameState(0)[1]))\n# grab result\n# C.setFrameState( IK.getConfiguration(1) )\nC.setFrameState(IK.getFrameState(0))",
"** KOMO::run solver:dense collisions:0 x-dim:25 T:1 k:1 phases:1 stepsPerPhase:1 tau:1 #timeSlices:2 #totalDOFs:25 #frames:358\n** optimization time:0.000305789 (kin:0.000238 coll:0.000149 feat:0 newton: 0.001415) setJointStateCount:3\n sos:0.000285026 ineq:0 eq:0.0270084\n(179, 7)\n(7,)\n"
]
],
[
[
"Let's solve some other problems, always creating a novel IK engine:\n\nThe relative position of `goal` in `pr2R` coordinates equals [0,0,-.2] (which is 20cm straight in front of the yellow blob)",
"_____no_output_____"
]
],
[
[
"C.setFrameState(X0)\nIK = C.komo_IK(False)\nIK.addObjective(type=ry.OT.eq,times=[1], feature=ry.FS.positionRel, frames=['goal','pr2R'], target=[0,0,-.2])\nIK.optimize()\nC.setFrameState(IK.getFrameState(0))",
"** KOMO::run solver:dense collisions:0 x-dim:25 T:1 k:1 phases:1 stepsPerPhase:1 tau:1 #timeSlices:2 #totalDOFs:25 #frames:358\n** optimization time:0.00105824 (kin:5.2e-05 coll:1.1e-05 feat:0 newton: 0.000124) setJointStateCount:12\n sos:0.00848536 ineq:0 eq:0.0341739\n"
]
],
[
[
"The distance between `pr2R` and `pr2L` is zero:",
"_____no_output_____"
]
],
[
[
"C.setFrameState(X0)\nIK = C.komo_IK(False)\nIK.addObjective(type=ry.OT.eq, times=[1], feature=ry.FS.distance, frames=['pr2L','pr2R'])\nIK.optimize()\nC.setFrameState(IK.getFrameState(0))",
"** KOMO::run solver:dense collisions:0 x-dim:25 T:1 k:1 phases:1 stepsPerPhase:1 tau:1 #timeSlices:2 #totalDOFs:25 #frames:358\n** optimization time:0.00069327 (kin:3.3e-05 coll:5e-06 feat:0 newton: 5.9e-05) setJointStateCount:6\n sos:0.00209253 ineq:0 eq:0.0149894\n"
]
],
[
[
"The 3D difference between the z-vector of `pr2R` and the z-vector of `goal`:",
"_____no_output_____"
]
],
[
[
"C.setFrameState(X0)\nIK = C.komo_IK(False)\nIK.addObjective(type=ry.OT.eq, times=[1], feature=ry.FS.vectorZDiff, frames=['pr2R', 'goal'])\nIK.optimize()\nC.setFrameState(IK.getFrameState(0))",
"** KOMO::run solver:dense collisions:0 x-dim:25 T:1 k:1 phases:1 stepsPerPhase:1 tau:1 #timeSlices:2 #totalDOFs:25 #frames:358\n** optimization time:0.00144349 (kin:0.000111 coll:2.9e-05 feat:0 newton: 0.000115) setJointStateCount:12\n sos:0.0163838 ineq:0 eq:0.0143332\n"
]
],
[
[
"The scalar product between the z-vector of `pr2R` and the z-vector of `goal` is zero:",
"_____no_output_____"
]
],
[
[
"C.setFrameState(X0)\nIK = C.komo_IK(False)\nIK.addObjective(type=ry.OT.eq, times=[1], feature=ry.FS.scalarProductZZ, frames=['pr2R', 'goal'])\nIK.optimize()\nC.setFrameState(IK.getFrameState(0))",
"** KOMO::run solver:dense collisions:0 x-dim:25 T:1 k:1 phases:1 stepsPerPhase:1 tau:1 #timeSlices:2 #totalDOFs:25 #frames:358\n** optimization time:0.000686185 (kin:7.1e-05 coll:3e-06 feat:0 newton: 4.2e-05) setJointStateCount:4\n sos:0.000248896 ineq:0 eq:0.00308733\n"
]
],
[
[
"etc etc",
"_____no_output_____"
],
[
"## More explanations\n\nAll methods to compute paths or configurations solve constrained optimization problems. To use them, you need to learn to define constrained optimization problems. Some definitions:\n\n* An objective defines either a sum-of-square cost term, or an equality constraint, or an inequality constraint in the optimization problem. An objective is defined by its type and its feature. The type can be `sos` (sum-of-squares), `eq`, or `ineq`, referring to the three cases.\n* A feature is a (differentiable mapping) from the decision variable (the full path, or robot configuration) to a feature space. If the feature space is, e.g., 3-dimensional, this defines 3 sum-of-squares terms, or 3 inequality, or 3 equality constraints, one for each dimension. For instance, the feature can be the 3-dim robot hand position in the 15th time slice of a path optimization problem. If you put an equality constraint on this feature, then this adds 3 equality constraints to the overall path optimization problem.\n* A feature is defined by the keyword for the feature map (e.g., `pos` for position), typically by a set of frame names that tell which objects we refer to (e.g., `pr2L` for the left hand of the pr2), optionally some modifiers (e.g., a scale or target, which linearly transform the feature map), and the set of configuration IDs or time slices the feature is to be computed from (e.g., `confs=[15]` if the feat is to be computed from the 15th time slice in a path optimization problem).\n* In path optimization problems, we often want to add objectives for a whole time interval rather than for a single time slice or specific configuration. E.g., avoid collisions from start to end. When adding objectives to the optimization problem we can specify whole intervals, with `times=[1., 2.]`, so that the objective is added to each configuration in this time interval.\n* Some features, especially velocity and acceleration, refer to a tuple of (consecutive) configurations. E.g., when you impose an acceleration feature, you need to specify `confs=[13, 14, 15]`. Or if you use `times=[1.,1.]`, the acceleration features is computed from the configuration that corresponds to time=1 and the two configurations *before*.\n* All kinematic feature maps (that depend on only one configuration) can be modified to become a velocity or acceleration features. E.g., the position feature map can be modified to become a velocity or acceleration feature.\n* The `sos`, `eq`, and `ineq` always refer to the feature map to be *zero*, e.g., constraining all features to be equal to zero with `eq`. This is natural for many features, esp. when they refer to differences (e.g. `posDiff` or `posRel`, which compute the relative position between two frames). However, when one aims to constrain the feature to a non-zero constant value, one can modify the objective with a `target` specification.\n* Finally, all features can be rescaled with a `scale` specification. Rescaling changes the costs that arise from `sos` objectives. Rescaling also has significant impact on the convergence behavior for `eq` and `ineq` constraints. As a guide: scale constraints so that if they *would* be treated as squared penalties (squaredPenalty optim mode, to be made accessible) convergence to reasonable approximate solutions is efficient. Then the AugLag will also converge efficiently to precise constraints.",
"_____no_output_____"
]
],
[
[
"# Designing a cylinder grasp",
"_____no_output_____"
],
[
"D=0\nC=0",
"_____no_output_____"
],
[
"import sys\nsys.path.append('../build') #rai/lib')\nimport numpy as np\nimport libry as ry",
"**ry-c++-log** /home/student/Desktop/KOMO/rai-python/rai/rai/ry/ry.cpp:init_LogToPythonConsole:34(0) initializing ry log callback** INFO:/home/student/Desktop/KOMO/rai-python/rai/rai/Core/util.cpp:initCmdLine:602(1) ** cmd line arguments: 'rai-pybind -python'\n** INFO:/home/student/Desktop/KOMO/rai-python/rai/rai/Core/util.cpp:initCmdLine:606(1) ** run path: '/home/student/Desktop/KOMO/rai-python/tutorials'\n** INFO:/home/student/Desktop/KOMO/rai-python/rai/rai/Core/graph.cpp:initParameters:1363(1) ** parsed parameters:\n{python,\nLGP/cameraFocus:[1, 0.5, 1],\nLGP/collisions:1,\nLGP/stopTime:300,\nLGP/stopSol:6,\nopt/maxStep:0.1}\n\n\n\n** INFO:/home/student/Desktop/KOMO/rai-python/rai/rai/ry/ry.cpp:init_LogToPythonConsole:34(0) initializing ry log callback\n"
],
[
"C = ry.Config()\nC.addFile('../rai-robotModels/pr2/pr2.g')\nC.addFile('../rai-robotModels/objects/kitchen.g')\nC.view()",
"_____no_output_____"
],
[
"C.setJointState([.7], [\"l_gripper_l_finger_joint\"])\nC.setJointState( C.getJointState() )",
"_____no_output_____"
],
[
"goal = C.addFrame(\"goal\")\ngoal.setShape(ry.ST.cylinder, [0,0,.2, .03])\ngoal.setColor([.5,1,1])\ngoal.setPosition([1.81,.5,1])\nX0 = C.getFrameState()",
"_____no_output_____"
],
[
"C.setFrameState(X0)\ngoal.setPosition([1.81,.5,1])\nIK = C.komo_IK(False)\nIK.addObjective(type=ry.OT.eq, times=[1],feature=ry.FS.positionDiff, frames=['pr2L', 'goal'], scale=[[1,0,0],[0,1,0]])\nIK.addObjective(type=ry.OT.ineq, times=[1], feature=ry.FS.positionDiff, frames=['pr2L', 'goal'], scale=[[0,0,1]], target=[0,0,.0005])\nIK.addObjective(type=ry.OT.ineq, times=[1], feature=ry.FS.positionDiff, frames=['pr2L', 'goal'], scale=[[0,0,-1]], target=[0,0,-.0005])\nIK.addObjective(type=ry.OT.sos, times=[1], feature=ry.FS.scalarProductZZ, frames=['pr2L', 'goal'], scale=[0.1])\nIK.addObjective(type=ry.OT.eq, times=[1], feature=ry.FS.scalarProductXZ, frames=['pr2L', 'goal'])\nIK.optimize()\nC.setFrameState(IK.getFrameState(0))\nIK.getReport()",
"** KOMO::run solver:dense collisions:0 x-dim:25 T:1 k:1 phases:1 stepsPerPhase:1 tau:1 #timeSlices:2 #totalDOFs:25 #frames:358\n** optimization time:0.00273175 (kin:0.000107 coll:2.6e-05 feat:0 newton: 0.00033) setJointStateCount:33\n sos:0.355635 ineq:0.0438169 eq:0.634637\n"
],
[
"IK.view()",
"_____no_output_____"
]
]
] | [
"markdown",
"code",
"markdown",
"code",
"markdown",
"code",
"markdown",
"code",
"markdown",
"code",
"markdown",
"code",
"markdown",
"code",
"markdown",
"code",
"markdown",
"code",
"markdown",
"code",
"markdown",
"code"
] | [
[
"markdown",
"markdown"
],
[
"code",
"code"
],
[
"markdown"
],
[
"code"
],
[
"markdown"
],
[
"code"
],
[
"markdown"
],
[
"code"
],
[
"markdown"
],
[
"code"
],
[
"markdown"
],
[
"code"
],
[
"markdown"
],
[
"code"
],
[
"markdown"
],
[
"code"
],
[
"markdown"
],
[
"code"
],
[
"markdown"
],
[
"code"
],
[
"markdown",
"markdown"
],
[
"code",
"code",
"code",
"code",
"code",
"code",
"code",
"code"
]
] |
e772eba41c591bb483bb80ddb303cae3fdf3bd74 | 32,418 | ipynb | Jupyter Notebook | Activity_1_Python_Fundamentals.ipynb | catherinedrio/Linear-Algebra_ChE_2nd-Sem-2021-2022 | ee00ebd5940b20de5cdf71a9cf1deeee9849bb8b | [
"Apache-2.0"
] | null | null | null | Activity_1_Python_Fundamentals.ipynb | catherinedrio/Linear-Algebra_ChE_2nd-Sem-2021-2022 | ee00ebd5940b20de5cdf71a9cf1deeee9849bb8b | [
"Apache-2.0"
] | null | null | null | Activity_1_Python_Fundamentals.ipynb | catherinedrio/Linear-Algebra_ChE_2nd-Sem-2021-2022 | ee00ebd5940b20de5cdf71a9cf1deeee9849bb8b | [
"Apache-2.0"
] | null | null | null | 22.991489 | 277 | 0.396662 | [
[
[
"<a href=\"https://colab.research.google.com/github/catherinedrio/Linear-Algebra_ChE_2nd-Sem-2021-2022/blob/main/Activity_1_Python_Fundamentals.ipynb\" target=\"_parent\"><img src=\"https://colab.research.google.com/assets/colab-badge.svg\" alt=\"Open In Colab\"/></a>",
"_____no_output_____"
],
[
"# Welcome to Python Fundamentals\nIn this module, we are going to establish or review our skills in Python programming. In this notebook we are going to cover:\n* Variables and Data Types \n* Operations\n* Input and Output Operations\n* Logic Control\n* Iterables\n* Functions",
"_____no_output_____"
],
[
"## Variable and Data Types",
"_____no_output_____"
]
],
[
[
"x = 1\na,b = 0, -1",
"_____no_output_____"
],
[
"type(x)",
"_____no_output_____"
],
[
"y = 1,0\ntype(y)",
"_____no_output_____"
],
[
"x = float(x)\ntype(x)",
"_____no_output_____"
],
[
"s,t,u =\"0\", \"1\", \"one\"\ntype(s)",
"_____no_output_____"
],
[
"s_int = int(s)\ns_int",
"_____no_output_____"
]
],
[
[
"##Operations\n",
"_____no_output_____"
],
[
"### Arithmetic\n",
"_____no_output_____"
]
],
[
[
"a,b,c,d = 2.0, -0.5, 0, -32",
"_____no_output_____"
],
[
"### Addition\nS = a+b\nS",
"_____no_output_____"
],
[
"### Subtraction\nD = b-d\nD ",
"_____no_output_____"
],
[
"### Multiplication\nP = a*d \nP",
"_____no_output_____"
],
[
"### Division\nQ = c/d \nQ",
"_____no_output_____"
],
[
"### Floor Division\nFq = a//b\nFq",
"_____no_output_____"
],
[
"### Exponentiation\nE = a**b\nE",
"_____no_output_____"
],
[
"### Modulo\nmod = d%a\nmod",
"_____no_output_____"
]
],
[
[
"## Assingment Operations",
"_____no_output_____"
]
],
[
[
"G, H, J, K = 0, 100, 2, 2",
"_____no_output_____"
],
[
"G += a\nG",
"_____no_output_____"
],
[
"H -= d \nH",
"_____no_output_____"
],
[
"J *= 2\nJ",
"_____no_output_____"
],
[
"K **= 3\nK",
"_____no_output_____"
]
],
[
[
"## Comparators",
"_____no_output_____"
]
],
[
[
"res_1, res_2, res_3 = 1, 2.0, \"1\"\ntrue_val = 1.0",
"_____no_output_____"
],
[
"## Equality\nres_1 == true_val",
"_____no_output_____"
],
[
"## Non-equality\nres_2 != true_val",
"_____no_output_____"
],
[
"## Inequality\nt1 = res_1 > res_2\nt2 = res_1 < res_2/2\nt3 = res_1 >= res_2/2\nt4 = res_1 <= res_2\nt1",
"_____no_output_____"
]
],
[
[
"## Logical",
"_____no_output_____"
]
],
[
[
"res_1 == true_val",
"_____no_output_____"
],
[
"res_1 is true_val",
"_____no_output_____"
],
[
"res_1 is not true_val",
"_____no_output_____"
],
[
"p, q = True, False\nconj = p and q \nconj",
"_____no_output_____"
],
[
"p, q = True, False\ndisj = p or q \ndisj",
"_____no_output_____"
],
[
"p, q = True, False\nnand = not(p and q)\nnand",
"_____no_output_____"
],
[
"p, q = True, False\nxor = (not p and q) or (p and not q)\nxor",
"_____no_output_____"
]
],
[
[
"## 1/0",
"_____no_output_____"
]
],
[
[
"print (\"Hello World\")",
"Hello World\n"
],
[
"cnt = 1",
"_____no_output_____"
],
[
"string = \"Hello World\"\nprint(string, \", Current run count is:\", cnt)\ncnt +=1",
"Hello World , Current run count is: 1\n"
],
[
"print(f\"{string}, Current count is {cnt}\")",
"Hello World, Current count is 2\n"
],
[
"sem_grade = 82.24356457461234\nname = \"cath\"\nprint(\"Hello {}, your semestral grade is: {}\".format(name, sem_grade))",
"Hello cath, your semestral grade is: 82.24356457461234\n"
],
[
"w_pg, w_mg, w_fg = 0.3, 0.3, 0.4\nprint(\"The weights of your semestral grades are:\\\n\\n\\t{:.2%} for Prelims\\\n\\n\\t{:.2%} for Midterms, and\\\n\\n\\t{:.2%} for Finals, \".format(w_pg, w_mg, w_fg))",
"The weights of your semestral grades are:\n\t30.00% for Prelims\n\t30.00% for Midterms, and\n\t40.00% for Finals, \n"
],
[
"x = input(\"enter a number: \")\nx",
"enter a number: 7\n"
],
[
"name = input(\"kimi no nawa: \")\npg = input(\"Enter prelim grade: \")\nmg = input(\"Enter midterm grade: \")\nfg = input(\"Enter finals grade: \")\nsem_grade = None\nprint(\"Hello {}, your semestral grade is: {}\". format (name, sem_grade))",
"kimi no nawa: Cath\nEnter prelim grade: 1.00\nEnter midterm grade: 1.00\nEnter finals grade: 1.00\nHello Cath, your semestral grade is: None\n"
]
],
[
[
"# Looping Statements",
"_____no_output_____"
],
[
"## While",
"_____no_output_____"
]
],
[
[
"## while loops\ni, j = 0, 10\nwhile(i<=j):\n print(f\"{i}\\t|\\t{j}\")\n i+=1",
"0\t|\t10\n1\t|\t10\n2\t|\t10\n3\t|\t10\n4\t|\t10\n5\t|\t10\n6\t|\t10\n7\t|\t10\n8\t|\t10\n9\t|\t10\n10\t|\t10\n"
]
],
[
[
"## For",
"_____no_output_____"
]
],
[
[
"# for(int i=0; i<10; i++){\n # printf(i)\n # }\n\ni=0\nfor i in range(11):\n print(i)",
"0\n1\n2\n3\n4\n5\n6\n7\n8\n9\n10\n"
],
[
"playlist = [\"Crazier\", \"Bahay-Kubo\", \"Happier\"]\nprint('Now Playing:\\n')\nfor song in playlist:\n print(song)",
"Now Playing:\n\nCrazier\nBahay-Kubo\nHappier\n"
]
],
[
[
"# Flow Control",
"_____no_output_____"
],
[
"## Conditions Statemnents",
"_____no_output_____"
]
],
[
[
"numeral1, numeral2 = 12, 12\nif(numeral1 == numeral2):\n print(\"Yey\")\nelif(numeral1>numeral2):\n print(\"Hoho\")\nelse:\n print(\"AWW\")\nprint(\"Hip hip\")",
"Yey\nHip hip\n"
]
],
[
[
"## Functions\n\n",
"_____no_output_____"
]
],
[
[
"[ ] # void DeleteUser(int userid){\n # delete(userid);\n # }\n\ndef delete_user (userid):\n print(\"Successfully deleted user: {}\". format(userid))\n\ndef delete_all_users ():\n print(\"Successfully deleted all users\")\n\nuserid = 202011844\ndelete_user(202011844)\ndelete_all_users()",
"Successfully deleted user: 202011844\nSuccessfully deleted all users\n"
],
[
"def add(addend1, addend2):\n print(\"I know how to add addend1 and addend2\")\n return addend1 + addend2\n\ndef power_of_base2(exponent):\n return 2**exponent\n\naddend1 = 5\naddend2 = 10\n\nexponent = 5\n\n#add(addend1, addend2)\npower_of_base2(exponent)",
"_____no_output_____"
]
],
[
[
"## Grade Calculator",
"_____no_output_____"
],
[
"Create a grade calculator that computes for the semestral grade of a course. Students could type their names, the name of the course, then their prelim, midterm, and final grade.\nThe program should print the semestral grade in 2 decimal points and should display the following emojis depending on the situation:\nhappy - when grade is greater thann 70.00\nlaughing - wen grade is exactly 70.00\nsad - when grade is below 70.00\n...\nhappy, lol, sad - \"\\U0001F600\", \"\\U0001F606\", \"\\U0001F62D\"",
"_____no_output_____"
]
],
[
[
"w_pg, w_mg, w_fg = 0.3, 0.3, 0.4\nname = input(\"Enter your name: \")\ncourse = input(\"Enter your course: \")\npg = float(input(\"Enter prelim grade: \"))\nmg = float(input(\"Enter midterm grade: \"))\nfg = float(input(\"Enter final grade: \"))\nsem_grade = (pg*w_pg)+(mg*w_mg)+(fg*w_fg)\nprint(\"Hello {} from {}, your semestral grade is: {}\" .format(name, course, round(sem_grade,2)))\nif(sem_grade > 70.00):\n print(\"\\U0001f600\")\nelif(sem_grade == 70.00):\n print(\"\\U0001F606\")\nelse:\n print(\"\\U0001F620\")",
"Enter your name: Catherine\nEnter your course: BS Chemical Engineering\nEnter prelim grade: 97\nEnter midterm grade: 98\nEnter final grade: 99\nHello Catherine from BS Chemical Engineering, your semestral grade is: 98.1\n😀\n"
]
]
] | [
"markdown",
"code",
"markdown",
"code",
"markdown",
"code",
"markdown",
"code",
"markdown",
"code",
"markdown",
"code",
"markdown",
"code",
"markdown",
"code",
"markdown",
"code",
"markdown",
"code",
"markdown",
"code"
] | [
[
"markdown",
"markdown",
"markdown"
],
[
"code",
"code",
"code",
"code",
"code",
"code"
],
[
"markdown",
"markdown"
],
[
"code",
"code",
"code",
"code",
"code",
"code",
"code",
"code"
],
[
"markdown"
],
[
"code",
"code",
"code",
"code",
"code"
],
[
"markdown"
],
[
"code",
"code",
"code",
"code"
],
[
"markdown"
],
[
"code",
"code",
"code",
"code",
"code",
"code",
"code"
],
[
"markdown"
],
[
"code",
"code",
"code",
"code",
"code",
"code",
"code",
"code"
],
[
"markdown",
"markdown"
],
[
"code"
],
[
"markdown"
],
[
"code",
"code"
],
[
"markdown",
"markdown"
],
[
"code"
],
[
"markdown"
],
[
"code",
"code"
],
[
"markdown",
"markdown"
],
[
"code"
]
] |
e772f422b4707f6efacf3fb6a7c4cd7c69319797 | 3,187 | ipynb | Jupyter Notebook | notebooks/Benchmark.ipynb | philippeller/Neurthino.jl | 69a19d02fbe87f5889f956bd3caeae6a1e7b09e3 | [
"MIT"
] | 4 | 2021-01-16T13:19:58.000Z | 2022-01-12T13:00:59.000Z | notebooks/Benchmark.ipynb | philippeller/Neurthino.jl | 69a19d02fbe87f5889f956bd3caeae6a1e7b09e3 | [
"MIT"
] | 7 | 2020-08-07T17:15:04.000Z | 2021-09-16T16:02:17.000Z | notebooks/Benchmark.ipynb | philippeller/Neurthino.jl | 69a19d02fbe87f5889f956bd3caeae6a1e7b09e3 | [
"MIT"
] | 3 | 2021-01-26T01:57:28.000Z | 2022-01-14T12:25:50.000Z | 23.262774 | 81 | 0.486665 | [
[
[
"empty"
]
]
] | [
"empty"
] | [
[
"empty"
]
] |
e772f501622f3bc4b3834bb4eac7f3ddd3ea7c00 | 4,756 | ipynb | Jupyter Notebook | examples/notebooks/nifti_read_example.ipynb | gml16/MONAI | e4c434bacf5b5c5c7222a50ea3e551b1d3f3d12c | [
"Apache-2.0"
] | null | null | null | examples/notebooks/nifti_read_example.ipynb | gml16/MONAI | e4c434bacf5b5c5c7222a50ea3e551b1d3f3d12c | [
"Apache-2.0"
] | null | null | null | examples/notebooks/nifti_read_example.ipynb | gml16/MONAI | e4c434bacf5b5c5c7222a50ea3e551b1d3f3d12c | [
"Apache-2.0"
] | null | null | null | 25.031579 | 131 | 0.534483 | [
[
[
"# Nifti Read Example\n\nThe purpose of this notebook is to illustrate reading Nifti files and iterating over patches of the volumes loaded from them.",
"_____no_output_____"
]
],
[
[
"%matplotlib inline\n\nimport os\nimport sys\nfrom glob import glob\nimport tempfile\nimport numpy as np\nimport matplotlib.pyplot as plt\nimport nibabel as nib\nimport torch\nfrom torch.utils.data import DataLoader\n\nimport monai\nfrom monai.data import NiftiDataset, GridPatchDataset, create_test_image_3d\nfrom monai.transforms import Compose, AddChannel, Transpose, ScaleIntensity, ToTensor, RandSpatialCrop\n\nmonai.config.print_config()",
"MONAI version: 0.1a1.dev8+6.gb3c5761.dirty\nPython version: 3.6.9 |Anaconda, Inc.| (default, Jul 30 2019, 19:07:31) [GCC 7.3.0]\nNumpy version: 1.18.1\nPytorch version: 1.4.0\nIgnite version: 0.3.0\n"
]
],
[
[
"Create a number of test Nifti files:",
"_____no_output_____"
]
],
[
[
"tempdir = tempfile.mkdtemp()\n\nfor i in range(5):\n im, seg = create_test_image_3d(128, 128, 128)\n \n n = nib.Nifti1Image(im, np.eye(4))\n nib.save(n, os.path.join(tempdir, 'im%i.nii.gz'%i))\n \n n = nib.Nifti1Image(seg, np.eye(4))\n nib.save(n, os.path.join(tempdir, 'seg%i.nii.gz'%i))",
"_____no_output_____"
]
],
[
[
"Create a data loader which yields uniform random patches from loaded Nifti files:",
"_____no_output_____"
]
],
[
[
"images = sorted(glob(os.path.join(tempdir, 'im*.nii.gz')))\nsegs = sorted(glob(os.path.join(tempdir, 'seg*.nii.gz')))\n\nimtrans = Compose([\n ScaleIntensity(),\n AddChannel(),\n RandSpatialCrop((64, 64, 64), random_size=False),\n ToTensor()\n]) \n\nsegtrans = Compose([\n AddChannel(),\n RandSpatialCrop((64, 64, 64), random_size=False),\n ToTensor()\n]) \n \nds = NiftiDataset(images, segs, transform=imtrans, seg_transform=segtrans)\n\nloader = DataLoader(ds, batch_size=10, num_workers=2, pin_memory=torch.cuda.is_available())\nim, seg = monai.utils.misc.first(loader)\nprint(im.shape, seg.shape)",
"torch.Size([5, 1, 64, 64, 64]) torch.Size([5, 1, 64, 64, 64])\n"
]
],
[
[
"Alternatively create a data loader which yields patches in regular grid order from loaded images:",
"_____no_output_____"
]
],
[
[
"imtrans = Compose([\n ScaleIntensity(),\n AddChannel(),\n ToTensor()\n]) \n\nsegtrans = Compose([\n AddChannel(),\n ToTensor()\n]) \n \nds = NiftiDataset(images, segs, transform=imtrans, seg_transform=segtrans)\nds = GridPatchDataset(ds, (64, 64, 64))\n\nloader = DataLoader(ds, batch_size=10, num_workers=2, pin_memory=torch.cuda.is_available())\nim, seg = monai.utils.misc.first(loader)\nprint(im.shape, seg.shape)",
"torch.Size([10, 1, 64, 64, 64]) torch.Size([10, 1, 64, 64, 64])\n"
],
[
"!rm -rf {tempdir}",
"_____no_output_____"
]
]
] | [
"markdown",
"code",
"markdown",
"code",
"markdown",
"code",
"markdown",
"code"
] | [
[
"markdown"
],
[
"code"
],
[
"markdown"
],
[
"code"
],
[
"markdown"
],
[
"code"
],
[
"markdown"
],
[
"code",
"code"
]
] |
e773004c28bb5cca655859633f36169f62ab87ef | 164,783 | ipynb | Jupyter Notebook | Chapter05/02_community_detection_algorithms.ipynb | Wapiti08/Graph-Machine-Learning | 51f9ad2f1fb87c9c1713b90ca98180bcc286a269 | [
"MIT"
] | 93 | 2021-05-26T18:36:42.000Z | 2022-03-31T13:02:30.000Z | Chapter05/02_community_detection_algorithms.ipynb | Wapiti08/Graph-Machine-Learning | 51f9ad2f1fb87c9c1713b90ca98180bcc286a269 | [
"MIT"
] | 5 | 2021-07-23T10:28:26.000Z | 2022-03-29T08:32:32.000Z | Chapter05/02_community_detection_algorithms.ipynb | Wapiti08/Graph-Machine-Learning | 51f9ad2f1fb87c9c1713b90ca98180bcc286a269 | [
"MIT"
] | 62 | 2021-05-26T00:13:39.000Z | 2022-03-30T01:42:33.000Z | 301.248629 | 60,132 | 0.932432 | [
[
[
"# Network Communities Detection ",
"_____no_output_____"
],
[
"In this notebook, we will explore some methods to perform a community detection using several algortihms. Before testing the algorithms, let us create a simple benchmark graph. ",
"_____no_output_____"
]
],
[
[
"%matplotlib inline\nfrom matplotlib import pyplot as plt",
"_____no_output_____"
],
[
"import numpy as np\nimport pandas as pd",
"_____no_output_____"
],
[
"import networkx as nx \nG = nx.barbell_graph(m1=10, m2=4) ",
"_____no_output_____"
]
],
[
[
"### Matrix Factorization ",
"_____no_output_____"
],
[
"We start by using some matrix factorization technique to extract the embeddings, which are visualized and then clustered traditional clustering algorithms. ",
"_____no_output_____"
]
],
[
[
"from gem.embedding.hope import HOPE \ngf = HOPE(d=4, beta=0.01) \ngf.learn_embedding(G) \nembeddings = gf.get_embedding() ",
"SVD error (low rank): 0.052092\n"
],
[
"from sklearn.manifold import TSNE",
"_____no_output_____"
],
[
"tsne = TSNE(n_components=2) \n\nemb2d = tsne.fit_transform(embeddings)",
"_____no_output_____"
],
[
"plt.plot(embeddings[:, 0], embeddings[:, 1], 'o', linewidth=0)",
"_____no_output_____"
]
],
[
[
"We start by using a GaussianMixture model to perform the clustering",
"_____no_output_____"
]
],
[
[
"from sklearn.mixture import GaussianMixture",
"_____no_output_____"
],
[
"gm = GaussianMixture(n_components=3, random_state=0) #.(embeddings)",
"_____no_output_____"
],
[
"labels = gm.fit_predict(embeddings)",
"_____no_output_____"
],
[
"colors = [\"blue\", \"green\", \"red\"]",
"_____no_output_____"
],
[
"nx.draw_spring(G, node_color=[colors[label] for label in labels])",
"_____no_output_____"
]
],
[
[
"### Spectral Clustering",
"_____no_output_____"
],
[
"We now perform a spectral clustering based on the adjacency matrix of the graph. It is worth noting that this clustering is not a mutually exclusive clustering and nodes may belong to more than one community",
"_____no_output_____"
]
],
[
[
"adj=np.array(nx.adjacency_matrix(G).todense())",
"_____no_output_____"
],
[
"from communities.algorithms import spectral_clustering\n\ncommunities = spectral_clustering(adj, k=3)",
"_____no_output_____"
]
],
[
[
"In the next plot we highlight the nodes that belong to a community using the red color. The blue nodes do not belong to the given community",
"_____no_output_____"
]
],
[
[
"plt.figure(figsize=(20, 5))\n\nfor ith, community in enumerate(communities):\n cols = [\"red\" if node in community else \"blue\" for node in G.nodes]\n plt.subplot(1,3,ith+1)\n plt.title(f\"Community {ith}\")\n nx.draw_spring(G, node_color=cols)",
"_____no_output_____"
]
],
[
[
"The next command shows the node ids belonging to the different communities",
"_____no_output_____"
]
],
[
[
"communities",
"_____no_output_____"
]
],
[
[
"### Non Negative Matrix Factorization ",
"_____no_output_____"
],
[
"Here, we again use matrix factorization, but now using the Non-Negative Matrix Factorization, and associating the clusters with the latent dimensions.",
"_____no_output_____"
]
],
[
[
"from sklearn.decomposition import NMF",
"_____no_output_____"
],
[
"nmf = NMF(n_components=2)",
"_____no_output_____"
],
[
"emb = nmf.fit_transform(adj)",
"/Users/deusebio/.pyenv/versions/3.8.6/envs/ml-book-5/lib/python3.8/site-packages/sklearn/decomposition/_nmf.py:312: FutureWarning: The 'init' value, when 'init=None' and n_components is less than n_samples and n_features, will be changed from 'nndsvd' to 'nndsvda' in 1.1 (renaming of 0.26).\n warnings.warn((\"The 'init' value, when 'init=None' and \"\n"
],
[
"plt.plot(emb[:, 0], emb[:, 1], 'o', linewidth=0)",
"_____no_output_____"
]
],
[
[
"By setting a threshold value of 0.01, we determine which nodes belong to the given community.",
"_____no_output_____"
]
],
[
[
"communities = [set(np.where(emb[:,ith]>0.01)[0]) for ith in range(2)]",
"_____no_output_____"
],
[
"plt.figure(figsize=(20, 5))\n\nfor ith, community in enumerate(communities):\n cols = [\"red\" if node in community else \"blue\" for node in G.nodes]\n plt.subplot(1,3,ith+1)\n plt.title(f\"Community {ith}\")\n nx.draw_spring(G, node_color=cols)",
"_____no_output_____"
]
],
[
[
"Although the example above does not show this, in general also this clustering method may be non-mutually exclusive, and nodes may belong to more than one community",
"_____no_output_____"
],
[
"### Louvain and Modularity Optimization",
"_____no_output_____"
],
[
"Here, we use the Louvain method, which is one of the most popular methods for performing community detection, even on fairly large graphs. As described in the chapter, the Louvain method basically optimize the partitioning (it is a mutually exclusing community detection algorithm), identifying the one that maximize the modularity score, meaning that nodes belonging to the same community are very well connected among themself, and weakly connected to the other communities. \n\n**Louvain, unlike other community detection algorithms, does not require to specity the number of communities in advance and find the best, optimal number of communities.**",
"_____no_output_____"
]
],
[
[
"from communities.algorithms import louvain_method\ncommunities = louvain_method(adj)",
"_____no_output_____"
],
[
"c = pd.Series({node: colors[ith] for ith, nodes in enumerate(communities) for node in nodes}).values\nnx.draw_spring(G, node_color=c)",
"_____no_output_____"
],
[
"communities",
"_____no_output_____"
]
],
[
[
"### Girvan Newman",
"_____no_output_____"
],
[
"The Girvan–Newman algorithm detects communities by progressively removing edges from the original graph. The algorithm removes the “most valuable” edge, traditionally the edge with the highest betweenness centrality, at each step. As the graph breaks down into pieces, the tightly knit community structure is exposed and the result can be depicted as a dendrogram.\n\n**BE AWARE that because of the betweeness centrality computation, this method may not scale well on large graphs**",
"_____no_output_____"
]
],
[
[
"from communities.algorithms import girvan_newman\ncommunities = girvan_newman(adj, n=2)",
"_____no_output_____"
],
[
"c = pd.Series({node: colors[ith] for ith, nodes in enumerate(communities) for node in nodes}).values\nnx.draw_spring(G, node_color=c)",
"_____no_output_____"
],
[
"communities",
"_____no_output_____"
]
]
] | [
"markdown",
"code",
"markdown",
"code",
"markdown",
"code",
"markdown",
"code",
"markdown",
"code",
"markdown",
"code",
"markdown",
"code",
"markdown",
"code",
"markdown",
"code",
"markdown",
"code"
] | [
[
"markdown",
"markdown"
],
[
"code",
"code",
"code"
],
[
"markdown",
"markdown"
],
[
"code",
"code",
"code",
"code"
],
[
"markdown"
],
[
"code",
"code",
"code",
"code",
"code"
],
[
"markdown",
"markdown"
],
[
"code",
"code"
],
[
"markdown"
],
[
"code"
],
[
"markdown"
],
[
"code"
],
[
"markdown",
"markdown"
],
[
"code",
"code",
"code",
"code"
],
[
"markdown"
],
[
"code",
"code"
],
[
"markdown",
"markdown",
"markdown"
],
[
"code",
"code",
"code"
],
[
"markdown",
"markdown"
],
[
"code",
"code",
"code"
]
] |
e773046a4ad91ba28cff74d91215d1a001c9ec81 | 59,316 | ipynb | Jupyter Notebook | models/ML Pipeline Preparation.ipynb | thiagofuruchima/disaster_message_classification | 3f11f1152f127d71c7ce8aeaa58393f6f2344c22 | [
"MIT"
] | 1 | 2021-03-22T21:42:53.000Z | 2021-03-22T21:42:53.000Z | models/ML Pipeline Preparation.ipynb | thiagofuruchima/disaster_message_classification | 3f11f1152f127d71c7ce8aeaa58393f6f2344c22 | [
"MIT"
] | null | null | null | models/ML Pipeline Preparation.ipynb | thiagofuruchima/disaster_message_classification | 3f11f1152f127d71c7ce8aeaa58393f6f2344c22 | [
"MIT"
] | null | null | null | 44.035635 | 341 | 0.319054 | [
[
[
"<a href=\"https://colab.research.google.com/github/thiagofuruchima/disaster_message_classification/blob/main/models/ML%20Pipeline%20Preparation.ipynb\" target=\"_parent\"><img src=\"https://colab.research.google.com/assets/colab-badge.svg\" alt=\"Open In Colab\"/></a>",
"_____no_output_____"
],
[
"# ML Pipeline Preparation\nFollow the instructions below to help you create your ML pipeline.\n### 1. Import libraries and load data from database.\n- Import Python libraries\n- Load dataset from database with [`read_sql_table`](https://pandas.pydata.org/pandas-docs/stable/generated/pandas.read_sql_table.html)\n- Define feature and target variables X and Y",
"_____no_output_____"
]
],
[
[
"# import libraries\nimport pandas as pd\nfrom sqlalchemy import create_engine\nfrom sklearn.model_selection import train_test_split, GridSearchCV\nfrom sklearn.multioutput import MultiOutputClassifier\nfrom sklearn.ensemble import RandomForestClassifier\nfrom sklearn.pipeline import Pipeline, FeatureUnion\nfrom sklearn.metrics import classification_report\nfrom sklearn.feature_extraction.text import CountVectorizer, TfidfTransformer\nfrom nltk.tokenize import word_tokenize\nfrom nltk.stem import WordNetLemmatizer\nimport pickle\n\nimport nltk",
"_____no_output_____"
],
[
"nltk.download(['punkt', 'wordnet', 'averaged_perceptron_tagger'])",
"[nltk_data] Downloading package punkt to /root/nltk_data...\n[nltk_data] Package punkt is already up-to-date!\n[nltk_data] Downloading package wordnet to /root/nltk_data...\n[nltk_data] Package wordnet is already up-to-date!\n[nltk_data] Downloading package averaged_perceptron_tagger to\n[nltk_data] /root/nltk_data...\n[nltk_data] Package averaged_perceptron_tagger is already up-to-\n[nltk_data] date!\n"
],
[
"# load data from database\nengine = create_engine('sqlite:///DISASTER.db')\ndf = pd.read_sql_table(\"CLEAN_MESSAGES\", engine)\nX = df['message']\nY = df.iloc[:,4:]\nX.head()",
"_____no_output_____"
]
],
[
[
"### 2. Write a tokenization function to process your text data",
"_____no_output_____"
]
],
[
[
"def tokenize(text):\n tokens = word_tokenize(text)\n lemmatizer = WordNetLemmatizer()\n\n clean_tokens = []\n for tok in tokens:\n clean_tok = lemmatizer.lemmatize(tok).lower().strip()\n clean_tokens.append(clean_tok)\n\n return clean_tokens\n",
"_____no_output_____"
]
],
[
[
"### 3. Build a machine learning pipeline\nThis machine pipeline should take in the `message` column as input and output classification results on the other 36 categories in the dataset. You may find the [MultiOutputClassifier](http://scikit-learn.org/stable/modules/generated/sklearn.multioutput.MultiOutputClassifier.html) helpful for predicting multiple target variables.",
"_____no_output_____"
]
],
[
[
"# create the NLP ML Pipeline\npipeline = Pipeline([\n ('vect', CountVectorizer(tokenizer=tokenize)),\n ('tfidf', TfidfTransformer()),\n ('clf', MultiOutputClassifier(RandomForestClassifier()))\n])",
"_____no_output_____"
]
],
[
[
"### 4. Train pipeline\n- Split data into train and test sets\n- Train pipeline",
"_____no_output_____"
]
],
[
[
"# Split the data in train and test sets\nX_train, X_test, Y_train, Y_test = train_test_split(X, Y)",
"_____no_output_____"
],
[
"# Fit the pipeline\r\npipeline.fit(X_train, Y_train)",
"_____no_output_____"
]
],
[
[
"### 5. Test your model\nReport the f1 score, precision and recall for each output category of the dataset. You can do this by iterating through the columns and calling sklearn's `classification_report` on each.",
"_____no_output_____"
]
],
[
[
"# Predict using the test data\r\nY_pred = pipeline.predict(X_test)",
"_____no_output_____"
],
[
"# Print the classification report for for each column\nfor i, column in enumerate(Y_train.columns):\n print(\"Columns: \", column)\n print(classification_report(Y_test.values[:,i], Y_pred[:,i]))\n print()",
"Columns: related\n precision recall f1-score support\n\n 0 0.76 0.26 0.39 1539\n 1 0.80 0.98 0.88 4967\n 2 1.00 0.04 0.08 48\n\n accuracy 0.80 6554\n macro avg 0.86 0.43 0.45 6554\nweighted avg 0.80 0.80 0.76 6554\n\n\nColumns: request\n precision recall f1-score support\n\n 0 0.89 0.99 0.94 5442\n 1 0.89 0.40 0.56 1112\n\n accuracy 0.89 6554\n macro avg 0.89 0.70 0.75 6554\nweighted avg 0.89 0.89 0.87 6554\n\n\nColumns: offer\n precision recall f1-score support\n\n 0 1.00 1.00 1.00 6527\n 1 0.00 0.00 0.00 27\n\n accuracy 1.00 6554\n macro avg 0.50 0.50 0.50 6554\nweighted avg 0.99 1.00 0.99 6554\n\n\nColumns: aid_related\n precision recall f1-score support\n\n 0 0.77 0.89 0.82 3873\n 1 0.79 0.61 0.69 2681\n\n accuracy 0.77 6554\n macro avg 0.78 0.75 0.76 6554\nweighted avg 0.78 0.77 0.77 6554\n\n\nColumns: medical_help\n precision recall f1-score support\n\n 0 0.93 1.00 0.96 6054\n 1 0.62 0.05 0.10 500\n\n accuracy 0.93 6554\n macro avg 0.77 0.52 0.53 6554\nweighted avg 0.90 0.93 0.90 6554\n\n\nColumns: medical_products\n precision recall f1-score support\n\n 0 0.95 1.00 0.97 6221\n 1 0.72 0.05 0.10 333\n\n accuracy 0.95 6554\n macro avg 0.84 0.53 0.54 6554\nweighted avg 0.94 0.95 0.93 6554\n\n\nColumns: search_and_rescue\n precision recall f1-score support\n\n 0 0.97 1.00 0.99 6358\n 1 0.86 0.03 0.06 196\n\n accuracy 0.97 6554\n macro avg 0.91 0.52 0.52 6554\nweighted avg 0.97 0.97 0.96 6554\n\n\nColumns: security\n precision recall f1-score support\n\n 0 0.98 1.00 0.99 6430\n 1 0.00 0.00 0.00 124\n\n accuracy 0.98 6554\n macro avg 0.49 0.50 0.50 6554\nweighted avg 0.96 0.98 0.97 6554\n\n\nColumns: military\n precision recall f1-score support\n\n 0 0.97 1.00 0.98 6350\n 1 0.75 0.03 0.06 204\n\n accuracy 0.97 6554\n macro avg 0.86 0.51 0.52 6554\nweighted avg 0.96 0.97 0.96 6554\n\n\nColumns: child_alone\n precision recall f1-score support\n\n 0 1.00 1.00 1.00 6554\n\n accuracy 1.00 6554\n macro avg 1.00 1.00 1.00 6554\nweighted avg 1.00 1.00 1.00 6554\n\n\nColumns: water\n precision recall f1-score support\n\n 0 0.95 1.00 0.97 6117\n 1 0.95 0.21 0.34 437\n\n accuracy 0.95 6554\n macro avg 0.95 0.60 0.66 6554\nweighted avg 0.95 0.95 0.93 6554\n\n\nColumns: food\n precision recall f1-score support\n\n 0 0.94 0.99 0.96 5843\n 1 0.83 0.48 0.61 711\n\n accuracy 0.93 6554\n macro avg 0.89 0.73 0.79 6554\nweighted avg 0.93 0.93 0.92 6554\n\n\nColumns: shelter\n precision recall f1-score support\n\n 0 0.93 1.00 0.96 5978\n 1 0.85 0.25 0.38 576\n\n accuracy 0.93 6554\n macro avg 0.89 0.62 0.67 6554\nweighted avg 0.92 0.93 0.91 6554\n\n\nColumns: clothing\n precision recall f1-score support\n\n 0 0.98 1.00 0.99 6442\n 1 0.67 0.05 0.10 112\n\n accuracy 0.98 6554\n macro avg 0.83 0.53 0.55 6554\nweighted avg 0.98 0.98 0.98 6554\n\n\nColumns: money\n precision recall f1-score support\n\n 0 0.98 1.00 0.99 6407\n 1 1.00 0.02 0.04 147\n\n accuracy 0.98 6554\n macro avg 0.99 0.51 0.51 6554\nweighted avg 0.98 0.98 0.97 6554\n\n\nColumns: missing_people\n precision recall f1-score support\n\n 0 0.99 1.00 0.99 6486\n 1 1.00 0.01 0.03 68\n\n accuracy 0.99 6554\n macro avg 0.99 0.51 0.51 6554\nweighted avg 0.99 0.99 0.98 6554\n\n\nColumns: refugees\n"
]
],
[
[
"### 6. Improve your model\nUse grid search to find better parameters. ",
"_____no_output_____"
]
],
[
[
"# Define GridSearch parameters\nparameters = {'clf__estimator__n_estimators': range(100,200,100),\n 'clf__estimator__min_samples_split': range(2,3)}\n\n# Instantiate GridSearch object\ncv = GridSearchCV(pipeline, param_grid=parameters, n_jobs=4)\n\n# Use GridSearch to find the best parameters\ncv.fit(X_train, Y_train)",
"_____no_output_____"
]
],
[
[
"### 7. Test your model\nShow the accuracy, precision, and recall of the tuned model. \n\nSince this project focuses on code quality, process, and pipelines, there is no minimum performance metric needed to pass. However, make sure to fine tune your models for accuracy, precision and recall to make your project stand out - especially for your portfolio!",
"_____no_output_____"
]
],
[
[
"# Predict using the trained model with the best parameters\r\nY_pred = cv.predict(X_test)",
"_____no_output_____"
],
[
"# Print the classification report for for each column\r\nfor i, column in enumerate(Y_train.columns):\r\n print(\"Columns: \", column)\r\n print(classification_report(Y_test.values[:,i], Y_pred[:,i]))\r\n print()",
"Columns: related\n precision recall f1-score support\n\n 0 0.77 0.25 0.38 1539\n 1 0.80 0.98 0.88 4967\n 2 1.00 0.04 0.08 48\n\n accuracy 0.80 6554\n macro avg 0.86 0.42 0.45 6554\nweighted avg 0.80 0.80 0.76 6554\n\n\nColumns: request\n precision recall f1-score support\n\n 0 0.89 0.99 0.94 5442\n 1 0.88 0.42 0.57 1112\n\n accuracy 0.89 6554\n macro avg 0.89 0.70 0.75 6554\nweighted avg 0.89 0.89 0.88 6554\n\n\nColumns: offer\n precision recall f1-score support\n\n 0 1.00 1.00 1.00 6527\n 1 0.00 0.00 0.00 27\n\n accuracy 1.00 6554\n macro avg 0.50 0.50 0.50 6554\nweighted avg 0.99 1.00 0.99 6554\n\n\nColumns: aid_related\n precision recall f1-score support\n\n 0 0.77 0.88 0.82 3873\n 1 0.79 0.63 0.70 2681\n\n accuracy 0.78 6554\n macro avg 0.78 0.76 0.76 6554\nweighted avg 0.78 0.78 0.77 6554\n\n\nColumns: medical_help\n precision recall f1-score support\n\n 0 0.93 1.00 0.96 6054\n 1 0.68 0.06 0.11 500\n\n accuracy 0.93 6554\n macro avg 0.80 0.53 0.54 6554\nweighted avg 0.91 0.93 0.90 6554\n\n\nColumns: medical_products\n precision recall f1-score support\n\n 0 0.95 1.00 0.97 6221\n 1 0.72 0.05 0.10 333\n\n accuracy 0.95 6554\n macro avg 0.84 0.53 0.54 6554\nweighted avg 0.94 0.95 0.93 6554\n\n\nColumns: search_and_rescue\n precision recall f1-score support\n\n 0 0.97 1.00 0.99 6358\n 1 0.80 0.02 0.04 196\n\n accuracy 0.97 6554\n macro avg 0.89 0.51 0.51 6554\nweighted avg 0.97 0.97 0.96 6554\n\n\nColumns: security\n precision recall f1-score support\n\n 0 0.98 1.00 0.99 6430\n 1 0.00 0.00 0.00 124\n\n accuracy 0.98 6554\n macro avg 0.49 0.50 0.50 6554\nweighted avg 0.96 0.98 0.97 6554\n\n\nColumns: military\n precision recall f1-score support\n\n 0 0.97 1.00 0.98 6350\n 1 0.65 0.05 0.10 204\n\n accuracy 0.97 6554\n macro avg 0.81 0.53 0.54 6554\nweighted avg 0.96 0.97 0.96 6554\n\n\nColumns: child_alone\n precision recall f1-score support\n\n 0 1.00 1.00 1.00 6554\n\n accuracy 1.00 6554\n macro avg 1.00 1.00 1.00 6554\nweighted avg 1.00 1.00 1.00 6554\n\n\nColumns: water\n precision recall f1-score support\n\n 0 0.95 1.00 0.97 6117\n 1 0.88 0.21 0.34 437\n\n accuracy 0.95 6554\n macro avg 0.91 0.60 0.65 6554\nweighted avg 0.94 0.95 0.93 6554\n\n\nColumns: food\n precision recall f1-score support\n\n 0 0.93 0.99 0.96 5843\n 1 0.86 0.42 0.56 711\n\n accuracy 0.93 6554\n macro avg 0.90 0.70 0.76 6554\nweighted avg 0.93 0.93 0.92 6554\n\n\nColumns: shelter\n precision recall f1-score support\n\n 0 0.93 1.00 0.96 5978\n 1 0.84 0.21 0.33 576\n\n accuracy 0.93 6554\n macro avg 0.88 0.60 0.65 6554\nweighted avg 0.92 0.93 0.91 6554\n\n\nColumns: clothing\n precision recall f1-score support\n\n 0 0.98 1.00 0.99 6442\n 1 0.73 0.07 0.13 112\n\n accuracy 0.98 6554\n macro avg 0.86 0.54 0.56 6554\nweighted avg 0.98 0.98 0.98 6554\n\n\nColumns: money\n precision recall f1-score support\n\n 0 0.98 1.00 0.99 6407\n 1 1.00 0.02 0.04 147\n\n accuracy 0.98 6554\n macro avg 0.99 0.51 0.51 6554\nweighted avg 0.98 0.98 0.97 6554\n\n\nColumns: missing_people\n precision recall f1-score support\n\n 0 0.99 1.00 0.99 6486\n 1 1.00 0.01 0.03 68\n\n accuracy 0.99 6554\n macro avg 0.99 0.51 0.51 6554\nweighted avg 0.99 0.99 0.98 6554\n\n\nColumns: refugees\n"
]
],
[
[
"### 8. Try improving your model further. Here are a few ideas:\n* try other machine learning algorithms\n* add other features besides the TF-IDF",
"_____no_output_____"
]
],
[
[
"# TODO: Model is taking too long to fit\r\n# I have to find a better engine to process it, \r\n# before testing new ideas",
"_____no_output_____"
]
],
[
[
"### 9. Export your model as a pickle file",
"_____no_output_____"
]
],
[
[
"# Save the model to pickl file\nwith open(\"DISASSTER_MODEL.pkl\", 'wb') as file:\n file.write(pickle.dumps(cv))",
"_____no_output_____"
]
],
[
[
"### 10. Use this notebook to complete `train.py`\nUse the template file attached in the Resources folder to write a script that runs the steps above to create a database and export a model based on a new dataset specified by the user.",
"_____no_output_____"
]
]
] | [
"markdown",
"code",
"markdown",
"code",
"markdown",
"code",
"markdown",
"code",
"markdown",
"code",
"markdown",
"code",
"markdown",
"code",
"markdown",
"code",
"markdown",
"code",
"markdown"
] | [
[
"markdown",
"markdown"
],
[
"code",
"code",
"code"
],
[
"markdown"
],
[
"code"
],
[
"markdown"
],
[
"code"
],
[
"markdown"
],
[
"code",
"code"
],
[
"markdown"
],
[
"code",
"code"
],
[
"markdown"
],
[
"code"
],
[
"markdown"
],
[
"code",
"code"
],
[
"markdown"
],
[
"code"
],
[
"markdown"
],
[
"code"
],
[
"markdown"
]
] |
e7731a5063f3b64b6909cf58e657652acb590b19 | 153,894 | ipynb | Jupyter Notebook | DSC 530 - Data Exploration and Analysis/ThinkStats2/solutions/chap06soln.ipynb | Hakuna-Patata/BU_MSDS_PTW | 4759cb2db3e63ae5722bd42771e4d228dfbc733d | [
"MIT"
] | null | null | null | DSC 530 - Data Exploration and Analysis/ThinkStats2/solutions/chap06soln.ipynb | Hakuna-Patata/BU_MSDS_PTW | 4759cb2db3e63ae5722bd42771e4d228dfbc733d | [
"MIT"
] | null | null | null | DSC 530 - Data Exploration and Analysis/ThinkStats2/solutions/chap06soln.ipynb | Hakuna-Patata/BU_MSDS_PTW | 4759cb2db3e63ae5722bd42771e4d228dfbc733d | [
"MIT"
] | null | null | null | 154.978852 | 23,260 | 0.904233 | [
[
[
"# Examples and Exercises from Think Stats, 2nd Edition\n\nhttp://thinkstats2.com\n\nCopyright 2016 Allen B. Downey\n\nMIT License: https://opensource.org/licenses/MIT\n",
"_____no_output_____"
]
],
[
[
"from __future__ import print_function, division\n\n%matplotlib inline\n\nimport numpy as np\n\nimport brfss\n\nimport thinkstats2\nimport thinkplot",
"_____no_output_____"
]
],
[
[
"I'll start with the data from the BRFSS again.",
"_____no_output_____"
]
],
[
[
"df = brfss.ReadBrfss(nrows=None)",
"_____no_output_____"
]
],
[
[
"Here are the mean and standard deviation of female height in cm.",
"_____no_output_____"
]
],
[
[
"female = df[df.sex==2]\nfemale_heights = female.htm3.dropna()\nmean, std = female_heights.mean(), female_heights.std()\nmean, std",
"_____no_output_____"
]
],
[
[
"`NormalPdf` returns a Pdf object that represents the normal distribution with the given parameters.\n\n`Density` returns a probability density, which doesn't mean much by itself.",
"_____no_output_____"
]
],
[
[
"pdf = thinkstats2.NormalPdf(mean, std)\npdf.Density(mean + std)",
"_____no_output_____"
]
],
[
[
"`thinkplot` provides `Pdf`, which plots the probability density with a smooth curve.",
"_____no_output_____"
]
],
[
[
"thinkplot.Pdf(pdf, label='normal')\nthinkplot.Config(xlabel='x', ylabel='PDF', xlim=[140, 186])",
"_____no_output_____"
]
],
[
[
"`Pdf` provides `MakePmf`, which returns a `Pmf` object that approximates the `Pdf`. ",
"_____no_output_____"
]
],
[
[
"pmf = pdf.MakePmf()\nthinkplot.Pmf(pmf, label='normal')\nthinkplot.Config(xlabel='x', ylabel='PDF', xlim=[140, 186])",
"_____no_output_____"
]
],
[
[
"If you have a `Pmf`, you can also plot it using `Pdf`, if you have reason to think it should be represented as a smooth curve.",
"_____no_output_____"
]
],
[
[
"thinkplot.Pdf(pmf, label='normal')\nthinkplot.Config(xlabel='x', ylabel='PDF', xlim=[140, 186])",
"_____no_output_____"
]
],
[
[
"Using a sample from the actual distribution, we can estimate the PDF using Kernel Density Estimation (KDE).\n\nIf you run this a few times, you'll see how much variation there is in the estimate.",
"_____no_output_____"
]
],
[
[
"thinkplot.Pdf(pdf, label='normal')\n\nsample = np.random.normal(mean, std, 500)\nsample_pdf = thinkstats2.EstimatedPdf(sample, label='sample')\nthinkplot.Pdf(sample_pdf, label='sample KDE')\nthinkplot.Config(xlabel='x', ylabel='PDF', xlim=[140, 186])",
"_____no_output_____"
]
],
[
[
"## Moments\n\nRaw moments are just sums of powers.",
"_____no_output_____"
]
],
[
[
"def RawMoment(xs, k):\n return sum(x**k for x in xs) / len(xs)",
"_____no_output_____"
]
],
[
[
"The first raw moment is the mean. The other raw moments don't mean much.",
"_____no_output_____"
]
],
[
[
"RawMoment(female_heights, 1), RawMoment(female_heights, 2), RawMoment(female_heights, 3)",
"_____no_output_____"
],
[
"def Mean(xs):\n return RawMoment(xs, 1)\n\nMean(female_heights)",
"_____no_output_____"
]
],
[
[
"The central moments are powers of distances from the mean.",
"_____no_output_____"
]
],
[
[
"def CentralMoment(xs, k):\n mean = RawMoment(xs, 1)\n return sum((x - mean)**k for x in xs) / len(xs)",
"_____no_output_____"
]
],
[
[
"The first central moment is approximately 0. The second central moment is the variance.",
"_____no_output_____"
]
],
[
[
"CentralMoment(female_heights, 1), CentralMoment(female_heights, 2), CentralMoment(female_heights, 3)",
"_____no_output_____"
],
[
"def Var(xs):\n return CentralMoment(xs, 2)\n\nVar(female_heights)",
"_____no_output_____"
]
],
[
[
"The standardized moments are ratios of central moments, with powers chosen to make the dimensions cancel.",
"_____no_output_____"
]
],
[
[
"def StandardizedMoment(xs, k):\n var = CentralMoment(xs, 2)\n std = np.sqrt(var)\n return CentralMoment(xs, k) / std**k",
"_____no_output_____"
]
],
[
[
"The third standardized moment is skewness.",
"_____no_output_____"
]
],
[
[
"StandardizedMoment(female_heights, 1), StandardizedMoment(female_heights, 2), StandardizedMoment(female_heights, 3)",
"_____no_output_____"
],
[
"def Skewness(xs):\n return StandardizedMoment(xs, 3)\n\nSkewness(female_heights)",
"_____no_output_____"
]
],
[
[
"Normally a negative skewness indicates that the distribution has a longer tail on the left. In that case, the mean is usually less than the median.",
"_____no_output_____"
]
],
[
[
"def Median(xs):\n cdf = thinkstats2.Cdf(xs)\n return cdf.Value(0.5)",
"_____no_output_____"
]
],
[
[
"But in this case the mean is greater than the median, which indicates skew to the right.",
"_____no_output_____"
]
],
[
[
"Mean(female_heights), Median(female_heights)",
"_____no_output_____"
]
],
[
[
"Because the skewness is based on the third moment, it is not robust; that is, it depends strongly on a few outliers. Pearson's median skewness is more robust.",
"_____no_output_____"
]
],
[
[
"def PearsonMedianSkewness(xs):\n median = Median(xs)\n mean = RawMoment(xs, 1)\n var = CentralMoment(xs, 2)\n std = np.sqrt(var)\n gp = 3 * (mean - median) / std\n return gp",
"_____no_output_____"
]
],
[
[
"Pearson's skewness is positive, indicating that the distribution of female heights is slightly skewed to the right.",
"_____no_output_____"
]
],
[
[
"PearsonMedianSkewness(female_heights)",
"_____no_output_____"
]
],
[
[
"## Birth weights\n\nLet's look at the distribution of birth weights again.",
"_____no_output_____"
]
],
[
[
"import first\n\nlive, firsts, others = first.MakeFrames()",
"_____no_output_____"
]
],
[
[
"Based on KDE, it looks like the distribution is skewed to the left.",
"_____no_output_____"
]
],
[
[
"birth_weights = live.totalwgt_lb.dropna()\npdf = thinkstats2.EstimatedPdf(birth_weights)\nthinkplot.Pdf(pdf, label='birth weight')\nthinkplot.Config(xlabel='Birth weight (pounds)', ylabel='PDF')",
"_____no_output_____"
]
],
[
[
"The mean is less than the median, which is consistent with left skew.",
"_____no_output_____"
]
],
[
[
"Mean(birth_weights), Median(birth_weights)",
"_____no_output_____"
]
],
[
[
"And both ways of computing skew are negative, which is consistent with left skew.",
"_____no_output_____"
]
],
[
[
"Skewness(birth_weights), PearsonMedianSkewness(birth_weights)",
"_____no_output_____"
]
],
[
[
"## Adult weights\n\nNow let's look at adult weights from the BRFSS. The distribution looks skewed to the right.",
"_____no_output_____"
]
],
[
[
"adult_weights = df.wtkg2.dropna()\npdf = thinkstats2.EstimatedPdf(adult_weights)\nthinkplot.Pdf(pdf, label='Adult weight')\nthinkplot.Config(xlabel='Adult weight (kg)', ylabel='PDF')",
"_____no_output_____"
]
],
[
[
"The mean is greater than the median, which is consistent with skew to the right.",
"_____no_output_____"
]
],
[
[
"Mean(adult_weights), Median(adult_weights)",
"_____no_output_____"
]
],
[
[
"And both ways of computing skewness are positive.",
"_____no_output_____"
]
],
[
[
"Skewness(adult_weights), PearsonMedianSkewness(adult_weights)",
"_____no_output_____"
]
],
[
[
"## Exercises",
"_____no_output_____"
],
[
"The distribution of income is famously skewed to the right. In this exercise, we’ll measure how strong that skew is.\nThe Current Population Survey (CPS) is a joint effort of the Bureau of Labor Statistics and the Census Bureau to study income and related variables. Data collected in 2013 is available from http://www.census.gov/hhes/www/cpstables/032013/hhinc/toc.htm. I downloaded `hinc06.xls`, which is an Excel spreadsheet with information about household income, and converted it to `hinc06.csv`, a CSV file you will find in the repository for this book. You will also find `hinc2.py`, which reads this file and transforms the data.\n\nThe dataset is in the form of a series of income ranges and the number of respondents who fell in each range. The lowest range includes respondents who reported annual household income “Under \\$5000.” The highest range includes respondents who made “\\$250,000 or more.”\n\nTo estimate mean and other statistics from these data, we have to make some assumptions about the lower and upper bounds, and how the values are distributed in each range. `hinc2.py` provides `InterpolateSample`, which shows one way to model this data. It takes a `DataFrame` with a column, `income`, that contains the upper bound of each range, and `freq`, which contains the number of respondents in each frame.\n\nIt also takes `log_upper`, which is an assumed upper bound on the highest range, expressed in `log10` dollars. The default value, `log_upper=6.0` represents the assumption that the largest income among the respondents is $10^6$, or one million dollars.\n\n`InterpolateSample` generates a pseudo-sample; that is, a sample of household incomes that yields the same number of respondents in each range as the actual data. It assumes that incomes in each range are equally spaced on a `log10` scale.",
"_____no_output_____"
]
],
[
[
"def InterpolateSample(df, log_upper=6.0):\n \"\"\"Makes a sample of log10 household income.\n\n Assumes that log10 income is uniform in each range.\n\n df: DataFrame with columns income and freq\n log_upper: log10 of the assumed upper bound for the highest range\n\n returns: NumPy array of log10 household income\n \"\"\"\n # compute the log10 of the upper bound for each range\n df['log_upper'] = np.log10(df.income)\n\n # get the lower bounds by shifting the upper bound and filling in\n # the first element\n df['log_lower'] = df.log_upper.shift(1)\n df.loc[0, 'log_lower'] = 3.0\n\n # plug in a value for the unknown upper bound of the highest range\n df.loc[41, 'log_upper'] = log_upper\n \n # use the freq column to generate the right number of values in\n # each range\n arrays = []\n for _, row in df.iterrows():\n vals = np.linspace(row.log_lower, row.log_upper, row.freq)\n arrays.append(vals)\n\n # collect the arrays into a single sample\n log_sample = np.concatenate(arrays)\n return log_sample\n",
"_____no_output_____"
],
[
"import hinc\nincome_df = hinc.ReadData()",
"_____no_output_____"
],
[
"log_sample = InterpolateSample(income_df, log_upper=6.0)",
"/home/downey/anaconda3/lib/python3.6/site-packages/ipykernel_launcher.py:26: DeprecationWarning: object of type <class 'numpy.float64'> cannot be safely interpreted as an integer.\n"
],
[
"log_cdf = thinkstats2.Cdf(log_sample)\nthinkplot.Cdf(log_cdf)\nthinkplot.Config(xlabel='Household income (log $)',\n ylabel='CDF')",
"_____no_output_____"
],
[
"sample = np.power(10, log_sample)",
"_____no_output_____"
],
[
"cdf = thinkstats2.Cdf(sample)\nthinkplot.Cdf(cdf)\nthinkplot.Config(xlabel='Household income ($)',\n ylabel='CDF')",
"_____no_output_____"
]
],
[
[
"Compute the median, mean, skewness and Pearson’s skewness of the resulting sample. What fraction of households report a taxable income below the mean? How do the results depend on the assumed upper bound?",
"_____no_output_____"
]
],
[
[
"# Solution\n\nMean(sample), Median(sample)",
"_____no_output_____"
],
[
"# Solution\n\nSkewness(sample), PearsonMedianSkewness(sample)",
"_____no_output_____"
],
[
"# Solution\n\n# About 66% of the population makes less than the mean\n\ncdf.Prob(Mean(sample))",
"_____no_output_____"
]
],
[
[
"All of this is based on an assumption that the highest income is one million dollars, but that's certainly not correct. What happens to the skew if the upper bound is 10 million?\n\nWithout better information about the top of this distribution, we can't say much about the skewness of the distribution.",
"_____no_output_____"
]
]
] | [
"markdown",
"code",
"markdown",
"code",
"markdown",
"code",
"markdown",
"code",
"markdown",
"code",
"markdown",
"code",
"markdown",
"code",
"markdown",
"code",
"markdown",
"code",
"markdown",
"code",
"markdown",
"code",
"markdown",
"code",
"markdown",
"code",
"markdown",
"code",
"markdown",
"code",
"markdown",
"code",
"markdown",
"code",
"markdown",
"code",
"markdown",
"code",
"markdown",
"code",
"markdown",
"code",
"markdown",
"code",
"markdown",
"code",
"markdown",
"code",
"markdown",
"code",
"markdown",
"code",
"markdown",
"code",
"markdown"
] | [
[
"markdown"
],
[
"code"
],
[
"markdown"
],
[
"code"
],
[
"markdown"
],
[
"code"
],
[
"markdown"
],
[
"code"
],
[
"markdown"
],
[
"code"
],
[
"markdown"
],
[
"code"
],
[
"markdown"
],
[
"code"
],
[
"markdown"
],
[
"code"
],
[
"markdown"
],
[
"code"
],
[
"markdown"
],
[
"code",
"code"
],
[
"markdown"
],
[
"code"
],
[
"markdown"
],
[
"code",
"code"
],
[
"markdown"
],
[
"code"
],
[
"markdown"
],
[
"code",
"code"
],
[
"markdown"
],
[
"code"
],
[
"markdown"
],
[
"code"
],
[
"markdown"
],
[
"code"
],
[
"markdown"
],
[
"code"
],
[
"markdown"
],
[
"code"
],
[
"markdown"
],
[
"code"
],
[
"markdown"
],
[
"code"
],
[
"markdown"
],
[
"code"
],
[
"markdown"
],
[
"code"
],
[
"markdown"
],
[
"code"
],
[
"markdown"
],
[
"code"
],
[
"markdown",
"markdown"
],
[
"code",
"code",
"code",
"code",
"code",
"code"
],
[
"markdown"
],
[
"code",
"code",
"code"
],
[
"markdown"
]
] |
e7731b25a73d440ea3243269a24da186513c42be | 43,662 | ipynb | Jupyter Notebook | 9.cross-validation.ipynb | qkrwjdan/dacon_news_topic_clasiification | a77c5b19348b6dcdd1c6d150627c909b4caae53d | [
"MIT"
] | 1 | 2022-01-18T05:03:46.000Z | 2022-01-18T05:03:46.000Z | 9.cross-validation.ipynb | qkrwjdan/dacon_news_topic_clasiification | a77c5b19348b6dcdd1c6d150627c909b4caae53d | [
"MIT"
] | null | null | null | 9.cross-validation.ipynb | qkrwjdan/dacon_news_topic_clasiification | a77c5b19348b6dcdd1c6d150627c909b4caae53d | [
"MIT"
] | null | null | null | 29.946502 | 451 | 0.53211 | [
[
[
"# 교차 검증 학습 \n \n모델을 학습할 때, 검증을 위해 우리는 train data와 validation data를 분리합니다. \n이 경우, validation data는 모델의 학습에 영향을 미치지 않습니다. \n따라서 모델이 학습하는 data의 수가 줄어들고, train data에 overfitting 됩니다. \n \n이를 해결하기 위해 train data와 validation data를 나누는 과정을 여러번 반복하고 \n다양한 데이터셋을 사용하여 모델을 학습하는 방법을 cross validation (교차 검증 학습) 이라고 합니다. \n \ncross validation을 사용할 경우 모든 데이터를 학습과 평가에 사용할 수 있다는 장점이 있지만 \n학습시간이 오래걸린다는 단점이 있습니다.. \n",
"_____no_output_____"
],
[
"cross validation에는 다양한 방법이 있지만 이번 노트북에서는 Stratified k-fold cross validation을 사용해보았습니다. \nstratified k-fold cross validation을 사용하면 \nLabel의 분포가 불균형한 데이터일 경우 Label의 갯수를 고려하여 train, validation data를 나눠줍니다. ",
"_____no_output_____"
],
[
"모델은 klue/bert-base 모델을 사용했습니다. ",
"_____no_output_____"
]
],
[
[
"import random\nfrom tqdm.notebook import tqdm, tnrange\nimport os\n\nimport numpy as np\nimport pandas as pd\nfrom transformers import AutoTokenizer, AutoModelForSequenceClassification\nfrom transformers import AdamW\nfrom transformers import get_linear_schedule_with_warmup\n\nfrom sklearn.model_selection import train_test_split\nfrom sklearn.model_selection import StratifiedKFold\nfrom sklearn.metrics import accuracy_score\n\nimport torch\nfrom torch import nn\nfrom torch.utils.data import Dataset,TensorDataset, DataLoader, RandomSampler\n\n\nif torch.cuda.is_available():\n print(\"사용가능한 GPU수 : \",torch.cuda.device_count())\n device = torch.device(\"cuda\")\nelse:\n print(\"CPU 사용\")\n device = torch.device(\"cpu\")",
"사용가능한 GPU수 : 1\n"
]
],
[
[
"Reproduction을 위한 Seed 고정 \n출처 : https://dacon.io/codeshare/2363?dtype=vote&s_id=0",
"_____no_output_____"
]
],
[
[
"RANDOM_SEED = 42\n\ndef seed_everything(seed: int = 42):\n random.seed(seed)\n np.random.seed(seed)\n os.environ[\"PYTHONHASHSEED\"] = str(seed)\n torch.manual_seed(seed)\n torch.cuda.manual_seed(seed) # type: ignore\n torch.backends.cudnn.deterministic = True # type: ignore\n torch.backends.cudnn.benchmark = True # type: ignore\n \nseed_everything(RANDOM_SEED)",
"_____no_output_____"
],
[
"model_checkpoint = \"klue/bert-base\"\nbatch_size = 32",
"_____no_output_____"
],
[
"dataset = pd.read_csv(\"data/train_data.csv\")\ntest = pd.read_csv(\"data/test_data.csv\")",
"_____no_output_____"
],
[
"tokenizer = AutoTokenizer.from_pretrained(model_checkpoint, use_fast=True)",
"_____no_output_____"
],
[
"def bert_tokenize(dataset,sent_key,label_key,tokenizer):\n if label_key is None :\n labels = [np.int64(0) for i in dataset[sent_key]]\n else :\n labels = [np.int64(i) for i in dataset[label_key]]\n \n sentences = tokenizer(dataset[sent_key].tolist(),truncation=True,padding=True)\n\n input_ids = sentences.input_ids\n token_type_ids = sentences.token_type_ids\n attention_mask = sentences.attention_mask\n \n return [input_ids, token_type_ids, attention_mask, labels]",
"_____no_output_____"
]
],
[
[
"sklearn의 StratifiedKFold를 불러오고 예측한 데이터를 저장할 수 있는 변수를 만듭니다. \n`StratifiedKFold()`에서 `n_split=5`는 5개의 train data와 validation data를 만들겠다는 이야기입니다. ",
"_____no_output_____"
]
],
[
[
"NUM_TEST_DATA = len(test)\nskf = StratifiedKFold(n_splits=5)\nfinal_test_pred = np.zeros([NUM_TEST_DATA,7])",
"_____no_output_____"
]
],
[
[
"parameter들을 정의합니다. ",
"_____no_output_____"
]
],
[
[
"lr = 2e-5\nadam_epsilon = 1e-8\nepochs = 3\nnum_warmup_steps = 0\nnum_labels = 7",
"_____no_output_____"
]
],
[
[
"`train()`, `evaluate()`, `predict()`를 정의합니다. ",
"_____no_output_____"
]
],
[
[
"def train(model,train_dataloader):\n train_loss_set = []\n learning_rate = []\n batch_loss = 0\n\n for step, batch in enumerate(tqdm(train_dataloader)):\n model.train()\n\n batch = tuple(t.to(device) for t in batch)\n b_input_ids, b_token_type_ids, b_input_mask, b_labels = batch\n\n outputs = model(b_input_ids, token_type_ids=b_token_type_ids, attention_mask=b_input_mask, labels=b_labels)\n loss = outputs[0]\n\n loss.backward()\n torch.nn.utils.clip_grad_norm_(model.parameters(), 1.0)\n\n optimizer.step()\n\n scheduler.step()\n optimizer.zero_grad()\n\n batch_loss += loss.item()\n\n avg_train_loss = batch_loss / len(train_dataloader)\n\n\n for param_group in optimizer.param_groups:\n print(\"\\n\\tCurrent Learning rate: \",param_group['lr'])\n learning_rate.append(param_group['lr'])\n\n train_loss_set.append(avg_train_loss)\n print(F'\\n\\tAverage Training loss: {avg_train_loss}')\n \ndef evaluate(model, validation_dataloader):\n # validation\n model.eval()\n eval_accuracy,nb_eval_steps = 0, 0\n\n for batch in tqdm(validation_dataloader):\n \n batch = tuple(t.to(device) for t in batch)\n b_input_ids, b_token_type_ids, b_input_mask, b_labels = batch\n \n with torch.no_grad():\n logits = model(b_input_ids, token_type_ids=b_token_type_ids, attention_mask=b_input_mask)\n \n logits = logits[0].to('cpu').numpy()\n label_ids = b_labels.to('cpu').numpy()\n\n pred_flat = np.argmax(logits, axis=1).flatten()\n labels_flat = label_ids.flatten()\n\n tmp_eval_accuracy = accuracy_score(labels_flat,pred_flat)\n\n eval_accuracy += tmp_eval_accuracy\n nb_eval_steps += 1\n\n print(F'\\n\\tValidation Accuracy: {eval_accuracy/nb_eval_steps}')\n \ndef predict(model, test_dataloader):\n pred = []\n model.eval()\n\n for batch in tqdm(test_dataloader):\n\n batch = tuple(t.to(device) for t in batch)\n b_input_ids, b_token_type_ids, b_input_mask, b_labels = batch\n\n with torch.no_grad():\n logits = model(b_input_ids, token_type_ids=b_token_type_ids, attention_mask=b_input_mask)\n logits = logits[0].to('cpu').numpy()\n\n for p in logits:\n pred.append(p)\n\n return pred",
"_____no_output_____"
]
],
[
[
"`StratifiedKFold()`의 `split()`함수를 사용하면 인자로 주어진 데이터를 train data와 validation data로 나눈 index를 돌려줍니다. DataFrame에 index를 사용하여 train data와 validation data를 나눌 수 있습니다. \n나눠진 데이터로 학습과 평가를 진행한 뒤 test data를 예측합니다. \n예측한 데이터는 최종 예측 데이터(`final_test_pred`)에 합쳐집니다. \n총 학습에 걸리는 시간은 한번 학습하는데 걸리는 시간 * `n_splits`로 넘겨준 수 ( 여기서는 5 )입니다. \n",
"_____no_output_____"
]
],
[
[
"for train_idx, validation_idx in skf.split(dataset[\"title\"],dataset[\"topic_idx\"]):\n \n dataset_train = pd.DataFrame()\n dataset_val = pd.DataFrame()\n \n dataset_train[\"title\"] = dataset[\"title\"][train_idx]\n dataset_train[\"topic_idx\"] = dataset[\"topic_idx\"][train_idx]\n \n dataset_val[\"title\"] = dataset[\"title\"][validation_idx]\n dataset_val[\"topic_idx\"] = dataset[\"topic_idx\"][validation_idx]\n \n train_inputs = bert_tokenize(dataset_train,\"title\",\"topic_idx\",tokenizer)\n validation_inputs = bert_tokenize(dataset_val,\"title\",\"topic_idx\",tokenizer)\n test_inputs = bert_tokenize(test,\"title\",None,tokenizer)\n \n for i in range(len(train_inputs)):\n train_inputs[i] = torch.tensor(train_inputs[i])\n\n for i in range(len(validation_inputs)):\n validation_inputs[i] = torch.tensor(validation_inputs[i])\n\n for i in range(len(test_inputs)):\n test_inputs[i] = torch.tensor(test_inputs[i])\n \n train_data = TensorDataset(*train_inputs)\n train_sampler = RandomSampler(train_data)\n train_dataloader = DataLoader(train_data,sampler=train_sampler,batch_size=batch_size)\n\n validation_data = TensorDataset(*validation_inputs)\n validation_sampler = RandomSampler(validation_data)\n validation_dataloader = DataLoader(validation_data,sampler=validation_sampler,batch_size=batch_size)\n\n test_data = TensorDataset(*test_inputs)\n test_dataloader = DataLoader(test_data,batch_size=batch_size)\n \n model = AutoModelForSequenceClassification.from_pretrained(model_checkpoint,num_labels=num_labels)\n model.zero_grad()\n \n model.to(device)\n\n optimizer = AdamW(model.parameters(), lr=lr,eps=adam_epsilon,correct_bias=False)\n scheduler = get_linear_schedule_with_warmup(optimizer,\n num_warmup_steps=num_warmup_steps,\n num_training_steps=len(train_dataloader)*epochs) \n \n for _ in tnrange(1,epochs+1,desc='Epoch'):\n print(\"<\" + \"=\"*22 + F\" Epoch {_} \"+ \"=\"*22 + \">\")\n # train\n train(model, train_dataloader)\n \n # validation\n evaluate(model, validation_dataloader)\n \n # predict\n pred = predict(model, test_dataloader)\n final_test_pred += pred\n \n ",
"Some weights of the model checkpoint at klue/bert-base were not used when initializing BertForSequenceClassification: ['cls.predictions.transform.LayerNorm.bias', 'cls.predictions.transform.dense.bias', 'cls.predictions.transform.LayerNorm.weight', 'cls.seq_relationship.weight', 'cls.predictions.decoder.bias', 'cls.predictions.transform.dense.weight', 'cls.seq_relationship.bias', 'cls.predictions.decoder.weight', 'cls.predictions.bias']\n- This IS expected if you are initializing BertForSequenceClassification from the checkpoint of a model trained on another task or with another architecture (e.g. initializing a BertForSequenceClassification model from a BertForPreTraining model).\n- This IS NOT expected if you are initializing BertForSequenceClassification from the checkpoint of a model that you expect to be exactly identical (initializing a BertForSequenceClassification model from a BertForSequenceClassification model).\nSome weights of BertForSequenceClassification were not initialized from the model checkpoint at klue/bert-base and are newly initialized: ['classifier.bias', 'classifier.weight']\nYou should probably TRAIN this model on a down-stream task to be able to use it for predictions and inference.\n"
]
],
[
[
"5번의 교차 학습동안 서로 다른 train, validation data를 통해 학습한 model이 예측한 값은 `final_test_pred`에 더해져 있습니다. \n이 예측값을 `argmax`하여 최종 예측값을 만들어냅니다. ",
"_____no_output_____"
]
],
[
[
"final_test_pred[:10]",
"_____no_output_____"
],
[
"len(final_test_pred)",
"_____no_output_____"
],
[
"total_pred = np.argmax(final_test_pred,axis = 1)\ntotal_pred[:10]",
"_____no_output_____"
],
[
"submission = pd.read_csv('data/sample_submission.csv')\nsubmission['topic_idx'] = total_pred\nsubmission.to_csv(\"results/klue-bert-base-kfold5.csv\",index=False)",
"_____no_output_____"
]
]
] | [
"markdown",
"code",
"markdown",
"code",
"markdown",
"code",
"markdown",
"code",
"markdown",
"code",
"markdown",
"code",
"markdown",
"code"
] | [
[
"markdown",
"markdown",
"markdown"
],
[
"code"
],
[
"markdown"
],
[
"code",
"code",
"code",
"code",
"code"
],
[
"markdown"
],
[
"code"
],
[
"markdown"
],
[
"code"
],
[
"markdown"
],
[
"code"
],
[
"markdown"
],
[
"code"
],
[
"markdown"
],
[
"code",
"code",
"code",
"code"
]
] |
e773265a22043c77a2ccdd165856115db2c038e2 | 9,581 | ipynb | Jupyter Notebook | 5.0-tl-pytorch.ipynb | titus-leistner/3dcv-students | 372f97205a8f8bc989f94e08dd95b180a26eef74 | [
"MIT"
] | 4 | 2020-04-21T21:40:13.000Z | 2022-02-13T18:18:13.000Z | 5.0-tl-pytorch.ipynb | titus-leistner/3dcv-students | 372f97205a8f8bc989f94e08dd95b180a26eef74 | [
"MIT"
] | 1 | 2022-02-03T11:24:07.000Z | 2022-02-03T11:24:07.000Z | 5.0-tl-pytorch.ipynb | titus-leistner/3dcv-students | 372f97205a8f8bc989f94e08dd95b180a26eef74 | [
"MIT"
] | 8 | 2020-04-22T10:24:27.000Z | 2022-01-13T16:25:52.000Z | 27.141643 | 155 | 0.488049 | [
[
[
"# Exercise 3",
"_____no_output_____"
],
[
"**Please Note**: We updated the requirements.txt\n\nPlease install the new requirements before editing this exercise.",
"_____no_output_____"
],
[
"## Import packages",
"_____no_output_____"
]
],
[
[
"import os\n\nfrom vll.utils.download import download_mnist\nimport numpy as np\nimport matplotlib.pyplot as plt\n\nimport skimage\nimport skimage.io\n\nimport torch\nimport torch.nn.functional as F\nfrom torchvision import transforms\n\nfrom models.mnist.simple_cnn import Net",
"_____no_output_____"
]
],
[
[
"## Task 1\n(2 points)\n\nIn this task, you will learn some basic tensor operations using the PyTorch library.\n\nReference for torch: https://pytorch.org/docs/stable/torch.html",
"_____no_output_____"
]
],
[
[
"# Create a numpy array that looks like this: [0, 1, 2, ..., 19]\narr = \n\n# Convert the numpy array to a torch tensor\ntensor = \nprint(tensor)\n\n# Create a tensor that contains random numbers.\n# It should have the same size like the numpy array.\n# Multiply it with the previous tensor.\nrand_tensor = \ntensor = \nprint(tensor)\n\n# Create a tensor that contains only 1s.\n# It should have the same size like the numpy array.\n# Substract it from the previous tensor.\ntensor = \nprint(tensor)\n\n# Get the 5th element using a index.\nelement = \nprint(element)\n\n# Create a tensor that contains only 0s.\n# It should have the same size like the numpy array.\n# Multiply it with the previous tensor without any assignment (in place).\n",
"_____no_output_____"
],
[
"# Load the image from the last exercise as RGB image.\nimage = \n\n# Convert the image to a tensor\nimage = \n\n# Print its shape\nprint(image.shape)\n\n# Flatten the image\nimage = \nprint(len(image))\n\n# Add another dimension resulting in a 1x78643 tensor\n\nprint(image.shape)\n\n# Revert the last action\n\nprint(image.shape)\n\n# Reshape the tensor, so that it has the original 2D dimensions\nimage = \nprint(image.shape)\n\n# Calculate the sum, mean and max of the tensor\nprint(torch.sum(image))\nprint(torch.mean(image))\nprint(torch.max(image))",
"_____no_output_____"
]
],
[
[
"## Task 2\n(2 points)\n\nUse Autograd to perform operations on a tensor and output then gradients.",
"_____no_output_____"
]
],
[
[
"# Create a random 2x2 tensor which requires gradients\nx = \nprint(x)\n\n# Create another tensor by adding 2.0\ny = \nprint(y)\n\n# Create a third tensor z = y^2\nz = \nprint(z)\n\n# Compute out as the mean of values in z\nout = \nprint(out)\n\n# Perform back propagation on out\n\n\n# Print the gradients dout/dx\n\n\n# Create a copy of y whithout gradients\ny2 = \nprint(y2.requires_grad)\n\n# Perform the mean operation on z\n# with gradients globally disabled\n",
"_____no_output_____"
]
],
[
[
"## Task 3\n(3 points)\n\nImplement a Dataset class for MNIST.",
"_____no_output_____"
]
],
[
[
"# We first download the MNIST dataset\ndownload_mnist()",
"_____no_output_____"
],
[
"class MNIST:\n \"\"\"\n Dataset class for MNIST\n \"\"\"\n\n def __init__(self, root, transform=None):\n \"\"\"\n root -- path to either \"training\" or \"testing\"\n \n transform -- transform (from torchvision.transforms)\n to be applied to the data\n \"\"\"\n # save transforms\n self.transform = transform\n \n # TODO: create a list of all subdirectories (named like the classes) \n # within the dataset root\n \n \n # TODO: create a list of paths to all images\n # with the ground truth label\n \n \n def __len__(self):\n \"\"\"\n Returns the lenght of the dataset (number of images)\n \"\"\"\n # TODO: return the length (number of images) of the dataset\n \n\n def __getitem__(self, index):\n \"\"\"\n Loads and returns one image as floating point numpy array\n \n index -- image index in [0, self.__len__() - 1]\n \"\"\"\n # TODO: load the ith image as an numpy array (dtype=float32)\n \n \n # TODO: apply transforms to the image (if there are any)\n \n \n # TODO: return a tuple (transformed image, ground truth)\n ",
"_____no_output_____"
]
],
[
[
"## Task 4\n(3 points)\n\nYou can now load a pretrained neural network model we provide.\nYour last task is to run the model on the MNIST test dataset, plot some example images with the predicted labels and compute the prediction accuracy.",
"_____no_output_____"
]
],
[
[
"def validate(model, data_loader):\n # TODO: Create a 10x10 grid of subplots\n \n \n model.eval()\n correct = 0 # count for correct predictions\n \n with torch.no_grad():\n for i, item in enumerate(data_loader):\n # TODO: unpack item into image and ground truth\n # and run network on them\n \n \n # TODO: get class with highest probability\n \n \n # TODO: check if prediction is correct\n # and add it to correct count\n \n \n # plot the first 100 images\n if i < 100:\n # TODO: compute position of ith image in the grid\n \n \n # TODO: convert image tensor to numpy array\n # and normalize to [0, 1]\n \n \n # TODO: make wrongly predicted images red\n \n \n # TODO: disable axis and show image\n \n \n # TODO: show the predicted class next to each image\n \n \n elif i == 100:\n plt.show()\n \n # TODO: compute and print the prediction accuracy in percent\n \n\n# create a DataLoader using the implemented MNIST dataset class\ndata_loader = torch.utils.data.DataLoader(\n MNIST('data/mnist/testing',\n transform=transforms.Compose([\n transforms.ToTensor(),\n transforms.Normalize((0.1307,), (0.3081,))\n ])),\n batch_size=1, shuffle=True)\n\n# create the neural network\nmodel = Net()\n\n# load the statedict from 'models/mnist/simple_cnn.pt'\nmodel.load_state_dict(torch.load('models/mnist/simple_cnn.pt'))\n\n# validate the model\nvalidate(model, data_loader)",
"_____no_output_____"
]
]
] | [
"markdown",
"code",
"markdown",
"code",
"markdown",
"code",
"markdown",
"code",
"markdown",
"code"
] | [
[
"markdown",
"markdown",
"markdown"
],
[
"code"
],
[
"markdown"
],
[
"code",
"code"
],
[
"markdown"
],
[
"code"
],
[
"markdown"
],
[
"code",
"code"
],
[
"markdown"
],
[
"code"
]
] |
e7732f1968635fb1a5ac163df5ef93ec78aaf57d | 25,074 | ipynb | Jupyter Notebook | 7.18.ipynb | Y-kiwi77/python1 | 187b1dfca3ba9666bb6a9826c7ea582eea27fa8a | [
"Apache-2.0"
] | null | null | null | 7.18.ipynb | Y-kiwi77/python1 | 187b1dfca3ba9666bb6a9826c7ea582eea27fa8a | [
"Apache-2.0"
] | null | null | null | 7.18.ipynb | Y-kiwi77/python1 | 187b1dfca3ba9666bb6a9826c7ea582eea27fa8a | [
"Apache-2.0"
] | null | null | null | 19.712264 | 497 | 0.422469 | [
[
[
"# 选择\n## 布尔类型、数值和表达式\n\n- 注意:比较运算符的相等是两个等号,一个等到代表赋值\n- 在Python中可以用整型0来代表False,其他数字来代表True\n- 后面还会讲到 is 在判断语句中的用发",
"_____no_output_____"
],
[
"## 字符串的比较使用ASCII值",
"_____no_output_____"
],
[
"## Markdown \n- https://github.com/younghz/Markdown",
"_____no_output_____"
],
[
"## EP:\n- <img src=\"../Photo/34.png\"></img>\n- 输入一个数字,判断其实奇数还是偶数",
"_____no_output_____"
]
],
[
[
"#除了bool(0)是false以外,其他数全是true\n#bool(0) 执行时也是false\n#if bool(1-1):\n# print(yes)\n#else:\n# print(no)\n#结果是打印 no\n ",
"_____no_output_____"
],
[
"b1=bool(4)\nprint(b1)",
"True\n"
],
[
"i=3\nif i==5:\n print('i=5')\nelse:\n print(\"i!=5\")\n",
"i!=5\n"
],
[
"i=eval(input(\"输入i\" ))\nif i==5:\n print('i=5')\nelse:\n print(\"i!=5\")",
"输入i3\ni!=5\n"
]
],
[
[
"## 产生随机数字\n- 函数random.randint(a,b) 可以用来产生一个a和b之间且包括a和b的随机整数",
"_____no_output_____"
],
[
"产生一个随机数,你去输入,如果你输入的数大于随机数,那么就告诉你太大了,反之,太小了,\n然后你一直输入,知道它满意为止",
"_____no_output_____"
]
],
[
[
"import random\na=random.randint(1,100)\nwhile 1:\n b=eval(input(\"比较数\"))\n if a>b:\n print(\"太小了\")\n if a<b:\n print(\"太大了\")\n if a==b:\n print(\"yes\")\n break",
"比较数50\n太小了\n比较数60\n太小了\n比较数70\n太小了\n比较数80\n太小了\n比较数90\n太小了\n比较数95\nyes\n"
]
],
[
[
"## 其他random方法\n- random.random 返回0.0到1.0之间前闭后开区间的随机浮点\n- random.randrange(a,b) 前闭后开",
"_____no_output_____"
],
[
"## EP:\n- 产生两个随机整数number1和number2,然后显示给用户,使用户输入数字的和,并判定其是否正确\n- 进阶:写一个随机序号点名程序",
"_____no_output_____"
]
],
[
[
"import random\na=random.randint(1,10)\nb=random.randint(1,10)\nc=a+b\nnumber=0\nwhile number<5:\n d=eval(input(\"和为?\"))\n if c>d:\n print(\"太小了\")\n if c<d:\n print(\"太大了\")\n if c==d:\n print(\"yes\")\n break\n number +=1\n",
"和为?15\n太大了\n和为?10\n太大了\n和为?5\n太小了\n和为?8\n太小了\n和为?9\n"
],
[
"import random\na=random.randint(1,10)\nb=random.randint(1,10)\nc=a+b\nfor i in range(5):\n d=eval(input(\"和为?\"))\n if c>d:\n print(\"太小了\")\n if c<d:\n print(\"太大了\")\n if c==d:\n print(\"yes\")\n break\n\n",
"和为?50\n太大了\n和为?10\n太大了\n和为?20\n太大了\n和为?30\n太大了\n和为?40\n太大了\n"
],
[
"#输入一个数字,把它拆分成因子\n#range(a,b) 从a按正序输出到b, a,b 可以是数字可以是变量。",
"_____no_output_____"
],
[
"a=eval(input(\"输入一个数\"))\nwhile number<a:\n ",
"_____no_output_____"
]
],
[
[
"## if语句\n- 如果条件正确就执行一个单向if语句,亦即当条件为真的时候才执行if内部的语句\n- Python有很多选择语句:\n> - 单向if \n - 双向if-else\n - 嵌套if\n - 多向if-elif-else\n \n- 注意:当语句含有子语句的时候,那么一定至少要有一个缩进,也就是说如果有儿子存在,那么一定要缩进\n- 切记不可tab键和space混用,单用tab 或者 space\n- 当你输出的结果是无论if是否为真时都需要显示时,语句应该与if对齐",
"_____no_output_____"
],
[
"## EP:\n- 用户输入一个数字,判断其实奇数还是偶数\n- 进阶:可以查看下4.5实例研究猜生日",
"_____no_output_____"
],
[
"## 双向if-else 语句\n- 如果条件为真,那么走if内部语句,否则走else内部语句",
"_____no_output_____"
]
],
[
[
"a=eval(input(\"数字\"))\nif a>2:\n if a%2==0:\n print(\"大于二的偶数\")\n else:\n print(\"大于二的奇数\")\nelse:\n print(\"不大于二\")",
"数字5\n大于二的奇数\n"
],
[
"a=input(\"有钱吗?\")\na1=\"有钱\"\nb1=\"帅\"\nc1=\"没有\"\nif a==a1: #字符串可以直接比较,不需要定义变量 \n b=input(\"帅不帅\")\n if b==b1:\n print(\"有没有老婆\")\n c=input(\"\")\n if c==c1:\n print(\"见一面\")\n else:\n print(\"滚\") \n else:\n print(\"回家等着吧\")\nelse:\n print(\"不大于二\")",
"有钱吗?有钱\n帅不帅帅\n有没有老婆\n有\n滚\n"
]
],
[
[
"## EP:\n- 产生两个随机整数number1和number2,然后显示给用户,使用户输入数字,并判定其是否正确,如果正确打印“you‘re correct”,否则打印正确错误",
"_____no_output_____"
],
[
"## 嵌套if 和多向if-elif-else\n",
"_____no_output_____"
]
],
[
[
"#出现一次elif,就要出现一次if\n#有点相似于else不能单独出现",
"_____no_output_____"
],
[
"a=input(\"有钱吗?\")\nif a==\"有\":\n b=input(\"帅不帅 \")\nelif b==\"不帅\":\n c=input(\"有老婆吗 \")\nelif c==\"没有\":\n print(\"结婚\")\nelse:\n print(\"滚\")",
"有钱吗?有\n帅不帅 不帅\n"
]
],
[
[
"## EP:\n- 提示用户输入一个年份,然后显示表示这一年的动物\n\n- 计算身体质量指数的程序\n- BMI = 以千克为单位的体重除以以米为单位的身高的平方\n",
"_____no_output_____"
]
],
[
[
"#多行同时输入 按住ALT 等鼠标变加号,下拉被选中的行,同时编写",
"_____no_output_____"
],
[
"year=eval(input(\"请输入年份\"))\nif year%12==0:\n print(\"猴\") \nelif year%12==1:\n print(\"鸡\")\nelif year%12==2:\n print(\"狗\")\nelif year%12==3:\n print(\"猪\")\nelif year%12==4:\n print(\"鼠\")\nelif year%12==5:\n print(\"牛\")\nelif year%12==6:\n print(\"虎\")\nelif year%12==7:\n print(\"兔\")\nelif year%12==8:\n print(\"龙\")\nelif year%12==9:\n print(\"蛇\")\nelif year%12==10:\n print(\"马\")\nelif year%12==11:\n print(\"羊\")\n",
"请输入年份1999\n兔\n"
],
[
"h=eval(input(\"请输入身高\"))\nw=eval(input(\"请输入体重\"))\nBMI=w/h/h\nif BMI<18.5:\n print(\"超轻\")\nelif 18.5<=BMI<25:\n print(\"标准\")\nelif 25<=BMI<30:\n print(\"超重\")\nelif 30<=30:\n print(\"痴肥\")",
"请输入身高1.69\n请输入体重47\n超轻\n"
]
],
[
[
"## 逻辑运算符\n",
"_____no_output_____"
],
[
"\n",
"_____no_output_____"
],
[
"## EP:\n- 判定闰年:一个年份如果能被4整除但不能被100整除,或者能被400整除,那么这个年份就是闰年\n- 提示用户输入一个年份,并返回是否是闰年\n- 提示用户输入一个数字,判断其是否为水仙花数",
"_____no_output_____"
]
],
[
[
"year=eval(input(\"请输入年份\"))\nif (year%100!=0) and (year%4==0):\n print(\"是闰年\")\nif year%400==0:\n print(\"是闰年\")\nelse:\n print(\"是平年\")",
"请输入年份2000\n是闰年\n"
],
[
"shu=eval(input(\"请输入一个数\"))\nbai=shu//100\nshi=shu//10\nshi1=shi%10\nge=shu%10\na=bai/bai\nb=shi1/shi1\n#已经知道是三位数了,不需要判断\n#c=ge/ge\n#d=a+b+c\n#e=bai**d+shi1**d+ge**d\ne=bai**3+shi1**3+ge**3\nif e==shu:\n print(\"是水仙花数\")\nelse:\n print(\"不是\")",
"请输入一个数371\n是水仙花数\n"
],
[
"shu=eval(input(\"请输入一个数\"))\nbai=shu//100\nshi=shu//10%10\nge=shu%10\nprint(bai,shi,ge)\nif bai**3+shi**3+ge**3==shu:\n print(shu)\nelse:\n print(\"不是\")",
"请输入一个数370\n3 7 0\n370\n"
],
[
"for i in range(100,999):\n bai=i//100\n shi=i//10\n shi1=shi%10\n ge=i%10\n e=bai**3+shi1**3+ge**3\n if e==i:\n print(i)",
"153\n370\n371\n407\n"
]
],
[
[
"## 实例研究:彩票\n",
"_____no_output_____"
],
[
"# Homework\n- 1\n",
"_____no_output_____"
]
],
[
[
"import math\na=eval(input(\"a\"))\nb=eval(input(\"b\"))\nc=eval(input(\"c\"))\npan=b**2-4*a*c\nif pan>0:\n print(\"两个根\")\nelif pan<0:\n print(\"没有根\")\nelse:\n print(\"有一个根\")",
"a1\nb2\nc3\n没有根\n"
]
],
[
[
"- 2\n",
"_____no_output_____"
]
],
[
[
"import random\na=random.randint(1,100)\nb=random.randint(1,100)\nc=a+b\nd=eval(input(\"和为?\"))\nif c==d:\n print(\"真\")\nelse:\n print(\"假\")",
"_____no_output_____"
]
],
[
[
"- 3\n",
"_____no_output_____"
]
],
[
[
"x=eval(input(\"今天是星期几?\"))\njth=eval(input(\"你想算几天以后\"))\nc=(x+jth)%7\nif c==0:\n print(\"今天是星期日\")\nelse:\n print(\"今天是星期\",c)",
"今天是星期几?5\n你想算几天以后7\n今天是星期 5\n"
]
],
[
[
"- 4\n",
"_____no_output_____"
]
],
[
[
"i=eval(input(\"请输入一个整数\"))\nc=eval(input(\"请输入一个整数\"))\nk=eval(input(\"请输入一个整数\"))\nlist1=[i,c,k]\nlist1.sort()\nprint(list1)",
"请输入一个整数5\n请输入一个整数1\n请输入一个整数9\n[1, 5, 9]\n"
]
],
[
[
"- 5\n",
"_____no_output_____"
]
],
[
[
"w1=eval(input(\"请输入包装\"))\nm1=eval(input(\"请输入重量\"))\nw2=eval(input(\"请输入包装\"))\nm2=eval(input(\"请输入重量\"))\nb1=w1*m1\nb2=w2*m2\nif b1>b2:\n print(\"b2更合适\")\nelse :\n print(\"b1更合适\")",
"请输入包装50\n请输入重量24.59\n请输入包装25\n请输入重量11.99\nb2更合适\n"
]
],
[
[
"- 6\n",
"_____no_output_____"
]
],
[
[
"mo1=eval(input(\"请输入月\"))\nyear1=eval(input(\"请输入年\"))\nif (year1%100!=0) and (year1%4==0) and year1%400==0:\n if mo1==2:\n print(year1,\"年\",mo1,\"月份\",\"有29天\") \nelse:\n if mo1==1:\n print(year1,\"年\",mo1,\"月份\",\"有31天\") \n elif mo1==2:\n print(year1,\"年\",mo1,\"月份\",\"有28天\") \n elif mo1==3:\n print(year1,\"年\",mo1,\"月份\",\"有31天\") \n elif mo1==4:\n print(year1,\"年\",mo1,\"月份\",\"有30天\") \n elif mo1==5:\n print(year1,\"年\",mo1,\"月份\",\"有31天\") \n elif mo1==6:\n print(year1,\"年\",mo1,\"月份\",\"有30天\") \n elif mo1==7:\n print(year1,\"年\",mo1,\"月份\",\"有31天\") \n elif mo1==8:\n print(year1,\"年\",mo1,\"月份\",\"有31天\") \n elif mo1==9:\n print(year1,\"年\",mo1,\"月份\",\"有30天\") \n elif mo1==10:\n print(year1,\"年\",mo1,\"月份\",\"有31天\") \n elif mo1==11:\n print(year1,\"年\",mo1,\"月份\",\"有30天\") \n elif mo1==12:\n print(year1,\"年\",mo1,\"月份\",\"有31天\") \n",
"请输入月2\n请输入年2001\n2001 年 2 月份 有28天\n"
]
],
[
[
"- 7\n",
"_____no_output_____"
]
],
[
[
"import random\nyingbi=random.randint(1,2)\ncai=eval(input(\"你猜猜\"))\nif yingbi==cai:\n print(\"正确\")\nelse:\n print(\"错误\")",
"你猜猜2\n错误\n"
]
],
[
[
"- 8\n",
"_____no_output_____"
]
],
[
[
"import random\ndian_nao=random.randint(0,2)\nren=eval(input(\"你要出什么?\"+\"石头=0 剪刀=2 布=1 \"))\nprint(dian_nao)\nif ren==dian_nao:\n print(\"平局\")\nelse:\n if ren==0 and dian_nao==2:\n print(\"赢了\")\n elif ren==2 and dian_nao==0:\n print(\"输了\")\n elif ren>dian_nao:\n print(\"赢了\")\n else:\n print(\"输了\")",
"你要出什么?石头=0 剪刀=2 布=1 0\n1\n输了\n"
]
],
[
[
"- 9\n",
"_____no_output_____"
]
],
[
[
"import math\nyear=eval(input(\"请输入年\"))\nm=eval(input(\"请输入月\"))\nq=eval(input(\"请输入日\"))\nif m==1:\n m=13\n year=year-1\nif m==2:\n m=14\n year=year-1\nh=(q+int(26*(m+1)/10)+int(year%100)+int(year%100/4)+int(year/100/4)+int(5*year/100))%7\nif h==0:\n print(\"今天是星期六\")\nif h==1:\n print(\"今天是星期日\")\nif h==2:\n print(\"今天是星期一\")\nif h==3:\n print(\"今天是星期二\")\nif h==4:\n print(\"今天是星期三\")\nif h==5:\n print(\"今天是星期四\")\nif h==6:\n print(\"今天是星期五\")",
"请输入年2019\n请输入月4\n请输入日29\n今天是星期一\n"
],
[
"a=3.7\nprint(int(a))\nh=(q+int(26*(m+1)/10)+int(year%100)+int(year%100/4)+int(year/100/4)+int(5*year/100)%7",
"3\n"
]
],
[
[
"- 10\n",
"_____no_output_____"
]
],
[
[
"import random\nhua=random.randint(1,4)\ndaxiao=random.randint(1,13)\nif hua==1:\n hua=\"红桃\"\nelif hua==2:\n hua=\"梅花\"\nelif hua==3:\n hua=\"方块\"\nelif hua==4:\n hua=\"黑桃\"\nif daxiao==1:\n daxiao=\"Ace\"\nelif daxiao==11:\n daxiao=\"Jack\"\nelif daxiao==12:\n daxiao=\"Queen\"\nelif daxiao==13:\n daxiao=\"King\"\nprint(\"这张牌是 \",hua,daxiao)\n\n \n \n ",
"这张牌是 方块 King\n"
]
],
[
[
"- 11\n",
"_____no_output_____"
]
],
[
[
"shu11=eval(input(\"请输入一个数\"))\nbai=shu11//100\nshi=shu11//10%10\nge=shu11%10\nif bai==ge:\n print(shu11,\"是回文数\")\nelse:\n print(\"不是回文数\")",
"请输入一个数123\n不是回文数\n"
]
],
[
[
"- 12\n",
"_____no_output_____"
]
],
[
[
"bian1=eval(input(\"请输入第一条边的边长\"))\nbian2=eval(input(\"请输入第二条边的边长\"))\nbian3=eval(input(\"请输入第三条边的边长\"))\nif bian1+bian2>bian3 and abs(bian1-bian2)<bian3:\n print(\"合理\")\nelse:\n print(\"不合理\")",
"请输入第一条边的边长1\n请输入第二条边的边长9\n请输入第三条边的边长1\n不合理\n"
],
[
"bian1=eval(input(\"请输入第一条边的边长\"))\nbian2=eval(input(\"请输入第二条边的边长\"))\nbian3=eval(input(\"请输入第三条边的边长\"))\nqing3=bian1+bian2\nqing2=bian1+bian3\nqing1=bian3+bian2\nq3=bian1-bian2\nq2=bian1-bian3\nq1=bian3-bian2\nif qing1>bian1 and qing2>bian2 and qing3>bian3 :\n print(\"合理\")\nelse:\n print(\"不合理\")",
"请输入第一条边的边长1\n请输入第二条边的边长1\n请输入第三条边的边长9\n不合理\n"
]
]
] | [
"markdown",
"code",
"markdown",
"code",
"markdown",
"code",
"markdown",
"code",
"markdown",
"code",
"markdown",
"code",
"markdown",
"code",
"markdown",
"code",
"markdown",
"code",
"markdown",
"code",
"markdown",
"code",
"markdown",
"code",
"markdown",
"code",
"markdown",
"code",
"markdown",
"code",
"markdown",
"code",
"markdown",
"code",
"markdown",
"code",
"markdown",
"code"
] | [
[
"markdown",
"markdown",
"markdown",
"markdown"
],
[
"code",
"code",
"code",
"code"
],
[
"markdown",
"markdown"
],
[
"code"
],
[
"markdown",
"markdown"
],
[
"code",
"code",
"code",
"code"
],
[
"markdown",
"markdown",
"markdown"
],
[
"code",
"code"
],
[
"markdown",
"markdown"
],
[
"code",
"code"
],
[
"markdown"
],
[
"code",
"code",
"code"
],
[
"markdown",
"markdown",
"markdown"
],
[
"code",
"code",
"code",
"code"
],
[
"markdown",
"markdown"
],
[
"code"
],
[
"markdown"
],
[
"code"
],
[
"markdown"
],
[
"code"
],
[
"markdown"
],
[
"code"
],
[
"markdown"
],
[
"code"
],
[
"markdown"
],
[
"code"
],
[
"markdown"
],
[
"code"
],
[
"markdown"
],
[
"code"
],
[
"markdown"
],
[
"code",
"code"
],
[
"markdown"
],
[
"code"
],
[
"markdown"
],
[
"code"
],
[
"markdown"
],
[
"code",
"code"
]
] |
e7733271d5914f9d701a3c71db8614214f04c601 | 17,842 | ipynb | Jupyter Notebook | Data Modeling with Cassandra/Project_1B_ Project_Template.ipynb | fernandofsilva/Data_Engineering-Udacity | 1d444cfd8ba40e28b2345c027c2eeaace3e534ae | [
"MIT"
] | null | null | null | Data Modeling with Cassandra/Project_1B_ Project_Template.ipynb | fernandofsilva/Data_Engineering-Udacity | 1d444cfd8ba40e28b2345c027c2eeaace3e534ae | [
"MIT"
] | null | null | null | Data Modeling with Cassandra/Project_1B_ Project_Template.ipynb | fernandofsilva/Data_Engineering-Udacity | 1d444cfd8ba40e28b2345c027c2eeaace3e534ae | [
"MIT"
] | 2 | 2021-08-05T18:29:47.000Z | 2021-08-06T00:52:06.000Z | 30.815199 | 210 | 0.470015 | [
[
[
"# Part I. ETL Pipeline for Pre-Processing the Files",
"_____no_output_____"
],
[
"## PLEASE RUN THE FOLLOWING CODE FOR PRE-PROCESSING THE FILES",
"_____no_output_____"
],
[
"#### Import Python packages ",
"_____no_output_____"
]
],
[
[
"# Import Python packages \nimport pandas as pd\nimport cassandra\nimport re\nimport os\nimport glob\nimport numpy as np\nimport json\nimport csv",
"_____no_output_____"
]
],
[
[
"#### Creating list of filepaths to process original event csv data files",
"_____no_output_____"
]
],
[
[
"# checking your current working directory\nprint(os.getcwd())\n\n# Get your current folder and subfolder event data\nfilepath = os.getcwd() + '/event_data'\n\n# Create a for loop to create a list of files and collect each filepath\nfor root, dirs, files in os.walk(filepath):\n \n# join the file path and roots with the subdirectories using glob\n file_path_list = glob.glob(os.path.join(root,'*'))\n #print(file_path_list)",
"/home/workspace\n"
]
],
[
[
"#### Processing the files to create the data file csv that will be used for Apache Casssandra tables",
"_____no_output_____"
]
],
[
[
"# initiating an empty list of rows that will be generated from each file\nfull_data_rows_list = [] \n \n# for every filepath in the file path list \nfor f in file_path_list:\n\n# reading csv file \n with open(f, 'r', encoding = 'utf8', newline='') as csvfile: \n # creating a csv reader object \n csvreader = csv.reader(csvfile) \n next(csvreader)\n \n # extracting each data row one by one and append it \n for line in csvreader:\n #print(line)\n full_data_rows_list.append(line) \n \n# uncomment the code below if you would like to get total number of rows \n#print(len(full_data_rows_list))\n# uncomment the code below if you would like to check to see what the list of event data rows will look like\n#print(full_data_rows_list)\n\n# creating a smaller event data csv file called event_datafile_full csv that will be used to insert data into the \\\n# Apache Cassandra tables\ncsv.register_dialect('myDialect', quoting=csv.QUOTE_ALL, skipinitialspace=True)\n\nwith open('event_datafile_new.csv', 'w', encoding = 'utf8', newline='') as f:\n writer = csv.writer(f, dialect='myDialect')\n writer.writerow(['artist','firstName','gender','itemInSession','lastName','length',\\\n 'level','location','sessionId','song','userId'])\n for row in full_data_rows_list:\n if (row[0] == ''):\n continue\n writer.writerow((row[0], row[2], row[3], row[4], row[5], row[6], row[7], row[8], row[12], row[13], row[16]))\n",
"_____no_output_____"
],
[
"# check the number of rows in your csv file\nwith open('event_datafile_new.csv', 'r', encoding = 'utf8') as f:\n print(sum(1 for line in f))",
"6821\n"
]
],
[
[
"# Part II. Complete the Apache Cassandra coding portion of your project. \n\n## Now you are ready to work with the CSV file titled <font color=red>event_datafile_new.csv</font>, located within the Workspace directory. The event_datafile_new.csv contains the following columns: \n- artist \n- firstName of user\n- gender of user\n- item number in session\n- last name of user\n- length of the song\n- level (paid or free song)\n- location of the user\n- sessionId\n- song title\n- userId\n\nThe image below is a screenshot of what the denormalized data should appear like in the <font color=red>**event_datafile_new.csv**</font> after the code above is run:<br>\n\n<img src=\"images/image_event_datafile_new.jpg\">",
"_____no_output_____"
],
[
"## Begin writing your Apache Cassandra code in the cells below",
"_____no_output_____"
],
[
"#### Creating a Cluster",
"_____no_output_____"
]
],
[
[
"# This should make a connection to a Cassandra instance your local machine \n# (127.0.0.1)\n\nfrom cassandra.cluster import Cluster\ncluster = Cluster()\n\n# To establish connection and begin executing queries, need a session\nsession = cluster.connect()",
"_____no_output_____"
]
],
[
[
"#### Create Keyspace",
"_____no_output_____"
]
],
[
[
"# TO-DO: Create a Keyspace\ntry:\n session.execute(\"\"\"\n CREATE KEYSPACE IF NOT EXISTS udacity \n WITH REPLICATION = \n { 'class' : 'SimpleStrategy', 'replication_factor' : 1 }\"\"\"\n)\n\nexcept Exception as e:\n print(e)",
"_____no_output_____"
]
],
[
[
"#### Set Keyspace",
"_____no_output_____"
]
],
[
[
"# TO-DO: Set KEYSPACE to the keyspace specified above\ntry:\n session.set_keyspace('udacity')\nexcept Exception as e:\n print(e)",
"_____no_output_____"
]
],
[
[
"### Now we need to create tables to run the following queries. Remember, with Apache Cassandra you model the database tables on the queries you want to run.",
"_____no_output_____"
],
[
"## Create queries to ask the following three questions of the data\n\n### 1. Give me the artist, song title and song's length in the music app history that was heard during sessionId = 338, and itemInSession = 4\n\n\n### 2. Give me only the following: name of artist, song (sorted by itemInSession) and user (first and last name) for userid = 10, sessionid = 182\n \n\n### 3. Give me every user name (first and last) in my music app history who listened to the song 'All Hands Against His Own'\n\n\n",
"_____no_output_____"
]
],
[
[
"# Creating table for query \"sessionId = 338, and itemInSession = 4\"\ncreate_table_query = \"\"\"\n CREATE TABLE IF NOT EXISTS session_library\n (\n session_id INT,\n item INT,\n artist TEXT,\n song_title TEXT,\n song_length FLOAT,\n PRIMARY KEY (session_id, item)\n );\n \"\"\"\ntry:\n session.execute(create_table_query)\nexcept Exception as e:\n print(e)",
"_____no_output_____"
],
[
"# CSV file\nfile = 'event_datafile_new.csv'\n\n# Insert data into table\nwith open(file, encoding = 'utf8') as f:\n csvreader = csv.reader(f)\n next(csvreader) # skip header\n for line in csvreader:\n insert_query = \"\"\"\n INSERT INTO session_library\n (session_id,\n item,\n artist,\n song_title,\n song_length)\n VALUES (%s, %s, %s, %s, %s);\n \"\"\"\n session.execute(insert_query, (int(line[8]), int(line[3]), line[0], line[9], float(line[5])))",
"_____no_output_____"
]
],
[
[
"#### Do a SELECT to verify that the data have been inserted into each table",
"_____no_output_____"
]
],
[
[
"query = \"\"\"\n SELECT artist,\n song_title,\n song_length\n FROM session_library\n WHERE session_id = %s\n AND item = %s\n \"\"\"\ntry:\n rows = session.execute(query, (338, 4))\nexcept Exception as e:\n print(e)\n \nfor row in rows:\n print (\"Artist:\", row.artist, \", Song:\", row.song_title, \", Song length:\", row.song_length)",
"Artist: Faithless , Song: Music Matters (Mark Knight Dub) , Song length: 495.30731201171875\n"
]
],
[
[
"### COPY AND REPEAT THE ABOVE THREE CELLS FOR EACH OF THE THREE QUESTIONS",
"_____no_output_____"
]
],
[
[
"# Creating table for query \"userid = 10, sessionid = 182\" sorted by item\ncreate_table_query = \"\"\"\n CREATE TABLE IF NOT EXISTS user_library\n (\n user_id TEXT,\n session_id INT,\n item INT,\n artist TEXT,\n song_title TEXT,\n first_name TEXT,\n last_name TEXT,\n PRIMARY KEY ((user_id, session_id), item)\n ); \n \"\"\"\ntry:\n session.execute(create_table_query)\nexcept Exception as e:\n print(e)\n\n# Insert data into table\nwith open(file, encoding = 'utf8') as f:\n csvreader = csv.reader(f)\n next(csvreader) # skip header\n for line in csvreader:\n insert_query = \"\"\"\n INSERT INTO user_library\n (user_id,\n session_id,\n item,\n artist,\n song_title,\n first_name,\n last_name)\n VALUES (%s, %s, %s, %s, %s, %s, %s);\n \"\"\"\n session.execute(insert_query, (line[10], int(line[8]), int(line[3]), line[0], line[9], line[1], line[4]))\n\n# Select the data\nquery = \"\"\"\n SELECT artist, \n song_title, \n first_name, \n last_name \n FROM user_library \n WHERE user_id =% s \n AND session_id = %s \n \"\"\"\ntry:\n rows = session.execute(query, (\"10\", 182))\nexcept Exception as e:\n print(e)\n \nfor row in rows:\n print (\"Artist:\", row.artist, \", Song:\", row.song_title, \", First name:\", row.first_name, \", Last name:\", row.last_name)",
"Artist: Down To The Bone , Song: Keep On Keepin' On , First name: Sylvie , Last name: Cruz\nArtist: Three Drives , Song: Greece 2000 , First name: Sylvie , Last name: Cruz\nArtist: Sebastien Tellier , Song: Kilometer , First name: Sylvie , Last name: Cruz\nArtist: Lonnie Gordon , Song: Catch You Baby (Steve Pitron & Max Sanna Radio Edit) , First name: Sylvie , Last name: Cruz\n"
],
[
"# Creating table for query \"song_title = All Hands Against His Own\"\ncreate_table_query = \"\"\"\n CREATE TABLE IF NOT EXISTS song_library \n (\n song_title TEXT, \n user_id TEXT,\n first_name TEXT,\n last_name TEXT,\n PRIMARY KEY (song_title, user_id)\n ); \n \"\"\"\ntry:\n session.execute(create_table_query)\nexcept Exception as e:\n print(e)\n\n# Insert data into table\nwith open(file, encoding = 'utf8') as f:\n csvreader = csv.reader(f)\n next(csvreader) # skip header\n for line in csvreader:\n insert_query = \"\"\"\n INSERT INTO song_library\n (song_title,\n user_id,\n first_name,\n last_name)\n VALUES (%s, %s, %s, %s);\n \"\"\"\n session.execute(insert_query, (line[9], line[10], line[1], line[4]))\n\n# Select the data\nquery = \"\"\"\n SELECT first_name, \n last_name \n FROM song_library \n WHERE song_title = %s\n \"\"\"\ntry:\n rows = session.execute(query, (\"All Hands Against His Own\",))\nexcept Exception as e:\n print(e)\n \nfor row in rows:\n print (\"First Name:\", row.first_name, \", Last Name:\", row.last_name,)",
"First Name: Jacqueline , Last Name: Lynch\nFirst Name: Tegan , Last Name: Levine\nFirst Name: Sara , Last Name: Johnson\n"
]
],
[
[
"### Drop the tables before closing out the sessions",
"_____no_output_____"
]
],
[
[
"## TO-DO: Drop the table before closing out the sessions",
"_____no_output_____"
],
[
"try:\n session.execute(\"DROP TABLE IF EXISTS session_library\")\n session.execute(\"DROP TABLE IF EXISTS user_library\")\n session.execute(\"DROP TABLE IF EXISTS song_library\")\nexcept Exception as e:\n print(e)",
"_____no_output_____"
]
],
[
[
"### Close the session and cluster connection¶",
"_____no_output_____"
]
],
[
[
"session.shutdown()\ncluster.shutdown()",
"_____no_output_____"
]
]
] | [
"markdown",
"code",
"markdown",
"code",
"markdown",
"code",
"markdown",
"code",
"markdown",
"code",
"markdown",
"code",
"markdown",
"code",
"markdown",
"code",
"markdown",
"code",
"markdown",
"code",
"markdown",
"code"
] | [
[
"markdown",
"markdown",
"markdown"
],
[
"code"
],
[
"markdown"
],
[
"code"
],
[
"markdown"
],
[
"code",
"code"
],
[
"markdown",
"markdown",
"markdown"
],
[
"code"
],
[
"markdown"
],
[
"code"
],
[
"markdown"
],
[
"code"
],
[
"markdown",
"markdown"
],
[
"code",
"code"
],
[
"markdown"
],
[
"code"
],
[
"markdown"
],
[
"code",
"code"
],
[
"markdown"
],
[
"code",
"code"
],
[
"markdown"
],
[
"code"
]
] |
e7733654bbff0e7ed6f54b90f78cce58bf3e626f | 42,405 | ipynb | Jupyter Notebook | Module 2.3_ LSTMs.ipynb | PacktPublishing/-Mastering-Keras | b1f97a2593660cf8ab8788004dde967674f0852a | [
"MIT"
] | 13 | 2020-01-14T07:02:58.000Z | 2021-08-15T01:51:33.000Z | Module 2.3_ LSTMs.ipynb | PacktPublishing/-Mastering-Keras | b1f97a2593660cf8ab8788004dde967674f0852a | [
"MIT"
] | 2 | 2020-12-19T15:49:12.000Z | 2021-01-20T12:45:17.000Z | Module 2.3_ LSTMs.ipynb | PacktPublishing/-Mastering-Keras | b1f97a2593660cf8ab8788004dde967674f0852a | [
"MIT"
] | 16 | 2019-12-24T18:16:48.000Z | 2022-01-27T23:08:45.000Z | 42,405 | 42,405 | 0.727273 | [
[
[
"## Module 2.3: Working with LSTMs in Keras (A Review)\n\nWe turn to implementing a type of recurrent neural network know as LSTM in the Keras functional API. In this module we will pay attention to:\n\n1. Using the Keras functional API for defining models.\n2. Mounting your Google drive to your Colab environment for file interface.\n3. Generating synthetic data from a LSTM and sequence seed.\n\nThose students who are comfortable with all these matters might consider skipping ahead.\n\nNote that we will not spend time tuning hyper-parameters: The purpose is to show how different techniques can be implemented in Keras, not to solve particular data science problems as optimally as possible. Obviously, most techniques include hyper-parameters that need to be tuned for optimal performance.",
"_____no_output_____"
],
[
"First we import required libraries.",
"_____no_output_____"
]
],
[
[
"import sys\nimport numpy\n\nfrom google.colab import drive\n\nfrom keras.models import Sequential\nfrom keras import Model\nfrom keras.optimizers import Adadelta\nfrom keras.layers import Dense,Dropout,LSTM,Input\nfrom keras.callbacks import ModelCheckpoint\nfrom keras.utils import np_utils",
"Using TensorFlow backend.\n"
]
],
[
[
"We will have a little fun and try to teach a neural network to write like Lewis Carroll, the author of Alice in Wonderland.\n\nNote, though, that the same technique can be used to model any sequential system, and generate simulations from seeds for such a system. Here the sequence are the characters written by Carroll during Alice in Wonderland, but it could be, for example, an industrial system that evolves in time. In that case, when we generate simulations of the system based on current and recent conditions we simulate the expected evolution of the system - something of great value!",
"_____no_output_____"
],
[
"We will use the [Project Gutenburg text file of Alice in Wonderland](https://www.gutenberg.org/files/11/11.txt). But we need to get the file into our colab environment and this takes some work.\n\nFirst, you need to place the file in your google drive. We will assume that you will place it in a folder called \"Mastering Keras Datasets\", and that you rename it \"Alice.txt\". If you don't, you will need to the file path used in the code.\n\nOnce you have done that, you will need to mount your google drive in Colab. Run the following code and complete the required authorizations.\n\nNote that you will need to mount your drive every time you use code from this tutorial.",
"_____no_output_____"
]
],
[
[
"# Note: You will need to mount your drive every time you \n# run code in this tutorial.\ndrive.mount('/content/drive')",
"Go to this URL in a browser: https://accounts.google.com/o/oauth2/auth?client_id=947318989803-6bn6qk8qdgf4n4g3pfee6491hc0brc4i.apps.googleusercontent.com&redirect_uri=urn%3Aietf%3Awg%3Aoauth%3A2.0%3Aoob&scope=email%20https%3A%2F%2Fwww.googleapis.com%2Fauth%2Fdocs.test%20https%3A%2F%2Fwww.googleapis.com%2Fauth%2Fdrive%20https%3A%2F%2Fwww.googleapis.com%2Fauth%2Fdrive.photos.readonly%20https%3A%2F%2Fwww.googleapis.com%2Fauth%2Fpeopleapi.readonly&response_type=code\n\nEnter your authorization code:\n··········\nMounted at /content/drive\n"
]
],
[
[
"Now we can load the file using code and prepare the data. We want to work with sequences of 100 characters as input data, and our target will be the next (101st) character.\n\nTo keep things simple, we will ignore upper/lower case character distinctions, and cast all alphabetical characters to lower case. To allow our model to work with these characters, we will encode them as integers. We will then normalize them to real numbers between 0 and 1 and add a dimension (we are working with a system with a single feature). Finally we will one-hot encode the target character (see previous module for discussion of one-hot encoding). This is not the only way to handle the data, but it is a simple one.\n\nWe will also return the unnormalized and non-reshaped X data, the number of characters found and an integer coding to character dictionary, all for use later.\n",
"_____no_output_____"
]
],
[
[
"def load_alice (\n rawTextFile=\"/content/drive/My Drive/Mastering Keras Datasets/Alice.txt\" \n ):\n # load ascii text and covert to lowercase\n raw_text = open(rawTextFile, encoding='utf-8').read()\n raw_text = raw_text.lower()\n # create mapping of unique chars to integers\n chars = sorted(list(set(raw_text)))\n char_to_int = dict((c, i) for i, c in enumerate(chars))\n int_to_char = dict((i, c) for i, c in enumerate(chars))\n # summarize the loaded data\n n_chars = len(raw_text)\n n_vocab = len(chars)\n print (\"Total Characters: \", n_chars)\n print (\"Total Vocab: \", n_vocab)\n # prepare the dataset of input to output pairs encoded as integers\n seq_length = 100\n dataX = []\n dataY = []\n for i in range(0, n_chars - seq_length, 1):\n \tseq_in = raw_text[i:i + seq_length]\n \tseq_out = raw_text[i + seq_length]\n \tdataX.append([char_to_int[char] for char in seq_in])\n \tdataY.append(char_to_int[seq_out])\n n_patterns = len(dataX)\n print (\"Total Patterns: \", n_patterns)\n # reshape X to be [samples, time steps, features]\n X = numpy.reshape(dataX, (n_patterns, seq_length, 1))\n # normalize\n X = X / float(n_vocab)\n # one hot encode the output variable\n Y = np_utils.to_categorical(dataY)\n return X,Y,dataX,n_vocab,int_to_char",
"_____no_output_____"
]
],
[
[
"Now lets load the data. X and Y are the input and target label datasets we will use in training. X_ is the un-reshaped X data for use later.",
"_____no_output_____"
]
],
[
[
"X,Y,X_,n_vocab,int_to_char = load_alice()",
"Total Characters: 163810\nTotal Vocab: 58\nTotal Patterns: 163710\n"
]
],
[
[
"You can play around below to look at the shape of the resulting X and Y arrays, as well as their contents. But they are no longer understandable character strings.",
"_____no_output_____"
]
],
[
[
"# Play around here to look at data characteristics",
"_____no_output_____"
]
],
[
[
"Now we define our LSTM using the Keras function API. We are going to make use of LSTM layers, and add a dropout layer for regularization.\n\nWe will pass the data to the model defining function so that we can read input and output dimensions of it, rather than hard coding them.\n\nFor comparison, a second version of the function is included showing how to use the sequential approach.",
"_____no_output_____"
]
],
[
[
"def get_model (X,Y):\n # define the LSTM model\n inputs=Input(shape=(X.shape[1],X.shape[2]),name=\"Input\")\n lstm1=LSTM(256, input_shape=(100,1),return_sequences=True)(inputs)\n drop1=Dropout(0.2)(lstm1)\n lstm2=LSTM(256)(drop1)\n drop2=Dropout(0.2)(lstm2)\n outputs=Dense(Y.shape[1], activation='softmax')(drop2)\n model=Model(inputs=inputs,outputs=outputs)\n return model\n\ndef get_model_sequential (X,Y):\n # define the LSTM model\n model = Sequential()\n model.add(LSTM(256, input_shape=(X.shape[1],X.shape[2]),return_sequences=True))\n model.add(Dropout(0.2))\n model.add(LSTM(256))\n model.add(Dropout(0.2))\n model.add(Dense(Y.shape[1], activation='softmax'))\n return model",
"_____no_output_____"
]
],
[
[
"We get our model.",
"_____no_output_____"
]
],
[
[
"model=get_model(X,Y)",
"_____no_output_____"
]
],
[
[
"Now we will define an optimizer and compile it. If you are unfamiliar with the different types of optimizers available in keras, I suggest you read the keras documentation [here](https://keras.io/optimizers/) and play around training the model with different alternatives.",
"_____no_output_____"
]
],
[
[
"opt=Adadelta()\n",
"_____no_output_____"
]
],
[
[
"And we compile our model with the optimizer ready for training. We use categorical crossentropy as our loss function as this is a good default choice for working with a multi-class categorical target variable (i.e. the next character labels).",
"_____no_output_____"
]
],
[
[
"model.compile(optimizer=opt,\n loss='categorical_crossentropy',\n metrics=['accuracy'])",
"_____no_output_____"
]
],
[
[
"Now we will make a function to fit the model. We will not do this very professionally (it is just a fun project), and so will not use any validation data. Rather, we will just run the training for a number of epoches - by default 20, though you can change this.\n\nWe will, though, use a ModelCheckpoint callback to save the best performing weights and load these into the model and the conclusion of the training. Note that training performance should normally improve with more epoches, so this is unlikely to improve performance. What we really want is to be able to load the best weights without having to redo the training process (see below)\n\nIf you want to, you are encouraged to alter the code in this tutorial to work with a training and validation set, and adjust the fit function below to incorporate an EarlyStopping callback based on performance on the validation data.\n\nWe have two one LSTM layer, we are dealing with sequences of length 100. So if we 'unroll' it, we have a network of 200 LSTM layers. And inside these layers are infact multiple internal layers setting up the LSTM architecture! So this is actually a pretty big network, and training will take some time (about 200 hours on the free Colab environment for 200 epochs). This is probably too much to conveniently run yourself.\n\nHere we have an example of how we could train it on Colab. Colab will eventually time out. The best thing to do is to save our weights file to our google drive, so we can load it at leisure later and resume training. This is what we will do. Remember that if you didn't use the default name for your folder in your google drive you should change the path string in the code.\n\nIn real life, you will also often want to save the state of the optimizer (so that it keeps its current learning rate, etc). You can do this by accessing and saving model.optimizer.get_state(). It is left as an exercise to implement this.\n\n*It is not expected that you train the network using this function - see below to load trained weights from your google drive.*",
"_____no_output_____"
]
],
[
[
"def fit_model (model,X,Y,epochs=100):\n # define the checkpoint callback\n filepath=\"/content/drive/My Drive/Mastering Keras Datasets/alice_best_weights.hdf5\" \n checkpoint = ModelCheckpoint(filepath, monitor='loss', verbose=1, \n save_best_only=True, mode='min')\n callbacks_list = [checkpoint]\n # fit the model\n model.fit(X, Y, epochs=epochs, batch_size=128, callbacks=callbacks_list)\n # load the best weights\n model.load_weights(filename)\n # return the final model\n return model\n",
"_____no_output_____"
]
],
[
[
"We would then fit (train) the model by calling the above function.\n\n*It is not expected that you train the network using this function - see below to load trained weights from your google drive.*",
"_____no_output_____"
]
],
[
[
"model=fit_model(model,X,Y,100)",
"Epoch 1/100\n163710/163710 [==============================] - 3246s 20ms/step - loss: 3.0840 - acc: 0.1663\n\nEpoch 00001: loss improved from inf to 3.08398, saving model to /content/drive/My Drive/Mastering Keras Datasets/alice_best_weights.hdf5\n"
]
],
[
[
"Here we will load saved weights. You can use the \"alice_best_weights.hdf5\" file that comes with the course - just place it in the same folder as the \"alice.txt\" file in your google drive. This file has been trained for 200 epoches, and gets a loss around 1.16.\n\nIf you train the network yourself, the best weights will be saved as \"alice_best_weights.hdf5\" in the same location as above. You can therefore use the same code in both cases.\n\nIn all cases remember to change the filepath if you are not using the default folder name.\n\nIf you are resuming this tutorial here in a new session, you should re-mount your Google drive using the earlier code, re-load the data, and then run this code block to load the weights into a new model. \n\nIf you want to train the model further, you will need to compile it with an optimizer.",
"_____no_output_____"
]
],
[
[
"model=get_model(X,Y)\nfilepath=\"/content/drive/My Drive/Mastering Keras Datasets/alice_best_weights.hdf5\"\nmodel.load_weights(filepath)",
"WARNING:tensorflow:From /usr/local/lib/python3.6/dist-packages/keras/backend/tensorflow_backend.py:541: The name tf.placeholder is deprecated. Please use tf.compat.v1.placeholder instead.\n\nWARNING:tensorflow:From /usr/local/lib/python3.6/dist-packages/keras/backend/tensorflow_backend.py:66: The name tf.get_default_graph is deprecated. Please use tf.compat.v1.get_default_graph instead.\n\nWARNING:tensorflow:From /usr/local/lib/python3.6/dist-packages/keras/backend/tensorflow_backend.py:4432: The name tf.random_uniform is deprecated. Please use tf.random.uniform instead.\n\nWARNING:tensorflow:From /usr/local/lib/python3.6/dist-packages/keras/backend/tensorflow_backend.py:148: The name tf.placeholder_with_default is deprecated. Please use tf.compat.v1.placeholder_with_default instead.\n\nWARNING:tensorflow:From /usr/local/lib/python3.6/dist-packages/keras/backend/tensorflow_backend.py:3733: calling dropout (from tensorflow.python.ops.nn_ops) with keep_prob is deprecated and will be removed in a future version.\nInstructions for updating:\nPlease use `rate` instead of `keep_prob`. Rate should be set to `rate = 1 - keep_prob`.\nWARNING:tensorflow:From /usr/local/lib/python3.6/dist-packages/keras/backend/tensorflow_backend.py:190: The name tf.get_default_session is deprecated. Please use tf.compat.v1.get_default_session instead.\n\nWARNING:tensorflow:From /usr/local/lib/python3.6/dist-packages/keras/backend/tensorflow_backend.py:197: The name tf.ConfigProto is deprecated. Please use tf.compat.v1.ConfigProto instead.\n\nWARNING:tensorflow:From /usr/local/lib/python3.6/dist-packages/keras/backend/tensorflow_backend.py:203: The name tf.Session is deprecated. Please use tf.compat.v1.Session instead.\n\nWARNING:tensorflow:From /usr/local/lib/python3.6/dist-packages/keras/backend/tensorflow_backend.py:207: The name tf.global_variables is deprecated. Please use tf.compat.v1.global_variables instead.\n\nWARNING:tensorflow:From /usr/local/lib/python3.6/dist-packages/keras/backend/tensorflow_backend.py:216: The name tf.is_variable_initialized is deprecated. Please use tf.compat.v1.is_variable_initialized instead.\n\nWARNING:tensorflow:From /usr/local/lib/python3.6/dist-packages/keras/backend/tensorflow_backend.py:223: The name tf.variables_initializer is deprecated. Please use tf.compat.v1.variables_initializer instead.\n\n"
]
],
[
[
"Now we can see if our network has mastered the art of writing like Lewis Carroll! Let's write a function to let us see, and then call it.",
"_____no_output_____"
]
],
[
[
"def write_like_Lewis_Carroll(model,X_,n_vocab,int_to_char):\n # pick a random seed...\n start = numpy.random.randint(0, len(X_)-1)\n # ... in order to decide which X datum to use to start\n pattern = X_[start]\n\n print (\"Seed:\")\n print (\"\\\"\", ''.join([int_to_char[value] for value in pattern]), \"\\\"\")\n # generate characters\n for i in range(1000):\n # We transform the integer mapping of the characters to\n # real numbers suitable for input into our model.\n x = numpy.reshape(pattern, (1, len(pattern), 1))\n x = x/float(n_vocab)\n # We use the model to estimate the probability distribution for\n # the next character\n prediction = model.predict(x, verbose=0)\n # We choose as the next character whichever the model thinks is most likely\n index = numpy.argmax(prediction)\n result = int_to_char[index]\n seq_in = [int_to_char[value] for value in pattern]\n sys.stdout.write(result)\n # We add the integer to our pattern... \n pattern.append(index)\n # ... and drop the earliest integer from our pattern.\n pattern = pattern[1:len(pattern)]\n print (\"\\nDone.\")",
"_____no_output_____"
],
[
"write_like_Lewis_Carroll(model,X_,n_vocab,int_to_char)",
"Seed:\n\" for it to speak with.\n\nalice waited till the eyes appeared, and then nodded. 'it's no use\nspeaking t \"\no see the mock turtle shat '\n\n'i should hiv tereat ' thought alice, 'i must be giederen seams to be a bonk,' she said to herself, 'it would be of very curious to onow what there was a sery dortut, and the ooral of that iss thin the cook and a large rister sha thought the was now one of the court.\nbut the dould not heve a little botrle of the thate with a things of tee the door, she could not hear the conlers on the coor with pisted so see it was she same sotnd and mook up and was that it was ouer the whnle shoiek, and the thought the was now a bot of ceain, and was domencd it voice and bookdrs shat the was nuire silent for a minute, and she was nooiing at the court.\n\n'i should hit tere things,' said the caterpillar.\n\n'well, perhaps you may bean the same siings tuertion,' the duchess said to the gryphon.\n\n'what i cen the thing,' said the caterpillar.\n\n'well, perhaps you may bean the same siings tuertion,' the mock turtle seplied,\n\n'that i man the mice,' said the caterpillar.\n\n'well, per\nDone.\n"
]
],
[
[
"If you run the above a few times, you will see that we have had some success - though we are still a long way from a good Alice in Wonderland simulator!\n\nHere is an extract from one simulation I ran:\n\n*'i should hit tere things,' said the caterpillar.*\n\n*'well, perhaps you may bean the same siings tuertion,' the duchess said to the gryphon.*\n\n*'what i cen the thing,' said the caterpillar.*\n\n*'well, perhaps you may bean the same siings tuertion,' the mock turtle seplied,*\n\n*'that i man the mice,' said the caterpillar.*\n\nWe have got to the point of basic sentence structure, quotations for speech, plausible characters given the context, etc. There remains misspellings, and occasional punctuation errors, and other issues. (And this was a good selection.) \n\nIn fact, you should be able to do much better. Trying with 500 time points (predicting the 501st character from the preceeding 500) and using a three layer LSTM will lead to major improvements. So would using more training data (multiple Lewis Carole books). You can see the performance achieved on a Shakespeare simulator [here](http://karpathy.github.io/2015/05/21/rnn-effectiveness/). \n\nIf you have time, consider it an exercise to try to improve this implementation to that level - but be warned, the suggested changes would lead to training time being about 7 times longer for the same number of epochs, and of course more epoches would be required as it would be a more complex model. Since it would have taken 100+ hours on the Colab environment (which disconnects after a time limit) this is really only an exercise for those with access to a powerful local environment. ",
"_____no_output_____"
]
]
] | [
"markdown",
"code",
"markdown",
"code",
"markdown",
"code",
"markdown",
"code",
"markdown",
"code",
"markdown",
"code",
"markdown",
"code",
"markdown",
"code",
"markdown",
"code",
"markdown",
"code",
"markdown",
"code",
"markdown",
"code",
"markdown",
"code",
"markdown"
] | [
[
"markdown",
"markdown"
],
[
"code"
],
[
"markdown",
"markdown"
],
[
"code"
],
[
"markdown"
],
[
"code"
],
[
"markdown"
],
[
"code"
],
[
"markdown"
],
[
"code"
],
[
"markdown"
],
[
"code"
],
[
"markdown"
],
[
"code"
],
[
"markdown"
],
[
"code"
],
[
"markdown"
],
[
"code"
],
[
"markdown"
],
[
"code"
],
[
"markdown"
],
[
"code"
],
[
"markdown"
],
[
"code"
],
[
"markdown"
],
[
"code",
"code"
],
[
"markdown"
]
] |
e77337591b3497cbfe9b0b5181c9aa8b1dee9a69 | 24,584 | ipynb | Jupyter Notebook | Model Training - Basic Model.ipynb | ravishchawla/QuorainsincereQuestions | 7e7905c38a5bdc54d195130e1f4d90840fed61e2 | [
"MIT"
] | null | null | null | Model Training - Basic Model.ipynb | ravishchawla/QuorainsincereQuestions | 7e7905c38a5bdc54d195130e1f4d90840fed61e2 | [
"MIT"
] | null | null | null | Model Training - Basic Model.ipynb | ravishchawla/QuorainsincereQuestions | 7e7905c38a5bdc54d195130e1f4d90840fed61e2 | [
"MIT"
] | 1 | 2020-06-06T20:22:26.000Z | 2020-06-06T20:22:26.000Z | 33.132075 | 282 | 0.526155 | [
[
[
"# Model Training - Basic Model\n\nIn this Notebook, we will go through building a basic PyTorch Model for Training, and training it to get results on our dataset.",
"_____no_output_____"
],
[
"### Imports\n\nIn this project, we will be using PyTorch for Deep Learning. NLP Pre-Processing, however, will be done using Keras's modules, because I prefer the implementation provided in the library. Instead of installing Keras, the relavant modules are imported in as scripts from GitHub.",
"_____no_output_____"
]
],
[
[
"import pandas as pd;\nimport numpy as np;\n\nimport torch;\nfrom torch import nn;\nfrom torch.utils.data import Dataset, DataLoader;\nimport torch.nn.functional as F;\nfrom sklearn.metrics import accuracy_score, f1_score, recall_score, precision_score;\n\nimport math;\nfrom numpy import save, load;\nimport keras_sequence_preprocessing as seq_preprocessing;\nimport keras_text_preprocessing as text_preprocessing;\n\nimport matplotlib.pyplot as plt;\n\nimport time;\n\nfrom PyTorchTools import EarlyStopping;",
"_____no_output_____"
],
[
"quora_train_text = pd.read_csv('data/augmented_quora_text.txt');",
"_____no_output_____"
],
[
"quora_train_text = quora_train_text.dropna()",
"_____no_output_____"
]
],
[
[
"### Word Embeddings\n\nWe have 2 different types of Word Embeddings we will try in this application: Glove and FastText. To use the specific embedding, run that cell and not the other, as both are loaded in with the same formatting.",
"_____no_output_____"
]
],
[
[
"embed_size = 300;",
"_____no_output_____"
],
[
"# GLOVE Embeddings\n\nembeddings_dict = {};\nwith open('../Embeddings/glove.6B/glove.6B.%dd.txt'%(embed_size), 'rb') as f:\n for line in f:\n values = line.split()\n word = values[0]\n vector = np.asarray(values[1:], \"float32\")\n embeddings_dict[word] = vector",
"_____no_output_____"
],
[
"# FASTTEXT Embeddings\n\nembeddings_dict = {};\nwith open('../Embeddings/crawl-%dd-2M.vec'%(embed_size), 'rb') as f:\n for line in f:\n splits = line.split();\n word = splits[0];\n vec = np.asarray(splits[1:], dtype='float32')\n \n embeddings_dict[word.decode()] = vec;",
"_____no_output_____"
]
],
[
[
"We build a Word Index from the embeddings. To quickly do this, we will simply be iterating over the dataset and assigning an integer value to each word.",
"_____no_output_____"
]
],
[
[
"word_index = {};\n\ntoken_num = 0;\nfor row in quora_train_text[['cleaned_text', 'target']].iterrows():\n text, label = row[1]\n \n tokens = [token for token in text.split(' ')];\n \n for token in tokens:\n if token not in word_index:\n word_index[token] = token_num;\n token_num = token_num + 1;",
"_____no_output_____"
],
[
"MAX_WORDS = 200000\nMAX_LEN = 70",
"_____no_output_____"
]
],
[
[
"Next, we encode the individual sentences into sequences of integers from the word index. Than Pad them to fixed lengths using post-sequence-padding.",
"_____no_output_____"
]
],
[
[
"def encode_sentences(sentence, word_index=word_index, max_words=MAX_WORDS):\n output = [];\n for token in sentence.split(' '):\n if (token in word_index) and (word_index[token] < max_words):\n output.append(word_index[token]);\n return output;",
"_____no_output_____"
],
[
"encoded_sentences = [encode_sentences(sent) for sent in quora_train_text['cleaned_text']]",
"_____no_output_____"
],
[
"encoded_lengths = [len(x) for x in encoded_sentences]",
"_____no_output_____"
],
[
"padded_sequences = seq_preprocessing.pad_sequences(encoded_sentences, maxlen=MAX_LEN, padding='post', truncating='post');",
"_____no_output_____"
]
],
[
[
"To do training / testing, we will divide the dataset into proper Training and Validation. 85% of the dataset for training, and the remaining 15% fo validation.",
"_____no_output_____"
]
],
[
[
"val_split = int(0.85 * len(quora_train_text));\n\ntrain_ds = padded_sequences[:val_split];\nval_ds = padded_sequences[val_split:];\n\ntrain_y = quora_train_text.iloc[:val_split]['target'].values;\nval_y = quora_train_text.iloc[val_split:]['target'].values;\n\ntrain_lens = encoded_lengths[:val_split];\nval_lens = encoded_lengths[val_split:];\n\nlen(train_ds), len(val_ds)",
"_____no_output_____"
]
],
[
[
"We build an Embeddings Matrix. Each row in the matrix is a vector from Glove / Fasttext.",
"_____no_output_____"
]
],
[
[
"vocab_size = min(MAX_WORDS, len(word_index))+1;\nembeddings_matrix = np.zeros((vocab_size, embed_size));\n\nfor word, posit in word_index.items():\n if posit >= vocab_size:\n break;\n \n vec = embeddings_dict.get(word);\n if vec is None:\n vec = np.random.sample(embed_size);\n embeddings_dict[word] = vec;\n \n embeddings_matrix[posit] = vec;",
"_____no_output_____"
],
[
"embeddings_tensor = torch.Tensor(embeddings_matrix)",
"_____no_output_____"
]
],
[
[
"Build a Data Loader to iterate over during the training process in a fixed batch size:",
"_____no_output_____"
]
],
[
[
"class QuoraDataset(Dataset):\n def __init__(self, encoded_sentences, labels, lengths):\n self.encoded_sentences = encoded_sentences;\n self.labels = labels;\n self.lengths = lengths;\n \n def __len__(self):\n return len(self.encoded_sentences);\n \n def __getitem__(self, index):\n x = self.encoded_sentences[index, :];\n x = torch.LongTensor(x);\n \n y = self.labels[index];\n y = torch.Tensor([y]);\n \n length = self.lengths[index];\n length = torch.Tensor([length]);\n \n return x, y, length;",
"_____no_output_____"
],
[
"train_dataset = QuoraDataset(train_ds, train_y, train_lens);\nval_dataset = QuoraDataset(val_ds, val_y, val_lens);",
"_____no_output_____"
],
[
"batch_size = 512;\n\ntrain_loader = DataLoader(train_dataset, batch_size=batch_size, shuffle=True);\nval_loader = DataLoader(val_dataset, batch_size=batch_size, shuffle=True);",
"_____no_output_____"
]
],
[
[
"## Creating a Model",
"_____no_output_____"
],
[
"The Torch Model will have the following architecture:\n\n1. Embeddings Layer\n2. 1st LSTM Layer\n2. 1st Dense Fully Connected Layer\n3. ReLU Activation\n4. 2nd LSTM Layer\n5. Global Max-Average Pooling Layer\n6. 2nd Dense Fully Connected Layer",
"_____no_output_____"
]
],
[
[
"class Model(nn.Module):\n def __init__(self, embedding_matrix, hidden_unit = 64):\n super(Model, self).__init__();\n vocab_size = embeddings_tensor.shape[0];\n embedding_dim = embeddings_tensor.shape[1];\n \n self.embedding_layer = nn.Embedding(vocab_size, embedding_dim);\n self.embedding_layer.weight = nn.Parameter(embeddings_tensor);\n self.embedding_layer.weight.requires_grad = True;\n \n self.lstm_1 = nn.LSTM(embedding_dim, hidden_unit, bidirectional=True);\n \n self.fc_1 = nn.Linear(hidden_unit*2, hidden_unit*2);\n \n self.lstm_2 = nn.LSTM(hidden_unit*2, hidden_unit, bidirectional=True);\n \n self.fc_2 = nn.Linear(hidden_unit * 2 * 2, 1);\n \n def forward(self, x):\n out = self.embedding_layer(x);\n \n out, _ = self.lstm_1(out);\n \n out = self.fc_1(out);\n \n out = torch.relu(out);\n \n out, _ = self.lstm_2(out);\n \n out_avg, out_max = torch.mean(out, 1), torch.max(out, 1)[0];\n out = torch.cat((out_avg, out_max), 1);\n \n out = self.fc_2(out);\n return out;",
"_____no_output_____"
],
[
"device = 'cuda' if torch.cuda.is_available() else 'cpu'\ndevice",
"_____no_output_____"
],
[
"model = Model(embeddings_tensor, 64);\nmodel = model.to(device);\nmodel",
"_____no_output_____"
]
],
[
[
"We use a Binary-Cross-Entropy Loss Function, and an Adam Optimizer with a 0.003 Learning Rate.",
"_____no_output_____"
]
],
[
[
"criterion = nn.BCEWithLogitsLoss();\noptimizer = torch.optim.Adam(lr=0.003, params = model.parameters());",
"_____no_output_____"
]
],
[
[
"## Model Training\n\nNow we write the methods to iterate over the data to train and evaluate our model.",
"_____no_output_____"
]
],
[
[
"def train(nn_model, nn_optimizer, nn_criterion, data_loader, val_loader = None, num_epochs = 5, print_ratio = 0.1, verbose=True):\n \n print_every_step = int(print_ratio * len(train_loader));\n \n if verbose:\n print('Training with model: ');\n print(nn_model);\n \n for epoch in range(num_epochs):\n\n epoch_time = time.time(); \n\n f1_scores_train = []\n\n # Enable Training for the model\n nn_model.train()\n running_loss = 0;\n\n all_ys = torch.tensor(data=[]).to(device);\n all_preds = torch.tensor(data=[]).to(device);\n\n for ite, (x, y, l) in enumerate(data_loader):\n init_time = time.time();\n\n # Convert our tensors to GPU tensors\n x = x.cuda()\n y = y.cuda()\n\n # Clear gradients\n nn_optimizer.zero_grad()\n\n # Forward Propagation and compute predictions\n preds = nn_model.forward(x, l)\n\n # Compute loss against actual values\n loss = nn_criterion(preds, y)\n\n # Add predictions and actuals into larger list for scoring\n all_preds = torch.cat([all_preds, preds]);\n all_ys = torch.cat([all_ys, y]);\n\n # Back Propagation and Updating weights\n loss.backward()\n nn_optimizer.step()\n\n running_loss = running_loss + loss.item();\n\n if ite % print_every_step == print_every_step-1:\n \n # Compute Sigmoid Activation and Prediction Probabilities\n preds_sigmoid = torch.sigmoid(all_preds).cpu().detach().numpy();\n \n # Compute Predictions over the Sigmoid base line\n all_preds = (preds_sigmoid > 0.5).astype(int);\n\n # Compute Metrics\n all_ys = all_ys.detach().cpu().numpy();\n\n f_score = f1_score(all_ys, all_preds);\n precision = precision_score(all_ys, all_preds);\n recall = recall_score(all_ys, all_preds);\n accuracy = accuracy_score(all_ys, all_preds);\n\n print('\\t[%d %5d %.2f sec] loss: %.3f acc: %.3f prec: %.3f rec: %.3f f1: %.3f'%(epoch+1, ite+1, time.time() - init_time, running_loss / 2000, accuracy, precision, recall, f_score))\n\n all_ys = torch.tensor(data=[]).to(device);\n all_preds = torch.tensor(data=[]).to(device);\n \n print('Epoch %d done in %.2f min'%(epoch+1, (time.time() - epoch_time)/60 ));\n\n if val_loader is not None:\n eval(nn_model, nn_criterion, val_loader);\n \n running_loss = 0.0;",
"_____no_output_____"
],
[
"def eval(nn_model, nn_criterion, data_loader):\n\n # Disable weight updates\n with torch.no_grad():\n\n # Enable Model Evaluation\n nn_model.eval()\n running_loss = 0;\n \n all_ys = torch.tensor(data=[]).to(device);\n all_preds = torch.tensor(data=[]).to(device);\n\n init_time = time.time();\n\n for ite, (x, y, l) in enumerate(data_loader):\n\n # Convert tensors to GPU tensors\n x = x.cuda()\n y = y.cuda()\n\n # Forward propagation to compute predictions\n preds = nn_model.forward(x, l)\n\n # Compute loss on these predictions\n loss = nn_criterion(preds, y)\n\n all_preds = torch.cat([all_preds, preds]);\n all_ys = torch.cat([all_ys, y]);\n\n running_loss = running_loss + loss.item();\n\n # Compute Sigmoid activation on the predictions, and derive predictions over the Sigmoid base line\n preds_sigmoid = torch.sigmoid(all_preds).cpu().detach().numpy();\n all_preds = (preds_sigmoid > 0.5).astype(int);\n\n # Compute metrics\n all_ys = all_ys.detach().cpu().numpy();\n f_score = f1_score(all_ys, all_preds);\n\n precision = precision_score(all_ys, all_preds);\n recall = recall_score(all_ys, all_preds);\n accuracy = accuracy_score(all_ys, all_preds);\n\n print('\\tEVAL: [%5d %.2f sec] loss: %.3f acc: %.3f prec: %.3f rec: %.3f f1: %.3f'%(ite+1, time.time() - init_time, running_loss / 2000, accuracy, precision, recall, f_score))",
"_____no_output_____"
]
],
[
[
"Running Training on the Model",
"_____no_output_____"
]
],
[
[
"train(model, optimizer, criterion, train_loader)",
"Training with model: \nModel(\n (embedding_layer): Embedding(100001, 100)\n (lstm_1): LSTM(100, 64, bidirectional=True)\n (fc_1): Linear(in_features=128, out_features=128, bias=True)\n (lstm_2): LSTM(128, 64, bidirectional=True)\n (fc_2): Linear(in_features=256, out_features=1, bias=True)\n)\n\t[1 356 0.13 sec] loss: 0.038 acc: 0.938 prec: 0.548 rec: 0.004 f1: 0.008\n\t[1 712 0.13 sec] loss: 0.069 acc: 0.940 prec: 0.615 rec: 0.104 f1: 0.178\n\t[1 1068 0.13 sec] loss: 0.098 acc: 0.942 prec: 0.629 rec: 0.180 f1: 0.279\n\t[1 1424 0.13 sec] loss: 0.125 acc: 0.943 prec: 0.615 rec: 0.207 f1: 0.310\n\t[1 1780 0.14 sec] loss: 0.152 acc: 0.944 prec: 0.617 rec: 0.231 f1: 0.336\n\t[1 2136 0.14 sec] loss: 0.179 acc: 0.945 prec: 0.640 rec: 0.255 f1: 0.365\n\t[1 2492 0.14 sec] loss: 0.205 acc: 0.946 prec: 0.650 rec: 0.277 f1: 0.389\n\t[1 2848 0.14 sec] loss: 0.231 acc: 0.946 prec: 0.635 rec: 0.272 f1: 0.381\n\t[1 3204 0.13 sec] loss: 0.256 acc: 0.947 prec: 0.652 rec: 0.288 f1: 0.399\n\t[1 3560 0.13 sec] loss: 0.281 acc: 0.947 prec: 0.648 rec: 0.306 f1: 0.416\nEpoch 1 done in 164.36\n\t[2 356 0.14 sec] loss: 0.023 acc: 0.950 prec: 0.689 rec: 0.364 f1: 0.476\n\t[2 712 0.14 sec] loss: 0.046 acc: 0.950 prec: 0.682 rec: 0.375 f1: 0.484\n\t[2 1068 0.14 sec] loss: 0.068 acc: 0.952 prec: 0.687 rec: 0.397 f1: 0.504\n\t[2 1424 0.14 sec] loss: 0.090 acc: 0.952 prec: 0.683 rec: 0.404 f1: 0.508\n\t[2 1780 0.14 sec] loss: 0.112 acc: 0.951 prec: 0.682 rec: 0.406 f1: 0.509\n\t[2 2136 0.14 sec] loss: 0.134 acc: 0.951 prec: 0.680 rec: 0.435 f1: 0.530\n\t[2 2492 0.14 sec] loss: 0.156 acc: 0.953 prec: 0.678 rec: 0.436 f1: 0.531\n\t[2 2848 0.14 sec] loss: 0.177 acc: 0.953 prec: 0.689 rec: 0.458 f1: 0.550\n\t[2 3204 0.14 sec] loss: 0.198 acc: 0.953 prec: 0.673 rec: 0.443 f1: 0.534\n\t[2 3560 0.14 sec] loss: 0.219 acc: 0.954 prec: 0.694 rec: 0.462 f1: 0.554\nEpoch 2 done in 164.47\n\t[3 356 0.14 sec] loss: 0.020 acc: 0.956 prec: 0.709 rec: 0.509 f1: 0.593\n\t[3 712 0.14 sec] loss: 0.039 acc: 0.956 prec: 0.708 rec: 0.513 f1: 0.595\n\t[3 1068 0.14 sec] loss: 0.059 acc: 0.955 prec: 0.697 rec: 0.500 f1: 0.582\n\t[3 1424 0.14 sec] loss: 0.079 acc: 0.956 prec: 0.700 rec: 0.498 f1: 0.582\n\t[3 1780 0.14 sec] loss: 0.099 acc: 0.956 prec: 0.705 rec: 0.504 f1: 0.588\n\t[3 2136 0.14 sec] loss: 0.119 acc: 0.957 prec: 0.706 rec: 0.499 f1: 0.585\n\t[3 2492 0.14 sec] loss: 0.139 acc: 0.956 prec: 0.700 rec: 0.492 f1: 0.578\n\t[3 2848 0.14 sec] loss: 0.158 acc: 0.957 prec: 0.707 rec: 0.514 f1: 0.595\n\t[3 3204 0.14 sec] loss: 0.177 acc: 0.958 prec: 0.704 rec: 0.523 f1: 0.600\n\t[3 3560 0.14 sec] loss: 0.196 acc: 0.957 prec: 0.709 rec: 0.505 f1: 0.590\nEpoch 3 done in 164.54\n\t[4 356 0.14 sec] loss: 0.018 acc: 0.959 prec: 0.722 rec: 0.544 f1: 0.620\n\t[4 712 0.14 sec] loss: 0.036 acc: 0.958 prec: 0.722 rec: 0.545 f1: 0.621\n\t[4 1068 0.14 sec] loss: 0.055 acc: 0.958 prec: 0.715 rec: 0.543 f1: 0.618\n\t[4 1424 0.14 sec] loss: 0.073 acc: 0.959 prec: 0.724 rec: 0.548 f1: 0.624\n\t[4 1780 0.13 sec] loss: 0.092 acc: 0.958 prec: 0.714 rec: 0.550 f1: 0.621\n\t[4 2136 0.14 sec] loss: 0.110 acc: 0.960 prec: 0.728 rec: 0.541 f1: 0.620\n\t[4 2492 0.14 sec] loss: 0.128 acc: 0.959 prec: 0.724 rec: 0.528 f1: 0.610\n\t[4 2848 0.14 sec] loss: 0.147 acc: 0.958 prec: 0.715 rec: 0.521 f1: 0.603\n\t[4 3204 0.13 sec] loss: 0.166 acc: 0.958 prec: 0.721 rec: 0.544 f1: 0.621\n\t[4 3560 0.14 sec] loss: 0.185 acc: 0.958 prec: 0.715 rec: 0.541 f1: 0.616\nEpoch 4 done in 163.86\n\t[5 356 0.14 sec] loss: 0.017 acc: 0.962 prec: 0.733 rec: 0.581 f1: 0.648\n\t[5 712 0.14 sec] loss: 0.034 acc: 0.961 prec: 0.734 rec: 0.568 f1: 0.640\n\t[5 1068 0.14 sec] loss: 0.052 acc: 0.961 prec: 0.734 rec: 0.566 f1: 0.639\n\t[5 1424 0.14 sec] loss: 0.070 acc: 0.960 prec: 0.729 rec: 0.578 f1: 0.644\n\t[5 1780 0.14 sec] loss: 0.088 acc: 0.960 prec: 0.726 rec: 0.565 f1: 0.635\n\t[5 2136 0.14 sec] loss: 0.106 acc: 0.961 prec: 0.738 rec: 0.580 f1: 0.650\n\t[5 2492 0.14 sec] loss: 0.123 acc: 0.960 prec: 0.722 rec: 0.564 f1: 0.633\n\t[5 2848 0.13 sec] loss: 0.141 acc: 0.961 prec: 0.735 rec: 0.567 f1: 0.640\n\t[5 3204 0.14 sec] loss: 0.159 acc: 0.960 prec: 0.730 rec: 0.561 f1: 0.634\n\t[5 3560 0.14 sec] loss: 0.177 acc: 0.960 prec: 0.734 rec: 0.562 f1: 0.637\nEpoch 5 done in 164.38\n"
],
[
"eval(model, criterion, val_loader)",
"\tEVAL: [ 764 16.99 sec] loss: 0.046 acc: 0.953 prec: 0.617 rec: 0.480 f1: 0.540\n"
]
],
[
[
"### The best training F1 score is **0.637** over 5 epochs and the evaluation F1 score is **0.540**",
"_____no_output_____"
]
]
] | [
"markdown",
"code",
"markdown",
"code",
"markdown",
"code",
"markdown",
"code",
"markdown",
"code",
"markdown",
"code",
"markdown",
"code",
"markdown",
"code",
"markdown",
"code",
"markdown",
"code",
"markdown",
"code",
"markdown"
] | [
[
"markdown",
"markdown"
],
[
"code",
"code",
"code"
],
[
"markdown"
],
[
"code",
"code",
"code"
],
[
"markdown"
],
[
"code",
"code"
],
[
"markdown"
],
[
"code",
"code",
"code",
"code"
],
[
"markdown"
],
[
"code"
],
[
"markdown"
],
[
"code",
"code"
],
[
"markdown"
],
[
"code",
"code",
"code"
],
[
"markdown",
"markdown"
],
[
"code",
"code",
"code"
],
[
"markdown"
],
[
"code"
],
[
"markdown"
],
[
"code",
"code"
],
[
"markdown"
],
[
"code",
"code"
],
[
"markdown"
]
] |
e7734da43b531d4e204453235ee02541795f1c2e | 2,596 | ipynb | Jupyter Notebook | Jupyter/.ipynb_checkpoints/CriandoFuncoes-checkpoint.ipynb | andersonkramer/Python | 7c9a5684c4789ccde303558c8b59c2fa582f36ee | [
"MIT"
] | null | null | null | Jupyter/.ipynb_checkpoints/CriandoFuncoes-checkpoint.ipynb | andersonkramer/Python | 7c9a5684c4789ccde303558c8b59c2fa582f36ee | [
"MIT"
] | null | null | null | Jupyter/.ipynb_checkpoints/CriandoFuncoes-checkpoint.ipynb | andersonkramer/Python | 7c9a5684c4789ccde303558c8b59c2fa582f36ee | [
"MIT"
] | null | null | null | 18.411348 | 107 | 0.469183 | [
[
[
"#Aula criando funções\n\ndef parabens():\n print(' Parabéns pra você\\n Nessa data querida\\n Muitas felicidades\\n Muitos anos de vida\\n')",
"_____no_output_____"
],
[
"#Rodando a função\n\nparabens()",
" Parabéns pra você\n Nessa data querida\n Muitas felicidades\n Muitos anos de vida\n\n"
],
[
"#funcao para ver se tem letra U na frase\n\ndef temletrau():\n frase = input('Digite uma frase: ')\n if 'u' in frase:\n print('Tem letra U')\n else:\n print('Não tem letra U')",
"_____no_output_____"
],
[
"temletrau()",
"Digite uma frase: Caraiu\nTem letra U\n"
],
[
"def somaquadrados(a,b):\n somaQ = a**2 + b**2\n print('A soma dos quadrados = {}'.format(somaQ))\n return somaQ",
"_____no_output_____"
],
[
"somaquadrados(2,3)",
"A soma dos quadrados = 13\n"
]
]
] | [
"code"
] | [
[
"code",
"code",
"code",
"code",
"code",
"code"
]
] |
e773551db332f6db2350032efaf2f70e7d072434 | 172,495 | ipynb | Jupyter Notebook | Bitcoin Data Viz_1.ipynb | anishs37/ML | afb6bcd46b19b682b7fd1afa3dc04587a8a50913 | [
"MIT"
] | null | null | null | Bitcoin Data Viz_1.ipynb | anishs37/ML | afb6bcd46b19b682b7fd1afa3dc04587a8a50913 | [
"MIT"
] | null | null | null | Bitcoin Data Viz_1.ipynb | anishs37/ML | afb6bcd46b19b682b7fd1afa3dc04587a8a50913 | [
"MIT"
] | null | null | null | 344.301397 | 43,908 | 0.925667 | [
[
[
"import pandas as pd\nimport matplotlib.pyplot as plt\n\ndf = pd.read_csv('https://drive.google.com/u/0/uc?id=1Fo_QyN4ttdz1muDHKgHtXVtZpXJ5ODoV&export=download')\ndf.head()",
"_____no_output_____"
],
[
"plt.figure(figsize = (12,5))\nplt.title(\"adjustedTxVolume Vs. Price\")\nplt.xlabel(\"adjustedTxVolume\")\nplt.ylabel(\"price\")\nplt.plot(df[\"adjustedTxVolume\"], df[\"price\"], \"o\")\nplt.savefig(\"adjustedTxVolume VS price.png\")\nplt.show()",
"_____no_output_____"
],
[
"plt.figure(figsize = (12,5))\nplt.title(\"adjustedTxVolume Vs. Price (X vs Y)\")\nplt.xlabel(\"adjustedTxVolume\")\nplt.ylabel(\"price\")\nplt.plot(df[\"adjustedTxVolume\"], df[\"price\"], \"o\")\nplt.savefig(\"adjustedTxVolume VS price (X vs Y).png\")\nplt.show()",
"_____no_output_____"
],
[
"import numpy as np\n\nplt.figure(figsize = (12,5))\nplt.title(\"adjustedTxVolume Vs. Price (X vs log Y)\")\nplt.xlabel(\"adjustedTxVolume\")\nplt.ylabel(\"log of price\")\nplt.plot(df[\"adjustedTxVolume\"], np.log(df[\"price\"]), \"o\")\nplt.savefig(\"adjustedTxVolume VS price (X vs log Y).png\")\nplt.show()",
"_____no_output_____"
],
[
"plt.figure(figsize = (12,5))\nplt.title(\"adjustedTxVolume Vs. Price (log X vs log Y)\")\nplt.xlabel(\"log of adjustedTxVolume\")\nplt.ylabel(\"log of price\")\nplt.plot(np.log(df[\"adjustedTxVolume\"]), np.log(df[\"price\"]), \"o\")\nplt.savefig(\"adjustedTxVolume VS price (log X vs log Y).png\")\nplt.show()",
"_____no_output_____"
],
[
"def calc_corr(a, b):\n a_std = np.std(a)\n a_mean = a.mean()\n a_final = (a - a_mean) / a_std\n\n b_std = np.std(b)\n b_mean = b.mean()\n b_final = (b - b_mean) / b_std\n\n r = (a_final * b_final).mean()\n return r\n\nx = \"adjustedTxVolume\"\ny = \"price\"\n\nx_raw = df[x].copy()\ny_raw = df[y].copy()\n\nlog_x = np.log(df[x]).copy()\nlog_y = np.log(df[y]).copy()\n\nprint(calc_corr(x_raw, y_raw))\nprint(calc_corr(x_raw, log_y))\nprint(calc_corr(log_x, log_y))",
"0.9142162621028266\n0.7771601812065383\n0.9520469542944037\n"
],
[
"log_x = np.log(df[x])\nlog_y = np.log(df[y])\n\nx_std = np.std(log_x)\nx_mean = log_x.mean()\nlog_x = (log_x - x_mean) / x_std\n\ny_std = np.std(log_y)\ny_mean = log_y.mean()\nlog_y = (log_y - y_mean) / y_std\n\nw_norm = (log_x * log_y).mean()\nb_norm = 0\n\nprint(w_norm)\nprint(b_norm)",
"0.9520469542944037\n0\n"
],
[
"plt.figure(figsize = (12,5))\n#plt.title(\"adjustedTxVolume Vs. Price Residual Plot\")\n#plt.xlabel(\"adjustedTxVolume\")\n#plt.ylabel(\"price\")\nplt.plot(log_x, log_y - (log_x * w_norm), \"o\")\nplt.savefig(\"adjustedTxVolume VS price residual plot.png\")\nplt.show()",
"_____no_output_____"
],
[
"log_x = np.log(df[x])\nlog_y = np.log(df[y])\n\nr = ((log_x * log_y).sum()) / (log_x.size)\nw = r * (np.std(log_y)/np.std(log_x))\nb = log_y - (w*log_x)\ny_hat = w*df[x] + b\n\nprint(y_hat)",
"0 3.800716e+09\n1 5.810843e+09\n2 6.853469e+09\n3 9.892807e+09\n4 7.018509e+09\n ... \n2030 1.571605e+11\n2031 3.157569e+11\n2032 4.753922e+11\n2033 2.675879e+11\n2034 2.062605e+11\nLength: 2035, dtype: float64\n"
]
]
] | [
"code"
] | [
[
"code",
"code",
"code",
"code",
"code",
"code",
"code",
"code",
"code"
]
] |
e77359ea7a04ac77f71df8a82baca7d70cdb2ce2 | 1,685 | ipynb | Jupyter Notebook | test/ipynb/groovy/AutotranslationBetweenKernels.ipynb | ssadedin/beakerx | 34479b07d2dfdf1404692692f483faf0251632c3 | [
"Apache-2.0"
] | 1 | 2018-10-16T18:59:59.000Z | 2018-10-16T18:59:59.000Z | test/ipynb/groovy/AutotranslationBetweenKernels.ipynb | ssadedin/beakerx | 34479b07d2dfdf1404692692f483faf0251632c3 | [
"Apache-2.0"
] | 1 | 2019-10-27T19:56:51.000Z | 2019-10-27T19:56:51.000Z | test/ipynb/groovy/AutotranslationBetweenKernels.ipynb | ssadedin/beakerx | 34479b07d2dfdf1404692692f483faf0251632c3 | [
"Apache-2.0"
] | null | null | null | 16.85 | 37 | 0.503858 | [
[
[
"empty"
]
]
] | [
"empty"
] | [
[
"empty"
]
] |
e7736a02e96a7e758e30a2d9c647fef47808f6aa | 15,642 | ipynb | Jupyter Notebook | surprise/source_code/DeepLearningIntro/Keras-RNN.ipynb | AlphaSunny/RecSys | 6e14a910ea810e2ec6501ee7a9a0ac9205e2232e | [
"MIT"
] | 73 | 2019-05-08T05:59:53.000Z | 2022-03-28T16:59:19.000Z | surprise/source_code/DeepLearningIntro/Keras-RNN.ipynb | AlphaSunny/RecSys | 6e14a910ea810e2ec6501ee7a9a0ac9205e2232e | [
"MIT"
] | 1 | 2021-02-07T18:01:54.000Z | 2021-02-23T12:26:16.000Z | surprise/source_code/DeepLearningIntro/Keras-RNN.ipynb | AlphaSunny/RecSys | 6e14a910ea810e2ec6501ee7a9a0ac9205e2232e | [
"MIT"
] | 43 | 2019-07-18T03:26:22.000Z | 2022-03-10T22:03:33.000Z | 28.082585 | 399 | 0.504667 | [
[
[
"# Recurring Neural Networks with Keras\n\n## Sentiment analysis from movie reviews\n\nThis notebook is inspired by the imdb_lstm.py example that ships with Keras. But since I used to run IMDb's engineering department, I couldn't resist!\n\nIt's actually a great example of using RNN's. The data set we're using consists of user-generated movie reviews and classification of whether the user liked the movie or not based on its associated rating.\n\nMore info on the dataset is here:\n\nhttps://keras.io/datasets/#imdb-movie-reviews-sentiment-classification\n\nSo we are going to use an RNN to do sentiment analysis on full-text movie reviews!\n\nThink about how amazing this is. We're going to train an artificial neural network how to \"read\" movie reviews and guess whether the author liked the movie or not from them.\n\nSince understanding written language requires keeping track of all the words in a sentence, we need a recurrent neural network to keep a \"memory\" of the words that have come before as it \"reads\" sentences over time.\n\nIn particular, we'll use LSTM (Long Short-Term Memory) cells because we don't really want to \"forget\" words too quickly - words early on in a sentence can affect the meaning of that sentence significantly.\n\nLet's start by importing the stuff we need:",
"_____no_output_____"
]
],
[
[
"from tensorflow.keras.preprocessing import sequence\nfrom tensorflow.keras.models import Sequential\nfrom tensorflow.keras.layers import Dense, Embedding\nfrom tensorflow.keras.layers import LSTM\nfrom tensorflow.keras.datasets import imdb",
"_____no_output_____"
]
],
[
[
"Now import our training and testing data. We specify that we only care about the 20,000 most popular words in the dataset in order to keep things somewhat managable. The dataset includes 5,000 training reviews and 25,000 testing reviews for some reason.",
"_____no_output_____"
]
],
[
[
"print('Loading data...')\n(x_train, y_train), (x_test, y_test) = imdb.load_data(num_words=20000)",
"Loading data...\n"
]
],
[
[
"Let's get a feel for what this data looks like. Let's look at the first training feature, which should represent a written movie review:",
"_____no_output_____"
]
],
[
[
"x_train[0]",
"_____no_output_____"
]
],
[
[
"That doesn't look like a movie review! But this data set has spared you a lot of trouble - they have already converted words to integer-based indices. The actual letters that make up a word don't really matter as far as our model is concerned, what matters are the words themselves - and our model needs numbers to work with, not letters.\n\nSo just keep in mind that each number in the training features represent some specific word. It's a bummer that we can't just read the reviews in English as a gut check to see if sentiment analysis is really working, though.\n\nWhat do the labels look like?",
"_____no_output_____"
]
],
[
[
"y_train[0]",
"_____no_output_____"
]
],
[
[
"They are just 0 or 1, which indicates whether the reviewer said they liked the movie or not.\n\nSo to recap, we have a bunch of movie reviews that have been converted into vectors of words represented by integers, and a binary sentiment classification to learn from.\n\nRNN's can blow up quickly, so again to keep things managable on our little PC let's limit the reviews to their first 80 words:",
"_____no_output_____"
]
],
[
[
"x_train = sequence.pad_sequences(x_train, maxlen=80)\nx_test = sequence.pad_sequences(x_test, maxlen=80)",
"_____no_output_____"
]
],
[
[
"Now let's set up our neural network model! Considering how complicated a LSTM recurrent neural network is under the hood, it's really amazing how easy this is to do with Keras.\n\nWe will start with an Embedding layer - this is just a step that converts the input data into dense vectors of fixed size that's better suited for a neural network. You generally see this in conjunction with index-based text data like we have here. The 20,000 indicates the vocabulary size (remember we said we only wanted the top 20,000 words) and 128 is the output dimension of 128 units.\n\nNext we just have to set up a LSTM layer for the RNN itself. It's that easy. We specify 128 to match the output size of the Embedding layer, and dropout terms to avoid overfitting, which RNN's are particularly prone to.\n\nFinally we just need to boil it down to a single neuron with a sigmoid activation function to choose our binay sentiment classification of 0 or 1.",
"_____no_output_____"
]
],
[
[
"model = Sequential()\nmodel.add(Embedding(20000, 128))\nmodel.add(LSTM(128, dropout=0.2, recurrent_dropout=0.2))\nmodel.add(Dense(1, activation='sigmoid'))",
"_____no_output_____"
]
],
[
[
"As this is a binary classification problem, we'll use the binary_crossentropy loss function. And the Adam optimizer is usually a good choice (feel free to try others.)",
"_____no_output_____"
]
],
[
[
"model.compile(loss='binary_crossentropy',\n optimizer='adam',\n metrics=['accuracy'])",
"_____no_output_____"
]
],
[
[
"Now we will actually train our model. RNN's, like CNN's, are very resource heavy. Keeping the batch size relatively small is the key to enabling this to run on your PC at all. In the real word of course, you'd be taking advantage of GPU's installed across many computers on a cluster to make this scale a lot better.\n\n## Warning\n\nThis will take a very long time to run, even on a fast PC! Don't execute the next block unless you're prepared to tie up your computer for an hour or more.",
"_____no_output_____"
]
],
[
[
"model.fit(x_train, y_train,\n batch_size=32,\n epochs=15,\n verbose=2,\n validation_data=(x_test, y_test))",
"C:\\Users\\Frank\\AppData\\Local\\Enthought\\Canopy\\edm\\envs\\User\\lib\\site-packages\\tensorflow\\python\\ops\\gradients_impl.py:100: UserWarning: Converting sparse IndexedSlices to a dense Tensor of unknown shape. This may consume a large amount of memory.\n \"Converting sparse IndexedSlices to a dense Tensor of unknown shape. \"\n"
]
],
[
[
"OK, let's evaluate our model's accuracy:",
"_____no_output_____"
]
],
[
[
"score, acc = model.evaluate(x_test, y_test,\n batch_size=32,\n verbose=2)\nprint('Test score:', score)\nprint('Test accuracy:', acc)",
"Test score: 0.9316869865119457\nTest accuracy: 0.80904\n"
]
],
[
[
"81% eh? Not too bad, considering we limited ourselves to just the first 80 words of each review.\n\nBut again - stop and think about what we just made here! A neural network that can \"read\" reviews and deduce whether the author liked the movie or not based on that text. And it takes the context of each word and its position in the review into account - and setting up the model itself was just a few lines of code! It's pretty incredible what you can do with Keras.",
"_____no_output_____"
]
]
] | [
"markdown",
"code",
"markdown",
"code",
"markdown",
"code",
"markdown",
"code",
"markdown",
"code",
"markdown",
"code",
"markdown",
"code",
"markdown",
"code",
"markdown",
"code",
"markdown"
] | [
[
"markdown"
],
[
"code"
],
[
"markdown"
],
[
"code"
],
[
"markdown"
],
[
"code"
],
[
"markdown"
],
[
"code"
],
[
"markdown"
],
[
"code"
],
[
"markdown"
],
[
"code"
],
[
"markdown"
],
[
"code"
],
[
"markdown"
],
[
"code"
],
[
"markdown"
],
[
"code"
],
[
"markdown"
]
] |
e77372271ad7b576666cac8246811861e27ac92b | 18,412 | ipynb | Jupyter Notebook | src/prj01.ipynb | hhk54250/20MA573-HHK | 2f60bda24e8ee77899f3b1a98739f2cfae0009ea | [
"MIT"
] | null | null | null | src/prj01.ipynb | hhk54250/20MA573-HHK | 2f60bda24e8ee77899f3b1a98739f2cfae0009ea | [
"MIT"
] | null | null | null | src/prj01.ipynb | hhk54250/20MA573-HHK | 2f60bda24e8ee77899f3b1a98739f2cfae0009ea | [
"MIT"
] | null | null | null | 135.382353 | 15,288 | 0.862753 | [
[
[
"<a href=\"https://colab.research.google.com/github/hhk54250/20MA573-HHK/blob/master/src/prj01.ipynb\" target=\"_parent\"><img src=\"https://colab.research.google.com/assets/colab-badge.svg\" alt=\"Open In Colab\"/></a>",
"_____no_output_____"
],
[
"# First Python Notebook project\n- we perform a simple code and upload to git\n\n- task\nwe will define a function of\n$$\\phi(x) = \\frac{1}{\\sqrt{2\\pi}} e^{-\\frac{x^2}{2}}$$\n\n\n\n",
"_____no_output_____"
]
],
[
[
"import numpy as np\n\ndef phi(x):\n out = 1./np.sqrt(2.*np.pi)*np.exp(-x**2/2.)\n return out",
"_____no_output_____"
],
[
"import matplotlib.pyplot as plt\n%matplotlib inline\n\nx_cod = np.linspace(-5,5,111)\ny_cod = phi(x_cod)\nplt.plot(x_cod,y_cod)",
"_____no_output_____"
],
[
"",
"_____no_output_____"
],
[
"",
"_____no_output_____"
]
]
] | [
"markdown",
"code"
] | [
[
"markdown",
"markdown"
],
[
"code",
"code",
"code",
"code"
]
] |
e7737278b9c9cc4d8c6a6510dd6d77d372dbb9d0 | 142,366 | ipynb | Jupyter Notebook | In-Class Notes/Logistic Regression/.ipynb_checkpoints/logistic regression_sparse-checkpoint.ipynb | cartemic/CHE-599-intro-to-data-science | a2afe72b51a3b9e844de94d59961bedc3534a405 | [
"MIT"
] | null | null | null | In-Class Notes/Logistic Regression/.ipynb_checkpoints/logistic regression_sparse-checkpoint.ipynb | cartemic/CHE-599-intro-to-data-science | a2afe72b51a3b9e844de94d59961bedc3534a405 | [
"MIT"
] | null | null | null | In-Class Notes/Logistic Regression/.ipynb_checkpoints/logistic regression_sparse-checkpoint.ipynb | cartemic/CHE-599-intro-to-data-science | a2afe72b51a3b9e844de94d59961bedc3534a405 | [
"MIT"
] | 2 | 2019-10-02T16:11:36.000Z | 2019-10-15T20:10:40.000Z | 283.59761 | 75,962 | 0.919117 | [
[
[
"empty"
]
]
] | [
"empty"
] | [
[
"empty"
]
] |
e77377771d9c6d3967dea6589e3632e379f3dde9 | 14,035 | ipynb | Jupyter Notebook | notebooks/bytopic/git-workflows/git/05_clones.ipynb | jukent/ncar-python-tutorial | 85c899e865c1861777e99764ef697219355e0585 | [
"CC-BY-4.0"
] | 38 | 2019-09-10T05:00:52.000Z | 2021-12-06T17:39:14.000Z | notebooks/bytopic/git-workflows/git/05_clones.ipynb | jukent/ncar-python-tutorial | 85c899e865c1861777e99764ef697219355e0585 | [
"CC-BY-4.0"
] | 60 | 2019-08-28T22:34:17.000Z | 2021-01-25T22:53:21.000Z | notebooks/bytopic/git-workflows/git/05_clones.ipynb | NCAR/ncar-pangeo-tutorial | 54d536d40cfaf6f8990c58edb438286c19d32a67 | [
"CC-BY-4.0"
] | 22 | 2019-08-29T18:11:57.000Z | 2021-01-07T02:23:46.000Z | 27.682446 | 1,313 | 0.583826 | [
[
[
"empty"
]
]
] | [
"empty"
] | [
[
"empty"
]
] |
e773a79b5b4903e0482c61dd035242e04102a2b3 | 9,451 | ipynb | Jupyter Notebook | notebook/Untitled3.ipynb | marvinren/aiops_gaussian_learnware | 47683546d6648a38bb71988c33f959cf7308376f | [
"Apache-2.0"
] | null | null | null | notebook/Untitled3.ipynb | marvinren/aiops_gaussian_learnware | 47683546d6648a38bb71988c33f959cf7308376f | [
"Apache-2.0"
] | null | null | null | notebook/Untitled3.ipynb | marvinren/aiops_gaussian_learnware | 47683546d6648a38bb71988c33f959cf7308376f | [
"Apache-2.0"
] | null | null | null | 23.686717 | 399 | 0.423659 | [
[
[
"import pandas as pd",
"_____no_output_____"
],
[
"df = pd.DataFrame({\"a\":[1,2,None], \"b\":[1,2,3]})",
"_____no_output_____"
],
[
"df[df.isnull().T.any()]",
"_____no_output_____"
],
[
"df.sort_values(by=['b'], ascending=False)",
"_____no_output_____"
],
[
"df.sort_index(ascending=False)",
"_____no_output_____"
],
[
"df = pd.DataFrame(data={'Animal': ['cat', 'penguin', 'dog',\n 'spider', 'snake'],\n 'Number_legs': [4, 2, 4, 8, 3]})\ndf[\"Number_legs\"].rank(method='max')",
"_____no_output_____"
],
[
"r = dict(list(df.groupby('Animal')['Number_legs']))\nr['cat']",
"_____no_output_____"
],
[
"df.groupby('Animal').agg([('Number_legs', mean)])",
"_____no_output_____"
]
]
] | [
"code"
] | [
[
"code",
"code",
"code",
"code",
"code",
"code",
"code",
"code"
]
] |
e773a94b8b94e05102e43c5933410df970b98f79 | 13,025 | ipynb | Jupyter Notebook | nturesell/algo/plot.ipynb | shinying/SA | 9473fc8fb987b8b1a4c8194f6e8311a27a00ca4b | [
"MIT"
] | null | null | null | nturesell/algo/plot.ipynb | shinying/SA | 9473fc8fb987b8b1a4c8194f6e8311a27a00ca4b | [
"MIT"
] | 1 | 2020-06-05T21:41:11.000Z | 2020-06-05T21:41:11.000Z | nturesell/algo/plot.ipynb | shinying/SA | 9473fc8fb987b8b1a4c8194f6e8311a27a00ca4b | [
"MIT"
] | null | null | null | 30.938242 | 207 | 0.52215 | [
[
[
"import plotly.tools as pt\nimport plotly.plotly as pp\nimport plotly.figure_factory as ff\nfrom datetime import datetime\nfrom datetime import timedelta\nfrom his.data import history_tasks\nfrom main import main\nfrom utils.utility import count_move_time\nimport numpy as np\nimport random\n# pt.set_credentials_file(username='hanjuTsai', api_key='XEOnjaC9Om7WcOwgbRqs')\npt.set_credentials_file(username='angyeahyeah6', api_key='heDVJdzx2KYVJAfpReWi')\ntasks = main(['','e']) \nimport numpy as np\nnumber_of_colors = 100\ncolor = [\"#\"+''.join([random.choice('0123456789ABCDEF') for j in range(number_of_colors)]) for i in range(number_of_colors)]",
"Dispatching 0 tasks with Efficient Greedy...\n"
],
[
"i = 0\ncolors = dict()\nfor task in tasks:\n# color = \"rgb({}, {}, {})\".format(random.randint(0, 255), random.randint(0, 255), random.randint(0, 255))\n color = 'rgb(128, 138, 135)'\n colors[str(task.id)] = color\ncolors['delay_time'] = 'rgb(255,153,51)'\ncolors['move_time'] = 'rgb(0,204,204)'\ncolors['work_time'] = 'rgb(128, 138, 135)'",
"_____no_output_____"
],
[
"# for i in tasks:\n# print(i.delay_time)",
"_____no_output_____"
],
[
"df = []\n# tasks.sort(key=lambda x: x.start_time + x.delay_time)\nfor task in tasks:\n df.append(dict(Task=str(task.ship.ship_id), Start = task.start_time, Finish = task.start_time + task.work_time, Resource=str(task.id)))\n df.append(dict(Task=str(task.ship.ship_id), Start = task.start_time - task.delay_time , Finish = task.start_time , Resource = 'delay_time'))",
"_____no_output_____"
],
[
"fig = ff.create_gantt(df, colors=colors, index_col='Resource', show_colorbar=True, group_tasks=True)\npp.iplot(fig, filename='task-gantt', world_readable=True)",
"High five! You successfully sent some data to your account on plotly. View your plot in your browser at https://plot.ly/~angyeahyeah6/0 or inside your plot.ly account where it is named 'task-gantt'\n"
],
[
"df = []\nfor task in tasks:\n for tug in task.tugs:\n df.append(dict(Task=str(tug.tug_id), Start = task.start_time, Finish = task.start_time + task.work_time, Resource= 'work_time'))\n df.append(dict(Task=str(tug.tug_id), Start = task.start_time - count_move_time(task.task_state, tug.pos, task.start) , Finish = task.start_time, Resource='move_time'))",
"_____no_output_____"
],
[
"fig = ff.create_gantt(df, group_tasks=True, show_colorbar=True , colors=colors, index_col='Resource',showgrid_x=True)\npp.iplot(fig, filename='tug-worktime-gantt', world_readable=True)",
"_____no_output_____"
],
[
"\n",
"_____no_output_____"
]
]
] | [
"code"
] | [
[
"code",
"code",
"code",
"code",
"code",
"code",
"code",
"code"
]
] |
e773aa721927cb3a6af34f7a4044b1369fdc0c06 | 9,838 | ipynb | Jupyter Notebook | Training/RegressionExampleComplete.ipynb | fermaat/afi_deep_learning_intro | f4f783168da9a161f187a9ae5dc4962ad6f3904f | [
"Apache-2.0"
] | null | null | null | Training/RegressionExampleComplete.ipynb | fermaat/afi_deep_learning_intro | f4f783168da9a161f187a9ae5dc4962ad6f3904f | [
"Apache-2.0"
] | 13 | 2020-02-22T18:42:13.000Z | 2022-02-10T01:34:21.000Z | Training/RegressionExampleComplete.ipynb | fermaat/afi_deep_learning_intro | f4f783168da9a161f187a9ae5dc4962ad6f3904f | [
"Apache-2.0"
] | null | null | null | 40.821577 | 1,070 | 0.614048 | [
[
[
"# Predicting house prices: a regression example\nAnother common type of machine-learning problem is regression, which consists of predicting a continuous value instead of a discrete label: for instance, predicting the temperature tomorrow, given meteorological data; or predicting the time that a software project will take to complete, given its specifications.\n\n## Dataset: The Boston Housing Price dataset \nWe’ll attempt to predict the median price of homes in a given Boston suburb in the mid-1970s, given data points about the suburb at the time, such as the crime rate, the local property tax rate, and so on. It has relatively few data points: only 506, split between 404 training samples and 102 test samples. And each feature in the input data (for example, the crime rate) has a different scale. For instance, some values are pro- portions, which take values between 0 and 1; others take values between 1 and 12, others between 0 and 100, and so on.",
"_____no_output_____"
]
],
[
[
"import os, time\nimport tensorflow as tf\nphysical_devices = tf.config.experimental.list_physical_devices('GPU')\ntf.config.experimental.set_memory_growth(physical_devices[0], True)\n\ntf.keras.backend.clear_session() \n\nfrom tensorflow.keras.datasets import boston_housing\n(train_data, train_targets), (test_data, test_targets) = boston_housing.load_data()\n# Let’s look at the data:\nprint (train_data.shape, test_data.shape) ",
"_____no_output_____"
],
[
"train_targets",
"_____no_output_____"
]
],
[
[
"The prices are typically between 10,000 and 50,000. If that sounds cheap, remember that this was the mid-1970s, and these prices aren’t adjusted for inflation.",
"_____no_output_____"
],
[
"## Preparing the data \nIt would be problematic to feed into a neural network values that all take wildly differ- ent ranges. The network might be able to automatically adapt to such heterogeneous data, but it would definitely make learning more difficult. A widespread best practice to deal with such data is to do feature-wise normalization: for each feature in the input divide by the standard deviation, so that the feature is centered around 0 and has a unit standard deviation. This is easily done in Numpy.\n",
"_____no_output_____"
]
],
[
[
"mean = train_data.mean(axis=0)\ntrain_data -= mean\nstd = train_data.std(axis=0)\ntrain_data /= std\ntest_data -= mean\ntest_data /= std",
"_____no_output_____"
]
],
[
[
"## Model Architecture \nBecause so few samples are available, you’ll use a very small network with two hidden layers, each with 64 units. In general, the less training data you have, the worse overfitting will be, and using a small network is one way to mitigate overfitting.\n",
"_____no_output_____"
]
],
[
[
"from tensorflow.keras import models\nfrom tensorflow.keras import layers\n\ndef build_model():\n model = models.Sequential()\n model.add(layers.Dense(64, activation='relu',\n input_shape=(train_data.shape[1],)))\n model.add(layers.Dense(64, activation='relu'))\n model.add(layers.Dense(1))\n model.compile(optimizer='rmsprop', loss='mse', metrics=['mae'])\n return model\n",
"_____no_output_____"
]
],
[
[
"### Validating your approach using K-fold validation\nTo evaluate your networ while you keep adjusting its parameters (such as the number of epochs used for training), you could split the data into a training set and a validation set, as you did in the previous examples. But because you have so few data points, the validation set would end up being very small (for instance, about 100 examples). As a consequence, the validation scores might change a lot depending on which data points you chose to use for validation and which you chose for training: the validation scores might have a high variance with regard to the validation split. This would prevent you from reliably evaluating your model. The best practice in such situations is to use K -fold cross-validation. It consists of splitting the available data into K partitions (typically K = 4 or 5), instantiating K identical models, and training each one on K – 1 partitions while evaluating on the remaining partition. The validation score for the model used is then the average of the K validation scores obtained. In terms of code, this is straightforward",
"_____no_output_____"
]
],
[
[
"import numpy as np\nk = 4\nnum_val_samples = len(train_data) // k\nnum_epochs = 100\nall_scores = []\nfor i in range(k):\n print('processing fold #', i)\n val_data = train_data[i * num_val_samples: (i + 1) * num_val_samples]\n val_targets = train_targets[i * num_val_samples: (i + 1) * num_val_samples]\n partial_train_data = np.concatenate([train_data[:i * num_val_samples], \n train_data[(i + 1) * num_val_samples:]],\n axis=0)\n partial_train_targets = np.concatenate([train_targets[:i * num_val_samples],\n train_targets[(i + 1) * num_val_samples:]],\n axis=0)\n\nmodel = build_model()\nmodel.fit(partial_train_data, partial_train_targets,\n epochs=num_epochs, batch_size=1, verbose=0)\nval_mse, val_mae = model.evaluate(val_data, val_targets, verbose=0)\nall_scores.append(val_mae)\nall_scores",
"_____no_output_____"
]
],
[
[
"Let's train the network for a little longer: 500 epochs",
"_____no_output_____"
]
],
[
[
"num_epochs = 500\nall_mae_histories = []\ndata from partition #k\nfor i in range(k): print('processing fold #', i)\n val_data = train_data[i * num_val_samples: (i + 1) * num_val_samples]\n val_targets = train_targets[i * num_val_samples: (i + 1) * num_val_samples]\n partial_train_data = np.concatenate([train_data[:i * num_val_samples],\n train_data[(i + 1) * num_val_samples:]],\n axis=0)\n\n partial_train_targets = np.concatenate([train_targets[:i * num_val_samples], \n train_targets[(i + 1) * num_val_samples:]],\n axis=0)\n\n model = build_model()\n history = model.fit(partial_train_data, partial_train_targets,\n validation_data=(val_data, val_targets),\n epochs=num_epochs, batch_size=1, verbose=0)\n mae_history = history.history['val_mean_absolute_error']\n all_mae_histories.append(mae_history)",
"_____no_output_____"
],
[
"average_mae_history = [np.mean([x[i] for x in all_mae_histories]) for i in range(num_epochs)]\nimport matplotlib.pyplot as plt\nplt.plot(range(1, len(average_mae_history) + 1), average_mae_history)\nplt.xlabel('Epochs')\nplt.ylabel('Validation MAE')\nplt.show()",
"_____no_output_____"
],
[
"def smooth_curve(points, factor=0.9):\n smoothed_points = []\n for point in points:\n if smoothed_points:\n previous = smoothed_points[-1]\n smoothed_points.append(previous * factor + point * (1 - factor))\n else:\n smoothed_points.append(point)\n return smoothed_points\nsmooth_mae_history = smooth_curve(average_mae_history[10:])\nplt.plot(range(1, len(smooth_mae_history) + 1), smooth_mae_history)\nplt.xlabel('Epochs')\nplt.ylabel('Validation MAE')\nplt.show()",
"_____no_output_____"
],
[
"model = build_model()\nmodel.fit(train_data, train_targets, epochs=80, batch_size=16, verbose=0)\ntest_mse_score, test_mae_score = model.evaluate(test_data, test_targets)",
"_____no_output_____"
]
]
] | [
"markdown",
"code",
"markdown",
"code",
"markdown",
"code",
"markdown",
"code",
"markdown",
"code"
] | [
[
"markdown"
],
[
"code",
"code"
],
[
"markdown",
"markdown"
],
[
"code"
],
[
"markdown"
],
[
"code"
],
[
"markdown"
],
[
"code"
],
[
"markdown"
],
[
"code",
"code",
"code",
"code"
]
] |
e773b38859f3208af574bfc37abcbd2d8e3a23f6 | 8,363 | ipynb | Jupyter Notebook | speedlimits.ipynb | kimsejin111/git_test | c0059e0597cf07607ed5bacc67433dca6249a137 | [
"MIT"
] | null | null | null | speedlimits.ipynb | kimsejin111/git_test | c0059e0597cf07607ed5bacc67433dca6249a137 | [
"MIT"
] | null | null | null | speedlimits.ipynb | kimsejin111/git_test | c0059e0597cf07607ed5bacc67433dca6249a137 | [
"MIT"
] | null | null | null | 29.139373 | 228 | 0.49169 | [
[
[
"<a href=\"https://colab.research.google.com/github/kimsejin111/git_test/blob/main/speedlimits.ipynb\" target=\"_parent\"><img src=\"https://colab.research.google.com/assets/colab-badge.svg\" alt=\"Open In Colab\"/></a>",
"_____no_output_____"
]
],
[
[
"from google.colab import drive\ndrive.mount('/content/gdrive')",
"_____no_output_____"
],
[
"import matplotlib.pyplot as plt\nimport numpy as np\nimport tensorflow as tf\nfrom tensorflow import keras\nfrom tensorflow.keras import layers\nfrom tensorflow.keras.models import Sequential\nimport PIL\nfrom PIL import Image\nimport time\nimport os\nos.environ['TF_CPP_MIN_LOG_LEVEL']='3'\nimport pathlib",
"_____no_output_____"
],
[
"data_dir='/content/gdrive/MyDrive/data'",
"_____no_output_____"
],
[
"data_dir=pathlib.Path(data_dir)\n\nbatch_size=32\nimage_height=180\nimage_width=180\n\nd_image_count = len(list(data_dir.glob('*/*.png')))\nprint(d_image_count)\n",
"_____no_output_____"
],
[
"train_ds=tf.keras.preprocessing.image_dataset_from_directory(\n data_dir,\n validation_split=0.2, # 왜 0.2로 하는거지?\n subset=\"training\",\n seed=123, # seed는 무얼 의미하는 거지?\n image_size=(image_height, image_width),\n batch_size=batch_size\n)\nval_ds=tf.keras.preprocessing.image_dataset_from_directory(\n data_dir,\n validation_split=0.2,\n subset=\"validation\",\n seed=123,\n image_size=(image_height, image_width),\n batch_size=batch_size\n)",
"_____no_output_____"
],
[
"class_names = train_ds.class_names\nplt.figure(figsize=(10,10))\nfor images, labels in train_ds.take(1): #take 함수는 무슨 함수일까\n for i in range(9):\n ax=plt.subplot(3,3,i+1)\n plt.imshow(images[i].numpy().astype(\"uint8\")) #numpy,imshow 는 무슨 함수일까\n plt.title(class_names[labels[i]])\n plt.axis(\"off\")\nplt.show()",
"_____no_output_____"
],
[
"AUTOTUNE =tf.data.experimental.AUTOTUNE\ntrain_ds=train_ds.cache().shuffle(1000).prefetch(buffer_size=AUTOTUNE)\nval_ds=val_ds.cache().prefetch(buffer_size=AUTOTUNE)",
"_____no_output_____"
],
[
"normaliztion_layer= layers.experimental.preprocessing.Rescaling(1./255)\n\nnormalized_ds=train_ds.map(lambda x,y:(normaliztion_layer(x),y))\nimage_batch,labels_batch = next(iter(normalized_ds)) #자동 반복 함수\nfirst_image = image_batch[0]\nprint(np.min(first_image), np.max(first_image))",
"_____no_output_____"
],
[
"data_augmentation = keras.Sequential(\n [\n layers.experimental.preprocessing.RandomFlip(\n \"horizontal\",\n input_shape=(\n image_height,\n image_width,\n 3)),\n layers.experimental.preprocessing.RandomRotation(0.1),\n layers.experimental.preprocessing.RandomZoom(0.1),\n ]\n)",
"_____no_output_____"
],
[
"num_classes=5\n\nmodel2 =Sequential([\n layers.experimental.preprocessing.Rescaling(1. / 255, input_shape=(image_height, image_width, 3)),\n layers.Conv2D(16, 3, padding='same', activation='relu'),\n layers.MaxPool2D(),\n layers.Conv2D(32, 3, padding='same', activation='relu'),\n layers.MaxPool2D(),\n layers.Conv2D(64, 3, padding='same', activation='relu'),\n layers.MaxPool2D(), # Pooling 필터링 개념으로 생각하자 해당 convolution에서 MAX값으로 필터링 하여 데이터 크기를 줄임\n layers.Dropout(0.2),\n layers.Flatten(), # convolution의 dimension을 줄여주는 함수\n layers.Dense(128, activation='relu'),\n layers.Dense(num_classes)\n])",
"_____no_output_____"
]
],
[
[
"",
"_____no_output_____"
]
],
[
[
"model2.compile(optimizer='adam',\n loss=tf.keras.losses.SparseCategoricalCrossentropy(from_logits=True),\n metrics=['accuracy'])",
"_____no_output_____"
],
[
"epochs=15\nhistory=model2.fit(\n train_ds,\n validation_data=val_ds,\n epochs=epochs,\n)",
"_____no_output_____"
],
[
"acc_E=history.history['accuracy']\nval_E_acc=history.history['val_accuracy']\n\nloss_E=history.history['loss']\nval_E_loss=history.history['val_loss']\n\nepochs_range= range(epochs)\n\nplt.figure(figsize=(8,8))\nplt.subplot(1,2,1)\nplt.plot(epochs_range,acc_E,label=\"Training ACC\")\nplt.plot(epochs_range,val_E_acc,label=\"Validation ACC\")\nplt.legend(loc='lower right')\nplt.title('Training and Validation Accuracy')\n\nplt.subplot(1,2,2)\nplt.plot(epochs_range,loss_E,label=\"Training Loss\")\nplt.plot(epochs_range,val_E_loss,label=\"Validation Loss\")\nplt.legend(loc='upper right')\nplt.title('Training and Validation Loss')\n\nplt.show()",
"_____no_output_____"
]
]
] | [
"markdown",
"code",
"markdown",
"code"
] | [
[
"markdown"
],
[
"code",
"code",
"code",
"code",
"code",
"code",
"code",
"code",
"code",
"code"
],
[
"markdown"
],
[
"code",
"code",
"code"
]
] |
e773c98b1ac973442e737d326a6926dc0a011e92 | 18,706 | ipynb | Jupyter Notebook | notebooks/user/pjayasundara/AEFI_with_uncertainty.ipynb | monash-emu/AuTuMN | fa3b81ef54cf561e0e7364a48f4ff96585dc3310 | [
"BSD-2-Clause-FreeBSD"
] | 14 | 2020-03-11T06:15:30.000Z | 2022-03-09T03:38:35.000Z | notebooks/user/pjayasundara/AEFI_with_uncertainty.ipynb | monash-emu/AuTuMN | fa3b81ef54cf561e0e7364a48f4ff96585dc3310 | [
"BSD-2-Clause-FreeBSD"
] | 96 | 2020-01-29T05:10:29.000Z | 2022-03-31T01:48:46.000Z | notebooks/user/pjayasundara/AEFI_with_uncertainty.ipynb | monash-emu/AuTuMN | fa3b81ef54cf561e0e7364a48f4ff96585dc3310 | [
"BSD-2-Clause-FreeBSD"
] | 10 | 2020-04-24T00:38:00.000Z | 2021-08-19T16:19:03.000Z | 39.464135 | 199 | 0.539666 | [
[
[
"# Import packages\nimport os\nfrom matplotlib import pyplot as plt\nimport pandas as pd\nimport math \n\nimport numpy as np\n\n# Import AuTuMN modules\nfrom autumn.settings import Models, Region\nfrom autumn.settings.folders import OUTPUT_DATA_PATH\nfrom autumn.tools.project import get_project\nfrom autumn.tools import db\nfrom autumn.tools.plots.calibration.plots import calculate_r_hats, get_output_from_run_id\nfrom autumn.tools.plots.uncertainty.plots import _plot_uncertainty, _get_target_values\nfrom autumn.tools.plots.plotter.base_plotter import COLOR_THEME\nfrom autumn.tools.plots.utils import get_plot_text_dict, change_xaxis_to_date, REF_DATE, ALPHAS, COLORS, _apply_transparency, _plot_targets_to_axis\nfrom autumn.models.covid_19.stratifications.agegroup import AGEGROUP_STRATA\n\nimport matplotlib.patches as mpatches\n\nfrom autumn.dashboards.calibration_results.plots import get_uncertainty_df",
"_____no_output_____"
],
[
"# Specify model details\nmodel = Models.COVID_19\nregion = Region.MALAYSIA\ndirname = \"2021-10-20\"",
"_____no_output_____"
],
[
"# get the relevant project and output data\nproject = get_project(model, region)\nproject_calib_dir = os.path.join(\n OUTPUT_DATA_PATH, \"calibrate\", project.model_name, project.region_name\n)\ncalib_path = os.path.join(project_calib_dir, dirname)\n# Load tables\nmcmc_tables = db.load.load_mcmc_tables(calib_path)\nmcmc_params = db.load.load_mcmc_params_tables(calib_path)\n\nuncertainty_df = get_uncertainty_df(calib_path, mcmc_tables, project.plots)\nscenario_list = uncertainty_df['scenario'].unique()\n\n# make output directories\noutput_dir = f\"{model}_{region}_{dirname}\"\nbase_dir = os.path.join(\"outputs\", output_dir)\nos.makedirs(base_dir, exist_ok=True)\ndirs_to_make = [\"MLE\", \"median\",\"csv_files\"]\nfor dir_to_make in dirs_to_make:\n os.makedirs(os.path.join(base_dir, dir_to_make), exist_ok=True)",
"_____no_output_____"
],
[
"titles = {\n \"notifications\": \"Daily number of notified Covid-19 cases\",\n \"infection_deaths\": \"Daily number of Covid-19 deaths\",\n \"accum_deaths\": \"Cumulative number of Covid-19 deaths\",\n \"incidence\": \"Daily incidence (incl. asymptomatics and undetected)\",\n \"hospital_occupancy\": \"Hospital beds occupied by Covid-19 patients\",\n \"icu_occupancy\": \"ICU beds occupied by Covid-19 patients\",\n \"new_hospital_admissions\": \"New hospital admissions\",\n \"cdr\": \"Proportion detected among symptomatics\",\n \"proportion_vaccinated\": \"Proportion vaccinated\",\n \"prop_incidence_strain_delta\": \"Proportion of Delta variant in new cases\"\n}\n\ndef plot_outputs(output_type, output_name, scenario_list, sc_linestyles, sc_colors, show_v_lines=False, x_min=590, x_max=775):\n\n # plot options\n title = titles[output_name]\n title_fontsize = 18\n label_font_size = 15\n linewidth = 3\n n_xticks = 10\n\n # initialise figure\n fig = plt.figure(figsize=(12, 8))\n plt.style.use(\"ggplot\")\n axis = fig.add_subplot()\n\n # prepare colors for ucnertainty\n n_scenarios_to_plot = len(scenario_list)\n uncertainty_colors = _apply_transparency(COLORS[:n_scenarios_to_plot], ALPHAS[:n_scenarios_to_plot])\n\n if output_type == \"MLE\":\n derived_output_tables = db.load.load_derived_output_tables(calib_path, column=output_name)\n for i, scenario in enumerate(scenario_list): \n linestyle = sc_linestyles[scenario]\n color = sc_colors[scenario]\n\n if output_type == \"MLE\":\n times, values = get_output_from_run_id(output_name, mcmc_tables, derived_output_tables, \"MLE\", scenario)\n axis.plot(times, values, color=color, linestyle=linestyle, linewidth=linewidth)\n elif output_type == \"median\":\n _plot_uncertainty(\n axis,\n uncertainty_df,\n output_name,\n scenario,\n x_max,\n x_min,\n [_, _, _, color],\n overlay_uncertainty=False,\n start_quantile=0,\n zorder=scenario + 1,\n linestyle=linestyle,\n linewidth=linewidth,\n )\n elif output_type == \"uncertainty\":\n scenario_colors = uncertainty_colors[i] \n _plot_uncertainty(\n axis,\n uncertainty_df,\n output_name,\n scenario,\n x_max,\n x_min,\n scenario_colors,\n overlay_uncertainty=True,\n start_quantile=0,\n zorder=scenario + 1,\n )\n else:\n print(\"Please use supported output_type option\")\n\n\n axis.set_xlim((x_min, x_max))\n axis.set_title(title, fontsize=title_fontsize)\n plt.setp(axis.get_yticklabels(), fontsize=label_font_size)\n plt.setp(axis.get_xticklabels(), fontsize=label_font_size)\n change_xaxis_to_date(axis, REF_DATE)\n plt.locator_params(axis=\"x\", nbins=n_xticks)\n\n if show_v_lines:\n release_dates = {}\n y_max = plt.gca().get_ylim()[1]\n linestyles = [\"dashdot\", \"solid\"]\n i = 0\n for time, date in release_dates.items():\n plt.vlines(time, ymin=0, ymax=y_max, linestyle=linestyles[i])\n text = f\"Lockdown relaxed on {date}\"\n plt.text(time - 5, .5*y_max, text, rotation=90, fontsize=11)\n i += 1\n \n return axis\n",
"_____no_output_____"
]
],
[
[
"# Scenario plots with single lines",
"_____no_output_____"
]
],
[
[
"output_names = [\"notifications\", \"icu_occupancy\"]\nscenario_x_min, scenario_x_max = 367, 920 \n\nsc_to_plot = [0, 1]\nlegend = [\"With vaccine\", \"Without vaccine\"]\nlift_time = 731\ntext_font = 14\n\nsc_colors = [COLOR_THEME[i] for i in scenario_list]\nsc_linestyles = [\"solid\"] * (len(scenario_list))\nfor output_type in [\"median\", \"MLE\"]:\n for output_name in output_names:\n plot_outputs(output_type, output_name, sc_to_plot, sc_linestyles, sc_colors, False, x_min=scenario_x_min, x_max=scenario_x_max)\n path = os.path.join(base_dir, output_type, f\"{output_name}.png\")\n plt.legend(labels=legend, fontsize=text_font, facecolor=\"white\")\n \n ymax = plt.gca().get_ylim()[1]\n plt.vlines(x=lift_time,ymin=0,ymax=1.05*ymax, linestyle=\"dashed\") # 31 Dec 2021\n \n plt.text(x=(scenario_x_min + lift_time) / 2., y=1.* ymax, s=\"Vaccination phase\", ha=\"center\", fontsize = text_font)\n \n plt.text(x=lift_time + 3, y=ymax, s=\"Restrictions lifted\", fontsize = text_font, rotation=90, va=\"top\")\n \n plt.savefig(path)\n ",
"_____no_output_____"
]
],
[
[
"# Make Adverse Effects figures",
"_____no_output_____"
]
],
[
[
"params = project.param_set.baseline.to_dict()\nae_risk = {\n \"AstraZeneca\": params[\"vaccination_risk\"][\"tts_rate\"],\n \"mRNA\": params[\"vaccination_risk\"][\"myocarditis_rate\"]\n}",
"_____no_output_____"
],
[
"agg_agegroups = [\"10_14\",\"15_19\", \"20_29\", \"30_39\", \"40_49\", \"50_59\", \"60_69\", \"70_plus\"]\ntext_font = 12\n \nvacc_scenarios = {\n \"mRNA\": 2,\n \"AstraZeneca\": 2,\n}\n\nadverse_effects = {\n \"mRNA\": \"myocarditis\",\n \"AstraZeneca\": \"thrombosis with thrombocytopenia syndrome\",\n}\n\nadverse_effects_short= {\n \"mRNA\": \"myocarditis\",\n \"AstraZeneca\": \"tts\",\n}\n\nleft_title = \"COVID-19-associated hospitalisations prevented\"\n\ndef format_age_label(age_bracket):\n if age_bracket.startswith(\"70\"):\n return \"70+\"\n else:\n return age_bracket.replace(\"_\", \"-\")\n \n\n\ndef make_ae_figure(vacc_scenario, log_scale=False):\n trimmed_df = uncertainty_df[\n (uncertainty_df[\"scenario\"]==vacc_scenarios[vacc_scenario]) & (uncertainty_df[\"time\"]==913)\n ] \n \n right_title = f\"Cases of {adverse_effects[vacc_scenario]}\"\n \n fig = plt.figure(figsize=(10, 4))\n plt.style.use(\"default\")\n axis = fig.add_subplot() \n \n h_max = 0\n delta_agegroup = 1.2 if log_scale else 4000 \n barwidth = .7\n text_offset = 0.5 if log_scale else 20\n unc_color = \"black\"\n unc_lw = 1.\n \n for i, age_bracket in enumerate(agg_agegroups):\n y = len(agg_agegroups) - i - .5\n plt.text(x=delta_agegroup / 2, y=y, s=format_age_label(age_bracket), ha=\"center\", va=\"center\", fontsize=text_font)\n \n # get outputs\n hosp_output_name = f\"abs_diff_cumulative_hospital_admissionsXagg_age_{age_bracket}\"\n ae_output_name = f\"abs_diff_cumulative_{adverse_effects_short[vacc_scenario]}_casesXagg_age_{age_bracket}\"\n \n prev_hosp_df = trimmed_df[trimmed_df[\"type\"] == hosp_output_name]\n prev_hosp_values = [ # median, lower, upper\n float(prev_hosp_df['value'][prev_hosp_df[\"quantile\"] == q]) for q in [0.5, 0.025, 0.975]\n ]\n log_prev_hosp_values = [math.log10(v) for v in prev_hosp_values]\n \n ae_df = trimmed_df[trimmed_df[\"type\"] == ae_output_name]\n ae_values = [ # median, lower, upper\n - float(ae_df['value'][ae_df[\"quantile\"] == q]) for q in [0.5, 0.975, 0.025]\n ] \n log_ae_values = [max(math.log10(v), 0) for v in ae_values]\n \n if log_scale:\n plot_h_values = log_prev_hosp_values\n plot_ae_values = log_ae_values\n else:\n plot_h_values = prev_hosp_values\n plot_ae_values = ae_values\n \n h_max = max(plot_h_values[2], h_max) \n \n origin = 0\n # hospital\n rect = mpatches.Rectangle((origin, y - barwidth/2), width=-plot_h_values[0], height=barwidth, facecolor=\"cornflowerblue\")\n axis.add_patch(rect) \n plt.hlines(y=y, xmin=-plot_h_values[1], xmax=-plot_h_values[2], color=unc_color, linewidth=unc_lw)\n \n disp_val = int(prev_hosp_values[0])\n plt.text(x= -plot_h_values[0] - text_offset, y=y + barwidth/2, s=int(disp_val), ha=\"right\", va=\"center\", fontsize=text_font*.7) \n \n min_bar_length = 0\n if not log_scale:\n min_bar_length = 0 if vacc_scenario == \"Astrazeneca\" else 0\n \n rect = mpatches.Rectangle((delta_agegroup + origin, y - barwidth/2), width=max(plot_ae_values[0], min_bar_length), height=barwidth, facecolor=\"tab:red\")\n axis.add_patch(rect)\n plt.hlines(y=y, xmin=delta_agegroup + origin + plot_ae_values[1], xmax=delta_agegroup + origin + plot_ae_values[2], color=unc_color, linewidth=unc_lw)\n \n disp_val = int(ae_values[0])\n plt.text(x=delta_agegroup + origin + max(plot_ae_values[0], min_bar_length) + text_offset, y=y + barwidth/2, s=int(disp_val), ha=\"left\", va=\"center\", fontsize=text_font*.7) \n\n # main title\n axis.set_title(f\"Benefit/Risk analysis with {vacc_scenario} vaccine\", fontsize = text_font + 2)\n \n # x axis ticks\n if log_scale:\n max_val_display = math.ceil(h_max)\n else:\n magnitude = 500\n max_val_display = math.ceil(h_max / magnitude) * magnitude \n \n # sub-titles \n plt.text(x= - max_val_display / 2, y=len(agg_agegroups) + .3, s=left_title, ha=\"center\", fontsize=text_font)\n plt.text(x= max_val_display / 2 + delta_agegroup, y=len(agg_agegroups) + .3, s=right_title, ha=\"center\", fontsize=text_font)\n \n if log_scale:\n ticks = range(max_val_display + 1)\n rev_ticks = [-t for t in ticks]\n rev_ticks.reverse() \n x_ticks = rev_ticks + [delta_agegroup + t for t in ticks]\n \n labels = [10**(p) for p in range(max_val_display + 1)]\n rev_labels = [l for l in labels]\n rev_labels.reverse()\n x_labels = rev_labels + labels \n x_labels[max_val_display] = x_labels[max_val_display + 1] = 0\n else:\n n_ticks = 6\n x_ticks = [-max_val_display + j * (max_val_display/(n_ticks - 1)) for j in range(n_ticks)] + [delta_agegroup + j * (max_val_display/(n_ticks - 1)) for j in range(n_ticks)]\n rev_n_ticks = x_ticks[:n_ticks]\n rev_n_ticks.reverse()\n x_labels = [int(-v) for v in x_ticks[:n_ticks]] + [int(-v) for v in rev_n_ticks]\n \n plt.xticks(ticks=x_ticks, labels=x_labels)\n \n # x, y lims\n axis.set_xlim((-max_val_display, max_val_display + delta_agegroup))\n axis.set_ylim((0, len(agg_agegroups) + 1)) \n \n # remove axes\n axis.set_frame_on(False)\n axis.axes.get_yaxis().set_visible(False)\n\n log_ext = \"_log_scale\" if log_scale else \"\" \n path = os.path.join(base_dir, f\"{vacc_scenario}_adverse_effects{log_ext}.png\") \n plt.tight_layout()\n plt.savefig(path, dpi=600)\n\nfor vacc_scenario in [\"mRNA\", \"AstraZeneca\"]:\n for log_scale in [False,True]:\n make_ae_figure(vacc_scenario, log_scale) \n ",
"_____no_output_____"
]
],
[
[
"# Counterfactual no vaccine scenario",
"_____no_output_____"
]
],
[
[
"output_type = \"uncertainty\"\noutput_names = [\"notifications\", \"icu_occupancy\", \"accum_deaths\"]\nsc_to_plot = [0, 1]\nx_min, x_max = 400, 670\nvacc_start = 426\nfor output_name in output_names:\n axis = plot_outputs(output_type, output_name, sc_to_plot, sc_linestyles, sc_colors, False, x_min=400, x_max=670)\n y_max = plt.gca().get_ylim()[1]\n plt.vlines(x=vacc_start, ymin=0, ymax=y_max, linestyle=\"dashdot\")\n plt.text(x=vacc_start - 5, y=.6 * y_max, s=\"Vaccination starts\", rotation=90, fontsize=12)\n \n path = os.path.join(base_dir, f\"{output_name}_counterfactual.png\") \n plt.tight_layout()\n plt.savefig(path, dpi=600)\n",
"_____no_output_____"
]
],
[
[
"# number of lives saved",
"_____no_output_____"
]
],
[
[
"today = 660 # 21 Oct\ndf = uncertainty_df[(uncertainty_df[\"type\"] == \"accum_deaths\") & (uncertainty_df[\"quantile\"] == 0.5) & (uncertainty_df[\"time\"] == today)]\n\nbaseline = float(df[df[\"scenario\"] == 0][\"value\"])\ncounterfact = float(df[df[\"scenario\"] == 1][\"value\"])\n\nprint(counterfact - baseline)",
"_____no_output_____"
]
]
] | [
"code",
"markdown",
"code",
"markdown",
"code",
"markdown",
"code",
"markdown",
"code"
] | [
[
"code",
"code",
"code",
"code"
],
[
"markdown"
],
[
"code"
],
[
"markdown"
],
[
"code",
"code"
],
[
"markdown"
],
[
"code"
],
[
"markdown"
],
[
"code"
]
] |
e773df1bb9a9a26ac772fbb0bfcc70e806063569 | 1,030,254 | ipynb | Jupyter Notebook | tutorials/Multimodal_VQA_Captum_Insights.ipynb | doc22940/captum | b414b9e8dbd3ec2e7722b339d12419f9c4538fc5 | [
"BSD-3-Clause"
] | 1 | 2021-03-06T23:30:39.000Z | 2021-03-06T23:30:39.000Z | tutorials/Multimodal_VQA_Captum_Insights.ipynb | doc22940/captum | b414b9e8dbd3ec2e7722b339d12419f9c4538fc5 | [
"BSD-3-Clause"
] | null | null | null | tutorials/Multimodal_VQA_Captum_Insights.ipynb | doc22940/captum | b414b9e8dbd3ec2e7722b339d12419f9c4538fc5 | [
"BSD-3-Clause"
] | null | null | null | 1,746.19322 | 1,010,344 | 0.957898 | [
[
[
"# Captum Insights for Visual Question Answering",
"_____no_output_____"
],
[
"This notebook provides a simple example for the [Captum Insights API](https://captum.ai/docs/captum_insights), which is an easy to use API built on top of Captum that provides a visualization widget.\n\nIt is suggested to first read the multi-modal [tutorial](https://captum.ai/tutorials/Multimodal_VQA_Interpret) with VQA that utilises the `captum.attr` API. This tutorial will skip over a large chunk of details for setting up the VQA model.\n\nAs with the referenced tutorial, you will need the following installed on your machine:\n\n- Python Packages: torchvision, PIL, and matplotlib\n- pytorch-vqa: https://github.com/Cyanogenoid/pytorch-vqa\n- pytorch-resnet: https://github.com/Cyanogenoid/pytorch-resnet\n- A pretrained pytorch-vqa model, which can be obtained from: https://github.com/Cyanogenoid/pytorch-vqa/releases/download/v1.0/2017-08-04_00.55.19.pth\n\nPlease modify the below section for your specific installation paths:",
"_____no_output_____"
]
],
[
[
"import sys, os\n\n# Replace the placeholder strings with the associated \n# path for the root of pytorch-vqa and pytorch-resnet respectively\nPYTORCH_VQA_DIR = os.path.realpath(\"../../pytorch-vqa\")\nPYTORCH_RESNET_DIR = os.path.realpath(\"../../pytorch-resnet\")\n\n# Please modify this path to where it is located on your machine\n# you can download this model from: \n# https://github.com/Cyanogenoid/pytorch-vqa/releases/download/v1.0/2017-08-04_00.55.19.pth\nVQA_MODEL_PATH = \"models/2017-08-04_00.55.19.pth\"\n\nassert(os.path.exists(PYTORCH_VQA_DIR))\nassert(os.path.exists(PYTORCH_RESNET_DIR))\nassert(os.path.exists(VQA_MODEL_PATH))\n\nsys.path.append(PYTORCH_VQA_DIR)\nsys.path.append(PYTORCH_RESNET_DIR)",
"_____no_output_____"
]
],
[
[
"Now, we will import the necessary modules to run the code in this tutorial. Please make sure you have the [prerequisites to run captum](https://captum.ai/docs/getting_started), along with the pre-requisites to run this tutorial (as described in the first section).",
"_____no_output_____"
]
],
[
[
"import numpy as np\nimport matplotlib.pyplot as plt\nfrom matplotlib.colors import LinearSegmentedColormap\nfrom PIL import Image\n\nimport torch\nimport torchvision\nimport torchvision.transforms as transforms\nimport torch.nn.functional as F\n\ntry:\n import resnet # from pytorch-resnet\nexcept:\n print(\"please provide a valid path to pytorch-resnet\")\n\ntry:\n from model import Net, apply_attention, tile_2d_over_nd # from pytorch-vqa\n from utils import get_transform # from pytorch-vqa\nexcept:\n print(\"please provide a valid path to pytorch-vqa\")\n \nfrom captum.insights import AttributionVisualizer, Batch\nfrom captum.insights.features import ImageFeature, TextFeature\nfrom captum.attr import TokenReferenceBase, configure_interpretable_embedding_layer, remove_interpretable_embedding_layer",
"_____no_output_____"
],
[
"# Let's set the device we will use for model inference\ndevice = torch.device(\"cuda:0\" if torch.cuda.is_available() else \"cpu\")",
"_____no_output_____"
]
],
[
[
"# VQA Model Setup\n\nLet's load the VQA model (again, please refer to the [model interpretation tutorial on VQA](https://captum.ai/tutorials/Multimodal_VQA_Interpret) if you want details)",
"_____no_output_____"
]
],
[
[
"saved_state = torch.load(VQA_MODEL_PATH, map_location=device)\n\n# reading vocabulary from saved model\nvocab = saved_state[\"vocab\"]\n\n# reading word tokens from saved model\ntoken_to_index = vocab[\"question\"]\n\n# reading answers from saved model\nanswer_to_index = vocab[\"answer\"]\n\nnum_tokens = len(token_to_index) + 1\n\n# reading answer classes from the vocabulary\nanswer_words = [\"unk\"] * len(answer_to_index)\nfor w, idx in answer_to_index.items():\n answer_words[idx] = w\n\nvqa_net = torch.nn.DataParallel(Net(num_tokens), device_ids=[0, 1])\nvqa_net.load_state_dict(saved_state[\"weights\"])\nvqa_net = vqa_net.to(device)",
"_____no_output_____"
],
[
" # for visualization to convert indices to tokens for questions\nquestion_words = [\"unk\"] * num_tokens\nfor w, idx in token_to_index.items():\n question_words[idx] = w",
"_____no_output_____"
]
],
[
[
"Let's modify the VQA model to use pytorch-resnet. Our model will be called `vqa_resnet`.",
"_____no_output_____"
]
],
[
[
"class ResNetLayer4(torch.nn.Module):\n def __init__(self):\n super().__init__()\n self.r_model = resnet.resnet152(pretrained=True)\n self.r_model.eval()\n self.r_model.to(device)\n self.buffer = None\n\n def save_output(module, input, output):\n self.buffer = output\n\n self.r_model.layer4.register_forward_hook(save_output)\n\n def forward(self, x):\n self.r_model(x)\n return self.buffer\n\nclass VQA_Resnet_Model(Net):\n def __init__(self, embedding_tokens):\n super().__init__(embedding_tokens)\n self.resnet_layer4 = ResNetLayer4()\n\n def forward(self, v, q, q_len):\n q = self.text(q, list(q_len.data))\n v = self.resnet_layer4(v)\n\n v = v / (v.norm(p=2, dim=1, keepdim=True).expand_as(v) + 1e-8)\n\n a = self.attention(v, q)\n v = apply_attention(v, a)\n\n combined = torch.cat([v, q], dim=1)\n answer = self.classifier(combined)\n return answer\n\nvqa_resnet = VQA_Resnet_Model(vqa_net.module.text.embedding.num_embeddings)\n\n# `device_ids` contains a list of GPU ids which are used for parallelization supported by `DataParallel`\nvqa_resnet = torch.nn.DataParallel(vqa_resnet, device_ids=[0, 1])\n\n# saved vqa model's parameters\npartial_dict = vqa_net.state_dict()\n\nstate = vqa_resnet.state_dict()\nstate.update(partial_dict)\nvqa_resnet.load_state_dict(state)\n\nvqa_resnet.to(device)\nvqa_resnet.eval()\n\n# This is original VQA model without resnet. Removing it, since we do not need it\ndel vqa_net\n\n# this is necessary for the backpropagation of RNNs models in eval mode\ntorch.backends.cudnn.enabled = False",
"_____no_output_____"
]
],
[
[
"# Input Utilities\n\nNow we will need some utility functions for the inputs of our model. \n\nLet's start off with our image input transform function. We will separate out the normalization step from the transform in order to view the original image.",
"_____no_output_____"
]
],
[
[
"image_size = 448 # scale image to given size and center\ncentral_fraction = 1.0\n\ntransform = get_transform(image_size, central_fraction=central_fraction)\ntransform_normalize = transform.transforms.pop()",
"/opt/homebrew/lib/python3.7/site-packages/torchvision/transforms/transforms.py:210: UserWarning: The use of the transforms.Scale transform is deprecated, please use transforms.Resize instead.\n warnings.warn(\"The use of the transforms.Scale transform is deprecated, \" +\n"
]
],
[
[
"Now for the input question, we will need an encoding function (to go from words -> indices):",
"_____no_output_____"
]
],
[
[
"def encode_question(question):\n \"\"\" Turn a question into a vector of indices and a question length \"\"\"\n question_arr = question.lower().split()\n vec = torch.zeros(len(question_arr), device=device).long()\n for i, token in enumerate(question_arr):\n index = token_to_index.get(token, 0)\n vec[i] = index\n return vec, torch.tensor(len(question_arr), device=device)",
"_____no_output_____"
]
],
[
[
"# Baseline Inputs ",
"_____no_output_____"
],
[
"The insights API utilises captum's attribution API under the hood, hence we will need a baseline for our inputs. A baseline is (typically) a neutral output to reference in order for our attribution algorithm(s) to understand which features are important in making a prediction (this is very simplified explanation, 'Remark 1' in the [Integrated Gradients paper](https://arxiv.org/pdf/1703.01365.pdf) has an excellent explanation on why they must be utilised).\n\nFor images and for the purpose of this tutorial, we will let this baseline be the zero vector (a black image).",
"_____no_output_____"
]
],
[
[
"def baseline_image(x):\n return x * 0",
"_____no_output_____"
]
],
[
[
"For sentences, as done in the multi-modal VQA tutorial, we will use a sentence composed of padded symbols.\n\nWe will also require to pass our model through the [`configure_interpretable_embedding_layer`](https://captum.ai/api/utilities.html?highlight=configure_interpretable_embedding_layer#captum.attr._models.base.configure_interpretable_embedding_layer) function, which separates the embedding layer and precomputes word embeddings. To put it simply, this function allows us to precompute and give the embedding vectors directly to our model, which will allow us to reference the words associated to particular embeddings (for visualization purposes).",
"_____no_output_____"
]
],
[
[
"interpretable_embedding = configure_interpretable_embedding_layer(\n vqa_resnet, \"module.text.embedding\"\n)\n\nPAD_IND = token_to_index[\"pad\"]\ntoken_reference = TokenReferenceBase(reference_token_idx=PAD_IND)\n\ndef baseline_text(x):\n seq_len = x.size(0)\n ref_indices = token_reference.generate_reference(seq_len, device=device).unsqueeze(\n 0\n )\n return interpretable_embedding.indices_to_embeddings(ref_indices).squeeze(0)\n\ndef input_text_transform(x):\n return interpretable_embedding.indices_to_embeddings(x)",
"../captum/attr/_models/base.py:168: UserWarning: In order to make embedding layers more interpretable they will\n be replaced with an interpretable embedding layer which wraps the\n original embedding layer and takes word embedding vectors as inputs of\n the forward function. This allows to generate baselines for word\n embeddings and compute attributions for each embedding dimension.\n The original embedding layer must be set\n back by calling `remove_interpretable_embedding_layer` function\n after model interpretation is finished.\n after model interpretation is finished.\"\"\"\n"
]
],
[
[
"# Using the Insights API\n\nFinally we have reached the relevant part of the tutorial.\n\nFirst let's create a utility function to allow us to pass data into the insights API. This function will essentially produce `Batch` objects, which tell the insights API what your inputs, labels and any additional arguments are.",
"_____no_output_____"
]
],
[
[
"def vqa_dataset(image, questions, targets):\n img = Image.open(image).convert(\"RGB\")\n img = transform(img).unsqueeze(0)\n\n for question, target in zip(questions, targets):\n q, q_len = encode_question(question)\n\n q = q.unsqueeze(0)\n q_len = q_len.unsqueeze(0)\n\n target_idx = answer_to_index[target]\n\n yield Batch(\n inputs=(img, q), labels=(target_idx,), additional_args=q_len\n )",
"_____no_output_____"
]
],
[
[
"Let's create our `AttributionVisualizer`, to do this we need the following:\n\n- A score function, which tells us how to interpret the model's output vector\n- Description of the input features given to the model\n- The data to visualize (as described above)\n- Description of the output (the class names), in our case this is our answer words",
"_____no_output_____"
],
[
"In our case, we want to produce a single answer output via softmax",
"_____no_output_____"
]
],
[
[
"def score_func(o):\n return F.softmax(o, dim=1)",
"_____no_output_____"
]
],
[
[
"The following function will convert a sequence of question indices to the associated question words for visualization purposes. This will be provided to the `TextFeature` object to describe text features.",
"_____no_output_____"
]
],
[
[
"def itos(input):\n return [question_words[int(i)] for i in input.squeeze(0)]",
"_____no_output_____"
]
],
[
[
"Let's define some dummy data to visualize using the function we declared earlier.",
"_____no_output_____"
]
],
[
[
"dataset = vqa_dataset(\"./img/vqa/elephant.jpg\", \n [\"what is on the picture\",\n \"what color is the elephant\",\n \"where is the elephant\" ],\n [\"elephant\", \"gray\", \"zoo\"]\n)",
"_____no_output_____"
]
],
[
[
"Now let's describe our features. Each feature requires an input transformation function and a set of baselines. As described earlier, we will use the black image for the image baseline and a padded sequence for the text baseline.\n\nThe input image will be transformed via our normalization transform (`transform_normalize`).\nOur input text will need to be transformed into embeddings, as it is a sequence of indices. Our model only accepts embeddings as input, as we modified the model with `configure_interpretable_embedding_layer` earlier.\n\nWe also need to provide how the input text should be transformed in order to be visualized, which will be accomplished through the `itos` function, as described earlier.",
"_____no_output_____"
]
],
[
[
"features = [\n ImageFeature(\n \"Picture\",\n input_transforms=[transform_normalize],\n baseline_transforms=[baseline_image],\n ),\n TextFeature(\n \"Question\",\n input_transforms=[input_text_transform],\n baseline_transforms=[baseline_text],\n visualization_transform=itos,\n ),\n]",
"_____no_output_____"
]
],
[
[
"Let's define our AttributionVisualizer object with the above parameters and our `vqa_resnet` model. ",
"_____no_output_____"
]
],
[
[
"visualizer = AttributionVisualizer(\n models=[vqa_resnet],\n score_func=score_func,\n features=features,\n dataset=dataset,\n classes=answer_words,\n)",
"_____no_output_____"
]
],
[
[
"And now we can visualize the outputs produced by the model.\n\nAs of writing this tutorial, the `AttributionVisualizer` class utilizes captum's implementation of [integrated gradients](https://captum.ai/docs/algorithms#integrated-gradients) ([`IntegratedGradients`](https://captum.ai/api/integrated_gradients.html)).",
"_____no_output_____"
]
],
[
[
"visualizer.render()",
"_____no_output_____"
],
[
"# show a screenshot if using notebook non-interactively\nfrom IPython.display import Image\nImage(filename='img/captum_insights_vqa.png')",
"_____no_output_____"
]
],
[
[
"Finally, since we are done with visualization, we will revert the change to the model we made with `configure_interpretable_embedding_layer`. To do this, we will invoke the `remove_interpretable_embedding_layer` function.",
"_____no_output_____"
]
],
[
[
"remove_interpretable_embedding_layer(vqa_resnet, interpretable_embedding)",
"_____no_output_____"
]
]
] | [
"markdown",
"code",
"markdown",
"code",
"markdown",
"code",
"markdown",
"code",
"markdown",
"code",
"markdown",
"code",
"markdown",
"code",
"markdown",
"code",
"markdown",
"code",
"markdown",
"code",
"markdown",
"code",
"markdown",
"code",
"markdown",
"code",
"markdown",
"code",
"markdown",
"code",
"markdown",
"code"
] | [
[
"markdown",
"markdown"
],
[
"code"
],
[
"markdown"
],
[
"code",
"code"
],
[
"markdown"
],
[
"code",
"code"
],
[
"markdown"
],
[
"code"
],
[
"markdown"
],
[
"code"
],
[
"markdown"
],
[
"code"
],
[
"markdown",
"markdown"
],
[
"code"
],
[
"markdown"
],
[
"code"
],
[
"markdown"
],
[
"code"
],
[
"markdown",
"markdown"
],
[
"code"
],
[
"markdown"
],
[
"code"
],
[
"markdown"
],
[
"code"
],
[
"markdown"
],
[
"code"
],
[
"markdown"
],
[
"code"
],
[
"markdown"
],
[
"code",
"code"
],
[
"markdown"
],
[
"code"
]
] |
e773f0959e1c9f3ef37ac8914fda5d8db3072229 | 9,116 | ipynb | Jupyter Notebook | notebooks/03.00-Widget_Basics.ipynb | mbektasbbg/tutorial | bff94e929d58b9c570201d81dd4e87d04b13cc70 | [
"BSD-3-Clause"
] | null | null | null | notebooks/03.00-Widget_Basics.ipynb | mbektasbbg/tutorial | bff94e929d58b9c570201d81dd4e87d04b13cc70 | [
"BSD-3-Clause"
] | null | null | null | notebooks/03.00-Widget_Basics.ipynb | mbektasbbg/tutorial | bff94e929d58b9c570201d81dd4e87d04b13cc70 | [
"BSD-3-Clause"
] | null | null | null | 22.620347 | 406 | 0.560663 | [
[
[
"# Simple Widget Introduction",
"_____no_output_____"
],
[
"## What are widgets?",
"_____no_output_____"
],
[
"Widgets are eventful python objects that have a representation in the browser, often as a control like a slider, textbox, etc.",
"_____no_output_____"
],
[
"## What can they be used for?",
"_____no_output_____"
],
[
"You can use widgets to build **interactive GUIs** for your notebooks. \nYou can also use widgets to **synchronize stateful and stateless information** between Python and JavaScript.",
"_____no_output_____"
],
[
"## Using widgets ",
"_____no_output_____"
],
[
"To use the widget framework, you need to import `ipywidgets`.",
"_____no_output_____"
]
],
[
[
"import ipywidgets as widgets",
"_____no_output_____"
]
],
[
[
"### repr",
"_____no_output_____"
],
[
"Widgets have their own display `repr` which allows them to be displayed using IPython's display framework. Constructing and returning an `IntSlider` automatically displays the widget (as seen below). Widgets are displayed inside the output area below the code cell. Clearing cell output will also remove the widget.",
"_____no_output_____"
]
],
[
[
"widgets.IntSlider()",
"_____no_output_____"
]
],
[
[
"### display()",
"_____no_output_____"
],
[
"You can also explicitly display the widget using `display(...)`.",
"_____no_output_____"
]
],
[
[
"from IPython.display import display\nw = widgets.IntSlider()\ndisplay(w)",
"_____no_output_____"
]
],
[
[
"### Multiple display() calls",
"_____no_output_____"
],
[
"If you display the same widget twice, the displayed instances in the front-end will remain in sync with each other. Try dragging the slider below and watch the slider above.",
"_____no_output_____"
]
],
[
[
"display(w)",
"_____no_output_____"
]
],
[
[
"## Why does displaying the same widget twice work?",
"_____no_output_____"
],
[
"Widgets are represented in the back-end by a single object. Each time a widget is displayed, a new representation of that same object is created in the front-end. These representations are called views.\n\n",
"_____no_output_____"
],
[
"## Widget properties",
"_____no_output_____"
],
[
"All of the IPython widgets share a similar naming scheme. To read the value of a widget, you can query its `value` property.",
"_____no_output_____"
]
],
[
[
"w = widgets.IntSlider()\ndisplay(w)",
"_____no_output_____"
],
[
"w.value",
"_____no_output_____"
]
],
[
[
"Similarly, to set a widget's value, you can set its `value` property.",
"_____no_output_____"
]
],
[
[
"w.value = 100",
"_____no_output_____"
]
],
[
[
"### Keys",
"_____no_output_____"
],
[
"In addition to `value`, most widgets share `keys`, `description`, and `disabled`. To see the entire list of synchronized, stateful properties of any specific widget, you can query the `keys` property. Generally you should not interact with properties starting with an underscore.",
"_____no_output_____"
]
],
[
[
"w.keys",
"_____no_output_____"
]
],
[
[
"### Shorthand for setting the initial values of widget properties",
"_____no_output_____"
],
[
"While creating a widget, you can set some or all of the initial values of that widget by defining them as keyword arguments in the widget's constructor (as seen below).",
"_____no_output_____"
]
],
[
[
"widgets.Text(value='Hello World!', disabled=True)",
"_____no_output_____"
]
],
[
[
"## Linking two similar widgets",
"_____no_output_____"
],
[
"If you need to display the same value two different ways, you'll have to use two different widgets. Instead of attempting to manually synchronize the values of the two widgets, you can use the `link` or `jslink` function to link two properties together (the difference between these is discussed in [Widget Events](08.00-Widget_Events.ipynb)). Below, the values of two widgets are linked together.",
"_____no_output_____"
]
],
[
[
"a = widgets.FloatText()\nb = widgets.FloatSlider()\ndisplay(a,b)\n\nmylink = widgets.link((a, 'value'), (b, 'value'))",
"_____no_output_____"
]
],
[
[
"### Unlinking widgets",
"_____no_output_____"
],
[
"Unlinking the widgets is simple. All you have to do is call `.unlink` on the link object. Try changing one of the widgets above after unlinking to see that they can be independently changed.",
"_____no_output_____"
]
],
[
[
"# mylink.unlink()",
"_____no_output_____"
]
],
[
[
"## `observe` changes in a widget value\n\nAlmost every widget can be observed for changes in its value that trigger a call to a function. The example below is the slider from the first notebook of the tutorial. \n\nThe `HTML` widget below the slider displays the square of the number.",
"_____no_output_____"
]
],
[
[
"slider = widgets.FloatSlider(\n value=7.5,\n min=5.0,\n max=10.0,\n step=0.1,\n description='Input:',\n)\n\n# Create non-editable text area to display square of value\nsquare_display = widgets.HTML(description=\"Square: \", value='{}'.format(slider.value**2))\n\n# Create function to update square_display's value when slider changes\ndef update_square_display(change):\n square_display.value = '{}'.format(change.new**2)\n \nslider.observe(update_square_display, names='value')\n\n# Put them in a vertical box\nwidgets.VBox([slider, square_display])",
"_____no_output_____"
]
]
] | [
"markdown",
"code",
"markdown",
"code",
"markdown",
"code",
"markdown",
"code",
"markdown",
"code",
"markdown",
"code",
"markdown",
"code",
"markdown",
"code",
"markdown",
"code",
"markdown",
"code",
"markdown",
"code"
] | [
[
"markdown",
"markdown",
"markdown",
"markdown",
"markdown",
"markdown",
"markdown"
],
[
"code"
],
[
"markdown",
"markdown"
],
[
"code"
],
[
"markdown",
"markdown"
],
[
"code"
],
[
"markdown",
"markdown"
],
[
"code"
],
[
"markdown",
"markdown",
"markdown",
"markdown"
],
[
"code",
"code"
],
[
"markdown"
],
[
"code"
],
[
"markdown",
"markdown"
],
[
"code"
],
[
"markdown",
"markdown"
],
[
"code"
],
[
"markdown",
"markdown"
],
[
"code"
],
[
"markdown",
"markdown"
],
[
"code"
],
[
"markdown"
],
[
"code"
]
] |
e773f6302331b46797f5cf874857c48b40b3abe0 | 1,043,485 | ipynb | Jupyter Notebook | analyses/.ipynb_checkpoints/altchain_temporal_study-checkpoint.ipynb | Gingeropolous/monero_archival_project | 2ecb90c8d566115df62308a4363acee4c006a4fe | [
"MIT"
] | 7 | 2019-03-16T20:42:48.000Z | 2022-01-27T08:04:08.000Z | analyses/.ipynb_checkpoints/altchain_temporal_study-checkpoint.ipynb | dginovker/archival_network | 720e9324e0a887783c18894ef0ee89849dc19f20 | [
"MIT"
] | 4 | 2018-05-30T22:10:41.000Z | 2018-06-01T19:16:31.000Z | analyses/.ipynb_checkpoints/altchain_temporal_study-checkpoint.ipynb | dginovker/archival_network | 720e9324e0a887783c18894ef0ee89849dc19f20 | [
"MIT"
] | 3 | 2019-03-11T00:36:41.000Z | 2021-03-07T02:15:12.000Z | 237.641767 | 256,644 | 0.883475 | [
[
[
"# Statistical study of alternative blocks/chains\n\nAnalysis by IsthmusCrypto for the [Monero Archival Project](https://github.com/mitchellpkt/monero_archival_project), a product of *#Noncesense-research-lab*\n\nContributors: [NeptuneResearch](https://github.com/neptuneresearch), [Nathan](https://github.com/neffmallon), [IsthmusCrypto](https://github.com/mitchellpkt)\n\nThis notebook investigates various phenomena related to mining of orphaned blocks and alt chains. This data was collected by a MAP archival node, running a customized daemon modified by NeptuneResearch.\n\nYou can jump to the **Results** section if you want to skip data import and cleaning/engineering.\n\n### Background\nSee CuriousInventor's 5-minute [non-technical introduction](https://www.youtube.com/watch?v=t5JGQXCTe3c) to Bitcoin, for a review of why forks in the blockchain can occur naturally, and how they are resolved by the concensus process.\n\nWe will see several instances of these benign latency-induced forks, along with different alt-chain events from different mechanisms.\n\nMonero aims for a 2-minute block time, by adjusting the 'difficulty' for the solutions. Since there is a heavy element of chance involved in mining, some intervals between blocks will be shorter/longer than 2-minutes.\n\n### Conventions\nThe data in this analysis is already separated into blocks that became the main chain (\"block0\") and blocks were part of abandoned chains (\"block1\"). The block0 data is not recorded at heights with an alternate block. (*note to self, check for exceptions*)\n\nThe \"random\" field exists to differentiate each time a copy of a block is received (e.g. multiple times from multiple peers). Being able to distinguish between instances is important for latency studies, but not for the scope of this notebook, so it is dropped and de-duped.",
"_____no_output_____"
],
[
"# Preliminaries",
"_____no_output_____"
],
[
"## Where are files saved?",
"_____no_output_____"
]
],
[
[
"block0s_relative_path = 'data_for_analysis/block0s.txt.backup' # without the earlier stuff tacked on\nblock1s_relative_path = 'data_for_analysis/block1s.txt'",
"_____no_output_____"
]
],
[
[
"## Import libraries",
"_____no_output_____"
]
],
[
[
"from copy import copy\nimport time\nimport datetime\nimport pandas as pd\nimport numpy as np\nimport matplotlib.pyplot as plt\nimport seaborn as sns\nimport scipy as sp",
"_____no_output_____"
]
],
[
[
"## Disable auto-scroll",
"_____no_output_____"
]
],
[
[
"%%javascript\nIPython.OutputArea.prototype._should_scroll = function(lines) {\n return false;\n}",
"_____no_output_____"
]
],
[
[
"# Import and pre-process data\n\nTwo separate pandas DataFrames are used, `b0s` for main chain, and `b1s` for blocks that were abandoned",
"_____no_output_____"
],
[
"### Read in from CSV",
"_____no_output_____"
]
],
[
[
"# Read in the raw data from CSV files\nb0s = pd.read_csv(block0s_relative_path)\nb1s = pd.read_csv(block1s_relative_path)",
"_____no_output_____"
]
],
[
[
"### Sort the rows by height",
"_____no_output_____"
]
],
[
[
"b0s = b0s.sort_values('block_height')\nb1s = b1s.sort_values('block_height')",
"_____no_output_____"
]
],
[
[
"### Glance at the data",
"_____no_output_____"
]
],
[
[
"display(b0s.describe())\ndisplay(b1s.describe())",
"_____no_output_____"
],
[
"b0s.head()",
"_____no_output_____"
]
],
[
[
"### De-dupe\n\nBecause the MAP nodes record *every* instance that a block was received, most heights contain multiple copies from different peers. Each copy is identical, and stamped with a different `block_random`\n\nFor the purposes of this analysis/notebook, we only need one copy of each block.\n\nTake a peek for current duplicaties:",
"_____no_output_____"
]
],
[
[
"b1s.head(20)",
"_____no_output_____"
]
],
[
[
"First we remove the `block_random` *column*, so that multiple copies become indistinguishable.",
"_____no_output_____"
]
],
[
[
"b0s.drop(['block_random'],1,inplace=True)\nb1s.drop(['block_random'],1,inplace=True)",
"_____no_output_____"
]
],
[
[
"Then drop the duplicate *rows*",
"_____no_output_____"
]
],
[
[
"b0s=b0s.drop_duplicates()\nb1s=b1s.drop_duplicates()\nb1s.head(20)",
"_____no_output_____"
]
],
[
[
"# Feature Engineering\n\nRather than looking at raw block timestamps, we'll want to study derived features like the time between blocks, alt chain lengths, etc.",
"_____no_output_____"
],
[
"## Generate difference columns\n\n`delta_time` is the timestamp difference between two blocks. The `merlin_block` label is applied when a block's miner-reported timestamp precedes the one in the block prior. `delta_height` marks the difference in height between subsequent rows of the DataFrame, used as an imperfect proxy for identifying breaks between different alt chains.",
"_____no_output_____"
]
],
[
[
"b0s['delta_time'] = b0s['block_time']-b0s['block_time'].shift()\nb1s['delta_time'] = b1s['block_time']-b1s['block_time'].shift()\n\nb0s['merlin_block'] = 0 # unnecessary?\nb1s['merlin_block'] = 0 # unnecessary?\nb0s['merlin_block'] = b0s['delta_time'].transform(lambda x: x < 0).astype(int)\nb1s['merlin_block'] = b1s['delta_time'].transform(lambda x: x < 0).astype(int)\n\nb0s['delta_height'] = b0s['block_height']-b0s['block_height'].shift()\nb1s['delta_height'] = b1s['block_height']-b1s['block_height'].shift()",
"_____no_output_____"
]
],
[
[
"### Replace delta_height != 1 to NaN\n\nThe first block in a alt chain (or following a gap in `b0s`) will have an anomalous `delta_time` and `delta_height`. We convert these to NaNs so that we can hang on to orphaned blocks and still have the start of alt chains included in our data set.",
"_____no_output_____"
]
],
[
[
"def mapper(x):\n if x == 1:\n return x\n else:\n return np.nan\n\nb0s.delta_height = b0s.delta_height.map(mapper, na_action='ignore')\nb0s.loc[b0s.delta_height.isnull(),('delta_time')] = np.nan\nb1s.delta_height = b1s.delta_height.map(mapper, na_action='ignore')\nb1s.loc[b1s.delta_height.isnull(),('delta_time')] = np.nan\nb1s.head(20)",
"_____no_output_____"
]
],
[
[
"### What are we left with?",
"_____no_output_____"
]
],
[
[
"print('Retained ' + str(len(b0s)) + ' main-chain blocks')\nprint('Retained ' + str(len(b1s)) + ' alt-chain blocks')",
"Retained 17925 main-chain blocks\nRetained 241 alt-chain blocks\n"
]
],
[
[
"## Label alt chains",
"_____no_output_____"
],
[
"### Initialize new labels and features:\n\n- `alt_chain_ID` assigns an arbitrary integer to identify each alt chain. NOTE: there is a (bad) implicit assumption here alternate blocks at two subsequent heights belong to the same chain. Will be fixed in versions with linked blocks.\n- `alt_chain_length` records the length of the alt chain (up to each point, not retroactively adjusted)\n- `alt_chain_time` records how long a given chain has been growing (based on spoofable miner-reported timestamp)\n- `terminal_block` labels the 'end' block in each alt chain. Subject to artifacts from the limitation noted for alt_chain_ID.",
"_____no_output_____"
]
],
[
[
"b1s['alt_chain_ID'] = 0\nb1s['alt_chain_length'] = b1s['block_height']-b1s['block_height'].shift() # how long did this alt-chain get?\nb1s['alt_chain_time'] = 0\nb1s['terminal_block']= 0 # is this the last block in the alt-chain?\n\nb1s = b1s.reset_index()\nb1s.drop(['index'], 1, inplace=True)\nb1s.loc[0,('alt_chain_length')] = 1 # since we don't know what preceded\n\nb1s.loc[b1s.delta_time.isnull(),('alt_chain_length')] = 1 # first block in chain\nb1s.head(20)",
"_____no_output_____"
]
],
[
[
"### Add new info\n\nCalculate accumulated alt chain length/time, and label terminal blocks.\n\nNote that initialization of field `alt_chain_length` produces some value > 1 for the first block, and = 1 for subsequent blocks. Below, this is converted into actual alt chain lengths.\n\nConvention: starting alt chains at length 0",
"_____no_output_____"
]
],
[
[
"alt_chain_counter = -1\n\n# Loop over rows = blocks\nfor index, row in b1s.iterrows():\n # If you want extra details:\n # print('index: ' + str(index) + ' this_row_val: ' + str(this_row_val))\n \n # Check whether this is the first block in the chain, or further down\n if str(row['delta_height']) == 'nan':\n # first block in the alt-chain\n b1s.loc[index,('alt_chain_length')] = 1\n b1s.loc[max(0,index-1),('terminal_block')] = 1 # if this is the first block, the last one was terminal on the previous chain\n alt_chain_counter += 1 # increment the counter\n b1s.loc[index,('alt_chain_ID')] = alt_chain_counter # mark the counter\n else:\n # subsequent block\n if index > 0:\n b1s.loc[index, ('alt_chain_length')] = b1s.alt_chain_length[index-1]+1\n delta_t_seconds = b1s.block_time[index] - b1s.block_time[index-1]\n b1s.loc[index, ('alt_chain_time')] = b1s.alt_chain_time[index-1] + delta_t_seconds\n b1s.loc[index, ('alt_chain_ID')] = alt_chain_counter\nb1s.head(20)",
"_____no_output_____"
]
],
[
[
"# Results",
"_____no_output_____"
],
[
"## General block interval study\n\nLet's take a look at the intervals between blocks, for both the main and alt chains. ",
"_____no_output_____"
],
[
"What is the *average* interval between blocks? ",
"_____no_output_____"
]
],
[
[
"b0_mean_time_s = np.mean(b0s.delta_time)\nb1_mean_time_s = np.mean(b1s.delta_time)\n\nprint('Main-chain blocks come with mean time: ' + str(round(b0_mean_time_s)) + ' seconds = ' + str(round(b0_mean_time_s/60,1)) + ' min')\nprint('alt-chain blocks come with mean time: ' + str(round(b1_mean_time_s)) + ' seconds = ' + str(round(b1_mean_time_s/60,1)) + ' min')",
"Main-chain blocks come with mean time: 120 seconds = 2.0 min\nalt-chain blocks come with mean time: 6254 seconds = 104.2 min\n"
]
],
[
[
"The main chain blocks are 2 minutes apart, on average. This is what we expect, and is a good validation.\n\nThe alt chain blocks come at VERY long intervals. The (not-representative) average is almost two hours! ",
"_____no_output_____"
],
[
"### Visualize block discovery time",
"_____no_output_____"
]
],
[
[
"fig = plt.figure(figsize=(10,10),facecolor='white')\nplt.style.use('seaborn-white')\nplt.subplots_adjust(left=None, bottom=None, right=None, top=None, wspace=None, hspace=0.4)\nplt.rcParams['ytick.labelsize'] = 20\nplt.rcParams['xtick.labelsize'] = 20\nplt.rcParams['font.size'] = 20\n\nax1 = fig.add_subplot(211)\nax1.set_xlabel('time (seconds)')\nax1.set_ylabel('occurrences')\nax1.set_title('interval between discoveries of main-chain blocks')\nplt.hist(b0s.delta_time[b0s.delta_time.notnull()], bins=range(0,600,10))\nax1.set_xlim((0,600))\nplt.axvline(x=120, c='red', linestyle=':', linewidth=5)\nplt.legend(('120 s = target block time','block interval histogram'))\n\nax2 = fig.add_subplot(212)\nplt.hist(b1s.delta_time[b1s.delta_time.notnull()], bins=range(0,50000,250))\nax2.set_xlabel('time (seconds)')\nax2.set_ylabel('occurrences')\nax2.set_title('interval between discoveries of sequential alt-chain blocks')\nax2.set_xlim((0,50000))\nplt.axvline(x=120, c='red', linestyle=':', linewidth=5)\nplt.legend(('120 s = target block time','block interval histogram'))\n\npass",
"_____no_output_____"
]
],
[
[
"### Observed wait times\n\n**Main chain:**\n\nThe top histogram (main chain) shows roughly the distribution that we would expect: long-tailed with a mean of 2 minutes. There seems to be some skew around 60 seconds, which is peculiar. \n\nIttay Eyal and Emin Gün Sirer [point out](http://hackingdistributed.com/2014/01/15/detecting-selfish-mining/) that \"One could detect [[selfish mining](https://arxiv.org/pdf/1311.0243.pdf)] by looking at the timestamps on successive blocks in the blockchain. Since mining is essentially an independent random process, we'd expect the interblock time gap to be exponentially distributed. Any deviation from this expectation would be suggestive of selfish mining\"\n\n**alt chains:**\n\nThe alt-chain histogram shows mining on an entirely different timescale. Blocks are released very belatedly, often hours behind the preceding height.\n\nNote the x-scale in seconds, and the fact that the majority of alt-chain blocks are minutes, hours, or days behind the preceding block. These blocks have NO chance of ever becoming the main chain. Something unusual is happening, and this figure captures multiple phenomena:\n\n- Most of the natural latency-induced forks would appear in the bin around 120 seconds (2 minutes)\n\n- Some of the single blocks with extreme times (e.g. ~ 50000 s = 1 month) are probably due to somebody solo mining a block by accident or for fun. \n\n- There are many strong chains with blocks on the order of that are too long (e.g. 15 blocks) for the first case, and have too much hashpower for the second case.",
"_____no_output_____"
],
[
"### Expected waiting times \n\nHow long do we expect to wait between blocks?? \n\n\"Events that occur independently with some average rate are modeled with a Poisson process. **The waiting times between k occurrences of the event are Erlang distributed.** The related question of the number of events in a given amount of time is described by the Poisson distribution.\" ... from [Wikipedia](https://en.wikipedia.org/wiki/Erlang_distribution). \n\n*Credit: Erlang analysis started by Nathan*",
"_____no_output_____"
]
],
[
[
"dt = b0s.delta_time[b0s.delta_time > 0]\nsns.distplot(dt, bins=np.linspace(0, 1000,100))\nmean = np.nanmean(dt)\nstddev = np.std(dt)\nlam = mean/stddev**2\nk = 2000\nx = range(10000)\nshift = 1975\ny = sp.stats.erlang.pdf(x, k, lam)\nx_plot = [xi-shift for xi in x]\nplt.plot(x_plot, y, color='r', label = \"Erlong\")\nplt.xlim(0, 750)\nplt.title(\"Distribution of times to solve block.\")\nplt.legend(('Observed MRT','Erlong'))\n#plt.savefig(\"dist\")\n#plt.figure()\n#plt.plot(x, sp.stats.erlang.pdf(x, k, lam, 100000), color='r', label = \"Erlong\")\npass",
"_____no_output_____"
]
],
[
[
"If we are correct that Erlang statistics theoretically describe the situation, there are two main reasons why the distribution does not match observations:\n- IsthmusCrypto used arbitrary (and presumably wrong) parameters to make the fit line up\n- This analysis used miner-reported timestamps (MRTs) which are known to be wrong (see Merlin blocks, below). The node-receipt timestamps (NRTs) will accurately reflect the block announcement intervals.",
"_____no_output_____"
],
[
"How many alternative blocks did we see that were single orphaned blocks, and how many blocks that were part of longer alternative chains.",
"_____no_output_____"
]
],
[
[
"orph_block_cut1 = copy(b1s[b1s.alt_chain_length==1])\norph_block_cut2 = copy(orph_block_cut1[orph_block_cut1.terminal_block==1])\n\nexperiment_time_d = (max(orph_block_cut2.block_time) - min(orph_block_cut2.block_time))/86400 # seconds per day\nexperiment_time_height = (max(orph_block_cut2.block_height) - min(orph_block_cut2.block_height))\n\nnum_of_orphans = len(orph_block_cut2)\nnum_alt_chain_blocks = len(b1s)-num_of_orphans\n\norphans_per_day = num_of_orphans/experiment_time_d\nheights_per_orphan = experiment_time_height/num_of_orphans\nheights_per_side_block = experiment_time_height/num_alt_chain_blocks\n\nprint('Experiment lasted for:' + str(round(experiment_time_d)) + ' days = ' + str(experiment_time_height) + ' heights')\nprint('Observed ' + str(num_of_orphans) + ' single orphan blocks')\nprint('Observed ' + str(num_alt_chain_blocks) + ' blocks assocated with longer alternative chains')\nprint('This corresponds to 1 natural orphan per ' + str(round(heights_per_orphan)) + ' heights')\nprint('This corresponds to 1 alt-chain-related block per ' + str(round(heights_per_orphan)) + ' heights')",
"Experiment lasted for:85 days = 60171 heights\nObserved 22 single orphan blocks\nObserved 219 blocks assocated with longer alternative chains\nThis corresponds to 1 natural orphan per 2735 heights\nThis corresponds to 1 alt-chain-related block per 2735 heights\n"
]
],
[
[
"Assuming that the longer side chains are a different phenomenon that does not impact the frequency of natural orphaned blocks, how often would we expect to see a triple block?",
"_____no_output_____"
]
],
[
[
"monero_blocks_per_day = 720\nheights_per_triple = heights_per_orphan*heights_per_side_block\ntriple_frequency_days = heights_per_triple/monero_blocks_per_day\nprint('Statistically we expect to see a triple block once per ' + str(round(heights_per_triple)) \n + ' blocks (' + str(round(triple_frequency_days)) + ' days)')\n",
"Statistically we expect to see a triple block once per 751463 blocks (1044 days)\n"
]
],
[
[
"\nprint('Observed: ' + str(number_of_orphans) + ' over the course of ' + str(round(experiment_time_d)) + ' days.')\nprint('This corresponds to ' + str(round(orphans_per_day,3)) + ' orphans per day.')\nprint('Over ' + str(experiment_time_height) + ' blocks, averaged:')\nprint(str(round(orphans_per_height,5)), ' orphans per height')\nprint(str(round(1/orphans_per_height)) + ' blocks per orphan')\n",
"_____no_output_____"
],
[
"### Merlin Blocks\n\nCheck it out, there appear to be time-traveling blocks in the main chain!\n\nThese plots are based off of the miner-reported timestamps (MRTs), which are spoofable. This phenomenon will probably disappear with node-receipt timestamps (NRTs)... ",
"_____no_output_____"
]
],
[
[
"fig = plt.figure(figsize=(10,5),facecolor='white', dpi=300)\nplt.style.use('seaborn-white')\nplt.rcParams['ytick.labelsize'] = 20\nplt.rcParams['xtick.labelsize'] = 20\nplt.rcParams['font.size'] = 20\nplt.hist(b0s.delta_time.dropna(), bins=np.linspace(-500,1000,100))\n\nplt.xlabel('time between blocks (seconds)')\nplt.ylabel('occurrences')\nplt.title('Block interval [according to miner timestamp] in sec')\nplt.axvline(x=120, c='red', linestyle=':', linewidth=5)\nplt.legend(('120 s = target block time','block interval histogram'))\npass\n\nprint(str(round(len(b0s.delta_time[b0s.delta_time<0])/len(b0s.delta_time)*100,2)) + '% of blocks on the main chain were delivered from the future.')",
"2.68% of blocks on the main chain were delivered from the future.\n"
]
],
[
[
"**Time-traveling blocks:**\n\nAbout 2.5% of blocks on the main chain arrive with a miner timestamp BEFORE the timestamp of the block prior. This conclusively shows that miner-reported timestamps cannot be trusted, and further analysis must rely on node-reported timestamps.",
"_____no_output_____"
],
[
"### Direction of time travel\n\nLet `M` be the height of a Merlin block, meaning that `[time(M) < time(M-1)]`. This could be caused by :\n- block `M-1` being mined with a false late timestamp\n- block `M` being mined with a false early timestamp\n\nLet's take a look at which it could be, by looking at the discovery times of the blocks before and after the Merlin blocks.",
"_____no_output_____"
]
],
[
[
"# Indexing\nM_block_inds = b0s.index[b0s.merlin_block== 1].tolist()\nM_parent_inds = [x - 1 for x in M_block_inds]\nM_child_inds = [x + 1 for x in M_block_inds]\n\nfig = plt.figure(figsize=(10,10))\nplt.style.use('seaborn-white')\nplt.subplots_adjust(left=None, bottom=None, right=None, top=None, wspace=None, hspace=0.4)\nplt.rcParams['ytick.labelsize'] = 20\nplt.rcParams['xtick.labelsize'] = 20\nplt.rcParams['font.size'] = 20\nplt.title('MRT block interval for Merlin blocks')\nplt.xlabel('block discovery time (s)')\nplt.ylabel('occurrences')\nplt.hist(b0s.delta_time[M_block_inds].dropna())\n\nfig = plt.figure(figsize=(10,10))\nplt.style.use('seaborn-white')\nplt.subplots_adjust(left=None, bottom=None, right=None, top=None, wspace=None, hspace=0.4)\nplt.rcParams['ytick.labelsize'] = 20\nplt.rcParams['xtick.labelsize'] = 20\nplt.rcParams['font.size'] = 18\nplt.title('MRT-based interval for heights before/after Merlin blocks')\nplt.xlabel('block discovery time (s)')\nplt.ylabel('occurrences')\n#plt.hist(b0s.delta_time.dropna(), alpha=0.5, bins=np.linspace(-500,500,500))\nbinnum = 20\nplt.hist(b0s.delta_time[M_parent_inds].dropna(), alpha=0.6, bins=np.linspace(-500,500,binnum)) # gets a different warning\nplt.hist(b0s.delta_time[M_child_inds].dropna(), alpha=0.6, bins=np.linspace(-500,500,binnum)) # gets a different warning\nplt.legend(('for height M-1', 'for height M+1'))\npass",
"_____no_output_____"
]
],
[
[
"From the bottom plot, we notice that the blocks *preceding* (according to height) Merlin blocks to have mostly arrived on schedule (since, qualitatively at least, the interval distribution for M-1 blocks matches the interval distribution for all blocks)\n\nHowever, many of the blocks *following* (according to height) Merlin blocks arrive conspicuously late...! \n\n**This suggests that many of the Merlin blocks appear to be time traveling because their miner-reported timestamp is too early** (not because the block at height M-1 was stamped too late)",
"_____no_output_____"
],
[
"### Fishing for multi-Merlans\n\nInterestingly, the above plot shows Merlin blocks that follow Merlin blocks (and are followed by Merlin blocks)! Let's fish some of these up for visual inspection, since this would mean multiple timestamps moving in reverse...",
"_____no_output_____"
]
],
[
[
"b0s_M = copy(b0s[b0s.merlin_block==1])\n\ndel b0s_M['delta_time']\n# b0s_M['delta_time'] = b0s_M['block_time']-b0s_M['block_time'].shift()\nb0s_M['delta_height'] = b0s_M['block_height']-b0s_M['block_height'].shift()\n\n################################################\n## Sticking a pin in this section for now.....\n## Not sure why 1589928 is flagged as a Merlin?",
"_____no_output_____"
]
],
[
[
"## Investigate alt chains\n\nLet's look at alt chains. The following plots will show how quickly each chain grew.",
"_____no_output_____"
],
[
"### How long do these alt chains persist?\n\nWe expect lots of alt chains with length 1 or 2 from natural causes. \n\nIs anybody out there dumping mining power into longer altchains? We'll conaltr 'longer' in terms of height (top plot), and in terms of time (bottom plot)",
"_____no_output_____"
]
],
[
[
"fig = plt.figure(figsize=(10,10))\nplt.style.use('seaborn-white')\nplt.subplots_adjust(left=None, bottom=None, right=None, top=None, wspace=None, hspace=0.4)\nplt.rcParams['ytick.labelsize'] = 20\nplt.rcParams['xtick.labelsize'] = 20\nplt.rcParams['font.size'] = 20\n\nax1 = fig.add_subplot(211)\nax1.set_xlabel('alt-chain length')\nax1.set_ylabel('frequency')\nax1.set_title('How long are the alt chains? (length)')\nplt.hist(b1s.alt_chain_length[b1s.terminal_block==1], bins = range(0,75))\nax1.autoscale(enable=True, axis='x', tight=True)\n\nax2 = fig.add_subplot(212)\nplt.hist(b1s.alt_chain_time[b1s.terminal_block==1],bins=range(0,65000,2500))\nax2.set_xlabel('time (seconds)')\nax2.set_ylabel('frequency')\nax2.set_title('How long are the alt chains? (time)')\nax2.autoscale(enable=True, axis='x', tight=True)\npass",
"_____no_output_____"
]
],
[
[
"Unexpectedly, there are lots of alt chains being mined 10, 20, and 30 blocks deep (top plot).\n\nSome of these futile alt chains are mined for weeks (bottom plot)\n\nHighly unnatural...",
"_____no_output_____"
],
[
"## A closer look at the growth of alt chains",
"_____no_output_____"
],
[
"It is time to individually inspect different alt chains, and see how fast they were produced. The plots below fingerprint the growth of each alt chain.\n\nEach chain is colored/numbered differently. Each point shows a single block: the x-axis position indicates how long alt chain has grown, and the y-axis position indicates the *cumulative* time that has gone into mining that particular alt chain (calculated as the difference between the timestamp on this block and its first block).\n\nThe speed with which a given entity can produce blocks for their altchain is proportional to their hash power. This can be used to identify distinct signatures of different phenomena or entities! Two different long alt chains that were mined on the same equipment will show up together on these plots, assuming that their hashrate hasn't changed between runs.\n\nThe red line shows 2-minutes per block, so any entity producing blocks near that speed can feasibly overtake (and become) the main chain. The further a alt chain is from the red line, the more astronomically improbable this becomes.",
"_____no_output_____"
]
],
[
[
"# Let's take a look at the first 20 blocks:\nmax_chain_length = 20\nmax_chain_time = 25000\nnorm_block_time = 120 # seconds\n\nfig = plt.figure(figsize=(8,8), dpi=100)\nplt.style.use('seaborn-white')\nplt.rcParams['ytick.labelsize'] = 15\nplt.rcParams['xtick.labelsize'] = 15\nplt.rcParams['font.size'] = 15\n\nplt.scatter(b1s.alt_chain_length, b1s.alt_chain_time+1, c=b1s.alt_chain_ID, cmap='tab20')\n#fig.suptitle('Looking at alt chain lengths and time, all blocks')\nplt.xlabel('Nth block of the alt chain')\nplt.ylabel('Accumulated time on this alt chain (seconds)')\nplt.xlim((0,max_chain_length))\nplt.ylim((0,max_chain_time))\nplt.title('Growth of alt chains')\n\nfor i, txt in enumerate(b1s.alt_chain_ID):\n # print(i)\n X = b1s.alt_chain_length[i]\n Y = b1s.alt_chain_time[i]\n S = b1s.alt_chain_ID[i]\n # print(\"X = \" + str(X), \" // Y = \" + str(Y) + \" // S = \" + str(S))\n if i > 0 and X <= max_chain_length and Y <= max_chain_time:\n plt.text(X,Y,S)\n \n# Add on a regular rate\nplt.plot((0,max_chain_length), (0, max_chain_length*norm_block_time), c='red', linewidth=4, linestyle=':')\npass",
"_____no_output_____"
]
],
[
[
"**Wow** there are several interesting things to note:\n\nSeveral of the alt chains, separated by weeks, show the exact same signature in hashrate (e.g. #5 and #11) and presumably were produced by the same equipment\n\nalt chain #10 produced the first 8 blocks at approximately 2 minutes per block! This could indicate two things:\n- A alt chain came within a razor's edge of overtaking the main chain\n- A alt chain DID overtake the main chain (!!!) so the original version is marked as \"alternate\" here\n\nSomething seems to cause the chains to lose steam about 7 or 8 blocks in. This could be a coincidence from looking at a small number of examples, but seems prominent in #5, #10, #11, #13. Equipment overheating??\n\nWho could have that much hash power? And these are all since July after we were supposedly rid of ASICs.\n\nAbsurdly, one of the alt chains was 70 blocks long, with an average of a four hour block discovery time. \n- To reliably mine at ~ 4 hr/block the entity must have around 1% of total network hashrate!\n- This single alt chain would have used around 40,000 EUR worth of energy! \n\nThat is not something that an unlucky amateur miner would accidentally overlook. Here's a zoomed-out version of the above plot:",
"_____no_output_____"
]
],
[
[
"fig = plt.figure(figsize=(4,4), dpi=100)\nplt.scatter(b1s.alt_chain_length, b1s.alt_chain_time+1, c=b1s.alt_chain_ID, cmap='tab10')\nfig.suptitle('Looking at alt chain lengths and time, all blocks')\nplt.xlabel('Nth block of the alt chain')\nplt.ylabel('Accumulated time (seconds)')\nplt.axis('tight')\npass",
"_____no_output_____"
]
],
[
[
"Let's look at these chains in terms of a (very rough and noisy) estimate of their hashpower, which is inversely proportional the length of time between discovering blocks.\n\nThis can be normalized by the network discovery time (average 120s) to the alt chain's hashrate relative to the network-wide hashrate. \n\n`fraction_of_network_hashrate = [(alt chain discovery time)/(normal time = 120 s)]^(-1)`\n\nThis is a **really** noisy proxy, given the element of chance in block discovery. However, it can be seen that some chains like #10 and #13 consistently perform as though they have a *significant* amount of hash power. Don't stare at this plot for too long, it needs smoothing and is better described by summary statistics that follow later.",
"_____no_output_____"
]
],
[
[
"# Let's take a look at the first 20 blocks:\nmax_chain_length = 20\nnorm_block_time = 120 # seconds\nmax_prop = 2\n\nfig = plt.figure(figsize=(8,8), dpi=100)\nplt.style.use('seaborn-white')\nplt.rcParams['ytick.labelsize'] = 15\nplt.rcParams['xtick.labelsize'] = 15\nplt.rcParams['font.size'] = 15\n\nplt.scatter(b1s.alt_chain_length, np.reciprocal(b1s.delta_time/norm_block_time), c=b1s.alt_chain_ID, cmap='tab20')\n\n#fig.suptitle('Looking at alt chain lengths and time, all blocks')\nplt.xlabel('Nth block of the alt chain')\nplt.ylabel('Hashrate relative to network total (seconds)')\nplt.xlim((0,max_chain_length))\nplt.ylim((0,max_prop))\nplt.title('Rough estimate of hashrate')\n\nfor i, txt in enumerate(b1s.alt_chain_ID):\n # print(i)\n X = b1s.alt_chain_length[i]\n Y = np.reciprocal(b1s.delta_time[i]/120)\n S = b1s.alt_chain_ID[i]\n # print(\"X = \" + str(X), \" // Y = \" + str(Y) + \" // S = \" + str(S))\n if i > 0 and X <= max_chain_length and Y <= max_prop and Y > 0:\n plt.text(X,Y,S)\n pass\n \n# Add on a regular rate\nplt.axhline(y=1, c='red', linestyle=':', linewidth=5)\npass",
"_____no_output_____"
]
],
[
[
"### Ratio of chain length to chain time\n\nFor each chain, we calculate the total chain_length/chain_time to produce the average blocks per second\n\nThis should be directly proportional to the hashrate being used to mine that chain",
"_____no_output_____"
]
],
[
[
"b1s_terminal = copy(b1s[b1s.terminal_block==1])\nb1s_terminal['average_speed'] = np.nan\nb1s_terminal['fraction_of_network_hashrate'] = np.nan\n\n\n\n# Loop over rows = blocks\nfor index, row in b1s_terminal.iterrows():\n if b1s_terminal.alt_chain_time[index] > 0:\n b1s_terminal.loc[index,('average_speed')] = b1s_terminal.alt_chain_length[index]/b1s_terminal.alt_chain_time[index]\n b1s_terminal.loc[index,('fraction_of_network_hashrate')] = b1s_terminal.average_speed[index]*120 # normalized against the usual 1/120 blocks/second\n \n \nfig = plt.figure(figsize=(10,10),facecolor='white')\nplt.style.use('seaborn-white')\nplt.subplots_adjust(left=None, bottom=None, right=None, top=None, wspace=None, hspace=0.4)\nplt.rcParams['ytick.labelsize'] = 20\nplt.rcParams['xtick.labelsize'] = 20\nplt.rcParams['font.size'] = 20\n\nplt.hist(b1s_terminal.average_speed.dropna(), bins=np.linspace(0,0.1,30))\nplt.xlabel('average mining speed (blocks per second)')\nplt.ylabel('occurrences')\nplt.title('')\nplt.axvline(x=(1/120), c='red', linestyle=':', linewidth=5)\n\nplt.legend(('1/120 blocks/second = network usual','average speed of each alt chain'))\n\npass",
"_____no_output_____"
]
],
[
[
"Almost all of the alternate chains have an average speed that is SLOWER than the main chain (to the left of red line).\n\nSome of the chains clocked in with speeds higher than the network average. Let's see if these are long chains that should have overtaken, or fluke statistics from short chains.",
"_____no_output_____"
]
],
[
[
"b1s_order = copy(b1s_terminal.sort_values(['average_speed'], ascending=False))\ndisplay(b1s_order.dropna()[['alt_chain_ID', 'alt_chain_length', 'alt_chain_time', 'average_speed', 'fraction_of_network_hashrate']])",
"_____no_output_____"
]
],
[
[
"As expected, the chains that clocked in faster than average were all just 2-block detours.\n\nLet's take a look without those",
"_____no_output_____"
]
],
[
[
"b1s_order = copy(b1s_order[b1s_order.alt_chain_length > 2])\ndisplay(b1s_order[['alt_chain_ID', 'alt_chain_length', 'alt_chain_time', 'average_speed', 'fraction_of_network_hashrate']])",
"_____no_output_____"
]
],
[
[
"### Surprising observations:\n\nNote that chain with ID 10 was 20 blocks long, and averaged production at a speed that would have required **13% of the total network hashrate.**\n\nChain ID 13 managed 18 blocks at a speed consistent with having **8% of the total network hashrate.**",
"_____no_output_____"
],
[
"## Comparison between chains\n\nNow we look whether an influx of hashrate onto an alt chain corresponds with loss of hashrate on the main chain.\n\nWe'll stick with looking at times, because taking the reciprocal makes a noisy function noisier",
"_____no_output_____"
]
],
[
[
"# Create plot\nfig = plt.figure()\n \nplt.scatter(b0s.block_height, b0s.delta_time)\nplt.scatter(b1s.block_height, b1s.delta_time)\n\n#plt.xlim(1580000, 1615000)\nplt.ylim(0, 1500)\nplt.title('Matplot scatter plot')\nplt.show()",
"_____no_output_____"
]
],
[
[
"..... This doesn't line up exactly right. Need to get b0 times during b1 stretches...",
"_____no_output_____"
],
[
"## Summarization of the alt chains\n\nQuickly spitting out some text data so I can cross-reference these alt chains against mining timing on the main chain.",
"_____no_output_____"
]
],
[
[
"verbose_text_output = 1\n\nif verbose_text_output:\n for i, x in enumerate(b1s.alt_chain_ID.unique()):\n try:\n #print('alt chain #' + str(i) + ' median time: ', + np.median(b1s.block_time[b1s.alt_chain_ID==i]))\n print('alt chain #' + str(i) + ' median time: ' + time.strftime('%Y-%m-%d %H:%M:%S', time.localtime(np.median(b1s.block_time[b1s.alt_chain_ID==i]))))\n print('\\t... started at height ' + str(min(b1s.block_height[b1s.alt_chain_ID==i])) + '\\n')\n except:\n pass",
"alt chain #0 median time: 2018-04-04 23:11:27\n\t... started at height 1545098\n\nalt chain #1 median time: 2018-04-06 03:24:11\n\t... started at height 1546000\n\nalt chain #2 median time: 2018-04-10 00:11:39\n\t... started at height 1547963\n\nalt chain #3 median time: 2018-04-29 15:03:35\n\t... started at height 1562061\n\nalt chain #4 median time: 2018-05-01 19:00:07\n\t... started at height 1563626\n\nalt chain #5 median time: 2018-05-07 07:36:20\n\t... started at height 1565690\n\nalt chain #6 median time: 2018-05-05 20:01:05\n\t... started at height 1566500\n\nalt chain #7 median time: 2018-05-06 10:06:00\n\t... started at height 1566908\n\nalt chain #8 median time: 2018-05-08 18:22:59\n\t... started at height 1568536\n\nalt chain #9 median time: 2018-05-17 01:15:02\n\t... started at height 1574526\n\nalt chain #10 median time: 2018-05-23 02:55:11\n\t... started at height 1578847\n\nalt chain #11 median time: 2018-05-25 03:13:18\n\t... started at height 1580196\n\nalt chain #12 median time: 2018-05-27 07:47:34\n\t... started at height 1581881\n\nalt chain #13 median time: 2018-05-29 08:53:28\n\t... started at height 1583266\n\nalt chain #14 median time: 2018-05-29 14:14:48\n\t... started at height 1583283\n\nalt chain #15 median time: 2018-05-29 12:35:05\n\t... started at height 1583284\n\nalt chain #16 median time: 2018-05-29 18:22:29\n\t... started at height 1583285\n\nalt chain #17 median time: 2018-05-30 21:51:04\n\t... started at height 1584464\n\nalt chain #18 median time: 2018-06-03 21:10:57\n\t... started at height 1587293\n\nalt chain #19 median time: 2018-06-07 11:25:10\n\t... started at height 1589926\n\nalt chain #20 median time: 2018-06-07 12:36:02\n\t... started at height 1589929\n\nalt chain #21 median time: 2018-06-08 10:59:40\n\t... started at height 1590614\n\nalt chain #22 median time: 2018-06-09 16:08:46\n\t... started at height 1591490\n\nalt chain #23 median time: 2018-06-10 03:44:43\n\t... started at height 1591844\n\nalt chain #24 median time: 2018-06-10 11:35:53\n\t... started at height 1592056\n\nalt chain #25 median time: 2018-06-10 20:11:19\n\t... started at height 1592321\n\nalt chain #26 median time: 2018-06-10 22:24:19\n\t... started at height 1592393\n\nalt chain #27 median time: 2018-06-11 12:05:59\n\t... started at height 1592780\n\nalt chain #28 median time: 2018-06-11 12:08:21\n\t... started at height 1592780\n\nalt chain #29 median time: 2018-06-12 10:41:18\n\t... started at height 1593453\n\nalt chain #30 median time: 2018-06-13 01:05:44\n\t... started at height 1593865\n\nalt chain #31 median time: 2018-06-13 01:07:11\n\t... started at height 1593865\n\nalt chain #32 median time: 2018-06-13 11:34:31\n\t... started at height 1594175\n\nalt chain #33 median time: 2018-06-15 06:42:39\n\t... started at height 1595491\n\nalt chain #34 median time: 2018-06-16 19:10:42\n\t... started at height 1596549\n\nalt chain #35 median time: 2018-06-17 14:29:53\n\t... started at height 1597199\n\nalt chain #36 median time: 2018-06-17 15:37:00\n\t... started at height 1597231\n\nalt chain #37 median time: 2018-06-17 15:37:13\n\t... started at height 1597232\n\nalt chain #38 median time: 2018-06-18 20:43:18\n\t... started at height 1598110\n\nalt chain #39 median time: 2018-06-21 12:29:33\n\t... started at height 1599986\n\nalt chain #40 median time: 2018-06-21 12:30:03\n\t... started at height 1599986\n\nalt chain #41 median time: 2018-06-21 12:30:41\n\t... started at height 1599987\n\nalt chain #42 median time: 2018-06-24 00:29:35\n\t... started at height 1601812\n\nalt chain #43 median time: 2018-06-24 05:21:28\n\t... started at height 1601963\n\nalt chain #44 median time: 2018-06-24 08:52:19\n\t... started at height 1602053\n\nalt chain #45 median time: 2018-06-25 13:26:38\n\t... started at height 1602963\n\nalt chain #46 median time: 2018-06-27 09:15:11\n\t... started at height 1604268\n\nalt chain #47 median time: 2018-06-27 09:16:17\n\t... started at height 1604268\n\nalt chain #48 median time: 2018-06-28 19:58:12\n\t... started at height 1605269\n\nalt chain #49 median time: 2018-06-29 06:02:34\n\t... started at height 1605586\n\nalt chain #50 median time: 2018-07-02 12:27:47\n\t... started at height 1607956\n\n"
]
],
[
[
"# Work in progress. Check back later for more excitement!",
"_____no_output_____"
],
[
"Ah, here's a bug to fix:\n\nNaNs in delta_time get marked as a `merlin_block` which is not true",
"_____no_output_____"
]
],
[
[
"b0s[b0s.merlin_block==1]",
"_____no_output_____"
]
]
] | [
"markdown",
"code",
"markdown",
"code",
"markdown",
"code",
"markdown",
"code",
"markdown",
"code",
"markdown",
"code",
"markdown",
"code",
"markdown",
"code",
"markdown",
"code",
"markdown",
"code",
"markdown",
"code",
"markdown",
"code",
"markdown",
"code",
"markdown",
"code",
"markdown",
"code",
"markdown",
"code",
"markdown",
"code",
"markdown",
"code",
"markdown",
"code",
"markdown",
"code",
"markdown",
"code",
"markdown",
"code",
"markdown",
"code",
"markdown",
"code",
"markdown",
"code",
"markdown",
"code",
"markdown",
"code",
"markdown",
"code",
"markdown",
"code",
"markdown",
"code",
"markdown",
"code",
"markdown",
"code"
] | [
[
"markdown",
"markdown",
"markdown"
],
[
"code"
],
[
"markdown"
],
[
"code"
],
[
"markdown"
],
[
"code"
],
[
"markdown",
"markdown"
],
[
"code"
],
[
"markdown"
],
[
"code"
],
[
"markdown"
],
[
"code",
"code"
],
[
"markdown"
],
[
"code"
],
[
"markdown"
],
[
"code"
],
[
"markdown"
],
[
"code"
],
[
"markdown",
"markdown"
],
[
"code"
],
[
"markdown"
],
[
"code"
],
[
"markdown"
],
[
"code"
],
[
"markdown",
"markdown"
],
[
"code"
],
[
"markdown"
],
[
"code"
],
[
"markdown",
"markdown",
"markdown"
],
[
"code"
],
[
"markdown",
"markdown"
],
[
"code"
],
[
"markdown",
"markdown"
],
[
"code"
],
[
"markdown",
"markdown"
],
[
"code"
],
[
"markdown"
],
[
"code"
],
[
"markdown",
"markdown"
],
[
"code"
],
[
"markdown",
"markdown"
],
[
"code"
],
[
"markdown",
"markdown"
],
[
"code"
],
[
"markdown",
"markdown"
],
[
"code"
],
[
"markdown",
"markdown",
"markdown"
],
[
"code"
],
[
"markdown"
],
[
"code"
],
[
"markdown"
],
[
"code"
],
[
"markdown"
],
[
"code"
],
[
"markdown"
],
[
"code"
],
[
"markdown"
],
[
"code"
],
[
"markdown",
"markdown"
],
[
"code"
],
[
"markdown",
"markdown"
],
[
"code"
],
[
"markdown",
"markdown"
],
[
"code"
]
] |
e773f670504cdf0afce44e6127b3cf23d79ed320 | 3,979 | ipynb | Jupyter Notebook | notebooks/multi-modal-data/00-Intro.ipynb | xjqbest/HugeCTR | 0b1c92d5e65891dfdd90d917bc6d520d0ca5d1e1 | [
"Apache-2.0"
] | 130 | 2021-10-11T11:55:28.000Z | 2022-03-31T21:53:07.000Z | notebooks/multi-modal-data/00-Intro.ipynb | PeterXingke/HugeCTR | d7552c4c5f93ff18ded961645cac82d5d8b5b785 | [
"Apache-2.0"
] | 72 | 2021-10-09T04:59:09.000Z | 2022-03-31T11:27:54.000Z | notebooks/multi-modal-data/00-Intro.ipynb | PeterXingke/HugeCTR | d7552c4c5f93ff18ded961645cac82d5d8b5b785 | [
"Apache-2.0"
] | 29 | 2021-11-03T22:35:01.000Z | 2022-03-30T13:11:59.000Z | 39.79 | 302 | 0.645137 | [
[
[
"# Copyright 2021 NVIDIA Corporation. All Rights Reserved.\n#\n# Licensed under the Apache License, Version 2.0 (the \"License\");\n# you may not use this file except in compliance with the License.\n# You may obtain a copy of the License at\n#\n# http://www.apache.org/licenses/LICENSE-2.0\n#\n# Unless required by applicable law or agreed to in writing, software\n# distributed under the License is distributed on an \"AS IS\" BASIS,\n# WITHOUT WARRANTIES OR CONDITIONS OF ANY KIND, either express or implied.\n# See the License for the specific language governing permissions and\n# limitations under the License.\n# ==============================================================================",
"_____no_output_____"
]
],
[
[
"# Training Recommender Systems on Multi-modal Data",
"_____no_output_____"
],
[
"## Overview\n\nRecommender systems are often trained on tabular data, containing numeric fields (such as item price, numbers of user's purchases) and categorical fields (such as user and item IDs).\n\nMulti-modal data refer to data types in other modalities, such as text, image and video. Such data can additionally provide rich inputs to and potentially improve the effectiveness of recommender systems.\n\nSeveral examples include:\n- Movie recommendation, where movie poster, plot and synopsis can be used.\n- Music recommendation, where audio features and lyric can be used.\n- Itinerary planning and attractions recommendation, where text (user profile, attraction description & review) and photos can be used.\n\nOften times, features from multi-modal data are extracted using domain-specific networks, such as ResNet for images and BERT for text data. These pretrained features, also called pretrained embeddings, are then combined with other trainable features and embeddings for the task of recommendation.",
"_____no_output_____"
],
[
"This series of notebooks demonstrate the use of multi-modal data (text, image) for the task of movie recommendation, using the Movielens-25M dataset.\n\n- [01-Download-Convert.ipynb](01-Download-Convert.ipynb): download and convert the raw data\n- [02-Data-Enrichment.ipynb](02-Data-Enrichment.ipynb): enrich the tabular data with image and text data \n- [03-Feature-Extraction-Poster.ipynb](03-Feature-Extraction-Poster.ipynb): extract image features from movie posters\n- [04-Feature-Extraction-Text.ipynb](04-Feature-Extraction-Text.ipynb): extract text features from movie synopsis\n- [05-Create-Feature-Store.ipynb](05-Create-Feature-Store.ipynb): create a combined feature store\n- [06-ETL-with-NVTabular.ipynb](06-ETL-with-NVTabular.ipynb): feature transform with NVTabular\n- [07-Training-with-HugeCTR.ipynb](07-Training-with-HugeCTR.ipynb): train model with HugeCTR, making use of pretrained embeddings.",
"_____no_output_____"
]
]
] | [
"code",
"markdown"
] | [
[
"code"
],
[
"markdown",
"markdown",
"markdown"
]
] |
e77403ff5e9de3319dc079dd98377525500cf22e | 706,012 | ipynb | Jupyter Notebook | docs/source/tutorials/linalg/linear_solvers_quickstart.ipynb | tskarvone/probnum | acc5fe55feeb9590c839a874ecf2534795c05454 | [
"MIT"
] | 1 | 2021-04-16T14:45:26.000Z | 2021-04-16T14:45:26.000Z | docs/source/tutorials/linalg/linear_solvers_quickstart.ipynb | tskarvone/probnum | acc5fe55feeb9590c839a874ecf2534795c05454 | [
"MIT"
] | 42 | 2021-03-08T07:20:40.000Z | 2022-03-28T05:04:48.000Z | docs/source/tutorials/linalg/linear_solvers_quickstart.ipynb | tskarvone/probnum | acc5fe55feeb9590c839a874ecf2534795c05454 | [
"MIT"
] | null | null | null | 263.92972 | 148,870 | 0.88088 | [
[
[
"# Linear Solvers Quickstart\n\nThis tutorial illustrates the basic usage and functionality of ProbNum's linear solver. \n\nIn particular:\n\n- Loading a random linear system from ProbNum's `problems.zoo`.\n- Solving the system with one of ProbNum's linear solvers.\n- Visualizing the return objects of the solver: These are distributions that describe the values of possible solutions and how probable they are.",
"_____no_output_____"
]
],
[
[
"import warnings\nwarnings.filterwarnings('ignore')\n\n# Make inline plots vector graphics instead of raster graphics\n%matplotlib inline\nfrom IPython.display import set_matplotlib_formats\n\nset_matplotlib_formats(\"pdf\", \"svg\")\n\n# Plotting\nimport matplotlib.pyplot as plt\nfrom matplotlib.colors import TwoSlopeNorm \n\nplt.style.use(\"../../probnum.mplstyle\")",
"_____no_output_____"
]
],
[
[
"## Linear Systems & Linear Solvers\n\nConsider a linear system of the form\n\n$$\nA \\mathbf{x} = \\mathbf{b}\n$$\n\nwhere $A\\in\\mathbb{R}^{n\\times n}$ is a symmetric positive definite matrix, $\\mathbf{b}\\in\\mathbb{R}^n$ is a vector and $\\mathbf{x}\\in\\mathbb{R}^n$ is the unknown solution of the linear system. \n\nSolving such a linear system is arguably one of the most fundamental computations in statistics, machine learning and scientific computation. Many problems can be reduced to the solution of one or many (large-scale) linear systems. Some examples include least-squares regression, kernel methods, second-order optimization, quadratic programming, Kalman filtering, linear differential equations and all Gaussian (process) inference. Here, we will solve such a system using one of ProbNum' *probabilistic linear solvers*.\n",
"_____no_output_____"
],
[
"## Loading a Test Problem with ProbNum's `problems.zoo` package\n\nWe begin by creating a random linear system. ProbNum lets you quickly generate test problems via its `problem.zoo` package. In particular we generate an $n=25$ dimensional symmetric positive definite random matrix $A$ using `random_spd_matrix` with a predefined eigenspectrum, as well as a normal random vector $\\mathbf{b}$.\n",
"_____no_output_____"
]
],
[
[
"import numpy as np\nfrom probnum.problems.zoo.linalg import random_spd_matrix\n\nrng = np.random.default_rng(42) # for reproducibility\nn = 25 # dimensionality\n\n# generate linear system\nspectrum = 10 * np.linspace(0.5, 1, n) ** 4\nA = random_spd_matrix(rng=rng, dim=n, spectrum=spectrum)\nb = rng.normal(size=(n, 1))\n\nprint(\"Matrix condition: {:.2f}\".format(np.linalg.cond(A)))\nprint(\"Eigenvalues: {}\".format(np.linalg.eigvalsh(A)))",
"Matrix condition: 16.00\nEigenvalues: [ 0.625 0.73585981 0.8608519 1.00112915 1.15788966 1.33237674\n 1.52587891 1.73972989 1.97530864 2.23403931 2.51739125 2.82687905\n 3.1640625 3.53054659 3.92798153 4.35806274 4.82253086 5.32317173\n 5.86181641 6.44034115 7.06066744 7.72476196 8.43463662 9.19234853\n 10. ]\n"
]
],
[
[
"Now we visualize the linear system.",
"_____no_output_____"
]
],
[
[
"# Plot linear system\nfig, axes = plt.subplots(\n nrows=1,\n ncols=4,\n figsize=(5, 3.5),\n sharey=True,\n squeeze=False,\n gridspec_kw={\"width_ratios\": [4, 0.25, 0.25, 0.25]},\n)\n\nvmax = np.max(np.hstack([A, b]))\nvmin = np.min(np.hstack([A, b]))\n\n# normalize diverging colobar, such that it is centered at zero\nnorm = TwoSlopeNorm(vmin=vmin, vcenter=0, vmax=vmax)\n\naxes[0, 0].imshow(A, cmap=\"bwr\", norm=norm)\naxes[0, 0].set_title(\"$A$\", fontsize=24)\naxes[0, 1].text(0.5, A.shape[0] / 2, \"$\\\\bm{x}$\", va=\"center\", ha=\"center\", fontsize=32)\naxes[0, 1].axis(\"off\")\naxes[0, 2].text(0.5, A.shape[0] / 2, \"$=$\", va=\"center\", ha=\"center\", fontsize=32)\naxes[0, 2].axis(\"off\")\naxes[0, 3].imshow(b, cmap=\"bwr\", norm=norm)\naxes[0, 3].set_title(\"$\\\\bm{b}$\", fontsize=24)\nfor ax in axes[0, :]:\n ax.set_xticks([])\n ax.set_yticks([])\nplt.tight_layout()\nplt.show()",
"_____no_output_____"
]
],
[
[
"## Solve the Linear System with ProbNum's Solver\n\nWe now use ProbNum's probabilistic linear solver `problinsolve` to estimate the solution vector $\\mathbf{x}$.\nThe algorithm iteratively chooses *actions* $\\mathbf{s}$ and makes linear *observations* $\\mathbf{y}=A \\mathbf{s}$ to update its belief over the solution $\\mathbf{x}$, the matrix $A$ and its inverse $H:=A^{-1}$. We do not run the solver to convergence here (which would require 25 iterations and yield an exact solution) but only run it for `maxiter`=10 iterations.",
"_____no_output_____"
]
],
[
[
"from probnum.linalg import problinsolve\n\n# Solve with probabilistic linear solver\nx, Ahat, Ainv, info = problinsolve(A=A, b=b, maxiter=10)\nprint(info)",
"{'iter': 10, 'maxiter': 10, 'resid_l2norm': 0.022193410186189838, 'trace_sol_cov': 27.593259516810043, 'conv_crit': 'maxiter', 'rel_cond': None}\n"
]
],
[
[
"## Visualization of Return Objects & Estimated Uncertainty \n\nThe solver returns random variables $\\mathsf{x}$, $\\mathsf{A}$, and $\\mathsf{H}:=\\mathsf{A}^{-1}$ (described by distributions) which are called `x`, `Ahat` and `Ainv` respectively in the cell above. \nThose distributions describe possible values of the solution $\\mathbf{x}$ of the linear system, the matrix $A$ and its inverse $H=A^{-1}$ respectively and how probable they are being considered by the solver. \nIn other words, the distributions describe the uncertainty the solvers still has about the value of their respective quantity. \nBelow we visualize those distributions. For all of them, the mean estimator $\\mathbb{E}(\\cdot)$ of the random variable can be used as a \"best guess\" for the true value of each quantity. In fact, the mean estimate of $\\hat{x}:=\\mathbb{E}(\\mathsf{x})$ in this particular tutorial coincides with the conjugate gradient solution (see e.g., [1, 2]). ",
"_____no_output_____"
],
[
"### Solution $\\mathsf{x}$\n\nThe solution object `x` is a normal distribution of size of the linear system (in this case $n=25$) which quantifies the uncertainty over the solution. The mean $\\mathbb{E}(\\mathsf{x})$ of the random variable is the \"best guess\" for $\\mathbf{x}$. The covariance matrix (of which we only print the first row below) quantifies possible deviation of the solution from the mean. We can also sample from the normal distribution to obtain possible solution vectors $\\mathsf{x}_1$, $\\mathsf{x}_2$, ...",
"_____no_output_____"
]
],
[
[
"x",
"_____no_output_____"
],
[
"x.mean",
"_____no_output_____"
],
[
"x.cov.todense()[0, :]",
"_____no_output_____"
],
[
"n_samples = 10\nx_samples = x.sample(rng=rng, size=n_samples)\nx_samples.shape",
"_____no_output_____"
]
],
[
[
"Furthermore, the standard deviations together with the mean $\\mathbb{E}(\\mathsf{x})$ yield credible intervals for each dimension (entry) of $\\mathbf{x}$. Credible intervals are a quick way to visualize the numerical uncertainty, but keep in mind that they only consider marginal (per element) distributions of $\\mathsf{x}$ and do not capture correlations between the entries of $\\mathsf{x}$.\n\nIn the plot below, the samples are drawn from the joint distribution of $\\mathsf{x}$ taking into account cross correlations between the entries. The error bars only show the marginal credible intervals.",
"_____no_output_____"
]
],
[
[
"plt.figure()\nplt.plot(x_samples[0, :], '.', color='gray', label='sample')\nplt.plot(x_samples[1:, :].T, '.', color='gray') \nplt.errorbar(np.arange(0, 25), x.mean, 1 * x.std, ls='none', \n label='68\\% credible interval')\nplt.plot(x.mean, 'o', color='C0', label='$\\mathbb{E}(\\mathsf{x})$')\nplt.xlabel(\"index of element in $\\mathsf{x}$\")\nplt.ylabel(\"value of element in $\\mathsf{x}$\")\nplt.legend()\nplt.show() ",
"_____no_output_____"
]
],
[
[
"Here are the credible intervals printed out:",
"_____no_output_____"
]
],
[
[
"x_true = np.linalg.solve(A, b)[:, 0]\nabs_err = abs(x.mean - x_true)\nrel_err = abs_err / abs(x.mean + x_true)\nprint(f\"Maximal absolute and relative error to mean estimate: {max(abs_err):.2e}, {max(rel_err):.2e}\")\nprint(f\"68% marginal credible intervals of the entries of x\")\nfor i in range(25):\n print(f\"element {i : >2}: {x.mean[i]: 0.2f} pm {1 * x.std[i]:.2f}\")",
"Maximal absolute and relative error to mean estimate: 4.84e-03, 9.16e-02\n68% marginal credible intervals of the entries of x\nelement 0: 0.43 pm 1.14\nelement 1: 0.16 pm 0.91\nelement 2: -0.96 pm 0.87\nelement 3: 0.33 pm 0.85\nelement 4: -0.01 pm 0.86\nelement 5: -0.78 pm 0.88\nelement 6: 0.72 pm 1.14\nelement 7: 0.13 pm 1.11\nelement 8: 0.23 pm 0.95\nelement 9: -0.16 pm 1.15\nelement 10: 0.55 pm 1.04\nelement 11: -0.59 pm 1.09\nelement 12: 0.31 pm 1.21\nelement 13: 0.04 pm 1.11\nelement 14: 0.78 pm 1.18\nelement 15: 0.28 pm 1.16\nelement 16: -1.30 pm 1.21\nelement 17: -0.30 pm 1.08\nelement 18: 0.13 pm 0.97\nelement 19: -0.22 pm 1.11\nelement 20: 0.95 pm 0.77\nelement 21: -1.15 pm 1.11\nelement 22: 0.42 pm 1.19\nelement 23: 0.09 pm 0.98\nelement 24: -1.22 pm 1.01\n"
]
],
[
[
"Generally, the uncertainty is a conservative estimate of the error, especially for small $n$, hence the credible intervals above as well as in the plot are quiet large. For large $n$ where uncertainty quantification matters more, the error bars are expected to fit the true uncertainty better.",
"_____no_output_____"
],
[
"### System Matrix $\\mathsf{A}$ and its Inverse $\\mathsf{H}$\n\nFor completeness, we also illustrate the random variables $\\mathsf{A}$ and $\\mathsf{H}$. These quantities are not needed to describe the solution $\\mathbf{x}$ and can usually be disregarded. However, in certain cases it may be of interest to acquire an approximate representation of $A$ or $H$, especially when it is infeasible to work with $A$ or $H$ directly due to their dimensionality. \n\nIndeed, it might sound confusing at first that the solver even constructs a belief about the matrix $A$ that initially defined the linear system, but keep in mind that linear solvers generally do not have nor require access to $A$ but only to a function handle of the matrix-vector product $\\mathcal{A}(\\mathbf{s}):=A\\mathbf{s}$, $\\mathcal{A}: \\mathbb{R}^n \\rightarrow \\mathbb{R}^n$. The matrix might for all practical purposes be unknown (in large liner systems it is often impossible to even construct it in memory).\n\n\nBoth return objects `A` and `Ainv` are matrix-valued normal distributions describing the random variables $\\mathsf{A}$ and $\\mathsf{H}$.\nWe plot the mean $\\mathbb{E}(\\mathsf{A})$ of $\\mathsf{A}$, two samples $\\mathsf{A}_1$ and $\\mathsf{A}_2$ as well as the ground truth $A$; analogously for $\\mathsf{H}$ below. The mean $\\mathbb{E}(\\mathsf{A})$ can be used as estimate for $A$, same for $\\mathbb{E}(\\mathsf{H})$ and $H$.",
"_____no_output_____"
]
],
[
[
"Ahat",
"_____no_output_____"
],
[
"Ainv",
"_____no_output_____"
],
[
"# Draw samples\nrng = np.random.default_rng(seed=42)\nAhat_samples = Ahat.sample(rng=rng, size=3)\nAinv_samples = Ainv.sample(rng=rng, size=3)\n\nvmax = np.max(np.hstack([A, b]))\nvmin = np.min(np.hstack([A, b]))\n\n# normalize diverging colobar, such that it is centered at zero\nnorm = TwoSlopeNorm(vmin=vmin, vcenter=0, vmax=vmax)\n\n# Plot A\nrvdict = {\n \"$A$\": A,\n \"$\\mathbb{E}(\\mathsf{A})$\": Ahat.mean.todense(),\n \"$\\mathsf{A}_1$\": Ahat_samples[0],\n \"$\\mathsf{A}_2$\": Ahat_samples[1],\n}\n\nfig, axes = plt.subplots(nrows=1, ncols=len(rvdict), figsize=(10, 3), sharey=True)\nfor i, (title, rv) in enumerate(rvdict.items()):\n axes[i].imshow(rv, cmap=\"bwr\", norm=norm)\n axes[i].set_axis_off()\n axes[i].title.set_text(title)\nplt.tight_layout()\n\nH = np.linalg.inv(A)\nvmax = np.max(H)\nvmin = np.min(H)\nnorm = TwoSlopeNorm(vmin=vmin, vcenter=0, vmax=vmax)\n\n# Plot H\nrvdict = {\n \"$H$\": H,\n \"$\\mathbb{E}(\\mathsf{H})$\": Ainv.mean.todense(),\n \"$\\mathsf{H}_1$\": Ainv_samples[0],\n \"$\\mathsf{H}_2$\": Ainv_samples[1],\n}\n\nfig, axes = plt.subplots(nrows=1, ncols=len(rvdict), figsize=(10, 3), sharey=True)\nfor i, (title, rv) in enumerate(rvdict.items()):\n axes[i].imshow(rv, cmap=\"bwr\", norm=norm)\n axes[i].set_axis_off()\n axes[i].title.set_text(title)\nplt.tight_layout()",
"_____no_output_____"
]
],
[
[
"Especially the mean estimates look fairly close to the true values $A$ and $H$, even though the solver only ran for `maxiter`$=10$ steps.\n\nSimilar to above, the uncertainty of the solver about these quantities is still relatively high by looking at the samples from $\\mathsf{A}$ and $\\mathsf{H}$.",
"_____no_output_____"
],
[
"## References\n\n[1] J. Wenger & P. Hennig, *Probabilistic Linear Solvers for Machine Learning*, 34th Conference on Neural Information Processing Systems (NeurIPS), 2020.\n\n[2] P. Hennig, *Probabilistic interpretation of linear solvers*, SIAM Journal on Optimization, 2015.",
"_____no_output_____"
]
]
] | [
"markdown",
"code",
"markdown",
"code",
"markdown",
"code",
"markdown",
"code",
"markdown",
"code",
"markdown",
"code",
"markdown",
"code",
"markdown",
"code",
"markdown"
] | [
[
"markdown"
],
[
"code"
],
[
"markdown",
"markdown"
],
[
"code"
],
[
"markdown"
],
[
"code"
],
[
"markdown"
],
[
"code"
],
[
"markdown",
"markdown"
],
[
"code",
"code",
"code",
"code"
],
[
"markdown"
],
[
"code"
],
[
"markdown"
],
[
"code"
],
[
"markdown",
"markdown"
],
[
"code",
"code",
"code"
],
[
"markdown",
"markdown"
]
] |
e77407521c018672ecb9fbad2c7c18f59da8947c | 944 | ipynb | Jupyter Notebook | TwoD array.ipynb | SriVinayA/numpy-100 | 01bc6294cc8482716285c51cc1beda2c325ab88a | [
"MIT"
] | null | null | null | TwoD array.ipynb | SriVinayA/numpy-100 | 01bc6294cc8482716285c51cc1beda2c325ab88a | [
"MIT"
] | null | null | null | TwoD array.ipynb | SriVinayA/numpy-100 | 01bc6294cc8482716285c51cc1beda2c325ab88a | [
"MIT"
] | null | null | null | 17.163636 | 49 | 0.463983 | [
[
[
"import numpy as np\nlist = [[1, 2, 3], [4, 5, 6], [7, 8, 9]]\narr=np.array(list)\nprint(arr)",
"[[1 2 3]\n [4 5 6]\n [7 8 9]]\n"
]
]
] | [
"code"
] | [
[
"code"
]
] |
e7740cd9f59025524ae5266aa74fa0ddb131152c | 63,474 | ipynb | Jupyter Notebook | Assignment 3_Tensorflow/CIFAR_10Xavier_initializer.ipynb | RajeshreeKale/CSYE | e440aa99152f0b0111b0f5b927091da590b6e3a7 | [
"MIT"
] | null | null | null | Assignment 3_Tensorflow/CIFAR_10Xavier_initializer.ipynb | RajeshreeKale/CSYE | e440aa99152f0b0111b0f5b927091da590b6e3a7 | [
"MIT"
] | null | null | null | Assignment 3_Tensorflow/CIFAR_10Xavier_initializer.ipynb | RajeshreeKale/CSYE | e440aa99152f0b0111b0f5b927091da590b6e3a7 | [
"MIT"
] | null | null | null | 47.546067 | 7,640 | 0.448735 | [
[
[
"# ASSIGNMENT 3\nUsing Tensorflow to build a CNN network for CIFAR-10 dataset. Each record is of size 1*3072. Building a CNN network to classify the data into the 10 classes.\n\n# Dataset\nCIFAR-10 dataset The CIFAR-10 dataset consists of 60000 32x32 colour images in 10 classes, with 6000 images per class. There are 50000 training images and 10000 test images.\n\nThe dataset is divided into five training batches and one test batch, each with 10000 images. The test batch contains exactly 1000 randomly-selected images from each class. The training batches contain the remaining images in random order, but some training batches may contain more images from one class than another. Between them, the training batches contain exactly 5000 images from each class.\n\nhttp://www.cs.utoronto.ca/~kriz/cifar.html\n\n# Installing pydrive",
"_____no_output_____"
]
],
[
[
"!pip install pydrive",
"Collecting pydrive\n\u001b[?25l Downloading https://files.pythonhosted.org/packages/52/e0/0e64788e5dd58ce2d6934549676243dc69d982f198524be9b99e9c2a4fd5/PyDrive-1.3.1.tar.gz (987kB)\n\r\u001b[K 1% |▎ | 10kB 15.9MB/s eta 0:00:01\r\u001b[K 2% |▋ | 20kB 4.9MB/s eta 0:00:01\r\u001b[K 3% |█ | 30kB 7.0MB/s eta 0:00:01\r\u001b[K 4% |█▎ | 40kB 4.4MB/s eta 0:00:01\r\u001b[K 5% |█▋ | 51kB 5.4MB/s eta 0:00:01\r\u001b[K 6% |██ | 61kB 6.4MB/s eta 0:00:01\r\u001b[K 7% |██▎ | 71kB 7.2MB/s eta 0:00:01\r\u001b[K 8% |██▋ | 81kB 8.0MB/s eta 0:00:01\r\u001b[K 9% |███ | 92kB 8.8MB/s eta 0:00:01\r\u001b[K 10% |███▎ | 102kB 7.1MB/s eta 0:00:01\r\u001b[K 11% |███▋ | 112kB 7.3MB/s eta 0:00:01\r\u001b[K 12% |████ | 122kB 9.5MB/s eta 0:00:01\r\u001b[K 13% |████▎ | 133kB 9.5MB/s eta 0:00:01\r\u001b[K 14% |████▋ | 143kB 16.9MB/s eta 0:00:01\r\u001b[K 15% |█████ | 153kB 17.1MB/s eta 0:00:01\r\u001b[K 16% |█████▎ | 163kB 17.0MB/s eta 0:00:01\r\u001b[K 17% |█████▋ | 174kB 16.7MB/s eta 0:00:01\r\u001b[K 18% |██████ | 184kB 17.1MB/s eta 0:00:01\r\u001b[K 19% |██████▎ | 194kB 17.1MB/s eta 0:00:01\r\u001b[K 20% |██████▋ | 204kB 40.7MB/s eta 0:00:01\r\u001b[K 21% |███████ | 215kB 20.9MB/s eta 0:00:01\r\u001b[K 22% |███████▎ | 225kB 20.7MB/s eta 0:00:01\r\u001b[K 23% |███████▋ | 235kB 20.4MB/s eta 0:00:01\r\u001b[K 24% |████████ | 245kB 20.2MB/s eta 0:00:01\r\u001b[K 25% |████████▎ | 256kB 20.3MB/s eta 0:00:01\r\u001b[K 26% |████████▋ | 266kB 19.7MB/s eta 0:00:01\r\u001b[K 27% |█████████ | 276kB 20.5MB/s eta 0:00:01\r\u001b[K 29% |█████████▎ | 286kB 20.6MB/s eta 0:00:01\r\u001b[K 30% |█████████▋ | 296kB 20.5MB/s eta 0:00:01\r\u001b[K 31% |██████████ | 307kB 21.9MB/s eta 0:00:01\r\u001b[K 32% |██████████▎ | 317kB 46.6MB/s eta 0:00:01\r\u001b[K 33% |██████████▋ | 327kB 48.1MB/s eta 0:00:01\r\u001b[K 34% |███████████ | 337kB 52.4MB/s eta 0:00:01\r\u001b[K 35% |███████████▎ | 348kB 49.0MB/s eta 0:00:01\r\u001b[K 36% |███████████▋ | 358kB 48.5MB/s eta 0:00:01\r\u001b[K 37% |████████████ | 368kB 51.2MB/s eta 0:00:01\r\u001b[K 38% |████████████▎ | 378kB 51.1MB/s eta 0:00:01\r\u001b[K 39% |████████████▋ | 389kB 51.3MB/s eta 0:00:01\r\u001b[K 40% |█████████████ | 399kB 30.2MB/s eta 0:00:01\r\u001b[K 41% |█████████████▎ | 409kB 29.4MB/s eta 0:00:01\r\u001b[K 42% |█████████████▋ | 419kB 29.1MB/s eta 0:00:01\r\u001b[K 43% |██████████████ | 430kB 28.6MB/s eta 0:00:01\r\u001b[K 44% |██████████████▎ | 440kB 28.4MB/s eta 0:00:01\r\u001b[K 45% |██████████████▋ | 450kB 27.5MB/s eta 0:00:01\r\u001b[K 46% |███████████████ | 460kB 27.5MB/s eta 0:00:01\r\u001b[K 47% |███████████████▎ | 471kB 27.9MB/s eta 0:00:01\r\u001b[K 48% |███████████████▋ | 481kB 27.7MB/s eta 0:00:01\r\u001b[K 49% |████████████████ | 491kB 27.6MB/s eta 0:00:01\r\u001b[K 50% |████████████████▎ | 501kB 44.8MB/s eta 0:00:01\r\u001b[K 51% |████████████████▋ | 512kB 39.1MB/s eta 0:00:01\r\u001b[K 52% |█████████████████ | 522kB 40.0MB/s eta 0:00:01\r\u001b[K 53% |█████████████████▎ | 532kB 41.6MB/s eta 0:00:01\r\u001b[K 54% |█████████████████▋ | 542kB 41.8MB/s eta 0:00:01\r\u001b[K 55% |██████████████████ | 552kB 48.3MB/s eta 0:00:01\r\u001b[K 57% |██████████████████▎ | 563kB 48.9MB/s eta 0:00:01\r\u001b[K 58% |██████████████████▋ | 573kB 48.4MB/s eta 0:00:01\r\u001b[K 59% |███████████████████ | 583kB 49.1MB/s eta 0:00:01\r\u001b[K 60% |███████████████████▎ | 593kB 49.5MB/s eta 0:00:01\r\u001b[K 61% |███████████████████▋ | 604kB 49.4MB/s eta 0:00:01\r\u001b[K 62% |████████████████████ | 614kB 61.6MB/s eta 0:00:01\r\u001b[K 63% |████████████████████▎ | 624kB 62.2MB/s eta 0:00:01\r\u001b[K 64% |████████████████████▋ | 634kB 62.4MB/s eta 0:00:01\r\u001b[K 65% |█████████████████████ | 645kB 59.9MB/s eta 0:00:01\r\u001b[K 66% |█████████████████████▎ | 655kB 55.2MB/s eta 0:00:01\r\u001b[K 67% |█████████████████████▋ | 665kB 50.8MB/s eta 0:00:01\r\u001b[K 68% |██████████████████████ | 675kB 49.8MB/s eta 0:00:01\r\u001b[K 69% |██████████████████████▎ | 686kB 50.1MB/s eta 0:00:01\r\u001b[K 70% |██████████████████████▋ | 696kB 50.2MB/s eta 0:00:01\r\u001b[K 71% |███████████████████████ | 706kB 49.6MB/s eta 0:00:01\r\u001b[K 72% |███████████████████████▎ | 716kB 50.1MB/s eta 0:00:01\r\u001b[K 73% |███████████████████████▋ | 727kB 50.3MB/s eta 0:00:01\r\u001b[K 74% |████████████████████████ | 737kB 49.5MB/s eta 0:00:01\r\u001b[K 75% |████████████████████████▎ | 747kB 52.0MB/s eta 0:00:01\r\u001b[K 76% |████████████████████████▋ | 757kB 56.1MB/s eta 0:00:01\r\u001b[K 77% |████████████████████████▉ | 768kB 61.6MB/s eta 0:00:01\r\u001b[K 78% |█████████████████████████▏ | 778kB 64.1MB/s eta 0:00:01\r\u001b[K 79% |█████████████████████████▌ | 788kB 63.8MB/s eta 0:00:01\r\u001b[K 80% |█████████████████████████▉ | 798kB 64.2MB/s eta 0:00:01\r\u001b[K 81% |██████████████████████████▏ | 808kB 64.1MB/s eta 0:00:01\r\u001b[K 82% |██████████████████████████▌ | 819kB 63.6MB/s eta 0:00:01\r\u001b[K 83% |██████████████████████████▉ | 829kB 63.7MB/s eta 0:00:01\r\u001b[K 85% |███████████████████████████▏ | 839kB 65.1MB/s eta 0:00:01\r\u001b[K 86% |███████████████████████████▌ | 849kB 65.2MB/s eta 0:00:01\r\u001b[K 87% |███████████████████████████▉ | 860kB 51.4MB/s eta 0:00:01\r\u001b[K 88% |████████████████████████████▏ | 870kB 48.5MB/s eta 0:00:01\r\u001b[K 89% |████████████████████████████▌ | 880kB 48.2MB/s eta 0:00:01\r\u001b[K 90% |████████████████████████████▉ | 890kB 47.9MB/s eta 0:00:01\r\u001b[K 91% |█████████████████████████████▏ | 901kB 46.6MB/s eta 0:00:01\r\u001b[K 92% |█████████████████████████████▌ | 911kB 47.0MB/s eta 0:00:01\r\u001b[K 93% |█████████████████████████████▉ | 921kB 46.3MB/s eta 0:00:01\r\u001b[K 94% |██████████████████████████████▏ | 931kB 46.3MB/s eta 0:00:01\r\u001b[K 95% |██████████████████████████████▌ | 942kB 46.1MB/s eta 0:00:01\r\u001b[K 96% |██████████████████████████████▉ | 952kB 45.6MB/s eta 0:00:01\r\u001b[K 97% |███████████████████████████████▏| 962kB 55.4MB/s eta 0:00:01\r\u001b[K 98% |███████████████████████████████▌| 972kB 58.7MB/s eta 0:00:01\r\u001b[K 99% |███████████████████████████████▉| 983kB 58.2MB/s eta 0:00:01\r\u001b[K 100% |████████████████████████████████| 993kB 19.8MB/s \n\u001b[?25hRequirement already satisfied: google-api-python-client>=1.2 in /usr/local/lib/python3.6/dist-packages (from pydrive) (1.6.7)\nRequirement already satisfied: oauth2client>=4.0.0 in /usr/local/lib/python3.6/dist-packages (from pydrive) (4.1.3)\nRequirement already satisfied: PyYAML>=3.0 in /usr/local/lib/python3.6/dist-packages (from pydrive) (3.13)\nRequirement already satisfied: uritemplate<4dev,>=3.0.0 in /usr/local/lib/python3.6/dist-packages (from google-api-python-client>=1.2->pydrive) (3.0.0)\nRequirement already satisfied: six<2dev,>=1.6.1 in /usr/local/lib/python3.6/dist-packages (from google-api-python-client>=1.2->pydrive) (1.11.0)\nRequirement already satisfied: httplib2<1dev,>=0.9.2 in /usr/local/lib/python3.6/dist-packages (from google-api-python-client>=1.2->pydrive) (0.11.3)\nRequirement already satisfied: pyasn1-modules>=0.0.5 in /usr/local/lib/python3.6/dist-packages (from oauth2client>=4.0.0->pydrive) (0.2.4)\nRequirement already satisfied: pyasn1>=0.1.7 in /usr/local/lib/python3.6/dist-packages (from oauth2client>=4.0.0->pydrive) (0.4.5)\nRequirement already satisfied: rsa>=3.1.4 in /usr/local/lib/python3.6/dist-packages (from oauth2client>=4.0.0->pydrive) (4.0)\nBuilding wheels for collected packages: pydrive\n Building wheel for pydrive (setup.py) ... \u001b[?25ldone\n\u001b[?25h Stored in directory: /root/.cache/pip/wheels/fa/d2/9a/d3b6b506c2da98289e5d417215ce34b696db856643bad779f4\nSuccessfully built pydrive\nInstalling collected packages: pydrive\nSuccessfully installed pydrive-1.3.1\n"
]
],
[
[
"# Creates connection",
"_____no_output_____"
]
],
[
[
"from pydrive.auth import GoogleAuth\nfrom pydrive.drive import GoogleDrive\nfrom google.colab import auth\nimport tensorflow as tf\nfrom oauth2client.client import GoogleCredentials",
"_____no_output_____"
]
],
[
[
"# Authenticating and creating the PyDrive client",
"_____no_output_____"
]
],
[
[
"auth.authenticate_user()\ngauth = GoogleAuth()\ngauth.credentials = GoogleCredentials.get_application_default()\ndrive = GoogleDrive(gauth)",
"_____no_output_____"
]
],
[
[
"# Getting ids of all the files in folder",
"_____no_output_____"
]
],
[
[
"file_list = drive.ListFile({'q': \"'1DCFFw2O6BFq8Gk0eYu7JT4Qn224BNoCt' in parents and trashed=false\"}).GetList()\nfor file1 in file_list:\n print('title: %s, id: %s' % (file1['title'], file1['id']))",
"title: data_batch_1, id: 11Bo2ULl9_aOQ761ONc2vhepnydriELiT\ntitle: data_batch_2, id: 1asFrGiOMdHKY-_KO94e1fLWMBN_Ke92I\ntitle: test_batch, id: 1Wyz_RdmoLe9r9t1rloap8AttSltmfwrp\ntitle: data_batch_3, id: 11ky6i6FSTGWJYOzXquELD4H-GUr49C4f\ntitle: data_batch_5, id: 1rmRytfjJWua0cv17DzST6PqoDFY2APa6\ntitle: data_batch_4, id: 1bb6TRjqNY5A0FsD_P7s3ssepMGWNW-Eh\n"
]
],
[
[
"# Importing libraries",
"_____no_output_____"
]
],
[
[
"import tensorflow as tf\nimport numpy as np\nimport pandas as pd\nimport matplotlib.pyplot as plt\nfrom IPython import display\nfrom sklearn.model_selection import train_test_split\nimport pickle\n%matplotlib inline",
"_____no_output_____"
]
],
[
[
"# Loading the data",
"_____no_output_____"
]
],
[
[
"def unpickle(file):\n with open(file, 'rb') as fo:\n dict = pickle.load(fo, encoding='bytes')\n return dict",
"_____no_output_____"
]
],
[
[
"# if file is zipped",
"_____no_output_____"
]
],
[
[
"zip_file = drive.CreateFile({'id': '11Bo2ULl9_aOQ761ONc2vhepnydriELiT'})\nzip_file.GetContentFile('data_batch_1')\n\nzip_file = drive.CreateFile({'id': '1asFrGiOMdHKY-_KO94e1fLWMBN_Ke92I'})\nzip_file.GetContentFile('data_batch_2')\n\nzip_file = drive.CreateFile({'id': '11ky6i6FSTGWJYOzXquELD4H-GUr49C4f'})\nzip_file.GetContentFile('data_batch_3')\n\nzip_file = drive.CreateFile({'id': '1bb6TRjqNY5A0FsD_P7s3ssepMGWNW-Eh'})\nzip_file.GetContentFile('data_batch_4')\n\nzip_file = drive.CreateFile({'id': '1rmRytfjJWua0cv17DzST6PqoDFY2APa6'})\nzip_file.GetContentFile('data_batch_5')\n\nzip_file = drive.CreateFile({'id': '1Wyz_RdmoLe9r9t1rloap8AttSltmfwrp'})\nzip_file.GetContentFile('test_batch')",
"_____no_output_____"
],
[
"data1 = unpickle(\"data_batch_1\")\ndata2 = unpickle(\"data_batch_2\")\ndata3 = unpickle(\"data_batch_3\")\ndata4 = unpickle(\"data_batch_4\")\ndata5 = unpickle(\"data_batch_5\")\n#label_data = unpickle('../input/batches.meta')[b'label_names']",
"_____no_output_____"
],
[
"labels1 = data1[b'labels']\ndata1 = data1[b'data'] * 1.0\nlabels2 = data2[b'labels']\ndata2 = data2[b'data'] * 1.0\nlabels3 = data3[b'labels']\ndata3 = data3[b'data'] * 1.0\nlabels4 = data4[b'labels']\ndata4 = data4[b'data'] * 1.0\nlabels5 = data5[b'labels']\ndata5 = data5[b'data'] * 1.0",
"_____no_output_____"
]
],
[
[
"# Combine the remaining four arrays to use as training data",
"_____no_output_____"
]
],
[
[
"X_tr = np.concatenate([data1, data2, data3, data4, data5], axis=0)\nX_tr = np.dstack((X_tr[:, :1024], X_tr[:, 1024:2048], X_tr[:, 2048:])) / 1.0\nX_tr = (X_tr - 128) / 255.0\nX_tr = X_tr.reshape(-1, 32, 32, 3)\n\ny_tr = np.concatenate([labels1, labels2, labels3, labels4, labels5], axis=0)",
"_____no_output_____"
]
],
[
[
"# Setting the number of classes",
"_____no_output_____"
]
],
[
[
"num_classes = len(np.unique(y_tr))\n\nprint(\"X_tr\", X_tr.shape)\nprint(\"y_tr\", y_tr.shape)",
"X_tr (50000, 32, 32, 3)\ny_tr (50000,)\n"
]
],
[
[
"# Importing the test data",
"_____no_output_____"
]
],
[
[
"test_data = unpickle(\"test_batch\")\n\nX_test = test_data[b'data']\nX_test = np.dstack((X_test[:, :1024], X_test[:, 1024:2048], X_test[:, 2048:])) / 1.0\nX_test = (X_test - 128) / 255.0\nX_test = X_test.reshape(-1, 32, 32, 3)\ny_test = np.asarray(test_data[b'labels'])",
"_____no_output_____"
]
],
[
[
"# Spliting into test and validation",
"_____no_output_____"
]
],
[
[
"X_te, X_cv, y_te, y_cv = train_test_split(X_test, y_test, test_size=0.5, random_state=1)\n\nprint(\"X_te\", X_te.shape)\nprint(\"X_cv\", X_cv.shape)\nprint(\"y_te\", y_te.shape)\nprint(\"y_cv\", y_cv.shape)",
"X_te (5000, 32, 32, 3)\nX_cv (5000, 32, 32, 3)\ny_te (5000,)\ny_cv (5000,)\n"
]
],
[
[
"# Batch generator",
"_____no_output_____"
]
],
[
[
"def get_batches(X, y, batch_size, crop=False, distort=True):\n # Shuffle X,y\n shuffled_idx = np.arange(len(y))\n np.random.shuffle(shuffled_idx)\n i, h, w, c = X.shape\n \n # Enumerate indexes by steps of batch_size\n for i in range(0, len(y), batch_size):\n batch_idx = shuffled_idx[i:i+batch_size]\n X_return = X[batch_idx]\n \n # optional random crop of images\n if crop:\n woff = (w - 24) // 4\n hoff = (h - 24) // 4\n startw = np.random.randint(low=woff,high=woff*2)\n starth = np.random.randint(low=hoff,high=hoff*2)\n X_return = X_return[:,startw:startw+24,starth:starth+24,:]\n \n # do random flipping of images\n coin = np.random.binomial(1, 0.5, size=None)\n if coin and distort:\n X_return = X_return[...,::-1,:]\n \n yield X_return, y[batch_idx]",
"_____no_output_____"
]
],
[
[
"# Configurations",
"_____no_output_____"
]
],
[
[
"epochs = 20 # how many epochs\nbatch_size = 128\nsteps_per_epoch = X_tr.shape[0] / batch_size",
"_____no_output_____"
]
],
[
[
"# Building the network\n\n\n## MODEL 7.13.4.6.7f\n\nModel description:\n\n* 7.6 - changed kernel reg rate to 0.01 from 0.1\n* 7.7 - optimize loss instead of ce 7.8 - remove redundant lambda, replaced scale in regularizer with lambda, changed lambda from 0.01 to 0.001\n* 7.9 - lambda 0 instead of 3\n* 7.9.1 - lambda 1 instead of 0\n* 7.9.2 - use lambda 2 instead of 1\n* 7.9.4f - use 3x3 pooling instead of 2x2\n* 7.11.6f - add batch norm after conv 5\n* 7.11.2f - raise lambda, add dropout after fc2\n* 7.12.2f - change fully connected dropout to 20%\n* 7.12.2.2g - change fc dropout to 25%, increase filters in last 2 conv layers to 192 from 128\n* 7.13.2.2f - change all pool sizes to 2x2 from 3x3\n7.13.3.6f - use different lambda for conv + fc layers",
"_____no_output_____"
]
],
[
[
"# Create new graph\ngraph = tf.Graph()\n# whether to retrain model from scratch or use saved model\ninit = True\nmodel_name = \"model_7.13.4.7.7l\"\n\nwith graph.as_default():\n # Placeholders\n X = tf.placeholder(dtype=tf.float32, shape=[None, 32, 32, 3])\n y = tf.placeholder(dtype=tf.int32, shape=[None])\n training = tf.placeholder(dtype=tf.bool)\n \n # create global step for decaying learning rate\n global_step = tf.Variable(0, trainable=False)\n\n # lambda 6\n lamC = 0.000050\n lamF = 0.0025000\n \n # learning rate j\n epochs_per_decay = 10\n starting_rate = 0.003\n decay_factor = 0.9\n staircase = True\n \n learning_rate = tf.train.exponential_decay(starting_rate, # start at 0.003\n global_step, \n steps_per_epoch * epochs_per_decay, # 100 epochs\n decay_factor, # 0.5 decrease\n staircase=staircase) \n \n # Small epsilon value for the BN transform\n epsilon = 1e-3\n \n with tf.name_scope('conv1') as scope:\n # Convolutional layer 1 \n conv1 = tf.layers.conv2d(\n X, # Input data\n filters=64, # 64 filters\n kernel_size=(5, 5), # Kernel size: 5x5\n strides=(1, 1), # Stride: 1\n padding='SAME', # \"same\" padding\n activation=None, # None\n kernel_initializer=tf.truncated_normal_initializer(stddev=5e-2, seed=10),\n kernel_regularizer=tf.contrib.layers.l2_regularizer(scale=lamC),\n name='conv1' \n )\n\n # try batch normalization\n bn1 = tf.layers.batch_normalization(\n conv1,\n axis=-1,\n momentum=0.99,\n epsilon=epsilon,\n center=True,\n scale=True,\n beta_initializer=tf.zeros_initializer(),\n gamma_initializer=tf.ones_initializer(),\n moving_mean_initializer=tf.zeros_initializer(),\n moving_variance_initializer=tf.ones_initializer(),\n training=training,\n name='bn1'\n )\n\n #apply relu\n conv1_bn_relu = tf.nn.relu(bn1, name='relu1')\n\n conv1_bn_relu = tf.layers.dropout(conv1_bn_relu, rate=0.1, seed=9, training=training)\n \n with tf.name_scope('conv2') as scope:\n # Convolutional layer 2\n conv2 = tf.layers.conv2d(\n conv1_bn_relu, # Input data\n filters=64, # 64 filters\n kernel_size=(5, 5), # Kernel size: 5x5\n strides=(1, 1), # Stride: 1\n padding='SAME', # \"same\" padding\n activation=None, # None\n kernel_initializer=tf.truncated_normal_initializer(stddev=5e-2, seed=8),\n kernel_regularizer=tf.contrib.layers.l2_regularizer(scale=lamC),\n name='conv2' # Add name\n )\n\n # try batch normalization\n bn2 = tf.layers.batch_normalization(\n conv2,\n axis=-1,\n momentum=0.9,\n epsilon=epsilon,\n center=True,\n scale=True,\n beta_initializer=tf.zeros_initializer(),\n gamma_initializer=tf.ones_initializer(),\n moving_mean_initializer=tf.zeros_initializer(),\n moving_variance_initializer=tf.ones_initializer(),\n training=training,\n name='bn2'\n )\n\n #apply relu\n conv2_bn_relu = tf.nn.relu(bn2, name='relu2')\n \n with tf.name_scope('pool1') as scope:\n # Max pooling layer 1\n pool1 = tf.layers.max_pooling2d(\n conv2_bn_relu, # Input\n pool_size=(2, 2), # Pool size: 3x3\n strides=(2, 2), # Stride: 2\n padding='SAME', # \"same\" padding\n name='pool1'\n )\n\n # dropout at 10%\n pool1 = tf.layers.dropout(pool1, rate=0.1, seed=1, training=training)\n\n with tf.name_scope('conv3') as scope:\n # Convolutional layer 3\n conv3= tf.layers.conv2d(\n pool1, # Input\n filters=96, # 96 filters\n kernel_size=(4, 4), # Kernel size: 4x4\n strides=(1, 1), # Stride: 1\n padding='SAME', # \"same\" padding\n activation=None, # None\n kernel_initializer=tf.truncated_normal_initializer(stddev=5e-2, seed=7),\n kernel_regularizer=tf.contrib.layers.l2_regularizer(scale=lamC),\n name='conv3' \n )\n\n bn3 = tf.layers.batch_normalization(\n conv3,\n axis=-1,\n momentum=0.9,\n epsilon=epsilon,\n center=True,\n scale=True,\n beta_initializer=tf.zeros_initializer(),\n gamma_initializer=tf.ones_initializer(),\n moving_mean_initializer=tf.zeros_initializer(),\n moving_variance_initializer=tf.ones_initializer(),\n training=training,\n name='bn3'\n )\n\n #apply relu\n conv3_bn_relu = tf.nn.relu(bn3, name='relu3')\n \n # dropout at 10%\n conv3_bn_relu = tf.layers.dropout(conv3_bn_relu, rate=0.1, seed=0, training=training)\n\n with tf.name_scope('conv4') as scope:\n # Convolutional layer 4\n conv4= tf.layers.conv2d(\n conv3_bn_relu, # Input\n filters=96, # 96 filters\n kernel_size=(4, 4), # Kernel size: 4x4\n strides=(1, 1), # Stride: 1\n padding='SAME', # \"same\" padding\n activation=None, \n kernel_initializer=tf.truncated_normal_initializer(stddev=5e-2, seed=1), \n kernel_regularizer=tf.contrib.layers.l2_regularizer(scale=lamC),\n name='conv4' \n )\n\n bn4 = tf.layers.batch_normalization(\n conv4,\n axis=-1,\n momentum=0.9,\n epsilon=epsilon,\n center=True,\n scale=True,\n beta_initializer=tf.zeros_initializer(),\n gamma_initializer=tf.ones_initializer(),\n moving_mean_initializer=tf.zeros_initializer(),\n moving_variance_initializer=tf.ones_initializer(),\n training=training,\n name='bn4'\n )\n\n #apply relu\n conv4_bn_relu = tf.nn.relu(bn4, name='relu4')\n \n # Max pooling layer 2 \n pool2 = tf.layers.max_pooling2d(\n conv4_bn_relu, # input\n pool_size=(2, 2), # pool size 2x2\n strides=(2, 2), # stride 2\n padding='SAME',\n name='pool2'\n )\n\n with tf.name_scope('conv5') as scope:\n # Convolutional layer 5\n conv5= tf.layers.conv2d(\n pool2, # Input\n filters=128, # 128 filters\n kernel_size=(3, 3), # Kernel size: 3x3\n strides=(1, 1), # Stride: 1\n padding='SAME', # \"same\" padding\n activation=None, \n kernel_initializer=tf.truncated_normal_initializer(stddev=5e-2, seed=2),\n kernel_regularizer=tf.contrib.layers.l2_regularizer(scale=lamC),\n name='conv5' \n )\n \n \n bn5 = tf.layers.batch_normalization(\n conv5,\n axis=-1,\n momentum=0.9,\n epsilon=epsilon,\n center=True,\n scale=True,\n beta_initializer=tf.zeros_initializer(),\n gamma_initializer=tf.ones_initializer(),\n moving_mean_initializer=tf.zeros_initializer(),\n moving_variance_initializer=tf.ones_initializer(),\n training=training,\n name='bn5'\n )\n \n # activation\n conv5_bn_relu = tf.nn.relu(bn5, name='relu5')\n\n # try dropout here\n conv5_bn_relu = tf.layers.dropout(conv5_bn_relu, rate=0.1, seed=3, training=training) \n\n with tf.name_scope('conv6') as scope:\n # Convolutional layer 6\n conv6= tf.layers.conv2d(\n conv5_bn_relu, # Input\n filters=128, # 128 filters\n kernel_size=(3, 3), # Kernel size: 3x3\n strides=(1, 1), # Stride: 1\n padding='SAME', # \"same\" padding\n activation=None, # None\n kernel_initializer=tf.truncated_normal_initializer(stddev=5e-2, seed=3), \n kernel_regularizer=tf.contrib.layers.l2_regularizer(scale=lamC),\n name='conv6' \n )\n\n bn6 = tf.layers.batch_normalization(\n conv6,\n axis=-1,\n momentum=0.9,\n epsilon=epsilon,\n center=True,\n scale=True,\n beta_initializer=tf.zeros_initializer(),\n gamma_initializer=tf.ones_initializer(),\n moving_mean_initializer=tf.zeros_initializer(),\n moving_variance_initializer=tf.ones_initializer(),\n training=training,\n name='bn6'\n )\n\n #apply relu\n conv6_bn_relu = tf.nn.relu(bn6, name='relu6')\n \n # Max pooling layer 3\n pool3 = tf.layers.max_pooling2d(\n conv6_bn_relu, # input\n pool_size=(2, 2), # pool size 2x2\n strides=(2, 2), # stride 2\n padding='SAME',\n name='pool3'\n )\n \n with tf.name_scope('flatten') as scope:\n # Flatten output\n flat_output = tf.contrib.layers.flatten(pool3)\n\n # dropout at 10%\n flat_output = tf.layers.dropout(flat_output, rate=0.1, seed=5, training=training)\n \n # Fully connected layer 1\n with tf.name_scope('fc1') as scope:\n fc1 = tf.layers.dense(\n flat_output, # input\n 1024, # 1024 hidden units\n activation=None, # None\n kernel_initializer=tf.variance_scaling_initializer(scale=2, seed=4),\n bias_initializer=tf.zeros_initializer(),\n kernel_regularizer=tf.contrib.layers.l2_regularizer(scale=lamF),\n name=\"fc1\"\n )\n \n bn7 = tf.layers.batch_normalization(\n fc1,\n axis=-1,\n momentum=0.9,\n epsilon=epsilon,\n center=True,\n scale=True,\n beta_initializer=tf.zeros_initializer(),\n gamma_initializer=tf.ones_initializer(),\n moving_mean_initializer=tf.zeros_initializer(),\n moving_variance_initializer=tf.ones_initializer(),\n training=training,\n name='bn7'\n )\n \n fc1_relu = tf.nn.relu(bn7, name='fc1_relu')\n \n # dropout at 25%\n fc1_do = tf.layers.dropout(fc1_relu, rate=0.25, seed=10, training=training)\n \n # Fully connected layer 2\n with tf.name_scope('fc2') as scope:\n fc2 = tf.layers.dense(\n fc1_do, # input\n 512, # 512 hidden units\n activation=None, # None\n kernel_initializer=tf.variance_scaling_initializer(scale=2, seed=5),\n bias_initializer=tf.zeros_initializer(),\n kernel_regularizer=tf.contrib.layers.l2_regularizer(scale=lamF),\n name=\"fc2\"\n )\n \n bn8 = tf.layers.batch_normalization(\n fc2,\n axis=-1,\n momentum=0.9,\n epsilon=epsilon,\n center=True,\n scale=True,\n beta_initializer=tf.zeros_initializer(),\n gamma_initializer=tf.ones_initializer(),\n moving_mean_initializer=tf.zeros_initializer(),\n moving_variance_initializer=tf.ones_initializer(),\n training=training,\n name='bn8'\n )\n \n fc2_relu = tf.nn.relu(bn8, name='fc2_relu')\n \n # dropout at 10%\n fc2_do = tf.layers.dropout(fc2_relu, rate=0.25, seed=11, training=training)\n \n # Output layer\n logits = tf.layers.dense(\n fc2_do, # input\n num_classes, # One output unit per category\n activation=None, # No activation function\n kernel_initializer=tf.contrib.layers.xavier_initializer(uniform=True,\n seed=6,dtype=tf.dtypes.float32),\n \n )\n \n # Kernel weights of the 1st conv. layer\n with tf.variable_scope('conv1', reuse=True):\n conv_kernels1 = tf.get_variable('kernel')\n \n # Mean cross-entropy\n mean_ce = tf.reduce_mean(tf.nn.sparse_softmax_cross_entropy_with_logits(labels=y, logits=logits))\n loss = mean_ce + tf.losses.get_regularization_loss()\n \n # Adam optimizer\n optimizer = tf.train.AdamOptimizer(learning_rate=learning_rate)\n \n # Minimize cross-entropy\n train_op = optimizer.minimize(loss, global_step=global_step)\n\n # Compute predictions and accuracy\n predictions = tf.argmax(logits, axis=1, output_type=tf.int32)\n is_correct = tf.equal(y, predictions)\n accuracy = tf.reduce_mean(tf.cast(is_correct, dtype=tf.float32))\n \n # add this so that the batch norm gets run\n extra_update_ops = tf.get_collection(tf.GraphKeys.UPDATE_OPS)\n \n # Create summary hooks\n tf.summary.scalar('accuracy', accuracy)\n tf.summary.scalar('cross_entropy', mean_ce)\n tf.summary.scalar('learning_rate', learning_rate)\n \n # Merge all the summaries and write them out to /tmp/mnist_logs (by default)\n merged = tf.summary.merge_all()",
"_____no_output_____"
]
],
[
[
"# CONFIGURE OPTIONS",
"_____no_output_____"
]
],
[
[
"init = True # whether to initialize the model or use a saved version\ncrop = False # do random cropping of images?\n\nmeta_data_every = 5\nlog_to_tensorboard = False\nprint_every = 1 # how often to print metrics\ncheckpoint_every = 1 # how often to save model in epochs\nuse_gpu = True # whether or not to use the GPU\nprint_metrics = True # whether to print or plot metrics, if False a plot will be created and updated every epoch\n\n# Placeholders for metrics\nif init:\n valid_acc_values = []\n valid_cost_values = []\n train_acc_values = []\n train_cost_values = []\n train_lr_values = []\n train_loss_values = []\n \n\nconfig = tf.ConfigProto()",
"_____no_output_____"
]
],
[
[
"# Trainig the model",
"_____no_output_____"
]
],
[
[
"with tf.Session(graph=graph, config=config) as sess:\n if log_to_tensorboard:\n train_writer = tf.summary.FileWriter('./logs/tr_' + model_name, sess.graph)\n test_writer = tf.summary.FileWriter('./logs/te_' + model_name)\n \n if not print_metrics:\n # create a plot to be updated as model is trained\n f, ax = plt.subplots(1,3,figsize=(20,5))\n \n # create the saver\n saver = tf.train.Saver()\n \n # If the model is new initialize variables, else restore the session\n if init:\n sess.run(tf.global_variables_initializer())\n else:\n saver.restore(sess, './model/cifar_'+model_name+'.ckpt')\n\n # Set seed\n np.random.seed(0)\n \n print(\"Training\", model_name, \"...\")\n \n # Train several epochs\n for epoch in range(epochs):\n # Accuracy values (train) after each batch\n batch_acc = []\n batch_cost = []\n batch_loss = []\n batch_lr = []\n \n # only log run metadata once per epoch\n write_meta_data = False\n \n for X_batch, y_batch in get_batches(X_tr, y_tr, batch_size, crop=crop, distort=True):\n if write_meta_data and log_to_tensboard:\n # create the metadata\n run_options = tf.RunOptions(trace_level=tf.RunOptions.FULL_TRACE)\n run_metadata = tf.RunMetadata()\n \n # Run training and evaluate accuracy\n _, _, summary, acc_value, cost_value, loss_value, step, lr = sess.run([train_op, extra_update_ops, merged, accuracy, mean_ce, loss, global_step, learning_rate], feed_dict={\n X: X_batch,\n y: y_batch,\n training: True\n },\n options=run_options,\n run_metadata=run_metadata)\n\n # Save accuracy (current batch)\n batch_acc.append(acc_value)\n batch_cost.append(cost_value)\n batch_lr.append(lr)\n batch_loss.append(loss_value)\n \n # write the summary\n train_writer.add_run_metadata(run_metadata, 'step %d' % step)\n train_writer.add_summary(summary, step)\n write_meta_data = False\n \n else:\n # Run training without meta data\n _, _, summary, acc_value, cost_value, loss_value, step, lr = sess.run([train_op, extra_update_ops, merged, accuracy, mean_ce, loss, global_step, learning_rate], feed_dict={\n X: X_batch,\n y: y_batch,\n training: True\n })\n\n # Save accuracy (current batch)\n batch_acc.append(acc_value)\n batch_cost.append(cost_value)\n batch_lr.append(lr)\n batch_loss.append(loss_value)\n \n # write the summary\n if log_to_tensorboard:\n train_writer.add_summary(summary, step)\n\n # save checkpoint every nth epoch\n if(epoch % checkpoint_every == 0):\n print(\"Saving checkpoint\")\n # save the model\n save_path = saver.save(sess, './model/cifar_'+model_name+'.ckpt')\n \n # Now that model is saved set init to false so we reload it\n init = False\n \n # init batch arrays\n batch_cv_acc = []\n batch_cv_cost = []\n batch_cv_loss = []\n \n # Evaluate validation accuracy with batches so as to not crash the GPU\n for X_batch, y_batch in get_batches(X_cv, y_cv, batch_size, crop=crop, distort=False):\n summary, valid_acc, valid_cost, valid_loss = sess.run([merged, accuracy, mean_ce, loss], feed_dict={\n X: X_batch,\n y: y_batch,\n training: False\n })\n\n batch_cv_acc.append(valid_acc)\n batch_cv_cost.append(valid_cost)\n batch_cv_loss.append(valid_loss)\n\n # Write average of validation data to summary logs\n if log_to_tensorboard:\n summary = tf.Summary(value=[tf.Summary.Value(tag=\"accuracy\", simple_value=np.mean(batch_cv_acc)),tf.Summary.Value(tag=\"cross_entropy\", simple_value=np.mean(batch_cv_cost)),])\n test_writer.add_summary(summary, step)\n step += 1\n \n # take the mean of the values to add to the metrics\n valid_acc_values.append(np.mean(batch_cv_acc))\n valid_cost_values.append(np.mean(batch_cv_cost))\n train_acc_values.append(np.mean(batch_acc))\n train_cost_values.append(np.mean(batch_cost))\n train_lr_values.append(np.mean(batch_lr))\n train_loss_values.append(np.mean(batch_loss))\n \n if print_metrics:\n # Print progress every nth epoch to keep output to reasonable amount\n if(epoch % print_every == 0):\n print('Epoch {:02d} - step {} - cv acc: {:.3f} - train acc: {:.3f} (mean) - cv cost: {:.3f} - lr: {:.5f}'.format(\n epoch, step, np.mean(batch_cv_acc), np.mean(batch_acc), np.mean(batch_cv_cost), lr\n ))\n else:\n # update the plot\n ax[0].cla()\n ax[0].plot(valid_acc_values, color=\"red\", label=\"Validation\")\n ax[0].plot(train_acc_values, color=\"blue\", label=\"Training\")\n ax[0].set_title('Validation accuracy: {:.4f} (mean last 3)'.format(np.mean(valid_acc_values[-3:])))\n \n # since we can't zoom in on plots like in tensorboard, scale y axis to give a decent amount of detail\n if np.mean(valid_acc_values[-3:]) > 0.85:\n ax[0].set_ylim([0.75,1.0])\n elif np.mean(valid_acc_values[-3:]) > 0.75:\n ax[0].set_ylim([0.65,1.0])\n elif np.mean(valid_acc_values[-3:]) > 0.65:\n ax[0].set_ylim([0.55,1.0])\n elif np.mean(valid_acc_values[-3:]) > 0.55:\n ax[0].set_ylim([0.45,1.0]) \n \n ax[0].set_xlabel('Epoch')\n ax[0].set_ylabel('Accuracy')\n ax[0].legend()\n \n ax[1].cla()\n ax[1].plot(valid_cost_values, color=\"red\", label=\"Validation\")\n ax[1].plot(train_cost_values, color=\"blue\", label=\"Training\")\n ax[1].set_title('Validation xentropy: {:.3f} (mean last 3)'.format(np.mean(valid_cost_values[-3:])))\n ax[1].set_xlabel('Epoch')\n ax[1].set_ylabel('Cross Entropy')\n ax[1].legend()\n \n ax[2].cla()\n ax[2].plot(train_lr_values)\n ax[2].set_title(\"Learning rate: {:.6f}\".format(np.mean(train_lr_values[-1:])))\n ax[2].set_xlabel(\"Epoch\")\n ax[2].set_ylabel(\"Learning Rate\")\n \n display.display(plt.gcf())\n display.clear_output(wait=True)\n \n # Print data every 50th epoch so I can write it down to compare models\n if (not print_metrics) and (epoch % 50 == 0) and (epoch > 1):\n if(epoch % print_every == 0):\n print('Epoch {:02d} - step {} - cv acc: {:.3f} - train acc: {:.3f} (mean) - cv cost: {:.3f} - lr: {:.5f}'.format(\n epoch, step, np.mean(batch_cv_acc), np.mean(batch_acc), np.mean(batch_cv_cost), lr\n )) \n \n # print results of last epoch\n print('Epoch {} - cv acc: {:.4f} - train acc: {:.4f} (mean) - cv cost: {:.3f}'.format(\n epochs, np.mean(batch_cv_acc), np.mean(batch_acc), np.mean(batch_cv_cost)\n ))\n \n # save the session\n save_path = saver.save(sess, './model/cifar_'+model_name+'.ckpt')\n \n # init the test data array\n test_acc_values = []\n \n # Check on the test data\n for X_batch, y_batch in get_batches(X_te, y_te, batch_size, crop=crop, distort=False):\n test_accuracy = sess.run(accuracy, feed_dict={\n X: X_batch,\n y: y_batch,\n training: False\n })\n test_acc_values.append(test_accuracy)\n \n # average test accuracy across batches\n test_acc = np.mean(test_acc_values)\n \n# show the plot\nplt.show()\n\n# print results of last epoch\nprint('Epoch {} - cv acc: {:.4f} - train acc: {:.4f} (mean) - cv cost: {:.3f}'.format(\n epochs, np.mean(batch_cv_acc), np.mean(batch_acc), np.mean(batch_cv_cost)\n ))",
"Training model_7.13.4.7.7l ...\nSaving checkpoint\nEpoch 00 - step 391 - cv acc: 0.514 - train acc: 0.480 (mean) - cv cost: 1.403 - lr: 0.00300\nSaving checkpoint\nEpoch 01 - step 782 - cv acc: 0.680 - train acc: 0.648 (mean) - cv cost: 0.928 - lr: 0.00300\nSaving checkpoint\nEpoch 02 - step 1173 - cv acc: 0.710 - train acc: 0.710 (mean) - cv cost: 0.902 - lr: 0.00300\nSaving checkpoint\nEpoch 03 - step 1564 - cv acc: 0.747 - train acc: 0.743 (mean) - cv cost: 0.733 - lr: 0.00300\nSaving checkpoint\nEpoch 04 - step 1955 - cv acc: 0.753 - train acc: 0.764 (mean) - cv cost: 0.740 - lr: 0.00300\nSaving checkpoint\nEpoch 05 - step 2346 - cv acc: 0.738 - train acc: 0.784 (mean) - cv cost: 0.802 - lr: 0.00300\nSaving checkpoint\nEpoch 06 - step 2737 - cv acc: 0.763 - train acc: 0.797 (mean) - cv cost: 0.731 - lr: 0.00300\nSaving checkpoint\nEpoch 07 - step 3128 - cv acc: 0.774 - train acc: 0.812 (mean) - cv cost: 0.689 - lr: 0.00300\nSaving checkpoint\nEpoch 08 - step 3519 - cv acc: 0.789 - train acc: 0.819 (mean) - cv cost: 0.624 - lr: 0.00300\nSaving checkpoint\nEpoch 09 - step 3910 - cv acc: 0.819 - train acc: 0.827 (mean) - cv cost: 0.553 - lr: 0.00270\nSaving checkpoint\nEpoch 10 - step 4301 - cv acc: 0.818 - train acc: 0.841 (mean) - cv cost: 0.564 - lr: 0.00270\nSaving checkpoint\nEpoch 11 - step 4692 - cv acc: 0.833 - train acc: 0.848 (mean) - cv cost: 0.510 - lr: 0.00270\nSaving checkpoint\nEpoch 12 - step 5083 - cv acc: 0.838 - train acc: 0.854 (mean) - cv cost: 0.503 - lr: 0.00270\nSaving checkpoint\nEpoch 13 - step 5474 - cv acc: 0.824 - train acc: 0.858 (mean) - cv cost: 0.555 - lr: 0.00270\nSaving checkpoint\nEpoch 14 - step 5865 - cv acc: 0.820 - train acc: 0.861 (mean) - cv cost: 0.532 - lr: 0.00270\nSaving checkpoint\nEpoch 15 - step 6256 - cv acc: 0.850 - train acc: 0.866 (mean) - cv cost: 0.455 - lr: 0.00270\nSaving checkpoint\nEpoch 16 - step 6647 - cv acc: 0.848 - train acc: 0.870 (mean) - cv cost: 0.476 - lr: 0.00270\nSaving checkpoint\nEpoch 17 - step 7038 - cv acc: 0.823 - train acc: 0.871 (mean) - cv cost: 0.551 - lr: 0.00270\nSaving checkpoint\nEpoch 18 - step 7429 - cv acc: 0.849 - train acc: 0.875 (mean) - cv cost: 0.458 - lr: 0.00270\nSaving checkpoint\nEpoch 19 - step 7820 - cv acc: 0.851 - train acc: 0.877 (mean) - cv cost: 0.501 - lr: 0.00243\nEpoch 20 - cv acc: 0.8510 - train acc: 0.8768 (mean) - cv cost: 0.501\nEpoch 20 - cv acc: 0.8510 - train acc: 0.8768 (mean) - cv cost: 0.501\n"
]
],
[
[
"# Scoring and Evaluating trained model",
"_____no_output_____"
]
],
[
[
"## MODEL 7.20.0.11g \nprint(\"Model : \", model_name)\n\nprint(\"Convolutional network accuracy (test set):\",test_acc, \" Validation Set\", valid_acc_values[-1])\n",
"Model : model_7.13.4.7.7l\nConvolutional network accuracy (test set): 0.84121096 Validation Set 0.8509766\n"
]
],
[
[
"# Results\nThe CIFAR-10 dataset for image classification model using convolutional neural network gave an accuracy of 84% for 20 epoochs. This was the model in which the parameters used were:\n\n* Activation Function: Rectified linear unit (ReLU)\n* Cost function: Cross-Entropy\n* No.of Epochs: 20\n* Gradient estimation: ADAM\n* Network Architecture:Number of layers: 12\n* Network initialization: xavier initializer\n\n\nI ran it for 20 epochs and got almost 84% accuracy. \nAfter changing the Network initialization, the model accuracy improved as the network initialization which is the xavier initializer which makes sure that the weights are ‘just right’, keeping the signal in a reasonable range of values through many layers. \n\nI ran it for 20 epochs and got almost 84.12% accuracy. It surely improved than the previous initializer which was a zero initializer.\n\nBased on the observations, network plateau of the network using the xavier network initializer that hits during the learning has no change in the accuracy\nThe learning rate changes during the epoochs and in a way improves the accuracy.\n\n# References\n[1] https://github.com/fchollet/keras/blob/master/examples/cifar10_cnn.py\n\n[2] https://www.tensorflow.org/api_docs/python/tf/contrib/layers/xavier_initializer\n\n[3] https://towardsdatascience.com/cifar-10-image-classification-in-tensorflow-5b501f7dc77c\n\n[4] https://www.kaggle.com/skooch/cifar-10-in-tensorflow/notebook\n\n[5] https://andyljones.tumblr.com/post/110998971763/an-explanation-of-xavier-initialization",
"_____no_output_____"
]
]
] | [
"markdown",
"code",
"markdown",
"code",
"markdown",
"code",
"markdown",
"code",
"markdown",
"code",
"markdown",
"code",
"markdown",
"code",
"markdown",
"code",
"markdown",
"code",
"markdown",
"code",
"markdown",
"code",
"markdown",
"code",
"markdown",
"code",
"markdown",
"code",
"markdown",
"code",
"markdown",
"code",
"markdown",
"code",
"markdown"
] | [
[
"markdown"
],
[
"code"
],
[
"markdown"
],
[
"code"
],
[
"markdown"
],
[
"code"
],
[
"markdown"
],
[
"code"
],
[
"markdown"
],
[
"code"
],
[
"markdown"
],
[
"code"
],
[
"markdown"
],
[
"code",
"code",
"code"
],
[
"markdown"
],
[
"code"
],
[
"markdown"
],
[
"code"
],
[
"markdown"
],
[
"code"
],
[
"markdown"
],
[
"code"
],
[
"markdown"
],
[
"code"
],
[
"markdown"
],
[
"code"
],
[
"markdown"
],
[
"code"
],
[
"markdown"
],
[
"code"
],
[
"markdown"
],
[
"code"
],
[
"markdown"
],
[
"code"
],
[
"markdown"
]
] |
e7740ddbd9a8071c3dbe86220c16e095ad7362db | 280,274 | ipynb | Jupyter Notebook | sampling-and-aliasing/notebooks/Sampling-and-reconstruction.ipynb | kjartan-at-tec/mr2007-computerized-control | 16e35f5007f53870eaf344eea1165507505ab4aa | [
"MIT"
] | 2 | 2020-11-07T05:20:37.000Z | 2020-12-22T09:46:13.000Z | sampling-and-aliasing/notebooks/Sampling-and-reconstruction.ipynb | alfkjartan/control-computarizado | 5b9a3ae67602d131adf0b306f3ffce7a4914bf8e | [
"MIT"
] | 4 | 2020-06-12T20:44:41.000Z | 2020-06-12T20:49:00.000Z | sampling-and-aliasing/notebooks/Sampling-and-reconstruction.ipynb | alfkjartan/control-computarizado | 5b9a3ae67602d131adf0b306f3ffce7a4914bf8e | [
"MIT"
] | 1 | 2019-09-25T20:02:23.000Z | 2019-09-25T20:02:23.000Z | 291.648283 | 81,978 | 0.915197 | [
[
[
"empty"
]
]
] | [
"empty"
] | [
[
"empty"
]
] |
e7741a541420ac6c282b8831ecf5bc825e7dbce2 | 101,290 | ipynb | Jupyter Notebook | [02 - Modeling]/dos ver 5.2/router fetch/wat-r8-mal.ipynb | chamikasudusinghe/nocml | d414da54e042d6f7505b81135882d6f1bd02f166 | [
"MIT"
] | null | null | null | [02 - Modeling]/dos ver 5.2/router fetch/wat-r8-mal.ipynb | chamikasudusinghe/nocml | d414da54e042d6f7505b81135882d6f1bd02f166 | [
"MIT"
] | null | null | null | [02 - Modeling]/dos ver 5.2/router fetch/wat-r8-mal.ipynb | chamikasudusinghe/nocml | d414da54e042d6f7505b81135882d6f1bd02f166 | [
"MIT"
] | null | null | null | 35.791519 | 118 | 0.219805 | [
[
[
"import pandas as pd",
"_____no_output_____"
],
[
"df = pd.read_csv('wat-mal.csv')\ndf = df.loc[df['router'] == 8]\ndf = df.drop(columns=['router'])\ndf.to_csv('wat-r8-mal.csv',index=False)",
"_____no_output_____"
],
[
"df = pd.read_csv('wat-r8-mal.csv')\ndf",
"_____no_output_____"
],
[
"timearr = []\ninterval = 99\ncount = 0\nfor index, row in df.iterrows():\n if row[\"timestamp\"]<=interval:\n count+=1\n else:\n timearr.append([interval+1,count])\n count=1\n interval+=100\ntimearr.append([interval+1,count])",
"_____no_output_____"
],
[
"countarr = []\nincrearr = []\nmaxarr = []\nfor i in range(len(timearr)):\n for cnt in range(timearr[i][1],0,-1):\n countarr.append(cnt)\n maxarr.append(timearr[i][1])\n increment = timearr[i][1] - cnt + 1\n increarr.append(increment)\nprint(len(countarr))",
"12949\n"
],
[
"df = df.assign(packet_count_decr=countarr)\ndf = df.assign(packet_count_incr=increarr)\ndf = df.assign(max_packet_count=maxarr)\ndf[\"packet_count_index\"] = df[\"packet_count_decr\"]*df[\"packet_count_incr\"]\ndf[\"packet_max_index\"] = df[\"packet_count_index\"]*df[\"max_packet_count\"]\ndf[\"port_index\"] = df[\"outport\"]*df[\"inport\"]\ndf[\"cache_coherence_flit_index\"] = df[\"cache_coherence_type\"]*df[\"flit_id\"]\ndf[\"flit_index\"] = df[\"cache_coherence_flit_index\"]*df[\"flit_type\"]\ndf[\"traversal_index\"] = df[\"flit_index\"]*df[\"traversal_id\"]\ndf[\"cache_coherence_vnet_index\"] = df[\"cache_coherence_type\"]*df[\"vnet\"]\ndf[\"vnet_vc_index\"] = df[\"vnet\"]*df[\"vc\"]\ndf[\"vnet_vc_cc_index\"] = df[\"vnet\"]*df[\"cache_coherence_vnet_index\"]\n\ndf.head(50)",
"_____no_output_____"
],
[
"#df[\"packet_types\"] = df[\"packet_type\"]\n#df=pd.get_dummies(df, prefix=['outport', 'inport', 'packet_type'], columns=['outport', 'inport','packet_type'])",
"_____no_output_____"
],
[
"df.dtypes",
"_____no_output_____"
],
[
"#df['inport_3'] = 0\n#df['outport_3'] = 0",
"_____no_output_____"
],
[
"df",
"_____no_output_____"
],
[
"df['target'] = 0\ndf",
"_____no_output_____"
],
[
"df.to_csv('wat-r8-mal.csv',index=False)",
"_____no_output_____"
]
]
] | [
"code"
] | [
[
"code",
"code",
"code",
"code",
"code",
"code",
"code",
"code",
"code",
"code",
"code",
"code"
]
] |
e774236df0b2e60b3729e5ea5e6f221411adb2c5 | 7,682 | ipynb | Jupyter Notebook | src/notebooks/downsampling from cool file.ipynb | nedo0shki/HiCPlus-PC | b237ef1d30f3362b58a7180a6e66af03d7fe468b | [
"MIT"
] | null | null | null | src/notebooks/downsampling from cool file.ipynb | nedo0shki/HiCPlus-PC | b237ef1d30f3362b58a7180a6e66af03d7fe468b | [
"MIT"
] | null | null | null | src/notebooks/downsampling from cool file.ipynb | nedo0shki/HiCPlus-PC | b237ef1d30f3362b58a7180a6e66af03d7fe468b | [
"MIT"
] | 1 | 2019-08-01T16:27:21.000Z | 2019-08-01T16:27:21.000Z | 29.775194 | 380 | 0.504686 | [
[
[
"import os\nimport sys\n#dir_path = os.path.dirname(os.path.realpath(__file__))\ndir_path = \"/Users/neda/HiCPlus_pytorch/src\"\nmodel_path = dir_path + \"/models\"\nutils_path = dir_path + \"/utils\"\nsys.path.insert(0, model_path)\nsys.path.insert(0, utils_path)\nimport model2\nimport utils\n\nimport numpy as np\nimport matplotlib.pyplot as plt\nimport pickle\nimport gzip\nfrom torch.utils import data\nimport torch\nimport torch.optim as optim\nfrom torch.autograd import Variable\nfrom time import gmtime, strftime\nimport torch.nn as nn\nfrom scipy.stats.stats import pearsonr\nimport argparse\nimport cooler\n\n\nargs = {\"low_res_cool_path\": \"/Users/neda/prostate-samples/PCa13266.multi-res.cool\"\n , \"high_res_cool_path\": \"/Users/neda/prostate-samples/HighRes-PCa13266.multi-res.cool\"\n , \"output_path\": \"/Users/neda/prostate-samples/PCa13266-down/\"\n , \"model_path\": \"/Users/neda/HiCPlus_pytorch/src/learned-networks\"\n , \"resolution\": \"10000\"\n , \"model_name\": \"chr1-17-PCa13266(up-wo-min)\"\n , \"chrN_min\": \"1\"\n , \"chrN_max\": \"17\"\n , \"frames_data_path\": \"/Users/neda/prostate-samples/divided-data\"\n , \"sample_name\": \"PCa13266\"}\n\nhigh_res_cool = cooler.Cooler(args['high_res_cool_path'] + '::/resolutions/' + str(args['resolution']))\np = high_res_cool.pixels()\ntotal_num_reads = sum(p[:,].iloc[:,2])\nlow_res_cool = cooler.Cooler(args['low_res_cool_path'] + '::/resolutions/' + str(args['resolution']))\np2 = low_res_cool.pixels()\nlow_total_num_reads = sum(p2[:,].iloc[:,2])\n\n# Obs1: when we fetch a specific chromosome it means first columns belong to regions in that chromosome but \n# second column regions are through whole genome\n# Obs2: reads are not considered twice in files, for example when we fetch chr2 pixels, there are not interactions \n# between chr2 and chr1 any more. \n# Obs3: number of intra reads: 125015861, whole reads: 153752070 (in low resolution sample)\n\nvec_of_prob = []\nfor chrName in high_res_cool.chromnames:\n vec_of_prob.extend(high_res_cool.matrix(balance = False, as_pixels = True).fetch(chrName).iloc[:,2])\nnum_inter_reads = total_num_reads - sum(vec_of_prob)\nvec_of_prob.append(num_inter_reads)\nvec_of_prob = [p/total_num_reads for p in vec_of_prob]\ndown_sampled_counts = np.random.multinomial(low_total_num_reads, vec_of_prob)\n\nif not os.path.exists(args['output_path']):\n os.makedirs(args['output_path'])\n\nstart_ind = 0\nfor chrName in high_res_cool.chromnames:\n chr_pixel = high_res_cool.matrix(balance = False, as_pixels = True).fetch(chrName)\n pixel_size = chr_pixel.shape[0]\n new_pixel = np.column_stack((chr_pixel.iloc[:,0],\n chr_pixel.iloc[:,1],\n down_sampled_counts[start_ind:start_ind+pixel_size]))\n start_ind = start_ind + pixel_size\n np.savetxt(args['output_path'] + chrName + \".txt\", new_pixel)\n\n\n\"\"\"\n\nnew_bins = high_res_cool.bins()\ncooler.create_cooler(cool_uri = \"/Users/neda/prostate-samples/PCa13266.down-sample.cool\", bins = new_bins, pixels = new_pixel)\n\"\"\"",
"_____no_output_____"
],
[
"## make frames\n\n",
"_____no_output_____"
],
[
"range(11,17)",
"_____no_output_____"
],
[
"a = range(11,17)\nprint([i for i in a])",
"[11, 12, 13, 14, 15, 16]\n"
],
[
"int(\"10000\") + 2",
"_____no_output_____"
]
]
] | [
"code"
] | [
[
"code",
"code",
"code",
"code",
"code"
]
] |
e774243021ea87341944300fcc3ea79bbc811c96 | 22,101 | ipynb | Jupyter Notebook | 04_dice.ipynb | jonathonfletcher/BiteSizeBayes | 6ef5c268deccdff3b3fa5fa6da6fca7945f3c38d | [
"MIT"
] | 116 | 2020-01-20T15:04:49.000Z | 2022-03-28T07:42:33.000Z | 04_dice.ipynb | jonathonfletcher/BiteSizeBayes | 6ef5c268deccdff3b3fa5fa6da6fca7945f3c38d | [
"MIT"
] | 5 | 2020-02-02T14:12:50.000Z | 2020-10-26T12:01:21.000Z | 04_dice.ipynb | jonathonfletcher/BiteSizeBayes | 6ef5c268deccdff3b3fa5fa6da6fca7945f3c38d | [
"MIT"
] | 28 | 2020-01-25T07:45:47.000Z | 2022-02-16T13:29:43.000Z | 29.745626 | 280 | 0.571332 | [
[
[
"# The Dice Problem",
"_____no_output_____"
],
[
"This notebook is part of [Bite Size Bayes](https://allendowney.github.io/BiteSizeBayes/), an introduction to probability and Bayesian statistics using Python.\n\nCopyright 2020 Allen B. Downey\n\nLicense: [Attribution-NonCommercial-ShareAlike 4.0 International (CC BY-NC-SA 4.0)](https://creativecommons.org/licenses/by-nc-sa/4.0/)",
"_____no_output_____"
],
[
"The following cell downloads `utils.py`, which contains some utility function we'll need.",
"_____no_output_____"
]
],
[
[
"from os.path import basename, exists\n\ndef download(url):\n filename = basename(url)\n if not exists(filename):\n from urllib.request import urlretrieve\n local, _ = urlretrieve(url, filename)\n print('Downloaded ' + local)\n\ndownload('https://github.com/AllenDowney/BiteSizeBayes/raw/master/utils.py')",
"_____no_output_____"
]
],
[
[
"If everything we need is installed, the following cell should run with no error messages.",
"_____no_output_____"
]
],
[
[
"import pandas as pd\nimport numpy as np\n\nfrom utils import values",
"_____no_output_____"
]
],
[
[
"## Review\n\n[In the previous notebook](https://colab.research.google.com/github/AllenDowney/BiteSizeBayes/blob/master/03_cookie.ipynb) we started with Bayes's Theorem, written like this:\n\n$P(A|B) = P(A) ~ P(B|A) ~/~ P(B)$\n\nAnd applied it to the case where we use data, $D$, to update the probability of a hypothesis, $H$. In this context, we write Bayes's Theorem like this:\n\n$P(H|D) = P(H) ~ P(D|H) ~/~ P(D)$\n\nAnd give each term a name:\n\n* $P(H)$ is the \"prior probability\" of the hypothesis, which represents how confident you are that $H$ is true prior to seeing the data,\n\n* $P(D|H)$ is the \"likelihood\" of the data, which is the probability of seeing $D$ if the hypothesis is true,\n\n* $P(D)$ is the \"total probability of the data\", that is, the chance of seeing $D$ regardless of whether $H$ is true or not.\n\n* $P(H|D)$ is the \"posterior probability\" of the hypothesis, which indicates how confident you should be that $H$ is true after taking the data into account.\n\nWe used Bayes's Theorem to solve a cookie-related problem, and I presented the Bayes table, a way to solve Bayesian problems more generally. I didn't really explain how it works, though. That's the goal of this notebook.\n\nI'll start by extending the table method to a problem with more than two hypotheses.",
"_____no_output_____"
],
[
"## More hypotheses\n\nOne nice thing about the table method is that it works with more than two hypotheses. As an example, let's do another version of the cookie problem.\n\nSuppose you have five bowls:\n\n* Bowl 0 contains no vanilla cookies.\n\n* Bowl 1 contains 25% vanilla cookies.\n\n* Bowl 2 contains 50% vanilla cookies.\n\n* Bowl 3 contains 75% vanilla cookies.\n\n* Bowl 4 contains 100% vanilla cookies.\n\nNow suppose we choose a bowl at random and then choose a cookie, and we get a vanilla cookie. What is the posterior probability that we chose each bowl?\n\nHere's a table that represents the five hypotheses and their prior probabilities:",
"_____no_output_____"
]
],
[
[
"import pandas as pd\n\ntable = pd.DataFrame()\ntable['prior'] = 1/5, 1/5, 1/5, 1/5, 1/5\ntable",
"_____no_output_____"
]
],
[
[
"The likelihood of drawing a vanilla cookie from each bowl is the given proportion of vanilla cookies:",
"_____no_output_____"
]
],
[
[
"table['likelihood'] = 0, 0.25, 0.5, 0.75, 1\ntable",
"_____no_output_____"
]
],
[
[
"Once we have priors and likelihoods, the remaining steps are always the same. We compute the unnormalized posteriors:",
"_____no_output_____"
]
],
[
[
"table['unnorm'] = table['prior'] * table['likelihood']\ntable",
"_____no_output_____"
]
],
[
[
"And the total probability of the data.",
"_____no_output_____"
]
],
[
[
"prob_data = table['unnorm'].sum()\nprob_data",
"_____no_output_____"
]
],
[
[
"Then divide through to get the normalized posteriors.",
"_____no_output_____"
]
],
[
[
"table['posterior'] = table['unnorm'] / prob_data\ntable",
"_____no_output_____"
]
],
[
[
"Two things you might notice about these results:\n\n1. One of the hypotheses has a posterior probability of 0, which means it has been ruled out entirely. And that makes sense: Bowl 0 contains no vanilla cookies, so if we get a vanilla cookie, we know it's not from Bowl 0.\n\n2. The posterior probabilities form a straight line. We can see this more clearly by plotting the results.",
"_____no_output_____"
]
],
[
[
"import matplotlib.pyplot as plt",
"_____no_output_____"
],
[
"table['posterior'].plot(kind='bar')\nplt.xlabel('Bowl #')\nplt.ylabel('Posterior probability');",
"_____no_output_____"
]
],
[
[
"**Exercise:** Use the table method to solve the following problem and plot the results as a bar chart.\n\n>The blue M&M was introduced in 1995. Before then, the color mix in a bag of plain M&Ms was (30% Brown, 20% Yellow, 20% Red, 10% Green, 10% Orange, 10% Tan). \n>\n>Afterward it was (24% Blue , 20% Green, 16% Orange, 14% Yellow, 13% Red, 13% Brown).\n>\n>A friend of mine has two bags of M&Ms, and he tells me that one is from 1994 and one from 1996. He won't tell me which is which, but he gives me one M&M from each bag. One is yellow and one is green. What is the probability that the yellow M&M came from the 1994 bag?\n\nHint: If the yellow came from 1994, the green must have come from 1996. By Theorem 2 (conjunction), the likelihood of this combination is (0.2)(0.2).",
"_____no_output_____"
]
],
[
[
"# Solution goes here",
"_____no_output_____"
],
[
"# Solution goes here",
"_____no_output_____"
]
],
[
[
"## Why does this work?\n\nNow I will explain how the table method works, making two arguments:\n\n1. First, I'll show that it makes sense to normalize the posteriors so they add up to 1.\n\n2. Then I'll show that this step is consistent with Bayes's Theorem, because the total of the unnormalized posteriors is the total probability of the data, $P(D)$.\n\nHere's the first argument. Let's start with Bayes's Theorem:\n\n$P(H|D) = P(H) ~ P(D|H)~/~P(D)$\n\nNotice that the denominator, $P(D)$, does not depend on $H$, so it is the same for all hypotheses. If we factor it out, we get:\n\n$P(H|D) \\sim P(H) ~ P(D|H)$\n\nwhich says that the posterior probabilities *are proportional to* the unnormalized posteriors. In other words, if we leave out $P(D)$, we get the proportions right, but not the total.",
"_____no_output_____"
],
[
"Then how do we figure out the total? Well, in this example we know that the cookie came from exactly one of the bowls. So the hypotheses are:\n\n* Mutually exclusive, that is, only one of them can be true, and\n\n* Collectively exhaustive, that is, at least one of them must be true.\n\nExactly one of the hypotheses must be true, so the posterior probabilities have to add up to 1. Most of the time, the unnormalized posteriors don't add up to 1, but when we divide through by the total, we ensure that the *normalized* posteriors do.\n\nThat's the first argument. I hope it makes some sense, but if you don't find it entirely satisfying, keep going.",
"_____no_output_____"
],
[
"## Rolling the dice\n\nBefore I can make the second argument, we need one more law of probability, which I will explain with a new example:\n\n> Suppose you have a 4-sided die and a 6-sided die. You choose one at random and roll it. What is the probability of getting a 1?\n\nTo answer that, I'll define two hypotheses and a datum:\n\n* $H_4$: You chose the 4-sided die.\n\n* $H_6$: You chose the 6-sided die.\n\n* $D$: You rolled a 1.",
"_____no_output_____"
],
[
"On a 4-sided die, the probability of rolling 1 is $1/4$; on a 6-sided die it is $1/6$. So we can write the conditional probabilities:\n\n$P(D|H_4) = 1/4$\n\n$P(D|H_6) = 1/6$\n\nAnd if the probability of choosing either die is equal, we know the prior probabilities:\n\n$P(H_4) = 1/2$\n\n$P(H_6) = 1/2$",
"_____no_output_____"
],
[
"But what is the total probability of the data, $P(D)$?\n\nAt this point your intuition might tell you that it is the weighted sum of the conditional probabilities:\n\n$P(D) = P(H_4)P(D|H_4) + P(H_6)P(D|H_6)$\n\nWhich is\n\n$P(D) = (1/2)(1/4) + (1/2)(1/6)$\n\nWhich is",
"_____no_output_____"
]
],
[
[
"(1/2)*(1/4) + (1/2)*(1/6)",
"_____no_output_____"
]
],
[
[
"And that's correct. But if your intuition did not tell you that, or if you would like to see something closer to a proof, keep going.",
"_____no_output_____"
],
[
"## Disjunction\n\nIn this example, we can describe the outcome in terms of logical operators like this:\n\n> The outcome is 1 if you choose the 4-sided die **and** roll 1 **or** you roll the 6-sided die **and** roll 1.\n\nUsing math notation, $D$ is true if:\n\n$(H_4 ~and~ D) ~or~ (H_6 ~and~ D)$\n\nWe've already seen the $and$ operator, also known as \"conjunction\", but we have not yet seen the $or$ operator, which is also known as \"disjunction\"?\n\nFor that, we a new rule, which I'll call **Theorem 4**:\n\n$P(A ~or~ B) = P(A) + P(B) - P(A ~and~ B)$",
"_____no_output_____"
],
[
"To see why that's true, let's take a look at the Venn diagram:\n\n<img width=\"200\" src=\"https://github.com/AllenDowney/BiteSizeBayes/raw/master/theorem4_venn_diagram.png\">\n\nWhat we want is the total of the blue, red, and purple regions. If we add $P(A)$ and $P(B)$, we get the blue and red regions right, but we double-count the purple region. So we have to subtract off one purple region, which is $P(A ~and~ B)$.",
"_____no_output_____"
],
[
"**Exercise:** Let's do a quick example using disjunction. \n\nA standard deck of playing cards contains 52 cards; \n\n* 26 of them are red, \n\n* 12 of them are face cards, and \n\n* 6 of them are red face cards.\n\nThe following diagram shows what I mean: the red rectangle contains the red cards; the blue rectangle contains the face cards, and the overlap includes the red face cards.\n\n<img width=\"500\"\n src=\"https://github.com/AllenDowney/BiteSizeBayes/raw/master/card_venn_diagram.png\">\n\n\nIf we choose a card at random, here are the probabilities of choosing a red card, a face card, and a red face card:",
"_____no_output_____"
]
],
[
[
"p_red = 26/52\np_face = 12/52\np_red_face = 6/52\n\np_red, p_face, p_red_face",
"_____no_output_____"
]
],
[
[
"Use Theorem 4 to compute the probability of choosing a card that is either red, or a face card, or both:",
"_____no_output_____"
]
],
[
[
"# Solution goes here",
"_____no_output_____"
]
],
[
[
"## Total probability\n\nIn the dice example, $H_4$ and $H_6$ are mutually exclusive, which means only one of them can be true, so the purple region is 0. Therefore:\n\n$P(D) = P(H_4 ~and~ D) + P(H_6 ~and~ D) - 0$\n\nNow we can use **Theorem 2** to replace the conjunctions with conditonal probabilities:\n\n$P(D) = P(H_4)~P(D|H_4) + P(H_6)~P(D|H_6)$\n\nBy a similar argument, we can show that this is true for any number of hypotheses. For example, if we add an 8-sided die to the mix, we can write:\n\n$P(D) = P(H_4)~P(D|H_4) + P(H_6)~P(D|H_6) + P(H_8)~P(D|H_8)$",
"_____no_output_____"
],
[
"And more generally, with any number of hypotheses $H_i$:\n\n$P(D) = \\sum_i P(H_i)~P(D|H_i)$\n\nWhich shows that the total probability of the data is the sum of the unnormalized posteriors.\n\nAnd that's why the table method works.",
"_____no_output_____"
],
[
"Now let's get back to the original question:\n\n> Suppose you have a 4-sided die and a 6-sided die. You choose one at random and roll it. What is the probability of getting a 1?\n\nWe can use a Bayes table to compute the answer. Here are the priors:",
"_____no_output_____"
]
],
[
[
"table = pd.DataFrame(index=['H4', 'H6'])\ntable['prior'] = 1/2, 1/2\ntable",
"_____no_output_____"
]
],
[
[
"And the likelihoods:",
"_____no_output_____"
]
],
[
[
"table['likelihood'] = 1/4, 1/6\ntable",
"_____no_output_____"
]
],
[
[
"Now we compute the unnormalized posteriors in the usual way:",
"_____no_output_____"
]
],
[
[
"table['unnorm'] = table['prior'] * table['likelihood']\ntable",
"_____no_output_____"
]
],
[
[
"And the total probability of the data:",
"_____no_output_____"
]
],
[
[
"prob_data = table['unnorm'].sum()\nprob_data",
"_____no_output_____"
]
],
[
[
"That's what we got when we solved the problem by hand, so that's good.",
"_____no_output_____"
],
[
"**Exercise:** Suppose you have a 4-sided, 6-sided, and 8-sided die. You choose one at random and roll it, what is the probability of getting a 1?\n\nDo you expect it to be higher or lower than in the previous example?",
"_____no_output_____"
]
],
[
[
"# Solution goes here",
"_____no_output_____"
],
[
"# Solution goes here",
"_____no_output_____"
]
],
[
[
"## Prediction and inference\n\nIn the previous section, we use a Bayes table to solve this problem:\n\n> Suppose you have a 4-sided die and a 6-sided die. You choose one at random and roll it. What is the probability of getting a 1?\n\nI'll call this a \"prediction problem\" because we are given a scenario and asked for the probability of a predicted outcome.\n\nNow let's solve a closely-related problem:\n\n> Suppose you have a 4-sided die and a 6-sided die. You choose one at random, roll it, and get a 1. What is the probability that the die you rolled is 4-sided?\n\nI'll call this an \"inference problem\" because we are given the outcome and asked to figure out, or \"infer\", which die was rolled.\n\nHere's a solution:",
"_____no_output_____"
]
],
[
[
"table = pd.DataFrame(index=['H4', 'H6'])\ntable['prior'] = 1/2, 1/2\ntable['likelihood'] = 1/4, 1/6\ntable['unnorm'] = table['prior'] * table['likelihood']\nprob_data = table['unnorm'].sum()\ntable['posterior'] = table['unnorm'] / prob_data\ntable",
"_____no_output_____"
]
],
[
[
"Given that the outcome is a 1, there is a 60% chance the die you rolled was 4-sided.\n\nAs this example shows, prediction and inference closely-related problems, and we can use the same methods for both.",
"_____no_output_____"
],
[
"**Exercise:** Let's add some more dice:\n\n1. Suppose you have a 4-sided, 6-sided, 8-sided, and 12-sided die. You choose one at random and roll it. What is the probability of getting a 1?\n\n2. Now suppose the outcome is a 1. What is the probability that the die you rolled is 4-sided? And what are the posterior probabilities for the other dice?",
"_____no_output_____"
]
],
[
[
"# Solution goes here",
"_____no_output_____"
],
[
"# Solution goes here",
"_____no_output_____"
]
],
[
[
"## Summary\n\nIn this notebook, I introduced a new law of probability, so now we have four:\n\n**Theorem 1** gives us a way to compute a conditional probability using a conjunction:\n\n$P(A|B) = \\frac{P(A~\\mathrm{and}~B)}{P(B)}$ \n\n**Theorem 2** gives us a way to compute a conjunction using a conditional probability:\n\n$P(A~\\mathrm{and}~B) = P(B) P(A|B)$\n\n**Theorem 3** gives us a way to get from $P(A|B)$ to $P(B|A)$, or the other way around:\n\n$P(A|B) = \\frac{P(A) P(B|A)}{P(B)}$\n\n**Theorem 4** gives us a way to compute a disjunction using a conjunction.\n\n$P(A ~or~ B) = P(A) + P(B) - P(A ~and~ B)$\n\nThen we used Theorems 2 and 4 to show that the sum of the unnormalized posteriors is the total probability of the data, which we wrote like this:\n\n$P(D) = \\sum_i P(H_i)~P(D|H_i)$\n\nThis conclusion is useful for two reasons:\n\n1. It provides a way to compute the probability of future data using prior probabilities and likelihoods, and\n\n2. It explains why the Bayes table method works.\n\n[In the next notebook](https://colab.research.google.com/github/AllenDowney/BiteSizeBayes/blob/master/05_test.ipynb) we will explore a famously useful application of Bayes's Theorem, medical testing.",
"_____no_output_____"
]
]
] | [
"markdown",
"code",
"markdown",
"code",
"markdown",
"code",
"markdown",
"code",
"markdown",
"code",
"markdown",
"code",
"markdown",
"code",
"markdown",
"code",
"markdown",
"code",
"markdown",
"code",
"markdown",
"code",
"markdown",
"code",
"markdown",
"code",
"markdown",
"code",
"markdown",
"code",
"markdown",
"code",
"markdown",
"code",
"markdown",
"code",
"markdown",
"code",
"markdown"
] | [
[
"markdown",
"markdown",
"markdown"
],
[
"code"
],
[
"markdown"
],
[
"code"
],
[
"markdown",
"markdown"
],
[
"code"
],
[
"markdown"
],
[
"code"
],
[
"markdown"
],
[
"code"
],
[
"markdown"
],
[
"code"
],
[
"markdown"
],
[
"code"
],
[
"markdown"
],
[
"code",
"code"
],
[
"markdown"
],
[
"code",
"code"
],
[
"markdown",
"markdown",
"markdown",
"markdown",
"markdown"
],
[
"code"
],
[
"markdown",
"markdown",
"markdown",
"markdown"
],
[
"code"
],
[
"markdown"
],
[
"code"
],
[
"markdown",
"markdown",
"markdown"
],
[
"code"
],
[
"markdown"
],
[
"code"
],
[
"markdown"
],
[
"code"
],
[
"markdown"
],
[
"code"
],
[
"markdown",
"markdown"
],
[
"code",
"code"
],
[
"markdown"
],
[
"code"
],
[
"markdown",
"markdown"
],
[
"code",
"code"
],
[
"markdown"
]
] |
e774247b99e8c96efb4d940628d2d15995afc7c1 | 12,639 | ipynb | Jupyter Notebook | tensorflow_privacy/privacy/membership_inference_attack/codelab.ipynb | LuluBeatson/privacy | 876b43b7a8e114f2194a9cd1328e0a61304d1cc5 | [
"Apache-2.0"
] | 1 | 2021-04-09T23:35:47.000Z | 2021-04-09T23:35:47.000Z | tensorflow_privacy/privacy/membership_inference_attack/codelab.ipynb | logangraham/privacy | d72e3400b76663489c8d41e6546929fd984f4a3a | [
"Apache-2.0"
] | null | null | null | tensorflow_privacy/privacy/membership_inference_attack/codelab.ipynb | logangraham/privacy | d72e3400b76663489c8d41e6546929fd984f4a3a | [
"Apache-2.0"
] | null | null | null | 35.108333 | 310 | 0.542606 | [
[
[
"##### Copyright 2020 The TensorFlow Authors.\n",
"_____no_output_____"
]
],
[
[
"#@title Licensed under the Apache License, Version 2.0 (the \"License\");\n# you may not use this file except in compliance with the License.\n# You may obtain a copy of the License at\n#\n# https://www.apache.org/licenses/LICENSE-2.0\n#\n# Unless required by applicable law or agreed to in writing, software\n# distributed under the License is distributed on an \"AS IS\" BASIS,\n# WITHOUT WARRANTIES OR CONDITIONS OF ANY KIND, either express or implied.\n# See the License for the specific language governing permissions and\n# limitations under the License.",
"_____no_output_____"
]
],
[
[
"# Assess privacy risks with TensorFlow Privacy Membership Inference Attacks",
"_____no_output_____"
],
[
"<table class=\"tfo-notebook-buttons\" align=\"left\">\n <td>\n <a target=\"_blank\" href=\"https://colab.research.google.com/github/tensorflow/privacy/blob/master/tensorflow_privacy/privacy/membership_inference_attack/codelab.ipynb\"><img src=\"https://www.tensorflow.org/images/colab_logo_32px.png\" />Run in Google Colab</a>\n </td>\n <td>\n <a target=\"_blank\" href=\"https://github.com/tensorflow/privacy/blob/master/tensorflow_privacy/privacy/membership_inference_attack/codelab.ipynb\"><img src=\"https://www.tensorflow.org/images/GitHub-Mark-32px.png\" />View source on GitHub</a>\n </td>\n</table>",
"_____no_output_____"
],
[
"##Overview\nIn this codelab we'll train a simple image classification model on the CIFAR10 dataset, and then use the \"membership inference attack\" against this model to assess if the attacker is able to \"guess\" whether a particular sample was present in the training set.",
"_____no_output_____"
],
[
"## Setup\nFirst, set this notebook's runtime to use a GPU, under Runtime > Change runtime type > Hardware accelerator. Then, begin importing the necessary libraries.",
"_____no_output_____"
]
],
[
[
"#@title Import statements.\nimport numpy as np\nfrom typing import Tuple, Text\nfrom scipy import special\n\nimport tensorflow as tf\nimport tensorflow_datasets as tfds\n\n# Set verbosity.\ntf.compat.v1.logging.set_verbosity(tf.compat.v1.logging.ERROR)\nfrom warnings import simplefilter\nfrom sklearn.exceptions import ConvergenceWarning\nsimplefilter(action=\"ignore\", category=ConvergenceWarning)\nsimplefilter(action=\"ignore\", category=FutureWarning)",
"_____no_output_____"
]
],
[
[
"Install TensorFlow Privacy.",
"_____no_output_____"
]
],
[
[
"!pip3 install git+https://github.com/tensorflow/privacy\n\nfrom tensorflow_privacy.privacy.membership_inference_attack import membership_inference_attack as mia",
"_____no_output_____"
]
],
[
[
"## Train a simple model on CIFAR10 with Keras.",
"_____no_output_____"
]
],
[
[
"dataset = 'cifar10'\nnum_classes = 10\nnum_conv = 3\nactivation = 'relu'\noptimizer = 'adam'\nlr = 0.02\nmomentum = 0.9\nbatch_size = 250\nepochs = 100 # Privacy risks are especially visible with lots of epochs.\n\n\ndef small_cnn(input_shape: Tuple[int],\n num_classes: int,\n num_conv: int,\n activation: Text = 'relu') -> tf.keras.models.Sequential:\n \"\"\"Setup a small CNN for image classification.\n\n Args:\n input_shape: Integer tuple for the shape of the images.\n num_classes: Number of prediction classes.\n num_conv: Number of convolutional layers.\n activation: The activation function to use for conv and dense layers.\n\n Returns:\n The Keras model.\n \"\"\"\n model = tf.keras.models.Sequential()\n model.add(tf.keras.layers.Input(shape=input_shape))\n\n # Conv layers\n for _ in range(num_conv):\n model.add(tf.keras.layers.Conv2D(32, (3, 3), activation=activation))\n model.add(tf.keras.layers.MaxPooling2D())\n\n model.add(tf.keras.layers.Flatten())\n model.add(tf.keras.layers.Dense(64, activation=activation))\n model.add(tf.keras.layers.Dense(num_classes))\n return model\n\n\nprint('Loading the dataset.')\ntrain_ds = tfds.as_numpy(\n tfds.load(dataset, split=tfds.Split.TRAIN, batch_size=-1))\ntest_ds = tfds.as_numpy(\n tfds.load(dataset, split=tfds.Split.TEST, batch_size=-1))\nx_train = train_ds['image'].astype('float32') / 255.\ny_train_indices = train_ds['label'][:, np.newaxis]\nx_test = test_ds['image'].astype('float32') / 255.\ny_test_indices = test_ds['label'][:, np.newaxis]\n\n# Convert class vectors to binary class matrices.\ny_train = tf.keras.utils.to_categorical(y_train_indices, num_classes)\ny_test = tf.keras.utils.to_categorical(y_test_indices, num_classes)\n\ninput_shape = x_train.shape[1:]\n\nmodel = small_cnn(\n input_shape, num_classes, num_conv=num_conv, activation=activation)\n\nprint('Optimizer ', optimizer)\nprint('learning rate %f', lr)\n\noptimizer = tf.keras.optimizers.SGD(lr=lr, momentum=momentum)\n\nloss = tf.keras.losses.CategoricalCrossentropy(from_logits=True)\nmodel.compile(loss=loss, optimizer=optimizer, metrics=['accuracy'])\nmodel.summary()\nmodel.fit(\n x_train,\n y_train,\n batch_size=batch_size,\n epochs=epochs,\n validation_data=(x_test, y_test),\n shuffle=True)\nprint('Finished training.')",
"_____no_output_____"
],
[
"#@title Calculate logits, probabilities and loss values for training and test sets.\n#@markdown We will use these values later in the membership inference attack to\n#@markdown separate training and test samples.\nprint('Predict on train...')\nlogits_train = model.predict(x_train, batch_size=batch_size)\nprint('Predict on test...')\nlogits_test = model.predict(x_test, batch_size=batch_size)\n\nprint('Apply softmax to get probabilities from logits...')\nprob_train = special.softmax(logits_train)\nprob_test = special.softmax(logits_test)\n\nprint('Compute losses...')\ncce = tf.keras.backend.categorical_crossentropy\nconstant = tf.keras.backend.constant\n\nloss_train = cce(constant(y_train), constant(prob_train), from_logits=False).numpy()\nloss_test = cce(constant(y_test), constant(prob_test), from_logits=False).numpy()",
"_____no_output_____"
]
],
[
[
"## Run membership inference attacks.",
"_____no_output_____"
]
],
[
[
"#@markdown We will now execute membership inference attack against the\n#@markdown previously trained CIFAR10 model. This will generate a number of\n#@markdown scores (most notably, attacker advantage and AUC for the membership\n#@markdown inference classifier). An AUC of close to 0.5 means that the attack\n#@markdown isn't able to identify training samples, which means that the model\n#@markdown doesn't have privacy issues according to this test. Higher values,\n#@markdown on the contrary, indicate potential privacy issues.\n\nlabels_train = np.argmax(y_train, axis=1)\nlabels_test = np.argmax(y_test, axis=1)\n\nresults_without_classifiers = mia.run_all_attacks(\n loss_train,\n loss_test,\n logits_train,\n logits_test,\n labels_train,\n labels_test,\n attack_classifiers=[],\n)\nprint(results_without_classifiers)\n\n# Note: This will take a while, since it also trains ML models to\n# separate train/test examples. If it's taking too looking, use\n# the `run_all_attacks` function instead.\nattack_result_summary = mia.run_all_attacks_and_create_summary(\n loss_train,\n loss_test,\n logits_train,\n logits_test,\n labels_train,\n labels_test,\n)[0]\n\nprint(attack_result_summary)",
"_____no_output_____"
]
],
[
[
"This is the end of the codelab! Feel free to change the parameters to see how the privacy risks change.",
"_____no_output_____"
]
]
] | [
"markdown",
"code",
"markdown",
"code",
"markdown",
"code",
"markdown",
"code",
"markdown",
"code",
"markdown"
] | [
[
"markdown"
],
[
"code"
],
[
"markdown",
"markdown",
"markdown",
"markdown"
],
[
"code"
],
[
"markdown"
],
[
"code"
],
[
"markdown"
],
[
"code",
"code"
],
[
"markdown"
],
[
"code"
],
[
"markdown"
]
] |
e7742b63b0825ac6728b6270a6c3137739ef9870 | 27,303 | ipynb | Jupyter Notebook | ipas/lookup_tables/ice_agg/create_lookuptables_newdb.ipynb | vprzybylo/IPAS | 9c9268097b9d7d02be1b14671b8fbfc1818e02c0 | [
"MIT"
] | null | null | null | ipas/lookup_tables/ice_agg/create_lookuptables_newdb.ipynb | vprzybylo/IPAS | 9c9268097b9d7d02be1b14671b8fbfc1818e02c0 | [
"MIT"
] | 7 | 2021-05-09T02:22:43.000Z | 2022-03-12T00:53:05.000Z | ipas/lookup_tables/ice_agg/create_lookuptables_newdb.ipynb | vprzybylo/IPAS | 9c9268097b9d7d02be1b14671b8fbfc1818e02c0 | [
"MIT"
] | null | null | null | 54.935614 | 229 | 0.469545 | [
[
[
"#Reloads modules to update any changes (after saving)\n#If a new method or object is created, autoreload doesn't work and the \n#kernel needs to be restarted\n%load_ext autoreload\n%autoreload 2\n%load_ext memory_profiler",
"_____no_output_____"
],
[
"import numpy as np\nimport pickle\nimport glob\nimport random\nimport pandas as pd\nimport matplotlib.pyplot as plt\nfrom dask import dataframe as dd\n\nimport sys\nsys.path.append('../../collection_from_db')\nsys.path.append('../../scripts')\nimport ipas\nimport batch_statistics",
"_____no_output_____"
],
[
"# Load data",
"_____no_output_____"
],
[
"orientation='flat'",
"_____no_output_____"
],
[
"f = open('../../instance_files/instance_db_iceagg_'+orientation, 'rb')\nresults = pickle.load(f)\nagg_as, agg_bs, agg_cs, phi2Ds, cplxs, dds= \\\n results['agg_as'], results['agg_bs'], results['agg_cs'], results['phi2Ds'], results['cplxs'], results['dds']\nf.close()\n\nf = open('../../instance_files/pulled_clusters_iceagg_'+orientation, 'rb')\npulled_clus = pickle.load(f)\nf.close()",
"_____no_output_____"
],
[
"agg_phi_bins = np.shape(agg_as)[0]\nagg_r_bins = np.shape(agg_as)[1]\nnclusters = np.shape(agg_as)[2]",
"_____no_output_____"
],
[
"%%time\n#read db \ndf = dd.read_parquet(\"/network/rit/lab/sulialab/share/IPAS_3radii/instance_files/parquet_files/*_\"+orientation+\"_*\", engine=\"pyarrow\").compute()",
"CPU times: user 4.05 s, sys: 3.67 s, total: 7.71 s\nWall time: 9.98 s\n"
],
[
"def shape(a,b,c):\n if (b-c) <= (a-b):\n return 'prolate'\n else:\n return 'oblate'",
"_____no_output_____"
],
[
"%%time \ndf['agg_r'] = np.power((np.power(df['a'], 2) * df['c']), (1./3.))\ndf = df[df['agg_r'] < 5000]\nvfunc = np.vectorize(shape)\ndf['shape'] = vfunc(df['a'], df['b'], df['c'])\ndf['agg_phi'] = df['c']/df['a']",
"CPU times: user 2.77 s, sys: 809 ms, total: 3.58 s\nWall time: 5.86 s\n"
],
[
"res, phi_bins = pd.qcut(df.agg_phi, 20, retbins=True)\n#print(phi_bins)\nphi_bin_labs = []\nall_r_bins= np.empty((len(phi_bins),len(phi_bins)))\nfor i in range(agg_phi_bins):\n phi_bin_labs.append('[%.3f-%.3f]' %(phi_bins[i],phi_bins[i+1]))\n #return a df that only queries within an aspect ratio bin\n df_phi = df[(df.agg_phi > phi_bins[i]) & (df.agg_phi < phi_bins[i+1])]\n #now break that aspect ratio bin into 20 equal r bins\n res, r_bins = pd.qcut(df_phi.agg_r, 20, retbins=True)\n all_r_bins[i,:] = r_bins\n",
"_____no_output_____"
],
[
"#find characteristic of gamma distribution for axis lengths\n#find mode from histogram bins for density change (multiple modes using statistics.mode())\n#all calculations are in the batch_statistics module in the scripts folder\n#takes a few mins to run\n\nagg_cs_ch = np.empty((agg_phi_bins, agg_r_bins), dtype=np.float64) \nagg_as_ch = np.empty((agg_phi_bins, agg_r_bins), dtype=np.float64) \nagg_as_mean = np.empty((agg_phi_bins, agg_r_bins), dtype=np.float64)\ndds_mode = np.empty((agg_phi_bins, agg_r_bins), dtype=np.float64)\n\nfor i in range(agg_phi_bins):\n for r in range(agg_r_bins):\n #print(i,r)\n for c, data in enumerate([agg_cs, agg_as, dds]):\n batch = batch_statistics.Batch(data[i,r,:])\n\n if c == 0:\n batch.fit_distribution()\n agg_cs_ch[i,r] = batch.gamma_ch\n \n if c == 1:\n batch.fit_distribution()\n agg_as_ch[i,r] = batch.gamma_ch\n agg_as_mean[i,r] = batch.mean\n \n if c == 2:\n batch.mode_of_hist()\n dds_mode[i,r] = batch.mode\n \n",
"_____no_output_____"
],
[
"#write to file for output as array:\nwith open(\"../../lookup_tables/ice_agg/newformat_minorax_ellipsoid_flat_ch_binedges.dat\",\"w\") as file1:\n with open(\"../../lookup_tables/ice_agg/newformat_majorax_ellipsoid_flat_ch_binedges.dat\",\"w\") as file2:\n with open(\"../../lookup_tables/ice_agg/newformat_majorax_ellipsoid_flat_mean_binedges.dat\",\"w\") as file3:\n with open(\"../../lookup_tables/ice_agg/newformat_dd_flat_mode_binedges.dat\",\"w\") as file4:\n\n file1.write('Ice-Agg collection for the flat orientation. \\n'\\\n 'Characteristic values taken from the peak of a fit \\n'\\\n 'gamma distribution from 300 aggregates. \\n'\\\n 'Minor axis taken as the smallest axis from the fit-ellipsoid \\n'\\\n 'Ranges are taken from the bin edges (maximum range) \\n'\\\n 'Order: agg phi min, agg phi max, agg r min, agg r max, \\n '\\\n 'monomer phi min, monomer phi max, monomer r min, monomer r max, value \\n')\n\n file2.write('Ice-Agg collection for the flat orientation. \\n'\n 'Characteristic values taken from the peak of a fit \\n'\\\n 'gamma distribution from 300 aggregates. \\n'\\\n 'Ranges are taken from the bin edges (maximum range) \\n'\\\n 'Order: agg phi min, agg phi max, agg r min, agg r max, \\n '\\\n 'monomer phi min, monomer phi max, monomer r min, monomer r max, value \\n')\n\n file3.write('Ice-Agg collection for the flat orientation. \\n'\n 'Mean value taken from the average across 300 aggregates. \\n'\\\n 'Major axis taken as the largest axis from the fit-ellipsoid \\n'\n 'Ranges are taken from the bin edges (maximum range) \\n'\\\n 'Order: agg phi min, agg phi max, agg r min, agg r max, \\n '\\\n 'monomer phi min, monomer phi max, monomer r min, monomer r max, value \\n')\n \n file4.write('Ice-Agg collection for the flat orientation. \\n'\n 'Mode from 300 aggregates. \\n' \\\n 'Characteristic value taken from the peak of a fit \\n'\\\n 'gamma distribution from 300 aggregates. \\n'\n 'Ranges are taken from the bin edges (maximum range) \\n'\\\n 'Order: agg phi min, agg phi max, agg r min, agg r max, \\n '\\\n 'monomer phi min, monomer phi max, monomer r min, monomer r max, value \\n')\n\n\n for i in range(agg_phi_bins):\n for r in range(agg_r_bins):\n listmonophi = [n.monophi for n in pulled_clus[i,r,:]]\n maxmonophi = max(listmonophi)\n minmonophi = min(listmonophi)\n listmonor = [n.monor for n in pulled_clus[i,r,:]]\n maxmonor = max(listmonor)\n minmonor = min(listmonor)\n\n #print(i,r)\n file1.write('%.3f %.3f %.3f %.3f %.3f %.3f %.3f %.3f %.3f \\n' %(phi_bins[i], phi_bins[i+1], all_r_bins[i,r], all_r_bins[i,r+1], minmonophi, maxmonophi, minmonor, maxmonor, agg_cs_ch[i,r]))\n file2.write('%.3f %.3f %.3f %.3f %.3f %.3f %.3f %.3f %.3f \\n' %(phi_bins[i], phi_bins[i+1], all_r_bins[i,r], all_r_bins[i,r+1], minmonophi, maxmonophi, minmonor, maxmonor, agg_as_ch[i,r]))\n file3.write('%.3f %.3f %.3f %.3f %.3f %.3f %.3f %.3f %.3f \\n' %(phi_bins[i], phi_bins[i+1], all_r_bins[i,r], all_r_bins[i,r+1], minmonophi, maxmonophi, minmonor, maxmonor, agg_as_mean[i,r]))\n file4.write('%.3f %.3f %.3f %.3f %.3f %.3f %.3f %.3f %.3f \\n' %(phi_bins[i], phi_bins[i+1], all_r_bins[i,r], all_r_bins[i,r+1], minmonophi, maxmonophi, minmonor, maxmonor, dds_mode[i,r]))\n \nfile1.close()\nfile2.close() \nfile3.close()\nfile4.close()",
"_____no_output_____"
],
[
"#write to file for output as array:\nwith open(\"../../lookup_tables/ice_agg/newformat_minorax_ellipsoid_flat_ch.dat\",\"w\") as file1:\n with open(\"../../lookup_tables/ice_agg/newformat_majorax_ellipsoid_flat_ch.dat\",\"w\") as file2:\n with open(\"../../lookup_tables/ice_agg/newformat_majorax_ellipsoid_flat_mean.dat\",\"w\") as file3:\n with open(\"../../lookup_tables/ice_agg/newformat_dd_flat_mode.dat\",\"w\") as file4:\n\n file1.write('Ice-Agg collection for the flat orientation. \\n'\\\n 'Characteristic values taken from the peak of a fit \\n'\\\n 'gamma distribution from 300 aggregates. \\n'\\\n 'Minor axis taken as the smallest axis from the fit-ellipsoid \\n'\\\n 'Ranges are taken from the aggregates being pulled from the db, \\n' \\\n 'not the bin edges (maximum range) \\n'\\\n 'Order: agg phi min, agg phi max, agg r min, agg r max, \\n '\\\n 'monomer phi min, monomer phi max, monomer r min, monomer r max, value \\n')\n\n file2.write('Ice-Agg collection for the flat orientation. \\n'\n 'Characteristic values taken from the peak of a fit \\n'\\\n 'gamma distribution from 300 aggregates. \\n'\\\n 'Ranges are taken from the aggregates being pulled from the db, \\n' \\\n 'not the bin edges (maximum range) \\n'\\\n 'Order: agg phi min, agg phi max, agg r min, agg r max, \\n '\\\n 'monomer phi min, monomer phi max, monomer r min, monomer r max, value \\n')\n\n file3.write('Ice-Agg collection for the flat orientation. \\n'\n 'Mean value taken from the average across 300 aggregates. \\n'\\\n 'Major axis taken as the largest axis from the fit-ellipsoid \\n'\n 'Ranges are taken from the aggregates being pulled from the db, \\n' \\\n 'not the bin edges (maximum range) \\n'\\\n 'Order: agg phi min, agg phi max, agg r min, agg r max, \\n '\\\n 'monomer phi min, monomer phi max, monomer r min, monomer r max, value \\n')\n \n file4.write('Ice-Agg collection for the flat orientation. \\n'\n 'Volume ratio of agg subtracted from volume ratio of new agg (Vagg/Vellipse)\\n'\\\n 'Mode from 300 aggregates. \\n'\\\n 'Ranges are taken from the aggregates being pulled from the db, \\n' \\\n 'not the bin edges (maximum range) \\n'\\\n 'Order: agg phi min, agg phi max, agg r min, agg r max, \\n '\\\n 'monomer phi min, monomer phi max, monomer r min, monomer r max, value \\n')\n\n\n for i in range(agg_phi_bins):\n for r in range(agg_r_bins):\n listmonophi = [n.monophi for n in pulled_clus[i,r,:]]\n maxmonophi = max(listmonophi)\n minmonophi = min(listmonophi)\n listmonor = [n.monor for n in pulled_clus[i,r,:]]\n maxmonor = max(listmonor)\n minmonor = min(listmonor)\n listaggphi = [n.c/n.a for n in pulled_clus[i,r,:]]\n maxaggphi = max(listaggphi)\n minaggphi = min(listaggphi)\n listaggr = [n.r for n in pulled_clus[i,r,:]]\n maxaggr = max(listaggr)\n minaggr = min(listaggr)\n #print(i,r)\n file1.write('%.3f %.3f %.3f %.3f %.3f %.3f %.3f %.3f %.3f \\n' %(minaggphi, maxaggphi, minaggr, maxaggr, minmonophi, maxmonophi, minmonor, maxmonor, agg_cs_ch[i,r]))\n file2.write('%.3f %.3f %.3f %.3f %.3f %.3f %.3f %.3f %.3f \\n' %(minaggphi, maxaggphi, minaggr, maxaggr, minmonophi, maxmonophi, minmonor, maxmonor, agg_as_ch[i,r]))\n file3.write('%.3f %.3f %.3f %.3f %.3f %.3f %.3f %.3f %.3f \\n' %(minaggphi, maxaggphi, minaggr, maxaggr, minmonophi, maxmonophi, minmonor, maxmonor, agg_as_mean[i,r]))\n file4.write('%.3f %.3f %.3f %.3f %.3f %.3f %.3f %.3f %.3f \\n' %(minaggphi, maxaggphi, minaggr, maxaggr, minmonophi, maxmonophi, minmonor, maxmonor, dds_mode[i,r]))\n \nfile1.close()\nfile2.close() \nfile3.close()\nfile4.close()",
"_____no_output_____"
],
[
"#write to file for output as array:\nwith open(\"../../lookup_tables/ice_agg/newformat_minorax_ellipsoid_rand_ch_binedges.dat\",\"w\") as file1:\n with open(\"../../lookup_tables/ice_agg/newformat_majorax_ellipsoid_rand_ch_binedges.dat\",\"w\") as file2:\n with open(\"../../lookup_tables/ice_agg/newformat_majorax_ellipsoid_rand_mean_binedges.dat\",\"w\") as file3:\n with open(\"../../lookup_tables/ice_agg/newformat_dd_rand_mode_binedges.dat\",\"w\") as file4:\n\n file1.write('Ice-Agg collection for the random orientation. \\n'\\\n 'Characteristic values taken from the peak of a fit \\n'\\\n 'gamma distribution from 300 aggregates. \\n'\\\n 'Minor axis taken as the smallest axis from the fit-ellipsoid \\n'\\\n 'Ranges are taken from the bin edges (maximum range) \\n'\\\n 'Order: agg phi min, agg phi max, agg r min, agg r max, \\n '\\\n 'monomer phi min, monomer phi max, monomer r min, monomer r max, value \\n')\n\n file2.write('Ice-Agg collection for the random orientation. \\n'\n 'Characteristic values taken from the peak of a fit \\n'\\\n 'gamma distribution from 300 aggregates. \\n'\\\n 'Ranges are taken from the bin edges (maximum range) \\n'\\\n 'Order: agg phi min, agg phi max, agg r min, agg r max, \\n '\\\n 'monomer phi min, monomer phi max, monomer r min, monomer r max, value \\n')\n\n file3.write('Ice-Agg collection for the random orientation. \\n'\n 'Mean value taken from the average across 300 aggregates. \\n'\\\n 'Major axis taken as the largest axis from the fit-ellipsoid \\n'\n 'Ranges are taken from the bin edges (maximum range) \\n'\\\n 'Order: agg phi min, agg phi max, agg r min, agg r max, \\n '\\\n 'monomer phi min, monomer phi max, monomer r min, monomer r max, value \\n')\n \n file4.write('Ice-Agg collection for the random orientation. \\n'\n 'Volume ratio of agg subtracted from volume ratio of new agg (Vagg/Vellipse)\\n'\\\n 'Mode from 300 aggregates. \\n'\\\n 'Ranges are taken from the bin edges (maximum range) \\n'\\\n 'Order: agg phi min, agg phi max, agg r min, agg r max, \\n '\\\n 'monomer phi min, monomer phi max, monomer r min, monomer r max, value \\n')\n\n\n for i in range(agg_phi_bins):\n for r in range(agg_r_bins):\n listmonophi = [n.monophi for n in pulled_clus[i,r,:]]\n maxmonophi = max(listmonophi)\n minmonophi = min(listmonophi)\n listmonor = [n.monor for n in pulled_clus[i,r,:]]\n maxmonor = max(listmonor)\n minmonor = min(listmonor)\n\n #print(i,r)\n file1.write('%.3f %.3f %.3f %.3f %.3f %.3f %.3f %.3f %.3f \\n' %(phi_bins[i], phi_bins[i+1], all_r_bins[i,r], all_r_bins[i,r+1], minmonophi, maxmonophi, minmonor, maxmonor, agg_cs_ch[i,r]))\n file2.write('%.3f %.3f %.3f %.3f %.3f %.3f %.3f %.3f %.3f \\n' %(phi_bins[i], phi_bins[i+1], all_r_bins[i,r], all_r_bins[i,r+1], minmonophi, maxmonophi, minmonor, maxmonor, agg_as_ch[i,r]))\n file3.write('%.3f %.3f %.3f %.3f %.3f %.3f %.3f %.3f %.3f \\n' %(phi_bins[i], phi_bins[i+1], all_r_bins[i,r], all_r_bins[i,r+1], minmonophi, maxmonophi, minmonor, maxmonor, agg_as_mean[i,r]))\n file4.write('%.3f %.3f %.3f %.3f %.3f %.3f %.3f %.3f %.3f \\n' %(phi_bins[i], phi_bins[i+1], all_r_bins[i,r], all_r_bins[i,r+1], minmonophi, maxmonophi, minmonor, maxmonor, dds_mode[i,r]))\n \nfile1.close()\nfile2.close() \nfile3.close()\nfile4.close()",
"_____no_output_____"
],
[
"#write to file for output as array: \nwith open(\"../../lookup_tables/ice_agg/newformat_minorax_ellipsoid_rand_ch.dat\",\"w\") as file1:\n with open(\"../../lookup_tables/ice_agg/newformat_majorax_ellipsoid_rand_ch.dat\",\"w\") as file2:\n with open(\"../../lookup_tables/ice_agg/newformat_majorax_ellipsoid_rand_mean.dat\",\"w\") as file3:\n with open(\"../../lookup_tables/ice_agg/newformat_dd_rand_mode.dat\",\"w\") as file4:\n\n file1.write('Ice-Agg collection for the random orientation. \\n'\\\n 'Characteristic values taken from the peak of a fit \\n'\\\n 'gamma distribution from 300 aggregates. \\n'\\\n 'Minor axis taken as the smallest axis from the fit-ellipsoid \\n'\\\n 'Ranges are taken from the aggregates being pulled from the db, \\n' \\\n 'not the bin edges (maximum range) \\n'\\\n 'Order: agg phi min, agg phi max, agg r min, agg r max, \\n '\\\n 'monomer phi min, monomer phi max, monomer r min, monomer r max, value \\n')\n\n file2.write('Ice-Agg collection for the random orientation. \\n'\n 'Characteristic values taken from the peak of a fit \\n'\\\n 'gamma distribution from 300 aggregates. \\n'\\\n 'Ranges are taken from the aggregates being pulled from the db, \\n' \\\n 'not the bin edges (maximum range) \\n'\\\n 'Order: agg phi min, agg phi max, agg r min, agg r max, \\n '\\\n 'monomer phi min, monomer phi max, monomer r min, monomer r max, value \\n')\n\n file3.write('Ice-Agg collection for the random orientation. \\n'\n 'Mean value taken from the average across 300 aggregates. \\n'\\\n 'Major axis taken as the largest axis from the fit-ellipsoid \\n'\n 'Ranges are taken from the aggregates being pulled from the db, \\n' \\\n 'not the bin edges (maximum range) \\n'\\\n 'Order: agg phi min, agg phi max, agg r min, agg r max, \\n '\\\n 'monomer phi min, monomer phi max, monomer r min, monomer r max, value \\n')\n \n file4.write('Ice-Agg collection for the random orientation. \\n'\n 'Volume ratio of agg subtracted from volume ratio of new agg (Vagg/Vellipse)\\n'\\\n 'Mode from 300 aggregates. \\n'\\\n 'Ranges are taken from the aggregates being pulled from the db, \\n' \\\n 'not the bin edges (maximum range) \\n'\\\n 'Order: agg phi min, agg phi max, agg r min, agg r max, \\n '\\\n 'monomer phi min, monomer phi max, monomer r min, monomer r max, value \\n')\n\n\n for i in range(agg_phi_bins):\n for r in range(agg_r_bins):\n listmonophi = [n.monophi for n in pulled_clus[i,r,:]]\n maxmonophi = max(listmonophi)\n minmonophi = min(listmonophi)\n listmonor = [n.monor for n in pulled_clus[i,r,:]]\n maxmonor = max(listmonor)\n minmonor = min(listmonor)\n listaggphi = [n.c/n.a for n in pulled_clus[i,r,:]]\n maxaggphi = max(listaggphi)\n minaggphi = min(listaggphi)\n listaggr = [n.r for n in pulled_clus[i,r,:]]\n maxaggr = max(listaggr)\n minaggr = min(listaggr)\n #print(i,r)\n file1.write('%.3f %.3f %.3f %.3f %.3f %.3f %.3f %.3f %.3f \\n' %(minaggphi, maxaggphi, minaggr, maxaggr, minmonophi, maxmonophi, minmonor, maxmonor, agg_cs_ch[i,r]))\n file2.write('%.3f %.3f %.3f %.3f %.3f %.3f %.3f %.3f %.3f \\n' %(minaggphi, maxaggphi, minaggr, maxaggr, minmonophi, maxmonophi, minmonor, maxmonor, agg_as_ch[i,r]))\n file3.write('%.3f %.3f %.3f %.3f %.3f %.3f %.3f %.3f %.3f \\n' %(minaggphi, maxaggphi, minaggr, maxaggr, minmonophi, maxmonophi, minmonor, maxmonor, agg_as_mean[i,r]))\n file4.write('%.3f %.3f %.3f %.3f %.3f %.3f %.3f %.3f %.3f \\n' %(minaggphi, maxaggphi, minaggr, maxaggr, minmonophi, maxmonophi, minmonor, maxmonor, dds_mode[i,r]))\n \nfile1.close()\nfile2.close() \nfile3.close()\nfile4.close()",
"_____no_output_____"
]
]
] | [
"code"
] | [
[
"code",
"code",
"code",
"code",
"code",
"code",
"code",
"code",
"code",
"code",
"code",
"code",
"code",
"code",
"code"
]
] |
e7743a84c3d3b4b22464316ee916b48701440c1f | 1,303 | ipynb | Jupyter Notebook | TensorFlow/TensorFlow Basics.ipynb | wuyuntao/deep-reinforcement-learning | e3bd0df136429b0576162e6747953a8ea8d7b4c6 | [
"MIT"
] | null | null | null | TensorFlow/TensorFlow Basics.ipynb | wuyuntao/deep-reinforcement-learning | e3bd0df136429b0576162e6747953a8ea8d7b4c6 | [
"MIT"
] | null | null | null | TensorFlow/TensorFlow Basics.ipynb | wuyuntao/deep-reinforcement-learning | e3bd0df136429b0576162e6747953a8ea8d7b4c6 | [
"MIT"
] | null | null | null | 17.849315 | 45 | 0.4835 | [
[
[
"import tensorflow as tf",
"_____no_output_____"
],
[
"a = tf.constant(5, name=\"input_a\")\nb = tf.constant(3, name=\"input_b\")\nc = tf.mul(a,b, name=\"mul_c\")\nd = tf.add(a,b, name=\"add_d\")\ne = tf.add(c,d, name=\"add_e\")\n\nsess = tf.Session()\nresult = sess.run(e)\nprint(result)",
"23\n"
]
]
] | [
"code"
] | [
[
"code",
"code"
]
] |
e7743b2ebc618d6bc3eab3db3cd9a54ae5cd25df | 20,769 | ipynb | Jupyter Notebook | bloc5/RechercheTextuelle/Recherche_textuelle.ipynb | frederic-junier/DIU-Junier | 6e3b23d06b69c992e03552f4626676e165f772e9 | [
"CC0-1.0"
] | null | null | null | bloc5/RechercheTextuelle/Recherche_textuelle.ipynb | frederic-junier/DIU-Junier | 6e3b23d06b69c992e03552f4626676e165f772e9 | [
"CC0-1.0"
] | null | null | null | bloc5/RechercheTextuelle/Recherche_textuelle.ipynb | frederic-junier/DIU-Junier | 6e3b23d06b69c992e03552f4626676e165f772e9 | [
"CC0-1.0"
] | null | null | null | 25.389976 | 161 | 0.445712 | [
[
[
"## Recherche naive par fenêtre glissante",
"_____no_output_____"
]
],
[
[
"def correspondance_motif(texte, motif,i):\n \"\"\"Recherche la correspondance de motif dans texte\n à partir de la position i\"\"\"\n if i + len(motif) > len(texte):\n return False\n for j in range(0, len(motif)):\n if motif[j] != texte[i + j]:\n return False\n return True\n\ndef recherche_motif_naive(texte, motif):\n \"\"\"Retourne la position où le motif a été trouvé par fenetre glissante\n ou -1 si le motif ne se trouve pas dans le texte\n Si n = len(texte) et m = len(motif), la complexité est en O((n-m)*m)\"\"\"\n for i in range(len(texte) - len(motif) + 1):\n if correspondance_motif(texte, motif,i):\n return i\n return -1",
"_____no_output_____"
]
],
[
[
"## Algorithme de Boyer-Moore",
"_____no_output_____"
],
[
"Sitographie :\n \n* [https://en.wikipedia.org/wiki/Boyer%E2%80%93Moore_string-search_algorithm](https://en.wikipedia.org/wiki/Boyer%E2%80%93Moore_string-search_algorithm)\n* [http://whocouldthat.be/visualizing-string-matching/](http://whocouldthat.be/visualizing-string-matching/)\n* [https://www.inf.hs-flensburg.de/lang/algorithmen/pattern/bmen.htm](https://www.inf.hs-flensburg.de/lang/algorithmen/pattern/bmen.htm)",
"_____no_output_____"
],
[
"### Règle du mauvais caractère",
"_____no_output_____"
]
],
[
[
"def mauvais_caractere(motif, alphabet):\n \"\"\"Retourne un dictionnaire avec pour chaque caractère de l'alphabet, le nombre de décalage \n à partir de la fin du motif avant de trouver ce caractère\n On ne compte pas la dernière lettre du motif et le décalage vaut m = len(motif)\"\n si on ne trouve pas le caractère\"\"\"\n m = len(motif)\n #mc = [0] * len(alphabet) \n mc = {c : 0 for c in alphabet} #j préfère utiliser un dictionnaire\n for c in alphabet:\n k = 1\n while k < m and c != motif[m - 1 - k]:\n k = k + 1\n mc[c] = k\n return mc",
"_____no_output_____"
],
[
"mauvais_caractere('GCAGAGAG', 'ACGT')",
"_____no_output_____"
],
[
"def correspondance_suffixe(motif, i, j):\n m = len(motif)\n if motif[j] != motif[i]:\n d = 1\n while i + d < m and motif[j + d] == motif[i + d]:\n d += 1\n return i + d == m\n return False\n \n\ndef comparaison_prefixe_suffixe(debut_suffixe, motif):\n index_prefixe = 0\n index_suffixe = debut_suffixe\n m = len(motif)\n while index_suffixe < m and motif[index_suffixe] == motif[index_prefixe]:\n index_prefixe += 1\n index_suffixe += 1\n return index_suffixe == m\n \ndef bon_suffixe(motif):\n m = len(motif)\n bs = [0] * m \n for i in range(m - 1, -1, -1): \n j = i - 1 \n while j >= 0 and not correspondance_suffixe(motif, i, j): \n j = j - 1 \n if j >= 0: #premier cas du bon suffixe : \n bs[i] = i - j \n else: # second cas du bon suffixe : rrecherche du début d'un suffixe/préfixe \n p = i + 1\n while p < m and not comparaison_prefixe_suffixe(p, motif):\n p = p + 1\n bs[i] = p\n return bs",
"_____no_output_____"
],
[
"bon_suffixe('GCAGAGAG')",
"_____no_output_____"
],
[
"bon_suffixe('ABABA')",
"_____no_output_____"
],
[
"bon_suffixe('AAA')",
"_____no_output_____"
],
[
"def boyer_moore(texte, motif, alphabet):\n #initialisation des longueurs\n n = len(texte)\n m = len(motif)\n #pré-traitement du motif\n bs = bon_suffixe(motif)\n mc = mauvais_caractere(motif, alphabet)\n print(bs, mc)\n #recherche du motif dans le texte\n i = 0 #indice dans le texte\n while i <= n - m:\n j = m - 1 #on lit le motif de droite à gauche\n while j >= 0 and motif[j] == texte[i+j]:\n j = j - 1\n if j < 0:\n print(f\"Motif trouvé en {i}\")\n #décalage du motif\n i = i + bs[0]\n else:\n #décalage du motif\n i = i + max(bs[j], mc[texte[i+j]] + j - m + 1)\n ",
"_____no_output_____"
],
[
"texte = \"GCATCGCAGAGAGTATACAGTACG\"\nmotif = \"GCAGAGAG\"\nalphabet = \"ACGT\"\nboyer_moore(texte, motif, alphabet)",
"[7, 7, 7, 2, 7, 4, 7, 1] {'A': 1, 'C': 6, 'G': 2, 'T': 8}\nMotif trouvé en 5\n"
],
[
"T = \"GCATCGCAGAGAGTATACAGTACG\"\nM = \"GCAGAGAG\"\nalphabet = \"ACGT\"\nboyer_moore(T, M, alphabet)",
"[7, 7, 7, 2, 7, 4, 7, 1] {'A': 1, 'C': 6, 'G': 2, 'T': 8}\nMotif trouvé en 5\n"
],
[
"bon_suffixe(M)",
"_____no_output_____"
],
[
"T='CBABABA'\nM='ABABA'\nalphabet = \"ACB\"\nprint(\"Mauvais caractère : \", mauvais_caractere(M, 'ABC'))\nprint(\"Bon suffixe : \", bon_suffixe(M))\nprint(f\"Recherche de {M} dans {T} avec Boyer-Moore\")\nboyer_moore(T, M, alphabet)",
"Mauvais caractère : {'A': 2, 'B': 1, 'C': 5}\nBon suffixe : [2, 2, 4, 4, 1]\nRecherche de ABABA dans CBABABA avec Boyer-Moore\n[2, 2, 4, 4, 1] {'A': 2, 'C': 5, 'B': 1}\nMotif trouvé en 2\n"
],
[
"bon_suffixe(\"TATATA\")",
"_____no_output_____"
],
[
"bon_suffixe(\"AAA\")",
"_____no_output_____"
]
],
[
[
"# Version du formateur",
"_____no_output_____"
]
],
[
[
"T = \"GCATCGCAGAGAGTATACAGTACG\"\nM = \"GCAGAGAG\"\n#M = \"CCGGTGAA\"\n#T = \"AAAAAAAAAAAAAAAAAAAA\"\n#M = \"AAAAAA\"\n#T = \"AAAAAAAAAAAAAAAAAAAA\"\n#M = \"ACGT\"\n#M = \"ACGCA\"\n\nn = len(T)\nm = len(M)",
"_____no_output_____"
],
[
"\n\nfor i in range(n-m+1):\n for j in range(m):\n if T[i+j] != M[j]: # on s'arrête dès qu'on voit une différence (mismatch)\n break\n if (j == (m-1)): # critère d'arrêt à (j == (m-1)) car j n'est pas incrémenté à la fin\n print(\"motif trouvé en \" + str(i))",
"motif trouvé en 5\n"
],
[
"\n\nnb_comp = 0 # nombre total de comparaisons\ni = 0\nwhile (i <= (n-m)):\n j = 0\n while (j < m) and (T[i+j] == M[j]): # on incrémente tant que c'est identique\n nb_comp += 1\n j = j + 1\n if (j == m): # on remarque que le critère d'arrêt est (j == m) ici\n print(\"motif trouvé en \" + str(i))\n else:\n nb_comp += 1 # pour ne pas oublier de compter les échecs de comparaison (mismatch)\n i = i + 1\nprint(\"Nombre total de comparaisons : \" + str(nb_comp))\n\n",
"motif trouvé en 5\nNombre total de comparaisons : 30\n"
]
],
[
[
"## Heuristique du Mauvais Caractère",
"_____no_output_____"
]
],
[
[
"symboles = [\"A\", \"C\", \"G\", \"T\"] # c'est l'alphabet\n \n# calcul préalable de MC\nMC = {}\nfor s in symboles: # on initialise à m par défaut (caractère introuvable dans le motif)\n MC[s] = m\nfor i in range(m-1): \n MC[M[i]] = m-i-1",
"_____no_output_____"
],
[
"MC",
"_____no_output_____"
],
[
"\n\nimport numpy as np\n\nnb_comp = 0 # nombre total de comparaisons\ni = 0\nwhile (i <= (n-m)):\n print(\"Position : \" + str(i)) \n j = m - 1 # on commence par la fin du motif\n while (j >= 0) and (T[i+j] == M[j]): # on incrémente tant que c'est identique\n #print(\"comp de \" + str(i+j) + \" et \" + str(j))\n nb_comp += 1\n j = j - 1\n if (j >= 0):\n nb_comp += 1\n i = i + np.max([1, MC[T[i+j]] + j - m + 1])\n else: # on remarque que le critère d'arrêt est à présent (j < 0)\n print(\"motif trouvé en \" + str(i))\n i = i + 1\n \nprint(\"Nombre total de comparaisons : \" + str(nb_comp))\n\n",
"Position : 0\nPosition : 1\nPosition : 5\nmotif trouvé en 5\nPosition : 6\nPosition : 14\nPosition : 15\nNombre total de comparaisons : 15\n"
]
],
[
[
"## Heuristique du Bon Suffixe (BS)",
"_____no_output_____"
]
],
[
[
"M = \"AAAA\"\nm = len(M)\n# calcul préalable de BS\n# (attention, il s'agit probablement de l'implémentation la moins efficace\n# mais peut-être la plus claire)\n\n# calcul du plus grand préfixe qui est également suffixe (mais pas M tout entier)\npref_suff = m\nfor i in range(m-1):\n if M[0:i+1] == M[m-(i+1):m]:\n pref_suff = m-(i+1)\nprint(pref_suff)\nBS = [pref_suff] * m\nBS[m-1] = 1 # cas particulier pour le dernier symbole de M\n# recherche du prochain motif le plus à droite\ni = m - 2\nwhile (i >= 0):\n # motif à rechercher\n MM = M[i+1:m]\n l_MM = len(MM)\n k = i\n # on cherche le motif \"à rebours\"\n while (k>=0):\n if (M[k:k+l_MM] == MM) and ((k==0) or (M[k-1]!=M[i])):\n print(\"à l'index \" + str(i) + \" : sous-motif \" + MM + \" trouvé en \" + str(k))\n BS[i] = i - k + 1\n break;\n k = k - 1\n i = i - 1",
"1\nà l'index 2 : sous-motif A trouvé en 0\nà l'index 1 : sous-motif AA trouvé en 0\nà l'index 0 : sous-motif AAA trouvé en 0\n"
],
[
"BS",
"_____no_output_____"
],
[
"import numpy as np\n\nnb_comp = 0 # nombre total de comparaisons\ni = 0\nwhile (i <= (n-m)):\n print(\"Position : \" + str(i))\n j = m - 1 # on commence par la fin du motif\n while (j >= 0) and (T[i+j] == M[j]): # on incrémente tant que c'est identique\n nb_comp += 1\n j = j - 1\n if (j >= 0):\n nb_comp += 1 \n i = i + BS[j]\n else:\n print(\"motif trouvé en \" + str(i))\n i = i + BS[0]\n\nprint(\"Nombre total de comparaisons : \" + str(nb_comp))",
"Position : 0\nPosition : 1\nPosition : 2\nPosition : 3\nPosition : 4\nPosition : 7\nPosition : 8\nPosition : 11\nPosition : 14\nPosition : 15\nPosition : 18\nNombre total de comparaisons : 16\n"
]
],
[
[
"## Boyer-Moore : mettre tout ça ensemble",
"_____no_output_____"
]
],
[
[
"import numpy as np\n\nnb_comp = 0 # nombre total de comparaisons\ni = 0\nwhile (i <= (n-m)):\n print(\"Position : \" + str(i))\n j = m - 1 # on commence par la fin du motif\n while (j >= 0) and (T[i+j] == M[j]): # on incrémente tant que c'est identique\n nb_comp += 1\n j = j - 1\n if (j >= 0):\n nb_comp += 1 \n i = i + np.max([BS[j], MC[T[i+j]] + j - m + 1]) \n else: \n print(\"motif trouvé en \" + str(i))\n i = i + BS[0]\nprint(\"Nombre total de comparaisons : \" + str(nb_comp))",
"Position : 0\nPosition : 8\nPosition : 11\nPosition : 18\nNombre total de comparaisons : 7\n"
]
],
[
[
"## Test",
"_____no_output_____"
]
],
[
[
"T='CBABABA'\nM='AEBBBA'\n\nn = len(T)\nm = len(M)\n\nsymboles = [\"A\", \"C\", \"B\"] # c'est l'alphabet\n \n# calcul préalable de MC\nMC = {}\nfor s in symboles: # on initialise à m par défaut (caractère introuvable dans le motif)\n MC[s] = m\nfor i in range(m-1): \n MC[M[i]] = m-i-1\n \n\n# calcul préalable de BS\n# (attention, il s'agit probablement de l'implémentation la moins efficace\n# mais peut-être la plus claire)\n\n# calcul du plus grand préfixe qui est également suffixe (mais pas M tout entier)\npref_suff = m\nfor i in range(m-1):\n if M[0:i+1] == M[m-(i+1):m]:\n pref_suff = m-(i+1)\nBS = [pref_suff] * m\nprint(pref_suff)\nBS[m-1] = 1 # cas particulier pour le dernier symbole de M\n# recherche du prochain motif le plus à droite\ni = m - 2\nwhile (i >= 0):\n # motif à rechercher\n MM = M[i+1:m]\n l_MM = len(MM)\n k = i\n # on cherche le motif \"à rebours\"\n while (k>=0):\n if (M[k:k+l_MM] == MM) and ((k==0) or (M[k-1]!=M[i])):\n #print(\"à l'index \" + str(i) + \" : sous-motif \" + MM + \" trouvé en \" + str(k))\n BS[i] = i - k + 1\n break;\n k = k - 1\n i = i - 1\n \nnb_comp = 0 # nombre total de comparaisons\ni = 0\nwhile (i <= (n-m)):\n print(\"Position : \" + str(i))\n j = m - 1 # on commence par la fin du motif\n while (j >= 0) and (T[i+j] == M[j]): # on incrémente tant que c'est identique\n nb_comp += 1\n j = j - 1\n if (j >= 0):\n nb_comp += 1 \n i = i + np.max([BS[j], MC[T[i+j]] + j - m + 1]) \n else: \n print(\"motif trouvé en \" + str(i))\n i = i + BS[0]\nprint(MC)\nprint(BS)\nprint(\"Nombre total de comparaisons : \" + str(nb_comp))",
"5\nPosition : 0\nPosition : 1\n{'A': 5, 'C': 6, 'B': 1, 'E': 4}\n[5, 5, 5, 5, 5, 1]\nNombre total de comparaisons : 4\n"
]
]
] | [
"markdown",
"code",
"markdown",
"code",
"markdown",
"code",
"markdown",
"code",
"markdown",
"code",
"markdown",
"code",
"markdown",
"code"
] | [
[
"markdown"
],
[
"code"
],
[
"markdown",
"markdown",
"markdown"
],
[
"code",
"code",
"code",
"code",
"code",
"code",
"code",
"code",
"code",
"code",
"code",
"code",
"code"
],
[
"markdown"
],
[
"code",
"code",
"code"
],
[
"markdown"
],
[
"code",
"code",
"code"
],
[
"markdown"
],
[
"code",
"code",
"code"
],
[
"markdown"
],
[
"code"
],
[
"markdown"
],
[
"code"
]
] |
e774449fa19526fd63c45e90c9814adaaeb2f823 | 8,557 | ipynb | Jupyter Notebook | examples/train_and_evaluate_save_restore.ipynb | 8ball030/tensortrade | 2308038d58c6f51dbe80db06b256163b9bf38bfd | [
"Apache-2.0"
] | 1 | 2021-01-02T18:55:42.000Z | 2021-01-02T18:55:42.000Z | examples/train_and_evaluate_save_restore.ipynb | 8ball030/tensortrade | 2308038d58c6f51dbe80db06b256163b9bf38bfd | [
"Apache-2.0"
] | null | null | null | examples/train_and_evaluate_save_restore.ipynb | 8ball030/tensortrade | 2308038d58c6f51dbe80db06b256163b9bf38bfd | [
"Apache-2.0"
] | null | null | null | 25.167647 | 132 | 0.497487 | [
[
[
"## Setup Data Fetching",
"_____no_output_____"
]
],
[
[
"import pandas as pd\nimport tensortrade.env.default as default\n\nfrom tensortrade.data.cdd import CryptoDataDownload\nfrom tensortrade.feed.core import Stream, DataFeed\nfrom tensortrade.oms.exchanges import Exchange\nfrom tensortrade.oms.services.execution.simulated import execute_order\nfrom tensortrade.oms.instruments import USD, BTC, ETH\nfrom tensortrade.oms.wallets import Wallet, Portfolio\nfrom tensortrade.agents import DQNAgent\nfrom ta import add_all_ta_features\n",
"_____no_output_____"
],
[
"# gather data\ndef get_feed(n_events=None):\n cdd = CryptoDataDownload()\n data = cdd.fetch(\"Bitstamp\", \"USD\", \"BTC\", \"1h\")\n data = add_all_ta_features(data, 'open', 'high', 'low', 'close', 'volume')\n \n if n_events is not None:\n data = data.iloc[n_events:]\n print(len(data))\n features = []\n for c in data.columns[2:]:\n s = Stream.source(list(data[c]), dtype=\"float\").rename(data[c].name)\n features += [s]\n feed = DataFeed(features)\n feed.compile() \n return data, feed\n\ndata, feed = get_feed()",
"/home/tom/.local/lib/python3.6/site-packages/ta/trend.py:768: RuntimeWarning:\n\ninvalid value encountered in double_scalars\n\n/home/tom/.local/lib/python3.6/site-packages/ta/trend.py:772: RuntimeWarning:\n\ninvalid value encountered in double_scalars\n\n"
],
[
"# Create environment\ndef create_env(config=None): \n bitstamp = Exchange(\"bitstamp\", service=execute_order)(\n Stream.source(list(data[\"close\"]), dtype=\"float\").rename(\"USD-BTC\")\n )\n\n portfolio = Portfolio(USD, [\n Wallet(bitstamp, 10000 * USD),\n Wallet(bitstamp, 10 * BTC)\n ])\n\n\n renderer_feed = DataFeed([\n Stream.source(list(data[\"date\"])).rename(\"date\"),\n Stream.source(list(data[\"open\"]), dtype=\"float\").rename(\"open\"),\n Stream.source(list(data[\"high\"]), dtype=\"float\").rename(\"high\"),\n Stream.source(list(data[\"low\"]), dtype=\"float\").rename(\"low\"),\n Stream.source(list(data[\"close\"]), dtype=\"float\").rename(\"close\"), \n Stream.source(list(data[\"volume\"]), dtype=\"float\").rename(\"volume\") \n ])\n\n\n env = default.create(\n portfolio=portfolio,\n action_scheme=\"simple\",\n reward_scheme=\"risk-adjusted\",\n feed=feed,\n renderer_feed=renderer_feed,\n renderer=default.renderers.FileLogger(),\n window_size=20\n )\n return env\n\nenv = create_env()",
"_____no_output_____"
]
],
[
[
"## Setup and Train DQN Agent",
"_____no_output_____"
]
],
[
[
"%load_ext autoreload\n%autoreload 2\n",
"The autoreload extension is already loaded. To reload it, use:\n %reload_ext autoreload\n"
],
[
"# create agent\ndef get_agent(env, agent_id=None):\n agent = DQNAgent(env)\n if agent_id is not None:\n agent.id = \"TEST_AGENT\"\n return agent\n\n\nagent = get_agent(env=env, agent_id=\"TEST_AGENT\")\n",
"_____no_output_____"
],
[
"# train the agent\n\nmean_reward = agent.train(n_steps=len(data) / 100,\n n_episodes=1,\n save_every=1\n )\n\nagent.save(\"./\")\n\nprint(mean_reward)",
"==== AGENT ID: TEST_AGENT ====\n-1120081.1682655017\n"
],
[
"# remove the agent\ndel agent",
"_____no_output_____"
],
[
"# we restore the agent\n\nagent = get_agent(env=env, agent_id=\"TEST_AGENT\")\n\nagent.restore(\"./policy_network__TEST_AGENT.hdf5\")\n",
"WARNING:tensorflow:No training configuration found in the save file, so the model was *not* compiled. Compile it manually.\n"
],
[
"# now we have restored our agent, we can save our model\nagent.save(\"./\")\n",
"_____no_output_____"
],
[
"# we reset the environment\n\ninitial_state = agent.env.reset()\n\ninitial_state",
"_____no_output_____"
],
[
"# predict our next action\n\nagent.get_action(state=initial_state)",
"_____no_output_____"
],
[
"env.action_space",
"_____no_output_____"
]
]
] | [
"markdown",
"code",
"markdown",
"code"
] | [
[
"markdown"
],
[
"code",
"code",
"code"
],
[
"markdown"
],
[
"code",
"code",
"code",
"code",
"code",
"code",
"code",
"code",
"code"
]
] |
e7744816057dbc2cd71602af5bd94335b6f84282 | 237,130 | ipynb | Jupyter Notebook | misc/jupyter_notebooks/17.10.27/scipy_examples.ipynb | iamaleksandra/scientific_python | 04be94e9451ed84e38dcbdc1281f11c96808c1d0 | [
"MIT"
] | 7 | 2017-10-27T21:23:42.000Z | 2022-02-10T14:56:02.000Z | misc/jupyter_notebooks/17.10.27/scipy_examples.ipynb | iamaleksandra/scientific_python | 04be94e9451ed84e38dcbdc1281f11c96808c1d0 | [
"MIT"
] | 3 | 2018-07-22T05:19:50.000Z | 2018-09-13T15:13:22.000Z | misc/jupyter_notebooks/17.10.27/scipy_examples.ipynb | iamaleksandra/scientific_python | 04be94e9451ed84e38dcbdc1281f11c96808c1d0 | [
"MIT"
] | 5 | 2017-10-23T10:35:50.000Z | 2020-12-16T11:37:40.000Z | 207.462817 | 58,623 | 0.86779 | [
[
[
"%autosave 10\n%matplotlib inline\nimport matplotlib.pyplot as plt\nimport numpy as np",
"_____no_output_____"
],
[
"from scipy import integrate\nintegral, error = integrate.quad(np.sin, 0, np.pi)\nprint(integral, error)\n\nt_ = np.linspace(0, 10, 1000)\ny_ = integrate.odeint(\n lambda y, t: [y[1], -y[0]-0.2*y[1]],\n [1, 0],\n t_,\n)\nplt.plot(t_, y_[:,0], label='x')\nplt.plot(t_, y_[:,1], label='v')\nplt.legend()\nplt.figure()\nplt.plot(y_[:,0], y_[:,1])",
"2.0 2.220446049250313e-14\n"
],
[
"from scipy import interpolate\n\ndef f(x):\n return np.exp(-0.5*x) * np.sin(x**2)\n\nx_ = np.sort(np.r_[\n 0,\n np.pi,\n np.random.uniform(0, np.pi, 20)\n])\n\ny_ = f(x_)\ninterp_func = interpolate.interp1d(x_, y_, kind='linear')\n\ncubic_spline = interpolate.CubicSpline(x_, y_)\nd_cubic_spline = cubic_spline.derivative()\ni_cubic_spline = cubic_spline.derivative(-1)\n\nx_long = np.linspace(0, np.pi, 1000)\nplt.plot(x_, y_, 'x', ms=7, label='points')\nplt.plot(x_long, f(x_long), label='origin')\nplt.plot(x_long, interp_func(x_long), label='linear')\nplt.plot(x_long, cubic_spline(x_long), label='cubic')\n# plt.plot(x_long, d_cubic_spline(x_long), label='derivative')\nplt.plot(x_long, i_cubic_spline(x_long), label='integral')\nplt.legend()",
"_____no_output_____"
],
[
"from scipy import interpolate\nx_ = np.sort(np.r_[\n 0,\n np.pi,\n np.random.uniform(0, np.pi, 20)\n])\ny_ = (np.exp(-x_**2/0.5)\n + np.random.uniform(-0.1, 0.1, size=x_.shape))\nx_long = np.linspace(0, np.pi, 1000)\nunivar_spline = interpolate.UnivariateSpline(x_, y_, k=5)\nplt.plot(x_, y_, 'x', ms=7, label='points')\nplt.plot(x_long, univar_spline(x_long), label='smooth')\nplt.legend()",
"_____no_output_____"
],
[
"from scipy import optimize\nresult = optimize.root(\n lambda x: x**2 - 2,\n 1.\n)\nprint(result)\nresult = optimize.brentq(\n lambda x: np.exp(x) - 1.5*x - 2,\n 0, 10,\n)\nprint(result)\nx = np.r_[0:2:100j]\nplt.plot(x, np.exp(x) - 1.5*x - 2)\nplt.grid()",
" fjac: array([[-1.]])\n fun: array([ -8.88178420e-16])\n message: 'The solution converged.'\n nfev: 8\n qtf: array([ 8.93144891e-10])\n r: array([-2.82842925])\n status: 1\n success: True\n x: array([ 1.41421356])\n1.417294180664143\n"
],
[
"from scipy import optimize\n\ndef f(x):\n return (-np.exp(-x[0]**2 - x[1]**2)\n * np.sin((x[0] + 2*x[1] + 1)))\n\nprint(optimize.minimize(f, [0,0]))\n\nX, Y = np.mgrid[-1:1:100j,-1:1:100j]\nZ = f(grid)\nplt.imshow(Z, extent=(-1,1,1,-1))\nplt.colorbar()",
" fun: -0.9544665458711115\n hess_inv: array([[ 0.82937944, -0.34123999],\n [-0.34123999, 0.31752228]])\n jac: array([ 1.49011612e-08, -7.45058060e-09])\n message: 'Optimization terminated successfully.'\n nfev: 24\n nit: 4\n njev: 6\n status: 0\n success: True\n x: array([ 0.08174717, 0.16349432])\n"
],
[
"from scipy import optimize\n# See lmfit module\ndef f(x, A, sigma, x0):\n return A * np.exp(-(x-x0)**2/(2*sigma))\n\nx_ = np.linspace(-2, 2, 10)\nmodel_p = (2, 1, -1)\ny_ = f(x_, *model_p) + np.random.uniform(-0.2, 0.2, size=x_.shape)\nfit_p, _ = optimize.curve_fit(f, x_, y_)\nx_long = np.linspace(-2,2,1000)\nplt.plot(x_long, f(x_long, *model_p), '-',\n x_, y_, 'x',\n x_long, f(x_long, *fit_p), '--')",
"_____no_output_____"
]
]
] | [
"code"
] | [
[
"code",
"code",
"code",
"code",
"code",
"code",
"code"
]
] |
e7745473c60f645af9b944c16a30528e6cf82bce | 1,110 | ipynb | Jupyter Notebook | VariantesMexico/SARS.ipynb | JManuelRG/sarscov2IPN | 2920dc50e1c337f28ea4e020ffce6f8bb5184e79 | [
"MIT"
] | null | null | null | VariantesMexico/SARS.ipynb | JManuelRG/sarscov2IPN | 2920dc50e1c337f28ea4e020ffce6f8bb5184e79 | [
"MIT"
] | null | null | null | VariantesMexico/SARS.ipynb | JManuelRG/sarscov2IPN | 2920dc50e1c337f28ea4e020ffce6f8bb5184e79 | [
"MIT"
] | null | null | null | 21.764706 | 238 | 0.482883 | [
[
[
"<a href=\"https://colab.research.google.com/github/JManuelRG/sarscov2IPN/blob/main/VariantesMexico/SARS.ipynb\" target=\"_parent\"><img src=\"https://colab.research.google.com/assets/colab-badge.svg\" alt=\"Open In Colab\"/></a>",
"_____no_output_____"
]
],
[
[
"",
"_____no_output_____"
]
],
[
[
"",
"_____no_output_____"
]
]
] | [
"markdown",
"code",
"markdown"
] | [
[
"markdown"
],
[
"code"
],
[
"markdown"
]
] |
e77468ab00407889c96dfedd9431f551360dcfae | 21,040 | ipynb | Jupyter Notebook | docs/downloads/code/letter-repetition/letter-repetition.ipynb | Ronak1958/blog | b477bda7641970ed1f1438994aa7a084c921b898 | [
"MIT"
] | 1 | 2019-09-11T03:30:30.000Z | 2019-09-11T03:30:30.000Z | docs/downloads/code/letter-repetition/letter-repetition.ipynb | Ronak1958/blog | b477bda7641970ed1f1438994aa7a084c921b898 | [
"MIT"
] | null | null | null | docs/downloads/code/letter-repetition/letter-repetition.ipynb | Ronak1958/blog | b477bda7641970ed1f1438994aa7a084c921b898 | [
"MIT"
] | null | null | null | 30.85044 | 134 | 0.336122 | [
[
[
"import pandas as pd \n\ndf = pd.read_csv('/usr/share/dict/words')\n# df = pd.read_csv('https://raw.githubusercontent.com/first20hours/google-10000-english/master/google-10000-english-usa.txt')\n# df = pd.read_csv('https://raw.githubusercontent.com/dwyl/english-words/master/words_alpha.txt')\n# df = pd.read_csv('http://www-personal.umich.edu/~jlawler/wordlist')\ndf.columns = ['Word']\ndf['Word'] = df['Word'].astype(str)\ndf.tail()",
"_____no_output_____"
],
[
"def num_letters(word):\n word = word.upper()\n letters = []\n for letter in word:\n if letter not in letters:\n letters.append(letter)\n return len(letters)\n \ndef len_word(word):\n return len(word)",
"_____no_output_____"
],
[
"df['Length'] = df['Word'].apply(len_word)\ndf['NLetters'] = df['Word'].apply(num_letters)\ndf['Ratio'] = df['NLetters'] / df['Length']",
"_____no_output_____"
],
[
"df[df['Word']=='senescence']",
"_____no_output_____"
],
[
"(df[df['Length']>3]\n .sort_values(by=['Ratio','Length','Word'], ascending=[True,False,True])\n .head(50)\n)",
"_____no_output_____"
]
]
] | [
"code"
] | [
[
"code",
"code",
"code",
"code",
"code"
]
] |
e7747235a68724cfeefe90d77cade0a7eec3dc92 | 1,770 | ipynb | Jupyter Notebook | examples/Coin Price.ipynb | sam2332/nbparameterise | e7e551a95c661d721bda37417088121d6653dcec | [
"MIT"
] | null | null | null | examples/Coin Price.ipynb | sam2332/nbparameterise | e7e551a95c661d721bda37417088121d6653dcec | [
"MIT"
] | null | null | null | examples/Coin Price.ipynb | sam2332/nbparameterise | e7e551a95c661d721bda37417088121d6653dcec | [
"MIT"
] | null | null | null | 22.125 | 228 | 0.519209 | [
[
[
"Coin Prices displays. Code cribbed from [this notebook](http://nbviewer.ipython.org/github/twiecki/financial-analysis-python-tutorial/blob/master/1.%20Pandas%20Basics.ipynb) by [Thomas Wiecki](https://github.com/twiecki).",
"_____no_output_____"
]
],
[
[
"COIN = ['bitcoin','eth','doge'] # Display names are stored in notebook metadata",
"_____no_output_____"
],
[
"import requests\ntry:\n headers = {\n 'X-Mboum-Secret': \"demo\"\n }\n res = requests.get(\n f\"https://mboum.com/api/v1/cr/crypto/coin/quote?key={COIN}\", \n headers=headers\n )\n data = res.json()['data']\n for key in data:\n print(key,\"\\t\", data[key])\nexcept Exception as e:\n print(e)",
"_____no_output_____"
]
]
] | [
"markdown",
"code"
] | [
[
"markdown"
],
[
"code",
"code"
]
] |
e774772eba5763e36063aeaf3d5b8282c5d72c32 | 315,082 | ipynb | Jupyter Notebook | Behavioural Cloning.ipynb | vedanshdwivedi/SDC-Self-Driving-Car- | 6d5106465175de235d97ec13e81c4ef02fa59906 | [
"MIT"
] | null | null | null | Behavioural Cloning.ipynb | vedanshdwivedi/SDC-Self-Driving-Car- | 6d5106465175de235d97ec13e81c4ef02fa59906 | [
"MIT"
] | null | null | null | Behavioural Cloning.ipynb | vedanshdwivedi/SDC-Self-Driving-Car- | 6d5106465175de235d97ec13e81c4ef02fa59906 | [
"MIT"
] | null | null | null | 377.796163 | 214,816 | 0.920916 | [
[
[
"import os\nimport ntpath\nimport numpy as np\nimport matplotlib.pyplot as plt\nimport keras\nimport cv2\nimport pandas as pd\nimport random\nimport matplotlib.image as mpimg\nfrom sklearn.utils import shuffle\nfrom sklearn.model_selection import train_test_split\nfrom keras.models import Sequential\nfrom keras.optimizers import Adam\nfrom keras.layers import Convolution2D, MaxPooling2D, Dropout, Flatten, Dense",
"_____no_output_____"
],
[
"datadir = 'data'\ncolumns = ['center', 'left', 'right', 'steering', 'throttle', 'reverse', 'speed']\ndata = pd.read_csv(os.path.join(datadir,'driving_log.csv'), names= columns)\npd.set_option('display.max_colwidth', -1)\ndata.head(5)",
"_____no_output_____"
],
[
"def path_leaf(path):\n head, tail = ntpath.split(path)\n return tail\n\ndata['center'] = data['center'].apply(path_leaf)\ndata['left'] = data['left'].apply(path_leaf)\ndata['right'] = data['right'].apply(path_leaf)\ndata.head(5)",
"_____no_output_____"
],
[
"num_bins = 25\nsamples_per_bin = 250\nhist, bins = np.histogram(data['steering'], num_bins)\ncenter = (bins[:-1] + bins[1:]) * 0.5\nplt.bar(center, hist, width=0.05)\nplt.plot((np.min(data['steering']), np.max(data['steering'])), (samples_per_bin, samples_per_bin))",
"_____no_output_____"
],
[
"remove_list = []\nfor j in range(num_bins):\n list_a = []\n for i in range(len(data['steering'])):\n if data['steering'][i] >= bins[j] and data['steering'][i] <= bins[j+1]:\n list_a.append(i) \n list_a = shuffle(list_a)\n list_a = list_a[samples_per_bin:]\n remove_list.extend(list_a)\n \n \nprint('removed : ' + str(len(remove_list)))\nprint('total : ' + str(len(data)))\ndata.drop(data.index[remove_list], inplace=True)\nprint('remaining : ' + str(len(data)))\n\nhist, _ = np.histogram(data['steering'], (num_bins))\nplt.bar(center, hist, width=0.05)\nplt.plot((np.min(data['steering']), np.max(data['steering'])), (samples_per_bin, samples_per_bin))",
"removed : 3119\ntotal : 4676\nremaining : 1557\n"
],
[
"print(data.iloc[1])\n\ndef load_img_steering(datadir, df):\n image_path = []\n steering = []\n for i in range(len(data)):\n indexed_data = data.iloc[i]\n center, left, right = indexed_data[0], indexed_data[1], indexed_data[2]\n image_path.append(os.path.join(datadir, center.strip()))\n steering.append(float(indexed_data[3]))\n image_paths = np.asarray(image_path)\n steerings = np.asarray(steering)\n return image_paths, steerings\n\nimage_paths, steerings = load_img_steering(datadir + '/IMG', data)",
"center center_2019_07_24_13_27_38_938.jpg\nleft left_2019_07_24_13_27_38_938.jpg \nright right_2019_07_24_13_27_38_938.jpg \nsteering 0 \nthrottle 0 \nreverse 0 \nspeed 7.78821e-05 \nName: 12, dtype: object\n"
],
[
"X_train, X_test, y_train, y_test = train_test_split(image_paths, steerings, test_size=0.2, random_state=87)\nprint('Training samples : {}\\n Test Samples : {}'.format(len(X_train), len(X_test)))",
"Training samples : 1245\n Test Samples : 312\n"
],
[
"fig, axes = plt.subplots(1, 2, figsize=(12,4))\naxes[0].hist(y_train, bins=num_bins, width=0.5, color='blue')\naxes[0].set_title('Training Set')\naxes[1].hist(y_test, bins=num_bins, width=0.5, color='red')\naxes[1].set_title('Test Set')",
"_____no_output_____"
],
[
"def img_preprocessing(img):\n img = mpimg.imread(img)\n img = img[60:140, :, :]\n img = cv2.cvtColor(img, cv2.COLOR_BGR2YUV)\n img = cv2.GaussianBlur(img, (3,3), 0)\n img = cv2.resize(img, (200, 66))\n img = img/255\n return img",
"_____no_output_____"
],
[
"image = image_paths[100]\noriginal_image = mpimg.imread(image)\npreprocessed_image = img_preprocessing(image)\n\nfig, axes = plt.subplots(1,2,figsize=(15,10))\nfig.tight_layout()\naxes[0].imshow(original_image)\naxes[1].imshow(preprocessed_image)\naxes[0].set_title('Original Image')\naxes[1].set_title('Preprocessed Image')",
"_____no_output_____"
],
[
"X_train = np.array(list(map(img_preprocessing, X_train)))\nX_test = np.array(list(map(img_preprocessing, X_test)))",
"_____no_output_____"
],
[
"plt.imshow(X_train[random.randint(0, len(X_train)-1)])\nplt.axis('off')\nprint(X_train.shape)",
"(1245, 66, 200, 3)\n"
],
[
"def nvidia_model():\n model = Sequential()\n model.add(Convolution2D(24, 5, 5, subsample=(2,2), input_shape=(66,200,3), activation='elu'))\n \n model.add(Convolution2D(36, 5, 5, subsample=(2,2), activation='elu'))\n model.add(Dropout(0.65))\n \n model.add(Convolution2D(48, 5, 5, subsample=(2,2), activation='elu'))\n model.add(Dropout(0.65))\n \n model.add(Convolution2D(64, 3, 3, activation='elu'))\n model.add(Dropout(0.65))\n \n model.add(Convolution2D(64, 3, 3, activation='elu'))\n model.add(Dropout(0.65))\n \n model.add(Flatten())\n \n model.add(Dense(100, activation='elu'))\n model.add(Dropout(0.65))\n \n model.add(Dense(50, activation='elu'))\n model.add(Dropout(0.65))\n \n model.add(Dense(10, activation='elu'))\n model.add(Dropout(0.65))\n \n model.add(Dense(1))\n \n optimizer = Adam(lr=1e-3)\n model.compile(loss = 'mse', optimizer=optimizer)\n return model",
"_____no_output_____"
],
[
"model = nvidia_model()\nprint(model.summary())",
"C:\\Users\\Vedansh\\Anaconda3\\lib\\site-packages\\ipykernel_launcher.py:3: UserWarning: Update your `Conv2D` call to the Keras 2 API: `Conv2D(24, (5, 5), input_shape=(66, 200, ..., activation=\"elu\", strides=(2, 2))`\n This is separate from the ipykernel package so we can avoid doing imports until\nC:\\Users\\Vedansh\\Anaconda3\\lib\\site-packages\\ipykernel_launcher.py:5: UserWarning: Update your `Conv2D` call to the Keras 2 API: `Conv2D(36, (5, 5), activation=\"elu\", strides=(2, 2))`\n \"\"\"\nC:\\Users\\Vedansh\\Anaconda3\\lib\\site-packages\\ipykernel_launcher.py:8: UserWarning: Update your `Conv2D` call to the Keras 2 API: `Conv2D(48, (5, 5), activation=\"elu\", strides=(2, 2))`\n \nC:\\Users\\Vedansh\\Anaconda3\\lib\\site-packages\\ipykernel_launcher.py:11: UserWarning: Update your `Conv2D` call to the Keras 2 API: `Conv2D(64, (3, 3), activation=\"elu\")`\n # This is added back by InteractiveShellApp.init_path()\nC:\\Users\\Vedansh\\Anaconda3\\lib\\site-packages\\ipykernel_launcher.py:14: UserWarning: Update your `Conv2D` call to the Keras 2 API: `Conv2D(64, (3, 3), activation=\"elu\")`\n \n"
],
[
"history = model.fit(X_train, y_train, epochs=30, validation_data=(X_test, y_test), batch_size = 100, verbose=1, shuffle=1)",
"Train on 1245 samples, validate on 312 samples\nEpoch 1/30\n1245/1245 [==============================] - 5s 4ms/step - loss: 11.6340 - val_loss: 0.0825\nEpoch 2/30\n1245/1245 [==============================] - 3s 2ms/step - loss: 3.1640 - val_loss: 0.0740\nEpoch 3/30\n1245/1245 [==============================] - 3s 2ms/step - loss: 1.6054 - val_loss: 0.0763\nEpoch 4/30\n1245/1245 [==============================] - 3s 2ms/step - loss: 1.1524 - val_loss: 0.0740\nEpoch 5/30\n1245/1245 [==============================] - 3s 2ms/step - loss: 0.9295 - val_loss: 0.0734\nEpoch 6/30\n1245/1245 [==============================] - 3s 2ms/step - loss: 0.7713 - val_loss: 0.0737\nEpoch 7/30\n1245/1245 [==============================] - 3s 2ms/step - loss: 0.6612 - val_loss: 0.0760\nEpoch 8/30\n1245/1245 [==============================] - 3s 2ms/step - loss: 0.6157 - val_loss: 0.0760\nEpoch 9/30\n1245/1245 [==============================] - 3s 2ms/step - loss: 0.5435 - val_loss: 0.0734\nEpoch 10/30\n1245/1245 [==============================] - 3s 2ms/step - loss: 0.5781 - val_loss: 0.0730\nEpoch 11/30\n1245/1245 [==============================] - 3s 2ms/step - loss: 0.4554 - val_loss: 0.0728\nEpoch 12/30\n1245/1245 [==============================] - 3s 2ms/step - loss: 0.4973 - val_loss: 0.0733\nEpoch 13/30\n1245/1245 [==============================] - 3s 2ms/step - loss: 0.4231 - val_loss: 0.0729\nEpoch 14/30\n1245/1245 [==============================] - 3s 2ms/step - loss: 0.4220 - val_loss: 0.0728\nEpoch 15/30\n1245/1245 [==============================] - 3s 2ms/step - loss: 0.3918 - val_loss: 0.0731\nEpoch 16/30\n1245/1245 [==============================] - 3s 2ms/step - loss: 0.3628 - val_loss: 0.0727\nEpoch 17/30\n1245/1245 [==============================] - 3s 2ms/step - loss: 0.3092 - val_loss: 0.0726\nEpoch 18/30\n1245/1245 [==============================] - 3s 2ms/step - loss: 0.3343 - val_loss: 0.0726\nEpoch 19/30\n1245/1245 [==============================] - 3s 2ms/step - loss: 0.3328 - val_loss: 0.0727\nEpoch 20/30\n1245/1245 [==============================] - 3s 2ms/step - loss: 0.3043 - val_loss: 0.0727\nEpoch 21/30\n1245/1245 [==============================] - 3s 2ms/step - loss: 0.2540 - val_loss: 0.0727\nEpoch 22/30\n1245/1245 [==============================] - 3s 2ms/step - loss: 0.2714 - val_loss: 0.0727\nEpoch 23/30\n1245/1245 [==============================] - 3s 2ms/step - loss: 0.2542 - val_loss: 0.0725\nEpoch 24/30\n1245/1245 [==============================] - 3s 2ms/step - loss: 0.2203 - val_loss: 0.0724\nEpoch 25/30\n1245/1245 [==============================] - 3s 2ms/step - loss: 0.2239 - val_loss: 0.0725\nEpoch 26/30\n1245/1245 [==============================] - 3s 2ms/step - loss: 0.2120 - val_loss: 0.0726\nEpoch 27/30\n1245/1245 [==============================] - 3s 2ms/step - loss: 0.2074 - val_loss: 0.0725\nEpoch 28/30\n1245/1245 [==============================] - 3s 2ms/step - loss: 0.2107 - val_loss: 0.0728\nEpoch 29/30\n1245/1245 [==============================] - 3s 2ms/step - loss: 0.1878 - val_loss: 0.0725\nEpoch 30/30\n1245/1245 [==============================] - 3s 2ms/step - loss: 0.1859 - val_loss: 0.0724\n"
],
[
"plt.plot(history.history['loss'])\nplt.plot(history.history['val_loss'])\nplt.legend(['training', 'validation'])\nplt.title('Loss')\nplt.xlabel('Epoch')",
"_____no_output_____"
],
[
"model.save('car.h5')",
"_____no_output_____"
]
]
] | [
"code"
] | [
[
"code",
"code",
"code",
"code",
"code",
"code",
"code",
"code",
"code",
"code",
"code",
"code",
"code",
"code",
"code",
"code",
"code"
]
] |
e774918476c6b989aaa67e6fc7ff3ad050df7d09 | 12,849 | ipynb | Jupyter Notebook | nbs/12_bug.ipynb | vtecftwy/unpackai | 5c6ff1ff141b15430ccf02cab34e9690ec168fda | [
"MIT"
] | 18 | 2021-08-30T00:15:06.000Z | 2022-01-28T01:41:16.000Z | nbs/12_bug.ipynb | vtecftwy/unpackai | 5c6ff1ff141b15430ccf02cab34e9690ec168fda | [
"MIT"
] | 75 | 2021-09-01T08:13:25.000Z | 2022-02-07T13:18:55.000Z | nbs/12_bug.ipynb | vtecftwy/unpackai | 5c6ff1ff141b15430ccf02cab34e9690ec168fda | [
"MIT"
] | 4 | 2021-08-30T03:22:28.000Z | 2021-11-13T12:48:06.000Z | 36.502841 | 1,020 | 0.508444 | [
[
[
"# Bug Helper\r\n> A friendly bug interceptor",
"_____no_output_____"
]
],
[
[
"# default_exp bug",
"_____no_output_____"
],
[
"# export\r\nfrom IPython.core.ultratb import AutoFormattedTB\r\nfrom traceback import format_exc\r\nfrom datetime import datetime\r\nfrom forgebox.html import list_group, list_group_kv, HTML\r\nimport html\r\nimport json\r\nimport base64\r\nfrom jinja2 import Template\r\nfrom unpackai.utils import STATIC\r\nimport logging\r\nfrom inspect import isfunction\r\nfrom typing import Union, Callable, Dict, Any\r\n",
"_____no_output_____"
],
[
"# export\r\ntry:\r\n ishell = get_ipython()\r\nexcept NameError as e:\r\n from IPython.testing.globalipapp import get_ipython\r\n ishell = get_ipython()",
"_____no_output_____"
]
],
[
[
"## BugBook\r\n> Collects the know bugs",
"_____no_output_____"
]
],
[
[
"# export\r\nclass BugBook(dict):\r\n \"\"\"\r\n A collection of bugs, and how to handle them\r\n \"\"\"\r\n\r\n def __init__(self, **kwargs):\r\n self.rules = dict(kwargs)\r\n\r\n def __repr__(self): return \"Bug Book\"\r\n\r\n def __getitem__(\r\n self, key\r\n ) -> Dict[str, Any]:\r\n if isfunction(key):\r\n return self.rules[key.__name__]\r\n return self[str(key)]\r\n\r\n def __setitem__(self,\r\n key: Union[str, Callable],\r\n value: Union[str, Callable]\r\n ) -> None:\r\n if type(key) == str:\r\n self.rules[key] = {\"key\": key,\r\n \"value\": value,\r\n \"keytype\": \"string\"}\r\n elif isfunction(key):\r\n self.rules[key.__name__] = {\"key\": key,\r\n \"value\": value,\r\n \"keytype\": \"function\"}\r\n else:\r\n self.rules[str(key)] = {\"key\": key, \"value\": value,\r\n \"keytype\": \"unknown\"}\r\n return\r\n\r\n def __call__(self, etype, evalue, tb):\r\n custom = None\r\n type_name = etype.__name__\r\n for d in self.rules.values():\r\n if d[\"keytype\"] == \"function\":\r\n if d['key'](etype, evalue, tb):\r\n custom = d[\"value\"]\r\n break\r\n if custom is None:\r\n if type_name in self.rules:\r\n custom = self.rules[type_name][\"value\"]\r\n if custom is None:\r\n return None\r\n else:\r\n if type(custom) == str:\r\n return custom\r\n elif isfunction(custom):\r\n return custom(etype, evalue, tb)\r\n else:\r\n logging.error(\r\n f\"{type(custom)} is not a valid type for bugbook\")\r\n return None",
"_____no_output_____"
]
],
[
[
"## Filter Error Rules",
"_____no_output_____"
]
],
[
[
"# export\r\n# functions that we can use as value of the rule\r\ndef module_not_found_message1(etype, evalue, tb):\r\n libname = str(evalue).replace(\"No module named \", \"\")[1:-1]\r\n return f'Library \"{libname}\" not installed, run a cell like \"pip install -q {libname}\"'\r\n\r\ndef module_not_found_message2(etype, evalue, tb):\r\n libname = str(evalue).replace(\"No module named \", \"\")[1:-1]\r\n return f'''\r\n Are you sure the library name <strong>{libname}</strong> is correct? <br>\r\n If so run \"pip install -q {libname}\" to install again📦 <br><br>\r\n Or ⏯ re-run the cell contains \"pip install ...\"\r\n '''\r\n\r\n# functions that we can use as key of the fule\r\ndef module_not_found_error_filter(etype, evalue, tb):\r\n if etype.__name__ == \"ModuleNotFoundError\":\r\n libname = str(evalue).replace(\"No module named \", \"\")[1:-1]\r\n if libname in [\"fastai\", \"unpackai\", \"transformers\",\"test_filter\"]:\r\n return True\r\n return False",
"_____no_output_____"
]
],
[
[
"## Assign filter to configuration",
"_____no_output_____"
]
],
[
[
"# export\r\nBUGBOOK = BugBook()\r\n\r\nBUGBOOK[\"ImportError\"] = \"Make sure all the libraries are installed for the required version🦮🐩\"\r\n\r\nBUGBOOK[\"SyntaxError\"] =\"\"\"\r\n<h5>There is a <strong>grammatical</strong> error in your python code</h5>\r\n<p>Please check the following</p>\r\n<p>Every '(' or '[' or '{' or '\"' or ' was closed with properly</p>\r\n<p>':' should be followed by a new line with 1 extra <strong>indent</strong> (4 spaces)</p>\r\n<p>or other grammatical errors, please check traceback below for clue, usually <strong>near ^ mark</strong></p>\r\n\"\"\"\r\n\r\nBUGBOOK[\"ModuleNotFoundError\"] = module_not_found_message2\r\n\r\nBUGBOOK[module_not_found_error_filter] = module_not_found_message1",
"_____no_output_____"
],
[
"# export\r\nitb = AutoFormattedTB(mode = 'Plain', tb_offset = 1)\r\n\r\ndef render_download_button(\r\n bytes_data:bytes,\r\n filename: str,\r\n description: str=\"Download\",\r\n color:str = \"default\"):\r\n\r\n \"\"\"\r\n Loads data from buffer into base64 payload\r\n embedded into a HTML button.\r\n Recommended for small files only.\r\n\r\n bytes_data: open file object ready for reading.\r\n A file like object with a read method.\r\n filename: str\r\n The name when it is downloaded.\r\n description: str\r\n The text that goes into the button.\r\n\r\n \"\"\"\r\n payload = base64.b64encode(bytes_data).decode()\r\n \r\n with open(STATIC/\"html\"/\"download_button.html\",\"r\") as f:\r\n temp = Template(f.read())\r\n \r\n download_button = temp.render(\r\n filename=filename,\r\n payload=payload,\r\n color=color,\r\n description=description)\r\n return download_button\r\n\r\ndef custom_exc(shell, etype, evalue, tb, tb_offset=None, ):\r\n \"\"\"\r\n A customize exception method\r\n That we can assign to the ishell kernel\r\n Arguments follow the format of default exeception function\r\n \"\"\"\r\n # gathering data on this error\r\n # the colorful traceback\r\n stb = itb.structured_traceback(etype, evalue, tb)\r\n sstb = itb.stb2text(stb)\r\n \r\n # the plain string of traceback\r\n traceback_string = format_exc()\r\n \r\n # input_history, sanitized(escape) for html\r\n input_history = list(html.escape(i)\r\n for i in ishell.history_manager.input_hist_parsed[-20:])\r\n \r\n # now time stamp\r\n now_full = datetime.now().strftime(\"%Y-%m-%d %H:%M:%S\")\r\n now = datetime.now().strftime(\"%m%d_%H%M%S\")\r\n \r\n error_data = {\r\n \"error_type_name\": etype.__name__,\r\n \"error_value\":str(evalue),\r\n \"traceback_string\":html.escape(traceback_string),\r\n \"timestamp\":now_full,\r\n \"input_history\":input_history,\r\n }\r\n \r\n # custom made error text\r\n msg = BUGBOOK(etype, evalue, tb)\r\n if msg is not None:\r\n error_data.update({\"msg\":msg})\r\n \r\n error_data = json.dumps(error_data, indent=2)\r\n \r\n # create an error report in html format\r\n # by rendering a jinja2 template with error_data\r\n with open(STATIC/\"html\"/\"bug\"/\"error_report.html\",\"r\") as f:\r\n temp = Template(f.read())\r\n \r\n error_report_page = temp.render(\r\n data = json.dumps(\r\n error_data,\r\n ))\r\n \r\n # create a mini error panel\r\n # a download button with embedded data\r\n download_button = render_download_button(\r\n error_report_page.encode(),\r\n filename=f\"npakai_{etype.__name__}_{now}.html\",\r\n description=\"🦋 Download Report\",\r\n color=\"success\")\r\n \r\n with open(STATIC/\"html\"/\"bug\"/\"error_tiny_page.html\", \"r\") as f:\r\n temp2 = Template(f.read())\r\n error_tiny_page = temp2.render(\r\n download_button=download_button,\r\n error_type_name=etype.__name__,\r\n msg=msg,\r\n error_value=str(evalue),\r\n )\r\n display(HTML(error_tiny_page))\r\n \r\n \r\n print(sstb)",
"_____no_output_____"
]
],
[
[
"## Assign our customized funtion",
"_____no_output_____"
]
],
[
[
"# export\r\n\r\n\r\nishell.set_custom_exc((Exception,), custom_exc)",
"_____no_output_____"
]
]
] | [
"markdown",
"code",
"markdown",
"code",
"markdown",
"code",
"markdown",
"code",
"markdown",
"code"
] | [
[
"markdown"
],
[
"code",
"code",
"code"
],
[
"markdown"
],
[
"code"
],
[
"markdown"
],
[
"code"
],
[
"markdown"
],
[
"code",
"code"
],
[
"markdown"
],
[
"code"
]
] |
e774958f68127b8911cc9d4f745b1e631ab46208 | 12,037 | ipynb | Jupyter Notebook | Chapter10/Causal Graphical Model.ipynb | PacktPublishing/Machine-Learning-Quick-Reference | 6dd8ce40265d683a64a186c1e9e3951764c530ae | [
"MIT"
] | 10 | 2019-02-08T00:27:29.000Z | 2022-02-11T22:12:08.000Z | Chapter10/Causal Graphical Model.ipynb | vidnk/Machine-Learning-Quick-Reference | 6dd8ce40265d683a64a186c1e9e3951764c530ae | [
"MIT"
] | null | null | null | Chapter10/Causal Graphical Model.ipynb | vidnk/Machine-Learning-Quick-Reference | 6dd8ce40265d683a64a186c1e9e3951764c530ae | [
"MIT"
] | 11 | 2019-02-08T00:27:35.000Z | 2021-08-10T14:24:31.000Z | 44.581481 | 133 | 0.506937 | [
[
[
"from causalgraphicalmodels import CausalGraphicalModel",
"_____no_output_____"
],
[
"Model = CausalGraphicalModel(\n nodes=[\"Job\", \"Smartwork\", \"Hardwork\", \"Reward\", \"Promotion\"],\n edges=[\n (\"Job\", \"Smartwork\"), \n (\"Job\", \"Hardwork\"), \n (\"Smartwork\", \"Reward\"),\n (\"Hardwork\", \"Reward\"), \n (\"Reward\", \"Promotion\")\n ]\n)\nModel.draw()",
"_____no_output_____"
],
[
"# get the distribution \nprint(Model.get_distribution())",
"P(Job)P(Hardwork|Job)P(Smartwork|Job)P(Reward|Smartwork,Hardwork)P(Promotion|Reward)\n"
],
[
"# get all the conditional independence relationships \nModel.get_all_independence_relationships()",
"_____no_output_____"
],
[
"\n# Let's intervene it by \"Reward\"\nIntervene = Model.do(\"Reward\")\nIntervene.draw()",
"_____no_output_____"
]
]
] | [
"code"
] | [
[
"code",
"code",
"code",
"code",
"code"
]
] |
Subsets and Splits
No community queries yet
The top public SQL queries from the community will appear here once available.